- TheFreeDictionary
- Word / Article
- Starts with
- Free toolbar & extensions
- Word of the Day
- Free content
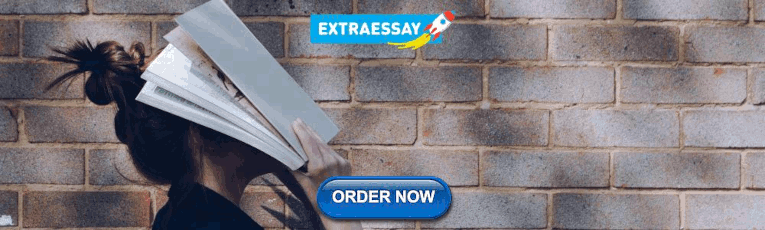
hy·poth·e·sis
Significance .
- ad hoc hypothesis
- alpha error
- alternative hypothesis
- APUD hypothesis
- Avogadro law
- Avogadro number
- Avogadro, Amadeo
- baby lung hypothesis
- background level
- Bayes theorem
- Bayesian hypothesis
- Beadle, George Wells
- bread mould
- chi squared test
- cohesion-tension hypothesis
- hypothalamic dysfunction
- hypothalamic fever
- hypothalamic infundibulum
- hypothalamic nucleus
- hypothalamic obesity
- hypothalamic sulcus
- hypothalamic-pituitary axis
- hypothalamic-pituitary-adrenal axis
- hypothalamocerebellar fibers
- hypothalamo-hypophyseal portal system
- hypothalamohypophysial
- hypothalamospinal fibers
- Hypothalamus
- hypothenar eminence
- hypothenar fascia
- hypothenar hammer syndrome
- hypothenar-hammer syndrome
- hypothermal
- hypothermia
- hypothermia blanket
- hypothermia therapy
- hypothermia treatment
- hypothermic anesthesia
- hypothermic circulatory arrest
- hypothesis test
- hypothesis to test
- hypothetic mean organism
- hypothetic mean strain
- hypothetical mean organism
- hypothrombinemia
- hypothromboplastinemia
- hypothymism
- hypothyroid
- hypothyroid dwarf
- hypothyroidism
- hypothyroxinemia
- hypotonia-cystinuria syndrome
- hypotonic duodenography
- hypotonic labor
- hypotonic saline
- hypotonic solution
- hypotonic uterine dysfunction
- hypotonicity
- hypotony maculopathy
- Hypothermia After Cardiac Arrest
- Hypothermia After Cardiac Arrest Registry
- Hypothermia, induced
- hypothermic
- Hypothermic machine perfusion
- hypothermicly
- Hypothèse Extraterrestre
- Hypothesis Driven Lexical Adaptation
- Hypothesis test
- Hypothesis testing
- hypothesis testing sampling
- Hypothesis-Based Testing
- Hypothesis-Oriented Algorithm for Clinicians
- hypothesise
- hypothesised
- hypothesiser
- hypothesisers
- hypothesises
- Facebook Share
- More from M-W
- To save this word, you'll need to log in. Log In
Definition of hypothesis
Did you know.
The Difference Between Hypothesis and Theory
A hypothesis is an assumption, an idea that is proposed for the sake of argument so that it can be tested to see if it might be true.
In the scientific method, the hypothesis is constructed before any applicable research has been done, apart from a basic background review. You ask a question, read up on what has been studied before, and then form a hypothesis.
A hypothesis is usually tentative; it's an assumption or suggestion made strictly for the objective of being tested.
A theory , in contrast, is a principle that has been formed as an attempt to explain things that have already been substantiated by data. It is used in the names of a number of principles accepted in the scientific community, such as the Big Bang Theory . Because of the rigors of experimentation and control, it is understood to be more likely to be true than a hypothesis is.
In non-scientific use, however, hypothesis and theory are often used interchangeably to mean simply an idea, speculation, or hunch, with theory being the more common choice.
Since this casual use does away with the distinctions upheld by the scientific community, hypothesis and theory are prone to being wrongly interpreted even when they are encountered in scientific contexts—or at least, contexts that allude to scientific study without making the critical distinction that scientists employ when weighing hypotheses and theories.
The most common occurrence is when theory is interpreted—and sometimes even gleefully seized upon—to mean something having less truth value than other scientific principles. (The word law applies to principles so firmly established that they are almost never questioned, such as the law of gravity.)
This mistake is one of projection: since we use theory in general to mean something lightly speculated, then it's implied that scientists must be talking about the same level of uncertainty when they use theory to refer to their well-tested and reasoned principles.
The distinction has come to the forefront particularly on occasions when the content of science curricula in schools has been challenged—notably, when a school board in Georgia put stickers on textbooks stating that evolution was "a theory, not a fact, regarding the origin of living things." As Kenneth R. Miller, a cell biologist at Brown University, has said , a theory "doesn’t mean a hunch or a guess. A theory is a system of explanations that ties together a whole bunch of facts. It not only explains those facts, but predicts what you ought to find from other observations and experiments.”
While theories are never completely infallible, they form the basis of scientific reasoning because, as Miller said "to the best of our ability, we’ve tested them, and they’ve held up."
- proposition
- supposition
hypothesis , theory , law mean a formula derived by inference from scientific data that explains a principle operating in nature.
hypothesis implies insufficient evidence to provide more than a tentative explanation.
theory implies a greater range of evidence and greater likelihood of truth.
law implies a statement of order and relation in nature that has been found to be invariable under the same conditions.
Examples of hypothesis in a Sentence
These examples are programmatically compiled from various online sources to illustrate current usage of the word 'hypothesis.' Any opinions expressed in the examples do not represent those of Merriam-Webster or its editors. Send us feedback about these examples.
Word History
Greek, from hypotithenai to put under, suppose, from hypo- + tithenai to put — more at do
1641, in the meaning defined at sense 1a
Phrases Containing hypothesis
- nebular hypothesis
- planetesimal hypothesis
- null hypothesis
- counter - hypothesis
- Whorfian hypothesis
Articles Related to hypothesis
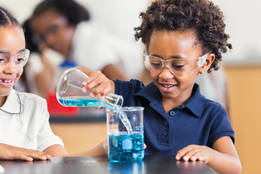
This is the Difference Between a...
This is the Difference Between a Hypothesis and a Theory
In scientific reasoning, they're two completely different things
Dictionary Entries Near hypothesis
hypothermia
hypothesize
Cite this Entry
“Hypothesis.” Merriam-Webster.com Dictionary , Merriam-Webster, https://www.merriam-webster.com/dictionary/hypothesis. Accessed 8 Apr. 2024.
Kids Definition
Kids definition of hypothesis, medical definition, medical definition of hypothesis, more from merriam-webster on hypothesis.
Nglish: Translation of hypothesis for Spanish Speakers
Britannica English: Translation of hypothesis for Arabic Speakers
Britannica.com: Encyclopedia article about hypothesis
Subscribe to America's largest dictionary and get thousands more definitions and advanced search—ad free!
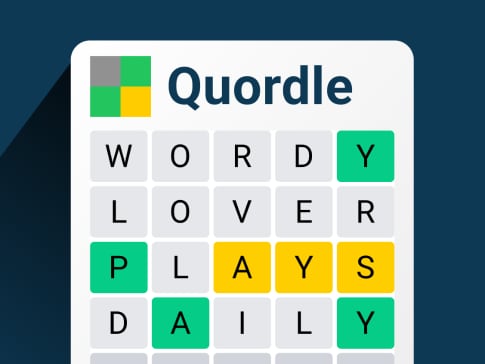
Can you solve 4 words at once?
Word of the day.
See Definitions and Examples »
Get Word of the Day daily email!
Popular in Grammar & Usage
The tangled history of 'it's' and 'its', more commonly misspelled words, why does english have so many silent letters, your vs. you're: how to use them correctly, every letter is silent, sometimes: a-z list of examples, popular in wordplay, the words of the week - apr. 5, 12 bird names that sound like compliments, 10 scrabble words without any vowels, 12 more bird names that sound like insults (and sometimes are), 8 uncommon words related to love, games & quizzes.
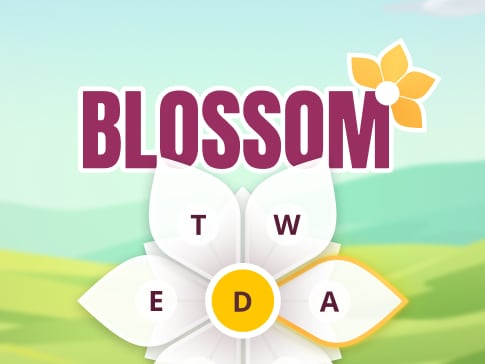
- Bipolar Disorder
- Therapy Center
- When To See a Therapist
- Types of Therapy
- Best Online Therapy
- Best Couples Therapy
- Best Family Therapy
- Managing Stress
- Sleep and Dreaming
- Understanding Emotions
- Self-Improvement
- Healthy Relationships
- Student Resources
- Personality Types
- Guided Meditations
- Verywell Mind Insights
- 2023 Verywell Mind 25
- Mental Health in the Classroom
- Editorial Process
- Meet Our Review Board
- Crisis Support
How to Write a Great Hypothesis
Hypothesis Format, Examples, and Tips
Kendra Cherry, MS, is a psychosocial rehabilitation specialist, psychology educator, and author of the "Everything Psychology Book."
:max_bytes(150000):strip_icc():format(webp)/IMG_9791-89504ab694d54b66bbd72cb84ffb860e.jpg)
Amy Morin, LCSW, is a psychotherapist and international bestselling author. Her books, including "13 Things Mentally Strong People Don't Do," have been translated into more than 40 languages. Her TEDx talk, "The Secret of Becoming Mentally Strong," is one of the most viewed talks of all time.
:max_bytes(150000):strip_icc():format(webp)/VW-MIND-Amy-2b338105f1ee493f94d7e333e410fa76.jpg)
Verywell / Alex Dos Diaz
- The Scientific Method
Hypothesis Format
Falsifiability of a hypothesis, operational definitions, types of hypotheses, hypotheses examples.
- Collecting Data
Frequently Asked Questions
A hypothesis is a tentative statement about the relationship between two or more variables. It is a specific, testable prediction about what you expect to happen in a study.
One hypothesis example would be a study designed to look at the relationship between sleep deprivation and test performance might have a hypothesis that states: "This study is designed to assess the hypothesis that sleep-deprived people will perform worse on a test than individuals who are not sleep-deprived."
This article explores how a hypothesis is used in psychology research, how to write a good hypothesis, and the different types of hypotheses you might use.
The Hypothesis in the Scientific Method
In the scientific method , whether it involves research in psychology, biology, or some other area, a hypothesis represents what the researchers think will happen in an experiment. The scientific method involves the following steps:
- Forming a question
- Performing background research
- Creating a hypothesis
- Designing an experiment
- Collecting data
- Analyzing the results
- Drawing conclusions
- Communicating the results
The hypothesis is a prediction, but it involves more than a guess. Most of the time, the hypothesis begins with a question which is then explored through background research. It is only at this point that researchers begin to develop a testable hypothesis. Unless you are creating an exploratory study, your hypothesis should always explain what you expect to happen.
In a study exploring the effects of a particular drug, the hypothesis might be that researchers expect the drug to have some type of effect on the symptoms of a specific illness. In psychology, the hypothesis might focus on how a certain aspect of the environment might influence a particular behavior.
Remember, a hypothesis does not have to be correct. While the hypothesis predicts what the researchers expect to see, the goal of the research is to determine whether this guess is right or wrong. When conducting an experiment, researchers might explore a number of factors to determine which ones might contribute to the ultimate outcome.
In many cases, researchers may find that the results of an experiment do not support the original hypothesis. When writing up these results, the researchers might suggest other options that should be explored in future studies.
In many cases, researchers might draw a hypothesis from a specific theory or build on previous research. For example, prior research has shown that stress can impact the immune system. So a researcher might hypothesize: "People with high-stress levels will be more likely to contract a common cold after being exposed to the virus than people who have low-stress levels."
In other instances, researchers might look at commonly held beliefs or folk wisdom. "Birds of a feather flock together" is one example of folk wisdom that a psychologist might try to investigate. The researcher might pose a specific hypothesis that "People tend to select romantic partners who are similar to them in interests and educational level."
Elements of a Good Hypothesis
So how do you write a good hypothesis? When trying to come up with a hypothesis for your research or experiments, ask yourself the following questions:
- Is your hypothesis based on your research on a topic?
- Can your hypothesis be tested?
- Does your hypothesis include independent and dependent variables?
Before you come up with a specific hypothesis, spend some time doing background research. Once you have completed a literature review, start thinking about potential questions you still have. Pay attention to the discussion section in the journal articles you read . Many authors will suggest questions that still need to be explored.
To form a hypothesis, you should take these steps:
- Collect as many observations about a topic or problem as you can.
- Evaluate these observations and look for possible causes of the problem.
- Create a list of possible explanations that you might want to explore.
- After you have developed some possible hypotheses, think of ways that you could confirm or disprove each hypothesis through experimentation. This is known as falsifiability.
In the scientific method , falsifiability is an important part of any valid hypothesis. In order to test a claim scientifically, it must be possible that the claim could be proven false.
Students sometimes confuse the idea of falsifiability with the idea that it means that something is false, which is not the case. What falsifiability means is that if something was false, then it is possible to demonstrate that it is false.
One of the hallmarks of pseudoscience is that it makes claims that cannot be refuted or proven false.
A variable is a factor or element that can be changed and manipulated in ways that are observable and measurable. However, the researcher must also define how the variable will be manipulated and measured in the study.
For example, a researcher might operationally define the variable " test anxiety " as the results of a self-report measure of anxiety experienced during an exam. A "study habits" variable might be defined by the amount of studying that actually occurs as measured by time.
These precise descriptions are important because many things can be measured in a number of different ways. One of the basic principles of any type of scientific research is that the results must be replicable. By clearly detailing the specifics of how the variables were measured and manipulated, other researchers can better understand the results and repeat the study if needed.
Some variables are more difficult than others to define. How would you operationally define a variable such as aggression ? For obvious ethical reasons, researchers cannot create a situation in which a person behaves aggressively toward others.
In order to measure this variable, the researcher must devise a measurement that assesses aggressive behavior without harming other people. In this situation, the researcher might utilize a simulated task to measure aggressiveness.
Hypothesis Checklist
- Does your hypothesis focus on something that you can actually test?
- Does your hypothesis include both an independent and dependent variable?
- Can you manipulate the variables?
- Can your hypothesis be tested without violating ethical standards?
The hypothesis you use will depend on what you are investigating and hoping to find. Some of the main types of hypotheses that you might use include:
- Simple hypothesis : This type of hypothesis suggests that there is a relationship between one independent variable and one dependent variable.
- Complex hypothesis : This type of hypothesis suggests a relationship between three or more variables, such as two independent variables and a dependent variable.
- Null hypothesis : This hypothesis suggests no relationship exists between two or more variables.
- Alternative hypothesis : This hypothesis states the opposite of the null hypothesis.
- Statistical hypothesis : This hypothesis uses statistical analysis to evaluate a representative sample of the population and then generalizes the findings to the larger group.
- Logical hypothesis : This hypothesis assumes a relationship between variables without collecting data or evidence.
A hypothesis often follows a basic format of "If {this happens} then {this will happen}." One way to structure your hypothesis is to describe what will happen to the dependent variable if you change the independent variable .
The basic format might be: "If {these changes are made to a certain independent variable}, then we will observe {a change in a specific dependent variable}."
A few examples of simple hypotheses:
- "Students who eat breakfast will perform better on a math exam than students who do not eat breakfast."
- Complex hypothesis: "Students who experience test anxiety before an English exam will get lower scores than students who do not experience test anxiety."
- "Motorists who talk on the phone while driving will be more likely to make errors on a driving course than those who do not talk on the phone."
Examples of a complex hypothesis include:
- "People with high-sugar diets and sedentary activity levels are more likely to develop depression."
- "Younger people who are regularly exposed to green, outdoor areas have better subjective well-being than older adults who have limited exposure to green spaces."
Examples of a null hypothesis include:
- "Children who receive a new reading intervention will have scores different than students who do not receive the intervention."
- "There will be no difference in scores on a memory recall task between children and adults."
Examples of an alternative hypothesis:
- "Children who receive a new reading intervention will perform better than students who did not receive the intervention."
- "Adults will perform better on a memory task than children."
Collecting Data on Your Hypothesis
Once a researcher has formed a testable hypothesis, the next step is to select a research design and start collecting data. The research method depends largely on exactly what they are studying. There are two basic types of research methods: descriptive research and experimental research.
Descriptive Research Methods
Descriptive research such as case studies , naturalistic observations , and surveys are often used when it would be impossible or difficult to conduct an experiment . These methods are best used to describe different aspects of a behavior or psychological phenomenon.
Once a researcher has collected data using descriptive methods, a correlational study can then be used to look at how the variables are related. This type of research method might be used to investigate a hypothesis that is difficult to test experimentally.
Experimental Research Methods
Experimental methods are used to demonstrate causal relationships between variables. In an experiment, the researcher systematically manipulates a variable of interest (known as the independent variable) and measures the effect on another variable (known as the dependent variable).
Unlike correlational studies, which can only be used to determine if there is a relationship between two variables, experimental methods can be used to determine the actual nature of the relationship—whether changes in one variable actually cause another to change.
A Word From Verywell
The hypothesis is a critical part of any scientific exploration. It represents what researchers expect to find in a study or experiment. In situations where the hypothesis is unsupported by the research, the research still has value. Such research helps us better understand how different aspects of the natural world relate to one another. It also helps us develop new hypotheses that can then be tested in the future.
Some examples of how to write a hypothesis include:
- "Staying up late will lead to worse test performance the next day."
- "People who consume one apple each day will visit the doctor fewer times each year."
- "Breaking study sessions up into three 20-minute sessions will lead to better test results than a single 60-minute study session."
The four parts of a hypothesis are:
- The research question
- The independent variable (IV)
- The dependent variable (DV)
- The proposed relationship between the IV and DV
Castillo M. The scientific method: a need for something better? . AJNR Am J Neuroradiol. 2013;34(9):1669-71. doi:10.3174/ajnr.A3401
Nevid J. Psychology: Concepts and Applications. Wadworth, 2013.
By Kendra Cherry, MSEd Kendra Cherry, MS, is a psychosocial rehabilitation specialist, psychology educator, and author of the "Everything Psychology Book."
Have a language expert improve your writing
Run a free plagiarism check in 10 minutes, generate accurate citations for free.
- Knowledge Base
Methodology
- How to Write a Strong Hypothesis | Steps & Examples
How to Write a Strong Hypothesis | Steps & Examples
Published on May 6, 2022 by Shona McCombes . Revised on November 20, 2023.
A hypothesis is a statement that can be tested by scientific research. If you want to test a relationship between two or more variables, you need to write hypotheses before you start your experiment or data collection .
Example: Hypothesis
Daily apple consumption leads to fewer doctor’s visits.
Table of contents
What is a hypothesis, developing a hypothesis (with example), hypothesis examples, other interesting articles, frequently asked questions about writing hypotheses.
A hypothesis states your predictions about what your research will find. It is a tentative answer to your research question that has not yet been tested. For some research projects, you might have to write several hypotheses that address different aspects of your research question.
A hypothesis is not just a guess – it should be based on existing theories and knowledge. It also has to be testable, which means you can support or refute it through scientific research methods (such as experiments, observations and statistical analysis of data).
Variables in hypotheses
Hypotheses propose a relationship between two or more types of variables .
- An independent variable is something the researcher changes or controls.
- A dependent variable is something the researcher observes and measures.
If there are any control variables , extraneous variables , or confounding variables , be sure to jot those down as you go to minimize the chances that research bias will affect your results.
In this example, the independent variable is exposure to the sun – the assumed cause . The dependent variable is the level of happiness – the assumed effect .
Prevent plagiarism. Run a free check.
Step 1. ask a question.
Writing a hypothesis begins with a research question that you want to answer. The question should be focused, specific, and researchable within the constraints of your project.
Step 2. Do some preliminary research
Your initial answer to the question should be based on what is already known about the topic. Look for theories and previous studies to help you form educated assumptions about what your research will find.
At this stage, you might construct a conceptual framework to ensure that you’re embarking on a relevant topic . This can also help you identify which variables you will study and what you think the relationships are between them. Sometimes, you’ll have to operationalize more complex constructs.
Step 3. Formulate your hypothesis
Now you should have some idea of what you expect to find. Write your initial answer to the question in a clear, concise sentence.
4. Refine your hypothesis
You need to make sure your hypothesis is specific and testable. There are various ways of phrasing a hypothesis, but all the terms you use should have clear definitions, and the hypothesis should contain:
- The relevant variables
- The specific group being studied
- The predicted outcome of the experiment or analysis
5. Phrase your hypothesis in three ways
To identify the variables, you can write a simple prediction in if…then form. The first part of the sentence states the independent variable and the second part states the dependent variable.
In academic research, hypotheses are more commonly phrased in terms of correlations or effects, where you directly state the predicted relationship between variables.
If you are comparing two groups, the hypothesis can state what difference you expect to find between them.
6. Write a null hypothesis
If your research involves statistical hypothesis testing , you will also have to write a null hypothesis . The null hypothesis is the default position that there is no association between the variables. The null hypothesis is written as H 0 , while the alternative hypothesis is H 1 or H a .
- H 0 : The number of lectures attended by first-year students has no effect on their final exam scores.
- H 1 : The number of lectures attended by first-year students has a positive effect on their final exam scores.
If you want to know more about the research process , methodology , research bias , or statistics , make sure to check out some of our other articles with explanations and examples.
- Sampling methods
- Simple random sampling
- Stratified sampling
- Cluster sampling
- Likert scales
- Reproducibility
Statistics
- Null hypothesis
- Statistical power
- Probability distribution
- Effect size
- Poisson distribution
Research bias
- Optimism bias
- Cognitive bias
- Implicit bias
- Hawthorne effect
- Anchoring bias
- Explicit bias
Here's why students love Scribbr's proofreading services
Discover proofreading & editing
A hypothesis is not just a guess — it should be based on existing theories and knowledge. It also has to be testable, which means you can support or refute it through scientific research methods (such as experiments, observations and statistical analysis of data).
Null and alternative hypotheses are used in statistical hypothesis testing . The null hypothesis of a test always predicts no effect or no relationship between variables, while the alternative hypothesis states your research prediction of an effect or relationship.
Hypothesis testing is a formal procedure for investigating our ideas about the world using statistics. It is used by scientists to test specific predictions, called hypotheses , by calculating how likely it is that a pattern or relationship between variables could have arisen by chance.
Cite this Scribbr article
If you want to cite this source, you can copy and paste the citation or click the “Cite this Scribbr article” button to automatically add the citation to our free Citation Generator.
McCombes, S. (2023, November 20). How to Write a Strong Hypothesis | Steps & Examples. Scribbr. Retrieved April 7, 2024, from https://www.scribbr.com/methodology/hypothesis/
Is this article helpful?
Shona McCombes
Other students also liked, construct validity | definition, types, & examples, what is a conceptual framework | tips & examples, operationalization | a guide with examples, pros & cons, what is your plagiarism score.
- - Google Chrome
Intended for healthcare professionals
- Access provided by Google Indexer
- My email alerts
- BMA member login
- Username * Password * Forgot your log in details? Need to activate BMA Member Log In Log in via OpenAthens Log in via your institution

Search form
- Advanced search
- Search responses
- Search blogs
- Introduction to...
Introduction to clinical reasoning
- Related content
- Peer review
- Alison Round , consultant in public health medicine 1
- 1 North and East Devon Health Authority, Southernhay East, Exeter
How do doctors make decisions? Alison Round explains some of the thought processes that lead to a diagnosis
Doctors make decisions all the time - what the problem is, what the diagnosis is, whether to do anything, what to do. What facts do doctors take into account when they come to a decision, and what processes do they use to decide on a course of action? Where does intuition come from? These are the basics of clinical reasoning. When decisions are made in conjunction with the patient, doctors need to have an understanding of the “building blocks” of their thinking in order to explain this to the patient and to explore areas where differences in values and opinion may occur.
In all fields, not just medicine, experts make decisions in very different ways from students or beginners. Traditional bedside teaching guides students to take a history and perform an examination before constructing a differential diagnosis. In real life, however, experienced doctors do not work like this. They utilise a number of shortcuts (heuristics), based on knowledge and previous experience, which enable them to work much more quickly and, in general, more accurately than students. 1 There are many advantages to heuristics, such as very rapid processing and an ability to handle complex information without overload. There are, however, also a number of biases incorporated in the heuristics that may lead to poor decision making. 2 This article aims to discuss the processes and biases, using making a diagnosis as an example, and considers how improvements could be made.
Clinical reasoning in differential diagnosis
Experts use three main methods, or a combination of these, in making a diagnosis. Probably the most common is the hypothetico-deductive approach. An initial hypothesis or hypotheses are generated very early during the initial presentation of the problem, from existing knowledge, associations, and experience. Further questions or examination are oriented towards supporting or refuting these first ideas. If an hypoth- esis is discarded, an alternative one is considered and treated in the same way. Several hypotheses can be actively considered at any one time. Both awareness of probabilities (prevalence) of disease and knowledge of causal pathways are important. 3
Pattern recognition is also common. A particular combination of symptoms, or even certain phrases used to describe a symptom, can suggest a diagnosis very strongly. People build up their own internal library of patterns on the basis of their experience and existing knowledge.
Finally, pathognomonic signs and symptoms exist where a particular finding almost guarantees a certain diagnosis. Ulnar deviation in rheumatoid arthritis, Kaiser-Fleischer rings in Wilson's disease, and the slow relaxing jerks of hypothyroidism are examples. Unfortunately, most of these findings are rare and of little help in day to day practice.
All diagnostic methods depend on breadth and depth of knowledge, but the application of knowledge is not as straightforward as it seems. The use of algorithms (following a structured guideline to reach a diagnosis) is not welcomed by many doctors, despite their accuracy and relative freedom from bias. Professionals may consider they have enough confidence in their unaided decision making, describing algorithms as too time consuming. 4
Biases in thinking
Several common features of thinking can occur during the clinical reasoning process. These are known as cognitive biases. Firstly, there is the difficulty in estimating probabilities accurately, giving undue weight to small samples, or overestimating the similarity between people or events (often known as representativeness bias). Secondly, there is a tendency to attribute too much weight to easily available information, or to an event that is easily remem- bered because of particularly salient features; an example is overestimation of the probability of death by lightning. Thirdly, some people, when asked to estimate the probability of an event, place the initial probability at too extreme a figure and then make insufficient adjustment for subsequent information. Finally, there is also a bias towards positive and confirming evidence at the expense of negative evidence.
The case studies illustrate these points. When you read case study 1 ( table ), imagine you are seeing a patient in an accident and emergency department, and think what is going through your mind at each point.
- View inline
When doctors are not certain about a diagnosis they search for more information, either from the history or examination or by performing investigations or tests. One important source of bias in handling information is incorrect application or interpretation of tests. Case study 2 ( box 1 ) considers these points (see below for answers).
Box 1: Case study 2
Estimate the probabilities for yourself. A 40 year old woman has intermittent chest pain, sometimes associated with exercise, for 6 weeks. She is worried about ischaemic heart disease as her cousin has just had a heart attack at the age of 47. History and examination are unhelpful. What do you estimate as the probability of her pain being angina? She now has an exercise electrocardiogram which shows positive changes after 5 minutes. What do you estimate the probability of angina to be after this? She also has a thallium scan, which is normal. What do you estimate the final probability of angina to be after these test results?
Bayes' theorem and its uses
Bayes' theorem is a simple formula which suggests how one should modify one's original idea in the light of new information. In the case above, the new information is in the form of test results. In order to work out how much modification is necessary with the positive exercise electrocardiogram, one needs to know what the chance of having a positive test result is when the disease is present and what the chance of having a positive test result is when the disease is absent. This gives an idea of the diagnostic value of a test, is called the likelihood ratio, and can be calculated from the sensitivity and specificity of tests (see box 2 ).
Box 2: Calculating likelihood ratios
In the example above, assume that the sensitivity of an exercise electrocardiogram is 60%, or 0.6, and the specificity is 91%, or 0.91, for the diagnosis of ischaemic heart disease. From these the likelihood ratio can be calculated. The formula for the likelihood ratio is
Sensitivity / 1-specificity
In this case the likelihood ratio is 0.6/0.09=6.7 In order to calculate the chance that disease is present when the test is positive, Bayes' theorem uses odds, rather than probabilities. (Odds range from 0 to infinity, probability is bounded between 0 and 1. A version of Bayes' theorem that uses probabilities is available, but is more complicated.) Chance of disease with positive test (posterior odds)=initial odds * likelihood ratio The prior probability of disease is 1%, or P=0.01. This needs to be converted to the prior odds of disease by using the formula “odds=P/1-P.” Here, prior odds are 0.0101. The posterior odds-the odds of disease after the test result is known-are:
0.0101*6.7=0.0677
Conversion of odds back to probabilities “probability=odds/(1+odds)” gives the final probability as 0.063 or 6.3%. As can be seen, when probabilities are small there is little difference between odds and probabilities, and a rough estimate can be made simply by multiplying the initial probability by the likelihood ratio. A similar calculation can be performed using the negative result of the thallium scan; a likelihood ratio for a negative test should be used.
The sensitivity of a test is equivalent to the chance of having a positive test result when the disease is present. The specificity of a test is equivalent to the chance of having a negative test result when the disease is absent, and therefore (12specificity) is the chance of having a positive test result when the disease is absent.
Bayes' theorem is perceived to be complicated and difficult to understand. If, however, the initial probability of disease can be estimated (which one does whether or not Bayes' theorem is being used), estimates of the sensitivity and specificity of tests can be used easily to arrive at a final (posterior) probability of a diagnosis, given a particular test result. Such data are available for many investigations, but the method is not generally taught when differential diagnosis is being discussed.
In the case study above, most people estimate the probabilities as about 5% initially, rising to about 70% with the positive exercise test and back to about 50% with a negative thallium scan. In fact the actual probabilities are 1%, 6%, and 2% respectively. So whereas the initial probability is not far wrong, the final estimate is markedly inaccurate. The positive exercise test seems much more important than it is, partly because the initial probability of disease is so low that more positive tests are false positives than true positives. What Bayes' theorem demonstrates is that for diseases that are initially unlikely, a positive test of reasonable sensitivity and specificity increases the absolute chance of disease by only a small amount. Conversely, for diseases where the prior probability is very high, a positive test may add very little, or a negative test may not substantially reduce the chances of disease. Box 3 shows some tips to improve clinical reasoning.
Box 3: Tips for improving clinical reasoning
Reflect on the reasons that make you consider a particular diagnosis in each case Estimate the initial probability as carefully as you can Ask what the sensitivity and specificity is when a test is being discussed Remember that negative results are just as important as positive ones
This article has covered only a basic introduction. There are several good texts that provide more information, such as Clinical Epidemiology by Sackett et al, and Professional Judgement , edited by Dowie et al. 5 6
For particularly interested readers, the publications by Elstein et al 7 and Kahnemann et al 8 are strongly recommended, as are articles discussing other theoretical approaches to clinical reasoning. 9
Originally published as: Student BMJ 2000;08:15
- ↵ Kassirer JP, Kuipers BJ, Gorry GA. Toward a theory of clinical expertise. Am J Med 1982 ; 73 : 251 –9. OpenUrl CrossRef PubMed Web of Science
- ↵ Tversky A, Kahnemann D. Judgement under uncertainty: heuristics and biases. Science 1974 ; 185 : 1124 –31. OpenUrl Abstract / FREE Full Text
- ↵ Kassirer JP. Diagnostic reasoning. Ann Intern Med 1989 ; 110 : 893 –900. OpenUrl CrossRef PubMed Web of Science
- ↵ Pearson SD, Goldman L, Garcia TB, Cook EF, Lee TH. Physician response to a prediction rule for the triage of emergency department patients with chest pain. J Gen Int Med 1994 9 ( 5 ): 241 –7. OpenUrl CrossRef PubMed Web of Science
- ↵ Sackett DL, Haynes RB, Guyatt GH, Tugwell P. Clinical epidemiology. A basic science for clinical medicine . Boston : Little, Brown , 1991
- ↵ Dowie J, Elstein A. Professional judgement. A reader in clinical decision making . Cambridge , Cambridge University Press , 1988 .
- ↵ Elstein AS, Shulman LS, Sprafka SA. Medical problem solving-an analysis of clinical reasoning . Cambridge, Massachusetts , Harvard University Press , 1978 .
- ↵ Kahnemann D, Tversky A, eds. Judgement under uncertainty: heuristics and biases . New York : Cambridge University Press , 1982 : 359 –69.
- ↵ Greenwood J. Theoretical approaches to the study of nurses' clinical reasoning: getting things clear. Contemp Nurse 1998 ; 7 : 110 –6. OpenUrl PubMed
- Privacy Policy
Buy Me a Coffee

Home » What is a Hypothesis – Types, Examples and Writing Guide
What is a Hypothesis – Types, Examples and Writing Guide
Table of Contents

Definition:
Hypothesis is an educated guess or proposed explanation for a phenomenon, based on some initial observations or data. It is a tentative statement that can be tested and potentially proven or disproven through further investigation and experimentation.
Hypothesis is often used in scientific research to guide the design of experiments and the collection and analysis of data. It is an essential element of the scientific method, as it allows researchers to make predictions about the outcome of their experiments and to test those predictions to determine their accuracy.
Types of Hypothesis
Types of Hypothesis are as follows:
Research Hypothesis
A research hypothesis is a statement that predicts a relationship between variables. It is usually formulated as a specific statement that can be tested through research, and it is often used in scientific research to guide the design of experiments.
Null Hypothesis
The null hypothesis is a statement that assumes there is no significant difference or relationship between variables. It is often used as a starting point for testing the research hypothesis, and if the results of the study reject the null hypothesis, it suggests that there is a significant difference or relationship between variables.
Alternative Hypothesis
An alternative hypothesis is a statement that assumes there is a significant difference or relationship between variables. It is often used as an alternative to the null hypothesis and is tested against the null hypothesis to determine which statement is more accurate.
Directional Hypothesis
A directional hypothesis is a statement that predicts the direction of the relationship between variables. For example, a researcher might predict that increasing the amount of exercise will result in a decrease in body weight.
Non-directional Hypothesis
A non-directional hypothesis is a statement that predicts the relationship between variables but does not specify the direction. For example, a researcher might predict that there is a relationship between the amount of exercise and body weight, but they do not specify whether increasing or decreasing exercise will affect body weight.
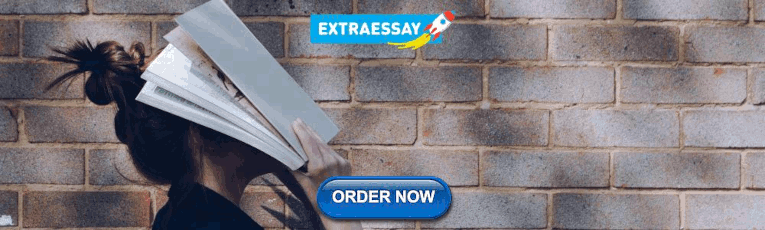
Statistical Hypothesis
A statistical hypothesis is a statement that assumes a particular statistical model or distribution for the data. It is often used in statistical analysis to test the significance of a particular result.
Composite Hypothesis
A composite hypothesis is a statement that assumes more than one condition or outcome. It can be divided into several sub-hypotheses, each of which represents a different possible outcome.
Empirical Hypothesis
An empirical hypothesis is a statement that is based on observed phenomena or data. It is often used in scientific research to develop theories or models that explain the observed phenomena.
Simple Hypothesis
A simple hypothesis is a statement that assumes only one outcome or condition. It is often used in scientific research to test a single variable or factor.
Complex Hypothesis
A complex hypothesis is a statement that assumes multiple outcomes or conditions. It is often used in scientific research to test the effects of multiple variables or factors on a particular outcome.
Applications of Hypothesis
Hypotheses are used in various fields to guide research and make predictions about the outcomes of experiments or observations. Here are some examples of how hypotheses are applied in different fields:
- Science : In scientific research, hypotheses are used to test the validity of theories and models that explain natural phenomena. For example, a hypothesis might be formulated to test the effects of a particular variable on a natural system, such as the effects of climate change on an ecosystem.
- Medicine : In medical research, hypotheses are used to test the effectiveness of treatments and therapies for specific conditions. For example, a hypothesis might be formulated to test the effects of a new drug on a particular disease.
- Psychology : In psychology, hypotheses are used to test theories and models of human behavior and cognition. For example, a hypothesis might be formulated to test the effects of a particular stimulus on the brain or behavior.
- Sociology : In sociology, hypotheses are used to test theories and models of social phenomena, such as the effects of social structures or institutions on human behavior. For example, a hypothesis might be formulated to test the effects of income inequality on crime rates.
- Business : In business research, hypotheses are used to test the validity of theories and models that explain business phenomena, such as consumer behavior or market trends. For example, a hypothesis might be formulated to test the effects of a new marketing campaign on consumer buying behavior.
- Engineering : In engineering, hypotheses are used to test the effectiveness of new technologies or designs. For example, a hypothesis might be formulated to test the efficiency of a new solar panel design.
How to write a Hypothesis
Here are the steps to follow when writing a hypothesis:
Identify the Research Question
The first step is to identify the research question that you want to answer through your study. This question should be clear, specific, and focused. It should be something that can be investigated empirically and that has some relevance or significance in the field.
Conduct a Literature Review
Before writing your hypothesis, it’s essential to conduct a thorough literature review to understand what is already known about the topic. This will help you to identify the research gap and formulate a hypothesis that builds on existing knowledge.
Determine the Variables
The next step is to identify the variables involved in the research question. A variable is any characteristic or factor that can vary or change. There are two types of variables: independent and dependent. The independent variable is the one that is manipulated or changed by the researcher, while the dependent variable is the one that is measured or observed as a result of the independent variable.
Formulate the Hypothesis
Based on the research question and the variables involved, you can now formulate your hypothesis. A hypothesis should be a clear and concise statement that predicts the relationship between the variables. It should be testable through empirical research and based on existing theory or evidence.
Write the Null Hypothesis
The null hypothesis is the opposite of the alternative hypothesis, which is the hypothesis that you are testing. The null hypothesis states that there is no significant difference or relationship between the variables. It is important to write the null hypothesis because it allows you to compare your results with what would be expected by chance.
Refine the Hypothesis
After formulating the hypothesis, it’s important to refine it and make it more precise. This may involve clarifying the variables, specifying the direction of the relationship, or making the hypothesis more testable.
Examples of Hypothesis
Here are a few examples of hypotheses in different fields:
- Psychology : “Increased exposure to violent video games leads to increased aggressive behavior in adolescents.”
- Biology : “Higher levels of carbon dioxide in the atmosphere will lead to increased plant growth.”
- Sociology : “Individuals who grow up in households with higher socioeconomic status will have higher levels of education and income as adults.”
- Education : “Implementing a new teaching method will result in higher student achievement scores.”
- Marketing : “Customers who receive a personalized email will be more likely to make a purchase than those who receive a generic email.”
- Physics : “An increase in temperature will cause an increase in the volume of a gas, assuming all other variables remain constant.”
- Medicine : “Consuming a diet high in saturated fats will increase the risk of developing heart disease.”
Purpose of Hypothesis
The purpose of a hypothesis is to provide a testable explanation for an observed phenomenon or a prediction of a future outcome based on existing knowledge or theories. A hypothesis is an essential part of the scientific method and helps to guide the research process by providing a clear focus for investigation. It enables scientists to design experiments or studies to gather evidence and data that can support or refute the proposed explanation or prediction.
The formulation of a hypothesis is based on existing knowledge, observations, and theories, and it should be specific, testable, and falsifiable. A specific hypothesis helps to define the research question, which is important in the research process as it guides the selection of an appropriate research design and methodology. Testability of the hypothesis means that it can be proven or disproven through empirical data collection and analysis. Falsifiability means that the hypothesis should be formulated in such a way that it can be proven wrong if it is incorrect.
In addition to guiding the research process, the testing of hypotheses can lead to new discoveries and advancements in scientific knowledge. When a hypothesis is supported by the data, it can be used to develop new theories or models to explain the observed phenomenon. When a hypothesis is not supported by the data, it can help to refine existing theories or prompt the development of new hypotheses to explain the phenomenon.
When to use Hypothesis
Here are some common situations in which hypotheses are used:
- In scientific research , hypotheses are used to guide the design of experiments and to help researchers make predictions about the outcomes of those experiments.
- In social science research , hypotheses are used to test theories about human behavior, social relationships, and other phenomena.
- I n business , hypotheses can be used to guide decisions about marketing, product development, and other areas. For example, a hypothesis might be that a new product will sell well in a particular market, and this hypothesis can be tested through market research.
Characteristics of Hypothesis
Here are some common characteristics of a hypothesis:
- Testable : A hypothesis must be able to be tested through observation or experimentation. This means that it must be possible to collect data that will either support or refute the hypothesis.
- Falsifiable : A hypothesis must be able to be proven false if it is not supported by the data. If a hypothesis cannot be falsified, then it is not a scientific hypothesis.
- Clear and concise : A hypothesis should be stated in a clear and concise manner so that it can be easily understood and tested.
- Based on existing knowledge : A hypothesis should be based on existing knowledge and research in the field. It should not be based on personal beliefs or opinions.
- Specific : A hypothesis should be specific in terms of the variables being tested and the predicted outcome. This will help to ensure that the research is focused and well-designed.
- Tentative: A hypothesis is a tentative statement or assumption that requires further testing and evidence to be confirmed or refuted. It is not a final conclusion or assertion.
- Relevant : A hypothesis should be relevant to the research question or problem being studied. It should address a gap in knowledge or provide a new perspective on the issue.
Advantages of Hypothesis
Hypotheses have several advantages in scientific research and experimentation:
- Guides research: A hypothesis provides a clear and specific direction for research. It helps to focus the research question, select appropriate methods and variables, and interpret the results.
- Predictive powe r: A hypothesis makes predictions about the outcome of research, which can be tested through experimentation. This allows researchers to evaluate the validity of the hypothesis and make new discoveries.
- Facilitates communication: A hypothesis provides a common language and framework for scientists to communicate with one another about their research. This helps to facilitate the exchange of ideas and promotes collaboration.
- Efficient use of resources: A hypothesis helps researchers to use their time, resources, and funding efficiently by directing them towards specific research questions and methods that are most likely to yield results.
- Provides a basis for further research: A hypothesis that is supported by data provides a basis for further research and exploration. It can lead to new hypotheses, theories, and discoveries.
- Increases objectivity: A hypothesis can help to increase objectivity in research by providing a clear and specific framework for testing and interpreting results. This can reduce bias and increase the reliability of research findings.
Limitations of Hypothesis
Some Limitations of the Hypothesis are as follows:
- Limited to observable phenomena: Hypotheses are limited to observable phenomena and cannot account for unobservable or intangible factors. This means that some research questions may not be amenable to hypothesis testing.
- May be inaccurate or incomplete: Hypotheses are based on existing knowledge and research, which may be incomplete or inaccurate. This can lead to flawed hypotheses and erroneous conclusions.
- May be biased: Hypotheses may be biased by the researcher’s own beliefs, values, or assumptions. This can lead to selective interpretation of data and a lack of objectivity in research.
- Cannot prove causation: A hypothesis can only show a correlation between variables, but it cannot prove causation. This requires further experimentation and analysis.
- Limited to specific contexts: Hypotheses are limited to specific contexts and may not be generalizable to other situations or populations. This means that results may not be applicable in other contexts or may require further testing.
- May be affected by chance : Hypotheses may be affected by chance or random variation, which can obscure or distort the true relationship between variables.
About the author
Muhammad Hassan
Researcher, Academic Writer, Web developer
You may also like

Data Collection – Methods Types and Examples

Delimitations in Research – Types, Examples and...

Research Process – Steps, Examples and Tips

Research Design – Types, Methods and Examples

Institutional Review Board – Application Sample...

Evaluating Research – Process, Examples and...
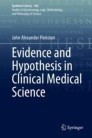
Evidence and Hypothesis in Clinical Medical Science pp 39–66 Cite as
Confirmation of Hypotheses in Clinical Medical Science
- John Alexander Pinkston 7
- First Online: 09 May 2020
164 Accesses
Part of the book series: Synthese Library ((SYLI,volume 426))
In this chapter I discuss the ways in which information is gathered and used to confirm typical hypotheses encountered in clinical medical science. I discuss three kinds of hypotheses, namely, therapeutic , etiologic , and diagnostic . Therapeutic hypotheses are those concerned with treatments or other interventions, and etiologic hypotheses are those concerned with disease causation. Diagnostic hypotheses are those considered by clinicians when making a diagnosis . Examples from the medical scientific literature are extensively used. Included are an example of a randomized clinical trial and an N of 1 study for therapeutic hypotheses, and cohort, case – control, and cross – sectional studies are used for etiologic hypotheses. Approaches to the confirmation of diagnostic hypotheses are illustrated using actual published cases with discussions of the various strategies that are employed. Some possible pitfalls that may occur in the confirmation process are briefly discussed.
This is a preview of subscription content, log in via an institution .
Buying options
- Available as PDF
- Read on any device
- Instant download
- Own it forever
- Available as EPUB and PDF
- Compact, lightweight edition
- Dispatched in 3 to 5 business days
- Free shipping worldwide - see info
- Durable hardcover edition
Tax calculation will be finalised at checkout
Purchases are for personal use only
The stratification referred to is a common method for attempting to control bias such as confounding. For example, by choosing controls with the same age structure (strata) as cases, any influence due to age imbalance would be minimized or eliminated.
For example, a person who had smoked 20 cigarettes daily for 10 years was classed as having smoked 10 cigarettes daily for 20 years.
Both equations contain a random error term that has been omitted.
For example, laboratory tests may be in error, or signs or symptoms may be evolving or misinterpreted.
A “pathognomonic” finding is one that can occur only with a single condition.
Additional references include Gill et al. ( 2005 ), Willis et al. ( 2013 ) , and Jain ( 2017 ).
Anderson, Kristin E., Thomas M. Mack, and Debra T. Silverman. 2006. Cancer of the pancreas. In Cancer epidemiology and prevention , ed. David Schottenfeld and Joseph F. Fraumeni Jr., 3rd ed., 721–762. Oxford: Oxford University Press.
Google Scholar
Berghmans, Ron, and Harry C. Schouten. 2011. Sir Karl Popper, swans, and the general practitioner. British Medical Journal 343: d5469.
Casey, Jonathan D., Daniel H. Solomon, Thomas A. Gaziano, Amy Leigh Miller, and Joseph Loscalzo. 2013. A patient with migrating polyarthralgias. New England Journal of Medicine 369: 75–80.
Checkoway, Harvey, Neil E. Pearce, and Douglas J. Crawford-Brown. 1989. Research methods in occupational epidemiology . Oxford: Oxford University Press.
Clavel, Françoise, Ellen Benhamou, Ariane Auquier, Michèle Tarayre, and Robert Flamant. 1989. Coffee, alcohol, smoking and cancer of the pancreas: A case-control study. International Journal of Cancer 43: 17–21.
Cox, D.R. 1972. Regression models and life-tables. Journal of the Royal Statistical Society, Series B 34: 187–220.
Dajani, Adnan S., Elia Ayoub, Fredrick Z. Bierman, Alan L. Bisno, Floyd W. Denny, David T. Durak, Patricia Ferrieri, et al. 1993. Guidelines for the diagnosis of rheumatic fever: Jones criteria, updated 1992. Circulation 87: 302–307.
Daling, Janet R., and Karen J. Sherman. 1996. Cancers of the vulva and vagina. In Cancer epidemiology and prevention , ed. David Schottenfeld and Joseph F. Fraumeni Jr., 2nd ed., 1117–1129. Oxford: Oxford University Press.
Doll, Richard, and A. Bradford Hill. 1950. Smoking and carcinoma of the lung. Preliminary report. British Medical Journal 221: 739–748.
Doll, Richard, Richard Peto, Jillian Boreham, and Isabelle Sutherland. 2004. Mortality in relation to smoking: 50 years’ observations on male British doctors. British Medical Journal 328: 1519–1528.
Eddy, David M., and Charles H. Clanton. 1982. The art of diagnosis. Solving the clinicopathological exercise. New England Journal of Medicine 306: 1263–1268.
Elstein, Arthur S., and Alan Schwarz. 2002. Clinical problem solving and diagnostic decision making: Selective review of the cognitive literature. British Medical Journal 324: 729–732.
Gill, Christopher J., Lora Sabin, and Christopher H. Schmid. 2005. Why clinicians are natural bayesians. British Medical Journal 330: 1080–1083.
Greenhalgh, Trisha. 2010. How to read a paper: The basics of evidence-based medicine . 4th ed. Chichester: Wiley.
Gutensohn, Nancy, Frederick P. Li, Ralph E. Johnson, and Philip Cole. 1975. Hodgkin’s disease, tonsillectomy, and family size. New England Journal of Medicine 292: 22–25.
Guyatt, Gordon, Sackett David, D. Wayne Taylor, John Chong, Robin Roberts, and Stewart Pugsley. 1986. Determining optimal therapy – Randomized trials in individual patients. New England Journal of Medicine 314: 889–892.
Harvey, A. McGehee. 1994. Foreword. In Differential diagnosis , ed. Jeremiah A. Barondess, Charles C.J. Carpenter, and A. McGehee Harvey. Philadelphia: Lea and Febiger.
Herbst, Arthur L., Howard Ulfelder, and David Poskanzer. 1971. Adenocarcinoma of the vagina. Association of maternal stilbestrol therapy with tumor appearance in young women. New England Journal of Medicine 284: 878–881.
Hoen, Bruno, Isabelle Béguinot, Christian Rabaud, Roland Jaussaud, Christine Selton-Suty, Thierry May, and Philippe Canton. 1996. The Duke criteria for diagnosing infective endocarditis are specific: Analysis of 100 patients with acute fever or fever of unknown origin. Clinical Infectious Diseases 23: 298–302.
Hosmer, David W., and Stanley Lemeshow. 1989. Applied logistic regression . New York: Wiley.
International Agency for Research on Cancer. 2004. Tobacco smoke and involuntary smoking , IARC monographs on the evaluation of carcinogenic risks to humans. Vol. 83. Lyon: IARC.
Jain, Bimal. 2017. The scientific nature of diagnosis. Diagnosis 4: 17–19.
Jones, T. Duckett. 1944. The diagnosis of rheumatic fever. Journal of the American Medical Association 126: 481–484.
Kaplan, E.L., and Paul Meier. 1958. Nonparametric estimation from incomplete observations. Journal of the American Statistical Association 53: 457–481.
Kassirer, Jerome P., John B. Wong, and Richard I. Kopelman. 2010. Learning clinical reasoning . 2nd ed. Baltimore: Lippincott Williams and Wilkins.
Kelsey, Jennifer L., W. Douglas Thompson, and Alfred S. Evans. 1986. Methods in observational epidemiology. Volume 10 of monographs in epidemiology and biostatistics , General ed. Abraham M. Lilienfeld. Oxford: Oxford University Press.
Li, Jennifer S., Daniel J. Sexton, Nathan Mick, Richard Nettles, Vance G. Fowler Jr., Thomas Ryan, Thomas Bashore, and G. Ralph Corey. 2000. Proposed modifications to the Duke criteria for the diagnosis of infective endocarditis. Clinical Infectious Diseases 30: 633–638.
MacMahon, Brian, Stella Yen, Dimitrios Trichopoulos, Kenneth Warren, and George Nardi. 1981. Coffee and cancer of the pancreas. New England Journal of Medicine 304: 630–633.
Mantel, Nathan, and William Haenszel. 1959. Statistical aspects of the analysis of data from retrospective studies of disease. Journal of the National Cancer Institute 22: 719–748.
Mayo, Deborah G. 1996. Error and the growth of experimental knowledge . Chicago: University of Chicago Press.
Miettinen, Olli S. 2001a. The modern scientific physician: 2. Medical science versus scientific medicine. Canadian Medical Association Journal 165: 591–592.
———. 2001b. The modern scientific physician: 3. Scientific diagnosis. Canadian Medical Association Journal 165: 781–782.
Morris, J.N., J.A. Heady, P.A.B. Raffle, C.G. Roberts, and J.W. Parks. 1953. Coronary heart-disease and physical activity of work. Lancet 2: 1053–1057.
Mueller, Nancy E. 1996. Hodgkin’s disease. In Cancer epidemiology and prevention , ed. David Schottenfeld and Joseph F. Fraumeni Jr., 2nd ed., 893–919. Oxford: Oxford University Press.
Mueller, Nancy, G. Marie Swanson, Chung-cheng Hsieh, and Philip Cole. 1987. Tonsillectomy and Hodgkin’s disease: Results from companion population-based studies. Journal of the National Cancer Institute 78: 1–5.
Rothman, Kenneth J., Sander Greenland, and Timothy L. Lash. 2008. Case-control studies. In Modern epidemiology , ed. Kenneth J. Rothman, Sander Greenland, and Timothy L. Lash, 3rd ed., 111–127. Philadelphia: Lippincott Williams and Wilkins.
Slone, Dennis, Samuel Shapiro, Lynn Rosenberg, David W. Kaufman, Stuart C. Hartz, Allen C. Rossi, Paul D. Stolley, and Olli S. Miettinen. 1978. Relation of cigarette smoking to myocardial infarction in young women. New England Journal of Medicine 298: 1273–1276.
Sox, Harold C., Marshall A. Blott, Michael C. Higgins, and Keith I. Martin. 2007. Medical decision making . Philadelphia: American College of Physicians.
Straus, Sharon E., Glasziou Paul, W. Scott Richardson, and R. Brian Haynes. 2011. Evidence-based medicine. How to practice and teach it . 4th ed. Edinburgh: Churchill Livingstone.
Thomas, Patrick R.M., and Anne S. Lindblad. 1988. Adjuvant postoperative radiotherapy and chemotherapy in rectal carcinoma: A review of the gastrointestinal tumor study group experience. Radiotherapy and Oncology 13: 245–252.
Ueshima, Hirotsugu, Takashi Shimamoto, Minoru Iida, Masamitsu Konishi, Masato Tanigaki, Mitsunori Doi, Katsuhiko Tsujioka, et al. 1984. Alcohol intake and hypertension among urban and rural Japanese populations. Journal of Chronic Diseases 37: 585–592.
Willis, Brian H., Helen Beebee, and Daniel S. Lasserson. 2013. Philosophy of science and the diagnostic process. Family Practice 30: 501–505.
Wynder, Ernest L., and Evarts A. Graham. 1950. Tobacco smoking as a possible etiologic factor in bronchiogenic carcinoma. Journal of the American Medical Association 143: 329–336.
Download references
Author information
Authors and affiliations.
Birmingham Radiological Group, Birmingham, AL, USA
John Alexander Pinkston
You can also search for this author in PubMed Google Scholar
Rights and permissions
Reprints and permissions
Copyright information
© 2020 Springer Nature Switzerland AG
About this chapter
Cite this chapter.
Pinkston, J.A. (2020). Confirmation of Hypotheses in Clinical Medical Science. In: Evidence and Hypothesis in Clinical Medical Science. Synthese Library, vol 426. Springer, Cham. https://doi.org/10.1007/978-3-030-44270-5_4
Download citation
DOI : https://doi.org/10.1007/978-3-030-44270-5_4
Published : 09 May 2020
Publisher Name : Springer, Cham
Print ISBN : 978-3-030-44269-9
Online ISBN : 978-3-030-44270-5
eBook Packages : Religion and Philosophy Philosophy and Religion (R0)
Share this chapter
Anyone you share the following link with will be able to read this content:
Sorry, a shareable link is not currently available for this article.
Provided by the Springer Nature SharedIt content-sharing initiative
- Publish with us
Policies and ethics
- Find a journal
- Track your research
What Is a Hypothesis? (Science)
If...,Then...
Angela Lumsden/Getty Images
- Scientific Method
- Chemical Laws
- Periodic Table
- Projects & Experiments
- Biochemistry
- Physical Chemistry
- Medical Chemistry
- Chemistry In Everyday Life
- Famous Chemists
- Activities for Kids
- Abbreviations & Acronyms
- Weather & Climate
- Ph.D., Biomedical Sciences, University of Tennessee at Knoxville
- B.A., Physics and Mathematics, Hastings College
A hypothesis (plural hypotheses) is a proposed explanation for an observation. The definition depends on the subject.
In science, a hypothesis is part of the scientific method. It is a prediction or explanation that is tested by an experiment. Observations and experiments may disprove a scientific hypothesis, but can never entirely prove one.
In the study of logic, a hypothesis is an if-then proposition, typically written in the form, "If X , then Y ."
In common usage, a hypothesis is simply a proposed explanation or prediction, which may or may not be tested.
Writing a Hypothesis
Most scientific hypotheses are proposed in the if-then format because it's easy to design an experiment to see whether or not a cause and effect relationship exists between the independent variable and the dependent variable . The hypothesis is written as a prediction of the outcome of the experiment.
- Null Hypothesis and Alternative Hypothesis
Statistically, it's easier to show there is no relationship between two variables than to support their connection. So, scientists often propose the null hypothesis . The null hypothesis assumes changing the independent variable will have no effect on the dependent variable.
In contrast, the alternative hypothesis suggests changing the independent variable will have an effect on the dependent variable. Designing an experiment to test this hypothesis can be trickier because there are many ways to state an alternative hypothesis.
For example, consider a possible relationship between getting a good night's sleep and getting good grades. The null hypothesis might be stated: "The number of hours of sleep students get is unrelated to their grades" or "There is no correlation between hours of sleep and grades."
An experiment to test this hypothesis might involve collecting data, recording average hours of sleep for each student and grades. If a student who gets eight hours of sleep generally does better than students who get four hours of sleep or 10 hours of sleep, the hypothesis might be rejected.
But the alternative hypothesis is harder to propose and test. The most general statement would be: "The amount of sleep students get affects their grades." The hypothesis might also be stated as "If you get more sleep, your grades will improve" or "Students who get nine hours of sleep have better grades than those who get more or less sleep."
In an experiment, you can collect the same data, but the statistical analysis is less likely to give you a high confidence limit.
Usually, a scientist starts out with the null hypothesis. From there, it may be possible to propose and test an alternative hypothesis, to narrow down the relationship between the variables.
Example of a Hypothesis
Examples of a hypothesis include:
- If you drop a rock and a feather, (then) they will fall at the same rate.
- Plants need sunlight in order to live. (if sunlight, then life)
- Eating sugar gives you energy. (if sugar, then energy)
- White, Jay D. Research in Public Administration . Conn., 1998.
- Schick, Theodore, and Lewis Vaughn. How to Think about Weird Things: Critical Thinking for a New Age . McGraw-Hill Higher Education, 2002.
- Null Hypothesis Definition and Examples
- Definition of a Hypothesis
- What Are the Elements of a Good Hypothesis?
- Six Steps of the Scientific Method
- What Are Examples of a Hypothesis?
- Understanding Simple vs Controlled Experiments
- Scientific Method Flow Chart
- Scientific Method Vocabulary Terms
- What Is a Testable Hypothesis?
- Null Hypothesis Examples
- What 'Fail to Reject' Means in a Hypothesis Test
- How To Design a Science Fair Experiment
- What Is an Experiment? Definition and Design
- Hypothesis Test for the Difference of Two Population Proportions
- How to Conduct a Hypothesis Test

- Cambridge Dictionary +Plus
Meaning of hypothesis in English
Your browser doesn't support HTML5 audio
- abstraction
- afterthought
- anthropocentrism
- anti-Darwinian
- exceptionalism
- foundation stone
- great minds think alike idiom
- non-dogmatic
- non-empirical
- non-material
- non-practical
- social Darwinism
- supersensible
- the domino theory
hypothesis | Intermediate English
Hypothesis | business english, examples of hypothesis, translations of hypothesis.
Get a quick, free translation!
Word of the Day
to add harmonies to a tune

Shoots, blooms and blossom: talking about plants

Learn more with +Plus
- Recent and Recommended {{#preferredDictionaries}} {{name}} {{/preferredDictionaries}}
- Definitions Clear explanations of natural written and spoken English English Learner’s Dictionary Essential British English Essential American English
- Grammar and thesaurus Usage explanations of natural written and spoken English Grammar Thesaurus
- Pronunciation British and American pronunciations with audio English Pronunciation
- English–Chinese (Simplified) Chinese (Simplified)–English
- English–Chinese (Traditional) Chinese (Traditional)–English
- English–Dutch Dutch–English
- English–French French–English
- English–German German–English
- English–Indonesian Indonesian–English
- English–Italian Italian–English
- English–Japanese Japanese–English
- English–Norwegian Norwegian–English
- English–Polish Polish–English
- English–Portuguese Portuguese–English
- English–Spanish Spanish–English
- English–Swedish Swedish–English
- Dictionary +Plus Word Lists
- English Noun
- Intermediate Noun
- Business Noun
- Translations
- All translations
Add hypothesis to one of your lists below, or create a new one.
{{message}}
Something went wrong.
There was a problem sending your report.

An official website of the United States government
The .gov means it’s official. Federal government websites often end in .gov or .mil. Before sharing sensitive information, make sure you’re on a federal government site.
The site is secure. The https:// ensures that you are connecting to the official website and that any information you provide is encrypted and transmitted securely.
- Publications
- Account settings
Preview improvements coming to the PMC website in October 2024. Learn More or Try it out now .
- Advanced Search
- Journal List
- v.19(7); 2019 Jul
Hypothesis tests
Associated data.
- • Hypothesis tests are used to assess whether a difference between two samples represents a real difference between the populations from which the samples were taken.
- • A null hypothesis of ‘no difference’ is taken as a starting point, and we calculate the probability that both sets of data came from the same population. This probability is expressed as a p -value.
- • When the null hypothesis is false, p- values tend to be small. When the null hypothesis is true, any p- value is equally likely.
Learning objectives
By reading this article, you should be able to:
- • Explain why hypothesis testing is used.
- • Use a table to determine which hypothesis test should be used for a particular situation.
- • Interpret a p- value.
A hypothesis test is a procedure used in statistics to assess whether a particular viewpoint is likely to be true. They follow a strict protocol, and they generate a ‘ p- value’, on the basis of which a decision is made about the truth of the hypothesis under investigation. All of the routine statistical ‘tests’ used in research— t- tests, χ 2 tests, Mann–Whitney tests, etc.—are all hypothesis tests, and in spite of their differences they are all used in essentially the same way. But why do we use them at all?
Comparing the heights of two individuals is easy: we can measure their height in a standardised way and compare them. When we want to compare the heights of two small well-defined groups (for example two groups of children), we need to use a summary statistic that we can calculate for each group. Such summaries (means, medians, etc.) form the basis of descriptive statistics, and are well described elsewhere. 1 However, a problem arises when we try to compare very large groups or populations: it may be impractical or even impossible to take a measurement from everyone in the population, and by the time you do so, the population itself will have changed. A similar problem arises when we try to describe the effects of drugs—for example by how much on average does a particular vasopressor increase MAP?
To solve this problem, we use random samples to estimate values for populations. By convention, the values we calculate from samples are referred to as statistics and denoted by Latin letters ( x ¯ for sample mean; SD for sample standard deviation) while the unknown population values are called parameters , and denoted by Greek letters (μ for population mean, σ for population standard deviation).
Inferential statistics describes the methods we use to estimate population parameters from random samples; how we can quantify the level of inaccuracy in a sample statistic; and how we can go on to use these estimates to compare populations.
Sampling error
There are many reasons why a sample may give an inaccurate picture of the population it represents: it may be biased, it may not be big enough, and it may not be truly random. However, even if we have been careful to avoid these pitfalls, there is an inherent difference between the sample and the population at large. To illustrate this, let us imagine that the actual average height of males in London is 174 cm. If I were to sample 100 male Londoners and take a mean of their heights, I would be very unlikely to get exactly 174 cm. Furthermore, if somebody else were to perform the same exercise, it would be unlikely that they would get the same answer as I did. The sample mean is different each time it is taken, and the way it differs from the actual mean of the population is described by the standard error of the mean (standard error, or SEM ). The standard error is larger if there is a lot of variation in the population, and becomes smaller as the sample size increases. It is calculated thus:
where SD is the sample standard deviation, and n is the sample size.
As errors are normally distributed, we can use this to estimate a 95% confidence interval on our sample mean as follows:
We can interpret this as meaning ‘We are 95% confident that the actual mean is within this range.’
Some confusion arises at this point between the SD and the standard error. The SD is a measure of variation in the sample. The range x ¯ ± ( 1.96 × SD ) will normally contain 95% of all your data. It can be used to illustrate the spread of the data and shows what values are likely. In contrast, standard error tells you about the precision of the mean and is used to calculate confidence intervals.
One straightforward way to compare two samples is to use confidence intervals. If we calculate the mean height of two groups and find that the 95% confidence intervals do not overlap, this can be taken as evidence of a difference between the two means. This method of statistical inference is reasonably intuitive and can be used in many situations. 2 Many journals, however, prefer to report inferential statistics using p -values.
Inference testing using a null hypothesis
In 1925, the British statistician R.A. Fisher described a technique for comparing groups using a null hypothesis , a method which has dominated statistical comparison ever since. The technique itself is rather straightforward, but often gets lost in the mechanics of how it is done. To illustrate, imagine we want to compare the HR of two different groups of people. We take a random sample from each group, which we call our data. Then:
- (i) Assume that both samples came from the same group. This is our ‘null hypothesis’.
- (ii) Calculate the probability that an experiment would give us these data, assuming that the null hypothesis is true. We express this probability as a p- value, a number between 0 and 1, where 0 is ‘impossible’ and 1 is ‘certain’.
- (iii) If the probability of the data is low, we reject the null hypothesis and conclude that there must be a difference between the two groups.
Formally, we can define a p- value as ‘the probability of finding the observed result or a more extreme result, if the null hypothesis were true.’ Standard practice is to set a cut-off at p <0.05 (this cut-off is termed the alpha value). If the null hypothesis were true, a result such as this would only occur 5% of the time or less; this in turn would indicate that the null hypothesis itself is unlikely. Fisher described the process as follows: ‘Set a low standard of significance at the 5 per cent point, and ignore entirely all results which fail to reach this level. A scientific fact should be regarded as experimentally established only if a properly designed experiment rarely fails to give this level of significance.’ 3 This probably remains the most succinct description of the procedure.
A question which often arises at this point is ‘Why do we use a null hypothesis?’ The simple answer is that it is easy: we can readily describe what we would expect of our data under a null hypothesis, we know how data would behave, and we can readily work out the probability of getting the result that we did. It therefore makes a very simple starting point for our probability assessment. All probabilities require a set of starting conditions, in much the same way that measuring the distance to London needs a starting point. The null hypothesis can be thought of as an easy place to put the start of your ruler.
If a null hypothesis is rejected, an alternate hypothesis must be adopted in its place. The null and alternate hypotheses must be mutually exclusive, but must also between them describe all situations. If a null hypothesis is ‘no difference exists’ then the alternate should be simply ‘a difference exists’.
Hypothesis testing in practice
The components of a hypothesis test can be readily described using the acronym GOST: identify the Groups you wish to compare; define the Outcome to be measured; collect and Summarise the data; then evaluate the likelihood of the null hypothesis, using a Test statistic .
When considering groups, think first about how many. Is there just one group being compared against an audit standard, or are you comparing one group with another? Some studies may wish to compare more than two groups. Another situation may involve a single group measured at different points in time, for example before or after a particular treatment. In this situation each participant is compared with themselves, and this is often referred to as a ‘paired’ or a ‘repeated measures’ design. It is possible to combine these types of groups—for example a researcher may measure arterial BP on a number of different occasions in five different groups of patients. Such studies can be difficult, both to analyse and interpret.
In other studies we may want to see how a continuous variable (such as age or height) affects the outcomes. These techniques involve regression analysis, and are beyond the scope of this article.
The outcome measures are the data being collected. This may be a continuous measure, such as temperature or BMI, or it may be a categorical measure, such as ASA status or surgical specialty. Often, inexperienced researchers will strive to collect lots of outcome measures in an attempt to find something that differs between the groups of interest; if this is done, a ‘primary outcome measure’ should be identified before the research begins. In addition, the results of any hypothesis tests will need to be corrected for multiple measures.
The summary and the test statistic will be defined by the type of data that have been collected. The test statistic is calculated then transformed into a p- value using tables or software. It is worth looking at two common tests in a little more detail: the χ 2 test, and the t -test.
Categorical data: the χ 2 test
The χ 2 test of independence is a test for comparing categorical outcomes in two or more groups. For example, a number of trials have compared surgical site infections in patients who have been given different concentrations of oxygen perioperatively. In the PROXI trial, 4 685 patients received oxygen 80%, and 701 patients received oxygen 30%. In the 80% group there were 131 infections, while in the 30% group there were 141 infections. In this study, the groups were oxygen 80% and oxygen 30%, and the outcome measure was the presence of a surgical site infection.
The summary is a table ( Table 1 ), and the hypothesis test compares this table (the ‘observed’ table) with the table that would be expected if the proportion of infections in each group was the same (the ‘expected’ table). The test statistic is χ 2 , from which a p- value is calculated. In this instance the p -value is 0.64, which means that results like this would occur 64% of the time if the null hypothesis were true. We thus have no evidence to reject the null hypothesis; the observed difference probably results from sampling variation rather than from an inherent difference between the two groups.
Table 1
Summary of the results of the PROXI trial. Figures are numbers of patients.
Continuous data: the t- test
The t- test is a statistical method for comparing means, and is one of the most widely used hypothesis tests. Imagine a study where we try to see if there is a difference in the onset time of a new neuromuscular blocking agent compared with suxamethonium. We could enlist 100 volunteers, give them a general anaesthetic, and randomise 50 of them to receive the new drug and 50 of them to receive suxamethonium. We then time how long it takes (in seconds) to have ideal intubation conditions, as measured by a quantitative nerve stimulator. Our data are therefore a list of times. In this case, the groups are ‘new drug’ and suxamethonium, and the outcome is time, measured in seconds. This can be summarised by using means; the hypothesis test will compare the means of the two groups, using a p- value calculated from a ‘ t statistic’. Hopefully it is becoming obvious at this point that the test statistic is usually identified by a letter, and this letter is often cited in the name of the test.
The t -test comes in a number of guises, depending on the comparison being made. A single sample can be compared with a standard (Is the BMI of school leavers in this town different from the national average?); two samples can be compared with each other, as in the example above; or the same study subjects can be measured at two different times. The latter case is referred to as a paired t- test, because each participant provides a pair of measurements—such as in a pre- or postintervention study.
A large number of methods for testing hypotheses exist; the commonest ones and their uses are described in Table 2 . In each case, the test can be described by detailing the groups being compared ( Table 2 , columns) the outcome measures (rows), the summary, and the test statistic. The decision to use a particular test or method should be made during the planning stages of a trial or experiment. At this stage, an estimate needs to be made of how many test subjects will be needed. Such calculations are described in detail elsewhere. 5
Table 2
The principle types of hypothesis test. Tests comparing more than two samples can indicate that one group differs from the others, but will not identify which. Subsequent ‘post hoc’ testing is required if a difference is found.
Controversies surrounding hypothesis testing
Although hypothesis tests have been the basis of modern science since the middle of the 20th century, they have been plagued by misconceptions from the outset; this has led to what has been described as a crisis in science in the last few years: some journals have gone so far as to ban p -value s outright. 6 This is not because of any flaw in the concept of a p -value, but because of a lack of understanding of what they mean.
Possibly the most pervasive misunderstanding is the belief that the p- value is the chance that the null hypothesis is true, or that the p- value represents the frequency with which you will be wrong if you reject the null hypothesis (i.e. claim to have found a difference). This interpretation has frequently made it into the literature, and is a very easy trap to fall into when discussing hypothesis tests. To avoid this, it is important to remember that the p- value is telling us something about our sample , not about the null hypothesis. Put in simple terms, we would like to know the probability that the null hypothesis is true, given our data. The p- value tells us the probability of getting these data if the null hypothesis were true, which is not the same thing. This fallacy is referred to as ‘flipping the conditional’; the probability of an outcome under certain conditions is not the same as the probability of those conditions given that the outcome has happened.
A useful example is to imagine a magic trick in which you select a card from a normal deck of 52 cards, and the performer reveals your chosen card in a surprising manner. If the performer were relying purely on chance, this would only happen on average once in every 52 attempts. On the basis of this, we conclude that it is unlikely that the magician is simply relying on chance. Although simple, we have just performed an entire hypothesis test. We have declared a null hypothesis (the performer was relying on chance); we have even calculated a p -value (1 in 52, ≈0.02); and on the basis of this low p- value we have rejected our null hypothesis. We would, however, be wrong to suggest that there is a probability of 0.02 that the performer is relying on chance—that is not what our figure of 0.02 is telling us.
To explore this further we can create two populations, and watch what happens when we use simulation to take repeated samples to compare these populations. Computers allow us to do this repeatedly, and to see what p- value s are generated (see Supplementary online material). 7 Fig 1 illustrates the results of 100,000 simulated t -tests, generated in two set of circumstances. In Fig 1 a , we have a situation in which there is a difference between the two populations. The p- value s cluster below the 0.05 cut-off, although there is a small proportion with p >0.05. Interestingly, the proportion of comparisons where p <0.05 is 0.8 or 80%, which is the power of the study (the sample size was specifically calculated to give a power of 80%).

The p- value s generated when 100,000 t -tests are used to compare two samples taken from defined populations. ( a ) The populations have a difference and the p- value s are mostly significant. ( b ) The samples were taken from the same population (i.e. the null hypothesis is true) and the p- value s are distributed uniformly.
Figure 1 b depicts the situation where repeated samples are taken from the same parent population (i.e. the null hypothesis is true). Somewhat surprisingly, all p- value s occur with equal frequency, with p <0.05 occurring exactly 5% of the time. Thus, when the null hypothesis is true, a type I error will occur with a frequency equal to the alpha significance cut-off.
Figure 1 highlights the underlying problem: when presented with a p -value <0.05, is it possible with no further information, to determine whether you are looking at something from Fig 1 a or Fig 1 b ?
Finally, it cannot be stressed enough that although hypothesis testing identifies whether or not a difference is likely, it is up to us as clinicians to decide whether or not a statistically significant difference is also significant clinically.
Hypothesis testing: what next?
As mentioned above, some have suggested moving away from p -values, but it is not entirely clear what we should use instead. Some sources have advocated focussing more on effect size; however, without a measure of significance we have merely returned to our original problem: how do we know that our difference is not just a result of sampling variation?
One solution is to use Bayesian statistics. Up until very recently, these techniques have been considered both too difficult and not sufficiently rigorous. However, recent advances in computing have led to the development of Bayesian equivalents of a number of standard hypothesis tests. 8 These generate a ‘Bayes Factor’ (BF), which tells us how more (or less) likely the alternative hypothesis is after our experiment. A BF of 1.0 indicates that the likelihood of the alternate hypothesis has not changed. A BF of 10 indicates that the alternate hypothesis is 10 times more likely than we originally thought. A number of classifications for BF exist; greater than 10 can be considered ‘strong evidence’, while BF greater than 100 can be classed as ‘decisive’.
Figures such as the BF can be quoted in conjunction with the traditional p- value, but it remains to be seen whether they will become mainstream.
Declaration of interest
The author declares that they have no conflict of interest.
The associated MCQs (to support CME/CPD activity) will be accessible at www.bjaed.org/cme/home by subscribers to BJA Education .
Jason Walker FRCA FRSS BSc (Hons) Math Stat is a consultant anaesthetist at Ysbyty Gwynedd Hospital, Bangor, Wales, and an honorary senior lecturer at Bangor University. He is vice chair of his local research ethics committee, and an examiner for the Primary FRCA.
Matrix codes: 1A03, 2A04, 3J03
Supplementary data to this article can be found online at https://doi.org/10.1016/j.bjae.2019.03.006 .
Supplementary material
The following is the Supplementary data to this article:
- Find a Lawyer
- Ask a Lawyer
- Research the Law
- Law Schools
- Laws & Regs
- Newsletters
- Justia Connect
- Pro Membership
- Basic Membership
- Justia Lawyer Directory
- Platinum Placements
- Gold Placements
- Justia Elevate
- Justia Amplify
- PPC Management
- Google Business Profile
- Social Media
- Justia Onward Blog
Q: What statutes define Opposition in response to Motion to Compel execution of authorization to release medical records?
When Defense files Motion to Compel execution of authorization to release medical records, Plaintiff responds with Opposition. What statutes define this Opposition?
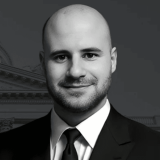
- Sacramento, CA
- Licensed in California
- (916) 704-3009
- Email Lawyer
- View Website
A: In California, the opposition to a motion to compel the execution of an authorization to release medical records is primarily governed by the California Code of Civil Procedure (CCP) and the California Evidence Code (Evid. Code). The key statutes that define the opposition are: 1. CCP § 2017.010 - General scope of discovery: This statute outlines the broad scope of discovery in California, allowing parties to obtain discovery regarding any matter, not privileged, that is relevant to the subject matter involved in the pending action. 2. CCP § 2025.480 - Motion to compel: This statute provides the basis for a party to file a motion to compel when another party fails to comply with discovery requests, including the execution of an authorization to release medical records. 3. Evid. Code § 990-1007 - Physician-patient privilege: These statutes define the physician-patient privilege, which protects confidential communications between a patient and their physician. The plaintiff may argue that the requested medical records are protected by this privilege. 4. Evid. Code § 994 - Exception to physician-patient privilege: This statute provides an exception to the physician-patient privilege when the patient's medical condition is at issue in the litigation. The defendant may argue that this exception applies, making the medical records discoverable. 5. CCP § 2031.060 - Objections to inspection demands: This statute outlines the grounds for objecting to inspection demands, which may include the authorization to release medical records. The plaintiff may base their opposition on these grounds, such as asserting that the request is unduly burdensome, oppressive, or seeks privileged information. In crafting the opposition, the plaintiff's attorney will typically cite these statutes and provide arguments as to why the motion to compel should be denied based on the specific facts of the case and the applicability of the physician-patient privilege or other objections under the California Code of Civil Procedure.
Justia Ask a Lawyer is a forum for consumers to get answers to basic legal questions. Any information sent through Justia Ask a Lawyer is not secure and is done so on a non-confidential basis only.
The use of this website to ask questions or receive answers does not create an attorney–client relationship between you and Justia, or between you and any attorney who receives your information or responds to your questions, nor is it intended to create such a relationship. Additionally, no responses on this forum constitute legal advice, which must be tailored to the specific circumstances of each case. You should not act upon information provided in Justia Ask a Lawyer without seeking professional counsel from an attorney admitted or authorized to practice in your jurisdiction. Justia assumes no responsibility to any person who relies on information contained on or received through this site and disclaims all liability in respect to such information.
Justia cannot guarantee that the information on this website (including any legal information provided by an attorney through this service) is accurate, complete, or up-to-date. While we intend to make every attempt to keep the information on this site current, the owners of and contributors to this site make no claims, promises or guarantees about the accuracy, completeness or adequacy of the information contained in or linked to from this site.
- Bankruptcy Lawyers
- Business Lawyers
- Criminal Lawyers
- Employment Lawyers
- Estate Planning Lawyers
- Family Lawyers
- Personal Injury Lawyers
- Estate Planning
- Personal Injury
- Business Formation
- Business Operations
- Intellectual Property
- International Trade
- Real Estate
- Financial Aid
- Course Outlines
- Law Journals
- US Constitution
- Regulations
- Supreme Court
- Circuit Courts
- District Courts
- Dockets & Filings
- State Constitutions
- State Codes
- State Case Law
- Legal Blogs
- Business Forms
- Product Recalls
- Justia Connect Membership
- Justia Premium Placements
- Justia Elevate (SEO, Websites)
- Justia Amplify (PPC, GBP)
- Testimonials
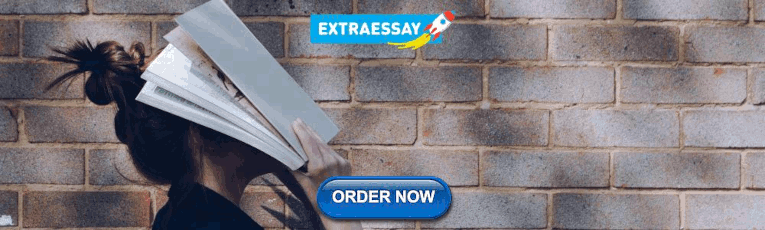
IMAGES
VIDEO
COMMENTS
hypothesis. (hī-pŏth′ĭ-sĭs) n. pl. hypothe·ses (-sēz′) 1. A tentative explanation for an observation, phenomenon, or scientific problem that can be tested by further investigation. 2. Something taken to be true for the purpose of argument or investigation; an assumption. 3. The antecedent of a conditional statement.
A snapshot analysis of citation activity of hypothesis articles may reveal interest of the global scientific community towards their implications across various disciplines and countries. As a prime example, Strachan's hygiene hypothesis, published in 1989,10 is still attracting numerous citations on Scopus, the largest bibliographic database ...
hypothesis: [noun] an assumption or concession made for the sake of argument. an interpretation of a practical situation or condition taken as the ground for action.
A medical hypothesis article has two main aims: to serve as a forum for theoretical work in medicine; and to facilitate the publication of potentially radical ideas. Medical hypotheses are particularly important in a field such as integrative medicine. ... A hypothesis is, by definition, unproven, and for every new hypothesis that proves to be ...
Medical providers often rely on evidence-based medicine to guide decision-making in practice. Often a research hypothesis is tested with results provided, typically with p values, confidence intervals, or both. Additionally, statistical or research significance is estimated or determined by the investigators. Unfortunately, healthcare providers may have different comfort levels in interpreting ...
The present paper attempts to put the P value in proper perspective by explaining different types of probabilities, their role in clinical decision making, medical research and hypothesis testing. Keywords: Hypothesis testing, P value, Probability. The clinician who wishes to remain abreast with the results of medical research needs to develop ...
What is a hypothesis and how can you write a great one for your research? A hypothesis is a tentative statement about the relationship between two or more variables that can be tested empirically. Find out how to formulate a clear, specific, and testable hypothesis with examples and tips from Verywell Mind, a trusted source of psychology and mental health information.
The specific group being studied. The predicted outcome of the experiment or analysis. 5. Phrase your hypothesis in three ways. To identify the variables, you can write a simple prediction in if…then form. The first part of the sentence states the independent variable and the second part states the dependent variable.
Experts use three main methods, or a combination of these, in making a diagnosis. Probably the most common is the hypothetico-deductive approach. An initial hypothesis or hypotheses are generated very early during the initial presentation of the problem, from existing knowledge, associations, and experience.
hypothesis. science. scientific hypothesis, an idea that proposes a tentative explanation about a phenomenon or a narrow set of phenomena observed in the natural world. The two primary features of a scientific hypothesis are falsifiability and testability, which are reflected in an "If…then" statement summarizing the idea and in the ...
Medical Hypotheses is a forum for ideas in medicine and related biomedical sciences. It will publish interesting and important theoretical papers that foster the diversity and debate upon which the scientific process thrives. The Aims and Scope of Medical Hypotheses are no different now from what was proposed by the founder of the journal, the ...
Hypothesis testing is the process used to evaluate the strength of evidence from the sample and provides a framework for making determinations related to the population, ie, it provides a method for understanding how reliably one can extrapolate observed findings in a sample under study to the larger population from which the sample was drawn ...
Definition: Hypothesis is an educated guess or proposed explanation for a phenomenon, based on some initial observations or data. It is a tentative statement that can be tested and potentially proven or disproven through further investigation and experimentation. Hypothesis is often used in scientific research to guide the design of experiments ...
In clinical medical science, typical therapeutic hypotheses might include hypotheses such as: H 0: Treatment A and treatment B give equivalent results. H 1: Treatment A is better than treatment B. Here, "treatment" could include a wide range of interventions, for example, drug therapy, radiation therapy, physical therapy, or some surgical ...
The hypothesis of Andreas Cellarius, showing the planetary motions in eccentric and epicyclical orbits.. A hypothesis (pl.: hypotheses) is a proposed explanation for a phenomenon.For a hypothesis to be a scientific hypothesis, the scientific method requires that one can test it. Scientists generally base scientific hypotheses on previous observations that cannot satisfactorily be explained ...
A hypothesis is a supposition or tentative explanation for (a group of) phenomena, (a set of) facts, or a scientific inquiry that may be tested, verified or answered by further investigation or methodological experiment. It is like a scientific guess. It's an idea or prediction that scientists make before they do experiments.
A hypothesis (plural hypotheses) is a proposed explanation for an observation. The definition depends on the subject. In science, a hypothesis is part of the scientific method. It is a prediction or explanation that is tested by an experiment. Observations and experiments may disprove a scientific hypothesis, but can never entirely prove one.
Formulating Hypotheses for Different Study Designs. Generating a testable working hypothesis is the first step towards conducting original research. Such research may prove or disprove the proposed hypothesis. Case reports, case series, online surveys and other observational studies, clinical trials, and narrative reviews help to generate ...
HYPOTHESIS definition: 1. an idea or explanation for something that is based on known facts but has not yet been proved…. Learn more.
HYPOTHESIS meaning: 1. an idea or explanation for something that is based on known facts but has not yet been proved…. Learn more.
hypothesis, something supposed or taken for granted, with the object of following out its consequences (Greek hypothesis, "a putting under," the Latin equivalent being suppositio ). Discussion with Kara Rogers of how the scientific model is used to test a hypothesis or represent a theory. Kara Rogers, senior biomedical sciences editor of ...
Read the latest articles of Medical Hypotheses at ScienceDirect.com, Elsevier's leading platform of peer-reviewed scholarly literature ... select article Hypothesis: Androgen receptor/Aurora A kinase/NF-κB signaling axis induce inflammatory response during polycystic ovarian syndrome progression ... A SbCO Index value may define risk for ...
A hypothesis test is a procedure used in statistics to assess whether a particular viewpoint is likely to be true. They follow a strict protocol, and they generate a 'p-value', on the basis of which a decision is made about the truth of the hypothesis under investigation.All of the routine statistical 'tests' used in research—t-tests, χ 2 tests, Mann-Whitney tests, etc.—are all ...
3. Evid. Code § 990-1007 - Physician-patient privilege: These statutes define the physician-patient privilege, which protects confidential communications between a patient and their physician. The plaintiff may argue that the requested medical records are protected by this privilege. 4. Evid.