Thank you for visiting nature.com. You are using a browser version with limited support for CSS. To obtain the best experience, we recommend you use a more up to date browser (or turn off compatibility mode in Internet Explorer). In the meantime, to ensure continued support, we are displaying the site without styles and JavaScript.
- View all journals
- ADVERTISEMENT FEATURE Advertiser retains sole responsibility for the content of this article
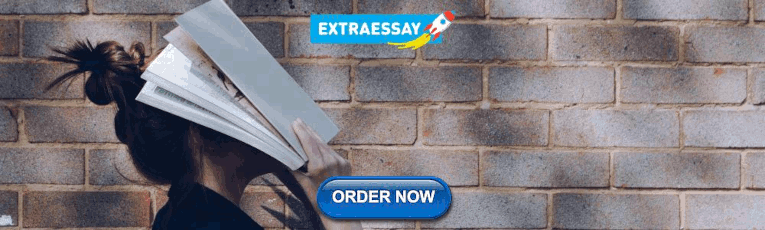
A direct view of DNA repair and beyond
Produced by
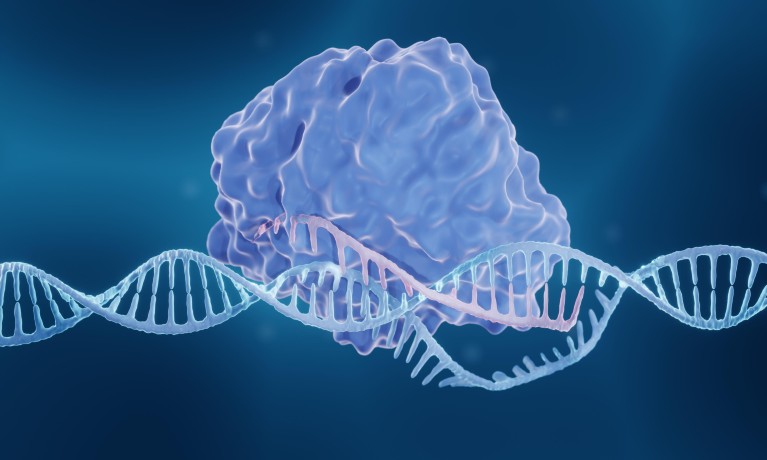
Dynamic single-molecule offers a direct view of protein interactions in real time. Credit: Artur Plawgo/ Getty Images
Guarding genes against damage is a crucial and complex job, so it’s to be expected that cells have several mechanisms to repair DNA breakages, each providing support under different circumstances.
For many of these pathways, scientists have long known the proteins involved. This knowledge came primarily from traditional biochemical assays, which identify the molecules that bind to DNA during repair, but cannot explain the intermediate and dynamic protein activity — often missed because of the heterogeneous assay outputs. It’s like knowing the ingredients for a dish but having no idea how they combine to produce the end result.
“Observing the dynamics of DNA-interacting proteins, rather than their static structure, can generate ground-breaking mechanistic insights,” says Andrea Candelli, chief scientific officer of life sciences company, LUMICKS.
The reward for unlocking the mysteries of DNA repair could be significant. For instance, women with defective versions of the BRCA repair proteins have a much higher risk of aggressive breast and ovarian cancers. These cancers instead rely on the PARP repair pathway, making this back-up pathway a solid target for anti-cancer treatments.
The key to building on this success for a wider range of cancers is understanding the delicate, fleeting interactions between proteins and DNA that drive complex repair processes. “The ability to visualize enzymes at a single molecule level has been transformative,” says geneticist, Simon Boulton from the Francis Crick Institute in London. He uses dynamic single-molecule imaging, which offers a chance to see activity in real time and get direct proof of the mechanisms at work.
“Single-molecule techniques deepen our understanding of disease through these mechanisms, which could yield new insights into cancer development and identify new targets for treatment," Candelli says.
Solving a 30-year mystery
Boulton became interested in dynamic single-molecule analysis when studying RAD51 — an enzyme regulated by BRCA2 to repair double-strand DNA breaks. Multiple RAD51 proteins bind along the length of broken single-stranded DNA to form filaments, which allows the damaged strands to be restored by lining up with their complete strands. Although RAD51 and similar proteins had been implicated in this process for three decades, how the filaments formed was a mystery.
“We essentially hit an impasse in understanding detailed mechanisms because we were relying on biochemical assays, which just give you an endpoint. They don’t really tell you about the intermediate stages and the dynamics of the system,” says Boulton.
The C-Trap dynamic single-molecule imaging system, produced by LUMICKS, allowed his team to see the activity in real time. The C-Trap uses optical tweezers to hold beads attached at either end of DNA strands, creating a rope bridge-like structure. With the DNA pinned in place, it is straightforward to trace the motion of fluorescently-labelled proteins under a microscope and view their activity.
This imaging showed that smaller proteins bind to the end of the filament — to stabilize the RAD51’s DNA binding, before detaching and repeating the process further along. This, in turn, allows the filament to grow 1 . “We would never have known that if we hadn’t seen these proteins act in real time at a single molecule level,” says Boulton. “This work was transformative: it explained findings from the last 30 years. Finally, we were able to nail how this complex works.”
What makes this imaging tool so unique?
Award-winning tech
The C-Trap system takes advantage of the power of optical tweezers, a biophysical technique that uses laser beams to control the movement of microscopic objects. This light-trapping approach was recognized by the 2018 Nobel Prize in Physics, although its adoption in molecular biology remained limited.
“Optical tweezers were very much in the realm of hardcore biophysics until recently,” says Boulton. But, even as a geneticist, he has found the C-Trap set-up easy to use: a unique yet simple tool. “It’s been designed so people who aren’t conventional biophysicists can quickly learn to use the system and start their experiments.”
The system is not limited to observing proteins at work. The versatility of the optical tweezers means that researchers can also manipulate and measure the DNA’s structure. When the DNA strands were stretched by the tweezers, Boulton could see the tension drop after RAD51 was added. As the protein helped to unwind the helix and make it longer, it resulted in the observed decreased force. Other researchers have used this method to understand how the accuracy of CRISPR/Cas9 gene editing changes as DNA is distorted under strain 2 . These insights could help to improve the process for more accurate editing in vivo .
“Nobody had seen that before”
Following success with RAD51, Boulton applied the same approach to another important DNA repair protein, HELQ. His lab’s previous work showed which other molecules HELQ interacts with, but not how. With the C-Trap, he could see individual molecules of HELQ travelling along single DNA strands, unwinding them from their usual helix shape. Surprisingly, HELQ travels much faster along the DNA when it forms a complex with RAD51, raising new questions about this process and bringing previously unknown functions to light 3 .
It also revealed that HELQ has another role: it can zip up two aligning strands of DNA to form the double helix. HELQ tethers to proteins that cover the single-stranded DNA, and then strips away this layer to facilitate the joining of complementary strands. This process is an alternative to the BRCA2-driven mechanism for repairing double-strand breaks in DNA and could be a useful target for new cancer therapies.
“It was really cool — nobody had seen that before,” says Boulton. “We could then show in cells that this activity is important in several DNA repair processes.”
Future insights
Analysing one molecule at a time provides a useful viewpoint into how proteins interact with DNA. Boulton is convinced that it represents an indispensable investment: “It’s definitely worth the time commitment to gain those dynamic insights.”
The C-Trap approach even works on one of the toughest targets — the BRCA2 protein itself. It is about 10 times the size of RAD51, which makes it difficult to work with. Purified BRCA2 has been available only since 2010, so the subject of just over a decade of biochemical investigation 4 .But now, Boulton can look at individual molecules, such as RAD51 or HELQ, interacting with DNA. “It’s a miracle we’ve been able to do this,” he says.
While this work is still preliminary, Boulton is clear that dynamic single-molecule imaging is leading to significant, previously unreachable discoveries. He hopes to unravel many more mysteries of the enzymes involved in DNA repair and beyond. “We’ve gained some interesting and unexpected insights into how BRCA2 behaves. That only came from direct visualization.”
Find out more about how dynamic single-molecule microscopy can advance your research at https://lumicks.com/applications/dna-binding-proteins/
Belan, O., et al. Mol Cell , 81 :1058–1073 (2021)
Google Scholar
Newton M., et al. Nat Struct & Mol Biol , 26 : 185–192 (2019)
Anand, R., et al. Nature , 601 : 268–273 (2022)
Jensen, RB., et al. Nature , 467 : 678–683 (2010)
Download references
Quick links
- Explore articles by subject
- Guide to authors
- Editorial policies
Loading metrics
Open Access
Peer-reviewed
Research Article
A quantitative modelling approach for DNA repair on a population scale
Roles Conceptualization, Data curation, Formal analysis, Investigation, Methodology, Software, Validation, Visualization, Writing – original draft, Writing – review & editing
Affiliation Université Paris-Saclay, CEA, CNRS, Institute for Integrative Biology of the Cell (I2BC), Gif-sur-Yvette, France

Roles Conceptualization, Data curation, Investigation, Resources, Supervision, Validation, Writing – original draft, Writing – review & editing
Roles Conceptualization, Formal analysis, Investigation, Methodology, Resources, Validation, Writing – original draft, Writing – review & editing
Roles Conceptualization, Funding acquisition, Investigation, Project administration, Resources, Supervision, Validation, Writing – original draft, Writing – review & editing
* E-mail: [email protected]
- Leo Zeitler,
- Cyril Denby Wilkes,
- Arach Goldar,
- Julie Soutourina
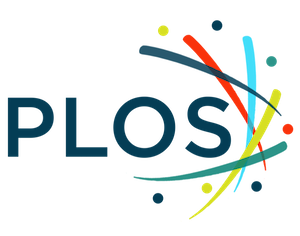
- Published: September 12, 2022
- https://doi.org/10.1371/journal.pcbi.1010488
- Peer Review
- Reader Comments
27 Oct 2023: The PLOS Computational Biology Staff (2023) Correction: A quantitative modelling approach for DNA repair on a population scale. PLOS Computational Biology 19(10): e1011602. https://doi.org/10.1371/journal.pcbi.1011602 View correction
The great advances of sequencing technologies allow the in vivo measurement of nuclear processes—such as DNA repair after UV exposure—over entire cell populations. However, data sets usually contain only a few samples over several hours, missing possibly important information in between time points. We developed a data-driven approach to analyse CPD repair kinetics over time in Saccharomyces cerevisiae . In contrast to other studies that consider sequencing signals as an average behaviour, we understand them as the superposition of signals from independent cells. By motivating repair as a stochastic process, we derive a minimal model for which the parameters can be conveniently estimated. We correlate repair parameters to a variety of genomic features that are assumed to influence repair, including transcription rate and nucleosome density. The clearest link was found for the transcription unit length, which has been unreported for budding yeast to our knowledge. The framework hence allows a comprehensive analysis of nuclear processes on a population scale.
Author summary
As DNA encodes our very identity, it has been subject to a plethora of studies over the last century. The advent of new technologies that permit rapid sequencing of large DNA and RNA samples opened doors to before unknown mechanisms and interactions on a genomic scale. This led to an in-depth analysis of several nuclear processes, including transcription of genes and lesion repair. However, the applied protocols do not allow a high temporal resolution. Quite the contrary, the experiments yield often only some few data signals over several hours. The details of the dynamics between time points are chiefly ignored, implicitly assuming that they straightforwardly transition from one to another. Here, we show that such an understanding can be flawed. We use the repair process of UV-induced DNA damage as an example to present a quantitative analysis framework that permits the representation of the entire temporal process. We subsequently describe how they can be linked to other heterogeneous data sets. Consequently, we evaluate a correlation to the whole kinetic process rather than to a single time point. Although the approach is exemplified using DNA repair, it can be readily applied to any other mechanism and sequencing data that represent a transition between two states, such as damaged and repaired .
Citation: Zeitler L, Denby Wilkes C, Goldar A, Soutourina J (2022) A quantitative modelling approach for DNA repair on a population scale. PLoS Comput Biol 18(9): e1010488. https://doi.org/10.1371/journal.pcbi.1010488
Editor: Carl Herrmann, Heidelberg University, GERMANY
Received: March 30, 2022; Accepted: August 12, 2022; Published: September 12, 2022
Copyright: © 2022 Zeitler et al. This is an open access article distributed under the terms of the Creative Commons Attribution License , which permits unrestricted use, distribution, and reproduction in any medium, provided the original author and source are credited.
Data Availability: All code that was used for the study is available on https://github.com/leoTiez/jmak .
Funding: LZ was supported by the CEA NUMERICS program, which has received funding from European Union’s Horizon 2020 research and innovation program under the Marie Sklodowska-Curie grant agreement No 800945. The work was supported by the Fondation ARC (PGA1 RF20170205342) and Comité Ile-de-France - La Ligue Nationale Contre le Cancer. The funders had no role in study design, data collection and analysis, decision to publish, or preparation of the manuscript.
Competing interests: The authors have declared that no competing interests exist.
This is a PLOS Computational Biology Methods paper.
Introduction
As DNA represents the hereditary unit of life, maintaining its integrity is vital for every organism’s survival. A large variety of different genotoxic factors have the potential to damage the molecular structure of DNA. Among others, it has been shown that UV light induces Cyclobutane Pyrimidine Dimers (CPDs). Nucleotide Excision Repair (NER) is an evolutionarily conserved repair mechanism in Saccharomyces cerevisiae that can remove a broad range of damage, including CPDs [ 1 ]. NER is conventionally divided into two subpathways. The first recognition mechanism is named Global-Genome Repair (GGR) and can be observed along the entire genome. DNA damage is recognised directly by protein association. There is evidence that protein loading is promoted through interactions with chromatin remodellers that change the nucleosome density or distribution [ 2 , 3 ]. The second pathway is restricted to actively transcribed regions; hence the name Transcription-Coupled Repair (TCR). Expressed genes exhibit quicker repair than silent downstream regions [ 4 ]. This promoted the assumption that TCR is more efficient than GGR, although constrained to the transcribed strand (TS) [ 5 , 6 ]. TCR is initiated by lesion-blocked RNA polymerase II (Pol II) which cannot continue elongation [ 7 ]. Thus, a potential link of TCR to transcription rate has been indicated by several studies [ 8 , 9 ]. After recognition, TCR and GGR use the same incision and nucleotide replacement mechanism. DNA is incised to either side of the lesion leaving an approximately 30-nucleotide gap, which is subsequently replaced and ligated (for a comprehensive description and analysis, see the review by [ 10 ]).
Our understanding of such processes in living cells has been largely enhanced by Next Generation Sequencing (NGS). It allows the identification of enriched loci of a selected property on a genome-wide scale. Among others, it has been applied to investigate the CPD repair mechanisms in vivo through analysing temporal changes of the damage distribution. [ 8 ] obtained high-resolution CPD-seq data that are often used as a benchmark reference (see for example [ 9 ]). Their analysis indicates that single nucleosomes and DNA-bound transcription factors have an impact on the CPD formation. Moreover, they point out that repair is seemingly influenced by the CPD position with respect to the nucleosomal dyad as well as the transcription rate of genes. Another major contribution has been done by [ 9 ]. Their protocol for eXcision Repair sequencing (XR-seq) revealed strong TCR at early time points which is followed by repair in non-transcribed regions. Furthermore, [ 11 ] and [ 12 ] utilised CPD data to compute repair rates in different areas, which indicated that the process is highly organised into genomic regions. By using GGR-deficient strains, they show that repair is changing globally when the subpathway is repressed. This is compared to the distribution of repair proteins and histone modifications.
Unfortunately, due to costs and constraints in the experimental protocol, NGS data sets contain barely more than a few time points over several hours. Consequently, previous studies could only derive limited conclusions, e.g. the absolute change at different loci. We argue that such an analysis ignores valuable information about the transitional process from one time point to another. Furthermore, it should be emphasised that sequencing signals are commonly understood as representing an average cell. We advocate an interpretation where the data is explained as the product of many independent cells. Thus, the repair dynamics are driven by non-interfering stochastic processes. Without assuming any specific molecular mechanism, we hypothesise that they are composed of two independent random variables namely accessibility to the lesion governing repair times and Brownian motions of proteins through the nucleus to find their target. It has been shown by several studies that proteins exhibit a range of different movements in the nucleus [ 13 – 18 ]. Diffusion has also been investigated and modelled in context of DNA repair for the Rad4-Rad23 complex [ 19 ]. Although protein movements have been used to understand specifics of NER kinetics, a framework to quantitatively describe population-based sequencing data is still lacking.
Our approach and main results can be summarised as follows. The sparse temporal resolution of NGS data sets makes it necessary to incorporate precise assumptions about the nature of the process in order to recover missing information. Here, we present a computational framework to analyse DNA repair kinetics. We derive a function to study CPD removal as a Poisson point process of independent cells. Since we do not impose any molecular mechanism, we obtain a simple and minimal representation. The parameters can be derived using the well-studied physical model for phase transitions, which is described in detail by Kolmogorov [ 20 ], Johnson and Mehl, [ 21 ], and Avrami [ 22 – 24 ] (KJMA model). It can be conveniently transformed to a linear regression problem and is therefore executable on almost any ordinary computer. A consequence that is implied by our repair model is that the observed change of CPDs is non-constant over time. To our understanding, this has not been explicitly incorporated in the analysis of NGS data. The model validity can be verified with independently probed XR-seq data [ 9 ]. We are able to recover particular aspects of the NER kinetics despite our broadly applicable assumptions. We ultimately use the framework to predict correlations with other nuclear processes. It is able to establish interrelationships that are supported by other studies such as nucleosome density [ 8 , 12 ] and transcription rate [ 8 ]. It is most surprising, however, that we find the strongest correlation with transcription unit (TU) length, which is a new finding for budding yeast to our knowledge. Interestingly, our model allows also an alternative understanding of the data in which repair positions grow as patterns in a population. Although the analysis has been demonstrated for DNA repair, it can be applied to any process that can be modelled by an irreversible binary state transition. The source code is available on GitHub: https://github.com/leoTiez/jmak [ 25 ].
Modelling DNA repair
In a single cell, CPD damage describes the mispairing of two adjacent pyrimidine nucleobases. Instead of establishing hydrogen bonds to the opposite strand, they cause two consecutive nucleotides to bind to each other. Consequently, there can be maximally one lesion per position. This results in a zero-one (i.e. damaged - repaired ) state space per position and per cell. During ongoing repair, lesions are removed, and positions change subsequently their state to repaired . It can be assumed that this process is stochastic and involves to some extent unpredictable noise. If we could repeatedly and independently measure the repair times for a single position in a single cell, we could distribute the measurements over a timeline ( Fig 1B ). This type of data can be investigated by a Poisson point process, which allows the derivation of a predictive function that expresses the probability of repair over time. If we would temporally discretise the data over larger bins, all repair time points within a bin were aggregated together. In the following, we assume that this is given by the change of the CPD-seq signal, as the amplitude decrease at an arbitrary position must explain the number of cells that have repaired their lesions. Consequently, the data represent the process over the entire cell population. We conjecture that the dynamics are independent between cells. The CPD-seq data can be therefore alternatively interpreted as a two-dimensional grid: one axis representing the cells and the other the nucleotide positions ( Fig 1C ). We understand NGS signals not as representing an average cell but the mutual effect of multiple independent cells.
- PPT PowerPoint slide
- PNG larger image
- TIFF original image
(A) The repair proteins’ search process (purple with arrow) to the lesion (red) can be understood as a Brownian motion. Repair happens after association with some delay, which we assume to be another random variable (waiting time). (B) If we could repeatedly measure the repair times for one position in a single cell individually, we could distribute the measurements over a timeline. This can be analysed by a stochastic point process. Binning the timeline should correspond to the observable change of CPDs in a given window. (C) We presume that this stochastic repair process happens independently in each cell. Therefore, CPD-seq data can be understood as an accumulation of several experiments. This allows an understanding of the signal as a two-dimensional grid. Since there can be only one lesion per position per cell, we understand the amplitude of the signal as a surrogate for the number of cells that contain a lesion at this locus (marked in green).
https://doi.org/10.1371/journal.pcbi.1010488.g001
Applying the model to the data
We formulated three expectations in order to prove the model validity. Firstly, we required that the estimated repair dynamics need to be in line with independently probed data; secondly, we thought it to be indispensable to recover NER-specific features that were not implicitly incorporated into the model; and lastly, Eq 3 needs to make verifiable predictions about other factors that influence repair. CPD data for the parameter estimation were taken from [ 8 ] (0, 20, 60, and 120 minutes after irradiation) and divided into different segments where λ( t ) was assumed to be spatially constant. We distinguish between TCR regions, which are the TS of genes that presumably exhibit TCR; the NTS of TCR regions; and non-TCR areas, which are composed of transcripts where the effect of TCR is not evident and intergenic regions (see S1 Appendix as well as S1 and S2 Figs). Moreover, TS and NTS of TCR regions were equally divided into start, centre, and end. Subsequently, CPD data was converted to represent repair using Eq 7 (in the following also called repair data). An example of the predicted repair dynamics is given in S3 Fig . We also compared the presented results with the analysis of a more traditional segmentation into TS and NTS of all genes as well as intergenic regions ( S3 Appendix ).
XR sequencing provides a snapshot of currently ongoing repair in the cell culture, and it therefore represents an independent angle on CPD removal. It should correspond to the derivative of Eq 3 (given in Eq 6 ). XR-seq signals were taken from [ 9 ] (5, 20, and 60 minutes after irradiation) and segmented as for the CPD-seq data. We assumed that a surrogate for ongoing repair can be additionally derived from the CPD-seq data themselves by calculating the damage decrease per time ( S4 Appendix Eq 4). This was used as a baseline value for the correlation between model and data. As we assumed a non-linear interrelationship, we used the distance correlation (DC) as a correlation measurement ( S4 Appendix ). Strikingly, the predicted repair rates correlate clearly better (DC = 0.441) than the actual data (DC = 0.209) (compare Fig 2A with 2B ; see S2 Table ). Moreover, the model predictions align fairly well with the XR data (exemplified in S4 Fig ). We hence surmise that Eq 3 is in agreement with independently probed data.
The values at 5 minutes are given in blue, 20 minutes are coloured yellow, and 60 minutes are green. The plots show that the distance correlation between prediction and XR-seq data is even higher than for the CPD-seq data. (A) Predicted repair rates with respect to the XR-seq data exhibit a considerably strong correlation (DC = 0.441). Predictions are given as the square root of the model prediction. This reduces the effect of increasing variance with larger derivatives. (B) The repair rates derive from the data as a function of XR-seq values shows a weaker correlation (DC = 0.209).
https://doi.org/10.1371/journal.pcbi.1010488.g002
Despite the fact that we model time-dependent repair, we nevertheless do not incorporate two (potentially competing) repair mechanisms, i.e. TCR and GGR. It is commonly presumed that CPD removal through TCR is quicker than by GGR [ 4 , 26 ]. Moreover, as GGR acts genome-wide, they are spatially non-exclusive for genes. Indeed, we can recover the cumulative effect when averaging the repair evolution for a group of segments, e.g. the start of TCR regions. The beginning of TCR areas is almost solely repaired by a single mechanism ( Fig 3A ). The contribution of this pathway is decreasing as a function of distance from the transcription start site (TSS). Instead, a later acting mechanism becomes increasingly observable ( Fig 3B and 3C ). Lastly, lesions in non-TCR regions are only detected by the late-acting process ( Fig 3D ). This is even the case despite the fact that non-TCR areas also include transcripts. We deduce that these two distinct pathways show the effect of TCR and GGR along the gene. We were therefore able to separate the effect of two distinct NER processes without involving any particular mechanism. Parameter distributions (i.e. m , τ , and θ ) that create these repair kinetics are exemplified in Fig 4 . Surprisingly, the NTS possesses different dynamics whilst not exhibiting any difference between start, centre, and end ( S5 Fig ). The average repair fraction is much lower than for all other areas ( θ ≈ 0.6 instead of ≈0.8). Moreover, we observe a subtle early increase of the derivative, indicating a larger presence of early repair in comparison to non-TCR regions. It is difficult to analyse this trend without additional experiments. It could simply be the impact of neighbouring overlapping regions. However, these results could equally point to different repair dynamics on the NTS.
Dashed lines give the mean whereas the shaded areas show the standard deviation. Blue and orange represent the repair fraction and the repair rate (derivative of the repair fraction), respectively. (A) The start of TCR regions is repaired early after irradiation, demonstrating the effect of TCR. (B) At the centre of TCR areas, we can observe the mutual effect of TCR (first peak) and GGR (second peak). (C) GGR’s contribution increases whilst the impact of TCR becomes less important towards the end of the gene. (D) Non-TCR regions are solely repaired by GGR. Therefore, repair is expected at later time points during the process.
https://doi.org/10.1371/journal.pcbi.1010488.g003
The x and y-axis give the values of m and θ , respectively. The size of the circles show 1/ τ : the larger the circle, the shorter the characteristic time. Significant interrelationships are marked with a red frame. (A) and (B) are coloured with respect to NET-seq data (pink/low to green/high) for the centre of TS and NTS; (C) and (D) indicate the nucleosome density (turquoise/low to brown/high) for non-TCR regions and the NTS centre; (E) and (F) show the distribution with respect to the TU length (red/small to blue/big) for the centre of TS and NTS.
https://doi.org/10.1371/journal.pcbi.1010488.g004
Lastly, we extended the analysis to make predictions about influencing factors for CPD repair in vivo . Previous studies published various measurements of different nuclear properties that could possibly interact with lesion removal dynamics. To assess the predictive power of our model, we opted to analyse a link to transcription rate [ 8 , 9 ] and nucleosome density as representing chromatin structure [ 8 , 12 , 27 ]. We also investigated a link to TU length and the relative distance to centromeres and telomeres as possible unreported affecting parameters. We used the NET-seq signal produced by [ 28 ] as a surrogate for transcription rate without UV irradiation. The TU length was measured by [ 29 ]. Nucleosome data after UV treatment were acquired by [ 12 ]. We excluded regions outside a reasonable parameter range from the subsequent analysis (see Methods and materials and S2 Appendix ; the number of removed regions is given in S1 Table ). Correlations with the model parameters were verified with a significance test, during which we compared a binary classification model with the performance of a random model (see Methods and materials and S5 Appendix for more information; the working of the classifier is explained in S6 Fig ). Interrelationships to other sequencing data are elaborated and discussed in S6 Appendix . All data distributions are given in S7 – S12 Figs. The results differed considerably depending on the genomic area and context ( S3 Table ). TCR has been repeatedly investigated with respect to transcription rate, and it is surmised to be positively correlated. Hence, higher transcription yields quicker repair, whereas low transcription results in slower lesion removal [ 8 , 9 ]. Our model confirms these findings ( Fig 4A and 4B ). Non-TCR segments and the start of the TS seem to be starkly influenced by the nucleosome density, whereas all other areas do not show a strong correlation ( Fig 4C and 4D ). The clearest results, however, were obtained by the TU length. Both, TS ( Fig 4E ) and NTS ( Fig 4F ) are clearly influenced. The TU length is therefore likely to be contributing to the lesion removal dynamics. This is an unreported finding for budding yeast to our knowledge. The developed quantitative framework has hence the potential to identify established as well as new interrelationships. Importantly, a correlation with the distance to telomeres and centromeres ( Eq 9 ) did not indicate a significant link. This shows that the applied method is selective for certain correlations ( S12 Fig ).
The few time points of NGS data sets require a temporal model to recover missing information between data samples. In this work, we developed a computational approach to describe the DNA repair kinetics on a population scale. We recover region-specific properties based on the genome-wide distribution of DNA damages. We assume a mixture of two stochastic processes (diffusion and lesion accessibility) that collectively explain the change of CPD data over time. Parameters of the derived equation can be estimated with the KJMA model, that is conveniently converted to a linear regression problem. This allowed the analysis of the temporal process as a whole rather than only comparing single time points. Importantly, it points out that the signal changes non-linearly over time. This is expected from a biological point of view, as TCR and GGR are commonly seen as acting within different time scales. However, it should be emphasised that it has not been incorporated in the analysis of temporal changes in sequencing data to our knowledge. The model therefore accounts specifically for dynamics on a population scale. Moreover, the derivative ( Eq 6 ) provides key information about active ongoing repair. It thus permits linking CPD-seq data—showing the DNA damage distribution over the genome—and XR-seq data of excised DNA fragments generated by repair. This provides strong support for the validity of the model. Even though Eq 3 represents repair only with one mechanism per region, the combined effect of TCR shortly after UV treatment and GGR at later time points can be recovered when considering the average over several areas. The model can be readily used to uncover interrelationships between repair parameters and genomic contexts. Our outcomes are consistent with known influencing factors such as transcription rate and nucleosome density. Remarkably, the clearest link was established between the repair dynamics within genes and their length. To our knowledge, this is an unreported finding for budding yeast. In the following sections, we discuss the relevance of our approach and results within the context of previous publications.
Applying the CPD repair model
Several studies proposed temporal models for UV-induced lesion repair on different levels of detail. [ 30 ] represented NER kinetics in human cells using a Markov-Chain Monte-Carlo approach. It explains the removal of 6–4 photoproducts on a single-cell scale through the random and reversible assembly of repair complexes. A similar model was proposed by [ 31 ]. Interestingly, though, they derive very different conclusions, as they suggested that random or pre-assembly of repair proteins is unfavourable. Despite a great level of detail of both models, they are incapable to make region specific predictions. Moreover, as both models are based on microscopy data, they do not explain temporal changes in genome-wide sequencing data on a population scale. A Monte Carlo approach to explain the damage distribution and subsequent repair induced by ionised irradiation in a single cell was proposed by [ 32 ]. It also incorporates the collective effect of NER and base excision repair (BER), therefore accounting for potentially competing mechanisms. It should be stressed that the lesion type is considerably different. Again, the predictions are location unspecific. [ 33 ] provided a different angle by presenting a protein-protein interaction landscape of NER components in yeast. Predictions about the repair efficiency in different regions were not established. To our knowledge, our model is the first that accounts for region specific changes in population-based data.
As there are only three time points, a data-driven machine learning model is prone to overfitting. In order to find a reasonable representation of the data, we incorporate explicit suppositions to derive Eq 3 . To be precise, we presume that repair times follow a Poisson point process, and the non-constant rate λ( t ) can be described by a mixture of protein diffusion and repair times. This restricts the trajectory to an S-shape. Our understanding of the process makes two independence assumptions. Firstly, there is non-interfering DNA repair between cells; and secondly, protein diffusion is independent of lesion accessibility or repair time.
It is important that the points in a Poisson process are (sufficiently) independent from each other [ 34 ]. The repair times that are distributed along the timeline (e.g. as in Fig 1B ) should hence symbolise values of independent stochastic variables. We find the presumption of non-interfering repair between cells trivial. Saccharomyces cerivisiae are single-cell organisms and should thus react independently to DNA damage. Moreover, yeast cultures were grown in rich medium after UV treatment, precluding any limitations for growth [ 8 ]. We conjecture the independence presumption to be reasonable.
The Poisson process is governed by the rate parameter λ( t ). It is presumed that nearby genomic positions posses similar rates. Therefore, λ( t ) should have a slow spatial variation, and a segmentation of the CPD-seq data into similar behaving areas is possible. A similar binning approach was applied by [ 8 ]. The simplest model sets λ to a constant and therefore independent of time. In such a setup, we would expect to observe the largest signal change right after irradiation which subsequently slows down ( S13A Fig ). This could indeed resemble the beginning of the TS of TCR regions ( Fig 3A ). However, the majority of areas exhibits the strongest repair rates between 20 and 60 minutes. We conclude that a non-constant λ( t ) provides a broader applicability for explaining CPD repair.
The simplest representation of an irreversible transition between two states is given by an S-shaped function which contains at most one inflection point. It is important to emphasise that this function is not necessarily inversely symmetric around this point, meaning that the left side can be differently shaped than the right side. These requirements are fulfilled by the KJMA model, which is therefore a sensible choice (see S7 Appendix and S13 Fig ).
Analysing genomic properties which influence repair kinetics
TCR has been identified as a rapid repair pathway on the TS. Intergenic regions and the NTS exhibit significantly slower lesion removal, which was demonstrated on the genomic scale in yeast and human cells [ 8 , 9 , 27 , 36 , 37 ]. It remains an unsolved quest to find an interrelationship between TCR efficiency and transcription rate. Whilst the two parameters are indeed assumed to be correlated [ 8 , 9 ], some studies point out that TCR repairs CPDs efficiently at nearly all genes including those with a low transcription [ 27 ]. An in-depth analysis is still missing, and there is no clear consensus on how transcription rate is affecting repair. In this work, we compared the model predictions to gene expression. Our analysis clearly shows a significant correlation on the TS and is therefore supporting the common assumption ( S7 Fig ).
As repair proteins need to recognise and repair lesions on the DNA, it is conjectured that chromatin organisation can significantly modulate the efficiency of CPD repair [ 8 , 12 , 27 ]. However, previous studies were mostly scrutinising the positioning of damage at nucleosomes. CPD removal was shown to be less efficient at the dyad of strongly positioned nucleosomes in yeast [ 8 ]. Moreover, GGR on the NTS was asymmetrically inhibited in yeast and human cells with respect to the position within the nucleosome [ 27 ]. Even though nucleosome occupancy after UV treatment was already previously probed, the potential relationship of these data with CPD repair was not directly addressed [ 12 ]. Our results demonstrate a significant correlation between repair and nucleosome density in non-TCR regions ( Fig 4C ). We also discovered a clear influence on the beginning of TCR areas ( S8 Fig ).
Unexpectedly, our outcomes show a strong correlation between TU length and repair. Differences in transcription shutdown and restart after UV treatment relative to gene size were previously reported for human cells [ 38 ]. Both transcription regulation and efficient repair are necessary to orchestrate an effective cellular response to UV light. The restart of transcription to pre-irradiation levels is an important step at the final stages. However, a direct evaluation of lesion removal with respect to gene size was not performed. To our knowledge, this is a new finding for CPD repair in yeast. Due to our data pre-processing ( Eq 7 ), we can rule out that the result derives only from the fact that larger areas have a greater potential to include more damage. This is true due to two reasons. Firstly, we normalised the CPD value in each bin (e.g. beginning of the TS) by the number of pyrimidine dimers in the sequence as described in [ 8 ]. Secondly, and more importantly, we want to point out that the quotient in Eq 7 lets any length dependence and normalisation of the binned data vanish. Therefore, the values become automatically comparable due to the design of Eq 7 . It should be mentioned though that the regions of interest can become rather small when segmenting the gene into subareas. Influence or noise from neighbouring areas cannot be excluded. However, due to the fact that the same result can be obtained with a different segmentation ( S3 Appendix and S9 Fig ), we presume that it represents a genuine feature of the CPD removal mechanisms in yeast cells.
Lastly, we investigated a potential link to the distance relative to the centromere and telomere depending on which was closer to the region of interest ( S12 Fig ). A link to repair has not been proposed to our knowledge, which made it an interesting property to produce verifiable model predictions.
In conclusion, our work opens interesting perspectives for future research on DNA repair mechanisms and influencing genomic factors. New experimental data with increased temporal resolution will help to refine the model and analysis. The approach can be similarly used for other organisms including human cells. Moreover, it can be readily applied to sequencing data of any nuclear process that can be represented as a two-state system, and it is not restricted to repair.
Introducing the repair space—An alternative understanding of the data
We have discussed the model in detail with respect to a stochastic point process. We want to provide an additional interpretation that is motivated by the physical implications of the KJMA model. Next to assuming independence between cells, we conjecture in the following also independent repair within each cell ( S8 Appendix ). Moreover, we presume that CPD data were converted by Eq 7 .
Considering the two-dimensional grid in Fig 1C , the independence assumptions above permit us to re-order repair positions to patterns. Nevertheless, we restrict the re-grouping to stay within areas of interest which are assumed to behave homogeneously. The growth of patterns in the virtual repair space reminds strongly of the phase transition in solids which is described by the KJMA model.
Methods and materials
Parameter estimation and derivative.
Data processing
All experimental data that were analysed in this study comes from public databases (see overview in Table 1 ). CPD-seq data was taken from [ 8 ]. It contains two time courses with samples taken at t 1 ∈ {0, 60}min and t 2 ∈ {0, 20, 120}min, respectively. The location of transcribed areas was taken from [ 29 ]. Data signals were partitioned into different segments, i.e. the TS and NTS of TCR regions as well as non-TCR areas. For the latter, we combined both strands to one group. Consequently, the linear regression problem in non-TCR regions was required to find the best representation for both strands.
https://doi.org/10.1371/journal.pcbi.1010488.t001
All other sequencing data that were used for the correlation analysis were averaged over the size of the area of interest. Start, centre, and end of TS and NTS were linked to the same value to smooth out the potential influence of noise. For example, all subregions of a TS were associated to the same transcription rate. Moreover, both strands were compared to the same data, e.g. the TS and the NTS were related to the same nucleosome density. We noticed that the NET-seq signal amplitude decreases as a function of distance from the TSS ( S15 Fig ). This could possibly induce a TU length-specific bias that is not removed by taking the average over the TU length. We could verify, however, that the NET-seq data strongly correlates with independently probed Pol2 ChIP-seq data [ 41 ] ( S16 and S17 Figs). We therefore assume that it reasonably represents transcription rate, whilst allowing a direct comparison to the results obtained by [ 9 ] ( S9 Appendix ).
With the exception of nucleosome density, all biological data values possess a biased distribution. They strongly peak around a low value but contain large positive tails. To remove a potential bias introduced by outliers, we limited our analysis to the lower 95th percentile. As this procedure was applied to all data (except nucleosome density), we did not introduce a bias towards a certain model. Rather, we improved comparability. The only exception is the MNase-seq signal, as it is approximately normally distributed. We consider that trimming could introduce a bias rather than removing one.
We divide only by half of the length since the maximal distance ( d mere = 1) to both centromere and telomere should be the middle between them two.
Correlating repair dynamics to genomic contexts
This was compared to a random baseline model, for which classes were randomly shuffled to a given parameter triple ( m , β , θ ) during training. Data { X , c } were arbitrarily partitioned into learning and testing data sets. Every experiment was independently repeated 100 times to reduce the effect of any potential bias. We consider an interrelationship to be important if the prediction error of the true function is significantly lower than the error of the random model ( p < 0.00001% of a one-sided t-test). Moreover, we require that 90% of the prediction errors are below E < 0.5, which is the expected outcome of an unbiased coin-flipping experiment. This significance must be found in three out of the five evaluated k to indicate an interrelationship.
Supporting information
S1 appendix. determining tcr regions..
https://doi.org/10.1371/journal.pcbi.1010488.s001
S2 Appendix. Discussing the Effect of Data Transformation and Selection.
https://doi.org/10.1371/journal.pcbi.1010488.s002
S3 Appendix. Dividing the Data into Genes versus Intergenic Regions.
https://doi.org/10.1371/journal.pcbi.1010488.s003
S4 Appendix. Explaining the Correlation Between XR-seq Data and Repair Rate.
https://doi.org/10.1371/journal.pcbi.1010488.s004
S5 Appendix. Discussing the k NN Approach.
https://doi.org/10.1371/journal.pcbi.1010488.s005
S6 Appendix. Analysing Repair Kinetics in Context of Abf1 and H2A.Z Distribution.
https://doi.org/10.1371/journal.pcbi.1010488.s006
S7 Appendix. Comparing the KJMA Model With Other Approximations.
https://doi.org/10.1371/journal.pcbi.1010488.s007
S8 Appendix. Discussing the Model in Context of the Physical KJMA Model.
https://doi.org/10.1371/journal.pcbi.1010488.s008
S9 Appendix. Investigating a Link Between Transcription Rate and TU length.
https://doi.org/10.1371/journal.pcbi.1010488.s009
S1 Table. The number of models per region, before and after applying the requirements for parameter ranges.
IGR abbreviates intergenic regions.
https://doi.org/10.1371/journal.pcbi.1010488.s010
S2 Table. The DC between XR-seq and repair predictions / data for different experimental configurations.
https://doi.org/10.1371/journal.pcbi.1010488.s011
S3 Table. Number of non-random interrelationships between model parameters and sequencing data over k .
The table gives the number of k NN models that could find a correlation between model parameters and genomic context. k ∈ {5, 10, 20, 50, 100}. We defined a link to be significant if at least three out of five k find a non-random interrelationship.—means that data was not used in the given configuration. NET denotes NET-seq data, ND is nucleosome density, and meres give the relative distance to centromeres or telomeres. Suffixes S, C, and E denote start, centre and end of an area. NTCR are non-TCR areas. IGR are intergenic/non-transcribed regions.
https://doi.org/10.1371/journal.pcbi.1010488.s012
S1 Fig. Scheme of the segmentation setup.
The circles represent the number of cells with ongoing repair in the region. The arrows indicate the region and direction of transcription. The results in the paper follow the TCR setup. Here, only the first gene is considered as TCR area which shows more efficient repair than intergenic regions within the first 20 minutes after UV irradiation. All other parts are labelled as non-TCR region. Therefore, it spans from the end of the first gene to the end of the second. The gene configuration ( S3 Appendix ) partitions the genome into the traditional notion of transcribed and intergenic regions. The TU positions were determined by [ 29 ].
https://doi.org/10.1371/journal.pcbi.1010488.s013
S2 Fig. Relative repair distribution over genomic areas.
Relative repair in non-transcribed regions is chiefly lower than 20% within the first 20 minutes (88.95%). Genic areas with stronger repair dynamics are thus likely supported by TCR. For all other transcripts, we cannot exclude the possibility that they are exclusively repaired by GGR.
https://doi.org/10.1371/journal.pcbi.1010488.s014
S3 Fig. Example for model predictions.
Data points are given by solid dots. The blue dashed line represents the repair fraction predicted by the model (left axis). The orange dashed line shows the derivative (right axis). (A) The SNF6 gene can be well approximated. (B) However, GEM1 exhibits no repair within the first 20 minutes, which results in a switch-like behaviour. (C) This is better understood when showing the data points after transformation according to Eq 5 . A linear regression is difficult since they do not align.
https://doi.org/10.1371/journal.pcbi.1010488.s015
S4 Fig. Example for model prediction and XR-seq data over time.
XR-seq data (points) and the predicted repair rate (dashed lines) are exemplified for genes BDH1 (orange) and BDH2 (blue). When re-scaling XR data and repair rate prediction between 0 and 1, both follow clearly similar trends. BDH2 has its largest XR-seq value at 20 minutes post-irradiation, whereas BDH1 shows biggest repair rates after 5 minutes. This is indeed captured by the model.
https://doi.org/10.1371/journal.pcbi.1010488.s016
S5 Fig. The evolution of repair along the NTS.
The average repair evolution for the NTS (blue dashed line) shows a much lower repair fraction (≈0.6) than the other areas. Moreover, the repair rate (orange dashed line) indicates repair at early time points. This could be caused by possible overlapping transcripts or by antisense-transcription-coupled repair. Shaded areas show the standard deviation. The repair trajectory is the same for (A) the beginning, (B) the centre, and (C) the end of the NTS.
https://doi.org/10.1371/journal.pcbi.1010488.s017
S6 Fig. Example of the learnt function between model parameters and genomic context.
(A) The learnt parameter distribution and the associated class for the true model after applying a principle component transformation. The x and y-axis give the first and second principle component, respectively. Red represent large genes, whereas blue shows low values. (B) The error distribution for the predictions follows the expected outline given by the learnt function in (A). The blue and red circles give values that were classified as short but were actually large and vice versa, respectively. White points are correctly classified. The right bar shows the error distribution along the colour axis, i.e. over estimated, correctly classified, and underestimated values from top to bottom. The lower histogram shows the distribution of overall correctly and incorrectly classified values. (C, D) The learnt parameter map and the error distribution of the random model.
https://doi.org/10.1371/journal.pcbi.1010488.s018
S7 Fig. Model parameters with respect to transcription rate.
Our results for the transcription rate support the hypothesis that it influences repair on the TS. The x and y-axis give the values of m and θ , respectively. The size of the circles show 1/ τ : the larger the circle, the shorter the characteristic time. Significant interrelationships are marked with a red frame.
https://doi.org/10.1371/journal.pcbi.1010488.s019
S8 Fig. Model parameters with respect to nucleosome density.
Nucleosome density is seemingly influencing repair in non-transcribed/non-TCR regions as well as the beginning of the TCR TS and the TS in the gene setup. The x and y-axis give the values of m and θ , respectively. The size of the circles show 1/ τ : the larger the circle, the shorter the characteristic time. Significant interrelationships are marked with a red frame.
https://doi.org/10.1371/journal.pcbi.1010488.s020
S9 Fig. Model parameters with respect to size.
The size is clearly influencing repair for both, TS and NTS in the TCR and gene configuration. The x and y-axis give the values of m and θ , respectively. The size of the circles show 1/ τ : the larger the circle, the shorter the characteristic time. Significant interrelationships are marked with a red frame.
https://doi.org/10.1371/journal.pcbi.1010488.s021
S10 Fig. Model parameters with respect to Abf1.
The results for Abf1 are more ambiguous. Although we can find a significant correlation to non-TCR regions as expected in the TCR setup, the picture is less clear for the gene configuration. The x and y-axis give the values of m and θ , respectively. The size of the circles show 1/ τ : the larger the circle, the shorter the characteristic time. Significant interrelationships are marked with a red frame.
https://doi.org/10.1371/journal.pcbi.1010488.s022
S11 Fig. Model parameters with respect to H2A.Z.
Similar to Abf1, the correlations with H2A.Z do not allow a straightforward interpretation. Whilst repair in all areas in the TCR configuration is seemingly linked to H2A.Z, this tends to be restricted to the TS and NTS in the gene setup. The x and y-axis give the values of m and θ , respectively. The size of the circles show 1/ τ : the larger the circle, the shorter the characteristic time. Significant interrelationships are marked with a red frame.
https://doi.org/10.1371/journal.pcbi.1010488.s023
S12 Fig. Model parameters with respect to cetromeres and telomeres.
With the exception of the TS in the gene setup, the distance to telomeres or centromeres (shortened with meres ) does not affect repair dynamics. The x and y-axis give the values of m and θ , respectively. The size of the circles show 1/ τ : the larger the circle, the shorter the characteristic time. Significant interrelationships are marked with a red frame.
https://doi.org/10.1371/journal.pcbi.1010488.s024
S13 Fig. Comparison of the KJMA model with other functional descriptions.
(A) A homogeneous Poisson repair process with λ( t ) = c has the strongest change in the beginning which subsequently flattens out. In most investigated regions, such a behaviour is not observed. (B) We compared the performance of different models to describe the data, which is exemplified for gene LDB16 ( YCL005W ). Black dots represent the repair data (converted CPD-seq data, see Eq 7 ), whereas the best fit of each model is given in dashed lines. (C) We applied the mean-squared error (MSE, S7 Appendix Eq 1) to compare the performance of the models with respect to the data. The KJMA model and the Hill equation perform undoubtedly better than simpler models like linear or logistic regression. Nevertheless, the Hill equation describes the data slightly yet significantly better. It should be emphasised though that the performance difference is marginal. Width of the shaded areas represents the number of genes that yielded the corresponding error, which is mirrored at the vertical line. The centre horizontal line with the corresponding numbers give the error median. The top and bottom horizontal lines show maximum and minimum, respectively. (D) Despite the fact that non-TCR regions are not expected to show an observable impact by TCR, the Hill equation indicates two mechanisms that act at different time points. Dashed lines give the mean whereas the shaded areas show the standard deviation. Together with the fact that there is no straightforward interpretation of the Hill equation in context of repair evolution, we conclude that Eq 3 is a sensible choice.
https://doi.org/10.1371/journal.pcbi.1010488.s025
S14 Fig. Example of the KJMA model.
The KJMA model includes two governing parameters (as the original model does not involve θ ), which are exemplified in (A) for m and (B) for τ . Eq 3 can be conviniently converted to a linear regression problem which is shown for the parameter settings of (A) in (C) and for the parameters of (B) in (D).
https://doi.org/10.1371/journal.pcbi.1010488.s026
S15 Fig. Example of sequencing data representing transcription rate.
The example of the NET-seq signal in comparison to the Pol2 ChIP-seq data probed by [ 41 ] shows that Pol2 exhibits a constant augmentation of the signal amplitude at transcribed regions, whereas NET-seq data decrease as a function of distance from the TSS. The Pol2 data is coloured in green, whereas NET-seq is given in blue (light blue represents the Watson, and dark blue is the Crick strand). The example is given for chromosome II around CHS2 and CHS3 .
https://doi.org/10.1371/journal.pcbi.1010488.s027
S16 Fig. Correlation between size and transcription rate.
The plots show the two-dimensional histogram distribution of TU length and different measurements of transcription rate. The number of genes per bin is given through the colour intensities and white numbers. The DC ( S4 Appendix Eq 3) per measurement is given in the title. Size and transcription rate data was divided into 50 equally sized bins, which is given by the x and y axis. We use the 95th percentile to remove strong outliers. (A) The histogram distribution of NET-seq transcription with respect to size reveals that smaller genes tend to have higher transcription rates than larger genes. (B) This link is weakened when considering Pol2 ChIP-seq data.
https://doi.org/10.1371/journal.pcbi.1010488.s028
S17 Fig. Correlation between NET-seq and Pol2 ChIP-seq data.
The plot shows the two-dimensional histogram distribution of NET-seq and Pol2 data. The number of genes per bin is given through the colour intensities and white numbers. Size and transcription rate data was divided into 50 equally sized bins, which is given by the x and y axis. NET-seq data and Pol2 ChIP-seq signal are strongly related (DC = 0.75, S4 Appendix Eq 3). As we consider only two groups of genes with respect to transcription, i.e. genes with a low (blue) or high transcription rate (red), we can confirm that the majority of regions fall into the same category.
https://doi.org/10.1371/journal.pcbi.1010488.s029
S18 Fig. Model predictions with respect to XR-seq data in the gene setup.
(A) The model predictions in the gene configuration are less correlated with the XR-seq data than in the TCR setup (DC = 0.241, S4 Appendix Eq 3). (B) When correlating the relative repair rate and the XR-seq data in the gene setup, the DC is as low as for the model predictions (DC = 0.231). Therefore, we assume that the weak linkage is due to the data segmentation.
https://doi.org/10.1371/journal.pcbi.1010488.s030
Acknowledgments
We thank Zoë Slattery for proofreading the manuscript.
- View Article
- PubMed/NCBI
- Google Scholar
- 25. Zeitler L. leoTiez/jmak: v1.0.0; 2022. Available from: https://doi.org/10.5281/zenodo.6669794 .
- 34. Daley DJ, Vere-Jones D, et al. An introduction to the theory of point processes: volume I: elementary theory and methods. Springer; 2003.
- 40. Christian JW. The theory of transformations in metals and alloys. Newnes; 2002.
- Search Menu
- Advance articles
- Author Guidelines
- Submission Site
- Open Access
- About Journal of Radiation Research
- About the Japanese Radiation Research Society
- About the Japanese Society for Radiation Oncology
- Editorial Board
- Advertising and Corporate Services
- Journals Career Network
- Self-Archiving Policy
- Dispatch Dates
- Terms and Conditions
- Journals on Oxford Academic
- Books on Oxford Academic
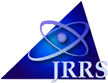
Article Contents
Introduction, acknowledgements, conflict of interest, morphogenesis-coupled dna repair - in mammalian embryogenesis, morphogenesis and dna double strand break (dsb) repair are carried out simultaneously to ensure normal development.
- Article contents
- Figures & tables
- Supplementary Data
Asao Noda, Morphogenesis-coupled DNA repair - in mammalian embryogenesis, morphogenesis and DNA double strand break (DSB) repair are carried out simultaneously to ensure normal development, Journal of Radiation Research , 2024;, rrae028, https://doi.org/10.1093/jrr/rrae028
- Permissions Icon Permissions
Embryonic development in higher organisms is an irreversible process controlled by a strict and predetermined genetic program. In their early embryo, dramatic changes called ‘embryo morphogenesis,’ which involve cell division and migration of cell populations, as well as three-dimensional tissue organization, occur precisely within a fixed time slot. This elaborate mechanism has fascinated developmental biologists for many years. However, excessive stress from the external environment can disrupt this process. For example, certain toxic chemicals and radiation can inhibit development or cause abnormal morphogenesis. During evolution, organisms have developed molecular mechanisms to deal with problems in all phases of cell metabolism. Thus, mechanisms must be present to deal with genomic damage during embryonic development, while maintaining a predetermined timetable. To date, damaged cells occurring during embryonic development were only considered to be eliminated by p53-dependent apoptosis to prevent the development of malformations. In this short review, I summarize recent findings on the mechanisms of double-strand break (DSB) repair (or DSB stress response) coupled with morphogenesis using regulatory proteins like chromodomain helicase DNA binding protein 7 (CHD7), Pax2 transactivation domain interacting protein (PTIP), co-factor of BRCA1 (COBRA1) and BRCT-repeat inhibitor of TERT expression (BRIT1) as examples.
Example 1: Chromodomain helicase DNA binding protein 7(CHD7)
Excessive radiation exposure can cause unrepaired DSBs in cell nuclei. These can be easily detected and quantified in apoptosis-resistant mesenchymal and stromal cells, such as fibroblasts [ 1 ]. In the process of screening for genes characteristic in cells bearing unrepaired DSBs, we discovered that CHD7 transcription was upregulated in these cells [ 2 ]. We also confirmed ATM-dependent phosphorylation of CHD7 protein and its accumulation in DSB foci protein complexes after radiation exposure. CHD7 is a transcription factor ubiquitously expressed in adult tissues; it is also known to control neural crest differentiation and morphogenesis during early development [ 3 ]. Neural crest cells are a migratory cell population transiently located in the border region between the neural plate and epidermis during early embryogenesis. They form neural, skeletal, epithelial and mesenchymal tissues and participate in several organogenesis processes, such as the development of craniofacial architecture, brain, and heart. The neural crest is thus called the ‘fourth germinal lobe’ [ 4 ]. CHD7 is expressed upstream of the transcription factors Slug, Twist, and Sox9 in the mesenchymal-epithelial transition of the neural crest and is essential for the formation of migratory neural crest cells [ 3 ]. It is also expressed upstream of semaphorin 3A (SEMA3A) and ephrin receptors and regulates the guidance of neuroaxonal elongation in 9.5- to 11.5-day mouse embryos [ 5 ]. CHD7 heterozygotes arising from de novo germline mutations show multiple developmental abnormalities in neurosensory organs, such as the eyes, mouth, nose, ears and brain, as well as heart malformations, indicating that gene haploinsufficiency induces fetal malformations. CHD7 is the major gene responsible for the Coloboma, Heart defect, Atresia choanae, Retarded growth and development, Genital abnormality, and Ear abnormality (CHARGE) congenital malformation syndrome in humans [ 6 , 7 ]. SEMA3A mutations are found in Kallmann syndrome, which resembles CHARGE [ 5 ]. Further, CHD7 is essential for neural crest induction from undifferentiated tissues. The fact that CHD7 localizes to the DSB site [ 8 ] and is phosphorylated in an ATM-dependent manner by switching its localization from promoter/enhancer binding to DSB binding [ 2 ] indicates that this transcription factor has both morphogenetic and DSB repair functions in the developing neural crest. The embryonic morphogenetic stage is highly sensitive to radiation, and excessive radiation induces malformations [ 9 ]. In mouse fetuses, induction of malformations peaks in 7- to 13-day embryos irradiated at 2 Gy. Although CHD7 is the most active during this period and drives morphogenesis, it may also drive concurrent repair in response to genomic stress. This coupling, or switching, between morphogenesis and DSB repair is attributed to ATM-dependent phosphorylation and the transient translocation of proteins to DSB sites. In case of excessive DNA damage or many cells bearing unrepairable damage, CHD7 remains at the DSB site for too long, thereby resulting in a reduction of its morphogenetic activity. Otherwise, cells with unrepaired DSBs could progress to mitotic arrest, preventing development. This is a plausible cause of radiation-induced fetal malformations and a good example of a morphogenetic transcription factor with DSB repair (response) function ( Fig. 1 ).

Decision of embryo with DSB damage.
Protein factors involved in morphogenesis-coupled DSB repair
NR: nuclear receptor; CHARGE: coloboma, heart defects, atresia choanae, growth retardation, genital abnormalities, and ear abnormalities
Example 2: Pax2 transactivation domain interacting protein (PTIP)
Paired Box Gene 2 ( Pax2 ) is a transcription factor that controls intermediate mesodermal differentiation. Pax2 KO mice are born without kidneys. They also lack the ureter, reproductive tract, oviduct, uterus, and vagina [ 10 , 11 ]. Moreover, Pax2 controls eye and inner ear formation—in KO neonates, the eye cup cannot be closed, the optic nerve cannot be semi-crossed and becomes fully crossed, and inner ear hearing is defective. Therefore, Pax2 is a causative gene for congenital malformations [ 12 , 13 ]. PTIP was discovered as a Pax2-binding protein and was thought to bind the transactivation domain of Pax2 to promote transcription [ 14 ]. During the initial stage of kidney primordium formation from the intermediate mesoderm, Pax2 remains activated in the absence of PTIP. However, in the late stages of kidney formation, Pax2-activated gene expression is suppressed in the absence of PTIP. PTIP KO causes embryonic lethality in 9.5-day embryos [ 15 ] and arrhythmia and glomerular disease in conditional KO adults [ 16 , 17 ]. PTIP is an adaptor protein carrying six BRCA1 C-terminal (BRCT) domains and is a protein that is typically associated with DSB foci [ 14 , 18 ]. During DSB repair, PTIP binds to 53BP1 on DSBs, transiently suppresses end-resection, and promotes nonhomologous end-joining [ 19 ]. This complex of PTIP and Pax2 is required in kidney development [ 20 ].
Example 3: Cofactor of BRCA1 (COBRA1)
COBRA1 is a BRCA1-binding protein [ 21 ] that promotes mammary gland development in a hormone-dependent manner [ 22 ]. Deletion of COBRA1 suppresses mammary gland development. However, when BRCA1 is also depleted (double KO), mammary gland development resumes (double negative shows somewhat positive), suggesting that the BRCA1/COBRA1 complex normally suppresses mammary gland development at the transcriptional level, timing its hormone-dependent activation [ 23 ]. As neither the RING nor BRCT domains are involved in the BRCA1-induced repression of COBRA1 function, the homologous recombination repair (HRR) function of BRCA1 and mammary gland development do not seem to be directly related. However, breast cancer may occur if the suppressive function of the BRCA1/COBRA1 complex is attenuated and abnormal mammary cell proliferation (hyperplasia) occurs owing to over-mobilization of BRCA1 to DSBs for HRR. In other words, this is another good example of morphogenesis coupled with DSB repair, considering that it normally regulates mammary gland development, while DSB repair occurs simultaneously in the event of damage.
Example 4: BRCT-repeat inhibitor of TERT expression/Microcephalin (BRIT1/MCPH1)
The transcription factor BRIT1 was initially identified as a negative regulator of hTERT gene expression during somatic cell differentiation [ 24 ]. BRIT1 controls the transcription of target genes by binding to TRF2 and E2F. BRIT1 was also found to be a typical DSB foci factor shortly after its discovery because BRIT1 encodes three BRCT domain repeats. Surprisingly, this gene is identical to MCPH1 , the causative gene for microcephaly [ 25 ], indicating that it binds to telomere ends and radiation-induced DSBs, as well as regulates brain development [ 26 ]. BRIT1/MCPH1 regulates brain development by controlling the division of neuroprogenitor cells and temporal–spatial processes via mitotic checkpoint regulation. Thus, this key transcription factor regulating brain development and cell division also acts as a DSB stress response factor during radiation exposure.
The above examples indicate that some of the key transcription factors controlling embryo development and differentiation are also involved in the DSB stress response or directly in DSB repair. They ensure normal development by simultaneously performing morphogenesis and DSB repair in the irreversible process of morphogenesis. Under excessive genome damage, the DSB response may reduce the driving force of morphogenesis owing to the mobilization of more proteins to the DSB sites, resulting in the induction of fetal malformations. The morphogenetic period of early development is a continuous biological process with a point of no return, in which the most complex life phenomena must occur precisely within a predetermined timeframe. To ensure this process, it is reasonable to assume that the key transcription factors have themselves acquired a DSB repair (DSB stress response) function during evolution. From this perspective, the p53 family of genes might broadly fall into the same category as p53, which is a stress-responsive transcription factor and malformation suppressor [ 27 , 28 ]. However, p53 is a negative regulator that removes damaged cells during embryogenesis, whereas the morphogenic factors described above are positive regulators that promote the recovery of damaged cells. Furthermore, it has been suggested that some of these regulatory factors may also play a role in carcinogenesis [ 13 , 29 ], as cancer could potentially result from a disruption in morphogenetic activity.
During evolution, organisms have successfully dealt with genomic damage during the most critical phases of life to maintain their continuity. This has led to the creation of proofreading mechanisms in DNA replication and the development of a transcription-coupled repair mechanism. Similarly, a mechanism must have been established for simultaneous morphogenesis and repair of genomic damage occurring in cells during early embryogenesis because of exposure to radiation or other stresses. Additional morphogenic factors with such DSB-stress response functions are expected to be identified in future.
The Radiation Effects Research Foundation (RERF), Hiroshima and Nagasaki, Japan is a public interest foundation funded by the Japanese Ministry of Health, Labour and Welfare and the US Department of Energy with the purpose of conducting research and studies for peaceful purposes on medical effects of radiation and associated diseases in humans, to contribute to the health and welfare of atomic bomb survivors and all humankind. The views of the authors do not necessarily reflect those of the funding agencies. This study is based on RERF research protocol A4-09 and the result of collaboration among laboratory staff.
The author declares that there is no conflict of interest.
Noda A , Hirai Y , Hamasaki K . et al. Unrepairable DNA double-strand breaks that are generated by ionizing radiation determine the fate of normal human cells . J Cell Sci 2012 ; 125 : 5280 – 7 . https://doi.org/10.1242/jcs.101006 .
Google Scholar
Noda A , Muramoto K , Mishima S . ATM-dependent phosphorylation of CHD7 regulates morphogenesis-coupled DSB stress response in fetal radiation exposure . Mol Biol Cell 2023 ; 34 : ar39 . https://doi.org/10.1091/mbc.E22-10-0450 .
Bajpai R , Chen DA , Rada-Iglesias A . et al. CHD7 cooperates with PBAF to control multipotent neural crest formation . Nature 2010 ; 463 : 958 – 62 . https://doi.org/10.1038/nature08733 .
Hall BK . The neural crest as a fourth germ layer and vertebrates as quadroblastic not triploblastic . Evol Dev 2000 ; 2 : 3 – 5 . https://doi.org/10.1046/j.1525-142x.2000.00032.x .
Schulz Y , Wehner P , Opitz L . et al. CHD7, the gene mutated in CHARGE syndrome, regulates genes involved in neural crest cell guidance . Hum Genet 2014 ; 133 : 997 – 1009 . https://doi.org/10.1007/s00439-014-1444-2 .
Vissers LE , van Ravenswaaij CM , Admiraal R . et al. Mutations in a new member of the chromodomain gene family cause CHARGE syndrome . Nat Genet 2004 ; 36 : 955 – 7 . https://doi.org/10.1038/ng1407 .
Zentner GE , Layman WS , Martin DM , Scacheri PC . Molecular and phenotypic aspects of CHD7 mutation in CHARGE syndrome . Am J Med Genet A 2010 ; 152A : 674 – 86 . https://doi.org/10.1002/ajmg.a.33323 .
Rother MB , Pellegrino S , Smith R . et al. CHD7 and 53BP1 regulate distinct pathways for the re-ligation of DNA double-strand breaks . Nat Commun 2020 ; 11 : 5775 . https://doi.org/10.1038/s41467-020-19502-5 .
Streffer C , Shore R , Konermann G . et al. Biological effects after prenatal irradiation (embryo and fetus). A report of the International Commission on Radiological Protection . Ann ICRP 2003 ; 33 : 5 – 206 .
Torres M , Gómez-Pardo E , Dressler GR , Gruss P . Pax-2 controls multiple steps of urogenital development . Development 1995 ; 121 : 4057 – 65 . https://doi.org/10.1242/dev.121.12.4057 .
Torres M , Gómez-Pardo E , Gruss P . Pax2 contributes to inner ear patterning and optic nerve trajectory . Development 1996 ; 122 : 3381 – 91 . https://doi.org/10.1242/dev.122.11.3381 .
Chi N , Epstein JA . Getting your Pax straight: Pax proteins in development and disease . Trends Genet 2002 ; 18 : 41 – 7 . https://doi.org/10.1016/S0168-9525(01)02594-X .
Robson EJ , He SJ , Eccles MR . A PANorama of PAX genes in cancer and development . Nat Rev Cancer 2006 ; 6 : 52 – 62 . https://doi.org/10.1038/nrc1778 .
Lechner MS , Levitan I , Dressler GR . PTIP, a novel BRCT domain-containing protein interacts with Pax2 and is associated with active chromatin . Nucleic Acids Res 2000 ; 28 : 2741 – 51 . https://doi.org/10.1093/nar/28.14.2741 .
Cho EA , Prindle MJ , Dressler GR . BRCT domain-containing protein PTIP is essential for progression through mitosis . Mol Cell Biol 2003 ; 23 : 1666 – 73 . https://doi.org/10.1128/MCB.23.5.1666-1673.2003 .
Stein AB , Jones TA , Herron TJ . et al. Loss of H3K4 methylation destabilizes gene expression patterns and physiological functions in adult murine cardiomyocytes . J Clin Invest 2011 ; 121 : 2641 – 50 . https://doi.org/10.1172/JCI44641 .
Lefevre GM , Patel SR , Kim D . et al. Altering a histone H3K4 methylation pathway in glomerular podocytes promotes a chronic disease phenotype . PLoS Genet 2010 ; 6 :e1001142. https://doi.org/10.1371/journal.pgen.1001142 .
Muñoz IM , Rouse J . Control of histone methylation and genome stability by PTIP . EMBO Rep 2009 ; 10 : 239 – 45 . https://doi.org/10.1038/embor.2009.21 .
Callen E , Zong D , Wu W . et al. 53BP1 enforces distinct pre- and post-resection blocks on homologous recombination . Mol Cell 2020 ; 77 : 26 – 38.e7 . https://doi.org/10.1016/j.molcel.2019.09.024 .
Ranghini EJ , Dressler GR . Evidence for intermediate mesoderm and kidney progenitor cell specification by Pax2 and PTIP dependent mechanisms . Dev Biol 2015 ; 399 : 296 – 305 . https://doi.org/10.1016/j.ydbio.2015.01.005 .
Nair SJ , Zhang X , Chiang HC . et al. Genetic suppression reveals DNA repair-independent antagonism between BRCA1 and COBRA1 in mammary gland development . Nat Commun 2016 ; 7 : 10913 . https://doi.org/10.1038/ncomms10913 .
Sun J , Blair AL , Aiyar SE , Li R . Cofactor of BRCA1 modulates androgen-dependent transcription and alternative splicing . J Steroid Biochem Mol Biol 2007 ; 107 : 131 – 9 . https://doi.org/10.1016/j.jsbmb.2007.05.031 .
Chiang HC , Zhang X , Zhao X . et al. Gene-specific genetic complementation between BRCA1 and Cobra1 during mouse mammary gland development . Sci Rep 2018 ; 8 : 2731 . https://doi.org/10.1038/s41598-018-21044-2 .
Lin SY , Rai R , Li K . et al. BRIT1/MCPH1 is a DNA damage responsive protein that regulates the BRCA1-Chk1 pathway, implicating checkpoint dysfunction in microcephaly . Proc Natl Acad Sci USA 2005 ; 102 : 15105 – 9 . https://doi.org/10.1073/pnas.0507722102 .
Jackson AP , Eastwood H , Bell SM . et al. Identification of microcephalin, a protein implicated in determining the size of the human brain . Am J Hum Genet 2002 ; 71 : 136 – 42 . https://doi.org/10.1086/341283 .
Peng G , Yim EK , Dai H . et al. BRIT1/MCPH1 links chromatin remodelling to DNA damage response . Nat Cell Biol 2009 ; 11 : 865 – 72 . https://doi.org/10.1038/ncb1895 .
Norimura T , Nomoto S , Katsuki M . et al. p53-dependent apoptosis suppresses radiation-induced teratogenesis . Nat Med 1996 ; 2 : 577 – 80 . https://doi.org/10.1038/nm0596-577 .
Van Nostrand JL , Brady CA , JungF H . et al. Inappropriate p53 activation during development induces features of CHARGE syndrome . Nature 2014 ; 514 : 228 – 32 . https://doi.org/10.1038/nature13585 .
Machando RAC , Schneider H , DeOcesano-Pereira C . et al. CHD7 promotes glioblastoma cell motility and invasiveness through transcriptional modulation of an invasion signature . Sci Rep 2019 ; 9 : 3952 . https://doi.org/10.1038/s41598-019-39564-w .
Email alerts
Citing articles via, affiliations.
- Online ISSN 1349-9157
- Copyright © 2024 The Japanese Radiation Research Society and Japanese Society for Radiation Oncology
- About Oxford Academic
- Publish journals with us
- University press partners
- What we publish
- New features
- Open access
- Institutional account management
- Rights and permissions
- Get help with access
- Accessibility
- Advertising
- Media enquiries
- Oxford University Press
- Oxford Languages
- University of Oxford
Oxford University Press is a department of the University of Oxford. It furthers the University's objective of excellence in research, scholarship, and education by publishing worldwide
- Copyright © 2024 Oxford University Press
- Cookie settings
- Cookie policy
- Privacy policy
- Legal notice
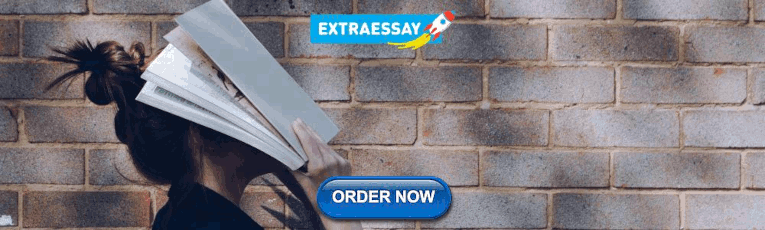
This Feature Is Available To Subscribers Only
Sign In or Create an Account
This PDF is available to Subscribers Only
For full access to this pdf, sign in to an existing account, or purchase an annual subscription.
MINI REVIEW article
Exploring the intersection of epigenetics, dna repair, and immunology from studies of icf syndrome, an inborn error of immunity.
- Department of Human Genetics, School of International Health, Graduate School of Medicine, The University of Tokyo, Tokyo, Japan
Immunodeficiency, centromeric instability, and facial anomalies (ICF) syndrome, a rare autosomal recessive disorder, manifests with hypoglobulinemia and chromosomal instability accompanied by DNA hypomethylation. Pathological variants in the DNMT3B , ZBTB24 , CDCA7 , or HELLS genes underlie its etiology. Activated lymphocytes from patients often display distinctive multiradial chromosomes fused via pericentromeric regions. Recent studies have provided deeper insights into how pathological variants in ICF-related proteins cause DNA hypomethylation and chromosome instability. However, the understanding of the molecular pathogenesis underlying immunodeficiency is still in its nascent stages. In the past half-decade, the roles of CDCA7, HELLS, and ZBTB24 in classical non-homologous end joining during double-strand DNA break repair and immunoglobulin class-switch recombination (CSR) have been unveiled. Nevertheless, given the decreased all classes of immunoglobulins in most patients, CSR deficiency alone cannot fully account for the immunodeficiency. The latest finding showing dysregulation of immunoglobulin signaling may provide a clue to understanding the immunodeficiency mechanism. While less common, a subgroup of patients exhibits T-cell abnormalities alongside B-cell anomalies, including reduced regulatory T-cells and increased effector memory T- and follicular helper T-cells. The dysregulation of immunoglobulin signaling in B-cells, the imbalance in T-cell subsets, and/or satellite RNA-mediated activation of innate immune response potentially explain autoimmune manifestations in a subset of patients. These findings emphasize the pivotal roles of ICF-related proteins in both B- and T-cell functions. ICF syndrome studies have illuminated many fundamental mechanisms. Further investigations will certainly continue to unveil additional mechanisms and their interplay.
1 Introduction
Immunodeficiency, centromeric instability, and facial anomalies (ICF) syndrome is a rare autosomal recessive disorder characterized by reduced immunoglobulin levels in the serum and recurrent, often fatal, respiratory and gastrointestinal infections of bacteria, viruses, fungi, and/or parasites ( 1 , 2 ). ICF patients possess naïve B-cells but lack memory B-cells and plasma cells in their peripheral blood. Centromeric instability manifests as stretched heterochromatin, chromosome breaks, and multiradial configurations involving the pericentromeric regions of chromosomes 1, 9, and 16, which are composed of highly repetitive satellite-2 or -3 (hSATII or hSATIII) repeats and exhibit hypomethylation in patients’ cells. While chromosomal rearrangements are primarily observed in T-cells, only a subset of ICF patients demonstrates apparent T-cell immunodeficiency. Additionally, ICF patients display facial anomalies and neurologic defects across a spectrum of severity.
To date, five genes have been reported to be mutated in ICF patients: DNA methyltransferase 3B ( DNMT3B ), zinc finger and BTB domain containing 24 ( ZBTB24 ), cell division cycle associated 7 ( CDCA7 ), helicase lymphoid specific ( HELLS , also known as LSH ), and Ubiquitin-like containing PHD and RING finger domains 1 ( UHRF1 , also known as ICBP90 or Np95 ) in type-1 (ICF1), -2 (ICF2), -3 (ICF3), -4 (ICF4), and atypical ICF patients, respectively ( 3 , 4 ). Briefly, DNMT3B functions as a de novo DNA methyltransferase, ZBTB24 serves as a transcriptional factor whose targets include CDCA7 , and CDCA7 and HELLS proteins constitute a chromatin remodeling complex. Because of the absence of immunodeficiency in the atypical case with hypomorphic variants in UHRF1 , an essential factor for maintenance of DNA methylation alongside DNMT1 ( 5 ), we focus on ICF1–4 in this mini-review.
2 Chromosome instability in ICF patients
Recent studies have unveiled that DNA hypomethylation patterns observed in patients’ cells can be broadly categorized into three types based on their underlying mechanisms. The first pattern arises from pathogenic variants in DNMT3B , causing impairment in DNA methylation establishment during post-implantation development, resulting in hypomethylation at distinct regions represented by pericentromeric and subtelomeric regions. The second pattern results from pathogenic variants in ZBTB24 , CDCA7 , and HELLS , disrupting replication-uncoupled maintenance DNA methylation. Consequently, specific regions, where chromatin remodeling by the CDCA7/HELLS complex is required, undergo hypomethylation. These hypomethylated regions typically exhibit characteristics of the B genomic compartment, heterochromatin, and/or late replicating regions ( 6 , 7 ), encompassing pericentromeric and centromeric regions, but not including subteromeric regions. The third pattern arises from pathogenic variants in UHRF1 , which could attenuate the efficiency of both replication-coupled and -uncoupled maintenance DNA methylation, resulting in a distinct hypomethylation pattern. The hypomethylated regions include pericentromeric, centromeric, and subtelomeric regions. Detailed molecular mechanisms regarding DNA methylation maintenance are described elsewhere ( 3 ).
Among the five ICF types, pericentromeric regions composed of hSATII or hSATIII repeats are commonly hypomethylated. Utilizing ICF model human embryonic kidney (HEK) 293 cells, in which ICF causative genes were knocked out via genome editing, we have demonstrated abnormal transcription of hSATII mRNA from the hypomethylated regions. This could cause unphysiological R-loops, leading to DNA double-strand breaks (DSBs) ( 8 ). This mechanism sheds light on the occurrence of chromosome instability in ICF patients’ cells. Notably, lymphoblastoid cells derived from ICF patients and the ICF model cells exhibit slower growth rates compared to control cells with an increase of apoptotic cells ( 9 ). The enlarged nucleus observed in the ICF model cells, along with a significant increase in centrosome numbers and size, likely due to excessive DSBs, suggests that these cells may undergo cell cycle slippages from G2 into G1 due to prolonged G2. While the ICF model cells manage to withstand these abnormalities and survive, primary cells may be more sensitive and undergo apoptosis under such severe stresses.
3 B-cell deficiency in ICF patients
By conducting a comprehensive analysis to identify interacting proteins of CDCA7, we discovered that CDCA7 interacts with Ku80 and DNA-dependent protein kinase (DNA-PK), both of which are involved in classical non-homologous end joining (c-NHEJ) in DSB repair, V(D)J recombination, and class-switch recombination (CSR) ( 10 ). Furthermore, we revealed that CDCA7 and HELLS assist Ku80 in accessing DSB sites and facilitate NHEJ possibly through their chromatin remodeling activity ( 9 ). Subsequently, Helfricht et al. demonstrated that ZBTB24 can directly facilitate c-NHEJ. They found that ZBTB24 stimulates auto-poly(ADP-ribosyl)ation of poly(ADP-ribose) polymerase 1 (PARP1) and subsequently recognizes the PAR chains on PARP1 to localize at DSB sites ( 11 ). This localization prevents degradation of PAR chains by PAR glycohydrolase (PARG), resulting in the facilitation of NHEJ via the PAR-dependent assembly of the DNA ligase 4 (LIG4)/X-ray repair cross-complementing 4 (XRCC4) complex at the DSB sites. Although PARP1 can be involved in c-NHEJ via stimulation of DNA-PK kinase activity by PARsylation and alternative NHEJ ( 12 ), their findings suggest that ZBTB24 promotes c-NHEJ. Hence, ZBTB24 can facilitate c-NHEJ directly via the interaction with PARP1 and indirectly through transcriptional activation of CDCA7 ( Figure 1 ).
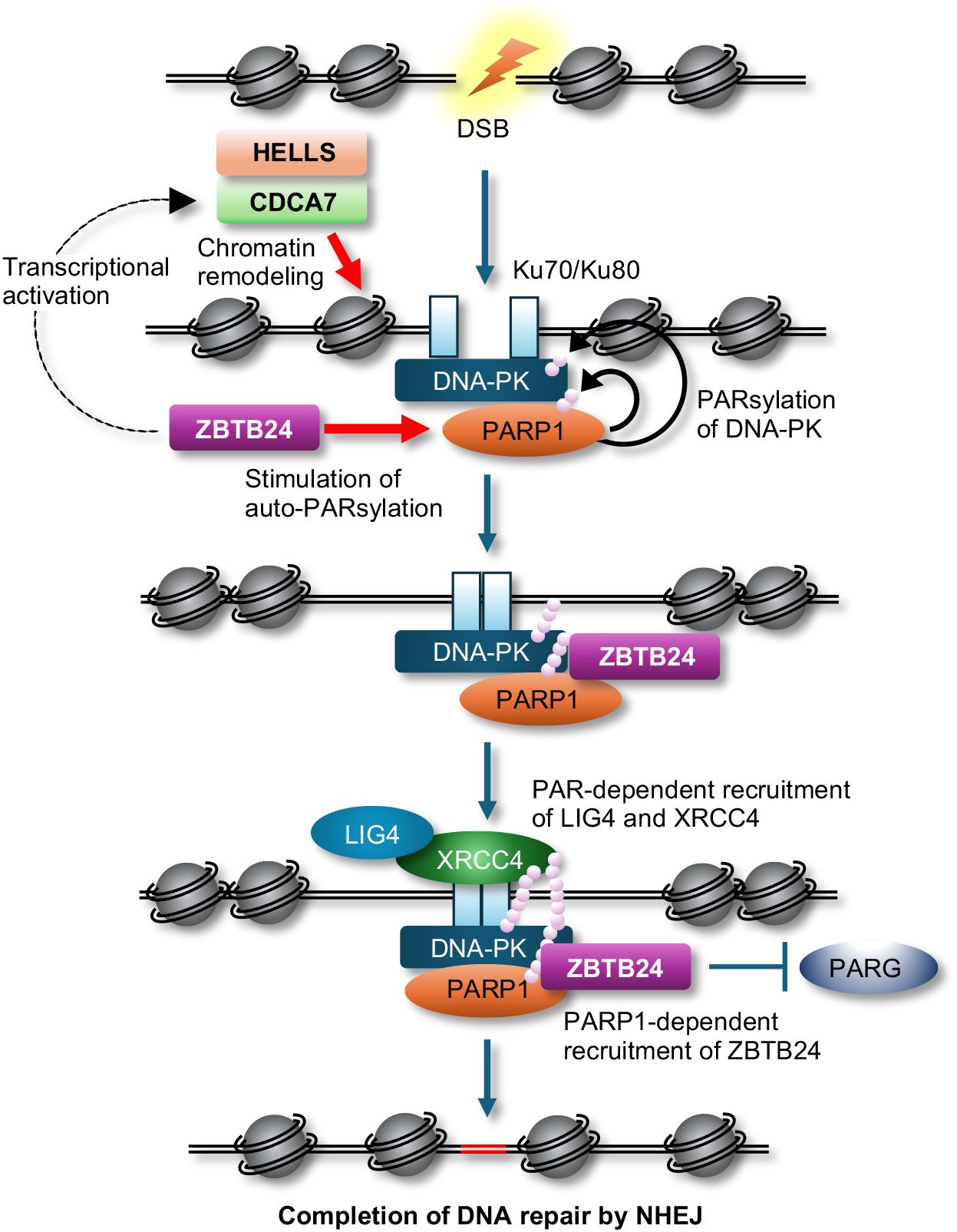
Figure 1 A possible model of c-NHEJ during DSB repair. The CDCA7 and HELLS complex assists Ku proteins and DNA-PK in accessing DSB sites possibly by their chromatin remodeling activity, while ZBTB24 enhances auto-PARsylation of PARP1, which stimulates kinase activity of DNA-PK via PARsylation. ZBTB24 recognizes the PAR chains on PARP1, protects the PAR chains from degradation by PARG, resulting in the facilitation of NHEJ by PAR-dependent assembly of the LIG4/XRCC4 complex at DSB sites.
It has been demonstrated that immunoglobulin CSR heavily relies on the c-NHEJ-mediated repair of activation-induced cytidine deaminase (AID)-induced DSBs ( 13 ). Recently, He et al. demonstrated that HELLS facilitates NHEJ in CSR in B-cells using the hematopoietic cell lineage-specific Hells conditional KO (cKO) mice ( 14 ). While serum IgM levels remain unchanged, IgG levels are significantly decreased in these mice, suggesting that HELLS aids in CSR from IgM to IgG in mice. Furthermore, they showed that HELLS is not required for the initiation of CSR, including induction of AID, chromatin accessibility at switch regions, and formation of DSBs, but is required for the completion of recombination. Around the same time, Helfricht et al. implicated the involvement of human ZBTB24 in the final stage of CSR by demonstrating that ICF2 patients possess almost unchanged numbers of unswitched memory B-cells but possess decreased numbers of switched B-cells in their peripheral blood ( 11 ). They also showed that V(D)J recombination and AID induction are intact in B-cells from ICF2 patients.
Here, the remaining largest question is that levels of all classes of immunoglobulins (IgM, IgG, and IgA) are frequently decreased in all types of ICF patients, which cannot be solely attributed to CSR deficiency. The latest findings by Ying et al. may provide a clue ( 15 ). They reported that plasma cells, along with all classes of immunoglobulins, are reduced in the hematopoietic cell lineage-specific Zbtb24 cKO mice, possibly due to increased phosphorylation levels of CD19 in B-cells, known to establish intrinsic B-cell signaling threshold. The Zbtb24 cKO mice are hypo- and hyper-responsive to T-independent type 2 (TI-2) and T-dependent (TD) antigens, respectively. They also discovered that the expression of interleukin 5 receptor subunit alpha ( Il5ra ) is elevated in Zbtb24 -deficient B-cells accompanied by drastic hypomethylation of its promoter, and increased IL-5Rα enhances phosphorylation of CD19. Their findings suggest that decreased natural antibodies due to impaired responses to TI-2 antigens including repetitive surface structures of encapsulated bacteria are likely the major cause of immunodeficiency, and abnormal responses to TD antigens may also contribute to ICF phenotype ( Figure 2 ). Two decades ago, Blanco-Betancourt et al. hypothesized that negative selection could be impaired in ICF B-cells because ICF naïve B-cells bear potentially autoreactive long heavy chain variable regions complementarity determining region 3 (V H CDR3), composed of full-length D H gene segments ( 16 ). Because human CD19 transgenic mice demonstrate elevated levels of autoantibodies alongside displaying enhanced immunoglobulin signaling ( 17 ), CD19 activation could explain why ICF B-cells often carry autoreactive immunoglobulins. In summary, while HELLS and ZBTB24 are crucial for CSR, the dysregulation of immunoglobulin signaling in ICF B-cells may have a more significant impact on B-cell abnormalities, potentially masking CSR deficiency in many ICF cases.
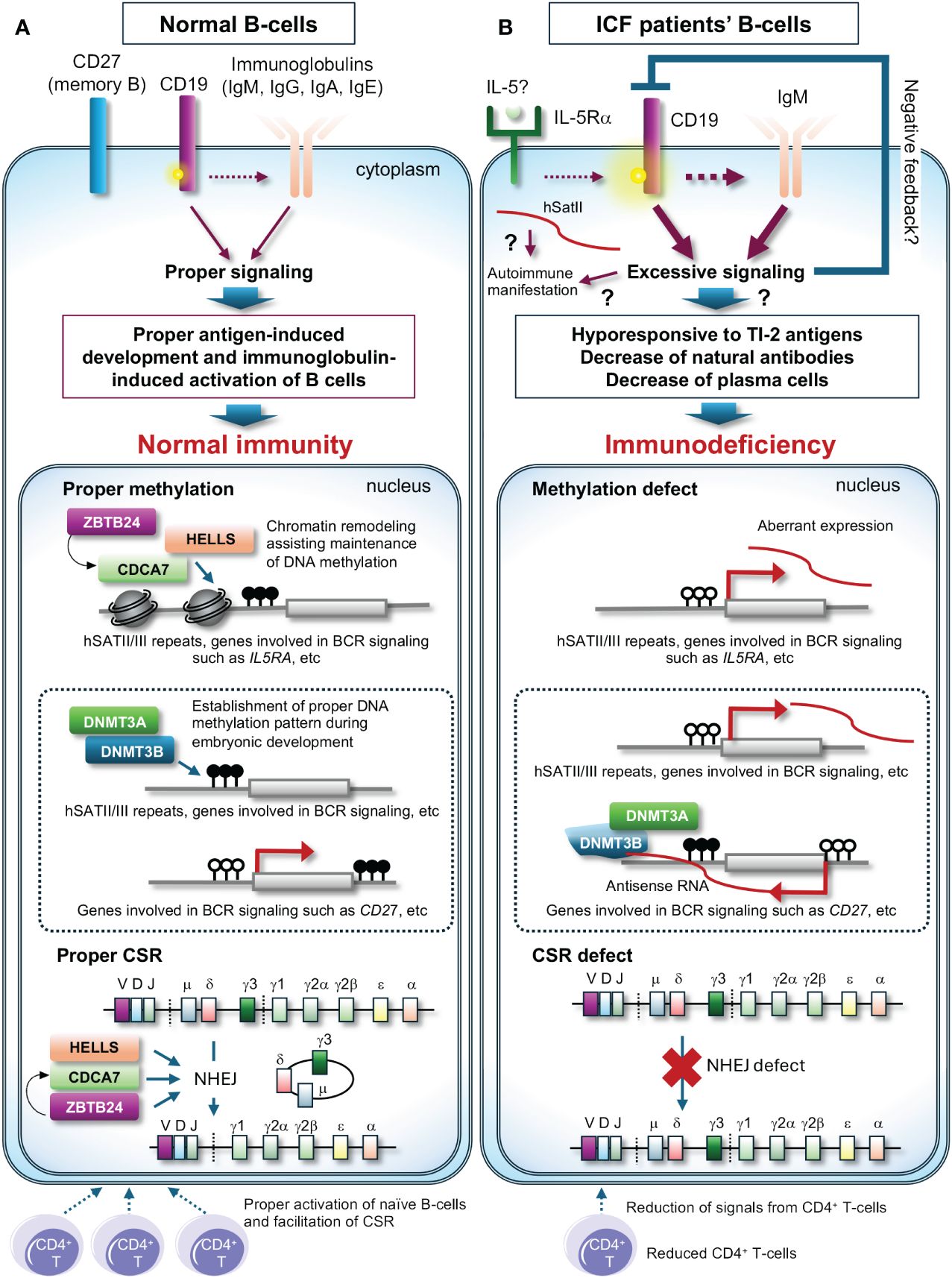
Figure 2 A possible molecular pathogenesis behind immunodeficiency of ICF patients. (A) In normal B-cells, class-switch recombination (CSR) properly occurs upon signals from CD4 + T-cells with the assistance of the CDCA7/HELLS complex and ZBTB24, and the promoter region of genes involved in immunoglobulin signaling possibly including the IL5RA promoter is likely highly methylated and maintained the status with the assistance of the CDCA7/HELLS complex, resulting in proper expression levels of these genes. In this status, phosphorylation levels of CD19 are normal, resulting in proper antigen-induced development and immunoglobulin-induced activation of B-cells. The proper methylation patterns are established by DNMT3B and/or DNMT3A during embryonic development. (B) In ICF patients’ B-cells, CSR is deficient, and the promoter regions of genes involved in immunoglobulin signaling possibly including the IL5RA promoter are hypomethylated, resulting in induced expression of these genes. High expression of IL-5Rα could facilitate phosphorylation of CD19 and the downstream signaling. This may induce negative feedback and/or cause hypo-responsiveness to T-independent type 2 (TI-2) antigens, a reduction of natural antibodies, and a decrease in plasma cells, resulting in immunodeficiency. In ICF1 cells, DNMT3B defects could cause aberrant methylation, resulting in aberrant expression of genes possibly including CD27 , a memory B-cell marker. Dysregulation of T cells may exacerbate immunodeficiency. White and black circles on the genes indicate unmethylated and methylated cytosines, respectively. ? indicates potential pathways contributing to the outcomes.
Another remaining question is how pathogenic variants in DNMT3B , which encodes a de novo DNA methyltransferase, affect immunoglobulin levels in ICF1 patients. An early attempt to clarify the molecular pathogenesis using murine models carrying pathogenic variants found in ICF1 patients has yielded a result slightly different from the expectation, as the models do not exhibit B-cell deficiency ( 18 ). A more recent comprehensive study by Gatto et al. demonstrated that many genes involved in the immunoglobulin signaling pathway are differentially expressed in ICF1 patients’ immortalized lymphoblast cell lines ( 19 ). Intriguingly, in the cell lines, expression of CD27 , a memory B-cell marker, is decreased alongside its promoter hyper-methylation possibly via recruitment of the DNMT3B/DNMT3A complex on its antisense transcript ( 19 ). They also detected increased alternative splicing events of CD45, a regulator of immunoglobulin and T-cell receptor signaling, in the cell lines ( 19 ). These transcriptional alterations mediated via aberrant DNA methylation and/or RNA binding ability of DNMT3B or its interacting proteins may explain decreased memory B-cells and plasma cells in ICF1 patients’ peripheral blood ( Figure 2 ), although this pioneering work needs to be confirmed using patients’ primary cells in the future.
4 T-cell deficiency and autoimmune manifestation in ICF patients: Possible involvement of regulatory T (Treg) cells in ICF pathogenesis
Since some ICF patients suffer from recurrent viral and/or fungal infections mostly with bacterial infections, ICF patients have been suspected of having concomitant T-cell immunodeficiency ( 1 , 2 ). Although the molecular mechanism remains elusive, a subset of ICF patients exhibit lymphopenia and/or an inverted CD4 + /CD8 + ratio due to the reduction of CD4 + T-cells, which potentially hampers activation of naïve B-cells and CSR ( Figure 2 ). It is also demonstrated that the number of apoptotic lymphocytes in mitogen-stimulated cells was significantly higher in patients than in controls ( 20 ). This could be attributed to the frequent occurrence of abnormal chromosomes in activated T-cells ( 1 ).
In addition to these abnormalities, recent studies have shown that Treg cells, which suppress immune response, and recent thymic emigrant T-cells (RTEs), which maintain T-cell diversity in the periphery, were decreased in many ICF patients, while effector memory CD4 + T-cells (CD4 + T EM ), which augments the immune response, and circulating follicular helper T-cells (cT FH ), which are increased in the periphery of patients with autoimmune diseases, were increased in the majority of ICF patients ( 2 , 21 , 22 ). While less common, approximately 10 to 20% of patients show autoimmune manifestations. The manifestations in ICF patients can be explained by activation of the immunoglobulin signaling in B-cells accompanied possibly by CD19 activation (see chapter 3) but reduced total numbers of Treg cells and/or increased CD4 + T EM cells and cT FH cells in patients may also contribute to these manifestations ( 23 , 24 ). Moreover, it has been reported that enhanced expression of pericentromeric repeats hSATII RNA in senescent and cancer cells traps CCCTC-binding factor (CTCF), resulting in the alteration of chromatin structure and subsequent activation of inflammatory genes ( 25 ). In addition to our finding, of which expression of hSATII is increased in ICF model cells ( 8 ), the innate immune response is activated by sensors of cytosolic RNA, which recognize aberrantly expressed satellite repeats from hypomethylated pericentromeric regions, in Zbtb24 knockout zebrafish ( 26 ). Hence, the increased expression of pericentromeric repeats can also contribute to the autoimmune manifestation ( Figure 2 ).
5 ICF murine models
The phenotype related to immune cells in murine ICF models slightly differs from ICF patients. The ICF1 murine models demonstrate increased apoptosis of thymocytes after birth, while B-cells remain normal ( 18 ). Although an ICF2 murine model lacking Zbtb24 specifically in the hematopoietic lineage displays similar phenotypes of ICF2 patients (see chapter 3), Zbtb24 null mice exhibit embryonic lethality, which contrasts with ICF2 patients, some of whom carry null variants ( 15 , 27 , 28 ). Similarly, Hells null mice exhibit perinatal lethality, despite some ICF4 patients having null variants ( 29 , 30 ). Another ICF4 murine model reconstituted with bone marrow from hematopoietic lineage - specific Hells cKO mice shows reduced B-cell numbers and skewed CD4 + T cell proportions ( 14 ). Considering the phenotypic differences among ICF patients and various murine models, ICF-related proteins could have similar but slightly different roles and/or different compensational proteins in murine and human tissues composed of various cell types, including niche cells in the bone marrow and the thymus. However, these observations highlight the potential involvement of ICF-related proteins in both human and murine B- and T-cell functions.
6 Conclusions
ICF studies have been uncovering key cellular regulatory mechanisms, spanning DNA methylation, DNA repair, and immune cell regulation. Since the understanding of immunodeficiency in ICF patients has just started, and facial anomalies and neurologic defects in ICF patients have not been well-studied, ICF studies will continuously contribute to further deepening insights into fundamental life mechanisms.
Author contributions
MU: Writing – original draft, Writing – review & editing.
The author(s) declare financial support was received for the research, authorship, and/or publication of this article. Japan Society for Promotion of Science KAKENHI Grant Number 13K05728 supported for the publication of this article.
Conflict of interest
The author declares that the research was conducted in the absence of any commercial or financial relationships that could be construed as a potential conflict of interest.
Publisher’s note
All claims expressed in this article are solely those of the authors and do not necessarily represent those of their affiliated organizations, or those of the publisher, the editors and the reviewers. Any product that may be evaluated in this article, or claim that may be made by its manufacturer, is not guaranteed or endorsed by the publisher.
1. Ehrlich M, Jackson K, Weemaes C. Immunodeficiency, centromeric region instability, facial anomalies syndrome (ICF). Orphanet J Rare Dis . (2006) 1:2. doi: 10.1186/1750–1172-1–2
PubMed Abstract | CrossRef Full Text | Google Scholar
2. Sterlin D, Velasco G, Moshous D, Touzot F, Mahlaoui N, Fischer A, et al. Genetic, cellular and clinical features of ICF syndrome: a french national survey. J Clin Immunol . (2016) 36:149–59. doi: 10.1007/s10875–016-0240–2
3. Unoki M. Chromatin remodeling in replication-uncoupled maintenance DNA methylation and chromosome stability: Insights from ICF syndrome studies. Genes Cells . (2021) 26:349–59. doi: 10.1111/gtc.12850
4. Unoki M, Velasco G, Kori S, Arita K, Daigaku Y, Yeung WKA, et al. Novel compound heterozygous mutations in UHRF1 are associated with atypical immunodeficiency, centromeric instability and facial anomalies syndrome with distinctive genome-wide DNA hypomethylation. Hum Mol Genet . (2023) 32:1439–56. doi: 10.1093/hmg/ddac291
5. Unoki M, Sasaki H. The UHRF protein family in epigenetics, development, and carcinogenesis. Proc Jpn Acad Ser B Phys Biol Sci . (2022) 98:401–15. doi: 10.2183/pjab.98.021
6. Velasco G, Grillo G, Touleimat N, Ferry L, Ivkovic I, Ribierre F, et al. Comparative methylome analysis of ICF patients identifies heterochromatin loci that require ZBTB24, CDCA7 and HELLS for their methylated state. Hum Mol Genet . (2018) 27:2409–24. doi: 10.1093/hmg/ddy130
7. Vukic M, Chouaref J, Della Chiara V, Dogan S, Ratner F, Hogenboom JZM, et al. CDCA7-associated global aberrant DNA hypomethylation translates to localized, tissue-specific transcriptional responses. Sci Adv . (2024) 10:eadk3384. doi: 10.1126/sciadv.adk3384
8. Unoki M, Sharif J, Saito Y, Velasco G, Francastel C, Koseki H, et al. CDCA7 and HELLS suppress DNA: RNA hybrid-associated DNA damage at pericentromeric repeats. Sci Rep . (2020) 10:17865. doi: 10.1038/s41598–020-74636–2
9. Unoki M, Funabiki H, Velasco G, Francastel C, Sasaki H. CDCA7 and HELLS mutations undermine nonhomologous end joining in centromeric instability syndrome. J Clin Invest . (2019) 129:78–92. doi: 10.1172/JCI99751
10. Castaneda-Zegarra S, Fernandez-Berrocal M, Tkachov M, Yao R, Upfold NLE, Oksenych V. Genetic interaction between the non-homologous end-joining factors during B and T lymphocyte development: In vivo mouse models. Scand J Immunol . (2020) 92:e12936. doi: 10.1111/sji.12936
11. Helfricht A, Thijssen PE, Rother MB, Shah RG, Du L, Takada S, et al. Loss of ZBTB24 impairs nonhomologous end-joining and class-switch recombination in patients with ICF syndrome. J Exp Med . (2020) 217:e20191688. doi: 10.1084/jem.20191688
12. Ray Chaudhuri A, Nussenzweig A. The multifaceted roles of PARP1 in DNA repair and chromatin remodelling. Nat Rev Mol Cell Biol . (2017) 18:610–21. doi: 10.1038/nrm.2017.53
13. Alt FW, Zhang Y, Meng FL, Guo C, Schwer B. Mechanisms of programmed DNA lesions and genomic instability in the immune system. Cell . (2013) 152:417–29. doi: 10.1016/j.cell.2013.01.007
14. He Y, Ren J, Xu X, Ni K, Schwader A, Finney R, et al. Lsh/HELLS is required for B lymphocyte development and immunoglobulin class switch recombination. Proc Natl Acad Sci U.S.A . (2020) 117:20100–08. doi: 10.1073/pnas.2004112117
15. Ying Z, Hardikar S, Plummer JB, Hamidi T, Liu B, Chen Y, et al. Enhanced CD19 activity in B cells contributes to immunodeficiency in mice deficient in the ICF syndrome gene Zbtb24. Cell Mol Immunol . (2023) 20:1487–98. doi: 10.1038/s41423-023-01106-w
16. Blanco-Betancourt CE, Moncla A, Milili M, Jiang YL, Viegas-Pequignot EM, Roquelaure B, et al. Defective B-cell-negative selection and terminal differentiation in the ICF syndrome. Blood . (2004) 103:2683–90. doi: 10.1182/blood-2003–08-2632
17. Inaoki M, Sato S, Weintraub BC, Goodnow CC, Tedder TF. CD19-regulated signaling thresholds control peripheral tolerance and autoantibody production in B lymphocytes. J Exp Med . (1997) 186:1923–31. doi: 10.1084/jem.186.11.1923
18. Ueda Y, Okano M, Williams C, Chen T, Georgopoulos K, Li E. Roles for Dnmt3b in mammalian development: a mouse model for the ICF syndrome. Development . (2006) 133:1183–92. doi: 10.1242/dev.02293
19. Gatto S, Gagliardi M, Franzese M, Leppert S, Papa M, Cammisa M, et al. ICF-specific DNMT3B dysfunction interferes with intragenic regulation of mRNA transcription and alternative splicing. Nucleic Acids Res . (2017) 45:5739–56. doi: 10.1093/nar/gkx163
20. Pezzolo A, Prigione I, Facchetti P, Castellano E, Viale M, Gimelli G, et al. T-cell apoptosis in ICF syndrome. J Allergy Clin Immunol . (2001) 108:310–2. doi: 10.1067/mai.2001.116863
21. Kamae C, Imai K, Kato T, Okano T, Honma K, Nakagawa N, et al. Clinical and immunological characterization of ICF syndrome in Japan. J Clin Immunol . (2018) 38:927–37. doi: 10.1007/s10875-018-0559-y
22. Bilgic Eltan S, Nain E, Catak MC, Ezen E, Sefer AP, Karimi N, et al. Evaluation of clinical and immunological alterations associated with ICF syndrome. J Clin Immunol . (2023) 44:26. doi: 10.1007/s10875–023-01620–6
23. McDaniel MM, Chawla AS, Jain A, Meibers HE, Saha I, Gao Y, et al. Effector memory CD4(+) T cells induce damaging innate inflammation and autoimmune pathology by engaging CD40 and TNFR on myeloid cells. Sci Immunol . (2022) 7:eabk0182. doi: 10.1126/sciimmunol.abk0182
24. Walker LSK. The link between circulating follicular helper T cells and autoimmunity. Nat Rev Immunol . (2022) 22:567–75. doi: 10.1038/s41577–022-00693–5
25. Miyata K, Imai Y, Hori S, Nishio M, Loo TM, Okada R, et al. Pericentromeric noncoding RNA changes DNA binding of CTCF and inflammatory gene expression in senescence and cancer. Proc Natl Acad Sci U.S.A . (2021) 118:e2025647118. doi: 10.1073/pnas.2025647118
26. Rajshekar S, Yao J, Arnold PK, Payne SG, Zhang Y, Bowman TV, et al. Pericentromeric hypomethylation elicits an interferon response in an animal model of ICF syndrome. Elife . (2018) 7:e39658. doi: 10.7554/eLife.39658
27. Wu H, Thijssen PE, de Klerk E, Vonk KK, Wang J, den Hamer B, et al. Converging disease genes in ICF syndrome: ZBTB24 controls expression of CDCA7 in mammals. Hum Mol Genet . (2016) 25:4041–51. doi: 10.1093/hmg/ddw243
28. de Greef JC, Wang J, Balog J, den Dunnen JT, Frants RR, Straasheijm KR, et al. Mutations in ZBTB24 are associated with immunodeficiency, centromeric instability, and facial anomalies syndrome type 2. Am J Hum Genet . (2011) 88:796–804. doi: 10.1016/j.ajhg.2011.04.018
29. Geiman TM, Muegge K. Lsh, an SNF2/helicase family member, is required for proliferation of mature T lymphocytes. Proc Natl Acad Sci U.S.A . (2000) 97:4772–7. doi: 10.1073/pnas.97.9.4772
30. Thijssen PE, Ito Y, Grillo G, Wang J, Velasco G, Nitta H, et al. Mutations in CDCA7 and HELLS cause immunodeficiency-centromeric instability-facial anomalies syndrome. Nat Commun . (2015) 6:7870. doi: 10.1038/ncomms8870
Keywords: ICF syndrome, epigenetics, DNA methylation, DNA repair, NHEJ, class-switch recombination, hypoglobulinemia, immunodeficiency
Citation: Unoki M (2024) Exploring the intersection of epigenetics, DNA repair, and immunology from studies of ICF syndrome, an inborn error of immunity. Front. Immunol. 15:1405022. doi: 10.3389/fimmu.2024.1405022
Received: 22 March 2024; Accepted: 30 April 2024; Published: 10 May 2024.
Reviewed by:
Copyright © 2024 Unoki. This is an open-access article distributed under the terms of the Creative Commons Attribution License (CC BY) . The use, distribution or reproduction in other forums is permitted, provided the original author(s) and the copyright owner(s) are credited and that the original publication in this journal is cited, in accordance with accepted academic practice. No use, distribution or reproduction is permitted which does not comply with these terms.
*Correspondence: Motoko Unoki, [email protected]
Disclaimer: All claims expressed in this article are solely those of the authors and do not necessarily represent those of their affiliated organizations, or those of the publisher, the editors and the reviewers. Any product that may be evaluated in this article or claim that may be made by its manufacturer is not guaranteed or endorsed by the publisher.
- Open access
- Published: 11 May 2024
Chemotherapy-induced PTEN-L secretion promotes the selection of PTEN-deficient tumor cells
- Ming Wang 1 na1 ,
- Zhenzhen Pan 1 na1 ,
- Xiaohan Yao 1 ,
- Xixi Duan 1 ,
- Jiajia Wan 1 ,
- Xiaohan Lou 1 ,
- Wenqing Li 1 ,
- Yan Yan 1 ,
- Lin Chen 1 ,
- Junfeng An 3 &
- Zhihai Qin 1
Journal of Experimental & Clinical Cancer Research volume 43 , Article number: 140 ( 2024 ) Cite this article
61 Accesses
Metrics details
PTEN loss has been identified in various tumor types and is linked to unfavorable clinical outcomes. In addition to PTEN mutation, multiple mechanisms contribute to PTEN loss during tumor development. However, the natural selection process of PTEN-deficient tumor cells remains unclear. Here, we aimed at further elucidating the role of PTEN-L in tumor progression.
PTEN knockout cell lines were generated using CRISPR/Cas9 technology. Ni-NTA affinity column chromatography was employed for PTEN-L purification. Tumor cell metastasis was evaluated in murine models and observed using the IVIS Spectrum Imaging System. RNA-sequencing, western blotting, PCR, flow cytometry, and cell proliferation assays were employed to investigate tumor cell dormancy and related mechanisms.
The chemotherapeutic drugs, cisplatin, paclitaxel, and doxorubicin, induced tumor cells to secrete PTEN-long (PTEN-L), which shields PTEN-deficient tumor cells from chemotherapy-induced apoptosis better than it shields PTEN-intact cells. Further investigation revealed that PTEN-L treatment induced dormancy in PTEN-null tumor cells, characterized by an increase in p16 and p27 levels, cell-cycle arrest, reduced cell proliferation, and enhanced DNA repair. Furthermore, PTEN-L treatment selectively promoted the accumulation and growth of PTEN-null tumor cells in the lungs of C57BL/6J mice, while evading immune surveillance. Mechanistically, PTEN-L induced dormancy in PTEN-null tumor cells by activating the p38 signaling pathway. Addition of a p38 inhibitor effectively reversed dormancy and growth of PTEN-deficient tumor cells in the lungs. We also demonstrated that PTEN expression played a pivotal role in determining the outcome of PTEN-L-mediated antitumor therapy.
Conclusions
In summary, PTEN-L was identified as a potent inducer of dormancy in PTEN-deficient tumor cells, which increased their efficient selection within the tumor microenvironment.
Genetic mutations play a pivotal role in malignant transformation and tumor progression. PTEN is among the most frequently mutated tumor suppressor genes, with documented instances of inactivation or deletion in a wide spectrum of tumors [ 1 ]. Intriguingly, deletion of a single copy of PTEN can lead to markedly reduced PTEN expression within tumor tissues. For instance, PTEN mutations have been reported in less than 5% of all breast cancer cases, while in 40% of these cases, PTEN expression is diminished or absent [ 2 ]. Extensive research has shown that non-genetic mechanisms, including epigenetic regulation, non-coding RNAs, phosphorylation, ubiquitination, and other post-translational modifications, contribute to PTEN loss [ 3 ]. However, how PTEN-deficient tumor cells survive and their natural selection process during tumor promotion and after therapy remain unclear.
PTEN-long (PTEN-L) is a translational variant of PTEN that contains an additional 173 amino acids at its N-terminus [ 4 ]. It is secreted from cells and can enter neighboring cells, facilitated by its distinctive sequence featuring six arginine residues. Notably, PTEN-L induces breast cancer cell death by counteracting the PI3K pathways [ 4 ]. Furthermore, PTEN-L treatment reduces the proliferation of HepG2 liver cancer cells while promoting apoptosis in these cells [ 5 ]. Despite the promising findings of studies investigating PTEN-L, its comprehensive antitumor effects have not been exhaustively elucidated in preclinical studies. Consequently, questions about PTEN-L function in various tumor types and its interaction with PTEN expression or PTEN-loss selection during tumor progression remain unresolved. Therefore, delving into the impact of PTEN-L on specific tumor types and unraveling the underlying mechanisms represent crucial steps in advancing preclinical studies of PTEN-L.
Tumor cell metastasis is the primary contributor to patient mortality. As the tumor cells travel from the primary tumor to distant organs, they must adapt their characteristics to endure immune attacks [ 6 ]. Zhang et al. revealed that primary tumor cells may lose PTEN expression upon dissemination into the brain, which is reversible upon exiting the brain microenvironment. Furthermore, reduced PTEN expression was linked to an unfavorable prognosis and a shorter time to brain recurrence [ 7 ]. These findings imply that PTEN expression plays a pivotal role in the fate of tumor cells during metastasis. Nevertheless, the role of PTEN-L in progression is yet to be explored, particularly in cases where PTEN-null cells were selected or PTEN expression was hindered in the metastatic microenvironment during the transfer from primary organs to metastatic sites.
The current study was aimed at elucidating the role of PTEN-L in tumor progression as well as the process by which PTEN-deficient tumor cells are naturally selected during tumor promotion.
Cell culture
The mouse mammary carcinoma cell line (EO771), mouse pancreatic carcinoma cell line (Pan02), and mouse immortalized embryonic fibroblasts (iMEFs) were maintained in our laboratory, while the mouse Lewis lung carcinoma cell line (LLC) was generously provided by Professor Yan Li’s group at the Academy of Military Medical Sciences in Beijing. The human malignant glioblastoma cell line U87MG was procured from ATCC (HTB-14). These cell lines were cultured in DMEM (Gibco, Carlsbad, CA, USA) supplemented with 10% FBS (Invigentech, Irvine, CA, USA), 100 IU/mL penicillin, and 100 mg/mL streptomycin (HyClone, Logan, UT, USA) at 37 °C with 5% CO2. Bone marrow-derived cells (BMDMs) were isolated from the tibia and femur of C57BL/6J mice and cultured in RPMI-1640 (Gibco) complete medium. The immortalized bone marrow-derived macrophages (iBMDMs) were cultured in RPMI-1640 complete medium.
The C57BL/6J mice (female, 10 weeks old, weighing 20–22 g) and severe combined immunodeficiency (SCID) mice (female, 12 weeks old, weighing 20–22 g) utilized in this research were procured from Charles River (Beijing, China) and accommodated at the Experimental Animal Center of Zhengzhou University. The mice were kept under a temperature range of 20–25 °C with a 12-hour light-dark cycle.
PTEN-L purification
PTEN-L purification followed established protocols, as previously published [ 8 ]. Recombinant plasmids with the PTEN-L sequence were introduced into Escherichia coli BL21, and the V451 strain, containing these plasmids, was induced for protein expression in 300 ml LB medium at 37 °C. Upon reaching an OD600 of approximately 0.6, 5 µM IPTG (I8070, Solarbio, Beijing, China) was added, and cultivation continued at 37 °C for 6 to 8 h. The harvested culture underwent purification using the His-tag Protein Purification Kit (P2226, Beyotime, Shanghai, China), with verification of purity through western blotting and Coomassie brilliant blue staining. Subsequently, the purified protein underwent procedures such as dialysis, concentration, and activity verification for use in subsequent experiments.
Cell proliferation assay
Tumor cells were plated at a density of 2000 cells per well in 96-well plates, followed by the addition of PTEN-L (1 µg/ml) once cells adhered. At 24 h, 48 h, and 72 h, 10% CCK8 (CK-04, Dojindo, Kumamoto, Japan) was introduced, and cells were incubated for 2 h at 37 °C. Absorbance values at 450 nm were subsequently quantified using a microplate reader (ThermoFisher Scientific, Waltham, MA, USA).
Cloning formation experiment
In the clone formation assay, 400 cells were initially seeded per well in a 6-well plate. The experimental group received 1 µg/ml of PTEN-L, while phosphate-buffered saline (PBS) served as the control. After ten days, the culture medium was discarded, and cells were washed with PBS. Subsequently, cells were fixed with 4% paraformaldehyde (PFA, Solarbio, Beijing, China) for 15 min at room temperature, followed by staining with 0.1% crystal violet (G1014, Servicebio, Wuhan, China) for 10 min. Excess dye was removed by washing with 1×PBS. Cell colony images were captured using the ChemiDoc MP imaging system from Bio-Rad, Hercules, CA, USA, and colony quantification was performed using Image J software.
To examine the impact of PTEN-L on tumor cells during chemotherapeutic drug treatment, cells were exposed to either conditional medium, PTEN-L (1 µg/ml), paclitaxel (PTX) at 100 nM (D8810, Solarbio), or the PTEN inhibitor SF1670 (HY-15,842, MCE, NJ, USA) at 300 nM. After 24 h, the Apoptosis Detection Kit (556,547, BD, Franklin Lakes, NJ, USA) was utilized for staining apoptotic and dead cells, and Annexin V + PI + cells were analyzed using flow cytometry (FACSCanto II, BD).
PTEN Knockout
PTEN knockout cell lines, including EO771-PTEN-KO, Pan02-PTEN-KO, LLC-PTEN-KO, iMEF-PTEN-KO, and iBMDM-PTEN-KO, were generated using CRISPR/Cas9 technology. The specific sgRNA sequences targeting PTEN were Pten-sgRNA-1-Forward: 5′- CACCGAGTCACAATTCCCAGTCAG-3′ and Pten-sgRNA-1-Reverse: 5′- AAACCTGACTGGGAATTGTGACTC-3′. The sgRNA cloning vector utilized was lentiCRISPR v2-AmCyan. Cells were transfected with plasmids containing the sgRNA sequences using Lipofectamine 3000 transfection reagent (L3000015, Invitrogen, Waltham, MA, USA). Single clones were screened, amplified, and subsequently employed in downstream experiments.
Tumor cell labeling
The EO771-PTEN-KO-GFP-Luc cell line was established through lentiviral transduction following the manufacturer’s protocol. Additionally, EO771-mCherry cells expressing red fluorescent protein (mCherry) were generated using the identical method. Lentivirus vectors were procured from Genechem (Shanghai, China), and the cell populations expressing the desired fluorescent proteins were subsequently isolated via cell sorting.
Flow cytometry
To assess the expression of p16 and p21 in tumor cells within lung tissue, single-cell suspensions were prepared by digesting lung tissue with collagenase D (LS004188, Worthington, Lakewood, New Jersey, USA). For intracellular staining of p16 and p21, cells were fixed and permeabilized using fixation/permeabilization buffer (00-5523-00, Invitrogen) for 10 min, followed by washing with 1× permeabilization washing buffer. The collected cells were blocked with 1% BSA in PBS for 30 min. Subsequently, primary antibodies p16 (1:100, 10883-1-AP, Proteintech, Wuhan, China) and p21 (1:100, sc-6246, Santa Cruz Biotechnology, Santa Cruz, CA, USA) were used to stain the cell suspensions at 4 °C for 30 min. Alexa Fluor 647-labeled rabbit anti-mouse IgG (H + L) (1:200, A21236, Invitrogen) and Alexa Fluor 647-labeled mouse anti-rabbit IgG (H + L) (1:200, A31573, Invitrogen) served as secondary antibodies for subsequent staining. Following all staining steps, cells were washed twice with 1× PBS. Data analysis was conducted using a BD FACSCanto II flow cytometer and FlowJo v10 software.
For the assessment of PTEN-L impact on immune cells in lung tissue, the tissue was digested and converted into a single-cell suspension. Specific primary antibodies were employed to stain distinct cell populations, including CD206 + macrophages (CD45 + F4/80 + CD206 + ), MHCII-expressing cells (CD45 + F4/80 + MHCII + ), myeloid-derived suppressor cells (MDSCs) (CD45 + CD11b + Gr-1 + ), CD4 T cells (CD45 + CD4 + ), CD8 T cells (CD45 + CD8 + ), B cells (CD45 + B220 + ), and NK cells (CD45 + NK1.1 + ). Antibodies used are listed in supplementary Table 1 .
Western blot
For cellular or tissue total protein extraction, cells were lysed in RIPA lysis buffer (P0013C, Beyotime, Shanghai, China) supplemented with phosphatase and protease inhibitors cocktail (P1049, Beyotime). Cell membrane proteins and cytoplasmic proteins were extracted using cell membrane protein and cytoplasmic protein extraction kits (P0033, Beyotime), while nuclear proteins were extracted using a nuclear protein extraction kit (P0028, Beyotime). Protein concentration was determined using the BCA protein assay kit (23,225, ThermoFisher Scientific) following the manufacturer’s instructions. Subsequently, 20 µg of protein samples were electrophoresed on a 10% SDS-PAGE gel (PG112, EpiZyme Biotechnology, Shanghai, China), transferred to a Hybridization Nitrocellulose filter membrane (HATF00010, Millipore, Billerica, MA, USA), and blocked at room temperature for 1 h in 1×TBS-T (TBS with 0.01% Tween-20) containing 5% skim milk. The membrane was then incubated with primary antibodies (supplementary Table 2) overnight on a shaker at 4 °C. After washing, the membrane was incubated with secondary antibody (AS014 or AS003, ABclonal, 1:3000) at room temperature for 1 h. Enhanced Chemiluminescent reagent (P10300, NCM Biotech) was applied to the membrane, and bands were visualized using the ChemiDoc MP imaging system (Bio-Rad, Hercules, CA, USA).
Total RNA was isolated from cells using Trizol reagent (9109, Takara, Tokyo, Japan), followed by reverse transcription into cDNA using the PrimeScript RT Master Mix (RR036A; Takara) in accordance with the manufacturer’s instructions. Real-time quantitative polymerase chain reaction (PCR) was conducted using the TB Green Premix Ex Taq II reagent (RR820A, Takara), and detection was performed using the Agilent Mx3005P instrument. RNA expression levels were determined using the 2^(-ΔΔCt) method, with β-actin serving as the reference gene for normalization. Primer sequences for mRNAs are provided in supplementary Table 3.
Animal experiments
To investigate the impact of PTEN-L on tumor cell metastasis in the lungs, 5 × 10 5 EO771-mock or EO771-PTEN-KO cells were injected into C57BL/6J mice, and 5 × 10 5 EO771-PTEN-KO cells were injected into SCID mice via the tail vein. On the third day, mice were intraperitoneally administered 4 mg/kg of PTEN-L, with PBS as a control. Lung tissues were collected for hematoxylin and eosin (HE) staining. Furthermore, to assess the influence of PTEN-L on PTEN-wide type or PTEN loss tumor cell metastasis, a total of 1 × 10 6 mCherry-labeled EO771 cells and GFP-Luc-labeled EO771-PTEN-KO cells (mixed at a 1:1 ratio) were injected into C57BL/6J mice via the tail vein. Tumor cell numbers in the lungs were detected by flow cytometry on days 1, 3, and 7, the mCherry and luciferin signals were observed after D-luciferin injection (150 mg/kg body weight). Fluorescence quantification was conducted using the IVIS Spectrum Imaging System (Caliper Life Sciences, Hopkinton, MA, USA) at day 21, and fluorescence intensity within regions of interest was analyzed using Living Image 4.4 software.
To study the impact of PTEN-L on lung macrophages, C57BL/6J mice were intraperitoneally injected with PTEN-L (4 mg/kg), with PBS as the negative control. After 48 h, macrophages from lung, spleen, liver, and brain tissues were analyzed using flow cytometry. To explore the effect of chemotherapy drugs on PTEN-L secretion, C57BL/6J mice were treated with DDP (5 mg/kg), PTX (10 mg/kg), or DOX (5 mg/kg). After 48 h, mice were euthanized, and PTEN-L levels in the liver, spleen, and lung were analyzed using western blot experiments.
To investigate the impact of p38 inhibition on tumor cell lung colonization, 5 × 10 5 EO771-PTEN-KO tumor cells were injected via the tail vein and treated with either 4 mg/kg PTEN-L, SB202190 (MCE, 5 mg/kg), or a combination. C57BL/6J mice were euthanized at day 21, and lung tissues were collected for HE staining. To determine if PTEN-L treatment could enhance the anti-tumor effect of chemotherapy, we established a subcutaneous tumor model by injecting 1 × 10 6 EO771-PTEN-KO cells in C57BL/6J mice. Once the tumor size reached 100 mm 3 on day 13, the mice were randomly divided into four groups ( n = 8 per group). The mice then received treatments of PTEN-L (4 mg/kg), PTX (10 mg/kg), or a combination thereof. Tumor sizes were determined using the formula: 1/2× length (L) × width (W) 2 . On day 25, lung tissues were collected for hematoxylin and eosin (HE) staining to assess metastasis.
To analyze the distinct mRNA expression profiles of EO771-PTEN-KO cells treated with PBS and PTEN-L (1 µg/ml, 3 h), RNA-sequencing was conducted using Illumina HiSeq 2500 (OE Biotech, Shanghai, China). DESeq2 software normalized gene counts in each sample, and differential protein-coding genes were selected based on fold change and significance tests. Differential gene expression with statistical significance was defined as p < 0.05.
Statistical analysis utilized GraphPad Prism 9 software, and data were expressed as mean ± standard deviation (SD). Unpaired t-test was employed for two-group comparisons, while one-way or two-way ANOVA was utilized for multiple comparisons. Statistical significance was indicated by asterisks (*), where * p < 0.05, ** p < 0.01, and *** p < 0.001.
Chemotherapy-induced PTEN-L secretion protects PTEN-null tumor cells from apoptosis
To investigate the distinct effects of PTEN-wild type or PTEN-null tumor cells, we initially established PTEN-knockout (KO) cell lines using the CRISPR-Cas-9 system in the tumor cell lines LLC, EO771, Pan02, iBMDMs, and iMEFs (Supplementary Fig. 1 A). PTEN-L was purified using a 6-His plasmid system. The purity of PTEN-L was confirmed using Coomassie brilliant blue staining and western blot (Supplementary Fig. 1 B). The cell permeability of PTEN-L was verified by detecting PTEN-L expression in the cell membrane, plasma, and nuclei of U87MG glioblastoma cells lacking PTEN expression (Supplementary Fig. 1 C). The phosphatase activity of PTEN-L was confirmed by its ability to reduce AKT phosphorylation (Supplementary Fig. 1 D).
When investigating the mechanism underlying PTEN-L secretion, we first found that cisplatin (DDP), paclitaxel (PTX), and doxorubicin (DOX) upregulated PTEN-L secretion in cell-conditioned media (CM) without reducing the basal level of PTEN/PTEN-L in the cell lysates (Fig. 1 A). Consistently, DDP, PTX, and DOX also significantly upregulated PTEN-L expression in the tissue lysates of lungs, but not in the brain, liver, or spleen (Fig. 1 B).
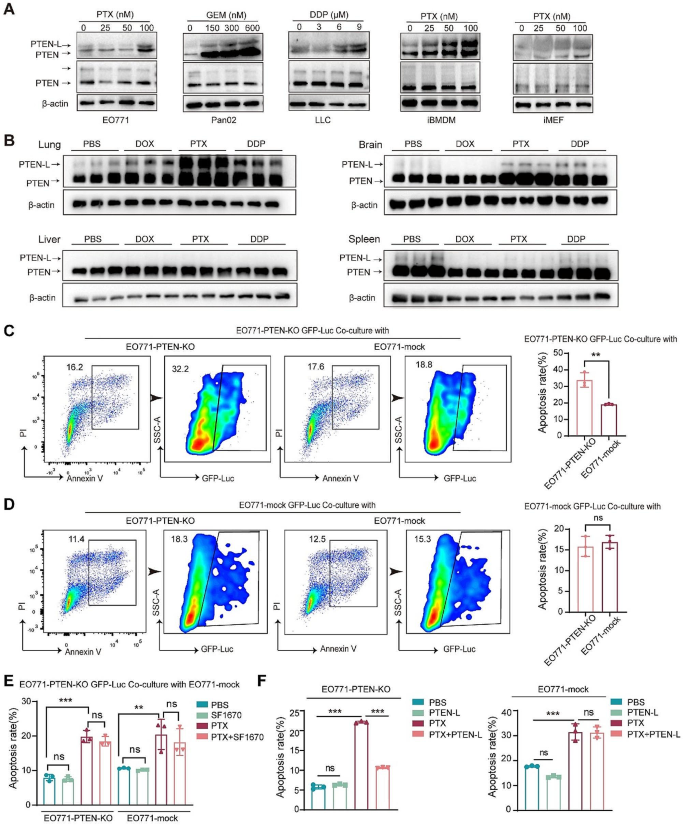
PTEN-L inhibits PTX-induced apoptosis in PTEN-null tumor cells. ( A ) EO771, Pan02, LLC, iBMDM, and MEF were subjected to 24-h treatments with PTX, GEM, and DDP at specified concentrations. Subsequently, cell conditioned media and extracts underwent immunoblotting with antibodies against PTEN, with representative images provided. β-actin served as the loading control for cell extracts. ( B ) C57BL/6J mice received treatments of DOX (5 mg/kg), PTX (10 mg/kg), or DDP (5 mg/kg) over a 48-h period. Western blot analysis was employed to detect PTEN/PTEN-L in tissue lysates from the lung, liver, brain, and spleen, with representative images presented. ( n = 3) ( C, D ) GFP-Luc labeled EO771-PTEN-KO cells (upper panel) and EO771-mock GFP-Luc cells (lower panel) were co-cultured with unlabeled EO771-mock or EO771-PTEN-KO cells at a 1:1 ratio. Post 24-h PTX (100 nM) treatment, the proportion of apoptotic GFP-Luc labeled cells was determined via flow cytometry. The gating strategy is depicted on the left, and the results are summarized in bar plots from three independent experiments (mean ± SD, Welch’s t-test). ( E ) EO771-PTEN-KO GFP-Luc cells were co-cultured with unlabeled EO771-mock cells at a 1:1 ratio and subsequently treated with PTX (100 nM) alone or in combination with the PTEN inhibitor SF1670 (300 nM) for 24 h. Apoptosis in both cell populations was assessed, and the results from three independent experiments are summarized in bar plots (Welch’s t-test). ( F ) EO771-PTEN-KO (left panel) and EO771-mock (right panel) cells were treated with PBS, PTEN-L (1 µg/ml), PTX (100 nM), or a combination thereof, for 24 h. The percentage of apoptotic cells was calculated ( n = 3); one-way ANOVA; ns: not significant
Next, we demonstrated that the supernatants of EO771 cells, iMEFs, and iBMDMs, which contained high amounts of PTEN-L after PTX stimulation, could protect EO771-PTEN-KO cells from apoptosis after DDP treatment (Supplementary Fig. 2 A-D). Similarly, EO771-mock cells could protect EO771-PTEN-KO cells from PTX induced apoptosis, which was nullified when the PTEN inhibitor, SF1670, was added (Fig. 1 C-E). Moreover, the purified PTEN-L had a similar protective effect against PTX-induced apoptosis in EO771-PTEN-KO tumor cells (Fig. 1 F). These results further confirmed that exogenous PTEN-L inhibited apoptosis in PTEN-null tumor cells during chemotherapy.
PTEN-L induces cell-cycle arrest in PTEN-null tumor cells
To demonstrate that PTEN-L protects PTEN-deficient tumor cells from apoptosis, we investigated the underlying mechanisms. Through RNA sequencing (RNA-seq) analysis, we identified 134 significantly altered genes in PTEN-L-treated EO771-PTEN-KO cells (Fig. 2 A). Tumor cells can counteract chemotherapy by evading cell-cycle arrest. Gene set enrichment analysis revealed significant enrichment of cell cycle-related genes (Fig. 2 B). Moreover, PTEN-L treatment markedly induced cell-cycle arrest by downregulating key enzymes in the cell cycle, particularly cyclins E1 and B1 (Fig. 2 C-D, Supplementary Fig. 3 A-B, G-H). Cell-cycle arrest was further validated by the reduced rate of tumor cell proliferation observed in all three PTEN-KO cell lines (Fig. 2 E, Supplementary Fig. 3 D, J). Additionally, the diminished colony formation rate suggested the decreased proliferative capacity of PTEN-null tumor cells upon PTEN-L treatment (Fig. 2 G, Supplementary Fig. 3 F, L). Importantly, PTEN-L treatment had no impact on the proliferation or colony formation of PTEN-mock tumor cells (Fig. 2 F, H, Supplementary Fig. 3 C, E, I, K).
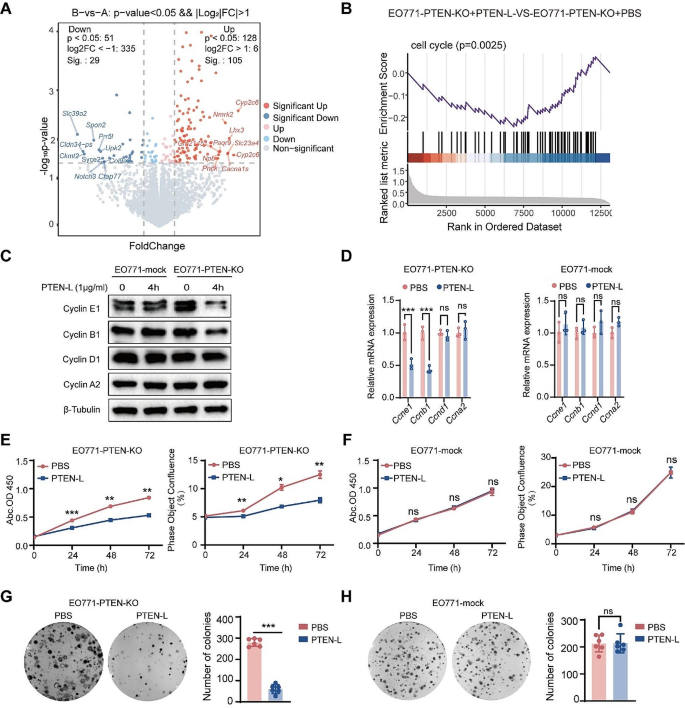
PTEN-L induces cell cycle arrest in PTEN-null tumor cells. A-B : EO771-PTEN-KO cells were administered PTEN-L (1 µg/ml) for 3 h. The RNA-seq volcano plot illustrates the differentially expressed genes, with red and blue dots signifying significantly upregulated or downregulated genes, respectively ( p < 0.05). ( B ) GSEA revealed a pronounced enrichment of cell cycle-related genes. ( C-D ) EO771-PTEN-KO and EO771-Mock cells were exposed to PTEN-L (1 µg/ml) for 4 h. The expression levels of cyclins, both at the protein and mRNA stages, were quantified via western blot (left) and RT-PCR (right) ( n = 3); Welch’s t-test. ( E-H ) Treatment of EO771-PTEN-KO and EO771-Mock cells with PTEN-L (1 µg/ml) led to analyses of cell proliferation using either the CCK8 assay (measuring OD450) or Incucyte (assessing cell confluence). ( G-H ) The cell fusion assay was employed to evaluate the cells’ colony formation potentials. Representative images are displayed on the left, and bar plots summarize the results from six independent experiments. Welch’s t-test; * p < 0.05, ** p < 0.01; *** p < 0.001; ns: not significant
PTEN-L selectively induces the colonization and growth of PTEN-null tumor cells in the lungs
We determined whether PTEN-L-induced cell-cycle arrest could lead to the selection of PTEN-null tumor cells in vivo. We established an in vivo tracking system by injecting a mixture of mCherry-labeled EO771-mock and GFP-Luc-labeled EO771-PTEN-KO cells into the tail vein (Fig. 3 A). PTEN-L treatment resulted in markedly increased GFP-Luc signals, whereas mCherry signals were largely unaffected (Fig. 3 B, C). The ratio of GFP-Luc/mCherry fluorescence was significantly elevated in the PTEN-L-treated lungs (Fig. 3 D).
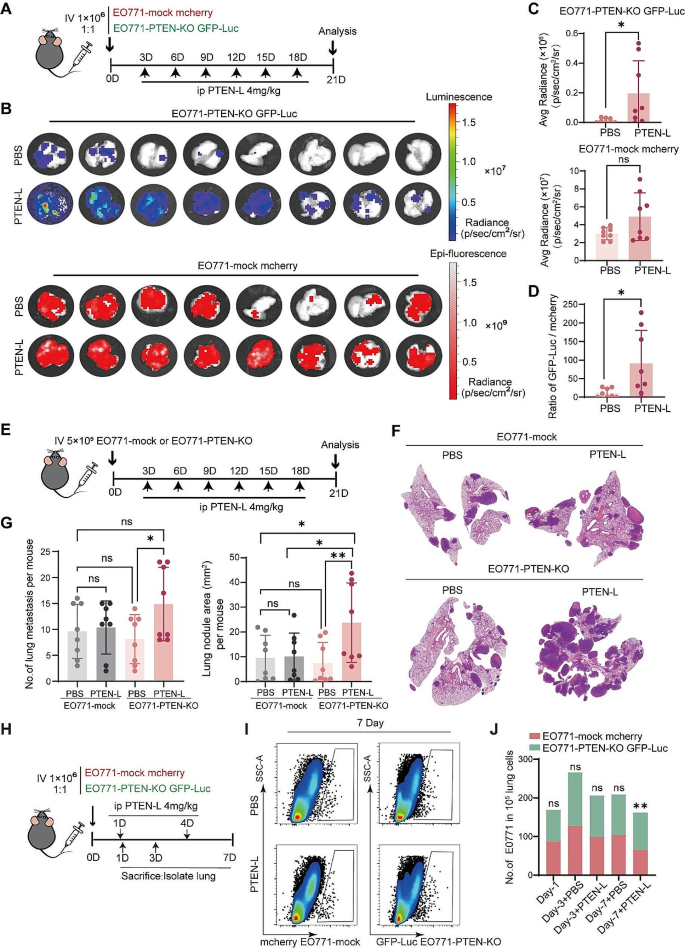
PTEN-L selectively induces PTEN-null tumor cells colonization and outgrowth in the lung. A-D : A total of 1*10 6 EO771-mock mCherry and EO771-PTEN-KO GFP-luc cells (mixed at a 1:1 ratio) were intravenously injected into C57BL/6J mice. PTEN-L (4 mg/kg) was administered every 3 d. ( A ) Schematic representation of the experimental design. ( B ) IVIS imaging displays the signals of GFP-Luc and mCherry in the lungs. ( C ) Fluorescence intensity quantification of EO771-PTEN-KO GFP-Luc (upper) and EO771-mCherry (lower) cells in lung tissues ( n = 8); Unpaired t-test. ( D ) Ratio of GFP-Luc to mCherry fluorescence signals. E-G: A total of 1*10 6 EO771-mock or EO771-PTEN-KO cells were injected into the tail vein of C57BL/6J mice, with PTEN-L (4 mg/kg) treatment every 3 d. ( E ) Schematic of the experimental design. ( F ) HE staining illustrates representative lung tissues from each group. ( G ) Quantitative analysis of the number (left) and total area (right) of lung metastatic nodules across four groups of mice ( n = 8); one-way ANOVA. * p < 0.05), ** p < 0.01. H-J : A total of 1*10 6 EO771-mock mCherry and EO771-PTEN-KO GFP-luc cells (mixed at a 1:1 ratio) were injected into the tail vein of C57BL/6J mice. PTEN-L (4 mg/kg) was administered on days 1 and 4, and mice were sacrificed on days 1, 3, and 7. Lung single-cell suspensions were analyzed via flow cytometry. ( H ) Schematic of the experimental design. ( I ) Images depicting the labeled EO771 tumor cells in each group. ( J ) Calculation of the relative ratio of EO771-PTEN-KO GFP-Luc to EO771 mCherry cells ( n = 5), two-way ANOVA, ** p < 0.01
To validate these findings and eliminate the potential confounding effects of fluorescent proteins on tumor cells, we established an in vivo lung metastasis model by injecting EO771-mock or EO771-PTEN-KO cells (Fig. 3 E). Conversely, PTEN-L treatment significantly increased the number and size of lung nodules in EO771-PTEN-KO cells but not in the PTEN-mock cells (Fig. 3 F, G). We also investigated the number of injected tumor cells in the lungs (Fig. 3 H). On days 1 and 3, there was no significant difference in the numbers of EO771-mock and EO771-PTEN-KO cells. However, the EO771-PTEN-KO/EO771-mock ratio increased significantly after PTEN-L treatment on day 7 (Fig. 3 I, J). This suggests that PTEN-L enhances the survival of PTEN-null tumor cells in the lungs during the early stages of tumor cell colonization.
PTEN-L-induced immune escape plays a major role in the selection of PTEN-null tumor cells
Immune attack is a key mechanism for eliminating tumor cells [ 9 ]. Further experiments confirmed that PTEN-L treatment significantly upregulated the expression of the key immune escape molecule PD-L1 in EO771-PTEN-KO cells (Fig. 4 A-C). In vivo experiments further confirmed that PTEN-L treatment enhances the expression of PD-L1 in EO771-PTEN-KO tumor cells within lung metastatic nodules following the treatment. (Supplementary Fig. 4 A). Furthermore, DNA repair plays a role in the immune surveillance of tumor cells by inhibiting the release of new antigens and immunogenic cell death [ 10 , 11 ]. We demonstrated that PTEN-L could confer protection against DNA damage in EO771-PTEN-KO cells by downregulating γ-H2AX, which responds to double-stranded DNA breaks (Fig. 4 C). This downregulation potentially prevents innate immune responses from targeting tumor cells. Moreover, excluding tumor cells, the PTEN-L treatment could also alter the phenotype of macrophages. PTEN-L treatment upregulated Mrc1 and Tgfb expression and downregulated Tnfa , Il6 , and Inos expression in BMDMs; (Supplementary Fig. 5 A). PTEN-L treatment may also prompt BMDMs to adopt an immunosuppressive phenotype characterized by the upregulation of markers such as F4/80, CD11b, and CD206 (Supplementary Fig. 5 B). In line with the findings, PTEN-L treatment was observed to significantly increase the population of F4/80 + CD206 + M2 macrophages within the pulmonary environment (Supplementary Fig. 5 C-F). To delve deeper into whether effective immunosurveillance can selectively promote the survival of PTEN-KO EO771 tumor cells in the lungs, the experiment was replicated using severe combined immunodeficiency (SCID) mice. However, PTEN-L treatment did not increase the number or size of EO771-PTEN-KO nodules in the lungs (Fig. 4 D-F). These results further suggested that immune cells play a major role in inducing the growth of PTEN-null tumor cells in the lungs.
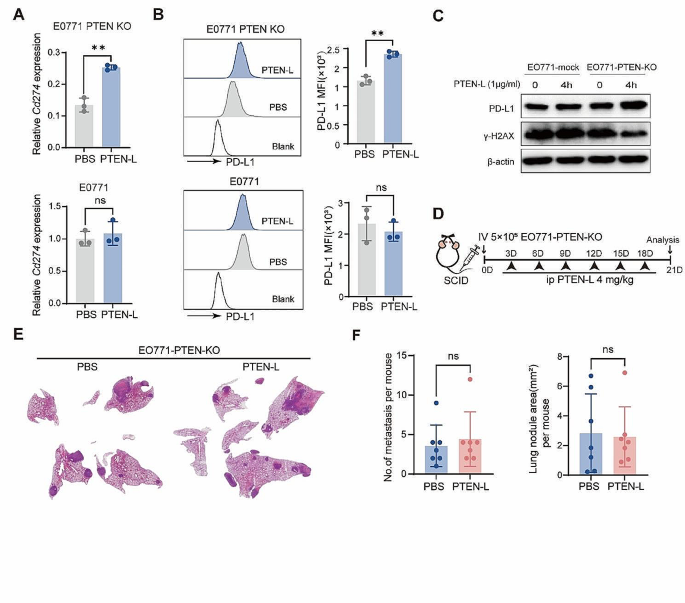
PTEN-L induces PTEN-null tumor cells immune escape. A-C : EO771-PTEN-KO (upper) and EO771-mock (lower) cells underwent PTEN-L treatment (1 µg/ml) for varying durations. ( A ) The experimental design is depicted schematically. ( B ) RT-PCR was employed to assess the mRNA expression of Cd274 . ( C ) The MFI of PD-L1 was quantified by flow cytometry 24 h post-treatment ( n = 3), Welch’s t-test. ( D ) Western blot analysis revealed the expression levels of PD-L1 and γ-H2AX following a 4-h treatment. D-F : A total of 5* 10 5 EO771-PTEN-KO cells were intravenously administered into the tail vein of SCID mice, followed by PTEN-L treatment (4 mg/kg) every 3 d. ( D ) The experimental setup is outlined. ( E ) HE staining showcases representative lung histopathology from two groups. ( F ) The number and area of lung metastatic lesions were analyzed statistically ( n = 7), Welch’s t-test
PTEN-L induces selection of PTEN-null tumor cells via p38 signaling activation
PTEN-L induces cell-cycle arrest, inhibits proliferation, enhances DNA repair, and confers chemoresistance and immune escape, all of which are characteristics of cell dormancy [ 12 ]. We confirmed that PTEN-L treatment significantly activated the major dormancy-related protein p27 in PTEN-null tumor cells at both the mRNA and protein levels but had no clear effect on PTEN-wild-type cells (Fig. 5 A, Supplementary Fig. 6 A-B). Similarly, PTEN-L treatment upregulated p16 and p21 in EO771-PTEN-KO cells in the lungs (Fig. 5 B-C). Further RNA-seq data revealed that the p38 signaling pathway, which regulates dormancy, was significantly enriched after PTEN-L treatment (Fig. 5 D). This was confirmed by the increased phosphorylation of p38 (Fig. 5 E, Supplementary Fig. 6 C-D). Moreover, SB202190, a p38 inhibitor, significantly reversed the PTEN-L-induced upregulation of p27 and PD-L1(Fig. 5 F), as well as the cell proliferation inhibition in EO771-PTEN-KO cells (Supplementary Fig. 6 E). Additionally, the combination of PTEN-L and SB202190 significantly inhibited the growth of EO771-PTEN-null tumor cells in the lungs (Fig. 5 G-I). These results confirmed that p38 inhibition suppressed PTEN-L-induced PTEN-null tumor cell proliferation in the lungs. Similarly, in PTEN inhibitor SF1670-treated EO771 cells, PTEN-L treatment significantly upregulated p38 activation and p27 expression (Fig. 5 J). This further suggests that PTEN loss or inactivation is essential for the activation of p38 signaling and tumor cell dormancy during PTEN-L treatment.
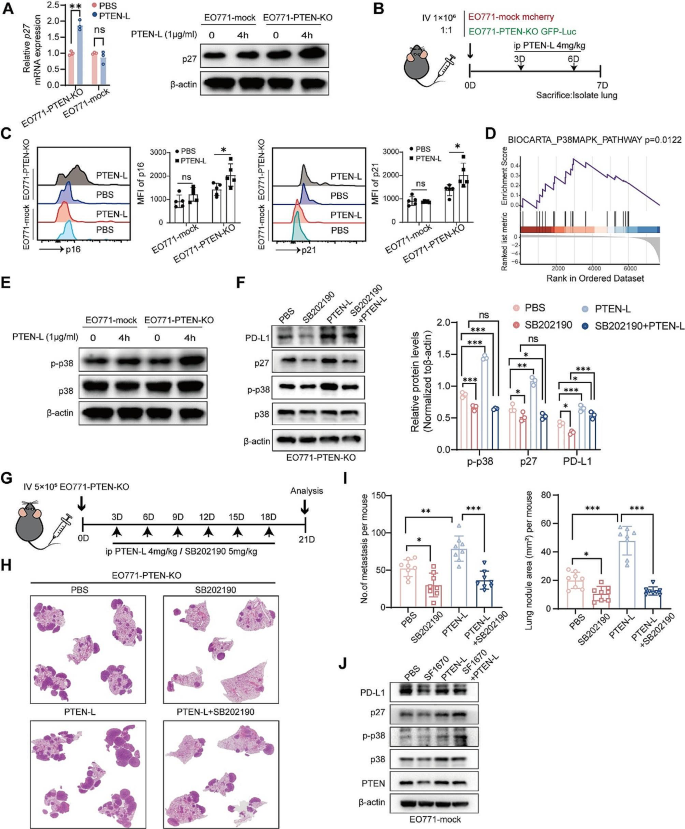
PTEN-L induces dormancy in PTEN-deficient tumor cells through the activation of p38. ( A ) EO771-PTEN-KO and EO771-mock cells were treated with PTEN-L (1 µg/ml) for various durations. The relative mRNA levels of p27 were detected using RT-PCR (left), and protein levels were assessed by western blot (right). ( B ) A total of 1* 10 6 EO771-mock mCherry and EO771-PTEN-KO GFP-luc cells (mixed at a 1:1 ratio) were injected into the tail vein of C57BL/6J mice. PTEN-L (4 mg/kg) was administered on days 3 and 6, and mice were sacrificed on day 7. The MFI of p16 and p21 was analyzed using flow cytometry. Repetitive histograms and bar plots are shown ( n = 5), two-way ANOVA, * p < 0.05. ( C ) GSEA revealed the enrichment of p38-MAPK and ERK signaling pathways in genes related to PTEN-L-treated EO771-PTEN-KO cells. ( D ) The expression of phosphorylated p38 protein in EO771-PTEN-KO or EO771-mock cells post PTEN-L treatment was validated by western blot. ( E ) Western blot analysis was conducted to examine the expression of PD-L1, p-p38, and p27 proteins in EO771-PTEN-KO cells treated with PTEN-L (1 µg/ml) and/or SB202190 (10 nM) for 4 h. Quantification results from three experiments are shown below; one-way ANOVA, * P < 0.05; ** P < 0.01; *** P < 0.001. F-H: A total of 5* 10 5 EO771-PTEN-KO cells were injected into the tail vein of C57BL/6J mice. Treatments with PTEN-L (4 mg/kg) and/or SB202190 (5 mg/kg) were administered intraperitoneally every 3 d. ( F ) Schematic of the experimental design. ( G ) Representative HE staining results of lung metastases from the four treatment groups. ( H ) Statistical analysis of the number of lung metastatic foci (left) and the total metastatic area (right) (( n = 8), one-way ANOVA. ( I ) Expression analysis of PD-L1, p-p38, and p27 in EO771 cells treated with the PTEN inhibitor SF1670 (300 nM), PTEN-L (1 µg/ml), or a combination thereof. Representative western blot images are provided
PTEN-L does not exhibit any antitumor effect on PTEN-null tumor cells in vivo
Having demonstrated that PTEN-L selectively induces lung colonization and growth of PTEN-null tumor cells, we next compared the effects of PTEN-L and chemotherapeutic drugs on tumor promotion. Therefore, we established a subcutaneous tumor model using EO771-PTEN-KO tumor cells. C57BL/6J mice were treated with either PTEN-L or PTX (Fig. 6 A). Surprisingly, in contrast to the previously reported antitumor effect of PTEN-L on 4T1 tumor cells [ 4 ], PTEN-L treatment did not inhibit the growth of EO771-PTEN-KO cells but led to a higher number of metastatic colonization in the lungs (Fig. 6 B-D).
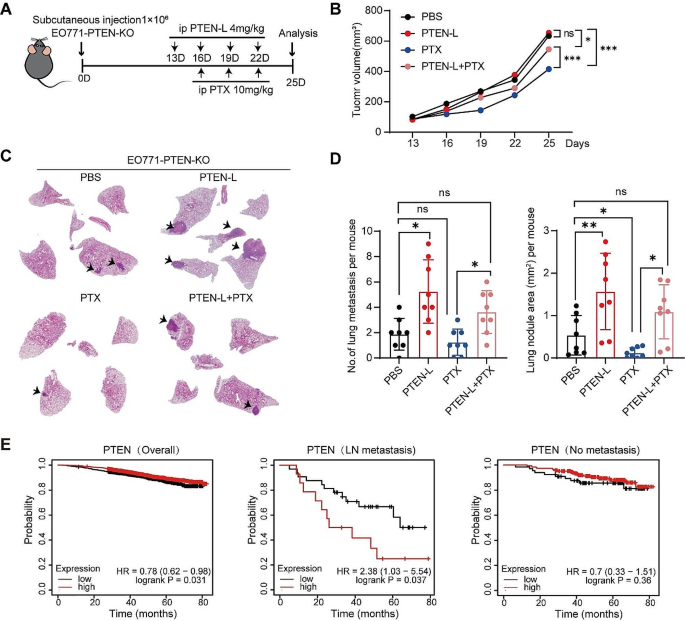
PTEN-L does not exhibit any anti-tumor effect on PTEN-null tumor cells in vivo. A-D : A total of 1*10 6 EO771-PTEN-KO cells were subcutaneously injected into mice. Treatments with PTEN-L (4 mg/kg) or a combination with PTX (10 mg/kg) were administered intraperitoneally every 3 d. ( A ) Schematic diagram of the experimental design. ( B ) Tumor volume measurements are presented. ( C ) The HE staining images illustrate the histopathological changes in lung tissues. ( D ) Bar plots depict the number (left) and area (right) of lung metastases across the four groups ( n = 8), mean ± SD; one-way ANOVA; * p < 0.05, ** p < 0.01. ( E ) Overall survival analysis of breast cancer patients based on PTEN expression, as sourced from the TCGA database. The analysis is segmented into: General survival based on PTEN expression (Left); Survival of patients with lymph node metastasis (Middle); and Survival of patients without lymph node metastasis (Right)
Finally, we analyzed the clinical relevance of PTEN expression in breast tumor metastasis. By examining The Cancer Genome Atlas dataset, we confirmed that PTEN expression was positively correlated with the overall survival of breast cancer patients, which is consistent with most existing literature [ 13 ]. However, in patients with lymph node metastasis, those with high PTEN expression had significantly shorter durations of survival, although this did not affect those without lymph node metastasis (Fig. 6 E). These results further suggest that PTEN-L may negatively impact the outcomes of tumor therapy, especially in PTEN-deficient tumor cells.
Loss of PTEN has been documented across diverse neoplastic entities and is positively correlated with malignant tumor progression [ 14 ]. Despite the presence of PTEN mutations, various mechanisms are known to contribute to the loss or downregulation of PTEN in tumor cells [ 15 ]. Nonetheless, a comprehensive understanding of the survival and selection of PTEN-deficient cells during tumor treatment has not been achieved yet. In this study, we demonstrated that the secreted PTEN subtype PTEN-L exerts distinct effects on both PTEN-wild-type and PTEN-deficient tumor cells. PTEN-L treatment induced dormancy-related characteristics in PTEN-null tumor cells, including cell-cycle arrest, proliferation inhibition, chemoresistance, and immune escape. These characteristics enabled PTEN-null tumor cells to survive and grow in distinct microenvironments. Thus, this study accentuates a PTEN-L-dependent mechanism governing PTEN-loss selection during tumor metastasis, thereby highlighting the pivotal influence of PTEN expression in tumor cells on the efficacy of PTEN-L-based antitumor therapy.
The principle of natural selection is a cornerstone of cancer progression, where cells endowed with advantageous genetic and epigenetic characteristics gain a proliferative advantage. The concept is well-documented in the literature [ 16 ]. As tumors evolve, the immune system engages in a relentless battle against cancerous cells. Yet, some cells develop strategies to elude immune surveillance, enabling their survival and proliferation in distant organs [ 17 ]. Our findings demonstrate that PTEN-deficient tumor cells can endure and establish themselves in the lungs post-PTEN-L treatment. Notably, the metastatic potential of PTEN-mock and PTEN-KO EO771 cells exhibits no disparity in immunodeficient mice, implying that natural selection is not the sole driver of PTEN-KO tumor cell metastasis. In contrast, PTEN-L treatment or the secretion of PTEN-L induced by chemotherapy seems to promote the survival and pulmonary colonization of PTEN-KO tumor cells. Further investigations reveal that PTEN-L treatment elevates PD-L1 expression and curtails DNA damage, which may shield against immune cell-mediated eradication. Such insights lead us to postulate that PTEN-L modulates the selective survival of PTEN-KO tumor cells.
PTEN mutations are contribute to tumor malignancy. Numerous studies have reported that PTEN is a tumor suppressor gene that inhibits tumor cell proliferation and progression. Patients with breast cancer and high PTEN expression showed significantly prolonged survival [ 18 ]. In alignment with the prevailing body of literature, our Kaplan–Meier plot analysis reveals that patients with low PTEN expression levels have a markedly reduced overall survival rate when compared to those with high PTEN expression levels, especially in cases without lymph node metastasis. Conversely, among patients with lymph node metastasis, a higher PTEN expression level correlates with decreased survival times. This suggests that elevated PTEN expression level does not necessarily bestow a survival benefit in the context of lymph node metastasis; instead, it may, in fact, aggravate tumor metastasis in certain cases.
Loss of PTEN has been reported in multiple tumor types. PTEN exhibits a high mutation rate in prostate cancers [ 19 ]. Notably, the frequency of PTEN deletion is 10–20% in primary prostate cancers but increases to 30–45% in metastatic tumors [ 20 ]. PTEN loss is considered an early prognostic marker of prostate cancer metastasis [ 19 ]. Moreover, it is associated with advanced tumor stages and resistance to therapy. However, a tumor-promoting function of PTEN has also been reported. Fortin et al. reported that PTEN loss prevents malignant transformation in pre-B acute lymphoblastic leukemia [ 21 ]. Similarly, PTEN can enhance the stability of gain-of-function P53 mutants, thus conferring tumor-promoting functions to glioma cells [ 22 ]. During this study, an unanticipated observation emerged, wherein PTEN-L treatment resulted in distinct cellular behaviors in PTEN-deficient and PTEN-expressing tumor cells. This underscores the potentially critical role of PTEN-L in the selection of PTEN-deficient tumor cells during tumor metastasis.
Numerous studies have highlighted the link between PTEN loss and essential aspects of tumor cell behavior through the activation of downstream signaling pathways, notably the PI3K-AKT-mTOR pathway [ 23 ]. In terms of strategies for treating tumors, several PI3K and AKT inhibitors have shown promise in preclinical studies. Furthermore, PTEN reactivation in tumor cells has emerged as an attractive avenue for potential therapies. Lee et al. reported the potent antitumor effects of indole-3-carbinol, a pharmacological inhibitor of WWP1, which were achieved by reactivating PTEN [ 24 ]. Additionally, the delivery of PTEN mRNA via nanoparticle-mediated methods has restored PTEN function in PTEN-mutated prostate cancer cells and effectively inhibited tumor growth [ 25 ]. Our findings showed that PTEN-L delivery could impede AKT activation and induce cell-cycle arrest, thus suggesting a potential pro-survival effect in tumors by evading immune attack. This effect was particularly pronounced in PTEN-null tumor cells.
Emerging evidence indicates that DNA damage and mutational burden on tumor cells influence T cell infiltration and the success of immunotherapy. It has been widely accepted that genomic instability activates immune responses via production of neoantigens [ 26 ]. PTEN plays a critical role in maintaining genomic integrity [ 27 ]. Shen et al. reported that genomic and chromosomal instability was significantly increased in PTEN-null mice, and PTEN promoted double-stranded DNA break repair by regulating Rad51 expression [ 28 ]. PTEN deficiency-induced stabilization of DNA binding protein 1 facilitates TNFα transcription [ 29 ]. Similarly, we found that γ-H2AX, a sensitive molecular marker of DNA damage, was highly expressed in PTEN-null EO771 tumor cells, but it was inhibited by PTEN-L treatment. This finding implies that PTEN-L plays a protective role against PTEN in the regulation of genomic integrity and DNA repair, albeit exclusively in PTEN-null tumor cells. The nuances of this distinction warrant further in-depth investigation.
Mutations in PTEN are frequently observed in immunoresistant cancers. Previous studies have reported that PTEN deficiency facilitates the establishment of an immunosuppressive environment that is unfavorable for effective antitumor immune responses [ 30 , 31 ]. However, the underlying mechanism remains unclear. PTEN loss is linked to a highly immunosuppressive tumor microenvironment as it mediates the accumulation of MDSCs, regulatory T cells, and M2 macrophages [ 32 , 33 ]. Here, we observed that PTEN-L treatment induced high PD-L1 expression in PTEN-null tumor cells, which may have contributed to the escape of T cell-mediated immunosurveillance. Therefore, it is speculated that PTEN-L protects PTEN-deficient tumor cells from immune attacks during tumor progression. In addition to tumor cells, we found that PTEN-L mediates the polarization of macrophages into immunosuppressive phenotypes both in vitro and in vivo. The increased number of CD206 + macrophages in the lungs may provide a suitable immunosuppressive environment for circulating tumor cell colonization. In the present study, selection for PTEN-L-treated PTEN-KO EO771 cells was not observed in SCID mice. SCID mice, characterized by their lack of T and B cells, are a model of immunodeficiency. Notably, macrophages in SCID mice demonstrate enhanced expression of the inducible nitric oxide synthase pathway [ 34 ]. The observation underscores the notion that the establishment of an immunosuppressive microenvironment contingent on PTEN-L dependence contributes significantly to the selection, subsequent colonization, and growth of PTEN-deficient tumor cells in the lungs.
PTEN/PTEN-L expression is tissue-dependent. Previous studies have reported that the brain, lymph nodes, lungs, and intestines exhibit higher PTEN-L (PTENa) expression [ 35 ]. PTEN-L is a secreted protein derived from PTEN-expressing cells. Although the mechanisms underlying PTEN-L production and secretion are not fully understood, PTEN/PTEN-L levels have been reported to differ between tissues. We found that the chemotherapy drugs DDP and PTX could increase the production of PTEN/PTEN-L in the lungs, but not in the liver and spleen. Similarly, these chemotherapeutic drugs upregulated PTEN-L secretion in the supernatant of PTEN-wild-type tumor cells. Moreover, we found that these chemotherapeutic drugs upregulated PTEN expression. It has been reported that PTEN can be secreted and transported via exosomes. Whether secreted exosomal PTEN has a similar effect on PTEN-null tumor cell dormancy and growth in the lungs requires further investigation.
Tumor cell dormancy is essential for the survival of tumor cells in a new environment and initiation of metastasis. Aged lung microenvironment enables melanoma cells to enter dormancy and efficiently initiate metastatic outgrowth in the lungs by inhibiting WNT5A [ 36 ]. In this study, we found that PTEN-L treatment induced dormancy in PTEN-null tumor cells; namely, it increased cell-cycle arrest and reduced cell proliferation. Cell-cycle arrest is known to play a key role in dormancy, causing resistance to therapy while adapting to a new microenvironment, and is believed to be a factor in the formation of metastatic tumors [ 37 ]. Additionally, reduced cell proliferation can protect tumor cells from immune attacks. Our results suggest that PTEN-L-treated PTEN-null EO771 tumor cells exhibit a higher ability to survive and grow in the lungs by activating the p38 signaling pathways. Moreover, targeting PTEN-L secretion or p38 activation can inhibit the survival and growth of PTEN-null tumor cells in the lungs. Further studies are required to investigate how dormant tumor cells are reawakened in the lungs.
In summary, the present study shows that PTEN-L safeguards the selection of PTEN-null tumor cells during the metastatic cascade by inducing p38-mediated tumor cell dormancy. When formulating therapeutic strategies involving PTEN-L delivery, it is imperative to consider PTEN expression and strategically focus on preventing PTEN-deficient tumor cell selection.
Data availability
The RNA sequencing data were deposited in the GEO database under the accession number GSE246600.
Abbreviations
Doxorubicin
Severe combined immunodeficiency
Paraformaldehyde
Gene set enrichment analysis
Milella M, Falcone I, Conciatori F, et al. PTEN: multiple functions in human malignant tumors. [J] Front Oncol. 2015;5:24.
PubMed Central Google Scholar
Zhang HY, Liang F, Jia ZL, et al. PTEN mutation, methylation and expression in breast cancer patients [J]. Oncol Lett. 2013;6(1):161–8.
Article PubMed PubMed Central Google Scholar
Correia NC, Gírio A, Antunes I, et al. The multiple layers of non-genetic regulation of PTEN tumour suppressor activity [J]. Eur J cancer (Oxford England: 1990). 2014;50(1):216–25.
Article CAS Google Scholar
Hopkins BD, Fine B, Steinbach N, et al. A secreted PTEN phosphatase that enters cells to alter signaling and survival [. J] Sci. 2013;341(6144):399–402.
CAS Google Scholar
Tan L, Xu Z, Mao Q, et al. Purified PTEN-Long induces Liver Cancer cells to Undergo Autophagy and apoptosis [J]. Front Surg. 2022;9:767611.
Fares J, Fares MY, Khachfe HH, et al. Molecular principles of metastasis: a hallmark of cancer revisited [J]. Signal Transduct Target Ther. 2020;5(1):28.
Zhang L, Zhang S, Yao J, et al. Microenvironment-induced PTEN loss by exosomal microRNA primes brain metastasis outgrowth [. J] Nat. 2015;527(7576):100–4.
Jochner MCE, An J, Lättig-Tünnemann G, et al. Unique properties of PTEN-L contribute to neuroprotection in response to ischemic-like stress [J]. Sci Rep. 2019;9(1):3183.
Kim SK, Cho SW. The Evasion Mechanisms of Cancer Immunity and Drug Intervention in the Tumor Microenvironment [J]. Frontiers in pharmacology , 2022, 13: 868695.
Germano G, Lamba S, Rospo G, et al. Inactivation of DNA repair triggers neoantigen generation and impairs tumour growth [. J] Nat. 2017;552(7683):116–20.
Nastasi C, Mannarino L, D’incalci M. DNA Damage Response Immune De?F [J] Int J Mol Sci, 2020, 21(20).
Santos-De-Frutos K, Djouder N. When dormancy fuels tumour relapse [J]. Commun Biology. 2021;4(1):747.
Article Google Scholar
Depowski PL, Rosenthal SI, Ross JS. Loss of expression of the PTEN gene protein product is associated with poor outcome in breast cancer [. J] Mod Pathology: Official J United States Can Acad Pathol Inc. 2001;14(7):672–6.
Hollander MC, Blumenthal GM, Dennis PA. PTEN loss in the continuum of common cancers, rare syndromes and mouse models [J]. Nat Rev Cancer. 2011;11(4):289–301.
Article CAS PubMed PubMed Central Google Scholar
Alvarez-Garcia V, Tawil Y, Wise HM, et al. Mechanisms of PTEN loss in cancer: it’s all about diversity [J]. Semin Cancer Biol. 2019;59:66–79.
Article CAS PubMed Google Scholar
Khong HT, Restifo NP. Natural selection of tumor variants in the generation of tumor escape phenotypes [J]. Nat Immunol. 2002;3(11):999–1005.
Peng L, Zhang Y, Wang Z, Immune. Responses against Disseminated Tumor Cells [J] Cancers (Basel), 2021, 13(11).
Carbognin L, Miglietta F, Paris I et al. Prognostic and predictive implications of PTEN in breast Cancer: unfulfilled promises but intriguing perspectives. [J] Cancers, 2019, 11(9).
Jamaspishvili T, Berman DM, Ross AE, et al. Clinical implications of PTEN loss in prostate cancer [. J] Nat Reviews Urol. 2018;15(4):222–34.
Zhang S, Yu D. PI(3)king apart PTEN’s role in cancer [J]. Clin cancer Research: Official J Am Association Cancer Res. 2010;16(17):4325–30.
Fortin J, Bassi C, Mak TW. PTEN enables the development of pre-B acute lymphoblastic leukemia [J]. Nat Med. 2016;22(4):339–40.
Li Y, Guessous F, Kwon S, et al. PTEN has tumor-promoting properties in the setting of gain-of-function p53 mutations [J]. Cancer Res. 2008;68(6):1723–31.
He Y, Sun MM, Zhang GG, et al. Targeting PI3K/Akt signal transduction for cancer therapy [J]. Signal Transduct Target Therapy. 2021;6(1):425.
Lee YR, Chen M, Lee JD et al. Reactivation of PTEN tumor suppressor for cancer treatment through inhibition of a MYC-WWP1 inhibitory pathway [J]. Science, 2019, 364(6441).
Islam MA, Xu Y, Tao W, et al. Restoration of tumour-growth suppression in vivo via systemic nanoparticle-mediated delivery of PTEN mRNA [J]. Nat Biomedical Eng. 2018;2(11):850–64.
Chen M, Linstra R, Van Vugt M. Genomic instability, inflammatory signaling and response to cancer immunotherapy [J]. Biochimica et biophysica acta Reviews on cancer , 2022, 1877(1): 188661.
Xie N, Shen G, Gao W, et al. Neoantigens: promising targets for cancer therapy [J]. Signal Transduct Target Therapy. 2023;8(1):9.
Shen WH, Balajee AS, Wang J, et al. Essential role for nuclear PTEN in maintaining chromosomal integrity [. J] Cell. 2007;128(1):157–70.
Zhao D, Lu X, Wang G, et al. Synthetic essentiality of chromatin remodelling factor CHD1 in PTEN-deficient cancer [. J] Nat. 2017;542(7642):484–8.
Murphy BM, Burd CE. The aging lung microenvironment awakens melanoma metastases [J]. Cancer Cell. 2022;40(8):815–7.
Vidotto T, Melo CM, Castelli E, et al. Emerging role of PTEN loss in evasion of the immune response to tumours [J]. Br J Cancer. 2020;122(12):1732–43.
Sosa MS, Bragado P, Aguirre-Ghiso JA. Mechanisms of disseminated cancer cell dormancy: an awakening field [J]. Nat Rev Cancer. 2014;14(9):611–22.
Cetintas VB, Batada NN. Is there a causal link between PTEN deficient tumors and immunosuppressive tumor microenvironment? [J] J Translational Med. 2020;18(1):45.
Mills CD, Kincaid K, Alt JM, et al. M-1/M-2 macrophages and the Th1/Th2 paradigm [J]. J Immunol. 2000;164(12):6166–73.
Sun Y, Lu D, Yin Y, et al. PTENα functions as an immune suppressor and promotes immune resistance in PTEN-mutant cancer [J]. Nat Commun. 2021;12(1):5147.
Fane ME, Chhabra Y, Alicea GM, et al. Stromal changes in the aged lung induce an emergence from melanoma dormancy [. J] Nat. 2022;606(7913):396–405.
Neophytou CM, Kyriakou TC, Papageorgis P. Mechanisms of metastatic Tumor Dormancy and implications for Cancer therapy [J]. Int J Mol Sci, 2019, 20(24).
Download references
Acknowledgements
We thank the Editage ( https://www.editage.cn/ ) for editing of the manuscript prior to submission.
This work was supported by the National Natural Science Foundation of China (Grant Nos. 82073231 to Z.H. Q.; 81901466 to M. W); Henan Province Scientific and Technological Research (Grant Nos. 232102520026 to M. W.).
Author information
Ming Wang and Zhenzhen Pan contributed equally to this work.
Authors and Affiliations
Medical Research Center, The First Affiliated Hospital of Zhengzhou University, Zhengzhou University, Zhengzhou, Henan, 450052, China
Ming Wang, Zhenzhen Pan, Xiaohan Yao, Xixi Duan, Jiajia Wan, Xiaohan Lou, Wenqing Li, Yan Yan, Lin Chen & Zhihai Qin
The first affiliated hospital of Henan University of science and technology, Luo Yang, China
Guangzhou DaAn Clinical Laboratory Center Co. Ltd, YunKang Group, Guangzhou, 510000, China
You can also search for this author in PubMed Google Scholar
Contributions
M. W. and Z.H Q. designed the study. M. W., Z.Z P. and X. C. interpreted the data and wrote the paper with input from all authors. M. W., Z.Z P., X.H Y., J.J W., X. H L. W.Q L. and L. C. performed all experiments, J.F A supplies the PTEN-L plasmid and offers guidance on the purification of PTEN-L.
Corresponding authors
Correspondence to Ming Wang or Zhihai Qin .
Ethics declarations
Ethics approval and consent to participate.
All animal experiments have been approved by the Ethics Committee of the First Affiliated Hospital of Zhengzhou University (Ethics Approval Number: 2022-KY-1206-001).
Consent for publication
Not applicable.
Conflict of interest
The authors declare no potential conflicts of interest.
Competing interests
Additional information, publisher’s note.
Springer Nature remains neutral with regard to jurisdictional claims in published maps and institutional affiliations.
Electronic supplementary material
Below is the link to the electronic supplementary material.
Supplementary Material 1
Rights and permissions.
Open Access This article is licensed under a Creative Commons Attribution 4.0 International License, which permits use, sharing, adaptation, distribution and reproduction in any medium or format, as long as you give appropriate credit to the original author(s) and the source, provide a link to the Creative Commons licence, and indicate if changes were made. The images or other third party material in this article are included in the article’s Creative Commons licence, unless indicated otherwise in a credit line to the material. If material is not included in the article’s Creative Commons licence and your intended use is not permitted by statutory regulation or exceeds the permitted use, you will need to obtain permission directly from the copyright holder. To view a copy of this licence, visit http://creativecommons.org/licenses/by/4.0/ . The Creative Commons Public Domain Dedication waiver ( http://creativecommons.org/publicdomain/zero/1.0/ ) applies to the data made available in this article, unless otherwise stated in a credit line to the data.
Reprints and permissions
About this article
Cite this article.
Wang, M., Pan, Z., Chu, X. et al. Chemotherapy-induced PTEN-L secretion promotes the selection of PTEN-deficient tumor cells. J Exp Clin Cancer Res 43 , 140 (2024). https://doi.org/10.1186/s13046-024-03059-y
Download citation
Received : 07 February 2024
Accepted : 29 April 2024
Published : 11 May 2024
DOI : https://doi.org/10.1186/s13046-024-03059-y
Share this article
Anyone you share the following link with will be able to read this content:
Sorry, a shareable link is not currently available for this article.
Provided by the Springer Nature SharedIt content-sharing initiative
Journal of Experimental & Clinical Cancer Research
ISSN: 1756-9966
- Submission enquiries: Access here and click Contact Us
- General enquiries: [email protected]

RSC Medicinal Chemistry
Research progress of anticancer drugs targeting cdk12.

* Corresponding authors
a School of Chemistry & Chemical Engineering, Qilu University of Technology (Shandong Academy of Sciences), 3501 Da Xue Road, Jinan, China E-mail: [email protected]
b School of Water Conservancy and Environment, University of Jinan, 336 Nanxinzhuang West Road, Jinan, China
Cyclin-dependent kinase 12 (CDK12) is a transcription-associated CDK that plays key roles in transcription, translation, mRNA splicing, the cell cycle, and DNA damage repair. Research has identified that high expression of CDK12 in organs such as the breast, stomach, and uterus can lead to HER2-positive breast cancer, gastric cancer and cervical cancer. Inhibiting high expression of CDK12 suppresses tumor growth and proliferation, suggesting that it is both a biomarker for cancer and a potential target for cancer therapy. CDK12 inhibitors can competitively bind the CDK12 hydrophobic pocket with ATP to avoid CDK12 phosphorylation, blocking subsequent signaling pathways. The development of CDK12 inhibitors is challenging due to the high homology of CDK12 with other CDKs. This review summarizes the research progress of CDK12 inhibitors, their mechanism of action and the structure–activity relationship, providing new insights into the design of CDK12 selective inhibitors.
Article information
Download citation, permissions.

Z. Yan, Y. Du, H. Zhang, Y. Zheng, H. Lv, N. Dong and F. He, RSC Med. Chem. , 2023, 14 , 1629 DOI: 10.1039/D3MD00004D
To request permission to reproduce material from this article, please go to the Copyright Clearance Center request page .
If you are an author contributing to an RSC publication, you do not need to request permission provided correct acknowledgement is given.
If you are the author of this article, you do not need to request permission to reproduce figures and diagrams provided correct acknowledgement is given. If you want to reproduce the whole article in a third-party publication (excluding your thesis/dissertation for which permission is not required) please go to the Copyright Clearance Center request page .
Read more about how to correctly acknowledge RSC content .
Social activity
Search articles by author, advertisements.

Cellular activity hints that recycling is in our DNA
New research shows that 'spliceosomes' might reinsert problematic gene sequences after removing them.
Although you may not appreciate them, or have even heard of them, throughout your body, countless microscopic machines called spliceosomes are hard at work. As you sit and read, they are faithfully and rapidly putting back together the broken information in your genes by removing sequences called "introns" so that your messenger RNAs can make the correct proteins needed by your cells.
Introns are perhaps one of our genome's biggest mysteries. They are DNA sequences that interrupt the sensible protein-coding information in your genes, and need to be "spliced out." The human genome has hundreds of thousands of introns, about 7 or 8 per gene, and each is removed by a specialized RNA protein complex called the "spliceosome" that cuts out all the introns and splices together the remaining coding sequences, called exons. How this system of broken genes and the spliceosome evolved in our genomes is not known.
Over his long career, Manny Ares, UC Santa Cruz distinguished professor of molecular, cellular, and developmental biology, has made it his mission to learn as much about RNA splicing as he can.
"I'm all about the spliceosome," Ares said. "I just want to know everything the spliceosome does -- even if I don't know why it is doing it."
In a new paper published in the journal Genes and Development , Ares reports on a surprising discovery about the spliceosome that could tell us more about the evolution of different species and the way cells have adapted to the strange problem of introns. The authors show that after the spliceosome is finished splicing the mRNA, it remains active and can engage in further reactions with the removed introns.
This discovery provides the strongest indication we have so far that spliceosomes could be able to reinsert an intron back into the genome in another location. This is an ability that spliceosomes were not previously believed to possess, but which is a common characteristic of "Group II introns," distant cousins of the spliceosome that exist primarily in bacteria.
The spliceosome and Group II introns are believed to share a common ancestor that was responsible for spreading introns throughout the genome, but while Group II introns can splice themselves out of RNA and then directly back into DNA, the "spliceosomal introns" that are found in most higher-level organisms require the spliceosome for splicing and were not believed to be reinserted back into DNA. However, Ares's lab's finding indicates that the spliceosome might still be reinserting introns into the genome today. This is an intriguing possibility to consider because introns that are reintroduced into DNA add complexity to the genome, and understanding more about where these introns come from could help us to better understand how organisms continue to evolve.
Building on an interesting discovery
An organism's genes are made of DNA, in which four bases, adenine (A), cytosine (C), guanine (G) and thymine (T) are ordered in sequences that code for biological instructions, like how to make specific proteins the body needs. Before these instructions can be read, the DNA gets copied into RNA by a process known as transcription, and then the introns in that RNA have to be removed before a ribosome can translate it into actual proteins.
The spliceosome removes introns using a two-step process that results in the intron RNA having one of its ends joined to its middle, forming a circle with a tail that looks like a cowboy's "lariat," or lasso. This appearance has led to them being named "lariat introns." Recently, researchers at Brown University who were studying the locations of the joining sites in these lariats made an odd observation -- some introns were actually circular instead of lariat shaped.
This observation immediately got Ares's attention. Something seemed to be interacting with the lariat introns after they were removed from the RNA sequence to change their shape, and the spliceosome was his main suspect.
"I thought that was interesting because of this old, old idea about where introns came from," Ares said. "There is a lot of evidence that the RNA parts of the spliceosome, the snRNAs, are closely related to Group II introns."
Because the chemical mechanism for splicing is very similar between the spliceosomes and their distant cousins, the Group II introns, many researchers have theorized that when the process of self-splicing became too inefficient for Group II introns to reliably complete on their own, parts of these introns evolved to become the spliceosome. While Group II introns were able to insert themselves directly back into DNA, however, spliceosomal introns that required the help of spliceosomes were not thought to be inserted back into DNA.
"One of the questions that was sort of missing from this story in my mind was, is it possible that the modern spliceosome is still able to take a lariat intron and insert it somewhere in the genome?" Ares said. "Is it still capable of doing what the ancestor complex did?"
To begin to answer this question, Ares decided to investigate whether it was indeed the spliceosome that was making changes to the lariat introns to remove their tails. His lab slowed the splicing process in yeast cells, and discovered that after the spliceosome released the mRNA that it had finished splicing introns from, it hung onto intron lariats and reshaped them into true circles. The Ares lab was able to reanalyze published RNA sequencing data from human cells and found that human spliceosomes also had this ability.
"We are excited about this because while we don't know what this circular RNA might do, the fact that the spliceosome is still active suggests it may be able to catalyze the insertion of the lariat intron back into the genome," Ares said.
If the spliceosome is able to reinsert the intron into DNA, this would also add significant weight to the theory that spliceosomes and Group II introns shared a common ancestor long ago.
Testing a theory
Now that Ares and his lab have shown that the spliceosome has the catalytic ability to hypothetically place introns back into DNA like their ancestors did, the next step is for the researchers to create an artificial situation in which they "feed" a DNA strand to a spliceosome that is still attached to a lariat intron and see if they can actually get it to insert the intron somewhere, which would present "proof of concept" for this theory.
If the spliceosome is able to reinsert introns into the genome, it is likely to be a very infrequent event in humans, because the human spliceosomes are in incredibly high demand and therefore do not have much time to spend with removed introns. In other organisms where the spliceosome isn't as busy, however, the reinsertion of introns may be more frequent. Ares is working closely with UCSC Biomolecular Engineering Professor Russ Corbett-Detig, who has recently led a systematic and exhaustive hunt for new introns in the available genomes of all intron-containing species that was published in the journal Proceedings of the National Academy of Sciences (PNAS) last year.
The paper in PNAS showed that intron "burst" events far back in evolutionary history likely introduced thousands of introns into a genome all at once. Ares and Corbett-Detig are now working to recreate a burst event artificially, which would give them insight into how genomes reacted when this happened.
Ares said that his cross-disciplinary partnership with Corbett-Detig has opened the doors for them to really dig into some of the biggest mysteries about introns that would probably be impossible for them to understand fully without their combined expertise.
"It is the best way to do things," Ares said. "When you find someone who has the same kind of questions in mind but a different set of methods, perspectives, biases, and weird ideas, that gets more exciting. That makes you feel like you can break out and solve a problem like this, which is very complex."
- Human Biology
- Back and Neck Pain
- Biochemistry Research
- New Species
- Evolutionary Biology
- Genetic code
- Human genome
- DNA microarray
Story Source:
Materials provided by University of California - Santa Cruz . Original written by Rose Miyatsu. Note: Content may be edited for style and length.
Journal Reference :
- Manuel Ares, Haller Igel, Sol Katzman, John P. Donohue. Intron lariat spliceosomes convert lariats to true circles: implications for intron transposition . Genes & Development , 2024; DOI: 10.1101/gad.351764.124
Cite This Page :
Explore More
- Fastest Rate of CO2 Rise Over Last 50,000 Years
- Like Dad and Like Mum...all in One Plant
- What Makes a Memory? Did Your Brain Work Hard?
- Plant Virus Treatment for Metastatic Cancers
- Controlling Shape-Shifting Soft Robots
- Brain Flexibility for a Complex World
- ONe Nova to Rule Them All
- AI Systems Are Skilled at Manipulating Humans
- Planet Glows With Molten Lava
- A Fragment of Human Brain, Mapped
Trending Topics
Strange & offbeat.
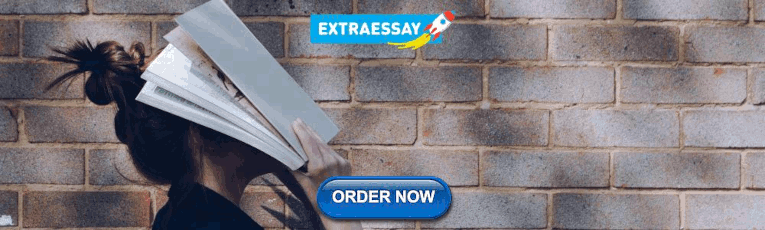
IMAGES
VIDEO
COMMENTS
All organisms possess molecular mechanisms that govern DNA repair and associated DNA damage response (DDR) processes. Owing to their relevance to human disease, most notably cancer, these ...
The Journey of DNA repair machinery system discovery. In recent years, the availability of high-quality data on DNA from in vivo and in vitro research reported in the literature, as well as ...
Research on DNA repair enzymes has been performed and studied since the 1970s [17,18]. The enzymes concerned in DNA restoration are methylguanine methyltransferase, ... In our review article, we summarized DNA repair enzymes that are responsible for fixing DNA damage, DNA repair databases that are helpful for researchers to study DNA damage and ...
Replication errors, DNA base mismatches and topoisomerase-DNA complexes. Every time a human cell replicates, approximately 3 X 10 9 bases are copied over by high fidelity replicative polymerases (δ and ε). However, a battery of other DNA polymerases (α, β, σ, γ, λ, REV1, ζ, η, ι, κ, θ, ν, μ, Tdt and PrimPol) can carry out lower fidelity DNA synthesis during DNA replication or ...
Produced by. Dynamic single-molecule offers a direct view of protein interactions in real time. Credit: Artur Plawgo/ Getty Images. Guarding genes against damage is a crucial and complex job, so ...
DNA Repair (DNAREP) is a journal focusing on DNA repair and cellular responses to DNA damage.DNA Repair publishes articles on genetic, cellular, biochemical, structural and molecular aspects of DNA repair. The journal welcomes articles describing databases, methods and new technologies supporting research on DNA repair and responses to DNA damage.
The DNA repair process is helped by the cell cycle checkpoint arrest. The DNA damage response must be rapid and occurs before transition to the next phase of the cell cycle. ... [C416/A25145], Radiation Research Unit at the Cancer Research UK City of London Centre Award [C7893/A28990], Royal Society Research Grant (RSG/R1/180431) and the Bill ...
The germ line is the major tissue where DNA repair and damage response pathways are studied. At the same time, DSBs occur naturally during meiosis and are processed by recombination pathways. The germ line makes up approximately half of the cells in the adult worm, providing a rich source for analyses.
Structure, function and evolution of the Helix-hairpin-Helix DNA glycosylase superfamily: Piecing together the evolutionary puzzle of DNA base damage repair mechanisms. C.H. Trasviña-Arenas, Merve Demir, Wen-Jen Lin, Sheila S. David. Article 103231.
Author summary As DNA encodes our very identity, it has been subject to a plethora of studies over the last century. The advent of new technologies that permit rapid sequencing of large DNA and RNA samples opened doors to before unknown mechanisms and interactions on a genomic scale. This led to an in-depth analysis of several nuclear processes, including transcription of genes and lesion repair.
To ensure the integrity and stability of genetic material, DNA damage repair mechanisms play a crucial role. Nucleotide excision repair, base excision repair, mismatch repair, double-strand break repair, and postreplication repair are the repair pathways for DNA damage. These mechanisms can recognize and repair DNA damage caused by various factors.
Morphogenesis-coupled DNA repair - in mammalian embryogenesis, morphogenesis and DNA double strand break (DSB) repair are carried out simultaneously to ensure normal development ... Corresponding author. Department of Molecular Biosciences, Radiation Effects Research Foundation, 5-2 Hijiyama-Park, Minami-Ku, Hiroshima 732-0815, Japan. Tel: 011 ...
Double Strand Breaks Repair. Among various DNA lesions, DSBs is the leading lethal damage that leads to cell death and genetic mutations. DNA-dependent protein kinase (DNA-PK), a member of the PI3K-related protein kinase (PIKK) family, is involved in DSBs repair pathway via non-homologous end joining (NHEJ) (Jette and Lees-Miller, 2015).It has been reported that DNA-PK activity plays a role in ...
AbstractExpression of protein arginine methyltransferase 5 (PRMT5) is highly positively correlated to DNA damage repair (DDR) and DNA replication pathway genes in many types of cancer cells, including ovarian and breast cancer. In the current study, we investigated whether pharmacologic inhibition of PRMT5 downregulates DDR/DNA replication pathway genes and sensitizes cancer cells to ...
CometChip enables parallel analysis of multiple DNA repair activities [DNA repair 106 (2021) 103176-103202] Jing Ge, Le P. Ngo, Simran Kaushal, Ian J. Tay, ... Bevin P. Engelward. Article 103677. View PDF. Previous vol/issue. Read the latest articles of DNA Repair at ScienceDirect.com, Elsevier's leading platform of peer-reviewed scholarly ...
Our study provides a global analysis of an entire DNA repair pathway and reveals the mechanism of SMC5/6 relocalization to damaged DNA in vertebrate cells. Cellular genomes are particularly susceptible to DNA damage during S phase of the cell cycle, when unrepaired lesions interfere with DNA replication.
R-loops and DNA damage repair. Prolonged accumulation of R-loops can ultimately result in DNA damage that requires repair (Hodroj et al., 2017, Song et al., 2017, Sridhara et al., 2017). A number of RNA helicases that possess DNA:RNA unwinding activity have been shown to have a role in the DNA repair process.
Research advances understanding of DNA repair. Date: March 8, 2022. Source: Florida State University. Summary: A researcher has made a discovery that alters our understanding of how the body's DNA ...
DNA damage is a critical factor contributing to genetic alterations, directly affecting human health, including developing diseases such as cancer and age-related disorders. DNA repair mechanisms play a pivotal role in safeguarding genetic integrity and preventing the onset of these ailments. Over the past decade, substantial progress and pivotal discoveries have been achieved in DNA damage ...
This article is part of the Research Topic Inborn Errors of Immunity (IEI) Breaking Immune Homeostasis and Tolerance: ... HELLS, and ZBTB24 in classical non-homologous end joining during double-strand DNA break repair and immunoglobulin class-switch recombination (CSR) have been unveiled. Nevertheless, given the decreased all classes of ...
This research was designed to prospect the mechanism and impact of glycyrrhizic acid (GA) on DNA damage repair and cisplatin (CP)-induced apoptosis of melanoma cells. First, human melanoma cell SK-MEL-28 was stimulated using GA for 24, 48, and 72 h. Then, the optimal treatment time and dosage were selected.
The cellular responses to DNA damage include processes that deal with its consequences (e.g. tolerance and apoptosis) as well as direct correction of the damage by DNA repair mechanisms, which may ...
Furthermore, DNA repair plays a role in the immune surveillance of tumor cells by inhibiting the release of new antigens and immunogenic cell death [10, 11]. We demonstrated that PTEN-L could confer protection against DNA damage in EO771-PTEN-KO cells by downregulating γ-H2AX, which responds to double-stranded DNA breaks (Fig. 4 C).
The review of Huang et al., 2022 [ 11] shows that the DNA damage response (DDR) is a valuable target for the development of anti-cancer therapies. Although deficiency in DDR genes induces genomic instability and facilitates cancer development, DDR can, conversely, also help cancer cells to resist therapy-induced DNA damage.
Dormancy may reduce the wear and tear on oocytes, but it also reduces their ability to repair the inevitable damage they sustain at the molecular level. Recently, in a study published in Cell, researchers discovered how mouse oocytes keep DNA safe from damaging proteins. The cells construct special compartments within themselves to temporarily ...
Cyclin-dependent kinase 12 (CDK12) is a transcription-associated CDK that plays key roles in transcription, translation, mRNA splicing, the cell cycle, and DNA damage repair. Research has identified that high expression of CDK12 in organs such as the breast, stomach, and uterus can lead to HER2-positive brea.
MLA. APA. Chicago. University of California - Santa Cruz. "Cellular activity hints that recycling is in our DNA." ScienceDaily. ScienceDaily, 10 May 2024. <www.sciencedaily.com / releases / 2024 ...