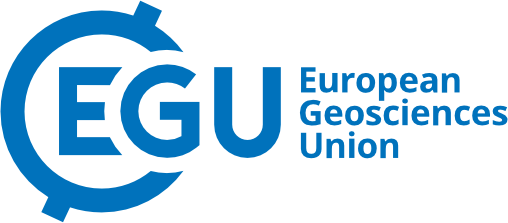
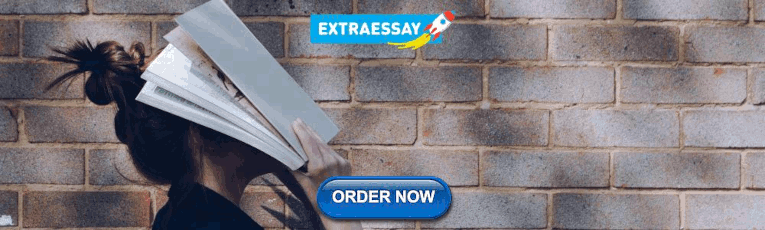
Article
- Volume 6, issue 1
- SOIL, 6, 35–52, 2020
- Peer review
- Related articles
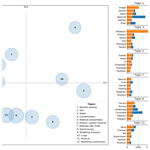
Machine learning and soil sciences: a review aided by machine learning tools
José padarian, budiman minasny, alex b. mcbratney.
The application of machine learning (ML) techniques in various fields of science has increased rapidly, especially in the last 10 years. The increasing availability of soil data that can be efficiently acquired remotely and proximally, and freely available open-source algorithms, have led to an accelerated adoption of ML techniques to analyse soil data. Given the large number of publications, it is an impossible task to manually review all papers on the application of ML in soil science without narrowing down a narrative of ML application in a specific research question. This paper aims to provide a comprehensive review of the application of ML techniques in soil science aided by a ML algorithm (latent Dirichlet allocation) to find patterns in a large collection of text corpora. The objective is to gain insight into publications of ML applications in soil science and to discuss the research gaps in this topic. We found that (a) there is an increasing usage of ML methods in soil sciences, mostly concentrated in developed countries, (b) the reviewed publications can be grouped into 12 topics, namely remote sensing, soil organic carbon, water, contamination, methods (ensembles), erosion and parent material, methods (NN, neural networks, SVM, support vector machines), spectroscopy, modelling (classes), crops, physical, and modelling (continuous), and (c) advanced ML methods usually perform better than simpler approaches thanks to their capability to capture non-linear relationships. From these findings, we found research gaps, in particular, about the precautions that should be taken (parsimony) to avoid overfitting, and that the interpretability of the ML models is an important aspect to consider when applying advanced ML methods in order to improve our knowledge and understanding of soil. We foresee that a large number of studies will focus on the latter topic.
- Article (PDF, 2353 KB)
- Article (2353 KB)
- Full-text XML

Padarian, J., Minasny, B., and McBratney, A. B.: Machine learning and soil sciences: a review aided by machine learning tools, SOIL, 6, 35–52, https://doi.org/10.5194/soil-6-35-2020, 2020.
The application of machine learning (ML) techniques in various fields of science has increased rapidly, especially in the last 10 years. Soil science research, in particular, pedometrics, has used statistical models to “learn” or understand from data how soil is distributed in space and time ( McBratney et al. , 2019 ) . The increasing availability of soil data that can be efficiently acquired remotely and proximally, and freely available open-source algorithms, have led to an accelerated adoption of ML techniques to analyse soil data. Several well-known ML applications in soils science include the prediction of soil types and properties via digital soil mapping (DSM) or pedotransfer functions and analysis of infrared spectral data to infer soil properties. Machine learning analysis of soil data is also used to draw conclusions on the controls of the distribution of the soil.
The definition of what constitutes ML is still contentious or sometimes mistaken. In this work, instead of adding a new argument to differentiate ML from statistical science, we will focus on the view of Jordan and Mitchell ( 2015 ) , where ML is “lying at the intersection of computer science and statistics”. With respect to artificial intelligence (AI), sometimes we have seen the terms ML and AI used interchangeably. This is understandable confusion since ML is a subset of AI, but not everything related to AI falls in the ML category (e.g. expert systems).
There are concerns that ML application ignores soil science knowledge ( Rossiter , 2018 ) and that the results could be misleading and wrong. Nevertheless, many would find that ML methods can help in the scientific process ( Mjolsness and DeCoste , 2001 ; Rudin and Wagstaff , 2014 ) : observations, empirical and theory-based model development, and simulations of soil processes ( Rossiter , 2018 ) . For example, exploration of high-dimensional infrared spectral data helps in understanding the horizonation designation in a soil profile ( Fajardo et al. , 2016 ) . The process of modelling and validation can be used to formulate a model to explain soil distribution ( Brungard et al. , 2015 ) . Modelling via ML can also be used to improve our understanding of the causes of soil variation. Results from ML models can inform on which environmental variables control soil distribution. New relationships revealed by ML analysis can help to stimulate ideas, generate hypotheses, and formulate future questions for research ( Ma et al. , 2019 ) .
This paper aims to provide a comprehensive review of the application of ML techniques in soil science. A quick Google Scholar search of “soil” and “machine learning” resulted in more than 70 000 items, with 16 000 items published in 2018. While we can narrow down a narrative of ML application in a specific research question, such as the application of ML in yield prediction in precision agriculture ( Chlingaryan et al. , 2018 ) or DSM, it is an impossible task to manually review all papers on the application of ML in soil science. One ML technique that has not been applied in soil science is topic modelling, a type of quantitative text mining method. Similar to what ML does to numerical data, topic modelling finds patterns in a large collection of text corpora ( Blei et al. , 2003 ; Blei , 2012 ) , and it has been used to study the evolution of various disciplines and topics ( Zhou et al. , 2006 ; Sugimoto et al. , 2011 ; Wu et al. , 2014 ) .
This paper uses topic modelling to analyse the trend in ML application in soil science. The objective is to gain insight into publications of ML applications in soil science; in particular, we will try to answer the following questions.
Who is using ML, and is the application of ML as ubiquitous as we think?
Which ML methods are commonly used and how often have they been used?
In which areas of soil sciences do we use ML, and how are they clustered and related?
Do advanced ML methods perform significantly better than linear or non-linear statistical approaches?
Can ML methods simulate soil processes in space and time?
Can we use ML methods to improve our knowledge and understanding of soil?
Throughout this review, we will refer to models as “simple” or “complex/advanced”, trusting in the readers' criteria. To illustrate that gradient between simple and complex, we considered a linear model (LM) with 2 variables to be simple compared to a LM with 100 variables; a classification and regression tree (CART) with 2 branches to be simple compared to a CART with 100 branches; and finally, a CART with 2 branches to be simple compared with a LM with 100 variables. We also hope that is clear for the reader that a model such as a deep convolutional neural network (CNN) has many parameters; hence, it is more complex than a CART model.
2.1 Article selection
In order to identify the primary group of articles, we used the term “soil `machine learning”' to perform a full-text search in databases from different publishers. We selected the publishers based on (a) our institution having access to full-text articles and (b) that they provide text-mining permission. We limited our search to the English-only literature, without fixing a specific time frame, and completing the search on 1 February 2019. After performing a screening for the relevance of the initial 3044 matches, we decided to narrow down the selection to the articles containing the word “soil” in their title, yielding a total of 322 articles. The final journal names and number of articles are shown in Table A1 in the Appendix.
2.2 Topic modelling
Topic modelling is a probabilistic ML method that aims to discover and annotate large archives of documents with thematic information Blei ( 2012 ) . By analysing the words contained in a set of documents, these topic modelling algorithms are capable of identifying common themes. These methods allow processing of an arbitrarily large number of articles, which can help to reduce part of the bias introduced by only selecting a manageable subset of documents or by manually assigning documents to topics.
In order to determine in which areas of soil sciences we use ML, we selected an algorithm commonly used in topic modelling called latent Dirichlet allocation (LDA) ( Blei et al. , 2003 ) to perform the task of allocating the articles to topics. LDA is a probabilistic model that assumes that a number of topics exist in a document collection and each topic is represented by a distribution of words. Each document is represented by a distribution over topics, and each word is a sample over each topic's vocabulary (Fig. 1 ). For more details about the LDA, we refer the reader to Blei ( 2012 ) .
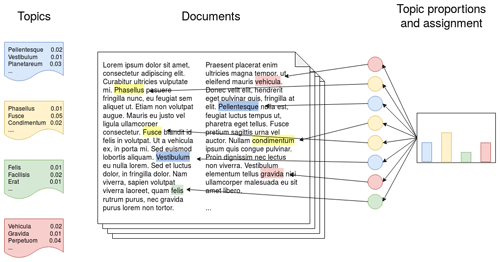
Figure 1 Collection of topics with distribution of words (left), document distribution over topics (histogram, right), and words sampled from the topics' vocabularies (circles). The topics, words, and assignment are for illustrative purposes. Adapted from Blei ( 2012 ) .
Before modelling the topics, we pre-processed the documents in order to reduce the noise of the unstructured texts. We (a) removed stop words (common words such as “from” and “are”), (b) generated bi- and tri-grams, which are groups of two or three words which commonly appear together in the text (e.g. “remote sensing”, “particle size distribution”), and (c) removed extremely uncommon (that appear in less than five documents) and common words (that appear in more than 50 % of the documents), which do not help to differentiate between topics.
The LDA algorithm is capable of learning different topics to which each document is assigned given the words that constitute it. The first challenge is to find the optimal number of topics, which has to be general enough to capture similarities between articles but with some degree of specificity in order to have a manageable and sensible group of topics. That balance between generality and specificity is key to generating topics that are semantically interpretable by a human ( Stevens et al. , 2012 ) . One of the measures that is highly correlated with human interpretation of the topics is topic coherence ( Stevens et al. , 2012 ) . We estimated a coherence measure proposed by Röder et al. ( 2015 ) (referenced as C V in their paper) for different models trained with an increasing number of topics, from 2 to 30. C V is an aggregated measure which combines a normalised point-wise mutual information coherence measure, cosine vector similarity, and a Boolean sliding window of size 110. It ranges from 0 to 1, 1 being the highest coherence. Other parameters of the LDA algorithm, such as the threshold of the probability above which a topic is considered, and the number of training iterations, were set to 0.2 and 1000, respectively, after performing a parameter grid search.
2.3 Text extraction
In order to identify the information required to answer our questions, we used a combination of named-entity recognition and rule-based matching. To extract the MODEL entities, we used a list of modelling methods from the Outline_of_machine_learning Wikipedia article in addition to other algorithms that are commonly used in soil sciences and that were not present in the list (e.g. Cubist). After extracting the MODEL entities, we proceeded to extract the abbreviations used to reference those models. In order to extract the abbreviations, we relied on the commonly seen pattern of writing model names followed by their corresponding abbreviation (e.g. “we used a random forest model (RF)”). By extracting the abbreviations we expected to discriminate between (a) models used to generate the results reported in the articles and (b) models mentioned to give context to the studies. Extracting abbreviations also allowed us to capture variations of models not present in our original list (e.g. BART for bagged regression trees in Fig. 2 ).
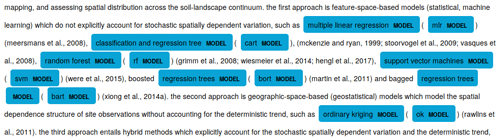
Figure 2 Excerpt from one of the reviewed articles showing named entities recognised as models. Note that the word “bagged” is not recognised, but the abbreviation “bart” is.
2.4 Implementation
We performed all our analysis in Python, using the libraries gensim v3.6.0 ( Řehůřek and Sojka , 2010 ) and the MALLET package ( McCallum , 2002 ) for the topic modelling and spacy v2.1.0a6 ( Matthew and Honnibal , 2017 ) for the named entity recognition.
3.1 Who is using machine learning methods?
The first questions related to the current status of the ML literature in soil sciences can be answered after correctly organising all the articles' metadata. Regarding the general usage of ML methods, in our review, we observed an expected increment in time in the number of publications using ML to model different aspects of soils (Fig. 3 ). This increment is most likely due to a combination of increasing computational power and accessibility to high-performance computers, increasing availability of data (e.g. remote sensing) ( Jordan and Mitchell , 2015 ) , and the increasing interest in “data science”. It is also confounded with the overall increase in the number of publications, which was estimated in 2015 at nearly 2.5 million new publications per year ( Ware and Mabe , 2015 ) .
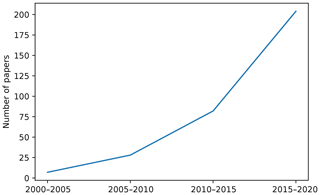
Figure 3 Distribution in time of the articles used in this review.
Besides the temporal trend in publishing, we were also interested in how ubiquitous the application of ML methods is. Fig. 4 shows the number of institutions per country (log 10 ) that appeared listed as affiliations in the analysed articles. ML techniques in the context of soil sciences are used in many countries around the world, but are mostly concentrated in developed countries. This is due to the inseparable relationship between science, technology, and development ( Sagasti , 1973 ) , which is also related to what is usually called the “digital divide” ( Rossiter , 2018 ) . Inter-institutional collaboration could be an important aspect of closing this gap ( Sonnenwald , 2007 ) . Similarly to what is happening in many disciplines ( Sonnenwald , 2007 ) , we observed an increase in the number of co-authors per article (Fig. 5 ), which might be a good sign if we avoid bad practices like “helicopter science” ( Minasny and Flantis , 2018 ) .
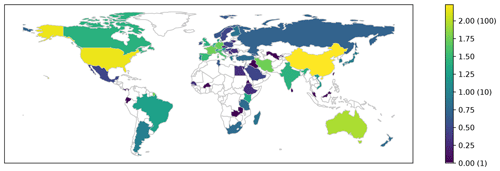
Figure 4 Total number (log 10 ) of institutions per country that participated in articles included in this review. Numbers between brackets are the real number of publications. Outlined countries have zero occurrences.
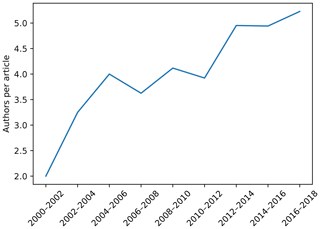
Figure 5 Evolution of the number of authors per publication. Mean values per time period.
The advance of a discipline is not only measured by the number of publications. Dissemination of knowledge is a key component of research and open access (OA) has been recognised as an optimal solution since it is in the best interests of all stakeholders involved in the process ( Björk , 2017 ) . In the application of ML in soil sciences, the proportion of OA publications is very low (Fig. 6 ). This number is in line with the overall OA presence in science ( Björk , 2017 ) but on the opposite side of the general trend in ML, where scientists prefer AO ( Hutson , 2018 ) .
3.2 Most used methods
From the huge variety of ML models available, we found over 100 different variants that have been applied in the soil sciences. From those, most have been applied experimentally in one or two papers, and just a handful are consistently used. Fig. 7 depicts the evolution of some selected models. There is an overall increase in the usage of all the models but, proportionally, it is possible to see a decrease in the usage of some models such as support vector machines (SVM), multivariate adaptive regression spline (MARS), and CART, giving way to more advanced alternatives such as random forest (RF). The adoption of the latter has an accelerated growth, and it has been used in a diversity of topics, including mapping and spectroscopy. The appearance of deep learning is also noticeable, which at the moment has only been used in a few publications related to mapping and spectroscopy.
3.3 Main topics
As we mentioned in Sect. 2.2 , in order to find the optimal number of topics present in the corpora, we trained models with an increasing number of topics (from 2 to 30) and we plotted the evolution of the C V coherence (Fig. 8 ). From this curve it is possible to select the number of topics that yield the highest coherence, which in this case is 12.
These 12 topics correspond to main soil areas detected by the LDA algorithm where ML is applied. We extracted the most relevant words for each of the 12 topics and we examined the titles of the more relevant papers to identify suitable “topic names”. The 12 identified areas were the following.
Articles heavily based on remote sensing ( Grunwald et al. , 2015 ; Xu et al. , 2017 ; Zhang et al. , 2018 b ) . Articles related to salinity were also assigned to this group since most of them use remote sensing techniques ( Khadim et al. , 2019 ; Zhang et al. , 2019 ) .
Articles related to soil organic carbon (SOC) cycles and dynamics and its relationship with the environment. Carbon stocks in different ecosystems, with particular emphasis on grasslands and topsoil ( Rial et al. , 2017 ; Liu et al. , 2018 ; Song et al. , 2018 ; Wang et al. , 2018 a ) .
Articles mostly focused on soil water content and its changes over time ( Ahmad et al. , 2010 ; Coopersmith et al. , 2014 ; Greifeneder et al. , 2018 ; Han et al. , 2018 ) . Other articles in this category are related to soil temperature and CO 2 fluxes ( Xing et al. , 2018 ; Oh et al. , 2019 ; Warner et al. , 2019 ; Zeynoddin et al. , 2019 ) . All these articles comprise measurements made by “stations”.
Articles addressing problems related to heavy metals, soil pollution, and bio-availability ( Costa et al. , 2017 ; Reeves et al. , 2018 ; Wu et al. , 2013 ) .
Articles with a focus on model ensembles such as RF ( Blanco et al. , 2018 ; Tziachris et al. , 2019 ) .
Articles focused on soil formation processes, specifically additions and losses by deposition and erosion, respectively ( Geissen et al. , 2007 ; Märker et al. , 2011 ; Martinez et al. , 2017 ) . Since soil formation depends on the parent material, articles aiming to characterise it were also included in this category ( Kheir et al. , 2008 ; Lacoste et al. , 2011 ) .
Articles with a focus on methods such as neural networks (NN) and SVM ( Kovačević et al. , 2010 ; Farfani et al. , 2015 ; Hanna et al. , 2007 ) .
This topic is related to proximal soil sensing covering different light wavelength sections, from microwave to infrared to gamma ( Heggemann et al. , 2017 ; Butler et al. , 2018 ; Xie and Li , 2018 ) .
Articles focused on the modelling, especially mapping, of categorical soil properties based on their relationship with environmental covariates ( Mansuy et al. , 2014 ; Camera et al. , 2017 ; Dharumarajan et al. , 2017 ; Massawe et al. , 2018 ) . In this category it is also possible to find articles related to the use of conventional soil maps, especially spatial disaggregation of polygons ( Subburayalu et al. , 2014 ; Vincent et al. , 2018 ; Flynn et al. , 2019 ) .
This group of articles focused not merely on soil, but also on its interaction within the soil–plant continuum. Water and nutrient availability in order to ensure crop yields is a key component of this topic ( Karandish and Šimŭnek , 2016 ; Ivushkin et al. , 2018 ; Khanal et al. , 2018 ; Leenaars et al. , 2018 ) .
Articles related to the physical properties of soils, including texture and bulk density ( Bondi et al. , 2018 ; Naderi-Boldaji et al. , 2019 ) , and how they affect aspects of soil such as water retention and flow ( Koestel and Jorda , 2014 ; Gao et al. , 2018 ) .
Articles focused on the modelling, especially mapping, of continuous soil properties based on their relationship with environmental covariates, from regional to continental scales ( Henderson et al. , 2005 ; Dai et al. , 2014 ; Poggio et al. , 2016 ; Padarian et al. , 2019 a ; Caubet et al. , 2019 ) . In this category it is also possible to find articles related to pedotransfer functions ( Dobarco et al. , 2019 ) .
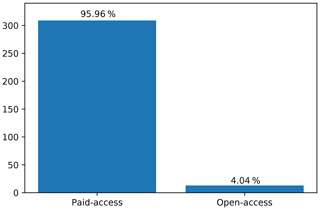
Figure 6 Number of articles published under paid and open access.
These topics are not completely independent and they share some commonalities. For instance, Fig. 9 shows an overlap between Topic 12 (Modelling continuous properties) and 9 (Modelling classes) since both are related to mapping using environmental covariables. Both topics are also related to Topic 3 (Water) since its articles usually have a spatial component. Something similar occurs between Topics 8 (Spectroscopy) and 1 (Remote sensing) since both are related to spectral data.
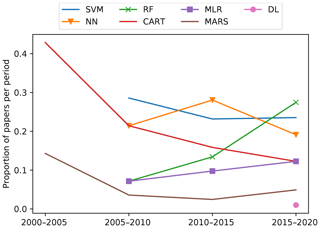
Figure 7 Evolution of model usage in time. SVM: support vector machines; NN: neural networks; RF: random forest; CART: classification and regression trees; MLR: multiple linear regression; MARS: multivariate adaptive regression spline; DL: deep learning
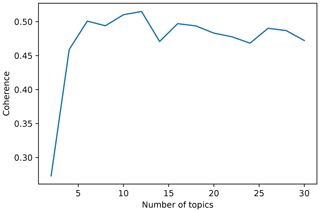
Figure 8 Coherence by number of topics used to train a LDA model.
Besides the shared features between topics, given that LDA is a probabilistic model, articles also contain features related to more than one topic; i.e. they talk about more than one topic (Fig. 10 ). For instance, many of the articles related to SOC are also related to soil modelling and mapping ( Deng et al. , 2018 ; Wang et al. , 2018 b ; Gomes et al. , 2019 ; Keskin et al. , 2019 ) .
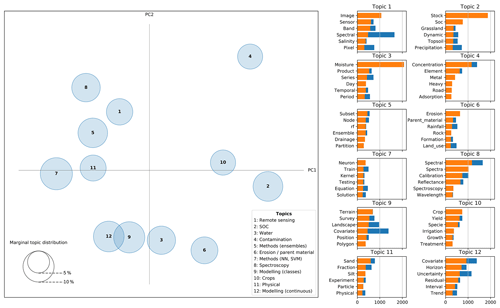
Figure 9 Inter-topic distance map. Dimension reduction via Jensen–Shannon divergence ( Lin , 1991 ) and principal coordinate analysis. Top-6 more relevant words per topic. Complete bars (blue + orange) correspond to overall term frequency and shaded bars (orange) correspond to term frequency within the selected topic.
3.4 Performance of machine learning models
Our review shows that more advanced modelling techniques usually yield better results compared with simpler approaches. In one of the more extensive comparisons, Sirsat et al. ( 2018 ) compared 76 different algorithms, where ensembles of extremely randomised regression trees ranked first when predicting soil fertility indices. Other comparative studies also showed a consistent higher performance of ML methods (NN, SVM, RF) over simpler approaches (principal component regression, partial least squares regression (PLSR), multiple linear regression (MLR), k -nearest neighbours) in applications such as spectroscopy ( Viscarra-Rossel and Behrens , 2010 ; Morellos et al. , 2016 ) and DSM ( Brungard et al. , 2015 ; Taghizadeh-Mehrjardi et al. , 2015 ; Camera et al. , 2017 ; Jeong et al. , 2017 ) . Most studies mention that the superiority of these algorithms is given by their capability to deal with complex non-linearities present in the data. Moreover, the better performance of more advanced ML methods is reported in studies related to the prediction of continuous properties and classes.
Regarding the connection between performance and model usage (Sect. 3.2 ), we observed that some simpler methods such as MLR, despite their lower performance compared to more advanced models, are very popular. This is expected for statistical models since they have a long tradition in science. On the other hand, we also observed a natural tendency of leaving some models behind despite being used for a long time. For instance, PLSR is very popular and has been used since the 1980–1990s but, when used in the studies included in this review (mostly published post the 2000s), very few studies use it as their main algorithm and, instead, it is used in comparative studies where it is outperformed by more advanced models.
It is worth noting that the final performance is not solely dependent on the selected modelling method. Advanced methods like NN have a big number of parameters to fit, especially in the context of deep learning. In order to correctly fit those parameters, from a computational and statistical point of view, the size of the dataset is an essential factor ( Jordan and Mitchell , 2015 ) . Padarian et al. ( 2019 b ) show that a deep CNN trained using a large dataset (around 20 000 soil samples) outperformed methods such as PLS and Cubist when predicting soil properties from spectral data. Using the same method but training on a significantly smaller dataset (390 soil samples), the CNN yielded the worst results.
There is no clear rule on how big a dataset should be, especially because it certainly depends on the complexity of the underlying problem, but the relationship between dataset size and performance has been shown in many studies, using what is usually known as “learning curves” ( Catlett , 1991 ; Shavlik et al. , 1991 ; Cortes et al. , 1994 ; Perlich et al. , 2003 ; Somarathna et al. , 2017 ) . During our review, we observed that the dataset size varied greatly depending on the ML methods (Fig. 11 ).
Considering that ML models could generate a similar solution to a linear model (e.g. a single-rule tree), it should not be a problem to use any method for any dataset size. However, the main difficulty is that training a complex ML model is not a trivial task, especially to avoid overfitting and to obtain a good generalisation, which becomes challenging in the presence of small datasets (i.e. training and test datasets). Even if a researcher can overcome the training process, it is probable that a simpler model can yield similar results.
3.5 Space–time modelling
Compared with the spatial component of soil variation, which is prominent in the topics found using the LDA algorithm (Sect. 3.3 ), the number of studies that address the spatio-temporal dynamics of soil properties using ML methods is still limited. Our findings agree with the review by Grunwald ( 2009 ) , who characterised studies covering the years 2007 and 2008. A big proportion of studies that deal with the temporal dynamics of soil properties are related to soil–water interactions, as shown in Topic 3 of our topic detection analysis (Fig. 9 ).
We found three main approaches to deal with the temporal variation of soil.
The studies generate models for a specific time step, including one or more predictors that vary in time to then apply that fitted model to another time step (e.g. Grinand et al. , 2017 ).
The studies model two or more time steps independently followed by a change analysis. For instance, Schillaci et al. ( 2017 a ) and Zhang et al. ( 2018 b ) subtracted the maps of the modelled properties from 2 different years to compute the change in SOC concentration and pH, respectively.
Studies that model the actual dynamics of a soil property based on some mechanistic or semi-mechanistic method. Stumpf et al. ( 2018 ) created yearly land-use covers for 8500 km 2 in Switzerland using a combination of Landsat 5–7–8 and field land-use observations in order to model the SOC dynamics based on the conversion regimes from their land-use sequence patterns ( Watson et al. , 2014 ) .
Despite there being ML algorithms that have the capacity to capture 4-D structures (e.g. convolutional recurrent neural networks), we did not find studies using ML to continuously model space and time simultaneously. We think the main reason is that soil observations are usually sparse in space–time ( Grunwald , 2016 ) and that it is not possible to fulfill the dataset size requirements of such models. That is the reason why we mostly find studies that use a mechanistic or semi-mechanistic approach.
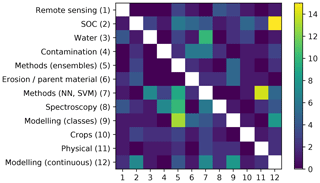
Figure 10 Co-occurrence between the two most likely topics per document. Values correspond to the number of papers.
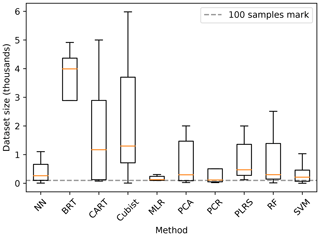
Figure 11 Boxplot of reported dataset sizes grouped by method. Outliers were removed.
3.6 Uncertainty assessment
Uncertainty assessment is an important requirement for any model, especially if the predictions are going to be used to guide decision-making. In this review, 24 % of the studies, among most topics, present uncertainty assessment or mention the importance of considering it (Fig. 12 ).
In this review, a major contributor to the promotion of uncertainty assessment in soil modelling is the GlobalSoilMap project ( Arrouays et al. , 2014 ) which, through specification developed by the DSM scientific community, recommends an uncertainty assessment of all their products. This is evident from Fig. 12 , where topics related to DSM show a relatively high proportion of articles mentioning or reporting uncertainty. In the GlobalSoilMap specifications, the proposed uncertainty assessment method is the use of bootstrapping when training the model ( Stine , 1985 ) , effectively making predictions with many models trained with subsets of the original data to then estimate the 90 % prediction interval (e.g. Castro-Franco et al. , 2017 ; Ma et al. , 2017 ). Another approach is the use of quantile regression ( Koenker and Bassett Jr. , 1978 ) to estimate the complete conditional distribution of the prediction. This method has been recently applied in some DSM studies ( Vaysse and Lagacherie , 2017 ; Sirsat et al. , 2018 ; Cao et al. , 2019 ) . Less common approaches are the use of the fuzzy k -means with the extragrades ( Tranter et al. , 2010 ) algorithm, which defines areas within the covariate space, with different levels of uncertainty, where a new observation (to be predicted) can be placed, and the use of Bayesian optimisation approaches ( Snoek et al. , 2015 ; Gal and Ghahramani , 2016 ) .
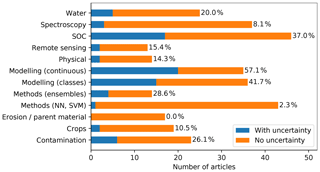
Figure 12 Number of articles per topic that report or mention “uncertainty”. Percentage represents the proportion of total articles per topic.
4.1 Interpretability
Based on our findings, it is possible to state that, in general, ML methods have shown superior performance over more traditional methods in terms of predictive power. We now address the last questions from the aims of this paper — does an advanced model provide new insights that improve our knowledge and understanding of soils?
In order for a human to understand the decisions made by the model, the model has to be interpretable. The motivations for interpretability are varied, including trust, causality, transferability, informativeness, and fairness (in ethical terms) ( Lipton , 2016 ) . In our review, researches usually associate advanced ML models with low interpretability. For instance, Brungard et al. ( 2015 ) assigned multiple models to different groups according to their complexity, with NN and SVM categorised as difficult to interpret compared to MLR or CART. Beguin et al. ( 2017 ) also mention the lower interpretability of ML models compared with an explicit geostatistical model. Because how to measure interpretability is usually not well defined, there are also contradictory opinions. For instance, RF is mostly considered in the category of low interpretability ( Brungard et al. , 2015 ; Were et al. , 2015 ; Taghizadeh-Mehrjardi et al. , 2016 ; Deng et al. , 2018 ) , but its use is also sometimes justified due to its ease of interpretability via the use of variables of importance ( Jeong et al. , 2017 ) .
It is important to clearly define the goal of a modelling exercise. If we want to obtain the model with the greatest accuracy in order to solve a specific problem, maybe interpretability should not be an important factor. If we consider a) that nature is a complex combination of non-linear phenomena and b) the limited capacity of humans to understand non-linear relationships ( Doherty and Balzer , 1988 ) , by requiring our model to have complete transparency we are limiting its capability. However, it is important to corroborate that the model is a valid generalisation of the studied phenomenon. If our goal is to obtain new insights, it is important to consider that interpretability goes hand in hand with prior knowledge and biases and that we could be optimising an algorithm to present misleading but plausible explanations ( Lipton , 2016 ) .
4.1.1 How can we increase interpretability?
A common conclusion reported by authors of the reviewed papers is that the selection of the most informative or relevant predictors before training the model can increase interpretability ( Xiong et al. , 2014 ; Prasad et al. , 2018 ; Wang et al. , 2018 a ; Keskin et al. , 2019 ) , although some authors do not recommend selection of predictors based on the researchers' knowledge since it could lead to biased and suboptimal model performance ( Brungard et al. , 2015 ; Keskin et al. , 2019 ) . This discordance leads to a large range in the number of the predictors used, with some extreme cases using more than 200 ( Xiong et al. , 2014 ; Keskin et al. , 2019 ) .
NN are some of the most performing models but, given the complexity of their operation, they are usually labelled as “black-box” models. In consequence, many authors have focused on trying to provide frameworks to interpret the knowledge extracted by these models. For instance, Bau et al. ( 2017 ) dissected a CNN to understand how different layers work and which features they favour by visualising their (neurons) activation map. Rauber et al. ( 2017 ) used the activation maps projected into a 2-D space in order to visualise and identify confusion zones, outliers, and clusters in the internal representations learned by the model.
In soil sciences, one of the reported methods to interpret ML models is to assess the importance of the variables used, usually derived from the number of times they have been used in the rules generated by tree-like models ( Henderson et al. , 2005 ; Martin et al. , 2014 ; Schillaci et al. , 2017 b ; Khanal et al. , 2018 ) . Another method to assess the relative influence of predictors in tree-like models is to estimate the average reduction of the error at each split of the tree, for all the predictors ( Friedman , 2001 ) . Another alternative, in the context of soil mapping, is to map the rules generated by the model to identify their spatial context or to map where important predictors were used ( Bui et al. , 2006 ) . For CNN, by feeding simulated data to a trained model, Ng et al. ( 2019 ) explored the most important wavelengths used when predicting multiple soil properties from soil spectral data using a sensitivity analysis. The logic behind their analysis is that modifying unimportant wavelengths should not affect the prediction. By plotting the variance for the predictions by wavelengths it is possible to unveil the most important areas of the spectrum (Fig. 13 ).
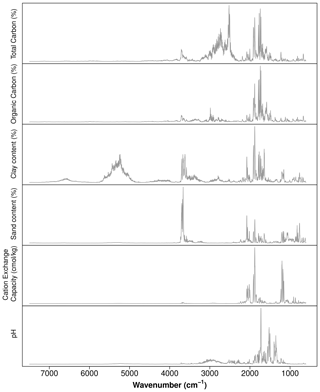
Figure 13 Sensitivity analysis of CNN model prediction as a function of wavelength. Average variance of predictions by wavelength. This analysis allows us to explore the most important wavelengths in a CNN model. Adapted from Ng et al. ( 2019 ) .
Interpretability is an important concept that should be revisited, since it is neither absolute nor static; hence, a specific model cannot be simply labelled as interpretable or not. Linear models can quickly become unintelligible as we add more variables ( Lou et al. , 2012 ) , and methods to better understand complex models such as NN are constantly being developed ( Bau et al. , 2017 ; Montavon et al. , 2018 ; Zhang et al. , 2018 a ) .
4.2 New good practices
Thanks to the effort of some groups to rescue soil legacy data ( Arrouays et al. , 2017 ) and cheaper and faster methods to analyse soil samples, there are more soil data available than ever before. This data availability not only allows us to use new ML algorithms, which usually require more observations, but also opens the door to new ways to train those models. An important part of model development is validation. The literature traditionally recommends that an independent, unseen (by the model) dataset should be used as validation ( Kohavi , 1995 ) . In practice, the data is usually partitioned into training and validation datasets. A more stable solution is the use of k cross-validation where the dataset is partitioned into k groups, where k −1 groups are used for training and 1 groups for validation, repeating the training k times, each with a different validation group. When data availability is a limitation, researchers resort to techniques such as n cross-validation or “leave-one-out” validation to make the most of the available data ( Stevens et al. , 2008 ; Pasini , 2015 ) .
A new generation of models based on NN has been introduced in the later years, which has revolutionised many fields. Deep learning (DL) models, consisting of multiple hidden layers of neurons, have many parameters (from hundreds to millions) which need to be fitted in the training process. This is the reason why they usually need access to large sample sizes. A second characteristic of these models is that they have a considerable number of hyper-parameters. Hyper-parameters are parameters that are not learned from the data during the training phase and include things like the number of iterations during the training, learning rate, layer parameters, or number of layers. A common practice when training DL models is to split the original dataset into three sub-datasets: training, validation, and test. The training dataset is used to learn the parameters, the validation dataset to compare models fitted with different hyper-parameters in order to find the optimal combination, and the test dataset as the independent, unseen data.
In soil sciences, ML algorithms are usually trained using the traditional train–validation split or cross-validation ( Keskin et al. , 2019 ; Liang et al. , 2019 ) , or even no validation ( Feng et al. , 2019 ) , except for some studies based on DL or with an engineering background (e.g. Reale et al. , 2018 ), including some of our publications on the use of DL for DSM ( Padarian et al. , 2019 c ) or soil spectroscopy ( Padarian et al. , 2019 b , a ) , which use a train–validation–test split. Considering the increasing size of datasets, we think soil scientists should transition towards the implementation of some DL practices such as dataset split and hyper-parameter optimisation ( Bergstra and Bengio , 2012 ; Snoek et al. , 2012 ) , not only for NN, but also for any algorithm that has hyper-parameters. Some potential candidates are random forest, Cubist, classification and regression trees, and support vector machines. Most of the implementations of these algorithms have sensible default hyper-parameters, but some studies report an important impact of them in their results ( Mutanga et al. , 2012 ; Lu et al. , 2018 ) . For general hyper-parameter tuning strategies, we refer the reader to Bergstra and Bengio ( 2012 ) for simple strategies such as grid or random search. For an in-depth report of hyper-parameter tuning and its effects in the context of random forest, we refer the reader to Probst et al. ( 2019 ) .
4.3 Commercial ML applications
This work explores the use of ML in soil sciences by exploring the current scientific literature, but use of ML extends beyond research and companies are very welcoming to this technology, especially in applications such as computer vision, speech recognition, natural language processing, and robot control ( Jordan and Mitchell , 2015 ) . It is not hard to imagine a commercial application of approaches such as soil properties prediction using vis-NIR spectroscopy, either in the laboratory or the field. While in research there are some transparency requirements, including describing the methods and data used, companies are usually very secretive about their methods since they are a trade secret that gives them a competitive advantage. Considering that lack of transparency, how can we be sure that the predictions of their models are good? There is not a unique answer, but it should include at least some uncertainty assessment (as discussed in Sect. 3.6 ) and information about the range of soils used during training.
In terms of reporting soil types coverage, different approaches can be applied. A simple, perhaps overconfident method can be to report the geographical extent from where the soil samples used during training were collected (e.g. Tomasella et al. , 2000 ; Børgesen and Schaap , 2005 ) or a broad soil classification based on the soil characteristics such as “sandy soils” (e.g Schaap and Bouten , 1996 and Shaw et al. , 2000 ). A better approach, based on the covariate space of the samples used during training, is fuzzy k -means with extragrades, which has the benefit of describing both coverage and uncertainty levels.
Even if uncertainty levels and coverage are reported, another factor to consider is how much we should trust in companies and their reports. Especially for applications involving public funding, but generally as a consumer protection measure, these type of products should be certifiable in the same way many soil laboratories are. A usual approach is the use of reference materials ( Dybczyński et al. , 1979 ; Pueyo et al. , 2001 ; Ahmed et al. , 2017 ) , which should be consistent with the model coverage reported. The properties measured in the reference materials should fall within the prediction interval produced by the model, with a confidence defined for each application.
Aided by a topic modelling approach, we were able to review the status of ML in soil sciences. We observed a general increase in the adoption of ML methods in time, mostly concentrated in developed countries. This gap is probably due to the link between science, technology, and development. We believe that proper inter-institutional collaboration plans should be put in place in order to close this gap.
By using topic modelling, we identified 12 categories of studies where ML is commonly used, namely remote sensing, soil organic carbon, water, contamination, methods (ensembles), erosion and parent material, methods (NN, SVM), spectroscopy, modelling (classes), crops, physical, and modelling (continuous). The final topic model successfully captured relationships between topics such as modelling of continuous and categorical soil properties, and water, given that all these topics share a spatial component.
We also found that advanced ML methods usually perform better than simpler approaches thanks to their capability to capture non-linear relationships. However, it is important to note that more advanced methods usually require more data and that some precautions should be taken in order to avoid obtaining misleading results. Considering parsimony is always advised, if only a small, simple dataset is available, we recommend using a simple model. This also applies to the number of predictors. In consequence, according to many authors of the reviewed articles, it is better to use meaningful predictors instead of relying on the model capabilities to “select the best variables” in order to improve interpretability.
Interpretability is an important aspect to consider when applying advanced ML methods in order to improve our knowledge and understanding of soil. Simpler methods (e.g. linear models) have been used for a long time and the way of interpreting them is well defined. More advanced methods (e.g. neural networks) are usually considered “black box” models, but that is just a reflection of the current research state and not because it is impossible to interpret them. During our review, we found studies that proposed some solutions to improve their interpretability, and we foresee that a large number of studies will focus on this topic.
Table A1 List of journals by publisher and number of articles that matched the term “soil `machine learning”' in a full-text search.
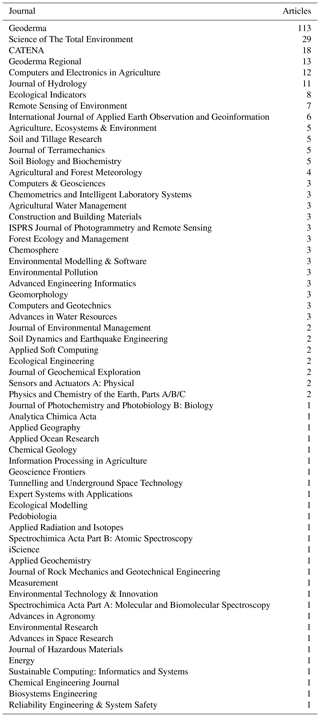
Download Print Version | Download XLSX
The data are not publicly available due to publisher restriction. A complete list of the publication DOIs used is available from the corresponding author on reasonable request.
JP conceived of and designed the study, performed the modelling and analysis, and wrote the first draft. All the authors contributed to generating and reviewing the subsequent versions of the manuscript.
The authors declare that they have no conflict of interest.
The authors acknowledge the University of Sydney HPC service at the University of Sydney for providing HPC resources that have contributed to the research results reported within this paper.
This paper was edited by Olivier Evrard and reviewed by two anonymous referees.
Ahmad, S., Kalra, A., and Stephen, H.: Estimating soil moisture using remote sensing data: A machine learning approach, Adv. Water Resour., 33, 69–80, 2010. a
Ahmed, O., Habbani, F. I., Mustafa, A., Mohamed, E., Salih, A., and Seedig, F.: Quality assessment statistic evaluation of X-ray fluorescence via NIST and IAEA standard reference materials, World Journal of Nuclear Science and Technology, 7, 121–128, 2017. a
Arrouays, D., Grundy, M. G., Hartemink, A. E., Hempel, J. W., Heuvelink, G. B., Hong, S. Y., Lagacherie, P., Lelyk, G., McBratney, A. B., McKenzie, N., Mendonca-Santos, M. d. L., Minasny, B., Montanarella, L., Odeh, I., Sanchez, P., Thompson, J., and Zhang, G.: GlobalSoilMap: Toward a fine-resolution global grid of soil properties, in: Advances in agronomy, Elsevier, 125, 93–134, 2014. a
Arrouays, D., Leenaars, J., Richer-de-Forges, A., Adhikari, K., Ballabio, C., Greve, M., Grundy, M., Guerrero, E., Hempel, J., Hengl, T., Heuvelink, G., Batjes, N., Carvalho, E., Hartemink, A., Hewitt, A., Hong, S., Krasilnikov, P., Lagacherie, P., Lelyk, G., Libohova, Z., Lilly, A., McBratney, A., McKenzie, N., Vasquez, G., Mulder, V., Minasny, B., Montanarella, L., Odeh, I., Padarian, J., Poggio, L., Roudier, P., Saby, N., Savin, I., Searle, R., Solbovoy, V., Thompson, J., Smith, S., Sulaeman, Y., Vintila, R., Rossel, R., Wilson, P., Zhang, G., Swerts, M., Oorts, K., Karklins, A., Feng, L., Navarro, A., Levin, A., Laktionova, T., Dell'Acqua, M., Suvannang, N., Ruam, W., Prasad, J., Patil, N., Husnjak, S., Pásztor, L., Okx, J., Hallett, S., Keay, C., Farewell, T., Lilja, H., Juilleret, J., Marx, S., Takata, Y., Kazuyuki, Y., Mansuy, N., Panagos, P., Liedekerke, M., Skalsky, R., Sobocka, J., Kobza, J., Eftekhari, K., Alavipanah, S., Moussadek, R., Badraoui, M., Silva, M., Paterson, G., da Gonçalves, M., Theocharopoulos, S., Yemefack, M., Tedou, S., Vrscaj, B., Grob, U., Kozák, J., Boruvka, L., Dobos, E., Taboada, M., Moretti, L., and Rodriguez, D.: Soil legacy data rescue via GlobalSoilMap and other international and national initiatives, Geo. Res. J., 14, 1–19, 2017. a
Bau, D., Zhou, B., Khosla, A., Oliva, A., and Torralba, A.: Network dissection: Quantifying interpretability of deep visual representations, in: Proceedings of the IEEE Conference on Computer Vision and Pattern Recognition, 6541–6549, 2017. a , b
Beguin, J., Fuglstad, G.-A., Mansuy, N., and Paré, D.: Predicting soil properties in the Canadian boreal forest with limited data: Comparison of spatial and non-spatial statistical approaches, Geoderma, 306, 195–205, 2017. a
Bergstra, J. and Bengio, Y.: Random search for hyper-parameter optimization, J. Mach. Learn. Res., 13, 281–305, 2012. a , b
Björk, B.-C.: Open access to scientific articles: a review of benefits and challenges, Int. Emerg. Med., 21, 247–253, 2017. a , b
Blanco, C. M. G., Gomez, V. M. B., Crespo, P., and Ließ, M.: Spatial prediction of soil water retention in a Páramo landscape: Methodological insight into machine learning using random forest, Geoderma, 316, 100–114, 2018. a
Blei, D. M.: Probabilistic topic models, Commun. ACM, 55, 77–84, 2012. a , b , c , d
Blei, D. M., Ng, A. Y., and Jordan, M. I.: Latent dirichlet allocation, J. Mach. Learn. Res., 3, 993–1022, 2003. a , b
Bondi, G., Creamer, R., Ferrari, A., Fenton, O., and Wall, D.: Using machine learning to predict soil bulk density on the basis of visual parameters: Tools for in-field and post-field evaluation, Geoderma, 318, 137–147, 2018. a
Børgesen, C. D. and Schaap, M. G.: Point and parameter pedotransfer functions for water retention predictions for Danish soils, Geoderma, 127, 154–167, 2005. a
Brungard, C. W., Boettinger, J. L., Duniway, M. C., Wills, S. A., and Edwards Jr., T. C.: Machine learning for predicting soil classes in three semi-arid landscapes, Geoderma, 239, 68–83, 2015. a , b , c , d , e
Bui, E. N., Henderson, B. L., and Viergever, K.: Knowledge discovery from models of soil properties developed through data mining, Ecol. Modell., 191, 431–446, 2006. a
Butler, B. M., O'Rourke, S. M., and Hillier, S.: Using rule-based regression models to predict and interpret soil properties from X-ray powder diffraction data, Geoderma, 329, 43–53, https://doi.org/10.1016/j.geoderma.2018.04.005 , 2018. a
Camera, C., Zomeni, Z., Noller, J. S., Zissimos, A. M., Christoforou, I. C., and Bruggeman, A.: A high resolution map of soil types and physical properties for Cyprus: A digital soil mapping optimization, Geoderma, 285, 35–49, 2017. a , b
Cao, B., Domke, G. M., Russell, M. B., and Walters, B. F.: Spatial modeling of litter and soil carbon stocks on forest land in the conterminous United States, Sci. Total Environ., 654, 94–106, 2019. a
Castro-Franco, M., Domenech, M. B., Borda, M. R., and Costa, J.: Spatial dataset of topsoil texture for the southern Argentine Pampas, Geoderma Regional, 12, 18–27, 2017. a
Catlett, J.: Mega induction: A test flight, in: Machine Learning Proceedings 1991, Proceedings of the Eighth International Conference, Evanston, Elsevier, Illinois, 596–599, 1991. a
Caubet, M., Dobarco, M. R., Arrouays, D., Minasny, B., and Saby, N. P.: Merging country, continental and global predictions of soil texture: Lessons from ensemble modelling in France, Geoderma, 337, 99–110, 2019. a
Chlingaryan, A., Sukkarieh, S., and Whelan, B.: Machine learning approaches for crop yield prediction and nitrogen status estimation in precision agriculture: A review, Comput. Electron. Agr., 151, 61–69, 2018. a
Coopersmith, E. J., Minsker, B. S., Wenzel, C. E., and Gilmore, B. J.: Machine learning assessments of soil drying for agricultural planning, Comput. Electron. Agr., 104, 93–104, 2014. a
Cortes, C., Jackel, L. D., Solla, S. A., Vapnik, V., and Denker, J. S.: Learning curves: Asymptotic values and rate of convergence, in: Advances in Neural Information Processing Systems, 327–334, 1994. a
Costa, J. G., Reigosa, M., Matías, J., and Covelo, E.: Soil Cd, Cr, Cu, Ni, Pb and Zn sorption and retention models using SVM: variable selection and competitive model, Sci. Total Environ., 593, 508–522, 2017. a
Dai, F., Zhou, Q., Lv, Z., Wang, X., and Liu, G.: Spatial prediction of soil organic matter content integrating artificial neural network and ordinary kriging in Tibetan Plateau, Ecol. Indic., 45, 184–194, 2014. a
Deng, X., Chen, X., Ma, W., Ren, Z., Zhang, M., Grieneisen, M. L., Long, W., Ni, Z., Zhan, Y., and Lv, X.: Baseline map of organic carbon stock in farmland topsoil in East China, Agr. Ecosyst. Environ., 254, 213–223, 2018. a , b
Dharumarajan, S., Hegde, R., and Singh, S.: Spatial prediction of major soil properties using Random Forest techniques-A case study in semi-arid tropics of South India, Geoderma Regional, 10, 154–162, 2017. a
Dobarco, M. R., Cousin, I., Le Bas, C., and Martin, M. P.: Pedotransfer functions for predicting available water capacity in French soils, their applicability domain and associated uncertainty, Geoderma, 336, 81–95, 2019. a
Doherty, M. E. and Balzer, W. K.: Cognitive feedback, in: Advances in psychology, Elsevier, 54, 163–197, 1988. a
Dybczyński, R., Tugsavul, A., and Suschny, O.: Soil-5, a new IAEA certified reference material for trace element determinations, Geostandard. Newslett., 3, 61–87, 1979. a
Fajardo, M., McBratney, A., and Whelan, B.: Fuzzy clustering of Vis–NIR spectra for the objective recognition of soil morphological horizons in soil profiles, Geoderma, 263, 244–253, 2016. a
Farfani, H. A., Behnamfar, F., and Fathollahi, A.: Dynamic analysis of soil-structure interaction using the neural networks and the support vector machines, Expert Syst. Appl., 42, 8971–8981, 2015. a
Feng, Y., Cui, N., Hao, W., Gao, L., and Gong, D.: Estimation of soil temperature from meteorological data using different machine learning models, Geoderma, 338, 67–77, 2019. a
Flynn, T., Rozanov, A., de Clercq, W., Warr, B., and Clarke, C.: Semi-automatic disaggregation of a national resource inventory into a farm-scale soil depth class map, Geoderma, 337, 1136–1145, 2019. a
Friedman, J. H.: Greedy function approximation: a gradient boosting machine, Ann. Stat., 29, 1189–1232, 2001. a
Gal, Y. and Ghahramani, Z.: Dropout as a bayesian approximation: Representing model uncertainty in deep learning, in: International conference on machine learning, 1050–1059, 2016. a
Gao, M., Li, H.-Y., Liu, D., Tang, J., Chen, X., Chen, X., Blöschl, G., and Leung, L. R.: Identifying the dominant controls on macropore flow velocity in soils: A meta-analysis, J. Hydrol., 567, 590–604, 2018. a
Geissen, V., Kampichler, C., López-de Llergo-Juárez, J., and Galindo-Acántara, A.: Superficial and subterranean soil erosion in Tabasco, tropical Mexico: development of a decision tree modeling approach, Geoderma, 139, 277–287, 2007. a
Gomes, L. C., Faria, R. M., de Souza, E., Veloso, G. V., Schaefer, C. E. G., and Fernandes Filho, E. I.: Modelling and mapping soil organic carbon stocks in Brazil, Geoderma, 340, 337–350, 2019. a
Greifeneder, F., Khamala, E., Sendabo, D., Wagner, W., Zebisch, M., Farah, H., and Notarnicola, C.: Detection of soil moisture anomalies based on Sentinel-1, Phys. Chem. Earth, Pt. A/B/C, 112, 75–82, 2018. a
Grinand, C., Le Maire, G., Vieilledent, G., Razakamanarivo, H., Razafimbelo, T., and Bernoux, M.: Estimating temporal changes in soil carbon stocks at ecoregional scale in Madagascar using remote-sensing, Int. J. Appl. Earth Obs., 54, 1–14, 2017. a
Grunwald, S.: Multi-criteria characterization of recent digital soil mapping and modeling approaches, Geoderma, 152, 195–207, 2009. a
Grunwald, S.: What do we really know about the space–time continuum of soil-landscapes?, in: Environmental Soil-Landscape Modeling, CRC Press, 16–49, 2016. a
Grunwald, S., Vasques, G. M., and Rivero, R. G.: Fusion of soil and remote sensing data to model soil properties, Adv. Agron., Elsevier, 131, 1–109, 2015. a
Han, J., Mao, K., Xu, T., Guo, J., Zuo, Z., and Gao, C.: A soil moisture estimation framework based on the cart algorithm and its application in china, J. Hydrol., 563, 65–75, 2018. a
Hanna, A. M., Ural, D., and Saygili, G.: Neural network model for liquefaction potential in soil deposits using Turkey and Taiwan earthquake data, Soil Dyn. Earthq. Eng., 27, 521–540, 2007. a
Heggemann, T., Welp, G., Amelung, W., Angst, G., Franz, S. O., Koszinski, S., Schmidt, K., and Pätzold, S.: Proximal gamma-ray spectrometry for site-independent in situ prediction of soil texture on ten heterogeneous fields in Germany using support vector machines, Soil Till. Res., 168, 99–109, https://doi.org/10.1016/j.still.2016.10.008 , 2017. a
Henderson, B. L., Bui, E. N., Moran, C. J., and Simon, D.: Australia-wide predictions of soil properties using decision trees, Geoderma, 124, 383–398, 2005. a , b
Hutson, M.: Boycott highlights AI's publishing rebellion, Science, 360, p. 699, 2018. a
Ivushkin, K., Bartholomeus, H., Bregt, A. K., Pulatov, A., Bui, E. N., and Wilford, J.: Soil salinity assessment through satellite thermography for different irrigated and rainfed crops, Int. J. Appl. Earth Obs., 68, 230–237, 2018. a
Jeong, G., Oeverdieck, H., Park, S. J., Huwe, B., and Ließ, M.: Spatial soil nutrients prediction using three supervised learning methods for assessment of land potentials in complex terrain, Catena, 154, 73–84, 2017. a , b
Jordan, M. I. and Mitchell, T. M.: Machine learning: Trends, perspectives, and prospects, Science, 349, 255–260, 2015. a , b , c , d
Karandish, F. and Šimŭnek, J.: A field-modeling study for assessing temporal variations of soil-water-crop interactions under water-saving irrigation strategies, Agr. Water Manage., 178, 291–303, 2016. a
Keskin, H., Grunwald, S., and Harris, W. G.: Digital mapping of soil carbon fractions with machine learning, Geoderma, 339, 40–58, 2019. a , b , c , d , e
Khadim, F. K., Su, H., Xu, L., and Tian, J.: Soil salinity mapping in Everglades National Park using remote sensing techniques and vegetation salt tolerance, Phys. Chem. Earth, Pt. A/B/C, 110, 31–50, 2019. a
Khanal, S., Fulton, J., Klopfenstein, A., Douridas, N., and Shearer, S.: Integration of high resolution remotely sensed data and machine learning techniques for spatial prediction of soil properties and corn yield, Comput. Electron. Agr., 153, 213–225, 2018. a , b
Kheir, R. B., Chorowicz, J., Abdallah, C., and Dhont, D.: Soil and bedrock distribution estimated from gully form and frequency: A GIS-based decision-tree model for Lebanon, Geomorphology, 93, 482–492, https://doi.org/10.1016/j.geomorph.2007.03.010 , 2008. a
Koenker, R. and Bassett Jr., G.: Regression quantiles, Econometrica: journal of the Econometric Society, 33–50, 1978. a
Koestel, J. and Jorda, H.: What determines the strength of preferential transport in undisturbed soil under steady-state flow?, Geoderma, 217, 144–160, 2014. a
Kohavi, R.: A study of cross-validation and bootstrap for accuracy estimation and model selection, in: Ijcai, Montreal, Canada, 14, 1137–1145, 1995. a
Kovačević, M., Bajat, B., and Gajić, B.: Soil type classification and estimation of soil properties using support vector machines, Geoderma, 154, 340–347, 2010. a
Lacoste, M., Lemercier, B., and Walter, C.: Regional mapping of soil parent material by machine learning based on point data, Geomorphology, 133, 90–99, https://doi.org/10.1016/j.geomorph.2011.06.026 , 2011. a
Leenaars, J. G., Claessens, L., Heuvelink, G. B., Hengl, T., González, M. R., van Bussel, L. G., Guilpart, N., Yang, H., and Cassman, K. G.: Mapping rootable depth and root zone plant-available water holding capacity of the soil of sub-Saharan Africa, Geoderma, 324, 18–36, 2018. a
Liang, Z., Chen, S., Yang, Y., Zhao, R., Shi, Z., and Rossel, R. A. V.: National digital soil map of organic matter in topsoil and its associated uncertainty in 1980's China, Geoderma, 335, 47–56, 2019. a
Lin, J.: Divergence measures based on the Shannon entropy, IEEE T. Inform. Theory, 37, 145–151, 1991. a
Lipton, Z. C.: The mythos of model interpretability, arXiv preprint arXiv:1606.03490, 2016. a , b
Liu, S., Yang, Y., Shen, H., Hu, H., Zhao, X., Li, H., Liu, T., and Fang, J.: No significant changes in topsoil carbon in the grasslands of northern China between the 1980s and 2000s, Sci. Total Environ., 624, 1478–1487, 2018. a
Lou, Y., Caruana, R., and Gehrke, J.: Intelligible models for classification and regression, in: Proceedings of the 18th ACM SIGKDD international conference on Knowledge discovery and data mining, ACM, 150–158, 2012. a
Lu, W., Lu, D., Wang, G., Wu, J., Huang, J., and Li, G.: Examining soil organic carbon distribution and dynamic change in a hickory plantation region with Landsat and ancillary data, Catena, 165, 576–589, 2018. a
Ma, Y., Minasny, B., and Wu, C.: Mapping key soil properties to support agricultural production in Eastern China, Geoderma Regional, 10, 144–153, 2017. a
Ma, Y., Minasny, B., Malone, B. P., and Mcbratney, A. B.: Pedology and digital soil mapping (DSM), Europ. J. Soil Sci., 70, 216–235, 2019. a
Mansuy, N., Thiffault, E., Paré, D., Bernier, P., Guindon, L., Villemaire, P., Poirier, V., and Beaudoin, A.: Digital mapping of soil properties in Canadian managed forests at 250 m of resolution using the k-nearest neighbor method, Geoderma, 235, 59–73, 2014. a
Märker, M., Pelacani, S., and Schröder, B.: A functional entity approach to predict soil erosion processes in a small Plio-Pleistocene Mediterranean catchment in Northern Chianti, Italy, Geomorphology, 125, 530–540, 2011. a
Martin, M., Orton, T., Lacarce, E., Meersmans, J., Saby, N., Paroissien, J., Jolivet, C., Boulonne, L., and Arrouays, D.: Evaluation of modelling approaches for predicting the spatial distribution of soil organic carbon stocks at the national scale, Geoderma, 223, 97–107, 2014. a
Martinez, G., Weltz, M., Pierson, F. B., Spaeth, K. E., and Pachepsky, Y.: Scale effects on runoff and soil erosion in rangelands: Observations and estimations with predictors of different availability, Catena, 151, 161–173, 2017. a
Massawe, B. H., Subburayalu, S. K., Kaaya, A. K., Winowiecki, L., and Slater, B. K.: Mapping numerically classified soil taxa in Kilombero Valley, Tanzania using machine learning, Geoderma, 311, 143–148, 2018. a
Matthew and Honnibal, M. I.: spaCy 2: Natural language understanding with Bloom embeddings, convolutional neural networks and incremental parsing, https://github.com/explosion/spaCy/ (last access: 5 February 2020), 2017. a
McBratney, A., de Gruijter, J., and Bryce, A.: Pedometrics timeline, Geoderma, 338, 568–575, 2019. a
McCallum, A. K.: MALLET: A Machine Learning for Language Toolkit, http://mallet.cs.umass.edu (last access: 5 February 2020), 2002. a
Minasny, B. and Flantis, D.: “Helicopter research”: who benefits from international studies in Indonesia?, https://theconversation.com/helicopter-research-who-benefits-from-international-studies-in-indonesia-102165 (last access: 29 April 2019), 2018. a
Mjolsness, E. and DeCoste, D.: Machine learning for science: state of the art and future prospects, Science, 293, 2051–2055, 2001. a
Montavon, G., Samek, W., and Müller, K.-R.: Methods for interpreting and understanding deep neural networks, Digit. Signal Process., 73, 1–15, 2018. a
Morellos, A., Pantazi, X.-E., Moshou, D., Alexandridis, T., Whetton, R., Tziotzios, G., Wiebensohn, J., Bill, R., and Mouazen, A. M.: Machine learning based prediction of soil total nitrogen, organic carbon and moisture content by using VIS-NIR spectroscopy, Biosyst. Eng., 152, 104–116, 2016. a
Mutanga, O., Adam, E., and Cho, M. A.: High density biomass estimation for wetland vegetation using WorldView-2 imagery and random forest regression algorithm, Int. J. Appl. Earth Obs., 18, 399–406, 2012. a
Naderi-Boldaji, M., Tekeste, M. Z., Nordstorm, R. A., Barnard, D. J., and Birrel, S. J.: A mechanical-dielectric-high frequency acoustic sensor fusion for soil physical characterization, Comput. Electron. Agr., 156, 10–23, 2019. a
Ng, W., Minasny, B., Montazerolghaem, M., Padarian, J., Ferguson, R., Bailey, S., and McBratney, A. B.: Convolutional neural network for simultaneous prediction of several soil properties using visible/near-infrared, mid-infrared, and their combined spectra, Geoderma, 352, 251-267, 2019. a , b
Oh, Y.-Y., Yun, S.-T., Yu, S., Kim, H.-J., and Jun, S.-C.: A novel wavelet-based approach to characterize dynamic environmental factors controlling short-term soil surface CO 2 flux: Application to a controlled CO2 release test site (EIT) in South Korea, Geoderma, 337, 76–90, 2019. a
Padarian, J., Minasny, B., and McBratney, A.: Transfer learning to localise a continental soil vis-NIR calibration model, Geoderma, 340, 279–288, 2019a. a , b
Padarian, J., Minasny, B., and McBratney, A.: Using deep learning to predict soil properties from regional spectral data, Geoderma Regional, 16, e00198, https://doi.org/10.1016/j.geodrs.2018.e00198 , 2019b. a , b
Padarian, J., Minasny, B., and McBratney, A. B.: Using deep learning for digital soil mapping, Soil, 5, 79–89, 2019c. a
Pasini, A.: Artificial neural networks for small dataset analysis, J. Thoracic Dis., 7, 953–960, 2015. a
Perlich, C., Provost, F., and Simonoff, J. S.: Tree induction vs. logistic regression: A learning-curve analysis, J. Mach. Learn. Res., 4, 211–255, 2003. a
Poggio, L., Gimona, A., Spezia, L., and Brewer, M. J.: Bayesian spatial modelling of soil properties and their uncertainty: The example of soil organic matter in Scotland using R-INLA, Geoderma, 277, 69–82, 2016. a
Prasad, R., Deo, R. C., Li, Y., and Maraseni, T.: Ensemble committee-based data intelligent approach for generating soil moisture forecasts with multivariate hydro-meteorological predictors, Soil Till. Res., 181, 63–81, 2018. a
Probst, P., Wright, M. N., and Boulesteix, A.-L.: Hyperparameters and tuning strategies for random forest, WIRES Data Min. Knowl., 9, e1301, https://doi.org/10.1002/widm.1301 , 2019. a
Pueyo, M., Rauret, G., Bacon, J., Gomez, A., Muntau, H., Quevauviller, P., and López-Sánchez, J.: A new organic-rich soil reference material certified for its EDTA-and acetic acid-extractable contents of Cd, Cr, Cu, Ni, Pb and Zn, following collaboratively tested and harmonised procedures, J. Environ. Monit., 3, 238–242, 2001. a
Rauber, P. E., Fadel, S. G., Falcao, A. X., and Telea, A. C.: Visualizing the hidden activity of artificial neural networks, IEEE T. Vis. Comput. Gr., 23, 101–110, 2017. a
Reale, C., Gavin, K., Librić, L., and Jurić-Kaćunić, D.: Automatic classification of fine-grained soils using CPT measurements and Artificial Neural Networks, Adv. Eng. Inform., 36, 207–215, 2018. a
Reeves, M. K., Perdue, M., Munk, L. A., and Hagedorn, B.: Predicting risk of trace element pollution from municipal roads using site-specific soil samples and remotely sensed data, Sci. Total Environ., 630, 578–586, 2018. a
Rial, M., Cortizas, A. M., Taboada, T., and Rodríguez-Lado, L.: Soil organic carbon stocks in Santa Cruz Island, Galapagos, under different climate change scenarios, Catena, 156, 74–81, 2017. a
Röder, M., Both, A., and Hinneburg, A.: Exploring the space of topic coherence measures, in: Proceedings of the eighth ACM international conference on Web search and data mining, ACM, 399–408, 2015. a
Rossiter, D. G.: Past, present & future of information technology in pedometrics, Geoderma, 324, 131–137, 2018. a , b , c
Rudin, C. and Wagstaff, K. L.: Machine learning for science and society, Mach. Learn., 95, 1–9, 2014. a
Sagasti, F. R.: Underdevelopment, science and technology: the point of view of the underdeveloped countries, Sci. Stud., 3, 47–59, 1973. a
Schaap, M. G. and Bouten, W.: Modeling water retention curves of sandy soils using neural networks, Water Resour. Res., 32, 3033–3040, 1996. a
Schillaci, C., Acutis, M., Lombardo, L., Lipani, A., Fantappie, M., Märker, M., and Saia, S.: Spatio-temporal topsoil organic carbon mapping of a semi-arid Mediterranean region: The role of land use, soil texture, topographic indices and the influence of remote sensing data to modelling, Sci. Total Environ., 601, 821–832, 2017a. a
Schillaci, C., Lombardo, L., Saia, S., Fantappiè, M., Märker, M., and Acutis, M.: Modelling the topsoil carbon stock of agricultural lands with the Stochastic Gradient Treeboost in a semi-arid Mediterranean region, Geoderma, 286, 35–45, 2017b. a
Shavlik, J. W., Mooney, R. J., and Towell, G. G.: Symbolic and neural learning algorithms: An experimental comparison, Mach. Learn., 6, 111–143, 1991. a
Shaw, J., West, L., Radcliffe, D., and Bosch, D.: Preferential flow and pedotransfer functions for transport properties in sandy Kandiudults, Soil Sci. Soc. Am. J., 64, 670–678, 2000. a
Sirsat, M., Cernadas, E., Fernández-Delgado, M., and Barro, S.: Automatic prediction of village-wise soil fertility for several nutrients in India using a wide range of regression methods, Comput. Electron. Agr., 154, 120–133, 2018. a , b
Snoek, J., Larochelle, H., and Adams, R. P.: Practical bayesian optimization of machine learning algorithms, Adv. Neur. In., 25, 2951–2959, 2012. a
Snoek, J., Rippel, O., Swersky, K., Kiros, R., Satish, N., Sundaram, N., Patwary, M., Prabhat, M., and Adams, R.: Scalable bayesian optimization using deep neural networks, Proceedings of the 32nd International Conference on Machine Learning, Int. Conf. Mach. Learn., 2171–2180, 2015. a
Somarathna, P., Minasny, B., and Malone, B. P.: More data or a better model? Figuring out what matters most for the spatial prediction of soil carbon, Soil Sci. Soc. Am. J., 81, 1413–1426, 2017. a
Song, X.-D., Yang, F., Ju, B., Li, D.-C., Zhao, Y.-G., Yang, J.-L., and Zhang, G.-L.: The influence of the conversion of grassland to cropland on changes in soil organic carbon and total nitrogen stocks in the Songnen Plain of Northeast China, Catena, 171, 588–601, 2018. a
Sonnenwald, D. H.: Scientific collaboration, Ann. Rev. Inf. Sci. Technol., 41, 643–681, 2007. a , b
Stevens, A., van Wesemael, B., Bartholomeus, H., Rosillon, D., Tychon, B., and Ben-Dor, E.: Laboratory, field and airborne spectroscopy for monitoring organic carbon content in agricultural soils, Geoderma, 144, 395–404, 2008. a
Stevens, K., Kegelmeyer, P., Andrzejewski, D., and Buttler, D.: Exploring topic coherence over many models and many topics, in: Proceedings of the 2012 Joint Conference on Empirical Methods in Natural Language Processing and Computational Natural Language Learning, Association for Computational Linguistics, 952–961, 2012. a , b
Stine, R. A.: Bootstrap prediction intervals for regression, J. Am. Stat. Assoc., 80, 1026–1031, 1985. a
Stumpf, F., Keller, A., Schmidt, K., Mayr, A., Gubler, A., and Schaepman, M.: Spatio-temporal land use d ynamics and soil organic carbon in Swiss agroecosystems, Agr. Ecosyst. Environ., 258, 129–142, 2018. a
Subburayalu, S., Jenhani, I., and Slater, B.: Disaggregation of component soil series on an Ohio County soil survey map using possibilistic decision trees, Geoderma, 213, 334–345, 2014. a
Sugimoto, C. R., Li, D., Russell, T. G., Finlay, S. C., and Ding, Y.: The shifting sands of disciplinary development: Analyzing North American Library and Information Science dissertations using latent Dirichlet allocation, J. Am. Soc. Inf. Sci. Tech., 62, 185–204, 2011. a
Taghizadeh-Mehrjardi, R., Nabiollahi, K., Minasny, B., and Triantafilis, J.: Comparing data mining classifiers to predict spatial distribution of USDA-family soil groups in Baneh region, Iran, Geoderma, 253, 67–77, 2015. a
Taghizadeh-Mehrjardi, R., Nabiollahi, K., and Kerry, R.: Digital mapping of soil organic carbon at multiple depths using different data mining techniques in Baneh region, Iran, Geoderma, 266, 98–110, 2016. a
Tomasella, J., Hodnett, M. G., and Rossato, L.: Pedotransfer Functions for the Estimation of Soil Water Retention in Brazilian Soils, Soil Sci. Soc. Am. J., 64, 327–338, 2000. a
Tranter, G., Minasny, B., and McBratney, A.: Estimating Pedotransfer Function Prediction Limits Using Fuzzy k-Means with Extragrades, Soil Sci. Soc. Am. J., 74, 1967–1975, 2010. a
Tziachris, P., Aschonitis, V., Chatzistathis, T., and Papadopoulou, M.: Assessment of spatial hybrid methods for predicting soil organic matter using DEM derivatives and soil parameters, Catena, 174, 206–216, 2019. a
Vaysse, K. and Lagacherie, P.: Using quantile regression forest to estimate uncertainty of digital soil mapping products, Geoderma, 291, 55–64, 2017. a
Vincent, S., Lemercier, B., Berthier, L., and Walter, C.: Spatial disaggregation of complex Soil Map Units at the regional scale based on soil-landscape relationships, Geoderma, 311, 130–142, 2018. a
Viscarra-Rossel, R. and Behrens, T.: Using data mining to model and interpret soil diffuse reflectance spectra, Geoderma, 158, 46–54, 2010. a
Řehůřek, R. and Sojka, P.: Software Framework for Topic Modelling with Large Corpora, in: Proceedings of the LREC 2010 Workshop on New Challenges for NLP Frameworks, ELRA, Valletta, Malta, 45–50, http://is.muni.cz/publication/884893/en (last access: 5 February 2020), 2010. a
Wang, B., Waters, C., Orgill, S., Cowie, A., Clark, A., Li Liu, D., Simpson, M., McGowen, I., and Sides, T.: Estimating soil organic carbon stocks using different modelling techniques in the semi-arid rangelands of eastern Australia, Ecol. Indic., 88, 425–438, 2018a. a , b
Wang, B., Waters, C., Orgill, S., Gray, J., Cowie, A., Clark, A., and Li Liu, D.: High resolution mapping of soil organic carbon stocks using remote sensing variables in the semi-arid rangelands of eastern Australia, Sci. Total Environ., 630, 367–378, 2018b. a
Ware, M. and Mabe, M.: The STM report: An overview of scientific and scholarly journal publishing, available at: https://www.stm-assoc.org/2015_02_20_STM_Report_2015.pdf (last access: 5 February 2020), 2015. a
Warner, D. L., Guevara, M., Inamdar, S., and Vargas, R.: Upscaling soil-atmosphere CO 2 and CH 4 fluxes across a topographically complex forested landscape, Agr. Forest Meteorol., 264, 80–91, 2019. a
Watson, S. J., Luck, G. W., Spooner, P. G., and Watson, D. M.: Land-use change: incorporating the frequency, sequence, time span, and magnitude of changes into ecological research, Front. Ecol. Environ., 12, 241–249, 2014. a
Were, K., Bui, D. T., Dick, Ø. B., and Singh, B. R.: A comparative assessment of support vector regression, artificial neural networks, and random forests for predicting and mapping soil organic carbon stocks across an Afromontane landscape, Ecol. Ind., 52, 394–403, 2015. a
Wu, G., Kechavarzi, C., Li, X., Wu, S., Pollard, S. J., Sui, H., and Coulon, F.: Machine learning models for predicting PAHs bioavailability in compost amended soils, Chem. Engin. J., 223, 747–754, 2013. a
Wu, Q., Zhang, C., Hong, Q., and Chen, L.: Topic evolution based on LDA and HMM and its application in stem cell research, J. Inf. Sci., 40, 611–620, 2014. a
Xie, X.-L. and Li, A.-B.: Identification of soil profile classes using depth-weighted visible-near-infrared spectral reflectance, Geoderma, 325, 90–101, https://doi.org/10.1016/j.geoderma.2018.03.029 , 2018. a
Xing, L., Li, L., Gong, J., Ren, C., Liu, J., and Chen, H.: Daily soil temperatures predictions for various climates in United States using data-driven model, Energy, 160, 430–440, 2018. a
Xiong, X., Grunwald, S., Myers, D. B., Kim, J., Harris, W. G., and Comerford, N. B.: Holistic environmental soil-landscape modeling of soil organic carbon, Environ. Modell. Softw., 57, 202–215, 2014. a , b
Xu, Y., Smith, S. E., Grunwald, S., Abd-Elrahman, A., and Wani, S. P.: Incorporation of satellite remote sensing pan-sharpened imagery into digital soil prediction and mapping models to characterize soil property variability in small agricultural fields, ISPRS J Photogramm., 123, 1–19, 2017. a
Zeynoddin, M., Bonakdari, H., Ebtehaj, I., Esmaeilbeiki, F., Gharabaghi, B., and Haghi, D. Z.: A reliable linear stochastic daily soil temperature forecast model, Soil Till. Res., 189, 73–87, 2019. a
Zhang, C., Mishra, D. R., and Pennings, S. C.: Mapping salt marsh soil properties using imaging spectroscopy, ISPRS J. Photogramm., 148, 221–234, 2019. a
Zhang, Q., Nian Wu, Y., and Zhu, S.-C.: Interpretable convolutional neural networks, in: Proceedings of the IEEE Conference on Computer Vision and Pattern Recognition, 8827–8836, 2018a. a
Zhang, Y., Sui, B., Shen, H., and Wang, Z.: Estimating temporal changes in soil pH in the black soil region of Northeast China using remote sensing, Comput. Electron. Agr., 154, 204–212, 2018b. a , b
Zhou, D., Ji, X., Zha, H., and Giles, C. L.: Topic evolution and social interactions: how authors effect research, in: Proceedings of the 15th ACM international conference on Information and knowledge management, ACM, 248–257, 2006. a
- Introduction
- Results and discussion
- General discussion
- Conclusions and recommendations
- Data availability
- Author contributions
- Competing interests
- Acknowledgements
- Review statement
Thank you for visiting nature.com. You are using a browser version with limited support for CSS. To obtain the best experience, we recommend you use a more up to date browser (or turn off compatibility mode in Internet Explorer). In the meantime, to ensure continued support, we are displaying the site without styles and JavaScript.
- View all journals
Soil microbiology articles from across Nature Portfolio
Soil microbiology is the scientific discipline that is concerned with the study of all biological aspects of the microorganisms (bacteria, archaea, viruses, fungi, parasites and protozoa) that exist in the soil environment. This is a subdiscipline of environmental microbiology.
Latest Research and Reviews
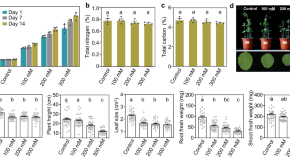
Purines enrich root-associated Pseudomonas and improve wild soybean growth under salt stress
Root-associated microbiota confers benefits to plant in responding to environmental stress. Here, the authors show that wild soybean secretes purines under salt stress, reshapes the microbiota and recruits Pseudomonas.
- Yanfen Zheng
- Cheng-Sheng Zhang
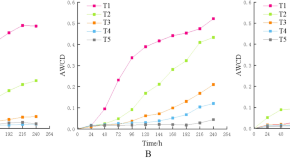
Vertical differences in carbon metabolic diversity and dominant flora of soil bacterial communities in farmlands
- Bufan Zheng
- Zhipeng Xiao
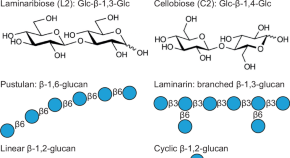
Structural and biochemical analysis of family 92 carbohydrate-binding modules uncovers multivalent binding to β-glucans
Carbohydrate binding modules (CBMs) are non-catalytic domains found within multi-modular carbohydrate-active enzymes like glycoside hydrolases. Here, the authors show the crystal structures of two CBM family 92 members, which use three different surface binding sites to bind to β-glucans.
- Meng-Shu Hao
- Scott Mazurkewich
- Lauren S. McKee
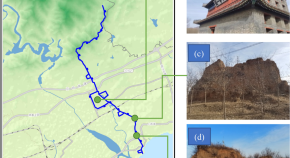
Experimental test and mechanism analysis of soil crust erosion resistance of rammed earth Great Wall in rainy season
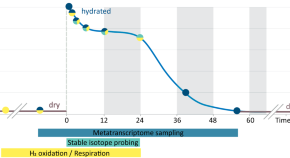
Survival and rapid resuscitation permit limited productivity in desert microbial communities
Prompt physiological reactivation after rainfall pulses may be key for microbial survival in arid ecosystems. Here, the authors use stable isotope tracers, single-cell NanoSIMS and metatranscriptomics to shed light on how desert biocrust microbial communities respond to rewetting.
- Stefanie Imminger
- Dimitri V. Meier
- Dagmar Woebken
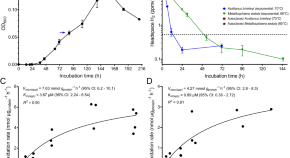
Trace gas oxidation sustains energy needs of a thermophilic archaeon at suboptimal temperatures
Diverse bacteria can use the low levels of hydrogen and carbon monoxide present in the air as energy sources for growth and survival. Here, Leung et al. show that ability is also found in thermophilic archaea of the order Sulfolobales .
- Pok Man Leung
- Rhys Grinter
- Chris Greening
News and Comment
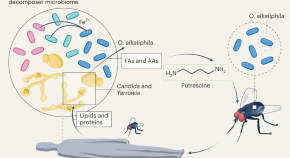
Decomposer communities are universal in death
Decomposer microbiomes are universal across cadavers regardless of environmental conditions, and they use complex cross-feeding and interkingdom interactions to break down organic matter.
- Michael S. Strickland
- Laurel Lynch
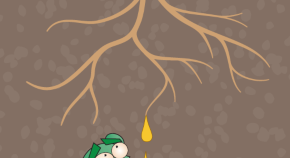
An intended leak
In this study, Liu et al. demonstrate that the T7SS of the rhizobacterium Bacillus velezensis SQR9 and its effector protein YukE cause iron leakage in plant roots to support root colonization.
- Agustina Taglialegna
Celebrating 15 years of The ISME Journal
- George A. Kowalchuk
- Mark J. Bailey
Viral regulators of responses to soil warming
This study set out to increase our understanding of how viruses might mediate responses of microbial communities to climate warming.
- Andrea Du Toit
Fungi feed bacteria for biodegradation
Khan et al. show that mycelial nutrients can promote bacterial biodegradation of hexachlorocyclohexane pesticide in nutrient-deprived habitats.
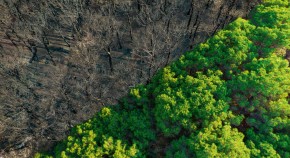
Soil microbiota takes the heat
Two studies report how soil microbiota adapts after wildfires and soil warming.
- Lucia Brunello
Quick links
- Explore articles by subject
- Guide to authors
- Editorial policies

You are using an outdated browser. This page doesn't support Internet Explorer 6, 7 and 8. Please upgrade your browser or activate Google Chrome Frame to improve your experience.
Special Issue
Current reviews in the soil sciences.
- Submit your abstract
- Submit your manuscript
- Participate
About this Special Issue
This special issue will publish high-quality scholarly review papers on key topics in the soil sciences. It aims to highlight recent advances in the field, whilst emphasizing important directions and new possibilities for future inquiries. We anticipate this will promote discussion in the soil science community that will translate to best practice applications in soil management, agriculture, forestry, conservation, and policy settings. The Reviews in Soil Science collection welcomes full-length, mini or systematic review papers. New articles will be added to this collection as they are published.
Keywords : soil management, agriculture, forestry, conservation
Issue Editors
Participating journals.
Manuscripts can be submitted to this Special Issue via the following journals:
- Demographics
No records found
total views article views article downloads topic views
Top countries
Top referring sites.
Advertisement
Soil science research in Brazilian terrestrial biomes: A review of evolution, collaboration, current topics, and impact
- Soils, Sec 3 • Remediation and Management of Contaminated or Degraded Lands • Research Article
- Published: 21 March 2024
Cite this article
- José de Souza Oliveira Filho ORCID: orcid.org/0000-0002-0788-1037 1 nAff2
94 Accesses
Explore all metrics
Brazilian territory is divided into six biomes (Amazon, Atlantic Forest, Cerrado, Caatinga, Pantanal, and Pampa) that represent important global biodiversity hotspots. Research into soil science in these environments has increased in recent years, but a quantitative and qualitative mapping of research into the soils of Brazilian biomes has never been carried out. In this study, research on soil science in the Brazilian biomes were analyzed to map the evolution, scientific collaboration, current status, research trends and impact over the last 50 years (1972–2022).
The database was obtained from the Core Collection of Web of Science. The data was quantitatively analyzed through descriptive statistics and linear regression using Microsoft Excel, and qualitatively using the bibliometric software VOSviewer.
A total of 11,231 papers were obtained. The results indicated a significant increase in soil science research across all biomes (polynomial growth), with greater emphasis for research in the Cerrado (R 2 = 0.9361), Amazon (R 2 = 0.9170) and Atlantic Forest (R 2 = 0.9104) biomes. Soil science research in the Amazon accounted for 47.0% of the research conducted in all Brazilian biomes. While in the Amazon (38.8%), Atlantic Forest (34.8%), and Pantanal (36.7%), most of the research was related to environmental sciences and ecology, in the Cerrado (47.4%), Caatinga (58.2%), and Pampa biome (48.9%), research in the field of agriculture was more significant. For all biomes, except for the Caatinga (a dry climate biome), a significant participation of international researchers and institutions was evident in the overall publications. This demonstrates a lower global interest in soil science research in the Brazilian dry ecosystem.
Soil science research in the Brazilian biomes is disproportionate, with greater interest in the soils of the larger forest biomes and less interest in the dry and flooded areas. The results of this study can be useful for the development of public policies, research and scientific collaboration aimed at soil conservation in Brazilian terrestrial biomes.
This is a preview of subscription content, log in via an institution to check access.
Access this article
Price includes VAT (Russian Federation)
Instant access to the full article PDF.
Rent this article via DeepDyve
Institutional subscriptions
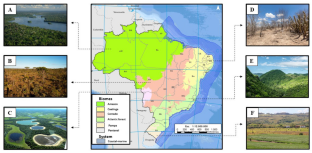
Similar content being viewed by others
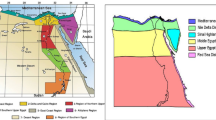
An overview of land degradation, desertification and sustainable land management using GIS and remote sensing applications
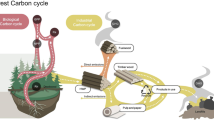
Forest Carbon Management: a Review of Silvicultural Practices and Management Strategies Across Boreal, Temperate and Tropical Forests
Agricultural Soil Degradation in Australia
Ab’Sáber AN (2003) Os domínios de natureza no Brasil: potencialidades paisagísticas. Ateliê Editorial, São Paulo. 153 p
Agnew D, Fryirs KA, Ralph TJ, Kobayashi T (2021) Soil carbon dynamics and aquatic metabolism of a wet–dry tropics wetland system. Wetl Ecol Manag 29:1–25. https://doi.org/10.1007/s11273-020-09745-w
Article CAS Google Scholar
Alho CJR (2008) Biodiversity of the Pantanal: response to seasonal flooding regime and to environmental degradation. Braz J Biol 68:957–966. https://doi.org/10.1590/S1519-69842008000500005
Article CAS PubMed Google Scholar
Alvarez R, Steinbach HS (2009) A review of the effects of tillage systems on some soil physical properties, water content, nitrate availability and crops yield in the Argentine Pampas. Soil Tillage Res 104:1–15. https://doi.org/10.1016/j.still.2009.02.005
Article Google Scholar
Alves LA, Tiecher TL, Flores JPM, Filippi D, Gatiboni LC, Bayer C, Pias OHC, Marquez AA, Bordignon V, Goulart RZ, Tiecher T (2021) Soil chemical properties and crop response to gypsum and limestone on a coarse-textured Ultisol under no-till in the Brazilian Pampa biome. Geoderma Reg 25:e00372. https://doi.org/10.1016/j.geodrs.2021.e00372
Alves KJ, Pylro VS, Nakayama CR, Vital VG, Taketani RG, Santos DG, Rodrigues JLM, Tsai SM, Andreote FD (2022) Methanogenic communities and methane emissions from enrichments of Brazilian Amazonia soils under land-use change. Microbiol Res 265:127178. https://doi.org/10.1016/j.micres.2022.127178
Barbosa DR, Pinheiro HS, Santos, (2019) Seasonal variability of trace elements by soil depth in a protected area. Floresta Ambient 26:e20170203. https://doi.org/10.1590/2179-8087.020317
Bolzani VS, Valli M, Pivatto M, Viegas C Jr (2012) Natural products from Brazilian biodiversity as a source of new models for medicinal chemistry. Pure Appl Chem 84:1837–1846. https://doi.org/10.1351/PAC-CON-12-01-11
Bonfim JA, Vasconcellos RLF, Gumiere T, Mescolotti DLC, Oehl F, Cardoso EJBN (2016) Diversity of arbuscular mycorrhizal fungi in a brazilian atlantic forest toposequence. Microb Ecol 71:164–177. https://doi.org/10.1007/s00248-015-0661-0
Article PubMed Google Scholar
Cabarello CB, Ruhoff A, Biggs T (2022) Land use and land cover changes and their impacts on surface-atmosphere interactions in Brazil: a systematic review. Sci Total Environ 808:152134. https://doi.org/10.1016/j.scitotenv.2021.152134
Cabarello CB, Biggs TW, Vergopolan N, West TAP, Ruhoff A (2023) Transformation of Brazil’s biomes: the dynamics and fate of agriculture and pasture expansion into native vegetation. Sci Total Environ 896:166323. https://doi.org/10.1016/j.scitotenv.2023.166323
Caló LO, Caldeira MVW, Silva CF, Camara R, Castro KC, Lima SS, Pereira MG, Aquino AM (2022) Epigeal fauna and edaphic properties as possible soil quality indicators in forest restoration areas in Espírito Santo. Brazil Acta Oecol 117:103870. https://doi.org/10.1016/j.actao.2022.103870
Campos JA, Silva DD, Moreira MC, Menezes Filho FCM (2021) Environmental fragility and land use capacity as instruments of environmental planning, Caratinga River basin. Brazil Environ Earth Sci 80:264. https://doi.org/10.1007/s12665-021-09553-2
Cardoso D et al (2017) Amazon plant diversity revealed by a taxonomically verified species list. Proc Natl Acad Sci USA 114:10695–10700. https://doi.org/10.1073/pnas.1706756114
Article CAS PubMed PubMed Central Google Scholar
Carvalho JLL, Avanzi JC, Silva MLN, Mello CR, Cerri CEP (2010) Potencial de sequestro de carbono em diferentes biomas do Brasil. Rev Bras Cienc Solo 34:277–289. https://doi.org/10.1590/S0100-06832010000200001
Castellón SEM, Cattanio JH, Berrêdo JF, Rollnic M, Ruivo ML, Noriega C (2022) Greenhouse gas fluxes in mangrove forest soil in an Amazon estuary. Biogeosciences 19:5483–5497. https://doi.org/10.5194/bg-19-5483-2022
Chaddad F, Mello FAO, Tayebi M, Safanelli JL, Campos LR, Amorim MTA, Sousa GPB, Ferreira TO, Ruiz F, Perlatti F, Greschuk LT, Rosin NA, Rosas JTF, Demattê JAM (2022) Impact of mining-induced deforestation on soil surface temperature and carbon stocks: a case study using remote sensing in the Amazon rainforest. J South Am Earth Sci 119:103983. https://doi.org/10.1016/j.jsames.2022.103983
Colman CB, Oliveira PTS, Almagro A, Soares-Filho BS, Rodrigues DBB (2019) Effects of climate and land-cover changes on soil erosion in brazilian pantanal. Sustainability 11:7053. https://doi.org/10.3390/su11247053
Conceição CG, Robaina AD, Peiter MX, Bem LHB, Ferreira LD, Parizi ARC (2022) Performance of the AquaCrop model for corn hybrids under different irrigation strategies. Rev Bras Eng Agríc Ambiental 26:441–450. https://doi.org/10.1590/1807-1929/agriambi.v26n6p441-450
Crespo C, Martínez RD, Wyngaard N, Divito G, Cuesta NM, Barbieri P (2022) Nitrogen diagnosis for double-cropped maize. Eur J Agron 140:126600. https://doi.org/10.1016/j.eja.2022.126600
Delgado-Baquerizo M et al (2020) The influence of soil age on ecosystem structure and function across biomes. Nat Commun 11:4721. https://doi.org/10.1038/s41467-020-18451-3
Dick M, Silva MA, Silva RRF, Ferreira OGL, Maia MS, Lima SF, Paiva Neto VB, Dewes H (2021) Environmental impacts of Brazilian beef cattle production in the Amazon, Cerrado, Pampa, and Pantanal biomes. J Clean Prod 311:127750. https://doi.org/10.1016/j.jclepro.2021.127750
Ellwanger JH, Nobre CA, Chies JAB (2023) Brazilian biodiversity as a source of power and sustainable development: a neglected opportunity. Sustainability 15:482. https://doi.org/10.3390/su15010482
Fabrizzi KP, García FO, Costa JL, Picone LI (2005) Soil water dynamics, physical properties and corn and wheat responses to minimum and no-tillage systems in the southern Pampas of Argentina. Soil Tillage Res 81:57–69. https://doi.org/10.1016/j.still.2004.05.001
Fearnside PM, Laurance W (2004) Tropical deforestation and greenhouse-gas emissions. Ecol Appl 14:982–986. https://doi.org/10.1890/03-5225
Florio EL, Nosetto MD (2022) A modeling approach to explore the influence of different crop rotations on water-table depths and crop yields in the Pampas. Soil Tillage Res 223:105496. https://doi.org/10.1016/j.still.2022.105496
Frene JP, Figuerola E, Gabbarini LA, Erijman L, Wall LG (2022) Impact of diversification and intensification of crop rotation (DICR) in soil bacterial microbiota in on-farm study after four and seven years. Appl Soil Ecol 179:104592. https://doi.org/10.1016/j.apsoil.2022.104592
FUNCEME-Fundação Cearense de Meteorologia e Recursos Hídricos (2012) Levantamento de reconhecimento de média intensidade dos solos - Mesorregião do Sul Cearense. http://www.funceme.br/wp-content/uploads/2019/02/LEVANTAMENTO_DE_RECONHECIMENTO_DOS_SOLOS.pdf . Accessed 25 July 2023
Giacomeli R, Carlesso R, Petry MT, Chechi L, Beutler NA, Fulaneti FS, Ferrazza CF (2022) Improving irrigation, crop, and soil management for sustainable soybean production in Southern Brazilian lowlands. Sci Agric 79:e20210115. https://doi.org/10.1590/1678-992X-2021-0115
Grace J, José JS, Meir P, Miranda HS, Montes RA (2006) Productivity and carbon fluxes of tropical savannas. J Biogeogr 33:387–400. https://doi.org/10.1111/j.1365-2699.2005.01448.x
Guimarães JRD, Meili M, Hylander LD, Silva EC, Roulet M, Mauro JBN, Lemos RA (2000) Mercury net methylation in five tropical flood plain regions of Brazil: high in the root zone of floating macrophyte mats but low in surface sediments and flooded soils. Sci Total Environ 261:99–107. https://doi.org/10.1016/S0048-9697(00)00628-8
Guo L, Nkoh JN, Xu R (2023) A critical review of the interactions of organic carbon components with soil minerals: Insight from bibliometric analysis of the environmental behaviors of heavy metal(loid)s. J Soils Sediments 23:2396–2416. https://doi.org/10.1007/s11368-023-03502-1
Haque KMS, Uddin M, Ampah JD, Haque MK, Hossen MS, Rokonuzzaman M, Hossain MY, Hossain MS, Rahman MZ (2023) Wildfres in Australia: a bibliometric analysis and a glimpse on ‘Black Summer’ (2019/2020) disaster. Environ Sci Pollut Res 30:73061–73086. https://doi.org/10.1007/s11356-023-27423-1
Hirsch JE (2005) An index to quantify an individual’s scientific research output. Proc Natl Acad Sci USA 102: 16.569–16.572. https://doi.org/10.1073/pnas.0507655102
Hoorn C, Wesselingh FP, Steege HT, Bermudez MA, Mora A, Sevink J, Sanmartín I, Sanchez-Meseguer A, Anderson CL, Figueiredo JP, Jaramillo C, Riff D, Negri FR, Hooghiemstra H, Lundberg J, Stadler T, Särkinen T, Antonelli A (2010) Amazonia through time: andean uplift, climate change, landscape evolution, and biodiversity. Science 330:927–931. https://doi.org/10.1126/science.1194585
IBGE-Instituto Brasileiro de Geografia e Estatística (2011) Evolução da Divisão Territorial do Brasil 1872–2010. Documentos para Disseminação (Memória Institucional 17): Rio de Janeiro, 261p. Available in: https://biblioteca.ibge.gov.br/index.php/biblioteca-catalogo?view=detalhes&id=284481 . Accessed 24 July 2023
IBGE-Instituto Brasileiro de Geografia e Estatística (2018) Resolução n. 1, de 28 de junho de 2018. [Área territorial oficial]. Available in: https://atlasescolar.ibge.gov.br/images/atlas/mapas_mundo/mundo_paises_mais_extensos.pdf . Accessed 24 July 2023
IBGE-Instituto Brasileiro de Geografia e Estatística (2019) Biomas e sistema costeiro-marinho do Brasil: compatível com a escala 1:250 000. IBGE: Rio de Janeiro. 164p. Available in: https://biblioteca.ibge.gov.br/index.php/biblioteca-catalogo?view=detalhes&id=2101676 . Accessed 24 July 2023
Jiang X, Sun Y, Qu Y, Zeng H, Yang J, Zhang K, Liu L (2023) The development and future frontiers of global ecological restoration projects in the twenty-first century: a systematic review based on scientometrics. Environ Sci Pollut Res 30:32230–32245. https://doi.org/10.1007/s11356-023-25615-3
Kauffman JB, Sanford RL Jr, Cummings DL, Salcedo IH, Sampaio EVSB (1997) Biomass and nutrient dynamics associated with slash fires in neotropical dry forests. Ecology 74:140–151. https://doi.org/10.2307/1939509
Kraemer FB, Catiglioni MG, Chagas CI, De Paula R, Sainz DS, De Gerónimo E, Aparicio V, Ferraro DO (2022) Pesticide dynamics in agroecosystems: assessing climatic and hydro-physical effects in a soybean cycle under no-tillage. Soil Tillage Res 223:105489. https://doi.org/10.1016/j.still.2022.105489
Leal IR, Silva JMC, Tabarelli M, Lacher TE Jr (2005) Changing the course of biodiversity conservation in the caatinga of northeastern Brazil. Conserv Biol 19:701–706. https://doi.org/10.1111/j.1523-1739.2005.00703.x
Lehmann J, Cravo MS, Zech W (2001) Organic matter stabilization in a Xanthic Ferralsol of the central Amazon as affected by single trees: chemical characterization of density, aggregate, and particle size fractions. Geoderma 99:147–168. https://doi.org/10.1016/S0016-7061(00)00070-7
Lehmann J, Silva Junior JP, Steiner C, Nehls T, Zech W, Glaser B (2003) Nutrient availability and leaching in an archaeological Anthrosol and a Ferralsol of the Central Amazon basin: fertilizer, manure and charcoal amendments. Plant Soil 249:343–357. https://doi.org/10.1023/A:1022833116184
Leite MFA, Liu B, Cardozo EG, Silva HR, Luz RL, Muchavisoy KHM, Moraes FHR, Rousseau GX, Kowalchuk G, Gehring C, Kuramae EE (2023) Microbiome resilience of amazonian forests: Agroforest divergence to bacteria and secondary forest succession convergence to fungi glob chang biol 29:1314–1327. https://doi.org/10.1111/gcb.16556
Li T, Cui L, Xu Z, Hu R, Joshi PK, Song X, Tang L, Xia A, Wang Y, Guo D, Zhu J, Hao Y, Song L (2021) Quantitative analysis of the research trends and areas in grassland remote sensing: A scientometrics analysis of web of science from 1980 to 2020. Remote Sens 13:1279. https://doi.org/10.3390/rs13071279
Li T, Cui L, Scotton M, Dong J, Xu Z, Che R, Tang L, Cai S, Wu W, Andreatta D, Wang Y, Song X, Hao Y, Cui X (2022a) Characteristics and trends of grassland degradation research. J Soils Sediments 22:1901–1912. https://doi.org/10.1007/s11368-022-03209-9
Li T, Cui L, Liu L, Wang H, Dong J, Wang F, Song X, Che R, Li C, Tang L, Xu Z, Wang Y, Du J, Hao Y, Cui X (2022b) Characteristics of nitrogen deposition research within grassland ecosystems globally and its insight from grassland microbial community changes in China. Front Plant Sci 13:947279. https://doi.org/10.3389/fpls.2022.947279
Article PubMed PubMed Central Google Scholar
Li T, Cui L, Liu L, Chen Y, Liu H, Song X, Xu Z (2023a) Advances in the study of global forest wildfires. J Soils Sediments 23:2654–2668. https://doi.org/10.1007/s11368-023-03533-8
Li X, Wen Z, Cui L, Chen Y, Li T, Liu H, Xu Z, Cui X, Song X (2023b) Quantitatively mapping the research status and trends of vegetation responses to climate change with bibliometric analysis. J Soils Sediments 23:2963–2979. https://doi.org/10.1007/s11368-023-03583-y
Liang B, Lehmann J, Solomon D, Kinyangi J, Grossman J, O’Neill N, Skjemstad JO, Thies J, Luizão FJ, Petersen J, Neves EG (2006) Black carbon increases cation exchange capacity in soils. Soil Sci Soc Am J 70:1719–1730. https://doi.org/10.2136/sssaj2005.0383
Lima FF, Duda GP, Medeiros E, Marques MC, Costa DP, Hammecker C, Santos UJ (2023) Land use regulates microbial biomass and activity in highly degraded soil from Brazilian dry tropical forest. Arch Agron Soil Sci 69:1519–1534. https://doi.org/10.1080/03650340.2022.2099845
Lombardo et at (2022) Evidence confirms an anthropic origin of Amazonian Dark Earths. Nat Commun 13:3444. https://doi.org/10.1038/s41467-022-31064-2
Lucas KRG, Antón A, Ventura UM, Andrade EP, Ralisch R (2021) Using the available indicators of potential biodiversity damage for Life Cycle Assessment on soybean crop according to Brazilian ecoregions. Ecol Indic 127:107809. https://doi.org/10.1016/j.ecolind.2021.107809
Malhi Y, Roberts T, Betts RA, Killeen TJ, Li W, Nobre CA (2008) Climate change, deforestation, and the fate of the amazon. Science 319:169–172. https://doi.org/10.1126/science.1146961
Matos AMS, Bonini CSB, Moreira BRA, Andreotti M, Heinrichs R, Silva DT, Souza JAL, Santos MA, Andrighetto C, Pavan GM, Barreto VCM, Neto AB (2022) Long-term integrated crop–livestock–forestry systems recover the structural quality of ultisol soil. Agronomy 12:2961. https://doi.org/10.3390/agronomy12122961
Medeiros AS, Santos TC, Maia SMF (2022) Effect of long-term and soil depth on soil organic carbon stocks after conversion from native vegetation to conventional tillage systems in Brazil. Soil Tillage Res 219:105336. https://doi.org/10.1016/j.still.2022.105336
Melo VF, Barros LS, Silva MCS, Veloso TGR, Senwo ZN, Matos KS, Nunes TKO (2021) Soil bacterial diversities and response to deforestation, land use and burning in North Amazon. Brazil Appl Soil Ecol 158:103775. https://doi.org/10.1016/j.apsoil.2020.103775
Meng L, Chambers J, Koven C, Pastorello G, Gimenez B, Jardine K, Tang Y, McDowell N, Negron-Juarez R, Longo M, Araújo A, Tomasella J, Fontes C, Mohan M, Higuchi N (2022) Soil moisture thresholds explain a shift from light-limited to water-limited sap velocity in the Central Amazon during the 2015–16 El Niño drought. Environ Res Lett 17:064023. https://doi.org/10.1088/1748-9326/ac6f6d
MMA-Ministério do Meio Ambiente (1999) First National Report for the Convention on Biological Diversity, Brazil. Brasília-DF: Secretaria de Biodiversidade e Florestas. 272p. Available in: https://www.cbd.int/doc/world/br/br-nr-01-p1-en.pdf . Accessed 04 Jan 2024
MMA-Ministério do Meio Ambiente (2011) Monitoramento do desmatamento dos biomas brasileiros por satélite: monitoramento do bioma Caatinga 2008–2009. Brasília-DF: Centro de Sensoriamento Remoto – IBAMA. 57p. Available in: https://www.acaatinga.org.br/wp-content/uploads/Conhe%C3%A7a_e_Conserve_a_Caatinga__Volume_1__O_Bioma_Caatinga.pdf . Accessed 24 July 2023
Mora KE, Burbano NM, Aburto F, Matus-Baeza F, Fernandez IJ, Cuevas PD, Dorner J, Dippold MA, Guzman CM (2023) Four decades in fires research – A bibliometric analysis about the impact on mineralogy and nutrients. CATENA 226:107065. https://doi.org/10.1016/j.catena.2023.107065
Morrás H, Kremer FB, Sainz D, Fernández P, Chagas C (2022) Soil structure and glyphosate fate under no-till management in the Pampa region. II. Glyphosate and AMPA persistence and spatial distribution in the long-term. A conceptual model. Soil Tillage Res 223:105471. https://doi.org/10.1016/j.still.2022.105471
Muylaert RL, Vancine MH, Bernardo R, Oshima JEF, Sobral-Souza T, Tonetti VR, Niebuhr BB, Ribeiro MC (2018) Uma nota sobre os limites territoriais da Mata Atlântica. Oecol Aust 22:302–311. https://doi.org/10.4257/oeco.2018.2203.09
Nepstad DC, Carvalho CR, Davidson EA (1994) The role of deep roots in the hydrological and carbon cycles of Amazonian forests and pastures. Nature 372:666–669. https://doi.org/10.1038/372666a0
Oliveira Filho JS (2020) A bibliometric analysis of soil research in Brazil 1989–2018. Geoderma Reg 23:e00345. https://doi.org/10.1016/j.geodrs.2020.e00345
Oliveira Filho JS (2023) How do climate and land-use affect soil carbon and nitrogen stocks and the chemical properties of floodplain soils in tropical drylands? CATENA 231:107289. https://doi.org/10.1016/j.catena.2023.107289
Oliveira Filho JS, Pereira MG (2021) Global soil science research on drylands: an analysis of research evolution, collaboration, and trends. J Soils Sediments 21:3856–3867. https://doi.org/10.1007/s11368-021-03036-4
Oliveira Filho JS, Pinheiro Junior CR, Pereira MG, Valladares GS, Camara R (2020) Sodification and solodization processes: pedogenesis or natural soil degradation? J South Am Earth Sci 104:102909. https://doi.org/10.1016/j.jsames.2020.102909
Oliveira Filho JS, Barrozo MVS, Bastos FH, Pereira MG (2023) Effects of climate and land-use on physicochemical properties of gneiss-derived soils in tropical drylands: evidence from northeastern Brazil. Geoderma Reg 33:e00639. https://doi.org/10.1016/j.geodrs.2023.e00639
Oliveira Filho JS, Pereira MG (2023) Is Environmental contamination a concern in global technosols? a bibliometric analysis. Water Air Soil Pollut 234:142. https://doi.org/10.1007/s11270-023-06171-5
Paula BV, Rozane DE, Santos EMH, Vitto BB, Hindersmann J, Antunes LEC, Nava G, Loss A, Melo GWB, Nicoloso FT, Brunetto G (2022) Nitrogen sources in young peach trees in the presence and absence of paspalum notatum co-cultivation. Agronomy 12:2669. https://doi.org/10.3390/agronomy12112669
Pedrinho A, Mendes LW, Barros FMR, Merloti LF, Martins MM, Cotta SR, Andreote FD, Tsai SM (2023) Impacts of deforestation and forest regeneration on soil bacterial communities associated with phosphorus transformation processes in the Brazilian Amazon region. Ecol Indic 146:109779. https://doi.org/10.1016/j.ecolind.2022.109779
Pereira CMR, Silva DKA, Ferreira ACA, Goto BT, Maia LC (2014) Diversity of arbuscular mycorrhizal fungi in Atlantic Forest areas under different land uses. Agric Ecosyst Environ 185:245–252. https://doi.org/10.1016/j.agee.2014.01.005
Pereira APA, Mendes LW, Oliveira FAZ, Antunes JEL, Melo VMM, Araujo ASF (2022) Land degradation affects the microbial communities in the Brazilian Caatinga biome. CATENA 211:105961. https://doi.org/10.1016/j.catena.2021.105961
Pinheiro Junior CR, Salvador CA, Tavares TR, Abreu MC, Fagundes HS, Almeida WS, Silva Neto EC, Anjos LHC, Pereira MG (2022) Lithic soils in the semi-arid region of Brazil: edaphic characterization and susceptibility to erosion. J Arid Land 14:56–69. https://doi.org/10.1007/s40333-022-0002-3
Pires GC, Denardin LGO, Silva LS, Freitas CM, Gonçalves EC, Camargo TA, Bremm C, Carvalho PCF, Souza ED (2022) System fertilization increases soybean yield through soil quality improvements in integrated crop-livestock system in tropical soils. J Soil Sci Plant Nutr 22:4487–4495. https://doi.org/10.1007/s42729-022-01050-0
Reale R, Ribas LC, Lindenkamp TCM (2022) Ecosystem services as a ballast to guide sustained economic growth by biodiversity conservation actions. J Clean Prod 358:131846. https://doi.org/10.1016/j.jclepro.2022.131846
Ribeiro S, Moreira LFB, Overbeck GE, Maltchik L (2021) Protected Areas of the Pampa biome presented land use incompatible with conservation purposes. J Land Use Sci 16:260–272. https://doi.org/10.1080/1747423X.2021.1934134
Richter L, Hernández AH, Pessôa GS, Arruda MAZ, Rezende-Filho AT, Almeida RB, Menezes HÁ, Valles V, Barbiero L, Fostier AH (2019) Dissolved arsenic in the upper Paraguay River basin and Pantanal wetlands. Sci Total Environ 687:917–928. https://doi.org/10.1016/j.scitotenv.2019.06.147
Rodrigues M, Soltangheisi A, Abdala DB, Ebuele VO, Thoss V, Withers PJA, Pavinato PS (2023) Long-term land use and tillage influence on phosphorus species in Brazilian Oxisols: A multi-technique assessment by chemical P fractionation, 31 P NMR and P K-edge XANES spectroscopies. Soil Tillage Res 229:105683. https://doi.org/10.1016/j.still.2023.105683
Rossi JB, Ruhoff A, Fleischmann AS, Laipelt L (2023) Drought propagation in brazilian biomes revealed by remote sensing. Remote Sens 15:454. https://doi.org/10.3390/rs15020454
Scarano FR, Ceotto P (2015) Brazilian atlantic forest: Impact, vulnerability, and adaptation to climate change. Biodivers Conserv 24:2319–2331. https://doi.org/10.1007/s10531-015-0972-y
Serafim ME, Mendes IC, Wu J, Ono FB, Zancanaro L, Valendorff JDP, Zeviani WM, Pierangeli MAP, Fan M, Lal R (2023) Soil physicochemical and biological properties in soybean areas under no-till Systems in the Brazilian Cerrado. Sci Total Environ 826:160674. https://doi.org/10.1016/j.scitotenv.2022.160674
Silva CEM (2009) Ordenamento Territorial no Cerrado brasileiro: da fronteira monocultora a modelos baseados na sociobiodiversidade. Desenvolv Meio Ambiente 19:89–109. https://doi.org/10.5380/dma.v19i0.16407
Silva JSV, Abdon MM (1998) Delimitação do Pantanal brasileiro e suas sub-regiões. Pesq Agrop Brasileira 33:1703–1711
Google Scholar
Silva LCR, Correa RS, Wright JL, Bomfim B, Hendricks L, Gavin DG, Muniz WA, Martins GC, Motta ACV, Barbosa JZ, Melo VF, Young SD, Broadley MR, Santos RV (2021) A new hypothesis for the origin of Amazonian Dark Earths. Nat Commun 12:127. https://doi.org/10.1038/s41467-020-20184-2
Silva RB, Rosa JS, Packer AP, Bento CB, Silva FAM (2022) A soil quality physical–chemical approach 30 years after land-use change from forest to banana plantation. Environ Monit Assess 194:482. https://doi.org/10.1007/s10661-022-10167-9
Silva IC, Rodríguez NL (2021) Formação territorial, economia e projetos de integração regional da Pan-Amazônia. Rev Tempo Mundo 27:19–43. https://doi.org/10.38116/rtm27art1
Sousa MG, Araujo JKS, Ferreira TO, Andrade GRP, Araújo Filho JC, Fracetto GGM, Santos JGB, Fracetto FJC, Lima GK, Souza Junior V (2021) Long-term effects of irrigated agriculture on Luvisol pedogenesis in semi-arid region, northeastern Brazil. CATENA 206:105529. https://doi.org/10.1016/j.catena.2021.105529
Van Eck NJ, Waltman L (2010) Software survey: VOSviewer, a computer program for bibliometric mapping. Scientometrics 84:523–538. https://doi.org/10.1007/s11192-009-0146-3
Van Eck NJ, Waltman L (2014) Visualizing bibliometric networks. In: Ding Y, Rousseau R, Wolfram D (eds) Measuring scholarly impact: Methods and practice. Springer, pp 285–320. https://doi.org/10.1007/978-3-319-10377-8_13
Chapter Google Scholar
Vasconcelos TC, Londe V, Velasquez E, Lavelle P, Rodrigues RR (2023) Atlantic Forest restoration recovers physical and biological properties in the short term, but not the general soil quality. Land Degrad Dev 34:1842–1858. https://doi.org/10.1002/ldr.4573
Viana JLM, Souza AF, Hernández AH, Elias LP, Eismann CE, Resende-Filho AT, Barbiero L, Menegario AA, Fostier AH (2022) In situ arsenic speciation at the soil/water interface of saline-alkaline lakes of the Pantanal, Brazil: A DGT-based approach. Sci Total Environ 804:150113. https://doi.org/10.1016/j.scitotenv.2021.150113
Viglizzo EF, Frank FC, Carreño LV, Jobbágys EG, Pereyra H, Clatt J, Pincén D, Ricard F (2011) Ecological and environmental footprint of 50 years of agricultural expansion in Argentina. Glob Chang Biol 17:959–973. https://doi.org/10.1111/j.1365-2486.2010.02293.x
Wang LQ, Ali A (2021) Climate regulates the functional traits – aboveground biomass relationships at a community-level in forests: a global meta-analysis. Sci Total Environ 761:143238. https://doi.org/10.1016/j.scitotenv.2020.143238
Zhou S, Williams AP, Lintner BR, Findell KL, Keenan TF, Zhang Y, Gentine P (2022) Diminishing seasonality of subtropical water availability in a warmer world dominated by soil moisture–atmosphere feedbacks. Nat Commun 13:5756. https://doi.org/10.1038/s41467-022-33473-9
Download references
Author information
José de Souza Oliveira Filho
Present address: Department of Soil Science, Federal University of Ceará, Fortaleza, Brazil
Authors and Affiliations
Research Institute of Meteorology and Water Resources, FUNCEME, Rui Barbosa avenue, 1246, Aldeota, Brazil
You can also search for this author in PubMed Google Scholar
Corresponding author
Correspondence to José de Souza Oliveira Filho .
Ethics declarations
Conflict of interest.
The author declares no competing interests.
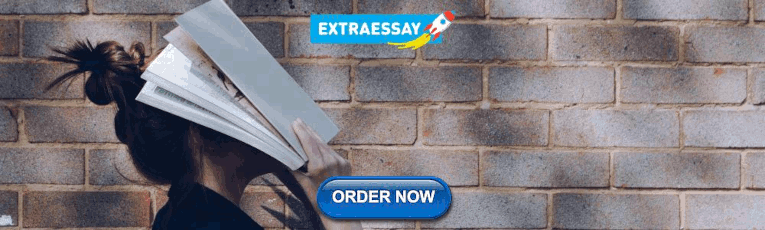
Additional information
Responsible editor: Claudio Colombo
Publisher's Note
Springer Nature remains neutral with regard to jurisdictional claims in published maps and institutional affiliations.
Rights and permissions
Springer Nature or its licensor (e.g. a society or other partner) holds exclusive rights to this article under a publishing agreement with the author(s) or other rightsholder(s); author self-archiving of the accepted manuscript version of this article is solely governed by the terms of such publishing agreement and applicable law.
Reprints and permissions
About this article
Oliveira Filho, J. Soil science research in Brazilian terrestrial biomes: A review of evolution, collaboration, current topics, and impact. J Soils Sediments (2024). https://doi.org/10.1007/s11368-024-03778-x
Download citation
Received : 24 September 2023
Accepted : 09 March 2024
Published : 21 March 2024
DOI : https://doi.org/10.1007/s11368-024-03778-x
Share this article
Anyone you share the following link with will be able to read this content:
Sorry, a shareable link is not currently available for this article.
Provided by the Springer Nature SharedIt content-sharing initiative
- Bibliometrics
- Ecosystem services
- Forest soils
- Soil research
- Find a journal
- Publish with us
- Track your research

- Cornell University Home
- College of Agriculture & Life Sciences Home
School of Integrative Plant Science

Research: Soil & Crop Sciences
The Section of Soil and Crop Sciences addresses the challenge of developing environmentally sustainable agricultural systems to produce food on regional, national, and international scales through three major program areas: Soil Science, Crop Science, and Environmental Information Systems.
Program Areas
Soil biogeochemistry.
Advance our understanding of biogeochemical cycles of carbon and nutrient elements in soil, providing important insight into regional and global element cycles which provide the basis for sustainable soil and land use management. Stabilization mechanisms of organic matter in soil nano-structures and the development of a biochar soil management technology that improves soil fertility, sequesters carbon and reduces off-site pollution. Research topics include: carbon sequestration in the context of climate change and black carbon dynamics; using synchrotron-based NEXAFS and FTIR for the micro- and nano-scale observation of biogeochemical cycles in soils; and the study of so-called Terra Preta de Indio (Amazonian Dark Earths), anthropogenic soils. Recent efforts include the combination of bio-energy and biochar applications to soil, which offer the opportunity to develop a carbon-negative energy technology which at the same time improves the environment.
Faculty Programs: Lehmann , Solomon
Soil Chemistry
Behavior of contaminants such as heavy metals at the soil-water interface in the environment, and soil health, as it is impacted by the contamination of soils by various waste materials, commercial fertilizers and manures. Protecting food crops from toxic metal contaminants, minimizing trace element deficiencies, and developing methods for testing and remediating contaminated soils. Use process-based measurements and state-of-the-art spectroscopic (e.g., XAS, FTIR, NMR), microscopic (e.g., TEM, SEM) and diffraction (e.g., XRD) methods to understand ecosystem element cycles and processes from the molecular to the field scale.
Faculty Programs: Martinez , McBride , Rutzke
Soil Fertility/Plant Nutrition
Assessment of nitrogen and phosphorus cycle processes in agricultural soils with respect to enhancing plant nutrient uptake. Specific research topics include: improve fertilizer N recovery by crops; the functions and dynamics of soil organic matter; appropriate practices for management of soil organic matter.
Faculty Programs: Lehmann
Soil Genesis/Classification/Pedology
Examination of the spatial distribution and variability of soil characteristics in urban and human influenced environments.
Faculty Programs: Russell-Anelli
Soil Ecology, Waste Management & Environmental Microbiology
Assessment of pathogens in composting systems; microbial diversity, and relationships between microbial diversity, environmental characteristics, and ecosystem processes; methods to assess soil biological quality, remediate degraded soils, and improve soil management practices.
Faculty Programs: Buckley , Thies , Bonhotal , Grantham
Soil Microbial Genomics & Evolutionary Biology
Examine the effects of agricultural management practices on soil ecology and soil microbial processes. Microbial diversity, and relationships between microbial diversity, environmental characteristics, and ecosystems processes; the development of soil health diagnostics; the impact of soil microbial diversity and community composition on agroecological function; and the use of environmental genomics to identify factors which impact bacterial population structure in soils at landscape and regional scales.
Faculty Programs: Buckley , Thies
Soil Physics/Environmental Biophysics
Examination of physical soil characteristics that contribute to soil fertility, soil quality, transport and exchange processes in soils. Research topics include: soil physical tests to determine soil health; examination of natural porous media; transport theories; the exchange processes in soils.
Faculty Programs: van Es
Crop Management: Grain, Seed, Forage and Bioenergy
Field experiments to develop management practices that improve forage quality, production, profitability and animal performance; best management practices for corn, soybeans, and other grain crops. Development of grass production and processing for a profitable and environmentally sound source of bioenergy. Evaluation of environmental, biotic, and management interactions that influence the growth, development, yield, and quality of crops. Management of crops for maximum economic returns and minimum soil erosion and nitrate losses with environmentally safe management practices.
Faculty Programs: Cherney , Ryan
Cropping Systems
Development of cover crops by identifying management strategies that maximize their benefits and minimize obstacles to their adoption. Diversifying crop rotations, exploring the impacts of conservation agriculture on soil health, and water management.
Faculty Programs: McDonald , Ryan , Hobbs
Crop Physiology and Molecular Biology
Mechanisms by which drought and other environmental stresses arrest sink-organ development (especially kernels and other storage organs), alter phytohormone levels and modify the expression of gene products involved in stress response and floral/seed set. Mechanisms used to detoxify heavy metals; genes involved in uptake, transport and sequestration of metals and interacting pathways.
Faculty Programs: Setter , Vatamaniuk
Weed Science
Integrated weed management involving ways that biotic and abiotic factors affect weed population and community dynamics, and seed dormancy. Invasive weed biology and biological control measures. Weed control recommendations, herbicide residues and resistant biotypes.
Faculty Programs: DiTommaso
Geospatial Sciences
Processes governing land-atmosphere interactions
Faculty Programs: Sun
Resource Inventory & Analysis
Inventory, spatial analysis, and digital map finishing capabilities for expediting the publication of modern soil surveys and environmental resource inventories in New York and northeastern USA.
Faculty Programs: Grantham , Hoskins
Environmental Modeling & Impact Assessment
Integration and analysis of resource inventory data for use in spatially-explicit models and risk assessments of environmental contamination in mixed use landscapes.
Faculty Programs: Woodbury
Space-Time Statistics
Examination of agronomic and environmental measurements at varying scales through the use of advanced statistical methods, including geostatistics and data mining.
soil mechanics Recently Published Documents
Total documents.
- Latest Documents
- Most Cited Documents
- Contributed Authors
- Related Sources
- Related Keywords
Surrogate Models in Rock and Soil Mechanics: Integrating Numerical Modeling and Machine Learning
Benefit of unsaturated soil mechanics approach on the modeling of early-age behavior of rammed earth building.
Rammed earth has the potential to reduce the carbon footprint and limit the energy consumption in the building sector due to its sustainable characteristics. Still, its use is not generalized due to a lack of understanding of the material behavior, notably its sensitivity to water. The coupled hydro-mechanical behavior has been recently studied in the framework of unsaturated soil mechanics, using suction as the parameter to represent the hydric state. This dependency of the mechanical behavior on the hydric state leads to uncertainty of the drying period required to progress in the construction process. Notably, the drying period before building the next floor is unknown. To determine the drying period, thermo-hydro-mechanical coupled finite element method simulations were carried out on a single wall by using the unsaturated soil mechanics approach and safety criterion recommendations from the practical guide for rammed earth construction in France. It was determined that it takes significant time for the construction of additional floor both in ‘summer-like’ and ‘winter-like’ environmental conditions, whereas the walls were far away from the ultimate failure state. Thus the drying periods were overestimated. It was concluded that the safety criterion from the practical guide is very conservative and drying periods can be reduced without significantly compromising the safety factor.
Architectural proposal for the rehabilitation and improvement of the houses of the city Yagúl housing unit, Oaxaca
This article presents the results of an investigation carried out in the Yagul city housing unit in the state of Oaxaca, in order to study the deterioration problems that have caused the abandonment of the houses, and in this context, to propose strategies that solve these problems in addition to improving the quality of life of the inhabitants. For this investigation, non-destructive tests were carried out on the houses of the place, such as: Concrete compression resistance test using a digital sclerometer, temperature tests with a thermographic camera and infrared digital thermometer, detection of reinforcements using a portable Pachometer and soil mechanics. The results obtained have allowed us to know the current state of the houses and based on these data, make an architectural proposal for the rehabilitation and improvement of said houses, generating a bioclimatic environment and comfort for its inhabitants.
The importance of geotechnical research in building projects: case study sustainable rural housing
When conceiving the project of a building, we understand that it will be interacting with the ground through a "soil-structure" system, and that it will be the appropriate interaction between the ground and the foundation that will guarantee functionality and stability from the geotechnical perspective as structural of the project. Today we identify buildings that present problems concerning structural stability due to a deficient geotechnical study that entails a negative impact from the point of view of safety, functionality and economics of the project. The professional in charge to determine the geotechnical conditions of a site is the Geotechnical Engineer or Engineer specializing in Soil Mechanics, in fact, it must be a professional with comprehensive training with knowledge in various disciplines such as geotechnics, structures, foundations and construction. The document with which we base ourselves to establish the guidelines to follow from an analysis and design perspective is the geotechnical study. This document also allows us to understand the possible threats to which the project will be at some point and how to carry out the analysis and design of the buildings, so that they can interact in perfect harmony with their surroundings. By means of a case study concerning the project of a sustainable rural house, we show the stages and elements that make up the report of the geotechnical study that was carried out, indicating the development, analysis and methodology of the study, as well as the various elements that comprise it.
Discussion on lode angle in advanced soil mechanics
Description of limit states in the subsurface layer of loosened subsoil in view of critical state soil mechanics.
The article aims to present an effective numerical method for the behaviour analysis and safety assessment of a subsurface layer of subsoil in the existing or predicted states of mining and post-mining deformations. Based on our own analytical record, using the equations of the Modified Cam-Clay model, the description of limit states in the subsurface layer of subsoil was validated, making it consistent with in situ observations. The said effect was demonstrated by comparing numerical analyses of the subsoil layer subjected to the limit state, using the Modified Cam-Clay (MCC) model and the Coulomb-Mohr model (C-M). The article also presents the applicability potential of the numerical analysis of the loosened subsoil layer for the assessment of protection elements (e.g., geo-matresses) used under linear structures in the areas subjected to mining and post-mining impacts.
Stability and Consolidation of Sediment Tailings Incorporating Unsaturated Soil Mechanics
Tailing dams are commonly used to safely store tailings without damaging the environment. Sand tailings (also called Sediment tailings) usually have a high water content and hence undergo consolidation during their placement. As the sediment tailings are usually placed above the ground water level, the degree of saturation and permeability of the sediment tailing is associated with the unsaturated condition due to the presence of negative pore-water pressure or suction. Current practices normally focus on the analyses saturated conditions. However, this consolidation process requires the flow of water between saturated and unsaturated zones to be considered. The objective of this study is to investigate the stability and consolidation of sediment tailings for the construction of road pillars considering the water flow between saturated and unsaturated zones. The scope of this study includes the unsaturated laboratory testing of sediments and numerical analyses of the road pillar. The results show that the analyses based on saturated conditions overestimate the time required to achieve a 90% degree of consolidation. The incorporation of the unsaturated soil properties is able to optimize the design of slopes for road pillars into steeper slope angles.
Abstract The title of the Conference XXI Conference of PhD Students and Young Scientists “Interdisciplinary topics in mining and geology” The location and the date of the conference virtual event - online conference, Poland, June 23-25, 2021 XXIth Conference of PhD Students and Young Scientists “Interdisciplinary topics in mining and geology” continues a series of events that started in 2000 at Wroclaw University of Science and Technology. Scientific programme of the Conference focuses on four thematic panels: 1. Mining Engineering: sustainable development, digitalisation in mining, problems of securing, protecting and using remnants of old mining works, underground mining, opencast mining, mineral processing, waste management, mining machinery, mine transport, economics in mining, mining aeronautics, ventilation and air conditioning in mines 2. Earth and Space Science: geology, hydrogeology, extraterrestrial resources, groundwater and medicinal waters, geotourism 3. Geoengineering: environmental protection, applied geotechnics, rock and soil mechanics, geohazards 4. Geoinformation: mining geodesy, GIS, photogrammetry and remote sensing, geodata modeling and analysis. List of Scientific Committee, Organizing Committee, Editorial Team are available in this pdf.
Electrical Resistivity Tomography (ERT) Monitoring for Landslides: Case Study in the Lantai Area, Yilan Taiping Mountain, Northeast Taiwan
Water saturation in the bedrock or colluvium is highly related to most landslide hazards, and rainfall is likely a crucial factor. The dynamic processes of onsite rock/soil mechanics could be revealed via monitoring using the electrical resistivity tomography (ERT) technique and Archie’s law. This study aims to investigate water saturation changes over time using time-lapse ERT images, providing a powerful method for monitoring landslide events. A fully automatic remote resistivity monitoring system was deployed to acquire hourly electrical resistivity data using a nontraditional hybrid array in the Lantai area of Yilan Taiping Mountain in Northeast Taiwan from 2019 to 2021. Six subzones in borehole ERT images were examined for the temporal and spatial resistivity variations, as well as possible pathways of the groundwater. Two representative cases of inverted electrical resistivity images varying with precipitation may be correlated with water saturation changes in the studied hillslope, implying the process of rainfall infiltration. Layers with decreased and increased electrical resistivity are also observed before sliding events. Accordingly, we suggest that high-frequency time-lapse ERT monitoring could play a crucial role in landslide early warning.
Detrainment and braking of snow avalanches interacting with forests
Abstract. Mountain forests provide natural protection against avalanches. They can both prevent avalanche formation in release zones and reduce avalanche mobility in runout areas. Although the braking effect of forests has been previously explored through global statistical analyses on documented avalanches, little is known about the mechanism of snow detrainment in forests for small and medium avalanches. In this study, we investigate the detrainment and braking of snow avalanches in forested terrain, by performing three-dimensional simulations using the Material Point Method (MPM) and a large strain elastoplastic snow constitutive model based on Critical State Soil Mechanics. First, the snow internal friction is evaluated using existing field measurements based on the detrainment mass, showing the feasibility of the numerical framework and offering a reference case for further exploration of different snow types. Then, we systematically investigate the influence of snow properties and forest parameters on avalanche characteristics. Our results suggest that, for both dry and wet avalanches, the detrainment mass decreases with the square of the avalanche front velocity before it reaches a plateau value. Furthermore, the detrainment mass significantly depends on snow properties. It can be as much as ten times larger for wet snow compared to dry snow. By examining the effect of forest configurations, it is found that forest density and tree diameter have cubic and square relations with the detrainment mass, respectively. The outcomes of this study may contribute to the development of improved formulations of avalanche–forest interaction models in popular operational simulation tools and thus improve hazard assessment for alpine geophysical mass flows in forested terrain.
Export Citation Format
Share document.
- HIRE A WRITER
- AUTHORISED AGENCIES

SOIL SCIENCE PROJECT TOPICS AND MATERIALS
Soil science project topics and materials for undergraduate and post graduate students. research project paper, seminar topics, proposals, titles, ideas and materials are available for dissertation, thesis and essay in soil science department. find below the list of research project topics for ond, hnd, bsc, pgd, msc and phd soil science students, pdf, doc., spatial variability of soil properties as influenced by parent materials in the cross river basin farm, itu, akwa ibom state., soil hydro-physical properties and precipitation concentration index in enhancing runoff under vetiver grass strips, phosphorus status of three of three wetland types in akwa ibom state, south eastern nigeria (ms.c), analyzed some suitable ways to design soil conservation measures which will solve the gulley erosion problem at uyo village road, uyo akwa ibom state, phosphorus status of three wetland types in akwa ibom state, south eastern nigeria (msc 5k), effects of simulated petroleum oil pollution on soil characteristic and growth parameters of jatropha in obio akpa, south-eastern nigeria (5k), influence of fertilization type and methods of application on nutrient uptake and yield of maize, improvement of bearing capacity of sandy soil by grouting, effect of n:p:k and poutry manure on population of rhizobia bacteria, growth and yield of cowpea., evaluation of the macro-nutrient status of the current field practical training (305) experimental farm-site, distribution of micronutrients in coastal plain sand parent material, effects of fertilizers on soil microbial diversity, growth and yield of two forage grasses ( panicum maximum and sorghum almum), effects of fertilizers on soil microbial diversity, growth and yield of two pasture grasses, a critical study of organoclays and bentonites, a survey on soil testing services awareness (a case study of mikang l.g.a. plateau state), the compressibility properties of soil in maryland area, enugu state, the effect of crude oil contaminated soil on the physical and biochemical properties of beans (phaseolus vulgaris), comparative effectiveness of organic manure and n.p.k fertilizer on soil properties and shoot dry matter yield of cassava ( manihot esculenta ), the effect of moringa oleifera leaf extract on the growth and yield of pepper (capsicum annum), micronutrients in broiler production in owerri north l.g.a. of imo state, the isolation and identification of nematode affecting tomatoes grown in some selected area of river kaduna in kaduna state, the impact of tillage practices on soil properties (a study of owerri north lga)., effect of different rates of poultry droppings and urea on the performance of (fluted pumpkin) telfairia occidentals in imo state polytechnic umuagwo, effects of fertilization on crop production and nutrient supplying capacity under rice-oilseed rape rotation system, improvement of bearing capacity of sandy soil by grouting, aggregate stability of nkpologu sandy loam soil under different soil and crop management systems, assessment of iron, zinc and anthropometric indices of preschool children in ozubulu, anambra state, nigeria, assessment of knowledge and practice of periconceptional folic acid supplementation among childbearing age women attending antenatal clinics in enugu nigeria, assessment of the feeding practices, vitamin a, iron, zinc and anthropometrics status of under-five children in orphanages of federal capital territory abuja, nigeria., assessment of the nutritional status, care and support of people living with hiv/aids in nsukka local government area, enugu state, comparative effects of lime, poultry manure and npk compound fertilizer on soil physicochemical properties and yield of maize in an ultisol of southeastern nigeria, chemical composition of processed tender leaves and husks of cowpea and organoleptic attributes of their soups as consumed in tiv communities, benue state, nigeria, consumption pattern of antioxidant rich foods by middle aged adults in enugu north local government area enugu state, nigeria, dietary management in national hospital abuja a case study, effect of different processing methods on the chemical composition of african yam bean (sphenostylis stenocarpa) flours and organoleptic characteristics of their gruels., effect of drying methods on the nutrient and phytochemical properties of some cultivated and wild leafy vegetables., effect of maternal nutrition knowledge and nutritional status on pregnancy outcome in rural and urban areas of ebonyi state, nigeria, evaluation of beta-carotene, iron and other micronutrient status of rats fed sun or shade-dried fluted pumpkin (telfaria occidentalis) leaves and its product, the leaf curd, effects of cover management practices on physical properties, runoff and erosion in nsukka sandy loam soil, evaluation of food potentials of tigernut tubers (cyperus esculentus) and its products (milk, coffee and wine), evaluation of nutritional status and dietary management of in-patient diabetics in university of nigeria teaching hospital, ituku-ozalla, enugu state, nigeria, food consumption pattern, anthropometric indices and micronutrient status of children aged 6 – 59 months in kaduna state, nigeria, identification, consumption pattern and nutrient composition of processed green leafy vegetables and their yam dishes as consumed in igbo-ukwu in anambra state, nigeria, iodine and nutritional status of primary school children in okpuje, nsukka lga, enugu state, nigeria., knowledge, attitude and adoption of appropriate feeding practices by mothers and anthropometric indices of their children in aguata local government area of anambra state, nigeria, micronutrient content and effects of jatropha curcas and brillantasia nitens leaves extracts in anaemia induced adult male albino rats, mineral profile of infant-fed composite complementary food prepared from miaze (zea mays), soybean (glycine max) and moringa oleifera leaves, morphology, characterization and classification of soils of api-river flood plain in opi, southeastern nigeria, nutrient and bioactive potentials of sphenostylis stenocarpa and glycine max and their effects on persistent physically induced stress of adult male wistar rats, nutrient composition and organoleptic attributes of fresh and sun-dried carica papaya l and solanum macrocarpon l fruit soups consumed by tiv, benue state, nutrient potential of wild and cultivated edible mushrooms and their possible use in fortifying snacks for pre-school and school children , nutrients and phytochemical composition of lesser – known vegetables – phyllantus nuriri and mucuna pruriens and their effects on iron and beta carotene status of rats, nutritional and iron status of pregnant women attending antenatal clinic at the university of nigeria teaching hospital, ituku ozalla, enugu, nutritional status of children living in ika north east local government area of delta state, phosphate mobilization by addition of organic acids in two soils of the southern guinea savanna of nigria, prevalence and influence of exercise on overweight and obese children 6-12 years in enugu south local government area of enugu state, nigeria, soil organic carbon sequestration potentials in aggregate fractions of cultivated and uncultivated soils of southeastern nigeria, studies on nutrient potentials of blends of treated aerial yam (dioscorea bulbifera) and cowpea (vignaunguiculata l. walp) fed adult albino rats, the chemical composition and organoleptic attributes of lesser-known vegetables as consumed in njikoka local government area, anambra state, nigeria., comparative effects of palm bunch waste and calcium carbonate on selected soil properties under white yam dioscorea rotundata cultivated in an ultisol, effect of crude oil pollution on soil characteristics and performance of maize zea mays, a comparative study on the corrosion of stainless steel in 1m hydrochloric acid using tamarindus indica (tamarind) and terminalia catappa (tropical almond) leaves extracts as inhibitors, effects of oxygenates on neem and tigernut oil methyl ester, an assessment of coliforms in the american univerity of nigeria water distribution system, analysis of heavy metals concentrations in commercially available fish from the yola-jimeta region, northeastern nigeria and public-health implications, assesment of bacteria found in the american university of nigeria (aun) facilities and potential health impacts, latest projects, studies on some aspects of anthracnose-blight-dieback complex of cultivars of grapevines (vitis spp.) in..., genetic variability studies of twenty potato genotypes, relationship of haemoglobin and potassium polymorphism with conformation, milk production and blood biochemical profiles..., adoption of agricultural innovations among members and non-members of women co-operative societies in oju..., small farmer credit with particular reference to nigeria, research department.
- ACCOUNTING PROJECT TOPICS AND MATERIALS 3553
- EDUCATION PROJECT TOPICS AND MATERIALS 3486
- ENGLISH AND LINGUISTIC PROJECT TOPICS AND MATERIALS 2939
- COMPUTER SCIENCE PROJECT TOPICS AND MATERIALS FINAL YEAR 1274
- BANKING AND FINANCE PROJECT TOPICS AND MATERIALS 1250
- BUSINESS ADMINISTRATION PROJECT TOPICS AND MATERIALS 1236
- EDUCATION FOUNDATION GUIDANCE AND COUNSELLING TOPICS AND MATERIALS 1045
- ZOOLOGY PROJECT TOPICS AND MATERIALS 1002
- MASS COMMUNICATION PROJECT TOPICS AND MATERIALS 1001
- ANIMAL SCIENCE PROJECT TOPICS AND MATERIALS 978
- LAW PROJECT TOPICS AND MATERIALS 896
- ARTS EDUCATION PROJECT TOPICS AND MATERIALS 844
- MARKETING PROJECT TOPICS AND MATERIALS 690
- AGRICULTURAL EXTENSION PROJECT TOPICS AND MATERIALS 676
- PUBLIC ADMINISTRATION PROJECT TOPICS AND MATERIALS 654
- POLITICAL SCIENCE PROJECT TOPICS AND MATERIALS 565
- ECONOMICS PROJECT TOPICS AND MATERIALS 457
- AGRICULTURAL SCIENCE PROJECT TOPICS AND MATERIALS 449
- LIBRARY SCIENCE PROJECT TOPICS AND MATERIALS 444
All undertaking works, records and reports posted on this website, modishproject.com are the property/copyright of their individual proprietors. They are for research reference/direction purposes and the works are publicly supported. Do not present another person’s work as your own to maintain a strategic distance from counterfeiting its results. Use it as a guide and not to duplicate the work in exactly the same words (verbatim). modishproject.com is a vault of exploration works simply like academia.edu, researchgate.net, scribd.com, docsity.com, coursehero and numerous different stages where clients transfer works. The paid membership on modishproject.com is a method by which the site is kept up to help Open Education. In the event that you see your work posted here, and you need it to be eliminated/credited, it would be ideal if you call us on +2348053692035 or send us a mail along with the web address linked to the work, to [email protected] . We will answer to and honor each solicitation. Kindly note notification it might take up to 24 - 48 hours to handle your solicitation.
EDITOR PICKS
Studies on some aspects of anthracnose-blight-dieback complex of cultivars of grapevines..., relationship of haemoglobin and potassium polymorphism with conformation, milk production and..., popular posts, accounting project topics, civil service in nigeria, top 5 best trusted research project topics and materials website in..., popular category.
- Terms of Use
- Browse Works
- Agriculture
Soil Science
Soil science research papers/topics, soil characterization, genesis, classification and land suitability evaluation of kulumsa subwatershed, arsi, ethiopia.
Abstract: Environmental factors such as topography, climate, geology, biotic factors and land-use activities are largely responsible for creating local differences in soil characteristics. These in turn create variations in the agricultural potential and management options of the soils that need detailed study in order to have adequate and comprehensive information and be able to solve site specific problems related to their sustainable use. However, there exists still a dearth of data in th...
EFFECT OF SOIL AND WATER CONSERVATION PRACTICES ON THE DYNAMICS OF SOIL BIOTA AND BIOMASS CARBON IN NORTHERN HIGHLANDS OF ETHIOPIA
Abstract: Soil and water conservation practices in Tigray, northern highlands of Ethiopia are given special emphasis for the restoration of degraded lands. However, information on the effect of SWC practices on the dynamics of soil biota is limited. This study was conducted with the objective of evaluating the effect of two decades long community-based soil and water conservation (CBSWC) practices, viz, stone terraces, exclosure with and without stone terraces which were evaluated against no...
SOIL CHARACTERIZATION AND MANAGEMENT OF ACID SOILS FOR FABA BEAN (Vicia faba L.) PRODUCTION IN YIKALO WATERSHED, NORTHWESTERN HIGHLANDS OF ETHIOPIA
Abstract: The morphological, physical and chemical properties of soils in Lay Gayint district have never been studied and scientifically classified to provide soil resource information. Besides, the area receives high amount of annual rainfall, and the soils develop acidity which is a critical issue requiring urgent attention in most highlands of Ethiopia due to its impact on crop production and productivity. Therefore, characterization and classification of soils in Yikalo subwatershed of L...
EXTRACTION AND CHARACTERIZATION OF CELLULASE FROM FOREST AND COMPOST SOIL FUNGAL ISOLATES AND EVALUATION OF ITS APPLICATION FOR DEGRADATION OF FINGER MILLET AND OAT STRAW
Abstract: Cellulases are complex hydrolytic enzymes working synergistically on the hydrolysis of cellulose to produce smaller sugar components including glucose units. These enzymes have tremendous environmental, industrial and agricultural applications including enhancement of the degradability of lignocellulosic materials. In this research fungal isolates were isolated from forest and compost soil samples and allowed to produce cellulases; and the extent to which the cellulases enhance the...
Prevalence and Intensity of Soil-Transmitted Helminth Parasitic Infections and their Associations with Anthropometric Measurements of School Children in Tulu Nageso Primary School, Oromia Reg
Abstract: Soil-transmitted helminth parasitic infections constitute major public health challenges among school children in developing tropical and sub-tropical countries. Therefore, the objective of the present study was to determine the prevalence and intensity of soil transmitted helminth parasitic infections and their associations with anthropometric measurements at Tulu Nageso Primary School of Oromia Region, Ethiopia. The design was cross sectional survey, involving 348 participants (1...
FLORISTIC COMPOSITION OF HOMEGARDEN AGROFORESTRY SYSTEM AND EFFECT OF INDIGENOUS TREE SPECIES COMPONENT ON SELECTED SOIL PHYSICOCHEMICAL PROPERTIES IN HABRO DISTRICT, OROMIA REGIONAL STATE, E
Abstract: Homegardens are one of the most important niche in which farmers feel confident to plant and maintain tree and shrub species. Homegardens floristic composition and its indigenous tree species that are used for soil fertility improvement in western Harerghe in general and Habro district have not been given much research attention. The study investigated the floristic composition of the homegarden agroforestry system in 36 homegardens, and assessed the effect of indigenous tree speci...
POTASSIUM FRACTIONATION AND ADSORPTION CHARACTERISTICS OF SOILS OF KAFTA HUMERA DISTRICT UNDER DIFFERENT LAND USES, WESTERN TIGRAY, ETHIOPIA
Abstract: Potassium in soil system found in different forms in different soil types and land use systems. Information on the forms and characteristics of potassium in different soils under different land use types is vital for the management of potassium fertility of the soils. This study was aimed at investigate the forms of potassium and its adsorption characteristics in soils of Kafta Humera district under different land use types. The bulk soil samples were collected randomly from adjace...
SOIL FERTILITY ASSESSMENT, MAPPING AND FERTILIZER TYPE RECOMMENDATION FOR FARTA, FOGERA, AND GONDAR ZURIA DISTRICTS IN NORTHWESTERN AMHARA REGION, ETHIOPIA
Abstract: Detailed characterization of agricultural landscapes and knowledge on soil spatial variability induced soil fertility differences are crucial for developing site specific, profitable and sustainable nutrient management. In view of this, landscape characterization and soil fertility assessment studies were conducted on the agricultural lands of the Amhara National Regional State, northwestern Ethiopia in the year 2014. Semi-structured questionnaire and personal observations were use...
IMPACT OF WATER TABLE DEPTH AND GROUNDWATER QUALITY ON OCCURRENCE OF SALT AFFECTED SOILS IN AMIBARA, MIDDLE AWASH, ETHIOPIA
Abstract: Soil salinity is an enormous problem for agriculture under irrigation as it affects the growth and development of plants in Ethiopia, the problem is widespread in the rift valley areas where middle Awash and Amibara irrigated farms are present. Therefore, this study was conducted to investigate the impact of depth of water table fluctuation on the occurrence of salt affected soil in Amibara irrigation scheme. Based on the existing piezometers points, additional ground water monitor...
EVALUATION OF SOIL ACIDITY AND EFFECTS OF AMENDMENTS ON SELECTED SOIL PROPERTIES AND YIELD OF MAIZE (Zea mays L.) IN EBANTU DISTRICT, WESTERN HIGHLANDS OF ETHIOPIA
Abstract: In highly weathered and leached soils, acid soil infertility is a major limitation to crop production. Three major experiments were conducted under laboratory, greenhouse, and field conditions to assess soil acidity status of surface soils under three land use types (forest, cultivated, and grazing lands), evaluate the effects of lime, vermicompost (VC), and chemical phosphorus (P) fertilizer on selected soil properties, and investigate the effects of the three amendments on select...
MODIFIED METHOD FOR PREDICTING PHOSPHATE DESORPTION KINETICS AND DYNAMICS FROM SOILS AND ITS RELATION WITH PLANT GROWTH
Abstract: Phosphorus (P) limits plant growth particularly in very strongly acidic soils. P availability to plant is a functional concept rather than measurable quantity. This research work was intended to synthesize nanocomposite material that can monitor soil P desorption kinetics and dynamics and relate to P uptake by maize (Zea mays L.). The binary oxide Fe-Al composite system, both in a crystalline and amorphous form was synthesized by sol-gel evaporation method and characterized by XRD,...
Soil Fertility Mapping of Agamsa Watershed in Habru District, Northeastern Ethiopia
Abstract: Declining soil fertility is the major challenge to boost crop production in Ethiopia. As a result, assessment of soil fertility status and mapping can help to design site specific soil fertility management techniques. Therefore, this study was conducted at Agamsa watershed in Habru District, Northeastern Ethiopia, with the objectives of assessing the soil fertility status of the watershed and mapping the spatial distribution of the selected soil fertility parameters. Field survey w...
Response of Faba Bean (Vicia faba L.) to Rhizobium Inoculation and Potassium Fertilizer Application on Nitisols at Wolaita Sodo, Southern Ethiopia
Abstract: Lack of effective indigenous soil rhizobia and low soil fertility including potassium deficiency are the major production constraints that limit the potential yield of faba bean in the study area. Hence, field experiment was conducted to evaluate the effect of Rhizobium inoculation and potassium fertilizer application on nodulation, yield components and yield of faba bean; to determine the potassium use efficiency; and to evaluate the economic feasibility of the treatments for faba...
SOYBEAN (Glycine max L.) RESPONSE TO LIME AND VERMICOMPOST AMELIORATION OF ACIDIC NITISOLS OF ASSOSA, NORTH WESTERN ETHIOPIA
Abstract: Acidic soils limit the production potential of crops because of low availability of basic cations and excess of hydrogen (H+) and aluminium (Al3+) in exchangeable forms. In the western part of the country such as Assosa and Wellega, soil acidity is a well-known problem limiting crop productivity. A field study was conducted to assess the effects of lime, vermicompost and their combinations on selected soil physic-chemical properties and responses of soybean grown on acidic Nitisols...
Assessment of Physicochemical Properties of Soil Under Different Land Use Types at Wuye Gose Sub-Watershed, North Shoa Zone of Oromia Region, Ethiopia
Abstract: ASSESSMENT OF PHYSICOCHEMICAL PROPERTIES OF SOIL UNDER DIFFERENT LAND USE TYPES AT WUYE GOSE SUBWATERSHED, NORTH SHOs loamy sand while it was sandy loam in homestead land and forest land. The BD ranged from 1.51 (GL) to 1.13 g/cm3 (HL) and TP varied 57.25 (HL) to 43.04% (GL). Soil water content at FC was ranged from (45.18) in homestead to (36.35%v) in grazing land. Permanent wilting point, in %v, was high (16.99) in homestead and forest to low (8.49) in grazing and cultivated land...
Soil science is the study of soil as a natural resource on the surface of the Earth including soil formation, classification and mapping; physical, chemical, biological, and fertility properties of soils; and these properties in relation to the use and management of soils. Browse Project topics in soil science. View academic research papers, thesis, seminars in Soil Science. Soil Science projects, thesis, seminars and termpapers topic and materials
Popular Papers/Topics
The impacts of agroforestry practices on soil fertility in rural areas, influence of nitrogen and phosphorus on nodulation and yield of soybean, effects of neem-based organic fertilizer, npk and their combinations on soil properties and growth of okra (abelmoschus esculentus) in a degraded ultisol of calabar, nigeria, nodulation of cowpea, the effects of soil degradation to sustainable agriculture in chongwe district, zambia, predictive mapping of soil properties for precision agriculture using geographic information system (gis) based geostatistics models, distribution of total and exchangeable potassium in the teaching and research farm of federal university of technology, minna, assessment of soil chemical degradation under kolanut production in imota, lagos state, addition of different levels of poultry manure on total heterotrophic bacteria in a cultivated soil, assessment of soil chemical degradation under kolanut production in imota lagos state, status and distribution of soil available micronutrients along a hillslope at ekpri ibami in akamkpa local government area of cross river state, nigeria, an assessment of some soil quality indicators in dekina local government area of kogi state a case study of abocho, egume, and ojikpadala, interaction between some soil physicochemical properties and weather variables on sub-humid tropical rainforest soils of cross river state, southeastern nigeria., effect of phosphate fertilizer application on manganese adsorption by some soils in borno state, land evaluation, characterization and classification of soil for the proposed oil palm plantation in ekpri ibami, akamkpa local government area, nigeria.
Privacy Policy | Refund Policy | Terms | Copyright | © 2024, Afribary Limited. All rights reserved.
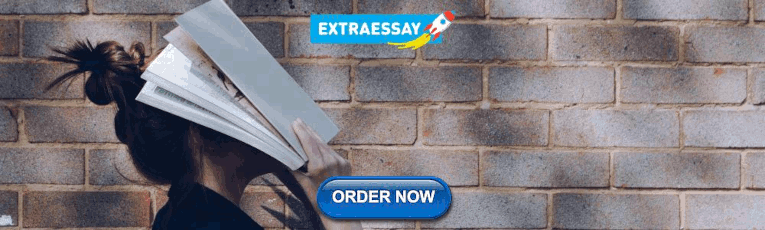
IMAGES
VIDEO
COMMENTS
A forum for excellence in soil science - from plant-soil interactions to mathematical modelling on soil processes - deepening our knowledge of the subject. ... Research Topics. Submission open Soil Tillage and Nitrogen Fertilization Methods for Sustainable Productivity of Industrial Crops. Dimitrios J Bilalis;
An open discussion board on issues related with Soil Science | Explore the latest full-text research PDFs, articles, conference papers, preprints and more on SOIL SCIENCE. Find methods information ...
Soil health and water quality nexus in sustainable agroecosystems (Soil Drainage Research) Soil health assessment across the ohio edge-of-field network (Soil Drainage Research) American society of agronomy, crop science society of america, and soil science society of america (asa, cssa, sssa) (Southeast Watershed Research)
The book is divided into five parts - soil science, soil physics, soil chemistry, soil biology and soil environment. The first part "Soil Science" serves as the introduction to the book and discusses some common topics such as soil formation, mineralogy, taxonomy, quality and analytical techniques. The second part "Soil Physics" is ...
This paper contains an analysis of the technical options in agriculture for reducing greenhouse-gas emissions and increasing sinks, arising from three distinct mechanisms: (i) increasing carbon ...
Past, current, and, presumably, future scientific literature has and will include studies related to soils. Soils are a vital life sphere that can generate indispensable resources and goods to supply natural and human ecosystems. 1,2 The numerous biogeochemical cycles and different interactions among spheres (atmosphere, geosphere, biosphere, anthroposphere, hydrosphere) all converge into the ...
This paper aims to provide a comprehensive review of the application of ML techniques in soil science aided by a ML algorithm (latent Dirichlet allocation) to find patterns in a large collection of text corpora. The objective is to gain insight into publications of ML applications in soil science and to discuss the research gaps in this topic.
Advances in Agronomy. Alfred E. Hartemink, in Advances in Agronomy, 2002 B. Funding and Scope Throughout past decades funding opportunities for fundamental soil research have been reduced (Mermut and Eswaran, 1997), and much soil research is externally funded with a strong problem-solving character.With this trend soil science has returned to where it started: little fundamental research and a ...
Lime and Nutrient Addition Affects the Dynamics and Fractions of Soil Carbon in a Short-term Incubation Study With 13 C-Labeled Wheat Straw. Coonan, Elizabeth C.; Kirkby, Clive A.; Kirkegaard, John A.; More. Soil Science. 184 (2):43-51, April 2019. Abstract.
This paper has summarized the research advances made over the past decade in soil science with respect to five critical areas. It is important to ask how this research has been utilized to drive sustainable development. Figure 1 presents a timeline of some of the global initiatives towards which soil scientists have contributed over the past ...
Soil microbiology is the scientific discipline that is concerned with the study of all biological aspects of the microorganisms (bacteria, archaea, viruses, fungi, parasites and protozoa) that ...
Search for more papers by this author. Lewis S. Rose, Lewis S. Rose. ... ESNR stakeholder views of the contribution of soil science focussed on fewer topics than those from agriculture (60% of all keywords) and highlighted soil organic matter, land management and ecosystem services as key areas to which soil science research has contributed ...
The following is a summary of current soil research priorities identified by federal funding agencies, including the U.S. Department of Agriculture National Institute of Food and Agriculture (NIFA) and the Natural Resource Conservation Service, the National Science Foundation (NSF), the Department of Energy, the Environmental Protection Agency, the National Institute of Environmental Health ...
In this paper, we present the outcomes of an initiative to identify priority research questions as a tool for guiding future soil science research. The collaborative approach involved four stages including (i) survey-based solicitation of questions; (ii) criteria-based screening of submitted candidate questions, (iii) criteria-based ranking of ...
Flowchart showing four-stage collaborative process adopted to identify 25 top priority research questions for soil science in the 21 st century. Chart showing number of candidate questions ...
This special issue will publish high-quality scholarly review papers on key topics in the soil sciences. It aims to highlight recent advances in the field, whilst emphasizing important directions and new possibilities for future inquiries. We anticipate this will promote discussion in the soil science community that will translate to best practice applications in soil management, agriculture ...
A total of 11,231 papers were obtained. The results indicated a significant increase in soil science research across all biomes (polynomial growth), with greater emphasis for research in the Cerrado (R2 = 0.9361), Amazon (R2 = 0.9170) and Atlantic Forest (R2 = 0.9104) biomes.
Soil & Crop Sciences Section. Research. The Section of Soil and Crop Sciences addresses the challenge of developing environmentally sustainable agricultural systems to produce food on regional, national, and international scales through three major program areas: Soil Science, Crop Science, and Environmental Information Systems.
2010-05-01. The fertility of soil is a capacity for ensuring plants by water, nutrients, air and capacity for making optimal conditions for growth and development of plants. The result of it is a yield. The main characteristic of fertility of soil is maintenance of humus. The humus is important part of organic matter.
Find the latest published documents for soil mechanics, Related hot topics, top authors, the most cited documents, and related journals ... topics in mining and geology" continues a series of events that started in 2000 at Wroclaw University of Science and Technology. Scientific programme of the Conference focuses on four thematic panels: 1 ...
Research project paper, seminar topics, proposals, titles, ideas and materials are available for dissertation, thesis and essay in Soil Science department. Find below the list of research project topics for OND, HND, BSC, PGD, MSC and PHD Soil Science students, Pdf, Doc.
Soil Science . Soil science is the study of soil as a natural resource on the surface of the Earth including soil formation, classification and mapping; physical, chemical, biological, and fertility properties of soils; and these properties in relation to the use and management of soils. Browse Project topics in soil science.
The soil. color (Wet method) of s oil varied from olive brown, olive, olive yellow, dark brown and. dark yellowish brown, sand, silt and clay percentage varied from sand - 50-65 %, silt -. 20 ...