Advertisement
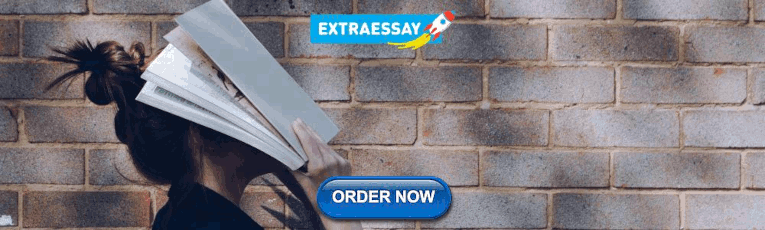
A survey of the literature: how scholars use text mining in Educational Studies?
- Published: 12 August 2022
- Volume 28 , pages 2071–2090, ( 2023 )
Cite this article
- Junhe Yang 1 , 2 ,
- Kinshuk 1 &
- Yunjo An 1
932 Accesses
3 Citations
1 Altmetric
Explore all metrics
The massive amount of text related to education provides rich information to support education in many aspects. In the meantime, the vast yet increasing volume of text makes it impossible to analyze manually. Text mining is a powerful tool to automatically analyze large-scaled texts and generate insights from the texts. However, many educational scholars are not fully aware of whether text mining is useful and how to use it in their studies. To address this problem, we reviewed the literature to examine the educational research that used text mining techniques. Specifically, we proposed an educational text mining workflow and focused on identifying the articles’ bibliographic information, research methodologies, and applications in alignment with the workflow. We selected 161 articles published in educational journals from 2015 to 2020. We find that text mining is becoming more popular and essential in educational research. The conclusion is that we can employ three steps (text source selection, text mining techniques application, and educational information discovery) to use text mining in educational studies. We also summarize different options in each step in this paper. Our work should help educational scholars better understand educational text mining and provide support information for future research in text mining for educational contexts.
This is a preview of subscription content, log in via an institution to check access.
Access this article
Price includes VAT (Russian Federation)
Instant access to the full article PDF.
Rent this article via DeepDyve
Institutional subscriptions
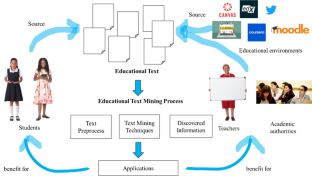
Similar content being viewed by others
Using Text Mining Techniques for Extracting Information from Research Articles
A Text Mining Based Literature Analysis for Learning Theories and Computer Science Education
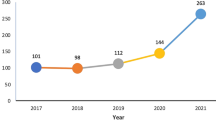
Text mining applied to distance higher education: A systematic literature review
Patrícia Takaki & Moisés Lima Dutra
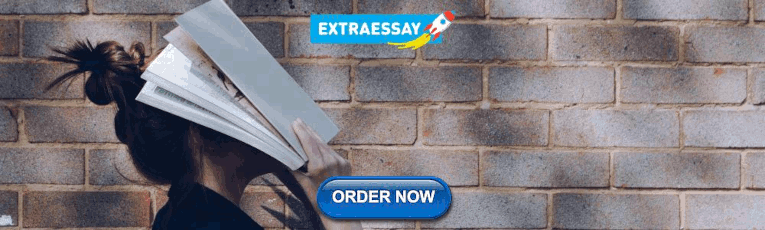
Data Availability
We make sure that all data and materials support our published claims and comply with field standards.
Akçapınar, G. (2015). How automated feedback through text mining changes plagiaristic behavior in online assignments. Computers & Education , 87 , 123–130. https://doi.org/10.1016/j.compedu.2015.04.007
Article Google Scholar
Arispe, M., Capucao, J., Relucio, F., & Maligat, D. E. Jr. (2019). Teachers’ sentiments to Bikol MTB-MLE: Using sentiment analysis and text mining techniques. International Journal of Research Studies in Education , 8 (4), 21–26. https://doi.org/10.5861/ijrse.2019.4906
Abuzir, Y. (2018). Innovative Model for Student Project Evaluation Based on Text Mining. International Journal of Research in Education and Science , 4 (2), 409–419. https://doi.org/10.21890/ijres.409481
Bayrak, T. (2020). A content analysis of top-ranked universities’ mission statements from five global regions. International Journal of Educational Development , 72 , 102130. https://doi.org/10.1016/j.ijedudev.2019.102130
Buenaño-Fernandez, D., González, M., Gil, D., & Luján-Mora, S. (2020). Text mining of open-ended questions in self-assessment of university teachers: An LDA topic modeling approach. Ieee Access : Practical Innovations, Open Solutions , 8 , 35318–35330
Bedenlier, S., Kondakci, Y., & Zawacki-Richter, O. (2018). Two decades of research into the internationalization of higher education: Major themes in the Journal of Studies in International Education (1997–2016). Journal of Studies in International Education , 22 (2), 108–135. https://doi.org/10.1177/1028315317710093
Baddam, S., Bingi, P., & Shuva, S. (2019). Student Evaluation of Teaching in Business Education: Discovering Student Sentiments Using Text Mining Techniques. e-Journal of Business Education and Scholarship of Teaching , 13 (3), 1–13
Google Scholar
Bozkurt, A., Koseoglu, S., & Singh, L. (2019). An analysis of peer reviewed publications on openness in education in half a century: Trends and patterns in the open hemisphere. Australasian Journal of Educational Technology , 35 (4), https://doi.org/10.14742/ajet.4252
Chong, S. W. (2019). A systematic review of written corrective feedback research in ESL/EFL contexts. Language Education & Assessment , 2 (2), 70–95. https://doi.org/10.29140/lea.v2n2.138
Chen, Z., Zhang, R., Xu, T., Yang, Y., Wang, J., & Feng, T. (2020). Emotional attitudes towards procrastination in people: A large-scale sentiment-focused crawling analysis. Computers in Human Behavior , 110 , 106391. https://doi.org/10.1016/j.chb.2020.106391
Chen, X., Zou, D., Cheng, G., & Xie, H. (2020). Detecting latent topics and trends in educational technologies over four decades using structural topic modeling: A retrospective of all volumes of Computers & Education. Computers & Education , 151 , 103855. https://doi.org/10.1016/j.compedu.2020.103855
Cronin, A., Intepe, G., Shearman, D., & Sneyd, A. (2019). Analysis using natural language processing of feedback data from two mathematics support centres. International Journal of Mathematical Education in Science and Technology , 50 (7), 1087–1103. https://doi.org/10.1080/0020739X.2019.1656831
Chee, K. N., Yahaya, N., Ibrahim, N. H., & Hasan, M. N. (2017). Review of mobile learning trends 2010- 2015: A meta-analysis. Journal of Educational Technology & Society , 20 (2), 113–126
Chung, K. S. K., & Paredes, W. C. (2015). Towards a social networks model for online learning & performance. Journal of Educational Technology & Society , 18 (3), 240–253
Çepni, S. B., & Demirel, E. T. (2016). Impact of Text-Mining and Imitating Strategies on Lexical Richness, Lexical Diversity and General Success in Second Language Writing. Turkish Online Journal of Educational Technology-TOJET , 15 (4), 61–68
Doleck, T., Basnet, R. B., Poitras, E. G., & Lajoie, S. P. (2015). Mining learner–system interaction data: implications for modeling learner behaviors and improving overlay models. Journal of Computers in Education , 2 (4), 421–447. https://doi.org/10.1007/s40692-015-0040-3
Elia, G., Solazzo, G., Lorenzo, G., & Passiante, G. (2019). Assessing learners’ satisfaction in collaborative online courses through a big data approach. Computers in Human Behavior , 92 , 589–599. https://doi.org/10.1016/j.chb.2018.04.033
Elena Gallagher, S., O’Dulain, M., O’Mahony, N., Kehoe, C., McCarthy, F., & Morgan, G. (2017). Instructor-provided summary infographics to support online learning. Educational Media International , 54 (2), 129–147. https://doi.org/10.1080/09523987.2017.1362795
Erkens, M., Bodemer, D., & Hoppe, H. U. (2016). Improving collaborative learning in the classroom: Text mining based grouping and representing. International Journal of Computer-Supported Collaborative Learning , 11 (4), 387–415. https://doi.org/10.1007/s11412-016-9243-5
Freak, A., & Miller, J. (2017). Magnifying pre-service generalist teachers’ perceptions of preparedness to teach primary school physical education. Physical Education and Sport Pedagogy , 22 (1), 51–70. https://doi.org/10.1080/17408989.2015.1112775
Fan, W., Wallace, L., Rich, S., & Zhang, Z. (2006). Tapping the power of text mining. Communications of the ACM , 49 (9), 76–82
Gašević, D., Joksimović, S., Eagan, B. R., & Shaffer, D. W. (2019). SENS: Network analytics to combine social and cognitive perspectives of collaborative learning. Computers in Human Behavior , 92 , 562–577. https://doi.org/10.1016/j.chb.2018.07.003
Geng, Z., Chen, G., Han, Y., Lu, G., & Li, F. (2020). Semantic relation extraction using sequential and tree-structured LSTM with attention. Information Sciences , 509 , 183–192
Gottipati, S., Shankararaman, V., & Lin, J. R. (2018). Text analytics approach to extract course improvement suggestions from students’ feedback. Research and Practice in Technology Enhanced Learning , 13 (1), 1–19
Gupta, V., & Lehal, G. S. (2009). A survey of text mining techniques and applications. Journal of emerging technologies in web intelligence , 1 (1), 60–76
Hotho, A., Nürnberger, A., & Paaß, G. (2005, May). A brief survey of text mining. In Ldv Forum (Vol. 20, No. 1, pp. 19–62)
Hyndman, B., Suesee, B., McMaster, N., Harvey, S., Jefferson-Buchanan, R., Cruickshank, V. … Pill, S. (2019). Physical education across the international media: A five-year analysis. Sport Education and Society . https://doi.org/10.1080/13573322.2019.1583640
Harvey, S., & Atkinson, O. (2017). One youth soccer coach’s maiden implementation of the tactical games model. Ágora para la Educación Física y el Deporte , 19 (2–3), 135–157
Howard, S. K., Yang, J., Ma, J., Maton, K., & Rennie, E. (2018). App clusters: Exploring patterns of multiple app use in primary learning contexts. Computers & Education , 127 , 154–164. https://doi.org/10.1016/j.compedu.2018.08.021
Hujala, M., Knutas, A., Hynninen, T., & Arminen, H. (2020). Improving the quality of teaching by utilizing written student feedback: A streamlined process. Computers & Education , 157 , 103965. https://doi.org/10.1016/j.compedu.2020.103965
Haynes, J. E., Miller, J. A., & Varea, V. (2016). Preservice generalist teachers enlightened approach to teaching physical education through teacher biography. Australian Journal of Teacher Education (Online) , 41 (3), 21–38. https://doi.org/10.14221/ajte.2016v41n3.2
Harvey, S., Curtner-Smith, M., & Kuklick, C. (2018). Influence of a models-based physical education teacher education program on the perspectives and practices of preservice teachers. Curriculum Studies in Health and Physical Education , 9 (3), 220–236. https://doi.org/10.1080/25742981.2018.1475246
Harvey, S., & Hyndman, B. (2018). An investigation into the reasons physical education professionals use Twitter. Journal of Teaching in Physical Education , 37 (4), 383–396. https://doi.org/10.1123/jtpe.2017-0188
Harvey, S., Pill, S., Hastie, P., & Wallhead, T. (2020). Physical education teachers’ perceptions of the successes, constraints, and possibilities associated with implementing the sport education model. Physical Education and Sport Pedagogy , 25 (5), 555–566. https://doi.org/10.1080/17408989.2020.1752650
Harvey, S., Carpenter, J. P., & Hyndman, B. P. (2020). Introduction to social media for professional development and learning in physical education and sport pedagogy. Journal of Teaching in Physical Education , 39 (4), 425–433
Intepe, G., & Shearman, D. (2020). Developing Statistical Understanding and Overcoming Anxiety via Drop-In Consultations.Statistics Education Research Journal, 19 (1)
Jo, T. (2019). Text mining. Studies in Big Data. Cham :. Springer International Publishing
Joo, S., & Cahill, M. (2018). Exploring research topics in the field of school librarianship based on text mining. School Libraries Worldwide , 24 (1), 15–28
Kim, D. H., & Pior, M. Y. (2018). A Study on the Mainstream of Real Estate Education with Core Term Analysis. Education Sciences , 8 (4), 182. https://doi.org/10.3390/educsci8040182
Koseoglu, S., & Bozkurt, A. (2018). An exploratory literature review on open educational practices. Distance education , 39 (4), 441–461. https://doi.org/10.1080/01587919.2018.1520042
Kagklis, V., Karatrantou, A., Tantoula, M., Panagiotakopoulos, C. T., & Verykios, V. S. (2015). A learning analytics methodology for detecting sentiment in student fora: A Case Study in Distance Education. European Journal of Open Distance and E-learning , 18 (2), 74–94
Liu, Q., Zhang, S., Wang, Q., & Chen, W. (2017). Mining online discussion data for understanding teachers reflective thinking. IEEE Transactions on Learning Technologies , 11 (2), 243–254
Martí-Parreño, J., Méndez‐Ibáñez, E., & Alonso‐Arroyo, A. (2016). The use of gamification in education: a bibliometric and text mining analysis. Journal of computer assisted learning , 32 (6), 663–676. https://doi.org/10.1111/jcal.12161
Machado, C. J. R., Maciel, A. M. A., Rodrigues, R. L., & Menezes, R. (2019). An approach for thematic relevance analysis applied to textual contributions in discussion forums. International Journal of Distance Education Technologies (IJDET) , 17 (3), 37–51
Ming, N. C., & Ming, V. L. (2015). Visualizing and Assessing Knowledge from Unstructured Student Writing.Technology, Instruction, Cognition & Learning, 10 (1)
Magnier-Watanabe, R., Watanabe, Y., Aba, O., & Herrig, H. (2017). Global virtual teams’ education: experiential learning in the classroom. On the Horizon , 25 (4), 267–285. https://doi.org/10.1108/OTH-02-2017-0007
Nuankaew, W., & Nuankaew, P. (2019). The study of the factors and development of educational model: The relationship between the learner context and the curriculum context in higher education. International Journal of Emerging Technologies in Learning (iJET) , 14 (21), 205–226
Okoye, K., Arrona-Palacios, A., Camacho-Zuñiga, C., Hammout, N., Nakamura, E. L., Escamilla, J., & Hosseini, S. (2020). Impact of students evaluation of teaching: a text analysis of the teachers qualities by gender. International Journal of Educational Technology in Higher Education , 17 (1), 1–27. https://doi.org/10.1186/s41239-020-00224-z
Okada, Y., Sawaumi, T., & Ito, T. (2017). Effects of Observing Model Video Presentations on Japanese EFL Learners’ Oral Performance.Electronic Journal of Foreign Language Teaching, 14 (2)
Poblete, C., Leguina, A., Masquiarán, N., & Carreño, B. (2019). Informal and non formal music experience: power, knowledge and learning in music teacher education in Chile. International Journal of Music Education , 37 (2), 272–285. https://doi.org/10.1177/0255761419836015
Park, A., Conway, M., & Chen, A. T. (2018). Examining thematic similarity, difference, and membership in three online mental health communities from Reddit: a text mining and visualization approach. Computers in human behavior , 78 , 98–112. https://doi.org/10.1016/j.chb.2017.09.001
Pei, B., Xing, W., & Lee, H. S. (2019). Using automatic image processing to analyze visual artifacts created by students in scientific argumentation. British Journal of Educational Technology , 50 (6), 3391–3404. https://doi.org/10.1111/bjet.12741
Peng, X., & Xu, Q. (2020). Investigating learners’ behaviors and discourse content in MOOC course reviews. Computers & Education , 143 , 103673. https://doi.org/10.1016/j.compedu.2019.103673
Pillutla, V. S., Tawfik, A. A., & Giabbanelli, P. J. (2020). Detecting the depth and progression of learning in massive open online courses by mining discussion data. Technology Knowledge and Learning , 25 (4), 881–898. https://doi.org/10.1007/s10758-020-09434-w
Poole, F., Clarke-Midura, J., Sun, C., & Lam, K. (2019). Exploring the pedagogical affordances of a collaborative board game in a dual language immersion classroom. Foreign Language Annals , 52 (4), 753–775
Romero, C., & Ventura, S. (2007). Educational data mining: A survey from 1995 to 2005. Expert systems with applications , 33 (1), 135–146. https://doi.org/10.1016/j.eswa.2006.04.005
Rodriguez-Andara, A., Río-Belver, R. M., Rodríguez-Salvador, M., & Lezama-Nicolás, R. (2018). Roadmapping towards sustainability proficiency in engineering education. International Journal of Sustainability in Higher Education , 19 (2), 413–438. https://doi.org/10.1108/IJSHE-06-2017-0079
Sukanya, M., & Biruntha, S. (2012, August). Techniques on text mining. In 2012 IEEE International Conference on Advanced Communication Control and Computing Technologies (ICACCCT) (pp. 269–271). IEEE. https://doi.org/10.1109/ICACCCT.2012.6320784
Song, D., Lin, H., & Yang, Z. (2007). Opinion mining in e-learning system. In 2007 IFIP international conference on network and parallel computing workshops (NPC 2007) (pp. 788-792). IEEE. https://doi.org/10.1109/NPC.2007.51
Sumathy, K. L., & Chidambaram, M. (2013). Text mining: concepts, applications, tools and issues-an overview.International Journal of Computer Applications, 80 (4)
Stupans, I., McGuren, T., & Babey, A. M. (2016). Student evaluation of teaching: A study exploring student rating instrument free-form text comments. Innovative Higher Education , 41 (1), 33–42. https://doi.org/10.1007/s10755-015-9328-5
Shen, W., & Zhang, S. (2018). Emotional Tendency Dictionary Construction for College Teaching Evaluation. International Journal of Emerging Technologies in Learning , 13 (11), https://doi.org/10.3991/ijet.v13i11.9605
Schiller, S. Z. (2016). CHAT for chat: Mediated learning in online chat virtual reference service. Computers in Human Behavior , 65 , 651–665. https://doi.org/10.1016/j.chb.2016.06.053
Tan, A. H. (1999, April). Text mining: The state of the art and the challenges. In Proceedings of the pakdd 1999 workshop on knowledge disocovery from advanced databases (Vol. 8, pp. 65–70). sn
Tseng, W. T. (2020). Mining Text in Online News Reports of COVID-19 Virus: Key Phrase Extractions and Graphic Modeling. English Teaching & Learning , 1–11. https://doi.org/10.1007/s42321-020-00070?2
Tawfik, A. A., Law, V., Ge, X., Xing, W., & Kim, K. (2018). The effect of sustained vs. faded scaffolding on students’ argumentation in ill-structured problem solving. Computers in Human Behavior , 87 , 436–449. https://doi.org/10.1016/j.chb.2018.01.035
Takagi, D., Hayashi, M., Iida, T., Tanaka, Y., Sugiyama, S., Nishizaki, H., & Morimoto, Y. (2019). Effects of dental students’ training using immersive virtual reality technology for home dental practice. Educational Gerontology , 45 (11), 670–680. https://doi.org/10.1080/03601277.2019.1686284
Tao, Y., & Xie, M. (2019). Technical Writing as a Supplement. In Restructuring Translation Education (pp. 145–156) . Springer, Singapore
Wang, Y., & Fikis, D. J. (2019). Common core state standards on Twitter: Public sentiment and opinion leaders. Educational Policy , 33 (4), 650–683. https://doi.org/10.1177/0895904817723739
Wang, S. (2017). Determinants of mobile apps downloads: A systematic literature review. In The European Conference on Information Systems Management (pp. 353–360). Academic Conferences International Limited
Wu, J. Y., Hsiao, Y. C., & Nian, M. W. (2020). Using supervised machine learning on large-scale online Forums to classify course-related Facebook messages in predicting learning achievement within the personal learning environment. Interactive Learning Environments , 28 (1), 65–80. https://doi.org/10.1080/10494820.2018.1515085
Wu, P., Yu, S., & Wang, D. (2018). Using a Learner-Topic Model for Mining Learner Interests in Open Learning. Educational Technology & Society , 21 (2), 192–204
Wu, F., & Lai, S. (2019). Linking prediction with personality traits: a learning analytics approach. Distance Education , 40 (3), 330–349. https://doi.org/10.1080/01587919.2019.1632170
Wook, M., Razali, N. A. M., Ramli, S., Wahab, N. A., Hasbullah, N. A., Zainudin, N. M., & Talib, M. L. (2019). Opinion mining technique for developing student feedback analysis system using lexicon- based approach (OMFeedback). Education and Information Technologies , 1–12. https://doi.org/10.1007/s10639-019-10073-7
Wook, M., Razali, N. A. M., Ramli, S., Wahab, N. A., Hasbullah, N. A., Zainudin, N. M., & Talib, M. L. (2020). Opinion mining technique for developing student feedback analysis system using lexicon-based approach (OMFeedback). Education and Information Technologies , 25 (4), 2549–2560
Xing, W., & Gao, F. (2018). Exploring the relationship between online discourse and commitment in Twitter professional learning communities. Computers & Education , 126 , 388–398. https://doi.org/10.1016/j.compedu.2018.08.010
Xie, K., Di Tosto, G., Lu, L., & Cho, Y. S. (2018). Detecting leadership in peer-moderated online collaborative learning through text mining and social network analysis. The Internet and Higher Education , 38 , 9–17. https://doi.org/10.1016/j.iheduc.2018.04.002
Yim, S., & Warschauer, M. (2017). Web-based collaborative writing in L2 contexts: Methodological insights from text mining. Language Learning & Technology , 21 (1), 146–165
Zanini, N., & Dhawan, V. (2015). Text Mining: An introduction to theory and some applications. Research Matters , 19 , 38–45
Zawacki-Richter, O., & Latchem, C. (2018). Exploring four decades of research in Computers & Education. Computers & Education , 122 , 136–152. https://doi.org/10.1016/j.compedu.2018.04.001
Zheng, J., Xing, W., & Zhu, G. (2019). Examining sequential patterns of self-and socially shared regulation of STEM learning in a CSCL environment. Computers & Education , 136 , 34–48. https://doi.org/10.1016/j.compedu.2019.03.005
Zuo, Z., Zhao, K., & Eichmann, D. (2017). The state and evolution of US iSchools: From talent acquisitions to research outcome. Journal of the Association for Information Science and Technology , 68 (5), 1266–1277. https://doi.org/10.1002/asi.23751
Zhang, K. (2015). Mining data from Weibo to WeChat: A comparative case study of MOOC communities on social media in China. International Journal on E-Learning , 14 (3), 305–329
Download references
Not applicable.
Author information
Authors and affiliations.
Department of Learning Technologies, University of North Texas, Denton, TX, USA
Junhe Yang, Kinshuk & Yunjo An
Department of Learning Technologies, University of North Texas, 3940 N. Elm St., Suite G150, UNT College of Information, Denton, TX, 76207, USA
You can also search for this author in PubMed Google Scholar
Corresponding author
Correspondence to Junhe Yang .
Ethics declarations
Conflict of interest.
The author declares no competing interests.
Additional information
Publisher’s note.
Springer Nature remains neutral with regard to jurisdictional claims in published maps and institutional affiliations.
Rights and permissions
Reprints and permissions
About this article
Yang, J., Kinshuk & An, Y. A survey of the literature: how scholars use text mining in Educational Studies?. Educ Inf Technol 28 , 2071–2090 (2023). https://doi.org/10.1007/s10639-022-11193-3
Download citation
Received : 05 September 2021
Accepted : 28 June 2022
Published : 12 August 2022
Issue Date : February 2023
DOI : https://doi.org/10.1007/s10639-022-11193-3
Share this article
Anyone you share the following link with will be able to read this content:
Sorry, a shareable link is not currently available for this article.
Provided by the Springer Nature SharedIt content-sharing initiative
- Educational text mining
- Literature review
- Educational technology
- Find a journal
- Publish with us
- Track your research
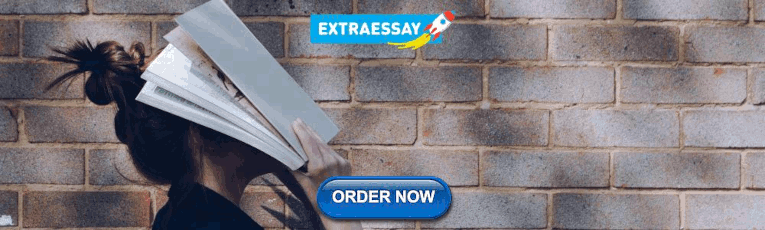
IMAGES
VIDEO
COMMENTS
analysis of text, extraction o f information, categorization, clustering, visualization, mining of data, and machine. learning. There are five basic text mining steps as under: Text mining steps ...
The book starts with a gentle introduction to text mining that presents the basic definitions and prepares the reader for the next chapters. In the second chapter we describe the core text mining operations in detail while providing examples for each operation. The third chapter serves as an introduction to text mining preprocess-ing techniques.
Therefore, this thesis aims to achieve two objectives: 1) constructing a text-mining pipeline that extracts solid-state synthesis datasets from scientific papers, and 2) imple- menting an interpretable machine-learning method to predict solid-state synthesis condi-
ABSTRACT. The objective of this thesis is to develop efficient text classification models to. classify text documents. In usual text mining algorithms, a document is represented as. a vector whose dimension is the number of distinct keywords in it, which can be very. large.
tion of a text, such as an interest rate announcement, before making it public. The remainder of the paper is organized as follows. The next section covers theoretical background behind text analysis and interpretation of text. Section3describes text extraction and Section4presents methodologies for cleaning and storing text data for text ...
Text mining is the task of extracting meaningful information from text, which has gained significant attentions in recent years. In this paper, we describe several of the most fundamental text mining tasks and techniques including text pre-processing, classification and clustering.
Text mining techniques that rely on word frequency counts to measure contextual, psychological, linguistic, or semantic concepts and constructs are among the most widely adopted approaches for computer-aided analysis of textual data in management-related research so far (Duriau et al., 2007; Short et al., 2010).
Text Summarization: Another common function needed in many text mining applications is to summarize the text documents in order to ob-tain a brief overview of a large text document or a set of documents on a topic. Summarization techniques generally fall into two categories. In extractive summarization, a summary consists of information units ex-
Effectively searching, managing and exploring the text data has become a main task. In this paper, we first represent an introduction to text mining and a probabilistic topic model Latent ...
What is Text Mining? • It is also referred as text data mining and is roughly equivalent to text analytics. • Process of extracting interesting and non-trivial knowledge from unstructured text. • Text analysis also involves following: • information retrieval • lexical analysis to study word frequency distribution
of 'analysing', as the aim is to draw useful information from the text data in order to build up new knowledge. To do this, given that data are now in a structured form, it is possible to make use of standard statistical procedures and techniques applied to text data that are now in structured form.3 Applications of Text Mining
The state-of-the-art text mining approaches and techniques used for analyzing transcripts and speeches, meeting transcripts, and academic journal articles, as well as websites, emails, blogs, and social media platforms, are investigated. Text mining in big data analytics is emerging as a powerful tool for harnessing the power of unstructured textual data by analyzing it to extract new ...
Text mining - a field located at the intersection of computer and information science, mathematics, and (computational) linguistics - promises not only to analyze large text corpora efficiently, but also to do so in a transparent and reproducible manner (Humphreys and Wang, 2018). As such, text min-
References (24) ... termed as Text Mining (Anisha et al., 2021) .Web scraping is an application of text mining, it is a technique for extracting useful data from huge amounts of data available on ...
This hands-on guide to text analytics using SAS provides detailed, step-by-step instructions and explanations on how to mine your text data for valuable insight, and how to collect, cleanse, organize, categorize, explore, and interpret it. Big data: It's unstructured, it's coming at you fast, and there's lots of it. In fact, the majority of big data is text-oriented, thanks to the ...
Text mining has been a powerful tool for information extraction, classification and prediction in multiple field. This thesis prototyped several text mining tools and their application in the sarcoma research field. Specifically, text data are fetched from PubMed around the topic of Sarcoma.
This requires exploitation of text mining capabilities. This thesis aims to investigate results of text classification applied on functional software requirements by creating a framework in R and making use of algorithms and techniques like k-nearest neighbors, support vector machine, and many others like boosting, bagging, maximum ...
Text mining is a data analysis technique that involves exploring and examining extensive amounts of unstructured text data. This process is facilitated by specialized software that can efficiently ...
Keywords: data mining, data and information extraction, full text articles, abstracts, postgraduate students, Nigeria. 1. Introduction. The burden of the postgraduate programme and the necessity to generate new knowledge, innovations, and research have brought the prerequisite for scholarly and scientific research to a mainstay.
Text mining is a powerful tool to automatically analyze large-scaled texts and generate insights from the texts. ... types of documents (such as conference proceedings, books, and thesis, etc.) offer valuable information as well, academic journal articles are considered as the high-quality and reliable resource for scholars. The language of the ...
The primary goals of this research are (1) Using text mining techniques for. identifying the topics of a scienti fic text related to ML research and developing a. hierarchical and evolutionary ...
Introduction: There's more to text mining than "Siri, find the good stuff for me." 3 Step 1: Diagnose and define the problem 4 Step 2: Figure out how text mining can help 4 Step 3: Communicate the goal and set expectations 5 Step 4: Make detailed plans 6 Step 5: Establish and maintain technical parameters 7