ORIGINAL RESEARCH article
Internet addiction and related clinical problems: a study on italian young adults.
- 1 Department of Neurosciences, University of Verona, Verona, Italy
- 2 Unit of Addiction Medicine, Department of Internal Medicine, Integrated University Hospital of Verona, Policlinico “G.B. Rossi”, Verona, Italy
- 3 Department of Medical Sciences and Public Health, University of Cagliari, Cagliari, Italy
- 4 Amici C.A.S.A. San Simone No Profit Association, Mantova, Italy
The considerable prominence of internet addiction (IA) in adolescence is at least partly explained by the limited knowledge thus far available on this complex phenomenon. In discussing IA, it is necessary to be aware that this is a construct for which there is still no clear definition in the literature. Nonetheless, its important clinical implications, as emerging in recent years, justify the lively interest of researchers in this new form of behavioral addiction. Over the years, studies have associated IA with numerous clinical problems. However, fewer studies have investigated what factors might mediate the relationship between IA and the different problems associated with it. Ours is one such study. The Italian version of the SCL-90 and the IAT were administered to a sample of almost 800 adolescents aged between 16 and 22 years. We found the presence of a significant association between IA and two variables: somatization (β = 7.80; p < 0.001) and obsessive-compulsive symptoms (β = 2.18; p < 0.05). In line with our hypothesis, the results showed that somatization predicted the relationship between obsessive-compulsive symptoms and IA (β = −2.75; t = −3.55; p < 0.001), explaining 24.5% of its variance (Δ R 2 = 1.2%; F = 12.78; p < 0.01). In addition, simple slopes analyses revealed that, on reaching clinical significance (+1 SD), somatization showed higher moderation effects in the relationship between obsessive-compulsive symptoms and IA (β = 6.13; t = 7.83; p < 0.001). These results appear to be of great interest due to the absence of similar evidence in the literature, and may open the way for further research in the IA field. Although the absence of studies in the literature does not allow us to offer an exhaustive explanation of these results, our study supports current addiction theories which emphasize the important function performed by the enteroceptive system, alongside the more cited reflexive and impulsive systems.
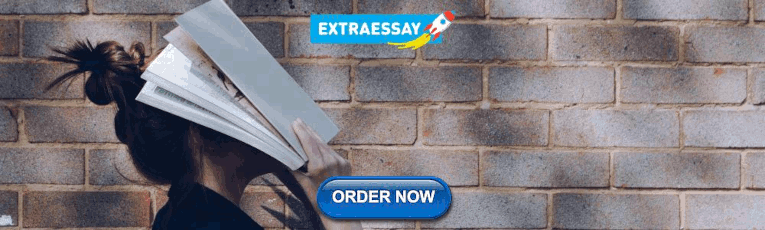
Introduction
Internet addiction (IA), also referred to as problematic , pathological , or compulsive Internet use , is a controversial concept in the research field. The frequent use of different terms to describe this new phenomenon, linked to the advent and growth of the Internet, leads to confusion over what it really consists of Tereshchenko and Kasparov (2019) .
Although researchers have yet to find a common definition of IA, it can be considered a “ non-chemical, behavioral addiction, which involves human-machine interaction ” ( Griffiths, 2000 ). Useful clinical criteria were proposed by Block (2008) , who associates IA with (a) increased feelings of anger, anxiety or sadness when the Internet is not accessible (craving); (b) the need to spend more hours on Internet devices in order to feel pleasure or cope with dysregulation of mood (tolerance); (c) poor school performance or vocational achievement; and (d) isolation or social withdrawal.
One aspect that researchers agree on is the importance of IA prevention in children and adolescents ( Lan and Lee, 2013 ). As with other forms of addiction, younger people are at greater risk of the negative effects of out-of-control Internet use ( Ko et al., 2008 ). In adolescence, distress is expressed in the form of behavioral agitation, somatic symptoms, boredom and an inclination to act ( Carlson, 2000 ), all modalities that facilitate the development of a coping strategy based on compulsive Internet use. This is a problem, given that 80% of adolescents use tablets or smartphones ( Fox and Duggan, 2013 ), whereas general population prevalence rates range from 0.8% (Italy) to 26.5% (Hong Kong) ( Kuss et al., 2014 ).
We currently know that IA is associated with symptoms of ADHD in teens ( Yoo et al., 2004 ), pathological gambling ( Phillips et al., 2012 ), depression ( Andreou and Svoli, 2013 ; Ho et al., 2014 ), anxiety ( Griffiths and Meredith, 2009 ; Zboralski et al., 2009 ), social phobia ( Carli et al., 2013 ; Gonzalez-Bueso et al., 2018 ), experiential avoidance ( Hayes et al., 1996 ; García-Oliva and Piqueras, 2016 ), obsessive-compulsive disorder (OCD) ( Jang et al., 2008 ; Cecilia et al., 2013 ), eating disorders ( Shapira et al., 2003 ; Bernardi and Pallanti, 2009 ), and sleep disorders ( Nuutinen et al., 2014 ; Tamura et al., 2017 ), as well as with relational conflicts ( Gundogar et al., 2012 ), aggression ( Cecilia et al., 2013 ), self-destructive behaviors ( Sasmaz et al., 2014 ), suicidal behaviors ( Durkee et al., 2016 ), physical health problems ( Sung et al., 2013 ), and chronic pain syndrome ( Wei et al., 2012 ). However, little is known about the factors potentially implicated in the etiopathogenesis of IA ( Tereshchenko and Kasparov, 2019 ).
Many of the most common symptoms of addiction and OCD are similar to each other, to the point that some authors define IA as compulsive computer use ( Kuss et al., 2014 ). However, there are also significant differences between the two sets of psychopathological symptoms. The obsessive-compulsive symptoms that characterize OCD can be described as recurring and persistent inappropriate thoughts (obsessions) that lead the individual to implement behaviors (compulsions) aimed at reducing the intensity of the distress deriving from these obsessive thoughts ( American Psychiatric Association [APA], 2013 ). Instead, the obsessive-compulsive symptoms reported in the context of addiction can also derive from positive thoughts about the object of the addiction, which drive the individual to seek and, in this case, engage in the activity in order to obtain gratification ( Robbins and Clark, 2015 ).
In this framework, the obsessive-compulsive component of IA can be considered in terms of (a) recurrent positive and negative thoughts (obsessions), associated, respectively, with the memory of the enjoyable experience of using the Internet, and with craving or withdrawal syndrome; and (b) instrumental behaviors (compulsion) geared toward seeking the former (positive reward) or reducing the discomfort associated with the latter (negative reward).
Adolescents with IA can be expected to display: (a) a lower ability to use reflexivity to manage their internal states; and (b) a greater propensity for impulsive behaviors to manage these states. This is the hypothesis recently proposed by Wei et al. (2017) to explain internet gaming disorder (IGD), a form of IA. However, alongside the presence of a hypoactive reflective system and an overactive impulsive system, these authors also hypothesize a dysregulation of the interoceptive awareness system, and suggest that this dysregulation increases the incentive salience of Internet use, as well as the feeling of craving deriving from its compulsive use ( Wei et al., 2017 ). This thesis could explain the relationship commonly observed between compulsive use of the Internet and somatization ( Yang et al., 2005 ).
Somatization is defined as the “ unconscious process of expressing psychological distress in the form of physical symptoms ” ( Nakkas et al., 2019 ), and it is commonly found among adolescents with IA. It is estimated that 9% of Internet-addicted adolescents display somatization ( Yang, 2001 ), reported in the literature to consist of somatic symptoms ( Potembska et al., 2019 ), chronic pain ( Wei et al., 2012 ; Fava et al., 2019 ), physical health problems ( Sung et al., 2013 ), and sleep disorders ( Tamura et al., 2017 ). Moreover, in late adolescence, the presence of somatization has been positively associated with the intensity of specific forms of IA, such as IGD ( Cerniglia et al., 2019 ). One study showed that higher somatization and interpersonal sensitivity scores predict problematic smartphone use ( Fırat et al., 2018 ). Ballespi et al. (2019) , illustrate that inability to mentalize is associated with a higher frequency of somatic complaints.
Although the involvement of somatization in the etiopathogenesis of IA is not yet clear, models recently advanced to explain the development of addiction assign it a primary role. In the triadic neurocognitive model of addiction ( Noël et al., 2013 ), for example, perception of the somatic state of the organism, governed by the insular cortex, is considered a factor that mediates the development of addiction. In fact, in the absence of cognitive processing of the bottom-up somatic signals mediated by this cerebral structure, the main symptoms of addiction suddenly disappear.
These data were recently confirmed by Naqvi et al. (2007) , who showed that absence of the somatic symptoms typical of craving and physical abstinence, induced by ischemic damage to the insula, allowed heavy smokers to give up smoking.
Somatization has been reported in association with IA in a college student population ( Alavi et al., 2011 ), and it has also been identified among the causal factors and predictors of IA among first-year college students ( Yao et al., 2013 ). Indeed, this latter study confirmed that students with somatization seem to have a greater tendency to develop IA. In addition, a study by Biby (1998) showed that higher somatization scores are linked to higher obsessive-compulsive tendency scores. Therefore, if a key role of somatic symptoms in modulating the activity of the reflexive and impulsive systems can be taken to explain the development of IA, it seems possible to hypothesize that the presence of obsessive-compulsive symptoms, commonly found in adolescents with IA ( Yen et al., 2008 ), may also be linked to the presence of somatization. In this sense, an additional hypothesis is that higher somatization in adolescents might exacerbate the effect of obsessive-compulsive symptoms on IA. Surprisingly, this hypothesis has not been investigated in the literature to date, although contemporary etiopathogenetic models suggest the importance of bottom-up somatic signals in addiction disorders ( Verdejo-García and Bechara, 2009 ). In fact, somatic symptoms may be linked to the presence of the same top-down processing of body signals related to craving or abstinence. According to the above hypothesis, these symptoms may upset the activity of the cognitive system, shifting it away from inhibitory control of Internet use, implemented by the reflexive system, toward compulsive behaviors, driven by the impulsive system ( Wei et al., 2017 ). In this way, high levels of somatization could both promote the development of IA and reinforce the relationship between obsessive-compulsive symptoms and IA.
In conclusion, our hypothesis is that somatization moderates the positive relationship between OC symptoms and IA. Specifically, the higher the level of somatization, the stronger the relationship.
Materials and Methods
Participants.
Participants were recruited from schools in the north of Italy. The study was presented during the participants’ classes. Students were invited to take part in a research study that aimed to investigate: drug use/abuse, gambling problems, alcohol use/abuse, mood. Students who provided informed consent were given two self-report instruments. All the participants were free to stop filling in the questionnaires at any time. Underage subjects needed parental permission to participate in this study.
The participants (57.7% females) ranged in age from 16 to 22 years (mean age 17.52 ± 1.15). All were third (35%), fourth (37%), or fifth grade (28%) Italian secondary school students.
Instruments
Symptom checklist 90—revised (scl-90-r; derogatis, 1994 ).
The Somatization (SOM;12 items) and Obsessive-Compulsive (OC; 10 items) subscales of the Italian version of the SCL-90-R were used. The participants used a five-point Likert scale, ranging from 0 (Not at all) to 4 (Extremely), to rate the extent to which they had experienced the listed symptoms during the past week. Cronbach’s alpha was 0.86 for SOM, and 0.82 for OC.
Internet Addiction Test (IAT; Young, 1998 )
This is a 20-item questionnaire on which respondents are asked to rate, on a five-point Likert scale, items investigating the degree to which their Internet use affects their daily routine, social life, productivity, sleeping patterns, and feelings. The minimum score is 20, and the maximum is 100; the higher the score, the greater the problems caused by Internet use. Young suggests that a score of 20–39 points is that of an average on-line user who has complete control over his/her Internet use; a score of 40–69 indicates frequent problems due to Internet use; and a score of 70–100 means that the individual’s Internet use is causing significant problems. Cronbach’s alpha was 0.88.
Socio-Demographics
The participants reported their age, gender, school and grade. In order to maintain privacy, no other personal information was requested.
Control Variables
The use of illicit drugs and gambling behavior were introduced as control variables. Specifically, the participants answered questions on their habits regarding any use of illicit drugs (cannabis, cocaine, heroin), alcohol consumption, and gambling activities, such as scratch cards, lottery tickets, football pools, new slot machines (VLTs) and video poker, betting on sporting or other events, poker and other card games.
Statistical Analyses
All the analyses were carried out using IBM SPSS Statistics 26.0 and AMOS ( Arbuckle, 2012 ). A series of confirmatory factor analyses (CFAs) was conducted to establish the discriminant validity of the scales. A full measurement model was initially tested, comparing it to a one-factor structure (in which all the items loaded into a common factor). The model fit was tested by using the comparative fit index (CFI), the incremental fit index (IFI), and the root-mean-square error of approximation (RMSEA). According to Kline (2008) and Byrne (2016) , the CFI and IFI values should have a cutoff value of ≥0.90, and the RMSEA a value of ≤ 0.08 to indicate a good fit of the model. Internal consistency of the constructs was evaluated using Cronbach’s alpha (α).
We tested the effects of somatization symptoms, obsessive-compulsive symptoms, and their interaction on IA by using the SPSS version of Hayes’s (2017) bootstrap-based PROCESS macro ( Hayes, 2012 , 2013 ; Model 1). All predictors were mean-centered prior to computing the interaction term and simple slopes were calculated at ± 1 SD. Age, sex, type of school, grade, use of illicit drugs, and gambling behaviors were included as covariates. To account for non-normality, analyses were performed with bootstrapping with 5,000 resamples.
Preliminary Analyses
Table 1 shows the means, standard deviations and internal consistencies obtained for each scale, and the correlations between the measures used in the current study.

Table 1. Descriptives of study variables ( n = 796).
Measurement Model
Prior to testing our hypothesis, we used CFAs to examine the convergent and discriminant validity of our study variables. The data were found to fit the measurement model: χ 2 (811) = 1150.99, p < 0.001, CFI = 0.90, TLI = 0.90, RMSEA = 0.035. All items loaded significantly on the intended latent factors.
Moderation Analysis
It was hypothesized that obsessive-compulsive symptoms would predict IA, depending on the somatization symptoms (moderation hypothesis). Regression analyses ( Table 2 ) conducted with the PROCESS macro (Model 1; Hayes, 2012 ) showed that obsessive-compulsive symptoms (β = 7.80, p < 0.001) and somatization symptoms (β = 2.18, p < 0.05) were related to IA after controlling for age, sex, grade, school, illicit drug use, and gambling behaviors. The moderation effect was significant t (788) = −3.55; p < 0.001 (β = −2.75, SE = 0.77, CI −4.27 to −1.2) and accounted for a significant portion of variance of IA [Δ R 2 = 1.2%; F ( 788 ) = 12.78 p < 0.01]. In this sense, increasing obsessive-compulsive symptoms predicted increased IA, but this effect was greatest at higher levels of somatization symptoms. The final model accounted for a total of 24.5% of the variance in IA.
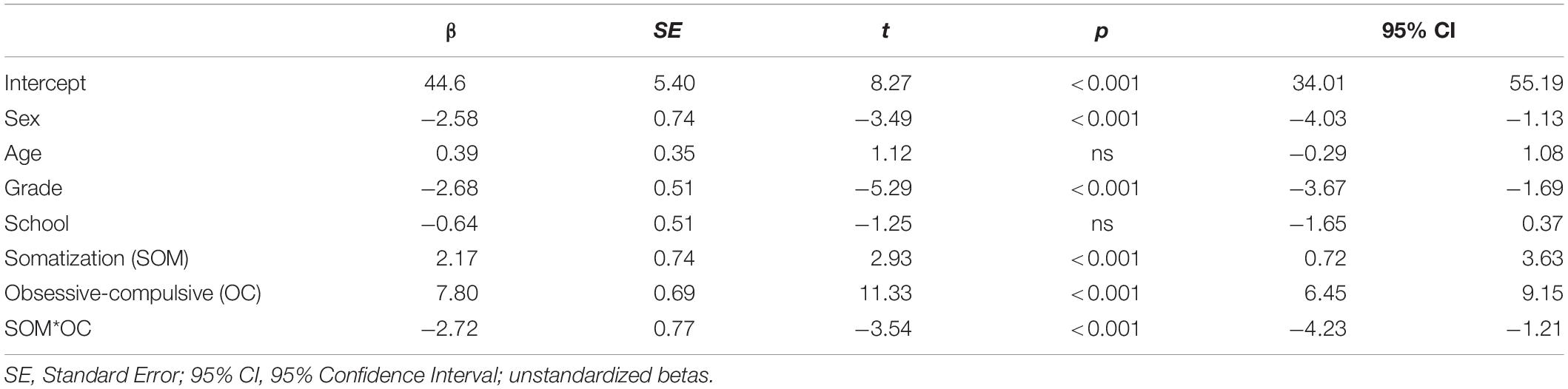
Table 2. Results of the moderation analysis.
Simple slopes analyses revealed that when somatization symptoms were low (−1 SD), there was a statistically significant effect of obsessive-compulsive symptoms on increased IA (β = 9.48, SE = 0.88, t = 10.80, p < 0.01). Furthermore, also when somatization symptoms were high (+1 SD), there was a significant effect of obsessive-compulsive symptoms on IA (β = 6.13, SE = 0.79, t = 7.83, p < 0.001). Simple slopes analyses ( Figure 1 ) revealed that when somatization symptoms were low.
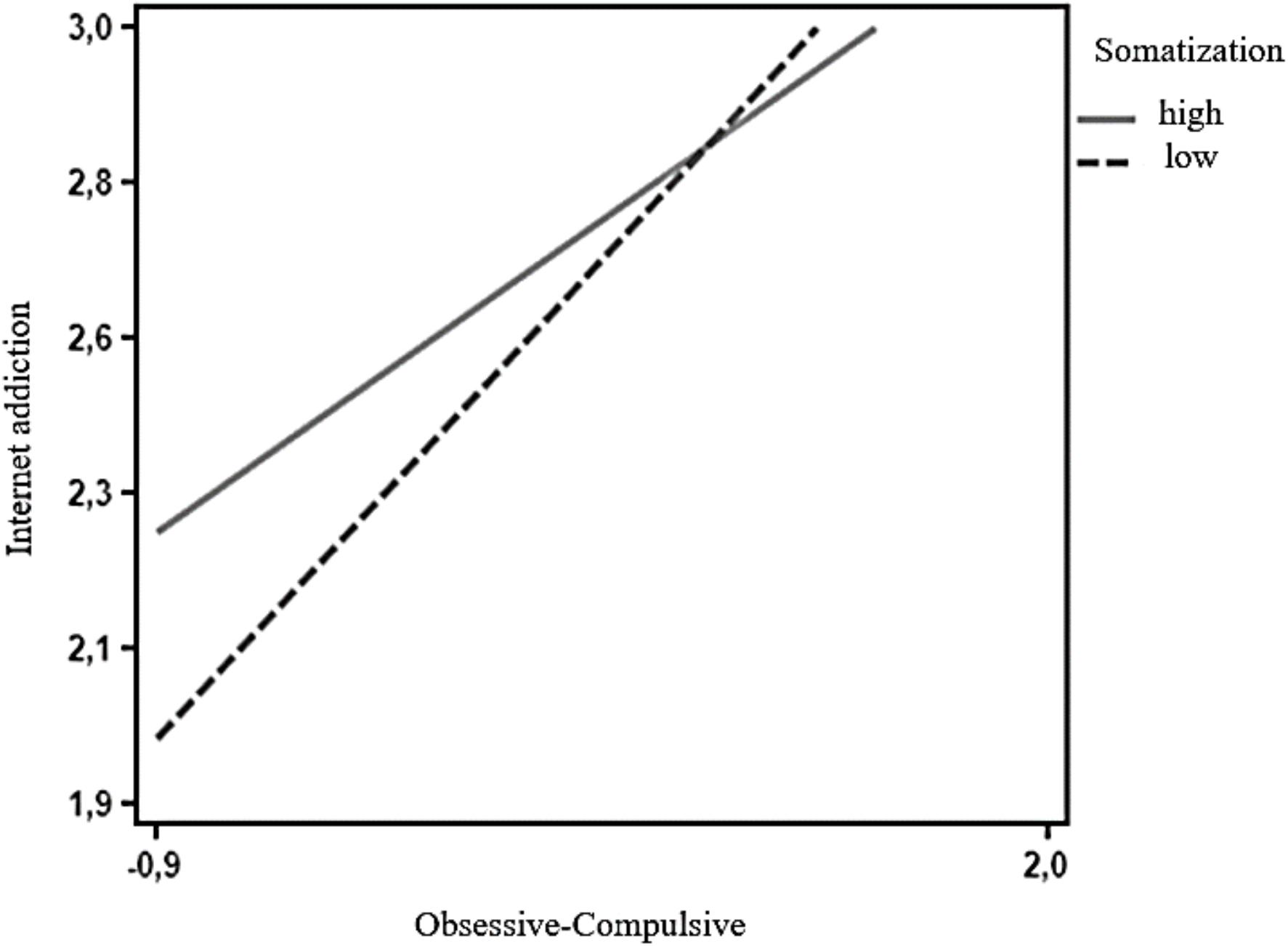
Figure 1. Interaction term for two levels of somatization: low (−1 SD) and high (+1 SD). Using the Johnson-Neyman technique ( Bauer and Curran, 2005 ), we identified the region where the effect of somatization symptoms on the relationship between OC and IA ceased to be statistically significant. Application of the Johnson-Neyman technique gave cutoff scores for somatization symptoms of below 1.81 and above 1.63.
The aim of this study was to increase current knowledge about the relationship between somatization symptoms, obsessive-compulsive symptoms, and IA in adolescents. Specifically, we hypothesized that the relationship between obsessive-compulsive symptoms and IA would be stronger at higher levels of somatization symptoms.
First, findings from our study suggest that obsessive-compulsive symptoms are associated with IA. These results are in line with prior research, which found that high levels of obsessive-compulsive symptoms are linked to higher IA risk ( Jang et al., 2008 ; Dong et al., 2011 ; Ko et al., 2012 ). Furthermore, IA has typically been described as a secondary condition resulting from various primary disorders, although findings in young adult samples have suggested that, within a range of psychopathologies, only obsessive-compulsive symptoms preceded IA ( Dong et al., 2011 ; Ko et al., 2012 ). The obsessive-compulsive symptoms observed in association with IA are similar to those of OCD, so much so that many researchers define IA as compulsive computer use ( Kuss et al., 2014 ). However, the obsessive-compulsive symptoms of OCD have been described as more ego-dystonic than those of IA ( Shapira et al., 2000 ). In general, the obsessive-compulsive symptoms of IA stem from recurring or persistent positive or negative thoughts (obsessions) that motivate the individual to implement behaviors (compulsions) intended to allow him/her to experience the hedonic satisfaction deriving from obtaining a positive reinforcement ( Robbins and Clark, 2015 ), or to reduce the distress typically associated with craving and abstinence states. IA may thus serve as a strategy for relieving pre-existing obsessive-compulsive psychopathology, a mechanism that, in turn, could actually reinforce the symptoms ( Ko et al., 2012 ). Similarly, this association could be further reinforced by underlying mechanisms shared by OC and IA behaviors ( Ko et al., 2012 ). Repetitive behavioral manifestations aimed at achieving immediate gratification or de-escalating the distress triggered by obsessive thoughts in order to improve one’s feelings are typical of addictions and compulsive behaviors ( Robbins and Clark, 2015 ). In the present study, the main effect of somatization symptoms on IA was in line with the findings of previous research ( Yang et al., 2005 ; Yen et al., 2008 ; Alavi et al., 2011 ; Yao et al., 2013 ). Somatization is conceptualized as a process that leads to translation of psycho-emotional distress into bodily discomfort ( Nakkas et al., 2019 ). Subjects with somatization disorders requiring inpatient treatment manifest deficits in both emotional awareness and Theory of Mind functioning. These deficits may underlie the phenomenon of somatization ( Subic-Wrana et al., 2010 ).
As regards our moderation hypothesis, we found that the relationship between obsessive-compulsive symptoms and IA was greatest at higher levels of somatization symptoms. Our results showed that in adolescents with higher somatization (+1 SD), the relationship between obsessive-compulsive symptoms and IA was stronger. To our knowledge, this is the first study that has investigated this relationship. Our results are in line with the triadic theory of addiction ( Noël et al., 2013 ), where somatization, as a major expression of the enteroceptive system, could hinder the management of normal emotional distress through problem-focused coping strategies based on reflexive system mentalization skills. This apparent partial impairment of the reflexive system’s capacity to regulate emotional distress could therefore lead adolescents to adopt emotion-focused coping strategies, such as ones related to implementation of the same obsessive-compulsive behaviors promoted by the impulsive system. Somatization could therefore impair the mentalization skills used by the reflexive system to inhibit compulsive behaviors driven by the impulsive system, predisposing the adolescent to develop IA. This could explain why obsessive-compulsive symptoms are often found in the literature as prodromes of IA development ( Dong et al., 2011 ; Ko et al., 2012 ), as well as why IA has typically been described as a secondary disorder resulting from a primary one, like obsessive-compulsive symptomatology ( Dong et al., 2011 ; Ko et al., 2012 ), a relationship that is confirmed in our study.
Our analyses were performed controlling for gender, age, grade, and school. Specifically, a significant gender difference emerged, as showed in previous studies ( Cao et al., 2011 ; Barke et al., 2012 ; Kuss et al., 2013 ). As showed in a study by Feng et al. (2019) , we have found a significant grade difference.
The present study has several limitations. First, the cross-sectional design used does not allow the identification of causal relationships among variables. We cannot definitively conclude that obsessive-compulsive symptoms cause IA and that this relationship depends on levels of somatization. Future studies should consider longitudinal data to overcome the cross-sectional limitations. A second, potential, limitation concerns the reliance on self-reported data, which might have caused common method bias. However, we ran the Harman’s single factor test, which suggested that common method bias did not affect the results of this study. A third limitation concerns mentalization ability. Good mentalization could be protective against somatization, but we did not measure it. Future research could explore this aspect through specific questionnaires.
Adolescence is an important period of physical and psychological development. From a clinical perspective, the results of this study show that somatization is an important moderation factor in adolescence. The incapacity to use coping strategies and mentalization strategies to counter negative emotions could increase the somatization effect. In adolescents, obsessive-compulsive symptoms can be moderated by somatization. In this period of development, it is very important to pay attention to bodily signals, as they can mask psychological problems. Obsessive-compulsive symptoms can be very invalidating, and they can be exacerbated by somatization. Teenagers seeking a coping response in technological devices are at considerable risk of developing pathological use of these devices.
In conclusion, somatization is an important aspect to consider when dealing with adolescent patients. It could be a moderation factor capable of exacerbating obsessive-compulsive symptoms or IA. This particular aspect needs more studies in the future.
Data Availability Statement
The raw data supporting the conclusions of this article will be made available by the authors, without undue reservation, to any qualified researcher.
Ethics Statement
The studies involving human participants were reviewed and approved by the CARU-Comitato di Approvazione per la Ricerca sull’Uomo, Università di Verona. Written informed consent to participate in this study was provided by the participants’ legal guardian/next of kin.
Author Contributions
FL, AR, and LZ were responsible for the study concept and design. SC, FC, and RM contributed to the data acquisition. IP assisted with the data analysis and interpretation of findings. AF, LZ, IP, and AC drafted the manuscript. All authors critically reviewed the content and approved the final version of the manuscript for publication.
Conflict of Interest
The authors declare that the research was conducted in the absence of any commercial or financial relationships that could be construed as a potential conflict of interest.
Alavi, S. S., Maracy, M. R., Jannatifard, F., and Eslami, M. (2011). The effect of psychiatric symptoms on the Internet addiction disorder in Isfahan’s university students. J. Res. Med. Sci. 16, 793–800.
Google Scholar
American Psychiatric Association [APA] (2013). Manuale Diagnostico e Statistico dei Disturbi Mentali, DSM-5 , 5th Edn. Milano: Tr. it. Raffaello Cortina.
Andreou, E., and Svoli, H. (2013). The association between internet user characteristics and dimensions of internet addiction among Greek adolescents. Int. J. Ment. Health Addict. 11, 139–148. doi: 10.1007/s11469-012-9404-3
CrossRef Full Text | Google Scholar
Arbuckle, J. L. (2012). IBM SPSS AMOS (Version 21.0) [Computer Program] . Chicago, IL: IBM.
Ballespi, S., Vives, J., Alonso, N., Sharp, C., Ramirez, M. S., Fonagy, P., et al. (2019). To know or not to know? Mentalization as protection from somatic complaints. PLoS One 14:e0215308. doi: 10.1371/journal.pone.0215308
PubMed Abstract | CrossRef Full Text | Google Scholar
Barke, A., Nyenhuis, N., and Kröner-Herwig, B. (2012). The German version of the internet addiction test: a validation study. Cyberpsychol. Behav. Soc. Netw. 15, 534–542. doi: 10.1089/cyber.2011.0616
Bauer, D. J., and Curran, P. J. (2005). Probing interactions in fixed and multilevel regression: inferential and graphical techniques. Multi. Behav. Res. 40, 373–400. doi: 10.1207/s15327906mbr4003_5
Bernardi, S., and Pallanti, S. (2009). Internet addiction: a descriptive clinical study focusing on comorbidities and dissociative symptoms. Compr. Psychiatry 50, 510–516. doi: 10.1016/j.comppsych.2008.11.011
Biby, E. L. (1998). The relationship between body dysmorphic disorder and depression, self-esteem, somatization, and obsessive-compulsive disorder. J. Clin. Psychol. 54, 489–499. doi: 10.1002/(sici)1097-4679(199806)54:4<489::aid-jclp10<3.0.co;2-b
Block, J. J. (2008). Issues for DSM-V: internet addiction. Am. J. Psychiatry 165, 306–307. doi: 10.1176/appi.ajp.2007.07101556
Byrne, B. M. (2016). Structural Equation Modeling With AMOS: Basic Concepts, Applications and Programming. London: Routledge.
Cao, H., Sun, Y., Wan, Y., Hao, J., and Tao, F. (2011). Problematic internet use in Chinese adolescents and its relation to psychoso- matic symptoms and life satisfaction. BMC Public Health 11:802. doi: 10.1186/1471-2458-11-802
Carli, V., Durkee, T., Wasserman, D., Hadlaczky, G., Despalins, R., Kramarz, E., et al. (2013). The association between pathological internet use and comorbid psychopathology: a systematic review. Psychopathology 46, 1–13. doi: 10.1159/000337971
Carlson, G. A. (2000). The challenge of diagnosing depression in childhood and adolescence. J. Affect. Disord. 61, S3–S8. doi: 10.1016/s0165-0327(00)00283-4
Cecilia, M. R., Mazza, M., Cenciarelli, S., Grassi, M., and Cofini, V. (2013). The relationshipbetween compulsive behavior and internet addiction. Styles Commun. 5, 24–31.
Cerniglia, L., Guicciardi, M., Sinatra, M., Macis, L., Simonelli, A., and Cimino, S. (2019). The use of digital technologies, impulsivity and psychopathological symptoms in adolescence. Behav. Sci. 9:82. doi: 10.3390/bs9080082
Derogatis, L. R. (1994). Symptom Checklist-90-R (SCL-90-R): Administration, Scoring and Proce- dures Manual , 3rd Edn. Minneapolis, MN: National Computer Systems.
Dong, G., Lu, Q., Zhou, H., and Zhao, X. (2011). Precursor or sequela: pathological disorders in people with Internet addiction disorder. PLoS One 6:e14703. doi: 10.1371/journal.pone.0014703
Durkee, T., Carli, V., Floderus, B., Wasserman, C., Sarchiapone, M., Apter, A., et al. (2016). Pathological internet use and risk-behaviors among European adolescents. Int. J. Environ. Res. Public Health 13, 1–17. doi: 10.3390/ijerph13030294
Fava, G. A., McEwen, B. S., Guidi, J., Gostoli, S., Offidani, E., and Sonino, N. (2019). Clinical characterization of allostatic overload. Psychoneuroendocrinology 108, 94–101. doi: 10.1016/j.psyneuen.2019.05.028
Feng, Y., Ma, Y., and Zhong, Q. (2019). ‘The relationship between adolescents’ stress and internet addiction: a mediated-moderation model’. Front. Psychol. 10:2248. doi: 10.3389/fpsyg.2019.02248
Fırat, S., Gül, H., Sertçelik, M., Gül, A., Gürel, Y., and Kılıç, B. G. (2018). The relationship between problematic smartphone use and psychiatric symptoms among adolescents who applied to psychiatry clinics. Psychiatry Res. 270, 97–103. doi: 10.1016/j.psychres.2018.09.015
Fox, S., and Duggan, M. (2013). Health Online, 2013. Washington, D.C: Pew Research Center.
García-Oliva, C., and Piqueras, J. A. (2016). Experiential avoidance and technological addictions in adolescents. J. Behav. Addict. 5, 293–303. doi: 10.1556/2006.5.2016.041
Gonzalez-Bueso, V., Santamaria, J. J., Fernandez, D., Merino, L., Montero, E., and Riba, J. (2018). Association between internet gaming disorder or pathological video-game use and comorbid psychopathology: a comprehensive review. Int. J. Environ. Res. Public Health 15:668. doi: 10.3390/ijerph15040668
Griffiths, M. (2000). Does internet and computer addiction exist? Some case study evidence. Cyberpsychol. Behav. 3, 211–218. doi: 10.1089/109493100316067
Griffiths, M. D., and Meredith, A. (2009). Videogame addiction and its treatment. J. Contemp. Psychotherapy 39, 247–253. doi: 10.1007/s10879-009-9118-4
Gundogar, A., Bakim, B., Ozer, O., and Karamustafalioglu, O. (2012). P-32 – The association between internet addiction, depression and ADHD among high school students. Eur. Psychiatry 27:1. doi: 10.1016/s0924-9338(12)74199-8
Hayes, A. F. (2012). PROCESS: A Versatile Computational Tool for Observed Variable Mediation, Moderation, and Conditional Process Modeling [White Paper] .
Hayes, A. F. (2013). An Introduction to Mediation, Moderation, and Conditional Process Analysis: A Regression-Based Approach . New York, NY: Guilford Press.
Hayes, A. F. (2017). An Introduction to Mediation, Moderation, and Conditional Process Analysis: A Regression-Based Approach , 2nd Edn. New York, NY: Guilford.
Hayes, S. C., Wilson, K. G., Gifford, E. V., Follette, V. M., and Strosahl, K. (1996). Experiential avoidance and behaviour disorder: a functional dimensional approach to diagnosis and treatment. J. Consul. Clin. Psychol. 64, 1152–1168. doi: 10.1037//0022-006x.64.6.1152
Ho, R. C., Zhang, M. W., Tsang, T. Y., Toh, A. H., Pan, F., and Lu, Y. (2014). The association between internet addiction and psychiatric co-morbidity: a meta-analysis. BMC Psychiatry 14:183. doi: 10.1186/1471-244X-14-183
Jang, K. S., Hwang, S. Y., and Choi, J. Y. (2008). Internet addiction and psychiatric symptoms among Korean adolescents. J. Sch. Health 78, 165–171. doi: 10.1111/j.1746-1561.2007.00279.x
Kline, R. B. (2008). Principles and Practice of Structural Equation Modeling , 2nd Edn. New York, NY: Guilford.
Ko, C.-H., Yen, J.-Y., Chen, C.-S., Chen, C.-C., and Yen, C.-F. (2008). Psychiatric comorbidity of Internet addiction in college students: an interview study. CNS Spectrums 13, 147–153. doi: 10.1017/s1092852900016308
Ko, C.-H., Yen, J.-Y., Yen, C.-F., Chen, C.-S., and Chen, C.-C. (2012). The association between Internet addiction and psychiatric disorder: a review of the literature. Eur. Psychiatry 27, 1–8. doi: 10.1016/j.eurpsy.2010.04.011
Kuss, D. J., Griffiths, M. D., and Binder, J. F. (2013). Internet addiction in students: prevalence and risk factors. Comput. Hum. Behav. 29, 959–966. doi: 10.1016/j.chb.2012.12.024
Kuss, D. J., Griffiths, M. D., Karila, L., and Billieux, J. (2014). Internet addiction: a systematic review of epidemiological research for the last decade. Curr. Pharm. Des. 20, 4026–4052. doi: 10.2174/13816128113199990617
Lan, C. M., and Lee, Y. H. (2013). The predictors of internet addiction behaviours for taiwanese elementary school students. Sch. Psychol. Int. 34, 648–657. doi: 10.1177/0143034313479690
Nakkas, C., Annen, H., and Brand, S. (2019). Somatization and coping in ethnic minority recruits. Milit. Med. 184, 11–12. doi: 10.1093/milmed/usz014
Naqvi, N. H., Rudrauf, D., Damasio, H., and Bechara, A. (2007). Damage to the insula disrupts addiction to cigarette smoking. Science 315, 531–534. doi: 10.1126/science.1135926
Noël, X., Brevers, D., and Bechara, A. (2013). A triadic neurocognitive approach to addiction for clinical interventions. Front. Psychiatry 4:179. doi: 10.3389/fpsyt.2013.00179
Nuutinen, T., Roos, E., Ray, C., Villberg, J., Valimaa, R., and Rasmussen, M. (2014). Computer use, sleep duration and health symptoms: a cross-sectional study of 15-year olds in three countries. Int. J. Public Health 59, 619–628. doi: 10.1007/s00038-014-0561-y
Phillips, J. G., Ogeil, R. P., and Blaszczynski, A. (2012). Electronic interests and behavioursassociated with gambling problems. Int. J. Ment. Health Addict. 10, 585–596. doi: 10.1007/s11469-011-9356-z
Potembska, E., Pawłowska, B., and Szymańska, J. (2019). Psychopathological symptoms in individuals at risk of Internet addiction in the context of selected demographic factors. Ann. Agric. Environ. Med. 26, 33–38. doi: 10.26444/aaem/81665
Robbins, T. W., and Clark, L. (2015). Behavioral addictions. Curr. Opin. Neurobiol. 30, 66–72. doi: 10.1016/j.conb.2014.09.005
Sasmaz, T., Oner, S., Kurt, A. O., Yapici, G., Yazici, A. E., Bugdayci, R., et al. (2014). Prevalence and risk factors of internet addiction in high school students. Eur. J. Public Health 24, 15–20. doi: 10.1093/eurpub/ckt051
Shapira, N. A., Goldsmith, T. D., Keck, P. E., Khosla, U. M., and McElroy, S. L. (2000). Psychiatric features of individuals with problematic internet use. J. Affect. Disord . 57, 267–272. doi: 10.1016/s0165-0327(99)00107-x
Shapira, N. A., Lessig, M. C., Goldsmith, T. D., Szabo, S. T., Lazoritz, M., Gold, M. S., et al. (2003). Problematic internet use: proposed classification and diagnostic criteria. Depress. Anxiety 17, 207–216. doi: 10.1002/da.10094
Subic-Wrana, C., Beutel, M. E., Knebel, A., and Lane, R. D. (2010). Theory of mind and emotional awareness deficits in patients with somatoform disorders. Psychosom. Med. 72, 404–411. doi: 10.1097/PSY.0b013e3181d35e83
Sung, J., Lee, J., Noh, H., Park, Y. S., and Ahn, E. J. (2013). Associations between the risk of internet addiction and problem behaviors among Korean adolescents. Korean J. Fam. Med. 34, 115–122. doi: 10.4082/kjfm.2013.34.2.115
Tamura, H., Nishida, T., Tsuji, A., and Sakakibara, H. (2017). Association between excessive use of mobile phone and insomnia and depression among Japanese adolescents. Int. J. Environ. Res. Public Health 14, 1–11.
Tereshchenko, S., and Kasparov, E. (2019). Neurobiological risk factors for the development of internet addiction in adolescents. Behav. Sci. 9:62. doi: 10.3390/bs9060062
Verdejo-García, A., and Bechara, A. (2009). A somatic-marker theory of addiction. Neuropharmacology 56, 48–62. doi: 10.1016/j.neuropharm.2008.07.035
Wei, H. T., Chen, M. H., Huang, P. C., and Bai, Y. M. (2012). The association between online gaming, social phobia, and depression: an internet survey. BMC Psychiatry 12:92. doi: 10.1186/1471-244X-12-92
Wei, L., Zhang, S., Turel, O., Bechara, A., and He, Q. (2017). A tripartite neurocognitive model of internet gaming disorder. Front. Psychiatry 8:285. doi: 10.3389/fpsyt.2017.00285
Yang, C. K. (2001). Sociopsychiatric characteristics of adolescents who use computers to excess. Acta Psychiatr. Scand. 104, 217–222. doi: 10.1034/j.1600-0447.2001.00197.x
Yang, C. K., Choe, B. M., Baity, M., Lee, J. H., and Cho, J. S. (2005). SCL-90-R and 16PF profiles of senior high school students with excessive internet use. Can. J. Psychiatry 50, 407–414. doi: 10.1177/070674370505000704
Yao, B., Han, W., Zeng, L., and Guo, X. (2013). Freshman year mental health symptoms and level of adaptation as predictors of internet addiction: a retrospective nested case-control study of male Chinese college students. Psychiatry Res. 210, 541–547. doi: 10.1016/j.psychres.2013.07.023
Yen, J. Y., Ko, C. H., Yen, C. F., Chen, S. H., Chung, W. L., and Chen, C. C. (2008). Psychiatric symptoms in adolescents with Internet addiction: comparison with substance use. Psychiatry Clin. Neurosci. 62, 9–16. doi: 10.1111/j.1440-1819.2007.01770.x
Yoo, H. J., Cho, S. C., Ha, J., Yune, S. K., Kim, S. J., Hwang, J., et al. (2004). Attention deficit hyperactivity symptoms and Internet addiction. Psychiatry Clin. Neurosci. 58, 487–494.
Young, K. S. (1998). Internet addiction: the emergence of a new clinical disorder. CyberPsychol. Behav. 1, 237–244. doi: 10.1089/cpb.1998.1.237
Zboralski, K., Orzechowska, A., Talarowska, M., Darmosz, A., Janiak, A., Janiak, M., et al. (2009). The prevalence of computer and internet addiction among pupils. Postępy Higieny i Medycyny Doświadczalnej 63, 8–12.
Keywords : somatization, internet addiction, adolescent, moderation, obssessive-compulsive disorder
Citation: Zamboni L, Portoghese I, Congiu A, Carli S, Munari R, Federico A, Centoni F, Rizzini AL and Lugoboni F (2020) Internet Addiction and Related Clinical Problems: A Study on Italian Young Adults. Front. Psychol. 11:571638. doi: 10.3389/fpsyg.2020.571638
Received: 11 June 2020; Accepted: 21 October 2020; Published: 10 November 2020.
Reviewed by:
Copyright © 2020 Zamboni, Portoghese, Congiu, Carli, Munari, Federico, Centoni, Rizzini and Lugoboni. This is an open-access article distributed under the terms of the Creative Commons Attribution License (CC BY) . The use, distribution or reproduction in other forums is permitted, provided the original author(s) and the copyright owner(s) are credited and that the original publication in this journal is cited, in accordance with accepted academic practice. No use, distribution or reproduction is permitted which does not comply with these terms.
*Correspondence: Lorenzo Zamboni, [email protected]
Disclaimer: All claims expressed in this article are solely those of the authors and do not necessarily represent those of their affiliated organizations, or those of the publisher, the editors and the reviewers. Any product that may be evaluated in this article or claim that may be made by its manufacturer is not guaranteed or endorsed by the publisher.
- Alzheimer's disease & dementia
- Arthritis & Rheumatism
- Attention deficit disorders
- Autism spectrum disorders
- Biomedical technology
- Diseases, Conditions, Syndromes
- Endocrinology & Metabolism
- Gastroenterology
- Gerontology & Geriatrics
- Health informatics
- Inflammatory disorders
- Medical economics
- Medical research
- Medications
- Neuroscience
- Obstetrics & gynaecology
- Oncology & Cancer
- Ophthalmology
- Overweight & Obesity
- Parkinson's & Movement disorders
- Psychology & Psychiatry
- Radiology & Imaging
- Sleep disorders
- Sports medicine & Kinesiology
- Vaccination
- Breast cancer
- Cardiovascular disease
- Chronic obstructive pulmonary disease
- Colon cancer
- Coronary artery disease
- Heart attack
- Heart disease
- High blood pressure
- Kidney disease
- Lung cancer
- Multiple sclerosis
- Myocardial infarction
- Ovarian cancer
- Post traumatic stress disorder
- Rheumatoid arthritis
- Schizophrenia
- Skin cancer
- Type 2 diabetes
- Full List »
share this!
April 11, 2024
This article has been reviewed according to Science X's editorial process and policies . Editors have highlighted the following attributes while ensuring the content's credibility:
fact-checked
trusted source
Depression in young people being driven by internet addiction, say researchers
by University of Derby
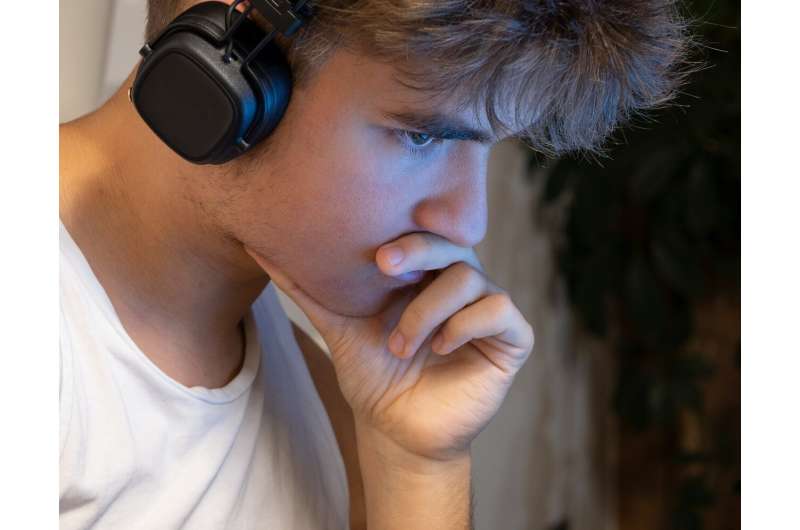
Spending excessive time online is causing daytime sleepiness and symptoms of depression in adolescents, a major international study led by researchers at the University of Derby has found.
Dr. Hassam Waheed, from the University's College of Business, Law and Social Sciences, worked with Dr. Peter Macaulay, Senior Lecturer in Psychology, and colleagues in Malaysia and Australia on a meta-analysis of 52 published studies. The results have been published in a report, " Caught in the web: a meta-analysis of internet addiction, excessive daytime sleepiness and depressive symptoms in adolescents ," in the journal Information Technology & People .
The study looked at adolescents aged between 10 and 19 years who are more prone to internet addiction given their transitional stage of physical and psychological development. It found that internet-induced daytime sleepiness displaces a host of activities that are known to be beneficial for maintaining mental health, such as day-to-day social interactions and physical activities. This in turn exacerbates their depressive symptoms .
Dr. Macaulay said, "One of the main issues we noticed was that time online is often unstructured; while there may be boundaries for some uses such as time playing games on a PlayStation, this isn't extended to other internet use."
Dr. Waheed added, "We live in an increasingly connected world and there are lots of positives of using the internet, for studies and recreational purposes. Our research supports existing efforts to promote awareness of the consequences of increased internet usage and time spent online, and calls for further action to consider maximizing offline opportunities and interactions."
Interventions recommended by the research team include:
- Encouraging good sleep practices, such as not using devices for 90 minutes before bedtime
- Ensuring devices are not being looked at during the night
- Encouraging more face-to-face interactions
- Supporting more active pursuits such as after-school clubs and sports
The team also advocate the use of focused time online, with an agreed time limit and divided between homework/study and recreation as necessary.
Dr. Macaulay explained, "Such focused time would also foster a healthier relationship with technology through mindful consumption."
At present the medical community is divided on whether internet addiction constitutes a mental disorder. The researchers believe that, with greater understanding of the negative implications of internet addiction, the study will help build the consensus for it to be recognized as a distinct mental disorder.
As part of this project, the researchers are also examining whether the use of virtual reality leads to deviant behaviors offline as well as interventions that could mitigate such behaviors.
Explore further
Feedback to editors

Researchers discover new therapeutic target for non-small cell lung cancer
9 hours ago

Immune cells carry a long-lasting 'memory' of early-life pain
10 hours ago

Cannabis legalization and rising sales have not contributed to increase in substance abuse, study finds

No negative impact from prolonged eye patching on child's development or family stress levels

COVID-19 booster immunity lasts much longer than primary series alone, study shows

Study finds that human neuron signals flow in one direction
11 hours ago

A common pathway in the brain that enables addictive drugs to hijack natural reward processing identified
12 hours ago

Scientists identify airway cells that sense aspirated water and acid reflux

Environment may influence metacognitive abilities more than genetics

Contracting RSV before age two can cause long-term lung changes and impairment
Related stories.

Is the internet addictive?
Oct 23, 2019

Researcher finds that pandemic led to increase in internet addiction among American adults
Dec 8, 2021

Parental support linked to teen internet addiction
Jan 23, 2023

Teen internet usage associated with stress and suicidal ideation
Mar 23, 2022

Study introduces new internet addiction spectrum. Where are you on the scale?
Oct 2, 2023

Expert claims internet addiction should be recognized as a disorder requiring treatment
Sep 18, 2017
Recommended for you

Novel cell therapy treatments offer promise to immune-compromised children
13 hours ago

Molecular atlas shows how the growth factor erythropoietin affects neurons
16 hours ago

Scientists uncover 95 regions of the genome linked to PTSD
21 hours ago
Let us know if there is a problem with our content
Use this form if you have come across a typo, inaccuracy or would like to send an edit request for the content on this page. For general inquiries, please use our contact form . For general feedback, use the public comments section below (please adhere to guidelines ).
Please select the most appropriate category to facilitate processing of your request
Thank you for taking time to provide your feedback to the editors.
Your feedback is important to us. However, we do not guarantee individual replies due to the high volume of messages.
E-mail the story
Your email address is used only to let the recipient know who sent the email. Neither your address nor the recipient's address will be used for any other purpose. The information you enter will appear in your e-mail message and is not retained by Medical Xpress in any form.
Newsletter sign up
Get weekly and/or daily updates delivered to your inbox. You can unsubscribe at any time and we'll never share your details to third parties.
More information Privacy policy
Donate and enjoy an ad-free experience
We keep our content available to everyone. Consider supporting Science X's mission by getting a premium account.
E-mail newsletter
A study of internet addiction and its effects on mental health: A study based on Iranian University Students
Affiliations.
- 1 Health Education and Health Promotion, Health Institute, Kermanshah University of Medical Sciences, Kermanshah, Iran.
- 2 Social Determinants in Health Promotion Research Center, Hormozgan Health Institute, Hormozgan University of Medical Sciences, Bandar Abbas, Iran.
- 3 Antai College of Economics and Management/School of Media and Communication, Shanghai Jiao Tong University, Shanghai-China.
- 4 Department of Health Care Services and Health Education, School of Health, Ardabil University of Medical Science, Ardabil, Iran.
- 5 Department of Anatomical Sciences, Medical School, Kermanshah University of Medical Sciences, Kermanshah, Iran.
- 6 Health Management and Economics Research Center, Iran University of Medical Sciences, Tehran, Iran.
- 7 Students Research Committee, Kermanshah University of Medical Sciences, Kermanshah, Iran.
- PMID: 33062738
- PMCID: PMC7530416
- DOI: 10.4103/jehp.jehp_148_20
Introduction: The Internet has drastically affected human behavior, and it has positive and negative effects; however, its excessive usage exposes users to internet addiction. The diagnosis of students' mental dysfunction is vital to monitor their academic progress and success by preventing this technology through proper handling of the usage addiction.
Materials and methods: This descriptive-analytical study selected 447 students (232 females and 215 males) of the first and second semesters enrolled at Kermanshah University of Medical Sciences, Iran, in 2018 by using Cochrane's sample size formula and stratified random sampling. The study applied Young's Internet Addiction Test and Goldberg General Health Questionnaire 28 for data collection. The study screened the data received and analyzed valid data set through the t -test and Pearson's correlation coefficient by incorporating SPSS Statistics software version 23.0.
Results: The results of the current study specified that the total mean score of the students for internet addiction and mental health was 3.81 ± 0.88 and 2.56 ± 0.33, correspondingly. The results revealed that internet addiction positively correlated with depression and mental health, which indicated a negative relationship ( P > 0.001). The multiple regression analysis results showed students' five significant vulnerability predictors toward internet addiction, such as the critical reason for using the Internet, faculty, depression, the central place for using the Internet, and somatic symptoms.
Conclusions: The study findings specified that students' excessive internet usage leads to anxiety, depression, and adverse mental health, which affect their academic performance. Monitoring and controlling students' internet addiction through informative sessions on how to use the Internet adequately is useful.
Keywords: Internet addiction; medical sciences; mental health; students; technology advancement.
Copyright: © 2020 Journal of Education and Health Promotion.

Internet Addiction
Reviewed by Psychology Today Staff
More a popular idea than a scientifically valid concept, internet addiction is the belief that people can become so dependent on using their mobile phones or other electronic devices that they lose control of their own behavior and suffer negative consequences. The harm is alleged to stem both from direct involvement with the device—something that has never been proven—and from the abandonment of other activities, such as studying, face-to-face socializing, or sleep.
- What Is Internet Addiction?
- Signs of Excessive Internet Use
- Internet Use and Mental Health
- What to Do About Internet Addiction
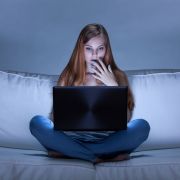
There is much debate in the scientific community about whether excessive internet use can be classified as a true addiction. In an addiction to substances such as drugs or alcohol , consumption ceases being pleasurable but continues and is difficult to escape even as the likelihood of harm to the body and life mounts. In the case of internet use, there is no clear point at which being online becomes non-pleasurable for most individuals. In part for this reason, behavioral "addictions," including using the internet, remain controversial: Experts debate where the line should be drawn between passionate absorption in any activity—say, devoting a lot of time to playing the cello or reading books—and being stuck in a rut of compulsivity that stops being useful and detrimentally affects other areas of life.
In preparing the current edition of the Diagnostic and Statistical Manual of Mental Disorders , psychiatrists and other experts debated whether to include internet addiction. They decided that there was not enough scientific evidence to support inclusion at this time, although the DSM-5 does recognize Internet Gaming Disorder as a condition warranting further study.
Most often, the word “addiction” is used in the colloquial sense. Common Sense Media finds that 59 percent of parents “feel” their kids are addicted to their mobile devices—just as 27 percent of the parents feel that they themselves are. Sixty-nine percent of parents say they check their own devices at least hourly, as do 78 percent of teens. Spending a lot of time on the internet is increasingly considered normal behavior, especially for adolescents. Much of their social activity has simply moved online. Like any new technology, the computer has changed the way everyone lives, learns, and communicates. It is possible to be online far too much, even though this does not constitute a true addiction in the eyes of most clinicians.
Internet content creators leverage the ways in which the brain works to rally consumers ' attention . One simple example: A perceived threat activates your fight-or-flight response, a part of the brain known as the Reticular Activating System mobilizes the body for action. So online content exploits potential dangers—violence, natural disaster, disease, etc.—to attract and hold your attention.
Problematic or excessive internet use can indeed pose a serious problem. It can displace such important needs as sleep, homework, and exercise, often a source of friction between parents and teens. It can have negative effects on real-life relationships.
The idea of internet addiction is a particular concern among parents, who worry about the harmful effects of screen time and often argue about device use with their children. According to a 2019 survey conducted by Common Sense Media, children aged 8 to 12 now spend 5 hours a day on digital devices, while teens clock more than 7 hours—not including schoolwork. Teen screen time is slowly ticking upward, and most teens take their phones to bed with them.
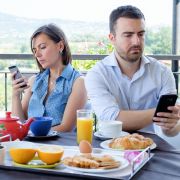
Whether classified as an addiction or not, heavy use of technology can be detrimental. It can impair focus, resulting in poor performance at school or work. Excessive internet consumption also makes it more difficult for people to communicate normally or to regulate their emotions. They spend less time on non-internet-related activities at the cost of relationships with friends, family, and significant others.
One way to assess whether you’re using the internet too much is to ask yourself if your basics needs (or your child’s, if they are the concern) are being met. Do you sleep enough, eat healthy, get enough exercise, enjoy the outdoors, and spend time socializing in-person? The real harm of screen time may lie in missed opportunities for growth and connection.
Excessive screen time can be particularly harmful to a developing brain: It decreases focus and attention span while increasing the need for more constant stimulation and instant gratification. Those who use the internet excessively may feel anxious if their access to their device gets restricted. They tend to be more impulsive and struggle to recognize facial and nonverbal cues in real life.
Internet use becomes a problem when people start substituting online connections for real, physical relationships. The effects of technology on relationships include increased isolation and loneliness . Defaulting to online communication also denies us the opportunity to hear someone’s voice and read their facial cues in-person; it can also lead to poorer outcomes and miscommunication. Experts recommend that we save the important conversations for when we can be face-to-face for just this reason.
Online content has been designed to elicit specific “checking habits,” which can result in distraction and poor performance at school or work. Constantly checking your smartphone or another device can also lead to relationship-sabotaging behaviors, like phubbing (snubbing loved ones for the instant gratification of checking the internet on your device). As more time is spent online, less is devoted to the natural pleasures of everyday life.
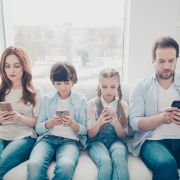
Excessive use of the internet is known to negatively impact a person’s mental health. It has been associated with mental health issues, such as loneliness, depression , anxiety , and attention-deficit/hyperactivity disorder. Research suggests that people are likely to use the internet more as an emotional crutch to cope with negative feelings instead of addressing them in proactive and healthy ways.
This is a subject of debate at present. While internet addiction is not in the DSM-V, it is clearly a behavior that negatively impacts mental health and cognition for many, and many struggle to cut back on their time online. The term "addiction" is often used as a shorthand for, “My child spends a lot of time on social media , texting friends, or playing video games, and I’m worried how it will affect his or her future development and success.” At the same time, many people label it a behavioral addiction, engaging reward circuitry seen in other problematic behaviors such as gambling.
Time online is also sometimes used as an escape from boredom or relief from loneliness or other unpleasantness. Occasionally, excessive screen time masks a state of depression or anxiety. In such cases, digital engagement becomes an attempt to remedy the feelings of distress caused by true mental health disorders that could likely benefit from professional or other attention.
Given how much people rely on technology to complete everyday tasks, from online schooling to paying bills to ordering food to keeping in touch with loved ones who are far away, it isn’t feasible to stop using the internet altogether. In most cases, the goal should be to reduce the time spent online. Many of those who’ve struggled to balance internet use with other activities recommend such simple “digital detox” measures as leaving devices in the kitchen or any other room but the bedroom at night. Cognitive behavioral therapy can also help address addiction-like behaviors, like constant checking habits.
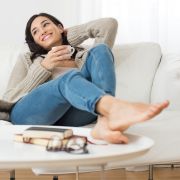
Amidst growing concerns about the increased amount of time people are spending online, the “digital detox” has become a popular way to cope. A digital detox involves temporarily abstaining from using devices, like computers and smartphones. Someone may go on a digital detox in order to re-engage with a passion or activity, focus more on in-person interactions, or break free of a pattern of compulsive or excessive use. Digital detoxes also allow more time for self-care that a person may have been neglecting in order to stay plugged into the internet, which can lead to lower stress levels and better sleep.
There is no one-size-fits-all answer. You may want to digitally detox if you notice that you’re experiencing sleep disruptions due to staying up late or waking up early to be on a device, if the internet is making you feel depressed, or if the constant need to be connected causes you stress. Other signs may include feeling anxious if you can’t locate your phone, having FOMO ( fear of missing out) if you’re not checking the internet or social media, struggling to focus without (or due to) constant checking behaviors, etc.
Unlike other detoxes where the goal is to abstain completely, digital detoxes are more flexible and tailored to the individual. It may not be possible due to work or personal obligations to shut your devices off entirely for long periods of time. If it’s time for a digital detox , there are some strategies you can try: Block off non-screen time during the day and/or night, set a “digital curfew” for using devices at night or on weekends, specify digital-free spaces in your home (e.g., the bedroom or dinner table), and use the additional time in fulfilling ways (e.g., socialize, rekindle old interests, volunteer, etc.).
Use the internet and social media with purpose; set time limits on your unstructured use to avoid going down long and unfulfilling rabbit holes. Take advantage of the extra free time you suddenly have. Spend more time socializing in-person and volunteer. Rekindle old interests or take up a new hobby. Go outside. Pay more attention to how you are feeling, both physically and emotionally.
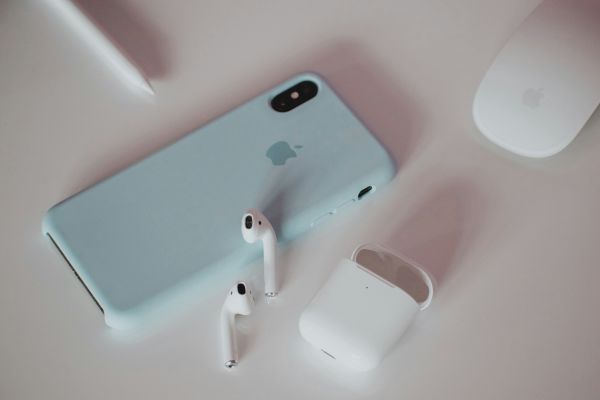
Dating apps are designed to help people find dates and develop long-term relationships. But they are also designed to keep users involved with the app.
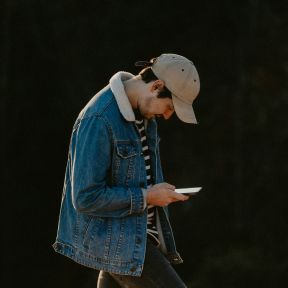
Encouraging young adults to tell their stories may help heal the sense of disconnection technology and the pandemic have created.
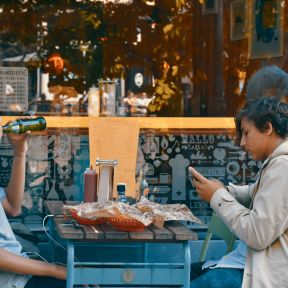
Smartphones bridge global connections yet chip away at the essence, and the joy, of face-to-face interactions.
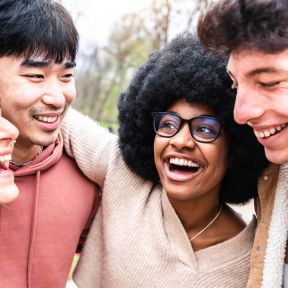
In a new study, teens report finding more benefits than harms online, yet they admit to feeling “happy” and “peaceful” when away from their smartphones.
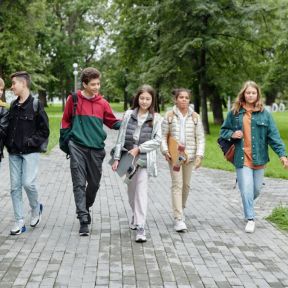
Today, more teens are at peace and happier when they are detached from their devices. Here are 5 ways to help parents create a smartphone contract to manage screen time.
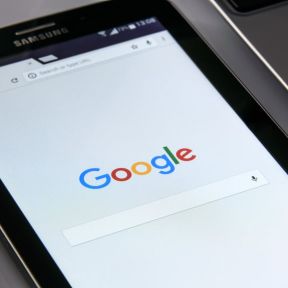
Personal Perspective: How the internet influences understanding mental illness.
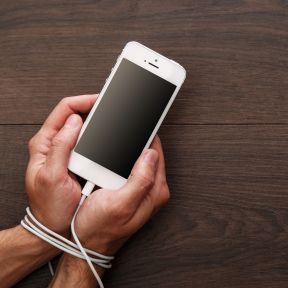
Take charge of your unhealthy smartphone use.
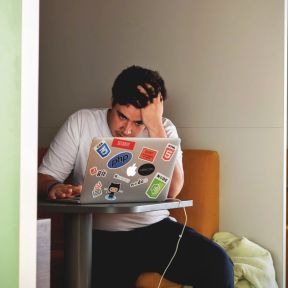
"Infinite" video games are designed to keep you hooked. Switching to "finite" games could help you find balance in your life.
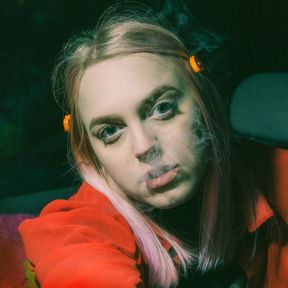
One of these addiction game-changers could save a loved one’s life. Or your own.
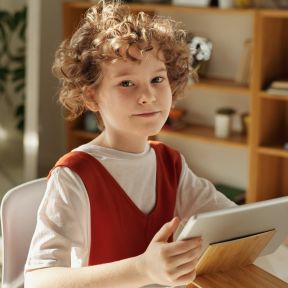
Most parenting blogs recommend setting a two-hour-per-day time limit on all screens for minors. Is that what's best for your children?
- Find a Therapist
- Find a Treatment Center
- Find a Psychiatrist
- Find a Support Group
- Find Teletherapy
- United States
- Brooklyn, NY
- Chicago, IL
- Houston, TX
- Los Angeles, CA
- New York, NY
- Portland, OR
- San Diego, CA
- San Francisco, CA
- Seattle, WA
- Washington, DC
- Asperger's
- Bipolar Disorder
- Chronic Pain
- Eating Disorders
- Passive Aggression
- Personality
- Goal Setting
- Positive Psychology
- Stopping Smoking
- Low Sexual Desire
- Relationships
- Child Development
- Therapy Center NEW
- Diagnosis Dictionary
- Types of Therapy
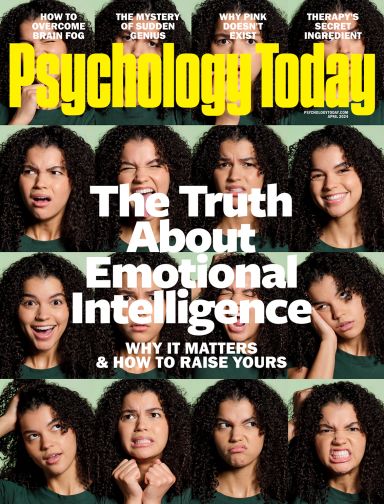
Understanding what emotional intelligence looks like and the steps needed to improve it could light a path to a more emotionally adept world.
- Coronavirus Disease 2019
- Affective Forecasting
- Neuroscience
- Open access
- Published: 15 April 2024
Social media addiction: associations with attachment style, mental distress, and personality
- Christiane Eichenberg 1 ,
- Raphaela Schneider 1 &
- Helena Rumpl 1
BMC Psychiatry volume 24 , Article number: 278 ( 2024 ) Cite this article
102 Accesses
Metrics details
Social media bring not only benefits but also downsides, such as addictive behavior. While an ambivalent closed insecure attachment style has been prominently linked with internet and smartphone addiction, a similar analysis for social media addiction is still pending. This study aims to explore social media addiction, focusing on variations in attachment style, mental distress, and personality between students with and without problematic social media use. Additionally, it investigates whether a specific attachment style is connected to social media addiction.
Data were collected from 571 college students (mean age = 23.61, SD = 5.00, 65.5% female; response rate = 20.06%) via an online survey administered to all enrolled students of Sigmund Freud PrivatUniversity Vienna. The Bergen Social Media Addiction Scale (BSMAS) differentiated between students addicted and not addicted to social media. Attachment style was gauged using the Bielefeld Partnership Expectations Questionnaire (BFPE), mental distress by the Brief Symptom Inventory (BSI-18), and personality by the Big Five Inventory (BFI-10).
Of the total sample, 22.7% of students were identified as addicted to social media. For personality, it was demonstrated that socially media addicted (SMA) students reported significantly higher values on the neuroticism dimension compared to not socially media addicted (NSMA) students. SMA also scored higher across all mental health dimensions—depressiveness, anxiety, and somatization. SMA more frequently exhibited an insecure attachment style than NSMA, specifically, an ambivalent closed attachment style. A two-step cluster analysis validated the initial findings, uncovering three clusters: (1) secure attachment, primarily linked with fewer occurrences of social media addiction and a lower incidence of mental health problems; (2) ambivalent closed attachment, generally associated with a higher rate of social media addiction and increased levels of mental health problems; and (3) ambivalent clingy attachment, manifesting a medium prevalence of social media addiction and a relatively equitable mental health profile.
Conclusions
The outcomes are aligned with previous research on internet and smartphone addiction, pointing out the relevance of an ambivalent closed attachment style in all three contexts. Therapeutic interventions for social media addiction should be developed and implemented considering these findings.
Peer Review reports
Introduction
Digital media have become ubiquitous. As of April 2023, 5.18 billion people worldwide use the Internet [ 1 ]. On average, global Internet users spend 6 h and 43 min online daily [ 2 ]. In 2023, social media platforms engage 4.8 billion users worldwide, a significant rise from 2.46 billion in 2017 [ 1 , 2 ]. These users spend an average of 2 h and 25 min on social networks each day and have, on average, 8.9 social media accounts [ 2 ]. Smartphones, now an essential device for many, are especially popular among the youth. Specifically, teenagers aged 14 to 24 access their phones approximately 214 times daily [ 3 ]. While social media networks have grown in importance, they also introduce challenges. Issues such as social media fatigue manifest in negative emotional responses like burnout, exhaustion, and frustration during social network activities [ 4 ]. Another possible negative consequence of social media activity is addictive behavior that is reported prior in the context of internet addiction.
Classification and definition of social media addiction
Digital media addictions, with a particular emphasis on social media addictions, are increasingly prevalent in psychotherapy, especially among younger demographics [ 5 , 6 ]. The concern for social media addiction is heightened among females, who show a higher propensity towards this addiction [ 7 , 8 ]. Despite its growing prevalence, social media addiction is yet to be fully acknowledged in diagnostic classification systems. The term “addiction” is therefore only used in this context for the sake of simplicity, as it is not yet officially recognized. The concept of ‘behavioral addiction,’ which characterizes excessive, rewarding behaviors leading to psychological addiction symptoms [ 9 ], is applicable here, though social media addiction still lacks distinct recognition in diagnostic manuals like the ICD and DSM. This gap highlights the need for more comprehensive research and understanding.
Prior research conforms mainly to differentiate between generalized and specific internet addictions [ 10 , 11 , 12 , 13 ]. The first means a multidimensional misuse of the internet using multiple internet functions, whereas the ladder aims a sole specific internet function (e.g., gaming, gambling, social media etc.) [ 13 , 14 ]. Social Media Addiction, encompassing variants like Facebook addiction and general addictive use of social networking sites (SNSs), is characterized as a maladaptive psychological dependency on SNSs, leading to behavioral addiction symptoms [ 15 , 16 , 17 ]. Currently, Social Media Addiction assessment relies on questionnaires like the Bergen Social Media Addiction Scale (BSMAS [ 18 ]),, which is momentarily the most widely used tool and applies criteria such as salience, mood modification, tolerance, withdrawal, conflict, and relapse [ 19 ] to evaluate addictive behaviors [ 10 ].
Prevalence rates and mental stress correlations of social media addiction
Data regarding the prevalence of social media addiction indicate a range between 1% and 18.7% [ 20 ]. However, the accuracy of these rates is debated. Cheng et al. [ 21 ] suggest that estimates of social media addiction are often either under- or overestimated. Their recent meta-analysis revealed prevalence rates ranging from 0 to 82%, a wide disparity stemming from differing theoretical frameworks and measurement instruments. Depending on the strictness of the classification system used, the researchers identified three mean prevalence benchmarks: 5%, 13%, and 25%. Frequently, individuals with problematic social media use also grapple with other mental health issues. Depression [ 20 , 22 ] and social anxiety [ 23 ] are commonly co-occurring disorders, as are challenges related to self-esteem (ibid.). Particularly, young women often feel dissatisfied with their bodies due to social media engagement. The frequent exposure to manipulated and idealized images of models or influencers fuels a comparison culture. As a result, many young women develop a desire to alter their appearance [ 24 ]. The number of “likes” they receive on platforms becomes a proxy for their self-worth, heavily influencing their self-esteem [ 25 ]. Several studies highlight that young adults spending over two hours daily on social media tend to exhibit higher rates of anxiety, depression, and sleep disturbances.
Personality traits and social media addiction
The personality trait neuroticism, and the “fear of missing out” or FOMO [ 26 ], have been identified as predictors of Social Media Addiction [ 27 ]. Conversely, extraversion’s link to social media use is debated. While some evidence suggests extraversion is not a significant factor [ 28 ], other research indicates extraverted individuals are more prone to social media use and potential addiction. Kuss & Griffiths [ 29 ] offer a more nuanced view in their literature review. According to them, extraverted individuals might use social media to augment their social interactions, i.e. they use social media in a positive manner to expand opportunities to interact with others in more ways. Introverted users, on the other hand, use social media to compensate for a perceived social deficit. For them, using social media is a way to connect with others in a way that they feel is not sufficiently possible in real life.
Attachment styles and social media addiction
Extensive research has been conducted on the association between insecure attachment and substance addictions [ 30 , 31 ]. The attachment system, which comprises secure, insecure, and disorganized categories, is a biologically and evolutionarily rooted motivational and behavioral system that operates through attachment figures [ 32 ]. Schuhler et al. [ 33 ] proposed a model elucidating the link between internet addiction and attachment, suggesting that addictive behaviors may arise as a means to compensate for attachment issues. From this perspective, digital addiction represents a flawed attempt to address early attachment deficiencies [ 33 , 34 ]. In a related vein, Brisch [ 35 ] introduced a model that positions the ‘reference object’ as central to the understanding of addictions. According to this model, the primary function of social media addiction isn’t to escape negative emotions, as is often the case with substance addictions. Instead, it’s seen as an excessive digitally-mediated social behavior aiming to substitute for insecure attachments. Supporting this, Eichenberg et al. [ 34 ] showed that insecure attachment style is correlated with problematic smartphone usage and problematic internet usage [ 36 ]. Notably, an ambivalently attached style was identified as particularly relevant in both contexts. A plethora of studies showed a link between social media addiction and attachment in general [ 37 , 38 , 39 , 40 , 41 , 42 , 43 ]. But the question arises whether the specific attachment style as has shown relevant for internet and smartphone addiction will also be prominent for social media addiction.
Research objectives and questions
The primary objective of this study is to explore whether an insecure attachment style correlates with addictive social media use, and to pinpoint which specific style is most relevant. While research has identified an ambivalent closed insecure attachment style as being significant in the context of internet and smartphone addiction, a detailed examination specific to social media addiction remains lacking.
Moreover, this study seeks to gather further information regarding the still emerging psychopathology, specifically focusing on the personality traits neuroticism and extraversion, as well as mental stress.
Mental health
The research questions will be, whether social media addicted students report higher levels of depression, anxiety, and somatization.
- Personality
Further, it will be explored whether neuroticism and extraversion influence an individual’s susceptibility to social media addiction.
Recruitment
A comprehensive survey ( N = 2846, response rate = 20.06%) was created with the SoSci Survey online survey tool [ 44 ] and was conducted among students at the Sigmund Freud PrivatUniversität in Vienna, Austria. The data collection took place from January to March 2021, resulting in a final sample of 571 respondents. To distribute the online questionnaire, the Study Service Centers from the faculties of psychology, psychotherapy, law, and medicine were approached. They were requested to email the link to the questionnaire, accompanied by a pre-written invitation text, to all actively enrolled students at the Sigmund Freud PrivatUniversität Vienna. Once the participants provided informed consent and completed the survey, they were redirected to a debriefing page. This page detailed the study’s objectives and offered the contact information of the researchers, in case the participants sought support related to the survey topics or had additional inquiries. The survey received approval from the Ethics Commission of the Faculty of Psychotherapy Science and the Faculty of Psychology of the Sigμund Freud University PrivatVienna. Recognizing the sensitive nature of the topic, paramount emphasis was placed on safeguarding the confidentiality of participants’ responses. Furthermore, participants had the liberty to opt out of the study at any juncture. Should they wish to have their data expunged, they could simply reach out to a researcher via email, referencing an unique anonymized code. This would enable the researcher to identify and delete the participant’s anonymized data.
Survey structure
The survey, created using Sosci-Survey, began with a brief that outlined the research rationale and the survey’s objectives. Participants affirmed their agreement with the study’s privacy policy through a checkbox.
Section 1 asked about socio-demographic factors, including age, gender, and study subject. Subsequently, it touched upon matters related to social media:
Services most used : Participants identified which social media services they frequently use, answered dichotomously (yes/no).
Usage frequency : Choices ranged from “less than 30 minutes” to “more than four hours per day” on a seven-point scale.
Social Media Importance : Participants rated from “very significant” to “not significant” on a four-point scale.
Purposes of Use : Employing a five-point scale, respondents indicated frequency, ranging from 1 (“never”) to 5 (“several times a day”).
Perceived downsides : Participants assessed their sentiments on a five-point scale from 1 (“not true at all”) to 5 (“completely true”).
In light of evidence suggesting a discrepancy between objective and self-reported usage time—where users often overestimate their screen time [ 45 ]—the survey did not deploy open-ended questions concerning usage duration. Instead, participants were presented with predefined categories to streamline their responses.
Section 2 incorporated standardized questionnaires to examine further social media addiction, mental distress, personality traits, and attachment styles.
Bergen social media addiction scale BSMAS [ 18 ]
The Bergen Social Media Addiction Scale (BSMAS) [ 18 ] categorizes users into two groups: those addicted to social media and those not addicted. All six items pertain to one’s experience with social media over the past 12 months. It employs a five-point scale, ranging from 1 (“very rarely”) to 5 (“very often”). The scale asks at the beginning of each item “How often during the last year have you…” and continues with “…spent a lot of time thinking about social media or planned use of social media?” (i.e., salience) or “…become restless or troubled if you have been prohibited from using social media?” (i.e., withdrawal). A higher BSMAS score indicates a heightened risk of social media addiction. As suggested by a substantial Hungarian study involving 6000 adolescents [ 20 ], a cutoff score of 19 out of 30 was adopted. The scale was repeatedly reported with high internal consistency, e.g., α = 0.97 [ 46 ] and α = 0.82 (at baseline) plus α = 0.86 (at follow-up) [ 10 ]. Chen et al. [ 10 ] confirm the single-factor structure of the scale, report only medium correlations with scales close to the construct (SABAS/smartphone addiction, IGDS-SF9/internet gaming disorder, r =.06 and 0.42), and showed invariance across three months among young adults. They presented a good test–retest reliability after three months ( ICC = 0.86, p <.001).
Brief symptom inventory BSI-18 [ 47 ]
The BSI-18 is a brief, reliable instrument for assessing mental stress. It contains the three subscales somatization, depression, and anxiety, comprising 6 items, as well as the Global Severity Index (GSI) including all 18 items. Response format of the 18 items is a five-point scale (0=”not at all” to 4=”very strong”). The scale asks at the beginning of a symptoms list: “How much have you had within the past 7 days…”. Examples for the symptoms on this list are “Nausea or upset stomach” for somatization, “Feelings of worthlessness” for depression”, and “Spells of terror or panic” for anxiety. The BSI-18 is the newest and shortest of the multidimensional versions of the Symptom Checklist 90-R. The BSI-18 assesses validly mental stress in both normal population [ 48 ] and clinical populations [ 49 ]. Confirmatory analyses confirm the three-factor structure [ 48 ]. Franke et al. [ 49 ] report good internal consistencies of the scales fear of rejection (BSI-18 (α (somatization) = 0,79, α (depression) = 0,84, α (anxiety) = 0,84, α (GSI) = 0,91).
Big five inventory BFI-10 [ 50 ]
The questionnaire is based on the Big Five personality traits model, also called OCEAN model that is the most widely used model for describing overall personality [ 51 ]. Theoretical background is the sedimentation hypothesis that assumes that every personality trait must be represented in language and, therefore, factor analyses were used to find universal personality dimensions [ 52 ]. Multiple analyses by various researchers resulted repeatedly in the OCEAN model, which consists of the five dimensions agreeableness, neuroticism, conscientiousness, openness to experience, and extraversion. The BFI-10 [ 50 ] contains 10 items, two for each of the five dimensions. The scale asks, “How well do the following statements describe your personality?” and starts a list of attitudes with “I see myself as someone who…“. Example answers are: “…does a thorough job” (i.e., conscientiousness) or “…is outgoing, sociable” (i.e., extraversion). Respondents answered a five-point rating scale from “does not apply at all” (1) to “applies completely” (5) for each item. Rammstedt und John [ 50 ] report moderate test–retest reliability after 6 weeks in a student sample (agreeableness: rtt = 0.58, neuroticism: rtt = 0.74, conscientiousness: rtt = 0.77, openness to experience: rtt = 0.72, extraversion: rtt = 0.84). In a representative sample, however, the retest coefficients are lower overall ranging from ( rtt =.62) for openness to experience to ( rtt =.49) for neuroticism [ 51 ]. Rammstedt et al. [ 51 ] report sufficient construct validity correlating the BFI-10 with the NEO-PI-R and factorial validity by conducting principal component analyses on a representative sample.
Bielefeld questionnaire on partnership expectations BFPE [ 53 ]
The BFPE operationalizes attachment styles of adults by recording self-reports on three scales: conscious need for care (8 items), fear of rejection (11 items), and readiness for self-disclosure (11 items) [ 53 ]. Example items are: “Knowing myself as I do, I can hardly imagine that my partner will appreciate me” (i.e., fear of rejection), “I prefer to talk with my partner about facts rather than about feelings” (i.e., readiness for self- disclosure), and “It’s important for me that my partner thinks of me often, even when we are not together” (i.e., conscious need for care). The first of the 31 items serves as an icebreaker item and is not evaluated. The degree of expression of each item is indicated on a 5-point scale (1= “does not apply at all” to 5 = “applies exactly”). From the aggregate scores of these scales, one of five attachment styles can be determined: secure, two variations of ambivalent/anxious (closed and clinging), and two variations of the avoidant style (closed and conditionally secure). For simplification purposes, these styles can be dichotomized into two primary categories: secure (which includes both secure and conditionally secure types) and insecure (encompassing avoidant-closed, ambivalent-clingy, and ambivalent-closed types). These distinct attachment styles emerged originally from cluster analysis research [ 53 ]. Höger and Buschkämper [ 53 ] report good internal consistencies of the scales fear of rejection (Cronbach’s α = 0.88), readiness for self-disclosure (Cronbach’s α = 0.89), and conscious need for care (Cronbach’s α = 0.77). The split-half reliabilities calculated according to Guttman and Spearman-Brown are also similarly good for the three scales (fear of rejection = 0.91, readiness for self-disclosure = 0.89, and conscious need for care = 0.77). A validation is based on a German translation of the “Adult Attachment Scale” (AAS [ 54 ]),.
Statistical analysis
The Statistical Package for the Social Sciences Program (SPSS version 27) was used for data input, processing, and statistical analyses. The participants were divided into social media addicted (SMA) and not addicted (NSMA) using the cut-off score according to Bányai et al. [ 20 ]. Additionally, the percentage of social media dependent students has been calculated. To evaluate differences between SMA and NSMA in social media usage, Mann-Whitney U tests for two independent samples were analyzed for differences in downsides of social media and usage purposes, and chi-square tests for differences in social media services, usage frequency, and social media importance, as the corresponding data were not normally distributed. Based on the data obtained with the BFPE, participants were allocated (see above) to the five attachment styles “secure,” “conditionally secure,” “ambivalent clingy,” “ambivalent closed,” and “avoidant closed.” Subsequently, the five attachment styles were dichotomized into the variables “secure” and “insecure” attachment styles. Subsequently, the five attachment styles were dichotomized into the variables “secure” and “insecure” attachment styles. Finally, using the chi-square tests, attachment styles and social media addiction were tested for significance differences. While chi-square tests provide valuable insights into individual associations, a two-step cluster analysis was conducted to gain a comprehensive understanding of how these variables collectively group participants. Two-step cluster analysis was chosen due to its capacity to handle both continuous and categorical variables. The number of clusters was determined based on the Schwarz Bayesian Criterion (BIC), and the selected model was further validated by examining the silhouette measure of cohesion and separation. Since gender and age are variables that could influence social media addiction, they were included in the cluster analysis to investigate their distribution over the resulting clusters. To maintain robustness of analyses, the non-binary gender category was omitted due to very small case number.
The total sample ( N = 571) consisted of 65.5% female students ( n = 374) 33.3% male students ( n = 190), and 1.2% those who did not wish to be defined by these two genders ( n = 7). Participants were between 18 and 60 years old ( M = 23.61 years, SD = 5.00, median = 23, modus = 22). The distribution of study subject was the following: medicine ( n = 344, 59.7%), psychology ( n = 121, 21.0%), psychotherapy ( n = 79, 13.7%), and law ( n = 32, 5.6%) (some students studied two subjects).
- Social media addiction
A total of 131 people (22.7% of the total sample) could be classified as addicted to social media. In addition, it was also relevant how genders were distributed between the two groups. Of the total number of participants classified as addicted participants ( N = 131), 79.39% were female, 19.08% male, and 1.53% non-binary. These values are to be contrasted with the group of not addicted ( N = 440), in which 61.36% were female, 37.5% male, and 1.14% non-binary.
Social media usage
Among the various social media platforms, “WhatsApp” was the predominant choice with 99.1% usage. It was trailed by “YouTube” at 91.2%, “Instagram” at 82.1%, “Facebook” at 66.9%, “Snapchat” at 63.7%, “Facebook Messenger” at 35.6%, “Pinterest” at 32.9%, and “Twitter” at 10.5%. In addressing frequency of use, a significant 91% indicated they access social media multiple times per day. Delving into the duration of daily usage: 12.8% were on for less than an hour, 25.6% used it for around an hour, 32.7% for two hours, 16.8% for three hours, and 12.1% devoted more than three hours. When participants were asked about the significance of social media, 8.9% viewed it as very important, 55.1% as important, 31.3% as less important, and a mere 4.7% as not important. Participants predominantly engaged with social media for “entertainment” ( M = 4.17, SD = 1.05), staying “up to date” ( M = 4.12, SD = 1.03), combating “boredom” ( M = 3.94, SD = 1.22), maintaining “contact with family” ( M = 3.86, SD = 1.2), and for “music” ( M = 3.55, SD = 1.4). They also sought “inspiration (e.g., fashion, interior)” with a mean score of ( M = 3.35, SD = 1.29). However, not all experiences were positive. Downsides associated with social media usage were led by “comparison with others” ( M = 3.19, SD = 1.3), followed by “dissatisfaction with own body” ( M = 2.55, SD = 1.38), “negative self-esteem in contact with influencers” ( M = 2.23, SD = 1.32), and encountering “insults, intrusive behavior” ( M = 1.88, SD = 1.3). Distinguishing between SMA and NSMA users, differences emerged in their consumption patterns (see for details Table 1 ). SMA users predominantly gravitated towards image-centric platforms such as “Instagram” (93.1% SMA vs. 78.9% NSMA) and “Pinterest” (46.6% SMA vs. 28.9% NSMA). Remarkably, SMA users expressed heightened concerns regarding the downsides “comparison with others” ( M = 4.06, SD = 1.03 for SMA vs. M = 2.94, SD = 1.26 for NSMA), “dissatisfaction with own body (when viewing idealized bodies online)” (M = 3.45, SD = 1.34 for SMA vs. M = 2.28, SD = 1.28 for NSMA), and “negative self-esteem in contact with influencers” ( M = 3.16, SD = 1.34 for SMA vs. M = 1.95, SD = 1.18 for NSMA). It became evident that SMA users faced enhanced negative repercussions, especially in terms of body perception when comparing themselves with images of others. In addition, SMA use social media as tool for more purposes than NSMA. Not addicted report here, to use social media only for contact with family and music equally often.
Attachment style
Since 12 participants did not completely fill in the BFPE, the number of participants regarding attachment is 559. Frequencies and percentages of each attachment style can be seen in Table 2 . A small part of the student population was securely bound ( n = 88, 15.7%) with the biggest part being insecurely bound ( n = 471, 84.3%). Secure attachment style (corrected residuals: 3.1) is related to a disproportionately higher number of NSMA and insecure attachment style (corrected residuals: 3.1) is related to a disproportionately higher number of SMA, χ² (1) = 9.28, p = .002, C = 0.13 (see Fig. 1 , see Table 3 ). The five individual attachment styles differ in the frequency distribution of social media addiction, χ² (4) = 30.75, p < .001, C = 0.24, with avoidant closed (corrected residuals:3.2) having disproportionately more NSMA, ambivalent closed (corrected residuals: 4.8) having disproportionately more SMA, and conditionally secure (corrected residuals: 2.4) having disproportionately more NSMA (see Fig. 2 ). So, findings show that participants with social media addiction had a significant higher likelihood to have an ambivalent closed attachment style.
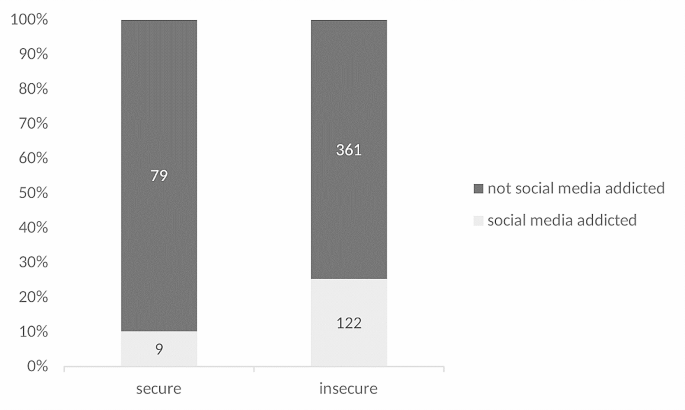
Relationship between attachment style and social media addiction. This stacked bar chart depicts the proportion of participants with ‘secure’ and ‘insecure’ attachment styles as determined by the Bielefeld Questionnaire on Partnership Expectations (BFPE). Attachment styles are defined by responses to three scales: conscious need for care, fear of rejection, and readiness for self-disclosure. These styles are subsequently dichotomized into ‘secure’ (including secure and conditionally secure styles) and ‘insecure’ (including avoidant-closed, ambivalent-clingy, and ambivalent-closed styles). Dark gray bars represent participants not addicted to social media, while light gray bars represent those with a self-reported addiction determined by the Bergen Social Media Addiction Scale (BSMAS). The numbers within the bars indicate the count of participants in each category
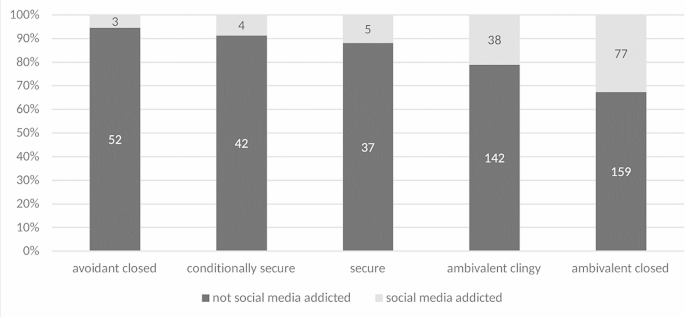
Distribution of five attachment styles and social media addiction. This bar chart visualizes the proportion of participants classified into five distinct attachment styles according to the Bielefeld Questionnaire on Partnership Expectations (BFPE) alongside their social media addiction status, as measured by the Bergen Social Media Addiction Scale (BSMAS). The attachment styles represented are ‘avoidant closed’, ‘conditionally secure’, ‘secure’, ‘ambivalent clingy’, and ‘ambivalent closed’. Dark gray bars indicate participants not identified as addicted to social media, while light gray bars represent those who meet the criteria for addiction according to the BSMAS. The numbers within the bars denote the count of participants corresponding to each category
Regarding extraversion, the total sample ( M = 3.58, SD = 0.92, modus = 5, Md = 3.5) is slightly but significantly less open-minded than a norm sample having same age and education ( M = 3.93, SD = 0.83, Rammstedt et al. 2012) ( t (570)=-9.23, p < .001) and regarding neuroticism, the sample ( M = 3.09, SD = 0.87, modus = 2.5, Md = 3) is significantly more neurotic than a comparable norm sample ( M = 2.25, SD = 0.69, Rammstedt et al. 2012) ( t (570) = 23.15, p < .001). Further, it was found that SMA ( M = 3.40, SD = 0.85) scored significantly higher than NSMA ( M = 3.00, SD = 0.85) on the dimension of neuroticism and thus could be classified as more emotionally unstable ( U = 20636.50, Z = -5.02, p < .001). However, on the dimension of extraversion, SMA ( M = 3.56, SD = 0.85) did not differ from NSMA ( M = 3.58, SD = 0.94) ( U = 28408.5, Z = − 0.25, p = .801).
- Mental distress
The total sample showed in comparison with a norm sample high levels of each of the three dimensions of depression ( M = 4.18; SD = 4.52 vs. M norm =1.27; SD norm =2.5, Franke et al. 2017) ( t (570) = 15.40, p < .001), anxiety ( M = 3.67; SD = 4.30, vs. M norm =1.09; SD norm =2.1, ibd.) ( t (570) = 14.35, p < .001), and somatization ( M = 2.23, SD = 3.00, vs. M norm =0.70; SD norm =14.8, ibd.) ( t (570) = 12.18, p < .001). Moreover, SMA reported still higher scores on all three scales of the BSI-18: depression (SMA M = 7.93, SD = 5.25, NSMA M = 3.06, SD = 3.59) ( U = 11,606, Z = -10.47, p < .001), anxiety (SMA M = 6.18, SD = 5.34, NSMA M = 2.92, SD = 3.61) ( U = 16,841, Z = -7.31, p < .001), and somatization (SMA M = 3.60, SD = 4.02, NSMA M = 1.82, SD = 2.48) ( U = 19,730, Z = -5.64, p < .001) than NSMA. Spitzer et al. (2011) reported BSI-18 patient scores relatively close to SMA scores for depression (mean scores ranging from 6.17 to 11.61) and anxiety (mean scores ranging from 6.26 to 9.51), but not for somatization (mean scores ranging from 6.47 to 6.90). It can therefore be assumed that students in this sample are generally more mentally stressed, with students who are addicted to social media being particularly mentally stressed. This finding could be explained due to the high distress and burden in the early phase of the COVID19 pandemic.
Two-step cluster analysis
The two-step cluster analysis suggested a three-cluster solution as the most appropriate fit. Evaluation of the centroids of continuous variables (Table 4 ) and frequencies of the categorical cluster composition (Table 5 ) result in the following clusters:
The Cluster ambivalent clingy attachment (ACA) ( N = 178) is relatively balanced in terms of extraversion, neuroticism, depression, anxiety, and somatization. They are uniquely characterized by the ambivalent clingy attachment style with a balanced representation of social media dependence.
The Cluster secure attachment (SA) ( N = 140) is characterized by individuals who are slightly extroverted, less neurotic, and show lower levels of depression, anxiety, and somatization. This cluster stands out due to its representation of secure and rather secure attachment styles and has the lowest proportion of individuals who are addicted to social media.
The Cluster ambivalent closed attachment (AVA) ( N = 231) is slightly introverted, more neurotic, and exhibits higher levels of depression and anxiety. Participants of this cluster are exclusively of the ambivalent closed attachment style, and a significant portion seems more susceptible to social media addiction.
For the validation of the derived clustering solution, the Bayesian Information Criterion (BIC) was employed as a model selection criterion to identify the optimal number of clusters. The BIC is advantageous in balancing the goodness of fit of the model against its complexity, penalizing models with more parameters to avoid overfitting. Various numbers of clusters were considered, ranging from 1 to 15, and the corresponding BIC values were calculated for each cluster solution. Table 6 presents the BIC values obtained for different cluster solutions. The BIC drops substantially from 1 cluster to 2 clusters, indicated by a change of -1180.384. There is a smaller but still notable drop from 2 clusters to 3 clusters, with a change of -605.464. After 3 clusters, the BIC drops more slowly, with smaller changes for each additional cluster. Even if the ratio for the change from 2 to 3 clusters is 0.512, the ratio of distance measures that indicates how distinct the clusters are from each other is for the 3-cluster solution still 1.780, which suggests that the 3-cluster solution is equally well-defined compared to the 2-cluster solution. Given this information, we opt for the 3-cluster solution, since the BIC drops more slowly beyond this point, suggesting diminishing returns in terms of model fit as more clusters are added and the 3-cluster solution offers a sufficient granular segmentation. The distribution of age (Table 4 ) and gender (Table 5 ) was relatively even.
Principal results
This study aimed to examine social media addiction with a focus on differences in attachment style, mental distress, and personality between students with and without social media addiction. For personality, it was shown that SMA had significantly higher values on the neuroticism dimension than NSMA, but they did not differ in the extraversion dimension. Thus, SMA can be classified as more emotionally unstable in comparison with NSMA. Further, SMA scored significantly higher on all three levels—depressiveness, anxiety, and somatization—than the group of NSMA, i.e., social media addicted users are comparatively more mentally stressed. At least for attachment style, the assumption that SMA are more likely to show an insecure attachment was confirmed here. In more detail, most SMA displayed an ambivalent closed attachment style. Two-step cluster analysis yielded a holistic insight into the collective grouping of cases by these variables. It corroborated the findings of the univariate analyses, revealing three predominant clusters, chiefly characterized by three attachment styles and varying levels of social media addiction: (a) secure attachment, predominantly associated with fewer instances of social media addiction and lower prevalence of mental health problems; (b) ambivalent closed attachment, typically marked by a higher frequency of social media addiction and elevated levels of mental health problems; and (c) ambivalent clingy attachment, presenting a moderate incidence of social media addiction and a relatively balanced mental health profile.
Prevalence rate of social media addiction (22.8%) lies within the literature reported prevalence of the used instrument (BSMAS), since Chen et al. [ 10 ] specify < 10–40% for the BSMAS. SMA differ from NSMA in their usage of social media, exhibiting higher values in usage frequency, time spent, and perceived importance. Notably, SMA are more active on image-oriented services such as “Instagram” and “Pinterest”. They also report higher levels of “comparison with others”, “dissatisfaction with their own body (especially when exposed to idealized online images)”, and “negative self-esteem when interacting with influencers”. This suggests that SMA may experience heightened negative body awareness when comparing themselves to online images. Moreover, SMA use social media for a broader range of purposes compared to NSMA.
SMA scored significantly higher on the neuroticism dimension than NSMA, suggesting that they tend to be more emotionally unstable and easily irritable. Conversely, no difference was observed in the extraversion dimension. Previous research supports the idea that internet-related addictions are linked to higher scores on the neuroticism dimension. Blackwell et al. [ 27 ] demonstrated that neuroticism predicts social media use. Moreover, a study by Müller [ 55 ] suggests that Internet addiction correlates with increased neuroticism scores. Interestingly, individuals with elevated neuroticism scores associate Internet topics with significantly stronger positive arousal compared to a healthy control group [ 56 ]. Social media addiction has also been positively linked to neuroticism [ 27 , 28 ], and individuals scoring high on this trait are drawn to social networks as they offer recognition and validation [ 27 ]. Marengo et al. [ 28 ] align with our findings by not observing a relationship between social media addiction and extraversion. The contrasting findings presented by Kuss and Griffiths [ 29 ] relate extraversion more to older individuals and openness more to younger ones. Given our primary focus on younger participants, our results are consistent with these observations.
SMA display significantly higher values for depression, anxiety, and somatization compared to NSMA, even considering the evident distress in the overall sample. This suggests that SMA may be mentally more strained than NSMA. Consequently, further evidence for the connection between mental disorders and internet-related addictions in terms of comorbidity was found in the present study. This augments the extant research on depression, anxiety, and internet addiction. Kırcaburun [ 57 ] also identified a significant positive relationship between depressive symptoms, internet use, and social media addiction. In his study, the level of depression in adolescents was indirectly influenced by social media addiction; addicts spent more time online, amplifying the risk of depressive symptoms. Similarly, Wu et al. [ 58 ] found that internet addiction correlates with depression in adolescents, exerting direct, mediated, and moderating effects on depression levels. For anxiety, there’s also documented evidence of a positive association with problematic social media consumption. Baltaci [ 23 ] highlighted social anxiety as a predictor for social media addiction among university students. Other studies have shown a positive correlation between internet addiction and general anxiety levels in students [ 59 , 60 ]. As for somatization, there’s a documented positive correlation with internet addiction in adolescents [ 61 , 62 , 63 ]. Research on somatization and smartphone addiction is somewhat limited [ 63 ]. Results here confirm the positive correlation adding to this research corpus also heightened somatic symptomatology in social media addicted students.
Users with an insecure attachment style are significantly more likely to exhibit social media addiction than those with a secure attachment style. These findings align with a substantial body of research that establishes a connection between insecure attachment styles and internet-related addictions. A systematic review has provided evidence linking insecure attachment styles with both internet addiction in general and social media addiction in particular [ 64 ]. Moreover, certain studies suggest that difficulties in relational behavior or the presence of insecure attachment styles can act as risk factors for smartphone addiction. For instance, Baek et al. [ 65 ] identified a correlation between attachment behavior (specifically internalization problems) and smartphone usage. Other research [ 66 , 67 ] has indicated a mediating effect of attachment style on smartphone addiction. Anxiously attached individuals showed patterns of self-regulation that directly influenced their susceptibility to smartphone addiction. While a secure attachment style offered a protective effect, an anxious attachment style increased vulnerability to addiction. In contrast, an avoidant attachment style didn’t significantly influence addiction development.
For social media addiction, several studies have highlighted its relationship with attachment. For instance, Hart et al. [ 37 ] demonstrated a link between dysfunctional attachment qualities and problematic social media use. A study involving Turkish students revealed that insecure attachment styles might serve as risk factors for social media addiction [ 38 ]. Conversely, secure attachment and high self-esteem can act as protective factors against such addiction [ 38 ]. Numerous studies have established a connection between an anxious attachment style and both heavy social media use [ 39 , 40 , 41 ] and addiction to it [ 42 ]. Specifically, Yaakobi and Goldenberg [ 43 ] identified a positive correlation between an anxious attachment style and the amount of time spent on social media. This same study found that an anxious attachment style negatively predicts the number of online friends. Oldmeadow et al. [ 41 ] also discovered a relationship between anxious attachment and seeking comfort on Facebook, noting an increase in Facebook usage, especially during negative emotional states.
Currently, no studies explore the relationship between an ambivalent closed attachment style and social media addiction. However, the findings in this study indicate that an ambivalent closed attachment style is significantly associated with social media addiction more frequently. These results are consistent with previous data suggesting this style is prevalent for internet-related addictions, as observed in the context of both smartphone [ 34 ] and internet [ 36 ] addictions. According to Höger and Buschkämper [ 53 ], individuals with an ambivalent attachment style exhibit an increased need for attention and concurrently face heightened acceptance issues. This pattern suggests heightened anxiety and a secondary hyperactivating (ambivalent) strategy (ibid.). It’s plausible that the social-compensatory component is particularly influential in this context when it comes to social media [ 34 ]. Individuals with an ambivalent-closed attachment style might turn to online platforms, especially social media, to mitigate their interpersonal relationship deficits (ibid.). The anonymity afforded by the internet allows a new representation of the self to be created, helping this group to compensate for feared problems of acceptance (ibid.). Based on the data, it appears this new representation of the self is often facilitated through image-focused platforms like “Instagram” and “Pinterest”. However, this may inadvertently expose SMA users to the pitfalls of social media, such as body dissatisfaction and reduced self-esteem when interacting with influencers. This dynamic could exacerbate their acceptance issues, perpetuating a detrimental cycle.
The ambivalent clinging and closed attachment styles differ primarily in their perceived willingness to open up. The former demonstrates a moderate willingness, allowing for the expression of strong attachment needs associated with the hyperactivated attachment system, while the latter exhibits a notably low willingness to open [ 53 ]. The findings presented in this study indicate that the degree of openness (for attachment) may play a crucial role in determining the severity of problematic user behavior. Specifically, the more receptive a user is to attachment, the less likely they are to exhibit addictive behaviors. Cluster analysis supports this interpretation. It identified three clusters with varying susceptibilities to social media addiction: those with secure attachment exhibit the lowest likelihood, those with ambivalent clingy attachment have a medium likelihood, and those with ambivalent closed attachment display the highest likelihood. This potential correlation warrants further exploration in subsequent research. Moreover, given that a mediating effect of mentalization between attachment style and both emotion dysregulation [ 68 ] and psychopathology [ 69 ] has been demonstrated, future research should delve deeper into exploring the relationships between mentalization, attachment style, and internet-related addictions.
Limitations
It should be noted that the data are based on self-reporting in an online survey. Response rate is comparable with other online-survey studies [ 70 ]. So, possible self-selection processes could be of importance since online surveys are prone to an inherent selection bias. Social media users may find it appealing to participate for trying to relativize the negative image of social media addiction. Further, the sample is due to the narrow age distribution and educational level not representative. Even if cluster analysis shows no noteworthy age distribution for the clusters, future research should collect sufficient case number for each age group or limit age to a homogenous group. Female students contributed disproportionately here. Which in turn can affect the prevalence of social media addiction since there is evidence that women are more prone to social media addiction [ 8 ]. Though, this gender bias has been frequently observed in online surveys [ 71 ]. Cluster analysis did not reveal any conspicuous distribution for gender either. Altogether, future studies with a broader recruitment strategy may provide more representative data and confirm discussed results. Further, it could be discussed that the design of the study is cross-sectional. Since there is evidence for differences in age, at least for personality dimensions, comparison of two points in time or more can corroborate data or reduce it to differences in generation cohort. Furthermore, since mental health is a key variable, future studies should check psychiatric history of participants.
This study enhances our understanding of how specific attachment problems could contribute to the development of social media addiction, reaffirming findings related to internet and smartphone addiction. It reveals that an avoidant closed attachment style, characterized by a pronounced need for attention, acceptance issues, and notably low openness for attachment, is frequently associated with this addiction. Such a deficit in openness may prompt compensatory behavior to satiate the intensive need for attention in the manageable environment of the digital world, where any conversation can be terminated with a click. This intense attention-seeking behavior seems to find satisfaction through image-centric services on social media, instigating negative comparative processes with others and potentially reinforcing acceptance issues in a self-perpetuating cycle, with mental stress being a substantial correlate.
To break this cycle, therapeutic interventions should consider these interrelations and specifically target critical areas. This could include conducting a thorough media anamnesis, educating about the effects of image-focused services and comparative processes, and establishing a robust and consistent therapeutic alliance—a cornerstone of successful addiction treatment [ 34 ]. The incorporation of attachment-oriented strategies is vital, as attachment-related aspects have yet to be integrated into existing internet addiction treatment protocols [ 34 , 36 ]. In addition, since research showed a good impact of whole school attachment-based interventions [ 72 ], prevention programs to combat digital addictions in schools and universities should also include content that promotes secure attachment behavior, especially to young people with a high need for attention, acceptance issues, and notably low openness for attachment. Beyond individual treatment, the implementation of these strategies has the potential to foster a healthier approach to digital media usage across society, thereby contributing to a more informed and mindful engagement with social media platforms, which can finally lead to a reduction in the prevalence and impact of social media addiction on a broader scale.
Data availability
The raw data supporting the conclusions of this article will be made available by the authors, without undue reservation.
Abbreviations
Big Five Inventory
Bielefelder Fragebogen zu Partnerschaftserwartungen
Brief Symptom Inventory
Bergen Social Media Addiction Scale
Not Social Media Addicted
Social Media Addicted
Statista. digital-population-worldwide. 2023. www.statista.com/statistics/617136/digital-population-worldwide . Accessed 14 Jun 2023.
Ahlgren M. 100 + Internet-Statistiken und Fakten zu 2022. 2022. www.websiterating.com/de/research/internet-statistics-facts/#references . Accessed 31 Aug 2022.
Scholz M, Studie. Wir nutzen unsere Smartphones 1.500 Mal pro Woche. mobile zeitgeist (We use our smartphones 1.500 times a week. Mobile zeitgeist). 2017. www.mobile-zeitgeist.com/studie-wir-nutzen-unsere-smartphones-1-500-mal-pro-woche/ . Accessed 18 Nov 2022.
Zheng H, Ling R. Drivers of social media fatigue: a systematic review. Telematics Inform. 2021;64:101696.
Article Google Scholar
Kapus K, Nyulas R, Nemeskeri Z, Zadori I, Muity G, Kiss J, et al. Prevalence and risk factors of internet addiction among Hungarian High School Students. IJERPH. 2021;18:6989.
Article PubMed PubMed Central Google Scholar
Rumpf HJ, Meyer C, Kreuzer A, John U, Vermulst A, Merkeerk G-J. Prävalenz der Internetabhängigkeit (PINTA). Bericht an das Bundesministerium für Gesundheit (prevalence of internet addiction (PINTA). Report to the federal ministry of health). 2011.
Baloğlu M, Şahin R, Arpaci I. A review of recent research in problematic internet use: gender and cultural differences. Curr Opin Psychol. 2020;36:124–9.
Article PubMed Google Scholar
Bischof G, Bischof A, Meyer C, John U, Rumpf HJ. Prävalenz Der Internetabhängigkeit– Diagnostik und Risikoprofile (PINTA-DIARI). Kompaktbericht an das Bundesministerium für Gesundheit (Prevalence of Internet Addiction - Diagnostics and Risk profiles (PINTA-DIARI). Compact report to the Federal Ministry of Health). Lübeck; 2013.
Grüßer-Sinopoli SM, Thalemann CN, Verhaltenssucht. Diagnostik, Therapie, Forschung. 1. Aufl. Bern: Huber; 2006.
Chen I-H, Strong C, Lin Y-C, Tsai M-C, Leung H, Lin C-Y, et al. Time invariance of three ultra-brief internet-related instruments: Smartphone Application-based addiction scale (SABAS), Bergen Social Media Addiction Scale (BSMAS), and the nine-item internet gaming disorder scale- short form (IGDS-SF9) (Study Part B). Addict Behav. 2020;101:105960.
Montag C, Bey K, Sha P, Li M, Chen Y, Liu W, et al. Is it meaningful to distinguish between generalized and specific internet addiction? Evidence from a cross-cultural study from G ermany, S weden, T aiwan and C hina. Asia-Pacific Psychiatry. 2015;7:20–6.
Brand M, Young KS, Laier C. Prefrontal control and internet addiction: a theoretical model and review of neuropsychological and neuroimaging findings. Front Hum Neurosci. 2014;8.
Griffiths M, Pontes H, Kuss D. Clinical psychology of internet addiction: a review of its conceptualization, prevalence, neuronal processes, and implications for treatment. NAN. 2015;11.
Davis RA. A cognitive-behavioral model of pathological internet use. Comput Hum Behav. 2001;17:187–95.
Cao X, Gong M, Yu L, Dai B. Exploring the mechanism of social media addiction: an empirical study from WeChat users. Internet Res. 2020;30:1305–28.
Chen A. From attachment to addiction: the mediating role of need satisfaction on social networking sites. Comput Hum Behav. 2019;98:80–92.
Turel O, Serenko A. The benefits and dangers of enjoyment with social networking websites. Eur J Inform Syst. 2012;21:512–28.
Andreassen CS, Billieux J, Griffiths MD, Kuss DJ, Demetrovics Z, Mazzoni E, et al. The relationship between addictive use of social media and video games and symptoms of psychiatric disorders: a large-scale cross-sectional study. Psychol Addict Behav. 2016;30:252–62.
Griffiths M. A ‘components’ model of addiction within a biopsychosocial framework. J Subst Use. 2005;10:191–7.
Bányai F, Zsila Á, Király O, Maraz A, Elekes Z, Griffiths MD, et al. Problematic social media use: results from a large-scale nationally representative adolescent sample. PLoS ONE. 2017;12:e0169839.
Cheng C, Lau YC, Chan L, Luk JW. Prevalence of social media addiction across 32 nations: meta-analysis with subgroup analysis of classification schemes and cultural values. Addict Behav. 2021;117:106845. https://doi.org/10.1016/j.addbeh.2021.106845 .
Pantic I. Online social networking and mental health. Cyberpsychology Behav Social Netw. 2014;17:652–7.
Ahi Evran University, Baltacı Ö. The predictive relationships between the social media addiction and social anxiety, loneliness, and happiness. IJPE. 2019;15:73–82.
Status of mind. Social media and young people ́s mental health. 2017.
Tiggemann M, Hayden S, Brown Z, Veldhuis J. The effect of Instagram likes on women’s social comparison and body dissatisfaction. Body Image. 2018;26:90–7.
Milyavskaya M, Saffran M, Hope N, Koestner R. Fear of missing out: prevalence, dynamics, and consequences of experiencing FOMO. Motiv Emot. 2018;42:725–37.
Blackwell D, Leaman C, Tramposch R, Osborne C, Liss M. Extraversion, neuroticism, attachment style and fear of missing out as predictors of social media use and addiction. Pers Indiv Differ. 2017;116:69–72.
Marengo D, Poletti I, Settanni M. The interplay between neuroticism, extraversion, and social media addiction in young adult Facebook users: testing the mediating role of online activity using objective data. Addict Behav. 2020;102:106150.
Kuss DJ, Griffiths MD. Online social networking and addiction—a review of the psychological literature. IJERPH. 2011;8:3528–52.
Borhani Y. Substance abuse and Insecure attachment styles: a relational study. lux. 2013;2:1–13.
Unterrainer HF, Hiebler-Ragger M, Rogen L, Kapfhammer HP. Sucht als Bindungsstörung. Nervenarzt. 2018;89:1043–8.
Article CAS PubMed Google Scholar
Ainsworth MDS. The Bowlby-Ainsworth attachment theory. Behav Brain Sci. 1978;1:436–8.
Schuhler P, Vogelgesang M, Petry J. Pathologischer PC-/Internetgebrauch: Krankheitsmodell, diagnostische und therapeutische Ansätze. Psychotherapeut. 2009;54:187–92.
Eichenberg C, Schott M, Schroiff A. Comparison of students with and without problematic smartphone use in light of attachment style. Front Psychiatry. 2019;10:681.
Brisch KH. Bindungsstörungen: von der Bindungstheorie zur Therapie. 17. Auflage. Stuttgart: Klett-Cotta; 2020.
Eichenberg C, Schott M, Decker O, Sindelar B. Attachment style and internet addiction: an online survey. J Med Internet Res. 2017;19:e170.
Hart J, Nailling E, Bizer GY, Collins CK. Attachment theory as a framework for explaining engagement with Facebook. Pers Indiv Differ. 2015;77:33–40.
Demircioğlu ZI, Köse AG. Mediating effects of self-esteem in the relationship between attachment styles and social media addiction among university students. 2020.
Jenkins-Guarnieri MA, Wright SL, Hudiburgh LM. The relationships among attachment style, personality traits, interpersonal competency, and Facebook use. J Appl Dev Psychol. 2012;33:294–301.
Liu H, Shi J, Liu Y, Sheng Z. The moderating role of attachment anxiety on social network site use intensity and social capital. Psychol Rep. 2013;112:252–65.
Oldmeadow JA, Quinn S, Kowert R. Attachment style, social skills, and Facebook use amongst adults. Comput Hum Behav. 2013;29:1142–9.
Eroglu Y. Interrelationship between attachment styles and Facebook addiction. J Educ Train Stud. 2016;4:150–60.
Google Scholar
Yaakobi E, Goldenberg J. Social relationships and information dissemination in virtual social network systems: an attachment theory perspective. Comput Hum Behav. 2014;38:127–35.
SoSci. Survey online survey tool.
Deng T, Kanthawala S, Meng J, Peng W, Kononova A, Hao Q, et al. Measuring smartphone usage and task switching with log tracking and self-reports. Mob Media Communication. 2019;7:3–23.
Monacis L, Palo VD, Griffiths MD, Sinatra M. Validation of the internet gaming disorder scale– short-form (IGDS9-SF) in an italian-speaking sample. J Behav Addictions. 2016;5:683–90.
Spitzer C, Hammer S, Löwe B, Grabe H, Barnow S, Rose M, et al. Die kurzform des brief symptom inventory (BSI– 18): erste Befunde zu den psychometrischen Kennwerten Der Deutschen version. Fortschr Neurol Psychiatr. 2011;79:517–23.
Franke GH, Jaeger S, Glaesmer H, Barkmann C, Petrowski K, Braehler E. Psychometric analysis of the brief symptom inventory 18 (BSI-18) in a representative German sample. BMC Med Res Methodol. 2017;17:14.
Franke GH, Ankerhold A, Haase M, Jäger S, Tögel C, Ulrich C, et al. Der Einsatz Des brief symptom inventory 18 (BSI-18) Bei psychotherapiepatienten. Psychother Psych Med. 2011;61:82–6.
Rammstedt B, John OP. Measuring personality in one minute or less: a 10-item short version of the big five inventory in English and German. J Res Pers. 2007;41:203–12.
Rammstedt B, Kemper CJ, Klein MC, Beierlein C, Kovaleva A. Eine Kurze Skala Zur Messung Der fünf Dimensionen Der Persönlichkeit: big-five-Inventory-10 (BFI-10). Mannheim: GESIS - Leibniz-Institut für Sozialwissenschaften; 2012.
Asendorpf JB, Neyer FJ. Psychologie Der Persönlichkeit. Berlin, Heidelberg: Springer Berlin Heidelberg; 2012.
Book Google Scholar
Höger D, Buschkämper S. Der Bielefelder Fragebogen zu Partnerschaftserwartungen. Z für Differentielle und Diagnostische Psychologie. 2002;23:83–98.
Collins NL, Read SJ. Adult attachment, working models, and relationship quality in dating couples. J Personal Soc Psychol. 1990;58:644–63.
Article CAS Google Scholar
Müller K. Wer ist gefährdet? Risikofaktoren für Internetsucht. Spielwiese internet. Berlin, Heidelberg: Springer Berlin Heidelberg; 2013. pp. 67–84.
Chapter Google Scholar
Wölfling K, Beutel M, Dreier M, Müller K. Risikofaktoren Von Verhaltenssucht: Explizite Persönlichkeitsfaktoren Und Implizite Assoziationsstärken Bei Pathologischem Glücksspiel Und Internetsucht. Suchttherapie. 2015;16:s–0035.
Kircaburun K, Self-Esteem. Daily internet use and social media addiction as predictors of depression among Turkish adolescents. J Educ Pract. 2016;7:64–72.
Wu AMS, Li J, Lau JTF, Mo PKH, Lau MMC. Potential impact of internet addiction and protective psychosocial factors onto depression among Hong Kong Chinese adolescents– direct, mediation and moderation effects. Compr Psychiatr. 2016;70:41–52.
Azher M, Khan RB, Salim M, Bilal M, Hussain A, Haseeb M, et al. The relationship between internet addiction and anxiety among students of University of Sargodha. Int J Humanit Social Sci. 2014;4:288–93.
Younes F, Halawi G, Jabbour H, El Osta N, Karam L, Hajj A, et al. Internet addiction and relationships with insomnia, anxiety, depression, stress and self-esteem in University students: a cross-sectional designed study. PLoS ONE. 2016;11:e0161126.
Cerutti R, Spensieri V, Amendola S, Presaghi F, Fontana A, Faedda N, et al. Sleep disturbances partially mediate the association between problematic internet use and somatic symptomatology in adolescence. Curr Psychol. 2021;40:4581–9.
Cerruti R, Spensieri V, Presaghi F, Valastro C, Fontana A, Guidetti V. An exploratory study on internet addiction, somatic symptoms and emotional and behavioral functioning in school-aged adolescents. Clin Neuropsychiatry. 2017.
Cerutti R, Presaghi F, Spensieri V, Valastro C, Guidetti V. The potential impact of internet and mobile use on headache and other somatic symptoms in adolescence. A Population-Based Cross‐Sectional Study. Headache. 2016;56:1161–70.
D’Arienzo MC, Boursier V, Griffiths MD. Addiction to social media and attachment styles: a systematic literature review. Int J Ment Health Addict. 2019;17:1094–118.
Baek HW, Shin YM, Shin KM. Emotional and behavioral problems related to smartphone overuse in elementary school children. J Korean Neuropsychiatr Assoc. 2014;53:320.
Kwan HC, Leung MT. The path model of parenting style, attachment style, self-regulation and smartphone addiction. Applied psychology. Concorde Hotel. Singapore: WORLD SCIENTIFIC; 2015. pp. 196–214.
Yelpaze İ. The mediator role of self-control in the relationship between insecure attachment styles and problematic smartphone use in adolescents. Turkish Psychol Couns Guidance J. 2020;10:457–73.
Parada-Fernández P, Herrero‐Fernández D, Oliva‐Macías M, Rohwer H. Analysis of the mediating effect of mentalization on the relationship between attachment styles and emotion dysregulation. Scandinavian J Psychol. 2021;62:312–20.
Santoro G, Midolo LR, Costanzo A, Schimmenti A. The vulnerability of insecure minds: the mediating role of mentalization in the relationship between attachment styles and psychopathology. Bull Menninger Clin. 2021;85:358–84.
Sax LJ, Gilmartin SK, Bryant AN. Assessing response rates and nonresponse bias in web and paper surveys. Res High Educt. 2003;44:409–32.
Jackob N, Schoen H, Zerback T, editors. Sozialforschung Im Internet. Wiesbaden: VS Verlag für Sozialwissenschaften; 2009.
Rose J, McGuire-Snieckus R, Gilbert L, McInnes K. Attachment aware schools: the impact of a targeted and collaborative intervention. Pastoral Care Educ. 2019;37:162–84.
Download references
Acknowledgements
Not applicable.
Author information
Authors and affiliations.
Faculty of Medicine, Institute of Psychosomatics, Sigmund Freud Private University, Freudplatz 3, Vienna, 1020, Austria
Christiane Eichenberg, Raphaela Schneider & Helena Rumpl
You can also search for this author in PubMed Google Scholar
Contributions
CE was involved in planning, supervising the work, and writing the manuscript. HR collected survey data and did the data curation. RS analyzed data and was involved in writing the manuscript.
Corresponding author
Correspondence to Raphaela Schneider .
Ethics declarations
Ethics approval and consent to participate.
The study involving human participants was reviewed and approved by the Ethics Commission of the Faculty of Psychotherapy Science and the Faculty of Psychology of the Sigmund Freud Private University Vienna. The patients/participants provided their written informed consent to participate in this study.
Consent for publication
Competing interests.
The authors declare no competing interests.
Additional information
Publisher’s note.
Springer Nature remains neutral with regard to jurisdictional claims in published maps and institutional affiliations.
Rights and permissions
Open Access This article is licensed under a Creative Commons Attribution 4.0 International License, which permits use, sharing, adaptation, distribution and reproduction in any medium or format, as long as you give appropriate credit to the original author(s) and the source, provide a link to the Creative Commons licence, and indicate if changes were made. The images or other third party material in this article are included in the article’s Creative Commons licence, unless indicated otherwise in a credit line to the material. If material is not included in the article’s Creative Commons licence and your intended use is not permitted by statutory regulation or exceeds the permitted use, you will need to obtain permission directly from the copyright holder. To view a copy of this licence, visit http://creativecommons.org/licenses/by/4.0/ . The Creative Commons Public Domain Dedication waiver ( http://creativecommons.org/publicdomain/zero/1.0/ ) applies to the data made available in this article, unless otherwise stated in a credit line to the data.
Reprints and permissions
About this article
Cite this article.
Eichenberg, C., Schneider, R. & Rumpl, H. Social media addiction: associations with attachment style, mental distress, and personality. BMC Psychiatry 24 , 278 (2024). https://doi.org/10.1186/s12888-024-05709-z
Download citation
Received : 31 January 2024
Accepted : 22 March 2024
Published : 15 April 2024
DOI : https://doi.org/10.1186/s12888-024-05709-z
Share this article
Anyone you share the following link with will be able to read this content:
Sorry, a shareable link is not currently available for this article.
Provided by the Springer Nature SharedIt content-sharing initiative
- Internet related addiction
- Insecure attachment style
BMC Psychiatry
ISSN: 1471-244X
- Submission enquiries: [email protected]
- General enquiries: [email protected]
Local news, paywall-free.
MinnPost’s timely reporting is available for free, all year round. But our work isn’t free to produce. Help sustain our nonprofit newsroom with a monthly donation today.

Nonprofit, independent journalism. Supported by readers.
Studies highlight impact of social media use on college student mental health
Share this:.
- Click to email a link to a friend (Opens in new window)
- Click to share on Facebook (Opens in new window)
- Click to share on Twitter (Opens in new window)
- Click to print (Opens in new window)

MinnPost’s Daily Newsletter
The latest on the politics and policy shaping Minnesota.
Delivered straight to your inbox.

Stay in the know.
MinnPost’s top stories delivered straight to your inbox Monday through Saturday.
When Kyle Palmberg set out to design a research study as the capstone project for his psychology major at St. Mary’s University of M i nnesota in Winona, he knew he wanted his focus to be topical and relevant to college students.
His initial brainstorming centered around the mental health impact of poor sleep quality.
“I wanted to look at college students specifically, to see the different ways that sleep quality can be harmed and how that can impact your mental health,” he said. As he reviewed the scientific literature, one variable kept appearing. “The topic that kept coming up was social media overuse,” he said. “It is such an important thing to my target demographic of college students.”
Palmberg, 22, grew up surrounded by social media. He’d heard plenty of warnings about the downsides of spending too much time online, and he’d seen many of his peers seemingly anchored to their phones, anxious or untethered if they had to put them down for more than a few minutes at a time.
“I think from my perspective as someone who’s been really interested in psychology as an academic discipline, social media addiction is also something I’ve been aware of personally,” Palmberg said. “I can tell within myself when things can become harmful or easy to misuse. I often see the hints of addictive behaviors in peers and coworkers.”
Palmberg found much of the published research on the topic inspiring, particularly a 2003 study on internet gambling addiction.
“They were looking at how internet gambling addiction permeates a person’s behavior,” he said. Palmberg hypothesized that there may be behavioral similarities between people addicted to online gambling and those addicted to social media.
“Social media provides this convenient platform for users to interact with others,” he said. “As users grow addicted, they learn that they can come back to that social platform more and more to get their needs met. The tolerance users have for gratifying that social need grows. Then they have to use social media more and more often to get those benefits.”
The negative impact of a growing dependence on social media is that time spent online takes away from real in-person interactions and reduces the time a person has available for basic personal care needs, like sleep and exercise, Palmberg said. This can ultimately have a negative impact on mental health.
“As a person builds a high tolerance for the use of social media it causes internal and external conflict,” he said. “You know it is wrong but you continue to use it. You relapse and struggle to stop using it.” Palmberg said that social media use can be a form of “mood modification. When a person is feeling down or anxious they can turn to it and feel better at least for a moment. They get a sense of withdrawal if they stop using it. Because of this negative side effect, it causes that relapse.”
Palmberg decided he wanted to survey college students about their social media use and devise a study that looked at connections between the different motivations for that use and potential for addictive behaviors. He ran his idea by his academic advisor, Molly O’Connor, associate professor of psychology at Saint Mary’s, who was intrigued by his topic’s clear connections to student life.

“We often notice social media addiction with our student population,” O’Connor said. She knew that Palmberg wouldn’t have a hard time recruiting study participants, because young people have first-hand experience and interest in the topic. “He’s looking at college students who are particularly vulnerable to that addiction. They are tuned into it and they are using it for coursework, socialization, entertainment, self-documentation.”
O’Connor said she and her colleagues at the university see signs of this addiction among many of their students.
“They’ll be on their phones during class when they are supposed to pay attention,” she said. “They can’t help themselves from checking when a notification comes through. They say they had trouble sleeping and you’ll ask questions about why and they’ll say they were scrolling on their phone before they went to bed and just couldn’t fall asleep.”
The entertainment-addiction connection
Once his study was given the go-ahead by his advisor and approved by the university for human-subjects research, Palmberg had two months to recruit participants.
To gather his research subjects, he visited classes and gave a short speech. Afterward, students were given an opportunity to sign up and provide their emails. Palmberg recruited 86 participants this way, and each was asked to fill out an anonymous survey about their social media habits.
Palmberg explained that the main framework of his study was to gain a deeper understanding of why college students use social media and the circumstances when it can become addictive and harmful to their mental health and well-being. He also hypothesized that perceived sleep quality issues would be connected to social media addiction.
After collecting the surveys, Palmberg said, “We essentially threw the data into a big spreadsheet. We worked with it, played with it, analyzed it.” He explained that his analysis focused on motivations for social media use, “including building social connections and self-documentation.”
What Palmberg discovered was that his subjects’ most popular motivation for social media use was for entertainment. While some participants listed other motivations, he said the most “statistically significant” motivation was entertainment.
“Not only was entertainment the most highly endorsed reason to use social media in the study,” Palmberg said, “for college students it was the only motivation we analyzed that was statistically connected to social media addiction and perceived stress. The entertainment motivation was also related to poor sleep quality.”
Mental Health & Addiction
A better way to deliver unexpected news, in her new book, ‘the rock in my throat,’ kao kalia yang shares her struggle with selective mutism, a community-based approach to suicide prevention.
He found connections between a reliance on social media for entertainment and addictive behaviors, like an inability to shut down apps or put a phone away for an extended period of time. “If a person is using social media for entertainment, they are more likely to be addicted to social media than someone who is not using it for entertainment,” Palmberg said.
The structures of popular social media platforms reinforce addictive behaviors, he said. “Current trends in social media lean more toward entertainment platforms like TikTok or Instagram. People are going on there just to pass time,” Palmberg said. These brief and repetitive formats encourage addiction, he said, because the dopamine high they create is short-lived, causing users to keep visiting to get those fleetingly positive feelings.
O’Connor supports Palmberg’s conclusions. A reliance on social media platforms for entertainment encourages addiction, she said. This is backed up by student behavior.
“My big takeaway was the interest in the entertainment variable was the key predictor of addiction. It’s not necessarily the students that are using it to communicate with each other, but the ones that say, ‘I need to kill time between classes,’ or, ‘I’m bored before bed,’ or, ‘I am trying to relieve stress after working on homework.’” The addictive aspect comes in, O’Connor said, “because users want to be entertained more and more. They are constantly looking for the next thing to talk about with their friends.”
Palmberg said he believes that not all social media use among college students has to be addictive. “It is important for people to view social media as not only something that can be harmful but also something that can be used as a tool. I like to emphasize with my study that it’s not all negative. It is more of an emphasis on moderation. It is possible to use social media responsibly. But just like almost anything, it can be addictive.”
An emphasis on digital well-being
Twice a year, in an effort to get out ahead of digital addiction, students at Gustavus Adolphus College in St. Peter are encouraged to take a deeper look at their social media use and its impact on their mental health. Charlie Potts, the college’s interim dean of students, heads the effort: It’s a clear match with his job and his research interests.

During the semiannual event, known as “Digital Well-Being Week,” Gustavus students learn about the potentially negative impact of social media overuse — as well as strategies for expanding their social networks without the help of technology.
Potts said that event has been held four times so far, and students now tell him they anticipate it.
“We’ve gotten to the point where we get comments from students saying, ‘It’s that time again,’” he said. Students say they appreciate the information and activities associated with Digital Well-Being Week, Potts continued, and they look forward to a week focused on spending less time with their phones.
“They remember that we put baskets on every table in the dining hall with a little card encouraging them to leave their phones there and instead focus on conversations with others,” he added. “We even include a card in the basket with conversation starters. Students are excited about it. They know the drill. It is something they like to do that feels good.”
Potts’ own academic research has focused on mental health and belonging. Each fall, he also heads up a campus-wide student survey focused on digital well-being and how to balance phone use with other aspects of mental and physical health.
In the survey, Potts said, “We ask students, ‘How much time do you spend every day on social media? How does it make you feel?’ Students are blown away when they see the number of hours that the average Gustie spends online. The vast majority are in the 4-7 hours a day on their phone range.”
The survey, which uses a motivational style of interviewing to help participants get at the root of why altering their social media behaviors may be valuable to their overall health and well-being, focuses on small changes that might reduce participants’ reliance on technology in favor of face-to-face interaction.
“We do a lot of conversations with students about strategies they could use,” Potts said. “Things like plugging your phone in across the room while you sleep, leaving it behind while you go to work out at the rec center, subtle changes like that. We also talk about mental health and mindfulness and how…you discern your values about what you are consuming and how that might affect you.”
Though Potts said he has encountered some resistance from students (“You roll with that and help them understand the value of that and think about how they are going to make that change,” he said), he’s also heard a lot of positive student feedback about his survey — and the twice-yearly focus on digital well-being.
“What we found with our students is they realize deep down that their relationship with their phones and social media was not having a positive impact on their life,” Potts said. “They knew change would be good but they didn’t know how to make change or who to talk to about that or what tools were at their disposal. These options help them understand how to do that.”

Andy Steiner
Andy Steiner is a Twin Cities-based writer and editor. Before becoming a full-time freelancer, she worked as senior editor at Utne Reader and editor of the Minnesota Women’s Press. Email her at [email protected] .
Thanks to our major sponsors

We've recently sent you an authentication link. Please, check your inbox!
Sign in with a password below, or sign in using your email .
Get a code sent to your email to sign in, or sign in using a password .
Enter the code you received via email to sign in, or sign in using a password .
Subscribe to our newsletters:
- Daily Newsletter MinnPost's top stories delivered to your inbox Monday through Saturday.
- Events & member benefits Be the first to know about opportunities around MinnPost membership & events.
Sign in with your email
Lost your password?
Try a different email
Send another code
Sign in with a password
Privacy Policy
Longitudinal Associations of Social Anxiety Trajectories with Internet-Related Addictive Behaviors Among College Students: A Five-Wave Survey Study
- Original Article
- Published: 12 April 2024
Cite this article
- Xiaomeng Li 1 ,
- Xiaoyan Chen 2 ,
- Ya Zhu 3 &
- Xuliang Shi ORCID: orcid.org/0000-0002-6234-5456 1
47 Accesses
Explore all metrics
The college stage is characterized by increased interpersonal interaction and intense social anxiety. Although the associations between social anxiety and internet-related addictive behaviors have been established, the question of whether the developmental trajectory of social anxiety is linked to subsequent internet-related addictive behaviors remains unexplored. Utilizing a longitudinal design combined with a person-centered approach, the current study examined the developmental trajectory of social anxiety among 3,861 students throughout their university years. Additionally, we explored the impacts of specific change patterns of social anxiety on subsequent internet-related addictive behaviors. The growth mixture modeling identified three distinct profiles of social anxiety: high-stable group ( n =515, 13.33%), high-decreasing group ( n =243, 6.30%), and low-decreasing group ( n =3103, 80.37%). Furthermore, the results of binary logistic regression analysis revealed that students in the high-decreasing and low-decreasing groups were less likely to experience smartphone addiction and Internet game addiction compared to those in the high-stable group. These findings underscore the importance of identifying individuals with a high risk for social anxiety and providing them with personalized and effective mental health services to mitigate their susceptibility of developing internet-related addictive behaviors.
Similar content being viewed by others
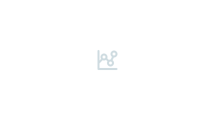
Social Media Use and Mental Health among Young Adults
Chloe Berryman, Christopher J. Ferguson & Charles Negy
Social Media Effects on Young Women’s Body Image Concerns: Theoretical Perspectives and an Agenda for Research
Richard M. Perloff
Understanding Social Anxiety Disorder in Adolescents and Improving Treatment Outcomes: Applying the Cognitive Model of Clark and Wells (1995)
Eleanor Leigh & David M. Clark
Avoid common mistakes on your manuscript.
The college years represent a critical period as students navigate the transition from late adolescence to emerging adulthood (Arnett, 2015 ; Arnett et al., 2014 ). During this periods, college students are exposed a wide range of social activities and are tasked with establishing new social relationships (Collins et al., 2018 ). However, for some students, the unfamiliarity of these social situations and environmental may trigger feelings of social anxiety. Therefore, it is not uncommon that up to 25% of college students report experiencing feelings of nervousness or anxiety when interacting with others (Melkam et al., 2023 ). Depending on the diagnostic threshold, the prevalence of social anxiety disorder among college students ranges from 11% to 33% (Baptista et al., 2012 ; Melkam et al., 2023 ; Reta et al., 2020 ), which is much higher than that of the general population (Brook & Willoughby, 2015 ). In addition, Meng et al. ( 2021 ) surveyed 2759 college students from China, and revealed that 33.38% of students have reported experiencing at least one social anxiety symptom. Furthermore, females are more likely to experience social anxiety disorder than males (Asher et al., 2017 ; Caballo et al., 2014 ). Individuals with social anxiety often have excessive and impaired fears in social and interpersonal situations and may actively avoid situations where they fear negative evaluation by others (American Psychiatric Association, 2013 ). Social anxiety among college students can be particularly problematic because it is related to other psychological and interpersonal challenges. Severe social anxiety can even result in substance abuse (Schry & White, 2013 ), depression (Stein et al., 2001 ), and suicidal ideation (Buckner et al., 2017 ).
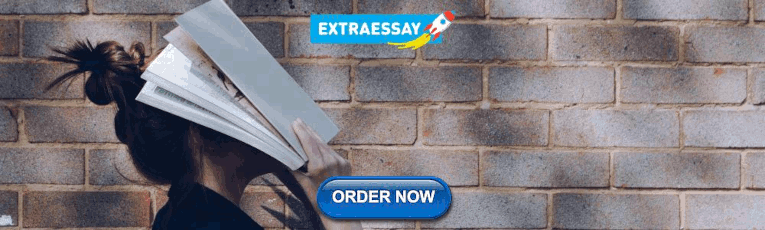
Developmental Trajectories of Social Anxiety
At the macro level, spanning across different age groups, the highly social environment of college campuses and the pressure to conform may increase the risk of social anxiety among college students. At the micro level, social anxiety may gradually decline as students mature and adapt to new environments. For example, a recent meta-analysis of longitudinal studies have found that a decline in social anxiety during emerging adulthood (B. Chen et al., 2023 ). However, most previous studies have relied on short-term longitudinal designs, variable-centered approaches and the Western population (Brook & Willoughby, 2015 ; Gautreau et al., 2015 ; Schry et al., 2016 ), paying less attention to the long-term dynamic changes in social anxiety experienced by college students over time. Therefore, a multiple-wave design to examine continuous trajectory of social anxiety across the university years is crucial from a developmental perspective.
Developmental theories of anxiety suggest that individuals can be categorized into subgroups based on their distinctive patterns of anxiety symptoms (Weems, 2008 ). Research on the taxonomy of social anxiety emphasizes the importance of examining heterogeneity in its development, as there may be significant differences between individuals with symptomatic problems, regardless of whether they meet diagnostic criteria for the disorder (Levinson et al., 2014 ). Growth mixture models (GMM), a person-centered approach, have been developed to separate a general population of individuals into subgroups characterized by qualitatively distinct patterns of change over time (Jung & Wickrama, 2008 ; Mo & Bodner, 2007 ). Existing research using GMM has provided evidence regarding the developmental trajectory of social anxiety (Branje et al., 2013 ; Broeren et al., 2013 ; Krygsman & Vaillancourt, 2022 ; Marmorstein et al., 2010 ; Miers et al., 2013 ). For instance, Marmorstein et al. ( 2010 ) followed a sample of 2,451 participants from childhood to early adolescence, and identified five trajectories of social anxiety symptoms: stable moderate group, stable high group, increasing symptom group, moderate decreasing symptom group, and high decreasing symptom group. Krygsman and Vaillancourt ( 2022 ) examined the heterogeneous development of social anxiety symptoms across childhood and adolescence, and found three distinct trajectories: high increasing group, moderate group, and low group. Similar results were found in two other studies (Branje et al., 2013 ; Miers et al., 2013 ).
Despite accumulating evidence demonstrating significant heterogeneity in social anxiety, the literature has several limitations. On the one hand, most previous studies have focused more on the developmental trajectory of social anxiety in children and adolescents, with less attention given to the college student. On the other hand, previous studies have predominantly relied on small samples from Western countries, overlooking the potential influence of cultural factors on the development of social anxiety. For example, collectivist countries tend to report higher levels of social anxiety and fear of blushing than individualist countries (Heinrichs et al., 2006 ).
Social Anxiety and Internet-Related Addictive Behaviors
College students are particularly susceptible to developing excessive reliance on smartphones or online games due to having large amounts of free time outside of class (Aljomaa et al., 2016 ; Borges et al., 2019 ). Social anxiety is an important predictor of the development of internet-related addictive behaviors, such as smartphone addiction and Internet game addiction (Gioia et al., 2022 ; Ran et al., 2022 ; Stănculescu & Griffiths, 2022 ). According to the compensatory Internet use theory, individuals with social anxiety may turn to the Internet as a substitute for face-to-face social and emotional connections, potentially leading to a dependence on online interactions (Kardefelt-Winther, 2014 ). More specifically, Internet-mediated communication can provide alternative virtual environments that allow highly socially anxious individuals to redirect most of their social interactions and alleviate their negative emotions associated with real-life social situations (Marino et al., 2020 ; Przepiorka et al., 2021 ). In addition to fulfilling social functions, smartphones and online games can serve as avoidance coping strategies (Elhai et al., 2018 ). Numerous studies have shown that social anxiety is indicative of distorted perceptions, such as low self-worth and excessive concern for negative feedback from others (Iancu et al., 2015 ; Morrison & Heimberg, 2013 ). According to the cognitive-behavioral model of pathological Internet use, individuals with negative emotions (such as social anxiety) are more prone to Internet addiction because they use the Internet as a coping strategy to regulate their negative emotions and escape from real-world troubles (Davis, 2001 ). Although previous studies have explored the relationship between social anxiety and internet-related addictive behaviors, most of these studies are cross-sectional. Moreover, it is still unclear whether there were differential associations between trajectories of social anxiety and internet-related addictive behaviors.
The Current Study
Using a prospective design and a person-centered approach, we aim to investigate the heterogeneous trajectory of social anxiety and further explore whether distinct patterns of social anxiety are differentially linked to subsequent internet-related addictive behaviors. Based on the literature and theories, we propose the existence of three or four trajectories of social anxiety in Chinese college students. Furthermore, we hypothesize that college students in the low-risk group (e.g., stable low group, decreasing symptom group) have a lower risk of internet-related addictive behaviors than those in the high-risk group (e.g., stable high group, increasing symptom group).
Participants and Procedure
Participants were recruited from a large-scale health-related cohort among Chinese college students. This cohort was a five-wave design from 2019 to 2021 and participants enrolled from three universities in Guangdong Province, China. The waves were conducted at the interval of 6 months, except for the second and the third wave, which had a 12-month interval. This longer interval between the second and the third waves was due to the outbreak of the COVID-19 pandemic, which resulted in students not returning to school during that period. Detailed sampling and data collection have been described in Fig. 1 . In brief, at the baseline (T 1 ), a total of 4352 freshmen were invited to complete an online-based mental health survey. After excluding the invalid questionnaires, a final sample of 4191 freshmen was identified for subsequent follow-up investigations. Of the participants at T 1 , 3985 (4.9% attrition rate), 3905 (6.8% attrition rate), 3892 (7.1% attrition rate), and 3957 (5.6% attrition rate) provided valid responses from T 2 to T 5 , respectively. The main reason for attrition was that students were absent from classes on the day of evaluation. To facilitate subsequent trajectory analysis, only participants who completed data collection for at least two waves were included in the present study ( N = 3861, M age = 19.12, SD = 0.98). All sociodemographic and other characteristics of the participants were summarized in Table S 1 (Supplemental Materials).
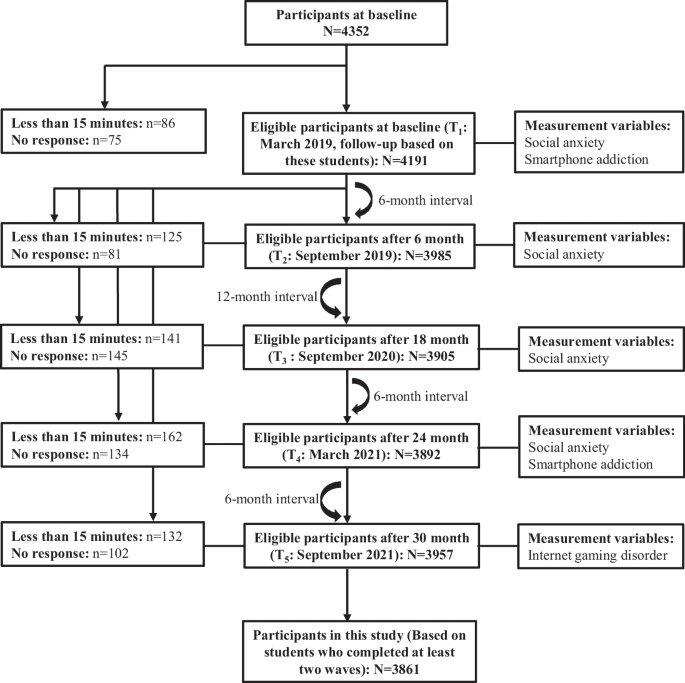
Sampling procedure for the current study. Notes in this figure: “less than 15 minutes” means that if the time it took to complete the survey was <15 min, the questionnaire was considered to be invalid
Before collecting data, we obtained permission from the principals of three schools and obtained an electronic version of the informed consent form from students. All participants were informed that their participation in the study was voluntary and that they could withdraw from the study at any time without repercussions. It took approximately 30 minutes to complete the questionnaires. The present study protocol was reviewed and approved by the Research Ethics Committee of the corresponding author’s institution.
Social Anxiety
Social anxiety was measured using the subscale of Screen for Adult Anxiety Related Disorders (SCAARED) (Angulo et al., 2017 ). The SCAARED is a self-assessment questionnaire with 44 items, which was adapted from the Screen for Children Anxiety Related Emotional Disorders (SCARED) (Birmaher et al., 1999 ). The SCAARED evaluated four types of anxiety disorders in DSM-5: panic disorder, generalized anxiety disorder, separation anxiety disorder, and social anxiety disorder. The Chinese version of the SCAARED was translated and revised by Wang et al. ( 2022 ). The social anxiety subscale consists of 7 items (e.g., “ It is hard for me to talk with people I don't know well ”). Each item was evaluated on a 3-point scale from 0 (not true or hardly ever true) to 2 (very true or often true). Item scores are summed to get a total score ranging from 0 to 14, and a cutoff score of 7 has been used to screen clinically probable social anxiety (Angulo et al., 2017 ). The SCAARED has shown good reliability and validity among Chinese adults (X. Chen et al., 2020 ). In this study, the Cronbach’s alpha coefficients were 0.86, 0.86, 0.86, and 0.90 at T 1 , T 2 , T 3 , and T 4 , respectively.
Smartphone Addiction
The Smartphone Addiction Scale-Short Version (SAS-SV) was used to measure smartphone addiction at T 1 and T 4 (Kwon et al., 2013 ). This scale comprises 10 items (e.g., “ Feeling impatient and fretful when I am not holding my smartphone ”) and each item is answered on a six-point scale ranging from 1 (strongly disagree) to 6 (strongly agree). Summing the scores on the ten items creates a total score ranging from 10 to 60, with a higher total score indicating a higher level of smartphone addiction. The scale developers proposed SAS-SV cut-off scores of ≥ 31 for males and ≥ 33 for females to identify individuals with potential smartphone addiction. The SAS-SV has demonstrated good psychometric properties in the Chinese population (Luk et al., 2018 ; Peng & Liang, 2023 ). In this study, the Cronbach’s alpha coefficients were 0.88 and 0.93 at T 1 and T 4 .
Internet Game Addiction
Internet game addiction was measured using the Internet Gaming Disorder Scale-Short-Form (IGDS9-SF) (Leung et al., 2020 ; Pontes & Griffiths, 2015 ). IGDS9-SF was developed according to the diagnostic criteria of IGD described in the DSM-5. This scale comprises 9 items (e.g., “ Do you feel more irritability, anxiety or even sadness when you try to either reduce or stop your gaming activity ”). All items were evaluated on a 5-point Likert scale ranging from 1 (never) to 5 (very often). Scores were summed, with higher scores indicating higher levels of Internet game addiction. A cutoff score of 21 has been recommended for identifying probable addictive behaviors (Monacis et al., 2016 ). The Chinese version of the IGDS9-SF has demonstrated good psychometric properties (Leung et al., 2020 ; Poon et al., 2021 ). In this study, the Cronbach’s alpha coefficient was 0.92 at T 5 .
Other Covariates
Other covariates were assessed at baseline, including gender (0 = female, 1 = male), age, the only child (0 = no, 1 = yes) and parental education levels (0 = less than nine years; 1= nine years or more).
Statistical Analyses
The primary analyses in this study included four steps. First, descriptive analyses and Pearson correlation analyses were conducted to examine main variables. Second, latent growth curve models (LGCM) were conducted to examine overall developmental trajectory of social anxiety. Third, growth mixture modeling (GMM) was used to identify distinct social anxiety trajectories across the four time points. Unconditional models with one- to five-class were tested for social anxiety. Intercepts, linear slope, and quadratic slope were estimated for each latent class. Full information maximum likelihood (FIML) estimation was applied to handle missing values (Giletta et al., 2015 ). Multiple criteria were applied to determine the optimal number of classes (Nylund et al., 2007 ), including lower information criteria fit indices (AIC, BIC, SSBIC), higher entropy values, likelihood ratio tests (LRTs; p < 0.05 implies c classes fit better than c-1), and a minimum of 5% of participants in each class. Furthermore, prior empirical evidence and theoretical considerations were taken into account to determine the final model (Nagin & Odgers, 2010 ). Fourth, binary logistic regression analysis was performed to examine whether social anxiety trajectories could prospectively predict internet-related addictive behaviors (i.e., smartphone addiction and Internet gaming disorder). Both crude and adjusted models were tested. All analyses in this study were performed using SPSS 21.0 and Mplus 8.3.
Attrition Analyses
The missing completely at random (MCAR) test was conducted on all variables across the five waves of the study. The results indicated that the data were not missing completely at random ( χ 2 = 223.05, df = 186, p < 0.05), which is common in longitudinal studies (Engels & Diehr, 2003 ). To further understand the missing data mechanism, the chi-square test and t test were conducted to compare demographic characteristics at baseline between participants who dropped out and those who participated in all waves. The results revealed that males ( χ 2 = 70.85, df = 1, p < 0.001) were more likely to drop out. No significant differences were found in other variables.
Sample Characteristics and Prevalence of Social Anxiety
Among the 3861 participants, 46.9% were females and 21.0% were only child. The average age at baseline was 19.12 years (ranging from 17 to 28, SD = 0.98). Regarding the prevalence of social anxiety, 23.0%, 20.3%, 14.8%, and 13.4% of college students reported probable social anxiety at four time points, respectively. Female college students reported higher prevalence rates of social anxiety than males at all time points (see Fig. 2 ). Table 1 provided zero-order correlations among the main variables. The results found that participants’ social anxiety at four time points were positively correlated with smartphone addiction and internet gaming disorder.
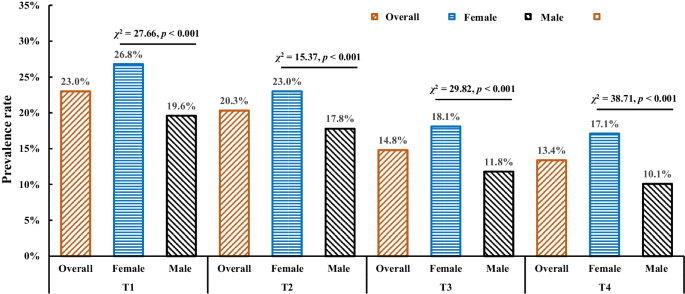
The prevalence of social anxiety in male and female college students across four timepoints
Identification of Overall Social Anxiety Trajectories
The results of latent growth curve models indicated that a quadratic model fit the data better (CFI = 0.998; TLI = 0.989; RMSEA = 0.062). The mean of the intercept (b = 5.41, SE = 0.05, p < 0.001), linear slope (b = -0.44, SE = 0.04, p < 0.001) and quadratic slope ( b = 0.03, SE = 0.01, p = 0.007) were statistically significant, indicating a significant downward trend in social anxiety levels among college students. Moreover, the variance of the intercept ( b = 8.28, SE = 0.36, p < 0.001), linear slope ( b = 1.17, SE = 0.28, p < 0.001) and quadratic slope ( b = 0.05, SE = 0.01, p < 0.001) were also significant, suggesting significant inter-individual differences in both the initial level and the change trend of social anxiety.
Identification of Distinct Social Anxiety Trajectories
As shown in Table 2 , model selection criteria were used to identify the optimal social anxiety trajectories. The four- and five-class models were not considered as the percentage of the smallest group was below 5% in both models (Nylund et al., 2007 ). The AIC, BIC and SSBIC all decrease from one- to four- class solution, which indicated improved model fit compared with base model. The significant LRT statistics produced by the three-class model supported accepting the hypothesis of a third trajectory. Additionally, the three-class model showed a higher entropy value than the two-class model. Based on these criteria mentioned above and the theoretical meaningfulness of group membership, the three-class model was finally chosen.
The final model included three trajectory groups (see Table 3 and Fig. 3 ). Specifically, the first trajectory group ( n =3103, 80.37%), labeled as the “ low-decreasing group ”, consisted of participants who reported low levels of social anxiety across four waves. The second trajectory group ( n =515, 13.33%), named the “ high-stable group ”, was characterized by participants’ social anxiety being above the clinical cutoff across all time points. The third trajectory group ( n =243, 6.30%) named the “ high-decreasing group ”, presented with an initial high level of social anxiety and gradually decreased over time.
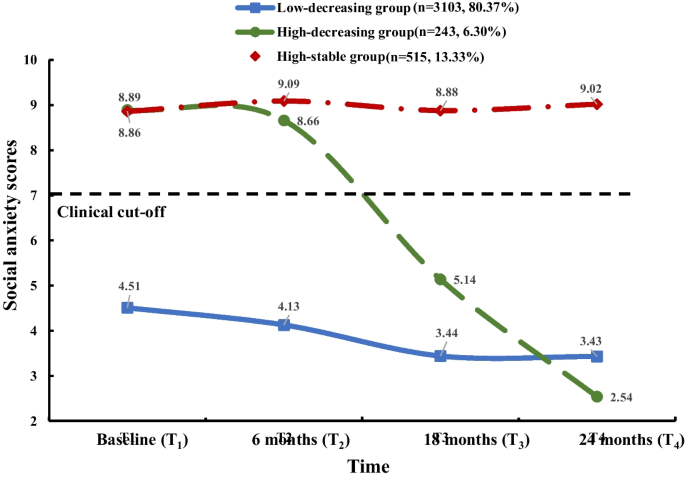
Trajectories of social anxiety in college students across four timepoints
Associations Between Social Anxiety Trajectories and Internet-Related Addictive Behaviors
Binary logistic regression analysis was conducted to examine whether social anxiety trajectories could prospectively predict internet-related addictive behaviors. As presented in Table 4 , after adjusting demographic information and smartphone addiction at T 1 , the results showed that, compared with high-stable group, individuals in low-decreasing group (OR = 0.26, 95%CI = 0.20-0.35) and high-decreasing group (OR = 0.15, 95%CI = 0.10-0.22) were less likely to experience smartphone addiction at T 4 . Furthermore, after adjusting for demographic information, we found that compared with high-stable group, individuals in low-decreasing group (OR = 0.15, 95%CI = 0.11-0.22) and high-decreasing group (OR = 0.20, 95%CI = 0.09-0.44) were also less likely to experience Internet game addiction at T 5 .
Utilizing a longitudinal design combined with a person-centered approach, this study conducted the first examination of the developmental trajectory of social anxiety and explored the impacts of specific change patterns of social anxiety on subsequent internet-related addictive behaviors. Three distinct trajectories of social anxiety were identified: low-decreasing group, high-decreasing group, and high-stable group. Compared with the high-stable group, individuals in low-decreasing group and high-decreasing group were less likely to develop subsequent smartphone addiction and Internet game addiction even after adjusting for other covariates.
The Prevalence of Social Anxiety
The present study has found a varying prevalence of probable social anxiety among college students, ranging from 13.4% to 23.0% at different time points. The high prevalence of social anxiety indicates the necessity to providing appropriate support for high-risk individuals. Additionally, our results found a significant downward trend in the prevalence of social anxiety, suggesting that the psychological adjustment of college students improves gradually throughout their university stage. In a previous meta-analysis, B. Chen et al. ( 2023 ) summarized the developmental trajectory of social anxiety from toddlerhood to young adulthood, highlighting a gradual decline in the average level of social anxiety during emerging adulthood (ages 18-25). With regard to gender differences, female students reported higher prevalence rates than males, which was in line with previous empirical studies (Asher et al., 2017 ; Caballo et al., 2014 ; MacKenzie & Fowler, 2013 ). The reasons for the higher prevalence rate in females are multifactorial. On the one hand, physiological differences such as genetic vulnerability, hormone and cortisol levels, may manifest in emotional and behavioral variations between females and males (McHenry et al., 2014 ; McLean & Anderson, 2009 ). These physiological differences can result in varying sensitivities towards stressors, making females more vulnerable to psychosocial stressors like interpersonal conflicts and increasing their likelihood of experiencing social anxiety than males. On the other hand, self-construal theory posits that females have stronger interpersonal orientations than males (Cross & Madson, 1997 ). Females are more likely to self-blame in relationship problems and exhibit greater concern about negative evaluations from peers (Rudolph, 2002 ). This emphasis on interpersonal relationships may contribute to females’ heightened susceptibility to social anxiety.
Distinct Developmental Trajectories of Social Anxiety
The present study highlights substantial individual variations in the trajectories of social anxiety among college students. Three distinct trajectories of social anxiety were found, namely, low-decreasing group , high-decreasing group , and high-stable group . First, the predominant pattern observed was low-decreasing group, indicating that the majority of college students possess effective social skills to engage in positive social interactions. Second, about 6.3% of students belonged to the high-decreasing group, showing a recovery pattern characterized by initially high levels of social anxiety that decreased significantly over time. This finding is consistent with a three-wave longitudinal study by Gao et al. ( 2021 ), which also identified a recovery group comprising 7.1% of participants experiencing a decline in anxiety symptoms from freshman to junior year. One possible explanation for this recovery pattern is that transition to college can often be a stressful experience for students, requiring them to navigate a new social environment and rebuild their social networks (Arnett, 2015 ; Zhao et al., 2023 ). These challenges and changes may exacerbate social anxiety. Although these students may initially struggle with adjustment difficulties upon entering university, they may develop increased adaptability, expand their social networks, and undergo psychological growth over time (Gale & Parker, 2014 ). This process can lead to improvements in the quality of interpersonal relationship quality and the acquisition of social support, ultimately resulting in a significant reduction in social anxiety. However, it is noteworthy that about 13.33% of participants displayed chronic social anxiety. Compared to other peers, these students may have higher levels of trait social anxiety. Trait anxiety is a personality trait that demonstrates consistency across different situations (Karasewich & Kuhlmeier, 2020 ). Individuals with trait social anxiety tend to engage in inhibitory and avoidant behaviors during social interactions. Early identification and targeted intervention programs are necessary for supporting these students.
Social Anxiety Trajectories and Subsequent Internet-Related Addictive Behaviors
Although a growing number of studies have explored the relationship between social anxiety and internet-related addictive behaviors (Gioia et al., 2022 ; Ran et al., 2022 ), limited investigations have examined whether distinct profiles or classes of social anxiety are differentially linked to subsequent internet-related addictive behaviors. The current study addressed this gap and revealed that the probability of internet-related addictive behaviors was significantly lower in the low-decreasing group and high-decreasing group compared to those in high-stable group. This suggests that the likelihood of engaging in addictive behaviors also decreases as social anxiety decreases. This result was consistent with our research hypothesis and extended the findings of the literature (Gao et al., 2021 ; Kim et al., 2022 ). For example, Gao et al. ( 2021 ) conducted a three-wave longitudinal survey and found that individuals in the low-stable anxiety group had a significantly lower probability of internet addiction compared to the recovery group and the escalating group.
These results support both the compensatory Internet use model (Kardefelt-Winther, 2014 ) and the cognitive-behavioral model of pathological Internet use (Davis, 2001 ). The Internet can provide comfort and reinforcement for individuals, positively reinforcing peer connections and negative reinforcing the avoidance of negative emotional states (Lavoie et al., 2023 ). On the one hand, college students with social anxiety may perceive the internet as a compensatory mechanism for the lack of social and emotional connections in their offline world. For them, smartphones or online games can serve as essential tools for maintaining current friendships (Valkenburg & Peter, 2007 ) and establishing new connections and social relationships (Marino et al., 2020 ). Research has shown that individuals with higher levels of social anxiety can perceive themselves as more successful through online communication, compared to face-to-face interactions (Shalom et al., 2015 ), all of which make them more susceptible to internet-related addictive behaviors (Sioni et al., 2017 ). On the other hand, internet-related addictive activities may represent coping mechanisms to alleviate symptoms of psychopathology and/or negative emotions (Blasi et al., 2019 ). Social networks and video games can help individuals escape from real-life stressors, problems, and feelings of isolation through online social interactions (Billieux et al., 2013 ). In contrast, individuals with low social anxiety are more inclined to engage in real-life socialization with peers and employ positive coping styles, thus maintaining a low probability of engaging in addictive behavior.
Clinical Implication
Given the heterogeneity of social anxiety, mental health practitioners in school settings should recognize the importance of long-term and continuous monitoring of social anxiety among college students. Early intervention for social anxiety, a modifiable risk factor, shows promise in improving internet-related addictive behaviors of college students. Numerous intervention studies have confirmed that cognitive behavioral therapy (CBT) can be a feasible approach for treating social anxiety (Goldin et al., 2016 ; Heimberg, 2002 ; Rodebaugh et al., 2004 ). CBT involves addressing unhelpful behaviors that contribute to maintaining social anxiety, such as avoidance, safety behaviors, and self-focus. In addition to traditional multi-stage psychotherapy, brief interventions can also serve as valuable alternatives or supplements, particularly in addressing the stigma associated with of mental health interventions and the limited resources available in higher education settings. For instance, a week-long imaginal exposure exercise conducted through a smartphone application has been confirmed effective in managing social anxiety (Schwob & Newman, 2023 ).
Strengths, Limitations, and Further Research
This study has several strengths, including the large sample size, longitudinal design, and utilization of a person-centered approach to investigate the relationship between social anxiety trajectories and internet-related addictive behaviors. However, several limitations should be acknowledged. First, all variables were assessed using self-reported questionnaires rather than clinical diagnosis to facilitate data collection from a large sample size. However, this reliance on self-report measures introduces the potential for response inaccuracies and social desirability bias. To enhance the reliability of future research, it would be advantageous to incorporate multiple assessment methods, such as clinical interviews and behavioral measures. Second, the measurement of IGD was only assessed at T 5 , which means that, IGD at baseline was not controlled for when examining the relationship between social anxiety trajectories and IGD. Cross-lagged panel model (CLPM) or random intercept cross-lagged panel model (RI-CLPM) can be used in future studies to explore the dynamic relationship between social anxiety and online-related addictive behaviors. Third, although the sample was large, the participants were limited to university students, which limited the generalizability of the current findings to other populations. Future research should aim to include more diverse and representative samples to replicate these findings. Finally, our data were collected during the COVID-19 pandemic. The participants were likely to experiencing reduced offline social interactions due to lockdown measures, which could have influenced their levels of social anxiety (Morrissette, 2021 ; Thompson et al., 2021 ).
Three distinct trajectories of social anxiety were found among Chinese college students, including a low-decreasing group (80.37%), a high-decreasing group (13.33%), and a high-stable group (6.30%). Additionally, compared to those in high-stable group, college students in low-decreasing group and high-decreasing group were less likely to develop internet-related addiction. These findings advance our understanding of the relationship between the developmental trajectory of social anxiety and internet-related addictive behaviors.
Data Availability
The dataset used and/or analyzed during the current study is available from the corresponding author on reasonable request.
Aljomaa, S. S., Al Qudah, M. F., Albursan, I. S., Bakhiet, S. F., & Abduljabbar, A. S. (2016). Smartphone addiction among university students in the light of some variables. Computers in Human Behavior, 61 , 155–164. https://doi.org/10.1016/j.chb.2016.03.041
Article Google Scholar
American Psychiatric Association. (2013). Diagnostic and statistical manual of mental disorders: DSM-5 . American Psychiatric Pub.
Book Google Scholar
Angulo, M., Rooks, B., Gill, M., Goldstein, T., Sakolsky, D., Goldstein, B., et al. (2017). Psychometrics of the screen for adult anxiety related disorders (SCAARED)- A new scale for the assessment of DSM-5 anxiety disorders. Psychiatry Research, 253 , 84–90. https://doi.org/10.1016/j.psychres.2017.02.034
Article PubMed PubMed Central Google Scholar
Arnett, J. J. (2015). College students as emerging adults: The developmental implications of the college context. Emerging Adulthood, 4 (3), 219–222. https://doi.org/10.1177/2167696815587422
Arnett, J. J., Žukauskienė, R., & Sugimura, K. (2014). The new life stage of emerging adulthood at ages 18–29 years: Implications for mental health. The Lancet Psychiatry, 1 (7), 569–576. https://doi.org/10.1016/S2215-0366(14)00080-7
Article PubMed Google Scholar
Asher, M., Asnaani, A., & Aderka, I. M. (2017). Gender differences in social anxiety disorder: A review. Clinical Psychology Review, 56 , 1–12. https://doi.org/10.1016/j.cpr.2017.05.004
Baptista, C. A., Loureiro, S. R., de Lima Osório, F., Zuardi, A. W., Magalhães, P. V., Kapczinski, F., et al. (2012). Social phobia in Brazilian university students: Prevalence, under-recognition and academic impairment in women. Journal of Affective Disorders, 136 (3), 857–861. https://doi.org/10.1016/j.jad.2011.09.022
Billieux, J., Van der Linden, M., Achab, S., Khazaal, Y., Paraskevopoulos, L., Zullino, D., & Thorens, G. (2013). Why do you play World of Warcraft? An in-depth exploration of self-reported motivations to play online and in-game behaviours in the virtual world of Azeroth. Computers in Human Behavior, 29 (1), 103–109. https://doi.org/10.1016/j.chb.2012.07.021
Birmaher, B., Brent, D. A., Chiappetta, L., Bridge, J., Monga, S., & Baugher, M. (1999). Psychometric Properties of the Screen for Child Anxiety Related Emotional Disorders (SCARED): A Replication Study. Journal of the American Academy of Child & Adolescent Psychiatry, 38 (10), 1230–1236. https://doi.org/10.1097/00004583-199910000-00011
Article CAS Google Scholar
Blasi, M. D., Giardina, A., Giordano, C., Coco, G. L., Tosto, C., Billieux, J., & Schimmenti, A. (2019). Problematic video game use as an emotional coping strategy: Evidence from a sample of MMORPG gamers. Journal of Behavioral Addictions, 8 (1), 25–34. https://doi.org/10.1556/2006.8.2019.02
Borges, G., Orozco, R., Benjet, C., Martínez Martínez, K. I., Contreras, E. V., Jiménez Pérez, A. L., et al. (2019). DSM-5 Internet gaming disorder among a sample of Mexican first-year college students. Journal of Behavioral Addictions, 8 (4), 714–724. https://doi.org/10.1556/2006.8.2019.62
Branje, S. J. T., Frijns, T., Hale, W. W., Meeus, W. H. J., Nelemans, S. A., Raaijmakers, Q. A. W., & van Lier, P. A. C. (2013). Heterogeneity in development of adolescent anxiety disorder symptoms in an 8-year longitudinal community study. Development and Psychopathology, 26 (1), 181–202. https://doi.org/10.1017/s0954579413000503
Broeren, S., Muris, P., Diamantopoulou, S., & Baker, J. R. (2013). The course of childhood anxiety symptoms: developmental trajectories and child-related factors in normal children. Journal of Abnormal Child Psychology, 41 (1), 81–95. https://doi.org/10.1007/s10802-012-9669-9
Brook, C. A., & Willoughby, T. (2015). The social ties that bind: Social anxiety and academic achievement across the university years. Journal of Youth and Adolescence, 44 (5), 1139–1152. https://doi.org/10.1007/s10964-015-0262-8
Buckner, J. D., Lemke, A. W., Jeffries, E. R., & Shah, S. M. (2017). Social anxiety and suicidal ideation: Test of the utility of the interpersonal-psychological theory of suicide. Journal of Anxiety Disorders, 45 , 60–63. https://doi.org/10.1016/j.janxdis.2016.11.010
Caballo, V. E., Salazar, I. C., Irurtia, M. J., Arias, B., & Hofmann, S. G. (2014). Differences in social anxiety between men and women across 18 countries. Personality and Individual Differences, 64 , 35–40. https://doi.org/10.1016/j.paid.2014.02.013
Chen, B., Huang, X., Niu, G., Sun, X., & Cai, Z. (2023). Developmental change and stability of social anxiety from toddlerhood to young adulthood: A three-level meta-analysis of longitudinal studies. Acta Psychologica Sinica, 55 (10), 1637–1652. https://doi.org/10.3724/sp.j.1041.2023.01637
Chen, X., Chen, J., Shi, X., Jiang, M., Li, Y., Zhou, Y., et al. (2020). Trajectories of maternal symptoms of posttraumatic stress disorder predict long-term mental health of children following the Wenchuan earthquake in China: A 10-year follow-up study. Journal of Affective Disorders, 266 , 201–206. https://doi.org/10.1016/j.jad.2020.01.084
Collins, J.-L., Thompson, K., Sherry, S. B., Glowacka, M., & Stewart, S. H. (2018). Drinking to cope with depression mediates the relationship between social avoidance and alcohol problems: A 3-wave, 18-month longitudinal study. Addictive Behaviors, 76 , 182–187. https://doi.org/10.1016/j.addbeh.2017.08.020
Cross, S. E., & Madson, L. (1997). Models of the self: Self-construals and gender. Psychological Bulletin, 122 (1), 5–37. https://doi.org/10.1037//0033-2909.122.1.5
Article CAS PubMed Google Scholar
Davis, R. A. (2001). A cognitive-behavioral model of pathological Internet use. Computers in Human Behavior, 17 (2), 187–195. https://doi.org/10.1016/s0747-5632(00)00041-8
Elhai, J. D., Tiamiyu, M., & Weeks, J. (2018). Depression and social anxiety in relation to problematic smartphone use. Internet Research, 28 (2), 315–332. https://doi.org/10.1108/IntR-01-2017-0019
Engels, J. M., & Diehr, P. (2003). Imputation of missing longitudinal data: a comparison of methods. Journal of Clinical Epidemiology, 56 (10), 968–976. https://doi.org/10.1016/s0895-4356(03)00170-7
Gale, T., & Parker, S. (2014). Navigating change: a typology of student transition in higher education. Studies in Higher Education, 39 (5), 734–753. https://doi.org/10.1080/03075079.2012.721351
Gao, T., Qin, Z., Hu, Y., Fei, J., Cao, R., Liang, L., et al. (2021). Trajectories of depression and anxiety in Chinese high school freshmen: Associations with Internet addiction. Journal of Affective Disorders, 286 , 180–186. https://doi.org/10.1016/j.jad.2021.02.074
Gautreau, C. M., Sherry, S. B., Mushquash, A. R., & Stewart, S. H. (2015). Is self-critical perfectionism an antecedent of or a consequence of social anxiety, or both? A 12-month, three-wave longitudinal study. Personality and Individual Differences, 82 , 125–130. https://doi.org/10.1016/j.paid.2015.03.005
Giletta, M., Prinstein, M. J., Abela, J. R. Z., Gibb, B. E., Barrocas, A. L., & Hankin, B. L. (2015). Trajectories of suicide ideation and nonsuicidal self-injury among adolescents in mainland China: Peer predictors, joint development, and risk for suicide attempts. Journal of Consulting and Clinical Psychology, 83 (2), 265–279. https://doi.org/10.1037/a0038652
Gioia, F., Colella, G. M., & Boursier, V. (2022). Evidence on problematic online gaming and social anxiety over the past ten years: A systematic literature review. Current Addiction Reports, 9 (1), 32–47. https://doi.org/10.1007/s40429-021-00406-3
Goldin, P. R., Morrison, A., Jazaieri, H., Brozovich, F., Heimberg, R., & Gross, J. J. (2016). Group CBT versus MBSR for social anxiety disorder: A randomized controlled trial. Journal of Consulting and Clinical Psychology, 84 (5), 427–437. https://doi.org/10.1037/ccp0000092
Heimberg, R. G. (2002). Cognitive-behavioral therapy for social anxiety disorder: current status and future directions. Biological Psychiatry, 51 (1), 101–108. https://doi.org/10.1016/s0006-3223(01)01183-0
Heinrichs, N., Rapee, R. M., Alden, L. A., Bögels, S., Hofmann, S. G., Ja, O., & Sakano, Y. (2006). Cultural differences in perceived social norms and social anxiety. Behaviour Research and Therapy, 44 (8), 1187–1197.
Iancu, I., Bodner, E., & Ben-Zion, I. Z. (2015). Self esteem, dependency, self-efficacy and self-criticism in social anxiety disorder. Comprehensive Psychiatry, 58 , 165–171. https://doi.org/10.1016/j.comppsych.2014.11.018
Jung, T., & Wickrama, K. A. S. (2008). An introduction to latent class growth analysis and growth mixture modeling. Social and Personality Psychology Compass, 2 (1), 302–317. https://doi.org/10.1111/j.1751-9004.2007.00054.x
Karasewich, T. A., & Kuhlmeier, V. A. (2020). Trait social anxiety as a conditional adaptation: A developmental and evolutionary framework. Developmental Review, 55 , 100886. https://doi.org/10.1016/j.dr.2019.100886
Kardefelt-Winther, D. (2014). A conceptual and methodological critique of internet addiction research: Towards a model of compensatory internet use. Computers in Human Behavior, 31 , 351–354. https://doi.org/10.1016/j.chb.2013.10.059
Kim, S., Champion, K. E., Gardner, L. A., Teesson, M., Newton, N. C., & Gainsbury, S. M. (2022). The directionality of anxiety and gaming disorder: An exploratory analysis of longitudinal data from an Australian youth population. Frontiers in Psychiatry, 13 , 1043490. https://doi.org/10.3389/fpsyt.2022.1043490
Krygsman, A., & Vaillancourt, T. (2022). Elevated social anxiety symptoms across childhood and adolescence predict adult mental disorders and cannabis use. Comprehensive Psychiatry, 115 , 152302. https://doi.org/10.1016/j.comppsych.2022.152302
Kwon, M., Kim, D., Cho, H., & Yang, S. (2013). The smartphone addiction scale: development and validation of a short version for adolescents. PloS one, 8 (12), e83558. https://doi.org/10.1371/journal.pone.0083558
Article CAS PubMed PubMed Central Google Scholar
Lavoie, C., Dufour, M., Berbiche, D., Therriault, D., & Lane, J. (2023). The relationship between problematic internet use and anxiety disorder symptoms in youth: Specificity of the type of application and gender. Computers in Human Behavior, 140 , 107604. https://doi.org/10.1016/j.chb.2022.107604
Leung, H., Pakpour, A. H., Strong, C., Lin, Y.-C., Tsai, M.-C., Griffiths, M. D., et al. (2020). Measurement invariance across young adults from Hong Kong and Taiwan among three internet-related addiction scales: Bergen Social Media Addiction Scale (BSMAS), Smartphone Application-Based Addiction Scale (SABAS), and Internet Gaming Disorder Scale-Short Form (IGDS-SF9) (Study Part A). Addictive Behaviors, 101 , 105969. https://doi.org/10.1016/j.addbeh.2019.04.027
Levinson, C. A., Kaplan, S. C., & Rodebaugh, T. L. (2014). Personality: Understanding the socially anxious temperament . In The Wiley Blackwell Handbook of Social Anxiety Disorder (pp. 111-132)
Luk, T. T., Wang, M. P., Shen, C., Wan, A., Chau, P. H., Oliffe, J., et al. (2018). Short version of the Smartphone Addiction Scale in Chinese adults: Psychometric properties, sociodemographic, and health behavioral correlates. Journal of Behavioral Addictions, 7 (4), 1157–1165. https://doi.org/10.1556/2006.7.2018.105
MacKenzie, M. B., & Fowler, K. F. (2013). Social anxiety disorder in the Canadian population: Exploring gender differences in sociodemographic profile. Journal of Anxiety Disorders, 27 (4), 427–434. https://doi.org/10.1016/j.janxdis.2013.05.006
Marino, C., Canale, N., Vieno, A., Caselli, G., Scacchi, L., & Spada, M. M. (2020). Social anxiety and Internet gaming disorder: The role of motives and metacognitions. Journal of Behavioral Addictions, 9 (3), 617–628. https://doi.org/10.1556/2006.2020.00044
Marmorstein, N. R., White, H., Chung, T., Hipwell, A., Stouthamer-Loeber, M., & Loeber, R. (2010). Associations between first use of substances and change in internalizing symptoms among girls: Differences by symptom trajectory and substance use type. Journal of Clinical Child & Adolescent Psychology, 39 (4), 545–558. https://doi.org/10.1080/15374416.2010.486325
McHenry, J., Carrier, N., Hull, E., & Kabbaj, M. (2014). Sex differences in anxiety and depression: Role of testosterone. Frontiers in Neuroendocrinology, 35 (1), 42–57. https://doi.org/10.1016/j.yfrne.2013.09.001
McLean, C. P., & Anderson, E. R. (2009). Brave men and timid women? A review of the gender differences in fear and anxiety. Clinical Psychology Review, 29 (6), 496–505. https://doi.org/10.1016/j.cpr.2009.05.003
Melkam, M., Segon, T., & Nakie, G. (2023). Social phobia of Ethiopian students: meta-analysis and systematic review. Systematic Reviews, 12 (1), 1–10. https://doi.org/10.1186/s13643-023-02208-2
Meng, T., He, Y., Zhang, Q., Yu, F., Zhao, L., Zhang, S., et al. (2021). Analysis of features of social anxiety and exploring the relationship between childhood major adverse experiences and social anxiety in early adulthood among Chinese college students. J Affect Disord, 292 , 614–622. https://doi.org/10.1016/j.jad.2021.05.105
Miers, A. C., Blöte, A. W., de Rooij, M., Bokhorst, C. L., & Westenberg, P. M. (2013). Trajectories of social anxiety during adolescence and relations with cognition, social competence, and temperament. Journal of Abnormal Child Psychology, 41 (1), 97–110. https://doi.org/10.1007/s10802-012-9651-6
Mo, W., & Bodner, T. E. (2007). Growth mixture modeling: Identifying and predicting unobserved subpopulations with longitudinal data. Organizational Research Methods, 10 (4), 635–656. https://doi.org/10.1177/1094428106289397
Monacis, L., Palo, V., & d., Griffiths, M. D., & Sinatra, M. (2016). Validation of the Internet Gaming Disorder Scale – Short-Form (IGDS9-SF) in an Italian-speaking sample. Journal of Behavioral Addictions, 5 (4), 683–690. https://doi.org/10.1556/2006.5.2016.083
Morrison, A. S., & Heimberg, R. G. (2013). Social anxiety and social anxiety disorder. Annual Review of Clinical Psychology, 9 (1), 249–274. https://doi.org/10.1146/annurev-clinpsy-050212-185631
Morrissette, M. (2021). School closures and social anxiety during the COVID-19 pandemic. Journal of the American Academy of Child & Adolescent Psychiatry, 60 (1), 6–7. https://doi.org/10.1016/j.jaac.2020.08.436
Nagin, D. S., & Odgers, C. L. (2010). Group-based trajectory modeling in clinical research. Annual Review of Clinical Psychology, 6 , 109–138. https://doi.org/10.1146/annurev.clinpsy.121208.131413
Nylund, K. L., Asparouhov, T., & Muthén, B. O. (2007). Deciding on the number of classes in latent class analysis and growth mixture modeling: A Monte Carlo simulation study. Structural Equation Modeling, 14 (4), 535–569. https://doi.org/10.1080/10705510701575396
Peng, M. M., & Liang, Z. (2023). Longitudinal associations between multiple types of adverse childhood experiences and depression trajectories in middle-aged and older Chinese adults: A growth mixture model. International Journal of Mental Health and Addiction . https://doi.org/10.1007/s11469-023-01188-7
Pontes, H. M., & Griffiths, M. D. (2015). Measuring DSM-5 internet gaming disorder: Development and validation of a short psychometric scale. Computers in Human Behavior, 45 , 137–143. https://doi.org/10.1016/j.chb.2014.12.006
Poon, L. Y. J., Tsang, H. W. H., Chan, T. Y. J., Man, S. W. T., Ng, L. Y., Wong, Y. L. E., et al. (2021). Psychometric properties of the Internet Gaming Disorder Scale–Short-Form (IGDS9-SF): Systematic review. Journal of Medical Internet Research, 23 (10), e26821. https://doi.org/10.2196/26821
Przepiorka, A., Błachnio, A., Cudo, A., & Kot, P. (2021). Social anxiety and social skills via problematic smartphone use for predicting somatic symptoms and academic performance at primary school. Computers & Education, 173 , 104286. https://doi.org/10.1016/j.compedu.2021.104286
Ran, G., Li, J., Zhang, Q., & Niu, X. (2022). The association between social anxiety and mobile phone addiction: A three-level meta-analysis. Computers in Human Behavior, 130 , 107198. https://doi.org/10.1016/j.chb.2022.107198
Reta, Y., Ayalew, M., Yeneabat, T., & Bedaso, A. (2020). Social anxiety disorder among undergraduate students of Hawassa university, college of medicine and health sciences, Ethiopia. Neuropsychiatric Disease and Treatment, 16 , 571–577. https://doi.org/10.2147/NDT.S235416
Rodebaugh, T. L., Holaway, R. M., & Heimberg, R. G. (2004). The treatment of social anxiety disorder. Clinical Psychology Review, 24 (7), 883–908. https://doi.org/10.1016/j.cpr.2004.07.007
Rudolph, K. D. (2002). Gender differences in emotional responses to interpersonal stress during adolescence. Journal of Adolescent Health, 30 (4), 3–13. https://doi.org/10.1016/s1054-139x(01)00383-4
Schry, A. R., Maddox, B. B., & White, S. W. (2016). Social anxiety and alcohol-related sexual victimization: A longitudinal pilot study of college women. Addictive Behaviors, 61 , 117–120. https://doi.org/10.1016/j.addbeh.2016.05.022
Schry, A. R., & White, S. W. (2013). Understanding the relationship between social anxiety and alcohol use in college students: A meta-analysis. Addictive Behaviors, 38 (11), 2690–2706. https://doi.org/10.1016/j.addbeh.2013.06.014
Schwob, J. T., & Newman, M. G. (2023). Brief imaginal exposure exercises for social anxiety disorder: A randomized controlled trial of a self-help momentary intervention app. Journal of Anxiety Disorders, 98 , 102749. https://doi.org/10.1016/j.janxdis.2023.102749
Shalom, J. G., Israeli, H., Markovitzky, O., & Lipsitz, J. D. (2015). Social anxiety and physiological arousal during computer mediated vs. face to face communication. Computers in Human Behavior, 44 , 202–208. https://doi.org/10.1016/j.chb.2014.11.056
Sioni, S. R., Burleson, M. H., & Bekerian, D. A. (2017). Internet gaming disorder: Social phobia and identifying with your virtual self. Computers in Human Behavior, 71 , 11–15. https://doi.org/10.1016/j.chb.2017.01.044
Stănculescu, E., & Griffiths, M. D. (2022). Social media addiction profiles and their antecedents using latent profile analysis: The contribution of social anxiety, gender, and age. Telematics and Informatics, 74 , 101879. https://doi.org/10.1016/j.tele.2022.101879
Stein, M. B., Fuetsch, M., Müller, N., Höfler, M., Lieb, R., & Wittchen, H.-U. (2001). Social anxiety disorder and the risk of depression: A prospective community study of adolescents and young adults. Archives of General Psychiatry, 58 (3), 251–256. https://doi.org/10.1001/archpsyc.58.3.251
Thompson, C., Mancebo, M. C., & Moitra, E. (2021). Changes in social anxiety symptoms and loneliness after increased isolation during the COVID-19 pandemic. Psychiatry Research, 298 , 113834. https://doi.org/10.1016/j.psychres.2021.113834
Valkenburg, P. M., & Peter, J. (2007). Online communication and adolescent well-being: Testing the stimulation versus the displacement hypothesis. Journal of Computer-Mediated Communication, 12 (4), 1169–1182. https://doi.org/10.1111/j.1083-6101.2007.00368.x
Wang, S., Shi, X., Zhu, Y., & Wang, Z. (2022). Factor structure of the Chinese version of the Screen for Adult Anxiety Related Disorders (SCARRED). Asian Journal of Psychiatry, 73 , 103159. https://doi.org/10.1016/j.ajp.2022.103159
Weems, C. F. (2008). Developmental trajectories of childhood anxiety: Identifying continuity and change in anxious emotion. Developmental Review, 28 (4), 488–502. https://doi.org/10.1016/j.dr.2008.01.001
Zhao, S., Zhang, Y., Yu, C., Zhang, H., Xie, M., Chen, P., & Lin, D. (2023). Trajectories of perceived stress among students in transition to college: Mindset antecedents and adjustment outcomes. Journal of Youth and Adolescence, 52 (9), 1873–1886. https://doi.org/10.1007/s10964-023-01788-5
Download references
Acknowledgements
The authors would like to thank all participants for their contribution to this study.
This study was supported from Education General Project of National Social Science Foundation of China in 2023 “Continuous monitoring of adolescents’ psychological adjustment after chronic stressful events and its intervention mechanisms” (Grant No. BBA230062).
Author information
Authors and affiliations.
College of Education, Hebei University, Baoding, China
Xiaomeng Li & Xuliang Shi
Department of Applied Social Sciences, The Hong Kong Polytechnic University, Hung Hom, Hong Kong
Xiaoyan Chen
Center for Mental Health Education and Counseling, Guangdong University of Science and Technology, Dongguan, China
You can also search for this author in PubMed Google Scholar
Corresponding author
Correspondence to Xuliang Shi .
Ethics declarations
Ethics approval.
The present study protocol was reviewed and approved by the Research Ethics Committee of the corresponding author’s institution. All participants provided informed consents before completing the questionnaires, and were paid after completing the whole questionnaires.
Conflict of Interest
The authors declare that they have no known competing financial interests or personal relationships that could have appeared to influence the work reported in this paper.
Additional information
Publisher’s note.
Springer Nature remains neutral with regard to jurisdictional claims in published maps and institutional affiliations.
Supplementary Information
(DOCX 16 kb)
Rights and permissions
Springer Nature or its licensor (e.g. a society or other partner) holds exclusive rights to this article under a publishing agreement with the author(s) or other rightsholder(s); author self-archiving of the accepted manuscript version of this article is solely governed by the terms of such publishing agreement and applicable law.
Reprints and permissions
About this article
Li, X., Chen, X., Zhu, Y. et al. Longitudinal Associations of Social Anxiety Trajectories with Internet-Related Addictive Behaviors Among College Students: A Five-Wave Survey Study. Int J Ment Health Addiction (2024). https://doi.org/10.1007/s11469-024-01300-5
Download citation
Accepted : 08 April 2024
Published : 12 April 2024
DOI : https://doi.org/10.1007/s11469-024-01300-5
Share this article
Anyone you share the following link with will be able to read this content:
Sorry, a shareable link is not currently available for this article.
Provided by the Springer Nature SharedIt content-sharing initiative
- Social anxiety
- Trajectories
- Addictive behaviors
- Longitudinal cohort
- College students
- Find a journal
- Publish with us
- Track your research

An official website of the United States government
The .gov means it’s official. Federal government websites often end in .gov or .mil. Before sharing sensitive information, make sure you’re on a federal government site.
The site is secure. The https:// ensures that you are connecting to the official website and that any information you provide is encrypted and transmitted securely.
- Publications
- Account settings
Preview improvements coming to the PMC website in October 2024. Learn More or Try it out now .
- Advanced Search
- Journal List
- ScientificWorldJournal
- v.2021; 2021

Internet Addiction Effect on Quality of Life: A Systematic Review and Meta-Analysis
Farzaneh noroozi.
1 Department of Health Promotion, School of Health, Shiraz University of Medical Sciences, Shiraz, Iran
Soheil Hassanipour
2 Cardiovascular Diseases Research Center, Department of Cardiology, Heshmat Hospital, School of Medicine, Guilan University of Medical Sciences, Rasht, Iran
Fatemeh Eftekharian
3 Institute of Health, Shiraz University of Medical Sciences, Shiraz, Iran
Kumars Eisapareh
Mohammad hossein kaveh.
4 Research Center for Health Sciences, Institute of Health, Department of Health Promotion, School of Health, Shiraz University of Medical Sciences, Shiraz, Iran
Associated Data
The data used to support the study are available from the corresponding author upon request.
Due to the use of different methodologies, tools, and measurements, the positive or negative impact of Internet use on human life quality is accompanied by a series of ambiguities and uncertainties. Therefore, in this study, a systematic review and meta-analysis are conducted regarding the effect of Internet addiction on the quality of life.
A systematic search of resources was conducted to investigate the effect of Internet addiction on the quality of life. The databases of PubMed, Cochrane Library, Scopus, Web of Science, Embase, and Science Direct were searched from January 1980 to July 2020. The articles were screened by two researchers in multiple levels in terms of the title, abstract, and full-text; then, final studies that met the inclusion criteria were retrieved and included in the study.
After searching the previously mentioned international databases, 3863 papers were found, 18 of which we included in the final analysis. Surveys indicated that people who had a high Internet addiction received lower scores of quality of life than those who were normal Internet users (OR = 2.45, 95% CI; 2.31–2.61, p < 0.001; I 2 = 85.23%, p < 0.001). Furthermore, There was a negative significant relationship between Internet addiction and quality of life in the psychological (OR = 0.56, 95% CI: 0.32–0.99, p =0.04, I 2 = 97.47%, p < 0.001), physical (OR = 0.58, 95% CI: 0.39–0.86, p =0.007, I 2 = 95.29%, p =0.001), and overall quality of life score (OR = 0.39, 95% CI: 0.27–0.55, p < 0.001, I 2 = 0.0%, p =0.746).
These findings illustrate that Internet addiction should be regarded as a major health concern and incorporated into health education and intervention initiatives.
1. Introduction
Among the different media types, the Internet is a recent achievement of mankind, a highly reachable global medium with an advanced modern communication technology capable of providing access to a wide range of information sources [ 1 , 2 ].
Although the Internet and its technologies have provided valuable opportunities in scientific, communicative, and economic aspects for human societies, its inappropriate and extreme application, mostly for recreational purposes, is a serious threat to the health and well-being of the human population, especially young people [ 3 ]. According to studies, the increasing demand for Internet technology is associated with major health, psychological, and social problems, overwhelming mental health, interactions, and communications. Researchers also believe that excessive use of the Internet and social networks can indicate stress, anxiety, and depression; indeed, the excessive use of these networks is a way to reduce negative emotions [ 4 ].
The Internet affects various dimensions of lifestyle, social interaction, and occupational performance in both positive and negative ways. As to its positive effects, people can solve most of their daily problems via the Internet. In terms of developing interpersonal relationships, it goes beyond the geographical boundaries. Further, it has become an important part of everyday lives by helping exchange information and personal or professional experience, carry out economic/commercial activities, reduce transportation costs and problems, and develop business and marketing activities [ 5 ].
Negative effects have also been reported as real physical communications are decreasing compared with online communications due to the power of new technologies in the development and transformation of social communications, leading to weaker social relationships in the real world [ 6 ]. Overall, the Internet and social networks are not only changing human relationships and interactive patterns but also create intense interactions and influence individual life [ 7 ].
Internet addiction (IA) is an extreme form of this phenomenon, an inability to avoid using the Internet that has adverse effects on various life aspects (e.g., interpersonal relationships and physical health) [ 8 ]. It is considered a disorder in the Diagnostic and Statistical Manual of Mental Disorders, 5th Edition (DSM-5) [ 8 , 9 ]. Estimation of the IA prevalence varies widely across countries (1.5% to 10.8%) [ 4 , 10 , 11 ]. Based on a meta-analysis result, its prevalence is 6% in 31 countries; the highest prevalence is 10.9% in the Middle East, and the lowest rate of 2.6% belongs to the north and west of Europe [ 8 ].
The studies of the IA show the reduction of life satisfaction in terms of family, friends, school, and living environment. References [ 12 – 14 ] have shown negative effects of IA on physical health aspects. As reported, the use of social networks causes insomnia, physical inactivity, and eye problems, as well as depression, social phobia, and hyperactivity disorders, in most users [ 12 , 15 ]. Based on a meta-analysis by Ho et al., IA is significantly associated with alcohol abuse, attention deficit, hyperactivity, depression, and anxiety [ 16 ].
As mentioned, the Internet has a great impact on various aspects of health. According to the World Health Organization (WHO), health is defined as a complete state of physical, mental, and social well-being [ 17 ]. Quality of life (QOL) is a comprehensive measure of health outcomes [ 18 ]. It is a multidimensional concept that includes understanding mental and objective conditions of individuals' life in their sociocultural and economic environment [ 19 ]. The effect of excessive Internet use on health can be found by examining the impact of IA on QOL.
Several studies have indicated that IA reduces QOL [ 20 ]. On the contrary, some other studies have shown an insignificant association between the use of the Internet and social networks with QOL [ 21 , 22 ]. For example, Ko et al. claim no relationship between QOL and moderate or intense Internet use [ 23 ]. A review study by Veenhoven, who examined IA and its derivatives, showed that the Internet increases QOL, and if the IA really exists, it can affect a relatively small percentage of an online population [ 24 ]. According to another review study, there is a positive relationship between using the Internet and computer and the QOL of the elderly [ 25 ]. Tran et al. have shown that the Internet can help people obtain a higher perceived QOL by promoting their work, education, and communication [ 26 ]. A cross-sectional study of college students found that the quality of life in daily users of social networking sites was higher than that of nondaily users [ 27 ].
On the other hand, a more in-depth study on types of applied programs on the Internet by individuals indicates the impact of a particular program on the individuals' mental well-being. In other words, spending time on programs involved with photo and video sharing is associated with higher levels of depression and anxiety; in contrast, using programs involved with book reading reduces depression and anxiety, thus increasing levels of mental well-being [ 28 ]. Researchers have also reported that people who spend much time online have lower perceived QOL due to the lack of long-term sleep, deteriorated physical health, difficulties in concentrating on work, and reduced intimacy with family members [ 29 , 30 ].
The association between the Internet and the quality of human life is accompanied by a series of ambiguities and uncertainties due to the wide range of its potential positive and negative effects. Possible reasons may be different methodologies and tools, leading to differences in the measurement of Internet use rates. The selection of a specific and agreed form of inappropriate use, namely, the IA, as an independent variable and the definition and measurement based on well-known tools and standards of QOL as a consequence can probably result in precise findings on their relationship. According to the previously mentioned considerations, a systematic review and meta-analysis are conducted on the impact of IA on QOL.
2. Materials and Methods
The present study was a systematic review and meta-analysis. A systematic search of resources was conducted by a librarian (L.E) to investigate whether IA affects the QOL (condition) of people (population) across the world (context).
The research method was based on the PRISMA checklist [ 31 ].
2.1. Data Sources and Search Strategy
The Web of Science, Scopus, Cochrane Library, Embase, Science Direct, and PubMed databases from Jan 1980 to Jul 2020 were searched to find English articles. Also, SID and Magiran databases were searched for Persian studies. The grey literature and ongoing studies were searched in OpenGrey and Google Scholar; further, ProQuest was searched for thesis, dissertations, and studies presented at conferences.
The search was performed using MESH and free keywords. The keywords selected for the search were “Internet addiction” and “quality of life.” After determining relevant keywords, searches were done on databases using associated keywords with “AND” and “OR” operators combined together to determine relevant terms and synonyms. Search strategy included the following keywords: “compulsive Internet,” “computer addict,” “cyber addict,” “excessive Internet use,” “Internet addict,” “Internet dependent,” “Internet disorder,” “Net addict,” “online addict,” “quality of life,” “life quality,” and “health related quality of life.” The PubMed advanced mesh search features used for example were: (((((((((((((“quality of life” [MeSH Terms]) OR (“value of life” [Title/Abstract])) AND (impact [Title/Abstract])) AND (“Internet addiction” [Title/Abstract])) OR (“problematic Internet use” [Title/Abstract]) OR (“online gaming addiction” [Title/Abstract])) OR (“game addiction” [Title/Abstract])) OR (“excessive Internet use” [Title/Abstract])) OR (“social media addiction” [Title/Abstract])) OR (“Internet dependency” [Title/Abstract])) OR (“pathological Internet use” [Title/Abstract])) OR (“computer addiction” [Title/Abstract])) OR (“social networking addiction” [Title/Abstract])) OR (“pornography addiction” [Title/Abstract])). The complete search strategy of other databases is in Supplementary File 1 .
The collected information entered EndNote, X7 (Thomson Reuters, Carlsbad, CA, USA), and duplicate papers were automatically deleted.
All cross-sectional, case-control, and cohort studies that examined the relationship between IA and QOL were searched.
2.2. Inclusion Criteria
- The study type had to be observational (cross-sectional, case-control, and cohort).
- The study was required to investigate the relation between IA and quality of life.
- The correlation level ( r ) between IA and quality of life had to be presented, or information based on which the correlation could be computed was required to be given.
- Papers had to be in English (due to the lack of translators for other languages) and Persian.
2.3. Exclusion Criteria
- The authors did not provide further information upon request, including the correlation level ( r ) between IA and QOL.
- Articles that had full texts written in non-English or non-Persian in spite of having abstracts in English or Persian were excluded.
- The study type was nonobservational (qualitative and interventional studies).
2.4. Study Selection
The selected articles were screened in multiple levels based on the title, abstract, and full-text; then, final studies that met the inclusion criteria were retrieved and included in the study. The initial search was conducted by two people. If there was unmatching between them, the team's supervisor (corresponding author) announced the final comment on that paper.
2.5. Articles' Quality Assessment
The STROBE checklist was used to check and control the quality of papers. This tool consists of 22 questions classified into “yes, no, and unclear.” It aims to assess the methodological quality of studies and strategies to identify bias in designs, implementations, and analyses in studies. During the evaluation process, papers with less than 50% of the inclusion criteria were excluded from the study [ 32 ].
2.6. Data Extraction and Quality Assessment
The information extracted from the articles was entered in the extraction form. Extracted data included: first author, year of publication, study name, country of study, sample size, sample characterization, age mean (SD), and study instrument.
2.7. Statistical Analysis
The heterogeneity between studies was examined by Cochran's test (with a significant level less than 0.1) and its composition using I 2 statistics (with a value greater than 50%). A random-effect model was used in the presence of heterogeneity, while a fixed-effect model was used in its absence. The odds ratio (OR) index, obtained from the comprehensive meta-analysis (CMA) software was used for comparing meta-analysis results. All analyses were done using the statistical CMA 2 software.
3.1. Search Results
Studies were reviewed and selected in three stages. At the first stage, 3863 papers from bases using keywords were retrieved and transferred to the reference management software (Endnote). Titles of papers were reviewed, and 1651 repetitive and 2178 irrelevant papers (to the main subject of research) were deleted. At the second stage, 34 papers associated with the main purpose of the project were selected by studying 2212 abstracts of the remaining papers. At the third stage, 14 studies were included in the final review by investigating the full text of 34 papers and considering inclusion criteria. The papers excluded at this stage were those with English abstract but non-English full text (two articles) and qualitative and interventional methodologies (9 articles), and not receiving the correlation level ( r ) between IA and QOL after communicating with authors (five articles). Finally, the results were evaluated using 18 papers eligible for inclusion in the study. Figure 1 shows the process of retrieving and selecting articles.

Flowchart of the included studies in systematic review.
3.2. Articles' Quality Assessment
All studies met more than 50% of the inclusion criteria (medium or high quality) and no studies were excluded during the evaluation process.
3.3. Characteristics of Included Studies
Table 1 presents the specifications of the articles investigated [ 33 ].
Data extraction results from studies.
3.4. Statistical Analysis
The meta-analysis results were divided into several sections in the present study: first, the comparison of QOL of ordinary people with IA people based on overall scores of QOL and each of its dimensions; second, the analysis of the relationship between the severity of IA and QOL based on r index and calculated OR index.
Due to the high heterogeneity of the analysis, the relationship between the severity of Internet addiction and each dimension of the quality of life, a power analysis was performed. The high power of the analysis for each dimension of the quality of life showed that the results of the study were not affected by heterogeneity.
3.5. Comparing the Quality of Life of Ordinary People with Internet Addicts
Four studies examined the overall scores of both groups. Based on the results, people with a high IA (779 participants) received lower scores of QOL than those with normal Internet use (2589 participants) (95% CI: 2.31–2.61; I 2 = 85.23%, p < 0.001).
Four studies examined other QOL dimensions. Based on the obtained results, people with severe IA received lower QOL scores than those with normal Internet use in terms of the environmental (95% CI: 1.65–2.08; I 2 = 22.45%, p =0.276), physical (95% CI: 2.44–2.93; I 2 = 0.0%, p =0.962), psychological (95% CI: 2.71–3.57; I 2 = 38.32%, p =0.182), and social dimensions (95% CI: 1.63–2.95; I 2 = 86.31%, p < 0.001). Figure 2 shows results of the Forest plot for comparison of the QOL of ordinary people with IA ( Figure 2 ).

Comparing the quality of life of ordinary people with that of internet addicts.
3.6. The Relationship between the Severity of Internet Addiction and Quality of Life
The research results indicated that IA is associated with a decrease in QOL. There was a negative significant relationship between the severity of IA and QOL in psychological (95% CI: 0.32–0.99; I 2 = 97.47%, p < 0.001) ( Figure 3 ), physical (95% CI: 0.39–0.86; I 2 = 95.29%, p =0.001) ( Figure 4 ), and overall QOL (95% CI: 0.27–0.55; I 2 = 92.7%, p < 0.001) ( Figure 5 ); however, no statistical significant reduction was observed in environmental (95% CI: 0.50–1.06; I 2 = 93.89%, p < 0.001) ( Figure 6 ) and social dimension (95% CI: 0.45–1.24; I 2 = 96.63%, p < 0.001) ( Figure 7 ).

The relationship between the severity of internet addiction and the quality of life in the psychological dimension.

The relationship between the severity of internet addiction and the quality of life in the physical dimension.

The relationship between the severity of internet addiction and the overall quality of life score.

The relationship between the severity of internet addiction and the quality of life in the environmental dimension.

The relationship between the severity of internet addiction and the quality of life in the social dimension.
Based on the results of the Egger ( p =(0/601)) and Begg test ( p =(0/945)), no publication bias was observed among studies due to the symmetry of the funnel plot ( Figure 8 ).

Funnel plot for assessing possible publication bias.
4. Discussion
Despite the increasing volume of research on the relationship between IA and QOL, no systematic review or meta-analysis has been conducted to summarize the findings to the best of the authors' knowledge. More specifically, the first study assessing IA and QOL has been published in 2013 [ 34 ]. Accordingly, the association between IA and sleep has been studied over the last seven years, and the cumulative evidence requires to be summarized. The present review is the first meta-analysis that uses empirical evidence from the past seven years to understand the association between IA and QOL. By a rigorous selection method using PRISMA guidelines, 18 studies with 11,097 participants were included in the present meta-analysis.
The high power of analysis for each dimension of quality of life showed that the results of the study were not affected by heterogeneity; one of the reasons could be the high number of samples in the study.
Meta-analysis results show differences in QOL based on Internet usage. As the results of four studies show that people with a high IA receive lower scores of QOL than those with normal Internet use (OR: 2.45, p < 0.001). This result was consistent with those of other studies in the field [ 35 – 38 ]; these results suggest that, in comparative studies, even after controlling some background variables affecting QOL, there are still significant independent correlations between IA and all aspects of QOL.
According to the obtained results of the meta-analysis (11studies), IA is associated with a decline in overall QOL (OR: 0.39; p < 0.001). This result, except in one [ 39 ] case, is consistent with the results of other studies included in the analysis [ 36 , 40 – 48 ]. In these studies, an Indian study had the smallest sample size which was 60 [ 39 ], and a Filipino study had the largest sample size, which was 1447 [ 40 ]. The studies are also conducted across 11 countries mostly located in Asia ( n = 11), followed by Europe ( n = 5) and the USA ( n = 2). Although the meta-analysis results in the present review are primarily derived from Asian populations, based on the Egger ( t : 0.539, p : 0.601) and Begg test ( z : 0.137, p : 0.190), no publication bias is observed among them. Additionally, with moderate- and high-quality studies using the STROBE checklist and standard measurement tools, the methodological concerns might have minimal impacts on the present findings.
The research results indicate a significant negative relationship between IA and QOL in the psychological (OR = 0.56, p =0.04) and physical dimensions (OR = 0.58, p =0.007). Different and sometimes contradictory results are reported in studies on the impact of IA on the QOL dimensions. For instance, in two studies by Solati et al. in Iran [ 44 ] and Kelley and Gruber in USA [ 34 ], IA decreased the QOL physical effect. Further, in a study by Lu et al. in China, IA reduced QOL in terms of physical, psychological, and environmental aspects [ 35 ]. Fatehi et al. [ 36 ] in Iran showed that IA decreased the QOL in physical, psychological, and social dimensions [ 36 ]. The results of three studies in Taiwan [ 37 ], China [ 49 ], and the USA [ 38 ] indicated that IA decreased the QOL in physical, social, psychological, and environmental aspects. In addition to a small number of cross-sectional studies, which make the comparison and deduction of causal relationships difficult, differences in contexts and ignorance of the underlying factors affecting the QOL dimensions (such as unemployment, chronic diseases, mental/psychological disorders (depression, negative feelings, and stress)) can be considered as reasons for the contradiction between results on the Internet impact on QOL dimensions [ 45 , 50 , 51 ].
On the other hand, a study conducted in Taiwan shows three specific IA manifestations (compulsive, interpersonal, health, and time management problems) to reduce the physical dimensions of QOL among college students. A possible explanation is that participants with higher compulsivity may have impaired control over Internet use, thereby developing the other two types of IA problems manifested through unhealthy lifestyles, such as poor diet and sleep deprivation, leading to lower physical QOL. Also, compulsivity concerning Internet use may cause poor mental health (depression, loneliness, anxiety, and stress), harming psychological HRQOL [ 37 ].
A longitudinal study in Hong Kong show that time management problem (staying online longer than originally intended) is considered the most common among the participants during the study period [ 52 ]. Such findings show the need for the implementation of IA intervention programs (time management, self-regulation, and self-efficacy) to prevent the deterioration of IA-related physical HRQOL.
4.1. Strengths and Limitations
Despite the increasing influence of the Internet in daily life, in the last eight years, no meta-analysis study has been conducted to investigate the effect of IA on QOL, and this study is the first study in this period.
The quality of the studies has been determined according to the information in the articles, and it is possible that the studies were of higher quality but did not provide all the information and as a result were in the group of medium-quality articles.
The study protocol was not registered before the start for this review and is considered as one of the limitations of the study because there is a concern that it may add to the possible bias over time.
5. Conclusion
According to the present review results, the Internet negatively affects overall QOL, physically and psychologically. Since the Internet meets the needs of information, entertainment, and social interactions, its use is an integral part of everyday human life (both work and leisure). Internet use can also trigger a compulsive need in a minority of individuals. These findings show that IA should be regarded as a major health concern and incorporated into health education and intervention initiatives. Also, further studies are suggested, in particular with a cohort and empirical design in different societies, using standardized methodologies and analytical reports that facilitate the comparison.
Acknowledgments
The authors acknowledge all the participants who were involved directly and indirectly in the study and provided professional, technical, and nontechnical support.
Abbreviations
Data availability, conflicts of interest.
There are no conflicts of interest regarding the publication of this study.
Supplementary Materials
The complete search strategy of other databases is provided as Supplementary File 1.
This paper is in the following e-collection/theme issue:
Published on 17.4.2024 in Vol 26 (2024)
This is a member publication of University College London (Jisc)
Twitter Analysis of Health Care Workers’ Sentiment and Discourse Regarding Post–COVID-19 Condition in Children and Young People: Mixed Methods Study
Authors of this article:

Original Paper
- Macarena Chepo 1 * , RN, BSN, MPH, PhD ;
- Sam Martin 2, 3 * , MSc, PhD ;
- Noémie Déom 2 , MSc ;
- Ahmad Firas Khalid 4 , MD, PhD ;
- Cecilia Vindrola-Padros 2 , BA, MA, PhD
1 School of Nursing, Universidad Andrés Bello, Santiago, Chile
2 Department of Targeted Intervention, University College London, London, United Kingdom
3 Oxford Vaccine Group, Churchill Hospital, University of Oxford, Oxford, United Kingdom
4 Canadian Institutes of Health Research Health System Impact Fellowship, Centre for Implementation Research, Ottawa Hospital Research Institute, Otawa, ON, Canada
*these authors contributed equally
Corresponding Author:
Sam Martin, MSc, PhD
Department of Targeted Intervention
University College London
Charles Bell House 43-45
Foley Street
London, W1W 7TY
United Kingdom
Phone: 44 (0)20 3108 3232
Email: [email protected]
Background: The COVID-19 pandemic has had a significant global impact, with millions of cases and deaths. Research highlights the persistence of symptoms over time (post–COVID-19 condition), a situation of particular concern in children and young people with symptoms. Social media such as Twitter (subsequently rebranded as X) could provide valuable information on the impact of the post–COVID-19 condition on this demographic.
Objective: With a social media analysis of the discourse surrounding the prevalence of post–COVID-19 condition in children and young people, we aimed to explore the perceptions of health care workers (HCWs) concerning post–COVID-19 condition in children and young people in the United Kingdom between January 2021 and January 2022. This will allow us to contribute to the emerging knowledge on post–COVID-19 condition and identify critical areas and future directions for researchers and policy makers.
Methods: From a pragmatic paradigm, we used a mixed methods approach. Through discourse, keyword, sentiment, and image analyses, using Pulsar and InfraNodus, we analyzed the discourse about the experience of post–COVID-19 condition in children and young people in the United Kingdom shared on Twitter between January 1, 2021, and January 31, 2022, from a sample of HCWs with Twitter accounts whose biography identifies them as HCWs.
Results: We obtained 300,000 tweets, out of which (after filtering for relevant tweets) we performed an in-depth qualitative sample analysis of 2588 tweets. The HCWs were responsive to announcements issued by the authorities regarding the management of the COVID-19 pandemic in the United Kingdom. The most frequent sentiment expressed was negative. The main themes were uncertainty about the future, policies and regulations, managing and addressing the COVID-19 pandemic and post–COVID-19 condition in children and young people, vaccination, using Twitter to share scientific literature and management strategies, and clinical and personal experiences.
Conclusions: The perceptions described on Twitter by HCWs concerning the presence of the post–COVID-19 condition in children and young people appear to be a relevant and timely issue and responsive to the declarations and guidelines issued by health authorities over time. We recommend further support and training strategies for health workers and school staff regarding the manifestations and treatment of children and young people with post–COVID-19 condition.
Introduction
More than 3 years after the outbreak of the COVID-19 pandemic [ 1 ], the social, political, and economic impact of this phenomenon has been more than significant, considering >700 million worldwide cases and nearly 7 million people’s deaths [ 2 ]. Given the scale of the phenomenon, it is imperative for all countries to thoroughly examine the lessons gleaned from the pandemic, particularly regarding a matter that has raised significant concern among the populace: the long-term effects experienced by individuals who have had COVID-19, spanning weeks, months, or even years after their initial infection [ 3 ]. This phenomenon, referred to as post–COVID-19 condition (or more commonly “long COVID”), warrants careful consideration and analysis [ 4 ].
There is increasing information regarding the clinical manifestation of this condition, particularly in the adult population. The worldwide prevalence has been estimated at approximately 50% to 70% in individuals hospitalized during acute COVID-19 infection and 10% to 12% in vaccinated cases [ 5 ]. While children and young people have a low likelihood of severe COVID-19 infection [ 6 ], the information available to date indicates that the presence of post–COVID-19 condition in this group may be as disabling as in adults, reaching a prevalence rate of 23.4% (range 3.7%-66.5%) [ 7 ].
An agreed definition by the World Health Organization indicates that post–COVID-19 condition in children and young people is a condition that occurs “in individuals with a history of confirmed or probable SARS-CoV-2 infection when experiencing symptoms lasting at least two months which initially occurred within three months of acute COVID-19” [ 8 ]. Post–COVID-19 condition strongly impacts daily functioning and can develop or continue after COVID-19 infection and may fluctuate or relapse over time [ 4 , 8 , 9 ].
Among the symptoms most frequently attributable to post–COVID-19 condition in children and young people are fatigue, altered smell or anosmia, and anxiety [ 8 ]. However, other symptoms have also been reported, such as sleep disturbances, difficulty in concentrating, abdominal pain, myalgia or arthralgia, earache or ringing in ears, mood swings, persistent chest pain, stomach pain, light sensitivity, diarrhea, heart palpitations, and skin lesions [ 8 , 10 ]. One of England’s most significant studies is the Children and Young People With Long COVID study by Stephenson et al [ 11 ]. This national research matched longitudinal and cohort studies in adolescent individuals aged 11 to 17 years and found the presence of symptoms in 35.4% of the adolescent individuals who tested positive at baseline and 8.3% who of the adolescent individuals who tested negative at baseline. A total of 3 months after testing, 66.5% of those who tested positive and 53.3% of those who tested negative had any symptoms [ 11 ]. However, Stephenson et al [ 12 ] recently indicated that in a 6-month follow-up, the prevalence of specific symptoms reported at the time of the polymerase chain reaction testing decreased over time, where, for example, the prevalence of chills, fever, myalgia, cough, and sore throat among those who tested positive decreased from 10% to 25% to <3%.
As research on the symptoms, prevalence, and treatment of post–COVID-19 condition in children and young people continues, it is essential to add to the literature by developing studies that determine the condition’s impact on this group, considering that they are experiencing a range of unwanted symptoms that disrupt their quality of life and that of their families.
Considering that listening to the voices of families and health workers could be helpful to broaden the knowledge achieved in post–COVID-19 condition in children and young people, a powerful tool could be social media, such as Twitter (subsequently rebranded as X). With >3729 million daily active users, Twitter has become one of the most important social platforms in the world [ 13 ]. People used Twitter during the COVID-19 pandemic for different purposes, such as world leaders communicating with citizens [ 14 , 15 ], organizations monitoring movement [ 16 ], scientists studying public discourse around the pandemic [ 17 , 18 ], and researchers performing sentiment analysis [ 19 - 21 ]. In the case of physicians and health care workers (HCWs), Twitter has been used to share and evaluate scientific evidence, guidelines, and technical advice [ 22 - 24 ] and track the course and burden of disease [ 25 ].
Using the social media monitoring platform Pulsar [ 26 ], we aimed to explore HCWs’ perceptions concerning post–COVID condition in children and young people in the United Kingdom between January 2021 and January 2022. We aimed to contribute to the emerging knowledge on post–COVID-19 condition in children and young people and identify critical areas and future directions for researchers and policy makers.
We considered a mixed methods approach to be a pragmatic research paradigm. We analyzed data by conducting a Collaborative and Digital Analysis of Big Qualitative Data in Time Sensitive Contexts (LISTEN) [ 27 ]. This mixed methods analysis consisted of iterative cycles intercalating team discussion and using digital text and discourse analytics tools to analyze related social media data [ 27 ]. We used the LISTEN method to perform quantitative and qualitative analyses of Twitter posts, extracted through the Pulsar platform [ 26 ], related to the experience of post–COVID-19 condition in children and young people in the United Kingdom (eg, phrases, words, hashtags, videos, and images), published between January 1, 2021, and January 31, 2022. We created an advanced Boolean search for keywords mentioning “long COVID” and corelated words, hashtags, and symptoms; furthermore, we filtered for user accounts who identified as HCWs in their Twitter biography description ( Multimedia Appendix 1 ).
Quantitative analysis of all tweets included the following: (1) engagement analysis, where we specifically measured reactions to posts, for example, a retweet, a share, or a comment or quote made toward a tweet; (2) sentiment and emotion analysis, where we measured the positive or negative sentiment in the words and tone of each post within the context of post–COVID-19 condition and HCW’s roles ( Multimedia Appendix 2 ); (3) emotion analysis, where we measured the emotions expressed in the tweets, classified as sadness, anger, disgust, fear, and joy; (4) frequency analysis, where we observed the frequency of keywords and themes in the data set; (5) segmentation analysis, where we measured the key connections or relationships between keywords and their frequent use in the same context; (6) demographic analysis, where we measured the occupation, gender (man or woman or nonbinary or unknown), and city of origin related to the users posting tweets; and (7) analyses, where we evaluated the most influential accounts and the most mentioned websites.
Big qualitative analysis was carried out through thematic discourse analysis of the data sample, using InfraNodus [ 28 ], specifically analyzing the key themes and topics of concern expressed throughout the data set. A codebook was constructed based on the mapping of themes agreed upon by 3 researchers (ND, SM, and MC; Multimedia Appendix 3 ).
The principal investigators (ND, AFK, SM, and MC) interpreted and analyzed the data collected, following the recommendations for rigorous research provided by Creswell and Poth [ 29 ]. Using the LISTEN method [ 27 ], we aimed to show that the integration of qualitative insights through thematic analysis with the quantitative backing of topic modeling can offer a comprehensive view of the discourse. This mixed methods approach allows us to capture the richness of qualitative data while leveraging the objectivity of quantitative measures. Our initial data harvest of the larger corpus data from the Pulsar platform captured 300,000 tweets; this data harvest helped to underpin the software’s sentiment analysis modeling of this specific data set, providing a robust quantitative foundation. The addition of further qualitative data analyses from a smaller qualitative sample allowed for an in-depth understanding of nuanced conversations, particularly when exploring new or complex phenomena such as post–COVID-19 condition in children and young people, with the provision of insights into the context, subtext, and sentiment behind the tweets offering valuable snapshots of public perception and discourse. We used an iterative mixed methods approach, iterating between team discussions and using digital analytics tools to discern relevant themes from the Twitter data corpus. Specifically, we used InfraNodus for thematic analysis, which incorporates a topic modeling script for analyzing and identifying key topics of concern with a data set and provides a structured and objective interpretation of the data. The coding process involved 3 independent researchers (MC, SM, and ND), each with expertise in health care, social network analysis, and digital global health. When initial coding disagreements arose, we meticulously tagged any queries and discussed the posts in question. These instances led to 3 structured meetings wherein the research team deliberated collaboratively to resolve conflicting interpretations. This approach resulted in an 81.99% (2122/2588) initial intercoder agreement rate for the tweets analyzed. For the remaining instances where consensus was not initially reached, the majority rule was applied to finalize theme codings. To quantify the reliability of our coding procedure, with 81.99% (2122/2588) of the tweets coded identically, we used the Cohen κ score, which provides a measure of interrater agreement adjusted for chance. Including the calculation of all variations, this score was calculated to be approximately κ=0.70, indicating good agreement among the coders.
Ethical Considerations
The study only collected data from publicly accessible social networks that have been anonymized by various means, particularly by replacing all usernames and links with anonymous text and summaries of tweets that have been edited, retaining the original message, avoiding direct quotations being identifiable, and ensuring that no information is provided on the identity of the individuals who posted the content studied on the platform.
Internet research requires researchers to carefully consider guidelines to determine whether ethics approval and informed consent are needed [ 30 ]. On the basis of the terms set out by the Research Ethics Committee at the University College London [ 31 ], the study was considered exempt from formal ethics approval for the following reasons: (1) study involving information freely available in the public domain, such as published biographies, newspaper accounts of an individual’s activities, and published minutes of a meeting, that although is considered personal under the Data Protection Act, would not require ethics review; and (2) study involving anonymized records and data sets in the public domain, such as data sets available through the Office for National Statistics or the UK Data Archive where appropriate permissions have already been obtained and it is not possible to identify individuals from the information provided.
Therefore, we anonymized all records and data sets collected during the study to make identification impossible. We removed social media usernames from the data samples. No direct or easily traceable quotes have been included. These measures align with best practices [ 32 - 35 ]. While this study was beyond the scope of the human ethics committee, we adhered to the principles of ethics: beneficence, nonmaleficence, autonomy, and justice [ 36 ]. We collected and analyzed data through secure encrypted servers via the Meltwater and InfraNodus platforms.
Audience Analysis
During the period from January 2021 to January 2022, we obtained 300,000 tweets from 936 accounts. After filtering for relevant posts (refer to inclusion and exclusion criteria in Multimedia Appendix 1 ), we analyzed a sample of 2588 tweets using mixed methods analysis. In terms of gender (man, woman, nonbinary, or unknown), 32.88% (851/2588) were female individuals, 23.49% (608/2588) were male individuals, and 43.59% (1128/2588) were unknown. According to the description given in the user’s biography, the most frequently self-reported terms were “NHS” (582/2588, 22.49%), “health” (230/2588, 8.89%), “medical” (168/2588, 6.49%), “nurse” (166/2588, 6.41%), “clinical” (160/2588, 6.18%), “mum” (158/2588, 6.11%), “doctor” (145/2588, 5.6%), and “GP” (145/2588, 5.6%). In terms of city, tweets came mainly from London (958/2588, 37.02%), Newcastle upon Tyne (326/2588, 12.6%), Redcar (160/2588, 6.18%), Manchester (140/2588, 5.41%), and Bradford (111/2588, 4.29%).
Regarding profession described in the user’s biography, the most frequently mentioned roles were nurses (176/2588, 6.8%); medical roles, for example, paramedic and nursing assistant (173/2588, 6.68%); clinical roles, for example, surgeon, physiotherapist, and anesthesiologist (160/2588, 6.18%); general practitioners (GPs), for example, hospital GP or local surgery GP (142/2588, 5.49%); and physician (140/2588, 5.41%). The most frequent organization affiliated with was the National Health Service (587/2588, 22.68%).
Most Influential Accounts
One of the accounts that generated the highest number of mentions and, therefore, some of the most influence, as they were the ones that talked the most about post–COVID-19 condition in children and young people, was the account for @longcovidkids (593/2588, 22.91% tweets), related to the most shared website longcovidkids.org [ 37 ] , an international UK-based charity for families and children living with post–COVID-19 condition. Although the account was created in October 2020, it was first mentioned in our data collection timeline on January 1, 2021. It offers web support services, funding, and research participation and represents children and young people living with post–COVID-19 condition in expert forums, research panels, health organizations, and parliamentary groups. The other most shared web pages were theguardian.com (the United Kingdom) [ 38 ], bbc.co.uk (the United Kingdom) [ 39 ], peoplewith.com (the United States) [ 40 ], and ncbi.nlm.nih.gov (the United States) [ 41 ]. This shows that in the United Kingdom, there was a mixed influence of UK and US link resources linked to HCW Twitter users in the United Kingdom.
Keyword Analysis
The volume of social media engagement in the discussion about the post–COVID-19 condition experience in children and young people in the United Kingdom reached 1400 posts, 1550 engagements, and 1.9 million impressions. Overall, comments were very responsive to government decisions regarding the vaccination program and school closures ( Multimedia Appendix 4 ). During the first peak of comments in January 2021, the amount of discourse expanded leading up to March 2021, when there were different announcements of school closures, and the guidelines were delivered regarding the priority groups of the vaccination program (frontline HCW and people aged >80 years first). The highest engagement was between June and July 2021, which coincides with the government announcement regarding the availability of vaccines for people aged >18 years. The third peak of comments occurred in September 2021, the same month the authorities announced the extension of the vaccination program to children aged 12 to 15 years.
Top Keywords Analysis
The top words in posts associated with children and young people’s experience of post–COVID-19 condition in the United Kingdom were “Children” (352/2588, 13.6%), “kids” (160/2588, 6.18%), “people” (158/2588, 6.11%), “Young” (148/2588, 5.72%), and “schools” (83/2588, 3.21%). The top hashtags were #longcovid (1387/2588, 53.59%), #longcovidkids (448/2588, 17.31%), #covid19 (370/2588, 14.3%), and #covid (176/2588, 6.8%).
Sentiment and Emotions Analysis
According to sentiment analysis, 99.38% (2572/2588) of the posts reflected negative sentiments and 0.62% (16/2588) reflected positive sentiments. Negative sentiments were mainly associated with comments on hospitalization figures related to the COVID-19 pandemic, criticism of pandemic mitigation policies, and vaccination of children and young people. Furthermore, positive sentiments mainly concerned acknowledgments around decreasing numbers of community support groups.
The primary emotions identified were as follows:
- Sadness (1752/2588, 67.7%), such as in the following tweet:
@[Username] Really upset, after my tough on-call last night. Hospitalisations are still going up, and Gov announcement minismises the effect of long-COVID in adults and children. It’s so hard to keep spirits up today. But we’ll try and continue doing our best in the NHS.
- Joy (367/2588, 14.18%), such as in the following tweet:
@[Username] It’s been an amazing day! [...] I’ve been able to share the experience I’ve gained treating children and adolescents with Long COVID over the last year.
- Fear (233/2588, 9%), as seen in the following tweet:
@[Username] It’s really urgent that young people get the message that they need to get vaccinated. Long COVID is ruining many people’s lives! It’s not a lie or hypochondria, there are real, physiological changes, please understand!
Segmentation Analysis
This analysis revealed the critical clusters of conversation around the main topics of concern within the discourse network around post–COVID-19 condition. Comments were distributed in 4 key conversation segments as follows:
- People, schools, and prevention (1734/2588, 67%): Most of the comments related to measures taken in terms of COVID-19 prevention in schools, concern about the risk of exposure, and sharing experiences of infection in schools.
- Health, adults, and impact (401/2588, 15.49%): Comments mainly reflected concerns and uncertainty about the long-term effect of post–COVID-19 condition on both children and young people and adults.
- Cases, virus, and risk (326/2588, 12.6%): Comments reflected worries about the associated risks and long-term consequences attributable to post–COVID-19 condition (in both adults and children and young people) and the constant mutation of the virus, which will create a permanent risk in the population.
- Months, distress, and symptoms (106/2588, 4.1%): Some HCWs used Twitter to share how children and young people experience post–COVID-19 condition and the extent of these symptoms. Some HCWs exemplified certain typical manifestations, such as fatigue.
Discourse Analysis by Theme
To better understand the topics discussed from the segmentation analysis, we performed a discourse analysis of the key co-occurring themes and topics of concern shared within discussions regarding post–COVID-19 condition in children and young people. The following themes emerged ( Textbox 1 ): concern or uncertainty for the future, school attendance, mask protection from COVID-19, vaccine uptake, infection rates, policy (support or skepticism), understanding and visualizing symptoms, child mental health, access to care, community support, and research ( Figures 1 and 2 ).
- Concern for the future or uncertainty (615/2588, 23.76% tweets): Most comments showed a concern for the future, focusing on shared statistics regarding the rate and spread of infection in children and young people and how this would affect future health outcomes. Furthermore, this group expressed concern regarding political decisions; the presence of illness in loved ones; the eventual overload and response capacity of the health system in the face of an increase in post–COVID-19 condition cases; and the need for training of health care workers (HCWs) to deal with comorbid, potentially long-term symptoms ( Figure 1 A).
- Schools (460/2588, 17.77% tweets): Comments aimed to promote vaccination policies for schoolchildren and flexible measures regarding teachers’ work and attendance, considering cases of people with prolonged symptoms. In addition, several tweets expressed dissatisfaction with school risk mitigation measures, such as the use of face masks and air filters ( Figure 1 B).
- Vaccine (386/2588, 14.9% tweets): Most tweets from this group showed their disapproval of the constant changes in the government’s decisions regarding schools and priority groups for vaccination. Between March and June 2021, the first set of tweets criticized the lack of priority in the vaccination program for schoolchildren and other at-risk groups (such as teachers). Once the authorities announced a vaccination program for schoolchildren aged 12 to 15 years ( Multimedia Appendix 4 ), most comments promoted vaccination for this group. A few comments (78/2588, 3.01%) shared concerns about the vaccine’s efficacy for children, based on the experiences of COVID-19 reinfection in adults despite having received the recommended initial doses. However, to a lesser extent (26/2588, 1%), there was a refusal to vaccinate children, citing fear of possible adverse effects. Nonetheless, it is worth noting that the community frequently refuted such comments ( Figure 1 C).
- Share statistics (334/2588, 12.91% tweets): Frequently, HCWs shared statistical data, such as the number of affected children and young people, the number of post–COVID-19 condition cases, and hospital admissions and deaths. Some of these data were used to validate the existence of the post–COVID-19 phenomenon or to express concern about it ( Figure 1 D).
- Policy (316/2588, 12.21% tweets): The comments were responsive to the policies emanating from the authorities over time ( Multimedia Appendix 4 ). There were 5 main criticisms, including changes in school closure or opening policies; HCWs question why the authorities ignore the evidence of post–COVID-19 cases in children and young people, leading them to question whether decision makers have sufficient training to control the pandemic adequately; the failure to include teachers and school workers in the COVID-19 vaccination program as well as the younger population; the lack of mitigation measures in schools, such as improvements in ventilation systems and mandatory use of masks; and the herd immunity as a plan in the government’s hidden agenda , that is, to promote work and activate the economy ( Figure 1 E).
- “Proof” (280/2588, 10.82% tweets): Most tweets in this group argued regarding the existence of children and young people with post–COVID-19 condition through pictures; statistics; scientific papers; and personal, family, and professional experiences ( Figure 1 F).
- Signs and symptoms (189/2588, 7.3% tweets): Among the symptoms described, chronic fatigue and exhaustion were the most frequent symptoms, which prevent normal activities. Other symptoms were respiratory: dyspnea, chronic cough, and shortness of breath; gastrointestinal: acute or intense abdominal pain, nausea, bloating, gastroparesis, and change in smell or taste; muscular: severe joint pain, “painful foot” and difficulty with physical activity; mental health: anxiety and low mood; topical: rash, skin rashes, and redness and pain in the eyes; and nonspecific symptoms, such as chest pain, heart palpitations, constant high body temperature, precocious puberty, hormonal changes, and erectile dysfunction ( Figure 2 A).
- Face masks (119/2588, 4.6% tweets): Face masks were widely promoted, especially in schools, because HCWs considered them as a practical and straightforward strategy to control the pandemic ( Figure 2 B).
- Skepticism (101/2588, 3.9% tweets): Comments showed reticence toward post–COVID-19 condition in children and young people. Some of the arguments focused on a perceived lack of clarity in the clinical manifestations and stressed the need to better differentiate the post–COVID-19 condition from other related symptomatologies, such as mood disorders (eg, depression and anxiety due to confinement). In contrast, several arguments agreed on the need for more scientific evidence, arguing that post–COVID-19 condition in children and young people are isolated. Other users claimed not to know of such cases instead of calling post–COVID-19 condition in children and young people SMS text message an exaggeration. In addition, several arguments favored releasing restrictions for children and young people, particularly arguments related to the use of masks, because of possible associated risks, for example, hypoxia ( Figure 2 C).
- Mental health (54/2588, 2.09% tweets): Symptoms attributable to mental health problems in children and young people were also a concern. For instance, HCWs mentioned sadness, fear of infecting their family, anxiety regarding sick parents, stress, night terrors, self-harm, and suicidal ideation. Furthermore, users discussed a perceived lack of specific support for children and young people and their families in situations such as hospitalization; prolonged COVID-19 condition; admission to intensive care; and death of a family member, schoolmate, or teacher, all situations that triggered permanent stress in these groups ( Figure 2 D).
- Community support or asking for advice (93/2588, 3.59% tweets): Some HCWs used Twitter to ask for guidance on a specific issue or share experiences of having post–COVID-19 condition or caring for children and young people or family members. Furthermore, they shared informative infographics provided by experts regarding post–COVID-19 condition in children and young people ( Figure 2 E).
- Access to health care or treatment (72/2588, 2.78% tweets): Some HCWs mentioned the lack of specialist (cardiology) support, concerns regarding prolonged National Health Service burnout, and criticisms regarding how follow-up was carried out concerning the relative symptomatology of children and young people with post–COVID-19 condition. At the same time, opening new centers for children and young people with post–COVID-19 condition generated different reactions. On the one hand, some HCWs recognized it as a substantial development, but on the other hand, some HCWs recognized it as proof of the existence of post–COVID-19 condition in children and young people, which raised concerns for the future ( Figure 2 F).
- Research (52/2588, 2% tweets): Under this theme, tweets largely promoted study on post–COVID-19 condition in children and young people or highlighted the need for further study on the subject ( Figure 2 G).
- Images (57/2588, 2.2% tweets): Images shared were primarily from scientific studies, including infographics (from organizations such as National Health Service or @LongCovidKids) and visualization of children and young people’s symptoms, such as rashes, COVID-19 toe, and joint pain. Most infographics shared by organizations (and not individuals), such as the organization LongCovidKids, were related to statistics, such as the number of children and young people with post–COVID-19 condition or the quantification of the type of symptoms experienced. Shared photographs tended to show the more “visually recognizable” symptoms of post–COVID-19 condition, such as skin lesions, rashes, or inflammation. The less visible symptoms, such as chronic fatigue and neurological issues, were represented with photographs of children and young people lying, sleeping under blankets, or duvets or on hospital beds ( Figure 2 H).

Principal Findings
Our primary objective was to explore HCWs’ perceptions concerning post–COVID-19 condition in children and young people in the United Kingdom between January 2021 and January 2022. Our findings indicated that comments made by HCWs on Twitter were responsive to announcements issued by authorities regarding the management of the COVID-19 pandemic in the United Kingdom and associated regulations on the operation of schools. The most frequent feelings and emotions were negative, mainly sadness. In turn, we identified relevant themes for HCWs, such as uncertainty or concern about the future; policies; and regulations for the prevention, management, and addressing both COVID-19 and post–COVID-19 condition in children and young people; vaccination; and the use of Twitter as a strategy to share scientific literature, management strategies, and clinical and personal experiences.
Concern from HCWs regarding the policies for addressing the COVID-19 pandemic in the children and young people in the United Kingdom (including vaccination and schools) was a recurring theme in our findings. Furthermore, concern regarding the side effects of the COVID-19 vaccine and how the vaccine might interact with preexisting physiological symptoms of post–COVID-19 condition in children and young people was a topic of discussion. Similarly, the constant change in policy making in the United Kingdom, as public health bodies and governments have tried to understand and adapt to the emergence of post–COVID-19 condition, have added to the strength of this ongoing debate [ 42 ]. The lack of up-to-date evidence on post–COVID-19 condition in children and young people prompted HCWs to rely on Twitter during the pandemic to communicate relevant information. Twitter has a broad audience reach; is used as a communication tool by politicians, health bodies, and other key influences; and facilitates real-time updates [ 43 ]. During the pandemic, HCWs, primarily those in frontline roles and local response coordination, have often been challenged to become credible spokespersons for pandemic information [ 44 ]. Such credibility directly influences public confidence and decision-making, ultimately determining the success or failure of a public health intervention [ 43 ].
Furthermore, failures in risk communication could explain the presence of uncertainty and negative feelings associated with school regulations. When people are upset, distressed, or fearful, they often do not trust the authority, decrease the perceived validity of the communication received, and find information processing difficult [ 45 ]. In this regard, Fotheringham et al [ 46 ] indicated that during 2020, school leaders in the United Kingdom faced pressures and challenges related to translating and enacting school policies, particularly with the perceived lack of agency shared by the government concerning being able to translate centrally issued guidelines. In turn, Tomson et al [ 47 ] reported that the pandemic has negatively impacted the well-being of leaders in all types of schools and across all demographic groups, affecting their ability to think clearly and solve work-related problems. Given that the protection and care of children and young people health during the COVID-19 pandemic ultimately rests with school leaders, the search for support strategies that focus on the needs of these groups becomes an urgent necessity.
Findings in Relation to Other Studies
Using Twitter’s information, this is one of the first studies to capture health professionals’ perceptions of prolonged COVID-19 in the children and young people in the United Kingdom. However, other studies have addressed post–COVID-19 condition on this social network. Callard and Peregov [ 48 ] reviewed how, through social platforms such as Twitter, patients made the persistence and heterogeneity of COVID-19 symptoms visible, thus catapulting the inclusion of post–COVID-19 condition as a relevant phenomenon in clinical and policy debates. In contrast, other authors in the last 2 years have explored on various platforms (including Twitter) the persistence of symptoms and emotional impact after months of suspected and confirmed diagnosis of COVID-19 [ 49 - 55 ], including the period of vaccination. Furthermore, others have explored web discussions regarding this phenomenon [ 56 ]. Several of these authors agree on a perceived lack of support and specific resources from governmental bodies, a lack of information or clarity in the instructions given, and the absence of formal mechanisms to allow the voices of patients and the community to be heard. The above point is critical as it highlights the gap between the needs of the population and the response provided by policy makers, which not only translates into a gap in access to health services but also limits citizen participation in decision-making on the issues that affect their own health and increases distrust toward regulations and instructions issued by the government.
Implications for Policy and Practice
Several policy recommendations and implications are targeted at various stakeholders to consider while implementing future policy guidelines to address post–COVID-19 health care delivery. First, policy makers should consider investing appropriate resources to collect data regarding post–COVID-19 condition in children and young people, specifically on the impact of COVID-19 on the mental health of children and young people. This implies working closely with researchers to streamline data collection and reporting on post–COVID-19 condition. Second, policy makers should consider providing a basic level of psychosocial support with access to quality mental health and psychosocial support services for HCWs, school staff, parents, and children and young people experiencing post–COVID-19 condition. This implies strengthening health systems, community-based programming, and mobilization. Policies must include documenting the impact of mental health and psychosocial support interventions and innovative approaches to be more widely disseminated and scaled up across different contexts and target population groups. Third, to address the criticism around frequent changes in school closure and opening policies, decision makers should develop clear, easy-to-understand school mitigation plans informed by the best available evidence. The plans should incorporate teachers, school workers, and parents to ensure all voices are included in the policy plan. Fourth, policy makers should adopt a shared decision-making approach incorporating HCWs in the decision-making process for managing the COVID-19 pandemic. Finally, government decision makers should set post–COVID-19 pandemic recovery policies informed from a health equity perspective and how this affects children and young people living with post–COVID-19 condition, factoring in childhood, family income, housing, domestic violence, access to health care, and racism.
In terms of the needed clearer road map for recommendations to support training strategies for HCWs and school staff regarding post–COVID-19 condition in children and young people, we have outlined the following 10 steps.
Step 1: Data Collection and Analysis
Our study underlines the critical need for comprehensive data on post–COVID-19 condition’s impact on the mental health of children and young people. As a first step, it is recommended that policy makers should allocate resources for the systematic collection and analysis of data on post–COVID-19 condition in children and young people, particularly focusing on mental health outcomes. These data should be used to identify the most prevalent symptoms and the most effective treatment strategies. In this context, it is recommended that experts emphasize the importance of early detection and medical consultation for mental health issues in children and young people diagnosed with post–COVID-19 condition, including mood changes, irritability, social withdrawal, memory problems, difficulty in concentrating, anxiety, depression, posttraumatic stress, school absenteeism, and suicidal ideation [ 57 , 58 ]. This entails working closely with researchers to streamline data collection and reporting on post–COVID-19 condition.
Step 2: Psychosocial Support Framework
It has been noted that globally, programs for managing post–COVID-19 condition in children and young people are heterogeneous, ranging from the use of physiotherapy, pediatric occupational therapy, and psychological support to interventions aimed at lifestyle modifications [ 59 ]. This diversity could impact differential outcomes in the treatment, recovery, and timely and effective rehabilitation of children and young people with post–COVID-19 condition. Upon analyzing the wider literature and the social media data in this study, it is recommended that a basic level of psychosocial support should be established. This would involve ensuring access to quality mental health services for HCWs, school staff, parents, and children and young people with post–COVID-19 condition. This framework should be integrated into the health system and community-based programming, emphasizing the mobilization of resources and strengthening of support networks. It is suggested that the psychosocial support framework should facilitate access to quality mental health services and support networks that are robust and responsive. Community engagement gleaned from further Twitter discourse analysis should be a helpful guide in the development of these services to ensure they meet the real and expressed needs of children and young people with post–COVID-19 condition. Practical examples of basic psychosocial support include using web support services; individual or group therapy sessions; school-based emotional support programs; and counseling sessions aimed at parents, family members, or school staff.
Step 3: Educational Mitigation Plans
The frequent policy changes around school closures highlight the necessity for stable and clear educational mitigation plans. It is recommended that these plans should be directly informed by the evidence collected and further analysis of sentiments and emotions surrounding post–COVID-19 condition in schools. Incorporating the viewpoints of teachers, parents, and school staff, as identified in our thematic analysis, will ensure that the mitigation strategies are comprehensive, feasible, and sensitive to the psychosocial impact on children and young people. School staff and policy makers should collaborate to develop clear, evidence-informed educational mitigation plans. These plans should be straightforward and involve teachers, school workers, and parents in their creation, ensuring a unified approach that considers the voices of all stakeholders.
Step 4: Shared Decision-Making in Health Care
In health care settings, the adoption of a shared decision-making model is crucial, enabling HCWs to actively contribute to the formulation of COVID-19 and post–COVID-19 policies. This inclusive approach ensures that frontline workers can provide valuable insights toward policy development. To facilitate this, the establishment of advisory committees composed of representatives from HCWs is recommended. This committee can convene regularly to deliberate on key decisions pertaining to the COVID-19 pandemic management, including prevention measures, resource distribution, and vaccination strategies. Such collaborative groups have demonstrated effectiveness in identifying priority needs within the context of a pandemic [ 60 ].
Step 5: Health Equity in Policy Setting
Post–COVID-19 recovery policies should be set with a health equity lens. This means considering factors such as family income, housing, domestic violence, access to health care, and racism and how these factors affect children and young people living with post–COVID-19 condition. Our findings emphasize the importance of framing post–COVID-19 recovery policies through a lens of health equity. The concerns raised by HCWs regarding the socioeconomic impacts, such as family income and access to health care, underline the need for policies that address not just the medical aspects of post–COVID-19 condition but also the social determinants of health. An equitable approach will ensure that children and young people from diverse backgrounds receive appropriate support.
Step 6: Documenting and Disseminating Interventions
It is vital to document the impact of mental health and psychosocial support interventions. In this context, it is crucial to implement innovative strategies to disseminate unbiased information about post–COVID-19 condition among health care professionals and educators working with children and young people, ensuring it reaches different contexts and populations. These strategies may include creating interactive multimedia resources, such as videos and mobile apps; organizing webinars; actively using social media; and forming web support groups. These groups will provide a space where patients, health care professionals, and educators can share their experiences and knowledge regarding post–COVID-19 condition. These actions will not only help reduce isolation and social stigma but also strengthen support for these groups considered vulnerable [ 61 ].
Step 7: Developing a Clear Communication Strategy
Policy makers must develop a clear communication strategy to address frequent policy changes and mitigate confusion. This strategy should be informed by the data collected and analysis conducted in Step 1. The data reveal a palpable sense of uncertainty and frustration due to frequent policy shifts, underscoring the need for a clear and consistent communication strategy. This strategy should be grounded in the evidence gathered from the health care community’s discourse and aim to minimize confusion by providing timely, transparent, and reliable information regarding post–COVID-19 policies and support services.
Step 8: Training and Support Strategies
On the basis of the findings of the comprehensive data analysis, specific training and support strategies should be developed for HCWs and school staff. These strategies should be focused on the practical aspects of identifying and managing post–COVID-19 condition in children and young people. For instance, training sessions could include practical workshops on recognizing post–COVID-19 symptoms in children and adolescents, conducting diagnostic assessments, and implementing appropriate treatment and support interventions.
Step 9: Continuous Feedback and Policy Adaptation
The continuous evolution of the post–COVID-19 phenomenon demands an iterative approach to policy making. On the basis of our study, we recommend establishing feedback mechanisms with HCWs and school staff to monitor the reception and effectiveness of implemented policies. This feedback, coupled with ongoing research, should inform policy adaptations to ensure they remain aligned with the evolving landscape of post–COVID-19 condition and its impact on children and young people.
Step 10: Evaluation and Research
Finally, there should be a commitment to ongoing evaluation and research. This will involve not only monitoring the implementation of the abovementioned steps but also supporting new research to fill any remaining gaps in understanding the long-term effects of COVID-19 on children and young people.
This sequence of steps is designed to be iterative and responsive, ensuring that the recommendations from the study are translated into concrete actions that adapt to emerging data and research findings.
Strengths and Limitations
A key strength of this study is that our social media analysis of post–COVID-19 condition contributes toward an emerging understanding of reported experiential, emotional, and practical dimensions of post–COVID-19 condition in children and young people specifically and questions of vaccine hesitancy in children and young people with post–COVID-19 condition. This is one of the few studies to collect HCWs’ perceptions regarding post–COVID-19 condition in children and young people in the United Kingdom using information from Twitter. We identify key areas that need considering attention and focus, such as the provision of psychosocial support with access to quality mental health resources to alleviate the impact of post–COVID-19 condition in children and young people and the development of clear post–COVID-19 pandemic recovery guidelines that are informed by health equity perspective, and how this affects children and young people living with post–COVID-19 condition.
One of the limitations this study acknowledges is the definition of post–COVID-19 condition in children and young people. When data were collected, the lack of consensus on the definition of post–COVID-19 condition in children and young people forced us to formulate a definition of post–COVID-19 condition in children and young people based on the available literature. Furthermore, this study is limited to the perceptions of people who used descriptors in their web biography attributable to HCWs; therefore, our results only represent some HCWs in the United Kingdom and those in other countries. In turn, this research collected data from Twitter only; therefore, further inquiry into HCWs’ perceptions of post–COVID-19 condition in children and young people required expanding to other data sources or social networks and including languages other than English. We acknowledge that demographic factors, geographic location, and individual daily activities of social media users can significantly influence language use and word choice, introducing potential biases in tweet-based data. Such biases are inherent in any analysis of social media content and can affect the generalizability of findings. For instance, our study relies on Twitter data, which do not encompass the full spectrum of global or the UK public opinion on post–COVID-19 condition in children and young people. While Twitter serves as a valuable platform for capturing real-time sentiments and experiences, it is not fully representative of all demographics and geographic regions. Our results may reflect the perspectives of more vocal or active social media users, which may not correspond to the silent majority or those without access to social media. In addition, the absence of geotagged information for many users limits our ability to conduct a more nuanced spatial analysis of the sentiments expressed.
Furthermore, our study is built upon the recognition that social media data may overrepresent certain demographic groups while underrepresenting others, such as the older population or those without reliable internet access. This skew can influence the apparent prevalence of certain views or experiences of post–COVID-19 condition. Moreover, individuals’ patterns of daily life, reflected in their social media use and content, contribute additional layers of complexity and potential bias to the discourse analyzed.
Consistent with scholarly precedents on the subject [ 62 , 63 ], our study acknowledges these biases as intrinsic limitations of social media–based research. Although our analysis did not control for these factors, we recognize their potential impact on our results. Future studies would benefit from incorporating a broader array of data sources, including interviews or focus groups, to provide a more representative and comprehensive understanding of post–COVID-19 condition in children and young people. This approach would complement our Twitter-based findings and help mitigate the biases inherent in social media data.
Conclusions
More than a year after the start of the COVID-19 pandemic, the perceptions described on Twitter by HCWs concerning the presence of post–COVID-19 condition in children and young people appear to be a relevant and timely issue as well as very responsive to the declarations and guidelines issued by the health authorities over time. The most prominent group within the discourse studied was the activist or lobbying organization @LongCovidKids, which shared the most tweets and images over the period studied. We recommend that future research focus on how web health activism is organized and carried out for children and young people with post–COVID-19 condition. Such a strategy would allow for a better understanding of the scope and impact of this phenomenon and how it can influence decision-making. Furthermore, we suggest different mitigation strategies, support, and training of HCWs and school staff regarding manifestations and treatment of post–COVID-19 condition in children and young people across all demographic areas.
Acknowledgments
The authors would like to thank the Rapid Research Evaluation and Assessment Lab, Department of Targeted Intervention, University College London, London, United Kingdom, whose support has been essential for developing this project.
Conflicts of Interest
None declared.
Filters used for the search strategy on Twitter.
Sentiment analysis framework: attitudes toward post–COVID-19 condition in children and young people.
Theme codebook: examples of tweets that fit into main themes tagged for mention of children and young people with post–COVID-19 condition.
Timeline of national governmental policies and guidelines regarding children and young people.
- World Health Organization. COVID-19 Public Health Emergency of International Concern (PHEIC) Global research and innovation forum. Global Research Collaboration for Infectious Disease Preparedness. Feb 12, 2020. URL: https://www.who.int/publications/m/item/covid-19-public-health-emergency-of-international-concern-(pheic)-global-research-and-inno vation-forum [accessed 2022-06-06]
- Johns Hopkins University of Medicine. COVID-19 Dashboard by the Center for Systems Science and Engineering (CSSE) at Johns Hopkins University (JHU). Coronavirus Resource Center. Jan 22, 2020. URL: https://coronavirus.jhu.edu/map.html [accessed 2023-06-09]
- Nature Editorial. Long COVID and kids: more research is urgently needed. Nature. Feb 08, 2022;602(7896):183. [ FREE Full text ] [ CrossRef ] [ Medline ]
- Long COVID or post-COVID conditions. Centers for Disease Control and Prevention. URL: https://www.cdc.gov/coronavirus/2019-ncov/long-term-effects/index.html [accessed 2023-06-09]
- Davis HE, McCorkell L, Vogel JM, Topol E. Long COVID: major findings, mechanisms and recommendations. Nat Rev Microbiol. Mar 13, 2023;21(3):133-146. [ FREE Full text ] [ CrossRef ] [ Medline ]
- Zimmermann P, Curtis N. Why is COVID-19 less severe in children? A review of the proposed mechanisms underlying the age-related difference in severity of SARS-CoV-2 infections. Arch Dis Child. Dec 01, 2020;106(5):429-439. [ FREE Full text ] [ CrossRef ] [ Medline ]
- Zheng YB, Zeng N, Yuan K, Tian SS, Yang YB, Gao N, et al. Prevalence and risk factor for long COVID in children and adolescents: a meta-analysis and systematic review. J Infect Public Health. Mar 07, 2023;16(5):660-672. [ FREE Full text ] [ CrossRef ] [ Medline ]
- World Health Organization. A clinical case definition for post COVID-19 condition in children and adolescents by expert consensus. World Health Organization. Feb 16, 2023. URL: https://www.who.int/publications/i/item/WHO-2019-nCoV-Post -COVID-19-condition-CA-Clinical-case-definition-2023-1 [accessed 2024-03-26]
- Stephenson T, Allin B, Nugawela MD, Rojas N, Dalrymple E, Pinto Pereira S, CLoCk Consortium, et al. Long COVID (post-COVID-19 condition) in children: a modified Delphi process. Arch Dis Child. Jun 17, 2022;107(7):674-680. [ FREE Full text ] [ CrossRef ] [ Medline ]
- Esposito S, Principi N, Azzari C, Cardinale F, Di Mauro G, Galli L, et al. Italian intersociety consensus on management of long Covid in children. Ital J Pediatr. Mar 09, 2022;48(1):1-9. [ FREE Full text ] [ CrossRef ] [ Medline ]
- Stephenson T, Pinto Pereira SM, Shafran R, de Stavola BL, Rojas N, McOwat K, CLoCk Consortium, et al. Physical and mental health 3 months after SARS-CoV-2 infection (long COVID) among adolescents in England (CLoCk): a national matched cohort study. Lancet Child Adolesc Health. Feb 08, 2022;6(4):230-239. [ FREE Full text ] [ CrossRef ] [ Medline ]
- Stephenson T, Pinto Pereira SM, Nugawela MD, McOwat K, Simmons R, Chalder T, et al. CLoCk Consortium. Long COVID-six months of prospective follow-up of changes in symptom profiles of non-hospitalised children and young people after SARS-CoV-2 testing: a national matched cohort study (The CLoCk) study. PLoS One. Mar 6, 2023;18(3):e0277704. [ FREE Full text ] [ CrossRef ] [ Medline ]
- Twitter users, stats, data and trends. Data Reportal. May 11, 2023. URL: https://datareportal.com/essential-twitter-stats#:~ :text=Based [accessed 2023-06-09]
- Rufai SR, Bunce C. World leaders' usage of Twitter in response to the COVID-19 pandemic: a content analysis. J Public Health (Oxf). Aug 18, 2020;42(3):510-516. [ FREE Full text ] [ CrossRef ] [ Medline ]
- Haman M. The use of Twitter by state leaders and its impact on the public during the COVID-19 pandemic. Heliyon. Nov 2020;6(11):e05540. [ FREE Full text ] [ CrossRef ] [ Medline ]
- Huang X, Li Z, Jiang Y, Li X, Porter D. Twitter reveals human mobility dynamics during the COVID-19 pandemic. PLoS One. Nov 10, 2020;15(11):e0241957. [ FREE Full text ] [ CrossRef ] [ Medline ]
- Wicke P, Bolognesi MM. Framing COVID-19: how we conceptualize and discuss the pandemic on Twitter. PLoS One. Sep 30, 2020;15(9):e0240010. [ FREE Full text ] [ CrossRef ] [ Medline ]
- Chang CH, Monselise M, Yang CC. What are people concerned about during the pandemic? detecting evolving topics about COVID-19 from Twitter. J Healthc Inform Res. Jan 17, 2021;5(1):70-97. [ FREE Full text ] [ CrossRef ] [ Medline ]
- Xue J, Chen J, Hu R, Chen C, Zheng C, Su Y, et al. Twitter discussions and emotions about the COVID-19 pandemic: machine learning approach. J Med Internet Res. Nov 25, 2020;22(11):e20550. [ FREE Full text ] [ CrossRef ] [ Medline ]
- Boon-Itt S, Skunkan Y. Public perception of the COVID-19 pandemic on Twitter: sentiment analysis and topic modeling study. JMIR Public Health Surveill. Nov 11, 2020;6(4):e21978. [ FREE Full text ] [ CrossRef ] [ Medline ]
- Valdez D, Ten Thij M, Bathina K, Rutter LA, Bollen J. Social media insights into US mental health during the COVID-19 pandemic: longitudinal analysis of Twitter data. J Med Internet Res. Dec 14, 2020;22(12):e21418. [ FREE Full text ] [ CrossRef ] [ Medline ]
- Rashid MA, Yip SW, Gill D, Arfeen Z. Sharing is caring: an analysis of #FOAMed Twitter posts during the COVID-19 pandemic. Postgrad Med J. Mar 14, 2022;98(1157):199-204. [ CrossRef ] [ Medline ]
- Ghosh P, Schwartz G, Narouze S. Twitter as a powerful tool for communication between pain physicians during COVID-19 pandemic. Reg Anesth Pain Med. Feb 21, 2021;46(2):187-188. [ CrossRef ] [ Medline ]
- Burgos LM, Gil Ramirez A, Utengen A, Thamman R. Use of Twitter during COVID-19 pandemic: an opportunity for continuing medical education in cardiology. Medicina (B Aires). 2020;80 Suppl 6:122-123. [ FREE Full text ] [ Medline ]
- Margus C, Brown N, Hertelendy AJ, Safferman MR, Hart A, Ciottone GR. Emergency physician Twitter use in the COVID-19 pandemic as a potential predictor of impending surge: retrospective observational study. J Med Internet Res. Jul 14, 2021;23(7):e28615. [ FREE Full text ] [ CrossRef ] [ Medline ]
- Pulsar TRAC- Audience intelligence platform and social listening tool. Pulsar. URL: https://www.pulsarplatform.com/solutions/pulsar-trac/ [accessed 2022-03-01]
- Vera San Juan N, Aceituno D, Djellouli N, Sumray K, Regenold N, Syversen A, et al. Mental health and well-being of healthcare workers during the COVID-19 pandemic in the UK: contrasting guidelines with experiences in practice. BJPsych Open. Dec 10, 2020;7(1):e15. [ FREE Full text ] [ CrossRef ] [ Medline ]
- Paranyushkin D. InfraNodus: generating insight using text network analysis. In: Proceedings of the World Wide Web Conference. 2019. Presented at: WWW '19; May 13-17, 2019; San Francisco, CA. [ CrossRef ]
- Creswell JW, Poth CN. Qualitative Inquiry and Research Design: Choosing Among Five Approaches 4th Edition. Thousand Oaks, CA. SAGE Publications, Inc; 2017.
- Eysenbach G, Till JE. Ethical issues in qualitative research on internet communities. BMJ. Nov 10, 2001;323(7321):1103-1105. [ FREE Full text ] [ CrossRef ] [ Medline ]
- UCL research ethics. University College London. URL: https://www.ucl.ac.uk/research-ethics/do-i-need-ethical-approval# When [accessed 2023-06-12]
- Martin S, Kilich E, Dada S, Kummervold PE, Denny C, Paterson P, et al. "Vaccines for pregnant women…?! Absurd" - Mapping maternal vaccination discourse and stance on social media over six months. Vaccine. Sep 29, 2020;38(42):6627-6637. [ CrossRef ] [ Medline ]
- Kummervold PE, Martin S, Dada S, Kilich E, Denny C, Paterson P, et al. Categorizing vaccine confidence with a transformer-based machine learning model: analysis of nuances of vaccine sentiment in Twitter discourse. JMIR Med Inform. Oct 08, 2021;9(10):e29584. [ FREE Full text ] [ CrossRef ] [ Medline ]
- Ahmed W, Bath PA, Demartini G. Using Twitter as a data source: an overview of ethical, legal, and methodological challenges. In: Woodfield K, editor. The Ethics of Online Research. Bingley, UK. Emerald Publishing Limited; Dec 12, 2017.
- Ayers JW, Caputi TL, Nebeker C, Dredze M. Don't quote me: reverse identification of research participants in social media studies. NPJ Digit Med. Aug 2, 2018;1(1):30. [ FREE Full text ] [ CrossRef ] [ Medline ]
- Varkey B. Principles of clinical ethics and their application to practice. Med Princ Pract. Jun 4, 2021;30(1):17-28. [ FREE Full text ] [ CrossRef ] [ Medline ]
- Long Covid Kids homepage. Long Covid Kids. URL: https://es.longcovidkids.org/ [accessed 2023-06-12]
- The Guardian homepage. The Guardian. URL: https://www.theguardian.com/uk [accessed 2023-06-12]
- Welcome to the BBC. BBC. URL: https://www.bbc.com/ [accessed 2023-06-12]
- People with health. PeopleWith. URL: https://peoplewith.com/ [accessed 2023-06-12]
- Welcome to NCBI. National Institutes of Health National Library of Medicine. URL: https://www.ncbi.nlm.nih.gov/ [accessed 2023-06-12]
- Edobor M. Trust and public discourse during the Covid‐19 pandemic. IPPR Progress Rev. Feb 15, 2021;27(4):354-360. [ CrossRef ]
- Nielsen RK, Fletcher R, Kalogeropoulos A, Simon F. Communications in the coronavirus crisis: lessons for the second wave. Reuters Institute for the Study of Journalism. Oct 27, 2020. URL: https://reutersinstitute.politics.ox.ac.uk/communications-coronavirus-crisis-lessons-second-wave [accessed 2024-03-26]
- Zohar T, Negev M, Sirkin M, Levine H. Trust in COVID-19 policy among public health professionals in Israel during the first wave of the pandemic: a cross-sectional study. Isr J Health Policy Res. Apr 11, 2022;11(1):20. [ FREE Full text ] [ CrossRef ] [ Medline ]
- Enria L, Waterlow N, Rogers NT, Brindle H, Lal S, Eggo RM, et al. Trust and transparency in times of crisis: results from an online survey during the first wave (April 2020) of the COVID-19 epidemic in the UK. PLoS One. Feb 16, 2021;16(2):e0239247. [ FREE Full text ] [ CrossRef ] [ Medline ]
- Fotheringham P, Harriott T, Healy G, Arenge G, Wilson E. Pressures and influences on school leaders navigating policy development during the COVID‐19 pandemic. Brit Educ Res J. Jul 30, 2021;48(2):201-227. [ CrossRef ]
- Thomson P, Greany T, Martindale N. The trust deficit in England: emerging research evidence about school leaders and the pandemic. J Educ Admin History. Sep 13, 2021;53(3-4):296-300. [ CrossRef ]
- Callard F, Perego E. How and why patients made long COVID. Soc Sci Med. Jan 2021;268:113426. [ FREE Full text ] [ CrossRef ] [ Medline ]
- Davis HE, Assaf GS, McCorkell L, Wei H, Low RJ, Re'em Y, et al. Characterizing long COVID in an international cohort: 7 months of symptoms and their impact. EClinicalMedicine. Aug 2021;38:101019. [ FREE Full text ] [ CrossRef ] [ Medline ]
- Miyake E, Martin S. Long Covid: online patient narratives, public health communication and vaccine hesitancy. Digit Health. Nov 29, 2021;7:20552076211059649. [ FREE Full text ] [ CrossRef ] [ Medline ]
- Matharaarachchi S, Domaratzki M, Katz A, Muthukumarana S. Discovering long COVID symptom patterns: association rule mining and sentiment analysis in social media tweets. JMIR Form Res. Sep 07, 2022;6(9):e37984. [ FREE Full text ] [ CrossRef ] [ Medline ]
- Bhattacharyya A, Seth A, Rai S. The effects of long COVID-19, its severity, and the need for immediate attention: analysis of clinical trials and Twitter data. Front Big Data. Dec 15, 2022;5:1051386. [ FREE Full text ] [ CrossRef ] [ Medline ]
- Samannodi M, Alwafi H, Naser AY, Al Qurashi AA, Qedair JT, Salawati E, et al. Determinants of post-COVID-19 conditions among SARS-CoV-2-infected patients in Saudi Arabia: a web-based cross-sectional study. Diseases. Aug 23, 2022;10(3):55. [ FREE Full text ] [ CrossRef ] [ Medline ]
- Santarossa S, Rapp A, Sardinas S, Hussein J, Ramirez A, Cassidy-Bushrow AE, et al. Understanding the #longCOVID and #longhaulers conversation on Twitter: multimethod study. JMIR Infodemiology. 2022;2(1):e31259. [ FREE Full text ] [ CrossRef ] [ Medline ]
- Déguilhem A, Malaab J, Talmatkadi M, Renner S, Foulquié P, Fagherazzi G, et al. Identifying profiles and symptoms of patients with long COVID in France: data mining infodemiology study based on social media. JMIR Infodemiology. Nov 22, 2022;2(2):e39849. [ FREE Full text ] [ CrossRef ] [ Medline ]
- Awoyemi T, Ebili U, Olusanya A, Ogunniyi KE, Adejumo AV. Twitter sentiment analysis of long COVID syndrome. Cureus. Jun 2022;14(6):e25901. [ FREE Full text ] [ CrossRef ] [ Medline ]
- Malone LA, Morrow A, Chen Y, Curtis D, de Ferranti SD, Desai M, et al. Multi-disciplinary collaborative consensus guidance statement on the assessment and treatment of postacute sequelae of SARS-CoV-2 infection (PASC) in children and adolescents. PM R. Oct 2022;14(10):1241-1269. [ FREE Full text ] [ CrossRef ] [ Medline ]
- Shachar-Lavie I, Shorer M, Segal H, Fennig S, Ashkenazi-Hoffnung L. Mental health among children with long COVID during the COVID-19 pandemic. Eur J Pediatr. Apr 14, 2023;182(4):1793-1801. [ FREE Full text ] [ CrossRef ] [ Medline ]
- Brackel CL, Noij LC, Vijverberg SJ, Legghe CL, Maitland-van der Zee AH, van Goudoever JB, et al. International care programs for pediatric post-COVID condition (long COVID) and the way forward. Pediatr Res. Jan 29, 2024. (forthcoming). [ CrossRef ] [ Medline ]
- Ripp J, Peccoralo L, Charney D. Attending to the emotional well-being of the health care workforce in a New York City health system during the COVID-19 pandemic. Acad Med. Aug 2020;95(8):1136-1139. [ FREE Full text ] [ CrossRef ] [ Medline ]
- Buonsenso D, Camporesi A, Morello R, De Rose C, Fracasso M, Chieffo DP, et al. Social stigma in children with long COVID. Children (Basel). Sep 07, 2023;10(9):1518. [ FREE Full text ] [ CrossRef ] [ Medline ]
- Mitchell L, Frank MR, Harris KD, Dodds PS, Danforth CM. The geography of happiness: connecting twitter sentiment and expression, demographics, and objective characteristics of place. PLoS One. May 29, 2013;8(5):e64417. [ FREE Full text ] [ CrossRef ] [ Medline ]
- Padilla JJ, Kavak H, Lynch CJ, Gore RJ, Diallo SY. Temporal and spatiotemporal investigation of tourist attraction visit sentiment on Twitter. PLoS One. Jun 14, 2018;13(6):e0198857. [ FREE Full text ] [ CrossRef ] [ Medline ]
Abbreviations
Edited by A Mavragani; submitted 20.06.23; peer-reviewed by R Gore, A Wahbeh; comments to author 02.11.23; revised version received 14.02.24; accepted 08.03.24; published 17.04.24.
©Macarena Chepo, Sam Martin, Noémie Déom, Ahmad Firas Khalid, Cecilia Vindrola-Padros. Originally published in the Journal of Medical Internet Research (https://www.jmir.org), 17.04.2024.
This is an open-access article distributed under the terms of the Creative Commons Attribution License (https://creativecommons.org/licenses/by/4.0/), which permits unrestricted use, distribution, and reproduction in any medium, provided the original work, first published in the Journal of Medical Internet Research, is properly cited. The complete bibliographic information, a link to the original publication on https://www.jmir.org/, as well as this copyright and license information must be included.
- Introduction
- Article Information
Data Sharing Statement
See More About
Sign up for emails based on your interests, select your interests.
Customize your JAMA Network experience by selecting one or more topics from the list below.
- Academic Medicine
- Acid Base, Electrolytes, Fluids
- Allergy and Clinical Immunology
- American Indian or Alaska Natives
- Anesthesiology
- Anticoagulation
- Art and Images in Psychiatry
- Artificial Intelligence
- Assisted Reproduction
- Bleeding and Transfusion
- Caring for the Critically Ill Patient
- Challenges in Clinical Electrocardiography
- Climate and Health
- Climate Change
- Clinical Challenge
- Clinical Decision Support
- Clinical Implications of Basic Neuroscience
- Clinical Pharmacy and Pharmacology
- Complementary and Alternative Medicine
- Consensus Statements
- Coronavirus (COVID-19)
- Critical Care Medicine
- Cultural Competency
- Dental Medicine
- Dermatology
- Diabetes and Endocrinology
- Diagnostic Test Interpretation
- Drug Development
- Electronic Health Records
- Emergency Medicine
- End of Life, Hospice, Palliative Care
- Environmental Health
- Equity, Diversity, and Inclusion
- Facial Plastic Surgery
- Gastroenterology and Hepatology
- Genetics and Genomics
- Genomics and Precision Health
- Global Health
- Guide to Statistics and Methods
- Hair Disorders
- Health Care Delivery Models
- Health Care Economics, Insurance, Payment
- Health Care Quality
- Health Care Reform
- Health Care Safety
- Health Care Workforce
- Health Disparities
- Health Inequities
- Health Policy
- Health Systems Science
- History of Medicine
- Hypertension
- Images in Neurology
- Implementation Science
- Infectious Diseases
- Innovations in Health Care Delivery
- JAMA Infographic
- Law and Medicine
- Leading Change
- Less is More
- LGBTQIA Medicine
- Lifestyle Behaviors
- Medical Coding
- Medical Devices and Equipment
- Medical Education
- Medical Education and Training
- Medical Journals and Publishing
- Mobile Health and Telemedicine
- Narrative Medicine
- Neuroscience and Psychiatry
- Notable Notes
- Nutrition, Obesity, Exercise
- Obstetrics and Gynecology
- Occupational Health
- Ophthalmology
- Orthopedics
- Otolaryngology
- Pain Medicine
- Palliative Care
- Pathology and Laboratory Medicine
- Patient Care
- Patient Information
- Performance Improvement
- Performance Measures
- Perioperative Care and Consultation
- Pharmacoeconomics
- Pharmacoepidemiology
- Pharmacogenetics
- Pharmacy and Clinical Pharmacology
- Physical Medicine and Rehabilitation
- Physical Therapy
- Physician Leadership
- Population Health
- Primary Care
- Professional Well-being
- Professionalism
- Psychiatry and Behavioral Health
- Public Health
- Pulmonary Medicine
- Regulatory Agencies
- Reproductive Health
- Research, Methods, Statistics
- Resuscitation
- Rheumatology
- Risk Management
- Scientific Discovery and the Future of Medicine
- Shared Decision Making and Communication
- Sleep Medicine
- Sports Medicine
- Stem Cell Transplantation
- Substance Use and Addiction Medicine
- Surgical Innovation
- Surgical Pearls
- Teachable Moment
- Technology and Finance
- The Art of JAMA
- The Arts and Medicine
- The Rational Clinical Examination
- Tobacco and e-Cigarettes
- Translational Medicine
- Trauma and Injury
- Treatment Adherence
- Ultrasonography
- Users' Guide to the Medical Literature
- Vaccination
- Venous Thromboembolism
- Veterans Health
- Women's Health
- Workflow and Process
- Wound Care, Infection, Healing
Get the latest research based on your areas of interest.
Others also liked.
- Download PDF
- X Facebook More LinkedIn
Grubbs JB , Kraus SW. Binge Drinking Among Sports Gamblers. JAMA Netw Open. 2024;7(4):e245473. doi:10.1001/jamanetworkopen.2024.5473
Manage citations:
© 2024
- Permissions
Binge Drinking Among Sports Gamblers
- 1 Department of Psychology, Center on Alcohol, Substance use, And Addictions, University of New Mexico, Albuquerque
- 2 Department of Psychology, University of Nevada, Las Vegas
Over the past 6 years, sports wagering has become accessible to most individuals in the US via mobile applications or websites. 1 Increasing evidence suggests that sports wagering is associated with greater substance use and misuse, particularly alcohol, and symptoms of alcohol use disorder. 2 - 4 Alcohol consumption is higher among sports gamblers, 3 and sports gamblers often use substances while gambling. 5 Sports gamblers tend to be more inclined toward risk taking, suggesting that sports gambling may be associated with more risky alcohol use behaviors. 4 - 6 Accordingly, we examined whether individuals who wager on sports in the US are at greater risk of binge use of alcohol.
This survey study was approved by the Bowling Green State University Institutional Review Board; informed consent was obtained from all participants. The study followed the AAPOR reporting guideline.
From March 17 to April 6, 2022, we collected a census-matched sample of US adults with an oversample of adults who wager on sports. Full information about this survey is available elsewhere. 1 Race and ethnicity data were collected because they are potential factors in sports gambling likelihood and binge drinking habits. Among those reporting any past year alcohol use, binge drinking was assessed via the National Institute on Drug Abuse Quick Screen, version 1.0, which asks how often respondents consumed an excess of alcohol at a single time (≥5 drinks for men; ≥4 for women). Participants responded on a scale of 1 (never) to 5 (daily or more). Sports betting status was assessed by asking participants whether they had placed bets on sporting events or esports or participated in daily fantasy sports over the past 12 months.
Statistical analyses were conducted in SPSS, version 28. We conducted 2-tailed χ 2 analyses for distributions of past year binge drinking frequency, followed by multinomial logistic regressions estimating binge drinking frequency; P < .05 was considered statistically significant.
A total of 4363 respondents were included (51.4% men, 46.4% women, and 2.2% nonbinary or other; mean [SD] age, 49.6 [16.2] years) ( Table 1 ). The national census-matched survey consisted of 2806 participants (mean [SD] age, 48.9 [17.2] years; 1365 [48.6%] men and 1441 [51.4%] women; response rate, 2806 of 3203 [87.6%]). The oversample of sports gamblers consisted of 1557 participants (mean [SD] age, 41.7 [15.3] years; 1043 [67.0%] men and 514 [33.0%] women; response rate, 1557 of 1978 [78.7%]), of whom 1474 reported past year sports betting. Additionally, in the national sample, 338 respondents (12.0%) indicated they had gambled on sports in the past 12 months, resulting in a total of 1812 sports gamblers ( Table 1 ). Sports gamblers were disproportionally likely to be men and younger. In these combined samples, 3267 respondents (74.9%) reported past year alcohol use.
Sports wagerers were disproportionately more likely to report binge drinking at monthly or greater frequency over the past 12 months and were also disproportionately less likely to report no binge drinking episodes in the past 12 months ( Table 1 ). Multinomial logistic regressions adjusted for age and race and ethnicity showed that sports gamblers were substantially more likely to report higher levels of binge drinking ( Table 2 ), suggesting that elevated risky drinking episodes among sports gamblers are not due to demographic differences.
In this survey study, binge drinking in both men and women was reported at greater frequency among sports wagering individuals compared with nongamblers and non–sports gamblers. This study is limited by its cross-sectional design and use of nonprobability polling methods. Regardless, with past research showing that sports gamblers are more likely to report symptoms of alcohol use disorder, our results suggest that individuals who wager on sports use alcohol in particularly risky ways. Given the rapid spread of sports wagering in the US over recent years, this finding highlights an immense need for ongoing research, particularly to examine how novel gambling technologies influence the prevalence, presentation, and prevention of alcohol use disorders and related harms.
Accepted for Publication: February 9, 2024.
Published: April 1, 2024. doi:10.1001/jamanetworkopen.2024.5473
Open Access: This is an open access article distributed under the terms of the CC-BY License . © 2024 Grubbs JB et al. JAMA Network Open .
Corresponding Author: Joshua B. Grubbs, PhD, Center for Alcohol, Substance Use, and Addiction, University of New Mexico, 2650 Yale Blvd SE, Albuquerque, NM 87106 ( [email protected] ).
Author Contributions: Drs Grubbs and Kraus had full access to all of the data in the study and takes responsibility for the integrity of the data and the accuracy of the data analysis.
Concept and design: Both authors.
Acquisition, analysis, or interpretation of data: Both authors.
Drafting of the manuscript: Both authors.
Critical review of the manuscript for important intellectual content: Both authors.
Statistical analysis: Both authors.
Obtained funding: Both authors.
Administrative, technical, or material support: Grubbs.
Conflict of Interest Disclosures: Dr Grubbs reported receiving research grant funding from the Problem Gambling Network of Ohio outside the submitted work. Dr Kraus reported receiving personal fees from New York Council on Problem Gambling, the International Center for Responsible Gaming, the California Council on Problem Gambling, and Massachusetts General Hospital Psychiatry Academy, serving as editor-in-chief for Taylor & Francis journals, and receiving Summer research support from the Nevada Project on Problem Gambling during the conduct of the study. No other disclosures were reported.
Funding/Support: This study was supported by grants from the International Center for Responsible Gaming, the Kindbridge Research Institute, and the Problem Gambling Network of Ohio.
Role of the Funder/Sponsor: The funders had no role in the design and conduct of the study; collection, management, analysis, and interpretation of the data; preparation, review, or approval of the manuscript; and decision to submit the manuscript for publication.
Data Sharing Statement: See the Supplement .
- Register for email alerts with links to free full-text articles
- Access PDFs of free articles
- Manage your interests
- Save searches and receive search alerts
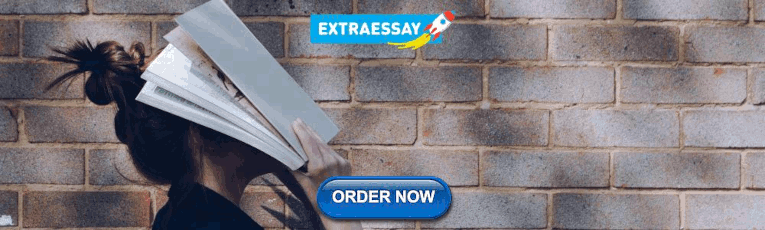
COMMENTS
In recent years (2017-2020) there has been an explosion of research on Internet addiction in young adults. In total, the meta-analysis consists of 30 studies with k = 37 samples from Europe, Asia, America and Oceania. The total sample of participants is 21,378, with 51.22% being male, 48.78% female (three studies do not provide data on the ...
Internet Addiction Disorder (IAD) ruins lives by causing neurological complications, psychological disturbances, and social problems. ... But due to the limitations of the study further research is necessary. THE TREATMENT OF INTERNET ADDICTION. There is a general consensus that total abstinence from the Internet should not be the goal of the ...
INTRODUCTION. Over the last 15 years, the number of Internet users has increased by 1000%[], and at the same time, research on addictive Internet use has proliferated.Internet addiction has not yet been understood very well, and research on its etiology and natural history is still in its infancy[].Currently, it is estimated that between 0.8% of young individuals in Italy[] and 8.8% of Chinese ...
Regarding the research design, the vast majority of studies on internet addiction are cross-sectional (n = 709; c = 85.84%), mostly gathering samples among individuals who may or may not fully represent attributes of the general population. Cross-sectional studies are primarily performed to estimate the prevalence and examine relevant risk ...
Purpose of Review This review describes recent research findings and contemporary viewpoints regarding internet addiction in adolescents including its nomenclature, prevalence, potential determinants, comorbid disorders, and treatment. Recent Findings Prevalence studies show findings that are disparate by location and vary widely by definitions being used. Impulsivity, aggression, and ...
Globally, Internet is a recognized form of leisure, but there are growing apprehensions about the increasing number of individuals developing an addiction to it. Recent research has focused on social issues associated with internet addiction (IA). However, the treatment of IA is currently unclear. This study aimed to explore the relationship between IA treatment outcomes and different ...
Empirical research has been produced on the topic of 'Internet Addiction' or 'Problematic Internet Use' (PIU) for more than 20 years, with a variety of theoretical approaches suggested by scholars to account for the behaviour. However, the discourse has been fraught with debate around construct definition, measurement, and validity.
Introduction. Internet addiction (IA), also referred to as problematic, pathological, or compulsive Internet use, is a controversial concept in the research field.The frequent use of different terms to describe this new phenomenon, linked to the advent and growth of the Internet, leads to confusion over what it really consists of Tereshchenko and Kasparov (2019).
Purposeof Review The prevalence of internet addiction and smartphone addiction has become a widespread global concern. Many studies have evaluated the impact of different interventions on these conditions; however, there is still a lack of integration of higher-level evidence. This review aims to assess the current evidence base of systematic reviews on the impact of different interventions on ...
Introduction. Given the ubiquity of the Internet, its evolving nature as a modern tool of society, and issues surrounding its excessive use and abuse by a minority of people, Internet addiction (IA) has become an increasingly important topic for dedicated research agendas from several scientific fields including psychology, psychiatry, and neuroscience.
Internet a ddiction during adolescence is a psychological phenomenon that has negative effects on mental health and. development. According to the results of the review, addiction is associated ...
1.1 Addiction and Internet Addiction Addiction is the compulsive abuse of a substance, but viewed by Morahan-martin (2 008) a s a neurobiological disorder.
Fourth, the development of theories of Internet addiction and research studies in this area has progressed slowly. Fifth, although general micro- and macro-theories can be used to understand Internet addiction, specific theories on Internet addiction are lacking. Sixth, while research shows correlates of Internet addiction in the ...
The study looked at adolescents aged between 10 and 19 years who are more prone to internet addiction given their transitional stage of physical and psychological development.
Despite the extensive usage of the internet, little is known about internet addiction among Americans during the pandemic. A valid and reliable questionnaire was deployed online via MTurk to recruit a national sample of adult Americans to understand the nature and extent of internet addiction. A total of 1305 individuals participated in the study where the majority were males (64%), whites (78 ...
Results: The results of the current study specified that the total mean score of the students for internet addiction and mental health was 3.81 ± 0.88 and 2.56 ± 0.33, correspondingly. The results revealed that internet addiction positively correlated with depression and mental health, which indicated a negative relationship (P > 0.001). The ...
In this clincial study, patients with internet addiction and either panic disorder or generalized anxiety disorder received medication for their anxiety and 10 sessions of modified CBT. All 39 patients showed improved anxiety and internet addiction scores reduced on average. [ PMC free article] [ PubMed] 75.••.
in to pattern and prevalence of internet addiction in university students. This study has also explored the association of internet addiction with depression and insomnia. Material and Methods: In this cross sectional study 954 subjects were enrolled who had been using internet for past 6 months. Information regarding pattern of use and socio demographic characteristics were recorded. Internet ...
Internet Use and Mental Health. Excessive use of the internet is known to negatively impact a person's mental health. It has been associated with mental health issues, such as loneliness ...
Since internet addiction is not officially recognized as a mental health condition, there's no clear consensus on symptoms. Research has identified a few key traits, however:. Excessive use.
Other studies have shown a positive correlation between internet addiction and general anxiety levels in students [59, 60]. As for somatization, there's a documented positive correlation with internet addiction in adolescents [61,62,63]. Research on somatization and smartphone addiction is somewhat limited . Results here confirm the positive ...
This study is a preliminary investigation of the extent of internet addiction in a management institute in India, where sampled were 300 students (first, second and third years' students). This ...
When Kyle Palmberg set out to design a research study as the capstone project for his psychology major at St. Mary's University of M i ... particularly a 2003 study on internet gambling addiction.
Background: The high prevalence of cannabis use among young adults poses substantial global health concerns due to the associated acute and long-term health and psychosocial risks. Digital modalities, including websites, digital platforms, and mobile apps, have emerged as promising tools to enhance the accessibility and availability of evidence-based interventions for young adults for cannabis ...
College students are particularly susceptible to developing excessive reliance on smartphones or online games due to having large amounts of free time outside of class (Aljomaa et al., 2016; Borges et al., 2019).Social anxiety is an important predictor of the development of internet-related addictive behaviors, such as smartphone addiction and Internet game addiction (Gioia et al., 2022; Ran ...
A systematic search of resources was conducted to investigate the effect of Internet addiction on the quality of life. The databases of PubMed, Cochrane Library, Scopus, Web of Science, Embase, and Science Direct were searched from January 1980 to July 2020. The articles were screened by two researchers in multiple levels in terms of the title ...
Background: The COVID-19 pandemic has had a significant global impact, with millions of cases and deaths. Research highlights the persistence of symptoms over time (post-COVID-19 condition), a situation of particular concern in children and young people with symptoms. Social media such as Twitter (subsequently rebranded as X) could provide valuable information on the impact of the post ...
Over the past 6 years, sports wagering has become accessible to most individuals in the US via mobile applications or websites. 1 Increasing evidence suggests that sports wagering is associated with greater substance use and misuse, particularly alcohol, and symptoms of alcohol use disorder. 2-4 Alcohol consumption is higher among sports gamblers, 3 and sports gamblers often use substances ...
Addiction is an SSA journal publishing peer-reviewed research reports on pharmalogical and behavioural addictions spanning many different disciplines. Abstract Background and aims Drug-related deaths in Scotland more than doubled between 2011 and 2020. ... Both studies aimed to estimate the size of the same underlying population, ...
There's a long journey to the publication of research findings. Scientists design experiments and studies to investigate questions about treatment or prevention, and follow certain scientific ...