- Open access
- Published: 06 June 2016
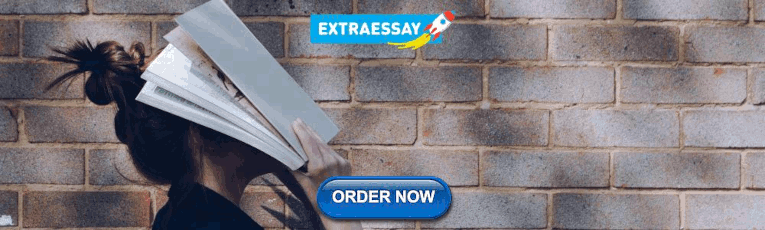
Operational research as implementation science: definitions, challenges and research priorities
- Thomas Monks 1
Implementation Science volume 11 , Article number: 81 ( 2015 ) Cite this article
31k Accesses
34 Citations
16 Altmetric
Metrics details
Operational research (OR) is the discipline of using models, either quantitative or qualitative, to aid decision-making in complex implementation problems. The methods of OR have been used in healthcare since the 1950s in diverse areas such as emergency medicine and the interface between acute and community care; hospital performance; scheduling and management of patient home visits; scheduling of patient appointments; and many other complex implementation problems of an operational or logistical nature.
To date, there has been limited debate about the role that operational research should take within implementation science. I detail three such roles for OR all grounded in upfront system thinking: structuring implementation problems, prospective evaluation of improvement interventions, and strategic reconfiguration. Case studies from mental health, emergency medicine, and stroke care are used to illustrate each role. I then describe the challenges for applied OR within implementation science at the organisational, interventional, and disciplinary levels. Two key challenges include the difficulty faced in achieving a position of mutual understanding between implementation scientists and research users and a stark lack of evaluation of OR interventions. To address these challenges, I propose a research agenda to evaluate applied OR through the lens of implementation science, the liberation of OR from the specialist research and consultancy environment, and co-design of models with service users.
Operational research is a mature discipline that has developed a significant volume of methodology to improve health services. OR offers implementation scientists the opportunity to do more upfront system thinking before committing resources or taking risks. OR has three roles within implementation science: structuring an implementation problem, prospective evaluation of implementation problems, and a tool for strategic reconfiguration of health services. Challenges facing OR as implementation science include limited evidence and evaluation of impact, limited service user involvement, a lack of managerial awareness, effective communication between research users and OR modellers, and availability of healthcare data. To progress the science, a focus is needed in three key areas: evaluation of OR interventions, embedding the knowledge of OR in health services, and educating OR modellers about the aims and benefits of service user involvement.
Peer Review reports
Operational research (OR) is the discipline of using models, either quantitative or qualitative, to aid decision-making in complex problems [ 1 ]. The practice of applied healthcare OR distinguishes itself from other model-based disciplines such as health economics as it is action research based where operational researchers participate collaboratively with those that work in or use the system to define, develop, and find ways to sustain solutions to live implementation problems [ 2 ]. The methods of OR have been used in healthcare since the 1950s [ 3 ] to analyse implementation problems in diverse areas such as emergency departments [ 4 – 6 ] and management policies for ambulance fleet [ 7 ]; acute stroke care [ 8 – 11 ], outpatient clinic waiting times [ 12 ], and locations [ 13 ]; cardiac surgery capacity planning [ 14 ]; the interface between acute and community care [ 15 ]; hospital performance [ 16 ]; scheduling and routing of nurse visits [ 17 ]; scheduling of patient appointments [ 18 ]; and many other complex implementation problems of an operational or logistical nature.
Implementation science is the study of methods to increase the uptake of research findings in healthcare [ 19 ]. Given the volume of OR research in healthcare implementation problems, it is remarkable that limited discussion of the discipline has occurred within the implementation science literature. A rare example of debate is given by Atkinson and colleagues [ 20 ] who introduce the notion of system science approaches for use in public health policy decisions. Their argument focused on two modelling methods, system dynamics and agent-based simulation, and the potential benefits they bring for disinvestment decisions in public health. To complement and extend this debate, I define the overlap between implementation science and OR. I have focused on the upfront role that OR takes when used as an implementation science tool. Although some detail of method is given, the full breath of OR is beyond the scope of this article; a detailed overview of all the methods can be found elsewhere [ 21 ]. I describe three roles for OR within implementation science: structuring an implementation problem, prospective evaluation of an intervention, and strategic reconfiguration of services. For each role, I provide a case study to illustrate the concepts described. I then describe the challenges for OR within implementation science at the organisational, interventional, and disciplinary levels. Given these challenges, I derive a research agenda for implementation science and OR.
OR to structure an implementation problem
The first role for OR in implementation science is to provide a mechanism for structuring an implementation problem. Within OR, problem structuring methods provide participatory modelling approaches to support stakeholders in addressing problems of high complexity and uncertainty [ 22 ]. These complex situations are often poorly defined and contain multiple actors with multiple perspectives and conflicting interests [ 23 ]. As such, they are unsuitable for quantitative approaches. Problem structuring methods aim to develop models that enable stakeholders to reach a shared understanding of their problem situation and commit to action(s) that resolve it [ 23 ]. Approaches might serve as a way to clearly define objectives for a quantitative modelling study [ 24 ], systematically identify the areas to intervene within a system [ 25 ], or may be an intervention to improve a system in its own right.
A case example—understanding patient flow in the mental health system
A mental health service provider in the UK provided treatment to patients via several specialist workforces. Here, I focus on two: psychology and psychiatric talking therapies (PPT) and recovering independent life (RIL) teams. Waiting times to begin treatment under these services were high (e.g. for RIL team median = 55 days, inter-quartile range = 40–95 days), and treatment could last many years once it had begun. The trust’s management team were eager to implement new procedures to help staff manage case load and hence reduce waiting times to prevent service users, here defined as patients, their families, and carers, from entering a crisis state due to diminishing health without treatment. Management believed that reasons for delays were more complex than lack of staff, but the exact details were unclear and there was much disagreement between the senior management. The implementation science intervention I detail was conducted as an OR problem structuring exercise.
A system dynamics (SD) model was constructed to aid management target their interventions. SD is a subset of system thinking—the process of understanding how things within a system influence one another within the whole. SD models can be either qualitative or quantitative. In this case, a purely qualitative model was created. Figure 1 illustrates stock and flow notation that is commonly used in SD. The example is the concept of a simple waiting list for a (generic) treatment. It can be explained as follows. General practitioners (GPs) refer service users to a waiting list at an average daily rate, while specialist clinicians treat according to how much daily treatment capacity they have. The variable waiting list is represented as a rectangular stock: an accumulation of patients. The waiting list stock is either depleted or fed by rate variables, referring and treating, represented as flows (pipes with valves) entering and leaving the stock. Figure 1 also contains two feedback loops that are illustrated by the curved lines. The first loop is related to the GP reluctance to refer to a service with a long waiting time. As the waiting list for a service increases in number, so does the average waiting time of service users and so does the pressure for GPs to consider an alternative service (lowering the daily referral rate). The second loop is related to specialist clinicians reacting to long waiting lists by creating a small amount of additional treatment capacity and increasing admission rates.
Example system thinking for a waiting list—stock and flow notation. Notation guide. Rectangles represent stocks which are acculations of quantity of interest; Pipes with valves represent flows which feed or deplete stocks; arrows represent how one aspect of a system positively or negatively influences another
A preliminary version of the SD model was created using a series of interviews with clinicians and managers from the three services. This was followed by a group model building workshop that involved all senior management. Group model building is a structured process that aims to create a shared mental model of a problem [ 26 ]. The workshop began with a nominal group exercise. The group were asked to individually write down what they believed were the key factors that affected patient waiting times. The group were specifically asked to focus on strategic issues as opposed to detailed process-based problems. After all individual results had been shared, the group were asked to (i) hypothesise how these factors influenced each other and (ii) propose any missing variables that may mediate influence. For example, available treatment capacity is reduced by non-clinical workload. Non-clinical workload is increased by several other factors (discussed below in results) and so on.
Figure 2 illustrates one of the qualitative SD models developed in collaboration with the mental health trust. It uses the same stock and flow notation illustrated in Fig. 1 . The model shown is focussed on the RIL teams. Several insights were gained in its construction. First, it was clear to all parties that that this was not a simple demand and treatment capacity problem. For example, a great deal of non-core work takes place due to monitoring of ‘discharged’ service users within social care. The fraction of service users who undergo monitoring is determined by the degree of trust between clinicians and social care teams. When trust is low, the fraction of service users monitored increases and vice versa. A similar soft issue can be found in the discharge of complex patients, i.e. those that require a combination of medication, management by GPs in the community, and social care input. In this case, there is a delay while GPs build confidence that it is appropriate for a patient to be discharged into their care. While this negotiation takes place, a patient still requires regular monitoring by a mental health clinician. Other systemic issues are also visible. For example, the long delays in beginning treatment lead to clinicians spending time contacting patients by phone before they were admitted. This all takes time and reinforces the delay cycle.
A simplified version of the RIL team patient flow model
The results of the modelling were used to inform where interventions could be targeted. For example, a more detailed qualitative SD study to identify the trust issues between clinicians, social services, and general practitioners.
OR as a tool for prospective evaluation
The second role of OR within implementation science is as a prospective evaluation tool. That is, to provide a formal assessment and appraisal of competing implementation options or choices before any actual implementation effort, commitment of resources or disinvestment takes place. Informally, this approach is often called what-if analysis [ 21 ]. A mathematical or computational model of a healthcare system is developed that predicts one or more measures of performance, for example, service waiting times, patients successfully treated, avoided mortality, or operating costs. The model can be set up to test and compare complex interventions to the status-quo. For example, decision makers may wish to compare the number of delayed transfers of care in a rehabilitation pathway before and after investment in services to prevent hospital admissions and disinvestment in rehabilitation in-patient beds. The approach has been applied widely in the areas outlined in the introduction to this article.
A case example—emergency medicine capacity planning
As a simple case example of prospective evaluation, consider the emergency department (ED) overcrowding problems faced by the United Kingdom’s (UK) National Health Service (NHS). The performance of NHS EDs is (very publically) monitored by recording the proportion of patients who can be seen and discharged from an ED within 4 hours of their arrival. The UK government has set a target that 95 % of service users must be processed in this time. In recent years, many NHS EDs have not achieved this benchmark. The reasons for this are complex and are not confined to the department [ 27 ] or even the hospital [ 15 ]. However, given the high public interest, many EDs are attempting to manage the demands placed on them by implementing initiatives to reduce waiting times and optimise their own processes.
Our case study took place at a large ‘underperforming’ hospital in the UK. The management team were divided in their view about how to reduce waiting times. One option was to implement a clinical decision-making unit (CDU). A CDU is a ward linked to the ED that provides more time for ED clinicians to make decisions about service users with complex needs. However, at times of high pressure, a CDU can also serve as buffer capacity between the ED and the main hospital. That is, a CDU provides space for service users at risk of breaching the 4-h target once admitted; service users are no longer at risk of breach. The question at hand was if a CDU were implemented, how many beds are required in order for the ED to achieve the 95 % benchmark?
Figure 3 illustrates the logic of a computer simulation model that was developed to evaluate the implementation of a CDU on ED waiting times. A computer simulation model is a simplified dynamic representation of the real system that in most cases is accompanied by an animation to help understanding. In this case, the simulation mimicked the flow of patients into an ED, their assessment, and treatment by clinicians and then flow out to different parts of the hospital or to leave the hospital entirely. The scope of the modelling included the hospital’s Acute Medical Unit (AMU) that admits medical patients from the ED. In Fig. 3 , the rectangular boxes represent processes, for example, assessment and treatment in the ED. The partitioned rectangles represent queues, for example, patient waiting for admission to the AMU. The model was set up to only admit patients to the CDU who had been in ED longer than 3.5 h and only then if there was a free bed. Once a patient’s CDU stay was complete, they would continue on their hospital journey as normal, i.e. discharged home, admitted to the AMU or admitted to another in-patient ward.
Emergency department and clinical decision-making unit model. Notation guide. Rectangles represent processes; partitioned rectangles represent queues; ellipses represent start and end points; arrows represent the direction of patient flow
In the model, the various departments and wards are conceptualised as stochastic queuing systems subject to constraints. This means that the variability we see in service user arrival and treatment rates (e.g. sudden bursts in arrivals combined with more complex and hence slower treatments) combined with limited cubicle and bed numbers results in queues. There are three reasons why prospective evaluation is appropriate for these systems. First, capacity planning for such complex systems based on average occupancy fails to take queuing into account and will substantially underestimate capacity requirements [ 28 ]. Second, the processing time, i.e. the time taken to transfer a patient to a ward and then to make a clinical decision, within a CDU is uncertain, although it is likely to be slower than the high pressure environment of the ED. Third, as the same ED and AMU clinicians must staff the CDU, the (negative or positive) impact on their respective processing times is uncertain.
The model developed was a discrete-event simulation [ 29 ] that mimics the variation in service user arrival and treatment rates in order to predict waiting times. The uncertainty in CDU processing time was treated as an unknown and varied in a sensitivity analysis . The limits of this analysis were chosen as 2 and 7 h on average, as these were observed in similar wards elsewhere.
The model predicted that the number of CDU beds would need to be between 30 and 70 in order to achieve the ED target (for reference, the ED had 10 cubicles for minor cases and 18 cubicles for major cases). This result illustrated that even if a decision was made in 2 h on average with no negative effect on ED or AMU processing time, the CDU would need to be at least the same size as the ED overall. It also highlighted that the CDU impact on ED performance was highly sensitive to processing time.
The benefit of evaluating the CDU implementation upfront was that it ruled the CDU out as a feasible intervention before any substantial resource had been mobilised to implement it. The hospital could not safely staff a 30-bedded CDU or indeed provide space for that size of ward. As such, the modelling helped the management team abandon their CDU plan and consider alternative solutions with minimal cost and no disruption to the service.
OR as a tool for strategic reconfiguration
The previous section described an implementation science approach to evaluate a small number of competing options at an operational level. In some instances, particularly in healthcare logistics and estate planning, a more strategic view of a system is needed to shortlist or choose options for reconfiguration. In such implementation problems, there may be a large number of options reaching into the hundreds, if not hundreds of thousands of competing alternatives. To analyse these problems, mathematical and computational optimization techniques are required. For example, if a provider of sexual health services wanted to consolidate community clinics from 50 to 20 and there are 100 candidate locations, then there are in the order of 10 20 configurations to consider. OR’s implementation science role is to provide tools that identify options that help meet a strategic objective. For example, this might be maintaining equitable patient access to services across different demographics groups or modes of transportation while increasing service quality and reducing cost.
A case example—where should TIA outpatient clinics be located?
As a simple exemplar, consider a rural region in the UK that provided a 7-day transient ischemic attack (TIA) service through outpatient clinics in the community. Clinics ran at five locations but with only one location open per day. Magnetic resonance imaging (MRI) was available at three locations. Service users attending clinics without imaging but who require access to an MRI make an additional journey to the closest location with imaging capacity.
Service users are booked into clinic appointments across the week as they are referred to the TIA service by their diagnosing clinician, typically the patients local GP or an attending emergency department physician. The diagnosing clinician risk stratifies service users as high or low risk of a major stroke. High-risk service users require to be seen within 24 h of symptom onset and low-risk patients within 7 days [ 30 ].
The healthcare providers had concerns that splitting the clinics across five sites increased the variation in care received by service users and wished to consolidate to one to three clinic locations. Hence, there were two complicating factors when assessing equitable access: how many locations and which ones. There were also concerns that one location—clinic X—on the coast of the region was extremely difficult for high-risk TIAs to reach on the same day as diagnosis. There would also be political implications for any closure at clinic X. In total, there were 25 combinations of clinics for the providers to consider for both the low- and high-risk TIA groups, i.e. 50 options to review.
A discrete-choice facility location model was developed to evaluate the consequences of different TIA clinic configurations and inform the decision-making process for the reconfiguration of the service. Location analysis is a specialised branch of combinatorial optimisation and involves solving for the optimal placement of a set of facilities in a region in order to minimise or maximise a measure of performance such transportation costs, travel time, or population coverage [ 31 ]. In this, case an analysis was conducted separately for high-risk and low-risk TIAs. The analysis of high-risk TIAs aimed to minimise the maximum travel time of a service user from their home location to the closest clinic (as these service users must be seen the same day). The low-risk analysis minimised the weighted average travel time to their closest clinic. The weighted average measure allows for locations with the highest level of demand to have the greatest impact on results, diminishing the impact of outlying points. In general, if there are n demand locations and on a given day the travel time x from locations i to the nearest clinic, then the weighted average travel \( \overline{x} \) time is given by the simple formula depicted in Eq. ( 1 ). Table 1 illustrates the use of the equation with two fictional locations. For each location, the number of patients who travel and the travel time for patients to a hospital is given. In the table, the weighted average is compared to the more familiar mean average.
The model demonstrated that clinics most central to the region were all good choices to provide equitable patient access. A three-clinic solution provided the most equitable solution for service users. The problematic clinic X on the coast of the region was not included in an optimal configuration; however, it could be included in a three-clinic solution without substantial effect on travel times if scheduled infrequently. This latter result allowed the decision makers to move on from the strategic debate about location and focus on the more detailed implementation issues of scheduling and capacity planning for clinics. This was again addressed upfront using a computer simulation study to evaluate a small number of competing options for scheduling the clinics.
Lessons for implementation science
Each of the three roles emphasises the use of OR to conduct implementation science upfront before any action to alter a care pathway or service has been taken. Many OR scholars argue that the benefit of constructing a model upfront is that it forces decision makers to move from a world of imprecise language to a world of a precise language (sometimes referred to as a common language [ 32 ]) and ultimately develop a shared understanding of the problem; although as I will argue later, there is very limited empirical evidence supporting this proposition. Such a shared understanding increases the likelihood if implementation will actually go ahead and importantly if it will be sustained or normalised.
It is important to emphasise that the three case studies illustrate the simpler end of what can be achieved in using OR for upfront implementation science. This is partly a stylistic choice in order to aid reader understanding, for example, many optimisation problems are hugely complex, but also because in my experience simpler models tend to be accepted and used more in healthcare. Simpler models also need less input data and hence can be built and run quickly.
Along with the three case studies, OR is in general grounded in the use of models to improve upfront decision-making in complex implementation problems. Although there is a significant overlap between OR and implementation research, there are differences. For example, OR would not provide the rich contextual information collected in a process evaluation.
Implementation science challenges for OR
Implementation science poses a number of challenges for OR. I propose that these lie at three levels: disciplinary, organisational, and interventional. Table 2 summarises these key challenges.
Challenges at a disciplinary level
This article describes three roles for OR within implementation science. An irony is that OR interventions themselves are poorly understood with barely any published evaluation of practice or impact [ 33 – 36 ]. Limited examples can be found in Monks et al. [ 37 ], Pagel et al. [ 38 ], and Brailsford et al. [ 39 ]. The explanation for this can be found at a disciplinary level. That is, academic OR is predominately driven and rewarded by the development of theory for modelling methodology as opposed to understanding interventions and the issues they raise for practice. As such, a discipline that promotes the use of evidence for decision-making in healthcare cannot confidently answer the question does OR in health work ? I am regularly challenged on this point by healthcare professionals.
A second disciplinary challenge is to systematically involve service users in the co-design of OR interventions. To date, evidence of service user involvement is limited (see Walsh and Holstick [ 40 ] for an example). There is also confusion between service users framed as research participants (typically treated as a data source to parameterise models with behavioural assumptions) and co-designers of research objectives and methods, although there has been an effort to clarify the important difference [ 41 ].
Challenges at the organisational level
The three roles of OR outlined above are widely applicable across healthcare implementation problems. However, before OR can be used within practice, users of the research, in this case, healthcare managers, clinicians and service users, must be aware of the approaches. This is currently a substantial barrier to a wide scale adoption in health services [ 42 – 44 ] and stands in stark contrast to domains such as manufacturing and defence where it is used frequently to generate evidence before action [ 45 ]. The implication of low awareness of OR in health is that it is often difficult to engage senior decision makers in the complex operational and logistical problems that matter the most for service users.
Challenges at an interventional level
Fifty years ago, Churchman and Schainblatt [ 46 ] wrote about a ‘dialectic of implementation’ in the journal management science. In this paper, the two authors advocated that a position of mutual understanding between a researcher and manager was necessary in order to implement results of a study. That is, the researcher must understand the manager’s position, values, and implementation problem in order to tackle the correct problem in the right way. The manager must understand the method that the researcher has applied, at least at the conceptual level, in order to scrutinise, challenge, and implement results. The concept of mutual understanding is an elegant one, but in practice, achieving it is a challenge for both sides. As a simple example from a researcher perspective, it is difficult to assess if the users of a model understand why a model is producing certain results [ 42 ]. That is, do users understand how the model works or are they simply accepting the results based on some heuristic, such as ‘these are the results I want’ or ‘I trust the person telling me the results’? Given the disciplinary challenge outlined above, to date, there is limited validated guidance about how to manage such complex interventions within OR.
The computer software used in the three case studies have been available for considerable time, but appropriate data to parameterise the quantitative models used to illustrate the second and third roles are potentially not collected routinely. All models require data from the system studied. The TIA clinic study had relatively low requirements: individual service user-level data detailing date of clinic attendance, clinic attended, the risk classification of patient, and a home location of the patient—much of which is collected routinely by a health system for financial reporting purposes. Simulation modelling studies such as that described in the emergency department case study have high data requirements, including fine-grained timings of processes such as triaging and doctor assessment. It is unlikely such data are collected routinely as they have no use in financial reporting.
An agenda for OR in implementation science
Given the organisational, interventional, and disciplinary issues outlined in the ‘ Implementation science challenges for OR ’ section, I propose the following agenda for OR within implementation science.
Priority 1: creating the evidence base
At the forefront of the research agenda is the need to evaluate the impact of OR on complex interventions. The focus here should be on the consumers of research as opposed to the modellers and the process they follow [ 47 , 48 ]. There is a need to understand how stakeholders make sense of an OR intervention and how the results of studies are used to assist decision-making. Recent research offers some promise in progressing this aim. PartiSim [ 49 ] is a participative modelling framework that aims to involve stakeholders in structured workshops throughout a simulation study. Structured frameworks like PartiSim provide an opportunity to study the user side of OR more efficiently, as the modelling steps are known upfront. Another area showing promise is the recent emergence of Behavioural OR [ 50 ]. One of the core aims of Behavioural OR is to analyse and understand the practice and impact of OR on context (e.g. [ 51 – 53 ]).
Priority 2: raising demand and the liberation of OR
Much of the challenge in the use of OR as an implementation science technique that I outline is rooted in the lack of organisational awareness and experience of the approach. But what if this challenge were to be resolved? To examine this further, consider a counterfactual world where all health service users, managers, and clinicians are well versed in the three implementation science roles of OR and all have free access to a substantial evidence base detailing the efficacy of the approach. In this world, where OR is an accepted implementation science approach, the constraint has now moved from demand to supply of modelling services. Current supply is predominately provided by the (relatively) small specialist consultancy and research communities. There is a great need to liberate OR from its roots as the tool of the ‘specialist’ and transfer knowledge to research users. Two initial efforts to achieve this priority include the Teaching Operational Research for Commissioning in Health (TORCH) in the UK [ 54 ] and the Research into Global Healthcare Tools (RIGHT) Project [ 55 ]. TORCH successfully developed a curriculum for teaching OR to commissioners, although it has yet to be implemented on a wide scale or evaluated. The RIGHT project developed a pilot web tool to enable healthcare providers select an appropriate OR approach to assist with an implementation problem. Both of these projects demonstrate preliminary efforts at liberating OR from the traditional paradigm of specialist delivery.
The liberation of OR has already taken place in some areas in the form of Community OR . The three case studies illustrated interventions where the collaboration puts the emphasis on a modeller to construct the model and provide results for the wider stakeholder group. Alternatively, service users could develop or make use of OR methods to analyse a problem themselves. Community OR changes the role of an operational researcher from a modeller to a facilitator in order to aid those from outside of OR to create appropriate systematic methodology to tackle important social and community-based issues. In a rare example of community OR in healthcare [ 40 ], two examples illustrate where service users take the lead. In the first example, users of mental health services used system methods to produce a problem structuring tool to evaluate the impact of service users on NHS decision-making. In the second example, service users developed and applied an idealised planning approach for the future structure of mental health services. These approaches are qualitative in nature but are systematic and in-line with an OR implementation science approach.
Priority 3: PPI education for OR modellers
The first two priorities listed might be considered long-term goals for the OR implementation science community. An immediate priority that is arguably achievable over the short term is Patient and Public Involvement (PPI) education for OR modellers. The co-design of healthcare models with decision makers is often held up as a critical success factor for modelling interventions [ 42 ]. For ethical and practical reasons, co-design of OR modelling interventions should also include service users [ 41 ]. Education need not be complicated and could at first be done through widely read OR magazines and a grass roots movement delivered through master degree courses.
Conclusions
Operational research offers improvement scientists and individuals who work in complex health systems the opportunity to do more upfront system thinking about interventions and change. OR's upfront role within implementation science aims to answer questions such as where best to target interventions, will such an intervention work even under optimistic assumptions, which options out of many should we implement, and should we consider de-implementing part of a service in favour of investing elsewhere. As OR becomes more widely adopted as an implementation science technique, evaluation of the method through the lens of implementation science itself becomes more necessary in order to generate an evidence base about how to effectively conduct OR interventions. It is also necessary to liberate OR from its traditional roots as a specialist tool.
Operational research (OR) is a mature discipline that has developed a significant volume of methodology to improve health services. OR offers implementation scientists the opportunity to do more upfront system thinking before committing resources and taking risks. OR has three roles within implementation science: structuring an implementation problem, upfront evaluation of implementation problems, and a tool for strategic reconfiguration of health services. Challenges facing OR as implementation science include limited evidence or evaluation of impact, limited service user involvement, a lack of managerial awareness, effective communication between research users and OR modellers, and availability of healthcare data. To progress the science, a focus is needed in three key areas: evaluation of OR interventions, transferring the knowledge of OR to health services, and educating OR modellers about the aims and benefits of service user involvement.
Abbreviations
AMU, Acute Medical Unit; CDU, clinical decision-making unit; ED, emergency department; GP, general practitioner; MRI, magnetic resonance imaging; NHS, National Health Service; OR, operational research (UK)/operations research (US); PPI, Patient and Public Involvement; PPT, psychology and psychiatric talking therapies; RIGHT, Research into Global Healthcare Tools; RIL, recovering independent life; SD, system dynamics; TIA, transient ischemic attack; TORCH, Teaching Operational Research for Commissioning in Health
Pitt M, Monks T, Crowe S, Vasilakis C. Systems modelling and simulation in health service design, delivery and decision making. BMJ Qual Saf. 2015. doi: 10.1136/bmjqs-2015-004430 .
PubMed Google Scholar
Ackoff RL. The future of operational research is past. J Oper Res Soc. 1979;30(2):93–104. doi: 10.2307/3009290 .
Article Google Scholar
Royston G. One hundred years of operational research in health—UK 1948-2048[star]. J Oper Res Soc. 2009;60(1):169–79.
Lane DC, Monefeldt C, Rosenhead JV. Looking in the wrong place for healthcare improvements: a system dynamics study of an accident and emergency department. J Oper Res Soc. 2000;51(5):518–31. doi: 10.2307/254183 .
Günal MM, Pidd M. Understanding target-driven action in emergency department performance using simulation. Emerg Med J. 2009;26(10):724–7. doi: 10.1136/emj.2008.066969 .
Article PubMed Google Scholar
Fletcher A, Halsall D, Huxham S, Worthington D. The DH accident and emergency department model: a national generic model used locally. J Oper Res Soc. 2007;58(12):1554–62.
Knight VA, Harper PR. Modelling emergency medical services with phase-type distributions. HS. 2012;1(1):58–68.
Google Scholar
Monks T, Pitt M, Stein K, James MA. Hyperacute stroke care and NHS England’s business plan. BMJ. 2014;348. doi: 10.1136/bmj.g3049 .
Monks T, Pitt M, Stein K, James M. Maximizing the population benefit from thrombolysis in acute ischemic stroke: a modeling study of in-hospital delays. Stroke. 2012;43(10):2706–11. doi: 10.1161/strokeaha.112.663187 .
Lahr MMH, van der Zee D-J, Luijckx G-J, Vroomen PCAJ, Buskens E. A simulation-based approach for improving utilization of thrombolysis in acute brain infarction. Med Care. 2013;51(12):1101–5. doi: 10.1097/MLR.0b013e3182a3e505 .
Monks T, Pearn K, Allen M. Simulating stroke care systems. In: Yilmaz L, et al, editors. Proceedings of the 2015 Winter Simulation Conference. Piscataway, New Jersey: IEEE; 2015. p. 1391–1402. doi: 10.1109/WSC.2015.7408262 .
Jun J, Jacobson S, Swisher J. Application of discrete-event simulation in health care clinics: a survey. J Oper Res Soc. 1999;50(2):109–23.
Harper PR, Shahani AK, Gallagher JE, Bowie C. Planning health services with explicit geographical considerations: a stochastic location–allocation approach. Omega. 2005;33(2):141–52. doi: 10.1016/j.omega.2004.03.011 .
Gallivan S, Utley M, Treasure T, Valencia O. Booked inpatient admissions and hospital capacity: mathematical modelling study. BMJ. 2002;324(7332):280–2. doi: 10.1136/bmj.324.7332.280 .
Article PubMed PubMed Central Google Scholar
Brailsford SC, Lattimer VA, Tarnaras P, Turnbull JC. Emergency and on-demand health care: modelling a large complex system. J Oper Res Soc. 2004;55(1):34–42.
Gunal MM. A guide for building hospital simulation models. Health Syst. 2012;1(1):17–25. doi: 10.1057/hs.2012.8 .
Bertels S, Fahle T. A hybrid setup for a hybrid scenario: combining heuristics for the home health care problem. Comput Oper Res. 2006;33(10):2866–90. doi: 10.1016/j.cor.2005.01.015 .
Gupta D, Denton B. Appointment scheduling in health care: challenges and opportunities. IIE Trans. 2008;40(9):800–19. doi: 10.1080/07408170802165880 .
Foy R et al. Implementation science: a reappraisal of our journal mission and scope. Implement Sci. 2015;10(1):1–7. doi: 10.1186/s13012-015-0240-2 .
Atkinson J-A, Page A, Wells R, Milat A, Wilson A. A modelling tool for policy analysis to support the design of efficient and effective policy responses for complex public health problems. Implement Sci. 2015;10(1):26.
Pitt M, Monks T, Allen M. Systems modelling for improving healthcare. In: Richards D, Rahm Hallberg I, editors. Complex interventions in health: an overview of research methods. London: Routledge; 2015.
Westcombe M, Alberto Franco L, Shaw D. Where next for PSMs—a grassroots revolution? J Oper Res Soc. 2006;57(7):776–8.
Mingers J, Rosenhead J. Problem structuring methods in action. Eur J Oper Res. 2004;152(3):530–54. http://dx.doi.org/10.1016/S0377-2217(03)00056-0 .
Kotiadis K, Mingers J. Combining PSMs with hard OR methods: the philosophical and practical challenges. J Oper Res Soc. 2006;57(7):856–67. doi: 10.1057/palgrave.jors.2602147 .
Penn ML, Kennedy AP, Vassilev II, Chew-Graham CA, Protheroe J, Rogers A, Monks T. Modelling self-management pathways for people with diabetes in primary care. BMC Fam Pract. 2015;16(1):1–10. doi: 10.1186/s12875-015-0325-7 .
Vennix JAM. Group model-building: tackling messy problems. Syst Dyn Rev. 1999;15(4):379–401.
Cooke MW, Wilson S, Halsall J, Roalfe A. Total time in English accident and emergency departments is related to bed occupancy. Emerg Med J. 2004;21(5):575–6. doi: 10.1136/emj.2004.015081 .
Article CAS PubMed PubMed Central Google Scholar
Utley M, Worthington D. Capacity planning. In: Hall R, editor. Handbook of Healthcare System Scheduling. New York: Springer; 2012.
Robinson S. Simulation: the practice of model development and use. London: Wiley; 2004.
National Institute of Clinical Excellence, Stroke. In: NICE Clinical Guideline, editor. Diagnosis and initial management of acute stroke and transient ischemic attack (TIA). 2008.
Smith HK, Harper PR, Potts CN, Thyle A. Planning sustainable community health schemes in rural areas of developing countries. Eur J Oper Res. 2009;193(3):768–77. doi: 10.1016/j.ejor.2007.07.031 .
Franco AL, Lord E. Understanding multi-methodology: evaluating the perceived impact of mixing methods for group budgetary decisions. Omega. 2010;39:362–72.
Katsaliaki K, Mustafee N. Applications of simulation within the healthcare context. J Oper Res Soc. 2011;62(8):1431–51.
Günal M, Pidd M. Discrete event simulation for performance modelling in health care: a review of the literature. J Simul. 2011;4:42–51.
Fone D et al. Systematic review of the use and value of computer simulation modelling in population health and health care delivery. J Public Health. 2003;25(4):325–35. doi: 10.1093/pubmed/fdg075 .
Brailsford SC, Harper PR, Patel B, Pitt M. An analysis of the academic literature on simulation and modelling in health care. J Simul. 2009;3(3):130–40.
Monks T, Pearson M, Pitt M, Stein K, James MA. Evaluating the impact of a simulation study in emergency stroke care. Oper Res Health Care. 2015;6:40–9. http://dx.doi.org/10.1016/j.orhc.2015.09.002 .
Pagel C et al. Real time monitoring of risk-adjusted paediatric cardiac surgery outcomes using variable life-adjusted display: implementation in three UK centres. Heart. 2013;99(19):1445–50. doi: 10.1136/heartjnl-2013-303671 .
Brailsford SC et al. Overcoming the barriers: a qualitative study of simulation adoption in the NHS. J Oper Res Soc. 2013;64(2):157–68.
Walsh M, Hostick T. Improving health care through community OR. J Oper Res Soc. 2004;56(2):193–201.
Pearson M et al. Involving patients and the public in healthcare operational research—the challenges and opportunities. Oper Res Health Care. 2013;2(4):86–9. http://dx.doi.org/10.1016/j.orhc.2013.09.001 .
Jahangirian M, Taylor SJE, Eatock J, Stergioulas LK, Taylor PM. Causal study of low stakeholder engagement in healthcare simulation projects. J Oper Res Soc. 2015;66(3):369–79. doi: 10.1057/jors.2014.1 .
Young T, Eatock J, Jahangirian M, Naseer A, Lilford R. Three critical challenges for modeling and simulation in healthcare. In: Simulation Conference (WSC), Proceedings of the 2009 Winter. 2009.
Seila AF, Brailsford S. Opportunities and challenges in health care simulation. In: Alexopoulos C, Goldsman D, Wilson JR, editors. Advancing the Frontiers of Simulation. US: Springer; 2009. p. 195–229.
Chapter Google Scholar
Jahangirian M, Eldabi T, Naseer A, Stergioulas LK, Young T. Simulation in manufacturing and business: a review. Eur J Oper Res. 2010;203(1):1–13. doi: 10.1016/j.ejor.2009.06.004 .
Churchman CW, Schainblatt AH. The researcher and the manager: a dialectic of implementation. Manag Sci. 1965;11(4):69–87. doi: 10.2307/2628012 .
Willemain TR. Model formulation: what experts think about and when. Oper Res. 1995;43(6):916–32. doi: 10.1287/opre.43.6.916 .
Pidd M, Woolley RN. A pilot study of problem structuring. J Oper Res Soc. 1980;31(12):1063–8. doi: 10.2307/2581818 .
Tako AA, Kotiadis K. PartiSim: a multi-methodology framework to support facilitated simulation modelling in healthcare. Eur J Oper Res. 2015;244(2):555–64. http://dx.doi.org/10.1016/j.ejor.2015.01.046 .
Franco LA, Hämäläinen RP. Behavioural operational research: returning to the roots of the OR profession. Eur J Oper Res. 2016;249(3):791–5. http://dx.doi.org/10.1016/j.ejor.2015.10.034 .
Gogi A, Tako AA, Robinson S. An experimental investigation into the role of simulation models in generating insights. Eur J Oper Res. 2016;249(3):931–44. http://dx.doi.org/10.1016/j.ejor.2015.09.042 .
Monks T, Robinson S, Kotiadis K. Learning from discrete-event simulation: exploring the high involvement hypothesis. Eur J Oper Res. 2014;235(1):195–205. http://dx.doi.org/10.1016/j.ejor.2013.10.003 .
Monks T, Robinson S, Kotiadis K. Can involving clients in simulation studies help them solve their future problems? A transfer of learning experiment. Eur J Oper Res. 2016;249(3):919–30. http://dx.doi.org/10.1016/j.ejor.2015.08.037 .
Pitt M, Davies R, Brailsford SC, Chausselet T, Harper PR, Worthington D, Pidd M, Bucci G. Developing competence in modelling and simulation for commissioning and strategic planning. A guide for commissioners. 2009 [cited 2016 07/01/2016]; Available from: http://mashnet.info/wp-content/files/CurriculumInModellingAndSimulation4Commissioning.pdf .
Naseer A, Eldabi T, Young TP. RIGHT: a toolkit for selecting healthcare modelling methods. J Sim. 2010;4(1):2–13.
Download references
Acknowledgements
This article presents independent research funded by the National Institute for Health Research (NIHR) Collaboration for Leadership in Applied Health Research and Care (CLAHRC) Wessex. The views expressed in this publication are those of the author(s) and not necessarily those of the National Health Service, the NIHR, or the Department of Health.
Case studies 1 and 3 were funded by NIHR CLAHRC South West Peninsula. Case study 3 used Selective Analytics MapPlace software.
Author’s contribution
TM developed the models described in the case studies, conceived the idea for debate, and wrote the paper.
Author’s information
TM leads the NIHR Collaboration in Leadership in Health Research and CLAHRC Wessex’s methodological hub where he conducts applied health service research in collaboration with the NHS. He is an operational researcher with experience in industry, the public sector, and academic research.
Competing interests
The author declares that he has no competing interest.
Author information
Authors and affiliations.
NIHR CLAHRC Wessex, Faculty of Health Sciences, University of Southampton, Southampton, UK
Thomas Monks
You can also search for this author in PubMed Google Scholar
Corresponding author
Correspondence to Thomas Monks .
Rights and permissions
Open Access This article is distributed under the terms of the Creative Commons Attribution 4.0 International License ( http://creativecommons.org/licenses/by/4.0/ ), which permits unrestricted use, distribution, and reproduction in any medium, provided you give appropriate credit to the original author(s) and the source, provide a link to the Creative Commons license, and indicate if changes were made. The Creative Commons Public Domain Dedication waiver ( http://creativecommons.org/publicdomain/zero/1.0/ ) applies to the data made available in this article, unless otherwise stated.
Reprints and permissions
About this article
Cite this article.
Monks, T. Operational research as implementation science: definitions, challenges and research priorities. Implementation Sci 11 , 81 (2015). https://doi.org/10.1186/s13012-016-0444-0
Download citation
Received : 19 March 2016
Accepted : 25 May 2016
Published : 06 June 2016
DOI : https://doi.org/10.1186/s13012-016-0444-0
Share this article
Anyone you share the following link with will be able to read this content:
Sorry, a shareable link is not currently available for this article.
Provided by the Springer Nature SharedIt content-sharing initiative
- Operational Research
- National Health Service
- Travel Time
- Service User
- System Dynamic Model
Implementation Science
ISSN: 1748-5908
- Submission enquiries: Access here and click Contact Us
- General enquiries: [email protected]

Understanding the Limitations of Operations Research
In this blog, we’ll dive into the various limitations of Operations Research.
Operations Research is a powerful tool that can help businesses and organizations optimize their operations and decision-making processes. However, like any tool, it has its limitations. Here are some of the key limitations of Operations Research:
- Assumptions: Operations Research relies heavily on making certain assumptions about the problem being studied. If these assumptions are incorrect or inaccurate, the results of the study may be unreliable or even misleading.
- Data Quality: Operations Research is only as good as the data it’s based on. If the data is incomplete, inaccurate, or outdated, the results of the study may be compromised.
- Human Factors: Operations Research models often don’t account for human factors such as emotions, biases, and preferences. This can lead to suboptimal solutions that don’t take into account the real-world human factors at play.
- Complexity: Some problems are simply too complex for Operations Research to handle effectively. As the complexity of the problem increases, the number of variables and constraints can quickly become overwhelming, making it difficult to find an optimal solution.
- Cost: Implementing the solutions proposed by Operations Research studies can be expensive, especially if significant changes need to be made to existing systems or processes.
Why are Limitations of Operations Research Important to Know?
As an MBA student studying Operations Research, it’s important to understand the limitations of the field. By understanding these limitations, you’ll be better equipped to identify when Operations Research is the appropriate tool to use and when other approaches may be more appropriate. Additionally, understanding the limitations of Operations Research will help you better interpret and evaluate the results of studies that rely on Operations Research.
Operations Research is a powerful tool that can help organizations optimize their decision-making processes. However, it’s important to understand the limitations of the field. By doing so, you’ll be better equipped to use Operations Research effectively and evaluate the results of studies that rely on it. Remember, no tool is perfect, and understanding its limitations is key to using it effectively.
How useful was this post?
Click on a star to rate it!
Average rating 0 / 5. Vote count: 0
No votes so far! Be the first to rate this post.
We are sorry that this post was not useful for you! 😔
Let us improve this post!
Tell us how we can improve this post?
Operations Research
1 Operations Research-An Overview
- History of O.R.
- Approach, Techniques and Tools
- Phases and Processes of O.R. Study
- Typical Applications of O.R
- Limitations of Operations Research
- Models in Operations Research
- O.R. in real world
2 Linear Programming: Formulation and Graphical Method
- General formulation of Linear Programming Problem
- Optimisation Models
- Basics of Graphic Method
- Important steps to draw graph
- Multiple, Unbounded Solution and Infeasible Problems
- Solving Linear Programming Graphically Using Computer
- Application of Linear Programming in Business and Industry
3 Linear Programming-Simplex Method
- Principle of Simplex Method
- Computational aspect of Simplex Method
- Simplex Method with several Decision Variables
- Two Phase and M-method
- Multiple Solution, Unbounded Solution and Infeasible Problem
- Sensitivity Analysis
- Dual Linear Programming Problem
4 Transportation Problem
- Basic Feasible Solution of a Transportation Problem
- Modified Distribution Method
- Stepping Stone Method
- Unbalanced Transportation Problem
- Degenerate Transportation Problem
- Transhipment Problem
- Maximisation in a Transportation Problem
5 Assignment Problem
- Solution of the Assignment Problem
- Unbalanced Assignment Problem
- Problem with some Infeasible Assignments
- Maximisation in an Assignment Problem
- Crew Assignment Problem
6 Application of Excel Solver to Solve LPP
- Building Excel model for solving LP: An Illustrative Example
7 Goal Programming
- Concepts of goal programming
- Goal programming model formulation
- Graphical method of goal programming
- The simplex method of goal programming
- Using Excel Solver to Solve Goal Programming Models
- Application areas of goal programming
8 Integer Programming
- Some Integer Programming Formulation Techniques
- Binary Representation of General Integer Variables
- Unimodularity
- Cutting Plane Method
- Branch and Bound Method
- Solver Solution
9 Dynamic Programming
- Dynamic Programming Methodology: An Example
- Definitions and Notations
- Dynamic Programming Applications
10 Non-Linear Programming
- Solution of a Non-linear Programming Problem
- Convex and Concave Functions
- Kuhn-Tucker Conditions for Constrained Optimisation
- Quadratic Programming
- Separable Programming
- NLP Models with Solver
11 Introduction to game theory and its Applications
- Important terms in Game Theory
- Saddle points
- Mixed strategies: Games without saddle points
- 2 x n games
- Exploiting an opponent’s mistakes
12 Monte Carlo Simulation
- Reasons for using simulation
- Monte Carlo simulation
- Limitations of simulation
- Steps in the simulation process
- Some practical applications of simulation
- Two typical examples of hand-computed simulation
- Computer simulation
13 Queueing Models
- Characteristics of a queueing model
- Notations and Symbols
- Statistical methods in queueing
- The M/M/I System
- The M/M/C System
- The M/Ek/I System
- Decision problems in queueing

- Embedded System
- Interview Q
- Send your Feedback to [email protected]
Help Others, Please Share

Learn Latest Tutorials

Transact-SQL

Reinforcement Learning

R Programming

React Native

Python Design Patterns

Python Pillow

Python Turtle

Preparation

Verbal Ability

Interview Questions

Company Questions
Trending Technologies

Artificial Intelligence

Cloud Computing

Data Science

Machine Learning

B.Tech / MCA

Data Structures

Operating System

Computer Network

Compiler Design

Computer Organization

Discrete Mathematics

Ethical Hacking

Computer Graphics

Software Engineering

Web Technology

Cyber Security

C Programming

Control System

Data Mining

Data Warehouse

Advantages & Limitations of Operations Research
Operations research is a robust tool and offers directions in making the best decisions possible given the data available.
Advantages of Operations Research
Better Systems: Often, an O.R. approach is initiated to analyze a particular problem of decision making such as best location for factories, whether to open a new warehouse, etc. It also helps in selecting economical means of transportation , jobs sequencing, production scheduling, replacement of old machinery, etc.
Better Control: The management of large organizations recognize that it is a difficult and costly affair to provide continuous executive supervision to every routine work. An O.R. approach may provide the executive with an analytical and quantitative basis to identify the problem area. The most frequently adopted applications in this category deal with production scheduling and inventory replenishment.
Better Decisions: O.R. models help in improved decision making and reduce the risk of making erroneous decisions. O.R. approach gives the executive an improved insight into how he makes his decisions.
"If you don't actively attack the risks, they will actively attack you." - Tom Gilb
Better Co-ordination: An operations-research-oriented planning model helps in co-ordinating different divisions of a company.
Limitations of Operations Research
Dependence on an Electronic Computer: O.R. techniques try to find out an optimal solution taking into account all the factors. In the modern society, these factors are enormous and expressing them in quantity and establishing relationships among these require voluminous calculations that can only be handled by computers.
Non-Quantifiable Factors: O.R. techniques provide a solution only when all the elements related to a problem can be quantified. All relevant variables do not lend themselves to quantification. Factors that cannot be quantified find no place in O.R. models.
Distance between Manager and Operations Researcher: O.R. being specialist's job requires a mathematician or a statistician, who might not be aware of the business problems. Similarly, a manager fails to understand the complex working of O.R. Thus, there is a gap between the two.
Money and Time Costs: When the basic data are subjected to frequent changes, incorporating them into the O.R. models is a costly affair. Moreover, a fairly good solution at present may be more desirable than a perfect O.R. solution available after sometime.
Implementation: Implementation of decisions is a delicate task. It must take into account the complexities of human relations and behaviour.
Operations Research Uses (Role)
Operations research plays an important role in almost all areas of business decisions. Some problems where operational research (OR) approach can be used:
1. Finance, Budgeting and Investments
- Credit policy analysis.
- Cash flow analysis.
- Dividend policies.
- Investment portfolios.
2. Marketing
- Product selection, timing, etc.
- Advertising media, budget allocation.
- Number of salesman required.
- Selection of product mix.
3. Purchasing, Procurement and Exploration
- Optimal buying and reordering.
- Replacement policies
4. Production Management
- Location and size of warehouses, factories, retail outlets, etc.
- Distribution policy.
- Loading and unloading facilities for trucks, etc.
- Production scheduling.
- Optimum product mix.
- Project scheduling and allocation of resources.
5. Personnel Management
- Selection of suitable personnel.
- Recruitment of employees.
- Assignment of jobs.
- Skills balancing.
6. Research and Development
- Project selection.
- Control of R&D projects.
- Reliability and alternative design.
O.R. is a relatively new academic discipline. It has its origin in World War II and soon became very popular throughout the world. Developing appropriate mathematical models for situations, processes, systems is the basic essence of O.R. study. Linear programming , dynamic programming , game theory , simulation , etc. are some of the models & techniques that are used in O.R. There is role for O.R. in almost all areas of business decisions.
Share This Article
Operations Research Simplified Back Next
Linear programming Simplex Method Transportation Problem Assignment Problem
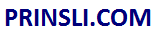
- [ March 6, 2024 ] 369 Universe Number : 369 कोड क्या है? 369 नंबर का क्या रहस्य है? ब्लॉग
- [ March 4, 2024 ] Save Banana Peels : प्रदूषण से लड़ने में कमाल कर सकता है केला TOP 10
- [ December 6, 2023 ] Difference Between Nebula and Galaxy : निहारिका और आकाशगंगा में क्या अंतर है? Knowledge
- [ December 1, 2023 ] वैज्ञानिकों ने खोजा एक नया ‘सौरमंडल’, 6 ग्रह एक लय में करते हैं अपने ‘सूर्य’ की परिक्रमा Science & Astronomy News
- [ November 23, 2023 ] What is Time : समय क्या है? समय आगे क्यों बढ़ता है? Knowledge
Advantages and Disadvantages of Operation Research
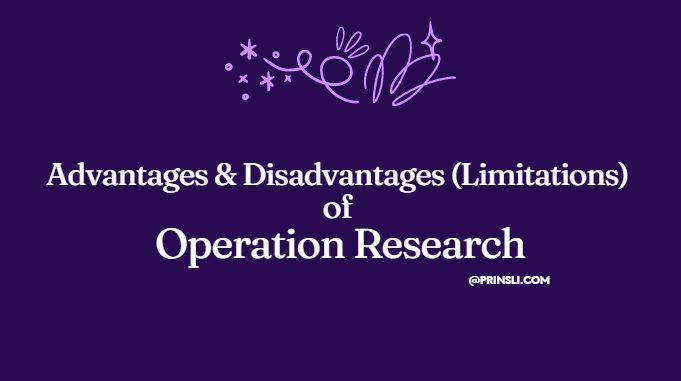
Advantages and Disadvantages (Limitations) of Operation Research:
In this article, we learn about the advantages and disadvantages (limitations) of Operation Research.
“Why do we need to learn Operations Research techniques is a question you might have.” Some reasons are as follows:
Since organisations are becoming more complex, the decisions you must make are becoming more difficult and challenging with large numbers of options, constant time pressure, and margin pressures. Environments are changing so rapidly that previous methods are no longer effective and sufficient. New enterprise applications and software are also producing enormous amounts of data, which can appear to be an extreme challenge to transform that data into knowledge and solutions. Making poor decisions now has higher costs.
Read Also: Approaches, Phases, Methodology Of OR / How OR works / Stages of Development of OR
♦ Advantages of Operation Research :
An O.R. approach helps in better decision-making and lowers the possibility of making erroneous decisions. Some advantages of Operation Research are as follows:
- An O.R. approach is frequently used to analyse a specific decision-making problem, such as the best location for factories, new warehouses etc. Locating a warehouse or depot that can deliver goods over shorter distances and at a lower cost is made easier with the help of operations research.
Read Also: Advantages of OR Approach in Decision-Making
- Operations Research helps to decide where to invest capital to grow.
- Operations research helps in forecasting sales for a new type of product that has never been marketed before.
- Operations research helps in deciding when and how much to discount.
- Operations research can help in deciding how much of a budget to spend on the Internet vs. traditional sales.
- Operations research aids in maximising the value of ERP (Enterprise Resource Planning), CRM (Customer Relationship Management), and other software systems.
- Operations research helps in the optimization of an investment portfolio, whether it contains financial securities or pharmaceutical product inventory.
Read Also: Scope / Applications of OR
- Using operations research, call centres can be run in the most efficient manner.
- Operations research helps in solving complex scheduling problems and it also helps in inventory replenishment problems.
- Using operations research, planning can be done to prevent potential terrorist attacks.
- When there is uncertainty about the weather and consumer demand, operations research is helpful when planting crops.
Read Also: Management Applications of OR
♦ Disadvantages (Limitations) of Operations Research :
Operations Research has a number of applications ; similarly, it also has certain limitations. These limitations are mostly related to the model building and money and time factors problems involved in its application. Some of them are as given below:
- These do not take into account qualitative and emotional factors.
- These are applicable to only specific categories of decision-making problems.
- These are required to be interpreted correctly.
- Due to conventional thinking, changes face a lot of resistance from workers and sometimes even from employers.
- Models are the only idealized representation of reality and not be regarded as absolute.
- The operations researcher’s job needs a mathematician or statistician, who might not be aware of the business problems. Similarly, a manager is unable to understand the complex nature of Operations Research. Thus there is a big gap between the two personnel.
- Operations analysis’s high cost is its most significant limitation. The operations investigation employs mathematical equations that necessitate the use of outstanding technology to create.
- The dependence on technology is another limitation of operations research. Computers are required to examine mathematical equations.
- The outcomes of operation research are often ideological, making them difficult to implement sometimes.
Read Also: Limitations of Operations Research in Decision Making
Read Also: Advantages and Disadvantages of a Model in Operation Research
(Source – Various books from college library)
Tags: limitation of operation research, advantages and disadvantages of operation research
Copyrighted Material © 2019 - 2024 Prinsli.com - All rights reserved
All content on this website is copyrighted. It is prohibited to copy, publish or distribute the content and images of this website through any website, book, newspaper, software, videos, YouTube Channel or any other medium without written permission. You are not authorized to alter, obscure or remove any proprietary information, copyright or logo from this Website in any way. If any of these rules are violated, it will be strongly protested and legal action will be taken.
- Click to share on Facebook (Opens in new window)
- Click to share on Twitter (Opens in new window)
- Click to share on LinkedIn (Opens in new window)
- Click to share on WhatsApp (Opens in new window)
- Click to share on Pinterest (Opens in new window)
- Click to share on Telegram (Opens in new window)
- Click to share on Tumblr (Opens in new window)
- Limitation of Operation Research
- operation research
- Operations Research
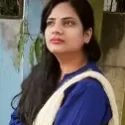
Related Articles
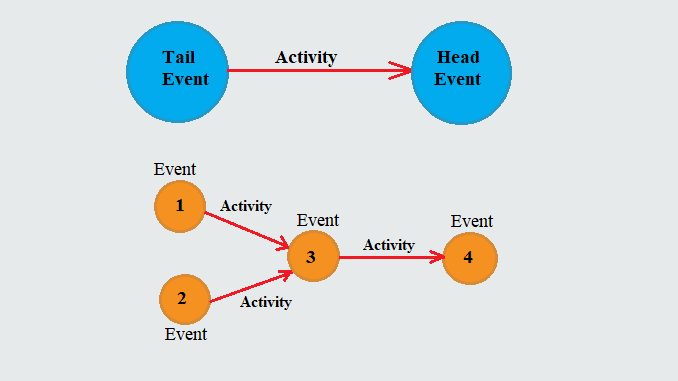
Difference between event and activity in Network Diagram
An event is that particular moment of time when a specific part of a project is to be completed, whereas an activity is the actual performance of a task. […]
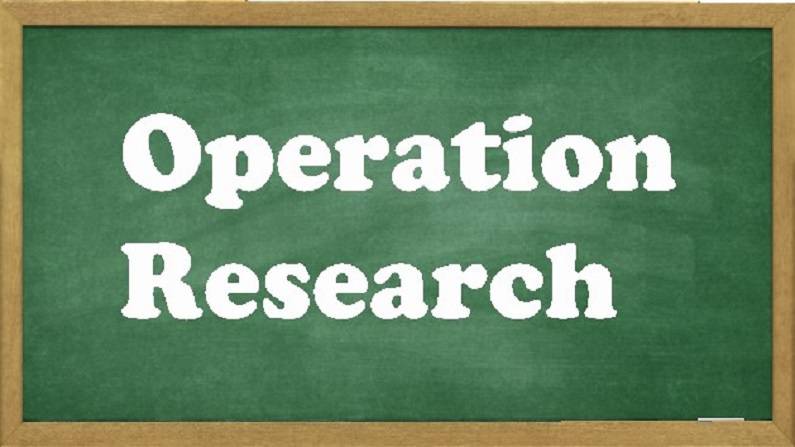
Evolution and Historical Development of Operation Research
The main origin of Operation Research was during the Second World-War, when there was a critical need to manage scarce … […]
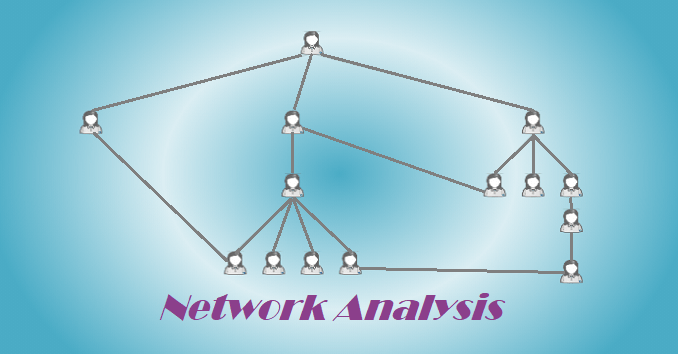
Objectives of Network Analysis in Operation Research
Followings are the main objectives of network analysis -To provide systematic approach in planning and scheduling. To minimize idle… […]
Be the first to comment
Leave a reply cancel reply.
Your email address will not be published.
Save my name, email, and website in this browser for the next time I comment.
Notify me of follow-up comments by email.
Notify me of new posts by email.
Copyright © 2019 - 2024 Prinsli.com - All rights reserved

An official website of the United States government
The .gov means it’s official. Federal government websites often end in .gov or .mil. Before sharing sensitive information, make sure you’re on a federal government site.
The site is secure. The https:// ensures that you are connecting to the official website and that any information you provide is encrypted and transmitted securely.
- Publications
- Account settings
Preview improvements coming to the PMC website in October 2024. Learn More or Try it out now .
- Advanced Search
- Journal List
- PeerJ Comput Sci

Approaches combining methods of Operational Research with Business Process Model and Notation: A systematic review
Hana tomaskova.
1 University of Hradec Kralove, Faculty of Informatics and Management, Hradec Kralove, Czech Republic
Gerhard-Wilhelm Weber
2 Faculty of Engineering Management, Poznan University of Technology, Poznan, Poland
3 Institute of Applied Mathematics, Middle East Technical University, Ankara, Turkey
Associated Data
The following information was supplied regarding data availability:
This article does not contain data or code because it is a literature review.
Business process modelling is increasingly used not only by the companies’ management but also by scientists dealing with process models. Process modeling is seldom done without decision-making nodes, which is why operational research methods are increasingly included in the process analyses.
This systematic literature review aimed to provide a detailed and comprehensive description of the relevant aspects of used operational research techniques in Business Process Model and Notation (BPMN) model.
The Web Of Science of Clarivate Analytics was searched for 128 studies of that used operation research techniques and business process model and notation, published in English between 1 January 2004 and 18 May 2020. The inclusion criteria were as follows: Use of Operational Research methods in conjunction with the BPMN, and is available in full-text format. Articles were not excluded based on methodological quality. The background information of the included studies, as well as specific information on the used approaches, were extracted.
In this research, thirty-six studies were included and considered. A total of 11 specific methods falling into the field of Operations Research have been identified, and their use in connection with the process model was described.
Operational research methods are a useful complement to BPMN process analysis. It serves not only to analyze the probability of the process, its economic and personnel demands but also for process reengineering.
Introduction
It has been more than 15 years since ‘Business Process Model and Notation’ or ‘Business Process Modelling Notation’ (BPMN) became the official notation for process modelling. During its lifetime, this notation has gained many users and, thanks to its user-friendliness, it is used in many areas. This wide usage has led to the interconnection and use of other technologies and methods. The fundamental problem of any complex process is decision making. Operational Research as a popular scientific approach is so often associated with procedural issues, making its connection to BPMN is more than natural. This article focuses on the analysis of the relationship between the Business Process Model and Notation (BPMN) process modelling and specific methods of Operational Research.
Business Process Modelling Notation was created by the Business Process Management Initiative (BPMI) as an open standards. It is very similar to flowcharts and Petri nets but offers much more sophisticated tools to describe and simulate behaviour. Silver (2009) stated that this approach is an ‘event-triggered behaviour,’ a description of the ‘something happened’ mode. Business Process Modelling is used to describe, recognize, re-engineer, or improve processes or practices, Tomaskova (2017) . Business Process Model and Notation (BPMN) is the language that is used to model business process steps from start to end. The notation was explicitly designed for wide-ranging use in process analysis, The Object Management Group (2011) . BPMN is both intelligible to non-specialists and allows a complicated processes between different participants to be represented. Another, very significant feature of BPMN is its ‘business-friendly’ orientation, which is essential for the company’s business and knowledge.
Operational Research (OR) is concerned with formulating, modelling, and solving a variety of decision-making situations to find the optimal solutions. The company’s philosophy and decide over business data are the most crucial management actions. The task of the manager is to select in the real system the problem to be analyzed and to formulate it precisely. The standard way of doing this involves the expression of the economic model and then the formulation of a mathematical model. It is necessary to build a simplified model of the real financial systems that only includes the essential elements that describe the formulated problem. The manager has to set the goal of the analysis and subsequent optimization. It is important to define all operations and processes that influence this goal, to describe all the factors, and to verbally express the relationships between the stated purpose and the mentioned processes and factors.
The article is divided into the following parts. The “Related works and background” section lists research articles that are relevant to a given combination of BPMN and OR areas and briefly. That part briefly provides essential information regarding the approaches that are fundamental to this systematic review. The “Research methodology” section describes a systematic search, i.e. entry conditions, exclusion criteria and limitations. The “Results” section presents the results of the analysis of articles fulfilling the requirements of the systematic review. We analyzed publications according to when they were published, their citations, the scientific areas covered, the cooperation of the authors and their keywords. Subsequently, we examined selected articles in terms of methodology, approach and research areas. In the “Discussion”, we focus on scientific gaps and future research. We present a research area where we expect an increase in publications, including their specific components. We also discuss the future development of applied methods and approaches. Finally, the “Conclusion” section summarizes the results and benefits of this study.
Related Works and Background
The background information and related works are listed in the paragraphs below. We first focused on process modelling and BPMN and then on OR and its essential methods and approaches.
Organizational processes and decision support can be captured in many ways, and for many areas, we can mention, for example: strategic management by: Maltz & Kohli (1996) , Certo (2003) , Tomaskova (2009) , Maresova (2010) , Tsakalidis et al. (2019) ; product development research and innovation implementation, see Repenning, 2002 , Garcia (2005) ; IT and economic analyzes see Shane & Cable (2002) , Dedrick, Gurbaxani & Kraemer (2003) , Krenek et al. (2014) , Tomaskova, Kuhnova & Kuca (2015) , Maresova, Tomaskova & Kuca (2016) , Tomaskova et al. (2016) , Maresova, Sobeslav & Krejcar (2017) , Cheng et al. (2019) , Tomaskova, Kopecky & Maresova (2019) , Tomaskova et al. (2019) , Kopecky & Tomaskova (2019) , Kopecky & Tomaskova (2020) ; different simulation approaches analysis, see Sterman (1994) , Kozlowski et al. (2013) , Cimler et al. (2018) or non-standard optimization techniques by: Gavalec & Tomaskova (2010) , Bacovsky, Gavalec & Tomaskova (2013) , Tomaskova & Gavalec (2013 , 2014 ), Gavalec, Plavka & Tomaskova (2014) , Gavalec, Mls & Tomaskova (2015) , Cimler et al. (2017) , Oudah, Jabeen & Dixon (2018) .
Some authors have attempted to provide a solution for process model analysis. For example Melao & Pidd (2000) discussed the strengths and limitations of the various modelling approaches used in business process transformation. The article by Glassey (2008) compares three process modelling processes used in case studies. The article by Sadiq & Orlowska (2000) analyze process models using graph reduction techniques. Other authors like Van der Aalst et al. (2007) , Krogstie, Sindre & Jorgensen (2006) use specific tools, frameworks and methods for process analysis and modelling.
Business process modelling
Today, process modelling and business process management (BPMN) have a significant impact. Process modelling is currently a mainly graphical representation of processes, e.g. in what order particular activities should be implemented and what inputs and outputs the processes require for proper functioning. The primary goal of process modelling is to increase the efficiency and effectiveness of the entire process as well as partial activities. Many business process modelling techniques have been proposed over the last decades, so the article Recker et al. (2009) comparatively assesses representational analyses of 12 popular process modelling techniques to provide insights into the extent to which they differ from each other. The review business process modelling literature and describe the leading process modelling techniques falling to and before 2004 are published in the articl Aguilar-Saven (2004) . The topic of visualization of business process model has been investigated in publication Dani, Freitas & Thom (2019) , where the authors performed a systematic literature review of the topic “visualization of business process models”. Kalogirou (2003) is a particularly fascinating article that illustrates how AI techniques might play an essential role in the modelling and prediction of the performance and control of the combustion process. Although BPM initially focused mainly on the industrial, service and business sectors, it has also appeared in other sectors in recent years. The popularity of BPMN has been confirmed by articles such as Zarour et al. (2019) , which presents the current state-of-the-art of BPN extensions. Publication De Ramon Fernandez, Ruiz Fernandez & Sabuco Garcia (2019) deals with the optimization of clinical processes.
Business process model and notation
Business process model and notation is a language for creating business process models Silver (2009) . Under the auspices of the Object Management Group (OMG), the Business Process Management Initiative (BPMI) created the BPMN as an open standard in 2004 by the first version 1.0. In 2005, BPMI merged with the Object Management Group (OMG), and the following year, the latter issued the BPMN specification document. In 2010, BPMN version 2.0 was developed, and the current version of BPMN 2.0.2 was released in December 2013. History of BPMN and notation development is a frequent topic of BPMN publications, we can mention Nisler & Tomaskova (2017) , Kocbek et al. (2015) , Chinosi & Trombetta (2012) , White (2008) , Van der Aalst, Adriansyah & Van Dongen (2012) and Recker (2012) . BPMN is similar to flowcharts and is based on the concept of Petri nets, but it is a more sophisticated and user-friendly language. The graphic form of BPMN makes it understandable even for non-experts. In BPMN, we distinguish several types of elements that we can use in modelling. The specific standards link these elements. In the base classification, we define four groups of items. These are Flow Objects, Connecting Objects, Swimlanes and Artifacts, see The Object Management Group (2011) .
Operational Reserach
Operational Research (OR) is the well-known approach of using analytical and advanced methods to help make the best possible decisions. As early as 1980, Article by authors Shannon, Long & Buckles (1980) presented the results of a survey of the perception of the usefulness and knowledge of the 12 OR methodologies commonly used in the practice of industrial engineering. The article by Dubey (2010) defines the relationship between OR and another branch of sciences. The article Gu, Goetschalckx & McGinnis (2010) presents a detailed survey of the research on warehouse design, performance evaluation, practical case studies, and computational support tools. The article Negahban & Smith (2014) provided a review of discrete event simulation publications with a particular focus on applications in manufacturing.
OR methods are often associated with new technologies. In article Sarac, Absi & Dauzère-Pérès (2010) , a state-of-the-art on RFID technology deployments in supply chains was given to analyze the impact on the supply chain performance. Xu, Wang & Newman (2011) , in their article, tries to identify future trends of computer-aided process planning (CAPP). Dynamic ride-share systems is investigated in the article Agatz et al. (2012) .
Linear programming
One of the most popular areas of OR in practice is linear programming (LP). The mathematical model of linear programming tasks contains a single linear purpose function, and the actual constraints of the problem are described only by linear equations and inequalities. These tasks are most often encountered in economic practices. Linear programming has been described in several books: Dantzig (1998) , Schrijver (1998) , Dorfman, Samuelson & Solow (1987) .
Multicriterial decision making
The solving of multi-criteria decision-making (MCDM) tasks comprises the search for optimal values of the unknowns, which are simultaneously assessed according to several often contradictory criteria. Thus, the mathematical model of multi-criteria decision problems contains several purpose functions. Depending on how the sets of decision variants are defined, we are talking about the tasks of multi-criteria linear programming or multi-criteria evaluation of options. A review of applications of Analytic Hierarchy Process in operational management is inverstigated in Subramanian & Ramanathan (2012) . The article Velasquez & Hester (2013) performs a literature review of common Multi-Criteria Decision Making methods. The authors present the results of a bibliometric-based survey on AHP and TOPSIS techniques in publication Zyoud & Fuchs-Hanusch (2017) .
Project planning
Project management tasks consist of several separate activities that are interdependent and may be run simultaneously. The most commonly used method is the so-called network analysis, where a network graph is created from the left chronologically arranged project activities representing the project life cycle. The longest possible path from the beginning to the end of the project is recorded by “the critical path”. The non-observance of this path will lead to a slowing down of the whole project, whose time duration is to be optimized. The optimistic, pessimistic, and most probable estimate of the implementation of the entire project is determined. The article Nutt (1983) relates the project planning process and implementation. Critical Path Method (CPM) is found in the article Jaafari (1984) , to be equally useful as a planning tool for linear or repetitive projects.
The Resource-Constrained Project Scheduling Problem (RCPSP) is a general problem in scheduling. The article Pellerin, Perrier & Berthaut (2020) examines the general tendency of moving from pure metaheuristic methods to solving the RCPSP to hybrid methods that rely on different metaheuristic strategies ( Cimr, Cimler & Tomaskova, 2018 ).
Nonlinear programming
Nonlinear programming is the case when the purpose function is not linear. Tasks then often have a large number of local extremes and often also have great difficulty finding them.
Dynamic programming
If constraints are functions of some parameter, which is most often time, we are talking about dynamic programming. This approach deals with the modelling of more complex multi-stage optimization problems divisible into related sub-problems. Depending on the time parameter, the system is always in one of the acceptable states during the process. At certain times it is necessary to choose from a set of possible decisions, which again results in the transition to the next state. We call the strategy a sequence of these states of the system and choices, looking for the course with the best valuation. Simulations are often used to model and analyze the operation of complex systems without realization and in less than real-time.
- Queuing theory is a type of dynamic programming task. It deals with streamlining the functioning of systems in which it is necessary to gradually serve all units whose requirements are continuously met on so-called service lines. The challenge is to find the most effective way to handle these requirements.
- Inventory management models address the issue of optimizing the supply process and the volume of inventory stored. Costs associated with ordering, issuing, and keeping stocks in stock should be minimized.
Stochastic programming
Stochastic programming deals with optimization problems in which they act as parameters of their constraints of random variables. Probabilistic calculus methods solve these problems, and their results have the character of random variables. Stochastic processes can also be ranked among tasks with the input data uncertainties. This approach is used to describe the behavior of systems evolving. We are talking about stochastic processes, a special case is the so-called Markov chains and Markov processes. Basic books on this topic are, for example: Kall, Wallace & Kall (1994) , Birge & Louveaux (2011) , Shapiro, Dentcheva & Ruszczyński (2014) .
Research Methodology
Kitchenham & Charters (2007) highlighted three essential elements for a systematic literary review: the determination of the research question(s), the organisation of an unbiased and extensive analysis of related publications, and the determination of precise criteria of inclusion and exclusion.
We identified three research questions:
- Research question 1 (R1): Greater adaptability of BPMN elements causes greater application of this notation in publications.
- Research question 2 (R2): The connection between BPMN and OR methods is most often applied to the business and economics areas.
- Research question 3 (R3): The queue theory is the most widely used method in BPMN processes.
The analysis process and criteria are given in the following relevant subsections.
Eligibility criteria
This study included publications listed in the Web Of Science (WOS) database of Clarivate Analytics that were published between 1 January 2004 and 18 May 2020. The year 2004 was selected as this is when BPMN was created by BPMI.
Exclusion criteria (EC) are:
- EC1 = The publication was published in a language other than English.
- EC2 = The full text of the publications was not available.
- EC3 = The publication did not coincide with the topic of systematic research.
- EC4 = BPMN was used only as a presentation tool and not as part of the research.
Information sources and search
The primary source of information for the study was the database Web Of Science (WOS) of Clarivate Analytics. An advanced search was performed for the search query mentiones below. The search was performed in the Topics (TS) section.
Especially, the CORE database with the indexes listed in Table 1 was selected. The search was performed for ‘All document types,’ ‘All languages’ and the years 2004–2020.
Study selection
The first step of the review process involved title and abstract screening, followed by a full-text review of the remaining articles. Two independent assessors verified the results of the title and abstract screening and the full-text review. One assessed the suitability of the results from the perspective of OR and the other from an IT perspective, i.e. whether it was BPMN notation and its use. Articles were included if they met all the following criteria: (i) they used an OR method, (ii) a BPMN model was used and (iii) the complete text was available in English (abstracts, commentaries, letters and unpublished data were excluded). Studies were not excluded based on their methodological quality.
The selected publications were examined from many perspectives, and each contribution was coded according to different criteria. This study aimed to enhance the discipline’s fundamental progress in understanding the link between OR methods and BPMN. The results of this study could encourage scientists to use OR methods for process analysis.
A limitation of this review was restricting the included articles to English-language publications that looked at process analysis using OR and BPMN published between 1 January 2004 and 18 May 2020. Relevant studies in other languages or published after 18 May 2020 may have been omitted.
Data collection process
Data was collected based on keywords selected from the article Lane, Mansour & Harpell (1993) , which analyzed the quantitative techniques of Operation Research. From this document, the 18 Operation Research methods were selected and listed in the Table 2 .
The results were further categorized as to whether they corresponded to the given keywords and their meaning. The main results of the systematic literature review were obtained by analyzing by the two main guidelines of PRISMA: Moher et al. (2009) and MECIR: Higgins et al. (2018) .
Synthesis of results
The individual studies were subjected to bibliometric analysis and then the studies were assessed according to the content and methods used. The bibliometric analysis describes and analyses up to date research. It aims at summarizing the latest progress in the field by quantitatively investigating the literature. This method provides a vast canvas of knowledge from the micro-level (institutes, researchers, and campuses) to the macro-level (countries and continents) Mryglod et al. (2013) . Frequency analysis was used to find the most common scientific areas, the countries with the most publications and the most common keywords. Science mapping was performing using the VOS viewer, Venn diagrams and bar and bubble graphs, Van Eck et al. (2010) , Cobo et al. (2011) .
The Venn/Euler diagram graphically represents the relationships of the largest set of keywords. Euler diagrams are considered to be an effective means of visualizing containment, intersection, and exclusion. The goal of this type of graph is to communicate scientific results visually. Leonhard Euler first popularized the principle of labeled closed curves in the article Euler (1775) Alternative names for Euler diagrams include ‘Euler circles.’ They can also be incorrectly called Venn diagrams. Venn diagrams require all possible curve intersections to be present, so can be seen as a subset of Euler diagrams, that is, every Venn diagram is a Euler diagram, but not every Euler diagram is a Venn diagram. John Venn introduced Venn diagrams a hundred years after Euler in the article Venn (1880) . Venn diagram is a schematic graph used in logic theory to depict collections of sets and represent their relationships.
The initial search resulted in 128 articles. After removing duplicates, 107 were left that underwent title and abstract screening. After screening, 61 articles remained that underwent full-text review. The final number of included articles for information abstraction was 36. Overview of the number of publications according to exclusion criteria is shown in Fig. 1 .

Eighteen keywords selected from the article by Lane, Mansour & Harpell (1993) were involved in the study. These keywords have been classified according to whether a publication meeting a study condition has been found for them. Only for 13 keywords were found a publication suitable for this study, as can be seen in Table 2
Categorization of publications based on the clarivate analytics
Journals and books covered by the Web of Science Core Collection were assigned to at least one Web of Science category. Each Web of Science category was mapped to one research area Clarivate Analytics (2019) . The research areas for the selected publications were:
- COMPUTER SCIENCE (CS)
- ENGINEERING (En)
- OPERATIONAL RESEARCH MANAGEMENT SCIENCE (OR)
- BUSINESS ECONOMICS (BE)
- ROBOTICS (Ro)
- AUTOMATION CONTROL SYSTEMS (ACS)
- TELECOMMUNICATIONS (Te)
- TRANSPORTATION (Tr)
We selected four main groups, for which we compiled a bar graph and a Venn diagram after analysis. We chose the number of four research areas for representation in the Venn diagram; four sets are still well arranged. Another argument was the number of publications in other areas, where the set "ROBOTICS" contains two documents and the sets ‘AUTOMATION CONTROL SYSTEMS,’ ‘TELECOMMUNICATIONS’ and ‘TRANSPORTATION’ each one document.
Bar graph on Fig. 2 is based on frequency analysis and contains the total number of publications in a given research area, their average number of citations, and the corresponding average number of pages per article. The graph shows the results by type of purpose. The first part shows the frequency of documents for each research areas. The second part focuses on the average number of citations, and the third shows the average number of pages per article.

The Venn diagram, in Fig. 3 , shows selected four research areas as sets, including their intersection areas. In a specific area, we also stated the relevant number of documents and their average number of citations.

This part of the bibliometric analysis showed us the answer to the research question R2. Although BPMN was explicitly designed for corporate analysis and economic analysis, and Operational Research focuses primarily on addressing managerial decisions, most publications were not in the field of business economics (BE). Surprisingly, this area actually has the fewest publications. The field of computer science had the most papers, and papers in the field of OR had the most citations. The field of BE had the most extended publications, however, i.e. the average number of pages per paper.
Result1: Research question R2—not confirmed.
Year of publication
Figure 4 illustrates the distribution over time of the selected publications with BPMN milestones. The BPMN versions adoption dates, taken from OMG.org (2018) , complements this figure.

The different BPMN versions brought more or fewer changes in notation. While the changes between BPMN 1.0 and BPMN 1.2 were rather consmetics, e.g. renaming ‘Rule’ elements to ‘Conditional’ or slight increasing the number of elements from 48 to 55. The arrival of BPMN 2.0 was a major breakthrough and represented the largest revision of BPMN since its inception. In this version, it is possible to create a new ‘Choreography model,’ ‘Collaborations model’ and ‘Conversation model’ in BPMN in addition to collaborative processes and internal (private) business processes. Events are now divided into ‘interrupted’ and ‘non-interrupted’ and ‘catching’ and ‘throwing.’ The message type is newly introduced, and the data object has three specifications. BPMN 2.0 contains 116 elements. BPMN 2.0.2 included only minor modifications in terms of typos.
Given the magnitude of changes between the different versions of the BPMN notation, the sharp increase in publications following the introduction of the BPMN 2.0 notation can be considered a confirmation of research question R1. It is very interesting that publications in the field of BE did not appear until 2017.
Result: Research question R1—confirmed.
The average number of citations of the analysed documents was 2.22. The first quartile was 0, and the third quartile was 3.75. The median was equal to 1 and data variability above the third quartile was limited to seven citations. We identified two outliers values: 12 citations for Hasic, De Smedt & Vanthienen (2018) and 15 citations for article Wu et al. (2015) .
Author analyses
Bibliometric analysis cannot be done without review by the authors. We focused on illustrating co-authorship. The total number of authors of publications selected for this study was 84: al achhab, m (1), aouina, zk (1), ayani, r (1), aysolmaz, b (1), bahaweres, rb (1), batoulis, k (1), ben ayed, ne (1), ben said, l (1), ben-abdallah, h (3), bisogno, s (1), bocciarelli, p (1), boukadi, k (1), braghetto, kr (1), burattin, a (1), calabrese, a (1), ceballos, hg (2), chien, cf (1), cho, sy (1), creese, s (1), cunha, p (1), d’ambrogio, a (1), d’ambrogio, sa (1), de lara, j (1), de smedt, j (2), demirors, o (1), duran, f (2), el hichami, o (1), el mohajir, b (1), ferreira, je (1), figl, k (1), fitriyah, a (1), flores-solorio, v (2), fookes, c (1), garcia-vazquez, jp (1), ghiron, nl (1), ghlala, r (1), gomez-martinez, e (1), hansen, z (1), hansen, znl (3), happa, j (1), hasic, f (2), herbert, lt (8), holm, g (1), iren, d (1), jacobsen, p (3), jobczyk, k (1), kamrani, f (1), khlif, w (2), kluza, k (1), ligeza, a (1), manuel vara, j (1), marcos, e (1), mazhar, s (1), mendling, j (1), mendoza morales, le (1), mengersen, k (1), monsalve, c (1), moradi, f (1), naoum, m (1), onggo, bss (1), pablo garcia, j (1), perez-blanco, f (1), pitchforth, j (1), proudlove, nc (1), rekik, m (1), rocha, c (2), rosemann, m (1), rozy, nf (1), salaun, g (2), sharp, r (4), sperduti, a (1), suchenia, a (1), tang, rz (1), tokdemir, g (1), tomaskova, h (1), vanden broucke, sklm (1), vanthienen, j (3), veluscek, m (1), villavicencio, m (1), vincent, jm (1), weske, m (1), wisniewski, p (1), wu, ppy (2), xie, y (1).
These authors formed different sized groups, as can be seen in Fig. 5 . We grouped the authors according to their co-authors’ collaborations with a curve connecting the co-authors. The size of the node of this connection corresponds to the number of documents by the given author. The colours used to distinguish the authors were created using the average years of the publication of their papers.

For the authors’ average publication years, the first quartile was 2015, the third quartile was 2018.5 and the median was 2017. The variability outside the lower and upper quartiles was given by 2011 and 2020. We identified one outlier value corresponding to the year 2009.
The most prominent groups were around the authors listed in Fig. 6 . This figure also contains the number of documents by the authors, their total number of citations and their average value.

According to this analysis Wu, P. Y. had the highest number of citations (7.5), followed by De Smedt, J. (7) and Hasic, F. (7). Herbert, L.T. had the most documents (8) and Tomaskova, H. had no co-author connections.
The authors were also analyzed in terms of their country or region affiliations. A total of 25 countries were identified and their location, including the number of relevant publications, are shown in Fig. 7 . The countries with the highest number of affiliated publications were Denmark (8) and Tunisia (4), followed by Belgium, France, Saudi Arabia, Italy and Spain, who all had three.

Keywords analysis
The keywords were categorized according to those identified by the published authors and the keywords PLUS assigned by Clarivate Analytics databases. The data in KeyWords Plus are words or phrases that frequently appear in the titles of an article’s references but do not appear in the title of the item itself. Based upon a special algorithm that is unique to Clarivate Analytics databases, KeyWords Plus enhances the power of cited-reference searching by searching across disciplines for all the articles that have cited references in common, more information is on the web link Clarivate Analytics (2018) . A total of 130 unique keywords and 46 unique KeyWords Plus keywords were found for selected publications.
A total of 130 author keywords were mentioned in the publications and a general view of their interconnection can be seen in Fig. 8 .

Below is a list of all author keywords with the number of the weight-link to other keywords: activity theory (4), affiliation (6), agent based model (4), agent-based systems engineering (3), airport passenger facilitation (8), atl (5), automated verification (4), bayesian network (4), bayesian networks (4), bpm (6), bpmn (60), bpmn business processes (4), bpmn extension (3), bpmn model restructuring (5), business process (18), business process automation (3), business process management (13), business process model (5), business process model measures (3), business process modelling notation (4), business process optimisation (5), business process outsourcing (3), business processes (3), cloud computing (3), clustering (5), communication theory (11), configurable reference model (8), consequence modelling and management (10), contextual factors (8), cycle time (4), decision making (15), decision mining (3), decision model and notation (3), decision modeling (4), decision modelling (5), dikw (11), discrete-event simulation (4), dmn (15), effort prediction model (3), engineering agent-based systems (3), engineering systems (6), enterprise risk management (4), eqn (5), evolutionary algorithm (2), evolutionary algorithms (5), facilitated modelling (4), fault tree analysis (6), fault tree generation (6), flow (8), formal risk analysis (6), genetic algorithm (3), healthcare (4), hierarchical clustering (6), incident response (11), integrated modelling (5), interviews (11), jeqn (5), knowledge discovery (6), knowledge management (11), knowledge rediscovery (6), licenses (11), maude (7), mc-dmn (5), mcdm (5), mda (5), metrics (5), model checking (4), model transformations (5), model-driven architecture (4), model-driven engineering (5), modelling (4), object modeling (4), optimisation (6), organizational mining (3), performance (5), performance evaluation (3), petri nets (5), pproduction optimisation (2), preference to criteria (5), prism (8), probability (2), process configuration (8), process enhancement (3), process chain network (5), process merging (8), process mining (6), process modeling (4), process modelling (5), project management (3), qualitative analysis (4), quantitative model checking (10), quantitative service analysis (6), quantitative workflow analysis (4), queues (3), queuing theory (4), reliability analysis and risk assessment methods (4), resource allocation (4), restructuring (6), rewriting logic (7), rules (5), safety assessment software tools (4), safety management and decision making (4), security (11), security operation center (11), sense-making (11), separation of concerns (5), service engineering (6), scheduling (4), simulation (4), simulations (3), social network (5), social network analysis (3), social network model (6), socio-technical systems (sts) (4), soundness (4), space-sensitive process model (8), statistical model checking (4), stocastic bpmn (2), stochastic automata network (3), stochastic bpmn (11), stochastic model checking (13), stochastic modeling and analysis (4), structural and semantic aspects (5), tacit knowledge (11), task analysis (11), task assignment (4), task model (4), timed automata (4), topsis (5), verification (2).
As you can see in the figure, most of the author’s keywords are directly or indirectly linked with the term ‘BPMN’, but there are also isolated groups. In the following text, we’ve listed separate keyword groups. We’ve added a year of publication, a number of citations, and a specific document to which the keywords belong.
- 2013; citations; (business process automation; business process model measures; effort prediction model; project management) Aysolmaz, Iren & Demirors (2013) .
- 2014; citation; (evolutionary algorithm; pproduction optimisation; stocastic bpmn) Herbert et al. (2014) ,
- 2015; citations; (agent based model; bayesian network; business process modelling notation; modelling; socio-technical systems (sts)) Wu et al. (2015) ,
- 2015; citation; (affiliation; bpm; hierarchical clustering; knowledge discovery; knowledge rediscovery; restructuring; social network model) Khlif & Ben-Abdallah (2015) ,
- 2016; citations; (bpmn extension; business process outsourcing; cloud computing; genetic algorithm) Rekik, Boukadi & Ben-Abdallah (2016) .
- 2017; citations; (bpmn model restructuring; clustering; metrics; rules; social network; structural and semantic aspects) Khlif, Ben-Abdallah & Ben Ayed (2017) .
- 2019; citations; (atl; business process model; model transformations; model-driven engineering; petri nets; process chain network) Gómez-Martnez et al. (2019) .
As mentioned above, there were only 46 KeyWords Plus keywords (the number of links to other keywords is given in parentheses after the keyword): accuracy (6), ambiguity (6), automation (3), bpmn (20), business process models (6), checking (6), cognitive effectiveness (7), communities (2), complex (0), confidence (6), context (9), critical path (9), decision-making (7), design (7), dimensions (7), distributed simulation (1), framework (8), functional size (2), group creativity (6), identification (9), implementation (5), information (6), integration (2), model (7), neural-network (7), organizational knowledge (1), patterns (6), performance (9), process execution (9), process models (9), productivity (2), quality (2), reality (2), reference models (2), resources (9), risk (6), science research (2), semantics (9), sensemaking (1), simulation (9), strategy (0), systems (6), tables (7), verification (15), web (1), workflow (9).
As can be seen in Fig. 9 , these keywords are far more separate from each other compared to the author’s keywords.

Classification of articles by methodology
Based on the expert assessment, we examined the documents regarding the methods and approaches used. We created seven groups corresponding to a method or approach that was an essential part of the publication: probabilistic models, Decision Model and Notation (DMN), dynamic task assignment problem, evolutionary and genetics algorithms, queuing theory, social networks and others. These groups were also based on keyword analysis, as some separate groups of copyright keywords belong to highly unique articles. We assigned each document to just one group. That is in contradiction to research areas, where one article can be attributed to more than one research area. The individual documents and their division between research areas and methodological groups can be seen in Table 3 . We further analyzed the documents regarding their years of publication and plotted a bubble graph ( Fig. 10 ) with the publication years on the x .axis and the methodological groups on the y -axis. The appropriate number of publications corresponding to the given year and the group is indicated in the respective bubble. This quantity is also graphically represented by the size of the given bubble.

The largest group consisted of 10 publications on DMN and BPMN. Given the initiate year of DMN, this is the most significant approach serving with BPMN. DMN 1.0 was made available to the public in September 2015, the OMG group released DMN 1.1 in June 2016, DMN 1.2 was released in January 2019 and the latest version of DMN 1.3 was released in March 2020. The latest version did not affect this systematic search; however, the growth of publications since 2017 (see Fig. 10 , for example, was undoubtedly be affected by the DMN update.
We only assigned four documents to the methodological group focused on queue theory (See Table 3 and Fig. 10 ). The specific articles are listed in the following section under the appropriate heading. As the largest group was the DMN and BPMN group, we can thus rule out research question R3.
Result: Research question R3—not confirmed.
The methods, techniques and approaches used in the included publications are listed in the following section.
Probabilistic models
The probabilistic model can be used to make decisions when the activity reaches an exclusive splitting gateway and the activity’s subject must decide between alternative actions. They can be used for predicting or deciding between alternative works based on desirable outcomes. Probabilistic models were presented in the following publications:
- Herbert & Sharp (2012) : Quantitative analysis of probabilistic BPMN workflows;
- Herbert & Sharp (2013) : Precise quantitative analysis of probabilistic business process model and notation workflows;
- Ceballos, Flores-Solorio & Garcia-Vazquez (2015) : Towards Probabilistic Decision Making on Human Activities modeled with Business Process Diagrams;
- Ceballos, Flores-Solorio & Pablo Garcia (2015) : A Probabilistic BPMN Normal Form to Model and Advise Human Activities;
- Naoum et al. (2016) : A probabilistic method for business process verification: Reachability, Liveness and deadlock detection,
there the (Causal) Bayesian Network or Markov Decision processes were used.
DMN and decision analysis
Decision Model and Notation (DMN) is an industry standard for modeling and executing decisions that are determined by business rules. The association of DMN and BPMN is now common practice:
- Batoulis & Weske (2017) : Soundness of decision-aware business processes,
- De Smedt et al. (2019) : Holistic discovery of decision models from process execution data,
- Durán, Rocha & Salaün (2019) : A rewriting logic approach to resource allocation analysis in business process models,
- Figl et al. (2018) : What we know and what we do not know about DMN,
- Ghlala, Aouina & Ben Said (2017) : MC-DMN: Meeting MCDM with DMN Involving Multi-criteria Decision-Making in Business Process
- Hasic, De Smedt & Vanthienen (2018) : Augmenting processes with decision intelligence: Principles for integrated modelling
- Cho, Happa & Creese (2020) : Capturing Tacit Knowledge in Security Operation Centers,
- Mazhar, Wu & Rosemann (2018) : Designing complex socio-technical process systems - the airport example,
- Suchenia et al. (2019) : Towards knowledge interoperability between the UML, DMN, BPMN and CMMN models
- Tomaskova (2018) : Modeling Business Processes for Decision-Making.
Both standards fall under OMG.
Dynamic task assignment approach
The study : A dynamic task assignment approach based on individual worklists for minimizing the cycle time of business processes by Xie, Chien & Tang (2016) develop a dynamic task assignment approach for minimizing the cycle time of business processes. The contribution of this article lies in developing a dynamic task assignment approach based on queuing theory, individual worklist model, and stochastic theory.
Evolutionary and genetic algorithms
The evolutionary algorithm was applied in the following publications:
- Herbert & Sharp (2014b) : Optimisation of BPMN business models via model checking;
- Herbert et al. (2014) : Evolutionary optimization of production materials workflow processes;
- Herbert, Hansen & Jacobsen (2015) : Using quantitative stochastic model checking tool to increase safety and improve efficiency in production processes;
- Herbert & Hansen (2016) : Restructuring of workflows to minimise errors via stochastic model checking: An automated evolutionary approach;
to optimize the BP diagram, thus looking for a more efficient process. Especially the publication: Specifying business process outsourcing requirements, Rekik, Boukadi & Ben-Abdallah (2016) , presented a genetic algorithm to identify most appropriate activities of a business process that should be outsourced.
Queuing theory
In the article: Comparative analysis of business process litigation using queue theory and simulation (case study: Religious courts of South Jakarta) Bahaweres, Fitriyah & Rozy (2017) , Onggo et al. (2018) . A BPMN extension to support discrete-event simulation for healthcare applications: an explicit representation of queues, attributes and data-driven decision points Onggo et al. (2018) and Gómez-Martnez et al. (2019) . Formal Support of Process Chain Networks using Model-driven Engineering and Petri nets Gómez-Martnez et al. (2019) , the authors use queuing theory and simulation to compare processes modeled in BPMN. In the article: Automated performance analysis of business processes Bocciarelli & D’Ambrogio (2012) , authors presented a BP performance model of EQN (Extended Queueing Network) type.
Social network
The publications below focus on the application of social network analysis metrics (SNA) to studies of biological interaction networks in informatics.
- Khlif & Ben-Abdallah (2015) : Semantic and structural performer clustering in BPMN models transformed into social network models;
- Khlif, Ben-Abdallah & Ben Ayed (2017) : A methodology for the semantic and structural restructuring of BPMN models.
Other approaches
The following publications were unique in their approaches. We can mention for example: Workflow fault tree generation through model checking by Herbert & Sharp (2014a) with FMEA analysis. An effort prediction model based on BPM measures for process by Aysolmaz, Iren & Demirors (2013) with Linear multiple regression analysis. Performance evaluation of business processes through a formal transformation to SAN by Braghetto, Ferreira & Vincent (2011) using Stochastic Automata Network. Estimating performance of a business process model by Kamrani et al. (2009) using a Task assignment approach. Formal verification of business processes as timed automata by Mendoza Morales, Monsalve & Villavicencio (2017) convert BPMN to Timed Automata and then perform standard Queuing analysis. Business models enhancement through discovery of roles by Burattin, Sperduti & Veluscek (2013) , there the authors have extended the process model to roles, specifically designed role-sharing algorithm. Stochastic analysis of BPMN with time in rewriting logic by Duran, Rocha & Salaun (2018) presents a rewriting logic executable specification of BPMN with time and extended with probabilities. SBAT: A STOCHASTIC BPMN ANALYSIS TOOL by Herbert, Hansen & Jacobsen (2014) presents SBAT, a tool framework for the modelling and analysis of complex business workflows and A framework for model integration and holistic modelling of socio-technical systems by Wu et al. (2015) presents a layered framework for the purposes of integrating different socio-technical systems (STS) models and perspectives into a whole-of-systems model.
We have identified several gaps in the research and issues that need to be addressed in future research. The main gaps concern the research area of business economics. We assumed that this area would be the main and most frequent for the combination of BPMN and OR methods. However, we found that this area could be affected by the absence of specific notation. The relevant publications were written only after the release of version DMN 1.1. The effect of DMN notation will be addressed in future research.
An unexpected gap was a solution to finance and human resources management through OR. We would like to introduce publications Savku & Weber (2018) and Graczyk-Kucharska et al. (2020) as the pioneering works. The first article added the problem of optimal consumption problem from cash flow with delay and regimes. The authors developed the general analytic model setting and methods for the solution by studying a stochastic optimal control problem using the tools of the maximum principle. They proved the necessary and sufficient maximum principles for a delayed jump-diffusion with regimes under full and partial information. The second publication focused on transversal competencies, which are sets of knowledge, skills and attitudes required for different positions and in different professions. The authors used the method of multivariate additive regression spline together with artificial neural networks to create a model describing the influence of various variables on the acceleration of the acquisition of transverse competencies.
We assume that future research will be influenced by simulation and prediction methods. This study showed the use of Agent-based modelling methods and discrete-event simulations, or probabilistic models and social networks, but neural networks or artificial intelligence methods appeared in any publication. Based on this study, we further expect the use of more sophisticated approaches and the effect of new techniques. At the same time, it is possible to extend process modelling to inaccurate data using Fuzzy methods.
This paper presented a systematic overview of publications using BPMN and OR methods in process analysis. We analyzed 108 articles, that were selected using the appropriate strings in the advanced search option of in the WOS database. The papers that met the conditions of the study were subjected to various analyzes and were briefly described.
The review showed that the processes modelled by BPMN can be extended or analyzed as probabilistic processes, queue theory, or role and task assignments. Alternatively, processes can be optimized using evolutionary or genetic algorithms. The research also highlighted the need to identify keywords in publications correctly. For example, less than two-thirds of the selected articles contained the keyword BPMN, even though all the documents used this notation. Most of the articles were so-called one-off publications. Only a small number of author teams developed their topic in further continuing publications. Due to this, the average number of citations is relatively low. Due to the average number of citations to the total number of publications in all research areas, documents falling into the field of Operational Research are outstanding; there is an average of seven citations per article.
We analyzed the publications by research area and found that there is great potential for the research area of business economics (BE). Only a few papers were associated with this area (five in total) but all of them had a higher than average number of citations. The first document we included in this research area was published in 2017, that is only in the last quarter of the examined publication years. This focus on BE may have been initiated by the introduction of DMN notation.
Among the authors, smaller collaborating groups around the world were been identified. That groups co-work within the framework of co-authorship and co-citations. We only identified one single-author publication.
The analysis of keywords showed a significant difference between the keywords assigned by the authors and the so-called KeyWords Plus keywords. While the former were almost completely connected across publications, the latter were significantly diversified.
We have pointed out that the introduction of BPMN 2.0 led to an increase in publications using this notation.
Acknowledgments
The authors thank the student M. Kopecký for support in the field of BPMN modeling.
Funding Statement
The research has been supported by a GACR 18-01246S and by the Faculty of Informatics and Management UHK Specific Research Project. The funders had no role in study design, data collection and analysis, decision to publish, or preparation of the manuscript.
Additional Information and Declarations
The author declares that they have no competing interests.
Hana Tomaskova conceived and designed the experiments, performed the experiments, analyzed the data, performed the computation work, prepared figures and/or tables, authored or reviewed drafts of the paper, and approved the final draft.
Gerhard-Wilhelm Weber analyzed the data, authored or reviewed drafts of the paper, and approved the final draft.
- What is Organizational Communication? Types, Importance, and How to Manage (PDF Included)
- What is Company Valuation? How to Calculate it? Objectives and Limitations [PDF Inside]
- Who are the Barefoot Entrepreneurs? Why They Struggle to Succeed?
- What is Departmentation? Types, Importance, Process
- Life Insurance: Types, Benefits, Common Terms, Myths, & Tips For Choosing Right Insurance

Operations Research: Evolution, Process, Characteristics, and Pros & Cons, [PDF Inside]
Operations research (OR) is a field of applied mathematics, engineering, and managemen t science that deals with the application of advanced analytical methods to help make better decisions . OR uses quantitative models and data analysis to address complex issues in a variety of fields, including business, government, and healthcare.
OR problems typically involve the optimization of some objective, such as minimizing cost or maximizing profit. OR models are often used to help make decisions about resource allocation, scheduling, and transportation.
What you are going to learn?
Evolution of Operations Research
The evolution of OR can be traced through four distinct phases:
- This was the period when OR was first developed and applied to military problems. During this time, OR practitioners developed many of the basic tools and techniques that are still used today, such as linear programming, queuing theory, and game theory.
- OR began to be applied to a wider range of problems in the 1950s and 1960s. This was due in part to the development of new computing technologies, which made it possible to solve more complex problems. During this time, OR also began to be taught in universities, which helped to increase the number of OR practitioners.
- OR reached a level of maturity in the 1970s and 1980s. During this time, OR practitioners focused on developing more specialized tools and techniques for solving specific problems . OR also began to be used in a wider range of industries, including healthcare, transportation, and manufacturing .
- OR has continued to evolve in the modern era. During this time, OR practitioners have made use of new technologies, such as artificial intelligence and big data, to solve even more complex problems. OR has also become more internationalized, with practitioners working in countries all over the world.
Today, OR is a well-established discipline with a wide range of applications. It is used by businesses, governments, and other organizations to improve efficiency, reduce costs, and make better decisions. OR is a valuable tool that can help organizations achieve their goals.
Here are some of the key developments that have shaped the evolution of OR:
- The development of new mathematical and statistical techniques
- The development of new computing technologies
- The increasing availability of data
- The growing recognition of the importance of decision-making
- The increasing globalization of business
These developments have enabled OR practitioners to solve more complex problems, work with more data, and have a greater impact on organizations. As a result, OR is now a widely used discipline that is helping organizations to improve their performance .
Characteristics of Operations Research

There are three essential characteristics of operations research:
- OR problems are typically viewed as systems, in which the parts are interrelated and interact with each other. This requires OR practitioners to take a holistic view of the problem and to consider the impact of their decisions on all parts of the system.
- OR problems are often too complex to be solved by a single individual. As a result, OR practitioners typically work in teams that include people with a variety of skills and backgrounds, such as mathematics, engineering, statistics, and computer science.
- OR practitioners use the scientific method to solve problems. This involves defining the problem, developing a model of the problem, solving the model, and testing the solution in the real world.
OR has been used to solve a wide variety of problems in a variety of industries, including:
- OR has been used to improve decision-making in areas such as production, inventory, scheduling, and marketing.
- OR has been used to improve decision-making in areas such as defense, transportation, and healthcare.
- OR has been used to improve decision-making in areas such as education, social welfare, and environmental protection.
OR is a valuable tool that can help organizations make better decisions. By using OR, organizations can improve their efficiency, effectiveness, and profitability.
Here are some additional characteristics of operations research:
- OR uses quantitative methods, such as mathematics, statistics, and computer science, to solve problems.
- OR practitioners often use mathematical models to represent real-world systems.
- OR practitioners use data to inform their decision-making.
- OR practitioners are often creative and innovative in their approach to solving problems.
- OR practitioners often work with other professionals, such as managers, engineers, and scientists, to solve problems.
Operations research is a growing field with a wide range of applications. As organizations continue to face complex and challenging problems OR will become an increasingly important tool for decision-making.
Process of Operations Research
The process of operations research (OR) can be broadly broken down into the following steps:
1. Problem Formulation
The first step is to identify and define the problem that needs to be solved. This involves understanding the goals of the organization, the constraints that are imposed on the solution, and the factors that influence the outcome.
The problem formulation step is critical to the success of the OR project. If the problem is not properly defined, it will be difficult to develop a model that accurately represents the problem.
2. Modeling
The next step is to develop a mathematical model of the problem. This involves representing the problem in a way that can be analyzed using mathematical techniques.
In this step, the OR practitioner’s skills and knowledge come into play. The model should be accurate, but it should also be simple enough to be solved.
3. Solution
The third step is to solve the model and obtain a solution to the problem. This can be done using a variety of mathematical techniques, such as linear programming, integer programming, and dynamic programming.
4. Sensitivity Analysis
The fourth step is to perform a sensitivity analysis on the solution. This involves examining how the solution changes in response to changes in the model’s parameters.
It is important because it helps to identify the factors that have the greatest impact on the solution. This information can be used to make decisions about how to implement the solution.
5. Implementation
The final step is to implement the solution. This involves putting the solution into practice and monitoring its effectiveness.
In this step, the solution is put into practice. This can be a challenging step, as there may be resistance to change from within the organization.
Operations Research Models
There are many different models used in operations research (OR). Some of the most common models include:
Linear programming:
Linear programming is a mathematical optimization technique that can be used to find the best solution to a problem when the objective function and all the constraints are linear. The linear programming model can be represented by the following equation:
maximize or minimize z = c1x1 + c2x2 + … + cnxn subject to a1x1 + a2x2 + … + anxn <= b x1 >= 0, x2 >= 0, …, xn >= 0
- z is the objective function
- c1, c2, …, cn are the coefficients of the objective function
- x1, x2, …, xn are the decision variables
- a1, a2, …, an are the coefficients of the constraints
- b is the right-hand side of the constraints
Integer Programming:
Integer programming is a type of linear programming where the decision variables must be integers. Integer programming is used to solve problems where the decision variables must be whole numbers, such as the number of machines to use or the number of workers to hire. The integer programming model can be represented by the following equation:
Maximize or minimize:
z = a1x1 + a2x2 + … + anxn
Subject to:
c1x1 + c2x2 + … + cnxn <= b
x1, x2, …, xn are integers
Dynamic Programming
Dynamic programming is a technique for solving problems that involve a sequence of decisions. Dynamic programming works by breaking the problem down into smaller subproblems and then solving the subproblems recursively. The dynamic programming model can be represented by the following set of equations:
V(n) = optimal value of the problem with n stages
V(n) = max(V(n-1) + f(n), V(n-2) + g(n), …, V(0) + h(n))
The models used in OR are constantly evolving as new techniques are developed and as the problems that need to be solved become more complex. By understanding the different types of models that are available, OR practitioners can select the best model for the specific problem that they are trying to solve.
Importance of Operations Research
Improved decision-making: .
OR can help organizations make better decisions by providing them with a systematic approach to problem-solving. OR techniques can be used to identify the best course of action, given the available information and constraints.
Increased efficiency:
OR can help organizations improve their efficiency by identifying ways to reduce costs, improve productivity, and optimize resource allocation. OR techniques can be used to identify bottlenecks and inefficiencies in production processes, transportation networks, and other systems.
Reduced risk:
OR can help organizations reduce risk by identifying and mitigating potential problems. OR techniques can be used to assess the likelihood of different events occurring and to develop contingency plans in case of unexpected events.
Increased innovation:
OR can help organizations develop new products and services, improve existing products and services, and enter new markets. OR techniques can be used to identify new opportunities, assess the feasibility of new ideas, and develop prototypes of new products and services.
Uses of Operation Research
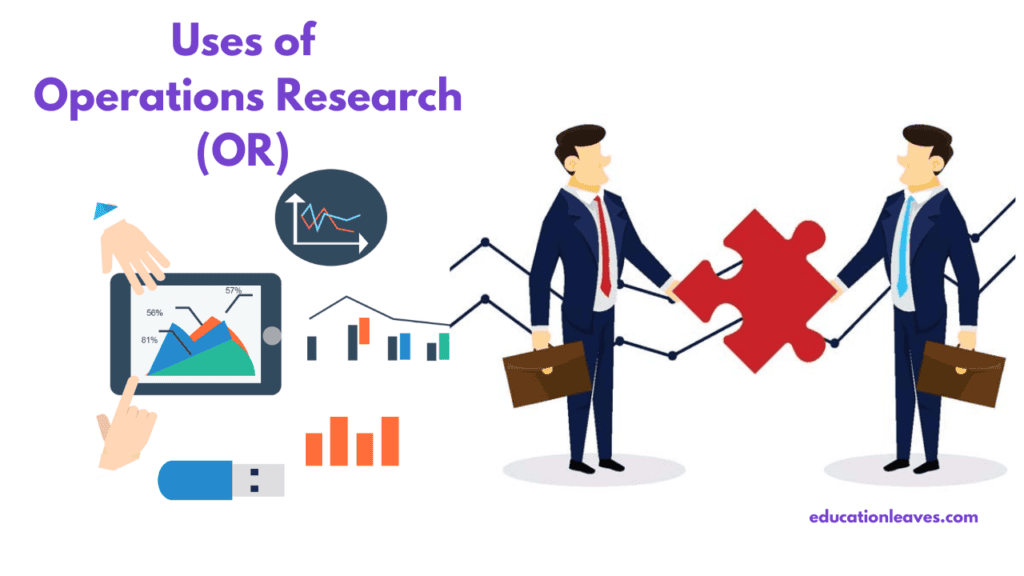
Operations research (OR) is a discipline that deals with the application of mathematical, statistical, and analytical techniques to help make better decisions. OR problems typically involve the allocation and control of limited resources, and they can arise in a wide variety of settings, including business, government, and the military.
OR practitioners use a variety of tools and techniques to solve problems, including:
Mathematical modeling:
This involves developing mathematical models of the problem that can be used to simulate different scenarios and evaluate the impact of different decisions.
Statistical analysis:
This involves collecting and analyzing data to identify trends and patterns that can be used to make better decisions.
Data mining:
This involves using statistical techniques to extract hidden patterns and trends from large datasets.
Computer simulation:
This involves using computers to create virtual models of real-world systems that can be used to test different hypotheses and solutions.
OR has been used to solve a wide variety of problems, including:
Optimizing production schedules:
OR can be used to help companies determine the best way to schedule production in order to minimize costs and maximize output.
Routing delivery vehicles:
OR can be used to help companies determine the best way to route delivery vehicles in order to minimize travel time and fuel costs.
Allocating resources:
OR can be used to help companies allocate resources, such as personnel and equipment, in order to maximize efficiency and minimize costs.
Managing risk:
OR can be used to help companies manage risk , such as the risk of stock market fluctuations or natural disasters.
OR is a powerful tool that can be used to improve decision-making in a wide variety of settings. By applying OR techniques, companies can save money, improve efficiency, and increase profits.
Nature and Scopes of Operations Research
The nature of OR is to be a problem-solving tool that can be used to improve the efficiency and effectiveness of organizations. OR can be used to solve a wide variety of problems, including:
- Production planning and scheduling
- Inventory management
- Transportation and logistics
- Financial planning
- Risk management
- Customer service
- Military operations
The scope of OR is broad and encompasses a wide range of industries and organizations. OR is used by businesses, government agencies, and non-profit organizations to improve their operations. OR has been used to solve problems in a variety of industries, including:
- Manufacturing
- Transportation
OR is a valuable tool for organizations that are looking to improve their performance. By using OR, organizations can make better decisions, improve efficiency, and reduce costs.
Here are some of the key benefits of using operations research:
- Improved decision-making: OR can help organizations make better decisions by providing them with a more systematic and analytical approach to problem-solving.
- Increased efficiency: OR can help organizations improve their efficiency by identifying and eliminating waste and inefficiency.
- Reduced costs: OR can help organizations reduce their costs by finding ways to save money on resources, such as materials, labor, and energy.
- Increased customer satisfaction: OR can help organizations improve customer satisfaction by providing them with better products and services, and by providing them with a more efficient and convenient way to do business.
If you are looking for a way to improve your organization’s performance, operations research is a valuable tool that you should consider using.
Concept of Optimizing
Optimization is a key concept in operations research. It is the process of finding the best possible solution to a problem, given a set of constraints. Optimization problems can be classified into two types:
- Deterministic optimization problems have known values for all of the variables in the problem.
- Stochastic optimization problems have some variables that have uncertain values.
There are a variety of methods that can be used to solve optimization problems. Some of the most common methods include:
- Linear programming is a method for solving deterministic optimization problems where the objective function and the constraints are linear.
- Nonlinear programming is a method for solving deterministic optimization problems where the objective function or the constraints are nonlinear.
- Stochastic programming is a method for solving stochastic optimization problems.
The choice of method for solving an optimization problem depends on the specific problem and the available resources.
In operations research, optimization is used to solve a wide variety of problems. Some of the most common applications of optimization in operations research include:
- Production planning
Optimization is a powerful tool that can be used to improve the efficiency and effectiveness of organizations. By using optimization, organizations can make better decisions, improve their operations, and reduce their costs.
Here are some examples of how optimization has been used in operations research:
- Production planning: A company can use optimization to determine the optimal production schedule for a product. This can help the company to reduce costs and improve efficiency.
- Inventory management: A company can use optimization to determine the optimal inventory levels for a product. This can help the company to reduce costs and improve customer service.
- Transportation and logistics: A company can use optimization to determine the optimal transportation and logistics network for its products. This can help the company to reduce costs and improve delivery times.
- Financial planning: A company can use optimization to determine the optimal investment portfolio for its shareholders. This can help the company to maximize shareholder returns.
- Risk management: A company can use optimization to determine the optimal risk management strategy for its operations. This can help the company to reduce the risk of financial losses.
- Customer service: A company can use optimization to determine the optimal customer service strategy for its customers. This can help the company to improve customer satisfaction and retention.
- Military operations: The military can use optimization to determine the optimal deployment of troops and resources for a military campaign. This can help the military to achieve its objectives with minimal casualties.
These are just a few examples of how optimization has been used in operations research. Optimization is a powerful tool that can be used to improve the efficiency and effectiveness of organizations in a wide variety of industries.
Vogel’s approximation method (VAM) of Transportation Problem
Vogel’s approximation method (VAM) is a heuristic algorithm for solving transportation problems. It is a greedy algorithm, which means that it makes local decisions that do not guarantee an optimal solution, but that often lead to good solutions.
VAM works by finding the cell with the highest penalty in each row and column. The penalty for a cell is the difference between the smallest cost in the row or column and the second smallest cost. The cell with the highest penalty in each row and column is then assigned a flow equal to the minimum of the supply or demand in that row or column.
VAM is a simple and efficient algorithm that can be used to solve transportation problems quickly. It is not guaranteed to find an optimal solution, but it often finds good solutions.
Here are the steps involved in Vogel’s approximation method:
1. Identify the two lowest costs in each row and column of the given cost matrix and then write the absolute row and column difference. These differences are called penalties.
2. Identify the row or column with the maximum penalty and assign the corresponding cell’s min(supply, demand). If two or more columns or rows have the same maximum penalty, then we can choose one among them as per our convenience.
3. Repeat steps 1 and 2 until all supply and demand is met.
Here is an example of how Vogel’s approximation method can be used to solve a transportation problem:
Source Destination Cost ——- ——– ——– A B 3 A C 4 B D 2 C D 5
Supply: 10 Demand: 12
The first step is to identify the two lowest costs in each row and column. The two lowest costs in row A are 3 and 4, the two lowest costs in column B are 2 and 5, and the two lowest costs in column C are 4 and 5. The absolute row and column differences are then calculated. The absolute row difference for row A is 1, the absolute row difference for column B is 3, and the absolute row difference for column C is 1. The row with the maximum penalty is column B, so the corresponding cell is assigned a flow of 10. The supply in column B is now 0, so it is removed from the problem.
The next step is to repeat steps 1 and 2. The two lowest costs in row A are now 4 and 5, the two lowest costs in column D are 2 and 5, and there are no costs in column C. The absolute row difference for row A is 1, the absolute row difference for column D is 3, and there is no row difference for column C. The row with the maximum penalty is column D, so the corresponding cell is assigned a flow of 2. The demand in column D is now 0, so it is removed from the problem.
The final step is to repeat steps 1 and 2 until all supply and demand is met. There is no supply or demand left, so the problem is solved. The optimal solution is to send 10 units from A to B and 2 units from A to D.
VAM is a simple and efficient algorithm for solving transportation problems. It is not guaranteed to find an optimal solution, but it often finds good solutions.
N.W. Corner’s method of Transportation Probloem
The Northwest Corner Method is a simple and efficient algorithm for solving transportation problems. It is a greedy algorithm, which means that it makes local decisions that do not guarantee an optimal solution, but that often lead to good solutions.
The Northwest Corner Method works by starting in the top left corner of the transportation matrix and assigning as much as possible to that cell. Then, it moves to the next cell in the row or column, whichever has the smallest remaining supply or demand, and repeats the process. This continues until all supply or demand is met.
The Northwest Corner Method is not guaranteed to find an optimal solution, but it often finds good solutions quickly. It is a good choice for problems with a small number of sources and destinations.
Here are the steps involved in the Northwest Corner Method:
- Start in the top left corner of the transportation matrix.
- Assign as much as possible to that cell.
- If the supply or demand in that cell is met, move on to the next cell in the row or column.
- If the supply or demand in that cell is not met, assign as much as possible to that cell.
- Repeat steps 3 and 4 until all supply or demand is met.
Here is an example of how the Northwest Corner Method can be used to solve a transportation problem:
The first step is to start in the top left corner of the transportation matrix. The top left corner is cell (A,B), so we assign as much as possible to that cell. The minimum of the supply in row A and the demand in column B is 10, so we assign 10 to cell (A,B).
The next step is to move to the next cell in the row or column, whichever has the smallest remaining supply or demand. In this case, the smallest remaining supply is in row A, so we move to cell (A,C). The minimum of the supply in row A and the demand in column C is 2, so we assign 2 to cell (A,C).
The next step is to repeat steps 3 and 4 until all supply or demand is met. In this case, there is still 8 units of supply in row A and 4 units of demand in column D. The minimum of 8 and 4 is 4, so we assign 4 to cell (A,D).
There is no supply or demand left, so the problem is solved. The optimal solution is to send 10 units from A to B, 2 units from A to C, and 4 units from A to D.
The Northwest Corner Method is a simple and efficient algorithm for solving transportation problems. It is not guaranteed to find an optimal solution, but it often finds good solutions quickly. It is a good choice for problems with a small number of sources and destinations.
Operations research is a broad field that uses mathematical, statistical, and computer-based techniques to solve complex problems in a variety of fields, including business, government, and healthcare. It is a powerful tool that can help organizations make better decisions, improve efficiency, and increase profits.
One of the key benefits of operations research is that it can help organizations to make better decisions. By using mathematical models and simulations, operations researchers can help organizations to understand the impact of different decisions on their operations. This can help organizations to make more informed decisions that are more likely to achieve their desired outcomes.
Another key benefit of operations research is that it can help organizations to improve efficiency. By identifying and eliminating waste in their operations, organizations can save money and improve their bottom line. Operations research can also help organizations to improve their customer service by reducing wait times and improving the quality of their products and services.
Finally, operations research can help organizations to increase profits. By optimizing their operations, organizations can increase their output and reduce their costs. This can lead to increased profits and a stronger bottom line.
Overall, operations research is a powerful tool that can help organizations to make better decisions, improve efficiency, and increase profits. If you are looking for a way to improve your organization’s performance, then operations research is a great place to start.
Download Operations Research (OR) PDF
- Formal Vs Informal Communication: A detailed comparison
- What is Managerial economics? Definition, Scopes, Nature of managerial economics (PDF)
You May Also Like

What is Just-In-Time? [PDF] | Definition, Process, Benefits, Disadvantages, and Example
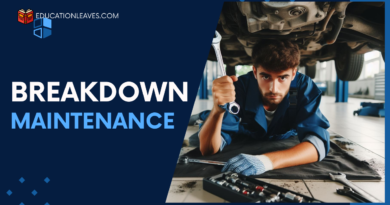
What is Breakdown maintenance? Disadvantages of Breakdown Maintenance, Causes of equipment breakdown.

What is Sales Forecasting? | Sales Forecasting Method, Types, Importance, and Elements | Sales Forecasting PDF
Leave a reply cancel reply.
Your email address will not be published. Required fields are marked *
Save my name, email, and website in this browser for the next time I comment.
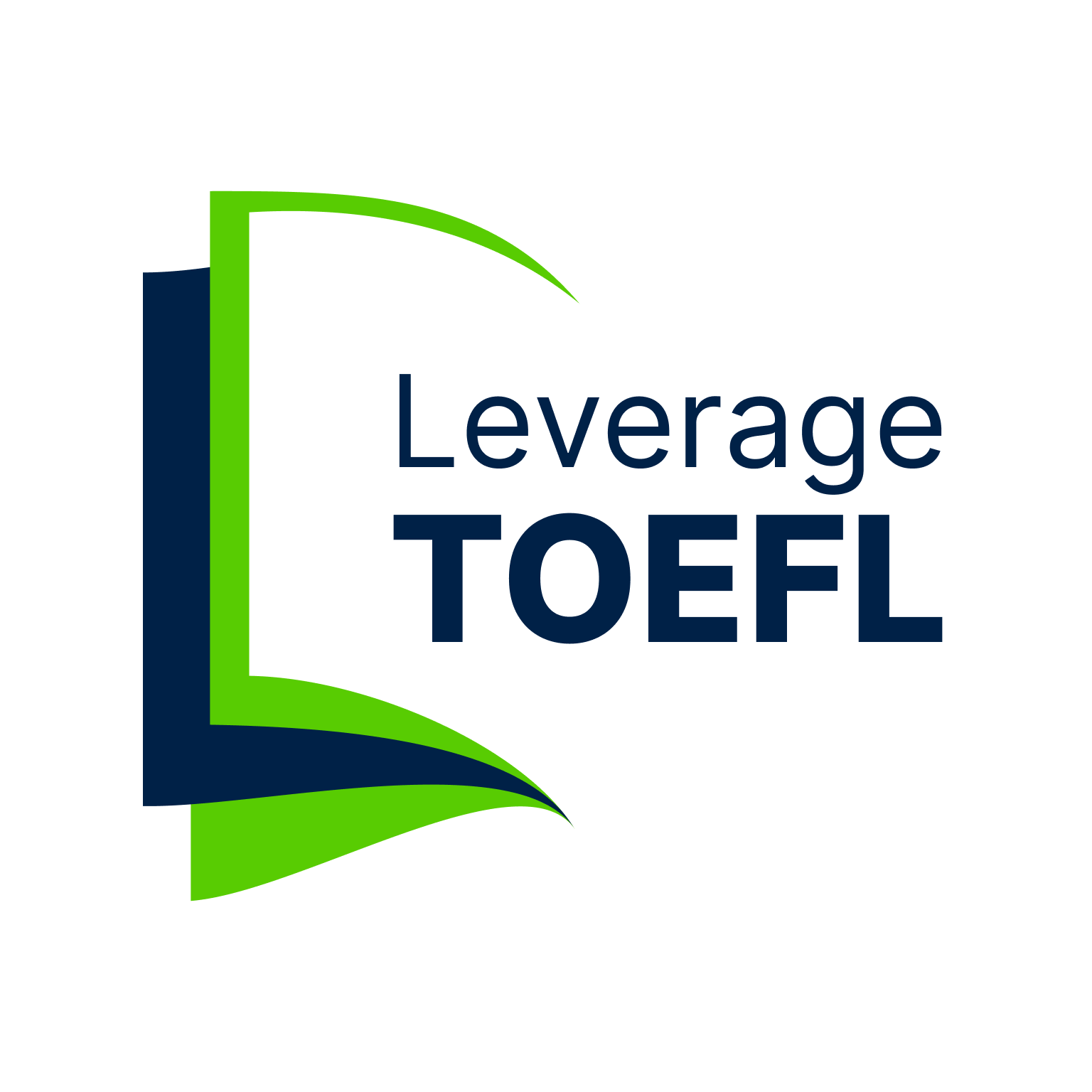
25,000+ students realised their study abroad dream with us. Take the first step today
Here’s your new year gift, one app for all your, study abroad needs, start your journey, track your progress, grow with the community and so much more.

Verification Code
An OTP has been sent to your registered mobile no. Please verify
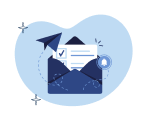
Thanks for your comment !
Our team will review it before it's shown to our readers.

Scope of Operation Research
- Updated on
- Aug 19, 2022
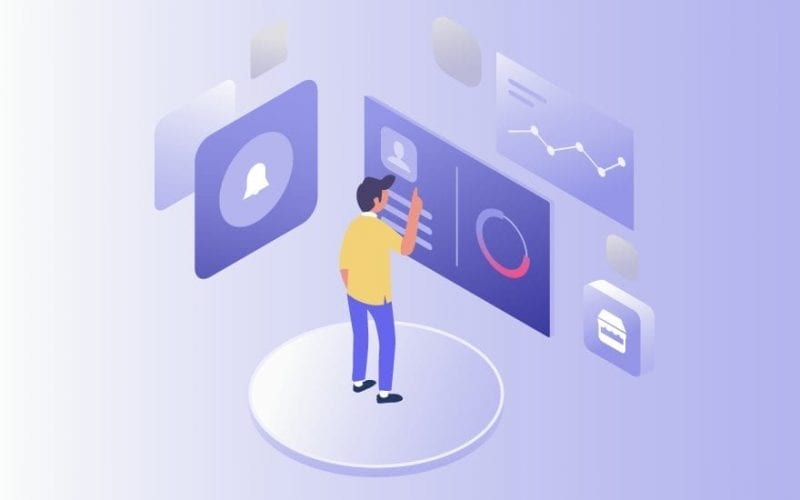
What is the scope of operational research? To answer this, we will first have to understand what operations research means. Operational Research can be simply formulated as the science of rational decision-making along with the study of the synthesis of intricate problematic situations. Thus, the nature of operations research is purely scientific as it aims at determining the systematic behaviour and hence optimizing the results. The scope and applications of operations research empower decision-making in those business aspects where there is a larger concern of allocation of scarce resources especially like capital, investment, labour, etc.
This Blog Includes:
Scope and application of operations research, nature of operations research, limitations of operations research, characteristics of operations research, phases of operation research, where is operation research used, methodologies, roles & responsibilities in operation research , defence services, agriculture, industrial sector, scope of operational research: job profiles .
Operation Research centrally focuses on simplifying complicated business problems into well-defined mathematical constructs and works specifying expected behaviour as well as goals. The key application of Operations Research is that it facilitates decision making in those aspects of a business where resource allocation is paramount, i.e. capital, labour, time and other business resources. Given that it is rooted in computer science and analytics, there is an immense scope of operation research in every business enterprise.
Here are the key areas of the scope and application of operations research:
- Healthcare Management and Hospital Administration
- Financial Management , Budgeting and Investments
- Government Development / Public Sector Units
- Energy and Environment
- Marketing and Revenue Management
- Telecommunication Networks
- Military Defences
- Supply Chain Management
- Purchasing / Procurement / Logistics
- National Plans / Budgets
Find out everything about Operations Management MBA !
When it comes to the nature of operations research, business experts and scholars describe it as a science as operations research takes a mathematical and scientific approach to decision-making and problem-solving. Let’s understand the nature of operations research through these key definitions:
- “Operations Research is a scientific approach to problem-solving for executive management.” – H.M Wagner
- “Operations Research is the systematic, method oriented study of the basic structure, characteristics, functions and relationships of an organization to provide the executive with a sound, scientific and quantitative basis for decision making.” – E.L. Arnoff and M.J. Netzorg
- “Operations Research is an aid for the executive in making his decision by providing him with the needed quantitative information based on the scientific method of analysis.” – C. Kittel
- “O.R. is an experimental and applied science devoted to observing, understanding and predicting the behavior of purposeful man-machine systems and operations research workers are actively engaged in applying this knowledge to practical problems in business, government and society.” – Operations Research Society of America
There are certain limitations and disadvantages of operations research that you must also know about. Here are the limitations of operations research:
- Operation Research can be costly in terms of implementation as well as using the best technology and tools for simulations.
- Operation Research also requires a team of analysts or experts to carry out every step of the process and thus needs a team of properly qualified professionals to find the best results and solutions.
- Operation Research also relies heavily on technology as software applications and tools play an imperative part in data analysis and predictions.
Given below are the 3 main characteristics of Operations Research, i.e. Optimization, Stimulation and Probability & Statistics.
Optimization The objective of Operations Research is to provide better performance in any given situation. The process of doing this involves a lot of data collection and analysis of possible outcomes. The process of optimization involves a critical analysis of all the available options and selecting the highly relevant ones.
Simulation Before you move on with selected options or methods to improve the overall result, you must be sure that the selected method is going to be beneficial. This part is ensured by the process of simulation.
Probability and Statistics Mathematical algorithms are the best way to evaluate potential risks and predict the possible outcomes beforehand. Thus, Operation Research involves intensive use of statistical and mathematical approach to cover all the circumstances.
There are three phases in an operation research study –
- Determining the operation and objectives
- It also includes determining the effectiveness of different steps, the type of problem, its origin and the causes behind it.
- Formulating the hypothesis and models
- Next step is analyzing the information collected and verifying the hypothesis.
- The last step in this phase is the production and generation of results and considering all the other alternatives.
- Giving recommendations for the solution to the problem including the assumptions, scope, limitations and other alternatives.
- Lastly, the solution has to be put to work.
Here are the most common problems that can be solved by Operation Research:
When operation research is carried out in a business enterprise, there are three major steps followed to complete the OR process. These steps are:
- Judgement : At this phase, the clarification of the problem happens. First, the problem is defined in a simple manner and then the objectives and ways for measuring the final results and success are also determined.
- Research : Once you have defined what the problem is, the research phase carries out the accumulation of data about the problem and then determining the hypothesis, validating the hypothesis and then developing the model(s) and thus making possible predictions.
- Action : After getting the predictions, results are incorporated and the necessary improvements and changes take place.
If you imagine yourself working in the field of Operational Research, there are many essential responsibilities that you will be expected to handle. Hence, you need to be well-versed with the qualities and attributes required to apprehend critical situations and problems. So, before moving to the scope of Operation Research, take a look at the following roles and responsibilities that you will be carried out under varied career profiles.
- To analyze and devise the plan using statistical analysis, predictive modelling, simulations and methods to exempt the organizations from business problems.
- To help organisational teams with in-depth research on various topics.
- Collect the required information for the company from co-workers and utilize it in situations to come up with decisions.
- Preparing reports by performing critical analyses of the key matrices.
- Support the company with advantageous financial decisions while comprehending the budgets.
Find our how to make a career in Operational Research !
Career Scope of Operation Research: Employment Areas
With a degree in Operation Research or its related field, candidates can enter many employment areas. These leading job destinations can carve your career and provide you with a high-end growth ahead. To help you understand the scope of operation research, let’s elaborate some of the top-notch job-sectors that you can explore.
- Logistics and Supply Chain Management
- Marketing Management
There are several departments in the defence services like administrations, training, supply etc. which involves in-depth knowledge of operations. To perform warfare tasks in the Army, Navy, Air Force with promptness, there is always a constant demand for candidates with a degree in operation research. Thus, in the defence services, there is a wide scope of operation research as candidates get the opportunity to work in a dynamic environment and under higher roles facilitating the formulation of policies and strategies.
Being brought up in a country like India where agriculture is a colossal part of the economy, we often think that this domain is only for farmers or rather scientists. But, with the dire need to ensure optimum utilization of resources, there is a greater scope of operation research in this field. With sky-rocketing population, operation research can ensure optimization in agriculture and potential graduates in this field can avail the opportunities to work as research assistants under this domain.
In the advent of globalization, the industrial sector is experiencing an upheaval across the globe. To frame and handle the ever-rising demands of the organizations, candidates with brainstorming abilities are selected for various profiles in operation research. You can also opt for a specialised role like an Operational Research Analyst, who is expected to tackle the upcoming discrepancies in a business.
Have a look at the Job Oriented Courses After MBA
In the above-mentioned sectors, there is a multitude of career profiles available for those wanting to kickstart their career in operation research. Below we have brought you some of the key job roles which depict the varied scope of operation research:
- Operations Analyst
- Research Analyst
- Operation Research Analyst
- Project Manager
- Data Analyst
- Consultant
- System Analyst
- Senior Analyst
- Operations Officer
- Project Analyst
- Quality Assurance Analyst
It is the research process from which management provides support for their decisions. While the name of this method is relatively new, the method used for this is not a new one. Operation Analysis is concerned with applying the concepts and techniques of science to strategic issues.
In its latest years of organized growth, O.R. Several research proceedings for the military, government and industry have been successfully resolved. In most developing countries in Asia and Africa, the underlying issue is that poverty and hunger should be removed as soon as possible. There is also a tremendous opportunity for economists , statisticians, managers, politicians and technicians working in a team to address this issue through an O.R. Approaching.
Over the next ten years, the employment of operational research analysts is expected to rise by 25 percent, far faster than the average for all occupations. As technology progresses and businesses are pursuing efficiency and cost savings, the market for analysis of operations research should continue to rise. Therefore, it is a good career option
Here are the advantages of Operation Research – 1. Effective Decisions 2. Better Coordination 3. Facilitates Control 4. Improves Productivity
Hence, we hope that this blog has helped you understand the career scope of operation research as well as an array of opportunities that you can discover in this specialised domain. If you are allured by the fascinating above-listed job profiles and want to start a career in this field, then you should definitely opt for an academic degree to understand the intricacies of this domain. Take the help of Leverage Edu ’s AI-based tool to browse through a plethora of specialised programs in this field and find the best course and university combination that can equip you with the required knowledge and exposure to establish your career in Operation Research.
Team Leverage Edu
Leave a Reply Cancel reply
Save my name, email, and website in this browser for the next time I comment.
Contact no. *
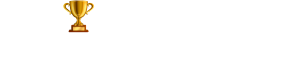
Leaving already?
8 Universities with higher ROI than IITs and IIMs
Grab this one-time opportunity to download this ebook
Connect With Us
25,000+ students realised their study abroad dream with us. take the first step today..

Resend OTP in

Need help with?
Study abroad.
UK, Canada, US & More
IELTS, GRE, GMAT & More
Scholarship, Loans & Forex
Country Preference
New Zealand
Which English test are you planning to take?
Which academic test are you planning to take.
Not Sure yet
When are you planning to take the exam?
Already booked my exam slot
Within 2 Months
Want to learn about the test
Which Degree do you wish to pursue?
When do you want to start studying abroad.
September 2024
January 2025
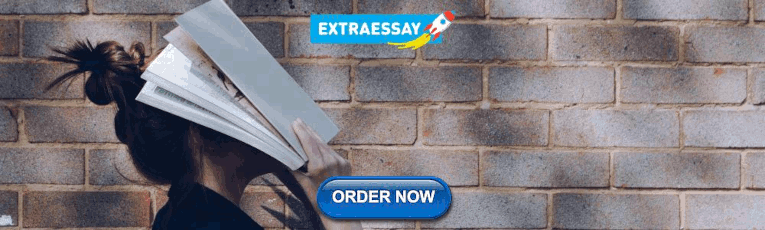
What is your budget to study abroad?
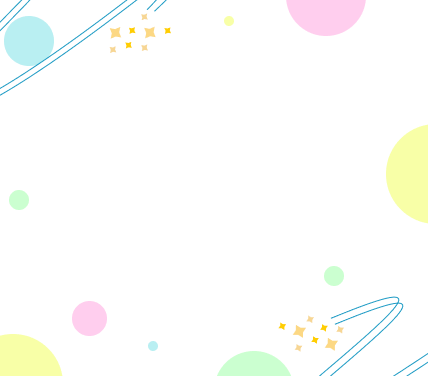
How would you describe this article ?
Please rate this article
We would like to hear more.
Operation Research: Definition, Scope and Techniques
After reading this article you will learn about:- 1. Meaning and Definition of Operation Research 2. Phases in Operation Research Study 3. Scope 4. Characteristics 5. Methodology 6. Models 7. Techniques 8. Applications 9. Limitations.
Meaning and Definition of Operation Research :
It is the method of analysis by which management receives aid for their decisions. Though the name of this method, Operation Research (O.R.) is relatively new, but the method used for this is not a new one. Operation Research is concerned with the application of the principles and the methods of science to the problems of strategy.
The subject of operation research was born during Second World War in U.K., and was used for military strategy. During World War II, a group of scientists, having representatives from mathematics, statistics, physical and social sciences were entrusted to the study of various military operations. This team was very successful and greatly contributed to the meticulous handling of entire operation and related problems of the operation.
The need for assigning such studies for operations arose because military strategies and their decisions become so important and costly and therefore, the best scientists, under the sponsorship of military organs were grouped together to provide quantitative information’s by adopting scientific techniques and methods for facilitating in taking decisions.
ADVERTISEMENTS:
After the World War II, it was started applying in the fields of industry, trade, agriculture, planning and various other fields of economy.
The operation research can be defined as:
Definitions:
(i) It is the application of scientific methods, techniques and tools to problems involving the operations of a system so as to provide those in the control of the system with optimum solutions to the problems.
(ii) Operation Research is a tool for taking decisions which searches for the optimum results in parity with the overall objectives and constraints of the organisation.
(iii) O.R. is a scientific method of providing executive department with a quantitative basis of decisions regarding the operations under their control.
(iv) O.R. is a scientific approach to problem solving for management.
(v) O.R. is an aid for executive in making his decisions by providing him with the needed quantitative information’s based on the scientific method of analysis.
(vi) O.R. is the application of modern methods of mathematical science to complex problems involving management of large systems of men, machines, materials, and money in industry, business, government and defence. The distinctive approach is to develop a scientific model of the system incorporating measurement of factors such as chance and risk, to predict and compare the outcome of alternative decisions, strategies or controls.
(vii) It is the application of the scientific methods by scientists and subject specialists to the study of the given operation. Its purpose is to give administration, a basis for predicting quantitatively the most effective results of an operation under given set of variable conditions and thereby to provide a sound basis for “decision-making”.
In fact in Operation Research, research techniques and scientific methods are employed for the analysis and also for studying the current or future problems. Thus, Operation Research offers alternative plans for a problem to the management for decisions.
Although it is very clear that operation research never make decisions for the management, instead the method presents management with a careful scientific and quantitative analysis of problem so that the management will be in a better position to make sounder decisions.
It can be used for solving different types of problems, such as:
i. Problems dealing with the waiting line, the arrival of units or persons requiring service.
ii. Problems dealing with the allocation of material or activities among limited facilities.
iii. Equipment replacement problems.
iv. Problems dealing with production processing i.e., production control and material shipment.
But it may be remembered that operation research never replaces a manager as decision maker. The ultimate and full responsibility for analysing all factors and making decision will be of the manager.
In the more wide sense, operation research does not deal with the everyday problems such as output by the one worker or machine capacity; instead it is concerned with the overall aspect of business operation such as something as the relationship between inventory, sales, production and scheduling. It may also deal with the overall flow of goods and services from plants to consumers.
The team doing operation research may have statisticians, psychologists, labour specialists, mathematicians and others depending upon the requirement for the problems.
Phases in Operation Research Study :
Since, the main objective of operation research is to provide better quantitative information’s for making decision. Now our aim is to learn how we can have better decisions.
The procedure for making decisions with the OR study generally involves the following phases:
(i) Judgment Phase:
i. Determination of operation.
ii. Determination of objectives.
iii. Determination of effectiveness of measures.
iv. Determination of type of problem, its origin and causes.
(ii) Research Phase:
i. Observation and data collection for better understanding of the problem.
ii. Formulation of relevant hypothesis and models.
iii. Analysis of available information and verification of hypothesis.
iv. Production and generation of results and consideration of alternatives.
(iii) Action Phase:
i. Recommendations for remedial action to those who first posed the problem, this includes the assumptions made, scope and limitations, alternative courses of action and their effect.
ii. Putting the solution to work: implementation.
Without OR, in many cases, we follow these phases in full, but in other cases, we leave important steps out. Judgment and subjective decision-making are not good enough. Thus industries look to operation research for more objective way to make decisions. It is found that method used should consider the emotional and subjective factors also.
For example, the skill and creative labour are important factors in our business and if management wants to have a new location, the management has to consider the personal feeling of the employees for the location which he chooses.
Scope of Operation Research:
In its recent years of organised development, O.R. has solved successfully many cases of research for military, the government and industry. The basic problem in most of the developing countries in Asia and Africa is to remove poverty and hunger as quickly as possible. So there is a great scope for economist, statisticians, administrators, politicians and technicians working in a team to solve this problem by an O.R. approach.
On the other hand, with the explosion of population and consequent shortage of food, every country is facing the problem of optimum allocation of land for various crops in accordance with climatic conditions and available facilities. The problem of optimal distribution of water from a resource like a canal for irrigation purposes is faced by developing country. Hence a good amount of scientific work can be done in this direction.
In the field of Industrial Engineering, there is a claim of problems, starting from the procurement of material to the despatch of finished products. Management is always interested in optimizing profits.
Hence in order to provide decision on scientific basis, O.R. study team considers various alternative methods and their effects on existing system. The O.R. approach is equally useful for the economists, administrators, planners, irrigation or agricultural experts and statisticians etc.
Operation research approach helps in operation management. Operation management can be defined as the management of systems for providing goods or services, and is concerned with the design and operation of systems for the manufacture, transport, supply or service. The operating systems convert the inputs to the satisfaction of customers need.
Thus the operation management is concerned with the optimum utilisation of resources i.e. effective utilisation of resources with minimum loss, under utilisation or waste. In other words, it is concerned with the satisfactory customer service and optimum resource utilisation. Inputs for an operating system may be material, machine and human resource.
O.R. study is complete only when we also consider human factors to the alternatives made available. Operation Research is done by a team of scientists or experts from different related disciplines.
For example, for solving a problem related to the inventory management, O.R. team must include an engineer who knows about stores and material management, a cost accountant a mathematician-cum-statistician. For large and complicated problems, the team must include a mathematician, a statistician, one or two engineers, an economist, computer programmer, psychologist etc.
Some of the problems which can be analysed by operations research are given hereunder:
1. Finance, Budgeting and Investment:
i. Cash flow analysis, long range capital requirement, investment portfolios, dividend policies,
ii. Claim procedure, and
iii. Credit policies.
2. Marketing:
i. Product selection, competitive actions,
ii. Number of salesmen, frequencies of calling on, and
iii. Advertising strategies with respect to cost and time.
3. Purchasing:
i. Buying policies, varying prices,
ii. Determination of quantities and timing of purchases,
iii. Bidding policies,
iv. Replacement policies, and
v. Exploitation of new material resources.
4. Production Management:
i. Physical distribution: Location and size of warehouses, distribution centres and retail outlets, distribution policies.
ii. Facilities Planning: Number and location of factories, warehouses etc. Loading and unloading facilities.
iii. Manufacturing: Production scheduling and sequencing stabilisation of production, employment, layoffs, and optimum product mix.
iv. Maintenance policies, crew size.
v. Project scheduling and allocation of resources.
5. Personnel Management:
i. Mixes of age and skills,
ii. Recruiting policies, and
iii. Job assignments.
6. Research and Development:
i. Areas of concentration for R&D.
ii. Reliability and alternate decisions.
iii. Determination of time-cost trade off and control of development projects.
Characteristics (Features) of Operation Research :
Main characteristics of operations research (O.R.) are follows:
(i) Inter-Disciplinary Team Approach:
This requires an inter-disciplinary team including individuals with skills in mathematics, statistics, economics, engineering, material sciences, computer etc.
(ii) Wholistic Approach to the System:
While evaluating any decision, the important interactions and their impact on the whole organisation against the functions originally involved are reviewed.
(iii) Methodological Approach:
O.R. utilises the scientific method to solve the problem
(iv) Objective Approach:
O.R. attempts to find the best or optimal solution to the problem under consideration, taking into account the goals of the organisation.
Methodology of Operation Research :
Operation Research, is a scientific approach for decision-making, and therefore must follow following steps:
1. Formulating the Problem:
The problem must be first clearly defined. It is common to start the O.R. study with tentative formulation of the problem, which is reformulated over and again during the study. The study must also consider economical aspects.
While formulating the O.R. study, analyists must analyse following major components:
(i) The environment:
Environment involves physical, social and economical factors which are likely to affect the problem under consideration. O.R. team or analysts must study the organisation contents including men, materials, machines, suppliers, consumers, competitors, the government and the public.
(ii) Decision-makers:
Operation analyst must study the decision-maker and his relationship to the problem at hand.
(iii) Objectives:
Considering the problem as whole, objectives should be defined.
(iv) Alternatives:
The O.R. study determines as to which alternative course of action is most effective to achieve the desired objectives. Expected reactions of the competitors to the alternative must also be considered.
2. Deriving Solution:
Models are used to determine the solution either by simulation or by mathematical analysis. Mathematical analysis for deriving optimum solution includes analytical or numerical procedure, and uses various branches of mathematics.
3. Testing the Model and Solution:
A properly formulated and correctly manipulated model is useful in predicting the effect of changes in control variables on the overall system effectiveness. The validity of the solution is checked by comparing the results with those obtained without using the model.
4. Establishing Controls over the Solution:
The solution derived from a model remains effective so long as the uncontrolled variables retain their values and the relationship. The solution goes out of control, if the values of one or more variables vary or relationship between them undergoes a change. In such circumstances the models need to be modified to take the changes into account.
5. Implementing the Solution:
Solution so obtained should be translated into operating procedure to make it easily understandable and applied by the concerned persons. After applying the solution to the system, O.R. group must study the response of the system to the changes made.
Operation Research Models :
Operation Research model is an idealised representation of the real life situation and represents one or more aspects of reality. Examples of operation research models are: a map, activity charts balance sheets, PERT network, break-even equation, economic ordering quantity equation etc. Objective of the model is to provide a means for analysing the behaviour of the system for improving its performance.
Classification of Models :
Models can be classified on the basis of following factors:
1. By degree of Abstraction:
i. Mathematical models.
ii. Language models.
2. By Function:
i. Descriptive models.
ii. Predictive models.
iii. Normative models for repetitive problems.
3. By Structure:
i. Physical models.
ii. Analogue (graphical) models.
iii. Symbolic or mathematical models.
4. By Nature of Environment:
i. Deterministic models.
ii. Probabilistic models.
5. By the Time Horizon:
i. Static models.
ii. Dynamic models.
Characteristics of a Good Model :
i. Assumptions should be simple and few.
ii. Variables should be as less as possible.
iii. It should be able to asscimilate the system environmental changes without change in its framework.
iv. It should be easy to construct.
Constructing the Model :
A mathematical model is a set of equations in which the system or problem is described. The equations represent objective function and constraints. Objective function is a mathematical expressions of objectives (cost or profit of the operation), while constraints are mathematical expressions of the limitations on the fulfillment of the objectives.
These expressions consist of controllable and uncontrollable variables.
The general form of a mathematical model is:
O = f (x i , y i )
where O = Objective function
x i = Controllable variables
y i = Uncontrollable variables
f = Relationship between O, and x i , y i .
Since model is only an approximation of the real situation, hence it may not include all the variables.
Simplification in Operation Research Models :
While constructing the model, efforts should be made to simplify them, but only up to the extent so that there is no significant loss of accuracy.
Some of the common simplifications are:
i. Omitting certain variables.
ii. Aggregating (or grouping) variables.
iii. Changing the nature of variables e.g., considering variables as constant or continuous.
iv. Changing relationship between variables i.e., considering them as linear or straight line.
v. Modify constraints.
Techniques of Operation Research :
Important techniques of Operation Research are being described hereunder:
(i) Inventory Control Models:
Operation Research study involves balancing inventory costs against one or more of the following costs:
i. Shortage costs.
ii. Ordering costs.
iii. Storage costs.
iv. Interest costs.
This study helps in taking decisions about:
i. How much to purchase.
ii. When to order.
iii. Whether to manufacture or to purchase i.e., make and buy decisions.
The most well-known use is in the form of Economic Order Quantity equation for finding economic lot size.
(ii) Waiting Line Models:
These models are used for minimising the waiting time and idle time together with the costs associated therewith.
Waiting line models are of two types:
(a) Queuing theory, which is applicable for determining the number of service facilities and/or the timing of arrivals for servicing.
(b) Sequencing theory which is applicable for determining the sequence of the servicing.
(iii) Replacement Models:
These models are used for determining the time of replacement or maintenance of item, which may either:
(i) Become obsolete, or
(ii) Become inefficient for use, and
(iii) Become beyond economical to repair or maintain.
(iv) Allocation Models:
These models are used to solve the problems arising when:
(a) There are number of activities which are to be performed and there are number of alternative ways of doing them,
(b) The resources or facilities are limited, which do not allow each activity to be performed in best possible way. Thus these models help to combine activities and available resources so as to optimise and get a solution to obtain an overall effectiveness.
(v) Competitive Strategies:
Such type of strategies are adopted where, efficiency of decision of one agency is dependent on the decision of another agency. Examples of such strategies are game of cards or chess, fixing of prices in a competitive market where these strategies are termed as “theory”.
(vi) Linear Programming Technique:
These techniques are used for solving operation problems having many variables subject to certain restrictions. In such problems, objectives are—profit, costs, quantities manufactured etc. whereas restrictions may be e.g. policies of government, capacity of the plant, demand of the product, availability of raw materials, water or power and storage capacity etc.
(vii) Sequencing Models:
These are concerned with the selection of an appropriate sequence of performing a series of jobs to be done on a service facility or machine so as to optimise some efficiency measure of performance of the system.
(viii) Simulation Models:
Simulation is an experimental method used to study behaviour over time.
(ix) Network Models:
This is an approach to planning, scheduling and controlling complex projects.
Applications of Operation Research :
These techniques are applied to a very wide range of problems.
Here only some of the common applications are being mentioned:
(i) Distribution or Transportation Problems:
In such problems, various centres with their demands are given and various warehouses with their stock positions are also known, then by using linear programming technique, we can find out most economical distribution of the products to various centres from various warehouses.
(ii) Product Mix:
These techniques can be applied to determine best mix of the products for a plant with available resources, so as to get maximum profit or minimum cost of production.
(iii) Production Planning:
These techniques can also be applied to allocate various jobs to different machines so as to get maximum profit or to maximise production or to minimise total production time.
(iv) Assignment of Personnel:
Similarly, this technique can be applied for assignment of different personnel with different aptitude to different jobs so as to complete the task within a minimum time.
(v) Agricultural Production:
We can also apply this technique to maximise cultivator’s profit, involving cultivation of number of items with different returns and cropping time in different type of lands having variable fertility.
(vi) Financial Applications:
Many financial decision making problems can be solved by using linear programming technique.
Some of them are:
(i) To select best portfolio in order to maximise return on investment out of alternative investment opportunities like bonds, stocks etc. Such problems are generally faced by the managers of mutual funds, banks and insurance companies.
(ii) In deciding financial mix strategies, involving the selection of means for financing firm, projects, inventories etc.
Limitations of Operations Research :
i. These do not take into account qualitative and emotional factors.
ii. These are applicable to only specific categories of decision-making problems.
iii. These are required to be interpreted correctly.
iv. Due to conventional thinking, changes face lot of resistance from workers and sometimes even from employer.
v. Models are only idealised representation of reality and not be regarded as absolute.
Related Articles:
- Term Paper on Operations Research | Techniques | Management
- Operations Research: History, Methodology and Applications
- Marketing Research: Definition, Process, Scope, Objectives, Types, Classification
- Industrial Marketing Research: Concept and Nature and Scope| Business Marketing
We use cookies
Privacy overview.

What is Operations Research (OR)? Definition, Concept, Characteristics, Tools, Advantages, Limitations, Applications and Uses
- Post last modified: 20 July 2022
- Reading time: 25 mins read
- Post category: Operations Research

What is Operations Research (OR)?
Operations Research (OR) may be defined as the science that aims for the application of analytical and numerical techniques along with information technology to solve organisational problems. There are various definitions of OR in the literature.
Table of Content
- 1 What is Operations Research (OR)?
- 2 Operations Research Definition
- 3 Concept of Operations Research
- 4 History of Operations Research
- 5.1 OR as a decision-making approach
- 5.2 OR as a scientific approach
- 5.3 OR as a computer-based approach
- 6 Objectives of Operations Research
- 7.1 Linear Programming
- 7.2 Simulation
- 7.3 Statistics
- 7.4 Assignment
- 7.5 Queuing Theory
- 7.6 Game Theory
- 7.7 Non-linear Programming
- 7.8 Dynamic Programming
- 7.9 Goal Programming
- 7.10 Network Scheduling
- 8.1 Increased productivity
- 8.2 Optimised outcomes
- 8.3 Better coordination
- 8.4 Lower failure risk
- 8.5 Improved control on the system
- 9.1 High costs
- 9.2 Dependence on technology
- 9.3 Reliance on experts
- 9.4 Unquantifiable factors
- 10.1 Resource distribution in projects
- 10.2 Project scheduling, monitoring and control
- 10.3 Production and facilities planning
- 10.4 Marketing
- 10.5 Personnel management
- 10.6 Supply chain management
Operations Research Definition
Some of the well-known operations research definitions are as:
Moarse and Kimbal (1946) defined OR as a scientific method of providing the executive department a quantitative basis for decision-making regarding the operations under their control.
According to Churchman, Ackoff and Arnoff (1957), OR is the application of scientific methods, techniques and tools to operational problems so as to provide those in control of the system an optimum solution to the problem.
McGraw-Hill Science & Technology Encyclopaedia states that OR is the application of scientific methods and techniques to decision-making problems.
Britannica Concise Encyclopaedia defines OR as the application of scientific methods to the management and administration of military, government, commercial, and industrial processes.
Decision-making problems arise when there are two or more alternative courses of action, each resulting in a different outcome. The goal of OR is to help select the alternative that will maximise the use of available resources and lead to the best possible outcome.
In this article, we introduce the topic of operation research that will allow students to gain an insight into the basic concepts of operations research. This will give them a better understanding of the upcoming chapters.
Concept of Operations Research
Decision-making is not a simple task in today’s socio-economic environment. Complex problems such as transportation, queuing, etc., are routinely presented and dealt with at the operational level. Moreover, higher attention is now being paid to a wide range of tactical and strategic problems.
Decision makers cannot afford to take decisions by simply taking their personal experiences or intuitions into account. Decisions made in the absence of suitable information can have seri- ous consequences. Being able to apply quantitative methods to deci- sion-making is, therefore, vital to decision-makers.
OR is a field of applied mathematics that makes use of analytical tools and mathematical models to solve problems and aid the management in decision-making. OR is an approach that allows decision makers to compare all possible courses of action, understand the likely outcomes and test the sensitivity of the solution to modifications or errors.
OR helps in making informed decisions, allocating optimal resource and improving the performance of systems. According to Ackoff (1965), the development (rather than the history) of OR as a science consists of the development of its methods, concepts, and techniques.
OR is neither a method nor a technique; it is or is becoming a science and as such is defined by a combination of the phenomena it studies.
History of Operations Research
The beginning of OR as a formal discipline can be traced back to 1937 when A.P. Rowe, Superintendent of the Bawdsey Research Station in the British Royal Air Force, sought British scientists to assist military leaders in the use of the recently developed radar system to detect enemy aircraft.
A few years later, the British Army and the Royal Navy also incorporated OR, again for assistance with the radar system. All three of Britain’s military services had set up formal OR teams by 1942. Similar developments took place in other countries (of which the most significant are those of the United States, in terms of further development of the discipline).
Once World War II ended, several British operations researchers relocated to government and industry. By the 1950s, the United States government and industry also incorporated OR programmes. In India, it was in 1949 when an Operation Research unit was set up at Regional Research Laboratory, Hyderabad, that OR came into being.
An OR team was also established at Defense Science Laboratory to resolve inventory, purchase and planning issues. The 1950s saw continual growth in the application of OR methods to non-defence activities in India. In 1953, the Indian Statistical Institute, Calcutta established an OR unit for national planning and survey-related issues. OR also became useful in the Indian Railways to resolve ticketing issues, train scheduling problems and so on.
Since then, OR, as a formal discipline, has expanded continuously in the last 70 years and is widely recognised as a central approach to decision-making in the management of different domains of an organisation.
With accessibility to faster and flexible computing facilities, OR has expanded further and is widely used in industry, finance, logistics, transportation, public health and government. One needsCharacteristics of Operations Research to bear in mind that OR is still a fairly new scientific discipline, despite its rapid evolution. This means that its methodology, tools and techniques, and applications still continue to grow rapidly.
Characteristics of Operations Research
OR aims to find the best possible solution for any problem. Its main goal is to help managers obtain a quantitative basis for decision-making. This results in increased efficiency, more control and better coordination in the organisation when fulfilling the required objectives.
OR is an interdisciplinary field involving mathematics and science. OR uses statistics, algorithms and mathematical modelling to provide the best possible solutions for complex problems. OR basically involves optimising the maxima or minima functions.
For example, a business problem could be the maximisation of profit, performance or yield or it could be related to minimising risk and loss. OR has various characteristics based on the different objectives for which it is used.
The characteristics of operations research (OR) are explained as follows:
OR as a decision-making approach
All organisations are faced with situations where they need to select the best available alterna- tive to solve a problem. OR techniques help managers in obtaining optimal solutions for their problems.
Additionally, OR techniques are also used by managers to understand the problems at hand in a better manner and make effective decisions. It is important to note that OR techniques help in improving the quality of decisions.
OR helps in finding bad answers to problems having worse answers. It means that for many problems, OR may not be able to give perfect replies but can help in improving the quality of decisions.
OR as a scientific approach
OR uses multiple scientific models along with tools and techniques to resolve complex problems while eliminating individual biasness. The scientific method involves observing and defining a problem, formulating and testing the hypothesis and analysing the results of the test. The results of the test determine whether the hypothesis should be accepted or rejected.
OR as an interdisciplinary approach: Since OR focuses on complex organisational problems, it includes expertise from different disciplines such as mathematics, economics, science and engineering. Having different experts ensures that the problem is analysed from different perspectives and alternative strategies are evolved for the selected problem.
Some of the complex problems that can be solved using OR include deciding or choosing optimal dividend policies, investment portfolio management, auditing, balance sheet and cash flow analysis, selection of product mix, marketing and export planning, advertising, media planning and packaging, procurement and exploration, optimal buying decisions, transportation planning, facilities planning, location and site selection, production cost and methods, assembly line, blending, purchasing and inventory control, etc.
OR as a systems approach: In OR, important interactions and their influence on the organisation as a whole are considered for decision-making. OR looks at problems from the perspective of the organisation:
- To determine the potential for enhancing the performance of the system as a whole
- To measure the impact of alterations in variables on the whole system
- To find reasons for the malfunctioning of the system as a whole
OR as a computer-based approach
OR solves business problems using mathematical models, manipulating large amount of data and performing computations on these large data sets. It is almost impossible to do such computations and manipulations manually. Therefore, most OR-based problems are solved using computers.
Objectives of Operations Research
Operations research in an organisation is responsible for managing and operating as efficiently as possible within the given resources and constraints. In case of complex problems as listed in the previous section, normal analysis does not work and in such cases, OR approach helps an organisation in reaching a viable solution.
OR is basically a problem-solving and decision-making tool used by organisations for enhancing their productivity and performance. Apart from this, certain other objectives of OR are as follows:
- Solving operational questions
- Solving queries related to resources’ operations such as human resource scheduling, machine and material scheduling, utilisation of funds, etc.
- Making informed decisions
- Improving the current systems
- Predicting all possible alternative outcomes
- Evaluating risks associated with each alternative
OR is needed for the following reasons:
- If the problem is a recurring one, it may make sense to create a model to make decision-making faster and better. OR provides a readymade model or process in such cases to help create a suitable model.
- OR provides an analytical, logical and quantitative basis to represent the problem
- OR models help in making sound decisions and decreases the risk of flawed decisions
Tools of Operations Research
OR is widely used in industries, businesses, governments, military establishments and agriculture. Most importantly, OR techniques are used by organisations. All the business decision areas, such as planning production and facilities, scheduling projects, minimising procurement costs, and selecting a product mix, which require optimisation of an objective, fall under the domain of operation research. OR uses a variety of tools to solve different business problems.
The most commonly used tools of OR are discussed below:
- Linear Programming
Organisations use the Linear Programming (LP) technique to determine the optimal solutions that may be defined as either most profitable or least cost solutions. Businesses use LP techniques to assign jobs to machines, select product mix, select advertising media, select an investment portfolio, etc.
Simulation is another important OR tool wherein an expert con- structs a model that replicates a real business scenario. Simulation is extremely useful in cases where actual market testing is risky or impossible due to various reasons such as high expenditure.
It has widely been used in a variety of probabilistic marketing situations. For example, finding the Net Present Value (NPV) distribution of the market introduction of a product.
Statistics allows an organisation to evaluate the risks present in all the domains of the business. It enables an organisation to predict future trends and thus makes informed business decisions. The OR team compares different trade-offs and chooses the best alternative.
For example, statistics is used in solving various real-life problems such as deterministic optimisation. Some of the problems where statistics serve as the primary vehicle for OR include decision theory, optimal strategies for search engine marketing, credit scoring, queuing theory, stochastic programming and inventory management.
The assignment method deals with the issue of how to allocate a fixed number of facilities to different tasks in the most optimal manner. The aim is to minimise the cost/time of completing a number of tasks by a number of agents (person or equipment). For example, assigning method can be used to assign specific workers to specific tasks.
Queuing Theory
If a problem involves queuing, the Queuing or Waiting Line theory is used. Using this tool, the expected number of people waiting in line, expected waiting time, expected idle time for the server and so on can be calculated. Queuing theory can be used to solve problems related to traffic congestion, repair and maintenance of broken machines, air traffic scheduling and control, scheduling bank counters, etc
Game Theory
Game theory is useful in decision-making in cases where there are one or more opponents (or players) with conflicting interests. Just as in a game, where the success of one person is influenced by the choices made by the opponent, in the game theory, the actions of all the players influence the outcomes.
For example, game theory is used for selecting war strategies and military decisions, bidding at auctions, negotiations, product pricing, stock market decisions, etc.
Non-linear Programming
Non-linear problems are similar to linear problems except that they have at least one non-linear function or constraint. Non-linear models become useful in cases where the objective function of some of the constraints is not linear in nature.
For instance, a non-linear programming is used for making optimal decisions in the production process, optimising fractionated protocols in cancer radiotherapy, training recur- rent neural networks in time series prediction problems, etc.
Dynamic Programming
Dynamic programming models deal with problems in which decisions need to be made over multiple stages in a sequence and the current decisions affect both present and future stages.
For example, dynamic programming is used by Google Maps to find the shortest path between a source and a destination. It is also used in networking to sequentially transfer data from one sender to various receivers.
Goal Programming
Goal programming tools allow organisations to handle multiple and incompatible objectives. These models are quite similar to linear programming models with the difference being that goal programming can have multiple objectives whereas linear programs have only one.
For example, goal programming can be applied to corporate budgeting, financial planning, working capital management, financing decisions, commercial bank management, accounting control, etc.
Network Scheduling
Network scheduling methods are useful in planning, scheduling and monitoring projects of large scales common in construction industry, information technology, etc.
For example, network scheduling is used for assembly line scheduling, inventory planning and control, launching new advertisement campaigns, installing new equipment, controlling projects, etc.
Advantages of Operations Research
The field of OR contains robust tools that can be applied in a variety of fields such as transportation, warehouse, production management, assignment of jobs, etc. The application of OR tools and techniques helps in making the best decisions with the available data.
There are many advantages of OR, as shown in Figure:
Increased productivity
OR helps in increasing the productivity of organisations to a huge extent. The use of OR for effective control of operations allows the managers to take informed decisions. Effective and precise decision-making leads to improvement in the productivity of an organisation.
OR tools also help increase the efficiency of various routine tasks in an organisation such as inventory control, workforce-related, business expansion, technology upgrades, installation etc. All these ultimately contribute towards productivity improvement.
Optimised outcomes
Management is responsible for making various important decisions about the organisation. OR tools can be used by the management to find out various alternative solutions to a problem and selecting the best solution. Selection is based on the profits accrued and costs incurred.
Better coordination
OR can be used to synchronise the objectives of different departments which results in achieving the goals of all departments. Managers belonging to different departments become aware of the common objectives of the organisation, which ensures that different departments coordinate towards achievement of the said goals.
For example, OR helps in coordinating the goals of the marketing department with the production department schedule.
Lower failure risk
Using OR tools and techniques, managers can find all the alternative solutions and risks associated with a given problem. Prior information with respect to all the possible risks helps in reducing the risks of failure.
Improved control on the system
Managers can apply OR to take better control of the work since it provides comprehensive information about any given course of action. Since OR informs managers about the expected outcome, they can determine what standards of performance need to be expected from employees.
They can compare the actual performance of the employees with the standard performance and, therefore, control them in a better manner. It also enables managers to prioritise tasks in terms of their importance.
Limitations of Operations Research
There is no doubt with respect to the practical utility and usability of OR and its applications in real life. However, OR also suffers from several limitations as shown in Figure:
High cost is one of the biggest limitations of OR. It not only needs expensive technology to create mathematical equations but also experts to perform simulations. Therefore, while OR does provide effective solutions to a particular problem, it comes with a high cost attached.
Dependence on technology
OR is heavily reliant on technology. Computers are generally needed to model and analyse OR problems. Since technology is quite costly as well as subject to failure, its use is severely restricted.
Reliance on experts
OR requires a team of experts from different fields to perform the assessments. Hiring multiple experts can be costly. In addition, maintaining good communication and coordination among experts and making all experts work together is a critical task.
Unquantifiable factors
It is known that OR tools are based on mathematical models that include various information based on quantifiable factors. It means that the efficacy of a solution provided by OR tool depends on quantifiable factors.
However, there are certain important unquantifiable factors that cannot be included in the models. When this happens, solutions can often be inexact, inaccurate and therefore, inefficient.
Applications and Uses of Operations Research in Management
The list of OR applications is notable, given its considerable involvement in various managerial and decision-making processes at several organisational levels. It can be applied in a wide range of industries to help with complex problems in planning, policy-making, scheduling, forecasting, resource allocation, process analysis, etc.
It may be employed by virtually any industry to determine the best solution to any problem. Various human activities that need optimisation of resources can use OR.
The following are some areas where OR may be applied:
Resource distribution in projects
Various OR tools are used to determine which resources are to be allocated to which activities. For instance, OR can help in determining the allocation of ‘n’ number of jobs among two machines. Similarly, OR can also be applied to determine and allocate materials, workforce, time and budget to projects.
Project scheduling, monitoring and control
OR is applied to activities involving scheduling, inventory control, improvement of workflow, elimination of bottlenecks, business process re-engineering, capacity planning and general operational planning.
OR tools such as the Critical Path Method (CPM) and Project Evaluation and Review Technique (PERT) are used for scheduling the different activities involved in a project. In addition, these tools are also used for continuous monitoring and control of the project.
Production and facilities planning
OR can be applied for activities involving site selection, factory size, facility planning, inventory forecasts, calculation of economic order quantities, computing reorder levels, maintenance policies, replacement policies, manpower planning, and assembly line scheduling, etc. All the important decisions and planning work related to facilities, manufacturing and maintenance can be completed using OR tools.
Application of OR can be done in budget allocation for advertising, choice of advertising media and product launch timing. For instance, how should a company allocate its budget for advertising a newly launched product on two TV channels, TV1 and TV2 within a given budget. A company may also use OR techniques to find out how many units of each product in a product mix should be produced to maximise demand.
Personnel management
OR also finds application in manpower planning, scheduling of training programs, wage administration, etc.
Finance and accounting: The application of OR in finance is concerned with effective capital planning, cash flow analysis, capital budgeting, credit policies, investment analysis and decisions, establishing costs for by-products and developing standard costs, portfolio management, risk management, etc.
Supply chain management
The application of OR in Supply Chain Management involves decision-making regarding the transportation of goods for the purpose of manufacturing and distribution. This further involves the selection of the shortest optimal routes so that the goods can be transported to maximum locations at minimum costs.
Business Ethics
( Click on Topic to Read )
- What is Ethics?
- What is Business Ethics?
- Values, Norms, Beliefs and Standards in Business Ethics
- Indian Ethos in Management
- Ethical Issues in Marketing
- Ethical Issues in HRM
- Ethical Issues in IT
- Ethical Issues in Production and Operations Management
- Ethical Issues in Finance and Accounting
- What is Corporate Governance?
- What is Ownership Concentration?
- What is Ownership Composition?
- Types of Companies in India
- Internal Corporate Governance
- External Corporate Governance
- Corporate Governance in India
- What is Enterprise Risk Management (ERM)?
- What is Assessment of Risk?
- What is Risk Register?
- Risk Management Committee
Corporate social responsibility (CSR)
- Theories of CSR
- Arguments Against CSR
- Business Case for CSR
- Importance of CSR in India
- Drivers of Corporate Social Responsibility
- Developing a CSR Strategy
- Implement CSR Commitments
- CSR Marketplace
- CSR at Workplace
- Environmental CSR
- CSR with Communities and in Supply Chain
- Community Interventions
- CSR Monitoring
- CSR Reporting
- Voluntary Codes in CSR
- What is Corporate Ethics?
Lean Six Sigma
- What is Six Sigma?
- What is Lean Six Sigma?
- Value and Waste in Lean Six Sigma
- Six Sigma Team
- MAIC Six Sigma
- Six Sigma in Supply Chains
- What is Binomial, Poisson, Normal Distribution?
- What is Sigma Level?
- What is DMAIC in Six Sigma?
- What is DMADV in Six Sigma?
- Six Sigma Project Charter
- Project Decomposition in Six Sigma
- Critical to Quality (CTQ) Six Sigma
- Process Mapping Six Sigma
- Flowchart and SIPOC
- Gage Repeatability and Reproducibility
- Statistical Diagram
- Lean Techniques for Optimisation Flow
- Failure Modes and Effects Analysis (FMEA)
- What is Process Audits?
- Six Sigma Implementation at Ford
- IBM Uses Six Sigma to Drive Behaviour Change
- Research Methodology
- What is Research?
- What is Hypothesis?
- Sampling Method
- Research Methods
- Data Collection in Research
- Methods of Collecting Data
- Application of Business Research
- Levels of Measurement
- What is Sampling?
- Hypothesis Testing
- Research Report
- What is Management?
- Planning in Management
- Decision Making in Management
- What is Controlling?
- What is Coordination?
- What is Staffing?
- Organization Structure
- What is Departmentation?
- Span of Control
- What is Authority?
- Centralization vs Decentralization
- Organizing in Management
- Schools of Management Thought
- Classical Management Approach
- Is Management an Art or Science?
- Who is a Manager?
Operations Research
- What is Operations Research?
- Operation Research Models
- Linear Programming Graphic Solution
- Linear Programming Simplex Method
- Linear Programming Artificial Variable Technique
Duality in Linear Programming
- Transportation Problem Initial Basic Feasible Solution
- Transportation Problem Finding Optimal Solution
- Project Network Analysis with Critical Path Method
Project Network Analysis Methods
Project evaluation and review technique (pert), simulation in operation research, replacement models in operation research.
Operation Management
- What is Strategy?
- What is Operations Strategy?
- Operations Competitive Dimensions
- Operations Strategy Formulation Process
- What is Strategic Fit?
- Strategic Design Process
- Focused Operations Strategy
- Corporate Level Strategy
- Expansion Strategies
- Stability Strategies
- Retrenchment Strategies
- Competitive Advantage
- Strategic Choice and Strategic Alternatives
- What is Production Process?
- What is Process Technology?
- What is Process Improvement?
- Strategic Capacity Management
- Production and Logistics Strategy
- Taxonomy of Supply Chain Strategies
- Factors Considered in Supply Chain Planning
- Operational and Strategic Issues in Global Logistics
- Logistics Outsourcing Strategy
- What is Supply Chain Mapping?
- Supply Chain Process Restructuring
- Points of Differentiation
- Re-engineering Improvement in SCM
- What is Supply Chain Drivers?
- Supply Chain Operations Reference (SCOR) Model
- Customer Service and Cost Trade Off
- Internal and External Performance Measures
- Linking Supply Chain and Business Performance
- Netflix’s Niche Focused Strategy
- Disney and Pixar Merger
- Process Planning at Mcdonald’s
Service Operations Management
- What is Service?
- What is Service Operations Management?
- What is Service Design?
- Service Design Process
- Service Delivery
- What is Service Quality?
- Gap Model of Service Quality
- Juran Trilogy
- Service Performance Measurement
- Service Decoupling
- IT Service Operation
- Service Operations Management in Different Sector
Procurement Management
- What is Procurement Management?
- Procurement Negotiation
- Types of Requisition
- RFX in Procurement
- What is Purchasing Cycle?
- Vendor Managed Inventory
- Internal Conflict During Purchasing Operation
- Spend Analysis in Procurement
- Sourcing in Procurement
- Supplier Evaluation and Selection in Procurement
- Blacklisting of Suppliers in Procurement
- Total Cost of Ownership in Procurement
- Incoterms in Procurement
- Documents Used in International Procurement
- Transportation and Logistics Strategy
- What is Capital Equipment?
- Procurement Process of Capital Equipment
- Acquisition of Technology in Procurement
- What is E-Procurement?
- E-marketplace and Online Catalogues
- Fixed Price and Cost Reimbursement Contracts
- Contract Cancellation in Procurement
- Ethics in Procurement
- Legal Aspects of Procurement
- Global Sourcing in Procurement
- Intermediaries and Countertrade in Procurement
Strategic Management
- What is Strategic Management?
- What is Value Chain Analysis?
- Mission Statement
- Business Level Strategy
- What is SWOT Analysis?
- What is Competitive Advantage?
- What is Vision?
- What is Ansoff Matrix?
- Prahalad and Gary Hammel
- Strategic Management In Global Environment
- Competitor Analysis Framework
- Competitive Rivalry Analysis
- Competitive Dynamics
- What is Competitive Rivalry?
- Five Competitive Forces That Shape Strategy
- What is PESTLE Analysis?
- Fragmentation and Consolidation Of Industries
- What is Technology Life Cycle?
- What is Diversification Strategy?
- What is Corporate Restructuring Strategy?
- Resources and Capabilities of Organization
- Role of Leaders In Functional-Level Strategic Management
- Functional Structure In Functional Level Strategy Formulation
- Information And Control System
- What is Strategy Gap Analysis?
- Issues In Strategy Implementation
- Matrix Organizational Structure
- What is Strategic Management Process?
Supply Chain
- What is Supply Chain Management?
- Supply Chain Planning and Measuring Strategy Performance
- What is Warehousing?
- What is Packaging?
- What is Inventory Management?
- What is Material Handling?
- What is Order Picking?
- Receiving and Dispatch, Processes
- What is Warehouse Design?
- What is Warehousing Costs?
You Might Also Like
Linear programming: artificial variable technique, linear programming: simplex method, project network analysis with critical path method, operation research models and modelling, linear programming: graphic solution, transportation problem: initial basic feasible solution, what is linear programming assumptions, properties, advantages, disadvantages, leave a reply cancel reply.
You must be logged in to post a comment.
World's Best Online Courses at One Place
We’ve spent the time in finding, so you can spend your time in learning
Digital Marketing
Personal growth.
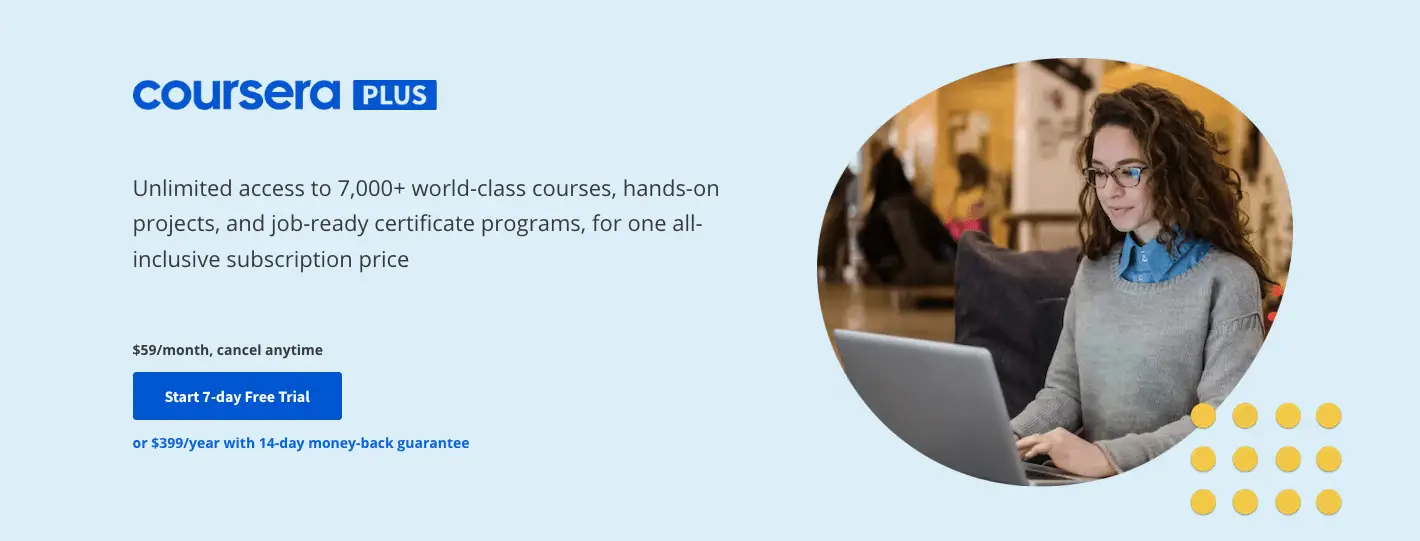
Development
Variational approach for a technology acquisition strategy model in context of the Internet of Things
- Original Research
- Published: 04 April 2024
Cite this article
- David Barilla ORCID: orcid.org/0000-0001-7072-1290 1 ,
- Alessandra Costa 1 , 2 ,
- Antonio Crupi 1 &
- Annamaria Barbagallo 3
In the era of highly dynamicity and uncertainty, it is of great importance to analyze the strategic decision process for companies’ technology acquisition between internal and external. In particular, the Internet of Things (IoT) has emerged as one of the crucial technologies that contribute to the resiliency and flexibility of companies willing to remain competitive in the markets. Despite the perceived advantages of IoT, it remains unclear what drive companies to adopt IoT in their businesses, due to strategic considerations about technology adoption and strategy selection. In that regard, this paper considers the relative payoffs among technology manufacturers, firms and demand markets, using game theory models and numerical analysis. Precisely by adopting a supply chain network perspective, our game theoretic representation assumes that technology manufacturers produce a technology that sell to firms, which in turn use the acquired technology to produce a commodity that is designed to be sold in the demand markets. Assuming that all the agents seek to maximize their profit functions, whereas the consumers want to satisfy their commodity requirement, in making their consumption decisions, the network structure of the problem is identified, the equilibrium conditions are derived, and the finite-dimensional variational inequality formulation is established. Finally, making use of the variational inequality theory, an existence and uniqueness theorem for the equilibrium distribution is obtained. The findings provide implications for competitive enterprises evaluating whether to adopt a new technology, identifying what conditions lead to win-win outcomes. In particular, firms must carefully consider the transaction costs both with technology manufacturers and demand markets, as key drivers of strategic considerations about the source of innovation. More precisely, when confronted with limited resources and the speed at which technology develop in the market, our findings suggest to companies to pursue a buy strategy, as it allows to gain time and benefit from lower innovation costs with respect to the alternative make strategy.
This is a preview of subscription content, log in via an institution to check access.
Access this article
Price includes VAT (Russian Federation)
Instant access to the full article PDF.
Rent this article via DeepDyve
Institutional subscriptions
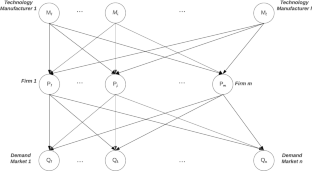
Abazi, B. (2016). An approach to the impact of transformation from the traditional use of ICT to the internet of things: How smart solutions can transform smes. IFAC-PapersOnLine, 49 (29), 148–151.
Article Google Scholar
Al-Khatib, A. W. (2023). The impact of industrial internet of things on sustainable performance: The indirect effect of supply chain visibility. Business Process Management Journal., 29 (5), 1607–1629.
Ali, S. M., Ashraf, M. A., Taqi, H. M. M., Ahmed, S., Rob, S. A., Kabir, G., & Paul, S. K. (2023). Drivers for internet of things (IoT) adoption in supply chains: Implications for sustainability in the post-pandemic era. Computers & Industrial Engineering, 183 , 109515.
Attia, T. M. (2019). Challenges and opportunities in the future applications of IoT technology.
Barbagallo, A., & Cojocaru, M.-G. (2009). Dynamic equilibrium formulation of the oligopolistic market problem. Mathematical and Computer Modelling, 49 (5–6), 966–976.
Barbagallo, A., & Mauro, P. (2012). Evolutionary variational formulation for oligopolistic market equilibrium problems with production excesses. Journal of Optimization Theory and Applications, 155 , 288–314.
Barbagallo, A., & Bianco, S. G. L. (2023). On the pseudo-monotonicity of tensor variational inequalities and applications. Pure and Applied Funtional Analysis, 8 , 1283–1300.
Google Scholar
Brem, A., & Elsner, R. (2018). Make-or-buy decisions on technology-intensive products: Insights from the consumer goods industry. International Journal of Innovation Management, 22 (06), 1850046.
Brem, A., Gerhard, D. A., & Voigt, K.-I. (2014). Strategic technological sourcing decisions in the context of timing and market strategies: An empirical analysis. International Journal of Innovation and Technology Management, 11 (03), 1450016.
Brous, P., Janssen, M., & Herder, P. (2020). The dual effects of the internet of things (IoT): A systematic review of the benefits and risks of IoT adoption by organizations. International Journal of Information Management, 51 , 101952.
Carcary, M., Maccani, G., Doherty, E., & Conway, G. (2018). Exploring the determinants of iot adoption: Findings from a systematic literature review. In Proceedings Perspectives in Business Informatics Research: 17th International Conference, BIR 2018 , Stockholm, Sweden, Sept. 24–26, 2018, pp. 113–125.
Cassiman, B. & Veugelers, R. (2002). Complementarity in the innovation strategy: internal R &D, external technology acquisition and cooperation.
Chen, Y.-J., Tomlin, B., & Wang, Y. (2017). Dual coproduct technologies: Implications for process development and adoption. Manufacturing & Service Operations Management, 19 (4), 692–712.
Cruz-Cazares, C., Bayona-Saez, C., & Garcia-Marco, T. (2013). Make, buy or both? R &D strategy selection. Journal of Engineering and Technology Management, 30 (3), 227–245.
Damanpour, F., Sanchez-Henriquez, F., & Chiu, H. H. (2018). Internal and external sources and the adoption of innovations in organizations. British Journal of Management, 29 (4), 712–730.
Falkenreck, C., Leszczyński, G., & Zieliński, M. (2023). What drives the successful launch of IoT-related business models? Journal of Business & Industrial Marketing, 38 (13), 180–194.
Fudenberg, D., & Tirole, J. (1985). Preemption and rent equalization in the adoption of new technology. The Review of Economic Studies, 52 (3), 383–401.
Garcia-Vega, M. (2006). Does technological diversification promote innovation?: An empirical analysis for European firms. Research Policy, 35 (2), 230–246.
Ghobakhloo, M., Hong, T. S., Sabouri, M. S., & Zulkifli, N. (2012). Strategies for successful information technology adoption in small and medium-sized enterprises. Information, 3 (1), 36–67.
Gopalakrishnan, S., & Bierly, P. (2001). Analyzing innovation adoption using a knowledge-based approach. Journal of Engineering and Technology management, 18 (2), 107–130.
Grenadier, S. R. (2000). Option exercise games: The intersection of real options and game theory. Journal of Applied Corporate Finance, 13 (2), 99–107.
Grimpe, C., & Kaiser, U. (2010). Balancing internal and external knowledge acquisition: The gains and pains from R &D outsourcing. Journal of Management Studies, 47 (8), 1483–1509.
He, H., Tang, H., & Guo, S. (2022). Technology acquisition strategy in the context of the internet of things using game theory. Technology Analysis & Strategic Management , pp. 1–17.
Heidarkhani, S., Barilla, D., & Caristi, G. (2023). Quasivariational inequalities for dynamic competitive economic equilibrium problems in discrete time. Decisions in Economics and Finance, 46 (1), 277–304.
Hitt, M. A., Ireland, R. D., Camp, S. M., & Sexton, D. L. (2001). Strategic entrepreneurship: Entrepreneurial strategies for wealth creation. Strategic Management Journal, 22 (6–7), 479–491.
Hoppe, H. C. (2000). Second-mover advantages in the strategic adoption of new technology under uncertainty. International Journal of Industrial Organization, 18 (2), 315–338.
Hu, B., Hu, M., & Yang, Y. (2017). Open or closed? Technology sharing, supplier investment, and competition. Manufacturing & Service Operations Management, 19 (1), 132–149.
Huisman, K. J., & Kort, P. M. (2004). Strategic technology adoption taking into account future technological improvements: A real options approach. European Journal of Operational Research, 159 (3), 705–728.
Jusoh, Y. Y., Abdullah, S., Ali, I. M., Noh, M. H. M., Mazlan, M. H., Bouh, C. S., & Sheng, T. Z. (2019). Adoption of agile software methodology among the smes developing an iot applications. In 2019 6th International Conference on Research and Innovation in Information Systems (ICRIIS) , pp. 1–6.
Katz, M. L., & Shapiro, C. (1986). Technology adoption in the presence of network externalities. Journal of Political Economy, 94 (4), 822–841.
Kaur, H. & Kumar, R. (2021). A survey on internet of things (IoT): Layer-specific, domain-specific and industry-defined architectures. In Advances in Computational Intelligence and Communication Technology: Proceedings of CICT 2019 , pp. 265–275. Springer.
Keser, C., Suleymanova, I., & Wey, C. (2012). Technology adoption in markets with network effects: Theory and experimental evidence. Information Economics and Policy, 24 (3–4), 262–276.
Kim, D. S., Vuong, P. T., & Khanh, P. D. (2016). Qualitative properties of strongly pseudomonotone variational inequalities. Optimization Letters, 10 , 1669–1679.
Li, M., Nguyen, B., & Yu, X. (2016). Competition vs. collaboration in the generation and adoption of a sequence of new technologies: A game theory approach. Technology Analysis & Strategic Management, 28 (3), 348–379.
Li, M., Nguyen, B., Yu, X., & Han, Y. (2018). Competition vs. collaboration: A four set game theory-innovation, collaboration, imitation, and “do nothing.” International Journal of Technology Management, 76 (3–4), 285–315.
Ma, M., Wang, P., & Chu, C.-H. (2013). Data management for internet of things: Challenges, approaches and opportunities. In 2013 IEEE International conference on green computing and communications and IEEE Internet of Things and IEEE cyber, physical and social computing , pp. 1144–1151.
Mamer, J. W. (1987). Monotone stopping games. Journal of Applied Probability, 24 (2), 386–401.
Mamer, J. W., & McCardle, K. F. (1987). Uncertainty, competition, and the adoption of new technology. Management Science, 33 (2), 161–177.
Mangasarian, O. (1965). Pseudo-convex functions. SIAM Journal on Control and Optimization, 3 (2), 281.
Milliou, C., & Petrakis, E. (2011). Timing of technology adoption and product market competition. International Journal of Industrial Organization, 29 (5), 513–523.
Mol, M. J., & Birkinshaw, J. (2014). The role of external involvement in the creation of management innovations. Organization Studies, 35 (9), 1287–1312.
Nagurney, A. (1999). Network economics: A variational inequality approach (2nd ed.). Kluwer Academic Publishers.
Book Google Scholar
Nash, J. F. (1950). Equilibrium points in n-person games. Proceedings of the National Academy of Sciences, 36 (1), 48–49.
Nash, J. F. (1951). Non-cooperative games. Annals of Mathematics, 54 , 286–295.
Nylander, S., Wallberg, A., & Hansson, P. (2017). Challenges for SMEs entering the IoT world: Success is about so much more than technology. pp. 1–7.
Pappas, N., Caputo, A., Pellegrini, M. M., Marzi, G., & Michopoulou, E. (2021). The complexity of decision-making processes and IoT adoption in accommodation SMEs. Journal of Business Research, 131 , 573–583.
Parra, D. T., & Guerrero, C. D. (2020). Technological variables for decision-making IoT adoption in small and medium enterprises. Journal of Information Systems Engineering and Management, 5 (4), em0124.
Patel, P., & Cassou, D. (2015). Enabling high-level application development for the internet of things. Journal of Systems and Software, 103 , 62–84.
Pavitt, K. (1998). Technologies, products and organization in the innovating firm: What Adam Smith tells us and Joseph Schumpeter doesn’t. Industrial and Corporate Change, 7 (3), 433–452.
Pimsakul, S., Samaranayake, P., & Laosirihongthong, T. (2021). Prioritizing enabling factors of IoT adoption for sustainability in supply chain management. Sustainability, 13 (22), 12890.
Reinganum, J. F. (1982). Strategic search theory. International Economic Review, 23 (1), 1–17.
Sabaei, D., Erkoyuncu, J., & Roy, R. (2015). A review of multi-criteria decision making methods for enhanced maintenance delivery. Procedia CIRP, 37 , 30–35.
Scarfo, A. (2014). Internet of things, the smart x enabler. In 2014 International Conference on Intelligent Networking and Collaborative Systems , pp. 569–574.
Schwandt, F. (2016). Internet of things (IoT) connected devices installed base worldwide from 2015 to 2025 (in billions). Statista .
Sharma, R., & Mishra, R. (2014). A review of evolution of theories and models of technology adoption. Indore Management Journal, 6 (2), 17–29.
Stanko, M. A., & Calantone, R. J. (2011). Controversy in innovation outsourcing research: Review, synthesis and future directions. R &D Management, 41 (1), 8–20.
Sullivan, Y., Fosso Wamba, S., & Dunaway, M. (2023). Internet of things and competitive advantage: A dynamic capabilities perspective. Journal of the Association for Information Systems, 24 (3), 745–781.
Svetina, A. C., & Prodan, I. (2008). How internal and external sources of knowledge contribute to firms’ innovation performance. Managing Global Transitions, 6 (3), 277–299.
Ulrich, K. T., & Ellison, D. J. (2005). Beyond make-buy: Internalization and integration of design and production. Production and Operations Management, 14 (3), 315–330.
Varaniūtė, V., Vitkauskaitė, E., & Tarutė, A. (2018). Peculiarities of iot-based business model transformations in SMEs. In Proceedings of The 18th International Conference on Electronic Business, International Consortium for Electronic Business , pp. 439–446
Veugelers, R., & Cassiman, B. (1999). Make and buy in innovation strategies: Evidence from Belgian manufacturing firms. Research Policy, 28 (1), 63–80.
Yan, B., Jin, Z., Liu, L., & Liu, S. (2018). Factors influencing the adoption of the internet of things in supply chains. Journal of Evolutionary Economics, 28 , 523–545.
Download references
Acknowledgements
The fourth author is member of Gruppo Nazionale per l’Analisi Matematica, la Probabilità e le loro Applicazioni (GNAMPA) of INdAM.
Not Applicable
Author information
Authors and affiliations.
Department of Economics, University of Messina, Via dei Verdi 75, 98123, Messina, Italy
David Barilla, Alessandra Costa & Antonio Crupi
Istituto Euro-Mediterraneo di Scienza e Tecnologia, Via Michele Miraglia 20, 90139, Palermo, Italy
Alessandra Costa
Department of Mathematics and Applications “R. Caccioppoli”, University of Naples Federico II, via Cintia, 80126, Naples, Italy
Annamaria Barbagallo
You can also search for this author in PubMed Google Scholar
Contributions
The authors contributed equally to this work.
Corresponding author
Correspondence to David Barilla .
Ethics declarations
Conflict of interest.
The authors have no relevant financial or non-financial interests to disclose.
Additional information
Publisher's note.
Springer Nature remains neutral with regard to jurisdictional claims in published maps and institutional affiliations.
Rights and permissions
Springer Nature or its licensor (e.g. a society or other partner) holds exclusive rights to this article under a publishing agreement with the author(s) or other rightsholder(s); author self-archiving of the accepted manuscript version of this article is solely governed by the terms of such publishing agreement and applicable law.
Reprints and permissions
About this article
Barilla, D., Costa, A., Crupi, A. et al. Variational approach for a technology acquisition strategy model in context of the Internet of Things. Ann Oper Res (2024). https://doi.org/10.1007/s10479-024-05946-1
Download citation
Received : 18 January 2024
Accepted : 13 March 2024
Published : 04 April 2024
DOI : https://doi.org/10.1007/s10479-024-05946-1
Share this article
Anyone you share the following link with will be able to read this content:
Sorry, a shareable link is not currently available for this article.
Provided by the Springer Nature SharedIt content-sharing initiative
- Oligopolistic market equilibrium problem
- Variational inequalities
- Technology acquisition
- Nash principle
- Digital technologies adoption
- Internet of Things
Mathematics Subject Classification
- Find a journal
- Publish with us
- Track your research
Thank you for visiting nature.com. You are using a browser version with limited support for CSS. To obtain the best experience, we recommend you use a more up to date browser (or turn off compatibility mode in Internet Explorer). In the meantime, to ensure continued support, we are displaying the site without styles and JavaScript.
- View all journals
- My Account Login
- Explore content
- About the journal
- Publish with us
- Sign up for alerts
- Open access
- Published: 26 March 2024
Australian human-induced native forest regeneration carbon offset projects have limited impact on changes in woody vegetation cover and carbon removals
- Andrew Macintosh ORCID: orcid.org/0000-0001-5700-7105 1 ,
- Don Butler ORCID: orcid.org/0000-0002-6019-1078 1 ,
- Pablo Larraondo 2 ,
- Megan C. Evans 3 ,
- Dean Ansell 1 ,
- Marie Waschka ORCID: orcid.org/0009-0001-4574-4834 1 ,
- Rod Fensham ORCID: orcid.org/0000-0003-3658-5867 4 ,
- David Eldridge ORCID: orcid.org/0000-0002-2191-486X 5 ,
- David Lindenmayer ORCID: orcid.org/0000-0002-4766-4088 1 ,
- Philip Gibbons 1 &
- Paul Summerfield 1
Communications Earth & Environment volume 5 , Article number: 149 ( 2024 ) Cite this article
11k Accesses
734 Altmetric
Metrics details
- Climate-change mitigation
- Environmental studies
Carbon offsets are a widely used climate policy instrument that can reduce mitigation costs and generate important environmental and social co-benefits. However, they can increase emissions if they lack integrity. We analysed the performance of one of the world’s largest nature-based offset types: human-induced regeneration projects under Australia’s carbon offset scheme. The projects are supposed to involve the human-induced regeneration of permanent even-aged native forests through changes in land management. We analysed 182 projects and found limited evidence of regeneration in credited areas. Changes in woody vegetation cover within the areas that have been credited also largely mirror changes in adjacent comparison areas, outside the projects, suggesting the observable changes are predominantly attributable to factors other than the project activities. The results add to the growing literature highlighting the practical limitations of offsets and the potential for offset schemes to credit abatement that is non-existent, non-additional and potentially impermanent.
Similar content being viewed by others
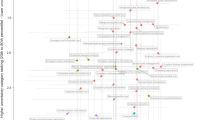
Expert review of the science underlying nature-based climate solutions
B. Buma, D. R. Gordon, … S. P. Hamburg
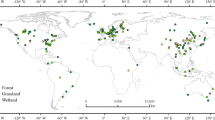
Meta-analysis shows the impacts of ecological restoration on greenhouse gas emissions
Tiehu He, Weixin Ding, … Quanfa Zhang
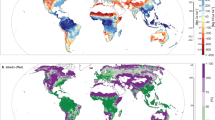
Accounting for albedo change to identify climate-positive tree cover restoration
Natalia Hasler, Christopher A. Williams, … Susan C. Cook-Patton
Introduction
Carbon offsets are a widely used climate policy instrument that are considered integral to government and corporate decarbonisation plans 1 , 2 , 3 . Under offset schemes, projects that reduce emissions relative to counterfactual baselines receive credits, which can be used by others to offset their emissions. The benefits of offsets include that they can reduce mitigation costs, generate important environmental and social co-benefits, and reduce political resistance to carbon pricing by lowering compliance costs for facilities with carbon liabilities 4 , 5 , 6 .
Whether the environmental and economic benefits of offsets materialise depends on the environmental integrity of the credits. If the credits lack integrity, offsets can facilitate increases in emissions and thereby work against greenhouse gas mitigation objectives. Carbon offsets are considered to have environmental integrity when there is high confidence they represent real, additional and permanent abatement 1 , 7 , 8 , 9 . In this context, ‘realness’ refers to the extent to which credits reflect carbon removals or emission reductions that are directly attributable to the project activities 1 , 9 , 10 . ‘Additionality’ requires that the credited removals or emission reductions would not have occurred without the incentive provided by the offset scheme 11 . Permanence relates exclusively to sequestration projects and requires credited removals to persist in relevant carbon stocks like vegetation and soils 9 , 11 , 12 .
Research on the integrity of carbon offsets has found material issues with the realness, additionality and permanence of credited abatement, raising questions about their effectiveness in assisting decarbonisation 13 , 14 , 15 , 16 , 17 , 18 , 19 , 20 , 21 , 22 , 23 , 24 , 25 . Similar issues have arisen with biodiversity offsets 26 , 27 .
Carbon offsets have been a central feature of climate policy in Australia for two decades. Under a provincial mandatory carbon trading scheme (the world’s first) that operated in New South Wales and the Australian Capital Territory between 2003 and 2012 covered facilities were allowed to use offsets from designated project types to meet their emission reduction obligations 28 . In late 2011, a national carbon offset scheme was introduced, which was relied upon as the main Australian Government mitigation policy between 2014 and 2022 29 . The object of the national offset scheme is to incentivise offset projects that help Australia meet its international greenhouse gas mitigation obligations 29 . Each credit issued under the scheme is supposed to represent abatement equivalent to one tonne of CO 2 .
The national offset scheme is now linked to a national mandatory carbon pricing instrument; the Safeguard Mechanism. As with the previous provincial carbon trading scheme, facilities covered by the Safeguard Mechanism can use credits issued under the offset scheme to meet their emission reduction obligations. There are no restrictions on the extent to which covered facilities can rely on offset credits to meet their obligations. The only relevant restriction is that the credits must come from projects registered under the national scheme.
The most popular project type under the national offset scheme is human-induced regeneration of permanent even-aged native forests (HIR) 30 . HIR projects received 37 million credits to June 2023, almost 30% of the issuances under the scheme 31 . The projects cover almost 42 million hectares, an area larger than Japan 31 . As of 30 June 2023, they were the world’s fifth largest nature-based offset type by credit issuances, and the largest when projects involving avoided emissions are excluded (Supplementary Fig. S 1 ).
Under the applicable rules (found in the ‘HIR method’), HIR projects should involve the human-induced regeneration of permanent even-aged native forests across the entirety of the areas that are credited (‘credited areas’) (Fig. 1 ) 30 . The projects do not involve planting or direct seeding. Regeneration must be induced by the project activities from ‘the germination of in situ seed, or the growth of in situ seedlings, rootstock or lignotuber’ 30 . The project activities can include reducing grazing pressure from livestock and feral animals, management of non-native plants, and cessation of clearing of native plant regrowth 30 , 32 , 33 .
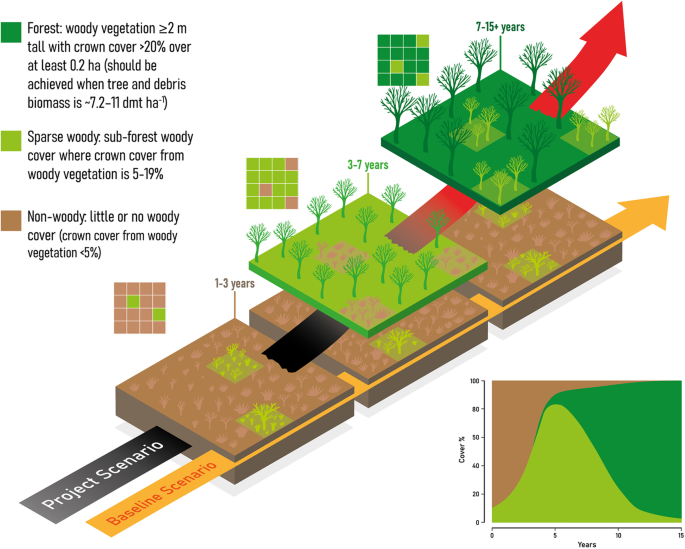
In the baseline scenario (yellow ribbon), clearing, grazing and/or weeds suppress regeneration of woody plants, ensuring the credited area has predominantly non-woody cover throughout the projection period. In the project scenario (black-red ribbon), the credited area initially has predominantly non-woody cover due to the effects of clearing, grazing and/or weeds. The project involves the removal or mitigation of these suppressors, which leads to even-aged forest regeneration. The credited area should transition from predominantly non-woody cover to predominantly sparse woody cover, and then to forest cover, and retain forest cover throughout the permanence period. In the regions where HIR projects are located, credited areas should have forest cover when tree and debris biomass reaches ~7.2–11 dry metric tonnes (dmt) per hectare.
Sequestration in HIR projects is not directly measured, it is estimated as the product of the size of the credited areas and sequestration per unit area, which is modelled using the Australian Government’s Full Carbon Accounting Model 34 . The model uses a simple tree yield formula to estimate above-ground biomass per hectare in regenerating forests 35 , 36 , 37 . It assumes credited areas start with little woody biomass and grow towards their maximum woody biomass potential under native vegetation. Maximum above-ground woody biomass potential ( M ) is modelled spatially using a range of biophysical parameters calibrated against measurements of intact native vegetation 38 . The most recent calibration of the tree yield formula estimates above-ground biomass in regeneration under average climate conditions after a years to be M.e (−23.81/a) (Supplementary Fig. S 2 ) 37 .
The above-ground biomass estimates from the model’s tree yield formula are partitioned into biomass and debris pools via standardised allocation ratios (e.g. root-shoot), and turnover and decomposition rates, to calculate carbon accumulation in live above- and below-ground biomass and debris 39 . The model includes a soil carbon module but it is not used for HIR projects; the projects are credited for increases only in live biomass and dead organic matter.
Most HIR projects are claiming to regenerate native forests by reducing grazing pressure from livestock and/or feral herbivores in arid and semi-arid ‘rangeland’ areas (<350 mm average annual rainfall) that have never been comprehensively cleared of native vegetation (Fig. 2 , Supplementary Fig. S 3 ). The location of the projects in uncleared rangelands (where there is often limited and highly variable rainfall) raises questions about the capacity of the credited areas to permanently support material additional woody biomass, and the realness, additionality and permanence of credited abatement 40 , 41 , 42 , 43 .
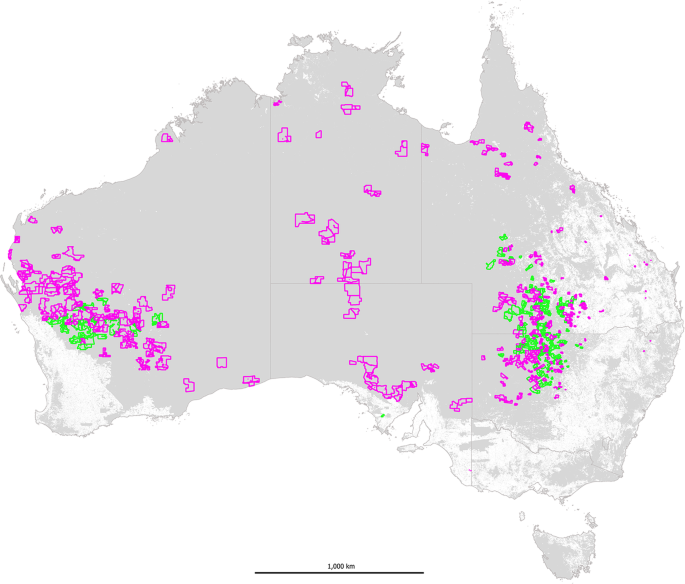
The 182 projects analysed in this paper in light green. Source: Australian Government. Area-based Emissions Reduction Fund (ERF) projects. Commonwealth of Australia, Canberra (2023); Department of Climate Change, Energy, the Environment and Water. National Vegetation Information System (NVIS) data products, version 6. Commonwealth of Australia, Canberra (2023); New South Wales Government. NSW State Vegetation Type Map. NSW Government, Sydney (2023); Geoscience Australia. GEODATA COAST 100K 2004. Commonwealth of Australia, Canberra (2023).
Plant growth is constrained by the availability of resources (water, nutrients, light etc.), which limit woody biomass potential under native vegetation 44 , 45 , 46 . In most of Australia’s uncleared rangelands, the key limiting resource is water and its availability depends on variable rainfall, which fluctuates over time-scales from months to decades 47 . Variations in rainfall and water availability drive changes in plant growth, including woody biomass 47 , 48 .
The primary way grazing could affect forest cover in uncleared areas is by impeding woody plant recruitment during recovery after periods of drought or fires, where cover has been lost through tree death 49 , 50 , 51 . For grazing to prevent the regeneration of forests in these circumstances, grazing intensity after a mortality event would need to be sufficiently intense to prevent recruitment and then be maintained over multiple decades to suppress subsequent recruitment. Grazing in Australia’s uncleared rangelands has been shown to have local, short-term effects on regeneration, but assessments over larger spatial and temporal scales show that grazing has not generally reduced tree cover 52 , and that the influence of grazing alone on woody plants is minimal compared to the effect of variable rainfall 53 , 54 , 55 .
This is illustrated through the well-documented increase in tree cover that occurred across substantial parts of Australia’s grazed eastern rangelands through the twentieth century, particularly following a series of La Niña events from the 1950s that brought above-average rainfall 56 , 57 , 58 , 59 . Similarly, more than 300,000 ha of secondary native forest is re-cleared annually in Australia in areas previously cleared for grazing 39 , 60 , 61 , typically on cycles of around 8–30 years 53 , 60 . This re-clearing would not be necessary if grazing was suppressing regeneration of native forests.
Because grazing does not have a material negative influence on tree cover in Australia’s rangelands, HIR projects are unlikely to regenerate permanent native forest through grazing control in uncleared areas. In some cases, reduced grazing could increase tree cover but, generally, any management-induced increases are likely to be relatively small and often short-lived (since droughts can remove excess biomass accumulated during wet times) 62 .
The modelling approach used to calculate abatement for HIR projects compounds the resulting integrity risks. Projects could be credited for forest regeneration that has not occurred or that does not persist. The HIR method also does not control for the over-riding impacts of rainfall on regeneration in the rangelands, creating a risk that projects will be credited for increases in tree cover that are mainly attributable to natural variations in rainfall rather than the project activities (i.e. non-additional) 42 , 43 .
Here we present the results of an analysis of HIR projects conducted using the Australian Government’s National Forest and Sparse Woody (NFSW) dataset (Version 7.0) 63 . The dataset provides Landsat-derived estimates of the spatial extent of three classes of woody vegetation cover across Australia over the period 1988 to 2022. The data are a near-annual time series in which 25 m grid cells are classified as either non-woody, sparse woody (sub-forest woody cover where crown cover is between 5–19%) or forest (woody vegetation ≥2 m tall with crown cover >20% over at least 0.2 ha).
The object of our analysis was to assess the performance of HIR projects using two metrics:
the extent of the increase in forest cover and ‘woody cover’ (areas with either forest or sparse woody cover) in the credited areas of HIR projects; and
the extent to which changes in forest and woody cover in the credited areas of HIR projects have mirrored trends in paired controls for each project, comprising 3 km wide buffer areas outside the project boundaries that exclude areas in other HIR projects (‘comparison areas’).
Metric (1) provides a proxy measure of the likely increases in woody biomass in the credited areas of HIR projects. When combined with data on credit issuances, it serves as an indicator of over-crediting risk (i.e. whether sequestered CO 2 is likely to be materially less than credited sequestration). Metric (2) provides a measure of the extent to which changes in forest and woody cover in the credited areas of HIR projects are additional to what would otherwise have occurred (i.e. attributable to the project activities or other factors such as rainfall variability). Together, metrics (1) and (2) provide a basis on which to draw conclusions about the extent to which HIR projects have helped Australia meet its international mitigation obligations, consistent with the scheme’s objectives 29 .
Published estimates of the accuracy of the classifications of pixels to forest, sparse or non-woody in the NFSW dataset suggest accuracy of 95% or more for forest and non-woody classes where no change is indicated, with lower confidence for classification of sparse woody pixels (~66%) 39 , 64 . Error rates are likely to be somewhat higher for classification of changes between years, but there is also no reason to expect biases in error between credited areas and comparison areas used in our analysis. Notably, the Australian Government relies on the NFSW dataset to estimate land sector emissions and removals in its greenhouse accounts 39 . Greenhouse gas outcomes from changes in tree cover in Australia’s rangelands are not accounted for in Australia’s greenhouse accounts if they are not detected in the NFSW dataset. The fact that the Australian Government relies on the NFSW dataset to track reforestation and revegetation for greenhouse accounting purposes justifies its use to assess outcomes from HIR projects.
All HIR projects whose credited area location data were published as of 22 June 2023 and that were registered in or before 2018 (providing at least four data points in the NFSW time series post registration) were included in the analysis, except where they were completely surrounded by other projects or the published spatial files were corrupt 65 , 66 . The projects ( n = 182) included in the analysis covered a combined area of 9.5 M ha, with their credited areas covering 3.4 M ha (Fig. 2 , Table 1 ). The projects in the sample were registered over the period 11 December 2013 to 30 November 2018, with most (75%) registered in 2015, 2016 and 2017 (Supplementary Fig. S 4 ).
Change in forest and sparse woody cover
The analysed projects received 27.4 million credits over the period from 11 December 2013 (when the first HIR project was registered) to 30 June 2022, suggesting a substantial proportion of the credited areas should have transitioned from non-woody cover to either sparse woody or forest cover because of the human-induced forest regeneration 31 . This has not occurred.
Almost 50% of the credited areas had sparse woody or forest cover when the projects were registered (median woody cover 46.5% (sd 22.5%), median forest cover 12.7% (sd 12.9%)). This is problematic as it indicates that most projects are seeking to regenerate permanent even-aged native forests on land that contained material amounts of pre-existing woody vegetation. Competition from the pre-existing woody vegetation is likely to limit additional forest regeneration.
Consistent with this, there was relatively little change in woody cover in the credited areas over the study period. Almost 80% of projects ( n = 143) experienced negative or negligible change in woody cover in the credited areas over the period from project registration to 2022 (Table 1 , see methods for definitions of negative, negligible and positive woody cover change). Despite the absence of positive woody cover change, these 143 projects received 22.9 million credits over the period 31 .
At an aggregate level, woody cover increased by a mere 0.8% (28,155 ha) across the 3.4 M ha credited area: forest cover increased by 3.6% (124,852 ha); and sparse woody cover decreased by −2.8% (96,697 ha) (Supplementary Fig. 5 ). By comparison, gains and losses in sparse woody cover alone across Australia averaged 2.2 M ha year −1 and −2.1 M ha year −1 respectively over the period 2013–14 to 2020–21 39 .
The modest gain in woody cover in the credited areas after project registration continued a trend that started in the late 2000s, before the HIR method was developed (Fig. 3 ). The increase in woody cover in the credited areas that pre-dates the method is difficult to reconcile with the premise of the projects: that grazing was previously suppressing regeneration and that, without the projects, it would not occur (Fig. 1 ).
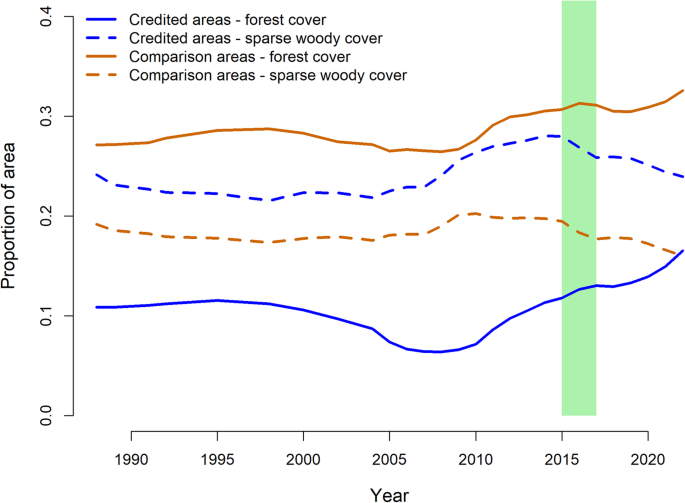
Source: Department of Climate Change, Energy, the Environment and Water. National Forest and Sparse Woody Vegetation Data (Version 7.0 - 2022 Release) (2023); Clean Energy Regulator. Emissions Reduction Fund project register. Commonwealth of Australia, Canberra (2023). The green bar shows when most (75%) of the HIR projects in the sample were registered (2015–2017).
There is a relationship between biomass in forest regeneration (above- and below-ground live biomass, litter, and dead wood) and crown cover in the forest systems where HIR projects are located 67 . This relationship suggests that forest cover (>20% crown cover) should be achieved when tree and debris biomass reaches 7.2 to 11 tonnes of dry matter per hectare, equivalent to 13.2–20.2 tCO 2 ha −1 67 . To 30 June 2022, estimated average credited sequestration in the 182 projects in the sample was 12.9 tCO 2 ha −1 (median 11.5 tCO 2 ha −1 , sd 8.9 tCO 2 ha −1 ) 31 . The estimated credited sequestration in 75 of these projects (41%) was ≥13.2 tCO 2 ha −1 (mean 21.6 tCO 2 ha −1 , median 20.8 tCO 2 ha −1 , sd 6.5 tCO 2 ha −1 ) 31 . This suggests that, based on the credits that have been issued, a substantial proportion of the total credited area should have already attained forest cover. However, for the 75 projects with credited sequestration ≥13.2 tCO 2 ha −1 , only 21% (188,880 ha) of the 898,680 ha total credited area had forest cover in 2022, and this was only a 1.8% (16,530 ha) increase relative to forest cover when the projects were registered (Fig. 4 , Supplementary Fig. S 6 ). There is a large apparent disparity between the credited and observed sequestration in the projects.
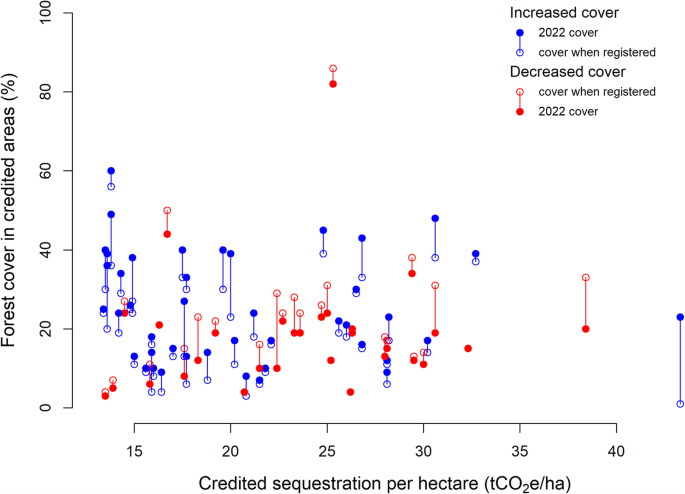
Note that under the HIR method, forest cover at project registration should be at or near 0% and reach 100% within ~10–15 years of when regeneration is modelled to have commenced. Source: Department of Climate Change, Energy, the Environment and Water. National Forest and Sparse Woody Vegetation Data (Version 7.0 − 2022 Release) (2023); Clean Energy Regulator. Emissions Reduction Fund project register. Commonwealth of Australia, Canberra (2023).
Change in woody cover relative to trends in external comparison areas
Changes in forest and sparse woody cover in credited areas were far more strongly correlated with changes in cover in comparison areas than to the timing of project registration. Table 2 presents standardised coefficients from hierarchical regression models predicting annual cover changes in credited areas as a function of cover changes in comparison areas and a variable indicating whether the year of observation was before or after project registration. The coefficients for comparison areas are many times larger than those for project registration. Project registration did have a statistically significant effect for forest cover change, but not for woody cover change. While statistically significant, the identified effect of project registration on forest cover was small, being equivalent to ~0.5% per year following project registration.
The extent to which changes in forest and sparse woody cover within credited areas have mirrored changes in comparison areas suggests the limited changes observed within the credited areas are largely non-additional. As shown in Figs. 3 , 5 (Supplementary Table S1 , Fig. S 7 ), there was a strong correlation between forest and sparse woody cover changes in the credited areas and comparison areas over the period before projects were first registered. Post registration, the correlation was maintained, suggesting factors other than the project activities (most likely rainfall variability) have been the dominant influence on woody cover changes.
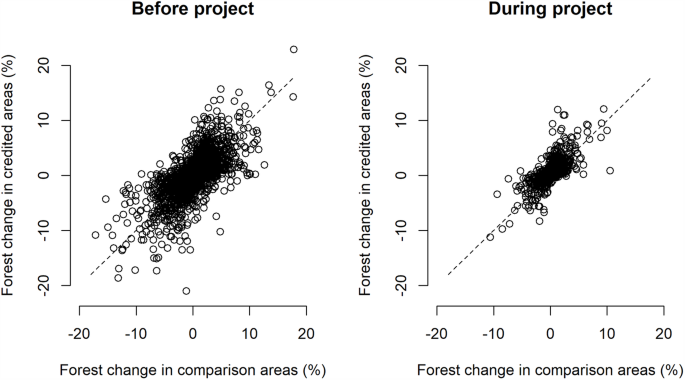
Dashed lines indicate 1:1. Source: Department of Climate Change, Energy, the Environment and Water. National Forest and Sparse Woody Vegetation Data (Version 7.0 − 2022 Release) (2023); Clean Energy Regulator. Emissions Reduction Fund project register. Commonwealth of Australia, Canberra (2023).
It is important to note that, while changes in forest and sparse woody cover relative to external comparison areas provide a useful indicator of the impact of project activities, they should not be construed as the only indicator of project effectiveness. HIR projects are credited on the basis that even-aged native forest is regenerating across the entirety of the credited area and that, within ~10–15 years of when regeneration is modelled to have commenced, all of the credited area will have forest cover. The modest gain in woody cover observed within credited areas, and small effect of project registration on forest cover change, suggest this is unlikely to occur.
Reforestation, avoided forest conversion and improved forest management have the potential to generate substantial amounts of low-cost abatement, while providing important biodiversity and other co-benefits 68 , 69 . Carbon offset schemes can incentivise these activities and reduce the economic cost of decarbonisation. However, the benefits of these nature-based offsets are contingent on offset projects being credited only for real, additional and permanent increases in relevant carbon stocks. Our findings suggest that HIR projects in Australia’s uncleared rangelands do not meet this requirement.
There was only a small positive overall increase in forest cover (3.6%), and negligible increase in combined sparse woody and forest cover (0.8%), across the combined 3.4 Mha credited area, where the 182 assessed projects are supposedly regenerating permanent even-aged native forests. Despite the absence of material increases in woody cover, the projects received 27.4 million credits over the study period 31 ; 22.9 million credits were issued to projects whose woody cover declined or was largely stagnant.
Given the levels of credited sequestration, the changes in woody cover should be readily apparent, beyond the levels of classification error in the underlying data, which is likely to be in the order of 5–10% 39 , 64 . A substantial proportion of the credited areas should have already attained forest cover and, at the very least, there should have been large increases in sparse woody cover that go well beyond changes observed in the external comparison areas. Neither has occurred.
Trends in forest and woody cover in the credited areas largely mirrored fluctuations in comparison areas, both before and after project registration. Regression models of changes in forest and woody cover in the credited areas identified far smaller effects for project registration than for cover changes in comparison areas. The results suggest the changes in forest and woody cover in the credited areas were largely non-additional, presumably because they reflect rainfall variability rather than responses to project activities 47 , 48 , 52 , 53 , 54 , 55 .
The small increases in forest and woody cover, and the small effect of project registration relative to variation in cover in the comparison areas, suggest HIR projects have done little to help Australia meet its international mitigation obligations, both in absolute terms and relative to credit issuances 39 . The underperformance is accentuated by the fact that, to date, the Australian Government alone has spent ~AU$300 million in purchasing credits from HIR projects and is contractually committed to purchase a further ~AU$1.2 billion 70 , 71 .
The results add to the growing literature highlighting the practical limitations of offsets and the potential for offset schemes to credit abatement that is non-existent, non-additional, and potentially impermanent 13 , 14 , 15 , 16 , 17 , 18 , 19 , 20 , 21 , 22 , 23 , 24 , 25 . They also serve as a reminder of why offsets are considered a high-risk policy instrument 10 , 72 , 73 .
Offsets are high-risk because of two factors: likelihood of error and the consequences when they occur. There is a high probability of error in the design and administration of the rules and processes that are intended to ensure credits are issued only for real, additional and permanent abatement. This is due to multiple factors, including the uncertainties associated with determining counterfactual baselines (what would net emissions have been within the project boundaries in the absence of the incentive provided by the scheme?) and the errors inherent in the measurement of emissions and removals from often dispersed sources and sinks 72 , 74 , 75 . Other pertinent factors that contribute to the likelihood of errors include the difficulty in overcoming adverse selection when seeking to exclude non-additional projects 74 , 76 , and the persistent tension within offset schemes to lower the stringency of measurement protocols to reduce transaction costs and thereby promote participation 77 .
Regardless of the cause, where errors occur and result in the issuance of low integrity credits, their use can lead to worse climate outcomes. This is because offsets are a permission to pollute, issued on the premise that the offset project has abated one tonne of emissions. Hence, when the credited abatement is not real, additional and permanent, offsets can enable an increase in emissions from a polluter with no offsetting emission reduction elsewhere.
The high-risk nature of offsets is why they are deprioritised in the ‘mitigation hierarchy’ that is often used in biodiversity-related regulatory approval processes 78 ; they are supposed to be a last resort reserved for when all other viable avoidance and mitigation options have been exhausted. The risk also provides the basis for the principle that offsets credits should only be used where there is high confidence the credits are likely to represent real, additional and permanent abatement 11 , 29 , 72 , 73 .
The root cause of the integrity issues with HIR projects is that credited areas have been allowed to be located in areas where native vegetation has not previously been comprehensively cleared, where the capacity to permanently increase forest carbon stocks is generally likely to be small, and in semi-arid and arid rangeland areas where there is substantial natural variability, which makes it difficult to separate the impacts of project activities from rainfall-induced changes 11 . The integrity problems with HIR projects have been compounded by the use of a modelled approach to the estimation of sequestration and allowing the model to be used in circumstances it was not calibrated for (i.e. to estimate regeneration on sites that contain material amounts of pre-existing woody vegetation) 30 , 37 .
Despite the risks, and the evidence of their limitations, carbon offsets are seen as indispensable by many policymakers; as evidenced through the Paris Agreement’s Article 6.4 Mechanism, the Carbon Offsetting and Reduction Scheme for International Aviation and other similar initiatives 8 , 79 . With the commitment to their continued use, the challenge for policymakers is to demonstrate that offset schemes can have integrity.
The experience with HIR projects provides two generalisable lessons. First, that sequestration-related offsets are inappropriate for use in situations where the relevant carbon stocks are likely to be at or near their maximum sustainable potentials and where natural variability in the stocks is materially larger than the likely effects of management change. Second, care needs to be taken where models are used to estimate carbon stocks to ensure they are applied appropriately and with due regard to the need for conservativism.
Australia’s experience with HIR projects also highlights the importance of transparency 11 , 80 . From January 2013 until June 2023, no data on the location of credited areas were published under the scheme, which shielded projects from scrutiny. At the time of writing, proponents were still not required to publish offset reports or audit reports, or information on how they have modelled sequestration. Proponents are also not required to undertake any direct measurements of biomass in HIR projects and, where biomass measurements are voluntarily undertaken, they are not required to be published. Effective offset schemes require constant scrutiny and critical assessment, including from third parties. This cannot be provided without the public release of all information that is necessary for the proper evaluation of the performance and integrity of offset projects 11 .
HIR projects included in sample
As at 10 December 2023, there were 469 registered HIR projects. Up until April 2023, it was unlawful for the Clean Energy Regulator to publish data on the location of credited areas. Following changes to the law in April 2023, the Regulator first published credited area location data for 223 HIR projects on 6 June 2023.
To be included in the sample for the analysis, projects had to have published credited area location data and at least 4 years of data in the NFSW time series post the year they were registered ( n = 191). This was to ensure there was a valid basis for determining the response of woody vegetation to the project activities. In addition, projects were excluded from the sample if they were completely surrounded by other projects ( n = 4). These projects were excluded because it was not possible to designate a valid comparison area in accordance with the method described below. A further five projects were removed because the spatial data on their credited areas were not useable.
Credited area location data
The Clean Energy Regulator publishes credited area data in vector format. To facilitate our analysis, the dataset was converted into raster format. Due to differences between the map projections of each dataset, credited area data were rasterised using 10-m resolution vs the 25-m of the NFSW dataset. This increase in resolution allowed for improving the accuracy of the masking operation around partially intercepted pixels. To perform this conversion we used the standard gdal_rasterize command from the GDAL library to generate a raster preserving the original projection of the vector dataset 81 .
Carbon credit issuances
Carbon credit issuance data were obtained from the ERF Project Register published by the Clean Energy Regulator 31 . The Register contains data on total issuances and total issuances by Australian financial year (1 July–30 June). Credit issuances were included in a calendar year only where they were issued prior to 30 June of the same year. This ensured conservative estimates of credit issuances for the purposes of making comparisons of project performance.
Relative size of HIR projects
The relative size of HIR projects was analysed using data from the registries of seven offset schemes for the period 2013–2023: the ACCU scheme; Clean Development Mechanism; Verified Carbon Standard (VCS, or Verra); Gold Standard; American Carbon Registry; Climate Action Reserve; and Plan Vivo. Data on credit issuances by project type are provided in Supplementary Fig. S 1 .
External comparison areas
HIR projects have an outer project boundary, which is typically the boundary of the property on which it is located. The credited areas lie within the project boundary. The comparison areas, which are used as paired controls for each project, comprised 3 km wide buffer areas around the outside of project boundaries, excluding areas that intersected with other HIR projects.
The use of these 3 km wide comparison areas is likely to overstate the relative effects of the projects on woody cover. This is due to the way the credited areas are delineated. Under the HIR method, credited areas must contain only areas that have the potential to achieve forest cover (woody vegetation ≥2 m tall with crown cover >20% at 0.2 ha scale). They are also not allowed to have forest cover at commencement. This results in credited areas having exclusions inside and around them, even when the areas are subject to the same project activities (i.e. grazing control) and lie within the same fenced areas. In contrast, the comparison areas are comprised of all land within the 3 km wide buffers, excluding other HIR projects.
The characteristics of the credited areas and comparison areas means that, where the same changes in tree cover occur, there is likely to be a greater proportionate increase (or decrease) in cover in the credited areas relative to the comparison areas. This approach was adopted to ensure conservative outputs and because of the practical difficulty associated with delineating areas that share the same characteristics as the areas included in the credited areas.
National forest and sparse woody dataset analysis
Changes in woody cover (forest and sparse woody cover) in the credited areas and comparison areas were analysed using the Australian Government’s NFSW dataset (Version 7.0) 63 . The dataset provides Landsat-derived estimates of the extents of three classes of woody vegetation cover across Australia over the period 1988–2022. The data are a near-annual time series in which 25 m grid cells are classified as either non-woody, sparse woody (sub-forest woody cover where crown cover is between 5–19%) or forest (woody vegetation ≥2 m tall with crown cover >20% over at least 0.2 ha).
The analysis was undertaken using Terrak.io, a geospatial analytics platform developed by Haizea Analytics. This platform builds upon Cloud infrastructure and can provide on-demand analytics on large satellite and climate datasets through an API. Users can rely on Terrak.io to generate maps or zonal statistics showing temporal trends for large numbers or areas, defined using custom vector polygons. This infrastructure was used to calculate zonal statistics on the frequency of forest, sparse woody, and non-woody cover pixels within each project’s credited areas and comparison areas.
Classifying project changes in forest and sparse woody cover since registration
To assess whether woody cover in the credited areas of each project (i.e. the proportion of pixels with forest or sparse woody cover) experienced negative, negligible and positive change since the projects were registered (i.e. the results presented in Table 1 ), simple linear regression models were fit for each project to the time-series of the forest and sparse woody percentages in their credited areas from the year of registration forward, with the percentage of each cover class as the response variable and year as the only independent variable.
Projects were classified as having increased woody cover if the slope of either of the fits for forest or sparse cover was greater than 0.25% per year, provided the slope for the fit to the other woody cover class (i.e. sparse if the forest fit has a positive slope >0.25%) was greater than −0.05% per year.
Tree cover was deemed to be negligible if the sum of the slopes from the linear models fit to the sparse and forest cover were greater than −0.25% per year, and they did not meet the ‘increased’ requirements.
Projects whose tree cover did not meet either the ‘increased’ or ‘negligible’ requirements were deemed to have decreasing cover.
Comparing cover trends in carbon estimation areas and adjacent comparison areas
Changes in woody cover were calculated from the time-series of NFSW data from 1988 to 2022 63 . The percentage of pixels within the credited areas and comparison area classified as forest in each year was subtracted from the percentage of forest pixels in the preceding time point in the time series. Most time steps were annual, including all from 2004 on, but some spanned two or more years (’89–’91, ’92–’95, ’95–’98, ’98–2000, 2000–’02 and ’02–’04).
Hierarchical linear regression models, built using the lme4 package 82 in R (4.3.0, R Core Team 2022) 83 , were used to model cover changes within credited areas as a function of cover changes in comparison areas (indicating responses to broader environmental drivers) and a binary variable indicating whether the interval over which cover changes occurred was before or after the year of project registration. Models were built to include random effects accommodating the numerous observations made for each project by fitting separate intercepts for projects, as well as coefficients for the two fixed-effects (cover change in comparison areas and project registration) and a higher level intercept (Eq. ( 1 ), in the syntax of lmer: project_cover_change ~ comparison_area_change + registration + (1|project_ID)). Statistical significance of predictors was assessed via the anova function, by comparing the full model to models with each predictor removed in turn.
The cover change variables for credited areas (response) and comparison areas (fixed effect 1) were standardised (centred and scaled) by subtracting the variable mean from each observation, and dividing by its standard deviation (Supplementary Table S2 ). The binary variable for project registration was not standardised (pre-registration = 0, post-registration = 1). This means that the coefficient for the comparison area predictor in each model is a measure of effect size, indicating the expected magnitude of change in the response credited area cover variable (in standard deviations) for a one standard deviation change in comparison area cover. The coefficient for project registration ( β 2 ) indicates the effect of project registration on year-to-year cover change in credited areas, again in units of standard deviation for the response variable, i.e. cover change in credited areas.
The strength of portfolio-scale correlations between the extent of each cover class (forest, sparse or woody) in the combined credited areas and in comparison areas, across the 182 projects (i.e. variables plotted in Fig. 3 ), was assessed using Pearson’s correlation coefficient from the cor.test function in R 83 . Correlation coefficients were also calculated for annual cover changes inside credited areas and in adjacent comparison areas, for both forest and woody cover classes (Supplementary Table S1 ).
Estimating credited sequestration
Credited sequestration was estimated using data from the ERF Project Register 31 . Total credit issuances to each project to 30 June 2022 were adjusted to account for relevant discounts (5% risk of reversal buffer and a 20% permanence period discount for projects with 25 year permanence periods). A uniform and conservative 0.5% deduction was made to account for fossil fuel use, based on Australian Government analysis of a sample of HIR projects that found average fuel use emissions were less than 0.02% of total project abatement 84 . The resulting estimates were converted from CO 2 to C using the atomic mass ratio, 44/12.
The approach used to compare credited sequestration to forest cover is conservative. Ideally, the comparison of forest cover to sequestration would be undertaken using the modelled sequestration for each project. This would ensure the estimates account for the fact that projects have been allowed to commence modelling regeneration before the projects were registered. Due to this, the amount of modelled sequestration across the projects is greater than the credited sequestration, accentuating the extent of relative underperformance. It was not possible to analyse the modelled sequestration because of transparency issues. Estimates of modelled sequestration are not published and no verified data are published on the modelling parameters used in HIR projects. At the time of writing, information about the choice of model calibration and modelling commencement dates had been published by the proponents of 63 HIR projects. However, the published data were incomplete (e.g. modelling points are not published) and unverified, rendering them unusable for these purposes.
Australian Government expenditure on carbon credits from HIR projects
We estimate that, to 4 December 2023, the Australian Government had spent ~AU$300 million in purchasing credits from HIR projects and was contractually committed to purchase a further ~AU$1.2 billion. The Australian Government does not publish data on carbon credit purchases or contracted credit prices by project. Due to this, our estimate of Australian Government expenditure on credits from HIR projects was based on the number of credits sold by each project to the Australian Government under Emissions Reduction Fund contracts, up until 4 December 2023 70 . Where contracts had multiple projects, the recorded credit sales were assumed to be sourced evenly from the contracted projects. Sale prices were assigned to each project based on the published weighted average carbon credit purchase price from the Emissions Reduction Fund auction at which the relevant project was contracted (range AU$10.23-AU$17.35) 71 . The estimate of the value of the remaining HIR credits contracted by the Australian Government was based on the number of credits originally contracted, less those delivered and the number of credits released or lapsed from delivery obligations 70 . The contracted prices assigned to each project were again based on the weighted average carbon credit purchase price from the Emissions Reduction Fund auction at which the relevant project was contracted 70 , 71 .
Data availability
The datasets generated during and/or analysed during the current study, including a summary of individual project data, are available on Figshare at: https://figshare.com/ [DOI: 10.6084/m9.figshare.25199786 and 10.6084/m9.figshare.25199789].
Code availability
Details of the hierarchical linear regression models developed in the study are provided above.
Schneider, L. & La Hoz Theuer, S. Environmental integrity of international carbon market mechanisms under the Paris Agreement. Clim. Policy 19 , 386–400 (2019).
Article Google Scholar
Black, R. et al. Taking stock: A global assessment of net zero targets. (Energy & Climate Intelligence Unit and Oxford Net Zero,United Kingdom, 2021).
Dawes, A. et al. Voluntary Carbon Markets: A Review of Global Initiatives and Evolving Models . (Center for Strategic and International Studies, Washington, DC, 2023).
Bushnell, J. The Economics of Carbon Offsets. in The Design and Implementation of U.S. Climate Policy (eds Don Fullerton and Catherine Wolfram) 197–209 (University of Chicago Press, 2010).
Kopp, R. Role of Offsets in Global and Domestic Climate Policy. In Proc. National Research Council, Modelling the Economics of Greenhouse Gas Mitigation: Summary of a Workshop 92–99 (The National Academies Press, Washington, DC, 2011).
Tavoni, M. et al. Forestry and the carbon market response to stabilize climate. Energy Policy 35 , 5346–5353 (2007).
Breidenich, C., Magraw, D., Rowley, A. & Rubin, J.W. The Kyoto Protocol to the United Nations Framework Convention on Climate Change. Am. J. Int. Law 92 , 315–331 (1998).
United Nations Framework Convention on Climate Change, Conference of the Parties. Adoption of the Paris Agreement, Decision 1/CP.21, paragraph 37 (2016).
Stubbs, M. et al. Agriculture and Forestry Offsets in Carbon Markets: Background and Selected Issues . (Congressional Research Service, Washington, DC, 2021).
Goodward, J., Kelly, A. The Bottom Line on Offsets. (World Resources Institute, Washington, DC, 2010).
Integrity Council for the Voluntary Carbon Market. Core Carbon Principles . (ICVCM, London, 2023).
Marland, G. et al. Accounting for sequestered carbon: the question of permanence. Environ. Sci. Policy 4 , 259–268 (2001).
Article CAS Google Scholar
Kollmuss, A. et al. Has Joint Implementation reduced GHG emissions? Lessons learned for the design of carbon market mechanisms . (Stockholm Environment Institute, Stockholm, 2015).
Calel, R. et al. Do Carbon Offsets Offset Carbon ? (Munich Society for the Promotion of Economic Research, Munich, 2021).
Cames, M. et al. How additional is the Clean Development Mechanism? Analysis of the application of current tools and proposed alternatives . (INFRAS and Stockholm Environment Institute, Zürich, 2016).
Passey, R. et al. The governance challenge for implementing effective market-based climate policies: a case study of The New South Wales Greenhouse Gas Reduction Scheme. Energy Policy 36 , 3009–3018 (2008).
Schneider, L. & Kollmuss, A. Perverse effects of carbon markets on HFC-23 and SF 6 abatement projects in Russia. Nat. Clim. Change 5 , 1061–1064 (2015).
Randazzo, R. et al. Improved assessment of baseline and additionality for forest carbon crediting. Ecol. Appl. 33 , e2817 (2023).
Guizar-Coutiño, A. et al. A global evaluation of the effectiveness of voluntary REDD+ projects at reducing deforestation and degradation in the moist tropics. Conserv. Biol. 36 , e13970 (2022).
Badgley, G. et al. California’s forest carbon offsets buffer pool is severely undercapitalized. Front. For. Glob. Change 5 , 930426 (2022).
Badgley, G. et al. Systematic over-crediting in California’s forest carbon offsets program. Glob. Change Biol. 28 , 1433–1445 (2022).
Coffield, S. et al. Using remote sensing to quantify the additional climate benefits of California forest carbon offset projects. Glob. Change Biol . 28 , 6789–6806 (2022).
West, T. A. P. et al. Overstated carbon emission reductions from voluntary REDD+ projects in the Brazilian Amazon. PNAS 117 , 24188–24194 (2020).
West, T. A. P. et al. Action needed to make carbon offsets from forest conservation work for climate change mitigation. Science 381 , 873–877 (2023).
Stapp, J. et al. Little evidence of management change in California’s forest offset program. Commun. Earth. Environ. 4 , 331 (2023).
Gibbons, P. et al. Outcomes from 10 years of biodiversity offsetting. Glob. Change Biol. 24 , e643–e654 (2018).
zu Ermgassen, S. et al. The ecological outcomes of biodiversity offsets under “no net loss” policies: a global review. Conserv. Lett. 12 , e12664 (2019).
NSW Independent Pricing and Regulatory Tribunal. NSW Greenhouse Reduction Scheme—Strengths, Weaknesses and Lessons Learned (IPART, Sydney, 2013).
Carbon Credits (Carbon Farming Initiative) Act 2011 (Cwlth).
Carbon Credits (Carbon Farming Initiative) (Human-Induced Regeneration of a Permanent Even-Aged Native Forest—1.1) Methodology Determination (2013).
Clean Energy Regulator. Emissions Reduction Fund project register . (Commonwealth of Australia, Canberra, 2023).
Evans, M. C. et al. Carbon farming via assisted natural regeneration as a cost-effective mechanism for restoring biodiversity in agricultural landscapes. Environ. Sci. Policy 50 , 114–129 (2015).
Evans, M. C. Effective incentives for reforestation: lessons from Australia’s carbon farming policies. Curr. Opin. Environ. Sustain. 32 , 38–45 (2018).
Richards, G. & Evens, D. Development of a carbon accounting model (FullCAM Vers. 1.0) for the Australian continent. Aust. For. 67 , 277–283 (2004).
Waterworth, R. et al. A generalised hybrid process-empirical model for predicting plantation forest growth. Forest Ecol. Manag. 238 , 231–243 (2007).
Paul, K. et al. Improved models for estimating temporal changes in carbon sequestration in above-ground biomass of mixed-species environmental plantings. Forest Ecol. Manag. 338 , 208–218 (2015).
Paul, K. & Roxburgh, S. Predicting carbon sequestration of woody biomass following land restoration. Forest Ecol. Manag. 460 , 117838 (2020).
Roxburgh, S. et al. A revised above-ground maximum biomass layer for the Australian continent. Forest Ecol. Manag. 432 , 264–275 (2019).
Department of Climate Change, Energy, the Environment and Water. National Inventory Report 2021 . (Commonwealth of Australia, Canberra, 2023).
Butler, D. et al. Australian National University (ANU)-University of New South Wales (UNSW) ERF research team submission to the Chubb Review. (Department of Climate Change, Energy, the Environment and Water, Canberra, 2022).
Wilson, R. et al. Why offsets are not a viable alternative to cutting emissions. (Climate Analytics, Berlin, 2023).
Australian Academy of Science. Review of Four Methods of Generating Australian Carbon Credit Units . (Australian Academy of Science, Canberra, 2022).
Wentworth Group of Concerned Scientists. Submission to the Independent Review of Australian Carbon Credit Units . (Department of Climate Change, Energy, the Environment and Water, Canberra, 2022).
Richards, G. & Brack, C. A continental biomass stock and stock change estimation approach for Australia. Aust. For. 67 , 284–288 (2004).
Morton, S. R. et al. A fresh framework for the ecology of arid Australia. J. Arid Environ. 75 , 313–329 (2011).
Fensham, R. J. et al. How does clay constrain woody biomass in drylands? Glob. Ecol. Biogeogr. 24 , 950–958 (2015).
Ma, X. et al. Drought rapidly diminishes the large net CO 2 uptake in 2011 over semi-arid Australia. Sci. Rep. 6 , 37747 (2016).
Fensham et al. Rainfall, landuse and woody vegetation cover in semi-arid Australian savannah. J. Ecol. 93 , 596–606 (2005).
Eldridge, D. J. et al. Impacts of shrub encroachment on ecosystem structure and functioning: towards a global synthesis. Ecol. Lett. 14 , 709–722 (2011).
Sankaran, M. et al. Native ungulates of diverse body sizes collectively regulate long-term woody plant demography and structure of a semi-arid savanna. J. Ecol. 101 , 1389–1399 (2013).
Travers, S. et al. Rabbits and livestock grazing alter the structure and composition of mid-storey plants in a wooded dryland. Agric. Ecosyst. Environ. 277 , 53–60 (2019).
Witt, G. B. Vegetation changes through the eyes of the locals: the ‘artificial wilderness’ in the mulga country of south-west Queensland. Rangel. J. 35 , 299–314 (2013).
Fensham, R. et al. Potential aboveground biomass in drought-prone forest used for rangeland pastoralism. Ecol. Appl. 22 , 894–908 (2012).
Landsberg, J. et al. Abundance and composition of plant species along grazing gradients in Australian rangelands. J. Appl. Ecol. 40 , 1008–1024 (2003).
Friedel, M. H. Discontinuous change in arid woodland and grassland vegetation along gradients of cattle grazing in central Australia. J. Arid Environ. 37 , 145–164 (1997).
Noble, J. C. T he Delicate and Noxious Scrub: CSIRO Studies on Native Tree and Shrub Proliferation in the Semi-Arid Woodlands of Eastern Australia . (CSIRO Publishing, Melbourne, 1997).
Burrows, W. H. Seeing the wood(land) for the trees—an individual perspective of Queensland woodland studies (1965-2005). Trop. Grasslands 36 , 202–217 (2002).
Google Scholar
Fensham, R. & Fairfax, R. Preliminary assessment of gidgee (Acacia cambagei) woodland thickening in the Longreach district, Queensland. The Rangel. J. 27 , 159–168 (2005).
Witt, B. et al. Is ‘vegetation thickening’ occurring in Queensland’s mulga lands—a 50-year aerial photographic analysis. Aust. J. Bot. 57 , 572–582 (2009).
Queensland Department of Environment and Science. Statewide Landcover and Trees Study: 2020–21 SLATS Report. (Queensland Government, Brisbane, 2023).
NSW Department of Planning and Environment. Results for NSW Vegetation Clearing 2021 . (NSW Government, Sydney, 2023).
Fensham, R. et al. To what extent is drought‐induced tree mortality a natural phenomenon? Glob. Ecol. Biogeogr. 28 , 365–373 (2019).
Department of Climate Change, Energy, the Environment and Water. National Forest and Sparse Woody Vegetation Data (Version 7.0 - 2022 Release) (Commonwealth of Australia, Canberra, 2023).
Department of the Environment and Energy. National Inventory Report 2017 . (Commonwealth of Australia, Canberra, 2019).
Macintosh, A. et al. HIR Project Data Summary. Figshare (2024). https://doi.org/10.6084/m9.figshare.25199786 .
Macintosh, A. et al. Forest & Sparse Woody Cover in HIR Projects. Figshare (2024). https://doi.org/10.6084/m9.figshare.25199789 .
Larmour, J. et al. Relating canopy cover and average height to the biomass of the stand. Report for the Department of the Environment and Energy . (CSIRO, Canberra, 2019).
Austin, K. et al. The economic costs of planting, preserving, and managing the world’s forests to mitigate climate change. Nat. Commun. 11 , 5946 (2020).
Nabuurs, G-J. et al. Agriculture, Forestry and Other Land Uses (AFOLU). In IPCC, 2022: Climate Change 2022: Mitigation of Climate Change. Contribution of Working Group III to the Sixth Assessment Report of the Intergovernmental Panel on Climate Change (eds. Shukla P. R. et al.) (Cambridge University Press, Cambridge, UK and New York, NY, USA 2022).
Clean Energy Regulator. Carbon abatement contract register . (Commonwealth of Australia, Canberra, 2023).
Clean Energy Regulator. Auction results . (Commonwealth of Australia, Canberra, 2023).
Gillenwater, M., Seres, S. The Clean Development Mechanism: A Review of the First International Offset Program . (Pew Center on Global Climate Change, Washington, DC, 2011).
United Nations Framework Convention on Climate Change, Conference of the Parties serving as the meeting of the Parties to the Kyoto Protocol (CMP). Modalities and procedures for a clean development mechanism as defined in Article 12 of the Kyoto Protocol, Decision 3/CMP.1, paragraph 45 (2005).
Bushnell, J. Adverse Selection and Emissions Offsets . Energy Institute at HAAS. Working Paper 222. (University of California, Berkeley, 2011).
Smith, P. et al. How to measure, report and verify soil carbon change to realize the potential of soil carbon sequestration for atmospheric greenhouse gas removal. Glob. Change Biol. 26 , 219–241 (2020).
Burke, P. J. Undermined by adverse selection: Australia’s direct action abatement subsidies. Econ. Papers 35 , 216–229 (2016).
Victor, D. The Politics and Economics of International Carbon Offsets. In Proc. National Research Council, Modelling the Economics of Greenhouse Gas Mitigation: Summary of a Workshop 132–142 (The National Academies Press, Washington, DC, (2011).
Arlidge, W. et al. A global mitigation hierarchy for nature conservation. BioScience 68 , 336–347 (2018).
International Civil Aviation Organization. Assembly Resolution A41-22: Consolidated statement of continuing ICAO policies and practices related to environmental protection—Carbon Offsetting and Reduction Scheme for International Aviation (CORSIA). (ICAO, Montreal, Canada, 2022).
Gupta, A. & Mason, M. Disclosing or obscuring? the politics of transparency in climate governance. Curr. Opin. Environ. Sustain. 18 , 82–90 (2016).
GDAL/OGR contributors. GDAL/OGR Geospatial Data Abstraction software Library. Open Source Geospatial Foundation (2022). URL https://gdal.org . https://doi.org/10.5281/zenodo.5884351 .
Bates, D. et al. Fitting linear mixed-effects models using lme4. J. Stat. Softw. 67 , 1–48 (2015).
R Core Team. R: A language and environment for statistical computing. R Foundation for Statistical Computing, Vienna, Austria (2022).
Emissions Reduction Assurance Committee. Review of the Human-Induced Regeneration and Native Forest from Managed Regrowth methods . (Commonwealth of Australia, Canberra, 2018).
Download references
Author information
Authors and affiliations.
The Australian National University, Canberra, ACT, Australia
Andrew Macintosh, Don Butler, Dean Ansell, Marie Waschka, David Lindenmayer, Philip Gibbons & Paul Summerfield
Haizea Analytics Pty Ltd, Canberra, ACT, Australia
Pablo Larraondo
University of New South Wales, Canberra, ACT, Australia
Megan C. Evans
University of Queensland, Brisbane, QLD, Australia
Rod Fensham
University of New South Wales, Sydney, NSW, Australia
David Eldridge
You can also search for this author in PubMed Google Scholar
Contributions
A.M., D.B., P.L. and M.E. initiated and designed the research, with input from D.A. and M.W. A.M. led the drafting, with input from all authors, except P.S. D.B. designed and performed the statistical analysis. P.L. processed and analysed the woody cover data. A.M. compiled and analysed data on projects and crediting. R.F., D.E., D.L. and P.G. provided input on drafting and the literature review. P.S. designed and illustrated Fig. 1 .
Corresponding authors
Correspondence to Andrew Macintosh or Don Butler .
Ethics declarations
Competing interests.
The authors declare the following competing interests. A.M. is a non-executive director of Paraway Pastoral Company Ltd. Paraway Pastoral Company Ltd has offset projects under Australia’s offset scheme. Paraway Pastoral Company Ltd does not have any human-induced regeneration projects. A.M., D.B., D.A. and M.W. advise public and private entities on environmental markets and Australia’s carbon offset scheme, including on the design of carbon offset methods. The remaining authors have no competing interests.
Peer review
Peer review information.
Communications Earth & Environment thanks Thales A. P. West, Michael Köhl and Shane Coffield for their contribution to the peer review of this work. Primary Handling Editors: Jinfeng Chang and Martina Grecequet. A peer review file is available.
Additional information
Publisher’s note Springer Nature remains neutral with regard to jurisdictional claims in published maps and institutional affiliations.
Supplementary information
Peer review file, supplementary information, rights and permissions.
Open Access This article is licensed under a Creative Commons Attribution 4.0 International License, which permits use, sharing, adaptation, distribution and reproduction in any medium or format, as long as you give appropriate credit to the original author(s) and the source, provide a link to the Creative Commons licence, and indicate if changes were made. The images or other third party material in this article are included in the article’s Creative Commons licence, unless indicated otherwise in a credit line to the material. If material is not included in the article’s Creative Commons licence and your intended use is not permitted by statutory regulation or exceeds the permitted use, you will need to obtain permission directly from the copyright holder. To view a copy of this licence, visit http://creativecommons.org/licenses/by/4.0/ .
Reprints and permissions
About this article
Cite this article.
Macintosh, A., Butler, D., Larraondo, P. et al. Australian human-induced native forest regeneration carbon offset projects have limited impact on changes in woody vegetation cover and carbon removals. Commun Earth Environ 5 , 149 (2024). https://doi.org/10.1038/s43247-024-01313-x
Download citation
Received : 22 September 2023
Accepted : 11 March 2024
Published : 26 March 2024
DOI : https://doi.org/10.1038/s43247-024-01313-x
Share this article
Anyone you share the following link with will be able to read this content:
Sorry, a shareable link is not currently available for this article.
Provided by the Springer Nature SharedIt content-sharing initiative
By submitting a comment you agree to abide by our Terms and Community Guidelines . If you find something abusive or that does not comply with our terms or guidelines please flag it as inappropriate.
Quick links
- Explore articles by subject
- Guide to authors
- Editorial policies
Sign up for the Nature Briefing newsletter — what matters in science, free to your inbox daily.

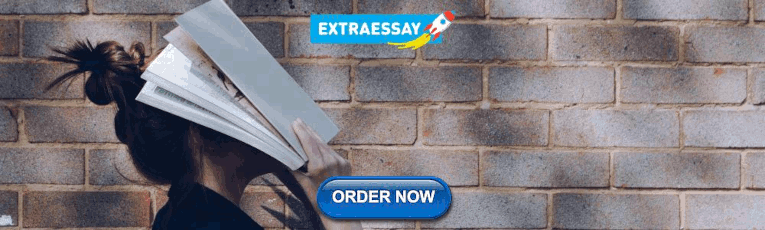
IMAGES
VIDEO
COMMENTS
Operational research (referred to as operations research in the USA) can be viewed as a collection of conceptual, mathematical, statistical, and computational modelling techniques used for the structuring, analysis, and solving of problems related to the design and operation of complex human systems. These techniques range from approaches that ...
Operational research (OR) is the discipline of using models, either quantitative or qualitative, to aid decision-making in complex implementation problems. The methods of OR have been used in healthcare since the 1950s in diverse areas such as emergency medicine and the interface between acute and community care; hospital performance; scheduling and management of patient home visits ...
Operations research refers to scientific methods (statistical and mathematical modeling, experiments, simulation, and optimization) applied to the solution of complex business problems. Operations ...
Operations research is an operations management strategy that uses mathematical methods to find the right decision among a series of potentials. It uses mathematical, computational, and scientific ...
operations research, application of scientific methods to the management and administration of organized military, governmental, commercial, and industrial processes.. Basic aspects. Operations research attempts to provide those who manage organized systems with an objective and quantitative basis for decision; it is normally carried out by teams of scientists and engineers drawn from a ...
30 1 Introduction. Operational research is a collection of modelling techniques used to structure, analyse, and solve problems related to the design and operation of complex human systems. While many argue that operational research should play a key role in improving healthcare services, staf may be largely unaware of its potential applications.
Here are some of the key limitations of Operations Research: Assumptions: Operations Research relies heavily on making certain assumptions about the problem being studied. If these assumptions are incorrect or inaccurate, the results of the study may be unreliable or even misleading. Data Quality: Operations Research is only as good as the data ...
The subject matter, operations research or management science (even though there may be philosophical differences, we use the two terms interchangeably), has been defined by many researchers in the field. Definitions range from "a scientific approach to decision making" to "the use of quantitative tools for systems that originate from real life," "scientific decision making," and ...
the name infers, operations research was clearly developed in light of the fact that the group was managing research on (military operations) [5]. The researchers examined the various problems and on the premise of quantitative investigation of operations recommended certain approaches which indicated noteworthy success.
Abstract. This paper is a retrospective look at 68 years of publication output of Operations Research, revealing changes in its publications, its authors, and their impact over time and how these ...
Operations Research is an expansive, diverse field that melds mathematics, statistics, and analytical methods to make informed, optimal decisions. From its roots in military operations to its widespread application across industries like logistics, healthcare, finance, and more, Operations Research is a testament to the power of analytical ...
O.R. models increase decision-making and lower the possibility of making bad decisions. The O.R. technique improves the executive's understanding of his decision-making process. Reduce chances of failure. Operations and research managers discover all potential solutions for a given problem with the aid of operations, reducing the risk of failure.
Throughout its history, Operational Research has evolved to include a variety of methods, models and algorithms that have been applied to a diverse and wide range of contexts. This encyclopedic ...
Advantages of Operations Research. Better Systems: Often, an O.R. approach is initiated to analyze a particular problem of decision making such as best location for factories, whether to open a new warehouse, etc. It also helps in selecting economical means of transportation, jobs sequencing, production scheduling, replacement of old machinery ...
speaking, an O.R. project comprises three steps: (1) building a model, (2) solving it, and. (3) implementing the results. The emphasis of this chapter is on the first and third steps. The second step typically involves specific methodologies or techniques, which could be.
Some advantages of Operation Research are as follows: An O.R. approach is frequently used to analyse a specific decision-making problem, such as the best location for factories, new warehouses etc. Locating a warehouse or depot that can deliver goods over shorter distances and at a lower cost is made easier with the help of operations research.
Operational Research (OR) is the well-known approach of using analytical and advanced methods to help make the best possible decisions. As early as 1980, Article by authors Shannon, Long & Buckles (1980) presented the results of a survey of the perception of the usefulness and knowledge of the 12 OR methodologies commonly used in the practice ...
Operations research (OR) is a field of applied mathematics, engineering, and management science that deals with the application of advanced analytical methods to help make better decisions.OR uses quantitative models and data analysis to address complex issues in a variety of fields, including business, government, and healthcare.
Operations research - Problem-Solving, Modeling, Analysis: Three essential characteristics of operations research are a systems orientation, the use of interdisciplinary teams, and the application of scientific method to the conditions under which the research is conducted. The systems approach to problems recognizes that the behaviour of any part of a system has some effect on the behaviour ...
The key application of Operations Research is that it facilitates decision making in those aspects of a business where resource allocation is paramount, i.e. capital, labour, time and other business resources. Given that it is rooted in computer science and analytics, there is an immense scope of operation research in every business enterprise.
Simulation involves evaluating outcomes of the operation research methods, adjusting factors or constraints to tailor solutions to business needs, performing implementation analysis, and finally suggesting the solution with the highest weightage from these factors. ... Though there may be some limitations of operations research, it is a ...
The operation research can be defined as: Definitions: (i) It is the application of scientific methods, techniques and tools to problems involving the operations of a system so as to provide those in the control of the system with optimum solutions to the problems. (ii) Operation Research is a tool for taking decisions which searches for the ...
Operations Research Definition. Some of the well-known operations research definitions are as: Moarse and Kimbal (1946) defined OR as a scientific method of providing the executive department a quantitative basis for decision-making regarding the operations under their control.. According to Churchman, Ackoff and Arnoff (1957), OR is the application of scientific methods, techniques and tools ...
Examples of desk research methods include but are not limited to: Literature review. Analyze findings from various types of literature, including medical journals, studies, academic papers, books, articles, online publications, and government agencies. Competitor analysis.
However, as for other technologies, benefits and limitations related to the adoption decision co-exist and need to be balanced (Scarfo, 2014; Ma et al., 2013). Indeed, IoT adoption impacts on organizations' practices and architecture, being able to boost both firms' technological progress and research and development (R &D) costs, thereby ...
Secondary research methods focus on analyzing existing data rather than collecting primary data. Common examples of secondary research methods include: Literature review. Researchers analyze and synthesize existing literature (e.g., white papers, research papers, articles) to find knowledge gaps and build on current findings. Content analysis.
The modelling approach used to calculate abatement for HIR projects compounds the resulting integrity risks. Projects could be credited for forest regeneration that has not occurred or that does ...