Jump to navigation

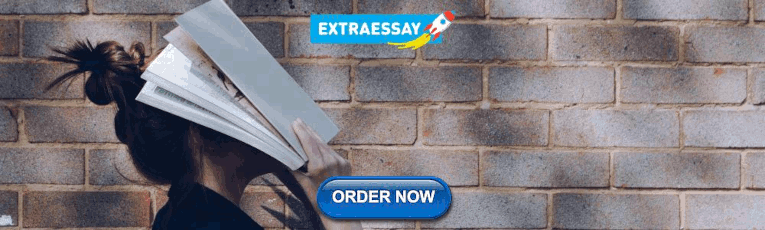
Cochrane Training
Chapter 21: qualitative evidence.
Jane Noyes, Andrew Booth, Margaret Cargo, Kate Flemming, Angela Harden, Janet Harris, Ruth Garside, Karin Hannes, Tomás Pantoja, James Thomas
Key Points:
- A qualitative evidence synthesis (commonly referred to as QES) can add value by providing decision makers with additional evidence to improve understanding of intervention complexity, contextual variations, implementation, and stakeholder preferences and experiences.
- A qualitative evidence synthesis can be undertaken and integrated with a corresponding intervention review; or
- Undertaken using a mixed-method design that integrates a qualitative evidence synthesis with an intervention review in a single protocol.
- Methods for qualitative evidence synthesis are complex and continue to develop. Authors should always consult current methods guidance at methods.cochrane.org/qi .
This chapter should be cited as: Noyes J, Booth A, Cargo M, Flemming K, Harden A, Harris J, Garside R, Hannes K, Pantoja T, Thomas J. Chapter 21: Qualitative evidence. In: Higgins JPT, Thomas J, Chandler J, Cumpston M, Li T, Page MJ, Welch VA (editors). Cochrane Handbook for Systematic Reviews of Interventions version 6.4 (updated August 2023). Cochrane, 2023. Available from www.training.cochrane.org/handbook .
21.1 Introduction
The potential contribution of qualitative evidence to decision making is well-established (Glenton et al 2016, Booth 2017, Carroll 2017). A synthesis of qualitative evidence can inform understanding of how interventions work by:
- increasing understanding of a phenomenon of interest (e.g. women’s conceptualization of what good antenatal care looks like);
- identifying associations between the broader environment within which people live and the interventions that are implemented;
- increasing understanding of the values and attitudes toward, and experiences of, health conditions and interventions by those who implement or receive them; and
- providing a detailed understanding of the complexity of interventions and implementation, and their impacts and effects on different subgroups of people and the influence of individual and contextual characteristics within different contexts.
The aim of this chapter is to provide authors (who already have experience of undertaking qualitative research and qualitative evidence synthesis) with additional guidance on undertaking a qualitative evidence synthesis that is subsequently integrated with an intervention review. This chapter draws upon guidance presented in a series of six papers published in the Journal of Clinical Epidemiology (Cargo et al 2018, Flemming et al 2018, Harden et al 2018, Harris et al 2018, Noyes et al 2018a, Noyes et al 2018b) and from a further World Health Organization series of papers published in BMJ Global Health, which extend guidance to qualitative evidence syntheses conducted within a complex intervention and health systems and decision making context (Booth et al 2019a, Booth et al 2019b, Flemming et al 2019, Noyes et al 2019, Petticrew et al 2019).The qualitative evidence synthesis and integration methods described in this chapter supplement Chapter 17 on methods for addressing intervention complexity. Authors undertaking qualitative evidence syntheses should consult these papers and chapters for more detailed guidance.
21.2 Designs for synthesizing and integrating qualitative evidence with intervention reviews
There are two main designs for synthesizing qualitative evidence with evidence of the effects of interventions:
- Sequential reviews: where one or more existing intervention review(s) has been published on a similar topic, it is possible to do a sequential qualitative evidence synthesis and then integrate its findings with those of the intervention review to create a mixed-method review. For example, Lewin and colleagues (Lewin et al (2010) and Glenton and colleagues (Glenton et al (2013) undertook sequential reviews of lay health worker programmes using separate protocols and then integrated the findings.
- Convergent mixed-methods review: where no pre-existing intervention review exists, it is possible to do a full convergent ‘mixed-methods’ review where the trials and qualitative evidence are synthesized separately, creating opportunities for them to ‘speak’ to each other during development, and then integrated within a third synthesis. For example, Hurley and colleagues (Hurley et al (2018) undertook an intervention review and a qualitative evidence synthesis following a single protocol.
It is increasingly common for sequential and convergent reviews to be conducted by some or all of the same authors; if not, it is critical that authors working on the qualitative evidence synthesis and intervention review work closely together to identify and create sufficient points of integration to enable a third synthesis that integrates the two reviews, or the conduct of a mixed-method review (Noyes et al 2018a) (see Figure 21.2.a ). This consideration also applies where an intervention review has already been published and there is no prior relationship with the qualitative evidence synthesis authors. We recommend that at least one joint author works across both reviews to facilitate development of the qualitative evidence synthesis protocol, conduct of the synthesis, and subsequent integration of the qualitative evidence synthesis with the intervention review within a mixed-methods review.
Figure 21.2.a Considering context and points of contextual integration with the intervention review or within a mixed-method review
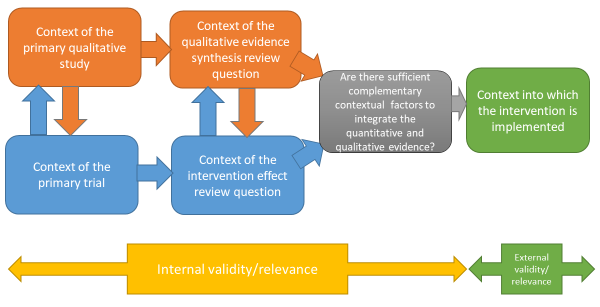
21.3 Defining qualitative evidence and studies
We use the term ‘qualitative evidence synthesis’ to acknowledge that other types of qualitative evidence (or data) can potentially enrich a synthesis, such as narrative data derived from qualitative components of mixed-method studies or free text from questionnaire surveys. We would not, however, consider a questionnaire survey to be a qualitative study and qualitative data from questionnaires should not usually be privileged over relevant evidence from qualitative studies. When thinking about qualitative evidence, specific terminology is used to describe the level of conceptual and contextual detail. Qualitative evidence that includes higher or lower levels of conceptual detail is described as ‘rich’ or ‘poor’. Associated terms ‘thick’ or ‘thin’ are best used to refer to higher or lower levels of contextual detail. Review authors can potentially develop a stronger synthesis using rich and thick qualitative evidence but, in reality, they will identify diverse conceptually rich and poor and contextually thick and thin studies. Developing a clear picture of the type and conceptual richness of available qualitative evidence strongly influences the choice of methodology and subsequent methods. We recommend that authors undertake scoping searches to determining the type and richness of available qualitative evidence before selecting their methodology and methods.
A qualitative study is a research study that uses a qualitative method of data collection and analysis. Review authors should include the studies that enable them to answer their review question. When selecting qualitative studies in a review about intervention effects, two types of qualitative study are available: those that collect data from the same participants as the included trials, known as ‘trial siblings’; and those that address relevant issues about the intervention, but as separate items of research – not connected to any included trials. Both can provide useful information, with trial sibling studies obviously closer in terms of their precise contexts to the included trials (Moore et al 2015), and non-sibling studies possibly contributing perspectives not present in the trials (Noyes et al 2016b).
21.4 Planning a qualitative evidence synthesis linked to an intervention review
The Cochrane Qualitative and Implementation Methods Group (QIMG) website provides links to practical guidance and key steps for authors who are considering a qualitative evidence synthesis ( methods.cochrane.org/qi ). The RETREAT framework outlines seven key considerations that review authors should systematically work through when planning a review (Booth et al 2016, Booth et al 2018) ( Box 21.4.a ). Flemming and colleagues (Flemming et al (2019) further explain how to factor in such considerations when undertaking a qualitative evidence synthesis within a complex intervention and decision making context when complexity is an important consideration.
Box 21.4.a RETREAT considerations when selecting an appropriate method for qualitative synthesis
21.5 Question development
The review question is critical to development of the qualitative evidence synthesis (Harris et al 2018). Question development affords a key point for integration with the intervention review. Complementary guidance supports novel thinking about question development, application of question development frameworks and the types of questions to be addressed by a synthesis of qualitative evidence (Cargo et al 2018, Harris et al 2018, Noyes et al 2018a, Booth et al 2019b, Flemming et al 2019).
Research questions for quantitative reviews are often mapped using structures such as PICO. Some qualitative reviews adopt this structure, or use an adapted variation of such a structure (e.g. SPICE (Setting, Perspective, Intervention or Phenomenon of Interest, Comparison, Evaluation) or SPIDER (Sample, Phenomenon of Interest, Design, Evaluation, Research type); (Cooke et al 2012). Booth and colleagues (Booth et al (2019b) propose an extended question framework (PerSPecTIF) to describe both wider context and immediate setting that is particularly suited to qualitative evidence synthesis and complex intervention reviews (see Table 21.5.a ).
Detailed attention to the question and specification of context at an early stage is critical to many aspects of qualitative synthesis (see Petticrew et al (2019) and Booth et al (2019a) for a more detailed discussion). By specifying the context a review team is able to identify opportunities for integration with the intervention review, or opportunities for maximizing use and interpretation of evidence as a mixed-method review progresses (see Figure 21.2.a ), and informs both the interpretation of the observed effects and assessment of the strength of the evidence available in addressing the review question (Noyes et al 2019). Subsequent application of GRADE CERQual (Lewin et al 2015, Lewin et al 2018), an approach to assess the confidence in synthesized qualitative findings, requires further specification of context in the review question.
Table 21.5.a PerSPecTIF Question formulation framework for qualitative evidence syntheses (Booth et al (2019b). Reproduced with permission of BMJ Publishing Group
21.6 Questions exploring intervention implementation
Additional guidance is available on formulation of questions to understand and assess intervention implementation (Cargo et al 2018). A strong understanding of how an intervention is thought to work, and how it should be implemented in practice, will enable a critical consideration of whether any observed lack of effect might be due to a poorly conceptualized intervention (i.e. theory failure) or a poor intervention implementation (i.e. implementation failure). Heterogeneity needs to be considered for both the underlying theory and the ways in which the intervention was implemented. An a priori scoping review (Levac et al 2010), concept analysis (Walker and Avant 2005), critical review (Grant and Booth 2009) or textual narrative synthesis (Barnett-Page and Thomas 2009) can be undertaken to classify interventions and/or to identify the programme theory, logic model or implementation measures and processes. The intervention Complexity Assessment Tool for Systematic Reviews iCAT_SR (Lewin et al 2017) may be helpful in classifying complexity in interventions and developing associated questions.
An existing intervention model or framework may be used within a new topic or context. The ‘best-fit framework’ approach to synthesis (Carroll et al 2013) can be used to establish the degree to which the source context (from where the framework was derived) resembles the new target context (see Figure 21.2.a ). In the absence of an explicit programme theory and detail of how implementation relates to outcomes, an a priori realist review, meta-ethnography or meta-interpretive review can be undertaken (Booth et al 2016). For example, Downe and colleagues (Downe et al (2016) undertook an initial meta-ethnography review to develop an understanding of the outcomes of importance to women receiving antenatal care.
However, these additional activities are very resource-intensive and are only recommended when the review team has sufficient resources to supplement the planned qualitative evidence syntheses with an additional explanatory review. Where resources are less plentiful a review team could engage with key stakeholders to articulate and develop programme theory (Kelly et al 2017, De Buck et al 2018).
21.6.1 Using logic models and theories to support question development
Review authors can develop a more comprehensive representation of question features through use of logic models, programme theories, theories of change, templates and pathways (Anderson et al 2011, Kneale et al 2015, Noyes et al 2016a) (see also Chapter 17, Section 17.2.1 and Chapter 2, Section 2.5.1 ). These different forms of social theory can be used to visualize and map the research question, its context, components, influential factors and possible outcomes (Noyes et al 2016a, Rehfuess et al 2018).
21.6.2 Stakeholder engagement
Finally, review authors need to engage stakeholders, including consumers affected by the health issue and interventions, or likely users of the review from clinical or policy contexts. From the preparatory stage, this consultation can ensure that the review scope and question is appropriate and resulting products address implementation concerns of decision makers (Kelly et al 2017, Harris et al 2018).
21.7 Searching for qualitative evidence
In comparison with identification of quantitative studies (see also Chapter 4 ), procedures for retrieval of qualitative research remain relatively under-developed. Particular challenges in retrieval are associated with non-informative titles and abstracts, diffuse terminology, poor indexing and the overwhelming prevalence of quantitative studies within data sources (Booth et al 2016).
Principal considerations when planning a search for qualitative studies, and the evidence that underpins them, have been characterized using a 7S framework from Sampling and Sources through Structured questions, Search procedures, Strategies and filters and Supplementary strategies to Standards for Reporting (Booth et al 2016).
A key decision, aligned to the purpose of the qualitative evidence synthesis is whether to use the comprehensive, exhaustive approaches that characterize quantitative searches or whether to use purposive sampling that is more sensitive to the qualitative paradigm (Suri 2011). The latter, which is used when the intent is to generate an interpretative understanding, for example, when generating theory, draws upon a versatile toolkit that includes theoretical sampling, maximum variation sampling and intensity sampling. Sources of qualitative evidence are more likely to include book chapters, theses and grey literature reports than standard quantitative study reports, and so a search strategy should place extra emphasis on these sources. Local databases may be particularly valuable given the criticality of context (Stansfield et al 2012).
Another key decision is whether to use study filters or simply to conduct a topic-based search where qualitative studies are identified at the study selection stage. Search filters for qualitative studies lack the specificity of their quantitative counterparts. Nevertheless, filters may facilitate efficient retrieval by study type (e.g. qualitative (Rogers et al 2018) or mixed methods (El Sherif et al 2016) or by perspective (e.g. patient preferences (Selva et al 2017)) particularly where the quantitative literature is overwhelmingly large and thus increases the number needed to retrieve. Poor indexing of qualitative studies makes citation searching (forward and backward) and the Related Articles features of electronic databases particularly useful (Cooper et al 2017). Further guidance on searching for qualitative evidence is available (Booth et al 2016, Noyes et al 2018a). The CLUSTER method has been proposed as a specific named method for tracking down associated or sibling reports (Booth et al 2013). The BeHEMoTh approach has been developed for identifying explicit use of theory (Booth and Carroll 2015).
21.7.1 Searching for process evaluations and implementation evidence
Four potential approaches are available to identify process evaluations.
- Identify studies at the point of study selection rather than through tailored search strategies. This involves conducting a sensitive topic search without any study design filter (Harden et al 1999), and identifying all study designs of interest during the screening process. This approach can be feasible when a review question involves multiple publication types (e.g. randomized trial, qualitative research and economic evaluations), which then do not require separate searches.
- Restrict included process evaluations to those conducted within randomized trials, which can be identified using standard search filters (see Chapter 4, Section 4.4.7 ). This method relies on reports of process evaluations also describing the surrounding randomized trial in enough detail to be identified by the search filter.
- Use unevaluated filter terms (such as ‘process evaluation’, ‘program(me) evaluation’, ‘feasibility study’, ‘implementation’ or ‘proof of concept’ etc) to retrieve process evaluations or implementation data. Approaches using strings of terms associated with the study type or purpose are considered experimental. There is a need to develop and test such filters. It is likely that such filters may be derived from the study type (process evaluation), the data type (process data) or the application (implementation) (Robbins et al 2011).
- Minimize reliance on topic-based searching and rely on citations-based approaches to identify linked reports, published or unpublished, of a particular study (Booth et al 2013) which may provide implementation or process data (Bonell et al 2013).
More detailed guidance is provided by Cargo and colleagues (Cargo et al (2018).
21.8 Assessing methodological strengths and limitations of qualitative studies
Assessment of the methodological strengths and limitations of qualitative research remains contested within the primary qualitative research community (Garside 2014). However, within systematic reviews and evidence syntheses it is considered essential, even when studies are not to be excluded on the basis of quality (Carroll et al 2013). One review found almost 100 appraisal tools for assessing primary qualitative studies (Munthe-Kaas et al 2019). Limitations included a focus on reporting rather than conduct and the presence of items that are separate from, or tangential to, consideration of study quality (e.g. ethical approval).
Authors should distinguish between assessment of study quality and assessment of risk of bias by focusing on assessment of methodological strengths and limitations as a marker of study rigour (what we term a ‘risk to rigour’ approach (Noyes et al 2019)). In the absence of a definitive risk to rigour tool, we recommend that review authors select from published, commonly used and validated tools that focus on the assessment of the methodological strengths and limitations of qualitative studies (see Box 21.8.a ). Pragmatically, we consider a ‘validated’ tool as one that has been subjected to evaluation. Issues such as inter-rater reliability are afforded less importance given that identification of complementary or conflicting perspectives on risk to rigour is considered more useful than achievement of consensus per se (Noyes et al 2019).
The CASP tool for qualitative research (as one example) maps onto the domains in Box 21.8.a (CASP 2013). Tools not meeting the criterion of focusing on assessment of methodological strengths and limitations include those that integrate assessment of the quality of reporting (such as scoring of the title and abstract, etc) into an overall assessment of methodological strengths and limitations. As with other risk of bias assessment tools, we strongly recommend against the application of scores to domains or calculation of total quality scores. We encourage review authors to discuss the studies and their assessments of ‘risk to rigour’ for each paper and how the study’s methodological limitations may affect review findings (Noyes et al 2019). We further advise that qualitative ‘sensitivity analysis’, exploring the robustness of the synthesis and its vulnerability to methodologically limited studies, be routinely applied regardless of the review authors’ overall confidence in synthesized findings (Carroll et al 2013). Evidence suggests that qualitative sensitivity analysis is equally advisable for mixed methods studies from which the qualitative component is extracted (Verhage and Boels 2017).
Box 21.8.a Example domains that provide an assessment of methodological strengths and limitations to determine study rigour
Adapted from Noyes et al (2019) and Alvesson and Sköldberg (2009)
21.8.1 Additional assessment of methodological strengths and limitations of process evaluation and intervention implementation evidence
Few assessment tools explicitly address rigour in process evaluation or implementation evidence. For qualitative primary studies, the 8-item process evaluation tool developed by the EPPI-Centre (Rees et al 2009, Shepherd et al 2010) can be used to supplement tools selected to assess methodological strengths and limitations and risks to rigour in primary qualitative studies. One of these items, a question on usefulness (framed as ‘how well the intervention processes were described and whether or not the process data could illuminate why or how the interventions worked or did not work’ ) offers a mechanism for exploring process mechanisms (Cargo et al 2018).
21.9 Selecting studies to synthesize
Decisions about inclusion or exclusion of studies can be more complex in qualitative evidence syntheses compared to reviews of trials that aim to include all relevant studies. Decisions on whether to include all studies or to select a sample of studies depend on a range of general and review specific criteria that Noyes and colleagues (Noyes et al (2019) outline in detail. The number of qualitative studies selected needs to be consistent with a manageable synthesis, and the contexts of the included studies should enable integration with the trials in the effectiveness analysis (see Figure 21.2.a ). The guiding principle is transparency in the reporting of all decisions and their rationale.
21.10 Selecting a qualitative evidence synthesis and data extraction method
Authors will typically find that they cannot select an appropriate synthesis method until the pool of available qualitative evidence has been thoroughly scoped. Flexible options concerning choice of method may need to be articulated in the protocol.
The INTEGRATE-HTA guidance on selecting methodology and methods for qualitative evidence synthesis and health technology assessment offers a useful starting point when selecting a method of synthesis (Booth et al 2016, Booth et al 2018). Some methods are designed primarily to develop findings at a descriptive level and thus directly feed into lines of action for policy and practice. Others hold the capacity to develop new theory (e.g. meta-ethnography and theory building approaches to thematic synthesis). Noyes and colleagues (Noyes et al (2019) and Flemming and colleagues (Flemming et al (2019) elaborate on key issues for consideration when selecting a method that is particularly suited to a Cochrane Review and decision making context (see Table 21.10.a ). Three qualitative evidence synthesis methods (thematic synthesis, framework synthesis and meta-ethnography) are recommended to produce syntheses that can subsequently be integrated with an intervention review or analysis.
Table 21.10.a Recommended methods for undertaking a qualitative evidence synthesis for subsequent integration with an intervention review, or as part of a mixed-method review (adapted from an original source developed by convenors (Flemming et al 2019, Noyes et al 2019))
21.11 Data extraction
Qualitative findings may take the form of quotations from participants, subthemes and themes identified by the study’s authors, explanations, hypotheses or new theory, or observational excerpts and author interpretations of these data (Sandelowski and Barroso 2002). Findings may be presented as a narrative, or summarized and displayed as tables, infographics or logic models and potentially located in any part of the paper (Noyes et al 2019).
Methods for qualitative data extraction vary according to the synthesis method selected. Data extraction is not sequential and linear; often, it involves moving backwards and forwards between review stages. Review teams will need regular meetings to discuss and further interrogate the evidence and thereby achieve a shared understanding. It may be helpful to draw on a key stakeholder group to help in interpreting the evidence and in formulating key findings. Additional approaches (such as subgroup analysis) can be used to explore evidence from specific contexts further.
Irrespective of the review type and choice of synthesis method, we consider it best practice to extract detailed contextual and methodological information on each study and to report this information in a table of ‘Characteristics of included studies’ (see Table 21.11.a ). The template for intervention description and replication TIDieR checklist (Hoffmann et al 2014) and ICAT_SR tool may help with specifying key information for extraction (Lewin et al 2017). Review authors must ensure that they preserve the context of the primary study data during the extraction and synthesis process to prevent misinterpretation of primary studies (Noyes et al 2019).
Table 21.11.a Contextual and methodological information for inclusion within a table of ‘Characteristics of included studies’. From Noyes et al (2019). Reproduced with permission of BMJ Publishing Group
Noyes and colleagues (Noyes et al (2019) provide additional guidance and examples of the various methods of data extraction. It is usual for review authors to select one method. In summary, extraction methods can be grouped as follows.
- Using a bespoke universal, standardized or adapted data extraction template Review authors can develop their own review-specific data extraction template, or select a generic data extraction template by study type (e.g. templates developed by the National Institute for Health and Clinical Excellence (National Institute for Health Care Excellence 2012).
- Using an a priori theory or predetermined framework to extract data Framework synthesis, and its subvariant ‘Best Fit’ Framework approach, involve extracting data from primary studies against an a priori framework in order to better understand a phenomenon of interest (Carroll et al 2011, Carroll et al 2013). For example, Glenton and colleagues (Glenton et al (2013) extracted data against a modified SURE Framework (2011) to synthesize factors affecting the implementation of lay health worker interventions. The SURE framework enumerates possible factors that may influence the implementation of health system interventions (SURE (Supporting the Use of Research Evidence) Collaboration 2011, Glenton et al 2013). Use of the ‘PROGRESS’ (place of residence, race/ethnicity/culture/language, occupation, gender/sex, religion, education, socioeconomic status, and social capital) framework also helps to ensure that data extraction maintains an explicit equity focus (O'Neill et al 2014). A logic model can also be used as a framework for data extraction.
- Using a software program to code original studies inductively A wide range of software products have been developed by systematic review organizations (such as EPPI-Reviewer (Thomas et al 2010)). Most software for the analysis of primary qualitative data – such as NVivo ( www.qsrinternational.com/nvivo/home ) and others – can be used to code studies in a systematic review (Houghton et al 2017). For example, one method of data extraction and thematic synthesis involves coding the original studies using a software program to build inductive descriptive themes and a theoretical explanation of phenomena of interest (Thomas and Harden 2008). Thomas and Harden (2008) provide a worked example to demonstrate coding and developing a new understanding of children’s choices and motivations to eating fruit and vegetables from included primary studies.
21.12 Assessing the confidence in qualitative synthesized findings
The GRADE system has long featured in assessing the certainty of quantitative findings and application of its qualitative counterpart, GRADE-CERQual, is recommended for Cochrane qualitative evidence syntheses (Lewin et al 2015). CERQual has four components (relevance, methodological limitations, adequacy and coherence) which are used to formulate an overall assessment of confidence in the synthesized qualitative finding. Guidance on its components and reporting requirements have been published in a series in Implementation Science (Lewin et al 2018).
21.13 Methods for integrating the qualitative evidence synthesis with an intervention review
A range of methods and tools is available for data integration or mixed-method synthesis (Harden et al 2018, Noyes et al 2019). As noted at the beginning of this chapter, review authors can integrate a qualitative evidence synthesis with an existing intervention review published on a similar topic (sequential approach), or conduct a new intervention review and qualitative evidence syntheses in parallel before integration (convergent approach). Irrespective of whether the qualitative synthesis is sequential or convergent to the intervention review, we recommend that qualitative and quantitative evidence be synthesized separately using appropriate methods before integration (Harden et al 2018). The scope for integration can be more limited with a pre-existing intervention review unless review authors have access to the data underlying the intervention review report.
Harden and colleagues and Noyes and colleagues outline the following methods and tools for integration with an intervention review (Harden et al 2018, Noyes et al 2019):
- Juxtaposing findings in a matrix Juxtaposition is driven by the findings from the qualitative evidence synthesis (e.g. intervention components related to the acceptability or feasibility of the interventions) and these findings form one side of the matrix. Findings on intervention effects (e.g. improves outcome, no difference in outcome, uncertain effects) form the other side of the matrix. Quantitative studies are grouped according to findings on intervention effects and the presence or absence of features specified by the hypotheses generated from the qualitative synthesis (Candy et al 2011). Observed patterns in the matrix are used to explain differences in the findings of the quantitative studies and to identify gaps in research (van Grootel et al 2017). (See, for example, (Ames et al 2017, Munabi-Babigumira et al 2017, Hurley et al 2018)
- Analysing programme theory Theories articulating how interventions are expected to work are analysed. Findings from quantitative studies, testing the effects of interventions, and from qualitative and process evaluation evidence are used together to examine how the theories work in practice (Greenhalgh et al 2007). The value of different theories is assessed or new/revised theory developed. Factors that enhance or reduce intervention effectiveness are also identified.
- Using logic models or other types of conceptual framework A logic model (Glenton et al 2013) or other type of conceptual framework, which represents the processes by which an intervention produces change provides a common scaffold for integrating findings across different types of evidence (Booth and Carroll 2015). Frameworks can be specified a priori from the literature or through stakeholder engagement or newly developed during the review. Findings from quantitative studies testing the effects of interventions and those from qualitative evidence are used to develop and/or further refine the model.
- Testing hypotheses derived from syntheses of qualitative evidence Quantitative studies are grouped according to the presence or absence of the proposition specified by the hypotheses to be tested and subgroup analysis is used to explore differential findings on the effects of interventions (Thomas et al 2004).
- Qualitative comparative analysis (QCA) Findings from a qualitative synthesis are used to identify the range of features that are important for successful interventions, and the mechanisms through which these features operate. A QCA then tests whether or not the features are associated with effective interventions (Kahwati et al 2016). The analysis unpicks multiple potential pathways to effectiveness accommodating scenarios where the same intervention feature is associated both with effective and less effective interventions, depending on context. QCA offers potential for use in integration; unlike the other methods and tools presented here it does not yet have sufficient methodological guidance available. However, exemplar reviews using QCA are available (Thomas et al 2014, Harris et al 2015, Kahwati et al 2016).
Review authors can use the above methods in combination (e.g. patterns observed through juxtaposing findings within a matrix can be tested using subgroup analysis or QCA). Analysing programme theory, using logic models and QCA would require members of the review team with specific skills in these methods. Using subgroup analysis and QCA are not suitable when limited evidence is available (Harden et al 2018, Noyes et al 2019). (See also Chapter 17 on intervention complexity.)
21.14 Reporting the protocol and qualitative evidence synthesis
Reporting standards and tools designed for intervention reviews (such as Cochrane’s MECIR standards ( http://methods.cochrane.org/mecir ) or the PRISMA Statement (Liberati et al 2009), may not be appropriate for qualitative evidence syntheses or an integrated mixed-method review. Additional guidance on how to choose, adapt or create a hybrid reporting tool is provided as a 5-point ‘decision flowchart’ ( Figure 21.14.a ) (Flemming et al 2018). Review authors should consider whether: a specific set of reporting guidance is available (e.g. eMERGe for meta-ethnographies (France et al 2015)); whether generic guidance (e.g. ENTREQ (Tong et al 2012)) is suitable; or whether additional checklists or tools are appropriate for reporting a specific aspect of the review.
Figure 21.14.a Decision flowchart for choice of reporting approach for syntheses of qualitative, implementation or process evaluation evidence (Flemming et al 2018). Reproduced with permission of Elsevier
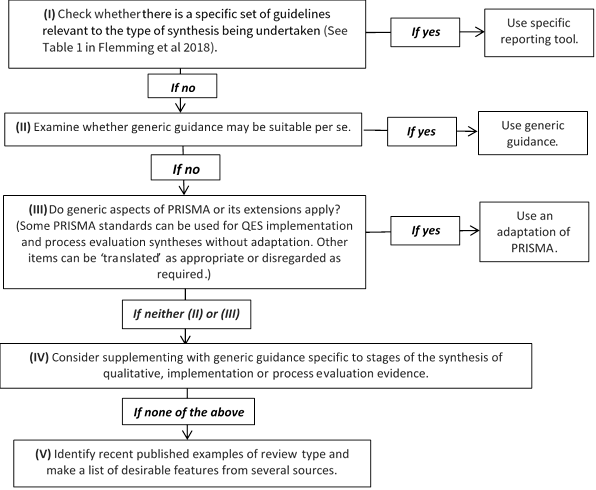
21.15 Chapter information
Authors: Jane Noyes, Andrew Booth, Margaret Cargo, Kate Flemming, Angela Harden, Janet Harris, Ruth Garside, Karin Hannes, Tomás Pantoja, James Thomas
Acknowledgements: This chapter replaces Chapter 20 in the first edition of this Handbook (2008) and subsequent Version 5.2. We would like to thank the previous Chapter 20 authors Jennie Popay and Alan Pearson. Elements of this chapter draw on previous supplemental guidance produced by the Cochrane Qualitative and Implementation Methods Group Convenors, to which Simon Lewin contributed.
Funding: JT is supported by the National Institute for Health Research (NIHR) Collaboration for Leadership in Applied Health Research and Care North Thames at Barts Health NHS Trust. The views expressed are those of the author(s) and not necessarily those of the NHS, the NIHR or the Department of Health.
21.16 References
Ames HM, Glenton C, Lewin S. Parents' and informal caregivers' views and experiences of communication about routine childhood vaccination: a synthesis of qualitative evidence. Cochrane Database of Systematic Reviews 2017; 2 : CD011787.
Anderson LM, Petticrew M, Rehfuess E, Armstrong R, Ueffing E, Baker P, Francis D, Tugwell P. Using logic models to capture complexity in systematic reviews. Research Synthesis Methods 2011; 2 : 33-42.
Barnett-Page E, Thomas J. Methods for the synthesis of qualitative research: a critical review. BMC Medical Research Methodology 2009; 9 : 59.
Benoot C, Hannes K, Bilsen J. The use of purposeful sampling in a qualitative evidence synthesis: a worked example on sexual adjustment to a cancer trajectory. BMC Medical Research Methodology 2016; 16 : 21.
Bonell C, Jamal F, Harden A, Wells H, Parry W, Fletcher A, Petticrew M, Thomas J, Whitehead M, Campbell R, Murphy S, Moore L. Public Health Research. Systematic review of the effects of schools and school environment interventions on health: evidence mapping and synthesis . Southampton (UK): NIHR Journals Library; 2013.
Booth A, Harris J, Croot E, Springett J, Campbell F, Wilkins E. Towards a methodology for cluster searching to provide conceptual and contextual "richness" for systematic reviews of complex interventions: case study (CLUSTER). BMC Medical Research Methodology 2013; 13 : 118.
Booth A, Carroll C. How to build up the actionable knowledge base: the role of 'best fit' framework synthesis for studies of improvement in healthcare. BMJ Quality and Safety 2015; 24 : 700-708.
Booth A, Noyes J, Flemming K, Gerhardus A, Wahlster P, van der Wilt GJ, Mozygemba K, Refolo P, Sacchini D, Tummers M, Rehfuess E. Guidance on choosing qualitative evidence synthesis methods for use in health technology assessment for complex interventions 2016. https://www.integrate-hta.eu/wp-content/uploads/2016/02/Guidance-on-choosing-qualitative-evidence-synthesis-methods-for-use-in-HTA-of-complex-interventions.pdf
Booth A. Qualitative evidence synthesis. In: Facey K, editor. Patient involvement in Health Technology Assessment . Singapore: Springer; 2017. p. 187-199.
Booth A, Noyes J, Flemming K, Gehardus A, Wahlster P, Jan van der Wilt G, Mozygemba K, Refolo P, Sacchini D, Tummers M, Rehfuess E. Structured methodology review identified seven (RETREAT) criteria for selecting qualitative evidence synthesis approaches. Journal of Clinical Epidemiology 2018; 99 : 41-52.
Booth A, Moore G, Flemming K, Garside R, Rollins N, Tuncalp Ö, Noyes J. Taking account of context in systematic reviews and guidelines considering a complexity perspective. BMJ Global Health 2019a; 4 : e000840.
Booth A, Noyes J, Flemming K, Moore G, Tuncalp Ö, Shakibazadeh E. Formulating questions to address the acceptability and feasibility of complex interventions in qualitative evidence synthesis. BMJ Global Health 2019b; 4 : e001107.
Candy B, King M, Jones L, Oliver S. Using qualitative synthesis to explore heterogeneity of complex interventions. BMC Medical Research Methodology 2011; 11 : 124.
Cargo M, Harris J, Pantoja T, Booth A, Harden A, Hannes K, Thomas J, Flemming K, Garside R, Noyes J. Cochrane Qualitative and Implementation Methods Group guidance series-paper 4: methods for assessing evidence on intervention implementation. Journal of Clinical Epidemiology 2018; 97 : 59-69.
Carroll C, Booth A, Cooper K. A worked example of "best fit" framework synthesis: a systematic review of views concerning the taking of some potential chemopreventive agents. BMC Medical Research Methodology 2011; 11 : 29.
Carroll C, Booth A, Leaviss J, Rick J. "Best fit" framework synthesis: refining the method. BMC Medical Research Methodology 2013; 13 : 37.
Carroll C. Qualitative evidence synthesis to improve implementation of clinical guidelines. BMJ 2017; 356 : j80.
CASP. Making sense of evidence: 10 questions to help you make sense of qualitative research: Public Health Resource Unit, England; 2013. http://media.wix.com/ugd/dded87_29c5b002d99342f788c6ac670e49f274.pdf .
Cooke A, Smith D, Booth A. Beyond PICO: the SPIDER tool for qualitative evidence synthesis. Qualitative Health Research 2012; 22 : 1435-1443.
Cooper C, Booth A, Britten N, Garside R. A comparison of results of empirical studies of supplementary search techniques and recommendations in review methodology handbooks: a methodological review. Systematic Reviews 2017; 6 : 234.
De Buck E, Hannes K, Cargo M, Van Remoortel H, Vande Veegaete A, Mosler HJ, Govender T, Vandekerckhove P, Young T. Engagement of stakeholders in the development of a Theory of Change for handwashing and sanitation behaviour change. International Journal of Environmental Research and Public Health 2018; 28 : 8-22.
Dixon-Woods M. Using framework-based synthesis for conducting reviews of qualitative studies. BMC Medicine 2011; 9 : 39.
Downe S, Finlayson K, Tuncalp, Metin Gulmezoglu A. What matters to women: a systematic scoping review to identify the processes and outcomes of antenatal care provision that are important to healthy pregnant women. BJOG: An International Journal of Obstetrics and Gynaecology 2016; 123 : 529-539.
El Sherif R, Pluye P, Gore G, Granikov V, Hong QN. Performance of a mixed filter to identify relevant studies for mixed studies reviews. Journal of the Medical Library Association 2016; 104 : 47-51.
Flemming K, Booth A, Hannes K, Cargo M, Noyes J. Cochrane Qualitative and Implementation Methods Group guidance series-paper 6: reporting guidelines for qualitative, implementation, and process evaluation evidence syntheses. Journal of Clinical Epidemiology 2018; 97 : 79-85.
Flemming K, Booth A, Garside R, Tuncalp O, Noyes J. Qualitative evidence synthesis for complex interventions and guideline development: clarification of the purpose, designs and relevant methods. BMJ Global Health 2019; 4 : e000882.
France EF, Ring N, Noyes J, Maxwell M, Jepson R, Duncan E, Turley R, Jones D, Uny I. Protocol-developing meta-ethnography reporting guidelines (eMERGe). BMC Medical Research Methodology 2015; 15 : 103.
France EF, Cunningham M, Ring N, Uny I, Duncan EAS, Jepson RG, Maxwell M, Roberts RJ, Turley RL, Booth A, Britten N, Flemming K, Gallagher I, Garside R, Hannes K, Lewin S, Noblit G, Pope C, Thomas J, Vanstone M, Higginbottom GMA, Noyes J. Improving reporting of Meta-Ethnography: The eMERGe Reporting Guidance BMC Medical Research Methodology 2019; 19 : 25.
Garside R. Should we appraise the quality of qualitative research reports for systematic reviews, and if so, how? Innovation: The European Journal of Social Science Research 2014; 27 : 67-79.
Glenton C, Colvin CJ, Carlsen B, Swartz A, Lewin S, Noyes J, Rashidian A. Barriers and facilitators to the implementation of lay health worker programmes to improve access to maternal and child health: qualitative evidence synthesis. Cochrane Database of Systematic Reviews 2013; 10 : CD010414.
Glenton C, Lewin S, Norris S. Chapter 15: Using evidence from qualitative research to develop WHO guidelines. In: Norris S, editor. World Health Organization Handbook for Guideline Development . 2nd. ed. Geneva: WHO; 2016.
Grant MJ, Booth A. A typology of reviews: an analysis of 14 review types and associated methodologies. Health Information and Libraries Journal 2009; 26 : 91-108.
Greenhalgh T, Kristjansson E, Robinson V. Realist review to understand the efficacy of school feeding programmes. BMJ 2007; 335 : 858.
Harden A, Oakley A, Weston R. A review of the effectiveness and appropriateness of peer-delivered health promotion for young people. London: Institute of Education, University of London; 1999.
Harden A, Thomas J, Cargo M, Harris J, Pantoja T, Flemming K, Booth A, Garside R, Hannes K, Noyes J. Cochrane Qualitative and Implementation Methods Group guidance series-paper 5: methods for integrating qualitative and implementation evidence within intervention effectiveness reviews. Journal of Clinical Epidemiology 2018; 97 : 70-78.
Harris JL, Booth A, Cargo M, Hannes K, Harden A, Flemming K, Garside R, Pantoja T, Thomas J, Noyes J. Cochrane Qualitative and Implementation Methods Group guidance series-paper 2: methods for question formulation, searching, and protocol development for qualitative evidence synthesis. Journal of Clinical Epidemiology 2018; 97 : 39-48.
Harris KM, Kneale D, Lasserson TJ, McDonald VM, Grigg J, Thomas J. School-based self management interventions for asthma in children and adolescents: a mixed methods systematic review (Protocol). Cochrane Database of Systematic Reviews 2015; 4 : CD011651.
Hoffmann TC, Glasziou PP, Boutron I, Milne R, Perera R, Moher D, Altman DG, Barbour V, Macdonald H, Johnston M, Lamb SE, Dixon-Woods M, McCulloch P, Wyatt JC, Chan AW, Michie S. Better reporting of interventions: template for intervention description and replication (TIDieR) checklist and guide. BMJ 2014; 348 : g1687.
Houghton C, Murphy K, Meehan B, Thomas J, Brooker D, Casey D. From screening to synthesis: using nvivo to enhance transparency in qualitative evidence synthesis. Journal of Clinical Nursing 2017; 26 : 873-881.
Hurley M, Dickson K, Hallett R, Grant R, Hauari H, Walsh N, Stansfield C, Oliver S. Exercise interventions and patient beliefs for people with hip, knee or hip and knee osteoarthritis: a mixed methods review. Cochrane Database of Systematic Reviews 2018; 4 : CD010842.
Kahwati L, Jacobs S, Kane H, Lewis M, Viswanathan M, Golin CE. Using qualitative comparative analysis in a systematic review of a complex intervention. Systematic Reviews 2016; 5 : 82.
Kelly MP, Noyes J, Kane RL, Chang C, Uhl S, Robinson KA, Springs S, Butler ME, Guise JM. AHRQ series on complex intervention systematic reviews-paper 2: defining complexity, formulating scope, and questions. Journal of Clinical Epidemiology 2017; 90 : 11-18.
Kneale D, Thomas J, Harris K. Developing and Optimising the Use of Logic Models in Systematic Reviews: Exploring Practice and Good Practice in the Use of Programme Theory in Reviews. PloS One 2015; 10 : e0142187.
Levac D, Colquhoun H, O'Brien KK. Scoping studies: advancing the methodology. Implementation Science 2010; 5 : 69.
Lewin S, Munabi-Babigumira S, Glenton C, Daniels K, Bosch-Capblanch X, van Wyk BE, Odgaard-Jensen J, Johansen M, Aja GN, Zwarenstein M, Scheel IB. Lay health workers in primary and community health care for maternal and child health and the management of infectious diseases. Cochrane Database of Systematic Reviews 2010; 3 : CD004015.
Lewin S, Glenton C, Munthe-Kaas H, Carlsen B, Colvin CJ, Gulmezoglu M, Noyes J, Booth A, Garside R, Rashidian A. Using qualitative evidence in decision making for health and social interventions: an approach to assess confidence in findings from qualitative evidence syntheses (GRADE-CERQual). PLoS Medicine 2015; 12 : e1001895.
Lewin S, Hendry M, Chandler J, Oxman AD, Michie S, Shepperd S, Reeves BC, Tugwell P, Hannes K, Rehfuess EA, Welch V, McKenzie JE, Burford B, Petkovic J, Anderson LM, Harris J, Noyes J. Assessing the complexity of interventions within systematic reviews: development, content and use of a new tool (iCAT_SR). BMC Medical Research Methodology 2017; 17 : 76.
Lewin S, Booth A, Glenton C, Munthe-Kaas H, Rashidian A, Wainwright M, Bohren MA, Tuncalp O, Colvin CJ, Garside R, Carlsen B, Langlois EV, Noyes J. Applying GRADE-CERQual to qualitative evidence synthesis findings: introduction to the series. Implementation Science 2018; 13 : 2.
Liberati A, Altman DG, Tetzlaff J, Mulrow C, Gøtzsche PC, Ioannidis JPA, Clarke M, Devereaux PJ, Kleijnen J, Moher D. The PRISMA statement for reporting systematic reviews and meta-analyses of studies that evaluate healthcare interventions: explanation and elaboration. BMJ 2009; 339 : b2700.
Moore G, Audrey S, Barker M, Bond L, Bonell C, Harderman W, et al. Process evaluation of complex interventions: Medical Research Council guidance. BMJ 2015; 350 : h1258.
Munabi-Babigumira S, Glenton C, Lewin S, Fretheim A, Nabudere H. Factors that influence the provision of intrapartum and postnatal care by skilled birth attendants in low- and middle-income countries: a qualitative evidence synthesis. Cochrane Database of Systematic Reviews 2017; 11 : CD011558.
Munthe-Kaas H, Glenton C, Booth A, Noyes J, Lewin S. Systematic mapping of existing tools to appraise methodological strengths and limitations of qualitative research: first stage in the development of the CAMELOT tool. BMC Medical Research Methodology 2019; 19 : 113.
National Institute for Health Care Excellence. NICE Process and Methods Guides. Methods for the Development of NICE Public Health Guidance . London: National Institute for Health and Care Excellence (NICE); 2012.
Newton BJ, Rothlingova Z, Gutteridge R, LeMarchand K, Raphael JH. No room for reflexivity? Critical reflections following a systematic review of qualitative research. Journal of Health Psychology 2012; 17 : 866-885.
Noblit GW, Hare RD. Meta-ethnography: synthesizing qualitative studies . Newbury Park: Sage Publications, Inc; 1988.
Noyes J, Hendry M, Booth A, Chandler J, Lewin S, Glenton C, Garside R. Current use was established and Cochrane guidance on selection of social theories for systematic reviews of complex interventions was developed. Journal of Clinical Epidemiology 2016a; 75 : 78-92.
Noyes J, Hendry M, Lewin S, Glenton C, Chandler J, Rashidian A. Qualitative "trial-sibling" studies and "unrelated" qualitative studies contributed to complex intervention reviews. Journal of Clinical Epidemiology 2016b; 74 : 133-143.
Noyes J, Booth A, Flemming K, Garside R, Harden A, Lewin S, Pantoja T, Hannes K, Cargo M, Thomas J. Cochrane Qualitative and Implementation Methods Group guidance series-paper 3: methods for assessing methodological limitations, data extraction and synthesis, and confidence in synthesized qualitative findings. Journal of Clinical Epidemiology 2018a; 97 : 49-58.
Noyes J, Booth A, Cargo M, Flemming K, Garside R, Hannes K, Harden A, Harris J, Lewin S, Pantoja T, Thomas J. Cochrane Qualitative and Implementation Methods Group guidance series-paper 1: introduction. Journal of Clinical Epidemiology 2018b; 97 : 35-38.
Noyes J, Booth A, Moore G, Flemming K, Tuncalp O, Shakibazadeh E. Synthesising quantitative and qualitative evidence to inform guidelines on complex interventions: clarifying the purposes, designs and outlining some methods. BMJ Global Health 2019; 4 (Suppl 1) : e000893.
O'Neill J, Tabish H, Welch V, Petticrew M, Pottie K, Clarke M, Evans T, Pardo Pardo J, Waters E, White H, Tugwell P. Applying an equity lens to interventions: using PROGRESS ensures consideration of socially stratifying factors to illuminate inequities in health. Journal of Clinical Epidemiology 2014; 67 : 56-64.
Oliver S, Rees R, Clarke-Jones L, Milne R, Oakley A, Gabbay J, Stein K, Buchanan P, Gyte G. A multidimensional conceptual framework for analysing public involvement in health services research. Health Expectations 2008; 11 : 72-84.
Petticrew M, Knai C, Thomas J, Rehfuess E, Noyes J, Gerhardus A, Grimshaw J, Rutter H. Implications of a complexity perspective for systematic reviews and guideline development in health decision making. BMJ Global Health 2019; 4 (Suppl 1) : e000899.
Rees R, Oliver K, Woodman J, Thomas J. Children's views about obesity, body size, shape and weight. A systematic review. London: EPPI-Centre, Social Science Research Unit, Institute of Education, University of London; 2009.
Rehfuess EA, Booth A, Brereton L, Burns J, Gerhardus A, Mozygemba K, Oortwijn W, Pfadenhauer LM, Tummers M, van der Wilt GJ, Rohwer A. Towards a taxonomy of logic models in systematic reviews and health technology assessments: A priori, staged, and iterative approaches. Research Synthesis Methods 2018; 9 : 13-24.
Robbins SCC, Ward K, Skinner SR. School-based vaccination: a systematic review of process evaluations. Vaccine 2011; 29 : 9588-9599.
Rogers M, Bethel A, Abbott R. Locating qualitative studies in dementia on MEDLINE, EMBASE, CINAHL, and PsycINFO: a comparison of search strategies. Research Synthesis Methods 2018; 9 : 579-586.
Sandelowski M, Barroso J. Finding the findings in qualitative studies. Journal of Nursing Scholarship 2002; 34 : 213-219.
Selva A, Sola I, Zhang Y, Pardo-Hernandez H, Haynes RB, Martinez Garcia L, Navarro T, Schünemann H, Alonso-Coello P. Development and use of a content search strategy for retrieving studies on patients' views and preferences. Health and Quality of Life Outcomes 2017; 15 : 126.
Shepherd J, Kavanagh J, Picot J, Cooper K, Harden A, Barnett-Page E, Jones J, Clegg A, Hartwell D, Frampton GK, Price A. The effectiveness and cost-effectiveness of behavioural interventions for the prevention of sexually transmitted infections in young people aged 13-19: a systematic review and economic evaluation. Health Technology Assessment 2010; 14 : 1-206, iii-iv.
Squires JE, Valentine JC, Grimshaw JM. Systematic reviews of complex interventions: framing the review question. Journal of Clinical Epidemiology 2013; 66 : 1215-1222.
Stansfield C, Kavanagh J, Rees R, Gomersall A, Thomas J. The selection of search sources influences the findings of a systematic review of people's views: a case study in public health. BMC Medical Research Methodology 2012; 12 : 55.
SURE (Supporting the Use of Research Evidence) Collaboration. SURE Guides for Preparing and Using Evidence-based Policy Briefs: 5 Identifying and Addressing Barriers to Implementing the Policy Options. Version 2.1, updated November 2011. https://epoc.cochrane.org/sites/epoc.cochrane.org/files/public/uploads/SURE-Guides-v2.1/Collectedfiles/sure_guides.html
Suri H. Purposeful sampling in qualitative research synthesis. Qualitative Research Journal 2011; 11 : 63-75.
Thomas J, Harden A, Oakley A, Oliver S, Sutcliffe K, Rees R, Brunton G, Kavanagh J. Integrating qualitative research with trials in systematic reviews. BMJ 2004; 328 : 1010-1012.
Thomas J, Harden A. Methods for the thematic synthesis of qualitative research in systematic reviews. BMC Medical Research Methodology 2008; 8 : 45.
Thomas J, Brunton J, Graziosi S. EPPI-Reviewer 4.0: software for research synthesis [Software]. EPPI-Centre Software. Social Science Research Unit, Institute of Education, University of London UK; 2010. https://eppi.ioe.ac.uk/CMS/Default.aspx?alias=eppi.ioe.ac.uk/cms/er4& .
Thomas J, O'Mara-Eves A, Brunton G. Using qualitative comparative analysis (QCA) in systematic reviews of complex interventions: a worked example. Systematic Reviews 2014; 3 : 67.
Tong A, Flemming K, McInnes E, Oliver S, Craig J. Enhancing transparency in reporting the synthesis of qualitative research: ENTREQ. BMC Medical Research Methodology 2012; 12 : 181.
van Grootel L, van Wesel F, O'Mara-Eves A, Thomas J, Hox J, Boeije H. Using the realist perspective to link theory from qualitative evidence synthesis to quantitative studies: broadening the matrix approach. Research Synthesis Methods 2017; 8 : 303-311.
Verhage A, Boels D. Critical appraisal of mixed methods research studies in a systematic scoping review on plural policing: assessing the impact of excluding inadequately reported studies by means of a sensitivity analysis. Quality & Quantity 2017; 51 : 1449-1468.
Walker LO, Avant KC. Strategies for theory construction in nursing . Upper Saddle River (NJ): Pearson Prentice Hall; 2005.
For permission to re-use material from the Handbook (either academic or commercial), please see here for full details.
Cochrane Colloquium Abstracts
Sensitivity analysis in systematic reviews of qualitative evidence: an example from a mixed-methods systematic review.
Article type Oral Year 2015 Vienna Authors Langer L 1 , Stewart R 1 , Winters N 1 1 University of Oxford , United Kingdom Abstract Background: The systematic synthesis of qualitative evidence is a recent development of systematic review methodology. Systematic reviews of qualitative evidence need to subscribe to the same methodological rigour and transparency that characterises reviews of quantitative evidence. As a result, reviewers should assess the sensitivity of the findings of any qualitative evidence synthesis to a priori defined variables. Objectives: To report on the methodological insights gained from a sensitivity analysis conducted as part of a qualitative evidence synthesis in a mixed-methods systematic review. Methods: We applied thematic synthesis to synthesise the findings from qualitative research in a mixed-methods systematic review. Prior to extracting themes from the primary research evidence, each study was subjected to a critical appraisal assessing the reliability of the study’s findings. After configuring findings in the thematic synthesis, the results from the synthesis were subjected to a sensitivity analysis. We assessed whether the synthesis results were sensitive to: 1) the exclusion of studies after the application of the critical appraisal tool; 2) the applied qualitative research methodologies; and 3) the inclusion of individual studies yielding a larger than average number of themes. Results: We found that the results of the synthesis were sensitive to the inclusion of individual studies that yielded a larger than average number of themes. We also established that the exclusion of studies after the application of the critical appraisal tool would have generated a number of themes not identified in the included evidence. Conclusions: To ensure the rigour and transparency of qualitative evidence syntheses, both a critical appraisal of the qualitative primary evidence, as well as a sensitivity analysis of the synthesis results, using a priori defined variables are required.

The Prevention and Treatment of Missing Data in Clinical Trials (2010)
Chapter: 5 principles and methods of sensitivity analyses, 5 principles and methods of sensitivity analyses.
This chapter concerns principles and methods for sensitivity analyses that quantify the robustness of inferences to departures from underlying assumptions. Unlike the well-developed literature on drawing inferences from incomplete data, the literature on the assessment of sensitivity to various assumptions is relatively new. Because it is an active area of research, it is more difficult to identify a clear consensus about how sensitivity analyses should be conducted. However, in this chapter we articulate a consensus set of principles and describe methods that respect those principles.
We begin by describing in some detail the difficulties posed by reliance on untestable assumptions. We then demonstrate how sensitivity to these assumptions can be represented and investigated in the context of two popular models, selection and pattern mixture models. We also provide case study illustrations to suggest a format for conducting sensitivity analyses, recognizing that these case studies cannot cover the broad range of types and designs of clinical trials. Because the literature on sensitivity analysis is evolving, the primary objective of this chapter is to assert the importance of conducting some form of sensitivity analysis and to illustrate principles in some simple cases. We close the chapter with recommendations for further research on specific aspects of sensitivity analysis methodology.
There are fundamental issues involved with selecting a model and assessing its fit to incomplete data that do not apply to inference from complete data. Such issues occur even in the missing at random (MAR)
case, but they are compounded under missing not at random (MNAR). We believe that, especially when the primary analysis assumes MAR, the fit of an MAR model can often be addressed by standard model-checking diagnostics, leaving the sensitivity analysis to MNAR models that deviate from MAR. This approach is suggested in order not to overburden the primary analysis. The discussion in Chapter 4 provides some references for model-checking of MAR models. In addition, with MAR missingness mechanisms that deviate markedly from missing completely at random (MCAR), as in the hypertension example in Chapter 4 , analyses with incomplete data are potentially less robust to violations of parametric assumptions than analyses with complete data, so checking them is even more critical.
The data can never rule out an MNAR mechanism, and when the data are potentially MNAR, issues of sensitivity to modeling asumptions are even more serious than under MAR. One approach could be to estimate from the available data the parameters of a model representing an MNAR mechanism. However, the data typically do not contain information on the parameters of the particular model chosen (Jansen et al., 2006).
In fact, different MNAR models may fit the observed data equally well but have quite different implications for the unobserved measurements and hence for the conclusions to be drawn from the respective analyses. Without additional information, one cannot usefully distinguish between such MNAR models based solely on their fit to the observed data, and so goodness-of-fit tools alone do not provide a relevant means of choosing between such models.
These considerations point to the necessity of sensitivity analysis. In a broad sense, one can define a sensitivity analysis as one in which several statistical models are considered simultaneously or in which a statistical model is further scrutinized using specialized tools, such as diagnostic measures. This rather loose and very general definition encompasses a wide variety of useful approaches.
A simple procedure is to fit a selected number of (MNAR) models, all of which are deemed plausible and have equivalent or nearly equivalent fit to the observed data; alternatively, a preferred (primary) analysis can be supplemented with a number of modifications. The degree to which conclusions (inferences) are stable across such analyses provides an indication of the confidence that can be placed in them.
Modifications to a basic model can be constructed in different ways. One obvious strategy is to consider various dependencies of the missing data process on the outcomes or the covariates. One can choose to supplement an analysis within the selection modeling framework, say, with one or several in the pattern mixture modeling framework, which explicitly models the missing responses at any given time given the previously observed responses. Alternatively, the distributional assumptions of the models can be altered.
The vast range of models and methods for handling missing data highlights the need for sensitivity analysis. Indeed, research on methodology has shifted from formulation of ever more complex models to methods for assessing sensitivity of specific models and their underlying assumptions. The paradigm shift to sensitivity analysis is, therefore, welcome. Prior to focused research on sensitivity, many methods used in practice were potentially useful but ad hoc (e.g., comparing several incompatible MNAR models to each other). Although informal sensitivity analyses are an indispensable step in the analysis of incomplete longitudinal data, it is desirable to have more formal frameworks within which to develop such analyses.
It is possible to assess model sensitivities of several different types, including sensitivity to: (a) distributional assumptions for the full data, (b) outlying or influential observations, and (c) assumptions about the missing data mechanism. Assessment of (a) can be partially carried out to the extent that one can compare observed and fitted values for the observables under the model specified for the full data. However, distributional assumptions for the missing data cannot be checked. Assessment of (b) can be used to identify observations that are outliers in the observed-data distribution or that may be driving weakly identified parts of an MNAR model (Molenberghs and Kenward, 2007). This chapter focuses on (c), sensitivity to assumptions about the missing data mechanism.
To focus ideas, we restrict consideration to follow-up randomized study designs with repeated measures. We consider the case in which interest is focused on treatment comparisons of visit-specific means of the repeated measures. With incomplete data, inference about the treatment arm means requires two types of assumptions: (i) untestable assumptions about the distribution of missing outcomes data, and (ii) testable assumptions about the distribution of observed outcomes. Recall that the full-data distribution, described in Chapter 4 , can be factored as

Type (i) assumptions are needed to estimate the distribution [ Y mis | Y obs , M , X ], while type (ii) assumptions are used, if necessary, to model the observables [ Y obs , M | X ] in a parsimonious way.
Type (i) assumptions are necessary to identify the treatment-specific means. Informally, a parameter is identified if one can write its estimator as a function that depends only on the observed data. When a parameter is not identified, it would not be possible to obtain a point estimate even if the sample size were infinite. It is therefore essential to conduct a sensitivity
analysis, whereby the data analysis is repeated under different type (i) assumptions, in order to clarify the extent to which the conclusions of the trial are dependent on unverifiable assumptions. The usefulness of a sensitivity analysis ultimately depends on the transparency and plausibility of the unverifiable assumptions. It is key that any sensitivity analysis methodology allow the formulation of these assumptions in a transparent and easy-to-communicate manner.
Ultimately, type (i) assumptions describe how missing outcomes are being “imputed” under a given model. A reasonable way to formulate these assumptions is in terms of the connection (or link) between the distributions of those having missing and those having observed outcomes but similar covariate profiles. Making this difference explicit is a feature of pattern mixture models. Examples discussed in this chapter illustrate both pattern mixture and selection modeling approaches.
In general, it is also necessary to impose type (ii) assumptions. An important consideration is that modeling assumptions of type (ii), which apply to the distribution of observed data , can be supported and scrutinized with standard model-checking techniques.
Broadly speaking, there are two approaches for combining type (i) and (ii) assumptions to draw inferences about the treatment-specific means: pattern mixture and selection modeling. To illustrate these approaches, the next four sections present four example designs of increasing complexity. The first two examples involve a single outcome, without and then with auxiliary data. These examples are meant to illustrate when and why the assumptions of type (i) and (ii) are needed. The third and fourth examples extend the designs to those with repeated measures, with monotone and non-monotone missing data, respectively, with and without auxiliary data.
Our examples are not meant to be prescriptive as to how every sensitivity analysis should be conducted, but rather to illustrate principles that can guide practice. Type (i) assumptions can only be justified on substantive grounds. As the clinical contexts vary between studies, so too will the specific form of the sensitivity analysis.
EXAMPLE: SINGLE OUTCOME, NO AUXILIARY DATA
We start with the simple case in which the trial records no baseline covariate data, and the only measurement to be obtained in the study is that of the outcome Y , taken at a specified time after randomization. We assume that the treatment-arm-specific means of Y form the basis for treatment comparisons and that in each arm there are some study participants on whom Y is missing. We let R = 1 if Y is observed and R = 0 otherwise.
Because estimation of each treatment arm mean relies solely on data from subjects assigned to that arm, the problem reduces to estimation of a mean E(Y) based on a random sample with Y missing in some units. Thus, formally, the problem is to estimate µ = E ( Y ) from the observed data, which comprises the list of indicators R , and the value of Y for those having R = 1.
The MAR assumption described in Chapter 4 is a type (i) assumption. In this setting, MAR means that, within each treatment arm, the distribution of Y among respondents (i.e., those with R = 1) is the same as that for nonrespondents (i.e., with R = 0).
This example illustrates several key ideas. First, it vividly illustrates the meaning of an untestable assumption. Let µ 1 = E ( Y | R = 1) denote the mean among respondents, µ 0 = E ( Y | R = 0) the mean among nonrespondents, and π = P ( R =1) the proportion of those responding. The full-data mean µ is a weighted average

but there is no information in the data about the value of µ 0 . Hence, any assumption one makes about the distribution for the nonrespondents will be untestable from the data available. In particular, the MAR assumption—that µ 1 = µ 0 —is untestable.
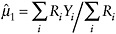
Third, this example is the simplest version of a pattern mixture model: the full-data distribution is written as a mixture—or weighted average—of the observed and missing data distributions. Under MAR, their means are equal. However, it is more typical to use pattern mixture models when the means are not assumed to be equal (MNAR).
By contrast, in the selection model approach, type (ii) assumptions are made in terms of how the probability of nonresponse relates to the possibly unobserved outcome. The full-data mean can be estimated using a weighted average of the observed outcomes, where the weights are individual-specific and correspond to the conditional probability of being observed given the observed outcome value. The reweighting serves to create a “pseudo
population” of individuals who are representative of the intended full-data sample of outcomes.
Importantly, there is a one-to-one relationship between the specification of a selection model and specification of a pattern-mixture model. The key distinction ultimately arises in how type (ii) assumptions are imposed. As it turns out, the two approaches generate equivalent estimators in this simple example, but for more complex models that rely on type (i) assumptions to model the observed data, that is not the case.
Pattern Mixture Model Approach
Because we are only interested in the mean of Y , it suffices to make assumptions about how the mean of Y among nonresponders links to the mean of Y among respondents. A simple way to accomplish this is by introducing a sensitivity parameter ∆ that satisfies µ 0 = µ 1 + ∆, or

It is easy to see that ∆ = µ o – µ 1 , the difference in means between respondents and nonrespondents. To accommodate general measurement scales, the model should be parameterized so that the sensitivity parameter satisfies an identity such as

where g ( ) is a function, specified by the data analyst, that is strictly increasing and maps values from the range of Y to the real line. The function g determines the investigator’s choice of scale for comparisons between the respondents’ and nonrespondents’ means and is often guided by the nature of the outcome.
For a continuous outcome, one might choose g ( u ) = u , which reduces to the simple contrast in means given by (3), where ∆ represents the difference in mean between nonrespondents and respondents.
For binary outcomes, a convenient choice is g ( u ) = log( u /(1– u )), which ensures that the µ 0 lies between 0 and 1. Here, ∆ is the log odds ratio comparing the odds of Y = 1 between respondents and nonrespondents.
Each value of ∆ corresponds to a different unverifiable assumption about the mean of Y in the nonrespondents. Any specific value of ∆ corresponds to an estimate of µ because µ can be written as the weighted average

To examine how inferences concerning µ depend on unverifiable assumptions about the missing data distribution, notice that µ is actually a function of ∆ in (5). Hence, one can proceed by generating an estimate of µ for each value of ∆ that is thought to be plausible. In this model, ∆ = 0 corresponds to MAR; hence, examining inferences about µ over a set or range for ∆ that includes ∆ = 0 will summarize the effects of departures from MAR on inferences about µ .
For fixed ∆, assumption (4) is of type (i). In this simple setting, type (ii) assumptions are not needed because µ 1 and π can be estimated with sample means, and no modeling is needed.
Finally, to test for treatment effects between two arms, one adopts a value ∆ 0 for the first arm and a value ∆ 1 for the second arm. One then estimates each mean separately under the adopted values of ∆ and conducts a Wald test that their difference is zero. To investigate how the conclusions depend on the adopted values of ∆, one repeats the testing over a range of plausible values for the pair (∆ 0, ∆ 1 ).
Selection Model Approach
A second option for conducting sensitivity analysis is to assume that one knows how the odds of nonresponse change with the values of the outcome Y . For example, one can assume that the log odds of nonresponse differs by α for those who differ by one unit on Y. This is equivalent to assuming that one knows the value of α (but not h ) in the logistic regression model

Models like (6) are called selection models because they model the probability of nonresponse (or selection) as a function of the outcome. Each unique value of α corresponds to a different unverifiable assumption about how the probability of nonresponse changes with the outcome.
The model in (6) is also equivalent to assuming that

Adopting a value of α is equivalent to adopting a known link between the distribution of the respondents and that of the nonrespondents, because one
cannot use the data to learn anything about the nonrespondent distribution or to check the value of α . Moreover, one cannot check two other important assumptions: that the log odds of nonresponse is linear in y and that the support of the distribution of Y among nonrespondents is the same as that among respondents (as implied by (7)).
Although not immediately apparent, once a value of α is adopted, one can estimate µ = E [ Y ] consistently. A sensitivity analysis consists of repeating the estimation of µ at different plausible values of α so as to assess the sensitivity of inferences about µ to assumptions about the missing data mechanism as encoded by α and model (6).
Estimation of µ relies on the identity
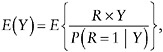
which suggests estimation of µ through inverse probability weighting (see below); in this case, the weights can depend on missing values of Y . The inverse probability weighting estimator is

where expit( u ) = logit –1 ( u ) = exp( u ) / {1 + exp( u )}. To compute ĥ , one solves the unbiased estimating equation

for h. 1 Analytic formulas for consistent standard error estimators are available (e.g., Rotnitzky et al., 1998), but bootstrap resampling can be used. Sensitivity analysis for tests of treatment effects proceeds by repeating the test over a set of plausible values for α , where different values of α can be chosen for each arm.
With the selection model approach described here we can conduct sensitivity analysis, not just about the mean but about any other component of the distribution of Y , for example, the median of Y . Just as in the preceding pattern mixture approach, the data structure in this setting is so simple that we need not worry about postulating type (ii) assumptions.
EXAMPLE: SINGLE OUTCOME WITH AUXILIARY DATA
We next consider a setting in which individuals are scheduled to have a measurement Y 0 at baseline, which we assume is never missing (this constitutes the auxiliary data), and a second measurement Y 1 at some specified follow-up time, which is missing in some subjects. We let R 1 = 1 if Y 1 is observed and R 1 = 0 otherwise. As in the preceding example, we limit our discussion to estimation of the arm-specific mean of Y 1 , denoted now by µ = E ( Y 1 ).
In this example, the type (i) MAR assumption states that, within each treatment group and within levels of Y 0 , the distribution of Y 1 among nonrespondents is the same as the distribution of Y 1 among respondents. That is,

In this and the next section, we demonstrate sensitivity analysis under MNAR. Under the pattern mixture approach one specifies a link between the distribution of Y 1 in the nonrespondents and respondents who share the same value of Y 0 . One can specify, for example, that

where η ( Y 0 ) = E ( Y 1 | R 1 = 1 , Y 0 ) and g is defined as in the example above.
Example: Continuous Values of Y Suppose Y 1 is continuous. One needs a specification of both the sensitivity analysis function g and the relationship between Y 1 and Y 0 , represented by η ( Y 0 ). A simple version of η is a regression of Y 1 on Y 0 ,

Now let g ( u ) = u as in the first example above. In this case, using (12), the mean of the missing Y 1 are imputed as regression predictions of Y 1 plus a shift ∆,

In this case, ∆ represents the baseline adjusted difference in the mean of Y 1 between nonrespondents and respondents. If ∆ > 0 (< 0), then for any fixed value of Y 0 , the mean of Y 1 among nonrespondents is ∆ units higher (lower) than the mean of Y 1 among respondents.
A few comments are in order for this example:
Model (12) assumes that mean differences do not depend on Y 0 . If one believes that they do, then one may choose a more complex version of the g function, such as

If this version is coupled with a linear regression for η ( Y 0 ), then both the slope and the intercept of that regression will differ for respondents and nonrespondents.
In general, any user-specified sensitivity function d ( Y 0 ,∆) can be posited, including the simple versions d ( Y 0 ,∆) = ∆ and d ( Y 0 ,∆) = ∆ 0 + ∆ 1 Y 0 . Importantly, no version of d ( Y 0 ,∆) can be checked using the observed data. The choice of d function is a type (i) assumption.
Likewise, more general choices can be made for the form of η ( Y 0 ), including versions that are nonlinear in Y 0 . The choice of η is a type (ii) assumption; it can be critiqued by standard goodness-of-fit procedures using the observed data.
Example: Binary Outcome Y If Y is binary, the functional form of g and η will need to be different than in the continuous case. Choosing g ( u ) = log( u /(1 + u ) implies that ∆ is the log odds ratio comparing the odds of Y 1 = 1 between respondents and nonrespondents, conditional on Y 0 . As with the continuous case, ∆ > 0 (∆ < 0) implies that, for every level of Y 0 , nonrespondents are more (less) likely to have Y 1 = 1 than respondents.
The function η ( Y 0 ), which describes E ( Y 1 | Y 0 , R = 1), should be specified in terms of a model that is appropriate for binary outcomes. For example, a simple logistic specification is

which is equivalent to writing
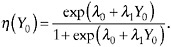
When Y 0 is binary, this model is saturated. But when Y 0 is continuous, or includes other auxiliary covariates, model choice for η will take on added importance.
Inference A sensitivity analysis to examine how inferences are impacted by the choice of ∆ consists of repeating the inference over a set or range of values of ∆ deemed to be plausible. It can proceed in the following manner:
Step 1. Specify models for η ( Y 0 ) and d ( Y 0 ,∆).

Step 3. The full-data mean µ = E ( Y 1 ) is

where expectations are taken over the distribution of Y 0 | R . Although the general formula looks complex, it is easily computed for a fixed value of ∆ once the model for η has been fit to data. Specifically,

Step 4. Standard errors are computed using bootstrap resampling.

intervals for treatment effect as a function of ∆. An illustration, computed using data from a diabetic neuropathy trial, appears in Figure 5-1 .
In parallel to the first example, with no auxiliary data, another way to postulate type (i) assumptions about the nature of selection bias is by postulating a model for the dependence of the probability of nonresponse on the (possibly missing) outcome Y 1 , within levels of Y 0 . For example, one can assume that, conditionally on Y 0 , each unit increase in Y 1 is associated with an increase of α in the log odds of nonresponse. That is,

or, equivalently,

where h ( Y 0 ) is an unknown function of Y 0 . This can also be written as

In this latter form, 2 one can see that the observed data have no information about α . The choice of α = 0 specifies that within levels of Y 0 , R 1 and Y 1 are independent (i.e., MAR). Values of α ≠ 0 reflect residual association between missingness and nonresponse after adjusting for Y 0 . 3
Analogous to the example with no auxiliary data, estimation of µ = E ( Y 1 ) relies on the identity
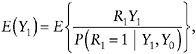
which suggests the inverse probability weighted (IPW) estimator
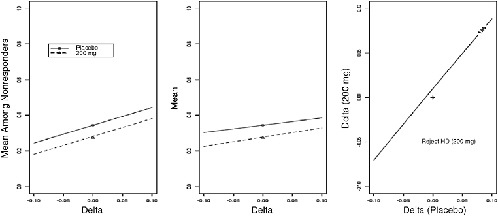
FIGURE 5-1 Pattern mixture sensitivity analysis. Left panel: plot of mean outcome among nonrespondents as a function of sensitivity parameter Δ, where Δ = 0 corresponds to MAR. Center panel: plot of full-data mean as function Δ. Right panel: contour of Z statistic for comparing placebo to active treatment, where Δ is varied separately by treatment.

where ĥ ( Y 0 ) is an estimator of h ( Y 0 ).
Unless Y 0 is discrete with a few levels, estimation of h ( Y 0 ) requires the assumption that h ( Y 0 ) takes a known form, such as h ( Y 0 ;γ ) = γ 0 + γ 1 Y 0 . (Note that if one adopts this model, one is assuming that the probability of response follows a logistic regression model on Y 0 and Y 1 with a given specified value for the coefficient α of Y 1 .) Specifying h ( Y 0 ) is a type (ii) assumption that is technically not needed to identify µ but is needed in practical situations involving finite samples.

Formulas for sandwich-type standard error estimators are available, but the bootstrap can also be used to compute standard error estimates. Hypothesis-testing sensitivity analysis is conducted in a manner similar to the one described in the example above with no auxiliary data.
As with the pattern mixture models, by repeating the estimation of µ at a set or interval of known α values, one can examine how different degrees of residual association between nonresponse and the outcome Y 1 affect inferences concerning E ( Y 1 ). A plot similar to the one constructed for the pattern mixture model is given in Figure 5-2 .
EXAMPLE: GENERAL REPEATED MEASURES SETTING
As the number of planned measurement occasions increases, the complexity of the sensitivity analysis grows because the number of missing data patterns grows. As a result, there can be limitless ways of specifying models.
Consider a study with K scheduled postbaseline visits. In the special case of monotone missing data, there are ( K + 1) patterns representing each of the visits at which a subject might last be seen, that is, 0,…, K . The
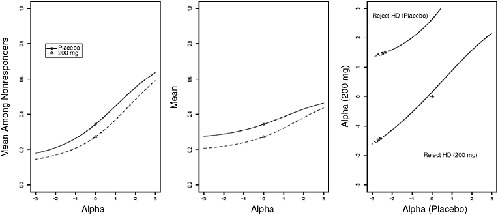
FIGURE 5-2 Selection model sensitivity analysis. Left panel: plot of mean outcome among nonrespondents as a function of sensitivity parameter α , where α = 0 corresponds to MAR. Center panel: plot of full-data mean as function of α . Right panel: contour of Z statistic for comparing placebo to active treatment where α is varied separately by treatment.
( K + 1) st pattern represent subjects with complete data, while the other K patterns represent those with varying degrees of missing data. In the general setting, there are many ways to specify pattern models—the models that link the distribution of missing outcomes to the distribution of observed outcomes within specified strata—and it is generally necessary to look for simplifications of the model structure.
For example, one could link the conditional (on a shared history of observed outcomes through visit k – 1) distribution of missing outcomes at visit k among those who were last seen at visit k – 1 to (a) the distribution of outcomes at visit k among those who complete the study, (b) the distribution of outcomes at visit k among those who are in the study through visit k, or (c) the distribution of outcomes at visit k among those who are last seen at visit k .

Monotone Missing Data

A Pattern Mixture Model Approach
As noted above, there are many pattern models that can be specified. Here, we discuss inference in one such model. Recall that both type (i) and type (ii) assumptions are needed. We first address type (i) specification, illustrating a way to link distributions with those having missing observations to those with observed data.
The general strategy is illustrated for the case K = 3, which relies on an assumption known as “nonfuture dependence” (Kenward et al., 2003). In simple terms, the nonfuture dependence assumption states that the probability of drop out at time L can only depend on observed data up to L and the possibly missing value of Y L , but not future values of L .
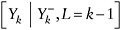
It can be shown that µ = E ( Y K ) can be estimated by a recursion algorithm, provided the following observed-data distributions are estimated:

and the following dropout probabilities

can also be estimated. Each is identified from observed data when missingness is monotone.
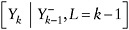
To be more concrete, suppose that the outcomes Y 0 ,…, Y 3 are continuous. One can assume regression models for each of (27) as follows,

This modeling of the observed data distribution comprises our type (i) assumptions. These can (and must) be checked using the observables.
Using type (ii) assumptions, the distributions of missing Y can be linked in a way similar to those for the first example above. For example,
those with L = 1 are missing Y 1 . One can link the observed-data regression E ( Y 1 | Y 0 , L ≥ 2) to the missing-data regression E ( Y 1 | Y 0 , L = 1) through

As with the previous cases, (33) is a type (ii) assumption and cannot be checked with data. Moreover, even using a simple structure like (33), the number of sensitivity parameters grows large very quickly with the number of repeated measures. Hence, it is important to consider simplifications, such as setting ∆ β = 0, assuming ∆ µ is equivalent across patterns, or some combination of the two.

A sensitivity analysis consists of estimating µ and its standard error repeatedly over a range of plausible values of specified ∆ parameters. For this illustration, setting ∆ = 0 implies MAR. 5
Selection Model

and is equivalent to assuming that after adjusting for the recorded history, the outcome to be measured at visit k + 1 is the only predictor of all future missing outcomes that is associated with the odds of dropping out between visits k and k + 1.
This last assumption, coupled with an assumption that quantifies the dependence of the odds in the right hand side on Y k +1 , suffices to identify µ = E ( Y K ): in fact, it suffices to identify E ( Y k ) for any k = 1,…, K . For example, one might assume

Assumptions (34) and (35) together are equivalent to specifying that

For instance, replacing in equation (36) the constant α with the function α 0 + α 1 Y k with α 0 and α 1 specified, encodes the belief that the residual association between dropping out between k and k + 1 and the outcome Y k +1 may be stronger for individuals with, say, higher (if α 1 > 0) values of the outcome at visit k . As an example, if Y k is a strong predictor of Y k +1 and that lower values of Y k +1 are preferable (e.g., HIV-RNA viral load), then it is reasonable to postulate that subjects with low values of Y k drop out for reasons unrelated to the drug efficacy (and, in particular, then to their outcome Y k +1 ) while subjects with higher values of Y k drop out for reasons related to drug efficacy and hence to their outcome Y k +1 .
Regardless of how the residual dependence is specified, µ can be expressed in terms of the distribution of the observed data, that is, it is identified. Estimation of µ = E [ Y K ] relies on the identity

This formula suggests that one can estimate µ with the IPW estimator

As with the selection approach of the two preceding examples, to estimate γ k, one cannot fit a logistic regression model because Y K+ 1 is missing when L = k . However, one can estimate it instead by solving the estimating equations

for γ , justified on similar grounds as the estimators of h functions in the previous examples.
Formula for sandwich-type standard error estimators are available (see Rotnitzky et al., 1997), but the bootstrap can also be used to compute standard error estimates. Sensitivity analysis with regard to hypothesis testing is conducted in a manner similar to the one described in the first example above.
Nonmonotone Missing Data
A typical way to analyze nonmonotone missing data is to treat the time of dropout as the key missing data variable and then to assume MAR within dropout pattern (or conditional on dropout time). The advantage of this approach is purely practical: It interpolates missing data under a specified model. That said, however, the current literature suggests that MAR within pattern does not easily correspond to realistic mechanisms for generating the data. This raises concern among members of the panel that nonmonotone dropouts may require more specialized methods for modeling the missing data mechanism, and accounting for departures from MAR.
This topic has not been deeply studied in the extant statistical literature, and in particular numerical studies are lacking. We recommend this as a key area of investigation that will: (a) examine the appropriateness of existing models and in particular the potential pitfalls of assuming MAR within missing data pattern; and (b) develop and apply novel, appropriate methods of model specification and sensitivity analysis to handle nonmonotone missing data patterns.
COMPARING PATTERN MIXTURE AND SELECTION APPROACHES
The main appeal of the selection model approach is that, since it models the probability of nonresponse rather than the distribution of outcomes, it can easily accommodate vectors of auxiliary factors with components that can be of all types, discrete, categorical, and continuous.
Two disadvantages of the selection approach as they relate to drawing inferences are (a) the inverse weighting estimation procedure, which can yield relatively inefficient inferences (i.e., large standard errors), and (b) that model checking of the type (ii) assumptions must be conducted for each unique value of the sensitivity analysis parameters. Formal model checking procedures have yet to be formally developed for this setting. The inefficiencies associated with the inverse weighting procedure are mitigated in settings with a sizable fraction of missing data, as the sampling variability is often of less concern than the range of type (i) assumptions that are entertained. To address (b), one should fit a highly flexible model for the h function in the selection model.
Another potential disadvantage of selection models relates to interpretation of the sensitivity parameter. Particularly for continuous measures, it may be difficult to interpret nonresponse rates on the odds scale and to specify reasonable ranges for the sensitivity parameter. Plots such as those shown in Figure 5-2 (above) can be helpful in understanding how values of the sensitivity parameter correspond to imputed means for the missing outcomes.
Advantages of the pattern mixture model include transparent interpretation of sensitivity parameters and straightforward model checking for the observed-data distribution. The sensitivity parameters are typically specified in terms of differences in mean between respondents and nonrespondents, which appeal directly to intuition and contributes to formulating plausible ranges for the parameter. Pattern mixture models also can be specified so that the fit to the observed data is identical across all values of the sensitivity parameters; hence, model checking will be straightforward and does not depend on the assumed missing data assumption.
Disadvantages of pattern mixture modeling include difficulties in including auxiliary information, which will generally require additional modeling. Computation of the weighted averages across patterns for models of large numbers of repeated measures also can become complex without significant simplifying assumptions.
TIME-TO-EVENT DATA
A major challenge in the analysis of time-to-event outcomes in randomized trials is to properly account for censoring that may be informative. Different approaches have been proposed in the research literature to address this issue. When no auxiliary prognostic factors are available, the general strategy has been to impose nonidentifiable assumptions concerning the dependence between failure and censoring times and then vary these assumptions in order to assess the sensitivity of inferences on the estimated survivor function. When prognostic factors are recorded, Robins and colleagues in a series of papers (Robins and Rotnitzky, 1992; Robins, 1993;
Robins and Finkelstein, 2000) proposed a general estimation strategy under the assumption that all measured prognostic factors that predict censoring are recorded in the database. Scharfstein and Robins (2002) proposed a method for conducting sensitivity analysis under the assumption that some but not all joint prognostic factors for censoring and survival are available. Their approach is to repeat inference under different values of a nonidentifiable censoring bias parameter that encodes the magnitude of the residual association between survival and censoring after adjusting for measured prognostic factors.
In randomized studies, censoring typically occurs for several reasons, some noninformative, others informative. For instance, in studies with staggered entry, the administrative end of the follow-up period typically induces noninformative censoring. However, loss to follow-up due to dropouts induces a competing censoring mechanism that is likely to be informative. Treatment discontinuation might induce yet another informative censoring process.
Under the Scharfstein and Robins methodology, the analyst specifies a range for the parameter encoding the residual dependence of the hazard of the minimum of competing censoring times on the censored outcome. However, this range might be rather difficult to specify if the reasons that each censoring might occur are quite different, more so if some censoring processes are informative and some are not. To ameliorate this problem in studies with staggered entry, one can eliminate the censoring by the administrative end of the follow-up period (typically a source of noninformative censoring) by restricting the follow-up period to a shorter interval in which (with probability) no subject is administratively censored. However, in doing so, one would lose valuable information on the survival experience of the study patients who remain at risk at the end of the reduced analysis interval. Rotnitzky et al. (2007) provide estimators of the survival function under separate models for the competing censoring mechanisms, including both informative and noninformative censoring. The methods can be used to exploit the data recorded throughout the entire follow-up period and, in particular, beyond the end of the reduced analysis interval discussed above.
DECISION MAKING
Even after model fitting and sensitivity analysis, investigators have to decide about how important the treatment effect is. Unfortunately, there is no scientific consensus on how to synthesize information from a sensitivity analysis into a single decision about treatment effect. At least three possibilities can be considered.
One possibility is to specify a plausible region for the sensitivity parameters and report estimates of the lower and upper bounds from this range. These
endpoints form bounds on the estimated treatment effect and would be used in place of point estimates. Accompanying these bounds would be a 95 percent confidence region. This procedure can be viewed as accounting for both sampling variability and variability due to model uncertainty (i.e., uncertainty about the sensitivity parameter value): see Molenberghs and Kenward (2007) for more detailed discussion and recommendations for computing a 95 percent confidence region.
A second possibility is to carry out inference under MAR and determine the set of sensitivity parameter values that would lead to overturning the conclusion from MAR. Results can be viewed as equivocal if the inference about treatment effects could be overturned for values of the sensitivity parameter that are plausible.
The third possibility is to derive a summary inference that averages over values of the sensitivity parameters in some principled fashion. This approach could be viewed as appropriate in settings in which reliable prior information about the sensitivity parameter value is known in advance.
Regardless of the specific approach taken to decision making, the key issue is weighting the results, either formally or informally, from both the primary analysis and each alternative analysis by assessing the reasonableness of the assumptions made in conjunction with each analysis. The analyses should be given little weight when the associated assumptions are viewed as being extreme and should be given substantial weight when the associated assumptions are viewed as being comparably plausible to those for the primary analysis. Therefore, in situations in which there are alternative analyses as part of the sensitivity analysis that support contrary inferences to that of the primary analysis, if the associated assumptions are viewed as being fairly extreme, it would be reasonable to continue to support the inference from the primary analysis.
RECOMMENDATION
Recommendation 15: Sensitivity analyses should be part of the primary reporting of findings from clinical trials. Examining sensitivity to the assumptions about the missing data mechanism should be a mandatory component of reporting.
We note that there are some often-used models for the analysis of missing data in clinical trials for which the form of a sensitivity analysis has not been fully developed in the literature. Although we have provided principles for the broad development of sensitivity analyses, we have not been prescriptive for many individual models. It is important that additional research be carried out so that methods to carry out sensitivity analyses for all of the standard models are available.
Randomized clinical trials are the primary tool for evaluating new medical interventions. Randomization provides for a fair comparison between treatment and control groups, balancing out, on average, distributions of known and unknown factors among the participants. Unfortunately, these studies often lack a substantial percentage of data. This missing data reduces the benefit provided by the randomization and introduces potential biases in the comparison of the treatment groups.
Missing data can arise for a variety of reasons, including the inability or unwillingness of participants to meet appointments for evaluation. And in some studies, some or all of data collection ceases when participants discontinue study treatment. Existing guidelines for the design and conduct of clinical trials, and the analysis of the resulting data, provide only limited advice on how to handle missing data. Thus, approaches to the analysis of data with an appreciable amount of missing values tend to be ad hoc and variable.
The Prevention and Treatment of Missing Data in Clinical Trials concludes that a more principled approach to design and analysis in the presence of missing data is both needed and possible. Such an approach needs to focus on two critical elements: (1) careful design and conduct to limit the amount and impact of missing data and (2) analysis that makes full use of information on all randomized participants and is based on careful attention to the assumptions about the nature of the missing data underlying estimates of treatment effects. In addition to the highest priority recommendations, the book offers more detailed recommendations on the conduct of clinical trials and techniques for analysis of trial data.
READ FREE ONLINE
Welcome to OpenBook!
You're looking at OpenBook, NAP.edu's online reading room since 1999. Based on feedback from you, our users, we've made some improvements that make it easier than ever to read thousands of publications on our website.
Do you want to take a quick tour of the OpenBook's features?
Show this book's table of contents , where you can jump to any chapter by name.
...or use these buttons to go back to the previous chapter or skip to the next one.
Jump up to the previous page or down to the next one. Also, you can type in a page number and press Enter to go directly to that page in the book.
Switch between the Original Pages , where you can read the report as it appeared in print, and Text Pages for the web version, where you can highlight and search the text.
To search the entire text of this book, type in your search term here and press Enter .
Share a link to this book page on your preferred social network or via email.
View our suggested citation for this chapter.
Ready to take your reading offline? Click here to buy this book in print or download it as a free PDF, if available.
Get Email Updates
Do you enjoy reading reports from the Academies online for free ? Sign up for email notifications and we'll let you know about new publications in your areas of interest when they're released.
Thank you for visiting nature.com. You are using a browser version with limited support for CSS. To obtain the best experience, we recommend you use a more up to date browser (or turn off compatibility mode in Internet Explorer). In the meantime, to ensure continued support, we are displaying the site without styles and JavaScript.
- View all journals
- My Account Login
- Explore content
- About the journal
- Publish with us
- Sign up for alerts
- Open access
- Published: 02 June 2020
A qualitative exploration of individual differences in wellbeing for highly sensitive individuals
- Becky A. Black ORCID: orcid.org/0000-0001-8421-0694 1 &
- Margaret L. Kern ORCID: orcid.org/0000-0003-4300-598X 1
Palgrave Communications volume 6 , Article number: 103 ( 2020 ) Cite this article
19k Accesses
11 Citations
38 Altmetric
Metrics details
- Health humanities
Cultures explicitly and implicitly create and reinforce social norms and expectations, which impact upon how individuals make sense of and experience their place within that culture. Numerous studies find substantial differences across a range of behavioral and cognitive indices between what have been called “Western, Educated, Industrialized, Rich, and Democratic (WEIRD)” societies and non-WEIRD cultures. Indeed, lay conceptions and social norms around wellbeing tend to emphasize social outgoingness and high-arousal positive emotions, with introversion and negative emotion looked down upon or even pathologized. However, this extravert-centric conception of wellbeing does not fit many individuals who live within WEIRD societies, and studies find that this mismatch can have detrimental effects on their wellbeing. There is a need to better understand how wellbeing is created and experienced by the large number of people for whom wellbeing manifests in alternative ways. This study investigated one such manifestation—the personality trait of sensory processing sensitivity (SPS)—qualitatively investigating how sensitive individuals experience and cultivate wellbeing within a WEIRD society. Twelve adults participated in semi-structured interviews. Findings suggest that highly sensitive individuals perceive that wellbeing arises from harmony across multiple dimensions. Interviewees emphasized the value of low-intensity positive emotion, self-awareness, self-acceptance, positive social relationships balanced by times of solitude, connecting with nature, contemplative practices, emotional self-regulation, practicing self-compassion, having a sense of meaning, and hope/optimism. Barriers of wellbeing included physical health issues and challenges with saying no to others. This study provides a rich idiographic representation of SPS wellbeing, highlighting diverse pathways, which can lead to wellbeing for individuals for whom wellbeing manifests in ways that contradict the broader social narratives in which they reside.
Similar content being viewed by others
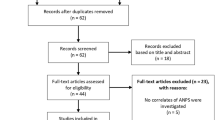
A meta-analysis on individual differences in primary emotional systems and Big Five personality traits
Davide Marengo, Kenneth L. Davis, … Christian Montag
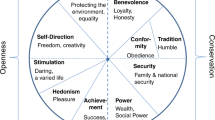
Well-being as a function of person-country fit in human values
Paul H. P. Hanel, Uwe Wolfradt, … Gregory R. Maio
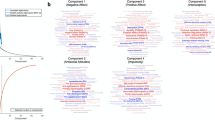
Antisocial and impulsive personality traits are linked to individual differences in somatosensory maps of emotion
Soren Wainio-Theberge & Jorge L. Armony
Introduction
Wellbeing is shaped by a wide range of factors, including our genes, early life experiences, personality, situations encountered, the choices we make, the behaviors we engage in, the social environments in which we live, and the intricate ways in which these factors interact (Eckersley et al., 2007 ). Beyond the individual, each of these factors intersect with and are influenced by culture. Culture can be described as “a network of knowledge that is produced, distributed, and reproduced among a collection of interconnected people” (Chiu and Chen, 2004 , p. 173). Culture creates, defines, and maintains the values, norms, attributes, and behaviors that are seen as appropriate (or not) for a collection of people (Benet-Martínez, 2006 ). The nature and experience of wellbeing “depends on how the concepts of ‘well’ and ‘being’ are defined and practiced” in any given society or culture (Kitayama and Markus, 2000 , p. 115).
Wellbeing is a growing area of interest worldwide (Delle Fave et al., 2013 ; Helliwell et al., 2016 ; The Treasury New Zealand Government, 2019 ). A range of theoretical and empirical wellbeing models, frameworks, measures, and interventions have emerged, providing a general understanding of what wellbeing is and how it can be improved. Although recent years have expanded wellbeing research and practice worldwide, much of this literature has occurred in what have been called “Western, Educated, Industrialized, Rich, and Democratic” (WEIRD) countries, including the US, the UK, and Australia (Henrich et al., 2010a , 2010b ). Numerous studies find significant differences between WEIRD and non-WEIRD societies across a range of behavioral and cognitive indices (e.g., Benet-Martínez, 2006 ; Disabato et al., 2020 ; Hendriks et al., 2019 ; Koopmann-Holm and Tsai, 2014 ; Laajaj et al., 2019 ; Lim, 2016 ; Tsai et al., 2006 ; Tsai et al., 2006 ; Tsai and Park, 2014 ; Wong et al., 2011 ). While most of the existing wellbeing models contend that wellbeing is nuanced and multi-dimensional, including eudaimonic and low-arousal dimensions, lay conceptions of—and social norms within—WEIRD societies tend to favor high-arousal emotions (Tsai, 2007 ), exemplified by extraverted, socially outgoing, high-energy individuals (Allik, 2005 ; Christopher and Hickinbottom, 2008 ; Davidson et al., 2015 ; Frawley, 2015 ; Fulmer et al., 2010 ; Lu and Gilmour, 2004 ; Uchida and Kitayama, 2009 ). Introversion (a personality characteristic) and negative emotions (a wellbeing characteristic) are looked down upon or even pathologized (e.g., Davidson et al., 2015 ; Fudjack, 2013 ). However, this extravert-centric conception of wellbeing does not fit many individuals who live within WEIRD societies, and this mismatch can have detrimental effects on these individuals’ wellbeing (Fulmer et al., 2010 ; Stephens et al., 2012 ). There is a need to better understand how happiness is created and experienced by the large number of people for whom wellbeing manifests in alternative ways.
Further, while influences of culture on personality (Diener et al., 2003 ; Triandis and Suh, 2002 ) and wellbeing (Diener, 2000 ; Disabato et al., 2016 ; Galinha et al., 2013 ; Mathews, 2012 ) are well recognized, studies often follow nomothetic approaches, focusing on identifying generalizable patterns and norms across numerous people. Less is known about idiographic experiences of individuals who might not fit those norms. This study investigated one such manifestation—the personality trait of sensory processing sensitivity (SPS)—qualitatively investigating how sensitive individuals experience and cultivate wellbeing within a WEIRD society.
Life within yet beyond social norms
Much of the research linking personality and wellbeing has been conducted with participants embedded within WEIRD countries (Disabato et al., 2020 ), where extravert-centric perceptions of wellbeing dominate (Fulmer et al., 2010 ). Yet the influence of these cultural norms on findings is mostly unacknowledged. Such studies often generalize findings, assuming universal notions of wellbeing (Zevnik, 2014 ), without recognizing the surrounding culture that values a particular type of wellbeing—one that does not apply to everyone within that culture (Christopher, 1999 ). One way of addressing this issue is to judiciously choose a select group of populations that can provide a test of universality (Henrich et al., 2010a ); the current qualitative study does just this.
Research indicates that when people are matched with their cultural environment, they experience higher rates of psychological wellbeing (Fulmer et al., 2010 ) and less negative emotion (Stephens et al., 2012 ) than those who feel a mismatch with the surrounding culture. When more extraverted individuals are part of a more extraverted culture, they experience greater wellbeing than introverted individuals within the same context (Fulmer et al., 2010 ). This means that the dominance of social norms can negatively impact upon individuals who experience wellbeing in different ways.
Indeed, well before the recent upsurge of interest in wellbeing-related research and topics, Henjum ( 1982 ) suggested that “it is not very complimentary to be called an introvert in our society” (referring to American society; p. 39). Echoing this sentiment, Eysenck ( 1983 ) posited that “happiness is a thing called stable extraversion” (p. 67). This still rings true today in WEIRD societies, where there appears to be a tacit approval of extraverted behaviors, with a corresponding negative judgment for introverted behaviors (Davidson et al., 2015 ; Fulmer et al., 2010 ). Indeed, studies in Australia, where the current study was conducted, show a distinctive cultural preference for extraversion (Lawn et al., 2018 ).
This dominant view of extraversion as desirable and preferable is accompanied by some potentially harmful consequences—such as the pathologizing of introversion. For instance, the International Statistical Classification of Diseases (ICD-9; World Health Organization, 1978 ) has for several decades (until the release of ICD-10) listed “introverted personality” (Code 301.21) and “introverted personality disorder of childhood” (Code 313.22) as personality disorders. Indeed, introversion has been equated with Autism Spectrum Disorder (Grimes, 2010 ) and with schizophrenia (McWilliams, 2006 ; Wells, 1964 ). Just a decade ago (Pierre, 2010 ; Steadman, 2008 ), proposals were advanced that introversion be listed as a personality disorder for the DSM-V (American Psychiatric Association, 2013 ). While this did not eventuate, several personality disorders include introverted traits as part of their diagnostic criteria (e.g., Avoidant Personality Disorder, Schizoid Personality Disorder (DSM-V, 2013). Notably, even though the DSM-V cautions practitioners to consider culture-related issues when diagnosing these disorders, this advice does not extend to acknowledging the extravert-centric culture from which the DSM emerged. Such debates highlight the need to advocate for the quieter members of our WEIRD societies—those who fall on the more introverted and sensitive end of these continuums.
Essentially, this means that introverted individuals (and other sensitive members of society) existing within WEIRD cultures may experience a poor fit with their wider cultural context. Indeed, studies suggest that introverted students feel out of place in extravert-centric classrooms (Davidson et al., 2015 ; Henjum, 1982 ). The socially accepted norms around ways of behaving and ways of being well in WEIRD cultures can leave introverts feeling invalidated (Fulmer et al., 2010 ), or feeling pressured to fit in and less authentic (Lawn et al., 2018 ), with implications for opportunities for—and experiences of—wellbeing.
Links between personality and wellbeing
Interest in human wellbeing has occurred across millennia (Crisp, 2014 ), and while recent years have brought considerable interest and focus on wellbeing, there remains little consensus on what wellbeing is, with robust discussions occurring on how to appropriately define and measure the construct (Cooke et al., 2016 ; Disabato et al., 2016 ; Goodman et al., 2017 ; Kern et al., 2019 ). For our purposes here, we focus on subjective aspects, or how individuals perceive their life (Huppert, 2014 ). We define wellbeing as a flexible state of high-quality psychosocial functioning—feeling good and doing well across a range of cognitive, emotional, and social domains.
Associations between wellbeing and personality have long been recognized. For example, trait-focused studies consistently find that extraversion and neuroticism link to positive (e.g., life satisfaction, happiness, positive affect) and negative (e.g., negative affect) wellbeing outcomes, respectively (DeNeve and Cooper, 1998 ; McCrae and Costa, 1991 ; Smillie, 2013 ; Smillie et al., 2015 ). Conscientiousness demonstrates moderate correlations with affective dimensions, with stronger associations with achievement and social competence dimensions (Friedman and Kern, 2014 ; Steel et al., 2008 ). Beyond trait-focused research, narrative studies find that coherence of one’s self narrative identity links to greater wellbeing (Baerger and McAdams, 1999 ), generativity, and psychosocial adaptation (McAdams and Guo, 2015 ). Interestingly, it appears that wellbeing benefits can arise not only from personality characteristics themselves, but also from engaging in behaviors that reflect those characteristics. For instance, studies find that acting in an extraverted manner increases positive affect and happiness levels, even for introverted individuals (Fleeson et al., 2002 ; Smillie et al., 2016 ; Sun et al., 2017 ; Zelenski et al., 2012 ).
Studies focused on personality and wellbeing have primarily taken a nomothetic approach, attempting to identify general associations across populations, while ignoring individual variations from the norms. Less is known about individual experiences of wellbeing, especially when those experiences run counter to social norms that define the outcomes studied.
Sensory processing sensitivity
The current study specifically focuses on experiences of wellbeing for people that are highly sensitive. Sensory processing sensitivity (SPS) is commonly characterized by a propensity for deep and complex processing of sensory information, easy over-arousal from sensory input (e.g., strong smells and tastes, sounds, temperatures), strong emotional reactivity and empathy, and increased awareness of subtleties in the environment (Aron and Aron, 1997 ). The trait is relatively continuously distributed, and individuals can be grouped into low, medium, and high levels of sensitivity (Lionetti et al., 2018 ; Pluess et al., 2018 ). High SPS is relatively common; recent studies estimate that ~25% of the general population can be classified as scoring high on SPS (Greven et al., 2019 ). Notably, although the trait appears to have some overlapping characteristics with introversion, ~30% of high-SPS individuals also score high on extraversion. Just as introversion is not highly valued in WEIRD cultures (Fulmer et al., 2010 ), sensitivity is not highly valued (Aron, 1996 , 2004 ), and high-SPS individuals living in Australia might feel a mismatch between their innate attributes and the culture in which they live (Aron, 2011 ).
Despite its prevalence, SPS is an under-researched trait, especially in relation to wellbeing. Existing SPS/wellbeing research associates high SPS with a range of maladaptive outcomes such as anxiety (Bakker and Moulding, 2012 ; Meredith et al., 2016 ), internalizing problems (Boterberg and Warreyn, 2016 ), depression (Brindle et al., 2015 ; Yano et al., 2019 ), social phobia (Neal et al., 2002 ), and low levels of life satisfaction (Booth et al., 2015 ), among others. Still, a few studies focused on functional aspects of SPS do exist, suggesting that high-SPS can also relate to positive outcomes (Black and Kern, 2020. Personality and flourishing: exploring Sensory Processing Sensitivity and wellbeing in an Australian adult population (unpublished manuscript); Sobocko and Zelenski, 2015 ; Yano et al., 2020 ).
The current study
Despite apparent mismatches between SPS characteristics and WEIRD socially accepted norms around behaviors and wellbeing, some highly sensitive persons (HSPs) do achieve high levels of wellbeing (Black and Kern, 2020. Personality and flourishing: exploring Sensory Processing Sensitivity and wellbeing in an Australian adult population (unpublished manuscript)). How do they do this? As promoting high levels of wellbeing is a goal worth pursuing for any community, organization, or government amongst its citizens (Hone et al., 2014 ), it is important to know what wellbeing looks like for individuals within society, not just relying on trends for the majority.
Following a nomothetic perspective, in an initial quantitative study (Black and Kern, 2020. Personality and flourishing: exploring Sensory Processing Sensitivity and wellbeing in an Australian adult population (unpublished manuscript)), 430 individuals completed a wellbeing survey that examined associations between a variety of wellbeing domains and sensitivity using existing wellbeing measurement tools. The study provided trends of SPS and wellbeing across a sizable number of individuals. Participants ranged both in terms of SPS scores and reported wellbeing across a range of dimensions. To better understand the experiences and perspectives of high-SPS individuals, the current study adds an idiographic exploration of how high-SPS individuals conceive of and experience wellbeing, using qualitative interviews with a subset of high-SPS individuals. As Hefferon et al. ( 2017 ) noted, qualitative approaches can “provide in-depth access to phenomena that links experiences and processes with emotions, thoughts, and behaviors” (p. 214), which can provide richer explorations of individual experiences than can be attained through quantitatively based measures alone. The current study aimed to investigate how high-SPS individuals living within an extravert-dominant social context conceive of and experience wellbeing.
Participants
The current study used a subset of respondents from the Wellbeing and Highly Sensitive Person study (Black and Kern, 2020. Personality and flourishing: exploring Sensory Processing Sensitivity and wellbeing in an Australian adult population (unpublished manuscript)), which included 430 individuals living in Australia. In the larger study, 152 participants scored high on wellbeing; of these, ~24% ( n = 37; 2 males, 34 females, 1 other gender) could be classified as high SPS. Of these 37 high-SPS/high-wellbeing respondents, 27 indicated willingness to potentially be interviewed, with interviews successfully conducted with 12 individuals (1 male, 11 females). Interviewees ranged in age from 19 to 69 and were of Asian ( n = 4) or White Australian ( n = 8) descent and reported good physical health. There were no significant differences in wellbeing and SPS scores between male and female, or between Asian and White Australian ethnicity interview participants. Half the participants were studying either full-time or part-time, and two thirds of participants were employed in full-time or part-time work.
As part of the larger study, participants completed the Highly Sensitive Person Scale (HSPS; Aron and Aron, 1997 ), the PERMA-Profiler (Butler and Kern, 2016 ), and the self-acceptance, autonomy, and personal growth sub-scales from the Psychological Wellbeing scales (PWB; Ryff and Singer, 1996 ). All 37 participants in the high-SPS/high-wellbeing group had relatively high scores compared to the rest of the larger sample on these measures.
The first author conducted interviews via videoconferencing, using a semi-structured questionnaire with 13 prompts (see Supplementary Information: https://doi.org/10.26188/5ea7b3d94f5b9 ). Interviews ranged from 25 to 110 min in length, with an average duration of 49 min (SD = 23.17).
Ethical considerations
All procedures were approved by the University of Melbourne’s Office of Research Ethics and Integrity. Participants were invited to participate in interviews via a personal email from the interviewer, which explained that participation was voluntary, and that all data would be treated confidentially. A copy of interview questions was also included in the email invitations. Participants were able to choose a suitable interview time via a secure online scheduling application, and informed consent was obtained before starting and recording each interview. To preserve anonymity, names and identifying information have been removed from what is reported here.
Data analysis
Recorded interviews were transcribed and analyzed by the first author, using an interpretative phenomenological analysis (IPA) process (Smith et al., 1999 ). NVivo 11 software (QSR International Pty Ltd, Released, 2015 ) was used to support analyses. IPA seeks to examine personal, lived experience and get as close to the participant’s personal world as possible (Smith, 1996 ). First, all interviews were transcribed. Second, each transcribed interview was sent to the interviewee for validation. Third, each transcript was read several times. Fourth, parts of the text that were relevant to wellbeing were highlighted. Fifth, key words were used to capture emerging theme titles, which were later designated as dimensions (Smith et al., 1999 ). Some dimensions followed the questions on the interview schedule, but new ones also emerged. Sixth, connections between dimensions were identified, and were clustered together to eliminate redundancy. Finally, the dimensions were grouped into key themes (Smith et al., 1999 ). Because of the nature of interview data, quotes can include multiple themes.
Limitations, boundaries, and potential for bias
For transparency, we note several limitations of our approach, boundaries of generalization, and characteristics of the authors that could impact upon the analyses and interpretations of the data.
Personality research has long struggled to reconcile competing agendas between nomothetic approaches and the idiographic study of people’s unique experiences in life (McAdams and Olsen, 2010 ). Our study addresses the misbalanced focus on nomothetic approaches by bringing an idiographic focus for a particular population, aiming to understand the perceptions and experiences of individuals, rather than identifying trends that capture the collective, focusing on depth rather than breadth. In qualitative interpretative phenomenological research (such as this), a sample size of 6–8 participants is considered acceptable for in-depth interviews (Eatough and Smith, 2017 ; Smith, 2017 ; Smith and Eatough, 2011 ; Smith et al., 2009 ). Our study included twelve participants, and thus aligns with these recommendations. However, due to the small sample size, the findings should not be generalized to all high-SPS individuals.
Further, while it might be useful to compare high-SPS and low-SPS experiences, such comparisons undermine the value of understanding high-SPS experiences in and of themselves. There may be something distinctive about high-SPS wellbeing, and the interviews might have some important insights for low or moderate SPS individuals as well. Such comparisons are beyond our scope here. Subsequent studies might consider the extent to which the results reported here are generalizable, distinctive, and useful.
As interviews were completed at a single period in time, participant responses and our analysis of those responses are impacted by the contemporary sociohistorical context. Although our interview group was demographically diverse, future studies should include a wider mix of participants of different genders, socio-economic backgrounds, ages, cultures, and historical periods in time.
Within the semi-structured interviews (see Supplementary Information), the interviewer noted several examples of wellbeing (e.g., positive emotions, absorption). The examples were included to encourage participants to think about positive aspects of wellbeing, rather than on mental illness and the lack of dysfunction. The interviewer ensured that participants understood that they were invited to explain wellbeing from their own perspective. However, it is possible that the prompts colored participants’ responses.
In addition, the authors have backgrounds in educational counseling, teaching, psychology, and wellbeing research. We both score high on SPS; while this may have influenced the interpretation of results, it also allowed the interviewer to respond to distinct cues provided by the participants.
As summarized in Table 1 , 32 dimensions were identified. Analysis of participants’ interviews revealed three key themes related to wellbeing: conceptions of wellbeing, how wellbeing is enabled, and barriers of experiencing wellbeing.
Conceptions of wellbeing
The first theme that emerged reflected how interviewees conceptualized wellbeing. All participants portrayed a multi-dimensional view, mentioning emotional, cognitive, physical, spiritual, and social/relational dimensions.
Eight participants highlighted that the emotional dimension was represented by low-arousal positive emotions, such as calmness, relaxation, and peacefulness, rather than the high-arousal emotions typical of WEIRD cultures. For example, one noted that wellbeing is when they “just feel relaxed and calm and peaceful,” and another participant shared “for me, it’s less exuberant happiness, and more the kind of contented, softer, and being comfortable in my space and with myself.”
Nine participants conceptualized wellbeing as a balance or harmony across multiple dimensions. For example, one participant described wellbeing as “a wheel with different segments, and I have to look after all the different segments in order for the wheel to roll.” Another participant noted:
“trying to have a balance of everything, so like physical health, eating well, having time to do things that you enjoy…like hobbies, and things like that outside work. Basically, finding a balance between those things.”
Participants commonly expressed harmonizing among psychosocial dimensions (e.g., “negative emotion, positive emotion, feelings of connectedness between people”). Others further included a cognitive dimension, reflecting self-knowledge and self-appreciation. For instance, one participant described wellbeing as “when you feel like the best self that you know you can be,” while another participant noted “being very comfortable in my space and with myself.” Another person included a spiritual dimension: “my relationship with God, and then also a sense of meaning in life.”
Enablers of wellbeing
The second main theme that emerged reflected strategies that enable high-SPS individuals to experience wellbeing. Aligned with seeing wellbeing as multi-dimensional in nature, interviewees suggested that many of the enablers impact across multiple wellbeing dimensions. For example, mindfulness was seen as contributing to emotional, cognitive, spiritual, and relational wellbeing. While we grouped enablers into different domains (emotional, cognitive, behavioral, social), this is simply for ease of communicating the results. Arguably, many of the enablers target multiple domains (e.g., contemplative practices can be emotional, cognitive, and behavioral in nature). Strategies generally focused on regulating emotions, cognitions, behaviors, and social processes. Participants also pointed to personal characteristics that may help buffer wellbeing.
Emotion-focused enablers
Eight interviewees shared how regulating their emotions helped improve their wellbeing. For example, one participant reported benefits in being able to take time in responding to negative stress and emotion. Another interviewee spoke of re-framing her internal dialog, suggesting her emotional self-regulation abilities had improved over time: “everyone has these moments and it’s not catastrophizing like I used to, but just being a bit more comfortable sitting with it, and thinking it’ll pass and it’ll work out.” One participant described feeling a sense of empowerment through choosing “the response that’s going to give you the outcome you want”, and another shared “I love feeling empowered to choose the way that I respond.”
Cognition-focused enablers
Most participants pointed to self-awareness and self-acceptance. Ten participants spoke to the importance of purposeful cognitive recognition of the self, including awareness of their own wellbeing needs. For instance, one participant noted, “I know the pathways that work for me, and I know the dosages on different days.” Others pointed to being aware of their needs and proactively acting upon those needs: “I’ve got to integrate some rest in there, or whatever I need”; and from another “I’m a lot more aware, physically, of the sensations of stress in my body.” One person noted the importance of “reminding myself to appreciate, you know, and to look at what’s there instead of what isn’t.” Self-awareness was beneficial when coupled with self-acceptance. For instance, one participant reflected on wellbeing as accepting one’s limitations and asking for assistance when needed: “part of that wellbeing is knowing when I need help and requesting it in an effective manner.”
All 12 interviewees believed that self-compassion was a significant contributor to their wellbeing. Several participants believed that self-compassion involved being kind to themselves through self-talk, such as using “self-talk like ‘this will pass’ and ‘it’s just a stressful time’ and ‘it’ll be okay’,” and telling oneself “I’ve been trying to do that with myself, treat myself the way I’d treat somebody I love.” One interviewee spoke of “being able to forgive myself for mistakes,” while another described self-compassion as being non-judgmental to the self: “I think compassion is a positive thing, and non-judgmental is neutral, I think. I’m just neutral to myself.” For some participants, their practice of self-compassion involved “being patient with myself,” and re-framing their internal dialog, for instance one participant shared, “I’m very conscious of the language that I use, so I’ve gotten rid of the word ‘should’.” For another participant, self-compassion was “very much about listening to what my body is telling me, but also being reflective on the way I respond to situations and respond to particular people.” One interviewee noted how practicing self-compassion helped alleviate her stress levels, “if you’re hard on yourself all the time, then you can never not be stressed.” Still, several participants noted that self-compassion was a work in progress. For example, one participant shared, “it’s something I have struggled with but I think that I’m getting better at it,” and another shared, “I think a lot of us can be hard on ourselves, but that’s one area I probably need to work on.”
Behavioral enablers
All participants reported the importance of engaging in regular activities such as physical, mental, and self-care behaviors. For example, one participant noted “every day I try to do something—it’s exercise or coming home and reading a book.” Another noted: “I do craft stuff at night. I give myself that hour to just not think about anything.” Others pointed to physical activity and exercise: “eating healthy food, and doing exercises every day” and “I think yoga and walking are incredibly important for me […] if I’m busy and I don’t get to walk one day I can survive, but my days with walking and yoga are so much better.”
These behavioral activities included exercise or movement, contemplative practices, and connecting with nature. For instance, half of the participants noted that connecting with nature was an important enabler of their wellbeing. Interestingly, all six participants mentioned being among trees as their “go-to” activity, for example, one participant shared how lovely it was walking through a nearby bush track “and looking at the way the leaves move on the trees.” Other participants shared a similar enjoyment of being amongst trees: “I’m still looking up at the trees and being mindful,” and from another: “I’ve got a creek trail behind me and I’ll go into the trees.” However, the theme of solitude permeated several areas, influencing participants’ emotional, cognitive, spiritual, and social/relational wellbeing.
All interviewees stated that including contemplative practices in their day was important for their wellbeing, and simultaneously expressed a desire to practice it more consistently. For some, their practice involved movement, such as walking mindfully (“my form would be walking in nature”) or running outdoors (“when I was running it was kind of a way for me to meditate”). Another movement-based practice shared by one interviewee was martial arts: “a meditative discipline is good—mine is tai chi.” Others noted that their mindful practice involved reciting a speaking prayer, with one participant sharing, “it’s something I do daily [before going to sleep], and occasionally during the day as well.” Other participants shared that they tended to employ their meditative practice in response to stressful situations: “I tend to do it more when I’m stressed or anxious,” or “I also try and do it when I’m stressed.”
Social enablers
Eleven interviewees indicated that close, supportive relationships helped bolster their wellbeing. For example, one participant described feeling very happy to have social connections to “confide in each other and to support my wellbeing,” and another interviewee shared her enjoyment of “sitting quietly and chatting with friends.” Another participant pointed to the significance of her close relationships: “it’s the sharing of life with people who love me, and I love them immensely.” Several participants specifically mentioned having a small, select circle of friends, with one interviewee noting she had “six dear friends.” Another participant pointed to the supportive nature of her friendship circle: “I don’t really like to be around people too much, but I have a close group of friends and they really help me get through life.” Another interviewee spoke of a preference for intimate interactions with friends, sharing “the last few times I’ve been out, it’s been more one-on-one with my friends.”
Notably, even as participants valued social relationships, all 12 interviewees reported that regular experiences of solitude were an important enabler of their wellbeing. For example, one participant noted that “solitude is something that’s very important for me to maintain some of my sanity and my energy.” Others emphasized that “alone time is very important” and that “it’s really important that I stay with myself.” Participants actively sought time alone, noting that “solitude is built into my everyday life” and “I do a lot of things with myself, just by myself.” Another noted “I’m quite happy to go away in the middle of the bush by myself and camp for a week with one of my dogs, and I’m in bliss.”
Participants spoke to the challenge of harmonizing a desire for social engagement with their need for solitude, especially for the eight more extraverted participants. One noted, “I get a lot of invitations, and I always feel obligated that I need to catch up with this person, and this person and this person […] but now I’m like, no, I’ve had one of those weeks when I need to go home.” Another contrasted loving times to connect with others, but also that “I think I would get really overwhelmed if I did not have that time where I could just turn away.” Participants insightfully understood their own nature, with one noting “I’m a gregarious and outgoing person […] I’m open and direct but I find social interactions exhausting.” Despite valuing other people, one participant shared “I always feel like I need a bit of time to myself, even away from my partner” and another noted “I really value talking [to my family] but I really value my solitude as well.”
Seven interviewees identified the need to consciously and deliberately choose with whom they spend time. These participants described how making more deliberate choices around their contact with others was beneficial for their wellbeing, for instance, “I’ve learned to let go of certain relationships that don’t improve my wellbeing.” These conscious choices extended to “[keeping] very select people in my life,” or to excluding people: “relationships that were having a negative influence on my mood or on my person I have just eliminated or cut down to a bare minimum, and I just see those people when I need to.”
Personal characteristics
Participants also spoke to several personal characteristics that they believed helped them experience wellbeing. Four interviewees spoke of being naturally optimistic and hopeful. Most expressed optimism/hope as a beneficial enabler of their wellbeing; for instance, “I have enormous hope and hope is so important,” and “I’m a naturally very positive person.” However, one interviewee noted some mixed feelings about being optimistic: “sometimes I think it’s good that I always think positively and optimistically, but sometimes I think that I’m not really in the world—I’m in my own world.”
Eight participants referred to meaning as important to their wellbeing and expressed through different areas of life. For instance, one interviewee revealed that a sense of meaning was elicited through religion/spirituality: “my religion is a satisfactory and adequate provider of meaning and purpose in my life.” Other participants spoke of deriving meaning through their work; for example, one interviewee noted “that’s why I do what I do, and I feel really clear about that, because that’s aligned with my meaning statement.” Another participant spoke of feeling a sense of meaning through helping others: “there’s no denying that sense of this is what I’m here for,” while another interviewee described meaning as a driving force in her life: “that’s the overarching thing.” Another area where meaning was embodied was through close relationships: “what provides the most meaning for me is the loving, supportive relationships that I have.”
Barriers of wellbeing
The third key theme to emerge was issues that challenge participants’ wellbeing. Two primary sub-themes emerged: physical health issues and being able to say “no”, along with a series of other issues that were particular to specific participants.
Physical health issues
Six interview participants shared how physical health concerns had been affecting their overall wellbeing, for instance, “I’m getting to the bottom of a few health issues I’ve had, and I think that’s really going to take [my wellbeing] to the next level,” and “I got some bronchial infection […] so I would really like to improve that.” Seven interviewees expressed a desire to improve their physical health in more general terms, “but with exercise I can’t seem to bring that together quite so well, so I find that a real challenge.”
The challenge of saying no
Eight participants specifically reported having difficulty in saying “no” to requests for their time. For example, participants noted: “I’m not very good at saying no, I think I like to please people. I definitely find that hard,” or “I’m so bad at this!” One interviewee pointed to the emotional discomfort inherent in this challenge: “if you always say yes to everything, (but) you feel bad if you say no.” This challenge was noted across work and social domains.
While being able to say “no” was perceived as a challenge, six interviewees shared how this ability had improved over time with ongoing practice, for instance one noted, “that’s something I definitely have to practice and I’m glad, because you do work better with a sharper knife,” and another: “that’s a work in progress but I would say that I’m pretty good at it and much better than I used to be.” One participant noted how through learning to say “no” she had also learnt “I actually don’t have to explain myself.” One participant pointed to this practice making a difference in her wellbeing: “I’ve gotten a lot better at that over the years, before I used to be like ‘oh, okay’ and just sacrifice my alone time for other people, but now it’s like nah!.” Other interviewees shared strategies that they had developed, such as “if I say no, I mean no; if I’m not sure, I’ll say no.” Another noted:
“I just use Spoon theory. It’s where you wake up every morning and you’ve got so many spoons, and it might take a spoon to have a shower, and a spoon to complete a certain task. And every morning when you wake up you don’t always have the same amount of spoons. So, I’ve learnt to say I don’t have the spoons for it.”
Social aspects also appeared in the challenge of saying “no.” Five participants expressed how saying “no” was especially challenging when it involved helping loved ones and close friends. For instance, one interviewee described not being able to take on more things, but still agreeing to help friends or family at a personal cost: “I maybe have to sacrifice my sleeping time, or I forget eating sometimes.” Another participant shared: “the only time I won’t say no when I really want to, is if it’s related to the kids.” One interviewee explained how saying “no” also involved saying “no” or “not yet” to herself: “I’m interested in a million different things and it’s really hard […] for me to say to myself well I can put that on my list, this isn’t the right time to do it, let’s just wait, stay focused.”
Other barriers
Participants also noted a variety of other barriers. One stress-inducing situation described by two interviewees was the sense of having too much on one’s plate. For example, one participant shared “I don’t deal with having to do a lot of things at the same time—that really stresses me out.” Similarly, another interviewee noted “if there’s one big simple task that takes 10 h, and ten smaller tasks that take 1 h each, I’d be a lot more stressed with the ten smaller tasks.” Other participants described having developed or adopted different strategies to help manage demands. For instance, one participant shared how she paced herself instead of “jumping in at the deep end and getting myself in a bit of a fix,” while another reported taking affirmative action in response to her stress: “I shift more toward getting goals achieved rather than focusing on reducing my stress, if I’m a bit stressed I may as well channel it into something productive.”
Another challenge mentioned by participants was having strong emotional responses. For instance, one participant shared: “[the other person] is fine in about thirty seconds, and it really impacts me for a long time, I feel really shaken up by it and it takes me a long time to calm down from things.” Another participant noted that their family “say to me that I’m always really emotional when I was young, and I’m still quite emotional now.” Additionally, two participants shared how strong feelings of empathy could impact their wellbeing at times. For instance, one interviewee reported feeling drained after being in crowded spaces: “I can go to a shopping center, and I need to get out after a while.” Another participant described being able to manage her empathetic responses through being alone “ because I don’t feel like I’m carrying other people’s stuff.”
Awareness of and interactions with mental health
Beyond the three main themes that emerged, additional insights emerged from participant reflections on their growing awareness of SPS and experiences with anxiety and depression. First, growing knowledge and awareness of the SPS trait seemed to increasingly be influencing participants’ wellbeing. For ten interviewees, their first awareness of SPS was through participation in the broader Wellbeing and Highly Sensitive Person study (Black and Kern, 2020. Personality and flourishing: exploring Sensory Processing Sensitivity and wellbeing in an Australian adult population (unpublished manuscript)). One interviewee expressed how learning about SPS had improved her wellbeing: “what has improved my wellbeing, being highly sensitive, is also the awareness of the positive aspects of the trait.” Participants anticipated that learning about the SPS trait would be beneficial to their wellbeing, noting for example, “it will probably alert me to take care of myself more,” another stated “this makes sense, and to be so much kinder to myself and respectful to myself,” while another shared “it will help me be more aware and not so hard on myself if I’m not coping in certain environments.” For some participants, their awareness and knowledge of SPS pointed to growing self-acceptance. For example, one interviewee shared having some prior negative perceptions about the trait, but that she had “learnt to recognize that it has positive qualities […] so I’ve learnt to accept that about myself and be more comfortable with it.” Another participant echoed this view: “knowing that sometimes how I’m feeling is due to the higher sensitivity and it’ll pass, and it’s natural, then it’s helped me separate out the anxiety from sensitivity.”
One participant directly pointed to the mismatch between their sensitivity and the extravert-centric Australian culture, noting “I don’t always like to be in a very loud environment, and the Australian culture is more people are outgoing than introverted, so I’m kind of changing myself to adapt to the culture.” Other interviewee accounts were less direct, but nonetheless suggestive of the mismatch between the surrounding culture and their sensitivity, reflecting self-blame. For example, “there’s like a desire to not be sometimes so sensitive. Cause it does make it hard, compared to some other people, to care less about certain things, and be more confident about certain things.” Another participant shared, “So that’s a challenge that I’ve always dealt with and always thought, well why don’t I have as much consistent energy as other people?[…] I’m definitely going to read up on it because that goes a long way to explain that. That would be a real relief for me.”
Second, nine interviewees shared how they had experienced anxiety and/or depression in the past. One participant noted for instance, “I’ve had poor wellbeing, a little bit of anxiety, and I’ve come to understand why,” and another shared “I went through a period where I had depression and anxiety, for about two to three years.” Interviewees also shared how they continued to utilize strategies that had assisted their recovery, for example, one reported “I’m pretty good at identifying warning signs […] and I’m much better at dealing with those warning signs early.”
As human beings, many of us seek wellbeing, yet the experience of wellbeing is not the same for everyone—it can be influenced by a range of individual and cultural factors (Khaw and Kern, 2014 ; Lawn et al., 2018 ; Sun et al., 2018 ). Predominant characteristics of high-SPS individuals (e.g., Aron, 1996 , 2004 , 2011 ) run counter to broader social discourses around wellbeing that dominate WEIRD cultures such as Australia. Indeed, SPS typically is portrayed as a negative characteristic that sets individuals up for a range of illbeing outcomes (e.g., Bakker and Moulding, 2012 ; Booth et al., 2015 ; Listou Grimen and Diseth, 2016 ; Meyerson et al., 2019 ). Yet despite this misfit, some high-SPS individuals do experience high levels of wellbeing ((Black and Kern, 2020. Personality and flourishing: exploring Sensory Processing Sensitivity and wellbeing in an Australian adult population (unpublished manuscript); Sobocko and Zelenski, 2015 ). Using semi-structured interviews, this study investigated idiographic conceptions and experiences of wellbeing within high-SPS individuals living in Australia. While the findings do not necessarily generalize to people beyond the study, these idiographic case studies provide insights into individual experiences of wellbeing, providing richer descriptions of how some individuals are able to successfully navigate clashes between their personality and the social context in which they live.
Idiographic conceptions of wellbeing
The past few decades have brought considerable theory, research, and practice focused on understanding and building subjective wellbeing. While some work has considered lay conceptions (e.g., Bharara et al., 2019 ; Ryff, 1989b ; Wong et al., 2011 ), many of the existing wellbeing models either are based upon academics’ theories (e.g., Huppert and So, 2013 ; Martela and Sheldon, 2019 ; Seligman, 2011 ), or arise from patterns across groups of people (e.g., Cummins, 1998 ; Diener et al., 1985 ). The former is inherently influenced by the cultural norms and experiences in which the academic resides, and the latter prioritizes consistent patterns at the expense of understanding individual experiences that may differ from the majority. The current study complements other approaches by adding depth and insights from the perspectives of individuals who identify with personality characteristics that sets them apart from the norm.
Notably, participants conceptualized wellbeing as multi-dimensional in nature, aligning with many contemporary models of wellbeing (Disabato et al., 2020 ; Huppert and So, 2013 ; Keyes, 2002 ; Rusk and Waters, 2015 ; Ryff, 1989a ; Seligman, 2011 ). Interestingly, participants emphasized the importance of balance or harmony across different dimensions. Similar perceptions of balancing and harmonizing wellbeing dimensions were noted in a study which investigated wellbeing perspectives of Australian young people and youth workers (Bourke and Geldens, 2007 ). The question of how much or how little of each wellbeing component might be needed (for people to thrive) generally goes unacknowledged in current wellbeing literature. Future studies might consider the extent to which balance and harmony are specific to highly sensitive individuals or might be true of other individuals as well.
In addition, several wellbeing enablers were spontaneously reported during interviews: balance/harmony between wellbeing dimensions; connecting with nature; low-intensity positive emotions; self-awareness; self-acceptance; and optimism and hope. These findings highlight the advantage of idiographic approaches (like the current study), which can provide valuable insights that are often not seen in quantitative studies.
Almost all existing models of subjective wellbeing include an emotional dimension, with scales specifically focusing on high-arousal emotions. Indeed, the positive emotion language on many wellbeing measures (e.g., PANAS; Watson et al., 1988 ) focuses on high-energy, high-intensity positive emotions (Lim, 2016 ). The language of these questions fails to incorporate the full range of positive emotions that are available to people, which can subtly imply that low-intensity positive emotions (e.g., contentment, peacefulness, calmness) are either not valid, or do not contribute to wellbeing. In contrast, participants suggested that low-intensity positive states (calm, peaceful, relaxed) are highly valued aspects of high-SPS individuals’ wellbeing. While our results cannot be generalized across broader populations, they suggest that to capture a full range of individual experiences, measures should include both high- and low-valence emotions, and not assume that a person lacks wellbeing simply because they are not excited, enthusiastic or joyful. Indeed, valuing and desiring low-intensity positive emotions, and meeting these ideal levels, has been shown more beneficial to health (predicting fewer physical health symptoms) than meeting ideal high-intensity emotion levels (Scheibe et al., 2013 ); our findings support this view.
Strategies for staying well despite cultural norms
Large surveys can provide average levels of wellbeing, which are often generalized across a population (Eckersley, 2016 ), but these surveys are unable to tell us the associated practices that people use to foster their wellbeing. Qualitative explorations—such as ours—provide rich data that can answer the what, how, and when of individual wellbeing (Hefferon et al., 2017 ). Our findings suggest that, within the extravert-dominant Australian culture, high-SPS individuals are able to instinctively create a unique constellation of “good things” that contribute to their wellbeing. Our findings can help to normalize a sensitive orientation and offer some measure of validation to high-SPS individuals who live within WEIRD countries.
By far, the most notable enabler of wellbeing was actively choosing solitude. In their initial series of studies into the SPS trait, Aron and Aron ( 1997 ) noted a preference among high-SPS individuals for time spent alone: our results support their findings and provide a foundation for linking this dimension with high-SPS wellbeing. Scholars and researchers have long discussed the benefits of solitary experiences for wellbeing (Merton, 1958 ; Montaigne, 1877 ; Zimmerman, 1799 ). Recent research further demonstrates the benefits of solitude for wellbeing (Leary et al., 2003 ; Leontiev, 2019 ; Long and Averill, 2003 ; Nguyen et al., 2018 ), yet this seemingly important wellbeing component does not appear in any of the commonly used wellbeing models or measures (e.g., Diener et al., 1985 ; Linton et al., 2016 ; Ryff, 1989a ). Future studies might benefit from focusing on these elements, identifying for whom, and under what conditions, they are useful.
Solitude may provide the time and space for self-awareness, self-acceptance, and self-compassion, aspects that participants pointed towards being helpful for wellbeing. Our findings are consistent with the literature that generally associates dispositional self-awareness with high levels of psychological wellbeing, and views it as a means of alleviating psychological distress (Gu et al., 2015 ; Sutton, 2016 ). Our results also align with existing research showing positive associations between self-acceptance and psychological wellbeing (Lindfors et al., 2006 ; Ryff, 1989a , 2014 ). Acknowledging and accepting both good and bad aspects of the self are key attributes of self-acceptance (Ryff, 1989a ) and these were reflected in participant reports. Cultivating self-compassion has been shown to positively predict wellbeing (Neff et al., 2007 ) and greater life satisfaction (Neff et al., 2008 ), and our findings lend support to this literature.
The experience of connecting with nature was important to at least half the interviewees, many of whom shared that being alone in nature was their preference, rather than in the company of others. This aligns with studies pointing to the restorative effect of nature on wellbeing (Korpela and Staats, 2014 ), and positive associations between wellbeing, mindfulness, and nature connectedness (Capaldi et al., 2014 ; Howell et al., 2011 ).
Participants also pointed to a complicated balance between using social relationships to support wellbeing, and when relationships became detrimental to their wellbeing. These themes align with research associating greater individual wellbeing with harmonious relationships (typified by high warmth and low levels of conflict; Sherman et al., 2006 ) and the fulfillment of the psychological need for relatedness (Patrick et al., 2007 ; Ryan and Deci, 2017 ). Conversely, conflicted (Sherman et al., 2006 ) or poor quality (Hartup and Stevens, 1999 ) relationships have been associated with low levels of individual wellbeing.
Highly sensitive individuals in WEIRD countries do not fit neatly within the wider social and cultural context (Aron, 1996 , 2004 ) in which they live, and high-SPS individuals may try to navigate social situations as expected, but find the situation overwhelming and oppressive, resulting in anxiety and self-blame. Interestingly, interview participants mostly did not explicitly mention feeling a mismatch between their innate sensitivity and the surrounding extravert-centric culture. However, when sharing their experiences around becoming aware of the SPS trait and its associated benefits, multiple responses did suggest this mismatch, particularly pointing to blaming themselves and wondering why they seem to be different than others. Indeed, learning about their sensitivity provided an explanation for these differences, which several interviewees found empowering. By understanding that the characteristics of SPS may impact how the person experiences and relates to the world, it can provide explanation and support a sense of autonomy. Future research might consider the role that identifying with high-SPS might have upon behaviors and perceptions of wellbeing.
Our participants identified physical health issues, the challenge of saying “no”, and the sense of having too much on one’s plate as barriers to their wellbeing. These perceived barriers are not unique to high-SPS individuals, especially the sense of feeling overwhelmed from too much to do (e.g., Bellezza et al., 2017 ; Leshed and Sengers, 2011 ; Skinner and Pocock, 2008 ). Future studies might benefit from further investigating factors that create a sense of overwhelm, and strategies that effectively decrease this sense for different individuals.
Our findings represent specific individuals living within a specific sociohistorical context. Future studies might benefit from considering other characteristics that could impact the experience of, enablers of, and barriers to wellbeing, for individuals who live in non-WEIRD sub-cultures or cultures, and/or for those who do not fit typical conceptualizations of the happy person. The specific causes and mechanisms of high-SPS wellbeing are beyond the scope of this study but could present a worthwhile focus for future research.
By combining multiple perspectives from individuals high in SPS, the current study provides a richer understanding of how some individuals—those who are high in SPS but also experience high levels of wellbeing—conceive of and create wellbeing. Participants pointed to the importance of considering multiple domains, and the balance amongst those domains, rather than emphasizing greater amounts of specific domains. Participants also pointed to the value of low-arousal positive emotions. They identified emotional, cognitive, behavioral, and social strategies that they engage to stay well despite social pressures. While results cannot be generalized to other groups, the combination of wellbeing contributors and enablers highlight a somewhat unique picture of wellbeing, offering an expanded view of what it means to feel good and function well. We suggest that the results provide key insights into how individuals live well, within the context of friction between their natural personality and the social, cultural, and historic context in which they live. As a whole, this study provides a richer, more sophisticated understanding of the lived experiences of—and pathways to—wellbeing for highly sensitive individuals.
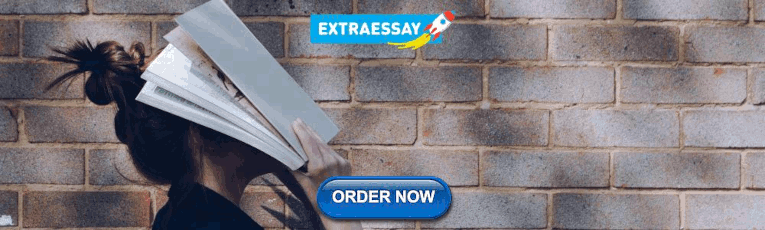
Data availability
The dataset from the 32 themes analyzed in the current study is available at: https://doi.org/10.26188/5e9950060e4d3
Allik J (2005) Personality dimensions across cultures. J Personal Disord 19(3):212–232
Article Google Scholar
American Psychiatric Association (2013) Diagnostic and statistical manual of mental disorders, 5th edn. American Psychiatric Association, Washington, DC
Book Google Scholar
Aron EN (1996) The highly sensitive person: how to thrive when the world overwhelms you. Broadway Books, New York
Google Scholar
Aron EN (2004) Revisiting Jung’s concept of innate sensitiveness. J Anal Psychol 49(3):337–367
Article PubMed Google Scholar
Aron EN (2011) Psychotherapy and the highly sensitive person: improving outcomes for that minority of people who are the majority of clients [electronic resource]. Taylor and Francis, Hoboken
Aron EN, Aron A (1997) Sensory-processing sensitivity and its relation to introversion and emotionality. J Personal Soc Psychol 73(2):345–368
Article CAS Google Scholar
Baerger DR, McAdams DP (1999) Life story coherence and its relation to psychological well-being. Narrative Inq 9(1):69–96. https://doi.org/10.1075/ni.9.1.05bae
Bakker K, Moulding R (2012) Sensory-processing sensitivity, dispositional mindfulness and negative psychological symptoms. Personal Individ Differences 53(3):341–346
Bellezza S, Paharia N, Keinan A (2017) Conspicuous consumption of time: when busyness and lack of leisure time become a status symbol. J Consum Res 44(1):118–138. https://doi.org/10.1093/jcr/ucw076
Benet-Martínez V (2006) Cross-cultural personality research: conceptual and methodological issues. In: Robins RW, Fraley C, Krueger RF (Eds.) Handbook of research methods in personality psychology. The Guilford Press, New York, NY, pp. 170–189
Bharara G, Duncan S, Jarden A, Hinckson E (2019) A prototype analysis of New Zealnd adolescents’ conceptualizaations of wellbeing. Int J Wellbeing 9(4):1–25. https://doi.org/10.5502/ijw.v9i4.975
Booth C, Standage H, Fox E (2015) Sensory-processing sensitivity moderates the association between childhood experiences and adult life satisfaction. Personal Individ Differences 87(Dec):24–29
Boterberg S, Warreyn P (2016) Making sense of it all: the impact of sensory processing sensitivity on daily functioning of children. Personal Individ Differences 92(Apr):80–86
Bourke L, Geldens P (2007) What does wellbeing mean? Yout Stud Aust 26(1):41–49
Brindle K, Moulding R, Bakker K, Nedeljkovic M (2015) Is the relationship between sensory processing sensitivity and negative affect mediated by emotional regulation? Aust J Psychol 67(4):214–221
Butler J, Kern ML (2016) The PERMA profiler: a brief multidimensional measure of flourishing. Int J Wellbeing 6(3):1–48. https://doi.org/10.5502/ijw.v6i3.1
Capaldi CA, Dopko RL, Zelenski JM (2014) The relationship between nature connectedness and happiness: a meta-analysis. Front Psychol 5(08 Sept):976. https://doi.org/10.3389/fpsyg.2014.00976
Article PubMed PubMed Central Google Scholar
Chiu CY, Chen J (2004) Symbols and interactions: application of the CCC Model to culture, language,and social identity. In: Ng SH, Candlin CN, Chiu CY (eds) Language matters: communication, culture, and identity. City University of Hong Kong Press, Hong Kong, pp. 155–182
Christopher JC (1999) Situating psychological well-being: exploring the cultural roots of its theory and research. J Counseling Dev 77(2):141–152. https://doi.org/10.1002/j.1556-6676.1999.tb02434.x
Christopher JC, Hickinbottom S (2008) Positive psychology, ethnocentrism, and the disguised ideology of individualism. Theory Psychol 18(5):563–589. https://doi.org/10.1177/0959354308093396
Cooke PJ, Melchert TP, Connor K (2016) Measuring well-being: a review of instruments. Counseling Psychologist 44(5):730–757
Crisp R (Ed.) (2014) Aristotle: Nicomachean Ethics. Cambridge University Press, Cambridge
Cummins RA (1998) The second approximation to an international standard for life satsifaction. Soc Indic Res 43(3):307–334. https://doi.org/10.1023/A:1006831107052
Davidson B, Gillies RA, Pelletier AL (2015) Introversion and medical student education: challenges for both students and educators. Teach Learn Med 27(1):99–104. https://doi.org/10.1080/10401334.2014.979183
Delle Fave A, Wissing M, Brdar I, Vella-Brodrick D, Freire T (2013) Cross-cultural perceptions of meaning and goals in adulthood: their roots and relation with happiness. In: Waterman A (ed.) The best within us: positive psychology perspectives on eudaimonia. APA, Washington DC, pp. 227–247
Chapter Google Scholar
DeNeve KM, Cooper H (1998) The happy personality: a meta analysis of 137 personality traits and subjective well-being. Psychological Bull 124(2):197–229
Diener E (2000) Subjective well-being: the science of happiness and a proposal for a national index. Am Psychologist 55(1):34–43
Diener E, Emmons RA, Larsen RJ, Griffin S (1985) The satisfaction with life scale. J Personal Assess 49(1):71–75
Diener E, Oishi S, Lucas RE (2003) Personality, culture, and subjective well-being: emotional and cognitive evaluations of life. Annu Rev Psychol 54:403–425
Disabato, DJ, Goodman, FR, & Kashdan, TB (2020). A hierarchical framework of well-being. PsyArXiv Database [Preprint], Dec 31. https://doi.org/10.31234/osf.io/5rhqj
Disabato DJ, Goodman FR, Kashdan TB, Short JL, Jarden A (2016) Different types of well-being? A cross-cultural examination of hedonic and eudaimonic well-being. Psychological Assess 28(5):471–482. https://doi.org/10.1037/pas0000209
Eatough V, Smith JA (2017) Interpretative phenomenological analysis. In: Willig C, Stainton-Rogers W (eds.) The SAGE handbook of qualitative research in psychology. SAGE Publications Ltd, London, pp. 193–209
Eckersley R (2016) Is the West really the best? Modernisation and the psychosocial dynamics of human progress and development. Oxf Dev Stud 44(3):349–365. https://doi.org/10.1080/13600818.2016.1166197
Eckersley R, Cahill H, Wierenga A, Wyn J (2007). Generations in dialogue about the future: the hopes and fears of young Australians. Retrieved from Weston, ACT
Eysenck HJ (1983) I do: your guide to a happy marriage. Century, London
Fleeson W, Malanos AB, Achille NM (2002) An intraindividual process approach to the relationship between extraversion and positive affect: is acting extraverted as “good” as being extraverted? J Personal Soc Psychol 83(6):1409–1422
Frawley A (2015) Happiness research: a review of critiques. Sociol Compass 9(1):62–77. https://doi.org/10.1111/soc4.12236
Friedman HS, Kern ML (2014) Personality, well-being, and health. Annu Rev Psychol 65:719–742
Fudjack SL (2013) Amidst a culture of noise silence is still golden: a sociocultural historical analysis of the pathologization of introversion. (Master of Social Work), Smith College, Northampton, Massachusetts
Fulmer CA, Gelfand MJ, Kruglanski AW, Kim-Prieto C, Pierro A, Higgins ET (2010) On “feeling right” in cultural contexts: how person-culture match affects self-esteem and subjective well-being. Psychological Sci 21(11):1563–1569
Galinha IC, Oishi S, Pereira C, Wirtz D, Esteves F (2013) The role of personality traits, attachment style, and satisfaction with relationships in the subjective well-being of Americans, Portuguese, and Mazambicans. J Cross-Cultural Psychol 44(3):416–437
Goodman FR, Disabato DJ, Kashdan TB, Kaufman SB (2017). Measuring well-being: a comparison of subjective well-being and PERMA. J Posit Psychol, Online(October), 1–12. https://doi.org/10.1080/17439760.2017.1388434
Greven CU, Lionetti F, Booth C, Aron EN, Fox E, Schendan HE, Homberg JR (2019) Sensory processing sensitivity in the context of environmental sensitivity: a critical review and development of research agenda. Neurosci Biobehav Rev 98(March):287–305. https://doi.org/10.1016/j.neubiorev.2019.01.009
Grimes JO (2010) Introversion and autism: a conceptual exploration of the placement of introversion on the austism spectrum. (Master of Arts), University of Central Florida, Orlando, FL
Gu J, Strauss C, Bond R, Cavanagh K (2015) How do mindfulness-based cognitive therapy and mindfulness-based stress reduction improve mental health and wellbeing? A systematic review and meta-analysis of mediation studies. Clin Psychol Rev 37(April):1–12
Article CAS PubMed Google Scholar
Hartup WW, Stevens N (1999) Friendships and adaptation across the life span. Curr Directions Psychological Sci 8(3):76–79
Hefferon K, Ashfield A, Waters L, Synard J (2017) Understanding optimal human functioning-The ‘call for qual’ in exploring human flourishing and well-being. J Posit Psychol 12(3):211–219
Helliwell J, Layard R, Sachs J (2016). World Happiness Report 2016, Update (vol. 1) Helliwell J, Layard R, Sachs J (eds.). Sustainable Development Solutions Network, New York
Hendriks T, Warren MA, Schotanus-Dijkstra M, Hassankhan A, Graafsma T, Bohlmeijer ET, de Jong J (2019) How WEIRD are positive psychology interventions? A bibliometric analysis of randomized controlled trials on the science of well-being. J Posit Psychol 14(4):489–501. https://doi.org/10.1080/17439760.2018.1484941
Henjum A (1982) Introversion: a misunderstood ‘individual difference’ among students. Educ Media Int 103(1):39–43
Henrich J, Heine SJ, Norenzayan, A (2010a). Most people are not WEIRD. Nature, 466(29). https://doi.org/10.1038/466029a
Henrich J, Heine SJ, Norenzayan A (2010b) The weirdest people in the world? Behav Brain Sci 33(2-3):61–83. https://doi.org/10.1017/S0140525X0999152X
Hone LC, Jarden A, Schofield GM, Duncan S (2014) Measuring flourishing: the impact of operational definitions on the prevalence of high levels of wellbeing. Int J Wellbeing 4(1):62–90
Howell AJ, Dopko RL, Passmore HA, Buro K (2011) Nature connectedness: associations with well-being and mindfulness. Personal Individ Differences 51(2):166–171. https://doi.org/10.1016/j.paid.2011.03.037
Huppert FA (2014) The state of wellbeing science. Concepts, measures, interventions, and policies. In: Huppert FA, Cooper CL (eds.) Wellbeing: a complete reference guide. Volume VI: interventions and policies to enhance wellbeing. Wiley Blackwell, Chichester, West Sussex, pp. 1–49
Huppert FA, So TTC (2013) Flourishing across Europe: application of a new conceptual framework for defining well-being. Soc Indic Res 110(3):837–861
Kern ML, Williams P, Spong C, Colla R, Sharma K, Downie A,… Oades, LG (2019). Systems informed positive psychology. J Posit Psychol, Online(12 July), 1–11. https://doi.org/10.1080/17439760.2019.1639799
Keyes CLM (2002) The mental health continuum: from languishing to flourishing in life. J Health Soc Behav 43(2):207–222. https://doi.org/10.2307/3090197
Khaw D, Kern ML (2014) A cross-cultural comparison of the PERMA model of well-being. Undergrad J Psychol Berkeley 8(Fall/Spring):10–23
Kitayama S, Markus HR (2000) The pursuit of happiness and the realization of sympathy: cultural patterns of self, social relations, and well-being. In: Diener E, Suh EM (eds.) Culture and subjective well-being. The MIT Press, Boston, pp. 113–161
Koopmann-Holm B, Tsai JL (2014) Focusing on the negative: cultural differences in expressions of sympathy. J Personal Soc Psychol 107(6):1092–1115
Korpela K, Staats H (2014) The restorative qualities of being alone with nature. In: Coplan RJ, Bowker JC (eds.) The handbook of solitude: psychological perspectives on social isolation, social withdrawal, and being alone. John Wiley & Sons, Ltd, West Sussex, UK, pp. 351–367
Laajaj R, Macours K, Pinzon Hernandez DA, Arias O, Gosling SD, Potter J, Vakis R (2019) Challenges to capture the big five personality traits in non-WEIRD populations. Sci Adv 5(7):eaaw5226. https://doi.org/10.1126/sciadv.aaw5226
Article PubMed PubMed Central ADS Google Scholar
Lawn RB, Slemp GR, Vella-Brodrick DA (2018). Quiet flourishing: the authenticity and well-being of trait introverts living in the West depends on extraversion-deficit beliefs. J Happiness Stud, Online First(01 October), 1–21. https://doi.org/10.1007/s10902-018-0037-5
Leary MR, Herbst KC, McCrary F (2003) Finding pleasure in solitary activities: desire for aloneness or disinterest in social contact? Personal Individ Differences 35(1):59–68. https://doi.org/10.1016/S0191-8869(02)00141-1
Leontiev D (2019). The dialectics of aloneness: positive vs. negative meaning and differential assessment. Counsell Psychol Quarter, Latest Articles(09 July), https://doi.org/10.1080/09515070.2019.1640186
Leshed G, Sengers P (2011). “I lie to myself that I have freedom in my own schedule: productivity tools and experiences of busyness. Paper presented at the CHI 2011, Vancouver, BC
Lim N (2016) Cultural differences in emotion: differences in emotional arousal level between the East and West. Integr Med Res 5(2):105–109
Lindfors P, Berntsson L, Lundberg U (2006) Factor structure of Ryff’s psychological well-being scales in Swedish female and male white-collar workers. Personal Individ Differences 40(6):1213–1222
Linton M-J, Dieppe P, Medina-Lara A (2016). Review of 99 self-report measures for assessing well-being in adults: exploring dimensions of well-being and developments over time. BMJ Open 6(e010641). https://doi.org/10.1136/bmjopen-2015-010641
Lionetti F, Aron A, Aron EN, Burns GL, Jagiellowicz J, Pluess M (2018) Dandelions, tulips and orchids: evidence for the existence of low-sensitive, medium-sensitive and high-sensitive individuals. Transl Psychiatry 8(24):1–11. https://doi.org/10.1038/s41398-017-0090-6
Listou Grimen H, Diseth A (2016) Sensory processing sensitivity: factors of the Highly Sensitive Person Scale and their relationships to personality and subjective health complaints. Percept Mot Skills 123(3):637–653
Long CR, Averill JR (2003) Solitude: an exploration of benefits of being alone. J Theory Soc Behav 33(1):21–44
Lu L, Gilmour R (2004) Culture and conceptions of happiness: individual oriented and social oriented SWB. J Happiness Stud 5(3):269–291
Martela F, Sheldon KM (2019). Clarifying the concept of well-being: psychological need satisfaction as the common core connecting eudaimonic and subjective well-being. Rev Gen Pscyhol, Online First(October), 1–17. https://doi.org/10.1177/1089268019880886
Mathews G (2012) Happiness, culture, and context. Int J Wellbeing 4(2):299–312. https://doi.org/10.5502/ijw.v2.i4.2
McAdams DP, Guo J (2015) Narrating the generative life. Psychological Sci 26(4):475–483. https://doi.org/10.1177/0956797614568318
McAdams DP, Olsen BD (2010) Personality development: continuity and change over the life course. Annu Rev Psychol 61(Jan):517–542. https://doi.org/10.1146/annurev.psych.093008.100507
McCrae RR, Costa PT (1991) Adding Liebe und Arbeit: the full Five-Factor model and well-being. Personal Soc Psychol Bull 17(2):227–232
McWilliams N (2006) Some thoughts about schizoid dynamics. Psychoanalytic Rev 93(1):1–24
Article MathSciNet Google Scholar
Meredith PJ, Bailey KJ, Strong J, Rappel G (2016) Adult attachment, sensory processing, and distress in healthy adults. Am J Occup Ther 70(1):1–8
Merton T (1958) Thoughts in solitude. Farrar, Straus, and Giroux, New York
Meyerson J, Gelkopf M, Eli I, Uziel N (2019). Burnout and professional quality of life among Israeli dentists: the role of sensory processing sensitivity. Int Dental J, Early View(Septmeber), https://doi.org/10.1111/idj.12523
Montaigne M (1877) Of solitude (C. Cotton, Trans.). In: Hazlitt WC (ed.) Essays of Michel de Montaigne. Stanford University Press, Stanford, CA (online)
Neal JA, Edelmann RJ, Glachan M (2002) Behavioural inhibition and symptoms of anxiety and depression: is there a specific relationship with social phobia? Br J Clin Psychol 41(4):361–374
Neff KD, Pisitsungkagarn K, Hsieh Y-P (2008) Self-compassion and self-construal in the United States, Thailand, and Taiwan. J Cross-Cultural Psychol 39(3):267–285. https://doi.org/10.1177/0022022108314544
Neff KD, Rude SS, Kirkpatrick KL (2007) An examination of self-compassion in relation to positive psychological functioning and personality traits. J Res Personal 41(4):908–916
Nguyen TT, Ryan RM, Deci EL (2018) Solitude as an approach to affective self-regulation. Personal Soc Psychol Bull 44(1):92–106
Patrick H, Knee CR, Canevello A, Lonsbary C (2007) The role of need fulfillment in relationship functioning and well-being: a self-determination theory perspective. J Personal Soc Psychol 92(3):434–457. https://doi.org/10.1037/0022-3514.92.3.434
Pierre J (2010) The borders of mental disorder in psychiatry and the DSM: past, present, and future. J Psychiatr Pract 16(6):375–386. https://doi.org/10.1097/01.pra.0000390756.37754.68
Pluess M, Assary E, Lionetti F, Lester KJ, Krapohl E, Aron EN, Aron A (2018) Environmental sensitivity in children: development of the Highly Sensitive Child Scale and identification of sensitivity groups. Developmental Psychol 54(1):51–70
QSR International Pty Ltd. (Released 2015). NVivo qualitative data analysis software (Version 11): QSR International Pty Ltd.
Rusk RD, Waters L (2015) A psycho-social system approach to well-being: empirically deriving the five domains of positive functioning. J Posit Psychol 10(2):141–152
Ryan RM, Deci EL (2017) Self-determination theory. Guilford Press, New York
Ryff CD (1989a) Happiness is everything, or is it? Explorations on the meaning of psychological well-being. J Personal Soc Psychol 57(6):1069–1081
Ryff CD (1989b) In the eye of the beholder: views of psychological well-being among middle-aged and older adults. Psychol Aging 4(2):195–210. https://doi.org/10.1037/0882-7974.4.2.195
Ryff CD (2014) Psychological well-being revisited: advances in the science and practice of eudaimonia. Psychother Psychosom 83(1):10–28. https://doi.org/10.1159/000353263
Ryff CD, Singer BH (1996) Psychological well-being: meaning, measurement, and implications for psychotherapy research. Psychother Psychosom 65(1):14–23
Scheibe S, English T, Tsai JL, Carstensen LL (2013) Striving to feel good: ideal affect, actual affect, and their correspondence across adulthood. Psychol Aging 28(1):160–171
Seligman M (2011) Flourish. A visionary new understanding of happiness and well-being. William Heinemann Random House Australia Pty Ltd, North Sydney
Sherman AM, Lansford JE, Volling BL (2006) Sibling relationships and best friendships in young adulthood: warmth, conflict, and well-being. Personal Relatsh 13(2):151–165. https://doi.org/10.1111/j.1475-6811.2006.00110.x
Skinner N, Pocock B (2008) Work-life conflict: is work time or work overload more important? Asia Pac J Hum Resour 46(3):303–315. https://doi.org/10.1177/1038411108095761
Smillie LD (2013) Why does it feel good to act like an extravert? Soc Personal Psychol Compass 7(12):878–887
Smillie LD, DeYoung CG, Hall PJ (2015) Clarifying the relation between extraversion and positive affect. J Personal 83(5):564–574. https://doi.org/10.1111/jopy.12138
Smillie LD, Wilt J, Kabbani R, Revelle W (2016) Making a mark on ones milieu: why it feels good to be and act extraverted. Personal Individ Differences 101:515. https://doi.org/10.1016/j.paid.2016.05.298
Smith JA (1996) Beyond the divide between cognition and discourse: using interpretative phenomenological analysis in health psychology. Psychol Health 11(2):261–271
Smith JA (2017) Interpretive phenomenological analysis: getting at lived experience. J Posit Psychol 12(3):303–304
Smith JA, Eatough V (2011) Interpretative phenomenological analysis. In: Lyons E, Coyle A (eds.) Analysing qualitative data in psychology. SAGE Publications Ltd, London, pp. 35–50
Smith JA, Flowers P, Larkin M (2009) Interpretative phenomenological analysis: theory, method and research. Sage, London
Smith JA, Jarman M, Osborn M (1999) Doing interpretative phenomenological analysis. In: Murray M, Chamberlain K (eds.) Qualitative health psychology. Sage, London, pp. 218–240
Sobocko K, Zelenski JM (2015) Trait sensory-processing sensitivity and subjective well-being: distinctive associations for different aspects of sensitivity. Personal Individ Differences 83(Sept):44–49. https://doi.org/10.1016/j.paid.2015.03.045
Steadman P (2008) ICD-11 and DSM-V: time to revisit the introversion/extroversion debate? BJ Psych Bull 32(6):233–233. https://doi.org/10.1192/pb.32.6.233
Steel P, Schmidt J, Shultz J (2008) Refining the relationship between personality and subjective well-being. Psychological Bull 134(1):138–161
Stephens NM, Townsend SSM, Markus HR, Phillips LT (2012) A cultural mismatch: independent cultural norms produce greater increases in cortisol and more negative emotions among first-generation college students. J Exp Soc Psychol 48(6):1389–1393. https://doi.org/10.1016/j.jesp.2012.07.008
Sun J, Kaufman SB, Smillie LD (2018) Unique associations between Big Five personality aspects and multiple dimensions of well-being. J Personal 86(2):158–172. https://doi.org/10.1111/jopy.12301
Sun J, Stevenson K, Kabbani R, Richardson B, Smillie LD (2017) The pleasure of making a difference: perceived social contribution explains the relation between extraverted behavior and positive affect. Emotion 17(5):794–810. https://doi.org/10.1037/emo0000273
Sutton A (2016) Measuring the effects of self-awareness: construction of the self-awareness outcomes questionnaire. Eur J Psychol 12(4):645–658. 10.5964%2Fejop.v12i4.1178
Article MathSciNet PubMed PubMed Central Google Scholar
The Treasury New Zealand Government. (2019). The Wellbeing Budget 2019. Wellington, NZ: The Treasury New Zealand Government Retrieved from https://treasury.govt.nz/publications/wellbeing-budget/wellbeing-budget-2019-html
Triandis HC, Suh EM (2002) Cultural influences on personality. Annu Rev Psychol 53(Feb):133–160. https://doi.org/10.1146/annurev.psych.53.100901.135200
Tsai JL (2007) Ideal affect: cultural causes and behavioral consequences. Perspect Psychological Sci 2(3):242–259. https://doi.org/10.1111/j.1745-6916.2007.00043.x
Tsai JL, Knutson B, Fung HH (2006) Cultural variation in affect valuation. J Personal Soc Psychol 90(2):288–307. https://doi.org/10.1037/0022-3514.90.2.288
Tsai JL, Levenson RW, McCoy K (2006) Cultural and temperamental variation in emotional response. Emotion 6(3):484–497
Tsai JL, Park B (2014) The cultural shaping of happiness. In: Gruber J, Moskowitz JT (eds.) Positive emotion: integrating the light sides and dark sides. Oxford University Press, New York, NY, pp. 345–362
Uchida Y, Kitayama S (2009) Happiness and unhappiness in East and West: themes and variations. Emotion 9(4):441–456. https://doi.org/10.1037/a0015634
Watson D, Clark LA, Tellegen A (1988) Development and validation of brief measures of positive and negative affect: the PANAS scales. J Personal Soc Psychol 54(6):1063–1070
Wells FL (1964) A note on numerical scores for introvert and extravert self-rating. J Abnorm Soc Psychol 21(3):316–318
Wong YJ, Ho RM, Li P, Shin M, Tsai P-C (2011) Chinese Singaporeans’ lay beliefs, adherence to Asian values, and subjective well-being. Personal Individ Differences 50(6):822–827. https://doi.org/10.1016/j.paid.2011.01.003
World Health Organization. (1978). ICD-9: International classification of diseases: Ninth revision. World Health Organization
Yano K, Kase T, Oishi K (2019). The effects of sensory-processing sensitivity and sense of coherence on depressive symptoms in university students. Health Psychol Open , July–December, 6:1–5
Yano K, Kase T, Oishi K (2020). Sensory processing sensitivity moderates the relationships between life skills and depressive tendencies in university students. Japan Psychol Res, Early View (April). https://doi.org/10.1111/jpr.12289
Zelenski JM, Santoro MS, Whelan DC (2012) Would introverts be better off if they acted more like extraverts? Exploring emotional and cognitive consequences of counterdispositional behavior. Emotion 12(2):290–303
Zevnik L (2014) Critical perspectives in happiness research. The birth of modern happiness. Springer, Heidelberg
Zimmerman JG (1799) Solitude considered, with respect to its influence upon the mind and the heart, 8th edn. C. Dilly, London
Download references
Acknowledgements
The first author was supported by an Australian Government Research Training Program (fee offset) Scholarship; and by a Melbourne Research Scholarship stipend bestowed by the University of Melbourne.
Author information
Authors and affiliations.
Melbourne Graduate School of Education, The University of Melbourne, Melbourne, VIC, Australia
Becky A. Black & Margaret L. Kern
You can also search for this author in PubMed Google Scholar
Corresponding author
Correspondence to Becky A. Black .
Ethics declarations
Competing interests.
The authors declare no competing interests.
Additional information
Publisher’s note Springer Nature remains neutral with regard to jurisdictional claims in published maps and institutional affiliations.
Rights and permissions
Open Access This article is licensed under a Creative Commons Attribution 4.0 International License, which permits use, sharing, adaptation, distribution and reproduction in any medium or format, as long as you give appropriate credit to the original author(s) and the source, provide a link to the Creative Commons license, and indicate if changes were made. The images or other third party material in this article are included in the article’s Creative Commons license, unless indicated otherwise in a credit line to the material. If material is not included in the article’s Creative Commons license and your intended use is not permitted by statutory regulation or exceeds the permitted use, you will need to obtain permission directly from the copyright holder. To view a copy of this license, visit http://creativecommons.org/licenses/by/4.0/ .
Reprints and permissions
About this article
Cite this article.
Black, B.A., Kern, M.L. A qualitative exploration of individual differences in wellbeing for highly sensitive individuals. Palgrave Commun 6 , 103 (2020). https://doi.org/10.1057/s41599-020-0482-8
Download citation
Received : 03 November 2019
Accepted : 29 April 2020
Published : 02 June 2020
DOI : https://doi.org/10.1057/s41599-020-0482-8
Share this article
Anyone you share the following link with will be able to read this content:
Sorry, a shareable link is not currently available for this article.
Provided by the Springer Nature SharedIt content-sharing initiative
This article is cited by
Socio-economic determinants of subjective wellbeing of indians in the post-liberalization era: evidence from the world value survey.
- Neha Kumari
- Naresh Chandra Sahu
Asia-Pacific Journal of Regional Science (2023)
Quick links
- Explore articles by subject
- Guide to authors
- Editorial policies

- Open access
- Published: 16 July 2013
A tutorial on sensitivity analyses in clinical trials: the what, why, when and how
- Lehana Thabane 1 , 2 , 3 , 4 , 5 ,
- Lawrence Mbuagbaw 1 , 4 ,
- Shiyuan Zhang 1 , 4 ,
- Zainab Samaan 1 , 6 , 7 ,
- Maura Marcucci 1 , 4 ,
- Chenglin Ye 1 , 4 ,
- Marroon Thabane 1 , 8 ,
- Lora Giangregorio 9 ,
- Brittany Dennis 1 , 4 ,
- Daisy Kosa 1 , 4 , 10 ,
- Victoria Borg Debono 1 , 4 ,
- Rejane Dillenburg 11 ,
- Vincent Fruci 12 ,
- Monica Bawor 13 ,
- Juneyoung Lee 14 ,
- George Wells 15 &
- Charles H Goldsmith 1 , 4 , 16
BMC Medical Research Methodology volume 13 , Article number: 92 ( 2013 ) Cite this article
273k Accesses
452 Citations
113 Altmetric
Metrics details
Sensitivity analyses play a crucial role in assessing the robustness of the findings or conclusions based on primary analyses of data in clinical trials. They are a critical way to assess the impact, effect or influence of key assumptions or variations—such as different methods of analysis, definitions of outcomes, protocol deviations, missing data, and outliers—on the overall conclusions of a study.
The current paper is the second in a series of tutorial-type manuscripts intended to discuss and clarify aspects related to key methodological issues in the design and analysis of clinical trials.
In this paper we will provide a detailed exploration of the key aspects of sensitivity analyses including: 1) what sensitivity analyses are, why they are needed, and how often they are used in practice; 2) the different types of sensitivity analyses that one can do, with examples from the literature; 3) some frequently asked questions about sensitivity analyses; and 4) some suggestions on how to report the results of sensitivity analyses in clinical trials.
When reporting on a clinical trial, we recommend including planned or posthoc sensitivity analyses, the corresponding rationale and results along with the discussion of the consequences of these analyses on the overall findings of the study.
Peer Review reports
The credibility or interpretation of the results of clinical trials relies on the validity of the methods of analysis or models used and their corresponding assumptions. An astute researcher or reader may be less confident in the findings of a study if they believe that the analysis or assumptions made were not appropriate. For a primary analysis of data from a prospective randomized controlled trial (RCT), the key questions for investigators (and for readers) include:
How confident can I be about the results?
Will the results change if I change the definition of the outcome (e.g., using different cut-off points)?
Will the results change if I change the method of analysis?
Will the results change if we take missing data into account? Will the method of handling missing data lead to different conclusions?
How much influence will minor protocol deviations have on the conclusions?
How will ignoring the serial correlation of measurements within a patient impact the results?
What if the data were assumed to have a non-Normal distribution or there were outliers?
Will the results change if one looks at subgroups of patients?
Will the results change if the full intervention is received (i.e. degree of compliance)?
The above questions can be addressed by performing sensitivity analyses—testing the effect of these “changes” on the observed results. If, after performing sensitivity analyses the findings are consistent with those from the primary analysis and would lead to similar conclusions about treatment effect, the researcher is reassured that the underlying factor(s) had little or no influence or impact on the primary conclusions. In this situation, the results or the conclusions are said to be “robust”.
The objectives of this paper are to provide an overview of how to approach sensitivity analyses in clinical trials. This is the second in a series of tutorial-type manuscripts intended to discuss and clarify aspects related to some key methodological issues in the design and analysis of clinical trials. The first was on pilot studies [ 1 ]. We start by describing what sensitivity analysis is, why it is needed and how often it is done in practice. We then describe the different types of sensitivity analyses that one can do, with examples from the literature. We also address some of the commonly asked questions about sensitivity analysis and provide some guidance on how to report sensitivity analyses.
Sensitivity Analysis
What is a sensitivity analysis in clinical research.
Sensitivity Analysis (SA) is defined as “a method to determine the robustness of an assessment by examining the extent to which results are affected by changes in methods, models, values of unmeasured variables, or assumptions” with the aim of identifying “results that are most dependent on questionable or unsupported assumptions” [ 2 ]. It has also been defined as “a series of analyses of a data set to assess whether altering any of the assumptions made leads to different final interpretations or conclusions” [ 3 ]. Essentially, SA addresses the “what-if-the-key-inputs-or-assumptions-changed”-type of question. If we want to know whether the results change when something about the way we approach the data analysis changes, we can make the change in our analysis approach and document the changes in the results or conclusions. For more detailed coverage of SA, we refer the reader to these references [ 4 – 7 ].
Why is sensitivity analysis necessary?
The design and analysis of clinical trials often rely on assumptions that may have some effect, influence or impact on the conclusions if they are not met. It is important to assess these effects through sensitivity analyses. Consistency between the results of primary analysis and the results of sensitivity analysis may strengthen the conclusions or credibility of the findings. However, it is important to note that the definition of consistency may depend in part on the area of investigation, the outcome of interest or even the implications of the findings or results.
It is equally important to assess the robustness to ensure appropriate interpretation of the results taking into account the things that may have an impact on them. Thus, it imperative for every analytic plan to have some sensitivity analyses built into it.
The United States (US) Food and Drug Administration (FDA) and the European Medicines Association (EMEA), which offer guidance on Statistical Principles for Clinical Trials, state that “it is important to evaluate the robustness of the results and primary conclusions of the trial.” Robustness refers to “the sensitivity of the overall conclusions to various limitations of the data, assumptions, and analytic approaches to data analysis” [ 8 ]. The United Kingdom (UK) National Institute of Health and Clinical Excellence (NICE) also recommends the use of sensitivity analysis in “exploring alternative scenarios and the uncertainty in cost-effectiveness results” [ 9 ].
How often is sensitivity analysis reported in practice?
To evaluate how often sensitivity analyses are used in medical and health research, we surveyed the January 2012 editions of major medical journals (British Medical Journal, New England Journal of Medicine, the Lancet, Journal of the American Medical Association and the Canadian Medical Association Journal) and major health economics journals (Pharmaco-economics, Medical Decision making, European Journal of Health Economics, Health Economics and the Journal of Health Economics). From every article that included some form of statistical analyses, we evaluated: i) the percentage of published articles that reported results of some sensitivity analyses; and ii) the types of sensitivity analyses that were performed. Table 1 provides a summary of the findings. Overall, the point prevalent use of sensitivity analyses is about 26.7% (36/135) —which seems very low. A higher percentage of papers published in health economics than in medical journals (30.8% vs. 20.3%) reported some sensitivity analyses. Among the papers in medical journals, 18 (28.1%) were RCTs, of which only 3 (16.6%) reported sensitivity analyses. Assessing robustness of the findings to different methods of analysis was the most common type of sensitivity analysis reported in both types of journals. Therefore despite their importance, sensitivity analyses are under-used in practice. Further, sensitivity analyses are more common in health economics research—for example in conducting cost-effectiveness analyses, cost-utility analyses or budget-impact analyses—than in other areas of health or medical research.
Types of sensitivity analyses
In this section, we describe scenarios that may require sensitivity analyses, and how one could use sensitivity analyses to assess the robustness of the statistical analyses or findings of RCTs. These are not meant to be exhaustive, but rather to illustrate common situations where sensitivity analyses might be useful to consider (Table 2 ). In each case, we provide examples of actual studies where sensitivity analyses were performed, and the implications of these sensitivity analyses.
Impact of outliers
An outlier is an observation that is numerically distant from the rest of the data. It deviates markedly from the rest of the sample from which it comes [ 14 , 15 ]. Outliers are usually exceptional cases in a sample. The problem with outliers is that they can deflate or inflate the mean of a sample and therefore influence any estimates of treatment effect or association that are derived from the mean. To assess the potential impact of outliers, one would first assess whether or not any observations meet the definition of an outlier—using either a boxplot or z-scores [ 16 ]. Second, one could perform a sensitivity analysis with and without the outliers.
In a cost–utility analysis of a practice-based osteopathy clinic for subacute spinal pain, Williams et al. reported lower costs per quality of life year ratios when they excluded outliers [ 17 ]. In other words, there were certain participants in the trial whose costs were very high, and were making the average costs look higher than they probably were in reality. The observed cost per quality of life year was not robust to the exclusion of outliers, and changed when they were excluded.
A primary analysis based on the intention-to-treat principle showed no statistically significant differences in reducing depression between a nurse-led cognitive self-help intervention program compared to standard care among 218 patients hospitalized with angina over 6 months. Some sensitivity analyses in this trial were performed by excluding participants with high baseline levels of depression (outliers) and showed a statistically significant reduction in depression in the intervention group compared to the control. This implies that the results of the primary analysis were affected by the presence of patients with baseline high depression [ 18 ].
Impact of non-compliance or protocol deviations
In clinical trials some participants may not adhere to the intervention they were allocated to receive or comply with the scheduled treatment visits. Non-adherence or non-compliance is a form of protocol deviation. Other types of protocol deviations include switching between intervention and control arms (i.e. treatment switching or crossovers) [ 19 , 20 ], or not implementing the intervention as prescribed (i.e. intervention fidelity) [ 21 , 22 ].
Protocol deviations are very common in interventional research [ 23 – 25 ]. The potential impact of protocol deviations is the dilution of the treatment effect [ 26 , 27 ]. Therefore, it is crucial to determine the robustness of the results to the inclusion of data from participants who deviate from the protocol. Typically, for RCTs the primary analysis is based on an intention-to-treat (ITT) principle—in which participants are analyzed according to the arm to which they were randomized, irrespective of whether they actually received the treatment or completed the prescribed regimen [ 28 , 29 ]. Two common types of sensitivity analyses can be performed to assess the robustness of the results to protocol deviations: 1) per-protocol (PP) analysis—in which participants who violate the protocol are excluded from the analysis [ 30 ]; and 2) as-treated (AT) analysis—in which participants are analyzed according to the treatment they actually received [ 30 ]. The PP analysis provides the ideal scenario in which all the participants comply, and is more likely to show an effect; whereas the ITT analysis provides a “real life” scenario, in which some participants do not comply. It is more conservative, and less likely to show that the intervention is effective. For trials with repeated measures, some protocol violations which lead to missing data can be dealt with alternatively. This is covered in more detail in the next section.
A trial was designed to investigate the effects of an electronic screening and brief intervention to change risky drinking behaviour in university students. The results of the ITT analysis (on all 2336 participants who answered the follow-up survey) showed that the intervention had no significant effect. However, a sensitivity analysis based on the PP analysis (including only those with risky drinking at baseline and who answered the follow-up survey; n = 408) suggested a small beneficial effect on weekly alcohol consumption [ 31 ]. A reader might be less confident in the findings of the trial because of the inconsistency between the ITT and PP analyses—the ITT was not robust to sensitivity analyses. A researcher might choose to explore differences in the characteristics of the participants who were included in the ITT versus the PP analyses.
A study compared the long-term effects of surgical versus non-surgical management of chronic back pain. Both the ITT and AT analyses showed no significant difference between the two management strategies [ 32 ]. A reader would be more confident in the findings because the ITT and AT analyses were consistent—the ITT was robust to sensitivity analyses.
Impact of missing data
Missing data are common in every research study. This is a problem that can be broadly defined as “missing some information on the phenomena in which we are interested” [ 33 ]. Data can be missing for different reasons including (1) non-response in surveys due to lack of interest, lack of time, nonsensical responses, and coding errors in data entry/transfer; (2) incompleteness of data in large data registries due to missing appointments, not everyone is captured in the database, and incomplete data; and (3) missingness in prospective studies as a result of loss to follow up, dropouts, non-adherence, missing doses, and data entry errors.
The choice of how to deal with missing data would depend on the mechanisms of missingness. In this regard, data can be missing at random (MAR), missing not at random (MNAR), or missing completely at random (MCAR). When data are MAR, the missing data are dependent on some other observed variables rather than any unobserved one. For example, consider a trial to investigate the effect of pre-pregnancy calcium supplementation on hypertensive disorders in pregnancy. Missing data on the hypertensive disorders is dependent (conditional) on being pregnant in the first place. When data are MCAR, the cases with missing data may be considered a random sample drawn from all the cases. In other words, there is no “cause” of missingness. Consider the example of a trial comparing a new cancer treatment to standard treatment in which participants are followed at 4, 8, 12 and 16 months. If a participant misses the follow up at the 8th and 16th months and these are unrelated to the outcome of interest, in this case mortality, then this missing data is MCAR. Reasons such as a clinic staff being ill or equipment failure are often unrelated to the outcome of interest. However, the MCAR assumption is often challenging to prove because the reason data is missing may not be known and therefore it is difficult to determine if it is related to the outcome of interest. When data are MNAR, missingness is dependent on some unobserved data. For example, in the case above, if the participant missed the 8th month appointment because he was feeling worse or the 16th month appointment because he was dead, the missingness is dependent on the data not observed because the participant was absent. When data are MAR or MCAR, they are often referred to as ignorable (provided the cause of MAR is taken into account). MNAR on the other hand, is nonignorable missingness. Ignoring the missingness in such data leads to biased parameter estimates [ 34 ]. Ignoring missing data in analyses can have implications on the reliability, validity and generalizability of research findings.
The best way to deal with missing data is prevention, by steps taken in the design and data collection stages, some of which have been described by Little et al. [ 35 ]. But this is difficult to achieve in most cases. There are two main approaches to handling missing data: i) ignore them—and use complete case analysis; and ii) impute them—using either single or multiple imputation techniques. Imputation is one of the most commonly used approaches to handling missing data. Examples of single imputation methods include hot deck, cold deck method, mean imputation, regression technique, last observation carried forward (LOCF) and composite methods—which uses a combination of the above methods to impute missing values. Single imputation methods often lead to biased estimates and under-estimation of the true variability in the data. Multiple imputation (MI) technique is currently the best available method of dealing with missing data under the assumption that data are missing at random (MAR) [ 33 , 36 – 38 ]. MI addresses the limitations of single imputation by using multiple imputed datasets which yield unbiased estimates, and also accounts for the within- and between-dataset variability. Bayesian methods using statistical models that assume a prior distribution for the missing data can also be used to impute data [ 35 ].
It is important to note that ignoring missing data in the analysis would be implicitly assuming that the data are MCAR, an assumption that is often hard to verify in reality.
There are some statistical approaches to dealing with missing data that do not necessarily require formal imputation methods. For example, in studies using continuous outcomes, linear mixed models for repeated measures are used for analyzing outcomes measured repeatedly over time [ 39 , 40 ]. For categorical responses or count data, generalized estimating equations [GEE] and random-effects generalized linear mixed models [GLMM] methods may be used [ 41 , 42 ]. In these models it is assumed that missing data are MAR. If this assumption is valid, then the complete-case analysis by including predictors of missing observations will provide consistent estimates of the parameter.
The choice of whether to ignore or impute missing data, and how to impute it, may affect the findings of the trial. Although one approach (ignore or impute, and if the latter, how to impute) should be made a priori, a sensitivity analysis can be done with a different approach to see how “robust” the primary analysis is to the chosen method for handling missing data.
A 2011 paper reported the sensitivity analyses of different strategies for imputing missing data in cluster RCTs with a binary outcome using the community hypertension assessment trial (CHAT) as an example. They found that variance in the treatment effect was underestimated when the amount of missing data was large and the imputation strategy did not take into account the intra-cluster correlation. However, the effects of the intervention under various methods of imputation were similar. The CHAT intervention was not superior to usual care [ 43 ].
In a trial comparing methotrexate with to placebo in the treatment of psoriatic arthritis, the authors reported both an intention-to-treat analysis (using multiple imputation techniques to account for missing data) and a complete case analysis (ignoring the missing data). The complete case analysis, which is less conservative, showed some borderline improvement in the primary outcome (psoriatic arthritis response criteria), while the intention-to-treat analysis did not [ 44 ]. A reader would be less confident about the effects of methotrexate on psoriatic arthritis, due to the discrepancy between the results with imputed data (ITT) and the complete case analysis.
Impact of different definitions of outcomes (e.g. different cut-off points for binary outcomes)
Often, an outcome is defined by achieving or not achieving a certain level or threshold of a measure. For example in a study measuring adherence rates to medication, levels of adherence can be dichotomized as achieving or not achieving at least 80%, 85% or 90% of pills taken. The choice of the level a participant has to achieve can affect the outcome—it might be harder to achieve 90% adherence than 80%. Therefore, a sensitivity analysis could be performed to see how redefining the threshold changes the observed effect of a given intervention.
In a trial comparing caspofungin to amphotericin B for febrile neutropoenic patients, a sensitivity analysis was conducted to investigate the impact of different definitions of fever resolution as part of a composite endpoint which included: resolution of any baseline invasive fungal infection, no breakthrough invasive fungal infection, survival, no premature discontinuation of study drug, and fever resolution for 48 hours during the period of neutropenia. They found that response rates were higher when less stringent fever resolution definitions were used, especially in low-risk patients. The modified definitions of fever resolution were: no fever for 24 hours before the resolution of neutropenia; no fever at the 7-day post-therapy follow-up visit; and removal of fever resolution completely from the composite endpoint. This implies that the efficacy of both medications depends somewhat on the definition of the outcomes [ 45 ].
In a phase II trial comparing minocycline and creatinine to placebo for Parkinson’s disease, a sensitivity analysis was conducted based on another definition (threshold) for futility. In the primary analysis a predetermined futility threshold was set at 30% reduction in mean change in Unified Parkinson’s Disease Rating Scale (UPDRS) score, derived from historical control data. If minocycline or creatinine did not bring about at least a 30% reduction in UPDRS score, they would be considered as futile and no further testing will be conducted. Based on the data derived from the current control (placebo) group, a new threshold of 32.4% (more stringent) was used for the sensitivity analysis. The findings from the primary analysis and the sensitivity analysis both confirmed that that neither creatine nor minocycline could be rejected as futile and should both be tested in Phase III trials [ 46 ]. A reader would be more confident of these robust findings.
Impact of different methods of analysis to account for clustering or correlation
Interventions can be administered to individuals, but they can also be administered to clusters of individuals, or naturally occurring groups. For example, one might give an intervention to students in one class, and compare their outcomes to students in another class – the class is the cluster. Clusters can also be patients treated by the same physician, physicians in the same practice center or hospital, or participants living in the same community. Likewise, in the same trial, participants may be recruited from multiple sites or centers. Each of these centers will represent a cluster. Patients or elements within a cluster often have some appreciable degree of homogeneity as compared to patients between clusters. In other words, members of the same cluster are more likely to be similar to each other than they are to members of another cluster, and this similarity may then be reflected in the similarity or correlation measure, on the outcome of interest.
There are several methods of accounting or adjusting for similarities within clusters, or “clustering” in studies where this phenomenon is expected or exists as part of the design (e.g., in cluster randomization trials). Therefore, in assessing the impact of clustering one can build into the analytic plans two forms of sensitivity analyses: i) analysis with and without taking clustering into account—comparing the analysis that ignores clustering (i.e. assumes that the data are independent) to one primary method chosen to account for clustering; ii) analysis that compares several methods of accounting for clustering.
Correlated data may also occur in longitudinal studies through repeat or multiple measurements from the same patient, taken over time or based on multiple responses in a single survey. Ignoring the potential correlation between several measurements from an individual can lead to inaccurate conclusions [ 47 ].
Here are a few references to studies that compared the outcomes that resulted when different methods were/were not used to account for clustering. Noteworthy, is the fact that the analytical approaches for cluster-RCTs and multi-site RCTs are similar.
Ma et al. performed sensitivity analyses of different methods of analysing cluster RCTs [ 48 ]. In this paper they compared three cluster-level methods (un-weighted linear regression, weighted linear regression and random-effects meta-regression) to six individual level analysis methods (standard logistic regression, robust standard errors approach, GEE, random effects meta-analytic approach, random-effects logistic regression and Bayesian random-effects regression). Using data from the CHAT trial, in this analysis, all nine methods provided similar results, re-enforcing the hypothesis that the CHAT intervention was not superior to usual care.
Peters et al. conducted sensitivity analyses to compare different methods—three cluster-level (un-weighted regression of practice log odds, regression of log odds weighted by their inverse variance and random-effects meta-regression of log odds with cluster as a random effect) and five individual-level methods (standard logistic regression ignoring clustering, robust standard errors, GEE, random-effects logistic regression and Bayesian random-effects logistic regression.)—for analyzing cluster randomized trials using an example involving a factorial design [ 13 ]. In this analysis, they demonstrated that the methods used in the analysis of cluster randomized trials could give varying results, with standard logistic regression ignoring clustering being the least conservative.
Cheng et al. used sensitivity analyses to compare different methods (six models for clustered binary outcomes and three models for clustered nominal outcomes) of analysing correlated data in discrete choice surveys [ 49 ]. The results were robust to various statistical models, but showed more variability in the presence of a larger cluster effect (higher within-patient correlation).
A trial evaluated the effects of lansoprazole on gastro-esophageal reflux disease in children from 19 clinics with asthma. The primary analysis was based on GEE to determine the effect of lansoprazole in reducing asthma symptoms. Subsequently they performed a sensitivity analysis by including the study site as a covariate. Their finding that lansoprazole did not significantly improve symptoms was robust to this sensitivity analysis [ 50 ].
In addition to comparing the performance of different methods to estimate treatment effects on a continuous outcome in simulated multicenter randomized controlled trials [ 12 ], the authors used data from the Computerization of Medical Practices for the Enhancement of Therapeutic Effectiveness (COMPETE) II [ 51 ] to assess the robustness of the primary results (based on GEE to adjust for clustering by provider of care) under different methods of adjusting for clustering. The results, which showed that a shared electronic decision support system improved care and outcomes in diabetic patients, were robust under different methods of analysis.
Impact of competing risks in analysis of trials with composite outcomes
A competing risk event happens in situations where multiple events are likely to occur in a way that the occurrence of one event may prevent other events from being observed [ 48 ]. For example, in a trial using a composite of death, myocardial infarction or stroke, if someone dies, they cannot experience a subsequent event, or stroke or myocardial infarction—death can be a competing risk event. Similarly, death can be a competing risk in trials of patients with malignant diseases where thrombotic events are important. There are several options for dealing with competing risks in survival analyses: (1) to perform a survival analysis for each event separately, where the other competing event(s) is/are treated as censored; the common representation of survival curves using the Kaplan-Meier estimator is in this context replaced by the cumulative incidence function (CIF) which offers a better interpretation of the incidence curve for one risk, regardless of whether the competing risks are independent; (2) to use a proportional sub-distribution hazard model (Fine & Grey approach) in which subjects that experience other competing events are kept in the risk set for the event of interest (i.e. as if they could later experience the event); (3) to fit one model, rather than separate models, taking into account all the competing risks together (Lunn-McNeill approach) [ 13 ]. Therefore, the best approach to assessing the influence of a competing risk would be to plan for sensitivity analysis that adjusts for the competing risk event.
A previously-reported trial compared low molecular weight heparin (LMWH) with oral anticoagulant therapy for the prevention of recurrent venous thromboembolism (VTE) in patients with advanced cancer, and a subsequent study presented sensitivity analyses comparing the results from standard survival analysis (Kaplan-Meier method) with those from competing risk methods—namely, the cumulative incidence function (CIF) and Gray's test [ 52 ]. The results using both methods were similar. This strengthened their confidence in the conclusion that LMWH reduced the risk of recurrent VTE.
For patients at increased risk of end stage renal disease (ESRD) but also of premature death not related to ESRD, such as patients with diabetes or with vascular disease, analyses considering the two events as different outcomes may be misleading if the possibility of dying before the development of ESRD is not taken into account [ 49 ]. Different studies performing sensitivity analyses demonstrated that the results on predictors of ESRD and death for any cause were dependent on whether the competing risks were taken into account or not [ 53 , 54 ], and on which competing risk method was used [ 55 ]. These studies further highlight the need for a sensitivity analysis of competing risks when they are present in trials.
Impact of baseline imbalance in RCTs
In RCTs, randomization is used to balance the expected distribution of the baseline or prognostic characteristics of the patients in all treatment arms. Therefore the primary analysis is typically based on ITT approach unadjusted for baseline characteristics. However, some residual imbalance can still occur by chance. One can perform a sensitivity analysis by using a multivariable analysis to adjust for hypothesized residual baseline imbalances to assess their impact on effect estimates.
A paper presented a simulation study where the risk of the outcome, effect of the treatment, power and prevalence of the prognostic factors, and sample size were all varied to evaluate their effects on the treatment estimates. Logistic regression models were compared with and without adjustment for the prognostic factors. The study concluded that the probability of prognostic imbalance in small trials could be substantial. Also, covariate adjustment improved estimation accuracy and statistical power [ 56 ].
In a trial testing the effectiveness of enhanced communication therapy for aphasia and dysarthria after stroke, the authors conducted a sensitivity analysis to adjust for baseline imbalances. Both primary and sensitivity analysis showed that enhanced communication therapy had no additional benefit [ 57 ].
Impact of distributional assumptions
Most statistical analyses rely on distributional assumptions for observed data (e.g. Normal distribution for continuous outcomes, Poisson distribution for count data, or binomial distribution for binary outcome data). It is important not only to test for goodness-of-fit for these distributions, but to also plan for sensitivity analyses using other suitable distributions. For example, for continuous data, one can redo the analysis assuming a Student-T distribution—which is symmetric, bell-shaped distribution like the Normal distribution, but with thicker tails; for count data, once can use the Negative-binomial distribution—which would be useful to assess the robustness of the results if over-dispersion is accounted for [ 52 ]. Bayesian analyses routinely include sensitivity analyses to assess the robustness of findings under different models for the data and prior distributions [ 58 ]. Analyses based on parametric methods—which often rely on strong distributional assumptions—may also need to be evaluated for robustness using non-parametric methods. The latter often make less stringent distributional assumptions. However, it is essential to note that in general non-parametric methods are less efficient (i.e. have less statistical power) than their parametric counter-parts if the data are Normally distributed.
Ma et al. performed sensitivity analyses based on Bayesian and classical methods for analysing cluster RCTs with a binary outcome in the CHAT trial. The similarities in the results after using the different methods confirmed the results of the primary analysis: the CHAT intervention was not superior to usual care [ 10 ].
A negative binomial regression model was used [ 52 ] to analyze discrete outcome data from a clinical trial designed to evaluate the effectiveness of a pre-habilitation program in preventing functional decline among physically frail, community-living older persons. The negative binomial model provided an improved fit to the data than the Poisson regression model. The negative binomial model provides an alternative approach for analyzing discrete data where over-dispersion is a problem [ 59 ].
Commonly asked questions about sensitivity analyses
Q: Do I need to adjust the overall level of significance for performing sensitivity analyses?
A: No. Sensitivity analysis is typically a re-analysis of either the same outcome using different approaches, or different definitions of the outcome—with the primary goal of assessing how these changes impact the conclusions. Essentially everything else including the criterion for statistical significance needs to be kept constant so that we can assess whether any impact is attributable to underlying sensitivity analyses.
Q: Do I have to report all the results of the sensitivity analyses?
A: Yes, especially if the results are different or lead to different a conclusion from the original results—whose sensitivity was being assessed. However, if the results remain robust (i.e. unchanged), then a brief statement to this effect may suffice.
Q: Can I perform sensitivity analyses posthoc?
A: It is desirable to document all planned analyses including sensitivity analyses in the protocol a priori . Sometimes, one cannot anticipate all the challenges that can occur during the conduct of a study that may require additional sensitivity analyses. In that case, one needs to incorporate the anticipated sensitivity analyses in the statistical analysis plan (SAP), which needs to be completed before analyzing the data. Clear rationale is needed for every sensitivity analysis. This may also occur posthoc .
Q: How do I choose between the results of different sensitivity analyses? (i.e. which results are the best?)
A: The goal of sensitivity analyses is not to select the “best” results. Rather, the aim is to assess the robustness or consistency of the results under different methods, subgroups, definitions, assumptions and so on. The assessment of robustness is often based on the magnitude, direction or statistical significance of the estimates. You cannot use the sensitivity analysis to choose an alternate conclusion to your study. Rather, you can state the conclusion based on your primary analysis, and present your sensitivity analysis as an example of how confident you are that it represents the truth. If the sensitivity analysis suggests that the primary analysis is not robust, it may point to the need for future research that might address the source of the inconsistency. Your study cannot answer the question which results are best? To answer the question of which method is best and under what conditions, simulation studies comparing the different approaches on the basis of bias, precision, coverage or efficiency may be necessary.
Q: When should one perform sensitivity analysis?
A: The default position should be to plan for sensitivity analysis in every clinical trial. Thus, all studies need to include some sensitivity analysis to check the robustness of the primary findings. All statistical methods used to analyze data from clinical trials rely on assumptions—which need to either be tested whenever possible, with the results assessed for robustness through some sensitivity analyses. Similarly, missing data or protocol deviations are common occurrences in many trials and their impact on inferences needs to be assessed.
Q: How many sensitivity analyses can one perform for a single primary analysis?
A: The number is not an important factor in determining what sensitivity analyses to perform. The most important factor is the rationale for doing any sensitivity analysis. Understanding the nature of the data, and having some content expertise are useful in determining which and how many sensitivity analyses to perform. For example, varying the ways of dealing with missing data is unlikely to change the results if 1% of data are missing. Likewise, understanding the distribution of certain variables can help to determine which cut points would be relevant. Typically, it is advisable to limit sensitivity analyses to the primary outcome. Conducting multiple sensitivity analysis on all outcomes is often neither practical, nor necessary.
Q: How many factors can I vary in performing sensitivity analyses?
A: Ideally, one can study the impact of all key elements using a factorial design—which would allow the assessment of the impact of individual and joint factors. Alternatively, one can vary one factor at a time to be able to assess whether the factor is responsible for the resulting impact (if any). For example, in a sensitivity analysis to assess the impact of the Normality assumption (analysis assuming Normality e.g. T-test vs. analysis without assuming Normality e.g. Based on a sign test) and outlier (analysis with and without outlier), this can be achieved through 2x2 factorial design.
Q: What is the difference between secondary analyses and sensitivity analyses?
A: Secondary analyses are typically analyses of secondary outcomes. Like primary analyses which deal with primary outcome(s), such analyses need to be documented in the protocol or SAP. In most studies such analyses are exploratory—because most studies are not powered for secondary outcomes. They serve to provide support that the effects reported in the primary outcome are consistent with underlying biology. They are different from sensitivity analyses as described above.
Q: What is the difference between subgroup analyses and sensitivity analyses?
A: Subgroup analyses are intended to assess whether the effect is similar across specified groups of patients or modified by certain patient characteristics [ 60 ]. If the primary results are statistically significant, subgroup analyses are intended to assess whether the observed effect is consistent across the underlying patient subgroups—which may be viewed as some form of sensitivity analysis. In general, for subgroup analyses one is interested in the results for each subgroup, whereas in subgroup “sensitivity” analyses, one is interested in the similarity of results across subgroups (ie. robustness across subgroups). Typically subgroup analyses require specification of the subgroup hypothesis and rationale, and performed through inclusion of an interaction term (i.e. of the subgroup variable x main exposure variable) in the regression model. They may also require adjustment for alpha—the overall level of significance. Furthermore, most studies are not usually powered for subgroup analyses.
Reporting of sensitivity analyses
There has been considerable attention paid to enhancing the transparency of reporting of clinical trials. This has led to several reporting guidelines, starting with the CONSORT Statement [ 61 ] in 1996 and its extensions [ http://www.equator-network.org ]. Not one of these guidelines specifically addresses how sensitivity analyses need to be reported. On the other hand, there is some guidance on how sensitivity analyses need to be reported in economic analyses [ 62 ]—which may partly explain the differential rates of reporting of sensitivity analyses shown in Table 1 . We strongly encourage some modifications of all reporting guidelines to include items on sensitivity analyses—as a way to enhance their use and reporting. The proposed reporting changes can be as follows:
In Methods Section: Report the planned or posthoc sensitivity analyses and rationale for each.
In Results Section: Report whether or not the results of the sensitivity analyses or conclusions are similar to those based on primary analysis. If similar, just state that the results or conclusions remain robust. If different, report the results of the sensitivity analyses along with the primary results.
In Discussion Section: Discuss the key limitations and implications of the results of the sensitivity analyses on the conclusions or findings. This can be done by describing what changes the sensitivity analyses bring to the interpretation of the data, and whether the sensitivity analyses are more stringent or more relaxed than the primary analysis.
Some concluding remarks
Sensitivity analyses play an important role is checking the robustness of the conclusions from clinical trials. They are important in interpreting or establishing the credibility of the findings. If the results remain robust under different assumptions, methods or scenarios, this can strengthen their credibility. The results of our brief survey of January 2012 editions of major medical and health economics journals that show that their use is very low. We recommend that some sensitivity analysis should be the default plan in statistical or economic analyses of any clinical trial. Investigators need to identify any key assumptions, variations, or methods that may impact or influence the findings, and plan to conduct some sensitivity analyses as part of their analytic strategy. The final report must include the documentation of the planned or posthoc sensitivity analyses, rationale, corresponding results and a discussion of their consequences or repercussions on the overall findings.
Abbreviations
- Sensitivity analysis
United States
Food and Drug Administration
European Medicines Association
United Kingdom
National Institute of Health and Clinical Excellence
Randomized controlled trial
Intention-to-treat
Per-protocol
Last observation carried forward
Multiple imputation
Missing at random
Generalized estimating equations
Generalized linear mixed models
Community hypertension assessment trial
Prostate specific antigen
Cumulative incidence function
End stage renal disease
Instrumental variable
Analysis of covariance
Statistical analysis plan
Consolidated Standards of Reporting Trials.
Thabane L, Ma J, Chu R, Cheng J, Ismaila A, Rios LP, Robson R, Thabane M, Giangregorio L, Goldsmith CH: A tutorial on pilot studies: the what, why and how. BMC Med Res Methodol. 2010, 10: 1-10.1186/1471-2288-10-1.
Article PubMed PubMed Central Google Scholar
Schneeweiss S: Sensitivity analysis and external adjustment for unmeasured confounders in epidemiologic database studies of therapeutics. Pharmacoepidemiol Drug Saf. 2006, 15 (5): 291-303. 10.1002/pds.1200.
Article PubMed Google Scholar
Viel JF, Pobel D, Carre A: Incidence of leukaemia in young people around the La Hague nuclear waste reprocessing plant: a sensitivity analysis. Stat Med. 1995, 14 (21–22): 2459-2472.
Article CAS PubMed Google Scholar
Goldsmith CH, Gafni A, Drummond MF, Torrance GW, Stoddart GL: Sensitivity Analysis and Experimental Design: The Case of Economic Evaluation of Health Care Programmes. Proceedings of the Third Canadian Conference on Health Economics 1986. 1987, Winnipeg MB: The University of Manitoba Press
Google Scholar
Saltelli A, Tarantola S, Campolongo F, Ratto M: Sensitivity Analysis in Practice: A Guide to Assessing Scientific Models. 2004, New York, NY: Willey
Saltelli A, Ratto M, Andres T, Campolongo F, Cariboni J, Gatelli D, Saisana M, Tarantola S: Global Sensitivity Analysis: The Primer. 2008, New York, NY: Wiley-Interscience
Hunink MGM, Glasziou PP, Siegel JE, Weeks JC, Pliskin JS, Elstein AS, Weinstein MC: Decision Making in Health and Medicine: Integrating Evidence and Values. 2001, Cambridge: Cambridge University Press
USFDA: International Conference on Harmonisation; Guidance on Statistical Principles for Clinical Trials. Guideline E9. Statistical principles for clinical trials. Federal Register, 16 September 1998, Vol. 63, No. 179, p. 49583. [ http://www.fda.gov/downloads/RegulatoryInformation/Guidances/UCM129505.pdf ],
NICE: Guide to the methods of technology appraisal. [ http://www.nice.org.uk/media/b52/a7/tamethodsguideupdatedjune2008.pdf ],
Ma J, Thabane L, Kaczorowski J, Chambers L, Dolovich L, Karwalajtys T, Levitt C: Comparison of Bayesian and classical methods in the analysis of cluster randomized controlled trials with a binary outcome: the Community Hypertension Assessment Trial (CHAT). BMC Med Res Methodol. 2009, 9: 37-10.1186/1471-2288-9-37.
Peters TJ, Richards SH, Bankhead CR, Ades AE, Sterne JA: Comparison of methods for analysing cluster randomized trials: an example involving a factorial design. Int J Epidemiol. 2003, 32 (5): 840-846. 10.1093/ije/dyg228.
Chu R, Thabane L, Ma J, Holbrook A, Pullenayegum E, Devereaux PJ: Comparing methods to estimate treatment effects on a continuous outcome in multicentre randomized controlled trials: a simulation study. BMC Med Res Methodol. 2011, 11: 21-10.1186/1471-2288-11-21.
Kleinbaum DG, Klein M: Survival Analysis – A-Self Learning Text. 2012, Springer, 3
Barnett V, Lewis T: Outliers in Statistical Data. 1994, John Wiley & Sons, 3
Grubbs FE: Procedures for detecting outlying observations in samples. Technometrics. 1969, 11: 1-21. 10.1080/00401706.1969.10490657.
Article Google Scholar
Thabane L, Akhtar-Danesh N: Guidelines for reporting descriptive statistics in health research. Nurse Res. 2008, 15 (2): 72-81.
Williams NH, Edwards RT, Linck P, Muntz R, Hibbs R, Wilkinson C, Russell I, Russell D, Hounsome B: Cost-utility analysis of osteopathy in primary care: results from a pragmatic randomized controlled trial. Fam Pract. 2004, 21 (6): 643-650. 10.1093/fampra/cmh612.
Zetta S, Smith K, Jones M, Allcoat P, Sullivan F: Evaluating the Angina Plan in Patients Admitted to Hospital with Angina: A Randomized Controlled Trial. Cardiovascular Therapeutics. 2011, 29 (2): 112-124. 10.1111/j.1755-5922.2009.00109.x.
Morden JP, Lambert PC, Latimer N, Abrams KR, Wailoo AJ: Assessing methods for dealing with treatment switching in randomised controlled trials: a simulation study. BMC Med Res Methodol. 2011, 11: 4-10.1186/1471-2288-11-4.
White IR, Walker S, Babiker AG, Darbyshire JH: Impact of treatment changes on the interpretation of the Concorde trial. AIDS. 1997, 11 (8): 999-1006. 10.1097/00002030-199708000-00008.
Borrelli B: The assessment, monitoring, and enhancement of treatment fidelity in public health clinical trials. J Public Health Dent. 2011, 71 (Suppl 1): S52-S63.
Article PubMed Central Google Scholar
Lawton J, Jenkins N, Darbyshire JL, Holman RR, Farmer AJ, Hallowell N: Challenges of maintaining research protocol fidelity in a clinical care setting: a qualitative study of the experiences and views of patients and staff participating in a randomized controlled trial. Trials. 2011, 12: 108-10.1186/1745-6215-12-108.
Ye C, Giangregorio L, Holbrook A, Pullenayegum E, Goldsmith CH, Thabane L: Data withdrawal in randomized controlled trials: Defining the problem and proposing solutions: a commentary. Contemp Clin Trials. 2011, 32 (3): 318-322. 10.1016/j.cct.2011.01.016.
Horwitz RI, Horwitz SM: Adherence to treatment and health outcomes. Arch Intern Med. 1993, 153 (16): 1863-1868. 10.1001/archinte.1993.00410160017001.
Peduzzi P, Wittes J, Detre K, Holford T: Analysis as-randomized and the problem of non-adherence: an example from the Veterans Affairs Randomized Trial of Coronary Artery Bypass Surgery. Stat Med. 1993, 12 (13): 1185-1195. 10.1002/sim.4780121302.
Montori VM, Guyatt GH: Intention-to-treat principle. CMAJ. 2001, 165 (10): 1339-1341.
CAS PubMed PubMed Central Google Scholar
Gibaldi M, Sullivan S: Intention-to-treat analysis in randomized trials: who gets counted?. J Clin Pharmacol. 1997, 37 (8): 667-672. 10.1002/j.1552-4604.1997.tb04353.x.
Porta M: A dictionary of epidemiology. 2008, Oxford: Oxford University Press, Inc, 5
Everitt B: Medical statistics from A to Z. 2006, Cambridge: Cambridge University Press, 2
Book Google Scholar
Sainani KL: Making sense of intention-to-treat. PM R. 2010, 2 (3): 209-213. 10.1016/j.pmrj.2010.01.004.
Bendtsen P, McCambridge J, Bendtsen M, Karlsson N, Nilsen P: Effectiveness of a proactive mail-based alcohol internet intervention for university students: dismantling the assessment and feedback components in a randomized controlled trial. J Med Internet Res. 2012, 14 (5): e142-10.2196/jmir.2062.
Brox JI, Nygaard OP, Holm I, Keller A, Ingebrigtsen T, Reikeras O: Four-year follow-up of surgical versus non-surgical therapy for chronic low back pain. Ann Rheum Dis. 2010, 69 (9): 1643-1648. 10.1136/ard.2009.108902.
McKnight PE, McKnight KM, Sidani S, Figueredo AJ: Missing Data: A Gentle Introduction. 2007, New York, NY: Guilford
Graham JW: Missing data analysis: making it work in the real world. Annu Rev Psychol. 2009, 60: 549-576. 10.1146/annurev.psych.58.110405.085530.
Little RJ, D'Agostino R, Cohen ML, Dickersin K, Emerson SS, Farrar JT, Frangakis C, Hogan JW, Molenberghs G, Murphy SA, et al: The Prevention and Treatment of Missing Data in Clinical Trials. New England Journal of Medicine. 2012, 367 (14): 1355-1360. 10.1056/NEJMsr1203730.
Article CAS PubMed PubMed Central Google Scholar
Little RJA, Rubin DB: Statistical Analysis with Missing Data. 2002, New York NY: Wiley, 2
Rubin DB: Multiple Imputation for Nonresponse in Surveys. 1987, John Wiley & Sons, Inc: New York NY
Schafer JL: Analysis of Incomplete Multivariate Data. 1997, New York: Chapman and Hall
Son H, Friedmann E, Thomas SA: Application of pattern mixture models to address missing data in longitudinal data analysis using SPSS. Nursing research. 2012, 61 (3): 195-203. 10.1097/NNR.0b013e3182541d8c.
Peters SA, Bots ML, den Ruijter HM, Palmer MK, Grobbee DE, Crouse JR, O'Leary DH, Evans GW, Raichlen JS, Moons KG, et al: Multiple imputation of missing repeated outcome measurements did not add to linear mixed-effects models. J Clin Epidemiol. 2012, 65 (6): 686-695. 10.1016/j.jclinepi.2011.11.012.
Zhang H, Paik MC: Handling missing responses in generalized linear mixed model without specifying missing mechanism. J Biopharm Stat. 2009, 19 (6): 1001-1017. 10.1080/10543400903242761.
Chen HY, Gao S: Estimation of average treatment effect with incompletely observed longitudinal data: application to a smoking cessation study. Statistics in medicine. 2009, 28 (19): 2451-2472. 10.1002/sim.3617.
Ma J, Akhtar-Danesh N, Dolovich L, Thabane L: Imputation strategies for missing binary outcomes in cluster randomized trials. BMC Med Res Methodol. 2011, 11: 18-10.1186/1471-2288-11-18.
Kingsley GH, Kowalczyk A, Taylor H, Ibrahim F, Packham JC, McHugh NJ, Mulherin DM, Kitas GD, Chakravarty K, Tom BD, et al: A randomized placebo-controlled trial of methotrexate in psoriatic arthritis. Rheumatology (Oxford). 2012, 51 (8): 1368-1377. 10.1093/rheumatology/kes001.
Article CAS Google Scholar
de Pauw BE, Sable CA, Walsh TJ, Lupinacci RJ, Bourque MR, Wise BA, Nguyen BY, DiNubile MJ, Teppler H: Impact of alternate definitions of fever resolution on the composite endpoint in clinical trials of empirical antifungal therapy for neutropenic patients with persistent fever: analysis of results from the Caspofungin Empirical Therapy Study. Transpl Infect Dis. 2006, 8 (1): 31-37. 10.1111/j.1399-3062.2006.00127.x.
A randomized, double-blind, futility clinical trial of creatine and minocycline in early Parkinson disease. Neurology. 2006, 66 (5)): 664-671.
Song P-K: Correlated Data Analysis: Modeling, Analytics and Applications. 2007, New York, NY: Springer Verlag
Pintilie M: Competing Risks: A Practical Perspective. 2006, New York, NY: John Wiley
Tai BC, Grundy R, Machin D: On the importance of accounting for competing risks in pediatric brain cancer: II. Regression modeling and sample size. Int J Radiat Oncol Biol Phys. 2011, 79 (4): 1139-1146. 10.1016/j.ijrobp.2009.12.024.
Holbrook JT, Wise RA, Gold BD, Blake K, Brown ED, Castro M, Dozor AJ, Lima JJ, Mastronarde JG, Sockrider MM, et al: Lansoprazole for children with poorly controlled asthma: a randomized controlled trial. JAMA. 2012, 307 (4): 373-381.
Holbrook A, Thabane L, Keshavjee K, Dolovich L, Bernstein B, Chan D, Troyan S, Foster G, Gerstein H: Individualized electronic decision support and reminders to improve diabetes care in the community: COMPETE II randomized trial. CMAJ: Canadian Medical Association journal = journal de l’Association medicale canadienne. 2009, 181 (1–2): 37-44.
Hilbe JM: Negative Binomial Regression. 2011, Cambridge: Cambridge University Press, 2
Forsblom C, Harjutsalo V, Thorn LM, Waden J, Tolonen N, Saraheimo M, Gordin D, Moran JL, Thomas MC, Groop PH: Competing-risk analysis of ESRD and death among patients with type 1 diabetes and macroalbuminuria. J Am Soc Nephrol. 2011, 22 (3): 537-544. 10.1681/ASN.2010020194.
Grams ME, Coresh J, Segev DL, Kucirka LM, Tighiouart H, Sarnak MJ: Vascular disease, ESRD, and death: interpreting competing risk analyses. Clin J Am Soc Nephrol. 2012, 7 (10): 1606-1614. 10.2215/CJN.03460412.
Lim HJ, Zhang X, Dyck R, Osgood N: Methods of competing risks analysis of end-stage renal disease and mortality among people with diabetes. BMC Med Res Methodol. 2010, 10: 97-10.1186/1471-2288-10-97.
Chu R, Walter SD, Guyatt G, Devereaux PJ, Walsh M, Thorlund K, Thabane L: Assessment and implication of prognostic imbalance in randomized controlled trials with a binary outcome–a simulation study. PLoS One. 2012, 7 (5): e36677-10.1371/journal.pone.0036677.
Bowen A, Hesketh A, Patchick E, Young A, Davies L, Vail A, Long AF, Watkins C, Wilkinson M, Pearl G, et al: Effectiveness of enhanced communication therapy in the first four months after stroke for aphasia and dysarthria: a randomised controlled trial. BMJ. 2012, 345: e4407-10.1136/bmj.e4407.
Spiegelhalter DJ, Best NG, Lunn D, Thomas A: Bayesian Analysis using BUGS: A Practical Introduction. 2009, New York, NY: Chapman and Hall
Byers AL, Allore H, Gill TM, Peduzzi PN: Application of negative binomial modeling for discrete outcomes: a case study in aging research. J Clin Epidemiol. 2003, 56 (6): 559-564. 10.1016/S0895-4356(03)00028-3.
Yusuf S, Wittes J, Probstfield J, Tyroler HA: Analysis and interpretation of treatment effects in subgroups of patients in randomized clinical trials. JAMA: the journal of the American Medical Association. 1991, 266 (1): 93-98. 10.1001/jama.1991.03470010097038.
Altman DG: Better reporting of randomised controlled trials: the CONSORT statement. BMJ. 1996, 313 (7057): 570-571. 10.1136/bmj.313.7057.570.
Mauskopf JA, Sullivan SD, Annemans L, Caro J, Mullins CD, Nuijten M, Orlewska E, Watkins J, Trueman P: Principles of good practice for budget impact analysis: report of the ISPOR Task Force on good research practices–budget impact analysis. Value Health. 2007, 10 (5): 336-347. 10.1111/j.1524-4733.2007.00187.x.
Pre-publication history
The pre-publication history for this paper can be accessed here: http://www.biomedcentral.com/1471-2288/13/92/prepub
Download references
Acknowledgements
This work was supported in part by funds from the CANNeCTIN programme.
Author information
Authors and affiliations.
Department of Clinical Epidemiology and Biostatistics, McMaster University, Hamilton, ON, Canada
Lehana Thabane, Lawrence Mbuagbaw, Shiyuan Zhang, Zainab Samaan, Maura Marcucci, Chenglin Ye, Marroon Thabane, Brittany Dennis, Daisy Kosa, Victoria Borg Debono & Charles H Goldsmith
Departments of Pediatrics and Anesthesia, McMaster University, Hamilton, ON, Canada
Lehana Thabane
Center for Evaluation of Medicine, St Joseph’s Healthcare Hamilton, Hamilton, ON, Canada
Biostatistics Unit, Father Sean O’Sullivan Research Center, St Joseph’s Healthcare Hamilton, Hamilton, ON, Canada
Lehana Thabane, Lawrence Mbuagbaw, Shiyuan Zhang, Maura Marcucci, Chenglin Ye, Brittany Dennis, Daisy Kosa, Victoria Borg Debono & Charles H Goldsmith
Population Health Research Institute, Hamilton Health Sciences, Hamilton, ON, Canada
Department of Psychiatry and Behavioral Neurosciences, McMaster University, Hamilton, ON, Canada
Zainab Samaan
Population Genomics Program, McMaster University, Hamilton, ON, Canada
GSK, Mississauga, ON, Canada
Marroon Thabane
Department of Kinesiology, University of Waterloo, Waterloo, ON, Canada
Lora Giangregorio
Department of Nephrology, Toronto General Hospital, Toronto, ON, Canada
Department of Pediatrics, McMaster University, Hamilton, ON, Canada
Rejane Dillenburg
Michael G. DeGroote School of Medicine, McMaster University, Hamilton, ON, Canada
Vincent Fruci
McMaster Integrative Neuroscience Discovery & Study (MiNDS) Program, McMaster University, Hamilton, ON, Canada
Monica Bawor
Department of Biostatistics, Korea University, Seoul, South Korea
Juneyoung Lee
Department of Clinical Epidemiology, University of Ottawa, Ottawa, ON, Canada
George Wells
Faculty of Health Sciences, Simon Fraser University, Burnaby, BC, Canada
Charles H Goldsmith
You can also search for this author in PubMed Google Scholar
Corresponding author
Correspondence to Lehana Thabane .
Additional information
Competing interests.
The authors declare that they have no competing interests.
Authors’ contributions
LT conceived the idea and drafted the outline and paper. GW, CHG and MT commented on the idea and draft outline. LM and SZ performed literature search and data abstraction. ZS, LG and CY edited and formatted the manuscript. MM, BD, DK, VBD, RD, VF, MB, JL reviewed and revised draft versions of the manuscript. All authors reviewed several draft versions of the manuscript and approved the final manuscript.
Rights and permissions
This article is published under license to BioMed Central Ltd. This is an Open Access article distributed under the terms of the Creative Commons Attribution License ( http://creativecommons.org/licenses/by/2.0 ), which permits unrestricted use, distribution, and reproduction in any medium, provided the original work is properly cited.
Reprints and permissions
About this article
Cite this article.
Thabane, L., Mbuagbaw, L., Zhang, S. et al. A tutorial on sensitivity analyses in clinical trials: the what, why, when and how. BMC Med Res Methodol 13 , 92 (2013). https://doi.org/10.1186/1471-2288-13-92
Download citation
Received : 11 December 2012
Accepted : 10 July 2013
Published : 16 July 2013
DOI : https://doi.org/10.1186/1471-2288-13-92
Share this article
Anyone you share the following link with will be able to read this content:
Sorry, a shareable link is not currently available for this article.
Provided by the Springer Nature SharedIt content-sharing initiative
- Clinical trials
BMC Medical Research Methodology
ISSN: 1471-2288
- General enquiries: [email protected]
9.7 Sensitivity analyses
The process of undertaking a systematic review involves a sequence of decisions. Whilst many of these decisions are clearly objective and non-contentious, some will be somewhat arbitrary or unclear. For instance, if inclusion criteria involve a numerical value, the choice of value is usually arbitrary: for example, defining groups of older people may reasonably have lower limits of 60, 65, 70 or 75 years, or any value in between. Other decisions may be unclear because a study report fails to include the required information. Some decisions are unclear because the included studies themselves never obtained the information required: for example, the outcomes of those who unfortunately were lost to follow-up. Further decisions are unclear because there is no consensus on the best statistical method to use for a particular problem.
It is desirable to prove that the findings from a systematic review are not dependent on such arbitrary or unclear decisions. A sensitivity analysis is a repeat of the primary analysis or meta-analysis, substituting alternative decisions or ranges of values for decisions that were arbitrary or unclear. For example, if the eligibility of some studies in the meta-analysis is dubious because they do not contain full details, sensitivity analysis may involve undertaking the meta-analysis twice: first, including all studies and second, only including those that are definitely known to be eligible. A sensitivity analysis asks the question, “Are the findings robust to the decisions made in the process of obtaining them?”.
There are many decision nodes within the systematic review process which can generate a need for a sensitivity analysis. Examples include:
Searching for studies:
Should abstracts whose results cannot be confirmed in subsequent publications be included in the review?
Eligibility criteria:
Characteristics of participants: where a majority but not all people in a study meet an age range, should the study be included?
Characteristics of the intervention: what range of doses should be included in the meta-analysis?
Characteristics of the comparator: what criteria are required to define usual care to be used as a comparator group?
Characteristics of the outcome: what time-point or range of time-points are eligible for inclusion?
Study design: should blinded and unblinded outcome assessment be included, or should study inclusion be restricted by other aspects of methodological criteria?
What data should be analysed?
Time-to-event data: what assumptions of the distribution of censored data should be made?
Continuous data: where standard deviations are missing, when and how should they be imputed? Should analyses be based on change scores or on final values?
Ordinal scales: what cut-point should be used to dichotomize short ordinal scales into two groups?
Cluster-randomized trials: what values of the intraclass correlation coefficient should be used when trial analyses have not been adjusted for clustering?
Cross-over trials: what values of the within-subject correlation coefficient should be used when this is not available in primary reports?
All analyses: what assumptions should be made about missing outcomes to facilitate intention-to-treat analyses? Should adjusted or unadjusted estimates of treatment effects used?
Analysis methods:
Should fixed-effect or random-effects methods be used for the analysis?
For dichotomous outcomes, should odds ratios, risk ratios or risk differences be used?
And for continuous outcomes, where several scales have assessed the same dimension, should results be analysed as a standardized mean difference across all scales or as mean differences individually for each scale?
Some sensitivity analyses can be pre-specified in the study protocol, but many issues suitable for sensitivity analysis are only identified during the review process where the individual peculiarities of the studies under investigation are identified. When sensitivity analyses show that the overall result and conclusions are not affected by the different decisions that could be made during the review process, the results of the review can be regarded with a higher degree of certainty. Where sensitivity analyses identify particular decisions or missing information that greatly influence the findings of the review, greater resources can be deployed to try and resolve uncertainties and obtain extra information, possibly through contacting trial authors and obtained individual patient data. If this cannot be achieved, the results must be interpreted with an appropriate degree of caution. Such findings may generate proposals for further investigations and future research.
Reporting of sensitivity analyses in a systematic review may best be done by producing a summary table. Rarely is it informative to produce individual forest plots for each sensitivity analysis undertaken.
Sensitivity analyses are sometimes confused with subgroup analysis. Although some sensitivity analyses involve restricting the analysis to a subset of the totality of studies, the two methods differ in two ways. First, sensitivity analyses do not attempt to estimate the effect of the intervention in the group of studies removed from the analysis, whereas in subgroup analyses, estimates are produced for each subgroup. Second, in sensitivity analyses, informal comparisons are made between different ways of estimating the same thing, whereas in subgroup analyses, formal statistical comparisons are made across the subgroups.

An official website of the United States government
The .gov means it’s official. Federal government websites often end in .gov or .mil. Before sharing sensitive information, make sure you’re on a federal government site.
The site is secure. The https:// ensures that you are connecting to the official website and that any information you provide is encrypted and transmitted securely.
- Publications
- Account settings
Preview improvements coming to the PMC website in October 2024. Learn More or Try it out now .
- Advanced Search
- Journal List
- Sage Choice

Sensitivity Analysis: A Method to Promote Certainty and Transparency in Nursing and Health Research
Fabrice i. mowbray.
1 Department of Health Research Methods, Evidence and Impact, McMaster University, Hamilton, Ontario, Canada
Donna Manlongat
2 College of Nursing, Wayne State University, Detroit, Michigan, United States
Meghna Shukla
Nursing and health researchers may be presented with uncertainty regarding the utilization or legitimacy of methodological or analytic decisions. Sensitivity analyses are purposed to gain insight and certainty about the validity of research findings reported. Reporting guidelines and health research methodologists have emphasized the importance of utilizing and reporting sensitivity analyses in clinical research. However, sensitivity analyses are underreported in nursing and health research. The aim of this methodological overview is to provide an introduction to the purpose, conduct, interpretation, and reporting of sensitivity analyses, using a series of simulated and contemporary case examples.
Nursing and health researchers share a common goal of producing robust study findings. Robust findings are insensitive to changes in methodological or analytic assumptions ( Thabane et al., 2013 ), and thus improve confidence in the inferences drawn and context-specific generalizability of study findings ( Delaney & Seeger, 2013 ). Sensitivity analyses are commonly conducted to gain insight and confidence in the decisions, methods, analytics, and measures used ( Thabane et al., 2013 ; Vandenbroucke et al., 2014 ). Health research methodologists and reporting guidelines have emphasized the value of conducting sensitivity analyses ( Hayden et al., 2013 ; Page et al., 2021 ; Vandenbroucke et al., 2014 ), though they are consistently underreported in clinical research ( Morris et al., 2014 ; Vandenbroucke et al., 2014 ). The aim of this paper is to provide an introductory and pragmatic overview for nursing and health researchers on the purpose, conduct, interpretation, and reporting, of sensitivity analysis using a series of simulated and contemporary case examples.
What is a sensitivity analysis?
Sensitivity analysis is a method used to evaluate the influence of alternative assumptions or analyses on the pre-specified research questions proposed ( Deeks et al., 2021 ; Schneeweiss, 2006 ; Thabane et al., 2013 ). In other words, a sensitivity analysis is purposed to evaluate the validity and certainty of the primary methodological or analytic strategy. Sensitivity analyses are most informative when there is an array of reasonable and differing assumptions ( Morris et al., 2014 ). To illustrate, envision a graduate nursing studentconcerned about the impact of outlier values on the statistical estimates of their regression model. To determine the influence of outlier values, they examine model estimates and associated measures of variance both with (pre-specified) and without outliers present in the analysis. The assumption in this scenario is that the few outlier values in the data have minimal influence on estimates if included in the analysis. If statistical estimates and corresponding measures of variance are similar between models, then confidence can be gained in the robustness of study findings with regard to outlier values ( El-Masri et al., 2020 ). In this scenario, it is recommended that authors report the change in estimates of interest, or lack thereof, with the secondary model included in the document's appendices.
Timing and benefits of conducting a sensitivity analysis
Sensitivity analyses are conducted after the study's primary analyses are completed and conclusions have been made on the results of the primary analysis ( Thabane et al., 2013 ). However, it is best to consider the use of sensitivity analyses during the protocol and study development stage to determine potential uncertainties in the study design after all variables and data analysis approaches have been selected (de Souza et al., 2016; Frey & Patil, 2002 ). Design of the primary research question should include a clear description of any planned sensitivity analyses, where applicable (e.g., covariate imbalance or protocol deviations) (de Souza et al., 2016). Therefore, the primary analysis should closely follow the pre-determined sensitivity analysis approach to address the impact of the previously identified concerns or uncertainties (de Souza et al., 2016).
There is utility in conducting sensitivity analyses on secondary research questions or post-hoc analytics, though these should be classified and reported as ‘ secondary analyses ’ to avoid confusion with the sensitivity analysis reported for the primary analysis ( Morris et al., 2014 ). This type of sensitivity analysis is often carried out to test the validity of arbitrary or unclear decisions made following protocol publication or data collection. For example, if there is uncertainty regarding the cut-off used to define an exposure or outcome, a sensitivity analysis could be conducted to determine if statistical or clinical significance changes when using a different proposed cut-off for these measures. Recently, a recent systematic review conducted a sensitivity analysis to determine if there was any difference in the choice of cut-off value used in the Clinical Frailty Scale to define frailty, and the influence it had on predicting survival after cardiac arrest ( Mowbray et al., 2021 ).
A lack of evidence-based guidelines or expert consensus regarding a particular methodological decision may necessitate this type of sensitivity analysis ( Deeks et al., 2021 ). If a post-hoc sensitivity analysis results in uncertainty about which statistical model to report, such as in an exploratory study, then it is recommended that the most biologically or theoretically plausible model be emphasized in the reports ( Morris et al., 2014 ).
Data screening, cleaning, and analysis, commonly reveal unanticipated barriers and findings, further highlighting the value of post-hoc sensitivity analyses ( Morris et al., 2014 ). However, these post-hoc analyses require a clear rationale and justification outlined in the Methods section of the manuscript, including an explanation of the need for the sensitivity analysis (de Souza et al., 2016). Regardless, whether sensitivity analyses are planned either a priori or post-hoc, both intend to answer: Are the inferences drawn from the data valid and reliable? This can be confirmed when consistency in results is noted between the primary analysis and sensitivity analysis ( Frey & Patil, 2002 ; Thabane et al., 2013 ).
Sensitivity analyses are not only beneficial for researchers and peer reviewers, but they also increase the reader's confidence in study findings by verifying and validating results when unable to meet or demonstrate ideal analytic conditions (de Souza et al., 2016; Frey & Patil, 2002 ). When a sensitivity analysis suggests that results are not robust or consistent (i.e., results differ greatly from the primary analysis), the researcher must take steps to further investigate the potential source of bias. This is particularly important in clinical research, where findings have the potential to influence health policy development, clinical practice, institutional protocols, and ultimately the care and safety of patients. By thoroughly considering and evaluating study assumptions, one gains confidence in translating and applying study findings to clinical and academic settings.
Case examples
Sensitivity analyses are conducted across a multitude of scientific disciplines and methodologies. To support knowledge retention and translation, we provide a series of simulated and contemporary case examples to display the utility and versatility of sensitivity analyses in nursing and health research. We provide three examples to showcase how sensitivity analyses can be used across multiple phases of the research process; missing data (data cleaning and screening), clustered data (statistical analysis), and meta-analysis (data synthesis).
Missing data
A common hurdle in clinical research is the presence of missing data ( El-Masri & Fox-Wasylyshyn, 2005 ). The analytic approach chosen when dealing with missing data must consider both the pattern and influence of missing data points (de Souza et al., 2016; El-Masri & Fox-Wasylyshyn, 2005 ). Complete-case analysis excludes patients with missing data on one or more variables from statistical estimates ( Little et al., 2012 ; Zhou, 2020 ). This method is not commonly recommended as its use can reduce statistical power and precision of estimates ( Little et al., 2012 ; Nakai et al., 2014 ; Zhu, 2014 ). More importantly, complete-case analysis has the potential to bias estimates if data are missing in a systematic fashion and thus not representative of the entire study cohort ( Moons et al., 2015 ). For example, older adults are commonly excluded from clinical trials given their greater propensity for poor health outcomes ( Herrera et al., 2010 ; Watts, 2012 ). However, if excluded from analyses, this can distort our understanding of the intervention or association of interest, considering they are the largest user of health services ( Gruneir et al., 2018 ; Rais et al., 2013 ).
Imputation methods are commonly recommended over complete-case analysis when appropriate (e.g., data missing completely at random), given the reasons previously mentioned. Multiple imputation methods are the preferred imputation technique, over single imputation, as they are more robust, though often more computationally intensive (de Souza et al., 2016; Nakai et al., 2014 ). Bearing in mind the importance of understanding the context and etiology of missing data ( Altman & Bland, 2007 ), one could conduct a sensitivity analysis to determine the influence of missing data by comparing the statistical estimates and model accuracy in a model with and without imputation (i.e., complete case analysis) ( Lee & Simpson, 2014 ). Study findings are considered robust if sensitivity analyses comparing the complete-case model and multiple imputation model yield similar results ( Lee & Simpson, 2014 ).
Systematic reviews and meta-analysis
Assessing the Risk of Bias (RoB) for studies included in a systematic review is a crucial step to determine the internal validity of individual studies, as well as how threats to validity influence synthesized estimates and reporting ( Patole, 2021 ; Sobieraj & Baker, 2021 ). To illustrate, imagine one is interested in conducting a systematic review with a meta-analysis aiming to pool data on the effect of enhanced discharge teaching for hospitalized older adults on follow-up with primary care. Figure 1 displays a forest plot with the five eligible studies, four with low RoB, and one with high RoB. To determine if the study with high RoB distorts our pooled estimate of enhanced discharge teaching, one could conduct a sensitivity analysis, in which the high RoB study is removed from the pooled analysis (see Figure 2 ), and both pooled estimates are compared. As one can see, removing the study with high RoB decreases the absolute risk difference of primary care follow-up by 8% (0.29 → 0.21). This indicates that the high RoB study overestimates the effect of enhanced discharge teaching on primary care follow-up, and thus is likely biasing pooled estimates. While we would typically not pool data with such a high level of heterogeneity (e.g., I 2 ) ( Foroutan et al., 2020 ), these figures are purely for educational purposes.

Forest plot with all eligible studies.

Forest plot with only low risk of bias studies.
Correlated (clustered) data
Often in clinical research, we deal with individuals who contribute multiple observations, whereas others may only contribute one ( Cleophas et al., 2012 ; Schober & Vetter, 2018 ; Senn et al., 2000 ). Emergency department visitation is an ideal example of this, where some patients may present for multiple repeat visits ( Seguin et al., 2018 ; Slankamenac et al., 2019 ). If one person ( John ) contributes five visits in a year, and another ( Roopa ) only contributes one visit for the year, analysts would need to take into consideration that John contributes approximately five times the information and weight to statistical estimates as Roopa , and thus John is said to have clustered (or correlated) data. Where clustered observations exist, pooling of these data points may be necessary to facilitate appropriate weighting of estimates and to best capture the variance both within, and between, clusters of data. The five observations contributed by John in the prior case example would be an example of one cluster of data (within-cluster variance). The difference in emergency outcomes between John and Roopa would be an example of between-cluster variance.
Methods exist to handle this clustering in the data, like multi-level modelling and generalized estimating equations (GEE), though the details of these methods are beyond the scope of this paper. With regard to sensitivity analyses, researchers may be interested in testing the influence of using a clustered statistical model versus a standard non-clustered model. If the model associations and measures of variance are similar, then one gains confidence in the decision to report the model without clustering. However, if a clinical or statistical difference is noted, then the authors would be encouraged to reported the clustered model as the primary model. It is recommended that the sensitivity analysis is mentioned in the Methods and Results sections of the manuscript or report, and any additional analyses are included as supplementary files to support reader decision-making.
Next steps for nursing and health researchers
The majority of medical journals reflect low usage of sensitivity analyses in most publications of clinical research ( Thabane et al., 2013 ) – the same can be assumed for nursing research. To increase consideration and use of sensitivity analyses in nursing research, nursing and clinical health programs should aim to include education about the utility and importance of sensitivity analyses in graduate level methodology and statistics courses. Students should be encouraged to understand threats to validity (e.g., selection bias, confounding), as this knowledge is necessary to limit and account for possible biases that may invalidate study findings ( Altman & Simera, 2010 ).
Both research and clinical faculty are likely to benefit from understanding the purpose, conduct, and interpretation of sensitivity analyses, as this technique can support the rigour and understanding of their work. Additionally, knowledge of sensitivity analyses can improve RoB assessments during literature appraisals and the peer review process. Encouraging nursing and health researchers to adhere to reporting guidelines (e.g., STROBE, PRISMA) is another strategy to indirectly draw attention to sensitivity analyses, among other analytic strategies (e.g., subgroup analysis) that may further benefit the conduct and reporting of clinical research.
Moving forward, nursing and health researchers should consider the use of sensitivity analyses during the study design phase, and include a priori sensitivity models into research protocols and registries. A discussion of the included sensitivity analyses should also be routinely inserted into funding applications to promote their use with the noble intention of results transparency. The inclusion of sensitivity analyses in these pre-study documents may demonstrate researchers’ thoughtfulness regarding analytic strategy to academic journal editors, funding agencies, and key stake holders.
Reporting the conduct and results of sensitivity analyses, either in-text or in a supplemental file, is recommended to allow readers to easily identify and evaluate any uncertainty in study findings (de Souza et al., 2016). Additionally, highlighting any post-hoc sensitivity analyses, as well as the reasoning for conducting them, is likely to improve contextual understanding of the study for both the researcher and reader. Clinical researchers are encouraged to highlight whether the sensitivity analysis improved certainty of their study findings. They are also encouraged to discuss how the analysis influenced their contextual understanding of the potential biases that influence the research question within the Discussion section of respective manuscripts (de Souza et al., 2016).
Sensitivity analyses can be used in a wide array of analytic scenarios to promote the transparency and validity of proposed research questions and methods. When there is possible uncertainty regarding study methods, analyses, measures, or decisions, conducting and reporting sensitivity analyses is beneficial for both researchers and readership alike. Nursing and other health researchers are encouraged to consider utilizing and reporting the value of sensitivity analyses during the study design phase.
The author(s) declared no potential conflicts of interest with respect to the research, authorship, and/or publication of this article.
Funding: The author(s) received no financial support for the research, authorship, and/or publication of this article.
ORCID iD: Fabrice I. Mowbray https://orcid.org/0000-0002-8844-5850
Sensitivity Analysis
- Reference work entry
- First Online: 01 January 2016
- pp 1379–1384
- Cite this reference work entry
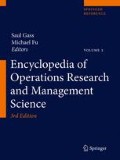
- Andres Redchuk 3 , 4 &
- David Ríos Insua 5
372 Accesses
This is a preview of subscription content, log in via an institution to check access.
Access this chapter
- Available as PDF
- Read on any device
- Instant download
- Own it forever
- Available as EPUB and PDF
- Durable hardcover edition
- Dispatched in 3 to 5 business days
- Free shipping worldwide - see info
Tax calculation will be finalised at checkout
Purchases are for personal use only
Institutional subscriptions
Berger, J., Betrò, B., Moreno, E., Pericchi, L., Ruggeri, F., Salinetti, G., & Wasserman, L. (eds.) (1996). Bayesian robustness . IMS Lecture Notes.
Google Scholar
French, S., & Ríos Insua, D. (2000). Statistical decision theory . London: Arnold.
Gal, T., & Greenberg, H. (1997). Advances in sensitivity analysis and parametric programming . Boston: Kluwer.
Book Google Scholar
Kadane, J., & Srinivasan, C. (1996). Bayesian robustness and stability. In Bayesian robustness, IMS Lecture Notes (Vol. 29, pp. 81–96).
Chapter Google Scholar
Ríos Insua, D. (1990). Sensitivity analysis in multiobjective decision making . New York: Springer.
Ríos Insua, D., & Ruggeri, F. (2000). Robust Bayesian analysis . New York: Springer.
Ríos Insua, D., Ruggeri, F., & Martin, J. (2000). Bayesian sensitivity analysis: A review. In A. Saltelli et al. (Eds.), Handbook on sensitivity analysis . New York: Wiley.
Ruggeri, F., & Wasserman, L. (1993). Infinitesimal sensitivity of posterior distributions. Canadian Journal of Statistics, 21 , 195–203.
Article Google Scholar
Saltelli, A., Chan, K., & Scott, M. (2000). Mathematical and statistical methods: Sensitivity analysis . New York: Wiley.
Saltelli, A., Tarantola, S., Campolongo, F., & Ratto, M. (2004). Sensitivity analysis in practice: A guide to assessing scientific models . New York: Wiley.
Walley, P. (1991). Statistical reasoning with imprecise probabilities . London: Chapman and Hall.
Download references
Author information
Authors and affiliations.
Department of Operations Research and Statistics, Universidad Rey Juan Carlos, Tulipan S/N, Mostoles, Madrid, Spain
Andres Redchuk
Facultad de Ciencias Empresariales, Universidad Autónoma de Chile, Santiago, Chile
Spanish Royal Academy of Sciences, Valverde 22, Madrid, Spain
David Ríos Insua
You can also search for this author in PubMed Google Scholar
Corresponding author
Correspondence to Andres Redchuk .
Editor information
Editors and affiliations.
Robert H. Smith School of Business, University of Maryland, College Park, MD, USA
Saul I. Gass
Robert H. Smith School of Business and Institute for Systems Research, University of Maryland, College Park, MD, USA
Michael C. Fu
Rights and permissions
Reprints and permissions
Copyright information
© 2013 Springer Science+Business Media New York
About this entry
Cite this entry.
Redchuk, A., Insua, D.R. (2013). Sensitivity Analysis. In: Gass, S.I., Fu, M.C. (eds) Encyclopedia of Operations Research and Management Science. Springer, Boston, MA. https://doi.org/10.1007/978-1-4419-1153-7_1155
Download citation
DOI : https://doi.org/10.1007/978-1-4419-1153-7_1155
Published : 23 January 2016
Publisher Name : Springer, Boston, MA
Print ISBN : 978-1-4419-1137-7
Online ISBN : 978-1-4419-1153-7
eBook Packages : Business and Economics
Share this entry
Anyone you share the following link with will be able to read this content:
Sorry, a shareable link is not currently available for this article.
Provided by the Springer Nature SharedIt content-sharing initiative
- Publish with us
Policies and ethics
- Find a journal
- Track your research
If you still have questions or prefer to get help directly from an agent, please submit a request. We’ll get back to you as soon as possible.
Please fill out the contact form below and we will reply as soon as possible.
- Economics, Finance, & Analytics
- Research, Quantitative Analysis, & Decision Science
Sensitivity Analysis - Explained
What is sensitivity analysis.

Written by Jason Gordon
Updated at June 22nd, 2023
- Marketing, Advertising, Sales & PR Principles of Marketing Sales Advertising Public Relations SEO, Social Media, Direct Marketing
- Accounting, Taxation, and Reporting Managerial & Financial Accounting & Reporting Business Taxation
- Professionalism & Career Development
- Law, Transactions, & Risk Management Government, Legal System, Administrative Law, & Constitutional Law Legal Disputes - Civil & Criminal Law Agency Law HR, Employment, Labor, & Discrimination Business Entities, Corporate Governance & Ownership Business Transactions, Antitrust, & Securities Law Real Estate, Personal, & Intellectual Property Commercial Law: Contract, Payments, Security Interests, & Bankruptcy Consumer Protection Insurance & Risk Management Immigration Law Environmental Protection Law Inheritance, Estates, and Trusts
- Business Management & Operations Operations, Project, & Supply Chain Management Strategy, Entrepreneurship, & Innovation Business Ethics & Social Responsibility Global Business, International Law & Relations Business Communications & Negotiation Management, Leadership, & Organizational Behavior
- Economics, Finance, & Analytics Economic Analysis & Monetary Policy Research, Quantitative Analysis, & Decision Science Investments, Trading, and Financial Markets Banking, Lending, and Credit Industry Business Finance, Personal Finance, and Valuation Principles
Sensitivity analysis, also referred to as simulation analysis, is a technique employed in financial modeling to determine how different values of a set of independent variables can influence a particular dependent variable under certain specific conditions and assumptions. It is used to ascertain how the overall uncertainty in the output of a mathematical model is affected by the various sources of uncertainty in its inputs. The application of sensitivity analysis spans a wide range of fields such as engineering, biology, environmental studies, social sciences, chemistry and economics. It is most often used in mathematical models where the output is an opaque function (i.e. one that cannot be subjected to an analysis) of several inputs.
Back to : RESEARCH, ANALYSIS, & DECISION SCIENCE
How is a Sensitivity Analysis Used?
Sensitivity analysis is popularly known as what-if analysis since the technique is used to measure varying outcomes using alternative assumptions and conditions across a range of independent variables. At the risk of oversimplification, sensitivity analysis can be said to observe changes in behavior for every change brought to the model. Sensitivity analysis can either be local or global. There are certain parameters that analysts need to be mindful of when undertaking such an activity. For starters, it is essential to determine the input variables for which the values will be altered during the analysis. Secondly, it is also necessary to ascertain how many variables will be affected at any given point in time. Thirdly, maximum and minimum values need to be assigned to all pertinent variables before the analysis commences. Lastly, analysts must scrutinize correlations between input and output and assign values to the combination accordingly. The values that can be altered include technical parameters, the number of activities and constraints, and the overall objective with respect to both the assumed risk as well as expected profits. Stipulated observations include the value of the objective with respect to the strategy, the values of the various decision variables, and the value of the objective function between two adopted strategies.
Steps in Sensitivity Analysis
Once the values of the input variables have been determined, sensitivity analysis can be performed in the following steps:
- Defining the base case output : The first step is to define the corresponding base case output for the base case input value for which the sensitivity is to be measured.
- Determining the new output value : During this step, we determine the value of the output for a new value of the input, given that the values of all other inputs are constant.
- Calculating the change : We then compute the percentage changes in the output as well as the input.
- Calculating sensitivity : This final step calculates sensitivity by dividing the percentage change in output by the percentage change in input.
Variations in Assumptions
Please enable JavaScript
Applications of Sensitivity Analysis
Sensitivity analysis has a wide variety of applications from something as trivial as planning a road trip to developing business models. Below are some of its most common applications.
- Sensitivity analysis is used in the study of Black Box Processes , which are processes that can be analyzed on the basis of their inputs and outputs, without having to determine the complexities of their inner workings.
- It is employed in Robust decision-making (RDM) frameworks in order to assess the robustness of the results of a model under epistemic situations that involve uncertainty.
- It is used in the development of evolved models by identifying and analyzing correlations between observations, inputs and forecasts.
- It is utilized in reducing uncertainty in models by identifying and omitting inputs that bring about significant uncertainty in the output.
- Sensitivity analysis is also commonly used as a tool for model simplification by identifying and omitting inputs that are redundant or do not have any significant effect on the output.
- It is used to generate sustainable, coherent as well as compelling recommendations that aim to enhance communication between modelers and decision makers.
Sensitivity analysis has become an integral part of Policy Impact Assessments (IAs) conducted by both national as well as international agencies.
Illustration of Sensitivity Analysis
Let us assume that a company C1 is involved in the manufacture and sale of snow plows. Joe, a sales analyst at the company is trying to understand the impact of an early advent of winter on total sales of snow plows. Company analysts have already determined that sales volume typically peaks during the last quarter of the year, i.e. during the months October through December. This increase in sales is driven by the anticipation of snowfall during late December, January and early February. However, Joe has determined from historical sales figures that during forecasts of early winter, snow plow sales have also peaked accordingly. For calendar years that have had snowfall 15 days earlier than usual, there has been a five percent rise in total sales volume. Based on this simple equation, Joe is able to construct a financial model as well as perform sensitivity analysis utilizing various what-if scenarios. According to Joes sensitivity analysis, whenever snowfall precedes the norm by 21, 15 and nine days, the total snow plow sales of C1 can also be expected to increase by seven, five and three percent respectively.
Related Articles
- Rescaled Range Analysis - Explained
- Variance Analysis - Explained
- Weighted Average - Explained
- Empirical Probability - Explained
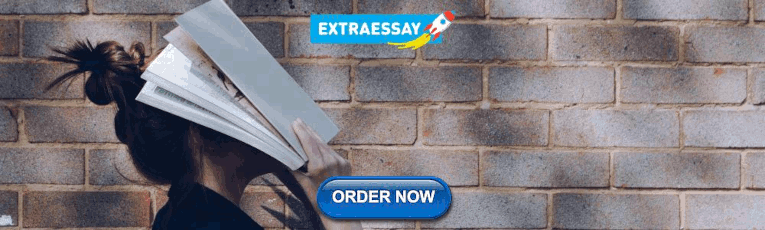
IMAGES
VIDEO
COMMENTS
What is a sensitivity analysis in clinical research? Sensitivity Analysis (SA) is defined as "a method to determine the robustness of an assessment by examining the extent to which results are affected by changes in methods, models, values of unmeasured variables, or assumptions" with the aim of identifying "results that are most dependent on questionable or unsupported assumptions" [].
In return for my sensitivity and attention to their pain, women willingly told their stories, including some disclosures they had previously never told. ... Hatchard J. (Eds.), Myths, methods, and messiness: Insights for qualitative research analysis (pp. 41-59). University of Bath. Google Scholar. Silverio S.A., Soulsby L.K. (2020). Turning ...
Sensitivity analysis is a method used to evaluate the influence of alternative assumptions or analyses on the pre-specified research questions proposed (Deeks et al., 2021; Schneeweiss, 2006; Thabane et al., 2013).In other words, a sensitivity analysis is purposed to evaluate the validity and certainty of the primary methodological or analytic strategy.
Evidence suggests that qualitative sensitivity analysis is equally advisable for mixed methods studies from which the qualitative component is extracted (Verhage and Boels 2017). Box 21.8.a Example domains that provide an assessment of methodological strengths and limitations to determine study rigour
Qualitative research is defined as "the study of the nature of ... This includes what is called reflexivity, i.e. sensitivity to the relationship between the researcher and the researched, including how contact was established and maintained, or the background and experience of the researcher(s) involved in data collection and analysis ...
Objectives: To report on the methodological insights gained from a sensitivity analysis conducted as part of a qualitative evidence synthesis in a mixed-methods systematic review. Methods: We applied thematic synthesis to synthesise the findings from qualitative research in a mixed-methods systematic review. Prior to extracting themes from the ...
The vast range of models and methods for handling missing data highlights the need for sensitivity analysis. Indeed, research on methodology has shifted from formulation of ever more complex models to methods for assessing sensitivity of specific models and their underlying assumptions. The paradigm shift to sensitivity analysis is, therefore ...
Sensitivity analysis examines the robustness of the result by conducting the analyses under a range of plausible assumptions about the methods, models, or data that differ from the assumptions ...
reported for the primary analysis (Morris et al., 2014). This type of sensitivity analysis is often carried out to test the val-idity of arbitrary or unclear decisions made following proto-col publication or data collection. For example, if there is uncertainty regarding the cut-off used to dene an exposure. fi.
Sensory processing sensitivity ... The SAGE handbook of qualitative research in psychology. SAGE Publications Ltd, London, pp. 193-209 ... NVivo qualitative data analysis software (Version 11 ...
comparative effectiveness research (CER), discusses assumptions that can be varied in a sensitivity analysis, and describes ways to implement a sensitivity analysis. All statistical models (and study results) are based on assumptions, and the validity of the inferences that can be drawn will often depend on the
Abstract. Sensitivity analysis provides users of mathematical and simulation models with tools to appreciate the dependency of the model output from model input and to investigate how important is each model input in determining its output. All application areas are concerned, from theoretical physics to engineering and socio-economics.
Sensitivity analyses play a crucial role in assessing the robustness of the findings or conclusions based on primary analyses of data in clinical trials. They are a critical way to assess the impact, effect or influence of key assumptions or variations—such as different methods of analysis, definitions of outcomes, protocol deviations, missing data, and outliers—on the overall conclusions ...
A sensitivity analysis is a repeat of the primary analysis or meta-analysis, substituting alternative decisions or ranges of values for decisions that were arbitrary or unclear. For example, if the eligibility of some studies in the meta-analysis is dubious because they do not contain full details, sensitivity analysis may involve undertaking ...
What is a sensitivity analysis? Sensitivity analysis is a method used to evaluate the influence of alternative assumptions or analyses on the pre-specified research questions proposed (Deeks et al., 2021; Schneeweiss, 2006; Thabane et al., 2013).In other words, a sensitivity analysis is purposed to evaluate the validity and certainty of the primary methodological or analytic strategy.
The resulting "pre-optimal" analysis is qualitative and provides sensitivity analysis results prior to solving the problem, which is an attractive feature for large-scale problems where the use of conventional parametric programming methods has a very high computational cost. Among the results discussed, the Ripple Theorem provides an upper ...
Sensitivity Analysis: A Method to Promote Certainty and Transparency in Nursing and Health Research June 2022 The Canadian journal of nursing research = Revue canadienne de recherche en sciences ...
Introduction. In operations research (OR), sensitivity analysis describes the methods and tools used to study how the output of a model varies with changes in the input data. The input data may refer to parameters affecting the objective functions and/or constraints or to the structure of the problem. Depending on the problem and model, the ...
They have been remapped onto the 30 km resolution research domain either by bilinear ... 3.2 Qualitative Sensitivity Analysis. Qualitative SA is often employed in preliminary uncertainty quantification experiments to reduce parameter dimensionality by screening out parameters that have negligible influences on model responses from all ...
Qualitative research methods are increasingly recognized for their importance in healthcare-related research, particularly in contextualizing social and cultural realities that impact human behavior (Al-Busaidi et al., 2008; Renjith et al., 2021).There is a growing interest in and acceptance of qualitative research approaches in the health sciences, both as stand-alone methodologies and ...
Sensitivity Analysis is used to understand the effect of a set of independent variables on some dependent variable under certain specific conditions. For example, a financial analyst wants to find out the effect of a company's net working capital on its profit margin. The analysis will involve all the variables that have an impact on the ...
Sensitivity analysis, also referred to as simulation analysis, is a technique employed in financial modeling to determine how different values of a set of independent variables can influence a particular dependent variable under certain specific conditions and assumptions. It is used to ascertain how the overall uncertainty in the output of a ...
Several researchers have highlighted the potential influence of the participants on the research process. Drawing upon fieldwork conducted in Fiji, Laverack and Brown (2003) noted how different cross-cultural dynamics between the researcher, interpreter and participants can influence the outcome and success of qualitative research. Laverack and Brown's (2003) experience demonstrated the need ...