Thank you for visiting nature.com. You are using a browser version with limited support for CSS. To obtain the best experience, we recommend you use a more up to date browser (or turn off compatibility mode in Internet Explorer). In the meantime, to ensure continued support, we are displaying the site without styles and JavaScript.
- View all journals
- My Account Login
- Explore content
- About the journal
- Publish with us
- Sign up for alerts
- Review Article
- Open access
- Published: 31 August 2023
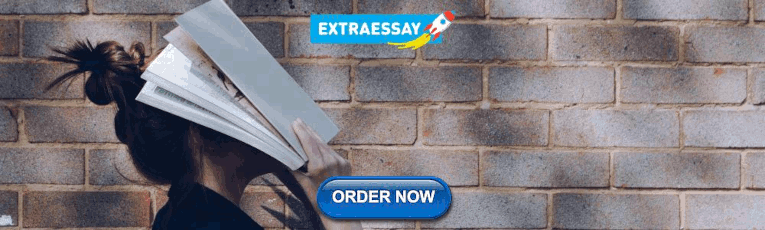
Artificial intelligence in ovarian cancer histopathology: a systematic review
- Jack Breen ORCID: orcid.org/0000-0002-9020-3383 1 ,
- Katie Allen 2 ,
- Kieran Zucker ORCID: orcid.org/0000-0003-4385-3153 3 ,
- Pratik Adusumilli ORCID: orcid.org/0000-0002-1567-9795 2 , 4 ,
- Andrew Scarsbrook ORCID: orcid.org/0000-0002-4243-032X 2 , 4 ,
- Geoff Hall 3 ,
- Nicolas M. Orsi 2 na1 &
- Nishant Ravikumar 1 na1
npj Precision Oncology volume 7 , Article number: 83 ( 2023 ) Cite this article
4375 Accesses
5 Citations
1 Altmetric
Metrics details
- Cancer imaging
- Ovarian cancer
- Translational research
This study evaluates the quality of published research using artificial intelligence (AI) for ovarian cancer diagnosis or prognosis using histopathology data. A systematic search of PubMed, Scopus, Web of Science, Cochrane CENTRAL, and WHO-ICTRP was conducted up to May 19, 2023. Inclusion criteria required that AI was used for prognostic or diagnostic inferences in human ovarian cancer histopathology images. Risk of bias was assessed using PROBAST. Information about each model was tabulated and summary statistics were reported. The study was registered on PROSPERO (CRD42022334730) and PRISMA 2020 reporting guidelines were followed. Searches identified 1573 records, of which 45 were eligible for inclusion. These studies contained 80 models of interest, including 37 diagnostic models, 22 prognostic models, and 21 other diagnostically relevant models. Common tasks included treatment response prediction (11/80), malignancy status classification (10/80), stain quantification (9/80), and histological subtyping (7/80). Models were developed using 1–1375 histopathology slides from 1–776 ovarian cancer patients. A high or unclear risk of bias was found in all studies, most frequently due to limited analysis and incomplete reporting regarding participant recruitment. Limited research has been conducted on the application of AI to histopathology images for diagnostic or prognostic purposes in ovarian cancer, and none of the models have been demonstrated to be ready for real-world implementation. Key aspects to accelerate clinical translation include transparent and comprehensive reporting of data provenance and modelling approaches, and improved quantitative evaluation using cross-validation and external validations. This work was funded by the Engineering and Physical Sciences Research Council.
Similar content being viewed by others
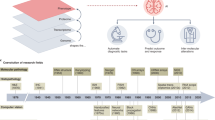
Artificial intelligence in histopathology: enhancing cancer research and clinical oncology
Artem Shmatko, Narmin Ghaffari Laleh, … Jakob Nikolas Kather
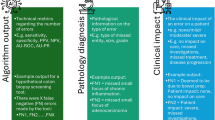
Understanding the errors made by artificial intelligence algorithms in histopathology in terms of patient impact
Harriet Evans & David Snead
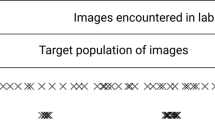
Recommendations on compiling test datasets for evaluating artificial intelligence solutions in pathology
André Homeyer, Christian Geißler, … Norman Zerbe
Introduction
Ovarian cancer is the eighth most common malignancy in women worldwide 1 . It is notoriously difficult to detect and diagnose, with ineffective screening 2 and non-specific symptoms similar to those caused by menopause 3 . Encompassing primary malignant tumours of the ovaries, fallopian tubes, and peritoneum, the disease has often started to spread within the abdomen at the time of diagnosis (FIGO 4 Stage 3). This typical late stage at diagnosis makes ovarian cancer a particularly deadly disease, with the 314,000 new cases diagnosed each year translating to 207,000 deaths per year globally 1 .
Most ovarian cancers are carcinomas (cancers of epithelial origin) which predominantly fall into five histological subtypes: high-grade serous, low-grade serous, clear cell, endometrioid, and mucinous. Non-epithelial ovarian cancers are much less common and include germ cell, sex cord-stromal, and mesenchymal tumours. Ovarian cancer subtypes differ morphologically and prognostically and have varying treatment options 5 . High-grade serous carcinoma is the most common form of ovarian cancer, accounting for approximately 70% of all cases 6 .
Histopathology, the examination of tissue specimens at the cellular level, is the gold standard for ovarian cancer diagnosis. Pathologists typically interpret tissue stained with haematoxylin and eosin (H&E), though interpretation can be a subjective, time-consuming process, with some tasks having a high level of inter-observer variation 7 , 8 , 9 . In the assessment of difficult cases, general pathologists may seek assistance from subspecialty gynaecological pathology experts, and/or use ancillary tests, such as immunohistochemistry (IHC). Referrals and ancillary testing can be essential to the accuracy of the diagnostic process but come at the cost of making it longer and more expensive. Worldwide, pathologists are in much greater demand than supply, with significant disparities in the number of pathologists between countries 10 , and with better-supplied countries still unable to meet demand 11 .
Traditionally, pathologists have analysed glass slides using a light microscope. However, the implementation of a digital workflow, where pathologists review scanned whole slide images (WSIs) using a computer, is becoming more common. While digital pathology uptake has likely been driven by efficiency benefits 12 , it has created an opportunity for the development of automated tools to assist pathologists. These tools often aim to improve the accuracy, efficiency, objectivity, and consistency of diagnosis. Such tools could help to alleviate the global workforce shortage of pathologists, increasing diagnostic throughput and reducing the demand for referrals and ancillary tests. This is an increasingly active area of research 13 and, for some malignancies, these systems are starting to achieve clinical utility 14 .
In this study, we systematically reviewed all literature in which artificial intelligence (AI) techniques (comprising both traditional machine learning (ML) and deep learning methods) were applied to digital pathology images for the diagnosis or prognosis of ovarian cancer. This included research that focused on a single diagnostic factor such as histological subtype and studies that performed computer-aided diagnostic tasks such as tumour segmentation. The review characterises the state of the field, describing which diagnostic and prognostic tasks have been addressed, and assessing factors relevant to the clinical utility of these methods, such as the risks of bias. Despite ovarian cancer being a particularly difficult disease to detect and diagnose, and the shortage of available pathologists, AI models have not yet been implemented in clinical practice for this disease. This review aims to provide insights and recommendations based on published literature to improve the clinical utility of future research, including reducing risks of bias, improving reproducibility, and increasing generalisability.
As shown in Fig. 1 , the literature searches returned a total of 1573 records, of which 557 were duplicates. Nine hundred and thirty records were excluded during the screening of titles and abstracts, and 41 were excluded based on full paper screening, including 3 records for which full articles could not be obtained. The remaining 45 studies were included in the review, of which 11 were conference papers and 34 were journal papers. All accepted studies were originally identified through searches of research databases, with no records from trial registries meeting the inclusion criteria. While the searches returned literature from as early as 1949, all of the research which met the inclusion criteria was published since 2010, with over 70% of the included literature published since 2020. Study characteristics are shown in Table 1 . The 45 accepted articles contained 80 models of interest, details of which are shown in Table 2 .
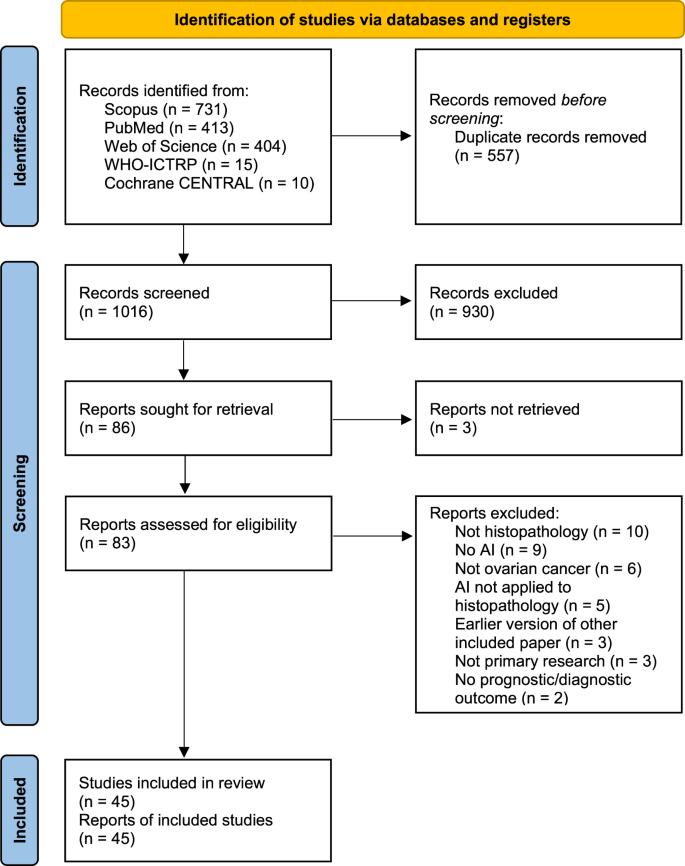
PRISMA 2020 flowchart of the study identification and selection process for the systematic review. Records were screened on titles and abstracts alone, and reports were assessed based on the full-text content. CENTRAL Central Register of Controlled Trials. WHO-ICTRP World Health Organisation International Clinical Trial Registry Platform.
Risk of bias assessment
The results of the PROBAST assessments are shown in Table 3 . While some studies contained multiple models of interest, none of these contained models with different risk of bias scores for any section of the PROBAST assessment, so one risk of bias analysis is presented per paper. All models showed either a high overall risk of bias (37/45) or an unclear overall risk of bias (8/45). Every high-risk model had a high-risk score in the analysis section (37/45), with several also being at high risk for participants (6/45), predictors (11/45), or outcomes (13/45). Less than half of the studies achieved a low risk of bias in any domain (21/45), with most low risks being found in the outcomes (16/45) and predictors (9/45) sections. Nearly all of the papers had an unclear risk of bias in at least one domain, most commonly the participants (36/45) and predictors (25/45) domains. Qualitative summaries are presented in Fig. 2 .
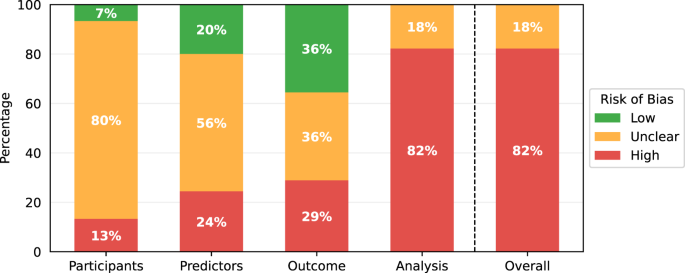
PROBAST risk of bias results summarised for the 45 papers included in this review.
Data synthesis results
Data in included literature.
The number of participants in internal datasets varied by orders of magnitude, with each study including 1–776 ovarian cancer patients, and one study including over 10,000 total patients across a range of 32 malignancies 15 . Most research only used data from the five most common subtypes of ovarian carcinoma, though one recent study included the use of sex cord-stromal tumours 16 . Only one study explicitly included any prospective data collection, and this was only for a small subset which was not used for external validation 17 .
As shown in Fig. 3 , the number of pathology slides used was often much greater than the number of patients included, with three studies using over 1000 slides from ovarian cancer patients 18 , 19 , 20 . In most of the studies, model development samples were WSIs containing resected or biopsied tissue (34/45), with others using individual tissue microarray (TMA) core images (5/45) or pre-cropped digital pathology images (3/45). Most studies used H&E-stained tissue (33/45) and others used a variety of IHC stains (11/45), with no two papers reporting the use of the same IHC stains. Some studies included multi-modal approaches, using genomics 17 , 21 , 22 , 23 , 24 , proteomics 21 , 24 , transcriptomics 24 , and radiomics 17 data alongside histopathological data.
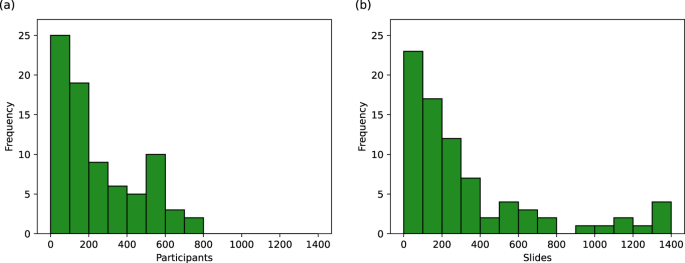
Histograms showing the number of a ovarian cancer patients and b ovarian cancer histopathology slides used in model development. Many of these values are uncertain due to incomplete reporting, as reflected in Table 2 .
The most commonly used data source was The Cancer Genome Atlas (TCGA) (18/45), a project from which over 30,000 digital pathology images from 33 malignancies are publicly available. The ovarian cancer subset, TCGA-OV 25 , contains 1481 WSIs from 590 cases of ovarian serous carcinoma (mostly, but not exclusively, high-grade), with corresponding genomic, transcriptomic, and clinical data. This includes slides from eight data centres in the United States, with most slides containing frozen tissue sections (1374/1481) rather than formalin-fixed, paraffin-embedded (FFPE) sections. Other recurring data sources were the University of British Columbia Ovarian Cancer Research Program (OVCARE) repository 26 , 27 , 28 , the Transcanadian study 29 , 30 , and clinical records at the Mayo Clinic 31 , 32 , Tri-Service General Hospital 33 , 34 , 35 , and Memorial Sloan Kettering Cancer Center 17 , 36 . All other researchers either used a unique data source (12/45) or did not report the provenance of their data (8/45). TCGA-OV, OVCARE, and the Transcanadian study are all multi-centre datasets. Aside from these, few studies reported the use of multi-centre data 17 , 24 , 28 , 37 , 38 , 39 . Only two studies reported the use of multiple slide scanners, with every slide scanned on one of two available scanners 27 , 28 . The countries from which data were sourced included Canada, China, Finland, France, Germany, Italy, Japan, the Netherlands, South Korea, Taiwan, the United Kingdom, and the United States of America.
Methods in included literature
There was a total of 80 models of interest in the 45 included papers, with each paper containing 1–6 such models. There were 37 diagnostic models, 22 prognostic models, and 21 other models predicting diagnostically relevant information. Diagnostic model outcomes included the classification of malignancy status (10/37), histological subtype (7/37), primary cancer type (5/37), genetic mutation status (4/37), tumour-stroma reaction level (3/37), grade (2/37), transcriptomic subtype (2/37), stage (1/37), microsatellite instability status (1/37), epithelial-mesenchymal transition status (1/37), and homologous recombination deficiency status (1/37). Prognostic models included the prediction of treatment response (11/23), overall survival (6/23), progression-free survival (3/23), and recurrence (2/23). The other models performed tasks that could be used to assist pathologists in analysing pathology images, including measuring the quantity/intensity of staining, generating segmentation masks, and classifying tissue/cell types.
A variety of models were used, with the most common types being convolutional neural network (CNN) (41/80), support vector machine (SVM) (10/80), and random forest (6/80). CNN architectures included GoogLeNet 40 , VGG16 19 , 32 , VGG19 26 , 28 , InceptionV3 33 , 34 , 35 , 38 , ResNet18 17 , 27 , 28 , 39 , 41 , 42 , ResNet34 43 , ResNet50 16 , 44 , 45 , ResNet182 36 , and MaskRCNN 32 . Novel CNNs typically used multiple standardised blocks involving convolutional, normalisation, activation, and/or pooling layers 22 , 46 , 47 , with two studies also including attention modules 20 , 35 . One study generated their novel architecture by using a topology optimisation approach on a standard VGG16 23 .
Most researchers split their original images into patches to be separately processed, with patch sizes ranging from 60×60 to 2048×2048 pixels, the most common being 512×512 pixels (19/56) and 256×256 pixels (12/56). A range of feature extraction techniques were employed, including both hand-crafted/pre-defined features (23/80) and features that were automatically learned by the model (51/80). Hand-crafted features included a plethora of textural, chromatic, and cellular and nuclear morphological features. Hand-crafted features were commonly used as inputs to classical ML methods, such as SVM and random forest models. Learned features were typically extracted using a CNN, which was often also used for classification.
Despite the common use of patches, most models made predictions at the WSI level (29/80), TMA core level (18/80), or patient level (6/80), requiring aggregation of patch-level information. Two distinct aggregation approaches were used, one aggregating before modelling and one aggregating after modelling. The former approach requires the generation of slide-level features before modelling, the latter requires the aggregation of patch-level model outputs to make slide-level predictions. Slide-level features were generated using summation 16 , averaging 21 , 24 , 36 , attention-based weighted averaging 20 , 41 , 42 , 44 , 45 , concatenation 15 , 30 , as well as more complex embedding approaches using Fisher vector encoding 29 and k-means clustering 48 . Patch-level model outputs were aggregated to generate slide-level predictions by taking the maximum 22 , 35 , median 43 , or average 23 , using voting strategies 27 , 34 , or using a random forest classifier 28 . These approaches are all examples of multiple instance learning (MIL), though few models of interest were reported using this terminology 22 , 41 , 42 , 44 .
Most studies included segmentation at some stage, with many of these analysing tumour/stain segmentation as a model outcome 32 , 36 , 37 , 47 , 49 , 50 , 51 , 52 , 53 , 54 . Some other studies used segmentation to determine regions of interest for further modelling, either simply separating tissue from background 15 , 18 , 44 , 45 , or using tumour segmentation to select the most relevant tissue regions 33 , 34 , 35 , 55 , 56 . One study also used segmentation to detect individual cells for classification 57 . Some studies also used segmentation in determining hand-crafted features relating to the quantity and morphology of different tissues, cells, and nuclei 17 , 18 , 21 , 24 , 30 , 31 .
While attention-based approaches have been applied to other malignancies for several years 58 , 59 , they were only seen in the most recent ovarian cancer studies 20 , 28 , 33 , 34 , 35 , 41 , 42 , 44 , 45 , and none of the methods included self-attention, an increasingly popular method for other malignancies 60 . Most models were deterministic, though hidden Markov trees 51 , probabilistic boosting trees 52 , and Gaussian mixture models 61 were also used. Aside from the common use of low-resolution images to detect and remove non-tissue areas, images were typically analysed at a single resolution, with only six papers including multi-magnification techniques in their models of interest. Four of these combined features from different resolutions for modelling 29 , 30 , 36 , 48 , and the other two used different magnifications for selecting informative tissue regions and for modelling 33 , 34 . Out of the papers for which it could be determined, the most common modelling magnifications were ×20 (35/41) and ×40 (7/41). Few models integrated histopathology data with other modalities (6/80). Multi-modal approaches included the concatenation of separately extracted uni-modal features before modelling 21 , 23 , 24 , the amalgamation of uni-modal predictions from separate models 17 , and a teacher–student approach where multiple modalities were used in model training but only histopathology data was used for prediction 22 .
Analysis in included literature
Analyses were limited, with less than half of the model outcomes being evaluated with cross-validation (39/80) and with very few externally validated using independent ovarian cancer data (7/80), despite small internal cohort sizes. Cross-validation methods included k -fold (22/39) with 3–10 folds, Monte Carlo (12/39) with 3–15 repeats, and leave-one-patient-out cross-validations (5/39). Some other papers included cross-validation on the training set to select hyperparameters but used only a small unseen test set from the same data source for evaluation. Externally validated models were all trained with WSIs, with validations either performed on TMA cores (2/7) or WSIs from independent data sources (5/7), with two of these explicitly using different scanners to digitise internal and external data 27 , 28 . Some reported methods were externally validated with data from non-ovarian malignancies, but none of these included ovarian cancer data in any capacity, so were not included in the review. However, there was one method which trained with only gastrointestinal tumour data and externally validated with ovarian tumour data 16 .
Most classification models were evaluated using accuracy, balanced accuracy, and/or area under the receiver operating characteristic curve (AUC), with one exception where only a p-value was reported measuring the association between histological features and transcriptomic subtypes based on a Kruskal–Wallis test 19 . Some models were also evaluated using the F1-score, which we chose not to tabulate (in Fig. 3) as the other metrics were reported more consistently. Survival model performance was typically reported using AUC, with other metrics including p-value, accuracy, hazard ratios, and C-index, which is similar to AUC but can account for censoring. Segmentation models were almost all evaluated differently from each other, with different studies reporting AUC, accuracy, Dice coefficient, intersection over union, sensitivity, specificity, and qualitative evaluations. Regression models were all evaluated using the coefficient of determination ( R 2 -statistic). For some models, performance was broken down per patient 39 , 61 , per subtype 16 , or per class 15 , 24 , 32 , 57 , without an aggregated, holistic measure of model performance.
The variability of model performance was not frequently reported (33/94), and when it was reported it was often incomplete. This included cases where it was unclear what the intervals represented (95% confidence interval, one standard deviation, variation, etc.), or not clear what the exact bounds of the interval were due to results being plotted but not explicitly stated. Within the entire review, there were only three examples in which variability was reported during external validation 27 , 38 , 39 , only one of which clearly reported both the bounds and the type of the interval 38 . No studies performed any Bayesian form of uncertainty quantification. Reported results are shown in Table 2 , though direct comparisons between the performance of different models should be treated with caution due to the diversity of data and validation methods used to evaluate different models, the lack of variability measures, the consistently high risks of bias, and the heterogeneity in reported metrics.
The vast majority of published research on AI for diagnostic or prognostic purposes in ovarian cancer histopathology was found to be at a high risk of bias due to issues within the analyses performed. Researchers often used a limited quantity of data and conducted analyses on a single train-test data split without using any methods to account for overfitting and model optimism (cross-validation, bootstrapping, external validation). These limitations are common in gynaecological AI research using other data types, with recent reviews pointing to poor clinical utility caused by predominantly retrospective studies using limited data 62 , 63 and limited methodologies with weak validation, which risk model performance being overestimated 64 , 65 .
The more robust analyses included one study in which several relevant metrics were evaluated using 10 repeats of Monte Carlo cross-validation on a set of 406 WSIs, with standard deviations reported for each metric 26 . Other positive examples included the use of both internal cross-validation and external validation for the same outcome, giving a more rigorous analysis 28 , 34 , 39 . While external validations were uncommon, those which were conducted offered a real insight into model generalisability, with a clear reduction in performance on all external validation sets except one 28 . The only study which demonstrated high generalisability included the largest training set out of all externally validated approaches, included more extensive data labelling than many similar studies, and implemented a combination of three colour normalisation approaches, indicating that these factors may benefit generalisability.
Studies frequently had an unclear risk of bias within the participants and predictors domains of PROBAST due to incomplete reporting. Frequently missing information included where the patients were recruited, how many patients were included, how many samples/images were used, whether any patients/images were excluded, and the methods by which tissue was processed and digitised. Reporting was often poor regarding open-access datasets. Only three papers were found to be at low risk of bias for participants, with these including clear and reasonable patient recruitment strategies and selection criteria, which can be seen as positive examples for other researchers 37 , 43 , 56 . Information about the predictors (histopathology images and features derived thereof) was generally better reported, but still often missed key details which meant that it was unclear whether all tissue samples were processed similarly to avoid risks of bias from visual heterogeneity. It was found that when patient characteristics were reported, they often showed a high risk of bias. Many studies included very small quantities of patients with specific differences from the majority (e.g. less than 20 patients with a different cancer subtype to the majority), causing a risk of spurious correlations and results which are not generalisable to the wider population.
Reporting was particularly sparse in studies which used openly accessible data, possibly indicating that AI-focused researchers were not taking sufficient time to understand these datasets and ensure their research was clinically relevant. For example, many of the researchers who used TCGA data included frozen tissue sections without commenting on whether this was appropriate, despite the fact that pathologists do not consider them to be of optimal diagnostic quality. One paper handled TCGA data more appropriately, with a clear explanation of the positives and negatives of the dataset, and entirely separate models for FFPE and frozen tissue slides 15 .
Sharing code can help to mitigate the effects of incomplete reporting and drastically improve reproducibility, but only 19 of the 45 papers did this, with some of these appearing to be incomplete or inaccessible. The better code repositories included detailed documentation to aid reproducibility, including environment set-up information 16 , 19 , overviews of included functions 17 , 36 , 42 , and code examples used to generate reported results 57 .
Two papers were found to have major discrepancies between the reported data and the study design, indicating much greater risks of bias than those seen in any other research 46 , 54 . In one paper 46 , it was reported that TCGA-OV data was used for subtyping with 5 classes, despite this dataset only including high-grade serous and low-grade serous carcinomas. In the other paper 54 , it was reported that TCGA-OV data was used for slide-level classification into ovarian cancer and non-ovarian cancer classes using PAS-stained tissue, despite TCGA-OV only containing H&E-stained ovarian cancer slides.
Limitations of the review
While the review protocol was designed to reduce biases and maximise the quantity of relevant research included, there were some limitations. This review is restricted to published literature in the English language, however, AI research may be published in other languages or made available as pre-prints without publication in peer-reviewed journals, making this review incomplete. While most of the review process was completed by multiple independent researchers, the duplicate detection was performed by only a single researcher, raising the possibility of errors in this step of the review process, resulting in incorrect exclusions. Due to the significant time gap between the initial and final literature searches (approximately 12 months), there may have been inconsistencies in interpretations, both for data extraction and risk of bias assessments. Finally, this review focused only on light microscopy images of human histopathology samples relating to ovarian cancer, so may have overlooked useful literature outside of this domain.
Development of the field
The field of AI in ovarian cancer histopathology diagnosis is rapidly growing, with more research published since the start of 2020 than in all preceding years combined. The earliest research, published between 2010 and 2013, used hand-crafted features to train classical ML methods such as SVMs. These models were used for segmentation 49 , 50 , 51 , 53 , malignancy classification 18 , 52 , grading 21 , and overall survival prediction 21 . Most of these early studies focused on IHC-stained tissue (5/7), which would be much less commonly used in subsequent research (6/38).
The field was relatively dormant in the following years, with only 6 papers published between 2014 and 2019, half of which had the same primary author 29 , 30 , 48 . These models still used traditional ML classifiers, though some used learned features rather than the traditional hand-crafted features. The models developed were used for histological subtyping 29 , 30 , 48 and cellular/tissue classification 40 , 57 , 66 .
Since 2020, there has been a much greater volume of research published, most of which has involved the use of deep neural networks for automatic feature extraction and classification, with a minority using traditional machine learning model 17 , 24 , 31 , 61 , 67 . Recent research has investigated a broader array of diagnostic outcomes, including the classification of primary cancer type 15 , 54 , mutation status 24 , 36 , 44 , homologous recombination deficiency status 42 , tumour–stroma reaction level 32 , transcriptomic subtypes 19 , 24 , microsatellite instability 24 , and epithelial-mesenchymal transition status 41 . Three additional prognostic outcomes have also been predicted in more recent literature—progression-free survival 17 , 43 , 56 , relapse 43 , 44 , and treatment response 19 , 22 , 33 , 34 , 35 .
Despite progress within a few specific outcomes, there was no obvious overall trend in the sizes of datasets used over time, either in terms of the number of slides or the number of participants. Similarly, there was no evidence that recent research included more rigorous internal validations, though external validations have been increasing in frequency—no research before 2021 included any external validation with ovarian cancer data, but seven studies published more recently did 16 , 24 , 27 , 28 , 34 , 38 , 39 . While these external validations were typically limited to small quantities of data, the inclusion of any external validation demonstrates progress from previous research. Such validations are essential to the clinical utility of these models as real-world implementation will require robustness to different sources of visual heterogeneity, with variation occurring across different data centres and within data centres over time. As this field continues to mature, we hope to see more studies conduct thorough validations with larger, high-quality independent datasets, including clearly reported protocols for patient recruitment and selection, pathology slide creation, and digitisation. This will help to reduce the biases, limited reproducibility, and limited generalisability identified in most of the existing research in this domain.
Current limitations and future recommendations
A large proportion of published work did not provide sufficient clinical and pathological information to assess the risk of bias. It is important that AI researchers thoroughly report data provenance to understand the extent of heterogeneity in the dataset, and to understand whether this has been appropriately accounted for in the study design. Modelling and analysis methods must also be thoroughly reported to improve reliability and reproducibility. Researchers may find it useful to refer to reporting checklists, such as transparent reporting of a multivariable prediction model for individual prognosis or diagnosis (TRIPOD) 68 , to ensure that they have understood and reported all relevant details of their studies. In many studies, it is not clear how AI would fit in the clinical workflow, or whether there are limitations in how these methods could be applied. AI researchers should ensure they understand the clinical context of their data and potential models before undertaking research to reduce bias and increase utility. Ideally, this will involve regular interactions with expert clinicians, including histopathologists and oncologists.
To further improve reproducibility, we recommend that researchers should make code and data available where possible. It is relatively easy to publish code and generate documentation to enhance usability, and there are few drawbacks to doing so when publishing research. Making data available is more often difficult due to data security requirements and the potential storage costs, but it can provide benefits beyond the primary research of the original authors. Digital pathology research in ovarian cancer is currently limited by the lack of openly accessible data, leading to over-dependence on TCGA, and causing many researchers to painstakingly collate similar but distinct datasets. These datasets often contain little of the heterogeneity seen in multi-centre, multi-scanner data, making it difficult for researchers to train robust models or assess generalisability. Where heterogeneous data is included, it often includes small quantities of data which are different to the majority, introducing risks of bias and confounding rather than helping to overcome these issues. TCGA-based studies are prone to this, with significant differences between TCGA slides originating from different data centres 69 , but with many of these centres only providing small quantities of data. Many researchers are reliant on open-access data, but there is a severe shortage of suitable open-access ovarian cancer histopathology data. Making such data available, with detailed protocols describing data creation, allows researchers to conduct more thorough analyses and significantly improve model generalisability and clinical implementability.
For AI to achieve clinical utility, it is essential that more robust validations are performed, especially considering the limitations of the available datasets. We recommend that researchers should always conduct thorough analyses, using cross-validation, bootstrapping, and/or external validations to ensure that results are robust and truly reflect the ability of their model(s) to generalise to unseen data, and are not simply caused by chance. This should include reporting the variability of results (typically in a 95% confidence interval), especially when comparing multiple models to help to distinguish whether one model is genuinely better than another or whether the difference is due to chance. Statistical tests can also be beneficial for these evaluations. Another option for capturing variability is Bayesian uncertainty quantification, which can be used to separate aleatoric (inherent) and epistemic (modelling) uncertainty.
Current literature in this field can be largely characterised as model prototyping with homogeneous retrospective data. Researchers rarely consider the reality of human-machine interaction, perhaps believing that these models are a drop-in replacement for pathologists. However, these models perform narrow tasks within the pathology pipeline and do not take into consideration the clinical context beyond their limited training datasets and siloed tasks. We believe these models would be more beneficial (and more realistic to implement) as assistive tools for pathologists, providing secondary opinions or novel ancillary information. While current research is typically focused on assessing model accuracy without any pathologist input, different study designs could be employed to better assess the real-world utility of these models as assistive tools. For example, usability studies could investigate which models are most accessible and most informative to pathologists in practice, and prospective studies could quantify any benefits to diagnostic efficiency and patient outcomes, and investigate the robustness of models in practice. Understanding the effects of AI on the efficiency of diagnosis is particularly important given the limited supply of pathologists worldwide. As such, this type of research will significantly benefit clinical translation.
Summary of recommendations
To improve clinical utility, researchers should understand their data and ensure planned research is clinically relevant before any modelling, ideally involving clinicians throughout the project. They should also consider different study designs, including usability studies and/or prospective studies. When evaluating models, researchers should conduct thorough analyses using cross-validation, external validation, and/or bootstrapping. When reporting research, researchers should clearly report the context of any histopathology data, including how patients were recruited/selected, and how tissue specimens were processed to generate digital pathology images. Finally, researchers should make all code openly accessible, and make data available where possible.
Literature search
Searches were conducted in three research databases, PubMed, Scopus and Web of Science, and two trial registries, Cochrane Central Register of Controlled Trials (CENTRAL) and the World Health Organisation International Clinical Trial Registry Platform (WHO-ICTRP). The research databases only include journals and conference proceedings which have undergone peer review, ensuring the integrity of included research. The initial searches were performed on 25/04/2022 and were most recently repeated on 19/05/2023. The search strategy was composed of three distinct aspects—artificial intelligence, ovarian cancer, and histopathology. For each aspect, multiple relevant terms were combined using the OR operator (e.g. “artificial intelligence” OR “machine learning”), and then these were combined using the AND operator to ensure that retrieved research met all three aspects. The widest possible set of search fields was used for each search engine except for Scopus, where restrictions were imposed to avoid searching within the citation list of each article, which is not an available field in the other search engines. The terms “ML” and “AI” were restricted to specific fields due to the diversity of their possible meanings. To ensure the most rigorous literature search possible, no restrictions were placed on the publication date or article type during searching.
Many AI approaches build on statistical models, such as logistic regression, which can blur the lines between disciplines. When conducting searches, a previously reported methodology was adopted 70 whereby typical AI approaches were searched by name (e.g. neural networks), and other methods were searched by whether the authors described their work as artificial intelligence . Full details of the search implementation for each database are provided in Supplementary Note 1 . The review protocol was registered with PROSPERO before the search results were screened for inclusion (CRD42022334730).
Literature selection
One researcher (J.B.) manually removed duplicate papers with the assistance of the referencing software EndNote X9 . Two researchers (J.B., K.A.) then independently screened articles for inclusion in two stages, the first based on title and abstract, the second based on full text. Disagreements were discussed and arbitrated by a third researcher (N.R. or N.M.O.). Trials in WHO-ICTRP do not have associated abstracts, so for these studies, only titles were available for initial screening.
The inclusion criteria required that research evaluated the use of at least one AI approach to make diagnostic or prognostic inferences on human histopathology images from suspected or confirmed cases of ovarian cancer. Studies were only included where AI methods were applied directly to the digital pathology images, or to features which were automatically extracted from the images. Fundamental tasks, such as segmentation and cell counting, were included as these could be used by pathologists for computer-aided diagnosis. Only conventional light microscopy images were considered, with other imaging modalities, such as fluorescence and hyperspectral imaging, excluded. Publications which did not include primary research were excluded (such as review papers). Non-English language articles and research where a full version of the manuscript was not accessible were excluded.
A model in an included study was considered to be a model of interest if it met the same inclusion criteria. Where multiple models were compared against the same outcome, the model of interest was taken to be the newly proposed model, with the best performing model during validation taken if this was unclear. If multiple model outcomes were assessed in the same study, a model of interest was taken for each model outcome, regardless of any similarity in modelling approaches. The same model outcome at different levels of precision (e.g. patch-level, slide-level, patient-level) were not considered to be different model outcomes. Models did not need to be entirely independent, for example, the output of one model of interest could have been used as the input of another model of interest on the condition that model performance was separately evaluated for each model.
The risk of bias was assessed for models of interest using the Prediction model Risk Of Bias ASsessment Tool (PROBAST) 71 , where risk of bias is the chance of reported results being distorted by limitations within the study design, conduct, and analysis. It includes 20 guiding questions which are categorised into four domains (participants, predictors, outcome, and analysis), which are summarised as either high-risk or low-risk, or unclear in the case that there is insufficient information to make a comprehensive assessment and none of the available information indicates a high risk of bias. As such, an unclear risk of bias does not indicate methodological flaws, but incomplete reporting.
The participants domain covers the recruitment and selection of participants to ensure the study population is consistent and representative of the target population. Relevant details include the participant recruitment strategy (when and where participants were recruited), the inclusion criteria, and how many participants were recruited.
The predictors domain covers the consistent definition and measurement of predictors, which in this field typically refers to the generation of digital pathology images. This includes methods for fixing, staining, scanning, and digitally processing tissue before modelling.
The outcome domain covers the appropriate definition and consistent determination of ground-truth labels. This includes the criteria used to determine diagnosis/prognosis, the expertise of any persons determining these labels, and whether labels are determined independently of any model outputs.
The analysis domain covers statistical considerations in the evaluation of model performance to ensure valid and not unduly optimistic results. This includes many factors, such as the number of participants in the test set with each outcome, the validation approaches used (cross-validation, external validation, bootstrapping, etc.), the metrics used to assess performance, and methods used to overcome the effects of censoring, competing risks/confounders, and missing data. The risks caused by some of these factors are interrelated, for example, the risk of bias from using a small dataset is somewhat mitigated by cross-validation, which increases the effective size of the test set and can be used to assess variability, reducing optimism in the results. Further, the risk caused by using a small dataset depends on the type of outcome being predicted, for example, more data is required for a robust analysis of 5-class classification than binary classification. There must also be sufficient data within all relevant patient subgroups, for example, if multiple subtypes of ovarian cancer are included, there must not be a subtype that is only represented by a few patients. Due to these interrelated factors, there are no strict criteria to determine the appropriate size of a dataset, though fewer than 50 samples per class or fewer than 100 samples overall is likely to be considered high-risk, and more than 1000 samples overall is likely to be considered low-risk.
Risks of bias often arise due to inconsistent methodologies. Inconsistency in the participants and predictors domains may cause heterogeneity in the visual properties of digital pathology slides which may lead to spurious correlations, either through random chance or systematic differences between subgroups in the dataset. Varied data may be beneficial during training to improve model generalisability when using large datasets, though this must be closely controlled to avoid introducing systematic confounding. Inconsistent determination of the outcome can mean that the results of a study are unreliable due to spurious correlations in the ground truth labels, or invalid due to incorrect determination of labels.
While PROBAST provides a framework to assess risks of bias, there is some level of subjectivity in the interpretation of signalling questions. As such, each model was analysed by three independent researchers (any of J.B., K.A., N.R., K.Z., N.M.O.), with at least one computer scientist and one clinician involved in the risk of bias assessment for each model. The PROBAST applicability of research analysis was not implemented as it is unsuitable for such a diverse array of possible research questions.
Data synthesis
Data extraction was performed independently by two researchers (J.B., K.A.) using a form containing 81 fields within the categories Overview , Data , Methods , Results , and Miscellaneous . Several of these fields were added or clarified during data extraction with the agreement of both researchers and retroactively applied to all accepted literature. The final data extraction form is available at www.github.com/scjjb/OvCaReview , and is summarised in Supplementary Table 1 .
Information was sought from full-text articles, as well as references and supplementary materials where appropriate. Inferences were made only when both researchers were confident that this gave the correct information, with disagreements resolved through discussion. Fields which could not be confidently completed were labelled as being unclear .
All extracted data were summarised in two tables, one each for study-level and model-level characteristics. Only models of interest were included in these tables. The term model outcome refers to the model output, whether this was a clinical outcome (diagnosis/prognosis), or a diagnostically relevant outcome that could be used for computer-aided diagnosis, such as tumour segmentation. The data synthesis did not include any meta-analysis due to the diversity of included methods and model outcomes. The PRISMA 2020 guidelines for reporting systematic reviews were followed, with checklists provided in Supplementary Tables 2 and 3 .
Data availability
The authors declare that the main data supporting the findings of this study are available within the article and its Supplementary Information files. Extra data are available from the corresponding author upon request.
Sung, H. et al. Global cancer statistics 2020: Globocan estimates of incidence and mortality worldwide for 36 cancers in 185 countries. CA Cancer J. Clin. 71 , 209–249 (2021).
PubMed Google Scholar
Menon, U. et al. Ovarian cancer population screening and mortality after long-term follow-up in the UK Collaborative Trial of Ovarian Cancer Screening (UKCTOCS): a randomised controlled trial. Lancet 397 , 2182–2193 (2021).
PubMed PubMed Central Google Scholar
Ebell, M. H., Culp, M. B. & Radke, T. J. A systematic review of symptoms for the diagnosis of ovarian cancer. Am. J. Prev. Med. 50 , 384–394 (2016).
Berek, J. S., Renz, M., Kehoe, S., Kumar, L. & Friedlander, M. Cancer of the ovary, fallopian tube, and peritoneum: 2021 update. Int. J. Gynecol. Obstet. 155 , 61–85 (2021).
Google Scholar
Köbel, M. et al. Ovarian carcinoma subtypes are different diseases: implications for biomarker studies. PLoS Med. 5 , e232 (2008).
Prat, J. Staging classification for cancer of the ovary, fallopian tube, and peritoneum. Int. J. Gynecol. Obstet. 124 , 1–5 (2014).
Matsuno, R. K. et al. Agreement for tumor grade of ovarian carcinoma: analysis of archival tissues from the surveillance, epidemiology, and end results residual tissue repository. Cancer Causes Control 24 , 749–757 (2013).
Köbel, M. et al. Ovarian carcinoma histotype determination is highly reproducible, and is improved through the use of immunohistochemistry. Histopathology 64 , 1004–1013 (2014).
Barnard, M. E. et al. Inter-pathologist and pathology report agreement for ovarian tumor characteristics in the nurses’ health studies. Gynecol. Oncol. 150 , 521–526 (2018).
Wilson, M. L. et al. Access to pathology and laboratory medicine services: a crucial gap. Lancet 391 , 1927–1938 (2018).
Royal College of Pathologists. Meeting pathology demand: histopathology workforce census. https://www.rcpath.org/static/952a934d-2ec3-48c9-a8e6e00fcdca700f/Meeting-Pathology-Demand-Histopathology-Workforce-Census-2018.pdf (2018).
Baidoshvili, A. et al. Evaluating the benefits of digital pathology implementation: time savings in laboratory logistics. Histopathology 73 , 784–794 (2018).
Stenzinger, A. et al. Artificial intelligence and pathology: from principles to practice and future applications in histomorphology and molecular profiling. Semin. Cancer Biol. 84 , 129–143 (2022).
CAS PubMed Google Scholar
Raciti, P. et al. Clinical validation of artificial intelligence–augmented pathology diagnosis demonstrates significant gains in diagnostic accuracy in prostate cancer detection. Arch. Pathol. Lab. Med. https://doi.org/10.5858/arpa.2022-0066-OA (2022).
Kalra, S. et al. Pan-cancer diagnostic consensus through searching archival histopathology images using artificial intelligence. npj Digit. Med. 3 , 31 (2020).
Meng, Z. et al. A deep learning-based system trained for gastrointestinal stromal tumor screening can identify multiple types of soft tissue tumors. Am. J. Pathol. 193 , 899–912 (2023).
Boehm, K. M. et al. Multimodal data integration using machine learning improves risk stratification of high-grade serous ovarian cancer. Nat. Cancer 3 , 723–733 (2022).
CAS PubMed PubMed Central Google Scholar
Kothari, S., Phan, J. H., Osunkoya, A. O. & Wang, M. D. Biological interpretation of morphological patterns in histopathological whole-slide images. In Proceedings of the ACM Conference on Bioinformatics, Computational Biology and Biomedicine 218–225 (ACM, 2012).
Yu, K. H. et al. Deciphering serous ovarian carcinoma histopathology and platinum response by convolutional neural networks. BMC Med. 18 , 1–14 (2020).
Liu, T., Su, R., Sun, C., Li, X. & Wei, L. EOCSA: Predicting prognosis of epithelial ovarian cancer with whole slide histopathological images. Expert Syst. Appl. 206 , 117643 (2022).
Poruthoor, A., Phan, J. H., Kothari, S. & Wang, M. D. Exploration of genomic, proteomic, and histopathological image data integration methods for clinical prediction. In 2013 IEEE China Summit and International Conference on Signal and Information Processing 259–263 (IEEE, 2013).
Yaar, A., Asif, A., Raza, S. E. A., Rajpoot, N. & Minhas, F. Cross-domain knowledge transfer for prediction of chemosensitivity in ovarian cancer patients. In Proceedings of the IEEE/CVF Conference on Computer Vision and Pattern Recognition Workshops 928–929 (IEEE, 2020).
Ghoniem, R. M., Algarni, A. D., Refky, B. & Ewees, A. A. Multi-modal evolutionary deep learning model for ovarian cancer diagnosis. Symmetry 13 , 643 (2021).
Zeng, H., Chen, L., Zhang, M., Luo, Y. & Ma, X. Integration of histopathological images and multi-dimensional omics analyses predicts molecular features and prognosis in high-grade serous ovarian cancer. Gynecol. Oncol. 163 , 171–180 (2021).
Holback, C. et al. The cancer genome atlas ovarian cancer collection (TCGA-OV) (version 4) [data set]. The Cancer Imaging Archive. https://wiki.cancerimagingarchive.net/pages/viewpage.action?pageId=7569497 (2016).
Levine, A. B. et al. Synthesis of diagnostic quality cancer pathology images by generative adversarial networks. J. Pathol. 252 , 178–188 (2020).
Boschman, J. et al. The utility of color normalization for ai-based diagnosis of hematoxylin and eosin-stained pathology images. J. Pathol. 256 , 15–24 (2022).
Farahani, H. et al. Deep learning-based histotype diagnosis of ovarian carcinoma whole-slide pathology images. Mod. Pathol. 35 , 1983–1990 (2022).
BenTaieb, A., Li-Chang, H., Huntsman, D. & Hamarneh, G. Automatic diagnosis of ovarian carcinomas via sparse multiresolution tissue representation. In Medical Image Computing and Computer-Assisted Intervention–MICCAI 2015: 18th International Conference, Munich, Germany, October 5-9, 2015, Proceedings, Part I 18 629–636 (Springer, 2015).
BenTaieb, A., Nosrati, M. S., Li-Chang, H., Huntsman, D. & Hamarneh, G. Clinically-inspired automatic classification of ovarian carcinoma subtypes. J. Pathol. Informatics 7 , 28 (2016).
Jiang, J. et al. Digital pathology-based study of cell- and tissue-level morphologic features in serous borderline ovarian tumor and high-grade serous ovarian cancer. J. Pathol. Informatics 12 , 24 (2021).
Jiang, J. et al. Computational tumor stroma reaction evaluation led to novel prognosis-associated fibrosis and molecular signature discoveries in high-grade serous ovarian carcinoma. Front. Med. 9 , 994467 (2022).
Wang, C.-W. et al. A weakly supervised deep learning method for guiding ovarian cancer treatment and identifying an effective biomarker. Cancers 14 , 1651 (2022).
Wang, C.-W. et al. Weakly supervised deep learning for prediction of treatment effectiveness on ovarian cancer from histopathology images. Comput. Med. Imaging Graphics 99 , 102093 (2022).
Wang, C.-W. et al. Interpretable attention-based deep learning ensemble for personalized ovarian cancer treatment without manual annotations. Comput. Med. Imaging Graphics 107 , 102233 (2023).
Ho, D. J. et al. Deep interactive learning-based ovarian cancer segmentation of h&e-stained whole slide images to study morphological patterns of brca mutation. J. Pathol. Informatics 14 , 100160 (2023).
Paijens, S. T. et al. Prognostic image-based quantification of cd8cd103 t cell subsets in high-grade serous ovarian cancer patients. Oncoimmunology 10 , 1935104 (2021).
Shin, S. J. et al. Style transfer strategy for developing a generalizable deep learning application in digital pathology. Comput. Methods Programs Biomed. 198 , 105815 (2021).
Mayer, R. S. et al. How to learn with intentional mistakes: Noisyensembles to overcome poor tissue quality for deep learning in computational pathology. Front. Med. 9 , 959068 (2022).
Du, Y. et al. Classification of tumor epithelium and stroma by exploiting image features learned by deep convolutional neural networks. Ann. Biomed. Eng. 46 , 1988–1999 (2018).
Hu, Y. et al. Predicting molecular traits from tissue morphology through self-interactive multi-instance learning. In Medical Image Computing and Computer Assisted Intervention–MICCAI 2022: 25th International Conference, Singapore, September 18–22, 2022, Proceedings, Part II 130–139 (Springer, 2022).
Lazard, T. et al. Deep learning identifies morphological patterns of homologous recombination deficiency in luminal breast cancers from whole slide images. Cell Rep. Med. 3 , 100872 (2022).
Yokomizo, R. et al. O3c glass-class: a machine-learning framework for prognostic prediction of ovarian clear-cell carcinoma. Bioinformatics Biol. Insights 16 , 11779322221134312 (2022).
Nero, C. et al. Deep-learning to predict brca mutation and survival from digital H&E slides of epithelial ovarian cancer. Int. J. Mol. Sci. 23 , 11326 (2022).
Wu, M. et al. Exploring prognostic indicators in the pathological images of ovarian cancer based on a deep survival network. Front. Genet. 13 , 1069673 (2023).
Kasture, K. R., Choudhari, D. & Matte, P. N. Prediction and classification of ovarian cancer using enhanced deep convolutional neural network. Int. J. Eng. Trends Technol. 70 , 310–318 (2022).
Kowalski, P. A., Błoniarz, J. & Chmura, Ł. Convolutional neural networks in the ovarian cancer detection. In Computational Intelligence and Mathematics for Tackling Complex Problems 2 55–64 (Springer, 2022).
BenTaieb, A., Li-Chang, H., Huntsman, D. & Hamarneh, G. A structured latent model for ovarian carcinoma subtyping from histopathology slides. Med. Image Anal. 39 , 194–205 (2017).
Dong, J., Li, J., Lu, J. & Fu, A. Automatic segmentation for ovarian cancer immunohistochemical image based on chroma criterion. In 2010 2nd International Conference on Advanced Computer Control , vol. 2 147–150 (IEEE, 2010).
Dong, J., Li, J., Fu, A. & Lv, H. Automatic segmentation for ovarian cancer immunohistochemical image based on YUV color space. In 2010 International Conference on Biomedical Engineering and Computer Science 1–4 (IEEE, 2010).
Signolle, N., Revenu, M., Plancoulaine, B. & Herlin, P. Wavelet-based multiscale texture segmentation: application to stromal compartment characterization on virtual slides. Signal Process. 90 , 2412–2422 (2010).
Janowczyk, A., Chandran, S., Feldman, M. & Madabhushi, A. Local morphologic scale: application to segmenting tumor infiltrating lymphocytes in ovarian cancer tmas. In Medical Imaging 2011: Image Processing, vol. 7962 827–840 (SPIE, 2011).
Janowczyk, A. et al. High-throughput biomarker segmentation on ovarian cancer tissue microarrays via hierarchical normalized cuts. IEEE Trans. Biomed. Eng. 59 , 1250–1252 (2012).
Ramasamy, S. & Kaliyaperumal, V. A hybridized channel selection approach with deep convolutional neural network for effective ovarian cancer prediction in periodic acid-Schiff-stained images. Concurrency Comput. Pract. Exp. 35 , e7568 (2023).
Gentles, L. et al. Integration of computer-aided automated analysis algorithms in the development and validation of immunohistochemistry biomarkers in ovarian cancer. J. Clin. Pathol. 74 , 469–474 (2021).
Laury, A. R., Blom, S., Ropponen, T., Virtanen, A. & Carpén, O. M. Artificial intelligence-based image analysis can predict outcome in high-grade serous carcinoma via histology alone. Sci. Rep. 11 , 19165 (2021).
Heindl, A. et al. Microenvironmental niche divergence shapes BRCA1-dysregulated ovarian cancer morphological plasticity. Nat. Commun. 9 , 3917 (2018).
Ilse, M., Tomczak, J. & Welling, M. Attention-based deep multiple instance learning. In International Conference on Machine Learning 2127–2136 (PMLR, 2018).
Lu, M. Y. et al. Data-efficient and weakly supervised computational pathology on whole-slide images. Nat. Biomed. Eng. 5 , 555–570 (2021).
He, K. et al. Transformers in medical image analysis: a review. Intell. Med. 3 , 59–78 (2022).
Elie, N. et al. Impact of automated methods for quantitative evaluation of immunostaining: towards digital pathology. Front. Oncol. 12 , 931035 (2022).
Shrestha, P. et al. A systematic review on the use of artificial intelligence in gynecologic imaging–background, state of the art, and future directions. Gynecol. Oncol. 166 , 596–605 (2022).
Zhou, J., Cao, W., Wang, L., Pan, Z. & Fu, Y. Application of artificial intelligence in the diagnosis and prognostic prediction of ovarian cancer. Comput. Biol. Med. 146 , 105608 (2022).
Fiste, O., Liontos, M., Zagouri, F., Stamatakos, G. & Dimopoulos, M. A. Machine learning applications in gynecological cancer: a critical review. Crit. Rev. Oncol. Hematol. 179 , 103808 (2022).
Xu, H.-L. et al. Artificial intelligence performance in image-based ovarian cancer identification: a systematic review and meta-analysis. EClinicalMedicine 53 , 101662 (2022).
Lorsakul, A. et al. Automated wholeslide analysis of multiplex-brightfield ihc images for cancer cells and carcinoma-associated fibroblasts. In Medical Imaging 2017: Digital Pathology, vol. 10140 41–46 (SPIE, 2017).
Salguero, J. et al. Selecting training samples for ovarian cancer classification via a semi-supervised clustering approach. In Medical Imaging 2022: Digital and Computational Pathology, vol. 12039 20–24 (SPIE, 2022).
Collins, G. S., Reitsma, J. B., Altman, D. G. & Moons, K. G. Transparent reporting of a multivariable prediction model for individual prognosis or diagnosis (tripod): the tripod statement. Ann. Intern. Med. 162 , 55–63 (2015).
Dehkharghanian, T. et al. Biased data, biased AI: deep networks predict the acquisition site of TCGA images. Diagn. Pathol. 18 , 1–12 (2023).
Dhiman, P. et al. Methodological conduct of prognostic prediction models developed using machine learning in oncology: a systematic review. BMC Med. Res. Methodol. 22 , 101 (2022).
Wolff, R. F. et al. Probast: a tool to assess the risk of bias and applicability of prediction model studies. Ann. Intern. Med. 170 , 51–58 (2019).
Köbel, M. et al. Diagnosis of ovarian carcinoma cell type is highly reproducible: a transcanadian study. Am. J. Surg. Pathol. 34 , 984–993 (2010).
Download references
Acknowledgements
There was no direct funding for this research. J.B. is supported by the UKRI Engineering and Physical Sciences Research Council (EPSRC) [EP/S024336/1]. K.A. and P.A. are supported by the Tony Bramall Charitable Trust. A.S. is supported by Innovate UK via the National Consortium of Intelligent Medical Imaging (NCIMI) [104688], Cancer Research UK [C19942/A28832] and Leeds Hospitals Charity [9R01/1403]. The funders had no role in influencing the content of this research. For the purpose of open access, the author has applied a Creative Commons Attribution (CC BY) licence to any Author Accepted Manuscript version arising from this submission.
Author information
These authors jointly supervised this work: Nicolas M. Orsi, Nishant Ravikumar.
Authors and Affiliations
Centre for Computational Imaging and Simulation Technologies in Biomedicine (CISTIB), School of Computing, University of Leeds, Leeds, UK
Jack Breen & Nishant Ravikumar
Leeds Institute of Medical Research at St James’s, School of Medicine, University of Leeds, Leeds, UK
Katie Allen, Pratik Adusumilli, Andrew Scarsbrook & Nicolas M. Orsi
Leeds Cancer Centre, St James’s University Hospital, Leeds, UK
Kieran Zucker & Geoff Hall
Department of Radiology, St James’s University Hospital, Leeds, UK
Pratik Adusumilli & Andrew Scarsbrook
You can also search for this author in PubMed Google Scholar
Contributions
J.B. created the study protocol with feedback and contributions from all other authors. J.B., K.A., K.Z., N.M.O., and N.R. performed the risk of bias assessments. J.B. and K.A. performed data extraction. J.B. analysed extracted data and wrote the manuscript, with feedback and contributions from all other authors.
Corresponding author
Correspondence to Jack Breen .
Ethics declarations
Competing interests.
G.H. receives research funding from IQVIA. N.M.O. receives research funding from 4D Path. The other authors declare no competing interests.
Additional information
Publisher’s note Springer Nature remains neutral with regard to jurisdictional claims in published maps and institutional affiliations.
Supplementary information
Supplemental material, rights and permissions.
Open Access This article is licensed under a Creative Commons Attribution 4.0 International License, which permits use, sharing, adaptation, distribution and reproduction in any medium or format, as long as you give appropriate credit to the original author(s) and the source, provide a link to the Creative Commons license, and indicate if changes were made. The images or other third party material in this article are included in the article’s Creative Commons license, unless indicated otherwise in a credit line to the material. If material is not included in the article’s Creative Commons license and your intended use is not permitted by statutory regulation or exceeds the permitted use, you will need to obtain permission directly from the copyright holder. To view a copy of this license, visit http://creativecommons.org/licenses/by/4.0/ .
Reprints and permissions
About this article
Cite this article.
Breen, J., Allen, K., Zucker, K. et al. Artificial intelligence in ovarian cancer histopathology: a systematic review. npj Precis. Onc. 7 , 83 (2023). https://doi.org/10.1038/s41698-023-00432-6
Download citation
Received : 31 March 2023
Accepted : 01 August 2023
Published : 31 August 2023
DOI : https://doi.org/10.1038/s41698-023-00432-6
Share this article
Anyone you share the following link with will be able to read this content:
Sorry, a shareable link is not currently available for this article.
Provided by the Springer Nature SharedIt content-sharing initiative
Quick links
- Explore articles by subject
- Guide to authors
- Editorial policies
Sign up for the Nature Briefing: Cancer newsletter — what matters in cancer research, free to your inbox weekly.

- Open access
- Published: 24 January 2023
Ovulation induction drug and ovarian cancer: an updated systematic review and meta-analysis
- Liang Yu 1 na1 ,
- Jiafan Sun 1 na1 ,
- Qiqin Wang 1 na1 ,
- Wennian Yu 1 na1 ,
- Anqi Wang 1 ,
- Shu Zhu 2 ,
- Wei Xu 2 &
- Xiuli Wang 2 , 3
Journal of Ovarian Research volume 16 , Article number: 22 ( 2023 ) Cite this article
2875 Accesses
1 Citations
8 Altmetric
Metrics details
To explore the association between ovulation induction drugs and ovarian cancer.
Systematic review and meta-analysis.
Not applicable.
Women without ovarian cancer who ever or never underwent ovarian induction.
Intervention(s)
An extensive electronic search of the following databases was performed: PubMed, EMBASE, MEDLINE, Google Scholar, Cochrane Library and CNKI, from inception until January 2022. A total of 34 studies fulfilled our inclusion criteria and were included in the final meta-analysis. The odds ratio (OR) and random-effects model were used to estimate the pooled effects. The Newcastle-Ottawa Scale was used to assess the quality of included studies. Funnel plots and Egger tests were used to assess publication bias.
Main outcomes
New diagnosed borderline ovarian tumor (BOT) and invasive ovarian cancer (IOC) between ovulation induction (OI) group and control (CT) group considering fertility outcome, OI cycles and specific OI drugs.
Primarily, there was no significant difference in the incidence of IOC and BOT between the OI and CT groups. Secondly, OI treatment did not increase the risk of IOC and BOT in the multiparous women, nor did it increase the risk of IOC in the nulliparous women. However, the risk of BOT appeared to be higher in nulliparous women treated with OI treatment. Thirdly, among women exposed to OI, the risk of IOC and BOT was higher in nulliparous women than in multiparous women. Fourthly, the risk of IOC did not increase with increasing OI cycles. Lastly, exposure to specific OI drugs also did not contribute to the risk of IOC and BOT.
Overall, OI treatment did not increase the risk of IOC and BOT in most women, regardless of OI drug type and OI cycle. However, nulliparous women treated with OI showed a higher risk of ovarian cancer, necessitating their rigorous monitoring and ongoing follow-up.
Introduction
Infertility affects more than 48.5 million couples worldwide [ 1 , 2 , 3 ]. It is emerging as a public health problem, driving the demand for assisted reproductive treatment [ 4 ]. Ovulation induction (OI) is a process in which the ovaries are drugged to stimulate the production of many follicles containing eggs, which usually begins early in the menstrual cycle. OI treatment is highly desirable, especially for isolated anovulatory infertility [ 5 ]. OI treatment is associated with ovarian hyper-stimulation and multiple follicular ovulations. As we know, ovulation is a common injurious process associated with an inflammatory response and destruction of ovarian epithelial cells [ 6 , 7 ]. According to the incessant ovulation and gonadotropin hypothesis, high levels of gonadotropin and excessive ovulation may engage patients into repeated cycles of injury, inducing inflammation and regeneration, which could potentially increase the risk of ovarian cancer by inducing somatic cell mutations [ 8 , 9 , 10 ]. Previous studies have debated whether OI could increase the risk of invasive ovarian cancer (IOC) and borderline ovarian tumors (BOT) [ 11 , 12 ]. Although most studies have concluded that OI does not contribute to the risk of IOC and BOT, some scholars still proposed that OI may be associated with them. Therefore, we performed this updated systematic review and meta-analysis to find out whether exposure to OI treatment significantly increases the risk of IOC and BOT.
Materials and methods
Search strategy.
The PRISMA guidelines were used for this study. A systematic literature search was then conducted in PubMed, EMBASE, MEDLINE, Google Scholar, Cochrane Library and CNKI, which included records up to January 2022. The main keywords included the following domains of Medical Subject Heading terms: “ ovulation induction “ and “ ovarian cancer “. The retrieval strategy adopted the combination of subject terms and free words. These terms were then combined with “AND” or “OR”. Also, to broaden the search, review articles were used to ensure that all relevant citations were identified and imported.
Study screening
Two independent researchers (YL and WQQ) simultaneously screened the titles, abstracts and full text of the literature according to the inclusion and exclusion criteria. Any disagreements were discussed and solved by consensus or third-party arbitration (ZS). The inclusion criteria were as follows: (1) Cohort studies and case-control studies with adequate samples; (2) Exposure to ovulation induction drugs such as clomiphene citrate (CC), gonadotrophin (GDT) and gonadotropin-releasing hormone analogs (GnRH-a); (3) Follow-up in the cohort study was sufficiently long to demonstrate treatment differences; (4) The study had a clear description of the exposure to OI drugs and essential information about enrolled patients;(5) The type of cancer included borderline ovarian tumor (BOT) or invasive ovarian cancer (IOC). The exclusion criteria were as follows: (1) Non-English or Non-Chinese literature; (2) Non-human studies; (3) Literature with incomplete data; (4) Duplicate and inaccessible literature.
Data extraction
Two independent researchers (YL and SJF) performed the data extraction after viewing the complete manuscripts of the eligible literature. Relevant data was input into separate spreadsheets and then cross-checked by each researcher to maintain the quality of the data. The data of bibliography (year and author), study design (sample size, study type, study duration and study location), outcome measures (cancer type and incidence of individual ovarian cancers in group) and other endpoint evaluation (fertility outcome, OI drug type and OI cycles) were extracted from each study. If necessary, discussions with the third-party arbitration (XW) would solve all disputes.
Quality evaluation
Two researchers (YL and YWN) independently assessed the quality of the literature by using the NOS scale (Newcastle-Ottawa Scale). The main components of the NOS scale included: patient selection, intergroup comparability and outcome measurement [ 13 ]. Disagreements were solved by consensus or third-party arbitration (WXL) when they appeared. A total score of more than 6 was considered to be of satisfactory quality [ 14 ].
Statistical analysis
Data aggregation and basic meta-analysis.
The meta-analysis was performed by using STATA 12.0. Binary variables were evaluated by odds ratio (OR) and its 95% confidence interval (95% CI). P < 0.05 was regarded as statistically significant.
Depending on heterogeneity, the appropriate model (random or fixed) was then selected to merge the outcome indicators [ 15 ]. The I 2 value less than 50% were deemed to be low heterogeneity, 51–75% were deemed to be moderate heterogeneity, and greater than 75% were deemed to be high heterogeneity [ 16 ]. If the I 2 value exceeded 50%, the random-effect model was chosen. Otherwise, if the I 2 value was less than 50%, both the random effects and fixed effects models were acceptable [ 17 ].
Assessment of publication bias
In principle, funnel plot analyses were performed to accompany meta-analyses involving more 10 studies and to judge the publication bias [ 18 ]. If there was no significant publication bias, the funnel plot was supposed to be symmetrical. A complementary approach for funnel plots was to perform Egger’s test to objectively measure bias [ 19 ].
Details of ethical approval
This meta-analysis was based on the data from published articles and independent of any patient participation. As such, institutional review board (IRB) approval was not required.
Study characteristics and quality evaluation
A flowchart detailing the process of identification and inclusion for the target literature was shown in Supplemental Material Fig. 1 . Three hundred seven articles were included in the initial screening phase. Of these articles, 42 articles met the criteria for full-text review. Finally, a total of 34 articles were included in the meta-analysis, 14 of which were case-control studies and 20 of which were cohort studies. The final meta-analysis included a total of 3,643,303 participants. All the included literature was of adequate quality. The quality evaluation of the included literature was presented in Supplemental Material Table S1 .
Part I: the risk of ovarian cancer between OI and CT group
Of the 34 studies, 12 reported BOT [ 12 , 20 , 21 , 22 , 23 , 24 , 25 , 26 , 27 , 28 , 29 , 30 ] and 30 reported IOC [ 11 , 12 , 20 , 21 , 23 , 24 , 25 , 26 , 29 , 31 , 32 , 33 , 34 , 35 , 36 , 37 , 38 , 39 , 40 , 41 , 42 , 43 , 44 , 45 , 46 , 47 , 48 , 49 , 50 , 51 ]. Basic information of the included studies was given in Supplemental Material Table S2 . For further study, we conducted subgroup analyses to assess the risk of IOC and BOT between groups according to study type.
The cancer risk between groups in case-control study
In the subgroup analysis of case-control studies, 12 studies reported IOC [ 11 , 23 , 29 , 32 , 36 , 37 , 38 , 39 , 40 , 41 , 42 , 43 ] and 5 studies reported BOT [ 23 , 27 , 28 , 29 , 30 ]. Among these studies, only 1 study showed a significantly higher risk of IOC in the OI group than in the CT group [ 11 ] and 3 studies showed a higher risk of BOT in the OI group than in the CT group [ 28 , 29 , 30 ]. Pooled result indicated that the risk of IOC (OR = 1.09, 95%CI: 0.88–1.35, I 2 = 54.9%, Table 1 , Fig. 1 A) and BOT (OR = 1.90, 95%CI: 0.89–4.09, I 2 = 73.4%, Table 1 , Fig. 1 B) did not show significant difference between groups.
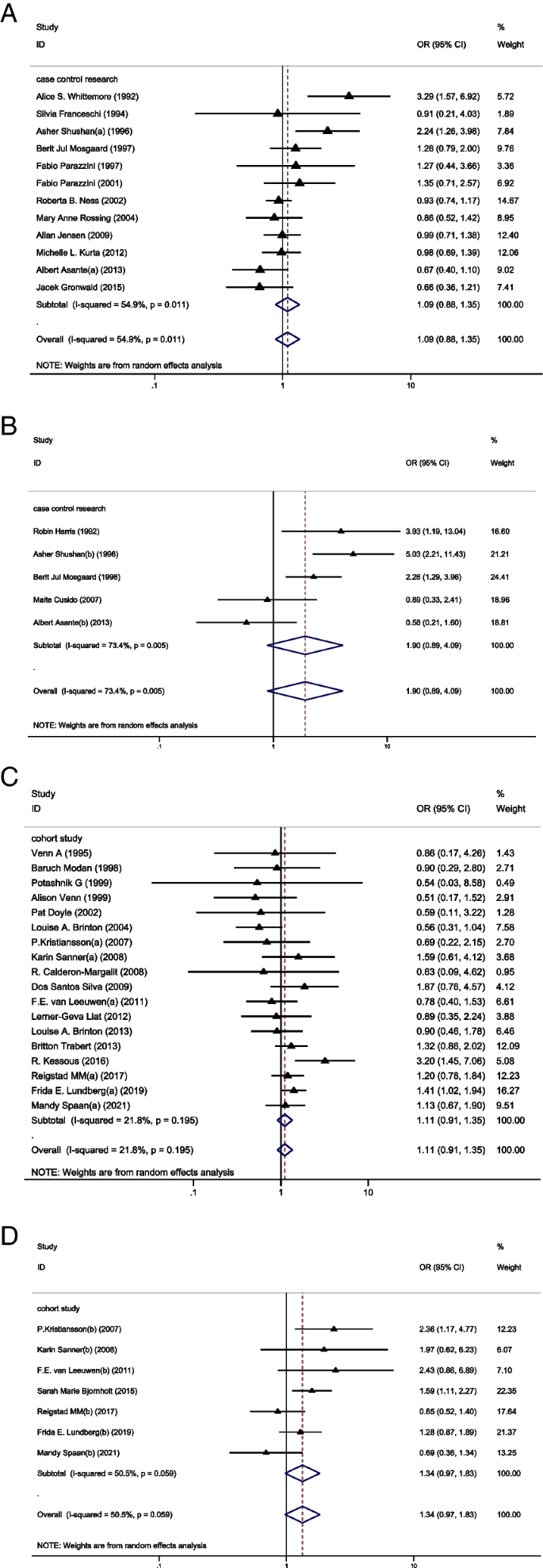
A Forest plot of IOC risk between OI group and CT group based on case-control studies; B Forest plot of IOC risk between OI group and CT group based on cohort studies; C Forest plot of BOT risk between OI group and CT group based on case-control studies; D Forest plot of BOT risk between OI group and CT group based on cohort studies
The cancer risk between groups in cohort study
In the subgroup analysis of cohort studies, 18 studies reported IOC [ 12 , 20 , 21 , 24 , 25 , 26 , 31 , 33 , 34 , 35 , 44 , 45 , 46 , 47 , 48 , 49 , 50 , 51 ] and 7 studies reported BOT [ 12 , 20 , 21 , 22 , 24 , 25 , 26 ]. Of these studies, 3 studies showed a higher risk of IOC [ 12 , 21 , 31 ] in the OI group than in the CT group and 3 studied showed a higher risk of BOT in the OI group than in the CT group [ 21 , 24 , 25 ]. Again, the results showed no significant difference between groups in the incidence of IOC (OR = 1.11, 95%CI: 0.91–1.35, I 2 = 21.8%, Table 1 , Fig. 1 C) and BOT (OR = 1.34, 95%CI: 0.97–1.83, I 2 = 50.5%, Table 1 , Fig. 1 D).
Part II: the incidence of ovarian cancer between OI and CT group according to fertility outcome
In this section, we sought to find out whether the multiparous and nulliparous women treated with OI presented an increased risk of ovarian tumors when compared to those who had not been treated with OI. Relevant data were presented in Supplemental Material Table S3 .
The cancer risk between groups in multiparous women
Firstly, 10 studies of IOC [ 11 , 12 , 34 , 36 , 37 , 38 , 40 , 41 , 42 , 50 ] and 3 studies of BOT [ 12 , 22 , 28 ] analyzed the risk of ovarian cancer in multiparous women with or without OI treatment. None of these studies demonstrated a higher risk for IOC and BOT in the OI group. Pooled result remained consistent, indicating that OI treatment did not increase the risk of IOC (OR = 0.83, 95%CI: 0.65–1.05, I 2 = 21.3%, Table 1 , Fig. 2 A) and BOT (OR = 1.17, 95%CI: 0.55–2.48, I 2 = 73.5%, Table 1 , Fig. 2 B) in multiparous women.
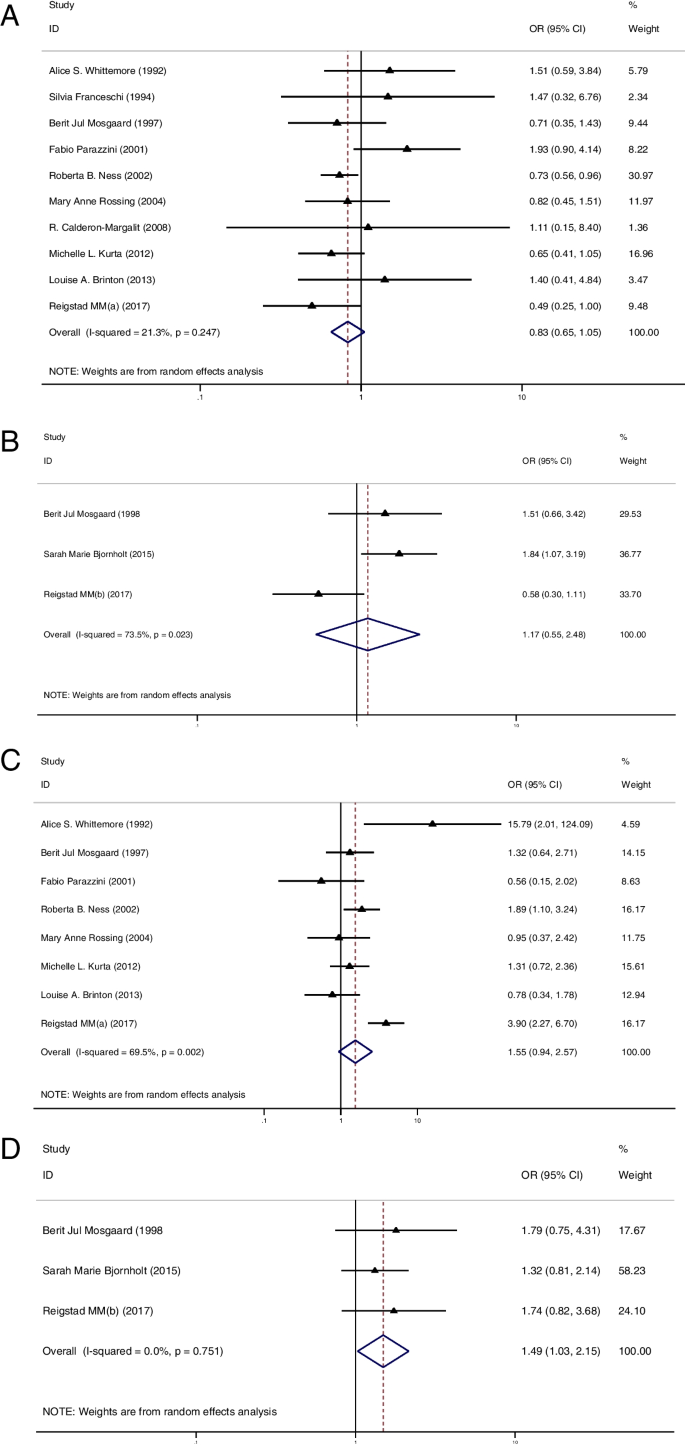
A Forest plot of IOC risk between OI group and CT group in multiparous women; B Forest plot of BOT risk between OI group and CT group in multiparous women; C Forest plot of IOC risk between OI group and CT group in nulliparous women; D Forest plot of BOT risk between OI group and CT group in nulliparous women
The cancer risk between groups in nulliparous women
In the second part, 8 studies of IOC [ 11 , 12 , 34 , 36 , 38 , 40 , 41 , 42 ] and 3 studies of BOT [ 12 , 22 , 28 ] reported the risk of ovarian cancer in nulliparous women with or without OI treatment. Of these studies, only 1 study showed a significantly higher risk of IOC in the OI group than in the CT group [ 11 ]. The summarized result for IOC showed no difference in cancer risk between groups (OR = 1.55, 95%CI: 0.94–2.57, I 2 = 69.5%, Table 1 , Fig. 2 C). Additionally, none of these studies reported a higher risk of BOT in the OI group. However, after pooled analysis, the risk of BOT appeared to be higher in nulliparous women treated with OI than in those nulliparous women who had not been treated with OI (OR = 1.49, 95%CI: 1.03–2.15, I 2 = 0%, Table 1 , Fig. 2 D).
Part III: the risk of ovarian cancer between the multiparous and nulliparous women in OI group
In this chapter, we attempted to figure out the differences in cancer risk between the multiparous and nulliparous woman in the OI group. Relevant data were presented in Supplemental Material Table S4 . In total, 8 studies of IOC [ 11 , 12 , 34 , 36 , 38 , 40 , 41 , 42 ] and 3 studies of BOT [ 12 , 22 , 28 ] reported on the risk of ovarian cancer in the nulliparous and multiparous women treated with OI. The summarized results showed a significantly higher risk of IOC (OR = 3.35, 95%CI: 2.10–5.34, I 2 = 52.2%, Table 1 , Fig. 3 A) and BOT (OR = 2.58, 95%CI: 1.76–3.79, I 2 = 0%, Table 1 , Fig. 3 B) in the nulliparous women treated with OI than in those multiparous women treated with OI.
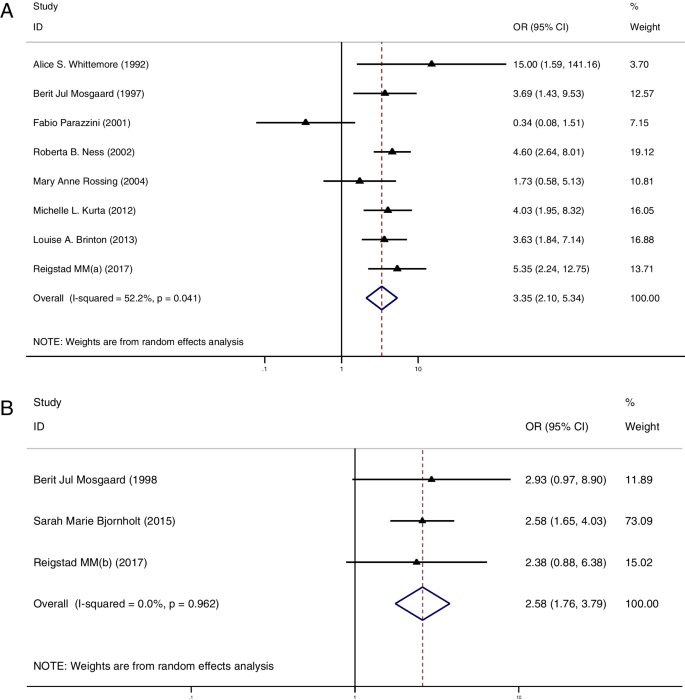
A Forest plot of IOC risk between nulliparous and multiparous women with OI treatment; B Forest plot of BOT risk between nulliparous and multiparous women with OI treatment
Part IV: the relationship between number of OI cycles and cancer risk
Then, we tried to find out whether cancer risk increased with more OI cycles. Totally, 8 studies provided relevant data for IOC [ 20 , 24 , 25 , 36 , 39 , 41 , 42 , 46 , 47 ]. Regrettably, data for BOT were not available for meta-analysis. Relevant data were presented in Supplemental Material Table S5 .
Using a cut-off of 3 cycles, we did not find a higher cancer risk in those women who received less than 3 cycles when compared to the CT group (OR = 1.05, 95%CI: 0.72–1.52, I 2 = 42.9%, Table 1 , Fig. 4 A). Meanwhile, we found a similar result in those women who received more than 3 cycles (OR = 0.98, 95%CI: 0.79–1.22, I 2 = 0%, Table 1 , Fig. 4 A). Using 6 cycles as a cut-off, those women who received less than 6 cycles did not present an increased cancer risk when compared to the CT group (OR = 0.85, 95%CI: 0.64–1.12, I 2 = 0%, Table 1 , Fig. 4 B) and a similar result was found in those women who received more than 6 OI cycles (OR = 0.88, 95%CI: 0.59–1.31, I 2 = 0%, Table 1 , Fig. 4 B). Lastly, using 12 cycles as a cut-off, we did not find a significantly increased cancer risk in those women who received less than 12 cycles when compared to the CT group (OR = 0.87, 95%CI: 0.69–1.10, I 2 = 0%, Table 1 , Fig. 4 C). Also, a similar result was found in those women who received more than 12 OI cycles (OR = 0.78, 95%CI: 0.49–1.22, I 2 = 0%, Table 1 , Fig. 4 C).
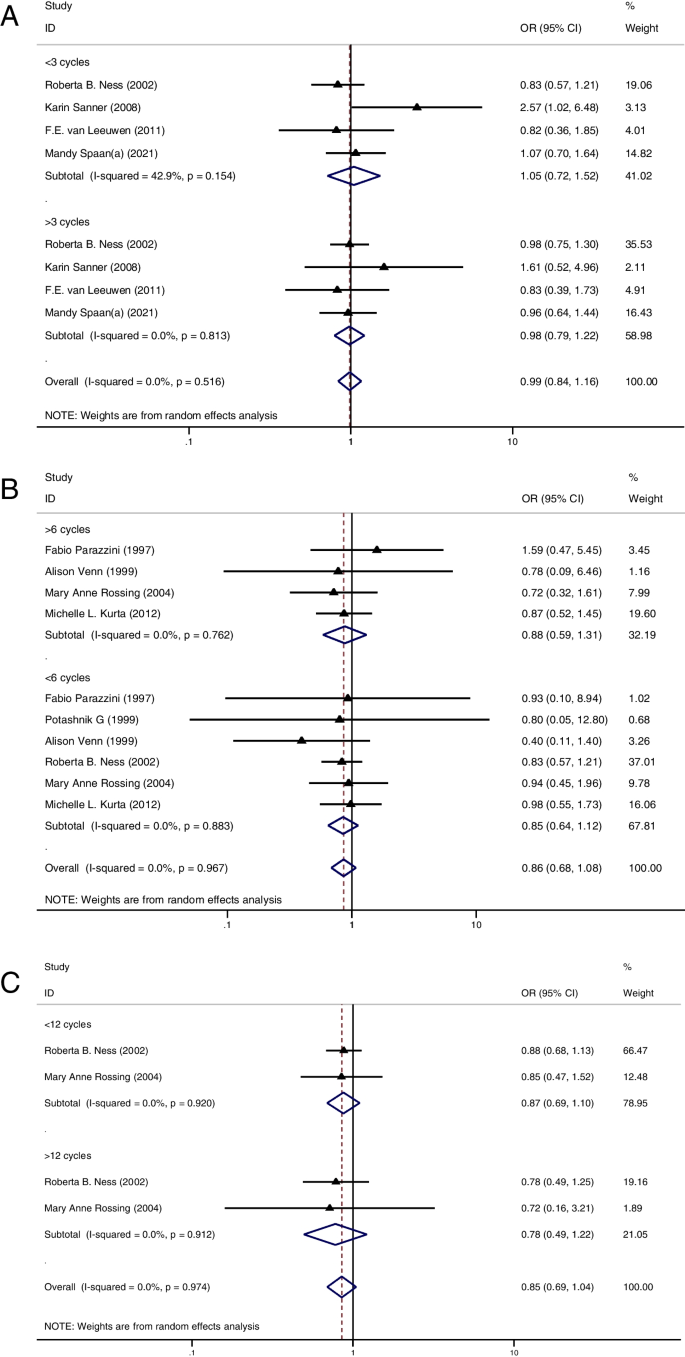
A Forest plot of IOC risk between OI group and CT group based on a cut-off value of 3 cycles; B Forest plot of IOC risk between OI group and CT group based on a cut-off value of 6 cycles; A Forest plot of IOC risk between OI group and CT group based on a cut-off value of 12 cycles
Part V: the relationship between specific OI treatment and cancer risk
At last, we wished to find out whether specific OI drugs were associated with an increased cancer risk. For further study, we divided the subjects into three groups according to the type of OI drug. These were the clomiphene citrate group (CC), the gonadotrophin group (GDT) and the gonadotropin-releasing hormone analog group (GnRH-a). Relevant data were provided in Supplemental Material Table S6 .
The relationship between CC and cancer risk
We firstly analyzed the relationship between CC and cancer risk. This part of analysis included 17 studies of IOC [ 25 , 29 , 35 , 36 , 38 , 41 , 42 , 43 , 45 , 46 , 47 , 50 , 51 ] and 7 studies of BOT [ 12 , 20 , 22 , 25 , 27 , 28 , 29 ]. Only 1 study reported a higher cancer risk in the CC group than in the CT group [ 12 ]. However, pooled results showed that the risk of IOC (OR = 1.01, 95%CI: 0.88–1.17, I 2 = 0%, Table 1 , Fig. 5 A) and BOT (OR = 1.32, 95%CI: 0.79–2.21, I 2 = 72.6%, Table 1 , Fig. 5 A) were not significantly higher in the CC group when compared to the CT group.
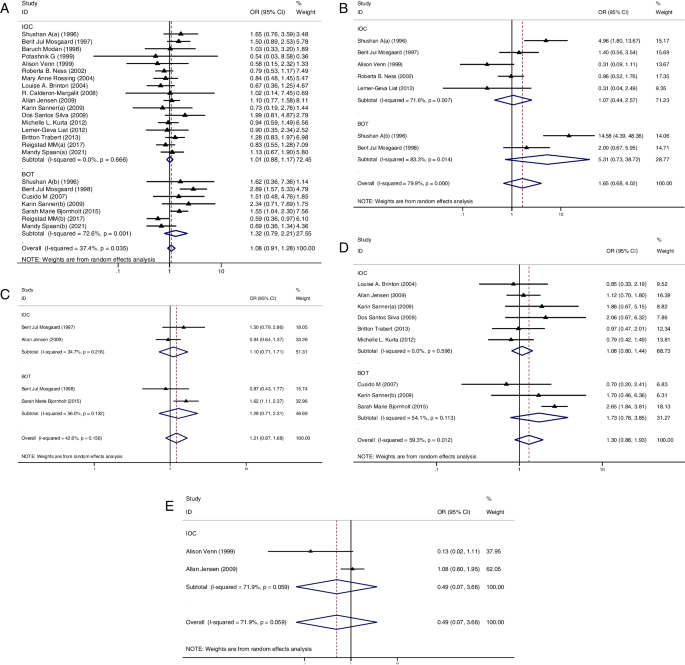
A Forest plot of IOC and BOT risk between CC group and CT group; B Forest plot of IOC and BOT risk between HMG group and CT group; C Forest plot of IOC and BOT risk between HCG group and CT group; D Forest plot of IOC and BOT risk between GDT group and CT group; E Forest plot of IOC risk between GnRH group and CT group
The relationship between GDT and cancer risk
Secondarily, we focused our attention on GDT and performed a subgroup analysis in this section. GDTs mainly consisted of human menopausal gonadotropin (HMG) and human chorionic gonadotropin (HCG). However, some studies did not further categorized GDT. For IOC, there were 5 studies of HMG [ 29 , 35 , 38 , 41 , 47 ], 2 studies of HCG [ 38 , 43 ] and 6 studies of unclassified GDT [ 25 , 33 , 36 , 43 , 49 , 51 ]. Only 1 study reported a higher cancer risk in HMG group than in the CT group [ 29 ]. Nevertheless, pooled results indicated that HMG (OR = 1.07, 95%CI: 0.44–2.57, I 2 = 71.6%, Table 1 , Fig. 5 B), HCG (OR = 1.10, 95%CI: 0.71–1.71, I 2 = 34.7%, Table 1 , Fig. 5 C) and unclassified GDT (OR = 1.08, 95%CI: 0.80–1.44, I 2 = 0%, Table 1 , Fig. 5 D) did not increase the cancer risk.
While for BOT, 2 studies of HMG [ 28 , 29 ], 2 studies of HCG [ 22 ] and 3 studies of GDT [ 22 , 25 , 27 ] were included in this part of analysis. Consistently, we found similar results that HMG (OR = 5.31, 95%CI: 0.73–38.72, I 2 = 83.3%, Table 1 , Fig. 5 B), HCG (OR = 1.28, 95%CI: 0.71–2.31, I 2 = 56%, Table 1 , Fig. 5 C) and unclassified GDT (OR = 1.73, 95%CI: 0.88–1.93, I 2 = 54.1%, Table 1 , Fig. 5 D) did not increase tumor risk.
The relationship between GnRH-a and cancer risk
Thirdly, we only found 2 studies which provided analyzable data on the relationship between the risk of IOC and GnRH-a [ 43 , 47 ]. Along the same lines, we did not find an increased risk of IOC (OR = 0.49, 95%CI: 0.07–3.66, I 2 = 71.9%, Table 1 , Fig. 5 E) in the GnRH-a group when compared to the CT group. However, it was regrettable that another meta-analysis focusing on the relationship between the risk of BOT and GnRH-a could not be performed due to lack of data.
Publication bias
In our analysis, funnel plot analysis and Egger regression analysis were performed to judge the publication bias of the included studies. Neither the funnel plot (Supplemental Material Fig. 2 A) nor the Egger test showed evidence of publication bias in our analysis (Supplemental Material Fig. 2 B).
The following points are currently discussed regarding the possible induction of ovarian cancer with the use of OI drugs: (1) the incessant ovulation hypothesis stated that ovarian epithelium could be destroyed and repaired during uninterrupted ovulation. When a sufficient amount of damage is caused, malignant transformation of ovarian epithelial cells will be triggered [ 8 ]. Furthermore, cancer risk had been found to decrease with increasing numbers of pregnancies and live births, longer duration of breastfeeding and use of oral contraceptives [ 52 , 53 , 54 , 55 , 56 , 57 ]. The effects of these anovulation factors confirmed the above observations. Thus, it is thought that the number of ovulatory cycles during the lifetime was associated with ovarian cancer risk, this finding has been observed in several animal models and epidemiological studies [ 58 , 59 , 60 , 61 , 62 ]. (2) The gonadotropin hypothesis suggested that excess gonadotropins could hyper-stimulate the ovaries and induce estrogen production. The amount of estrogen secreted in one gonadotropin-stimulated cycle was equivalent to the total production of natural cycles over a two-year period [ 63 ]. Meanwhile, there appeared to be growing evidence that estrogen conferred increased ovarian cancer risk [ 64 , 65 , 66 ]. Thus, gonadotropin-induced elevated estrogen levels might promote the malignant transformation of normal ovarian epithelium [ 63 , 67 ]. The above hypotheses had been tested in hen models but not in humans [ 68 , 69 ]. Therefore, speculation regarding the relationship between the use of ovulation induction drug use and ovarian cancer development continues.
Research in this area was based on cohort studies, case series and case-control studies. And, there were still no randomized controlled trials regarding the relationship between ovulation inducing drugs and ovarian cancer due to ethical issues, the relatively low incidence of ovarian cancer and recall bias after ovulation induction [ 70 ]. This updated systematic review and meta-analysis was based on cohort studies and case-control studies. Some of included studies provided supportive evidence that OI treatment might increase the risk of IOC. However, according to subgroup analysis based on study type, we found no convincing evidence that OI treatment could induce an increased risk of IOC. Compared with previous systematic reviews [ 71 , 72 ], we expanded our search to include more recent studies in our analysis and obtained consistent results.
BOTs are morphologically similar to IOCs and follow a similar pathogenesis [ 73 , 74 ]. As the etiology of BOT was still unknown, it was difficult to explain the possible causal relationship between infertility and OI drugs. In our analysis, no significant increased risk of BOT was found following OI treatment, which appeared to contradict the increasing risk of BOT reported by Barcroft et.al (OR = 1.69, 95%CI: 1.27–2.25). With more studies included in our pooled analysis, we used further subgroup analyses based on study type to circumvent the heterogeneity issues caused by retrospective studies. Ultimately, the results of subgroup analysis were highly consistent in that OI treatment did not increase the risk of BOT.
In addition, during the review of the literature, we found several studies supporting that OI treatment could induce an increased risk of ovarian cancer in the nulliparous women [ 11 , 12 ]. A cumulative analysis conducted by Whittemore et.al indicated an increased risk of ovarian cancer (OR = 27.0, 95%CI: 2.3–315.6) in the nulliparous women who ever received OI treatment. Reigstad et.al also noted a greater increased risk of ovarian cancer (HR 2.49, 95% CI 1.30 to 4.78) in the nulliparous women treated with OI. As we know, parity was known as an established protective factor for ovarian cancer [ 75 ]. Previous studies have shown that the greatest reduction in ovarian cancer risk was associated with the first pregnancy and each subsequent pregnancy could also reduce the risk of ovarian cancer [ 11 , 75 , 76 ]. This protective mechanism has been attributed to anovulation, reduced gonadotropin production and increased progesterone levels [ 77 ]. Hence, whether OI treatment would increase the ovarian cancer risk in nulliparous and multiparous women remained controversial. Therefore, we conducted supplementary analyses based on fertility outcomes in light of the above questions. Among the multiparous women, we did not find a higher risk of IOC and BOT in the OI group than in the CT group. Similarly, among the nulliparous women, OI treatment also did not increase the risk of IOC. However, an increased risk of BOT was found in the nulliparous women treated with OI when compared to those nulliparous women who had not been treated with OI. Nonetheless, none of these included studies initially reported a higher risk of BOT in nulliparous women treated with OI. Based on a review of the included studies, this finding might be due to a lack of ovulatory pause caused by pregnancy and exposure to ovarian hyper-stimulation [ 8 , 9 , 10 , 12 ]. Notably, the BOTs were generally seen in younger women [ 78 , 79 , 80 , 81 ]. Hence, the above association might also be due to a diagnostic bias occurring in young nulliparous women who might pursue medical attention and undergo intensive monitoring [ 41 ].
Rodriguez et.al previously found that infertility itself might increase ovarian cancer risk without concomitant exposure to OI drugs [ 82 ]. A current meta-analysis based on nine prospective cohort studies also suggested that infertility in women was associated with an increased risk of ovarian cancer [ 83 ]. Moreover, a number of diseases that cause infertility, including polycystic ovary syndrome (PCOS) and endometriosis, had been found to be associated with ovarian cancer development. Previous studies had indicated that the genetic and epigenetic profile of patients with PCOS was similar to that of ovarian cancer [ 84 ]. Further, the risk of ovarian cancer, particularly serous borderline ovarian tumor, was shown to be increased in patients with PCOS [ 85 , 86 , 87 ]. We also found that ovarian clear cell carcinoma and endometrioid carcinoma were most often associated with ovarian endometriosis in previous studies [ 88 , 89 ]. Thus, infertility itself might be an independent risk factor for ovarian cancer [ 90 ]. In parallel, whether there existed a difference in cancer risk between nulliparous and multiparous women treated with OI was under discussion, as it was difficult to separate OI treatment from infertility as a risk factor for ovarian cancer. In our analysis, we used OI exposure as a control variable to evaluate the relationship between infertility and ovarian cancer and found that the nulliparous women treated with OI showed a higher risk of IOC and BOT than those multiparous women treated with OI. Nieto et.al performed a retrospective study of ovarian cancer in first-degree relatives of infertile patients and showed an increased risk of ovarian cancer in infertile patients who failed to conceive despite receiving OI treatment, which supported our findings [ 91 ]. Rizzuto et.al also noted that the risk of BOT was slightly higher in nulliparous women treated with OI than in multiparous women [ 72 ]. In summary, we believed that there was a necessity to conduct a rigorous medical follow-up in those nulliparous patients treated with OI. Consistent with previous studies, the vast majority of patients were found within 5 years after ovulation induction [ 92 , 93 , 94 , 95 , 96 , 97 , 98 ]. In our analysis, the included cohort studies had a follow-up period of more than 5 years, which in our opinion is sufficient to detect ovarian cancer. Therefore, follow-up periods longer than 5 years should be considered.
Indeed, it would be arbitrary to diagnose the relationship between OI and ovarian cancer solely based on the history of OI exposure. According to incessant ovulation hypothesis, more ovulatory cycles appeared to be associated with a higher risk of developing ovarian cancer [ 75 , 99 , 100 ]. Whether such a cumulative effect exists remained controversial. After reviewing previous studies, we found no meta-analysis reported an association between OI cycles and the risk of ovarian cancer. Thereby, we performed a further subgroup analysis based on OI cycles, which was the focal point of our analysis. In our analysis, we used 3, 6 and 12 OI cycles as cut-off points, respectively. Compared to the control population, we found no correlation between increasing OI cycles and increased cancer risk. Unfortunately, the data for BOT in this aspect were not available for meta-analysis.
In accession, several studies had reported the risk of individual ovarian cancers due to specific OI drug exposure [ 12 , 29 , 101 ]. Consequently, for further study, we performed subgroup analyses according to the type of OI drug to assess whether specific OI drugs would increase the risk of ovarian cancer. CC was the most common drug to induce ovulation, especially in patients with ovulatory disturbances [ 102 ]. Reigstad et.al reported an increased risk of cancer in nulliparous women exposed to CC (HR = 2.5, 95%CI: 1.3–4.8). Rossing et.al also reported an increased ovarian tumor risk in women exposed to CC (SIR = 2.5, 95%CI: 1.3–4.5). A current meta-analysis conducted by Barcroft et.al supported the view mentioned above, which concluded that the exposure to CC was associated with a significant increased cancer risk (OR = 1.40, 95%CI: 1.10–1.77). However, in our meta- analysis, we included additional studies but did not find an increased cancer risk in those women exposed to CC. GDTs were also commonly used in women with proven hypopituitarism and in women who were not sensitive to CC [ 103 , 104 ]. Shan et.al reported a slight increased ovarian cancer risk in women exposed to HMG (OR = 3.95, 95%CI: 1.3–12.2). While in our study, we found that GDTs were not associated with an increased risk of IOC and BOT. GnRH-a was introduced in anovulatory women, which could reproduce spontaneous menstrual cycle and induce ovulation [ 105 , 106 ]. Our findings indicated that GnRH-a did not increase the risk of IOC. Due to the lack of the data on BOT risk in women exposed to GnRH-a, further meta-analysis could not be performed. In summary, CC, GDT and GnRH-a were proven to be safe for OI treatment without increasing ovarian tumor risk.
Most of our findings were generally consistent with previous studies on this topic [ 71 , 72 , 107 , 108 ]. A new study was included in this latest update of the systematic review and meta-analysis compared to previous studies in this area. This study provides new data on the risk of BOT and IOC to CC exposure. To assess the impact of the latest studies on the outcome of this update, an additional sensitivity analysis was conducted. The sensitivity analysis without the latest study did not change the results that exposure to CC did not increase the risk of IOC (OR = 1.05, 95%CI: 0.89–1.21) and BOT (OR = 1.73, 95%CI: 0.96–2.50). And this latest study made the results more reliable. The results of the sensitivity analysis were shown in Supplemental Material Table S7 . To summarize, OI treatment was relatively safe and cancer risk was not increased more cycles of OI and specific OI drugs. However, for those nulliparous women treated with OI, they appeared to have a higher tumor risk. Therefore, rigorous monitoring and sufficiently long follow-up were necessary for these women.
Strengths and limitations of the study
This study included 34 studies from around the world and provided an up-to-date meta-analysis to explore the potential impact of OI treatment on ovarian cancer risk. The inclusion and exclusion criteria for this systematic review and meta-analysis had been made more rigorous. In addition, the included studies were updated and the process of meta-analysis was made more rigorous. In our analysis, lessons learned from previous studies were incorporated and further subgroup analyses were conducted based on study type, tumor type, parity, OI cycle and specific OI drugs. Of note, this meta-analysis was the first study to evaluate the relationship between the OI cycles and ovarian cancer.
However, our study still had some objective shortcomings. Firstly, further work should focus more attention on patient demographics and specific data including drug combinations, cycles of use, use dosage and administration methods. Secondly, loss of follow-up existed in included studies in our analysis and retrospective studies were always considered to be lower quality evidence due to the presence of recall bias. We needed more large and long-term prospective cohort studies with careful follow-up. Thus, follow-up process needed to be improved. Last but not least, the formation of symbiotic relationships between cancer registries and fertility services should be encouraged to link fertility data with cancer information. Communication and collaboration between fertility services should also be encouraged in order to collect adequate data. We believe that further exploration in this area will facilitate the further development of reproductive science.
Conclusions
OI treatment did not increase risk of ovarian cancer, regardless of treatment regimen and treatment cycle. However, nulliparous women treated with OI might have an increased risk of BOT compared to the nulliparous women not treated with OI. Meanwhile, nulliparous women treated with OI appeared to have a higher risk of IOC and BOT than multiparous women treated with OI. In view of the above, OI treatment was relatively safe but those nulliparous women treated with OI must be followed up rigorously.
Availability of data and materials
This meta-analysis was based on the data from the published articles and independent of any patient involvement. All the data will be made available to the editors of the journal for review or query upon.
Abbreviations
- Ovulation induction
- Borderline ovarian tumor
- Invasive ovarian cancer
Clomiphene citrate
- Gonadotrophin
- Gonadotropin-releasing hormone analogues
Datta J, Palmer MJ, Tanton C, Gibson LJ, Jones KG, Macdowall W, et al. Prevalence of infertility and help seeking among 15 000 women and men. Hum Reprod. 2016;31(9):2108–18.
Article CAS Google Scholar
Madziyire MG, Magwali TL, Chikwasha V, Mhlanga T. The causes of infertility in women presenting to gynaecology clinics in Harare, Zimbabwe; a cross sectional study. Fertil Res Practice. 2021;7(1):1.
Article Google Scholar
GBD 2017 Population and Fertility Collaborators. Population and fertility by age and sex for 195 countries and territories, 1950-2017: a systematic analysis for the global burden of disease study 2017. Lancet (London, England). 2018;392(10159):1995–2051.
Boivin J, Bunting L, Collins JA, Nygren KG. International estimates of infertility prevalence and treatment-seeking: potential need and demand for infertility medical care. Hum Reprod. 2007;22(6):1506–12.
Wang R, Kim BV, van Wely M, Johnson NP, Costello MF, Zhang H, et al. Treatment strategies for women with WHO group II anovulation: systematic review and network meta-analysis. BMJ (Clinical research ed). 2017;356:j138.
Google Scholar
Meirow D, Schenker JG. The link between female infertility and cancer: epidemiology and possible aetiologies. Hum Reprod Update. 1996;2(1):63–75.
Lundberg FE, Iliadou AN, Rodriguez-Wallberg K, Gemzell-Danielsson K, Johansson ALV. The risk of breast and gynecological cancer in women with a diagnosis of infertility: a nationwide population-based study. Eur J Epidemiol. 2019;34(5):499–507.
Fathalla MF. Incessant ovulation--a factor in ovarian neoplasia? Lancet (London, England). 1971;2(7716):163.
Cramer DW, Welch WR. Determinants of ovarian cancer risk. II. Inferences regarding pathogenesis. J Natl Cancer Inst. 1983;71(4):717–21.
CAS Google Scholar
Mertens-Walker I, Baxter RC, Marsh DJ. Gonadotropin signalling in epithelial ovarian cancer. Cancer Lett. 2012;324(2):152–9.
Whittemore AS, Harris R, Itnyre J. Characteristics relating to ovarian cancer risk: collaborative analysis of 12 US case-control studies. II. Invasive epithelial ovarian cancers in white women. Collaborative Ovarian Cancer Group. Am J Epidemiol. 1992;136(10):1184–203.
Reigstad MM, Storeng R, Myklebust T, Oldereid NB, Omland AK, Robsahm TE, et al. Cancer risk in women treated with fertility drugs according to parity status-a registry-based cohort study. Cancer Epidemiol Biomarkers Prev. 2017;26(6):953–62.
Stang A. Critical evaluation of the Newcastle-Ottawa scale for the assessment of the quality of nonrandomized studies in meta-analyses. Eur J Epidemiol. 2010;25(9):603–5.
Yamada T, Shojima N, Yamauchi T, Kadowaki T. J-curve relation between daytime nap duration and type 2 diabetes or metabolic syndrome: a dose-response meta-analysis. Sci Rep. 2016;6:38075.
Cumpston M, Li T, Page MJ, Chandler J, Welch VA, Higgins JP, et al. Updated guidance for trusted systematic reviews: a new edition of the Cochrane handbook for systematic reviews of interventions. Cochrane Database Syst Rev. 2019;10:Ed000142.
Siddaway AP, Wood AM, Hedges LV. How to do a systematic review: a best practice guide for conducting and reporting narrative reviews, meta-analyses, and meta-syntheses. Annu Rev Psychol. 2019;70:747–70.
Higgins JP, Thompson SG, Deeks JJ, Altman DG. Measuring inconsistency in meta-analyses. BMJ (Clinical research ed). 2003;327(7414):557–60.
Zhang Y, Ren T, Li H, Zhu L, Tang Q. Effectiveness and safety of acupuncture for anxiety disorder of coronavirus disease 2019: a protocol of systematic review and meta-analysis. Medicine. 2020;99(38):e22177.
Song F, Gilbody S. Bias in meta-analysis detected by a simple, graphical test. Increase in studies of publication bias coincided with increasing use of meta-analysis. BMJ (Clinical research ed). 1998;316(7129):471.
Spaan M, van den Belt-Dusebout AW, Lambalk CB, van Boven HH, Schats R, Kortman M, et al. Long-term risk of ovarian cancer and borderline tumors after assisted reproductive technology. J Natl Cancer Inst. 2021;113(6):699–709.
Lundberg FE, Johansson ALV, Rodriguez-Wallberg K, Gemzell-Danielsson K, Iliadou AN. Assisted reproductive technology and risk of ovarian cancer and borderline tumors in parous women: a population-based cohort study. Eur J Epidemiol. 2019;34(11):1093–101.
Bjørnholt SM, Kjaer SK, Nielsen TS, Jensen A. Risk for borderline ovarian tumours after exposure to fertility drugs: results of a population-based cohort study. Hum Reprod. 2015;30(1):222–31.
Asante A, Leonard PH, Weaver AL, Goode EL, Jensen JR, Stewart EA, et al. Fertility drug use and the risk of ovarian tumors in infertile women: a case-control study. Fertil Steril. 2013;99(7):2031–6.
van Leeuwen FE, Klip H, Mooij TM, van de Swaluw AM, Lambalk CB, Kortman M, et al. Risk of borderline and invasive ovarian tumours after ovarian stimulation for in vitro fertilization in a large Dutch cohort. Hum Reprod. 2011;26(12):3456–65.
Sanner K, Conner P, Bergfeldt K, Dickman P, Sundfeldt K, Bergh T, et al. Ovarian epithelial neoplasia after hormonal infertility treatment: long-term follow-up of a historical cohort in Sweden. Fertil Steril. 2009;91(4):1152–8.
Kristiansson P, Björ O, Wramsby H. Tumour incidence in Swedish women who gave birth following IVF treatment. Hum Reprod. 2007;22(2):421–6.
Cusidó M, Fábregas R, Pere BS, Escayola C, Barri PN. Ovulation induction treatment and risk of borderline ovarian tumors. Gynecol Endocrinol. 2007;23(7):373–6.
Mosgaard BJ, Lidegaard O, Kjaer SK, Schou G, Andersen AN. Ovarian stimulation and borderline ovarian tumors: a case-control study. Fertil Steril. 1998;70(6):1049–55.
Shushan A, Paltiel O, Iscovich J, Elchalal U, Peretz T, Schenker JG. Human menopausal gonadotropin and the risk of epithelial ovarian cancer. Fertil Steril. 1996;65(1):13–8.
Harris R, Whittemore AS, Itnyre J. Characteristics relating to ovarian cancer risk: collaborative analysis of 12 US case-control studies. III. Epithelial tumors of low malignant potential in white women. Collaborative Ovarian Cancer Group. Am J Epidemiol. 1992;136(10):1204–11.
Kessous R, Davidson E, Meirovitz M, Sergienko R, Sheiner E. The risk of female malignancies after fertility treatments: a cohort study with 25-year follow-up. J Cancer Res Clin Oncol. 2016;142(1):287–93.
Gronwald J, Glass K, Rosen B, Karlan B, Tung N, Neuhausen SL, et al. Treatment of infertility does not increase the risk of ovarian cancer among women with a BRCA1 or BRCA2 mutation. Fertil Steril. 2016;105(3):781–5.
Trabert B, Lamb EJ, Scoccia B, Moghissi KS, Westhoff CL, Niwa S, et al. Ovulation-inducing drugs and ovarian cancer risk: results from an extended follow-up of a large United States infertility cohort. Fertil Steril. 2013;100(6):1660–6.
Brinton LA, Trabert B, Shalev V, Lunenfeld E, Sella T, Chodick G. In vitro fertilization and risk of breast and gynecologic cancers: a retrospective cohort study within the Israeli Maccabi Healthcare Services. Fertil Steril. 2013;99(5):1189–96.
Lerner-Geva L, Rabinovici J, Olmer L, Blumstein T, Mashiach S, Lunenfeld B. Are infertility treatments a potential risk factor for cancer development? Perspective of 30 years of follow-up. Gynecol Endocrinol. 2012;28(10):809–14.
Kurta ML, Moysich KB, Weissfeld JL, Youk AO, Bunker CH, Edwards RP, et al. Use of fertility drugs and risk of ovarian cancer: results from a U.S.-based case-control study. Cancer Epidemiol Biomarkers Prev. 2012;21(8):1282–92.
Franceschi S, La Vecchia C, Negri E, Guarneri S, Montella M, Conti E, et al. Fertility drugs and risk of epithelial ovarian cancer in Italy. Hum Reprod. 1994;9(9):1673–5.
Mosgaard BJ, Lidegaard O, Kjaer SK, Schou G, Andersen AN. Infertility, fertility drugs, and invasive ovarian cancer: a case-control study. Fertil Steril. 1997;67(6):1005–12.
Parazzini F, Negri E, La Vecchia C, Moroni S, Franceschi S, Crosignani PG. Treatment for infertility and risk of invasive epithelial ovarian cancer. Hum Reprod. 1997;12(10):2159–61.
Parazzini F, Pelucchi C, Negri E, Franceschi S, Talamini R, Montella M, et al. Use of fertility drugs and risk of ovarian cancer. Hum Reprod. 2001;16(7):1372–5.
Ness RB, Cramer DW, Goodman MT, Kjaer SK, Mallin K, Mosgaard BJ, et al. Infertility, fertility drugs, and ovarian cancer: a pooled analysis of case-control studies. Am J Epidemiol. 2002;155(3):217–24.
Rossing MA, Tang MT, Flagg EW, Weiss LK, Wicklund KG. A case-control study of ovarian cancer in relation to infertility and the use of ovulation-inducing drugs. Am J Epidemiol. 2004;160(11):1070–8.
Jensen A, Sharif H, Frederiksen K, Kjaer SK. Use of fertility drugs and risk of ovarian cancer: Danish population based cohort study. BMJ (Clinical research ed). 2009;338:b249.
Venn A, Watson L, Lumley J, Giles G, King C, Healy D. Breast and ovarian cancer incidence after infertility and in vitro fertilisation. Lancet (London, England). 1995;346(8981):995–1000.
Modan B, Ron E, Lerner-Geva L, Blumstein T, Menczer J, Rabinovici J, et al. Cancer incidence in a cohort of infertile women. Am J Epidemiol. 1998;147(11):1038–42.
Potashnik G, Lerner-Geva L, Genkin L, Chetrit A, Lunenfeld E, Porath A. Fertility drugs and the risk of breast and ovarian cancers: results of a long-term follow-up study. Fertil Steril. 1999;71(5):853–9.
Venn A, Watson L, Bruinsma F, Giles G, Healy D. Risk of cancer after use of fertility drugs with in-vitro fertilisation. Lancet (London, England). 1999;354(9190):1586–90.
Doyle P, Maconochie N, Beral V, Swerdlow AJ, Tan SL. Cancer incidence following treatment for infertility at a clinic in the UK. Hum Reprod. 2002;17(8):2209–13.
Brinton LA, Lamb EJ, Moghissi KS, Scoccia B, Althuis MD, Mabie JE, et al. Ovarian cancer risk after the use of ovulation-stimulating drugs. Obstet Gynecol. 2004;103(6):1194–203.
Calderon-Margalit R, Friedlander Y, Yanetz R, Kleinhaus K, Perrin MC, Manor O, et al. Cancer risk after exposure to treatments for ovulation induction. Am J Epidemiol. 2009;169(3):365–75.
Silva Idos S, Wark PA, McCormack VA, Mayer D, Overton C, Little V, et al. Ovulation-stimulation drugs and cancer risks: a long-term follow-up of a British cohort. Br J Cancer. 2009;100(11):1824–31.
Hunn J, Rodriguez GC. Ovarian cancer: etiology, risk factors, and epidemiology. Clin Obstet Gynecol. 2012;55(1):3–23.
Lee AW, Rosenzweig S, Wiensch A, Ramus SJ, Menon U, Gentry-Maharaj A, et al. Expanding our understanding of ovarian cancer risk: the role of incomplete pregnancies. J Natl Cancer Inst. 2021;113(3):301–8.
Babic A, Sasamoto N, Rosner BA, Tworoger SS, Jordan SJ, Risch HA, et al. Association between breastfeeding and ovarian cancer risk. JAMA Oncol. 2020;6(6):e200421.
Sung HK, Ma SH, Choi JY, Hwang Y, Ahn C, Kim BG, et al. The effect of breastfeeding duration and parity on the risk of epithelial ovarian cancer: a systematic review and meta-analysis. J Prev Med Public Health = Yebang Uihakhoe Chi. 2016;49(6):349–66.
Li DP, Du C, Zhang ZM, Li GX, Yu ZF, Wang X, et al. Breastfeeding and ovarian cancer risk: a systematic review and meta-analysis of 40 epidemiological studies. Asian Pac J Cancer Prev. 2014;15(12):4829–37.
Karlsson T, Johansson T, Höglund J, Ek WE, Johansson Å. Time-dependent effects of oral contraceptive use on breast, ovarian, and endometrial cancers. Cancer Res. 2021;81(4):1153–62.
Jemal A, Siegel R, Ward E, Murray T, Xu J, Thun MJ. Cancer statistics, 2007. CA Cancer J Clin. 2007;57(1):43–66.
Bray F, Loos AH, Tognazzo S, La Vecchia C. Ovarian cancer in Europe: cross-sectional trends in incidence and mortality in 28 countries, 1953-2000. Int J Cancer. 2005;113(6):977–90.
Beral V, Doll R, Hermon C, Peto R, Reeves G. Ovarian cancer and oral contraceptives: collaborative reanalysis of data from 45 epidemiological studies including 23,257 women with ovarian cancer and 87,303 controls. Lancet (London, England). 2008;371(9609):303–14.
Fathalla MF. Incessant ovulation and ovarian cancer - a hypothesis re-visited. Facts, Views Vision ObGyn. 2013;5(4):292–7.
Treviño LS, Buckles EL, Johnson PA. Oral contraceptives decrease the prevalence of ovarian cancer in the hen. Cancer Prev Res (Phila). 2012;5(2):343–9.
Fishel S, Jackson P. Follicular stimulation for high tech pregnancies: are we playing it safe? BMJ (Clinical research ed). 1989;299(6694):309–11.
Folsom AR, Anderson JP, Ross JA. Estrogen replacement therapy and ovarian cancer. Epidemiology. 2004;15(1):100–4.
Glud E, Kjaer SK, Thomsen BL, Høgdall C, Christensen L, Høgdall E, et al. Hormone therapy and the impact of estrogen intake on the risk of ovarian cancer. Arch Intern Med. 2004;164(20):2253–9.
Liu Y, Ma L, Yang X, Bie J, Li D, Sun C, et al. Menopausal hormone replacement therapy and the risk of ovarian cancer: a meta-analysis. Front Endocrinol. 2019;10:801.
Gjorgoska M, Rižner TL. Estrogens and the Schrödinger's cat in the ovarian tumor microenvironment. Cancers. 2021;13(19):5011.
Mocka EH, Stern RA, Fletcher OJ, Anderson KE, Petitte JN, Mozdziak PE. Chemoprevention of spontaneous ovarian cancer in the domestic hen. Poult Sci. 2017;96(6):1901–9.
Pal P, Starkweather KN, Hales KH, Hales DB. A review of principal studies on the development and treatment of epithelial ovarian cancer in the laying hen Gallus gallus. Comp Med. 2021;71(4):271–84.
Beltsos AN, Odem RR. Ovulation induction and ovarian malignancy. Semin Reprod Endocrinol. 1996;14(4):367–74.
Barcroft JF, Galazis N, Jones BP, Getreu N, Bracewell-Milnes T, Grewal KJ, et al. Fertility treatment and cancers-the eternal conundrum: a systematic review and meta-analysis. Hum Reprod. 2021;36(4):1093–107.
Rizzuto I, Behrens RF, Smith LA. Risk of ovarian cancer in women treated with ovarian stimulating drugs for infertility. Cochrane Database Syst Rev. 2019;6(6):Cd008215.
Link CJ Jr, Reed E, Sarosy G, Kohn EC. Borderline ovarian tumors. Am J Med. 1996;101(2):217–25.
Buis CC, van Leeuwen FE, Mooij TM, Burger CW. Increased risk for ovarian cancer and borderline ovarian tumours in subfertile women with endometriosis. Hum Reprod. 2013;28(12):3358–69.
Moorman PG, Calingaert B, Palmieri RT, Iversen ES, Bentley RC, Halabi S, et al. Hormonal risk factors for ovarian cancer in premenopausal and postmenopausal women. Am J Epidemiol. 2008;167(9):1059–69.
Tung KH, Wilkens LR, Wu AH, McDuffie K, Nomura AM, Kolonel LN, et al. Effect of anovulation factors on pre- and postmenopausal ovarian cancer risk: revisiting the incessant ovulation hypothesis. Am J Epidemiol. 2005;161(4):321–9.
Merritt MA, De Pari M, Vitonis AF, Titus LJ, Cramer DW, Terry KL. Reproductive characteristics in relation to ovarian cancer risk by histologic pathways. Hum Reprod. 2013;28(5):1406–17.
Kehoe S, Powell J. Long-term follow-up of women with borderline ovarian tumors. Int J Gynaecol Obstet. 1996;53(2):139–43.
Barnhill DR, Kurman RJ, Brady MF, Omura GA, Yordan E, Given FT, et al. Preliminary analysis of the behavior of stage I ovarian serous tumors of low malignant potential: a Gynecologic Oncology Group Study. J Clin Oncol. 1995;13(11):2752–6.
Li Y, Cui H, Shen DH, Zhao Y, Wei LH, Qian HN. Clinical and pathological features of borderline ovarian tumors. Zhonghua Fu Chan Ke Za Zhi. 2003;38(2):81–4.
Sun Y, Xu J, Jia X. The diagnosis, treatment, prognosis and molecular pathology of borderline ovarian tumors: current status and perspectives. Cancer Manag Res. 2020;12:3651–9.
Rodriguez C, Tatham LM, Calle EE, Thun MJ, Jacobs EJ, Heath CW Jr. Infertility and risk of fatal ovarian cancer in a prospective cohort of US women. Cancer Causes Control. 1998;9(6):645–51.
Jiang YT, Gong TT, Zhang JY, Li XQ, Gao S, Zhao YH, et al. Infertility and ovarian cancer risk: evidence from nine prospective cohort studies. Int J Cancer. 2020;147(8):2121–30.
Jiao J, Sagnelli M, Shi B, Fang Y, Shen Z, Tang T, et al. Genetic and epigenetic characteristics in ovarian tissues from polycystic ovary syndrome patients with irregular menstruation resemble those of ovarian cancer. BMC Endocr Disord. 2019;19(1):30.
Yin W, Falconer H, Yin L, Xu L, Ye W. Association between polycystic ovary syndrome and cancer risk. JAMA Oncol. 2019;5(1):106–7.
Harris HR, Titus LJ, Cramer DW, Terry KL. Long and irregular menstrual cycles, polycystic ovary syndrome, and ovarian cancer risk in a population-based case-control study. Int J Cancer. 2017;140(2):285–91.
Olsen CM, Green AC, Nagle CM, Jordan SJ, Whiteman DC, Bain CJ, et al. Epithelial ovarian cancer: testing the ‘androgens hypothesis’. Endocr Relat Cancer. 2008;15(4):1061–8.
Vargas-Hernández VM. Endometriosis as a risk factor for ovarian cancer. Cir Cir. 2013;81(2):163–8.
Králíčková M, Laganà AS, Ghezzi F, Vetvicka V. Endometriosis and risk of ovarian cancer: what do we know? Arch Gynecol Obstet. 2020;301(1):1–10.
Brinton LA, Lamb EJ, Moghissi KS, Scoccia B, Althuis MD, Mabie JE, et al. Ovarian cancer risk associated with varying causes of infertility. Fertil Steril. 2004;82(2):405–14.
Nieto JJ, Rolfe KJ, MacLean AB, Hardiman P. Ovarian cancer and infertility: a genetic link? Lancet (London, England). 1999;354(9179):649.
Hull ME, Kriner M, Schneider E, Maiman M. Ovarian cancer after successful ovulation induction: a case report. J Reprod Med. 1996;41(1):52–4.
Abboud J, Attieh E, Atallah D, Kessrouani A, Chaoul G. Three cases of ovarian cancer after ovulation induction for infertility. Contraception, fertilite, sexualite (1992). 1997;25(1):64–5
Wang Xia ZS, XiuLi W. The rapid development of bilateral ovarian krukenberg tumor after ovarian stimulation: a case report and literature review. J Int Obstet Gynecol. 2021;48(4):477–80.
Adewole IF, Babarinsa IA, Thomas JO, Ajayi AB. Ovarian cancer associated with ovulation induction: a case report. Afr J Med Med Sci. 1997;26(3–4):203–4.
Artini PG, Fasciani A, Cela V, Battaglia C, de Micheroux AA, D'Ambrogio G, et al. Fertility drugs and ovarian cancer. Gynecol Endocrinol. 1997;11(1):59–68.
Jeremic K, Gojnic M, Milenković V, Petković S, Stojnić J, Lazović G, et al. Treatment for infertility and risk of invasive epithelial ovarian cancer--a case report. Clin Exp Obstet Gynecol. 2006;33(3):190–1.
Milenković V, Sparić R, Dokić M, Petković S, Atanacković J. Ovarian cancer after in vitro fertilization. Srp Arh Celok Lek. 2004;132(9–10):331–3.
Shkolnik K, Tadmor A, Ben-Dor S, Nevo N, Galiani D, Dekel N. Reactive oxygen species are indispensable in ovulation. Proc Natl Acad Sci U S A. 2011;108(4):1462–7.
Sowamber R, Nelson O, Dodds L, DeCastro V, Paudel I, Milea A, et al. Integrative transcriptome analyses of the human fallopian tube: Fimbria and ampulla-site of origin of serous carcinoma of the ovary. Cancers. 2020;12(5):1090.
Rossing MA, Daling JR, Weiss NS, Moore DE, Self SG. Ovarian tumors in a cohort of infertile women. N Engl J Med. 1994;331(12):771–6.
Bansal S, Goyal M, Sharma C, Shekhar S. Letrozole versus clomiphene citrate for ovulation induction in anovulatory women with polycystic ovarian syndrome: a randomized controlled trial. Int J Gynaecol Obstet. 2021;152(3):345–50.
Zolton JR, Lindner PG, Terry N, DeCherney AH, Hill MJ. Gonadotropins versus oral ovarian stimulation agents for unexplained infertility: a systematic review and meta-analysis. Fertil Steril. 2020;113(2):417–25.e1.
Sarhan A, Beydoun H, Jones HW Jr, Bocca S, Oehninger S, Stadtmauer L. Gonadotrophin ovulation induction and enhancement outcomes: analysis of more than 1400 cycles. Reprod Biomed Online. 2011;23(2):220–6.
Weiss JM, Ludwig M, Ortmann O, Diedrich K. GnRH antagonists in the treatment of infertility. Ann Med. 2003;35(7):512–22.
Filicori M, Cognigni GE. Ovulation induction with pulsatile gonadotropin releasing hormone: missing in action. Fertil Steril. 2018;109(4):621–2.
Kashyap S, Moher D, Fung MF, Rosenwaks Z. Assisted reproductive technology and the incidence of ovarian cancer: a meta-analysis. Obstet Gynecol. 2004;103(4):785–94.
Li LL, Zhou J, Qian XJ, Chen YD. Meta-analysis on the possible association between in vitro fertilization and cancer risk. Int J Gynecol Cancer. 2013;23(1):16–24.
Download references
This work was supported by Jiangsu Provincial Commission of Health and Family Planning scientific research project (H2018017), Jiangsu Provincial Women’s and Children’s Health key talent project (RC201709).
Author information
Liang Yu, Jiafan Sun, Qiqin Wang and Wennian Yu contributed equally to this work.
Authors and Affiliations
The First Affiliated Hospital of Nanjing Medical University, The First Clinical Medical College of Nanjing Medical University, Nanjing, 210029, China
Liang Yu, Jiafan Sun, Qiqin Wang, Wennian Yu & Anqi Wang
Department of Gynecology, Jiangsu Province Hospital, The First Affiliated Hospital of Nanjing Medical University,The first clinical medical college of Nanjing Medical University, Nanjing, 210029, China
Shu Zhu, Wei Xu & Xiuli Wang
Department of Gynecology, Jiangsu Province Hospital, the First Affiliated Hospital of Nanjing Medical University, Nanjing, 210036, China
You can also search for this author in PubMed Google Scholar
Contributions
YL & SJF performed the database search. YL & WQQ collected the data. YL performed the analysis and wrote the manuscript. ZS & XW supplemented the database search, polished the language of the article and provided third party arbitration. WXL provided guidance on research directions. All the authors participated in this analysis and approved the final version of the manuscript.
Corresponding author
Correspondence to Xiuli Wang .
Ethics declarations
Ethics approval and consent to participate, consent for publication.
All authors agreed publication of the manuscript.
Competing interests
The authors declare no financial, personal, intellectual and professional conflicts of interest.
Additional information
Publisher’s note.
Springer Nature remains neutral with regard to jurisdictional claims in published maps and institutional affiliations.
Supplementary Information
Additional file 1: supplemental material fig. 1..
The flowchart of systematic search and screening process.
Additional file 2: Supplemental Material Fig. 2.
(A) Funnel plot of all the included studies; (B) Egger’s regression test of all the included studies.
Additional file 3: Supplementary Table S1a.
Quality evaluation of case-control study assessed by the Newcastle-Ottawa Scale. Supplementary Table S1b. Quality evaluation of cohort study assessed by the Newcastle-Ottawa Scale.
Additional file 4: Supplementary Table S2
. ovarian cancer study characteristics.
Additional file 5: Supplementary Table S3a
. Ovarian tumor in the nulliparous women. Supplementary Table S3b . Ovarian tumor in the multiparous women.
Additional file 6: Supplementary Table S4
. Ovarian tumor between the nulliparous and multiparous group.
Additional file 7: Supplementary Table S5a
. Ovarian tumor and stimulation cycles(< 3 cycles). Supplementary Table S5b . Ovarian tumor and stimulation cycles(≥3 cycles). Supplementary Table S5c . Ovarian tumor and stimulation cycles(< 6 cycles). Supplementary Table S5d . Ovarian tumor and stimulation cycles(≥6 cycles). Supplementary Table S5e . Ovarian tumor and stimulation cycles(< 12 cycles). Supplementary Table S5f . Ovarian tumor and stimulation cycles(≥12 cycles).
Additional file 8: Supplementary Table S6a
. Ovarian tumor in women who ever received CC. Supplementary Table S6b . Ovarian tumor in women who ever received HMG. Supplementary Table S6c . Ovarian tumor in women who ever received HCG. Supplementary Table S6d . Ovarian tumor in women who ever received GDT. Supplementary Table S6e . Ovarian tumor in women who ever received GnRH-a.
Additional file 9: Supplementary Table S7
. Sensitivity analyses for the novel study during this update.
Rights and permissions
Open Access This article is licensed under a Creative Commons Attribution 4.0 International License, which permits use, sharing, adaptation, distribution and reproduction in any medium or format, as long as you give appropriate credit to the original author(s) and the source, provide a link to the Creative Commons licence, and indicate if changes were made. The images or other third party material in this article are included in the article's Creative Commons licence, unless indicated otherwise in a credit line to the material. If material is not included in the article's Creative Commons licence and your intended use is not permitted by statutory regulation or exceeds the permitted use, you will need to obtain permission directly from the copyright holder. To view a copy of this licence, visit http://creativecommons.org/licenses/by/4.0/ . The Creative Commons Public Domain Dedication waiver ( http://creativecommons.org/publicdomain/zero/1.0/ ) applies to the data made available in this article, unless otherwise stated in a credit line to the data.
Reprints and permissions
About this article
Cite this article.
Yu, L., Sun, J., Wang, Q. et al. Ovulation induction drug and ovarian cancer: an updated systematic review and meta-analysis. J Ovarian Res 16 , 22 (2023). https://doi.org/10.1186/s13048-022-01084-z
Download citation
Received : 17 May 2022
Accepted : 17 December 2022
Published : 24 January 2023
DOI : https://doi.org/10.1186/s13048-022-01084-z
Share this article
Anyone you share the following link with will be able to read this content:
Sorry, a shareable link is not currently available for this article.
Provided by the Springer Nature SharedIt content-sharing initiative
- Ovarian stimulation
- Meta-analysis
Journal of Ovarian Research
ISSN: 1757-2215
- General enquiries: [email protected]
Advertisement
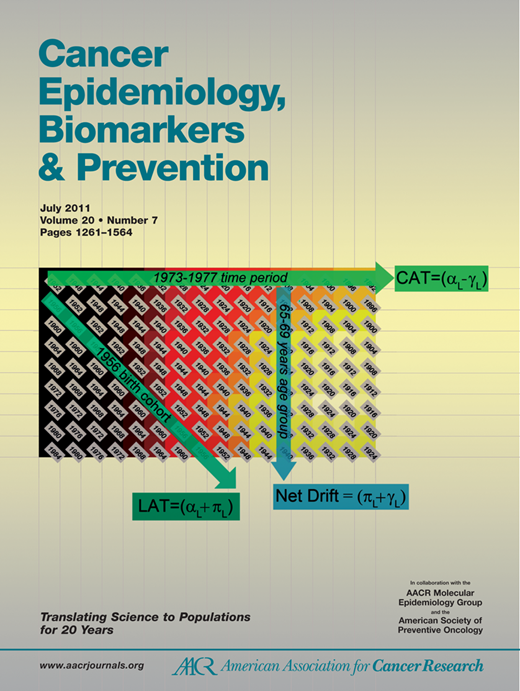
- Previous Article
- Next Article
Introduction
Disclosure of potential conflicts of interest, acknowledgments, does exposure to asbestos cause ovarian cancer a systematic literature review and meta-analysis.
- Split-Screen
- Article contents
- Figures & tables
- Supplementary Data
- Peer Review
- Open the PDF for in another window
- Get Permissions
- Cite Icon Cite
- Search Site
- Version of Record July 6 2011
- Proof June 21 2011
- Accepted Manuscript May 24 2011
Alison Reid , Nick de Klerk , Arthur W. (Bill) Musk; Does Exposure to Asbestos Cause Ovarian Cancer? A Systematic Literature Review and Meta-analysis. Cancer Epidemiol Biomarkers Prev 1 July 2011; 20 (7): 1287–1295. https://doi.org/10.1158/1055-9965.EPI-10-1302
Download citation file:
- Ris (Zotero)
- Reference Manager
Introduction: The asbestos and ovarian cancer relationship is not well understood because of small numbers of women exposed to asbestos, small numbers of cases, and misclassification of peritoneal mesothelioma as ovarian cancer on death certificates. The aim of this study was to conduct a meta-analysis to quantify the evidence that exposure to asbestos causes ovarian cancer.
Methods: Fourteen cohort and two case-control studies were identified in Medline searches from 1950 to 2008.
Results: Statistically significant excess mortality was reported in four of the cohort studies, all of which determined their outcomes from the death certificate. Peritoneal mesotheliomas were reported in these studies, two of which reexamined pathology specimens and reported disease misclassification. Exposure-response relationships were inconsistent. When all studies were included in a meta-analysis, the effect size was 1.75 (95% CI, 1.45–2.10) attenuating to 1.29 (95% CI, 0.97–1.73) in studies with confirmed ovarian cancers.
Conclusion: Taken without further analysis, women thought to have ovarian cancer had an increased rate in the meta-analysis if reporting having been exposed to asbestos, compared with reference populations. This result may have occurred because of disease misclassification. Cancer Epidemiol Biomarkers Prev; 20(7); 1287–95. ©2011 AACR .
In May 2009, a summary of the latest assessment of the carcinogenicity of metals, arsenic, dusts, and fibers, including asbestos, by the International Agency for Research on Cancer (IARC) Monograph Working Group was published in the Lancet Oncology ( 1 ). For the first time, the evidence was declared sufficient in humans to show that exposure to asbestos causes cancer of the ovary ( 2 ). It has long been established that exposure to asbestos causes malignant mesothelioma, lung cancer, and asbestosis, as well as “benign” pleural diseases. Excess mortality and incidence of these diseases have been shown repeatedly in cohorts of occupationally exposed workers and exposure–response relationships have shown a clear causal relationship between asbestos exposure and mesothelioma, lung cancer, and asbestosis ( 3–6 ). However, the IARC Monograph that will provide the evidence supporting the sufficient ruling has not yet been published.
The relationship between asbestos exposure and ovarian cancer is not as well understood as that of asbestos-related diseases. Studies that have examined this issue have been limited for 2 major reasons:
Small numbers of cases: Much fewer women than men have been exposed to asbestos, particularly in more heavily exposed occupational settings where relative risks are higher. Although many women in epidemiologic studies have had domestic or general environmental exposure, levels have generally been relatively low so that risks and hence numbers of cases have also been few.
Difficulties with diagnosis: Many of the studies that have reported excess ovarian cancer following asbestos exposure have examined mortality from ovarian cancer and used the cause of death as listed on the death certificate to identify the cause of death. The accuracy of death certificates has been questioned repeatedly ( 7, 8 ), particularly in relation to asbestos-related diseases ( 9 ). Pleural mesothelioma has a long history of being misreported on death certificates most often being labeled as lung or pleural cancer ( 3, 9, 10 ). It has been particularly difficult to distinguish between peritoneal mesothelioma and ovarian serous carcinoma. Immunohistochemical tests to aid in the identification of mesothelioma cells became available in 1996–1997 with the introduction of calretinin. Since then, many new markers have become available, although none of these are useful in distinguishing between ovarian cancer and peritoneal mesothelioma. A recent review of the value of immunohistochemistry to distinguish between peritoneal mesothelioma and serous carcinoma of the ovary and peritoneum concluded that “positive serous carcinoma markers, by and large, have a higher degree of sensitivity and specificity in assisting in discriminating between these malignancies than the positive mesothelioma markers. From a practical point of view, a combination of MOC-31 (or BER-EP4), estrogen receptors, and calretinin immunostaining should allow a clear distinction to be made between epithelioid peritoneal mesotheliomas and serous carcinomas in most cases ( 11 ).”
Accordingly, peritoneal mesothelioma has often been listed on the death certificate as stomach, colon, or ovarian cancer or carcinomatosis ( 9, 12–14 ).
However, there are biologically plausible reasons as to why exposure to asbestos may cause ovarian cancer. Asbestos fibers have been found in the ovaries of women who were exposed to asbestos in the Norwegian pulp and paper industry and also among women whose household contacts worked with asbestos ( 15, 16 ), although possible sample contamination cannot be ruled out. The mode of distribution of the fibers through the body following inhalation is not well understood. The fibers may migrate across the diaphragm through the peritoneal cavity and penetrate the ovaries. Animal studies have observed asbestos fibers within the cytoplasm of epithelial and interstitial cells within 24 hours after brief inhalation ( 17 ). Once fibers have entered the interstitium, they then have access to the vascular and lymphatic systems. Fibers in the lymphatic system can be channeled to the visceral pleura and subsequently to the pleural cavity. Mechanical irritation leading to fibrosis or to cancer, or “frustrated phagocytosis” (where the macrophage is damaged because it is unable to digest the whole asbestos fiber because of its length), thus leading to the production of hydroxyl radicals and reactive oxygen species that induce cell injury ( 18, 19 ), are 2 mechanisms by which the fibers may cause cancer once they reach the ovary. Experiments in which 10 g of tremolite mixed with 400 mL of water was injected intraperitoneally into mice, hamsters, guinea pigs, and rabbits showed that in 2 of 10 rabbits and 2 of 16 guinea pigs, the abnormality that developed in the epithelium of the ovary resembled lesions observed in early human ovarian cancers ( 20 ). “Overall, the available evidence in favor or against any of these mechanisms leading to the development of lung cancer and mesothelioma in either animals or humans is evaluated as weak” ( 21 ).
The aims of this study were ( 1 ) to review the epidemiologic studies that have reported effect estimates for ovarian cancer incidence or mortality in women following exposure to asbestos and ( 2 ) to conduct a meta-analysis of those studies to quantify whether that exposure to asbestos causes ovarian cancer.
Studies were identified through a systematic review of the literature available on MEDLINE from 1950 to December 2008. The database was searched using combinations of the search terms “women” or “females” or “girls” and “asbestos” or “fibres” or “dust” or “crocidolite” or “chrysotile” or “amosite” or “occupational exposure” or “environmental exposure” or “household exposure” or “neighbourhood exposure” or “residential exposure” or “locational exposure” or “domestic exposure” or “familial exposure” or “exposure” with one of the following outcomes “cancer” or “mortality” or “death” or “neoplasms” or “ovarian cancer.” Any cohort or case–control study that examined women and asbestos exposure and was published in English was included. Studies were also identified from references listed in published articles. Case reports were not included.
Summary effect estimates were examined using the metan suite of commands in Stata 10.1 ( 22, 23 ). Models that assumed that the study populations were all relatively homogenous (fixed effects) and models that assumed that the true exposure-related risks in each study vary randomly (random effects) were examined and both are reported. All studies described in Table 1 were included in the meta-analysis. Expected deaths [based on the number of observed deaths and the standardized mortality ratios (SMR)] and 95% Confidence Intervals (95% CI) were calculated for the study of Polish women diagnosed with asbestosis, which did not include them in their published article ( 24 ). Forest plots were produced automatically as part of the metan suite of commands in Stata 10.1.
Cohort and case–control studies of women exposed occupationally or environmentally to asbestos that examine ovarian cancer mortality or incidence as an outcome
a Lung and pleural cancers combined (number of mesotheliomas listed on death certificates in this cohort).
b Standardized proportionate mortality ratio.
c Peritoneal cancers.
d Pleural cancers.
e Medium/high exposure.
f Among women who worked in asbestos yarn and cloth production areas (high exposure).
g Fibers include asbestos, talc, and fiberglass.
Fourteen cohort ( 3, 12, 24–34 ) and 2 case–control ( 35, 36 ) studies of women exposed to asbestos in their jobs or from their general environment and that examined ovarian cancer incidence or mortality as an outcome were identified from the literature ( Table 1 ). Four of the cohort studies had been reported on several times over their years of follow-up: In each case, only the latest report is shown in the table and included in the meta-analysis ( 3, 12, 26, 28 ).
Generally, the number of cases of ovarian cancer reported in the cohort studies was small, ranging from 1 case [among Polish women diagnosed with asbestosis ( 24 ) and Turin textile workers ( 29 )] to 12 cases reported among Leyland crocidolite gas mask workers ( 25 ). However, 5,072 cancer cases were reported among the whole Finnish female working population born between 1906 and 1945 in which exposure to asbestos was determined from a job exposure matrix ( 33 ). The case–control studies had more cases than the cohort studies: 69 cases reported some respiratory exposure to asbestos and 18 cases reported relatives with occupational asbestos exposure among participants of the Johns Hopkins study. Among Norwegian pulp and paper mill workers, there were 6 cases who had worked in areas where they were likely to have been exposed to asbestos.
Statistically significant excess mortality or incidence of cancer of the ovary was reported in 4 of the 14 cohort studies. SMRs and their 95% CIs in these 4 studies ranged from 4.77 (95% CI, 2.18–9.06) to 2.27 (95% CI, 1.04–4.32; refs. 3, 25, 27, 28 ). Of the remaining 10 cohort studies, 5 reported a tendency to excess mortality, although SMR estimates were unstable, ranging from 2.61 (95% CI, 0.85–6.09) to 1.42 (95% CI, 0.71–2.54). Women who worked in asbestos yarn and cloth production in a Polish asbestos cement products factory had a statistically significant excess mortality from ovarian cancer [SMR = 3.76 (95% CI, 1.38–8.18)], although the association was not significant over all the female factory workers ( 12, 25, 26, 30, 34 ). Five studies reported ovarian cancer incidence or mortality around the same population as their reference populations ( 24, 29, 31–33 ). Both case–control studies reported a nonsignificant excess incidence with asbestos exposure. Women with relatives with occupational asbestos exposure reported a nonsignificant but 3-fold risk of ovarian cancer, although the overall risk for all women in that study was close to unity ( 35, 36 ).
The type of asbestos to which the women were exposed was crocidolite (blue asbestos—the most mesotheliogenic of the asbestos fibers; ref. 37 ) only in 3 cohorts ( 12, 25, 31 ), chrysotile (white asbestos) only in 2 ( 25, 29 ), chrysotile and crocidolite in 5 cohorts ( 3, 26–28, 32 ), mixed fibers including crocidolite in 1 cohort ( 30 ), and 4 studies (including the 2 case–control studies) did not report the fiber type ( 24, 34–36 ). The remaining study of economically active Finnish women born between 1906 and 1945 had their probability of asbestos exposure determined on the basis of their job titles, as reported in the 1970 census and a job exposure matrix (the FINJEM; ref. 38 ): the types of asbestos were not distinguished, although anthophyllite was mined and widely used throughout Finland ( 39 ). Four of the studies that reported a significant excess risk of ovarian cancer reported exposure to crocidolite or chrysotile and crocidolite.
Small numbers of cases or lack of exposure information inhibited the examination of exposure–response relationships and mortality or incidence of ovarian cancer. Nevertheless, 3 studies ( 3, 14, 28 ) examined SMRs by category of exposure (low/medium/high), duration of employment (years), or latency (time between first exposure to asbestos and onset of disease), and 1 examined ovarian cancer incidence and quantitative asbestos exposure characteristics by using a nested case–control design and conditional logistic regression ( 31 ). Among East London factory workers, there was a significant overall excess of ovarian cancers, but when examined by category and duration of exposure, the trend was not significant ( P = 0.18). However, the excess was significant among women who had “severe” exposure for more than 2 years ( 3 ). A nonstatistically significant exposure trend with duration of exposure was observed among Italian factory workers; 0 cases: <1 year, SMR = 2.4, 1–4 years; 0 cases: 5–9 years, SMR = 2.7, 10–19 years; SMR = 2.8, 20–29 years; and SMR = 2.9, ≥30 years ( 28 ). Exposure–response relationships were examined in an earlier report of the Nottingham gas mask workers. Mortality from ovarian cancer was higher among women exposed for more than 1 year [observed/expected (O/E) 3/0.95] than among those exposed for less than 1 year (O/E 2/1.13; ref. 14 ). Several quantitative measures of asbestos exposure, including intensity (f/mL), duration of employment or residence, and time since first exposure, were not associated with the incidence of ovarian cancer among the Wittenoom women ( 31 ).
Twelve of the cohort studies listed in Table 1 examined mortality from ovarian cancer and relied on the cause of death as listed on the death certificate. In addition, cases of peritoneal mesothelioma were observed in 8 of these studies, suggesting that misclassification of peritoneal mesothelioma as ovarian cancer may have occurred.
Cases of peritoneal mesothelioma were reported in 4 of the studies that reported a statistically significant excess mortality from ovarian cancer ( 3, 25, 27, 28 ). Two of these studies attempted to confirm the diagnosis of ovarian cancer pathologically. Among East London factory workers, ovarian cancer was confirmed in 2 cases with material available (from a total of 4 ovarian cancers up to 1968; ref. 13 ). Two cases listed as carcinomatosis were confirmed as ovarian cancers, and 1 case listed as ovarian cancer was determined to be a peritoneal mesothelioma ( 13 ). The latest follow-up to 1980 reported 9 cases of ovarian cancer, but it is not clear how many of these were confirmed histologically ( 3 ). Among Italian asbestos cement workers, 7 of 9 cases were confirmed as ovarian cancers ( 28 ). Three cohort studies reported a statistically significant excess rate of ovarian cancer but did not reexamine ovarian cancer pathology specimens. One of these (Italian women compensated for asbestosis; ref. 27 ) had more cases of peritoneal mesothelioma than ovarian cancer, suggesting that misclassification may have occurred. Among Leyland gas mask workers exposed to crocidolite, there were 2 cases of peritoneal mesothelioma and 12 cases of ovarian cancer. The authors suggested that ovarian cancers might have been peritoneal mesotheliomas misclassified ( 25 ). There were no reported cases of peritoneal mesothelioma among Polish asbestos cement products factory workers compared with 8 cases of ovarian cancer ( 34 ). Misclassification of disease is important to the internal validity of these studies, as with small numbers of cases of ovarian cancer, any misclassification could overestimate (or underestimate) the reported association with asbestos exposure.
Five studies that did not find a statistically significant excess rate of ovarian cancer reexamined ovarian cancer pathology where available. In an earlier report on the Nottingham crocidolite gas mask workers, of 6 ovarian cancer deaths, 2 were confirmed as ovarian cancers and 1 was determined to be a peritoneal mesothelioma. Material was not available on the other 3 cases ( 14 ). The latest report of this cohort by McDonald and colleagues could not further review ovarian cancer pathology as only cause of death codes were available ( 12 ). Among the Wittenoom women, specimens from 9 cases of ovarian cancer were available for reexamination from a total of 16 cases. All 9 cases were confirmed as ovarian cancers ( 31 ). Similarly, all cases of ovarian cancer were confirmed among Finnish women defined as exposed to asbestos from a job exposure matrix as well as the 2 case–control studies ( 33, 35, 36 ).
Confounding and independent risk factors for ovarian cancer have not been addressed well in most studies, predominantly because they have been retrospective studies of occupational cohorts and limited data on potential confounders were available. Only 3 of the studies (the 2 case–control studies and the cohort of economically active Finnish women) assessed any of the following variables: age at menarche or menopause, late first pregnancy and age at first delivery, use of oral contraceptives, or tubal ligation, all known to be independent risk factors for ovarian cancer. Although few studies have collected data on these other risk factors, this may not have influenced the findings significantly, as they are unlikely to be associated with asbestos exposure and therefore are not likely to confound any association.
Loss to follow-up was a significant problem for 3 studies; all reporting more than 20% loss to follow-up ( 3, 31 ), with the highest reporting 33% ( 12 ). In an attempt to overcome this loss, one of the studies stopped accruing person-years at risk for those lost to follow-up from the date they were last known to be alive, thus underestimating person-years at risk and overestimating the SMR ( 31 ). Berry and colleagues did not state how they censored those lost to follow-up ( 3 ), but in an earlier report, Newhouse and colleagues censored those lost to follow-up on their last date of employment, so underestimating person years at risk and thus overestimating the SMR ( 13 ). McDonald and colleagues presented 2 sets of results, 1 for the complete cohort and 1 for a subset with more complete follow-up. SMRs were slightly larger among the more complete subset than those for the whole cohort ( 12 ). High loss to follow-up may over- or underestimate the risk of disease following exposure to asbestos, depending on the type of censoring method used to account for the loss to follow-up.
The meta-analysis that examined all studies showed a 75% excess risk of ovarian cancer in women who had been exposed to asbestos ( Table 2 ). The effect size was similar between the models that assumed no heterogeneity between the studies (fixed effects) and those that assumed the exposure-related risks differed randomly between the studies (random effects). Figure 1 shows the corresponding forest plot for both the fixed- and random-effects models for all studies combined. The analyses were repeated for all cohort studies only and case–control studies only and similar effects were observed, although the effect was not statistically significant in the case–control studies ( Table 2 ). When only those studies that confirmed their ovarian cancer pathology were included in a meta-analysis, the effect estimate declined, although remained statistically significant. The effect declined again and was not statistically significant when those studies that examined cancer incidence were included in a meta-analysis. These 4 studies did not rely on cause of death information from the death certificates to classify their cases.
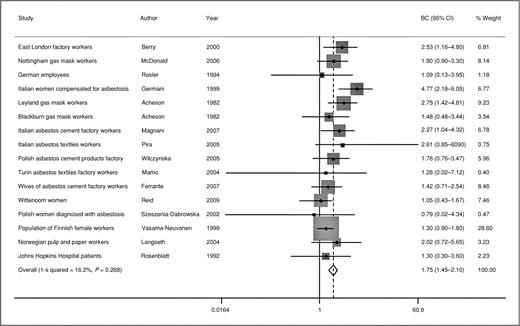
Forest plot of fixed summary effect for ovarian cancer and asbestos exposure.
Summary statistics for asbestos exposure and incidence or mortality from ovarian cancer
A meta-analysis conducted on 9 of the cohort studies ( 12, 24, 26–31, 34 ) that also reported SMRs and 95% CIs (or provided enough information so that they could be calculated) for mesothelioma gave a fixed-effects size of 70.9 (95% CI, 61.4–82.0) and random-effects size of 63.2 (95% CI, 41.9–95.3; data not shown). Figure 2 shows the relationship between standardized mortality or incidence ratios for mesothelioma and ovarian cancer for the 9 cohort studies that examined both mesothelioma and ovarian cancer included in the mesothelioma meta-analysis. There is clearly no relationship between the two. When the exposure is sufficient to have caused mesothelioma, there is no corresponding increased risk in ovarian cancer.
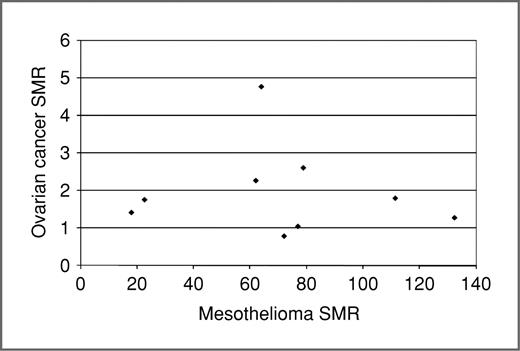
Relationship between mesothelioma and ovarian cancer-standardized mortality ratios for 9 cohort studies presented in Table 1 ( 3–6, 9, 27, 28, 31, 36 )
Taken without further analysis, women thought to have ovarian cancer had an increased rate in the meta-analysis if reporting having been exposed to asbestos, compared with reference populations. This result was obtained when all studies were included in the meta-analysis and again when only those studies that had reexamined ovarian cancer pathology were included. Only the meta-analysis of those studies that reported ovarian cancer incidence (i.e., those studies that did not rely on cause of death certification to classify their cases of ovarian cancer) did not observe a significant excess risk.
In the studies that did not examine ovarian cancer pathology, or confirmed cases of mesothelioma from a cancer or mesothelioma registry, misclassification of the cause of death in some cases is likely to have occurred, given that misclassification was reported in those studies that did reexamine cancer pathology specimens. Misclassification may result in an underestimate of peritoneal mesothelioma and an overestimate of ovarian cancer or the converse. Among women, peritoneal mesothelioma may be more likely to be classified as ovarian, colon, or stomach cancer, rather than a rare occupational cancer. The cohort study referred to in the Lancet summarizing the IARC reclassification (women gas mask workers) did not reexamine pathology ( 25 ). An examination of cancer incidence (and its use of cancer registration rather than death certification for disease outcome data) may have produced different results. Notably, the meta-analysis on the 2 case–control and 2 cohort studies that examined ovarian cancer incidence did not report a statistically significant excess risk of ovarian cancer.
The IARC makes its determinations of cancer causality (if an observed association between an exposure and a disease is causal) by using Bradford Hill's suggested hierarchy of criteria ( 40, 41 ). The first of these was the strength of the association. In this review, the greatest risk of ovarian cancer was observed among Italian women compensated for asbestosis. Their risk was almost 5-fold compared with their reference population (SMR = 4.77). However, their SMR for peritoneal mesothelioma was 40.9 and for pleural mesothelioma was 64.0, between 8 and 13 times larger than that observed for ovarian cancer ( 27 ). The effect size from the meta-analysis for ovarian cancer ranged from 1.29 among those studies that examined cancer incidence to 1.85 for all cohort studies. The effect size for mesothelioma was 70.9 (95% CI, 61.4–82.0). Clearly, the effect size for mesothelioma, a disease known to be caused by exposure to asbestos, is much larger than that for ovarian cancer. Also, if there was misclassification of mesothelioma and ovarian cancer, then some relationship between the 2 SMRs shown in Figure 2 is likely to have been observed. Similarly, if there was an exposure–response relationship, then some relationship between the SMRs should have been observed. Another explanation for the lack of correlation between mesothelioma and ovarian cancer SMRs is that asbestos exposure does not cause ovarian cancer.
Hill's second criterion for causality was consistency—that the observed association been repeated in different people, places, and times ( 40 ). The present study has shown that 4 of 14 cohort studies reported a statistically significant excess rate for ovarian cancer among women exposed to asbestos. Of the remaining 10 studies, 5 reported a tendency to excess but failed to reach statistical significance and 5 reported rates that were similar to those of their reference populations. Strong evidence of consistency was not observed among these studies, although no study reported any protective effect.
Also included in Hill's criteria for causation was biological gradient or demonstration of an exposure–response relationship ( 40 ). In the studies presented in this article, examination of exposure–response relationships was limited because of the small numbers of cases of ovarian cancer. Most of the studies were limited by small numbers of women both in terms of the number of women exposed to asbestos and the subsequent small numbers of ovarian cancers. However, where exposure–response relationships were examined, they were inconsistent. No study showed a statistically significant trend of ovarian cancer with degree of asbestos exposure. In addition, there was no evidence of a significant trend across studies as grouped exposure increased (see Fig. 2 ).
Other Hill's criteria are temporality (which is met because disease follows exposure) and specificity (which Hill largely discounts: It is known that asbestos causes more than one disease). Plausibility, coherence, and analogy are all satisfied, and experiment is not really applicable.
Taken without further analysis, women thought to have ovarian cancer had an increased rate in the meta-analysis if reporting having been exposed to asbestos, compared with reference populations. However, this finding may result from the methods used to identify the ovarian cancer cases. Where disease outcome was identified from the cause of death as listed on the death certificate, given the small numbers of ovarian cancer cases in each study, even misclassification of 1 cancer may exert a large impact on the exposure effect. The meta-analysis of those studies that examined ovarian cancer as determined on the death certificate reported an excess risk. In contrast, no significant excess risk was reported among those studies that examined the incidence of ovarian cancer where cases were ascertained from a cancer registry. The IARC Monograph that contains the evidence supporting its sufficient ruling that asbestos exposure causes ovarian cancer is not yet in the public domain. However, the authors of this article suggest that the IARC decision to determine asbestos exposure as a cause of ovarian cancer was premature and not wholly supported by the evidence. Meta-analysis techniques cannot account or adjust for the quality of the data contained in the original studies that are used in the meta-analysis. If the original data contain errors of classification, then errors are built into the meta-analysis.
No potential conflicts of interest were disclosed.
The authors thank Jan Sleith, Nola Olsen, Robin Mina, National Health and Medical Research Council, and JEM Foundation.
Citing articles via
Email alerts.
- Online First
- Online ISSN 1538-7755
- Print ISSN 1055-9965
AACR Journals
- Blood Cancer Discovery
- Cancer Discovery
- Cancer Epidemiology, Biomarkers & Prevention
- Cancer Immunology Research
- Cancer Prevention Research
- Cancer Research
- Cancer Research Communications
- Clinical Cancer Research
- Molecular Cancer Research
- Molecular Cancer Therapeutics
- Info for Advertisers
- Information for Institutions/Librarians

- Privacy Policy
- Copyright © 2023 by the American Association for Cancer Research.
This Feature Is Available To Subscribers Only
Sign In or Create an Account
Featured Clinical Reviews
- Screening for Atrial Fibrillation: US Preventive Services Task Force Recommendation Statement JAMA Recommendation Statement January 25, 2022
- Evaluating the Patient With a Pulmonary Nodule: A Review JAMA Review January 18, 2022
- Download PDF
- Share X Facebook Email LinkedIn
- Permissions
Cancer Stage Compared With Mortality as End Points in Randomized Clinical Trials of Cancer Screening : A Systematic Review and Meta-Analysis
- 1 Genomic Epidemiology Branch, International Agency for Research on Cancer, Lyon, France
- 2 Department of Respiratory Medicine, Leeds Teaching Hospitals, St James’s University Hospital, Leeds, United Kingdom
- 3 Leeds Institute of Health Sciences, University of Leeds, Leeds, United Kingdom
- 4 Division of Public Health Sciences, Fred Hutchinson Cancer Center, Seattle, Washington
- 5 Center for Early Detection Advanced Research, Knight Cancer Institute, Portland, Oregon
- Editorial Late-Stage Cancer End Points to Speed Cancer Screening Clinical Trials—Not So Fast Peter B. Bach, MD, MAPP JAMA
Question Compared with the end point of cancer-specific mortality, is incidence of late-stage cancer a suitable alternative end point in randomized clinical trials of cancer screening?
Findings In this systematic review and meta-analysis that included 41 randomized clinical trials of cancer screening, correlation between the reduction in stage III and IV cancer and the reduction in cancer-specific mortality varied by cancer type. The correlation was high in trials that screened for ovarian (Pearson ρ = 0.99) and lung (Pearson ρ = 0.92) cancers, moderate for breast cancer (Pearson ρ = 0.70), and weak for colorectal (Pearson ρ = 0.39) and prostate (Pearson ρ = −0.69) cancers.
Meaning In randomized clinical trials of cancer screening, the correlation between reductions in late-stage cancer and cancer-specific mortality varied meaningfully by cancer type. The end point of late-stage cancer may be an appropriate alternative to cancer-specific mortality for randomized clinical trials of screening for some types of cancer, but not for others.
Importance Randomized clinical trials of cancer screening typically use cancer-specific mortality as the primary end point. The incidence of stage III-IV cancer is a potential alternative end point that may accelerate completion of randomized clinical trials of cancer screening.
Objective To compare cancer-specific mortality with stage III-IV cancer as end points in randomized clinical trials of cancer screening.
Design, Setting, and Participants This meta-analysis included 41 randomized clinical trials of cancer screening conducted in Europe, North America, and Asia published through February 19, 2024. Data extracted included numbers of participants, cancer diagnoses, and cancer deaths in the intervention and comparison groups. For each clinical trial, the effect of screening was calculated as the percentage reduction between the intervention and comparison groups in the incidence of participants with cancer-specific mortality and stage III-IV cancer.
Exposures Randomization to a cancer screening test or to a comparison group in a clinical trial of cancer screening.
Main Outcomes and Measures End points of cancer-specific mortality and incidence of stage III-IV cancer were compared using Pearson correlation coefficients with 95% CIs, linear regression, and fixed-effects meta-analysis.
Results The included randomized clinical trials tested benefits of screening for breast (n = 6), colorectal (n = 11), lung (n = 12), ovarian (n = 4), prostate (n = 4), and other cancers (n = 4). Correlation between reductions in cancer-specific mortality and stage III-IV cancer varied by cancer type ( I 2 = 65%; P = .02). Correlation was highest for trials that screened for ovarian (Pearson ρ = 0.99 [95% CI, 0.51-1.00]) and lung (Pearson ρ = 0.92 [95% CI, 0.72-0.98]) cancers, moderate for breast cancer (Pearson ρ = 0.70 [95% CI, −0.26 to 0.96]), and weak for colorectal (Pearson ρ = 0.39 [95% CI, −0.27 to 0.80]) and prostate (Pearson ρ = −0.69 [95% CI, −0.99 to 0.81]) cancers. Slopes from linear regression were estimated as 1.15 for ovarian cancer, 0.75 for lung cancer, 0.40 for colorectal cancer, 0.28 for breast cancer, and −3.58 for prostate cancer, suggesting that a given magnitude of reduction in incidence of stage III-IV cancer produced different magnitudes of change in incidence of cancer-specific mortality ( P for heterogeneity = .004).
Conclusions and Relevance In randomized clinical trials of cancer screening, incidence of late-stage cancer may be a suitable alternative end point to cancer-specific mortality for some cancer types, but is not suitable for others. These results have implications for clinical trials of multicancer screening tests.
- Editorial Late-Stage Cancer End Points to Speed Cancer Screening Clinical Trials—Not So Fast JAMA
Read More About
Feng X , Zahed H , Onwuka J, et al. Cancer Stage Compared With Mortality as End Points in Randomized Clinical Trials of Cancer Screening : A Systematic Review and Meta-Analysis . JAMA. Published online April 07, 2024. doi:10.1001/jama.2024.5814
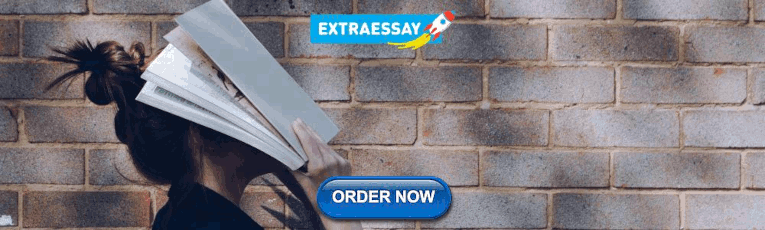
Manage citations:
© 2024
Artificial Intelligence Resource Center
Cardiology in JAMA : Read the Latest
Browse and subscribe to JAMA Network podcasts!
Others Also Liked
Select your interests.
Customize your JAMA Network experience by selecting one or more topics from the list below.
- Academic Medicine
- Acid Base, Electrolytes, Fluids
- Allergy and Clinical Immunology
- American Indian or Alaska Natives
- Anesthesiology
- Anticoagulation
- Art and Images in Psychiatry
- Artificial Intelligence
- Assisted Reproduction
- Bleeding and Transfusion
- Caring for the Critically Ill Patient
- Challenges in Clinical Electrocardiography
- Climate and Health
- Climate Change
- Clinical Challenge
- Clinical Decision Support
- Clinical Implications of Basic Neuroscience
- Clinical Pharmacy and Pharmacology
- Complementary and Alternative Medicine
- Consensus Statements
- Coronavirus (COVID-19)
- Critical Care Medicine
- Cultural Competency
- Dental Medicine
- Dermatology
- Diabetes and Endocrinology
- Diagnostic Test Interpretation
- Drug Development
- Electronic Health Records
- Emergency Medicine
- End of Life, Hospice, Palliative Care
- Environmental Health
- Equity, Diversity, and Inclusion
- Facial Plastic Surgery
- Gastroenterology and Hepatology
- Genetics and Genomics
- Genomics and Precision Health
- Global Health
- Guide to Statistics and Methods
- Hair Disorders
- Health Care Delivery Models
- Health Care Economics, Insurance, Payment
- Health Care Quality
- Health Care Reform
- Health Care Safety
- Health Care Workforce
- Health Disparities
- Health Inequities
- Health Policy
- Health Systems Science
- History of Medicine
- Hypertension
- Images in Neurology
- Implementation Science
- Infectious Diseases
- Innovations in Health Care Delivery
- JAMA Infographic
- Law and Medicine
- Leading Change
- Less is More
- LGBTQIA Medicine
- Lifestyle Behaviors
- Medical Coding
- Medical Devices and Equipment
- Medical Education
- Medical Education and Training
- Medical Journals and Publishing
- Mobile Health and Telemedicine
- Narrative Medicine
- Neuroscience and Psychiatry
- Notable Notes
- Nutrition, Obesity, Exercise
- Obstetrics and Gynecology
- Occupational Health
- Ophthalmology
- Orthopedics
- Otolaryngology
- Pain Medicine
- Palliative Care
- Pathology and Laboratory Medicine
- Patient Care
- Patient Information
- Performance Improvement
- Performance Measures
- Perioperative Care and Consultation
- Pharmacoeconomics
- Pharmacoepidemiology
- Pharmacogenetics
- Pharmacy and Clinical Pharmacology
- Physical Medicine and Rehabilitation
- Physical Therapy
- Physician Leadership
- Population Health
- Primary Care
- Professional Well-being
- Professionalism
- Psychiatry and Behavioral Health
- Public Health
- Pulmonary Medicine
- Regulatory Agencies
- Reproductive Health
- Research, Methods, Statistics
- Resuscitation
- Rheumatology
- Risk Management
- Scientific Discovery and the Future of Medicine
- Shared Decision Making and Communication
- Sleep Medicine
- Sports Medicine
- Stem Cell Transplantation
- Substance Use and Addiction Medicine
- Surgical Innovation
- Surgical Pearls
- Teachable Moment
- Technology and Finance
- The Art of JAMA
- The Arts and Medicine
- The Rational Clinical Examination
- Tobacco and e-Cigarettes
- Translational Medicine
- Trauma and Injury
- Treatment Adherence
- Ultrasonography
- Users' Guide to the Medical Literature
- Vaccination
- Venous Thromboembolism
- Veterans Health
- Women's Health
- Workflow and Process
- Wound Care, Infection, Healing
- Register for email alerts with links to free full-text articles
- Access PDFs of free articles
- Manage your interests
- Save searches and receive search alerts
- Open access
- Published: 13 April 2024
Associations between transport modes and site-specific cancers: a systematic review and meta-analysis
- Win Thu 1 ,
- Alistair Woodward 1 ,
- Alana Cavadino 1 &
- Sandar Tin Tin 1 , 2
Environmental Health volume 23 , Article number: 39 ( 2024 ) Cite this article
44 Accesses
Metrics details
Physical inactivity is a global public health problem. A practical solution would be to build physical activity into the daily routine by using active modes of transport. Choice of transport mode can influence cancer risk through their effects on levels of physical activity, sedentary time, and environmental pollution. This review synthesizes existing evidence on the associations of specific transport modes with risks of site-specific cancers.
Relevant literature was searched in PubMed, Embase, and Scopus from 1914 to 17th February 2023. For cancer sites with effect measures available for a specific transport mode from two or more studies, random effects meta-analyses were performed to pool relative risks (RR) comparing the highest vs. lowest activity group as well as per 10 Metabolic Equivalent of Task (MET) hour increment in transport-related physical activity per week ( ∼ 150 min of walking or 90 min of cycling).
27 eligible studies (11 cohort, 15 case-control, and 1 case-cohort) were identified, which reported the associations of transport modes with 10 site-specific cancers. In the meta-analysis, 10 MET hour increment in transport-related physical activity per week was associated with a reduction in risk for endometrial cancer (RR: 0.91, 95% CI: 0.83–0.997), colorectal cancer (RR: 0.95, 95% CI: 0.91–0.99) and breast cancer (RR: 0.99, 95% CI: 0.89–0.996). The highest level of walking only or walking and cycling combined modes, compared to the lowest level, were significantly associated with a 12% and 30% reduced risk of breast and endometrial cancers respectively. Cycling, compared to motorized modes, was associated with a lower risk of overall cancer incidence and mortality.
Active transport appears to reduce cancer risk, but evidence for cancer sites other than colorectum, breast, and endometrium is currently limited.
Peer Review reports
Introduction
Physical inactivity is a global public health problem, contributing to substantial disease and economic burden worldwide [ 1 , 2 ]. With rapid changes in technology, lifestyle, and habitual environment, people have been less active and more sedentary over the past few decades. Globally, about 1 in 4 adults were not active, i.e., did not meet the World Health Organization (WHO) recommendation of engaging at least 150–300 min of moderate-intensity or 75–150 min of vigorous-intensity aerobic physical activity per week [ 3 ], but the prevalence varied widely within and across countries [ 4 ]. If the current trends continue, it is unlikely that the WHO’s target to reduce physical inactivity by 10% in 2025 will be met.
One practical solution would be to build physical activity into the daily routine by using active modes of transport [ 5 ]. Walking and cycling have been shown to improve health (mainly all-cause mortality, cardiovascular disease, diabetes, and cancer) [ 6 ] and also provide social, economic and environmental benefits [ 7 , 8 ]. Car use, on the other hand, contributes to a significant proportion of daily sedentary time, and the situation is worsening with increasing traffic congestion/delays [ 9 ]; it has been associated with an increased risk of obesity and related outcomes [ 10 ]. Further, exposure to environmental pollutants such as nitrogen dioxide and/or particulate matter could differ across different road users [ 11 ], while it has been shown to increase the risk of certain cancers, particularly lung cancer [ 12 ].
While there is ample evidence linking leisure time physical activity or physical activity in general with a reduced risk for a number of cancer sites [ 13 , 14 ], and sedentary behavior in general with an increased risk [ 15 ], the findings may not be directly applicable to transport-related activity because the context and correlates of activity as well as its frequency, duration and intensity are likely to be different across different domains. We therefore reviewed the existing literature that reported the associations between transport modes and risks of site-specific cancers.
A systematic literature review and meta-analysis was conducted and reported according to the PRISMA guideline (Supplementary file S1 ). The review was not registered.
Search strategy and study selection
Relevant literature was searched from 1914 to 17th February 2023 in PubMed, Scopus, and Embase databases using the relevant search terms such as walking, cycling, car, public transport, commute and cancers. Site-specific cancers known to be associated with physical activity and body weight such as breast, colon, liver, esophageal adenocarcinoma and those associated with environmental factor such as lung and melanoma of skin were also searched (Supplementary file S2 ). The reference lists of systematic reviews on physical activity and cancers were also reviewed. Studies were included if they (1) used cohort, case-control, case-cohort or experimental design, (2) assessed transport modes such as walking, cycling, public transport or car use as the exposures of interest, (3) investigated one or more site-specific cancers, overall cancer incidence and/or mortality as the outcome(s), (4) reported effect measures associated with transport modes, and (5) published the full article in English. Studies that used cross-sectional design or mathematical modeling to estimate health impacts at the population level were excluded. Details of excluded studies after full text review, together with the reasons for exclusion, were provided in the Supplementary file S3 . WT conducted the search and selection, and STT oversaw the process.
Data extraction and study quality assessment
Information about title, first author, year of publication, study name (if available), country, study design, sample size, age range of the participants, follow-up duration (for cohort and case-cohort studies), data collection tool, measurement units for exposure(s), data sources for outcome(s), site-specific cancer assessed, effect measures, and confounders adjusted were extracted in a standardized data collection spreadsheet. The study quality was evaluated using the Newcastle-Ottawa Scale (NOS) [ 16 ], which scores the cohort and case-control studies based on three domains: selection of study groups, comparability of the groups and ascertainment of exposure (case-control studies) or outcome (cohort studies). For the second domain, a point was awarded for adjustment of Body Mass Index (BMI) - to evaluate the direct vs. indirect (through BMI) effect of physical activity on cancer risk, and another point for adjustment of physical activities from other domains - to isolate the effects of transport-related physical activity from other activities. A maximum of nine points were awarded, with a higher score indicating better quality [ 16 ]. For case-cohort studies, the NOS scale for cohort studies was used. WT conducted the data extraction and quality assessment, and STT oversaw the process.
Data analysis
For cancer sites with effect measures available for a specific transport mode from two or more studies, meta-analyses were performed using random effects models. The analyses compared the highest level of active transport such as walking, cycling or mixed mode with the lowest level as reported in the individual studies. Where necessary, the reference category for exposure was changed to the lowest group to facilitate pooling of the risks [ 17 ]. The pooled relative risks (RRs) and 95% CI were presented for breast, endometrial, colorectal and testicular cancers, and overall cancer mortality.
For studies that reported time or MET as measurement units, the dose-response effects were estimated using the trend estimation method proposed by Greenland and Longnecker [ 18 ]. The reported time spent for each mode/category was converted to MET hours (see Supplementary file S4 for conversion values and formulas used). For studies that only reported estimates for categorical exposures, study-specific slopes were calculated from the natural logs of the reported risk estimates across categories and risk estimates per unit change were then estimated. The pooled results were presented per 10 Metabolic Equivalent of Task (MET) hour increment in transport-related physical activity per week ( ∼ 150 min of walking or 90 min of cycling) to align with the WHO’s physical activity recommendation [ 3 ]. This approach enabled us to pool risk estimates from a large number of studies irrespective of how the exposures were assessed (e.g., walking and cycling separately or combined) or categorised. The results were presented for breast, endometrial, colorectal, prostate cancers, and overall cancer mortality.
Meta-analysis was not conducted for the studies that compared active and non-active modes in relation to overall cancer incidence and mortality due to the potential overlap of the study samples.
For meta-analyses involving four or more studies, publication bias was assessed through the visual inspection of funnel plots, Begg’s rank correlation test, and Egger’s regression test for asymmetry. If significant associations were observed, sensitivity analyses were conducted by removing one study at a time from the initial meta-analysis to test the robustness of the results. Where possible, sub-group analyses were performed to assess variability of summary effects across population groups (Western vs. Asian), study design (cohort vs. case-control), measurement units (time vs. MET) and adjustment for BMI (yes vs. no). Metafor [ 19 ] and dosresmeta [ 20 ] R packages were used for meta-analysis and trend estimation. All authors have access to the data.
Of the 11,829 records identified, 27 unique studies (total 34 publications) were included, of which 22 studies (28 records) contributed to the meta-analyses (Fig. 1 ). There were four publications from the Netherlands Cohort Study which reported endometrial [ 21 ], ovarian [ 22 ], prostate [ 23 ], and colorectal [ 24 ] cancers, three publications from United Kingdom Biobank which reported lung [ 25 ], breast and colon [ 26 ], and overall cancer incidence and mortality [ 27 ], two publications from Shanghai Women’s Health Study which reported breast [ 28 ] and overall cancer mortality [ 29 ], and two publications from National Institutes of Health - American Association of Retired Persons Diet and Health Study which reported breast [ 30 ] and endometrial [ 31 ] cancers. Of the included studies, 20 compared the risks between the highest and lowest levels of active transport (e.g., walking, cycling, walking and cycling) and two compared the risk between active and non-active commuting modes. The majority used case-control design ( n = 15), followed by cohort ( n = 11) and case-cohort ( n = 1) designs. Most of the studies were conducted in North America, mainly in the United States (US) ( n = 7), followed by Europe ( n = 5), China ( n = 5), United Kingdom ( n = 4), Australia ( n = 2) and the remaining four studies were from India, Iran, Brazil and Nigeria. (Table 1 )
Almost half of the studies assessed walking and cycling combined, i.e., did not provide the risk estimates for each mode ( n = 13), while others assessed walking and cycling separately ( n = 8), or assessed only one mode (walking: n = 3 and cycling: n = 3). Most studies quantified active transport in terms of time spent (e.g., minutes per day, hours per week) ( n = 14) or MET ( n = 7), but others assessed it in terms of activity status (e.g., yes, no) ( n = 3), or in comparison to car or motorized mode ( n = 2), and distance ( n = 1) (Supplementary file S5 ). The studies reported the risks associated with ten site-specific cancers, most commonly breast ( n = 12), endometrial ( n = 5), and colorectal ( n = 4) cancers (Fig. 2 ). Cancer cases were identified through cancer registries, death registries, pathological reports, or hospital or medical records (Supplementary file S5 ). The NOS score for cohort studies ranged from 5 to 9, with an average score of 6.5, and the score for case-control studies ranged from 4 to 7, with an average score of 5.6 (Table 1 , detailed scoring in Supplementary file S6 , S7 ).
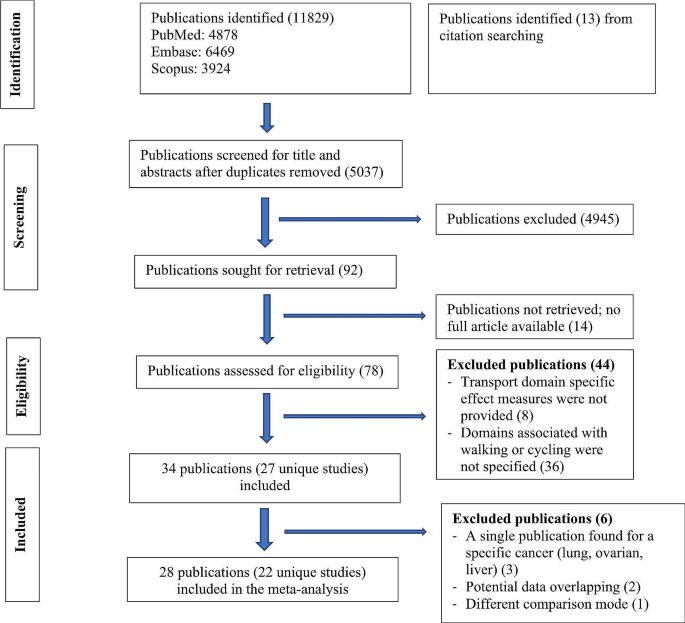
Flow diagram for study selection
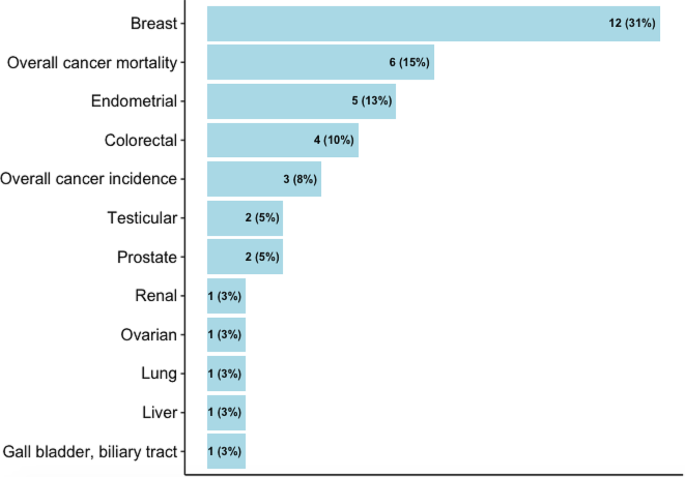
Cancers reported in the studies
Active transport studies
The pooled results were presented for breast, endometrial, colorectal, testicular and prostate cancers, and overall cancer mortality (Fig. 3 ). For other cancers where only one study was identified, the results from the individual study were presented.
Breast cancer
In the meta-analysis of six studies comparing the highest vs. lowest activity group, an inverse association was observed for walking (RR: 0.88, 95% CI: 0.78–0.98), a borderline inverse association for cycling (RR: 0.90, 95% CI: 0.77–1.05) and no significant association for walking and cycling combined (RR: 0.97, 95% CI: 0.84–1.12). 10 MET hour increment in transport-related physical activity per week ( ∼ 150 min of walking or 90 min of cycling) was associated with a marginally reduced risk (RR: 0.99, 95% CI: 0.97–0.996). (Fig. 3 , detailed forest plots in the supplementary file S9 )
Endometrial cancer
The meta-analysis of four studies indicated that walking and cycling combined was associated with a reduced risk of endometrial cancer (RR comparing highest vs. lowest: 0.70, 95% CI: 0.56–0.87; RR per 10 MET hour increment in activity per week: 0.91, 95% CI: 0.83–0.997). (Fig. 3 , detailed forest plots in S9 )
Colorectal cancer
In the meta-analysis of two studies, walking and cycling combined was associated with a reduced risk of colorectal cancer (RR comparing highest vs. lowest: 0.89, 95% CI: 0.78–1.01; RR per 10 MET hour increment in activity per week: 0.95, 95% CI: 0.91–0.99) (Fig. 3 , detailed forest plot in S9 ).
Testicular cancer
In the meta-analysis of two studies, there was no significant association between cycle commuting in adolescence and testicular germ cell cancer (RR comparing highest vs. lowest: 1.23, 95% CI: 0.71–2.13). (Fig. 3 , detailed forest plot in S9 )
Prostate cancer
10 MET hour increment per week for transport related physical activity was associated with a reduced risk of prostate cancer (RR: 0.96, 95% CI: 0.88–1.04) (Fig. 3 , detailed forest plot in S9 ).
Ovarian cancer
Only a case-cohort study assessed the relationship of walking and cycling combined mode with ovarian cancer risk, and reported no significant association (Supplementary file S5 ) [ 22 ].
Liver, gallbladder and biliary tract cancers
A cohort study reported a significant association of commuting physical activity with a reduced risk of gallbladder and biliary tract cancers in women (HR: 0.51, 95% CI: 0.28–0.94) but not in men (HR: 0.92, 95% CI: 0.61–1.37); there was no significant association with liver cancer in both sexes (supplementary file S5 ) [ 49 ].
Renal cancer
A case-control study assessed the association of walking and cycling with risk of renal cell carcinoma in white and black participants in the ages of 20s and 50s, and reported a significant association in the white participants in their 20s (OR comparing lowest vs. highest: 1.42, 95% CI: 1.10–1.83) but not in the black counterparts; the associations were also not significant in both groups in their 50s. (Supplementary file S5 ) [ 50 ].
Overall cancer mortality
In the meta-analyses of two studies, there was an inverse association for cycling only (RR comparing highest vs. lowest: 0.60, 95% CI: 0.34–1.04) and walking and cycling combined (RR: 0.98, 95% CI: 0.86–1.12), and also per 10 MET hour increment in activity per week (RR: 0.97, 95% CI: 0.92–1.01). (Fig. 3 , detailed forest plots in S9 )
In sub-group analyses, similar associations were observed between walking and breast cancer risk in terms of study design (cohort, case-control), population (western vs. Asian), measurement unit (time vs. MET), menopausal status (premenopausal and postmenopausal) and adjustment of BMI (yes vs. no); however, the associations were stronger in studies that adjusted for physical activity from other domains (Supplementary file S10 ). In the leave-one-out analyses assessing walking and breast cancer risk, the results were sensitive to effect sizes from some studies, but this was not the case for walking and cycling combined mode and endometrial cancer (Supplementary file S11 ). There was no evidence for funnel plot asymmetry; Egger’s regression tests and Begg’s ranks correlation tests were not significant (Supplementary file S12 ).
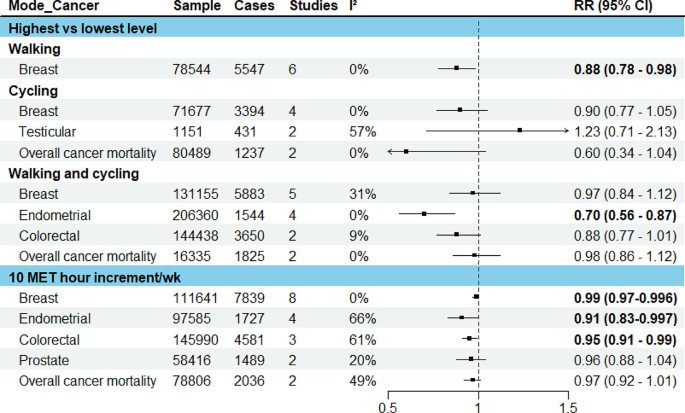
Results of meta-analysis for active transport studies. RE = a random-effects model, MET = Metabolic Equivalent of Task, I 2 = I 2 statistics for heterogeneity, RR = Summary relative risk
Studies comparing active vs. non-active modes for commuting
Four eligible publications were identified, of which three used the data from UK Biobank [ 25 , 26 , 27 ], one used the UK census data [ 51 ]. Three reported the associations for overall cancer incidence and mortality, and one reported the risk associated with lung cancer (Fig. 4 ). In the study that assessed lung cancer using the data from UK Biobank, when compared to automobile only mode, active modes did not show a significant association whereas frequent use of public transport (≥ 5 trips per week) was associated with an increased risk of lung cancer (HR: 1.58, 95% CI: 1.08–2.33) [ 25 ] (Fig. 4 ). In another UK Biobank study, no significant associations were observed for breast and colon cancers, and overall cancer incidence and mortality when more active patterns of commuting (walking, cycling, public transport, either alone or in combination with car) were compared to car only mode [ 26 ].
The results of two studies [ 27 , 51 ] that assessed overall cancer incidence and mortality were not combined as the outcome data was extracted from the same national cancer registry with an overlapped time frame (1991–2011 and 2007–2014), although the exposure information came from different sources (census and UK Biobank). In these studies, compared to private motorized mode or non-active mode, cycling was inversely associated with overall cancer incidence and mortality. Walking and public transport were also inversely associated with overall cancer incidence in the study that used the census data [ 51 ].
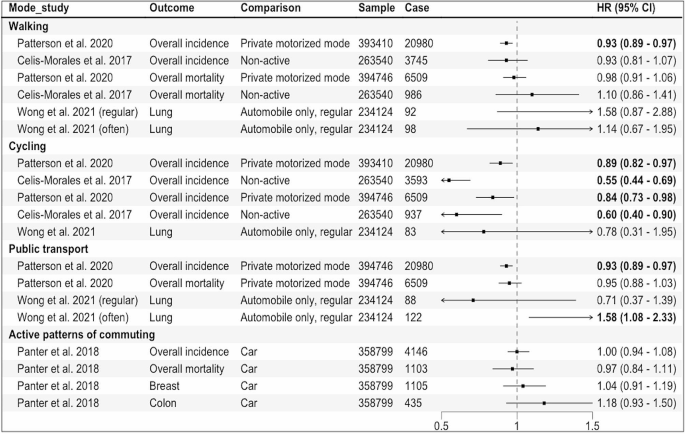
Results of the individual studies comparing active vs. non-active modes for commuting. Private motorized mode = car or motorcycle, Non-active = car or public transport, Active patterns of commuting = any other patterns including walking, cycling, public transport, either alone or in combination with car, HR = Hazard Ratio, regular:1–4, often: ≥5 work-bound trips/week
This review identified 27 studies (34 publications) that reported the associations of specific transport modes, mainly active transport modes, with risks of ten site-specific cancers along with overall cancer incidence and mortality. The most frequently studied cancer sites were breast, endometrium, and colorectum; our meta-analysis showed a reduction in risk of these cancers (1%, 9% and 5%, respectively) per 10 MET hour per week increment in transport-related physical activity ( ∼ 150 min of walking or 90 min of cycling).
We found an inverse association between active transport and risks of breast and endometrial cancers, with similar magnitude of risk reduction observed in previous systematic reviews on physical activity in general [ 55 , 56 ]. While obesity is known to increase post-menopausal but not pre-menopausal breast cancer risk [ 57 ], we found similar results by menopausal status. In contrast, an earlier review did not find any significant association between walking in general and risk of pre- or post-menopausal breast cancer [ 58 ], possibly because compared to walking for transport, walking for leisure or at home generally uses lower energy [ 59 ], and therefore may have less effect on body weight.
The inverse association of active transport with colorectal cancer risk observed in this review is also consistent with the findings from existing reviews on transport-related physical activity [ 60 ] as well as physical activity in general [ 61 ]. While physical activity in general or for leisure has also been associated with a reduced risk of many other cancer sites including liver, gastric, renal and lung [ 13 , 14 ], the evidence related to transport-related physical activity is currently limited.
Mechanisms linking physical activity with specific cancer sites have been proposed, including its effects on sex hormones (breast, endometrial and prostate cancers), insulin sensitivity, glucose metabolism and adipokines (obesity-related cancers), and inflammation and immune function (most cancers) [ 62 ]. For colorectal cancer, another potential mechanism is reduced contact time between carcinogens and bowel mucosa cells due to exercise-induced intestinal mobility [ 63 ].
The overall quality of the included studies, evaluated by NOS score, ranged from 4 to 9, and in general, cohort studies tend to have higher scores compared to case-control studies. The common criteria the studies did not meet include: inadequate exposure assessment, loss to follow-up (cohort studies) and low response rates (case-control studies). While we were not able to undertake subgroup analyses by NOS score due to the limited number of studies available, our subgroup analyses by study design showed similar associations between walking and breast cancer in cohort vs. case-control studies.
To our knowledge, this review represents the first systematic attempt to synthesize the existing evidence on specific transport modes and site-specific cancers. We provided mode-specific summary effects where possible and calculated the dose-response effects for transport-related physical activity, in line with WHO physical activity recommendation. When interpreting the findings, some limitations need to be considered. First, the review may not have included some eligible studies published in languages other than English. Second, due to the limited number of available studies, we were not able to pool the results separately for cohort and case-control studies; however, we conducted sub-group analyses by study design where possible. We were not able to evaluate the non-linear relationship between transport-related physical activity and the risks of site-specific cancers. While a recent systematic review on breast and colon cancers reported a linear relationship with physical activity [ 64 ], others suggested a non-linear relationship between physical activity and cancer risk [ 65 , 66 ]. Further, variations in measurement and categorization of the exposure across the studies make direct comparison of the results between different modes (e.g., walking vs. cycling) difficult. Finally, the majority of the studies included were conducted in high income countries in Europe, UK, and North America, limiting the generalizability of the findings to other populations and low and middle income countries where urbanization and motorization are mainly taking place [ 67 ].
Our findings suggest that transport choices may influence cancer risk, particularly of obesity-related cancers such as breast, colon and endometrial cancers. Breast cancer is the most common cancer in women globally, with an estimated over 2 million new cases (11.7% of all new cases) in 2020, while colon cancer stood at fourth place (over 1 million cases, 6% of total cases) [ 68 ]. The incidence of endometrial cancer also seems to be increasing in many countries particularly in younger women. Our findings indicate that the risks of these cancers can be reduced by meeting the WHO physical activity recommendation through active commuting ( ∼ 150 min of walking or 90 min of cycling per week). Yet, the current evidence is limited in relation to other cancer sites, underlying mechanisms, and potential environmental influences, requiring further exploration.
Given heterogeneity in exposure measurements in the existing studies, harmonizing choice of the assessment tool (e.g., using International Physical Activity Questionnaires that can capture information about all four physical activity domains including transport modes), and reporting the dose-response estimates for each transport mode such as walking and cycling separately rather than a combined mode would enhance comparability of results and provide mode-specific effects. Repeated or regular assessments of exposures/transport modes used throughout the study duration would capture changes and their potential impact on outcomes in cohort studies. Importantly, more research is needed in low and middle-income settings to generate context-specific evidence.
In conclusion, active transport modes appear to reduce cancer risk, but evidence for cancer sites other than colorectum, breast and endometrium is currently limited.
Data availability
No datasets were generated or analysed during the current study.
Lee IM, Shiroma EJ, Lobelo F, Puska P, Blair SN, Katzmarzyk PT. Effect of physical inactivity on major non-communicable diseases worldwide: an analysis of burden of disease and life expectancy. Lancet. 2012;380(9838):219–29. https://doi.org/10.1016/S0140-6736(12)61031-9 .
Article Google Scholar
Ding D, Lawson KD, Kolbe-Alexander TL, et al. The economic burden of physical inactivity: a global analysis of major non-communicable diseases. Lancet. 2016;388(10051):1311–24. https://doi.org/10.1016/S0140-6736(16)30383-X .
World Health Organization. Global status report on physical activity 2022.; 2022.
Guthold R, Stevens GA, Riley LM, Bull FC. Worldwide trends in insufficient physical activity from 2001 to 2016: a pooled analysis of 358 population-based surveys with 1·9 million participants. Lancet Global Health. 2018;6(10):e1077–86. https://doi.org/10.1016/S2214-109X(18)30357-7 .
Berrigan D, Troiano RP, McNeel T, DiSogra C, Ballard-Barbash R. Active transportation increases adherence to activity recommendations. Am J Prev Med. 2006;31(3):210–6. https://doi.org/10.1016/j.amepre.2006.04.007 .
Dinu M, Pagliai G, Macchi C, Sofi F. Active commuting and multiple Health outcomes: a systematic review and Meta-analysis. Sports Med. 2019;49(3):437–52. https://doi.org/10.1007/s40279-018-1023-0 .
Boniface S, Scantlebury R, Watkins SJ, Mindell JS. Health implications of transport: evidence of effects of transport on social interactions. J Transp Health. 2015;2(3):441–6. https://doi.org/10.1016/j.jth.2015.05.005 .
Higgins PAT. Exercise-based transportation reduces oil dependence, carbon emissions and obesity. Envir Conserv. 2005;32(3):197–202. https://doi.org/10.1017/S037689290500247X .
Article CAS Google Scholar
World Health Organization. Global Status Report on Road Safety 2018: Summary.; 2018.
Sugiyama T, Chandrabose M, Homer AR, Sugiyama M, Dunstan DW, Owen N. Car use and cardiovascular disease risk: systematic review and implications for transport research. J Transp Health. 2020;19:100930. https://doi.org/10.1016/j.jth.2020.100930 .
Panchal R, Panagi M, May HR, et al. Personal air pollution exposure during morning commute car and active transport journeys. J Transp Health. 2022;26:101365. https://doi.org/10.1016/j.jth.2022.101365 .
Turner MC, Andersen ZJ, Baccarelli A, et al. Outdoor air pollution and cancer: an overview of the current evidence and public health recommendations. CA Cancer J Clin. 2020;70(6):460–79. https://doi.org/10.3322/caac.21632 .
Moore SC, Lee IM, Weiderpass E, et al. Association of leisure-time physical activity with risk of 26 types of Cancer in 1.44 million adults. JAMA Intern Med. 2016;176(6):816. https://doi.org/10.1001/jamainternmed.2016.1548 .
Rezende LFMD, Sá THD, Markozannes G, et al. Physical activity and cancer: an umbrella review of the literature including 22 major anatomical sites and 770 000 cancer cases. Br J Sports Med. 2018;52(13):826–33. https://doi.org/10.1136/bjsports-2017-098391 .
Hermelink R, Leitzmann MF, Markozannes G, et al. Sedentary behavior and cancer–an umbrella review and meta-analysis. Eur J Epidemiol. 2022;37(5):447–60. https://doi.org/10.1007/s10654-022-00873-6 .
Wells G, Shea B, O’Connell D et al. The Newcastle-Ottawa Scale (NOS) for assessing the quality of nonrandomised studies in meta-analyses. The Newcastle-Ottawa Scale (NOS) for assessing the quality of nonrandomised studies in meta-analyses. https://www.ohri.ca/programs/clinical_epidemiology/oxford.asp .
Taylor K. Wanting a particular reference category in categorical risk data. Data extraction tips for meta-analysis. https://www.cebm.ox.ac.uk/resources/data-extraction-tips-meta-analysis/reference-category-risk-data .
Greenland S, Longnecker MP. Methods for Trend Estimation from Summarized Dose-Response Data, with applications to Meta-Analysis. Am J Epidemiol. 1992;135(11):1301–9. https://doi.org/10.1093/oxfordjournals.aje.a116237 .
Viechtbauer W. Conducting Meta-analyses in R with the metafor Package. J Stat Soft. 2010;36(3). https://doi.org/10.18637/jss.v036.i03 .
Crippa A, Orsini N. Multivariate Dose-Response Meta-Analysis: The dosresmeta R Package. J Stat Soft. 2016;72(Code Snippet 1). https://doi.org/10.18637/jss.v072.c01 .
Schouten LJ, Goldbohm RA, van den Brandt PA, Anthropometry. Physical activity, and Endometrial Cancer Risk: results from the Netherlands Cohort Study. JNCI J Natl Cancer Inst. 2004;96(21):1635–8. https://doi.org/10.1093/jnci/djh291 .
Biesma RG, Schouten LJ, Dirx MJM, Goldbohm RA, van den Brandt PA. Physical activity and risk of ovarian Cancer: results from the Netherlands Cohort Study (the Netherlands). Cancer Causes Control. 2006;17(1):109–15. https://doi.org/10.1007/s10552-005-0422-3 .
Zeegers MPA, Dirx MJM, van den Brandt PA. Physical activity and the risk of prostate Cancer in the Netherlands Cohort Study, results after 9.3 years of follow-up. Cancer Epidemiol Biomarkers Prev. 2005;14(6):1490–5. https://doi.org/10.1158/1055-9965.EPI-04-0771 .
Simons CCJM, Hughes LAE, van Engeland M, Goldbohm RA, van den Brandt PA, Weijenberg MP. Physical activity, occupational sitting time, and Colorectal Cancer Risk in the Netherlands Cohort Study. Am J Epidemiol. 2013;177(6):514–30. https://doi.org/10.1093/aje/kws280 .
Wong JYY, Jones RR, Breeze C, et al. Commute patterns, residential traffic-related air pollution, and lung cancer risk in the prospective UK Biobank cohort study. Environ Int. 2021;155:106698. https://doi.org/10.1016/j.envint.2021.106698 .
Panter J, Mytton O, Sharp S, et al. Using alternatives to the car and risk of all-cause, cardiovascular and cancer mortality. Heart. 2018;104(21):1749–55. https://doi.org/10.1136/heartjnl-2017-312699 .
Celis-Morales CA, Lyall DM, Welsh P, et al. Association between active commuting and incident cardiovascular disease, cancer, and mortality: prospective cohort study. BMJ Published Online April. 2017;19:j1456. https://doi.org/10.1136/bmj.j1456 .
Pronk A, Ji BT, Shu XO, et al. Physical activity and breast cancer risk in Chinese women. Br J Cancer. 2011;105(9):1443–50. https://doi.org/10.1038/bjc.2011.370 .
Matthews CE, Jurj AL, Shu Xo, et al. Influence of Exercise, walking, Cycling, and overall nonexercise physical activity on Mortality in Chinese women. Am J Epidemiol. 2007;165(12):1343–50. https://doi.org/10.1093/aje/kwm088 .
George SM, Irwin ML, Matthews CE, et al. Beyond recreational physical activity: examining Occupational and Household Activity, Transportation Activity, and sedentary behavior in relation to postmenopausal breast Cancer Risk. Am J Public Health. 2010;100(11):2288–95. https://doi.org/10.2105/AJPH.2009.180828 .
Gierach GL, Chang SC, Brinton LA, et al. Physical activity, sedentary behavior, and endometrial cancer risk in the NIH-AARP Diet and Health Study. Int J Cancer. 2009;124(9):2139–47. https://doi.org/10.1002/ijc.24059 .
Luoto R, Latikka P, Pukkala E, Hakulinen T, Vihko V. The effect of physical activity on breast cancer risk: a cohort study of 30,548 women. Eur J Epidemiol. 2000;16(10):973–80. https://doi.org/10.1023/A:1010847311422 .
Gomes MLB, Pinto SS, Domingues MR. Physical activity and breast Cancer: a case-control study in Southern Brazil. Nutr Cancer. 2022;74(1):149–57. https://doi.org/10.1080/01635581.2021.1880607 .
Azubuike SO, Hayes L, Sharp L, Alabi A, Oyesegun RA, McNally R. Physical activity and the risk of breast cancer among Nigerian women. Cancer Epidemiol. 2022;78:102163. https://doi.org/10.1016/j.canep.2022.102163 .
Si S, Boyle T, Heyworth J, Glass DC, Saunders C, Fritschi L. Lifetime physical activity and risk of breast cancer in pre-and post-menopausal women. Breast Cancer Res Treat. 2015;152(2):449–62. https://doi.org/10.1007/s10549-015-3489-x .
Mathew A, Gajalakshmi V, Rajan B, et al. Physical activity levels among urban and rural women in south India and the risk of breast cancer: a case–control study. Eur J Cancer Prev. 2009;18(5):368–76. https://doi.org/10.1097/CEJ.0b013e32832e1c46 .
Steindorf K. Case-control study of physical activity and breast Cancer risk among Premenopausal women in Germany. Am J Epidemiol. 2003;157(2):121–30. https://doi.org/10.1093/aje/kwf181 .
John EM, Horn-Ross PL, Koo J. Lifetime physical activity and breast cancer risk in a multiethnic population: the San Francisco Bay area breast cancer study. Cancer Epidemiol Biomarkers Prev. 2003;12(11 Pt 1):1143–52.
Google Scholar
Matthews CE, Shu XO, Jin F, et al. Lifetime physical activity and breast cancer risk in the Shanghai breast Cancer Study. Br J Cancer. 2001;84(7):994–1001. https://doi.org/10.1054/bjoc.2000.1671 .
Marcus PM, Newman B, Moorman PG, et al. Physical activity at age 12 and adult breast cancer risk (United States). Cancer Causes Control. 1999;10(4):293–302. https://doi.org/10.1023/A:1008971417282 .
Friberg E, Mantzoros CS, Wolk A. Physical activity and risk of Endometrial Cancer: a Population-based prospective cohort study. Cancer Epidemiol Biomarkers Prev. 2006;15(11):2136–40. https://doi.org/10.1158/1055-9965.EPI-06-0465 .
John EM, Koo J, Horn-Ross PL. Lifetime physical activity and risk of Endometrial Cancer. Cancer Epidemiol Biomarkers Prev. 2010;19(5):1276–83. https://doi.org/10.1158/1055-9965.EPI-09-1316 .
Matthews CE, Xu WH, Zheng W, et al. Physical activity and risk of Endometrial Cancer: a report from the Shanghai Endometrial Cancer Study. Cancer Epidemiol Biomarkers Prev. 2005;14(4):779–85. https://doi.org/10.1158/1055-9965.EPI-04-0665 .
Mahmood S, English DR, MacInnis RJ, et al. Domain-specific physical activity and the risk of colorectal cancer: results from the Melbourne Collaborative Cohort Study. BMC Cancer. 2018;18(1):1063. https://doi.org/10.1186/s12885-018-4961-x .
Hou L. Commuting physical activity and risk of Colon cancer in Shanghai, China. Am J Epidemiol. 2004;160(9):860–7. https://doi.org/10.1093/aje/kwh301 .
Littman AJ, Doody DR, Biggs ML, Weiss NS, Starr JR, Schwartz SM. Physical activity in adolescence and testicular germ cell cancer risk. Cancer Causes Control. 2009;20(8):1281–90. https://doi.org/10.1007/s10552-009-9347-6 .
Coldman AJ, Elwood JM, Gallagher RP. Sports activities and risk of testicular cancer. Br J Cancer. 1982;46(5):749–56. https://doi.org/10.1038/bjc.1982.267 .
Hosseini M, SeyedAlinaghi S, Mahmoudi M, McFarland W. A case-control study of risk factors for prostate cancer in Iran. Acta Med Iran. 2010;48(1):61–6.
Pang Y, Lv J, Kartsonaki C, et al. Association of physical activity with risk of hepatobiliary diseases in China: a prospective cohort study of 0.5 million people. Br J Sports Med. 2021;55(18):1024–33. https://doi.org/10.1136/bjsports-2020-102174 .
Xiao Q, Liao L, Matthews CE, et al. Physical activity and renal cell carcinoma among black and white americans: a case-control study. BMC Cancer. 2014;14(1):707. https://doi.org/10.1186/1471-2407-14-707 .
Patterson R, Panter J, Vamos EP, Cummins S, Millett C, Laverty AA. Associations between commute mode and cardiovascular disease, cancer, and all-cause mortality, and cancer incidence, using linked Census data over 25 years in England and Wales: a cohort study. Lancet Planet Health. 2020;4(5):e186–94. https://doi.org/10.1016/S2542-5196(20)30079-6 .
Sahlqvist S, Goodman A, Simmons RK, et al. The association of cycling with all-cause, cardiovascular and cancer mortality: findings from the population-based EPIC-Norfolk cohort. BMJ Open. 2013;3(11):e003797. https://doi.org/10.1136/bmjopen-2013-003797 .
Autenrieth CS, Baumert J, Baumeister SE, et al. Association between domains of physical activity and all-cause, cardiovascular and cancer mortality. Eur J Epidemiol. 2011;26(2):91–9. https://doi.org/10.1007/s10654-010-9517-6 .
Batty GD, Shipley MJ, Marmot M, Smith GD. Physical activity and cause-specific mortality in men: further evidence from the Whitehall study. Eur J Epidemiol. 2001;17(9):863–9. https://doi.org/10.1023/A:1015609909969 .
Wu Y, Zhang D, Kang S. Physical activity and risk of breast cancer: a meta-analysis of prospective studies. Breast Cancer Res Treat. 2013;137(3):869–82. https://doi.org/10.1007/s10549-012-2396-7 .
Moore SC, Gierach GL, Schatzkin A, Matthews CE. Physical activity, sedentary behaviours, and the prevention of endometrial cancer. Br J Cancer. 2010;103(7):933–8. https://doi.org/10.1038/sj.bjc.6605902 .
García-Estévez L, Cortés J, Pérez S, Calvo I, Gallegos I, Moreno-Bueno G. Obesity and breast Cancer: a paradoxical and controversial relationship influenced by Menopausal Status. Front Oncol. 2021;11:705911. https://doi.org/10.3389/fonc.2021.705911 .
Chan DSM, Abar L, Cariolou M, et al. World Cancer Research Fund International: continuous update project—systematic literature review and meta-analysis of observational cohort studies on physical activity, sedentary behavior, adiposity, and weight change and breast cancer risk. Cancer Causes Control. 2019;30(11):1183–200. https://doi.org/10.1007/s10552-019-01223-w .
Compedium of Physical Activities. 17-Walking. Accessed August 3. 2023. https://sites.google.com/site/compendiumofphysicalactivities/Activity-Categories/walking?authuser=0 .
Mahmood S, MacInnis RJ, English DR, Karahalios A, Lynch BM. Domain-specific physical activity and sedentary behaviour in relation to colon and rectal cancer risk: a systematic review and meta-analysis. Int J Epidemiol. 2017;46(6):1797–813. https://doi.org/10.1093/ije/dyx137 .
Samad AKA, Taylor RS, Marshall T, Chapman MAS. A meta-analysis of the association of physical activity with reduced risk of colorectal cancer. Colorect Dis. 2005;7(3):204–13. https://doi.org/10.1111/j.1463-1318.2005.00747.x .
McTiernan A. Mechanisms linking physical activity with cancer. Nat Rev Cancer. 2008;8(3):205–11. https://doi.org/10.1038/nrc2325 .
Peters HPF. Potential benefits and hazards of physical activity and exercise on the gastrointestinal tract. Gut. 2001;48(3):435–9. https://doi.org/10.1136/gut.48.3.435 .
Diao X, Ling Y, Zeng Y, et al. Physical activity and cancer risk: a dose-response analysis for the global burden of Disease Study 2019. Cancer Commun. 2023;43(11):1229–43. https://doi.org/10.1002/cac2.12488 .
Garcia L, Pearce M, Abbas A, et al. Non-occupational physical activity and risk of cardiovascular disease, cancer and mortality outcomes: a dose–response meta-analysis of large prospective studies. Br J Sports Med. 2023;57(15):979–89. https://doi.org/10.1136/bjsports-2022-105669 .
Li T, Wei S, Shi Y, et al. The dose–response effect of physical activity on cancer mortality: findings from 71 prospective cohort studies. Br J Sports Med. 2016;50(6):339–45. https://doi.org/10.1136/bjsports-2015-094927 .
Unite, Nations. Department of Economic and Social Affair. World urbanization prospects: the 2018 revision. United Nations; 2019.
Sung H, Ferlay J, Siegel RL, Cancer, et al. J Clin. 2021;71(3):209–49. https://doi.org/10.3322/caac.21660 .
Download references
Acknowledgements
STT is supported by Sir Charles Hercus Health Research Fellowship from the Health Research Council of New Zealand (Ref: 23/051).
STT is supported by Sir Charles Hercus Health Research Fellowship from the Health Research Council of New Zealand (Ref: 23/051). The funder had no role in the study design, data collection, data analysis, data interpretation, writing of the report, approval of the manuscript, or decision to submit the manuscript for publication.
Author information
Authors and affiliations.
Epidemiology and Biostatistics, School of Population Health, University of Auckland, Auckland, New Zealand
Win Thu, Alistair Woodward, Alana Cavadino & Sandar Tin Tin
Cancer Epidemiology Unit, Oxford Population Health, University of Oxford, Oxford, UK
Sandar Tin Tin
You can also search for this author in PubMed Google Scholar
Contributions
STT, AW, WT designed the study. STT supervised the study. WT conducted literature search, data analysis and wrote the original draft with critical inputs from STT, AC, and AW. All authors had full access to all the data in the study and had final responsibility for the decision to submit for publication. More than one author has directly accessed and verified the underlying data reported in the manuscript.
Corresponding author
Correspondence to Sandar Tin Tin .
Ethics declarations
Ethics approval and consent to participate.
Not applicable.
Consent for publication
Competing interests.
The authors declare no competing interests.
Additional information
Publisher’s note.
Springer Nature remains neutral with regard to jurisdictional claims in published maps and institutional affiliations.
Electronic supplementary material
Below is the link to the electronic supplementary material.
Supplementary Material 1
: Supplementary file S1 PRISMA checklist. Supplementary file S2 Literature search strategy. Supplementary file S3 List of excluded full texts with reasons. Supplementary file S4 Metabolic Equivalent of Task (MET) values used and MET hour per week conversion formulas. Supplementary file S5 Measurement units, effect measures and covariates included in the studies. Supplementary file S6 Newcastle-Ottawa Score of the studies (cohort studies). Supplementary file S7 Newcastle-Ottawa Score of the studies (case control studies). Supplementary file S8 Risks estimates used in the meta-analyses (separate excel sheet). Supplementary file S9 Forest plots. Supplementary file S10 Sub-group and covariates adjustment analyses. Supplementary file S11 Sensitivity analysis. Supplementary file S12 Funnel plots
Rights and permissions
Open Access This article is licensed under a Creative Commons Attribution 4.0 International License, which permits use, sharing, adaptation, distribution and reproduction in any medium or format, as long as you give appropriate credit to the original author(s) and the source, provide a link to the Creative Commons licence, and indicate if changes were made. The images or other third party material in this article are included in the article’s Creative Commons licence, unless indicated otherwise in a credit line to the material. If material is not included in the article’s Creative Commons licence and your intended use is not permitted by statutory regulation or exceeds the permitted use, you will need to obtain permission directly from the copyright holder. To view a copy of this licence, visit http://creativecommons.org/licenses/by/4.0/ . The Creative Commons Public Domain Dedication waiver ( http://creativecommons.org/publicdomain/zero/1.0/ ) applies to the data made available in this article, unless otherwise stated in a credit line to the data.
Reprints and permissions
About this article
Cite this article.
Thu, W., Woodward, A., Cavadino, A. et al. Associations between transport modes and site-specific cancers: a systematic review and meta-analysis. Environ Health 23 , 39 (2024). https://doi.org/10.1186/s12940-024-01081-3
Download citation
Received : 20 November 2023
Accepted : 08 April 2024
Published : 13 April 2024
DOI : https://doi.org/10.1186/s12940-024-01081-3
Share this article
Anyone you share the following link with will be able to read this content:
Sorry, a shareable link is not currently available for this article.
Provided by the Springer Nature SharedIt content-sharing initiative
- Transport modes
- Active transport
- Site-specific cancers
- Systematic review
- Meta-analysis
Environmental Health
ISSN: 1476-069X
- General enquiries: [email protected]
- Open access
- Published: 11 April 2024
Anatomical variants of the intercostobrachial nerve and its preservation during surgery, a systematic review and meta-analysis
- Roberto Cirocchi 1 ,
- Matteo Matteucci 2 ,
- Justus Randolph 3 ,
- Francesca Duro 1 ,
- Luca Properzi 1 ,
- Stefano Avenia 1 ,
- Bruno Amato 4 ,
- Ruggiero Iandoli 5 ,
- Giovanni Tebala 6 ,
- Carlo Boselli 1 ,
- Piero Covarelli 1 &
- Paolo Sapienza 7
World Journal of Surgical Oncology volume 22 , Article number: 92 ( 2024 ) Cite this article
89 Accesses
Metrics details
The anatomic variants of the intercostobrachial nerve (ICBN) represent a potential risk of injuries during surgical procedure such as axillary lymph node dissection and sentinel lymph node biopsy in breast cancer and melanoma patients. The aim of this systematic review and meta-analysis was to investigate the different origins and branching patterns of the intercostobrachial nerve also providing an analysis of the prevalence, through the analysis of the literature available up to September 2023.
Materials and methods
The protocol for this study was registered on PROSPERO (ID: CRD42023447932), an international prospective database for reviews. The PRISMA guideline was respected throughout the meta-analysis. A systematic literature search was performed using PubMed, Scopus and Web of Science. A search was performed in grey literature through google.
We included a total of 23 articles (1,883 patients). The prevalence of the ICBN in the axillae was 98.94%. No significant differences in prevalence were observed during the analysis of geographic subgroups or by study type (cadaveric dissections and in intraoperative dissections). Only five studies of the 23 studies reported prevalence of less than 100%. Overall, the PPE was 99.2% with 95% Cis of 98.5% and 99.7%. As expected from the near constant variance estimates, the heterogeneity was low, I 2 = 44.3% (95% CI 8.9%−65.9%), Q = 39.48, p = .012. When disaggregated by evaluation type, the difference in PPEs between evaluation types was negligible. For cadaveric dissection, the PPE was 99.7% (95% CI 99.1%–100.0%) compared to 99.0% (95% CI 98.1%–99.7%).
Conclusions
The prevalence of ICBN variants was very high. The dissection of the ICBN during axillary lymph-node harvesting, increases the risk of sensory disturbance. The preservation of the ICBN does not modify the oncological radicality in axillary dissection for patients with cutaneous metastatic melanoma or breast cancer. Therefore, we recommend to operate on these patients in high volume center to reduce post-procedural pain and paresthesia associated with a lack of ICBN variants recognition.
Introduction
The Intercostobrachial nerve (ICBN) emerges from the second intercostal space and it traverses the axilla horizontally [ 1 , 2 ]. Then it perforates the deep fascia of the arm, providing the sensory supply to the upper medial region of the arm [ 3 ]. The anatomy of ICBN represents a potential risk of unintentional injuries during routine surgical procedures such as axillary lymph node dissection (ALND) and sentinel lymph node biopsy (SLNB) [ 4 ].
Breast cancer stands as the most prevalent malignant in women throughout the world, affecting almost 12% of women in Western countries [ 5 ]. The ICBN seems to be frequently damaged during mastectomy procedures and other techniques such as ALND (Axillary Lymph Node Dissection) and SLNB (Sentinel Lymph Node Biopsy); some studies suggest that injuring ICBN could play a role in Persistent Pain After Breast Cancer Treatment (PPBCT) and reduction of sensory function in the affected area [ 1 , 3 ]. Additionally, the possibility of injury of the ICBN should also be considered in axillary surgery for melanoma [ 6 ].
Researchers have demonstrated that ICBN preservation significantly reduce post-procedural paresthesia and improve quality of life after the treatment for breast cancer and axillary surgery for melanoma. In fact, damaging ICBN or one of its primary branches could represents a cause of dysesthesia, paresthesia and chronic pain in these patients. Surgeons need to be accurate during the exploration of the axillary region, as the initial divisions and the connections between the ICBN and the brachial plexus may be damaged [ 3 ]. The configuration of the ICBN has an important variability, showing multiple points of origin, patterns of division, and connections with other branches. If surgeons could know the real frequency, the characteristics of these division and the origin models of ICBN they could reduce the ICBN lesions and the post operatory morbility [ 3 , 4 ].
The aims of this systematic review and meta-analysis are to assess the prevalence, the different origins and branching patterns of the intercostobrachial nerve, analyzing the literature available up to the present day.
Search strategy
The protocol for this study was registered on PROSPERO (ID: CRD42023447932), an international prospective database for reviews.
The PRISMA (preferred reporting items for systematic reviews and meta-analyses) guideline was respected throughout the meta-analysis [ 7 ]. The identification of articles to be included in the meta-analysis was carried out with searches up to September 2023 in the following databases: PubMed, Scopus and Web of Science. The search strategy performed in PubMed is presented as follows: nerve and Intercostobrachial or nervus intercostobrachialis. No date or language restrictions were applied. Identification of additional studies eligible for the meta-analysis was performed by searching the references of all included articles.
Evaluation of inclusion criteria
Eligibility for inclusion in this systematic review and meta-analysiss was performed by M.M. and R.C. All intraoperative or cadaveric studies reporting extractable prevalence data on the origin or branching of ICBN were included. Exclusion criteria included: case reports, case series, letters to the editor, or conference abstracts.
Data extraction
Data from the included studies were extracted by R.C. and M.M. For each article, the following information have been extracted: first author and year of publication, nation of study, sample size (number of patients and number of axillae analyzed), time of enrollment, type of study, type of evaluation (cadaveric or surgical), type of surgical treatment, prevalence of ICBN, origin of ICBN, mode of branching of ICBN. All selected full text articles were evaluated by quality assessment and analysis of the risk of bias using the Anatomical Quality Assurance (AQUA) [Henry, B.M. et al. 2017 [ 8 ]].
Study identification
The initial literature search identified 497 articles. Following the removal of duplicates and primary screening, 43 articles were assessed as full text for eligibility in the meta-analysis records. Finally, we included a total of 23 articles [ 2 , 3 , 4 , 6 , 9 , 10 , 11 , 12 , 13 , 14 , 15 , 16 , 17 , 18 , 19 , 20 , 21 , 22 , 23 , 24 , 25 , 26 , 27 ] selected by eliminating articles with incomplete information and articles that used different classification (Fig. 1 ).
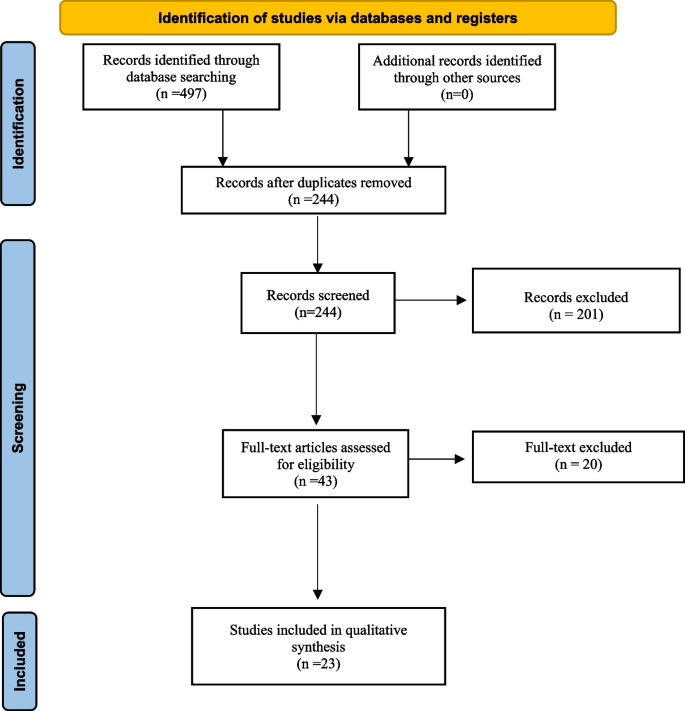
Prisma flow diagram of studies included
Characteristics of the included studies
The systematic review and meta-analysis include twenty-three studies published between 1999 and 2023. Six studies were conducted on cadaveric samples [ 9 , 15 , 16 , 23 , 27 , 28 ], and seventeen were performed intraoperatively [ 2 , 3 , 4 , 6 , 10 , 11 , 12 , 13 , 14 , 17 , 18 , 19 , 20 , 21 , 22 , 24 , 25 , 26 ]. The studies exhibit a broad geographic distribution, with 10 studies from Asia [ 3 , 4 , 9 , 10 , 11 , 12 , 16 , 18 , 21 , 28 ], 6 from Europe [ 6 , 14 , 19 , 20 , 25 , 26 ], 4 from South America [ 2 , 15 , 22 , 24 ], and one each from North America [ 23 ], Africa [ 17 ], and Australia [ 27 ]. Among the twenty-three studies, 1,636 patients were included, and 1,883 axillae were evaluated (494 from cadaveric dissections and 1,389 from intraoperative dissections): 765 from Asians, 570 from Europeans, 318 from South Americans, 200 from North Americans, 30 from Africans, and 28 from Australians (Table 1 ).
Quality valutation of the studies included
The AQUA tool probes for potential risk of bias in 5 studies domains (objectives and subject characteristics, study design, methodology characterization, descriptive anatomy and reporting of results) (Henry, B.M. et al. 2017 [ 8 ]). The risk of bias within each domain is normally categorized as “Low”, “High” or “Unclear”. Twenty-two of the studies included showed low risk in domain one (Objectives and Subject characteristics), ten studies showed high risk of bias in domain three (Methodology characterization), mainly because there is an important reduction of possibility of studying anatomy during an intervention. A summary of the assessment of quality and risk of bias by the AQUA tool is displayed in the Fig. 2 .
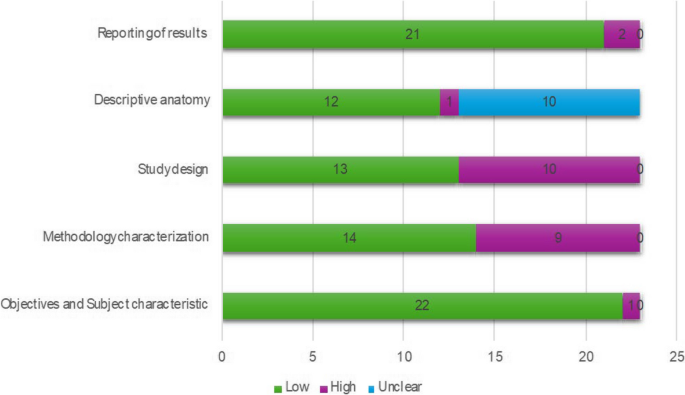
Assessment of quality and risk of bias by the AQUA tool
Statistical methods
For the primary outcome—prevalence of the ICBN—pooled prevalence estimates (PPES) and their 95% confidence intervals are reported using MetaXL software (V. 5.3). We used a DerSimonian and Larid random effects model with a double arcsin transformation, normalized prevalence, and a 0.5 continuity correction. Heterogeneity was investigated through the I 2 statistics, Cochrane’s Q statistic, and a visual analysis of forest plots and funnel plots. In addition, we examined evaluation type (cadaveric dissection or intraoperative dissection) and geographic region of the first author’s affiliation (Africa, Americas, Asia, Oceania, or Europe) as factors. A leave-one-out sensitivity analysis was conducted to examine the effect of outlying studies. Funnel plots and Doi plots were used to investigate possible sources of bias—including publication bias. The leave-one-out sensitively analyses yielded PPEs from 99.3% with the Andersen 2014 [ 19 ] study excluded to 99.1% with the Loukas et al. 2016 [ 23 ] study excluded.
There was great variety in the outcome categories that authors used in the secondary outcomes: this is the reason why a single multicategory pooled prevalence estimate was not possible for any secondary outcomes. Furthermore, it was not feasible to report on the PPEs for tens of secondary outcomes if each of the outcome categories were reported as individual binary outcomes. Therefore, we descriptively report the secondary outcomes using only raw, marginal proportions.
Primary outcome
Prevalence of the icbn.
All studies reported data on the prevalence of the ICBN (1,883 axillae). The overall total prevalence of the ICBN in the axillae was 98.94% (1,863 ICBN). No significant differences in prevalence were observed during the analysis of geographic subgroups [99.35% in Asians (760 ICBN), 97.54% in Europeans (556 ICBN), 99.68% in South Americans (317 IBCN), 100% in North Americans (200 IBCN), 100% in Africans (30 ICBN), and 100% in Australians (28 IBCN)] or by study type [99.8% (93.75–100%) in cadaveric dissections (493 ICBN) and 98.63% (94.69–100%) in intraoperative dissections (1,370 ICBN)] (Fig. 3 ).
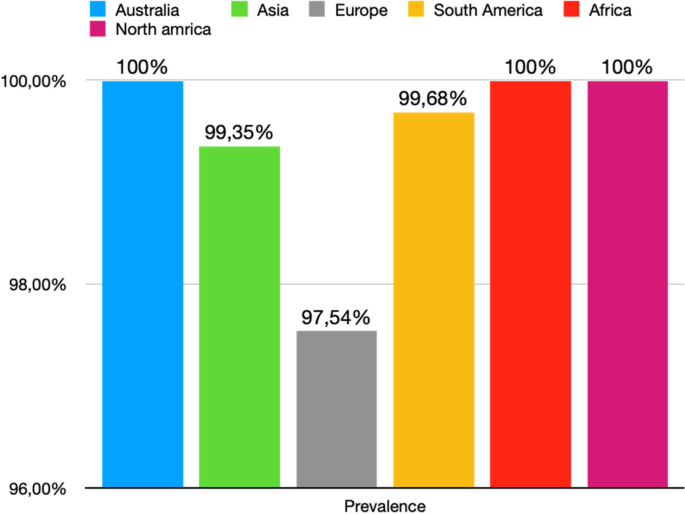
Prevalence of ICBN for continent
Figure 4 is a forest plot shows that the prevalence estimates for the ICBN had a near constant variance of 100%. Only five studies of the 23 studies reported prevalences of less than 100%. Overall, the PPE was 99.2% with 95% CIs of 98.5% and 99.7%. As expected from the near constant variance estimates, the heterogeneity was low, I 2 = 44.3% (95% CI 8.9%–65.9%), Q = 39.48, p = .012. The Doi plot showed minor asymmetry (Fig. 5 ) and the funnel plot (Fig. 6 ) as indicative of an outcome with near constant variance. Rather than a random display of data points within a funnel pattern, there was a line of studies with a prevalence of 100% and the other data points to the left of that line. When disaggregated by evaluation type, the difference in PPEs between evaluation types was negligible (Fig. 7 ). For cadaveric dissection, the PPE was 99.7% (95% CI 99.1%–100.0%) compared to 99.0% (95% CI 98.1%–99.7%). There were differences in PPEs between subgroups was also negligible for geographic region (Fig. 8 ).: Africa = 99.2% (95% CI 94.3%–100.0%), Americas = 99.7% (95% CI 99.0%–100.0%), Asia = 99.3% (95% CI 98.6%–99.9%), Oceania = 99.2% (95% CI 93.9%–100.0%), and Europe = 98.4% (95% CI 95.9%–100.0%).
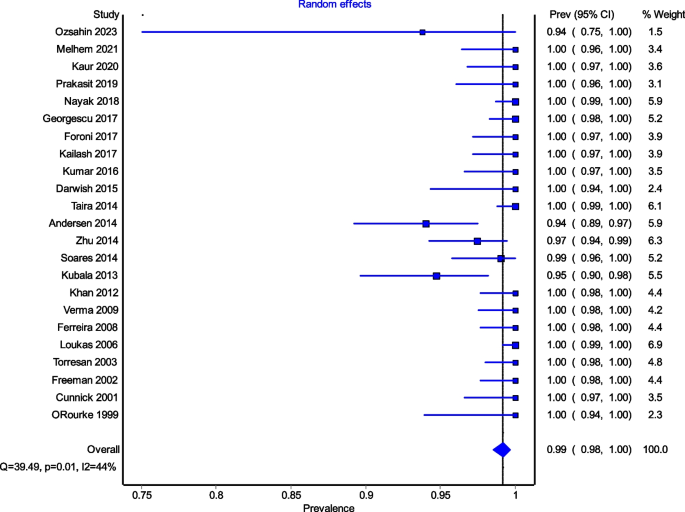
Prevalence estimates for the ICBN
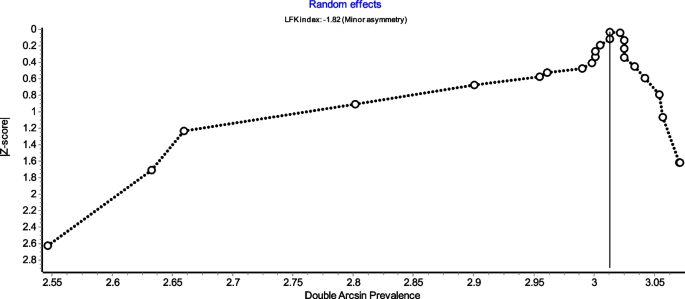
Funnel plot
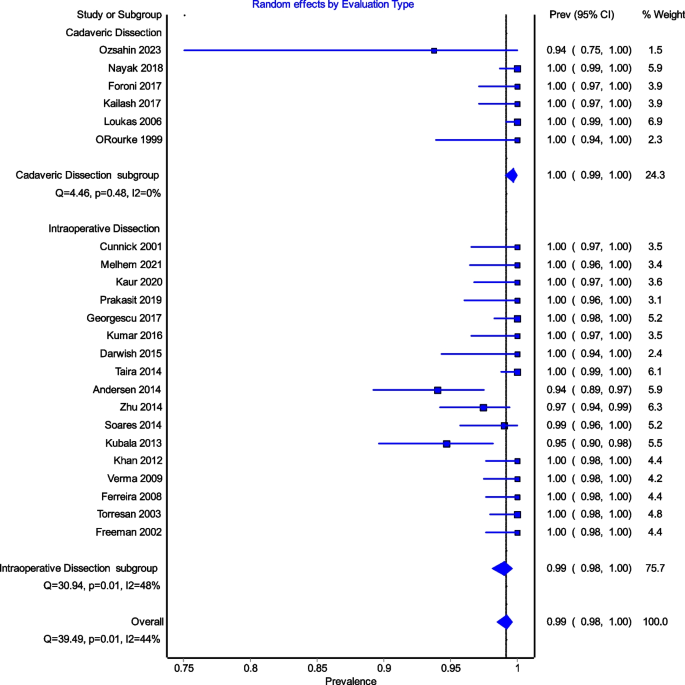
Random effects by Evaluation type
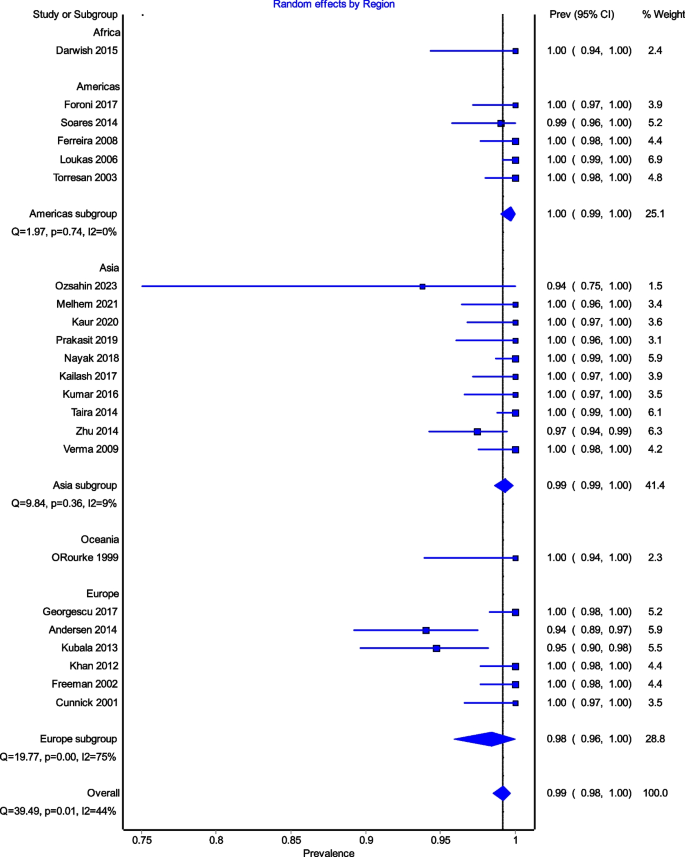
Random effects by Region
Secondary outcomes
Origin of the intercostobrachial nerve.
Eight studies (747 ICBN) reported data on the origin of the ICBN. The most common origin was at the T2 vertebral level, accounting for 81.79% of cases (611 ICBN), followed by T2-T3 at 8.17% (61 ICBN), T3 at 4.55% (34 ICBN), and T1-T2 at 2.9% (24 ICBN); much rarer origins were T1 at 1.07% (8 ICBN) and T1, T2, T3 at 1.2% (9 ICBN). To further clarify, combined origins were indicated when two separate roots were observed to merge into a common ICBN (Table 2 ). A total of 12 studies (1,060 ICBNs) reported data on the ICBN branching pattern. Table 3 provides detailed information on the ramifications of the ICBN. The ICBN most appeared as a single trunk in 51.6% of cases (547 ICBN), followed by the bifurcation pattern at 29.71% (315 IBCN), and the multiple branch pattern at 14.81% (157 IBCN). Subgroup analysis did not reveal significant differences.
Surgical analysis
Intraoperative preservation of the intercostobrachial nerve.
Sixteen studies (1,311 ICBN) reported data on the preservation of the ICBN during axillary dissection for breast cancer (15 studies) and melanoma (1 study). It was found that the ICBN was completely preserved in 63.39% (34-95.65%) (831 ICBN) and partially preserved in 4.27% (15–29%) (56 ICBN). Only three studies reported the reasons for ICBN division (52 ICBN): accidental injury (53.85%, 28 ICBN), necessity dissection due to nerve involvement in lymph node clusters (30.77%, 16 ICBN), necessity dissection due to the nerve hindering proper access to the axillary cavity (15.38%, 8 ICBN).
Sensorial analysis
Pain in patients with intercostobrachial nerve section.
Postoperative pain was assessed at the time of discharge (7 studies, 209 ICBN) and after 3 months (4 studies, 92 ICBN). Postoperative pain was present in 38.75% at discharge and 46.74% after 3 months from the procedure (Fig. 9 ).
Hypoesthesia in patients with intercostobrachial nerve section
The decrease in postoperative sensitivity was evaluated at the time of discharge (5 studies, 169 ICBN) and after 3 months (4 studies, 105 ICBN). Postoperative hypoesthesia was present in 62.13% at the time of discharge and 51.42% after 3 months from the procedure (Fig. 9 ).
Paresthesia in patients with intercostobrachial nerve section
The presence of postoperative paresthesia was assessed at the time of discharge (9 studies, 283 ICBN) and after 3 months (2 studies, 42 ICBN). Postoperative paresthesia was present in 40.99% at the time of discharge and 10.04% after 3 months from the procedure (Fig. 9 ).
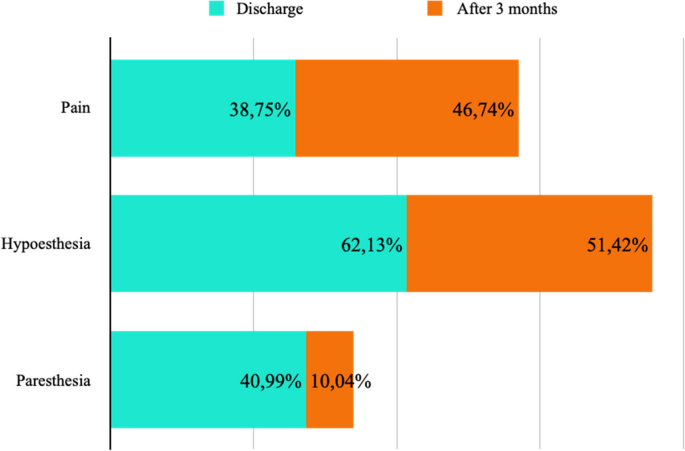
Patients with ICBN section
Pain in patients with intact intercostobrachial nerve
Postoperative pain was assessed at the time of discharge (7 studies, 288 ICBN) and after 3 months (4 studies, 103 ICBN). Postoperative pain was present in 31.59% at discharge and 12.62% after 3 months (Fig. 10 ).
Hypoesthesia in patients with intact intercostobrachial nerve
The decrease in postoperative sensitivity was evaluated at the time of discharge (5 studies, 228 ICBN) and after 3 months (4 studies, 123 ICBN). Postoperative hypoesthesia was present in 45.17% at discharge and 18.70% after 3 months (Fig. 10 ).
Paresthesia in patients with intact intercostobrachial nerve
The presence of postoperative paresthesia was assessed at the time of discharge (8 studies, 321 ICBN) and after 3 months (3 studies, 57 ICBN). Postoperative paresthesia was present in 29.6% at discharge and 22.8% after 3 months (Fig. 10 ).
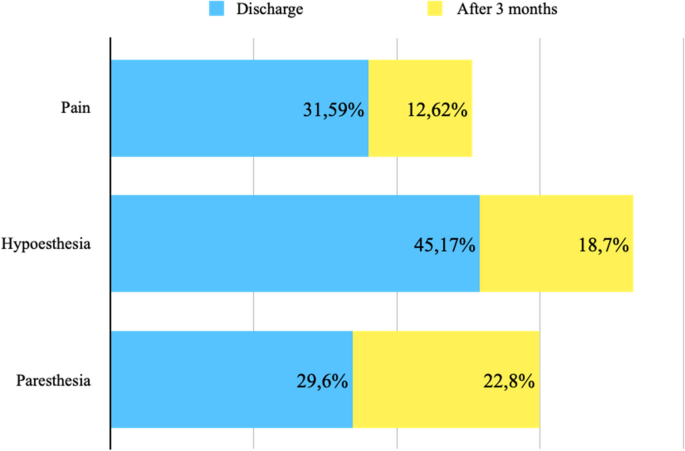
Patients with ICBN preservation
The intercostobrachial nerve (ICBN) is an anatomical structure with a high variability in origin and branching pattern. The ICBN is a branch of the second intercostal nerve: therefore, special attention should be paid to the area of the second intercostal space, where the origin of the ICBN is most likely (90.6%). However, the ICBN could present occasional contribution also from the third intercostal nerve and it can be identified in an anterior position during the exposure of the thoracic and thoracodorsal long nerves. The ICBN is at high risk of injury during operative procedures into the axilla. ICBN fiber’s injury has been associated with post-procedural pain, paresthesia and with a reduction of the quality of life.
The evaluation of prevalence of ICBN might have an impact on surgical plan and patient outcomes: in fact, lymphedema, motor and/or sensor alterations of the arm have an impact on the quality of life of patients who underwent to operative procedure into the axillary region. Moderate or severe post-operative pain is experienced by 50% of patients after breast surgery (Andersen; Besic).
The ICBN should always be preserved. However, current guidelines do not provide specific recommendations regarding the preservation or sacrifice of the ICBN during axillary lymph-node dissection or sentinel lymph-node biopsy. A study conducted by Henry et al. in 2017 [ 29 ] showed that the ICBN is a variable structure at risk for injury during operative procedures of the axilla and due to the postoperative pain and paresthesia experienced by patients following injury, surgeons need to exercise caution and need to preserve the ICBN. Another study, conducted by Warrier et al. in 2014 [ 30 ] showed that the incidence of sensory disorder was lower in case of preservation of ICBN compared with the division of the ICBN.
On the other hand, in three different studies, conducted respectively by Abdullah et al. 1998 [ 31 ], Salmon et al. 1998 [ 32 ] and Torresan et al. 2003 [ 24 ], showed that patients who presented sensory distur in the immediate post-operative period may have a resolution of the symptoms and patients who may not notice any sensory disturbance initially may develop it at a later stage.
The ICBN can be damaged for a variety of reasons, from traction to transection. In addition, damaged nerves can develop neuromas that can further complicate the patient’ symptoms.
Our systematic review and meta-analysis showed that the overall prevalence of ICBN was 98.94% and that more frequently it exists as a single trunk (51.6%) originating from the T2 vertebral level (81.79%). The branching subgroups are differently based on the type of the study (cadaveric or operational). In fact, we noticed that the ICBNs examined in cadavers (99.8%) had a bifurcation rate more than double than those evaluated during operation (98.63%).
From these differences we deduced two different hypotheses. Firstly, the limited intraoperative field of view and the inability to freely dissect tissue without consequences may limit the in vivo identification of the nerve branches. These differences may help to explain the reason why post-operative complications might be present even if nerves are successfully identified and protected. Kumar et al. 2016 [ 3 ] noted that, six months after surgery, 20% of patients who had successfully preserved ICBN still had numbness and paresthesia. The ICBN may also be damaged due to stretching by retractors or other intraoperative stresses on nerve fibers. Secondly, ICBN might have a propensity to bifurcate unequally when analyzing cadaveric or intraoperative studies (63.4% unequal bifurcation versus 36.6% equal bifurcation). Therefore, surgeons can easily identify the larger trunk, but a failure to identify the smaller branch puts it at high risk of operative injury.
Our meta-analysis was limited by several factors related to inconsistent reporting and small sample sizes. Some studies presented the origins of the first or third intercostal nerve as separate data. It was unclear whether these origins were duplications or simply contributing fibers and therefore were excluded from the present analysis. In addition, we observed a high heterogeneity which might have the result of an inherent variable nature of the ICBN course. Another limitation of our study is the lack of data regarding the preservation of the intercostobrachial nerve during SLNB. However, larger studies are needed to demonstrate this observation.
Furthermore, in our analysis, cadaveric dissection rather than intraoperative dissection was more specific in the demonstration of multiple branching pattern. In fact, anatomical data retrieved from operative field were less accurate. Probably this rate discrepancy was associated to reporting errors.
In general, the studies did not provide information on factors such as sex and laterality, which could allow further subgroup analyses. Most studies have been conducted on women; however, Loukas et al. 2006 [ 23 ] found no gender differences. Limited data from regions such as Africa, North America, Oceania, and South America precluded analysis of other regions besides Europe and Asia. Many studies have a lack of detailed data on the branching site or symmetry of post-division branches.
Future studies need to be carried out to further elucidate the behavior of the terminal branching of the ICBN and its anastomoses with the brachial plexus. There is also the possibility of studying the use of landmarks or relationships with adjacent structures to be able to intraoperatively identify the nerves. Structures such as the lateral thoracic vein discussed in O’Rourke et al. 1999 [ 27 ] could be a potential candidate. Integration with future research will provide valuable information for surgeons and, ideally, lead to more positive outcomes for patients undergoing surgeries in the axillary region. In fact, ICBN neuralgia and post-operative pain syndrome can be successfully managed with loco-regional anesthesia techniques, but the primary goal should be the overall reduction of their incidence.
An accurate knowledge of the anatomy of axillary region is crucial to reduce nerve’ injuries. Another possible strategy for minimizing nerve’ injuries during surgery could be the ultrasound guided identification of ICBN before surgery. In fact, in a study conducted by Feigl et al. the ICBN blocks with ultrasound guided anterior approach to intercostal nerves was evaluated to supplement the axillary block. The sonographic identification of ICBN and its possible anatomical variations may be useful to reduce ICBN’s injuries [ 33 ].
Furthermore, the group with intact ICBN have a higher percentage of paresthesia (32.8%) after three months than the group with ICBN section. We were unable to explain this result, but we think that this pain might be similar to groin pain after inguinal hernia repair [ 34 ]. For this reason, some surgeons suggest to perform the inguinal nerve neurectomy in all patient underwent open mesh hernia repair [ 35 ].
The prevalence of ICBN is very high, for this reason during axillary dissection a small operative theatre is associated at the inability to freely dissect tissue without a reduction of post-procedural pain and paresthesia. The division of the ICBN during axillary lymph node dissection, increases the risk of sensory disturbance such as hyposensitivity of the arm. The preservation of the ICBN do not modify the oncological radicality in axillary dissection for patients with cutaneous metastatic melanoma or breast cancer.
Availability of data and materials
The data of the article can be accessed by requesting the corresponding author.
Mewa Kinoo S, Singh B. Complex regional pain syndrome of the breast and chest wall. Breast J. 2016;22(3):366–8.
Article PubMed Google Scholar
Soares EW. Anatomical variations of the axilla. Springerplus. 2014;3:306.
Article PubMed PubMed Central Google Scholar
Kumar P, Meena RN, Sheikh BH, Belliappa V, Pais AV, et al. Intercostobrachial nerve - anatomical considerations and its importance in carcinoma breast of female patients. Ann Surg Perioper Care. 2016;1(2):1013.
Google Scholar
Zhu JJ, Liu XF, Zhang PL, Yang JZ, Wang J, Qin Y, Zhang GL, et al. Anatomical information for intercostobrachial nerve preservation in axillary lymph node dissection for breast cancer. Genet Mol Res. 2014;13(4):9315–23.
Article CAS PubMed Google Scholar
DeSantis CE, Ma J, Gaudet MM, Newman LA, Miller KD, Goding Sauer A, et al. Breast cancer statistics, 2013. CA Cancer J Clin. 2014;64(1):52–62.
Kubala O, Prokop J, Jelínek P, Ostruszka P, Tošenovský J, Ihnát P, et al. Anatomicko-chirurgická studie průběhu interkostobrachiálních nervů (ICBN) v axile při exenteraci I. a II. etáže axily u karcinomu prsu a maligního melanomu [Anatomic-surgical study of intercostobrachial nerve (ICBN) course in axilla during I. and II. Level of axilla clearance in breast cancer and malignant melanoma]. Rozhl Chir. 2013;92(6):320–9. Czech.
CAS PubMed Google Scholar
Page MJ, McKenzie JE, Bossuyt PM, et al. The PRISMA 2020 statement: an updated guideline for reporting systematic reviews. BMJ. 2021;372:n71.
Henry BM, Tomaszewski KA, Ramakrishnan PK, et al. Development of the Anatomical Quality Assessment (AQUA) Tool for the quality assessment of anatomical studies included in meta-analyses and systematic reviews. Clin Anat. 2017;30:6–13.
Özşahin MK, Kaynak G, Afacan MY, et al. Anatomical variations of intercostobrachial nerve: a potential candidate for neurotization after traumatic median nerve injury? Ulus Travma Acil Cerrahi Derg. 2023;29:22–9.
PubMed Central Google Scholar
Melhem J, Amarin M, Odeh G, Al-Bustami N, Al-Lauzy H, Ayoub R. Intercostobrachial nerve (ICBN) preservation versus sacrifice in axillary dissection: randomized controlled trial. Am J Clin Oncol. 2021;44(5):206–9.
Kaur N, Kumar R, Jain A, et al. Sensory changes and postmastectomy pain following preservation of intercostobrachial nerve in breast cancer surgery: a prospective randomized study. Indian J Surg Oncol. 2021;12(1):108–13.
Chirappapha P, Kongdan Y, Vassanasiri W, Ratchaworapong K, Sukarayothin T, Supsamutchai C, et al. Evaluation the effect of preserving intercostobrachial nerve in axillary dissection for breast cancer patient. Gland Surg. 2019;8(6):599–608.
Soubhagya Ranjan Nayak, Smita Singh Banerjee. Anatomic variations of the extrathoracic course of the intercostobrachial nerve and its clinical significance. Gland Surg. 2019;8(6):599–608.
Orsolya HB, Coros MF, Stolnicu S, Georgescu R, et al. Does the surgical management of the intercostobrachial nerve influence the Postoperatory Paresthesia of the upper limb and life quality in breast cancer patients? Chirurgia (Bucur). 2017;112(4):436–42.
Foroni L, Siqueira MG, Martins RS, Oliveira GP. The intercostobrachial nerve as a sensory donor for hand reinnervation in brachial plexus reconstruction is a feasible technique and may be useful for restoring sensation. Arq Neuropsiquiatr. 2017;75(7):439–45.
Kailash D, Balkund S, Priyadharshini. A study on variations of intercostobrachial nerve. MedPulse – Int J Anat 2017;4(2):14–6.
Article Google Scholar
Darwish A, Mlees A, El-Gendy M, Elghazeery M. Preservation of lateral thoracic vein and intercostobrachial nerve in breast conservative surgery. Ain Shams J Surg. 2015;8(1):53–60.
Taira N, Shimozuma K, Ohsumi S, et al. Impact of preservation of the intercostobrachial nerve during axillary dissection on sensory change and health-related quality of life 2 years after breast cancer surgery. Breast Cancer. 2014;21(2):183–90.
Andersen KG, Aasvang EK, Kroman N, Kehlet H. Intercostobrachial nerve handling and pain after axillary lymph node dissection for breast cancer. Acta Anaesthesiol Scand. 2014;58(10):1240–8.
Khan A, Chakravorty A, Gui GP. In vivo study of the surgical anatomy of the axilla. Br J Surg. 2012;99(6):871–7.
Verma S, Kala R, Bhargava G, Yadav R, Singh R, Maurya P, et al. Evaluation of the role of preservation of the intercostobrachial nerve on the post-mastectomy pain syndrome in breast cancer patients of North India. Internet J Surg 23. 2009;23:2.
Ferreira BP, Soares. Morbidade cirúrgica pós-biópsia de linfonodo sentinela e esvaziamento axilar: estudo comparativo em mulheres com e sem preservação do nervo intercostobraquial. Rev Assoc Med Bras. 2008;54(6):517–21.
Loukas M, Hullett J, Louis RG Jr, Holdman S, Holdman D. The gross anatomy of the extrathoracic course of the intercostobrachial nerve. Clin Anat. 2006;19(2):106–11.
Torresan RZ, Cabello C, Conde DM, Brenelli HB. Impact of the preservation of the intercostobrachial nerve in axillary lymphadenectomy due to breast cancer. Breast J. 2003;9(5):389–92.
Freeman SR, Washington SJ, Pritchard T, Barr L, Baildam AD, Bundred NJ. Long term results of a randomised prospective study of preservation of the intercostobrachial nerve. Eur J Surg Oncol. 2003;29(3):213–5.
Cunnick GH, Upponi S, Wishart GC. Anatomical variants of the intercostobrachial nerve encountered during axillary dissection. Breast. 2001;10(2):160–2.
O’Rourke MG, Tang TS, Allison SI, Wood W. The anatomy of the extrathoracic intercostobrachial nerve. Aust N Z J Surg. 1999;69(12):860–4.
Nayak SR, Banerjee SS. Anatomic variations of the extrathoracic course of the intercostobrachial nerve and its clinical significance. Asian J Med Sci. 2018;9(5):77–80.
Henry BM, Graves MJ, Pękala JR, Sanna B, Hsieh WC, Tubbs RS, et al. Origin, branching, and communications of the intercostobrachial nerve: a meta-analysis with implications for Mastectomy and axillary lymph node dissection in breast cancer. Cureus. 2017;9(3):e1101.
PubMed PubMed Central Google Scholar
Warrier S, Hwang S, Koh CE, Shepherd H, Mak C, Carmalt H, et al. Preservation or division of the intercostobrachial nerve in axillary dissection for breast cancer: meta-analysis of randomised controlled trials. Breast. 2014;23(4):310–6.
Abdullah TI, Iddon J, Barr L, Baildam AD, Bundred NJ. Prospective randomized controlled trial of preservation of the intercostobrachial nerve during axillary node clearance for breast cancer. Br J Surg. 1998;85(10):1443–5.
Salmon RJ, Ansquer Y, Asselain B. Preservation versus section of intercostal-brachial nerve (IBN) in axillary dissection for breast cancer–a prospective randomized trial. Eur J Surg Oncol. 1998;24(3):158–61.
Feigl GC, Litz RJ, Marhofer P. Anatomy of the brachial plexus and its implications for daily clinical practice: regional anesthesia is applied anatomy. Reg Anesth Pain Med. 2020;45(8):620–7.
Charalambous MP, Charalambous CP. Incidence of chronic groin pain following open mesh inguinal hernia repair, and effect of elective division of the ilioinguinal nerve: meta-analysis of randomized controlled trials. Hernia. 2018;22(3):401–9.
Cirocchi R, Sutera M, Fedeli P, Anania G, Covarelli P, Suadoni F, Boselli C, Carlini L, Trastulli S, D’Andrea V, Bruzzone P. Ilioinguinal nerve neurectomy is better than preservation in Lichtenstein Hernia repair: a systematic literature review and meta-analysis. World J Surg. 2021;45(6):1750–60.
Download references
Acknowledgements
We would like to thank all the participants who contributed to the study.
We do not have any funding.
Author information
Authors and affiliations.
Department of Medicine and Surgery, University of Perugia, Perugia, 06132, Italy
Roberto Cirocchi, Francesca Duro, Luca Properzi, Stefano Avenia, Carlo Boselli & Piero Covarelli
Department of Medicine and Surgery, University of Milan, Milan, 20122, Italy
Matteo Matteucci
Georgia Baptist College of Nursing, Mercer University, Atlanta, GA, 30341, USA
Justus Randolph
Department of Public Health, University of Naples “Federico II”, Naples, 80131, Italy
Bruno Amato
Department of General Surgery, P.O Frangipane Ariano Irpino, Avellino, 83031, Italy
Ruggiero Iandoli
Department of Digestive and Emergency Surgery, AOSP of Terni, Terni, 05100, Italy
Giovanni Tebala
Department of Surgery, “Sapienza“ University of Rome, Roma, 00161, Italy
Paolo Sapienza
You can also search for this author in PubMed Google Scholar
Contributions
R.C., M.M. and P.S. contributed to the design of study, conducted study and drafted the initial manuscript. G.T. and L.P., B.A., F.D revised manuscript. R.A. contributed to data collection. J.R. assisted in analyzing data and interpreting the data. P.C. and C.B. contributed to perform surgery and supervision. All authors read and approved the final manuscript.
Corresponding author
Correspondence to Roberto Cirocchi .
Ethics declarations
Ethics approval and consent to participate.
Not applicable.
Consent for publication
Competing interests.
The authors declare no competing interests.
Additional information
Publisher’s note.
Springer Nature remains neutral with regard to jurisdictional claims in published maps and institutional affiliations.
Supplementary Information
Supplementary material 1., rights and permissions.
Open Access This article is licensed under a Creative Commons Attribution 4.0 International License, which permits use, sharing, adaptation, distribution and reproduction in any medium or format, as long as you give appropriate credit to the original author(s) and the source, provide a link to the Creative Commons licence, and indicate if changes were made. The images or other third party material in this article are included in the article's Creative Commons licence, unless indicated otherwise in a credit line to the material. If material is not included in the article's Creative Commons licence and your intended use is not permitted by statutory regulation or exceeds the permitted use, you will need to obtain permission directly from the copyright holder. To view a copy of this licence, visit http://creativecommons.org/licenses/by/4.0/ . The Creative Commons Public Domain Dedication waiver ( http://creativecommons.org/publicdomain/zero/1.0/ ) applies to the data made available in this article, unless otherwise stated in a credit line to the data.
Reprints and permissions
About this article
Cite this article.
Cirocchi, R., Matteucci, M., Randolph, J. et al. Anatomical variants of the intercostobrachial nerve and its preservation during surgery, a systematic review and meta-analysis. World J Surg Onc 22 , 92 (2024). https://doi.org/10.1186/s12957-024-03374-w
Download citation
Received : 16 November 2023
Accepted : 28 March 2024
Published : 11 April 2024
DOI : https://doi.org/10.1186/s12957-024-03374-w
Share this article
Anyone you share the following link with will be able to read this content:
Sorry, a shareable link is not currently available for this article.
Provided by the Springer Nature SharedIt content-sharing initiative
World Journal of Surgical Oncology
ISSN: 1477-7819
- General enquiries: [email protected]
Vitamin D and Ovarian Cancer: Systematic Review of the Literature with a Focus on Molecular Mechanisms
Affiliations.
- 1 University Clinic for Gynaecology and Obstetrics, University Medical Centre Maribor, Ljubljanska 5, 2000 Maribor, Slovenia.
- 2 Department of Oncology, University Medical Centre Maribor, Ljubljanska 5, 2000 Maribor, Slovenia.
- PMID: 32024052
- PMCID: PMC7072673
- DOI: 10.3390/cells9020335
Vitamin D is a lipid soluble vitamin involved primarily in calcium metabolism. Epidemiologic evidence indicates that lower circulating vitamin D levels are associated with a higher risk of ovarian cancer and that vitamin D supplementation is associated with decreased cancer mortality. A vast amount of research exists on the possible molecular mechanisms through which vitamin D affects cancer cell proliferation, cancer progression, angiogenesis, and inflammation. We conducted a systematic review of the literature on the effects of vitamin D on ovarian cancer cell.
Keywords: ovarian cancer; vitamin D; vitamin D receptor; vitamin D response elements.
Publication types
- Systematic Review
- Epithelial-Mesenchymal Transition
- Immunomodulation
- Ovarian Neoplasms / epidemiology
- Ovarian Neoplasms / genetics*
- Ovarian Neoplasms / immunology
- Ovarian Neoplasms / pathology
- Receptors, Calcitriol / metabolism
- Vitamin D / pharmacology*
- Receptors, Calcitriol

An official website of the United States government
The .gov means it’s official. Federal government websites often end in .gov or .mil. Before sharing sensitive information, make sure you’re on a federal government site.
The site is secure. The https:// ensures that you are connecting to the official website and that any information you provide is encrypted and transmitted securely.
- Publications
- Account settings
Preview improvements coming to the PMC website in October 2024. Learn More or Try it out now .
- Advanced Search
- Journal List
- HHS Author Manuscripts

A Systematic Review of Ovarian Cancer and Fear of Recurrence
Melissa ozga.
Memorial Sloan Kettering Cancer Center
Carol Aghajanian
Shannon myers-virtue.
Temple University & Rutgers Cancer Institute of New Jersey
Glynnis McDonnell
Memorial Sloan Kettering Cancer Center & Saint John’s University
Sabrina Jhanwar
Shira hichenberg, isabel sulimanoff.
To assess demographic, medical and psychological factors that are associated with fear of recurrence (FCR) in ovarian cancer patients.
We searched PubMed, EMBASE, Cochrane, CINAHL, and PsycINFO. For PubMed, a search using Medical Subject Headings (MeSH) was run, as well as a textword search from 1990 to July 2014. Search terms that were used consisted of ovarian terms, fear terms, and recurrence/progression themes. Title and abstract reviews were conducted by two independent reviewers to determine eligibility, and discrepancies were decided by a third reviewer. Full-text reviews of potentially eligible articles were conducted by the review team, which met regularly to ensure the reliability of eligibility ratings across all articles.
Fifteen articles met our inclusion criteria. Nine were quantitative studies that utilized a cross-sectional design, and six studies consisted of three qualitative studies, two small intervention studies, and one study that utilized content analysis to explore written correspondence among ovarian cancer patients. FCR was reported as a significant concern for both older and younger women at both early and advanced stages. Women were distressed about recurrence at various times during their treatment and post-treatment. FCR was noted to be prevalent around cancer follow-up examinations. Many women report not receiving adequate support for recurrence. FCR was also shown to be linked in some way to hopelessness, faith/spirituality, and PTSD. FCR was also linked to patients’ anxiety about death and dying and the uncertainty of the future of their medical health.
Conclusions
This review demonstrates that FCR is prevalent in the ovarian cancer population. Cancer recurrence fears are not adequately assessed or treated. More information is needed on the factors that may be related to women’s fears about recurrence in the ovarian cancer population. In addition, a validated measure of FCR among ovarian cancer patients as well as a treatment intervention are needed.
Introduction
The American Cancer Society estimated that 21,980 women will be newly diagnosed with ovarian cancer in the United Stated in 2014. Ovarian cancer has the highest mortality rate of any other gynecological cancer ( NCI, 2013 ). It is the fifth leading cause of cancer death among women in the United States (Spiegel 2014). The majority of women are diagnosed at advanced stage. While survival rates vary depending upon stage of diagnosis, the 5-year relative survival rate for all stages of 44% ( ACS, 2014 ). The disease course for ovarian cancer is often difficult. Initial treatments often include surgery and chemotherapy which may cause significant physical, psychological, and social side effects (Holland 2010). Women who undergo successful initial treatment then require additional follow-up care to monitor for recurrence. Follow-up care generally includes ongoing physical examinations, CT scans, and blood tests (e.g., Ca-125). 1 It is estimated that between 70% and 75% of women diagnosed with ovarian cancer will experience a recurrence ( Armstrong et al., 2006 ; Sugimoto & Thomas 1998 ). Therefore, fear of cancer recurrence (FCR) is commonly reported among ovarian cancer patients ( Shinn et al., 2009 ; Steginga & Dunn, 1997 ; Wenzel et al., 2002 ). While FCR has been recognized as a common challenge for ovarian cancer patients, there has not been a comprehensive summary of the research on FCR in this population.
Cancer Recurrence
Cancer recurrence has been defined as the return of cancer after completing treatment and having a length of time during which the cancer has not been detected ( ACS, 2014 ). The period in which no cancer is detected is typically referred to by physicians as “no evidence of disease” (NED) or in “remission.” Recurrence after a period of remission differs from cancer progression, which is when an existing cancer spreads or worsens. It can be difficult to differentiate between recurrence and progression, especially when there is only a short time period between the completion of treatment without evidence of disease and the cancer returning, because there is no specific timeline to define a true remission. A recurrence or progression of the cancer will, in turn, lead to more work-ups and treatments, and will most likely evoke many emotions and questions.
Fear of Cancer Recurrence
While there is not a universal definition of fear of cancer recurrence (FCR), it is often defined as: “fear that the cancer will return or progress in the same place or a different part of the body” ( Simard et al., 2010 ; Simard et al., 2013 ; Vickburg, 2003). FCR has been recognized as a common concern among cancer patients ( Baker et al., 2005 ). Indeed, FCR has received growing attention in research with adult cancer survivors. There have been several literature reviews on FCR (Crist et al., 2012; Koch et al. 2012; Lee-Jones et al., 1997 ), including a systematic review which provided an overview of quantitative research conducted over the past twenty years on FCR among adult cancer survivors of various cancer diagnoses ( Simard et al., 2013 ). The authors concluded that cancer survivors consider FCR to be one of their greatest concerns and that many survivors’ psychosocial needs are not met when it comes to FCR, suggesting that it is an important area for future research.
There are limitations to the existing FCR research. These limitations include: a lack of well-validated measures of FCR ( Simard et al., 2013 ; Thewes et al., 2012 ), few longitudinal studies ( Simard et al., 2013 ), and majority of studies focusing on breast cancer patients ( Lee-Jones et al. 1997 ). Indeed, a recent review found that 42 of the 130 studies on FCR focused specifically on breast cancer ( Simard et al., 2013 ). Therefore, current research on FCR may not be generalizable to individuals with other types of cancer. Another 37 of the studies reported in the review included a mixed sample of cancer sites ( Simard et al., 2013 ). This is also problematic because there are significant differences between recurrence rates for different cancer types, which may influence the degree of FCR.
Fear of Cancer Recurrence and Ovarian Cancer
The natural tendency of ovarian cancer is of recurrence and remission ( ACS 2014 ). The most common histopathological type of ovarian cancer is epithelial, accounting for 90% of the cases. ( McCorkle 2003 ) Approximately 80% of patients diagnosed with ovarian epithelial cancer will relapse after standard first-line treatment, which includes platinum-based and taxane-based chemotherapy ( NCI 2014 ). Given the disease course, prognosis, and recurrence rates associated with ovarian cancer (NCI and ACS), FCR may be a particularly important area to understand when working with this population. Fear of recurrence has been identified as an ovarian cancer-specific symptom and a patient reported outcome that is prevalent and severe. In all cancer types worry and anxiety are prevalent. However, in ovarian cancer, fear of recurrence or fear of progression reflects the high likelihood that the cancer will recur. Additionally, the frequent follow-up testing that is standard after treatment for ovarian cancer may elevate FCR. ( Donovan et al., 2014 ) Many ovarian cancer patients identify Ca-125 levels with their disease status (Hamilton, 1999), which can be distressing. Indeed, there is evidence that anxiety when getting Ca- 125 testing is common ( Mirabeau-Beale et al., 2009 ) and preoccupation with Ca- 125 is associated with increased depression and anxiety symptoms ( Parker et al., 2006 ).
There has been increased attention to the importance of quality of life and psychological well-being among ovarian cancer patients (McCorckle et al. 2003). Some studies have suggested that while many ovarian cancer survivors report few unmet physical needs, they endorse significant unmet psychological needs ( Matulonis et al., 2008 ). Therefore, there is a need to better understand factors which may impact psychological well-being in this population, such as FCR. The literature on psychological adjustment among ovarian cancer survivors has suggested that FCR is an important issue that many women face (Hamilton, 1999). It is a commonly reported concern by ovarian cancer survivors ( Matulonis et al., 2008 ). Researchers suggest similar levels of FCR between early and advanced stage ovarian cancer patients ( Mirabeau-Beale et al., 2009 ), indicating its prevalence across various stages of ovarian cancer.
Overall, the evidence suggests that FCR is an important issue among ovarian cancer patients. However, there has not been a synthesis of the research on this topic. There is a need to better understand FCR among ovarian cancer patients. This information will guide further research and aid in the development of interventions aimed at assisting ovarian cancer patients coping with FCR. The purpose of this systematic review was 1) to identify research studies that have explored FCR among ovarian cancer patients, 2) to characterize FCR in this population in terms of prevalence and specific concerns, 3) to identify demographic, medical, and psychological factors associated with FCR and 4) to discuss the implications for treatment interventions and clinical practice.
Comprehensive literature searches were conducted in five databases for English language references between 1990 and July 2014. The databases searched were: PubMed, EMBASE, Cochrane, CINAHL, and PsycINFO. For PubMed, a search using Medical Subject Headings (MeSH) was run, as well as a textword search. For EMBASE, a search using Emtree (EMBASE vocabulary) terms was run, as well as a textword search. For Cochrane, a search using MeSH terms was run, as well as a textword search. For CINAHL, a search using CINAHL vocabulary terms was run, as well as a textword search. For PsycINFO, a search using PsycINFO vocabulary terms was run, as well as a textword search. The search strategy had three components, which were linked together using the AND operator: 1) ovarian terms, 2) fear terms, and 3) recurrence/progression terms. Table 1 contains the complete key word search strategy and Table 2 contains the complete MeSH search strategy.
After records were added to the EndNote library, the total figures on 7-22-14 were:
After duplicates were removed, the total figures on 7-23-14 were:
The number of duplicates removed was 431.
All search results were combined in a bibliographic management tool (EndNote) and duplicates were eliminated using the capabilities in EndNote. Any duplicates missed by the software were eliminated manually. Title and abstract reviews were conducted by two independent reviewers to determine eligibility, and discrepancies were decided by a third reviewer. Full-text reviews of potentially eligible articles were conducted by the review team, which met regularly to ensure the reliability of eligibility ratings across all articles.
A total of 15 articles met our inclusion criteria. Nine were quantitative studies that utilized a cross-sectional design. Of those nine studies, three studies utilized the same larger database to conduct separate analyses on specific subgroups of ovarian cancer patients based on age. An additional three of the quantitative studies utilized a larger database to conduct separate analyses on specific subgroups of ovarian cancer patients based on stage of diagnosis. All other quantitative studies utilized separate samples. The remaining six studies consisted of three qualitative studies, including interviews with ovarian cancer patients, two small intervention studies that did not target FCR specifically, but reported ovarian cancer patients’ FCR in findings, and one study that utilized content analysis to explore written correspondence among ovarian cancer patients. The majority of studies relied upon open-ended questions to glean information about FCR. One study utilized the Fear of Relapse/Recurrence Scale. Table 3 provides information specific to the sample, procedures, measures and FCR findings identified in each of these studies.
Characterization of FCR among Ovarian Cancer Patients
The methods of assessing FCR varied widely across studies, resulting in rates ranging from 22% ( Wenzel et al., 2002 ) to 80% ( Cesario et al., 2010 ) of ovarian cancer patients reporting FCR. Among the survey-based studies, approximately half of ovarian cancer patients report ever experiencing FCR and slightly less than half reported that they did not receive adequate help coping with FCR. For example, a large study (N = 315) conducted by Fitch and colleagues (1999) found that 54% of Canadian women with ovarian cancer reported FCR and 43% reported inadequate help for coping with this fear. Sub-studies from this survey divided the sample into older women (≥ 60 years) and younger women (≤ 45 years). Among older women (N = 146), 43% reported FCR since diagnosis, 22% reported FCR within the past month, and 37% reported receiving adequate support to manage this fear ( Fitch et al., 2001 ). Among younger women (N = 39), 64% reported FCR since diagnosis, 46% reported FCR in the past month, and 18% reported receiving adequate help for this problem ( Fitch et al., 2000 ). A second cross-sectional survey by Fitch and colleagues (2010) found that FCR was the top-reported unmet need among women with ovarian cancer, with 78% (N=50) listing it as an unmet need.
Kornblith and colleagues (2010) administered the Fear of Relapse/Recurrence Scale ( Greenberg et al., 1997 ) and reported a mean score of 2.5 among women diagnosed with advanced ovarian cancer at least 3 years previously who were currently relapse-free (N=42). The mean scored indicated uncertainty about greater FCR. However, the majority of women agreed or strongly agreed with statements regarding FCR (66.7%). In similar studies, FCR was reported in 56% of early stage ovarian cancer survivors ( Matulonis et al., 2008 ; Mirablaeu-Beale et al., 2009) and 48% of advanced stage cancer survivors. Among long-term, early stage ovarian cancer survivors (> 5 years) the prevalence of FCR was slightly lower, with 22% reporting FCR ( Wenzel et al., 2002 ). Cox and colleagues (2008) found that 33% of women who participated in a pilot study of nurse-led, phone-based follow-up care (N=52) discussed FCR during their calls with the nurse.
In terms of specific recurrence concerns, many studies identified concerns related to health and the future, as well as evidence that these concerns are significantly challenging for women. For example, Kornblith and colleagues (2010) found that 63.4% of the women they surveyed reported uncertainty to strong agreement with the statement that they would experience a relapse within 5 years, 54.8% reported worrying about their health, and 40.5% reported feeling unsure about the future (40.5%). Stewart and colleagues (2001) assessed views about recurrence among relapse-free ovarian cancer survivors (N=200). Of those women, 45% believed their risk of recurrence was less than average, 28.5% believed their risk to be average, 23% perceived above average risk, and 3.5% stated not to know their risk. In terms of time spent thinking about recurrence, 35% reported hardly ever thinking about the possibility of recurrence, 28% thought of it at least monthly, 24.5% at least weekly, and 12% at least daily. In terms of specific fears, 53% were afraid of what their futures held for them and 12% were very afraid of dying of ovarian cancer. Similarly, Wenzel and colleagues (2002) found that 10% of long-term ovarian cancer survivors said that FCR or death was one of their greatest problems during treatment and 14% indicated that it was currently their greatest challenge after all of this time.
The qualitative studies all noted FCR as a predominant theme that emerged among ovarian cancer patients. For example, an on-line qualitative survey of women diagnosed with ovarian cancer (N = 360) found that approximately 80% of women expressed worry about recurrence at some point. Women described recurrence as being denied a future. The most common response to a question about worry in all age groups was a single-word response: Recurrence ( Cesario et al., 2010 ). Qualitative interviews with ovarian cancer patients yielded four themes, two of which focused on recurrence: waiting for recurrence and facing the diagnosis of recurrence. Women who had experienced recurrence (N=11) reported feeling shock and devastation, despite awareness of the statistics regarding the prevalence of ovarian cancer recurrence ( Howell et al., 2003 ). Reb (2007) conducted Interviews with women diagnosed with stage III or IV ovarian cancer within the past years and had completed an initial course of chemotherapy and had no evidence of recurrence (N=20) suggested significant fear and anxiety related to recurrence ( Reb, 2007 ). Negative communications with their health care providers about these topics was associated with greater anxiety and less hopefulness. Several women reported recurrence and death as their greatest fear. Content analysis performed on women’s correspondence with the founding editor of Conversations! The International Newsletter for those Fighting Ovarian Cancer found that for women in remission, letters about stress and anxiety were more common than letters celebrating survival ( Ferrell et al., 2003 ). Similar to previously mentioned findings, women expressed that fears of recurrence dominated their lives due to the awareness that recurrent ovarian cancer is common and indicates a poorer prognosis. For those who experienced a recurrence, letters focused on the distress caused by recurrence and the need to accept ovarian cancer as a chronic condition.
Factors Associated with FCR among Ovarian Cancer Patients
Few studies specifically explored demographic, medical, or psychological factors associated with FCR. Qualitative studies found that FCR was often heightened during follow-up appointments and the end of active treatment ( Cesario et al., 2010 ; Ferrell et al., 2003 ; Reb, 2007 ). In written correspondence, women indicated that ending active treatment triggered new fears and anxieties because they were not actively fighting the disease ( Ferrell et al., 2003 ). Similarly, in qualitative interviews women stated that they found the end of treatment to be distressing because they felt that they were no longer under the protection of the treatment ( Reb, 2007 ). Women also reported feeling anxiety about recurrence prior to follow-up appointments ( Reb, 2007 ). Stewart and colleagues (2001) found that 62.5% reported high to extreme anxiety at cancer check-ups and Mirabeau- Beale and colleagues found that 50% were anxious when tested for Ca-125. There is some evidence that greater FCR is associated with less favorable psychosocial outcomes. For example, greater FCR correlated with lower scores on spirituality, greater negative impact of cancer, lower positive well-being, and more PTSD symptoms ( Kornblith et al., 2010 ; Matulonis et al., 2008 ).
In order to understand how women with ovarian cancer are affected by FCR of their cancer a systematic review of literature was carried out. Findings showed strong evidence that FCR is a problem amongst this population and research in this area is lacking.
General Trends and Unmet Needs
There were several general trends found in the literature. Most notably, FCR is a significant concern among women diagnosed with ovarian cancer. FCR is reported by women across various ages and disease stage. It is reported by women during treatment, after treatment has ended, and when faced with a recurrence of ovarian cancer. Factors that appeared to be associated with the reported FCR were cancer follow-up examinations and tests, such as the Ca-125 test. Another notable trend was that many women report not receiving adequate support in regard to FCR. Some women have developed their own strategies for coping with distress around recurrence, but this may not be true of all women diagnosed with ovarian cancer. The lack of adequate support regarding FCR is further concerning in light of the trend indicating that FCR is associated with psychosocial outcomes such as hopelessness, faith/spirituality, PTSD, anxiety about death and dying, and uncertainty about one’s future medical health.
While there were several trends noted in the literature, there were also limitations in the extant literature. First, it is not clear whether the current literature represents an adequate breadth of cultural and demographic diversity. Much of the literature was based on English or French speaking women and the samples were majority Caucasian. Given this information, it is inconclusive whether the findings in these studies can be generalized to more diverse samples. Second, much of the literature focused on women who had survived two or more years after diagnosis. As ovarian cancer is a diagnosis with poor prognosis and is rapidly fatal, many women may be excluded in these samples. Furthermore, the literature may not be adequately capturing FCR during the time period following diagnosis or initially completing treatment. Third, there is a lack of consistent use of a valid measure of FCR. The majority of studies utilized open-ended questions to assess FCR or focused on general psychosocial issues rather than specifically assessing FCR. Therefore, conclusions about the prevalence and severity of FCR in this population cannot be made. Fourth, the majority of studies did not explore the impact of FCR on a woman’s quality of life or psychosocial functioning. Finally, the literature does not provide sufficient information on the assessment and treatment of FCR among women with ovarian cancer. It is not clear how, or if, medical providers are assessing FCR among patients. It is also unclear what type of support is provided or available to women experiencing FCR. Moreover, it is unclear what type of support would be most effective.
Future Directions
This review demonstrates that FCR is prevalent in the ovarian cancer population and that it is often not adequately addressed or treated. The review also highlights significant limitations in the research on FCR among ovarian cancer patients. These general trends and limitations suggest important areas for future research and practice. First, there is a need for further research on FCR in this population, particularly among more diverse samples and among women who are more recently diagnosed. We also recommend longitudinal research that can examine the trajectory of FCR over time, the relationship between FCR and psychosocial outcomes, and factors that play a role in the maintenance of FCR. Second, there is a need for better assessment of FCR in this population, for both research and clinical purposes. A validated measure of FCR among ovarian cancer patients is needed to better understand FCR in this population. This would allow for more conclusive information on the prevalence of FCR. Additionally, it is suggested that assessment not only include the presence of FCR, but also the severity of FCR. It is important to differentiate FCR that is normal and expected from a level of FCR which may be having a significant impact on a women’s overall functioning. There are existent measures of FCR that have been utilized with broad cancer populations which may be adapted for use in this population. For example, the Concern about Recurrence Scale (CARS) has been validated among breast cancer patients (Vickburg, 2003). The CARS assesses overall fears, as well as including subscales that assess FCR in specific domains of worry (e.g., health, death, womanhood). There are additional measures of FCR that have demonstrated promising psychometric properties in other cancer populations which may inform assessment of FCR among ovarian cancer patients, such as the Fear of Relapse/Recurrence Scale ( Greenberg et al., 1997 ), the Fear of Recurrence Questionnaire ( Northouse, 1981 ), and the Fear of Cancer Recurrence Inventory ( Simard et al., 2013 ). In addition to the need for validated measures for research purposes, there is a need for clinical assessment of FCR by providers. Since FCR is a significant concern in this population, it may be important for providers to assess FCR in order to provide adequate support or to refer patients to further support services. Brief measures, such as the overall fear index of the CARS, which consists of xx items or the 5-item Fear of Relapse/Recurrence Scale may be considered. Alternately, providers may consider a brief one-question assessment of FCR, similar to the Distress Thermometer ( NCCN, 2007 ) that is often used in oncology settings. Further research is needed in the assessment of FCR in this population in order to identify those at risk and refer to the appropriate treatment. Finally, given the reported FCR and the lack of adequate support reported in the existing studies, there is a need for the development and implementation of interventions that address FCR among ovarian cancer patients. Treatment interventions aimed at ovarian cancer specific FCR do not currently exist. However, there are existing interventions that have been applied across broad cancer populations which may inform intervention among this population. For example, Humphris and colleagues developed a structured psychological intervention to address fears of recurrence among head and neck cancer patients (Humprhis, 2008). The intervention, which included cognitive-behavioral therapy techniques aimed at helping patients adjust to fears, showed initial promise and acceptability by patients. Another intervention developed by Butow and colleagues (2013) utilized mindfulness-based techniques and meta-cognitive therapy techniques to help patients change the way they regulate and respond to FCR. Herschbach and colleagues (2010) evaluated two types of group therapy, cognitive-behavioral therapy and supportive-expressive therapy, aimed at reducing fear of progression among individuals with various cancer diagnoses. Fear of progression decreased in both intervention groups compared to a control group, suggesting that these therapeutic techniques might be beneficial in addressing FCR specifically among ovarian cancer patients. Indeed, there is evidence that interventions that include cognitive-behavioral techniques, such as cognitive restructuring, coping skills to manage emotional reactions, and behavioral tasks, have led to improved general psychological functioning among gynecological cancer patients ( Manne et al., 2007 ). Therefore, these techniques may be incorporated into interventions designed to specifically target FCR among ovarian cancer patients.
In conclusion, this systematic review highlighted the relevance of FCR among ovarian cancer patients, as well as the need for further research and clinical recommendations in this area. Future efforts should focus on the assessment of FCR in this population and the development of appropriate interventions. The development of a validated scale to assess FCR would enable quantitative analysis which could be utilized by various physicians and psychosocial providers who, in turn, could make appropriate referrals for treatment and interventions. Interventions that specifically target FCR in the ovarian cancer population is currently an undeveloped area. Further research aimed at development of an assessment tool/ intervention and accurate timing of implementing these constructs may allow us to identify and treat woman with ovarian cancer most at risk for FCR and those most disabled by these fears. Decreasing cancer recurrence fears in this population may lead to better adherence to cancer surveillance and improved quality of life.
1 Ca-125 is a protein that is found more in ovarian cancer cells then in other cells. It is a blood test used to monitor those who have ovarian cancer. The test is usually performed every 3 months during chemotherapy treatment and after the treatment has ended.
Contributor Information
Melissa Ozga, Memorial Sloan Kettering Cancer Center.
Carol Aghajanian, Memorial Sloan Kettering Cancer Center.
Shannon Myers-Virtue, Temple University & Rutgers Cancer Institute of New Jersey.
Glynnis McDonnell, Memorial Sloan Kettering Cancer Center & Saint John’s University.
Sabrina Jhanwar, Memorial Sloan Kettering Cancer Center.
Shira Hichenberg, Memorial Sloan Kettering Cancer Center.
Isabel Sulimanoff, Memorial Sloan Kettering Cancer Center.
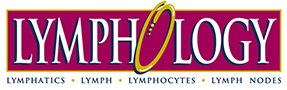
Login | Register
- Author Guidelines
- Submissions
- Editorial Team
- View Harvard Citation Style
- View Vancouver Citation Style
- View APA Citation Style
- Download RIS
- Download BibTeX
UNDERREPORTING AND UNDERREPRESENTATION OF RACIAL AND ETHNIC MINORITY PATIENTS IN LYMPHEDEMA CLINICAL TRIALS: A SYSTEMATIC REVIEW
- M. J. Escobar-Domingo
- V.P. Bustos
- J.E. Fanning
- J. Foppiani
- A. Hernandez-Alvarez
The generalizability of findings from Clinical Trials (CTs) investigating lymphedema treatment modalities requires an accurate representation of the target population. This study aims to evaluate racial and ethnic reporting and representation in lymphedema CTs. A comprehensive systematic literature search was conducted during May 2023 using multiple databases, following the PRISMA guidelines. All CTs published from 2018 to 2023 were included. A total of 84 articles were included in this review, from which 6,546 participants were included in the analysis. Seventy-four (88.1%) articles addressed secondary lymphedema, of which 60 (81.1%) were related to breast cancer. Only 12 (13%) of CTs reported at some extend race or ethnicity. Of these, five (41.6%) reported race and two (16.6%) reported ethnicity according to FDA guidelines. White race had the highest pooled prevalence (80%; 95% CI 72-86%; I2=90%), followed by Black (7%; 95% CI 2- 15%; I2= 94.3%) and Asian (4%; 95% CI 1-8%; I2= 89.9%). In studies reporting ethnicity, participants were predominantly non-Hispanic (92.1%; 95% CI 90 - 94%). There is an underreporting and underrepresentation of racial and ethnic minorities among lymphedema CTs, limiting their generalizability. It is imperative to future development of strategies to enhance diversity in the study sample.
Keywords: Lymphedema, Ethnicity, Racial Groups, Minority Groups, Breast Cancer Lymphedema
Escobar-Domingo, M. & Bustos, V. & Fanning, J. & Foppiani, J. & Kim, E. & Hernandez-Alvarez, A. & Lin, S. & Singhal, D. & Lee, B., (2024) “UNDERREPORTING AND UNDERREPRESENTATION OF RACIAL AND ETHNIC MINORITY PATIENTS IN LYMPHEDEMA CLINICAL TRIALS: A SYSTEMATIC REVIEW”, Lymphology 56(2), 41-60. doi: https://doi.org/10.2458/lymph.6064
Downloads: Download PDF
2 Downloads
Published on 14 apr 2024, peer reviewed, creative commons attribution-noncommercial-noderivs 4.0, harvard-style citation.
Escobar-Domingo, M , Bustos, V , Fanning, J , Foppiani, J , Kim, E , Hernandez-Alvarez, A , Lin, S , Singhal, D & Lee, B. (2024) 'UNDERREPORTING AND UNDERREPRESENTATION OF RACIAL AND ETHNIC MINORITY PATIENTS IN LYMPHEDEMA CLINICAL TRIALS: A SYSTEMATIC REVIEW', Lymphology . 56(2) :41-60. doi: 10.2458/lymph.6064
Show: Vancouver Citation Style | APA Citation Style
Vancouver-Style Citation
Escobar-Domingo, M , Bustos, V , Fanning, J , Foppiani, J , Kim, E , Hernandez-Alvarez, A , Lin, S , Singhal, D & Lee, B. UNDERREPORTING AND UNDERREPRESENTATION OF RACIAL AND ETHNIC MINORITY PATIENTS IN LYMPHEDEMA CLINICAL TRIALS: A SYSTEMATIC REVIEW. Lymphology. 2024 4; 56(2) :41-60. doi: 10.2458/lymph.6064
Show: Harvard Citation Style | APA Citation Style
APA-Style Citation
Escobar-Domingo, M Bustos, V Fanning, J Foppiani, J Kim, E Hernandez-Alvarez, A Lin, S Singhal, D & Lee, B. (2024, 4 14). UNDERREPORTING AND UNDERREPRESENTATION OF RACIAL AND ETHNIC MINORITY PATIENTS IN LYMPHEDEMA CLINICAL TRIALS: A SYSTEMATIC REVIEW. Lymphology 56(2) :41-60. doi: 10.2458/lymph.6064
Show: Harvard Citation Style | {% trans 'Vancouver Citation Style' %}
Non Specialist Summary
This article has no summary
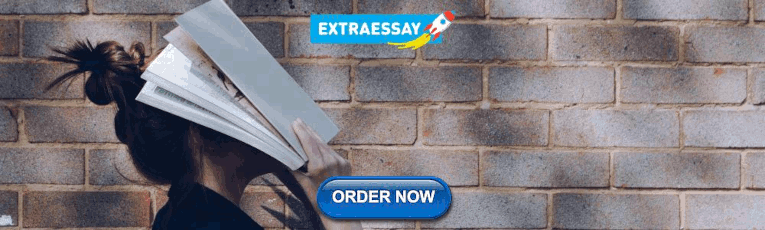
IMAGES
VIDEO
COMMENTS
Abstract. Aim: To review safety and efficacy outcomes in studies of first-line maintenance therapies for advanced ovarian cancer.Methods: A systematic literature review was performed (27 February 2020) to identify clinical outcomes including progression-free survival (PFS), overall survival (OS) and Grade ≥3 adverse events.Results: Overall 50 references met prespecified criteria; 18 studies ...
Of all the gynecologic tumors, ovarian cancer (OC) is known to be the deadliest. Advanced‐stages of OC are linked with high morbidity and low survival rates despite the immense amount of research in the field. ... Targeted therapy in ovarian cancer. A comprehensive systematic review of literature. Anticancer Res. 2017; 37 (6):2809‐2815 ...
The search strategy included the use of Mesh terms and keywords related to subject and study design (ovarian; ovary; cancer; carcinoma; neoplasm; tumor; Malignancy; review; systematic review; systematic literature review; meta-analysis). The detailed search strategy for the Medline can be found in the supplementary, Table 1 S. The reference ...
A comprehensive systematic literature search was performed to identify all published systematic reviews and meta-analysis on associated factors with ovarian cancer. Web of Science, Cochrane Library databases, and Google Scholar were searched up to 17th January 2020.
Mohammad Reza Alivand. Cellular & Molecular Biology Letters (2021) Recent preclinical and clinical research has led to exciting advances related to high-grade serous ovarian cancer, from examining ...
The risk of developing ovarian cancer is decreased by the use of oral contraceptives, higher parity, tubal sterilisation and removal of the ovaries, and increased by the use of hormones during menopause . We performed a systematic review of the literature on molecular mechanisms through which vitamin D can influence ovarian cancer cells.
A systematic review of publications on ... In this Review, we examine the literature supporting the role of surgery in the management of advanced ovarian cancer. ... Y. L. et al. Risk-reducing ...
Background/aim: We aimed to identify the most effectual groups of targeted therapies for ovarian cancer in recent clinical trials. Materials and methods: A systematic literature review has been gathered on an inventive study design that comprises of five steps. This involves search for pertinent publications from 2010 to date in various accessible medical data bases, usage of inclusion and ...
Evidence Review A systematic review of the literature was performed on March 4, 2022, to identify studies examining salpingectomy for ovarian cancer prevention. This review was performed according to the Preferred Reporting Items for Systematic Reviews and Meta-analyses (PRISMA) 2020 statement. Four databases were selected: PubMed via the ...
Data synthesis results Data in included literature. The number of participants in internal datasets varied by orders of magnitude, with each study including 1-776 ovarian cancer patients, and ...
Aim: To review safety and efficacy outcomes in studies of first-line maintenance therapies for advanced ovarian cancer.Methods: A systematic literature review was performed (27 February 2020) to identify clinical outcomes including progression-free survival (PFS), overall survival (OS) and Grade ≥3 adverse events.Results: Overall 50 references met prespecified criteria; 18 studies evaluated ...
Objective To explore the association between ovulation induction drugs and ovarian cancer. Design Systematic review and meta-analysis. Setting Not applicable. Patient(s) Women without ovarian cancer who ever or never underwent ovarian induction. Intervention(s) An extensive electronic search of the following databases was performed: PubMed, EMBASE, MEDLINE, Google Scholar, Cochrane Library and ...
Therefore, we conducted a systematic review to assess the strength of the evidence with regard to the claim that higher (vs lower) vitamin D-related exposures are associated with a reduction in the risk for ovarian cancer occurrence or mortality.
Ovarian cancer is a highly aggressive ovarian tumor. Because ovarian cancer rarely observes specific clinical manifestations or signs in the early stage, it is easy to be overlooked in the early stage and cannot be diagnosed. Studies have shown that only 20-25% of patients can be detected and diagnosed in the early stages of ovarian cancer .
Objectives: This analysis aimed to better define the relationship between progression-free survival and overall survival in adult patients with ovarian cancer (including fallopian tube or primary peritoneal cancer) following primary cytoreductive surgery or interval cytoreductive surgery. Methods: A systematic literature review was carried out across the Medline, Embase, and Cochrane Central ...
Abstract. Introduction: The asbestos and ovarian cancer relationship is not well understood because of small numbers of women exposed to asbestos, small numbers of cases, and misclassification of peritoneal mesothelioma as ovarian cancer on death certificates. The aim of this study was to conduct a meta-analysis to quantify the evidence that exposure to asbestos causes ovarian cancer.Methods ...
The purpose of this research was to review the HRQoL measures used in understanding ovarian cancer (OC) treated with poly(ADP) ribose polymerase inhibitors (PARPi). PCN222 Health Related Quality of Life (HRQoL) Review in Patients with Ovarian Cancer: A Systematic Literature Review (SLR) - Value in Health
We conducted a systematic review of the literature on the effects of vitamin D on ovarian cancer cell. Vitamin D is a lipid soluble vitamin involved primarily in calcium metabolism. Epidemiologic evidence indicates that lower circulating vitamin D levels are associated with a higher risk of ovarian cancer and that vitamin D supplementation is ...
Key Points. Question Compared with the end point of cancer-specific mortality, is incidence of late-stage cancer a suitable alternative end point in randomized clinical trials of cancer screening?. Findings In this systematic review and meta-analysis that included 41 randomized clinical trials of cancer screening, correlation between the reduction in stage III and IV cancer and the reduction ...
Only a case-cohort study assessed the relationship of walking and cycling combined mode with ovarian cancer risk, and reported no significant ... Abar L, Cariolou M, et al. World Cancer Research Fund International: continuous update project—systematic literature review and meta-analysis of observational cohort studies on physical activity ...
Opportunistic salpingectomy (OS) for the primary prevention of ovarian cancer is performed by gynecologists. Advocates have suggested expanding its use to other surgical specialties. General surgeons are the other group to routinely perform intraperitoneal operations in women and could play a role in ovarian cancer prevention. Herein, we review the current evidence and perioperative factors ...
Methods. A systematic literature review was carried out across the Medline, Embase, and Cochrane Central databases on 7 July 2020 (date limits 1 January 2011 to 7 July 2020) to identify studies with the following eligibility criteria: clinical trials/observational studies including >200 patients with ovarian cancer aged ≥18 years, evaluating overall survival/progression-free survival ...
Efficacy and safety of PARP inhibitors combined with antiangiogenic agents in the maintenance treatment of ovarian cancer: a systematic review and meta-analysis with trial sequential analysis of randomized controlled trials ... An exhaustive search of literature was undertaken using multiple databases, including PubMed, Web of Science, Embase ...
Methods: A comprehensive systematic review of the literature was conducted. PubMed, Embase and Cochrane Library databases were used. Prospero (ID number: CRD42024505274). Results: Out of the 152 studies identified, 63 were included, which involved 73 patients. ... Endometriosis-associated ovarian cancer appears to occur in younger women with a ...
Abstract aims at systematic review of meticulously synthesize existing research in mutations in the park 2 gene, linked to ovarian and breast cancer, and its role in Parkinson's disease (PD) and expression of synucleins in breast and ovarian cancer (OC) preneoplastic ovarian lesions, explore the association between a PARK2 germline variant and epithelial OC.
Aim: To review safety and efficacy outcomes in studies of first-line maintenance therapies for advanced ovarian cancer. Methods: A systematic literature review was performed (27 February 2020) to identify clinical outcomes including progression-free survival (PFS), overall survival (OS) and Grade ≥3 adverse events. Results: Overall 50 references met prespecified criteria; 18 studies ...
The anatomic variants of the intercostobrachial nerve (ICBN) represent a potential risk of injuries during surgical procedure such as axillary lymph node dissection and sentinel lymph node biopsy in breast cancer and melanoma patients. The aim of this systematic review and meta-analysis was to investigate the different origins and branching patterns of the intercostobrachial nerve also ...
A vast amount of research exists on the possible molecular mechanisms through which vitamin D affects cancer cell proliferation, cancer progression, angiogenesis, and inflammation. We conducted a systematic review of the literature on the effects of vitamin D on ovarian cancer cell.
The purpose of this systematic review was 1) to identify research studies that have explored FCR among ovarian cancer patients, 2) to characterize FCR in this population in terms of prevalence and specific concerns, 3) to identify demographic, medical, and psychological factors associated with FCR and 4) to discuss the implications for ...
The generalizability of findings from Clinical Trials (CTs) investigating lymphedema treatment modalities requires an accurate representation of the target population. This study aims to evaluate racial and ethnic reporting and representation in lymphedema CTs. A comprehensive systematic literature search was conducted during May 2023 using multiple databases, following the PRISMA guidelines.