Speech Production
- Reference work entry
- First Online: 01 January 2015
- pp 1493–1498
- Cite this reference work entry
- Laura Docio-Fernandez 3 &
- Carmen García Mateo 4
1261 Accesses
3 Altmetric
Sound generation; Speech system
Speech production is the process of uttering articulated sounds or words, i.e., how humans generate meaningful speech. It is a complex feedback process in which hearing, perception, and information processing in the nervous system and the brain are also involved.
Speaking is in essence the by-product of a necessary bodily process, the expulsion from the lungs of air charged with carbon dioxide after it has fulfilled its function in respiration. Most of the time, one breathes out silently; but it is possible, by contracting and relaxing the vocal tract, to change the characteristics of the air expelled from the lungs.
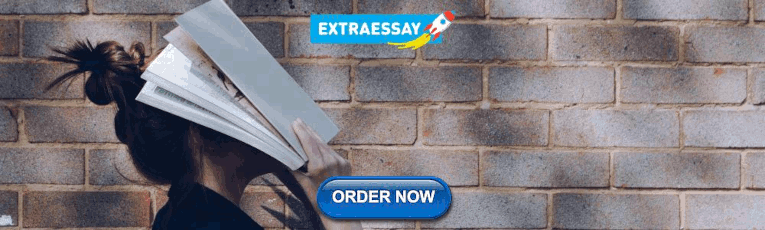
Introduction
Speech is one of the most natural forms of communication for human beings. Researchers in speech technology are working on developing systems with the ability to understand speech and speak with a human being.
Human-computer interaction is a discipline concerned with the design, evaluation, and implementation...
This is a preview of subscription content, log in via an institution to check access.
Access this chapter
Subscribe and save.
- Get 10 units per month
- Download Article/Chapter or eBook
- 1 Unit = 1 Article or 1 Chapter
- Cancel anytime
- Available as PDF
- Read on any device
- Instant download
- Own it forever
- Available as EPUB and PDF
- Durable hardcover edition
- Dispatched in 3 to 5 business days
- Free shipping worldwide - see info
Tax calculation will be finalised at checkout
Purchases are for personal use only
Institutional subscriptions
T. Hewett, R. Baecker, S. Card, T. Carey, J. Gasen, M. Mantei, G. Perlman, G. Strong, W. Verplank, Chapter 2: Human-computer interaction, in ACM SIGCHI Curricula for Human-Computer Interaction ed. by B. Hefley (ACM, 2007)
Google Scholar
G. Fant, Acoustic Theory of Speech Production , 1st edn. (Mouton, The Hague, 1960)
G. Fant, Glottal flow: models and interaction. J. Phon. 14 , 393–399 (1986)
R.D. Kent, S.G. Adams, G.S. Turner, Models of speech production, in Principles of Experimental Phonetics , ed. by N.J. Lass (Mosby, St. Louis, 1996), pp. 2–45
T.L. Burrows, Speech Processing with Linear and Neural Network Models (1996)
J.R. Deller, J.G. Proakis, J.H.L. Hansen, Discrete-Time Processing of Speech Signals , 1st edn. (Macmillan, New York, 1993)
Download references
Author information
Authors and affiliations.
Department of Signal Theory and Communications, University of Vigo, Vigo, Spain
Laura Docio-Fernandez
Atlantic Research Center for Information and Communication Technologies, University of Vigo, Pontevedra, Spain
Carmen García Mateo
You can also search for this author in PubMed Google Scholar
Editor information
Editors and affiliations.
Center for Biometrics and Security, Research & National Laboratory of Pattern Recognition, Institute of Automation, Chinese Academy of Sciences, Beijing, China
Departments of Computer Science and Engineering, Michigan State University, East Lansing, MI, USA
Anil K. Jain
Rights and permissions
Reprints and permissions
Copyright information
© 2015 Springer Science+Business Media New York
About this entry
Cite this entry.
Docio-Fernandez, L., García Mateo, C. (2015). Speech Production. In: Li, S.Z., Jain, A.K. (eds) Encyclopedia of Biometrics. Springer, Boston, MA. https://doi.org/10.1007/978-1-4899-7488-4_199
Download citation
DOI : https://doi.org/10.1007/978-1-4899-7488-4_199
Published : 03 July 2015
Publisher Name : Springer, Boston, MA
Print ISBN : 978-1-4899-7487-7
Online ISBN : 978-1-4899-7488-4
eBook Packages : Computer Science Reference Module Computer Science and Engineering
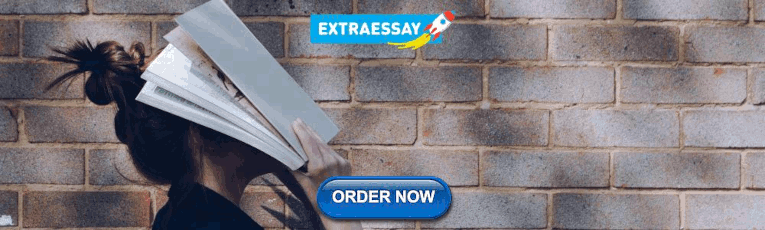
Share this entry
Anyone you share the following link with will be able to read this content:
Sorry, a shareable link is not currently available for this article.
Provided by the Springer Nature SharedIt content-sharing initiative
- Publish with us
Policies and ethics
- Find a journal
- Track your research
All Subjects
Psychology of Language
Study guides for every class, that actually explain what's on your next test, speech production, from class:.
Speech production is the process by which humans generate spoken language through the coordinated action of the brain, vocal tract, and articulators. It involves several stages, including conceptualization, formulation, and articulation, allowing individuals to express thoughts and ideas verbally. This complex mechanism is closely tied to the brain's lateralization of language function, indicating how specific regions contribute to generating speech, and also relates to understanding how we perceive speech through motor actions.
congrats on reading the definition of speech production . now let's actually learn it.
5 Must Know Facts For Your Next Test
- Speech production typically involves a sequence of cognitive processes: thinking about what to say, organizing those thoughts into grammatical sentences, and finally articulating them using vocal mechanisms.
- Broca's Area is crucial for speech production; damage to this area can lead to difficulties in forming grammatically correct sentences, known as Broca's aphasia.
- The left hemisphere of the brain is predominantly responsible for speech production in right-handed individuals, highlighting the importance of lateralization in language function.
- Feedback loops from hearing one's own speech can influence production, allowing speakers to make adjustments in real-time for clarity and fluency.
- Neuroscientific studies using imaging techniques show that specific brain networks are activated during speech production tasks, demonstrating the complexity of language generation.
Review Questions
- Lateralization significantly impacts speech production by designating specific areas of the brain, particularly in the left hemisphere, for language-related tasks. Broca's Area plays a key role in forming words and sentences, while Wernicke's Area is involved in understanding language. This division helps streamline the processes of conceptualization and articulation by distributing tasks across specialized regions, leading to more efficient communication.
- The motor theory of speech perception suggests that understanding spoken language is closely linked to the listener's ability to produce those sounds themselves. According to this theory, when people hear speech, they mentally simulate the motor actions required to produce those sounds. This connection between perception and production highlights how our brain encodes linguistic information not just as abstract symbols but as actual motor programs, creating a dynamic interplay between speaking and listening.
- Advancements in neuroimaging techniques, like fMRI and PET scans, have provided deeper insights into the neural mechanisms underlying speech production. These technologies allow researchers to observe which brain areas activate during specific language tasks. This has revealed a more intricate network than previously thought, involving not only Broca's Area but also regions responsible for auditory feedback and emotional tone. Such findings enhance our understanding of how speech is produced and processed by illustrating the collaborative efforts of various brain regions during communication.
Related terms
The physical process of producing speech sounds using the mouth, tongue, and vocal cords.
A region in the frontal lobe of the brain associated with speech production and language processing.
The study of the sounds of human speech, including how they are produced and perceived.
" Speech production " also found in:
Subjects ( 1 ).
- AP Psychology
© 2024 Fiveable Inc. All rights reserved.
Ap® and sat® are trademarks registered by the college board, which is not affiliated with, and does not endorse this website..
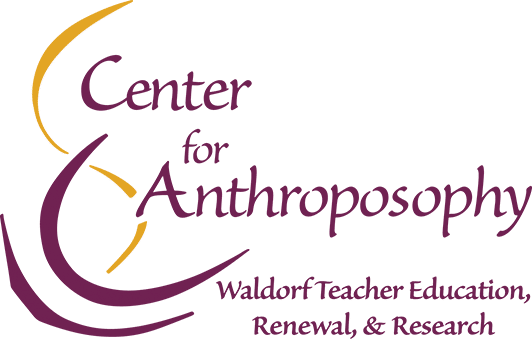
Introductory Programs
Teacher Training
Professional Development
Attending a Residency
Wilton, NH USA
Keene, NH USA
CfA's Op Ed
Developing the art of imagination: why creative speech formation is an essential part of teacher training.
by Debra Z. Spitulnik
Every morning, our classes begin with a morning verse that Steiner gave to the teachers. No matter what age we teach, we then incorporate into our classes some form of verses, poetry, movement, music, review, new material, story, instructions. We speak all the time, either individually or in chorus. Creative Speech formation makes us better teachers: clear in our instructions, enlivened in our storytelling, soulful in our poetry, and objective in our experiences. We can listen more deeply and understand why a student doesn’t understand. We can experience their spirit and soul in words because we have done it for ourselves.
Only when working out of Creative Speech formation in our teaching is our ego sense fully engaged. A Creative Speech class addresses every aspect of what one needs in order to be an excellent Waldorf teacher – curriculum, classroom management, self-development, and artistic skills – which is why teacher training centers provide classes in Creative Speech.
To deepen our understanding of how to effectively teach Creative Speech to teachers, I began a Pedagogical Speech Study with 15 participating teachers in the fall of 2019. In March 2020, everything changed and the schools closed due to the pandemic-induced quarantine. What now, as schools scrambled to provide classes in a way never thought possible in Waldorf education? How could we do this knowing how important direct interaction and engagement are to the child – and to the teacher? Where do we find the strengths in this endeavor? Pedagogical speech is one of those strengths, as teachers must bring curriculum in an enlivening way, even (or especially) when teaching remotely. This sudden, radical change in the educational environment brought an entirely new way of studying and learning about the importance of Creative Speech in training teachers.
To those who understand the sense of speech The world reveals itself as image. Rudolf Steiner, The Genius of Language
It is our job as teachers to imbue our speech with imagination, to allow our students to hear and learn with their whole being. Children don’t hear words, they feel and experience images. When we touch their soul, integrating the intellect with feeling, they learn. We never want our students to say, as this first grader did: “When I listen to an audiobook or a story being told, it is so boring because there are no pictures – just words words words.”
As educators, we use words incessantly, hardly taking a breath. This can literally take our breath away, and with it any power to create and form images. How can “the world reveal itself as image” through the sense of speech, and how can this be useful in the classroom?
In Creative Speech, the student learns to sculpt sounds and words to create a picture. For example, the word “dust” can be experienced by moving through each sound to create “dust” as an image so real that the speaker and listener can see, feel, touch, live it. Steiner was very clear that this must be done to enliven sounds, words, sentences, whole texts. If the teacher speaks through enlivened speech, whether giving directions, telling a story, speaking in a faculty meeting, or with parents, the audience will understand and listen in a newly active, engaged way.
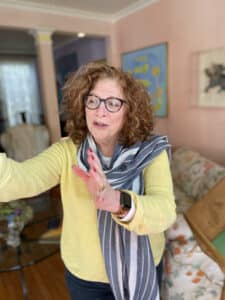
Another important aspect of this process is creating objective images. When images are held too closely or sympathetically – held inside by the speaker rather than projected outward – the listener cannot experience the image properly. The speaker must project the images to the listener as though they were shown on a screen in 3D. Without this outward projection, the spoken words and images become tied to a person rather than the spiritual, etheric reality. When the speaker is too inward-focused, listeners are not free to create their own relationship to the spoken word. When the words are filled with an inner gesture and inner experience, yet still spoken objectively, listeners are free to create their own relationship with the text, and students learn, becoming part of the learning process.
Learning this skill is particularly hard for adults. All we experience in the age of individual consciousness is that “I am important and the center of the world,” or “You are an individual and important.” However, communicating the objective picture that we can experience between or beyond us is much more difficult. Because adults use abstract thinking as their primary learning mode, it is not an easy or intuitive jump to experience that objectivity; just to try it can be confusing. However, once adult learners “hear” it in a classmate’s speech and experience the difference, they are excited and work to do it themselves.
“Speech has enhanced my storytelling, especially the images that I bring to my students. At first I was more conscious of my speech, trying so much that it felt worse, but as I keep working with speech I have experienced the depth and breadth of a story in an objective way and am able to give that to the children.” (PSSP – Pedagogical Speech Study participant)
Speech formation enhances storytelling, a major component of Waldorf education, so a pillar of Waldorf teacher training must be strengthening and enlivening the way we tell a story. When we tell a story that we have experienced , not just heard, our listeners experience it too. When we tell a story we have read through our intellect alone, it becomes cold, sharp, and linear. The students may like it, but they are not fully engaged, and neither is the teacher. Modern research confirms that human beings’ experiences are not just about what they themselves do, but also what they hear and see others doing (Patzlaff, 2019). They make it their own. The study shows that young children do not remember the difference between what they have done and what they see or hear others doing . According to Palzlaff, the activity of doing a task, and the inner gesture of speaking that task, i.e., “chop, chop, choppity – chop,” helps the child understand language. The movement, gesture, and word take place in the child’s sense organs and are recapitulated in their own language or movement.
To those who hear the Soul of speech The world unfolds its true being. Rudolf Steiner, The Genius of Language
“I am not conscious of when I am “doing speech,” but it is clear to me when I am not, and when the students are not.” (PSSP – Pedagogical Speech Study participant)
When listening to another’s speech, the other’s true being is revealed: their thoughts, feelings, and actions are exposed. This is why speaking in public has been cited as one of the most frightening activities, and why adults find Creative Speech classes challenging. By requiring the speaker to look within himself to find the image and project it outward, something of his personal experiences and feelings is exposed. But when adult learners are willing and able to be vulnerable with this learning, their ego becomes engaged and their capacity as a listener is enhanced. We now listen not just for the words, but for the gesture, images, and experience behind them, and we can understand one another. This builds community, empathy, trust, and respect; and it can also be very frightening, for reasons discussed below.
In the classroom, we are constantly assessing our students in various ways. By listening to different aspects of their speech, we can get a glimpse of their struggles and strengths. Can they articulate properly? What sounds do they or don’t they hear? Can they communicate through images and does their spoken language make sense? If teachers do not develop these capacities fully through their own Creative Speech work, what we hear may be merely abstract, ignoring a key component of the students’ being.
To those that live in the Spirit depths of speech The world bestows Wisdom’s strength. Rudolf Steiner, The Genius of Language
“When I am not breathing properly, my words get stuck or jumbled and the students get breathless. My teaching of the material then is unclear, and the students misbehave or get breathless themselves. My ego is not engaged, and then I have even less control of the classroom and the material.” (PSSP – Pedagogical Speech Study participant)
The human spirit lives in speech, which points in the direction of the future while teaching of the past and living in the present. Breath is the spirit of life, and with the breath of our speech, we can create the atmosphere of our classroom’s spirit. In his first lectures to teachers, Rudolf Steiner explained that our main task is to teach children to breathe (Steiner, Foundations of Human Experience) . If we teachers are not using our breath with the healthy rhythms of our heartbeats and lungs, we will not be able to discern the proper breathing rhythm of a lesson, with its aspects of expansion and contraction. With Creative Speech training the teacher learns to use the breath artistically, experiencing the expansion, contraction, and rhythm of texts in a way that enhances their speaking.
Before our recent experiences with recording lessons and providing videos for our students due to the quarantine, we rarely had the opportunity to listen to ourselves. This has now changed, giving us greater opportunity to observe how we can improve the breath and images in our speech.
When I listen to the recording, I can hear when I do not articulate properly, or when I haven’t breathed into the word to create a moment or an experience – an image. (PSSP – Pedagogical Speech Study participant)
Throughout my work in training teachers and in our ongoing study, I have seen the lack of breath in participants’ speech, especially when I observe their teaching. Creative Speech formation teaches us how to regulate our breath to a healthy rhythm without constantly being conscious of it. When we learn to speak the way Steiner indicates, we sculpt the sounds carefully, holding the image of the word as we begin to articulate its sounds. As the formed sounds move on the stream of breath, our image of the word moves along as well, and the words combined with the inner gesture and image touch the listener’s soul.
This difference between vowels and consonants is not intuitive, but lies at the foundation of Creative Speech. Vowels have a musical quality; consonants are expressions of the outer world and have a sculptural quality. We can form pictures and shapes with consonants, which relate to the elements: earth, air, water, fire. We cannot form pictures with vowels, but no true word can be spoken without them. They are in some ways the heart of a word, the magical quality that brings it breath and life. If someone lives too much in vowel sounds, the sympathy in their speech is often extreme and uncomfortable. Too much breath or too much reliance on vowels can make the speaker seem ungrounded and not genuine. Conversely, if someone speaks more strongly through consonants, their presentations can be experienced as harsh. It is important in andragogy to balance these forces in the teacher so the child’s soul is not imposed on by our speech.
“All vowels express inner soul stirrings that live in the sympathy we have towards things. Consonants are related to things and sounds outside ourselves. Consonants live in antipathy – they sound from the object without. In speech, we have a genuine synthesis, a true uniting of the human being “(Steiner, Practical Advice to Teachers ).
“In transposing gestures into sounds, we learn by means of the consonants to imitate inwardly outer processes; and in the vowel, we give form to inner experiences of the soul. In speech, the inner and the outer unite. Human nature, itself homogeneous, understands how to bring this about” (Steiner, Human Values in Education ).
To those who can lovingly dwell on speech
It will grant its own creative might.
Thus, I will turn my heart, my mind
Towards the spirit and soul of Words,
And in my love for them
For the first time, I will
Feel MySelf complete.
— Rudolf Steiner
“By focusing on and improving my speech, it helps me to keep the curriculum living and imaginative, enabling me to reach the students and affect their ability to learn.” (PSSP – Pedagogical Speech Study participant)
A teacher’s voice impacts the quality of a student’s articulation, modulation, breath, and above all, engagement. Steiner emphasized the importance of surrounding children with elevated speech during the day for healthy development. “…[D]on’t forget that children have to speak in class; good speaking is part of the instruction given at school” ( Speech and Drama Course , p. 30). In today’s overstimulating world, where children are bombarded with electronic hum, the quality of the teacher’s speech is more important than ever before. Perhaps the antidote to the degradation of students’ linguistic experience and development is innovating new techniques as we deepen Steiner’s indications for Creative Speech.
Young children imitate the teacher’s speech and find this fun; they like to play with sounds and words. If they don’t know something, they are excited and curious to learn. Adult learners are the opposite. Adults’ egos are already formed; their ability to move into a more intimate and precarious realm is hampered by their biographies and life experiences. Everyone has their history that guides them to become a Waldorf teacher, and that history is evident in their speech. Creative Speech comes directly from the astral body modified by the ego or “I” (Steiner, Speech and Drama ), therefore it reveals adults’ deep feelings, their etheric and astral bodies, their ego strength . Animals make sounds that convey needs; in human beings, this ability is raised and transformed by the ego. When adults begin to transform their speech, becoming conscious of form, inner movement, strength, or weakness, they feel vulnerable. This presents a challenge to teacher training students because while they are learning the Waldorf approach to pedagogy, child development, and curriculum, they are required to address some internal challenges they may not have addressed fully in their past. Clearing away those cobwebs creates space.
In addition, the adult student is expected to memorize words and sentences. Because memory and rhythm live in the etheric realm, its strength or weakness also becomes apparent during a Creative Speech class. Sometimes the student awakens to this in a conscious manner for the first time; often it is difficult. When the adult learner rises to the challenge, the result is a more well-rounded and gifted teacher. This is challenging and exciting, for the adult then has the opportunity to take up different aspects of their being and transform them, both for themselves and for the students they will teach. We are asking the adult learner to transform their already incarnated ego and give that away to another being while keeping it for themselves.
Great self-development can happen through speech, and frequently it does. But when the adult has no idea what to expect and thinks she is taking a speech class simply to learn how to tell a story or recite poetry, it can be a shock. Our task as teachers of Creative Speech to adults is to imbue ourselves with love, warmth, and knowledge for our subject and humanity, while still leaving the student free to discover the joys and struggles of finding their own authentic voice. The only way to truly find that as a teacher is to penetrate our astral and etheric bodies with our ego, to enlighten and lift ourselves out of the daily physical world to our higher self. Only then can we take up our task as teachers to help facilitate the ego incarnation of our students.
“In speech, the ego works as a puppeteer might, controlling the three other sheaths of the human being. When the ego is present, the astral is contained in a healthy way, managed. Speech strengthens the ego by awakening the individuality and sense of self. The more we participate in speech formation, the more the ego is called on to bring out this individuality. The spark of spirit that strikes the ego intensifies. Speech formation demands that the ego manipulate and move the etheric, astral, and physical bodies. In this way, the ego is strengthened and brought into the body” (D. Spitulnik, 1995) .
Note: This article is adapted from a contribution to a collection of essays by Torin Finser under the title A Call to Teach: In Service of Waldorf Teacher Education and Lifelong Learning (Hudson, NY: Waldorf Publications, 2020).
Debra Spitulnik ’s deep grounding in and her love for the art of Creative Speech and for the beauty and richness of Waldorf Education stem from her training as a speech artist and her years of class teaching. Debra is a Creative Speech teacher for the Washington Waldorf School, Center for Anthroposophy, and the Waldorf Teacher Education Program at Antioch University New England. Education: Goetheanum Diploma in the Arts of Speech and Drama, PerformInternational; M.Ed., Waldorf Education, Antioch University New England; B.S., Special and Elementary Education, Syracuse University .
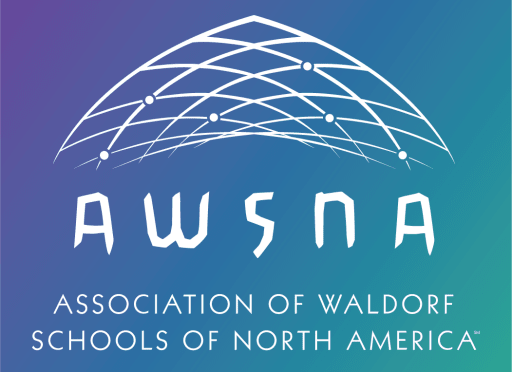
We are affiliated with...

Copyright © 2022 Center for Anthroposophy, all rights reserved.
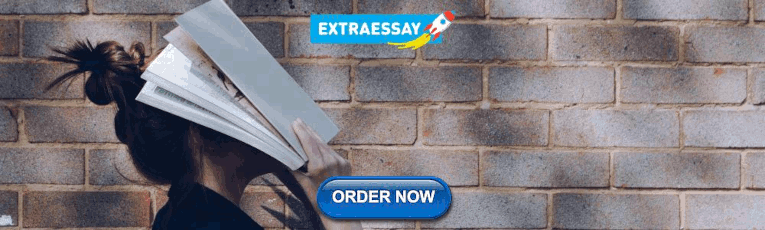
IMAGES
VIDEO
COMMENTS
The document discusses different methods of speech delivery: reading from a manuscript, memorized speeches, impromptu speeches, and extemporaneous speeches. It provides guidelines for each method, such as maintaining a normal speech rate, using hand gestures, and having a structure like thesis-argument-support-summary when speaking impromptu.
Speech production is the process by which thoughts are translated into speech. This includes the selection of words , the organization of relevant grammatical forms, and then the articulation of the resulting sounds by the motor system using the vocal apparatus .
Speech production is the process of uttering articulated sounds or words, i.e., how humans generate meaningful speech. It is a complex feedback process in which hearing, perception, and information processing in the nervous system and the brain are also involved.
Speech production is the process by which humans generate spoken language through the coordinated action of the brain, vocal tract, and articulators. It involves several stages, including conceptualization, formulation, and articulation, allowing individuals to express thoughts and ideas verbally.
In Creative Speech, the student learns to sculpt sounds and words to create a picture. For example, the word “dust” can be experienced by moving through each sound to create “dust” as an image so real that the speaker and listener can see, feel, touch, live it.
Our study investigated the role of creativity in second language (L2) speech production using a picture narrative and an open-ended argumentative task administered to 60 Japanese-speaking learners of English.
Language production is often described as the process of turning thoughts into speech: If you want to communicate a certain thought, or message, you must turn it into a verbal utterance that others can understand.
Speech production refers to the complex process of articulating sounds and words. It involves hearing, perception, and information processing by the brain and nervous system. Phonology and...
In a cognitive approach, creativity can be viewed as a purely cognitive construct (Simonton, 2012), and thus has been defined as the ability to produce novel ideas, logical solu-tions, and rationalized plans in response to a specific problem (Guilford, 1959).
•The ability to produce novel ideas, logical solutions and rationalized plans in response to a specific problem . (Guilford, 1959) •Two sub-components. ØDivergent thinking skills: To produce different ideas flexibly in response to a given problem: Fluency(number of ideas), Flexibility.