2020 Theses Doctoral
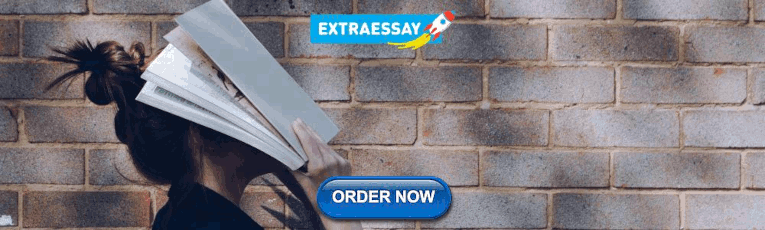
Design and Analysis of Smart Building Envelope Materials and Systems
Lin, Qiliang
As the largest consumer of electricity, the buildings sector accounts for about 76% of electricity use and 40% of all U.S. primary energy use and associated greenhouse gas (GHG) emissions. Research shows that a potential energy saving of 34.78% could be achieved by the smart buildings comparing to conventional buildings. Therefore, a smart management of building sectors becomes significantly important to achieve the optimal interior comfort with minimal energy expenditure. The ability of adaptation to the dynamic environments is considered the central aspect in smart building systems, which can be segmented into the passive adaptation and the active adaptation. The passive adaptation refers to the designs that do not change with the dynamic environment but improve the building overall performance by the integration of originally separated components, or by the application of advanced engineering materials. The active adaptation refers to the building management system (BMS) that actively responds and evolves with the changing environment, through the continuous monitoring of the surroundings via the sensor network, and the smart response through the controlling algorithms in the central controlling unit. This Ph.D. dissertation focuses on developing materials and systems for the smart building envelope, including a photovoltaic integrated roof with passive adaptation, and self-powered window systems with active responses environment. As the skin of a building, the building envelope provides the first level resistance towards air, water, heat, light and noise, which makes it the ideal target for the passive adaptation to the environments, as well as the perfect sensing location in the building management system for the active adaptation. This dissertation starts with a discussion of the building integrated photovoltaic thermal (BIPVT) roofing panel, including the fabrication, performance demonstration, and micromechanics-based theoretical modeling. The panel is structurally supported by a functionally graded material (FGM) panel made with high-density polyethylene as the matrix and aluminum particles as reinforcement. It prevents the heat from entering the building and directs the heat to the water tubes embedded inside the panel for the thermal energy harvesting, such that the overall energy efficiency is significantly improved. The design, fabrication and performance of the system is discussed, and an innovative non-destructive analysis method is developed to captures the authentic particle distribution of the FGM. As the main structural component, functionally graded material is comprehensively tested and modeled in elastic, thermoelastic, elastoplastic, and thermo-elastoplastic performance, based on the equivalent inclusion based method. An ensemble average approach was used to convert the particles’ interaction in the microscope to the averaged relation in the macroscope, such that both particle to matrix influence and particle to particle pair-wise interactions are characterized. The idea of the equivalent inclusion method extends to the plastic modeling of the FGM, by formulating an ensemble average form of the matrix stress norm in the macroscale that incorporate the local disturbance of particle reinforcement in the microscale. The accuracy of the proposed algorithm is verified and validated by comparing with another theory in homogeneous composite and experiments, respectively. To the best of the author’s knowledge, no prior theoretical algorithm has been proposed for the elastoplastic modeling of functionally graded materials. Therefore, the proposed algorithm can be used as a foundation and reference for further investigation and industry prediction of graded composites. Based on the theoretical modeling of the mechanical properties, a high order plate theory is also proposed in this dissertation to study for the thermo-mechanical performance of the FGM panel, to provide structural design guideline for the BIPVT panels. The shearing and bending behaviors are decomposed, solved independently, and combined to formulate the final solution. The shear strain components are assumed to follow a parabolic variation across the thickness, while the bending components follow the solution from classical plate theory. Closed-form solutions for the circular panel under different loadings are provided, with verification by comparing to other models and validation to experiments. Two smart window systems are proposed and demonstrated in this dissertation to actively monitor the building environment with active responses, and energy harvesting techniques are investigated to harvest energy from ambient environment the eternal power supply to the system. The thermoelectric powered wireless sensor network (TPWSN) platform is first demonstrated and discussed, where the energy is harvested from the temperature difference across the window frame. The TPWSN sits completely inside the window/façade frame with no compromise of the outlook and continuously monitors the building environment for the optimal control of the building energy consumption and indoor comfort. The energy harvesting technique grants eternal battery lifetime and significantly simplifies the installation and maintenance of the system with considerable saving of time and cost. In addition, the platform provides energy to various types of sensors for different kinds of sensing needs and store the data to the Google cloud for permanent storage and advanced analytics. The thermoelectric powered system works well for the sensors and microcontrollers but fails to provide enough power to the actuators. A novel sun-powered smart window blinds (SPSWB) system is designed, prototyped, and tested in this dissertation with solar energy harvesting on window blinds which provides enough power for the actuators. The thin-film photovoltaic cells are attached on one side of slats for energy harvesting and a PVdF-HFP coating is attached on the other side for the passive cooling. The voltage regulation and battery management systems are designed and tested, where a stable 55% energy efficiency from the PV into the battery has been achieved. The automatic control of the window blinds is accomplished with the help of sensors and a microcontroller. The energy equilibrium analysis is proposed and demonstrated with the local solar data to incorporate the influence of local weather conditions and solar zenith angle, from which we demonstrated that much more power than needed can be harvested. The abundant energy harvested validates the feasibility and the robustness of the system and proves its wide application potentials to various sensors and applications. In conclusion, both passive and active adaptations to the environment are investigated to build up the next generation of smart building envelope systems. The building integrated photovoltaic thermal roof is designed, fabricated, tested, and modeled in detail, which provides structural support to the external loads and improves the energy efficiency of buildings. The smart window/façade systems serve as a platform for various sensors and actuators via the energy harvesting from the ambient environment, and could significantly improve the energy expenditure with minimal impact of internal comfort.
- Civil engineering
- Electrical engineering
- Architecture and energy conservation
- Smart materials
- Building-integrated photovoltaic systems
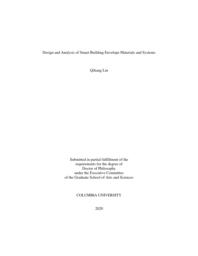
More About This Work
- DOI Copy DOI to clipboard
The Evolution of Smart Buildings: An Industrial Perspective of the Development of Smart Buildings in the 2010s
Marson, M. 2022. The Evolution of Smart Buildings: An Industrial Perspective of the Development of Smart Buildings in the 2010s. PhD thesis University of Westminster Architecture & Cities https://doi.org/10.34737/vvqx9
Related outputs
The human connection to an intelligent building Marson, M. and McAllister, J. 2021. The human connection to an intelligent building. Intelligent Buildings International. 13 (3), pp. 162-175. https://doi.org/10.1080/17508975.2021.1872480
The misleading simplicity of designing an intelligent city Marson, M. and Goncharov, K. 2021. The misleading simplicity of designing an intelligent city. Intelligent Buildings International. 13 (3), pp. 176-186. https://doi.org/10.1080/17508975.2020.1856636
Building Energy Management Systems Yang, T., Clements-Croome, D. and Marson, M. 2017. Building Energy Management Systems. in: Encyclopedia of Sustainable Technologies Elsevier.
Planning and Design Scenarios for Liveable Cities Clements-Croome, D., Marson, M., Yang, T. and Airaksinen, M. 2017. Planning and Design Scenarios for Liveable Cities. in: Encyclopedia of Sustainable Technologies Elsevier. pp. 81-97
Permalink - https://westminsterresearch.westminster.ac.uk/item/vvqx9/the-evolution-of-smart-buildings-an-industrial-perspective-of-the-development-of-smart-buildings-in-the-2010s
Usage statistics

UCL Energy Institute
- Partnerships

4-year PhD studentship in Smart Buildings Design and Digital Engineering
8 April 2022
The Energy Resilience and the Built Environment Centre for Doctoral Training and offering a 4 year studentship at UCL in partnership with AHMM investigating Smart Buildings Design and Digital Engineering.
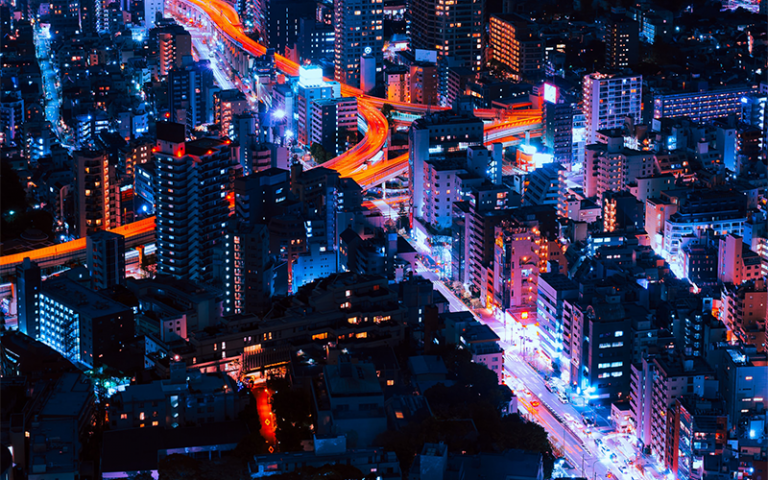
Key information
- Supervisors : Professor Dejan Mumovic , Dr Esfand Burman and Dr Craig Robertson
- Stipend : The studentship will cover UK course fees and an enhanced tax-free stipend of approx. £19,000 per year for 4 years along with a substantial budget for research, travel, and centre activities.
- Fees :ERBE CDT has very limited funding for applicants requiring coverage of overseas fees. We advise all interested applicants to be familiar with the changes to EU and International Eligibility for EPSRC/UKRI funded studentships
- Dates : 4 years from September 2022
- Deadline : Sunday 8 May 2022, 23:59 (UK time)
Project description
Carbon positive large developments take the concept of carbon-neutral design one step further by producing more energy on-site than the building requires to operate. Smart technologies are a crucial part of carbon positive design, and Smart Readiness Indices have been developed to indicate how suitable a building is for smart operation, e.g. adapting its operation to the needs of the occupant, optimising energy efficiency and overall performance and adapting its operation in reaction to signals from the grid (energy flexibility). These features will become an integral part of any industry-standard planning method that details each step of the process in a building construction project and will significantly influence early architectural decision making.
Understanding unintended consequences of full integration of Smart Readiness Indices in the 2020 RIBA Plan of Work and modifications required to support design decision making will be one of the key challenges for successful design, construction, and operation of carbon positive large developments.
Studentship aims
This PhD project aims to develop a decision-making framework for integration of smart readiness indices in the early architectural design process while endorsing energy design hierarchy.
Case studies of real building designs will be used to develop and ground the method. The student will investigate the most suitable approach but we envisage the following aspects:
a) Use of Smart Readiness Index (SRI) calculator to determine various “smartness” design options
b) Building performance simulation to determine operational performance and systems’ requirements of various “smartness” options,
c) Sensitivity and optimisation analysis,
d) Social research, for example multi criteria decision analysis (MCDA) workshops coupled with quantitative Analytic Hierarchy Process (AHP), to understand unintended consequences of energy flexibility and control strategies on early architectural design making especially RIBA stages 1-4.
How to apply
Please submit a pre-application by email to the UCL ERBE Centre Manager ( [email protected] ) with Subject Reference: 4-year PhD studentship in Smart Buildings Design and Digital Engineering.
The application should include all of the following: 1) A covering letter clearly stating why you are applying and how your interests and experience relate to this project, and your understanding of eligibility according to these guidelines: EU and International Eligibility for EPSRC/UKRI funded studentships 2) CV 3) Complete the CDT EPSRC fees background and EDI questionnaire via the linked Microsoft Forms.
Only shortlisted applicants will be invited for an interview.
- For the interview shortlisted candidates will be asked to show proof of their degree certificate(s) and transcript(s) of degree(s), and proof of their fees eligibility.
- The interview panel will consist of consist of the project’s academic supervisors at UCL, and a representative of the ERBE CDT Academic management The interview will include a short presentation from the candidate on their ideas of how to approach this PhD project.
Following the interview, the successful candidate will be invited to make a formal application to the UCL Research Degree programme for ERBE CDT.
Deadline for applications: Sunday, 8th May 2022 @ 23:59 (UK time) Interviews week commencing: TBC
For further details about the admission process, please contact: [email protected]
For any further details regarding the project, contact Professor Dejan Mumovic, [email protected]
You will be undertaking this project:
- In UCL at the main (Bloomsbury) campus as part of the new EPSRC-SFI Centre for Doctoral Training in Energy Resilience and the Built Environment (ERBE CDT). This is a collaboration between UCL, Loughborough University and Marine and Renewable Energy Ireland (MaREI). For more information please see https://erbecdt.ac.uk
- AHMM’s London office is a short commuting distance from UCL’s Bloomsbury campus.
Photo by Pawel Nolbert on Unsplash
Related News

Smart Buildings
Curious about what to expect when coming to campus? Please visit our Campus Learning page.
Learn about the digital technology and innovation that are changing the way we design, build, and use buildings forever. Create dynamic, responsive, and efficient spaces in which future generations will thrive. Everything in our buildings is, or soon will be, connected to the Internet. Explore how to build a networked intelligent building and how to take advantage of advanced analytics to increase its performance.
The way we learn has been rapidly altered by digital technologies. The fourth Industrial Revolution (or industry 4.0) is reshaping how business, institutions, and governments interact, collaborate, do business, and grow in a world full of economic, environmental, and social challenges. The real estate industry is scrambling to catch up with the digital revolution and technology era. “Smart Buildings” in itself is not a new term, but in today’s world it is taking on new meaning.
The buildings in which we work, live, learn, and play need to change accordingly in order to deliver productive, safe, secure, and energy-efficient spaces suited for today’s world. The time has come to incorporate the same digital capabilities that are changing our lives into our built environment, making for dynamic, responsive, and future-ready buildings.
A networked digital building is safe, secure, and can interact with its surrounding and occupants. These buildings can be personalized to the needs of each user. They are green, efficient, and cheaper to build and operate. The interaction of tenants with building systems and sensors provides a new paradigm through which we view our built environment. The greatest challenge we face is that the future is already here. Thus, our planning, design, construction, and building operations must quickly adapt to accommodate the changing possibilities and expectations.
The digital trends and lessons learned in this Smart Buildings program are grounded in a discussion around examples of real estate companies who have embraced digital technologies to help re-imagine their properties and deliver next-generation experiences and services to users/tenants.
After completing this program, participants will appreciate the transformation taking place and understand the impact of digital technology on design, construction, systems, lifecycle cost, environmental sustainability, and the future.
- Learn about the digital capabilities that change how we think about real estate and impact how we design, build, and operate our future buildings.
- Analyze operational considerations for the new built environment, with impact on cost, environment, quality, and user experiences.
- Articulate the impact of Smart Buildings on process, design, and construction.
- Learn how to create a digital transformation roadmap that will positively impact your future designs/buildings and increase your competitiveness in an increasingly digital world.
Architects, Analysts, Investors, Designers, Planners, Engineers, Contractors, Developers, Hoteliers, Landlords, Investors, Brokers, Realtors, Real Estate Agents, Retailers
Instructors

Rick Huijbregts
Principal, Oranje Consulting

Bill MacGowan
Director, Smart Building Digitization, Cisco Canada
Past Offering
Advertisement
Machine learning for energy consumption prediction and scheduling in smart buildings
- Research Article
- Published: 30 January 2020
- Volume 2 , article number 297 , ( 2020 )
Cite this article
- Safae Bourhnane ORCID: orcid.org/0000-0002-1785-5326 1 ,
- Mohamed Riduan Abid 2 ,
- Rachid Lghoul 2 ,
- Khalid Zine-Dine 3 ,
- Najib Elkamoun 1 &
- Driss Benhaddou 4
17k Accesses
66 Citations
6 Altmetric
Explore all metrics
Predicting energy consumption in Smart Buildings (SB), and scheduling it, is crucial for deploying Energy-efficient Management Systems. Most important, this constitutes a key aspect in the promising Smart Grids technology, whereby loads need to be predicted and scheduled in real-time to cope for the strongly coupled variance between energy demand and cost. Several approaches and models have been adopted for energy consumption prediction and scheduling. In this paper, we investigated available models and opted for machine learning. Namely, we use Artificial Neural Networks (ANN) along with Genetic Algorithms. We deployed our models in a real-world SB testbed. We used CompactRIO for ANN implementation. The proposed models are trained and validated using real-world data collected from a PV installation along with SB electrical appliances. Though our model exhibited a modest prediction accuracy, which is due to the small size of the data set, we strongly recommend our model as a blue-print for researchers willing to deploy real-world SB testbeds and investigate machine learning as a promising venue for energy consumption prediction and scheduling.
Similar content being viewed by others
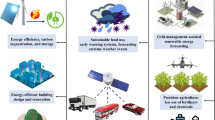
Artificial intelligence-based solutions for climate change: a review
Lin Chen, Zhonghao Chen, … Pow-Seng Yap
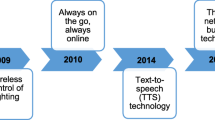
AI-big data analytics for building automation and management systems: a survey, actual challenges and future perspectives
Yassine Himeur, Mariam Elnour, … Abbes Amira
A Discussion of Mixed Integer Linear Programming Models of Thermostatic Loads in Demand Response
Avoid common mistakes on your manuscript.
1 Introduction
Smart Grids (SG) have emerged as a solution to the increasing demand on energy worldwide. The grid refers to the traditional electrical grid that is a collection of transmission lines, substations, and other components that make sure energy is delivered from the power plant to the home or business [ 1 ]. The smartness in the SG resides in the two-way communication between the utility and the customers, in addition to the sensing along the lines. The main components of a SG are controls, computers, automations, in addition to other new technologies that are working together to accommodate for the quick increase in the energy demand. The SG has many benefits among which we state: more efficient energy transmission, improving security, reducing peak demand which helps with the decrease of electricity rates, etc. SG are also known by the use of renewable energy sources.
The prediction and scheduling are two of the main pillars of efficient Energy Management Systems (EMS). EMSs are very crucial for the well-functioning of the SG. They are responsible for managing the power flux within the SG elements in order to minimize the costs and optimize the quality [ 2 ].
The prediction of the energy consumed by different appliances is one of the building blocks of the concept of SGs. The energy consumption can be seen as a nonlinear time series with a number of complex factors [ 3 ]. With many renewable energy sources used in the SGs, the energy prediction methods are getting more and more accurate, and hence, the prediction becomes a crucial part in the efficient planning of the entire SG.
There are different approaches that are used for the prediction of the energy consumption. The most popular ones use machine learning (ML).
Machine learning (ML) is one of the growing technical fields that merge between computer science and statistics. It tackles the issue of building computers that learn through experiences and hence provide more improved algorithms. ML keeps witnessing advances thanks to the new algorithms and the availability of online data, in addition to the accessibility of the computing power [ 4 ]. Artificial Neural Networks (ANN) are one of the ML algorithms are widely used in this context.
ANNs have seen light in the early 1940s but have not been widely used until lately. They became very popular thanks to the outstanding results they offer. They are very powerful with large datasets which gives the neural network enough data to train the model. In brief, ANNs are inspired by the way the brain processes information. They build an informational processing model that mimics the work of the neurons in the brain [ 5 ]. Their ability to learn quickly is what makes ANNs very powerful. This learning is done through an information flow that goes in two directions. Patterns from the training dataset are given to the ANN through the input neurons, then goes through the hidden layers and arrives to the output neurons.
Genetic Algorithms (GA) are considered the best solution for task and operation scheduling. They emerged from the research of Mr. John Holland conducted at the University of Michigan in 1960. However, it took them almost 30 years to become popular. The main purpose of GA is to solve complex problems where deterministic algorithms are considered an expensive solution. The Travelling Salesman Problem or the Knapsack problem are cases in point [ 6 ].
ANN and GA models are usually implemented in commodity computers or lately in Raspberry Pis. The NI CompactRIO is considered as a good alternative for deploying the ANN algorithms.
NI CompactRIO is a high-performance embedded controller with Input/Output modules. It has two targets: a real-time controller chassis, and an FPGA module. It includes a microprocessor to implement control algorithms and offer a support of a large pool of frequencies. The FPGA module is mainly used to accommodate for the high speed of certain modules and even certain programs. It deals with the data streaming from the I/O modules attached to the CompactRIO. The FPGA module is brought by Xilinx Virtex.
The CompactRIO is programmable using a specific graphical programming language named LabVIEW. This latter allows a better visualization of the data and an intuitive and easy way to implement control approaches.
In this paper, we are training an ANN model to predict the energy consumed by different appliances in a building. The model is developed in Python programming language but interfaced with LabVIEW for a potential integration in the NI CompactRIO.
The rest of the paper is organized as follows: Sect. 2 presents the scope of the research project under which this work is done. Section 3 contains the background of our work. In Sect. 4 , we present the implementation steps and discussing the results obtained. Then, we conclude and present our future work in Sect. 5 .
2 Literature review
A lot of work has been carried on in this area by different researchers in the community.
Authors in [ 7 ] are presenting a structure of a home energy management system to determine the best day-ahead scheduling for the different appliances. This scheduling is based on the hour price and the peak power-limiting-based demand response strategies. In addition to that, they introduced a realistic test-case in order to validate their schedule. The test showed a significant drop in the energy consumed by the different appliances thanks to the schedule they designed.
Sou Kin Cheong et al. presented in [ 8 ] a scheduling method for smart home appliances based on mixed integer linear programming. Furthermore, they took into consideration the expected duration and peak power consumption of the appliances. Based on a previously defined tariff, the proposed schedule achieved about 47% of cost saving. Furthermore, the authors demonstrated that very good solutions can be obtained using very little computational power.
Regarding the energy consumption prediction, a good amount of work has been published representing different attempts to predict the energy consumed by different appliances. Elkonomou presented in [ 9 ] a prediction method based on artificial neural network. In order to select the best architecture, the multilayer perceptron model was used to make a set of tests to select the one with the best generalization. Actual data about input and output was used in the training, validation, and testing process.
Authors in [ 10 ] are stating the fact that the building energy consumption prediction is crucial for efficient energy planning and management. To do the prediction, they are presenting a model that is data-driven and that allows for the energy consumption prediction. The review shows that the area of energy consumption prediction has a good amount of gaps that require more research to be filled: the prediction of long-term energy consumption, the prediction of energy consumed within residential buildings, and the prediction of energy consumed by the lighting in buildings. The lack of research in these areas can be due to the relatively small amount of data that is available.
3 Project scope
The work done in this paper falls under the scope of a research project named MiGrid.
The project mainly aims at creating a holistic testbed platform that couples smart buildings and renewable energy storage and production. The general architecture of the project is depicted in the Fig. 1 [ 11 ].
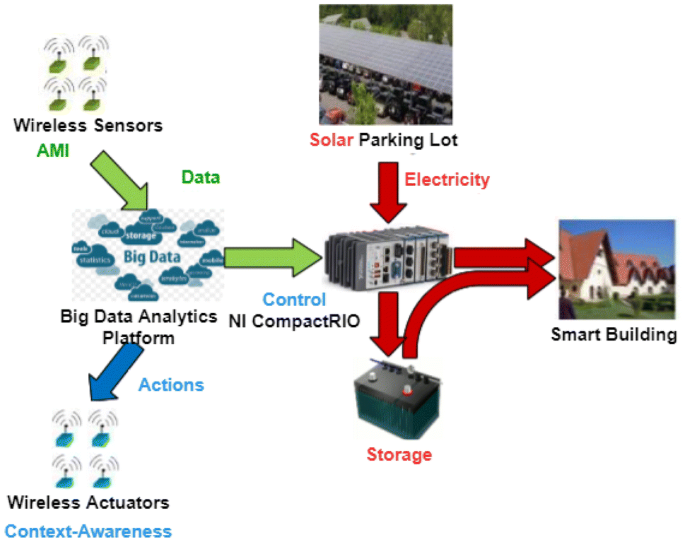
MiGrid general architecture
The testbed has six main components:
Wireless sensors This refers to a set of wirelessly connected sensors forming a Wireless Sensor Network. This is responsible for sensing data in a specific context.
Wireless actuators These are wirelessly connected actuators that are supposed to translate electrical signals into mechanical actions to act on appliances.
Big data analytics platform This is a platform that takes care of the processing and the storage of data stemming from the entire SG.
NI CompactRIO It is considered as the main controller of the system. It decides on whether to inject the energy produced to the grid, store it, or use it to power loads.
Storage device This consists of a lithium battery that is supposed to store the excess of energy and make it available for use when needed.
Solar parking lot The main renewable energy source in the system.
4 Background
4.1 smart grids.
By definition, a SG is nothing but an electrical grid that integrates a two-way communication system. In traditional electrical systems, electricity flows in one direction form the power plant to the home or business. The SG comes with an improvement to the network that resides in the on-the-spot feedback about the operations, power interruptions, and electricity consumption. This feedback is given back to the power plant and other operators [ 12 ].
The SG has the ability to tune itself to provide a better state of performance, and a better quality of the energy delivered. In addition to that, the SG is capable of anticipating problems and disturbances. Hence, the SG will allow for a more efficient transmission of electricity, lower kilowatt price, and quicker restoration.
The SG has three main components that are presented in Fig. 2 .
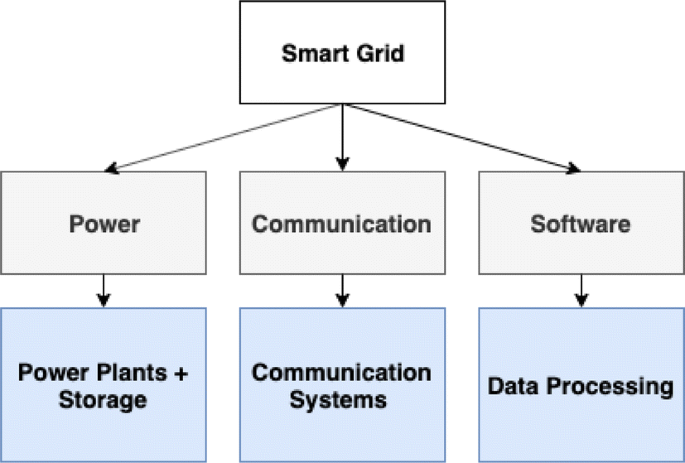
Smart grid’s components
Physical power assets This consists of the power lines, transformers, etc. The information given by these assets is used by the smart meters and wireless devices, in addition to microgeneration and storage devices.
Physical communication assets These are mainly the access and transport network (e.g. fiber) in addition to switches and routers. The main end users for this component are home area network.
Software and applications This is mainly about the distributed data processing (on-site, off-site, and virtualized). This includes the management of the grid, the load balancing, IT security, and other roles.
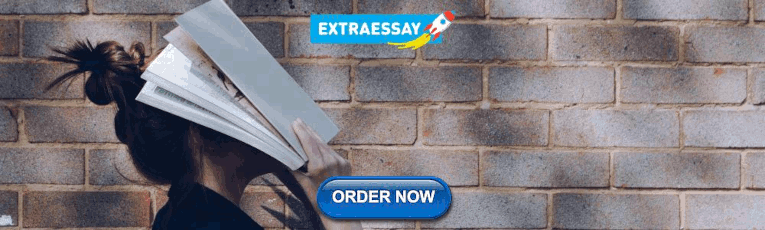
4.2 Energy management systems
EMSs are supposed to reduce energy consumption up to 20–30%. They are a set of connected hardware and software that have the following tasks:
Monitoring Collection of energy consumption related information in order to establish the basis and clarify the changes in targets.
Control Putting in place control algorithms in order to correct any deviations from the target.
The new EMS definition shows to be more people-centric than the older one. It engages users through connecting their actions with the energy consumption. The EMS gets the users involved by displaying their real-time energy consumption. Making the consumers aware of their daily energy usage can have a huge impact on their consuming behaviors [ 13 ].
EMSs are also well known for managing Microgrids whereby they have to process large amounts of data stemming from different sources. EMSs are supposed to use that data to control and monitor the Microgrid. Customers also can benefit from the EMS as it can provide them useful information inferred from the data generated by sensors (e.g. energy consumption, electricity prices, etc.) [ 14 ].
4.3 Machine learning
Machine learning is one of artificial intelligence (AI) applications. It involves providing systems with the ability to automatically learn and improve based on experiences without previous programming. This learning process is based on the exploration of large amounts of data.
It begins by closely observing the data to look for patterns that would help making better decisions. ML has a set of algorithms categorized as follows [ 15 ]:
Supervised machine learning algorithms It applies what was learnt from past data to predict future events. The system provides, after sufficient training, targets for new inputs. This algorithm has the possibility to compare the predicted output to the actual one.
Unsupervised machine learning algorithms It is used when the output needed to train the model is not classified or labeled. In other words, it infers a structure or pattern from hidden data.
Semi-supervised machine learning algorithms These algorithms fall in between the previous two categories as they use both labeled and unlabeled data to train models.
4.4 Time series forecasting methods
Several methods can be used in the forecasting of time series data. Classical time series are somehow sophisticated and perform well on a wide range of problems. In this section, we will be exploring the different methods existing.
4.4.1 Autoregression (AR)
The Autoregression model (AR) is a model that predicts the behavior by learning from previous ones. It works well with data that is related to entries that precedes it and that follows it. To create the model, only the past data is used, hence the name autoregression. The process of creating the model is nothing but a linear regression between the current data and the past data.
The AR model is a stochastic model that has a certain degree of uncertainty and randomness associated to it. This means that the model developed and tested will never deliver a 100% accuracy. It gets only close enough to be used in prediction scenarios.
AR models are also referred to as conditional models, Markov models, or transitions.
AR(p) models are autoregressive models where specific values are used to predict other values. The p in AR(p) is called the order, i.e. AR (1) is a regression model that is only interested in values that are one period apart. Eventually, models of second and third orders are related to values of two or three periods apart.
4.4.2 Moving average
A moving average (MA) is a well-known indicator that helps smoothing out actions by reducing the noise from random data. It is considered a trend-following because it is based on past data.
There are two main moving averages that are widely used: the simple moving average, and the exponential moving average. The simple moving average is the simple average of a security over a defined number of time slots. The exponential moving average focuses on the recent data entries and gives them higher weights. The most common applications of the MA are related to trend direction. This includes determining support and resistance levels.
Moving averages are useful enough by themselves, but they can also be combined with other technical indicators to deliver better results [ 16 ].
4.4.3 Autoregression integrated moving average
Autoregression integrated moving average (ARIMA) models are considered the most general class of time series forecasting models that can be used in conjunction with nonlinear alterations (e.g. logging).
There are two main models of ARIMA: seasonal, and non-seasonal models.
The main applications of this model are in areas of short-term forecasting which requires at least 40 past data points. It gives better results when the data used is stable over time with a minimum number of outliers.
4.4.4 Artificial neural networks
Artificial neural networks (ANN) are widely used in Machine Learning in general and in time series data forecasting in particular. ANNs are brain inspired and they intend to replicate the way that humans learn. They mainly consist of input and output layers in addition to hidden layers. ANNs are very powerful in finding patterns that are complex and numerous for humans to extract.
There are multiple types on ANNs, each one is used in specific scenarios and has a specific degree of complexity. The basic type is called feedforward neural network in which information travels in one direction (from input to output). Another widely used type is the recurrent neural network where data can flow in all directions. This type is used with complex tasks such as learning handwriting, face, and language recognition. Furthermore, convolutional neural networks and Boltzmann machine networks, and many others are used to solve machine learning problems.
ANNs learn in the same way that humans do: from past experiences. They require data to learn, and the more data they get, the more accurate the prediction. Data used to train ANNs is usually divided into three subsets: the training set which helps with establishing the required weights, the validation data set which helps fin-tuning the model, and the test set to see if the predicted output matches the actual output.
The main challenge that faces ANNs is the time needed to train the model, in addition to the required compute power that is needed for complex tasks. Furthermore, ANNs are considered black boxes that are fed with data to produce a certain output [ 17 ].
According to [ 18 ], neural networks perform better than many forecasting methods. Hence, we are opting for ANNs to develop our prediction model for energy consumption.
4.5 Genetic algorithms for tasks scheduling
A GA is a search method that is inspired by the theory of natural evolution. It mimics the natural selection process by selecting the fittest elements for the production of the next generation.
The natural selection process starts by identifying the fittest elements from the initial population. These elements produce what is called the offspring which inherits the characteristics of the parents and will be given to the next generation. If the fitness of the parents is good, the offspring will be better and hence the generations will survive. The same process keeps iterating until a new generation with the fittest elements is formed. This concept can be applied to search problems: a set of solutions is taken into consideration and the best solution is selected [ 19 ].
The GA goes through five phases:
Initial Population: Each process starts with a set of elements (also referred to as individuals) called population. Each element of the population is an actual solution to the problem.
Fitness Function It determines how fit each element is (i.e. its ability to compete with others). The function assigns a fitness score on which the probability of selection is based.
Selection In this phase, the fittest elements are selected, and their genes are passed to the next generation.
Crossover This is considered the most significant phase of the entire process. It consists of randomly choosing a crossover point from the genes of the parents to be mated.
Mutation In the offspring formed, some random genes can be subject to a mutation. This means that some of the bits in the string can be flipped.
The algorithm keeps executing until the population converges (starts producing offspring that is similar to the previous ones). Then, we can say that the GA has given a set of solutions to the problem.
The GA cycle is represented in the loop in Fig. 3 .
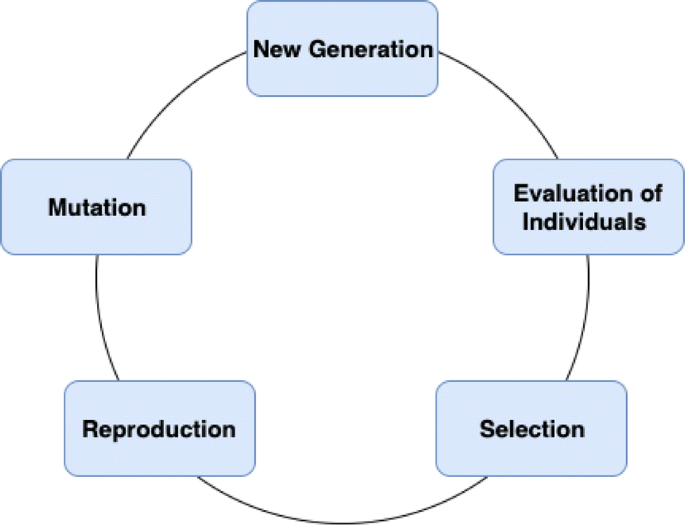
Genetic algorithm cycle
5 Implementation and results
5.1 data set.
To develop and train the model, we used a real-world dataset available online [ 20 ].
Some of the dataset’s attributes are shown in the list below:
Timestamp it shows the date and time when the energy consumption was recorded.
Main_i the energy consumption of the main room.
Ac the energy consumption of the air conditioner.
Living_rm the energy consumed by the living room appliances.
Fridge the energy consumption of the fridge.
Microwave the energy consumed by the microwave.
Furnace energy consumed by the heaters.
For our model, we used only data about the AC, fridge, furnace, and microwave. A sample of the data used is shown in Table 1 .
5.2 Artificial neural network model
To build the prediction model, we opted for a feedforward artificial neural network with two input neurons, ten hidden neurons and one output neuron.
The ANN we are using is depicted in Fig. 4 .
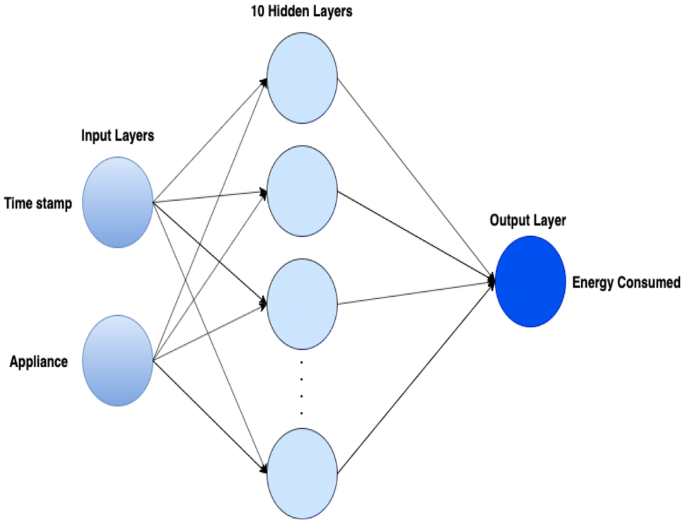
The model designed has as input the time stamp when the energy consumption has been recorded, and the appliance. For our case, we will be assigning a unique ID to each appliance and then feed it to the neural network. The appliances’ IDs are shown in the next table (Table 2 ).
The output layer of the model designed consists only of the energy consumed by each appliance.
Our input data is a 10 × 2 matrix (10 being the number of hidden neurons, and 2 being the number if input neurons), and the output data is a 10 × 1 matrix (1 being the number of output neurons).
After the first iteration, our model looks like Fig. 5 .
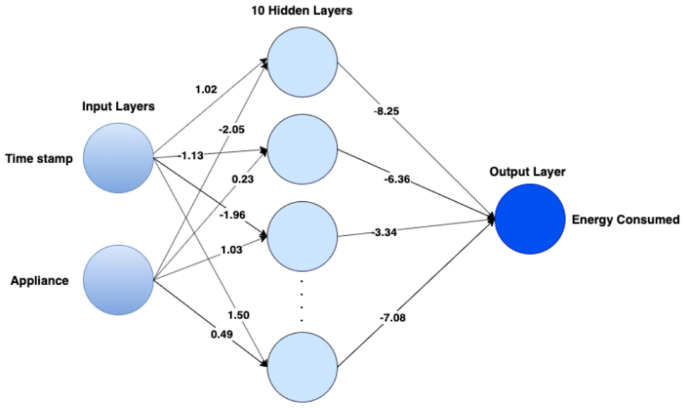
ANN after first iteration
To calculate the error of our model, we represented the loss function by using the mean sum squared loss. The loss value obtained through the training iterations is represented in Fig. 6 .
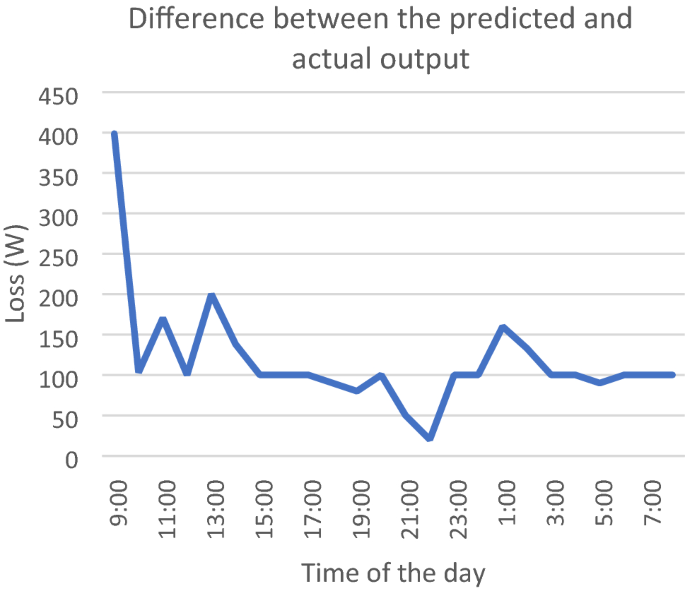
ANN model loss
The loss is found to be between 2.5% and 36% of the actual output.
The actual and predicted outputs are plotted in the Fig. 7 .
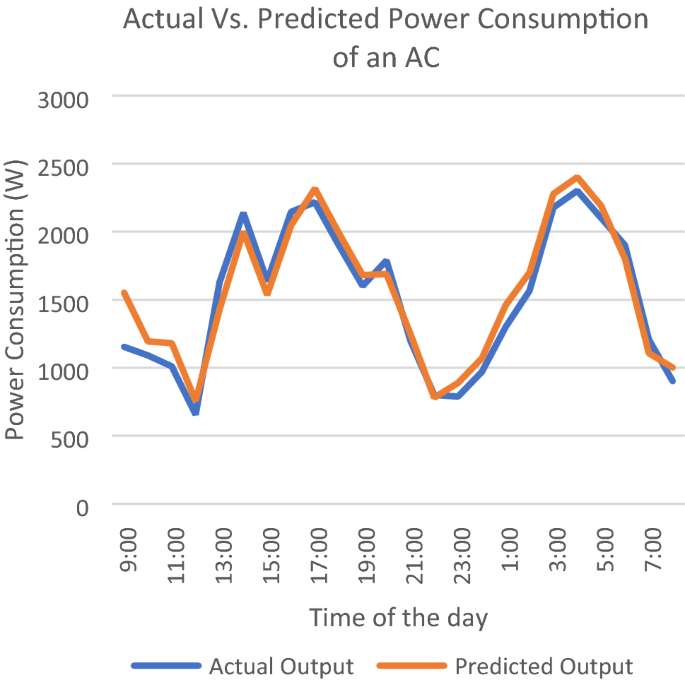
Actual versus predicted output
The study in [ 21 ] used artificial neural networks for the prediction of Greek long-term energy consumption. For this purpose, the authors made use of the multilayer perceptron model was investigated in order to test the different available architectures, and eventually select the optimal one. Furthermore, other prediction methods have been used, namely the linear regression and the support vector machine model. These models were compared to the ANN in terms of the accuracy.
The ANN model described showed a highest accuracy among all the tested prediction methods. The percentage error of the model was about 2%. This is mainly due to the high quality of the data used to train and validate the model. While we used data from an online source, the authors of this work have collected real records which had a great impact on the model output.
5.2.1 Genetic algorithm model
The GA model we are using takes into consideration the four appliances mentioned previously in this paper (i.e. AC, Fridge, Furnace, and Microwave).
Each appliance has a specific start time, working duration, and eventually a specific end time. These parameters are described in the diagram below (Fig. 8 ).
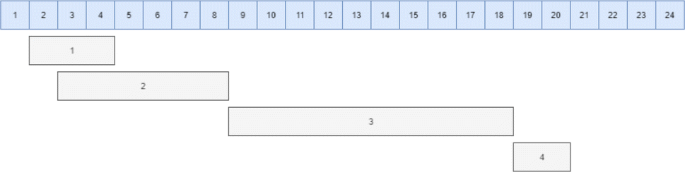
Appliances’ schedule
According to the diagram above, we have four resources that need to be scheduled daily. By scheduled, we mean for each appliances or resource, the start and end time need to be determined for each day (Table 3 ).
The data fed to the GA is represented as follows:
The GA model used is scored-based. Each combination is assigned a score which determines how accurate the schedule is. Hence the best schedule should have a score equal or closer to 0.
After training the model, we have the following schedule.
Start_times [0, 4, 8, 12, 16].
Appliances [1, 2, 2, 3, 4].
5.2.2 Deployment in CompactRIO using LabVIEW
Programming CompactRIO with LabVIEW is done through what is called a Virtual Instrument (VI). It has two components: a front panel and a block diagram. The front panel contains the design part of the VI. This means it includes buttons, labels, and indicators. The block diagram shows the actual wiring of the different components in the instrument.
Since we are integrating python with LabVIEW, we are following the architecture in Fig. 9 . The VI is to be deployed later on the CompactRIO controller.
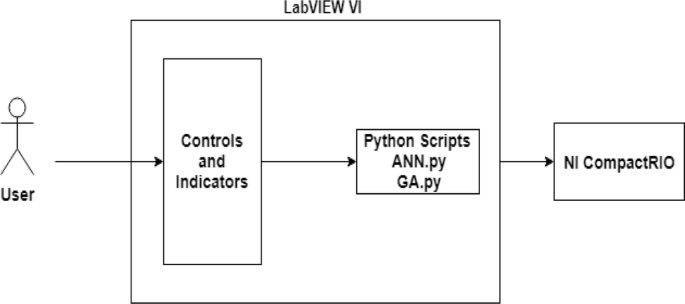
Program architecture
In the architecture presented above, the user is supposed to provide the program with input (mainly the time and the appliance ID for the energy consumption prediction). As a standalone python application, the input is given through the system arguments. After the integration with the LabVIEW VI, the input is given to the application through numeric controls implemented in the VI.
The inputs are then used by the python scripts through a LabVIEW library called “exec”.
Once the scripts finish execution, the results are shown in a numeric indicator.
The front panel of the VI used for this purpose is shown in Fig. 10 .
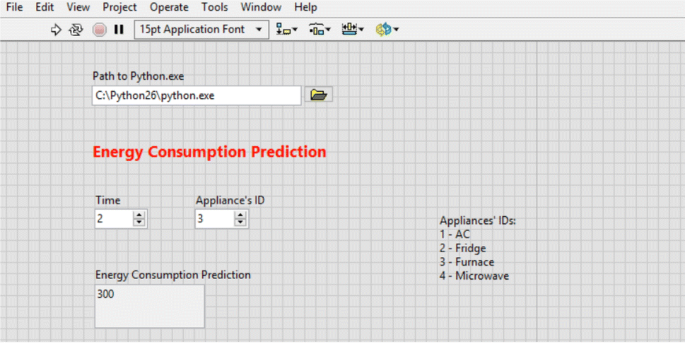
Front panel of the VI
In the front panel of the VI, we have two numerical controls where the user enters the time and the ID of the appliance. In addition to that, we introduced a numerical indicator that displays the predicted energy consumed at the time entered for a specific appliance.
The block diagram of the VI is shown in Fig. 11 .
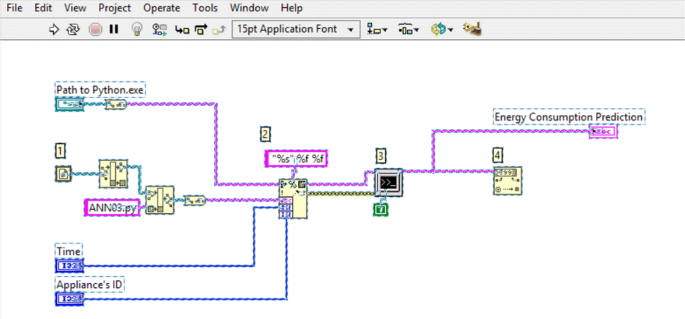
Block diagram of the VI
The VI has four main components. We are describing them below:
Build path to Python script, which is expected to be in the same folder as this VI. The Python script could be moved, but this VI would have to reflect that change.
Create command line argument, in the form of pythonpath scriptpath int32 int32. Quotation marks are used for the path, in case there are spaces in the path.
Execute the argument. The run in minimized, so that the command window does not quickly appear and disappear.
Convert the string output from Python to an int.
6 Discussion
As you may have noticed from the results, the models did not show a very high accuracy compared to other existing models. The loss of our ANN model is relatively high which makes it a non-reliable method for the energy consumption prediction. This is mainly due to the quality of data used. Our data did not go through any preprocessing.
Also, as ANNs need to learn from the data provided, our dataset did not help the ANN to learn and hence the final model was poor.
There is a huge ongoing debate that is taking place between practitioners on where to go for ANNs or statistical methods for the prediction. The article in [ 22 ] contains a study that compares ANNs to regression with a medium to large dataset of patients (size > 200). According to the review, ANN outperformed the regression method in 36% of the cases and was outperformed in 14% of them. However, as the dataset size increased (size > 5000), regression showed a better performance.
Authors in [ 23 ] have conducted a comparative study between ANNs and statistical methods in the prediction of consumer’s behavior. As results, they found that ANNs constantly win the battle against other methods. Due to the flexibility of the models, they were able to perform better with both known and unknown choice rules. The ANN model used in this study is iterative, this implies that the model learns complex attributes about consumers.
ANNs are different from other statistical methods in the was that they do not expect any relationship between the input and output variables. However, the model overfitting can represent a real problem when conceiving an ANN model. Hence, one needs to make sure that the data fed to the model is preprocessed.
7 Conclusion and future work
In this paper, we delineated the details of deploying a machine-learning-based model for energy consumption prediction and scheduling in Smart Buildings. The presented model can serve as a roadmap for deploying real-world SB testbeds, and thus paving the way towards grounded research around Smart Buildings for Smart Grids.
We integrated the models using python in a LabVIEW program by creating a VI instance that allows the user to select an appliance, along with the time of the day, and then compute relevant predicted consumption using our ANN-based model. The models did not show very high accuracy as the dataset used was not that big enough for training and validation. When looking, and analyzing, similar models in the literature using ANNs for prediction, we came to the conclusion that ANNs are not always the best and they can be outperformed by basic statistical methods, regression being a case in point. Nevertheless, this remains an ideal venue to investigate for researchers seeking machine learning as long as they can afford good data, ideally a big one.
As a future work, we intend to collect further data from our testbed, and get some relevant big data as well, in order to tailor better models. In our testbed, we are intending to further gather PV production data, energy consumption of different appliances, and the context data (i.e. motion, temperature, humidity, etc.). Last, but not least, we will deepen our investigation of existing prediction methods by namely incorporating statistical approaches as well.
SmartGrids.gov, What is the Smart Grid? [En ligne]. https://www.smartgrid.gov/the_smart_grid/smart_grid.html . [Accès le 22 April 2019]
Arcos-Aviles Diego et al (2016) Fuzzy logic-based energy management system design for residential grid-connected microgrids. IEEE Trans Smart Grids 9(12):530–543
Google Scholar
Mocanu Elena et al (2016) Unsupervised energy prediction in a Smart Grid context using reinforcement cross-building transfer learning. Energy Build 116:646–655
Article Google Scholar
Jordan I et al (2015) Machine learnig: trends, perspective, and prospects. Science 349(16245):255–260
Article MathSciNet Google Scholar
Dacombe J (2017) An introduction to artificial neural networks (with example), 23 Oct 2017. [Online]. https://medium.com/@jamesdacombe/an-introduction-to-artificial-neural-networks-with-example-ad459bb6941b . Accessed 22 Apr 2019
Sablier (2019) Genetic algorithms [Online]. https://www.codingame.com/playgrounds/334/genetic-algorithms/history . Accessed 1 May 2019
Paterakis NG, Erdinç O, Bakirtzis AG, Catalão JPS (2015) Optimal household appliances scheduling under day-ahead pricing and load-shaping demand response strategies. IEEE Trans Ind Inform 11(16):1509–1519
Cheong SK et al (2011) Scheduling smart home appliances using mixed integer linear programming. In: 50th IEEE conference on decision and control and European control conference, IEEE. https://doi.org/10.1109/cdc.2011.6161081
Ekonomou L (2010) Green long-term energy consumption prediction using artificial neural network. Energy 35(12):512–517
Amasyali K et al (2018) A review of data-driven building energy consumption prediction studies. Renew Sustain Energy Rev 81(11):1192–1205
Abid MR, Lghoul R, Benhaddou D (2017) ICT for renewable energy integration into smart buildings: IoT and big data approach. In: IEEE Africon, pp 883–888. https://doi.org/10.1109/afrcon.2017.8095594
Marc Lanllanilla SW (2013) What is a smart grid? LiveScience, 12 Dec 2013 [Online]. https://www.livescience.com/41920-smart-grid.html . Accessed 23 Apr 2019
Atin (2013) Energy managment system, 17 Sept 2013 [Online]. http://www.sensegrow.com/article/what-energy-management-system . Accessed 25 Nov 2019
Talei H, Essaidi M, Benhaddou D (2018) An end to end real time architecture for analyzing and clustering time series data: case of an energy management system. In: 6th international renewable and sustainable energy conference (IRSEC), 2018
Expert System (2017) What is machine learning? A definition, 3 Mar 2017 [Online]. https://expertsystem.com/machine-learning-definition/ . Accessed 3 Dec 2019
Hayes A (2019) Moving average (MA), 04 Apr 2019 [Online]. https://www.investopedia.com/terms/m/movingaverage.asp . Accessed 27 Apr 2019
Dormehl L (2019) What is an artificial neural network? Here’s everything you need to know. Digital Trends, 05 Jan 2019 [Online]. https://www.digitaltrends.com/cool-tech/what-is-an-artificial-neural-network/ . Accessed 27 Apr 2019
Hill T et al (1996) Neural network models for time series forecasts. Manag Sci 42(17):1082–1092
Mallawaarachchi V (2017) Introduction to genetic algorithms—including example code, 08 July 2017 [Online]. https://towardsdatascience.com/introduction-to-genetic-algorithms-including-example-code-e396e98d8bf3 . Accessed 01 May 2019
Ewood (2016) Home energy consumption, 05 Aug 2016 [Online]. https://data.world/ewood/home-energy-consumption/workspace/file?filename=download+%288%29.csv# . Accessed 27 Apr 2019
Ekonomou L (2009) Greek long-term energy consumption prediction using artificial neural network. Energy 35(1):512–517
Sargent DJ (2001) Comparison of artificial neural networks with other statistical approaches. Cancer 91(S8):1636–1642
West Patricia M et al (1997) A comparative analysis of neural networks and statistical methods for predicting consumer choice. Mark Sci 16(14):370–391
Download references
Acknowledgment
This work is sponsored by US-NAS/USAID under the PEER Cycle 5 project grant# 5-398, entitled ‘Towards Smart Microgrid: Renewable Energy Integration into Smart Buildings”.
Author information
Authors and affiliations.
LAROSERI Lab., Faculty of Sciences, Chouaib Doukkali University, El Jadida, Morocco
Safae Bourhnane & Najib Elkamoun
School of Science and Engineering, Al Akhawayn University, Ifrane, Morocco
Mohamed Riduan Abid & Rachid Lghoul
Mohammed V University, FSR, Rabat, Morocco
Khalid Zine-Dine
School of Engineering and Technology, University of Houston, Houston, USA
Driss Benhaddou
You can also search for this author in PubMed Google Scholar
Corresponding author
Correspondence to Safae Bourhnane .
Ethics declarations
Conflict of interest.
The authors declare that they have no conflict of interest.
Additional information
Publisher's note.
Springer Nature remains neutral with regard to jurisdictional claims in published maps and institutional affiliations.
Rights and permissions
Reprints and permissions
About this article
Bourhnane, S., Abid, M.R., Lghoul, R. et al. Machine learning for energy consumption prediction and scheduling in smart buildings. SN Appl. Sci. 2 , 297 (2020). https://doi.org/10.1007/s42452-020-2024-9
Download citation
Received : 14 October 2019
Accepted : 09 January 2020
Published : 30 January 2020
DOI : https://doi.org/10.1007/s42452-020-2024-9
Share this article
Anyone you share the following link with will be able to read this content:
Sorry, a shareable link is not currently available for this article.
Provided by the Springer Nature SharedIt content-sharing initiative
- Smart Grids
- Smart buildings
- Renewable energy
- Find a journal
- Publish with us
- Track your research
- DUT Open Scholar
- Faculty of Engineering and Built Environment
- Theses and dissertations (Engineering and Built Environment)
Files in This Item:
Page view(s), download(s).
Google Scholar TM
Items in DSpace are protected by copyright, with all rights reserved, unless otherwise indicated.
Select language

PhD Dissertation: Smart Urban Governance: Governing Cities in the “Smart” Era
Building a smart city requires “smart” governance approaches, including new government structures, new relationships, and new processes. My doctoral research conceptualizes smart urban governance that can achieve better governance processes and outcomes in the “smart” era and investigates the role that ICT can play in smart urban governance. The conceptualization of smart urban governance responds to arguments that alternatives to corporate-led, technocratic smart governance should take the urban into account and be more aware of how urban issues and the relevant smart solution are socially produced. It explores the role of the situated agents and their contribution to promoting a demand-driven smart governance. It also highlights a sociotechnical approach to ICT development and implementation. In other words, it is necessary to understand why smart technologies are designed, developed, and implemented as an appropriate answer to the perceived and constructed problems. Finally, smart urban governance highlights the importance of contextual factors for finding solutions to urban challenges. We therefore propose smart urban governance as a sociotechnical way of governing cities in the smart era by starting with the urban issue at stake, promoting demand-driven governance modes, and shaping technological intelligence more socially, given the specific context.
- Share on LinkedIn
- Share on Facebook
Utrecht University Heidelberglaan 8 3584 CS Utrecht The Netherlands Tel. +31 (0)30 253 35 50
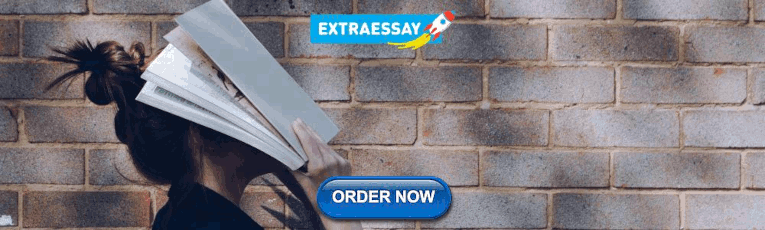
IMAGES
VIDEO
COMMENTS
Q1 Introduction to Smart Building devices. Smart Buildings are intelligent buildings that are using adaptable control systems, regenerative materials, and intelligent construction. To achieve the optimal combination of overall comfort and energy consumption, smart buildings use computer and IoT technologies.
As the largest consumer of electricity, the buildings sector accounts for about 76% of electricity use and 40% of all U.S. primary energy use and associated greenhouse gas (GHG) emissions. Research shows that a potential energy saving of 34.78% could be achieved by the smart buildings comparing to conventional buildings. Therefore, a smart management of building sectors becomes significantly ...
The thesis is composed of three parts. The first chapter presents the methodology of the thesis. It presents the architecture and construction of a novel smart building system that could monitor and control buildings use safely and optimally and could be easily used in buildings management. It includes nine modules that inter-communicate.
PhD program in Electronics Engineering Advanced energy management strategies for HVAC systems in smart buildings Doctoral thesis by: Enric Sala Cardoso Thesis advisors: Dr. Luis Romeral Dr. Miguel Delgado Prieto Department of Electronics Engineering Terrassa, September, 2019 .
5 Department of Mechanical Engineering, Mississippi State University, Starkville, MS 39759, USA. * Correspondence: [email protected] (K.L.); [email protected] (H.C.) Abstract: The building ...
This thesis studies the economic impact of Smart, Connected and Green buildings upon rents and transaction prices. Using numerous data sources, we identify buildings that offer at least one so-called "smart" amenity and link them with the building's achieved rent and transactions prices as well as to other so-called "innovation" amenities, like ...
The Evolution of Smart Buildings: An Industrial Perspective of the Development of Smart Buildings in the 2010s: Type: PhD thesis: Authors: Marson, M. Abstract: Over the course of the 2010s, specialist research bodies have failed to provide a holistic view of the changes in the prominent reason (as driven by industry) for creating a smart building.
Dissertation: Smart Buildings and Digital Engineering; Doctoral Research (PhD) Our doctoral researchers cover a broad range of topics in the broader context of our UCL Institute for Environmental Design and Engineering vision and themes, looking at factors related to both the physical environment and people.
demands of customers in managing the loads and DERs within the smart homes and buildings from both financial and environmental points of view. Thus, the Energy Management System (EMS), acting as the 'manager' in the smart homes and buildings, will afford the challenging responsibilities in helping customers optimise the operation
an application can be written once and deployed on 100s or 1000s of buildings. This thesis evaluates the challenges with existing metadata of sensors in smart-buildings and proposes ways to normalize it to uniform standard that would allow scalable (write-once and deploy everywhere) application development.
information made possible by smart devices. This creates an opportunity to enhance productivity and communication among stakeholders of the construction industry. Firstly, this thesis explored the concept of smart devices. Secondly, the drivers, challenges and Critical Success Factors for implementing smart devices were investigated.
ENERGY OPTIMIZATIONS FOR SMART BUILDINGS AND SMART GRIDS. A Dissertation Presented by ADITYA K. MISHRA Approved as to style and content by: Prashant Shenoy, Chair Jim Kurose, Member ... James Allan, Chair College of Information and Computer Sciences. ACKNOWLEDGMENTS. The process of PhD is challenging in many ways, and it would have been di cult ...
Smart technologies and controls are becoming increasingly important not only in research and development (R&D) but also in industrial and commercial domains, leading to a steady growth in their ...
How to apply. Please submit a pre-application by email to the UCL ERBE Centre Manager ([email protected]) with Subject Reference: 4-year PhD studentship in Smart Buildings Design and Digital Engineering.The application should include all of the following: 1) A covering letter clearly stating why you are applying and how your interests and experience relate to this project, and your ...
Smart Buildings. Campus Learning. Learn about the digital technology and innovation that are changing the way we design, build, and use buildings forever. Create dynamic, responsive, and efficient spaces in which future generations will thrive. Everything in our buildings is, or soon will be, connected to the Internet. Explore how to build a ...
Predicting energy consumption in Smart Buildings (SB), and scheduling it, is crucial for deploying Energy-efficient Management Systems. Most important, this constitutes a key aspect in the promising Smart Grids technology, whereby loads need to be predicted and scheduled in real-time to cope for the strongly coupled variance between energy demand and cost. Several approaches and models have ...
1. The Impact of Using Smart Architecture on Architectural Creativity. Basma S. Saad, Essam Eldin B. Abou Al- Einen and Faysal M. Abo El-Azm. ABSTRACT. Smart Architecture is considered as the most ...
Therefore, this dissertation seeks to asseeement of the adoption of smart building concepts in the Nigerian construction industry. The research poses the following questions: 1) What is the awareness level of construction professionals in the adoption of the smart building concept (SBCs) in the Nigerian construction industry?
Building a smart city requires "smart" governance approaches, including new government structures, new relationships, and new processes. My doctoral research conceptualizes smart urban governance that can achieve better governance processes and outcomes in the "smart" era and investigates the role that ICT can play in smart urban governance.
I create 1) mathematical models of integrated energy systems; 2) computational tools to design and operate them in new ways; and 3) software prototypes to conduct real-world efficiency and flexibility experiments with cyber-physical systems. I wrote my PhD dissertation in Stanford's Energy Science & Engineering department, advised by Profs.
The distinctive paper is devoted to contemporary problems of numerical modelling of unique structures, buildings and facilities and corresponding directions of activity of Scientific Research ...
Ali Saad studied Architecture at TU Berlin and UPC Barcelona with grants from the German National Academic Foundation and the European Commission. Between 2004 and 2007 he was assistant professor, lecturer and research fellow at the TU Berlin conducting research and teaching on the transformation of Post-war New Towns in Europe, the Middle East and South Asia, and a consultant to the giz in ...
This talk will draw from Otálvaro-Hormillosa's book, Erotic Resistance: The Struggle for the Soul of San Francisco (University of California Press, 2024) and explore the overlapping cultures of psychedelics, drag, erotic performance, and social justice movements that were unique to San Francisco during this time period. Gigi Otálvaro-Hormillosa, Ph.D. is an interdisciplinary artist, writer ...
Get directions to Yaroslavskoye shosse, 1 and view details like the building's postal code, description, photos, and reviews on each business in the building Yaroslavskoye shosse, 1 Coordinates :