Thank you for visiting nature.com. You are using a browser version with limited support for CSS. To obtain the best experience, we recommend you use a more up to date browser (or turn off compatibility mode in Internet Explorer). In the meantime, to ensure continued support, we are displaying the site without styles and JavaScript.
- View all journals
- My Account Login
- Explore content
- About the journal
- Publish with us
- Sign up for alerts
- Open access
- Published: 13 March 2024
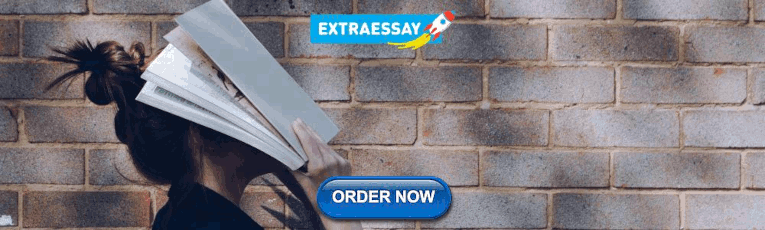
Observational reinforcement learning in children and young adults
- Julia M. Rodriguez Buritica ORCID: orcid.org/0000-0003-4232-2238 1 , 2 nAff10 ,
- Ben Eppinger 1 , 3 , 4 , 5 ,
- Hauke R. Heekeren 1 , 6 ,
- Eveline A. Crone 7 , 8 , 9 &
- Anna C. K. van Duijvenvoorde ORCID: orcid.org/0000-0001-9213-8522 8 , 9
npj Science of Learning volume 9 , Article number: 18 ( 2024 ) Cite this article
980 Accesses
3 Altmetric
Metrics details
- Social neuroscience
Observational learning is essential for the acquisition of new behavior in educational practices and daily life and serves as an important mechanism for human cognitive and social-emotional development. However, we know little about its underlying neurocomputational mechanisms from a developmental perspective. In this study we used model-based fMRI to investigate differences in observational learning and individual learning between children and younger adults. Prediction errors (PE), the difference between experienced and predicted outcomes, related positively to striatal and ventral medial prefrontal cortex activation during individual learning and showed no age-related differences. PE-related activation during observational learning was more pronounced when outcomes were worse than predicted. Particularly, negative PE-coding in the dorsal medial prefrontal cortex was stronger in adults compared to children and was associated with improved observational learning in children and adults. The current findings pave the way to better understand observational learning challenges across development and educational settings.
Similar content being viewed by others
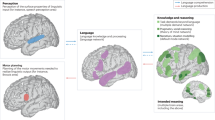
The language network as a natural kind within the broader landscape of the human brain
Evelina Fedorenko, Anna A. Ivanova & Tamar I. Regev
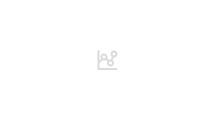
Uniquely human intelligence arose from expanded information capacity
Jessica F. Cantlon & Steven T. Piantadosi
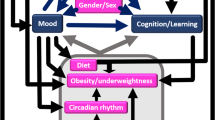
Effect of sleep and mood on academic performance—at interface of physiology, psychology, and education
Kosha J. Mehta
Introduction
Numerous findings indicated that others have a strong impact on learning and decision-making 1 , 2 , 3 , 4 , 5 , 6 . For instance, we may be swayed by the opinions of others to adjust our norms on acceptable behavior. Alternatively, others could be considered a source of information, as they can provide us with valuable information about our environment. Learning from observing others’s behaviors and outcomes may have benefits in dangerous or novel environments, in which observational learning allows us to learn from the actions and outcomes of others without having to engage in these (potentially hazardous) behaviors ourselves. Social situations lend themselves well for observational learning. That is, learning from others is ubiquitous on playgrounds, in schools and other social environments, in which we have the opportunity to observe others behaviors and subsequent outcomes without necessarily participating ourselves. However, compared to learning from own experiences, the developmental mechanisms underlying observational learning are poorly understood.
Several studies have examined how learning from own outcomes changes with age 7 , 8 . Many studies observed that adults typically outperform children during instrumental learning. That is, adults learn faster than younger ages and choose the most rewarding option more often. This is thought to be related to a developmental improvement in cognitive control, including behaviors such as sustained attention and working memory, which would benefit learning. It is yet unclear how a social observational context influences this adult advantage. That is, children are shown to be highly sensitive to the example of others, quickly copying behavior, particulary of their own peers 9 . On the other hand, a previous observational learning study showed that children may not process and use information of others as efficiently as young adults in their learning and decision making 10 . These age-related differences in observational learning have been related to differences in temporal processing of observed outcomes using electro-encephalogram (EEG) 9 , 10 . Children, ages 8–10, showed larger electrophysiological responses when observing peers as compared to adults 5 , but compared to adults their electrophysiological responses did not change according to their learning 6 , and they could not yet benefit in accuracy from observed information as much as adults. What remains unresolved in the current literature are the functional and computational processes supporting observational learning as compared to individual learning across development. In this study, we therefore applied a model-based neuroimaging approach to observational learning across development, by combining reinforcement learning (RL) modeling and functional magnetic resonance imaging (fMRI) in a children’s and adult age group.
Computational models of reinforcement learning haves been successfully applied to understand reinforcement learning in adults and children 5 , 11 , 12 , 13 , 14 , 15 , 16 , 17 , 18 . In these models, learning is driven by prediction errors, which reflect the mismatch between expected, Q a (t), and received outcomes, r(t), per trial (t). Whenever an outcome is better (worse) than expected, the model will generate a positive (negative) prediction error. Prediction errors are thought of as important learning signals, and are shown to scale with activity of midbrain dopamine neurons 19 , 20 , 21 , 22 . The weight in which prediction errors drive learning behavior is quantified by learning rates. High learning rates allow prediction errors to quickly change the value of choice options. Low learning rates result in slower updating and therefore a long-term integration of outcomes in the value of choice options. In addition, RL models typically include a temperature parameter that specifies how precisely one’s choices discriminate between the value of choice options, and thus controls the specificity in choice behavior. Previous research involving reinforcement learning models has yielded mixed results regarding age-related differences in positive and negative learning rates 8 . Recent reviews, however, indicate that choice specificity tends to consistently increase with advancing age 7 , 8 .
In contrast to individual learning situations, observational learning is a richer learning environment. For instance, behavior can be updated twice: First, by an experiential prediction-error (as in standard reinforcement learning) and second by an observational prediction-error. Where the first is generated by one’s own outcomes, the latter is generated by the outcomes of an observed other. Neuroimaging studies examining the underlying neural mechanisms have suggested neural systems may be partly overlapping and partly specific for learning from social and non-social outcomes 5 , 16 , 23 , 24 . That is, whereas prediction errors in various learning paradigms have been represented in brain regions such as the ventral striatum and ventral medial prefrontal cortex (vmPFC) 11 , 25 , 26 , 27 , prediction errors in other brain regions may be more specialized for social learning. For instance, social learning has been related specifically to the dorsal medial PFC (dmPFC) 28 , 29 and anterior cingulate cortex (ACC) 11 , 12 with the latter representing other-related reward-processing, and information on the consequences of actions of others 24 , 30 . In addition, social learning has been related to brain regions involved in mentalizing and modeling of others, such as the dmPFC, temporal-parietal junction (TPJ), and posterior superior temporal sulcus (pSTS) 23 . Recent studies highlighted the role of mentalizing particularly in situations in which individuals are confronted with opposing preferences and in which the mental state (goals, preferences, beliefs, or intentions) of another person needs to be inferred 14 , 28 , 31 . This mentalizing network is also thought to track trial-level updating during strategic social interactions 12 , 32 .
Until now, few studies have examined the computational and neural correlates of age-related differences in social and non-social reinforcement learning. We build on this previous work and extend this with the current study: Here, we aim to examine age-related differences in the neural correlates of observational and individual prediction errors. Including both observational and individual learning allows us to examine age-related differences in both learning situations, as well as to compare these learning situations directly. To do so, we used functional magnetic resonance imaging (fMRI) and a computational modeling approach. Thirty children (8–10-year-olds, 18 female) and 30 young adults (18–20-year-olds, 16 female) participated in this study. For the youngest ages we focused on middle childhood, including children between 8 and 10 years. In this way we captured an important phase of age-related differences in controlling responses towards positive and negative feedback 33 , and we built on previous EEG work on observational learning 9 , 10 .
Before the start of the experiment, participants met an age-matched and same-sex peer who was introduced as the observed other player in the task. Participants performed a probabilistic observational learning paradigm 9 , 10 , 11 (see Fig. 1 ). In this task, participants made repeated choices between pairs (i.e., 8 in total per pair) of stimuli, where one stimulus was associated with a high probability of reward (80% gains, 20% losses) and the other was associated with a low probability of reward (20% gains, 80% losses). Before participants could choose, they observed the other player choosing between the same two stimuli. We manipulated the amount of observable information of the other player across two learning conditions with each three runs (each with 2 stimulus pairs per learning condition; thus 32 trials per run): In the individual learning condition participants received no information about the behavior of the other individual; in the observational learning condition they could observe the other player’s actions and outcomes before making their own choice. With this setup, we can better understand how observed information of others is used for one’s own learning. The four stimulus pairs (two per condition) per run were presented intermixed. To better understand and explain age-related differences in learning in this task, we used a double update Q-learning algorithm 11 that captures learning from both other’s and own outcomes. Note that just in the observational learning condition, Q-values could be updated in the observational stage as in the individual learning no outcome was displayed (see Fig. 1 ; and Methods). Here, we included two independent learning rates (α pos , α neg ), separately for each valence (positive, negative) of the outcome for both learning conditions. A higher learning rate means a quicker updating of expected values and thereby faster learning. For both stages and conditions, the probability of choosing option a from a stimulus pair (ab) was computed using an inverse softmax function 34 (see Methods for further details), were we estimate the inverse temperature ( β )) indicating the specificity of the subject to differences in Q-values. Since β is the inverse temperature, this means that higher values indicate a less deviations from optimal choice behavior (i.e., a greater choice specificity). For the fMRI analyses we used trial-level calculated prediction errors when presented with own (action phase in IL condition; Fig. 1 ) and others (observational phase in OL condition; Fig. 1 ) outcomes derived from the median of the parameter estimates (i.e., α pos , α neg and β ) per age group (Fig. 2 ). Using a model-based parametric fMRI approach, we examined which brain regions correlated with prediction error activation.
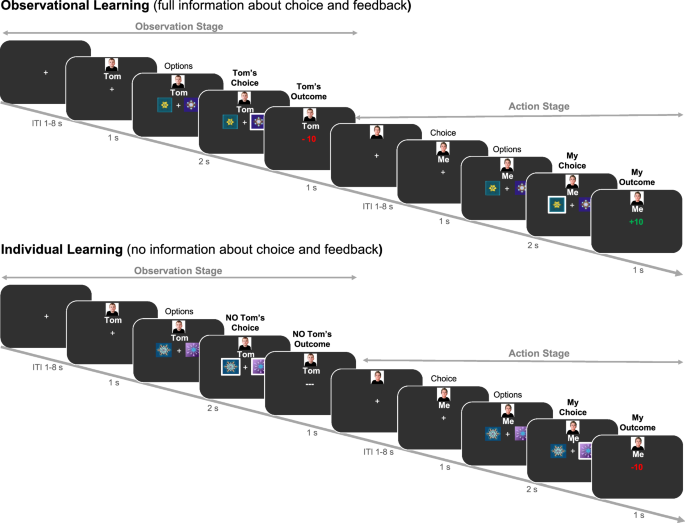
Example of the trial procedure for the observation learning and individual learning condition.
Given our previous developmental work, we expected that both age-groups benefitted from the additional observational information, although adults are expected to outperform children in both conditions and are expected to learn faster than children 10 . Based on work in adults, we expect that RL-models can be used to describe individual and observational learning across development, although during observational learning choice values will be updated twice 11 . Given previous mixed findings on age-related differences in learning rates, we had no clear predictions here, but expected that the inverse temperature parameter is sensitive to age-related differences 8 . Based on previous developmental work, prediction-error activation of own and observed outcomes is expected to be related to striatal and medial prefrontal cortex activation across development 9 , 10 . Observational learning is expected to relate to the medial PFC including the ACC and dmPFC 5 , 11 , 12 , 23 , 24 , 30 , and mentalizing regions such as the TPJ 14 , 28 , 31 . Finally, whereas both age groups may benefit from observational compared to individual learning, we expected greater neural differentiation between own and observed outcomes in adults compared to children 10 , 35 , reflective of greater efficiency of learning which is increased in observational situations. Taken together, we include a computational approach to examine the neural associations and age-related differences in observational versus individual learning between children and adults.
Performance differences in observational and individual learning
One participant (child) did not finish the learning session in the scanner and was not included in further analyses (see Methods). Both age groups selected the correct option (rewarded 80% of the time) more frequently than the incorrect option (rewarded 20% of the time) and showed accuracies above chance level in both learning conditions (four t- tests against chance level per condition and per age group; all p ’s <0.001). Performance was correlated between the observational and individual learning condition (Persons’ r = 0.46, p < 0.001), indicating that individuals who performed well in the observational condition, also performed well in the individual learning condition. Descriptively, 47 (24 children and 23 adults) out of 59 subjects performed more accurately in the observational than the individual condition, suggesting that most participants benefitted from the additional observable information.
To test age group and condition effects on learning we analyzed choice behavior (averaged across runs) using a mixed-effects general linear model (controlling for intelligence) with the between subject predictor age group (children, adults) and the within subject predictors learning condition (individual, observational) and trial number (1:8), as well as all interaction terms (see Methods). The results of this analysis (see Supplementary Table 1 for full model output and Fig. 2a for visualization) showed significant main effects of learning condition (β = 0.12, t = 5.2, p < 0.001) and trial number (β = 0.04, t = 3.0, p = 0.005), as well as a significant learning condition x trial number interaction (β = −0.04, t = -2.2, p = 0.026). Post-hoc comparisons per condition revealed that participants improved across trials in the individual learning condition (β = 0.06, t = 6.3, p < 0.001), while performance remained stable in the observational condition (see Fig. 2a ; β = 0.004, t = 0.43, p = 0.671). This suggests that learning from observing others resulted in relatively high-performance levels early in the learning process, while individual learning showed a more gradual improvement across trials. We also found age-related differences: including a significant main effect of age group (β = 0.1, t = 3.7, p < 0.001), as well as a significant age group x trial number interaction, β = 0.04, t = 2.2, p = 0.031. Post-hoc comparisons per age group revealed that adults improved across trials (β = 0.05, t = 4.6, p < 0.001), while children showed limited improvement (see Fig. 2a ; β = 0.18, t = 1.75, p = 0.08). This indicates that adults generally demonstrated greater accuracy improvement across trials compared to children. No significant age by learning condition interactions was observed ( p = .9). Thus, altough adults overall outperformed children, the age groups benefitted to a similar degree from the additional observable information (see Fig. 2a ).
To ensure that differences in learning conditions were not solely due to varying information levels provided to participants (i.e., more information in observational than individual learning condition), we conducted an additional mixed-model analysis. This analysis focused on an equal amount of information in each learning condition, comparing trials 1–4 in the observational learning condition (including 1/3/5/7 observed/received outcomes) to trial 2,4,6, and 8 in the individual learning condition (including 1/3/5/7 received outcomes). The results align with our main analysis, indicating better performance in the observational learning condition compared to the individual learning condition (main effect condition: β = 0.06, t = 5.03, p < 0.001), across both age groups (age x condition interaction: p = 0.8).
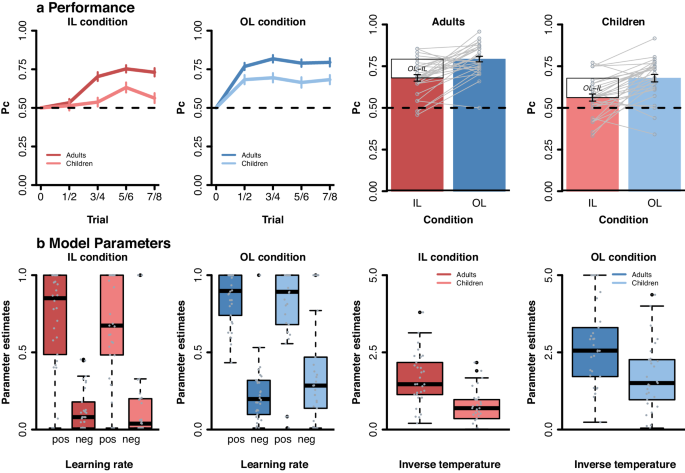
a Proportion correct choice (Pc). Pc displayed separately for the two age groups (adults, children) and learning conditions (individual (IL), observational (OL)). Data were averaged into four bins (across eight trials). Error bars reflect the SEM. Grey lines reflect individual learning differences between IL and OL condition. b Parameter estimates of the best fitting model across age groups. Learning rate (i.e., alpha pos and alpha neg) and inverse temperature (i.e., beta) per age group and condition (individual learning (IL) and observational learning (OL)).
Computational parameter differences in individual and observational learning
Next, we examined age and learning condition effects on computational parameters (i.e., α pos , α neg and β ; see Methods). Higher learning rates indicate that recent choice outcomes have a stronger effect on future choices than less recent choice outcomes. The inverse temperature indicates participants’ sensitivity to differences in these choice values. Here, the higher the beta parameter, the less stochastic choice behavior was.
Robust mixed-effects analyses were used to test main and interaction effects of age group, condition (IL, OL), and valence (Pos, Neg; only for learning rates; see Fig. 2b and Supplementary Table 2 ). Findings showed that positive and negative learning rates differed significantly across learning conditions (β = 0.75, t = 3.4, p < 0.001, main effect of valence) with both learning rates were higher for positive (median = 0.78) than negative outcomes (median = 0.2). No significant main effect of condition, age, or any interactions with age and condition were observed (all p’ s > 0.7). A similar analysis on the inverse temperature parameter (see Fig. 2b and Supplementary Table 3 ) showed that participants followed choice values more optimally in the observational (median = 2.14) than individual learning (median = 1.03) condition (main effect condition, β = 0.95, t = 7.4, p < 0.001). A main effect of age group showed that children (median = 1.13) were less optimal in their choice behavior than adults (median = 2.18; β = 0.91, t = 4.5, p < 0.001). No age x condition effect was observed ( p = 0.9).
Age group and condition differences in prediction-error activation
We analyzed neural activation related to prediction error coding (entered as a signed continuous trial-by-trial predictor) when participants received their own outcomes (Fig. 1 , individual learning condition action phase) and others’ outcomes (Fig. 1 , observational learning condition observational phase). We initially examined whether there were brain regions that responded differently to prediction errors in individual learning and observational learning conditions. Results showed that individual compared to observational prediction errors were more strongly related to activation in the vmPFC ( p FWE’s < 0.05), the left lateral PFC ( p FWE’s < 0.05), the bilateral striatum, and bilateral parietal cortex ( p FWE’s < 0.001; see Fig. 3 ; and Supplementary Table 4 ). No brain regions correlated stronger to observational than individual prediction errors (all p FWE’s > 0.05).
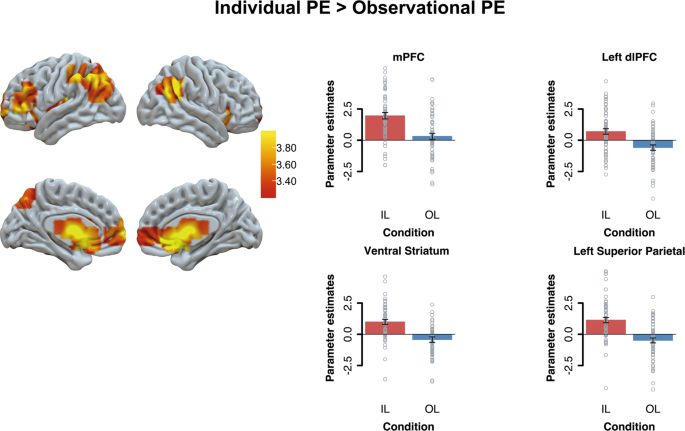
Results are displayed at Family-Wise Error (FWE) cluster-corrected p < 0.05, with an initial cluster forming threshold of p < 0.001. For visualization we extracted the beta-values from the whole-brain condition effect from regions of interest. Since our activation spanned multiple subcortical anatomical regions, the functional activation from the subcortical cluster is overlaid with a nucleus accumbens (ventral striatum) anatomical mask.
Next, we examined with a whole-brain ANOVA whether this difference in prediction error activation between individual and observational learning conditions was sensitivity to age group differences. An age group × learning condition interaction was observed in the left TPJ/inferior parietal cortex ( p FWE < 0.05; learning condition (IL > OL) × age (adults > children) contrast). Follow-up tests per age group showed that the TPJ/ inferior parietal cortex differently responded to individual and observational prediction errors in adults compared to children: That is, prediction errors related to increased TPJ activation when other’s outcomes were worse than expected and to increased activation when own outcomes were better than expected in adults ( t (29) = 5.07, p < 0.001). This differentiation was not observed in in children ( p = 0.15; see Fig. 4 ; and Supplementary Table 5 ).
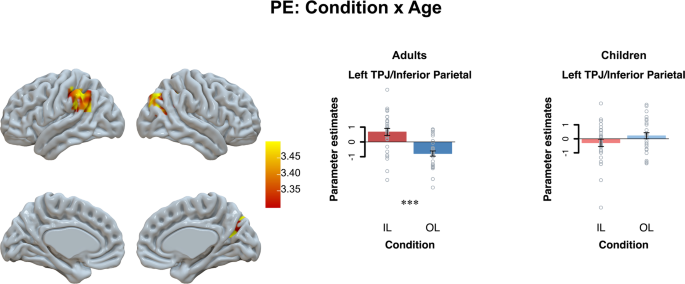
Results are displayed at Family-Wise Error (FWE) cluster-corrected p < 0.05, with an initial cluster forming threshold of p < 0.001. For visualization we extracted the beta-values from the whole brain interaction effect of learning condition × age. Significant differences of follow-up tests are marked here (*** p < 0.001).
Age effects in observational and individual prediction error activation
We first used a whole-brain F -test ( p FWE < 0.05). to examine both positive correlations with prediction error activation (i.e., regions where activation increased with larger positive prediction errors) and negative correlations with prediction error activation (i.e., regions where activation increased with larger negative prediction errors) within each learning condition. The results revealed that in the observational learning condition, prediction errors were associated with activation in the right lateral PFC, right inferior parietal, and right insula (see Fig. 5a and Supplementary Table 6 ). Similarly, in the individual learning condition, a comparable whole-brain analysis indicated that prediction errors were positively correlated with activation in the ventral medial prefrontal cortex, striatum, and parietal cortex (see Fig. 5b and Supplementary Table 7 ).
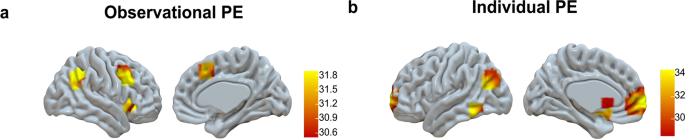
a observational PE activation and b individual PE activation, displayed based on a whole-brain F -test (pFWE <0.05) Observational PE activation showed negative correlations with brain activation (i.e., greater negative PEs resulted in larger activation, see bar charts of extracted beta-values Supplementary Fig. 1 ). Individual PE activation showed positive correlations with brain activation (i.e., greater positive PEs resulted in larger activation, see bar charts of extracted beta-values Supplementary Fig. 1 ).
Since our focus was on age differences, we then compared age groups in prediction error-associated brain activation for the observational and individual learning conditions. A whole-brain t -test comparing children versus adults revealed that observational prediction errors were more strongly represented in adults than in children in the dmPFC, dorsolateral PFC, right inferior parietal cortex, and right insula (see Fig. 6 and Supplementary Table 8 ). As can be seen in Fig. 6 , more negative prediction errors resulting in stronger PE-related activation. We also compared age groups in the IL condition, but no significant age differences were observed.
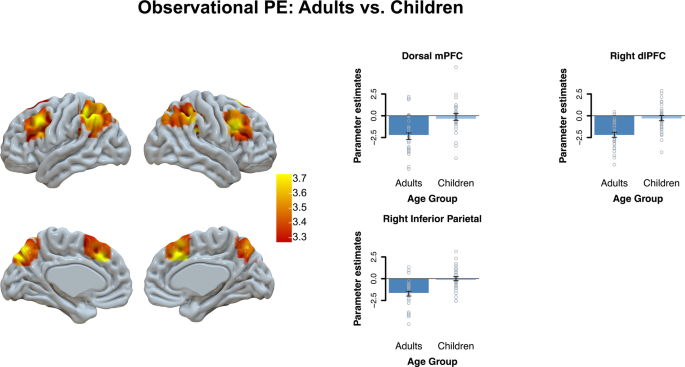
This figure displays age-group differences in observational PEs with a whole-brain t -test at qFDR < 0.05 with a primary voxel-wise threshold of p < 0.001. For visualization we extracted the beta-values from the whole-brain effects per age-group. Error bars reflect the SEM.
Brain-behavioral relations: relating learning performance to prediction error activation
Lastly, we investigated the extent to which age differences in learning behavior were associated with prediction-error-related brain activation. To achieve this, we extracted parameter estimates from clusters responsive to both individual and observational learning conditions (as shown in Fig. 5 ), as well as clusters indicating an age-by-condition interaction (i.e., TPJ; as shown in Fig. 4 ). We employed a linear regression analysis for each region of interest to examine the relationship between prediction error activation and learning accuracy in both individual learning and observational learning conditions. Additionally, we included interactions with age groups to assess whether this relationship varied across different age groups (further details can be found in the Methods section and Supplementary Table 9 ). To account for multiple linear regressions, we applied an FDR correction across all behavior-brain regression analyses.
Learning accuracy in the observational learning condition was found to be correlated with observation prediction-error activation in the dmPFC (β = −1.12, t = −3.79, p < 0.001; see Fig. 7 ) and the right dlPFC ( p = 0.037; see Supplementary Fig. 2a ), but not with the right inferior parietal cortex or the right insula ( p ’s > 0.596; see Supplementary Table 9 for further details). Only the brain-behavior relationship in the dmPFC (not dlPFC) remained significant after multiple comparison correction. This association between dmPFC activation and task performance was consistent across age groups (performance × age group: p = 0.558). Thus, improved learning in the observational learning condition was linked to a stronger prediction-error response in the dmPFC (see Fig. 7 ) for both children and adults. It is important to note that individual learning performance was included as a covariate in this regression analysis, indicating that this association appears to be specific to observational learning performance (Supplementary Table 9 ).
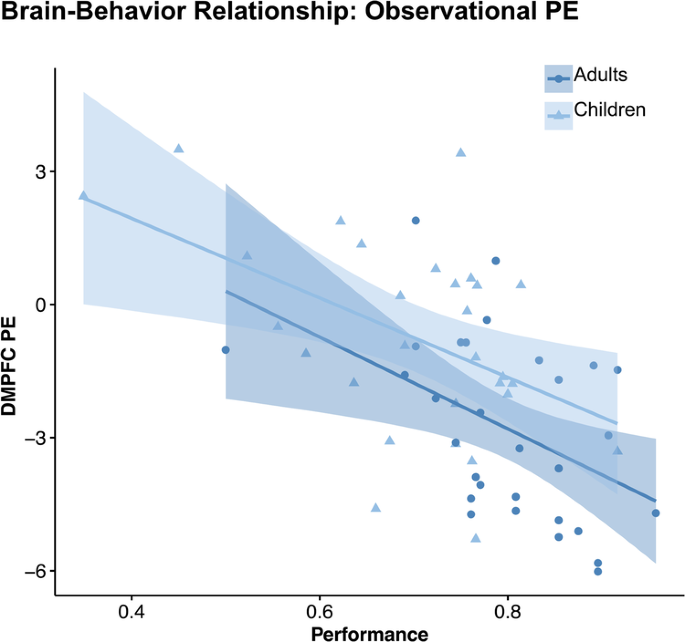
Scatter plot showing that more negative PE-related activation in the dmPFC was related to better performance during observational learning for both age groups. Shaded areas reflect 95% confidence intervals.
Learning accuracy in the individual learning condition, after controlling for observational learning performance, was associated with individual prediction error activation in the left parietal cortex (β = 0.98, p = .007; see Supplementary Fig. 2b ). However, this relationship did not survive multiple comparison correction. No significant relationships were observed between individual learning accuracy and activation in the vmPFC or striatal regions, nor in the right inferior parietal/TPJ ( p ’s > 0.076; Supplementary Table 9 ).
In this study we examined the behavioral and neural mechanisms underlying observational and individual reinforcement learning in 8–10-year-old children and young adults. Overall, we found that adults compared to children showed faster learning, better performance, and they were more optimally following the value of choice options across learning conditions (see Fig. 2 ). As expected, both age groups benefitted from observing other’s choices and outcomes. However, in contrast to our expectations, adults and children benefited to a similar degree from observing others behavior during learning and learning in observational versus individual conditions did not vary across development. To better understand the computational and neurobiological processes underlying learning we used reinforcement learning (RL) model in combination with fMRI. We observed that behavioral updating (as indicated by learning rates derived from the RL model fitting) did not differ across condition, and age, and both conditions and age groups showed higher learning rates from positive compared to negative feedback in both learning conditions. Choice behavior in children was generally less value-driven than in adults, and therefore showed more random, or potentially more exploratory, choice behavior. In addition, choice behavior was more value-driven in the observational than individual learning. Model-based parametric fMRI analyses complemented these behavioral insights. Observational and individual prediction errors were reflected in partly distinct (vmPFC, striatum), and (for adults) in partly overlapping brain regions, such as the temporal-parietal junction. Age-related differences were observed in observational prediction error coding in the dorsal medial prefrontal cortex, dorsal-lateral prefrontal cortex, parietal cortex, and insula cortex; regions closely related to cognitive control, social cognition, and social learning 24 , 30 . Moreover, only observational prediction error signals in the dmPFC correlated with observational compared to individual learning performance in both children and adults. This finding underlines the relevance of the dmPFC when learning from others.
Our behavioral results supported previously observed age-related differences in instrumental learning. In line with other developmental studies adults outperformed children in both individual and observational learning conditions. Children learned slower than adults 10 , 36 , 37 , 38 , 39 , 40 and their choice behavior was more stochastic and less value-driven 41 , 42 . This decrease in stochasticity across age is one of the most consistent findings observed in reinforcement learning 7 , 8 . Potentially, this may relate to an increase in maintaining sustained attention, or improved working memory, accelerated by a developmental improvement in cognitive control. Alternatively, children may be more stochastic because they are more explorative than adults. Theoretical work suggested that higher exploration provides children with more learning opportunities and could allow them to quickly discover changes in environments 43 . With the current task design, stochasticity and exploration cannot be easily distinguished. Whether age-related differences in stochasticity are valuable for exploration in social- and non-social learning environments, should be addressed in future studies. Interestingly, our behavioral findings indicate that both children and adults can learn from observing other’s outcomes: All ages increase their updating after positive and negative feedback during observational learning, and all age groups benefit in performance in observational compared to individual learning. The adults’ advantage in reinforcement learning may therefore be reflected in more value-driven choice behavior.
Our brain-based results complement and extend these behavioral findings. First, our results showed age-related differences in the temporal-parietal region. Unexpectedly, this region was sensitive to observational and individual prediction errors in adults (although differently signed), but not in children. In adults, prediction errors correlated positively with brain activation in the TPJ during individual learning (indicating greater positive prediction errors resulted in greater activation), and negatively with brain activation during observational learning (indicating greater negative prediction errors resulted in greater activation). This supports findings supports prior findings that the TPJ is associated with other’s and one’s own associations 44 , self-other distinctions 45 and that it can be linked in a valence-specific way to social and self-related prediction errors. However, in the current study prediction error related activation in the TPJ did not relate to age-related differences in behavioral performance. Future research will therefore need to replicate and extend these findings on the role of the TPJ in instrumental learning.
Second, our findings showed that observational prediction errors were related to neural activation in frontal regions including the dorsal medial PFC and dorsolateral PFC. and that that these relations were stronger for adults compared to children. Our finding that the medial prefrontal region is related to observational prediction error signaling in adults and children concurs with a broader framework, which links the ACC and dmPFC to a variety of social learning and decision-making skills 24 , 30 , such as outcome prediction error for confederate’s advice 31 , mentalizing 32 , 46 , and egocentric and allocentric outcomes of social decisions 24 , 47 . It has also been suggested that the role of different sub-regions of the mPFC may be more specific than previously recognized, and a more rostral part of the anterior cingulate cortex gyrus and dorsomedial prefrontal regions could be particularly specialized for observational prediction errors 5 , 23 , 24 , 28 , 48 , 49 . In addition, we observed that prediction-error activation in dmPFC related to performance in the observational learning condition in both children and adults (see Fig. 7 ). This may indicate an important role of the dmPFC for behavioral improvements in observational learning across age, although this does not necessarily relate to the observed age-related improvement in instrumental learning. Interestingly, prediction-error activation in the observational learning condition mostly increased when outcomes for others were worse than expected (see also 11 ). This finding may be interpreted in multiple ways. Possibly, the current setup led to an experienced competitiveness between the observer and observed individual, although it was explicitly instructed that participants were neither in competition nor dependent on the behavior of the observed other (e.g. 11 , 50 ,). Alternatively, seeing others lose may simply be a stronger learning signal. These directional effects were present across frontoparietal regions, indicating that these regions may be jointly involved in social comparison processes.
Finally, individual learning situations resulted in specific prediction-error related activation in the vmPFC, the left lateral PFC, the bilateral striatum, and bilateral parietal cortex (see Fig. 3 ) 10 , 35 , 51 . This supports previous findings and highlights there may be limited age-related differences per se in prediction error activation 27 , 40 . Whether adolescence is a period of heightened activation in reward-related learning environments as suggested by other studies 52 , 53 , 54 , is something that future research using samples with a wider, and more continuous, age range should disentangle.
Although our results confirmed several age-related findings from previous studies on individual and observational learning 9 , 10 , some of the results were unexpected. For instance, in contrast to previous findings on observational learning 10 , we did not find age-related performance differences between learning conditions. It seems viable that differences to results of previous studies arise because of the administered paradigm to make the task amenable for fMRI. For instance, in the previous EEG-studies the task was more complex in terms of learning conditions (i.e., another third condition was included) and more difficult as the timing was faster (i.e., no jitters were included). Moreover, when administering a social and non-social learning conditions an important question is to what extent findings are specific to social learning or reflective of more general learning processes 48 . In our experimental setup children and adults learn indirectly from others. To create a social learning setting, we informed participants that they could learn from another participant they met before the experiment, and they observe the other’s photo and name during the experiment. Varying the age of the other participant, in a previous study 9 , using the same social learning setting and task, we demonstrated that similarity in age (same-aged child vs. young adult) between the observed player and the observer influenced both behavioral and neural responses in 8-10 year olds. Building on these findings and because participants judged others as being similar to themselves (see Methods) we argue that it is likely that the participants perceived the observational learning conditions as social learning conditions rather than conditions in which they simply received only more information. Based on these considerations we would like to a few recommendations for future developmental studies on observational learning:
First, future studies should take the social context into account in which children learn “indirect” observational learning (e.g., observing others’ choices and outcomes in absence of the other player) in comparison to direct observational learning (e.g., “directly” observing others’ choices and outcomes in presence of the other player). Secondly, it is important to compare active individual learning to observational learning, where participants learn purely passively from observed information (i.e., without the ability to evaluate this information immediately after the observational phase on a trial-by-trial basis, as was done in this study). This allows for a more direct comparison of both learning processes based on a similar amount of information, which is not achievable in the current task design. Finally, we observed that our age groups differed in fluid intelligence. Children showed higher age-normed intelligence scores than adults, which may have influenced age-related differences in performance and neural activation. Although controlling for intelligence in our behavioral analyses did not change our main findings, future studies may address to what extent task difficulties and intelligence relate to age-related differences in observational learning. For instance, learning condition differences associated with prediction error activation might be related to greater task difficulty and higher cognitive load when learning from own outcomes. This could be linked to higher neural activation as task difficulty increases 55 , 56 , 57 .
To summarize, our findings show that learning from the outcomes of others, particularly when outcomes were worse than expected, was related to neural activation in the dmPFC, dlPFC and temporal-parietal activation which was more pronounced in adults than children. Here, only the dmPFC related to performance in observational learning for both children and adults. These findings confirm and extend the functional role of the medial PFC to a social observational learning context and specify its functional relevance for social learning for children and adults.
Participants
Thirty 18–20-year-old adults and 30 8–10-year-old children participated in the study. Data of one child was excluded due to inability to complete the task. For occasional occurring head motion (Framewise displacements >0.5) volumes with motion were flagged and were not included in regressors of interest but instead modeled by nuisance regressors (i.e., censored), number of censored volumes regressors varied between 2–12 (<10% of volumes). The final sample consisted of 30 adults (Age Mean (SD) = 19.45 (0.86); 16 female) and 29 children (Age Mean (SD) = 9.71 (0.89); 18 female). All participants were right-handed, had normal or corrected-to-normal vision, were screened for MRI contra-indications, and had no neurological or psychological disorders. Prior to the experiment we obtained informed written consent from the participants and both parents (in case of children). The study was approved by the Ethics Committee of the Leiden University Medical Center (LUMC). All anatomical MRI scans were reviewed and cleared by a radiologist from the radiology department of the LUMC. No anomalous findings were reported.
Subjects participated in one experimental session in which we assessed psychometric covariate measures not specific to the current study, and observational learning performance inside the MR scanner. Participants were recruited through local advertisements and received a compensation. Participants’ intelligence (IQ) was estimated with the subsets ‘similarities’ and ‘block design’ of the Wechsler Intelligence Scale for Children, third edition (WISC-III 58 ). For both age groups, estimated IQs were in the normal to high range (Adults: Mean IQ (SD) = 106.67 (8.82), Range = 87.5–122.5; Children: Mean IQ (SD) = 112.76 (11.96), Range = 87.5–132.5). IQ did differ between age groups: Children showed higher IQ scores than adults ( F (1, 57) = 4.952, p = 0.03, η p 2 = 0.08). IQ is controlled for in all behavioral analyses.
Experimental design
We used a probabilistic reward-based observational learning paradigm 9 , 11 (see Fig. 1 ; controlled by PsychToolBox-3 59 ). Participants were asked to choose one out of two abstract stimuli 60 . One stimulus was associated with a high probability of receiving reward (80% gains, 20% losses) and one associated with a low reward probability (20% gains, 80% losses). Before participants could choose, they observed an age- and gender-matched peer (who they met before starting the task) choosing between the same two abstract stimuli. Participants were told that the other player had already performed the task and that they could observe the recorded choices. In order to assess the credibility of our social manipulation we assessed participants perception of the other player at the end of the experiment. Almost all participants (93%) reported that they paid attention to the other player and on a 7-point Likert-scale (see Supplementary Fig. 3 ), that watching the other helped them for learning and that they judged the other as highly similar to themselves, reliable and believable ( p ’s < 0.001; one-sampled Wilcoxon tests for non-parametric data against 3.5). Age groups did not differ in their rating (unpaired Wilcoxon tests for non-parametric data; p ’s > 0.06). However, unbeknownst to participants the observed choices were computer generated using a RL model (see Supplementary Fig. 4 ). The computer-controlled behavior of the model players was associated with the objective percentage of probabilistic positive or negative outcomes associated with each of the stimuli (see supplementary files 9 , 10 ). The amount of observable information of the other player was manipulated across two learning conditions: Observational learning (observing both, the other player’s actions and outcomes; short OL) and individual learning (observing neither actions nor outcomes of the other player; short IL). In each condition, the trials followed the general structure of an observational phase that was followed by an action phase. That is, in the OL condition the participant would be first presented with a fixation cross for a variable amount of time per trial (not shown in Fig. 1 ). This jitter varied exponentially from 1 s to 8 s and was followed by a picture of the other person (i.e., precue of 1 s) and the presentation of a stimulus pair. By pressing a button with the ring finger of the right hand the other’s choices were revealed. Responses had to be given within a 2 s window and indicated by a white selection frame (i.e., 2 s – response time; see Fig. 1 ), which was followed by a 1 s outcome display representing the outcome of the other’s choice. Then, the action phase started with a fixation cross (i.e., jitter of 1–8 s) and then participants viewed his/her own picture (1 s), after which the same stimulus pair was presented. Participants could choose either the left or the right stimulus by pressing a button with the index or middle finger of the right hand. Again, responses had to be given within a 2 s window, which was followed by a 1 s outcome display. If no response was given within 2 s, in either the observational or the action phase of any condition the words “too slow” were presented on the screen. This happened rarely for adults ( M trials = 2.41, SD = 2.27) and children ( M trials = 6.89, SD = 5.54).
The IL condition followed the same timing and structure as the OL condition and participants also pressed a button when stimuli were presented in the observational phase, yet no choice (i.e., in this case both possible choices were surrounded by a white selection frame) or outcome information of the other were presented. Each condition was associated with two unique stimulus pairs per run and the order of conditions was mixed. In total, every pair was presented for eight trials per run (resulting in 32 trials and four abstract stimulus pairs per run). The four stimulus pairs (two per condition) per run were presented intermixed. Participants played in total three runs of approximately nine minutes each with 48 trials per learning condition (resulting in 96 trials with 16 abstract stimulus pairs in total). For five participants one run was excluded in further analyses because of high occasional motion (>10% of censored volumes per run). Participants were instructed to earn as many points as possible (as indicated by receiving a positive outcome signal) but were also informed that it was not possible to gain points on every trial, clarifying the probabilistic nature of the task. Before performing the task in the MRI, participants practiced the task for one run length.
Reinforcement learning models
Learning action-outcome-contingencies can be computationally captured using RL models 18 . During RL learning the discrepancy between expected outcome on trial (t), Q a (t), and the actually received outcome on trial (t), r(t), is called prediction error (PE):
If outcomes are better (worse) than expected, the model will generate a positive (negative) PE, which is used to increase (decrease) the predicted value, Q a ( t ), associated with the chosen option a in the current trial t . Positive and negative outcomes received in each trial are used to update the predicted values of both options a (Eq. ( 2 )) and b (Eq. ( 3 )) 61 , 62 :
Thus, for the value of the unchosen option b (Eq. ( 3 )) the counterfactural outcome is taken into account. The impact of the PE’s on forming new expectations is scaled by the learning rate α . A high learning rate (~1) indicates that a new experience (i.e., PE) has a stronger impact on future predictions whereas a low learning rate (~0) means that a PE only weakly influences the expected value and thereby choice behavior. Based on previous developmental studies, we included two independent learning rates for positive (α pos ) and negative outcomes (α neg ) to describe the learning behavior in the individual condition across development 40 , 63 .
For the OL condition, we extended the RL-algorithm used in the IL condition to describe social influences during learning: Learning from other’s choices and outcomes (see supplementary files for model comparisons and model recovery) was best captured by using a dual-update model, one updating phase for the observational (Eq. ( 4 )) and one for action stage (Eq. ( 5 )) as described in 64 .
Similarly, to the RL models used in the individual condition we included two independent learning rates (α pos , α neg ) in the OL condition. A higher learning rate thus means a quicker updating of expected values and thereby faster learning. For both stages and conditions, the probability of choosing option a from a stimulus pair (ab) was computed using a softmax function (Eq. ( 6 )) 34 :
The probability of selecting option a is influenced by the expected value Q of option a in trial t divided by the sum of the expected values of all possible options ( a and b ). The β parameter in this equation reflects the sensitivity of the subject to differences in expected value. Here, a lower β parameter indicates more stochastic responding.
For model comparisons we evaluated a set of alternative RL-algorithms (i.e., baseline RL-model with one learning rate (α), model with separate learning rates for positive (α pos ) and negative outcomes and (α neg ) 40 , 65 and a model with separate learning rates for observational and action stage; see supplementary files, Supplementary Table 10 and Supplementary Fig. 5 ). All learning rates (α, α pos and α neg ) and the noise parameter ( β ) per condition were individually estimated by fitting the model predictions to participants’ choices (see supplementary files for further details on the model fitting procedure). The β parameters were fit with constraints between [0 5]. The α parameters were constrained between [0 1]. For model selection purposes, we computed the Bayesian information criterion (BIC) across all subjects for the different models, where lower BIC values indicate better fit (see ref. 66 ). For both learning conditions (i.e., IL and OL) the best fitting model across all subjects included two independent learning rates (α pos , α neg ) and the noise parameter ( β ) (see supplementary files for further details and S2 for an overview of all model comparisons and parameter estimates per model). For the model-based fMRI analyses we used the median parameter estimates per age group (Fig. 2 ) of the best fitting model per learning condition to calculate trial-by-trial PEs 11 , 62 , 67 , 68 , 69 . In imaging analyses PEs were scaled and mean-centered.
To explore the validity of the RL models and the model selection procedure, we performed 1) model and 2) parameter recovery analyses (see supplementary files and Supplementary Fig. 6 for further details). As part of quality control, we further performed simulations using the individual parameter estimates for each subject for the best fitting model. The simulations indicated that the best fitting model per condition was able to capture learning on a trial-by-trial level in each age group (see supplementary files for further details and Supplementary Fig. 7 ).
Behavioral data analysis
Choice behavior was analyzed using a mixed-effects generalized linear model as implemented in the lme4 package in R 70 . Accuracy (proportion of optimal choice) was averaged across runs and modeled using the between-subjects predictor age group and the within-subjects predictors learning condition (IL, OL) and trial number (1:8).
Mixed effects model formula:
We treated the between-subjects predictor age group as a fixed effects factor, whereas all within-subjects’ predictors of interest were treated as fixed and random effects at the individual subject level. We additionally included intelligence as predictor to control for age differences in intelligence. The categorical predictors were contrast-coded, the continuous predictor trial was mean-centered. Regression weights (beta values), z-values and corresponding p-values are reported (see Supplementary Table 1 ).
The learning rates and inverse temperature of the best fitting models per conditions across age were significantly non-normally distributed ( p ’s < 0.05). Thus, we used robust mixed effects model to test for age and condition effects on learning rates and inverse temperature, respectively as implemented in the robustlmm package (see Supplementary Tables 2 and 3 ). Note that due to convergence problems we use a simpler fixed and random effect structure in these models (see Eq. ( 8 )).
Mixed effects model formula (example):
Finally, we assessed whether behavior per condition (i.e., accuracy) related to condition-specific prediction-error activation. We used multiple linear regression models predicting PE activation by proportion of optimal choice (accuracy), age, and their interaction, controlling for learning in the other condition, and intelligence. The categorical predictors were contrast-coded, the continuous predictor trial was mean-centered. Regression weights (beta values), z-values and corresponding p-values are reported (see supplementary files and Supplementary Table 9 ). P -values in brain-behavioral analyses were considered significant when evaluated against an FDR-corrected threshold that included all effects across the multiple ROIs (8) that were examined.
Multiple linear models’ formula (example):
MRI data acquisition
MRI data were acquired with a standard whole-head coil using a 3-T Philips Achieva scanner. T2*-weighted echoplanar images (EPIs) were obtained during three functional runs, in which the first two volumes were discarded to allow for equilibration of T1 saturation effects. Volumes covered the whole brain (38 slices; 2.75 mm slice thickness; ascending acquisition) and were acquired every 2200 ms (TE = 30 ms). A high resolution T1-weighted anatomical scan was included at the end of the imaging protocol (140 slices; TR = 9.76 ms; TE = 4.59 ms; flip angle = 8°; FOV = 224 × 177.33 × 168 mm; in-plane resolution = 0.875 × 0.875 mm; slice thickness = 2 mm). Visual stimuli were projected onto a screen that was visible for participants via a mirror attached to the head coil. Before the experiment, children were trained with a mock-scanning procedure. All participants were reminded during the session not to move during scanning, and head motion was restricted by using foam padding.
fMRI preprocessing and model specification
Data preprocessing and analysis were conducted using SPM8 (Welcome Department of Cognitive Neurology, London). Images were corrected for differences in timing of slice acquisition, followed by rigid body motion correction. The T1 structural image was co-registered to the functional images and segmented according to gray matter, white matter, and cerebrospinal fluid. Functional images were then spatially normalized using the normalization parameters obtained from the segmentation procedure. The normalization algorithm used a 12-parameter affine transformation together with a nonlinear transformation involving cosine basis functions. During normalization the data was re-sampled to 3-mm cubic voxels. Templates were based on the MNI305 stereotaxic space 71 . Functional volumes were smoothed with a 6-mm full-width at half maximum isotropic Gaussian kernel. Statistical analyses were performed on individual subjects’ data using the General Linear Model (GLM).
To investigate the neural responses to own and other’s outcomes and PE’s, we modeled in separate regressors the onset of the choice stimuli with the reaction time as the duration in the observational and the action phase for both conditions. Choice value, derived from the reinforcement learning model, was included as a parametric modulator of the choice regressor in the observational (OL), and of the choice regressor in the action phase (OL, IL). The onset of the outcome was modeled with a stick function. Separate outcome regressors were created for own and other’s outcomes in the OL condition, and for own and no-outcomes in the IL condition. In addition, three outcome regressors (own and other’s outcomes in the OL, and own outcomes in the IL condition) included a parametric modulation of trial-wise PE’s derived from the reinforcement learning model. Trials in which participants did not respond on time and censored motion trials were modeled separately as regressors of no interest. Finally, 6 head-motion parameters were included as nuisance regressors.
Our main analyses include the comparison between own outcomes in the IL (action phase), and other’s outcomes in the OL condition (observational phase, see Fig. 1 ). For completeness we include whole-brain maps of the non-modulated feedback event in Supplementary Fig. 8 . As a control analysis to investigate whether substantial differences would arise when considering one’s own outcomes in the IL and OL conditions, we compared PE-related activation for self-outcomes in both conditions (further details can be found in the supplementary files and Supplementary Fig. 9 ). It is important to note that PE activation patterns largely overlapped, and no significant differences were observed. We chose to use data from the IL condition exclusively to maintain a similar number of trials entered into the analysis. Also, the action phase in the IL condition is less affected by the information from the observational phase.
Unless stated otherwise, whole-brain results comparing learning conditions were considered significant if they exceeded an FWE cluster-corrected threshold of p < 0.05, with an initial threshold of p < 0.001. Age-related differences were tested with an FDR cluster-corrected threshold of p < 0.05, with an initial threshold of p < 0.001. We used the MarsBaR toolbox 72 for SPM8 to extract beta-values from cluster of activation observed in our contrasts of interest, which were used in subsequent correlations with performance and parameter estimates.
Reporting summary
Further information on research design is available in the Nature Research Reporting Summary linked to this article.
Data availability
The data that support the findings of this study can be found in the Leiden Repository ( https://doi.org/10.34894/W4WMPZ ).
Code availability
All relevant R codes can be found in the Leiden Repository ( https://doi.org/10.34894/W4WMPZ ).
Albert, D., Chein, J. & Steinberg, L. The Teenage Brain: Peer Influences on Adolescent Decision Making. Curr. Dir. Psychol. Sci. 22 , 114–120 (2013).
Article PubMed PubMed Central Google Scholar
Blakemore, S.-J. The social brain in adolescence. Nat. Rev. Neurosci. 9 , 267–277 (2008).
Article CAS PubMed Google Scholar
Chein, J., Albert, D., O’Brien, L., Uckert, K. & Steinberg, L. Peers increase adolescent risk taking by enhancing activity in the brain’s reward circuitry. Dev. Sci. 14 , F1–F10 (2011).
Steinberg, L. et al. Age differences in sensation seeking and impulsivity as indexed by behavior and self-report: Evidence for a dual systems model. Dev. Psychol. 44 , 1764–1778 (2008).
Article PubMed Google Scholar
Olsson, A., Knapska, E. & Lindström, B. The neural and computational systems of social learning. Nat. Rev. Neurosci. 21 , 197–212 (2020).
Towner, A. V., Kock, A. A., Stopforth, C., Hurwitz, D. & Elwen, S. H. Direct observation of killer whales preying on white sharks and evidence of a flight response. Ecology 104 , e3875 (2023).
Topel, S. et al. Expecting the unexpected: a review of learning under uncertainty across development. Cogn. Affect. Behav. Neurosci. 23 , 718–738 (2023).
Nussenbaum, K. & Hartley, C. A. Reinforcement learning across development: What insights can we draw from a decade of research? Dev. Cogn. Neurosci. 40 , 100733 (2019).
Rodriguez Buritica, J. M., Eppinger, B., Schuck, N. W., Heekeren, H. R. & Li, S.-C. Electrophysiological correlates of observational learning in children. Dev. Sci. 19 , 699–709 (2016).
Rodriguez Buritica, J. M., Heekeren, H. R., Li, S.-C. & Eppinger, B. Developmental differences in the neural dynamics of observational learning. Neuropsychologia 119 , 12–23 (2018).
Burke, C. J., Tobler, P. N., Baddeley, M. & Schultz, W. Neural mechanisms of observational learning. Proc. Natl Acad. Sci. 107 , 14431–14436 (2010).
Article CAS PubMed PubMed Central ADS Google Scholar
Hill, M. R., Boorman, E. D. & Fried, I. Observational learning computations in neurons of the human anterior cingulate cortex. Nat. Commun. 7 , 12722 (2016).
Aquino, T. G. et al. Value-Related Neuronal Responses in the Human Amygdala during Observational Learning. J. Neurosci. 40 , 4761–4772 (2020).
Article CAS PubMed PubMed Central Google Scholar
Charpentier, C. J., Iigaya, K. & O’Doherty, J. P. A Neuro-computational Account of Arbitration between Choice Imitation and Goal Emulation during Human Observational Learning. Neuron 106 , 687–699.e7 (2020).
Hackel, L. M. & Amodio, D. M. Computational neuroscience approaches to social cognition. Curr. Opin. Psychol. 24 , 92–97 (2018).
Lockwood, P. L. & Klein-Flügge, M. C. Computational modelling of social cognition and behaviour—a reinforcement learning primer. Soc. Cogn. Affect. Neurosci . nsaa040, https://doi.org/10.1093/scan/nsaa040 (2020).
Zhang, L. & Gläscher, J. A brain network supporting social influences in human decision-making. Sci. Adv. 6 , eabb4159 (2020).
Article PubMed PubMed Central ADS Google Scholar
Sutton, R. S. & Barto, A. G. Reinforcement learning: an introduction (MIT Press, 1998).
Schultz, W. Dopamine reward prediction-error signalling: a two-component response. Nat. Rev. Neurosci. 17 , 183–195 (2016).
Schultz, W. & Dickinson, A. Neuronal Coding of Prediction Errors. Annu. Rev. Neurosci. 23 , 473–500 (2000).
Diederen, K. M. J., Spencer, T., Vestergaard, M. D., Fletcher, P. C. & Schultz, W. Adaptive Prediction Error Coding in the Human Midbrain and Striatum Facilitates Behavioral Adaptation and Learning Efficiency. Neuron 90 , 1127–1138 (2016).
Schultz, W. Reward prediction error. Curr. Biol. 27 , R369–R371 (2017).
Joiner, J., Piva, M., Turrin, C. & Chang, S. W. Social learning through prediction error in the brain. NPJ Sci. Learn. 2 , 1–9 (2017).
Article Google Scholar
Apps, M. A. J. & Sallet, J. Social Learning in the Medial Prefrontal Cortex. Trends Cogn. Sci. 21 , 151–152 (2017).
Sul, J. H., Kim, H., Huh, N., Lee, D. & Jung, M. W. Distinct Roles of Rodent Orbitofrontal and Medial Prefrontal Cortex in Decision Making. Neuron 66 , 449–460 (2010).
Lockwood, P. L., Apps, M. A. J., Valton, V., Viding, E. & Roiser, J. P. Neurocomputational mechanisms of prosocial learning and links to empathy. Proc. Natl Acad. Sci. 113 , 9763–9768 (2016).
Westhoff, B., Blankenstein, N. E., Schreuders, E., Crone, E. A. & van Duijvenvoorde, A. C. K. Increased Ventromedial Prefrontal Cortex Activity in Adolescence Benefits Prosocial Reinforcement Learning. Dev. Cogn. Neurosci. 52 , 101018 (2021).
Collette, S., Pauli, W. M., Bossaerts, P. & O’Doherty, J. Neural computations underlying inverse reinforcement learning in the human brain. eLife 6 , e29718 (2017).
Yoshida, K., Saito, N., Iriki, A. & Isoda, M. Social error monitoring in macaque frontal cortex. Nat. Neurosci. 15 , 1307–1312 (2012).
Apps, M. A. J., Rushworth, M. F. S. & Chang, S. W. C. The Anterior Cingulate Gyrus and Social Cognition: Tracking the Motivation of Others. Neuron 90 , 692–707 (2016).
Behrens, T. E. J., Hunt, L. T. & Rushworth, M. F. S. The Computation of Social Behavior. Science 324 , 1160–1164 (2009).
Article CAS PubMed ADS Google Scholar
Hampton, A. N., Bossaerts, P. & O’Doherty, J. P. Neural correlates of mentalizing-related computations during strategic interactions in humans. Proc. Natl Acad. Sci. 105 , 6741–6746 (2008).
Achterberg, M. et al. The neural and behavioral correlates of social evaluation in childhood. Dev. Cogn. Neurosci. 24 , 107–117 (2017).
O’Doherty, J. P. Reward representations and reward-related learning in the human brain: insights from neuroimaging. Curr. Opin. Neurobiol. 14 , 769–776 (2004).
Burnside, R., Fischer, A. G. & Ullsperger, M. The feedback‐related negativity indexes prediction error in active but not observational learning. Psychophysiology , e13389, https://doi.org/10.1111/psyp.13389 (2019).
van Duijvenvoorde, A. C. K., Zanolie, K., Rombouts, S. A. R. B., Raijmakers, M. E. J. & Crone, E. A. Evaluating the Negative or Valuing the Positive? Neural Mechanisms Supporting Feedback-Based Learning across Development. J. Neurosci. 28 , 9495–9503 (2008).
Eppinger, B., Mock, B. & Kray, J. Developmental differences in learning and error processing: Evidence from ERPs. Psychophysiology 46 , 1043–1053 (2009).
Crone, E. A., Jennings, J. R. & Van der Molen, M. W. Developmental Change in Feedback Processing as Reflected by Phasic Heart Rate Changes. Dev. Psychol. 40 , 1228–1238 (2004).
Hämmerer, D., Li, S.-C., Müller, V. & Lindenberger, U. Life Span Differences in Electrophysiological Correlates of Monitoring Gains and Losses during Probabilistic Reinforcement Learning. J. Cogn. Neurosci. 23 , 579–592 (2011).
van den Bos, W., Cohen, M. X., Kahnt, T. & Crone, E. A. Striatum–Medial Prefrontal Cortex Connectivity Predicts Developmental Changes in Reinforcement Learning. Cereb. Cortex 22 , 1247–1255 (2012).
Decker, J. H., Lourenco, F. S., Doll, B. B. & Hartley, C. A. Experiential reward learning outweighs instruction prior to adulthood. Cogn. Affect. Behav. Neurosci. 15 , 310–320 (2015).
Thompson-Schill, S. L., Ramscar, M. & Chrysikou, E. G. Cognition Without Control: When a Little Frontal Lobe Goes a Long Way. Curr. Dir. Psychol. Sci. 18 , 259–263 (2009).
Gopnik, A. Childhood as a solution to explore–exploit tensions. Philos. Trans. R. Soc. B Biol. Sci. 375 , 20190502 (2020).
Lockwood, P. L. et al. Neural mechanisms for learning self and other ownership. Nat. Commun. 9 , 4747 (2018).
Decety, J. & Lamm, C. The Role of the Right Temporoparietal Junction in Social Interaction: How Low-Level Computational Processes Contribute to Meta-Cognition. Neuroscientist 13 , 580–593 (2007).
Frith, C. D. & Frith, U. The Neural Basis of Mentalizing. Neuron 50 , 531–534 (2006).
Lee, D. & Seo, H. Neural Basis of Strategic Decision Making. Trends Neurosci. 39 , 40–48 (2016).
Lockwood, P. L., Apps, M. A., Roiser, J. P. & Viding, E. Encoding of Vicarious Reward Prediction in Anterior Cingulate Cortex and Relationship with Trait Empathy. J. Neurosci. 35 , 13720–13727 (2015).
Dunne, S. & O’Doherty, J. P. Insights from the application of computational neuroimaging to social neuroscience. Curr. Opin. Neurobiol. 23 , 387–392 (2013).
Fliessbach, K. et al. Social Comparison Affects Reward-Related Brain Activity in the Human Ventral Striatum. Science 318 , 1305–1308 (2007).
Bellebaum, C., Kobza, S., Thiele, S. & Daum, I. It Was Not M. Y. Fault: Event-Related Brain Potentials in Active and Observational Learning from Feedback. Cereb. Cortex 20 , 2874–2883 (2010).
Davidow, J. Y., Foerde, K., Galván, A. & Shohamy, D. An Upside to Reward Sensitivity: The Hippocampus Supports Enhanced Reinforcement Learning in Adolescence. Neuron 92 , 93–99 (2016).
Cohen, J. R. et al. A unique adolescent response to reward prediction errors. Nat. Neurosci. 13 , 669–671 (2010).
Galvan, A. et al. Earlier Development of the Accumbens Relative to Orbitofrontal Cortex Might Underlie Risk-Taking Behavior in Adolescents. J. Neurosci. 26 , 6885–6892 (2006).
Gould, R. L., Brown, R. G., Owen, A. M., ffytche, D. H. & Howard, R. J. FMRI BOLD response to increasing task difficulty during successful paired associates learning. NeuroImage 20 , 1006–1019 (2003).
Lewandowska, M., Piatkowska-Janko, E., Bogorodzki, P., Wolak, T. & Szelag, E. Changes in fMRI BOLD response to increasing and decreasing task difficulty during auditory perception of temporal order. Neurobiol. Learn. Mem. 94 , 382–391 (2010).
Sunaert, S., Van Hecke, P., Marchal, G. & Orban, G. A. Attention to Speed of Motion, Speed Discrimination, and Task Difficulty: An fMRI Study. NeuroImage 11 , 612–623 (2000).
Wechsler, D. WISC-3: Wechsler intelligence scale for children: manual (Psychological Corporation, 1991).
Brainard, D. H. The Psychophysics Toolbox. Spat. Vis. 10 , 433–436 (1997).
Windell, O. Vector Snowflake Application. Retrieved September 7, 2012, from http://www.evilmadscientist.com/2008/vector-snowflake-application/ (2008).
Hampton, A. N. & O’Doherty, J. P. Decoding the neural substrates of reward-related decision making with functional MRI. Proc. Natl Acad. Sci. 104 , 1377–1382 (2007).
Gläscher, J. P. & O’Doherty, J. P. Model‐based approaches to neuroimaging: combining reinforcement learning theory with fMRI data. WIREs Cogn. Sci. 1 , 501–510 (2010).
Kahnt, T. et al. Dorsal Striatal–midbrain Connectivity in Humans Predicts How Reinforcements Are Used to Guide Decisions. J. Cogn. Neurosci. 21 , 1332–1345 (2009).
Apps, M. A. J., Lesage, E. & Ramnani, N. Vicarious Reinforcement Learning Signals When Instructing Others. J. Neurosci. 35 , 2904–2913 (2015).
Palminteri, S., Kilford, E. J., Coricelli, G. & Blakemore, S.-J. The Computational Development of Reinforcement Learning during Adolescence. PLOS Comput. Biol. 12 , e1004953 (2016).
Farrell, S. & Lewandowsky, S. Computational modeling of cognition and behavior , (Cambridge University Press, 2018).
Gläscher, J., Daw, N., Dayan, P. & O’Doherty, J. P. States versus Rewards: Dissociable Neural Prediction Error Signals Underlying Model-Based and Model-Free Reinforcement Learning. Neuron 66 , 585–595 (2010).
O’Doherty, J. P., Hampton, A. & Kim, H. Model-Based fMRI and Its Application to Reward Learning and Decision Making. Ann. N. Y. Acad. Sci. 1104 , 35–53 (2007).
Article PubMed ADS Google Scholar
Wilson, R. C. & Niv, Y. Is Model Fitting Necessary for Model-Based fMRI? PLOS Comput. Biol. 11 , e1004237 (2015).
Bates, D., Mächler, M., Bolker, B. & Walker, S. Fitting Linear Mixed-Effects Models Using lme4. J. Stat. Softw. 67 , 1–48 (2015).
Cocosco, C. A., Kollokian, V., Kwan, R. K.-S., Pike, G. B. & Evans, A. C. Brainweb: Online interface to a 3D MRI simulated brain database (Citeseer, 1997).
Brett, M., Anton, J., Valabregue, R. & Poline, J. Region of interest analysis using an SPM toolbox. NeuroImage 16 , 497 (2002).
Google Scholar
Download references
Acknowledgements
We thank Chris Burke and Geert-Jan Will for valuable input; Iris Koele, Laura Steinmann, and Sibel Altikulaç for help with data collection and project management; Stella Berboth for help with manuscript preparation and Rasmus Bruckner for help with the computational modeling. This work is supported by the Social Resilience and Security program (Leiden University) and by the Netherlands Organization for Scientific Research (NWO) (Open Research Area 464-15-176) awarded to A.C.K.v.D.; by a PhD DAAD Stipend and CIC (Center for International Cooperation FU Berlin) awarded to JRB and the German Research Foundation (DFG) (SFB 940/2 B7) awarded to B.E.
Open Access funding enabled and organized by Projekt DEAL.
Author information
Julia M. Rodriguez Buritica
Present address: Department of Education and Psychology, Freie Universität Berlin, Berlin, Germany
Authors and Affiliations
Department of Psychology, University of Greifswald, Greifswald, Germany
Julia M. Rodriguez Buritica, Ben Eppinger & Hauke R. Heekeren
Berlin School of Mind and Brain & Department of Psychology, Humboldt University of Berlin, Berlin, Germany
Department of Education and Psychology, Freie Universität Berlin, Berlin, Germany
Ben Eppinger
Department of Psychology, Concordia University, Montreal, Canada
Department of Psychology, Technische Universität Dresden, Dresden, Germany
Executive University Board, Universität Hamburg, Hamburg, Germany
Hauke R. Heekeren
Department of Psychology, Education and Child Studies, Erasmus University Rotterdam, Rotterdam, Netherlands
Eveline A. Crone
Institute of Psychology, Leiden University, Leiden, The Netherlands
Eveline A. Crone & Anna C. K. van Duijvenvoorde
Leiden Institute for Brain and Cognition, Leiden, The Netherlands
You can also search for this author in PubMed Google Scholar
Contributions
J.M.R.B., B.E. and A.C.K.vD. designed the research. J.M.R.B., and A.C.K. vD. performed the experiments and J.M.R.B., and A.C.K.vD. analyzed data. J.M.R.B., B.E., A.C.K.v.D., H.R.H. and E.A.C. interpreted findings and wrote the manuscript.
Corresponding authors
Correspondence to Julia M. Rodriguez Buritica or Anna C. K. van Duijvenvoorde .
Ethics declarations
Competing interests.
The authors declare no competing interests.
Additional information
Publisher’s note Springer Nature remains neutral with regard to jurisdictional claims in published maps and institutional affiliations.
Supplementary information
Supplementary files, reporting summary, rights and permissions.
Open Access This article is licensed under a Creative Commons Attribution 4.0 International License, which permits use, sharing, adaptation, distribution and reproduction in any medium or format, as long as you give appropriate credit to the original author(s) and the source, provide a link to the Creative Commons licence, and indicate if changes were made. The images or other third party material in this article are included in the article’s Creative Commons licence, unless indicated otherwise in a credit line to the material. If material is not included in the article’s Creative Commons licence and your intended use is not permitted by statutory regulation or exceeds the permitted use, you will need to obtain permission directly from the copyright holder. To view a copy of this licence, visit http://creativecommons.org/licenses/by/4.0/ .
Reprints and permissions
About this article
Cite this article.
Rodriguez Buritica, J.M., Eppinger, B., Heekeren, H.R. et al. Observational reinforcement learning in children and young adults. npj Sci. Learn. 9 , 18 (2024). https://doi.org/10.1038/s41539-024-00227-9
Download citation
Received : 15 June 2022
Accepted : 21 February 2024
Published : 13 March 2024
DOI : https://doi.org/10.1038/s41539-024-00227-9
Share this article
Anyone you share the following link with will be able to read this content:
Sorry, a shareable link is not currently available for this article.
Provided by the Springer Nature SharedIt content-sharing initiative
Quick links
- Explore articles by subject
- Guide to authors
- Editorial policies
Sign up for the Nature Briefing newsletter — what matters in science, free to your inbox daily.

- Bipolar Disorder
- Therapy Center
- When To See a Therapist
- Types of Therapy
- Best Online Therapy
- Best Couples Therapy
- Best Family Therapy
- Managing Stress
- Sleep and Dreaming
- Understanding Emotions
- Self-Improvement
- Healthy Relationships
- Student Resources
- Personality Types
- Guided Meditations
- Verywell Mind Insights
- 2023 Verywell Mind 25
- Mental Health in the Classroom
- Editorial Process
- Meet Our Review Board
- Crisis Support
How Observational Learning Affects Behavior
Kendra Cherry, MS, is a psychosocial rehabilitation specialist, psychology educator, and author of the "Everything Psychology Book."
:max_bytes(150000):strip_icc():format(webp)/IMG_9791-89504ab694d54b66bbd72cb84ffb860e.jpg)
- Influential Factors
- Pros and Cons
Observational learning describes the process of learning by watching others, retaining the information, and then later replicating the behaviors that were observed.
There are a number of learning theories, such as classical conditioning and operant conditioning , that emphasize how direct experience, reinforcement, or punishment can lead to learning. However, a great deal of learning happens indirectly.
For example, think about how a child may watch adults waving at one another and then imitates these actions later on. A tremendous amount of learning happens through this process. In psychology , this is referred to as observational learning.
Observational learning is sometimes called shaping, modeling, and vicarious reinforcement. While it can take place at any point in life, it tends to be the most common during childhood.
It also plays an important role in the socialization process. Children learn how to behave and respond to others by observing how their parent(s) and/or caregivers interact with other people.
Verywell / Brianna Gilmartin
History of Observational Learning
Psychologist Albert Bandura is the researcher most often associated with learning through observation. He and others have demonstrated that we are naturally inclined to engage in observational learning.
Studies suggest that imitation with social understanding tends to begin around 2 years old, but will vary depending on the specific child. In the past, research has claimed that newborns are capable of imitation, but this likely isn't true, as newborns often react to stimuli in a way that may seem like imitation, but isn't.
Basic Principles of Social Learning Theory
If you've ever made faces at a toddler and watched them try to mimic your movements, then you may have witnessed how observational learning can be such an influential force. Bandura's social learning theory stresses the power of observational learning.
Bobo Doll Experiment
Bandura's Bobo doll experiment is one of the most famous examples of observational learning. In the Bobo doll experiment , Bandura demonstrated that young children may imitate the aggressive actions of an adult model. Children observed a film where an adult repeatedly hit a large, inflatable balloon doll and then had the opportunity to play with the same doll later on.
Children were more likely to imitate the adult's violent actions when the adult either received no consequences or when the adult was rewarded. Children who saw the adult being punished for this aggressive behavior were less likely to imitate them.
Observational Learning Examples
The following are instances that demonstrate observational learning has occurred.
- A child watches their parent folding the laundry. They later pick up some clothing and imitate folding the clothes.
- A young couple goes on a date to an Asian restaurant. They watch other diners in the restaurant eating with chopsticks and copy their actions to learn how to use these utensils.
- A child watches a classmate get in trouble for hitting another child. They learn from observing this interaction that they should not hit others.
- A group of children play hide-and-seek. One child joins the group and is not sure what to do. After observing the other children play, they quickly learn the basic rules and join in.
Stages of Observational Learning
There are four stages of observational learning that need to occur for meaningful learning to take place. Keep in mind, this is different than simply copying someone else's behavior. Instead, observational learning may incorporate a social and/or motivational component that influences whether the observer will choose to engage in or avoid a certain behavior.
For an observer to learn, they must be in the right mindset to do so. This means having the energy to learn, remaining focused on what the model is engaging in, and being able to observe the model for enough time to grasp what they are doing.
How the model is perceived can impact the observer's level of attention. Models who are seen being rewarded for their behavior, models who are attractive, and models who are viewed as similar to the observer tend to command more focus from the observer.
If the observer was able to focus on the model's behavior, the next step is being able to remember what was viewed. If the observer is not able to recall the model's behavior, they may need to go back to the first stage again.
Reproduction
If the observer is able to focus and retains the information, the next stage in observational learning is trying to replicate it. It's important to note that every individual will have their own unique capacity when it comes to imitating certain behaviors, meaning that even with perfect focus and recall, some behaviors may not be easily copied.
In order for the observer to engage in this new behavior, they will need some sort of motivation . Even if the observer is able to imitate the model, if they lack the drive to do so, they will likely not follow through with this new learned behavior.
Motivation may increase if the observer watched the model receive a reward for engaging in a certain behavior and the observer believes they will also receive some reward if they imitate said behavior. Motivation may decrease if the observer had knowledge of or witnessed the model being punished for a certain behavior.
Influences on Observational Learning
According to Bandura's research, there are a number of factors that increase the likelihood that a behavior will be imitated. We are more likely to imitate:
- People we perceive as warm and nurturing
- People who receive rewards for their behavior
- People who are in an authoritative position in our lives
- People who are similar to us in age, sex, and interests
- People we admire or who are of a higher social status
- When we have been rewarded for imitating the behavior in the past
- When we lack confidence in our own knowledge or abilities
- When the situation is confusing, ambiguous, or unfamiliar
Pros and Cons of Observational Learning
Observational learning has the potential to teach and reinforce or decrease certain behaviors based on a variety of factors. Particularly prevalent in childhood, observational learning can be a key part of how we learn new skills and learn to avoid consequences.
However, there has also been concern about how this type of learning can lead to negative outcomes and behaviors. Some studies, inspired by Bandura's research, focused on the effects observational learning may have on children and teenagers.
For example, previous research drew a direct connection between playing certain violent video games and an increase in aggression in the short term. However, later research that focused on the short- and long-term impact video games may have on players has shown no direct connections between video game playing and violent behavior.
Similarly, research looking at sexual media exposure and teenagers' sexual behavior found that, in general, there wasn't a connection between watching explicit content and having sex within the following year.
Another study indicated that if teenagers age 14 and 15 of the same sex consumed sexual media together and/or if parents restricted the amount of sexual content watched, the likelihood of having sex was lower. The likelihood of sexual intercourse increased when opposite-sex peers consumed sexual content together.
Research indicates that when it comes to observational learning, individuals don't just imitate what they see and that context matters. This may include who the model is, who the observer is with, and parental involvement.
Uses for Observational Learning
Observational learning can be used in the real world in a number of different ways. Some examples include:
- Learning new behaviors : Observational learning is often used as a real-world tool for teaching people new skills. This can include children watching their parents perform a task or students observing a teacher engage in a demonstration.
- Strengthening skills : Observational learning is also a key way to reinforce and strengthen behaviors. For example, if a study sees another student getting a reward for raising their hand in class, they will be more likely to also raise their hand the next time they want to ask a question.
- Minimizing negative behaviors : Observational learning also plays an important role in reducing undesirable or negative behaviors. For example, if you see a coworker get reprimanded for failing to finish a task on time, it means that you may be more likely to finish your work more quickly.
A Word From Verywell
Observational learning can be a powerful learning tool. When we think about the concept of learning, we often talk about direct instruction or methods that rely on reinforcement and punishment . But, a great deal of learning takes place much more subtly and relies on watching the people around us and modeling their actions. This learning method can be applied in a wide range of settings including job training, education, counseling, and psychotherapy .
Jones SS. The development of imitation in infancy. Philosophical Transactions of the Royal Society B: Biological Sciences . 2009;364(1528):2325-2335. doi:10.1098/rstb.2009.0045
Bandura A. Social Learning Theory . Prentice Hall; 1977.
Kühn S, Kugler DT, Schmalen K, Weichenberger M, Witt C, Gallinat J. Does playing violent video games cause aggression? A longitudinal intervention study. Mol Psychiatry . 2019;24(8):1220-1234. doi:10.1038/s41380-018-0031-7
Gottfried JA, Vaala SE, Bleakley A, Hennessy M, Jordan A. Does the effect of exposure to TV sex on adolescent sexual behavior vary by genre? Communication Research . 2013;40(1):73-95. doi:10.1177/0093650211415399
Parkes A, Wight D, Hunt K, Henderson M, Sargent J. Are sexual media exposure, parental restrictions on media use and co-viewing TV and DVDs with parents and friends associated with teenagers’ early sexual behaviour? Journal of Adolescence . 2013;36(6):1121-1133. doi:10.1016/j.adolescence.2013.08.019
The impact of interactive violence on children . U.S. Senate Hearing 106-1096. March 21, 2000.
Anderson CA, Dill KE. Video games and aggressive thoughts, feelings, and behavior in the laboratory and in life . J Pers Soc Psychol. 2000;78(4):772-790. doi:10.1037/0022-3514.78.4.772
Collins RL, Elliott MN, Berry SH, et al. Watching sex on television predicts adolescent initiation of sexual behavior . Pediatrics . 2004;114(3):e280-9. dloi:10.1542/peds.2003-1065-L
By Kendra Cherry, MSEd Kendra Cherry, MS, is a psychosocial rehabilitation specialist, psychology educator, and author of the "Everything Psychology Book."
6.4 Observational Learning (Modeling)
Learning objectives.
By the end of this section, you will be able to:
- Define observational learning
- Discuss the steps in the modeling process
- Explain the prosocial and antisocial effects of observational learning
Previous sections of this chapter focused on classical and operant conditioning, which are forms of associative learning. In observational learning , we learn by watching others and then imitating, or modeling, what they do or say. For instance, have you ever gone to YouTube to find a video showing you how to do something? The individuals performing the imitated behavior are called models . Research suggests that this imitative learning involves a specific type of neuron, called a mirror neuron (Hickock, 2010; Rizzolatti, Fadiga, Fogassi, & Gallese, 2002; Rizzolatti, Fogassi, & Gallese, 2006).
Humans and other animals are capable of observational learning. For example, in a study of social learning in chimpanzees, researchers gave juice boxes with straws to two groups of captive chimpanzees. The first group dipped the straw into the juice box, and then sucked on the small amount of juice at the end of the straw. The second group sucked through the straw directly, getting much more juice. When the first group, the “dippers,” observed the second group, “the suckers,” what do you think happened? All of the “dippers” in the first group switched to sucking through the straws directly. By simply observing the other chimps and modeling their behavior, they learned that this was a more efficient method of getting juice (Yamamoto, Humle, and Tanaka, 2013).
Imitation is sometimes called the highest form of flattery. But consider Claire’s experience with observational learning. Claire’s nine-year-old son, Jay, was getting into trouble at school and was defiant at home. Claire feared that Jay would end up like her brothers, two of whom were in prison. One day, after yet another bad day at school and another negative note from the teacher, Claire, at her wit’s end, beat her son with a belt to get him to behave. Later that night, as she put her children to bed, Claire witnessed her four-year-old daughter, Anna, take a belt to her teddy bear and whip it. Claire was horrified, realizing that Anna was imitating her mother. It was then that Claire knew she wanted to discipline her children in a different manner.
Link to Learning
Are chimps smarter than children? Watch this video showing chimps and children performing tasks and contemplate who performed the task better. How about quicker?
Like Tolman, whose experiments with rats suggested a cognitive component to learning, psychologist Albert Bandura’s ideas about learning were different from those of strict behaviorists. Bandura and other researchers proposed a brand of behaviorism called social learning theory, which took cognitive processes into account. According to Bandura , pure behaviorism could not explain why learning can take place in the absence of external reinforcement. He felt that internal mental states must also have a role in learning and that observational learning involves much more than imitation. In imitation, a person simply copies what the model does. Observational learning is much more complex. According to Lefrançois (2012) there are several ways that observational learning can occur:
- You learn a new response. After watching your coworker get chewed out by your boss for coming in late, you start leaving home 10 minutes earlier so that you won’t be late.
- You choose whether or not to imitate the model depending on what you saw happen to the model. Remember Julian and his father? When learning to surf, Julian might watch how his father pops up successfully on his surfboard and then attempt to do the same thing. On the other hand, Julian might learn not to touch a hot stove after watching his father get burned on a stove.
- You learn a general rule that you can apply to other situations.
Bandura identified three kinds of models: live, verbal, and symbolic. A live model demonstrates a behavior in person, as when Ben stood up on his surfboard so that Julian could see how he did it. A verbal instructional model does not perform the behavior, but instead explains or describes the behavior, as when a soccer coach tells his young players to kick the ball with the side of the foot, not with the toe. A symbolic model can be fictional characters or real people who demonstrate behaviors in books, movies, television shows, video games, or Internet sources ( Figure 6.17 ).
Latent learning and modeling are used all the time in the world of marketing and advertising. This Ford commercial starring Derek Jeter played for months across the New York, New Jersey, and Connecticut areas. Jeter was an award-winning baseball player for the New York Yankees. The commercial aired in a part of the country where Jeter is an incredibly well-known athlete. He is wealthy, and considered very loyal and good looking. What message are the advertisers sending by having him featured in the ad? How effective do you think it is?
Steps in the Modeling Process
Of course, we don’t learn a behavior simply by observing a model. Bandura described specific steps in the process of modeling that must be followed if learning is to be successful: attention, retention, reproduction, and motivation. First, you must be focused on what the model is doing—you have to pay attention. Next, you must be able to retain, or remember, what you observed; this is retention. Then, you must be able to perform the behavior that you observed and committed to memory; this is reproduction. Finally, you must have motivation. You need to want to copy the behavior, and whether or not you are motivated depends on what happened to the model. If you saw that the model was reinforced for their behavior, you will be more motivated to copy them. This is known as vicarious reinforcement . On the other hand, if you observed the model being punished, you would be less motivated to copy them. This is called vicarious punishment . For example, imagine that four-year-old Allison watched her older sister Kaitlyn playing in their mother’s makeup, and then saw Kaitlyn get a time out when their mother came in. After their mother left the room, Allison was tempted to play in the make-up, but she did not want to get a time-out from her mother. What do you think she did? Once you actually demonstrate the new behavior, the reinforcement you receive plays a part in whether or not you will repeat the behavior.
Bandura researched modeling behavior, particularly children’s modeling of adults’ aggressive and violent behaviors (Bandura, Ross, & Ross, 1961). He conducted an experiment with a five-foot inflatable doll that he called a Bobo doll. In the experiment, children’s aggressive behavior was influenced by whether the teacher was punished for her behavior. In one scenario, a teacher acted aggressively with the doll, hitting, throwing, and even punching the doll, while a child watched. There were two types of responses by the children to the teacher’s behavior. When the teacher was punished for her bad behavior, the children decreased their tendency to act as she had. When the teacher was praised or ignored (and not punished for her behavior), the children imitated what she did, and even what she said. They punched, kicked, and yelled at the doll.
Watch this video clip about the famous Bobo doll experiment to see a portion of the experiment and an interview with Albert Bandura.
What are the implications of this study? Bandura concluded that we watch and learn, and that this learning can have both prosocial and antisocial effects. Prosocial (positive) models can be used to encourage socially acceptable behavior. Parents in particular should take note of this finding. If you want your children to read, then read to them. Let them see you reading. Keep books in your home. Talk about your favorite books. If you want your children to be healthy, then let them see you eat right and exercise, and spend time engaging in physical fitness activities together. The same holds true for qualities like kindness, courtesy, and honesty. The main idea is that children observe and learn from their parents, even their parents’ morals, so be consistent and toss out the old adage “Do as I say, not as I do,” because children tend to copy what you do instead of what you say. Besides parents, many public figures, such as Martin Luther King, Jr. and Mahatma Gandhi, are viewed as prosocial models who are able to inspire global social change. Can you think of someone who has been a prosocial model in your life?
The antisocial effects of observational learning are also worth mentioning. As you saw from the example of Claire at the beginning of this section, her daughter viewed Claire’s aggressive behavior and copied it. Research suggests that this may help to explain why victims of abuse often grow up to be abusers themselves (Murrell, Christoff, & Henning, 2007). In fact, about 30% of child abuse victims become abusive parents (U.S. Department of Health & Human Services, 2013). We tend to do what we know. Children who grow up witnessing their parents deal with anger and frustration through violent and aggressive acts often learn to behave in that manner themselves.
Some studies suggest that violent television shows, movies, and video games may also have antisocial effects ( Figure 6.18 ) although further research needs to be done to understand the correlational and causational aspects of media violence and behavior. Some studies have found a link between viewing violence and aggression seen in children (Anderson & Gentile, 2008; Kirsch, 2010; Miller, Grabell, Thomas, Bermann, & Graham-Bermann, 2012). These findings may not be surprising, given that a child graduating from high school has been exposed to around 200,000 violent acts including murder, robbery, torture, bombings, beatings, and rape through various forms of media (Huston et al., 1992). Not only might viewing media violence affect aggressive behavior by teaching people to act that way in real life situations, but it has also been suggested that repeated exposure to violent acts also desensitizes people to it. Psychologists are working to understand this dynamic.
View this video about the connection between violent video games and violent behavior to learn more.
What Do You Think?
Violent media and aggression.
Does watching violent media or playing violent video games cause aggression? Albert Bandura's early studies suggested television violence increased aggression in children, and more recent studies support these findings. For example, research by Craig Anderson and colleagues (Anderson, Bushman, Donnerstein, Hummer, & Warburton, 2015; Anderson et al., 2010; Bushman et al., 2016) found extensive evidence to suggest a causal link between hours of exposure to violent media and aggressive thoughts and behaviors. However, studies by Christopher Ferguson and others suggests that while there may be a link between violent media exposure and aggression, research to date has not accounted for other risk factors for aggression including mental health and family life (Ferguson, 2011; Gentile, 2016). What do you think?
As an Amazon Associate we earn from qualifying purchases.
This book may not be used in the training of large language models or otherwise be ingested into large language models or generative AI offerings without OpenStax's permission.
Want to cite, share, or modify this book? This book uses the Creative Commons Attribution License and you must attribute OpenStax.
Access for free at https://openstax.org/books/psychology-2e/pages/1-introduction
- Authors: Rose M. Spielman, William J. Jenkins, Marilyn D. Lovett
- Publisher/website: OpenStax
- Book title: Psychology 2e
- Publication date: Apr 22, 2020
- Location: Houston, Texas
- Book URL: https://openstax.org/books/psychology-2e/pages/1-introduction
- Section URL: https://openstax.org/books/psychology-2e/pages/6-4-observational-learning-modeling
© Jan 6, 2024 OpenStax. Textbook content produced by OpenStax is licensed under a Creative Commons Attribution License . The OpenStax name, OpenStax logo, OpenStax book covers, OpenStax CNX name, and OpenStax CNX logo are not subject to the Creative Commons license and may not be reproduced without the prior and express written consent of Rice University.
- Search Menu
- Advance articles
- Author Guidelines
- Submission Site
- About Social Work Research
- National Association of Social Workers
- Editorial Board
- Advertising and Corporate Services
- Journals Career Network
- Self-Archiving Policy
- Dispatch Dates
- Journals on Oxford Academic
- Books on Oxford Academic

- < Previous
Determining the Effectiveness of Behavior Skills Training and Observational Learning on Classroom Behaviors: A Case Study
- Article contents
- Figures & tables
- Supplementary Data
Thea Ervin, Alyssa N Wilson, Brandy R Maynard, Tracy Bramblett, Determining the Effectiveness of Behavior Skills Training and Observational Learning on Classroom Behaviors: A Case Study, Social Work Research , Volume 42, Issue 2, June 2018, Pages 106–117, https://doi.org/10.1093/swr/svy005
- Permissions Icon Permissions
Approximately three to four students in an average classroom engage in disruptive behaviors that interfere with normal academic and social development. School social work interventions to prevent and reduce challenging behaviors in the classroom can be used to improve behavior and academic success; however, there is a lack of research on classroom-based interventions social workers can deliver. The current study used a single-subject multiple-baseline across-classrooms design to examine the effects of behavior skills training (BST) paired with observational learning of students’ engagement in and responses to disruptive behavior in the classroom setting. Six students (ages eight through 18) with emotional and behavioral disorders were randomly selected as models ( n = 2) or observers ( n = 4). During training, each model was trained to ignore, walk away, or engage in a calming strategy when peers engaged in disruption, while observers watched. Using a concurrent multiple-baseline across-classrooms design, student engagement in disruptive behavior and response to peers’ disruptive behavior were observed before and after BST across classrooms. All students demonstrated an increase in appropriately responding to disruptive behavior following BST, and instances of disruptive behavior decreased.
Email alerts
Citing articles via.
- Recommend to your Library
Affiliations
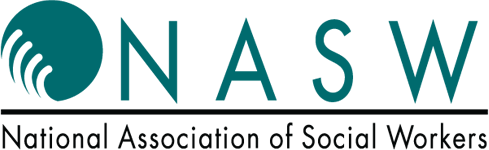
- Online ISSN 1545-6838
- Print ISSN 1070-5309
- Copyright © 2024 National Association of Social Workers
- About Oxford Academic
- Publish journals with us
- University press partners
- What we publish
- New features
- Open access
- Institutional account management
- Rights and permissions
- Get help with access
- Accessibility
- Advertising
- Media enquiries
- Oxford University Press
- Oxford Languages
- University of Oxford
Oxford University Press is a department of the University of Oxford. It furthers the University's objective of excellence in research, scholarship, and education by publishing worldwide
- Copyright © 2024 Oxford University Press
- Cookie settings
- Cookie policy
- Privacy policy
- Legal notice
This Feature Is Available To Subscribers Only
Sign In or Create an Account
This PDF is available to Subscribers Only
For full access to this pdf, sign in to an existing account, or purchase an annual subscription.
Observational Learning
- Reference work entry
- First Online: 01 January 2021
- pp 3207–3212
- Cite this reference work entry
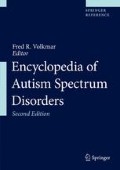
- Amanda P. Laprime 2 , 3 &
- Ryan Knighton 2 , 4
25 Accesses
This is a preview of subscription content, log in via an institution to check access.
Access this chapter
- Available as PDF
- Read on any device
- Instant download
- Own it forever
- Available as EPUB and PDF
- Durable hardcover edition
- Dispatched in 3 to 5 business days
- Free shipping worldwide - see info
Tax calculation will be finalised at checkout
Purchases are for personal use only
Institutional subscriptions
References and Reading
Bandura, A., & Huston, A. C. (1961). Identification as a process of incidental learning. Journal of Abnormal and Social Psychology, 63 , 311–318.
PubMed Google Scholar
Bandura, A., Ross, D., & Ross, S. A. (1963). Vicarious reinforcement and imitative learning. Journal of Abnormal and Social Psychology, 67 , 601–607.
Google Scholar
Birnbrauer, J. S. (1976). Mental retardation. In H. Leitenberg (Ed.), Handbook of behavior modification and behavior therapy (pp. 361–404). Englewood Cliffs, NJ: Prentice Hall.
Birnbrauer, J. S., Hopkins, N. R., & Kauffman, J. M. (1981). The effects of vicarious prompting on attentive behavior of children with behavior dis-orders. Child Behavior Therapy, 3 , 27–41.
Brody, G. H., Lahey, B. B., & Combs, M. L. (1978). Effects of intermittent modeling on observation learning. Journal of Applied Behavior Analysis, 11 , 87–90.
PubMed PubMed Central Google Scholar
Bruzek, J. L., & Thompson, R. H. (2007). Antecedent effects of observing peer play. Journal of Applied Behavior Analysis, 40 , 327–331.
Catania, A. C. (2007). Learning (4th ed.). Cornwall-on-Hudson: Sloan.
Detguchi, H. (1984). Observational learning from a radical behavioristic viewpoint. The Behavior Analyst, 7 , 83–95.
Gladstone, B. W., & Cooley, J. (1979). Behavioral similarity as a reinforcer for preschool children. Journal of the Experimental Analysis of Behavior, 23 , 357–368.
Goldstein, H., & Mousetis, L. (1989). Generalized language learning by children with severe mental retardation: Effects of peers’ expressive modeling. Journal of Applied Behavior Analysis, 22 , 245–259.
Greer, R. D., & Singer-Dudek, J. (2008). The emergence of conditioned reinforcement from observation. Journal of the Experimental Analysis of Behavior, 89 , 15–29.
Greer, R., Dudek-Singer, J., & Gautreaux, G. (2006). Observational learning. International Journal of Psychology, 41 (6), 486–499.
Griffen, A. K., Wolery, M., & Schuster, J. W. (1992). Triadic instruction of chained food preparation responses: Acquisition and observational learning. Journal of Applied Behavior Analysis, 25 , 193–204.
Kazdin, A. E. (1973). The effect of vicarious reinforcement on attentive behavior in the classroom. Journal of Applied Behavior Analysis, 6 , 71–78.
Leaf, J. B., Oppenhenheim-Leaf, M., Leaf, R., Courtemanche, A. B., Taubman, M., McEachin, J., Sheldon, J., & Sherman, J. A. (2012). Observational effects on the preferences of children with autism. Journal of Applied Behavior Analysis, 45 , 473–483.
MacDonald, J., & Ahearn, W. H. (2015). Teaching observational learning to children with autism. Journal of Applied Behavior Analysis, 48 (4), 800–816.
Masia, C. L., & Chase, P. (1997). Vicarious learning revisited: A contemporary behavior analytic interpretation. Journal of Behavior Therapy and Experimental Psychiatry, 28 , 41–51.
Miller, N., & Dollard, J. (1941). Social learning and imitation . New Haven: Yale University Press.
Nikopoulos, C. K., & Keenan, M. (2003). Promoting social initiation in children with autism using video modeling. Behavioral Interventions, 18 (2), 87–108.
Ramirez, J., & Rehfeldt, R. A. (2009). Observational learning and the emergence of symmetry relations in teaching Spanish vocabulary words to typically developing children. Journal of Applied Behavior Analysis, 42 , 801–805.
Rehfeldt, R. A., Latimore, D., & Stromer, R. (2003). Observational learning and the formation of classes of reading skills by individuals with autism and other developmental disabilities. Research in Developmental Disabilities, 24 , 333–358.
Singer-Dudek, J., Oblak, M., & Greer, R. D. (2011). Establishing books as conditioned reinforcers for preschool children as a function of an observational intervention. Journal of Applied Behavior Analysis, 44 , 421–434.
Smith, T. (2001). Discrete trial training in the treatment of autism. Focus on Autism and Other Disabilities, 16 , 86–92.
Taylor, B. A., DeQuinzio, J. A., & Stine, J. (2012). Increasing observational learning of children with autism: A preliminary analysis. Journal of Applied Behavior Analysis, 45 , 815–820.
Werts, M. G., Caldwell, N. K., & Wolery, M. (1996). Peer modeling of response chains: Observational learning by students with disabilities. Journal of Applied Behavior Analysis, 29 , 53–66.
Williams, J. H., Whiten, A., & Singh, T. (2004). A systematic review of action imitation in autistic spectrum disorder. Journal of Autism and Developmental Disorders, 34 , 285–299.
Download references
Author information
Authors and affiliations.
The Center for Children with Special Needs, Glastonbury, CT, USA
Amanda P. Laprime & Ryan Knighton
University of Rochester Medical Center, Rochester, NY, USA
Amanda P. Laprime
Newton Public Schools, Newton, MA, USA
Ryan Knighton
You can also search for this author in PubMed Google Scholar
Corresponding author
Correspondence to Amanda P. Laprime .
Editor information
Editors and affiliations.
Yale University, New Haven, CT, USA
Fred R. Volkmar
Rights and permissions
Reprints and permissions
Copyright information
© 2021 Springer Nature Switzerland AG
About this entry
Cite this entry.
Laprime, A.P., Knighton, R. (2021). Observational Learning. In: Volkmar, F.R. (eds) Encyclopedia of Autism Spectrum Disorders. Springer, Cham. https://doi.org/10.1007/978-3-319-91280-6_102283
Download citation
DOI : https://doi.org/10.1007/978-3-319-91280-6_102283
Published : 14 March 2021
Publisher Name : Springer, Cham
Print ISBN : 978-3-319-91279-0
Online ISBN : 978-3-319-91280-6
eBook Packages : Behavioral Science and Psychology Reference Module Humanities and Social Sciences Reference Module Business, Economics and Social Sciences
Share this entry
Anyone you share the following link with will be able to read this content:
Sorry, a shareable link is not currently available for this article.
Provided by the Springer Nature SharedIt content-sharing initiative
- Publish with us
Policies and ethics
- Find a journal
- Track your research
Bandura’s Bobo Doll Experiment on Social Learning
Saul Mcleod, PhD
Editor-in-Chief for Simply Psychology
BSc (Hons) Psychology, MRes, PhD, University of Manchester
Saul Mcleod, PhD., is a qualified psychology teacher with over 18 years of experience in further and higher education. He has been published in peer-reviewed journals, including the Journal of Clinical Psychology.
Learn about our Editorial Process
Olivia Guy-Evans, MSc
Associate Editor for Simply Psychology
BSc (Hons) Psychology, MSc Psychology of Education
Olivia Guy-Evans is a writer and associate editor for Simply Psychology. She has previously worked in healthcare and educational sectors.
During the 1960s, Albert Bandura conducted a series of experiments on observational learning , collectively known as the Bobo doll experiments. Two of the experiments are described below:
Bandura (1961) conducted a controlled experiment study to investigate if social behaviors (i.e., aggression) can be acquired by observation and imitation.
Bandura, Ross, and Ross (1961) tested 36 boys and 36 girls from the Stanford University Nursery School aged between 3 to 6 years old.
The researchers pre-tested the children for how aggressive they were by observing the children in the nursery and judged their aggressive behavior on four 5-point rating scales.
It was then possible to match the children in each group so that they had similar levels of aggression in their everyday behavior. The experiment is, therefore, an example of a matched pairs design .
To test the inter-rater reliability of the observers, 51 of the children were rated by two observers independently, and their ratings were compared. These ratings showed a very high-reliability correlation (r = 0.89), which suggested that the observers had a good agreement about the behavior of the children.
A lab experiment was used, in which the independent variable (the type of model) was manipulated in three conditions:
- Aggressive model is shown to 24 children
- Non-aggressive model is shown to 24 children
- No model is shown (control condition) – 24 children
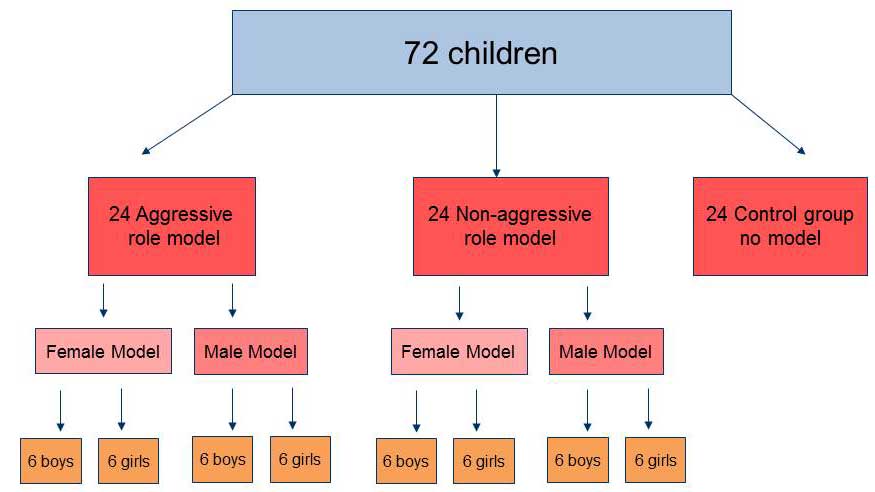
Stage 1: Modeling
In the experimental conditions, children were individually shown into a room containing toys and played with some potato prints and pictures in a corner for 10 minutes while either:
- 24 children (12 boys and 12 girls) watched a male or female model behaving aggressively towards a toy called a “Bobo doll”. The adults attacked the Bobo doll in a distinctive manner – they used a hammer in some cases, and in others threw the doll in the air and shouted “Pow, Boom.”
- Another 24 children (12 boys and 12 girls) were exposed to a non-aggressive model who played in a quiet and subdued manner for 10 minutes (playing with a tinker toy set and ignoring the bobo-doll).
- The final 24 children (12 boys and 12 girls) were used as a control group and not exposed to any model at all.
Stage 2: Aggression Arousal
All the children (including the control group) were subjected to “mild aggression arousal.” Each child was (separately) taken to a room with relatively attractive toys.
As soon as the child started to play with the toys, the experimenter told the child that these were the experimenter’s very best toys and she had decided to reserve them for the other children.
Stage 3: Test for Delayed Imitation
- The next room contained some aggressive toys and some non-aggressive toys. The non-aggressive toys included a tea set, crayons, three bears and plastic farm animals. The aggressive toys included a mallet and peg board, dart guns, and a 3 foot Bobo doll.
- The child was in the room for 20 minutes, and their behavior was observed and rated though a one-way mirror. Observations were made at 5-second intervals, therefore, giving 240 response units for each child.
- Other behaviors that didn’t imitate that of the model were also recorded e.g., punching the Bobo doll on the nose.
- Children who observed the aggressive model made far more imitative aggressive responses than those who were in the non-aggressive or control groups.
- There was more partial and non-imitative aggression among those children who had observed aggressive behavior, although the difference for non-imitative aggression was small.
- The girls in the aggressive model condition also showed more physically aggressive responses if the model was male, but more verbally aggressive responses if the model was female. However, the exception to this general pattern was the observation of how often they punched Bobo, and in this case the effects of gender were reversed.
- Boys were more likely to imitate same-sex models than girls. The evidence for girls imitating same-sex models is not strong.
- Boys imitated more physically aggressive acts than girls. There was little difference in verbal aggression between boys and girls.
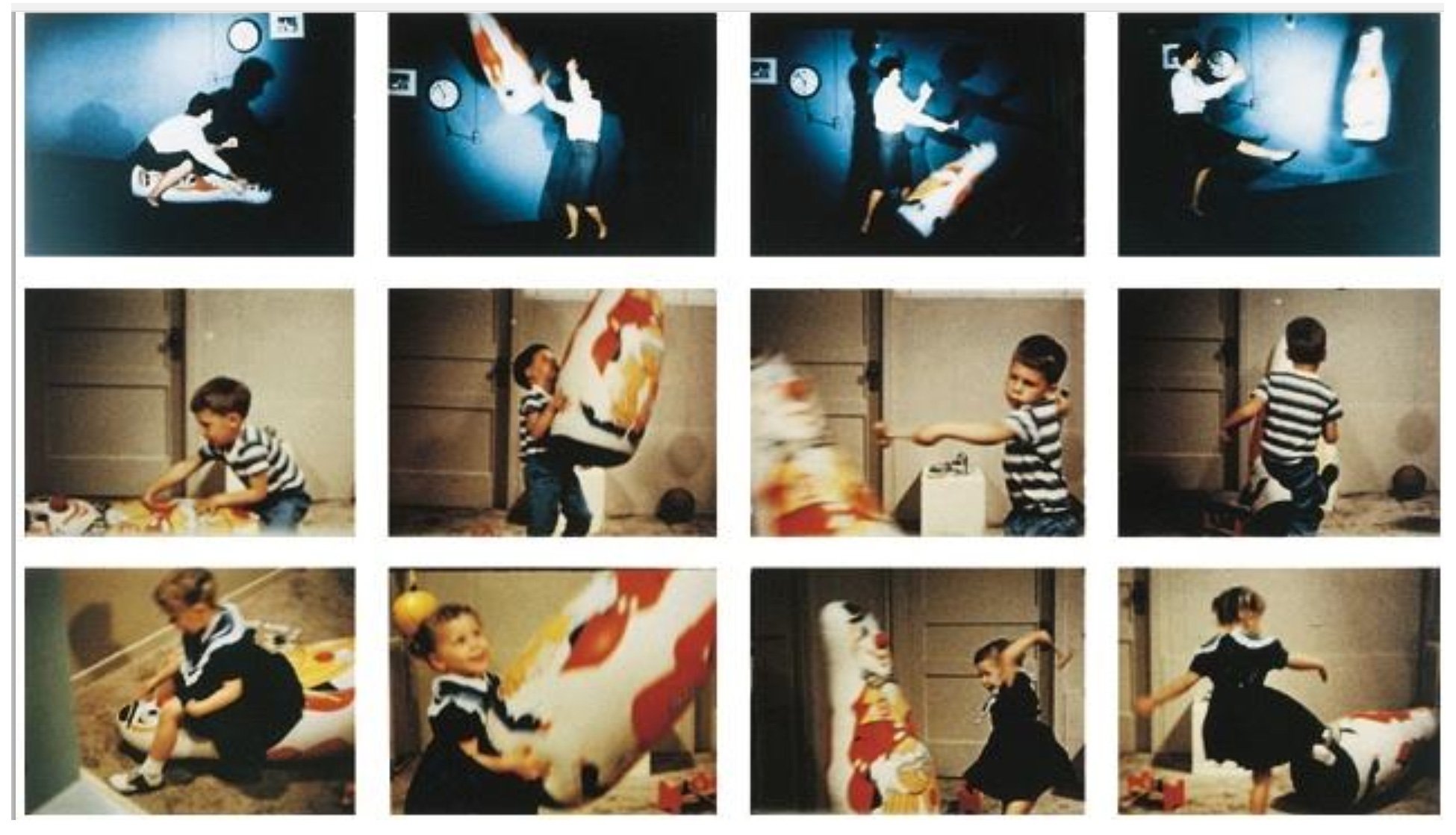
Bobo doll experiment demonstrated that children are able to learn social behavior such as aggression through the process of observation learning, through watching the behavior of another person. The findings support Bandura’s (1977) Social Learning Theory .
This study has important implications for the effects of media violence on children.
There are three main advantages of the experimental method .
- Experiments are the only means by which cause and effect can be established. Thus, it could be demonstrated that the model did have an effect on the child’s subsequent behavior because all variables other than the independent variable are controlled.
- It allows for precise control of variables. Many variables were controlled, such as the gender of the model, the time the children observed the model, the behavior of the model, and so on.
- Experiments can be replicated. Standardized procedures and instructions were used, allowing for replicability. In fact, the study has been replicated with slight changes, such as using video, and similar results were found (Bandura, 1963).
Limitations of the procedure include:
- Many psychologists are very critical of laboratory studies of imitation – in particular, because they tend to have low ecological validity. The situation involves the child and an adult model, which is a very limited social situation and there is no interaction between the child and the model at any point; certainly the child has no chance to influence the model in any way.
- Also, the model and the child are strangers. This, of course, is quite unlike “normal” modeling, which often takes place within the family.
- Cumberbatch (1990) found that children who had not played with a Bobo Doll before were five times as likely to imitate the aggressive behavior than those who were familiar with it; he claims that the novelty value of the doll makes it more likely that children will imitate the behavior.
- A further criticism of the study is that the demonstrations are measured almost immediately. With such snapshot studies, we cannot discover if such a single exposure can have long-term effects.
- It is possible to argue that the bobo doll experiment was unethical. For example, there is the problem of whether or not the children suffered any long-term consequences as a result of the study. Although it is unlikely, we can never be certain.
Vicarious Reinforcement Bobo Doll Study
An observer’s behavior can also be affected by the positive or negative consequences of a model’s behavior.
So we not only watch what people do, but we watch what happens when they do things. This is known as vicarious reinforcement. We are more likely to imitate behavior that is rewarded and refrain from behavior that is punished.
Bandura (1965) used a similar experimental set up to the one outlined above to test vicarious reinforcement. The experiment had different consequences for the model’s aggression to the three groups of children.
One group saw the model’s aggression being rewarded (being given sweets and a drink for a “championship performance,” another group saw the model being punished for the aggression (scolded), and the third group saw no specific consequences (control condition).
When allowed to enter the playroom, children in the reward and control conditions imitated more aggressive actions of the model than did the children in the punishment condition.
The children in the model punished group had learned the aggression by observational learning, but did not imitate it because they expected negative consequences.
Reinforcement gained by watching another person is known as vicarious reinforcement.
Bandura, A. (1965). Influence of models” reinforcement contingencies on the acquisition of imitative responses . Journal of personality and social psychology, 1(6) , 589.
Bandura, A., Ross, D. & Ross, S.A. (1961). Transmission of aggression through imitation of aggressive models . Journal of Abnormal and Social Psychology , 63, 575-82.
Bandura, A., Ross, D., & Ross, S. A. (1963). Imitation of film-mediated aggressive models . The Journal of Abnormal and Social Psychology , 66(1), 3.
Bandura, A. (1977). Social Learning Theory . Englewood Cliffs, NJ: Prentice Hall.
Further Information
- Bandura’s Social Learning Theory
- Bobo Doll Study Summary
- BBC Radio 4 Programme: The Bobo Doll
- Bobo Doll Summary PowerPoint

Click through the PLOS taxonomy to find articles in your field.
For more information about PLOS Subject Areas, click here .
Loading metrics
Open Access
Peer-reviewed
Research Article
Learning by Observation: Insights from Williams Syndrome
Contributed equally to this work with: Francesca Foti, Deny Menghini
* E-mail: [email protected]
Affiliations Department of Developmental and Social Psychology, University “Sapienza” of Rome, Rome, Italy, IRCCS Santa Lucia Foundation, Rome, Rome, Italy
Affiliation Child Neuropsychiatry Unit, Neuroscience Department, “Children’s Hospital Bambino Gesu’”, Rome, Rome, Italy
Affiliations School of Movement Sciences (DiSIST), University of Naples “Parthenope”, Naples, Italy, IRCCS Santa Lucia Foundation, Rome, Rome, Italy
Affiliation Department of Developmental and Social Psychology, University “Sapienza” of Rome, Rome, Italy
Affiliations Department of Psychology, University “Sapienza” of Rome, Rome, Italy, IRCCS Santa Lucia Foundation, Rome, Rome, Italy
- Francesca Foti,
- Deny Menghini,
- Laura Mandolesi,
- Francesca Federico,
- Stefano Vicari,
- Laura Petrosini
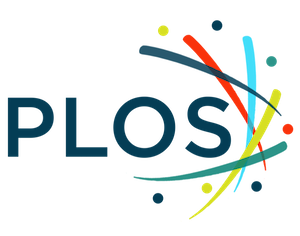
- Published: January 10, 2013
- https://doi.org/10.1371/journal.pone.0053782
- Reader Comments
Observing another person performing a complex action accelerates the observer’s acquisition of the same action and limits the time-consuming process of learning by trial and error. Observational learning makes an interesting and potentially important topic in the developmental domain, especially when disorders are considered. The implications of studies aimed at clarifying whether and how this form of learning is spared by pathology are manifold. We focused on a specific population with learning and intellectual disabilities, the individuals with Williams syndrome. The performance of twenty-eight individuals with Williams syndrome was compared with that of mental age- and gender-matched thirty-two typically developing children on tasks of learning of a visuo-motor sequence by observation or by trial and error. Regardless of the learning modality, acquiring the correct sequence involved three main phases: a detection phase, in which participants discovered the correct sequence and learned how to perform the task; an exercise phase, in which they reproduced the sequence until performance was error-free; an automatization phase, in which by repeating the error-free sequence they became accurate and speedy. Participants with Williams syndrome beneficiated of observational training (in which they observed an actor detecting the visuo-motor sequence) in the detection phase, while they performed worse than typically developing children in the exercise and automatization phases. Thus, by exploiting competencies learned by observation, individuals with Williams syndrome detected the visuo-motor sequence, putting into action the appropriate procedural strategies. Conversely, their impaired performances in the exercise phases appeared linked to impaired spatial working memory, while their deficits in automatization phases to deficits in processes increasing efficiency and speed of the response. Overall, observational experience was advantageous for acquiring competencies, since it primed subjects’ interest in the actions to be performed and functioned as a catalyst for executed action.
Citation: Foti F, Menghini D, Mandolesi L, Federico F, Vicari S, Petrosini L (2013) Learning by Observation: Insights from Williams Syndrome. PLoS ONE 8(1): e53782. https://doi.org/10.1371/journal.pone.0053782
Editor: Tricia A. Thornton-Wells, Vanderbilt University, United States of America
Received: July 12, 2012; Accepted: December 4, 2012; Published: January 10, 2013
Copyright: © 2013 Foti et al. This is an open-access article distributed under the terms of the Creative Commons Attribution License, which permits unrestricted use, distribution, and reproduction in any medium, provided the original author and source are credited.
Funding: This work was supported by the Fondation Jérôme Lejeune to L.M. The funders had no role in study design, data collection and analysis, decision to publish, or preparation of the manuscript.
Competing interests: The authors have declared that no competing interests exist.
Introduction
In humans and other animals new competencies may be learned through active experience and through observation of others’ experience [1] , [2] . Observing another person performing a complex action accelerates the observer’s acquisition of the same action and limits the time-consuming process of learning by trial and error [3] – [5] . Indeed, observational learning does not just involve copying an action and requires that the observer transforms the observation into an action as similar as possible to the model in terms of the goal to be reached and the motor strategies to be applied [5] – [10] .
Observational learning is already present at birth [5] , [11] – [13] and it is crucial for developing complex abilities such as language, social responsiveness, use of instruments to get things done [9] , [14] . Thus, in children, learning new competencies by observing adults or peers is a central process in cognitive development [15] .
By using an innovative task based on learning to detect a visuo-motor sequence, we demonstrated that in the presence of dyslexia the ability to learn by observation a previously observed visuo-motor sequence was markedly impaired, while the ability to detect a correct sequence by trial and error was preserved [16] . In the present research we focused on a population with learning as well as intellectual disability (ID), the Williams syndrome (WS) whose well-known neuropsychological profile with specific points of strengths and weaknesses allowed singling out cognitive processes working as learning went by. WS individuals show severely impaired visuo-spatial processing, planning and implicit learning [17] – [22] , while they exhibit relatively preserved perception of the visual characteristics of objects and face recognition [23] . WS individuals have specific difficulty in maintaining visuo-spatial information in working memory and in performing long-term memory tasks [24] , [25] , consistently with a deficit of dorsal stream. Considering that the visuo-motor task to be learned by observation required to translate visual information into action, specific function of dorsal stream network [26] , [27] , WS individuals appear to be the ideal participants to investigate the cognitive processes involved in the observational learning. Performances of a group of WS individuals were compared with those of a mental age- and gender-matched group of typically developing (TD) children on a task requiring the learning of a visuo-motor sequence. The participants learned the sequence either by performing the task after observing an actor detect the sequence of correct items by trial and error (observational training) or by actually performing the task by trial and error ( Fig. 1 ).
- PPT PowerPoint slide
- PNG larger image
- TIFF original image
Condition 1: Learning by Trial and Error followed by Observational Learning: participants detected a sequence by trial and error (TE1), then they observed an actor detecting a sequence different from the one they had previously detected (observational training) and, finally, they reproduced the observed sequence (OBS2). Condition 2: Observational Learning followed by Learning by Trial and Error: participants were submitted to an observational training, then they reproduced the observed sequence (OBS1) and, finally, detected by trial and error a different sequence they had never observed (TE2). The incorrect positions touched by the actor during the observational training are evidenced in grey. S: starting point; F: final point.
https://doi.org/10.1371/journal.pone.0053782.g001
Materials and Methods
Participants.
Twenty-eight WS participants and 32 TD children (used as controls) matching the WS individuals for mental age (MA) have been examined in the present study constituted by two experimental conditions: Learning by Trial and Error followed by Observational Learning (Condition 1); Observational Learning followed by Learning by Trial and Error (Condition 2) ( Table 1 ). Only WS individuals with mental age (MA) of at least 5 years were included in the present research because participants with inferior MA did not succeed in completing the task. No significant differences in chronological age (CA), MA and IQ ( P always >0.2) among participants performing Conditions 1 and 2 were found ( Table 2 ).
https://doi.org/10.1371/journal.pone.0053782.t001
https://doi.org/10.1371/journal.pone.0053782.t002
The clinical diagnosis of WS was confirmed by fluorescence in situ hybridization (FISH) genetic investigation, which showed the characteristic deletion on chromosome band 7q11.23. WS participants were part of a larger pool of individuals with learning disabilities attending the Children’s Hospital Bambino Gesù of Rome for clinical and rehabilitative follow-up. All of them lived at home with their families. The parents of all individuals who participated in the study provided written informed consent. This study was approved by the Ethic Committee of the Children’s Hospital Bambino Gesù of Rome and conducted according to the Helsinki declaration.
WS individuals were tested in a quiet room at the Children’s Hospital Bambino Gesù. TD children were individually tested in a quiet room at their schools.
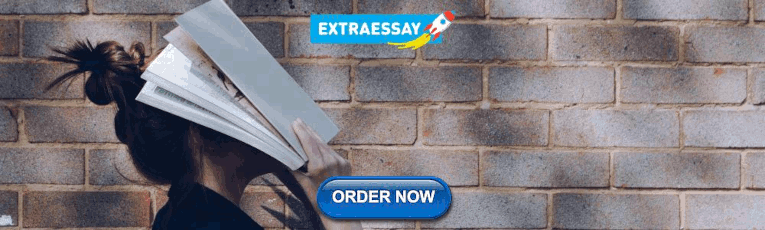
Intelligence Evaluation and Neuropsychological Assessment
In the present study, the brief version of the Leiter International Performance Scale–Revised [28] was employed (four out of 10 subtests: Figure Ground, Form Completion, Sequential Order and Repeated Patterns). The brief IQ and the corresponding mental ages were computed. Visuo-motor integration [29] , visuo-spatial perception [30] and memory [31] were assessed ( Table 3 ).
https://doi.org/10.1371/journal.pone.0053782.t003
Experimental Procedure
Each participant was sat in front of a computer touch screen (distance 60 cm). In both Conditions, the experimenter acting as the actor (F.F.) was sat near the participant. A 8×8 black matrix appeared on the touch screen. The subject was asked to find a hidden sequence of “correct” squares prepared in advance by the experimenters. The sequence was composed of 10 adjacent spatial positions in the matrix, which formed a “snake-like” pattern ( Fig.1 ). To explain the task to each participant the experimenter used the same verbal instructions: “You have to find a route formed by ten squares. When you touch a correct square it will be turned grey and you will hear a sound; conversely, if you touch a wrong square, it will be turned red. In this case, you have to find a new grey square. You have to start the route each time you find a new correct square. After finding the whole route, you have to re-touch it three times without making lighted red squares”. The participants started touching a grey square, which was the first element of the sequence and was always lit up. In the search for the second correct square, the participants had to touch one of the four squares bordering the grey square by moving in the matrix vertically or horizontally, but never diagonally. Each touched square (correct or incorrect) was lit up for 500 ms and then lighted off again; thus, no trace of the performed sequence remained on the screen. In learning the sequence by trial and error, the participants tried to find the correct sequence immediately after the verbal instructions. Conversely, in the observational learning task after the verbal instructions the participants observed the experimenter while she detected a 10-item sequence by trial and error (observational training). The experimenter performed the task by always making the same errors in the same positions, so that all participants observed the same pattern of correct and incorrect touches. Two minutes after the end of the observational training the participants were required to actually reproduce the observed correct sequence.
The tasks involved three phases: the Detection Phase (DP) that ended once the participants found the tenth correct position; the Exercise Phase (EP) in which they had to repeat the 10-item sequence until their performance was error-free; the Automatization Phase (AP) that ended when the correct sequence was repeated three consecutive times without errors.
Error parameters: DP errors, calculated as the number of incorrect items touched in detecting the ten correct positions; EP repetitions, calculated as the number of replications needed to reach the error-free performance. Time parameters: AP times (in msec), calculated as the time spent carrying out each of the three repetitions of the sequence.
Analysis of Error
To assess the kind of error further parameters were taken into account considering the two phases DP and EP together: the number of sequence errors , as touching a “correct” square in “wrong” moment (e.g. touching E7 before than F7); side-by-side errors , as touching the squares bordering the “correct” sequence (e.g. E8); illogical errors , as touching any other square (e.g. B5); perseverations, as consecutively touching the same item or a fixed sequence of items. Furthermore, in the task of observational learning we calculated the number of imitative errors , as touching the same squares wrongly touched by the actor during the observational training (e.g. F4) ( Fig.1 ).
Condition 1: Learning by Trial and Error Followed by Observational Learning
Fourteen WS and 16 TD individuals ( Table 1 ) firstly detected a sequence by Trial and Error (TE1) and, after ten minutes from task end, they were submitted to the observational training. After two minutes, participants were required to actually reproduce the observed sequence (OBS2). There was no fixed time limit for executing the task.
A pilot study was conducted to verify if the two sequences arranged to be used as “TE” and “OBS” sequences did not differ as to degree of difficulty. Six TD children [3 M] of mental age 6.10±0.3 detected the two different sequences by trial and error; presentation order was randomized among participants. Errors made in detecting each sequence were calculated by one-way ANOVA with repeated measures. The analysis failed to reveal any significant difference between sequences ( F (1,5) = 0.63, P = 0.46), confirming sequences of the same difficulty.
Condition 2: Learning by Observation Followed by Learning by Trial and Error
Fourteen WS and 16 TD individuals ( Table 1 ) first observed the experimenter detect a sequence (OBS1) and then actually reproduce it. After ten minutes from task end, they detected a different sequence by trial and error (TE2). Thus, the difference of the two conditions was that participants reproduced a sequence learned by observation after (Condition 1) or before (Condition 2) the detection of a different sequence by trial and error.
To evaluate mental representative mapping abilities, at the end of the reproduction of the sequence participants were required to draw the arrangement of the sequence on a 8×8 matrix sketched on a paper sheet. Thus, any participant drew the arrangement of two sequences, one learned by observation and the other one by trial and error. Mapping abilities were evaluated by tabulating the variable “errors” into three categories: “no error”, “one error” and “more than one error”.
Attentional Task
The sustained attentional abilities of all participants were tested. Participants sat in front of a computer monitor and were required to put their left index fingers on the A key of the keyboard and to put their right index fingers on the L key. The visual stimulus was a grey circle presented on monitor center for a duration varying from 1400 (short) to 2600 (long) msec in steps of 200 msec in a randomized order. Participants were submitted to a brief training in which they were instructed to judge 20 stimuli as short or long and to press the A or L keys, respectively. In the testing phase the participants had to judge the duration of 70 stimuli (10 stimuli of each of the 7 durations) and to press the A or L keys as quickly as possible after the stimulus appeared. The computer program recorded reaction times (with 1-ms resolution) and accuracy of the response. The responses were then analyzed by clustering them in blocks of ten (regardless of stimulus duration) (i.e. 1–10, 11–20, 21–30….61–70).
Statistical Analyses
The data were first tested for normality (Shapiro-Wilk normality test) and homoscedasticity (Levene test) and then compared by using two-way, three-way or four-way analyses of variance (ANOVAs). The two-way ANOVAs were performed by applying the mixed model for independent variable (group) and repeated measures (error, square or block). Three-way ANOVAs (group×condition×task) were performed on most parameters, while the four-way ANOVA on the three AP times was performed by applying the mixed model for independent variables (group, condition and task) and repeated measures (time). These analyses were followed by post-hoc multiple comparisons using Newman–Keuls test. In evaluating mapping abilities the error categories were analyzed by Chi-Square.
Because the 28 WS participants were differently aged (N = 9 age range: 8;9–14;1; N = 10 age range: 14;9–19;9; N = 9 age range: 22;9–35;3), we verified the sample homogeneity by comparing the performances of three differently aged WS sub-groups on three main parameters of the learning tasks they performed (DP errors; EP repetitions and AP times) by using MANOVAs. These analyses revealed no significant difference among WS sub-groups’ performances. Namely, in the tasks of learning by trial and error (TE1–TE2), the MANOVA revealed a not significant sub-group effect (F (2,25) = 0.12, P = 0.88) and a significant parameter effect (F (2,50) = 154.54, P <0.0001). The interaction was not significant (F (4,50) = 0.13, P = 0.96). In the tasks of observational learning (OBS1–OBS2) the MANOVA also revealed a not significant sub-group effect (F (2,25) = 0.47, P = 0.62) and a significant parameter effect (F (2,50) = 85.46, P <0.0001). The interaction was not significant (F (4,50) = 0.47, P = 0.75). Thus, we pooled together the 28 differently aged WS individuals.
All statistical analyses were performed by using Statistica 8.0 for Windows and the significance level was established at P< 0.05.
Learning Tasks
WS participants performed a number of DP errors not significantly different from TD children after the observational trainings (OBS1–OBS2) and were significantly impaired in detecting the sequence by trial and error in TE1 compared with any other intra- or inter-group condition ( Fig. 2A ), as revealed by post-hoc comparisons (always P <0.001) on the second-order interaction (F (1,56) = 8.37, P = 0.0054) of the three-way ANOVA (group×condition×task).
DP: Detection Phase; EP: Exercise Phase; AP: Automatization Phase. Data are expressed as mean ± SEM. The asterisks indicate the significance level of post hoc comparisons between groups (*** P <0.001).
https://doi.org/10.1371/journal.pone.0053782.g002
In EP, when individuals repeated the sequence until their performance was error-free, WS participants needed a significantly higher number of repetitions in comparison to TD children regardless of condition (1 or 2) and trial (OBS or TE), as revealed by the group effect (F (1,56) = 9.58, P = 0.0030) of the three-way ANOVA ( Fig. 2B ). The analysis of the three AP times revealed that although all participants exhibited significantly reduced times as the task went by (F (2,112) = 27.62, P <0.00001), WS individuals were significantly slower than TD children (F (1,56) = 10.37, P = 0.0021), revealing a difficulty in automatizing the sequence ( Fig. 2C ).
In TE1, although WS and TD participants did not differ in the number of illogical errors, WS individuals exhibited values of sequence, side-by-side and perseverative errors higher than TD children, as revealed by post-hoc comparisons made on the interaction (F (3,84) = 3.14, P = 0.029) of the two-way ANOVA (group×kind of error) ( Fig. 3 ). The highest number of sequence errors of WS individuals was found in E7 and F7 squares when a change of strategy was required (i.e. after an error re-starting the sequence from the first item rather than continuing along on the “snake”) ( Fig. 1 ), as revealed by post-hoc comparisons made on the interaction (F (9,252) = 1.96, P = 0.044) of the two-way ANOVA (group×square) ( Fig. 4 ). As for side-by-side errors, the high number of errors of WS individuals was due to their significantly more frequent touching of a wrong square when a change of direction was required (squares: D7, F6, E1) ( Fig. 1 ), as revealed by post-hoc comparisons made on the interaction (F (27,756) = 2.42, P <0.0001) of the two-way ANOVA (group×square) ( Fig. 4 ).
Data are expressed as mean ± SEM. The asterisks indicate the significance level of post hoc comparisons between groups (* P <0.05; ** P <0.005; *** P <0.001).
https://doi.org/10.1371/journal.pone.0053782.g003
On the right, the chromatic scale indicates the sum of incorrectly touched items (brown and blue denote maximal and minimal values, respectively). S: starting point; F: final point.
https://doi.org/10.1371/journal.pone.0053782.g004
The analysis of error in the remaining tasks (OBS2, OBS1 and TE2) revealed no significant difference between groups, even if significant difference among kind of errors was found (always P <0.00001) ( Fig. 3 ).
Mapping Abilities
Mental representative mapping abilities of the participants were evaluated by having them draw the arrangement of sequences they had just performed. No significant difference among categories of errors and between groups was found in any sequence ( P at least >0.4).
Two-way ANOVAs (group×block) on reaction times or response accuracy of the WS and TD groups revealed no attentional decay in both groups, as indicated by not significant difference in the reaction times in the seven blocks (F (6,348) = 1.55, P = 0.15). A similar result was obtained when response accuracy was analyzed (F (6,348) = 1.80, P = 0.10). Notably, a significant difference was found between WS and TD groups on reaction times (F (1,58) = 13.52, P = 0.00051), given WS participants pressed the keys at the appearance of the stimulus more quickly than TD children ( Fig. 5 ).
Data are expressed as mean ± SEM.
https://doi.org/10.1371/journal.pone.0053782.g005
Our study adopted a matched-group design to determine whether the learning performance of WS individuals was above or below that expected given their general level of intellectual functioning indexed as MA. However, although this design is one the most commonly employed measures of matching in ID research, we are aware that it has limitations in respect to ID-matched control group design that takes into account the cognitive profile of the specific pathology. Nevertheless, even the ID-matched control group cannot be taken as a guarantee of normative group, due cognitive profiles among different etiological groups with ID exhibit different peaks and troughs [32] . In an attempt to overcome the difficulties in matching individuals of different groups on any one particular measure it has been proposed the use of regression techniques that take the factors related to task performance into account [32] . However, this measure requires specific statistical properties of the data (as homogeneity of regression slopes or sample size), hardly available in studies on population affected by rare genetic conditions as WS.
The present study documented as WS participants significantly beneficiated of observational training as TD MA-matched children. This was true specifically in the DPs of learning tasks, while as for EPs and APs, in all tasks regardless of presentation order (1 or 2) or learning modalities (OBS or TE), WS participants performed significantly worse than TD children. The powerfully positive effect of observational training was present not only in reproducing the previously observed sequences (OBS1 and OBS2) but also affected the subsequent detection of a sequence by trial and error (TE2). However, the practice effect, inevitably present in any second task, potentially could affect performances.
Since WS individuals exhibit difficulties in maintaining visuo-spatial information in working memory and in performing spatial long-term memory tasks ( Table 3 ) [20] , [24] , [25] , their heavily impaired performances in all EPs appear linked to spatial working memory deficits and difficulties in bringing together the short sequences detected during DP, in maintaining them in working memory to recall the whole sequence trace and in monitoring the correct execution of the sequence. These findings indicate that the observational training exerts beneficial effects mainly on the acquisition of strategies to be applied.
In both Conditions, WS participants displayed AP times longer than TD children, even if progressively diminishing as the task went by. This finding was not a consequence of the fine motor deficits usually reported in WS individuals. In fact, consistently with Vicari et al. (2007) [22] , the reaction times exhibited by WS group in the Attentional Task were even shorter than those of TD group. Thus, the longer WS times were related to deficits in automatization processes increasing efficiency and speed of the response to reach highest levels of performance [33] . Automatizing skills is mainly linked to the functions of sub-cortical structures, as the cerebellum and basal ganglia and to their bidirectional interconnections with cortical structures [34] , [35] , [36] , [37] . The cause of automatization and procedural deficits of WS individuals could be their remarkable hypoplasia of the basal ganglia [38] and the disproportionate enlargement of the cerebellum [39] , [40] , [41] , [42] , [43] . Indeed, in WS individuals skill-learning abilities are impaired, as revealed by their performance in Tower of London test [21] , Serial Reaction Time task [22] and Radial or Multiple Reward Mazes [44] , [45] , [46] .
By analyzing the kind of errors, some remarks can be made. First of all, both groups made a very low number of illogical errors, thus suggesting all participants similarly managed task fundamentals ( Fig. 3 and 4 ). As for imitative errors, no difference between groups was found, thus suggesting participants did imitate but did not hyperimitate [47] . Conversely, WS individuals made more sequence and side-by-side errors than TD children in TE1, particularly when a change of direction was required. Errors in stopping the more easy “keep-straight” response and performing the more demanding “turn-left” response resulted by the WS difficulty in suppressing a previously correct but then inappropriate response. Correctly responding requires executive control processes based on frontal lobe function, as response inhibition, cognitive flexibility and attentional shifting [48] , [49] , [50] , [51] . WS individuals are impaired in spatial planning, working memory, cognitive flexibility and inhibiting well-learned responses become inappropriate to the situation [52] . Indeed, the executive function deficits that impaired WS performance dramatically reduced after the observational training, once more indicating the teaching power of the observation. WS participants made perseverative errors that could result from difficulties in withholding the inappropriate repetition of a response despite knowing that it was not the correct one. This is an important component of top-down executive control. Notably, perseverations may be symptom not only of prefrontal dysfunction but also of cerebellar and basal ganglia damage provoking “frontal-like” cognitive deficits [34] , [53] , [54] , [55] , [56] , [57] .
The prevalently frontal processes require a modulation in more posterior brain systems, via the attention networks. Basic aspects of attentional processing are selective spatial attention that allows maintaining the focus of processing between spatial locations, and the attentional processing that allows a kind of “selection for action” [58] , [59] , [60] . Namely, the action of reaching the right square required attentional modulation to plan, select and initiate the appropriate behavior, to direct it toward the selected goal, and to inhibit actions inappropriate for the current goal. Because many brain structures that are part of the attention networks are included within the dorsal stream network [27] , it is not surprising that WS participants performed more errors when behavioral inhibition and attentive shifting were required but no help from observing the actor was provided [61] . The “dorsal-stream vulnerability” in WS [62] is manifested not only in the spatial and visual processing occurring within the occipital and parietal areas but also in the processing of spatial information by frontal control systems, as reported in an fMRI study [63] .
At the end of testing, mental representative mapping abilities were evaluated by drawing the arrangement of squares just discovered. WS and TD participants were similarly able to represent the shape of the “snake”. This finding is consistent with the observation that WS individuals exhibit no difficulty in mentally visualizing objects without spatially manipulating them [25] , [64] and supports the indication that the present learning protocols encompassed requests of visual imagery.
If observative experience functions as a catalyst for executed action, it can be advanced that observing a sequence prior to experiencing it primes subjects’ interest in the actions to be performed to detect rules and sequence. In fact, the present results indicate that the observation of action has a strong impact on action memory. The influence of action perception on action production requires cross-modal information be coordinated. Action and perception share the distal reference and are coded in a common representational medium [65] , so that perceiving an action activates the corresponding motor representation within the observer automatically and without conscious effort [66] , [67] , [68] .
The close interplay between observation and execution of actions found in the present study is supported by studies providing evidence of a striking overlap in the brain systems recruited for one’s own action, observation of others’ action and imitation of action [69] . In particular, when imitation is aimed at learning novel actions, the activation of the “core circuit for imitation” [70] involving the inferior frontal gyrus, the inferior parietal lobule and the superior temporal sulcus seems to be integrated with activation of the dorso-lateral and ventro-medial prefrontal cortex, for selection of motor acts and error prediction [71] , of the premotor areas, for motor preparation [70] , [72] , [73] as well as of cerebellar areas, whether or not it is accompanied by actual motor acts [74] , [75] , [76] .
The existence of direct feed-forward connections from perceptual to motor processes allows observation sculpting motor abilities by exploiting the functional overlapping between perception and action systems. It has been suggested that observation of actions engages motor-related processes similar to those of actual execution, promoting the development of an efference copy of the descending motor commands, which in combination with a forward model provides a prediction of sensory consequences [77] , [78] , [79] , [80] . Thus, action observation, efficiently translated into the matching motor representation, powerfully activates the feed-forward predictive processes, so that learning does occur. Notably, even in WS individuals the beneficial effect of observation was evident although linked only to the DP. Action observation seems to result in an amelioration of frontal functions, as motor strategy planning, decision-making processes or response inhibition needed to guide planned sequential actions. Thus, observational training allows the acquisition of the strategies to be applied to identify and learn the visuo-spatial sequence. Notably, when the observation did not play any role (as in the DP of TE1), frontal deficits markedly affected WS performance. However, it has to be underlined that as far as the observational training was beneficial, in WS individuals it did not succeed in smoothing out the deficits in processing visuo-spatial information mainly linked to their repeatedly described dorsal stream vulnerability [62] .
Acknowledgments
We would like to thank the individuals with Williams syndrome and their parents for making this study possible. Moreover, we wish to thank Dr. P. Pani for his kind and competent help in analyzing data.
Author Contributions
Conceived and designed the experiments: F. Foti DM LM F. Frederico SV LP. Performed the experiments: F. Foti. Analyzed the data: F. Foti DM LP. Contributed reagents/materials/analysis tools: DM LM SV LP. Wrote the paper: F. Foti DM LP.
- View Article
- Google Scholar
- 12. Nadel J, Butterworth G (1998) Imitation in infancy. Cambridge: Cambridge University Press.
- 13. Nadel J (2002) The imitative mind. In Meltzoff A Prinz W editors. Cambridge: Cambridge University Press. 42–62.
- 28. Roid GH, Miller LJ (2002) Leiter – R, Leiter International Performance Scale–Revised. Giunti O.S. Organizzazioni Speciali, Firenze.
- 29. Beery KE (2000) VMI: Developmental test of visual-motor integration. Italian Adaptation. Organizzazioni Speciali, Firenze.
- 30. Hammill D, Pearson N, Voress J (1994) TPV. Test di percezione visiva e integrazione visuo-motoria. Erickson, Trento.
- 31. Vicari S (2007) In O. S. Giunti (Ed.), PROMEA: Prove di memoria e apprendimento. Firenze: Organizzazioni Speciali.
- 60. Hoffstaedter F, Grefkes C, Zilles K, Eickhoff SB (2012) The “What” and “When” of Self-Initiated Movements. Cereb Cortex In press .
36 Observational Learning (Modeling)
[latexpage]
Learning Objectives
By the end of this section, you will be able to:
- Define observational learning
- Discuss the steps in the modeling process
- Explain the prosocial and antisocial effects of observational learning
Previous sections of this chapter focused on classical and operant conditioning, which are forms of associative learning. In observational learning , we learn by watching others and then imitating, or modeling, what they do or say. The individuals performing the imitated behavior are called models . Research suggests that this imitative learning involves a specific type of neuron, called a mirror neuron (Hickock, 2010; Rizzolatti, Fadiga, Fogassi, & Gallese, 2002; Rizzolatti, Fogassi, & Gallese, 2006).
Humans and other animals are capable of observational learning. As you will see, the phrase “monkey see, monkey do” really is accurate ( [link] ). The same could be said about other animals. For example, in a study of social learning in chimpanzees, researchers gave juice boxes with straws to two groups of captive chimpanzees. The first group dipped the straw into the juice box, and then sucked on the small amount of juice at the end of the straw. The second group sucked through the straw directly, getting much more juice. When the first group, the “dippers,” observed the second group, “the suckers,” what do you think happened? All of the “dippers” in the first group switched to sucking through the straws directly. By simply observing the other chimps and modeling their behavior, they learned that this was a more efficient method of getting juice (Yamamoto, Humle, and Tanaka, 2013).
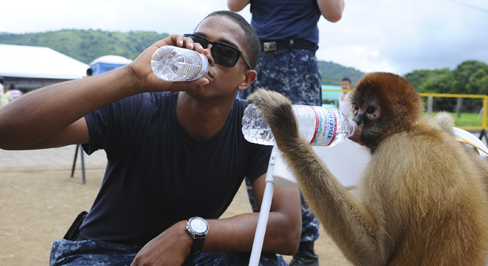
Imitation is much more obvious in humans, but is imitation really the sincerest form of flattery? Consider Claire’s experience with observational learning. Claire’s nine-year-old son, Jay, was getting into trouble at school and was defiant at home. Claire feared that Jay would end up like her brothers, two of whom were in prison. One day, after yet another bad day at school and another negative note from the teacher, Claire, at her wit’s end, beat her son with a belt to get him to behave. Later that night, as she put her children to bed, Claire witnessed her four-year-old daughter, Anna, take a belt to her teddy bear and whip it. Claire was horrified, realizing that Anna was imitating her mother. It was then that Claire knew she wanted to discipline her children in a different manner.
Like Tolman, whose experiments with rats suggested a cognitive component to learning, psychologist Albert Bandura’s ideas about learning were different from those of strict behaviorists. Bandura and other researchers proposed a brand of behaviorism called social learning theory, which took cognitive processes into account. According to Bandura , pure behaviorism could not explain why learning can take place in the absence of external reinforcement. He felt that internal mental states must also have a role in learning and that observational learning involves much more than imitation. In imitation, a person simply copies what the model does. Observational learning is much more complex. According to Lefrançois (2012) there are several ways that observational learning can occur:
Bandura identified three kinds of models: live, verbal, and symbolic. A live model demonstrates a behavior in person, as when Ben stood up on his surfboard so that Julian could see how he did it. A verbal instructional model does not perform the behavior, but instead explains or describes the behavior, as when a soccer coach tells his young players to kick the ball with the side of the foot, not with the toe. A symbolic model can be fictional characters or real people who demonstrate behaviors in books, movies, television shows, video games, or Internet sources ( [link] ).
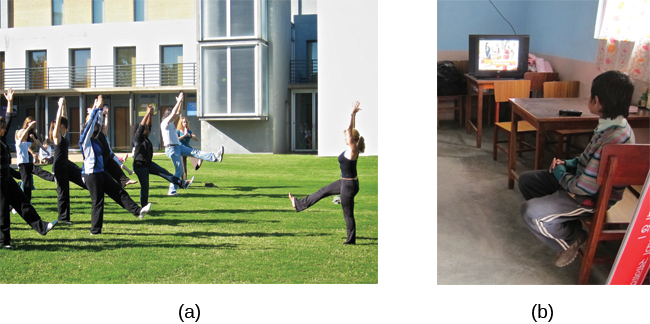
Latent learning and modeling are used all the time in the world of marketing and advertising. This commercial played for months across the New York, New Jersey, and Connecticut areas, Derek Jeter, an award-winning baseball player for the New York Yankees, is advertising a Ford. The commercial aired in a part of the country where Jeter is an incredibly well-known athlete. He is wealthy, and considered very loyal and good looking. What message are the advertisers sending by having him featured in the ad? How effective do you think it is?
STEPS IN THE MODELING PROCESS
Of course, we don’t learn a behavior simply by observing a model. Bandura described specific steps in the process of modeling that must be followed if learning is to be successful: attention, retention, reproduction, and motivation. First, you must be focused on what the model is doing—you have to pay attention. Next, you must be able to retain, or remember, what you observed; this is retention. Then, you must be able to perform the behavior that you observed and committed to memory; this is reproduction. Finally, you must have motivation. You need to want to copy the behavior, and whether or not you are motivated depends on what happened to the model. If you saw that the model was reinforced for her behavior, you will be more motivated to copy her. This is known as vicarious reinforcement . On the other hand, if you observed the model being punished, you would be less motivated to copy her. This is called vicarious punishment . For example, imagine that four-year-old Allison watched her older sister Kaitlyn playing in their mother’s makeup, and then saw Kaitlyn get a time out when their mother came in. After their mother left the room, Allison was tempted to play in the make-up, but she did not want to get a time-out from her mother. What do you think she did? Once you actually demonstrate the new behavior, the reinforcement you receive plays a part in whether or not you will repeat the behavior.
Bandura researched modeling behavior, particularly children’s modeling of adults’ aggressive and violent behaviors (Bandura, Ross, & Ross, 1961). He conducted an experiment with a five-foot inflatable doll that he called a Bobo doll. In the experiment, children’s aggressive behavior was influenced by whether the teacher was punished for her behavior. In one scenario, a teacher acted aggressively with the doll, hitting, throwing, and even punching the doll, while a child watched. There were two types of responses by the children to the teacher’s behavior. When the teacher was punished for her bad behavior, the children decreased their tendency to act as she had. When the teacher was praised or ignored (and not punished for her behavior), the children imitated what she did, and even what she said. They punched, kicked, and yelled at the doll.
Watch this video clip to see a portion of the famous Bobo doll experiment, including an interview with Albert Bandura.
What are the implications of this study? Bandura concluded that we watch and learn, and that this learning can have both prosocial and antisocial effects. Prosocial (positive) models can be used to encourage socially acceptable behavior. Parents in particular should take note of this finding. If you want your children to read, then read to them. Let them see you reading. Keep books in your home. Talk about your favorite books. If you want your children to be healthy, then let them see you eat right and exercise, and spend time engaging in physical fitness activities together. The same holds true for qualities like kindness, courtesy, and honesty. The main idea is that children observe and learn from their parents, even their parents’ morals, so be consistent and toss out the old adage “Do as I say, not as I do,” because children tend to copy what you do instead of what you say. Besides parents, many public figures, such as Martin Luther King, Jr. and Mahatma Gandhi, are viewed as prosocial models who are able to inspire global social change. Can you think of someone who has been a prosocial model in your life?
The antisocial effects of observational learning are also worth mentioning. As you saw from the example of Claire at the beginning of this section, her daughter viewed Claire’s aggressive behavior and copied it. Research suggests that this may help to explain why abused children often grow up to be abusers themselves (Murrell, Christoff, & Henning, 2007). In fact, about 30% of abused children become abusive parents (U.S. Department of Health & Human Services, 2013). We tend to do what we know. Abused children, who grow up witnessing their parents deal with anger and frustration through violent and aggressive acts, often learn to behave in that manner themselves. Sadly, it’s a vicious cycle that’s difficult to break.
Some studies suggest that violent television shows, movies, and video games may also have antisocial effects ( [link] ) although further research needs to be done to understand the correlational and causational aspects of media violence and behavior. Some studies have found a link between viewing violence and aggression seen in children (Anderson & Gentile, 2008; Kirsch, 2010; Miller, Grabell, Thomas, Bermann, & Graham-Bermann, 2012). These findings may not be surprising, given that a child graduating from high school has been exposed to around 200,000 violent acts including murder, robbery, torture, bombings, beatings, and rape through various forms of media (Huston et al., 1992). Not only might viewing media violence affect aggressive behavior by teaching people to act that way in real life situations, but it has also been suggested that repeated exposure to violent acts also desensitizes people to it. Psychologists are working to understand this dynamic.
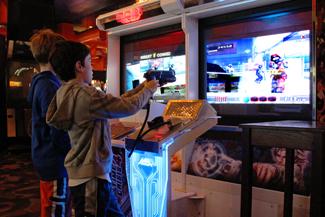
View this video to hear Brad Bushman, a psychologist who has published extensively on human aggression and violence, discuss his research.
According to Bandura, learning can occur by watching others and then modeling what they do or say. This is known as observational learning. There are specific steps in the process of modeling that must be followed if learning is to be successful. These steps include attention, retention, reproduction, and motivation. Through modeling, Bandura has shown that children learn many things both good and bad simply by watching their parents, siblings, and others.
Review Questions
The person who performs a behavior that serves as an example is called a ________.
In Bandura’s Bobo doll study, when the children who watched the aggressive model were placed in a room with the doll and other toys, they ________.
- ignored the doll
- played nicely with the doll
- played with tinker toys
- kicked and threw the doll
Which is the correct order of steps in the modeling process?
- attention, retention, reproduction, motivation
- motivation, attention, reproduction, retention
- attention, motivation, retention, reproduction
- motivation, attention, retention, reproduction
Who proposed observational learning?
- Ivan Pavlov
- John Watson
- Albert Bandura
- B. F. Skinner
Critical Thinking Questions
What is the effect of prosocial modeling and antisocial modeling?
Prosocial modeling can prompt others to engage in helpful and healthy behaviors, while antisocial modeling can prompt others to engage in violent, aggressive, and unhealthy behaviors.
Cara is 17 years old. Cara’s mother and father both drink alcohol every night. They tell Cara that drinking is bad and she shouldn’t do it. Cara goes to a party where beer is being served. What do you think Cara will do? Why?
Cara is more likely to drink at the party because she has observed her parents drinking regularly. Children tend to follow what a parent does rather than what they say.
Personal Application Question
What is something you have learned how to do after watching someone else?

Share This Book
- Increase Font Size

An official website of the United States government
The .gov means it’s official. Federal government websites often end in .gov or .mil. Before sharing sensitive information, make sure you’re on a federal government site.
The site is secure. The https:// ensures that you are connecting to the official website and that any information you provide is encrypted and transmitted securely.
- Publications
- Account settings
Preview improvements coming to the PMC website in October 2024. Learn More or Try it out now .
- Advanced Search
- Journal List
- Aust Prescr
- v.41(3); 2018 Jun

Observational studies and their utility for practice
Julia fm gilmartin-thomas.
2 Research Department of Practice and Policy, University College London, School of Pharmacy, London
1 Department of Epidemiology and Preventive Medicine, School of Public Health and Preventive Medicine, Monash University, Melbourne
Ingrid Hopper
Randomised controlled clinical trials are the best source of evidence for assessing the efficacy of drugs. Observational studies provide critical descriptive data and information on long-term efficacy and safety that clinical trials cannot provide, at generally much less expense.
Observational studies include case reports and case series, ecological studies, cross-sectional studies, case-control studies and cohort studies. New and ongoing developments in data and analytical technology, such as data linkage and propensity score matching, offer a promising future for observational studies. However, no study design or statistical method can account for confounders and bias in the way that randomised controlled trials can.
Clinical registries are gaining importance as a method to monitor and improve the quality of care in Australia. Although registries are a form of cohort study, clinical trials can be incorporated into them to exploit the routine follow-up of patients to capture relevant outcomes.
Introduction
Observational studies involve the study of participants without any forced change to their circumstances, that is, without any intervention. 1 Although the participants’ behaviour may change under observation, the intent of observational studies is to investigate the ‘natural’ state of risk factors, diseases or outcomes. For drug therapy, a group of people taking the drug can be compared to people not taking the drug.
The main types of observational studies used in health research, their purpose and main strengths and limitations are shown in the Table . 2 - 8
Their purpose may be descriptive, analytical or both.
- Descriptive studies are primarily designed to describe the characteristics of a studied population.
- Analytical studies seek to address cause-and-effect questions.
Case reports and case series
Case reports and case series are strictly speaking not studies. However, they serve a useful role in describing new or notable events in detail. These events often warrant further formal investigation. Examples include reports of unexpected benefits or adverse events, such as a case report describing the use of high-dose quetiapine in treatment-resistant schizophrenia after intolerance to clozapine developed 9 and a case report of a medication error involving lookalike packaging. 10
Ecological studies
Ecological studies are based on analysis of aggregated data at group levels (for example populations), and do not involve data on individuals. These data can be analysed descriptively, but not definitively for causation. Typical examples include studies that examine patterns of drug use over time. One example is the comparison of the use of non-steroidal anti-inflammatory drugs and COX-2 inhibitors in Australia and Canada. 11 Sometimes ecological studies describe associations between drugs and outcomes, such as changes in the rates of upper gastrointestinal haemorrhage after the introduction of COX-2 inhibitors. 12 However, because individual-level data are not presented, causality is at best only implied in ecological studies. The 'ecological fallacy' refers to the error of assuming that associations observed in ecological studies are causal when they are not.
Cross-sectional studies
Cross-sectional studies collect data at a single point in time for each single individual, but the actual data collection may take place over a period of time or on more than one occasion. There is no longitudinal follow-up of individuals. Cross-sectional studies represent the archetypal descriptive study. 1 Typically, they provide a profile of a population of interest, which may be broad, like the Australian Health Survey undertaken intermittently by the Australian Bureau of Statistics, 13 or focused on specific populations, such as older Australians. 14
Case-control studies
Case-control studies focus on determining risk factors for an outcome of interest (such as a disease or a drug’s adverse effect) that has already occurred. 5
- those who already have the outcome (cases)
- those who do not have the outcome (controls), who are often matched to the cases to make them similar and reduce bias.
Second, data on previous exposure to selected risk factors are collected and compared to see if these risk factors are more (or less) common among cases versus controls. Case-control studies are useful for studying the risk factors of rare outcomes, as there is no need to wait for these to occur. Multiple risk factors can be studied, but each case-control study can involve only one outcome. 5 One example explored the relationship between the use of antiplatelet and anticoagulant drugs (risk factor) and the risk of hospitalisation for bleeding (outcome) in older people with a history of stroke. 15 Another case-control study explored the risk factors for the development of flucloxacillin-associated jaundice (outcome). 16
Cohort studies
Cohort studies compare outcomes between or among subgroups of participants defined on the basis of whether or not they are exposed to a particular risk or protective factor (defined as an exposure). They provide information on how these exposures are associated with changes in the risk of particular downstream outcomes. Compared to case-control studies, cohort studies take individuals with exposures and look for outcomes, rather than taking those with outcomes and looking for exposures. Cohort studies are longitudinal, that is they involve follow-up of a cohort of participants over time. This follow-up can be prospective or retrospective. Retrospective cohort studies are those for which follow-up has already occurred. They are typically used to estimate the incidence of outcomes of interest, including the adverse effects of drugs.
Cohort studies provide a higher level of evidence of causality than case-control studies because temporality (the explicit time relationship between exposures and outcomes) is preserved. They also have the advantage of not being limited to a single outcome of interest. Their main disadvantage, compared to case-control studies, has been that longitudinal data are more expensive and time-consuming to collect. However, with the availability of electronic data, it has become easier to collect longitudinal data.
One prospective cohort study explored the relationship between the continuous use of antipsychotic drugs (exposure) and mortality (outcome) and hospitalisation (outcome) in older people. 17 In another older cohort, a retrospective study was used to explore the relationship between long-term treatment adherence (exposure) and hospital readmission (outcome). 18
Observational studies versus randomised controlled trials
Compared to randomised controlled trials, observational studies are relatively quick, inexpensive and easy to undertake. Observational studies can be much larger than randomised controlled trials so they can explore a rare outcome. They can be undertaken when a randomised controlled trial would be unethical. However, observational studies cannot control for bias and confounding to the extent that clinical trials can. Randomisation in clinical trials remains the best way to control for confounding by ensuring that potential confounders (such as age, sex and comorbidities) are evenly matched between the groups being compared. In observational studies, adjustment for potential confounders can be undertaken, but only for a limited number of confounders, and only those that are known. Randomisation in clinical trials also minimises selection bias, while blinding (masking) controls for information bias. Hence, for questions regarding drug efficacy, randomised controlled trials provide the most robust evidence.
New and upcoming developments
New methods of analysis and advances in technology are changing the way observational studies are performed.
Clinical registries
Clinical registries are essentially cohort studies, and are gaining importance as a method to monitor and improve the quality of care. 19 These registries systematically collect a uniform longitudinal dataset to evaluate specific outcomes for a population that is identified by a specific disease, condition or exposure. This allows for the identification of variations in clinical practice 20 and benchmarking across practitioners or institutions. These data can then be used to develop initiatives to improve evidence-based care and patient outcomes. 21
An example of a clinical registry in Australia is the Australian Rheumatology Association Database, 22 which collects data on the biologic disease-modifying antirheumatic drugs used for inflammatory arthritis. Clinical data from treating specialists are combined with patient-reported quality of life data and linked to national databases such as Medicare and the National Death Index. This registry has provided insight into the safety and efficacy of drugs and their effect on quality of life. It was used by the Pharmaceutical Benefits Advisory Committee to assess cost-effectiveness of these drugs. 23
Another example is the Haemostasis Registry. It was used to determine the thromboembolic adverse effects of off-label use of recombinant factor VII. 24
Clinical registries can also be used to undertake clinical trials which are nested within the registry architecture. Patients within a registry are randomised to interventions and comparators of interest. Their outcome data are then collected as part of the routine operation of the registry. The key advantages are convenience, reduced costs and greater representativeness of registry populations as opposed to those of traditional clinical trials.
One of the first registry-based trials was nested within the SWEDEHEART registry. 25 This prospectively examined manual aspiration of thrombus at the time of percutaneous coronary intervention in over 7000 patients. 26 The primary endpoint of all-cause mortality was ascertained through linkage to another Swedish registry. The cost of the trial was estimated to be US$400 000, which was a fraction of the many millions that a randomised controlled trial would have cost.
Propensity score matching
Even without randomising people within cohorts, methods have emerged in recent years that allow for less biased comparisons of two or more subgroups. Propensity score matching is a way to assemble two or more groups for comparison so that they appear like they had been randomised to an intervention or a comparator. 27 In short, the method involves logistic regression analyses to determine the likelihood (propensity) of each person within a cohort being on the intervention, and then matching people who were on the intervention to those who were not on the basis of propensity scores. Outcomes are then compared between the groups. Propensity score analysis of a large cohort of patients with relapsing remitting multiple sclerosis found that natalizumab was superior to interferon beta and glatiramer acetate in terms of improved outcomes. 28
Data technology
Increasing sophistication in techniques for data collection will lead to ongoing improvements in the capacity to undertake observational studies (and also clinical trials). Data linkage already offers a convenient way to capture outcomes, including retrospectively. However, ethical considerations must be taken into account, such as the possibility that informed consent might be required before linking data. Machine learning will soon allow for easy analyses of unstructured text (such as free text entries in an electronic prescription). 29 Patient-reported outcome measures are important and in future will be greatly facilitated by standardised, secure hardware and software platforms that allow for their capture, processing and analyses.
While clinical trials remain the best source of evidence regarding the efficacy of drugs, observational studies provide critical descriptive data. Observational studies can also provide information on long-term efficacy and safety that is usually lacking in clinical trials. New and ongoing developments in data and analytical technology offer a promising future for observational studies in pharmaceutical research.
Conflict of interest: Julia Gilmartin-Thomas is a Dementia research development fellow with the National Health and Medical Research Council (NHMRC) - Australian Research Council (ARC). Ingrid Hopper is supported by an NHMRC Early Career Fellowship.
Academia.edu no longer supports Internet Explorer.
To browse Academia.edu and the wider internet faster and more securely, please take a few seconds to upgrade your browser .
Enter the email address you signed up with and we'll email you a reset link.
- We're Hiring!
- Help Center
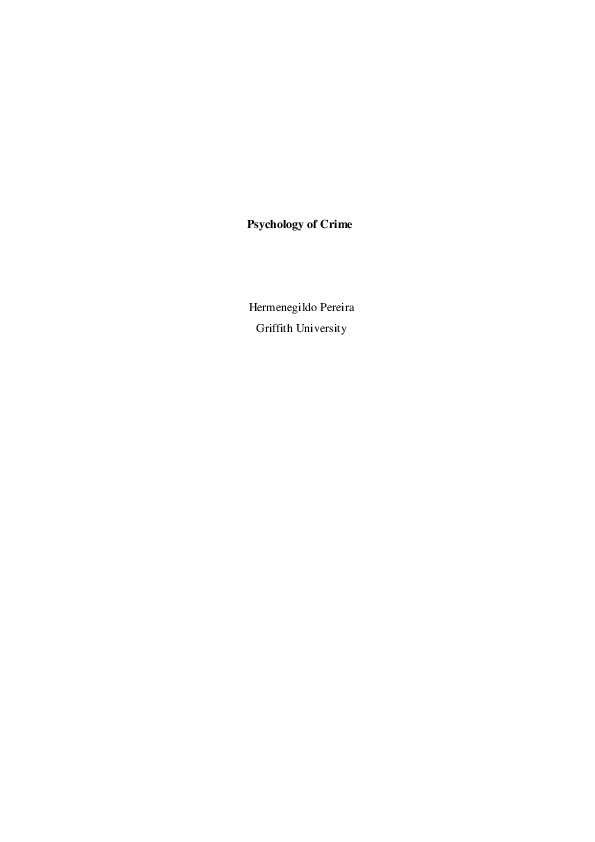
Case Study based on Albert Banduras theory

Related Papers
International Journal of Advanced Research (IJAR)
Aman Tiwari
This research is set out to carefully analyze the psyche of the criminals and to identify commonality in their behaviour irrespective of the demographic aspect. The research aims at analyzing criminal law theories and their practical usage in the modern scenario. Crime is defined as an act of deviance from socially accepted norms translated as criminal code. Countries all around the world have a definite set of criminal code compromising principles of morality and ethics as per their unique culture and society. But in practicality, these principles have failed to culminate the desired result of prevention of crime upon implementation by the traditional methods of enforcement. Deviance relates to the subjectivity of society. Considering morality as a subjective aspect would propagate deviance among individuals formed out of different circumstances than the majority. Hence, crime is often committed by the minority upon the majority in a society. The ultimate aim of the research is to identify the principle of causality in relation to crime and eventually portraying an effective approach for the prevention of crime.
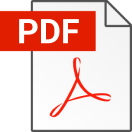
Hande Sahin
— Crime is a complex phenomenon and therefore has many definitions. At the same time it is a major problem for the entire world. The crime rates and also juvenile crime has increased over the world. Therefore especially social sciences focus on subject of crime. There are also so many theories about crime. Each of them focuses on a different aspect of the crime. This study focuses on the social aspect of crime. The subject of this study is the relationship between crime and education. The study aims to reveal the crime can be learned. Learning theory is a functional to discuss all these aims. The main assumption of the study is that crime can be learned with process of socialization like other social phenomenon.Failed and inadequate socialization are key phenomenon in this process. People learn criminal behaviour through interaction with intimate person. Or they can commit crime because they don't know correct norms of society because of limited education. The method of the study is that nine master and three doctoral theses, four extensive field researches and government statistics about juvenile delinquency in Turkey have been evaluated related to the subject of the study. Their data has been collected. Some results have been achieved: The education level of juvenile offenders and their families are too low. The number of offenders in families and relatives of these children is quite.
Julius A Adinoyi
Nadia Rahaman
Aggressive Behavior
Anthony Mawson
Karen moore
Diana Shlyapnikova
Through many centuries, criminal behavior was mistakenly explained mostly with biological defects of the individual. It was believed, that abnormal actions, negatively affected society, were preferably conducted by those, who suffered from serious physical deviations in development. However, as the science was progressing, scholars have begun searching other possible causes of the formation of antisocial behavior and psychology began to actively explore this area. Nowadays, it is known, that incorrect parenting style, negative influence of environment, and formation of improper role models may significantly result inner conflicts and thus, led to the abnormal behavior. In this essay, we would further examine, how these aspects can influence the behavior of individuals and lead to criminal conduct, illustrating it with examples from biographies of famous criminals to show how concepts can be applied in reality. First of all, it is necessary to draw a distinction between biological and psychological factors, influencing the behavior of individual. Under biological factors we mean physical anomalies, such as neurotransmitter dysfunction, bradygenesis, and other problems of neural development, genetically predisposed or caused by trauma or injury. Under psychological factors we mean mental disorders, and deviation in education and mental development of the individual. However, despite the fact that the differences seem obvious, both areas are interconnected between deeply. As we know, the connection of nature and nature is rooted into every individual. As the course textbook states " everything psychological is simultaneously biological " (Meyers, 1999, Chapter 2, p.47). Thus, various theories, designed to explain the emerging and development of deviant behavior, combine both approaches. For example, theory of personality and crime by Hans J. Eysenck, British psychologist, states that each individual has innate hereditary predisposition towards asocial behavior, which discloses in certain circumstances. Thus, " criminal behavior is the result of an interaction between certain environmental conditions and features of the nervous system " (Bartol & Bartol, 2005). However, Eysenck does not say, that deviant behavior inborn, but may be caused by the compound of heredity and environment. It is not itself, or criminality that is innate; it is certain peculiarities of the central and autonomic nervous system that react with the environment, with upbringing, and many other environmental factors to increase the probability that a given person would act in a certain antisocial manner (Eysenck & Gudjonsson, 1989)
Journal of emerging technologies and innovative research
trimurthi rao
IJPUBLICATION
Srinivas Katherasala
Juvenile delinquency is an enormous problem in India by which most of the youth ruin their lives. On account of adolescent wrongdoing and relate issues youth, their families and the whole society endure numerous fallouts. Besides the fact that the issue influences the survivors of the wrongdoing; it additionally influences the adolescent reprobates family, The most significant result of violations perpetrated by adolescents conveys due to financial and mental issues which consider their relatives and the general public. The primary goal of this paper is to concentrate on the frequency of adolescent misconduct regarding mental viewpoints. The adolescent who carry out serious wrongdoings challenge their future to fight apparent maltreatments that have been executed against them. "Current cultures appear to see youngsters in a fairly irresolute way. The last many years of the 20th century gave us two or three telling models from a few nations. From one perspective, the kids and adolescents are seen as requiring care and security. Assuming that they become delinquent, instructive measures are believed to be the fitting in the event that not vital response to the issues they are causing or may experience the ill effects of. This is the overall disposition among everybody in most of nations on the planet, insofar as the offenses committed by youths don't rise steeply in numbers and stay frivolous or moderate in quality.
Cumhuriyet Medical Journal
RELATED PAPERS
Shekhar Gupta
Journal of the Brazilian Chemical Society
Yahya Pasdar
Rev. bras. educ. …
Ana Claudia Bortolozzi Maia
Jean-hugues Chauchat
mohamed afifi
World Journal of Dentistry
shakila nazir
Arquivos Brasileiros de Oftalmologia
Leopoldo Magacho
ECONOMICS AND INNOVATION MANAGEMENT
Zikri Zakaria
Indian Journal of Gender Studies
Deepita Chakravarty
JOSÉ GREGORIO PEREIRA ARIAS
Chemical research in toxicology
Ana Coimbra
Graciela Hamame
Biomath Communications
DANIEL IKHU-OMOREGBE
Zerrin Sungur
andrea tintori
Wang Xiaofei
Lancet (London, England)
Pedro Orduñez
IOP Conference Series: Earth and Environmental Science
Reza Zubaedah
Jürgen Schüttler
Research Square (Research Square)
Dheeraj Dhiman
International Journal of Pharmaceutical Chemistry and Analysis
Abdul Kader Mohiuddin
Tecnología Química
Electo E . Silva Lora
Frontiers in Communication
Slavka Demuthova
Victoria Vargas Downing
RELATED TOPICS
- We're Hiring!
- Help Center
- Find new research papers in:
- Health Sciences
- Earth Sciences
- Cognitive Science
- Mathematics
- Computer Science
- Academia ©2024
- Open access
- Published: 16 April 2024
PheSeq, a Bayesian deep learning model to enhance and interpret the gene-disease association studies
- Xinzhi Yao 1 , 2 ,
- Sizhuo Ouyang 1 , 2 ,
- Yulong Lian 3 ,
- Qianqian Peng 1 , 2 ,
- Xionghui Zhou 1 , 2 ,
- Feier Huang 4 ,
- Xuehai Hu 1 , 2 ,
- Feng Shi 3 &
- Jingbo Xia ORCID: orcid.org/0000-0002-7285-588X 1 , 2
Genome Medicine volume 16 , Article number: 56 ( 2024 ) Cite this article
128 Accesses
10 Altmetric
Metrics details
Despite the abundance of genotype-phenotype association studies, the resulting association outcomes often lack robustness and interpretations. To address these challenges, we introduce PheSeq, a Bayesian deep learning model that enhances and interprets association studies through the integration and perception of phenotype descriptions. By implementing the PheSeq model in three case studies on Alzheimer’s disease, breast cancer, and lung cancer, we identify 1024 priority genes for Alzheimer’s disease and 818 and 566 genes for breast cancer and lung cancer, respectively. Benefiting from data fusion, these findings represent moderate positive rates, high recall rates, and interpretation in gene-disease association studies.
In the scenario of genotype-phenotype association, the association significance usually comes from a good variety of sequence analysis experiments in the form of a p -value, e.g., GWAS [ 1 ], PheWAS [ 2 ], RNA-seq [ 3 ], and MeRIP-seq [ 4 ]. While p -value data provide a genome-wide significance for each genotype-phenotype association, the characteristics of high density and uncertainty make it a fine-grained but weak signal. The uncertainty of the p -value and the rigorous significance threshold setting [ 5 , 6 ] make it challenging to obtain robust association results, and in the meantime, the interpretations of the results are largely unclear [ 7 ].
In recent years, a big trend has emerged in the field of deep learning (DL), focusing on diverse multi-omics data including genome, transcriptome, epigenome, proteome, exposome, and microbiome [ 8 ]. Commonly employed deep learning techniques have been widely utilized for feature extraction, integrated analysis, and robust predictive modeling across various life omics datasets. However, this research directs its primary attention to the perceptual aspects of deep learning methods pertaining to gene-disease association, particularly those derived from textual evidence and network structures. The primary objective of this study is to enhance the robustness and interpretability of gene-disease associations through the integration of external text and network data, thus augmenting the findings obtained from single-omic sequence analysis. In this regard, it is necessary to fuse association information from two different modalities, the association significance ( p -values) and DL-generated phenotype descriptions (embeddings), through a data fusion strategy.
DL models have shown a strong ability to perceive semantic interpretation in texts and the topological structure of networks in association studies [ 9 , 10 ]. Benefiting from its powerful perception ability, DL plays a role in interpreting the text or network data for associations and making the association prediction evidence-supportive and ontology-normalized [ 11 , 12 ]. The Human Phenotype Ontology (HPO) was initially published in 2008 [ 13 ] with the goal of integrating phenotypic data for translational research and diagnostics, and it maintains a stable rate of update [ 14 , 15 , 16 ]. In recent years, the range of HPO has been extended from rare to common human disease [ 17 ]. Today, HPO terms are extended to a broader range of diseases [ 18 ], as well as specific diseases like cancer, as it permits the tagging and curation of the underlying phenotypes that are associated with variants described in the literature [ 19 ]. Galer et al. [ 20 ] used the terms defined in HPO, performed a DL-based semantic similarity analysis, and associated clinical features with distinct genetic etiologies. Greene et al. [ 21 ] merged HPO-coded profiles with functional gene-specific information and successfully identified several true gene-disease associations among a large collection of genome-sequenced and HPO-coded cases with rare diseases. Peterson et al. [ 22 ] derived HPO-based phenotype descriptions from patients’ clinical notes and used DL-based means to prioritize disease-associated patients. James et al. [ 23 ] systematically integrated clinical phenotype data with genotype information, and leveraged HPO-based patient phenotype and variant data into clinical variant prioritization.
In addition to data perception, the ultimate objective of this study is to comprehend the interrelationships between two distinct modalities of association information. To achieve the goal of association inference, one commonly used algorithmic option is the Bayesian network framework [ 24 , 25 , 26 ]. This framework is rooted in a statistical model that effectively captures and models the uncertainty of observations, enabling inference of the hidden relational dependencies within the data. With its Bayesian nature, a Bayesian network treats the data in the form of distribution and regards data relation dependencies as conditional probability [ 27 ]. Under a Bayesian framework, a Bayesian network learns the data relationship, unveils the potential conditional dependencies, and achieves relational modeling among observations, thus making it a strong tool in association investigation [ 28 ]. Shaw and Campbell [ 29 ] used the Bayesian network to combine gene variation frequency with biological annotations and developed a variation interpretation model. Dai [ 30 ] proposed IGESS, a Bayesian network framework, to model the distribution dependency of the p -value in GWAS and trait output, and improved the accuracy of risk variant inference. De et al. [ 31 ] proposed a Bayesian network model that aggregated inputs from multiple variant prioritization algorithms with genomic and clinical database annotations and prioritized potentially damaged genes and candidate diseases. Zhou [ 32 ] applied another Bayesian network framework to investigate the potential dependencies between GWAS summary data and mutation descriptors from the literature and reconstructed the observation and their dependencies to promote the inference of gene-disease associations. By using the Bayesian network, all of the above works facilitated conditional dependency modeling in genotype-phenotype association studies, but they ignored the perception of the data semantics as done by DL methods, thus imposing a limitation on result interpretation.
Recently, there has been a trend of hybrid strategy that combines DL with a Bayesian network, known as Bayesian deep learning (BDL), formed by a series of works [ 33 , 34 , 35 ]. Inheriting the Bayesian idea of traditional Bayesian neural networks (BNN), [ 36 , 37 ] BDL uses the probabilistic graphical model (PGM) in the Bayesian network to model the uncertainty and relational dependence among data but integrates a DL perception module into the probabilistic graphical model through a hinge variable. A general BDL framework involves a DL perception module and a PGM inference module, which extracts high-quality semantic representations upon the observations and investigates the potential dependencies among the data. Adam [ 38 ] used a variational autoencoder (VAE) module to perceive a semantic representation from the integrated omics data including transcriptomic and proteomic data. The observation representation is then input into a Bayesian network to assist the inference of the hidden relationships among the single cell and its corresponding omics data. This work also demonstrates an intuition that the perception of omics data using DL can boost the performance of higher-level inference and in turn, the feedback from the inference process also enhances the perceptual power.
All the above advances suggest that a BDL framework not only perceives the data feature representation but also infers the hidden relationships among the data. Therefore, BDL encourages the effective synergy of DL and Bayesian network, and supports conditional dependence modeling in the genotype-phenotype association study.
This study presents a novel Bayesian deep learning model named PheSeq, which aims to bridge the phenotype descriptions with association significance in gene-disease associations. To achieve this, PheSeq trains a 96-layer deep learning module to perceive the phenotype descriptions in the literature and network, incorporates association significance within a Bayesian network framework, learns the inherent dependencies among associations through data fusion techniques, and ultimately discovers novel gene-disease observations. As a result, PheSeq offers an interpretable high-level inference for novel gene-disease associations
The PheSeq model is employed in three distinct gene-disease association case studies. The first case study involves the use of GWAS summary data for AD, the second case study utilizes transcriptomic data for breast cancer (BC), and the third one employs methylation data for lung cancer (LC). We collect p -values for sequence analysis under three distinct cases to obtain association significance between gene and disease. Simultaneously, we collect phenotype descriptions for each gene-disease pair in literature and network and employ a computational pipeline to generate phenotypic embedding for the gene-disease pair. Our model, utilizing data fusion learning, integrates association information from two different modalities, resulting in a more comprehensive recommendation of gene-disease associations. The findings indicate that PheSeq produces prioritized genes with a moderately positive rate when compared to traditional single sequence analysis. In the case of AD, the percentage of prioritized significant genes over background GWAS genes is 5.6%, which represents a substantial improvement over the low positive rate of 1.7% observed in the GWAS experiment. Similarly, PheSeq filters 2.3% of genes in LC methylation data and 0.75% in BC transcriptome data, compared to the respective positive rates of 4.7% and 2.7% in the conventional sequence analysis. In all three case studies, the top 50 prioritized genes include over half that is consistent with previously known gene-disease associations recorded in the DISEASES database [ 39 ]. In addition, it is worth noting that a significant proportion of prioritized genes in AD cases, specifically 90% (45 out of 50), can be readily interpreted with supporting evidence obtained from GWAS experiments or established databases.
The contribution of the PheSeq model is twofold. Firstly, it employs a data fusion strategy to improve the study of gene-disease associations by combining p -value data and phenotypic embeddings. Additionally, it utilizes phenotype descriptions to interpret the associations. Results in case studies show that PheSeq obtains a moderate positive rate and high recall rate, benefiting from the data fusion strategy. In addition, The model derives a vast dataset of association evidence, making it possible for the interpretation and exploration of gene-disease associations.
Data collection for sequence analysis, literature, and network data in three case studies
To investigate the efficacy and robustness of the PheSeq model, three diseases characterized by distinct pathological features are selected as case studies. Relevant sequence analysis and literature data are collected for each of them.
The three selected diseases, along with their corresponding sequence analysis data, represent a broad spectrum of gene-pathology associations among patient populations. Alzheimer’s disease (AD), a prototypical genetic disorder, is analyzed using genome-wide association study (GWAS) summary data to elucidate the significance of single nucleotide polymorphisms (SNPs) in genetic inheritance. Breast cancer (BC), a typical cancer type that has been extensively studied, has comprehensive and representative expression profile data for association research. We therefore employ transcriptome data for sequence analysis. Additionally, the etiology of lung cancer (LC) is more complex, influenced by environmental factors and epigenetics. Therefore, methylation data is chosen as the representative sequence analysis data.
For the sequence analysis, the AD-related GWAS summary data were collected from GCST002245 [ 40 ] on the GWAS Catalog. The transcriptome data were generated using the Agilent G4502A_07_3 platform, and the methylation data from the Human Methylation 450 platform were retrieved from TCGA [ 41 ]. Then, the association significance for each gene was obtained, and the Manhattan-style plot of the above results is shown in Fig. 1 .
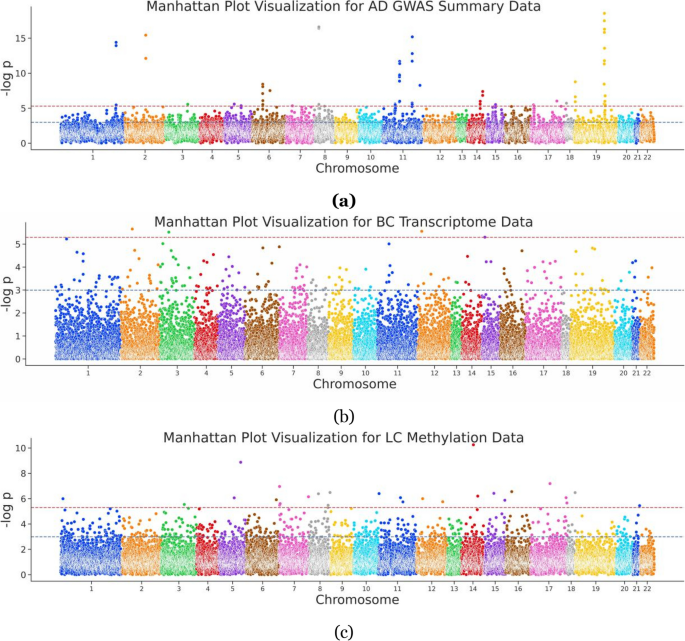
Manhattan plot of sequence analysis data. a AD GWAS, depicting 18,157 genes visualized in a Manhattan plot with \(-\log\) p value represented along a vertical line and chromosome position on the x -axis; b BC sequence analysis incorporating transcriptomic data, 17,374 genes encompassed; c LC sequence analysis integrating methylation data, involving 24,578 genes
In detail, for AD, GWAS summary data were downloaded from summary data of the international genomics of Alzheimer’s project (IGAP) [ 40 ] ( https://www.niagads.org/datasets/ng00036 ), which performed a two-stage GWAS on individuals of European ancestry on 7,055,881 SNPs, and 23 genes were proposed to include the AD-related SNPs. In accordance with standard conventions [ 42 ], we adhered to a straightforward practice in retrieving genes located ± 100 kb of the SNP site by using Bedtools, thus assigning association significance to corresponding genes. For BC, the transcriptome data from TCGA [ 41 ] were collected (Platform: AgilentG4502A_07_3), which includes 597 cancer samples and 64 healthy samples. The two-sample T -test was used to calculate the differential expression for each gene in the patient population and the normal population, thus obtaining the association significance for each gene. For LC data, 862 samples with prognostic information were collected from TCGA (Platform: HumanMethylation450). A prognostic analysis was performed by combining the prognostic data of each patient. A Cox regression was performed to infer the correlation between the methylation site and the prognostic risk, and the association significance for each methylation site was assigned to the corresponding gene. In total, GWAS in AD covers 18,157 genes and assigns p -value to each of them. Meanwhile, the count of genes in sequence analysis upon BC and LC is 17,374 and 24,578, respectively (Table 1 ).
To collect phenotype descriptions, disease-related literature was downloaded on a PubMed and PMC scale. Specifically, we gathered the full names of diseases (Alzheimer’s disease, breast cancer, and lung cancer) and their corresponding MeSH terms. After downloading the PMID and PMCID list, the PubTator API was then utilized to retrieve all available abstracts and full-text articles. To ensure the relevance of the literature to the diseases, keyword matching was employed as an additional filtering step. Specifically, we required that the full name or abbreviation of the disease is mentioned at least 3 or 5 times within the abstract or full text respectively. In total, 24,440 pieces of literature were obtained within the AD topic, mentioning 14,261 genes. Likewise, 55,638 and 81,463 pieces of literature were obtained for BC and LC, covering 10,498 and 20,460 genes, respectively (Table 1 ).
In addition, network data were collected to capture structural information of genes. Specifically, the protein-protein interaction (PPI) network data for Homo sapiens were gathered from the STRING database [ 43 ], and the filtering of PPIs was performed by applying a confidence threshold of 0.7, following the guidelines provided by the STRING database. As a result, a PPI network with 359,776 edges was obtained. After mapping protein IDs to gene Entrez ID, 15,131 unique genes were included in this network.
A phenotypic embedding generation pipeline
For a given gene-disease pair, a phenotypic embedding generation pipeline is proposed to process concept and sentence embeddings from literature and process graph embedding from network data.
When processing sentences which contain a given gene, we first annotate three types of phenotype description phrases, including biological process terms, phenotypic terms, and disease terms. These terms are then normalized by gene ontology (GO), HPO, and MeSH, respectively. In detail, OGER++ [ 44 ] is used to annotate and normalize the GO terms, PhenoTagger [ 45 ] is for the HPO terms, and PubTator is used for the gene and disease mentions. Subsequently, sentences that address phenotype description of the gene-disease association are filtered by a biomedical event extraction model [ 32 ] on AGAC corpus [ 46 ]. This model detects the biomedical events in texts, including molecular physiological activity, cell physiological activity, and interactions. Altogether, concepts and sentences for each gene are encoded by BioBERT [ 47 ] and put into a deep neural network to generate concept and sentence embeddings.
When processing network that contains a specific gene, we locate the gene within the STRING network and then apply a graph embedding method. The proposed pipeline provides a diverse range of options for embedding computation, including node2vec [ 48 ], Mashup [ 49 ], BioPlex 3.0 [ 50 , 51 ], HuRI, and drug-target network [ 50 , 52 ], and struct2vec [ 53 ]. In the case studies, we primarily adhere to Yue’s guideline [ 54 ] and employ struct2vec to compute the graph embedding.
Finally, a dynamic meta-embedding method, proposed by Douwe [ 55 ], is used to compute an average weight of the bio-concept embedding, sentence embedding, and graph embedding for each association. The resulting integration of these distinct embedding modalities aims to enable a robust phenotypic embedding representation of each gene-disease association.
Furthermore, to facilitate user observation of the quality of their embedding data, the corresponding visualization tools are also provided in this pipeline, and the details are given in the Additional file 1 .
PheSeq, the proposed data fusion model
Motive of data fusion.
In this section, we introduce mathematical notations to illustrate the motive and setting of the model.
For a given disease d , the gene-disease association data include p -value data \(P_g\) and embedding data \(L_g\) for gene g , each of which is collected from multi-omics sequence analysis and text/network representation learning, respectively. The left side of the figure presents association data from two perspectives: the Manhattan plot for \(P_g\) from the sequence analysis and the embedded semantic space plot for \(L_g\) from the embedding generation pipeline. The Manhattan plot serves as a standard graphical representation of the association significance p -values between genes and diseases in sequence analysis, where the chromosome position for each g is placed on the x -axis, while the significance of \(P_g\) is stated as \(-\log p\) along the y -axis. In the embedded semantic space plot, each point signifies the phenotypic embedding associated with g and d . Typically, genes with similar semantic associations cluster together. Both the Manhattan plot and the semantic space plot provide visualizations of two modalities of data pertaining to gene-disease associations.
Generally, the p -value threshold or semantic similarity is applied in sequence analysis or representation learning. For example, a false discovery rate such as the Bonferroni or Benjamini test [ 56 ] is applied as a strict threshold for \(P_g\) -based significant analysis, while 0.05 is regarded as an empirical but less strict threshold, as shown in Fig. 2 a. Therefore, the threshold of the p -value needs to be considered in terms of the data congruence with the phenotype description. Intuitively, from a view of semantic similarity, embedding data \(L_g\) with similar significant or non-significant association semantics is prone to forming a cluster in a semantic space. As illustrated in Fig. 2 a, under the guidance of a color-coding scheme, the synergy and fusion of data from these two modalities can be observed.
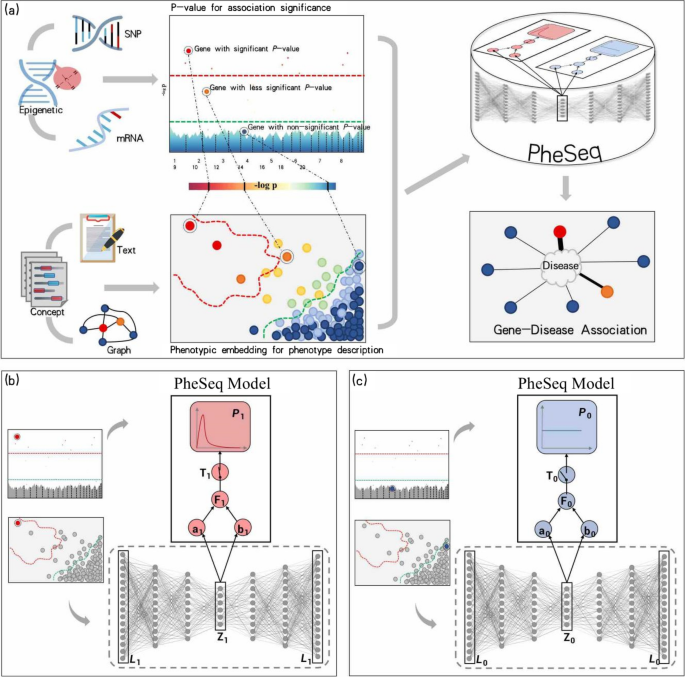
The framework of PheSeq. a General model input in PheSeq involves p -values for association significance in sequence analysis and phenotypic embeddings for phenotype description from texts or graphs. The associations with p -values are graphically depicted in a Manhattan-style plot. A threshold line with a strict criterion (red line) or a less strict criterion (green line) is then applied. Concurrently, a DL perception module learns the association description of gene-disease association from text or graph. Genes exhibiting significant association descriptions tend to aggregate in the top-left region of the semantic space, as shown in the figure. Analogous patterns emerge in other scenarios. Finally, PheSeq learns the data distributions and performs data fusion for gene-disease associations. b / c Data fusion of association significance and phenotype description for a significant/non-significant gene-disease association by PheSeq. For each gene-disease association, two distinct types of observations, denote as L for phenotypic embedding and P for p -value, are considered for data fusion. Both sets of observations are input into the PGM inference module, facilitating the learning of dependency relationships among them in conjunction with latent variables. The phenotypic embedding L is initially processed through the DL perception module for semantic training, resulting in the generation of high-quality embeddings denoted as Z . The latent variable T serves a pivotal role in synchronizing the phenotypic embedding data with the p -value data, the latter adhering to a beta distribution indicative of a predisposition toward “small- p -value.” In addition, another latent variable F functions as an association score, establishing connnections among model parameters. Conceptually, the switch mechanism activates when both the association significance and phenotype description align, effectively bridging the above heterogeneous data modalities. Part c shows the converse situation, wherein the data indicate non-significance for the gene-disease association. In this case, a uniform distribution is employed to characterize the distribution of the p -value. The remaining configurations of the model remain consistent
Model setting
Under a BDL framework, we propose a generative model, PheSeq, to uncover underlying gene-disease associations by bridging two types of heterogeneous association data. The data comprise the phenotype description data in texts and graphs, along with association significance data derived from sequence analysis, collectively unveiling associations between genes and diseases.
PheSeq consists of two modules, i.e., a DL module for the perception task and a PGM module for the inference task. The DL module consists of a 96-layer deep neural network, designed to perceive semantic interpretation in texts and the network topology structures related to the phenotype description of associations. In the meantime, the PGM module models the association significance with inherent uncertainty via a random variable setting and captures the distribution dependency among phenotype description and association significance. Finally, data fusion of the heterogeneous association data is performed in a BDL framework, whose inherent attribute is a generative model. This framework then generates novel association significance by establishing a connection between the two types of heterogeneous association data. In summary, PheSeq learns the data congruence of phenotype description and association significance, leverages the collective power of the heterogeneous data, and enables the inference of novel gene-disease associations.
PheSeq encompasses two algorithmic variations based on the distinction in pheotypic embedding input. Specifically, it includes a Static-PheSeq model designed for a predefined set of embedding data and a Dynamic-PheSeq model tailored for a set of flexible and learnable embedding data. The Static-PheSeq model assumes that the embedding data are already well-learned to represent the source data, so a fixed deep-learned representation is fed into a Bayesian network and captures the potential relations and dependencies among the genotype-phenotype data. Meanwhile, the Dynamic-PheSeq model involves both phenotypic embedding and p -value into a BDL framework, and the embedding data and all the PGM parameters are adjusted dynamically.
Embedding computation and p -value modeling for gene-disease association
In the DL perception module, a neural network \(V_{\theta }(\cdot )\) is introduced to perceive the association description and learn a semantic representation. In the Static-PheSeq model, \(V_{\theta }(\cdot )\) is implemented by the BioBERT deep network to generate a fixed representation. Instead, \(V_{\theta }(\cdot )\) is implemented by a VAE neural network in the Dynamic-PheSeq.
To model the gene-disease association, two latent variables are imported into the PGM inference module. First, a latent variable \(F=\{F_g\}\) represents the score of the gene-disease association and follows the beta distribution with \(a_g\) and \(b_g\) as parameters, i.e., \(F_g\sim Beta(a_g, b_g)\) . Another latent variable \(T=\{T_g\}\sim Bernoulli(F_g)\) plays a “switch” role in synchronizing the heterogeneous association data. It takes a binary value, where 1 indicates a g - d association (as shown in Fig. 2 b) and 0 indicates a non-association (as shown in Fig. 2 c).
Modeling the uncertainty and prior of the p -value has long been a research issue [ 57 , 58 ]. Parker and Rothenberg [ 59 ] found that any distribution on the interval [0, 1] can be modeled as a mixture of individual beta distributions. Allison [ 60 ] chose a standard two-parameter beta distribution for the p -value as it allows for the flexible modeling of shapes on the unit interval and demonstrated its effectiveness in p -value from microarray data. Xiang [ 61 ] further justified the precision of the parameter estimated obtained by fitting a mixture beta-uniform distribution to a p -value distribution. Hu [ 62 ] presented empirical evidence that a standard beta distribution can accurately capture the shape of the true density of the p -value. Zhou [ 32 ] adopted a beta-uniform approximation approach within the Bayesian network framework to approximate the actual p -value obtained from GWAS.
Therefore, in the PheSeq model, a mixture beta-uniform distribution is selected as the prior of p -value and we assume
When the switch is on ( \(T_g=1\) ), \(P_g\) follows a beta distribution, \(Beta(\alpha _g, 1)\) . Here, \(\alpha _g\) is generated by \(\Phi\) and prone to be close to zero, thus leading to a significant association between gene g and disease. Conversely, when the switch is off ( \(T_g=0\) ), \(P_g\) follows a uniform distribution of U (0, 1) and makes it a high chance to sample a less significant p -value.
In summary, all the variables used in PheSeq are:
The BDL framework for Static-PheSeq model solving
In the view of the generative model, the optimization goal of Static-PheSeq is to maximize the log-likelihood of the p -value and random variables conditioned on phenotype descriptions. Since the DL module is fixed in Static-PheSeq when tuning the PGM module, it is straightforward to obtain the parameter iterations via the standard maximum likelihood estimate (MLE) computation.
Based on the distribution dependencies provided in Fig. 2 b and c, the logarithm of the joint probability equals to
Considering the variational sampling of the latent variable, the expectation of the logarithm of the joint pdf, \(L(\Phi ) = E_{q(T, F)}[\log p(P, T, F | Z, \Theta )]\) , is set as the loss function. A Monte Carlo estimation leads to \(L_{MC}(\Phi ) = \log p(P, T^*, F^* | Z, \Theta )\) , where \(T^*, F^* \sim q^*(T, F)\) . As \(\nabla _\Phi L(\Phi ) \approx \nabla _\Phi L_{MC}(\Phi )\) , the noisy estimate of the gradient with respect to the neural network parameters, \(\Phi\) , is
The gradient computation leads to the optimization iteration in MLE. Here, \(\Psi (x)=\Gamma ^\prime (x)/ \Gamma (x)\) is the digamma function. Eventually, a gradient ascent iteration, \(\Phi ^{(t+1)}=\Phi ^{(t)} + \eta \nabla _\Phi L(\Phi )\) , is adopted, where \(\eta\) is the learning rate.
In Static-PheSeq, the model parameters are mainly derived by formula ( 2 ). The iteration ends when these parameters achieve convergence. The implementation of Static-PheSeq is concluded in Algorithm 1, “MLE for Static-PheSeq,” and the complete proof is given in Additional file 2 . The effectiveness of the model is then evaluated in the selected case studies.
The BDL framework for Dynamic-PheSeq model solving
Compared with Static-PheSeq, the main difference in Dynamic-PheSeq is the importing of the learnable embedding data \(Z_g\) , which is encoded by the description data \(L_g\) in a variational autoencoder (VAE) framework. It should be noted that the model parameters, a and b , are relevant to the input learned embedding Z and the neural network with parameter \(\Phi\) , thus denoting it as \(a(\Phi , Z)\) and \(b(\Phi , Z)\) , respectively.
Since \(Z_g\) and \(P_g\) need to be learned jointly in Dynamic-Pheseq, a maximum a posteriori (MAP) estimation and MLE for Bayesian network optimization are applied in the model solving. Here, the optimization goal is to maximize the evidence lower bound (ELBO), which is obtained by computing the expectation of the logarithm of evidence, w.r.t. posterior of all latent variables, i.e.,
where, \(q_\theta (Z_g |L_g)\) denotes an approximation for the posterior pdf of embedding \(Z_g\) generated from the DL perception module, and \(\mathbb{K}\mathbb{L}(\cdot \Vert \cdot )\) refers to the Kullback-Leibler divergence between two pdfs.
Subsequently, MAP with respect to \(q_\theta (Z_g |L_g)\) is applied to maximize the objective for \(\{F_g\}\) , where \(\theta =\{w_{\{1...L\} }, b_{\{1...L\} } \}\) is the parameters of the DL neural network, and \(w_l\) and \(b_l\) are the weight and bias of the \(l^{th}\) layer, respectively.
By using the block coordinate ascent, direct computation yields the iteration formula for \(F_g\) , i.e., \(F_g \leftarrow (T_g+a_g-1)/(a_g+ b_g -1)\) . Considering only the terms related to \(Z_g\) , the Monte Carlo estimation is used to simplify the expectation calculation.
where \(Z_g^{(n)}=\mu _g+ \sigma _g+ \varepsilon _g^{(n)}\) , and \(\varepsilon _g ^{(n)} \sim N(0, I_K)\) , and \(\{1, 2, \cdots , N\}\) is the index for Monte Carlo sampling.
Given a fixed \(F_g\) in the PGM, the gradient computation with respect to \(\mu _g\) and \(\sigma _g\) suffices to optimize the DL perception module.
From ( 3 ), optimization of the weights and bias in DL perception modules is performed through a conventional backpropagation process. The gradient ascent iteration, \(\mu _g^{(t+1)} = \mu _g^{(t)} + \eta \nabla _{\mu _g} L(\Theta , \theta ; Z_g)\) and \(\sigma _g^{(t+1)} = \sigma _g^{(t)} + \eta \nabla _{\sigma _g} L(\Theta , \theta ; Z_g)\) , is applied, where \(\eta\) is the learning rate.
A prerequisite of MLE computation is to compute the joint probability of all observations and latent variables conditioning on parameters set, \(\Theta\) . From the graphical model in Fig. 2 b and c, we have the logarithm of the joint probability
Similar to Static-PheSeq, the expectation of the logarithm of the joint probability w.r.t. posterior distribution of the latent variables is set up as the loss function, i.e., \(L(\Phi ) = E_{q(T, F)}[\log p(P, T, F, Z, L|\Theta )]\)
The gradient computation w.r.t. \(\Phi\) considers the back-propagation flows through the model parameters a , b , and \(\alpha\) , and the iteration formula is the same as the Static-PheSeq in ( 2 ). The implementation of Dynamic-PheSeq is concluded in Algorithm 2, “MAP-MLE for Dynamic-PheSeq,” and the complete proof is given in Additional file 2 .
The results section is organized based on the following logic. Firstly, we present the obtained association significance data and phenotype description data for three different case studies, visualizing the distribution consistency required for data fusion. Secondly, we evaluate the data fusion results of the PheSeq model, including its performance on the reference dataset DISEASES [ 39 ] and a quantitative comparison analysis with results obtained from a single sequence analysis method. Subsequently, we provide an overall comparative observation of the predictions of PheSeq and a single sequence analysis method. Following that, we analyze the positive impact imposed by phenotype description in the PheSeq model. Subsequently, considering that PheSeq incorporates prior knowledge from the literature, we design ablation study to assess PheSeq’s predictive capability by removing prior knowledge. Simultaneously, we conduct a horizontal comparison between PheSeq and several other data fusion methods, comparing the differences in data modalities and data integration strategies. Finally, we develop a phenotype description network to exemplify and showcase the results.
Data visualization for association significance and phenotype description
In the context of three distinct case studies, a total of 24,440 AD-related literature, 55,638 BC-related literature, and 81,463 LC-related literature are fed into the phenotypic embedding generation pipeline. This yields 18,157 gene-AD pairs, 17,374 gene-BC pairs, and 24,578 gene-LC pairs, respectively. We visually represent these associations within the cubic grid in the graphical presentation in Fig. 3 . Leveraging the inherent principles of semantic computation, gene-disease pairs with similar phenotypic descriptions are anticipated to exhibit proximity within this embedding space.
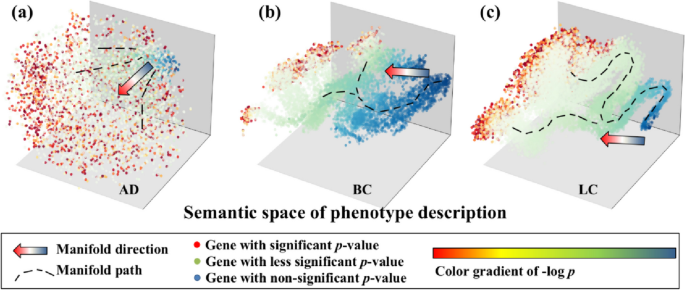
View of data congruence in three case studies. a 3-D semantic representation of AD genes; b BC genes with 3-D representation; c LC genes with 3-D representation. With the color gradient representing the significance level by a single sequence analysis, genes after the phenotypic embedding computation are projected onto a 3-D semantic space. Intuitively, the significant and less significant disease-associated genes are distinguished along the manifold direction based on their phenotypic embeddings. The observation suggests the high data quality of association significance and phenotype description, which supports the subsequent data fusion
To observe the data congruence of the phenotypic embedding and p -value from sequence analysis, we employ a color-coding scheme to visualize the congruence in the distribution of two distinct modalities of data. Here, each gene is colored in a gradient ranging from red to blue, with color intensity denotes the level of statistical significance associated with the p -value of the corresponding gene.
In this figure, the congruence in data distribution between association significance and phenotype description is readily discernible through distinct data partitioning and segmentation. Specifically, genes exhibiting significant p -values (depicted as red dots) tend to disperse across the outer regions of the 3-D manifold space along the manifold path. Conversely, genes with non-significant p -values (represented by green-blue dots) manifest discernible partitioning and segmentation along a distinct direction within the manifold space.
In summary, the significance of p -values aligns with the clustering trend observed in phenotypic embedding. This suggests the potential and rationale for merging embedding data with significant p -values to prioritize disease-related genes. Furthermore, this fusion-based approach has the potential to deepen our understanding of gene-disease associations with the aid of phenotype descriptions.
Evaluation of the predicted genes by PheSeq
After feeding the association significance data and phenotype description data into the PheSeq model in AD, BC, and LC cases, model iterations ran data fusion processes and generated new p -values for gene-disease associations. Upon the generation of the association significance for each gene after PheSeq implementation, abundant novel gene-disease associations were subsequently suggested.
Comparison of prioritized genes by PheSeq and sequence analysis methods
As illustrated in Table 2 , the number of genes predicted by the PheSeq model for AD is 1024. This accounts for 5.6% of the 18,157 background GWAS genes, thereby establishing a moderate ratio when compared to the low positive rate of 1.7% obtained from the GWAS experiment. Similarly, the PheSeq model prioritizes 818 BC genes with a positive rate of 4.7%, which is comparatively higher than the positive rate of 2.7% obtained from the transcriptome experiment utilizing AgilentG4502A_07_3. Furthermore, 566 genes are prioritized for LC, and the resulting positive rate of 2.3% is significantly higher than the positive rate of 0.75% in the methylation experiment with Human Methylation 450.
PheSeq yields newly predicted genes for the association study, of which, a good portion are overlapped ones with single sequence analysis, and the rest are newly recalled ones. In summary, for AD, 236 genes out of 1024 prioritized genes overlap with the GWAS experiment. Similarly, 68 out of 566 genes in LC and 347 out of 818 genes in BC overlap with the methylation experiment and transcriptome experiment, respectively. Furthermore, PheSeq recalls 768, 471, and 498 novel significant genes for AD, BC, and LC, respectively.
Evaluation by the benchmark dataset
To evaluate the prioritization result, a benchmark database, DISEASES [ 39 ], is referenced, which integrates 36,448 gene-disease associations from three resources with increasing reliability, i.e., “Text mining,” “Experiments,” and “Knowledge.” Among them, the “Text mining” results are retrieved by text co-occurrence, and “Experiments” collect GWAS databases like target illumination GWAS analytics (TIGA), Catalogue of Somatic Mutations in Cancer (COSMIC), and DistiLD, while the “Knowledge” results involve general gene-disease association databases like AmyCo, MedlinePlus, and UniProtKB.
In AD, three mutually overlapping sources in DISEASES, Text mining, Experiment, and Knowledge, encompass 315, 339, and 26 significant genes, respectively, corresponding to 624 AD genes in total. Among these, PheSeq achieves 128, 48, and 17 gene hits in the three sources, respectively, contributing to 151 significant AD genes in total. The result reveals a relatively higher recall rate, i.e., 17/26, in the Knowledge source, lower in the Experiment source (48/339), and intermediate in the Text mining source (128/315).
The recall rate in BC and LC is higher than that in AD. In BC, the three sources contain 176, 344, and 29 LC genes, contributing to 533 LC genes. The recall rate for each source is 72/176, 73/344, and 19/29. The overall recall rate is 159/533, whereas in LC, the overall recall rate is 342/669. PheSeq hit 119 out of 297 genes in the Text mining source, 239 out of 391 genes in the Experiment source, and 21 out of 25 genes in the Knowledge source. In brief, though it still misses many hits in DISEASES, PheSeq obtains a reasonable recall rate.
By observing the Top 50 genes prioritized by PheSeq (Fig. 4 ), it is concluded that over half of them have been recorded in the DISEASES database, of which 26/50 for AD, 33/50 for BC, and 36/50 for LC. The high coverage reveals that PheSeq well replicates the associations in the benchmark database. In comparison, the record of significant genes by sequence analysis is relatively scarce within the top 50. It is noted that DISEASES covers a good portion of the significant genes by sequence analysis. Among 311 significant AD genes through sequence analysis, 233 are encompassed in the DISEASES database. In the cases of sequence analysis experiments for BC and LC, 424 out of 470 significant BC genes and 137 out of 184 significant LC genes are cataloged in the DISEASES database.
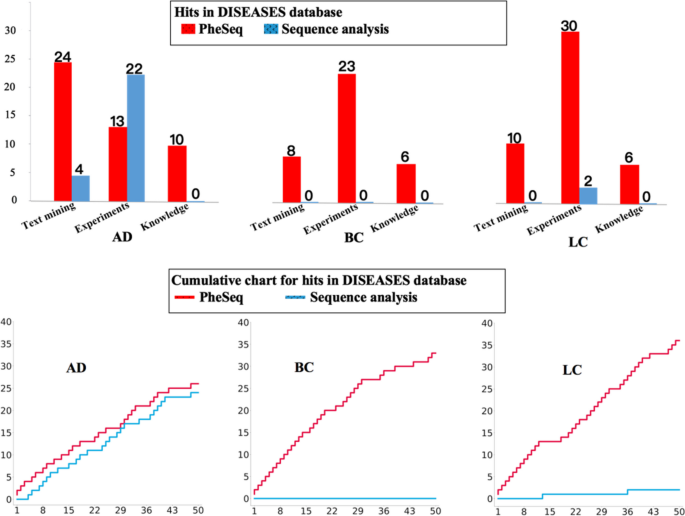
The top prioritized genes from PheSeq and sequence analysis in the DISEASES database. The hits plot and cumulative charts in DISEASES serve to compare the recall rate of PheSeq and sequence analysis methods
Interestingly, PheSeq replicates the associations in the three DISEASES resources with different coverage rates. Among them, 24 genes in the AD prediction have been recorded in the “Text mining” part, while the number for BC and LC is 20 and 10. Comparatively, LC obtains higher recall in the “Experiments” part. The results also suggest that it is hard for a single source of data to recover gene-disease associations, and PheSeq is capable of fusing the heterogeneous data to achieve better data comprehension.
Investigation of top prioritized AD-associated genes
Taking AD as an example, the top 5 genes with and without GWAS supports are investigated. Being the Top 5, MAPT, PSEN1, APP, APOE, and GRN are known vital ones in the AD pathological hypothesis. In detail, MAPT encodes the Tau protein, and its hyper-phosphorylation forms the neurogenic fiber tangles in neurons and leads to neuronal apoptosis [ 63 ]. Moreover, mutations in PSEN1 and PSEN2 have an impact on an APP-cleaving enzyme, \(\gamma\) -secretase, thus regulating APP expression. Meanwhile, the accumulation of Abeta, an APP-encoded protein, forms the fibrillar amyloid plaques in the brain and impairs the ability of spatial learning and memory, which is a known direct cause of AD [ 64 ]. Being the most widely studied AD-associated gene, APOE is known to cause neuro-inflammation among AD patients by affecting the microglia [ 65 ]. In addition, GRN is a causal gene for frontotemporal dementia, a neurodegenerative disease [ 66 ].
Investigation of the top 50 genes leads to a discovery that a good trade-off leverages association significance and phenotype description and helps to infer the potential associations. First, 24 out of 50 prioritized PheSeq genes pass the IGAP GWAS significant test. They both carry significant p -values and supportive semantic evidence. For example, TREM106B, with a significant p -value of 9.53E−14, wins 50 hits in the literature. In addition, 18 out of 50 prioritized genes pass the significant test in the GWAS dataset EFO_0000249. Among them are GRN, TMEM106B, SPI1, CR1, and PICALM from GCST90044699; SORL1 and SQSTM1 from GCST002245; CLU and ABCA7 from GCST90012877; MAPT from GCST90038452; APP from GCST012182; APOE from GCST009019; TREM2 from GCST005549; TTR from GCST007319; FUS from GCST007320; TOMM40 from GCST000682; BIN1 from GCST005922; and ACE from GCST90013835.
Second, for the rest of the 32 genes that do not exist in the GWAS Catalog, 4 of them are included in the known database. In detail, PSEN1 and PSEN2 are both in UniProtKB and MedlinePlus, while SNCA and CSK3B are in UniProtKB. Eventually, for the 28 genes that are not reported by GWAS or known databases, 23 of them are suggested to be AD-related with confirmed phenotype description.
To compare the global prioritization results between PheSeq and sequence analysis, a cumulative chart for database hits for the top 50 filtered genes is given in Fig. 5 b.
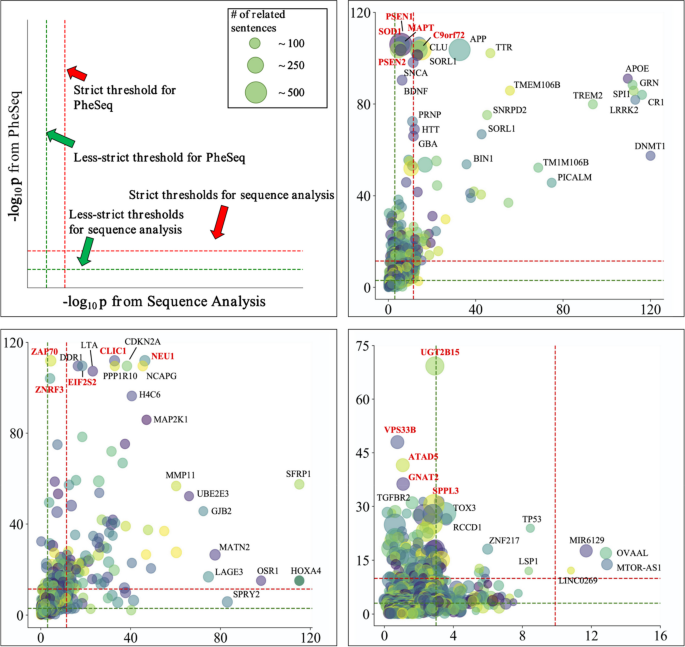
The \(-\log p\) plots of overlapping and recalled genes after applying PheSeq and sequence analysis in AD, BC, and LC. a Layout of the \(-\log p\) plot. The x -axis and y -axis denote the \(-\log\) p value from the sequence analysis and the PheSeq model respectively. The red line refers to a strict threshold line such as Benjamini FDR in our case, and the green line refers to a less strict threshold line such as \(-\log 0.005\) in our case. Genes are labeled when overlapped in PheSeq and sequence analysis or recalled by PheSeq. b The \(-\log p\) plot of significance for both PheSeq and sequence analysis in AD. Five genes are marked in red, i.e., MAPT, PSEN1, C9orf72, SOD1, and PSEN2. All of them are PheSeq recalled genes, which obtain high significance in PheSeq but obtain less or limited significance in GWAS. c The \(-\log p\) plot in BC. Five PheSeq recalled genes are chosen and marked in red, i.e., NEU1, ZAP70, EIF2S2, ZNRF3, and CLIC11. These genes obtain comparatively higher significance in PheSeq than that in sequence analysis. d The \(-\log p\) plot in LC. The five marked genes are UGT2B15, VPS33B, ATAD5, GNAT2, and SPPL3. All five genes show strong significance in PheSeq but limited significance in sequence analysis
Overall, the results suggest that the PheSeq model effectively leverages the synergy among heterogeneous association data, alleviating the limitations of using single-source association significance data.
Genes with significance in PheSeq and single-omics sequence analysis: a comparative observation
It is noted that the sequence analysis used in comparative experiments may introduce errors, particularly when considering the inherent instability of results obtained from single-omics sequence analyses. Consequently, the sequence analysis unavoidably overlooks certain known significant associations and may erroneously produce false positive results. PheSeq, on the other hand, aims to reduce the error by data fusion. Therefore, a comparative analysis is performed for both types of experiments.
To display and further investigate each overlapping and recalled gene by considering its significance value both on PheSeq and the sequence analysis, \(-\log p\) plots for all significant genes in AD, BC, and LC are given in Fig. 5 . In this figure, the horizontal \(-\log p\) axis refers to the association significance obtained by sequence analysis, while the vertical \(-\log p\) axis corresponds to the generated p -value by PheSeq. In addition, the size of the circle for each gene reflects the count of the phenotype descriptions related to the gene (Fig. 5 a).
Intuitively, these figures offer a means by which to investigate the genes with overlapped significance both in sequence analysis and phenotype description. In particular, the plot is separated into sections by threshold lines. The genes with overlapped significance genes are located in the top right corner of the plot, which pass the significance test in sequence analysis and in the meantime carry sufficient association semantics. Meanwhile, the newly reported significant genes by PheSeq are located in the top left section of the plot, which may show less or limited significance in sequence analysis.
In the context of AD, genes with overlapping associations, including APOE, GRN, LRRK2, and SPI1, are visually presented in the top right corner (Fig. 5 b), all of which pass the significance test in GWAS and possess sufficient AD-relevance association semantic by PheSeq. Furthermore, genes with less significance in the sequence analysis, e.g., PSEN1, SOD1, MAPT, C9orf72, and PSEN2, are displayed in the top left section. Among the four, PSEN1 and PSEN2 are known AD-related genes, reported in AlzGene [ 67 ], while C9orf72 and SOD1 are known to be relevant to neurogenetic disease and possess AD-relevant literature support in GeneCards [ 68 ].
In BC, overlapped genes, such as SFRP1, HOXA4, and OSR1 genes, are clearly displayed (Fig. 5 c). The location of these genes in the figures show that these genes possess both significance in sequence analysis and PheSeq. Again, we are focusing on the recalled genes by PheSeq. Here, PheSeq recalled less significant genes in sequence analysis such as NEU1, ZAP70, EIF2S2, ZNRF3, and CLIC1, while all of which possess strong significance in PheSeq. The literature review as well shows the relevance of BC to these genes, e.g., NEU1 [ 69 ], ZAP70 [ 70 ], EIF2S2 [ 71 ], ZNRF3 [ 72 ], and CLIC1 [ 73 ].
Similar observations are carried on for LC, where overlapped genes such as MIR6129, OVAAL, MTOR-AS1, and LINC0269 are displayed in the top right corner of Fig. 5 d. Meanwhile, the top left part of the figure indicates PheSeq-recalled genes. The literature review again shows the relevance of LC to these genes, e.g., UGT2B15 [ 74 ], VPS33B [ 75 ], ATAD5 [ 76 ], GNAT2 [ 68 ], and SPPL3 [ 77 ].
In conclusion, association study results obtained by PheSeq and a single sequence analysis can be simultaneously observed by the above figures. In addition, this figure enables specific investigation on overlapped genes or newly recalled genes by PheSeq after data fusion. The analysis in the three cases suggests that the genes recalled by Pheseq may not be directly associated with the target disease, but they with a high chance exhibit relevance via database or literature review.
Impact of phenotype description on PheSeq with association interpretability
PheSeq incorporates rich semantic information within its data fusion framework, and it leverages the synergy between the sequence analysis and association descriptions. As a result, PheSeq retrieves a vast dataset of phenotype sentences and bio-concepts for interpreting the prioritized gene-disease association. In summary, 14,084 phenotype sentences are utilized by PheSeq to support 1024 prioritized genes in AD. With an average of 13 phenotype sentences per gene, this dataset includes 1849 GO terms and 1351 HPO terms. In BC and LC, 2250 and 10,440 phenotype sentences are obtained, respectively, with each gene associated with an average of 9 and 10 phenotype sentences. More details on the validation of embedding quality, and the statistic of phenotype description are provided in Additional file 3 .
Actually, the PheSeq model prioritizes the gene-disease associations by perceiving corresponding description descriptions. As per observation, genes recalled by PheSeq generally possess pertinent phenotype descriptions. Taking MAPT in AD as an example, it is known to be relevant to the etiology of AD by the widely accepted Tau protein hypothesis, although it fails to pass the significance test in GWAS. As can be observed from Table 3 , the most frequently cited phenotype descriptions related to MAPT include “Neurofibrillary tangles” (HP:0002185), “Hyperphosphorylation” (GO:0048151), “Cognitive impairment” (0100543), “Microtubule binding” (GO:0008017), “Long-term synaptic potentiation” (GO:0060291), and “Microtubule polymerization potentiation” (GO:0046784). According to the Tau protein hypothesis, hyperphosphorylation of the Tau protein leads to its aggregation, ultimately disrupting microtubule stability and resulting in the formation of neurofibrillary tangles―a hallmark pathological feature of AD. The observation shows that the top ranked associated phenotype descriptions are highly relevant and supportive for the MAPT-AD association.
We further investigated four such genes, namely PSEN1, c9orf72, SOD1, and PSEN2, all of which displayed robust significance in PheSeq, despite exhibiting less or limited significance in sequence analysis.
Table 3 presents examples and statistics of the phenotype descriptions including bio-concepts and sentences. Except for C9orf72, the rest of them are all recalled ones by PheSeq. Here, frequently mentioned bio-concepts include “Senile plaques” (HP:0100256), “Neurofibrillary tangles” (HP:0002185), “Hippocampal atrophy” (HP:0410170), “Abnormality of mitochondrial metabolism” (HP:0003287), and “Inflammatory response” (GO: 0006954). These phenotype descriptions are known to be relevant to AD, thus suggesting a potential gene list for further AD-gene association investigations.
Similarly, an inquiry is undertaken regarding NEU1, ZAP70, EIF2S2, ZNRF3, and CLIC1 in BC. Remarkably, these genes exhibit significant importance in PheSeq analysis, despite showing relatively modest significance in sequence analysis.
In accordance with the aforementioned observations in the AD case, phenotype descriptions with high association relevance are derived. Specifically, bio-concepts such as “Angiogenesis” (GO:00001525), “Cytokine production” (GO:0001816), “Epidermal growth factor-activated receptor activity” (GO:0005006), “Aldehyde dehydrogenase [NAD(P)+] activity” (GO:0004030), and “Wnt signaling pathway” (GO:0016055) are frequently mentioned.
Meanwhile, UGT2B15, VPS33B, ATAD5, GNAT2, and SPPL3 exhibit a significant impact on PheSeq in LC and win corresponding literature support [ 68 , 74 , 75 , 76 , 77 ], despite not meeting the reference threshold in sequence analysis. Consistent with previous observations in AD and BC cases, these genes are commonly associated with LC-relevant phenotypes, including “Low-density lipoprotein particle receptor activity” (GO:0005041), “Fibroblast growth factor-activated receptor activity” (GO:0005007), “GDP-dissociation inhibitor activity” (GO:0005092), “Goodpasture-antigen-binding protein kinase activity” (GO:0033868), and “Transforming growth factor beta receptor binding” (GO:0005160).
In summary, these results indicate that PheSeq underscores the disease-specific phenotype descriptions and incorporate them with sequence analysis significance. Remarkably, PheSeq holds particular importance in situations where a single sequence analysis may elicit systematic bias and flawed predictions of crucial genes. In such instances, PheSeq serves as an effective tool for establishing a connection between phenotype descriptions and association significance in sequence analysis and helps to recall the significant genes.
Impact of prior knowledge on PheSeq with association prediction: an ablation study
In the aforementioned analysis, we compare the performance of PheSeq with that of a single sequence analysis in three distinct case studies. It is essential to note that, as a data fusion method, PheSeq inherently incorporates prior knowledge from literature and networks. Consequently, PheSeq is a model integrating prior knowledge and holds an inherent advantage over conventional sequence analysis models. In this section, we conduct an ablation study to evaluate how prior knowledge is incorporated into the PheSeq model. We systematically remove specific prior information and rerun the entire prediction process to assess the impact accordingly.
Based on the publication dates of omics data, we exclude all literature data beyond those time points. Specifically, for AD, the literature cutoff date is set at October 27, 2013. Correspondingly, for BC and LC, the respective dates are January 28, 2016. Consequently, this approach results in a significant compression of the prior knowledge derived from the literature. In the original experiments, the literature on AD covers 14,261 genes; however, with the cutoff set on October 27, 2013, only 1017 genes are now covered. In the case of BC, the gene coverage decreases from 10,498 to 3,399, and in LC, the reduction rate is greater, dropping from 20,460 to 749 genes.
PheSeq in the ablation setting predicts 391 significant genes associated with AD, 1398 significant genes associated with BC, and 172 ones with LC. Despite the relatively limited inclusion of prior literature knowledge for these genes, the results in Table 4 clearly demonstrate two patterns. First, predicted significant genes typically carry a higher proportion of literature knowledge. For instance, among the 391 key AD genes, each gene, on average, possesses 21.17 literature references, 31.80 pieces of related sentence evidence, and 11.54 core concepts, whereas in the corresponding non-significant genes, these values are only 2.32, 3.28, and 2.90, respectively. Second, due to the preservation of PPI data in prior knowledge, prioritized genes are more likely to be adjacent to other significant ones. For instance, among the 391 AD significant genes, statistical analysis of information from their top 10 neighbors reveals an average of 5.54 significant genes per gene, with a cumulative literature count of 115.35, a sentence evidence count of 173.22, and a concept count of 62.86. In contrast, for non-significant genes, the number of significant genes among their top 10 neighbors decreases to 3.13, with corresponding literature, sentence, and concept counts of 3.08, 4.36, and 3.87, respectively. The two patterns are observed as well in BC and LC case studies.
In short, significant genes exhibit extensive prior knowledge, either encompassing abundant literature in historical data or demonstrating strong associations with significant disease-related genes in PPI networks.
Taking PICALM as an example, this gene is notably associated with a substantial amount of AD literature. As of the end of 2023, a total of 264 publications are available for PICALM, with 112 publications retained before the cutoff in 2013. This abundance of literature contributes to PICALM being identified as a significant gene with a high probability in the ablation study conducted by PheSeq. Similarly, ESR1 in LC also maintains a considerable literature count, totaling 132 publications by the end of 2023 and retaining 54 publications before the cutoff in the preceding years of 2016.
In AD, GBA emerges as the gene exhibiting the strongest association in the PPI network. Its neighbors, such as UGCG, PSAP, GALC, and SGMS2, are all linked to known AD pathological processes and exhibit significant p -values in sequence analysis, namely 0.045, 0.029, 0.00056, and 0.0014, respectively. This significantly increases the likelihood of PheSeq identifying GBA as a significant gene.
Similarly, in BC, the NEU1 gene is strongly linked to several significant genes in the PPI network, including GLB1 (4.11e−11), ARSA (5.15e−05), and GAL3ST1 (1.99e−13). This, in turn, leads to PheSeq maintaining positive predictions for these genes in the ablation study.
In summary, the observed patterns in the ablation experiments indicate that despite the extensive removal of literature prior knowledge, the predicted significant genes still predominantly retain both literature and network priors. This in turn aligns with the initial purpose of data fusion.
Furthermore, we evaluate the predictive capacity of PheSeq with removed prior literature knowledge, and the top 50 significant genes with the cumulative charts in DISEASES are shown in Fig. 6 a. The yellow line represents the ablation method where literature priors are excluded, while the red line corresponds to the original PheSeq method. In the cumulative line plot, it is observable that the yellow line consistently remains below the red line. This result indicates a significant decline in the predictive capability of PheSeq when a substantial amount of literature priors is removed, and it aligns with the data fusion concept in PheSeq.
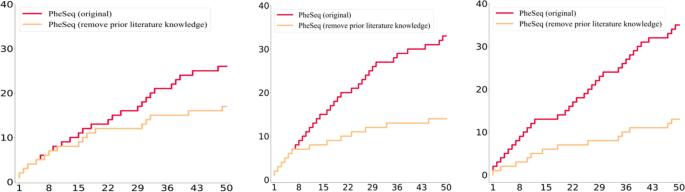
The top 50 significant genes with cumulative charts in DISEASES in the ablation study
Comparison of other data fusion models
As a representative data fusion algorithm, PheSeq combines two distinct types of association information: sequence analysis data and embedding data. When addressing gene-disease associations, there are diverse strategies for data incorporation and model selection within data fusion algorithms. Even when examining the same disease, variations in results among different fusion methods can arise due to the use of diverse data modalities. Figure 7 illustrates the overlap of significant genes under various methodologies. As depicted in the figure, achieving a high degree of overlap between different methods is challenging, regardless of the number of significant genes predicted by each approach.
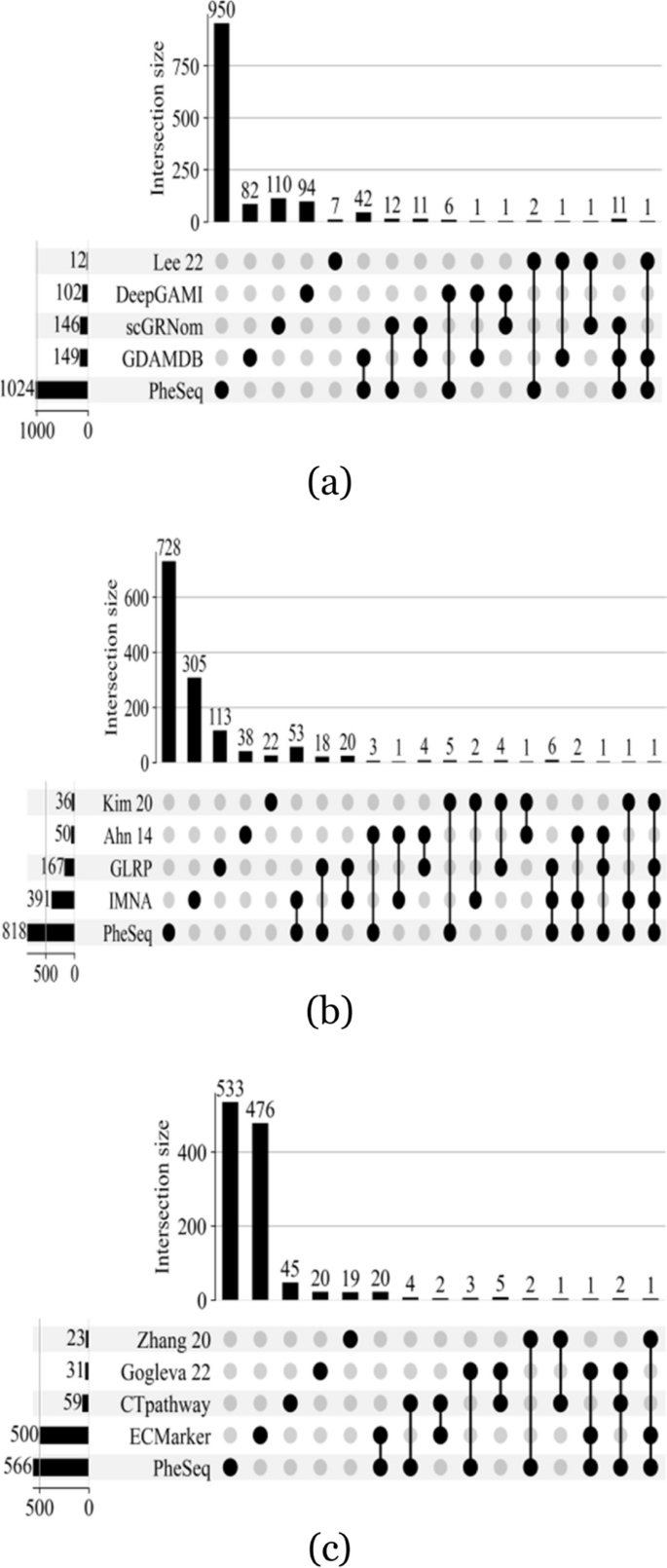
Overlap of significant genes from different data fusion methods on gene-disease associations. a AD. b BC. c LC
Nevertheless, conducting a comparative analysis of outcomes from various data fusion methods, including PheSeq, remains crucial for obtaining a comprehensive evaluation of PheSeq’s performance. As shown in Table 5 , different methods cover various data modalities, including data from GWAS, gene expression, gene regulatory network (GRN), expression quantitative trait loci (eQTL) high-throughput chromosome conformation capture (Hi-C), copy number alteration (CNA), literature, and protein-protein interaction. The number of significant genes varies across methods, with Lee et al. [ 78 ] having the lowest at 12 and PheSeq having the highest at 1024 in AD. In BC, Kim et al. [ 79 ] report the lowest count at 35 while PheSeq has the highest count at 818. In LC, Zhang et al. [ 80 ] have the lowest at 23 whereas PheSeq exhibits the highest at 566. This likely reflects differences in the identification of significant genes when using different methods and data modalities.
There are three main types of data fusion strategies used in machine learning; early (data-level), intermediate (joint-level), and late (decision-level) [ 89 , 90 ]. In the early data fusion algorithms, data from various sources, once fully collected, are mapped to a unified data space through vectorization methods such as concatenation or addition. Subsequently, a machine learning model is employed for knowledge-based decision-making. Researches [ 78 , 80 , 81 , 83 , 84 , 85 , 88 ] fall into this scope. In contrast, intermediate data fusion algorithms often utilize a series of models within a step-wise set, where different models handle distinct stages of data, ultimately completing data fusion and knowledge-based decision-making within a single pipeline. This type of algorithm includes researches [ 79 , 82 , 86 , 87 ]. Late data fusion algorithms, on the other hand, involve the simultaneous processing of data from different sources by various models, achieving integrated decision-making. Although the selected comparative experiments only represent a small portion of the data fusion methods for three case studies, it is suggested that early and intermediate data fusion methods remain predominant, and late data fusion methods are relatively less frequently employed. GDAMDB [ 32 ] and PheSeq stand as representatives of late data fusion methods, utilizing Bayesian networks to learn the distribution relationships among data variables, offering interpretable fusion decisions.
In addition, the interpretation approaches vary widely among these methods. While some methods rely on enrichment analysis and pathway analysis, others incorporate more sophisticated techniques such as interpretable neural networks or recommendation systems. Additionally, some methods do not explicitly specify their interpretation approach. This diversity highlights the complexity of interpreting integrated data and underscores the need for tailored approaches based on the specific objectives of each study.
Finally, we utilize DISEASES as the external dataset referenced to compare the performance of predictive capacity among these methods. As detailed in the rightmost column of the table, PheSeq exhibits superior predictive performance in BC and LC, outperforming other methods in precision and recall. For instance, PheSeq recalls 159 DISEASES genes out of 818 predicted significant genes. Both the amount and the ratio are greater than the rest methods. In AD, while PheSeq recalls 151 DISEASES genes, this is attributed to its larger overall prediction quantity. Conversely, Lee et al. [ 78 ] and GDAMDB [ 32 ] demonstrate higher precision, with GDAMDB displaying notably high recall values. This also underscores the advantages of the late data fusion approach.
In summary, PheSeq stands out as a late data fusion algorithm in the context of gene-disease associations, predominantly employing phenotype descriptions extracted from literature to enhance the interpretive aspects of the obtained results.
Association interpretation in a visualized phenotype description network
Benefiting from the good amount of phenotype description and sentence support, we derive abundant phenotype descriptions for gene-disease associations. To summarize all the PheSeq-prioritized genes with the collected bio-concepts and sentences, a visualized phenotype description network is built for AD, BC, and LC, separately. In the network, the significant genes (both from PheSeq and sequence analysis) and the bio-concepts are treated as nodes, and a gene-concept edge is linked when a sentence description addressing the association is available. The network is released in a user-friendly webpage Footnote 1 , while the pipeline of the network construction is introduced in Additional file 3 .
The network offers diverse patterns of association interpretations that serve to enhance the comprehension of the mechanisms that underlie gene-disease associations.
Pattern 1. GO enrichment analysis
The network enables GO enrichment analysis. Here, four gene sets are shown in Fig. 8 a, b, c with GO terms corresponding to apoptosis [ 91 ], mitophagy [ 92 ], chemical synaptic transmission [ 93 ], and long-term synaptic potentiation [ 94 ]. In Fig. 8 a, 24 genes are linked with the “Apoptotic process” (GO:0006915), supported by 133 pieces of sentence evidence. In total, the 24 genes consist of 7 ones from GWAS, 17 ones from PheSeq, and 5 ones that overlapped. After applying the hypergeometric test, the gene set is significantly enriched in “Negative regulation of neuron apoptotic process” (GO:0043524) with an association significance of 3.4305E-12 and “Positive regulation of apoptotic process” (GO:0043065) with an association significance of 1.8137E−06. The results confirm the relevance of these 24 genes to the “apoptosis process.” Moreover, all the GO-linked genes in Fig. 8 b,c pass the corresponding GO enrichment test.
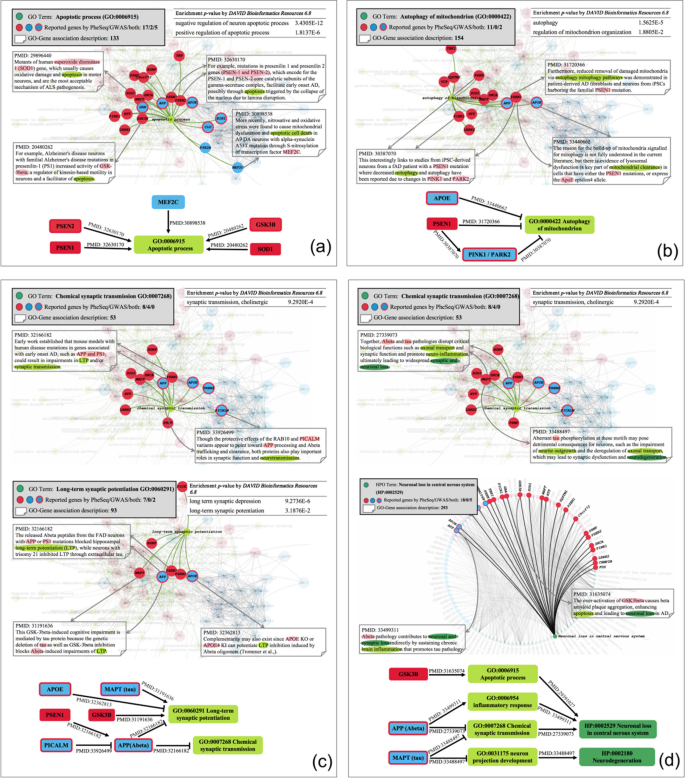
Association interpretation in the visualized phenotype description network for AD. a Observation of the gene-phenotype links. There are 19 significant PheSeq genes linked with the phenotype term “apoptosis process” in AD, and sequence analysis provides 7 significant links. b Gene-gene interaction through genes with shared phenotype descriptions. APOE and PSEN1 inhibit the autophagy of mitochondria directly, as reported in PMID:33440662 [ 95 ] and PMID:31720366 [ 96 ]. Meanwhile, PSNE1 inhibits this biological process by affecting PINK1 and PARK2. c Multiple GO terms lead to complex gene pathological pathways. PSEN1 and GSK3B are exclusively found in PheSeq, while the other four, i.e., APOE, MAPT, APP, and PICALM are both found by PheSeq and IGAP GWAS. All of them interact with each other and lead to two biological processes, long-term synaptic potentiation, and synaptic transmission. d Links between genes and GO or HPO interpret a multi-level pathology mechanism. By tracing two HPO terms, i.e., neuronal loss in the central nervous system and neurodegeneration, to GO terms and their linked genes, multi-level links are formulated. Three genes are included in these links, in which GSK3B is exclusively found by PheSeq, while APP and MAPT are separately found by PheSeq and IGAP GWAS. These links unveil a cascade mechanism that starts from gene involvement in multiple biological processes and ends in two phenotypic processes
Pattern 2. Link genes from two sources
The inclusion of significant genes identified by both PheSeq and sequence analysis provides avenues for further investigation into pathological mechanisms. Taking the five genes in Fig. 8 a as an example, MEF2C is a GWAS-reported gene, the S-nitrosylation of which causes mitochondrial dysfunction and apoptotic cell death in neurons. Furthermore, PheSeq prioritized genes such as PSEN1, PSEN2, SOD1, and GSK3B are also added to the linking graph. Among them, PSEN1 and PSEN2 are known AD-related genes, the mutation of which contributes to the clinical syndrome of early-onset AD (EOAD) through apoptosis. In addition, SOD1 and GSK3B both trigger apoptosis in neurons. Evidence in literature (PMID: 32006534 [ 97 ]) indicates that mutations in PSEN1 increase the activity of GSK3B, cause apoptosis, and facilitate AD. These observations imply that all the linked genes are related to apoptosis and AD pathology.
Pattern 3. Hybrid gene-phenotype associations
The network facilitates hybrid investigation of gene-phenotype associations. First, exploring genes that share phenotype descriptions holds the potential to reveal gene-gene interactions. As shown in Fig. 8 b, APOE and PSEN1 inhibit the autophagy of mitochondria directly, as reported in PMID:33440662 [ 95 ] and PMID:31720366 [ 96 ]. Meanwhile, PSNE1 inhibits this biological process by affecting PINK1 and PARK2. In detail, PMID:31720366 [ 96 ] claims that PSEN1 mutation reduces the removal of damaged mitochondria via autophagy mitophagy pathways, PMID:30387070 [ 98 ] claims that patients with a PSEN1 mutation where there is decreased mitophagy and autophagy have been reported due to changes in PINK1 and PARK2, and PMID:33440662 [ 95 ] figures out the PSEN1 mutations or expresses the APOE which induces the lysosomal dysfunction, which is a key part of the mitochondrial clearance. After combining the three pieces of evidence, the interaction between these genes is inferred. Mutations in the PSEN1 induce changes in PINK and PARK2, which induces lysosomal dysfunction, thus causing mitochondrial accumulation by inhibiting mitophagy in iPSC-derived neurons of AD patients.
Second, an integrative analysis of multiple GO terms leads to the discovery of complex gene pathological pathways. For instance, sentence evidence from PMID:32166182 [ 99 ], 33926499 [ 100 ], 31191636 [ 101 ], and 32362813 [ 102 ] in Fig. 8 c curates the pathway information: the released Abeta peptides from the FAS neurons with APP or PSEN1 mutations causes synaptic inhibition, such as long-term potentiation (LTP) blockade and neurotransmission defects. Meanwhile, the Abeta protein encoded by APP plays a crucial role in this pathway. In more detail, APOE potentiates LTP inhibition induced by Abeta oligomers. In addition, the genetic deletion of tau protein, as well as GSK3B inhibition, blocks Abeta-induced impairments of LTP. Eventually, PICALM variants appear to cause Abeta trafficking and clearance, thereby protecting the synaptic function and neurotransmission.
Third, exploring links between genes and GO or HPO can enhance the understanding of a comprehensive pathology mechanism across multiple levels. As shown in Fig. 8 d, GSK3B, APP, and MAPT induce two clinical phenotypes in AD pathology, neuronal loss, and neurodegeneration, by affecting four molecular-level physiological processes, including the apoptotic process, inflammatory response, synaptic transmission, and neuron projection development.
Finally, an evidence-supported gene-GO network contributes to integrating the findings, pinpointing vital disease-associated genes. For instance, PSEN1 is linked with a good variety of GO terms, which make the PSEN1-centric gene-GO links illuminative. Figure 8 a, b ,c shows that PSEN1 facilitates early-onset AD possibly through apoptosis triggered by the collapse of the nucleus due to lamina disruption. In addition, PSEN1 reduces the removal of damaged mitochondria via autophagy mitophagy pathways in patient-derived AD fibroblasts and neurons from iPSCs. Furthermore, PSEN1 mutation blocks hippocampal LTP by promoting the release of Abeta peptides in FAD neurons. Altogether, the network is beneficial for a comprehensive understanding of the different mechanisms that PSEN1 plays in the AD process.
Interestingly, although GSK3B has not been reported to be AD-related, Fig. 8 c, d shows that it is involved in two AD pathological pathways. One is the increased activity of GSK3B induced by PSEN1, followed by facilitated apoptosis. The other is the genetic deletion of tau protein mediates the inhibition of GSK3B, thereby blocking Abeta-induced impairments of LTP.
Pattern 4. Association augments with auxiliary PPI info
Considering the rich PPI information encompassed within the data modalities integrated by PheSeq, we also incorporate the representation of PPI connections in the visualized phenotype description network. The black edges between genes in the network represent PPI information sourced from the STRING database. Previous experiments demonstrate that, in the absence of literature data, PPI links crucially contribute to graph embedding, aiding PheSeq in retrieving relevant significant genes. Additional file 3 provides examples of the hub or common gene nodes in the PPI network, which link to other significant gene neighbors in PPI connections. Examples suggest that the observation of auxiliary PPI attributes provides augmented mechanistic insights for a given gene-disease association.
In short, the visualized phenotype description network contributes to addressing gene association in an interpretable manner and provides further potential to unveil the disease pathology mechanism.
In the present day, the co-existence of sequence analysis outcomes and textual resources has emerged as an increasingly pervasive practice. In light of this trend, data fusion of the above heterogeneous data holds considerable promise for advancing comprehensive data fusion techniques.
The scenario focused on in this research is such a case when a rich resource of p -values and descriptive texts are available, both of which form a pair of heterogeneous association datasets supporting the discovery of the gene-disease associations.
The PheSeq model effectively integrates the advantages of two types of data by leveraging the heterogeneous synergy in a Bayesian deep learning framework. PheSeq specifically utilizes the DL perception module to generate high-quality embedding representations from phenotype descriptions. Additionally, it makes use of the Bayesian network to effectively model the uncertainty of observation and infer the inherent dependence relations among gene-disease associations.
PheSeq takes advantage of the interpretability nature of the phenotype descriptions. The use of bio-concepts and sentence evidence further improves the interpretability of PheSeq results. Moreover, the knowledge inference patterns shown in Fig. 8 suggest that only when literature and sequence information are well integrated can the model unveil hidden in-depth mechanisms out of the network.
In addition to a promising data fusion idea, PheSeq also encourages certain concerns for further exploration of gene-disease associations.
First, PheSeq does not functionas a predictive algorithm solely focused on achieving absolute confidence in association prioritization. Instead, our primary objective is to addressthe inherent limitations of inference derived from single-omics sequence analysis. Therefore, we adopt a data fusion approach to facilitate interpretable novel associations.
Second, the prioritized gene-disease association needs to be investigated with a methodical approach. As evident in sequence analysis, depending solely on statistical significance and employing stringent cutoff criteria may result in high false negatives. As shown in Fig. 5 , the prioritization of PheSeq does not always align with the sequence analysis. The significant p -value may be discarded due to missing embedding support, and the non-significant p -value may be recalled due to supportive embedding. Fortunately, the PheSeq model provides strong evidence traceability, which enables further validation or investigations of genes of concern by checking the evidence support, even if the gene has lower rankings.
Third, considerations are needed when applying PheSeq in a general genotype-phenotype association study. For example, the appropriate thresholding strategy is needed to evaluate the significance of associations after the sequence analysis. In addition, appropriate benchmarks datasets, such as DISEASES used in our cases, are used for the sake of evaluation. Furthermore, the inconsistency of association significance and phenotype description needs to be investigated ahead of the model implementation.
In conclusion, this research performs a worth-trying attempt in heterogeneous association data fusion This framework successfully bridges the phenotype description perception and p -value uncertainty inference. The association significance is utilized as a fine-grained weak signal for the association significance. Overall, it is an inspiring idea to unveil genotype-phenotype associations and investigate the potential relation dependency through data perception, data fusion, and probabilistic inference in a novel Bayesian framework.
Availability of data and materials
The authors claim that all datasets on which the conclusions of the paper rely are deposited in publicly available repositories.
The AD GWAS summary data of IGAP can be downloaded from https://www.niagads.org/system/tdf/public_docs/IGAP_summary_statistics.zip?file=1 [ 103 ]. The link for BC transcriptome data is https://tcga-xena-hub.s3.us-east-1.amazonaws.com/download/TCGA.BRCA.sampleMap/AgilentG4502A_07_3.gz [ 104 ]. The link for LC prognostic data is https://tcga-xena-hub.s3.us-east-1.amazonaws.com/download/TCGA.LUNG.sampleMap/HumanMethylation450.gz [ 104 ]. The three processed sequence analysis data can be downloaded from https://github.com/bionlp-hzau/PheSeq/tree/main/HeterogeneousData/P-ValeData [ 105 ].
For the edge files of gene-diseases association networks mentioned in this work, STRING-PPI can be downloaded at https://github.com/xiangyue9607/BioNEV/tree/master/data/STRING_PPI [ 43 ]. The PPI network node2vec_PPI can be downloaded at https://github.com/xiangyue9607/BioNEV/tree/master/data/node2vec_PPI [ 48 ]. In addition, Mashup_PPI, is downloadable by https://github.com/xiangyue9607/BioNEV/tree/master/data/Mashup_PPI [ 49 ]. The link to BioPlax 3.0 is https://bioplex.hms.harvard.edu/interactions.php [ 51 ], HuRI can be downloaded from http://www.interactome-atlas.org/download [ 52 ], and the drug-target networks can be downloaded from https://github.com/bionlp-hzau/PheSeq/blob/main/HeterogeneousData/EmbeddingData/GraphData/SupplementaryGraphEmbedding/edge_supp_dir/41587_2007_BFnbt1338_MOESM6_ESM.xls [ 106 ]. The pre-computed embedding files for all six networks can be downloaded from https://github.com/bionlp-hzau/PheSeq/tree/main/HeterogeneousData/EmbeddingData/GraphData/SupplementaryGraphEmbedding [ 105 ].
The literature annotation files containing gene and phenotype descriptions are available for downloading at https://drive.google.com/file/d/1EjqsiFvT4acuSmv FkfWuSRZ2DIfdYvjJ/view?usp=sharing [ 107 ].
The text annotation files and pre-computed embedding files of 32 pan-cancers can be downloaded from http://lit-evi.hzau.edu.cn/PheSeq/more-diseases [ 108 ].
The source codes and example data are publicly available at https://github.com/bionlp-hzau/PheSeq/tree/main [ 105 ].
A user-friendly web page ( http://lit-evi.hzau.edu.cn/PheSeq [ 108 ]) provides the visualized phenotype description network and all the phenotype description and association significance data in three case studies. In addition, pre-computed embedding representation and association description for 32 specific cancer types is also provided.
For a wide range of users who are aiming to investigate an aimed disease by using PheSeq, data observation is suggested by the proposed visualization methods. The data formatting, code pipeline, and result analysis are suggested in Additional file 3 . Additional file 2 : Appendix A.0.3.
Webpage of the visualized phenotype description network. http://lit-evi.hzau.edu.cn/PheSeq [ 108 ]
Ruigrok YM, Rinkel GJ. From GWAS to the clinic: risk factors for intracranial aneurysms. Genome Med. 2010;2(9):1–4.
Article Google Scholar
Verma A, Lucas A, Verma SS, Zhang Y, Josyula N, Khan A, et al. PheWAS and beyond: the landscape of associations with medical diagnoses and clinical measures across 38,662 individuals from Geisinger. Am J Hum Genet. 2018;102(4):592–608.
Article CAS PubMed PubMed Central Google Scholar
Roberts A, Pachter L. RNA-Seq and find: entering the RNA deep field. Genome Med. 2011;3(11):1–4.
Article CAS Google Scholar
Xiong F, Wang R, Lee JH, Li S, Chen SF, Liao Z, et al. RNA m6A modification orchestrates a LINE-1-host interaction that facilitates retrotransposition and contributes to long gene vulnerability. Cell Res. 2021;31(8):861–85.
Nuzzo R. Statistical errors. Nature. 2014;506(7487):150.
Article CAS PubMed Google Scholar
Mezlini AM, Das S, Goldenberg A. Finding associations in a heterogeneous setting: statistical test for aberration enrichment. Genome Med. 2021;13(1):68.
Zhao J, Cheng F, Jia P, Cox N, Denny JC, Zhao Z. An integrative functional genomics framework for effective identification of novel regulatory variants in genome-phenome studies. Genome Med. 2018;10(1):1–15.
Kang M, Ko E, Mersha TB. A roadmap for multi-omics data integration using deep learning. Brief Bioinforma. 2022;23(1):bbab454.
Shen WX, Zeng X, Zhu F, Qin C, Tan Y, Jiang YY, et al. Out-of-the-box deep learning prediction of pharmaceutical properties by broadly learned knowledge-based molecular representations. Nat Mach Intell. 2021;3(4):334–43.
Zha Y, Chong H, Qiu H, Kang K, Dun Y, Chen Z, et al. Ontology-aware deep learning enables ultrafast and interpretable source tracking among sub-million microbial community samples from hundreds of niches. Genome Med. 2022;14(1):43.
Wang F, Lei X, Liao B, Wu FX. Predicting drug–drug interactions by graph convolutional network with multi-kernel. Brief Bioinforma. 2022;23(1):bbab511.
Wang LL, Lo K. Text mining approaches for dealing with the rapidly expanding literature on COVID-19. Brief Bioinforma. 2021;22(2):781–99.
Robinson PN, Köhler S, Bauer S, Seelow D, Horn D, Mundlos S. The Human Phenotype Ontology: a tool for annotating and analyzing human hereditary disease. Am J Hum Genet. 2008;83(5):610–5.
Robinson PN, Mundlos S. The human phenotype ontology. Clin Genet. 2010;77(6):525–34.
Köhler S, Carmody L, Vasilevsky N, Jacobsen JOB, Danis D, Gourdine JP, et al. Expansion of the Human Phenotype Ontology (HPO) knowledge base and resources. Nucleic Acids Res. 2019;47(D1):D1018–27.
Article PubMed Google Scholar
Gargano MA, Matentzoglu N, Coleman B, Addo-Lartey EB, Anagnostopoulos AV, Anderton J, et al. The Human Phenotype Ontology in 2024: phenotypes around the world. Nucleic Acids Res. 2024;52(D1):D1333–46.
Groza T, Köhler S, Moldenhauer D, Vasilevsky N, Baynam G, Zemojtel T, et al. The human phenotype ontology: semantic unification of common and rare disease. Am J Hum Genet. 2015;97(1):111–24.
Köhler S, Gargano M, Matentzoglu N, Carmody LC, Lewis-Smith D, Vasilevsky NA, et al. The human phenotype ontology in 2021. Nucleic Acids Res. 2021;49(D1):D1207–17.
Krysiak K, Danos AM, Saliba J, McMichael JF, Coffman AC, Kiwala S, et al. CIViCdb 2022: evolution of an open-access cancer variant interpretation knowledgebase. Nucleic Acids Res. 2023;51(D1):D1230–41.
Galer PD, Ganesan S, Lewis-Smith D, McKeown SE, Pendziwiat M, Helbig KL, et al. Semantic similarity analysis reveals robust gene-disease relationships in developmental and epileptic encephalopathies. Am J Hum Genet. 2020;107(4):683–97.
Greene D, BioResource N, Richardson S, Turro E. Phenotype similarity regression for identifying the genetic determinants of rare diseases. Am J Hum Genet. 2016;98(3):490–9.
Peterson B, Hernandez EJ, Hobbs C, Malone Jenkins S, Moore B, Rosales E, et al. Automated prioritization of sick newborns for whole genome sequencing using clinical natural language processing and machine learning. Genome Med. 2023;15(1):1–9.
James RA, Campbell IM, Chen ES, Boone PM, Rao MA, Bainbridge MN, et al. A visual and curatorial approach to clinical variant prioritization and disease gene discovery in genome-wide diagnostics. Genome Med. 2016;8:1–17.
Ruklisa D, Ware JS, Walsh R, Balding DJ, Cook SA. Bayesian models for syndrome-and gene-specific probabilities of novel variant pathogenicity. Genome Med. 2015;7:1–16.
Kleftogiannis D, Kalnis P, Bajic VB. Progress and challenges in bioinformatics approaches for enhancer identification. Brief Bioinforma. 2016;17(6):967–79.
Hawe JS, Saha A, Waldenberger M, Kunze S, Wahl S, Müller-Nurasyid M, et al. Network reconstruction for trans acting genetic loci using multi-omics data and prior information. bioRxiv. 2020;2020–05.
Mourad R, Sinoquet C, Leray P. Probabilistic graphical models for genetic association studies. Brief Bioinforma. 2012;13(1):20–33.
Sharma M, Jha IP, Chawla S, Pandey N, Chandra O, Mishra S, et al. Associating pathways with diseases using single-cell expression profiles and making inferences about potential drugs. Brief Bioinforma. 2022;23(4):bbac241.
Shaw CA, Campbell IM. Variant interpretation through Bayesian fusion of frequency and genomic knowledge. Genome Med. 2015;7(1):1–3.
Dai M, Ming J, Cai M, Liu J, Yang C, Wan X, et al. IGESS: a statistical approach to integrating individual-level genotype data and summary statistics in genome-wide association studies. Bioinformatics. 2017;33(18):2882–9.
De La Vega FM, Chowdhury S, Moore B, Frise E, McCarthy J, Hernandez EJ, et al. Artificial intelligence enables comprehensive genome interpretation and nomination of candidate diagnoses for rare genetic diseases. Genome Med. 2021;13:1–19.
Google Scholar
Zhou K, Wang Y, Bretonnel Cohen K, Kim JD, Ma X, Shen Z, et al. Bridging heterogeneous mutation data to enhance disease gene discovery. Brief Bioinforma. 2021;22(5):bbab079.
Wang H, Wang N, Yeung DY. Collaborative deep learning for recommender systems. In: In: Proceedings of the 21th ACM SIGKDD international conference on knowledge discovery and data mining. New York: Association for Computing Machinery; 2015. p. 1235–44.
Chapter Google Scholar
Wang H, Yeung DY. Towards Bayesian deep learning: a framework and some existing methods. IEEE Trans Knowl Data Eng. 2016;28(12):3395–408.
Li X, She J. Collaborative variational autoencoder for recommender systems. In: Proceedings of the 23rd ACM SIGKDD international conference on knowledge discovery and data mining. 2017. pp. 305–14.
Neal RM. Connectionist learning of belief networks. Artif Intell. 1992;56(1):71–113.
Dj M. A practical Bayesian framework for backprop networks. Neural Comput. 1992;3:448–72.
Gayoso A, Steier Z, Lopez R, Regier J, Nazor KL, Streets A, et al. Joint probabilistic modeling of single-cell multi-omic data with totalVI. Nat Methods. 2021;18(3):272–82.
Grissa D, Junge A, Oprea TI, Jensen LJ. DISEASES 2.0: a weekly updated database of disease–gene associations from text mining and data integration. Database. 2022;2022:baac019.
Lambert JC, Ibrahim-Verbaas CA, Harold D, Naj AC, Sims R, Bellenguez C, et al. Meta-analysis of 74,046 individuals identifies 11 new susceptibility loci for Alzheimer’s disease. Nat Genet. 2013;45(12):1452–8.
Weinstein JN, Collisson EA, Mills GB, Shaw KR, Ozenberger BA, Ellrott K, et al. The cancer genome atlas pan-cancer analysis project. Nat Genet. 2013;45(10):1113–20.
Article PubMed PubMed Central Google Scholar
Liu H, Zhou J, Tian W, Luo C, Ecker JR. DNA methylation atlas of the mouse brain at single-cell resolution. Nature. 2020;598(7879):120–8.
Szklarczyk D, Gable AL, Nastou KC, Lyon D, Kirsch R, Pyysalo S, et al. The STRING database in 2021: customizable protein-protein networks, and functional characterization of user-uploaded gene/measurement sets. Nucleic Acids Res. 2021;49(D1):D605–12.
Furrer L, Jancso A, Colic N, Rinaldi F. OGER++: hybrid multi-type entity recognition. J Cheminformatics. 2019;11(1):1–10.
Luo L, Yan S, Lai PT, Veltri D, Oler A, Xirasagar S, et al. PhenoTagger: a hybrid method for phenotype concept recognition using human phenotype ontology. Bioinformatics. 2021;37(13):1884–90.
Wang Y, Zhou K, Gachloo M, Xia J. An overview of the active gene annotation corpus and the BioNLP OST 2019 AGAC track tasks. In: In: Proceedings of The 5th workshop on BioNLP open shared tasks. Hong Kong: Association for Computational Linguistics; 2019. p. 62–71.
Lee J, Yoon W, Kim S, Kim D, Kim S, So CH, et al. BioBERT: a pre-trained biomedical language representation model for biomedical text mining. Bioinformatics. 2020;36(4):1234–40.
Grover A, Leskovec J. node2vec: scalable feature learning for networks. In: Proceedings of the 22nd ACM SIGKDD international conference on Knowledge discovery and data mining. New York: Association for Computing Machinery; 2016. p. 855–64.
Choi Y, Chiu CYI, Sontag D. Learning low-dimensional representations of medical concepts. AMIA Summits Transl Sci Proc. 2016;2016:41.
PubMed PubMed Central Google Scholar
Ratajczak F, Joblin M, Hildebrandt M, Ringsquandl M, Falter-Braun P, Heinig M. Speos: an ensemble graph representation learning framework to predict core gene candidates for complex diseases. Nat Commun. 2023;14(1):7206.
Huttlin EL, Bruckner RJ, Navarrete-Perea J, Cannon JR, Baltier K, Gebreab F, et al. Dual proteome-scale networks reveal cell-specific remodeling of the human interactome. Cell. 2021;184(11):3022–40.
Luck K, Kim DK, Lambourne L, Spirohn K, Begg BE, Bian W, et al. A reference map of the human binary protein interactome. Nature. 2020;580(7803):402–8.
Ribeiro LFR, Saverese PHP, Figueiredo DR. struc2vec : learning node representations from structural identity. In: Proceedings of the 23rd ACM SIGKDD International Conference on Knowledge Discovery and Data Mining. Halifax: ACM; 2017. pp. 385–94. https://doi.org/10.1145/3097983.3098061 .
Yue X, Wang Z, Huang J, Parthasarathy S, Moosavinasab S, Huang Y, et al. Graph embedding on biomedical networks: methods, applications and evaluations. Bioinformatics. 2020;36(4):1241–51.
Kiela D, Wang C, Cho K. Dynamic Meta-Embeddings for Improved Sentence Representations. In: In Proceedings of the 2018 Conference on Empirical Methods in Natural Language Processing. Brussels: Association for Computational Linguistics; 2018. p. 1466–77.
Glickman ME, Rao SR, Schultz MR. False discovery rate control is a recommended alternative to Bonferroni-type adjustments in health studies. J Clin Epidemiol. 2014;67(8):850–7.
Yang Q, Flanders WD, Moonesinghe R, Ioannidis JP, Guessous I, Khoury MJ. Using lifetime risk estimates in personal genomic profiles: estimation of uncertainty. Am J Hum Genet. 2009;85(6):786–800.
Fine RS, Pers TH, Amariuta T, Raychaudhuri S, Hirschhorn JN. Benchmarker: an unbiased, association-data-driven strategy to evaluate gene prioritization algorithms. Am J Hum Genet. 2019;104(6):1025–39.
Parker R, Rothenberg R. Identifying important results from multiple statistical tests. Stat Med. 1988;7(10):1031–43.
Allison DB, Gadbury GL, Heo M, Fernández JR, Lee CK, Prolla TA, et al. A mixture model approach for the analysis of microarray gene expression data. Comput Stat Data Anal. 2002;39(1):1–20.
Xiang Q, Edwards J, Gadbury GL. Interval estimation in a finite mixture model: modeling P-values in multiple testing applications. Comput Stat Data Anal. 2006;51(2):570–86.
Hu X, Gadbury GL, Xiang Q, Allison DB. Illustrations on using the distribution of a P-value in high dimensional data analyses. Adv Appl Stat Sci. 2010;1(2):191.
Neitzel J, Franzmeier N, Rubinski A, Dichgans M, Brendel M, Malik R, et al. KL-VS heterozygosity is associated with lower amyloid-dependent tau accumulation and memory impairment in Alzheimer’s disease. Nat Commun. 2021;12(1):1–12.
Chávez-Gutiérrez L, Bammens L, Benilova I, Vandersteen A, Benurwar M, Borgers M, et al. The mechanism of \(\gamma\) -secretase dysfunction in familial Alzheimer disease. EMBO J. 2012;31(10):2261–74.
Riemenschneider M, Schwarz S, Wagenpfeil S, Diehl J, Müller U, Förstl H, et al. A polymorphism of the brain-derived neurotrophic factor (BDNF) is associated with Alzheimer’s disease in patients lacking the Apolipoprotein E \(\varepsilon\) 4 allele. Mol Psychiatry. 2002;7(7):782–5.
Jin SC, Pastor P, Cooper B, Cervantes S, Benitez BA, Razquin C, et al. Pooled-DNA sequencing identifies novel causative variants in PSEN1, GRN and MAPT in a clinical early-onset and familial Alzheimer’s disease Ibero-American cohort. Alzheimers Res Ther. 2012;4(4):1–9.
Bertram L, McQueen MB, Mullin K, Blacker D, Tanzi RE. Systematic meta-analyses of Alzheimer disease genetic association studies: the AlzGene database. Nat Genet. 2007;39(1):17–23.
Safran M, Dalah I, Alexander J, Rosen N, Iny Stein T, Shmoish M, et al. GeneCards Version 3: the human gene integrator. Database 2010;2010:baq020.
Garbar C, Mascaux C, Giustiniani J, Salesse S, Debelle L, Antonicelli F, et al. Autophagy is decreased in triple-negative breast carcinoma involving likely the MUC1-EGFR-NEU1 signalling pathway. Int J Clin Exp Pathol. 2015;8(5):4344.
Lin YP, Cheng YJ, Huang JY, Lin HC, Yang BC. Zap70 controls the interaction of talin with integrin to regulate the chemotactic directionality of T-cell migration. Mol Immunol. 2010;47(11–12):2022–9.
Gatza ML, Silva GO, Parker JS, Fan C, Perou CM. An integrated genomics approach identifies drivers of proliferation in luminal-subtype human breast cancer. Nat Genet. 2014;46(10):1051–9.
Xu X, Zhang M, Xu F, Jiang S. Wnt signaling in breast cancer: biological mechanisms, challenges and opportunities. Mol Cancer. 2020;19:1–35.
Xia J, Wang Q, Ju F, Luo X, Wang F, Zhou Y, et al. Chloride intracellular channel 1 is a potential biomarker for breast cancer. Breast Cancer Targets Ther. 2022;2022:247–58.
Yao X, Zhang H, Tang S, Zheng X, Jiang L. Bioinformatics analysis to reveal potential differentially expressed long non-coding RNAs and genes associated with tumour metastasis in lung adenocarcinoma. OncoTargets Ther. 2020;13:3197–207.
Liu J, Wen Y, Liu Z, Liu S, Xu P, Xu Y, et al. VPS33B modulates c-Myc/p53/miR-192-3p to target CCNB1 suppressing the growth of non-small cell lung cancer. Mol Ther Nucleic Acids. 2021;23:324–35.
Li J, Bi L, Sun Y, Lu Z, Lin Y, Bai O, et al. RETRACTION: text mining and network analysis of molecular interaction in non-small cell lung cancer by using natural language processing. Mol Biol Rep. 2014;41(12):324–35.
Bentham R, Litchfield K, Watkins TB, Lim EL, Rosenthal R, Martínez-Ruiz C, et al. Using DNA sequencing data to quantify T cell fraction and therapy response. Nature. 2021;597(7877):555–60.
Lee B, Yao X, Shen L, Initiative ADN. Integrative analysis of summary data from GWAS and eQTL studies implicates genes differentially expressed in Alzheimer’s disease. BMC Genomics. 2022;23(Suppl 4):414.
Kim HY, Choi HJ, Lee JY, Kong G. Cancer Target Gene Screening: a web application for breast cancer target gene screening using multi-omics data analysis. Brief Bioinforma. 2020;21(2):663–75.
Zhang H, Jin Z, Cheng L, Zhang B. Integrative analysis of methylation and gene expression in lung adenocarcinoma and squamous cell lung carcinoma. Front Bioeng Biotechnol. 2020;8:3.
Chandrashekar PB, Alatkar S, Wang J, Hoffman GE, He C, Jin T, et al. DeepGAMI: deep biologically guided auxiliary learning for multimodal integration and imputation to improve genotype-phenotype prediction. Genome Med. 2023;15(1):88.
Jin T, Rehani P, Ying M, Huang J, Liu S, Roussos P, et al. scGRNom: a computational pipeline of integrative multi-omics analyses for predicting cell-type disease genes and regulatory networks. Genome Med. 2021;13(1):95.
Ahn T, Park T, et al. Pathway-driven discovery of rare mutational impact on cancer. BioMed Res Int. 2014;2014.
Chereda H, Bleckmann A, Menck K, Perera-Bel J, Stegmaier P, Auer F, et al. Explaining decisions of graph convolutional neural networks: patient-specific molecular subnetworks responsible for metastasis prediction in breast cancer. Genome Med. 2021;13:1–16.
Chen YX, Chen H, Rong Y, Jiang F, Chen JB, Duan YY, et al. An integrative multi-omics network-based approach identifies key regulators for breast cancer. Comput Struct Biotechnol J. 2020;18:2826–35.
Gogleva A, Polychronopoulos D, Pfeifer M, Poroshin V, Ughetto M, Martin MJ, et al. Knowledge graph-based recommendation framework identifies drivers of resistance in EGFR mutant non-small cell lung cancer. Nat Commun. 2022;13(1):1667.
Liu H, Yuan M, Mitra R, Zhou X, Long M, Lei W, et al. CTpathway: a CrossTalk-based pathway enrichment analysis method for cancer research. Genome Med. 2022;14(1):1–20.
Jin T, Nguyen ND, Talos F, Wang D. ECMarker: interpretable machine learning model identifies gene expression biomarkers predicting clinical outcomes and reveals molecular mechanisms of human disease in early stages. Bioinformatics. 2021;37(8):1115–24.
Lahat D, Adali T, Jutten C. Multimodal data fusion: an overview of methods, challenges, and prospects. Proc IEEE. 2015;103(9):1449–77.
Kline A, Wang H, Li Y, Dennis S, Hutch M, Xu Z, et al. Multimodal machine learning in precision health: a scoping review. npj Digit Med. 2022;5(1):171.
LaFerla FM, Tinkle BT, Bieberich CJ, Haudenschild CC, Jay G. The Alzheimer’s A \(\beta\) peptide induces neurodegeneration and apoptotic cell death in transgenic mice. Nat Genet. 1995;9(1):21–30.
Cen X, Chen Y, Xu X, Wu R, He F, Zhao Q, et al. Pharmacological targeting of MCL-1 promotes mitophagy and improves disease pathologies in an Alzheimer’s disease mouse model. Nat Commun. 2020;11(1):1–13.
Peña-Bautista C, Torres-Cuevas I, Baquero M, Ferrer I, García L, Vento M, et al. Early neurotransmission impairment in non-invasive Alzheimer disease detection. Sci Rep. 2020;10(1):1–9.
Qi Y, Klyubin I, Ondrejcak T, Hu NW, Rowan MJ. Enduring glucocorticoid-evoked exacerbation of synaptic plasticity disruption in male rats modelling early Alzheimer’s disease amyloidosis. Neuropsychopharmacology. 2021;46(12):2170–9.
Bell SM, Barnes K, De Marco M, Shaw PJ, Ferraiuolo L, Blackburn DJ, et al. Mitochondrial dysfunction in Alzheimer’s disease: a biomarker of the future? Biomedicines. 2021;9(1):63.
Trushina E. Alzheimer’s disease mechanisms in peripheral cells: promises and challenges. Alzheimers Dement Transl Res Clin Interv. 2019;5:652–60.
Lauretti E, Dincer O, Praticò D. Glycogen synthase kinase-3 signaling in Alzheimer’s disease. Biochim Biophys Acta (BBA) Mol Cell Res. 2020;1867(5):118664.
Rowland HA, Hooper NM, Kellett KA. Modelling sporadic Alzheimer’s disease using induced pluripotent stem cells. Neurochem Res. 2018;43(12):2179–98.
Bliss T, Collingridge G. Persistent memories of long-term potentiation and the N-methyl-d-aspartate receptor. Brain and neuroscience advances. 2019;3:2398212819848213.
Seto M, Weiner RL, Dumitrescu L, Hohman TJ. Protective genes and pathways in Alzheimer’s disease: moving towards precision interventions. Mol Neurodegener. 2021;16(1):29.
Jaworski T, Banach-Kasper E, Gralec K. GSK-3 \(\beta\) at the intersection of neuronal plasticity and neurodegeneration. Neural Plast. 2019;2019:1–14.
Perdigão C, Barata MA, Araújo MN, Mirfakhar FS, Castanheira J, Guimas Almeida C. Intracellular trafficking mechanisms of synaptic dysfunction in Alzheimer’s disease. Front Cell Neurosci. 2020;14:72.
Wightman DP, Jansen IE, Savage JE, Shadrin AA, Bahrami S, Holland D, et al. A genome-wide association study with 1,126,563 individuals identifies new risk loci for Alzheimer’s disease. Nat Genet. 2021;53(9):1276–82.
Goldman MJ, Craft B, Hastie M, Repečka K, McDade F, Kamath A, et al. Visualizing and interpreting cancer genomics data via the Xena platform. Nat Biotechnol. 2020;38(6):675–8.
Yao X, Ouyang S, Lian Y, Peng Q, Zhou X, Huang F, et al. Github repository for the code and data used in PheSeq. 2023. https://github.com/bionlp-hzau/PheSeq . Accessed 28 Feb 2022.
Yıldırım MA, Goh KI, Cusick ME, Barabasi AL, Vidal M. Drug-target network. Nat Biotechnol. 2007;25(10):1119–27.
Yao X, Ouyang S, Lian Y, Peng Q, Zhou X, Huang F, et al. Gene and phenotype literature annotation dataset used in PheSeq. 2023. https://drivegoogle.com/file/d/1EjqsiFvT4acuSmvFkfWuSRZ2DIfdYvjJ/view?usp=sharing . Accessed 3 Feb 2024.
Yao X, Ouyang S, Lian Y, Peng Q, Zhou X, Huang F, et al. Webpage of the visualized phenotype description network. 2023. https://lit-evi.hzau.edu.cn/PheSeq . Accessed 26 Nov 2021.
Download references
Acknowledgements
The authors would like to express their gratitude for many instructive discussions with Dr. Shichao Liu and Prof. Li Li.
The work is funded by the Fundamental Research Funds for the Central Universities (No. 2662021JC008, No. 2662022XXYJ001), and the Major Project of Hubei Hongshan Laboratory (No.2022HSZD031).
Author information
Authors and affiliations.
College of Informatics, Hubei Key Laboratory of Agricultural Bioinformatics, Huazhong Agricultural University, Wuhan, China
Xinzhi Yao, Sizhuo Ouyang, Qianqian Peng, Xionghui Zhou, Xuehai Hu & Jingbo Xia
Hubei Key Laboratory of Agricultural Bioinformatics, Huazhong Agricultural University, Wuhan, China
College of Science, Huazhong Agricultural University, Wuhan, China
Yulong Lian & Feng Shi
College of Life Science and Technology, Huazhong Agricultural University, Wuhan, China
Feier Huang
You can also search for this author in PubMed Google Scholar
Contributions
XY collected the data, performed the analysis, and wrote the paper; SO, YL, and QP collected the data and performed the analysis; XZ and FH performed the analysis; XH and FS contributed the tools; JX conceived and designed the analysis, performed the analysis, and wrote the paper.
Corresponding author
Correspondence to Jingbo Xia .
Ethics declarations
Ethics approval and consent to participate.
Not applicable.
Consent for publication
Competing interests.
The authors declare no competing interests.
Additional information
Publisher's note.
Springer Nature remains neutral with regard to jurisdictional claims in published maps and institutional affiliations.
Supplementary Information
Additional file 1..
Usage Guideline of PheSeq Code. This file provides detailed parameter descriptions and command line usage instructions for all scripts involved in the PheSeq.
Additional file 2.
Model Solution and Implementation. This file contains comprehensive algorithmic solutions implemented in PheSeq, detailing the models and their implementations.
Additional file 3.
Phenotype Description by PheSeq and A Visualized Phenotype Description Network for AD, BC, and LC. This file includes the embedding visualization of phenotype descriptions utilized in PheSeq. Additionally, it also introduces the web service for visualizing the phenotype description network mentioned in the paper.
Rights and permissions
Open Access This article is licensed under a Creative Commons Attribution 4.0 International License, which permits use, sharing, adaptation, distribution and reproduction in any medium or format, as long as you give appropriate credit to the original author(s) and the source, provide a link to the Creative Commons licence, and indicate if changes were made. The images or other third party material in this article are included in the article's Creative Commons licence, unless indicated otherwise in a credit line to the material. If material is not included in the article's Creative Commons licence and your intended use is not permitted by statutory regulation or exceeds the permitted use, you will need to obtain permission directly from the copyright holder. To view a copy of this licence, visit http://creativecommons.org/licenses/by/4.0/ . The Creative Commons Public Domain Dedication waiver ( http://creativecommons.org/publicdomain/zero/1.0/ ) applies to the data made available in this article, unless otherwise stated in a credit line to the data.
Reprints and permissions
About this article
Cite this article.
Yao, X., Ouyang, S., Lian, Y. et al. PheSeq, a Bayesian deep learning model to enhance and interpret the gene-disease association studies. Genome Med 16 , 56 (2024). https://doi.org/10.1186/s13073-024-01330-7
Download citation
Received : 26 July 2023
Accepted : 02 April 2024
Published : 16 April 2024
DOI : https://doi.org/10.1186/s13073-024-01330-7
Share this article
Anyone you share the following link with will be able to read this content:
Sorry, a shareable link is not currently available for this article.
Provided by the Springer Nature SharedIt content-sharing initiative
- Data fusion
- Embedding data
- Associated genes
Genome Medicine
ISSN: 1756-994X
- Submission enquiries: [email protected]
- General enquiries: [email protected]
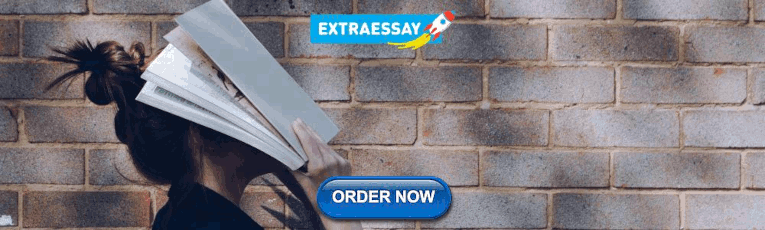
IMAGES
VIDEO
COMMENTS
The Role of Modeling . An early and longstanding aim of the observational learning literature is to understand the role of modeling in behavior change (e.g., Bandura & Huston, 1961; Bandura & McDonald, 1963; Bandura, Ross, & Ross, 1961).For example, an early study examined how the incidental behaviors of an experimenter might be acquired in the context of learning another task (Bandura & Huston).
First, future studies should take the social context into account in which children learn "indirect" observational learning (e.g., observing others' choices and outcomes in absence of the ...
Bobo Doll Experiment. Bandura's Bobo doll experiment is one of the most famous examples of observational learning. In the Bobo doll experiment, Bandura demonstrated that young children may imitate the aggressive actions of an adult model. Children observed a film where an adult repeatedly hit a large, inflatable balloon doll and then had the ...
However, little is known about the actual behavior of learners while carrying out observation tasks. In this case study, students' learning activities when processing observation tasks are closely ...
Participants. Ninety right-handed students (45 males and 45 females; mean age = 20.5 years; SD = 0.9 years) from the Département de kinésiologie at the Université de Montréal participated in this experiment. The participants were naive to the purpose of the study and had no prior experience with the task, and all participants were self-declared as being right-handed.
Observational learning involves acquiring skills or new or changed behaviors through watching the behavior of others. The person or actor performing the action that the observational learner replicates is called a model. The educational psychologist Albert Bandura was the first to recognize observational learning through his Bobo Doll experiment.
Bandura described specific steps in the process of modeling that must be followed if learning is to be successful: attention, retention, reproduction, and motivation. First, you must be focused on what the model is doing—you have to pay attention. Next, you must be able to retain, or remember, what you observed; this is retention.
The current study used a single-subject multiple-baseline across-classrooms design to examine the effects of behavior skills training (BST) paired with observational learning of students' engagement in and responses to disruptive behavior in the classroom setting.
Observational learning has proved to be effective with learners of various ages and in various school subjects, including writing. However, little is known about the actual behavior of learners while carrying out observation tasks. In this case study, students' learning activities when processing observa- tion tasks are closely analyzed: six students thought aloud while observing sets of ...
The procedures of an observational learning intervention vary based on the environment, the model, and the skill being taught. As previously described, observational learning interventions all include the steps of (1) observing someone else perform a behavior, (2) observing the consequence for performing the behavior (i.e., reinforcement), and (3) performing the behavior (MacDonald and Ahearn ...
Young infants can easily imitate, but only older infants demonstrate observational learning. In one study, a group of 14-month-old infants saw a novel act (e.g., the adult actor used his head to turn on a light panel). When encountering the same panel after a 1-week delay, 67% of infants used their head to turn on the light panel. ...
The current study used a single-subject multiplebaseline across-classrooms design to examine the effects of behavior skills training (BST) paired with observational learning of students ...
After his seminal work, more studies continue to advance our understanding of human social learning. For instance, observational learning is regarded as one of the prominent verbal behavior ...
In social learning theory, Albert Bandura (1977) agrees with the behaviorist learning theories of classical conditioning and operant conditioning. However, he adds two important ideas: Mediating processes occur between stimuli & responses. Behavior is learned from the environment through the process of observational learning.
During the 1960s, Albert Bandura conducted a series of experiments on observational learning, collectively known as the Bobo doll experiments.Two of the experiments are described below: Aim. Bandura (1961) conducted a controlled experiment study to investigate if social behaviors (i.e., aggression) can be acquired by observation and imitation.. Sample ...
An observational study is used to answer a research question based purely on what the researcher observes. There is no interference or manipulation of the research subjects, and no control and treatment groups. These studies are often qualitative in nature and can be used for both exploratory and explanatory research purposes.
Observational learning makes an interesting and potentially important topic in the developmental domain, especially when disorders are considered. ... The implications of studies aimed at clarifying whether and how this form of learning is spared by pathology are manifold. ... Knoblich G (2005) The case for motor involvement in perceiving ...
In observational learning, we learn by watching others and then imitating, or modeling, what they do or say. The individuals performing the imitated behavior are called models. Research suggests that this imitative learning involves a specific type of neuron, called a mirror neuron (Hickock, 2010; Rizzolatti, Fadiga, Fogassi, & Gallese, 2002 ...
The current article investigated the effects of direct and observational learning of auditory matching protocol on two twin boys' articulation. During the intervention, Max received direct instruction on matching auditory stimuli using the Sounds the Same app, while his twin Ryan learned through observing his brother's responses and the consequences he received. A multiple probe design was ...
A case study is one of the most commonly used methodologies of social research. This article attempts to look into the various dimensions of a case study research strategy, the different epistemological strands which determine the particular case study type and approach adopted in the field, discusses the factors which can enhance the effectiveness of a case study research, and the debate ...
Observational studies include case reports and case series, ecological studies, cross-sectional studies, case-control studies and cohort studies. New and ongoing developments in data and analytical technology, such as data linkage and propensity score matching, offer a promising future for observational studies. ... Machine learning will soon ...
based studies, observation methods were incorporated from the outset in the design of the research. However, as conventional case study models, such as Yin (2014), do not distinguish observation data from other types of data collection in terms of their unique significance and poten-tial, we modified Yin's CSR method. This observation-
Since the study case was presented as a brief discussion, it is expected that some plausible assumptions are added to fill in the gaps. Bandura's observational learning theory was selected in recognition to its relevance to this particular case of a family prone with domestic violence, criminal record, sexual abuse and alcoholism.
Despite the abundance of genotype-phenotype association studies, the resulting association outcomes often lack robustness and interpretations. To address these challenges, we introduce PheSeq, a Bayesian deep learning model that enhances and interprets association studies through the integration and perception of phenotype descriptions. By implementing the PheSeq model in three case studies on ...