
An official website of the United States government
The .gov means it’s official. Federal government websites often end in .gov or .mil. Before sharing sensitive information, make sure you’re on a federal government site.
The site is secure. The https:// ensures that you are connecting to the official website and that any information you provide is encrypted and transmitted securely.
- Publications
- Account settings
Preview improvements coming to the PMC website in October 2024. Learn More or Try it out now .
- Advanced Search
- Journal List
- Scand J Child Adolesc Psychiatr Psychol

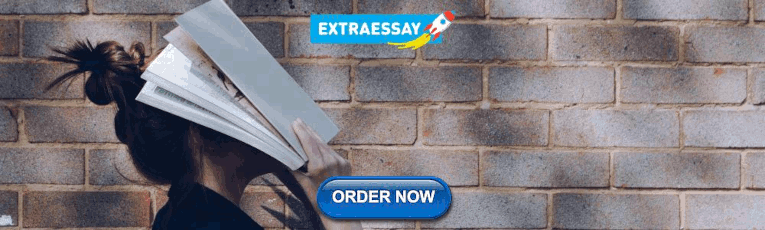
Cyberbullying: relationship with developmental variables and cyber victimization ▪
Gülendam akgül.
1 Yenimahalle Science and Art Center, Republic of Turkey Ministry of National Education, Ankara, Turkey
Müge Artar
2 Faculty of Educational Sciences, Ankara University, Ankara, Turkey
This study has been presented as oral presentation in VIth International Eurasian Educational Research Congress, Ankara, Turkey
Background and objective:
Cyberbullying is increasingly turning into a significant problem for children and adolescents due to its adverse psychological and academic outcomes. In the present study, the protective and risk factors for cyberbullying has been investigated. One of the aims of the study was to examine the relationship between peer relations, negative emotion regulation strategies, and cyberbullying. The successful identity development process is thought to influence both cyberbullying behaviors as well as adolescents’ peer relations and emotion regulation. Also, cyber victimization is seen as a risk factor for cyberbullying. The second aim of the study is to investigate the causal relationship between cyber victimization and cyberbullying.
The study is a descriptive research in which both cross-sectional and longitudinal data were used. In the cross-sectional part of the study, 1,151 adolescents have participated, and the data of the second wave was obtained from 322 of them four months later. Data were analyzed through structural equation modeling (SEM) and hierarchical regression analyses.
Results and conclusion:
According to the results of SEM, good peer relations predicted less cyberbullying. The expressive repression explained the cyberbullying through peer relationships. For identity development, contrary to expectations, commitment dimension of identity seemed to be positively related to more cyberbullying and so did higher reconsideration of commitment. Cross-lagged panel analyses revealed that Time 1 cyber victimization predicted Time 2 cyberbullying. Given the pattern of cross-lagged relationships, it was tentatively inferred that cyber victimization was the temporal precursor to cyberbullying. The results of the study have implications for the prevention of cyberbullying.
Introduction
The technology is gradually having an important impact on adolescents, as online communication becomes a significant part of their social life ( 1 ). At the same time, bullying, that is aggressive repetitive behaviors against a defenseless victim ( 2 ), has found a new medium, online environments. Cyberbullying is defined as “an aggressive, intentional act carried out by a group or individual, using electronic forms of contact, repeatedly and overtime against a victim who cannot easily defend him or herself” ( 3 ). In other words, cyberbullying is the act of bullying others while cyber victimization is being subjected to others’ bullying acts. Since it has adverse psychological consequences ( 4 - 7 ), academic outcomes ( 8 - 10 ), and increasing rates ( 11 ) more research is needed to understand the causal factors for cyberbullying.
One of the most critical psychosocial and developmental characteristics of adolescents is identity development. According to Erikson ( 12 ), identity brings in adolescents “purpose and direction” in life, and provides a sense of “inner sameness and continuity” over time and place. Although many researchers ( 13 , 14 ) investigated this concept, the research recently has been focusing on the process by which identity develops. One of the process-oriented identity models was the three-dimensional identity model of identity formation ( 15 ). It focuses on three processes in ideological (i.e. education) and interpersonal (i.e. relationship with best friend) area. The first process, commitment, refers to making permanent choices in life and the sense of confidence in these choices. In-depth exploration, the second process, refers to the more detailed examination of choices adolescents made, questioning them, and striving for more in-depth information about the choices. The last process, which is the reconsideration of commitment, means that adolescents are not happy with their choices and look for alternatives. As a result of these processes, adolescents dynamically form their identity and discover who they are.
The identity formation process, which is intensive during the adolescence period, makes adolescents more vulnerable to online risks. Valkenburg and Peter ( 16 ) emphasized that adolescents could face cyberbullying and unwanted sexual demands. Intense interest in sexuality during this period could result in being subjected to sexting, a kind of cyberbullying ( 17 ). Finally, identity exploration in online environments was found to be related to cyberbullying ( 18 ). Especially adolescents with “identity fluidity”, i.e. assuming different online identities, had higher rates of cyberbullying. In short, the process of identity development confronts adolescents with higher online risks. On the other hand, a well-developed identity that is making commitments and exploring them protect them from risky behaviors ( 19 - 21 ). No study so far has investigated the protective effect of identity development on cyberbullying. On basis of the literature findings which revealed the protective role of a well-developed identity in different kinds of risky behaviors, it may be possible that adolescents with higher commitment and in-depth exploration would engage in less cyberbullying behaviors.
It is essential to find out the cause of cyberbullying. One of the theories of cyberbullying, general strain theory (GST), states that strain factors cause cyberbullying and there are three primary sources of strain in negative relationships with others ( 22 ). These are the prevention of someone from achieving positively valued goals, removing or threatening to remove positively valued stimuli, and present or threaten to present someone with dangerous or negatively valued stimuli. For example, adolescents who think that they cannot achieve the deserved success, who lost one of their friends in an accident or who have to move to another city, could experience strain. Anger and similar negative emotions, which result from a negative relationship with others, lead to aggressive behaviors in adolescents. In social media, being a cyber victim is also a source of strain. An adolescent who is excluded from a group in social media, threatened to post personal information online or denigrated experiences strains.
The strains cause negative emotions of which anger has particular importance for GST since it arouses revenge and activates the person. In a study in three different European countries, anger was found to be the most expressed emotion when the emotional effects of varying bullying behaviors were examined ( 23 ).
In the case of cyberbullying, GST identified many causes for cyberbullying behaviors. Traditional victimization, for example, was found to be an essential source of strain in terms of cyberbullying ( 24 ). Adolescents who were subjected to traditional victimization engaged in more cyberbullying behavior than those who were not, which meant that they acted out their anger online. This study also found that other sources of stress, such as parental stress (conflicts between parents and adolescents), studying for the university entrance exam, and financial burdens (insufficient pocket money) caused cyberbullying behaviors. Similarly, Lianos and McGrath ( 25 ) investigated the effects of being a cyber victim, perceived social support, academic stress, and financial stress. They found that participants, who experienced more of these strains, felt more anger and which in turn lead to more cyberbullying behaviors. Parental relationships, peer relationships, peer rejection, school, and homework stress were investigated as the causes of strains for adolescents in another study ( 26 ). The results revealed that these strain factors significantly predicted cyberbullying behaviors.
Peer relations are one of the strain factors proposed by GST. During the adolescence period, the importance of parents increasingly declines ( 27 ), while the time spent with peers shows a sharp increment ( 28 ). They strive for independence from the family and support from their friends ( 29 ). When the relationship between peer relations and cyberbullying examined, adolescents who perceived their friends as reliable, supportive, and caring were less likely to engage in cyberbullying behaviors ( 1 ). Besides, adolescents who had low social support from peers ( 30 ), who had a lesser attachment to their peers ( 31 ) and who perceived less help and support from them ( 32 ), were found to engage in more cyberbullying behaviors. The patterns of peer relationships, quality of friendship, and behavioral adjustment in real life predicted peer relations in online environments ( 33 ). Peer relations also have a buffering role in the victimization of cyberbullying ( 34 ).
Anger is an important motivator for many aggressive behaviors and cyberbullying. Yet not all adolescents who experience strains engage in cyberbullying. The reason why some better cope with their negative emotions could be related to emotion regulation strategies. Emotion regulation is an internal or external process that includes monitoring, evaluating, and changing the intensity, timing and emotional responses to reach a goal ( 35 ). It determines which emotions people will have, how will they experience and express them ( 36 ). There are mainly two types of emotion regulation strategies ( 37 ). In cognitive reappraisal, the person tries to reevaluate the emotional impact or meaning of the situation, which arouses the emotion. It is carried out during the production phase of emotions. In expressive suppression, the person actively and consciously tries to suppress or inhibit the behavioral expression of emotions. It is achieved after the production of emotion. The former is associated with positive well-being, more positive emotions, less negative emotions and better interpersonal relationships, whereas the latter is associated with depression, negative mood ( 38 - 40 ), less positive emotions and more negative emotions ( 36 ). In other words, while the cognitive reappraisal is accepted as a better strategy for emotion regulation, the expressive repression is recognized as a more negative strategy.
The research on the relationship between emotion regulation and cyberbullying is usually derived from the research on traditional bullying. It has been known that emotion regulation is related to aggressive behaviors and traditional bullying ( 41 , 42 ). Both bullies and victims have deficiencies in their emotion regulation strategies ( 43 ). There are fewer studies on the relationship between cyberbullying and emotion regulation. It was reported that cyberbullies and cyberbully/cyber victims were not successful at expressing their emotions and they experience their negative emotions more intensively when compared to those who were unrelated to bullying or who were cyber victims ( 44 ). Moreover, adolescents who had deficiencies in positive emotion regulation strategies like cognitive reappraisal did more cyberbullying ( 45 ). Emotion regulation strategies influence not only cyberbullying but also cyber victimization behaviors. Adolescents with non-adaptive emotion regulation strategies, such as self-blame, other-blame, rumination and catastrophizing, were found to engage in more cyberbullying behaviors longitudinally than those with adaptive emotion regulation strategies ( 46 ). Also, cyber victims who use positive emotion regulation strategies (i.e. such as putting into perspective, positive refocusing, positive reappraisal, acceptance and refocusing on planning) improved better than those using negative ones ( 47 ). Eventually, emotion regulation strategies, particularly negative ones like expressive repression, are expected to be related to positively with cyberbullying.
To sum up, based on the literature, peer relations are expected to be related to cyberbullying behaviors. In the presence of poor relationships with peers and expressive repression, one of the negative emotion regulation strategies, adolescents would have difficulty in managing negative emotions and are expected to engage in more cyberbullying. In this context, identity development in the interpersonal relationship area is a broader concept influencing peer relations, emotion regulation strategies and cyberbullying. Figure Figure1 1 depicts the proposed model about the relationship between these variables.

Proposed Cross-Sectional Model about the Relationship between Dimensions of Identity Development, Peer Relations, Emotion Regulation Strategies and Cyberbullying
Based on the research on aggressive behaviors, exposure to aggressive behaviors is associated with performing similar acts later ( 48 ). Most of the theories which help to the understanding of cyberbullying, concentrate on the importance of exposure to cyberbullying behaviors. General aggression theory, based on social learning and social cognitive theories, was proposed to explain the relationship between aggressive behaviors and violence in media ( 49 ). When applied to cyberbully, exposure to cyberbullying is accepted as one of the factors for the emergence of similar behaviors ( 50 ). Similarly, the Barlett and Gentile cyberbullying model ( 51 ) explain how cyberbullies continue to be cyberbullies. The theory emphasizes the importance of learning in the maintenance of these behaviors. Cyberbullying experiences lead to the beliefs of anonymity. The cyberbully eventually learns that the harmful act can be done anonymously and develop a positive attitude. The theory is thought to be incomplete in the sense that it did not take into account that the same learning is likely for cyber victims. In other words, cyber victims just as cyberbullies, could learn that the act of cyberbullying often goes undetected. Hence, they are more likely to engage in similar cyberbullying behaviors.
The relationship between cyberbullying and cyber victimization is clearly demonstrated in many cross-sectional studies ( 52 - 57 ). Some studies reported the co-occurrence of cyberbullying and cyber victimization as high as 80% ( 58 , 59 ), whereas others did not report such a relationship ( 60 ). Li ( 61 ) founded that the best predictor of cyber victimization was cyberbullying behavior. Besides, the transition between being a cyberbully and the cyber victim is thought to be easy due to the nature of the online environment ( 62 ). The longitudinal relationship has been investigated in fewer studies. Barlett and Gentile ( 51 ) investigated the cross-lagged relationship between two variables with two months interval. They reported that there was a strong relationship between two variables, both cross-sectionally and longitudinally. Another study also said that being a cyber victim was significantly associated with being a cyberbully six months later yet it did not significantly predict the cyberbullying behaviors ( 63 ). Still, another study tested if cyber victims turns out to be cyberbullies ( 57 ). The results revealed that cyberbullying behaviors were not significantly predicted from three months earlier cyber victimization, despite the significant correlation between them. Similar results were obtained in a study with a one-year interval ( 64 ). In short, the cyber victimization and cyberbullying were longitudinally associated with each other despite the lack of any causal evidence.
The ambiguity regarding the causal relationship between cyber victimization and cyberbullying involvement can be overcome with cross-lagged panel models that allow describing reciprocal relationships between variables ( 65 - 68 ). In these models, measurement of the same variables has taken at two or more points in time. Both the directional influences of variables (cross-lags) and the stability of each variable over time can be estimated. Thus, identification of cross-lagged effects between cyberbullying and cyber victimization will elucidate the relationship between these variables. Also, it will reveal whether cyber victims learn to cyberbully through exposure.
Thus, the present study has two aims. First, it aims to investigate the relationship between peer relations, emotion regulation and cyberbullying cross-sectionally, and to examine the role of identity development on cyberbullying and other variables. A cross-sectional model (Figure (Figure1) 1 ) will test the relationship between expressive repression, peer relations and cyberbullying. The direct relationship between identity development and cyberbullying, in addition to the indirect relationship through peer relations and expressive repression will also be revealed in this model. Second, the study aims to explain the causal relationship between cyberbullying and cyber victimization. A cross-lagged panel model will be applied to reveal the causal precedence of them, in addition to the stability of them across two waves. The cross-lagged relationship from Time 1 (T1) cyber victimization to Time 2 (T2) cyberbullying would be larger than the cross-lagged relationship from T1 cyberbullying to T2 cyber victimization.
The investigation was conducted using a standardized survey during class hours, with permission from the Ethical Committee (Ankara University), Ministry of National Education and informed consent from parents and students. Students were informed about the opportunity to refuse participation, although none of them declined. Before giving the questionnaires, the students were told to write the school numbers if they would like to participate in the second part of the study. Data were collected from the same sample twice with four months interval.
Participants
Total 1,389 high school students between the ages of 13-20, attending different types of high schools (9th-12th grades) in Ankara in the 2017-2018 educational year participated in the first wave of the study. Of the sample, 155 cases were not included in the sample because of the missing data or response pattern (same response to all questions). The remaining cases were examined in terms of missing cases and multivariate outliers, 55 cases with more than 5% missing, and 28 univariate/multivariate outliers were removed from the sample. The final sample consisted of 1,151 adolescents (648 ([6.3%] female) from various types of high schools (28.8% Academic High School, 18% Social Sciences School, 48.5% Vocational High School and 4.7% vocational education center). The mean age was 15.23 (SD = 1.08).
Data for the second wave were obtained at the end of the second semester. Only 500 students from the first sample were reached because of the high absenteeism. Data for 156 cases were not paired due to the lack of school numbers. After the analysis of missing cases and outliers, a total of 344 students (232 [72%] female) took part in the second wave of the study. Students had an average age of 15.25 years (SD=1.11).
Independent samples t -test indicated that participants who provided data for both waves did not differ from those who provided data only for the first wave in terms of initial cyber victimizations scores ( t (1,109) = 1.04, p > 0.05) and mean age ( t (1,098) = −0.76, p > 0.05). However, cyberbullying scores of those who attend the both wave (M = 13.05, SD = 4.38) are significantly higher ( t (1,110) = 3.09, p < 0.05) than those who attend only the first wave (M = 12.20, SD = 3.42). This should be taken into account when interpreting the current findings. χ 2 tests indicated that participants who attend the second wave were significantly more likely to be female ( χ 2 (1, N =1,206) = 54.44, p < 0.05). However, the gender difference was not expected to distort the results since no analyses focused on the effect of gender.
Revised cyberbullying inventory-II (RCBI-II)
Cyberbullying were measured through RCBI-II. It is a 10-item questionnaire, was developed by Erdur-Baker and Kavşut ( 69 ) and revised by Topcu and Erdur-Baker ( 70 ). It assessed frequencies of participants’ cyberbullying and cyber victimization behaviors on a four-point Likert scale (never, once, two-three times, more than three times) ( α = 0.69). The cyber victimization refers to the total scores of the cyber victimization section of the inventory (“It happened to me” column). The cyberbullying refers to the total scores of cyberbullying section (“I did it” column).
Utrecht-management of identity commitments scale (U-MICS)
It was developed ( 14 ) to measure adolescents’ identity development process and adapted into Turkish ( 71 ). It consisted of 26 items, three dimensions, commitment ( α = 0.90), in-depth exploration ( α = 0.77), and reconsideration of commitment ( α = 0.82) in two areas; education and interpersonal relations. The items are designed using a five-point Likert scale ranging from completely untrue ( 1 ), to completely true ( 5 ). For the present study, the relational part of the scale (13 items) was employed.
Friendship qualities scale
It examined the friendship qualities of adolescents ( 72 ) and adapted to the Turkish ( 73 ). It has 22 items and five dimensions, help ( α = 0.89) companionship ( α = 0.68), conflict ( α = 0.69), security ( α = 0.72) and closeness( α = 0.87) The respondents are asked to think about one of their close friends and answer the questions using a five-point Likert scale ranging from completely untrue ( 1 ) to completely true ( 5 ). The Cronbach’s α for the whole scale was 0.87.
Emotion regulation scale
The scale, initially developed by Gross and John ( 74 ) and adapted into Turkish ( 75 ), aimed to measure the individual differences in emotion regulation using ten items rated on a six-point Likert type scale from completely true to completely untrue. It has two dimensions, cognitive reappraisal ( α = 0.64) and emotional repression ( α = 0.70). The latter was used to measure repressive emotion in the present study.
The proposed model was analyzed by the structural equation modeling (SEM) in LISREL 8.71. Before the analysis, the data were examined for the assumptions of SEM. The two-stage approach in which the measurement and the structural models are tested separately was preferred in SEM ( 76 ). Sample size, missing values, outliers, multicollinearity, linearity and homoscedasticity assumptions were met. Since the data did not meet the assumptions of normality, robust maximum likelihood with asymptotic covariance matrices was preferred as suggested by Jöreskog, Olsson and Wallentin, ( 77 ). Model-data fit were evaluated by using Satorra-Bentler χ 2 /df, root mean square error of approximation (RMSEA), standardized root mean square residual (SRMSR), comparative fit index (CFI) and Tucker Lewis index (TLI). The acceptable fit values are less than 5 for Satorra-Bentler χ 2 /df, less than 0.08 for RMSEA, less than 0.05 for SRMSR, greater than 0.90 for CFI and TLI ( 78 ).
To compute the standard coefficients of the relationships in the cross-lagged panel model, two hierarchic regression analyses were employed (SPSS 24.0). Before the analysis, data were checked for the assumptions of the regression analysis. In each regression analysis, time one measures of predicted variables were controlled by being entered in the first block of analysis.
Test of the cross-sectional model of cyberbullying
Correlations between the variables in the study, mean and standard deviations are presented in Table Table1. 1 . According to the table, the variables of the study has small to medium correlations with each other.
The descriptive statistics and correlations between variables
First of all a measurement model was tested using six latent variables (cyberbullying, emotional repression, peer relations, and three dimensions of interpersonal identity) and 31 observed variables. An acceptable model fit was obtained (SB χ 2 = 1,646.64, df = 419, SB χ 2 /df = 3.92, RMSEA = 0.05, SRMR = 0.059, TLI = 0.94, CFI = 0.94).Then, the proposed structural model was tested. The non-significant paths between in-depth exploration-cyberbullying ( t = −0.50, β = −0.02); in-depth exploration-peer relations ( t = −0.22, β = −0.01); reconsideration of commitment-emotional repression ( t = −1.94, β = −0.09) and emotional repression-cyberbullying ( t = 1.49, β = 0.10) were removed from the model. The resulting model produced acceptable fit values (SB χ 2 = 1,651.21, df = 423, SB χ 2 /df = 390, RMSEA = 0.50, SRMR = 0.058, TLI = 0.94, CFI = 0.95). The standard coefficients of the tested cross-sectional model were presented in Figure Figure2 2 .

Standardized Coefficient of Cross-Sectional Model of Cyberbullying ( p > 0.05)
According to the model, the most critical variable affecting cyberbullying was peer relations ( β = −0.53, t = −9.08, p < 0.001). There was a negative relationship between peer relations and cyberbullying behaviors. In other words, in the presence of good peer relations, adolescents engaged in less cyberbullying.
The expressive repression explained the cyberbullying through peer relationships. The relationship between expressive repression and peer relations was positive ( β = 0.58, t = −9.08, p < 0.001). Adolescents who repressed their emotions more reported better relationships with their friends than those who did not.
The second aim was to observe the effects of identity development directly on cyberbullying and indirectly through peer relations and expressive repression. According to the structural model, of the three dimensions of identity development, commitment ( β = 0.21, t = 3.92, p < 0.01) and reconsideration of commitment ( β = 0.15, t = 3.59, p < 0.05) had positive effects on cyberbullying. Contrary to expectations, adolescents with higher commitments who made their permanent choices in the relational area seemed to do more cyberbullying. Those with reconsidering their commitments and unhappy with their relations were, as expected, more likely to do cyberbullying acts. Commitment also had positive effects on expressive repression ( β = 0.30, t = 7.71 p < 0.01) and peer relations ( β = 0.32, t =8.07, p < 0.05). Adolescents with higher commitments used a repressive strategy to regulate their emotions and they had better friendship quality. In-depth exploration was found to be related to expressive repression ( β = 0.15, t = 4.37, p < 0.01) which meant that adolescents who deeply examined their choices, just like those with higher commitments, repressed their emotions more. Finally, by contrast with expectations, adolescents who were reconsidering their commitments, seemed to have better relations with their friends ( β = 0.10, t = 2.95, p < 0.05).
Test of the cross-lagged panel model
In terms of descriptive statistics, the mean scores for cyber victimization at Time 1 ( x ¯ = 13.26 , SD=3.99) and at Time 2 ( x ¯ = 13.24 , SD = 3.84) were found to be higher than cyberbullying at Time 1( x ¯ = 12.66 , SD = 3.56) and at Time 2 ( x ¯ = 12.49 , SD = 3.72). There were significant positive moderate correlations between the variables of the study. The descriptive statistics and correlations are presented in Table Table2 2 .
In order to understand the longitudinal relationship between cyberbullying and cyber victimization, cross-lagged panel design analyses were conducted. Two hierarchical regression analyses were conducted to compute the standard coefficients between the cross-lagged relationships between T1 and T2 measures of cyber victimization and cyberbullying. First, the assumptions of the regression were tested. Data with more than 5% missing cases (12 cases) and multivariate outliers (16 cases) were omitted. The analyses were performed with the remaining 322 cases, which is a large enough sample size ( 79 ). The medium correlations between variables, tolerance (0.71), variance inflation factor (1.41) and condition index (11.80) ensured multicollinearity, residual, and scatter plots indicated the assumptions of normality ( 79 ). The results are presented in Table Table3. 3 . The findings of the cross-lagged panel design were presented in Figure Figure3 3 .
The hierarchic regression analysis results

The cross-lagged panel model of cyber victimization and cyberbullying with four months interval.
Non-significant paths are dotted. * p < 0.05, ** p < 0.01, *** p < 0.001
In the first analysis, after controlling for T1 cyber victimization ( β =0.51**, t=8.98, R 2 =0.27, p <0.001), T1 cyberbullying did not significantly predicted T2 cyber victimization ( β =0.02, t =0.34, ∆ R 2 =0.00, p >0.001). Being a cyber victim was predicted from four months earlier cyber victimization but not from cyberbullying. In the second hierarchical regression analysis, the effects of T1 cyberbullying and T1 cyber victimization on T2 cyberbullying behaviors were analyzed. This time after controlling for T1 cyberbullying ( β =0.35, t =6.07, R 2 =0.23, p <0.001), T1 cyber victimization significantly predicted T2 cyberbullying behaviors ( β =0.24, t =4.16, ∆ R 2 =0.04, p <0.001).
Moreover, the results of the analysis also revealed that both cyberbullying and cyber victimization were stable across time (0.35 and 0.51, respectively). The pattern of significant (T1 cyber victimization to T2 cyberbullying of 0.24) and non-significant (T1 cyberbullying to T2 cyber victimization of 0.02) standard coefficients of cross-lagged paths allows the inference of temporal precedence of cyber victimization to cyberbullying.
The study aimed to reveal the cross-sectional relationship between the developmental variables and cyberbullying, in addition to finding out the cross-lagged relationship between cyberbullying and cyber victimization. The results showed that having good peer relations were related to less cyberbullying behavior. Expressive repression, as a negative emotion regulation strategy, is positively associated with peer relations. In other words, adolescents who repressed their emotions more had better relationships with their peers, and those with better peer relations did less cyberbullying. Surprisingly, adolescents with higher identity commitment (successful identity) and adolescents with higher reconsidering their commitment (unsuccessful identity) did more cyberbullying. Thus contrary to expectations, a successful identity process did not protect adolescents from engaging cyberbullying behaviors. Another aim was to look at the causal relationship between cyberbullying and cyber victimization. The results supported that cyber victims turned out to cyberbullies with time by showing that being a cyber victim was related to cyberbullying four months later.
One of the aims of the study was to test the relationship between peer relations, expressive repression and cyberbullying. Peer relations were found to have a negative relationship with cyberbullying. This finding is consistent with the expectation of GST, which accepts peer relationships as a source of strain. Peer relations, by itself, were seen as a reason for cyberbullying ( 80 ). Moreover, patterns of a face-to-face relationship with peers were repeated in online environments ( 33 ). The finding that adolescents who were excluded by their friends were more likely to engage in externalizing behaviors ( 81 ), also explains that those with better friendship involved in less cyberbullying.
Since expressive repression is a negative emotion regulation strategy, it was expected to correlate with cyberbullying positively. The results showed that it has an indirect effect on cyberbullying through peer relationships. Once again, it stressed the importance of peer relations. In spite of using negative emotion regulation, they had good relationships with peers that, in turn, protected them from misbehaving in online environments. Expressive repression was found to be correlated with peer relations positively, contrary to the literature. Actually, this strategy was connected to problems in interpersonal relations ( 82 ). But the finding differed according to culture. In Asian cultures, repressing emotions were not perceived as unfavorable as in European cultures. Turkish culture might be more alike in Asian cultures in terms of emotional expression. Especially in terms of negative emotions, repression rather than the expression of emotion seemed preferable for a better interpersonal relationship.
Another aim was to explore the relationship between the dimensions of identity development and cyberbullying. As expected, the reconsideration of commitment had a weak positive correlation with cyberbullying. This dimension of identity means that the adolescent is unhappy with the present relations and in a search process. It is associated with more psychosocial problems ( 83 , 84 ). Therefore, they can experience issues with their friends in online environments. Due to the cross-sectional design of this model, it could also be possible that the adolescent who engages in online cyberbullying activities would be more likely to reconsider their current relationships and search for new friends.
On the contrary to expectations, the findings revealed a weak positive relationship between the commitment dimension of identity development and cyberbullying. It was stated no study so far seemed to investigate this relationship in the literature. However, a well-developed identity was expected to protect adolescents from cyberbullying, a kind of risky behavior ( 19 ). Adolescents tended to hide their real names, ages, or gender in online environments ( 85 ). The nature of the cyber environment does not allow the individuals to detect the identity of the bully and usually, cyberbullying is unpunished ( 86 , 87 ). As a result, adolescents would calculate fewer risks online, threatening someone or spread rumors or visual materials about a friend. Thus, the protective effects of identity may not work in cyber environments. Another explanation for this finding would be related to the possibilities which the online environment presented to adolescents. The cyber environment is a place where they can communicate with others, socialize, express themselves and test different identities ( 17 ). Sexual exploration, one of the subjects attract their attention, is more accessible and perceived as less risky in online environments ( 16 ). Also, social media provide them with feedback about their identity trials ( 88 ). More engagement in online environments for identity search could result in more developed identity, i.e. more commitment and more cyberbullying behaviors.
Results indicated that T1 cyber victimization predicted T2 cyberbullying, whereas T1 cyberbullying did not predict T2 cyber victimization. Consequently, it appears that being a cyber victim precedes being a cyberbully in time. In other words, cyber victims learn to cyberbully through exposure. The finding is important in terms of explaining cyberbullying behaviors. Both General Aggression Theory and Barlett and Gentile’s Cyberbullying Model posit that exposure to cyberbullying is vital in the emergence of cyberbullying behaviors, although the two theories explain the mechanism differently. The former relies on social learning principles, whereas the latter stresses the importance of anonymity beliefs. The current results provided support for both theories. However, the mechanisms through which cyber victims become cyberbullies are still vague.
In addition to cross-lagged relationships between cyber victimization and cyberbullying, the lagged relationships, as shown by significant autoregressive correlations revealed that both variables were stable over time. This finding is consistent with previous studies that reported significant longitudinal associations ( 51 , 63 ). It is seen that bullying or being victimized in the cyber environment is not a one-time event; it persists if not intervened. This result uncovers the need for prevention.
The prior longitudinal studies have provided additional support for the causal relationship between T1 cyber victimization and T2 cyberbullying, with different time intervals changing from three months to one year ( 57 , 63 , 64 ). These studies did not differ from the current research in terms of the sample characteristics. They reported significant correlations similar to the present study. However, in none of these studies, cyberbullying was significantly predicted from prior cyber victimization.
The results correspond to those of Den Hamer and Konjin ( 46 ), who investigated the effects of emotion regulation strategies on cyber victimization and cyberbullying relationships longitudinally. They reported that cyber victims turn into cyberbullies, especially if they are not good at regulating their emotions. The results are inconsistent with those reported by Barlett and Gentile ( 51 ), who similarly investigated cross-lagged correlations between cyberbullying and cyber victimization. They found both cross-lagged relationships significant; even the relationship from T1 cyberbullying to T2 cyber victimization was higher than the vice versa. The difference might be due to the sample, which consisted of university students. Also, the shorter time interval between waves (two months) in that study might be another factor for the difference. Unfortunately, both studies had two waves. As Kearney ( 67 ) suggested, the lag between measurements should be enough to observe the effects of variables. Thus, it would be better to include more than two waves to see the effects. The findings also revealed that both cyber victimization and cyberbullying are stable over time. The result is also consistent with prior research.
Making causal inferences as a result of cross-lagged panel analysis is a disputable subject in the literature. Some researchers argue that they cannot be accepted as causal models, but they are still powerful for starting a discussion on the causal effect of one variable on another ( 65 ). It is challenging to reveal cause-effect relationships through experimental designs in developmental researches. Tyagi and Singh ( 66 ) claim that these models can give a clue about the cause-effect relationship between the variable in cases where it is impossible to use real experimental designs. In either case, this study presents important findings on the temporal relations between cyberbullying and cyber victimization.
Given the increasing importance of cyber environments for identity search during adolescence, the present research will contribute to filling the gap in the literature on the relationship between identity development and cyberbullying. Future research could explore a causal relationship with longitudinal models because the cross-sectional nature of the present study does not allow to make an inference about whether a well-developed identity caused more cyberbullying behaviors or not. Additionally, more research is needed, which unveils why and how cyber victims do more cyberbullying through the inclusion of possible emotions such as anger or revenge, as stated by GST.
Clinical significance
Overall, this study demonstrated the importance of peer relations and cyber victimization for cyberbullying. Adolescents with difficulty in peer relationships are more vulnerable to engage in cyberbullying, thus being a clear target for prevention programs in educational settings. Moreover, the finding that children and adolescents who experienced cyber victimization are more likely to cyberbully in the future has also implications for prevention. Preventive efforts should focus on increasing awareness on how to respond to cyber victimization. Alternative responses to cyberbullying would help them to fight against cyberbullying. The research and practice should more focus on interventions for cyber victims who are prone to be cyberbullied after some time.
Conflicts of interest
The authors declare no conflicts of interests.
- Open access
- Published: 22 December 2021
Cyberbullying detection: advanced preprocessing techniques & deep learning architecture for Roman Urdu data
- Amirita Dewani ORCID: orcid.org/0000-0002-3816-3644 1 ,
- Mohsin Ali Memon ORCID: orcid.org/0000-0003-2638-4252 1 &
- Sania Bhatti ORCID: orcid.org/0000-0002-0887-8083 1
Journal of Big Data volume 8 , Article number: 160 ( 2021 ) Cite this article
10k Accesses
25 Citations
1 Altmetric
Metrics details
Social media have become a very viable medium for communication, collaboration, exchange of information, knowledge, and ideas. However, due to anonymity preservation, the incidents of hate speech and cyberbullying have been diversified across the globe. This intimidating problem has recently sought the attention of researchers and scholars worldwide and studies have been undertaken to formulate solution strategies for automatic detection of cyberaggression and hate speech, varying from machine learning models with vast features to more complex deep neural network models and different SN platforms. However, the existing research is directed towards mature languages and highlights a huge gap in newly embraced resource poor languages. One such language that has been recently adopted worldwide and more specifically by south Asian countries for communication on social media is Roman Urdu i-e Urdu language written using Roman scripting. To address this research gap, we have performed extensive preprocessing on Roman Urdu microtext. This typically involves formation of Roman Urdu slang- phrase dictionary and mapping slangs after tokenization. We have also eliminated cyberbullying domain specific stop words for dimensionality reduction of corpus. The unstructured data were further processed to handle encoded text formats and metadata/non-linguistic features. Furthermore, we performed extensive experiments by implementing RNN-LSTM, RNN-BiLSTM and CNN models varying epochs executions, model layers and tuning hyperparameters to analyze and uncover cyberbullying textual patterns in Roman Urdu. The efficiency and performance of models were evaluated using different metrics to present the comparative analysis. Results highlight that RNN-LSTM and RNN-BiLSTM performed best and achieved validation accuracy of 85.5 and 85% whereas F1 score was 0.7 and 0.67 respectively over aggression class.
Introduction
Cyberbullying (aka hate speech, cyberaggression and toxic speech) is a critical social problem plaguing today’s Internet users typically youth and lead to severe consequences like low self-esteem, anxiety, depression, hopelessness and in some cases causes lack of motivation to be alive, ultimately resulting in death of a victim [ 1 ]. Cyberbullying incidents can occur via various modalities. For example, it can take the form of sharing/ posting offensive video content or uploading violent images or sharing the pictures without permission of the owner etc. However, cyberbullying via textual content is far more common [ 2 ]. In Pakistan, the usage of internet, smartphones and social media has increasingly become prevalent these days and the very frequent users are youngsters. According to a report, more than 65% of all the users lie between 18 and 29, and typically women are more susceptible and unprotected. People often use offensive language, use hate speech, and become aggressive to bully celebrities, leaders, women and an individual [ 3 ]. In Pakistan, victims have reported life disturbing and annoying experiences and most of the victims are educated youngsters (age group of 21–30 years) [ 4 ]. The traffic in cyberspace has escalated significantly during covid-19 pandemic. A report “COVID 19 and Cyber Harassment”, released by DRF in 2020 highlights a great rise in the number of cyberbullying and harassment cases during the pandemic. The complaints registered with DRF’s Cyber Harassment Helpline were surged by 189% [ 5 ].
Recently, Roman Urdu language has been a contemporary trend and a viable language for communication on different social networking platforms. Urdu is national and official language of Pakistan and predominant among most communities across different regions. A survey statistic in [ 6 ] affirms that 300 million people are speaking Urdu language and approximately 11 million speakers are in Pakistan from which maximum users switched to Roman Urdu language for the textual communication, typically on social media. It is linguistically rich and morphologically complex language [ 7 ]. Roman Urdu language is highly variant with respect to word structures, writing styles, irregularities, and grammatical compositions. It is deficit of standard lexicon and available resources and hence become extremely challenging when performing NLP tasks.
An elaboration of script of Urdu instances and Roman Urdu is given in Table 1 . Instances highlighted are describing anti-social behavior.
This paper addresses toxicity/cyberbullying detection problem in Roman Urdu language using deep learning techniques and advanced preprocessing methods including usage of lexicons/resource that are typically developed to accomplish this work. Intricacies in analyzing the structure and patterns behind these typical aggressive behaviors, typically in a newly adopted language, and forming it as a comprehensive computational task is very complicated. The major contributions of this study are formation of a slang and contraction mapping procedure along with slang lexicon for Roman Urdu language and development of hybrid deep neural network models to capture complex aggression and bullying patterns.
The rest of the paper is organized as follows: Review of existing literature is presented in " Related Work " Section. " Problem statement " Section states research gap and gives formal definition of the addressed problem. " Methodology " Section describes the steps of research methodology and techniques and models used for the experimentations. Advanced preprocessing steps applied on Roman Urdu data are elaborated in " Data Preprocessing on Roman Urdu microtext " section. Implementation of proposed model architecture and hyperparameter settings are discussed in " Experimental Setup " section. " Results and Discussion " Section highlights and discusses study results and finally " Conlusion " Section concludes the research work and provides future research directions.
Related work
Due to the accretion of social media communication and adverse effects arising from its darker side on users, the field of automatic cyberbullying detection has become an emerging and evolving research trend [ 8 ]. Research work in [ 9 ] presents cyberbullying detection algorithm for textual data in English language. It is considered as one of the pioneers and highly cited research. They divided the task in text-classification sub problems related to sensitive topics and collected 4500 textual comments on controversial YouTube videos. This study implemented Naive Bayes, SVM and J48 binary and multiclass classifiers using general and specific feature sets. Study contributed in [ 10 ] applied deep learning architectures on Kaggle dataset and conducted experimental analysis to determine the effectiveness and performance of deep learning algorithms LSTM, BiLSTM, RNN and GRU in detecting antisocial behavior. Authors in [ 11 ] extracted data from four platforms i-e Twitter, YouTube, Wikipedia, and Reddit for developing an online hate classifier in English language using different classification techniques. Research carried out in [ 12 ] developed an automated approach to detect toxicity and unethical behavior in online communication using word embeddings and varying neural network layers. They suggested that LSTM layers and mimicked word embedding can uncover such behavior with good accuracy level.
Few of the studies in recent years has been contributed by researchers on other languages apart from English. Research work in [ 13 ] is unique and has gathered textual data from Instagram and twitter in Turkish language. They have implemented Naïve Bayes Multinomial, SVM, KNN and decision trees for cyberbullying detection along with Chi-square and information gain (IG) for feature selection. Work accomplished in [ 14 ] also addresses the problem of cyberaggression in Turkish language. The work extends comparison of different machine learning algorithms and found optimal results using Light Gradient Boosting Model. Van Hee, Cynthia, et al. in [ 15 ] proposed cyberbullying detection scheme for Dutch language. This is the first study on Dutch social media. Data was collected from ASKfm where users can ask and answer questions. The research uses default parameter settings for un-optimized linear kernel SVM based on n-grams and keyword system to identify bullying traces. F1 score for Dutch language was 61%. Problem of Arabic language cyberbullying detection was addressed and accomplished in [ 16 ]. This study used Dataiku DSS and WEKA for ML tasks. The data was scrapped from facebook and twitter. The study concluded that even though the detection approach was not comparable with the other studies in English language but overall Naive Bayes and SVM yield reasonable performance. Research work in [ 17 ] by Gomez-Adorno, Helena, et al. proposed automatic aggression detection for Spanish tweets. Several types of n-grams and linguistically motivated patterns were used but the best run could only achieve F1 score of 42.85%. Studies presented in [ 18 , 19 , 20 ] are based on automatic detection of cyberbullying content in German language. Research conducted in [ 18 ] proposed an approach based on SVM, CNN and ensemble model using unigram, bigrams and character N-grams for categorizing offensive tweets in German language. Research presented in [ 21 ] attempted for the very first time to identify bullying traces in Indonesian language. Association Rule mining and FP growth text mining were used to identify trends for bullying patterns in Jakarta and Surabaya cities using social media text. This baseline study on Indonesian language was further extended by Nurrahmi, Hani et al. in [ 22 ]. Study in [ 23 ] made first attempt to develop a corpus of code-mixed data considering Hindi and English language. They proposed a scheme for hate speech detection using N-grams and lexical features. An ensemble approach by combining the predictions of Convolutional Neural Network (CNN) and SVM algorithms were used for identifying such patterns. The weighted F1 score for Hindi language ranged between 0.37 and 0.55 for different experiments [ 24 ]. In the year 2019, Association for computational linguistics initiated the project for automatic detection of cyberbullying in Polish language [ 25 ]. Research conducted in [ 26 ] attempted to uncover cyberbullying patterns in Bengali language implementing passive aggressive, SVM and logistic regression. The optimum accuracy achieved was 78.1%. Recently, work contributed in [ 27 ] presented first study in Roman Urdu using lexicon based approach. The dataset was highly skewed comprising of only 2.2% toxic data. As according to [ 28 ], biased sampling and measurement errors are highly prone to classification errors when working on such datasets. Moreover, pattern detection based on predefined bullying and non-bullying lexicons were shortcomings of this study.
For automated detection of complex cyberbullying patterns, studies contributed by different scholars employ supervised, unsupervised, hybrid and deep learning models, vast feature engineering techniques, corpora, and social media platforms. However, the existing literature is mainly oriented towards unstructured data in English language. Some recent studies and projects have been initiated in other languages as discussed previously. To the best of our knowledge and literature review, no detailed work has been contributed in Roman Urdu to systematically analyze cyberbullying detection phenomenon using advanced preprocessing techniques (involving the usage of Roman Urdu resources) and deep learning approaches under different configurations.
Problem statement
The escalated usage of social networking sites and freedom of speech has given optimal ground to individuals across all demographics for cyberbullying and cyberaggression. This leaves drastic and noticeable impacts on behavior of a victim, ranging from disturbance in emotional wellbeing and isolation from society to more severe and deadly consequences [ 29 ]. Automatic Cyberbullying detection has remained very challenging task since social media content is in natural language and is usually posted in unstructured free-text form leaving behind the language norms, rules, and standards. Evidently, there exists a substantial number of research studies which primarily focus on discovering cyberbullying textual patterns over diverse social media platforms as discussed previously in literature review section. However, most of the detection schemes and automated approaches formulated are for resource-rich and mature languages spoken worldwide. Roman Urdu is typically spoken in South Asia and is a highly resource deficient language. Hence this research puts novel efforts to propose data pre-processing techniques on Roman Urdu scripting and develop deep learning-based hybrid models for automated cyberbullying detection in Roman Urdu language. The outcomes of this study, if implemented, will assist cybercrime centers and investigation agencies for monitoring social media contents and in making cyberspace secure and safer place for all segments of society.
Methodology
The research methodology is depicted in Fig. 1 .
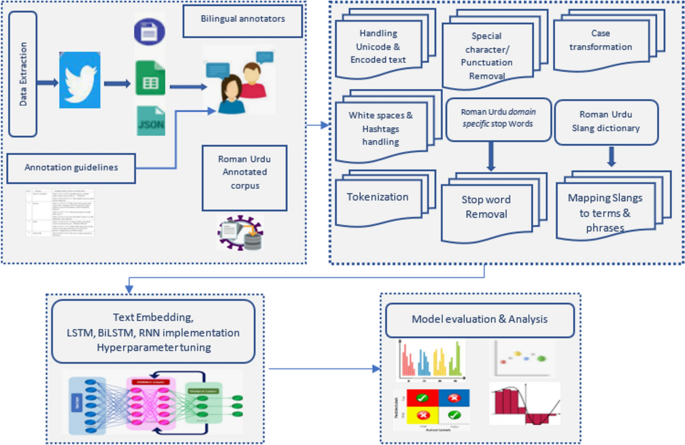
Proposed research methodology
The development of hate speech/cyberbullying corpus with minor skew and automated development of domain specific roman Urdu stop words is published in our previous work [ 30 ]. The work details formation of computational linguist resources. Further steps of methodology are discussed in subsequent sections. The Deep Neural Network (DNN) based techniques and models used for the experimentations are detailed below.
Model description
Recurrent neural networks (rnn).
RNN [ 31 ] has been applied in literature for successive time series applications with temporal dependencies. An unfolded RNN can handle processing of current data by utilizing past data. Meanwhile, RNN has the issue of training long-term dependencies. This has been addressed by one of the RNN variant.
Long short-term memory networks (LSTM)
LSTM has been employed as an advanced version of RNN network. It resolves the shortcoming of RNN by applying memory cells also known as hidden layer units. Memory cells are controlled through three gates named as: input gate, output gate and forget gate. They have the self-connections which store the temporal state of network [ 31 ]. Input and output gates address and control the flow of information from memory cell input and output to rest of the network. The forget gate, usually called as a remember vector, transfers the information with higher weights from previous neuron to the next neuron. The forget gate is added to the memory cell. The information resides in memory depending upon the high activation results; the information will be stored in memory cell iff the input unit has high activation. However, the information will be transferred to next neuron if the output unit has high activation. Otherwise, input information with high weights resides in memory cell [ 31 ].
Mathematically, LSTM network can be described as [ 32 ]:
where W h ∈ R m × d and U h ∈ R m × m indicates weight matrices, x t denotes the current word embedding, b h ∈ R m refers to bias term, whereas f(x ) is a non-linear function.
LSTM has more complex architecture including hidden states and tends to remember information for either short or long term. The hidden state [ 33 ] of LSTM is computed as follows:
where f t denotes the forget gate, i t refers to the input gate, c t denotes the cell state, o t is the output gate, h t is the regular hidden state, σ indicates sigmoid function, and ◦ is the Hadamard product.
Bidirectional Long short-term memory networks (BiLSTM)
In the traditional recurrent neural network model and LSTM model, the propagation of information is only in forward direction. This results in computation of an output vector only based on the current input at time t and the output of the previous unit. The back propagation of information in network is achieved by merging two bidirectional recurrent neural network (BiRNN) and LSTM units, one for forward direction and one for backward direction. This helps in capturing contextual information and enhances learning ability [ 34 ].
In bidirectional LSTM, outputs of two LSTM networks are stacked together. The first LSTM is a regular sequence starting from the starting of the paragraph, while the second LSTM is a standard sequence, and the series of inputs are fed in the opposite order. The first hidden state is denoted by ht forward whereas second LSTM unit’s hidden state is denoted by ht backward . After processing data, the final state ht Bilstm is computed by concatenating the two hidden states as given in Eq. 3 .
where ⊕ denotes a concatenation operator.
Convolutional neural networks (CNN)
Convolution neural networks (aka CNN), originally incorporated for image processing tasks, have become very efficacious in different NLP and text classification applications. The network identifies correlations and patterns of data via their feature maps. Information about local structure of data is extracted by applying multiple filters with different dimensions [ 35 ].
Data preprocessing on Roman Urdu microtext
Big social media data in Roman Urdu language is inconsistent, incomplete, or precise, missing in certain behaviors or trends, and is likely to incorporate many errors. Roman Urdu users highly deviate language norms while communicating on social media. Hence data preprocessing is immensely significant. Some major data preprocessing steps applied on Roman Urdu microtext are detailed below.
Handling Unicode and encoded text formats
Unicode scheme provides every character in natural language text a unique code from 0 to 0 × 10FFFF. The uncleaned Roman Urdu data comprised of special symbols, emojis, and other typical stray characters represented using Unicode. We used Unicode transformation type 8 encoding to convert the data. This data was converted and handled using re and string modules in python.
Text cleaning
Text cleaning is essential step to eliminate or at least reduce noise from Microtext. This step comprised of case transformation, removal of punctuations and URLs, elimination of additional white spaces, exclusion of hashtags, digits & special character removal and removal of metadata/non-linguistic features.
Tokenization
Tokenization is immensely essential phase of text processing. It is the process of generating tokens by splitting textual content into words, phrases, or other meaningful parts. It is generally a form of text segmentation [ 36 ]. Tokenization was performed using Keras tokenizer to prepare the text for implementing deep learning networks.
Filtering stop words
Stop words are non-semantic division of text in natural language. The necessity that stop words should be eliminated from text is that they make the text higher dimensional with redundant features which are less significant for analysts. Removing stop words reduces the dimensionality of term space [ 37 ]. Development of domain specific stop words in Roman Urdu language automatically using statistical techniques and bilingual experts’ input, comprising of 173 words is detailed in our previous work [ 30 ]. Insignificant Roman Urdu words were typically articles such as ek (ایک), conjunctions and pronouns such as tum (تم), tumhara (تمھارا), us (اُس), wo/who (وہ), usko (اُسکو), preposition such as main (میں), pe (پے), par (پر), demonstratives such as ye (یے), inko (انکو), yahan(یہاں), and interrogatives such as kahan (کہاں), kab (کب), kisko (کس کو), kiski (کس کی) etc. Stop words were removed from Roman Urdu corpus, leaving behind the index terms which are important.
Mapping slangs and contractions
Existing libraries, APIs and toolkits in python language primarily support preprocessing functions for English and other mature languages. They can be partially used for Roman Urdu language. Moreover, most of the communication in Roman Urdu comprises of bully terms being used as slangs. High dimensional textual data also suppress significant features. Hence contraction mapping is mandatory for dimensionality reduction and to capture complex bullying patterns. Currently, Pycontractions Library [ 38 ] only supports English contraction mapping process. To address this problem, the study developed data slang mapping process. To map slangs to original terms and phrases in Roman Urdu language, we created Slang lexicon in Roman Urdu (SLRU) which also included Roman Urdu abuses and offensive terms used as a norm by Roman Urdu users. SLRU is in the form of a dictionary. It comprises of the key: value pairs, where key is the slang and value is its equivalent Roman Urdu phrase/term such as “AFIK”: “Jahan tak mujhay pata hai”, ASAP: “Jitna jaldi ho sakay”, “tbh”: “Sach main” and so on. The process of slang mapping is detailed in Fig. 2 .
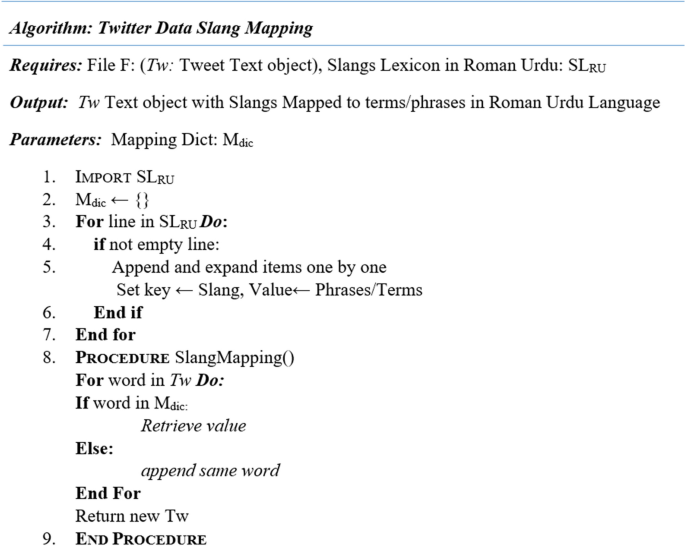
Mapping process for slangs in Roman Urdu
The results of mapping process are highlighted in Fig. 3 .

Mapping on Roman Urdu Data
Experimental setup
This section discusses implementation of proposed neural network architecture and all hyper-parameter settings. All the experiments were performed on 11 Gen, core i7, 4 cores, 8 logical microprocessors, with 2.8 GHz processor speed, 256 GB Solid State Drive and python version 3.8, 64 bits.
Proposed model implementation and hyper-parameter settings
All models were implemented and trained in Keras; a high-level neural network API that works with open-source machine learning framework called TensorFlow [ 39 ]. All the implementation was accomplished using PyCharm. The optimal parameters and results were achieved through repeated experimentations.
Data was split into training and testing datasets. The data split was 0.8 for training and 0.2 for testing i-e 80% of data instances were used for training and 20% were holdout for testing and validation purpose. The sets were made using shuffled array. This allows model to learn over different data instances. Moreover, it helps to uncover reliability of model and consistency of results over repeated executions. Random state is also generated using numpy.random [ 40 ] for random sampling during splitting of data to ensure reproducible splits.
Textual input data must be integer encoded. In RNN-LSTM architecture, a sequential model was created. Initially an Embedding layer was added to the network and textual Roman Urdu data was provided as an input. Embedding layer embedded high dimensional text data in low dimensional vector space for generating dense vector representation of data. Embedding was formed using 2000 features and 128 embed dimensions. The experiment was initially executed on 20 epochs and 50 batch size. The batch size was based on the fact that model was having single lstm layer, and comparatively took lower training and validation time per step. The execution time for each epoch was approximately 10 ms. SpatialDropout1D was used with rate of 0.3. It helped to regularize the activations and maintain effective learning rate of the model. For updating network weights iteratively, this work uses binary cross entropy loss function and Adam optimizer. Sigmoid activation function was also implemented. It is denoted by f(x) and is defined as:
The Spatial Dropout layer was implemented instead of a simple Dropout. The major reason being was to retain the context of textual data established by neighboring words. Dropping random words (except for stop words, which were already removed during preprocessing step) can highly affect the context of uttered sentences and ultimately the performance of model. We incorporated two hidden dense layers denoted by D 1 and D 2 . The output of each hidden layer was computed to get the final output for cyberbullying text detection.
Keras tokenizer was used to accomplish pre-tokenization of all the data required for the implementation of RNN-biLSTM model. We created a sequential model with Embedding layer having 2000 maximum features. Subsequently a biLSTM layer comprising of two LSTM layers, one to read sequence in forward direction and other in backward direction, each with 64 units was added. Hidden layer (H 1 ) was formed using sigmoid activation function. For down sampling the feature maps, Dropout layer was added with 0.2 dropout rate. Moreover, we used 128 batch size to utilize low to moderate computational resources while still not slowing down the training process. Batch size highlights number of samples processed by model before updating of internal parameters. To combat overfitting, we added second dropout layer with rate of 0.25. Adam optimization was used with learning rate of 0.01 since batch size was not too small. For this model, we used binary cross entropy loss function. As the Epochs increase, the generalization ability of the model improves. However, too many epochs also lead to the problem of overfitting. The model was executed over different number of Epochs and average execution time for each epoch was 13 to 15 ms. The performance of model stabilized over 20 Epochs, above which the improvement was almost negligible.
In CNN model, initially the sentence was transformed into matrix where each row of matrix represented word vectors representation of data. We used 1000 features and 32 dimensions. Two convolutional filters were applied with 8 and 16 filters and 3 kernel size. Each filter was used to perform one dimensional convolution on word embeddings. Both Layers were 1D in nature. We set two dropout layers with dropout rate of 0.25 to improve generalization ability of developed model. Hidden layers with Relu and sigmoid activation functions were used. To extract most salient and prominent features, global maximum pooling layers were used with pool size = 2. Flatten layer was created after convolutional layers to flatten the output of the previous layer to a single long feature vector. The experiment was simulated over different Epochs. However, results got stable at 30 epochs.
Results and discussion
Empirical evaluation of cyberbullying detection scheme performance in Roman Urdu and experimental setups is accomplished via accuracy, precision, recall, and f1 measure metrics.
All the implemented models were executed several times over number of epochs to get consistency in evaluation parameters until it was a minor difference of ± 0.1. The results for LSTM are depicted in Fig. 4 . To ensure results validity and reliability, for a comparatively less skewed dataset, F1 measure (i-e a harmonic mean of precision and recall) is used as an evaluation metric. Furthermore, we have also reported macro and weighted average scores across all the classes. The evaluation results of RNN-LSTM are given in Fig. 4 .
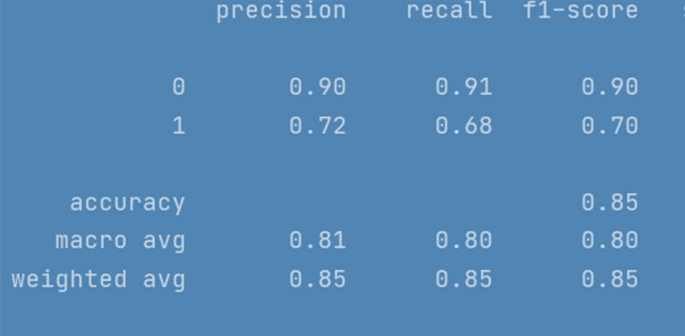
RNN-LSTM evaluation Results
F1 score for RNN-LSTM over cyberbullying class was only 70%, however for non-cyberbullying class, score was 90%. We observed that nearly all the instances of majority class of non-cyber bullying are correctly classified by this model. The experimental simulation depicting model accuracy and validation accuracy during training and validation phases, before and after stabilization of evaluation parameters is represented in Figs. 5 and 6 respectively.
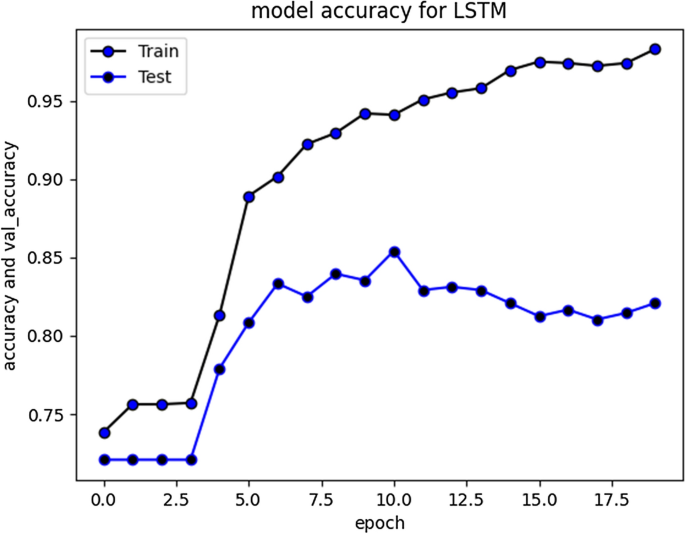
RNN-LSTM Model accuracy graph for 20 epochs
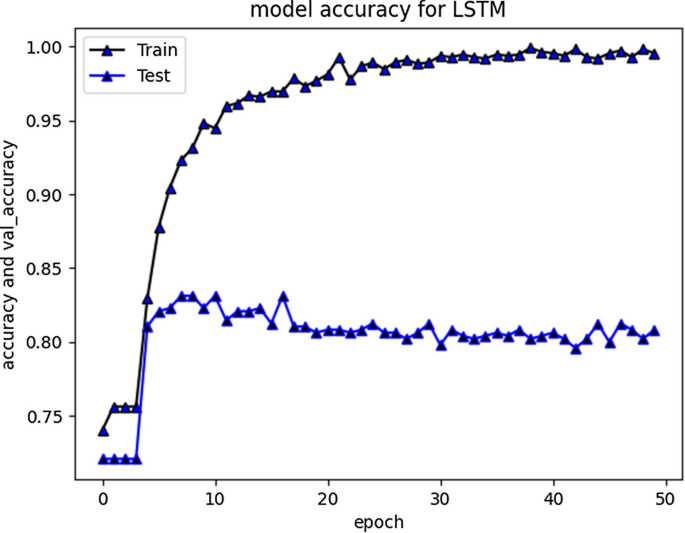
RNN-LSTM Model accuracy graph for 50 epochs
The accuracy improved over subsequent epochs. However, after 20 epochs it got stabilized. The average accuracy produced by this model was 93.5% during training and 85.5% during validation. Overall curve variation is indicating that no overfitting problem arise. The model loss during training and validation loss during testing over 20 and 50 epochs is shown in Figs. 7 and 8 respectively. The cross-entropy loss considered during configuration over different epochs converged well, thus indicating optimal model performance.
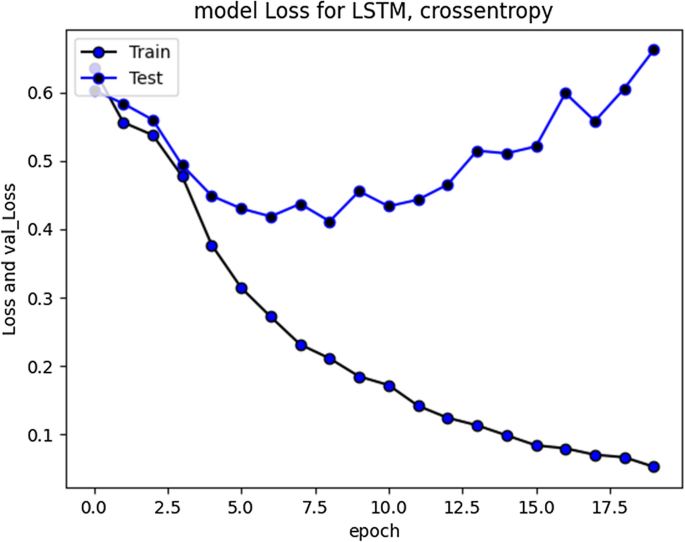
RNN-LSTM Model loss plot- Binary Cross entropy for 20 epochs
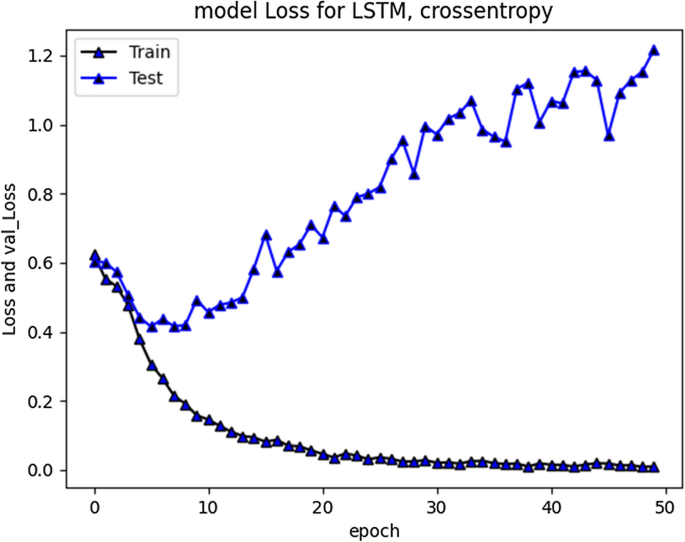
RNN-LSTM Model loss plot- Binary Cross entropy for 50 epochs
The evaluation results of RNN-BILSTM model over 20 epochs are given in Fig. 9 .
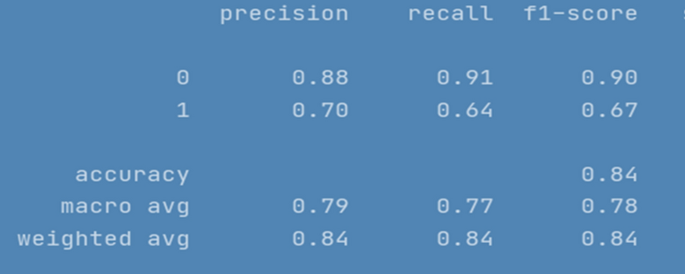
RNN-BiLSTM evaluation Results
RNN-LSTM also performed reasonably well for cyberbullying detection task on Roman Urdu data. F1 score for non-cyberbullying content prediction was 90% whereas for cyberbullying content, the score was 67% only. This indicates that model erroneously classified/misclassified some of the aggressive class instances and TN rate was at average. Figs. 10 and 11 are depicting model accuracy and validation accuracy for RNN-BiLSTM.
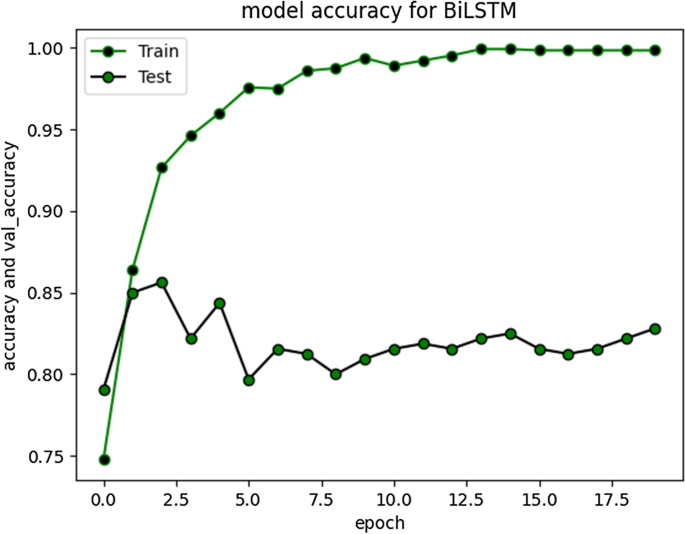
RNN-biLSTM Model accuracy plot for 20 epochs
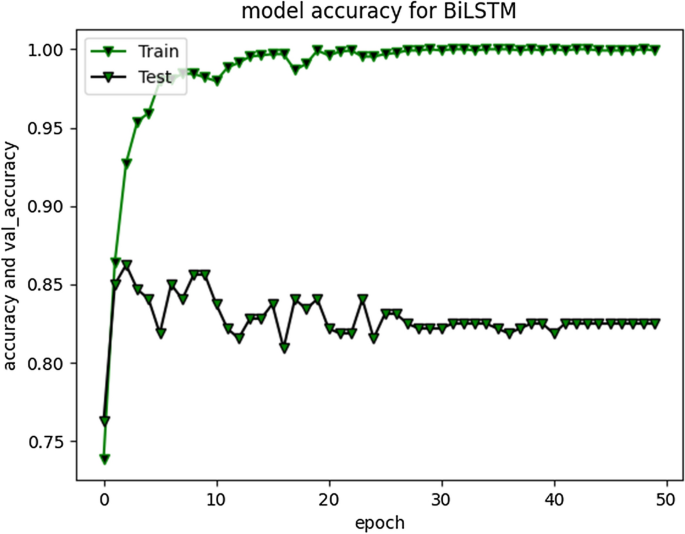
RNN-biLSTM Model accuracy plot for 50 epochs
The accuracy improved highly during training process up to 20 epochs. Overall average accuracy was 97% in training and 85% on validation set. 20% of the data was used for as a validation set, as stated earlier. During experimentation, we identified that accuracy of our model is not improving after a specific point i-e after 20 Epochs. The trivial variations can be clearly visualized from the graph in Fig. 9 . Model loss and validation loss during training and testing process for RNN-BiLSTM over 20 and 50 epochs is given in Figs. 12 and 13 respectively.
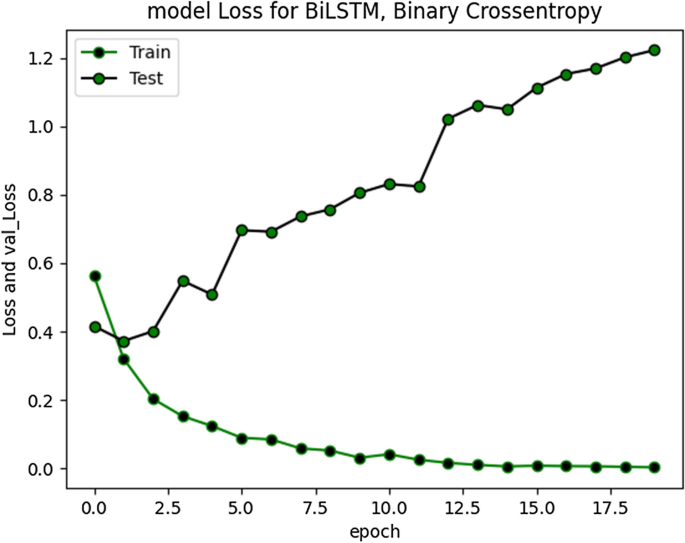
RNN-BiLSTM Model loss plot- Binary Cross entropy for 20 epochs
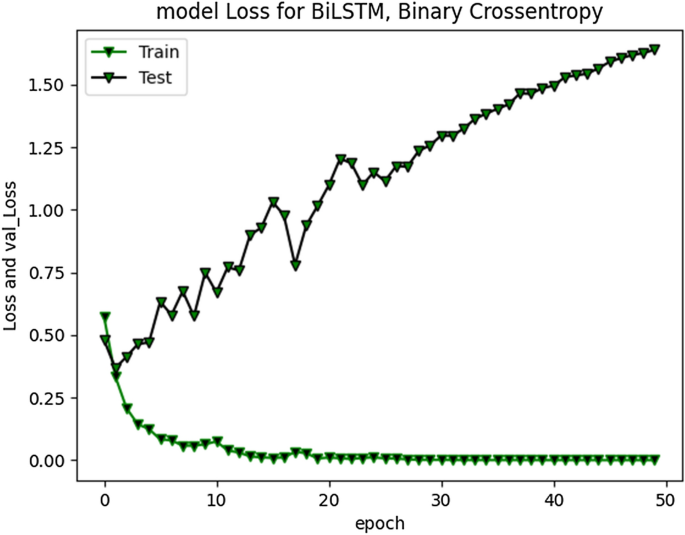
RNN-BiLSTM Model loss plot- Binary Cross entropy for 50 epochs
The cross-entropy loss was minimal (approximately 1.2), indicating good prediction capability of developed model.
Figure 14 represents the evaluation results for CNN model.
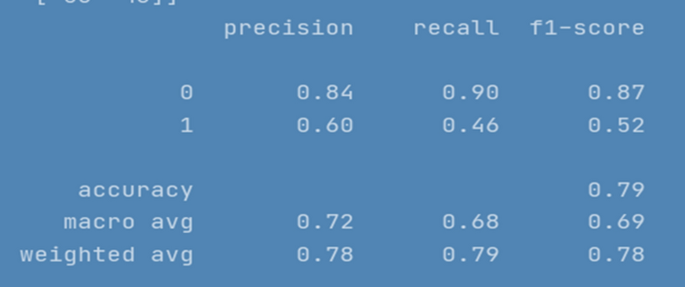
CNN model evaluation Results
CNN performed well for prediction of non-cyberbullying content, providing F1 score of 87%. However, model did not yield good efficiency for categorizing cyberbullying class, producing f1-score of 52%. The repeated experiments performed for CNN showed continuous improvements up to 30 Epochs. Figure 15 depicts model accuracy and validation accuracy. The experimental simulation over 50 epochs only shown minor improvements as represented in Fig. 16 . The average execution time for Epoch was 9 ms each. The training accuracy of 98% was achieved over different executions whereas model produced 85% validation accuracy.
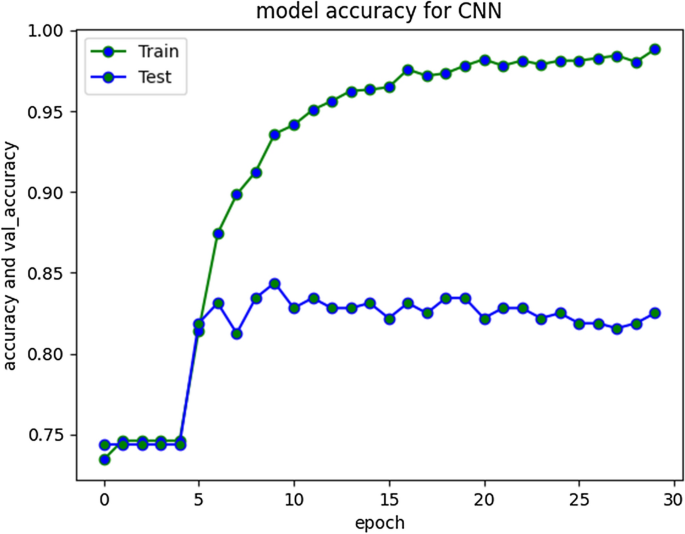
CNN Model accuracy plot for 30 epochs
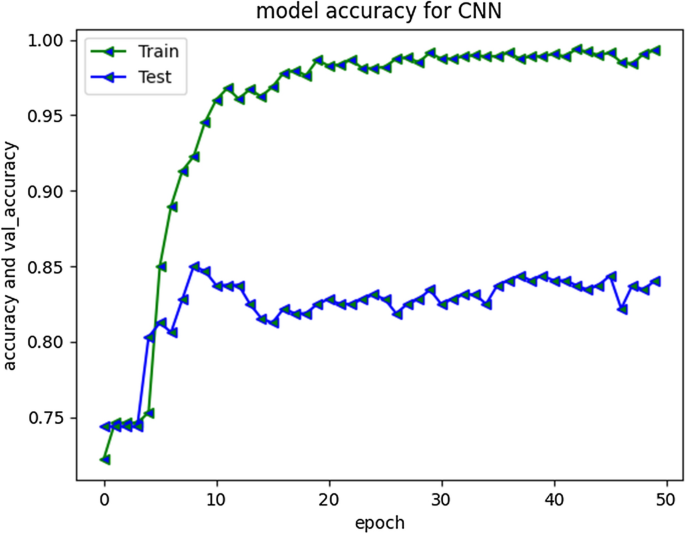
CNN Model accuracy plot for 50 epochs
CNN model loss and validation loss results at 30 and 50 epochs are presented in Fig. 17 and 18 respectively. The loss was minimal during training and converged. During validation the loss increased and diverged indicating only moderate performance over unseen instances typically from aggressive class.
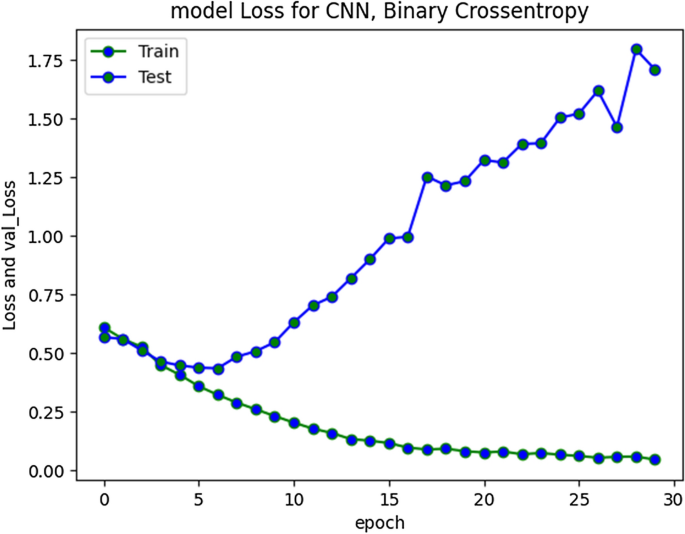
CNN Model loss plot- Binary Cross entropy for 30 epochs
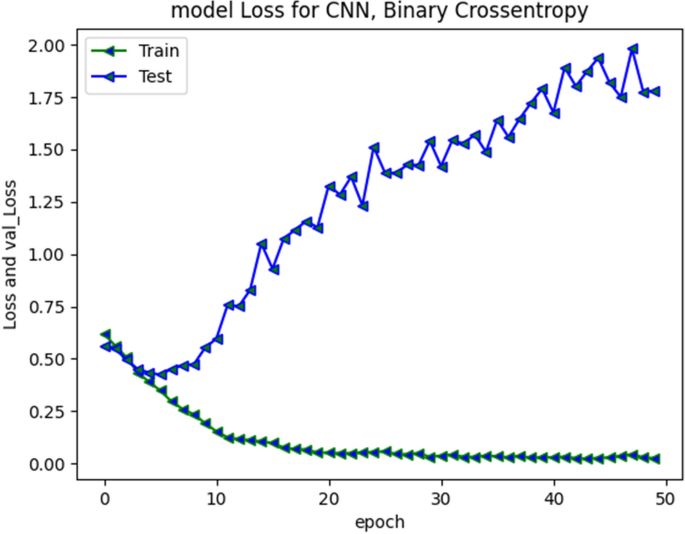
CNN Model loss plot- Binary Cross entropy for 50 epochs
The compiled model results indicating evaluation measures at stabilized epochs are depicted in Table 2 .
Cyberbullying has become an alarming social threat for today’s youth and has recently gained huge attention from research community. This research has addressed the problem of cyberbullying detection in Roman Urdu Language. Since Roman Urdu is highly resource deficient language, having different writing patterns, word structures, and irregularities thus making this work a challenging task. In this work we have presented advanced preprocessing techniques mainly a slang mapping mechanism, domain specific stop word removal, handling encoded formats and formulation of deep learning architecture to detect cyberbullying patterns in Roman Urdu language. We created experiments with vast parameters to build optimal classifier for cyberbullying tweets. The results highlighted that RNN-LSTM and RNN-BiLSTM with concatenation of forward and backward units provided better performance in 20 Epochs as compared to CNN. The existing work can be extended in numerous ways. The future studies can focus on development of ensemble models to uncover harassing and hate speech patterns. Moreover, the incorporation of context-specific features and handling of morphological variations might produce better results.
Availability of data and materials
The used raw dataset in this research is not publicly available. The data that support the findings of this research work are available from the corresponding author, on valid request due to privacy and ethical restrictions.
Abbreviations
Recurrent neural network
Long-short term memory
Bidirectional long-short term memory
Convolutional neural network
Social networking
Right to left
Left to right
True negative
Hellfeldt K, López-Romero L, Andershed H. Cyberbullying and psychological well-being in young adolescence: the potential protective mediation effects of social support from family, friends, and teachers. Int J Environ Res Public Health. 2020;17(1):45.
Article Google Scholar
Dadvar M. Experts and machines united against cyberbullying [PhD thesis]. University of Twente. 2014.
Magsi H, Agha N, Magsi I. Understanding cyber bullying in Pakistani context: causes and effects on young female university students in Sindh province. New Horiz. 2017;11(1):103.
Google Scholar
Qureshi SF, Abbasi M, Shahzad M. Cyber harassment and women of Pakistan: analysis of female victimization. J Bus Soc Rev Emerg Econ. 2020;6(2):503–10.
S. Irfan Ahmed, Cyber bullying doubles during pandemic. https://www.thenews.com.pk/tns/detail/671918-cyber-bullying-doubles-during-pandemic . Accessed 24 Aug 2020.
Shahroz M, Mushtaq MF, Mehmood A, Ullah S, Choi GS. RUTUT: roman Urdu to Urdu translator based on character substitution rules and unicode mapping. IEEE Access. 2020;8:189823–41.
Mehmood F, Ghani MU, Ibrahim MA, Shahzadi R, Mahmood W, Asim MN. A precisely xtreme-multi channel hybrid approach for roman urdu sentiment analysis. IEEE Access. 2020;8:192740–59.
Alotaibi M, Alotaibi B, Razaque A. A multichannel deep learning framework for cyberbullying detection on social media. Electronics. 2021;10(21):2664.
Dinakar K, Reichart R, Lieberman H. Modeling the detection of textual cyberbullying. In: 5th international AAAI conference on weblogs and social media. 2011.
Iwendi C, Srivastava G, Khan S, Maddikunta PKR. Cyberbullying detection solutions based on deep learning architectures. Multimed Syst. 2020. https://doi.org/10.1007/s00530-020-00701-5 .
Salminen J, Hopf M, Chowdhury SA, Jung S, Almerekhi H, Jansen BJ. Developing an online hate classifier for multiple social media platforms. Hum Cent Comput Inf Sci. 2020;10(1):1–34.
Dessì D, Recupero DR, Sack H. An assessment of deep learning models and word embeddings for toxicity detection within online textual comments. Electronics. 2021;10(7):779.
S. A. Özel, E. Saraç, S. Akdemir, and H. Aksu, Detection of cyberbullying on social media messages in Turkish, In: 2017 International Conference on Computer Science and Engineering (UBMK), 2017, pp. 366–370.
E. C. Ates, E. Bostanci, and M. S. Guzel, Comparative Performance of Machine Learning Algorithms in Cyberbullying Detection: Using Turkish Language Preprocessing Techniques, arXiv Prepr. arXiv2101.12718, 2021.
Van Hee C, et al. Automatic detection of cyberbullying in social media text. PLoS ONE. 2018;13(10):e0203794.
Haidar B, Chamoun M, Serhrouchni A. A multilingual system for cyberbullying detection: Arabic content detection using machine learning. Adv Sci Technol Eng Syst J. 2017;2(6):275–84.
Gómez-Adorno H, Bel-Enguix G, Sierra G, Sánchez O, Quezada D. A machine learning approach for detecting aggressive tweets in Spanish, In: IberEval@ SEPLN. 2018. pp. 102–107.
X. Bai, F. Merenda, C. Zaghi, T. Caselli, and M. Nissim, RuG at GermEval: Detecting Offensive Speech in German Social Media, in 14th Conference on Natural Language Processing KONVENS 2018, 2018, p. 63.
B. Birkeneder, J. Mitrovic, J. Niemeier, L. Teubert, and S. Handschuh, upInf—Offensive Language Detection in German Tweets, In: Proceedings of the GermEval 2018 Workshop, 2018, pp. 71–78.
J. M. Schneider, R. Roller, P. Bourgonje, S. Hegele, and G. Rehm, Towards the Automatic Classification of Offensive Language and Related Phenomena in German Tweets, In: 14th Conference on Natural Language Processing KONVENS 2018, 2018, p. 95.
H. Margono, X. Yi, and G. K. Raikundalia, Mining Indonesian cyber bullying patterns in social networks, In: Proceedings of the Thirty-Seventh Australasian Computer Science Conference-Volume 147, 2014, pp. 115–124.
H. Nurrahmi and D. Nurjanah, Indonesian Twitter Cyberbullying Detection using Text Classification and User Credibility, In: 2018 International Conference on Information and Communications Technology (ICOIACT), 2018, pp. 543–548.
A. Bohra, D. Vijay, V. Singh, S. S. Akhtar, and M. Shrivastava, A Dataset of Hindi-English Code-Mixed Social Media Text for Hate Speech Detection, In: Proceedings of the Second Workshop on Computational Modeling of People’s Opinions, Personality, and Emotions in Social Media, 2018, pp. 36–41.
A. Roy, P. Kapil, K. Basak, and A. Ekbal, An ensemble approach for aggression identification in english and hindi text, In: Proceedings of the First Workshop on Trolling, Aggression and Cyberbullying (TRAC-2018), 2018, pp. 66–73.
Association for Computational Linguistics. https://www.aclweb.org/portal/content/deadline-extension-first-task-automatic-cyberbullying-detection-polish-language . Accessed 09 May 2019.
Ghosh R, Nowal S, Manju G. Social media cyberbullying detection using machine learning in bengali language. Int J Eng Res Technol. 2021. https://doi.org/10.1109/ICECE.2018.8636797 .
Talpur KR, Yuhaniz SS, Sjarif NNBA, Ali B. Cyberbullying detection in Roman Urdu language using lexicon based approach. J Crit Rev. 2020;7(16):834–48. https://doi.org/10.31838/jcr.07.16.109 .
J. Brownlee, Imbalanced Classification, December 23, 2019. https://machinelearningmastery.com . Accessed 10 May 2021.
Arif M. A systematic review of machine learning algorithms in cyberbullying detection: future directions and challenges. J Inf Secur Cybercrimes Res. 2021;4(1):1–26.
A. Dewani, M. Ali Memon, and S. Bhatti, Development of Computational Linguistic Resources for Automated Detection of Textual Cyberbullying Threats in Roman Urdu Language, 3C TIC. Cuad. Desarro. Apl. a las TIC, 101–121., p. 17, 2021.
Shahid F, Zameer A, Muneeb M. Predictions for COVID-19 with deep learning models of LSTM, GRU and Bi-LSTM. Chaos Solitons Fractals. 2020;140:110212.
M. Cliche, “BB_twtr at SemEval-2017 task 4: Twitter sentiment analysis with CNNs and LSTMs,” arXiv Prepr. arXiv1704.06125, 2017.
W. Zaremba, I. Sutskever, and O. Vinyals, Recurrent neural network regularization, arXiv Prepr. arXiv1409.2329, 2014.
Xu G, Meng Y, Qiu X, Yu Z, Wu X. Sentiment analysis of comment texts based on BiLSTM. Ieee Access. 2019;7:51522–32.
S. Minaee, E. Azimi, and A. Abdolrashidi, Deep-sentiment: Sentiment analysis using ensemble of cnn and bi-lstm models, arXiv Prepr. arXiv1904.04206, 2019.
Uysal AK, Gunal S. The impact of preprocessing on text classification. Inf Process Manag. 2014;50(1):104–12.
Vijayarani S, Ilamathi MJ, Nithya M. Preprocessing techniques for text mining-an overview. Int J Comput Sci Commun Networks. 2015;5(1):7–16.
Pycontractions 2.0.1. https://pypi.org/project/pycontractions/ . Accessed 17 Nov 2021.
API Documentation. https://www.tensorflow.org/api_docs . Accessed 21 Oct 2020.
NumPy. https://numpy.org/ . Accessed 18 Nov 2021.
Download references
Acknowledgements
We would like to thank Institute of Information and Communication Technology, Mehran University of Engineering & Technology, for providing resources and funding, necessary to accomplish this research work.
This research has been performed at Institute of Information and Communication Technology, Mehran University of Engineering and Technology, Pakistan and is fully funded under MUET funds for postgraduate students.
Author information
Authors and affiliations.
Institute of Information and Communication Technologies, Department of Software Engineering, Mehran University of Engineering & Technology, Jamshoro, Sindh, Pakistan
Amirita Dewani, Mohsin Ali Memon & Sania Bhatti
You can also search for this author in PubMed Google Scholar
Contributions
Corresponding author is the main contributor of this work from conception, drafting, algorithms development and implementation to analysis of results. Other authors are research supervisors who provided valuable guidance for work and content improvement. All authors read and approved the final manuscript.
Corresponding author
Correspondence to Amirita Dewani .
Ethics declarations
Ethics approval and consent to participate.
Not applicable.
Consent for publication
Competing interests.
The authors declare that they have no competing or conflicts of interest to report regarding the present study.
Additional information
Publisher's note.
Springer Nature remains neutral with regard to jurisdictional claims in published maps and institutional affiliations.
Rights and permissions
Open Access This article is licensed under a Creative Commons Attribution 4.0 International License, which permits use, sharing, adaptation, distribution and reproduction in any medium or format, as long as you give appropriate credit to the original author(s) and the source, provide a link to the Creative Commons licence, and indicate if changes were made. The images or other third party material in this article are included in the article's Creative Commons licence, unless indicated otherwise in a credit line to the material. If material is not included in the article's Creative Commons licence and your intended use is not permitted by statutory regulation or exceeds the permitted use, you will need to obtain permission directly from the copyright holder. To view a copy of this licence, visit http://creativecommons.org/licenses/by/4.0/ .
Reprints and permissions
About this article
Cite this article.
Dewani, A., Memon, M.A. & Bhatti, S. Cyberbullying detection: advanced preprocessing techniques & deep learning architecture for Roman Urdu data. J Big Data 8 , 160 (2021). https://doi.org/10.1186/s40537-021-00550-7
Download citation
Received : 03 September 2021
Accepted : 10 December 2021
Published : 22 December 2021
DOI : https://doi.org/10.1186/s40537-021-00550-7
Share this article
Anyone you share the following link with will be able to read this content:
Sorry, a shareable link is not currently available for this article.
Provided by the Springer Nature SharedIt content-sharing initiative
- Advanced preprocessing
- Deep learning
- Hate speech detection
- Cyberbullying
Advertisement
The effect of the cyberbullying awareness program on adolescents’ awareness of cyberbullying and their coping skills
- Published: 03 August 2022
- Volume 42 , pages 24208–24222, ( 2023 )
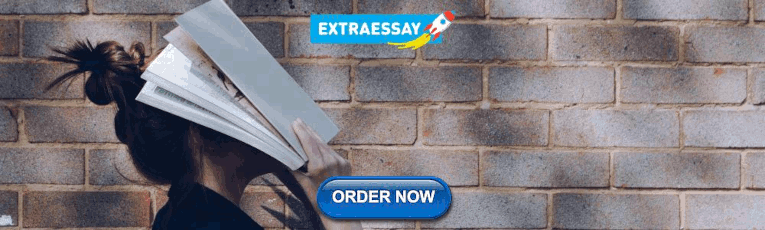
Cite this article
- Yeşim Yurdakul 1 &
- Aynur BÜTÜN AYHAN ORCID: orcid.org/0000-0003-3306-9672 2
5376 Accesses
2 Citations
Explore all metrics
Due to the prevalence of cyberbullying in adolescence and its association with a number of negative psychosocial consequences, there is a need to develop programs to prevent this phenomenon. In this study, the aim was to examine the effect of the Cyberbullying Awareness Program on adolescents’ awareness of cyberbullying and their coping skills. A total of 38 adolescents were included in the study, where 17 adolescents were assigned to the intervention group and 21 to the control group. The mean age of the adolescents was 13.8 (SD = 0.44). The Cyberbullying Awareness Program was administered to the intervention group in 10 sessions. The Cyberbullying Awareness Scale for Adolescents and Coping with Cyberbullying Scale were used as data collection tools in the study. As a result of the study, it was determined that the Cyberbullying Awareness Program was effective in increasing the awareness level of the adolescents in the intervention group about cyberbullying, as well the development of their skills to cope with cyberbullying. In line with the results of the study, suggestions are presented to educators and policy makers. It is recommended that policy makers include cyberbullying prevention programs in their national curriculums in order to increase the awareness of adolescents about cyberbullying and improve their coping skills, and these programs should be implemented by educators to children and adolescents nationwide.
Similar content being viewed by others
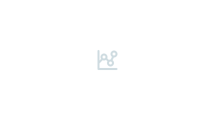
The Effects of Psychological Resilience, Self-efficacy and Metacognition on Cyberbullying Among Adolescents
Merve Güçlü-Aydogan, Pınar Ünal-Aydın & Orkun Aydın
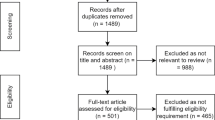
Cyberbullying Awareness Intervention in Digital and Non-digital Environment for Youth: Current Knowledge
Willone Lim, Bee Theng Lau & Fakir M Amirul Islam
Cyberbullying and Bullying: Impact on Psychological Symptoms and Well-Being
Marina Carvalho, Cátia Branquinho & Margarida Gaspar de Matos
Avoid common mistakes on your manuscript.
Introduction
Combined with the rapid development and widespread use of information and communication technologies, the fact that today’s adolescents belong to a generation that is growing up with technology is a situation that presents both advantages and disadvantages for educators and parents. Although Information and Communication Technologies (ICT) provide adolescents with advantages such as access to information and improved interpersonal relations, inappropriate use of ICT presents many dangers. In particular, the social interaction opportunities offered by the Internet and mobile phones allow bullying behaviors to go beyond the physical boundaries of the school environment and facilitate bullying in the virtual environment (Cross et al., 2016 ). Cyberbullying is defined as the aggressive and deliberate actions performed by a group or individual repeatedly over time, using electronic communication forms, towards a victim who is unable to defend him/herself (Smith et al., 2008 ).
Cyberbullying, which is one of the risks that arises as a result of the negative use of social media and the Internet, is accepted as an important public health problem as a result of its high prevalence (Ridell et al., 2018 ; Espelage et al., 2018 ). Studies conducted in various countries emphasize that cyberbullying is a common phenomenon during adolescence (Calvete et al., 2010 ; Schneider et al., 2012 ; Garaigordobil, 2015 ; Gimenez Gualdo et al., 2015 ; Pereira et al., 2016 ; Brochado et al., 2017 ). It is stated that the prevalence of cyberbullying among adolescents varies between 1% and 41%, whereas the prevalence of cyber-victimization ranges between 3% and 72% (Selkie et al., 2016 ). Similar results have been found in studies conducted in Turkey, and it is stated that cyberbullying is common in adolescence. Research results indicated that 25.5% of adolescents experience cyber victimization and 40.8% engage in cyberbullying themselves (Topçu & Erdur Baker, 2016 ; Beyazıt et al., 2019 ).
Although there is no physical interaction between the bully and the victim in cyber bullying acts, cyber victimization negatively affects the development and life of adolescents emotionally, psychologically, academically and socially. The psychological and emotional aspects of cyber victimization include depression, depressive symptoms and anxiety (Perren et al., 2010 ; Wang et al., 2011 ; Kowalski & Limber, 2013 ; Landoll et al., 2015 ), stress (Kubiszewksi et al., 2015 ), suicide and suicidal ideation (Hinduja & Patchin, 2010 ; Bonana & Hymel, 2013 ), low self-perception (Brewer & Kerslake, 2015 ; Urano et al., 2020 ), and substance abuse risk (Díaz & Fite, 2019 ). Depending on the psychological and emotional effects of cyber victimization, psychosomatic symptoms are observed in cyber victims (Li et al., 2019 ). Although cyberbullying occurs outside the school environment, unlike traditional bullying, it is stated that cyber victimization negatively affects perceived school climate, academic achievement and school engagement (Bayar & Uçanok, 2012 ; Wigderson & Lynch, 2013 ; Cross et al., 2015 ). Cyber victimization also negatively affects important social development processes such as socialization and peer relationships related to adolescence. It is stated that cyber victims experience problems such as avoiding making new friends and distrusting people (Crosslin & Crosslin, 2014 ). It has been determined that cyber victimization in adolescence is negatively related to perceived social support and social competence (Olenik-Shemesh & Heiman, 2016 ). Studies have shown that cyberbullying also has negative consequences for perpetrators. It is stated that engagement in cyberbullying perpetration is associated with high levels of depressive symptoms and depression (Wang et al., 2011 ; Camphell et al., 2012 ). Engaging in cyberbullying as a victim or perpetrator is a predictor of both depressive symptoms and suicidal ideation (Bonana & Hymel, 2013 ). Cyberbullying perpetration is associated with perceived difficulties, hyperactivity, conduct problems, low prosocial behavior, substance use, headache, and not feeling safe at school (Sourander et al., 2010 ). Cyberbullying perpetration is negatively associated with perceived social support and positively associated with proactive aggressiveness (Calvete et al., 2010 ).
It is claimed that the negative effects of cyber victimization are related to the coping strategy adopted by the victims. In other words, it is stated that the negative emotions experienced by the victims who adopt an ineffective coping strategy are more intense (Jacobs et al., 2014 ; Elipe et al., 2017 ; Beyazıt et al., 2021 ). For example, seeking social support from family and peers, one of the strategies used to cope with cyberbullying, reduces depressive symptoms, while confronting the bully increases the likelihood that victims will experience depression (Machmutow et al., 2012 ). It is stated that when the majority of adolescents experience cyber victimization, they do nothing (Li, 2010 ) and ignore the bullying (Smith et al., 2008 ), even though they experience negative feelings. Avoidant focused strategies such as ignoring and the adolescent thinking that they cannot do anything increase the prevalence of cyberbullying and cause negative consequences that harm the mental health of adolescents (Hoff & Mitchell, 2009 ). The coping styles of adolescents with cyberbullying are suggested to be related to their emotion regulation skills. Adolescents who are cyber victims use ineffective coping strategies such as retaliation, ignoring, and accepting the situation when they cannot adaptively regulate the negative emotions they experience as a result of cyberbullying (Jacobs et al., 2014 ; Den Hamer & Konijn, 2016 ; Trompeter et al., 2018 ; Arato et al., 2022 ). In addition to emotion regulation skills, online security skills (i.e. managing online privacy, ensuring personal security), in other words digital literacy skills, are an important factor in the use of effective coping strategies with cyberbullying (Vandoninck et al., 2013 ). Equipping potential and actual victims of cyberbullying with effective coping strategies requires interventions based on both theory and evidence (Jacobs et al., 2014 ).
Considering the negative effects of cyberbullying in adolescence, it is important to examine the motivations of adolescents to exhibit cyberbullying in order to prevent cyberbullying. It is stated that cyberbullying is performed for reasons such as entertainment, jokes, revenge, jealousy, retaliation, moral disengagement, pro-bullying attitudes, to attain high levels of peer status and to display their technological skills, and that some adolescents do not characterize cyberbullying as bullying (Smith et al., 2008 ; Topçu et al., 2008 ; Vandebosch & Van Cleemput, 2008 ; Wright, 2017 ; Yurdakul & Bütün Ayhan, 2021 ). Aggressive behavior can sometimes occur as a result of the motivation to attain a higher social status within the peer group.The non-anonymous display of cyberbullying in a particular social group may result from the desire to attain high levels of social status (Wright, 2017 ). However, the relationship between traditional bullying and cyberbullying suggests that some people may engage in cyberbullying as a way of retaliating for traditional bullying victimization (Hemphill et al., 2012 ; Kowalski et al., 2014 ). Individuals who are unable to retaliate in face-to-face situations due to the lack of physical strength, shyness or low self-esteem may feel more comfortable online (Law et al., 2012 ). The reason why adolescents claim that the reason that cyberbullying is only perpetrated for entertainment and joking could also be due to moral disengagement. Moral disengagement is used to decrease the seriousness of the consequences of the bullying behavior and to provide justification for it (e.g., by saying it was only a joke) (Bandura, 1999 ; Hymel & Bonanno, 2014 ). According to Pornari & Wood ( 2010 ), moral disengagement is a predictor of cyberbullying perpetration. Perpetrators may engage in moral disengagement such that their aggressive actions are more well-intentioned, the consequences are less harmful, or the bullying is caused by the victim’s deserving behavior (Bauman, 2010 ; Lazuras et al., 2013 ; Kowalski et al., 2014 ). Perpetrators feel less guilt or shame as a result of ignoring or distorting the consequences of their harmful behavior (Pornari & Wood, 2010 ). Due to the lack of face-to-face communication in the virtual environment, cyberbullies or bystanders who witness cyberbullying cannot directly observe the negative effects of cyberbullying acts on the victim. This situation causes cyberbullies or bystanders to have a lack of empathy with the victim and to be less aware of the consequences of cyberbullying compared to traditional bullying (Solanje & Smith, 2008 ; Steffgen et al., 2011 ). Antisocial behaviors such as cyberbullying are considered to be the result of an individual’s lack of awareness of the negative consequences of their own behavior. This lack of empathy and awareness of the consequences of one’s own actions can be exacerbated in the cyber world, where there is often no direct contact with the victim (Baldry et al., 2018 ). It is stated that interacting anonymously on the Internet leads to low self-awareness in individuals and may cause them to react impulsively and aggressively towards other people in the online environment (Arıcak et al., 2008 ). Adolescents’ awareness of the consequences of their cyberbullying behaviors is thought to be an important factor in shaping their anti-bullying attitudes. According to Theory of Planned Behavior (Ajzen, 1991 ), the strongest predictor of the intention to engage in cyberbullying is the attitudes towards cyberbullying (Heirman & Walrave, 2012 ). It is emphasized that attitudes are influenced by the knowledge and beliefs that individuals have about a behavior. Positive attitudes towards a behavior increase the tendency to exhibit the behavior. Accordingly, adolescents’ positive attitudes towards cyberbullying, such as jokes and entertainment, increase their cyberbullying tendency (Ajzen, 1991 ; Heirman & Walrave, 2012 ; Barlett et al., 2014 ). In addition to reducing their cyberbullying tendencies, anti-bullying behaviors in adolescents are effective in terms of supporting a victim when they witness cyberbullying and thus ending the bullying by reducing the rewards of the bullies (Williford et al., 2013 ). In this respect, it is important to focus on transforming the positive attitudes of adolescents towards cyberbullying such as jokes and entertainment into anti-bullying attitudes aimed at preventing cyberbullying in adolescence. It is thought that the reason why adolescents state that cyberbullying is done for reasons such as entertainment and jokes and that they do not describe these behaviors as bullying (Vandebosch & Van Cleemput, 2008 ) is due to their low level of awareness about the negative consequences of cyberbullying.
Increasing the awareness of adolescents about cyberbullying is seen as a priority in studies conducted on methods of preventing and intervening in cyberbullying, which negatively affects adolescents (Ayas et al., 2015 ). In addition, the fact that adolescents are knowledgeable and aware of the possible risks of cyberbullying that they may encounter in the online environment is decisive in terms of taking preventive measures to protect and cope with cyberbullying (Altundağ & Ayas, 2020 ). Considering the prevalence and negative effects of cyberbullying in adolescence, studies are needed to prevent cyberbullying and to develop effective coping skills that enable adolescents to be less affected by the negative consequences of cyberbullying. It is seen that programs to prevent cyberbullying are implemented in adolescence in various countries around the world (e.g., KiVA, Media Heroes Program). The results of programs that focus on supporting adolescents’ ability to empathize with victims, develop anti-bullying attitudes, and increase their level of knowledge about Internet risks and safety are promising. Prevention programs also assist by providing strategies that help victims protect themselves against victimization (Williford et al., 2013 ; Wölfer et al., 2014 ; Cross et al., 2016 ). It has been determined that the KiVA program, which focuses on supporting the skills of empathizing with victims and increasing the bystanders’ punishing attitudes of the cyberbullies, is effective in preventing cyberbullying. The KiVa Program focuses on increasing the empathy, self-efficacy, and antibullying attitudes of bystanders, who are neither perpetrators nor victims. The aim of the program is to enable bystanders to show that they are opposed to bullying and to make them support victims rather than encourage bullies (Williford et al., 2013 ). In another study, it was found that the Media Heroes Program was effective in preventing cyberbullying by supporting adolescents’ ability to empathize with victims and increasing their awareness of Internet safety and risks (Wölfer et al., 2014 ). The theoretical basis of the Media Heroes Program, which was developed to prevent cyberbullying in adolescence, is based on the Theory of Planned Behavior. The program aims to change attitudes and beliefs towards cyberbullying by providing information on the definition of cyberbullying acts, online security options, legal rights and the impact of cyberbullying on the victim. In addition, the program aims to promote empathy with the victim and perspective-taking skills (Wölfer et al., 2014 ; Schultze-Krumbholz et al., 2016 ).
Developing a cyberbullying prevention program in accordance with the society’s cultural characteristics and education system is seen as an important factor in the effectiveness of the program. It is noteworthy that in Turkey, cyberbullying prevention and intervention programs are mostly carried out in the form of group therapy targeting adolescents who are cyberbullies or cyber victims, and they are applied to adolescents studying at high school (Tanrıkulu, 2013 ; Özbay, 2017 ; Akyüz & Koç, 2020 ). The fact that cyberbullying programs in Turkey target cyberbullies and cyber victims suggests that they are applied to intervene after the cyberbullying behavior has been exhibited.Considering the effects of social norms and bystanders on cyberbullying behaviors, there is a need for preventive programs that target bystanders as well as victims and bullies, while the aim should be to prevent cyberbullying before it occurs.In addition, most of the cyberbullying prevention and intervention programs in Turkey are targeted at high school students. Considering the research results (Ayas & Horzum, 2012 ; Camphell et al., 2012 ) showing that the prevalence of cyberbullying peaks in adolescents in the 13–14 age group, it is thought that this age group is particularly at risk in terms of the negative effects of cyberbullying. In this respect, it can be said that the high school period is potentially too late to prevent and minimize the negative consequences of cyberbullying, and younger age groups should be targeted for early intervention. Some programs developed to prevent cyberbullying in Turkey include other topics (e.g., abuse, peer pressure, respect, honesty, tolerance) besides cyberbullying (Peker, 2013 ; Nedim-Bal & Kahraman, 2015 ). It is thought that the focus of these programs moves away from cyberbullying and remains superficial in terms of the prevention of such behavior. In this study, the Cyberbullying Awareness Program was developed in order to increase the awareness of adolescents about cyberbullying and to improve their coping skills. The Cyberbullying Awareness Program differs from other programs in that it targets secondary school students, its content is specific to cyberbullying, and it focuses on the anti-bullying attitudes of bystanders as well as perpetrators and victims. In addition, the program has a preventive nature as it aims to prevent cyberbullying before it occurs.
The present study includes the theoretical background, structure, content and evaluation of the Cyberbullying Awareness Program.
Cyberbullying awareness program
The Cyberbullying Awareness Program targets secondary school students. The program is a modularized, school-based and theoretically-based cyberbullying prevention program.It is built on certain theoretical foundations as well as research results on cyberbullying. The theoretical background of the program is based on the Theory of Planned Behavior (Ajzen, 1991 ) and Social Cognitive Theory (Bandura, 1989 ). The reason why the program builds on these theoretical approaches is that these models successfully explain aggressive behavior and cyberbullying (Heirman & Welrave, 2012 ; Wölfer et al., 2014 ; Williford et al., 2013 ).
According to Theory of Planned Behavior (TPB), an individual’s knowledge and beliefs about a behavior shape her/his attitudes towards that behavior. Attitudes towards behavior determine the tendency to exhibit the behavior. The strongest predictor of cyberbullying intention was stated as positive attitudes towards cyberbullying.Other components of TPB are subjective norms and perceived behavioral control. Subjective norms refer to the social pressure that an individual perceives to perform the behavior. When the individual thinks that significant others will react negatively to the cyberbullying conduct, this will reduce their motivation to engage in such behavior. Perceived behavioral control refers to the fact that adolescents who perceive cyberbullying as easy to perform are more inclined to exhibit these behaviors (Ajzen, 1991 ; Heirman & Walrave, 2012 ). In this context, increasing the awareness of adolescents about the negative consequences of cyberbullying is considered to be an important factor in the formation of negative attitudes towards cyberbullying and thus reducing their cyberbullying tendencies.According to Social Cognitive Theory, it is stated that bullying behaviors are motivated by the desire to seek social reputation and status in peer groups. Bystanders who witness bullying can contribute to the perpetuation of bullying by providing bullies with the social reputation they seek and reinforcing their actions. Bystander support for victims can reduce bullies’ motivation to commit bullying acts by reducing the rewards they receive for their status seeking and social reputation. It is stated that the way that bystanders react to bullying is effective in maintaining or ending the bullying (Williford et al., 2013 ). It is stated that when a bystander is aware of the harmful consequences of bullying for the victim, they intervene in the bullying (Shultz et al., 2014 ).
Based on these theoretical models, the Cyberbullying Awareness Program focuses on the attitudes and knowledge levels of adolescents towards cyberbullying. There are two reasons for focusing on adolescents’ attitudes towards cyberbullying in order to prevent cyberbullying. First, adolescents’ anti-cyberbullying attitudes can reduce their motivations and tendencies towards cyberbullying. Secondly, the fact that adolescents have anti-cyberbullying attitudes may be effective in reducing their support for perpetrators and thus shaping the perpetrators’ subjective norms. The program aims to raise the awareness of adolescents about cyberbullying act, the negative effects of cyberbullying on the victim and legal rights. It is thought that increasing the knowledge and awareness levels of adolescents about cyberbullying will be effective in the development of their attitudes towards cyberbullying. In addition, the program includes the development of social skills such as empathy, social and virtual communication skills, as well as social responsibility. In this context, the Cyberbullying Awareness Program aims to prevent cyberbullying by focusing on adolescents’ awareness of the negative consequences of cyberbullying for victims, ability to empathize with the victim, and anti-bullying attitudes. It is thought that increasing the awareness level of adolescents about the harmful consequences of cyberbullying will be effective in terms of taking preventive measures to protect against cyberbullying in the Internet environment. In addition, the program aims to develop adolescents’ skills, including helping and coping strategies, when faced with cyberbullying behaviors directed towards themselves or others on the Internet.
After the literature review and the theoretical basis were completed, the content of the Cyberbullying Awareness Program was created. The content, learning methods, educational environment and activities of the Cyberbullying Awareness Program, which was prepared in accordance with a child-centered design, were prepared in a way that was designed to ensure the active participation of adolescents. The content of the program is based on an active learning and active participation approach. In parallel with this approach, adolescents took part in the program implementation process not as passive listeners, but as active participants who could shape their own learning.During the program, adolescents were asked to participate in group discussions, explain their views, and make connections between their experiences and the topics in the program. In this direction, active learning methods such as question-answer, discussion, game, case study, role playing, animation, mind map, brainstorming, story formation, station, and still image were used in the program.
Objectives and hypotheses of the study
The aim of the present study was to examine the effect of the Cyberbullying Awareness Program on adolescents’ awareness of cyberbullying and their coping skills.It has been hypothesized that the Cyberbullying Awareness Program will reduce the cyberbullying tendencies of adolescents, while also enabling them to improve their awareness about and protect themselves from cyberbullying (H1). It has also been hypothesized that the Cyberbullying Awareness Program will improve the skill and competence level of the intervention group in effective coping strategies from pre to post test (H2). It was also hypothesized that the effect of the program would continue when it was retested (follow-up test) three weeks after the completion of the program (H3).
Participants
The study participants comprised 38 adolescents studying in the eighth grade of secondary schools affiliated to the Provincial Directorate of National Education in the city center of Antalya/Turkey in the 2019–2020 academic year. In the study, before starting the implementation of the program, the “Cyberbullying Awareness Scale for Adolescents” and “Coping with Cyberbullying Scale” were applied to determine the extent of the participants’ awareness and coping skills regarding cyberbullying (Pre-test). In the study, a pre-test was administered to a total of 47 adolescents, where 22 adolescents were in the intervention group and 25 adolescents in the control group. After the pre-test application was completed, the Cyber Bullying Awareness Program was administered to the intervention group in 10 sessions, two days a week for five weeks. After the program implementation was completed, the post-test was administered to 17 adolescents in the intervention group and 21 adolescents in the control group. During the program and post-test application, five adolescents in the intervention group and four adolescents in the control group could not participate in the post-test application due to school changes and absenteeism. Thus, the study was completed with a total of 38 adolescents (17 in the intervention group and 21 in the control group). The follow-up test was administered to 17 adolescents in the intervention group three weeks after the post-test. In the study, 41.2% (n = 7) of the adolescents in the intervention group were girls, 58.8% (n = 10) were boys, 29.4% (n = 5) were 13 years old, and 70.6% of them (n = 12) were 14 years old (Mean age = 13.8 years, SD = 0.56). Of the adolescents in the control group, 47.6% (n = 10) were girls, 52.4% (n = 11) were boys, 9.5% (n = 2) were 13 years old, and 90.5% (n = 19) were 14 years old (Mean age = 13.9 years, SD = 0.30).
Individual information form
In the study, the Individual Information Form prepared by the researcher was used to determine the demographic characteristics of the adolescents and their families. The Individual Information Form consists of questions about age, gender and whether the adolescent has been involved in cyberbullying before (as a victim, perpetrator or bully/victim).
Cyberbullying awareness scale for adolescents
In the study, the Cyberbullying Awareness Scale for Adolescents developed by Yurdakul ( 2020 ) was used to determine the awareness levels of adolescents about cyberbullying. The Cyberbullying Awareness Scale for Adolescents consists of three sub-dimensions namely ‘‘Cyberbullying Tendency, Coping with Cyberbullying and Protection from Cyberbullying’’, with a total of 21 items. Total points are not taken from the scale and each sub-dimension is scored within itself. The items in the scale are scored as “I completely disagree (1), I do not agree (2), I am indecisive (3), I agree (4), I completely agree (5)”. The Cyberbullying Tendency sub-dimension consists of 11 items (e.g., “I enjoy making comments that make fun of my friends below photos shared on social media” ) and possible scores range between 11 and 55 points. Increased scores obtained from the Cyberbullying Tendency sub-dimension indicate that the adolescents have positive attitudes towards cyberbullying and that the cyberbullying tendency is high. The Coping with Cyberbullying sub-dimension consists of five items (e.g., “I have information about the institutions to whom I can complain if I am exposed to disturbing behavior on the Internet”) and possible scores vary between 5 and 25. An increase in the scores obtained in the Coping with Cyberbullying sub-dimension indicates that the level of knowledge and awareness of adolescents about coping with cyberbullying is high. The Protection from Cyberbullying sub-dimension consists of five items (e.g., “I consider that people can communicate by hiding their real identities on the Internet”) and the scores vary between 5 and 25. An increase in the Protection from Cyberbullying sub-dimension shows that adolescents have a high level of awareness regarding how they can protect themselves from cyberbullying. The Cronbach’s alpha coefficient of the Cyberbullying Tendency sub-dimension of the Cyberbullying Awareness Scale for Adolescents is 0.80, the Cronbach’s alpha coefficient of the Coping with Cyberbullying sub-dimension is 0.76, and the Cronbach’s alpha coefficient of the Protection from Cyberbullying sub-dimension is 0.63 (Yurdakul, 2020 ). In the present study, the Cronbach’s alpha coefficient of the Cyberbullying Tendency sub-dimension is 0.82, the Cronbach’s alpha coefficient of the Coping with Cyberbullying sub-dimension is 0.70, and the Cronbach’s alpha coefficient of the Protection from Cyberbullying sub-dimension is 0.71.
Coping with cyberbullying scale
The scale was originally developed by Koç et al. ( 2016 ) to determine the strategies used by adolescents in the 15–18 age group to cope with cyberbullying. The scale consists of 19 items in four dimensions: Increasing Cognitive Security and Confidentiality (7 items), Seeking Help (5 items), Avoidance and Ignoring (5 items), and Information Searching (2 items). The Cronbach’s alpha coefficient for the Increasing Cognitive Security and Confidentiality sub-dimension is 0.65, the Seeking Help sub-dimension Cronbach’s alpha coefficient is 0.76, the Avoidance and Ignoring sub-dimension Cronbach’s alpha coefficient is 0.73, and the Cronbach’s alpha coefficient of the Information Searching sub-dimension is 0.63. The Cronbach’s alpha coefficient for the overall scale in the original study is 0.75 (Koç et al., 2016 ).
The Coping with Cyberbullying scale was adapted for use with adolescents in the 12–14 age group. The secondary school form of the Coping with Cyberbullying Scale consists of three sub-dimensions and 17 items: ‘‘Seeking Help and Information, Increasing Cognitive Security and Confidentiality, and Avoiding and Ignoring’’. Items in this scale are graded as “I completely disagree (1), I do not agree (2), I am indecisive (3), I agree (4), I completely agree (5)”. Total points are not taken from the scale and each sub-dimension is scored within itself. The Seeking Help and Information sub-dimension consists of nine items (e.g., “I would like help from my friends”) . Scores from the Seeking Help and Information sub-dimension range from 9 to 45 points, and high scores from this dimension indicate that adolescents use coping strategies for seeking help and information when faced with cyberbullying. The Increasing Cognitive Security and Confidentiality sub-dimension consists of four items (e.g., ‘’I will remove you from my friend list’’) . Scores from the sub-dimension of Increasing Cognitive Security and Confidentiality vary between 4–20 points, and high scores from this dimension indicate that adolescents use technological solutions as coping strategies more frequently when faced with cyberbullying. The Avoidance and Ignoring sub-dimension consists of four items (e.g., “I delete incoming messages without reading them” ). Scores from the Avoidance and Ignoring sub-dimension range from 4–20 points, and high scores from this dimension indicate that adolescents prefer avoidant coping strategies more frequently when faced with cyberbullying. It has been determined that the Cronbach’s alpha coefficient of the Seeking Help and Information sub-dimension is .78, the Cronbach’s alpha coefficient of the Increasing Cognitive Security and Confidentiality sub-dimension is .56, and the Cronbach’s alpha coefficient of the Avoidance and Ignoring sub-dimension is .47 (Yurdakul, 2020 ). In the present study, the Cronbach’s alpha coefficient for the overall scale is .75.
In the study, a quasi-experimental design was used, including the measurements of the intervention and control groups and pre test-post test-follow-up test. In the research, the independent variable is the Cyberbullying Awareness Program, while the dependent variables are the adolescents’ awareness of cyberbullying and their ability to cope with cyberbullying.
Firstly, ethics committee approval (No. 56786525-050.04.04/ 67,272) and institutional permission were obtained from Ankara University and the Antalya Provincial Directorate of National Education, respectively. Permission was obtained from the scale authors to use the Coping with Cyberbullying Scale in the study. After obtaining the necessary permissions, the Cyberbullying Awareness Program was developed. In the program development process, a literature review was firstly conducted and cyberbullying prevention and intervention programs developed and implemented around the world for adolescents were examined in terms of content, method and application. Studies on cyberbullying and theories and models for cyberbullying were also examined. The theoretical background of the program is based on the Theory of Planned Behavior (Ajzen, 1991 ) and Social Cognitive Theory (Bandura, 1989 ). The prepared Cyberbullying Awareness Program was presented to six experts to obtain their opinions, which included five faculty members in the fields of child development, guidance and psychological counseling, measurement and evaluation as well as a school counsellor. The opinions and suggestions of the experts were evaluated, necessary arrangements were made and the final version of the program was created. The Cyberbullying Awareness Program consists of 14 group sessions, each of which lasts 40 min, and is planned to be implemented for 7 weeks, two sessions per week ( Appendix 1). After the program was developed, interventionand control groups were formed.
While forming the participant groups, a list of secondary schools in the central districts was obtained from the Provincial Directorate of National Education. Data on the socioeconomic level of schools were obtained from the Turkish Statistical Institute (TSI). According to the opinions of the Provincial Directorate of National Education and TSI data, secondary schools in the middle socioeconomic level were determined. By examining the websites of the schools determined to be in the middle socioeconomic level, schools with an average class size of 20–25 students were identified. Two schools were randomly chosen from the selected schools. The reason for including two different schools in the study was so that classes that would form the intervention and control groups would not be affected by each other during the program implementation process. For this reason, the classes that made up the intervention and control groups were selected from different schools. The reason why the maximum number of students in the classes that would form intervention and control groups was fixed at 25 was to ensure the active participation of all participants in the program implementation process, to enable each participant to express their feelings, thoughts and opinions, and for the researcher to manage the program implementation process effectively. It was considered that if there were more than 25 students in the groups, this could have prevented the participants in the intervention group from actively participating in the program implementation process and would have made it difficult for the researcher to control the education process. Among the two determined secondary schools, one was randomly selected as the intervention group and the other as the control group. The implementation process was planned by interviewing the school administrators of the selected schools. In the secondary school chosen as the intervention group, the class that would form the intervention group was determined by drawing lots from among three eighth grade classes. In the school determined as the control group, the class that would form the control group was determined by drawing lots among the eight eighth grade classes.The inclusion criteria for the adolescents were having normal development, no previous participation in a cyberbullying prevention program and willingness to participate in the study. All of the adolescents in the classes determined as the intervention group and control group showed normal development and volunteered to participate in the study.
First of all, the adolescents in the intervention and control group and their families were informed about the purpose of the study, data collection tools, and the Cyberbullying Awareness Program by the researcher. In addition, it was stated to the adolescents and their families that the data collected within the scope of the research would remain confidential and would only be used for scientific study purposes. Consent forms were obtained from the adolescents who volunteered to participate in the study as well as their families. The Cyberbullying Awareness Scale for Adolescents and the Coping with Cyberbullying Scale were administered to adolescents in the intervention and control groups as a pre-test on February 3, 2020. After the pre-test application was completed, the Cyberbullying Awareness Program began to be administered to the adolescents in the intervention group. The Cyberbullying Awareness Program was prepared in 14 sessions and the plan was to apply the program to the intervention group for seven weeks, two days a week, one class hour a day (40 min). When the program was in progress, it was only possible to apply 10 sessions to the intervention group, as the Ministry of National Education suspended its educational activities on 13 March 2020 due to the COVID-19 epidemic. Therefore, the last four sessions of the program could not be implemented. No application was made to the control group. Due to the suspension of education and training activities as a result of the pandemic and the lockdown of individuals under the age of 20, the post-test application was made online for the intervention and control groups. In this direction, the measurement tools were first made into an online form by the researcher. Two different online message groups with adolescents were created by the teachers of the classes that formed the intervention and control groups. The researcher joined both message groups and made the necessary explanations to the adolescents. The measurement tools prepared as an online form were sent to the adolescents. The final test application was completed in March 2020. The post-test was administered to 17 adolescents in the intervention group and 21 adolescents in the control group. Five adolescents in the intervention group could not be included in the post-test application due to school changes and absenteeism during the program implementation process, and four adolescents in the control group were excluded due to the inability to reach them for the application of the post-test. Consequently, the post-test application was carried out with a total of 38 adolescents. Three weeks after the completion of the post-test application, the follow-up test was administered to the adolescents in the intervention group. The follow-up test application to the intervention group was made online due to the ongoing measures implemented within the scope of the COVID-19 outbreak. The follow-up test was completed in April 2020. During the research process, the ethical rules stated in the 1964 Declaration of Helsinki were followed.
Data analysis
SPSS 25 Program was used in the analysis of the data obtained within the scope of the research. In the analysis of the data, the data set was first examined. It was determined that there were no missing data and univariate outliers in the data set (|z| < 3). A normality test was performed as the preliminary analysis. Whether the data were normally distributed or not was determined by examining the Kurtosis and Skewness coefficients. When the kurtosis and skewness coefficients were examined, it was determined that the values were between − 1.65 and 3.02. In order to ensure the normal distribution assumption, the skewness and kurtosis coefficients are expected to be between − 1 and + 1 (George & Mallery, 2019 ). According to the results obtained, it was determined that the data deviated from normal distribution. For this reason, non-parametric methods were preferred for statistical analysis of the data, and therefore, the Wilcoxon Signed-Rank Test was used.
Analysis results of adolescents’ awareness of Cyberbullying
The mean and standard deviations of the pre-test, post-test and follow-up test scores of the adolescents in the intervention and control groups related to the Cyberbullying Awareness Scale for Adolescents sub-dimensions are shown in Table 1 .
The Wilcoxon Signed Rank Test results regarding the comparison of the pre-test, post-test and follow-up test scores of the Cyberbullying Awareness Scale for Adolescents of the adolescents in the intervention and control groups are given in Table 2 .
When the pre-test and post-test scores are compared in Table 2 , it can be observed that there are significant differences for all measures in the intervention group (as shown by the Wilcoxon signed-rank test results). In regard to the control group, no significant differences were found.
There is a significant difference between the pre-test and post-test scores of the Cyberbullying Tendency sub-dimension in the intervention group ( z= - 2.56; p < . 05). When the Cyberbullying Tendency pre-test and post-test scores of the intervention group are compared, it is seen that the post-test scores ( M = 17.94; SD = 6.19) are significantly lower than the pre-test scores ( M = 20.71; SD = 7.53).The application of the Cyberbullying Awareness Program led to a significant decrease in the cyberbullying tendencies of the adolescents in the intervention group. A significant difference can also be observed between the pre-test and post-test scores of the adolescents in the intervention group in the Coping with Cyberbullying ( z= -2.03; p < .05) and Protection from Cyberbullying ( z= -2.30; p < . 05) subdimensions. When the pre-test and post-test scores of the adolescents in the intervention group Coping with Cyberbullying sub-dimension are compared, it can be seen that the post-test scores ( M = 20.35; SD = 3.48) are significantly higher than the pre-test scores ( M = 17.00.; SD = 3.98). When the pre-test and post-test scores of the adolescents in the intervention group in the Protection from Cyberbullyingsub-dimension are compared, it can be seen that the post-test scores ( M = 21.53; SD = 2.81) are significantly higher than the pre-test scores ( M = 18.94; SD = 4.19). The Cyberbullying Awareness Program generated a significant increase in the scores for coping with cyberbullying and protection from cyberbullying of the adolescents in the intervention group. In terms of the differences between the pre-test and post-test scores, when the effect size values of the intervention group were examined according to the scores of the Cyberbullying Awareness Scale for Adolescents, it was found that the Tendency to Cyberbullying (0.44), Coping with Cyberbullying (0.35) and Protection from Cyberbullying (0.40), it is seen that the partial eta square values in the sub-dimensions are at a moderate level.
When the post-test scores and the scores of the test applied taken three weeks later (the follow-up test scores) of the intervention group are compared, it can be seen that there are no significant differences.
Analysis results of adolescents’ coping skills with cyberbullying
The mean and standard deviations of the pre-test, post-test and follow-up test scores of the adolescents in the intervention and control groups regarding the Coping with Cyberbullying Scale sub-dimensions are given in Table 3 .
The Wilcoxon Signed Rank Test results regarding the comparison of the pre-test, post-test and follow-up test scores of the adolescents in the intervention and control groups regarding the Coping with Cyberbullying Scale sub-dimensions are given in Table 4 :
When Table 4 is examined, it is seen that in the intervention group there is a significant difference between the pre-test and post-test scores of Seeking for Help and Information ( z=- 2.14, p < . 05; partial η2 = 0.37) and Increasing of Cognitive Security and Confidentiality ( z=- 2.28, p < . 05; partial η2 = 0.39) sub-dimensions. When the pre-test and post-test scores of the adolescents in the intervention group in the Seeking for Help and Information sub-dimension are compared, it is seen that the post-test scores ( M = 31.77; SD = 7.01) are significantly higher than the pre-test scores ( M = 27.94 ; SD = 8.29). When the pre-test and post-test scores of the adolescents in the intervention group in the Increasing Cognitive Security and Confidentiality sub-dimension are compared, it is seen that the post-test scores ( M = 16.82 ; SD = 2.63) are significantly higher than the pre-test scores ( M = 14.41; SD = 3.41). The Cyberbullying Awareness Program caused a significant increase in the Seeking for Help and Information and Increasing Cognitive Security and Confidentiality scores of the adolescents in the intervention group.In regard to the Avoidance and Ignore sub-dimensions, no significant differences were found ( z = -0.59; p > .05). In this respect, it can be stated that the Cyberbullying Awareness Program did not cause a significant effect on the Avoidance and Ignoring sub-dimension scores of the adolescents in the intervention group. When the effect size value is examined according to the sub-dimensions of the Coping with Cyberbullying Scale, it is seen that the partial eta square values in the sub-dimensions of Seeking Help and Information (0.37) and Increasing Cognitive Security and Confidentiality (0.39) are moderate. In regard to the control group, no significant differences were found.
When the post and follow-up test scores of intervention group are compared, it is seen that there are no significant differences.It was determined that the effect of the Cyberbullying Awareness Program continued three weeks after the program was completed.
In the study, the aim was to examine the effect of the Cyberbullying Awareness Program on the awareness of adolescents about cyberbullying and their coping skills. For this purpose, 10 sessions of the Cyberbullying Awareness Program were applied to the intervention group, two days a week for five weeks. Our results have shown that the Cyberbullying Awareness Program reduced the tendency of adolescents towards cyberbullying, and increased their awareness levels for coping with and protecting themselves from cyberbullying (H1). Also, the results of the present study have demonstrated that the Cyberbullying Awareness Program was effective in improving the knowledge and competence levels of the intervention group in terms of effective strategies for coping with cyberbullying (H2). The effect of the program continued when a re-test was administered three weeks after (follow-up test) the completion of the program (H3). No significant differences were observed in the control group.
Firstly, we hypothesized that the Cyberbullying Awareness Program would reduce the cyberbullying tendency of adolescents and improve their awareness of coping with and protecting themselves from cyberbullying. Our results confirmed hypothesis (H1). The most important predictor of the intention to show cyberbullying behaviors, in other words, of cyberbullying tendency, is attitudes towards cyberbullying. It is stated that adolescents who have positive attitudes towards cyberbullying have a high tendency towards cyberbullying. Adolescents’ awareness levels and beliefs about the consequences of cyberbullying shape their attitudes (Heirman & Walrave, 2012 ). Due to the lack of face-to-face communication on the Internet and the fact that cyberbullying acts do not leave a physical trace on the victims, the negative effects experienced by the victims as a result of cyberbullying cannot be directly observed by the bullies and bystanders. This situation causes the bullies and bystanders to be unable to empathize with the victim in cyberbullying acts, and to be less or not aware of the consequences of cyberbullying compared to traditional bullying (Solanje & Smith, 2008 ). Studies have shown that adolescents state that cyberbullying is performed for entertainment and jokes and that the victims should not experience negative emotions (Topçu et al., 2008 ; Vandebosch & Van Cleemput, 2008 ). It is thought that the positive attitudes and perceptions of adolescents towards cyberbullying, such as jokes and entertainment, are due to their low awareness of the negative consequences of cyberbullying. According to Kowalski et al. ( 2014 ), the level of awareness of adolescents about the negative consequences of cyberbullying will affect their perception, motivation and attitudes towards cyberbullying behaviors and thus their cyberbullying tendencies. It is thought that enabling them to empathize with the victims will be effective in reducing their motivation and tendency towards cyberbullying. In addition,the results of the present study showed that the Cyberbullying Awareness Program is effective in protecting adolescents from cyberbullying by assisting them with avoiding threatening and risky behaviors in the Internet environment, and in improving their awareness of effective coping skills that will enable them to be less affected by the consequences of cyberbullying when they encounter such behavior.
We hypothesized that the Cyberbullying Awareness Program would improve the knowledge and competence levels of adolescents regarding effective strategies for coping with cyberbullying. Our results partially supported hypothesis (H2). In this direction, the Cyberbullying Awareness Program applied to the intervention group was effective in improving the coping skills of adolescents in terms of seeking social support and information as well as their ability to use technological solutions when faced with cyberbullying. However, we found that the implemented program was not effective in developing the adolescents’ understanding that the use of avoidant strategies in coping with cyberbullying is ineffective. It is thought that this result of the research might be due to the inability to implement the last four sessions of the Cyberbullying Awareness Program as a result of the interruption of educational activities caused by the COVID-19 pandemic.
Adolescents use various coping strategies when they encounter cyberbullying online (Arıcak et al., 2008 ; Smith et al., 2008 ; Raskuskas & Huynh, 2015 ). It is stated that the coping strategy used when faced with cyberbullying reduces or strengthens the negative effects of cyberbullying (Machmutow et al., 2012 ; Jacobs et al., 2014 ). Also, previous research has revealed that the negative effects of cyberbullying on adolescents’ lives are related to the coping strategy used, and that adolescents who use ineffective coping strategies are more likely to experience the negative effects of cyberbullying more intensely (Jacobs et al., 2014 ; Elipe et al., 2017 ). It is stated that the strategy of seeking help and information by asking support from family, peers or an adult in coping with cyberbullying is effective in reducing depressive symptoms, which is one of the psychological effects of cyberbullying. However, retaliation and communicating with the bully increase the probability of experiencing depression (Machmutow et al., 2012 ). According to Li ( 2010 ), some adolescents do not consider cyberbullying acts as bullying or do not react because they are concerned that the situation will deteriorate. Some adolescents, on the other hand, think that they can do nothing and try to ignore the bullying, although they experience negative emotional effects when they experience cyber victimization (Li, 2010 ). The fact that adolescents do not know how to deal effectively with cyberbullying and adopt avoidant-focused coping strategies increases cyberbullying acts and causes adolescents to reach dangerous levels that harm their psychological and emotional well-being (Hoff & Mitchell, 2009 ). Increasing the level of knowledge of adolescents on effective coping methods with cyberbullying is considered important in terms of protecting them from the negative effects of cyberbullying.
In Turkey as well as various other countries, it is seen that the programs developed purely to improve the skills of adolescents to cope with cyberbullying are limited, but the cyberbullying prevention programs do include content aimed at improving coping skills indirectly. Cyberbullying prevention programs include content aimed at developing indirect coping skills such as peer support, the importance of safe Internet use, conflict resolution skills, empathy, and responsibility (Del Rey et al., 2012 ; Williford et al., 2013 ; Wölfer et al., 2014 ; Nedim Bal & Kahraman, 2015 ; Chaux et al., 2016 ; Cross et al., 2016 ). Similar results were obtained in the programs aimed at improving adolescents’ coping skills with cyberbullying. It has been revealed that a program for school counselors focused on coping with and raising awareness about cyberbullying was effective in the development of teachers’ skills in coping with cyberbullying (Atundağ & Ayas, 2020 ). The ConRed Program, which was developed to prevent cyberbullying in adolescence, increased adolescents’ awareness of the strategies they could use for controlling the content they shared on the Internet, hiding personal information on the Internet, and online security measures, thus improving their coping skills with respect to technological solutions. The Media Heroes Program was developed in Germany in order to increase the awareness of adolescents about the legal consequences of cyberbullying and to develop their skills in helping and coping strategies. It was determined that the program is effective in terms of the development of empathy, perspective taking, self-esteem skills of adolescents and in preventing cyberbullying behaviors (Wölfer et al., 2014 ; Chaux et al., 2016 ).
Studies conducted in various countries have revealed that cyberbullying behaviors are common among adolescents (Selkie et al., 2016 ; Baldry et al., 2018 ). It is emphasized that cyberbullying has negative effects on the life and development of adolescents in terms of psychological, academic and social aspects. It is thought that the negative effects of cyberbullying will also negatively affect critical developmental processes such as gaining identity, autonomy, belonging to a group and gaining approval, as well as academic success in adolescence. It is stated that the fact that adolescents often describe cyberbullying acts as jokes and entertainment (Vandebosch & Van Cleemput, 2008 ; Kowalski et al., 2014 ) and do not perceive them to be bullying is due to their low level of awareness about the negative consequences of cyberbullying.Adolescents’ evaluation of cyberbullying as a positive behavior increases their motivation and tendencies towards cyberbullying (Heirman & Walrave, 2012 ). In this context, increasing awareness regarding cyberbullying and the negative consequences of such behavior plays an important role in preventing cyberbullying in adolescence. In addition, increasing the level of awareness about the negative effects of cyberbullying is considered as an important factor for adolescents to take preventive measures to protect themselves from and cope with cyberbullying. Today, the prevalence of cyberbullying reaches a peak in adolescents aged 13–14, due to the ease of access to technological tools, the frequency of Internet use and the high rate of participation in social media (Ayas & Horzum, 2012 ; Camphell et al., 2012 ). Considering that this age group is at risk of cyberbullying and the negative effects of cyberbullying stem from the adopted coping methods, it is thought that it is important for adolescents to acquire the knowledge and skills required for effective coping strategies against cyberbullying. Considering that Internet-based communication applications are increasingly prevalent in the lives of adolescents, there is a need for programs designed to increase adolescents’ awareness of cyberbullying and to develop their skills to deal with this problem effectively. Our results have shown that the Cyberbullying Awareness Program is effective in increasing the awareness levels of adolescents about cyberbullying and developing their skills to cope with cyberbullying.
Limitations
Although the results obtained within the scope of the study make important contributions to the literature, they should be evaluated within the scope of the limitations of the study. The results obtained from the research are limited to 38 adolescents who were studying in the eighth grade of a secondary school in the middle socio-economic level in the city center of Antalya/Turkey. While developing the Cyberbullying Awareness Program, the developmental characteristics of adolescents were taken into account and activities that included active learning methods were created. The research was carried out with a small group (N = 38) in order to ensure the active participation of all participants in the program implementation process and for the researcher to direct the implementation process. The Cyberbullying Awareness Program developed within the scope of the study should be implemented in larger samples to provide more evidence for the effectiveness of the program. Whether the participants had experienced cyberbullying before (as a bully or victim) was not used as an inclusion criterion in the study. Since the previous experiences of the participants are likely to affect the results of the research, the inclusion criteria of the participants should include whether they have experienced cyberbullying or not. The research was started shortly before the COVID-19 pandemic began to impact Turkey. Although the Cyberbullying Awareness Program was planned to consist of 14 sessions, only 10 sessions could be implemented due to the interruption of education activities caused by the Covid-19 pandemic. The results obtained in the study are limited to the 10 sessions of the program. By applying the entire Cyberbullying Awareness Program, the effect of adolescents’ awareness of cyberbullying and their ability to cope with cyberbullying should be examined. Another limitation of the study is that different data collection methods were used in the research process. In the study, the pre-test application was conducted face-to-face with the adolescents, but the post-test and follow-up test were conducted online due to the imposition of the lockdown as part of the pandemic measures.We also did not have information about how the different data collection settings (offline and online, pre-COVID and during COVID) could have influenced our participants, their answers and their cyberbullying experiences.In the present study, all of the Cronbch’s alpha values were found to be higher than 0.70. However in the original studies, the Cronbach’s alpha values of some sub-dimensions of the scales were lower than 0.70. Therefore, we suggest that in future studies, the Cronbach’s alpha values of these measures should be assessed and interpreted cautiously.
Conclusions
When the findings of this study were examined, it was determined that the Cyberbullying Awareness Program was effective in reducing the cyberbullying tendencies of adolescents and developing their skills to cope with cyberbullying.The results obtained in the study have shown that the level of cyberbullying awareness is an important factor in preventing cyberbullying. TheCyberbullying Awareness Program has a preventive nature as it focuses on preventing cyberbullying before it occurs. The program reduces the cyberbullying tendencies of adolescents by improving their anti-cyberbullying attitudes and also helps them develop strategies to help the victim and protect themselves against victimization.In line with the purpose of helping schools to help themselves, the Cyberbullying Awareness Program provides schools with a practical cyberbullying prevention strategy by enabling teachers to repeatedly implement the program in classrooms. Although the prevalence of cyberbullying reaches its peak in middle adolescence, it can also be seen at younger ages. Considering that younger children’s technological competence is limited, an additional version of the program is needed. Therefore, our main suggestion for future studies is that the Cyberbullying Awareness Program, which has been demonstrated to be effective within the scope of this research, should be adapted to the younger age group. In addition, considering the prevalence and negative effects of cyberbullying in adolescence, it is recommended that cyberbullying prevention programs be added to the national curriculum by policy makers and implemented by teachers across the country.
Data Availability
The datasets generated during and/or analysed during the current study are available in the [ www.osf.io .] repository, [ https://osf.io/4szp3/ ]
Ajzen, I. (1991). The theory of planned behavior. Organizational Behavior and Human Decision Processes , 50 (2), 179–211
Google Scholar
Akyüz, A., & Koç, Z. (2020). Effect of empathy-oriented cyberbullying psychoeducation program on cyberbullying and empathy level of 9th and 10th grade students. GUJGEF , 40 (1), 75–111. [in Turkish]
Altundağ, Y., & Ayas, T. (2020). Effectiveness of the whole school-based program for equipping high school counselors with strategies of coping with cyberbullying and cyberbullying awareness. Education and Science , 45 (201), 109–123
Arató, N., Zsidó, A. N., Rivnyák, A., Péley, B., & Lábadi, B. (2022). Risk and protective factors in cyberbullying: the role of family, social support and emotion regulation. International Journal of Bullying Prevention , 4 (2), 160–173
Arıcak, T., Siyahhan, S., Uzunhasanoğlu, A., Sarıbeyoğlu, S., Çıplak, S., Yılmaz, N., & Memmedov, C. (2008). Cyberbullying among Turkish Adolescents. Cyberpsychology & Behavior , 11 (3), 253–261
Ayas, T., & Horzum, M. B. (2012). On being cyber bully and victim among primary school students. Elementary Education Online , 11 (2), 369–380
Ayas, T., Aydın, F., & Horzum, M. (2015). Cyberbullying awareness scale: A validity and realiablity study. Online Journal Of Technology Addiction & Cyberbullying , 2 (2), 38–51. [in Turkish]
Baldry, A. C., Blaya, C., & Farrington, D. P. (2018). International perspectives on cyberbullying: Prevalence, risk factors and interventions . Palgrave Macmillan
Bandura, A. (1989). Social cognitive theory. In R. Vasta (Ed.), Annals of child development. Vol.6. Six theories of child development (pp. 1–60). JAI Press
Bandura, A. (1999). Moral disengagement in the perpetration of inhumanities. Personality and Social Psychology Review , 3 (3), 193–209
PubMed Google Scholar
Barlett, C. P., Gentile, D. A., Anderson, C. A., Suzuki, K., Sakamoto, A., Yamaoka, A., & Katsura, R. (2014). Cross-cultural differences in cyberbullying behavior: A short-term longitudinal study. Journal of Cross-Cultural Psychology , 45 (2), 300–313
Bauman, S. (2010). Cyberbullying in a rural intermediate school: An exploratory study. Journal of Early Adolescence , 30 , 803–833
Bayar, Y., & Uçanok, Z. (2012). School social climate and traditional / cyberbullying: The mediational role of peer perception. Turkish Journal of Psychology , 27 (70), 101–114. [in Turkish]
Beyazıt, U., Aslan, B., Yılmaz, Z., & Bütün Ayhan, A. (2019). The relationship between parents’ neglect of their children and their children’s internet addiction and cyberbullying behaviors. Turkish Studies Educational Sciences , 14 (3), 993–1008. [in Turkish]
Beyazıt, U., Yurdakul, Y., & Bütün Ayhan, A. (2021). The Turkish adaptation of a measure to assess the impacts of cyberbullying in adolescents: The Cybervictimization Emotional Impact Scale. International Journal of Social and Humanities Sciences (IJSHS) , 5 (2), 35–52
Bonanno, R. A., & Hymel, S. (2013). Cyber bullying and internalizing difficulties: Above and beyond the impact of traditional forms of bullying. Journal of Youth and Adolescence , 42 (5), 685–697
Brewer, G., & Kerslake, J. (2015). Cyberbullying, self-esteem, empathy and loneliness. Computers in Human Behavior , 48 , 255–260
Brochado, S., Soares, S., & Fraga, S. (2017). A scoping review on studies of cyberbullying prevalence among adolescents. Trauma Violence & Abuse , 18 (5), 523–531
Calvete, E., Orue, I., Estévez, A., Villardón, L., & Padilla, P. (2010). Cyberbullying in adolescents: Modalities and aggressors’ profile. Computers in HumanBehavior , 26 , 1128–1135
Campbell, M., Spears, B., Slee, P. T., Butler, D., & Kift, S. (2012). Victims’ perceptions of bullying: Traditional and cyber and the psychosocial correlates of their victimisation. Emotional and Behavioural Difficulties , 17 , 389–401
Chaux, E., Velásquez, A. M., Schultze Krumbholz, A., & Scheithauer, H. (2016). Effects of the cyberbullying prevention program media heroes (Medienhelden) on traditional bullying. Aggressive Behavior , 42 (2), 157–165
Cross, D., Lester, L., & Barnes, A. (2015). A longitudinal study of the social and emotional predictors and consequences of cyber and traditional bullying victimisation. International Journal of Public Health , 60 (2), 207–217
Cross, D., Shaw, T., Hadwen, K., Cardoso, P., Slee, P., Roberts, C., Thomas, L., & Barnes, A. (2016). Longitudinal impact of the Cyber Friendly Schools program on adolescents’ cyberbullying behavior. Aggressive Behavior , 42 (2), 166–180
Crosslin, K., & Crosslin, M. (2014). Cyberbullying at a Texas University: A mixed methods approach to examining online aggression. Texas Public Health Journal , 66 (3), 26–31
Del Rey, A. R., Casas, J. A., & Ortega-Ruiz, R. (2012). The ConRed Program, an evidence-based practice. Comunicar: Revista Científica de Comunicación y Educación , 20 (39), 129–138
Den Hamer, A. H., & Konijn, E. A. (2016). Can emotion regulation serve as a tool in combating cyberbullying? Personality and Individual Differences , 102 , 1–6
Díaz, K. I., & Fite, P. J. (2019). Cyber victimization and its association with substance use, anxiety, and depression symptoms among middle school youth. Child & Youth Care Forum , 48 (4), 529–544
Elipe, P., Mora-Merchan, J. A., & Nacimiento, L. (2017). Development and validation of an ınstrument to assess the ımpact of cyberbullying: The cybervictimization emotional impact scale. Cyberpsychology Behavior and Social Networking , 20 (8), 479–485
Espelage, D. L., Hong, J. S., & Valido, A. (2018). Cyberbullying in the United States. In A. C. Baldry, C. Blaya, & P. Farrington (Eds.), International Perspectives on Cyberbullying Prevalence, Risk Factors and Interventions (pp. 65–100). Palgrave Macmillan
Garaigordobil, M. (2015). Cyberbullying in adolescents and youth in the Basque country: Prevalence of cybervictims, cyberaggressors, and cyberobservers. Journal of Youth Studies , 18 , 569–582
George, D., & Mallery, P. (2019). IBM SPSS statistics 23 step by step: A simple guide and reference . Routledge
Gimenez Gualdo, A. M., Hunter, S. C., Durkin, K., Arnaiz, P., & Maquilón, J. J. (2015). The emotional impact of cyberbullying: Differences in perceptions and experiences as a function of role. Computers & Education , 82 , 228–235
Heirman, W., & Walrave, M. (2012). Predicting adolescent perpetration in cyberbullying: An application of the theory of planned behavior. Psicothema , 24 (4), 614–620
Hemphill, S. A., Kotevski, A., Tollit, M., Smith, R., Herrenkohl, T. I., Toumbourou, J. W., & Catalano, R. F. (2012). Longitudinal predictors of cyber and traditional bullying perpetration in Australian secondary school students. Journal of Adolescent Health , 51 , 59–65
Hinduja, S., & Patchin, J. W. (2010). Bullying, cyberbullying, and suicide. Archives of Suicide Research , 14 , 206–221
Hoff, D. L., & Mitchell, S. N. (2009). Cyberbullying: Causes, effects, and remedies. Journal of Educational Administration , 47 (5), 652–665
Hymel, S., & Bonanno, R. A. (2014). Moral disengagement processes in bullying. Theory into Practice , 53 (4), 278–285
Jacobs, N. C., Dehue, F., Vollink, T., & Lechner, L. (2014). Determinants of adolescents’ ineffective and improved coping with cyberbullying: A Delphi study. Journal of Adolescence , 37 (4), 373–385
Koç, M., Horzum, M. B., Ayas, T., Aydın, F., Özbay, A., & Uğur, E. (2016). Coping with cyberbullying scale: Study of reliability and validity. Sakarya University Journal of Education , 6 (3), 116–128. [in Turkish]
Kowalski, R. M., & Limber, S. P. (2013). Psychological, physical, and academic correlates of cyberbullying and traditional bullying. Journal of Adolescent Health , 53 (1), 13–20
Kowalski, R. M., Giumetti, G. W., Schroeder, A. N., & Lattanner, M. R. (2014). Bullying in the digital age: A critical review and meta-analysis of cyberbullying research among youth. Psychological Bulletin , 140 (4), 1073–1137
Kubiszewski, V., Fontaine, R., Potard, C., & Auzoult, L. (2015). Does cyberbullying overlap with school bullying when taking modality of involvement into account? Computers in Human Behavior , 43 , 49–57
Landoll, R. R., Greca, L., Lai, A. M., Chan, B. S., S. F., & Herge, W. M. (2015). Cyber victimization by peers: Prospective associations with adolescent social anxiety and depressive symptoms. Journal of Adolescence , 42 , 77–86
Law, D. M., Shapka, J. D., Domene, J. F., & Gagné, M. H. (2012). Are cyberbullies really bullies? An investigation of reactive and proactive online aggression. Computers in Human Behavior , 28 , 664–672
Lazuras, L., Barkoukis, V., Ourda, D., & Tsorbatzoudis, H. (2013). A process model of cyberbullying in adolescence. Computers in Human Behavior , 29 , 881–887
Li, J., Sidibe, A. M., Shen, X., & Hesketh, T. (2019). Incidence, risk factors and psychosomatic symptoms for traditional bullying and cyberbullying in Chinese adolescents. Children and Youth Services Review , 107 , 104511
Li, Q. (2010). Cyberbullying in high schools: A study of students’ behaviors and beliefs about this new phenomenon. Journal of Aggression Maltreatment & Trauma , 19 (4), 372–392
Machmutow, K., Perren, S., Sticca, F., & Alsaker, F. D. (2012). Peer victimisation and depressive symptoms: Can specific coping strategies buffer the negative impact of cybervictimisation. Emotional and Behavioral Difficulties , 17 , 403–420
Nedim Bal, P., & Kahraman, S. (2015). The effect of cyber bullying sensibility improvement group training program on gifted students. Journal of Gifted Education Research , 3 (1), 48–57
Olenik-Shemesh, D., & Heiman, T. (2016). Cyberbullying victimization in adolescents as related to body esteem, social support, and social self-efficacy. The Journal of Genetic Psychology , 178 (1), 28–43
Özbay, A. (2017). The effect of solution-focused psychoeducation program on the psychological symptoms and cyber-victimization of adolescents who are cyber-bullied . (Doctoral Dissertation). Sakarya University, Sakarya. [in Turkish]
Peker, A. (2013). Investigation of effect of human values-oriented psycho-training programme on problematic internet use and cyber bullying . (Doctoral Dissertation). Sakarya University, Sakarya. [in Turkish]
Pereira, F., Spitzberg, B., & Matos, M. (2016). Cyber-harassment victimization in Portugal: Prevalence, fear and help-seeking among adolescents. Computers in Human Behavior , 62 , 136–146
Perren, S., Dooley, J., Shaw, T., & Cross, D. (2010). Bullying in school and cyberspace: Associations with depressive symptoms in Swiss and Australian adolescents. Child and Adolescent Psychiatry and Mental Health , 4 (28), 1–10
Pornari, C. D., & Wood, J. (2010). Peer and cyber aggression in secondary school students: The role of moral disengagement, hostile attribution bias, and outcome expectancies. Aggressive Behavior , 36 (2), 81–94
Raskauskas, J., & Huynh, A. (2015). The process of coping with cyberbullying: A systematic review. Aggression and Violent Behavior , 23 , 118–125
Ridell, J., Pepler, D., & Craig, W. (2018). Cyberbullying in Canada. In A. C. Baldry, C. Blaya, & P. Farrington (Eds.), International Perspectives on Cyberbullying Prevalence, Risk Factors and Interventions (pp. 39–64). Palgrave Macmillan
Schneider, S. K., O’Donnell, L., Stueve, A., & Coulter, R. W. S. (2012). Cyberbullying, school bullying, and psychological distress: A regional census of high school students. American Journal of Public Health , 102 , 171–177
PubMed PubMed Central Google Scholar
Schultze-Krumbholz, A., Schultze, M., Zagorscak, P., Wölfer, R., & Scheithauer, H. (2016). Feeling cybervictims’ pain—The effect of empathy training on cyberbullying. Aggressive Behavior , 42 (2), 147–156
Selkie, E. M., Fales, J. L., & Moreno, M. A. (2016). Cyberbullying prevalence among US middle and high school–aged adolescents: A systematic review and quality assessment. Journal of Adolescent Health , 58 (2), 125–133
Shultz, E., Heilman, R., & Hart, K. J. (2014). Cyber-bullying: An exploration of bystander behavior and motivation.Cyberpsychology: Journal of Psychosocial Research on Cyberspace, 8(4)
Slonje, R., & Smith, P. K. (2008). Cyberbullying: Another main type of bullying? Scandinavian Journal of Psychology , 49 , 147–154
Smith, P. K., Mahdavi, J., Carvalho, M., Fisher, S., Russell, S., & Tippett, N. (2008). Cyberbullying: Its nature and impact in secondary school pupils. Journal of Child Psychology and Psychiatry , 49 (4), 376–385
Sourander, A., Klomek, A. B., Ikonen, M., Lindroos, J., Luntamo, T., Koskelainen, M., Ristkari, T., & Helenius, H. (2010). Psychosocial risk factors associated with cyberbullying among adolescents: A population-based study. Archives of General Psychiatry , 67 (7), 720–728
Steffgen, G., König, A., Pfetsch, J., & Melzer, A. (2011). Are cyberbullies less empathic? Adolescents’ cyberbullying behavior and empathic responsiveness. Cyberpsychology Behavior and Social Networking , 14 (11), 643–648
Tanrıkulu, T. (2013). The analysis of variables about cyber bullying and the effect of an intervention program with tendency to reality therapy on cyber bullying behaviors . (Doctoral Dissertation). Sakarya University, Sakarya. [in Turkish]
Topçu, Ç., & Erdur-Baker, Ö. (2016). Help seeking behaviors of bullying victims and resources for help. Ege Eğitim Dergisi , 17 (1), 127–145. [in Turkish]
Topçu, Ç., Erdur-Baker, Ö., & Çapa-Aydın, Y. (2008). Examination of cyberbullying experiences among Turkish students from different school types. Cyberpsychology & Behavior , 11 (6), 643–648
Trompeter, N., Bussey, K., & Fitzpatrick, S. (2018). Cyber victimization and internalizing difficulties: The mediating roles of coping self-efficacy and emotion dysregulation. Journal Of Abnormal Child Psychology , 46 , 1129–1139
Urano, Y., Takizawa, R., Ohka, M., Yamasaki, H., & Shimoyama, H. (2020). Cyber bullying victimization and adolescent mental health: The differential moderating effects of intrapersonal and interpersonal emotional competence. Journal of Adolescence , 80 , 182–191
Vandebosch, H., & Van Cleemput, K. (2008). Cyberbullying among youngsters: Profiles of bullies and victims. New Media & Society , 11 , 1349–1371
Vandoninck, S., d’Haenens, L., & Roe, K. (2013). Online risks: Coping strategies of less resilient children and teenagers across Europe. Journal of Children and Media , 7 (1), 60–78
Wang, J., Nansel, T. R., & Iannotti, R. J. (2011). Cyber and traditional bullying: Differential association with depression. Journal of Adolescent Health , 48 , 415–417
Wigderson, S., & Lynch, M. (2013). Cyber- and traditional peer victimization: Unique relationships with adolescent well-being. Psychology of Violence , 3 (4), 297–309
Williford, A., Elledge, L. C., Boulton, A. J., Depaolis, K. J., Little, T. D., & Salmivalli, C. (2013). Effects of the KiVa antibullying program on cyberbullying and cybervictimization frequency among Finnish youth. Journal of Clinical Child & Adolescent Psychology , 42 (6), 820–833
Wölfer, R., Krumbholz, S., Zagorscak, A., Jakel, P., Gobel, A., K., & Scheithauer, H. (2014). Prevention 2.0: Targeting cyberbullying @ school. Prevention Science , 15 , 879–887
Wright, M. F. (2017). Adolescents’ perceptions of popularity-motivated behaviors, characteristics, and relationships in cyberspace and cyber aggression: the role of gender. Cyberpsychology Behavior and Social Networking , 20 (6), 355–361
Yurdakul (2020). Examination of the effect of cyber bullying awareness program on adolescents’ awareness of cyberbullying and coping skills with cyberbullying . (Doctoral Dissertation). Ankara University, Ankara. [in Turkish]
Yurdakul, Y., & Bütün Ayhan, A. (2021). Cyber Victimization in Adolescence: A qualitative study. Children and Youth Services Review , 128 , 106139
Download references
The author(s) received no financial support for the research, authorship, and/or publication of this article.
Author information
Authors and affiliations.
Akdeniz University, Kumluca Health Sciences Faculty, Child Development Department, Antalya, Turkey
Yeşim Yurdakul
Faculty of Health Sciences, Child Development Department, Ankara University, Ankara, Turkey
Aynur BÜTÜN AYHAN
You can also search for this author in PubMed Google Scholar
Contributions
All authors contributed in the development and implementation of the program.
Corresponding author
Correspondence to Aynur BÜTÜN AYHAN .
Ethics declarations
Conflict of interest.
On behalf of all authors, the corresponding author states that there is no conflict of interest.
Ethics approval
This study was performed in line with the principles of the Declaration of Helsinki. Approval was granted by the Ethics Committee of Ankara University (Date. 27 September 2018/ No. 56786525-050.04.04/ 67272).
Consent to participate
Written informed consent was obtained from the parents.
Additional information
Publisher’s note.
Springer Nature remains neutral with regard to jurisdictional claims in published maps and institutional affiliations.
Cyberbullying Awareness Program
Rights and permissions.
Reprints and permissions
About this article
Yurdakul, Y., AYHAN, A.B. The effect of the cyberbullying awareness program on adolescents’ awareness of cyberbullying and their coping skills. Curr Psychol 42 , 24208–24222 (2023). https://doi.org/10.1007/s12144-022-03483-3
Download citation
Received : 19 September 2021
Revised : 09 July 2022
Accepted : 11 July 2022
Published : 03 August 2022
Issue Date : October 2023
DOI : https://doi.org/10.1007/s12144-022-03483-3
Share this article
Anyone you share the following link with will be able to read this content:
Sorry, a shareable link is not currently available for this article.
Provided by the Springer Nature SharedIt content-sharing initiative
- Adolescence
- Coping with cyberbullying
- Cyberbullying
- Find a journal
- Publish with us
- Track your research
Experts and Machines United Against Cyberbullying
Research output : Thesis › PhD Thesis - Research UT, graduation UT
- METIS-304975
- Information Retrieval
- Sentiment Analysis
- Cyberbullying
- Expert Systems
Access to Document
- 10.3990/1.9789036537391
- thesis_M_Dadvar Final published version, 1.25 MB
- DISSERTATION_84_eprint Final published version, 2.22 MB
T1 - Experts and Machines United Against Cyberbullying
AU - Dadvar, M.
N1 - SIKS Dissertation series no. 2014-37
PY - 2014/9/12
Y1 - 2014/9/12
N2 - One form of online misbehaviour which has deeply affected society with harmful consequences is known as cyberbullying. Cyberbullying can simply be defined as an intentional act that is conducted through digital technology to hurt someone. Cyberbullying is a widely covered topic in the social sciences. There are many studies in which the problem of cyberbullying has been introduced and its origins and consequences have been explored in detail. There are also studies which have investigated the intervention and prevention strategies and have proposed guidelines for parents and adults in this regard. However, studies on the technical dimensions of this topic are relatively rare. In this research the overall goal was to bridge the gap between social science approaches and technical solutions. In order to be able to suggest solutions that could contribute to minimizing the risk and impact of cyberbullying we have investigated the phenomenon of cyberbullying from different angles. We have thoroughly studied the origin of cyberbullying and its growth over time, as well as the role of technology in the emergence of this type of virtual behaviour and in the potential for reducing the extent of the social concern it raises. First we introduced a novel outlook towards the cyberbullying phenomenon. We looked into the gradual changes which have occurred in relationships and social communication with the emergence of the Internet. We argued that one should look at virtual environments as virtual communities, because the human needs projected on these environments, the relationships, human concerns and misbehaviour have the same nature as in real-life societies. Therefore, to make virtual communities safe, we need to take safety measures and precautions that are similar to the ones that are common in non-virtual communities. We derived the assumption that if cyberbullying is recognized and treated as a social problem and not just seen as some random mischief conducted by individuals with the use of technology, the methods for handling its consequences are likely to be more realistic, effective and comprehensive. This part of our study led to the conviction that for combating cyberbullying, behavioural and psychological studies, and the study of technical solutions should go hand in hand. One of the main limitations that we faced when we started our research was the lack of a comprehensive dataset for cyberbullying studies. We needed a dataset which included real instances of bullying incidents. Moreover, it was essential for our studies to also have the demographic information of the social media users as well as the history of their activities. We started our preliminary experiments using a dataset that was collected from MySpace forums. This dataset did not meet all the requirements for our experiment, namely in terms of size and sufficiency of information. Therefore we developed our own YouTube dataset, with the aim to encompass extensive information about the users and their activities as well as larger numbers of bullying comments. We collected information on user activities and posted textual comments as well as personal and demographic details of the users involved. Detecting a bullying comment or post at the earliest possible moment in time can substantially decrease the negative effects of cyberbullying incidents. We started our experiments by showing that besides the conventional features used for text mining methods such as sentiment analysis and specifically bullying detection, more personal features, in this experiment gender, can improve the accuracy of the detection models. As expected the models which were optimized accordingly resulted in a more accurate classification. The improved outcome motivated us to look into other personal features as well, such as age and the writing style of users. By adding more personal information, the previous classification results were outperformed and the detection accuracy enhanced even further. In the last experiment we made use of experts’ knowledge to identify potential bully users in social networks. To better understand and interpret the intentions underlying the online activities of users of social media, we decided to incorporate human reasoning and knowledge into a bulliness rating system by developing a Multi-Criteria Evaluation System. Moreover, to have more sources of information and to make use of the potential of both human and machine, we designed a hybrid approach, incorporating machine learning models on top of the expert system. The hybrid approach reached an optimum model which outperformed the results obtained from the machine learning models and the expert system individually. Our hybrid model illustrates the added value of integrating technical solutions with insights from the social sciences for the first time. As argued in this thesis, the integration of social studies into a software-enhanced monitoring workflow could pave the way towards the tackling of this kind of online misbehaviour. The ideas and algorithms proposed for fulfilling this purpose can be a stepping stone for future research in this direction. The work carried out is also a demonstration of the added value of frameworks for text categorization, sentiment mining and user profiling in applications addressing societal issues. This work can be viewed as a contribution to the more general societal challenge of increasing the level of cybersecurity, in particular for the younger generations of social network users. By turning the internet into a safer place for children, the chances increase that they will be able to benefit from the informational richness that it also offers.
AB - One form of online misbehaviour which has deeply affected society with harmful consequences is known as cyberbullying. Cyberbullying can simply be defined as an intentional act that is conducted through digital technology to hurt someone. Cyberbullying is a widely covered topic in the social sciences. There are many studies in which the problem of cyberbullying has been introduced and its origins and consequences have been explored in detail. There are also studies which have investigated the intervention and prevention strategies and have proposed guidelines for parents and adults in this regard. However, studies on the technical dimensions of this topic are relatively rare. In this research the overall goal was to bridge the gap between social science approaches and technical solutions. In order to be able to suggest solutions that could contribute to minimizing the risk and impact of cyberbullying we have investigated the phenomenon of cyberbullying from different angles. We have thoroughly studied the origin of cyberbullying and its growth over time, as well as the role of technology in the emergence of this type of virtual behaviour and in the potential for reducing the extent of the social concern it raises. First we introduced a novel outlook towards the cyberbullying phenomenon. We looked into the gradual changes which have occurred in relationships and social communication with the emergence of the Internet. We argued that one should look at virtual environments as virtual communities, because the human needs projected on these environments, the relationships, human concerns and misbehaviour have the same nature as in real-life societies. Therefore, to make virtual communities safe, we need to take safety measures and precautions that are similar to the ones that are common in non-virtual communities. We derived the assumption that if cyberbullying is recognized and treated as a social problem and not just seen as some random mischief conducted by individuals with the use of technology, the methods for handling its consequences are likely to be more realistic, effective and comprehensive. This part of our study led to the conviction that for combating cyberbullying, behavioural and psychological studies, and the study of technical solutions should go hand in hand. One of the main limitations that we faced when we started our research was the lack of a comprehensive dataset for cyberbullying studies. We needed a dataset which included real instances of bullying incidents. Moreover, it was essential for our studies to also have the demographic information of the social media users as well as the history of their activities. We started our preliminary experiments using a dataset that was collected from MySpace forums. This dataset did not meet all the requirements for our experiment, namely in terms of size and sufficiency of information. Therefore we developed our own YouTube dataset, with the aim to encompass extensive information about the users and their activities as well as larger numbers of bullying comments. We collected information on user activities and posted textual comments as well as personal and demographic details of the users involved. Detecting a bullying comment or post at the earliest possible moment in time can substantially decrease the negative effects of cyberbullying incidents. We started our experiments by showing that besides the conventional features used for text mining methods such as sentiment analysis and specifically bullying detection, more personal features, in this experiment gender, can improve the accuracy of the detection models. As expected the models which were optimized accordingly resulted in a more accurate classification. The improved outcome motivated us to look into other personal features as well, such as age and the writing style of users. By adding more personal information, the previous classification results were outperformed and the detection accuracy enhanced even further. In the last experiment we made use of experts’ knowledge to identify potential bully users in social networks. To better understand and interpret the intentions underlying the online activities of users of social media, we decided to incorporate human reasoning and knowledge into a bulliness rating system by developing a Multi-Criteria Evaluation System. Moreover, to have more sources of information and to make use of the potential of both human and machine, we designed a hybrid approach, incorporating machine learning models on top of the expert system. The hybrid approach reached an optimum model which outperformed the results obtained from the machine learning models and the expert system individually. Our hybrid model illustrates the added value of integrating technical solutions with insights from the social sciences for the first time. As argued in this thesis, the integration of social studies into a software-enhanced monitoring workflow could pave the way towards the tackling of this kind of online misbehaviour. The ideas and algorithms proposed for fulfilling this purpose can be a stepping stone for future research in this direction. The work carried out is also a demonstration of the added value of frameworks for text categorization, sentiment mining and user profiling in applications addressing societal issues. This work can be viewed as a contribution to the more general societal challenge of increasing the level of cybersecurity, in particular for the younger generations of social network users. By turning the internet into a safer place for children, the chances increase that they will be able to benefit from the informational richness that it also offers.
KW - METIS-304975
KW - Information Retrieval
KW - EWI-25014
KW - Sentiment Analysis
KW - Cyberbullying
KW - Expert Systems
KW - IR-91720
U2 - 10.3990/1.9789036537391
DO - 10.3990/1.9789036537391
M3 - PhD Thesis - Research UT, graduation UT
SN - 978-90-365-3739-1
PB - University of Twente
CY - Enschede
The nature and impact of cyberbullying among South African youth : an explanatory analysis
Master Thesis
Permanent link to this Item
Supervisors, journal title, link to journal, journal issn, volume title.
University of Cape Town
Description
Payne, A. 2015. The nature and impact of cyberbullying among South African youth : an explanatory analysis. University of Cape Town.
Collections
DSpace software copyright © 2002-2024 LYRASIS
- " class="ru"> " class="language-link">Russian
PhD Thesis: Of all Types of Bullying, Cyberbullying is Most Devastating to Well-Being of Adolescents
On 26 June, Lauraliisa Mark from the Tallinn University School of Governance, Law and Society will defend her doctoral thesis, which studied the suicidal thoughts and depressive feelings among Estonian youth.
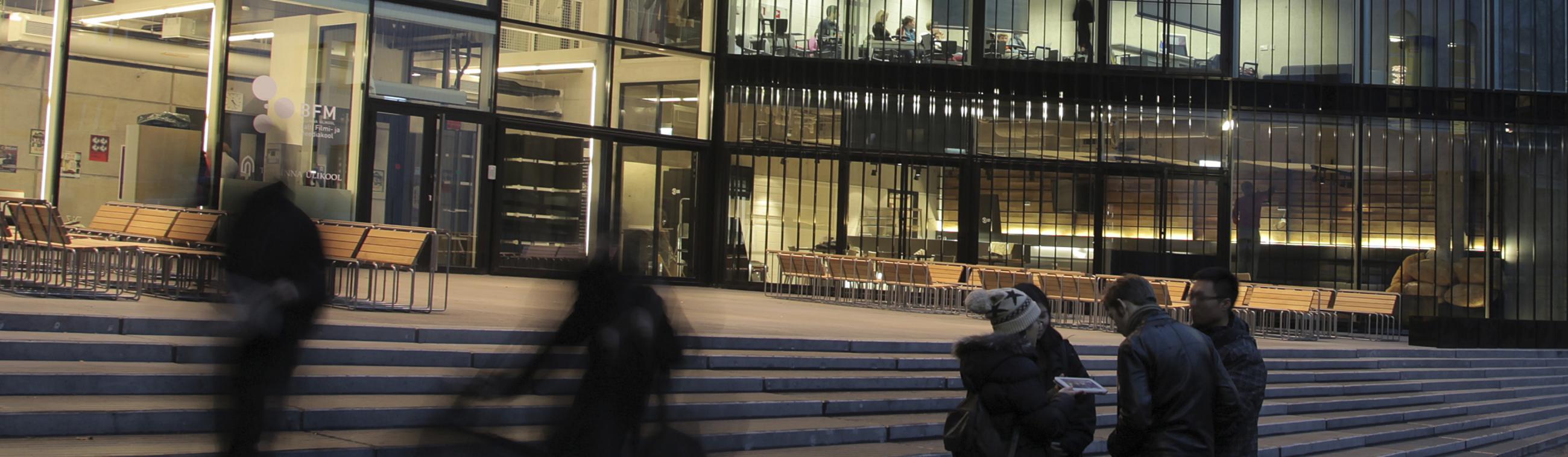
According to the study, in the past 12 months, 13% of students in grades 7-9 had had suicidal thoughts, and 26% had felt depression.
According to the World Health Organisation, suicide is one of the main causes of death among 15-29-year-olds. At the same time, among 15-19-year-olds in Estonia, the rate of suicide is one of the highest in the world. “In my thesis, I studied the spread of suicidal thoughts and depressive feelings among the pupils in grades 5-9, and analysed the relation between suicidal thoughts, depressive feelings, and risk behaviour,” Mark explained and added that depression is one of the most common mental disorders to lead to suicide.
“Even though risk behaviour is often seen as a normal part of being young, all risk behaviours analysed in this thesis (early sexual activity, regular smoking, alcohol use, getting into physical fights, bullying) were potential sources of suicidal ideas and/or depressive feelings,” Mark stated. For example, being sexually active at 13 years old or earlier was four times (among boys) to eight times (among girls) more likely to lead to suicidal thoughts. “The earlier a person became sexually active, the more often they would also consume alcohol, smoke, fight, bully, and have worse estimation toward their health,” she added.
“In the case of multiple risk factors appearing simultaneously among 15-year-olds, the emergence of suicidal thoughts increased multiple times; in the case of four or five factors, the probability increased by more than 10 times,” she said and added that ease of communication with parents, especially mothers, was also an important factor. “When the young person had difficulties talking to their mother, the probability of suicidal thoughts increased five times. The ease of communication with parents was an important buffer in stopping suicidal thoughts,” Mark added.
Both being bullied and bullying was seen as a result of severe disturbances in mental well-being. Among boys, the biggest disturbances in well-being came from being bullied, girls were equally disturbed while being bullied and/or bullying. Among all groups, cyberbullying turned out to be the most devastating form of bullying.
The results show that risk behaviour should be seen as a sign of danger in the mental health of adolescents, not just a trait of their age. Especially in cases when risk behaviour tends to cumulate. “The risk behaviour of young people can and should be used to recognise and prevent possible mental health problems. In addition to minimising risk behaviour, bullying in schools must also be contained. Research-based methods of school bullying must be applied as soon as possible, continuously, and they should incorporate all parties of bullying (including onlookers),” Mark said.
The PhD thesis “Depressive Feelings and Suicidal Ideation Among Estonian Adolescents and Associations with Selected Risk Behaviours” was defended on 26 June at Tallinn University. The supervisors were Professors Airi Värnik and Merike Sisask from Tallinn University. The opponents were Professor Rory O’Connor from University of Glasgow and Associate Professor Dagmar Kutsar from University of Tartu.
You can access the full text of the thesis via the Tallinn University Academic Library e-vault ETERA .
Related news
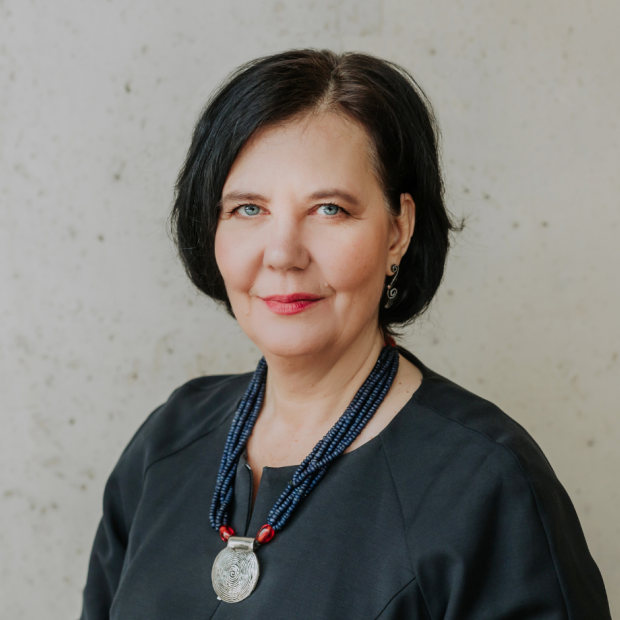
Press Release
Support groups for loved ones of people diagnosed with dementia are helpful in getting necessary information and social support for care
Merle Varik, from the School of Governance, Law and Society, defended her doctoral thesis on 22 February 2022. The thesis is entitled "Empowering Informal Caregivers of People with Dementia Through Su...

Doctoral studies
Doctoral dissertation: Participation in adult learning requires multilevel support – important is the role of the individual, the workplace as well as the country-specific institutions
On 28 January 2022 at 17.00, Eve-Liis Roosmaa from the School of Governance, Law and Society will defend her doctoral thesis “Adult education and training: a comparative perspective of participation p...

Doctoral thesis explores feminist threads in Johannes Semper’s oeuvre
On 24 January 2022 at 14.30, Merlin Kirikal from Tallinn University School of Humanities defends her doctoral thesis ““I broke open to life”: representing modern gender and bodies in Johannes Semper’s...
The results of doctoral thesis mapped coping mechanisms for academic burnout among middle school students in Estonia
At 10:00 on 16 December 2021, Kati Vinter, doctoral student of Tallinn University’s School of Educational Sciences, will defend her thesis “Academic burnout among middle-school students in Estonia: An...
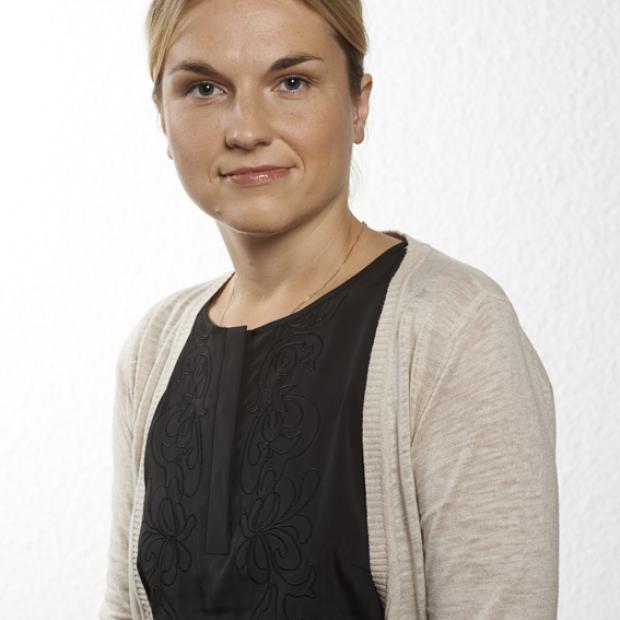
Doctoral dissertation: The mental health of children is influenced by the context and the ratings affected by the subjectivity of the assessor
At 16:00 on 15 December 2021, Karin Streimann from Tallinn University’s School of Governance, Law and Society will defend her doctoral thesis “Preventing Mental Health Problems in Elementary Schools: ...

Doctoral thesis: Research into creative thinking is vital for preservation of humanity
At 12 o’clock on 14 December 2021, Elen Lotman of Baltic Film, Media and Arts School will defend her doctoral thesis, "Experiential Heuristics in Fiction Film Cinematography“ („Kogemuslikud heuristika...
Finished Papers
Who is an essay writer? 3 types of essay writers
Will i get caught if i buy an essay.
The most popular question from clients and people on the forums is how not to get caught up in the fact that you bought an essay, and did not write it yourself. Students are very afraid that they will be exposed and expelled from the university or they will simply lose their money, because they will have to redo the work themselves.
If you've chosen a good online research and essay writing service, then you don't have to worry. The writers from the firm conduct their own exploratory research, add scientific facts and back it up with the personal knowledge. None of them copy information from the Internet or steal ready-made articles. Even if this is not enough for the client, he can personally go to the anti-plagiarism website and check the finished document. Of course, the staff of the sites themselves carry out such checks, but no one can forbid you to make sure of the uniqueness of the article for yourself.
Thanks to the privacy policy on web platforms, no one will disclose your personal data and transfer to third parties. You are completely safe from start to finish.
Original Drafts
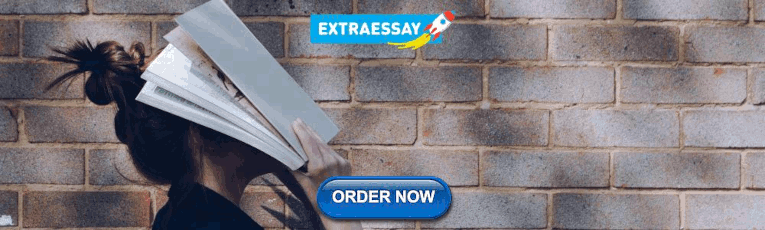
IMAGES
VIDEO
COMMENTS
To the Graduate Council: I am submitting herewith a dissertation written by Michelle Pearl Black entitled "Cyberbullying, Bullying, and Victimization among Adolescents: Rates of Occurrence, Internet Use and
Introduction. Bullying has been considered "one of the most outstanding topics in educational research" (Espinosa, Citation 2018), a public health problem among children and adolescents (Chester et al., Citation 2015), and also a reason for concern in schools and communities (Bradshaw, Citation 2015).According to the PISA 2018 report, on average, 23% of students reported being bullied at ...
bullying incident and 25% an educator2 (Patchin, 2018). Additionally, the Pew Research Center found that 60% of teenagers feel that parents are doing an excellent or good job in addressing cyberbullying — a statistic significantly higher than positive assessments of, for instance, social media companies (33%) or elected officials (20%) (Anderson,
Cyber bullying and sexting are new and emerging phenomena. There has been a number of news stories and police reports published about cyber incidents in different parts of the world. Research in the area of cyber bullying has utilized online, telephone, and in-person surveys. Cyber bullying studies conducted in the United States have
1. Introduction. Cyberbullying is defined as the electronic posting of mean-spirited messages about a person (such as a student) often done anonymously (Merriam-Webster, 2017).Most of the investigations of cyberbullying have been conducted with students in elementary, middle and high school who were between 9 and 18 years old.
THE RELATIONSHIP OF BULLYING AND CYBERBULLYING TO SOCIAL AND EMOTIONAL LEARNING AND THE IMPACT ON STUDENT ENGAGEMENT Sandy Larson, B.S., M.S. ... This dissertation would not be successful without you. A special "thank you" to Dr. Brad Hunt for all the encouragement and check ins! Lastly, to my UNT Cohort Family. ...
A second applied extension of our work is that parents, and other caregivers, can help reduce cyberbullying. Our central thesis is that cyberbullying is a learned behavior through the BGCM lens. Thus, parents, peers, school counselors, etc. can help reduce the likelihood of cyberbullying by disrupting the learning germane to cyberbullying.
In terms of descriptive statistics, the mean scores for cyber victimization at Time 1 ( x¯ = 13.26, SD=3.99) and at Time 2 ( x¯ = 13.24, SD = 3.84) were found to be higher than cyberbullying at Time 1 ( x¯ = 12.66, SD = 3.56) and at Time 2 ( x¯ = 12.49, SD = 3.72). There were significant positive moderate correlations between the variables ...
School bullying and cyberbullying are complex phenomena, and a range of methodological approaches is thus needed to understand their complexity (Pellegrini & Bartini, 2000; Thornberg, 2011). Indeed, over-relying on quantitative meth-ods limits understanding of the contexts and experiences of bullying (Hong & Espelage, 2012; Patton et al., 2017).
Dadvar M. Experts and machines united against cyberbullying [PhD thesis]. University of Twente. 2014. Magsi H, Agha N, Magsi I. Understanding cyber bullying in Pakistani context: causes and effects on young female university students in Sindh province. ... Mining Indonesian cyber bullying patterns in social networks, In: Proceedings of the ...
Cyberbullying (CB) is an electronic form of bullying [3, 4] ... Victor Chang received PhD in Computer Science from University of Southampton, UK. He is currently a Professor in Data Science and Information Systems at Teesside University, Middlesbrough, UK. He is the Conference Chair of 4 international conferences, Associate Editor of IEEE TII ...
While the prevalence of cyberbullying along with its effects have been researched and identified, the theoretical underpinnings for determining why young people engage in these behaviours has been under researched. ... PhD thesis, University of Glasgow. Full text available as: PDF Download (3MB) Printed Thesis Information: https://eleanor.lib ...
cyberbullying, in which individuals or groups of individuals use the media to inflict emotional distress on. other individuals (Bocij 2004). According to a rece nt study of 743 teenager s and ...
The Established and the Outsiders: Cyberbullying as an Exclusionary Process Cindy L. Corliss B.A, M.Ed, M.Sc Thesis Submitted in Fulfilment of the Degree of
Cyberbullying is a virtual form (e.g., textual or image) of bullying or harassment, also known as online bullying. Cyberbullying detection is a pressing need in today's world, as the prevalence of ...
Bullying can be in the forms of physical attacks, name-calling and more subtle. ways such as social isolation, direct bullying involving open attacks and threats. on a victim features the imbalance of power and aggressive nature of school. bullying, which may lead to more detrimental outcomes (p. 3). Bullying is often.
The analysis of variables about cyber bullying and the effect of an intervention program with tendency to reality therapy on cyber bullying behaviors. (Doctoral Dissertation). Sakarya University, Sakarya. [in Turkish] Topçu, Ç., & Erdur-Baker, Ö. (2016). Help seeking behaviors of bullying victims and resources for help.
One form of online misbehaviour which has deeply affected society with harmful consequences is known as cyberbullying. Cyberbullying can simply be defined as an intentional act that is conducted through digital technology to hurt someone. ... M3 - PhD Thesis - Research UT, graduation UT. SN - 978-90-365-3739-1. PB - University of Twente. CY ...
Cyberbullying, bullying via electronic media and communication, is on the increase. It has been thrust to the forefront of the public agenda, with concerns about the psychological and health impacts resulting from online victimisation. ... This dissertation proposes an alternate definition which does not rely upon repetition as a major ...
While bullying within schools has been around and studied over several decades, the emergence of cyberbullying is a relatively new cultural phenomenon. Since the term cyberbullying was first coined in 1999, society has become more attentive to the use and abuse of the internet and text messaging. However, a major problem that exists with
Chief Academic Officer and Provost Sue Subocz, Ph.D. Walden University 2020 Abstract. Teachers' Perceptions on Bullying and School Bullying Policy Enforcement. by. Angelena Elizabeth Clagon. Dissertation Submitted in Partial Fulfillment. of the Requirements for the Degree of. Doctor of Philosophy.
Research-based methods of school bullying must be applied as soon as possible, continuously, and they should incorporate all parties of bullying (including onlookers)," Mark said. The PhD thesis "Depressive Feelings and Suicidal Ideation Among Estonian Adolescents and Associations with Selected Risk Behaviours" was defended on 26 June at ...
Phd Thesis On Cyberbullying, Ceremony Silko Essays, Tribal Tourism Essay, Cite Paragraphs In Essay, Essay Tentang Diri Sendiri Untuk Kuliah, Aron Culotta Thesis, Custom Paper Editor For Hire Us. fpea-org. 4.7 stars - 1621 reviews. Phd Thesis On Cyberbullying -.