Thank you for visiting nature.com. You are using a browser version with limited support for CSS. To obtain the best experience, we recommend you use a more up to date browser (or turn off compatibility mode in Internet Explorer). In the meantime, to ensure continued support, we are displaying the site without styles and JavaScript.
- View all journals
- My Account Login
- Explore content
- About the journal
- Publish with us
- Sign up for alerts
- Open access
- Published: 09 October 2023
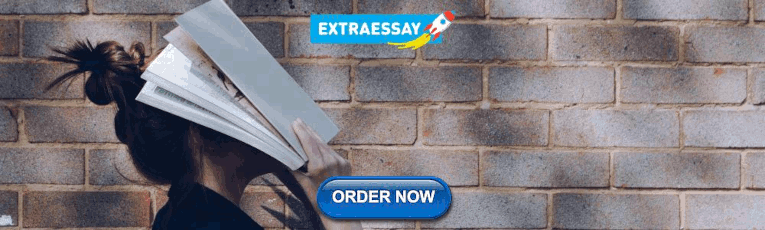
Sign language recognition using the fusion of image and hand landmarks through multi-headed convolutional neural network
- Refat Khan Pathan 1 ,
- Munmun Biswas 2 ,
- Suraiya Yasmin 3 ,
- Mayeen Uddin Khandaker ORCID: orcid.org/0000-0003-3772-294X 4 , 5 ,
- Mohammad Salman 6 &
- Ahmed A. F. Youssef 6
Scientific Reports volume 13 , Article number: 16975 ( 2023 ) Cite this article
12k Accesses
5 Citations
Metrics details
- Computational science
- Image processing
Sign Language Recognition is a breakthrough for communication among deaf-mute society and has been a critical research topic for years. Although some of the previous studies have successfully recognized sign language, it requires many costly instruments including sensors, devices, and high-end processing power. However, such drawbacks can be easily overcome by employing artificial intelligence-based techniques. Since, in this modern era of advanced mobile technology, using a camera to take video or images is much easier, this study demonstrates a cost-effective technique to detect American Sign Language (ASL) using an image dataset. Here, “Finger Spelling, A” dataset has been used, with 24 letters (except j and z as they contain motion). The main reason for using this dataset is that these images have a complex background with different environments and scene colors. Two layers of image processing have been used: in the first layer, images are processed as a whole for training, and in the second layer, the hand landmarks are extracted. A multi-headed convolutional neural network (CNN) model has been proposed and tested with 30% of the dataset to train these two layers. To avoid the overfitting problem, data augmentation and dynamic learning rate reduction have been used. With the proposed model, 98.981% test accuracy has been achieved. It is expected that this study may help to develop an efficient human–machine communication system for a deaf-mute society.
Similar content being viewed by others
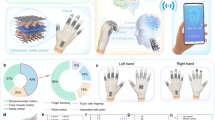
AI enabled sign language recognition and VR space bidirectional communication using triboelectric smart glove
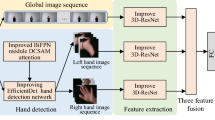
Improved 3D-ResNet sign language recognition algorithm with enhanced hand features
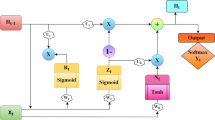
An integrated mediapipe-optimized GRU model for Indian sign language recognition
Introduction.
Spoken language is the medium of communication between a majority of the population. With spoken language, it would be workable for a massive extent of the population to impart. Nonetheless, despite spoken language, a section of the population cannot speak with most of the other population. Mute people cannot convey a proper meaning using spoken language. Hard of hearing is a handicap that weakens their hearing and makes them unfit to hear, while quiet is an incapacity that impedes their talking and makes them incapable of talking. Both are just handicapped in their hearing or potentially, therefore, cannot still do many other things. Communication is the only thing that isolates them from ordinary people 1 . As there are so many languages in the world, a unique language is needed to express their thoughts and opinions, which will be understandable to ordinary people, and such a language is named sign language. Understanding sign language is an arduous task, an ability that must be educated with training.
Many methods are available that use different things/tools like images (2D, 3D), sensor data (hand globe 2 , Kinect sensor 3 , neuromorphic sensor 4 ), videos, etc. All things are considered due to the fact that the captured images are excessively noisy. Therefore an elevated level of pre-processing is required. The available online datasets are already processed or taken in a lab environment where it becomes easy for recent advanced AI models to train and evaluate, causing prone to errors in real-life applications with different kinds of noises. Accordingly, it is a basic need to make a model that can deal with noisy images and also be able to deliver positive results. Different sorts of methods can be utilized to execute the classification and recognition of images using machine learning. Apart from recognizing static images, work has been done in depth-camera detecting and video processing 5 , 6 , 7 . Various cycles inserted in the system were created utilizing other programming languages to execute the procedural strategies for the final system's maximum adequacy. The issue can be addressed and deliberately coordinated into three comparable methodologies: initially using static image recognition techniques and pre-processing procedures, secondly by using deep learning models, and thirdly by using Hidden Markov Models.
Sign language guides this part of the community and empowers smooth communication in the community of people with trouble talking and hearing (deaf and dumb). They use hand signals along with facial expressions and body activities to cooperate. Yet, as a global language, not many people become familiar with communication via sign language gestures 8 . Hand motions comprise a significant part of communication through signing vocabulary. At the same time, facial expressions and body activities assume the jobs of underlining the words and phrases communicated by hand motions. Hand motions can be static or dynamic 9 , 10 . There are methodologies for motion discovery utilizing the dynamic vision sensor (DVS), a similar technique used in the framework introduced in this composition. For example, Arnon et al. 11 have presented an event-based gesture recognition system, which measures the event stream utilizing a natively event-based processor from International Business Machines called TrueNorth. They use a temporal filter cascade to create Spatio-temporal frames that CNN executes in the event-based processor, and they reported an accuracy of 96.46%. But in a real-life scenario, corresponding background situations are not static. Therefore the stated power saving process might not work properly. Jun Haeng Lee et al. 12 proposed a motion classification method with two DVSs to get a stereo-vision system. They used spike neurons to handle the approaching occasions with the same real-life issue. Static hand signals are also called hand acts and are framed in different shapes and directions of hands without speaking to any movement data. Dynamic hand motions comprise a sequence of hand stances with related movement information 13 . Using facial expressions, static hand images, and hand signals, communication through signing gives instruments to convey similarly as if communicated in dialects; there are different kinds of communication via gestures as well 14 .
In this work, we have applied a fusion of traditional image processing with extracted hand landmarks and trained on a multi-headed CNN so that it could complement each other’s weights on the concatenation layer. The main objective is to achieve a better detection rate without relying on a traditional single-channel CNN. This method has been proven to work well with less computational power and fewer epochs on medical image datasets 15 . The rest of the paper is divided into multiple sections as literature review in " Literature review " section, materials and methods in " Materials and methods " section with three subsections: dataset description in Dataset description , image pre-processing in " Pre-processing of image dataset " and working procedure in " Working procedure ", result analysis in " Result analysis " section, and conclusion in " Conclusion " section.
Literature review
State-of-the-art techniques centered after utilizing deep learning models to improve good accuracy and less execution time. CNNs have indicated huge improvements in visual object recognition 16 , natural language processing 17 , scene labeling 18 , medical image processing 15 , and so on. Despite these accomplishments, there is little work on applying CNNs to video classification. This is halfway because of the trouble in adjusting the CNNs to join both spatial and fleeting data. Model using exceptional hardware components such as a depth camera has been used to get the data on the depth variation in the image to locate an extra component for correlation, and then built up a CNN for getting the results 19 , still has low accuracy. An innovative technique that does not need a pre-trained model for executing the system was created using a capsule network and versatile pooling 11 .
Furthermore, it was revealed that lowering the layers of CNN, which employs a greedy way to do so, and developing a deep belief network produced superior outcomes compared to other fundamental methodologies 20 . Feature extraction using scale-invariant feature transform (SIFT) and classification using Neural Networks were developed to obtain the ideal results 21 . In one of the methods, the images were changed into an RGB conspire, the data was developed utilizing the movement depth channel lastly using 3D recurrent convolutional neural networks (3DRCNN) to build up a working system 5 , 22 where Canny edge detection oriented FAST and Rotated BRIEF (ORB) has been used. ORB feature detection technique and K-means clustering algorithm used to create the bag of feature model for all descriptors is described, but the plain background, easy to detect edges are totally dependent on edges; if the edges give wrong info, the model may fall accuracy and become the main problem to solve.
In recent years, utilizing deep learning approaches has become standard for improving the recognition accuracy of sign language models. Using Faster Region-based Convolutional Neural Network (Faster-RCNN) 23 , a CNN model is applied for hand recognition in the data image. Rastgoo et al. 24 proposed a method where they cropped an image properly, used fusion between RGB and depth image (RBM), added two noise types (Gaussian noise + salt n paper noise), and prepared the data for training. As a naturally propelled deep learning model, CNNs achieve every one of the three phases with a single framework that is prepared from crude pixel esteems to classifier yields, but extreme computation power was needed. Authors in ref. 25 proposed 3D CNNs where the third dimension joins both spatial and fleeting stamps. It accepts a few neighboring edges as input and performs 3D convolution in the convolutional layers. Along with them, the study reported in 26 followed similar thoughts and proposed regularizing the yields with high-level features, joining the expectations of a wide range of models. They applied the developed models to perceive human activities and accomplished better execution in examination than benchmark methods. But it is not sure it works with hand gestures as they detected face first and thenody movement 27 .
On the other hand, the Microsoft and Leap Motion companies have developed unmistakable approaches to identify and track a user’s hand and body movement by presenting Kinect and the leap motion controller (LMC) separately. Kinect recognizes the body skeleton and tracks the hands, whereas the LMC distinguishes and tracks hands with its underlying cameras and infrared sensors 3 , 28 . Using the provided framework, Sykora et al. 7 utilized the Kinect system to catch the depth data of 10 hand motions to classify them using a speeded-up robust features (SURF) technique that came up to an 82.8% accuracy, but it cannot test on more extensive database and modified feature extraction methods (SIFT, SURF) so it can be caused non-invariant to the orientation of gestures. Likewise, Huang et al. 29 proposed a 10-word-based ASL recognition system utilizing Kinect by tenfold cross-validation with an SVM that accomplished a precision pace of 97% using a set of frame-independent features, but the most significant problem in this method is segmentation.
The literature summarizes that most of the models used in this application either depend on a single variable or require high computational power. Also, their dataset choice for training and validating the model is in plain background, which is easier to detect. Our main aim is to show how to reduce the computational power for training and the dependency of model training on one layer.
Materials and methods
Dataset description.
Using a generalized single-color background to classify sign language is very common. We intended to avoid that single color background and use a complex background with many users’ hand images to increase the detection complexity. That’s why we have used the “ASL Finger Spelling” dataset 30 , which has images of different sizes, orientations, and complex backgrounds of over 500 images per sign (24 sign total) of 4 users (non-native to sign language). This dataset contains separate RGB and depth images; we have worked with the RGB images in this research. The photos were taken in 5 sessions with the same background and lighting. The dataset details are shown in Table 1 , and some sample images are shown in Fig. 1 .
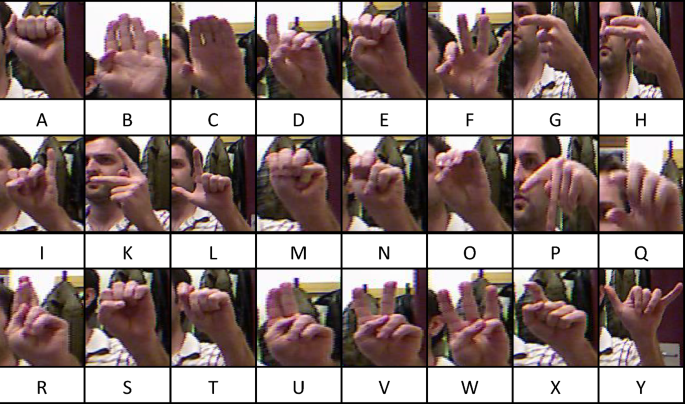
Sample images from a dataset containing 24 signs from the same user.
Pre-processing of image dataset
Images were pre-processed for two operations: preparing the original image training set and extracting the hand landmarks. Traditional CNN has one input data channel and one output channel. We are using two input data channels and one output channel, so data needs to be prepared for both inputs individually.
Raw image processing
In raw image processing, we have converted the images from RGB to grayscale to reduce color complexity. Then we used a 2D kernel matrix for sharpening the images, as shown in Fig. 2 . After that, we resized the images into 50 × 50 pixels for evaluation through CNN. Finally, we have normalized the grayscale values (0–255) by dividing the pixel values by 255, so now the new pixel array contains value ranges (0–1). The primary advantage of this normalization is that CNN works faster in the (0–1) range rather than other limits.
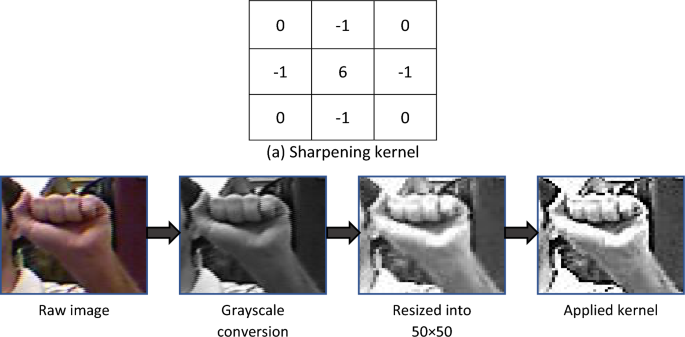
Raw image pre-processing with ( a ) sharpening kernel.
Hand landmark detection
Google’s hand landmark model has an input channel of RGB and an image size of (224 × 224 × 3). So, we have taken the RGB images, converted pixel values into float32, and resized all the images into (256 × 256 × 3). After applying the model, it gives 21 coordinated 3-dimensional points. The landmark detection process is shown in Fig. 3 .
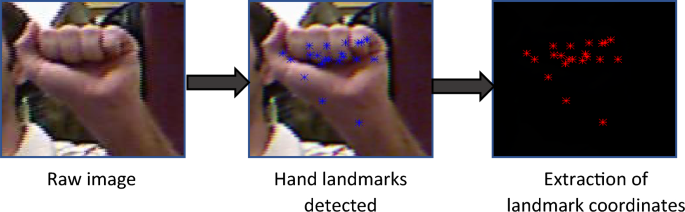
Hand landmarks detection and extraction of 21 coordinates.
Working procedure
The whole work is divided into two main parts, one is the raw image processing, and another one is the hand landmarks extraction. After both individual processing had been completed, a custom lightweight simple multi-headed CNN model was built to train both data. Before processing through a fully connected layer for classification, we merged both channel’s features so that the model could choose between the best weights. This working procedure is illustrated in Fig. 4 .
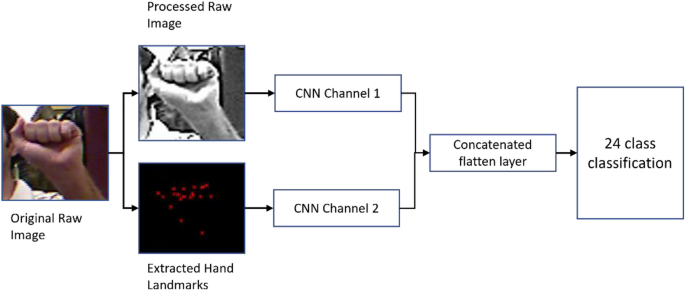
Flow diagram of working procedure.
Model building
In this research, we have used multi-headed CNN, meaning our model has two input data channels. Before this, we trained processed images and hand landmarks with two separate models to compare. Google’s model is not best for “in the wild” situations, so we needed original images to complement the low faults in Google’s model. In the first head of the model, we have used the processed images as input and hand landmarks data as the second head’s input. Two-dimensional Convolutional layers with filter size 50, 25, kernel (3, 3) with Relu, strides 1; MaxPooling 2D with pool size (2, 2), batch normalization, and Dropout layer has been used in the hand landmarks training side. Besides, the 2D Convolutional layer with filter size 32, 64, 128, 512, kernel (3, 3) with Relu; MaxPooling 2D with pool size (2, 2); batch normalization and dropout layer has been used in the image training side. After both flatten layers, two heads are concatenated and go through a dense, dropout layer. Finally, the output dense layer has 24 units with Softmax activation. This model has been compiled with Adam optimizer and MSE loss for 50 epochs. Figure 5 illustrates the proposed CNN architecture, and Table 2 shows the model details.
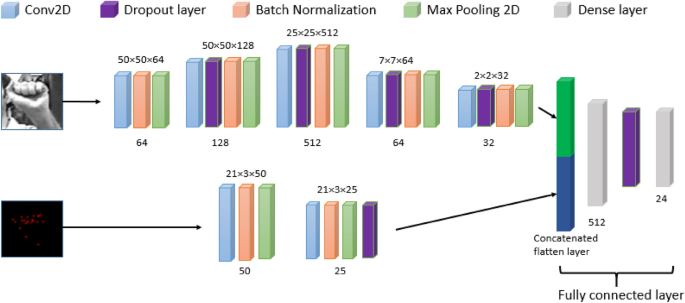
Proposed multi-headed CNN architecture. Bottom values are the number of filters and top values are output shapes.
Training and testing
The input images were augmented to generate more difficulty in training so that the model could not overfit. Image Data Generator did image augmentation with 10° rotation, 0.1 zoom range, 0.1 widths and height shift range, and horizontal flip. Being more conscious about the overfitting issues, we have used dynamic learning rates, monitoring the validation accuracy with patience 5, factor 0.5, and a minimum learning rate of 0.00001. For training, we have used 46,023 images, and for testing, 19,725 images. For 50 epochs, the training vs testing accuracy and loss has been shown in Fig. 6 .
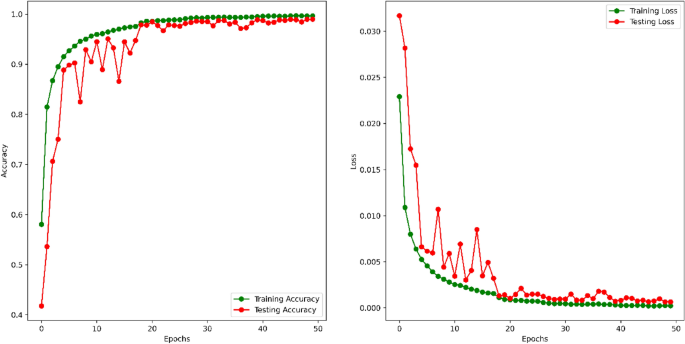
Training versus testing accuracy and loss for 50 epochs.
For further evaluation, we have calculated the precision, recall, and F1 score of the proposed multi-headed CNN model, which shows excellent performance. To compute these values, we first calculated the confusion matrix (shown in Fig. 7 ). When a class is positive and also classified as so, it is called true positive (TP). Again, when a class is negative and classified as so, it is called true negative (TN). If a class is negative and classified as positive, it is called false positive (FP). Also, when a class is positive and classified as not negative, it is called false negative (FN). From these, we can conclude precision, recall, and F1 score like the below:
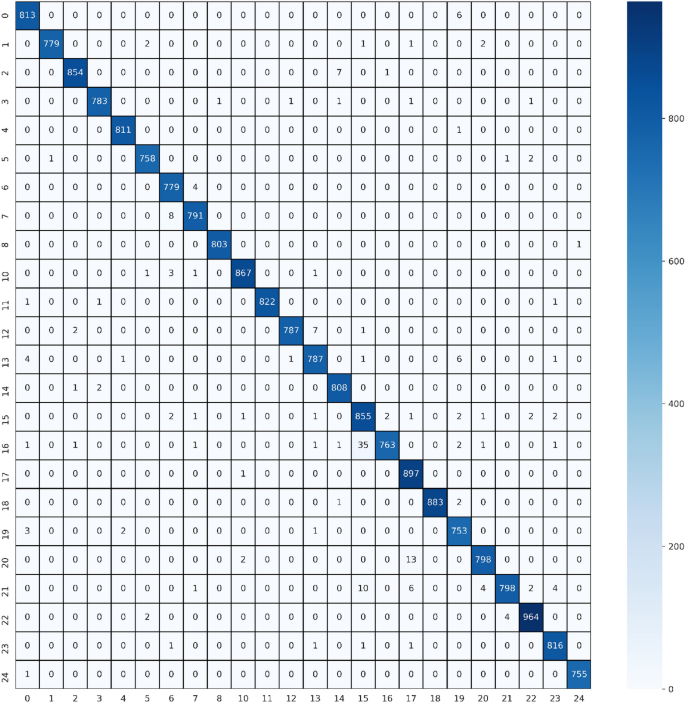
Confusion matrix of the testing dataset. Numerical values in X and Y axis means the sequential letters from A = 0 to Y = 24, number 9 and 25 is missing because dataset does not have letter J and Z.
Precision: Precision is the ratio of TP and total predicted positive observation.
Recall: It is the ratio of TP and total positive observations in the actual class.
F1 score: F1 score is the weighted average of precision and recall.
The Precision, Recall, and F1 score for 24 classes are shown in Table 3 .
Result analysis
In human action recognition tasks, sign language has an extra advantage as it can be used to communicate efficiently. Many techniques have been developed using image processing, sensor data processing, and motion detection by applying different dynamic algorithms and methods like machine learning and deep learning. Depending on methodologies, researchers have proposed their way of classifying sign languages. As technologies develop, we can explore the limitations of previous works and improve accuracy. In ref. 13 , this paper proposes a technique for acknowledging hand motions, which is an excellent part of gesture-based communication jargon, because of a proficient profound deep convolutional neural network (CNN) architecture. The proposed CNN design disposes of the requirement for recognition and division of hands from the captured images, decreasing the computational weight looked at during hand pose recognition with classical approaches. In our method, we used two input channels for the images and hand landmarks to get more robust data, making the process more efficient with a dynamic learning rate adjustment. Besides in ref 14 , the presented results were acquired by retraining and testing the sign language gestures dataset on a convolutional neural organization model utilizing Inception v3. The model comprises various convolution channel inputs that are prepared on a piece of similar information. A capsule-based deep neural network sign posture translator for an American Sign Language (ASL) fingerspelling (posture) 20 has been introduced where the idea concept of capsules and pooling are used simultaneously in the network. This exploration affirms that utilizing pooling and capsule routing on a similar network can improve the network's accuracy and convergence speed. In our method, we have used the pre-trained model of Google to extract the hand landmarks, almost like transfer learning. We have shown that utilizing two input channels could also improve accuracy.
Moreover, ref 5 proposed a 3DRCNN model integrating a 3D convolutional neural network (3DCNN) and upgraded completely associated recurrent neural network (FC-RNN), where 3DCNN learns multi-methodology features from RGB, motion, and depth channels, and FCRNN catch the fleeting data among short video clips divided from the original video. Consecutive clips with a similar semantic significance are singled out by applying the sliding window way to deal with a section of the clips on the whole video sequence. Combining a CNN and traditional feature extractors, capable of accurate and real-time hand posture recognition 26 where the architecture is assessed on three particular benchmark datasets and contrasted and the cutting edge convolutional neural networks. Extensive experimentation is directed utilizing binary, grayscale, and depth data and two different validation techniques. The proposed feature fusion-based CNN 31 is displayed to perform better across blends of approval procedures and image representation. Similarly, fusion-based CNN is demonstrated to improve the recognition rate in our study.
After worldwide motion analysis, the hand gesture image sequence was dissected for keyframe choice. The video sequences of a given gesture were divided in the RGB shading space before feature extraction. This progression enjoyed the benefit of shaded gloves worn by the endorsers. Samples of pixel vectors representative of the glove’s color were used to estimate the mean and covariance matrix of the shading, which was sectioned. So, the division interaction was computerized with no user intervention. The video frames were converted into color HSV (Hue-SaturationValue) space in the color object tracking method. Then the pixels with the following shading were distinguished and marked, and the resultant images were converted to a binary (Gray Scale image). The system identifies image districts compared to human skin by binarizing the input image with a proper threshold value. Then, at that point, small regions from the binarized image were eliminated by applying a morphological operator and selecting the districts to get an image as an applicant of hand.
In the proposed method we have used two-headed CNN to train the processed input images. Though the single image input stream is widely used, two input streams have an advantage among them. In the classification layer of CNN, if one layer is giving a false result, it could be complemented by the other layer’s weight, and it is possible that combining both results could provide a positive outcome. We used this theory and successfully improved the final validation and test results. Before combining image and hand landmark inputs, we tested both individually and acquired a test accuracy of 96.29% for the image and 98.42% for hand landmarks. We did not use binarization as it would affect the background of an image with skin color matched with hand color. This method is also suitable for wild situations as it is not entirely dependent on hand position in an image frame. A comparison of the literature and our work has been shown in Table 4 , which shows that our method overcomes most of the current position in accuracy gain.
Table 5 illustrates that the Combined Model, while having a larger number of parameters and consuming more memory, achieves the highest accuracy of 98.98%. This suggests that the combined approach, which incorporates both image and hand landmark information, is effective for the task when accuracy is priority. On the other hand, the Hand Landmarks Model, despite having fewer parameters and lower memory consumption, also performs impressively with an accuracy of 98.42%. But it has its own error and memory consumption rate in model training by Google. The Image Model, while consuming less memory, has a slightly lower accuracy of 96.29%. The choice between these models would depend on the specific application requirements, trade-offs between accuracy and resource utilization, and the importance of execution time.
This work proposes a methodology for perceiving the classification of sign language recognition. Sign language is the core medium of communication between deaf-mute and everyday people. It is highly implacable in real-world scenarios like communication, human–computer interaction, security, advanced AI, and much more. For a long time, researchers have been working in this field to make a reliable, low cost and publicly available SRL system using different sensors, images, videos, and many more techniques. Many datasets have been used, including numeric sensory, motion, and image datasets. Most datasets are prepared in a good lab condition to do experiments, but in the real world, it may not be a practical case. That’s why, looking into the real-world situation, the Fingerspelling dataset has been used, which contains real-world scenarios like complex backgrounds, uneven image shapes, and conditions. First, the raw images are processed and resized into a 50 × 50 size. Then, the hand landmark points are detected and extracted from these hand images. Making images goes through two processing techniques; now, there are two data channels. A multi-headed CNN architecture has been proposed for these two data channels. Total data has been augmented to avoid overfitting, and dynamic learning rate adjustment has been done. From the prepared data, 70–30% of the train test spilled has been done. With the 30% dataset, a validation accuracy of 98.98% has been achieved. In this kind of large dataset, this accuracy is much more reliable.
There are some limitations found in the proposed method compared with the literature. Some methods might work with low image dataset numbers, but as we use the simple CNN model, this method requires a good number of images for training. Also, the proposed method depends on the hand landmark extraction model. Other hand landmark model can cause different results. In raw image processing, it is possible to detect hand portions to reduce the image size, which may increase the recognition chance and reduce the model training time. Hence, we may try this method in future work. Currently, raw image processing takes a good amount of training time as we considered the whole image for training.
Data availability
The dataset used in this paper (ASL Fingerspelling Images (RGB & Depth)) is publicly available at Kaggle on this URL: https://www.kaggle.com/datasets/mrgeislinger/asl-rgb-depth-fingerspelling-spelling-it-out .
Anderson, R., Wiryana, F., Ariesta, M. C. & Kusuma, G. P. Sign language recognition application systems for deaf-mute people: A review based on input-process-output. Proced. Comput. Sci. 116 , 441–448. https://doi.org/10.1016/j.procs.2017.10.028 (2017).
Article Google Scholar
Mummadi, C. et al. Real-time and embedded detection of hand gestures with an IMU-based glove. Informatics 5 (2), 28. https://doi.org/10.3390/informatics5020028 (2018).
Hickeys Kinect for Windows - Windows apps. (2022). Accessed 01 January 2023. https://learn.microsoft.com/en-us/windows/apps/design/devices/kinect-for-windows
Rivera-Acosta, M., Ortega-Cisneros, S., Rivera, J. & Sandoval-Ibarra, F. American sign language alphabet recognition using a neuromorphic sensor and an artificial neural network. Sensors 17 (10), 2176. https://doi.org/10.3390/s17102176 (2017).
Article ADS PubMed PubMed Central Google Scholar
Ye, Y., Tian, Y., Huenerfauth, M., & Liu, J. Recognizing American Sign Language Gestures from Within Continuous Videos. In 2018 IEEE/CVF Conference on Computer Vision and Pattern Recognition Workshops (CVPRW) , 2145–214509 (IEEE, 2018). https://doi.org/10.1109/CVPRW.2018.00280 .
Ameen, S. & Vadera, S. A convolutional neural network to classify American Sign Language fingerspelling from depth and colour images. Expert Syst. 34 (3), e12197. https://doi.org/10.1111/exsy.12197 (2017).
Sykora, P., Kamencay, P. & Hudec, R. Comparison of SIFT and SURF methods for use on hand gesture recognition based on depth map. AASRI Proc. 9 , 19–24. https://doi.org/10.1016/j.aasri.2014.09.005 (2014).
Sahoo, A. K., Mishra, G. S. & Ravulakollu, K. K. Sign language recognition: State of the art. ARPN J. Eng. Appl. Sci. 9 (2), 116–134 (2014).
Google Scholar
Mitra, S. & Acharya, T. “Gesture recognition: A survey. IEEE Trans. Syst. Man Cybern. Part C 37 (3), 311–324. https://doi.org/10.1109/TSMCC.2007.893280 (2007).
Rautaray, S. S. & Agrawal, A. Vision based hand gesture recognition for human computer interaction: A survey. Artif. Intell. Rev. 43 (1), 1–54. https://doi.org/10.1007/s10462-012-9356-9 (2015).
Amir A. et al A low power, fully event-based gesture recognition system. In 2017 IEEE Conference on Computer Vision and Pattern Recognition (CVPR) , 7388–7397 (IEEE, 2017). https://doi.org/10.1109/CVPR.2017.781 .
Lee, J. H. et al. Real-time gesture interface based on event-driven processing from stereo silicon retinas. IEEE Trans. Neural Netw. Learn Syst. 25 (12), 2250–2263. https://doi.org/10.1109/TNNLS.2014.2308551 (2014).
Article PubMed Google Scholar
Adithya, V. & Rajesh, R. A deep convolutional neural network approach for static hand gesture recognition. Proc. Comput. Sci. 171 , 2353–2361. https://doi.org/10.1016/j.procs.2020.04.255 (2020).
Das, A., Gawde, S., Suratwala, K., & Kalbande, D. Sign language recognition using deep learning on custom processed static gesture images. In 2018 International Conference on Smart City and Emerging Technology (ICSCET) , 1–6 (IEEE, 2018). https://doi.org/10.1109/ICSCET.2018.8537248 .
Pathan, R. K. et al. Breast cancer classification by using multi-headed convolutional neural network modeling. Healthcare 10 (12), 2367. https://doi.org/10.3390/healthcare10122367 (2022).
Article PubMed PubMed Central Google Scholar
Lecun, Y., Bottou, L., Bengio, Y. & Haffner, P. Gradient-based learning applied to document recognition. Proc. IEEE 86 (11), 2278–2324. https://doi.org/10.1109/5.726791 (1998).
Collobert, R., & Weston, J. A unified architecture for natural language processing. In Proceedings of the 25th international conference on Machine learning—ICML ’08 , 160–167 (ACM Press, 2008). https://doi.org/10.1145/1390156.1390177 .
Farabet, C., Couprie, C., Najman, L. & LeCun, Y. Learning hierarchical features for scene labeling. IEEE Trans. Pattern Anal. Mach. Intell. 35 (8), 1915–1929. https://doi.org/10.1109/TPAMI.2012.231 (2013).
Xie, B., He, X. & Li, Y. RGB-D static gesture recognition based on convolutional neural network. J. Eng. 2018 (16), 1515–1520. https://doi.org/10.1049/joe.2018.8327 (2018).
Jalal, M. A., Chen, R., Moore, R. K., & Mihaylova, L. American sign language posture understanding with deep neural networks. In 2018 21st International Conference on Information Fusion (FUSION) , 573–579 (IEEE, 2018).
Shanta, S. S., Anwar, S. T., & Kabir, M. R. Bangla Sign Language Detection Using SIFT and CNN. In 2018 9th International Conference on Computing, Communication and Networking Technologies (ICCCNT) , 1–6 (IEEE, 2018). https://doi.org/10.1109/ICCCNT.2018.8493915 .
Sharma, A., Mittal, A., Singh, S. & Awatramani, V. Hand gesture recognition using image processing and feature extraction techniques. Proc. Comput. Sci. 173 , 181–190. https://doi.org/10.1016/j.procs.2020.06.022 (2020).
Ren, S., He, K., Girshick, R., & Sun, J. Faster r-cnn: Towards real-time object detection with region proposal networks. Adv. Neural Inf. Process Syst. , 28 (2015).
Rastgoo, R., Kiani, K. & Escalera, S. Multi-modal deep hand sign language recognition in still images using restricted Boltzmann machine. Entropy 20 (11), 809. https://doi.org/10.3390/e20110809 (2018).
Jhuang, H., Serre, T., Wolf, L., & Poggio, T. A biologically inspired system for action recognition. In 2007 IEEE 11th International Conference on Computer Vision , 1–8. (IEEE, 2007) https://doi.org/10.1109/ICCV.2007.4408988 .
Ji, S., Xu, W., Yang, M. & Yu, K. 3D convolutional neural networks for human action recognition. IEEE Trans. Pattern Anal. Mach. Intell. 35 (1), 221–231. https://doi.org/10.1109/TPAMI.2012.59 (2013).
Huang, J., Zhou, W., Li, H., & Li, W. sign language recognition using 3D convolutional neural networks. In 2015 IEEE International Conference on Multimedia and Expo (ICME) , 1–6 (IEEE, 2015). https://doi.org/10.1109/ICME.2015.7177428 .
Digital worlds that feel human Ultraleap. Accessed 01 January 2023. Available: https://www.leapmotion.com/
Huang, F., & Huang, S. Interpreting american sign language with Kinect. Journal of Deaf Studies and Deaf Education, [Oxford University Press] , (2011).
Pugeault, N., & Bowden, R. Spelling it out: Real-time ASL fingerspelling recognition. In 2011 IEEE International Conference on Computer Vision Workshops (ICCV Workshops) , 1114–1119 (IEEE, 2011). https://doi.org/10.1109/ICCVW.2011.6130290 .
Rahim, M. A., Islam, M. R. & Shin, J. Non-touch sign word recognition based on dynamic hand gesture using hybrid segmentation and CNN feature fusion. Appl. Sci. 9 (18), 3790. https://doi.org/10.3390/app9183790 (2019).
“ASL Alphabet.” Accessed 01 Jan, 2023. https://www.kaggle.com/grassknoted/asl-alphabet
Download references
Funding was provided by the American University of the Middle East, Egaila, Kuwait.
Author information
Authors and affiliations.
Department of Computing and Information Systems, School of Engineering and Technology, Sunway University, 47500, Bandar Sunway, Selangor, Malaysia
Refat Khan Pathan
Department of Computer Science and Engineering, BGC Trust University Bangladesh, Chittagong, 4381, Bangladesh
Munmun Biswas
Department of Computer and Information Science, Graduate School of Engineering, Tokyo University of Agriculture and Technology, Koganei, Tokyo, 184-0012, Japan
Suraiya Yasmin
Centre for Applied Physics and Radiation Technologies, School of Engineering and Technology, Sunway University, 47500, Bandar Sunway, Selangor, Malaysia
Mayeen Uddin Khandaker
Faculty of Graduate Studies, Daffodil International University, Daffodil Smart City, Birulia, Savar, Dhaka, 1216, Bangladesh
College of Engineering and Technology, American University of the Middle East, Egaila, Kuwait
Mohammad Salman & Ahmed A. F. Youssef
You can also search for this author in PubMed Google Scholar
Contributions
R.K.P and M.B, Conceptualization; R.K.P. methodology; R.K.P. software and coding; M.B. and R.K.P. validation; R.K.P. and M.B. formal analysis; R.K.P., S.Y., and M.B. investigation; S.Y. and R.K.P. resources; R.K.P. and M.B. data curation; S.Y., R.K.P., and M.B. writing—original draft preparation; S.Y., R.K.P., M.B., M.U.K., M.S., A.A.F.Y. and M.S. writing—review and editing; R.K.P. and M.U.K. visualization; M.U.K. and M.B. supervision; M.B., M.S. and A.A.F.Y. project administration; M.S. and A.A.F.Y, funding acquisition.
Corresponding author
Correspondence to Mayeen Uddin Khandaker .
Ethics declarations
Competing interests.
The authors declare no competing interests.
Additional information
Publisher's note.
Springer Nature remains neutral with regard to jurisdictional claims in published maps and institutional affiliations.
Rights and permissions
Open Access This article is licensed under a Creative Commons Attribution 4.0 International License, which permits use, sharing, adaptation, distribution and reproduction in any medium or format, as long as you give appropriate credit to the original author(s) and the source, provide a link to the Creative Commons licence, and indicate if changes were made. The images or other third party material in this article are included in the article's Creative Commons licence, unless indicated otherwise in a credit line to the material. If material is not included in the article's Creative Commons licence and your intended use is not permitted by statutory regulation or exceeds the permitted use, you will need to obtain permission directly from the copyright holder. To view a copy of this licence, visit http://creativecommons.org/licenses/by/4.0/ .
Reprints and permissions
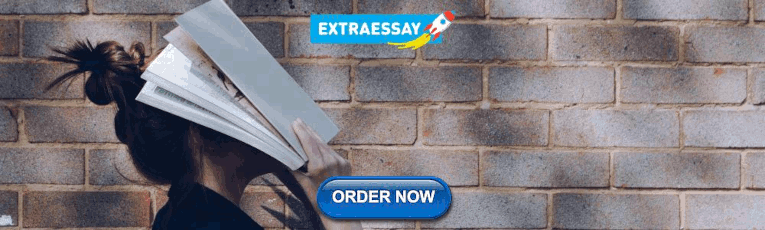
About this article
Cite this article.
Pathan, R.K., Biswas, M., Yasmin, S. et al. Sign language recognition using the fusion of image and hand landmarks through multi-headed convolutional neural network. Sci Rep 13 , 16975 (2023). https://doi.org/10.1038/s41598-023-43852-x
Download citation
Received : 04 March 2023
Accepted : 29 September 2023
Published : 09 October 2023
DOI : https://doi.org/10.1038/s41598-023-43852-x
Share this article
Anyone you share the following link with will be able to read this content:
Sorry, a shareable link is not currently available for this article.
Provided by the Springer Nature SharedIt content-sharing initiative
This article is cited by
Boxing behavior recognition based on artificial intelligence convolutional neural network with sports psychology assistant.
- Yuanhui Kong
- Zhiyuan Duan
Scientific Reports (2024)
Using LSTM to translate Thai sign language to text in real time
- Werapat Jintanachaiwat
- Kritsana Jongsathitphaibul
- Thitirat Siriborvornratanakul
Discover Artificial Intelligence (2024)
By submitting a comment you agree to abide by our Terms and Community Guidelines . If you find something abusive or that does not comply with our terms or guidelines please flag it as inappropriate.
Quick links
- Explore articles by subject
- Guide to authors
- Editorial policies
Sign up for the Nature Briefing: AI and Robotics newsletter — what matters in AI and robotics research, free to your inbox weekly.

Research of a Sign Language Translation System Based on Deep Learning
Ieee account.
- Change Username/Password
- Update Address
Purchase Details
- Payment Options
- Order History
- View Purchased Documents
Profile Information
- Communications Preferences
- Profession and Education
- Technical Interests
- US & Canada: +1 800 678 4333
- Worldwide: +1 732 981 0060
- Contact & Support
- About IEEE Xplore
- Accessibility
- Terms of Use
- Nondiscrimination Policy
- Privacy & Opting Out of Cookies
A not-for-profit organization, IEEE is the world's largest technical professional organization dedicated to advancing technology for the benefit of humanity. © Copyright 2024 IEEE - All rights reserved. Use of this web site signifies your agreement to the terms and conditions.
Recognition of Indian Sign Language (ISL) Using Deep Learning Model
- Published: 28 September 2021
- Volume 123 , pages 671–692, ( 2022 )
Cite this article
- Sakshi Sharma 1 &
- Sukhwinder Singh 1
1252 Accesses
21 Citations
Explore all metrics
An efficient sign language recognition system (SLRS) can recognize the gestures of sign language to ease the communication between the signer and non-signer community. In this paper, a computer-vision based SLRS using a deep learning technique has been proposed. This study has primary three contributions: first, a large dataset of Indian sign language (ISL) has been created using 65 different users in an uncontrolled environment. Second, the intra-class variance in dataset has been increased using augmentation to improve the generalization ability of the proposed work. Three additional copies for each training image are generated in this paper, by using three different affine transformations. Third, a novel and robust model using Convolutional Neural Network (CNN) have been proposed for the feature extraction and classification of ISL gestures. The performance of this method is evaluated on a self-collected ISL dataset and publicly available dataset of ASL. For this total of three datasets have been used and the achieved accuracy is 92.43, 88.01, and 99.52%. The efficiency of this method has been also evaluated in terms of precision, recall, f-score, and time consumed by the system. The results indicate that the proposed method shows encouraging performance compared with existing work.
This is a preview of subscription content, log in via an institution to check access.
Access this article
Price includes VAT (Russian Federation)
Instant access to the full article PDF.
Rent this article via DeepDyve
Institutional subscriptions
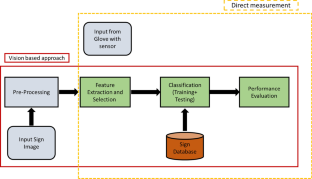
Similar content being viewed by others
A Study for Sign Language Detection Using Deep Learning Methods
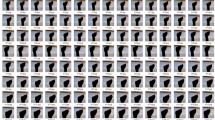
Sign language detection using convolutional neural network
Hybrid Sign Language Learning Approach Using Multi-scale Hierarchical Deep Convolutional Neural Network (MDCnn)
Availability of data and material.
The authors declare that no data or material was taken illegally. However, publically available dataset was taken for implementation. The dataset generated in the study are available from corresponding author on request base only after copyrights are reserved.
Census of India 2011: Disabled population. Available: http://enabled.in/wp/census-of-india-2011-disabledpopulation . Accessed 30 Apr 2021.
Johnson, J. E., & Johnson, R. J. (2008). Assessment of regional language varieties in indian sign language. SIL International, Dallas, Texas, vol 2008, (pp. 1–121).
Kumar, D. A., Sastry, A. S. C. S., Kishore, P. V. V., & Kumar, E. K. (2018). 3D sign language recognition using spatio temporal graph kernels. Journal of King Saud University-Computer and Information Sciences.
Sharma, S., & Singh, S. (2020). Vision-based sign language recognition system: A Comprehensive Review. In: IEEE International Conference on Inventive Computation Technologies (ICICT), (pp. 140–144).
Sharma, S., & Singh, S. (2021). Vision-based hand gesture recognition using deep learning for the interpretation of sign language. Expert Systems with Applications, 182 , 115657.
Article Google Scholar
Cheok, M. J., Omar, Z., & Jaward, M. H. (2019). A review of hand gesture and sign language recognition techniques. International Journal of Machine Learning and Cybernetics, 10 (1), 131–153.
Gangrade, J., & Bharti, J. (2020). Vision-based hand gesture recognition for indian sign language using convolution neural network. IETE Journal of Research, 1–10.
Sharma, S., & Singh, S. (2019). An analysis of reversible data hiding algorithms for encrypted domain. In: 2019 Third International conference on I-SMAC (IoT in Social, Mobile, Analytics and Cloud) (I-SMAC), (pp. 644–648). IEEE.
2021, ISL Dictionary Launch, Indian Sign Language Research and Training centre (ISLRTC). Available: http://www.islrtc.nic.in/isl-dictionary-launch . Accessed 30 Apr 2021.
Kakoty, N. M., & Sharma, M. D. (2018). Recognition of sign language alphabets and numbers based on hand kinematics using A data glove. Procedia Computer Science, 133 , 55–62.
Suri, K., & Gupta, R. (2019). Convolutional neural network array for sign language recognition using wearable IMUs. In: 2019 6th International Conference on Signal Processing and Integrated Networks (SPIN), (pp. 483–488).
Rewari, H., Dixit, V., Batra, D., & Hema, N. (2018). Automated sign language interpreter. In: Eleventh International Conference on Contemporary Computing (IC3), (pp. 1–5).
Chong, T. W., & Kim, B. J. (2020). American sign language recognition system using wearable sensors with deep learning approach. The Journal of the Korea Institute of Electronic Communication Sciences, 15 (2), 291–298.
Google Scholar
Gupta, R., & Kumar, A. (2020). Indian sign language recognition using wearable sensors and multi-label classification. Computers & Electrical Engineering, 90 , 106898.
Das, S. P., Talukdar, A. K., & Sarma, K. K. (2015). Sign language recognition using facial expression. Procedia Computer Science, 58 , 210–216.
Tripathi, K., & Nandi, N. B. G. (2015). Continuous Indian sign language gesture recognition and sentence formation. Procedia Computer Science, 54 , 523–531.
Lee, G. C., Yeh, F. H., & Hsiao, Y. H. (2016). Kinect-based Taiwanese sign-language recognition system. Multimedia Tools and Applications, 75 (1), 261–279.
Ansari, Z. A., & Harit, G. (2016). Nearest neighbour classification of Indian sign language gestures using kinect camera. Sadhana, 41 (2), 161–182.
Article MathSciNet Google Scholar
Beena, M. V., Namboodiri, M. A., & Dean, P. G. (2017). Automatic sign language finger spelling using convolution neural network: Analysis. International Journal of Pure and Applied Mathematics, 117 (20), 9–15.
Kumar, E. K., Kishore, P. V. V., Sastry, A. S. C. S., Kumar, M. T. K., & Kumar, D. A. (2018). Training CNNs for 3-D sign language recognition with color texture coded joint angular displacement maps. IEEE Signal Processing Letters, 25 (5), 645–649.
Müller, M., Röder, T., Clausen, M., Eberhardt, B., Krüger, B., & Weber, A. (2007). Documentation mocap database hdm05.
Rao, G. A., & Kishore, P. V. V. (2018). Selfie video based continuous Indian sign language recognition system. Ain Shams Engineering Journal, 9 (4), 1929–1939.
Xie, B., He, X., & Li, Y. (2018). RGB-D static gesture recognition based on convolutional neural network. The Journal of Engineering, 1515–1520.
Pugeault, N., & Bowden, R. (2011). Spelling it out: Real-time ASL fingerspelling recognition. In: IEEE International Conference on Computer Vision Workshops (ICCV workshops), (pp. 1114–1119).
Elpeltagy, M., Abdelwahab, M., Hussein, M. E., Shoukry, A., Shoala, A., & Galal, M. (2018). Multi-modality-based Arabic sign language recognition. IET Computer Vision, 12 (7), 1031–1039.
Ibrahim, N. B., Selim, M. M., & Zayed, H. H. (2018). An automatic arabic sign language recognition system (ArSLRS). Journal of King Saud University-Computer and Information Sciences, 30 (4), 470–477.
Kumar, P., Roy, P. P., & Dogra, D. P. (2018). Independent bayesian classifier combination based sign language recognition using facial expression. Information Sciences, 428 , 30–48.
Jose, H., & Julian, A. (2019). Tamil sign language translator—An assistive system for hearing-and speech-impaired people. In: Information and Communication Technology for Intelligent Systems, Springer, (pp. 249–257).
Ferreira, P. M., Cardoso, J. S., & Rebelo, A. (2019). On the role of multimodal learning in the recognition of sign language. Multimedia Tools and Applications, 78 (8), 10035–10056.
Sruthi, C. J., & Lijiya, A. (2019). Signet: A deep learning based indian sign language recognition system. In: 2019 International Conference on Communication and Signal Processing (ICCSP), (pp. 596–600).
Wadhawan, A., & Kumar, P. (2020). Deep learning-based sign language recognition system for static signs. Neural Computing and Applications, 1–12.
Kumar, A., & Kumar, R. (2021). A novel approach for ISL alphabet recognition using Extreme Learning Machine. International Journal of Information Technology, 13 (1), 349–357.
Sharma, A., Sharma, N., Saxena, Y., Singh, A., & Sadhya, D. (2020). Benchmarking deep neural network approaches for Indian Sign Language recognition. Neural Computing and Applications, 1–12.
Szegedy, C., Liu, W., Jia, Y., Sermanet, P., Reed, S., Anguelov, D., & Rabinovich, A. (2015). Going deeper with convolutions. In: Proceedings of the IEEE Conference on Computer Vision and Pattern Recognition, (pp. 1–9).
Simonyan, K., & Zisserman, A. (2014). Very deep convolutional networks for large-scale image recognition. arXiv preprint. arXiv:1409.1556.
Szegedy, C., Vanhoucke, V., Ioffe, S., Shlens, J., & Wojna, Z. (2016). Rethinking the inception architecture for computer vision. In: Proceedings of the IEEE Conference on Computer Vision and Pattern Recognition, (pp. 2818–2826).
Fukushima, K. (1980). A self-organizing neural network model for a mechanism of pattern recognition unaffected by shift in position. Biological Cybernetics, 36 , 193–202.
Rahman, M. M., Islam, M. S., Sassi, R., & Aktaruzzaman, M. (2019). Convolutional neural networks performance comparison for handwritten bengali numerals recognition. SN Applied Sciences, 1 (12), 1–11.
LeCun, Y., Bottou, L., Bengio, Y., & Haffner, P. (1998). Gradient-based learning applied to document recognition. Proceedings of the IEEE, 86 (11), 2278–2324.
Perez, L., & Wang, J. (2017). The effectiveness of data augmentation in image classification using deep learning. arXiv preprint. arXiv:1712.04621.
Triesch, J., & Von Der Malsburg, C. (2001). A system for person-independent hand posture recognition against complex backgrounds. IEEE Transactions on Pattern Analysis and Machine Intelligence, 23 (12), 1449–1453.
Rokade, Y. I., & Jadav, P. M. (2017). Indian sign language recognition system. International Journal of engineering and Technology, 9 (3), 189–196.
Kaur, J., & Krishna, C. R. (2019). An efficient Indian sign language recognition system using sift descriptor. International Journal of Engineering and Advanced Technology (IJEAT), 8(6).
Kumar, D. A., Kishore, P. V. V., Sastry, A. S. C. S., & Swamy, P. R. G. (2016). Selfie continuous sign language recognition using neural network. In: 2016 IEEE Annual India Conference (INDICON), (pp. 1–6).
Dour, G., & Sharma, S. (2016). Recognition of alphabets of indian sign language by Sugeno type fuzzy neural network. Pattern Recognit Lett, 30 , 737–742.
Athira, P. K., Sruthi, C. J., & Lijiya, A. (2019). A signer independent sign language recognition with co-articulation elimination from live videos: An indian scenario. Journal of King Saud University-Computer and Information Sciences.
Just, A., Rodriguez, Y., & Marcel, S. (2006). Hand posture classification and recognition using the modified census transform. In: 7th International Conference on Automatic Face and Gesture Recognition (FGR06), (pp. 351–356).
Kelly, D., McDonald, J., & Markham, C. (2010). A person independent system for recognition of hand postures used in sign language. Pattern Recognition Letters, 31 (11), 1359–1368.
Dahmani, D., & Larabi, S. (2014). User-independent system for sign language finger spelling recognition. Journal of Visual Communication and Image Representation, 25 (5), 1240–1250.
Kaur, B., Joshi, G., & Vig, R. (2017). Identification of ISL alphabets using discrete orthogonal moments. Wireless Personal Communications, 95 (4), 4823–4845.
Sahoo, J. P., Ari, S., & Ghosh, D. K. (2018). Hand gesture recognition using DWT and F-ratio based feature descriptor. IET Image Processing, 12 (10), 1780–1787.
Joshi, G., Vig, R., & Singh, S. (2018). DCA-based unimodal feature-level fusion of orthogonal moments for Indian sign language dataset. IET Computer Vision, 12 (5), 570–577.
Download references
Authors declare that no funding was received for this research work.
Author information
Authors and affiliations.
ECE Department, Punjab Engineering College (Deemed to be University), Chandigarh, India
Sakshi Sharma & Sukhwinder Singh
You can also search for this author in PubMed Google Scholar
Corresponding author
Correspondence to Sakshi Sharma .
Ethics declarations
Conflict of interest.
The authors declares that they have no conflict of interest.
Additional information
Publisher's note.
Springer Nature remains neutral with regard to jurisdictional claims in published maps and institutional affiliations.
Rights and permissions
Reprints and permissions
About this article
Sharma, S., Singh, S. Recognition of Indian Sign Language (ISL) Using Deep Learning Model. Wireless Pers Commun 123 , 671–692 (2022). https://doi.org/10.1007/s11277-021-09152-1
Download citation
Accepted : 16 September 2021
Published : 28 September 2021
Issue Date : March 2022
DOI : https://doi.org/10.1007/s11277-021-09152-1
Share this article
Anyone you share the following link with will be able to read this content:
Sorry, a shareable link is not currently available for this article.
Provided by the Springer Nature SharedIt content-sharing initiative
- Indian sign language
- Sign language recognition system
- Gesture recognition
- Convolutional neural network
- Deep learning
- Data augmentation
- Find a journal
- Publish with us
- Track your research
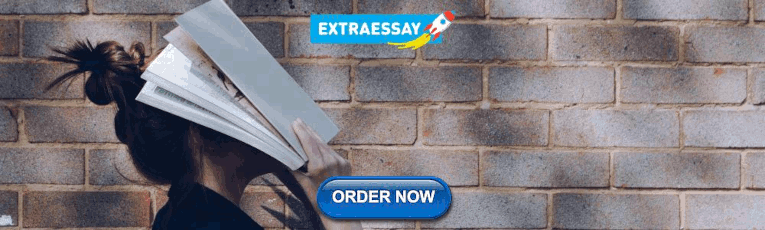
IMAGES
VIDEO
COMMENTS
Abstract. A real time sign language detector is a significant step forward in improving communication between the deaf and the general population. We are pleased to showcase the creation and ...
The three countries play a key role in advancing sign language recognition research, with India leading worldwide. India led with 123 publications over the past two decades, covering 15.4%of the total global publications. ... and joining the edges. Edge detection techniques reviewed in this paper are Robert edge detector, Sobel edge detector ...
Sign Language Recognition is a breakthrough for communication among deaf-mute society and has been a critical research topic for years. ... paper is divided ... Sign Language Detection Using SIFT ...
Sign language is a predominant form of communication among a large group of society. The nature of sign languages is visual, making them distinct from spoken languages. Unfortunately, very few able people can understand sign language making communication with the hearing-impaired infeasible. Research in the field of sign language recognition (SLR) can help reduce the barrier between deaf and ...
People with hearing impairments are found worldwide; therefore, the development of effective local level sign language recognition (SLR) tools is essential. We conducted a comprehensive review of automated sign language recognition based on machine/deep learning methods and techniques published between 2014 and 2021 and concluded that the current methods require conceptual classification to ...
A machine can understand human activities, and the meaning of signs can help overcome the communication barriers between the inaudible and ordinary people. Sign Language Recognition (SLR) is a fascinating research area and a crucial task concerning computer vision and pattern recognition. Recently, SLR usage has increased in many applications, but the environment, background image resolution ...
The remainder of this paper is organized as follows. Section 2 includes a brief review of Deep Learning algorithms. Section 3 presents a taxonomy of the sign language recognition area. Hand sign language, face sign language, and human sign language literature are reviewed in Sections 4, 5, and 6, respectively.Section 7 presents the recent models in continuous sign language recognition.
This research paper presents a comprehensive study employing five distinct deep learning models to recognize hand gestures for the American Sign Language (ASL) alphabet. ... motion detection, feature extraction, and vocabulary size in a real ... E.S.M.; Luqman, H. A comprehensive survey and taxonomy of sign language research. Eng. Appl. Artif ...
convey the meaning; We recognize this language as a sign language. Sign language recognition (SLR) is challenging and complex, and many research opportunities are available with Dr. M. MADHIARASAN was with the Department of Computer Sci-ence and Engineering, Indian Institute of Technology Roorkee, Roor-kee, Uttarakhand, India - 247667 ...
Abstract: Sign Language Detection has become crucial and effective for humans and research in this area is in progress and is one of the applications of Computer Vision. Earlier works included detection using static signs with the help of a simple deep learning-based Convolutional Neural Network. This proposal is based on continuous detection of image frames in real-time using action detection ...
Section 3 describes the methodology for implementing an isolated SLR system for real-time sign language detection and recognition, which involves pre-processing, feature extraction, training, and testing steps. This research paper proposes a feedback-based learning methodology using these options, based on a combination of LSTM and GRU: (1) a ...
Sign language is a system of communication using visual gestures and signs. Hearing impaired people and the deaf and dumb community use sign language as their only means of communication. Understanding sign language is so much difficult for a normal person. Therefore, the minority group has always faced many difficulties in communicating with the general population. In this research paper, we ...
Convolutional Neural network (CNN) was used to analyze sign language detection and create a workable system that might be used in the future. Neural networks have had a significant impact on the development of sign language identification, as well as the role that machine learning plays in this process, as well as the feasibility and efficiency of a python program in this area.
From previous published papers, it has been observed that very limited amount of work has been done on the survey of the sign language recognition as shown in Table 3.Khan et al. [] reviewed sign language components and the challenges and research issues have been discussed.Kausar and Javed [] presented a survey of current research trends and the challenges faced by the researchers.
Therefore, this paper studies hand locating and sign language recognition of common sign language based on neural network, and the main research contents include: 1. A hand locating network based on the Faster R-CNN is established to recognize the sign language video or the part of the hand in the picture, and the result of recognition is ...
double-handed gestures but they are not real-time. In this paper, we propose a method to create an Indian Sign Language dataset using a webcam and then using transfer learning, train a TensorFlow model to create a real-time Sign Language Recognition system. The system achieves a good level of accuracy even with a limited size dataset. Keywords:
This work designs and implements a model which detects real-time sign language using deep learning and uses a YOLOv8 model to train the dataset, which consists of Indian sign Language having9991 images of 26 alphabets and 9 numeric digits. Sign language is commonly used by speech and hearing-disabled people as a way of exchanging thoughts, emotions, feelings, and needs. There are multiple sign ...
An efficient sign language recognition system (SLRS) can recognize the gestures of sign language to ease the communication between the signer and non-signer community. In this paper, a computer-vision based SLRS using a deep learning technique has been proposed. This study has primary three contributions: first, a large dataset of Indian sign language (ISL) has been created using 65 different ...
International Journal of Research Publication and Reviews, Vol 4, no 4, pp 2495-2499, April 2023 International Journal of Research Publication and Reviews Journal homepage: www.ijrpr.com ISSN 2582-7421 Real Time Sign Language Detection Using Yolov5 Algorithm Mr. D. Chiranjeevulu1, J. Tejasri2, I. Sasi Kala3, K. Hemanth4, N. Rakesh5