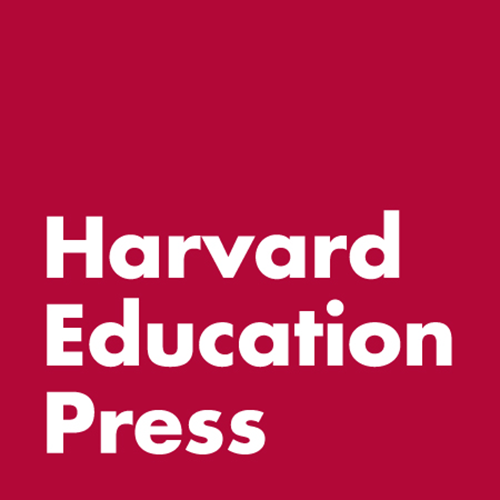
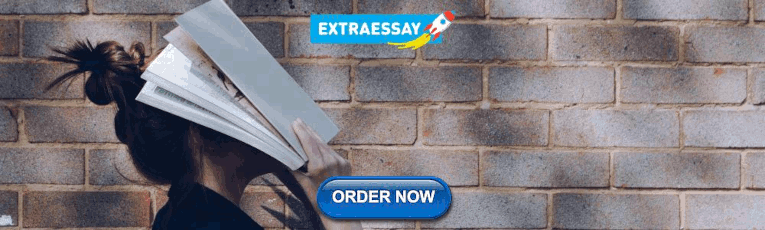
On The Site
Harvard educational review.
Edited by Maya Alkateb-Chami, Jane Choi, Jeannette Garcia Coppersmith, Ron Grady, Phoebe A. Grant-Robinson, Pennie M. Gregory, Jennifer Ha, Woohee Kim, Catherine E. Pitcher, Elizabeth Salinas, Caroline Tucker, Kemeyawi Q. Wahpepah
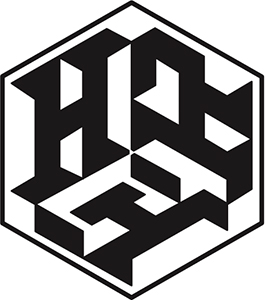
Individuals
Institutions.
- Read the journal here
Journal Information
- ISSN: 0017-8055
- eISSN: 1943-5045
- Keywords: scholarly journal, education research
- First Issue: 1930
- Frequency: Quarterly
Description
The Harvard Educational Review (HER) is a scholarly journal of opinion and research in education. The Editorial Board aims to publish pieces from interdisciplinary and wide-ranging fields that advance our understanding of educational theory, equity, and practice. HER encourages submissions from established and emerging scholars, as well as from practitioners working in the field of education. Since its founding in 1930, HER has been central to elevating pieces and debates that tackle various dimensions of educational justice, with circulation to researchers, policymakers, teachers, and administrators.
Our Editorial Board is composed entirely of doctoral students from the Harvard Graduate School of Education who review all manuscripts considered for publication. For more information on the current Editorial Board, please see here.
A subscription to the Review includes access to the full-text electronic archives at our Subscribers-Only-Website .
Editorial Board
2023-2024 Harvard Educational Review Editorial Board Members
Maya Alkateb-Chami Development and Partnerships Editor, 2023-2024 Editor, 2022-2024 [email protected]
Maya Alkateb-Chami is a PhD student at the Harvard Graduate School of Education. Her research focuses on the role of schooling in fostering just futures—specifically in relation to language of instruction policies in multilingual contexts and with a focus on epistemic injustice. Prior to starting doctoral studies, she was the Managing Director of Columbia University’s Human Rights Institute, where she supported and co-led a team of lawyers working to advance human rights through research, education, and advocacy. Prior to that, she was the Executive Director of Jusoor, a nonprofit organization that helps conflict-affected Syrian youth and children pursue their education in four countries. Alkateb-Chami is a Fulbright Scholar and UNESCO cultural heritage expert. She holds an MEd in Language and Literacy from Harvard University; an MSc in Education from Indiana University, Bloomington; and a BA in Political Science from Damascus University, and her research on arts-based youth empowerment won the annual Master’s Thesis Award of the U.S. Society for Education Through Art.
Jane Choi Editor, 2023-2025
Jane Choi is a second-year PhD student in Sociology with broad interests in culture, education, and inequality. Her research examines intra-racial and interracial boundaries in US educational contexts. She has researched legacy and first-generation students at Ivy League colleges, families served by Head Start and Early Head Start programs, and parents of pre-K and kindergarten-age children in the New York City School District. Previously, Jane worked as a Research Assistant in the Family Well-Being and Children’s Development policy area at MDRC and received a BA in Sociology from Columbia University.
Jeannette Garcia Coppersmith Content Editor, 2023-2024 Editor, 2022-2024 [email protected]
Jeannette Garcia Coppersmith is a fourth-year Education PhD student in the Human Development, Learning and Teaching concentration at the Harvard Graduate School of Education. A former public middle and high school mathematics teacher and department chair, she is interested in understanding the mechanisms that contribute to disparities in secondary mathematics education, particularly how teacher beliefs and biases intersect with the social-psychological processes and pedagogical choices involved in math teaching. Jeannette holds an EdM in Learning and Teaching from the Harvard Graduate School of Education where she studied as an Urban Scholar and a BA in Environmental Sciences from the University of California, Berkeley.
Ron Grady Editor, 2023-2025
Ron Grady is a second-year doctoral student in the Human Development, Learning, and Teaching concentration at the Harvard Graduate School of Education. His central curiosities involve the social worlds and peer cultures of young children, wondering how lived experience is both constructed within and revealed throughout play, the creation of art and narrative, and through interaction with/production of visual artifacts such as photography and film. Ron also works extensively with educators interested in developing and deepening practices rooted in reflection on, inquiry into, and translation of the social, emotional, and aesthetic aspects of their classroom ecosystems. Prior to his doctoral studies, Ron worked as a preschool teacher in New Orleans. He holds a MS in Early Childhood Education from the Erikson Institute and a BA in Psychology with Honors in Education from Stanford University.
Phoebe A. Grant-Robinson Editor, 2023-2024
Phoebe A. Grant-Robinson is a first year student in the Doctor of Education Leadership(EdLD) program at the Harvard Graduate School of Education. Her ultimate quest is to position all students as drivers of their destiny. Phoebe is passionate about early learning and literacy. She is committed to ensuring that districts and school leaders, have the necessary tools to create equitable learning organizations that facilitate the academic and social well-being of all students. Phoebe is particularly interested in the intersection of homeless students and literacy. Prior to her doctoral studies, Phoebe was a Special Education Instructional Specialist. Supporting a portfolio of more than thirty schools, she facilitated the rollout of New York City’s Special Education Reform. Phoebe also served as an elementary school principal. She holds a BS in Inclusive Education from Syracuse University, and an MS in Curriculum and Instruction from Pace University.
Pennie M. Gregory Editor, 2023-2024
Pennie M. Gregory is a second-year student in the Doctor of Education Leadership (EdLD) program at the Harvard Graduate School of Education. Pennie was born in Incheon, South Korea and raised in Gary, Indiana. She has decades of experience leading efforts to improve outcomes for students with disabilities first as a special education teacher and then as a school district special education administrator. Prior to her doctoral studies, Pennie helped to create Indiana’s first Aspiring Special Education Leadership Institute (ASELI) and served as its Director. She was also the Capacity Events Director for MelanatED Leaders, an organization created to support educational leaders of color in Indianapolis. Pennie has a unique perspective, having worked with members of the school community, with advocacy organizations, and supporting state special education leaders. Pennie holds an EdM in Education Leadership from Marian University.
Jennifer Ha Editor, 2023-2025
Jen Ha is a second-year PhD student in the Culture, Institutions, and Society concentration at the Harvard Graduate School of Education. Her research explores how high school students learn to write personal narratives for school applications, scholarships, and professional opportunities amidst changing landscapes in college access and admissions. Prior to doctoral studies, Jen served as the Coordinator of Public Humanities at Bard Graduate Center and worked in several roles organizing academic enrichment opportunities and supporting postsecondary planning for students in New Haven and New York City. Jen holds a BA in Humanities from Yale University, where she was an Education Studies Scholar.
Woohee Kim Editor, 2023-2025
Woohee Kim is a PhD student studying youth activists’ civic and pedagogical practices. She is a scholar-activist dedicated to creating spaces for pedagogies of resistance and transformative possibilities. Shaped by her activism and research across South Korea, the US, and the UK, Woohee seeks to interrogate how educational spaces are shaped as cultural and political sites and reshaped by activists as sites of struggle. She hopes to continue exploring the intersections of education, knowledge, power, and resistance.
Catherine E. Pitcher Editor, 2023-2025
Catherine is a second-year doctoral student at Harvard Graduate School of Education in the Culture, Institutions, and Society program. She has over 10 years of experience in education in the US in roles that range from special education teacher to instructional coach to department head to educational game designer. She started working in Palestine in 2017, first teaching, and then designing and implementing educational programming. Currently, she is working on research to understand how Palestinian youth think about and build their futures and continues to lead programming in the West Bank, Gaza, and East Jerusalem. She holds an EdM from Harvard in International Education Policy.
Elizabeth Salinas Editor, 2023-2025
Elizabeth Salinas is a doctoral student in the Education Policy and Program Evaluation concentration at HGSE. She is interested in the intersection of higher education and the social safety net and hopes to examine policies that address basic needs insecurity among college students. Before her doctoral studies, Liz was a research director at a public policy consulting firm. There, she supported government, education, and philanthropy leaders by conducting and translating research into clear and actionable information. Previously, Liz served as a high school physics teacher in her hometown in Texas and as a STEM outreach program director at her alma mater. She currently sits on the Board of Directors at Leadership Enterprise for a Diverse America, a nonprofit organization working to diversify the leadership pipeline in the United States. Liz holds a bachelor’s degree in civil engineering from the Massachusetts Institute of Technology and a master’s degree in higher education from the Harvard Graduate School of Education.
Caroline Tucker Co-Chair, 2023-2024 Editor, 2022-2024 [email protected]
Caroline Tucker is a fourth-year doctoral student in the Culture, Institutions, and Society concentration at the Harvard Graduate School of Education. Her research focuses on the history and organizational dynamics of women’s colleges as women gained entry into the professions and coeducation took root in the United States. She is also a research assistant for the Harvard and the Legacy of Slavery Initiative’s Subcommittee on Curriculum and the editorial assistant for Into Practice, the pedagogy newsletter distributed by Harvard University’s Office of the Vice Provost for Advances in Learning. Prior to her doctoral studies, Caroline served as an American politics and English teaching fellow in London and worked in college advising. Caroline holds a BA in History from Princeton University, an MA in the Social Sciences from the University of Chicago, and an EdM in Higher Education from the Harvard Graduate School of Education.
Kemeyawi Q. Wahpepah Co-Chair, 2023-2024 Editor, 2022-2024 [email protected]
Kemeyawi Q. Wahpepah (Kickapoo, Sac & Fox) is a fourth-year doctoral student in the Culture, Institutions, and Society concentration at the Harvard Graduate School of Education. Their research explores how settler colonialism is addressed in K-12 history and social studies classrooms in the United States. Prior to their doctoral studies, Kemeyawi taught middle and high school English and history for eleven years in Boston and New York City. They hold an MS in Middle Childhood Education from Hunter College and an AB in Social Studies from Harvard University.
Submission Information
Click here to view submission guidelines .
Contact Information
Click here to view contact information for the editorial board and customer service .
Subscriber Support
Individual subscriptions must have an individual name in the given address for shipment. Individual copies are not for multiple readers or libraries. Individual accounts come with a personal username and password for access to online archives. Online access instructions will be attached to your order confirmation e-mail.
Institutional rates apply to libraries and organizations with multiple readers. Institutions receive digital access to content on Meridian from IP addresses via theIPregistry.org (by sending HER your PSI Org ID).
Online access instructions will be attached to your order confirmation e-mail. If you have questions about using theIPregistry.org you may find the answers in their FAQs. Otherwise please let us know at [email protected] .
How to Subscribe
To order online via credit card, please use the subscribe button at the top of this page.
To order by phone, please call 888-437-1437.
Checks can be mailed to Harvard Educational Review C/O Fulco, 30 Broad Street, Suite 6, Denville, NJ 07834. (Please include reference to your subscriber number if you are renewing. Institutions must include their PSI Org ID or follow up with this information via email to [email protected] .)
Permissions
Click here to view permissions information.
Article Submission FAQ
Submissions, question: “what manuscripts are a good fit for her ”.
Answer: As a generalist scholarly journal, HER publishes on a wide range of topics within the field of education and related disciplines. We receive many articles that deserve publication, but due to the restrictions of print publication, we are only able to publish very few in the journal. The originality and import of the findings, as well as the accessibility of a piece to HER’s interdisciplinary, international audience which includes education practitioners, are key criteria in determining if an article will be selected for publication.
We strongly recommend that prospective authors review the current and past issues of HER to see the types of articles we have published recently. If you are unsure whether your manuscript is a good fit, please reach out to the Content Editor at [email protected] .
Question: “What makes HER a developmental journal?”
Answer: Supporting the development of high-quality education research is a key tenet of HER’s mission. HER promotes this development through offering comprehensive feedback to authors. All manuscripts that pass the first stage of our review process (see below) receive detailed feedback. For accepted manuscripts, HER also has a unique feedback process called casting whereby two editors carefully read a manuscript and offer overarching suggestions to strengthen and clarify the argument.
Question: “What is a Voices piece and how does it differ from an essay?”
Answer: Voices pieces are first-person reflections about an education-related topic rather than empirical or theoretical essays. Our strongest pieces have often come from educators and policy makers who draw on their personal experiences in the education field. Although they may not present data or generate theory, Voices pieces should still advance a cogent argument, drawing on appropriate literature to support any claims asserted. For examples of Voices pieces, please see Alvarez et al. (2021) and Snow (2021).
Question: “Does HER accept Book Note or book review submissions?”
Answer: No, all Book Notes are written internally by members of the Editorial Board.
Question: “If I want to submit a book for review consideration, who do I contact?”
Answer: Please send details about your book to the Content Editor at [email protected].
Manuscript Formatting
Question: “the submission guidelines state that manuscripts should be a maximum of 9,000 words – including abstract, appendices, and references. is this applicable only for research articles, or should the word count limit be followed for other manuscripts, such as essays”.
Answer: The 9,000-word limit is the same for all categories of manuscripts.
Question: “We are trying to figure out the best way to mask our names in the references. Is it OK if we do not cite any of our references in the reference list? Our names have been removed in the in-text citations. We just cite Author (date).”
Answer: Any references that identify the author/s in the text must be masked or made anonymous (e.g., instead of citing “Field & Bloom, 2007,” cite “Author/s, 2007”). For the reference list, place the citations alphabetically as “Author/s. (2007)” You can also indicate that details are omitted for blind review. Articles can also be blinded effectively by use of the third person in the manuscript. For example, rather than “in an earlier article, we showed that” substitute something like “as has been shown in Field & Bloom, 2007.” In this case, there is no need to mask the reference in the list. Please do not submit a title page as part of your manuscript. We will capture the contact information and any author statement about the fit and scope of the work in the submission form. Finally, please save the uploaded manuscript as the title of the manuscript and do not include the author/s name/s.
Invitations
Question: “can i be invited to submit a manuscript how”.
Answer: If you think your manuscript is a strong fit for HER, we welcome a request for invitation. Invited manuscripts receive one round of feedback from Editors before the piece enters the formal review process. To submit information about your manuscript, please complete the Invitation Request Form . Please provide as many details as possible. The decision to invite a manuscript largely depends on the capacity of current Board members and on how closely the proposed manuscript reflects HER publication scope and criteria. Once you submit the form, We hope to update you in about 2–3 weeks, and will let you know whether there are Editors who are available to invite the manuscript.
Review Timeline
Question: “who reviews manuscripts”.
Answer: All manuscripts are reviewed by the Editorial Board composed of doctoral students at Harvard University.
Question: “What is the HER evaluation process as a student-run journal?”
Answer: HER does not utilize the traditional external peer review process and instead has an internal, two-stage review procedure.
Upon submission, every manuscript receives a preliminary assessment by the Content Editor to confirm that the formatting requirements have been carefully followed in preparation of the manuscript, and that the manuscript is in accord with the scope and aim of the journal. The manuscript then formally enters the review process.
In the first stage of review, all manuscripts are read by a minimum of two Editorial Board members. During the second stage of review, manuscripts are read by the full Editorial Board at a weekly meeting.
Question: “How long after submission can I expect a decision on my manuscript?”
Answer: It usually takes 6 to 10 weeks for a manuscript to complete the first stage of review and an additional 12 weeks for a manuscript to complete the second stage. Due to time constraints and the large volume of manuscripts received, HER only provides detailed comments on manuscripts that complete the second stage of review.
Question: “How soon are accepted pieces published?”
Answer: The date of publication depends entirely on how many manuscripts are already in the queue for an issue. Typically, however, it takes about 6 months post-acceptance for a piece to be published.
Submission Process
Question: “how do i submit a manuscript for publication in her”.
Answer: Manuscripts are submitted through HER’s Submittable platform, accessible here. All first-time submitters must create an account to access the platform. You can find details on our submission guidelines on our Submissions page.
Education →
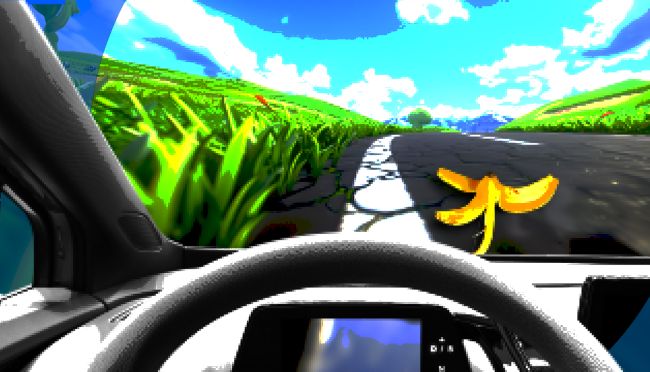
- 26 Mar 2024
- Research & Ideas
How Humans Outshine AI in Adapting to Change
Could artificial intelligence systems eventually perform surgeries or fly planes? First, AI will have to learn to navigate shifting conditions as well as people do. Julian De Freitas and colleagues pit humans against machines in a video game to study AI's current limits and mine insights for the real world.
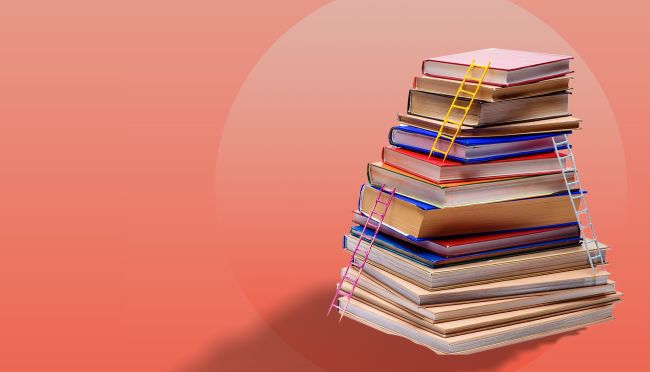
- 12 Mar 2024
Publish or Perish: What the Research Says About Productivity in Academia
Universities tend to evaluate professors based on their research output, but does that measure reflect the realities of higher ed? A study of 4,300 professors by Kyle Myers, Karim Lakhani, and colleagues probes the time demands, risk appetite, and compensation of faculty.
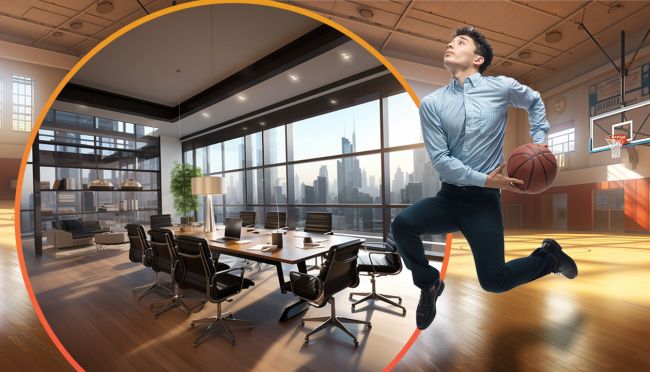
- 25 Jan 2024
Being a Team Player: Why College Athletes Succeed in Business
Forget rocks for jocks. A study by Paul Gompers of more than 400,000 Ivy League athletes probes how the rigors of college sports can help people climb the corporate ladder faster and into higher-paying positions.
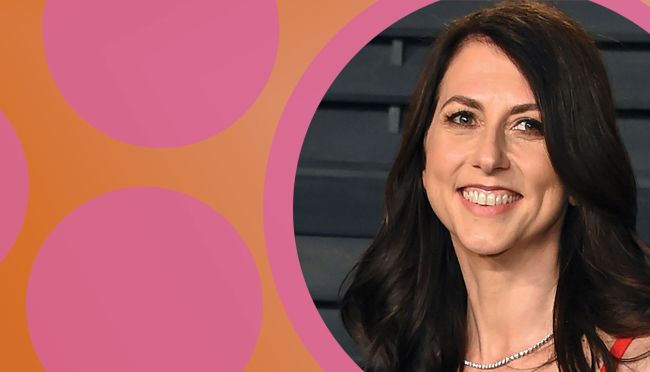
- 19 Dec 2023
$15 Billion in Five Years: What Data Tells Us About MacKenzie Scott’s Philanthropy
Scott's hands-off approach and unparalleled pace—helping almost 2,000 organizations and counting—has upended the status quo in philanthropy. While her donations might seem scattershot, an analysis of five years of data by Matthew Lee, Brian Trelstad, and Ethan Tran highlights clear trends and an emerging strategy.
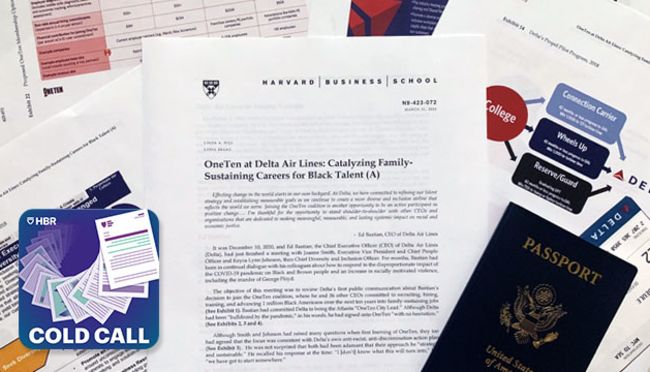
- 21 Nov 2023
- Cold Call Podcast
Cold Call: Building a More Equitable Culture at Delta Air Lines
In December 2020 Delta Air Lines CEO Ed Bastian and his leadership team were reviewing the decision to join the OneTen coalition, where he and 36 other CEOs committed to recruiting, hiring, training, and advancing one million Black Americans over the next ten years into family-sustaining jobs. But, how do you ensure everyone has equal access to opportunity within an organization? Professor Linda Hill discusses Delta’s decision and its progress in embedding a culture of diversity, equity, and inclusion in her case, “OneTen at Delta Air Lines: Catalyzing Family-Sustaining Careers for Black Talent.”
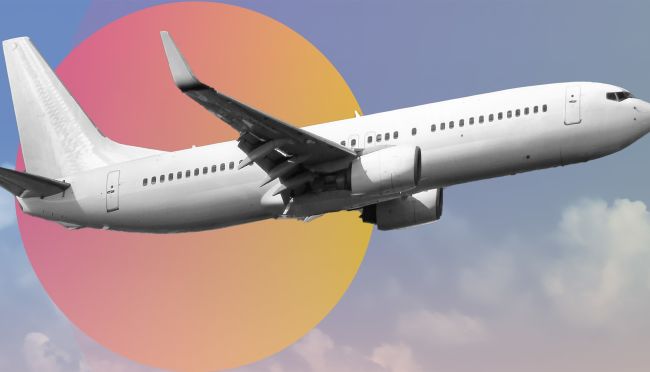
- 16 Oct 2023
Advancing Black Talent: From the Flight Ramp to 'Family-Sustaining' Careers at Delta
By emphasizing skills and expanding professional development opportunities, the airline is making strides toward recruiting and advancing Black employees. Case studies by Linda Hill offer an inside look at how Delta CEO Ed Bastian is creating a more equitable company and a stronger talent pipeline.
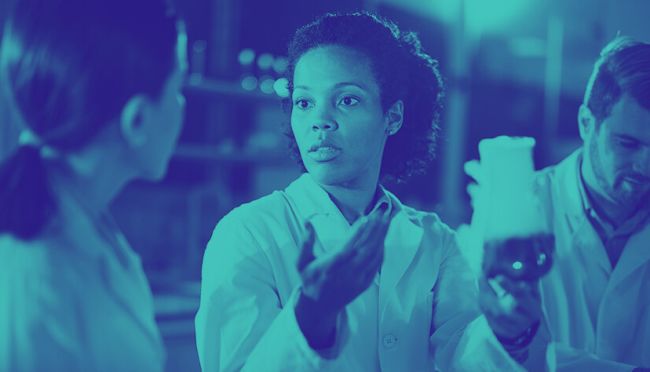
- 26 Jul 2023
STEM Needs More Women. Recruiters Often Keep Them Out
Tech companies and programs turn to recruiters to find top-notch candidates, but gender bias can creep in long before women even apply, according to research by Jacqueline Ng Lane and colleagues. She highlights several tactics to make the process more equitable.
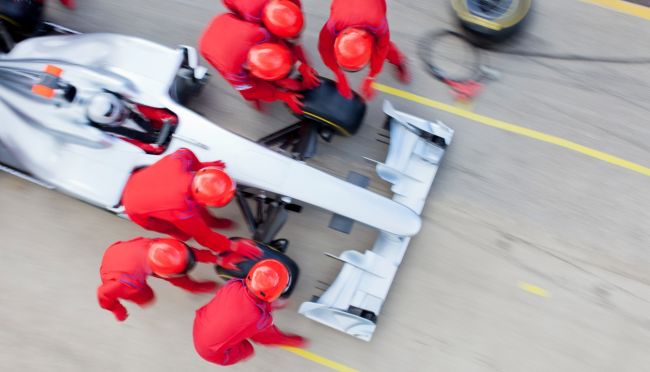
- 14 Jun 2023
Four Steps to Building the Psychological Safety That High-Performing Teams Need
Struggling to spark strategic risk-taking and creative thinking? In the post-pandemic workplace, teams need psychological safety more than ever, and a new analysis by Amy Edmondson highlights the best ways to nurture it.
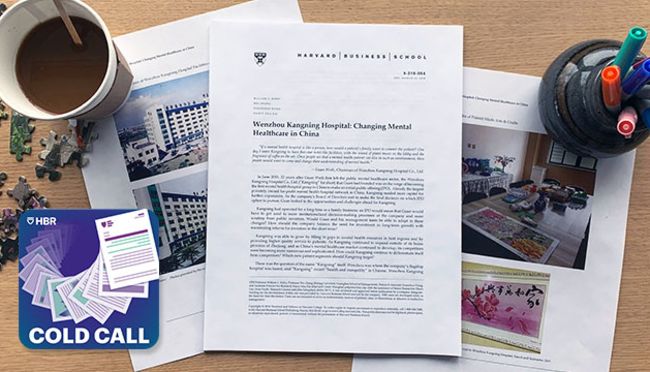
- 23 May 2023
The Entrepreneurial Journey of China’s First Private Mental Health Hospital
The city of Wenzhou in southeastern China is home to the country’s largest privately owned mental health hospital group, the Wenzhou Kangning Hospital Co, Ltd. It’s an example of the extraordinary entrepreneurship happening in China’s healthcare space. But after its successful initial public offering (IPO), how will the hospital grow in the future? Harvard Professor of China Studies William C. Kirby highlights the challenges of China’s mental health sector and the means company founder Guan Weili employed to address them in his case, Wenzhou Kangning Hospital: Changing Mental Healthcare in China.
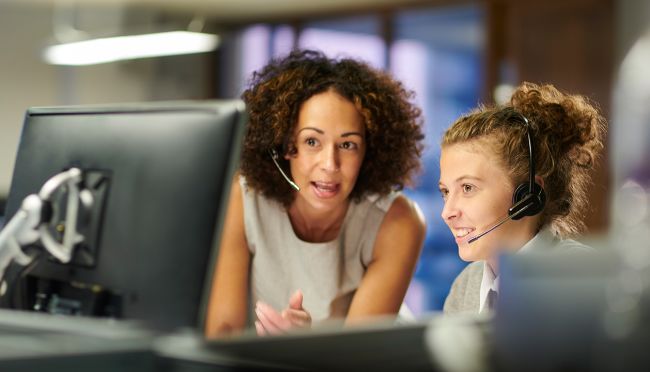
- 28 Feb 2023
Can Apprenticeships Work in the US? Employers Seeking New Talent Pipelines Take Note
What if the conventional college-and-internship route doesn't give future employees the skills they need to build tomorrow's companies? Research by Joseph Fuller and colleagues illustrates the advantages that apprenticeships can provide to employees and young talent.
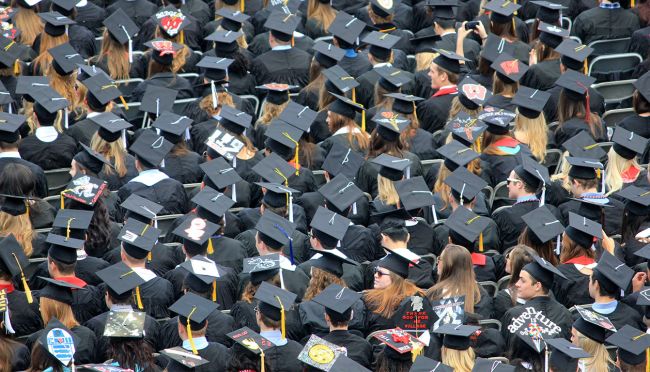
- 15 Aug 2022
University of the Future: Finding the Next World Leaders in Higher Ed
Which universities will step into the void as American colleges decline? In the book Empires of Ideas, William Kirby explores how the history of higher education in the US, China, and Germany might shape its future.
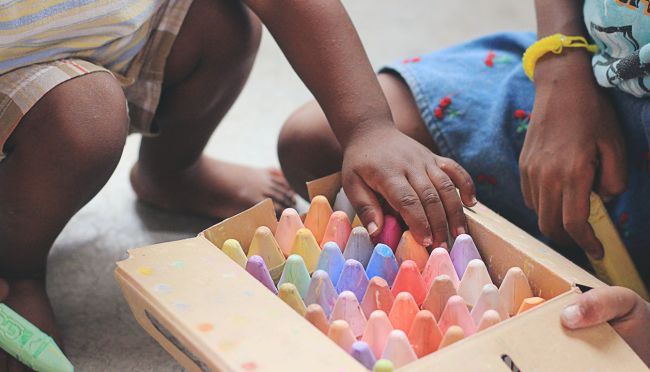
- 11 Aug 2022
When Parents Tell Kids to ‘Work Hard,’ Do They Send the Wrong Message?
It takes more than grit to succeed in a world rife with systemic inequity. So why don't we tell children that? Research by Ashley Whillans and colleagues shows how honest talk about social barriers could empower kids to break them down.
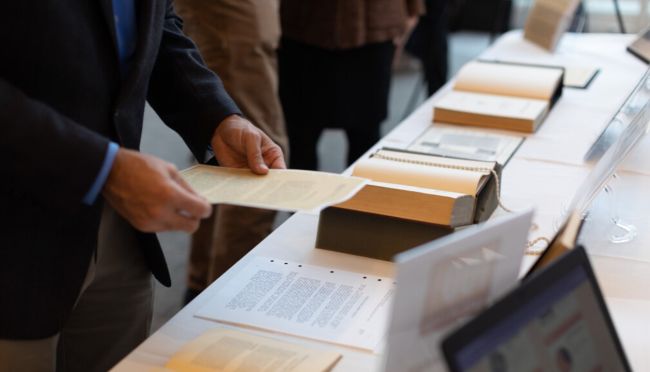
- 02 May 2022
- What Do You Think?
Can the Case Method Survive Another Hundred Years?
The case method pioneered by Harvard Business School has weathered a hundred years of controversy and criticism. However, is the approach the best way to teach people to lead in a world that demands more agility and adaptability? James Heskett asks. Open for comment; 0 Comments.
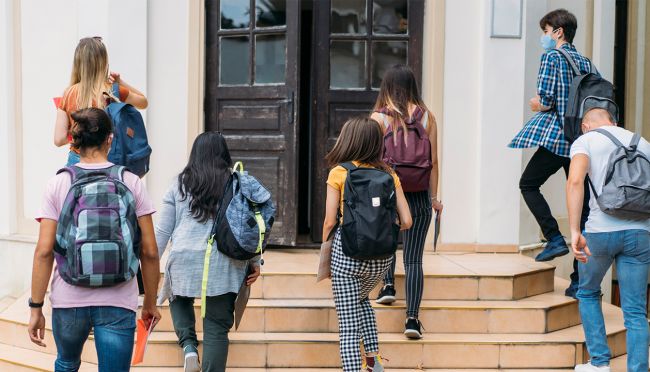
- 18 Nov 2021
5 Principles for Scaling Change from IBM’s High School Innovation
P-TECH has bolstered graduation rates for students of color while creating a new tech hiring pipeline. Rosabeth Moss Kanter and program architect Stanley Litow discuss the social impact lessons for other organizations. Open for comment; 0 Comments.
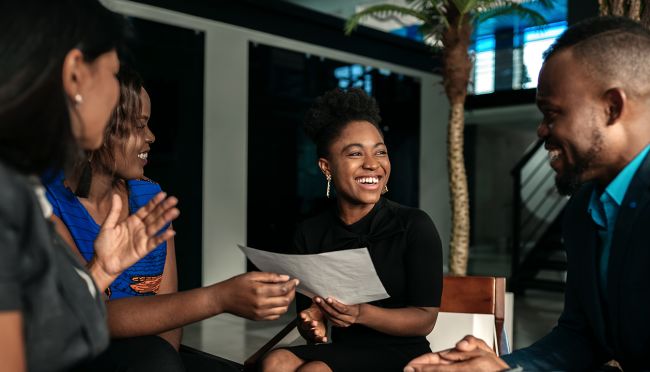
- 09 Aug 2021
OneTen: Creating a New Pathway for Black Talent
A new organization aims to help 1 million Black Americans launch careers in the next decade, expanding the talent pool. Rawi E. Abdelal, Katherine Connolly Baden, and Boris Groysberg explain how. Open for comment; 0 Comments.
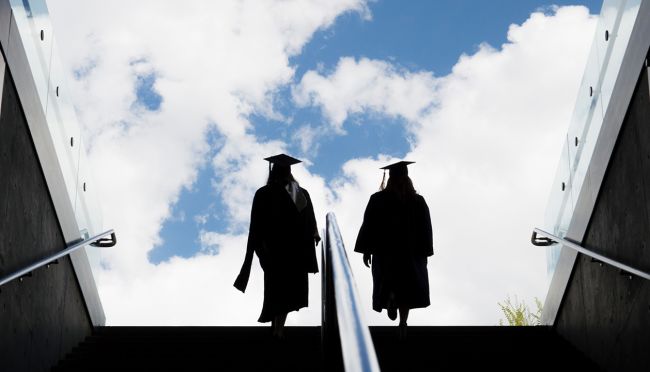
- 19 May 2021
Why America Needs a Better Bridge Between School and Career
As the COVID-19 pandemic wanes, America faces a critical opportunity to close gaps that leave many workers behind, say Joseph Fuller and Rachel Lipson. What will it take? Open for comment; 0 Comments.
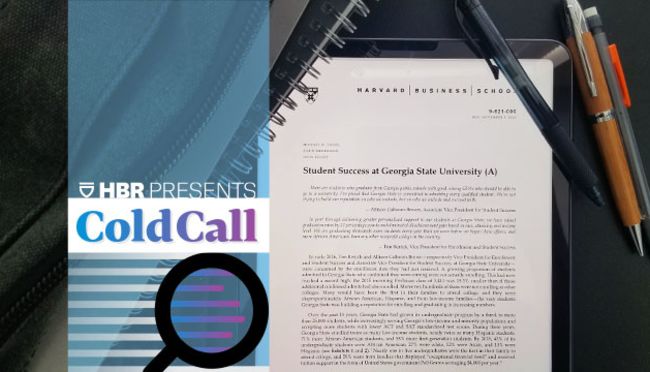
- 18 May 2021
How Georgia State University Increased Graduation Rates
Georgia State University was facing a growing "summer melt" problem, where nearly 20 percent of incoming students never actually enrolled. The university used a data-based approach to retain students of all racial, ethnic, and socioeconomic backgrounds and help them graduate. Professor Mike Toffel> and Harvard Advanced Leadership Initiative fellow Robin Mendelson discuss what the university learned about improving student success, while scaling its efforts to help other universities, in their case, “Student Success at Georgia State University.” Open for comment; 0 Comments.
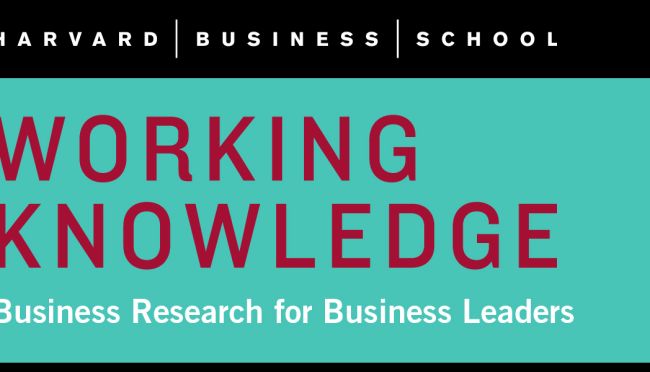
- 13 Apr 2021
- Working Paper Summaries
Population Interference in Panel Experiments
In panel experiments, units are exposed to different interventions over time. This article introduces a unifying framework for studying panel experiments with population interference, in which a treatment assigned to one experimental unit affects another experimental unit's outcome. Findings have implications for fields as diverse as education, economics, and public health.
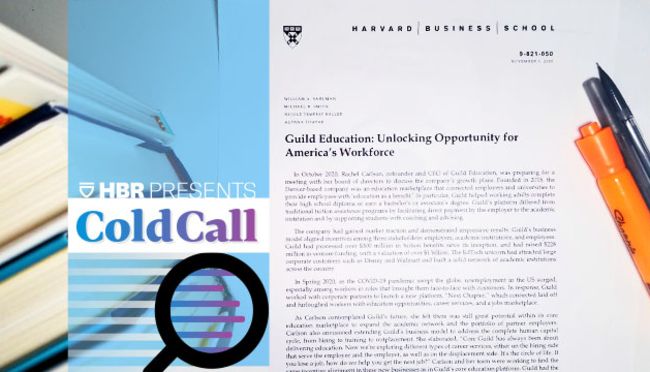
- 23 Mar 2021
Managing Future Growth at an Innovative Workforce Education Startup
Guild Education is an education marketplace that connects employers and universities to provide employees with “education as a benefit.” Now CEO and co-founder Rachel Carlson must decide how to manage the company’s future growth. Professor Bill Sahlman discusses this unique startup and Carlson’s plans for its growth in his case, “Guild Education: Unlocking Opportunity for America's Workforce.” Open for comment; 0 Comments.
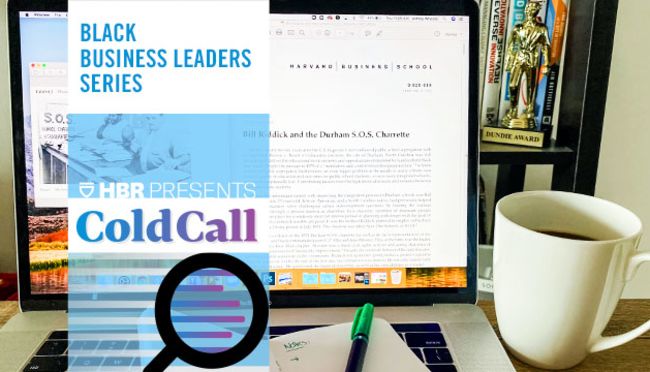
- 02 Feb 2021
Using Empathy and Curiosity to Overcome Differences
Bill Riddick, an African-American community leader and counselor, must find a way to bridge the divide between Black and white community leaders, who are on opposing sides of school integration in Durham, North Carolina, in 1971. Harvard Business School professor Francesca Gino and Jeffrey Huizinga explain how empathy and curiosity can foster understanding in divisive situations in their case, “Bill Riddick and the Durham S.O.S. Charrette.” Open for comment; 0 Comments.
Click through the PLOS taxonomy to find articles in your field.
For more information about PLOS Subject Areas, click here .
Loading metrics
Open Access
Peer-reviewed
Research Article
Do open educational resources improve student learning? Implications of the access hypothesis
Roles Conceptualization, Formal analysis, Investigation, Methodology, Project administration, Software, Visualization, Writing – original draft, Writing – review & editing
* E-mail: [email protected]
Affiliation OpenStax, Rice University, Houston, Texas, United States of America

Roles Conceptualization, Writing – review & editing
Roles Conceptualization, Formal analysis, Writing – review & editing
Roles Supervision, Writing – review & editing
- Phillip J. Grimaldi,
- Debshila Basu Mallick,
- Andrew E. Waters,
- Richard G. Baraniuk
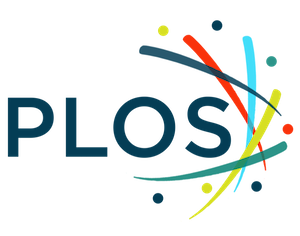
- Published: March 6, 2019
- https://doi.org/10.1371/journal.pone.0212508
- Reader Comments
Open Educational Resources (OER) have been lauded for their ability to reduce student costs and improve equity in higher education. Research examining whether OER provides learning benefits have produced mixed results, with most studies showing null effects. We argue that the common methods used to examine OER efficacy are unlikely to detect positive effects based on predictions of the access hypothesis. The access hypothesis states that OER benefits learning by providing access to critical course materials, and therefore predicts that OER should only benefit students who would not otherwise have access to the materials. Through the use of simulation analysis, we demonstrate that even if there is a learning benefit of OER, standard research methods are unlikely to detect it.
Citation: Grimaldi PJ, Basu Mallick D, Waters AE, Baraniuk RG (2019) Do open educational resources improve student learning? Implications of the access hypothesis. PLoS ONE 14(3): e0212508. https://doi.org/10.1371/journal.pone.0212508
Editor: James A.L. Brown, National University Ireland Galway, IRELAND
Received: December 20, 2018; Accepted: February 5, 2019; Published: March 6, 2019
Copyright: © 2019 Grimaldi et al. This is an open access article distributed under the terms of the Creative Commons Attribution License , which permits unrestricted use, distribution, and reproduction in any medium, provided the original author and source are credited.
Data Availability: Data used in this report were generated via code, and are available on GitHub ( https://github.com/openstax/oer-simulation-study ).
Funding: Authors PJG, DBM, and AEW are employees of OpenStax, a non-profit OER textbook publisher based out of Rice University. RGB is the founder. OpenStax provided support in the form of full or partial salaries for authors PJG, DBM, AEW, & RGB, but did not have any additional role in the study design, data collection and analysis, decision to publish, or preparation of the manuscript. The specific roles of these authors are articulated in the ‘author contributions’ section.
Competing interests: Authors PJG, DBM, and AEW are employees of OpenStax, a non-profit OER textbook publisher based out of Rice University. RGB is the founder of OpenStax. This does not alter our adherence to PLOS ONE policies on sharing data and materials.
Introduction
The textbook has long been a critical component of the education system at all levels. In addition to providing a scaffold for content discussed in a course, textbooks have historically been the primary learning resource for students. For a variety of market-based reasons, the price of textbooks has risen dramatically over the last two decades, outpacing the price increases of all goods and services by almost four times [ 1 ]. Within higher education, these price increases ultimately fall on the students, who are responsible for procuring their own course materials. In response to these price trends, many educators have turned to open educational resources (OER) [ 2 , 3 ]. While OER refers to any educational resource that is openly licensed and freely distributed, for the purposes of this document we will limit our discussion to OER textbooks. Over the last decade, OER has risen dramatically in popularity. According to OpenStax, the leading producer of OER textbooks, adoption of OER textbooks has saved students an estimated $500 million dollars since 2012 [ 4 ]. Moreover, recent survey data [ 5 ] suggest that OER textbooks now rival commercial textbooks in terms of overall market share. More importantly, textbook prices appear to have recently leveled off for the first time in three decades, an effect which is partially attributed to increased competition from OER alternatives [ 6 ].
While the OER movement has been successful in reducing the cost of educational materials, many have wondered whether adoption of OER affords additional benefits, such as improved student learning outcomes [ 7 ]. This question has motivated a flurry of empirical research comparing the grades of students who used OER textbooks to students who used a commercial textbook (for a recent review, see [ 8 ]). Overall, this research has produced somewhat mixed results. Several studies have found no significant differences between OER and traditional textbooks on student grades [ 9 – 12 ]. Occasionally, however, negative or positive effects are found. One study [ 13 ] found no significant difference in regular exam scores, but did find a benefit of OER adoption on a specialized exam score. Another study [ 14 ] compared OER and traditional texts across seven high school classes and found a negative effect of OER in two classes, and no significant difference in the other five classes. In a study comparing OER and a commercial textbook across fifteen courses [ 15 ] a negative effect of OER was found in one course, a positive effect of OER in five courses, and a non-significant difference in the remaining nine courses. A six-semester study comparing OER to non-OER [ 16 ] observed a negative effect in two semesters, and a positive effect in one semester. However, a later analysis revealed these effects were likely artifacts of confounding variables. A study comparing digital and print OER books to traditional print text across three course exams [ 17 ] found a positive effect of digital OER on only one exam. A large scale evaluation of OER [ 18 ] found positive effects of OER adoption on student grades. It is worth noting that this research varies considerably in terms of quality and rigor. Nearly all used quasi-experimental designs, and some failed to control for possible confounding variables (e.g., [ 18 ]; see [ 19 ] for a discussion). Nevertheless, the important thing to note is that the majority of comparisons in the literature find null effects of OER adoption on learning outcomes.
Why do most comparisons of OER to traditional materials fail to find a positive effect of OER? On one hand, the primary goal of OER is to offer an alternative to commercial textbooks that are comparable in quality, but free and openly licensed. Assuming an OER textbook is no different in quality, then there are no meaningful differences to explain effects on learning outcomes. License and cost certainly should not affect learning at a cognitive level. In this sense, the frequency of null effects is expected. On the other hand, the price of a textbook can affect whether a student decides to purchase a textbook, and a student cannot learn from a textbook they do not have. If we reasonably assume that having a textbook is better for student learning than not having a textbook, these students would then be at a learning disadvantage. Thus, adoption of OER would be effective as a learning intervention because it ensures that all students have access to the textbook, and would therefore result in better learning outcomes (for similar discussion, see [ 8 , 15 , 18 ]). We refer to this idea as the access hypothesis .
If access is the primary mechanism for how OER might affect learning outcomes, then we can see that current research approaches are not well suited for detecting an effect of OER adoption. In most educational research, an intervention is expected to impact all students who receive the intervention, and its impact is measured by comparing students who receive the intervention to students in a control condition. However, the access hypothesis predicts that an OER intervention should only affect a subset of students—specifically those who would not otherwise have access to the textbook. Students who are willing or able to purchase the textbook should not be affected. Yet, every study that has evaluated OER efficacy to date has treated OER as any other intervention, specifically by comparing an entire sample of students who received the OER intervention to a sample of students who did not. Indeed, this is the approach recommended by the most active researchers of the field [ 20 ]. The problem with this approach is that the effect of the intervention is washed out by students who are not expected to be affected by the intervention. To draw an analogy, the current research approach in OER is the equivalent of measuring the effect of a pain relieving drug on a sample of people who are mostly not in pain. In this sense, we should not expect to observe effects of an OER intervention, even if we believe that having access to a textbook is beneficial to learning.
If the impact of OER is measured across an entire sample of students, then it is necessary for researchers to consider the textbook access rates prior to implementation of OER. Past research reveals some insights as to what the expected textbook access rates are in a typical classroom. A recent survey of over 22,000 Florida students enrolled in public universities and colleges found that close to 66.5% of students reported not purchasing a textbook at some point in their academic career [ 21 ]. While this statistic is concerning, the data are limited in that they do not indicate what the access rates are in any given classroom. Just because a student avoids purchasing a textbook once does not mean they will repeat the behavior for all of their classes. Indeed, more targeted research reveals that access rates can be very high. A survey of 824 University of Colorado at Boulder Physics students [ 22 ] found that 97% purchased the required texts. Another survey of 1023 students at an undisclosed university across a range of introductory level science courses [ 23 ] found that 96% of students reported purchasing their required texts. A survey of 162 students in a political science course [ 12 ] found a 98% access rate. We can imagine that if an OER intervention were conducted on these samples, it would be very difficult to observe a positive effect because the existing access rates are already so high. Of course, we cannot expect access rates to be high in every classroom. An internal survey at Virginia State University [ 24 ] reported that only 47% of students purchased textbooks. Unfortunately, they did not report how many students were included in this sample. Regardless, it is fair to say that the rate of textbook access will vary across contexts and student populations. As we will see, the access rate of any given population can have a profound effect on the results of research aimed at evaluating the impact of OER adoption.
In this paper, we argue that the standard approach taken in past research on OER efficacy is severely limited in its functional ability to properly evaluate the impact of OER. This functional limitation is controlled by the existing textbook access rate prior to an OER intervention. In order to formally illustrate this point, we conducted a series of simulated experiments designed to mimic a typical study on OER effectiveness. We used these simulated experiments to measure the likelihood of a standard OER efficacy study to correctly reject the null hypothesis (i.e., statistical power [ 25 ]). A simulation study is useful because we can examine the expected results of an experiment in perfectly controlled conditions. Most real world educational research is plagued by instructor artifacts, confounding variables, and random differences between groups. Moreover, it is incredibly difficult to implement a randomized control trial with real students. In a simulation, we do not have to worry about any of these constraints. A simulation is also necessary in this case, because traditional power analysis does not allow us to vary the number of students who might be affected by an intervention, as is predicted by the access hypothesis. The primary goal of these simulations was to examine the influence of access rate on statistical power in a typical study of OER effectiveness, and make inferences about the likelihood of detecting a positive effect of OER adoption in a real world study. We then apply this model to evaluate existing studies that have already been conducted.
Simulation Study
For each simulated experiment, we first generated a sample of n student scores s from a normal distribution s ∼ N ( μ , σ 2 ), truncated between 0 and 100. These scores represented the final grade of each student in the course on a 100 point scale, where μ was the sample mean and σ was the sample standard deviation. Second, students were randomly determined to have access to the textbook at a rate of a , and not have access at a rate of 1 − a . Third, students were randomly assigned to either an OER or Non-OER condition with the constraint that both conditions must have an equal size. Fourth, in order to simulate the effect of access, we decreased the score of the students in the Non-OER condition who were previously determined to not have access to the textbook. The scores of students in the OER condition were unaffected, representing the fact that all of these students now have access to the book. The magnitude of the score decrease was equivalent to dσ . The parameter d represents the effect size [ 26 ] of having access to a textbook. Finally, we fit a regression model that predicted student score by condition, and tested the condition coefficient against 0 by using a standard t-test. An overview of the simulation is shown in Fig 1 .
- PPT PowerPoint slide
- PNG larger image
- TIFF original image
For each experiment, a sample of student scores were generated, and students were determined to have access or not to the textbook. Students were then randomly assigned to either an OER or Non-OER condition. The effect of access was simulated by reducing scores for students determined to not have access, but only in the Non-OER condition. Lastly, a statistical test the OER and Non-OER conditions was performed. See text for more information.
https://doi.org/10.1371/journal.pone.0212508.g001
Parameter values.
For determining the value of n , we wanted to use similar sample sizes as studies that have examined OER in past research. Of the 42 direct comparisons of OER and non-OER materials on course grade [ 9 , 12 , 14 – 18 , 27 , 28 ], 95% involved sample sizes smaller than 5000 students. Thus, we examined levels of n between 100 and 5,000. We address sample sizes larger than 5,000 later in this report.
For generating our sample distribution, we set μ to 70 and σ to 20. We chose these values because we felt they were representative of a typical classroom, and similar to those we have observed in past research. However, because the effect of an intervention is measured by relative differences in scores, the actual values used here do not have much influence on the outcome of the simulation.
For the a parameter, which represents the proportion of students who are expected to have access to the textbook, we wanted to examine access rates that would be expected in a typical college classroom. We examined 6 different levels of a − 40%, 50%, 60%, 70%, 80%, and 90%. This range is likely to cover most student populations that might appear in OER research.
The d parameter represents the effect of having access to a textbook versus not having access to a textbook. We anticipated the literature would provide a clear direction for setting this parameter. To our surprise, despite the ubiquity of textbooks in higher education, there are few studies examining the effects of textbooks in general (both OER and non-OER) on learning outcomes. To our knowledge, there are no experimental studies that would afford calculation of a reasonable effect size of textbook usage. There are some correlational studies that at least show positive relationships between textbooks and learning. One study [ 22 ] reported moderate correlations between the amount of reading assignments a student completed and their final course grade for conceptual physics courses ( r = .45), but no correlation for calculus based physics ( r = .07). Another study [ 23 ] found that students who reported regularly reading their textbook had higher grades than students who read their textbooks only occasionally. However, they also found no difference between students who never read their books and those who read regularly. A positive relationship between student grades and engagement was found between student grades and engagement with a digital textbook, even after accounting for general student aptitude [ 29 ]. In sum, these studies show at the very least that use of the textbook can be beneficial to learning. We concluded that if there is an effect of textbook access on learning, it is likely to be small. Thus, we set d to a value of 0.25, which is considered to be the minimum effect size necessary for an educational intervention to be substantively important [ 30 ].
For each level of n and a , we repeated the experiment 10,000 times and recorded the p -values of each experiment. Statistical power was computed as the proportion of studies with p -values lower than α . All simulations were conducted using R [ 31 ], and based on code presented in [ 32 ]. The full code used for the simulations is available on GitHub ( https://github.com/openstax/oer-simulation-study ).
We examined the proportion of simulated experiments that rejected the null hypothesis at the standard α of.05 (i.e., power). The results are shown on Fig 2 . As is the case of all experiments where samples are drawn from normal distributions, the probability of success increases with n [ 25 ]. However, we also see that access rate ( a ) plays a strong influence on the ability to detect the effect of OER. When access is very low, experiments have a much higher likelihood of correctly rejecting the null hypothesis with smaller n . This makes sense, because there are more student in the sample that can be impacted by the intervention. However, as a increases, it pulls the probability of success down. Indeed, when a is large, it requires very large numbers of students to detect a significant effect. To illustrate the strength of this relationship, an OER experiment with 10,000 students will have a 89.3% chance of success when the access rate is 70%. However, the same 10,000 student experiment conducted on a sample with an access of 80% will only have a 56.5% success rate. The situation gets considerably worse when the access rate is 90%. This experiment would only have a 19% success rate. The fact that an experiment with 10,000 students would have such a low chance of correctly rejecting the null hypothesis demonstrates the influential role of access rate.
A study sample’s access rate to textbooks prior to adopting OER can severely hinder the likelihood of detecting an effect of OER, even at large sample sizes.
https://doi.org/10.1371/journal.pone.0212508.g002
Examination of past studies
The results of these simulations beg the question—how are we to interpret previous studies that have examined the effects of OER interventions on learning outcomes? To this end, we used the simulation procedure described previously to conceptually replicate prior studies on OER efficacy, with the goal of estimating the probability that such a study would have detected an effect of OER, given the reported sample sizes used in those studies at different levels of access.
Selection of research.
From the literature, we were able to find 42 direct comparisons of OER to traditional materials, across 9 publications [ 9 , 12 , 14 – 18 , 27 , 28 ]. We did not include a study or comparison if tests of statistical significance were not reported. Further, we only included comparisons that used a continuous performance metric as their dependent variable (i.e., grades on a 0-4 scale or test scores). Comparisons that used non-performance based dependent variables (e.g., drop or withdrawal) were not included, as they are not suitable for use as measures of learning. Some studies (e.g., [ 15 ]) examined both grades and pass rates separately, which is a dichotomous version of grade (i.e., C- or better.). As an aside, it is not clear to us why both measures are sometimes used, as the measures are likely highly correlated. In cases where both measures were used, we only included comparisons on course grade. We did not examine studies that only examined pass rates, because these studies use non-parametric statistics which are not applicable to the power analysis we conducted. Also, several studies conducted both an analysis which collapsed across different courses or semesters, and then conducted separate analyses for each of these levels [ 16 , 27 ]. In these cases, we only included each separate analysis, but not the overall analyses. In the case of [ 18 ], they collapsed across multiple courses without conducting separate analysis for those courses. In this case, we only included the overall analysis. The complete list of comparisons is shown on Table 1 .
https://doi.org/10.1371/journal.pone.0212508.t001
Simulation power analysis.
For each of the prior comparisons, we conducted 10,000 simulations using the same sample sizes reported by the authors. Since there is no way of determining the true access rate of the samples used in these comparisons, we used a range of a values (40%, 60%, and 80%). All other assumptions of the prior simulations were the same ( μ = 70, σ = 20, d = .25, α = .05), with one exception. We noted that many of the prior studies under consideration had imbalanced numbers of Non-OER and OER students, typically with far more Non-OER students than OER students. Rather than assuming equal sample sizes like in the previous simulations, we matched the sample size allocation ratio of the comparison study in the simulations. For example, the study in [ 15 ] reported one comparison with 4615 students, but 4531 in the Non-OER condition, and 84 students in the OER condition. In our simulations of this study, we drew samples of 4615 students, and allocated 98.2% to the Non-OER condition, and 1.8% to OER condition.
The estimated power for each comparison, for access rates of 40%, 60%, and 80%, are shown on the far right columns of Table 1 . We can see that for most comparisons, even under the most optimistic of scenarios (i.e., 40% access), the expected likelihood that the comparison would yield a positive significant effect of OER is very small. Only the comparisons which had very large sample sizes had substantial power at the 40% access level [ 16 , 18 ], though even some of these comparisons had low power at 80% access rates. Note that for many studies, power is so low at the 80% access level that the probability of correctly rejecting the null hypothesis is just as likely as falsely rejecting a true null hypothesis ( α = .05)! Thus, if there was an 80% access rate, these experiments were just as likely to detect a real effect of OER as they were to detect a false effect of OER. Interestingly, one comparison [ 15 ] had low power even with a sample size over 4000 students. This was due to the extreme imbalance of students in the Non-OER and OER conditions.
Given the results of this power analysis, we can determine the expected number of comparisons that should have correctly rejected the null hypothesis by summing the power values for each level of access. Across the 42 reported comparisons, we would only expect to observe significant effects of OER 18, 11.5, or 5.2 times, for the 40%, 60%, and 80% access rates, respectively. Note that only 9 of the 42 comparisons on Table 1 found positive effects of OER on learning outcomes. Even though this number seems very low, the results of the simulation power analysis demonstrate that this is well aligned with what should be expected, even if there is a real effect of OER. Of course, our power estimates assume perfect conditions. These real world studies have many confounding factors to contend with, so it is likely that the real power of these studies was even lower than what we estimated. In this case, it is possible that the number of significant effects found so far could even be higher than what would be expected.
Over the last decade, there has been a fair amount of research examining whether the adoption of OER textbooks improves student learning outcomes relative to commercial textbooks. The majority of this research has found no significant difference between OER and commercial texts when measuring learning performance. We have argued that one possible reason why most tests of OER efficacy fail stems from the predictions of the access hypothesis. The access hypothesis, formally introduced by us, states that OER might improve learning outcomes relative to traditional course materials by improving access to the textbook. Therefore, an OER intervention should only affect a subset of students who would not have access to the textbook. Through the use of simulated power experiments, we have demonstrated that the textbook access rate of a research sample prior to the intervention has profound effects on statistical power. As the access rate of a sample increases, the power of an experiment decreases dramatically. If the access rate is high, even studies with very large sample sizes should produce null results most of the time.
Overall, our analysis helps to provide better context to the studies that have examined OER efficacy. Even under ideal conditions, detecting positive effects of OER should be extremely difficult. The fact that most studies have found null effects is not surprising; in fact, these null effects are expected. Furthermore, our results stress the importance of being skeptical of studies that report positive effects of OER interventions. This is especially true if the study used relatively small sample sizes. In our simulation experiments, even comparisons with 1000 students are more likely to discover null effects than positive ones, even with access rates at the low end of the scale.
Implications for OER research
These results have several implications for future research in OER. First, we recommend that researchers attempt to measure textbook access rates in their student population prior to implementation of OER. If access rates are very high, it is important to consider that the likelihood of detecting an effect on learning outcomes should be very low. The effect of access rates should be considered when interpreting null results.
Second, it is critical that future research works towards determining the true effect size of textbook access on learning. Determining the true effect size will afford far more reliable power calculations, and more importantly, enable more meaningful interpretation of research studies. For instance, a high powered study that produces a null result is more meaningful than a low powered study that produces a null result, because the null result is unexpected in the case of a high powered study. Unfortunately, accurate measures of power require a reliable measure of effect size, and the vast majority of studies on OER efficacy do not report enough statistics in their analyses for computation of effect size estimates. It is critical that researchers report all relevant test statistics, p-values, sample sizes, means, and measures of dispersion. We encourage reviewers and editors of future research insist that authors report these measures.
Finally, it is common for OER researchers to conduct comparisons without making an explicit hypothesis or prediction. Hypotheses and predictions are critical, because they help guide research designs and interpretation of results. In the case of the access hypothesis, having an explicit mechanism makes it clear that the intervention should only affect some students. We cannot help but wonder if so many low power null effects would have been published had the access hypothesis been formally proposed earlier.
Potential theoretical mechanisms for OER efficacy
In this paper, we have discussed access as being the primary mechanism for why OER might improve learning. It is certainly possible that adoption of OER could affect learning outcomes in other ways. One idea is that the open nature of OER affords the ability to teach in ways that are not possible given the constraints of closed source materials [ 7 ]. Another idea is that OER may provide better or worse quality than the commercial counterpart (e.g., [ 17 ]). However, as mentioned previously, these ideas are rarely expressed as a formal hypothesis, and the mechanisms are rarely tested as part of the research. One exception is the work of [ 17 ], which compared learning outcomes from an OER and commercial textbook, but also examined the perceived quality and readability of the books. While differences in perceived quality and readability were observed, these differences did not translate into strong benefits to learning [ 17 ]. It should be noted that other mechanisms would not be subject to the same power constraints as access, as these mechanisms would presumably affect all students in the study. Thus, detecting quality difference effects, for example, should require far fewer students than access effects.
With regards to the access hypothesis, we made the assumption throughout this paper that students who have access to the textbook would use the textbook in effective ways. Of course, access is not a guarantee for learning. A student with access to a textbook could easily choose to ignore it or engage in ineffective learning strategies. These students are no better off with a textbook than they were without one. This fact creates a general boundary condition on the ability for access alone to affect learning. Practically speaking, the effect of access on learning depends critically on usage after access to the materials is supplied. If students engage with the book in ineffective ways, then access will be an irrelevant factor. To this end, we simply caution readers that while access is an important step towards improving learning, it not sufficient.
Limitations
It is important to point out that our simulated experiments provide only a proxy measure of statistical power. In particular, these simulations estimate power under an unrealistically optimistic experimental scenario. The situation only gets more difficult in real world studies, which have instructor effects, student effects, and other confounds to control for. These variables only add noise to the data, and reduce this probability of success even further. Thus, a researcher hoping to estimate their statistical power with a real-world data should understand that their actual power will be lower than those shown in Fig 2 .
Another limitation of our analysis of past research studies is that we assumed an effect size d of 0.25, rather than computing the observed effect sizes post hoc. Unfortunately, as previously mentioned, the vast majority of research we reviewed did not report sufficient statistics to conduct such analysis. If the true effect size of OER adoption is larger, then these studies may have had considerably more statistical power than what we estimated. To this end, we conducted a supplemental analysis which estimates the minimum effect size required in order for an OER study of varying sample sizes and access rates to achieve an acceptable level of power. This analysis is explained in detail in S1 Appendix , and the results shown on S1 Fig . Should additional research become available that suggests the effect size is different than the one we used, S1 Fig can be used to determine whether power of these past studies was adequate. Also, we remind readers that the source code of our analysis is available such that anyone rerun our analysis with varying levels of d .
Relevance to educational research
While it is tempting to think that the research failings discussed in this paper are unique to OER, the reality is that these failings are the result of a common mistake in educational research (and even social science research more broadly). Specifically, that mistake is overgeneralizing the influence of an experimental variable without critically considering the context in which that variable is manipulated. The importance of contextual factors was articulated decades ago by [ 33 , 34 ], who noted the fragile nature of many of the most landmark findings in memory research (e.g., levels of processing [ 35 ]). In particular, it was observed that minor changes to an experimental design could completely change the outcome of a manipulation. The critical insight of [ 33 ] was that variables not manipulated by the experimenter are just as important as the ones that were manipulated. The materials used, the final assessment, the types of students, and the interactions among all these factors were all critically important. Thus, if one wants to understand whether an intervention affects learning, they need to be aware of the context in which that intervention is taking place.
Of course, researchers and practitioners are naturally compelled to focus only on variables of interest in isolation. To illustrate, one of the most influential studies in education is a meta-analysis of over 800 factors that affect student learning [ 36 ]. While compiling such an extensive list of factors is quite the achievement, in our view, it presents an unrealistic view about the nature of learning. It leads one to a misplaced belief that certain techniques are better than others. However, even the strongest factors listed by [ 36 ] could quite easily be rendered ineffective by applying them to certain topics, certain populations of students, or certain outcome measures. For example, it is well known that effectiveness of an educational strategy or intervention can depend on the prior aptitude of individual’s in a study [ 37 ]. The very existence of such interactions prevents us as a field from ever discovering “laws” of human learning [ 38 ] or making broad sweeping claims about any intervention. In sum, the effectiveness of any educational intervention will almost always depend on the context in which it is implemented.
Failing to consider the importance of context can lead to poor study design and misleading conclusions. In this paper, we discussed the importance of student access in moderating the effectiveness of OER. Past researchers assumed OER would have a general effect on learning and failed to context influences, which lead to a dearth of under powered and ill designed studies. A similar analogue comes from the oft maligned enterprise of media comparison studies [ 39 – 42 ]. Media comparison studies typically evaluate student learning from a standard instructional strategy delivered on different types of “media” (e.g., computer vs. paper). Like OER, most of these studies have produced null results, and have been vehemently criticized for decades as being without merit [ 39 ]. Indeed, [ 39 ] took a strong stance that media is only a vehicle for delivering instructional strategies, and that media itself will never influence learning. While this is often true, others [ 41 , 42 ] have argued that many media comparison studies employed standardized research designs that were not well suited to measure the unique mechanisms afforded by media evaluated in the study. [ 43 ] reviews a wide variety of media studies which reveal the nuances of when media can have a meaningful influence on student outcomes. Thus, by carefully considering the context in which an intervention occurs and is evaluated and devising appropriate hypothesis to test, one can design studies that effectively and appropriately measure the unique merits of the intervention.
Conclusions
The goal of educational research is to answer important questions about education through scientific analysis. However, studies that are not grounded in theory or lack statistical power do not provide meaningful insights for answering these questions. On the contrary, such studies only muddy the waters, and move us further from determining the truth. Despite the large number of studies that have been conducted on OER efficacy, these studies unfortunately do not provide much information about the potential impacts of OER on student learning. While the large number of null effects may suggest that OER adoption may not provide much benefit to student learning, the reality is these studies do not provide much insight, because they were incapable of detecting positive effects even if they did exist. As it currently stands, the question of whether OER affects student learning remains unanswered.
Supporting information
S1 appendix. supplemental simulation analysis..
https://doi.org/10.1371/journal.pone.0212508.s001
S1 Fig. Minimum effect size required to detect an effect of OER at 80% success rate as a function of access rate and sample size.
For a given value of a , the minimum value of d necessary to detect an effect of OER is very sensitive to sample sizes n below 1000. Conversely, for a given value of n , the minimum value of d is extremely sensitive to the access rate.
https://doi.org/10.1371/journal.pone.0212508.s002
Acknowledgments
The authors would like to thank Micaela McGlone for her project management support on this project.
- 1. Perry MJ. Chart of the Day: The Astronomical Rise in College Textbook Prices vs. Consumer Prices and Recreational Books; 2016. Retrieved from http://www.aei.org/publication/chart-of-the-day-the-astronomical-rise-in-college-textbook-prices-vs-consumer-prices-and-recreational-books/ .
- View Article
- Google Scholar
- PubMed/NCBI
- 4. Ruth D. 48 Percent of Colleges, 2.2 Million Students Using Free OpenStax Textbooks This Year; 2018. Retrieved from: https://openstax.org/press/48-percent-colleges-22-million-students-using-free-openstax-textbooks-year .
- 5. Seaman JE, Seaman J. Opening the Textbook: Educational Resources in U.S. Higher Education, 2017; 2017. Retrieved from https://www.onlinelearningsurvey.com/reports/openingthetextbook2017.pdf .
- 6. Perry MJ. Wednesday Afternoon Links; 2017. Retrieved from http://www.aei.org/publication/wednesday-afternoon-links-30/ .
- 9. Allen G, Guzman-Alvarez A, Molinaro M, Larsen DS. Assessing the Impact and Efficacy of the Open-Access ChemWiki Textbook Project; 2015. Retrieved from https://library.educause.edu/resources/2015/1/assessing-the-impact-and-efficacy-of-the-openaccess-chemwiki-textbook-project .
- 10. Bowen WG, Chingos MM, Lack KA, Nygren TI. Interactive Learning Online at Public Universities: Evidence from Randomized Trials; 2012. Retrieved from http://mitcet.mit.edu/wpcontent/uploads/2012/05/BowenReport-2012.pdf .
- 14. Robinson TJ. The Effects of Open Educational Resource Adoption on Measures of Post-Secondary Student Success [Unpublished Dissertation]. Brigham Young University; 2015.
- 20. Hilton J, Wiley D, Fischer L, Nyland R. Guidebook to Research on Open Educational Resources Adoption. Retrieved from http://openedgroup.org/wp-content/uploads/2016/08/OER-Research-Guidebook.pdf ; 2016.
- 21. Florida Virtual Campus. 2016 Student Textbook and Course Materials Survey; 2016. Retrieved from http://www.openaccesstextbooks.org/pdf/2016_Florida_Student_Textbook_Survey.pdf .
- 26. Cohen J. Statistical Power Analysis for the Behavioural Sciences. 2nd ed. Hillsdale, NJ: Erlbaum; 1988.
- 30. Institute of Education Sciences, U S Department of Education. What Works Clearinghouse, What Works Clearinghouse Procedures Handbook (Version 4.0). Retrieved from http://whatworks.ed.gov ; 2017, October.
- 31. R Core Team. R: A Language and Environment for Statistical Computing; 2017. Available from: https://www.R-project.org/ .
- 32. Coppock A. Power Analysis Simulations in R; 2013. Retrieved from http://egap.org/content/power-analysis-simulations-r .
- 33. Jenkins JJ. Four points to remember: a tetrahedral model of memory experiments. In: Craik FM, Cermak L, editors. Levels of Processing in Human Memory. Hillsdale, NJ: Erlbaum; 1979. p. 429–446.
- 36. Hattie J. Visible learning: A synthesis of over 800 meta-analyses relating to achievement. Abingdon, Oxon: Routledge; 2008.
- 37. Cronbach L, Snow R. Aptitudes and Instructional Methods: A Handbook for Research on Interactions. Oxford, England: Irvington; 1977.
Greater Good Science Center • Magazine • In Action • In Education
Parenting & Family Articles & More
Our best education articles of 2020, readers and editors pick the most interesting and insightful articles from the past year about teaching, learning, and the keys to well-being at school..
In February of 2020, we launched the new website Greater Good in Education , a collection of free, research-based and -informed strategies and practices for the social, emotional, and ethical development of students, for the well-being of the adults who work with them, and for cultivating positive school cultures. Little did we know how much more crucial these resources would become over the course of the year during the COVID-19 pandemic.
Now, as we head back to school in 2021, things are looking a lot different than in past years. Our most popular education articles of 2020 can help you manage difficult emotions and other challenges at school in the pandemic, all while supporting the social-emotional well-being of your students.
In addition to these articles, you can also find tips, tools, and recommended readings in two resource guides we created in 2020: Supporting Learning and Well-Being During the Coronavirus Crisis and Resources to Support Anti-Racist Learning , which helps educators take action to undo the racism within themselves, encourage their colleagues to do the same, and teach and support their students in forming anti-racist identities.
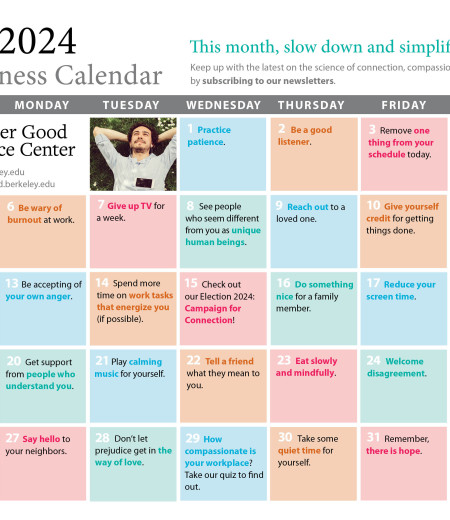
Here are the 10 best education articles of 2020, based on a composite ranking of pageviews and editors’ picks.
Can the Lockdown Push Schools in a Positive Direction? , by Patrick Cook-Deegan: Here are five ways that COVID-19 could change education for the better.
How Teachers Can Navigate Difficult Emotions During School Closures , by Amy L. Eva: Here are some tools for staying calm and centered amid the coronavirus crisis.
Six Online Activities to Help Students Cope With COVID-19 , by Lea Waters: These well-being practices can help students feel connected and resilient during the pandemic.
Help Students Process COVID-19 Emotions With This Lesson Plan , by Maurice Elias: Music and the arts can help students transition back to school this year.
How to Teach Online So All Students Feel Like They Belong , by Becki Cohn-Vargas and Kathe Gogolewski: Educators can foster belonging and inclusion for all students, even online.
How Teachers Can Help Students With Special Needs Navigate Distance Learning , by Rebecca Branstetter: Kids with disabilities are often shortchanged by pandemic classroom conditions. Here are three tips for educators to boost their engagement and connection.
How to Reduce the Stress of Homeschooling on Everyone , by Rebecca Branstetter: A school psychologist offers advice to parents on how to support their child during school closures.
Three Ways to Help Your Kids Succeed at Distance Learning , by Christine Carter: How can parents support their children at the start of an uncertain school year?
How Schools Are Meeting Social-Emotional Needs During the Pandemic , by Frances Messano, Jason Atwood, and Stacey Childress: A new report looks at how schools have been grappling with the challenges imposed by COVID-19.
Six Ways to Help Your Students Make Sense of a Divisive Election , by Julie Halterman: The election is over, but many young people will need help understanding what just happened.
Train Your Brain to Be Kinder (video), by Jane Park: Boost your kindness by sending kind thoughts to someone you love—and to someone you don’t get along with—with a little guidance from these students.
From Othering to Belonging (podcast): We speak with john a. powell, director of the Othering & Belonging Institute, about racial justice, well-being, and widening our circles of human connection and concern.
About the Author
Greater good editors, you may also enjoy.

This article — and everything on this site — is funded by readers like you.
Become a subscribing member today. Help us continue to bring “the science of a meaningful life” to you and to millions around the globe.
Publications
Academic articles, employer learning and the dynamics of returns to universities: evidence from chinese elite education during university expansion, contexts of convenience: generalizing from published evaluations of school finance policies, some evolving issues in k–12 education, global universal basic skills: current deficits and implications for world development, performance information and personnel decisions in the public sector: the case of school principals, academic mobility in u.s. public schools: evidence from nearly 3 million students, incidence and outcomes of school finance litigation: 1968-2021, u.s. school finance: resources and outcomes, long-run trends in the u.s. ses-achievement gap, patience, risk-taking, and human capital investment across countries, residential location and education in the united states, the political economy of ilsas in education: the role of knowledge capital in economic growth, addressing cross-national generalizability in educational impact evaluation, united states: the uphill schools’ struggle, a quantitative look at the economic impact of the european union’s educational goals, education, knowledge capital, and economic growth, quality education and economic development, education production functions, the value of smarter teachers: international evidence on teacher cognitive skills and student performance, knowledge capital and economic growth, testing, accountability and the american economy, volkswirtschaftliche folgen von bildungsarmut: was ein entwicklungsziel „grundkompetenzen für alle“ erreichen könnte, education and the growth-equity trade-off, missouri's economic future lies with school reform, economic gains from educational reform by us states, knowledge capital and aggregate income differences: development accounting for u.s. states, defining productivity in education: issues and illustrations, coping with change: international differences in the returns to skills, general education, vocational education, and labor-market outcomes over the life-cycle, school resources and student achievement: a review of cross-country economic research, dynamic effects of teacher turnover on the quality of instruction, skills, mobility, and growth, will more higher education improve economic growth, knowledge capital, growth, and the east asian miracle, school human capital and teacher salary policies, land use controls, fiscal zoning, and the local provision of education, why standard measures of human capital are misleading, the economic impact of educational quality, economics of education, returns to skills around the world: evidence from piaac, is location fate distributional aspects of schooling, institutional structures of the education system and student achievement: a review of cross-country economic research, borrowing constraints, college aid, and intergenerational mobility, school resources and educational outcomes in developing countries: a review of the literature from 1990 to 2010, economic growth in developing countries: the role of human capital, schools and location: tiebout, alonso, and governmental finance policy, financing schools, does school autonomy make sense everywhere panel estimates from pisa, do better schools lead to more growth cognitive skills, economic outcomes, and causation.
- Research Guides
- Vanderbilt University Libraries
- Peabody Library
- PreK-12 Education (Teaching & Learning) Research
- Finding Scholarly Articles
PreK-12 Education (Teaching & Learning) Research: Finding Scholarly Articles
- About Research
- Education Reference
- Finding Books
- Finding Statistics and Data
- Citing Sources (APA) This link opens in a new window
- Peabody Library Test Collection This link opens in a new window
- Curriculum Materials Center This link opens in a new window
- Academic Standards
- Praxis Study Guides
What is a Scholary, Academic, or Peer-Reviewed Journal?
A scholarly, academic, or peer-reviewed journal is a published collection of articles written by scholars in the field. The articles present original research and undergo a peer-review process before publication. Before the article can be published, it is vetted by other scholars for quality and accuracy according to the journal's editorial standards (i.e. the peer-review process). To find these articles, you can browse by journal title listed in the Scholarly Journals in Education (listed by subject) below. To do a targeted search on a topic, you will want to search in the databases listed below.
Browse the Latest Research in Education
Associations & research centers.
Professional associations and research centers are good sites to find research-based information outside of the typical publication stream. Scholars and practitioners in the field usually produce the content found on these sites.
- International Literacy Association
- National Council for the Social Studies
- National Council of Teachers of English
- National Council of Teachers of Mathematics
- National Science Teachers Association
Databases for finding Journal Articles
The following databases will help you find scholarly articles on education topics:
- Education Full Text This link opens in a new window Articles, periodicals, yearbooks, series, and supplements on special education, educational tests, adult education, multicultural/ethnic education, teaching methods, continuing education, literacy standards, multicultural/ethnic education, etc. more... less... Coverage: 1929 to present. Full text begins 1996. User Limit: 4.
- ERIC (ProQuest version) This link opens in a new window Journal articles, conferences, meetings, government documents, theses, dissertations, reports, audiovisual media, bibliographies, directories, books and monographs on education-related literature. more... less... Coverage: 1966 to present.
- Child Care and Early Education Research Connections This link opens in a new window Research in child care and early education and promotion of that research in policy making.
Depending on your topic, you may need to broaden your search to include research from other areas such as psychology or history. Here are databases that include scholarly research adjacent to education:
- America: History and Life This link opens in a new window Index of literature covering the history and culture of the United States and Canada. Journal articles, book titles, book reviews, and dissertations. more... less... Coverage: 1910 to present. User Limit: 6.
- Google Scholar Vanderbilt Login This link opens in a new window Use this link to seamlessly access VU resources via Google Scholar both on and off campus. You can also adjust your Google Scholar settings to display the Vanderbilt Findit@VU link to appear in search results and allow for access from on or off-campus. Access Google Scholar settings by visiting this page (https://scholar.google.com/scholar_settings). Click library links on the left hand menu. in the search box, search for Vanderbilt and select: “Vanderbilt University Library – Findit@VU” (with uppercase F), Click Save. more... less... Vendor Tutorials: Add VU to your Google profile - https://scholar.google.com/scholar_settings
- Linguistics and Language Behavior Abstracts (LLBA) This link opens in a new window Abstracts and indexing of all areas of linguistics. Applied linguistics, psycholinguistics, history and philosophy of language, syntax and morphology, lexicography, sociolinguistics, nonverbal communication, phonetics, learning disabilities, hearing and speech physiology. more... less... Coverage: 1973 to present.
- ProQuest This link opens in a new window Search all ProQuest databases simultaneously. Includes arts, humanities, social sciences, news, and science and technology.
- PsycINFO This link opens in a new window APA PsycINFO is a comprehensive indexing and abstracts of the international psychological literature. Journals, articles, books, dissertations, etc. Subjects include: applied psychology, communication systems, developmental psychology, educational psychology, experimental human and animal psychology, personality, psychological and physical disorders, physiological psychology and neuroscience, professional personnel and issues, psychometrics and statistics, social psychology, social processes and issues, sports psychology and leisure, treatment and prevention. more... less... Coverage: 1800s to present.
- Web of Science: Social Sciences Citation Index (SSCI) This link opens in a new window Social sciences journals, and science and technical journals related to the social sciences. Complete bibliographic data, author abstracts, and references cited. more... less... Coverage: 1900-present.
Scholarly Journals in Education
- ELA and Literacy Education
- Math Education
- Music Education
- Science Education
- Social Studies Education
- Annals of Dyslexia
- Early Childhood Research Quarterly
- Educational Researcher
- Elementary School Journal
- Gifted Child Quarterly
- Harvard Educational Review
- Journal of Adolescent & Adult Literacy
- Journal of Early Childhood Literacy
- Journal of Educational Psychology
- Journal of Learning Disabillities
- Journal of Literacy Research
- Journal of Research in Reading
- Language Arts
- Literacy, Research & Instruction
- Reading & Writing Quarterly
- Reading Research Quarterly
- Reading Teacher
- Research in the Teaching of English
- Scientific Studies of Reading
- Voices From the Middle
- Yearbook of the Literacy Research Association Prior to 2011, this title was known as 'Yearbook of the National Reading Conference'. Copies of the earlier years are on the shelves with the newer volumes.
- American Educational Research Journal
- Cognition & Instruction
- Educational Studies in Mathematics
- Journal for Research in Mathematics Education
- Journal of Curriculum Studies
- Journal of Mathematical Behavior
- Journal of Mathematics Education at Teachers College
- Journal of the Learning Sciences
- Journal of Urban Mathematics Education
- Mathematical Thinking & Learning
- Teachers College Record
- Urban Education
- ZDM = International reviews on mathematical education
These following journals do not have empirical research but could have articles on related topics and cite empirical research:
- Mathematics Teacher
- Mathematics Teaching in the Middle School
- British Journal of Music Education (BJME)
- Bulletin of the Council for Research in Music Education.
- Contributions to Music Education
- General Music Today
- International Journal of Music Education (IJME)
- International Journal of Research in Choral Singing
- Journal of Band Research
- Journal of General Music Education
- Journal of Music, Technology, and Education
- Journal of Research in Music Education (JRME)
- Music Educators Journal
- Update: Applications of Research in Music Education
- International Journal of Science Education
- Journal of Research in Science Teaching
- Journal of Science Teacher Education
- School Science & Mathematics
- Science & Children
- Science Scope
- Critical Education
- The Geography Teacher
- The Journal of Geography
- Journal of Social Studies Research
- Learning for Democracy
- Social Education
- The Social Studies
- Social Studies and the Young Learner
- Social Studies Research and Practice
- Theory & Research in Social Education
- << Previous: Finding Books
- Next: Finding Statistics and Data >>

- Last Updated: Dec 7, 2023 2:08 PM
- URL: https://researchguides.library.vanderbilt.edu/education
Advertisement
Artificial Intelligence in Education (AIEd): a high-level academic and industry note 2021
- Original Research
- Open access
- Published: 07 July 2021
- Volume 2 , pages 157–165, ( 2022 )
Cite this article
You have full access to this open access article
- Muhammad Ali Chaudhry ORCID: orcid.org/0000-0003-0154-2613 1 &
- Emre Kazim 2
39k Accesses
52 Citations
24 Altmetric
Explore all metrics
In the past few decades, technology has completely transformed the world around us. Indeed, experts believe that the next big digital transformation in how we live, communicate, work, trade and learn will be driven by Artificial Intelligence (AI) [ 83 ]. This paper presents a high-level industrial and academic overview of AI in Education (AIEd). It presents the focus of latest research in AIEd on reducing teachers’ workload, contextualized learning for students, revolutionizing assessments and developments in intelligent tutoring systems. It also discusses the ethical dimension of AIEd and the potential impact of the Covid-19 pandemic on the future of AIEd’s research and practice. The intended readership of this article is policy makers and institutional leaders who are looking for an introductory state of play in AIEd.
Similar content being viewed by others
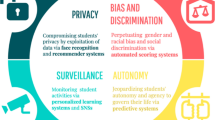
Artificial intelligence in education: Addressing ethical challenges in K-12 settings
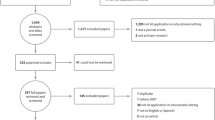
Systematic review of research on artificial intelligence applications in higher education – where are the educators?
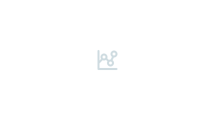
Evolution and Revolution in Artificial Intelligence in Education
Avoid common mistakes on your manuscript.
1 Introduction
Artificial Intelligence (AI) is changing the world around us [ 42 ]. As a term it is difficult to define even for experts because of its interdisciplinary nature and evolving capabilities. In the context of this paper, we define AI as a computer system that can achieve a particular task through certain capabilities (like speech or vision) and intelligent behaviour that was once considered unique to humans [ 54 ]. In more lay terms we use the term AI to refer to intelligent systems that can automate tasks traditionally carried out by humans. Indeed, we read AI within the continuation of the digital age, with increased digital transformation changing the ways in which we live in the world. With such change the skills and knowhow of people must reflect the new reality and within this context, the World Economic Forum identified sixteen skills, referred to as twenty-first century skills necessary for the future workforce [ 79 ]. This includes skills such as technology literacy, communication, leadership, curiosity, adaptability, etc. These skills have always been important for a successful career, however, with the accelerated digital transformation of the past 2 years and the focus on continuous learning in most professional careers, these skills are becoming necessary for learners.
AI will play a very important role in how we teach and learn these new skills. In one dimension, ‘AIEd’ has the potential to dramatically automate and help track the learner’s progress in all these skills and identify where best a human teacher’s assistance is needed. For teachers, AIEd can potentially be used to help identify the most effective teaching methods based on students’ contexts and learning background. It can automate monotonous operational tasks, generate assessments and automate grading and feedback. AI does not only impact what students learn through recommendations, but also how they learn, what are the learning gaps, which pedagogies are more effective and how to retain learner’s attention. In these cases, teachers are the ‘human-in-the-loop’, where in such contexts, the role of AI is only to enable more informed decision making by teachers, by providing them predictions about students' performance or recommending relevant content to students after teachers' approval. Here, the final decision makers are teachers.
Segal et al. [ 58 ] developed a system named SAGLET that utilized ‘human-in-the-loop’ approach to visualize and model students’ activities to teachers in real-time enabling them to intervene more effectively as and when needed. Here the role of AI is to empower the teachers enabling them to enhance students’ learning outcomes. Similarly, Rodriguez et al. [ 52 ] have shown how teachers as ‘human-in-the-loop’ can customize multimodal learning analytics and make them more effective in blended learning environments.
Critically, all these achievements are completely dependent on the quality of available learner data which has been a long-lasting challenge for ed-tech companies, at least until the pandemic. Use of technology in educational institutions around the globe is increasing [ 77 ], however, educational technology (ed-tech) companies building AI powered products have always complained about the lack of relevant data for training algorithms. The advent and spread of Covid in 2019 around the world pushed educational institutions online and left them at the mercy of ed-tech products to organize content, manage operations, and communicate with students. This shift has started generating huge amounts of data for ed-tech companies on which they can build AI systems. According to a joint report: ‘Shock to the System’, published by Educate Ventures and Cambridge University, optimism of ed-tech companies about their own future increased during the pandemic and their most pressing concern became recruitment of too many customers to serve effectively [ 15 ].
Additionally, most of the products and solutions provided by ed-tech start-ups lack the quality and resilience to cope with intensive use of several thousands of users. Product maturity is not ready for huge and intense demand as discussed in Sect. “ Latest research ” below. We also discuss some of these products in detail in Sect. “ Industry’s focus ” below. How do we mitigate the risks of these AI powered products and who monitors the risk? (we return to this theme in our discussion of ethics—Sect. “ Ethical AIEd ”).
This paper is a non-exhaustive overview of AI in Education that presents a brief survey of the latest developments of AI in Education. It begins by discussing different aspects of education and learning where AI is being utilized, then turns to where we see the industry’s current focus and then closes with a note on ethical concerns regarding AI in Education. This paper also briefly evaluates the potential impact of the pandemic on AI’s application in education. The intended readership of this article is the policy community and institutional executives seeking an instructive introduction to the state of play in AIEd. The paper can also be read as a rapid introduction to the state of play of the field.
2 Latest research
Most work within AIEd can be divided into four main subdomains. In this section, we survey some of the latest work in each of these domains as case studies:
Reducing teachers’ workload: the purpose of AI in Education is to reduce teachers’ workload without impacting learning outcomes
Contextualized learning for students: as every learner has unique learning needs, the purpose of AI in Education is to provide customized and/or personalised learning experiences to students based on their contexts and learning backgrounds.
Revolutionizing assessments: the purpose of AI in Education is to enhance our understanding of learners. This not only includes what they know, but also how they learn and which pedagogies work for them.
Intelligent tutoring systems (ITS): the purpose of AI in Education is to provide intelligent learning environments that can interact with students, provide customized feedback and enhance their understanding of certain topics
2.1 Reducing teachers’ workload
Recent research in AIEd is focusing more on teachers than other stakeholders of educational institutions, and this is for the right reasons. Teachers are at the epicenter of every learning environment, face to face or virtual. Participatory design methodologies ensure that teachers are an integral part of the design of new AIEd tools, along with parents and learners [ 45 ]. Reducing teachers’ workload has been a long-lasting challenge for educationists, hoping to achieve more affective teaching in classrooms by empowering the teachers and having them focus more on teaching than the surrounding activities.
With the focus on online education during the pandemic and emergence of new tools to facilitate online learning, there is a growing need for teachers to adapt to these changes. Importantly, teachers themselves are having to re-skill and up-skill to adapt to this age, i.e. the new skills that teachers need to develop to fully utilize the benefits of AIEd [ 39 ]. First, they need to become tech savvy to understand, evaluate and adapt new ed-tech tools as they become available. They may not necessarily use these tools, but it is important to have an understanding of what these tools offer and if they share teachers’ workload. For example, Zoom video calling has been widely used during the pandemic to deliver lessons remotely. Teachers need to know not only how to schedule lessons on Zoom, but also how to utilize functionalities like breakout rooms to conduct group work and Whiteboard for free style writing. Second, teachers will also need to develop analytical skills to interpret the data that are visualized by these ed-tech tools and to identify what kind of data and analytics tools they need to develop a better understanding of learners. This will enable teachers to get what they exactly need from ed-tech companies and ease their workload. Third, teachers will also need to develop new team working, group and management skills to accommodate new tools in their daily routines. They will be responsible for managing these new resources most efficiently.
Selwood and Pilkington [ 61 ] showed that the use of Information and Communication Technologies (ICT) leads to a reduction in teachers’ workload if they use it frequently, receive proper training on how to use ICT and have access to ICT in home and school. During the pandemic, teachers have been left with no options other than online teaching. Spoel et al. [ 76 ] have shown that the previous experience with ICT did not play a significant role in how they dealt with the online transition during pandemic. Suggesting that the new technologies are not a burden for teachers. It is early to draw any conclusions on the long-term effects of the pandemic on education, online learning and teachers’ workload. Use of ICT during the pandemic may not necessarily reduce teacher workload, but change its dynamics.
2.2 Contextualized learning for students
Every learner has unique learning contexts based on their prior knowledge about the topic, social background, economic well-being and emotional state [ 41 ]. Teaching is most effective when tailored to these changing contexts. AIEd can help in identifying the learning gaps in each learner, offer content recommendations based on that and provide step by step solutions to complex problems. For example, iTalk2Learn is an opensource platform that was developed by researchers to support math learning among students between 5 and 11 years of age [ 22 ]. This tutor interacted with students through speech, identified when students were struggling with fractions and intervened accordingly. Similarly, Pearson has launched a calculus learning tool called AIDA that provides step by step guidance to students and helps them complete calculus tasks. Use of such tools by young students also raises interesting questions about the illusion of empathy that learners may develop towards such educational bots [ 73 ].
Open Learner Models [ 12 , 18 ] have been widely used in AIEd to facilitate learners, teachers and parents in understanding what learners know, how they learn and how AI is being used to enhance learning. Another important construct in understanding learners is self-regulated learning [ 10 , 68 ]. Zimmerman and Schunk [ 85 ] define self-regulated learning as learner’s thoughts, feelings and actions towards achieving a certain goal. Better understanding of learners through open learner models and self-regulated learning is the first step towards contextualized learning in AIEd. Currently, we do not have completely autonomous digital tutors like Amazon’s Alexa or Apple’s Siri for education but domain specific Intelligent Tutoring Systems (ITS) are also very helpful in identifying how much students know, where they need help and what type of pedagogies would work for them.
There are a number of ed-tech tools available to develop basic literacy skills in learners like double digit division or improving English grammar. In future, AIEd powered tools will move beyond basic literacy to develop twenty-first century skills like curiosity [ 49 ], initiative and creativity [ 51 ], collaboration and adaptability [ 36 ].
2.3 Revolutionizing assessments
Assessment in educational context refers to ‘any appraisal (or judgement or evaluation) of a student’s work or performance’ [ 56 ]. Hill and Barber [ 27 ] have identified assessments as one of the three pillars of schooling along with curriculum and learning and teaching. The purpose of modern assessments is to evaluate what students know, understand and can do. Ideally, assessments should take account of the full range of student abilities and provide useful information about learning outcomes. However, every learner is unique and so are their learning paths. How can standardized assessment be used to evaluate every student, with distinct capabilities, passions and expertise is a question that can be posed to broader notions of educational assessment. According to Luckin [ 37 ] from University College London, ‘AI would provide a fairer, richer assessment system that would evaluate students across a longer period of time and from an evidence-based, value-added perspective’.
AIAssess is an example of an intelligent assessment tool that was developed by researchers at UCL Knowledge lab [ 38 , 43 ]. It assessed students learning math and science based on three models: knowledge model, analytics model and student model. Knowledge component stored the knowledge about each topic, the analytics component analyzed students’ interactions and the student model tracked students’ progress on a particular topic. Similarly, Samarakou et al. [ 57 ] have developed an AI assessment tool that also does qualitative evaluation of students to reduce the workload of instructors who would otherwise spend hours evaluating every exercise. Such tools can be further empowered by machine learning techniques such as semantic analysis, voice recognition, natural language processing and reinforcement learning to improve the quality of assessments.
2.4 Intelligent tutoring systems (ITS)
An intelligent tutoring system is a computer program that tries to mimic a human teacher to provide personalized learning to students [ 46 , 55 ]. The concept of ITS in AIEd is decades old [ 9 ]. There have always been huge expectations from ITS capabilities to support learning. Over the years, we have observed that there has been a significant contrast between what ITS were envisioned to deliver and what they have actually been capable of doing [ 4 ].
A unique combination of domain models [ 78 ], pedagogical models [ 44 ] and learner models [ 20 ] were expected to provide contextualized learning experiences to students with customized content, like expert human teachers [ 26 , 59 , 65 ],. Later, more models were introduced to enhance students' learning experience like strategy model, knowledge-base model and communication model [ 7 ]. It was expected that an intelligent tutoring system would not just teach, but also ensure that students have learned. It would care for students [ 17 ]. Similar to human teachers, ITS would improve with time. They would learn from their experiences, ‘understand’ what works in which contexts and then help students accordingly [ 8 , 60 ].
In recent years, ITS have mostly been subject and topic specific like ASSISTments [ 25 ], iTalk2Learn [ 23 ] and Aida Calculus. Despite being limited in terms of the domain that a particular intelligent tutoring system addresses, they have proven to be effective in providing relevant content to students, interacting with students [ 6 ] and improving students’ academic performance [ 18 , 41 ]. It is not necessary that ITS would work in every context and facilitate every teacher [ 7 , 13 , 46 , 48 ]. Utterberg et al. [78] showed why teachers have abandoned technology in some instances because it was counterproductive. They conducted a formative intervention with sixteen secondary school mathematics teachers and found systemic contradictions between teachers’ opinions and ITS recommendations, eventually leading to the abandonment of the tool. This highlights the importance of giving teachers the right to refuse AI powered ed-tech if they are not comfortable with it.
Considering a direct correlation between emotions and learning [ 40 ] recently, ITS have also started focusing on emotional state of students while learning to offer a more contextualized learning experience [ 24 ].
2.5 Popular conferences
To reflect on the increasing interest and activity in the space of AIEd, some of the most popular conferences in AIEd are shown in Table 1 below. Due to the pandemic all these conferences will be available virtually in 2021 as well. The first international workshop on multimodal artificial intelligence in education is being organized at AIEd [74] conference to promote the importance of multimodal data in AIEd.
3 Industry’s focus
In this section, we introduce the industry focus in the area of AIEd by case-studying three levels of companies start-up level, established/large company and mega-players (Amazon, Cisco). These companies represent different levels of the ecosystem (in terms of size).
3.1 Start-ups
There have been a number of ed-tech companies that are leading the AIEd revolution. New funds are also emerging to invest in ed-tech companies and to help ed-tech start-ups in scaling their products. There has been an increase in investor interest [ 21 ]. In 2020 the amount of investment raised by ed-tech companies more than doubled compared to 2019 (according to Techcrunch). This shows another dimension of pandemic’s effect on ed-tech. With an increase in data coming in during the pandemic, it is expected that industry’s focus on AI powered products will increase.
EDUCATE, a leading accelerator focused on ed-tech companies supported by UCL Institute of Education and European Regional Development Fund was formed to bring research and evidence at the centre of product development for ed-tech. This accelerator has supported more than 250 ed-tech companies and 400 entrepreneurs and helped them focus on evidence-informed product development for education.
Number of ed-tech companies are emerging in this space with interesting business models. Third Space Learning offers maths intervention programs for primary and secondary school students. The company aims to provide low-cost quality tuition to support pupils from disadvantaged backgrounds in UK state schools. They have already offered 8,00,000 h of teaching to around 70,000 students, 50% of who were eligible for free meals. Number of mobile apps like Kaizen Languages, Duolingo and Babbel have emerged that help individuals in learning other languages.
3.2 Established players
Pearson is one of the leading educational companies in the world with operations in more than 70 countries and more than 22,000 employees worldwide. They have been making a transition to digital learning and currently generate 66% of their annual revenue from it. According to Pearson, they have built world’s first AI powered calculus tutor called Aida which is publicly available on the App Store. But, its effectiveness in improving students’ calculus skills without any human intervention is still to be seen.
India based ed-tech company known for creating engaging educational content for students raised investment at a ten billion dollar valuation last year [ 70 ]. Century tech is another ed-tech company that is empowering learning through AI. They claim to use neuroscience, learning science and AI to personalize learning and identifying the unique learning pathways for students in 25 countries. They make more than sixty thousand AI powered smart recommendations to learners every day.
Companies like Pearson and Century Tech are building great technology that is impacting learners across the globe. But the usefulness of their acclaimed AI in helping learners from diverse backgrounds, with unique learning needs and completely different contexts is to be proven. As discussed above, teachers play a very important role on how their AI is used by learners. For this, teacher training is vital to fully understand the strengths and weaknesses of these products. It is very important to have an awareness of where these AI products cannot help or can go wrong so teachers and learners know when to avoid relying on them.
In the past few years, the popularity of Massive Online Open Courses (MOOCS) has grown exponentially with the emergence of platforms like Coursera, Udemy, Udacity, LinkedIn Learning and edX [ 5 , 16 , 28 ]. AI can be utilized to develop a better understanding of learner behaviour on MOOCS, produce better content and enhance learning outcomes at scale. Considering these platforms are collecting huge amounts of data, it will be interesting to see the future applications of AI in offering personalized learning and life-long learning solutions to their users [ 81 ].
3.3 Mega-players
Seeing the business potential of AIEd and the kind of impact it can have on the future of humanity, some of the biggest tech companies around the globe are moving into this space. The shift to online education during the pandemic boosted the demand for cloud services. Amazon’s AWS (Amazon Web Services) as a leader in cloud services provider facilitated institutions like Instituto Colombiano para la Evaluacion de la Educacion (ICFES) to scale their online examination service for 70,000 students. Similarly, LSE utilized AWS to scale their online assessments for 2000 students [ 1 , 3 ].
Google’s CEO Sunder Pichai stated that the pandemic offered an incredible opportunity to re-imagine education. Google has launched more than 50 new software tools during the pandemic to facilitate remote learning. Google Classroom which is a part of Google Apps for Education (GAFE) is being widely used by schools around the globe to deliver education. Research shows that it improves class dynamics and helps with learner participation [ 2 , 29 , 62 , 63 , 69 ].
Before moving onto the ethical dimensions of AIEd, it is important to conclude this section by noting an area that is of critical importance to processing industry and services. Aside from these three levels of operation (start-up, medium, and mega companies), there is the question of development of the AIEd infrastructure. As Luckin [41] points out, “True progress will require the development of an AIEd infrastructure. This will not, however, be a single monolithic AIEd system. Instead, it will resemble the marketplace that has been developed for smartphone apps: hundreds and then thousands of individual AIEd components, developed in collaboration with educators, conformed to uniform international data standards, and shared with researchers and developers worldwide. These standards will enable system-level data collation and analysis that help us learn much more about learning itself and how to improve it”.
4 Ethical AIEd
With a number of mishaps in the real world [ 31 , 80 ], ethics in AI has become a real concern for AI researchers and practitioners alike. Within computer science, there is a growing overlap with the broader Digital Ethics [ 19 ] and the ethics and engineering focused on developing Trustworthy AI [ 11 ]. There is a focus on fairness, accountability, transparency and explainability [ 33 , 82 , 83 , 84 ]. Ethics in AI needs to be embedded in the entire development pipeline, from the decision to start collecting data till the point when the machine learning model is deployed in production. From an engineering perspective, Koshiyama et al. [ 35 ] have identified four verticals of algorithmic auditing. These include performance and robustness, bias and discrimination, interpretability and explainability and algorithmic privacy.
In education, ethical AI is crucial to ensure the wellbeing of learners, teachers and other stakeholders involved. There is a lot of work going on in AIEd and AI powered ed-tech tools. With the influx of large amounts of data due to online learning during the pandemic, we will most likely see an increasing number of AI powered ed-tech products. But ethics in AIEd is not a priority for most ed-tech companies and schools. One of the reasons for this is the lack of awareness of relevant stakeholders regarding where AI can go wrong in the context of education. This means that the drawbacks of using AI like discrimination against certain groups due to data deficiencies, stigmatization due to reliance on certain machine learning modelling deficiencies and exploitation of personal data due to lack of awareness can go unnoticed without any accountability.
An AI wrongly predicting that a particular student will not perform very well in end of year exams or might drop out next year can play a very important role in determining that student’s reputation in front of teachers and parents. This reputation will determine how these teachers and parents treat that learner, resulting in a huge psychological impact on that learner, based on this wrong description by an AI tool. One high-profile case of harm was in the use of an algorithm to predict university entry results for students unable to take exams due to the pandemic. The system was shown to be biased against students from poorer backgrounds. Like other sectors where AI is making a huge impact, in AIEd this raises an important ethical question regarding giving students the freedom to opt out of AI powered predictions and automated evaluations.
The ethical implications of AI in education are dependent on the kind of disruption AI is doing in the ed-tech sector. On the one hand, this can be at an individual level for example by recommending wrong learning materials to students, or it can collectively impact relationships between different stakeholders such as how teachers perceive learners’ progress. This can also lead to automation bias and issues of accountability [ 67 ] where teachers begin to blindly rely on AI tools and prefer the tool’s outcomes over their own better judgement, whenever there is a conflict.
Initiatives have been observed in this space. For example, Professor Rose Luckin, professor of learner centered design at University College London along with Sir Anthony Seldon, vice chancellor of the University of Buckingham and Priya Lakhani, founder and CEO of Century Tech founded the Institute of Ethical AI in Education (IEAIEd) [ 72 ] to create awareness and promote the ethical aspects of AI in education. In its interim report, the institute identified seven different requirements for ethical AI to mitigate any kind of risks for learners. This included human agency and oversight to double-check AI’s performance, technical robustness and safety to prevent AI going wrong with new data or being hacked; diversity to ensure similar distribution of different demographics in data and avoid bias; non-discrimination and fairness to prevent anyone from being unfairly treated by AI; privacy and data governance to ensure everyone has the right to control their data; transparency to enhance the understanding of AI products; societal and environmental well-being to ensure that AI is not causing any harm and accountability to ensure that someone takes the responsibility for any wrongdoings of AI. Recently, the institute has also published a framework [ 71 ] for educators, schools and ed-tech companies to help them with the selection of ed-tech products with various ethical considerations in mind, like ethical design, transparency, privacy etc.
With the focus on online learning during the pandemic, and more utilization of AI powered ed-tech tools, risks of AI going wrong have increased significantly for all the stakeholders including ed-tech companies, schools, teachers and learners. A lot more work needs to be done on ethical AI in learning contexts to mitigate these risks, including assessment balancing risks and opportunities.
UNESCO published ‘Beijing Consensus’ on AI and Education that recommended member states to take a number of actions for the smooth and positively impactful integration of AI with education [ 74 ]. International bodies like EU have also recently published a set of draft guidelines under the heading of EU AI Act to ban certain uses of AI and categorize some as ‘high risk’ [ 47 ].
5 Future work
With the focus on online education due to Covid’19 in the past year, it will be consequential to see what AI has to offer for education with vast amounts of data being collected online through Learning Management Systems (LMS) and Massive Online Open Courses (MOOCS).
With this influx of educational data, AI techniques such as reinforcement learning can also be utilized to empower ed-tech. Such algorithms perform best with the large amounts of data that was limited to very few ed-tech companies in 2021. These algorithms have achieved breakthrough performance in multiple domains including games [ 66 ], healthcare [ 14 ] and robotics [ 34 ]. This presents a great opportunity for AI’s applications in education for further enhancing students’ learning outcomes, reducing teachers’ workloads [ 30 ] and making learning personalized [ 64 ], interactive and fun [ 50 , 53 ] for teachers and students.
With a growing number of AI powered ed-tech products in future, there will also be a lot of research on ethical AIEd. The risks of AI going wrong in education and the psychological impact this can have on learners and teachers is huge. Hence, more work needs to be done to ensure robust and safe AI products for all the stakeholders.
This can begin from the ed-tech companies sharing detailed guidelines for using AI powered ed-tech products, particularly specifying when not to rely on them. This includes the detailed documentation of the entire machine learning development pipeline with the assumptions made, data processing approaches used and the processes followed for selecting machine learning models. Regulators can play a very important role in ensuring that certain ethical principles are followed in developing these AI products or there are certain minimum performance thresholds that these products achieve [ 32 ].
6 Conclusion
AIEd promised a lot in its infancy around 3 decades back. However, there are still a number of AI breakthroughs required to see that kind of disruption in education at scale (including basic infrastructure). In the end, the goal of AIEd is not to promote AI, but to support education. In essence, there is only one way to evaluate the impact of AI in Education: through learning outcomes. AIEd for reducing teachers’ workload is a lot more impactful if the reduced workload enables teachers to focus on students’ learning, leading to better learning outcomes.
Cutting edge AI by researchers and companies around the world is not of much use if it is not helping the primary grade student in learning. This problem becomes extremely challenging because every learner is unique with different learning pathways. With the recent developments in AI, particularly reinforcement learning techniques, the future holds exciting possibilities of where AI will take education. For impactful AI in education, learners and teachers always need to be at the epicenter of AI development.
About Amazon.: Helping 7,00,000 students transition to remote learning. https://www.aboutamazon.com/news/community/helping-700-000-students-transition-to-remote-learning (2020)
Al-Maroof, R.A.S., Al-Emran, M.: Students acceptance of google classroom: an exploratory study using PLS–SEM approach. Int. J. Emerg. Technol Learn. (2018). https://doi.org/10.3991/ijet.v13i06.8275
Article Google Scholar
Amazon Web Services, Inc. (n.d.).: Amazon Web Services, Inc. https://pages.awscloud.com/whitepaper-emerging-trends-in-education.html (2020)
Baker, R.S.: Stupid tutoring systems, intelligent humans. Int. J. Artif. Intell. Educ. 26 (2), 600–614 (2016)
Baturay, M.H.: An overview of the world of MOOCs. Procedia. Soc. Behav. Sci. 174 , 427–433 (2015)
Baylari, A., Montazer, G.A.: Design a personalized e-learning system based on item response theory and artificial neural network approach. Expert. Syst. Appl. 36 (4), 8013–8021 (2009)
Beck, J., Stern, M., Haugsjaa, E.: Applications of AI in education. Crossroads 3 (1), 11–15 (1996). https://doi.org/10.1016/j.eswa.2008.10.080
Beck, J.E.: Modeling the Student with Reinforcement Learning. Proceedings of the Machine learning for User Modeling Workshop at the Sixth International Conference on User Modeling (1997)
Beck, J.E., Woolf, B.P., Beal, C.R.: ADVISOR: A machine learning architecture for intelligent tutor construction. Proceedings of the 7th National Conference on Artificial Intelligence, New York, ACM, 552–557 (2000)
Boekaerts, M.: Self-regulated learning: where we are today. Int. J. Educ. Res. 31 (6), 445–457 (1999)
Brundage, M., Avin, S., Wang, J., Belfield, H., Krueger, G., Hadfield, G., Maharaj, T.: Toward trustworthy AI development: mechanisms for supporting verifiable claims. arXiv preprint arXiv:2004.07213 (2020)
Bull, S., Kay, J.: Open learner models. In: Nkambou, R., Bourdeau, J., Mizoguchi, R. (eds.) Studies in computational intelligence, pp. 301–322. Springer, Berlin (2010)
Google Scholar
Cunha-Perez, C., Arevalillo-Herraez, M., Marco-Gimenez, L., Arnau, D.: On incorporating affective support to an intelligent tutoring system: an empirical study. IEEE. R. Iberoamericana. De. Tecnologias. Del. Aprendizaje. 13 (2), 63–69 (2018)
Callaway, E.: “It will change everything”: DeepMind’s AI makes gigantic leap in solving protein structures. Nature. https://www.nature.com/articles/d41586-020-03348-4 . (2020)
Cambridge University Press and Educate Ventures. Shock to the system: lessons from Covid-19 Volume 1: Implications and recommendations. https://www.cambridge.org/pk/files/1616/1349/4545/Shock_to_the_System_Lessons_from_Covid19_Volume_1.pdf (2021). Accessed 12 Apr 2021
Deng, R., Benckendorff, P., Gannaway, D.: Progress and new directions for teaching and learning in MOOCs. Comput. Educ. 129 , 48–60 (2019)
Erümit, A.K., Çetin, İ: Design framework of adaptive intelligent tutoring systems. Educ. Inf. Technol. 25 (5), 4477–4500 (2020)
Fang, Y., Ren, Z., Hu, X., Graesser, A.C.: A meta-analysis of the effectiveness of ALEKS on learning. Educ. Psychol. 39 (10), 1278–1292 (2019)
Floridi, L.: Soft ethics, the governance of the digital and the general data protection regulation. Philos. Trans. R. Soc. A. Math. Phys. Eng. Sci. 376 (2133), 20180081 (2018)
Goldstein, I.J.: The genetic graph: a representation for the evolution of procedural knowledge. Int. J. Man. Mach. Stud. 11 (1), 51–77 (1979)
Goryachikh, S.P., Sozinova, A.A., Grishina, E.N., Nagovitsyna, E.V.: Optimisation of the mechanisms of managing venture investments in the sphere of digital education on the basis of new information and communication technologies: audit and reorganisation. IJEPEE. 13 (6), 587–594 (2020)
Grawemeyer, B., Gutierrez-Santos, S., Holmes, W., Mavrikis, M., Rummel, N., Mazziotti, C., Janning, R.: Talk, tutor, explore, learn: intelligent tutoring and exploration for robust learning, p. 2015. AIED, Madrid (2015)
Hansen, A., Mavrikis, M.: Learning mathematics from multiple representations: two design principles. ICTMT-12, Faro (2015)
Hasan, M.A., Noor, N.F.M., Rahman, S.S.A., Rahman, M.M.: The transition from intelligent to affective tutoring system: a review and open issues. IEEE Access (2020). https://doi.org/10.1109/ACCESS.2020.3036990
Heffernan, N.T., Heffernan, C.L.: The ASSISTments ecosystem: building a platform that brings scientists and teachers together for minimally invasive research on human learning and teaching. Int. J. Artif. Intell. Educ. (2014). https://doi.org/10.1007/s40593-014-0024-x
Heffernan, N.T., Koedinger, K.R.: An intelligent tutoring system incorporating a model of an experienced human tutor. Proceedings of the 6th International Conference on Intelligent Tutoring Systems, 2363, p 596–608, (2002)
Hill, P., Barber, M.: Preparing for a Renaissance in Assessment. Pearson, London (2014)
Hollands, F.M., Tirthali, D.: Why do institutions offer MOOCs? Online Learning 18 (3), 3 (2014)
Iftakhar, S.: Google classroom: what works and how. J. Educ. Soc. Sci. 3 (1), 12–18 (2016)
Iglesias, A., Martínez, P., Aler, R., Fernández, F.: Reinforcement learning of pedagogical policies in adaptive and intelligent educational systems. Knowl. Based. Syst. 22 (4), 266–270 (2009)
Johnson, D.G., Verdicchio, M.: AI, agency and responsibility: the VW fraud case and beyond. Ai. Soc. 34 (3), 639–647 (2019)
Kazim, E., Denny, D.M.T., Koshiyama, A.: AI auditing and impact assessment: according to the UK information commissioner’s office. AI. Ethics. 1 , 1–10 (2021)
Kazim, E., Koshiyama, A.: A High-Level Overview of AI Ethics. SSRN J (2020). https://doi.org/10.2139/ssrn.3609292
Kober, J., Bagnell, J.A., Peters, J.: Reinforcement learning in robotics: a survey. Int. J. Robot. Res. 32 (11), 1238–1274 (2013)
Koshiyama, A., Kazim, E., Treleaven, P., Rai, P., Szpruch, L., Pavey, G., Ahamat, G., Leutner, F., Goebel, R., Knight, A., Adams, J., Hitrova, C., Barnett, J., Nachev, P., Barber, D., Chamorro-Premuzic, T., Klemmer, K., Gregorovic, M., Khan, S., Lomas, E.: Towards algorithm auditing a survey on managing legal ethical and technological risks of AI, ML and associated algorithms. SSRN J (2021). https://doi.org/10.2139/ssrn.3778998
LaPierre, J.: How AI Enhances Collaborative Learning. Filament Games (2018). https://www.filamentgames.com/blog/how-ai-enhances-collaborative-learning/ . Accessed 12 Apr 2021
Luckin, R.: Towards artificial intelligence-based assessment systems. Nat. Hum. Behav. (2017). https://doi.org/10.1038/s41562-016-0028
Luckin, R., du Boulay, B.: Int. J. Artif. Intell. Educ. 26 , 416–430 (2016)
Luckin, R., Holmes, W., Griffiths, M., Pearson, L.: Intelligence Unleashed An argument for AI in Education. https://static.googleusercontent.com/media/edu.google.com/en//pdfs/Intelligence-Unleashed-Publication.pdf (2016)
Barron-Estrada M.L., Zatarain-Cabada, R., Oramas-Bustillos, R., Gonzalez-Hernandez, F.: Sentiment analysis in an affective intelligent tutoring system. Proc. IEEE 17th Int. Conf. Adv. Learn. Technol. (ICALT), Timisoara pp. 394–397 2017.
Ma, W., Adesope, O., Nesbit, J.C., Liu, Q.: Intelligent tutoring systems and learning outcomes: a meta-analysis. J. Educ. Psychol. 106 (4), 901–918 (2014)
Makridakis, S.: The forthcoming Artificial Intelligence (AI) revolution: Its impact on society and firms. Futures 90 , 46–60 (2017)
Mavrikis, M.: Int. J. Artif. Intell. Tools. 19 , 733–753 (2010)
Merrill, D.C., Reiser, B.J., Ranney, M., Trafton, J.G.: Effective tutoring techniques: a comparison of human tutors and intelligent tutoring systems. J. Learn. Sci. 2 (3), 277–305 (1992)
Moeini, A.: Theorising Evidence-Informed Learning Technology Enterprises: A Participatory Design-Based Research Approach. Doctoral dissertation, UCL University College London, London, (2020)
Mohamed, H., Lamia, M.: Implementing flipped classroom that used an intelligent tutoring system into learning process. Comput. Educ. 124 , 62–76 (2018). https://doi.org/10.1016/j.compedu.2018.05.011
Mueller, B.: The Artificial Intelligence Act: A Quick Explainer. [online] Center for Data Innovation (2021). https://datainnovation.org/2021/05/the-artificial-intelligence-act-a-quick-explainer/ . Accessed 12 Apr 2021
Murray, M.C., Pérez, J.: Informing and performing: A study comparing adaptive learning to traditional learning. Inform. Sci. J. 18 , 111–125 (2015)
Oudeyer, P-Y.: Computational Theories of Curiosity-Driven Learning. https://arxiv.org/pdf/1802.10546.pdf (2018)
Park, H.W., Grover, I., Spaulding, S., Gomez, L., Breazeal, C.: A model-free affective reinforcement learning approach to personalization of an autonomous social robot companion for early literacy education. AAAI. 33 (1), 687–694 (2019)
Resnick, M., Robinson, K.: Lifelong kindergarten: cultivating creativity through projects, passion, peers, and play. MIT press, Cambridge (2017)
Book Google Scholar
Rodríguez-Triana, M.J., Prieto, L.P., Martínez-Monés, A., Asensio-Pérez, J.I. and Dimitriadis, Y.: The teacher in the loop: Customizing multimodal learning analytics for blended learning. In Proceedings of the 8th international conference on learning analytics and knowledge. pp 417–426 (2018)
Rowe, J.P., Lester, J.C.: Improving student problem solving in narrative-centered learning environments: a modular reinforcement learning framework. In International Conference on Artificial Intelligence in Education. pp. 419–428. Springer, Cham (2015)
Russell, S.J., Norvig, P., Davis, E.: Artificial intelligence: a modern approach. Prentice Hall, Upper Saddle River (2010)
MATH Google Scholar
Jiménez, S., Juárez-Ramírez, R., Castillo, V.H., Licea, G., Ramírez-Noriega, A., Inzunza, S.: A feedback system to provide affective support to students. Comput. Appl. Eng. Educ. 26 (3), 473–483 (2018)
Sadler, D.R.: Formative assessment in the design of instructional systems. Instr. Sci. 18 , 119–144 (1989)
Samarakou, M., Fylladitakis, E., Prentakis, P., Athineos, S.: Implementation of artificial intelligence assessment in engineering laboratory education. https://files.eric.ed.gov/fulltext/ED557263.pdf (2014). Accessed 24 Feb 2021
Segal, A., Hindi, S., Prusak, N., Swidan, O., Livni, A., Palatnic, A., Schwarz, B.: Keeping the teacher in the loop: Technologies for monitoring group learning in real-time. In International Conference on Artificial Intelligence in Education. pp. 64–76. Springer, Cham (2017)
Self, J. A. (1990). Theoretical foundations of intelligent tutoring systems. J. Artif. Intell
Self, J.A.: The defining characteristics of intelligent tutoring systems research: ITSs care, precisely. IJAIEd. 10 , 350–364 (1998)
Selwood, I., Pilkington, R.: Teacher workload: using ICT to release time to teach. Educ. Rev. 57 (2), 163–174 (2005)
Shaharanee, I.N.M., Jamil, J.M. and Rodzi, S.S.M.:æ Google classroom as a tool for active learning. AIP Conference Proceedings, 1761 (1), pp. 020069, AIP Publishing LLC, College Park (2016)
Shaharanee, I.N.M., Jamil, J.M., Rodzi, S.S.M.: The application of Google Classroom as a tool for teaching and learning. J. Telecommun. Electron. Comp. Eng. 8 (10), 5–8 (2016)
Shawky, D., Badawi, A.: Towards a personalized learning experience using reinforcement learning. In: Hassanien, A.E. (ed.) Machine learning paradigms Theory and application, pp. 169–187. Springer (2019)
Shute, V.J. (1991). Rose garden promises of intelligent tutoring systems: blossom or thorn. NASA, Lyndon B. Johnson Space Center, Fourth Annual Workshop on Space Operations Applications and Research (SOAR 90) . Available at: https://ntrs.nasa.gov/citations/19910011382 . Accessed 4 July 2021
Silver, D., Huang, A., Maddison, C.J., Guez, A., Sifre, L., Van Den Driessche, G., Schrittwieser, J., Antonoglou, I., Panneershelvam, V., Lanctot, M., Dieleman, S.: Mastering the game of Go with deep neural networks and tree search. Nature 529 (7587), 484–489 (2016)
Skitka, L.J., Mosier, K., Burdick, M.D.: Accountability and automation bias. Int. J. Hum. Comput. Stud. 52 (4), 701–717 (2000)
Steenbergen-Hu, S., Cooper, H.: A meta-analysis of the effectiveness of intelligent tutoring systems on K–12 students’ mathematical learning. J. Educ. Psychol. 105 (4), 970–987 (2013)
Sudarsana, I.K., Putra, I.B., Astawa, I.N.T., Yogantara, I.W.L.: The use of google classroom in the learning process. J. Phys. Conf. Ser 1175 (1), 012165 (2019)
TechCrunch. Indian education startup Byju’s is fundraising at a $10B valuation. https://techcrunch.com/2020/05/01/indian-education-startup-byjus-is-fundraising-at-a-10b-valuation/ (2020). Accessed 12 Apr 2021
The Institute for Ethical AI in Education The Ethical Framework for AI in Education (IEAIED). https://fb77c667c4d6e21c1e06.b-cdn.net/wp-content/uploads/2021/03/The-Ethical-Framework-for-AI-in-Education-Institute-for-Ethical-AI-in-Education-Final-Report.pdf (2021). Accessed 12 Apr 2021
The Institute for Ethical AI in Education The Ethical Framework for AI in Education (n.d.). Available at: https://www.buckingham.ac.uk/wp-content/uploads/2021/03/The-Institute-for-Ethical-AI-in-Education-The-Ethical-Framework-for-AI-in-Education.pdf . Accessed 4 July 2021
Tisseron, S., Tordo, F., Baddoura, R.: Testing Empathy with Robots: a model in four dimensions and sixteen ítems. Int. J. Soc. Robot. 7 (1), 97–102 (2015)
UNESCO. Artificial intelligence in education. UNESCO. https://en.unesco.org/artificial-intelligence/education . (2019). Accessed 12 Apr 2021
Utterberg Modén, M., Tallvid, M., Lundin, J., Lindström, B.: Intelligent Tutoring Systems: Why Teachers Abandoned a Technology Aimed at Automating Teaching Processes. In: Proceedings of the 54th Hawaii International Conference on System Sciences, Maui, p. 1538 (2021)
van der Spoel, I., Noroozi, O., Schuurink, E., van Ginkel, S.: Teachers’ online teaching expectations and experiences during the Covid19-pandemic in the Netherlands. Eur. J. Teach. Educ. 43 (4), 623–638 (2020)
Weller, M.: Twenty years of EdTech. Educa. Rev. Online. 53 (4), 34–48 (2018)
Wenger, E.: Artificial intelligence and tutoring systems. Morgan Kauffman, Los Altos (1987)
World Economic Forum and The Boston Consulting Group. New vision for education unlocking the potential of technology industry agenda prepared in collaboration with the Boston consulting group. http://www3.weforum.org/docs/WEFUSA_NewVisionforEducation_Report2015.pdf (2015). Accessed 12 Apr 2021
Yampolskiy, R.V., Spellchecker, M.S.: Artificial intelligence safety and cybersecurity: a timeline of AI failures. arXiv:1610.07997 (2016)
Yu, H., Miao, C., Leung, C., White, T.J.: Towards AI-powered personalization in MOOC learning. Npj. Sci. Learn. 2 (1), 1–5 (2017)
Yu, H., Shen, Z., Miao, C., Leung, C., Lesser, V.R., Yang, Q.: Building ethics into artificial intelligence. arXiv:1812.02953 (2018)
Zemel, R., Wu Y., Swersky, K., Pitassi, T., Dwork, C.: Learning fair representations. In: International Conference on Machine Learning, pp. 325–333 (2013)
Zhang, Y., Liao, Q.V., Bellamy, R.K.E.: Effect of confidence and explanation on accuracy and trust calibration in AI-assisted decision making. In: Proceedings of the 2020 Conference on Fairness, Accountability, and Transparency. arXiv:2001.02114 (2020)
Zimmerman, B.J., Schunk, D.H.: Handbook of Self-Regulation of Learning and Performance. Routledge, Oxfordshire (2011)
Download references
Author information
Authors and affiliations.
Artificial Intelligence at University College, London, UK
Muhammad Ali Chaudhry
Department of Computer Science, University College, London, UK
You can also search for this author in PubMed Google Scholar
Corresponding author
Correspondence to Muhammad Ali Chaudhry .
Additional information
Publisher's note.
Springer Nature remains neutral with regard to jurisdictional claims in published maps and institutional affiliations.
Rights and permissions
Open Access This article is licensed under a Creative Commons Attribution 4.0 International License, which permits use, sharing, adaptation, distribution and reproduction in any medium or format, as long as you give appropriate credit to the original author(s) and the source, provide a link to the Creative Commons licence, and indicate if changes were made. The images or other third party material in this article are included in the article's Creative Commons licence, unless indicated otherwise in a credit line to the material. If material is not included in the article's Creative Commons licence and your intended use is not permitted by statutory regulation or exceeds the permitted use, you will need to obtain permission directly from the copyright holder. To view a copy of this licence, visit http://creativecommons.org/licenses/by/4.0/ .
Reprints and permissions
About this article
Chaudhry, M.A., Kazim, E. Artificial Intelligence in Education (AIEd): a high-level academic and industry note 2021. AI Ethics 2 , 157–165 (2022). https://doi.org/10.1007/s43681-021-00074-z
Download citation
Received : 25 April 2021
Accepted : 17 June 2021
Published : 07 July 2021
Issue Date : February 2022
DOI : https://doi.org/10.1007/s43681-021-00074-z
Share this article
Anyone you share the following link with will be able to read this content:
Sorry, a shareable link is not currently available for this article.
Provided by the Springer Nature SharedIt content-sharing initiative
- Artificial Intelligence
- Machine learning
- Learning science
- Artificial Intelligence in Education (AIEd)
- Intelligent Tutoring Systems (ITS)
- Find a journal
- Publish with us
- Track your research
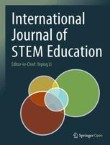
- Search by keyword
- Search by citation
Page 1 of 10
When perceived similarity overrides demographic similarity: examining influences on STEM students’ developmental mentor networks
While dyadic faculty–mentored relationship research currently saturates the mentoring literature, recent developments suggest the need for a broader consideration of a student's mentor network. Research taking...
- View Full Text
Science teacher identity research: a scoping literature review
Science teacher identity significantly influences teacher professional development, practices, and attitudes, which in turn impacts student learning outcomes. With an increased number of studies on science tea...
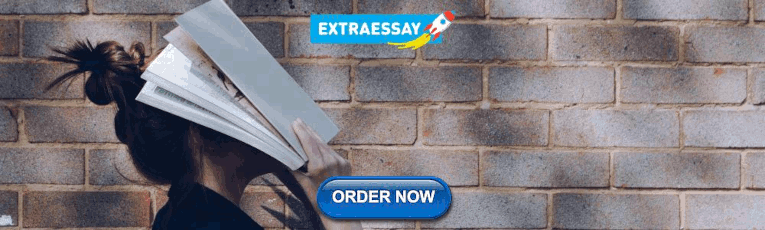
Beyond performance, competence, and recognition: forging a science researcher identity in the context of research training
Studying science identity has been useful for understanding students’ continuation in science-related education and career paths. Yet knowledge and theory related to science identity among students on the path...
Prior experiences as students and instructors play a critical role in instructors’ decision to adopt evidence-based instructional practices
There has been a growing interest in characterizing factors influencing teaching decisions of science, technology, engineering, and mathematics (STEM) instructors in order to address the slow uptake of evidenc...
Promoting STEM learning perseverance through recognizing communal goals: understanding the impact of empathy and citizenship
Previous research has indicated that placing emphasis on communal goals within the field of science, technology, engineering, and mathematics (STEM) education can yield beneficial learning outcomes. However, t...
Correction: Pre-service elementary teachers’ science and engineering teaching self-efficacy and outcome expectancy: exploring the impacts of efficacy source experiences through varying course modalities
The original article was published in International Journal of STEM Education 2024 11 :4
Beyond STEM attrition: changing career plans within STEM fields in college is associated with lower motivation, certainty, and satisfaction about one’s career
Research and policy often focus on reducing attrition from educational trajectories leading to careers in science, technology, engineering, and mathematics (STEM), but many students change career plans within STE...
Systemic advantage has a meaningful relationship with grade outcomes in students’ early STEM courses at six research universities
Large introductory lecture courses are frequently post-secondary students’ first formal interaction with science, technology, engineering, and mathematics (STEM) disciplines. Grade outcomes in these courses ar...
Using collaborative autoethnography to investigate mentoring relationships for novice engineering education researchers
The National Science Foundation Research Initiation in Engineering Formation (RIEF) program aims to increase research capacity in the field by providing funding for technical engineering faculty to learn to co...
Students’ perspectives on the ‘STEM belonging’ concept at A-level, undergraduate, and postgraduate levels: an examination of gender and ethnicity in student descriptions
Women and ethnic minorities have historically been underrepresented in some STEM fields. It is therefore important to understand the factors influencing students’ persistence in STEM fields, and what STEM belo...
Strategies and difficulties during students’ construction of data visualizations
Data visualizations transform data into visual representations such as graphs, diagrams, charts and so forth, and enable inquiries and decision-making in many professional fields, as well as in public and econ...
Characteristics of departments with high-use of active learning in introductory STEM courses: implications for departmental transformation
It is well established in the literature that active learning instruction in introductory STEM courses results in many desired student outcomes. Yet, regular use of high-quality active learning is not the norm...
Single-paper meta-analyses of the effects of spaced retrieval practice in nine introductory STEM courses: is the glass half full or half empty?
Undergraduate STEM instructors want to help students learn and retain knowledge for their future courses and careers. One promising evidence-based technique that is thought to increase long-term memory is spac...
Effects of a first-year undergraduate engineering design course: survey study of implications for student self-efficacy and professional skills, with focus on gender/sex and race/ethnicity
Students’ academic self-efficacy maximizes likelihood for success and retention, yet prior research suggests that historically underrepresented (minoritized) undergraduate students in higher education and in c...
The effects of educational robotics in STEM education: a multilevel meta-analysis
Educational robotics, as emerging technologies, have been widely applied in the field of STEM education to enhance the instructional and learning quality. Although previous research has highlighted potentials ...
Can training and apprentice programs in STEM increase worker life satisfaction and optimism?
Despite the significant relationship between life satisfaction and education, less is known about the connection between life satisfaction and informal learning in the context of training and apprenticeship pr...
Gender patterns in engineering PhD teaching assistant evaluations corroborate role congruity theory
The body of work regarding gender bias in academia shows that female instructors are often rated lower by students than their male counterparts. Mechanisms are complex and intersectional and often associated w...
Pre-service elementary teachers’ science and engineering teaching self-efficacy and outcome expectancy: exploring the impacts of efficacy source experiences through varying course modalities
Teacher efficacy is one of the most influential components for effective instruction, highlighting the importance of providing preservice teachers (PSTs) with opportunities to learn how to teach engineering du...
The Correction to this article has been published in International Journal of STEM Education 2024 11 :16
The implementation of peer assessment as a scaffold during computer-supported collaborative inquiry learning in secondary STEM education
Computer-supported collaborative inquiry learning (CSCiL) has been proposed as a successful learning method to foster scientific literacy. This research aims to bridge the knowledge gap surrounding the role of...
Persisting in tough times across Hong Kong, mainland China, and the Philippines: grit, achievement goal orientation, and science engagement
Past studies of grit's educational benefits, such as science engagement, showed mixed results across cultures. So, we elaborated the prior model of grit (perseverance of effort, consistency of interest) with adap...
STEM learning opportunities and career aspirations: the interactive effect of students’ self-concept and perceptions of STEM professionals
Students’ positive perceptions of scientists or engineers have been reported to be positively related to their science, technology, engineering, and mathematics (STEM) career aspirations. However, other resear...
Nine years of development in establishing the journal as a learning and research hub in STEM education
From August 2014 to July 2023, the International Journal of STEM Education went through nine publication cycle years. In this editorial, I provide a brief summary of the journal’s development up to and including ...
Using intensive longitudinal methods to quantify the sources of variability for situational engagement in science learning environments
Situational engagement in science is often described as context-sensitive and varying over time due to the impact of situational factors. But this type of engagement is often studied using data that are collec...
STEM education institutional change projects: examining enacted approaches through the lens of the Four Categories of Change Strategies Model
Enacting STEM education reform is a complex task and there are a variety of approaches that might be selected by change agents. When working on an institutional change project to impact multiple parts of the S...
ChatGPT and its ethical implications for STEM research and higher education: a media discourse analysis
With the increasing demand brought on by the beginning of the fourth industrial revolution in the period of post-digital education and bio-digital technology, artificial intelligence (AI) has played a pivotal ...
Motivational climate predicts effort and achievement in a large computer science course: examining differences across sexes, races/ethnicities, and academic majors
The motivational climate within a course has been shown to be an important predictor of students’ engagement and course ratings. Because little is known about how students’ perceptions of the motivational clim...
Exploring the multifaceted roles of mathematics learning in predicting students' computational thinking competency
There exist shared competencies between computational thinking (CT) and mathematics, and these two domains also mutually benefit from various teaching approaches. However, the linkages between mathematics and ...
Correction: How are primary school computer science curricular reforms contributing to equity? Impact on student learning, perception of the discipline, and gender gaps
The original article was published in International Journal of STEM Education 2023 10 :60
Authentic STEM education through modelling: an international Delphi study
The literature asserts that science, technology, engineering, and mathematics (STEM) education needs to be authentic. Although models and modelling provide a basis from which to increase authenticity by bridgi...
Integrating artificial intelligence into science lessons: teachers’ experiences and views
In the midst of digital transformation, schools are transforming their classrooms as they prepare students for a world increasingly automated by new technologies, including artificial intelligence (AI). During...
How are primary school computer science curricular reforms contributing to equity? Impact on student learning, perception of the discipline, and gender gaps
Early exposure to Computer Science (CS) and Computational Thinking (CT) for all is critical to broaden participation and promote equity in the field. But how does the introduction of CS and CT into primary sch...
The Correction to this article has been published in International Journal of STEM Education 2023 10 :63
Gender differences in high school students’ interest in STEM careers: a multi-group comparison based on structural equation model
Females are underrepresented in Science, Technology, Engineering, and Mathematics (STEM) fields all over the world. To encourage more girls to choose STEM majors and careers, it is critical to increase their i...
Gender gap in STEM pathways: the role of secondary curricula in a highly differentiated school system—the case of Chile
STEM fields are instrumental in increasing the technological and innovative capacity of the economy. As women are underrepresented in the STEM workforce, diverse strategies have been implemented to boost their...
Teamwork dynamics in the context of large-size software development courses
Effectively facilitating teamwork experiences, particularly in the context of large-size courses, is difficult to implement. This study seeks to address the challenges of implementing effective teamwork experi...
The role of media in influencing students’ STEM career interest
Digital media are pervasive in the lives of young people and provide opportunities for them to learn about STEM. Multiple theories argue that the STEM media environment may shape how youth see a STEM career in...
Validity, acceptability, and procedural issues of selection methods for graduate study admissions in the fields of science, technology, engineering, and mathematics: a mapping review
This review presents the first comprehensive synthesis of available research on selection methods for STEM graduate study admissions. Ten categories of graduate selection methods emerged. Each category was cri...
Game-based learning in computer science education: a scoping literature review
Using games in education has the potential to increase students’ motivation and engagement in the learning process, gathering long-lasting practical knowledge. Expanding interest in implementing a game-based a...
Possibilities and pitfalls of practitioners in trying to apply change theory as viewed through the lens of Reinholz, White, and Andrews “Change theory in STEM higher education: a systematic review”
The original article was published in International Journal of STEM Education 2021 8 :37
Precision education via timely intervention in K-12 computer programming course to enhance programming skill and affective-domain learning objectives
In the realm of Science, Technology, Engineering, and Mathematic (STEM) education, computer programming stands as a vital discipline, amalgamating cross-disciplinary knowledge and fostering the capacity to sol...
Limited or complete? Teaching and learning conceptions and instructional environments fostered by STEM teaching versus research faculty
An instructor’s conceptions of teaching and learning contribute to the establishment of learning environments that may benefit or hinder student learning. Previous studies have defined the continuum of teachin...
Embracing a culture of talk: STEM teachers’ engagement in small-group discussions about photovoltaics
Small-group discussions are well established as an effective pedagogical tool to promote student learning in STEM classrooms. However, there are a variety of factors that influence how and to what extent K-12 ...
Math anxiety affects career choices during development
Links between math anxiety and the choice of a math-intensive career might change over development and differ by gender. The study included three research populations: primary school ( N = 87, 48 females, mean age...
Exploring senior engineering students’ engineering identity: the impact of practice-oriented learning experiences
Engineering identity reflects students' acceptance and recognition of engineering, which has a great influence on their willingness to enter and stay in the engineering field. Existing studies have shown that ...
Fostering computational thinking through unplugged activities: A systematic literature review and meta-analysis
Unplugged activities as a low-cost solution to foster computational thinking (CT) skills seem to be a trend in recent years. However, current evidence of the effectiveness of unplugged activities in promoting ...
Pathways of opportunity in STEM: comparative investigation of degree attainment across different demographic groups at a large research institution
We used an opportunity gap framework to analyze the pathways through which students enter into and depart from science, technology, engineering, and mathematics (STEM) degrees in an R1 higher education institu...
How well-intentioned white male physicists maintain ignorance of inequity and justify inaction
We present an analysis of interviews with 27 self-identified progressive white-male physics faculty and graduate students discussing race and gender in physics. White cis men dominate most STEM fields and are ...
The relation of representational competence and conceptual knowledge in female and male undergraduates
Representational competence is commonly considered a prerequisite for the acquisition of conceptual knowledge, yet little exploration has been undertaken into the relation between these two constructs. Using a...
A meta-analytic investigation of the impact of middle school STEM education: where are all the students of color?
Integrated science, technology, engineering, and mathematics (STEM) education initiatives are becoming an increasingly popular approach to narrow the opportunity gap among underrepresented minority (i.e., Blac...
The effect of embedded structures on cognitive load for novice learners during block-based code comprehension
Coding has become an integral part of STEM education. However, novice learners face difficulties in processing codes within embedded structures (also termed nested structures). This study aimed to investigate ...
Applicant qualifications and characteristics in STEM faculty hiring: an analysis of faculty and administrator perspectives
The lack of racial diversity in science, technology, engineering, and mathematics (STEM) disciplines is perhaps one of the most challenging issues in the United States higher education system. The issue is no...
- Discover article collections
- Editorial Board
- Sign up for article alerts and news from this journal
This journal is indexed by
- Social Sciences Citation Index (SSCI)
- EBSCO Discovery Service
- Google Scholar
- OCLC WorldCat Discovery Service
- ProQuest Advanced Technologies & Aerospace Database
- ProQuest Computer Science
- ProQuest Environmental Science
- ProQuest Engineering
- ProQuest Agricultural & Environmental Science Database
- ProQuest Biological Science Database
- ProQuest Central
- ProQuest Earth Atmospheric & Aquatic Science Database
- ProQuest Education Database
- ProQuest Materials Science & Engineering Database
- ProQuest Natural Science Collection
- ProQuest SciTech Premium Collection
- ProQuest Social Science Collection
- ProQuest Technology Collection
- ProQuest-ExLibris Summon
- ProQuest-ExLibris Primo
- UGC-CARE List (India)
- TD Net Discovery Service
- Science Citation Index Expanded (SciSearch)
- Norwegian Register for Scientific Journals and Series
- Journal Citation Reports/Social Sciences Edition
- Journal Citation Reports/Science Edition
- Current Contents / Social & Behavioral Sciences
- Chinese Academy of Sciences (CAS) - GoOA
Annual Journal Metrics
2022 Citation Impact 6.7 - 2-year Impact Factor 7.0 - 5-year Impact Factor 3.617 - SNIP (Source Normalized Impact per Paper) 1.668 - SJR (SCImago Journal Rank)
2023 Speed 8 days submission to first editorial decision for all manuscripts (Median) 224 days submission to accept (Median)
2023 Usage 1,774,317 downloads 819 Altmetric mentions
- More about our metrics
Check out institutional waivers and discounts
Need help with APC funding?
We offer a free open access support service to make it easier for you to discover and apply for article-processing charge (APC) funding.
Hot topics in STEM Education - Infographics
Find us on facebook.
- ISSN: 2196-7822 (electronic)
Artificial Intelligence and Education: A Reading List
A bibliography to help educators prepare students and themselves for a future shaped by AI—with all its opportunities and drawbacks.
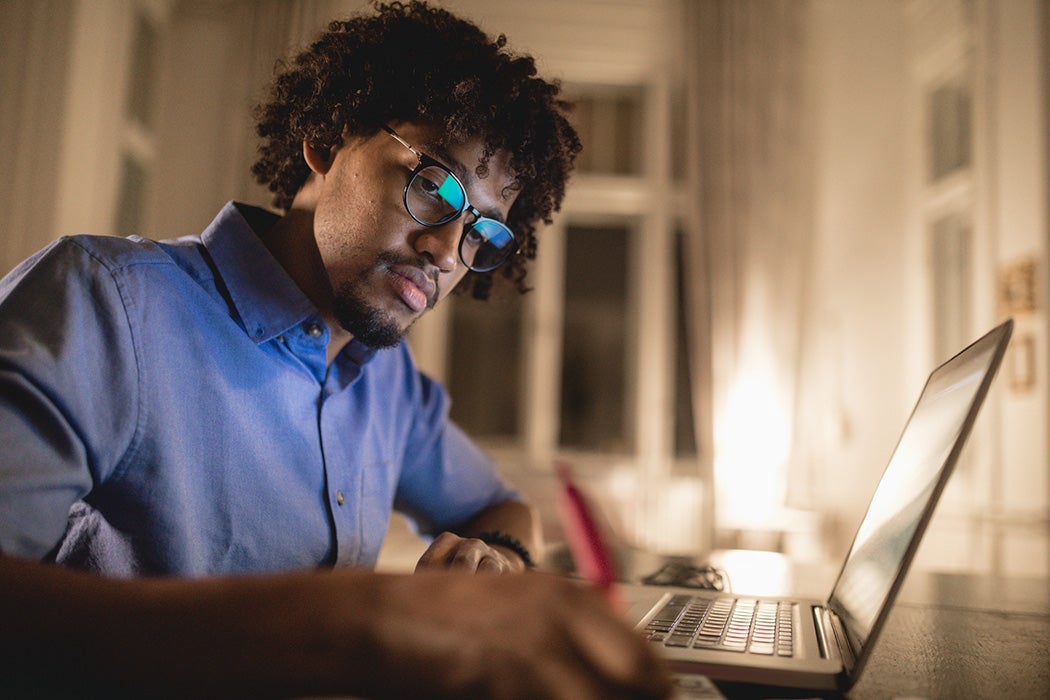
How should education change to address, incorporate, or challenge today’s AI systems, especially powerful large language models? What role should educators and scholars play in shaping the future of generative AI? The release of ChatGPT in November 2022 triggered an explosion of news, opinion pieces, and social media posts addressing these questions. Yet many are not aware of the current and historical body of academic work that offers clarity, substance, and nuance to enrich the discourse.
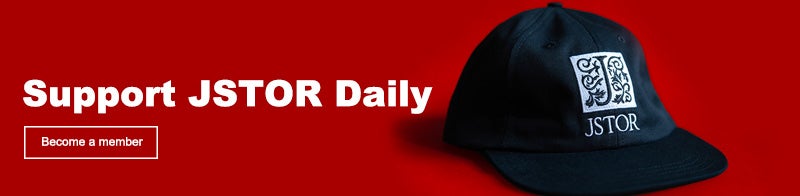
Linking the terms “AI” and “education” invites a constellation of discussions. This selection of articles is hardly comprehensive, but it includes explanations of AI concepts and provides historical context for today’s systems. It describes a range of possible educational applications as well as adverse impacts, such as learning loss and increased inequity. Some articles touch on philosophical questions about AI in relation to learning, thinking, and human communication. Others will help educators prepare students for civic participation around concerns including information integrity, impacts on jobs, and energy consumption. Yet others outline educator and student rights in relation to AI and exhort educators to share their expertise in societal and industry discussions on the future of AI.
Nabeel Gillani, Rebecca Eynon, Catherine Chiabaut, and Kelsey Finkel, “ Unpacking the ‘Black Box’ of AI in Education ,” Educational Technology & Society 26, no. 1 (2023): 99–111.
Whether we’re aware of it or not, AI was already widespread in education before ChatGPT. Nabeel Gillani et al. describe AI applications such as learning analytics and adaptive learning systems, automated communications with students, early warning systems, and automated writing assessment. They seek to help educators develop literacy around the capacities and risks of these systems by providing an accessible introduction to machine learning and deep learning as well as rule-based AI. They present a cautious view, calling for scrutiny of bias in such systems and inequitable distribution of risks and benefits. They hope that engineers will collaborate deeply with educators on the development of such systems.
Jürgen Rudolph, Samson Tan, and Shannon Tan, “ ChatGPT: Bullshit Spewer or the End of Traditional Assessments in Higher Education? ” The Journal of Applied Learning and Teaching 6, no. 1 (January 24, 2023).
Jürgen Rudolph et al. give a practically oriented overview of ChatGPT’s implications for higher education. They explain the statistical nature of large language models as they tell the history of OpenAI and its attempts to mitigate bias and risk in the development of ChatGPT. They illustrate ways ChatGPT can be used with examples and screenshots. Their literature review shows the state of artificial intelligence in education (AIEd) as of January 2023. An extensive list of challenges and opportunities culminates in a set of recommendations that emphasizes explicit policy as well as expanding digital literacy education to include AI.
Emily M. Bender, Timnit Gebru, Angela McMillan-Major, and Shmargaret Shmitchell, “ On the Dangers of Stochastic Parrots: Can Language Models Be Too Big? 🦜 ,” FAccT ’21: Proceedings of the 2021 ACM Conference on Fairness, Accountability, and Transparency (March 2021): 610–623.
Student and faculty understanding of the risks and impacts of large language models is central to AI literacy and civic participation around AI policy. This hugely influential paper details documented and likely adverse impacts of the current data-and-resource-intensive, non-transparent mode of development of these models. Bender et al. emphasize the ways in which these costs will likely be borne disproportionately by marginalized groups. They call for transparency around the energy use and cost of these models as well as transparency around the data used to train them. They warn that models perpetuate and even amplify human biases and that the seeming coherence of these systems’ outputs can be used for malicious purposes even though it doesn’t reflect real understanding.
The authors argue that inclusive participation in development can encourage alternate development paths that are less resource intensive. They further argue that beneficial applications for marginalized groups, such as improved automatic speech recognition systems, must be accompanied by plans to mitigate harm.
Erik Brynjolfsson, “ The Turing Trap: The Promise & Peril of Human-Like Artificial Intelligence ,” Daedalus 151, no. 2 (2022): 272–87.
Erik Brynjolfsson argues that when we think of artificial intelligence as aiming to substitute for human intelligence, we miss the opportunity to focus on how it can complement and extend human capabilities. Brynjolfsson calls for policy that shifts AI development incentives away from automation toward augmentation. Automation is more likely to result in the elimination of lower-level jobs and in growing inequality. He points educators toward augmentation as a framework for thinking about AI applications that assist learning and teaching. How can we create incentives for AI to support and extend what teachers do rather than substituting for teachers? And how can we encourage students to use AI to extend their thinking and learning rather than using AI to skip learning?
Kevin Scott, “ I Do Not Think It Means What You Think It Means: Artificial Intelligence, Cognitive Work & Scale ,” Daedalus 151, no. 2 (2022): 75–84.
Brynjolfsson’s focus on AI as “augmentation” converges with Microsoft computer scientist Kevin Scott’s focus on “cognitive assistance.” Steering discussion of AI away from visions of autonomous systems with their own goals, Scott argues that near-term AI will serve to help humans with cognitive work. Scott situates this assistance in relation to evolving historical definitions of work and the way in which tools for work embody generalized knowledge about specific domains. He’s intrigued by the way deep neural networks can represent domain knowledge in new ways, as seen in the unexpected coding capabilities offered by OpenAI’s GPT-3 language model, which have enabled people with less technical knowledge to code. His article can help educators frame discussions of how students should build knowledge and what knowledge is still relevant in contexts where AI assistance is nearly ubiquitous.
Laura D. Tyson and John Zysman, “ Automation, AI & Work ,” Daedalus 151, no. 2 (2022): 256–71.
How can educators prepare students for future work environments integrated with AI and advise students on how majors and career paths may be affected by AI automation? And how can educators prepare students to participate in discussions of government policy around AI and work? Laura Tyson and John Zysman emphasize the importance of policy in determining how economic gains due to AI are distributed and how well workers weather disruptions due to AI. They observe that recent trends in automation and gig work have exacerbated inequality and reduced the supply of “good” jobs for low- and middle-income workers. They predict that AI will intensify these effects, but they point to the way collective bargaining, social insurance, and protections for gig workers have mitigated such impacts in countries like Germany. They argue that such interventions can serve as models to help frame discussions of intelligent labor policies for “an inclusive AI era.”
Todd C. Helmus, Artificial Intelligence, Deepfakes, and Disinformation: A Primer (RAND Corporation, 2022).
Educators’ considerations of academic integrity and AI text can draw on parallel discussions of authenticity and labeling of AI content in other societal contexts. Artificial intelligence has made deepfake audio, video, and images as well as generated text much more difficult to detect as such. Here, Todd Helmus considers the consequences to political systems and individuals as he offers a review of the ways in which these can and have been used to promote disinformation. He considers ways to identify deepfakes and ways to authenticate provenance of videos and images. Helmus advocates for regulatory action, tools for journalistic scrutiny, and widespread efforts to promote media literacy. As well as informing discussions of authenticity in educational contexts, this report might help us shape curricula to teach students about the risks of deepfakes and unlabeled AI.
William Hasselberger, “ Can Machines Have Common Sense? ” The New Atlantis 65 (2021): 94–109.
Students, by definition, are engaged in developing their cognitive capacities; their understanding of their own intelligence is in flux and may be influenced by their interactions with AI systems and by AI hype. In his review of The Myth of Artificial Intelligence: Why Computers Can’t Think the Way We Do by Erik J. Larson, William Hasselberger warns that in overestimating AI’s ability to mimic human intelligence we devalue the human and overlook human capacities that are integral to everyday life decision making, understanding, and reasoning. Hasselberger provides examples of both academic and everyday common-sense reasoning that continue to be out of reach for AI. He provides a historical overview of debates around the limits of artificial intelligence and its implications for our understanding of human intelligence, citing the likes of Alan Turing and Marvin Minsky as well as contemporary discussions of data-driven language models.
Gwo-Jen Hwang and Nian-Shing Chen, “ Exploring the Potential of Generative Artificial Intelligence in Education: Applications, Challenges, and Future Research Directions ,” Educational Technology & Society 26, no. 2 (2023).
Gwo-Jen Hwang and Nian-Shing Chen are enthusiastic about the potential benefits of incorporating generative AI into education. They outline a variety of roles a large language model like ChatGPT might play, from student to tutor to peer to domain expert to administrator. For example, educators might assign students to “teach” ChatGPT on a subject. Hwang and Chen provide sample ChatGPT session transcripts to illustrate their suggestions. They share prompting techniques to help educators better design AI-based teaching strategies. At the same time, they are concerned about student overreliance on generative AI. They urge educators to guide students to use it critically and to reflect on their interactions with AI. Hwang and Chen don’t touch on concerns about bias, inaccuracy, or fabrication, but they call for further research into the impact of integrating generative AI on learning outcomes.
Weekly Newsletter
Get your fix of JSTOR Daily’s best stories in your inbox each Thursday.
Privacy Policy Contact Us You may unsubscribe at any time by clicking on the provided link on any marketing message.
Lauren Goodlad and Samuel Baker, “ Now the Humanities Can Disrupt ‘AI’ ,” Public Books (February 20, 2023).
Lauren Goodlad and Samuel Baker situate both academic integrity concerns and the pressures on educators to “embrace” AI in the context of market forces. They ground their discussion of AI risks in a deep technical understanding of the limits of predictive models at mimicking human intelligence. Goodlad and Baker urge educators to communicate the purpose and value of teaching with writing to help students engage with the plurality of the world and communicate with others. Beyond the classroom, they argue, educators should question tech industry narratives and participate in public discussion on regulation and the future of AI. They see higher education as resilient: academic skepticism about former waves of hype around MOOCs, for example, suggests that educators will not likely be dazzled or terrified into submission to AI. Goodlad and Baker hope we will instead take up our place as experts who should help shape the future of the role of machines in human thought and communication.
Kathryn Conrad, “ Sneak Preview: A Blueprint for an AI Bill of Rights for Education ,” Critical AI 2.1 (July 17, 2023).
How can the field of education put the needs of students and scholars first as we shape our response to AI, the way we teach about it, and the way we might incorporate it into pedagogy? Kathryn Conrad’s manifesto builds on and extends the Biden administration’s Office of Science and Technology Policy 2022 “Blueprint for an AI Bill of Rights.” Conrad argues that educators should have input into institutional policies on AI and access to professional development around AI. Instructors should be able to decide whether and how to incorporate AI into pedagogy, basing their decisions on expert recommendations and peer-reviewed research. Conrad outlines student rights around AI systems, including the right to know when AI is being used to evaluate them and the right to request alternate human evaluation. They deserve detailed instructor guidance on policies around AI use without fear of reprisals. Conrad maintains that students should be able to appeal any charges of academic misconduct involving AI, and they should be offered alternatives to any AI-based assignments that might put their creative work at risk of exposure or use without compensation. Both students’ and educators’ legal rights must be respected in any educational application of automated generative systems.
Support JSTOR Daily! Join our new membership program on Patreon today.
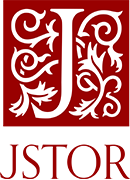
JSTOR is a digital library for scholars, researchers, and students. JSTOR Daily readers can access the original research behind our articles for free on JSTOR.
Get Our Newsletter
More stories.
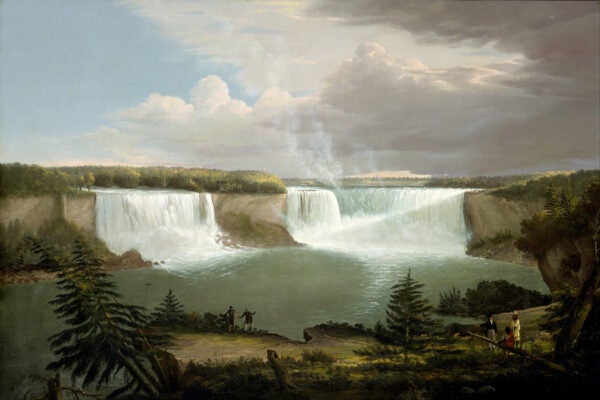
- The Fashionable Tour : or, The First American Tourist Guidebook
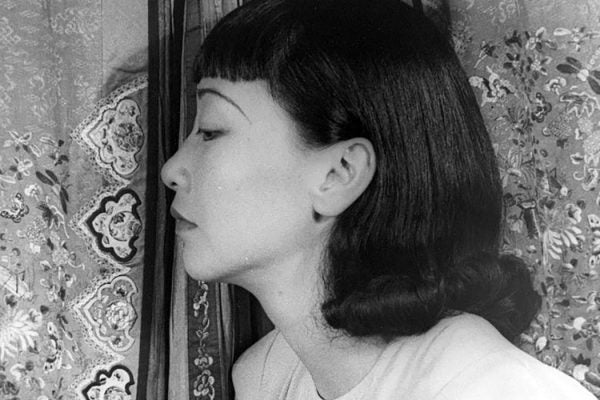
- Celebrating Asian American and Pacific Islander Heritage Month
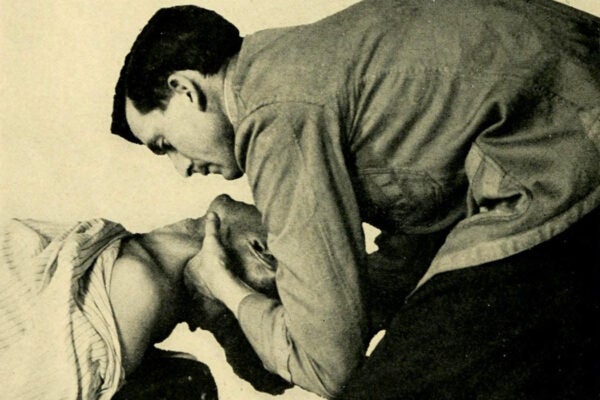
The Metaphysical Story of Chiropractic
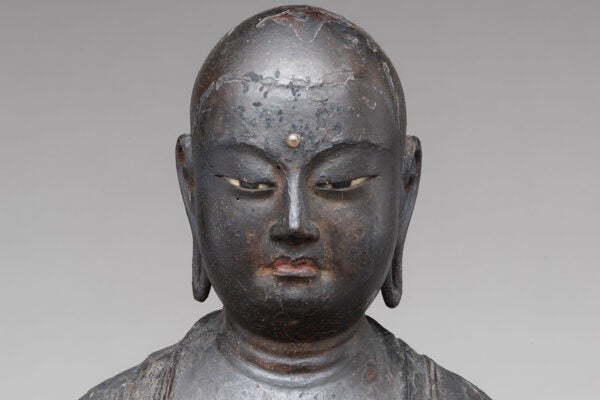
A Bodhisattva for Japanese Women
Recent posts.
- The Development of Central American Film
- Remembering Maud Lewis
- Rice, Famine, and the Seven Wonders of the World
Support JSTOR Daily
Sign up for our weekly newsletter.
- Share on twitter
- Share on facebook
These are the 20 most popular academic papers of 2016
Alternative metrics find that research from barack obama received the most online attention this year.
- Share on linkedin
- Share on mail
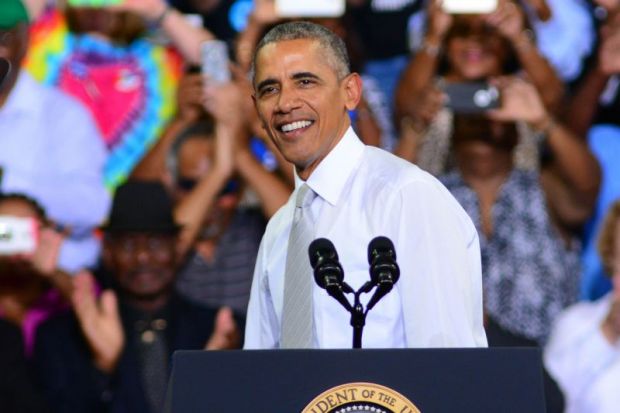
Barack Obama’s research on US healthcare reform – the first academic article to be published by a sitting US president – has topped a list of the most popular online papers of the year.
The article, “United States health care reform: progress to date and next steps”, published in the Journal of the American Medical Association in July, took pole position in a top 100 list compiled by Altmetric, a London start-up that tracks and analyses the online activity around scholarly literature.
The paper from the outgoing US president resulted in the highest Altmetric “attention score” ever tracked, 8,063, compared with a score of 4,912 for the second-most popular article.
The term “altmetrics” describes the practice of rating papers using “alternative metrics”, such as mentions on social media networking sites, rather than, for example, citations in other journals.
Other research that caught the public’s attention in 2016 includes an article on medical errors being the third leading cause of death in the US (second), a paper on mastering the board game Go (ninth), and a report finding that the ease with which we tell lies grows with repetition (18th).
The top 20 most popular academic papers of 2016
- United States health care reform: progress to date and next steps
- Medical error – the third leading cause of death in the US
- Observation of gravitational waves from a binary black hole merger
- Evidence for a distant giant planet in the solar system
- Sugar industry and coronary heart disease research: a historical analysis of internal industry documents
- Zika virus and birth defects – reviewing the evidence for causality
- The association between income and life expectancy in the United States, 2001-2014
- Effect of wearable technology combined with a lifestyle intervention on long-term weight loss
- Mastering the game of Go with deep neural networks and tree search
- The new world atlas of artificial night sky brightness
- Evidence for a limit to human lifespan
- The terrorist inside my husband’s brain
- The antibody aducanumab reduces Aβ plaques in Alzheimer’s disease
- Trends in adult body-mass index in 200 countries from 1975 to 2014
- Contribution of Antarctica to past and future sea-level rise
- Does physical activity attenuate, or even eliminate, the detrimental association of sitting time with mortality?
- Human commensals producing a novel antibiotic impair pathogen colonization
- The brain adapts to dishonesty
- The third international consensus definitions for sepsis and septic shock (sepsis-3)
- Zika virus associated with microcephaly
The full top 100 list, along with more information about the methodology, is available at Altmetric.com .
Register to continue
Why register?
- Registration is free and only takes a moment
- Once registered, you can read 3 articles a month
- Sign up for our newsletter
Or subscribe for unlimited access to:
- Unlimited access to news, views, insights & reviews
- Digital editions
- Digital access to THE’s university and college rankings analysis
Already registered or a current subscriber? Login
Related articles

Don’t throw out the baby with the metrics bathwater
Peer review is the least bad system we have for assessing quality, but metrics can help to determine attention and impact, says Euan Adie
Reader's comments (1)
You might also like.
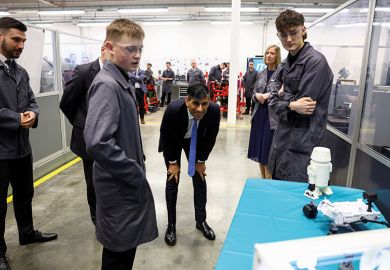
Asia University Rankings 2024: results announced
Updated methodology reveals previously underappreciated research strength in some Asian countries, reports Rosa Ellis
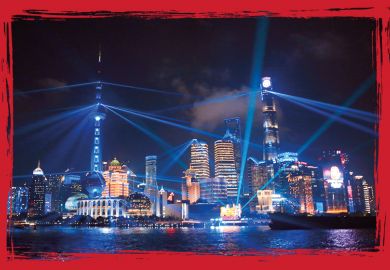
Asia University Rankings 2024: revelations of research strength
Our revised rankings methodology shows Asian research is stronger than appreciated, as participation and competition rises across a dynamic region writes Ellie Bothwell
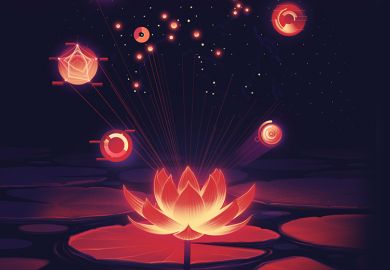
Asia University Rankings 2024: in charts
Continent’s elite shuffle positions, while more universities from West and South Asia feature
Featured jobs
Librarians/Admins
- EBSCOhost Collection Manager
- EBSCO Experience Manager
- EBSCO Connect
- Start your research
- EBSCO Mobile App
Clinical Decisions Users
- DynaMed Decisions
- Dynamic Health
- Waiting Rooms
- NoveList Blog
Education Full Text
Education Full Text is a research database for education students, professionals and policymakers. It includes full-text education journals that cover the essentials of education and related fields of study, including in-depth coverage of special education.
Education Full Text offers a selection of 260 active full-text journals covering the essentials of education and related fields of study. With a journal retail value of $39,186.13, it is the fourth largest database within the Education Source family.
Strengthen Exposure to Open Access Journals
Education Full Text includes rigorous curation and indexing of open access (OA) journals, which has resulted in a growing collection of 693 active global OA journals. Once validated and certified for inclusion, these OA journals are treated with high-quality subject indexing and sophisticated, precise/accurate full-text linking.
NOTE: EBSCOhost databases and EBSCO Discovery Service generate a lot more referrals for DOAJ than any other online platform .
Unique Full-Text Journals
Education Full Text includes 151 active full-text journals not available in any version of Academic Search.
The Highest Quality Subject Indexing
EBSCO has the premier and most highly regarded scholarly vocabularies curated by subject matter experts, covering all disciplines and major publishers.
EBSCO believes in breaking down barriers to information through Enhanced Subject Precision (ESP) mapping, bridging the gap between content and end users through inclusion of natural languages.
EBSCO databases support all learning types through textual and visual subject browse and information literacy training through subject access points in more than 30 languages. Watch video to learn more .
Education Full Text includes 2,069 active indexed and abstracted journals. 1,861 of them are peer-reviewed.
Customers also bought:
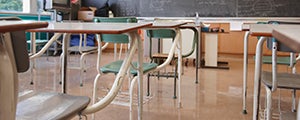
Updated Biden administration rules will soon affect students across US: What to know

Students and school employees across America will be impacted in the fall by new changes to a rule that affects all federally-funded schools.
Practically, it's a sweeping update to how schools will have to handle sex discrimination and abuse cases. Politically, it's a part of a power struggle between the Trump and Biden administrations.
What's new? The Biden administration released a new set of rules this week overhauling the Trump administration's Title IX rules – which gave more rights to alleged perpetrators of sexual assault and harassment. The new rules also stipulate further protections for LGBTQ+ students as well as parenting and pregnant students.
What is Title IX? Title IX is a civil rights law that bans sex discrimination against students, employees and others at public schools, colleges and universities that receive federal funding.
What were some of the old rules? For cases of sexual assault, they stipulated that schools only had to investigate claims that met a certain threshold of sex discrimination and were made through a formal reporting process. It also raised the bar of proof for sexual misconduct on college campuses. They prohibited investigations of cases that occurred off campus.
Why does the change matter? Critics argued the Trump-era rules prevented people accused of sexual harassment, assault or discrimination from facing repercussions. Supporters contended the rules rightly strengthened due-process protections for accused students or faculty members.
Biden's new Title IX rules will affect people on the nation's school campuses starting in August.
What happened this week?
The Biden administration's new set of guidance overhauls Trump-era rules that in part narrowed which and how schools could investigate sex discrimination cases. President Joe Biden in his 2020 presidential campaign vowed to overturn the changes made under Education Secretary Betsy DeVos.
The new rules expand the definition of sexual assault and harassment. That means schools could investigate more cases of discrimination, abuse or harassment filed by people on school campuses under the scope of the law.
College student survivors will no longer be required to attend live hearings or go through cross examinations. And people will be given the right to "prompt and equitable grievance procedures," the rule reads.
LGBTQ+ students will be guaranteed protection under the law if they are discriminated against for their gender identity or sexual orientation.
Pregnant and parenting students who might receive unwanted sexual attention, shame or punishment at schools will also be granted more protections from sex discrimination in the admissions process and on campus.
“These final regulations build on the legacy of Title IX by clarifying that all our nation’s students can access schools that are safe, welcoming, and respect their rights," said U.S. Secretary of Education Miguel Cardona.
Who is impacted by the changes to Title IX?
The rule changes have a wide range of effects on students, among the most notable examples:
- Sexual assault survivors : The new rules will have sweeping effects on survivors of sexual misconduct and those accused of crimes. Among the changes: The definition of sexual assault will be expanded in K-12 schools and colleges.
- LGBTQ+ students : Biden's Title IX update stipulates protections from sex discrimination based gender identity for the first time.
- Pregnant and parenting students : The new regulations extend the definition of "sex-based harassment" to include pregnant people on campuses.
Ruling on transgender student participation in sports remains unsettled
The Biden administration did not rule on whether transgender and nonbinary students can participate on the sports teams that align with their gender.
The administration released a proposed rule in April 2023 that said schools and colleges largely could not ban nonbinary and transgender students from sports teams in the new Title IX rules.
Contact Kayla Jimenez at [email protected] . Follow her on X at @kaylajjimenez.
Columbia University president takes heat at congressional antisemitism hearing
- Medium Text
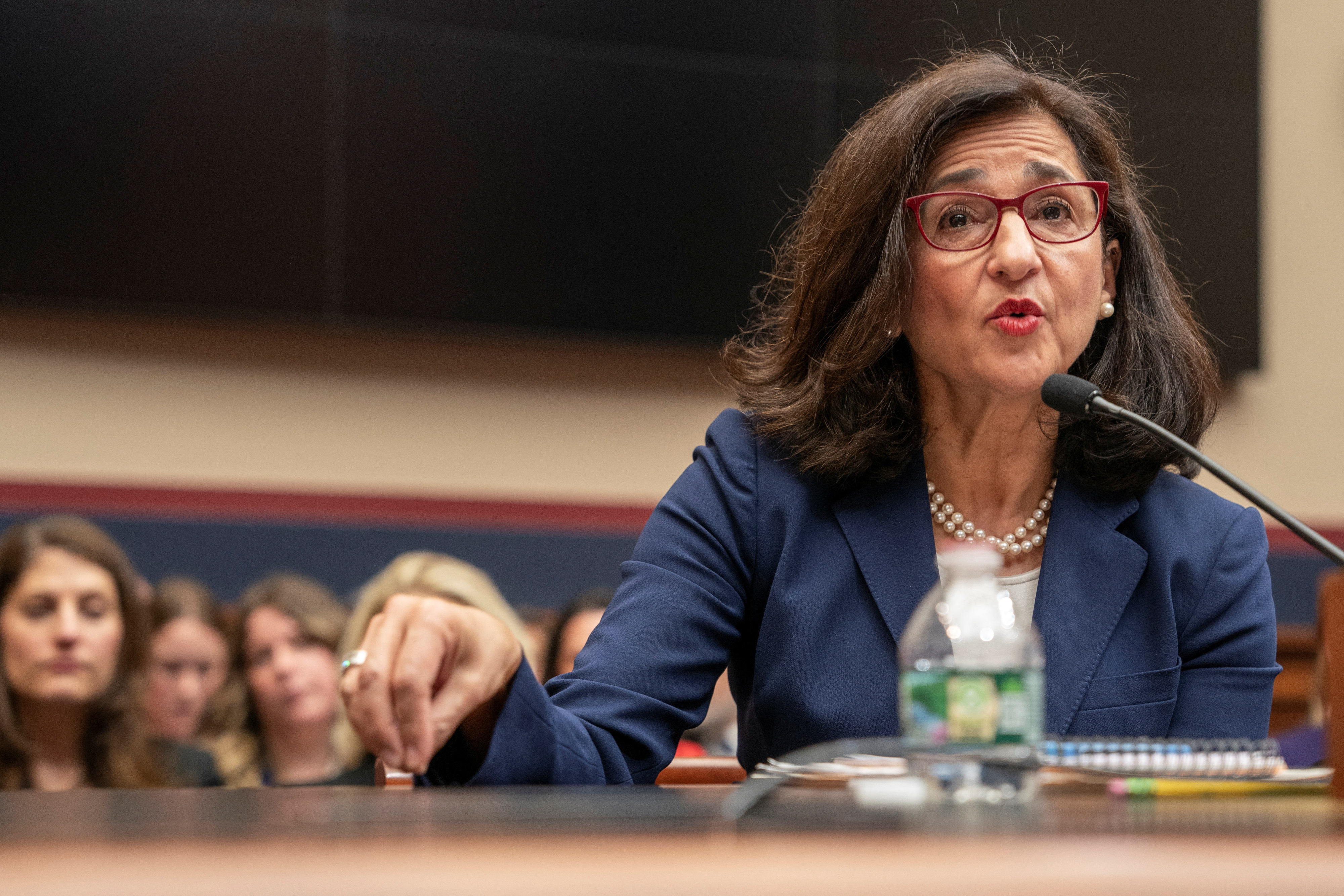
Sign up here.
Reporting by Gabriella Borter; editing by Jonathan Oatis
Our Standards: The Thomson Reuters Trust Principles. New Tab , opens new tab

Thomson Reuters
Gabriella Borter is a reporter on the U.S. National Affairs team, covering cultural and political issues as well as breaking news. She has won two Front Page Awards from the Newswomen’s Club of New York - in 2020 for her beat reporting on healthcare workers during the COVID-19 pandemic, and in 2019 for her spot story on the firing of the police officer who killed Eric Garner. The latter was also a Deadline Club Awards finalist. She holds a B.A. in English from Yale University and joined Reuters in 2017.
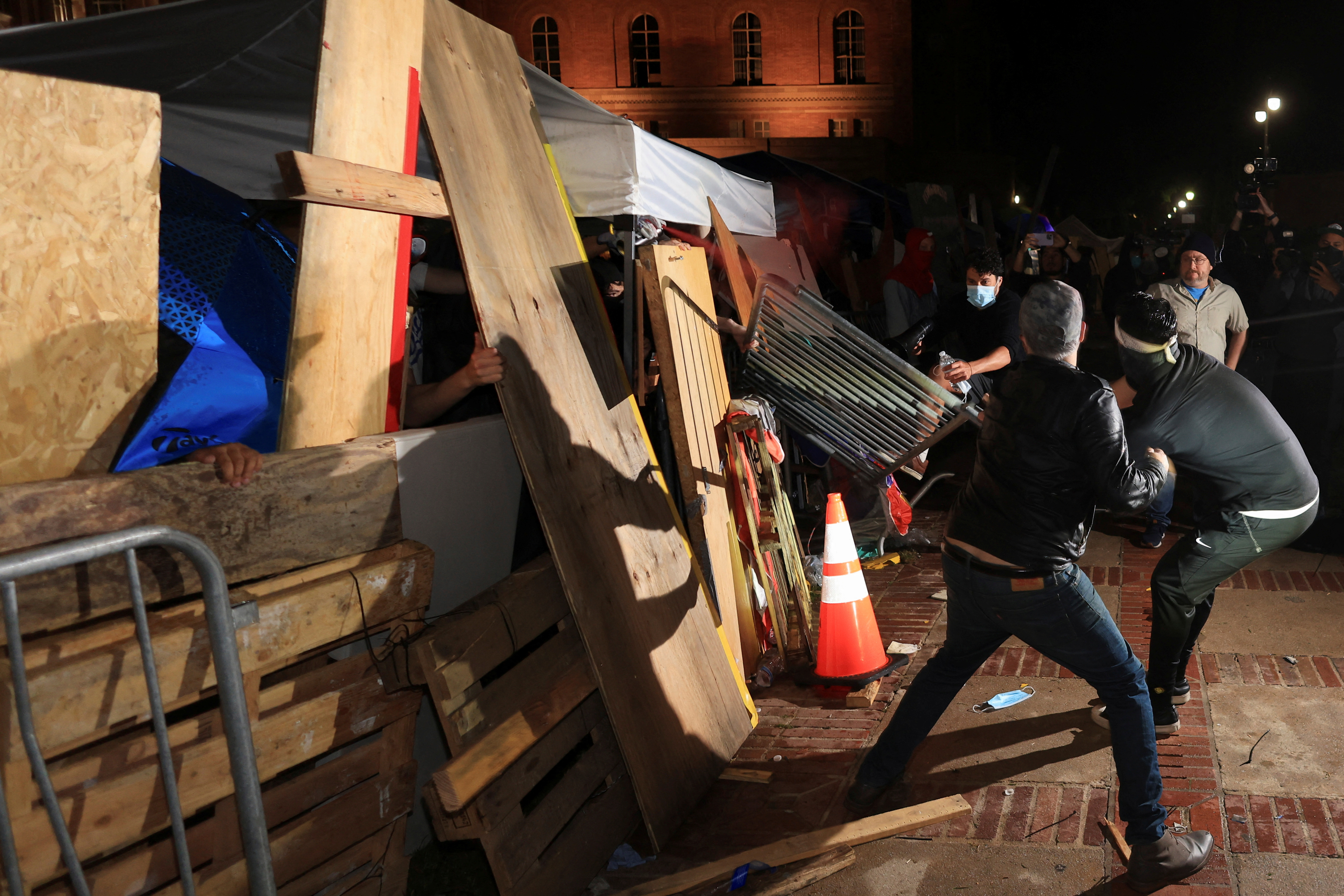
World Chevron
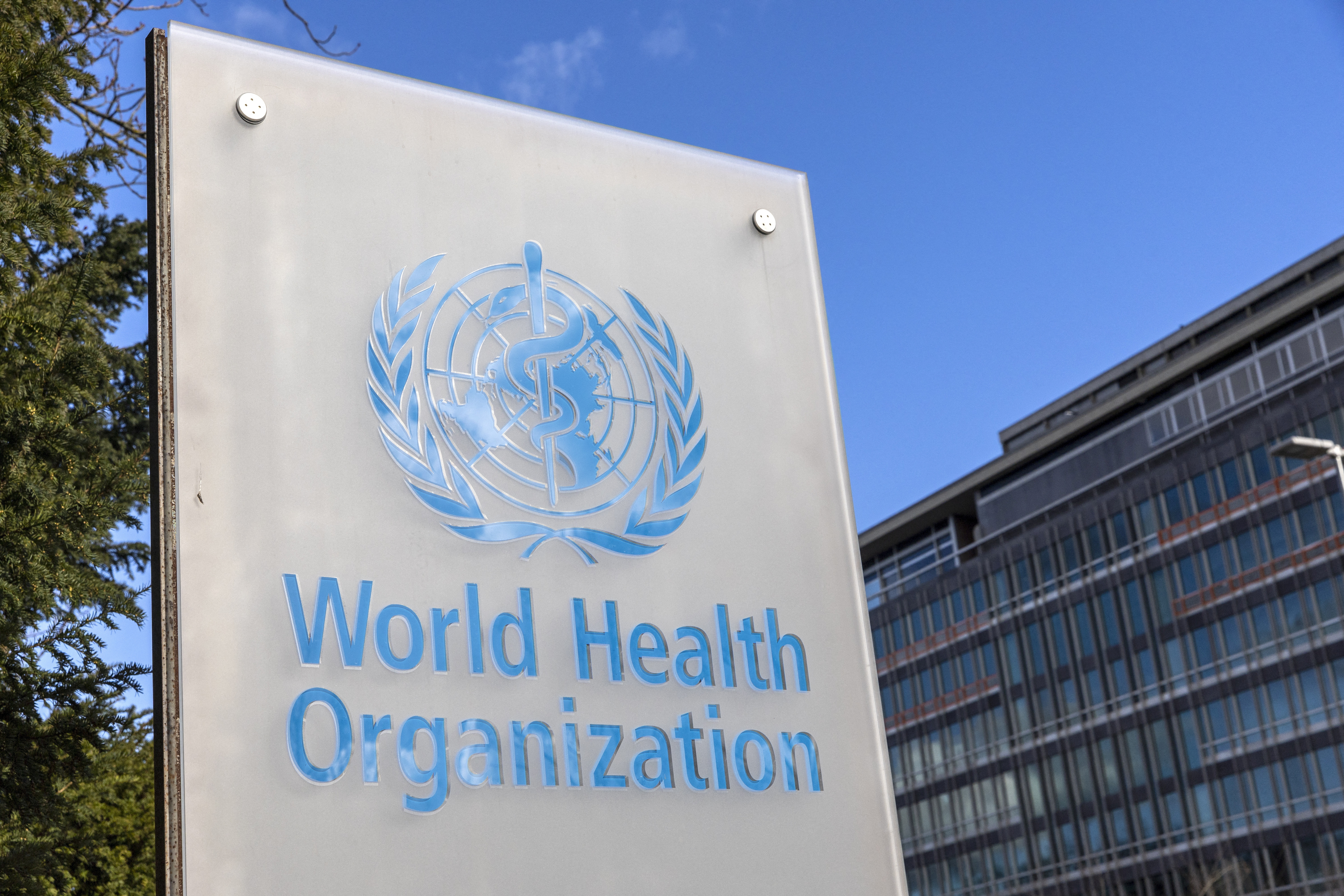
Taiwan says may be hard to attend WHO assembly, Blinken offers support
It will be hard for Taiwan to attend this year's World Health Organization annual assembly, and it hopes more countries will support its presence, the island's foreign minister said on Thursday after the United States pressed for an invite.
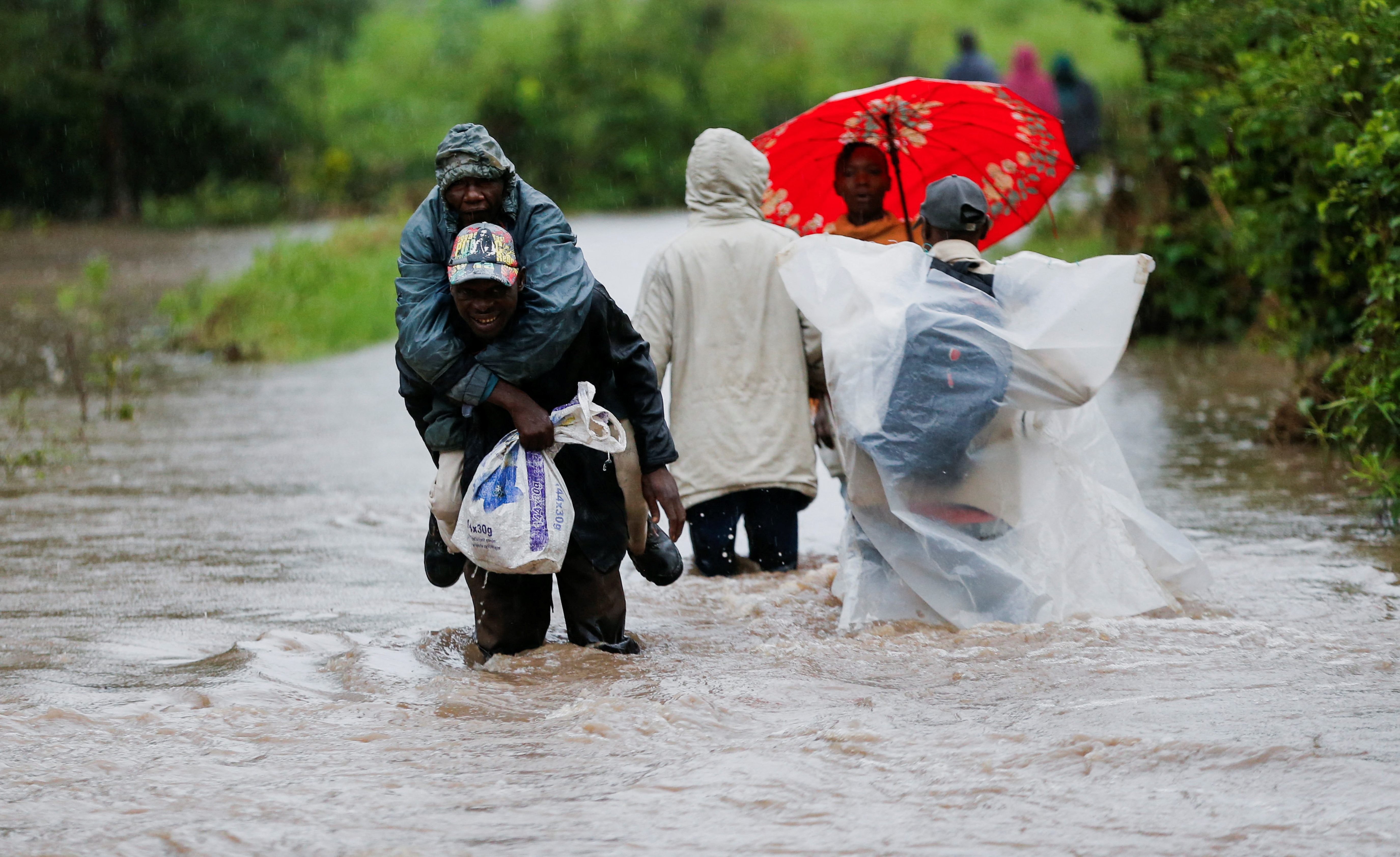
Solomon Islands lawmakers selected a new prime minister in a secret ballot on Thursday, choosing foreign minister Jeremiah Manele, who has pledged to continue the Pacific Island nation's foreign policy that saw it grow close to China.
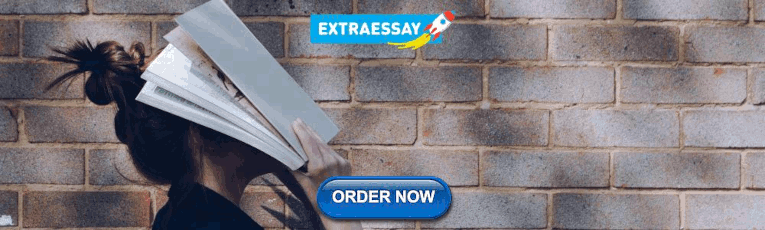
IMAGES
VIDEO
COMMENTS
4 issues/year. 0195-6744. 1549-6511. 2.5. Ranked #123 out of 269 "Education & Educational Research" journals. 3.3. Ranked #414 out of 1,469 "Education" journals. The American Journal of Education seeks to bridge and integrate the intellectual, methodological, and substantive diversity of educational scholarship and to encourage a ...
The Harvard Educational Review (HER) is a scholarly journal of opinion and research in education. The Editorial Board aims to publish pieces from interdisciplinary and wide-ranging fields that advance our understanding of educational theory, equity, and practice. HER encourages submissions from established and emerging scholars, as well as from ...
Google Scholar provides a simple way to broadly search for scholarly literature. Search across a wide variety of disciplines and sources: articles, theses, books, abstracts and court opinions. ... Advanced search. Find articles. with all of the words. with the exact phrase. with at least one of the words. without the words. where my words occur ...
Beyond Text: Learning through Arts-Based Research 2021 Beyond the Academic Gateway: Looking back on the Tenure-Track Journey ... Curriculum Inquiry in South African Higher Education: Some scholarly affirmations and challenges 2011 A Curriculum of Fear: Homeland Security in U.S. Public Schools 2016 ...
The American Educational Research Journal (AERJ) is the flagship journal of AERA, with articles that advance the empirical, theoretical, and methodological understanding of education and learning. It publishes original peer-reviewed analyses spanning the field of education research across all subfields and disciplines and all levels of analysis, all levels of education throughout the life span ...
This article introduces a unifying framework for studying panel experiments with population interference, in which a treatment assigned to one experimental unit affects another experimental unit's outcome. Findings have implications for fields as diverse as education, economics, and public health. 23 Mar 2021.
Using the Universal Design for Learning (UDL) (CAST, Inc., 2012) principles as a guide, technology can increase access to, and representation of, content, provide students with a variety of ways to communicate and express their knowledge, and motivate student learning through interest and engagement.
Furthermore, digital platforms provide students with reliable and high-quality data from their PC, anywhere and anytime. Aside from information resources, technology in education allows students to contact academic professionals worldwide. Technology in education is the most significant revolution in teaching that will ever witness.
Open Educational Resources (OER) have been lauded for their ability to reduce student costs and improve equity in higher education. Research examining whether OER provides learning benefits have produced mixed results, with most studies showing null effects. We argue that the common methods used to examine OER efficacy are unlikely to detect positive effects based on predictions of the access ...
Journal of Education. The oldest educational publication in the country, the Journal of Education's mission is to disseminate knowledge that informs practice in PK-12, higher, and professional education. A refereed publication, the Journal offers … | View full journal description. This journal is a member of the Committee on Publication ...
Here are the 10 best education articles of 2020, based on a composite ranking of pageviews and editors' picks. Can the Lockdown Push Schools in a Positive Direction?, by Patrick Cook-Deegan: Here are five ways that COVID-19 could change education for the better. How Teachers Can Navigate Difficult Emotions During School Closures, by Amy L. Eva: Here are some tools for staying calm and ...
Academic Articles. Displaying 1 - 50 of 190 Academic Articles. Employer Learning and the Dynamics of Returns to Universities: Evidence from Chinese Elite Education during University Expansion ... Economics of Education, 2nd Edition, London: Academic Press, pp. 171-182. 2020. Download. Education Production Functions. in Steve Bradley and Colin ...
A scholarly, academic, or peer-reviewed journal is a published collection of articles written by scholars in the field. The articles present original research and undergo a peer-review process before publication. Before the article can be published, it is vetted by other scholars for quality and accuracy according to the journal's editorial ...
In the past few decades, technology has completely transformed the world around us. Indeed, experts believe that the next big digital transformation in how we live, communicate, work, trade and learn will be driven by Artificial Intelligence (AI) [83]. This paper presents a high-level industrial and academic overview of AI in Education (AIEd). It presents the focus of latest research in AIEd ...
With the rapid increase in the number of scholarly publications on STEM education in recent years, reviews of the status and trends in STEM education research internationally support the development of the field. For this review, we conducted a systematic analysis of 798 articles in STEM education published between 2000 and the end of 2018 in 36 journals to get an overview about developments ...
View Full Text View PDF Nine years of development in establishing the journal as a learning and research hub in STEM education. From August 2014 to July 2023, the International Journal of STEM Education went through nine publication cycle years. In this editorial, I provide a brief summary of the journal's development up to and including ...
Selectively indexed journals contain an average of 50-79% education-related articles and are critical to topic area coverage; ERIC applies a manual article-by-article selection process and indexes only the articles that conform to the standard and criteria outlined in the ERIC selection policy.
The Importance of Education. Education is an important issue in one's life. It is the key to success in the future, and t o. have many opportunities in our life. Education has many advantages ...
ERIC is an online library of education research and information, sponsored by the Institute of Education Sciences (IES) of the U.S. Department of Education.
Educators' considerations of academic integrity and AI text can draw on parallel discussions of authenticity and labeling of AI content in other societal contexts. Artificial intelligence has made deepfake audio, video, and images as well as generated text much more difficult to detect as such.
Academic writing is a formal style of writing used in universities and scholarly publications. You'll encounter it in journal articles and books on academic topics, and you'll be expected to write your essays, research papers, and dissertation in academic style. Academic writing follows the same writing process as other types of texts, but ...
Other research that caught the public's attention in 2016 includes an article on medical errors being the third leading cause of death in the US (second), a paper on mastering the board game Go (ninth), and a report finding that the ease with which we tell lies grows with repetition (18th). The top 20 most popular academic papers of 2016
Education Full Text. $531,528.74. $378,968.20. $338,622.61. $39,186.13. Education Full Text offers a selection of 260 active full-text journals covering the essentials of education and related fields of study. With a journal retail value of $39,186.13, it is the fourth largest database within the Education Source family.
President Joe Biden in his 2020 presidential campaign vowed to overturn the changes made under Education Secretary Betsy DeVos. The new rules expand the definition of sexual assault and harassment.
Columbia University President Nemat "Minouche" Shafik testifies before a House Education and the Workforce Committee hearing on "Columbia University's Response to Antisemitism," on Capitol Hill in ...
Police officers in riot gear arrested dozens of pro-Palestinian demonstrators at Columbia University in Manhattan on Tuesday night and cleared a building that protesters had seized about 20 hours ...