Navigation Menu
Search code, repositories, users, issues, pull requests..., provide feedback.
We read every piece of feedback, and take your input very seriously.
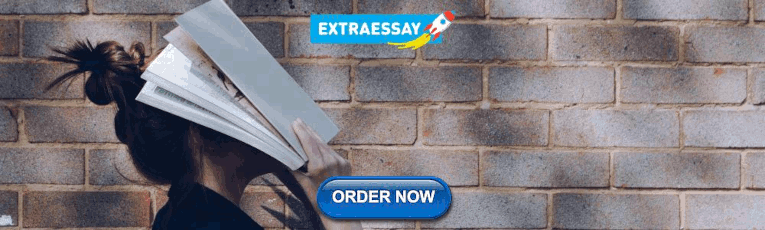
Saved searches
Use saved searches to filter your results more quickly.
To see all available qualifiers, see our documentation .
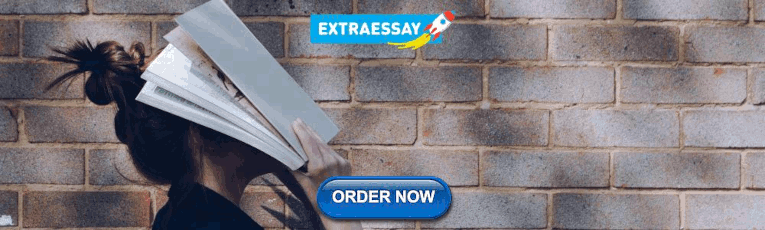
COMMENTS
This study focuses on employing artificial intelligence and computer vision analysis to automatically identify aggregates quarries from satellite images within continental …
5034 benchmarks • 1517 tasks • 3271 datasets • 53695 papers with code.
This comprehensive review paper aims to delve into the intricate evolution, methodologies, applications, challenges, and future trajectories of Computer Vision.
Deep learning has been overwhelmingly successful in computer vision (CV), natural language processing, and video/speech recognition. In this paper, our focus is on CV. …
Subjects: Computer Vision and Pattern Recognition (cs.CV); Artificial Intelligence (cs.AI); Graphics (cs.GR); Robotics (cs.RO)
Here are my top 10 of the most interesting research papers of the year in computer vision, in case you missed any of them. In short, it is basically a curated list of the latest breakthroughs in AI and CV with a clear video …
Abstract: Detecting objects remains one of computer vision and image understanding applications’ most fundamental and challenging aspects. Significant advances in object …
This review paper provides a brief overview of some of the most significant deep learning schemes used in computer vision problems, that is, Convolutional Neural Networks, …