Thank you for visiting nature.com. You are using a browser version with limited support for CSS. To obtain the best experience, we recommend you use a more up to date browser (or turn off compatibility mode in Internet Explorer). In the meantime, to ensure continued support, we are displaying the site without styles and JavaScript.
- View all journals
- Explore content
- About the journal
- Publish with us
- Sign up for alerts
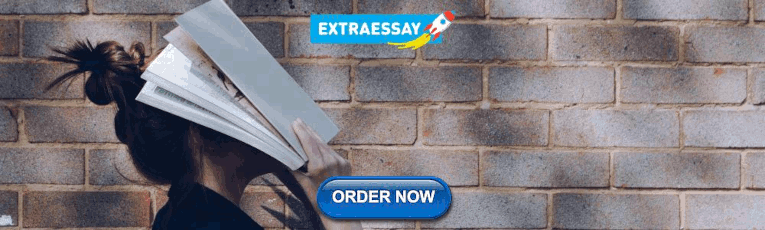
Behavioural genetics articles within Nature Genetics
Article 20 November 2023 | Open Access
Multi-ancestry genome-wide association study of cannabis use disorder yields insight into disease biology and public health implications
Multi-ancestry genome-wide association meta-analyses identify risk loci for cannabis use disorder. Genomic structural equation modeling and genetic correlation analyses show overlap with several other traits, including impulsivity and psychopathology.
- Daniel F. Levey
- , Marco Galimberti
- & Joel Gelernter
News & Views | 13 July 2023
Leveraging family relatedness to detect participation bias in genetic studies
Identifying the ways that a study sample is not representative is essential for maximizing the generalizability of findings to the population. A new method proposes discerning non-representativeness in large-scale genetic studies by comparing the genotypes of closely related participants.
- Mark J. Adams
Technical Report 13 July 2023 | Open Access
Studying the genetics of participation using footprints left on the ascertained genotypes
A new methodology to study participation genetics via analyzing shared and unshared genotypes is applied to the UK Biobank, suggesting that participating in genetic studies is a complex trait with a meaningful genetic component.
- Stefania Benonisdottir
- & Augustine Kong
Article 12 June 2023 | Open Access
Rare coding variants in CHRNB2 reduce the likelihood of smoking
An exome-wide association study of six smoking phenotypes in up to 749,459 individuals identifies associations of rare coding variants in CHRNB2 that may reduce the likelihood of smoking.
- Veera M. Rajagopal
- , Kyoko Watanabe
- & Giovanni Coppola
Research Highlight | 12 May 2023
Shared genetics of substance use disorders
Research Highlight | 13 January 2023
Mapping dog behavior
Article | 26 September 2022
Identification of shared and differentiating genetic architecture for autism spectrum disorder, attention-deficit hyperactivity disorder and case subgroups
Cross-disorder genetic association analyses identify five loci differentiating attention-deficit hyperactivity disorder (ADHD) and autism spectrum disorder (ASD). Individuals diagnosed with both ADHD and ASD are double-loaded with genetic risk for both disorders.
- Manuel Mattheisen
- , Jakob Grove
- & Anders D. Børglum
Article 22 August 2022 | Open Access
Gene–environment correlations across geographic regions affect genome-wide association studies
Analyses of gene–environment correlations across geographic regions for 56 complex traits in UK Biobank suggest that both passive and active sources of gene–environment correlation affect genetic association signals.
- Abdel Abdellaoui
- , Conor V. Dolan
- & Michel G. Nivard
Research Highlight | 11 July 2022
Keeping the beat
Article 02 June 2022 | Open Access
Genetic correlates of phenotypic heterogeneity in autism
Comprehensive factor analysis of core diagnostic features provides insights into the complex genetic architecture underlying phenotypic heterogeneity in autism.
- Varun Warrier
- , Xinhe Zhang
- & Simon Baron-Cohen
Article | 02 June 2022
A phenotypic spectrum of autism is attributable to the combined effects of rare variants, polygenic risk and sex
Integrated analyses in a large collection of families provide insights into the combined effects of rare variants and polygenic risk on autism spectrum disorder.
- Danny Antaki
- , James Guevara
- & Jonathan Sebat
Article | 11 April 2022
Exome sequencing in bipolar disorder identifies AKAP11 as a risk gene shared with schizophrenia
Exome sequencing analysis of 13,933 individuals with bipolar disorder finds enrichment of ultra-rare protein-truncating variants in constrained genes. Combined analysis with schizophrenia exome data identifies AKAP11 as a risk gene for both disorders.
- Duncan S. Palmer
- , Daniel P. Howrigan
- & Benjamin M. Neale
World View | 04 April 2022
Exploring the epigenetics of resilience
To do good science, we need to include diverse perspectives, work across disciplines and think outside the box while reminding ourselves that our goal as scientists is to serve humanity. I am sharing my story to encourage others to trust their gut feelings and to have the courage to see what everyone sees, but think what no one has thought.
- Rana Dajani
Article 31 March 2022 | Open Access
Polygenic prediction of educational attainment within and between families from genome-wide association analyses in 3 million individuals
A genome-wide association study in ~3 million individuals identifies 3,952 independent variants associated with educational attainment. A polygenic index explains 12–16% of variance for this trait and contributes to risk prediction for ten diseases.
- & Alexander I. Young
Comment | 06 September 2021
FoGS provides a public FAQ repository for social and behavioral genomic discoveries
Here we introduce ‘FAQs on Genomic Studies’ (FoGS), an open-access repository of explanatory documents that accompany genomic analyses in social and behavioral genomics. For fields such as social and behavioral genomics that are shaped by an ugly history and uncertain future, socially and ethically responsible research and research communication are crucial. FoGS amplifies one such approach towards responsible research communication.
- Daphne Oluwaseun Martschenko
- , Benjamin W. Domingue
- & Sam Trejo
Article | 22 April 2021
Genetic analyses identify widespread sex-differential participation bias
Genetic analyses identify widespread sex-differential participation bias in population-based studies and show how this bias can lead to incorrect inferences. These findings highlight new challenges for association studies as sample sizes continue to grow.
- Nicola Pirastu
- , Mattia Cordioli
- & Andrea Ganna
Article | 19 August 2019
A global overview of pleiotropy and genetic architecture in complex traits
Systematic analyses of large-scale genome-wide association data provide an overview of pleiotropy and genetic architecture for hundreds of human complex traits and diseases.
- Kyoko Watanabe
- , Sven Stringer
- & Danielle Posthuma
Article | 14 January 2019
Genome-wide association analyses of risk tolerance and risky behaviors in over 1 million individuals identify hundreds of loci and shared genetic influences
A genetic study identifies hundreds of loci associated with risk tolerance and risky behaviors, finds evidence of substantial shared genetic influences across these phenotypes, and implicates genes involved in neurotransmission.
- Richard Karlsson Linnér
- , Pietro Biroli
- & Jonathan P. Beauchamp
Article | 23 July 2018
Gene discovery and polygenic prediction from a genome-wide association study of educational attainment in 1.1 million individuals
Gene discovery and polygenic predictions from a genome-wide association study of educational attainment in 1.1 million individuals.
- James J. Lee
- , Robbee Wedow
- & David Cesarini
Analysis | 15 May 2017
Polygenic transmission disequilibrium confirms that common and rare variation act additively to create risk for autism spectrum disorders
Elise Robinson and colleagues present the polygenic transmission disequilibrium test (pTDT) for evaluating transmission of polygenic risk in family-based study designs. The authors apply pTDT to a cohort of autism spectrum disorder (ASD) families and find that common polygenic variation acts additively with de novo variants to contribute to ASD risk.
- Daniel J Weiner
- , Emilie M Wigdor
- & Elise B Robinson
Article | 13 March 2017
Disruption of the ATXN1–CIC complex causes a spectrum of neurobehavioral phenotypes in mice and humans
Huda Zoghbi and colleagues report that loss of the ATXN1–CIC protein complex in the developing mouse forebrain results in hyperactivity and defects in learning and memory. Loss of Cic in specific brain regions causes social interaction defects, and patients with de novo CIC mutations present signs of hyperactivity, autism spectrum disorder and intellectual disability.
- Hsiang-Chih Lu
- , Qiumin Tan
- & Huda Y Zoghbi
Letter | 30 January 2017
Whole-genome analysis of introgressive hybridization and characterization of the bovine legacy of Mongolian yaks
Ivica Medugorac, Aurélien Capitan and colleagues use high-density SNP genotyping and whole-genome sequencing to infer bovine haplotypes in the genomes of 76 Mongolian yaks. They show that these introgressed regions are enriched for genes involved in nervous system development and function, supporting the idea that introgressive hybridization contributed to the improvement of yak management and breeding.
- Ivica Medugorac
- , Alexander Graf
- & Aurélien Capitan
Letter | 19 December 2016
Genome-wide association analyses of sleep disturbance traits identify new loci and highlight shared genetics with neuropsychiatric and metabolic traits
Richa Saxena and colleagues report genome-wide association analyses of sleep disturbance traits in the UK Biobank cohort. They discover loci associated with insomnia symptoms and excessive daytime sleepiness and identify genetic correlations with several neuropsychiatric and metabolic traits.
- Jacqueline M Lane
- , Jingjing Liang
- & Richa Saxena
Letter | 05 December 2016
Genome-wide analyses for personality traits identify six genomic loci and show correlations with psychiatric disorders
Chi-Hua Chen and colleagues report a GWAS for five personality traits and identify four loci associated with extraversion and two associated with neuroticism at genome-wide significance. They find that the five personality traits are genetically correlated and identify genetic correlations between personality traits and psychiatric disorders.
- , David A Hinds
- & Chi-Hua Chen
Article | 31 October 2016
Genome-wide analysis identifies 12 loci influencing human reproductive behavior
Melinda Mills, Nicola Barban, Harold Snieder, Marcel den Hoed and colleagues perform a meta-analysis of data from over 300,000 individuals for age at first birth and number of children ever born. They identify 12 significant loci that associate with these traits, providing insights into the genetic basis of human reproductive behavior.
- Nicola Barban
- , Rick Jansen
- & Melinda C Mills
Article | 18 April 2016
Genetic variants associated with subjective well-being, depressive symptoms, and neuroticism identified through genome-wide analyses
Daniel Benjamin, Meike Bartels, Philipp Koellinger and colleagues report a genome-wide association meta-analysis of subjective well-being, depressive symptoms and neuroticism. The study leverages a large sample size together with genetic correlations between the phenotypes to identify, with high confidence, loci associated with each phenotype.
- , Bart M L Baselmans
Editorial | 01 January 2016
A diamond in the ruff
Letter 16 November 2015 | Open Access
A supergene determines highly divergent male reproductive morphs in the ruff
Terry Burke, Mark Blaxter, David Lank and colleagues report a reference genome sequence of the ruff and analysis of the three distinct male morphs of this bird species. They identify a ‘supergene’ consisting of a fixed inversion in two of the morphs and identify candidate reproductive trait genes in this region.
- Clemens Küpper
- , Michael Stocks
- & Terry Burke
Structural genomic changes underlie alternative reproductive strategies in the ruff ( Philomachus pugnax )
Leif Andersson and colleagues report the genome sequence of the ruff, a bird species with three male morphs with different reproductive strategies. Satellite and faeder morphs differ from the common independent morph by a 4.5-Mb inversion that occurred approximately 3.8 million years ago, and multiple genetic changes within this inverted region are associated with the satellite and faeder morphs.
- Sangeet Lamichhaney
- , Guangyi Fan
- & Leif Andersson
Research Highlights | 25 February 2015
Long-term memory genes in C. elegans
- Brooke LaFlamme
Research Highlights | 27 March 2014
Two pathways for courtship behavior
Research Highlights | 26 September 2013
Hedgehog agonist for Down syndrome
- Pamela Feliciano
Research Highlights | 27 February 2012
Sexually dimorphic behavior genes
News & Views | 01 May 2010
Chipping away at the genetics of smoking behavior
Three large consortia present comprehensive analyses that identify genetic factors influencing smoking initiation, intensity and cessation. The genetic architecture of these three phases of smoking behavior appears to be largely distinct.
- Christopher I Amos
- , Margaret R Spitz
- & Paul Cinciripini
Letter | 25 April 2010
Genome-wide meta-analyses identify multiple loci associated with smoking behavior
The Tobacco and Genetics Consortium report meta-analyses of several smoking phenotypes across 16 cohorts including 74,053 individuals. They identify three loci associated with cigarettes smoked per day.
- Helena Furberg
- , YunJung Kim
- & Patrick F Sullivan
Browse broader subjects
Quick links.
- Explore articles by subject
- Guide to authors
- Editorial policies


Behavioural genetics methods

Vol: 3 (2006) > Issue: 1 (January)
Primer | DOI: 10.1038/s43586-022-00191-x
- John D. Bower School of Population Health, University of Mississippi Medical Center, Jackson, MS, USA
- Department of Child and Adolescent Psychiatry & Social Care, Amsterdam UMC, Amsterdam, Netherlands
- Department of Psychology, University of Minnesota Twin Cities, Minneapolis, MN, USA
- School of Applied Sciences, University of Mississippi, University, MS, USA
- Department of Clinical Developmental Psychology, Vrije Universiteit, Amsterdam, Netherlands

The question of why people show individual differences in their behaviours and capacities has intrigued researchers for centuries. Behaviour genetics offers us various methods to address this question. The answers are interesting for a range of
The question of why people show individual differences in their behaviours and capacities has intrigued researchers for centuries. Behaviour genetics offers us various methods to address this question. The answers are interesting for a range of research fields, varying from medicine to psychology, economics and neuroscience. Starting with twin and family studies in the late 1970s, the field of behaviour genetics has rapidly developed by applying molecular genetic techniques next to, and sometimes combined with, family data. The overarching conclusion at this point in time is that all measured human traits are to some extent heritable, and that many genetic variants, with each exerting a small effect, explain this heritability. Against this backdrop, we offer readers who might be less familiar with behaviour genetics a brief Primer on the topic. Sitting atop our list of goals is to be a resource for scholars interested in applying the widely useful techniques of the field to their particular specialty, regardless of what that might be.
Figures ( 0 ) & Videos ( 0 )
Fig. 1 : Three common twin family models.
Fig. 2 : Correlations in cognitive ability between twin pairs.
Fig. 3 : Example raw and standardized variance path diagrams.
Fig. 4 : A cross-lagged longitudinal MZ discordant twin design.
Fig. 5 : The Wilson effect for cognitive ability.
Fig. 6 : Estimates of within-family shrinkage for GWAS predictors across a range of phenotypes from a within-sibship study 52 .
Experimental Specifications
Other keywords.

Citations (9)
Associated articles, supplementary information.
- Supplementary Information
- Turkheimer, E. Three laws of behavior genetics and what they mean. Curr. Dir. Psychol. Sci. 9 , 160–164 (2000).
- Harden, K. P. “Reports of my death were greatly exaggerated”: behavior genetics in the postgenomic era. Annu. Rev. Psychol. 72 , 37–60 (2021).
- Barnes, J. C. et al. Demonstrating the validity of twin research in criminology. Criminology 52 , 588–626 (2014).
- Friedman, N. P., Banich, M. T. & Keller, M. C. Twin studies to GWAS: there and back again. Trends Cognit. Sci. 25 , 855–869 (2021).
- Polderman, T. J. et al. Meta-analysis of the heritability of human traits based on fifty years of twin studies. Nat. Genet. 47 , 702–709 (2015).
- Chabris, C. F., Lee, J. J., Cesarini, D., Benjamin, D. J. & Laibson, D. I. The fourth law of behavior genetics. Curr. Dir. Psychol. Sci. 24 , 304–312 (2015).
- Visscher, PeterM. et al. Assumption-free estimation of heritability from genome-wide identity-by-descent sharing between full siblings. PLoS Genet. 2 , e41 (2006).
- Plomin, R., DeFries, J. C. & Loehlin, J. C. Genotype–environment interaction and correlation in the analysis of human behavior. Psychol. Bull. 84 , 309–322 (1997).
- Scarr, S. & McCartney, K. How people make their own environments: a theory of genotype–environment effects. Child. Dev. 54 , 424 (1983).
- Fowler-Finn, K. D. & Boutwell, B. B. Using variation in heritability estimates as a test of G × E in behavioral research: a brief research note. Behav. Genet. 49 , 340–346 (2019).
- Tucker-Drob, E. M. & Bates, T. C. Large cross-national differences in gene × socioeconomic status interaction on intelligence. Psychol. Sci. 27 , 138–149 (2016).
- Loehlin, J., Corley, R., Reynolds, C. & Wadsworth, S. Heritability × SES interaction for IQ: is it present in US adoption studies? Behav. Genet. 52 , 1–8 (2022).
- Heath, A. C., Kendler, K. S., Eaves, L. J. & Markell, D. The resolution of cultural and biological inheritance: informativeness of different relationships. Behav. Genet. 15 , 439–465 (1985).
- Truett, K. R. et al. A model system for analysis of family resemblance in extended kinships of twins. Behav. Genet. 24 , 35–49 (1994).
- Keller, M. C. et al. Modeling extended twin family data I: description of the Cascade model. Twin Res. Hum. Genet. 12 , 8–18 (2009).
- Pettersson, E. et al. Genetic influences on eight psychiatric disorders based on family data of 4 408 646 full and half-siblings, and genetic data of 333 748 cases and controls. Psychol. Med. 49 , 1166–1173 (2019).
- Lawlor, D. A., Tilling, K. & Davey Smith, G. Triangulation in aetiological epidemiology. Int. J. Epidemiol. 45 , 1866–1886 (2016).
- Yang, J. et al. Common SNPs explain a large proportion of the heritability for human height. Nat. Genet. 42 , 565–569 (2010).
- Young, A. I. Solving the missing heritability problem. PLoS Genet. 15 , e1008222 (2019).
- Young, A. I. Discovering missing heritability in whole-genome sequencing data. Nat. Genet. 54 , 224–226 (2022).
- Bulik-Sullivan, B. et al. An atlas of genetic correlations across human diseases and traits’. Nat. Genet. 47 , 1236–1241 (2015).
- Burgess, S., Butterworth, A. & Thompson, S. G. Mendelian randomization analysis with multiple genetic variants using summarized data. Genet. Epidemiol. 37 , 658–665 (2013).
- Falconer, D. S. Introduction to quantitative genetics (Longman Group, 1960).
- Falconer, D. S. & Mackay, T. F. C. Quantitative Genetics (Longman Group, 1996).
- Martin, N. & Eaves, L. The genetical analysis of covariance structure. Heredity 38 , 79–95 (1977).
- Neale, M. C. et al. OpenMx 2.0: extended structural equation and statistical modeling. Psychometrika 81 , 535–549 (2016).
- Burt, S. A., McGue, M. & Iacono, W. G. Nonshared environmental mediation of the association between deviant peer affiliation and adolescent externalizing behaviors over time: results from a cross-lagged monozygotic twin differences design. Dev. Psychol. 45 , 1752–1760 (2009).
- Bartels, M., Boomsma, D. I., Hudziak, J. J., van Beijsterveldt, T. C. E. M. & van den Oord, E. J. C. G. Twins and the study of rater (dis)agreement. Psychol. Meth. 12 , 451–466 (2007); erratum 13 , 170 (2008).
- Nivard, M. G., Middeldorp, C. M., Dolan, C. V. & Boomsma, D. I. Genetic and environmental stability of neuroticism from adolescence to adulthood. Twin Res. Hum. Genet. 18 , 746–754 (2015).
- Visscher, P. M. et al. 10 years of GWAS discovery: biology, function, and translation. Am. J. Hum. Genet. 101 , 5–22 (2017).
- Willoughby, E. & Lee, J. in The Cambridge Handbook of Intelligence and Cognitive Neuroscience (eds Barbey, A., Karama, S., & Haier, R.) 349–364 (Cambridge Univ. Press, 2021).
- Dudbridge, F. Power and predictive accuracy of polygenic risk scores. PLoS Genet. 9 , e1003348 (2013).
- Plomin, R. & von Stumm, S. Polygenic scores: prediction versus explanation. Mol. Psychiatry 27 , 49–52 (2022).
- Li, M. X. et al. A major gene model of adult height is suggested in Chinese. J. Hum. Genet. 49 , 148–153 (2004).
- Roberts, D. F., Billewicz, W. Z. & McGregor, I. A. Heritability of stature in a West African population. Ann. Hum. Genet. 42 , 15–24 (1978).
- Tarnoki, A. D., Tarnoki, D. L. & Molnar, A. A. Past, present and future of cardiovascular twin studies. Cor Vasa 56 , e486–e493 (2014).
- Pechlivanis, S. et al. Risk prediction for coronary heart disease by a genetic risk score — results from the Heinz Nixdorf Recall study. BMC Med. Genet. 21 , 178 (2020).
- Yang, R. et al. A healthy lifestyle mitigates the risk of heart disease related to type 2 diabetes: a prospective nested case–control study in a nationwide Swedish twin cohort. Diabetologia 64 , 530–539 (2021).
- McGue, M., Osler, M. & Christensen, K. Causal inference and observational research: the utility of twins. Perspect. Psychol. Sci. 5 , 546–556 (2010).
- Lemvigh, C. et al. The relative and interactive impact of multiple risk factors in schizophrenia spectrum disorders: a combined register-based and clinical twin study. Psychol. Med. (2021). https://doi.org/10.1017/S0033291721002749
- Squarcina, L., Fagnani, C., Bellani, M., Altamura, C. A. & Brambilla, P. Twin studies for the investigation of the relationships between genetic factors and brain abnormalities in bipolar disorder. Epidemiol. Psychiatr. Sci. 25 , 515–520 (2016).
- Bouchard, T. J. & McGue, M. Genetic and environmental influences on human psychological differences. J. Neurobiol. 54 , 4–45 (2003).
- Wilson, R. S. The Louisville twin study: developmental synchronies in behavior. Child. Dev. 54 , 298–316 (1983).
- Bouchard, T. J., Lykken, D. T., McGue, M., Segal, N. L. & Tellegen, A. Sources of human psychological differences: the minnesota study of twins reared apart. Science. 250 , 223–228 (1990).
- Bouchard, T. The Wilson effect: the increase in heritability of IQ with age. Twin Res. Hum. Genet. 16 , 923–930 (2013).
- Deary, I. J., Johnson, W. & Houlihan, L. M. Genetic foundations of human intelligence. Hum. Genetics. 126 , 215–232 (2009).
- Jang, K. L., Livesley, W. J. & Vernon, P. A. Heritability of the big five personality dimensions and their facets: a twin study. J. Pers. 64 , 577–591 (1996).
- Tellegen, A. & Niels, G. W. Exploring personality through test construction: development of the multidimensional personality questionnaire. SAGE Handb. Pers. Theory Assess. 2 , 261–292 (2008).
- Vukasović, T. & Bratko, D. Heritability of personality: a meta-analysis of behavior genetic studies. Psychol. Bull. 141 , 769–785 (2015).
- Krueger, R. F., South, S., Johnson, W. & Iacono, W. The heritability of personality is not always 50%: gene–environment interactions and correlations between personality and parenting. J. Pers. 76 , 1485–1522 (2008).
- Matteson, L. K., McGue, M. & Iacono, W. G. Shared environmental influences on personality: a combined twin and adoption approach. Behav. Genet. 43 , 491–504 (2013).
- Howe, L. J. et al. Within-sibship genome-wide association analyses decrease bias in estimates of direct genetic effects. Nat. Genet. 54 , 581–592 (2022).
- Lee, J. J. et al. Gene discovery and polygenic prediction from a genome-wide association study of educational attainment in 1.1 million individuals. Nat. Genet. 50 , 1112–1121 (2018).
- Kong, A. et al. The nature of nurture: effects of parental genotypes. Science 359 , 424–428 (2018).
- Belsky, D. W. et al. Genetic analysis of social-class mobility in five longitudinal studies. Proc. Natl Acad. Sci. USA 115 , E7275–E7284 (2018).
- Bates, T. C. et al. Social competence in parents increases children’s educational attainment: replicable genetically-mediated effects of parenting revealed by non-transmitted DNA. Twin Res. Hum. Genet. 22 , 1–3 (2019).
- Willoughby, E. A., McGue, M., Iacono, W. G., Rustichini, A. & Lee, J. J. The role of parental genotype in predicting offspring years of education: evidence for genetic nurture. Mol. Psychiatry 26 , 3896–3904 (2021).
- Loehlin, J. C., Horn, J. M. & Willerman, L. in Intelligence, Heredity, and Environment (eds Sternberg, R. J., & Grigorenko, E. L.) 105–125 (Cambridge Univ. Press, 1997).
- Cadoret, R. J. Adoption Studies. Alcohol Health Res. World 19 , 195–200 (1995).
- Rhea, S. A., Bricker, J. B., Corley, R. P., DeFries, J. C. & Wadsworth, S. J. Design utility and history of the Colorado Adoption Project: examples involving adjustment interactions. Adopt. Q. 16 , 17–39 (2013).
- Willoughby, E. A., McGue, M., Iacono, W. G. & Lee, J. J. Genetic and environmental contributions to IQ in adoptive and biological families with 30-year-old offspring. Intelligence 88 , 101579 (2021).
- Baker, M. Reproducibility crisis? Nature 533 , 26 (2016).
- Fanelli, D. Opinion: is science really facing a reproducibility crisis, and do we need it to? Proc. Natl Acad. Sci. USA 115 , 2628–2631 (2018).
- Plomin, R., DeFries, J. C., Knopik, V. S. & Neiderhiser, J. M. Top 10 replicated findings from behavioral genetics. Perspect. Psychol. Sci. 11 , 3–23 (2016).
- Chabris, C. F. et al. Most reported genetic associations with general intelligence are probably false positives. Psychol. Sci. 23 , 1314–1323 (2012).
- Johnson, E. C. et al. No evidence that schizophrenia candidate genes are more associated with schizophrenia than noncandidate genes. Biol. Psychiatry 82 , 702–708 (2017).
- Border, R. et al. No support for historical candidate gene or candidate gene-by-interaction hypotheses for major depression across multiple large samples. Am. J. Psychiatry 176 , 376–387 (2019).
- Lin, X. Learning lessons on reproducibility and replicability in large scale genome-wide association studies. Harvard Data Sci. Rev. (2020). https://doi.org/10.1162/99608f92.33703976
- Røysamb, E., & Tambs, K. The beauty, logic and limitations of twin studies. Nor. Epidemiol . 26 , 35–46 (2016).
- van Dongen, J., Slagboom, P. E., Draisma, H. H., Martin, N. G. & Boomsma, D. I. The continuing value of twin studies in the omics era. Nat. Rev. Genet. 13 , 640–653 (2012).
- Wilson, S. et al. Minnesota Center for Twin and Family Research. Twin Res. Hum. Genet. 22 , 746–752 (2019).
- Hindorff, L. A. et al. Prioritizing diversity in human genomics research. Nat. Rev. Genet. 19 , 175–185 (2017).
- Mills, M. C. & Rahal, C. A scientometric review of genome-wide association studies. Commun. Biol. 2 , 1–11 (2019).
- George, S., Duran, N. & Norris, K. A systematic review of barriers and facilitators to minority research participation among African Americans, Latinos, Asian Americans, and Pacific Islanders. Am. J. Public Health (2014). https://doi.org/10.2105/AJPH.2013.301706
- Wojcik, G. L. et al. Genetic analyses of diverse populations improves discovery for complex traits. Nature 570 , 514–518 (2019).
- Martin, A. R. et al. Human demographic history impacts genetic risk prediction across diverse populations. Am. J. Hum. Genet. 100 , 635–649 (2017).
- Giannakopoulou, O. et al. The genetic architecture of depression in individuals of East Asian ancestry: a genome-wide association study. JAMA Psychiatry 78 , 1258–1269 (2021).
- Meng, X. et al. Multi-ancestry GWAS of major depression aids locus discovery, fine-mapping, gene prioritisation, and causal inference. Preprint at bioRxiv (2022). https://doi.org/10.1101/2022.07.20.500802
- Martin, A. R. et al. Clinical use of current polygenic risk scores may exacerbate health disparities. Nat. Genet. 51 , 584–591 (2019).
- Bigdeli, T. B. et al. Genetic effects influencing risk for major depressive disorder in China and Europe. Transl. Psychiatry 7 , e1074 (2017).
- Bigdeli, T. B. et al. Contributions of common genetic variants to risk of schizophrenia among individuals of African and Latino ancestry. Mol. Psychiatry 25 , 2455–2467 (2020).
- Hyttinen, V., Kaprio, J., Kinnunen, L., Koskenvuo, M. & Tuomilehto, J. Genetic liability of type 1 diabetes and the onset age among 22,650 young Finnish twin pairs: a nationwide follow-up study. Diabetes 52 , 1052–1055 (2003).
- Snieder, H. et al. HbA1c levels are genetically determined even in type 1 diabetes: evidence from healthy and diabetic twins. Diabetes 50 , 2858–2863 (2001).
- McAdams, T. A., Rijsdijk, F. V., Zavos, H. M. & Pingault, J. B. Twins and causal inference: leveraging nature’s experiment. Cold Spring Harb. Perspect. Med. 11 , a039552 (2021).
- Alberg, A. J., Brock, M. V. & Samet, J. M. in Murray & Nadel’s Textbook of Respiratory Medicine 6th edn, Ch. 52, 927–939 (Saunders Elsevier, 2016).
- Poulton, R., Moffitt, T. E. & Silva, P. A. The Dunedin multidisciplinary health and development study: overview of the first 40 years, with an eye to the future. Soc. Psychiatry Psychiatr. Epidemiol., 50 , 679–693 (2015).
- Krüger, O., Korsten, P. & Hoffman, J. I. in J. Call, G. M. Burghardt, I. M. Pepperberg, C. T. Snowdon, & T. Zentall (Eds.), APA handbook of comparative psychology: Basic concepts, methods, neural substrate, and behavior (eds Call, J., Burghardt, G. M., Pepperberg, I. M., Snowdon, C. T. & Zentall, T.) 365–379 (American Psychological Association, 2017).
- Turkheimer, E. & Harden, K. P. in Handbook of Research Methods in Social and Personality Psychology (eds Reis, H. T. & Judd, C. M.) 159–187 (Cambridge Univ. Press, 2014).
- Okbay, A. et al. Polygenic prediction of educational attainment within and between families from genome-wide association analyses in 3 million individuals. Nat. Genet. 54 , 437–449 (2022).
- Marouli, E. et al. Rare and low-frequency coding variants alter human adult height. Nature 542 , 186190 (2017).
- Wainschtein, P. Assessing the contribution of rare variants to complex trait heritability from whole-genome sequence data. Nat. Genet. 54 , 263–273 (2022).
- Wang, Z., Gerstein, M. & Snyder, M. RNA-seq: a revolutionary tool for transcriptomics. Nat. Rev. Genet. 10 , 57–63 (2009).
- Gupta, S. et al. Transcriptome analysis reveals dysregulation of innate immune response genes and neuronal activity-dependent genes in autism. Nat. Commun. 5 , 5748 (2014).
- Pers, T.H. et al. Biological interpretation of genome-wide association studies using predicted gene functions. Nat. Commun. 6 , 5890 (2015).
- de Leeuw, C. A., Mooij, J. M., Heskes, T. & Posthuma, D. MAGMA: generalized gene-set analysis of GWAS data. PLoS Comput. Biol. 11 , 1–19 (2015).
- Neale, M. C. & Cardon, L. R. Methodology for Genetic Studies of Twins and Families . (Kluwer Academic/Plenum, 1992).
- Vitaro, F., Brendgen, M. & Arseneault, L. Methods and measures: the discordant MZ-twin method: one step closer to the holy grail of causality. Int. J. Behav. Dev. 33 , 376–382 (2009).
- Keller, M. C., Medland, S. E. & Duncan, L. E. Are extended twin family designs worth the trouble? A comparison of the bias, precision, and accuracy of parameters estimated in four twin family models. Behav. Genet. 40 , 377–393 (2010).
- McAdams, T. A. et al. Revisiting the children-of-twins design: improving existing models for the exploration of intergenerational associations. Behav. Genet. 48 , 397–412 (2018).
- Scarr, S. & Weinberg, R. A. The Minnesota Adoption Studies: genetic differences and malleability. Child. Dev. 54 , 260–267 (1983).
- Murphy, K. et al. Twins Research Australia: a new paradigm for driving twin research. Twin Res. Hum. Genet. 22 , 438–445 (2019).
- Otta, E. et al. The University of São Paulo Twin Panel: current status and prospects for Brazilian twin studies in behavioral research. Twin Res. Hum. Genet. 22 , 467–474 (2019).
- Huang et al. The Chinese National Twin Registry: a unique data source for systems epidemiology of complex disease. Twin Res. Hum. Genet. 22 , 482–485 (2019).
- Pedersen et al. The Danish Twin Registry: an updated overview. Twin Res. Hum. Genet. 22 , 499–507 (2020).
- Bjerregaard-Andersen et al. The Guinea-Bissau Twin Registry update: a platform for studying twin mortality and metabolic disease. Twin Res. Hum. Genet. 22 , 554–560 (2019).
- Gharipour et al. Isfahan Twins Registry (ITR): an invaluable platform for epidemiological and epigenetic studies: design and methodology of ITR. Twin Res. Hum. Genet. 22 , 579–582 (2019).
- Ligthart et al. The Netherlands Twin Register: longitudinal research based on twin and twin-family designs. Twin Res. Hum. Genet. 22 , 623–636 (2019).
- Rimfeld et al. Twins Early Development Study: a genetically sensitive investigation into behavioral and cognitive development from infancy to emerging adulthood. Twin Res. Hum. Genet. 22 , 508–513 (2019).
- Pingault, J. B. et al. Using genetic data to strengthen causal inference in observational research. Nat. Rev. Genet. 19 , 566–580 (2018).
- Lee, J. J. Correlation and causation in the study of personality. Eur. J. Personal. 26 , 372–412 (2012).
- Vilhjámsson, B. J. et al. Modeling linkage disequilibrium increases accuracy of polygenic risk scores. Am. J. Hum. Genet. 97 , 576–592 (2015).
- de Vlaming, R. & Groenen, P. J. F. The current and future use of ridge regression for prediction in quantitative genetics. BioMed. Res. Int. 2015 , 1–18 (2015).
- Vattikuti, S., Lee, J. J., Chang, C. C., Hsu, S. D. H. & Chow, C. C. Applying compressed sensing to genome-wide association studies. GigaScience 3 , 10 (2014).
- Lello, L. et al. Accurate genomic prediction of human height. Genetics 210 , 477–497 (2018).
- Allegrini, A. G. et al. Genomic prediction of cognitive traits in childhood and adolescence. Mol. Psychiatry 24 , 819–827 (2019).
Advertisement
Overview of Behavioral Genetics Research for Family Researchers
Affiliation.
- 1 Department of Psychology, N218 Elliot Hall, 75 East River Road, Minneapolis, MN 55108 ( [email protected] ).
- PMID: 24073018
- PMCID: PMC3780434
- DOI: 10.1111/jftr.12013
This article provides an overview of the methods, assumptions, and key findings of behavioral genetics methodology for family researchers with a limited background. We discuss how family researchers can utilize and contribute to the behavioral genetics field, particularly in terms of conducting research that seeks to explain shared environmental effects. This can be done, in part, by theoretically controlling for genetic confounds in research that seeks to determine cause-and-effect relationships among family variables and individual outcomes. Gene-environment correlation and interaction are especially promising areas for the family researcher to address. Given the methodological advancements in the field, we also briefly comment on new methods in molecular genetics for studying psychological mental health disorders.
Keywords: Behavior genetics; genetic relatedness; human development; shared environment.
Grants and funding
- T32 MH017069/MH/NIMH NIH HHS/United States
- Alzheimer's disease & dementia
- Arthritis & Rheumatism
- Attention deficit disorders
- Autism spectrum disorders
- Biomedical technology
- Diseases, Conditions, Syndromes
- Endocrinology & Metabolism
- Gastroenterology
- Gerontology & Geriatrics
- Health informatics
- Inflammatory disorders
- Medical economics
- Medical research
- Medications
- Neuroscience
- Obstetrics & gynaecology
- Oncology & Cancer
- Ophthalmology
- Overweight & Obesity
- Parkinson's & Movement disorders
- Psychology & Psychiatry
- Radiology & Imaging
- Sleep disorders
- Sports medicine & Kinesiology
- Vaccination
- Breast cancer
- Cardiovascular disease
- Chronic obstructive pulmonary disease
- Colon cancer
- Coronary artery disease
- Heart attack
- Heart disease
- High blood pressure
- Kidney disease
- Lung cancer
- Multiple sclerosis
- Myocardial infarction
- Ovarian cancer
- Post traumatic stress disorder
- Rheumatoid arthritis
- Schizophrenia
- Skin cancer
- Type 2 diabetes
- Full List »
share this!
April 9, 2024
This article has been reviewed according to Science X's editorial process and policies . Editors have highlighted the following attributes while ensuring the content's credibility:
fact-checked
peer-reviewed publication
Mouse study uncovers how altered gene expression can induce autism
by Juntendo University Research Promotion Center
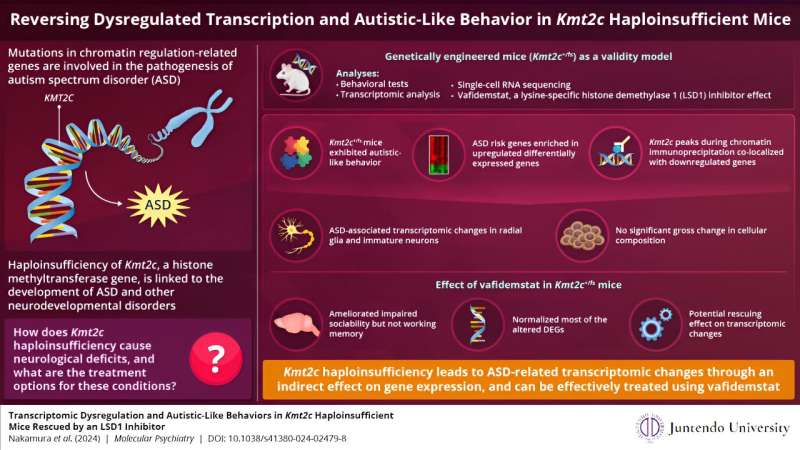
Autism spectrum disorder (ASD) encompasses neurodevelopmental conditions where patients display repetitive behavior and impaired sociality. Genetic factors have been shown to influence the development of ASD. Additionally, recent studies have shown that the genes involved in chromatin modification and gene transcription are involved in the pathogenesis of ASD.
Among the many genes implicated in this process, the gene KMT2C (lysine methyltransferase 2c), which codes for a catalytic unit of H3K4 (histone H3 lysine 4) methyltransferase complex, has been identified to be associated with the development of autism and other neurodevelopmental disorders.
Previous studies have shown that haploinsufficiency (a condition where, of the two copies of the gene, only one remains functional) of KMT2C is a risk factor for ASD and other neurodevelopmental disorders. However, the molecular mechanism through which the loss-of-function mutation in KMT2C leads to these conditions remains unclear.
To address this knowledge gap, researchers from Juntendo University, RIKEN, and the University of Tokyo in Japan aimed to provide answers to these questions in a benchmark study published in the journal Molecular Psychiatry on 26 March 2024. The research team included Professor Tadafumi Kato from the Department of Psychiatry and Behavioral Science at Juntendo University Graduate School of Medicine, Dr. Takumi Nakamura and Dr. Atsushi Takata from the RIKEN Center for Brain Science, and Professor Takashi Tsuboi from Graduate School of Arts and Sciences, The University of Tokyo.
To get to the bottom of KMT2C's role in ASD pathogenesis, the team developed and analyzed genetically engineered strain mice (Kmt2c +/fs ) having a frameshift mutation that models the KMT2C haploinsufficiency. They then performed various behavioral analyses, in which they observed that the mutant mice exhibited lower sociality, inflexibility, auditory hypersensitivity, and cognitive impairments, which are all ASD-related symptoms.
Next, they performed transcriptomic and epigenetic profiling to understand the basis of the molecular changes observed in the mutant mice. What they discovered was remarkable: the genes associated with increased ASD risk showed higher expression in these mutant mice. Dr. Takata says, "This was somewhat unexpected. KMT2C mediates H3K4 methylation, which is thought to activate gene expression , and thereby KMT2C haploinsufficiency was expected to cause reduced expression of target genes."
To gain mechanistic insights into their finding, the researchers carried out chromatin immunoprecipitation, a technique to determine the location on the DNA where the protein interacts with it. They found an overlap between KMT2C and the differentially expressed genes exhibiting reduced expression, suggesting that KMT2C haploinsufficiency leads to ASD-related transcriptomic changes through an indirect effect on gene expression.
To identify the cell types that contribute more to the pathological changes seen in the mutant mice, the researchers performed single-cell RNA sequencing of newborn mice brains. They observed that the altered genes associated with ASD risk were predominant in undifferentiated radial glial cells. However, a gross change in the cell composition was not observed, implying that the transcriptomic dysregulation does not severely impact cell fate.
Finally, the researchers tested the effects of vafidemstat, a brain penetrant inhibitor of LSD1 (lysine-specific histone demethylase 1A), that could ameliorate histone methylation abnormalities. They found that vafidemstat improved the social deficits in the mutant mice and had an exceptional rescuing effect by changing the expression levels of the differentially expressed genes to their normal expression level. This finding showed that vafidemstat is a valid drug for mutant mice and can potentially help restore the normal transcriptomic state.
What sets this discovery apart is that it challenges the commonly held belief that ASD disability may not be cured and demonstrates the efficacy of vafidemstat in improving ASD-like phenotypes. The results open doors to future research to strengthen the foundation for the pharmacologic treatment of ASD and other neurodevelopmental disorders.
Prof. Kato concludes, "Our research shows that drugs similar to vafidemstat may be generalizable to multiple categories of psychiatric disorders."
Explore further
Feedback to editors

Study finds that efforts to help low-income Americans by buying up their medical debt aren't going as planned
4 minutes ago

Clinical trial finds nasal spray safely treats recurrent abnormal heart rhythms
14 minutes ago

Dubin-Johnson Syndrome in twins linked to novel genetic mutations

Novel mechanism supports antitumor response and T-cell survival

Study reveals mechanism linking heart disease to cancer development

Transdifferentiation with RNA sequencing aids diagnosis of genetic disorders
2 hours ago

Researchers develop statistical method for genetic mapping of autoimmune diseases
3 hours ago

New atlas of mRNA variants captures inner workings of the brain
4 hours ago

Study suggests light physical activity as a child is key to reducing risk of type 2 diabetes

No link between acetaminophen use during pregnancy and cognitive risks, says large sibling study
5 hours ago
Related Stories

Cause of metastasis in prostate cancer discovered
Apr 4, 2022

SUV39H2: A direct genetic link to autism spectrum disorders
Jul 19, 2021

Set of genetic markers in lung cancer identified
Apr 14, 2021

Longitudinal characterization of behavioral, morphological and transcriptomic changes in a tauopathy mouse model
Nov 15, 2023

Pathological mechanism of intellectual disability caused by haploinsufficiency may be a disorder of excitatory synapses
Mar 28, 2023

Altering the behavior of living mice by editing genes in the brain
Dec 4, 2023
Recommended for you

Targeting RAS proteins may prevent relapse in acute myeloid leukemia
6 hours ago
Let us know if there is a problem with our content
Use this form if you have come across a typo, inaccuracy or would like to send an edit request for the content on this page. For general inquiries, please use our contact form . For general feedback, use the public comments section below (please adhere to guidelines ).
Please select the most appropriate category to facilitate processing of your request
Thank you for taking time to provide your feedback to the editors.
Your feedback is important to us. However, we do not guarantee individual replies due to the high volume of messages.
E-mail the story
Your email address is used only to let the recipient know who sent the email. Neither your address nor the recipient's address will be used for any other purpose. The information you enter will appear in your e-mail message and is not retained by Medical Xpress in any form.
Newsletter sign up
Get weekly and/or daily updates delivered to your inbox. You can unsubscribe at any time and we'll never share your details to third parties.
More information Privacy policy
Donate and enjoy an ad-free experience
We keep our content available to everyone. Consider supporting Science X's mission by getting a premium account.
E-mail newsletter

An official website of the United States government
The .gov means it’s official. Federal government websites often end in .gov or .mil. Before sharing sensitive information, make sure you’re on a federal government site.
The site is secure. The https:// ensures that you are connecting to the official website and that any information you provide is encrypted and transmitted securely.
- Publications
- Account settings
Preview improvements coming to the PMC website in October 2024. Learn More or Try it out now .
- Advanced Search
- Journal List
- v.2(4); 2022 Dec
- PMC10242940

The next 10 years of behavioural genomic research
Robert plomin.
1 King's College London, Institute of Psychiatry, Psychology and Neuroscience, London UK
Associated Data
Data sharing not applicable to this article as no datasets were generated or analysed during the current study.
The explosion caused by the fusion of quantitative genetics and molecular genetics will transform behavioural genetic research in child and adolescent psychology and psychiatry.
Although the fallout has not yet settled, the goal of this paper is to predict the next 10 years of research in what could be called behavioural genomics .
I focus on three research directions: the genetic architecture of psychopathology, causal modelling of gene‐environment interplay, and the use of DNA as an early warning system.
Eventually, whole‐genome sequencing will be available for all newborns, which means that behavioural genomics could potentially be applied ubiquitously in research and clinical practice.

Three transformative developments for behavioural genomic research in the next 10 years are described. First, behavioural genomic research will use dimensional measures to reveal the hierarchical genetic architecture of psychopathology. A second direction for behavioural genomic research will be to investigate causa models of GE interplay. Third, polygenic scores will be used as an early warning system in childhood to predict profiles of adult psychopathology, which will eventually transform clinical practice.
- During the next 10 years, the fusion of quantitative genetics and molecular genetics will transform behavioural genetic research.
- Behavioural genomics will reveal the genetic architecture of psychopathology.
- Causal modelling of GE interplay will be a focus of behavioural genomic research.
- Polygenic scores will be used in childhood to predict profiles of adult psychopathology.
To appreciate the next 10 years of behavioural genetic research, it is useful to begin by looking back at the past decade when the two worlds of genetics, quantitative genetics and molecular genetics, fused in a synthesis that could be labelled behavioural genomics . Although the two worlds of genetics were both born more than a century ago, they went their separate ways for most of the century. Following on from Francis Galton ( 1865 ), quantitative genetics focused on complex traits such as behavioural traits that were presumed to be influenced by many genes of small effect (Fisher, 1918 ). A century of quantitative genetic research showed that all behavioural traits, including psychiatric disorders, are substantially heritable, with an average heritability of 50% (Polderman et al., 2015 ). In contrast, understanding Gregor Mendel's mechanisms of heredity (Mendel, 1866 ) was the goal of molecular genetics, which focused on single‐gene disorders. The goal of molecular genetics was to identify the chromosomal location of these genes and to understand how they worked.
The two fields converged as advances in molecular genetics made it possible to go beyond single‐gene effects to investigate complex traits influenced by many genes. The origins of this development began in the 1970s with the ability to sequence DNA's nucleotide bases. By the turn of the 21 st century, these techniques identified the sequence of most of the three billion nucleotide base pairs in the human genome. This led eventually to the discovery of millions of inherited differences in DNA sequence. In the 1990s, thousands of studies reported associations between behavioural traits and variants in ‘candidate’ genes, typically neurotransmitter genes thought to be involved in behavioural pathways. Candidate‐gene studies genotyped only a few DNA variants because genotyping was expensive and slow. However, these studies failed to replicate (e.g., Border et al., 2019 ; Chabris et al., 2012 ) – they were genomics' contribution to the replication crisis, committing every sin in the catalogue of questionable research practices (Ritchie, 2021 ).
In 1996, the idea emerged that association studies could be made systematic if thousands of DNA variants across the genome were genotyped (Risch & Merikangas, 1996 ). However, genome‐wide association (GWA) seemed a dream because few DNA variants had been identified and genotyping was expensive and slow. In 2003, the Human Genome Project published the sequence of most of the 3 billion steps in the spiral staircase of DNA and soon discovered millions of variants in DNA sequence. The problem of the expense of genotyping each DNA variant was solved in the mid‐2000s by the DNA microarray, which can genotype hundreds of thousands of DNA variants for an individual quickly, accurately, and inexpensively. These arrays are called SNP chips because they genotype the most common type of DNA variant, a single nucleotide polymorphism (SNP), and because they are analogous to silicon chips in computers. In the next 10 years, SNP chips will be replaced by whole‐genome sequencing in which the sequence of the four‐letter alphabet of DNA will be assessed for the three billion nucleotide base pairs in the genome, thus uncovering all inherited DNA differences between individuals, not just SNPs.
The SNP chip paved the way for GWA analyses. In 2007, the first major GWA analysis included 2000 cases for each of seven major disorders and compared SNP allele frequencies for these cases versus controls (The Wellcome Trust Case Control Consortium, 2007 ). Replicable associations were found but they were few in number and small in effect size. Hundreds of GWA reports appeared over the next decade with similar results across the behavioural and biological sciences (Visscher et al., 2017 ), including childhood behaviour problems such as ADHD (Demontis et al., 2019 ), autism (Grove et al., 2019 ), and anorexia nervosa (Watson et al., 2019 ). These findings led to the realisation that the biggest effect sizes were much smaller than anyone anticipated – risk ratios were less than 1.1 for case‐control studies and the variance explained for dimensional traits was less than 0.1%. This meant that complex traits were extremely polygenic and that thousands of SNPs would need to be identified to account for heritability. It also meant that huge sample sizes would be needed to detect these miniscule effects.
Genome‐wide association and the ensuing recognition that the heritability of behavioural traits is caused by extreme polygenicity brought the two worlds of genetics together during the past decade, creating a synthesis that could be designated behavioural genomics . Energy unleashed from this fusion will fuel research in child and adolescent psychology and psychiatry during the next 10 years and beyond. Most of this energy comes from two new categories of tools that will play a major role in research in the next 10 years.
First, instead of using the specialised samples of twins and adoptees to estimate the heritability of traits and genetic correlations between them, behavioural genomics provides two different methods to estimate heritability and genetic correlation. The first method, called Genome‐wide Complex Trait Analysis (GCTA or GREML), uses SNP chip genotyping data for samples of at least several thousand unrelated individuals (Yang et al., 2011 ). For each pair of individuals, GCTA compares the pair's overall SNP similarity to their similarity on a trait. Although each pair of unrelated individuals' SNP similarity can only vary from only −2.5% to +2.5% (which excludes pairs who are even fifth‐degree relatives), across samples of thousands of individuals, this provides millions of pair‐by‐pair comparisons, which produces a powerful estimate of heritability. This estimate is called SNP heritability because it is limited to heritability estimated by the SNPs on the SNP chip. Genetic correlations are estimated by comparing each pair's SNP similarity to their cross‐trait similarity.
SNP heritabilities are about 25% for psychopathology, which is about half the heritability estimates from twin studies (Cross‐Disorder Group of the Psychiatric Genomics Consortium, 2013b ). This ‘missing heritability’ occurs because SNP heritability is limited to the common SNPs genotyped on current SNP chips, which also creates a ceiling for discovery in GWA research. Most SNPs are not common, and rare SNPs appear to be responsible for much of the missing heritability, at least for height (Wainschtein et al., 2022 ).
The second method, called LD score regression, estimates heritability and genetic correlations just using summary statistics from GWA studies rather than requiring SNP chip data for each individual as in GTCA (Bulik‐Sullivan et al., 2015 ). The essence of the method is the regression of association effect sizes of SNPs on their distance apart on a chromosome (linkage disequilibrium) because SNPs closer together on a chromosome should show similar effect sizes for true associations. LD score regression yields SNP heritability estimates similar to GCTA.
GCTA and LD score regression both document missing heritability. However, when it comes to genetic correlations, GCTA and LD score regression yield results similar to twin estimates. In other words, there is no ‘missing genetic covariance’, for reasons explained elsewhere (Trzaskowski et al., 2013 ). LD score regression has been combined with structural equation modelling from twin analyses to model the genetic structure of genetic correlations among multiple traits, a method called Genomic Structural Equation Modelling (Genomic SEM) (Grotzinger et al., 2019 ).
The second category of tools is polygenic scores. Although the heritability of complex traits is due to many SNPs of small effect, it is possible to add up these small effects weighted by each SNP's effect size from GWA summary statistics to create polygenic scores (Allegrini et al., 2022 ; Wray et al., 2021 ). Polygenic scores for any GWA target trait can be created in any sample of unrelated individuals for whom GWA genotype data are available. It should be noted that, although huge samples are needed for genome‐wide association studies, a polygenic score that predicts 10% of the variance only needs a sample size of 60 to detect its effect with 80% power ( p = 0.05, one‐tailed). Polygenic scores are the topic of the third section of this paper.
Because the fallout has not yet settled from the fusion of the two worlds of genetics, it is difficult to predict research directions during the next 10 years. What is clear is that this will be an exciting time for behavioural genomic research (Larsson, 2021a ). I chose three substantive issues that I predict will stand out as major areas of research during the next 10 years: the genetic architecture of psychopathology, causal modelling of gene‐environment interplay, and the use of DNA as an early warning system. My aim was to make provocative rather than pedestrian predictions, and ones that are sufficiently specific to have their validity evaluated in 10 years' time.
Although the pace of advances in behavioural genomics is exhilarating, it should be noted that most of the major discoveries so far in behavioural genetics have come from quantitative genetic studies using twin and adoption designs that originated a century ago (Plomin et al., 2016 ). Quantitative genetic research will continue to make important contributions because it estimates total genetic influence and is intrinsically as much about the environment as it is about genetics. It is heartening to see that results from behavioural genomics generally confirm those from quantitative genetics.
THE GENETIC ARCHITECTURE OF PSYCHOPATHOLOGY
In the next 10 years, behavioural genomic research will move beyond documenting the inadequacies of current nosology to reveal the genetic architecture of psychopathology in childhood, adolescence, and adulthood.
Psychiatry has been having a crisis of confidence about its classification of disorders, which is based on symptoms (Zachar & Kendler, 2017 ). Attempts to re‐classify disorders on the basis of presumed causes such as neural processes have produced a welter of findings about biomarkers but no breakthroughs in terms of re‐classification (Pacheco et al., 2022 ). Genetics is different because, unlike other biomarkers whose correlations with behavioural traits cannot be interpreted causally, genetics has a unique causal status in that there can be no backward causation. That is, events in the environment, behaviour or the brain cannot change inherited DNA differences (Plomin & von Stumm, 2022 ).
Genetics is not everything – it accounts for about half of the variance for psychopathological traits and all other behavioural traits (Polderman et al., 2015 ) – but it is almost everything systematic about psychopathology (Plomin, 2018 ). For psychopathology, the nongenetic half of the variance is not due to systematic effects of family environment shared by children growing up in the same family. The salient environmental influences are nonshared and seem to be unsystematic, perhaps idiosyncratic and stochastic (Gidziela, Malanchini, et al., 2022 ).
For these reasons, it makes sense scientifically to focus on the genetic architecture of psychopathology, even though causes are not necessarily related to cures and the impact on treatment remains to be seen. Although phenotypic structures reflect genetic structures for cognitive abilities and personality, domains whose structures were built psychometrically from the ground up, the genetic architecture of psychopathology so far looks very different from current symptom‐based diagnoses, whose origins are more historical than empirical.
In this section, I will provide a brief overview of how genetic and genomic research has revealed the inadequacies of current nosology, despite a circularity in that this research has necessarily relied on current diagnoses. I will then consider how research in the next 10 years can reveal the genetic architecture of psychopathology.
GENETIC CORRELATIONS BETWEEN DISORDERS
For decades, genetic research has revealed dramatic examples of the inadequacy of current nosology. One of the oldest examples from twin studies is that anxiety and depression show genetic correlations near 1.0, indicating that these two disorders do not differ genetically (Middeldorp et al., 2005 ). Another example is that the first GWA studies of psychopathology, which focused on the psychotic disorders of schizophrenia and bipolar disorder, found that many of the same SNPs were associated with both disorders (The International Schizophrenia Consortium, 2009 ). This finding was shocking because schizophrenia and bipolar disorder are among the oldest and most distinctive diagnoses and, at that time, were differentiated in the first tier of DSM‐4 diagnosis so that people could not receive both diagnoses.
A great advantage of behavioural genomic analysis is that LD score regression (Bulik‐Sullivan et al., 2015 ) can estimate genetic correlations between disorders from GWA summary statistics without the need to assess the disorders in the same individuals or to have access to genotyping data for individuals. I will focus on the most recent report of this type (Grotzinger et al., 2022 ), which replicates and extends previous research (e.g., Bulik‐Sullivan et al., 2015 ; Cross‐Disorder Group of the Psychiatric Genomics Consortium, 2013a ; Lee et al., 2019 ; Selzam et al., 2018 ), including twin studies (Martin et al., 2018 ). Genetic correlations were estimated from LD score regression using case‐control GWA summary statistics for 11 psychiatric disorders with the largest GWA samples (See Figure 1 .).

Patterns of genetic correlations for nine psychiatric disorders derived from GWA summary statistics (LD score regression). Derived from results reported by Grotzinger et al. ( 2022 ). To simplify the presentation, two disorders in the Grotzinger et al. analysis – problematic alcohol use and Tourette syndrome – are not depicted here because they are largely independent of the other disorders.
The highest genetic correlation, 0.87, was found between major depressive disorder (MDD) and anxiety disorders (ANX). Also confirming previous research, the genetic correlation between schizophrenia (SCZ) and bipolar disorder (BIP) was 0.68. For the childhood disorders of attention‐deficit/hyperactivity disorder (ADHD) and autism (AUT), the genetic correlation was 0.38. Another cluster involves anorexia nervosa (AN) and obsessive‐compulsive disorder (OCD), which correlate genetically 0.46. A surprising finding was that post‐traumatic stress disorder (PTSD) correlated strongly with MDD (0.75), ANX (0.58), and ADHD (0.78). In summary, in line with previous research, there is a great deal of genetic overlap between disorders.
Genomic SEM was used to factor analyse these genetic correlations. These analyses identified four broad factors that correspond to the clusters of genetic correlations indicated by the patterns of genetic correlations illustrated in Figure 1 : internalising (MDD and ANX), psychotic (SCZ and BIP), developmental (ADHD and AUT), and compulsive (AN and OCD). PTSD loaded on both the internalising and developmental factors.
A second important behavioural genomic discovery is that, even beyond these four genetic clusters, there is a positive manifold among all 11 disorders. Of the 55 pairwise genetic correlations, all but six were statistically significant and the average genetic correlation for the 55 correlations was 0.28. For example, ADHD and AUT correlated genetically with SCZ (0.20, 0.25, respectively), BIP (0.19, 0.13), MDD (0.47, 0.40), and ANX (0.36, 0.35), in addition to genetic correlations of 0.78 and 0.47 with PTSD.
This genetic overlap among disorders reflects a transdiagnostic factor that has been called p (Caspi & Moffitt, 2018 ), analogous to g , the general factor of cognitive ability. Phenotypic research during the past decade shows substantial comorbidity among disorders in adulthood (Caspi et al., 2014 ; Lahey et al., 2012 ; Wright et al., 2013 ) and childhood (Allegrini, Cheesman, et al., 2020 ; Sallis et al., 2019 ). Genetic and genomic studies have shown that this ubiquitous comorbidity is mostly genetic in origin (McLaughlin et al., 2012 ; Pettersson et al., 2016 ; Selzam et al., 2018 ).
Behavioural genomic analyses provide the strongest evidence for a genetic p factor among psychiatric disorders (Waszczuk et al., 2020 ) but not for neurological disorders (Anttila et al., 2018 ). The Grotzinger et al. ( 2022 ) Genomic SEM analysis tested the fit of a hierarchical model of p, analogous to the widely accepted hierarchical model of g. In the hierarchical model, the latent variable of p extracts genetic covariance among the four latent factors illustrated in Figure 1 . The hierarchical model fit the data well, and the p factor explained 30–55% of the genetic variance in the four factors. The p factor has a broad reach, yielding substantial genetic correlations with diverse behavioural traits including personality (agreeableness, −0.73; neuroticism, 0.78; subjective wellbeing, −0.75), lifetime symptoms (depression, 0.94; mania, 0.87, psychosis, 0.90, stress‐related disorders, 0.91), suicide attempts (0.67), family relationship satisfaction (−0.58), deprivation (0.54), insomnia (0.42) and cannabis use (0.43).
Thus, despite analysing genetic correlations for putatively distinct disorders diagnosed according to current nosology, genetic overlap is the rule rather than the exception. In other words, currently nosology does not reflect the genetic architecture of psychopathology.
Disorders are dimensions
A second finding with far‐reaching implications for the genetic architecture of psychopathology is that common disorders are dimensions. That is, there are no genetically distinct disorders, just continuous dimensions (Plomin et al., 2009 ). A dimensional approach does not denigrate the clinical and societal problems at the extremes of these dimensions nor the clinical necessity of identifying these extremes. The point is that there is nothing to be gained scientifically by reifying the extremes of normal dimensions as aetiologically distinct categories.
Much phenotypic evidence supports this view that disorders are dimensions (Krueger et al., 2018 ). Genetic support comes from twin studies which report that diagnosed disorders yield substantial genetic correlations with corresponding quantitative traits (Geschwind & Flint, 2015 ; Martin et al., 2018 ; Taylor et al., 2019 ).
Behavioural genomic research provides definitive evidence in two ways. The first is so obvious that it is easy to overlook its importance: Polygenic scores derived from case‐control GWA studies of diagnosed disorders are perfectly normally distributed, showing no indication of a breakpoint or threshold for disorder (See Figure 2 .). Although these normal distributions necessarily follow from the central limit theorem of probability, as polygenic scores are used to predict genetic risk for psychopathology, it will be impossible to ignore the fact that this risk is continuous.

Distributions of polygenic scores for schizophrenia, bipolar disorder and depression for 10,346 individuals in the Twins Early Development Study (Rimfeld et al., 2019 ). Polygenic scores were standardised with a mean of 0 and a standard deviation of 1 because of the varying ranges of the polygenic scores. The polygenic scores were created for individuals in TEDS using summary statistics from the following case‐control GWA meta‐analyses: schizophrenia (Pardiñas et al., 2018 ), bipolar disorder (Mullins et al., 2021 ), and depression (Howard et al., 2019 ).
More specific support for the view that disorders are dimensions comes from behavioural genomic research that shows substantial genetic correlations between diagnosed disorders and quantitative traits (Martin et al., 2018 ). For example, the genetic correlation between ADHD diagnosis and quantitative traits, for which comparable measures exist for diagnosis and traits, exceeds 0.90 (Demontis et al., 2019 ). A polygenic score derived from a case‐control GWA analysis of ADHD also predicts ADHD symptoms in a community sample (Taylor et al., 2019 ). Major depressive disorder yields genetic correlations greater than 0.90 with quantitative measures of depressive symptoms (Anttila et al., 2018 ; Wray et al., 2018 ). Comparisons for schizophrenia and bipolar disorder are more difficult because comparable trait measures are less straightforward (Martin et al., 2018 ).
Categorical models have been less dominant in child psychopathology than in adulthood (Caspi & Moffitt, 2018 ), due in part to the high frequency of behaviour problems in childhood and the availability of dimensional measures of behaviour problems. This suggests that research in childhood could be in the forefront of research revealing the genetic architecture of psychopathology.
Revealing the genetic architecture of psychopathology
Genetic correlations between diagnoses and evidence that common disorders are dimensions clearly demonstrate that current diagnoses do not reflect the genetic architecture of psychopathology. In the next 10 years, behavioural genomic research will move beyond documenting the inadequacies of current nosology to reveal the genetic architecture of psychopathology.
The major roadblock is that most GWA studies have used case‐control designs that are based on traditional diagnostic nosology, which makes it difficult to go beyond this nosology because these studies are limited to the specific diagnosis for which cases were selected. In the near term, GWA studies will consider symptoms of diagnoses within case‐control studies. However, this is limited by the same circularity – for example, if a diagnosis requires three symptoms, it is not possible to use such cases to assess the genetic associations between the three symptoms.
The sound way to reveal the genetic architecture of behavioural problems is to build it from the ground up, using batteries of behavioural problems assessed as dimensions in samples of unrelated individuals. A starting point is the phenotypic structure of psychopathology. An important example is the Hierarchical Taxonomy of Psychopathology (HiTOP) project (Kotov et al., 2017 ), whose goal is to group symptoms phenotypically using dimensional measures. Emerging from this research is a hierarchical model with a p factor on top of broad factors of externalising, internalising and psychotic experiences. The extent to which the phenotypic structure of symptoms coincides with the genetic structure is an open, but empirical, issue (Lahey et al., 2021 ; Waszczuk et al., 2020 ). In the meantime, it would be useful to sharpen up genomic studies of specific disorders by correcting for p using a technique called GWAS‐by‐subtraction (Demange et al., 2021 ).
Instead of focussing on traits viewed as symptoms of current diagnoses of behaviour problems, a more radical approach is to consider problems as the extremes of normal dimensions of personality (Widiger, 2011 ), such as the extremes of activity, attention, impulsivity, shyness, fearfulness, and anger. One advantage of a personality perspective is that, unlike psychopathology, the architecture of personality has been a focus of psychometric research from the beginning, leading to a model of adult personality dominated by the ‘Big 5’ factors of OCEAN (openness to experience, conscientiousness, extraversion, agreeableness, and neuroticism). A general p‐like factor has also been proposed (van der Linden et al., 2010 ). In childhood, it would be worth revisiting temperament theories from decades ago, which focus on early appearing personality traits (Goldsmith et al., 1987 ; Shiner et al., 2012 ). For example, activity level is not well represented in measures of adult personality, but it is impossible to ignore activity level in childhood (Buss & Plomin, 1984 ).
Another advantage of a personality perspective is that personality traits, unlike measures of symptoms, are normally distributed, as are polygenic scores. These normal distributions will draw attention to both ends of the distribution. Measures of symptoms presuppose that one end of the distribution is the problem – for example, too much activity and insufficient attention. However, for most personality distributions, the other extreme can also be problematic, but these are ignored because they are not as disruptive – too little activity or too much attention. The normal distribution of polygenic scores will foster research on the both the low and high ends of the distribution.
During the next 10 years, I predict that the outlines of the genetic architecture of psychopathology in childhood, adolescence, and adulthood will be revealed by behavioural genomic research, unconstrained by current nosology. Research to date suggests that the structure at each age is hierarchical with p on top, and that it will be built from dimensional measures, with psychopathology defined as the quantitative extremes of these dimensions.
Although the structure of psychopathology is likely to differ in childhood, adolescence and adulthood, another important research direction is to capitalise on the fact that inherited DNA sequence variants do not change during development so that it is possible to identify traits in childhood that best predict adult psychopathology (Akingbuwa et al., 2020 ; Allegrini, Cheesman, et al., 2020 ; Gidziela, Rimfeld, et al., 2022 ; Riglin et al., 2022 ). In other words, this suggests that, in addition to outlining the genetic structure of psychopathology in childhood, the genetic structure of psychopathology in adulthood could be used in childhood to explore what could be called the predictive structure of childhood psychopathology. For example, p in adulthood, which will be different from p in childhood, could be used to probe the predictive structure of p in childhood. The third section of this paper considers this direction for research in greater detail.
The clinical utility of knowing the genetic structure of psychopathology remains to be seen, although exciting advances are looming such as transdiagnostic treatments (Caspi & Moffitt, 2018 ) and quantitative approaches to treatment rather than ‘cures’. Turning the question of clinical utility around, what is to be gained clinically by pretending that current disorders are aetiologically distinct and that they are dichotomous rather than dimensional? Regardless of its clinical utility, the scientific value of an aetiologically accurate architecture seems beyond doubt.
CAUSAL MODELLING OF GE INTERPLAY
In the next 10 years, a major direction for behavioural genomic research will be causal modelling of GE interplay.
A major advance in quantitative genetics was to go beyond estimating genetic and environmental influence to investigate their interplay, which includes interactions (GxE) and correlations (rGE) between genetic and environmental effects. Traditional quantitative genetic methods, such as twin and adoption studies, are only able to provide indirect glimpses of GE interplay (Plomin et al., 1977 ). An overview of three subsequent stages in research on GE interplay is instructive because it shows why GE interplay will be a focus of behavioural genomic research during the next 10 years.
The first stage incorporated measures of the environment in quantitative genetic designs. For GxE, twin studies found examples in which heritability of psychopathology differed as a function of the environment, a very limited form of GxE (Dick, 2011 ), which might be called heritability × E interaction to highlight its limitation. Adoption studies offered wider scope for GxE studies because the genotype of adopted children can be inferred from their biological parents and the environment can be assessed in the adoptive home. Examples have been reported in which the effect of this genetic index on childhood psychopathology was moderated by characteristics of the adoptive home (Knopik et al., 2017 ).
The main finding from this first stage of research on GE interplay was about the importance of rGE rather than GxE: Measures of the environment widely used in psychology show significant and substantial genetic influence, about 25% on average (Kendler & Baker, 2007 ), called the nature of nurture (Plomin & Bergeman, 1991 ). Furthermore, correlations between environmental measures and children's outcomes were shown to be mediated genetically, about 50% on average (Avinun & Knafo, 2014 ; Plomin, 1994 ). These findings documented the importance of rGE. Moreover, twin and adoption studies can distinguish passive rGE in which parents pass on family environments correlated with their genotypes, evocative rGE in which parents respond to the children's genetic propensities, and active rGE in which children modify or create environments correlated with their genetic propensities (Plomin, 1994 ). In general, evidence for passive rGE was found for cognitive traits. Causal modelling of rGE was enabled by designs that include parents of twins and children of twin parents (McAdams et al., 2014 ) and by longitudinal data in which time is used to leverage causality (Berry & Willoughby, 2017 ).
The second stage of research on GE interplay incorporated measured genotypes in candidate genes in addition to measured environments. Hundreds of reports of GxE appeared but most failed to replicate (Dick et al., 2015 ). Some correlations between candidate genes and environmental measures also indicated rGE, but these too had a poor track record for replication (Jaffee & Price, 2007 ). The fundamental problem with candidate‐gene research is lack of power: we now know that single DNA variants hardly ever account for as much as 0.1% of the variance in the population. Candidate‐gene studies were vastly underpowered to detect such effect sizes, and as a result most reported associations were false positives (Border et al., 2019 ; Chabris et al., 2012 ; Duncan & Keller, 2011 ).
The third stage of research on GE interplay is behavioural genomics, which exploits GWA genotyping data for hundreds of thousands of SNPs on SNP chips from large samples. Much of this research attempts to confirm the existence of GxE and rGE using strategies similar to the candidate‐gene era but substituting polygenic scores for candidate genes (Plomin & Viding, 2022 ). It will be important to avoid repeating the questionable research practices that led to failures to replicate in the candidate‐gene era. Systematic multivariate studies will help (Allegrini, Karhunen, et al., 2020 ), and replication will be key.
The focus of this section is on methods that have enabled new possibilities for causal modelling of GE interplay (Pingault et al., 2018 ). The overall goal of causal modelling in relation to GE interplay is to disentangle ‘direct’ effects of genes and environments from rGE.
Mendelian randomisation
Mendelian randomisation is a method for causal modelling that uses genetics to identify causal effects of modifiable environmental factors on outcomes rather than investigating rGE itself (Davey Smith & Ebrahim, 2003 ). The strongest proof of causality is an experiment with random assignment to conditions, as in randomised controlled trials, but this is often precluded by ethical issues. Rather than randomising participants into different levels of treatment, Mendelian randomisation capitalises on the fact that individuals are randomised by genotypes because genotypes are randomly allocated from parents to offspring. If genotypes are strongly associated with an environmental factor (exposure) as well as with an outcome, the genetic ‘instrument’ can be used as a proxy for randomly assigned exposure as it affects the outcome. A path model can isolate the causal effect of the exposure if the model meets demanding assumptions: the genetic instrument is correlated with the outcome exclusively through its effect on the exposure and is not correlated with confounding factors that influence the correlation between the exposure and the outcome.
Mendelian randomisation was initially applied to single‐gene effects and dichotomous ‘exposures’ and outcomes, which are conditions not relevant to the complexity of psychopathology, but it has been extended to polygenic scores and quantitative environmental factors and outcomes (Pingault et al., 2018 ). However, the assumptions of Mendelian randomisation are much more daunting with polygenic scores which are embedded in a complex web of rGE (Koellinger & de Vlaming, 2019 ; Krapohl et al., 2017 ). Although the method has been reported to identify some modifiable risks, for example, for depression (Choi et al., 2020 ), as yet no strong causal paths have been discovered for psychopathology (Richmond & Davey Smith, 2022 ).
Mendelian randomisation analyses will be added to many behavioural genomic studies of GE interplay during the next 10 years. However, I predict that few modifiable environmental risks will be found and replicated for psychopathology because of the complex web of rGE.
Back to families
Although the ability of GWA to identify genetic effects in large samples of unrelated individuals is its strength, returning to family data enriches causal modelling of GE interplay. GWA analyses based on unrelated individuals and the polygenic scores derived from them include rGE effects as well as between‐family effects such as ethnicity, socioeconomic status (SES) and assortative mating. Finding that polygenic scores correlate with traits within families moves a step closer to causal genetic effects because it controls for all between‐family effects. That is, if the sibling with the higher polygenic score has a higher trait score than the other sibling, the association between the polygenic score and the trait cannot be due to between‐family effects. Finding within‐family correlations eliminates passive rGE effects because both siblings passively receive their genes and environments from their parents. Within‐sibship analyses also eliminate all other between‐family effects such as SES and assortative mating because siblings also share these effects. Although within‐sibship effects have been called ‘direct effects’, they only control for passive rGE, not evocative or active rGE.
To the extent that polygenic score correlations for unrelated individuals exceed within‐family polygenic score correlations this indicates the effect of between‐family factors. So far, it appears that between‐family effects contribute to polygenic score prediction for cognitive traits, which are impacted by SES and assortative mating, but not for behaviour problems (Selzam et al., 2019 ). A novel approach directly assesses within‐sibship effects by conducting GWA analyses of sibling differences rather than individual differences among unrelated individuals (Howe et al., 2022 ).
Adding parental GWA genotypes to create trios consisting of two parents and a child can further tease apart rGE. The first research in this area showed that parental polygenic scores predict their children's traits independent of the children's polygenic scores (Bates et al., 2018 ; Kong et al., 2018 ). These effects have been labelled ‘indirect effects’ or ‘genetic nurture’, but again only passive rGE is controlled in these analyses. Such indirect effects have so far only been found for cognitive traits, not behaviour problems (Willoughby et al., 2021 ) and it has been suggested that these effects of ‘genetic nurture’ are actually between‐family stratification effects (Nivard et al., 2022 ).
These analyses do not specify which environmental factors are responsible, but measured environments can be incorporated in this approach. For example, ADHD polygenic scores of mothers and their children both correlate with household chaos, indicating rGE contributions from both mothers and children (Agnew‐Blais et al., 2022 ). However, children's ADHD polygenic scores continue to correlate with household chaos after controlling for mothers' ADHD polygenic scores. This controls for passive rGE effects and suggests that children contribute to household chaos by evocative or active rGE. Research like this incorporating environmental measures is needed to go beyond passive rGE to investigate the more general processes of evocative and active rGE, although the major hurdle here is that current measures of the environment are not well suited to assess evocative rGE and especially active rGE.
Causal modelling of GE interplay using polygenic scores is limited by their effect size in predicting behaviour. In contrast, GCTA (Yang et al., 2011 ) estimates heritability and genetic correlations by comparing overall SNP differences from GWA genotyping with phenotypic differences pair by pair for thousands of individuals. GCTA can be used in these ways to investigate GE interplay (Choi et al., 2022 ). Combined with trios, GCTA can also separate direct and indirect genetic effects on behaviour problems (Eilertsen et al., 2022 ).
Given the torrent of new methods and opportunities to apply them, causal inference about GE interplay is certain to be a focal area of behavioural genomic research during the next 10 years.
USING POLYGENIC SCORES AS AN EARLY WARNING SYSTEM
In the next 10 years, polygenic scores for psychopathology will predict more than 10% of the variance and will be used in childhood to predict profiles of adult vulnerabilities.
As indicated in the previous section, extracting causality from essentially correlational data is difficult, despite the advances in behavioural genomic methods to tease apart GE interplay in causal models (Pingault et al., 2022 ). However, polygenic scores can be used to predict behavioural traits without knowing anything about intervening causal processes. The goal of prediction is to account for as much variance as possible without regard for explanation (Larsson, 2021a ). It has been argued that, from the perspective of prediction, it is not even necessary to disentangle the extent to which the prediction is due to rGE, assortative mating, or population stratification (Plomin & von Stumm, 2022 ).
Polygenic scores have a unique causal status because, as noted earlier, correlations between polygenic scores and behaviour are not subject to backward causation. This means that polygenic scores that predict adult psychopathology can be used in infancy as an early warning system to predict psychopathology in adulthood. In other words, polygenic score predictions are the same for DNA from an infant and from an adult. Although behaviour problems in childhood and adolescence are important in their own right, predicting adult psychopathology in childhood will be a focus for research in the next 10 years because of its clinical implications for intervention and prevention (Larsson, 2021b ).
Figure 3 shows polygenic score heritability estimates for eight psychiatric disorders with the largest GWA meta‐analyses. The average polygenic score heritability estimate is 4%, ranging from 1% for anxiety and anorexia to 8% for bipolar depression. However, these are logistic regressions that discriminate cases and controls to estimate heritability of liability, which is a hypothetical construct that assumes a normal distribution of risk underlying the diagnosis and does not translate directly to variance explained in the population.

Liability heritability estimates for eight psychiatric disorders based on polygenic scores (PGS h 2 ), SNP chip GWA genotypes (SNP h 2 ), and family/twin h 2 . PGS h 2 is the liability variance predicted by the polygenic score (usually Nagelkerke’s R 2 ). SNP h 2 is the liability variance estimated from GWA summary statistics using LD score regression. Family h 2 is the liability model‐fitting estimate from family or twin data. PGS h 2 references: schizophrenia (SCZ; Trubetskoy et al., 2022 ); bipolar disorder (BIP; Stahl et al., 2019 ); major depressive disorder (MDD; Howard et al., 2019 ); autism (Grove et al., 2019 ); attention deficit/hyperactivity disorder (ADHD; Demontis et al., 2019 ); obsessive‐compulsive disorder (OCD; Heinzel et al., 2021 ); anxiety (Purves et al., 2020 ); anorexia (Watson et al., 2019 ). SNP h 2 references: (Grotzinger et al., 2022 ). Family h 2 references: SCZ, BIP, MDD (Song et al., 2015 ); autism (Colvert et al., 2015 ); ADHD (Larsson et al., 2014 ); OCD (Mataix‐Cols et al., 2013 ); anxiety (Song et al., 2015 ); anorexia (Bulik et al., 2010 ).
The 10% target
During the next 10 years, I predict that polygenic scores will explain more than 10% of the variance of psychopathology. I hope by that time psychopathology will be assessed dimensionally so that the target of predicting 10% of the variance in the population will be clearly in view rather than obfuscated by liability statistics based on case‐control data.
The ability to predict 10% of the variance is a watershed in three ways. First, predicting 10% of the variance is off the scale of most predictions in the behavioural sciences. However, this is often overlooked because of the lingering preoccupation with statistical significance rather than subjecting findings to the harsh spotlight of effect size.
Second, polygenic scores with such effect sizes predict large differences at the extremes. Using the most recent polygenic score for schizophrenia that predicts 7% of the liability as an example (Trubetskoy et al., 2022 ), individuals in the highest centile are 39 times more likely to be diagnosed as schizophrenic as compared to individuals in the lowest centile of the polygenic score. The top centile is 5.6 times more likely to be diagnosed when compared to the remaining 99% of individuals.
Third, effect sizes of this magnitude are large enough to be ‘perceptible to the naked eye of a reasonably sensitive observer’ (Cohen, 1988 , p. 26). Nonetheless, 10% of the variance is equivalent to a correlation of 0.32, an oval‐shaped scatterplot that reflects the limitations of the probabilistic nature of prediction when correlations are less than 1.0.
Reaching the 10% target
Predicting 10% of the variance is a conservative target because polygenic scores in the cognitive realm already predict more than 10% of the variance for the quantitative traits of intelligence (Plomin & von Stumm, 2018 ), educational attainment (Okbay et al., 2022 ), and educational achievement (Allegrini et al., 2019 ).
Figure 3 indicates that there is plenty of headroom for increasing the predictive power of polygenic scores for psychopathology. The ultimate ceiling for polygenic score prediction is twin heritability, but the current ceiling is SNP heritability because GWA results and the polygenic scores derived from them rely on the common SNPs assessed on extant SNP chips. On average for these eight disorders, polygenic score heritability is only one‐fifth of the SNP heritability. This missing heritability gap can be narrowed through the brute force of larger GWA case‐control studies, but a bigger pay‐off is likely to come from GWA research using dimensional measures that correspond to the genetic architecture of psychopathology. Support for the hierarchical dimensional approach comes from a recent GWA study of a broad factor of externalising behaviours using Genomic SEM that yielded a polygenic score that predicted 10% of the variance (Karlsson Linnér et al., 2021 ). Another analytic strategy is to use longitudinal assessments that can capture the dynamic nature of psychopathology and capitalise on the fact that genetics is largely responsible for age‐to‐age continuity (Gidziela, Rimfeld, et al., 2022 ).
The second type of missing heritability is the gap between SNP heritability and estimates of heritability using family and twin designs. Figure 3 shows that, on average, SNP heritability is only 37% of family and twin estimates of heritability. Narrowing this missing heritability gap can substantially raise the ceiling for polygenic score prediction. This will require different technologies such as whole‐genome sequencing, which can increase SNP heritability by adding rarer DNA variants to the common variants assessed on current SNP chips (Wainschtein et al., 2022 ).
Implications and applications
Polygenic scores are already transforming research in developmental psychology. They will democratise genomics by making it possible for all researchers to add genomics to their programme of research, which will produce novel findings that permeate research and clinical practice. No longer are special samples of twins or adoptees needed for genomic analysis, just DNA. Behavioural assessment is not even needed because polygenic scores can be used as genetic proxies. For example, the genetics of cognitive abilities can be brought to bear without costly assessment using polygenic scores for cognitive ability. Finally, polygenic scores can be employed as genetic predictors in any moderately sized sample of unrelated individuals. As noted earlier, a polygenic score that predicts 10% of the variance only needs a sample size of 60 to detect its effect with 80% power. These are the reasons why all major longitudinal cohort studies have obtained DNA.
The most exciting application of polygenic scores will be to transform clinical work from symptoms to causes, from treatment to prediction and prevention, from one‐size‐fits‐all interventions to individually tailored interventions based on treatment response, and, as highlighted earlier, from qualitative diagnoses to quantitative dimensions (Plomin, 2018 ). For these reasons, during the next 10 years, as polygenic scores become more predictive, they will begin to make a difference clinically as they are used in childhood to predict profiles of vulnerabilities for adult psychopathology.
‘I am almost certain that complete genome sequencing will become part of newborn screening in the next few years…. It is likely that within a few decades people will look back on our current circumstance with a sense of disbelief that we screened for so few conditions’ (Collins, 2010, p. 50). This prediction was made by Francis Collins, who directed the Human Genome Project and then served as director of the US National Institutes of Health until 2021. There are signs that his prediction is belatedly becoming true. For example, it has been reported that by 2025 China expects to conduct whole‐genome sequencing on half of its 10 million babies born each year (Metzl, 2019 ). It is not a question of whether infants are genotyped at birth – for decades, newborns in most countries have been screened for a few single‐gene mutations. The cost of this screening is comparable to genotyping on a SNP chip, but rather than switching to SNP chips, whole‐genome sequencing seems inevitable as the cost continues to drop, perhaps to £100 (Pennisi, 2022 ), because whole‐genome sequencing captures all DNA variation. Newborn screening focuses on single‐gene mutations for medical disorders but polygenic scores will surely be incorporated as they become more predictive of problems later in life. Also inevitable is the use of polygenic scores in prenatal screening, at least for couples undergoing in vitro fertilisation (von Stumm & Plomin, 2021 ).
Although difficult ethical complications of newborn genotyping need to be addressed, a practical implication for developmental researchers is that genotype data could potentially be available for all children, without the need to obtain DNA or genotype it. That is, once children are genotyped on a SNP chip or by whole‐genome sequencing, their genotypes could be used to create any polygenic score. Thus, developmental researchers and clinicians in the future may be able to add polygenic scores to their research at no cost other than analytic costs of creating polygenic scores, which are becoming routinised.
Another direction for research during the next 10 years is to create more predictive polygenic scores by moving beyond Eurocentric samples to more diverse samples (Peterson et al., 2019 ). This effort has begun, for example, with the US project All of Us, which was launched in 2018 and plans to enrol more than one million people of diverse ancestry ( https://allofus.nih.gov/about ), and the UK project, Our Future Health , which plans whole‐genome sequencing for 5 million individuals of diverse ancestry ( https://ourfuturehealth.org.uk/ ).
The outpouring of opportunities created by behavioural genomics is unparallelled in the behavioural sciences, as illustrated by the three transformative developments for research in the next 10 years outlined here. Understanding the genetic architecture of psychopathology, investigating genetic and environmental causal paths, and using polygenic scores as an early warning system will also eventually transform clinical practice.
Allele frequency Population frequency of a DNA variant.
Candidate genes A gene whose function suggests that it might be associated with a trait.
DNA Deoxyribonucleic acid, the double‐stranded molecule that encodes genetic information. The two strands are held together by hydrogen bonds between two of the four nucleotide bases, with adenine bonded to thymine and cytosine bonded to guanine.
DNA microarray Miniature slides with hundreds of thousands of short single‐stranded DNA sequences that serve as probes to detect SNPs. Commonly called SNP chip.
Genetic correlation A statistic indexing the extent to which genetic influences on one trait are correlated with genetic influences on another trait independent of the heritabilities of the traits.
Genome‐wide association (GWA) An association study that assesses DNA variants throughout the genome, typically for SNPs assessed on DNA microarrays.
Genome‐wide complex trait analysis (GCTA) Estimates SNP heritability and genetic correlations explained by all SNPs for a trait rather than testing the association between any particular SNP and the trait.
Genomic structural equation modelling (Genomic SEM) A statistical framework for modelling multivariate genetic variance structures.
Genotyping Assessing an individual's pair of alleles at a particular locus.
Heritability The proportion of phenotypic variance among individuals that can be attributed to inherited DNA differences in a particular population.
Human genome All three billion DNA nucleotide base pairs in our species, packaged in 23 pairs of chromosomes.
Linkage disequilibrium (LD) score regression Estimating SNP heritability and genetic correlations from GWA summary statistics by regressing association effect sizes of SNPs on their distance apart on a chromosome (linkage disequilibrium).
Missing heritability The difference between variance explained by polygenic scores (PGS heritability) and family estimates of heritability. Another type of missing heritability is the difference between PGS heritability and SNP heritability, which creates a ceiling for PGS heritability.
Molecular genetics The investigation of the effects of specific genes at the level of DNA.
Nature of nurture Genetic influence on measures of the environment and on the covariance between environmental measures and behavioural traits.
Nonshared environment Environmental influences that do not contribute to resemblance between family members.
Nucleotide base A single step in the spiral staircase of the DNA double helix consisting of hydrogen bonds between two of the four nucleotide bases, with adenine bonding to thymine and cytosine bonding to guanine. The DNA code is a sequence of three nucleotide base pairs that codes for one of the 20 amino acids, which are the building blocks of proteins.
Polygenic A trait influenced by many genes.
Polygenic score A genetic index of a trait for an individual that is the sum across the genome of thousands of the individual's increasing alleles associated with the trait, usually weighted by the effect size of each SNP's association with the trait, based on GWA summary statistics for the trait.
Quantitative genetics A theory of multiple‐gene influences that, together with environmental variation, result in in quantitative (continuous) distributions of traits. Quantitative genetic methods such as twin and adoption methods estimate genetic and environmental contributions to variance of traits and covariance between traits in a population.
Shared environment Environmental influences that make family members similar.
Single‐gene disorder Caused by variants in a single gene, which yields Mendelian patterns of inheritance.
Single‐nucleotide polymorphism (SNP) The most common type of DNA variant which involves a difference in a single nucleotide.
SNP heritability Heritability estimated directly from DNA differences (SNPs) between individuals.
Whole‐genome sequencing Determining the complete sequence of nucleotide base pairs for a genome.
AUTHOR CONTRIBUTION
Robert Plomin : Conceptualization, Writing – original draft.
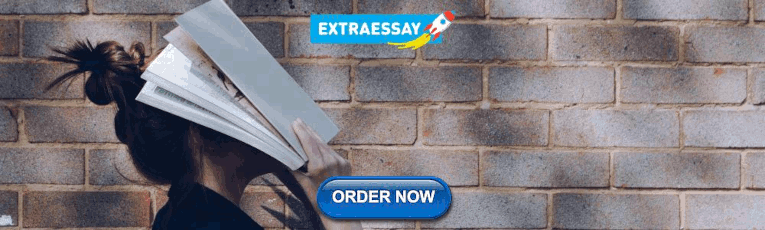
CONFLICTS OF INTEREST
The author has declared that he has no competing or potential conflicts of interest.
ETHICAL CONSIDERATIONS
Not applicable to this article.
ACKNOWLEDGEMENTS
We gratefully acknowledge the ongoing contribution of the participants in the Twins Early Development Study (TEDS) and their families. TEDS is supported by a programme grant from the UK Medical Research Council (Grant Nos. MR/V012878/1 and previously MR/M021475/1), with additional support from the US National Institutes of Health (Grant No. AG046938).
Plomin, R. (2022). The next 10 years of behavioural genomic research . JCPP Advances , 2 ( 4 ), e12112. 10.1002/jcv2.12112 [ CrossRef ] [ Google Scholar ]
DATA AVAILABILITY STATEMENT
- Agnew‐Blais, J. , Wetz, J. , Arseneault, L. , Belsky, D. , Danese, A. , Pingault, J.‐B. , Polanczyk, G. , Sugden, K. , Williams, B. , & Moffitt, T. (2022). Mother’s and children’s ADHD genetic risk, household chaos and children’s ADHD symptoms: A gene‐environment correlation study . Journal of Child Psychology and Psychiatry , 63 ( 10 ), 1153–1163. [ PMC free article ] [ PubMed ] [ Google Scholar ]
- Akingbuwa, W. A. , Hammerschlag, A. R. , Jami, E. S. , Allegrini, A. G. , Karhunen, V. , Sallis, H. , Ask, H. , Askeland, R. B. , Baselmans, B. , Diemer, E. , Hagenbeek, F. A. , Havdahl, A. , Hottenga, J.‐J. , Mbarek, H. , Rivadeneira, F. , Tesli, M. , van Beijsterveldt, C. , Breen, G. , Lewis, C. M. , … Bipolar Disorder and Major Depressive Disorder Working Groups of the Psychiatric Genomics Consortium . (2020). Genetic associations between childhood psychopathology and adult depression and associated traits in 42 998 individuals: A meta‐analysis . JAMA Psychiatry , 77 ( 7 ), 715. 10.1001/jamapsychiatry.2020.0527 [ PMC free article ] [ PubMed ] [ CrossRef ] [ Google Scholar ]
- Allegrini, A. G. , Baldwin, J. R. , Barkhuizen, W. , & Pingault, J. (2022). Research review: A guide to computing and implementing polygenic scores in developmental research . Journal of Child Psychology and Psychiatry , 63 ( 10 ), 1111–1124. 10.1111/jcpp.13611 [ PMC free article ] [ PubMed ] [ CrossRef ] [ Google Scholar ]
- Allegrini, A. G. , Cheesman, R. , Rimfeld, K. , Selzam, S. , Pingault, J. , Eley, T. C. , & Plomin, R. (2020). The p factor: Genetic analyses support a general dimension of psychopathology in childhood and adolescence . Journal of Child Psychology and Psychiatry , 61 ( 1 ), 30–39. 10.1111/jcpp.13113 [ PMC free article ] [ PubMed ] [ CrossRef ] [ Google Scholar ]
- Allegrini, A. G. , Karhunen, V. , Coleman, J. R. I. , Selzam, S. , Rimfeld, K. , von Stumm, S. , Pingault, J.‐B. , & Plomin, R. (2020). Multivariable G‐E interplay in the prediction of educational achievement . PLoS Genetics , 16 ( 11 ), e1009153. 10.1371/journal.pgen.1009153 [ PMC free article ] [ PubMed ] [ CrossRef ] [ Google Scholar ]
- Allegrini, A. G. , Selzam, S. , Rimfeld, K. , von Stumm, S. , Pingault, J. B. , & Plomin, R. (2019). Genomic prediction of cognitive traits in childhood and adolescence . Molecular Psychiatry , 24 ( 6 ), 819–827. 10.1038/s41380-019-0394-4 [ PMC free article ] [ PubMed ] [ CrossRef ] [ Google Scholar ]
- Anttila, V. , The Brainstorm Consortium , Bulik‐Sullivan, B. , Finucane, H. K. , Walters, R. K. , Bras, J. , Duncan, L. , Escott‐Price, V. , Falcone, G. J. , Gormley, P. , Malik, R. , Patsopoulos, N. A. , Ripke, S. , Wei, Z. , Yu, D. , Lee, P. H. , Turley, P. , Grenier‐Boley, B. , Chouraki, V. , … Neale, B. M . (2018). Analysis of shared heritability in common disorders of the brain . Science , 360 ( 6395 ), eaap8757. 10.1126/science.aap8757 [ PMC free article ] [ PubMed ] [ CrossRef ] [ Google Scholar ]
- Avinun, R. , & Knafo, A. (2014). Parenting as a reaction evoked by children’s genotype: A meta‐analysis of children‐as‐twins studies . Personality and Social Psychology Review , 18 ( 1 ), 87–102. 10.1177/1088868313498308 [ PubMed ] [ CrossRef ] [ Google Scholar ]
- Bates, T. C. , Maher, B. S. , Medland, S. E. , McAloney, K. , Wright, M. J. , Hansell, N. K. , Kendler, K. S. , Martin, N. G. , & Gillespie, N. A. (2018). The nature of nurture: Using a virtual‐parent design to test parenting effects on children’s educational attainment in genotyped families . Twin Research and Human Genetics , 21 ( 2 ), 73–83. 10.1017/thg.2018.11 [ PubMed ] [ CrossRef ] [ Google Scholar ]
- Berry, D. , & Willoughby, M. T. (2017). On the practical interpretability of cross‐lagged panel models: Rethinking a developmental workhorse . Child Development , 88 ( 4 ), 1186–1206. 10.1111/cdev.12660 [ PubMed ] [ CrossRef ] [ Google Scholar ]
- Border, R. , Johnson, E. C. , Evans, L. M. , Smolen, A. , Berley, N. , Sullivan, P. F. , & Keller, M. C. (2019). No Support for historical candidate gene or candidate gene‐by‐interaction hypotheses for major depression across multiple large samples . American Journal of Psychiatry , 176 ( 5 ), 376–387. 10.1176/appi.ajp.2018.18070881 [ PMC free article ] [ PubMed ] [ CrossRef ] [ Google Scholar ]
- Bulik‐Sullivan, B. , ReproGen Consortium , Psychiatric Genomics Consortium , Genetic Consortium for Anorexia Nervosa of the Wellcome Trust Case Control Consortium 3 , Finucane, H. K. , Anttila, V. , Gusev, A. , Day, F. R. , Loh, P.‐R. , Duncan, L. , Perry, J. R. B. , Patterson, N. , Robinson, E. B. , Daly, M. J. , Price, A. L. , Neale, B. M. , & Neale, B. M . (2015). An atlas of genetic correlations across human diseases and traits . Nature Genetics , 47 ( 11 ), 1236–1241. 10.1038/ng.3406 [ PMC free article ] [ PubMed ] [ CrossRef ] [ Google Scholar ]
- Bulik, C. M. , Thornton, L. M. , Root, T. L. , Pisetsky, E. M. , Lichtenstein, P. , & Pedersen, N. L. (2010). Understanding the relation between anorexia nervosa and bulimia nervosa in a Swedish National Twin Sample . Biological Psychiatry , 67 ( 1 ), 71–77. 10.1016/j.biopsych.2009.08.010 [ PMC free article ] [ PubMed ] [ CrossRef ] [ Google Scholar ]
- Buss, A. H. , & Plomin, R. (1984). Temperament: Early developing personality traits . Psychology Press. [ Google Scholar ]
- Caspi, A. , Houts, R. M. , Belsky, D. W. , Goldman‐Mellor, S. J. , Harrington, H. , Israel, S. , Meier, M. H. , Ramrakha, S. , Shalev, I. , Poulton, R. , & Moffitt, T. E. (2014). The p factor: One general psychopathology factor in the structure of psychiatric disorders? Clinical Psychological Science , 2 ( 2 ), 119–137. 10.1177/2167702613497473 [ PMC free article ] [ PubMed ] [ CrossRef ] [ Google Scholar ]
- Caspi, A. , & Moffitt, T. E. (2018). All for one and one for all: Mental disorders in one dimension . American Journal of Psychiatry , 175 ( 9 ), 831–844. 10.1176/appi.ajp.2018.17121383 [ PMC free article ] [ PubMed ] [ CrossRef ] [ Google Scholar ]
- Chabris, C. F. , Hebert, B. M. , Benjamin, D. J. , Beauchamp, J. , Cesarini, D. , van der Loos, M. , Johannesson, M. , Magnusson, P. K. E. , Lichtenstein, P. , Atwood, C. S. , Freese, J. , Hauser, T. S. , Hauser, R. M. , Christakis, N. , & Laibson, D. (2012). Most reported genetic associations with general intelligence are probably false positives . Psychological Science , 23 ( 11 ), 1314–1323. 10.1177/0956797611435528 [ PMC free article ] [ PubMed ] [ CrossRef ] [ Google Scholar ]
- Choi, K. W. , Stein, M. B. , Nishimi, K. M. , Ge, T. , Coleman, J. R. I. , Chen, C.‐Y. , Ratanatharathorn, A. , Zheutlin, A. B. , Dunn, E. C. , 23andMe Research Team , Major Depressive Disorder Working Group of the Psychiatric Genomics Consortium , Breen, G. , Koenen, K. C. , & Smoller, J. W . (2020). An exposure‐wide and Mendelian randomization approach to identifying modifiable factors for the prevention of depression . American Journal of Psychiatry , 177 ( 10 ), 944–954. 10.1176/appi.ajp.2020.19111158 [ PMC free article ] [ PubMed ] [ CrossRef ] [ Google Scholar ]
- Choi, K. W. , Wilson, M. , Ge, T. , Kandola, A. , Patel, C. J. , Lee, S. H. , & Smoller, J. W. (2022). Integrative analysis of genomic and exposomic influences on youth mental health . Journal of Child Psychology and Psychiatry , 63 ( 10 ), 1196–1205. 10.1111/jcpp.13664 [ PMC free article ] [ PubMed ] [ CrossRef ] [ Google Scholar ]
- Cohen, J. (1988). Statistical power analysis for the behavioral sciences (2nd ed.). Erlbaum Associates. [ Google Scholar ]
- Colvert, E. , Tick, B. , McEwen, F. , Stewart, C. , Curran, S. R. , Woodhouse, E. , Gillan, N. , Hallett, V. , Lietz, S. , Garnett, T. , Ronald, A. , Plomin, R. , Rijsdijk, F. , Happé, F. , & Bolton, P. (2015). Heritability of autism spectrum disorder in a UK population‐based twin sample . JAMA Psychiatry , 72 ( 5 ), 415–423. 10.1001/jamapsychiatry.2014.3028 [ PMC free article ] [ PubMed ] [ CrossRef ] [ Google Scholar ]
- Cross‐Disorder Group of the Psychiatric Genomics Consortium . (2013a). Identification of risk loci with shared effects on five major psychiatric disorders: A genome‐wide analysis . The Lancet , 381 ( 9875 ), 1371–1379. 10.1016/S0140-6736(12)62129-1 [ PMC free article ] [ PubMed ] [ CrossRef ] [ Google Scholar ]
- Cross‐Disorder Group of the Psychiatric Genomics Consortium . (2013b). Genetic relationship between five psychiatric disorders estimated from genome‐wide SNPs . Nature Genetics , 45 ( 9 ), 984–994. 10.1038/ng.2711 [ PMC free article ] [ PubMed ] [ CrossRef ] [ Google Scholar ]
- Davey Smith, G. , & Ebrahim, S. (2003). ‘Mendelian randomization’: Can genetic epidemiology contribute to understanding environmental determinants of disease? International Journal of Epidemiology , 32 ( 1 ), 1–22. 10.1093/ije/dyg070 [ PubMed ] [ CrossRef ] [ Google Scholar ]
- Demange, P. A. , Malanchini, M. , Mallard, T. T. , Biroli, P. , Cox, S. R. , Grotzinger, A. D. , Tucker‐Drob, E. M. , Abdellaoui, A. , Arseneault, L. , van Bergen, E. , Boomsma, D. I. , Caspi, A. , Corcoran, D. L. , Domingue, B. W. , Harris, K. M. , Ip, H. F. , Mitchell, C. , Moffitt, T. E. , Poulton, R. , … Nivard, M. G. (2021). Investigating the genetic architecture of noncognitive skills using GWAS‐by‐subtraction . Nature Genetics , 53 ( 1 ), 35–44. 10.1038/s41588-020-00754-2 [ PMC free article ] [ PubMed ] [ CrossRef ] [ Google Scholar ]
- Demontis, D. , ADHD Working Group of the Psychiatric Genomics Consortium (PGC) , Early Lifecourse & Genetic Epidemiology (EAGLE) Consortium, 23andMe Research Team , Walters, R. K. , Martin, J. , Mattheisen, M. , Als, T. D. , Agerbo, E. , Baldursson, G. , Belliveau, R. , Bybjerg‐Grauholm, J. , Bækvad‐Hansen, M. , Cerrato, F. , Chambert, K. , Churchhouse, C. , Dumont, A. , Eriksson, N. , Gandal, M. , … Neale, B. M. (2019). Discovery of the first genome‐wide significant risk loci for attention deficit/hyperactivity disorder . Nature Genetics , 51 ( 1 ), 63–75. 10.1038/s41588-018-0269-7 [ PMC free article ] [ PubMed ] [ CrossRef ] [ Google Scholar ]
- Dick, D. M. (2011). Gene‐environment interaction in psychological traits and disorders . Annual Review of Clinical Psychology , 7 ( 1 ), 383–409. 10.1146/annurev-clinpsy-032210-104518 [ PMC free article ] [ PubMed ] [ CrossRef ] [ Google Scholar ]
- Dick, D. M. , Agrawal, A. , Keller, M. C. , Adkins, A. , Aliev, F. , Monroe, S. , Hewitt, J. K. , Kendler, K. S. , & Sher, K. J. (2015). Candidate gene–environment interaction research: Reflections and recommendations . Perspectives on Psychological Science , 10 ( 1 ), 37–59. 10.1177/1745691614556682 [ PMC free article ] [ PubMed ] [ CrossRef ] [ Google Scholar ]
- Duncan, L. E. , & Keller, M. C. (2011). A critical review of the first 10 years of candidate gene‐by‐environment interaction research in psychiatry . American Journal of Psychiatry , 168 ( 10 ), 1041–1049. 10.1176/appi.ajp.2011.11020191 [ PMC free article ] [ PubMed ] [ CrossRef ] [ Google Scholar ]
- Eilertsen, E. M. , Cheesman, R. , Ayorech, Z. , Roysamb, E. , Pingault, J.‐B. , Njolstad, P. , Andreassen, O. , Havdahl, A. , McAdams, T. , & Torvik, F. A. (2022). On the importance of parenting in externalizing disorders: An evaluation of indirect genetic effects in families . Journal of Child Psychology and Psychiatry , 63 ( 10 ), 1186–1195. [ PMC free article ] [ PubMed ] [ Google Scholar ]
- Fisher, R. A. (1918). The correlation between relatives on the supposition of Mendelian inheritance . Transactions of the Royal Society of Edinburgh , 52 , 399–433. [ Google Scholar ]
- Galton, F. (1865). Hereditary talent and character . Macmillan’s Magazine , 12 ( 157–166 ), 318–327. [ Google Scholar ]
- Geschwind, D. H. , & Flint, J. (2015). Genetics and genomics of psychiatric disease . Science , 349 ( 6255 ), 1489–1494. 10.1126/science.aaa8954 [ PMC free article ] [ PubMed ] [ CrossRef ] [ Google Scholar ]
- Gidziela, A. , Malanchini, M. , Rimfeld, K. , McMillan, A. , Ronald, A. , Pike, A. , Asbury, K. , Eley, T. C. , von Stumm, S. , & Plomin, R. (2022). Explaining the influence of nonshared environment (NSE) on symptoms of behaviour problems from preschool to adulthood: Mind the missing NSE gap . Journal of Child Psychology and Psychiatry (in press). [ PMC free article ] [ PubMed ] [ Google Scholar ]
- Gidziela, A. , Rimfeld, K. , Malanchini, M. , Allegrini, A. G. , McMillan, A. , Selzam, S. , Ronald, A. , Viding, E. , Stumm, S. , Eley, T. C. , & Plomin, R. (2022). Using DNA to predict behaviour problems from preschool to adulthood . Journal of Child Psychology and Psychiatry , 63 ( 7 ), 781–792. 10.1111/jcpp.13519 [ PubMed ] [ CrossRef ] [ Google Scholar ]
- Goldsmith, H. H. , Buss, A. H. , Plomin, R. , Rothbart, M. K. , Thomas, A. , Chess, S. , Hinde, R. A. , & McCall, R. B. (1987). Roundtable: What is temperament? Four approaches . Child Development , 58 ( 2 ), 505–529. [ PubMed ] [ Google Scholar ]
- Grotzinger, A. D. , Mallard, T. T. , Akingbuwa, W. A. , Ip, H. F. , Adams, M. J. , Lewis, C. M. , McIntosh, A. M. , Grove, J. , Dalsgaard, S. , Lesch, K.‐P. , Strom, N. , Meier, S. M. , Mattheisen, M. , Børglum, A. D. , Mors, O. , Breen, G. , iPSYCHMattheisen, M. , Mors, O. , … Nivard, M. G. (2022). Genetic architecture of 11 major psychiatric disorders at biobehavioral, functional genomic and molecular genetic levels of analysis . Nature Genetics , 54 ( 5 ), 548–559. 10.1038/s41588-022-01057-4 [ PMC free article ] [ PubMed ] [ CrossRef ] [ Google Scholar ]
- Grotzinger, A. D. , Rhemtulla, M. , de Vlaming, R. , Ritchie, S. J. , Mallard, T. T. , Hill, W. D. , Ip, H. F. , Marioni, R. E. , McIntosh, A. M. , Deary, I. J. , Koellinger, P. D. , Harden, K. P. , Nivard, M. G. , & Tucker‐Drob, E. M. (2019). Genomic structural equation modelling provides insights into the multivariate genetic architecture of complex traits . Nature Human Behaviour , 3 ( 5 ), 513–525. 10.1038/s41562-019-0566-x [ PMC free article ] [ PubMed ] [ CrossRef ] [ Google Scholar ]
- Grove, J. , Autism Spectrum Disorder Working Group of the Psychiatric Genomics Consortium , BUPGEN , Major Depressive Disorder Working Group of the Psychiatric Genomics Consortium , 23andMe Research Team , Ripke, S. , Als, T. D. , Mattheisen, M. , Walters, R. K. , Won, H. , Pallesen, J. , Agerbo, E. , Andreassen, O. A. , Anney, R. , Awashti, S. , Belliveau, R. , Bettella, F. , Buxbaum, J. D. , Bybjerg‐Grauholm, J. , … Børglum, A. D . (2019). Identification of common genetic risk variants for autism spectrum disorder . Nature Genetics , 51 ( 3 ), 431–444. 10.1038/s41588-019-0344-8 [ PMC free article ] [ PubMed ] [ CrossRef ] [ Google Scholar ]
- Heinzel, S. , Kaufmann, C. , Grützmann, R. , Klawohn, J. , Riesel, A. , Bey, K. , Heilmann‐Heimbach, S. , Weinhold, L. , Ramirez, A. , Wagner, M. , & Kathmann, N. (2021). Polygenic risk for obsessive‐compulsive disorder (OCD) predicts brain response during working memory task in OCD, unaffected relatives, and healthy controls . Scientific Reports , 11 ( 1 ), 18914. 10.1038/s41598-021-98333-w [ PMC free article ] [ PubMed ] [ CrossRef ] [ Google Scholar ]
- Howard, D. M. , Adams, M. J. , Clarke, T.‐K. , Hafferty, J. D. , Gibson, J. , Shirali, M. , Coleman, J. R. I. , Hagenaars, S. P. , Ward, J. , Wigmore, E. M. , Alloza, C. , Shen, X. , Barbu, M. C. , Xu, E. Y. , Whalley, H. C. , Marioni, R. E. , Porteous, D. J. , Davies, G. , Deary, I. J. , … McIntosh, A. M. (2019). Genome‐wide meta‐analysis of depression identifies 102 independent variants and highlights the importance of the prefrontal brain regions . Nature Neuroscience , 22 ( 3 ), 343–352. 10.1038/s41593-018-0326-7 [ PMC free article ] [ PubMed ] [ CrossRef ] [ Google Scholar ]
- Howe, L. J. , Nivard, M. G. , Morris, T. T. , Hansen, A. F. , Rasheed, H. , Cho, Y. , Chittoor, G. , Ahlskog, R. , Lind, P. A. , Palviainen, T. , van der Zee, M. D. , Cheesman, R. , Mangino, M. , Wang, Y. , Li, S. , Klaric, L. , Ratliff, S. M. , Bielak, L. F. , Nygaard, M. , … Davies, N. M. (2022). Within‐sibship genome‐wide association analyses decrease bias in estimates of direct genetic effects . Nature Genetics , 54 ( 5 ), 581–592. 10.1038/s41588-022-01062-7 [ PMC free article ] [ PubMed ] [ CrossRef ] [ Google Scholar ]
- Jaffee, S. R. , & Price, T. S. (2007). Gene–environment correlations: A review of the evidence and implications for prevention of mental illness . Molecular Psychiatry , 12 ( 5 ), 432–442. 10.1038/sj.mp.4001950 [ PMC free article ] [ PubMed ] [ CrossRef ] [ Google Scholar ]
- Karlsson Linnér, R. , Mallard, T. T. , Barr, P. B. , Sanchez‐Roige, S. , Madole, J. W. , Driver, M. N. , Poore, H. E. , de Vlaming, R. , Grotzinger, A. D. , Tielbeek, J. J. , Johnson, E. C. , Liu, M. , Rosenthal, S. B. , Ideker, T. , Zhou, H. , Kember, R. L. , Pasman, J. A. , Verweij, K. J. H. , Liu, D. J. , … Dick, D. M. (2021). Multivariate analysis of 1.5 million people identifies genetic associations with traits related to self‐regulation and addiction . Nature Neuroscience , 24 ( 10 ), 1367–1376. 10.1038/s41593-021-00908-3 [ PMC free article ] [ PubMed ] [ CrossRef ] [ Google Scholar ]
- Kendler, K. S. , & Baker, J. H. (2007). Genetic influences on measures of the environment: A systematic review . Psychological Medicine , 37 ( 05 ), 615–626. 10.1017/S0033291706009524 [ PubMed ] [ CrossRef ] [ Google Scholar ]
- Knopik, V. S. , Neiderhiser, J. M. , DeFries, J. C. , & Plomin, R. (2017). Behavioral genetics (7th ed.). Worth Publishers, Macmillan Learning. [ Google Scholar ]
- Koellinger, P. D. , & de Vlaming, R. (2019). Mendelian randomization: The challenge of unobserved environmental confounds . International Journal of Epidemiology , 48 ( 3 ), 665–671. 10.1093/ije/dyz138 [ PMC free article ] [ PubMed ] [ CrossRef ] [ Google Scholar ]
- Kong, A. , Thorleifsson, G. , Frigge, M. L. , Vilhjalmsson, B. J. , Young, A. I. , Thorgeirsson, T. E. , Benonisdottir, S. , Oddsson, A. , Halldorsson, B. V. , Masson, G. , Gudbjartsson, D. F. , Helgason, A. , Bjornsdottir, G. , Thorsteinsdottir, U. , & Stefansson, K. (2018). The nature of nurture: Effects of parental genotypes . Science , 359 ( 6374 ), 424–428. 10.1126/science.aan6877 [ PubMed ] [ CrossRef ] [ Google Scholar ]
- Kotov, R. , Krueger, R. F. , Watson, D. , Achenbach, T. M. , Althoff, R. R. , Bagby, R. M. , Brown, T. A. , Carpenter, W. T. , Caspi, A. , Clark, L. A. , Eaton, N. R. , Forbes, M. K. , Forbush, K. T. , Goldberg, D. , Hasin, D. , Hyman, S. E. , Ivanova, M. Y. , Lynam, D. R. , Markon, K. , … Zimmerman, M. (2017). The hierarchical taxonomy of psychopathology (HiTOP): A dimensional alternative to traditional nosologies . Journal of Abnormal Psychology , 126 ( 4 ), 454–477. 10.1037/abn0000258 [ PubMed ] [ CrossRef ] [ Google Scholar ]
- Krapohl, E. , Hannigan, L. J. , Pingault, J.‐B. , Patel, H. , Kadeva, N. , Curtis, C. , Breen, G. , Newhouse, S. J. , Eley, T. C. , O’Reilly, P. F. , & Plomin, R. (2017). Widespread covariation of early environmental exposures and trait‐associated polygenic variation . Proceedings of the National Academy of Sciences , 114 ( 44 ), 11727–11732. 10.1073/pnas.1707178114 [ PMC free article ] [ PubMed ] [ CrossRef ] [ Google Scholar ]
- Krueger, R. F. , Kotov, R. , Watson, D. , Forbes, M. K. , Eaton, N. R. , Ruggero, C. J. , Simms, L. J. , Widiger, T. A. , Achenbach, T. M. , Bach, B. , Bagby, R. M. , Bornovalova, M. A. , Carpenter, W. T. , Chmielewski, M. , Cicero, D. C. , Clark, L. A. , Conway, C. , DeClercq, B. , DeYoung, C. G. , … Zimmermann, J. (2018). Progress in achieving quantitative classification of psychopathology . World Psychiatry , 17 ( 3 ), 282–293. 10.1002/wps.20566 [ PMC free article ] [ PubMed ] [ CrossRef ] [ Google Scholar ]
- Lahey, B. B. , Applegate, B. , Hakes, J. K. , Zald, D. H. , Hariri, A. R. , & Rathouz, P. J. (2012). Is there a general factor of prevalent psychopathology during adulthood? Journal of Abnormal Psychology , 121 ( 4 ), 971–977. 10.1037/a0028355 [ PMC free article ] [ PubMed ] [ CrossRef ] [ Google Scholar ]
- Lahey, B. B. , Moore, T. M. , Kaczkurkin, A. N. , & Zald, D. H. (2021). Hierarchical models of psychopathology: Empirical support, implications, and remaining issues . World Psychiatry , 20 ( 1 ), 57–63. 10.1002/wps.20824 [ PMC free article ] [ PubMed ] [ CrossRef ] [ Google Scholar ]
- Larsson, H. (2021a). Quantitative and molecular genetics of child and adolescent mental health disorders: Recent advances, knowledge gaps and directions for future research . JCPP Advances , 1 ( 1 ). 10.1111/jcv2.12014 [ CrossRef ] [ Google Scholar ]
- Larsson, H. (2021b). Causation and prediction in child and adolescent mental health research . JCPP Advances , 1 ( 2 ). 10.1002/jcv2.12026 [ CrossRef ] [ Google Scholar ]
- Larsson, H. , Chang, Z. , D’Onofrio, B. M. , & Lichtenstein, P. (2014). The heritability of clinically diagnosed attention deficit hyperactivity disorder across the lifespan . Psychological Medicine , 44 ( 10 ), 2223–2229. 10.1017/S0033291713002493 [ PMC free article ] [ PubMed ] [ CrossRef ] [ Google Scholar ]
- Lee, P. H. , Anttila, V. , Won, H. , Feng, Y.‐C. A. , Rosenthal, J. , Zhu, Z. , Tucker‐Drob, E. M. , Nivard, M. G. , Grotzinger, A. D. , Posthuma, D. , Wang, M. M.‐J. , Yu, D. , Stahl, E. A. , Walters, R. K. , Anney, R. J. L. , Duncan, L. E. , Ge, T. , Adolfsson, R. , Banaschewski, T. , … Smoller, J. W. (2019). Genomic relationships, novel loci, and pleiotropic mechanisms across eight psychiatric disorders . Cell , 179 ( 7 ), 1469–1482.e11. 10.1016/j.cell.2019.11.020 [ PMC free article ] [ PubMed ] [ CrossRef ] [ Google Scholar ]
- Martin, J. , Taylor, M. J. , & Lichtenstein, P. (2018). Assessing the evidence for shared genetic risks across psychiatric disorders and traits . Psychological Medicine , 48 ( 11 ), 1759–1774. 10.1017/S0033291717003440 [ PMC free article ] [ PubMed ] [ CrossRef ] [ Google Scholar ]
- Mataix‐Cols, D. , Boman, M. , Monzani, B. , Rück, C. , Serlachius, E. , Långström, N. , & Lichtenstein, P. (2013). Population‐based, multigenerational family clustering study of obsessive‐compulsive disorder . JAMA Psychiatry , 70 ( 7 ), 709–717. 10.1001/jamapsychiatry.2013.3 [ PubMed ] [ CrossRef ] [ Google Scholar ]
- McAdams, T. A. , Neiderhiser, J. M. , Rijsdijk, F. V. , Narusyte, J. , Lichtenstein, P. , & Eley, T. C. (2014). Accounting for genetic and environmental confounds in associations between parent and child characteristics: A systematic review of children‐of‐twins studies . Psychological Bulletin , 140 ( 4 ), 1138–1173. 10.1037/a0036416 [ PubMed ] [ CrossRef ] [ Google Scholar ]
- McLaughlin, K. A. , Gadermann, A. M. , Hwang, I. , Sampson, N. A. , Al‐Hamzawi, A. , Andrade, L. H. , Angermeyer, M. C. , Benjet, C. , Bromet, E. J. , Bruffaerts, R. , Caldas‐de‐Almeida, J. M. , de Girolamo, G. , de Graaf, R. , Florescu, S. , Gureje, O. , Haro, J. M. , Hinkov, H. R. , Horiguchi, I. , Hu, C. , … Kessler, R. C. (2012). Parent psychopathology and offspring mental disorders: Results from the WHO world mental health Surveys . British Journal of Psychiatry , 200 ( 4 ), 290–299. 10.1192/bjp.bp.111.101253 [ PMC free article ] [ PubMed ] [ CrossRef ] [ Google Scholar ]
- Mendel, G. (1866). Versuche ueber Pflanzenhybriden . Verhandllungen Des Naturforschhunden Vereines in Bruenn , 4 , 3–47. [ Google Scholar ]
- Metzl, J. F. (2019). Hacking Darwin: Genetic engineering and the future of humanity . Sourcebooks. [ Google Scholar ]
- Middeldorp, C. M. , Cath, D. C. , Van Dyck, R. , & Boomsma, D. I. (2005). The co‐morbidity of anxiety and depression in the perspective of genetic epidemiology. A review of twin and family studies . Psychological Medicine , 35 ( 5 ), 611–624. 10.1017/S003329170400412X [ PubMed ] [ CrossRef ] [ Google Scholar ]
- Mullins, N. , Forstner, A. J. , O’Connell, K. S. , Coombes, B. , Coleman, J. R. I. , Qiao, Z. , Als, T. D. , Bigdeli, T. B. , Børte, S. , Bryois, J. , Charney, A. W. , Drange, O. K. , Gandal, M. J. , Hagenaars, S. P. , Ikeda, M. , Kamitaki, N. , Kim, M. , Krebs, K. , Panagiotaropoulou, G. , … Andreassen, O. A. (2021). Genome‐wide association study of more than 40,000 bipolar disorder cases provides new insights into the underlying biology . Nature Genetics , 53 ( 6 ), 817–829. 10.1038/s41588-021-00857-4 [ PMC free article ] [ PubMed ] [ CrossRef ] [ Google Scholar ]
- Nivard, M. G. , Belsky, D. , Harden, K. P. , Baier, T. , Ystrom, E. , & Lyngstad, T. H. (2022). Neither nature nor nurture: Using extended pedigree data to elucidate the origins of indirect genetic effects on offspring educational outcomes . [Preprint]. PsyArXiv. 10.31234/osf.io/bhpm5 [ CrossRef ] [ Google Scholar ]
- Okbay, A. , Wu, Y. , Wang, N. , Jayashankar, H. , Bennett, M. , Nehzati, S. M. , Sidorenko, J. , Kweon, H. , Goldman, G. , Gjorgjieva, T. , Jiang, Y. , Hicks, B. , Tian, C. , Hinds, D. A. , Ahlskog, R. , Magnusson, P. K. E. , Oskarsson, S. , Hayward, C. , Campbell, A. , … Young, A. I. (2022). Polygenic prediction of educational attainment within and between families from genome‐wide association analyses in 3 million individuals . Nature Genetics , 54 ( 4 ), 437–449. 10.1038/s41588-022-01016-z [ PMC free article ] [ PubMed ] [ CrossRef ] [ Google Scholar ]
- Pacheco, J. , Garvey, M. A. , Sarampote, C. S. , Cohen, E. D. , Murphy, E. R. , & Friedman‐Hill, S. R. (2022). Annual research review: The contributions of the RDoC research framework on understanding the neurodevelopmental origins, progression and treatment of mental illnesses . Journal of Child Psychology and Psychiatry , 63 ( 4 ), 360–376. 10.1111/jcpp.13543 [ PMC free article ] [ PubMed ] [ CrossRef ] [ Google Scholar ]
- Pardiñas, A. F. , GERAD1 Consortium , CRESTAR Consortium , Holmans, P. , Pocklington, A. J. , Escott‐Price, V. , Ripke, S. , Carrera, N. , Legge, S. E. , Bishop, S. , Cameron, D. , Hamshere, M. L. , Han, J. , Hubbard, L. , Lynham, A. , Mantripragada, K. , Rees, E. , MacCabe, J. H. , McCarroll, S. A. , … Walters, J. T. R . (2018). Common schizophrenia alleles are enriched in mutation‐intolerant genes and in regions under strong background selection . Nature Genetics , 50 ( 3 ), 381–389. 10.1038/s41588-018-0059-2 [ PMC free article ] [ PubMed ] [ CrossRef ] [ Google Scholar ]
- Pennisi, E. (2022). Upstart DNA sequencers could be a ‘game changer’ . Science , 376 ( 6599 ), 1257–1258. 10.1126/science.add4867 [ PubMed ] [ CrossRef ] [ Google Scholar ]
- Peterson, R. E. , Kuchenbaecker, K. , Walters, R. K. , Chen, C.‐Y. , Popejoy, A. B. , Periyasamy, S. , Lam, M. , Iyegbe, C. , Strawbridge, R. J. , Brick, L. , Carey, C. E. , Martin, A. R. , Meyers, J. L. , Su, J. , Chen, J. , Edwards, A. C. , Kalungi, A. , Koen, N. , Majara, L. , … Duncan, L. E. (2019). Genome‐wide association studies in ancestrally diverse populations: Opportunities, methods, pitfalls, and recommendations . Cell , 179 ( 3 ), 589–603. 10.1016/j.cell.2019.08.051 [ PMC free article ] [ PubMed ] [ CrossRef ] [ Google Scholar ]
- Pettersson, E. , Larsson, H. , & Lichtenstein, P. (2016). Common psychiatric disorders share the same genetic origin: A multivariate sibling study of the Swedish population . Molecular Psychiatry , 21 ( 5 ), 717–721. 10.1038/mp.2015.116 [ PubMed ] [ CrossRef ] [ Google Scholar ]
- Pingault, J. , Allegrini, A. G. , Odigie, T. , Frach, L. , Baldwin, J. R. , Rijsdijk, F. , & Dudbridge, F. (2022). Research review: How to interpret associations between polygenic scores, environmental risks, and phenotypes . Journal of Child Psychology and Psychiatry , 63 ( 10 ), 1125–1139. 10.1111/jcpp.13607 [ PMC free article ] [ PubMed ] [ CrossRef ] [ Google Scholar ]
- Pingault, J.‐B. , O’Reilly, P. F. , Schoeler, T. , Ploubidis, G. B. , Rijsdijk, F. , & Dudbridge, F. (2018). Using genetic data to strengthen causal inference in observational research . Nature Reviews Genetics , 19 ( 9 ), 566–580. 10.1038/s41576-018-0020-3 [ PubMed ] [ CrossRef ] [ Google Scholar ]
- Plomin, R. (1994). Genetics and experience: The interplay between nature and nurture . Sage Publications. [ Google Scholar ]
- Plomin, R. (2018). Blueprint: How DNA makes us who we are . Allen Lane/Plenum Press. [ Google Scholar ]
- Plomin, R. , & Bergeman, C. S. (1991). The nature of nurture: Genetic influence on “environmental” measures . Behavioral and Brain Sciences , 14 ( 3 ), 373–386. 10.1017/S0140525X00070278 [ CrossRef ] [ Google Scholar ]
- Plomin, R. , DeFries, J. C. , Knopik, V. S. , & Neiderhiser, J. M. (2016). Top 10 replicated findings from behavioral genetics . Perspectives on Psychological Science , 11 ( 1 ), 3–23. 10.1177/1745691615617439 [ PMC free article ] [ PubMed ] [ CrossRef ] [ Google Scholar ]
- Plomin, R. , DeFries, J. C. , & Loehlin, J. C. (1977). Genotype‐environment interaction and correlation in the analysis of human behavior . Psychological Bulletin , 84 ( 2 ), 309–322. 10.1037/0033-2909.84.2.309 [ PubMed ] [ CrossRef ] [ Google Scholar ]
- Plomin, R. , Haworth, C. M. A. , & Davis, O. S. P. (2009). Common disorders are quantitative traits . Nature Reviews Genetics , 10 ( 12 ), 872–878. 10.1038/nrg2670 [ PubMed ] [ CrossRef ] [ Google Scholar ]
- Plomin, R. , & Viding, E. (2022). Will genomics revolutionise research on gene‐environment interplay? Journal of Child Psychology and Psychiatry , 63 , 1214–1218. [ PMC free article ] [ PubMed ] [ Google Scholar ]
- Plomin, R. , & von Stumm, S. (2018). The new genetics of intelligence . Nature Reviews Genetics , 19 ( 3 ), 148–159. 10.1038/nrg.2017.104 [ PMC free article ] [ PubMed ] [ CrossRef ] [ Google Scholar ]
- Plomin, R. , & von Stumm, S. (2022). Polygenic scores: Prediction versus explanation . Molecular Psychiatry , 27 ( 1 ), 49–52. 10.1038/s41380-021-01348-y [ PMC free article ] [ PubMed ] [ CrossRef ] [ Google Scholar ]
- Polderman, T. J. C. , Benyamin, B. , de Leeuw, C. A. , Sullivan, P. F. , van Bochoven, A. , Visscher, P. M. , & Posthuma, D. (2015). Meta‐analysis of the heritability of human traits based on fifty years of twin studies . Nature Genetics , 47 ( 7 ), 702–709. 10.1038/ng.3285 [ PubMed ] [ CrossRef ] [ Google Scholar ]
- Purves, K. L. , Coleman, J. R. I. , Meier, S. M. , Rayner, C. , Davis, K. A. S. , Cheesman, R. , Bækvad‐Hansen, M. , Børglum, A. D. , Wan Cho, S. , Jürgen Deckert, J. , Gaspar, H. A. , Bybjerg‐Grauholm, J. , Hettema, J. M. , Hotopf, M. , Hougaard, D. , Hübel, C. , Kan, C. , McIntosh, A. M. , Mors, O. , … Eley, T. C. (2020). A major role for common genetic variation in anxiety disorders . Molecular Psychiatry , 25 ( 12 ), 3292–3303. 10.1038/s41380-019-0559-1 [ PMC free article ] [ PubMed ] [ CrossRef ] [ Google Scholar ]
- Richmond, R. C. , & Davey Smith, G. (2022). Mendelian randomization: Concepts and scope . Cold Spring Harbor Perspectives in Medicine , 12 ( 1 ), a040501. 10.1101/cshperspect.a040501 [ PMC free article ] [ PubMed ] [ CrossRef ] [ Google Scholar ]
- Riglin, L. , Tobarra‐Sanchez, E. , Stergiakouli, E. , Havdahl, A. , Tilling, K. , O’Donovan, M. , Nigg, J. , Langley, K. , & Thapar, A. (2022). Early manifestations of genetic liability for ADHD, autism and schizophrenia at ages 18 and 24 months . JCPP Advances . 10.1002/jcv2.12093 [ PMC free article ] [ PubMed ] [ CrossRef ] [ Google Scholar ]
- Rimfeld, K. , Malanchini, M. , Spargo, T. , Spickernell, G. , Selzam, S. , McMillan, A. , Dale, P. S. , Eley, T. C. , & Plomin, R. (2019). Twins Early Development Study: A genetically sensitive investigation into behavioral and cognitive development from infancy to emerging adulthood . Twin Research and Human Genetics , 22 ( 6 ), 508–513. 10.1017/thg.2019.56 [ PMC free article ] [ PubMed ] [ CrossRef ] [ Google Scholar ]
- Risch, N. , & Merikangas, K. (1996). The future of genetic studies of complex human diseases . Science (New York, N.Y.) , 273 ( 5281 ), 1516–1517. 10.1126/science.273.5281.1516 [ PubMed ] [ CrossRef ] [ Google Scholar ]
- Ritchie, S. (2021). Science fictions: Exposing fraud, bias, negligence and hype in science . Vintage. [ Google Scholar ]
- Sallis, H. , Szekely, E. , Neumann, A. , Jolicoeur‐Martineau, A. , van IJzendoorn, M. , Hillegers, M. , Greenwood, C. M. T. , Meaney, M. J. , Steiner, M. , Tiemeier, H. , Wazana, A. , Pearson, R. M. , & Evans, J. (2019). General psychopathology, internalising and externalising in children and functional outcomes in late adolescence . Journal of Child Psychology and Psychiatry , 60 ( 11 ), 1183–1190. 10.1111/jcpp.13067 [ PMC free article ] [ PubMed ] [ CrossRef ] [ Google Scholar ]
- Selzam, S. , Coleman, J. R. I. , Caspi, A. , Moffitt, T. E. , & Plomin, R. (2018). A polygenic p factor for major psychiatric disorders . Translational Psychiatry , 8 ( 1 ), 205. 10.1038/s41398-018-0217-4 [ PMC free article ] [ PubMed ] [ CrossRef ] [ Google Scholar ]
- Selzam, S. , Ritchie, S. J. , Pingault, J.‐B. , Reynolds, C. A. , O’Reilly, P. F. , & Plomin, R. (2019). Comparing within‐ and between‐family polygenic score prediction . The American Journal of Human Genetics , 105 ( 2 ), 351–363. 10.1016/j.ajhg.2019.06.006 [ PMC free article ] [ PubMed ] [ CrossRef ] [ Google Scholar ]
- Shiner, R. L. , Buss, K. A. , McClowry, S. G. , Putnam, S. P. , Saudino, K. J. , & Zentner, M. (2012). What Is Temperament Now? Assessing Progress in Temperament Research on the Twenty‐Fifth Anniversary of Goldsmith et al (pp. 436–444). Child Development Perspectives. 10.1111/j.1750-8606.2012.00254.x [ CrossRef ] [ Google Scholar ]
- Song, J. , Bergen, S. E. , Kuja‐Halkola, R. , Larsson, H. , Landén, M. , & Lichtenstein, P. (2015). Bipolar disorder and its relation to major psychiatric disorders: A family‐based study in the Swedish population . Bipolar Disorders , 17 ( 2 ), 184–193. 10.1111/bdi.12242 [ PubMed ] [ CrossRef ] [ Google Scholar ]
- Stahl, E. A. , eQTLGen Consortium , BIOS Consortium , the Bipolar Disorder Working Group of the Psychiatric Genomics Consortium , Breen, G. , Forstner, A. J. , McQuillin, A. , Ripke, S. , Trubetskoy, V. , Mattheisen, M. , Wang, Y. , Coleman, J. R. I. , Gaspar, H. A. , de Leeuw, C. A. , Steinberg, S. , Pavlides, J. M. W. , Trzaskowski, M. , Byrne, E. M. , Pers, T. H. , … Sklar, P . (2019). Genome‐wide association study identifies 30 loci associated with bipolar disorder . Nature Genetics , 51 ( 5 ), 793–803. 10.1038/s41588-019-0397-8 [ PMC free article ] [ PubMed ] [ CrossRef ] [ Google Scholar ]
- Taylor, M. J. , Martin, J. , Lu, Y. , Brikell, I. , Lundström, S. , Larsson, H. , & Lichtenstein, P. (2019). Association of genetic risk factors for psychiatric disorders and traits of these disorders in a Swedish population twin sample . JAMA Psychiatry , 76 ( 3 ), 280–289. 10.1001/jamapsychiatry.2018.3652 [ PMC free article ] [ PubMed ] [ CrossRef ] [ Google Scholar ]
- The International Schizophrenia Consortium . (2009). Common polygenic variation contributes to risk of schizophrenia and bipolar disorder . Nature , 460 ( 7256 ), 748–752. 10.1038/nature08185 [ PMC free article ] [ PubMed ] [ CrossRef ] [ Google Scholar ]
- The Wellcome Trust Case Control Consortium . (2007). Genome‐wide association study of 14,000 cases of seven common diseases and 3,000 shared controls . Nature , 447 ( 7145 ), 661–678. 10.1038/nature05911 [ PMC free article ] [ PubMed ] [ CrossRef ] [ Google Scholar ]
- Trubetskoy, V. , Pardiñas, A. F. , Qi, T. , Panagiotaropoulou, G. , Awasthi, S. , Bigdeli, T. B. , Bryois, J. , Chen, C.‐Y. , Dennison, C. A. , Hall, L. S. , Lam, M. , Watanabe, K. , Frei, O. , Ge, T. , Harwood, J. C. , Koopmans, F. , Magnusson, S. , Richards, A. L. , Sidorenko, J. , … van Os, J. (2022). Mapping genomic loci implicates genes and synaptic biology in schizophrenia . Nature , 604 ( 7906 ), 502–508. 10.1038/s41586-022-04434-5 [ PMC free article ] [ PubMed ] [ CrossRef ] [ Google Scholar ]
- Trzaskowski, M. , Davis, O. S. P. , DeFries, J. C. , Yang, J. , Visscher, P. M. , & Plomin, R. (2013). DNA evidence for strong genome‐wide pleiotropy of cognitive and learning abilities . Behavior Genetics , 43 ( 4 ), 267–273. 10.1007/s10519-013-9594-x [ PMC free article ] [ PubMed ] [ CrossRef ] [ Google Scholar ]
- van der Linden, D. , teNijenhuis, J. , & Bakker, A. B. (2010). The general factor of personality: A meta‐analysis of big five intercorrelations and a criterion‐related validity study . Journal of Research in Personality , 44 ( 3 ), 315–327. 10.1016/j.jrp.2010.03.003 [ CrossRef ] [ Google Scholar ]
- Visscher, P. M. , Wray, N. R. , Zhang, Q. , Sklar, P. , McCarthy, M. I. , Brown, M. A. , & Yang, J. (2017). 10 Years of GWAS discovery: Biology, function, and translation . The American Journal of Human Genetics , 101 ( 1 ), 5–22. 10.1016/j.ajhg.2017.06.005 [ PMC free article ] [ PubMed ] [ CrossRef ] [ Google Scholar ]
- von Stumm, S. , & Plomin, R. (2021). Using DNA to predict intelligence . Intelligence , 86 , 101530. 10.1016/j.intell.2021.101530 [ CrossRef ] [ Google Scholar ]
- Wainschtein, P. , Jain, D. , Zheng, Z. , TOPMed Anthropometry Working Group , Aslibekyan, S. , Becker, D. , Bi, W. , Brody, J. , Carlson, J. C. , Correa, A. , Du, M. M. , Fernandez‐Rhodes, L. , Ferrier, K. R. , Graff, M. , Guo, X. , He, J. , Heard‐Costa, N. L. , Highland, H. M. , Hirschhorn, J. N. , … Visscher, P. M . (2022). Assessing the contribution of rare variants to complex trait heritability from whole‐genome sequence data . Nature Genetics , 54 ( 3 ), 263–273. 10.1038/s41588-021-00997-7 [ PMC free article ] [ PubMed ] [ CrossRef ] [ Google Scholar ]
- Waszczuk, M. A. , Eaton, N. R. , Krueger, R. F. , Shackman, A. J. , Waldman, I. D. , Zald, D. H. , Lahey, B. B. , Patrick, C. J. , Conway, C. C. , Ormel, J. , Hyman, S. E. , Fried, E. I. , Forbes, M. K. , Docherty, A. R. , Althoff, R. R. , Bach, B. , Chmielewski, M. , DeYoung, C. G. , Forbush, K. T. , … Kotov, R. (2020). Redefining phenotypes to advance psychiatric genetics: Implications from hierarchical taxonomy of psychopathology . Journal of Abnormal Psychology , 129 ( 2 ), 143–161. 10.1037/abn0000486 [ PMC free article ] [ PubMed ] [ CrossRef ] [ Google Scholar ]
- Watson, H. J. , Anorexia Nervosa Genetics Initiative , Eating Disorders Working Group of the Psychiatric Genomics Consortium , Yilmaz, Z. , Thornton, L. M. , Hübel, C. , Coleman, J. R. I. , Gaspar, H. A. , Bryois, J. , Hinney, A. , Leppä, V. M. , Mattheisen, M. , Medland, S. E. , Ripke, S. , Yao, S. , Giusti‐Rodríguez, P. , Hanscombe, K. B. , Purves, K. L. , Adan, R. A. H. , … Bulik, C. M . (2019). Genome‐wide association study identifies eight risk loci and implicates metabo‐psychiatric origins for anorexia nervosa . Nature Genetics , 51 ( 8 ), 1207–1214. 10.1038/s41588-019-0439-2 [ PMC free article ] [ PubMed ] [ CrossRef ] [ Google Scholar ]
- Widiger, T. A. (2011). Personality and psychopathology . World Psychiatry , 10 ( 2 ), 103–106. 10.1002/j.2051-5545.2011.tb00024.x [ PMC free article ] [ PubMed ] [ CrossRef ] [ Google Scholar ]
- Willoughby, E. A. , McGue, M. , Iacono, W. G. , Rustichini, A. , & Lee, J. J. (2021). The role of parental genotype in predicting offspring years of education: Evidence for genetic nurture . Molecular Psychiatry , 26 ( 8 ), 3896–3904. 10.1038/s41380-019-0494-1 [ PMC free article ] [ PubMed ] [ CrossRef ] [ Google Scholar ]
- Wray, N. R. , Lin, T. , Austin, J. , McGrath, J. J. , Hickie, I. B. , Murray, G. K. , & Visscher, P. M. (2021). From basic science to clinical application of polygenic risk scores: A Primer . JAMA Psychiatry , 78 ( 1 ), 101. 10.1001/jamapsychiatry.2020.3049 [ PubMed ] [ CrossRef ] [ Google Scholar ]
- Wray, N. R. , eQTLGen , 23andMe , the Major Depressive Disorder Working Group of the Psychiatric Genomics Consortium , Ripke, S. , Mattheisen, M. , Trzaskowski, M. , Byrne, E. M. , Abdellaoui, A. , Adams, M. J. , Agerbo, E. , Air, T. M. , Andlauer, T. M. F. , Bacanu, S.‐A. , Bækvad‐Hansen, M. , Beekman, A. F. T. , Bigdeli, T. B. , Binder, E. B. , Blackwood, D. R. H. , … Sullivan, P. F . (2018). Genome‐wide association analyses identify 44 risk variants and refine the genetic architecture of major depression . Nature Genetics , 50 ( 5 ), 668–681. 10.1038/s41588-018-0090-3 [ PMC free article ] [ PubMed ] [ CrossRef ] [ Google Scholar ]
- Wright, A. G. C. , Krueger, R. F. , Hobbs, M. J. , Markon, K. E. , Eaton, N. R. , & Slade, T. (2013). The structure of psychopathology: Toward an expanded quantitative empirical model . Journal of Abnormal Psychology , 122 ( 1 ), 281–294. 10.1037/a0030133 [ PMC free article ] [ PubMed ] [ CrossRef ] [ Google Scholar ]
- Yang, J. , Manolio, T. A. , Pasquale, L. R. , Boerwinkle, E. , Caporaso, N. , Cunningham, J. M. , de Andrade, M. , Feenstra, B. , Feingold, E. , Hayes, M. G. , Hill, W. G. , Landi, M. T. , Alonso, A. , Lettre, G. , Lin, P. , Ling, H. , Lowe, W. , Mathias, R. A. , Melbye, M. , … Visscher, P. M. (2011). Genome partitioning of genetic variation for complex traits using common SNPs . Nature Genetics , 43 ( 6 ), 519–525. 10.1038/ng.823 [ PMC free article ] [ PubMed ] [ CrossRef ] [ Google Scholar ]
- Zachar, P. , & Kendler, K. S. (2017). The philosophy of nosology . Annual Review of Clinical Psychology , 13 ( 1 ), 49–71. 10.1146/annurev-clinpsy-032816-045020 [ PubMed ] [ CrossRef ] [ Google Scholar ]
Behavioral Genetics Research Paper
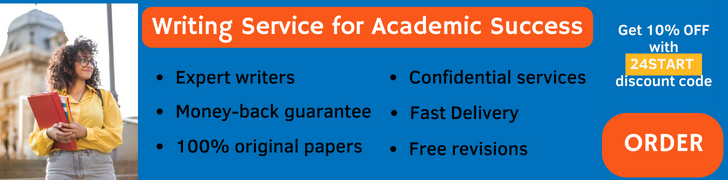
View sample behavioral genetics research paper. Browse research paper examples for more inspiration. If you need a psychology research paper written according to all the academic standards, you can always turn to our experienced writers for help. This is how your paper can get an A! Feel free to contact our writing service for professional assistance. We offer high-quality assignments for reasonable rates.
The field of behavior genetics is concerned with four issues in any animal species, including humans. These issues are (a) whether individual differences in a behavior are due to the effects of genes, (b) what the genes are that do or can effect individual differences in a behavior, (c) how genes and their interactions with the environment affect the development of a behavior, and (d) what genetic changes are involved in the evolution of a behavior. Although some aspects of the genetics of behavior have been investigated in a variety of organisms (see Ehrman & Parsons, 1981), most studies have been with four species. These are nematodes ( Caenorhabditis elegans ), fruit flies ( Drosophila melanogaster ), mice ( Mus musculus ) and humans ( Homo sapiens ).
Academic Writing, Editing, Proofreading, And Problem Solving Services
Get 10% off with 24start discount code.
The writings of two Victorians, Charles Darwin and his cousin Francis Galton, influenced different paths in the field of behavior genetics. One is concerned with the causes of variation in human behaviors, especially cognition, personality, psychopathology, and addictions; this path is derived largely from the works of Galton. The other is concerned with the genetics of adaptive behaviors in animals and humans;this path is derived largely from the writings of Darwin; it is the main focus of this review. In each section of this research paper, the relevant genetics are considered first and then their application to some exemplar behaviors is described.
The structure of the genetic material called DNA(deoxyribonucleic acid) was first proposed by Watson and Crick (1953a). Implicit in the structure of DNAare the mechanisms for how genes are replicated, how they mutate, and what they do (Watson & Crick, 1953b). This discovery has had a profound effect on the biological sciences and is beginning to have one on psychology and the other behavioral sciences. The potential impact on the behavioral sciences was described in a seminal paper by Ginsburg (1958) that was published just 5 years after those by Watson and Crick on the structure and function of DNA. Ginsburg proposed that genetics was a tool for the study of behavior in four ways. First, it is a tool to dissect behavior into its natural units. Second, it is a tool to study the neural and other mechanisms of behavior. Third, it is a tool to study the effects of the environment on behavior. Fourth, it is a tool to study the evolution of behavior.
The Heritability of Behavior
The methods of quantitative genetics (see Falconer & McKay, 1997) are used to assess the relative roles of genes and environment in individual differences in behavior. In animals, inbreeding, selective breeding, and crossbreeding are used, and in animals and humans, the resemblance among relatives and nonrelatives is assessed. Basically, these methods allow the phenotypic variance to be partitioned into genetic and environmental components. This can be expressed as the ratio of genotypic to phenotypic variance; this ratio is known as the heritability of the trait. This ratio can vary from zero to one. The value is specific to the population in which it is measured, and its estimate always has an error term dependent on sample size. The following are considered in this section as examples: mating speed in fruit flies, aggression in stickleback fish, nest building in mice, and body weight in humans.
Mating Speed in Fruit Flies
Manning (1961) selectively bred for fast and slow mating speeds based on the time from being introduced to the mating chamber to the start of copulation. From 50 pairs of flies, the 10 fastest and10 slowest pairs were selected. From these pairs, two fast-mating and two slow-mating lines were established, and a randomly bred control line was also maintained. After 25 generations of selective breeding, the mean mating speed was about 3 min in the fast line and 80 min in the slow line. The heritability computed from the response to selective breeding was .30, which demonstrates low to moderate genetic variability for this behavior. This study also demonstrated that the genes for variability in mating speed and general activity are not the same.
Aggression in Three-Spine Stickleback Fish
In stickleback fish, juveniles and adults of both sexes attack other species members, and attacks by adults of both sexes are usually territorial. Bakker (1994) selected for territorial attacks in males and females from a population of stickleback fish living in a fresh water stream in the Netherlands. High, low, and control lines were developed. For adult male attacks, there was no change in the high line across the three generations of selective breeding, but there was a decrease in the low line over the three generations. For adult female attacks, there was an increase in the high and a decrease in the low lines over the three generations. Where heritability could be calculated, it ranged from .29 to .64 (moderate to high genetic contribution to variation). It may be concluded from these results that (a) there is genetic variability for these behaviors in the natural population, (b) there may have been more intense selection on male than female attacks, and (c) some of the genes for variability in male and female attacks may not be the same.
Nest Building in Mice
Mice build nests as adaptations to the cold. In one study, mice were selectively bred for the size of their nests as measured by grams of cotton used to build a nest (Lynch, 1994). The foundation population was a randomly bred heterogeneous stock, and there were two high, two low, and two control lines. After 15 generations of selection, the heritability computed from the response to selection was .28, which is moderate. There have been more than 48 generations of selection. Thus, there is genetic variability for this trait in the foundation population. Studies with natural populations of mice show that there is less genetic variability in mice from Maine than from Florida; this finding is consistent with there being more selection in Maine for thermoregulatory nest size than in Florida.
Body Weight in Humans
The regulation of body weight is an adaptive trait; this regulation has behavioral components, such as eating and exercise. The correlation for body weight of identical twins raised together is .80 for identical twins reared together and .72 for those raised apart, whereas it is .43 for fraternal twins (Grilo & Pogue-Geille 1981). The correlation for biological parents and adopted siblings is .23, similar to that for nonadoptive parents and offspring (.26). Also, the correlations for adoptive parent and child or adoptive siblings are essentially zero. These findings are consistent with a heritability of .70 for body weight and with nonshared rather than shared environmental effects on individual differences in body weight. The shared environment is that which differs from one family to another; the nonshared environment is conceived of as the portion of trait variance not explained by genetics or shared environment. For many individual differences in behavioral or mental traits of humans, most of the variance is due to genetic and nonshared environmental effects (Plomin, DeFries, McClearn, & McGuffin, 2000).
It is now firmly established that individual differences in every studied behavioral trait in any animal species, including humans, is a function of both genetic and environmental variability. That is to say, for no trait studied is the heritability either zero or one in outbred populations. The interesting issues now are (a) what are the genes that do or can affect a trait’s variability, (b) how they interact with each other and the environment in the trait’s development, and (c) what the genetic mechanisms are of species diversity in and evolution of behavioral adaptations. These issues are beginning to be understood with the aid of molecular genetics.
The Genome Projects
An individual’s nuclear genome consists of the DNAfound on all the chromosomes in the nucleus of its cells; there is one molecule of DNA for each chromosome. The goal of the genome projects is to determine the sequence of the nucleotide bases—adenine, cytosine, guanine, or thymine (A, C, G, orT)—of the nuclear genome of one or more individuals in a species. This has been done for C. elegans with a genome size of 100 Mb (megabases), D. melanogaster with a genome size of 165 Mb, and Homo sapiens with a genome size of 3,300 Mb; it is nearing completion for M. musculus with a genome size 3,300 Mb.After the entire sequence is known for a species, it is possible to estimate the number of proteincoding genes in the genome; this now appears to be about 35,000 for humans. Also, the amino acid sequence in each protein can be deduced from the coding nucleotide triplets in the gene’s structural region. Other sequences of a proteincoding gene bind proteins known as transcription factors . These factors and sequences together are involved in controlling when and where a gene is transcribed as RNA (ribonucleic acid). The transcribed RNA is processed into a messenger RNA (mRNA), and the mRNA is then translated into the sequences of amino acids in its protein. Many DNA sequences, however, appear not to be transcribed nor to regulate transcription; in mice and humans, these make up about 98% of the nuclear genome.
There is also DNA in the mitochondria; this DNA codes for the amino acid sequence of some of the proteins involved in energy metabolism. This DNA has been sequenced for many organisms. There are neurological effects of variants of these mitochondrial genes (Wallace, 1999), and these genes may also have behavioral effects.
Identifying The Genes
Geneswitheffectsonbehaviorinmicecanbedetectedbymutagenizing one allele of a gene (Takahashi, Pinto, &Vitaterna, 1994). There are then two copies (variants or alleles) of the gene, and the homozygotes for the two variants can be compared for differences in one or more behaviors. One mutagenesis approach targets a specific gene creating an allele that does not produce a functional protein. These are the so-called knockout mutants. To delete a specific gene, the DNA sequence of the gene must be already known, and the chromosomallocationofthegenemayormaynotbeknown.Knockouts with effects on mouse behavior are reviewed in Nelson and Young (1998) and Crawley (2000). This approach is illustrated in the following section for genes with effects on mouse aggression.Another approach exposes male mice of an inbred strain to a chemical mutagen, such as N-ethyl-N-nitrosourea (ENU), with the goal of finding most—if not all—the genes that can cause variation in a trait such as a behavior. Dominant mutants would be detected in the first generation of progeny, and recessive mutants would be detected in subsequent generations of progeny. The chromosomal location and the DNA sequenceofeachmutantgenewithabehavioraleffectarethen determined. There are large-scale behavioral mutagenesis projects at the Jackson Laboratory, Northwestern University, andThe University ofTennessee Health Sciences Center.This approach is illustrated later in this research paper with the circadian rhythm gene known as Clock. Also, both mutagenesis approaches have also been used with nematodes (Segalat, 1999) and fruit flies (Jallon, 1999).
Genes with effects on behavior can also be detected by chromosomally mapping genes with existing allelic variants (see Segalat, 1999, for nematodes; see Sokolowski, 1999, for fruit flies; see Belknap, Dubay, Crabbe, & Buck, 1997, for mice; and see Plomin & Crabbe, 2000, for humans). Single genevariantswithlargeeffectcanbemappedasillustratedfor the foraging gene in Drosophila. Polygenic variants can also be mapped; later in the paper, this is illustrated in mice for quantitative trait loci (QTLs) with effects on emotionality. A goal in mapping these is to eventually identify the actual protein coding genes with effects on a trait’s variation. The markersthatareusedforgenemappingtoaregionofachromosome are usually DNA variants (Plomin et al., 2000; Plomin & Crabbe, 2000). These include restriction fragment length polymorphisms (RFLPs), simple sequence length polymorphisms (SSLPs), and single nucleotide polymorphisms (SNPs). These markers have two advantages in mapping: Many of them are closely spaced on the linkage map of each chromosome, and they have no effect on the measured traits. Another approach is to associate DNA variants of candidate genes with behavior, as is illustrated for a human personality trait later in this research paper. The selection of candidate genes often depends on hypotheses about the neural and other mechanisms involved in a behavior.
Another approach to identifying genes with potential effects on behavior is to look for quantitative differences in gene expression in brains of genotypically or phenotypically different individuals. Genes that differ across these individuals in level of brain mRNA are candidates for ones with behavioral effects. DNAmicrochips can be used to determine what genes are being expressed in a tissue (e.g., as a region of brain) and how the level of expression differs between individuals of different genotype or phenotype (Nisenbaum, 2002). A single microchip can be used to assay for thousands of genes simultaneously. The assay is based on specific hybridization between some of the DNAof a gene and of that gene’s mirror image or complimentary DNA (cDNA), which is synthesized from the gene’s messenger RNA.
Knockout Mutants and Aggression in Mice
There is more than one type of aggression in mice and other animals (Maxson, 1992, 1999). The research with knockout mutantshasfocusedonaggressivebehaviorknownas offense, which has the adaptive function of obtaining and retaining resources such as space, food, and mates. It is also characterized by specific motor patterns and attack targets. About 25 genes witheffectsonoffenseinmaleshavebeenidentified(Maxson, Roubetoux, Guillot, & Goldman, 2001; Miczek, Maxson, Fish, & Faccidomo, 2001); most of these have been identified with knockouts. Many of the genes act on either hormone or neurotransmitter systems, and it has recently been suggested that many such genes ultimately act on offense by affecting one or more of the serotonergic neurotransmitter systems (Nelson & Chiavegatto, 2001).
The effect of a knockout on a trait is determined by comparing mice homozygous for the knockout allele with those homozygous for the normal or functional allele. In evaluating results with knockouts, there are several methodological concerns (Nelson, 1997):
- To avoid maternal effects, the mother of the two genotypes must be the same, and the offspring should be the result of the mating of a heterozygous female to a heterozygous male.
- Some knockout strain pairs are coisogenic, differing only in the normal and mutant alleles of a single gene, but others are only congenic —they differ not only in the mutant and normal alleles of gene of interest, but also in alleles of genes linked to it. For congenic strains, any differences may be due to the genes linked to the knockout rather than due to the knockout itself, as discussed by Gerlai (1996).
- Often the knockout is made in one inbred strain, such as one of the 129 inbred strains and then transferred to another strain. Sometimes the effect of a knockout seen in one strain background is not detected in another. For example, the knockout for the NOS-1 (nitric oxide synthase-1) gene increases attacks is lost after many generations of backcrossing to C57BL6 inbred strain mice (LeRoy et al., 2000).
- For many knockouts, the mutant gene is present from the time of conception. Thus, it was not possible to tell when or where in the mouse the gene was expressed with consequent behavioral effect. Recently, techniques have been developed that permit tissue- and temporal-specific knockouts in mice (Tsien, 1999). Similar techniques are available for elegans (Seglant, 1999) and Drosophila (Jallon, 1999).
Saturation Mutagenesis and Circadian Rhythms in Mice
Knockouts mutagenize a specific gene. Exposure to a chemical mutagen, such as ENU, in theory can mutagenize all the genes that can cause a trait to vary (Takahashi et al., 1994). This approach was first used in Drosophila (see review by Benzer, 1971); mutants on the X chromosome were detected for circadian rhythms, courtship, and learning-memory. Saturation mutagenesis has also been used with nematodes (Seglant, 1999). This approach was first used in mice to screen for mutants with effects on circadian rhythms.
Circadian rhythms in mice can be measured by observing their running in a wheel. Mice normally run at night in a 12 light, 12 dark schedule, with precise onset of locomotor activity. Even when maintained in constant darkness, mice (and all other animals examined) display this cycle of activity that deviates only slightly from the 24-hour pattern observed during a light-dark cycle. Because these rhythms persist in constant conditions as well as other evidence, it is well accepted that these rhythms are generated from within the organism. Male mice were treated with ENU and their progeny were screened for dominant mutations affecting circadian rhythms (Vitaterna et al., 1994). One mouse out of 300 had a circadian period that was 1 hour longer than normal. This mouse had a semidominant mutation that was named Clock . When initially placed in constant darkness, homozygous Clock mice have long circadian periods of 27 to 28 hours. After about 2 weeks in constant darkness, the homozygous Clock mice have a complete loss of circadian rhythms. Intraspecic mapping crosses were used to show that the Clock gene is located on Chromosome 5 of the mouse. It was then possible to positionally clone the DNAand thereby to identify the protein of this gene (King et al., 1997). Clock encodes a member of the basic-helix-loop-helix (bHLH) PAS family of transcription factors, and it has a key role in the regulating the genetics mechanism of the biological clock (Allada, White, So, Hall, & Rosbash, 2001; King & Takahashi, 2000). The role of Clock in circadian rhythms was confirmed with transgenic rescue (Antoch et al., 1997); for this, the normal allele of Clock was inserted into the Clock mutants. These mice have normal circadian rhythms.
Natural Variants and Foraging in Fruit Flies
There is a polymorphism in the foraging behavior of larval Drosophila (Sokolowski & Riedl, 1999). Rovers have longer foraging trails on food than do sitters. This difference is not seen in the absence of food. These variants occur in natural populations of flies; in these, there are about 70% rovers and 30% sitters. Breeding experiments showed that this polymorphism is due primarily to allelic differences in a single gene with a large effect, but that this trait is also influenced by other genes that each contribute small effects. Chromosomal analyseslocalizedthegene,whichwascalled for, tothesecondchromosome. Because minor genes and the environment affect the distribution of rover and sitter phenotypes, a lethal tagging technique was used to map for to Region 24 of the polytene chromosome map. Chromosome rearrangements were used to further map for to region 24A3-5 of the polytene chromosome map containing about 150 to 125 kb (kilobases) of DNA. It is now known that the for gene is the same as the gene dg2 , which encodes a Drosophila cGMP/cGMP-dependent protein kinase (PKG). Insertion of transposon elements into dg2 caused a change from rover to sitter phenotypes; removal of the transposon elements caused the behavior to revert back to rover.
Genome-Wide Scan to Map QTLs for Emotionality in Mice
When confronted with a novel and unexpected situation, such as an open field, mice may freeze, defecate and urinate, or simply explore the new environment (Broadhurst, 1960). These behaviors, singly or in combination, are often used as measures of emotionality. In mice, negative correlations between defecation and ambulation are fairly general, although the association is affected somewhat by environmental variables such as light or noise (Archer, 1973). To some extent, the relation also depends on the strain, sex, and early experience of the subjects.
In open-field tests, C57BL6 inbred strain of mice are much more active than BALB/c inbred strain of mice. These strains were crossed to obtain an F3 generation, which was the base population for selective breeding for open-field activity. Replicate high and low lines were selectively bred over 30 generations for open-field ambulation (DeFries, Gervais, & Thomas, 1978). Two unselected control lines were also bred for the 30 generations. After 30 generations of selection, there was a threefold difference between the high and low lines in ambulation, and there was no overlap in distribution of ambulation scores between the high and low lines. The defecation scores of the low lines were seven times higher than those of the high lines. Based on the response to selection, the heritability was .26 for ambulation and .11 for defecation, and the genetic correlation for ambulation and defecation scores was .86.
An F3 of one of these high and low lines was bred to map QTLs for the strain differences (Flint et al., 1995). The most active and least active mice were screened for 84 DNAmarkers for which there were two alleles, and these 84 markers were spread across the 20 chromosomes of mice; this is known as a genome-wide scan. These were used to determine the chromosomal region (QTLs) associated with the openfield activity. Significant QTLs were found on Chromosomes 1, 4, 12, 15, 17, and 18. These six QTLs accounted for 26% of the phenotypic variance. There are several issues in evaluating such genome-wide scans to localize QTLs associated with a behavior. These are
- Because the association of the behavior with many DNA markers is tested, there is a risk of false positives (for mice, see Belknap et al., 1997; for humans, see Plomin & Crabbe, 2000). For this reason, QTLs should be confirmed by additional studies.
- The QTL is often a large region on a chromosome consisting of millions of base pairs of DNA and hundreds of genes. In order to positionally clone or identify positional candidates for the gene or genes underlying the QTL, the map distance between the QTL to the markers needs to be greatly reduced. The following illustrates one approach to resolving these issues (Talbot et al., 1999). For open-field behavior, the most active 20% and least active 20% of 751 mice of an heterogenous stock derived from an eight-way cross were screened for SSLPs closely spaced together in a 20 cM (centiMorgan) region of Chromosome 1. This not only confirmed that there is a QTLon Chromosome 1 with an effect on ambulation, but it also mapped the QTL more precisely.
Candidate Genes: Association of D4 Receptor Gene and Novelty Seeking in Humans
Novelty seeking is one of four personality traits in Cloninger’s theory of temperament development (Cloninger, Svrakic, & Przybeck, 1993). His theory predicts that genes acting on dopaminergic transmission would affect individual differences in novelty seeking. Associations between allelic variants of the dopamine D4 receptor (DRD4) were assessed in unselected samples (Benjamin et al., 1996; Ebstein et al., 1995). The seven alleles for the DRD4 receptor gene vary in the number of a 48-bp (base pair) repeat. In both studies, individuals with the longer alleles (6–8 repeats) had higher novelty seeking scores than did those with the shorter alleles (2–5 repeats). It may be that those with the longer alleles are dopamine deficient and seek novelty in order to increase dopamine release. Regardless, these variations in the D4DR gene account for about 4% of the phenotypic variation in novelty seeking.
The aforementioned study is a candidate gene rather than whole genome scan approach for finding genes with effects on individual differences in behavior; this type of approach presents the following methodological concerns:
- For association studies using population samples of humans, there may be artificial correlations between a candidate gene and a trait that are due to ethnic differences in frequency of the alleles of a candidate gene. This does not occur with within-family designs for association between a candidate gene and a trait. The association between length of repeat of the D4DR receptor and novelty seeking was also obtained in a within-family design.
- It is possible that an association may be a false positive. Hence, there should be independent confirmations of each reported association between a genetic variant and individual differences in behavior. There have been many replications of the association between length of repeat of the D4DR receptor and novelty (Plomin & Caspi, 1998). But there also have been a few failures to replicate this finding. This is likely to occur when the genetic variant has small effects on individual differences in the trait, as is the case for the effect of D4DR variants on novelty seeking.
Differences in Expression of Genes in the Brain
The technique of using DNAmicrochips is illustrated here for genes expressed in brain areas of two strains of mice. The inbred strains of mice are C57BL6 and 129SVEv, and the brain regions are midbrain, cerebellum, hippocampus, amygdala, entorhinal cortex, and cerebral cortex (Sandberg et al., 2000). Expression of 7,000 mouse genes were detected with the DNA microchip. Twenty-four of these were differentially expressed in all brain regions of the two strains, and 73 were differentially expressed in at least one of the brain regions of the two strains. These genes may be candidates for known behavioral differences between these strains. There are two methodological issues with regard to this approach.
- The microchip DNA arrays will not detect genes with low levels of mRNA. It is currently limited to detecting genes expressed at a relative abundance of 1/100000 mRNAs.
- There may be false positives with this technique. For this reason, findings on gene expression should be conformed with other techniques for detecting mRNAs such as Northern blots, RT-PCR (reverse transcriptase polymerase chain reaction), or instu
This technique can also be used to study gene expression from human postmortem tissue. For example, it has been used to suggest that there may be differences between individuals with schizophrenia and normally functioning individuals in brain expression of two genes involved in synaptic function (Mirnics, Middleton, Lewis, & Levitt, 2001). But because premortem and postmortem factors can influence the findings with these tissues, these and similar results should be interpreted with caution, and genes implicated with this approach in phenotypic differences should be confirmed with other approaches. For example, one of the genes detected by microchip hybridization to be differentially expressed in the brains of normal and schizophrenic individuals are in a region on Chromosome 1 associated with the risk for schizophrenia.
Eventually, most (if not all) the genes that do or can cause variation in behaviors of nematodes, flies, and mice will be known, as will those that cause variation in human behavior. Because every gene does not affect every behavior in a species, behaviors can be grouped by the genes that cause them to vary. This would be the basis for a behavioral taxonomy based on gene effects as proposed by Ginsburg in 1958. Also, as genes with behavioral effects are identified in one species, they may be useful in two ways in the search for genes with behavioral effects in other species. First, DNA hybridization techniques can be used to search for homologous genes— ones similar in base pair sequence—in two species. For example, the period gene, which affects circadian rhythms, was first identified and sequenced in flies. The DNA sequence of the period gene of flies was then used to see whether there were homologous genes in mice. This approach identified three period genes in mice. Second, the sequences of genes on chromosome segments are conserved in mammals. These homology maps can be used to suggest the chromosomal location in humans of a gene or QTL mapped in mice. For example, the QTL on Chromosome 1 with effects on emotionality of mice would be located in a specific region of human Chromosome 1.
Developmental Genetics of Behavior
Protein coding genes of eukaryotes essentially have two parts, the structural regions and the regulatory regions. The sequence of base pairs in the structural region codes for the sequence of amino acids in its protein. It serves as a template for synthesis of RNA; this is transcription. This RNA is first processed and the resulting mRNA is translated into the sequences of amino acids in the protein. The amino acid sequence of the protein is a determinant of its function. The base pair sequences in the regulatory region of a gene bind proteins known as transcription factors. Together, these determine when and where in the individual a gene is transcribed or expressed. In mice and humans, about 2% of the nuclear genome codes for proteins, and in mice about half of these genes are transcribed in adult brain. Further information on molecular genetics can be found in Lewin (1997) and on developmental genetics in Gilbert (2000).
In this context, three issues in developmental genetics of behavior are considered. These issues concern identifying (a) the pathway from DNA to a behavior, (b) the interaction of genes or epistasis and behavior, and (c) the effects of the interaction between genes and environments on behavior.
Pathways From the Gene to the Behavior
The initial step is to know the DNA sequences of a gene, thereby identifying the amino acid sequence of its protein. These sequences aid in identifying the cellular function of the gene. After this is known, the question becomes how varying that protein has behavioral effects.
For example, the gene for the enzyme nitric oxide synthase-1 (NOS-1) was knocked out. The homozygotes missing the enzyme in neurons are more aggressive than are those having the enzyme; these mice lack the gaseous neurotransmitter nitric oxide (NO; Nelson et al., 1995). The next steps would be to determine how the lack of NO increases aggression and how the presence of NO decreases aggression. It has recently been shown that the knockout mice have reduced serotonin (5-HT) turnover and are deficient in 5HT 1A and 5HT 1B receptor function (Chiavegatto et al., 2001). It remains to be determined just how the presence and absence of NO affects the serotonin system and just how it in turn effects aggression. However, there is much evidence that the serotonin system is a major player in regulating aggressive behavior.
Gene Interactions and Behavior
Although it is possible to trace the effect of some individual genes from DNA to behavior, it is becoming increasingly clear that the effects of alleles of one gene on behavior depend on alleles of other genes. The interaction of the alleles of different genes is known as epistasis. The following is an example: The Y chromosomes of DBA1 and C57BL10 inbred mouse strains mice can differ in their effects on mouse aggression (Maxson, Trattner, & Ginsburg, 1979). This difference only occurs, however, if all or half the autosomal genes are from DBA1; it does not occur if all the autosomal genes are from C57BL10. Also, these types of interactions are often detected when knockout mutants in mice are transferred from one genetic background to another. For example, the knockout for the NOS-1 gene that increases attacks in one genetic background is lost after generations of backcrossing to C57BL6 mice (LeRoy et al., 2000).
In these examples with mice, the interacting genes are not known; however, interactions of pairs of genes are now being investigated in fruit flies. Epistatic interactions have been shown for recessive mutants with effects on olfaction (Fedorowicz,Fry,Arholt,&McKay,1998).Othersarelooking at how allelic substitutions in a gene affect patterns of expression of many genes as a way to identify systems of interacting genes (Wahlsten, 1999). DNA microchips are used in this research. Greenspan (2001) has suggested that these systems of interactions may be very complex—networks of different genes can have the same behavioral effects and networks of the same genes can have different behavioral effects.
Genes, Environment, and Behavior
Effects of genes on behavior are dependent on the environment, just as effects of the environment on behavior are dependent on genes. For example, experience has effects on gene expression. Some of these are due to effects of experiences on levels of steroid hormones and thereby on gene expression in neurons. Others are due to effects of experience on synaptic transmission, which thereby affect gene expression in neurons. In this section, the effects of experience on gene expression are described for the circadian clock in mice; maternal care and pup development in rats; learning of birdsong; and long-term memory in mollusks, in fruit flies, and in mice.
If mice are kept in constant darkness, their circadian rhythm for wheel-running activity shows a cycle of about 24 hours, but the onset of activity drifts with each day in total darkness. The active period is known as subjective day, and the inactive period is known as subjective night. When mice and other rodents are exposed to light during the subjective night, the onset time for activity is shifted and the transcription of c-fos and several other immediate early genes in the suprachiasmatic nucleus (SCN) is induced (Kronhauser, Mayo, & Takahashi, 1996). Immediate early genes such as c-fos code for transcription factors that regulate the expression of other genes. It is believed that the expression of these genes resets the circadian clock in the SCN.
When crouching over their pups in arched-back nursing (ABN), mother rats lick and groom (LG) their pups (Meaney, 2001). This licking subsequently affects gene expression in the brains and the behavioral responses to stress of adult rats. There is variation in how much a mother rat licks and grooms her pups. As adults, pups with more LG have higher levels of corticotropin-releasing factor (CRF) mRNA in the hypothalamus and of glucocorticoid receptor mRNA in the hippocampus than do those with less LG. As adults, those with high-LG mothers are less fearful than are those with low-LG mothers. Also, females that had high-LG mothers lick their own pups more than do those that have low-LG mothers. This may represent one type of mechanism for nongenomic transfer of behavior across generations.
In some birds, there is a sensitive period for song learning. If at that time canaries or zebra finches are exposed to the song oftapedtutorsoftheirownspecies,theimmediateearlygenes, Zenk and c-fos are induced in the forebrain structures NCM (caudal part of the neostriatum) and cHV (hyperstriatum ventral; Mello, Vicario, & Clayton, 1992). These regions appear to be involved in song learning and not in song production. This increase does not occur when each species is exposed to the song of another species or to simple bursts of sound.
Long-term memory for sensitization and classical conditioning in the mollusk Aplysia, classical conditioning in fruit flies, and spatial learning in mice involve changes in gene expression in the brain (Squire & Kandel, 2000). In each of these, the neural events of learning activate the transcription factor CREB (cyclic adenosine monophosphate response element binding protein); this then turns on other genes that cause changes in structure and function of nerve cells involved in long-term memory. For example, fruit flies are trained to associate an odor with an electric shock and another odor with the absence of an electric shock (Dubnau & Tully, 1998). The memory for these associations is tested by allowing them to choose between chambers with the two different odors. Flies have short-term memory lasting less than an hour and long-term memory lasting 24 hours or more. Flies were engineered to make CREB in response to heat shock. In non-heat-shocked flies, a single odor and electric shock pairing produces short-term but not long-term memory, whereas in heat-shocked flies, a single odor and electric shock pairing also produces long-term memory. Conversely, flies were engineered to make a protein that blocks transcription by CREB. Induction of this protein by heat shock blocked long-term but not short-term memory.
Genes are being used as Ginsburg (1958) proposed as tools to study the brain mechanisms of behavior and the effects of the environment on the development of behavior. It is of interest not only that the protein products of genes are involved in both, but also that the expression of genes are involved in both; this implies that although we can experimentally separate and manipulate genes and environment, they are in fact two sides of the same coin. For this reason, we should look beyond the old and tired nature-nurture controversy.
The Genetics of Behavioral Evolution
The evolution of behavioral adaptations is due to effects of natural selection on gene frequency. Details on population genetics may be found in Falconer and McKay (1997) and on molecular evolution in Page and Holmes (1998). In behavior genetics, the goal is to identify the genes and the changes in them that are the basis of the evolution of species differences in behavioral adaptations. Three examples are discussed in this section: the evolution of courtship song in fruit flies, the evolution of mating systems in voles, and the evolution of color vision in mammals. Respectively, these examples illustrate behavioral evolution due to change in the structural part of a gene, change in the regulatory part of the gene, and gene duplication with changes in both parts of the gene.
Evolution of the Courtship Song in Fruit Flies
Courtship by male fruit flies consists of several motor patterns. In one of these patterns, the male vibrates a wing; the sound produced is known as the courtship song. In D. melanogaster, variants of the period (per) gene affect this song. One of these abolishes the song; it is known as per 01 . Others change the timing of the wing vibrations and thereby the quality of the song. The song also varies among fruit fly species; furthermore, females of a species may prefer to mate with males singing their species song. This species variation is in part due to variation in the per gene (Colot, Hall, & Robash, 1998). When a cloned copy of the D. similans per gene was inserted into the genome of D. melanaogaster per 01 , the male courtship song is restored but it resembles that of D. similans ratherthanthatof D.melanogaster (Wheeleret al., 1991). Further analysis suggested that four or fewer amino acid substitution in a nonconserved region of the per protein may control the species difference in courtship song.
Evolution of Mating Systems in Voles
Prairie voles are monogamous and montane voles are polygamous.These two species also differ in partner preference, parenting, social contact, and aggression (DeVries, Taymans, & Carter, 1997). These species differences are due—at least in part—to species variants of an arginine vasopressin receptor (V1aR) gene (Young, Winslow, Nilsen, & Insel, 1997). The structural part of the gene is the same in the two species, but the regulatory regions differ between the two species. As a consequence, there is species variation in the expression of mRNA in regions of the forebrain and therefore in the forebrain distribution of the V1aR. Several lines of evidence suggest that this between-species neural variation may be involved in the between-species behavioral variation:
- Injectionofargininevasopressin(AVP)intothebrainfacilitates aggression in prairie voles and not in montane voles, whereas it facilitates auto-grooming in montane but not in prairie voles. Also, partner preference in prairie voles is blocked by brain injection of anAVPantagonist and facilitated by brain injection ofAVP(Winslow et al., 1993).
- There are mice with a transgene for the prairie vole V1aR gene(Young,Nilsen,Waymire,MacGregor,&Insel,1999). These mice have the same brain distribution of this receptor as prairie voles, and these mice exhibit increased affiliative behavior after brain injection of arginine vasopressin.
- Aviral vector was used to insert another copy of the V1aR into neurons of a region of the basal ganglia in prairie voles (Pitkow et al., 2001). This increased V1aR binding in this region of the basal ganglia, and these males exhibited increased anxiety, affiliative behavior, and partner preference.
Evolution of Color Vision in Mammals
Most old world monkeys, great apes, and humans can discriminate between red and green, whereas all other mammals cannot (Bowmaker, 1991); this is—at least in part—due to the former’s having three types of cones in the retina and the latter’s having two types of cones. Each cone has its own opsin. Opsins are the proteins that confer spectral sensitivity on the visual receptors. Old world monkeys, great apes, and humans—but not other mammals—have cones with an opsin that is sensitive to the red end of the spectrum (Nathans, Thomas, & Hogness, 1986; Nathans, Merbs, Sung, Weitz, & Wang, 1992). The gene for the opsin sensitive to the red end of the spectrum is on the X chromosome of old world monkeys, great apes, and humans, and the gene for the opsin sensitive to the green region of the spectrum is on the X chromosome of all mammals. About 30 million years ago, the gene for the red opsin arose by gene duplication from the gene for the green opsin.At first, there were two green opsin genes. Then mutations accumulated in the structural region of one of these genes, changing its spectral sensitivity from green to red. There are seven amino acid differences between the red and the green opsins that appear to be critical for the difference in their spectral sensitivity. Changes in the DNA sequence of regulatory elements are likely; for example, the red opsin is synthesized in one cone and the green in another. Thus, after gene duplication, there are accumulated mutations in both the structural and regulatory regions of the second copy of the gene such that it came to code for a new protein with new expression patterns. This evolution by gene duplication has occurred many other times for other genes. For example, all the ion channel genes arose by gene duplications in common with the evolution of most of the serotonin receptor genes (Smith, 1991). Such genes are in families with similar DNA sequences. It is very likely that there were also behavioral consequences of these gene duplications.
It has been suggested that many genes are usually involved in the evolution of adaptations and speciation. But sometimes, as described previously, a single gene contributes most to the evolution of a trait. Also, it is possible for these genes to identify the DNAvariant with effect on the behavior that has been subject to natural selection. In time, the molecular basis of many species differences in behavior and their evolution will be understood. For example, we will know just what are the sequence and functions of the 2% of DNA that differs between chimpanzees and humans and how this difference contributes to the species differences in behaviors.
Future Directions
The completion of the respective genome projects in nematodes, flies, mice, and humans will make it possible to identify all of the protein coding genes of these species as well as whereandwhenthegenesaretranscribed,andthenewprotein initiative will eventually identify the structural conformation as well as metabolic or cellular function of each protein. This will greatly ease the task of identifying all the genes that can and do cause a behavior to vary in these four species, as well as that of tracing the pathways from gene to behavior. The great challenge will then be to understand how genes interact with each other, how they interact with the environment in the developmentandexpressionofbehaviors,andhowtheyrelate to behavioral evolution.
The study of the genetics of behaviors in animals can and should be for more than just the development of models relevant to human behaviors. The genetics of animal behaviors should also be researched in order to discover general principles relating genes to behavior across animal species and to have a comparative genetics of adaptive behaviors within related species. For this, there will need to be genome projects in other taxonomic groups; such work is already taking place on bees and other insects, many farm animals, domestic dogs, domesticcats,otherrodents,andmanyprimates;Ibelievethat this process represents the future of behavior genetics.
Bibliography:
- Archer, J. (1973). Tests of emotionality on rats and mice: A review. Animal Behavior, 21, 205–235.
- Allada, R., White, N. E., So, W. V., Hall, J. C., & Rosbash, M. (1998). A mutant Drosophila homolog of mammalian Clock disrupts circadian rhythms and transcription of period and timeless. Cell, 93, 781–804.
- Antoch, M. P., Song, E. J., Chang,A. M., Vitaterna, M. H., Zhao, Y., Wilsbacher, L. D., Sangoram, A. M., King, D. P., Pinto, L. H., & Takahashi,J.S.(1997).Functionalidentificationofthemousecircadian Clock gene by transgenic BAC rescue. Cell, 89, 655–667.
- Bakker, Th. C. M. (1994). Evolution of aggressive behavior in the threespine stickelback. In M. A. Bell & S. A. Foster (Eds.), The evolutionary biology of the threespine stickleback (pp. 345–380). Oxford, UK: Oxford University Press.
- Belknap, J. K., Dubay, C., Crabbe, J. C., & Buck, K. J. (1997). Mapping quantitative trait loci for behavioral traits in the mouse. In K. Blum & E. P. Npbel (Eds.), Handbook of psychiatric genetics (pp. 435–453). Boca Raton, FL: CRC Press.
- Benjamin, J., Li, L., Patterson, C., Greenburg, B. D., Murphy, D. L., &Hamer,D.H.(1996).Populationandfamilialassociation between the D4 dopamine receptor gene and measures of novelty seeking. Nature Genetics, 12, 81–84.
- Benzer, S. (1971). From genes to behavior. Journal of the American Medical Association, 218, 1015–1022.
- Bowmaker, J. K. (1991).The evolution of vertebrate visual pigments and photoreceptors. In J. R. Cronly-Dilllon & R. L. Gregory (Eds.), Vision and visual dysfunction: Vol. 2. Evolution of the eye and visual systems (pp. 63–81). Boca Raton, FL: CRC Press.
- Broadhurst,P.L.(1960).Experimentsinpsychogenetics:Application of biometrical genetics to the inheritance of behavior. In H. J. Eysenck(Ed.), Experimentsinpersonality:Vol.1.Psychogenetics and psychopharmacology (pp. 1–102). London: Routledge.
- Chiavegatto, S., Dawson, V. L., Mamounas, L. A., Koliatsos, V. E., Dawson, T. M., & Nelson, R. J. (2001). Brain serotonin dysfunction accounts for aggression in male mice lacking neuronal nitric oxide synthase. Proceedings of the National Academy of Sciences, USA, 98, 1277–1281.
- Cloninger, C. R., Svrakic, D. M., & Przybeck, T. R. (1993). A psychobiological model of temperament and character. Archives of General Psychiatry, 50, 975–990.
- Colot, H. V., Hall, J. C., & Robash, M. (1988). Interspecific comparison of the period gene of Drosophila reveals large blocks of non-conserved coding DNA. European Molecular Biology Organization Journal, 7, 3929–3937.
- Crawley, J. N. (2000). What’s wrong with my mouse? New York: Wiley-Liss.
- DeFries, J. C., Gervais, M. C., & Thomas, E. A. (1978). Response to 30 generations of selection for open-field activity in laboratory mice. Behavior Genetics, 8, 3–13.
- DeVries, A. C., Taymans, S. E., & Carter, C. S. (1997). Social modulation of corticosteroid responses in male prairie voles. Annals of the New York Academy of Sciences, USA, 807, 494–497.
- Dubnau, J., & Tully, T. (1998). Gene discovery in Drosophila: New insights for learning and memory. Annual Review of Neuroscience, 21, 407–444.
- Ehrman, L., & Parsons, P. A. (1981). Behavior genetics and evolution. New York: McGraw-Hill.
- Ebstien, R. B., Novick, O., Umansky, R., Priel, B., Osher, Y., Blaine, D., Benett, E. R., Nemanov, R., Katz, M., & Belmaker, R. H. (1995). Dopamine D4 receptor (D4DR) exon III repeat polymorphism associated with the human personality trait of novelty seeking. Nature Genetics, 12, 78–80.
- Falconer, D. S., & McKay, T. F. C. (1997). Introduction to quantitative genetics (4th ed.). Harlow, UK: Longman.
- Fedorowicz, G. M., Fry, J. D., Arholt, R., & McKay, T. F. (1998). Epistatic interaction between smell-impaired loci in Drosophila melanogaster. Genetics, 148, 1885–1891.
- Flint, J., Corely, R., DeFries, J. C., Fulker, D. W., Gray, J. A., Miller, S., & Collins, A. C. (1995). A simple genetic basis for a complex psychological trait in laboratory mice. Science, 269, 1432–1435.
- Gerlai, R. (1996). Gene-targeting studies of mammalian behavior: Is it the mutation or the background genotype? Trends in Neuroscience, 19, 177–181.
- Gilbert, S. F. (2000). Developmental biology (6th ed.). Sunderland, USA: Sinauer.
- Ginsburg, B. E. (1958). Genetics as a tool in the study of behavior. Perspectives in Biology and Medicine, 1, 397–424.
- Greenspan, R. J. (2001). The flexible genome. Nature Reviews Genetics, 2, 383–387.
- Grilo, C. M., & Pogue-Geille, M. F. (1991). The nature of environmental influences on weight and obesity: A behavior genetic analysis. Psychological Bulletin, 10, 520–537.
- Jallon, J.-M. (1999). Genetic analysis of Drosophila sexual behavior. In B. C. Jones & P. Mormede (Eds.), Neurobehavioral genetics: Methods and applications (pp. 365–372). Boca Raton, FL: CRC Press.
- King, D. P., & Takahashi, J. S. (2000). Molecular genetics of circadian rhythms in mammals. Annual Review of Neuroscience, 23, 713–742.
- King, D. P., Zhao, Y., Sangoram, A. M., Wilsbacher, L. D., Tanaka, ,Antoch,M.P.,Steeves,T.D.L.,Vitaterna,M.H.,Kornhauser, J. M., Lowery, P. L., Turek, F. W., & Takahashi, J. S. (1997). Positional cloning of the mouse circadian Clock gene. Cell, 89, 641–643.
- Kronhauser, J. M., Mayo, K. E., & Takahashi, J. S. (1996). Light, immediate early-genes, and circadian rhythms. Behavior Genetics, 26, 221–240.
- LeRoy, I., Pothion, S., Mortaud, S., Chabert, C., Nicolas, L., Cherfouh, A., & Roubertoux, P. L. (2000). Loss of aggression after transfer onto a C57BL/6J background in mice carrying a targeted disruption of the neuronal nitric oxide synthase gene. Behavior Genetics, 30, 367–373.
- Lewin, B. (1997). Genes (Vol. 6). Oxford, UK: Oxford University Press.
- Lynch, C. B. (1994). Evolutionary inferences from genetic analyses of cold adaptation in laboratory and wild populations of the house mouse. In C. R. B. Boake (Ed.), Quantitative genetic studies of behavioral evolution (pp. 278–301). Chicago: University of Chicago Press.
- Manning, A. (1961). The effect of artificial selection for mating speed in Drosophila melanogaster. Animal Behavior, 9, 82–92.
- Maxson, S. C. (1992). Methodological issues in genetic analyses of an agonistic behavior (offense) in mice. In D. Goldowitz, D. Wahlsten, & R. Wimer (Eds.), Techniques for the analysis of brain and behavior: Focus on the mouse (pp. 349–373). New York: Elsevier.
- Maxson, S. C. (1999). Aggression: Concepts and methods relevant to genetic analyses in mice and humans. In B. C. Jones & P. Mormede (Eds.), Neurobehavioral genetics: Methods and applications (pp. 293–300). Boca Raton, FL: CRC Press.
- Maxson, S. C., Roubetoux, P. L., Guillot, P.-V., & Goldman, D. (2001). The genetics of aggression: From mice to humans. In M. Martinez (Ed.), Prevention and control of aggression and the impact on its victims (pp. 71–81). New York: Kluwer.
- Maxson, S. C., Trattner, A., & Ginsburg (1979). Interaction of Y-chromosomal and autosomal genes in the development of aggression in mice. Behavior Genetics, 9, 219–226.
- Meaney, M. J. (2001). Maternal care, gene expression and the transmission of individual differences in stress reactivity across generations. Annual Review of Neuroscience, 24, 1161–1192.
- Mello, C., Vicario, D. S., & Clayton, D. F. (1992). Song presentation induces gene expression in the songbird forebrain. Proceedings of the National Academy of Sciences, USA, 89, 6819–6822.
- Miczek, K. A., Maxson, S. C., Fish, E. W., & Faccidomo, S. (2001). Aggressive behavioral phenotypes in mice. Behavioral Brain Research, 125, 167–181.
- Mirnics, K., Middleton, F. A., Lewis, D. A., & Levitt, P. (2001). Analysis of complex brain disorders with gene expression microarrays: Schizophrenia as a disease of the synapse. Trends in Neuroscience, 24, 479–486.
- Nathans, J., Merbs, S. L., Sung, C.-H., Weitz, C. J., & Wang, Y. (1992). Molecular genetics of human visual pigments. Annual Review of Genetics, 26, 403–424.
- Nathans, J., Thomas, D., & Hogness, D. S. (1986). Molecular genetics of human color vision: The genes encoding blue, green, and red pigments. Science, 232, 193–202.
- Nelson, R. J. (1997). The use of genetic “knock-out” mice in behavioral endocrinology research. Hormones and Behavior, 31, 188–196.
- Nelson, R. J., & Chiavegatto, S. (2001). Molecular basis of aggression. Trends in Neuroscience, 24, 713–719.
- Nelson, R. J., Demas, G. E., Huang, P., Fishman, M. C., Dawson, V., Dawson, T. M., & Snyder, S. H. (1995). Behavioural abnormalities in male mice lacking neuronal nitric oxide synthase. Nature, 378, 383–386.
- Nelson, R. J., & Young, K. A. (1998). Behavior in mice with targeted disruption of single genes. Neuroscience and Biobehavioral Reviews, 22, 453–462.
- Nisenbaum, L. K. (2002). The ultimate chip shot: Can microarray technology deliver for neuroscience? Genes, Brain, and Behavior, 1, 27–34.
- Page, R. D. M., & Holmes, E. C. (1998). Molecular evolution: A phylogenetic approach. Malden: Blackwell.
- Pitkow, L. J., Sharer, C. A., Ren, X., Insel, T. R., Terwilliger, E. F., & Young, L. J. (2001). Facilitation of affiliation and pair-bond formationbyvasopressinreceptorgenetransferintotheventralforebrain of a monogamous vole. Journal of Neuroscience, 21, 7392–7396.
- Plomin, R., & Caspi, A. (1998). DNA and personality. European Journal of Personality, 12, 387–407.
- Plomin, R., & Crabbe, J. (2000). DNA. Psychological Bulletin, 126, 806–828.
- Plomin, R., DeFries, J. C., McClearn, G. E., & McGuffin, P. (2000). Behavioral genetics (4th ed.). New York: Worth.
- Sandberg, R., Yasuda, R., Pankratz, D. G., Carter, T. A., Del Rio, J. A., Wodicka, L., Mayford, M., Lockhart, D., & Barlow, C. (2000). Regional and strain-specific gene expression mapping in the adult mouse brain. Proceedings of the National Academy of Sciences, USA, 97, 11039–11043.
- Segalat, L. (1999). Genetic analysis of behavior in the nematode, Caenorhabditis elegans. In B. C. Jones & P. Mormede (Eds.), Neurobehavioral genetics: Methods and applications (pp. 373– 381). Boca Raton, FL: CRC Press.
- Smith, C. U. M. (1991). Elements of molecular neurobiology (2nd ed.). New York: Wiley.
- Sokolowski, M. B. (1999). Genetic analysis of food search behavior in the fruit fly, Drosphilia melanogaster. In B. C. Jones & P. Mormede (Eds.), Neurobehavioral genetics: Methods and applications (pp. 357–364). Boca Raton, FL: CRC Press.
- Sokolowski, M. B., & Riedl, C. A. L. (1999). Behavior-genetic and molecularanalysisofnaturallyoccurringvariationin Drospophila larval foraging behavior. In W. E. Crusio & R. T. Gerlai (Eds.), Handbook of molecular-genetic techniques for brain and behavior research (pp. 496–511).Amsterdam: Elsevier.
- Squire, L. R., & Kandel, E. R. (2000). Memory: From mind to molecules. New York: Scientific American Library.
- Takahashi, J. S., Pinto, L. H., & Vitaterna, M. H. (1994). Forward and reverse genetic approaches to behavior in the mouse. Science, 264, 1724–1732.
- Talbot,C.J.,Nicod,A.,Cherny,S.S.,Fulker,D.W.,Collins,A.C.,& Flint, J. (1999). High resolution mapping of quantitative trait loci in outbred mice. Nature Genetics, 21, 305–308.
- Tsien, J. Z. (1999). Brain region-specific and temporally restricted gene knockout using the Cre recombinase system. In W. E. Crusio & R. T. Gerlai (Eds.), Handbook of molecular-genetic techniques for brain and behavior research (pp. 282–290). Amsterdam: Elsevier.
- Vitaterna, M. H., King, D. P., Chang, A. M., Kornhauser, J. M., Lowrey, P. L., McDonald, J. D., Dove, W. F., Turek, F. W., & Takahashi, J. S. (1994). Mutagenesis and mapping of a mouse gene, Clock, essential for circadian behavior. Science, 264, 719–725.
- Wahlsten, D. (1999). Single-gene influences on brain and behavior. Annual Review of Psychology, 50, 599–624.
- Wallace, D. C. (1999). Mitochondrial diseases of man and mouse. Science, 238, 1482–1488.
- Watson, J. D., & Crick, F. H. C. (1953a). Molecular structure of nucleic acids: A structure for deoxyribose nucleic acid. Nature, 171, 737–738.
- Watson, J. D., & Crick, F. H. C. (1953b). Genetical implications of the structure of deoxyribosenucleic acid. Nature, 171, 964–967.
- Winslow, J., Hastings, N., Carter, C. S., Harbaugh, C., & Insel, T. R. (1993). A role of central vasopressin in pair bonding in monogamous prairie voles. Nature, 365, 545–548.
- Wheeler, D. A., Kyriacou, C. P., Greenacre, M. L., Yu, Q., Rutila, J. E., Rosbash, M., & Hall, J. C. (1991). Molecular transfer of a species-specific behavior from Drospohila simulans to Drosophila melanogaster. Science, 251, 1082–1085.
- Young, L. J., Nilsen, R., Waymire, K. G., MacGregor, G. R., & Insel, T. R. (1999). Increased affiliative response in vassopressin mice expressing the V 1 a receptor from a monogamous vole. Nature, 400, 766–768.
- Young, L. J., Winslow, J. T., Nilsen, R., & Insel, T. R. (1997). Species differences in V 1 a receptor gene expression in monogamous and nonmanogamous voles: Behavioral consequences. Behavioral Neuroscience, 111, 599–605.
ORDER HIGH QUALITY CUSTOM PAPER

An International Journal Devoted to Research in the Inheritance of Behavior
Volumes and issues
Volume 54 january - march 2024 jan - mar 2024.
- Issue 2 March 2024
Special Issue: IDEA: Inclusion, Diversity, Equality, and Access in Behavioral and Quantitative Genetic Investigations
Volume 53 February - November 2023 Feb - Nov 2023
- Issue 5-6 November 2023
- Issue 4 July 2023
Special Issue: ABCD Behavior Genetics: Twin, family, and genomic studies using the Adolescent Brain Cognitive Development (ABCD) study dataset
- Issue 2 March 2023
- Issue 1 February 2023
Volume 52 January - November 2022 Jan - Nov 2022
- Issue 6 November 2022
- Issue 4-5 September 2022
- Issue 3 May 2022
- Issue 2 March 2022
- Issue 1 January 2022
Volume 51 January - November 2021 Jan - Nov 2021
- Issue 6 November 2021
Special Issue on: Developmental behavior genetics and externalizing psychopathology
- Issue 4 July 2021
Special Issue: The International Workshop on Statistical Genetic Methods for Human Complex Traits
- Issue 2 March 2021
- Issue 1 January 2021
Volume 50 January - November 2020 Jan - Nov 2020
- Issue 6 November 2020
- Issue 5 September 2020
Special Issue: The Genetic Architecture of Neurodevelopmental Disorders
- Issue 3 May 2020
- Issue 2 March 2020
- Issue 1 January 2020
Volume 49 January - November 2019 Jan - Nov 2019
- Issue 6 November 2019
- Issue 5 September 2019
- Issue 4 July 2019
- Issue 3 May 2019
Special Issue: Genetics and Human Agency: The Philosophy of Behavior Genetics
- Issue 1 January 2019
Volume 48 January - November 2018 Jan - Nov 2018
- Issue 6 November 2018
- Issue 5 September 2018
- Issue 4 July 2018
- Issue 3 May 2018
- Issue 2 March 2018
- Issue 1 January 2018
Volume 47 January - November 2017 Jan - Nov 2017
- Issue 6 November 2017
- Issue 5 September 2017
- Issue 4 July 2017
- Issue 3 May 2017
- Issue 2 March 2017
- Issue 1 January 2017
Volume 46 January - November 2016 Jan - Nov 2016
- Issue 6 November 2016
- Issue 5 September 2016
- Issue 4 July 2016
Special Issue: The Importance of the Prenatal Environment in Behavioral Genetics
- Issue 2 March 2016
Special Issue: Gene-Environment Interplay In Adulthood
Volume 45 January - November 2015 Jan - Nov 2015
Special Issue: Reviving the Louisville Twin Study: A Special Section in Honor of Adam P. Matheny, Jr.
- Issue 5 September 2015
- Issue 4 July 2015
Special Issue: Gene-Hormone Interplay
- Issue 2 March 2015
- Issue 1 January 2015
Volume 44 January - November 2014 Jan - Nov 2014
Special Issue: A Festschrift for John Loehlin
- Issue 5 September 2014
- Issue 4 July 2014
Special Issue: A Festschrift for Lindon J. Eaves
- Issue 2 March 2014
- Issue 1 January 2014
Volume 43 January - November 2013 Jan - Nov 2013
- Issue 6 November 2013
- Issue 5 September 2013
- Issue 4 July 2013
- Issue 3 May 2013
- Issue 2 March 2013
- Issue 1 January 2013
Volume 42 January - November 2012 Jan - Nov 2012
- Issue 6 November 2012
- Issue 5 September 2012
- Issue 4 July 2012
- Issue 3 May 2012
- Issue 2 March 2012
- Issue 1 January 2012
Volume 41 January - November 2011 Jan - Nov 2011
Special Issue: A Festchrift for Benson E Ginsburg
- Issue 5 September 2011
- Issue 4 July 2011
Special Issue: From Molecules to Behavior: Lessons the Study of Rare Genetic Disorders
- Issue 2 March 2011
Learning Disabilities
Volume 40 January - November 2010 Jan - Nov 2010
Special issue:A festskrift in honor of the career contributions of Gerald E. McClearn, Guest Editor: Gerald E. McClearn
- Issue 5 September 2010
- Issue 4 July 2010
- Issue 3 May 2010
Special issue: Pathways between Genes, Brain and Behavior; Guest Editors: Kristen C. Jacobson and William S. Kremen
- Issue 1 January 2010
Volume 39 January - November 2009 Jan - Nov 2009
- Issue 6 November 2009
- Issue 5 September 2009
Special Issue: Genetics of High Cognitive Ability Guest Editors: Robert Plomin and Claire Haworth
- Issue 3 May 2009
- Issue 2 March 2009
- Issue 1 January 2009
Volume 38 January - November 2008 Jan - Nov 2008
- Issue 6 November 2008
- Issue 5 September 2008
- Issue 4 July 2008
- Issue 3 May 2008
- Issue 2 March 2008
- Issue 1 January 2008
Volume 37 January - November 2007 Jan - Nov 2007
- Issue 6 November 2007
- Issue 5 September 2007
- Issue 4 July 2007
- Issue 3 May 2007
- Issue 2 March 2007
- Issue 1 January 2007
Volume 36 January - November 2006 Jan - Nov 2006
- Issue 6 November 2006
- Issue 5 September 2006
- Issue 4 July 2006
- Issue 3 May 2006
- Issue 2 March 2006
- Issue 1 January 2006
Volume 35 January - November 2005 Jan - Nov 2005
- Issue 6 November 2005
- Issue 5 September 2005
- Issue 4 July 2005
- Issue 3 May 2005
- Issue 2 March 2005
- Issue 1 January 2005
Volume 34 January - November 2004 Jan - Nov 2004
- Issue 6 November 2004
- Issue 5 September 2004
- Issue 4 July 2004
- Issue 3 May 2004
Variance Components Methods for Mapping Quantitative Trait Loci
- Issue 1 January 2004
Volume 33 January - November 2003 Jan - Nov 2003
Selective Breeding Programs with Rats: Part II
- Issue 4 July 2003
- Issue 3 May 2003
- Issue 1 January 2003
Volume 32 January - November 2002 Jan - Nov 2002
- Issue 6 November 2002
Selective Breeding Programs with Rats
- Issue 4 July 2002
- Issue 3 May 2002
- Issue 2 March 2002
- Issue 1 January 2002
Volume 31 January - November 2001 Jan - Nov 2001
- Issue 6 November 2001
- Issue 5 September 2001
- Issue 4 July 2001
- Issue 3 May 2001
- Issue 2 March 2001
- Issue 1 January 2001
Volume 30 January - November 2000 Jan - Nov 2000
- Issue 6 November 2000
- Issue 5 September 2000
- Issue 4 July 2000
- Issue 3 May 2000
- Issue 2 March 2000
- Issue 1 January 2000
Volume 29 January - November 1999 Jan - Nov 1999
- Issue 6 November 1999
- Issue 5 September 1999
- Issue 4 July 1999
- Issue 3 May 1999
- Issue 2 March 1999
- Issue 1 January 1999
Volume 28 January - November 1998 Jan - Nov 1998
- Issue 6 November 1998
- Issue 5 September 1998
- Issue 4 July 1998
- Issue 3 May 1998
- Issue 2 March 1998
- Issue 1 January 1998
Volume 27 January - November 1997 Jan - Nov 1997
- Issue 6 November 1997
- Issue 5 September 1997
- Issue 4 July 1997
- Issue 3 May 1997
- Issue 2 March 1997
- Issue 1 January 1997
Volume 26 January - November 1996 Jan - Nov 1996
- Issue 6 November 1996
- Issue 5 September 1996
- Issue 4 July 1996
- Issue 3 May 1996
- Issue 2 March 1996
- Issue 1 January 1996
Volume 25 January - November 1995 Jan - Nov 1995
- Issue 6 November 1995
- Issue 5 September 1995
- Issue 4 July 1995
- Issue 3 May 1995
- Issue 2 March 1995
- Issue 1 January 1995
Volume 24 January - November 1994 Jan - Nov 1994
- Issue 6 November 1994
- Issue 5 September 1994
- Issue 4 July 1994
- Issue 3 May 1994
- Issue 2 March 1994
- Issue 1 January 1994
Volume 23 January - November 1993 Jan - Nov 1993
- Issue 6 November 1993
- Issue 5 September 1993
- Issue 4 July 1993
- Issue 3 May 1993
- Issue 2 March 1993
- Issue 1 January 1993
Volume 22 January - November 1992 Jan - Nov 1992
- Issue 6 November 1992
- Issue 5 September 1992
- Issue 4 July 1992
- Issue 3 May 1992
- Issue 2 March 1992
- Issue 1 January 1992
Volume 21 January - November 1991 Jan - Nov 1991
- Issue 6 November 1991
- Issue 5 September 1991
- Issue 4 July 1991
- Issue 3 May 1991
- Issue 2 March 1991
- Issue 1 January 1991
Volume 20 January - November 1990 Jan - Nov 1990
- Issue 6 November 1990
- Issue 5 September 1990
- Issue 4 July 1990
- Issue 3 May 1990
- Issue 2 March 1990
- Issue 1 January 1990
Volume 19 January - November 1989 Jan - Nov 1989
- Issue 6 November 1989
- Issue 5 September 1989
- Issue 4 July 1989
- Issue 3 May 1989
- Issue 2 March 1989
- Issue 1 January 1989
Volume 18 January - November 1988 Jan - Nov 1988
- Issue 6 November 1988
- Issue 5 September 1988
- Issue 4 July 1988
- Issue 3 May 1988
- Issue 2 March 1988
- Issue 1 January 1988
Volume 17 January - November 1987 Jan - Nov 1987
- Issue 6 November 1987
- Issue 5 September 1987
- Issue 4 July 1987
- Issue 3 May 1987
- Issue 2 March 1987
- Issue 1 January 1987
Volume 16 January - November 1986 Jan - Nov 1986
- Issue 6 November 1986
- Issue 5 September 1986
- Issue 4 July 1986
- Issue 3 May 1986
- Issue 2 March 1986
- Issue 1 January 1986
Volume 15 January - November 1985 Jan - Nov 1985
- Issue 6 November 1985
- Issue 5 September 1985
- Issue 4 July 1985
- Issue 3 May 1985
- Issue 2 March 1985
- Issue 1 January 1985
Volume 14 January - November 1984 Jan - Nov 1984
- Issue 6 November 1984
- Issue 5 September 1984
- Issue 4 July 1984
- Issue 3 May 1984
- Issue 2 March 1984
- Issue 1 January 1984
Volume 13 January - November 1983 Jan - Nov 1983
- Issue 6 November 1983
- Issue 5 September 1983
- Issue 4 July 1983
- Issue 3 May 1983
- Issue 2 March 1983
- Issue 1 January 1983
Volume 12 February - November 1982 Feb - Nov 1982
- Issue 6 November 1982
- Issue 5 September 1982
- Issue 4 July 1982
- Issue 3 May 1982
- Issue 2 March 1982
- Issue 1 February 1982
Volume 11 January - November 1981 Jan - Nov 1981
- Issue 6 November 1981
- Issue 5 September 1981
- Issue 4 July 1981
- Issue 3 May 1981
- Issue 2 March 1981
- Issue 1 January 1981
Volume 10 January - November 1980 Jan - Nov 1980
- Issue 6 November 1980
- Issue 5 September 1980
- Issue 4 July 1980
- Issue 3 May 1980
- Issue 2 March 1980
- Issue 1 January 1980
Volume 9 January - November 1979 Jan - Nov 1979
- Issue 6 November 1979
- Issue 5 September 1979
- Issue 4 July 1979
- Issue 3 May 1979
- Issue 2 March 1979
- Issue 1 January 1979
Volume 8 January - November 1978 Jan - Nov 1978
- Issue 6 November 1978
- Issue 5 September 1978
- Issue 4 July 1978
- Issue 3 May 1978
- Issue 2 March 1978
- Issue 1 January 1978
Volume 7 January - November 1977 Jan - Nov 1977
- Issue 6 November 1977
- Issue 5 September 1977
- Issue 4 July 1977
- Issue 3 May 1977
- Issue 2 March 1977
- Issue 1 January 1977
Volume 6 March - October 1976 Mar - Oct 1976
- Issue 4 October 1976
- Issue 3 July 1976
- Issue 2 April 1976
- Issue 1 March 1976
Volume 5 January - October 1975 Jan - Oct 1975
- Issue 4 October 1975
- Issue 3 July 1975
- Issue 2 April 1975
- Issue 1 January 1975
Volume 4 March - December 1974 Mar - Dec 1974
- Issue 4 December 1974
- Issue 3 September 1974
- Issue 2 June 1974
- Issue 1 March 1974
Volume 3 March - December 1973 Mar - Dec 1973
- Issue 4 December 1973
- Issue 3 September 1973
- Issue 2 June 1973
- Issue 1 March 1973
Volume 2 March - December 1972 Mar - Dec 1972
- Issue 4 December 1972
- Issue 2-3 June 1972
- Issue 1 March 1972
Volume 1 February - August 1970 Feb - Aug 1970
- Issue 3-4 August 1970
- Issue 2 May 1970
- Issue 1 February 1970
- Find a journal
- Publish with us
- Track your research
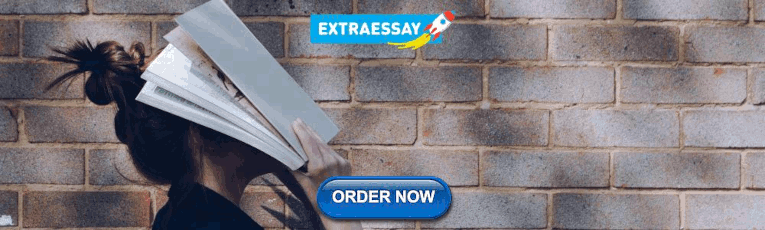
IMAGES
VIDEO
COMMENTS
Atom. RSS Feed. Behavioural genetics is the interdisciplinary effort to establish causal links between genes and animal (including human) behavioural traits and neural mechanisms. Methods used ...
Introduction. Although the history of heredity and behavior can be traced back to ancient times (Loehlin 2009), the first human behavioral genetic research was reported in the 1920s, which applied quantitative genetic twin and adoption designs to assess genetic influence on newly developed measures of intelligence.The 1920s also marked the beginning of single-gene research that led to ...
For fields such as social and behavioral genomics that are shaped by an ugly history and uncertain future, socially and ethically responsible research and research communication are crucial. FoGS ...
A Look at the Methods in Quantitative Behavioral Genetics. Typically, one sees something like Figure 1 in quantitative behavioral genetics papers. Behavioral geneticists would describe this figure as a phenotype being "decomposed" into three basic categories: additive genetic (A), shared environmental (C), and nonshared environmental (E) influences.
In the current study, the ethical challenges face by genetic studies that examine genetic variations contributing to antisocial behavior were discussed in this study by taking the Middle East and North Africa (MENA) researchers as an example. Go to: 2.1. Study Design. In the current study, a descriptive qualitative approach was used to explore ...
Bidirectional Causal Associations Between Same-Sex Attraction and Psychological Distress: Testing Moderation and Mediation Effects. Behavior Genetics is a leading journal concerned with the genetic analysis of behavioral traits. The journal offers the most current original research on ...
Insights In Behavioral and Psychiatric Genetics: 2022. We are now entering the third decade of the 21st Century, and, especially in the last years, the achievements made by scientists have been exceptional, leading to major advancements in the fast-growing field of Genetics. Frontiers has organized a series of Research Topics to highlight the ...
Using variation in heritability estimates as a test of G × E in behavioral research: a brief research note. Behav. Genet. 49, 340-346 (2019) ... we offer readers who might be less familiar with behaviour genetics a brief Primer on the topic. Sitting atop our list of goals is to be a resource for scholars interested in applying the widely ...
Overview. Behavior Genetics is a leading journal concerned with the genetic analysis of behavioral traits. The journal offers the most current original research on the inheritance and evolution of behavioral characteristics in humans and other species. Contributions focus on both the application of various genetic perspectives to the study of ...
Showcasing behavioral genetics in sex research with case studies . ... For more detailed discussions of these topics, readers may wish to refer to Acevedo . et al. (2020) ...
A century after the first twin and adoption studies of behavior in the 1920s, this review looks back on the journey and celebrates milestones in behavioral genetic research. After a whistle-stop tour of early quantitative genetic research and the parallel journey of molecular genetics, the travelogue focuses on the last fifty years. Just as quantitative genetic discoveries were beginning to ...
Applying Quantitative Genetic Methods to Primate Social Behavior. PubMed Central. Brent, Lauren J. N. 2013-01-01. Increasingly, behavioral ecologists have applied quantitative genetic methods to investigate the evolution of behaviors in wild animal populations. The promise of quantitative genetics in unmanaged populations opens the door for simultaneous analysis of inheritance, phenotypic ...
The recent advancements and developments reached in Behavioral and Psychiatric Genetics, especially over the past year, have been exceptional and are the result of the great achievements made by researchers and scientists thus far.In order to explore this fast-growing field, Frontiers is launching a new series of Research Topics focusing on the evolution of methods, processes, techniques and ...
Objectives We argue that research in the psychological sciences testing evolutionarily informed questions could benefit considerably from more frequent use of techniques common in behavior genetics. Methods We review some of the reasons why data and analytical strategies in behavior genetics confer certain advantages over more traditional forms of data analysis. In particular, we focus on the ...
Finally, we note that four of the top-10 findings (2, 7, 8 and 9) are about environmental influences rather than genetic influences. By using genetically sensitive designs such as twin studies, behavioral genetics has revealed almost as much about the environment as about genetics. 1. All psychological traits show significant and substantial ...
T32 MH017069/MH/NIMH NIH HHS/United States. This article provides an overview of the methods, assumptions, and key findings of behavioral genetics methodology for family researchers with a limited background. We discuss how family researchers can utilize and contribute to the behavioral genetics field, particularly in terms of conducting rese ….
Recent Advancements in Musculoskeletal Regenerative Medicine. Elizabeth Vinod. Csaba Matta. Boopalan Ramasamy. 225 views. The most cited genetics and heredity journal, which advances our understanding of genes from humans to plants and other model organisms. It highlights developments in the function and variability o...
Researchers from Japan have developed a new mouse model to study the molecular mechanism behind the ability of KMT2C to cause autism spectrum disorder. They also showed that vafidemstat has a ...
Polygenic scores are the topic of the third section of this paper. Because the fallout has not yet settled from the fusion of the two worlds of genetics, it is difficult to predict research directions during the next 10 years. What is clear is that this will be an exciting time for behavioural genomic research (Larsson, 2021a). I chose three ...
The articles included in this first of issue of Behavior Genetics devoted to topics of inclusion, diversity, equity, and access offer additional discussion of and solutions to the challenges around making quantitative genetic studies and the genomics workforce more diverse. ... The final group of papers presents behavior genetic research that ...
View sample behavioral genetics research paper. Browse research paper examples for more inspiration. If you need a psychology research paper written according to all the academic standards, you can always turn to our experienced writers for help. This is how your paper can get an A! Feel free to contact our writing service for professional assistance.
Mind-body medicine and its impacts on psychological networks, quality of life, and health - Volume II. Steffen Schulz. Dirk Cysarz. Georg Seifert. Frauke Musial. 5,224 views. 6 articles. Part of the world's most cited neuroscience journal series, this journal highlights research in all species that advances our understanding of the neural ...
Volume 51 January - November 2021. Issue 6 November 2021. Issue 5 September 2021. Special Issue on: Developmental behavior genetics and externalizing psychopathology. Issue 4 July 2021. Issue 3 May 2021. Special Issue: The International Workshop on Statistical Genetic Methods for Human Complex Traits. Issue 2 March 2021.