
An official website of the United States government
The .gov means it’s official. Federal government websites often end in .gov or .mil. Before sharing sensitive information, make sure you’re on a federal government site.
The site is secure. The https:// ensures that you are connecting to the official website and that any information you provide is encrypted and transmitted securely.
- Publications
- Account settings
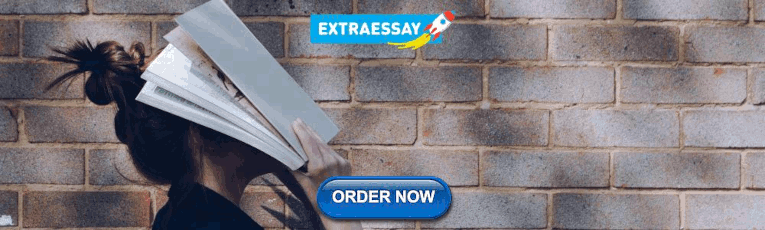
Trending Articles
- Global burden and strength of evidence for 88 risk factors in 204 countries and 811 subnational locations, 1990-2021: a systematic analysis for the Global Burden of Disease Study 2021. GBD 2021 Risk Factors Collaborators. Lancet. 2024. PMID: 38762324
- A modern way to teach and practice manual therapy. Kerry R, et al. Chiropr Man Therap. 2024. PMID: 38773515 Free PMC article. Review.
- Burden of disease scenarios for 204 countries and territories, 2022-2050: a forecasting analysis for the Global Burden of Disease Study 2021. GBD 2021 Forecasting Collaborators. Lancet. 2024. PMID: 38762325
- Oncogenic fatty acid oxidation senses circadian disruption in sleep-deficiency-enhanced tumorigenesis. Peng F, et al. Cell Metab. 2024. PMID: 38772364
- Dupilumab for COPD with Blood Eosinophil Evidence of Type 2 Inflammation. Bhatt SP, et al. N Engl J Med. 2024. PMID: 38767614
Latest Literature
- Am J Clin Nutr (1)
- Cochrane Database Syst Rev (2)
- J Biol Chem (6)
- Nature (52)
- PLoS One (110)
- Proc Natl Acad Sci U S A (15)
- Science (45)
NCBI Literature Resources
MeSH PMC Bookshelf Disclaimer
The PubMed wordmark and PubMed logo are registered trademarks of the U.S. Department of Health and Human Services (HHS). Unauthorized use of these marks is strictly prohibited.
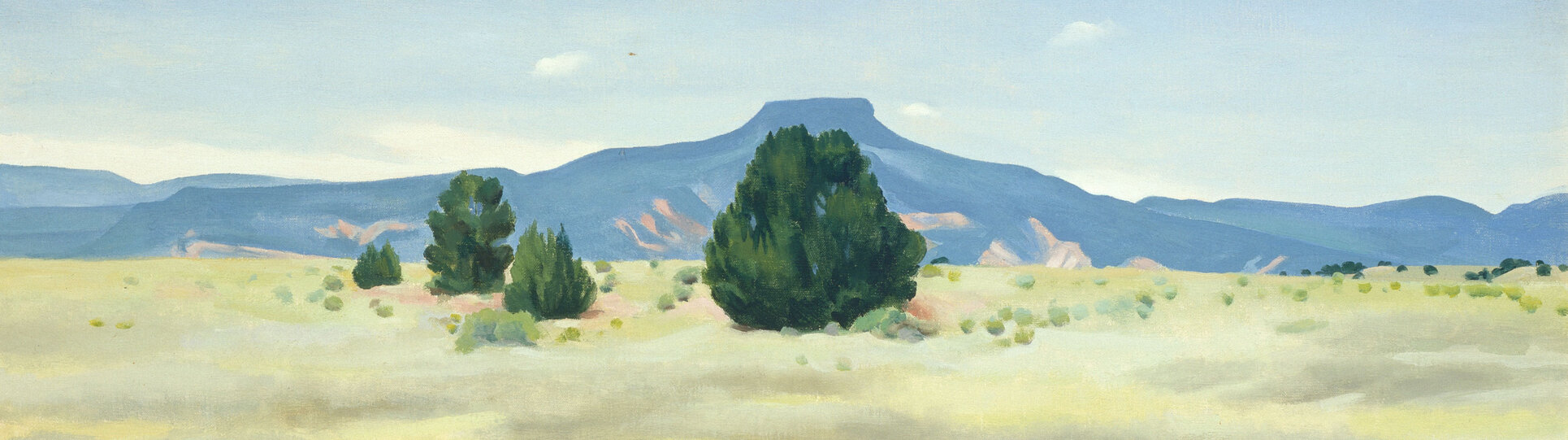
Explore millions of high-quality primary sources and images from around the world, including artworks, maps, photographs, and more.
Explore migration issues through a variety of media types
- Part of The Streets are Talking: Public Forms of Creative Expression from Around the World
- Part of The Journal of Economic Perspectives, Vol. 34, No. 1 (Winter 2020)
- Part of Cato Institute (Aug. 3, 2021)
- Part of University of California Press
- Part of Open: Smithsonian National Museum of African American History & Culture
- Part of Indiana Journal of Global Legal Studies, Vol. 19, No. 1 (Winter 2012)
- Part of R Street Institute (Nov. 1, 2020)
- Part of Leuven University Press
- Part of UN Secretary-General Papers: Ban Ki-moon (2007-2016)
- Part of Perspectives on Terrorism, Vol. 12, No. 4 (August 2018)
- Part of Leveraging Lives: Serbia and Illegal Tunisian Migration to Europe, Carnegie Endowment for International Peace (Mar. 1, 2023)
- Part of UCL Press
Harness the power of visual materials—explore more than 3 million images now on JSTOR.
Enhance your scholarly research with underground newspapers, magazines, and journals.
Explore collections in the arts, sciences, and literature from the world’s leading museums, archives, and scholars.
Skip to main page content
- AUTHOR INFO
- Institution: Google Indexer
- Sign In via User Name/Password

Call for Papers – Long-read sequencing
Top 10 Most Read Articles
Creative Commons Licensing
Open Access Publishing
Current Issue : April 2024
Advance online articles.
Last updated: May 22, 2024
August 1991 - April 2024
GR in the News
Author information, e-mail alerts & rss feeds, free online issue, job opportunities, in this issue.
Genome Research is an international, continuously published, peer-reviewed journal that features outstanding original research providing novel insights into the genome biology of all organisms, including significant advances in genomic medicine. The journal also provides high-quality reviews and perspectives written by respected leaders in the field and reports cutting-edge computational biology and high-throughput methodologies.

- Author Info
Copyright © 2024 by Cold Spring Harbor Laboratory Press
- Print ISSN: 1088-9051
- Online ISSN: 1549-5469
For an alternate route to Genome Research use this URL: http://intl-genome.cshlp.org [ More Information ]

Thank you for visiting nature.com. You are using a browser version with limited support for CSS. To obtain the best experience, we recommend you use a more up to date browser (or turn off compatibility mode in Internet Explorer). In the meantime, to ensure continued support, we are displaying the site without styles and JavaScript.
- View all journals
- Explore content
- About the journal
- Publish with us
- Sign up for alerts
- Published: 14 May 2024
2023 summer warmth unparalleled over the past 2,000 years
- Jan Esper ORCID: orcid.org/0000-0003-3919-014X 1 , 2 ,
- Max Torbenson ORCID: orcid.org/0000-0003-2720-2238 1 &
- Ulf Büntgen 2 , 3 , 4
Nature ( 2024 ) Cite this article
6165 Accesses
3406 Altmetric
Metrics details
We are providing an unedited version of this manuscript to give early access to its findings. Before final publication, the manuscript will undergo further editing. Please note there may be errors present which affect the content, and all legal disclaimers apply.
- Climate change
- Palaeoclimate
Including an exceptionally warm Northern Hemisphere (NH) summer 1 ,2 , 2023 has been reported as the hottest year on record 3-5 . Contextualizing recent anthropogenic warming against past natural variability is nontrivial, however, because the sparse 19 th century meteorological records tend to be too warm 6 . Here, we combine observed and reconstructed June-August (JJA) surface air temperatures to show that 2023 was the warmest NH extra-tropical summer over the past 2000 years exceeding the 95% confidence range of natural climate variability by more than half a degree Celsius. Comparison of the 2023 JJA warming against the coldest reconstructed summer in 536 CE reveals a maximum range of pre-Anthropocene-to-2023 temperatures of 3.93°C. Although 2023 is consistent with a greenhouse gases-induced warming trend 7 that is amplified by an unfolding El Niño event 8 , this extreme emphasizes the urgency to implement international agreements for carbon emission reduction.
This is a preview of subscription content, access via your institution
Access options
Access Nature and 54 other Nature Portfolio journals
Get Nature+, our best-value online-access subscription
24,99 € / 30 days
cancel any time
Subscribe to this journal
Receive 51 print issues and online access
185,98 € per year
only 3,65 € per issue
Rent or buy this article
Prices vary by article type
Prices may be subject to local taxes which are calculated during checkout
Similar content being viewed by others
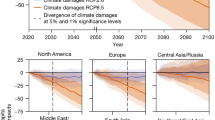
The economic commitment of climate change
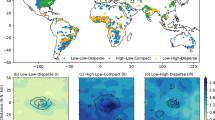
Urban development pattern’s influence on extreme rainfall occurrences
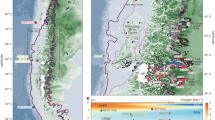
Near-synchronous Northern Hemisphere and Patagonian Ice Sheet variation over the last glacial cycle
Author information, authors and affiliations.
Department of Geography, Johannes Gutenberg University, Mainz, Germany
Jan Esper & Max Torbenson
Global Change Research Institute of the Czech Academy of Sciences, Brno, Czech Republic
Jan Esper & Ulf Büntgen
Department of Geography, University of Cambridge, Cambridge, United Kingdom
Ulf Büntgen
Department of Geography, Masaryk University, Brno, Czech Republic
You can also search for this author in PubMed Google Scholar
Corresponding author
Correspondence to Jan Esper .
Rights and permissions
Reprints and permissions
About this article
Cite this article.
Esper, J., Torbenson, M. & Büntgen, U. 2023 summer warmth unparalleled over the past 2,000 years. Nature (2024). https://doi.org/10.1038/s41586-024-07512-y
Download citation
Received : 16 January 2024
Accepted : 02 May 2024
Published : 14 May 2024
DOI : https://doi.org/10.1038/s41586-024-07512-y
Share this article
Anyone you share the following link with will be able to read this content:
Sorry, a shareable link is not currently available for this article.
Provided by the Springer Nature SharedIt content-sharing initiative
By submitting a comment you agree to abide by our Terms and Community Guidelines . If you find something abusive or that does not comply with our terms or guidelines please flag it as inappropriate.
Quick links
- Explore articles by subject
- Guide to authors
- Editorial policies
Sign up for the Nature Briefing newsletter — what matters in science, free to your inbox daily.

- [email protected] / [email protected]
- Create Account
- Join to Connect:

This paper is in the following e-collection/theme issue:
Published on 22.5.2024 in Vol 26 (2024)
AI Quality Standards in Health Care: Rapid Umbrella Review
Authors of this article:

- Craig E Kuziemsky 1 , BSc, BCom, PhD ;
- Dillon Chrimes 2 , BSc, MSc, PhD ;
- Simon Minshall 2 , BSc, MSc ;
- Michael Mannerow 1 , BSc ;
- Francis Lau 2 , BSc, MSc, MBA, PhD
1 MacEwan University, Edmonton, AB, Canada
2 School of Health Information Science, University of Victoria, Victoria, BC, Canada
Corresponding Author:
Craig E Kuziemsky, BSc, BCom, PhD
MacEwan University
10700 104 Avenue
Edmonton, AB, T5J4S2
Phone: 1 7806333290
Email: [email protected]
Background: In recent years, there has been an upwelling of artificial intelligence (AI) studies in the health care literature. During this period, there has been an increasing number of proposed standards to evaluate the quality of health care AI studies.
Objective: This rapid umbrella review examines the use of AI quality standards in a sample of health care AI systematic review articles published over a 36-month period.
Methods: We used a modified version of the Joanna Briggs Institute umbrella review method. Our rapid approach was informed by the practical guide by Tricco and colleagues for conducting rapid reviews. Our search was focused on the MEDLINE database supplemented with Google Scholar. The inclusion criteria were English-language systematic reviews regardless of review type, with mention of AI and health in the abstract, published during a 36-month period. For the synthesis, we summarized the AI quality standards used and issues noted in these reviews drawing on a set of published health care AI standards, harmonized the terms used, and offered guidance to improve the quality of future health care AI studies.
Results: We selected 33 review articles published between 2020 and 2022 in our synthesis. The reviews covered a wide range of objectives, topics, settings, designs, and results. Over 60 AI approaches across different domains were identified with varying levels of detail spanning different AI life cycle stages, making comparisons difficult. Health care AI quality standards were applied in only 39% (13/33) of the reviews and in 14% (25/178) of the original studies from the reviews examined, mostly to appraise their methodological or reporting quality. Only a handful mentioned the transparency, explainability, trustworthiness, ethics, and privacy aspects. A total of 23 AI quality standard–related issues were identified in the reviews. There was a recognized need to standardize the planning, conduct, and reporting of health care AI studies and address their broader societal, ethical, and regulatory implications.
Conclusions: Despite the growing number of AI standards to assess the quality of health care AI studies, they are seldom applied in practice. With increasing desire to adopt AI in different health topics, domains, and settings, practitioners and researchers must stay abreast of and adapt to the evolving landscape of health care AI quality standards and apply these standards to improve the quality of their AI studies.
Introduction
Growth of health care artificial intelligence.
In recent years, there has been an upwelling of artificial intelligence (AI)–based studies in the health care literature. While there have been reported benefits, such as improved prediction accuracy and monitoring of diseases [ 1 ], health care organizations face potential patient safety, ethical, legal, social, and other risks from the adoption of AI approaches [ 2 , 3 ]. A search of the MEDLINE database for the terms “artificial intelligence” and “health” in the abstracts of articles published in 2022 alone returned >1000 results. Even by narrowing it down to systematic review articles, the same search returned dozens of results. These articles cover a wide range of AI approaches applied in different health care contexts, including such topics as the application of machine learning (ML) in skin cancer [ 4 ], use of natural language processing (NLP) to identify atrial fibrillation in electronic health records [ 5 ], image-based AI in inflammatory bowel disease [ 6 ], and predictive modeling of pressure injury in hospitalized patients [ 7 ]. The AI studies reported are also at different AI life cycle stages, from model development, validation, and deployment to evaluation [ 8 ]. Each of these AI life cycle stages can involve different contexts, questions, designs, measures, and outcomes [ 9 ]. With the number of health care AI studies rapidly on the rise, there is a need to evaluate the quality of these studies in different contexts. However, the means to examine the quality of health care AI studies have grown more complex, especially when considering their broader societal and ethical implications [ 10 - 13 ].
Coiera et al [ 14 ] described a “replication crisis” in health and biomedical informatics where issues regarding experimental design and reporting of results impede our ability to replicate existing research. Poor replication raises concerns about the quality of published studies as well as the ability to understand how context could impact replication across settings. The replication issue is prevalent in health care AI studies as many are single-setting approaches and we do not know the extent to which they can be translated to other settings or contexts. One solution to address the replication issue in AI studies has been the development of a growing number of AI quality standards. Most prominent are the reporting guidelines from the Enhancing the Quality and Transparency of Health Research (EQUATOR) network [ 15 ]. Examples include the CONSORT-AI (Consolidated Standards of Reporting Trials–Artificial Intelligence) extension for reporting AI clinical trials [ 16 ] and the SPIRIT-AI (Standard Protocol Items: Recommendations for Interventional Trials–Artificial Intelligence) extension for reporting AI clinical trial protocols [ 17 ]. Beyond the EQUATOR guidelines, there are also the Minimum Information for Medical AI Reporting standard [ 18 ] and the Minimum Information About Clinical Artificial Intelligence Modeling checklist [ 19 ] on the minimum information needed in published AI studies. These standards mainly focus on the methodological and reporting quality aspects of AI studies to ensure that the published information is rigorous, complete, and transparent.
Need for Health Care AI Standards
However, there is a shortcoming of standard-driven guidance that spans the entire AI life cycle spectrum of design, validation, implementation, and governance. The World Health Organization has published six ethical principles to guide the use of AI [ 20 ] that cover (1) protecting human autonomy; (2) promoting human well-being and safety and the public interest; (3) ensuring transparency, explainability, and intelligibility; (4) fostering responsibility and accountability; (5) ensuring inclusiveness and equity; and (6) promoting AI that is responsive and sustainable. In a scoping review, Solanki et al [ 21 ] operationalized health care AI ethics through a framework of 6 guidelines that spans the entire AI life cycle of data management, model development, deployment, and monitoring. The National Health Service England has published a best practice guide on health care AI on how to get it right that encompasses a governance framework, addressing data access and protection issues, spreading the good innovation, and monitoring uses over time [ 22 ]. To further promote the quality of health care AI, van de Sande et al [ 23 ] have proposed a step-by-step approach with specific AI quality criteria that span the entire AI life cycle from development and implementation to governance.
Despite the aforementioned principles, frameworks, and guidance, there is still widespread variation in the quality of published AI studies in the health care literature. For example, 2 systematic reviews of 152 prediction and 28 diagnosis studies have shown poor methodological and reporting quality that have made it difficult to replicate, assess, and interpret the study findings [ 24 , 25 ]. The recent shifts beyond study quality to broader ethical, equity, and regulatory issues have also raised additional challenges for AI practitioners and researchers on the impact, transparency, trustworthiness, and accountability of the AI studies involved [ 13 , 26 - 28 ]. Increasingly, we are also seeing reports of various types of AI implementation issues [ 2 ]. There is a growing gap between the expected quality and performance of health care AI that needs to be addressed. We suggest that the overall issue is a lack of awareness and of the use of principles, frameworks, and guidance in health care AI studies.
This rapid umbrella review addressed the aforementioned issues by focusing on the principles and frameworks for health care AI design, implementation, and governance. We analyzed and synthesized the use of AI quality standards as reported in a sample of published health care AI systematic review articles. In this paper, AI quality standards are defined as guidelines, criteria, checklists, statements, guiding principles, or framework components used to evaluate the quality of health care AI studies in different domains and life cycle stages. In this context, quality covers the trustworthiness, methodological, reporting, and technical aspects of health care AI studies. Domains refer to the disciplines, branches, or areas in which AI can be found or applied, such as computer science, medicine, and robotics. The findings from this review can help address the growing need for AI practitioners and researchers to navigate the increasingly complex landscape of AI quality standards to plan, conduct, evaluate, and report health care AI studies.
With the increasing volume of systematic review articles that appear in the health care literature each year, an umbrella review has become a popular and timely approach to synthesize knowledge from published systematic reviews on a given topic. For this paper, we drew on the umbrella review method in the typology of systematic reviews for synthesizing evidence in health care by MacEntee [ 29 ]. In this typology, umbrella reviews are used to synthesize multiple systematic reviews from different sources into a summarized form to address a specific topic. We used a modified version of the Joanna Briggs Institute (JBI) umbrella review method to tailor the process, including developing of an umbrella review protocol, applying a rapid approach, and eliminating duplicate original studies [ 30 ]. Our rapid approach was informed by the practical guide to conducting rapid reviews in the areas of database selection, topic refinement, searching, study selection, data extraction, and synthesis by Tricco et al [ 31 ]. A PRISMA (Preferred Reporting Items for Systematic Reviews and Meta-Analyses) flow diagram of our review process is shown in Figure 1 [ 32 ]. A PRISMA checklist is provided in Multimedia Appendix 1 [ 32 ].

Objective and Questions
The objective of this rapid umbrella review was to examine the use of AI quality standards based on a sample of published health care AI systematic reviews. Specifically, our questions were as follows:
- What AI quality standards have been applied to evaluate the quality of health care AI studies?
- What key quality standard–related issues are noted in these reviews?
- What guidance can be offered to improve the quality of health care AI studies through the incorporation of AI quality standards?
Search Strategy
Our search strategy focused on the MEDLINE database supplemented with Google Scholar. Our search terms consisted of “artificial intelligence” or “AI,” “health,” and “systematic review” mentioned in the abstract (refer to Multimedia Appendix 2 for the search strings used). We used the .TW search field tag as it searches on title and abstract as well as fields such as abstract, Medical Subject Heading terms, and Medical Subject Heading subheadings. Our rationale to limit the search to MEDLINE with simple terms was to keep the process manageable, recognizing the huge volume of health care AI–related literature reviews that have appeared in the last few years, especially on COVID-19. One author conducted the MEDLINE and Google Scholar searches with assistance from an academic librarian. For Google Scholar, we restricted the search to the first 100 citations returned.
Inclusion Criteria
We considered all English-language systematic review articles published over a 36-month period from January 1, 2020, to December 31, 2022. The review could be any type of systematic review, meta-analysis, narrative review, qualitative review, scoping review, meta-synthesis, realist review, or umbrella review as defined in the review typology by MacEntee [ 29 ]. The overarching inclusion criteria were AI and health as the focus. To be considered for inclusion, the review articles must meet the following criteria:
- Each original study in the review is described, where an AI approach in the form of a model, method, algorithm, technique, or intervention is proposed, designed, implemented, or evaluated within a health care context to address a particular health care problem or topic area.
- We define AI as a simulation of the approximation of human intelligence in machines that comprises learning, reasoning, and logic [ 33 ]. In that approximation, AI has different levels of adaptivity and autonomy. Weak AI requires supervision or reinforced learning with human intervention to adapt to the environment, with low autonomous interaction. Strong AI is highly adaptive and highly autonomous via unsupervised learning, with no human intervention.
- We looked through all the articles, and our health care context categorization was informed by the stated settings (eg, hospital) and purpose (eg, diagnosis) mentioned in the included reviews.
- The review can include all types of AI approaches, such as ML, NLP, speech recognition, prediction models, neural networks, intelligent robotics, and AI-assisted and automated medical devices.
- The review must contain sufficient detail on the original AI studies, covering their objectives, contexts, study designs, AI approaches, measures, outcomes, and reference sources.
Exclusion Criteria
We excluded articles if any one of the following applied:
- Review articles published before January 1, 2020; not accessible in web-based format; or containing only an abstract
- Review articles in languages other than English
- Earlier versions of the review article with the same title or topic by the same authors
- Context not health care–related, such as electronic commerce or smart manufacturing
- The AI studies not containing sufficient detail on their purpose, features, or reference sources
- Studies including multiple forms of digital health technologies besides AI, such as telehealth, personal health records, or communication tools
Review Article Selection
One author conducted the literature searches and retrieved the citations after eliminating duplicates. The author then screened the citation titles and abstracts against the inclusion and exclusion criteria. Those that met the inclusion criteria were retrieved for full-text review independently by 2 other authors. Any disagreements in final article selection were resolved through consensus between the 2 authors or with a third author. The excluded articles and the reasons for their exclusion were logged.
Quality Appraisal
In total, 2 authors applied the JBI critical appraisal checklist independently to appraise the quality of the selected reviews [ 30 ]. The checklist has 11 questions that allow for yes , no , unclear , or not applicable as the response. The questions cover the areas of review question, inclusion criteria, search strategy and sources, appraisal criteria used, use of multiple reviewers, methods of minimizing data extraction errors and combining studies, publication bias, and recommendations supported by data. The reviews were ranked as high, medium, and low quality based on their JBI critical appraisal score (≥0.75 was high quality, ≥0.5 and <0.75 was medium quality, and <0.5 was low quality). All low-quality reviews were excluded from the final synthesis.
Data Extraction
One author extracted data from selected review articles using a predefined template. A second author validated all the articles for correctness and completeness. As this review was focused on AI quality standards, we extracted data that were relevant to this topic. We created a spreadsheet template with the following data fields to guide data extraction:
- Author, year, and reference: first author last name, publication year, and reference number
- URL: the URL where the review article can be found
- Objective or topic: objective or topic being addressed by the review article
- Type: type of review reported (eg, systematic review, meta-analysis, or scoping review)
- Sources: bibliographic databases used to find the primary studies reported in the review article
- Years: period of the primary studies covered by the review article
- Studies: total number of primary studies included in the review article
- Countries: countries where the studies were conducted
- Settings: study settings reported in the primary studies of the review article
- Participants: number and types of individuals being studied as reported in the review article
- AI approaches: the type of AI model, method, algorithm, technique, tool, or intervention described in the review article
- Life cycle and design: the stage or design of the AI study in the AI life cycle in the primary studies being reported, such as requirements, design, implementation, monitoring, experimental, observational, training-test-validation, or controlled trial
- Appraisal: quality assessment of the primary studies using predefined criteria (eg, risk of bias)
- Rating: quality assessment results of the primary studies reported in the review article
- Measures: performance criteria reported in the review article (eg, mortality, accuracy, and resource use)
- Analysis: methods used to summarize the primary study results (eg, narrative or quantitative)
- Results: aggregate findings from the primary studies in the review article
- Standards: name of the quality standards mentioned in the review article
- Comments: issues mentioned in the review article relevant to our synthesis
Removing Duplicate AI Studies
We identified all unique AI studies across the selected reviews after eliminating duplicates that appeared in them. We retrieved full-text articles for every tenth of these unique studies and searched for mention of AI quality standard–related terms in them. This was to ensure that all relevant AI quality standards were accounted for even if the reviews did not mention them.
Analysis and Synthesis
Our analysis was based on a set of recent publications on health care AI standards. These include (1) the AI life cycle step-by-step approach by van de Sande et al [ 23 ] with a list of AI quality standards as benchmarks, (2) the reporting guidelines by Shelmerdine et al [ 15 ] with specific standards for different AI-based clinical studies, (3) the international standards for evaluating health care AI by Wenzel and Wiegand [ 26 ], and (4) the broader requirements for trustworthy health care AI across the entire life cycle stages by the National Academy of Medicine (NAM) [ 8 ] and the European Union Commission (EUC) [ 34 ]. As part of the synthesis, we created a conceptual organizing scheme drawing on published literature on AI domains and approaches to visualize their relationships (via a Euler diagram) [ 35 ]. All analyses and syntheses were conducted by one author and then validated by another to resolve differences.
For the analysis, we (1) extracted key characteristics of the selected reviews based on our predefined template; (2) summarized the AI approaches, life cycle stages, and quality standards mentioned in the reviews; (3) extracted any additional AI quality standards mentioned in the 10% sample of unique AI studies from the selected reviews; and (4) identified AI quality standard–related issues reported.
For the synthesis, we (1) mapped the AI approaches to our conceptual organizing scheme, visualized their relationships with the AI domains and health topics found, and described the challenges in harmonizing these terms; (2) established key themes from the AI quality standard issues identified and mapped them to the NAM and EUC frameworks [ 8 , 34 ]; and (3) created a summary list of the AI quality standards found and mapped them to the life cycle phases by van de Sande et al [ 23 ].
Drawing on these findings, we proposed a set of guidelines that can enhance the quality of future health care AI studies and described its practice, policy, and research implications. Finally, we identified the limitations of this rapid umbrella review as caveats for the readers to consider. As health care, AI, and standards are replete with industry terminologies, we used the acronyms where they are mentioned in the paper and compiled an alphabetical acronym list with their spelled-out form at the end of the paper.
Summary of Included Reviews
We found 69 health care AI systematic review articles published between 2020 and 2022, of which 35 (51%) met the inclusion criteria. The included articles covered different review types, topics, settings, numbers of studies, designs, participants, AI approaches, and performance measures (refer to Multimedia Appendix 3 [ 36 - 68 ] for the review characteristics). We excluded the remaining 49% (34/69) of the articles because they (1) covered multiple technologies (eg, telehealth), (2) had insufficient detail, (3) were not specific to health care, or (4) were not in English (refer to Multimedia Appendix 4 for the excluded reviews and reasons). The quality of these reviews ranged from JBI critical appraisal scores of 1.0 to 0.36, with 49% (17/35) rated as high quality, 40% (14/35) rated as moderate quality, and 6% (2/35) rated as poor quality ( Multimedia Appendix 5 [ 36 - 68 ]). A total of 6% (2/35) of the reviews were excluded for their low JBI scores [ 69 , 70 ], leaving a sample of 33 reviews for the final synthesis.
Regarding review types, most (23/33, 70%) were systematic reviews [ 37 - 40 , 45 - 51 , 53 - 57 , 59 - 64 , 66 , 67 ], with the remaining being scoping reviews [ 36 , 41 - 44 , 52 , 58 , 65 , 68 ]. Only 3% (1/33) of the reviews were meta-analyses [ 38 ], and another was a rapid review [ 61 ]. Regarding health topics, the reviews spanned a wide range of specific health conditions, disciplines, areas, and practices. Examples of conditions were COVID-19 [ 36 , 37 , 49 , 51 , 56 , 62 , 66 ], mental health [ 48 , 65 , 68 ], infection [ 50 , 59 , 66 ], melanoma [ 57 ], and hypoglycemia [ 67 ]. Examples of disciplines were public health [ 36 , 37 , 56 , 66 ], nursing [ 42 , 43 , 61 ], rehabilitation [ 52 , 64 ], and dentistry [ 55 , 63 ]. Areas included mobile health and wearables [ 41 , 52 , 54 , 65 ], surveillance and remote monitoring [ 51 , 61 , 66 ], robotic surgeries [ 47 ], and biobanks [ 39 ]. Practices included diagnosis [ 37 , 47 , 49 , 58 , 59 , 62 ], prevention [ 47 ], prediction [ 36 , 38 , 49 , 50 , 57 ], disease management [ 41 , 46 , 47 , 58 ], and administration [ 42 ]. Regarding settings, less than half (12/33, 36%) were explicit in their health care settings, which included multiple sources [ 36 , 42 , 43 , 50 , 54 , 61 ], hospitals [ 45 , 49 ], communities [ 44 , 51 , 58 ], and social media groups [ 48 ]. The number of included studies ranged from 794 on COVID-19 [ 49 ] to 8 on hypoglycemia [ 67 ]. Regarding designs, most were performance assessment studies using secondary data sources such as intensive care unit [ 38 ], imaging [ 37 , 62 , 63 ], and biobank [ 39 ] databases. Regarding participants, they included patients, health care providers, educators, students, simulated cases, and those who use social media. Less than one-third of the reviews (8/33, 24%) mentioned sample sizes, which ranged from 11 adults [ 44 ] to 1,547,677 electronic medical records [ 40 ] (refer to Multimedia Appendix 3 for details).
Regarding AI approaches, there were >60 types of AI models, methods, algorithms, tools, and techniques mentioned in varying levels of detail across the broad AI domains of computer science, data science with and without NLP, and robotics. The main AI approaches were ML and deep learning (DL), with support vector machine, convolutional neural network, neural network, logistic regression, and random forest being mentioned the most (refer to the next section for details). The performance measures covered a wide range of metrics, such as diagnostic and prognostic accuracies (eg, sensitivity, specificity, accuracy, and area under the curve) [ 37 - 40 , 46 - 48 , 53 , 57 , 59 , 63 , 67 ], resource use (eg, whether an intensive care unit stay was necessary, length of stay, and cost) [ 37 , 58 , 62 ], and clinical outcomes (eg, COVID-19 severity, mortality, and behavior change) [ 36 , 37 , 49 , 56 , 62 , 65 ]. A few reviews (6/33, 18%) focused on the extent of the socioethical guidelines addressed [ 44 , 51 , 55 , 58 , 66 , 68 ]. Regarding life cycle stages, different schemes were applied, including preprocessing and classification [ 48 , 57 ], data preparation-preprocessing [ 37 , 38 ], different stages of adoption (eg, knowledge, persuasion, decision making, implementation) [ 44 ], conceptual research [ 42 ], model development [ 36 , 37 , 40 , 42 , 45 , 46 , 50 - 56 , 58 - 64 , 66 , 67 ], design [ 43 ], training and testing [ 38 , 42 , 45 , 50 - 53 , 58 , 61 - 64 ], validation [ 36 - 38 , 40 , 45 , 46 , 50 , 51 , 53 , 55 , 56 , 58 - 64 , 67 ], pilot trials [ 65 ], public engagement [ 68 ], implementation [ 42 , 44 , 60 - 62 , 66 , 68 ], confirmation [ 44 ], and evaluation [ 42 , 43 , 53 , 60 - 62 , 65 ] (refer to Multimedia Appendix 3 for details). It is worth noting that the period covered for our review did not include any studies on large language models (LLMs). LLM studies became more prevalent in the literature in the period just after our review.
Use of Quality Standards in Health Care AI Studies
To make sense of the different AI approaches mentioned, we used a Euler diagram [ 71 ] as a conceptual organizing scheme to visualize their relationships with AI domains and health topics ( Figure 2 [ 36 , 41 - 43 , 47 , 48 , 51 - 54 , 56 - 58 , 60 , 62 , 65 , 67 ]). The Euler diagram shows that AI broadly comprised approaches in the domains of computer science, data science with and without NLP, and robotics that could be overlapping. The main AI approaches were ML and DL, with DL being a more advanced form of ML through the use of artificial neural networks [ 33 ]. The diagram also shows that AI can exist without ML and DL (eg, decision trees and expert systems). There are also outliers in these domains with borderline AI-like approaches mostly intended to enhance human-computer interactions, such as social robotics [ 42 , 43 ], robotic-assisted surgery [ 47 ], and exoskeletons [ 54 ]. The health topics in our reviews spanned the AI domains, with most falling within data science with or without NLP. This was followed by computer science mostly for communication or database and other functional support and robotics for enhanced social interactions that may or may not be AI driven. There were borderline AI approaches such as programmed social robotics [ 42 , 43 ] or AI-enhanced social robots [ 54 ]. These approaches focus on AI enabled social robotic programming and did not use ML or DL. Borderline AI approaches also included virtual reality [ 60 ] and wearable sensors [ 65 , 66 , 68 ].
Regarding AI life cycle stages, we harmonized the different terms used in the original studies by mapping them to the 5 life cycle phases by van de Sande et al [ 23 ]: 0 (preparation), I (model development), II (performance assessment), III (clinical testing), and IV (implementation). Most AI studies in the reviews mapped to the first 3 life cycle phases by van de Sande et al [ 23 ]. These studies would typically describe the development and performance of the AI approach on a given health topic in a specific domain and setting, including their validation, sometimes done using external data sets [ 36 , 38 ]. A small number of reviews reported AI studies that were at the clinical testing phase [ 60 , 61 , 66 , 68 ]. A total of 7 studies were described as being in the implementation phase [ 66 , 68 ]. On the basis of the descriptions provided, few of the AI approaches in the studies in the AI reviews had been adopted for routine use in clinical settings [ 66 , 68 ] with quantifiable improvements in health outcomes (refer to Multimedia Appendix 6 [ 36 - 68 ] for details).
Regarding AI quality standards, only 39% (13/33) of the reviews applied specific AI quality standards in their results [ 37 - 40 , 45 , 46 , 50 , 54 , 58 , 59 , 61 , 63 , 66 ], and 12% (4/33) mentioned the need for standards [ 55 , 63 , 68 ]. These included the Prediction Model Risk of Bias Assessment Tool [ 37 , 38 , 58 , 59 ], Newcastle-Ottawa Scale [ 39 , 50 ], Critical Appraisal and Data Extraction for Systematic Reviews of Prediction Modeling Studies [ 38 , 59 ], Transparent Reporting of a Multivariable Prediction Model for Individual Prognosis or Diagnosis–Machine Learning Extension [ 50 ], levels of evidence [ 61 ], Critical Appraisal Skills Program Clinical Prediction Rule Checklist [ 40 ], Mixed Methods Appraisal Tool [ 66 ], and CONSORT-AI [ 54 ]. Another review applied 7 design justice principles as the criteria to appraise the quality of their AI studies [ 68 ]. There were also broader-level standards mentioned. These included the European Union ethical guidelines for trustworthy AI [ 44 ]; international AI standards from the International Organization for Standardization (ISO); and AI policy guidelines from the United States, Russia, and China [ 46 ] (refer to Multimedia Appendix 6 for details). We updated the Euler diagram ( Figure 2 [ 36 , 41 - 43 , 47 , 48 , 51 - 54 , 56 - 58 , 60 , 62 , 65 , 67 ]) to show in red the health topics in reviews with no mention of specific AI standards.

Of the 178 unique original AI studies from the selected reviews that were examined, only 25 (14%) mentioned the use of or need for specific AI quality standards (refer to Multimedia Appendix 7 [ 36 - 68 ] for details). They were of six types: (1) reporting—COREQ (Consolidated Criteria for Reporting Qualitative Research), Strengthening the Reporting of Observational Studies in Epidemiology, Standards for Reporting Diagnostic Accuracy Studies, PRISMA, and EQUATOR; (2) data—Unified Medical Language System, Food and Drug Administration (FDA) Adverse Event Reporting System, MedEx, RxNorm, Medical Dictionary for Regulatory Activities, and PCORnet; (3) technical—ISO-12207, FDA Software as a Medical Device, EU-Scholarly Publishing and Academic Resources Coalition, Sensor Web Enablement, Open Geospatial Consortium, Sensor Observation Service, and the American Medical Association AI recommendations; (4) robotics—ISO-13482 and ISO and TC-299; (5) ethics—Helsinki Declaration and European Union AI Watch; and (6) regulations—Health Insurance Portability and Accountability Act (HIPAA) and World Health Organization World Economic Forum. These standards were added to the list of AI quality standards mentioned by review in Multimedia Appendix 6 .
A summary of the harmonized AI topics, approaches, domains, the life cycle phases by van de Sande et al [ 23 ], and quality standards derived from our 33 reviews and 10% of unique studies within them is shown in Table 1 .
a Borderline AI approaches in the AI domains are identified with (x) .
b Italicized entries are AI quality standards mentioned only in the original studies in the reviews.
c CNN: convolutional neural network.
d SVM: support vector machine.
e RF: random forest.
f DT: decision tree.
g LoR: logistic regression.
h NLP: natural language processing.
i Phase 0: preparation before model development; phase I: AI model development; phase II: assessment of AI performance and reliability; phase III: clinical testing of AI; and phase IV: implementing and governing AI.
j AB: adaptive boosting or adaboost.
k ARMED: attribute reduction with multi-objective decomposition ensemble optimizer.
l BE: boost ensembling.
m BNB: Bernoulli naïve Bayes.
n PROBAST: Prediction Model Risk of Bias Assessment Tool.
o TRIPOD: Transparent Reporting of a Multivariable Prediction Model for Individual Prognosis or Diagnosis.
p FDA-SaMD: Food and Drug Administration–Software as a Medical Device.
q STROBE: Strengthening the Reporting of Observational Studies in Epidemiology.
r ICU: intensive care unit.
s ANN-ELM: artificial neural network extreme learning machine.
t ELM: ensemble machine learning.
u LSTM: long short-term memory.
v ESICULA: super intensive care unit learner algorithm.
w CHARMS: Checklist for Critical Appraisal and Data Extraction for Systematic Reviews of Prediction Modeling Studies.
x SFCN: sparse fully convolutional network.
y NOS: Newcastle-Ottawa scale.
z ANN: artificial neural network.
aa EN: elastic net.
ab GAM: generalized additive model.
ac CASP: Critical Appraisal Skills Programme.
ad mHealth: mobile health.
ae DL: deep learning.
af FL: federated learning.
ag ML: machine learning.
ah SAR: socially assistive robot.
ai CDSS: clinical decision support system.
aj COREQ: Consolidated Criteria for Reporting Qualitative Research.
ak ISO: International Organization for Standardization.
al EU-SPARC: Scholarly Publishing and Academic Resources Coalition Europe.
am AMS: Associated Medical Services.
an BICMM: Bayesian independent component mixture model.
ao BNC: Bayesian network classifier.
ap C4.5: a named algorithm for creating decision trees.
aq CPH: Cox proportional hazard regression.
ar IEC: international electrotechnical commission.
as NIST: National Institute of Standards and Technology.
at OECD-AI: Organisation for Economic Co-operation and Development–artificial intelligence.
au AUC: area under the curve.
av BCP-NN: Bayesian classifier based on propagation neural network.
aw BCPNN: Bayesian confidence propagation neural network.
ax BNM: Bayesian network model.
ay TRIPOD-ML: Transparent Reporting of a Multivariable Prediction Model for Individual Prognosis or Diagnosis–Machine Learning.
az FAERS: Food and Drug Administration Adverse Event Reporting System.
ba MedDRA: Medical Dictionary for Regulatory Activities.
bb MADE1.0: Medical Artificial Intelligence Data Set for Electronic Health Records 1.0.
bc ANFIS: adaptive neuro fuzzy inference system.
bd EML: ensemble machine learning.
be cTAKES: clinical Text Analysis and Knowledge Extraction System.
bf CUI: concept unique identifier.
bg KM: k-means clustering.
bh UMLS: Unified Medical Language System.
bi 3DQI: 3D quantitative imaging.
bj ACNN: attention-based convolutional neural network.
bk LASSO: least absolute shrinkage and selection operator.
bl MCRM: multivariable Cox regression model.
bm MLR: multivariate linear regression.
bn CNN-TF: convolutional neural network using Tensorflow.
bo IRRCN: inception residual recurrent convolutional neural network.
bp IoT: internet of things.
bq NVHDOL: notal vision home optical-based deep learning.
br HIPAA: Health Insurance Portability and Accountability Act.
bs BC: Bayesian classifier.
bt EM: ensemble method.
bu PRISMA: Preferred Reporting Items for Systematic Reviews and Meta-Analyses.
bv RCT: randomized controlled trial.
bw ROBINS-I: Risk of Bias in Non-Randomised Studies of Interventions.
bx DSP: deep supervised learning.
by NN: neural network.
bz SPIRIT: Standard Protocol Items: Recommendations for Interventional Trials.
ca ABS: agent based simulation.
cb LiR: linear regression.
cc TOPSIS: technique for order of preference by similarity to ideal solution.
cd ABC: artificial bee colony.
ce DCNN: deep convolutional neural network.
cf AL: abductive learning.
cg AR: automated reasoning.
ch BN: Bayesian network.
ci COBWEB: a conceptual clustering algorithm.
cj CH: computer heuristic.
ck AR-HMM: auto-regressive hidden Markov model.
cl MLoR: multivariate logistic regression.
cm ITS: intelligent tutoring system.
cn AMA: American Medical Association.
co APS: automated planning and scheduling.
cp ES: expert system.
cq SWE: software engineering.
cr OGC: open geospatial consortium standard.
cs SOS: start of sequence.
ct BiGAN: bidirectional generative adversarial network.
cu ADA-NN: adaptive dragonfly algorithms with neural network.
cv F-CNN: fully convolutional neural network.
cw FFBP-ANN: feed-forward backpropagation artificial neural network.
cx AFM: adaptive finite state machine.
cy ATC: anatomical therapeutic chemical.
cz AFC: active force control.
da FDA: Food and Drug Administration.
db MMAT: Mixed Methods Appraisal Tool.
dc STARD: Standards for Reporting of Diagnostic Accuracy Study.
dd VR: virtual reality.
de EU: European Union.
df EQUATOR: Enhancing the Quality and Transparency of Health Research.
dg WHO-WEF: World Health Organization World Economic Forum.
dh CCC: concordance correlation coefficient.
di IEEE: Institute of Electrical and Electronics Engineers.
There were also other AI quality standards not mentioned in the reviews or their unique studies. They included guidelines such as the do no harm road map, Factor Analysis of Information Risk, HIPAA, and the FDA regulatory framework mentioned by van de Sande et al [ 23 ]; AI clinical study reporting guidelines such as Clinical Artificial Intelligence Modeling and Minimum Information About Clinical Artificial Intelligence Modeling mentioned by Shelmerdine et al [ 15 ]; and the international technical AI standards such as ISO and International Electrotechnical Commission 22989, 23053, 23894, 24027, 24028, 24029, and 24030 mentioned by Wenzel and Wiegand [ 26 ].
With these additional findings, we updated the original table of AI standards in the study by van de Sande et al [ 23 ] showing crucial steps and key documents by life cycle phase ( Table 2 ).
a Italicized references are original studies cited in the reviews, and references denoted with the footnote t are those cited in our paper but not present in any of the reviews.
b AI: artificial intelligence.
c FDA: Food and Drug Administration.
d ECLAIR: Evaluate Commercial AI Solutions in Radiology.
e FHIR: Fast Healthcare Interoperability Resources.
f FAIR: Findability, Accessibility, Interoperability, and Reusability.
g PROBAST: Prediction Model Risk of Bias Assessment Tool.
h HIPAA: Health Insurance Portability and Accountability Act.
i OOTA: Office of The Assistant Secretary.
j GDPR: General Data Protection Regulation.
k EU: European Union.
l WMA: World Medical Association.
m WEF: World Economic Forum.
n SORMAS: Surveillance, Outbreak Response Management and Analysis System.
o WHO: World Health Organization.
p ML: machine learning.
q TRIPOD: Transparent Reporting of a multivariable prediction model for Individual Prognosis Or Diagnosis.
r TRIPOD-ML: Transparent Reporting of a multivariable prediction model for Individual Prognosis Or Diagnosis—Machine Learning.
s CLAIM: Checklist for Artificial Intelligence in Medical Imaging.
t References denoted with the footnote t are those cited in our paper but not present in any of the reviews.
u CHARMS: Checklist for Critical Appraisal and Data Extraction for Systematic Reviews of Prediction Modeling Studies.
v PRISMA-DTA: Preferred Reporting Items for Systematic Reviews and Meta-Analyses of Diagnostic Test Accuracy.
w MI-CLAIM: Minimum Information About Clinical Artificial Intelligence Modeling.
x MINIMAR: Minimum Information for Medical AI Reporting.
y NOS: Newcastle-Ottawa Scale.
z LOE: level of evidence.
aa MMAT: Mixed Methods Appraisal Tool.
ab CASP: Critical Appraisal Skills Programme.
ac STARD: Standards for Reporting of Diagnostic Accuracy Studies.
ad COREQ: Consolidated Criteria for Reporting Qualitative Research.
ae MADE1.0: Model Agnostic Diagnostic Engine 1.0.
af DECIDE-AI: Developmental and Exploratory Clinical Investigations of Decision-Support Systems Driven by Artificial Intelligence.
ag SPIRIT-AI: Standard Protocol Items: Recommendations for Interventional Trials–Artificial Intelligence.
ah CONSORT-AI: Consolidated Standards of Reporting Trials–Artificial Intelligence.
ai RoB 2: Risk of Bias 2.
aj ROBINS-I: Risk of Bias in Non-Randomised Studies of Interventions.
ak RCT: randomized controlled trial.
al STROBE: Strengthening the Reporting of Observational Studies in Epidemiology.
am AI-ML: artificial intelligence–machine learning.
an TAM: Technology Acceptance Model.
ao SaMD: Software as a Medical Device.
ap IMDRF: International Medical Device Regulators Forum.
aq EQUATOR: Enhancing the Quality and Transparency of Health Research.
ar NIST: National Institute of Standards and Technology.
as OECD: Organisation for Economic Co-operation and Development.
at AMA: American Medical Association.
au CCC: Computing Community Consortium.
av ISO: International Organization for Standardization.
aw IEEE: Institute of Electrical and Electronics Engineers.
ax OGC: Open Geospatial Consortium.
ay SWE: Sensor Web Enablement.
az SOS: Sensor Observation Service.
ba IEC: International Electrotechnical Commission.
bb FAERS: Food and Drug Administration Adverse Event Reporting System.
bc MedDRA: Medical Dictionary for Regulatory Activities.
bd UMLS: Unified Medical Language System.
be R&D: research and development.
bf SPARC: Scholarly Publishing and Academic Resources Coalition.
bg TC: technical committee.
Quality Standard–Related Issues
We extracted a set of AI quality standard–related issues from the 33 reviews and assigned themes based on keywords used in the reviews ( Multimedia Appendix 8 [ 36 - 68 ]). In total, we identified 23 issues, with the most frequently mentioned ones being clinical utility and economic benefits (n=10); ethics (n=10); benchmarks for data, model, and performance (n=9); privacy, security, data protection, and access (n=8); and federated learning and integration (n=8). Table 3 shows the quality standard issues by theme from the 33 reviews. To provide a framing and means of conceptualizing the quality-related issues, we did a high-level mapping of the issues to the AI requirements proposed by the NAM [ 8 ] and EUC [ 20 ]. The mapping was done by 2 of the authors, with the remaining authors validating the results. Final mapping was the result of consensus across the authors ( Table 4 ).
a AI: artificial intelligence.
b SDOH: social determinants of health.
a B5-1: key considerations in model development; T6-2: key considerations for institutional infrastructure and governance; and T6-3: key artificial intelligence tool implementation concepts, considerations, and tasks.
b 1—human agency and oversight; 2—technical robustness and safety; 3—privacy and data governance; 4—transparency; 5—diversity, nondiscrimination, and fairness; 6—societal and environmental well-being; and 7—accountability.
c N/A: not applicable.
d Themes not addressed.
e SDOH: social determinants of health.
We found that all 23 quality standard issues were covered in the AI frameworks by the NAM and EUC. Both frameworks have a detailed set of guidelines and questions to be considered at different life cycle stages of the health care AI studies. While there was consistency in the mapping of the AI issues to the NAM and EUC frameworks, there were some differences across them. Regarding the NAM, the focus was on key aspects of AI model development, infrastructure and governance, and implementation tasks. Regarding the EUC, the emphasis was on achieving trustworthiness by addressing all 7 interconnected requirements of accountability; human agency and oversight; technical robustness and safety; privacy and data governance; transparency; diversity, nondiscrimination, and fairness; and societal and environmental well-being. The quality standard issues were based on our analysis of the review articles, and our mapping was at times more granular than the issues from the NAM and EUC frameworks. However, our results showed that the 2 frameworks do provide sufficient terminology for quality standard–related issues. By embracing these guidelines, one can enhance the buy-in and adoption of the AI interventions in the health care system.
Principal Findings
Overall, we found that, despite the growing number of health care AI quality standards in the literature, they are seldom applied in practice, as is shown in a sample of recently published systematic reviews of health care AI studies. Of the reviews that mentioned AI quality standards, most were used to ensure the methodological and reporting quality of the AI studies involved. At the same time, the reviews identified many AI quality standard–related issues, including those broader in nature, such as ethics, regulations, transparency, interoperability, safety, and governance. Examples of broader standards mentioned in a handful of reviews or original studies are the ISO-12207, Unified Medical Language System, HIPAA, FDA Software as a Medical Device, World Health Organization AI governance, and American Medical Association augmented intelligence recommendations. These findings reflect the evolving nature of health care AI, which has not yet reached maturity or been widely adopted. There is a need to apply appropriate AI quality standards to demonstrate the transparency, robustness, and benefits of these AI approaches in different AI domains and health topics while protecting the privacy, safety, and rights of individuals and society from the potential unintended consequences of such innovations.
Another contribution of our study was a conceptual reframing for a systems-based perspective to harmonize health care AI. We did not look at AI studies solely as individual entities but rather as part of a bigger system that includes clinical, organizational, and societal aspects. Our findings complement those of recent publications, such as an FDA paper that advocates for a need to help people understand the broader system of AI in health care, including across different clinical settings [ 72 ]. Moving forward, we advocate for AI research that looks at how AI approaches will mature over time. AI approaches evolve through different phases of maturity as they move from development to validation to implementation. Each phase of maturity has different requirements [ 23 ] that must be assessed as part of evaluating AI approaches across domains as the number of health care applications rapidly increases [ 73 ]. However, comparing AI life cycle maturity across studies was challenging as there were a variety of life cycle terms used across the reviews, making it hard to compare life cycle maturity in and across studies. To address this issue, we provided a mapping of life cycle terms from the original studies but also used the system life cycle phases by van de Sande et al [ 23 ] as a common terminology for AI life cycle stages. A significant finding from the mapping was that most AI studies in our selected reviews were still at early stages of maturity (ie, model preparation, development, or validation), with very few studies progressing to later phases of maturity such as clinical testing and implementation. If AI research in health systems is to evolve, we need to move past single-case studies with external data validation to studies that achieve higher levels of life cycle maturity, such as clinical testing and implementation over a variety of routine health care settings (eg, hospitals, clinics, and patient homes and other community settings).
Our findings also highlighted that there are many AI approaches and quality standards used across domains in health care AI studies. To better understand their relationships and the overall construct of the approach, our applied conceptual organizing scheme for harmonized health care characterizes AI studies according to AI domains, approaches, health topics, life cycle phases, and quality standards. The health care AI landscape is complex. The Euler diagram shows multiple AI approaches in one or more AI domains for a given health topic. These domains can overlap, and the AI approaches can be driven by ML, DL, or other types (eg, decision trees, robotics). This complexity is expected to increase as the number of AI approaches and range of applications across all health topics and settings grows over time. For meaningful comparison, we need a harmonized scheme such as the one described in this paper to make sense of the multitude of AI terminology for the types of approaches reported in the health care AI literature. The systems-based perspective in this review provides the means for harmonizing AI life cycles and incorporating quality standards through different maturity stages, which could help advance health care AI research by scaling up to clinical validation and implementation in routine practice. Furthermore, we need to move toward explainable AI approaches where applications are based on clinical models if we are to move toward later stages of AI maturity in health care (eg, clinical validation, and implementation) [ 74 ].
Proposed Guidance
To improve the quality of future health care AI studies, we urge AI practitioners and researchers to draw on published health care AI quality standard literature, such as those identified in this review. The type of quality standards to be considered should cover the trustworthiness, methodological, reporting, and technical aspects. Examples include the NAM and EUC AI frameworks that address trustworthiness and the EQUATOR network with its catalog of methodological and reporting guidelines identified in this review. Also included are the Minimum Information for Medical AI Reporting guidelines and technical ISO standards (eg, robotics) that are not in the EQUATOR. Components that should be standardized are the AI ethics, approaches, life cycle stages, and performance measures used in AI studies to facilitate their meaningful comparison and aggregation. The technical standards should address such key design features as data, interoperability, and robotics. Given the complexities of the different AI approaches involved, rather than focusing on the underlying model or algorithm design, one should compare their actual performance based on life cycle stages (eg, degree of accuracy in model development or assessment vs outcome improvement in implementation). The summary list of the AI quality standards described in this paper is provided in Multimedia Appendix 9 for those wishing to apply them in future studies.
Implications
Our review has practice, policy, and research implications. For practice, better application of health care AI quality standards could help AI practitioners and researchers become more confident regarding the rigor and transparency of their health care AI studies. Developers adhering to standards may help make AI approaches in domains less of a black box and reduce unintended consequences such as systemic bias or threats to patient safety. AI standards may help health care providers better understand, trust, and apply the study findings in relevant clinical settings. For policy, these standards can provide the necessary guidance to address the broader impacts of health care AI, such as the issues of data governance, privacy, patient safety, and ethics. For research, AI quality standards can help advance the field by improving the rigor, reproducibility, and transparency in the planning, design, conduct, reporting, and appraisal of health care AI studies. Standardization would also allow for the meaningful comparison and aggregation of different health care AI studies to expand the evidence base in terms of their performance impacts, such as cost-effectiveness, and clinical outcomes.
Limitations
Despite our best effort, this umbrella review has limitations. First, we only searched for peer-reviewed English articles with “health” and “AI” as the keywords in MEDLINE and Google Scholar covering a 36-month period. It is possible to have missed relevant or important reviews that did not meet our inclusion criteria. Second, some of the AI quality standards were only published in the last few years, at approximately the same time when the AI reviews were conducted. As such, it is possible for AI review and study authors to have been unaware of these standards or the need to apply them. Third, the AI standard landscape is still evolving; thus, there are likely standards that we missed in this review (eg, Digital Imaging and Communications in Medicine in pattern recognition with convolutional neural networks [ 75 ]). Fourth, the broader socioethical guidelines are still in the early stages of being refined, operationalized, and adopted. They may not yet be in a form that can be easily applied when compared with the more established methodological and reporting standards with explicit checklists and criteria. Fifth, our literature review did not include any literature reviews on LLMs [ 76 ], and we know there are reviews of LLMs published in 2023 and beyond. Nevertheless, our categorization of NLP could coincide with NLP and DL in our Euler diagram, and furthermore, LLMs could be used in health care via approved chatbot applications at an early life cycle phase, for example, using decision trees first to prototype the chatbot as clinical decision support [ 77 ] before advancing it in the mature phase toward a more robust AI solution in health care with LLMs. Finally, only one author was involved in screening citation titles and abstracts (although 2 were later involved in full-text review of all articles that were screened in), and there is the possibility that we erroneously excluded an article on the basis of title and abstract. Despite these limitations, this umbrella review provided a snapshot of the current state of knowledge and gaps that exist with respect to the use of and need for AI quality standards in health care AI studies.
Conclusions
Despite the growing number of AI standards to assess the quality of health care AI studies, they are seldom applied in practice. With the recent unveiling of broader ethical guidelines such as those of the NAM and EUC, more transparency and guidance in health care AI use are needed. The key contribution of this review was the harmonization of different AI quality standards that could help practitioners, developers, and users understand the relationships among AI domains, approaches, life cycles, and standards. Specifically, we advocate for common terminology on AI life cycles to enable comparison of AI maturity across stages and settings and ensure that AI research scales up to clinical validation and implementation.
Acknowledgments
CK acknowledges funding support from a Discovery Grant from the Natural Sciences and Engineering Research Council of Canada (RGPIN/04884-2019). The authors affirm that no generative artificial intelligence tools were used in the writing of this manuscript.
Authors' Contributions
CK contributed to conceptualization (equal), methodology (equal), data curation (equal), formal analysis (equal), investigation (equal), and writing—original draft (lead). DC contributed to conceptualization (equal), methodology (equal), data curation (equal), formal analysis (equal), investigation (equal), and visualization (equal). SM contributed to conceptualization (equal), methodology (equal), data curation (equal), formal analysis (equal), investigation (equal), and visualization (equal). MM contributed to conceptualization (equal), methodology (equal), data curation (equal), formal analysis (equal), and investigation (equal). FL contributed to conceptualization (equal), methodology (lead), data curation (lead), formal analysis (lead), investigation (equal), writing—original draft (equal), visualization (equal), project administration (lead), and supervision (lead).
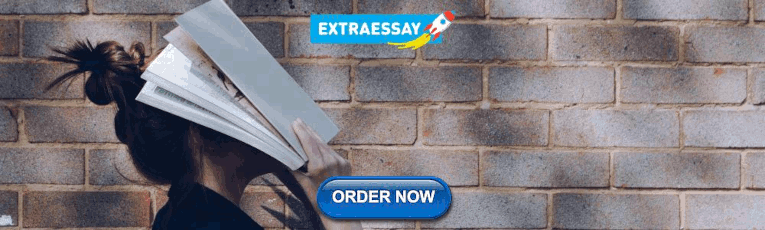
Conflicts of Interest
None declared.
PRISMA (Preferred Reporting Items for Systematic Reviews and Meta-Analyses) checklist.
PubMed search strings.
Characteristics of the included reviews.
List of excluded reviews and reasons.
Quality of the included reviews using Joanna Briggs Institute scores.
Health care artificial intelligence reviews by life cycle stage.
Quality standards found in 10% of unique studies in the selected reviews.
Quality standard–related issues mentioned in the artificial intelligence reviews.
Summary list of artificial intelligence quality standards.
- Saleh L, Mcheick H, Ajami H, Mili H, Dargham J. Comparison of machine learning algorithms to increase prediction accuracy of COPD domain. In: Proceedings of the 15th International Conference on Enhanced Quality of Life and Smart Living. 2017. Presented at: ICOST '17; August 29-31, 2017:247-254; Paris, France. URL: https://doi.org/10.1007/978-3-319-66188-9_22 [ CrossRef ]
- Gerke S, Minssen T, Cohen IG. Ethical and legal challenges of artificial intelligence-driven healthcare. Artif Intell Healthc. 2020:295-336. [ FREE Full text ] [ CrossRef ]
- Čartolovni A, Tomičić A, Lazić Mosler E. Ethical, legal, and social considerations of AI-based medical decision-support tools: a scoping review. Int J Med Inform. May 2022;161:104738. [ CrossRef ] [ Medline ]
- Das K, Cockerell CJ, Patil A, Pietkiewicz P, Giulini M, Grabbe S, et al. Machine learning and its application in skin cancer. Int J Environ Res Public Health. Dec 20, 2021;18(24):13409. [ FREE Full text ] [ CrossRef ] [ Medline ]
- Elkin P, Mullin S, Mardekian J, Crowner C, Sakilay S, Sinha S, et al. Using artificial intelligence with natural language processing to combine electronic health record's structured and free text data to identify nonvalvular atrial fibrillation to decrease strokes and death: evaluation and case-control study. J Med Internet Res. Nov 09, 2021;23(11):e28946. [ FREE Full text ] [ CrossRef ] [ Medline ]
- Kawamoto A, Takenaka K, Okamoto R, Watanabe M, Ohtsuka K. Systematic review of artificial intelligence-based image diagnosis for inflammatory bowel disease. Dig Endosc. Nov 2022;34(7):1311-1319. [ FREE Full text ] [ CrossRef ] [ Medline ]
- Anderson C, Bekele Z, Qiu Y, Tschannen D, Dinov ID. Modeling and prediction of pressure injury in hospitalized patients using artificial intelligence. BMC Med Inform Decis Mak. Aug 30, 2021;21(1):253. [ FREE Full text ] [ CrossRef ] [ Medline ]
- Matheny M, Israni ST, Ahmed M, Whicher D. Artificial Intelligence in Health Care: The Hope, the Hype, the Promise, the Peril. Washington, DC. National Academy of Medicine; 2019.
- Park Y, Jackson GP, Foreman MA, Gruen D, Hu J, Das AK. Evaluating artificial intelligence in medicine: phases of clinical research. JAMIA Open. Oct 2020;3(3):326-331. [ FREE Full text ] [ CrossRef ] [ Medline ]
- Yang C, Kors JA, Ioannou S, John LH, Markus AF, Rekkas A, et al. Trends in the conduct and reporting of clinical prediction model development and validation: a systematic review. J Am Med Inform Assoc. Apr 13, 2022;29(5):983-989. [ FREE Full text ] [ CrossRef ] [ Medline ]
- Van Calster B, Wynants L, Timmerman D, Steyerberg EW, Collins GS. Predictive analytics in health care: how can we know it works? J Am Med Inform Assoc. Dec 01, 2019;26(12):1651-1654. [ FREE Full text ] [ CrossRef ] [ Medline ]
- Kelly CJ, Karthikesalingam A, Suleyman M, Corrado G, King D. Key challenges for delivering clinical impact with artificial intelligence. BMC Med. Oct 29, 2019;17(1):195. [ FREE Full text ] [ CrossRef ] [ Medline ]
- Yin J, Ngiam KY, Teo HH. Role of artificial intelligence applications in real-life clinical practice: systematic review. J Med Internet Res. Apr 22, 2021;23(4):e25759. [ FREE Full text ] [ CrossRef ] [ Medline ]
- Coiera E, Ammenwerth E, Georgiou A, Magrabi F. Does health informatics have a replication crisis? J Am Med Inform Assoc. Aug 01, 2018;25(8):963-968. [ FREE Full text ] [ CrossRef ] [ Medline ]
- Shelmerdine SC, Arthurs OJ, Denniston A, Sebire NJ. Review of study reporting guidelines for clinical studies using artificial intelligence in healthcare. BMJ Health Care Inform. Aug 23, 2021;28(1):e100385. [ FREE Full text ] [ CrossRef ] [ Medline ]
- Liu X, Rivera SC, Moher D, Calvert MJ, Denniston AK, SPIRIT-AI and CONSORT-AI Working Group. Reporting guidelines for clinical trial reports for interventions involving artificial intelligence: the CONSORT-AI extension. BMJ. Sep 09, 2020;370:m3164-m3148. [ FREE Full text ] [ CrossRef ] [ Medline ]
- Rivera SC, Liu X, Chan AW, Denniston AK, Calvert MJ, SPIRIT-AI and CONSORT-AI Working Group. Guidelines for clinical trial protocols for interventions involving artificial intelligence: the SPIRIT-AI extension. BMJ. Sep 09, 2020;370:m3210. [ FREE Full text ] [ CrossRef ] [ Medline ]
- Hernandez-Boussard T, Bozkurt S, Ioannidis JP, Shah NH. MINIMAR (MINimum information for medical AI reporting): developing reporting standards for artificial intelligence in health care. J Am Med Inform Assoc. Dec 09, 2020;27(12):2011-2015. [ FREE Full text ] [ CrossRef ] [ Medline ]
- Norgeot B, Quer G, Beaulieu-Jones BK, Torkamani A, Dias R, Gianfrancesco M, et al. Minimum information about clinical artificial intelligence modeling: the MI-CLAIM checklist. Nat Med. Sep 2020;26(9):1320-1324. [ FREE Full text ] [ CrossRef ] [ Medline ]
- Ethics and governance of artificial intelligence for health: WHO guidance. Licence: CC BY-NC-SA 3.0 IGO. World Health Organization. URL: https://apps.who.int/iris/bitstream/handle/10665/341996/9789240029200-eng.pdf [accessed 2024-04-05]
- Solanki P, Grundy J, Hussain W. Operationalising ethics in artificial intelligence for healthcare: a framework for AI developers. AI Ethics. Jul 19, 2022;3(1):223-240. [ FREE Full text ] [ CrossRef ]
- Joshi I, Morley J. Artificial intelligence: how to get it right: putting policy into practice for safe data-driven innovation in health and care. National Health Service. 2019. URL: https://transform.england.nhs.uk/media/documents/NHSX_AI_report.pdf [accessed 2024-04-05]
- van de Sande D, Van Genderen ME, Smit JM, Huiskens J, Visser JJ, Veen RE, et al. Developing, implementing and governing artificial intelligence in medicine: a step-by-step approach to prevent an artificial intelligence winter. BMJ Health Care Inform. Feb 19, 2022;29(1):e100495. [ FREE Full text ] [ CrossRef ] [ Medline ]
- Andaur Navarro CL, Damen JA, Takada T, Nijman SW, Dhiman P, Ma J, et al. Completeness of reporting of clinical prediction models developed using supervised machine learning: a systematic review. BMC Med Res Methodol. Jan 13, 2022;22(1):12. [ FREE Full text ] [ CrossRef ] [ Medline ]
- Yusuf M, Atal I, Li J, Smith P, Ravaud P, Fergie M, et al. Reporting quality of studies using machine learning models for medical diagnosis: a systematic review. BMJ Open. Mar 23, 2020;10(3):e034568. [ FREE Full text ] [ CrossRef ] [ Medline ]
- Wenzel MA, Wiegand T. Towards international standards for the evaluation of artificial intelligence for health. In: Proceedings of the 2019 ITU Kaleidoscope: ICT for Health: Networks, Standards and Innovation. 2019. Presented at: ITU K '19; December 4-6, 2019:1-10; Atlanta, GA. URL: https://ieeexplore.ieee.org/abstract/document/8996131 [ CrossRef ]
- Varghese J. Artificial intelligence in medicine: chances and challenges for wide clinical adoption. Visc Med. Dec 2020;36(6):443-449. [ FREE Full text ] [ CrossRef ] [ Medline ]
- Nyarairo M, Emami E, Abbasgholizadeh S. Integrating equity, diversity and inclusion throughout the lifecycle of artificial intelligence in health. In: Proceedings of the 13th Augmented Human International Conference. 2022. Presented at: AH '22; May 26-27, 2022:1-4; Winnipeg, MB. URL: https://dl.acm.org/doi/abs/10.1145/3532530.3539565 [ CrossRef ]
- MacEntee MI. A typology of systematic reviews for synthesising evidence on health care. Gerodontology. Dec 06, 2019;36(4):303-312. [ CrossRef ] [ Medline ]
- Aromataris E, Fernandez R, Godfrey C, Holly C, Khalil H, Tungpunkom P. Umbrella reviews. In: Aromataris E, Lockwood C, Porritt K, Pilla B, Jordan Z, editors. JBI Manual for Evidence Synthesis. Adelaide, South Australia. Joanna Briggs Institute; 2020.
- Tricco AC, Langlois EV, Straus SE. Rapid reviews to strengthen health policy and systems: a practical guide. Licence CC BY-NC-SA 3.0 IGO. World Health Organization. 2017. URL: https://apps.who.int/iris/handle/10665/258698 [accessed 2024-04-05]
- Page MJ, McKenzie JE, Bossuyt PM, Boutron I, Hoffmann TC, Mulrow CD, et al. The PRISMA 2020 statement: an updated guideline for reporting systematic reviews. BMJ. Mar 29, 2021;372:n71. [ FREE Full text ] [ CrossRef ] [ Medline ]
- Russell S, Norvig P. Artificial Intelligence: A Modern Approach. 4th edition. London, UK. Pearson Education; 2021.
- Ethics guidelines for trustworthy AI. European Commission, Directorate-General for Communications Networks, Content and Technology. URL: https://data.europa.eu/doi/10.2759/346720 [accessed 2024-04-05]
- Lloyd N, Khuman AS. AI in healthcare: malignant or benign? In: Chen T, Carter J, Mahmud M, Khuman AS, editors. Artificial Intelligence in Healthcare: Recent Applications and Developments. Singapore, Singapore. Springer; 2022:1-46.
- Abd-Alrazaq A, Alajlani M, Alhuwail D, Schneider J, Al-Kuwari S, Shah Z, et al. Artificial intelligence in the fight against COVID-19: scoping review. J Med Internet Res. Dec 15, 2020;22(12):e20756. [ FREE Full text ] [ CrossRef ] [ Medline ]
- Adamidi ES, Mitsis K, Nikita KS. Artificial intelligence in clinical care amidst COVID-19 pandemic: a systematic review. Comput Struct Biotechnol J. 2021;19:2833-2850. [ FREE Full text ] [ CrossRef ] [ Medline ]
- Barboi C, Tzavelis A, Muhammad LN. Comparison of severity of illness scores and artificial intelligence models that are predictive of intensive care unit mortality: meta-analysis and review of the literature. JMIR Med Inform. May 31, 2022;10(5):e35293. [ FREE Full text ] [ CrossRef ] [ Medline ]
- Battineni G, Hossain MA, Chintalapudi N, Amenta F. A survey on the role of artificial intelligence in biobanking studies: a systematic review. Diagnostics (Basel). May 09, 2022;12(5):1179. [ FREE Full text ] [ CrossRef ] [ Medline ]
- Bertini A, Salas R, Chabert S, Sobrevia L, Pardo F. Using machine learning to predict complications in pregnancy: a systematic review. Front Bioeng Biotechnol. Jan 19, 2021;9:780389. [ FREE Full text ] [ CrossRef ] [ Medline ]
- Bhatt P, Liu J, Gong Y, Wang J, Guo Y. Emerging artificial intelligence-empowered mHealth: scoping review. JMIR Mhealth Uhealth. Jun 09, 2022;10(6):e35053. [ FREE Full text ] [ CrossRef ] [ Medline ]
- Buchanan C, Howitt ML, Wilson R, Booth RG, Risling T, Bamford M. Predicted influences of artificial intelligence on the domains of nursing: scoping review. JMIR Nurs. Dec 17, 2020;3(1):e23939. [ FREE Full text ] [ CrossRef ] [ Medline ]
- Buchanan C, Howitt ML, Wilson R, Booth RG, Risling T, Bamford M. Predicted influences of artificial intelligence on nursing education: scoping review. JMIR Nurs. Jan 28, 2021;4(1):e23933. [ FREE Full text ] [ CrossRef ] [ Medline ]
- Chew HS, Achananuparp P. Perceptions and needs of artificial intelligence in health care to increase adoption: scoping review. J Med Internet Res. Jan 14, 2022;24(1):e32939. [ FREE Full text ] [ CrossRef ] [ Medline ]
- Choudhury A, Renjilian E, Asan O. Use of machine learning in geriatric clinical care for chronic diseases: a systematic literature review. JAMIA Open. Oct 2020;3(3):459-471. [ FREE Full text ] [ CrossRef ] [ Medline ]
- Choudhury A, Asan O. Role of artificial intelligence in patient safety outcomes: systematic literature review. JMIR Med Inform. Jul 24, 2020;8(7):e18599. [ FREE Full text ] [ CrossRef ] [ Medline ]
- Eldaly AS, Avila FR, Torres-Guzman RA, Maita K, Garcia JP, Serrano LP, et al. Artificial intelligence and lymphedema: state of the art. J Clin Transl Res. Jun 29, 2022;8(3):234-242. [ FREE Full text ] [ Medline ]
- Le Glaz A, Haralambous Y, Kim-Dufor DH, Lenca P, Billot R, Ryan TC, et al. Machine learning and natural language processing in mental health: systematic review. J Med Internet Res. May 04, 2021;23(5):e15708. [ FREE Full text ] [ CrossRef ] [ Medline ]
- Guo Y, Zhang Y, Lyu T, Prosperi M, Wang F, Xu H, et al. The application of artificial intelligence and data integration in COVID-19 studies: a scoping review. J Am Med Inform Assoc. Aug 13, 2021;28(9):2050-2067. [ FREE Full text ] [ CrossRef ] [ Medline ]
- Hassan N, Slight R, Weiand D, Vellinga A, Morgan G, Aboushareb F, et al. Preventing sepsis; how can artificial intelligence inform the clinical decision-making process? A systematic review. Int J Med Inform. Jun 2021;150:104457. [ CrossRef ] [ Medline ]
- Huang JA, Hartanti IR, Colin MN, Pitaloka DA. Telemedicine and artificial intelligence to support self-isolation of COVID-19 patients: recent updates and challenges. Digit Health. May 15, 2022;8:20552076221100634. [ FREE Full text ] [ CrossRef ] [ Medline ]
- Kaelin VC, Valizadeh M, Salgado Z, Parde N, Khetani MA. Artificial intelligence in rehabilitation targeting the participation of children and youth with disabilities: scoping review. J Med Internet Res. Nov 04, 2021;23(11):e25745. [ FREE Full text ] [ CrossRef ] [ Medline ]
- Kirk D, Catal C, Tekinerdogan B. Precision nutrition: a systematic literature review. Comput Biol Med. Jun 2021;133:104365. [ FREE Full text ] [ CrossRef ] [ Medline ]
- Loveys K, Prina M, Axford C, Domènec Ò, Weng W, Broadbent E, et al. Artificial intelligence for older people receiving long-term care: a systematic review of acceptability and effectiveness studies. Lancet Healthy Longev. Apr 2022;3(4):e286-e297. [ FREE Full text ] [ CrossRef ] [ Medline ]
- Mörch CM, Atsu S, Cai W, Li X, Madathil SA, Liu X, et al. Artificial intelligence and ethics in dentistry: a scoping review. J Dent Res. Dec 01, 2021;100(13):1452-1460. [ CrossRef ] [ Medline ]
- Payedimarri AB, Concina D, Portinale L, Canonico M, Seys D, Vanhaecht K, et al. Prediction models for public health containment measures on COVID-19 using artificial intelligence and machine learning: a systematic review. Int J Environ Res Public Health. Apr 23, 2021;18(9):4499. [ FREE Full text ] [ CrossRef ] [ Medline ]
- Popescu D, El-Khatib M, El-Khatib H, Ichim L. New trends in melanoma detection using neural networks: a systematic review. Sensors (Basel). Jan 10, 2022;22(2):496. [ FREE Full text ] [ CrossRef ] [ Medline ]
- Abbasgholizadeh Rahimi S, Légaré F, Sharma G, Archambault P, Zomahoun HT, Chandavong S, et al. Application of artificial intelligence in community-based primary health care: systematic scoping review and critical appraisal. J Med Internet Res. Sep 03, 2021;23(9):e29839. [ FREE Full text ] [ CrossRef ] [ Medline ]
- Sahu P, Raj Stanly EA, Simon Lewis LE, Prabhu K, Rao M, Kunhikatta V. Prediction modelling in the early detection of neonatal sepsis. World J Pediatr. Mar 05, 2022;18(3):160-175. [ FREE Full text ] [ CrossRef ] [ Medline ]
- Sapci AH, Sapci HA. Artificial intelligence education and tools for medical and health informatics students: systematic review. JMIR Med Educ. Jun 30, 2020;6(1):e19285. [ FREE Full text ] [ CrossRef ] [ Medline ]
- Seibert K, Domhoff D, Bruch D, Schulte-Althoff M, Fürstenau D, Biessmann F, et al. Application scenarios for artificial intelligence in nursing care: rapid review. J Med Internet Res. Nov 29, 2021;23(11):e26522. [ FREE Full text ] [ CrossRef ] [ Medline ]
- Syeda HB, Syed M, Sexton KW, Syed S, Begum S, Syed F, et al. Role of machine learning techniques to tackle the COVID-19 crisis: systematic review. JMIR Med Inform. Jan 11, 2021;9(1):e23811. [ FREE Full text ] [ CrossRef ] [ Medline ]
- Talpur S, Azim F, Rashid M, Syed SA, Talpur BA, Khan SJ. Uses of different machine learning algorithms for diagnosis of dental caries. J Healthc Eng. Mar 31, 2022;2022:5032435-5032413. [ FREE Full text ] [ CrossRef ] [ Medline ]
- Vélez-Guerrero MA, Callejas-Cuervo M, Mazzoleni S. Artificial intelligence-based wearable robotic exoskeletons for upper limb rehabilitation: a review. Sensors (Basel). Mar 18, 2021;21(6):2146. [ FREE Full text ] [ CrossRef ] [ Medline ]
- Welch V, Wy TJ, Ligezka A, Hassett LC, Croarkin PE, Athreya AP, et al. Use of mobile and wearable artificial intelligence in child and adolescent psychiatry: scoping review. J Med Internet Res. Mar 14, 2022;24(3):e33560. [ FREE Full text ] [ CrossRef ] [ Medline ]
- Zhao IY, Ma YX, Yu MW, Liu J, Dong WN, Pang Q, et al. Ethics, integrity, and retributions of digital detection surveillance systems for infectious diseases: systematic literature review. J Med Internet Res. Oct 20, 2021;23(10):e32328. [ FREE Full text ] [ CrossRef ] [ Medline ]
- Zheng Y, Dickson VV, Blecker S, Ng JM, Rice BC, Melkus GD, et al. Identifying patients with hypoglycemia using natural language processing: systematic literature review. JMIR Diabetes. May 16, 2022;7(2):e34681. [ FREE Full text ] [ CrossRef ] [ Medline ]
- Zidaru T, Morrow EM, Stockley R. Ensuring patient and public involvement in the transition to AI-assisted mental health care: a systematic scoping review and agenda for design justice. Health Expect. Aug 12, 2021;24(4):1072-1124. [ FREE Full text ] [ CrossRef ] [ Medline ]
- Khan ZF, Alotaibi SR. Applications of artificial intelligence and big data analytics in m-Health: a healthcare system perspective. J Healthc Eng. 2020;2020:8894694. [ FREE Full text ] [ CrossRef ] [ Medline ]
- Sarker S, Jamal L, Ahmed SF, Irtisam N. Robotics and artificial intelligence in healthcare during COVID-19 pandemic: a systematic review. Rob Auton Syst. Dec 2021;146:103902. [ FREE Full text ] [ CrossRef ] [ Medline ]
- Herrmann H. The arcanum of artificial intelligence in enterprise applications: toward a unified framework. J Eng Technol Manag. Oct 2022;66:101716. [ CrossRef ]
- Wu E, Wu K, Daneshjou R, Ouyang D, Ho DE, Zou J. How medical AI devices are evaluated: limitations and recommendations from an analysis of FDA approvals. Nat Med. Apr 05, 2021;27(4):582-584. [ CrossRef ] [ Medline ]
- Bohr A, Memarzadeh K. The rise of artificial intelligence in healthcare applications. In: Bohr A, Memarzadeh K, editors. Artificial Intelligence in Healthcare. New York, NY. Academic Press; 2020:25-57.
- Jacobson FL, Krupinski EA. Clinical validation is the key to adopting AI in clinical practice. Radiol Artif Intell. Jul 01, 2021;3(4):e210104. [ FREE Full text ] [ CrossRef ] [ Medline ]
- Tran K, Bøtker JP, Aframian A, Memarzadeh K. Artificial intelligence for medical imaging. In: Bohr A, Memarzadeh K, editors. Artificial Intelligence in Healthcare. New York, NY. Academic Press; 2020:143-161.
- Hassija V, Chamola V, Mahapatra A, Singal A, Goel D, Huang K, et al. Interpreting black-box models: a review on explainable artificial intelligence. Cogn Comput. Aug 24, 2023;16(1):45-74. [ FREE Full text ] [ CrossRef ]
- Chrimes D. Using decision trees as an expert system for clinical decision support for COVID-19. Interact J Med Res. Jan 30, 2023;12:e42540. [ FREE Full text ] [ CrossRef ] [ Medline ]
Abbreviations
Edited by S Ma, T Leung; submitted 19.11.23; peer-reviewed by K Seibert, K Washington, X Yan; comments to author 21.03.24; revised version received 03.04.24; accepted 04.04.24; published 22.05.24.
©Craig E Kuziemsky, Dillon Chrimes, Simon Minshall, Michael Mannerow, Francis Lau. Originally published in the Journal of Medical Internet Research (https://www.jmir.org), 22.05.2024.
This is an open-access article distributed under the terms of the Creative Commons Attribution License (https://creativecommons.org/licenses/by/4.0/), which permits unrestricted use, distribution, and reproduction in any medium, provided the original work, first published in the Journal of Medical Internet Research, is properly cited. The complete bibliographic information, a link to the original publication on https://www.jmir.org/, as well as this copyright and license information must be included.
ISU Writing Program
About the Grassroots Writing Research Journal
Gwrj quick facts.
Two issues each year: Fall and Spring
Published by: the Writing Program at Illinois State University
Current issue available at: ISU’s Redbird Spirit Shop bookstores
Primary text for: ENG 101, ENG 101A10, ENG 145 at ISU
Past issues: available for free online
Accepting submissions: anytime
Started in: 2010
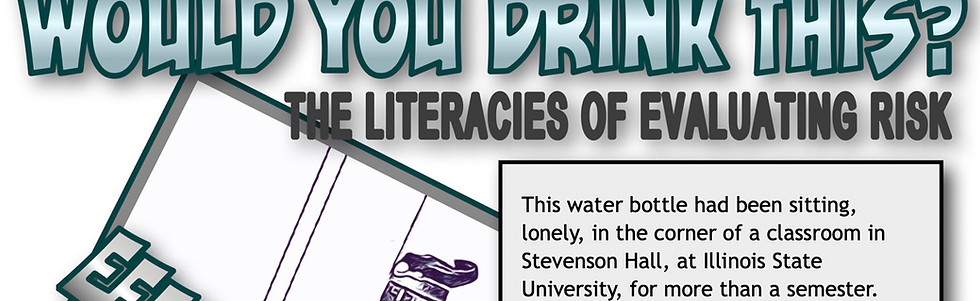
GWRJ Mission
The Grassroots Writing Research Journal (GWRJ) is dedicated to publishing writing research by everyday writers like you–like all of us.
We are committed to publishing GWRJ authors who are undergraduate writing students, graduate student teachers, university faculty members, high school teachers and students, middle school teachers and students—anyone! Because we are all writers and writing researchers.
We encourage people to write about any range of subjects that intersect with writing research. You might describe how you practice writing in your lives, how writing works toward specific writer and community goals, how you’re learning writing as a complex activity over time, and how writing makes things happen in your worlds.
We look forward to receiving submissions for genres we often publish (like full-length GWRJ articles), and we are always excited to experiment with new genres and media whether they’re some we’ve created recently (graphic shorts, writing co-interviews) or ones we hope you might create and share with us.
- Election 2024
- Entertainment
- Newsletters
- Photography
- Personal Finance
- AP Investigations
- AP Buyline Personal Finance
- AP Buyline Shopping
- Press Releases
- Israel-Hamas War
- Russia-Ukraine War
- Global elections
- Asia Pacific
- Latin America
- Middle East
- Election Results
- Delegate Tracker
- AP & Elections
- Auto Racing
- 2024 Paris Olympic Games
- Movie reviews
- Book reviews
- Personal finance
- Financial Markets
- Business Highlights
- Financial wellness
- Artificial Intelligence
- Social Media
Daily marijuana use outpaces daily drinking in the US, a new study says
Millions of people in the U.S. report using marijuana daily or nearly every day and those people now outnumber those who say they are daily or nearly-daily drinkers of alcohol., according to the study by Carnegie Mellon University.
FILE - Marijuana plants are displayed at a shop in San Francisco, Monday, March 20, 2023. Daily and near-daily marijuana use is now more common than similar levels of high-frequency drinking in the U.S., according to an analysis of survey data over four decades, according to research published Wednesday, May 22, 2024, in the journal Addiction. (AP Photo/Jeff Chiu, File)ff
- Copy Link copied
For the first time, the number of Americans who use marijuana just about every day has surpassed the number who drink that often, a shift some 40 years in the making as recreational pot use became more mainstream and legal in nearly half of U.S. states.
In 2022, an estimated 17.7 million people reported using marijuana daily or near-daily compared to 14.7 million daily or near-daily drinkers, according an analysis of national survey data . In 1992, when daily pot use hit a low point, less than 1 million people said they used marijuana nearly every day.
Alcohol is still more widely used, but 2022 was the first time this intensive level of marijuana use overtook daily and near-daily drinking, said the study’s author, Jonathan Caulkins, a cannabis policy researcher at Carnegie Mellon University.
“A good 40% of current cannabis users are using it daily or near daily, a pattern that is more associated with tobacco use than typical alcohol use,” Caulkins said.
The research, based on data from the National Survey on Drug Use and Health, was published Wednesday in the journal Addiction. The survey is a highly regarded source of self-reported estimates of tobacco, alcohol and drug use in the United States.
AP AUDIO: Daily marijuana use outpaces daily drinking in the US, a new study says
AP correspondent Haya Panjwani reports on new trends in daily and near daily marijuana use.
From 1992 to 2022, the per capita rate of reporting daily or near-daily marijuana use increased 15-fold. Caulkins acknowledged in the study that people may be more willing to report marijuana use as public acceptance grows, which could boost the increase.
Most states now allow medical or recreational marijuana, though it remains illegal at the federal level. In November, Florida voters will decide on a constitutional amendment allowing recreational cannabis, and the federal government is moving to reclassify marijuana as a less dangerous drug.
Research shows that high-frequency users are more likely to become addicted to marijuana, said Dr. David A. Gorelick, a psychiatry professor at the University of Maryland School of Medicine, who was not involved in the study.
The number of daily users suggests that more people are at risk for developing problematic cannabis use or addiction, Gorelick said.
“High frequency use also increases the risk of developing cannabis-associated psychosis,” a severe condition where a person loses touch with reality, he said.
The Associated Press Health and Science Department receives support from the Howard Hughes Medical Institute’s Science and Educational Media Group. The AP is solely responsible for all content.
- Open access
- Published: 17 May 2024
Metabolic landscape and pathogenic insights: a comprehensive analysis of high ovarian response in infertile women undergoing in vitro fertilization
- Ling-Ling Ruan 1 , 2 ,
- Xing-Yu Lv 6 ,
- Yu-Lin Hu 6 ,
- Ming-Xing Chen 2 ,
- Jing-Tang 9 ,
- Zhao-Hui Zhong 2 ,
- Mei-Hua Bao 7 ,
- Li-Juan Fu 2 , 7 ,
- Xin Luo 8 ,
- Shao-Min Yu 3 ,
- Qi Wan 4 , 5 , 6 &
- Yu-Bin Ding 1 , 2
Journal of Ovarian Research volume 17 , Article number: 105 ( 2024 ) Cite this article
142 Accesses
1 Altmetric
Metrics details
In the realm of assisted reproduction, a subset of infertile patients demonstrates high ovarian response following controlled ovarian stimulation (COS), with approximately 29.7% facing the risk of Ovarian Hyperstimulation Syndrome (OHSS). Management of OHSS risk often necessitates embryo transfer cancellation, leading to delayed prospects of successful pregnancy and significant psychological distress. Regrettably, these patients have received limited research attention, particularly regarding their metabolic profile. In this study, we aim to utilize gas chromatography-mass spectrometry (GC-MS) to reveal these patients’ unique serum metabolic profiles and provide insights into the disease’s pathogenesis.
We categorized 145 infertile women into two main groups: the CON infertility group from tubal infertility patients and the Polycystic Ovary Syndrome (PCOS) infertility group. Within these groups, we further subdivided them into four categories: patients with normal ovarian response (CON-NOR group), patients with high ovarian response and at risk for OHSS (CON-HOR group) within the CON group, as well as patients with normal ovarian response (PCOS-NOR group) and patients with high ovarian response and at risk for OHSS (PCOS-HOR group) within the PCOS group. Serum metabolic profiles were analyzed using GC-MS. The risk criteria for OHSS were: the number of developing follicles > 20, peak Estradiol (E2) > 4000pg/mL, and Anti-Müllerian Hormone (AMH) levels > 4.5ng/mL.
The serum metabolomics analysis revealed four different metabolites within the CON group and 14 within the PCOS group. Remarkably, 10-pentadecenoic acid emerged as a discernible risk metabolite for the CON-HOR, also found to be a differential metabolite between CON-NOR and PCOS groups. cysteine and 5-methoxytryptamine were also identified as risk metabolites for the PCOS-HOR. Furthermore, KEGG analysis unveiled significant enrichment of the aminoacyl-tRNA biosynthesis pathway among the metabolites differing between PCOS-NOR and PCOS-HOR.
Our study highlights significant metabolite differences between patients with normal ovarian response and those with high ovarian response and at risk for OHSS within both the tubal infertility control group and PCOS infertility group. Importantly, we observe metabolic similarities between patients with PCOS and those with a high ovarian response but without PCOS, suggesting potential parallels in their underlying causes.
Introduction
The latest report from the World Health Organization (WHO) highlights a statistic: approximately 17.5% of adults globally, equivalent to one in six patients, grapple with infertility [ 1 ]. Assisted reproductive technology (ART) emerges as a potent solution to help this demographic achieve pregnancy [ 2 ]. At the core of ART lies controlled ovarian stimulation (COS), which employs ovulation-stimulating drugs to simultaneously foster the simultaneous development of multiple ovarian follicles, facilitating the retrieval of numerous mature oocytes [ 2 ]. However, the pursuit of a high ovarian response presents a formidable challenge: mitigating the risk of ovarian hyperstimulation syndrome (OHSS). This iatrogenic complication manifests as ovarian cystic enlargement, heightened vascular permeability, and fluid extravasation into the third space, potentially leading to severe consequences such as acute respiratory distress, anuria/acute renal failure, thromboembolism, etc [ 3 ]. Current estimates indicate the incidence of moderate to severe OHSS in patients undergoing in vitro fertilization (IVF) at 1-5% [ 4 ]. Although various strategies have been employed to reduce OHSS incidence, consensus remains elusive due to divergent efficacy and safety outcomes [ 4 , 5 ].
Concurrently, when COS technology is employed to augment oocyte numbers, some patients may experience high ovarian response, with 29.7% of these patients being at high risk of developing OHSS (Supplementary Fig. 1 ). For this high-risk group of OHSS, clinicians will generally cancel embryo transfer to minimize the occurrence of late-onset OHSS in them [ 6 , 7 ]. This decision not only prolongs the path to successful conception but also poses a mental and physical challenge for the patient. However, there has been insufficient research attention directed towards this specific group, as the majority of studies have predominantly focused on individuals already diagnosed with OHSS. Therefore, urgent investigation is warranted to delineate differences between these patients and those with normal ovarian response, elucidate the underlying physiological and pathological mechanisms, and provide clues for targeted care in the future.
Metabolomics has proven valuable in exploring disease etiology and understanding normal physiological conditions [ 8 , 9 , 10 ]. Recent studies have unveiled notable differences in maternal serum levels of niacin and niacinamide metabolic-pathway-related substances between women with poor ovarian response and control groups [ 11 ]. Simultaneously, changes in maternal serum glutamic acid, aspartate, and 1-methylnicotinamide levels were significantly associated with improved symptoms in women with PCOS [ 12 ]. In cases of OHSS symptoms, researchers identified elevated follicular fluid levels of mannitol and pyruvate alongside decreased levels of L-carnitine and creatinine [ 13 ]. Additionally, investigations into women undergoing IVF revealed correlations between the majority of amino acids in serum and the number of mature oocytes [ 14 ]. Despite these advancements, the metabolic profiles of patients who exhibit high ovarian response and are at risk for OHSS remain unknown.
Given the prevalence of OHSS risk among a significant number of patients with PCOS and the close association between PCOS and reproductive issues [ 15 ], it is imperative to investigate the pathogenic mechanisms within the PCOS population. This exploration is essential for enhancing OHSS incidence management and improving pregnancy success rates despite the apparent effectiveness of infertility treatments/strategies, including IVF as the final step [ 16 ]. Furthermore, while some studies have identified abnormal expression of unsaturated fatty acid metabolites in follicular fluid as a risk factor for OHSS in PCOS patients using metabolomics [ 17 ], it remains unclear whether the presence of abnormally expressed metabolites in maternal serum is also associated with high ovarian response in PCOS patients.
Considering the intricate connection among high ovarian response, PCOS, and OHSS, this study aims to investigate the serum metabolic profiles of patients who exhibit high ovarian response and are at risk for OHSS within the tubal infertility and PCOS framework. The objective is to elucidate the metabolic profiles of this frequently overlooked population, thereby offering a valuable reference point for further research on the pathological mechanisms.
Materials and methods
Participants.
This study obtained ethical approval from both Sichuan Jinxin Xinan Women & Children’s Hospital (No. 2021014) and the Ethics Committee of Chongqing Medical University (No. 2021060). Informed consent was acquired from all participants, adhering to the principles outlined in the Declaration of Helsinki. The proportion of different ovarian responses following COS in ART was conducted utilizing data from 19,240 participants who underwent ART for the first time between January 2021 and December 2022. This data was sourced from the electronic database of Sichuan Jinxin Xinan Women & Children’s Hospital. The metabolomics investigation focused on participants from the “CYART Cohort,” a study group established at Sichuan Jinxin Xinan Women and Children ’ s Hospital in southwest China [ 18 ]. From December 2021 to December 2022, serum samples from 145 participants were selected for metabolic analysis based on specific inclusion and exclusion criteria, which are outlined as follows:
Inclusion criteria: (1) Ovulation-Stimulating Regimen: GnRH antagonist regimen. (2) Embryo Transfer Cycle Specification: the first embryo transfer cycle. (3) Age between 20 and 35 years, (4) Body Mass Index (BMI) ≤ 28 kg/m². (5) Infertility factors in the CON group: Tubal factors. (6) The diagnosis of PCOS is based on the “Chinese Polycystic Ovary Syndrome Diagnosis and Treatment Guide,” established by the Endocrinology Group of the Gynecology and Obstetrics Branch of the Chinese Medical Association in 2018 [ 19 ]. These diagnostic criteria are derived from the Rotterdam criteria [ 20 ].
Exclusion criteria: (1) History of ovarian surgery, including procedures such as cyst dissection and oophorectomy. (2) Hormone therapy within three months before treatment. (3) Contraindications to ovulation induction therapy. (4) Other systemic abnormalities, including genetic, endocrine, infectious and autoimmune diseases.
Subsequently, these 145 participants were categorized into two main groups based on clinical diagnosis: The control group (CON, n = 80) comprised patients clinically diagnosed with tubal factor infertility during IVF. The PCOS group ( n = 65) consisted of patients clinically diagnosed with PCOS infertility during IVF. Within the CON group, we further subdivided them into two categories: The normal response group (CON-NOR, n = 40) comprised patients diagnosed with tubal infertility who exhibited a normal ovarian response during IVF (10–15 oocytes after COS). The high response group (CON-HOR, n = 40) comprised patients diagnosed with tubal infertility who exhibited a high ovarian response and were at risk for OHSS during IVF (> 15 oocytes after COS). Similarly, within the PCOS group, we subdivided them into two categories: The normal response group (PCOS-NOR, n = 26) comprised patients diagnosed with PCOS infertility who exhibited a normal ovarian response during IVF (10–15 oocytes after COS). The high response group (PCOS-HOR, n = 39) comprised patients diagnosed with PCOS infertility who exhibited a high ovarian response and were at risk for OHSS during IVF (> 15oocytes after COS). The risk criteria for OHSS were: the number of developing follicles > 20, peak Estradiol (E2) > 4000pg/mL, and Anti-Müllerian Hormone (AMH) levels > 4.5ng/mL.
GnRH antagonist regimen
Between the second and fourth days of the menstrual period, gonadotropin (Gn) medications (such as Urofollitropin, Menotropins, Gonal F, Puregon, Fostimon, Menopur, Kim Sai Heng) were administered at a dosage ranging from 100 to 300 IU/day, with close monitoring of follicle development. Dosage adjustments to the Gn were made as necessary. Upon reaching a dominant follicle diameter of ≥ 12–14 mm or on gonadotropin days 5–6, daily subcutaneous injections of 0.25 mg of gonadotropin-releasing hormone (GnRH) antagonists (ganirelix acetate) were initiated and continued until the day of ovulation trigger.
The reference standards for triggering ovulation can be determined based on the size and quantity of target follicles and the levels of E2, LH, and progesterone. Ovulation triggering is initiated when there are three dominant follicles with a diameter of ≥ 17 mm each or two dominant follicles with a diameter of ≥ 18 mm each, while also considering the progesterone and estradiol levels. Two options are available for the triggering process: recombinant hCG at 250 µg or triptorelin acetate at 0.2 mg can be utilized.
Serum collection
All serum samples were obtained on the day of the ovulation trigger. Fasting venous blood was carefully collected into tubes, followed by centrifugation at 3500 rpm for 10 min at 4 ℃. The isolated serum was then subpackaged into new EP tubes, rapidly frozen in liquid nitrogen, and stored in a -80 °C refrigerator for long-term preservation until further analysis.
Serum sample preparation and derivatization for GC-MS analysis
Thawing was conducted in an ice bath to ensure the quality of serum samples. A 150 µl aliquot of serum was then mixed with 50 µl 4 M NaOH, 4 µl internal standard (10 mM 2,3,3,3-D4-alanine, Sigma, USA), and 200 µl methanol. After a 15-minute incubation at room temperature, the mixture underwent centrifugation at 12,000 rpm for 15 min. Following centrifugation, 300 µl of the supernatant was carefully transferred to a new glass test tube, where 34 µl pyridine (Sigma, USA) was added and thoroughly mixed. Next, 20 µl methyl chloroformate (Sigma, USA) was added twice, with a vortex mix for 30 s after each addition. Subsequently, 400 µl of chloroform and 400 µl of NaHCO 3 solution were sequentially added, with a vortex mix for 10 s after each addition. To facilitate phase separation, the blended liquid was centrifuged at 2000 rpm for 10 min, and the upper water and intermediate protein layers were carefully discarded. Following this step, sodium sulfate, similar to mung beans, was added to the lowest chloroform layer for water absorption. Finally, 200 µl of the resulting liquid phase, containing derivatized metabolites, was transferred into the GS automatic sampler sample bottle for subsequent analysis.
2.5 Instrumentation and parameters for metabolomic profiling
This study utilized an Agilent Intuvo 9000 gas chromatograph coupled to an Agilent MSD5977B mass spectrometer detector employing electron-impact ionization(70 eV) to analyze derivatized metabolites. Metabolite separation was achieved using a BD-1701 gas phase capillary column (30 m × 0.25 mm × 0.25 μm film thickness, Agilent Technologies).
The GC inlet was configured in splitless mode, with the injector temperature set at 290 °C. Helium was the carrier gas at a flow rate of 1.0 ml/min. The GC oven temperature followed a gradient protocol, starting at 45 °C and increasing to 180 °C at a rate of 9.0 °C/min, then to 220 °C at 40.0 °C/min, followed by an increase to 240 °C at 40.0 °C/min, and finally reaching 280 °C at 80.0 °C/min. The temperature settings for the auxiliary, MS quadrupole, MS source, and guard chip were 250 °C, 230 °C, 150 °C, and 280 °C, respectively. Mass detection occurred within the range of 50 μm to 550 μm, with a scan speed of 1.562 µs after a solvent delay of 5.5 min.
Metabolite deconvolution was facilitated by the Automatic Mass Spectrometry Deconvolution & Identification system software. Metabolite identification relied on comparing MS fragmentation patterns, specifically the mass-to-charge ratio and relative strength of the mass spectrum, alongside respective GC retention times, referencing an in-house MS library constructed with chemical standards. Any remaining putative compounds were identified using a commercial NIST mass spectral library. Relative concentrations of metabolites were extracted using a MassOmics R-based script through the peak height of the most abundant fragment ion mass.
Absolute quantification of metabolite concentration
Each metabolite analyzed was paired with a corresponding chemical standard for quantification. Chemical compound standards, including typical amino acids, fatty acids, and glucose metabolites, were utilized for this purpose. A standard curve for these metabolites was established based on the peak height corresponding to their concentrations. Subsequently, the concentration of metabolites detected in serum samples was normalized by the internal standard and quantified according to the standard curve established previously.
Quality control
Quality Control (QC) samples were incorporated into this study to ensure data quality and mitigate batch-to-batch variations. Preparing QC samples involved mixing samples of the same volume to be tested and subjecting them to the same pre-treatment method as the samples under investigation. Subsequently, the processed QC samples were introduced into the GC-MS system alongside the test samples for detection and analysis. The operation followed the protocol of inserting a processed 20 µl QC sample after every 15 test samples. Each metabolite’s relative standard deviation (RSD) was computed during the data processing stage. Metabolites with an RSD > 30% were excluded from further data analysis.
Data processing and statistical analysis
The Human Metabolome Database (HMDB) and PubChem were referenced to retrieve and further identify the detected metabolites in this study. Subsequently, all metabolite concentration values underwent normalization through Metaboanalyst 5.0 ( www.metaboanalyst.ca ). The differential metabolites were identified through a combined approach utilizing the False Discovery Rate (FDR) from the t-test and the Variable Importance in Projection (VIP) value based on the Orthogonal Partial Least Squares Discriminant Analysis (OPLS-DA) model. The threshold criteria were VIP > 1.0, P value < 0.05, and FDR < 0.2. The Kyoto Encyclopedia of Genes and Genomes (KEGG) analysis was conducted to elucidate the potential pathways involved. Binary logistic regression models, adjusted for age, BMI, and AMH as covariates, were utilized to estimate odds ratios (ORs) and 95% confidence intervals (CIs) for the relationship between differential metabolites and the risk of HOR. Metabolites with significant associations ( P value < 0.05) were identified as related to HOR risk within the defined groups. Spearman correlation analysis examined the associations between metabolites and clinical characteristics. Correlations with coefficients (R2) ≥ 0.2 or R2 ≤ -0.2 and P value < 0.05 were deemed statistically significant. All statistical analyses and visualizations were executed using the R software.
Clinical characteristics of participants
In this study, 145 infertile patients were categorized into two main groups: 80 in the CON group and 65 in the PCOS group. Within the CON group, there were two subgroups: CON-NOR ( n = 40) and CON-HOR( n = 40). Similarly, within the PCOS group, there were two subgroups: PCOS-NOR ( n = 26) and PCOS-HOR ( n = 39).
The clinical characteristics are depicted in Fig. 1 A and Supplementary Table 1 . Notably, within the CON group, the CON-HOR subgroup displayed lower basal serum FSH levels than the CON-NOR subgroup. However, no such difference was observed between the two PCOS subgroups. Furthermore, no significant differences were found between NOR and HOR patients in both CON and PCOS groups regarding other characteristics such as age, BMI, basal serum E2 levels and basal P levels. Additionally, HOR patients in both groups demonstrated significantly higher levels of various characteristics, including E2 level on hCG day, the number of follicles ≥ 14 mm and ≥ 17 mm in diameter on hCG day, as well as the number of follicles, oocytes retrieved, mature oocytes, and MII oocytes compared to the NOR patients.
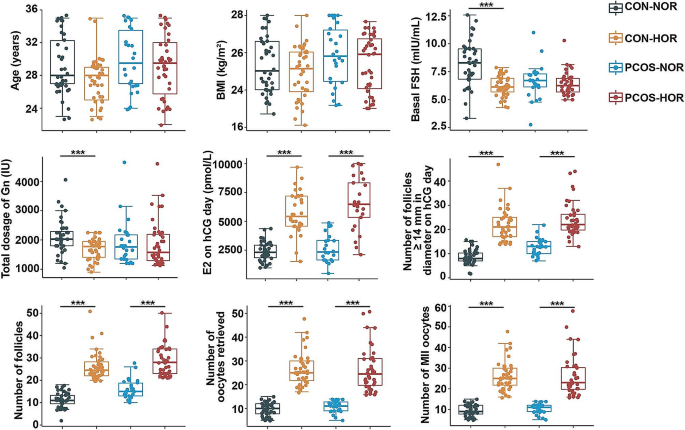
Comparative Analysis of Clinical Characteristics
Comparative analysis of clinical characteristics among different subgroups was conducted using the Wilcoxon rank-sum test. The legend indicates key abbreviations: CON (Tubal infertility), PCOS (Polycystic Ovarian Syndrome), NOR (Normal Ovarian Response), HOR (High Ovarian Response and at risk for OHSS), BMI (Body Mass Index), FSH (Follicle-Stimulating Hormone), E2 (Estradiol), Gn (Gonadotropin), and hCG (Human Chorionic Gonadotropin). Statistical significance was set at P < 0.05 (* P < 0.05; ** P < 0.01; *** P < 0.001)
Metabolic landscape within subgroups
A total of 125 different metabolites were identified in this study. Principal Component Analysis (PCA) initially indicated no significant difference in metabolic profiles between NOR and HOR patients in CON and PCOS groups (data not presented). To further verify the differences between NOR and HOR patients, we proceeded with OPLS-DA analysis to maximize the differences between subgroups in the model. The results demonstrated a significant divergence between NOR and HOR patients within the CON and PCOS groups (Supplementary Fig. 2 ).
In the CON group, four metabolites exhibited significant differences between the CON-NOR and CON-HOR subgroups ( P < 0.05, FDR < 0.2, VIP > 1): 10-pentadecenoic acid, glycine oxidized, glutathione, and N-acetyl-L-leucine (Fig. 2 A and Supplementary Table 2 ). Similarly, in the PCOS group, 14 metabolites serum metabolites displayed significant variances between the PCOS-NOR and PCOS-HOR subgroups ( P < 0.05, FDR < 0.2, VIP > 1). Among these metabolites, eight were amino acid metabolites, including glycine, N-acetyl-L-leucine, sarcosine, tyrosine, beta-alanine, ornithine, alanine, and valine, with lower levels observed in the PCOS-HOR subgroup (Fig. 2 and Supplementary Table 3 ). Furthermore, oxidized glutathione, another amino acid metabolite, was elevated in the PCOS-HOR subgroup. Additionally, tricarboxylic acid cycle (TCA) metabolites, such as succinic acid and fumaric acid, showed significantly lower levels in the PCOS-HOR subgroup compared to the PCOS-NOR subgroup. Similarly, fatty acid metabolites such as (10E,12Z)-octadecadienoic acid (C18_2n-10) and tryptamine metabolites like 5-methoxytryptamine were notably elevated in the PCOS- HOR subgroup compared to the PCOS-NOR subgroup.
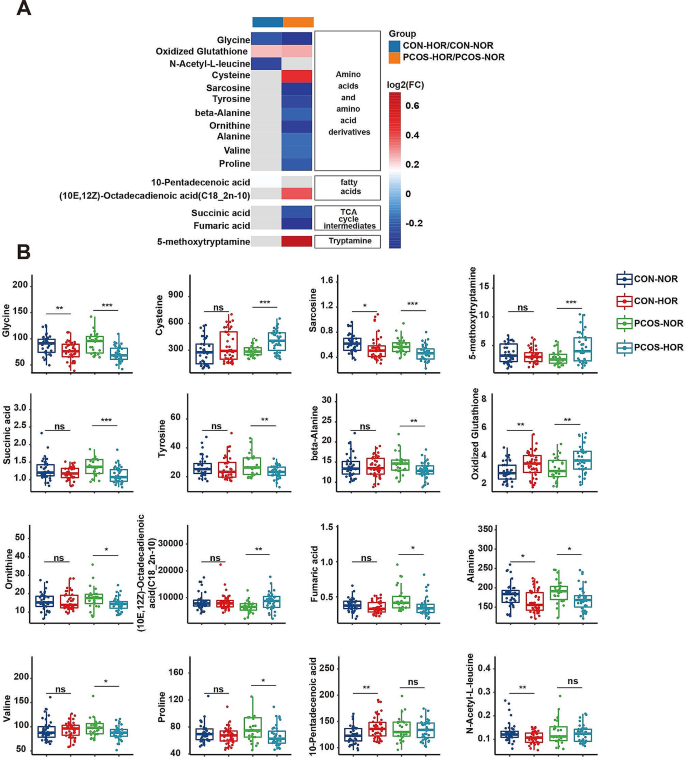
Differential metabolic profiles in serum of HOR patients
( A ) Heatmap illustrating the detected differential metabolites across each group, indicating the ratio of metabolite levels in subgroup comparisons. The color gradient reflects concentration disparities, with red indicating higher concentrations in the HOR group compared to the NOR group and blue representing lower concentrations. ( B ) The box and scatter plots display the concentrations of differential metabolites across various subgroups. Statistical significance was set at P < 0.05 (* P < 0.05; ** P < 0.01; *** P < 0.001), while ‘ns’ denotes non-significance
Interestingly, glycine and oxidized glutathione emerged as differentiated metabolites between NOR and HOR patients in both the CON and PCOS groups. Specifically, glycine levels consistently trended lower in HOR patients compared to NOR patients across both groups, while oxidized glutathione levels consistently trended higher in HOR patients (Fig. 2 ).
KEGG enrichment analysis of key metabolic signatures in all participants
We conducted a KEGG enrichment analysis on the identified differential metabolites. In the CON group, where only four metabolites displayed significant differences between the CON-NOR and CON-HOR subgroups, the decision was made to discontinue the KEGG analysis for this group. However, for the PCOS-NOR and PCOS-HOR subgroups, encompassing 14 differential metabolites, KEGG analysis revealed significant enrichment across several pivotal pathways, namely aminoacyl-tRNA biosynthesis, glutathione metabolism, and pantothenate and CoA biosynthesis (Fig. 3 ). These pathways are crucial for maintaining essential cellular processes and physiological functions. Specifically, aminoacyl-tRNA biosynthesis is vital for protein synthesis [ 21 ], glutathione metabolism plays a key role in cellular defense against oxidative stress [ 22 ], and pantothenate and CoA biosynthesis is critical for energy metabolism and the TCA cycle [ 23 ].
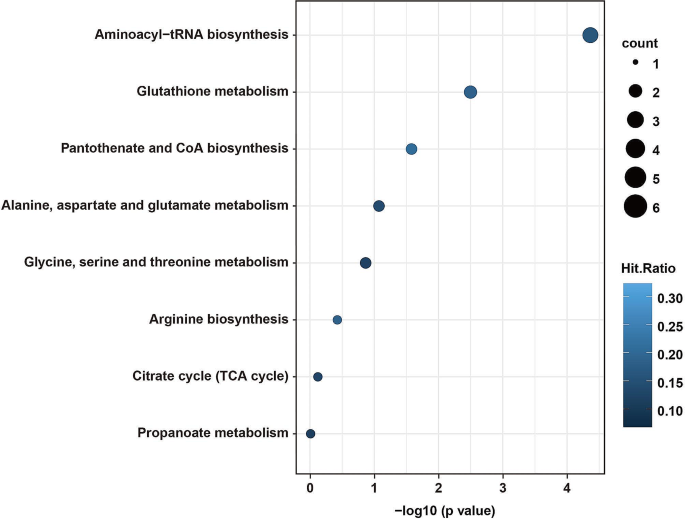
KEGG pathway enrichment analysis of differential metabolites in PCOS subgroup
KEGG pathway enrichment analysis of differential metabolites identified in the comparison between the PCOS-NOR and PCOS-HOR subgroups. The vertical axis represents distinct metabolic pathways, while the horizontal axis indicates the Holm-adjusted P value. The circle size reflects the number of Hits, and the color indicates Hits.Ratio
Identification of HOR Risk-Associated metabolites
After thorough adjustments for age, BMI, and AMH using binary logistic regression, a specific differential metabolite emerged as significantly associated with CON-HOR risk in the CON group. Specifically, 10-pentadecenoic acid demonstrated an increased risk of CON-HOR by 1.16-fold (95% CI 1.02–1.31; P =0.020) with each standard deviation (SD) increment (Fig. 4 and Supplemental Table 4 ).
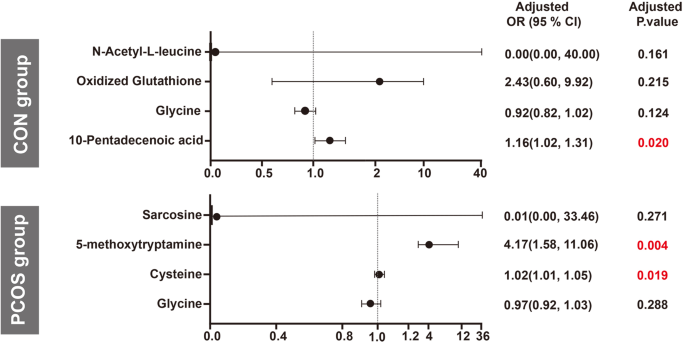
Associations between differential metabolites for HOR: adjusted OR (95% CIs) from binary logistic regression analysis
aOR: adjusted odds ratio; CI: confidence interval
In the PCOS group, we observed 14 differential metabolites between the PCOS-NOR and PCOS-HOR subgroups. Due to the limitations in sample size, we prioritized metabolites for further analysis based on the VIP scores. Specifically, we focused on the top four metabolites ( P < 0.001, FDR < 0.1) for subsequent binary logistic regression analysis. Notably, after adjustments for age, BMI, and AMH, our findings revealed that the risk of PCOS-HOR escalated by 1.02 (95% CI 1.01–1.05; P =0.019) with each SD increase in cysteine levels and 4.17 (95% CI 1.58–11.16; P =0.004) with each SD increase in 5-methoxytryptamine levels (Fig. 4 and Supplemental Table 5 ).
Correlations of Differential Metabolite with Clinical Characteristics in NOR and HOR Subgroups of CON and PCOS
Four differential metabolites differed between the CON-NOR and CON-HOR subgroups in the CON group. These four metabolites showed significant correlations with the number of oocytes retrieved and MII oocytes. However, none correlated with the total dose of Gn (Fig. 5 and Supplemental Tables 6 – 7 ).
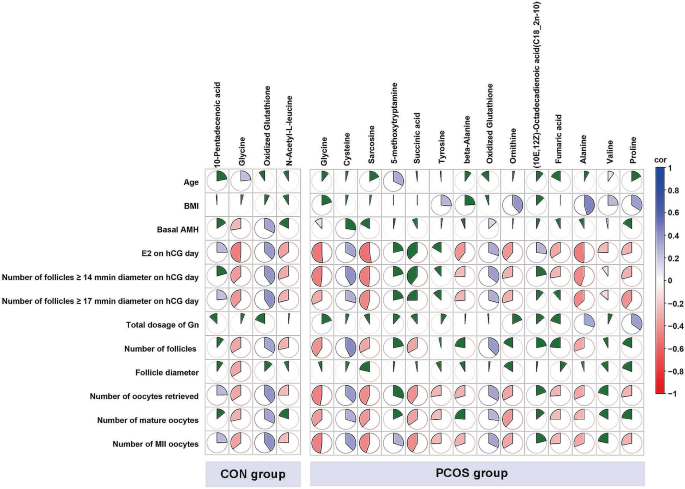
Spearman correlation analysis between differential metabolites and clinical characteristics
Positive correlations are denoted in blue, while negative correlations are depicted in red. The size of each sector corresponds to the correlation coefficient. The green-filled sectors represent correlations lacking statistical significance
Within the PCOS group, 14 metabolites differed significantly between the PCOS-NOR and PCOS-HOR subgroups. Among these, excluding (10E,12Z)-octadecadienoic acid (C18_2n-10) and valine, the remaining 12 metabolites demonstrated notable correlations with the number of oocytes retrieved and MII oocytes. Noteworthy is that only alanine and proline displayed significant correlations with the total dosage of Gn, while the others showed no such correlation (Fig. 5 and Supplemental Tables 8 – 9 ).
Interestingly, glycine and oxidized glutathione emerged as differential metabolites between NOR and HOR patients in both the CON and PCOS groups. In both groups, glycin displayed significant negative correlations with basal AMH levels, E2 levels on hCG day, the number of follicles ≥ 14 mm and ≥ 17 mm in diameter on hCG Day, as well as the number of oocytes retrieved, mature oocytes, and MII oocytes. Conversely, oxidized glutathione demonstrated significant positive correlations with these clinical characteristics (Fig. 5 and Supplemental Tables 6 – 9 ).
3.6. Unveiling Shared metabolic signatures: insights into PCOS and HOR Pathogenesis
To investigate potential similarities in metabolic profiles between PCOS and HOR, we analyzed metabolite changes within the PCOS group. Our findings revealed 29 metabolites identified as differential between the PCOS and CON-NOR groups ( P < 0.05, FDR < 0.2, VIP > 1, Supplementary Table 10 ). Notably, three of these metabolites—10-pentadecenoic acid, glycine, and oxidized glutathione—were also identified as differentially expressed between the CON-NOR and CON-HOR groups (Supplemental Fig. 3A ). Further analysis demonstrated that these three metabolites exhibited significant upregulation in both the PCOS and CON-HOR groups relative to the CON-NOR group (Fig. 6 ). This consistent alteration suggests a parallel in metabolic profiles between PCOS and HOR, hinting at a potential shared etiological similarity between the two conditions.
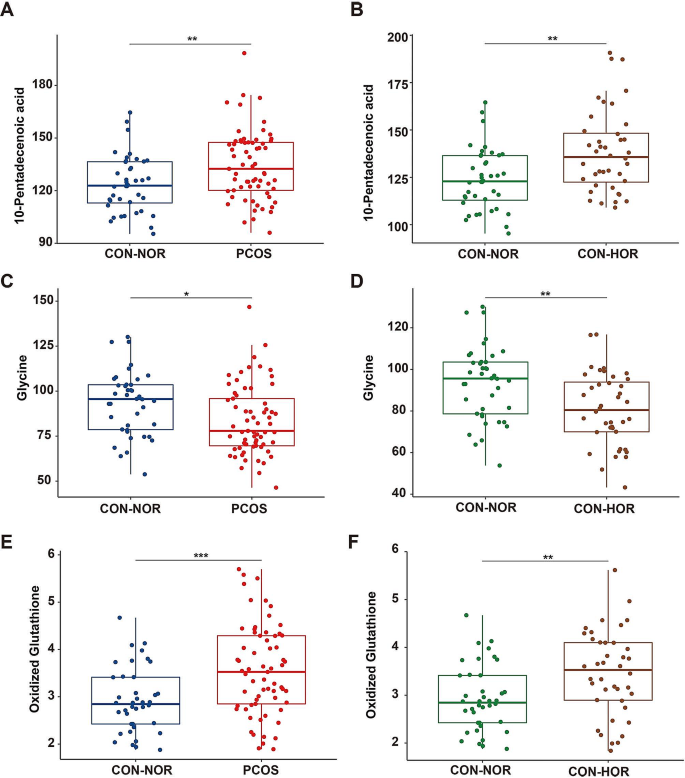
Comparative metabolite profiling in PCOS and CON subgroups
The box plot and scatter plot illustrate the concentrations of differential metabolite between PCOS and CON subgroups. Panels ( A , C , E ) display the metabolite concentrations between the CON-NOR and PCOS groups, while panels ( B , D , F ) show the metabolite concentrations between the CON-NOR and CON-HOR subgroups
Furthermore, KEGG pathway analysis of these metabolites revealed significant enrichment in aminoacyl-tRNA biosynthesis and glutathione metabolism pathways (Supplementary Fig. 3B ). These findings support earlier KEGG pathway analysis of differential metabolites in PCOS subgroups, indicating a close link between these pathways and the development of PCOS and PCOS-HOR.
In this study, we utilized GC-MS to investigate the serum metabolic profiles of patients with normal ovarian response and those exhibiting high ovarian response and at risk for OHSS in tubal and PCOS infertility. Our analysis revealed the identification of 4 and 14 differential metabolites between these two ovarian response patient subsets in each infertility background, respectively. Additionally, we observed a certain degree of similarity in the metabolic profiles between PCOS patients and those exhibiting high ovarian response and at risk for OHSS.
Previous studies have highlighted significant differences in amino acid and lipid metabolites within the follicular fluid of OHSS patients compared to control groups [ 13 , 17 , 24 ]. Similarly, multiple lipid components in the serum or follicular fluid of PCOS patients exhibit alterations [ 25 , 26 ], particularly noticeable in obese individuals with PCOS, where lipid abnormalities are most pronounced [ 27 ]. In line with these findings, our study also revealed abnormal elevations in several lipid metabolites, including myristic acid (C14_0), 10-pentadecenoic acid, lignoceric acid (C24_0), nervonic acid (C24_1n-9c), and hexanoic acid (C6_0), within the serum of PCOS group when compared to CON-NOR group.
Furthermore, some researchers explored the metabolic profiles of populations exhibiting different ovarian responses. Mu et al. [ 28 ] discovered that elevated glycine levels are associated with a heightened response to COS. Interestingly, our study contradicts this finding, as we observed lower glycine levels in the HOR patients across both infertility backgrounds. Additionally, glycine also showed negative correlations with several clinical characteristics, including levels of AMH, the number of oocytes retrieved, mature oocytes, and MII oocytes. The propensity for ovarian hyperstimulation significantly escalates in PCOS patients following COS stimulation [ 15 , 29 ]. Several metabolomic studies on PCOS patients have consistently documented significantly reduced glycine levels compared to controls [ 30 , 31 , 32 ], which aligns with our results of lower glycine levels in the PCOS group compared to the CON-NOR group. Furthermore, research has indicated heightened glycine expression in women with diminished ovarian reserve (DOR) [ 33 ], which is correlated with a poor response to ovarian stimulation [ 34 ]. However, it is noteworthy that a research has found a positive correlation between glycine and one marker of ovarian reserve, the antral follicle count (AFC) [ 35 ]. Considering these diverse research findings, the role of glycine in ovarian response remains unclear. Future studies should incorporate larger sample sizes from various regions and employ more rigorous experimental designs to elucidate the relationship between glycine and ovarian response.
Hood et al. [ 14 ] discovered that most fatty acids and amino acids in the serum metabolome correlate with the number of mature oocytes. Our study echoes these findings, as most differential metabolites between NOR and HOR patients are within the amino acid class. Moreover, these differential metabolites, including alanine, cysteine, glycine, ornithine, glutathione oxide, sarcosine, and tyrosine, demonstrate significant associations with the number of mature oocytes. This alignment underscores the importance of amino acid metabolism in modulating ovarian response and highlights its potential as a target for interventions to optimize oocyte yield.
Furthermore, our study revealed a significant enrichment of differential metabolites in the aminoacyl-tRNA biosynthesis pathway, both between PCOS-NOR and PCOS-HOR groups, and between PCOS and CON-NOR groups. This finding aligns with the investigation by Li et al., who focused on metabolic profiles in patients with DOR [ 36 ]. In their study, researchers noted a similar trend, wherein the metabolites distinguishing the DOR patients from patients with normal ovarian reserve were prominently associated with the aminoacyl-tRNA biosynthesis pathway [ 36 ]. The aminoacyl-tRNA biosynthesis pathway plays a pivotal role in cellular processes by coupling amino acids with their corresponding tRNA molecules, forming aminoacyl-tRNA complexes crucial for protein synthesis [ 21 , 37 ]. This fundamental process ensures the precise integration of amino acids into nascent polypeptide chains, thereby dictating proteins’ ultimate structure and function. These findings imply a potential link between the aminoacyl-tRNA biosynthesis pathway and reproductive disorders like PCOS and DOR. Nevertheless, further investigation is required to ascertain whether this pathway influences the onset of these conditions through its impact on protein synthesis.
In summary, this study identified the serum metabolic profiles of patients exhibiting high ovarian response and at risk for OHSS. However, several limitations should be acknowledged. Firstly, the relatively small overall sample size and the sole reliance on samples from a single medical institution limit the generalizability of our findings. Furthermore, this constraint impedes randomization and stratification of the sample, necessitating caution in interpreting our results. Secondly, to mitigate potential confounding effects, we applied stringent inclusion criteria for participant recruitment, restricting the age range to 20–35 years and setting a BMI threshold of ≤ 28 kg/m² for both groups. Hence, our study did not include older individuals or those with a higher BMI, and therefore, our findings may not fully represent the metabolic profile of the entire PCOS patients. Thirdly, our results offer an overview of metabolic aspects in PCOS patients but lack the detailed information required to identify different phenotypes within the patients. As a result, we are unable to characterize the unique metabolic profiles associated with these phenotypes.
Conclusions
Utilizing GC-MS technology, we described the metabolic profiles of specific patients who exhibit high ovarian response and are at risk for OHSS within both the tubal infertility group and the PCOS infertility group. The discovery of risk metabolites in these unique patients provides clues to studying their pathological mechanisms. Further, similarities in metabolic profiles between patients with PCOS and high ovarian response suggest a potential common underlying cause, urging more research into their connection.
Data Availability
To access the data supporting the findings of this study, kindly contact the corresponding author. They will be pleased to provide the necessary information upon request.
Abbreviations
Ovarian hyperstimulation syndrome
Tubal factor infertility
- Polycystic ovary syndrome
Normal ovarian response
High ovarian response and at risk for OHSS
Gas chromatography-mass spectrometry
In vitro fertilization
Kyoto Encyclopedia of Genes and Genomes
Organization WH. Infertility Prevalence Estimates: 1990–2021. 2023.
Jain M, Singh M. Assisted Reproductive Technology (ART) Techniques. StatPearls . Treasure Island (FL) ineligible companies. Disclosure: Manvinder Singh declares no relevant financial relationships with ineligible companies.2023.
Timmons D, Montrief T, Koyfman A, Long B. Ovarian hyperstimulation syndrome: a review for emergency clinicians. Am J Emerg Med. 2019;37(8):1577–84.
Article PubMed Google Scholar
Palomba S, Costanzi F, Nelson SM, Caserta D, Humaidan P. Interventions to prevent or reduce the incidence and severity of ovarian hyperstimulation syndrome: a systematic umbrella review of the best clinical evidence. Reprod Biol Endocrinol. 2023;21(1):67.
Article CAS PubMed PubMed Central Google Scholar
Palomba S, Costanzi F, Nelson SM, Besharat A, Caserta D, Humaidan P. Beyond the Umbrella: a systematic review of the interventions for the Prevention of and reduction in the incidence and severity of ovarian hyperstimulation syndrome in patients who Undergo in Vitro fertilization treatments. Int J Mol Sci. 2023;24:18.
Article Google Scholar
Schirmer DA 3rd, Kulkarni AD, Zhang Y, Kawwass JF, Boulet SL, Kissin DM. Ovarian hyperstimulation syndrome after assisted reproductive technologies: trends, predictors, and pregnancy outcomes. Fertil Steril. 2020;114(3):567–78.
Article PubMed PubMed Central Google Scholar
Lainas GT, Lainas TG, Sfontouris IA, et al. A decision-making algorithm for performing or cancelling embryo transfer in patients at high risk for ovarian hyperstimulation syndrome after triggering final oocyte maturation with hCG. Hum Reprod Open. 2020;2020(3):hoaa013.
Wishart DS. Metabolomics for investigating physiological and pathophysiological processes. Physiol Rev. 2019;99(4):1819–75.
Article CAS PubMed Google Scholar
Wu Y, Yang L, Wu X, et al. Identification of the hub genes in polycystic ovary syndrome based on disease-associated molecule network. FASEB J. 2023;37(7):e23056.
Norman BP, Davison AS, Hughes JH, et al. Metabolomic studies in the inborn error of metabolism alkaptonuria reveal new biotransformations in tyrosine metabolism. Genes Dis. 2022;9(4):1129–42.
Song H, Qin Q, Yuan C, Li H, Zhang F, Fan L. Metabolomic profiling of poor ovarian response identifies potential predictive biomarkers. Front Endocrinol (Lausanne). 2021;12:774667.
Ding X, Deng Y, Wang Y, et al. Serum metabolomic profiling reveals potential biomarkers in assessing the management of women with polycystic ovary syndrome: a randomized controlled trial. Chin Med J (Engl). 2022;135(1):79–85.
Wu Z, Fang L, Liu B, Jia Q, Cheng JC, Sun YP. Biomarkers identification in follicular fluid of women with OHSS by using UPLC-MS method. Front Endocrinol (Lausanne). 2023;14:1131771.
Hood RB, Liang D, Tan Y, et al. Serum and follicular fluid metabolome and markers of ovarian stimulation. Hum Reprod. 2023;38(11):2196–207.
Palomba S. Is fertility reduced in ovulatory women with polycystic ovary syndrome? An opinion paper. Hum Reprod. 2021;36(9):2421–8.
Palomba S. The progression of intensity and complexity of treatment as a cornerstone of the management of polycystic ovary syndrome-related infertility. Fertil Steril. 2024;121(2):252–3.
Sun Y, Hao L, Han W, et al. Intrafollicular fluid metabolic abnormalities in relation to ovarian hyperstimulation syndrome: follicular fluid metabolomics via gas chromatography-mass spectrometry. Clin Chim Acta. 2023;538:189–202.
Li XF, Zhang YJ, Yao YL et al. The association of post-embryo transfer SARS-CoV-2 infection with early pregnancy outcomes in in vitro fertilization: a prospective cohort study. Am J Obstet Gynecol 2023.
Endocrinology S, Expert Panel CSoO, Gyneocology CMA. [Chinese guideline for diagnosis and management of polycystic ovary syndrome]. Zhonghua Fu Chan Ke Za Zhi. 2018;53(1):2–6.
Google Scholar
Legro RS, Arslanian SA, Ehrmann DA, et al. Diagnosis and treatment of polycystic ovary syndrome: an endocrine Society clinical practice guideline. J Clin Endocrinol Metab. 2013;98(12):4565–92.
Ibba M, Soll D. Aminoacyl-tRNA synthesis. Annu Rev Biochem. 2000;69:617–50.
Diaz-Vivancos P, de Simone A, Kiddle G, Foyer CH. Glutathione–linking cell proliferation to oxidative stress. Free Radic Biol Med. 2015;89:1154–64.
Ma T, Liu T, Xie P, et al. UPLC-MS-based urine nontargeted metabolic profiling identifies dysregulation of pantothenate and CoA biosynthesis pathway in diabetic kidney disease. Life Sci. 2020;258:118160.
Gao Y, Li J, Fan S, Chen P, Huang M, Bi H. Lipid Analysis of Follicular Fluids by UHPLC-ESI-HRMS discovers potential biomarkers for ovarian hyperstimulation syndrome. Front Endocrinol (Lausanne). 2022;13:895116.
Palomba S, Daolio J, La Sala GB. Oocyte competence in women with polycystic ovary syndrome. Trends Endocrinol Metab. 2017;28(3):186–98.
Guan SY, Liu YY, Guo Y, Shen XX, Liu Y, Jin HX. Potential biomarkers for clinical outcomes of IVF cycles in women with/without PCOS: searching with metabolomics. Front Endocrinol (Lausanne). 2022;13:982200.
Ozegowska K, Plewa S, Mantaj U, Pawelczyk L, Matysiak J. Serum metabolomics in PCOS Women with different body Mass Index. J Clin Med. 2021;10:13.
Mu X, Pei ML, Zhu F, Shi JZ, Liu P. Serum metabolomic signature predicts ovarian response to controlled stimulation. Horm Metab Res. 2022;54(9):625–32.
Fischer D, Reisenbuchler C, Rosner S, Haussmann J, Wimberger P, Goeckenjan M, Avoiding OHSS. Controlled ovarian low-dose stimulation in women with PCOS. Geburtshilfe Frauenheilkd. 2016;76(6):718–26.
Zhao Y, Fu L, Li R, et al. Metabolic profiles characterizing different phenotypes of polycystic ovary syndrome: plasma metabolomics analysis. Bmc Med. 2012;10:153.
Ye Z, Zhang C, Wang S, et al. Amino acid signatures in relation to polycystic ovary syndrome and increased risk of different metabolic disturbances. Reprod Biomed Online. 2022;44(4):737–46.
Unni SN, Lakshman LR, Vaidyanathan K, Subhakumari KN, Menon NL. Alterations in the levels of plasma amino acids in polycystic ovary syndrome–A pilot study. Indian J Med Res. 2015;142(5):549–54.
Lu X, Lv X, Dong X, et al. Increased serine synthesis in cumulus cells of young infertile women with diminished ovarian reserve. Hum Reprod. 2023;38(9):1723–32.
Hu Q, Hong L, Nie M, et al. The effect of dehydroepiandrosterone supplementation on ovarian response is associated with androgen receptor in diminished ovarian reserve women. J Ovarian Res. 2017;10(1):32.
Al Rashid K, Taylor A, Lumsden MA, Goulding N, Lawlor DA, Nelson SM. Association of the functional ovarian reserve with serum metabolomic profiling by nuclear magnetic resonance spectroscopy: a cross-sectional study of ~ 400 women. Bmc Med. 2020;18(1):247.
Li J, Zhang Z, Wei Y, Zhu P, Yin T, Wan Q. Metabonomic analysis of follicular fluid in patients with diminished ovarian reserve. Front Endocrinol (Lausanne). 2023;14:1132621.
Ibba M, Soll D. The renaissance of aminoacyl-tRNA synthesis. EMBO Rep. 2001;2(5):382–7.
Download references
Acknowledgements
We sincerely thank Dr Ting-Li Han and Miss Yang Yang from the Department of Obstetrics at the First Affiliated Hospital of Chongqing Medical University for their invaluable guidance and assistance in establishing the metabolomics methods and analyzing data. Their expertise and support were instrumental in making this work possible. Additionally, we would like to thank all the participants of this study and the medical staff for their valuable contributions.
This work received partial support from the National Key Research and Development Program of China (no. 2023YFC2705900), the National Natural Science Foundation of China (nos. 82171664, 81971391, 82171668), and the Natural Science Foundation of Chongqing Municipality of China (nos. CSTB2022NSCQ-LZX0062, CSTB2023TIAD-KPX0052).
Author information
Authors and affiliations.
Department of Obstetrics and Gynecology, Women and Children’s Hospital of Chongqing Medical University, No. 23 Central Park North Road, Yubei District, Chongqing, 401147, PR China
Ling-Ling Ruan & Yu-Bin Ding
Joint International Research Laboratory of Reproduction and Development of the Ministry of Education of China, School of Public Health, Chongqing Medical University, Chongqing, 400016, China
Ling-Ling Ruan, Ming-Xing Chen, Zhao-Hui Zhong, Li-Juan Fu & Yu-Bin Ding
Department of Obstetrics and Gynecology, the People’s Hospital of Yubei District, No. 23 Central Park North Road, Chongqing, 401120, China
Shao-Min Yu
Department of Obstetrics and Gynecology, West China Second Hospital, Sichuan University, Chengdu, 610041, China
Key Laboratory of Birth Defects and Related Diseases of Women and Children (Sichuan University), Ministry of Education, Chengdu, 610041, China
The Reproductive Center, Sichuan Jinxin Xinan Women & Children’s Hospital, Chengdu, Sichuan, 610011, China
Xing-Yu Lv, Yu-Lin Hu & Qi Wan
Department of Pharmacology, Academician Workstation, Changsha Medical University, Changsha, 410219, China
Mei-Hua Bao & Li-Juan Fu
Department of Obstetrics and Gynecology, the First Affiliated Hospital of Chongqing Medical University, Chongqing, 400016, China
Department of Bioinformatics, School of Basic Medicine, Chongqing Medical University, Chongqing, 400016, People’s Republic of China
You can also search for this author in PubMed Google Scholar
Contributions
Y.-B. D., QW and S.-M. Y.: Conceptualization and Design. L.-L. R., X.-Y. L., Y.-L. H., and M.-X. C.: Data Acquisition, Analysis, and Interpretation. L.-L. R.: Manuscript Writing. JT, Z.-H. Z., M.-H. B., and L.-J. F.: m. L.-L. R., and XL: Statistical Analysis. Y.-B. D. and XL: Funding acquisition.
Corresponding authors
Correspondence to Shao-Min Yu , Qi Wan or Yu-Bin Ding .
Ethics declarations
Ethics approval and consent to participate.
This study received ethical approval from Sichuan Jinxin Xinan Women & Children’s Hospital (No. 2021014) and the Ethics Committee of Chongqing Medical University (No. 2021060). Informed consent was obtained from all participants, adhering to the principles of the Declaration of Helsinki.
Consent for publication
Not Applicable.
Conflict of interest
The authors have no conflict of interest to declare.
Competing interests
The authors declare no known competing financial interests or personal relationships that could influence the work reported in this article.
Additional information
Publisher’s note.
Springer Nature remains neutral with regard to jurisdictional claims in published maps and institutional affiliations.
Electronic supplementary material
Below is the link to the electronic supplementary material.
Supplementary Material 1
Rights and permissions.
Open Access This article is licensed under a Creative Commons Attribution 4.0 International License, which permits use, sharing, adaptation, distribution and reproduction in any medium or format, as long as you give appropriate credit to the original author(s) and the source, provide a link to the Creative Commons licence, and indicate if changes were made. The images or other third party material in this article are included in the article’s Creative Commons licence, unless indicated otherwise in a credit line to the material. If material is not included in the article’s Creative Commons licence and your intended use is not permitted by statutory regulation or exceeds the permitted use, you will need to obtain permission directly from the copyright holder. To view a copy of this licence, visit http://creativecommons.org/licenses/by/4.0/ . The Creative Commons Public Domain Dedication waiver ( http://creativecommons.org/publicdomain/zero/1.0/ ) applies to the data made available in this article, unless otherwise stated in a credit line to the data.
Reprints and permissions
About this article
Cite this article.
Ruan, LL., Lv, XY., Hu, YL. et al. Metabolic landscape and pathogenic insights: a comprehensive analysis of high ovarian response in infertile women undergoing in vitro fertilization. J Ovarian Res 17 , 105 (2024). https://doi.org/10.1186/s13048-024-01411-6
Download citation
Received : 11 January 2024
Accepted : 10 April 2024
Published : 17 May 2024
DOI : https://doi.org/10.1186/s13048-024-01411-6
Share this article
Anyone you share the following link with will be able to read this content:
Sorry, a shareable link is not currently available for this article.
Provided by the Springer Nature SharedIt content-sharing initiative
- High ovarian response
Journal of Ovarian Research
ISSN: 1757-2215
- General enquiries: [email protected]
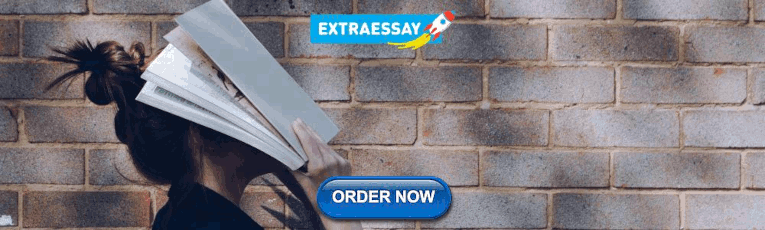
COMMENTS
Helicobacter pylori colonizes over 50% of people worldwide. Biofilm formation through penetrating gastric mucus and resistance acquired by H. pylori markedly reduces the efficacy of traditional antibiotics. The present triple therapy and bismuth-based quadruple therapy inevitably causes intestinal flora disturbance and fails to address the ...
Literature review as a research methodology: An overview and guidelines. Journal of Business Research, Volume 104. Prácticas sexuales de chicos y chicas españoles de 14-24 años de edad. Gaceta Sanitaria, Volume 22, Issue 6. Understanding the role of digital technologies in education: A review. Sustainable Operations and Computers, Volume 3
First published in 1869, Nature is the world's leading multidisciplinary science journal. Nature publishes the finest peer-reviewed research that drives ground-breaking discovery, and is read by ...
Theory and algorithms for application domains. Machine learning theory. Markov decision processes. Reinforcement learning. Sequential decision making. Database theory. Database query processing and optimization (theory) Randomness, geometry and discrete structures. Computational geometry.
Science is a leading outlet for scientific news, commentary, and cutting-edge research. Through its print and online incarnations, Science reaches an estimated worldwide readership of more than one million. Science 's authorship is global too, and its articles consistently rank among the world's most cited research. mission & scope.
Scientific Reports has a 2-year impact factor of 4.6 (2022), and is the 5th most-cited journal in the world, with more than 738,000 citations in 2022*. *2023 Journal Citation Reports® Science ...
14 May 2024 By . Katie Langin; LGBTQ Ph.D. graduates will soon be counted in key U.S. survey. 9 May 2024 By . ... The strength of Science and its online journal sites rests with the strengths of its community of authors, who provide cutting-edge research, incisive scientific commentary, and insights on what's important to the scientific world ...
All journal articles featured in Journal of Research in Childhood Education vol 14 issue 1
PubMed is a comprehensive database of biomedical literature from various sources, including MEDLINE, life science journals, and online books. You can search for citations, access full text content, and explore topics related to health, medicine, and biology. PubMed also provides advanced search options and tools for researchers and clinicians.
Sage empowers researchers, librarians and readers through: Gold and Green Open Access publishing options. Open access agreements. Author support and information. LEARN MORE. Explore the content of our microsites focusing on various topics from across all Sage journals. Subscription and open access journals from Sage, the world's leading ...
Elsevier Journal Finder helps you find journals that could be best suited for publishing your scientific article. Journal Finder uses smart search technology and field-of-research specific vocabularies to match your paper's abstract to scientific journals.
Harness the power of visual materials—explore more than 3 million images now on JSTOR. Enhance your scholarly research with underground newspapers, magazines, and journals. Explore collections in the arts, sciences, and literature from the world's leading museums, archives, and scholars. JSTOR is a digital library of academic journals ...
A total of 21.4% of patients in the andexanet group and 21.1% of patients in the usual-care group had a history of stroke, 10.7% and 14.5%, respectively, had a history of myocardial infarction, 7. ...
The journal publishes cutting-edge research articles and short reports, spanning the entire spectrum of the science of psychology. This journal is the source for the latest findings in cognitive, social, developmental, and health psychology, as well as behavioral neuroscience and biopsychology. ... First published May 14, 2024.
Creativity Research Journal, Volume 14, Issue 3-4 (2002) See all volumes and issues. Vol 36, 2024 Vol 35, 2023 Vol 34, 2022 Vol 33, 2021 Vol 32, 2020 Vol 31, 2019 Vol 30, 2018 Vol 29, 2017 Vol 28, 2016 Vol 27, 2015 Vol 26, 2014 Vol 25, 2013 Vol 24, 2012 Vol 23, 2011 Vol 22, 2010 Vol 21, 2009 Vol 20, 2008 Vol 19, 2007 Vol 18, 2006 Vol 17, 2005 ...
Genome Research is an international, continuously published, peer-reviewed journal that features outstanding original research providing novel insights into the genome biology of all organisms, including significant advances in genomic medicine. The journal also provides high-quality reviews and perspectives written by respected leaders in the field and reports cutting-edge computational ...
Creativity Research Journal Volume 14, 2002 - Issue 3-4. Submit an article Journal homepage. 4,153 Views 179 CrossRef citations to date 0. Altmetric Original Articles. The Potential Role of the Physical Environment in Fostering Creativity. Janetta Mitchell McCoy & ...
Cold chain packaging faces high levels of uncertainty due to its complex nature and dynamic environments during transportation, and the importance of safety and risk management. This study aims to propose a risk assessment model for cold chain packaging based on a fuzzy Bayesian Network. A case study on vaccine cold chain shipping containers is conducted for the illustration of the risk ...
The New England Journal of Medicine (NEJM) is a weekly general medical journal that publishes new medical research and review articles, and editorial opinion on a wide variety of topics of ...
Grassroots Writing Research Journal 15.1. The Grassroots Writing Research Journal current issue is only available through Illinois State University bookstores, not through other vendors. You can get a copy from ISU's Redbird Spirit Shop at two locations: on North Street and in the Bone Student Center. If one bookstore is sold out, contact the ...
Here, we combine observed and reconstructed June-August (JJA) surface air temperatures to show that 2023 was the warmest NH extra-tropical summer over the past 2000 years exceeding the 95% ...
Acceptance Letter Journal of MCU Buddhapanya Review Print ISSN: 2465-5503 Database Thai-Journal Citation Index Centre 1 (TCI 1) Accepted in publication as of March 15, 2024 Topic: The ...
IJSRP, Volume 14, Issue 4, April 2024 Edition [ISSN 2250-3153] All listed papers are published after full consent of respective author or co-author (s). For any discussion on research subject or research matter, the reader should directly contact to undersigned authors. Authors who paid publication fee after 3rd April, their paper will publish ...
Access 160+ million publications and connect with 25+ million researchers. Join for free and gain visibility by uploading your research.
The Grassroots Writing Research Journal editorial team is proud to announce the 28th publication of the journal. GWRJ 15.1 shares literate activity research on BeReal, naming, notebooking, internships, stickers, internet browser tabs as profiles, and so much more.
Health care AI quality standards were applied in only 39% (13/33) of the reviews and in 14% (25/178) of the original studies from the reviews examined, mostly to appraise their methodological or reporting quality. Only a handful mentioned the transparency, explainability, trustworthiness, ethics, and privacy aspects. ... Interactive Journal of ...
The Grassroots Writing Research Journal (GWRJ) is dedicated to publishing writing research by everyday writers like you-like all of us. Who. We are committed to publishing GWRJ authors who are undergraduate writing students, graduate student teachers, university faculty members, high school teachers and students, middle school teachers and ...
The survey is a highly regarded source of self-reported estimates of tobacco, alcohol and drug use in the United States. In 2022, an estimated 17.7 million people reported using marijuana daily or near-daily compared to 14.7 million daily or near-daily drinkers, according to the study. From 1992 to 2022, the per capita rate of reporting daily ...
Background In the realm of assisted reproduction, a subset of infertile patients demonstrates high ovarian response following controlled ovarian stimulation (COS), with approximately 29.7% facing the risk of Ovarian Hyperstimulation Syndrome (OHSS). Management of OHSS risk often necessitates embryo transfer cancellation, leading to delayed prospects of successful pregnancy and significant ...