
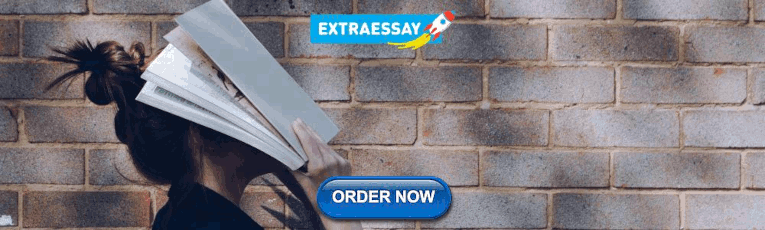
Systematic Review
- Library Help
- What is a Systematic Review (SR)?
Steps of a Systematic Review
- Framing a Research Question
- Developing a Search Strategy
- Searching the Literature
- Managing the Process
- Meta-analysis
- Publishing your Systematic Review
Forms and templates

Image: David Parmenter's Shop
- PICO Template
- Inclusion/Exclusion Criteria
- Database Search Log
- Review Matrix
- Cochrane Tool for Assessing Risk of Bias in Included Studies
• PRISMA Flow Diagram - Record the numbers of retrieved references and included/excluded studies. You can use the Create Flow Diagram tool to automate the process.
• PRISMA Checklist - Checklist of items to include when reporting a systematic review or meta-analysis
PRISMA 2020 and PRISMA-S: Common Questions on Tracking Records and the Flow Diagram
- PROSPERO Template
- Manuscript Template
- Steps of SR (text)
- Steps of SR (visual)
- Steps of SR (PIECES)
Adapted from A Guide to Conducting Systematic Reviews: Steps in a Systematic Review by Cornell University Library
Source: Cochrane Consumers and Communications (infographics are free to use and licensed under Creative Commons )
Check the following visual resources titled " What Are Systematic Reviews?"
- Video with closed captions available
- Animated Storyboard
- << Previous: What is a Systematic Review (SR)?
- Next: Framing a Research Question >>
- Last Updated: Mar 4, 2024 12:09 PM
- URL: https://lib.guides.umd.edu/SR
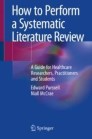
- © 2020
How to Perform a Systematic Literature Review
A Guide for Healthcare Researchers, Practitioners and Students
- Edward Purssell ORCID: https://orcid.org/0000-0003-3748-0864 0 ,
- Niall McCrae ORCID: https://orcid.org/0000-0001-9776-7694 1
School of Health Sciences, City, University of London, London, UK
You can also search for this author in PubMed Google Scholar
Florence Nightingale Faculty of Nursing Midwifery & Palliative Care, King’s College London, London, UK
- Presents a logical approach to systematic literature reviewing
- offers a corrective to flawed guidance in existing books
- An accessible but intellectually stimulating guide with illuminating examples and analogies
75k Accesses
27 Citations
10 Altmetric
- Table of contents
About this book
Authors and affiliations, about the authors, bibliographic information.
- Publish with us
Buying options
- Available as EPUB and PDF
- Read on any device
- Instant download
- Own it forever
- Compact, lightweight edition
- Dispatched in 3 to 5 business days
- Free shipping worldwide - see info
Tax calculation will be finalised at checkout
Other ways to access
This is a preview of subscription content, log in via an institution to check for access.
Table of contents (11 chapters)
Front matter, introduction.
- Edward Purssell, Niall McCrae
A Brief History of the Systematic Review
The aim and scope of a systematic review: a logical approach, searching the literature, screening search results: a 1-2-3 approach, critical appraisal: assessing the quality of studies, reviewing quantitative studies: meta-analysis and narrative approaches, reviewing qualitative studies and metasynthesis, reviewing qualitative and quantitative studies and mixed-method reviews, meaning and implications: the discussion, making an impact: dissemination of results, back matter.
The systematic review is a rigorous method of collating and synthesizing evidence from multiple studies, producing a whole greater than the sum of parts. This textbook is an authoritative and accessible guide to an activity that is often found overwhelming. The authors steer readers on a logical, sequential path through the process, taking account of the different needs of researchers, students and practitioners. Practical guidance is provided on the fundamentals of systematic reviewing and also on advanced techniques such as meta-analysis. Examples are given in each chapter, with a succinct glossary to support the text.
This up-to-date, accessible textbook will satisfy the needs of students, practitioners and educators in the sphere of healthcare, and contribute to improving the quality of evidence-based practice. The authors will advise some freely available or inexpensive open source/access resources (such as PubMed, R and Zotero) to help students how to perform a systemic review, in particular those with limited resources.
- Methodology
- Evidence-based practice
Edward Purssell
Florence Nightingale Faculty of Nursing Midwifery & Palliative Care, King’s College London, London, UK
Niall McCrae
Dr. Niall McCrae teaches mental health nursing and research methods at the Florence Nightingale Faculty of Nursing, Midwifery & Palliative Care at King’s College London. His research interests are dementia, depression, the impact of social media on younger people, and the history of mental health care. Niall has written two previous books: The Moon and Madness (Imprint Academic, 2011) and The Story of Nursing in British Mental Hospitals: Echoes from the Corridors (Routledge, 2016). He is a regular writer for Salisbury Review magazine.
In partnershipPurssell and McCrae have written several papers on research methodology and literature reviewing for healthcare journals. Both have extensive experience of teaching literature reviewing at all academic levels, and explaining complex concepts in a way that is accessible to all
Book Title : How to Perform a Systematic Literature Review
Book Subtitle : A Guide for Healthcare Researchers, Practitioners and Students
Authors : Edward Purssell, Niall McCrae
DOI : https://doi.org/10.1007/978-3-030-49672-2
Publisher : Springer Cham
eBook Packages : Medicine , Medicine (R0)
Copyright Information : The Editor(s) (if applicable) and The Author(s), under exclusive license to Springer Nature Switzerland AG 2020
Softcover ISBN : 978-3-030-49671-5 Published: 05 August 2020
eBook ISBN : 978-3-030-49672-2 Published: 04 August 2020
Edition Number : 1
Number of Pages : VII, 188
Number of Illustrations : 7 b/w illustrations, 12 illustrations in colour
Topics : Nursing Research , Nursing Education , Research Skills
Policies and ethics
- Find a journal
- Track your research
Jump to navigation

Cochrane Cochrane Interactive Learning
Cochrane interactive learning, module 1: introduction to conducting systematic reviews, about this module.
Part of the Cochrane Interactive Learning course on Conducting an Intervention Review, this module introduces you to what systematic reviews are and why they are useful. This module describes the various types and preferred format of review questions, and outlines the process of conducting systematic reviews.
45-60 minutes
What you can expect to learn (learning outcomes).
This module will teach you to:
- Recognize features of systematic reviews as a research design
- Recognize the importance of using rigorous methods to conduct a systematic review
- Identify the types of review questions
- Identify the elements of a well-defined review question
- Understand the steps in a systematic review
Authors, contributors, and how to cite this module
Module 1 has been written and compiled by Dario Sambunjak, Miranda Cumpston and Chris Watts, Cochrane Central Executive Team .
A full list of acknowledgements, including our expert advisors from across Cochrane, is available at the end of each module page.
This module should be cited as: Sambunjak D, Cumpston M, Watts C. Module 1: Introduction to conducting systematic reviews. In: Cochrane Interactive Learning: Conducting an intervention review. Cochrane, 2017. Available from https://training.cochrane.org/interactivelearning/module-1-introduction-conducting-systematic-reviews .
Update and feedback
The module was last updated on September 2022.
We're pleased to hear your thoughts. If you have any questions, comments or feedback about the content of this module, please contact us .
Have a language expert improve your writing
Run a free plagiarism check in 10 minutes, generate accurate citations for free.
- Knowledge Base
Methodology
- Systematic Review | Definition, Example, & Guide
Systematic Review | Definition, Example & Guide
Published on June 15, 2022 by Shaun Turney . Revised on November 20, 2023.
A systematic review is a type of review that uses repeatable methods to find, select, and synthesize all available evidence. It answers a clearly formulated research question and explicitly states the methods used to arrive at the answer.
They answered the question “What is the effectiveness of probiotics in reducing eczema symptoms and improving quality of life in patients with eczema?”
In this context, a probiotic is a health product that contains live microorganisms and is taken by mouth. Eczema is a common skin condition that causes red, itchy skin.
Table of contents
What is a systematic review, systematic review vs. meta-analysis, systematic review vs. literature review, systematic review vs. scoping review, when to conduct a systematic review, pros and cons of systematic reviews, step-by-step example of a systematic review, other interesting articles, frequently asked questions about systematic reviews.
A review is an overview of the research that’s already been completed on a topic.
What makes a systematic review different from other types of reviews is that the research methods are designed to reduce bias . The methods are repeatable, and the approach is formal and systematic:
- Formulate a research question
- Develop a protocol
- Search for all relevant studies
- Apply the selection criteria
- Extract the data
- Synthesize the data
- Write and publish a report
Although multiple sets of guidelines exist, the Cochrane Handbook for Systematic Reviews is among the most widely used. It provides detailed guidelines on how to complete each step of the systematic review process.
Systematic reviews are most commonly used in medical and public health research, but they can also be found in other disciplines.
Systematic reviews typically answer their research question by synthesizing all available evidence and evaluating the quality of the evidence. Synthesizing means bringing together different information to tell a single, cohesive story. The synthesis can be narrative ( qualitative ), quantitative , or both.
Receive feedback on language, structure, and formatting
Professional editors proofread and edit your paper by focusing on:
- Academic style
- Vague sentences
- Style consistency
See an example
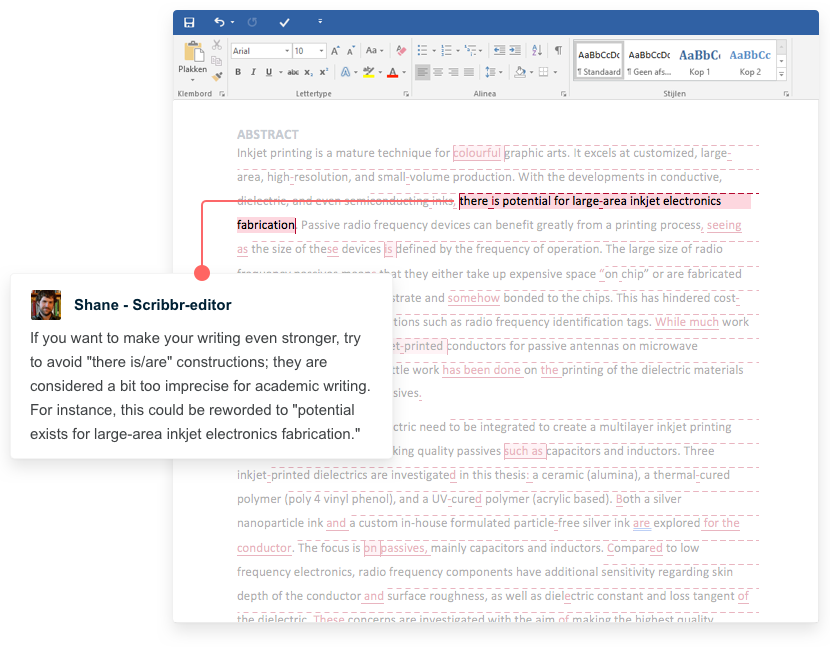
Systematic reviews often quantitatively synthesize the evidence using a meta-analysis . A meta-analysis is a statistical analysis, not a type of review.
A meta-analysis is a technique to synthesize results from multiple studies. It’s a statistical analysis that combines the results of two or more studies, usually to estimate an effect size .
A literature review is a type of review that uses a less systematic and formal approach than a systematic review. Typically, an expert in a topic will qualitatively summarize and evaluate previous work, without using a formal, explicit method.
Although literature reviews are often less time-consuming and can be insightful or helpful, they have a higher risk of bias and are less transparent than systematic reviews.
Similar to a systematic review, a scoping review is a type of review that tries to minimize bias by using transparent and repeatable methods.
However, a scoping review isn’t a type of systematic review. The most important difference is the goal: rather than answering a specific question, a scoping review explores a topic. The researcher tries to identify the main concepts, theories, and evidence, as well as gaps in the current research.
Sometimes scoping reviews are an exploratory preparation step for a systematic review, and sometimes they are a standalone project.
A systematic review is a good choice of review if you want to answer a question about the effectiveness of an intervention , such as a medical treatment.
To conduct a systematic review, you’ll need the following:
- A precise question , usually about the effectiveness of an intervention. The question needs to be about a topic that’s previously been studied by multiple researchers. If there’s no previous research, there’s nothing to review.
- If you’re doing a systematic review on your own (e.g., for a research paper or thesis ), you should take appropriate measures to ensure the validity and reliability of your research.
- Access to databases and journal archives. Often, your educational institution provides you with access.
- Time. A professional systematic review is a time-consuming process: it will take the lead author about six months of full-time work. If you’re a student, you should narrow the scope of your systematic review and stick to a tight schedule.
- Bibliographic, word-processing, spreadsheet, and statistical software . For example, you could use EndNote, Microsoft Word, Excel, and SPSS.
A systematic review has many pros .
- They minimize research bias by considering all available evidence and evaluating each study for bias.
- Their methods are transparent , so they can be scrutinized by others.
- They’re thorough : they summarize all available evidence.
- They can be replicated and updated by others.
Systematic reviews also have a few cons .
- They’re time-consuming .
- They’re narrow in scope : they only answer the precise research question.
The 7 steps for conducting a systematic review are explained with an example.
Step 1: Formulate a research question
Formulating the research question is probably the most important step of a systematic review. A clear research question will:
- Allow you to more effectively communicate your research to other researchers and practitioners
- Guide your decisions as you plan and conduct your systematic review
A good research question for a systematic review has four components, which you can remember with the acronym PICO :
- Population(s) or problem(s)
- Intervention(s)
- Comparison(s)
You can rearrange these four components to write your research question:
- What is the effectiveness of I versus C for O in P ?
Sometimes, you may want to include a fifth component, the type of study design . In this case, the acronym is PICOT .
- Type of study design(s)
- The population of patients with eczema
- The intervention of probiotics
- In comparison to no treatment, placebo , or non-probiotic treatment
- The outcome of changes in participant-, parent-, and doctor-rated symptoms of eczema and quality of life
- Randomized control trials, a type of study design
Their research question was:
- What is the effectiveness of probiotics versus no treatment, a placebo, or a non-probiotic treatment for reducing eczema symptoms and improving quality of life in patients with eczema?
Step 2: Develop a protocol
A protocol is a document that contains your research plan for the systematic review. This is an important step because having a plan allows you to work more efficiently and reduces bias.
Your protocol should include the following components:
- Background information : Provide the context of the research question, including why it’s important.
- Research objective (s) : Rephrase your research question as an objective.
- Selection criteria: State how you’ll decide which studies to include or exclude from your review.
- Search strategy: Discuss your plan for finding studies.
- Analysis: Explain what information you’ll collect from the studies and how you’ll synthesize the data.
If you’re a professional seeking to publish your review, it’s a good idea to bring together an advisory committee . This is a group of about six people who have experience in the topic you’re researching. They can help you make decisions about your protocol.
It’s highly recommended to register your protocol. Registering your protocol means submitting it to a database such as PROSPERO or ClinicalTrials.gov .
Step 3: Search for all relevant studies
Searching for relevant studies is the most time-consuming step of a systematic review.
To reduce bias, it’s important to search for relevant studies very thoroughly. Your strategy will depend on your field and your research question, but sources generally fall into these four categories:
- Databases: Search multiple databases of peer-reviewed literature, such as PubMed or Scopus . Think carefully about how to phrase your search terms and include multiple synonyms of each word. Use Boolean operators if relevant.
- Handsearching: In addition to searching the primary sources using databases, you’ll also need to search manually. One strategy is to scan relevant journals or conference proceedings. Another strategy is to scan the reference lists of relevant studies.
- Gray literature: Gray literature includes documents produced by governments, universities, and other institutions that aren’t published by traditional publishers. Graduate student theses are an important type of gray literature, which you can search using the Networked Digital Library of Theses and Dissertations (NDLTD) . In medicine, clinical trial registries are another important type of gray literature.
- Experts: Contact experts in the field to ask if they have unpublished studies that should be included in your review.
At this stage of your review, you won’t read the articles yet. Simply save any potentially relevant citations using bibliographic software, such as Scribbr’s APA or MLA Generator .
- Databases: EMBASE, PsycINFO, AMED, LILACS, and ISI Web of Science
- Handsearch: Conference proceedings and reference lists of articles
- Gray literature: The Cochrane Library, the metaRegister of Controlled Trials, and the Ongoing Skin Trials Register
- Experts: Authors of unpublished registered trials, pharmaceutical companies, and manufacturers of probiotics
Step 4: Apply the selection criteria
Applying the selection criteria is a three-person job. Two of you will independently read the studies and decide which to include in your review based on the selection criteria you established in your protocol . The third person’s job is to break any ties.
To increase inter-rater reliability , ensure that everyone thoroughly understands the selection criteria before you begin.
If you’re writing a systematic review as a student for an assignment, you might not have a team. In this case, you’ll have to apply the selection criteria on your own; you can mention this as a limitation in your paper’s discussion.
You should apply the selection criteria in two phases:
- Based on the titles and abstracts : Decide whether each article potentially meets the selection criteria based on the information provided in the abstracts.
- Based on the full texts: Download the articles that weren’t excluded during the first phase. If an article isn’t available online or through your library, you may need to contact the authors to ask for a copy. Read the articles and decide which articles meet the selection criteria.
It’s very important to keep a meticulous record of why you included or excluded each article. When the selection process is complete, you can summarize what you did using a PRISMA flow diagram .
Next, Boyle and colleagues found the full texts for each of the remaining studies. Boyle and Tang read through the articles to decide if any more studies needed to be excluded based on the selection criteria.
When Boyle and Tang disagreed about whether a study should be excluded, they discussed it with Varigos until the three researchers came to an agreement.
Step 5: Extract the data
Extracting the data means collecting information from the selected studies in a systematic way. There are two types of information you need to collect from each study:
- Information about the study’s methods and results . The exact information will depend on your research question, but it might include the year, study design , sample size, context, research findings , and conclusions. If any data are missing, you’ll need to contact the study’s authors.
- Your judgment of the quality of the evidence, including risk of bias .
You should collect this information using forms. You can find sample forms in The Registry of Methods and Tools for Evidence-Informed Decision Making and the Grading of Recommendations, Assessment, Development and Evaluations Working Group .
Extracting the data is also a three-person job. Two people should do this step independently, and the third person will resolve any disagreements.
They also collected data about possible sources of bias, such as how the study participants were randomized into the control and treatment groups.
Step 6: Synthesize the data
Synthesizing the data means bringing together the information you collected into a single, cohesive story. There are two main approaches to synthesizing the data:
- Narrative ( qualitative ): Summarize the information in words. You’ll need to discuss the studies and assess their overall quality.
- Quantitative : Use statistical methods to summarize and compare data from different studies. The most common quantitative approach is a meta-analysis , which allows you to combine results from multiple studies into a summary result.
Generally, you should use both approaches together whenever possible. If you don’t have enough data, or the data from different studies aren’t comparable, then you can take just a narrative approach. However, you should justify why a quantitative approach wasn’t possible.
Boyle and colleagues also divided the studies into subgroups, such as studies about babies, children, and adults, and analyzed the effect sizes within each group.
Step 7: Write and publish a report
The purpose of writing a systematic review article is to share the answer to your research question and explain how you arrived at this answer.
Your article should include the following sections:
- Abstract : A summary of the review
- Introduction : Including the rationale and objectives
- Methods : Including the selection criteria, search method, data extraction method, and synthesis method
- Results : Including results of the search and selection process, study characteristics, risk of bias in the studies, and synthesis results
- Discussion : Including interpretation of the results and limitations of the review
- Conclusion : The answer to your research question and implications for practice, policy, or research
To verify that your report includes everything it needs, you can use the PRISMA checklist .
Once your report is written, you can publish it in a systematic review database, such as the Cochrane Database of Systematic Reviews , and/or in a peer-reviewed journal.
In their report, Boyle and colleagues concluded that probiotics cannot be recommended for reducing eczema symptoms or improving quality of life in patients with eczema. Note Generative AI tools like ChatGPT can be useful at various stages of the writing and research process and can help you to write your systematic review. However, we strongly advise against trying to pass AI-generated text off as your own work.
If you want to know more about statistics , methodology , or research bias , make sure to check out some of our other articles with explanations and examples.
- Student’s t -distribution
- Normal distribution
- Null and Alternative Hypotheses
- Chi square tests
- Confidence interval
- Quartiles & Quantiles
- Cluster sampling
- Stratified sampling
- Data cleansing
- Reproducibility vs Replicability
- Peer review
- Prospective cohort study
Research bias
- Implicit bias
- Cognitive bias
- Placebo effect
- Hawthorne effect
- Hindsight bias
- Affect heuristic
- Social desirability bias
A literature review is a survey of scholarly sources (such as books, journal articles, and theses) related to a specific topic or research question .
It is often written as part of a thesis, dissertation , or research paper , in order to situate your work in relation to existing knowledge.
A literature review is a survey of credible sources on a topic, often used in dissertations , theses, and research papers . Literature reviews give an overview of knowledge on a subject, helping you identify relevant theories and methods, as well as gaps in existing research. Literature reviews are set up similarly to other academic texts , with an introduction , a main body, and a conclusion .
An annotated bibliography is a list of source references that has a short description (called an annotation ) for each of the sources. It is often assigned as part of the research process for a paper .
A systematic review is secondary research because it uses existing research. You don’t collect new data yourself.
Cite this Scribbr article
If you want to cite this source, you can copy and paste the citation or click the “Cite this Scribbr article” button to automatically add the citation to our free Citation Generator.
Turney, S. (2023, November 20). Systematic Review | Definition, Example & Guide. Scribbr. Retrieved April 9, 2024, from https://www.scribbr.com/methodology/systematic-review/
Is this article helpful?
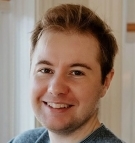
Shaun Turney
Other students also liked, how to write a literature review | guide, examples, & templates, how to write a research proposal | examples & templates, what is critical thinking | definition & examples, what is your plagiarism score.

An official website of the United States government
The .gov means it’s official. Federal government websites often end in .gov or .mil. Before sharing sensitive information, make sure you’re on a federal government site.
The site is secure. The https:// ensures that you are connecting to the official website and that any information you provide is encrypted and transmitted securely.
- Publications
- Account settings
Preview improvements coming to the PMC website in October 2024. Learn More or Try it out now .
- Advanced Search
- Journal List
- PMC10248995

Guidance to best tools and practices for systematic reviews
Kat kolaski.
1 Departments of Orthopaedic Surgery, Pediatrics, and Neurology, Wake Forest School of Medicine, Winston-Salem, NC USA
Lynne Romeiser Logan
2 Department of Physical Medicine and Rehabilitation, SUNY Upstate Medical University, Syracuse, NY USA
John P. A. Ioannidis
3 Departments of Medicine, of Epidemiology and Population Health, of Biomedical Data Science, and of Statistics, and Meta-Research Innovation Center at Stanford (METRICS), Stanford University School of Medicine, Stanford, CA USA
Associated Data
Data continue to accumulate indicating that many systematic reviews are methodologically flawed, biased, redundant, or uninformative. Some improvements have occurred in recent years based on empirical methods research and standardization of appraisal tools; however, many authors do not routinely or consistently apply these updated methods. In addition, guideline developers, peer reviewers, and journal editors often disregard current methodological standards. Although extensively acknowledged and explored in the methodological literature, most clinicians seem unaware of these issues and may automatically accept evidence syntheses (and clinical practice guidelines based on their conclusions) as trustworthy.
A plethora of methods and tools are recommended for the development and evaluation of evidence syntheses. It is important to understand what these are intended to do (and cannot do) and how they can be utilized. Our objective is to distill this sprawling information into a format that is understandable and readily accessible to authors, peer reviewers, and editors. In doing so, we aim to promote appreciation and understanding of the demanding science of evidence synthesis among stakeholders. We focus on well-documented deficiencies in key components of evidence syntheses to elucidate the rationale for current standards. The constructs underlying the tools developed to assess reporting, risk of bias, and methodological quality of evidence syntheses are distinguished from those involved in determining overall certainty of a body of evidence. Another important distinction is made between those tools used by authors to develop their syntheses as opposed to those used to ultimately judge their work.
Exemplar methods and research practices are described, complemented by novel pragmatic strategies to improve evidence syntheses. The latter include preferred terminology and a scheme to characterize types of research evidence. We organize best practice resources in a Concise Guide that can be widely adopted and adapted for routine implementation by authors and journals. Appropriate, informed use of these is encouraged, but we caution against their superficial application and emphasize their endorsement does not substitute for in-depth methodological training. By highlighting best practices with their rationale, we hope this guidance will inspire further evolution of methods and tools that can advance the field.
Supplementary Information
The online version contains supplementary material available at 10.1186/s13643-023-02255-9.
Part 1. The state of evidence synthesis
Evidence syntheses are commonly regarded as the foundation of evidence-based medicine (EBM). They are widely accredited for providing reliable evidence and, as such, they have significantly influenced medical research and clinical practice. Despite their uptake throughout health care and ubiquity in contemporary medical literature, some important aspects of evidence syntheses are generally overlooked or not well recognized. Evidence syntheses are mostly retrospective exercises, they often depend on weak or irreparably flawed data, and they may use tools that have acknowledged or yet unrecognized limitations. They are complicated and time-consuming undertakings prone to bias and errors. Production of a good evidence synthesis requires careful preparation and high levels of organization in order to limit potential pitfalls [ 1 ]. Many authors do not recognize the complexity of such an endeavor and the many methodological challenges they may encounter. Failure to do so is likely to result in research and resource waste.
Given their potential impact on people’s lives, it is crucial for evidence syntheses to correctly report on the current knowledge base. In order to be perceived as trustworthy, reliable demonstration of the accuracy of evidence syntheses is equally imperative [ 2 ]. Concerns about the trustworthiness of evidence syntheses are not recent developments. From the early years when EBM first began to gain traction until recent times when thousands of systematic reviews are published monthly [ 3 ] the rigor of evidence syntheses has always varied. Many systematic reviews and meta-analyses had obvious deficiencies because original methods and processes had gaps, lacked precision, and/or were not widely known. The situation has improved with empirical research concerning which methods to use and standardization of appraisal tools. However, given the geometrical increase in the number of evidence syntheses being published, a relatively larger pool of unreliable evidence syntheses is being published today.
Publication of methodological studies that critically appraise the methods used in evidence syntheses is increasing at a fast pace. This reflects the availability of tools specifically developed for this purpose [ 4 – 6 ]. Yet many clinical specialties report that alarming numbers of evidence syntheses fail on these assessments. The syntheses identified report on a broad range of common conditions including, but not limited to, cancer, [ 7 ] chronic obstructive pulmonary disease, [ 8 ] osteoporosis, [ 9 ] stroke, [ 10 ] cerebral palsy, [ 11 ] chronic low back pain, [ 12 ] refractive error, [ 13 ] major depression, [ 14 ] pain, [ 15 ] and obesity [ 16 , 17 ]. The situation is even more concerning with regard to evidence syntheses included in clinical practice guidelines (CPGs) [ 18 – 20 ]. Astonishingly, in a sample of CPGs published in 2017–18, more than half did not apply even basic systematic methods in the evidence syntheses used to inform their recommendations [ 21 ].
These reports, while not widely acknowledged, suggest there are pervasive problems not limited to evidence syntheses that evaluate specific kinds of interventions or include primary research of a particular study design (eg, randomized versus non-randomized) [ 22 ]. Similar concerns about the reliability of evidence syntheses have been expressed by proponents of EBM in highly circulated medical journals [ 23 – 26 ]. These publications have also raised awareness about redundancy, inadequate input of statistical expertise, and deficient reporting. These issues plague primary research as well; however, there is heightened concern for the impact of these deficiencies given the critical role of evidence syntheses in policy and clinical decision-making.
Methods and guidance to produce a reliable evidence synthesis
Several international consortiums of EBM experts and national health care organizations currently provide detailed guidance (Table (Table1). 1 ). They draw criteria from the reporting and methodological standards of currently recommended appraisal tools, and regularly review and update their methods to reflect new information and changing needs. In addition, they endorse the Grading of Recommendations Assessment, Development and Evaluation (GRADE) system for rating the overall quality of a body of evidence [ 27 ]. These groups typically certify or commission systematic reviews that are published in exclusive databases (eg, Cochrane, JBI) or are used to develop government or agency sponsored guidelines or health technology assessments (eg, National Institute for Health and Care Excellence [NICE], Scottish Intercollegiate Guidelines Network [SIGN], Agency for Healthcare Research and Quality [AHRQ]). They offer developers of evidence syntheses various levels of methodological advice, technical and administrative support, and editorial assistance. Use of specific protocols and checklists are required for development teams within these groups, but their online methodological resources are accessible to any potential author.
Guidance for development of evidence syntheses
Notably, Cochrane is the largest single producer of evidence syntheses in biomedical research; however, these only account for 15% of the total [ 28 ]. The World Health Organization requires Cochrane standards be used to develop evidence syntheses that inform their CPGs [ 29 ]. Authors investigating questions of intervention effectiveness in syntheses developed for Cochrane follow the Methodological Expectations of Cochrane Intervention Reviews [ 30 ] and undergo multi-tiered peer review [ 31 , 32 ]. Several empirical evaluations have shown that Cochrane systematic reviews are of higher methodological quality compared with non-Cochrane reviews [ 4 , 7 , 9 , 11 , 14 , 32 – 35 ]. However, some of these assessments have biases: they may be conducted by Cochrane-affiliated authors, and they sometimes use scales and tools developed and used in the Cochrane environment and by its partners. In addition, evidence syntheses published in the Cochrane database are not subject to space or word restrictions, while non-Cochrane syntheses are often limited. As a result, information that may be relevant to the critical appraisal of non-Cochrane reviews is often removed or is relegated to online-only supplements that may not be readily or fully accessible [ 28 ].
Influences on the state of evidence synthesis
Many authors are familiar with the evidence syntheses produced by the leading EBM organizations but can be intimidated by the time and effort necessary to apply their standards. Instead of following their guidance, authors may employ methods that are discouraged or outdated 28]. Suboptimal methods described in in the literature may then be taken up by others. For example, the Newcastle–Ottawa Scale (NOS) is a commonly used tool for appraising non-randomized studies [ 36 ]. Many authors justify their selection of this tool with reference to a publication that describes the unreliability of the NOS and recommends against its use [ 37 ]. Obviously, the authors who cite this report for that purpose have not read it. Authors and peer reviewers have a responsibility to use reliable and accurate methods and not copycat previous citations or substandard work [ 38 , 39 ]. Similar cautions may potentially extend to automation tools. These have concentrated on evidence searching [ 40 ] and selection given how demanding it is for humans to maintain truly up-to-date evidence [ 2 , 41 ]. Cochrane has deployed machine learning to identify randomized controlled trials (RCTs) and studies related to COVID-19, [ 2 , 42 ] but such tools are not yet commonly used [ 43 ]. The routine integration of automation tools in the development of future evidence syntheses should not displace the interpretive part of the process.
Editorials about unreliable or misleading systematic reviews highlight several of the intertwining factors that may contribute to continued publication of unreliable evidence syntheses: shortcomings and inconsistencies of the peer review process, lack of endorsement of current standards on the part of journal editors, the incentive structure of academia, industry influences, publication bias, and the lure of “predatory” journals [ 44 – 48 ]. At this juncture, clarification of the extent to which each of these factors contribute remains speculative, but their impact is likely to be synergistic.
Over time, the generalized acceptance of the conclusions of systematic reviews as incontrovertible has affected trends in the dissemination and uptake of evidence. Reporting of the results of evidence syntheses and recommendations of CPGs has shifted beyond medical journals to press releases and news headlines and, more recently, to the realm of social media and influencers. The lay public and policy makers may depend on these outlets for interpreting evidence syntheses and CPGs. Unfortunately, communication to the general public often reflects intentional or non-intentional misrepresentation or “spin” of the research findings [ 49 – 52 ] News and social media outlets also tend to reduce conclusions on a body of evidence and recommendations for treatment to binary choices (eg, “do it” versus “don’t do it”) that may be assigned an actionable symbol (eg, red/green traffic lights, smiley/frowning face emoji).
Strategies for improvement
Many authors and peer reviewers are volunteer health care professionals or trainees who lack formal training in evidence synthesis [ 46 , 53 ]. Informing them about research methodology could increase the likelihood they will apply rigorous methods [ 25 , 33 , 45 ]. We tackle this challenge, from both a theoretical and a practical perspective, by offering guidance applicable to any specialty. It is based on recent methodological research that is extensively referenced to promote self-study. However, the information presented is not intended to be substitute for committed training in evidence synthesis methodology; instead, we hope to inspire our target audience to seek such training. We also hope to inform a broader audience of clinicians and guideline developers influenced by evidence syntheses. Notably, these communities often include the same members who serve in different capacities.
In the following sections, we highlight methodological concepts and practices that may be unfamiliar, problematic, confusing, or controversial. In Part 2, we consider various types of evidence syntheses and the types of research evidence summarized by them. In Part 3, we examine some widely used (and misused) tools for the critical appraisal of systematic reviews and reporting guidelines for evidence syntheses. In Part 4, we discuss how to meet methodological conduct standards applicable to key components of systematic reviews. In Part 5, we describe the merits and caveats of rating the overall certainty of a body of evidence. Finally, in Part 6, we summarize suggested terminology, methods, and tools for development and evaluation of evidence syntheses that reflect current best practices.
Part 2. Types of syntheses and research evidence
A good foundation for the development of evidence syntheses requires an appreciation of their various methodologies and the ability to correctly identify the types of research potentially available for inclusion in the synthesis.
Types of evidence syntheses
Systematic reviews have historically focused on the benefits and harms of interventions; over time, various types of systematic reviews have emerged to address the diverse information needs of clinicians, patients, and policy makers [ 54 ] Systematic reviews with traditional components have become defined by the different topics they assess (Table 2.1 ). In addition, other distinctive types of evidence syntheses have evolved, including overviews or umbrella reviews, scoping reviews, rapid reviews, and living reviews. The popularity of these has been increasing in recent years [ 55 – 58 ]. A summary of the development, methods, available guidance, and indications for these unique types of evidence syntheses is available in Additional File 2 A.
Types of traditional systematic reviews
Both Cochrane [ 30 , 59 ] and JBI [ 60 ] provide methodologies for many types of evidence syntheses; they describe these with different terminology, but there is obvious overlap (Table 2.2 ). The majority of evidence syntheses published by Cochrane (96%) and JBI (62%) are categorized as intervention reviews. This reflects the earlier development and dissemination of their intervention review methodologies; these remain well-established [ 30 , 59 , 61 ] as both organizations continue to focus on topics related to treatment efficacy and harms. In contrast, intervention reviews represent only about half of the total published in the general medical literature, and several non-intervention review types contribute to a significant proportion of the other half.
Evidence syntheses published by Cochrane and JBI
a Data from https://www.cochranelibrary.com/cdsr/reviews . Accessed 17 Sep 2022
b Data obtained via personal email communication on 18 Sep 2022 with Emilie Francis, editorial assistant, JBI Evidence Synthesis
c Includes the following categories: prevalence, scoping, mixed methods, and realist reviews
d This methodology is not supported in the current version of the JBI Manual for Evidence Synthesis
Types of research evidence
There is consensus on the importance of using multiple study designs in evidence syntheses; at the same time, there is a lack of agreement on methods to identify included study designs. Authors of evidence syntheses may use various taxonomies and associated algorithms to guide selection and/or classification of study designs. These tools differentiate categories of research and apply labels to individual study designs (eg, RCT, cross-sectional). A familiar example is the Design Tree endorsed by the Centre for Evidence-Based Medicine [ 70 ]. Such tools may not be helpful to authors of evidence syntheses for multiple reasons.
Suboptimal levels of agreement and accuracy even among trained methodologists reflect challenges with the application of such tools [ 71 , 72 ]. Problematic distinctions or decision points (eg, experimental or observational, controlled or uncontrolled, prospective or retrospective) and design labels (eg, cohort, case control, uncontrolled trial) have been reported [ 71 ]. The variable application of ambiguous study design labels to non-randomized studies is common, making them especially prone to misclassification [ 73 ]. In addition, study labels do not denote the unique design features that make different types of non-randomized studies susceptible to different biases, including those related to how the data are obtained (eg, clinical trials, disease registries, wearable devices). Given this limitation, it is important to be aware that design labels preclude the accurate assignment of non-randomized studies to a “level of evidence” in traditional hierarchies [ 74 ].
These concerns suggest that available tools and nomenclature used to distinguish types of research evidence may not uniformly apply to biomedical research and non-health fields that utilize evidence syntheses (eg, education, economics) [ 75 , 76 ]. Moreover, primary research reports often do not describe study design or do so incompletely or inaccurately; thus, indexing in PubMed and other databases does not address the potential for misclassification [ 77 ]. Yet proper identification of research evidence has implications for several key components of evidence syntheses. For example, search strategies limited by index terms using design labels or study selection based on labels applied by the authors of primary studies may cause inconsistent or unjustified study inclusions and/or exclusions [ 77 ]. In addition, because risk of bias (RoB) tools consider attributes specific to certain types of studies and study design features, results of these assessments may be invalidated if an inappropriate tool is used. Appropriate classification of studies is also relevant for the selection of a suitable method of synthesis and interpretation of those results.
An alternative to these tools and nomenclature involves application of a few fundamental distinctions that encompass a wide range of research designs and contexts. While these distinctions are not novel, we integrate them into a practical scheme (see Fig. Fig.1) 1 ) designed to guide authors of evidence syntheses in the basic identification of research evidence. The initial distinction is between primary and secondary studies. Primary studies are then further distinguished by: 1) the type of data reported (qualitative or quantitative); and 2) two defining design features (group or single-case and randomized or non-randomized). The different types of studies and study designs represented in the scheme are described in detail in Additional File 2 B. It is important to conceptualize their methods as complementary as opposed to contrasting or hierarchical [ 78 ]; each offers advantages and disadvantages that determine their appropriateness for answering different kinds of research questions in an evidence synthesis.

Distinguishing types of research evidence
Application of these basic distinctions may avoid some of the potential difficulties associated with study design labels and taxonomies. Nevertheless, debatable methodological issues are raised when certain types of research identified in this scheme are included in an evidence synthesis. We briefly highlight those associated with inclusion of non-randomized studies, case reports and series, and a combination of primary and secondary studies.
Non-randomized studies
When investigating an intervention’s effectiveness, it is important for authors to recognize the uncertainty of observed effects reported by studies with high RoB. Results of statistical analyses that include such studies need to be interpreted with caution in order to avoid misleading conclusions [ 74 ]. Review authors may consider excluding randomized studies with high RoB from meta-analyses. Non-randomized studies of intervention (NRSI) are affected by a greater potential range of biases and thus vary more than RCTs in their ability to estimate a causal effect [ 79 ]. If data from NRSI are synthesized in meta-analyses, it is helpful to separately report their summary estimates [ 6 , 74 ].
Nonetheless, certain design features of NRSI (eg, which parts of the study were prospectively designed) may help to distinguish stronger from weaker ones. Cochrane recommends that authors of a review including NRSI focus on relevant study design features when determining eligibility criteria instead of relying on non-informative study design labels [ 79 , 80 ] This process is facilitated by a study design feature checklist; guidance on using the checklist is included with developers’ description of the tool [ 73 , 74 ]. Authors collect information about these design features during data extraction and then consider it when making final study selection decisions and when performing RoB assessments of the included NRSI.
Case reports and case series
Correctly identified case reports and case series can contribute evidence not well captured by other designs [ 81 ]; in addition, some topics may be limited to a body of evidence that consists primarily of uncontrolled clinical observations. Murad and colleagues offer a framework for how to include case reports and series in an evidence synthesis [ 82 ]. Distinguishing between cohort studies and case series in these syntheses is important, especially for those that rely on evidence from NRSI. Additional data obtained from studies misclassified as case series can potentially increase the confidence in effect estimates. Mathes and Pieper provide authors of evidence syntheses with specific guidance on distinguishing between cohort studies and case series, but emphasize the increased workload involved [ 77 ].
Primary and secondary studies
Synthesis of combined evidence from primary and secondary studies may provide a broad perspective on the entirety of available literature on a topic. This is, in fact, the recommended strategy for scoping reviews that may include a variety of sources of evidence (eg, CPGs, popular media). However, except for scoping reviews, the synthesis of data from primary and secondary studies is discouraged unless there are strong reasons to justify doing so.
Combining primary and secondary sources of evidence is challenging for authors of other types of evidence syntheses for several reasons [ 83 ]. Assessments of RoB for primary and secondary studies are derived from conceptually different tools, thus obfuscating the ability to make an overall RoB assessment of a combination of these study types. In addition, authors who include primary and secondary studies must devise non-standardized methods for synthesis. Note this contrasts with well-established methods available for updating existing evidence syntheses with additional data from new primary studies [ 84 – 86 ]. However, a new review that synthesizes data from primary and secondary studies raises questions of validity and may unintentionally support a biased conclusion because no existing methodological guidance is currently available [ 87 ].
Recommendations
We suggest that journal editors require authors to identify which type of evidence synthesis they are submitting and reference the specific methodology used for its development. This will clarify the research question and methods for peer reviewers and potentially simplify the editorial process. Editors should announce this practice and include it in the instructions to authors. To decrease bias and apply correct methods, authors must also accurately identify the types of research evidence included in their syntheses.
Part 3. Conduct and reporting
The need to develop criteria to assess the rigor of systematic reviews was recognized soon after the EBM movement began to gain international traction [ 88 , 89 ]. Systematic reviews rapidly became popular, but many were very poorly conceived, conducted, and reported. These problems remain highly prevalent [ 23 ] despite development of guidelines and tools to standardize and improve the performance and reporting of evidence syntheses [ 22 , 28 ]. Table 3.1 provides some historical perspective on the evolution of tools developed specifically for the evaluation of systematic reviews, with or without meta-analysis.
Tools specifying standards for systematic reviews with and without meta-analysis
a Currently recommended
b Validated tool for systematic reviews of interventions developed for use by authors of overviews or umbrella reviews
These tools are often interchangeably invoked when referring to the “quality” of an evidence synthesis. However, quality is a vague term that is frequently misused and misunderstood; more precisely, these tools specify different standards for evidence syntheses. Methodological standards address how well a systematic review was designed and performed [ 5 ]. RoB assessments refer to systematic flaws or limitations in the design, conduct, or analysis of research that distort the findings of the review [ 4 ]. Reporting standards help systematic review authors describe the methodology they used and the results of their synthesis in sufficient detail [ 92 ]. It is essential to distinguish between these evaluations: a systematic review may be biased, it may fail to report sufficient information on essential features, or it may exhibit both problems; a thoroughly reported systematic evidence synthesis review may still be biased and flawed while an otherwise unbiased one may suffer from deficient documentation.
We direct attention to the currently recommended tools listed in Table 3.1 but concentrate on AMSTAR-2 (update of AMSTAR [A Measurement Tool to Assess Systematic Reviews]) and ROBIS (Risk of Bias in Systematic Reviews), which evaluate methodological quality and RoB, respectively. For comparison and completeness, we include PRISMA 2020 (update of the 2009 Preferred Reporting Items for Systematic Reviews of Meta-Analyses statement), which offers guidance on reporting standards. The exclusive focus on these three tools is by design; it addresses concerns related to the considerable variability in tools used for the evaluation of systematic reviews [ 28 , 88 , 96 , 97 ]. We highlight the underlying constructs these tools were designed to assess, then describe their components and applications. Their known (or potential) uptake and impact and limitations are also discussed.
Evaluation of conduct
Development.
AMSTAR [ 5 ] was in use for a decade prior to the 2017 publication of AMSTAR-2; both provide a broad evaluation of methodological quality of intervention systematic reviews, including flaws arising through poor conduct of the review [ 6 ]. ROBIS, published in 2016, was developed to specifically assess RoB introduced by the conduct of the review; it is applicable to systematic reviews of interventions and several other types of reviews [ 4 ]. Both tools reflect a shift to a domain-based approach as opposed to generic quality checklists. There are a few items unique to each tool; however, similarities between items have been demonstrated [ 98 , 99 ]. AMSTAR-2 and ROBIS are recommended for use by: 1) authors of overviews or umbrella reviews and CPGs to evaluate systematic reviews considered as evidence; 2) authors of methodological research studies to appraise included systematic reviews; and 3) peer reviewers for appraisal of submitted systematic review manuscripts. For authors, these tools may function as teaching aids and inform conduct of their review during its development.
Description
Systematic reviews that include randomized and/or non-randomized studies as evidence can be appraised with AMSTAR-2 and ROBIS. Other characteristics of AMSTAR-2 and ROBIS are summarized in Table 3.2 . Both tools define categories for an overall rating; however, neither tool is intended to generate a total score by simply calculating the number of responses satisfying criteria for individual items [ 4 , 6 ]. AMSTAR-2 focuses on the rigor of a review’s methods irrespective of the specific subject matter. ROBIS places emphasis on a review’s results section— this suggests it may be optimally applied by appraisers with some knowledge of the review’s topic as they may be better equipped to determine if certain procedures (or lack thereof) would impact the validity of a review’s findings [ 98 , 100 ]. Reliability studies show AMSTAR-2 overall confidence ratings strongly correlate with the overall RoB ratings in ROBIS [ 100 , 101 ].
Comparison of AMSTAR-2 and ROBIS
a ROBIS includes an optional first phase to assess the applicability of the review to the research question of interest. The tool may be applicable to other review types in addition to the four specified, although modification of this initial phase will be needed (Personal Communication via email, Penny Whiting, 28 Jan 2022)
b AMSTAR-2 item #9 and #11 require separate responses for RCTs and NRSI
Interrater reliability has been shown to be acceptable for AMSTAR-2 [ 6 , 11 , 102 ] and ROBIS [ 4 , 98 , 103 ] but neither tool has been shown to be superior in this regard [ 100 , 101 , 104 , 105 ]. Overall, variability in reliability for both tools has been reported across items, between pairs of raters, and between centers [ 6 , 100 , 101 , 104 ]. The effects of appraiser experience on the results of AMSTAR-2 and ROBIS require further evaluation [ 101 , 105 ]. Updates to both tools should address items shown to be prone to individual appraisers’ subjective biases and opinions [ 11 , 100 ]; this may involve modifications of the current domains and signaling questions as well as incorporation of methods to make an appraiser’s judgments more explicit. Future revisions of these tools may also consider the addition of standards for aspects of systematic review development currently lacking (eg, rating overall certainty of evidence, [ 99 ] methods for synthesis without meta-analysis [ 105 ]) and removal of items that assess aspects of reporting that are thoroughly evaluated by PRISMA 2020.
Application
A good understanding of what is required to satisfy the standards of AMSTAR-2 and ROBIS involves study of the accompanying guidance documents written by the tools’ developers; these contain detailed descriptions of each item’s standards. In addition, accurate appraisal of a systematic review with either tool requires training. Most experts recommend independent assessment by at least two appraisers with a process for resolving discrepancies as well as procedures to establish interrater reliability, such as pilot testing, a calibration phase or exercise, and development of predefined decision rules [ 35 , 99 – 101 , 103 , 104 , 106 ]. These methods may, to some extent, address the challenges associated with the diversity in methodological training, subject matter expertise, and experience using the tools that are likely to exist among appraisers.
The standards of AMSTAR, AMSTAR-2, and ROBIS have been used in many methodological studies and epidemiological investigations. However, the increased publication of overviews or umbrella reviews and CPGs has likely been a greater influence on the widening acceptance of these tools. Critical appraisal of the secondary studies considered evidence is essential to the trustworthiness of both the recommendations of CPGs and the conclusions of overviews. Currently both Cochrane [ 55 ] and JBI [ 107 ] recommend AMSTAR-2 and ROBIS in their guidance for authors of overviews or umbrella reviews. However, ROBIS and AMSTAR-2 were released in 2016 and 2017, respectively; thus, to date, limited data have been reported about the uptake of these tools or which of the two may be preferred [ 21 , 106 ]. Currently, in relation to CPGs, AMSTAR-2 appears to be overwhelmingly popular compared to ROBIS. A Google Scholar search of this topic (search terms “AMSTAR 2 AND clinical practice guidelines,” “ROBIS AND clinical practice guidelines” 13 May 2022) found 12,700 hits for AMSTAR-2 and 1,280 for ROBIS. The apparent greater appeal of AMSTAR-2 may relate to its longer track record given the original version of the tool was in use for 10 years prior to its update in 2017.
Barriers to the uptake of AMSTAR-2 and ROBIS include the real or perceived time and resources necessary to complete the items they include and appraisers’ confidence in their own ratings [ 104 ]. Reports from comparative studies available to date indicate that appraisers find AMSTAR-2 questions, responses, and guidance to be clearer and simpler compared with ROBIS [ 11 , 101 , 104 , 105 ]. This suggests that for appraisal of intervention systematic reviews, AMSTAR-2 may be a more practical tool than ROBIS, especially for novice appraisers [ 101 , 103 – 105 ]. The unique characteristics of each tool, as well as their potential advantages and disadvantages, should be taken into consideration when deciding which tool should be used for an appraisal of a systematic review. In addition, the choice of one or the other may depend on how the results of an appraisal will be used; for example, a peer reviewer’s appraisal of a single manuscript versus an appraisal of multiple systematic reviews in an overview or umbrella review, CPG, or systematic methodological study.
Authors of overviews and CPGs report results of AMSTAR-2 and ROBIS appraisals for each of the systematic reviews they include as evidence. Ideally, an independent judgment of their appraisals can be made by the end users of overviews and CPGs; however, most stakeholders, including clinicians, are unlikely to have a sophisticated understanding of these tools. Nevertheless, they should at least be aware that AMSTAR-2 and ROBIS ratings reported in overviews and CPGs may be inaccurate because the tools are not applied as intended by their developers. This can result from inadequate training of the overview or CPG authors who perform the appraisals, or to modifications of the appraisal tools imposed by them. The potential variability in overall confidence and RoB ratings highlights why appraisers applying these tools need to support their judgments with explicit documentation; this allows readers to judge for themselves whether they agree with the criteria used by appraisers [ 4 , 108 ]. When these judgments are explicit, the underlying rationale used when applying these tools can be assessed [ 109 ].
Theoretically, we would expect an association of AMSTAR-2 with improved methodological rigor and an association of ROBIS with lower RoB in recent systematic reviews compared to those published before 2017. To our knowledge, this has not yet been demonstrated; however, like reports about the actual uptake of these tools, time will tell. Additional data on user experience is also needed to further elucidate the practical challenges and methodological nuances encountered with the application of these tools. This information could potentially inform the creation of unifying criteria to guide and standardize the appraisal of evidence syntheses [ 109 ].
Evaluation of reporting
Complete reporting is essential for users to establish the trustworthiness and applicability of a systematic review’s findings. Efforts to standardize and improve the reporting of systematic reviews resulted in the 2009 publication of the PRISMA statement [ 92 ] with its accompanying explanation and elaboration document [ 110 ]. This guideline was designed to help authors prepare a complete and transparent report of their systematic review. In addition, adherence to PRISMA is often used to evaluate the thoroughness of reporting of published systematic reviews [ 111 ]. The updated version, PRISMA 2020 [ 93 ], and its guidance document [ 112 ] were published in 2021. Items on the original and updated versions of PRISMA are organized by the six basic review components they address (title, abstract, introduction, methods, results, discussion). The PRISMA 2020 update is a considerably expanded version of the original; it includes standards and examples for the 27 original and 13 additional reporting items that capture methodological advances and may enhance the replicability of reviews [ 113 ].
The original PRISMA statement fostered the development of various PRISMA extensions (Table 3.3 ). These include reporting guidance for scoping reviews and reviews of diagnostic test accuracy and for intervention reviews that report on the following: harms outcomes, equity issues, the effects of acupuncture, the results of network meta-analyses and analyses of individual participant data. Detailed reporting guidance for specific systematic review components (abstracts, protocols, literature searches) is also available.
PRISMA extensions
PRISMA, Preferred Reporting Items for Systematic Reviews and Meta-Analyses
a Note the abstract reporting checklist is now incorporated into PRISMA 2020 [ 93 ]
Uptake and impact
The 2009 PRISMA standards [ 92 ] for reporting have been widely endorsed by authors, journals, and EBM-related organizations. We anticipate the same for PRISMA 2020 [ 93 ] given its co-publication in multiple high-impact journals. However, to date, there is a lack of strong evidence for an association between improved systematic review reporting and endorsement of PRISMA 2009 standards [ 43 , 111 ]. Most journals require a PRISMA checklist accompany submissions of systematic review manuscripts. However, the accuracy of information presented on these self-reported checklists is not necessarily verified. It remains unclear which strategies (eg, authors’ self-report of checklists, peer reviewer checks) might improve adherence to the PRISMA reporting standards; in addition, the feasibility of any potentially effective strategies must be taken into consideration given the structure and limitations of current research and publication practices [ 124 ].
Pitfalls and limitations of PRISMA, AMSTAR-2, and ROBIS
Misunderstanding of the roles of these tools and their misapplication may be widespread problems. PRISMA 2020 is a reporting guideline that is most beneficial if consulted when developing a review as opposed to merely completing a checklist when submitting to a journal; at that point, the review is finished, with good or bad methodological choices. However, PRISMA checklists evaluate how completely an element of review conduct was reported, but do not evaluate the caliber of conduct or performance of a review. Thus, review authors and readers should not think that a rigorous systematic review can be produced by simply following the PRISMA 2020 guidelines. Similarly, it is important to recognize that AMSTAR-2 and ROBIS are tools to evaluate the conduct of a review but do not substitute for conceptual methodological guidance. In addition, they are not intended to be simple checklists. In fact, they have the potential for misuse or abuse if applied as such; for example, by calculating a total score to make a judgment about a review’s overall confidence or RoB. Proper selection of a response for the individual items on AMSTAR-2 and ROBIS requires training or at least reference to their accompanying guidance documents.
Not surprisingly, it has been shown that compliance with the PRISMA checklist is not necessarily associated with satisfying the standards of ROBIS [ 125 ]. AMSTAR-2 and ROBIS were not available when PRISMA 2009 was developed; however, they were considered in the development of PRISMA 2020 [ 113 ]. Therefore, future studies may show a positive relationship between fulfillment of PRISMA 2020 standards for reporting and meeting the standards of tools evaluating methodological quality and RoB.
Choice of an appropriate tool for the evaluation of a systematic review first involves identification of the underlying construct to be assessed. For systematic reviews of interventions, recommended tools include AMSTAR-2 and ROBIS for appraisal of conduct and PRISMA 2020 for completeness of reporting. All three tools were developed rigorously and provide easily accessible and detailed user guidance, which is necessary for their proper application and interpretation. When considering a manuscript for publication, training in these tools can sensitize peer reviewers and editors to major issues that may affect the review’s trustworthiness and completeness of reporting. Judgment of the overall certainty of a body of evidence and formulation of recommendations rely, in part, on AMSTAR-2 or ROBIS appraisals of systematic reviews. Therefore, training on the application of these tools is essential for authors of overviews and developers of CPGs. Peer reviewers and editors considering an overview or CPG for publication must hold their authors to a high standard of transparency regarding both the conduct and reporting of these appraisals.
Part 4. Meeting conduct standards
Many authors, peer reviewers, and editors erroneously equate fulfillment of the items on the PRISMA checklist with superior methodological rigor. For direction on methodology, we refer them to available resources that provide comprehensive conceptual guidance [ 59 , 60 ] as well as primers with basic step-by-step instructions [ 1 , 126 , 127 ]. This section is intended to complement study of such resources by facilitating use of AMSTAR-2 and ROBIS, tools specifically developed to evaluate methodological rigor of systematic reviews. These tools are widely accepted by methodologists; however, in the general medical literature, they are not uniformly selected for the critical appraisal of systematic reviews [ 88 , 96 ].
To enable their uptake, Table 4.1 links review components to the corresponding appraisal tool items. Expectations of AMSTAR-2 and ROBIS are concisely stated, and reasoning provided.
Systematic review components linked to appraisal with AMSTAR-2 and ROBIS a
CoI conflict of interest, MA meta-analysis, NA not addressed, PICO participant, intervention, comparison, outcome, PRISMA-P Preferred Reporting Items for Systematic Review and Meta-Analysis Protocols, RoB risk of bias
a Components shown in bold are chosen for elaboration in Part 4 for one (or both) of two reasons: 1) the component has been identified as potentially problematic for systematic review authors; and/or 2) the component is evaluated by standards of an AMSTAR-2 “critical” domain
b Critical domains of AMSTAR-2 are indicated by *
Issues involved in meeting the standards for seven review components (identified in bold in Table 4.1 ) are addressed in detail. These were chosen for elaboration for one (or both) of two reasons: 1) the component has been identified as potentially problematic for systematic review authors based on consistent reports of their frequent AMSTAR-2 or ROBIS deficiencies [ 9 , 11 , 15 , 88 , 128 , 129 ]; and/or 2) the review component is judged by standards of an AMSTAR-2 “critical” domain. These have the greatest implications for how a systematic review will be appraised: if standards for any one of these critical domains are not met, the review is rated as having “critically low confidence.”
Research question
Specific and unambiguous research questions may have more value for reviews that deal with hypothesis testing. Mnemonics for the various elements of research questions are suggested by JBI and Cochrane (Table 2.1 ). These prompt authors to consider the specialized methods involved for developing different types of systematic reviews; however, while inclusion of the suggested elements makes a review compliant with a particular review’s methods, it does not necessarily make a research question appropriate. Table 4.2 lists acronyms that may aid in developing the research question. They include overlapping concepts of importance in this time of proliferating reviews of uncertain value [ 130 ]. If these issues are not prospectively contemplated, systematic review authors may establish an overly broad scope, or develop runaway scope allowing them to stray from predefined choices relating to key comparisons and outcomes.
Research question development
a Cummings SR, Browner WS, Hulley SB. Conceiving the research question and developing the study plan. In: Hulley SB, Cummings SR, Browner WS, editors. Designing clinical research: an epidemiological approach; 4th edn. Lippincott Williams & Wilkins; 2007. p. 14–22
b Doran, GT. There’s a S.M.A.R.T. way to write management’s goals and objectives. Manage Rev. 1981;70:35-6.
c Johnson BT, Hennessy EA. Systematic reviews and meta-analyses in the health sciences: best practice methods for research syntheses. Soc Sci Med. 2019;233:237–51
Once a research question is established, searching on registry sites and databases for existing systematic reviews addressing the same or a similar topic is necessary in order to avoid contributing to research waste [ 131 ]. Repeating an existing systematic review must be justified, for example, if previous reviews are out of date or methodologically flawed. A full discussion on replication of intervention systematic reviews, including a consensus checklist, can be found in the work of Tugwell and colleagues [ 84 ].
Protocol development is considered a core component of systematic reviews [ 125 , 126 , 132 ]. Review protocols may allow researchers to plan and anticipate potential issues, assess validity of methods, prevent arbitrary decision-making, and minimize bias that can be introduced by the conduct of the review. Registration of a protocol that allows public access promotes transparency of the systematic review’s methods and processes and reduces the potential for duplication [ 132 ]. Thinking early and carefully about all the steps of a systematic review is pragmatic and logical and may mitigate the influence of the authors’ prior knowledge of the evidence [ 133 ]. In addition, the protocol stage is when the scope of the review can be carefully considered by authors, reviewers, and editors; this may help to avoid production of overly ambitious reviews that include excessive numbers of comparisons and outcomes or are undisciplined in their study selection.
An association with attainment of AMSTAR standards in systematic reviews with published prospective protocols has been reported [ 134 ]. However, completeness of reporting does not seem to be different in reviews with a protocol compared to those without one [ 135 ]. PRISMA-P [ 116 ] and its accompanying elaboration and explanation document [ 136 ] can be used to guide and assess the reporting of protocols. A final version of the review should fully describe any protocol deviations. Peer reviewers may compare the submitted manuscript with any available pre-registered protocol; this is required if AMSTAR-2 or ROBIS are used for critical appraisal.
There are multiple options for the recording of protocols (Table 4.3 ). Some journals will peer review and publish protocols. In addition, many online sites offer date-stamped and publicly accessible protocol registration. Some of these are exclusively for protocols of evidence syntheses; others are less restrictive and offer researchers the capacity for data storage, sharing, and other workflow features. These sites document protocol details to varying extents and have different requirements [ 137 ]. The most popular site for systematic reviews, the International Prospective Register of Systematic Reviews (PROSPERO), for example, only registers reviews that report on an outcome with direct relevance to human health. The PROSPERO record documents protocols for all types of reviews except literature and scoping reviews. Of note, PROSPERO requires authors register their review protocols prior to any data extraction [ 133 , 138 ]. The electronic records of most of these registry sites allow authors to update their protocols and facilitate transparent tracking of protocol changes, which are not unexpected during the progress of the review [ 139 ].
Options for protocol registration of evidence syntheses
a Authors are advised to contact their target journal regarding submission of systematic review protocols
b Registration is restricted to approved review projects
c The JBI registry lists review projects currently underway by JBI-affiliated entities. These records include a review’s title, primary author, research question, and PICO elements. JBI recommends that authors register eligible protocols with PROSPERO
d See Pieper and Rombey [ 137 ] for detailed characteristics of these five registries
e See Pieper and Rombey [ 137 ] for other systematic review data repository options
Study design inclusion
For most systematic reviews, broad inclusion of study designs is recommended [ 126 ]. This may allow comparison of results between contrasting study design types [ 126 ]. Certain study designs may be considered preferable depending on the type of review and nature of the research question. However, prevailing stereotypes about what each study design does best may not be accurate. For example, in systematic reviews of interventions, randomized designs are typically thought to answer highly specific questions while non-randomized designs often are expected to reveal greater information about harms or real-word evidence [ 126 , 140 , 141 ]. This may be a false distinction; randomized trials may be pragmatic [ 142 ], they may offer important (and more unbiased) information on harms [ 143 ], and data from non-randomized trials may not necessarily be more real-world-oriented [ 144 ].
Moreover, there may not be any available evidence reported by RCTs for certain research questions; in some cases, there may not be any RCTs or NRSI. When the available evidence is limited to case reports and case series, it is not possible to test hypotheses nor provide descriptive estimates or associations; however, a systematic review of these studies can still offer important insights [ 81 , 145 ]. When authors anticipate that limited evidence of any kind may be available to inform their research questions, a scoping review can be considered. Alternatively, decisions regarding inclusion of indirect as opposed to direct evidence can be addressed during protocol development [ 146 ]. Including indirect evidence at an early stage of intervention systematic review development allows authors to decide if such studies offer any additional and/or different understanding of treatment effects for their population or comparison of interest. Issues of indirectness of included studies are accounted for later in the process, during determination of the overall certainty of evidence (see Part 5 for details).
Evidence search
Both AMSTAR-2 and ROBIS require systematic and comprehensive searches for evidence. This is essential for any systematic review. Both tools discourage search restrictions based on language and publication source. Given increasing globalism in health care, the practice of including English-only literature should be avoided [ 126 ]. There are many examples in which language bias (different results in studies published in different languages) has been documented [ 147 , 148 ]. This does not mean that all literature, in all languages, is equally trustworthy [ 148 ]; however, the only way to formally probe for the potential of such biases is to consider all languages in the initial search. The gray literature and a search of trials may also reveal important details about topics that would otherwise be missed [ 149 – 151 ]. Again, inclusiveness will allow review authors to investigate whether results differ in gray literature and trials [ 41 , 151 – 153 ].
Authors should make every attempt to complete their review within one year as that is the likely viable life of a search. (1) If that is not possible, the search should be updated close to the time of completion [ 154 ]. Different research topics may warrant less of a delay, for example, in rapidly changing fields (as in the case of the COVID-19 pandemic), even one month may radically change the available evidence.
Excluded studies
AMSTAR-2 requires authors to provide references for any studies excluded at the full text phase of study selection along with reasons for exclusion; this allows readers to feel confident that all relevant literature has been considered for inclusion and that exclusions are defensible.
Risk of bias assessment of included studies
The design of the studies included in a systematic review (eg, RCT, cohort, case series) should not be equated with appraisal of its RoB. To meet AMSTAR-2 and ROBIS standards, systematic review authors must examine RoB issues specific to the design of each primary study they include as evidence. It is unlikely that a single RoB appraisal tool will be suitable for all research designs. In addition to tools for randomized and non-randomized studies, specific tools are available for evaluation of RoB in case reports and case series [ 82 ] and single-case experimental designs [ 155 , 156 ]. Note the RoB tools selected must meet the standards of the appraisal tool used to judge the conduct of the review. For example, AMSTAR-2 identifies four sources of bias specific to RCTs and NRSI that must be addressed by the RoB tool(s) chosen by the review authors. The Cochrane RoB-2 [ 157 ] tool for RCTs and ROBINS-I [ 158 ] for NRSI for RoB assessment meet the AMSTAR-2 standards. Appraisers on the review team should not modify any RoB tool without complete transparency and acknowledgment that they have invalidated the interpretation of the tool as intended by its developers [ 159 ]. Conduct of RoB assessments is not addressed AMSTAR-2; to meet ROBIS standards, two independent reviewers should complete RoB assessments of included primary studies.
Implications of the RoB assessments must be explicitly discussed and considered in the conclusions of the review. Discussion of the overall RoB of included studies may consider the weight of the studies at high RoB, the importance of the sources of bias in the studies being summarized, and if their importance differs in relationship to the outcomes reported. If a meta-analysis is performed, serious concerns for RoB of individual studies should be accounted for in these results as well. If the results of the meta-analysis for a specific outcome change when studies at high RoB are excluded, readers will have a more accurate understanding of this body of evidence. However, while investigating the potential impact of specific biases is a useful exercise, it is important to avoid over-interpretation, especially when there are sparse data.
Synthesis methods for quantitative data
Syntheses of quantitative data reported by primary studies are broadly categorized as one of two types: meta-analysis, and synthesis without meta-analysis (Table 4.4 ). Before deciding on one of these methods, authors should seek methodological advice about whether reported data can be transformed or used in other ways to provide a consistent effect measure across studies [ 160 , 161 ].
Common methods for quantitative synthesis
CI confidence interval (or credible interval, if analysis is done in Bayesian framework)
a See text for descriptions of the types of data combined in each of these approaches
b See Additional File 4 for guidance on the structure and presentation of forest plots
c General approach is similar to aggregate data meta-analysis but there are substantial differences relating to data collection and checking and analysis [ 162 ]. This approach to syntheses is applicable to intervention, diagnostic, and prognostic systematic reviews [ 163 ]
d Examples include meta-regression, hierarchical and multivariate approaches [ 164 ]
e In-depth guidance and illustrations of these methods are provided in Chapter 12 of the Cochrane Handbook [ 160 ]
Meta-analysis
Systematic reviews that employ meta-analysis should not be referred to simply as “meta-analyses.” The term meta-analysis strictly refers to a specific statistical technique used when study effect estimates and their variances are available, yielding a quantitative summary of results. In general, methods for meta-analysis involve use of a weighted average of effect estimates from two or more studies. If considered carefully, meta-analysis increases the precision of the estimated magnitude of effect and can offer useful insights about heterogeneity and estimates of effects. We refer to standard references for a thorough introduction and formal training [ 165 – 167 ].
There are three common approaches to meta-analysis in current health care–related systematic reviews (Table 4.4 ). Aggregate meta-analyses is the most familiar to authors of evidence syntheses and their end users. This standard meta-analysis combines data on effect estimates reported by studies that investigate similar research questions involving direct comparisons of an intervention and comparator. Results of these analyses provide a single summary intervention effect estimate. If the included studies in a systematic review measure an outcome differently, their reported results may be transformed to make them comparable [ 161 ]. Forest plots visually present essential information about the individual studies and the overall pooled analysis (see Additional File 4 for details).
Less familiar and more challenging meta-analytical approaches used in secondary research include individual participant data (IPD) and network meta-analyses (NMA); PRISMA extensions provide reporting guidelines for both [ 117 , 118 ]. In IPD, the raw data on each participant from each eligible study are re-analyzed as opposed to the study-level data analyzed in aggregate data meta-analyses [ 168 ]. This may offer advantages, including the potential for limiting concerns about bias and allowing more robust analyses [ 163 ]. As suggested by the description in Table 4.4 , NMA is a complex statistical approach. It combines aggregate data [ 169 ] or IPD [ 170 ] for effect estimates from direct and indirect comparisons reported in two or more studies of three or more interventions. This makes it a potentially powerful statistical tool; while multiple interventions are typically available to treat a condition, few have been evaluated in head-to-head trials [ 171 ]. Both IPD and NMA facilitate a broader scope, and potentially provide more reliable and/or detailed results; however, compared with standard aggregate data meta-analyses, their methods are more complicated, time-consuming, and resource-intensive, and they have their own biases, so one needs sufficient funding, technical expertise, and preparation to employ them successfully [ 41 , 172 , 173 ].
Several items in AMSTAR-2 and ROBIS address meta-analysis; thus, understanding the strengths, weaknesses, assumptions, and limitations of methods for meta-analyses is important. According to the standards of both tools, plans for a meta-analysis must be addressed in the review protocol, including reasoning, description of the type of quantitative data to be synthesized, and the methods planned for combining the data. This should not consist of stock statements describing conventional meta-analysis techniques; rather, authors are expected to anticipate issues specific to their research questions. Concern for the lack of training in meta-analysis methods among systematic review authors cannot be overstated. For those with training, the use of popular software (eg, RevMan [ 174 ], MetaXL [ 175 ], JBI SUMARI [ 176 ]) may facilitate exploration of these methods; however, such programs cannot substitute for the accurate interpretation of the results of meta-analyses, especially for more complex meta-analytical approaches.
Synthesis without meta-analysis
There are varied reasons a meta-analysis may not be appropriate or desirable [ 160 , 161 ]. Syntheses that informally use statistical methods other than meta-analysis are variably referred to as descriptive, narrative, or qualitative syntheses or summaries; these terms are also applied to syntheses that make no attempt to statistically combine data from individual studies. However, use of such imprecise terminology is discouraged; in order to fully explore the results of any type of synthesis, some narration or description is needed to supplement the data visually presented in tabular or graphic forms [ 63 , 177 ]. In addition, the term “qualitative synthesis” is easily confused with a synthesis of qualitative data in a qualitative or mixed methods review. “Synthesis without meta-analysis” is currently the preferred description of other ways to combine quantitative data from two or more studies. Use of this specific terminology when referring to these types of syntheses also implies the application of formal methods (Table 4.4 ).
Methods for syntheses without meta-analysis involve structured presentations of the data in any tables and plots. In comparison to narrative descriptions of each study, these are designed to more effectively and transparently show patterns and convey detailed information about the data; they also allow informal exploration of heterogeneity [ 178 ]. In addition, acceptable quantitative statistical methods (Table 4.4 ) are formally applied; however, it is important to recognize these methods have significant limitations for the interpretation of the effectiveness of an intervention [ 160 ]. Nevertheless, when meta-analysis is not possible, the application of these methods is less prone to bias compared with an unstructured narrative description of included studies [ 178 , 179 ].
Vote counting is commonly used in systematic reviews and involves a tally of studies reporting results that meet some threshold of importance applied by review authors. Until recently, it has not typically been identified as a method for synthesis without meta-analysis. Guidance on an acceptable vote counting method based on direction of effect is currently available [ 160 ] and should be used instead of narrative descriptions of such results (eg, “more than half the studies showed improvement”; “only a few studies reported adverse effects”; “7 out of 10 studies favored the intervention”). Unacceptable methods include vote counting by statistical significance or magnitude of effect or some subjective rule applied by the authors.
AMSTAR-2 and ROBIS standards do not explicitly address conduct of syntheses without meta-analysis, although AMSTAR-2 items 13 and 14 might be considered relevant. Guidance for the complete reporting of syntheses without meta-analysis for systematic reviews of interventions is available in the Synthesis without Meta-analysis (SWiM) guideline [ 180 ] and methodological guidance is available in the Cochrane Handbook [ 160 , 181 ].
Familiarity with AMSTAR-2 and ROBIS makes sense for authors of systematic reviews as these appraisal tools will be used to judge their work; however, training is necessary for authors to truly appreciate and apply methodological rigor. Moreover, judgment of the potential contribution of a systematic review to the current knowledge base goes beyond meeting the standards of AMSTAR-2 and ROBIS. These tools do not explicitly address some crucial concepts involved in the development of a systematic review; this further emphasizes the need for author training.
We recommend that systematic review authors incorporate specific practices or exercises when formulating a research question at the protocol stage, These should be designed to raise the review team’s awareness of how to prevent research and resource waste [ 84 , 130 ] and to stimulate careful contemplation of the scope of the review [ 30 ]. Authors’ training should also focus on justifiably choosing a formal method for the synthesis of quantitative and/or qualitative data from primary research; both types of data require specific expertise. For typical reviews that involve syntheses of quantitative data, statistical expertise is necessary, initially for decisions about appropriate methods, [ 160 , 161 ] and then to inform any meta-analyses [ 167 ] or other statistical methods applied [ 160 ].
Part 5. Rating overall certainty of evidence
Report of an overall certainty of evidence assessment in a systematic review is an important new reporting standard of the updated PRISMA 2020 guidelines [ 93 ]. Systematic review authors are well acquainted with assessing RoB in individual primary studies, but much less familiar with assessment of overall certainty across an entire body of evidence. Yet a reliable way to evaluate this broader concept is now recognized as a vital part of interpreting the evidence.
Historical systems for rating evidence are based on study design and usually involve hierarchical levels or classes of evidence that use numbers and/or letters to designate the level/class. These systems were endorsed by various EBM-related organizations. Professional societies and regulatory groups then widely adopted them, often with modifications for application to the available primary research base in specific clinical areas. In 2002, a report issued by the AHRQ identified 40 systems to rate quality of a body of evidence [ 182 ]. A critical appraisal of systems used by prominent health care organizations published in 2004 revealed limitations in sensibility, reproducibility, applicability to different questions, and usability to different end users [ 183 ]. Persistent use of hierarchical rating schemes to describe overall quality continues to complicate the interpretation of evidence. This is indicated by recent reports of poor interpretability of systematic review results by readers [ 184 – 186 ] and misleading interpretations of the evidence related to the “spin” systematic review authors may put on their conclusions [ 50 , 187 ].
Recognition of the shortcomings of hierarchical rating systems raised concerns that misleading clinical recommendations could result even if based on a rigorous systematic review. In addition, the number and variability of these systems were considered obstacles to quick and accurate interpretations of the evidence by clinicians, patients, and policymakers [ 183 ]. These issues contributed to the development of the GRADE approach. An international working group, that continues to actively evaluate and refine it, first introduced GRADE in 2004 [ 188 ]. Currently more than 110 organizations from 19 countries around the world have endorsed or are using GRADE [ 189 ].
GRADE approach to rating overall certainty
GRADE offers a consistent and sensible approach for two separate processes: rating the overall certainty of a body of evidence and the strength of recommendations. The former is the expected conclusion of a systematic review, while the latter is pertinent to the development of CPGs. As such, GRADE provides a mechanism to bridge the gap from evidence synthesis to application of the evidence for informed clinical decision-making [ 27 , 190 ]. We briefly examine the GRADE approach but only as it applies to rating overall certainty of evidence in systematic reviews.
In GRADE, use of “certainty” of a body of evidence is preferred over the term “quality.” [ 191 ] Certainty refers to the level of confidence systematic review authors have that, for each outcome, an effect estimate represents the true effect. The GRADE approach to rating confidence in estimates begins with identifying the study type (RCT or NRSI) and then systematically considers criteria to rate the certainty of evidence up or down (Table 5.1 ).
GRADE criteria for rating certainty of evidence
a Applies to randomized studies
b Applies to non-randomized studies
This process results in assignment of one of the four GRADE certainty ratings to each outcome; these are clearly conveyed with the use of basic interpretation symbols (Table 5.2 ) [ 192 ]. Notably, when multiple outcomes are reported in a systematic review, each outcome is assigned a unique certainty rating; thus different levels of certainty may exist in the body of evidence being examined.
GRADE certainty ratings and their interpretation symbols a
a From the GRADE Handbook [ 192 ]
GRADE’s developers acknowledge some subjectivity is involved in this process [ 193 ]. In addition, they emphasize that both the criteria for rating evidence up and down (Table 5.1 ) as well as the four overall certainty ratings (Table 5.2 ) reflect a continuum as opposed to discrete categories [ 194 ]. Consequently, deciding whether a study falls above or below the threshold for rating up or down may not be straightforward, and preliminary overall certainty ratings may be intermediate (eg, between low and moderate). Thus, the proper application of GRADE requires systematic review authors to take an overall view of the body of evidence and explicitly describe the rationale for their final ratings.
Advantages of GRADE
Outcomes important to the individuals who experience the problem of interest maintain a prominent role throughout the GRADE process [ 191 ]. These outcomes must inform the research questions (eg, PICO [population, intervention, comparator, outcome]) that are specified a priori in a systematic review protocol. Evidence for these outcomes is then investigated and each critical or important outcome is ultimately assigned a certainty of evidence as the end point of the review. Notably, limitations of the included studies have an impact at the outcome level. Ultimately, the certainty ratings for each outcome reported in a systematic review are considered by guideline panels. They use a different process to formulate recommendations that involves assessment of the evidence across outcomes [ 201 ]. It is beyond our scope to describe the GRADE process for formulating recommendations; however, it is critical to understand how these two outcome-centric concepts of certainty of evidence in the GRADE framework are related and distinguished. An in-depth illustration using examples from recently published evidence syntheses and CPGs is provided in Additional File 5 A (Table AF5A-1).
The GRADE approach is applicable irrespective of whether the certainty of the primary research evidence is high or very low; in some circumstances, indirect evidence of higher certainty may be considered if direct evidence is unavailable or of low certainty [ 27 ]. In fact, most interventions and outcomes in medicine have low or very low certainty of evidence based on GRADE and there seems to be no major improvement over time [ 202 , 203 ]. This is still a very important (even if sobering) realization for calibrating our understanding of medical evidence. A major appeal of the GRADE approach is that it offers a common framework that enables authors of evidence syntheses to make complex judgments about evidence certainty and to convey these with unambiguous terminology. This prevents some common mistakes made by review authors, including overstating results (or under-reporting harms) [ 187 ] and making recommendations for treatment. This is illustrated in Table AF5A-2 (Additional File 5 A), which compares the concluding statements made about overall certainty in a systematic review with and without application of the GRADE approach.
Theoretically, application of GRADE should improve consistency of judgments about certainty of evidence, both between authors and across systematic reviews. In one empirical evaluation conducted by the GRADE Working Group, interrater reliability of two individual raters assessing certainty of the evidence for a specific outcome increased from ~ 0.3 without using GRADE to ~ 0.7 by using GRADE [ 204 ]. However, others report variable agreement among those experienced in GRADE assessments of evidence certainty [ 190 ]. Like any other tool, GRADE requires training in order to be properly applied. The intricacies of the GRADE approach and the necessary subjectivity involved suggest that improving agreement may require strict rules for its application; alternatively, use of general guidance and consensus among review authors may result in less consistency but provide important information for the end user [ 190 ].
GRADE caveats
Simply invoking “the GRADE approach” does not automatically ensure GRADE methods were employed by authors of a systematic review (or developers of a CPG). Table 5.3 lists the criteria the GRADE working group has established for this purpose. These criteria highlight the specific terminology and methods that apply to rating the certainty of evidence for outcomes reported in a systematic review [ 191 ], which is different from rating overall certainty across outcomes considered in the formulation of recommendations [ 205 ]. Modifications of standard GRADE methods and terminology are discouraged as these may detract from GRADE’s objectives to minimize conceptual confusion and maximize clear communication [ 206 ].
Criteria for using GRADE in a systematic review a
a Adapted from the GRADE working group [ 206 ]; this list does not contain the additional criteria that apply to the development of a clinical practice guideline
Nevertheless, GRADE is prone to misapplications [ 207 , 208 ], which can distort a systematic review’s conclusions about the certainty of evidence. Systematic review authors without proper GRADE training are likely to misinterpret the terms “quality” and “grade” and to misunderstand the constructs assessed by GRADE versus other appraisal tools. For example, review authors may reference the standard GRADE certainty ratings (Table 5.2 ) to describe evidence for their outcome(s) of interest. However, these ratings are invalidated if authors omit or inadequately perform RoB evaluations of each included primary study. Such deficiencies in RoB assessments are unacceptable but not uncommon, as reported in methodological studies of systematic reviews and overviews [ 104 , 186 , 209 , 210 ]. GRADE ratings are also invalidated if review authors do not formally address and report on the other criteria (Table 5.1 ) necessary for a GRADE certainty rating.
Other caveats pertain to application of a GRADE certainty of evidence rating in various types of evidence syntheses. Current adaptations of GRADE are described in Additional File 5 B and included on Table 6.3 , which is introduced in the next section.
Concise Guide to best practices for evidence syntheses, version 1.0 a
AMSTAR A MeaSurement Tool to Assess Systematic Reviews, CASP Critical Appraisal Skills Programme, CERQual Confidence in the Evidence from Reviews of Qualitative research, ConQual Establishing Confidence in the output of Qualitative research synthesis, COSMIN COnsensus-based Standards for the selection of health Measurement Instruments, DTA diagnostic test accuracy, eMERGe meta-ethnography reporting guidance, ENTREQ enhancing transparency in reporting the synthesis of qualitative research, GRADE Grading of Recommendations Assessment, Development and Evaluation, MA meta-analysis, NRSI non-randomized studies of interventions, P protocol, PRIOR Preferred Reporting Items for Overviews of Reviews, PRISMA Preferred Reporting Items for Systematic Reviews and Meta-Analyses, PROBAST Prediction model Risk Of Bias ASsessment Tool, QUADAS quality assessment of studies of diagnostic accuracy included in systematic reviews, QUIPS Quality In Prognosis Studies, RCT randomized controlled trial, RoB risk of bias, ROBINS-I Risk Of Bias In Non-randomised Studies of Interventions, ROBIS Risk of Bias in Systematic Reviews, ScR scoping review, SWiM systematic review without meta-analysis
a Superscript numbers represent citations provided in the main reference list. Additional File 6 lists links to available online resources for the methods and tools included in the Concise Guide
b The MECIR manual [ 30 ] provides Cochrane’s specific standards for both reporting and conduct of intervention systematic reviews and protocols
c Editorial and peer reviewers can evaluate completeness of reporting in submitted manuscripts using these tools. Authors may be required to submit a self-reported checklist for the applicable tools
d The decision flowchart described by Flemming and colleagues [ 223 ] is recommended for guidance on how to choose the best approach to reporting for qualitative reviews
e SWiM was developed for intervention studies reporting quantitative data. However, if there is not a more directly relevant reporting guideline, SWiM may prompt reviewers to consider the important details to report. (Personal Communication via email, Mhairi Campbell, 14 Dec 2022)
f JBI recommends their own tools for the critical appraisal of various quantitative primary study designs included in systematic reviews of intervention effectiveness, prevalence and incidence, and etiology and risk as well as for the critical appraisal of systematic reviews included in umbrella reviews. However, except for the JBI Checklists for studies reporting prevalence data and qualitative research, the development, validity, and reliability of these tools are not well documented
g Studies that are not RCTs or NRSI require tools developed specifically to evaluate their design features. Examples include single case experimental design [ 155 , 156 ] and case reports and series [ 82 ]
h The evaluation of methodological quality of studies included in a synthesis of qualitative research is debatable [ 224 ]. Authors may select a tool appropriate for the type of qualitative synthesis methodology employed. The CASP Qualitative Checklist [ 218 ] is an example of a published, commonly used tool that focuses on assessment of the methodological strengths and limitations of qualitative studies. The JBI Critical Appraisal Checklist for Qualitative Research [ 219 ] is recommended for reviews using a meta-aggregative approach
i Consider including risk of bias assessment of included studies if this information is relevant to the research question; however, scoping reviews do not include an assessment of the overall certainty of a body of evidence
j Guidance available from the GRADE working group [ 225 , 226 ]; also recommend consultation with the Cochrane diagnostic methods group
k Guidance available from the GRADE working group [ 227 ]; also recommend consultation with Cochrane prognostic methods group
l Used for syntheses in reviews with a meta-aggregative approach [ 224 ]
m Chapter 5 in the JBI Manual offers guidance on how to adapt GRADE to prevalence and incidence reviews [ 69 ]
n Janiaud and colleagues suggest criteria for evaluating evidence certainty for meta-analyses of non-randomized studies evaluating risk factors [ 228 ]
o The COSMIN user manual provides details on how to apply GRADE in systematic reviews of measurement properties [ 229 ]
The expected culmination of a systematic review should be a rating of overall certainty of a body of evidence for each outcome reported. The GRADE approach is recommended for making these judgments for outcomes reported in systematic reviews of interventions and can be adapted for other types of reviews. This represents the initial step in the process of making recommendations based on evidence syntheses. Peer reviewers should ensure authors meet the minimal criteria for supporting the GRADE approach when reviewing any evidence synthesis that reports certainty ratings derived using GRADE. Authors and peer reviewers of evidence syntheses unfamiliar with GRADE are encouraged to seek formal training and take advantage of the resources available on the GRADE website [ 211 , 212 ].
Part 6. Concise Guide to best practices
Accumulating data in recent years suggest that many evidence syntheses (with or without meta-analysis) are not reliable. This relates in part to the fact that their authors, who are often clinicians, can be overwhelmed by the plethora of ways to evaluate evidence. They tend to resort to familiar but often inadequate, inappropriate, or obsolete methods and tools and, as a result, produce unreliable reviews. These manuscripts may not be recognized as such by peer reviewers and journal editors who may disregard current standards. When such a systematic review is published or included in a CPG, clinicians and stakeholders tend to believe that it is trustworthy. A vicious cycle in which inadequate methodology is rewarded and potentially misleading conclusions are accepted is thus supported. There is no quick or easy way to break this cycle; however, increasing awareness of best practices among all these stakeholder groups, who often have minimal (if any) training in methodology, may begin to mitigate it. This is the rationale for inclusion of Parts 2 through 5 in this guidance document. These sections present core concepts and important methodological developments that inform current standards and recommendations. We conclude by taking a direct and practical approach.
Inconsistent and imprecise terminology used in the context of development and evaluation of evidence syntheses is problematic for authors, peer reviewers and editors, and may lead to the application of inappropriate methods and tools. In response, we endorse use of the basic terms (Table 6.1 ) defined in the PRISMA 2020 statement [ 93 ]. In addition, we have identified several problematic expressions and nomenclature. In Table 6.2 , we compile suggestions for preferred terms less likely to be misinterpreted.
Terms relevant to the reporting of health care–related evidence syntheses a
a Reproduced from Page and colleagues [ 93 ]
Terminology suggestions for health care–related evidence syntheses
a For example, meta-aggregation, meta-ethnography, critical interpretative synthesis, realist synthesis
b This term may best apply to the synthesis in a mixed methods systematic review in which data from different types of evidence (eg, qualitative, quantitative, economic) are summarized [ 64 ]
We also propose a Concise Guide (Table 6.3 ) that summarizes the methods and tools recommended for the development and evaluation of nine types of evidence syntheses. Suggestions for specific tools are based on the rigor of their development as well as the availability of detailed guidance from their developers to ensure their proper application. The formatting of the Concise Guide addresses a well-known source of confusion by clearly distinguishing the underlying methodological constructs that these tools were designed to assess. Important clarifications and explanations follow in the guide’s footnotes; associated websites, if available, are listed in Additional File 6 .
To encourage uptake of best practices, journal editors may consider adopting or adapting the Concise Guide in their instructions to authors and peer reviewers of evidence syntheses. Given the evolving nature of evidence synthesis methodology, the suggested methods and tools are likely to require regular updates. Authors of evidence syntheses should monitor the literature to ensure they are employing current methods and tools. Some types of evidence syntheses (eg, rapid, economic, methodological) are not included in the Concise Guide; for these, authors are advised to obtain recommendations for acceptable methods by consulting with their target journal.
We encourage the appropriate and informed use of the methods and tools discussed throughout this commentary and summarized in the Concise Guide (Table 6.3 ). However, we caution against their application in a perfunctory or superficial fashion. This is a common pitfall among authors of evidence syntheses, especially as the standards of such tools become associated with acceptance of a manuscript by a journal. Consequently, published evidence syntheses may show improved adherence to the requirements of these tools without necessarily making genuine improvements in their performance.
In line with our main objective, the suggested tools in the Concise Guide address the reliability of evidence syntheses; however, we recognize that the utility of systematic reviews is an equally important concern. An unbiased and thoroughly reported evidence synthesis may still not be highly informative if the evidence itself that is summarized is sparse, weak and/or biased [ 24 ]. Many intervention systematic reviews, including those developed by Cochrane [ 203 ] and those applying GRADE [ 202 ], ultimately find no evidence, or find the evidence to be inconclusive (eg, “weak,” “mixed,” or of “low certainty”). This often reflects the primary research base; however, it is important to know what is known (or not known) about a topic when considering an intervention for patients and discussing treatment options with them.
Alternatively, the frequency of “empty” and inconclusive reviews published in the medical literature may relate to limitations of conventional methods that focus on hypothesis testing; these have emphasized the importance of statistical significance in primary research and effect sizes from aggregate meta-analyses [ 183 ]. It is becoming increasingly apparent that this approach may not be appropriate for all topics [ 130 ]. Development of the GRADE approach has facilitated a better understanding of significant factors (beyond effect size) that contribute to the overall certainty of evidence. Other notable responses include the development of integrative synthesis methods for the evaluation of complex interventions [ 230 , 231 ], the incorporation of crowdsourcing and machine learning into systematic review workflows (eg the Cochrane Evidence Pipeline) [ 2 ], the shift in paradigm to living systemic review and NMA platforms [ 232 , 233 ] and the proposal of a new evidence ecosystem that fosters bidirectional collaborations and interactions among a global network of evidence synthesis stakeholders [ 234 ]. These evolutions in data sources and methods may ultimately make evidence syntheses more streamlined, less duplicative, and more importantly, they may be more useful for timely policy and clinical decision-making; however, that will only be the case if they are rigorously reported and conducted.
We look forward to others’ ideas and proposals for the advancement of methods for evidence syntheses. For now, we encourage dissemination and uptake of the currently accepted best tools and practices for their development and evaluation; at the same time, we stress that uptake of appraisal tools, checklists, and software programs cannot substitute for proper education in the methodology of evidence syntheses and meta-analysis. Authors, peer reviewers, and editors must strive to make accurate and reliable contributions to the present evidence knowledge base; online alerts, upcoming technology, and accessible education may make this more feasible than ever before. Our intention is to improve the trustworthiness of evidence syntheses across disciplines, topics, and types of evidence syntheses. All of us must continue to study, teach, and act cooperatively for that to happen.
Acknowledgements
Michelle Oakman Hayes for her assistance with the graphics, Mike Clarke for his willingness to answer our seemingly arbitrary questions, and Bernard Dan for his encouragement of this project.
Authors’ contributions
All authors participated in the development of the ideas, writing, and review of this manuscript. The author(s) read and approved the final manuscript.
The work of John Ioannidis has been supported by an unrestricted gift from Sue and Bob O’Donnell to Stanford University.
Declarations
The authors declare no competing interests.
This article has been published simultaneously in BMC Systematic Reviews, Acta Anaesthesiologica Scandinavica, BMC Infectious Diseases, British Journal of Pharmacology, JBI Evidence Synthesis, the Journal of Bone and Joint Surgery Reviews , and the Journal of Pediatric Rehabilitation Medicine .
Publisher’ s Note
Springer Nature remains neutral with regard to jurisdictional claims in published maps and institutional affiliations.
- Open access
- Published: 23 August 2022
Prognostic risk factors for moderate-to-severe exacerbations in patients with chronic obstructive pulmonary disease: a systematic literature review
- John R. Hurst 1 ,
- MeiLan K. Han 2 ,
- Barinder Singh 3 ,
- Sakshi Sharma 4 ,
- Gagandeep Kaur 3 ,
- Enrico de Nigris 5 ,
- Ulf Holmgren 6 &
- Mohd Kashif Siddiqui 3
Respiratory Research volume 23 , Article number: 213 ( 2022 ) Cite this article
6365 Accesses
20 Citations
42 Altmetric
Metrics details
Chronic obstructive pulmonary disease (COPD) is a leading cause of morbidity and mortality worldwide. COPD exacerbations are associated with a worsening of lung function, increased disease burden, and mortality, and, therefore, preventing their occurrence is an important goal of COPD management. This review was conducted to identify the evidence base regarding risk factors and predictors of moderate-to-severe exacerbations in patients with COPD.
A literature review was performed in Embase, MEDLINE, MEDLINE In-Process, and the Cochrane Central Register of Controlled Trials (CENTRAL). Searches were conducted from January 2015 to July 2019. Eligible publications were peer-reviewed journal articles, published in English, that reported risk factors or predictors for the occurrence of moderate-to-severe exacerbations in adults age ≥ 40 years with a diagnosis of COPD.
The literature review identified 5112 references, of which 113 publications (reporting results for 76 studies) met the eligibility criteria and were included in the review. Among the 76 studies included, 61 were observational and 15 were randomized controlled clinical trials. Exacerbation history was the strongest predictor of future exacerbations, with 34 studies reporting a significant association between history of exacerbations and risk of future moderate or severe exacerbations. Other significant risk factors identified in multiple studies included disease severity or bronchodilator reversibility (39 studies), comorbidities (34 studies), higher symptom burden (17 studies), and higher blood eosinophil count (16 studies).
Conclusions
This systematic literature review identified several demographic and clinical characteristics that predict the future risk of COPD exacerbations. Prior exacerbation history was confirmed as the most important predictor of future exacerbations. These prognostic factors may help clinicians identify patients at high risk of exacerbations, which are a major driver of the global burden of COPD, including morbidity and mortality.
Chronic obstructive pulmonary disease (COPD) is the third leading cause of death worldwide [ 1 ]. Based upon disability-adjusted life-years, COPD ranked sixth out of 369 causes of global disease burden in 2019 [ 2 ]. COPD exacerbations are associated with a worsening of lung function, and increased disease burden and mortality (of those patients hospitalized for the first time with an exacerbation, > 20% die within 1 year of being discharged) [ 3 ]. Furthermore, patients with COPD consider exacerbations or hospitalization due to exacerbations to be the most important disease outcome, having a large impact on their lives [ 4 ]. Therefore, reducing the future risk of COPD exacerbations is a key goal of COPD management [ 5 ].
Being able to predict the level of risk for each patient allows clinicians to adapt treatment and patients to adjust their lifestyle (e.g., through a smoking cessation program) to prevent exacerbations [ 3 ]. As such, identifying high-risk patients using measurable risk factors and predictors that correlate with exacerbations is critical to reduce the burden of disease and prevent a cycle of decline encompassing irreversible lung damage, worsening quality of life (QoL), increasing disease burden, high healthcare costs, and early death.
Prior history of exacerbations is generally thought to be the best predictor of future exacerbations; however, there is a growing body of evidence suggesting other demographic and clinical characteristics, including symptom burden, airflow obstruction, comorbidities, and inflammatory biomarkers, also influence risk [ 6 , 7 , 8 , 9 ]. For example, in the prospective ECLIPSE observational study, the likelihood of patients experiencing an exacerbation within 1 year of follow-up increased significantly depending upon several factors, including prior exacerbation history, forced expiratory volume in 1 s (FEV 1 ), St. George’s Respiratory Questionnaire (SGRQ) score, gastroesophageal reflux, and white blood cell count [ 9 ].
Many studies have assessed predictors of COPD exacerbations across a variety of countries and patient populations. This systematic literature review (SLR) was conducted to identify and compile the evidence base regarding risk factors and predictors of moderate-to-severe exacerbations in patients with COPD.
- Systematic literature review
A comprehensive search strategy was designed to identify English-language studies published in peer-reviewed journals providing data on risk factors or predictors of moderate or severe exacerbations in adults aged ≥ 40 years with a diagnosis of COPD (sample size ≥ 100). The protocol is summarized in Table 1 and the search strategy is listed in Additional file 1 : Table S1. Key biomedical electronic literature databases were searched from January 2015 until July 2019. Other sources were identified via bibliographic searching of relevant systematic reviews.
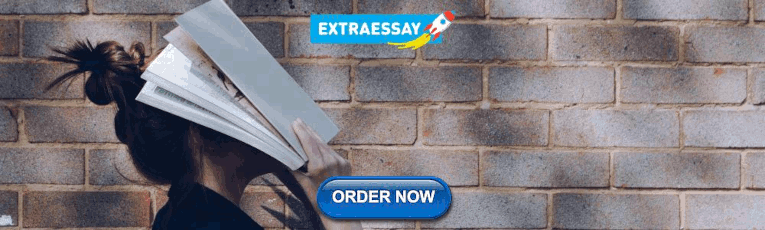
Study selection process
Implementation and reporting followed the recommendations and standards of the Preferred Reporting Items for Systematic reviews and Meta-analyses (PRISMA) statement [ 10 ]. An independent reviewer conducted the first screening based on titles and abstracts, and a second reviewer performed a quality check of the excluded evidence. A single independent reviewer also conducted the second screening based on full-text articles, with a quality check of excluded evidence performed by a second reviewer. Likewise, data tables of the included studies were generated by one reviewer, and another reviewer performed a quality check of extracted data. Where more than one publication was identified describing a single study or trial, data were compiled into a single entry in the data-extraction table to avoid double counting of patients and studies. One publication was designated as the ‘primary publication’ for the purposes of the SLR, based on the following criteria: most recently published evidence and/or the article that presented the majority of data (e.g., journal articles were preferred over conference abstracts; articles that reported results for the full population were preferred over later articles providing results of subpopulations). Other publications reporting results from the same study were designated as ‘linked publications’; any additional data in the linked publications that were not included in the primary publication were captured in the SLR. Conference abstracts were excluded from the SLR unless they were a ‘linked publication.’
Included studies
A total of 5112 references (Fig. 1 ) were identified from the database searches. In total, 76 studies from 113 publications were included in the review. Primary publications and ‘linked publications’ for each study are detailed in Additional file 1 : Table S2, and study characteristics are shown in Additional file 1 : Table S3. The studies included clinical trials, registry studies, cross-sectional studies, cohort studies, database studies, and case–control studies. All 76 included studies were published in peer-reviewed journals. Regarding study design, 61 of the studies were observational (34 retrospective observational studies, 19 prospective observational studies, four cross-sectional studies, two studies with both retrospective and prospective cohort data, one case–control study, and one with cross-sectional and longitudinal data) and 15 were randomized controlled clinical trials.
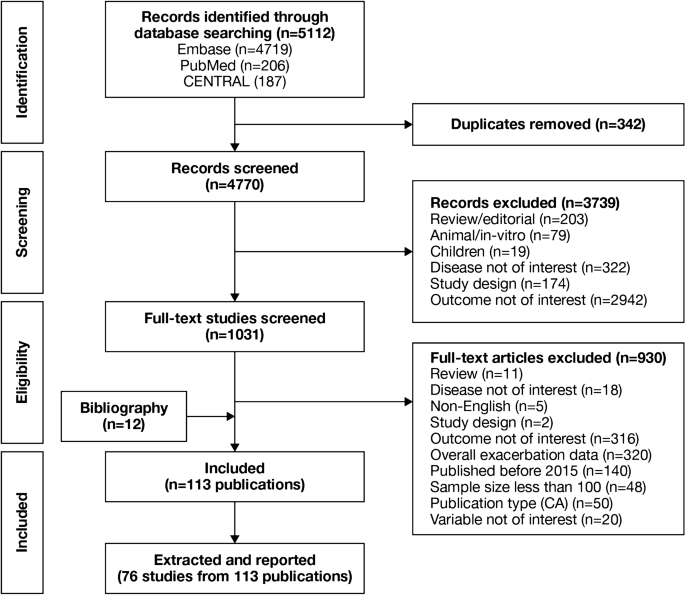
PRISMA flow diagram of studies through the systematic review process. CA conference abstract, CENTRAL Cochrane Central Register of Controlled Trials, PRISMA Preferred Reporting Items for Systematic Reviews and Meta-Analyses
Of the 76 studies, 16 were conducted in North America (13 studies in the USA, two in Canada, and one in Mexico); 26 were conducted in Europe (seven studies in Spain, four in the UK, three in Denmark, two studies each in Bulgaria, the Netherlands, and Switzerland, and one study each in Sweden, Serbia, Portugal, Greece, Germany, and France) and 17 were conducted in Asia (six studies in South Korea, four in China, three in Taiwan, two in Japan, and one study each in Singapore and Israel). One study each was conducted in Turkey and Australia. Fifteen studies were conducted across multiple countries.
The majority of the studies (n = 54) were conducted in a multicenter setting, while 22 studies were conducted in a single-center setting. The sample size among the included studies varied from 118 to 339,389 patients.
Patient characteristics
A total of 75 studies reported patient characteristics (Additional file 1 : Table S4). The mean age was reported in 65 studies and ranged from 58.0 to 75.2 years. The proportion of male patients ranged from 39.7 to 97.6%. The majority of included studies (85.3%) had a higher proportion of males than females.
Exacerbation history (as defined per each study) was reported in 18 of 76 included studies. The proportion of patients with no prior exacerbation was reported in ten studies (range, 0.1–79.5% of patients), one or fewer prior exacerbation in ten studies (range, 46–100%), one or more prior exacerbation in eight studies (range, 18.4–100%), and two or more prior exacerbations in 12 studies (range, 6.1–55.0%).
Prognostic factors of exacerbations
A summary of the risk factors and predictors reported across the included studies is provided in Tables 2 and 3 . The overall findings of the SLR are summarized in Figs. 2 and 3 .
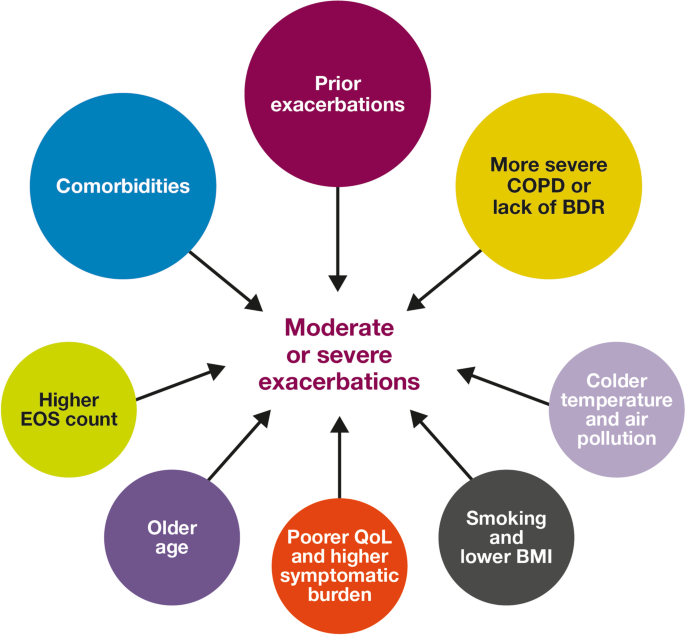
Risk factors for moderate-to-severe exacerbations in patients with COPD. Factors with > 30 supporting studies shown as large circles; factors with ≤ 30 supporting studies shown as small circles and should be interpreted cautiously. BDR bronchodilator reversibility, BMI body mass index, COPD chronic obstructive pulmonary disease, EOS eosinophil, QoL quality of life
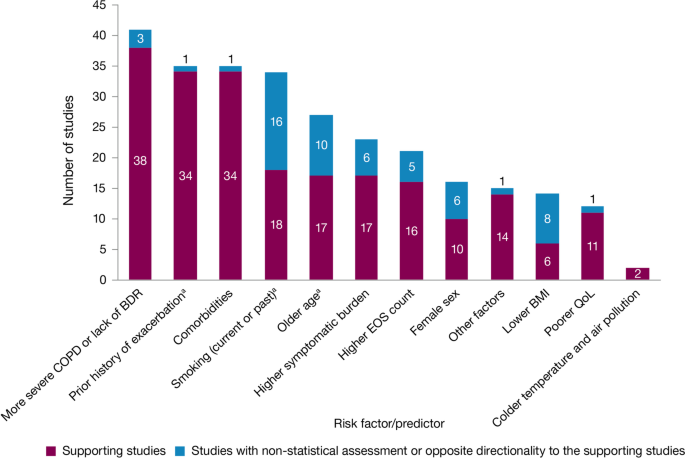
Summary of risk factors for exacerbation events. a Treatment impact studies removed. BDR bronchodilator reversibility, BMI body mass index, COPD chronic obstructive pulmonary disease, EOS eosinophil, QoL quality of life
Exacerbation history within the past 12 months was the strongest predictor of future exacerbations. Across the studies assessing this predictor, 34 out of 35 studies (97.1%) reported a significant association between history of exacerbations and risk of future moderate-to-severe exacerbations (Table 3 ). Specifically, two or more exacerbations in the previous year or at least one hospitalization for COPD in the previous year were identified as reliable predictors of future moderate or severe exacerbations. Even one moderate exacerbation increased the risk of a future exacerbation, with the risk increasing further with each subsequent exacerbation (Fig. 4 ). A severe exacerbation was also found to increase the risk of subsequent exacerbation and hospitalization (Fig. 5 ). Patients experiencing one or more severe exacerbations were more likely to experience further severe exacerbations than moderate exacerbations [ 11 , 12 ]. In contrast, patients with a history of one or more moderate exacerbations were more likely to experience further moderate exacerbations than severe exacerbations [ 11 , 12 ].
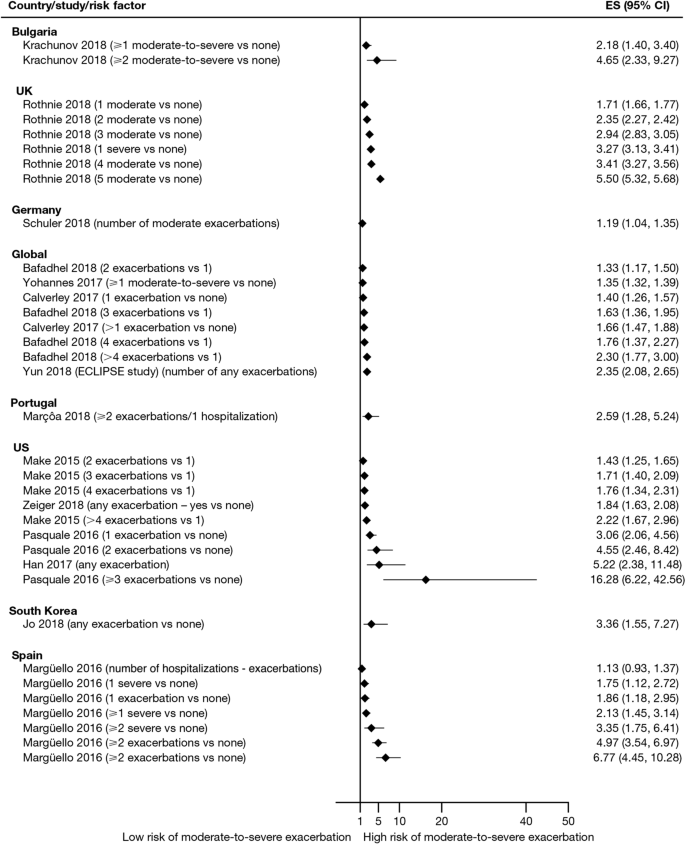
Exacerbation history as a risk factor for moderate-to-severe exacerbations. Yun 2018 included two studies; the study from which data were extracted (COPDGene or ECLIPSE) is listed in parentheses. CI confidence interval, ES effect size
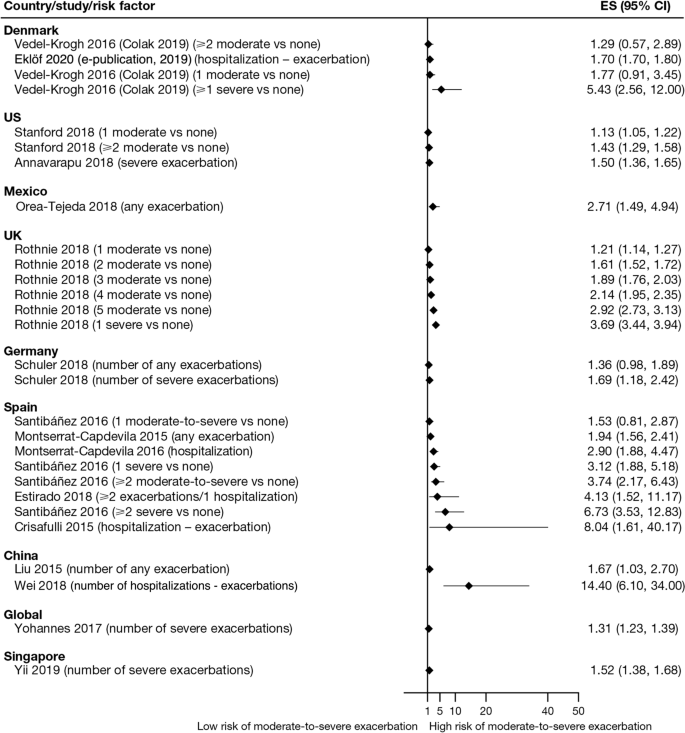
Exacerbation history as a risk factor for severe exacerbations. Where data have been extracted from a linked publication rather than the primary publication, the linked publication is listed in parentheses. CI confidence interval, ES , effect size
Overall, 35 studies assessed the association of comorbidities with the risk of exacerbation. All studies except one (97.1%) reported a positive association between comorbidities and the occurrence of moderate-to-severe exacerbations (Table 3 ). In addition to the presence of any comorbidity, specific comorbidities that were found to significantly increase the risk of moderate-to-severe exacerbations included anxiety and depression, cardiovascular comorbidities, gastroesophageal reflux disease/dyspepsia, and respiratory comorbidities (Fig. 6 ). Comorbidities that were significant risk factors for severe exacerbations included cardiovascular, musculoskeletal, and respiratory comorbidities, diabetes, and malignancy (Fig. 7 ). Overall, the strongest association between comorbidities and COPD readmissions in the emergency department was with cardiovascular disease. The degree of risk for both moderate-to-severe and severe exacerbations also increased with the number of comorbidities. A Dutch cohort study found that 88% of patients with COPD had at least one comorbidity, with hypertension (35%) and coronary heart disease (19%) being the most prevalent. In this cohort, the comorbidities with the greatest risk of frequent exacerbations were pulmonary cancer (odds ratio [OR] 1.85) and heart failure (OR 1.72) [ 7 ].
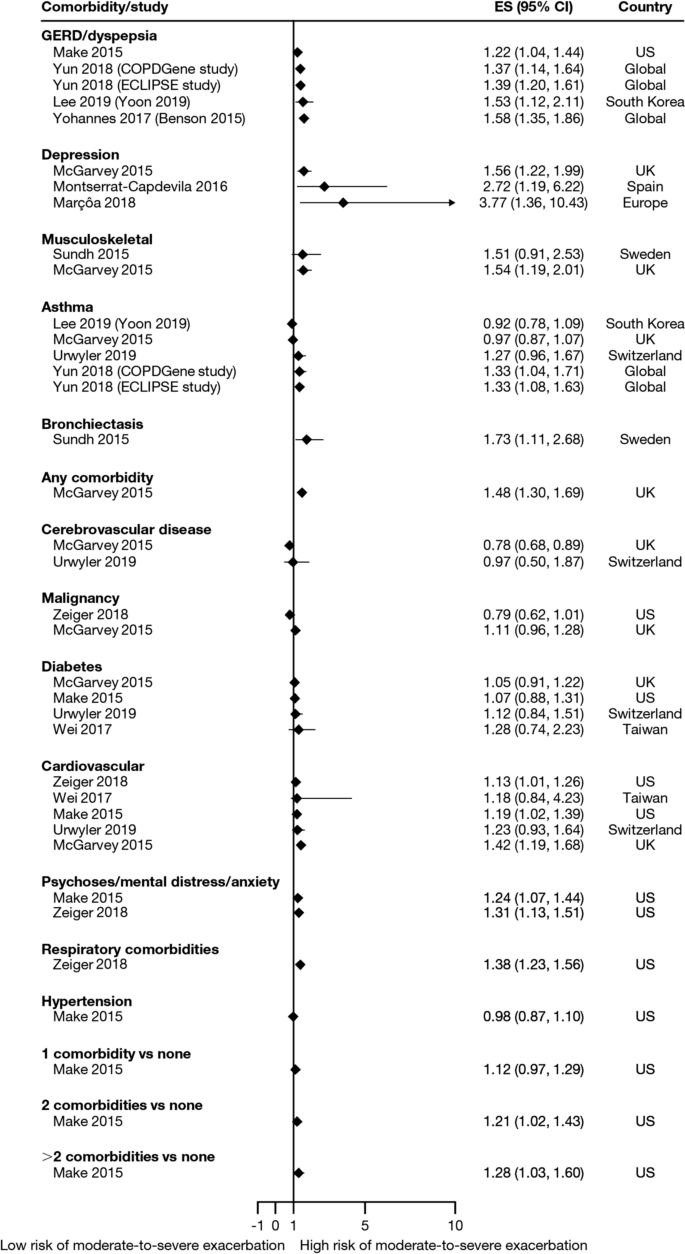
Comorbidities as risk factors for moderate-to-severe exacerbations. Yun 2018 included two studies; the study from which data were extracted (COPDGene or ECLIPSE) is listed in parentheses. Where data have been extracted from a linked publication rather than the primary publication, the linked publication is listed in parentheses. CI confidence interval, ES effect size, GERD gastroesophageal disease
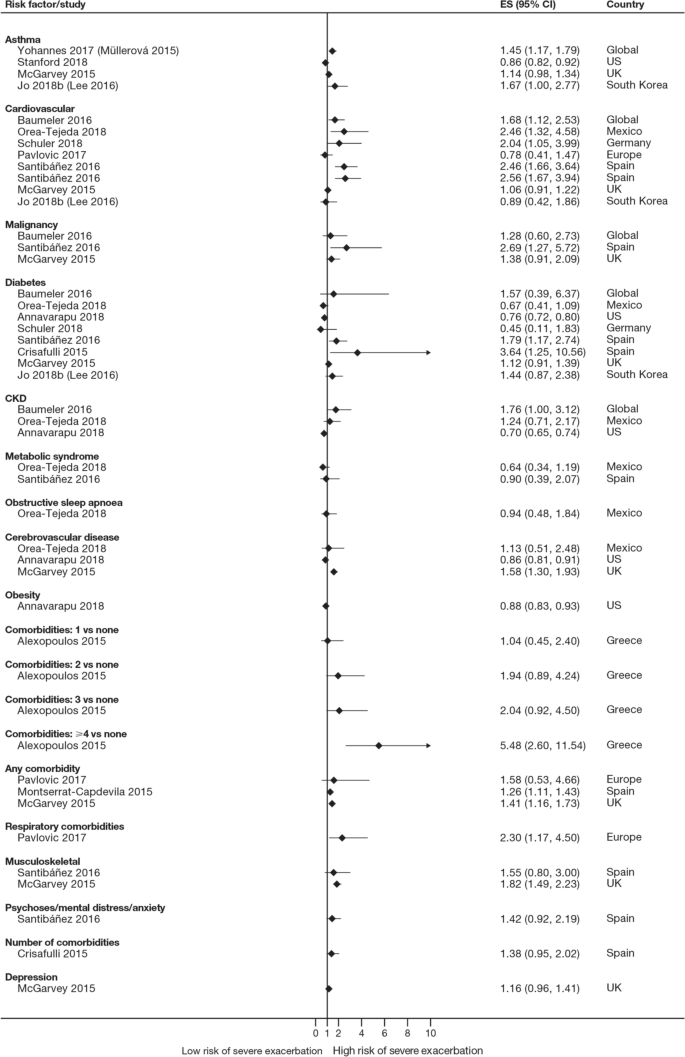
Comorbidities as risk factors for severe exacerbations. Where data have been extracted from a linked publication rather than the primary publication, the linked publication is listed in parentheses. CI confidence interval, CKD , chronic kidney disease, ES effect size
The majority of studies assessing disease severity or bronchodilator reversibility (39/41; 95.1%) indicated a significant positive relation between risk of future exacerbations and greater disease severity, as assessed by greater lung function impairment (in terms of lower FEV 1 , FEV 1 /forced vital capacity ratio, or forced expiratory flow [25–75]/forced vital capacity ratio) or more severe Global Initiative for Chronic Obstructive Lung Disease (GOLD) class A − D, and a positive relationship between risk of future exacerbations and lack of bronchodilator reversibility (Table 3 , Figs. 8 and 9 ).
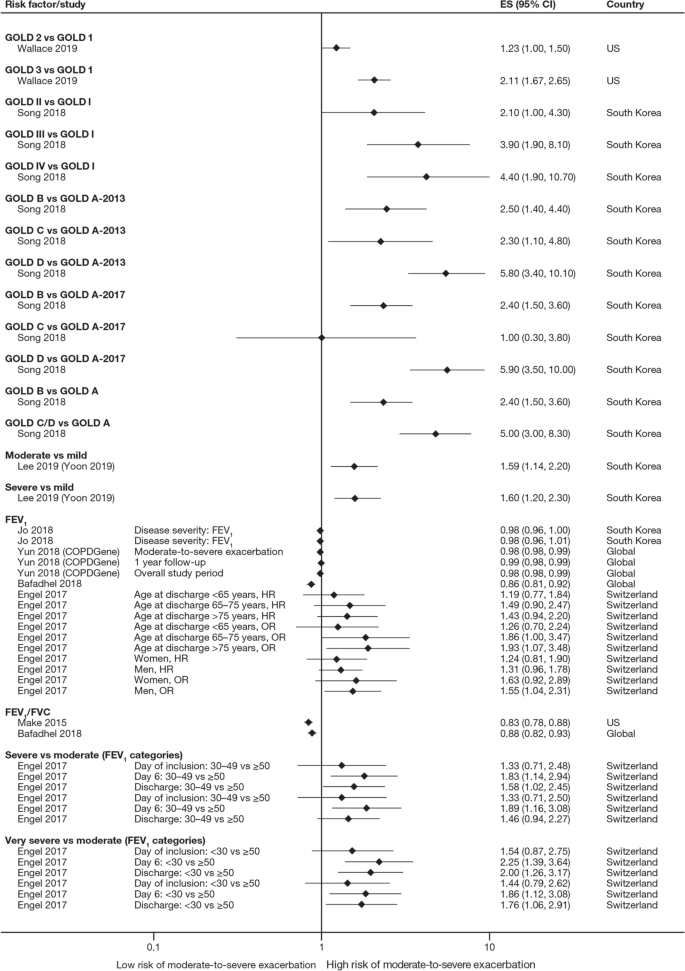
Disease severity as a risk factor for moderate-to-severe exacerbations. Yun 2018 included two studies; the study from which data were extracted (COPDGene or ECLIPSE) is listed in parentheses. Where data have been extracted from a linked publication rather than the primary publication, the linked publication is listed in parentheses. CI confidence interval, ES effect size, FEV 1 f orced expiratory volume in 1 s, FVC , forced vital capacity, GOLD Global Initiative for Obstructive Lung Disease, HR hazard ratio, OR odds ratio
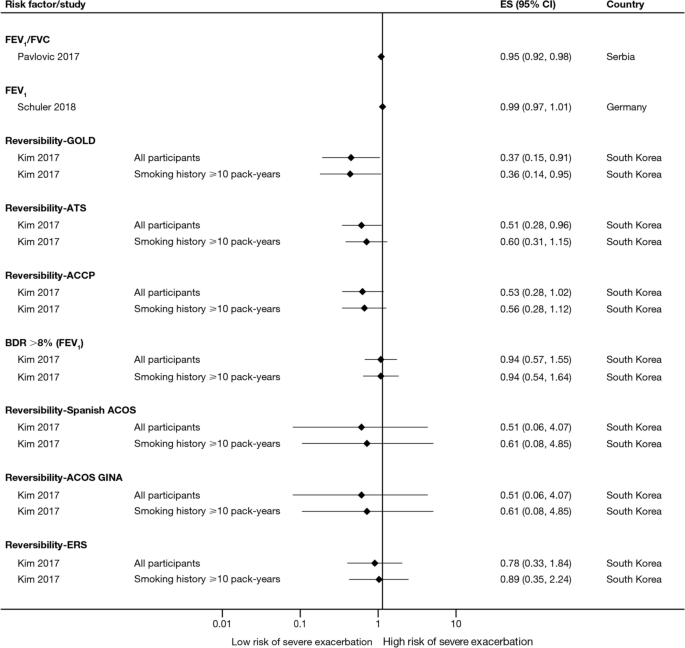
Disease severity and BDR as risk factors for severe exacerbations. ACCP American College of Chest Physicians, ACOS Asthma-COPD overlap syndrome, ATS American Thoracic Society, BDR bronchodilator reversibility, CI confidence interval, ERS European Respiratory Society, ES effect size, FEV 1 forced expiratory volume in 1 s, FVC forced vital capacity, GINA Global Initiative for Asthma, GOLD Global Initiative for Obstructive Lung Disease
Of 21 studies assessing the relationship between blood eosinophil count and exacerbations (Table 3 ), 16 reported estimates for the risk of moderate or severe exacerbations by eosinophil count. A positive association was observed between higher eosinophil count and a higher risk of moderate or severe exacerbations, particularly in patients not treated with an inhaled corticosteroid (ICS); however, five studies reported a significant positive association irrespective of intervention effects. The risk of moderate-to-severe exacerbations was observed to be positively associated with various definitions of higher eosinophil levels (absolute counts: ≥ 200, ≥ 300, ≥ 340, ≥ 400, and ≥ 500 cells/mm 3 ; % of blood eosinophil count: ≥ 2%, ≥ 3%, ≥ 4%, and ≥ 5%). Of note, one study found reduced efficacy of ICS in lowering moderate-to-severe exacerbation rates for current smokers versus former smokers at all eosinophil levels [ 13 ].
Of 12 studies assessing QoL scales, 11 (91.7%) studies reported a significant association between the worsening of QoL scores and the risk of future exacerbations (Table 3 ). Baseline SGRQ [ 14 , 15 ], Center for Epidemiologic Studies Depression Scale (for which increased scores may indicate impaired QoL) [ 16 ], and Clinical COPD Questionnaire [ 17 , 18 ] scores were found to be associated with future risk of moderate and/or severe COPD exacerbations. For symptom scores, six out of eight studies assessing the association between moderate-to-severe or severe exacerbations with COPD Assessment Test (CAT) scores reported a significant and positive relationship. Furthermore, the risk of moderate-to-severe exacerbations was found to be significantly higher in patients with higher CAT scores (≥ 10) [ 15 , 19 , 20 , 21 ], with one study demonstrating that a CAT score of 15 increased predictive ability for exacerbations compared with a score of 10 or more [ 18 ]. Among 15 studies that assessed the association of modified Medical Research Council (mMRC) scores with the risk of moderate-to-severe or severe exacerbation, 11 found that the risk of moderate-to-severe or severe exacerbations was significantly associated with higher mMRC scores (≥ 2) versus lower scores. Furthermore, morning and night symptoms (measured by Clinical COPD Questionnaire) were associated with poor health status and predicted future exacerbations [ 17 ].
Of 36 studies reporting the relationship between smoking status and moderate-to-severe or severe exacerbations, 22 studies (61.1%) reported a significant positive association (Table 3 ). Passive smoking was also significantly associated with an increased risk of severe exacerbations (OR 1.49) [ 20 ]. Of note, three studies reported a significantly lower rate of moderate-to-severe exacerbations in current smokers compared with former smokers [ 22 , 23 , 24 ].
A total of 14 studies assessed the association of body mass index (BMI) with the occurrence of frequent moderate-to-severe exacerbations in patients with COPD. Six out of 14 studies (42.9%) reported a significant negative association between exacerbations and BMI (Table 3 ). The risk of moderate and/or severe COPD exacerbations was highest among underweight patients compared with normal and overweight patients [ 23 , 25 , 26 , 27 , 28 ].
In the 29 studies reporting an association between age and moderate or severe exacerbations, more than half found an association of older age with an increased risk of moderate-to-severe exacerbations (58.6%; Table 3 ). Four of these studies noted a significant increase in the risk of moderate-to-severe or severe exacerbations for every 10-year increase in age [ 25 , 26 , 29 , 30 ]. However, 12 studies reported no significant association between age and moderate-to-severe or severe exacerbation risk.
Sixteen out of 33 studies investigating the impact of sex on exacerbation risk found a significant association (48.5%; Table 3 ). Among these, ten studies reported that female sex was associated with an increased risk of moderate-to-severe exacerbations, while six studies showed a higher exacerbation risk in males compared with females. There was some variation in findings by geographic location and exacerbation severity (Additional file 2 : Figs. S1 and S2). Notably, when assessing the risk of severe exacerbations, more studies found an association with male sex compared with female sex (6/13 studies vs 1/13 studies, respectively).
Both studies evaluating associations between exacerbations and environmental factors reported that colder temperature and exposure to major air pollution (NO 2 , O 3 , CO, and/or particulate matter ≤ 10 μm in diameter) increased hospital admissions due to severe exacerbations and moderate-to-severe exacerbation rates [ 31 , 32 ].
Four studies assessed the association of 6-min walk distance with the occurrence of frequent moderate-to-severe exacerbations (Table 3 ). One study (25.0%) found that shorter 6-min walk distance (representing low physical activity) was significantly associated with a shortened time to severe exacerbation, but the effect size was small (hazard ratio 0.99) [ 33 ].
Five out of six studies assessing the relationship between race or ethnicity and exacerbation risk reported significant associations (Table 3 ). Additionally, one study reported an association between geographic location in the US and exacerbations, with living in the Northeast region being the strongest predictor of severe COPD exacerbations versus living in the Midwest and South regions [ 34 ].
Overall, seven studies assessed the association of biomarkers with risk of future exacerbations (Table 3 ), with the majority identifying significant associations between inflammatory biomarkers and increased exacerbation risk, including higher C-reactive protein levels [ 8 , 35 ], fibrinogen levels [ 8 , 30 ], and white blood cell count [ 8 , 15 , 16 ].
This SLR has identified several demographic and clinical characteristics that predict the future risk of COPD exacerbations. Key factors associated with an increased risk of future moderate-to-severe exacerbations included a history of prior exacerbations, worse disease severity and bronchodilator reversibility, the presence of comorbidities, a higher eosinophil count, and older age (Fig. 2 ). These prognostic factors may help clinicians identify patients at high risk of exacerbations, which are a major driver of the burden of COPD, including morbidity and mortality [ 36 ].
Findings from this review summarize the existing evidence, validating the previously published literature [ 6 , 9 , 23 ] and suggesting that the best predictor of future exacerbations is a history of exacerbations in the prior year [ 8 , 11 , 12 , 13 , 14 , 16 , 17 , 18 , 19 , 20 , 21 , 22 , 23 , 26 , 29 , 34 , 35 , 37 , 38 , 39 , 40 , 41 , 42 , 43 , 44 , 45 , 46 , 47 , 48 , 49 , 50 , 51 , 52 , 53 , 54 , 55 , 56 , 57 , 58 , 59 , 60 ]. In addition, the effect size generally increased with the number of prior exacerbations, with a stronger effect observed with prior severe versus moderate exacerbations. This effect was observed across regions, including in Europe and North America, and in several global studies. This relationship represents a vicious circle, whereby one exacerbation predisposes a patient to experience future exacerbations and leading to an ever-increasing disease burden, and emphasizes the importance of preventing the first exacerbation event through early, proactive exacerbation prevention. The finding that prior exacerbations tended to be associated with future exacerbations of the same severity suggests that the severity of the underlying disease may influence exacerbation severity. However, the validity of the traditional classification of exacerbation severity has recently been challenged [ 61 ], and further work is required to understand relationships with objective assessments of exacerbation severity.
In addition to exacerbation history, disease severity and bronchodilator reversibility were also strong predictors for future exacerbations [ 8 , 14 , 16 , 18 , 19 , 20 , 22 , 23 , 24 , 26 , 28 , 29 , 33 , 37 , 40 , 43 , 44 , 45 , 46 , 48 , 50 , 51 , 52 , 56 , 59 , 62 , 63 , 64 , 65 , 66 , 67 , 68 , 69 , 70 , 71 , 72 , 73 , 74 , 75 , 76 , 77 , 78 ]. The association with disease severity was noted in studies that used GOLD disease stages 1–4 and those that used FEV 1 percent predicted and other lung function assessments as continuous variables. Again, this risk factor is self-perpetuating, as evidence shows that even a single moderate or severe exacerbation may almost double the rate of lung function decline [ 79 ]. Accordingly, disease severity and exacerbation history may be correlated. Margüello et al. concluded that the severity of COPD could be associated with a higher risk of exacerbations, but this effect was partly determined by the exacerbations suffered in the previous year [ 23 ]. It should be noted that FEV 1 is not recommended by GOLD for use as a predictor of exacerbation risk or mortality alone due to insufficient precision when used at the individual patient level [ 5 ].
Another factor that should be considered when assessing individual exacerbation risk is the presence of comorbidities [ 7 , 14 , 16 , 18 , 19 , 20 , 21 , 22 , 24 , 25 , 26 , 27 , 28 , 30 , 33 , 34 , 35 , 40 , 41 , 44 , 45 , 46 , 47 , 48 , 51 , 52 , 53 , 54 , 56 , 58 , 59 , 63 , 64 , 73 , 74 , 76 , 77 , 80 , 81 , 82 , 83 , 84 , 85 ]. Comorbidities are common in COPD, in part due to common risk factors (e.g., age, smoking, lifestyle factors) that also increase the risk of other chronic diseases [ 7 ]. Significant associations were observed between exacerbation risk and comorbidities, such as anxiety and depression, cardiovascular disease, diabetes, and respiratory comorbidities. As with prior exacerbations, the strength of the association increased with the number of comorbidities. Some comorbidities that were found to be associated with COPD exacerbations share a common biological mechanism of systemic inflammation, such as cardiovascular disease, diabetes, and depression [ 86 ]. Furthermore, other respiratory comorbidities, including asthma and bronchiectasis, involve inflammation of the airways [ 87 ]. In these patients, optimal management of comorbidities may reduce the risk of future COPD exacerbations (and improve QoL), although further research is needed to confirm the efficacy of this approach to exacerbation prevention. As cardiovascular conditions, including hypertension and coronary heart disease, are the most common comorbidities in people with COPD [ 7 ], reducing cardiovascular risk may be a key goal in reducing the occurrence of exacerbations. For other comorbidities, the mechanism for the association with exacerbation risk may be related to non-biological factors. For example, in depression, it has been suggested that the mechanism may relate to greater sensitivity to symptom changes or more frequent physician visits [ 88 ].
There is now a growing body of evidence reporting the relationship between blood eosinophil count and exacerbation risk [ 8 , 13 , 14 , 20 , 37 , 48 , 52 , 56 , 59 , 60 , 62 , 89 , 90 , 91 , 92 , 93 , 94 , 95 , 96 , 97 , 98 , 99 ]. Data from many large clinical trials (SUNSET [ 89 ], FLAME [ 96 ], WISDOM [ 98 ], IMPACT [ 13 ], TRISTAN [ 99 ], INSPIRE [ 99 ], KRONOS [ 91 ], TRIBUTE [ 48 ], TRILOGY [ 52 ], TRINITY [ 56 ]) have also shown relationships between treatment, eosinophil count, and exacerbation rates. Evidence shows that eosinophil count, along with other effect modifiers (e.g., exacerbation history), can be used to predict reductions in exacerbations with ICS treatment. Identifying patients most likely to respond to ICS should contribute to personalized medicine approaches to treat COPD. One challenge in drawing a strong conclusion from eosinophil counts is the choice of a cut-off value, with a variety of absolute and percentage values observed to be positively associated with the risk of moderate-to-severe exacerbations. The use of absolute counts may be more practical, as these are not affected by variations in other immune cell numbers; however, there is a lack of consensus on this point [ 100 ].
Across the studies examined, associations between sex and the risk of moderate and/or severe exacerbations were variable [ 14 , 16 , 18 , 20 , 21 , 22 , 23 , 24 , 26 , 27 , 28 , 29 , 37 , 40 , 42 , 44 , 45 , 46 , 47 , 48 , 51 , 52 , 56 , 58 , 59 , 63 , 73 , 74 , 77 , 80 , 83 , 84 , 85 ]. A greater number of studies showed an increased risk of exacerbations in females compared with males. In contrast, some studies failed to detect a relationship, suggesting that country-specific or cultural factors may play a role. A majority of the included studies evaluated more male patients than female patients; to further elucidate the relationship between sex and exacerbations, more studies in female patients are warranted. Over half of the studies that assessed the relationship between age and exacerbation risk found an association between increasing age and increasing risk of moderate-to-severe COPD exacerbations [ 14 , 16 , 18 , 20 , 21 , 22 , 23 , 24 , 26 , 27 , 28 , 29 , 33 , 40 , 42 , 44 , 45 , 47 , 51 , 52 , 54 , 56 , 63 , 73 , 74 , 77 , 80 , 83 , 85 ].
Our findings also suggested that patients with low BMI have greater risk of moderate and/or severe exacerbations. The mechanism underlying this increased risk in underweight patients is poorly understood; however, loss of lean body mass in patients with COPD may be related to ongoing systemic inflammation that impacts skeletal muscle mass [ 101 , 102 , 103 ].
A limitation of this SLR, that may have resulted in some studies with valid results being missed, was the exclusion of non-English-language studies and the limitation by date; however, the search strategy was otherwise broad, resulting in the review of a large number of studies. The majority of studies captured in this SLR were from Europe, North America, and Asia. The findings may therefore be less generalizable to patients in other regions, such as Africa or South America. Given that one study reported an association between geographic location within different regions of the US and exacerbations [ 34 ], it is plausible that risk of exacerbations may be impacted by global location. As no formal meta-analysis was planned, the assessments are based on a qualitative synthesis of studies. A majority of the included studies looked at exposures of certain factors (e.g., history of exacerbations) at baseline; however, some of these factors change over time, calling into question whether a more sophisticated statistical analysis should have been conducted in some cases to consider time-varying covariates. Our results can only inform on associations, not causation, and there are likely bidirectional relationships between many factors and exacerbation risk (e.g., health status). Finally, while our review of the literature captured a large number of prognostic factors, other variables such as genetic factors, lung microbiome composition, and changes in therapy over time have not been widely studied to date, but might also influence exacerbation frequency [ 104 ]. Further research is needed to assess the contribution of these factors to exacerbation risk.
This SLR captured publications up to July 2019. However, further studies have since been published that further support the prognostic factors identified here. For example, recent studies have reported an increased risk of exacerbations in patients with a history of exacerbations [ 105 ], comorbidities [ 106 ], poorer lung function (GOLD stage) [ 105 ], higher symptomatic burden [ 107 ], female sex [ 105 ], and lower BMI [ 106 , 108 ].
In summary, the literature assessing risk factors for moderate-to-severe COPD exacerbations shows that there are associations between several demographic and disease characteristics with COPD exacerbations, potentially allowing clinicians to identify patients most at risk of future exacerbations. Exacerbation history, comorbidities, and disease severity or bronchodilator reversibility were the factors most strongly associated with exacerbation risk, and should be considered in future research efforts to develop prognostic tools to estimate the likelihood of exacerbation occurrence. Importantly, many prognostic factors for exacerbations, such as symptom burden, QoL, and comorbidities, are modifiable with optimal pharmacologic and non-pharmacologic treatments or lifestyle modifications. Overall, the evidence suggests that, taken together, predicting and reducing exacerbation risk is an achievable goal in COPD.
Availability of data and materials
The datasets used and/or analyzed during the current study are available from the corresponding author on reasonable request.
Abbreviations
Body mass index
COPD Assessment Test
Chronic obstructive pulmonary disease
Forced expiratory volume in 1 s
Global Initiative for Chronic Obstructive Lung Disease
Inhaled corticosteroid
Modified Medical Research Council
Quality of life
St. George’s Respiratory Questionnaire
World Health Organization. The top 10 causes of death. 2018. https://www.who.int/news-room/fact-sheets/detail/the-top-10-causes-of-death . Accessed 22 Jul 2020.
GBD 2019 Diseases and Injuries Collaborators. Global burden of 369 diseases and injuries in 204 countries and territories, 1990–2019: a systematic analysis for the Global Burden of Disease Study 2019. The Lancet. 2020;396:1204–22.
Article Google Scholar
Hurst JR, Skolnik N, Hansen GJ, Anzueto A, Donaldson GC, Dransfield MT, Varghese P. Understanding the impact of chronic obstructive pulmonary disease exacerbations on patient health and quality of life. Eur J Intern Med. 2020;73:1–6.
Article PubMed Google Scholar
Zhang Y, Morgan RL, Alonso-Coello P, Wiercioch W, Bała MM, Jaeschke RR, Styczeń K, Pardo-Hernandez H, Selva A, Ara Begum H, et al. A systematic review of how patients value COPD outcomes. Eur Respir J. 2018;52:1800222.
Global Initiative for Chronic Obstructive Lung Disease. 2022 GOLD Report. Global strategy for the diagnosis, management and prevention of COPD. 2022. https://goldcopd.org/2022-gold-reports-2/ . Accessed 02 Feb 2022.
Müllerová H, Shukla A, Hawkins A, Quint J. Risk factors for acute exacerbations of COPD in a primary care population: a retrospective observational cohort study. BMJ Open. 2014;4: e006171.
Article PubMed PubMed Central Google Scholar
Westerik JAM, Metting EI, van Boven JFM, Tiersma W, Kocks JWH, Schermer TR. Associations between chronic comorbidity and exacerbation risk in primary care patients with COPD. Respir Res. 2017;18:31.
Vedel-Krogh S, Nielsen SF, Lange P, Vestbo J, Nordestgaard BG. Blood eosinophils and exacerbations in chronic obstructive pulmonary disease. The Copenhagen General Population Study. Am J Respir Crit Care Med. 2016;193:965–74.
Hurst JR, Vestbo J, Anzueto A, Locantore N, Müllerová H, Tal-Singer R, Miller B, Lomas DA, Agusti A, Macnee W, et al. Susceptibility to exacerbation in chronic obstructive pulmonary disease. N Engl J Med. 2010;363:1128–38.
Article CAS PubMed Google Scholar
Moher D, Liberati A, Tetzlaff J, Altman DG, The PRISMA Group. Preferred reporting items for systematic reviews and meta-analyses: the PRISMA statement. PLoS Med. 2009;6:e1000097.
Çolak Y, Afzal S, Marott JL, Nordestgaard BG, Vestbo J, Ingebrigtsen TS, Lange P. Prognosis of COPD depends on severity of exacerbation history: a population-based analysis. Respir Med. 2019;155:141–7.
Rothnie KJ, Müllerová H, Smeeth L, Quint JK. Natural history of chronic obstructive pulmonary disease exacerbations in a general practice-based population with chronic obstructive pulmonary disease. Am J Respir Crit Care Med. 2018;198:464–71.
Pascoe S, Barnes N, Brusselle G, Compton C, Criner GJ, Dransfield MT, Halpin DMG, Han MK, Hartley B, Lange P, et al. Blood eosinophils and treatment response with triple and dual combination therapy in chronic obstructive pulmonary disease: analysis of the IMPACT trial. Lancet Respir Med. 2019;7:745–56.
Yun JH, Lamb A, Chase R, Singh D, Parker MM, Saferali A, Vestbo J, Tal-Singer R, Castaldi PJ, Silverman EK, et al. Blood eosinophil count thresholds and exacerbations in patients with chronic obstructive pulmonary disease. J Allergy Clin Immunol. 2018;141:2037-2047.e10.
Yoon HY, Park SY, Lee CH, Byun MK, Na JO, Lee JS, Lee WY, Yoo KH, Jung KS, Lee JH. Prediction of first acute exacerbation using COPD subtypes identified by cluster analysis. Int J Chron Obstruct Pulmon Dis. 2019;14:1389–97.
Article CAS PubMed PubMed Central Google Scholar
Yohannes AM, Mulerova H, Lavoie K, Vestbo J, Rennard SI, Wouters E, Hanania NA. The association of depressive symptoms with rates of acute exacerbations in patients with COPD: results from a 3-year longitudinal follow-up of the ECLIPSE cohort. J Am Med Dir Assoc. 2017;18:955-959.e6.
Tsiligianni I, Metting E, van der Molen T, Chavannes N, Kocks J. Morning and night symptoms in primary care COPD patients: a cross-sectional and longitudinal study. An UNLOCK study from the IPCRG. NPJ Prim Care Respir Med. 2016;26:16040.
Jo YS, Yoon HI, Kim DK, Yoo CG, Lee CH. Comparison of COPD Assessment Test and Clinical COPD Questionnaire to predict the risk of exacerbation. Int J Chron Obstruct Pulmon Dis. 2018;13:101–7.
Marçôa R, Rodrigues DM, Dias M, Ladeira I, Vaz AP, Lima R, Guimarães M. Classification of Chronic Obstructive Pulmonary Disease (COPD) according to the new Global Initiative for Chronic Obstructive Lung Disease (GOLD) 2017: comparison with GOLD 2011. COPD. 2018;15:21–6.
Han MK, Quibrera PM, Carretta EE, Barr RG, Bleecker ER, Bowler RP, Cooper CB, Comellas A, Couper DJ, Curtis JL, et al. Frequency of exacerbations in patients with chronic obstructive pulmonary disease: an analysis of the SPIROMICS cohort. Lancet Respir Med. 2017;5:619–26.
Yii ACA, Loh CH, Tiew PY, Xu H, Taha AAM, Koh J, Tan J, Lapperre TS, Anzueto A, Tee AKH. A clinical prediction model for hospitalized COPD exacerbations based on “treatable traits.” Int J Chron Obstruct Pulmon Dis. 2019;14:719–28.
McGarvey L, Lee AJ, Roberts J, Gruffydd-Jones K, McKnight E, Haughney J. Characterisation of the frequent exacerbator phenotype in COPD patients in a large UK primary care population. Respir Med. 2015;109:228–37.
Margüello MS, Garrastazu R, Ruiz-Nuñez M, Helguera JM, Arenal S, Bonnardeux C, León C, Miravitlles M, García-Rivero JL. Independent effect of prior exacerbation frequency and disease severity on the risk of future exacerbations of COPD: a retrospective cohort study. NPJ Prim Care Respir Med. 2016;26:16046.
Engel B, Schindler C, Leuppi JD, Rutishauser J. Predictors of re-exacerbation after an index exacerbation of chronic obstructive pulmonary disease in the REDUCE randomised clinical trial. Swiss Med Wkly. 2017;147: w14439.
PubMed Google Scholar
Benson VS, Müllerová H, Vestbo J, Wedzicha JA, Patel A, Hurst JR. Evaluation of COPD longitudinally to identify predictive surrogate endpoints (ECLIPSE) investigators. Associations between gastro-oesophageal reflux, its management and exacerbations of chronic obstructive pulmonary disease. Respir Med. 2015;109:1147–54.
Santibáñez M, Garrastazu R, Ruiz-Nuñez M, Helguera JM, Arenal S, Bonnardeux C, León C, García-Rivero JL. Predictors of hospitalized exacerbations and mortality in chronic obstructive pulmonary disease. PLoS ONE. 2016;11: e0158727.
Article PubMed PubMed Central CAS Google Scholar
Jo YS, Kim YH, Lee JY, Kim K, Jung KS, Yoo KH, Rhee CK. Impact of BMI on exacerbation and medical care expenses in subjects with mild to moderate airflow obstruction. Int J Chron Obstruct Pulmon Dis. 2018;13:2261–9.
Alexopoulos EC, Malli F, Mitsiki E, Bania EG, Varounis C, Gourgoulianis KI. Frequency and risk factors of COPD exacerbations and hospitalizations: a nationwide study in Greece (Greek Obstructive Lung Disease Epidemiology and health ecoNomics: GOLDEN study). Int J Chron Obstruct Pulmon Dis. 2015;10:2665–74.
PubMed PubMed Central Google Scholar
Liu D, Peng SH, Zhang J, Bai SH, Liu HX, Qu JM. Prediction of short term re-exacerbation in patients with acute exacerbation of chronic obstructive pulmonary disease. Int J Chron Obstruct Pulmon Dis. 2015;10:1265–73.
Müllerová H, Maselli DJ, Locantore N, Vestbo J, Hurst JR, Wedzicha JA, Bakke P, Agusti A, Anzueto A. Hospitalized exacerbations of COPD: risk factors and outcomes in the ECLIPSE cohort. Chest. 2015;147:999–1007.
de Miguel-Díez J, Hernández-Vázquez J, López-de-Andrés A, Álvaro-Meca A, Hernández-Barrera V, Jiménez-García R. Analysis of environmental risk factors for chronic obstructive pulmonary disease exacerbation: a case-crossover study (2004–2013). PLoS ONE. 2019;14: e0217143.
Krachunov II, Kyuchukov NH, Ivanova ZI, Yanev NA, Hristova PA, Borisova ED, Popova TP, Pavlov PS, Nikolova PT, Ivanov YY. Impact of air pollution and outdoor temperature on the rate of chronic obstructive pulmonary disease exacerbations. Folia Med (Plovdiv). 2017;59:423–9.
Article CAS Google Scholar
Baumeler L, Papakonstantinou E, Milenkovic B, Lacoma A, Louis R, Aerts JG, Welte T, Kostikas K, Blasi F, Boersma W, et al. Therapy with proton-pump inhibitors for gastroesophageal reflux disease does not reduce the risk for severe exacerbations in COPD. Respirology. 2016;21:883–90.
Annavarapu S, Goldfarb S, Gelb M, Moretz C, Renda A, Kaila S. Development and validation of a predictive model to identify patients at risk of severe COPD exacerbations using administrative claims data. Int J Chron Obstruct Pulmon Dis. 2018;13:2121–30.
Crisafulli E, Torres A, Huerta A, Méndez R, Guerrero M, Martinez R, Liapikou A, Soler N, Sethi S, Menéndez R. C-reactive protein at discharge, diabetes mellitus and ≥1 hospitalization during previous year predict early readmission in patients with acute exacerbation of chronic obstructive pulmonary disease. COPD. 2015;12:311–20.
Bollmeier SG, Hartmann AP. Management of chronic obstructive pulmonary disease: a review focusing on exacerbations. Am J Health Syst Pharm. 2020;77:259–68.
Bafadhel M, Peterson S, De Blas MA, Calverley PM, Rennard SI, Richter K, Fagerås M. Predictors of exacerbation risk and response to budesonide in patients with chronic obstructive pulmonary disease: a post-hoc analysis of three randomised trials. Lancet Respir Med. 2018;6:117–26.
Calverley PM, Anzueto AR, Dusser D, Mueller A, Metzdorf N, Wise RA. Treatment of exacerbations as a predictor of subsequent outcomes in patients with COPD. Int J Chron Obstruct Pulmon Dis. 2018;13:1297–308.
Calverley PM, Tetzlaff K, Dusser D, Wise RA, Mueller A, Metzdorf N, Anzueto A. Determinants of exacerbation risk in patients with COPD in the TIOSPIR study. Int J Chron Obstruct Pulmon Dis. 2017;12:3391–405.
Eklöf J, Sørensen R, Ingebrigtsen TS, Sivapalan P, Achir I, Boel JB, Bangsborg J, Ostergaard C, Dessau RB, Jensen US, et al. Pseudomonas aeruginosa and risk of death and exacerbations in patients with chronic obstructive pulmonary disease: an observational cohort study of 22 053 patients. Clin Microbiol Infect. 2020;26:227–34.
Estirado C, Ceccato A, Guerrero M, Huerta A, Cilloniz C, Vilaró O, Gabarrús A, Gea J, Crisafulli E, Soler N, Torres A. Microorganisms resistant to conventional antimicrobials in acute exacerbations of chronic obstructive pulmonary disease. Respir Res. 2018;19:119.
Fuhrman C, Moutengou E, Roche N, Delmas MC. Prognostic factors after hospitalization for COPD exacerbation. Rev Mal Respir. 2017;34:1–18.
Krachunov I, Kyuchukov N, Ivanova Z, Yanev NA, Hristova PA, Pavlov P, Glogovska P, Popova T, Ivanov YY. Stability of frequent exacerbator phenotype in patients with chronic obstructive pulmonary disease. Folia Med (Plovdiv). 2018;60:536–45.
Make BJ, Eriksson G, Calverley PM, Jenkins CR, Postma DS, Peterson S, Östlund O, Anzueto A. A score to predict short-term risk of COPD exacerbations (SCOPEX). Int J Chron Obstruct Pulmon Dis. 2015;10:201–9.
Montserrat-Capdevila J, Godoy P, Marsal JR, Barbé F. Predictive model of hospital admission for COPD exacerbation. Respir Care. 2015;60:1288–94.
Montserrat-Capdevila J, Godoy P, Marsal JR, Barbé F, Galván L. Risk factors for exacerbation in chronic obstructive pulmonary disease: a prospective study. Int J Tuberc Lung Dis. 2016;20:389–95.
Orea-Tejeda A, Navarrete-Peñaloza AG, Verdeja-Vendrell L, Jiménez-Cepeda A, González-Islas DG, Hernández-Zenteno R, Keirns-Davis C, Sánchez-Santillán R, Velazquez-Montero A, Puentes RG. Right heart failure as a risk factor for severe exacerbation in patients with chronic obstructive pulmonary disease: prospective cohort study. Clin Respir J. 2018;12:2635–41.
Papi A, Vestbo J, Fabbri L, Corradi M, Prunier H, Cohuet G, Guasconi A, Montagna I, Vezzoli S, Petruzzelli S, et al. Extrafine inhaled triple therapy versus dual bronchodilator therapy in chronic obstructive pulmonary disease (TRIBUTE): a double-blind, parallel group, randomised controlled trial. Lancet. 2018;391:1076–84.
Lipson DA, Barnhart F, Brealey N, Brooks J, Criner GJ, Day NC, Dransfield MT, Halpin DMG, Han MK, Jones CE, et al. Once-daily single-inhaler triple versus dual therapy in patients with COPD. N Engl J Med. 2018;378:1671–80.
Pasquale MK, Xu Y, Baker CL, Zou KH, Teeter JG, Renda AM, Davis CC, Lee TC, Bobula J. COPD exacerbations associated with the modified Medical Research Council scale and COPD assessment test among Humana Medicare members. Int J Chron Obstruct Pulmon Dis. 2016;11:111–21.
Schuler M, Wittmann M, Faller H, Schultz K. Including changes in dyspnea after inpatient rehabilitation improves prediction models of exacerbations in COPD. Respir Med. 2018;141:87–93.
Singh D, Papi A, Corradi M, Pavlišová I, Montagna I, Francisco C, Cohuet G, Vezzoli S, Scuri M, Vestbo J. Single inhaler triple therapy versus inhaled corticosteroid plus long-acting β 2 -agonist therapy for chronic obstructive pulmonary disease (TRILOGY): a double-blind, parallel group, randomised controlled trial. Lancet. 2016;388:963–73.
Søgaard M, Madsen M, Løkke A, Hilberg O, Sørensen HT, Thomsen RW. Incidence and outcomes of patients hospitalized with COPD exacerbation with and without pneumonia. Int J Chron Obstruct Pulmon Dis. 2016;11:455–65.
Stanford RH, Nag A, Mapel DW, Lee TA, Rosiello R, Schatz M, Vekeman F, Gauthier-Loiselle M, Merrigan JFP, Duh MS. Claims-based risk model for first severe COPD exacerbation. Am J Manag Care. 2018;24:e45–53.
Stanford RH, Lau MS, Li Y, Stemkowski S. External validation of a COPD risk measure in a commercial and medicare population: the COPD treatment ratio. J Manag Care Spec Pharm. 2019;25:58–69.
Vestbo J, Papi A, Corradi M, Blazhko V, Montagna I, Francisco C, Cohuet G, Vezzoli S, Scuri M, Singh D. Single inhaler extrafine triple therapy versus long-acting muscarinic antagonist therapy for chronic obstructive pulmonary disease (TRINITY): a double-blind, parallel group, randomised controlled trial. Lancet. 2017;389:1919–29.
Wei X, Ma Z, Yu N, Ren J, Jin C, Mi J, Shi M, Tian L, Gao Y, Guo Y. Risk factors predict frequent hospitalization in patients with acute exacerbation of COPD. Int J Chron Obstruct Pulmon Dis. 2018;13:121–9.
Whalley D, Svedsater H, Doward L, Crawford R, Leather D, Lay-Flurrie J, Bosanquet N. Follow-up interviews from The Salford Lung Study (COPD) and analyses per treatment and exacerbations. NPJ Prim Care Respir Med. 2019;29:20.
Zeiger RS, Tran TN, Butler RK, Schatz M, Li Q, Khatry DB, Martin U, Kawatkar AA, Chen W. Relationship of blood eosinophil count to exacerbations in chronic obstructive pulmonary disease. J Allergy Clin Immunol Pract. 2018;6:944-954.e945.
Vogelmeier CF, Kostikas K, Fang J, Tian H, Jones B, Morgan CL, Fogel R, Gutzwiller FS, Cao H. Evaluation of exacerbations and blood eosinophils in UK and US COPD populations. Respir Res. 2019;20:178.
Celli BR, Fabbri LM, Aaron SD, Agusti A, Brook R, Criner GJ, Franssen FME, Humbert M, Hurst JR, O’Donnell D, et al. An updated definition and severity classification of COPD exacerbations: the Rome proposal. Am J Respir Crit Care Med. 2021;204:1251–8.
Adir Y, Hakrush O, Shteinberg M, Schneer S, Agusti A. Circulating eosinophil levels do not predict severe exacerbations in COPD: a retrospective study. ERJ Open Research. 2018;4:00022–2018.
Bartels W, Adamson S, Leung L, Sin DD, van Eeden SF. Emergency department management of acute exacerbations of chronic obstructive pulmonary disease: factors predicting readmission. Int J Chron Obstruct Pulmon Dis. 2018;13:1647–54.
Kim V, Zhao H, Regan E, Han MK, Make BJ, Crapo JD, Jones PW, Curtis JL, Silverman EK, Criner GJ, COPDGene Investigators. The St. George’s Respiratory Questionnaire definition of chronic bronchitis may be a better predictor of COPD exacerbations compared with the classic definition. Chest. 2019;156:685–95.
Abston E, Comellas A, Reed RM, Kim V, Wise RA, Brower R, Fortis S, Beichel R, Bhatt S, Zabner J, et al. Higher BMI is associated with higher expiratory airflow normalised for lung volume (FEF25-75/FVC) in COPD. BMJ Open Respir Res. 2017;4: e000231.
Emura I, Usuda H, Satou K. Appearance of large scavenger receptor A-positive cells in peripheral blood: a potential risk factor for severe exacerbation of chronic obstructive pulmonary disease. Pathol Int. 2019;69:187–92.
Erol S, Sen E, Gizem Kilic Y, Yousif A, Akkoca Yildiz O, Acican T, Saryal S. Does the 2017 revision improve the ability of GOLD to predict risk of future moderate and severe exacerbation? Clin Respir J. 2018;12:2354–60.
Han MZ, Hsiue TR, Tsai SH, Huang TH, Liao XM, Chen CZ. Validation of the GOLD 2017 and new 16 subgroups (1A–4D) classifications in predicting exacerbation and mortality in COPD patients. Int J Chron Obstruct Pulmon Dis. 2018;13:3425–33.
Huang TH, Hsiue TR, Lin SH, Liao XM, Su PL, Chen CZ. Comparison of different staging methods for COPD in predicting outcomes. Eur Resp J. 2018;51:1700577.
Jung YH, Lee DY, Kim DW, Park SS, Heo EY, Chung HS, Kim DK. Clinical significance of laryngopharyngeal reflux in patients with chronic obstructive pulmonary disease. Int J Chron Obstruct Pulmon Dis. 2015;10:1343–51.
CAS PubMed PubMed Central Google Scholar
Kim J, Kim WJ, Lee CH, Lee SH, Lee MG, Shin KC, Yoo KH, Lee JH, Lim SY, Na JO, et al. Which bronchodilator reversibility criteria can predict severe acute exacerbation in chronic obstructive pulmonary disease patients? Respir Res. 2017;18:107.
Kobayashi S, Hanagama M, Ishida M, Sato H, Ono M, Yamanda S, Yamada M, Aizawa H, Yanai M. Clinical characteristics and outcomes in Japanese patients with COPD according to the 2017 GOLD classification: the Ishinomaki COPD Network Registry. Int J Chron Obstruct Pulmon Dis. 2018;13:3947–55.
Lee SH, Lee JH, Yoon HI, Park HY, Kim TH, Yoo KH, Oh YM, Jung KS, Lee SD, Lee SW. Change in inhaled corticosteroid treatment and COPD exacerbations: an analysis of real-world data from the KOLD/KOCOSS cohorts. Respir Res. 2019;20:62.
Pavlovic R, Stefanovic S, Lazic Z, Jankovic S. Factors associated with the rate of COPD exacerbations that require hospitalization. Turk J Med Sci. 2017;47:134–41.
Song JH, Lee CH, Um SJ, Park YB, Yoo KH, Jung KS, Lee SD, Oh YM, Lee JH, Kim EK, Kim DK. Clinical impacts of the classification by 2017 GOLD guideline comparing previous ones on outcomes of COPD in real-world cohorts. Int J Chron Obstruct Pulmon Dis. 2018;13:3473–84.
Sundh J, Johansson G, Larsson K, Lindén A, Löfdahl CG, Sandström T, Janson C. The phenotype of concurrent chronic bronchitis and frequent exacerbations in patients with severe COPD attending Swedish secondary care units. Int J Chron Obstruct Pulmon Dis. 2015;10:2327–34.
Urwyler P, Hussein NA, Bridevaux PO, Chhajed PN, Geiser T, Grendelmeier P, Zellweger LJ, Kohler M, Maier S, Miedinger D, et al. Predictive factors for exacerbation and reexacerbation in chronic obstructive pulmonary disease: an extension of the Cox model to analyze data from the Swiss COPD cohort. Multidiscip Respir Med. 2019;14:7.
Wallace AE, Kaila S, Bayer V, Shaikh A, Shinde MU, Willey VJ, Napier MB, Singer JR. Health care resource utilization and exacerbation rates in patients with COPD stratified by disease severity in a commercially insured population. J Manag Care Spec Pharm. 2019;25:205–17.
Halpin DMG, Decramer M, Celli BR, Mueller A, Metzdorf N, Tashkin DP. Effect of a single exacerbation on decline in lung function in COPD. Respir Med. 2017;128:85–91.
Bade BC, DeRycke EC, Ramsey C, Skanderson M, Crothers K, Haskell S, Bean-Mayberry B, Brandt C, Bastian LA, Akgün KM. Sex differences in veterans admitted to the hospital for chronic obstructive pulmonary disease exacerbation. Ann Am Thorac Soc. 2019;16:707–14.
Iyer AS, Bhatt SP, Dransfield M, Kinney G, Holm K, Wamboldt FS, Hanania N, Martinez C, Regan E, Foreman MG, et al. Psychological distress prospectively predicts severe exacerbations in smokers with and without airflow limitation—a longitudinal follow-up study of the COPDGene cohort [abstract]. Am J Respir Crit Care Med. 2017. https://doi.org/10.1164/ajrccm-conference.2017.195.1_MeetingAbstracts.A4709 .
Diamond M, Zhao H, Armstrong HF, Morrison M, Bailey KL, Carretta EE, Criner GJ, Han MK, Bleeker E, Cooper CB, et al. Anxiety and depression, either alone or in combination, are associated with respiratory exacerbations in smokers with and without COPD [abstract]. Am J Respir Crit Care Med. 2017;195:1615–31.
Google Scholar
Lau CS, Siracuse BL, Chamberlain RS. Readmission After COPD Exacerbation Scale: determining 30-day readmission risk for COPD patients. Int J Chron Obstruct Pulmon Dis. 2017;12:1891–902.
Pikoula M, Quint JK, Nissen F, Hemingway H, Smeeth L, Denaxas S. Identifying clinically important COPD sub-types using data-driven approaches in primary care population based electronic health records. BMC Med Inform Decis Mak. 2019;19:86.
Wei YF, Tsai YH, Wang CC, Kuo PH. Impact of overweight and obesity on acute exacerbations of COPD—subgroup analysis of the Taiwan Obstructive Lung Disease cohort. Int J Chron Obstruct Pulmon Dis. 2017;12:2723–9.
Barnes PJ, Celli BR. Systemic manifestations and comorbidities of COPD. Eur Resp J. 2009;33:1165–85.
Polverino E, Dimakou K, Hurst J, Martinez-Garcia MA, Miravitlles M, Paggiaro P, Shteinberg M, Aliberti S, Chalmers JD. The overlap between bronchiectasis and chronic airway diseases: state of the art and future directions. Eur Respir J. 2018;52:1800328.
Xu W, Collet JP, Shapiro S, Lin Y, Yang T, Platt RW, Wang C, Bourbeau J. Independent effect of depression and anxiety on chronic obstructive pulmonary disease exacerbations and hospitalizations. Am J Respir Crit Care Med. 2008;178:913–20.
Chapman KR, Hurst JR, Frent SM, Larbig M, Fogel R, Guerin T, Banerji D, Patalano F, Goyal P, Pfister P, et al. Long-term triple therapy de-escalation to indacaterol/glycopyrronium in patients with chronic obstructive pulmonary disease (SUNSET): a randomized, double-blind, triple-dummy clinical trial. Am J Respir Crit Care Med. 2018;198:329–39.
Couillard S, Larivée P, Courteau J, Vanasse A. Eosinophils in COPD exacerbations are associated with increased readmissions. Chest. 2017;151:366–73.
Ferguson GT, Rabe KF, Martinez FJ, Fabbri LM, Wang C, Ichinose M, Bourne E, Ballal S, Darken P, DeAngelis K, et al. Triple therapy with budesonide/glycopyrrolate/formoterol fumarate with co-suspension delivery technology versus dual therapies in chronic obstructive pulmonary disease (KRONOS): a double-blind, parallel-group, multicentre, phase 3 randomised controlled trial. Lancet Respir Med. 2018;6:747–58.
Ko FWS, Chan KP, Ngai J, Ng SS, Yip WH, Ip A, Chan TO, Hui DSC. Blood eosinophil count as a predictor of hospital length of stay in COPD exacerbations. Respirology. 2019;25:259–66.
MacDonald MI, Osadnik CR, Bulfin L, Hamza K, Leong P, Wong A, King PT, Bardin PG. Low and high blood eosinophil counts as biomarkers in hospitalized acute exacerbations of COPD. Chest. 2019;156:92–100.
Müllerová H, Hahn B, Simard EP, Mu G, Hatipoğlu U. Exacerbations and health care resource use among patients with COPD in relation to blood eosinophil counts. Int J Chron Obstruct Pulmon Dis. 2019;14:683–92.
Bafadhel M, Greening NJ, Harvey-Dunstan TC, Williams JEA, Morgan MD, Brightling CE, Hussain SF, Pavord ID, Singh SJ, Steiner MC. Blood eosinophils and outcomes in severe hospitalised exacerbations of COPD. Chest. 2016;150:320–8.
Roche N, Chapman KR, Vogelmeier CF, Herth FJF, Thach C, Fogel R, Olsson P, Patalano F, Banerji D, Wedzicha JA. Blood eosinophils and response to maintenance chronic obstructive pulmonary disease treatment. Data from the FLAME trial. Am J Respir Crit Care Med. 2017;195:1189–97.
Vestbo J, Vogelmeier CF, Small M, Siddall J, Fogel R, Kostikas K. Inhaled corticosteroid use by exacerbations and eosinophils: a real-world COPD population. Int J Chron Obstruct Pulmon Dis. 2019;14:853–61.
Watz H, Tetzlaff K, Wouters EFM, Kirsten A, Magnussen H, Rodriguez-Roisin R, Vogelmeier C, Fabbri LM, Chanez P, Dahl R, et al. Blood eosinophil count and exacerbations in severe chronic obstructive pulmonary disease after withdrawal of inhaled corticosteroids: a post-hoc analysis of the WISDOM trial. Lancet Respir Med. 2016;4:390–8.
Pavord ID, Lettis S, Locantore N, Pascoe S, Jones PW, Wedzicha JA, Barnes NC. Blood eosinophils and inhaled corticosteroid/long-acting beta-2 agonist efficacy in COPD. Thorax. 2016;71:118–25.
Singh D. Predicting corticosteroid response in chronic obstructive pulmonary disease. Blood eosinophils gain momentum. Am J Respir Crit Care Med. 2017;196:1098–100.
Vestbo J, Prescott E, Almdal T, Dahl M, Nordestgaard BG, Andersen T, Sørensen TIA, Lange P. Body mass, fat-free body mass, and prognosis in patients with chronic obstructive pulmonary disease from a random population sample: findings from the Copenhagen City Heart Study. Am J Respir Crit Care Med. 2006;173:79–83.
Agustí AGN, Noguera A, Sauleda J, Sala E, Pons J, Busquets X. Systemic effects of chronic obstructive pulmonary disease. Eur Respir J. 2003;21:347–60.
Agustí AGN, Sauleda J, Miralles C, Gomez C, Togores B, Sala E, Batle S, Busquets X. Skeletal muscle apoptosis and weight loss in chronic obstructive pulmonary disease. Am J Respir Crit Care Med. 2002;166:485–9.
Labaki WW, Martinez FJ. Time to understand the infrequency of the frequent exacerbator phenotype in COPD. Chest. 2018;153:1087–8.
Hartley BF, Barnes NC, Lettis S, Compton CH, Papi A, Jones P. Risk factors for exacerbations and pneumonia in patients with chronic obstructive pulmonary disease: a pooled analysis. Respir Res. 2020;21:5.
Kim Y, Kim YJ, Kang YM, Cho WK. Exploring the impact of number and type of comorbidities on the risk of severe COPD exacerbations in Korean Population: a Nationwide Cohort Study. BMC Pulm Med. 2021;21:151.
Mackay AJ, Kostikas K, Roche N, Frent SM, Olsson P, Pfister P, Gupta P, Patalano F, Banerji D, Wedzicha JA. Impact of baseline symptoms and health status on COPD exacerbations in the FLAME study. Respir Res. 2020;21:93.
Smulders L, van der Aalst A, Neuhaus EDET, Polman S, Franssen FME, van Vliet M, de Kruif MD. Decreased risk of COPD exacerbations in obese patients. COPD. 2020;17:485–91.
Battisti WP, Wager E, Baltzer L, Bridges D, Cairns A, Carswell CI, Citrome L, Gurr JA, Mooney LA, Moore BJ, et al. Good publication practice for communicating company-sponsored medical research: GPP3. Ann Intern Med. 2015;163:461–4.
Putcha N, Barr RG, Han M, Woodruff PG, Bleecker ER, Kanner RE, Martinez FJ, Tashkin DP, Rennard SI, Breysse P, et al. Understanding the impact of passive smoke exposure on outcomes in COPD [abstract]. Am J Respir Crit Care Med. 2015;191:411–20.
Wu Z, Yang D, Ge Z, Yan M, Wu N, Liu Y. Body mass index of patients with chronic obstructive pulmonary disease is associated with pulmonary function and exacerbations: a retrospective real world research. J Thorac Dis. 2018;10:5086–99.
Download references
Acknowledgements
Medical writing support, under the direction of the authors, was provided by Julia King, PhD, and Sarah Piggott, MChem, CMC Connect, McCann Health Medical Communications, funded by AstraZeneca in accordance with Good Publication Practice (GPP3) guidelines [ 109 ].
This study was supported by AstraZeneca.
Author information
Authors and affiliations.
UCL Respiratory, University College London, London, WC1E 6BT, UK
John R. Hurst
Division of Pulmonary and Critical Care, University of Michigan, Ann Arbor, MI, USA
MeiLan K. Han
Formerly of Parexel International, Mohali, India
Barinder Singh, Gagandeep Kaur & Mohd Kashif Siddiqui
Parexel International, Mohali, India
Sakshi Sharma
Formerly of AstraZeneca, Cambridge, UK
Enrico de Nigris
AstraZeneca, Gothenburg, Sweden
Ulf Holmgren
You can also search for this author in PubMed Google Scholar
Contributions
The authors have made the following declaration about their contributions. JRH and MKH made substantial contributions to the interpretation of data; BS, SS, GK, and MKS made substantial contributions to the acquisition, analysis, and interpretation of data; EdN and UH made substantial contributions to the conception and design of the work and the interpretation of data. All authors contributed to drafting or critically revising the article, have approved the submitted version, and agree to be personally accountable for their own contributions and to ensure that questions related to the accuracy or integrity of any part of the work, even ones in which the author was not personally involved, are appropriately investigated, resolved, and the resolution documented in the literature. All authors read and approved the final manuscript.
Corresponding author
Correspondence to John R. Hurst .
Ethics declarations
Ethics approval and consent to participate.
Not applicable.
Consent for publication
Competing interests.
JRH reports consulting fees from AstraZeneca; speaker fees from AstraZeneca, Chiesi, Pfizer, and Takeda; and travel support from GlaxoSmithKline and AstraZeneca. MKH reports assistance with conduction of this research and publication from AstraZeneca; personal fees from Aerogen, Altesa Biopharma, AstraZeneca, Boehringer Ingelheim, Chiesi, Cipla, DevPro, GlaxoSmithKline, Integrity, Medscape, Merck, Mylan, NACE, Novartis, Polarean, Pulmonx, Regeneron, Sanofi, Teva, Verona, United Therapeutics, and UpToDate; either in kind research support or funds paid to the institution from the American Lung Association, AstraZeneca, Biodesix, Boehringer Ingelheim, the COPD Foundation, Gala Therapeutics, the NIH, Novartis, Nuvaira, Sanofi, and Sunovion; participation in Data Safety Monitoring Boards for Novartis and Medtronic with funds paid to the institution; and stock options from Altesa Biopharma and Meissa Vaccines. BS, GK, and MKS are former employees of Parexel International. SS is an employee of Parexel International, which was funded by AstraZeneca to conduct this analysis. EdN is a former employee of AstraZeneca and previously held stock and/or stock options in the company. UH is an employee of AstraZeneca and holds stock and/or stock options in the company.
Additional information
Publisher's note.
Springer Nature remains neutral with regard to jurisdictional claims in published maps and institutional affiliations.
Supplementary Information
Additional file1: table s1..
Search strategies. Table S2. List of included studies with linked publications. Table S3. Study characteristics across the 76 included studies. Table S4. Clinical characteristics of the patients assessed across the included studies.
Additional file 2: Fig. S1.
Sex (male vs female) as a risk factor for moderate-to-severe exacerbations. Fig. S2. Sex (male vs female) as a risk factor for severe exacerbations.
Rights and permissions
Open Access This article is licensed under a Creative Commons Attribution 4.0 International License, which permits use, sharing, adaptation, distribution and reproduction in any medium or format, as long as you give appropriate credit to the original author(s) and the source, provide a link to the Creative Commons licence, and indicate if changes were made. The images or other third party material in this article are included in the article's Creative Commons licence, unless indicated otherwise in a credit line to the material. If material is not included in the article's Creative Commons licence and your intended use is not permitted by statutory regulation or exceeds the permitted use, you will need to obtain permission directly from the copyright holder. To view a copy of this licence, visit http://creativecommons.org/licenses/by/4.0/ . The Creative Commons Public Domain Dedication waiver ( http://creativecommons.org/publicdomain/zero/1.0/ ) applies to the data made available in this article, unless otherwise stated in a credit line to the data.
Reprints and permissions
About this article
Cite this article.
Hurst, J.R., Han, M.K., Singh, B. et al. Prognostic risk factors for moderate-to-severe exacerbations in patients with chronic obstructive pulmonary disease: a systematic literature review. Respir Res 23 , 213 (2022). https://doi.org/10.1186/s12931-022-02123-5
Download citation
Received : 02 March 2022
Accepted : 20 July 2022
Published : 23 August 2022
DOI : https://doi.org/10.1186/s12931-022-02123-5
Share this article
Anyone you share the following link with will be able to read this content:
Sorry, a shareable link is not currently available for this article.
Provided by the Springer Nature SharedIt content-sharing initiative
- Exacerbations
- Comorbidities
- Hospitalization
Respiratory Research
ISSN: 1465-993X
- General enquiries: [email protected]
How to Conduct a Systematic Review: A Narrative Literature Review
Affiliations.
- 1 Psychiatry, Mount Sinai Chicago.
- 2 Psychiatry, KVC Prairie Ridge Hospital.
- 3 Department of Psychiatry, Bronx Lebanon Hospital Icahn School of Medicine at Mount Sinai, Bronx, NY.
- 4 Psychiatry, Suny Upstate Medical University, Syracuse, NY.
- PMID: 27924252
- PMCID: PMC5137994
- DOI: 10.7759/cureus.864
Systematic reviews are ranked very high in research and are considered the most valid form of medical evidence. They provide a complete summary of the current literature relevant to a research question and can be of immense use to medical professionals. Our goal with this paper is to conduct a narrative review of the literature about systematic reviews and outline the essential elements of a systematic review along with the limitations of such a review.
Keywords: meta-analysis; narrative literature review; prisma checklist; systematic reviews.
Publication types
- Open access
- Published: 03 April 2024
Capturing artificial intelligence applications’ value proposition in healthcare – a qualitative research study
- Jasmin Hennrich 1 ,
- Eva Ritz 2 ,
- Peter Hofmann 1 , 4 &
- Nils Urbach 1 , 3
BMC Health Services Research volume 24 , Article number: 420 ( 2024 ) Cite this article
939 Accesses
Metrics details
Artificial intelligence (AI) applications pave the way for innovations in the healthcare (HC) industry. However, their adoption in HC organizations is still nascent as organizations often face a fragmented and incomplete picture of how they can capture the value of AI applications on a managerial level. To overcome adoption hurdles, HC organizations would benefit from understanding how they can capture AI applications’ potential.
We conduct a comprehensive systematic literature review and 11 semi-structured expert interviews to identify, systematize, and describe 15 business objectives that translate into six value propositions of AI applications in HC.
Our results demonstrate that AI applications can have several business objectives converging into risk-reduced patient care, advanced patient care, self-management, process acceleration, resource optimization, and knowledge discovery.
We contribute to the literature by extending research on value creation mechanisms of AI to the HC context and guiding HC organizations in evaluating their AI applications or those of the competition on a managerial level, to assess AI investment decisions, and to align their AI application portfolio towards an overarching strategy.
Peer Review reports
Applications based on artificial intelligence (AI) have the potential to transform the healthcare (HC) industry [ 1 ]. AI applications can be characterized as applications or agents with capabilities that typically demand intelligence [ 2 , 3 ]. In our context, we understand AI as a collection of technological solutions from the field of applied computer science, in which algorithms are trained on medical and HC data to perform tasks that are normally associated with human intelligence (i.e., medical decision-making) [ 4 ]. AI is not a single type of technology, instead, it encompasses a diverse array of technologies spread across various application areas in HC, such as diagnostics (e.g., [ 5 ], biomedical research (e.g., [ 6 ], clinical administration (e.g., [ 7 ], therapy (e.g., [ 8 ], and intelligent robotics (e.g., [ 9 ]. These areas are expected to benefit from AI applications’ capabilities, such as accuracy, objectivity, rapidity, data processing, and automation [ 10 , 11 ]. Accordingly, AI applications are said to have the potential to drive business value and enhance HC [ 12 ], paving the way for transformative innovations in the HC industry [ 13 ]. There are already many promising AI use cases in HC that are expected to improve patient care and create value for HC organizations. For instance, AI applications can advance the quality of patient care by supporting radiologists with more accurate and rapid diagnosis, compensating for humans’ limitations (e.g., data processing speeds) and weaknesses (e.g., inattention, distraction, and fatigue) [ 10 , 14 ]. Klicken oder tippen Sie hier, um Text einzugeben.While the use of AI applications in HC has the overarching goal of creating significant value for patients through improved care, they also come with the potential for business value creation and the opportunity for HC organizations to gain a competitive edge (e.g., [ 15 , 16 ]).
Despite the promised advantages, AI applications’ implementation is slow, and the full realization of their potential within the HC industry is yet to be achieved [ 11 , 17 ]. With just a handful of practical examples of AI applications in the HC industry [ 13 , 18 ], the adoption of AI applications is still in its infancy. The AI in Healthcare Survey Report stated that in 2021, only 9% of respondents worldwide have reached a sophisticated adoption of AI Models, while 32% of respondents are still in the early stages of adopting AI models. According to the survey, the majority of HC organizations (60%) are not actively considering AI as a solution, or they are currently evaluating AI use cases and experimenting with the implementation [ 19 ]. Nevertheless, HC startups are increasingly entering the market [ 20 ], pressuring incumbent HC organizations to evaluate and adopt AI applications. Existing studies already investigate AI technologies in various use cases in HC and provide insights on how to design AI-based services [ 21 ], explain in detail the technical functions and capabilities of AI technologies [ 10 , 11 ], or take on a practical perspective with a focus on concrete examples of AI applications [ 14 ]. However, to foster the adoption of AI applications, HC organizations should understand how they can unfold AI applications’ capabilities into business value to ensure effective investments. Previous studies on the intersection of information systems and value creation have expressed interest into how organizations can actually gain value through the use of technology and thus, enhance their adoption [ 22 , 23 ]. However, to the best of our knowledge, a comprehensive investigation of the value creation of AI applications in the context of HC from a managerial level is currently missing. Thus, our study aims to investigate AI applications’ value creation and capture mechanisms in the specific HC context by answering the following question: How can HC organizations create and capture AI applications’ value?
We conduct a systematic literature analysis and semi structured expert interviews to answer this research question. In the systematic literature analysis, we identify and analyze a heterogeneous set of 21 AI use cases across five different HC application fields and derive 15 business objectives and six value propositions for HC organizations. We then evaluate and refine the categorized business objectives and value propositions with insights from 11 expert interviews. Our study contributes to research on the value creation mechanism of AI applications in the HC context. Moreover, our results have managerial implications for HC organizations since they can draw on our results to evaluate AI applications, assess investment decisions, and align their AI application portfolio toward an overarching strategy.
In what follows, this study first grounds on relevant work to gain a deeper understanding of the underlying constructs of AI in HC. Next, we describe our qualitative research method by describing the process of data collection and analysis, followed by our derived results on capturing AI applications’ value proposition in HC. Afterward, we discuss our results, including this study’s limitations and pathways for further research. Finally, we summarize our findings and their contribution to theory and practice in the conclusion.
Relevant work
In the realm of AI, a thorough exploration of its key subdiscipline, machine learning (ML), is essential [ 24 , 25 ]. ML is a computational model that learns from data without explicitly programming the data [ 24 ] and can be further divided into supervised, unsupervised, and reinforcement learning [ 26 ]. In supervised learning, the machine undergoes training with labeled data, making it well-suited for tasks involving regression and classification problems [ 27 ]. In contrast, unsupervised learning is designed to automatically identify patterns within unlabeled datasets [ 28 ], with its primary utility lying in the extraction of features [ 11 ]. Reinforcement learning, characterized as a method of systematic experimentation or trial and error, involves a situated agent taking specific actions and observing the rewards it gains from those actions, facilitating the learning of behavior in a given environment [ 29 ]. The choice of which type of ML will be used in the different application areas depends on the specific problem, the availability of labeled data, and the nature of the desired outcome.
In recent years, the rapid advances in AI have triggered a revolution in various areas, with numerous impressive advantages. In the financial sector, AI applications can significantly improve security by detecting anomalies and preventing fraud [ 30 ]. Within education, AI has emerged as a powerful tool for tailoring learning experiences, aiming to enhance engagement, understanding, and retention [ 31 ]. In the energy market, the efficacy of AI extends to fault detection and diagnosis in building energy systems, showcasing its robust capabilities in ensuring system integrity [ 32 ]. Moreover, the HC industry is expected to be a promising application area for AI applications. The HC sector is undergoing a significant transformation due to the increasing adoption of digital technologies, with AI technologies at the forefront of this shift. The increasing relevance of AI technologies in HC is underlined by a growing and multidisciplinary stream of AI research, as highlighted by Secinaro et al. [ 33 ]. Taking a closer look at the different application areas in HC, AI applications offer promising potential, as demonstrated by the following exemplary AI use cases. In diagnosis, AI applications can identify complex patterns in medical image data more accurately, resulting in precise and objective disease recognition. This can improve patient safety by reducing the risks of misinterpretation [ 5 ]. Another use case can be found in biomedical research. For example, AI technology is commonly used for de novo drug design. AI can rapidly browse through molecule libraries to detect nearly \({10}^{60}\) drug-like molecules, accelerating the drug development process [ 6 ]. Furthermore, AI applications are used in clinical administration. They enable optimized operation room capacities by automating the process and by including information about absence or waiting times, as well as predicting interruptions [ 34 ]. Furthermore, AI applications are used in therapy by predicting personalized medication dosages. As this helps to reduce the mortality risk, it leads to enhanced patient outcomes and quality of care [ 35 ]. Intelligent prostheses by which patients can improve interactions are another use case. The AI algorithm continuously detects and classifies myoelectric signal patterns to predict movements, leading to reduced training expenditure and more self-management by the patient [ 36 ]. In summary, envisioning that AI applications successfully address persisting challenges, such as lack of transparency (e.g., [ 37 ], bias (e.g., [ 38 ], privacy concerns, and trust issues (e.g., [ 39 ], the potential of AI applications is vast. The conceivable benefits extend to individual practitioners and HC organizations, including hospitals, enabling them to harness AI applications for creating business value and ultimately enhancing competitiveness. Thereby, we follow Schryen’s (p. 141) revisited definition of business value of technologies: “the impact of investments on the multidimensional performance and capabilities of economic entities at various levels, complemented by the ultimate meaning of performance in the economic environment” [ 40 ]. His perspective includes all kinds of tangible value (such as an increase in productivity or reduced costs) to intangible value (such as service innovation or customer satisfaction), as well as internal value for the HC organizations and external value for stakeholders, shareholders, and customers. To create business value, it is essential to have a clear understanding of how the potential of AI applications can be captured. The understanding of how information systems, in general, create value is already covered in the literature. For example, Badakhshan et al. [ 31 ] focus on how process mining can pave the way to create business value. Leidner et al. [ 32 ] examine how enterprise social media adds value for new employees, and Lehrer et al. [ 33 ] answer the question of how big data analytics can enable service. There are also studies focusing on the value creation of information systems in the context of HC. For instance, the study by Haddad and Wickramasinghe [ 41 ] shows that information technology in HC can capture value by improving the quality of HC delivery, increasing safety, or offering additional services. Strong et al. [ 42 ] analyze how electronic health records afford value for HC organizations and determine goal-oriented actions to capture this potential. There is even literature on how machine learning adds value within the discipline of radiology (e.g., [ 43 ].
However, these studies either do not address the context of HC, consider technologies other than AI or information systems in general, or focus only on a small area of HC (e.g., radiology) and a subset of AI technology (e.g., machine learning). Although these studies deliver valuable insights into the value creation of information systems, a comprehensive picture of how HC organizations can capture business value with AI applications is missing.
To answer our research question, we adopted a qualitative inductive research design. This research design is consistent with studies that took a similar perspective on how technologies can create business value [ 44 ]. In conducting our structured literature review, we followed the approach of Webster and Watson [ 45 ] and included recommendations of Wolfswinkel et al. [ 46 ] when considering the inclusion and exclusion criteria. We started by collecting relevant data on different successful AI use cases across five application areas in HC. Siggelkow [ 47 ] argued that use cases are able to provide persuasive arguments for causal relationships. In an initial literature screening, we identified five promising application domains focusing on AI applications for patients and HC providers: disease diagnostics (DD) (e.g., [ 5 ], biomedical research (BR) (e.g., [ 6 ], clinical administration (CA) (e.g., [ 7 ], therapy (T) (e.g., [ 8 ], and intelligent robotics (IR) (e.g., [ 9 ]. Second, to sample AI use cases, we aimed to collect a heterogeneous set of AI use cases within these application domains and consider the heterogeneity in AI applications, underlying data, innovation types, and implementation stages when selecting 21 AI use cases for our in-depth analysis. The AI use case and an exemplary study for each use case are listed in Table 1 .
After sampling the AI use cases, we used PubMed to identify papers for each use case. PubMed is recognized as a common database for biomedical and medical research for HC topics in the information systems domain (e.g., [ 62 , 63 ]. Our search included journal articles, clinical conferences, clinical studies, and comparative studies in English as of 2010. Based on the AI use case sample, we derived a search string based on keywords [ 45 ] considering titles and abstracts by following Shepherd et al. [ 62 ] guidelines. It was aimed to narrow and specific selection to increase data collection replicability for the use cases. Boolean operators (AND, OR) are used to improve results by combining search terms [ 62 ].
((artificial intelligence AND (radiology OR (cancer AND imaging) OR (radiology AND error) OR (cancer AND genomics) OR (speech AND cognitive AND impairment) OR (voice AND parkinson) OR EEG OR (facial AND analysis) OR (drug AND design) OR (Drug AND Biomarker) OR De-identification OR Splicing OR (emergency AND triage) OR (mortality AND prediction) OR (operating AND room) OR text summarization OR (artificial AND pancreas) OR vasopressor OR Chatbot OR (myoelectric prosthesis) OR (automated surgery task) OR (surgery AND workflow)))
The initial search led to 877 results (see Fig. 1 ). After title screening, we eliminated 516 papers that are not relevant (i.e., not covering a specific AI application, only including the description of AI algorithm, or not including a managerial perspective and the value created by AI applications). We further excluded 162 papers because their abstract is not concurrent with any specific use case (e.g., because they were literature reviews on overarching topics and did not include a specific AI application). We screened the remaining 199 papers for eligibility through two content-related criteria. First, papers need to cover an AI use case’s whole value proposition creation path, including information on data, algorithms, functions, competitive advantage, and business value of a certain AI application. The papers often only examine how a certain application works but lack the value proposition perspective, which leads to the exclusion of 63 articles. Second, we removed 89 papers that do not match any of our use cases. This step led to a remaining set of 47 relevant papers. During a backward-forward search according to Webster and Watson [ 45 ] and Levy and Ellis [ 64 ], we additionally included 35 papers. We also incorporated previous and subsequent clinical studies of the same researcher, resulting in an additional six papers. The final set contains 88 relevant papers describing the identified AI use cases, whereby at least three papers describe each AI use case.
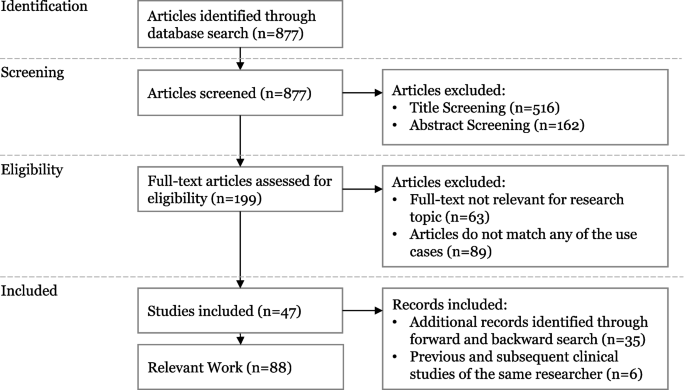
Search strategy
In the second step, we engaged in open, axial, and selective coding of the AI use cases following analysis techniques of grounded theory [ 65 ]. We focused on extracting business objectives, detailing how each AI application drives value. We documented these for each AI use case by recording codes of business objectives and value propositions and assigning relationships among the open codes. For example, from the following text passage of Berlyand et al. [ 56 ], who investigate the use case CA1: “Rapidly interpreting clinical data to classify patients and predict outcomes is paramount to emergency department operations, with direct impacts on cost, efficiency, and quality of care”, we derived the code rapid task execution.
After analyzing the AI use cases, we revised the documented tuples to foster consistency and comparability. Then, we iteratively coded the identified tuples by relying on selective coding techniques which is a process to identify and refine categories at a highly generalizable degree [ 65 ]. In all 14 coding iterations, one author continuously compares, relates, and associates categories and properties and discusses the coding results with another author. We modified some tuples during the coding process in two ways. First, we equalized small phrasing disparities for homogenous and refined wording. Second, we carefully adjusted the tuples regarding coherency. Finally, we reviewed the coding schema for internal validity through a final comparison with the data [ 66 ]. Then, we set the core variables “business objectives” and “value propositions”. We refer to business objectives as improvements through implementing the technology that drives a value proposition. We define value proposition as the inherent commitment to deliver reciprocal value to the organization, its customers, and/or partners [ 67 ].
In the third step following Schultze and Avital [ 68 ], we conducted semi structured expert interviews to evaluate and refine the value propositions and business objectives. We developed and refined an interview script following the guidelines of Meyers and Newman [ 69 ] for qualitative interviews. An additional file shows the used interview script (see Additional file 1 ). We conducted expert sampling to select suitable interviewees [ 70 ]. Due to the interdisciplinarity of the research topic, we chose experts in the two knowledge areas, AI and HC. In the process of expert selection, we ensured that interviewees possessed a minimum of two years of experience in their respective fields. We aimed for a well-balanced mix of diverse professions and positions among the interviewees. Additionally, for those with a primary background in HC, we specifically verified their proficiency and understanding of AI, ensuring a comprehensive perspective across the entire expert panel. Table 2 provides an overview of our expert sample. The interviewees were recruited in the authors’ networks and by cold calling. Identified experts were first contacted by email, including some brief information regarding the study. If there was no response within two weeks, they were contacted again by telephone to arrange an interview date. In total, we conducted 11 interviews that took place in a time range between 40 and 75 min. The expert interviews are transcribed verbatim using the software f4. As a coding aid, we use the software MAXQDA—a tool for qualitative data analysis which is frequently used in the analyses of qualitative data in the HC domain (e.g., [ 38 , 71 , 72 ]).
To systematically decompose how HC organizations can realize value propositions from AI applications, we identified 15 business objectives and six value propositions (see Fig. 2 ). These business objectives and value propositions resulted from analyzing the collected data, which we derived from the literature and refined through expert interviews. In the following, we describe the six value propositions and elaborate on how the specific AI business objectives can result in value propositions. This will be followed by a discussion of the results in the discussion of the paper.
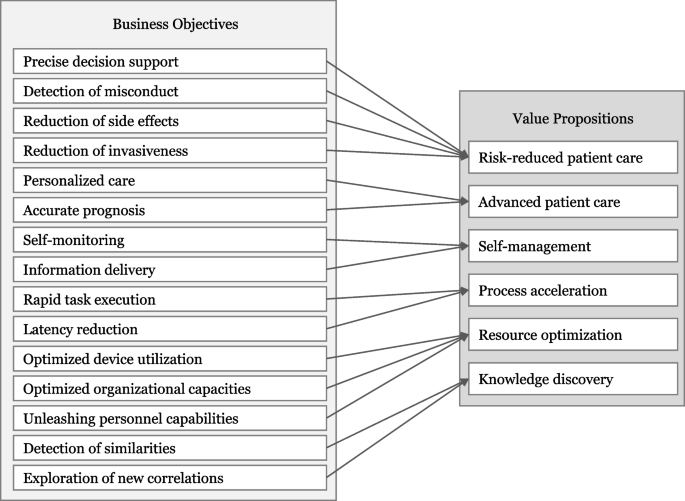
Business objectives and value propositions risk-reduced patient care
This value proposition follows business objectives that may identify and reduce threats and adverse factors during medical procedures. HC belongs to a high-risk domain since there are uncertain external factors (E4), including physicians’ fatigue, distractions, or cognitive biases [ 73 , 74 ]. AI applications can reduce certain risks by enabling precise decision support, detecting misconduct, reducing emergent side effects, and reducing invasiveness.
Precise decision support stems from AI applications’ capability to integrate various data types into the decision-making process, gaining a sophisticated overview of a phenomenon. Precise knowledge about all uncertainty factors reduces the ambiguity of decision-making processes [ 49 ]. E5 confirms that AI applications can be seen as a “perceptual enhancement”, enabling more comprehensive and context-based decision support. Humans are naturally prone to innate and socially adapted biases that also affect HC professionals [ 14 ]. Use Case CA1 highlights how rapid decision-making by HC professionals during emergency triage may lead to overlooking subtle yet crucial signs. AI applications can offer decision support based on historical data, enhancing objectivity and accuracy [ 56 ].
Detection of misconduct is possible since AI applications can map and monitor clinical workflows and recognize irregularities early. In this context, E10 highlights that “one of the best examples is the interception of abnormalities.” For instance, AI applications can assist in allocating medications in hospitals (Use case T2). Since HC professionals can be tired or distracted in medication preparation, AI applications may avoid serious consequences for patients by monitoring allocation processes and patients’ reactions. Thus, AI applications can reduce abuse and increase safety.
Reduction of emergent side effects is enabled by AI applications that continuously monitor and process data. If different treatments and medications are combined during a patient’s clinical pathway, it may cause overdosage or evoke co-effects and comorbidities, causing danger for the patient [ 75 ]. AI applications can prevent these by detecting and predicting these effects. For instance, AI applications can calculate the medication dosage for the individual and predict contraindications (Use case T2) [ 76 ]. E3 adds that the reduction of side effects also includes “cross-impacts between medications or possible symptoms that only occur for patients of a certain age or disease.” Avoidable side effects can thus be detected at an early stage, resulting in better outcomes.
Reduction of invasiveness of medical treatments or surgeries is possible by allowing AI applications to compensate for and overcome human weaknesses and limitations. During surgery, AI applications can continuously monitor a robot’s position and accurately predict its trajectories [ 77 ]. Intelligent robots can eliminate human tremors and access hard-to-reach body parts [ 60 ]. E2 validates, “a robot does not tremble; a robot moves in a perfectly straight line.” The precise AI-controlled movement of surgical robots minimizes the risk of injuring nearby vessels and organs [ 61 ]. Use cases DD5 and DD7 elucidate how AI applications enable new methods to perform noninvasive diagnoses. Reducing invasiveness has a major impact on the patient’s recovery, safety, and outcome quality.
Advanced patient care
Advanced patient care follows business objectives that extend patient care to increase the quality of care. One of HC’s primary goals is to provide the most effective treatment outcome. AI applications can advance patient care as they enable personalized care and accurate prognosis.
Personalized care can be enabled by the ability of AI technologies to integrate and process individual structured and unstructured patient data to increase the compatibility of patient and health interventions. For instance, by analyzing genome mutations, AI applications precisely assess cancer, enabling personalized therapy and increasing the likelihood of enhancing outcome quality (Use case DD4). E11 sums up that “we can improve treatment or even make it more specific for the patient. This is, of course, the dream of healthcare”. Use case T1 exemplifies how the integration of AI applications facilitates personalized products, such as an artificial pancreas. The pancreas predicts glucose levels in real time and adapts insulin supplementation. Personalized care allows good care to be made even better by tailoring care to the individual.
Accurate prognosis is achieved by AI applications that track, combine, and analyze HC data and historical data to make accurate predictions. For instance, AI applications can precisely analyze tumor tissue to improve the stratification of cancer patients. Based on this result, the selection of adjuvant therapy can be refined, improving the effectiveness of care [ 48 ]. Use case DD6 shows how AI applications can predict seizure onset zones to enhance the prognosis of epileptic seizures. In this context, E10 adds that an accurate prognosis fosters early and preventive care.
Self-management
Self-management follows the business objectives that increase disease controllability through the support of intelligent medical products. AI applications can foster self-management by self-monitoring and providing a new way of delivering information.
Self-monitoring is enhanced by AI applications, which can automatically process frequently measured data. There are AI-based chatbots, mobile applications, wearables, and other medical products that gather periodic data and are used by people to monitor themselves in the health context (e.g., [ 78 , 79 ]. Frequent data collection of these products (e.g., using sensors) enables AI applications to analyze periodic data and become aware of abnormalities. While the amount of data rises, the applications can improve their performance continuously (E2). Through continuous tracking of heartbeats via wearables, AI applications can precisely detect irregularities, notify their users in the case of irregularities, empower quicker treatment (E2), and may reduce hospital visits (E9). Self-monitoring enhances patient safety and allows the patient to be more physician-independent and involved in their HC.
Information delivery to the patient is enabled by AI applications that give medical advice adjusted to the patient’s needs. Often, patients lack profound knowledge about their anomalies. AI applications can contextualize patients’ symptoms to provide anamnesis support and deliver interactive advice [ 59 ]. While HC professionals must focus on one diagnostic pathway, AI applications can process information to investigate different diagnostic branches simultaneously (E5). Thus, these applications can deliver high-quality information based on the patient’s feedback, for instance, when using an intelligent conversational agent (use case T3). E4 highlights that this can improve doctoral consultations because “the patient is already informed and already has information when he comes to talk to doctors”.
Process acceleration
Process acceleration comprises business objectives that enable speed and low latencies. Speed describes how fast one can perform a task, while latency specifies how much time elapses from an event until a task is executed. AI applications can accelerate processes by rapid task execution and reducing latency.
Rapid task execution can be achieved by the ability of AI applications to process large amounts of data and identify patterns in a short time. In this context, E4 mentions that AI applications can drill diagnosis down to seconds. For instance, whereas doctors need several minutes for profound image-based detection, AI applications have a much faster report turnaround time (use case DD1). Besides, rapid data processing also opens up new opportunities in drug development. AI applications can rapidly browse through molecule libraries to detect nearly 10^60 molecules, which are synthetically available (use case BR1). This immense speed during a discovery process has an essential influence on the business potential and can enormously decrease research costs (E10).
Latency reduction can be enabled by AI technologies monitoring and dynamically processing information and environmental factors. By continuously evaluating vital signs and electrocardiogram records, AI applications can predict the in-house mortality of patients in real time [ 57 ]. The AI application can detect an increased mortality risk faster than HC professionals, enabling a more rapid emergency intervention. In this case, AI applications decrease the time delay between the cause and the reaction, which positively impacts patient care. E7 emphasizes the importance of short latencies: “One of the most important things is that the timeframe between the point when all the data is available, and a decision has been made, […] must be kept short.”
Resource optimization
Resource optimization follows the business objectives that manage limited resources and capacities. The HC industry faces a lack of sufficient resources, especially through a shortage of specialists (E8), which in turn negatively influences waiting times. AI applications can support efficient resource allocation by optimizing device utilization, organizational capacities and unleashing personnel capabilities.
Optimized device utilization can be enhanced by AI applications that track, analyze, and precisely predict load of times of medical equipment in real-time. For instance, AI applications can maximize X-Ray or magnetic resonance tomography device utilization (use case CA3). Besides, AI applications can enable a dynamic replanning of device utilization by including absence or waiting times and predicting interruptions. Intelligent resource optimization may include various key variables (e.g., the maximized lifespan of a radiation scanner) [ 48 ]. Optimized device utilization reduces the time periods when the device is not utilized, and thus, losses are made.
Optimized organizational capacities are possible due to AI applications breaking up static key performance indicators and finding more dynamic measuring approaches for the required workflow changes (E5, E10). The utilization of capacities in hospitals relies on various known and unknown parameters, which are often interdependent [ 80 ]. AI applications can detect and optimize these dependencies to manage capacity. An example is the optimization of clinical occupancy in the hospital (use case CA3), which has a strong impact on cost. E5 adds that the integration of AI applications may increase the reliability of planning HC resources since they can predict capacity trends from historical occupancy rates. Optimized planning of capacities can prevent capacities from remaining unused and fixed costs from being offset by no revenue.
Unleashing personnel capabilities is enabled by AI applications performing analytical and administrative tasks, relieving caregivers’ workload (E8, E10, E11). E7 validates that “our conviction is […] that administrational tasks generate the greatest added value and benefit for doctors and caregivers.” Administrative tasks include the creation of case summaries (use case CA4) or automated de-identification of private health information in electronic health records (use case BR2) [ 54 ]. E8 says that resource optimization enables “more time for direct contact with patients.”
Knowledge discovery
Knowledge discovery follows the business objectives that increase perception and access to novel and previously unrevealed information. AI applications might synthesize and contextualize medical knowledge to create uniform or equalized semantics of information (E5, E11). This semantics enables a translation of knowledge for specific users.
Detection of similarities is enabled by AI applications identifying entities with similar features. AI applications can screen complex and nonlinear databases to identify reoccurring patterns without any a priori understanding of the data (E3). These similarities generate valuable knowledge, which can be applied to enhance scientific research processes such as drug development (use case BR1). In drug development, AI applications can facilitate ligand-based screening to detect new active molecules based on similarities compared with already existing molecular properties. This increases the effectiveness of drug design and reduces risks in clinical trials [ 6 ].
Exploration of new correlations is facilitated by AI applications identifying relationships in data. In diagnostics, AI applications can analyze facial photographs to accurately identify genotype–phenotype correlations and, thus, increase the detection rate of rare diseases (use case DD7). E8 states the potential of AI applications in the field of knowledge discovery: “Well, if you are researching in any medical area, then everybody aims to understand and describe phenomena because science always demands a certain causation.” However, it is crucial to develop transparent and intelligible inferences that are comprehensible for HC professionals and researchers. Exploring new correlations improves diagnoses of rare diseases and ensures earlier treatment.
After describing each business objective and value proposition, we summarize the AI use cases’ contributions to the value propositions in Table 3 .
By revealing 15 business objectives that translate into six value propositions, we contribute to the academic discourse on the value creation of AI (e.g. [ 81 ] and provide prescriptive knowledge on AI applications' value propositions in the HC domain. Our discourse also emphasizes that our findings are not only relevant to the field of value creation research but can also be helpful for adoption research. The value propositions we have identified can be a good starting point to accelerate the adoption of AI in HC, as the understanding of potential value propositions that we foster could mitigate some of the current obstacles to the adoption of AI applications in HC. For example, our findings may help to mitigate the obstacle “added value”, which is presented in the study by Hennrich et al.38 [ 38 ] as users’ concerns that AI might create more burden than benefits.
Further, we deliver valuable implications for practice and provide a comprehensive picture of how organizations in the context of HC can achieve business value with AI applications from a managerial level, which has been missing until now. We guide HC organizations in evaluating their AI applications or those of the competition to assess AI investment decisions and align their AI application portfolio toward an overarching strategy. These results will foster the adoption of AI applications as HC organizations can now understand how they can unfold AI applications’ capabilities into business value. In case a hospital’s major strategy is to reduce patient risks due to limited personal capacities, it might be beneficial for them to invest in AI applications that reduce side effects by calculating medication dosages (use case T2). If an HC organization currently faces issues with overcrowded emergency rooms, the HC organization might acquire AI applications that increase information delivery and help patients decide if and when they should visit the hospital (use case T3) to increase patients’ self-management and, in turn, improve triage. Besides, our findings also offer valuable insights for AI developers. Addressing issues such as transparency and the alignment of AI applications with the needs of HC professionals is crucial. Adapting AI solutions to the specific requirements of the HC sector ensures responsible integration and thus the realization of the expected values.
A closer look at the current challenges in the HC sector reveals that new solutions to mitigate them and improve value creation are needed. Given that a nurse, for example, dedicates a substantial 25% of their working hours to administrative tasks [ 17 ], the rationale behind the respondents’ (E7) recognition of “the greatest added value” in utilizing AI applications for administrative purposes becomes evident. The potential of AI applications in streamlining administrative tasks lies in creating additional time for meaningful patient interactions. Acknowledging the significant impact of the doctor-patient interpersonal relationship on both the patient’s well-being and the processes of diagnosis and healing, as elucidated by Buck et al. [ 82 ] in their interview study, the physicians interviewed emphasized that the mere presence of the doctor in the same room often alleviates the patient’s problems. Consequently, it becomes apparent that the intangible value of AI applications plays a crucial role in the context of HC and is an important factor in the investment decision as to where an AI application should be deployed.
The interviews also indicate that the special context of the HC sector leads to concerns regarding the use of AI applications. For example, one interviewee emphasized a fundamental characteristic of medical staff by pointing out that physicians have a natural desire to understand all phenomena (E8). AI applications, however, are currently struggling with the challenge of transparency. This challenge is described by the so-called black box problem, a phenomenon that makes it impossible to decipher the underlying algorithms that lead to a particular recommendation [ 37 ]. The lack of transparency and the resulting lack of intervention options for medical staff can lead to incorrect decisions by the AI application, which may cause considerable damage. Aware of these risks, physicians are currently struggling with trust issues in AI applications [ 72 ]. The numerous opportunities for value creation through AI applications in HC are offset by the significant risk of causing considerable harm to patients if the technology is not yet fully mature. Ultimately, it remains essential to keep in mind that there are many ethical questions to be answered [ 83 ], and AI applications are still facing many obstacles [ 38 ] that must be overcome in order to realize the expected values and avoid serious harm. One important first step in mitigating the obstacles is disseminating the concerns and risks to relevant stakeholders, emphasizing the urgency for collaborative scientific and public monitoring efforts [ 84 ]. However, keeping these obstacles in mind, by providing prescriptive knowledge, we enhance the understanding of AI’s value creation paths in the HC industry and thus help to drive AI integration forward. For example, looking at the value proposition risk reduced patient care , we demonstrate that this value proposition is determined by four business objectives: precise decision support , detection of misconduct, reduction of side effects, and reduction of invasiveness . Similarly, the AI application’s capability to analyze data more accurately in diagnosis (use case DD1) enables the business objective precise decision support , thereby reducing risks in patient care. Another mechanism can be seen, for example, considering the business objective task execution , which leads to the value proposition process acceleration . The ability of AI applications to rapidly analyze large amounts of data and recognize patterns in biomedical research (use case BR1) allows a faster drug development process.
Further research
By investigating the value creation mechanism of AI applications for HC organizations, we not only make an important contribution to research and practice but also create a valuable foundation for future studies. While we have systematically identified the relations between the business objectives and value propositions, further research is needed to investigate how the business objectives themselves are determined. While the examination of AI capabilities was not the primary research focus, we found first evidence in the use cases that indicates AI technology’s unique capabilities (e.g., to make diagnoses accurate, faster, and more objective) that foster one or several business objectives (e.g., rapid task execution, precise decision support) and unlock one or several value propositions (e.g., Risk-reduced patient care, process acceleration ). In subsequent research, we aim to integrate these into the value creation mechanism by identifying which specific AI capabilities drive business objectives, thereby advancing the understanding of how AI applications in HC create value propositions.
Limitations
This study is subject to certain limitations of methodological and conceptual nature. First, while our methodological approach covers an in-depth analysis of 21 AI use cases, extending the sample of AI use cases would foster the generalizability of the results. This is especially important regarding the latest developments on generative AI and its newcoming use cases. However, our results demonstrate that these AI use cases already provide rich information to derive 15 business objectives, which translate into six value propositions. Second, while many papers assume the potential of AI applications to create value propositions, only a few papers explicitly focus on the value creation and capture mechanisms. To compensate for this paucity of appropriate papers, we used 11 expert interviews to enrich and evaluate the results. Besides, these interviews ensured the practical relevance and reliability of the derived results. Third, we acknowledge limitations of conceptual nature. Our study predominantly takes an optimistic perspective on AI applications in medicine. While we discuss the potential benefits and value propositions in detail, it is important to emphasize that there are still significant barriers and risks currently associated with AI applications that need to be addressed before the identified values can be realized. Furthermore, our investigation is limited because we derive the expected value of AI applications without having extensive real-world use cases to evaluate. It is important to emphasize that our findings are preliminary, and critical reassessment will be essential as the broader implementation of AI applications in medical practice progresses. These limitations emphasize the need for ongoing research and monitoring to understand the true value of AI applications in HC fully.
Conclusions
This study aimed to investigate how AI applications can create value for HC organizations. After elaborating on a diverse and comprehensive set of AI use cases, we are confident that AI applications can create value by making HC, among others, more precise, individualized, self-determined, faster, resource-optimized, and data insight-driven. Especially with regard to the mounting challenges of the industry, such as the aging population and the resulting increase in HC professionals’ workloads, the integration of AI applications and the expected benefits have become more critical than ever. Based on the systematic literature review and expert interviews, we derived 15 business objectives that translate into the following six value propositions that describe how HC organizations can capture the value of AI applications: risk-reduced patient care, advanced patient care, self-management, process acceleration, resource optimization, and knowledge discovery .
By presenting and discussing our results, we enhance the understanding of how HC organizations can unlock AI applications’ value proposition. We provide HC organizations with valuable insights to help them strategically assess their AI applications as well as those deployed by competitors at a management level. Our goal is to facilitate informed decision-making regarding AI investments and enable HC organizations to align their AI application portfolios with a comprehensive and overarching strategy. However, even if various value proposition-creating scenarios exist, AI applications are not yet fully mature in every area or ready for widespread use. Ultimately, it remains essential to take a critical look at which AI applications can be used for which task at which point in time to achieve the promised value. Nonetheless, we are confident that we can shed more light on the value proposition-capturing mechanism and, therefore, support AI application adoption in HC.
Availability of data and materials
The datasets analyzed during the current study are available from the corresponding author on reasonable request.
Abbreviations
Artificial Intelligence
Machine Learning
Fogel AL, Kvedar JC. Artificial intelligence powers digital medicine. NPJ Digit Med. 2018;1:5.
Article PubMed PubMed Central Google Scholar
Rai A, Constantinides P, Sarker S. Next-Generation Digital Platforms: Toward Human–AI Hybrid. Manag Inf Syst Q. 2019;43(1):iii–ix.
Google Scholar
Russell S, Norvig P. Artificial Intelligence: A Modern Approach. Pearson Education Limited; 2016.
He J, Baxter SL, Xu J, Xu J, Zhou X, Zhang K. The practical implementation of artificial intelligence technologies in medicine. Nat Med. 2019;25(1):30–6.
Article CAS PubMed PubMed Central Google Scholar
Hosny A, Parmar C, Quackenbush J, Schwartz LH, Aerts HJWL. Artificial intelligence in radiology. Nat Rev Cancer. 2018;18(8):500–10.
Kadurin A, Aliper A, Kazennov A, Mamoshina P, Vanhaelen Q, Khrabrov K, et al. The cornucopia of meaningful leads: Applying deep adversarial autoencoders for new molecule development in oncology. Oncotarget. 2017;8(7):10883–90.
Article PubMed Google Scholar
Rezazade Mehrizi MH, van Ooijen P, Homan M. Applications of artificial intelligence (AI) in diagnostic radiology: a technography study. Eur Radiol. 2020;31:1805–11.
Dankwa-Mullan I, Rivo M, Sepulveda M, Park Y, Snowdon J, Rhee K. Transforming Diabetes Care Through Artificial Intelligence: The Future Is Here. Popul Health Manag. 2019;22(3):229–42.
Bohr A, Memarzadeh K. The rise of artificial intelligence in healthcare applications. In: Artificial Intelligence in Healthcare. Elsevier; 2020. p. 25–60.
Yu K-H, Beam AL, Kohane IS. Artificial Intelligence in Healthcare. Nat Biomed Eng. 2018;2(10):719–31.
Jiang F, Jiang Y, Zhi H, Dong Y, Li H, Ma S, et al. Artificial intelligence in healthcare: past, present and future. Stroke Vasc Neurol. 2017;2(4):230–43.
Gilvary C, Madhukar N, Elkhader J, Elemento O. The Missing Pieces of Artificial Intelligence in Medicine. Trends Pharmacol Sci. 2019;40(8):555–64.
Article CAS PubMed Google Scholar
Fernández E. Innovation in Healthcare: Harnessing New Technologies. Journal of the Midwest Association for Information Systems 2017; (2). Available from: http://aisel.aisnet.org/jmwais/vol2017/iss2/8 .
Topol EJ. Deep medicine: How artificial intelligence can make healthcare human again. 1st ed. New York: Basic Books; 2019.
Ngiam KY, Khor IW. Big data and machine learning algorithms for health-care delivery. Lancet Oncol. 2019;20(5):e262–73.
Plastino E, Purdy M. Game changing value from artificial intelligence: eight strategies. Strategy Leadership. 2018;46(1):16–22.
Article Google Scholar
Davenport T, Kalakota R. The potential for artificial intelligence in healthcare. Future Healthcare J. 2019;6(2):94–8.
Meskó B, Görög M. A short guide for medical professionals in the era of artificial intelligence. NPJ Digit Med. 2020;3:126.
Statista. What is the stage of AI adoption in your organization?; 2021. Available from: https://www.statista.com/statistics/1225955/stage-of-ai-adoption-in-healthcare-worldwide/ . Cited 2024 Feb. 8
Garbuio M, Lin N. Artificial Intelligence as a Growth Engine for Health Care Startups: Emerging Business Models. Calif Manage Rev. 2019;61(2):59–83.
Väänänen A, Haataja K, Vehviläinen-Julkunen K, Toivanen P. AI in healthcare A narrative review. F1000Res. 2021;10:6.
Kim H-W, Chan HC, Gupta S. Value-based Adoption of Mobile Internet: An empirical investigation. Decis Support Syst. 2007;43(1):111–26.
Lin TC, Wu S, Hsu JSC, Chou YC. The integration of value-based adoption and expectation–confirmation models An example of IPTV continuance intention. Decision Support Systems. 2012;54(1):63–75.
Jordan MI, Mitchell TM. Machine learning: Trends, perspectives, and prospects. Science. 2015;349(6245):255–60.
LeCun Y, Bengio Y, Hinton G. Deep learning. Nature. 2015;521(7553):436–44.
Hamet P, Tremblay J. Artificial intelligence in medicine. Metab Clin Exp. 2017;69S:S36–40.
Lidströmer N, Aresu F, Ashrafian H. Basic Concepts of Artificial Intelligence Primed for Clinicians In Artificial Intelligence in Medicine. Cham: Springer; 2022. p. 3–20.
Choi RY, Coyner AS, Kalpathy-Cramer J, Chiang MF, Campbell JP. Introduction to machine learning, neural networks, and deep learning. Transl Vis Sci Technol. 2020;9(2):1–12.
Lorenz U. Reinforcement Learning: Aktuelle Ansätze verstehen - mit Beispielen in Java und Greenfoot. Berlin, Heidelberg: Springer Berlin Heidelberg; 2020.
Kunduru AR. Artificial intelligence advantages in cloud fintech application security. Central Asian J Mathematical Theory Comp Sci. 2023;4(8):48–53.
Zhai X, Chu X, Chai CS, Jong MSY, Istenic A, Spector M, et al. A Review of Artificial Intelligence (AI) in Education from 2010 to 2020. Complexity. 2021;2021:1–18.
Zhao Y, Li T, Zhang X, Zhang C. Artificial intelligence-based fault detection and diagnosis methods for building energy systems: Advantages, challenges and the future. Renew Sustain Energy Rev. 2019;109:85–101.
Secinaro S, Calandra D, Secinaro A, Muthurangu V, Biancone P. The role of artificial intelligence in healthcare: a structured literature review. BMC Med Inform Decis Mak. 2021;21(1):125.
Bellini V, Guzzon M, Bigliardi B, Mordonini M, Filippelli S, Bignami E. Artificial intelligence a new tool in operating room management role of machine learning models in operating room optimization. J Med Syst. 2019;44(1):20.
Komorowski M, Celi LA, Badawi O, Gordon AC, Faisal AA. The Artificial Intelligence Clinician learns optimal treatment strategies for sepsis in intensive care. Nat Med. 2018;24(11):1716–20.
Roland T. Motion Artifact Suppression for Insulated EMG to Control Myoelectric Prostheses. Sensors (Basel). 2020;20(4):1031.
Amann J, Blasimme A, Vayena E, Frey D, Madai VI. Explainability for artificial intelligence in healthcare: a multidisciplinary perspective. BMC Med Inform Decis Mak. 2020;20(1):310.
Hennrich J, Fuhrmann H, Eymann T. Accelerating the Adoption of Artificial Intelligence Technologies in Radiology: A Comprehensive Overview on Current Obstacles. Proceedings of the 57th Hawaii International Conference on System Sciences 2024.
Asan O, Bayrak AE, Choudhury A. Artificial Intelligence and Human Trust in Healthcare: Focus on Clinicians. J Med Internet Res. 2020;22(6):e15154.
Schryen G. Revisiting IS business value research: what we already know, what we still need to know, and how we can get there. Eur J Inf Syst. 2013;22(2):139–69.
Haddad P, Wickramasinghe N. Conceptualizing Business Value of IT in Healthcare to Design Sustainable e-Health Solutions. Proceedings of Americas Conference on Information Systems 2014.
Strong DM, Volkoff O, Johnson SA, Pelletier LR, Tulu B, Bar-On I, et al. A Theory of Organization-EHR Affordance Actualization. J Assoc Inf Syst. 2014;15(2):53–85.
Hofmann P, Oesterle S, Rust P, Urbach N. Machine Learning Approaches Along the Radiology Value Chain - Rethinking Value Propositions. Proceedings of the European Conference on Information Systems 2019.
Badakhshan P, Wurm B, Grisold T, Geyer-Klingeberg J, Mendling J, Vom Brocke J. Creating business value with process mining. J Strateg Inf Syst. 2022;31(4):101745.
Webster J, Watson RT. Analyzing the Past to Prepare for the Future Writing a Literature Review. MIS Quarterly. 2002;26(2):xiii–xxiii.
Wolfswinkel JF, Furtmueller E, Wilderom CPM. Using Grounded Theory as a Method for Rigorously Reviewing Literature. Eur J Inf Syst. 2013;22(1):45–55.
Siggelkow N. Persuasion With Case Studies. AMJ. 2007;50(1):20–4.
Lakhani P, Prater AB, Hutson RK, Andriole KP, Dreyer KJ, Morey J, et al. Machine Learning in Radiology: Applications Beyond Image Interpretation. J Am Coll Radiol. 2018;15(2):350–9.
Low S-K, Zembutsu H, Nakamura Y. Breast cancer: The translation of big genomic data to cancer precision medicine. Cancer Sci. 2018;109(3):497–506.
Altay EV, Alatas B. Association analysis of Parkinson disease with vocal change characteristics using multi-objective metaheuristic optimization. Med Hypotheses. 2020;141:109722.
Abbasi B, Goldenholz DM. Machine learning applications in epilepsy. Epilepsia. 2019;60(10):2037–47.
Qin B, Quan Q, Wu J, Liang L, Li D. Diagnostic performance of artificial intelligence to detect genetic diseases with facial phenotypes: A protocol for systematic review and meta analysis. Medicine (Baltimore). 2020;99(27):e20989.
Zhavoronkov A, Mamoshina P, Vanhaelen Q, Scheibye-Knudsen M, Moskalev A, Aliper A. Artificial intelligence for aging and longevity research: Recent advances and perspectives. Ageing Res Rev. 2019;49:49–66.
Meystre SM, Friedlin FJ, South BR, Shen S, Samore MH. Automatic de-identification of textual documents in the electronic health record - a review of recent research. BMC Med Res Methodol. 2010;10(70):1–6.
Rhine CL, Neil C, Glidden DT, Cygan KJ, Fredericks AM, Wang J, et al. Future directions for high-throughput splicing assays in precision medicine. Hum Mutat. 2019;40(9):1225–34.
Berlyand Y, Raja AS, Dorner SC, Prabhakar AM, Sonis JD, Gottumukkala RV, et al. How artificial intelligence could transform emergency department operations. Am J Emerg Med. 2018;36(8):1515–7.
Kwon J-M, Kim K-H, Jeon K-H, Park J. Deep learning for predicting in-hospital mortality among heart disease patients based on echocardiography. Echocardiography. 2019;36(2):213–8.
Yang M, Li C, Shen Y, Wu Q, Zhao Z, Chen X. Hierarchical Human-Like Deep Neural Networks for Abstractive Text Summarization. IEEE Trans Neural Netw Learn Syst. 2021;32(6):2744–57.
Hernandez JPT. Network diffusion and technology acceptance of a nurse chatbot for chronic disease self-management support a theoretical perspective. J Med Invest. 2019;66(1.2):24–30.
Bhandari M, Zeffiro T, Reddiboina M. Artificial Intelligence and Robotic Surgery: Current Perspective and Future Directions. Curr Opin Urol. 2020;30(1):48–54.
Padoy N. Machine and deep learning for workflow recognition during surgery. Minim Invasive Ther Allied Technol. 2019;28(2):82–90.
Shepherd M, Abidi SSR, Gao Q, Chen Z, Qi Q, Finley GA. Information Systems and Health Care IX: Accessing Tacit Knowledge and Linking It to the Peer-Reviewed Literature. CAIS 2006; 17.
Wilson EV, Wang W, Sheetz SD. Underpinning a Guiding Theory of Patient-Centered E-Health. CAIS. 2014;34(1):16.
Levy Y, Ellis TJ. A systems approach to conduct an effective literature review in support of information systems research. Informing Sci J. 2006;9:181–211.
Corbin JM, Strauss AL. Basics of qualitative research: Techniques and procedures for developing grounded theory. Fourth edition. Thousand Oaks, Kalifornien: SAGE; 2015.
Glaser BG, Strauss A. Discovery of grounded theory: Strategies for qualitative research. Routledge; 1967.
Feldman S, Horan T. The dynamics of information collaboration: a case study of blended IT value propositions for health information exchange in disability determination. J Assoc Inf Syst. 2011;12(2):189–207.
Schultze U, Avital M. Designing interviews to generate rich data for information systems research. Inf Organ. 2011;21(1):1–16.
Myers MD, Newman M. The qualitative interview in IS research: examining the craft. Inf Organ. 2007;17(1):2–26.
Bhattacherjee A. Social Science Research: Principles, Methods, and Practices. Textbooks Collection 2012; 3.
Moulaei K, Sheikhtaheri A, Fatehi F, Shanbehzadeh M, Bahaadinbeigy K. Patients’ perspectives and preferences toward telemedicine versus in-person visits: a mixed-methods study on 1226 patients. BMC Med Inform Decis Mak. 2023;23(1):261.
Buck C, Hennrich J, Kauffmann A-L. Artificial Intelligence in Radiology – A Qualitative Study on Imaging Specialists’ Perspectives. Proceedings of the 42nd International Conference on Information Systems 2021.
Degnan AJ, Ghobadi EH, Hardy P, Krupinski E, Scali EP, Stratchko L, et al. Perceptual and interpretive error in diagnostic radiology-causes and potential solutions. Acad Radiol. 2019;26(6):833–45.
Pesapane F, Codari M, Sardanelli F. Artificial intelligence in medical imaging: threat or opportunity? Radiologists again at the forefront of innovation in medicine. Eur Radiol Exp. 2018;2(1):35.
Schinkel M, Paranjape K, Nannan Panday RS, Skyttberg N, Nanayakkara PWB. Clinical applications of artificial intelligence in sepsis: A narrative review. Comput Biol Med. 2019;115:103488.
Shamim Nemati, Mohammad Ghassemi, Gari Clifford. Optimal medication dosing from suboptimal clinical examples: A deep reinforcement learning approach. International Conference of the IEEE Engineering in Medicine and Biology Society 2016. Available from: http://ieeexplore.ieee.org/servlet/opac?punumber=7580725 .
Padoy N, Hager GD. Human-Machine Collaborative Surgery Using Learned Methods. Proceedings of IEEE International Conference on Robotics and Automation 2011:5285–92.
Nadarzynski T, Miles O, Cowie A, Ridge D. Acceptability of artificial intelligence (AI)-led chatbot services in healthcare: A mixed-methods study. Digit Health. 2019;5:2055207619871808.
PubMed PubMed Central Google Scholar
Trevitt S, Simpson S, Wood A. Artificial Pancreas device systems for the closed-loop control of type 1 diabetes: what systems are in development? J Diabetes Sci Technol. 2016;10(3):714–23.
Luo L, Zhang F, Yao Y, Gong R, Fu M, Xiao J. Machine learning for identification of surgeries with high risks of cancellation. Health Informatics J. 2018;26(1):141–55.
Shollo A, Hopf K, Thiess T, Müller O. Shifting ML value creation mechanisms: a process model of ML value creation. J Strateg Inf Syst. 2022;31(3):101734.
Buck C, Doctor E, Hennrich J, Jöhnk J, Eymann T. General Practitioners’ Attitudes Toward Artificial Intelligence-Enabled Systems: Interview Study. J Med Internet Res. 2022;24(1):e28916.
Bennett SJ. Transmuting values in artificial intelligence: investigating the motivations and contextual constraints shaping the ethics of artificial intelligence practitioners; 2023.
Baumgartner R, Arora P, Bath C, Burljaev D, Ciereszko K, Custers B, et al. Fair and equitable AI in biomedical research and healthcare: Social science perspectives. Artif Intell Med. 2023;144:102658.
Download references
Acknowledgements
The authors thank all physicians who participated in this study.
Open Access funding enabled and organized by Projekt DEAL.
Author information
Authors and affiliations.
FIM Research Institute for Information Management, University of Bayreuth, Branch Business and Information Systems Engineering of the Fraunhofer FIT, Wittelsbacherring 10, 95444, Bayreuth, Germany
Jasmin Hennrich, Peter Hofmann & Nils Urbach
University St. Gallen, Dufourstrasse 50, 9000, St. Gallen, Switzerland
Faculty Business and Law, Frankfurt University of Applied Sciences, Nibelungenplatz 1, 60318, Frankfurt Am Main, Germany
Nils Urbach
appliedAI Initiative GmbH, August-Everding-Straße 25, 81671, Munich, Germany
Peter Hofmann
You can also search for this author in PubMed Google Scholar
Contributions
JH initially composed the introduction, background, and discussion sections; PH contributed to the analysis design, supported data analysis, and critically reviewed the manuscript; ER conducted data collection and analysis and produced the initial draft of the methodology section; NU edited and critically reviewed the manuscript and provided guidance. All authors read and approved the final manuscript.
Corresponding author
Correspondence to Jasmin Hennrich .
Ethics declarations
Ethics approval and consent to participate.
The study was based on original data. The authors confirm that all methods were carried out in accordance with relevant guidelines and regulations and confirm that informed consent was obtained from all participants. Ethics approval was granted by the Ethics Committee of the University of Bayreuth (Application-ID 23–032).
Consent for publication
Not applicable.
Competing interests
The authors declare no competing interests.
Additional information
Publisher’s note.
Springer Nature remains neutral with regard to jurisdictional claims in published maps and institutional affiliations.
Supplementary Information
Supplementary material 1., rights and permissions.
Open Access This article is licensed under a Creative Commons Attribution 4.0 International License, which permits use, sharing, adaptation, distribution and reproduction in any medium or format, as long as you give appropriate credit to the original author(s) and the source, provide a link to the Creative Commons licence, and indicate if changes were made. The images or other third party material in this article are included in the article's Creative Commons licence, unless indicated otherwise in a credit line to the material. If material is not included in the article's Creative Commons licence and your intended use is not permitted by statutory regulation or exceeds the permitted use, you will need to obtain permission directly from the copyright holder. To view a copy of this licence, visit http://creativecommons.org/licenses/by/4.0/ . The Creative Commons Public Domain Dedication waiver ( http://creativecommons.org/publicdomain/zero/1.0/ ) applies to the data made available in this article, unless otherwise stated in a credit line to the data.
Reprints and permissions
About this article
Cite this article.
Hennrich, J., Ritz, E., Hofmann, P. et al. Capturing artificial intelligence applications’ value proposition in healthcare – a qualitative research study. BMC Health Serv Res 24 , 420 (2024). https://doi.org/10.1186/s12913-024-10894-4
Download citation
Received : 26 October 2023
Accepted : 25 March 2024
Published : 03 April 2024
DOI : https://doi.org/10.1186/s12913-024-10894-4
Share this article
Anyone you share the following link with will be able to read this content:
Sorry, a shareable link is not currently available for this article.
Provided by the Springer Nature SharedIt content-sharing initiative
- Artificial intelligence
- Value propositions
- Business objectives
BMC Health Services Research
ISSN: 1472-6963
- General enquiries: [email protected]
Loading metrics
Open Access
Peer-reviewed
Research Article
Mathematical models of drug-resistant tuberculosis lack bacterial heterogeneity: A systematic review
Roles Conceptualization, Data curation, Formal analysis, Investigation, Methodology, Project administration, Validation, Visualization, Writing – original draft, Writing – review & editing
* E-mail: [email protected]
Affiliations Department of Infectious Disease Epidemiology, Faculty of Epidemiology and Population Health, London School of Hygiene and Tropical Medicine, London, United Kingdom, Centre for Mathematical Modelling of Infectious Diseases, London School of Hygiene and Tropical Medicine, London, United Kingdom, Antimicrobial Resistance Centre, London School of Hygiene and Tropical Medicine, London, United Kingdom, Tuberculosis Centre, London School of Hygiene and Tropical Medicine, London, United Kingdom

Roles Data curation, Validation, Writing – review & editing
Roles Data curation, Writing – review & editing
Roles Conceptualization, Writing – review & editing
Affiliation UCL Centre for Clinical Microbiology, Division of Infection & Immunity, Royal Free Campus, University College London, London, United Kingdom
Roles Conceptualization, Data curation, Formal analysis, Investigation, Methodology, Supervision, Validation, Visualization, Writing – original draft, Writing – review & editing
- Naomi M. Fuller,
- Christopher F. McQuaid,
- Martin J. Harker,
- Chathika K. Weerasuriya,
- Timothy D. McHugh,
- Gwenan M. Knight
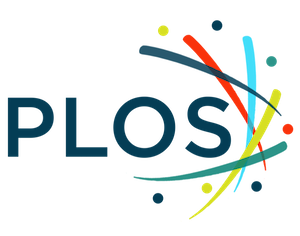
- Published: April 10, 2024
- https://doi.org/10.1371/journal.ppat.1011574
- Peer Review
- Reader Comments
This is an uncorrected proof.
Drug-resistant tuberculosis (DR-TB) threatens progress in the control of TB. Mathematical models are increasingly being used to guide public health decisions on managing both antimicrobial resistance (AMR) and TB. It is important to consider bacterial heterogeneity in models as it can have consequences for predictions of resistance prevalence, which may affect decision-making. We conducted a systematic review of published mathematical models to determine the modelling landscape and to explore methods for including bacterial heterogeneity. Our first objective was to identify and analyse the general characteristics of mathematical models of DR-mycobacteria, including M . tuberculosis . The second objective was to analyse methods of including bacterial heterogeneity in these models. We had different definitions of heterogeneity depending on the model level. For between-host models of mycobacterium, heterogeneity was defined as any model where bacteria of the same resistance level were further differentiated. For bacterial population models, heterogeneity was defined as having multiple distinct resistant populations. The search was conducted following PRISMA guidelines in five databases, with studies included if they were mechanistic or simulation models of DR-mycobacteria. We identified 195 studies modelling DR-mycobacteria, with most being dynamic transmission models of non-treatment intervention impact in M . tuberculosis (n = 58). Studies were set in a limited number of specific countries, and 44% of models (n = 85) included only a single level of “multidrug-resistance (MDR)”. Only 23 models (8 between-host) included any bacterial heterogeneity. Most of these also captured multiple antibiotic-resistant classes (n = 17), but six models included heterogeneity in bacterial populations resistant to a single antibiotic. Heterogeneity was usually represented by different fitness values for bacteria resistant to the same antibiotic (61%, n = 14). A large and growing body of mathematical models of DR-mycobacterium is being used to explore intervention impact to support policy as well as theoretical explorations of resistance dynamics. However, the majority lack bacterial heterogeneity, suggesting that important evolutionary effects may be missed.
Author summary
The emergence of drug-resistant tuberculosis (DR-TB), where the causative bacterium Mycobacterium tuberculosis is resistant to key antibiotics such as rifampicin and isoniazid, poses a significant threat to TB control efforts. To gain a broader understanding of the challenges surrounding DR-TB, mathematical models are increasingly being employed to estimate the impact of interventions, effectiveness of treatment, and to predict the evolution of drug-resistance. However, pragmaticism surrounding model construction often means that important aspects, such as bacterial heterogeneity, are overlooked. We undertook a systematic review of the existing DR-mycobacterium modelling literature, with the specific aim of capturing methods for including bacterial heterogeneity. Our analysis revealed that most models of drug-resistance in mycobacteria primarily focus on intervention strategies and cost-effectiveness analyses, with minimal attention to bacterial heterogeneity. Where heterogeneity is included it mostly consisted of different fitness costs for resistance.
Citation: Fuller NM, McQuaid CF, Harker MJ, Weerasuriya CK, McHugh TD, Knight GM (2024) Mathematical models of drug-resistant tuberculosis lack bacterial heterogeneity: A systematic review. PLoS Pathog 20(4): e1011574. https://doi.org/10.1371/journal.ppat.1011574
Editor: Mark Robert Davies, University of Melbourne, AUSTRALIA
Received: July 25, 2023; Accepted: March 25, 2024; Published: April 10, 2024
Copyright: © 2024 Fuller et al. This is an open access article distributed under the terms of the Creative Commons Attribution License , which permits unrestricted use, distribution, and reproduction in any medium, provided the original author and source are credited.
Data Availability: All relevant data are within the manuscript and its Supporting Information files.
Funding: This research and NMF was funded by the Biotechnology and Biological Sciences Research Council through the London Interdisciplinary Doctoral Training Programme (BBSRC LIDO, https://www.lido-dtp.ac.uk ) at the London School of Hygiene and Tropical Medicine (LSHTM) in partnership with University College London (UCL), Grant code - BB/M009513/1. CFM was funded for other work by Bill and Melinda Gates Foundation (TB MAC OPP1135288, INV-059518, https://www.gatesfoundation.org ) and Unitaid (20193–3-ASCENT, https://unitaid.org/calls/#en ). CKW was supported by a grant from the Bill and Melinda Gates Foundation (INV-001754, https://www.gatesfoundation.org ). GMK was supported by Medical Research Council UK, https://www.ukri.org/opportunity/career-development-award/ (MR/ W026643/1). The views expressed are those of the authors and not necessarily those of the BBSRC, LIDO, LSHTM or UCL. The funders had no role in study design, data collection and analysis, decision to publish, or preparation of the manuscript.
Competing interests: The authors have declared that no competing interests exist.
Introduction
Drug-resistant (DR-) strains of Mycobacterium tuberculosis ( M . tuberculosis ) are an urgent threat to the control of tuberculosis disease (TB) globally. For TB, the backbone antibiotics of standard therapy are rifampicin and isoniazid. In 2021, multidrug-resistant (combined rifampicin and isoniazid resistance) or rifampicin-resistant tuberculosis (MDR/RR-TB) caused an estimated 450,000 cases globally [ 1 ].
Routinely collected antimicrobial resistance (AMR) data use microbiological definitions of resistance, which are guided by threshold cut-offs for phenotypic resistance, resulting in discrete categorisations. For TB, these categorisations are further grouped with strains being classified as drug-susceptible (DS-), multidrug- or rifampicin-resistant- (MDR/RR-), pre-extensively-drug (pre-XDR) resistant (MDR plus resistance to a fluoroquinolone) or XDR- resistant (MDR plus resistance to a fluoroquinolone and a Group A drug) [ 1 ]. The MDR/RR grouping is based on the knowledge that isoniazid resistance is commonly acquired prior to rifampicin resistance and the wider prevalence of rifampicin-resistance testing through genotypic testing, making clinical management of RR- and MDR-TB similar [ 2 , 3 ]. These definitions are sufficient for patient care decision-making that does not need to account for the spectrum of phenotypic resistance levels (for example, those below the threshold for successful treatment) or any other bacterial characteristics (such as types of resistance-conferring mutations). However, bacterial populations are often highly diverse with a spectrum of characteristics. Hence, resistance categories will also have a high degree of bacterial heterogeneity, such as variation in transmission fitness between strains with the same phenotypic resistance, which affects the rate at which M . tuberculosis spreads between individuals.
Several important insights into the evolution of DR-TB, its emergence and spread, and the control of resistant bacteria more broadly have been generated by mathematical models. Some examples are the predominance of primary rather than acquired resistance, the effectiveness of TB surveillance for controlling DR-TB, and the potential impact of controlling HIV on reducing TB transmission [ 4 – 7 ]. Most mathematical models of AMR have typically adopted binary ( e . g . resistant versus susceptible) categorisations. When bacterial heterogeneity is included in mathematical models, the predicted public health outcomes can be different from those when bacterial heterogeneity is ignored [ 8 ]. We may lose subtlety in model outputs when modelling antibiotic treatment as a selective pressure if the traits allowing for bacterial heterogeneity are not included. Models may miss key dynamics, such as competition between strains and antibiotic effectiveness against strains with varying resistance levels, and be at risk of incorrectly predicting the effectiveness of a treatment intervention. As Trauer et al. (2018) point out, strain diversity, virulence and fitness costs have implications for the trajectory of drug resistance in TB [ 9 ]. Decisions as to what to include in a model will depend on the questions being asked, the selective pressures modelled, and the time-frame studied. Assessing this balance in model design between detailed and generalised parameters to allow a pragmatic approach for public health interventions can often prove challenging. Hence, assessing the extent to which bacterial heterogeneity has been included in existing models that predict intervention impact for DR-TB control is highly important.
Previous systematic reviews have explored the landscape of mathematical models of AMR [ 7 , 10 ] and TB [ 11 – 14 ], with up to 43 DR-TB transmission and 52 within-host studies being found prior to 2016. To our knowledge, only one expert review from 2009 focused on mathematical models of DR-TB [ 4 ], emphasising the useful insights from modelling but also highlighting important knowledge gaps in the economics, biological impact of mutations and ability to control DR-TB. To date, there is little evidence on how bacterial heterogeneity is incorporated into DR-TB models and little evidence of the effect this would have on model outcomes.
Mycobacteria predominantly develop antibiotic resistance via mutation [ 15 ], resulting in different patterns of resistance dynamics to other bacterial genera. Mycobacterial species other than M . tuberculosis can often be used as experimental or theoretical models for M . tuberculosis and are also responsible for a clinical burden [ 16 – 18 ]. They are often used to understand the resistance dynamics of M . tuberculosis [ 19 , 20 ].
We aimed to support future modelling of interventions against DR-TB by systematically surveying the characteristics of mathematical models of mycobacteria, of which we expect the M . tuberculosis species to dominate due to its substantial clinical burden. Our secondary objective was categorising the amount and type of bacterial heterogeneity included in mathematical models of DR-mycobacteria. We envisaged two broad settings of papers to be included in this review, within-host and between-host transmission models. This was noted by Cohen et al. (2009), a previous review of the DR-TB modelling literature [ 4 ], where “between-host” models refer to models on the human population scale. Since 2009, there has been an increase in models of bacterial populations set in the laboratory. As the populations captured will be similar to within-host models, we combined laboratory models and within-host models and collectively called them “bacterial population” models.
The aims, dynamics and model structure of between-host models differ considerably from bacterial population models, namely by transmission of the pathogen and populations included, making them difficult to compare. Therefore, we defined heterogeneity differently for bacterial populations and between-host models to compare methods within these categories and gain a clearer picture of bacterial heterogeneity modelling. At the between-host level, we were interested in capturing those models that went beyond capturing resistance phenotypes but included any added dimension of bacterial variation, including what may affect survival, such as fitness effects. Models of bacterial populations that captured any resistance variation were included; distinct populations of resistant bacteria needed to be modelled, which differed in their parameter values (e.g. growth rate or mutation rate).
Our review consisted of two stages of selection and data analysis. In Stage 1 of the review, our aim was to identify and analyse the general characteristics of mathematical models pertaining to drug-resistant (DR-) mycobacteria, such as model type and aim. In Stage 2 of the review, our focus was to identify mathematical models of DR-mycobacteria that specifically incorporated the concept of bacterial heterogeneity, as elucidated by the definition in the inclusion and exclusion criteria section.
Search strategy
The systematic review was designed and conducted following the PRISMA reporting protocol to search and review mathematical modelling papers of DR-mycobacteria [ 21 ]. The search terms consisted of those relevant to [ 1 ] “mycobacteria”, [ 2 ] “mathematical modelling”, and [ 3 ] “antibiotic resistance” ( S1 Text ). The search was conducted in five databases (Medline, Embase, Global Health, Web of Science and Scopus) initially on January 22nd, 2021, and then repeated on April 1st, 2022. Duplicates were removed before screening.
Inclusion and exclusion criteria
The screening process of the papers adhered to predefined inclusion and exclusion criteria ( Table 1 ). Initially, the titles and abstracts of the papers were screened to identify mathematical models specifically pertaining to DR-mycobacteria, followed by a full-text screening for inclusion in Stage 1. Finally, another round of full-text screening was carried out on the remaining papers to identify those appropriate for Stage 2 of the study.
- PPT PowerPoint slide
- PNG larger image
- TIFF original image
https://doi.org/10.1371/journal.ppat.1011574.t001
Mathematical models were defined as mechanistic models or simulation models reproducing a mathematically described scenario of DR-mycobacteria or of individuals carrying DR-mycobacteria. We excluded statistical analyses, such as regression models or risk analysis; molecular modelling (those focused on molecular structure of chemical compounds) or those only focused on drug development; models of drug-resistance that only used mycobacteria as an example or discussion point unless results for DR-mycobacteria were specifically included.
We split models into two groupings: “between-host” and “bacterial population” models, with the differences in their model scale, structure, and aims, resulting in a different bacterial heterogeneity definition. A “between-host” model was classed as a heterogenous model when strains infecting a human population resistant to the same drug varied in another characteristic such as fitness, rates of compensatory mutation evolution or associated treatment recovery rates. These characteristics were extracted during the full-text extraction stage. “Bacterial population” models included both within-host and models of bacterial populations capturing dynamics measured in laboratory or experimental conditions. A bacterial population model was classed as a heterogeneous model when there were distinct resistant strains captured which had different parameter values such as fitness, mutation rates and metabolic states. These parameter differences were extracted during the full-text extraction stage.
Selection and extraction: Stage 1
Title and abstract screening were performed for every paper by at least two authors (NMF, GMK, CFM, MJH and CKW) to determine if the paper likely included a mathematical model of DR-mycobacteria. High-level data extraction from these screened papers that continued to match the criteria for Stage 1 upon full-text screening provided a landscape analysis of DR-mycobacteria models. DR-mycobacteria models can address multiple aims with various methods, but they will have a common theme, such as parameter estimation or evaluation of the impact of interventions. We extracted information from the models to categorise and classify them into five categories, focusing on the main theme of the model. 1) model setting (such as geographic location), 2) model aims (7 categories of; non-treatment i nterventions that did not explore antibiotic usage (with and without cost-effectiveness), treatment interventions (with and without cost-effectiveness), parameter estimation, burden estimation or theoretical), 3) model type (7 categories of; bacterial dynamics, decision analytic, PK/PD, state transition (with and without a statistical component) or transmission (with or without an operational or state transition component), 4) mycobacterial species and 5) resistance classifications (such as MDR or XDR) ( S2 Text ). We extracted resistance classifications based on what the authors defined in their papers, as current resistance definitions are continuously updated. A resistance class is defined as a model stratification whereby strains (or the populations including them) are grouped across multiple antibiotic resistances (i.e. MDR could here be a single “resistance class” but represents resistance to multiple antibiotic agents). We only extracted which antibiotics were modelled in papers if their resistance was also considered. This extraction was performed by NMF and GMK, with discussions to resolve any conflicts.
Selection and extraction: Stage 2
For Stage 2, full-text screening of the Stage 1 papers was performed by three authors (NMF, GMK, CFM) to determine the models with bacterial heterogeneity, with subsequent discussions and consensus to resolve any discrepancies. NMF performed full-text extraction and data analysis of the extracted data from these papers ( S2 Table ). Stage 2 extracted data on the methods used to model heterogeneity, types of heterogeneity included, data sources and the effect of resistance inclusion (such as resistance effects on disease progression) ( S3 Text ).
After the removal of duplicates, 3,180 papers were identified ( Fig 1 ). Following a title and abstract screening, 372 papers remained for full-text screening. 195 papers were found to fulfil our Stage 1 criteria having a model of DR-mycobacteria strains ( S1 Table ). Of these papers, only 23 were found to meet the requirements of bacterial heterogeneity in mathematical models of DR-mycobacteria ( S2 Table ).
https://doi.org/10.1371/journal.ppat.1011574.g001
Stage 1 Results: DR-mycobacteria model landscape
Most models of mycobacteria were of M . tuberculosis (190 papers/97%) with HIV (59 papers) and diabetes mellitus (5 papers) often included. There was a rapid increase in the number of papers published on DR-mycobacterium from 2005 onwards ( S1 Fig ).
Settings captured
119 papers aimed to model a specific geographical location, typically at the national level ( Fig 2A and S3 Table ). This reflects the settings with the highest MDR-TB incidence but also highlights some countries that are not being focused on ( Fig 2B ). Of the 117 papers, 82 covered a single national analysis and 35 covered different countries. Other geographical locations included 7 models with a global focus, whilst 6 models covered regions with 4 models of Southeast Asia [ 22 – 24 ], and 1 of Eastern Europe [ 25 ] and 1 of the Asia-Pacific [ 26 ].
(a) Countries captured in models of DR-mycobacteria. Note: some models include outputs for multiple countries, therefore this image represents all countries modelled, not the total number of models. (b) From the WHO Global Tuberculosis Report 2022 [ 1 ], the 10 countries with the highest estimated MDR/RR-TB incidence are given with number of models in brackets. The colours in the table match the corresponding colours of the country in part (a). Map layer made with Natural Earth, free vector and raster map data @ naturalearthdata.com .
https://doi.org/10.1371/journal.ppat.1011574.g002
Model aims and types
Of the seven distinct categories of study aim found ( Fig 3 ), non-treatment interventions without cost-effectiveness considered (n = 45, 23%) was the most common. Transmission models (n = 129, 67%) were the most common model type used for all model aims, except for “treatment interventions with cost-effectiveness”, which mostly used state transition models ( Fig 3 ). As would be expected, PK/PD models were used almost exclusively for “treatment interventions”, with one model being used for parameter estimation. Six models used a combination of methods: transmission and state transition [ 27 , 28 ], transmission and operational [ 29 ]and state transition and statistical [ 30 – 32 ]. “Bacterial dynamics” type models were used for “treatment interventions”, “theoretical” and “parameter estimation” aims only. “Decision analytic” type models were used for all aims other than “theoretical” and “parameter estimation”.
The model type (colours) definitions can be summarised as follows: [ 1 ] Bacterial dynamics: Capture bacterial populations without considering between-host transmission. [ 2 ]; Decision analytic: Track cohorts of human individuals through treatment or diagnostic pathways without ongoing transmission. [ 3 ] Pharmacokinetic/pharmacodynamic (PK/PD): Focus on drug concentrations and their effects in vivo, incorporating parameters related to bacterial populations. [ 4 ] State Transition: Involve individuals or populations transitioning between different disease states, with the force of infection as a static input parameter. [ 5 ] Statistical: inference-based models of collected or population data. [ 6 ] Transmission: Dynamically account for the spread of bacteria between individuals or populations. [ 7 ] Operational models: simulation of patient pathways and treatment or diagnostic procedures. The model aim (x axis) definitions can be summarised as follows: (1) Non-treatment Interventions: Model the impact of interventions not related to changes in antibiotic usage or treatment without considering economic aspects. (2) Non-treatment Interventions + cost-effectiveness: Model the impact of interventions not related to changes in antibiotic usage or treatment while considering their economic impact. (3) Treatment interventions: Model interventions related to changes in antibiotic usage. (4) Treatment interventions + cost-effectiveness: Model interventions related to changes in antibiotic usage while considering their economic impact. (5) Parameter estimation: Estimate parameters by comparing to data, trends, or varying model structures or components. (6) Burden estimation models: Quantify the number of individuals potentially infected with DR-mycobacteria. (7) Theoretical models: Theoretically explore interactions between susceptible and resistant strains. Note: "CE" stands for cost-effectiveness. For full details of aim and model type see S2 Text .
https://doi.org/10.1371/journal.ppat.1011574.g003
Resistance categories
Most models of DR-mycobacteria capture resistance to fewer than three antibiotics. Six models considered all possible combinations of resistance to several antibiotics (‘*’, Fig 4 ). Of 16 models to capture four or more resistances at once, 11 of these models included antibiotic resistance as stepwise accumulation of resistance [ 22 , 30 , 33 – 41 ] and 5 models only included mono-resistance of resistance to multiple antibiotics [ 20 , 42 – 45 ].
Each coloured cell represents a specific combination of resistances included in a model, with the size of the cell representing how many models included this combination of resistances. “Single” and “Multiple” sections refer to the number of antibiotic resistances included in a model, with “Multiple” referring to models that captured resistance to more than one antibiotic. "*" indicates the model included all possible combinations of antibiotic resistance listed. A = INH, RIF, MDR/RR, MOX, PZA, BDQ, PA, RIF + MOX, RIF + PZA, B = INH, RIF, MDR/RR, AMI, MOX, BDQ, RIF + MOX, RIF + AMI, RIF + BDQ, C = INH, RIF, MDR/RR, XDR, MDR + FQ, MDR + SLInject, D = INH, RIF, MDR/RR, XDR, Pre-XDR. Antibiotic abbreviations as follows: AMI = amikacin, BDQ = bedaquiline, CLR = clarithromycin, ETM = ethambutol, FQ = undefined fluoroquinolone, MOX = moxifloxacin, PA = pretomanid, PZA = pyrazinamide, STR = streptomycin, INH = isoniazid, RIF = rifampicin, MDR/RR = multidrug resistant/rifampicin resistant, XDR = extensively drug-resistant, SLInject = second line injectable antibiotic (from WHO guidelines 2014). S1 Fig shows all resistance categories per 195 models.
https://doi.org/10.1371/journal.ppat.1011574.g004
Overall, for stage 1, most models included a resistance class of MDR/RR-TB (129 papers/67%, Fig 4 ) with 85 models that chose to model only a single resistance class of MDR/RR-TB alongside DS-TB ( Fig 4 ). 40/195 models included isoniazid resistance ( Fig 4 ) with 27/40 also including MDR/RR-TB. 21/195 models included rifampicin resistance separate from MDR with 15/21 including isoniazid and rifampicin resistance as mono-resistances that developed into MDR with 6/15 models including the development of XDR-TB. Of 18 models that modelled XDR, 16 included MDR/RR, while two did not [ 46 , 47 ]. Out of the first-line antibiotics used to treat TB, isoniazid (n = 40) and rifampicin (n = 27) resistance were modelled the most, followed by pyrazinamide (n = 8) and then ethambutol (n = 5) resistance. Pyrazinamide resistance was often found to be modelled alongside rifampicin and/or isoniazid resistance with only 3 models including resistance to all 4 first-line antibiotics, 2 with mono-resistances and 1 with a combination of all 4 resistances [ 33 , 37 , 42 ] ( Fig 4 ).
41 theoretical models included resistance to a non-named antibiotic ( S1 Table ). One of these explored differences in drug action (bacteriostatic or bactericidal [ 48 ], and two explored antibiotic persistence [ 49 , 50 ] ( S1 Fig ). There were 38 theoretical modelling studies ( S1 Fig ) capturing “drug resistance”, with four of these models exploring firstly hypothetical and then antibiotic-specific resistance ( S1 Table ).
Stage 2 Results: Heterogeneous models
We found 23 models with bacterial heterogeneity—15 bacterial population and 8 between-host models ( S2 Table ) [ 8 , 20 , 33 , 34 , 37 , 43 – 45 , 48 , 49 , 51 – 63 ]. The distribution of model aims that these papers fall into were different from Stage 1 with 13 “parameter estimation”, 8 “treatment interventions”, 1 “theoretical”, and 1 “non-treatment intervention”. 12 of the 23 models modelled the immune system.
Bacterial population models
The fifteen bacterial population models mostly captured multiple resistance classes (n = 13) ( Fig 5 and S2 Table ). One other considered a single resistance class of isoniazid only in an M . tuberculosis population and explored deterministically the impact of antibiotic exposure on resistance dominance with or without heterogeneity in fitness and mutation distributions [ 52 ]. Including heterogeneity in fitness and mutation distributions was also the most common method for exploring variation in models with multiple resistance classes. This was true both for stochastic and deterministic model structures [ 33 , 43 , 51 , 57 , 59 , 62 ], though one deterministic model only explored differences in mutation rates [ 43 ]. Four models additionally explored the impact of variation in growth rates induced by different metabolic states [ 20 , 34 , 45 , 60 ], with one model including fitness variation too [ 45 ].
https://doi.org/10.1371/journal.ppat.1011574.g005
Different clearance rates were used in 2 models, a PK/PD model and a bacterial dynamics model to differentiate between two resistant bacterial strains with the aim of determining the most effective treatment combination [ 48 , 58 ].
One model did not include AMR as a direct resistance to an antibiotic, but instead as persistence [ 49 ]. This was modelled as non-replicating bacterial populations and antibiotics had little to no effect on these bacterial populations. The model implemented heterogeneity by including fast and slow-growing bacteria.
Between-host models
All eight between-host models were compartmental models. Six of these models explored the impact of including a distribution of fitness costs affecting transmission resulting from resistance-conferring mutations to prevalence of either a single [ 8 , 53 , 55 , 56 ] or multiple resistance classes [ 54 , 63 ]. Four of these six models were deterministic [ 53 , 55 , 56 , 63 ], with Knight et al. (2015) exploring a stochastic version in the supplementary materials [ 8 ]. Blower et al. (2004) explored a stochastic model that included heterogeneity by modelling strains of M . tuberculosis with different fitness rates but also cure, treatment, detection, and resistance mutation rates. The model aimed to estimate MDR-TB prevalence [ 54 ].
Two stochastic models were classified as heterogeneous as they included resistance compartments stratified with different resistant genotypes [ 44 , 61 ]. These papers had different aims: Kendall et al. [ 44 ] explored the impact of high and low levels of moxifloxacin resistance on treatment regimens and drug susceptibility testing. Pecerska et al. [ 61 ] estimated the fitness cost of MDR-TB with and without pyrazinamide resistance from a genetic data set.
Use of data derived from the literature
All Stage 2 papers used at least one parameter sourced from existing literature, so no models were entirely theoretical. Some models used a primary data set that was collected from experiments or a population study [ 20 , 49 , 52 , 58 , 59 , 61 ]. Data types used were experimental (83%), epidemiological (26%), clinical (4%), genetic (4%) and WHO data (30%). All bacterial population models used experimental data, with one paper also including clinical data [ 37 ]. Between-host models used a combination of experimental, epidemiological, and WHO data, with one using only genetic data.
Acquired or primary resistance and discrete resistance
All models with heterogeneity represented resistance as discrete categories, such as MDR/RR-TB, with no models including resistance as a spectrum. 6/8 between-host heterogenous models modelled resistance as both primary and acquired and two models had no primary resistance, with acquired resistance only [ 44 , 63 ].
Resistance effects in models
Resistance affected the ability of M . tuberculosis to transmit in 6/8 between-host heterogenous models, with resistant strains usually having a lower value for the transmission coefficient or fitness parameter than the susceptible strain.
Resistance affected disease progression in all models except Knight et al. (2015) [ 8 ]. For bacterial population models, this was defined as different growth rates. For between-host models, this was included as a separate disease progression parameter for resistant strains [ 54 , 55 , 63 ], different relapse rates for patients with resistant bacteria [ 44 ], different associated mortality rates for each resistant strain [ 61 ], variance in cross-immunity by resistant strain [ 53 ], or different natural history pathways for resistant strains [ 56 ].
13/23 models assumed resistance affected operational parameters. In nine, resistance reduced treatment efficacy [ 8 , 44 , 45 , 53 – 56 , 61 , 63 ], with one also including different diagnostic (GeneXpert rapid nucleic acid amplification test for M . tuberculosis ) sensitivity parameters for each resistant strain [ 44 ]. Four bacterial population models had a different antibiotic kill rate [ 48 , 49 , 58 , 60 ], with one including different clinical conversion factors [ 49 ].
Our review of the mathematical modelling landscape of drug resistance in mycobacteria has revealed a growing body of work mostly using transmission dynamic models to explore intervention impact. We found that a minority (33%) explore resistances other than MDR/RR-TB. Few models account for the known heterogeneity that exists in bacterial populations. Where heterogeneity was captured in both bacterial population and between-host models, it was mostly through a variation in the model-specific fitness parameter (with the definition of fitness varying broadly from being related to transmission, ability to cause disease or speed of bacterial growth).
Our Stage 1 landscape analysis found that several high MDR-TB burden countries (e.g. Pakistan, Nigeria, Ukraine, and Myanmar) are underrepresented in the English DR-TB literature. Increasing modelling of DR-TB in specific countries may aid understanding of epidemiology in the specific country and increase the global understanding of DR-TB, as well as improve estimates of intervention efficacy and hence design of context-specific interventions. This is highly relevant when considering that, as has been found for models of M . tuberculosis in general [ 11 , 64 ], most models aimed to estimate the impact of public health interventions. Transmission models were used more than any other type of model across all categories, except for the category of "treatment interventions + cost-effectiveness”, where state transition models were most used. This indicates that most modellers are interested in modelling M . tuberculosis at a between-human host population scale.
MDR-TB was the most common category of resistance modelled (67% of DR-mycobacterium models)—an expected result linked to the historical importance of this as a clinical treatment threshold and reflected in most data collection [ 1 , 3 ]. Mono-isoniazid resistance was more commonly modelled than explicit mono-rifampicin resistance, with 27 models capturing the pathway from isoniazid resistance developing into MDR-TB. XDR-TB was not considered without MDR-TB other than by two papers by Basu et al. (2008, 2009), who were interested in the burden and interventions specific to XDR-TB [ 46 , 47 ]. XDR-TB was often treated as a final state of resistance in modelling systems, with no further resistance being acquired. This reflects the historic clinical decision-making pathway (susceptible or MDR or XDR) and that XDR-TB is resistant to a large number of anti-TB antibiotics. However, there is a great variation in DR-TB and the pathways that may lead to each level of it. Understanding this variation in DR-TB will drive improvements in treatment success by identifying which antibiotics will be most effective and, therefore improve patient outcomes.
Rifampicin and isoniazid resistance were the most modelled mono-resistances, followed by pyrazinamide and ethambutol, reflecting first-line treatments and prophylaxis for TB and data availability. Testing for pyrazinamide and ethambutol resistance is typically reserved for reference settings, and there is widespread use of GeneXpert (Cepheid 6/10-colour instrument), which tests for rifampicin resistance. Only 21% of models (n = 41) captured resistances beyond these four drugs. This will need to be expanded as we move into a period with many more treatment options–constructing, parameterising, and exploring mathematical models of other antibiotic resistances is vitally needed to optimise future treatment and TB control interventions, as well as to explore evolutionary pathways. For example, we found only two papers which explicitly modelled resistance to bedaquiline [ 44 , 45 ], whilst two new treatment regimens containing bedaquiline were approved by the WHO in 2022 [ 65 ].
Models that capture non-specific DR-TB can be useful in the absence of data or to explore broad trends. We found 45 models in this category and found that these theoretical or non-specific systems were used to understand under what constraints DR-TB would dominate over DS-TB or explored the efficacy of a theoretical intervention.
When designing a model to answer a specific question such as the impact of a public health intervention, a balance needs to be struck between designing a detailed or generalised model to allow for a pragmatic approach. This pragmatism is likely the reason for our stage 2 results that revealed few models including bacterial heterogeneity. This is despite several models showing how heterogeneity in transmission fitness can affect DR-TB prevalence estimates [ 8 , 54 – 56 ]. Or how including multiple levels of resistance to one antibiotic can affect treatment outcomes [ 44 , 61 ]. Authors cannot capture all the subtlety of antibiotics as a selection pressure without including the related resistance dynamics and from this the population diversity it fosters. Mathematically, it can be difficult to include complexities in all aspects, for example, population mixing, and often there is little context-specific data on bacterial heterogeneity to inform models. However, if authors want to understand the risk of antibiotic resistance developing under a new treatment regimen it should follow that those resistances are then included in predictions. Some nuance may be beneficial in results that are only achievable with models that include bacterial heterogeneity, such as in Basu et al. (2008) where their conclusions suggested that a weaker immune response to a DR-TB infection with high fitness levels leads to higher DR-TB prevalence in HIV-positive and -negative populations [ 53 ].
Interestingly, we found that all models included resistance in a small number of discrete compartments, with no near-continuous distributions of resistance. Biologically speaking, resistance exists across a spectrum with strains having a range of minimum inhibitory concentrations, but for therapeutic and diagnostic uses they are classified with discrete values. Modelling resistance at multiple possible sub-levels would enable new research questions to be posed about pathways to evolution and competition due to multiple resistant levels. To our knowledge, such a question has not yet been asked regarding M . tuberculosis .
We found that transmission fitness levels, by contrast to resistance levels, were commonly allowed to vary across a distribution within resistant populations, likely reflecting the available historical data pointing to fitness differences between TB strains [ 66 ]. This contrasts with the lack of data linking resistant strain variation with treatment outcomes such as failure or recovery. Including such fitness effects is a relatively easy single-parameter effect within standard transmission dynamic or bacterial dynamics models and is commonly included in models of drug resistance outside of M . tuberculosis [ 7 ].
In this review, we identified 190 published papers which included drug-resistant strains of M . tuberculosis , a further 5 with a drug-resistant non-tuberculosis mycobacteria species, and 1 including both M . tuberculosis and M . marinum . Our update on the literature shows an increasing trend to model DR-TB.
The limitations of our review included that we conducted the search for English language articles when a substantial burden of DR-TB is found in non-English speaking settings such as Eastern Europe [ 1 ]. We did not capture which antibiotics were explored in the models as our focus was on the resistance captured nor time horizons for each model. Our stage 1 analysis only extracted high-level information as our main interest was the bacterial heterogeneity in stage 2. Future work could use this baseline set of literature to explore how resistance is modelled in the natural history of tuberculosis.
We encourage future modellers to consider if the bacterial component of their research question would benefit from the inclusion of bacterial heterogeneity. By not including it, models miss key features of bacterial populations, such as competition or treatment efficacy differences between strains and may, for example, under or overestimate the degree by which an intervention might increase resistance or prevalence of DR-TB.
We were unable to provide a comprehensive review of how resistance was included in Stage 1 models due to the lack of model information provided in many papers such as parameter tables, model diagrams or equations. Future mathematical models should aim for clear model reporting as suggested by the WHO [ 67 ] and Bennett et al. (2012) for transparency and to enable reproducible research [ 68 ].
In this review, we identified 195 drug-resistant mycobacteria mathematical models, with 190 DR-TB models and 23 models including bacterial heterogeneity. This has provided us with an understanding of how resistant mycobacterial species have been modelled, in terms of geographical settings, model aims and types, resistances modelled and further insights into the inclusion of bacterial heterogeneity. However, we found that bacterial heterogeneity was often ignored despite evidence of its importance at the population level. Balancing pragmaticism with biological reality when building mathematical models is vital within the fundamental evolutionary dynamics of AMR.
Supporting information
S1 text. search strings..
https://doi.org/10.1371/journal.ppat.1011574.s001
S2 Text. Details of extraction table for stage 1.
https://doi.org/10.1371/journal.ppat.1011574.s002
S3 Text. Details of extraction table for stage 2.
https://doi.org/10.1371/journal.ppat.1011574.s003
S1 Fig. Heatmap of all resistance categories in stage 1 models.
Heatmap of resistances included per DR-TB model (n = 195) indicates a lack of diversity in resistances modelled, with MDR/RR-TB featuring in over half of all 195 models. Each coloured line indicates a model (y axis) included in stage 1 (purple) or stage 2 (orange). The graph groups models into specific (captures resistance to a named antibiotic), non-specific (defined resistance that are not specific to an antibiotic) and hypothetical (captures antibiotic resistance not linked to a named drug). Antibiotic acronyms as follows: AMI = amikacin, BDQ = bedaquiline, CLR = clarithromycin, ETM = ethambutol, FQ = undefined fluroquinolone, LZD = linezolid, MOX = moxifloxacin, PA = pretomanid, PZA = pyrazinamide, STR = streptomycin, INH = isoniazid, RIF = rifampicin, MDR/RR = multidrug resistant/ rifampicin resistant, XDR = extensively drug-resistant, SLInject = second line injectable antibiotic (from WHO guidelines 2014), another 1st line = rifampicin, ethambutol, or pyrazinamide. Index links to paper number in S1 Table .
https://doi.org/10.1371/journal.ppat.1011574.s004
S2 Fig. Plot of number of publications over time.
https://doi.org/10.1371/journal.ppat.1011574.s005
S1 Table. Extraction table results from stage 1.
https://doi.org/10.1371/journal.ppat.1011574.s006
S2 Table. Extraction table results from stage 2.
https://doi.org/10.1371/journal.ppat.1011574.s007
S3 Table. Geographic settings in models.
https://doi.org/10.1371/journal.ppat.1011574.s008
Acknowledgments
We would like to thank the support of library staff at the London School of Hygiene and Tropical Medicine. Thank you for the guidance and advice for this work from Quentin Leclerc and Alastair Clements.
- 1. World Health Organisation. Global tuberculosis report 2022 [Internet]. 2022 Oct. Available from: https://www.who.int/publications/i/item/9789240061729
- 2. World Health Organisation. WHO announces updated definitions of extensively drug-resistant tuberculosis [Internet]. 2021 [cited 2021 Jan 27]. Available from: https://www.who.int/news/item/27-01-2021-who-announces-updated-definitions-of-extensively-drug-resistant-tuberculosis
- 3. World Health Organisation. Definitions and reporting framework for tuberculosis–2013 revision: updated December 2014 and January 2020. 2013. Report No.: No. WHO/HTM/TB/2013.2.
- View Article
- PubMed/NCBI
- Google Scholar
- 65. World Health Organisation. WHO consolidated guidelines on tuberculosis. Module 4: treatment—drug-resistant tuberculosis treatment, 2022 update. 2022 Dec.
- 67. World Health Organization. Guidance for country-level TB modelling [Internet]. Geneva: World Health Organization; 2018 [cited 2023 Jun 6]. 37 p. Available from: https://apps.who.int/iris/handle/10665/274279
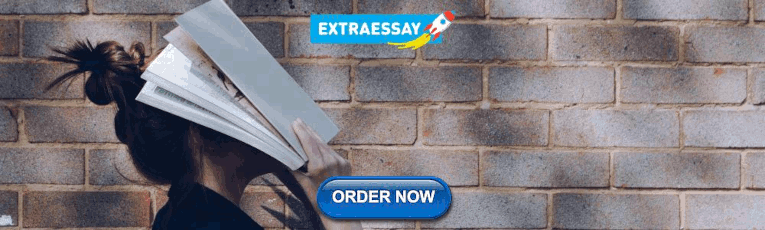
IMAGES
VIDEO
COMMENTS
Method details Overview. A Systematic Literature Review (SLR) is a research methodology to collect, identify, and critically analyze the available research studies (e.g., articles, conference proceedings, books, dissertations) through a systematic procedure [12].An SLR updates the reader with current literature about a subject [6].The goal is to review critical points of current knowledge on a ...
The best reviews synthesize studies to draw broad theoretical conclusions about what a literature means, linking theory to evidence and evidence to theory. This guide describes how to plan, conduct, organize, and present a systematic review of quantitative (meta-analysis) or qualitative (narrative review, meta-synthesis) information.
Literature reviews establish the foundation of academic inquires. However, in the planning field, we lack rigorous systematic reviews. In this article, through a systematic search on the methodology of literature review, we categorize a typology of literature reviews, discuss steps in conducting a systematic literature review, and provide suggestions on how to enhance rigor in literature ...
Image by TraceyChandler. Steps to conducting a systematic review. Quick overview of the process: Steps and resources from the UMB HSHSL Guide. YouTube video (26 min); Another detailed guide on how to conduct and write a systematic review from RMIT University; A roadmap for searching literature in PubMed from the VU Amsterdam; Alexander, P. A. (2020).
Reasons for inclusion and exclusion should be recorded. Step 3: Assessing the quality of studies. Study quality assessment is relevant to every step of a review. Question formulation (Step 1) and study selection criteria (Step 2) should describe the minimum acceptable level of design.
The systematic review is a rigorous method of collating and synthesizing evidence from multiple studies, producing a whole greater than the sum of parts. This textbook is an authoritative and accessible guide to an activity that is often found overwhelming.
Systematic reviews address a need for health decision makers to be able to access high quality, relevant, accessible and up-to-date information. Systematic reviews aim to minimize bias through the use of pre-specified research questions and methods that are documented in protocols, and by basing their findings on reliable research.
This module will teach you to: Recognize features of systematic reviews as a research design. Recognize the importance of using rigorous methods to conduct a systematic review. Identify the types of review questions. Identify the elements of a well-defined review question. Understand the steps in a systematic review.
Our goal with this paper is to conduct a narrative review of the literature about systematic reviews and outline the essential elements of a systematic review along with the limitations of such a review. Keywords: systematic reviews, meta-analysis, narrative literature review, prisma checklist. A literature review provides an important insight ...
The objective of this article is to provide guidance on how to conduct systematic literature review. By surveying publications on the methodology of literature review, we summarize the typology of literature review, describe the procedures for conducting the review, and provide tips to planning scholars.
Systematic review vs. literature review. A literature review is a type of review that uses a less systematic and formal approach than a systematic review. Typically, an expert in a topic will qualitatively summarize and evaluate previous work, without using a formal, explicit method. ... To conduct a systematic review, you'll need the ...
The meticulous nature of the systematic review research methodology differentiates a systematic review from a narrative review (literature review or authoritative review). This paper provides a brief step by step summary of how to conduct a systematic review, which may be of interest for clinicians and researchers. References, . . ; ...
The meticulous nature of the systematic review research methodology differentiates a systematic review from a narrative review (literature review or authoritative review). This paper provides a brief step by step summary of how to conduct a systematic review, which may be of interest for clinicians and researchers.
Abstract. Performing a literature review is a critical first step in research to understanding the state-of-the-art and identifying gaps and challenges in the field. A systematic literature review is a method which sets out a series of steps to methodically organize the review. In this paper, we present a guide designed for researchers and in ...
A systematic literature review is a method which sets out a series of steps to methodically organize the review. In this paper, we present a guide designed for researchers and in particular early-stage researchers in the computer-science field. The contribution of the article is the following:•Clearly defined strategies to follow for a ...
Librarians are experts trained in literature searching and systematic review methodology. Ask us a question or partner with a librarian to save time and improve the quality of your review. Our comparison chart detailing two tiers of partnership provides more information on how librarians can collaborate with and contribute to systematic review ...
A systematic review is a literature review that gathers all of the available evidence matching pre-specified eligibility criteria to answer a specific research question. It uses explicit, systematic methods, documented in a protocol, to minimize bias, provide reliable findings, and inform decision-making. ¹.
The purpose of this article is to understand the important steps involved in conducting a systematic review of all kinds of clinical studies. We conducted a narrative review of the literature about systematic reviews with a special focus on articles that discuss conducting reviews of randomized controlled trials. We discuss
A systematic literature review of parent influencer research and a future research agenda. ... Systematic reviews to support evidence-based medicine: How to appraise, conduct and publish reviews, CRC Press) and Stern's framework (1994, A revised communication model for advertising: Multiple dimensions of the source, the message, ...
Choice of an appropriate tool for the evaluation of a systematic review first involves identification of the underlying construct to be assessed. For systematic reviews of interventions, recommended tools include AMSTAR-2 and ROBIS for appraisal of conduct and PRISMA 2020 for completeness of reporting.
This systematic literature review (SLR) was conducted to identify and compile the evidence base regarding risk factors and predictors of moderate-to-severe exacerbations in patients with COPD. ... SS is an employee of Parexel International, which was funded by AstraZeneca to conduct this analysis. EdN is a former employee of AstraZeneca and ...
A systematic review was conducted of the literature published between 2010 and 2023 in the PsycINFO, ERIC, Education, and Psychology databases. An initial 1176 studies were reviewed by abstract, of which 485 were read in full text, leading to the selection and analysis of 22 studies.
Systematic reviews are ranked very high in research and are considered the most valid form of medical evidence. They provide a complete summary of the current literature relevant to a research question and can be of immense use to medical professionals. Our goal with this paper is to conduct a narra …
Performing a literature review is a critical first step in research to understanding the state-of-the-art and identifying gaps and challenges in the field. A systematic literature review is a method which sets out a series of steps to methodically organize the review. In this paper, we present a guide designed for researchers and in particular early-stage researchers in the computer-science field.
We conduct a systematic literature analysis and semi structured expert interviews to answer this research question. In the systematic literature analysis, we identify and analyze a heterogeneous set of 21 AI use cases across five different HC application fields and derive 15 business objectives and six value propositions for HC organizations.
This systematic review aims to assess the effectiveness of such interventions in reducing inappropriate antibiotic use among antibiotic providers and users in healthcare and community settings. Methods We will conduct a systematic literature search across multiple databases and grey literature sources. We will include studies which evaluate the ...
We undertook a systematic review of the existing DR-mycobacterium modelling literature, with the specific aim of capturing methods for including bacterial heterogeneity. Our analysis revealed that most models of drug-resistance in mycobacteria primarily focus on intervention strategies and cost-effectiveness analyses, with minimal attention to ...
A systematic review of the literature was also conducted. (3) Results: This is the largest published series of TMC dislocations in children and adolescents. Patients included a 12-year-old girl treated conservatively with a poor quickDASH; a 9-year-old girl treated surgically with the Eaton-Littler technique for a new dislocation with a ...