We will keep fighting for all libraries - stand with us!
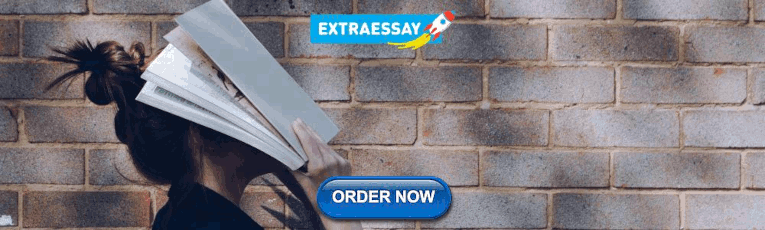
Internet Archive Audio
- This Just In
- Grateful Dead
- Old Time Radio
- 78 RPMs and Cylinder Recordings
- Audio Books & Poetry
- Computers, Technology and Science
- Music, Arts & Culture
- News & Public Affairs
- Spirituality & Religion
- Radio News Archive
- Flickr Commons
- Occupy Wall Street Flickr
- NASA Images
- Solar System Collection
- Ames Research Center
- All Software
- Old School Emulation
- MS-DOS Games
- Historical Software
- Classic PC Games
- Software Library
- Kodi Archive and Support File
- Vintage Software
- CD-ROM Software
- CD-ROM Software Library
- Software Sites
- Tucows Software Library
- Shareware CD-ROMs
- Software Capsules Compilation
- CD-ROM Images
- ZX Spectrum
- DOOM Level CD

- Smithsonian Libraries
- FEDLINK (US)
- Lincoln Collection
- American Libraries
- Canadian Libraries
- Universal Library
- Project Gutenberg
- Children's Library
- Biodiversity Heritage Library
- Books by Language
- Additional Collections
- Prelinger Archives
- Democracy Now!
- Occupy Wall Street
- TV NSA Clip Library
- Animation & Cartoons
- Arts & Music
- Computers & Technology
- Cultural & Academic Films
- Ephemeral Films
- Sports Videos
- Videogame Videos
- Youth Media
Search the history of over 866 billion web pages on the Internet.
Mobile Apps
- Wayback Machine (iOS)
- Wayback Machine (Android)
Browser Extensions
Archive-it subscription.
- Explore the Collections
- Build Collections
Save Page Now
Capture a web page as it appears now for use as a trusted citation in the future.
Please enter a valid web address
- Donate Donate icon An illustration of a heart shape
Heuristics : intelligent search strategies for computer problem solving
Bookreader item preview, share or embed this item, flag this item for.
- Graphic Violence
- Explicit Sexual Content
- Hate Speech
- Misinformation/Disinformation
- Marketing/Phishing/Advertising
- Misleading/Inaccurate/Missing Metadata
![[WorldCat (this item)] [WorldCat (this item)]](https://archive.org/images/worldcat-small.png)
plus-circle Add Review comment Reviews
716 Previews
13 Favorites
DOWNLOAD OPTIONS
No suitable files to display here.
EPUB and PDF access not available for this item.
IN COLLECTIONS
Uploaded by Tracey Gutierres on April 26, 2013
Follow the author

Image Unavailable

- To view this video, download Flash Player
Heuristics: Intelligent Search Strategies for Computer Problem Solving Hardcover – Jan. 1 1753
- Language English
- Publisher Addison-Wesley
- Publication date Jan. 1 1753
- Dimensions 17.15 x 1.91 x 24.13 cm
- ISBN-10 0201055945
- ISBN-13 978-0201055948
- See all details
Customers who bought this item also bought

Product details
- Publisher : Addison-Wesley (Jan. 1 1753)
- Language : English
- ISBN-10 : 0201055945
- ISBN-13 : 978-0201055948
- Item weight : 680 g
- Dimensions : 17.15 x 1.91 x 24.13 cm
- #158 in AI Heuristic & Constrained Search
- #615 in Business Operations Research
- #624 in Operations Research (Books)
About the author
Judea pearl.
Discover more of the author’s books, see similar authors, read author blogs and more
Customer reviews
- Sort reviews by Top reviews Most recent Top reviews
Top reviews from Canada
Top reviews from other countries.

- Amazon and Our Planet
- Investor Relations
- Press Releases
- Amazon Science
- Sell on Amazon
- Supply to Amazon
- Become an Affiliate
- Protect & Build Your Brand
- Sell on Amazon Handmade
- Advertise Your Products
- Independently Publish with Us
- Host an Amazon Hub
- Amazon.ca Rewards Mastercard
- Shop with Points
- Reload Your Balance
- Amazon Currency Converter
- Amazon Cash
- Shipping Rates & Policies
- Amazon Prime
- Returns Are Easy
- Manage your Content and Devices
- Recalls and Product Safety Alerts
- Customer Service
- Conditions of Use
- Privacy Notice
- Interest-Based Ads
- Amazon.com.ca ULC | 40 King Street W 47th Floor, Toronto, Ontario, Canada, M5H 3Y2 |1-877-586-3230

- Computing & Internet
- Computer Science
- AI & Machine Learning

Download the free Kindle app and start reading Kindle books instantly on your smartphone, tablet or computer – no Kindle device required .
Read instantly on your browser with Kindle for Web.
Using your mobile phone camera - scan the code below and download the Kindle app.

Follow the author

Image Unavailable

- To view this video download Flash Player
Heuristics: Intelligent Search Strategies for Computer Problem Solving Hardcover – 1 Jan. 1984
- Print length 399 pages
- Language English
- Publisher Addison Wesley Longman Publishing Co
- Publication date 1 Jan. 1984
- Dimensions 17.15 x 1.91 x 24.13 cm
- ISBN-10 0201055945
- ISBN-13 978-0201055948
- See all details
Product details
- Publisher : Addison Wesley Longman Publishing Co (1 Jan. 1984)
- Language : English
- Hardcover : 399 pages
- ISBN-10 : 0201055945
- ISBN-13 : 978-0201055948
- Dimensions : 17.15 x 1.91 x 24.13 cm
- 185,793 in Reference (Books)
About the author
Judea pearl.
Discover more of the author’s books, see similar authors, read author blogs and more
Customer reviews
Customer Reviews, including Product Star Ratings, help customers to learn more about the product and decide whether it is the right product for them.
To calculate the overall star rating and percentage breakdown by star, we don’t use a simple average. Instead, our system considers things like how recent a review is and if the reviewer bought the item on Amazon. It also analyses reviews to verify trustworthiness.
- Sort reviews by Top reviews Most recent Top reviews
Top reviews from United Kingdom
Top reviews from other countries.

- UK Modern Slavery Statement
- Sustainability
- Amazon Science
- Sell on Amazon
- Sell on Amazon Business
- Sell on Amazon Handmade
- Sell on Amazon Launchpad
- Supply to Amazon
- Protect and build your brand
- Associates Programme
- Fulfilment by Amazon
- Seller Fulfilled Prime
- Advertise Your Products
- Independently Publish with Us
- Host an Amazon Hub
- › See More Make Money with Us
- Instalments by Barclays
- Amazon Platinum Mastercard
- Amazon Classic Mastercard
- Amazon Currency Converter
- Payment Methods Help
- Shop with Points
- Top Up Your Account
- Top Up Your Account in Store
- COVID-19 and Amazon
- Track Packages or View Orders
- Delivery Rates & Policies
- Amazon Prime
- Returns & Replacements
- Manage Your Content and Devices
- Recalls and Product Safety Alerts
- Amazon Mobile App
- Customer Service
- Accessibility
- Conditions of Use & Sale
- Privacy Notice
- Cookies Notice
- Interest-Based Ads Notice

Download the free Kindle app and start reading Kindle books instantly on your smartphone, tablet or computer – no Kindle device required .
Read instantly on your browser with Kindle for Web.
Using your mobile phone camera, scan the code below and download the Kindle app.

Follow the author

Image Unavailable

- To view this video download Flash Player
Heuristics: Intelligent Search Strategies for Computer Problem Solving Hardcover – Import, 1 January 1984
Return policy.
Tap on the category links below for the associated return window and exceptions (if any) for returns.
10 Days Returnable
You can return if you receive a damaged, defective or incorrect product.
10 Days, Refund
Returnable if you’ve received the product in a condition that is damaged, defective or different from its description on the product detail page on Amazon.in.
Refunds will be issued only if it is determined that the item was not damaged while in your possession, or is not different from what was shipped to you.
Movies, Music
Not returnable, musical instruments.
Wind instruments and items marked as non-returnable on detail page are not eligible for return.
Video Games (Accessories and Games)
You can ask for a replacement or refund if you receive a damaged, defective or incorrect product.
Mobiles (new and certified refurbished)
10 days replacement, mobile accessories.
This item is eligible for free replacement/refund, within 10 days of delivery, in an unlikely event of damaged, defective or different/wrong item delivered to you. Note: Please keep the item in its original condition, with MRP tags attached, user manual, warranty cards, and original accessories in manufacturer packaging. We may contact you to ascertain the damage or defect in the product prior to issuing refund/replacement.
Power Banks: 10 Days; Replacement only
Screen guards, screen protectors and tempered glasses are non-returnable.
Used Mobiles, Tablets
10 days refund.
Refunds applicable only if it has been determined that the item was not damaged while in your possession, or is not different from what was shipped to you.
Mobiles and Tablets with Inspect & Buy label
2 days refund, tablets (new and certified refurbished), 7 days replacement.
This item is eligible for free replacement, within 7 days of delivery, in an unlikely event of damaged or different item delivered to you. In case of defective, product quality related issues for brands listed below, customer will be required to approach the brands’ customer service center and seek resolution. If the product is confirmed as defective by the brand then customer needs to get letter/email confirming the same and submit to Amazon customer service to seek replacement. Replacement for defective products, products with quality issues cannot be provided if the brand has not confirmed the same through a letter/email. Brands -HP, Lenovo, AMD, Intel, Seagate, Crucial
Please keep the item in its original condition, with brand outer box, MRP tags attached, user manual, warranty cards, CDs and original accessories in manufacturer packaging for a successful return pick-up. Before returning a Tablet, the device should be formatted and screen lock should be disabled.
For few products, we may schedule a technician visit to your location. On the basis of the technician's evaluation report, we will provide resolution.
This item is eligible for free replacement, within 7 days of delivery, in an unlikely event of damaged, defective or different item delivered to you.
Please keep the item in its original condition, with brand outer box, MRP tags attached, user manual, warranty cards, CDs and original accessories in manufacturer packaging for a successful return pick-up.
Used Laptops
Software products that are labeled as not returnable on the product detail pages are not eligible for returns.
For software-related technical issues or installation issues in items belonging to the Software category, please contact the brand directly.
Desktops, Monitors, Pen drives, Hard drives, Memory cards, Computer accessories, Graphic cards, CPU, Power supplies, Motherboards, Cooling devices, TV cards & Computing Components
All PC components, listed as Components under "Computers & Accessories" that are labeled as not returnable on the product detail page are not eligible for returns.
Digital Cameras, camera lenses, Headsets, Speakers, Projectors, Home Entertainment (new and certified refurbished)
Return the camera in the original condition with brand box and all the accessories Product like camera bag etc. to avoid pickup cancellation. We will not process a replacement if the pickup is cancelled owing to missing/damaged contents.
Return the speakers in the original condition in brand box to avoid pickup cancellation. We will not process a replacement if the pickup is cancelled owing to missing/ damaged box.
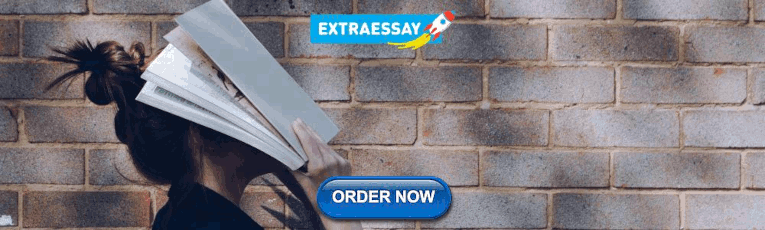
10 Days, Replacement
Speakers (new and certified refurbished), home entertainment.
This item is eligible for free replacement, within 10 days of delivery, in an unlikely event of damaged, defective or different/wrong item delivered to you.
Note: Please keep the item in its original condition, with MRP tags attached, user manual, warranty cards, and original accessories in manufacturer packaging for a successful return pick-up.
For TV, we may schedule a technician visit to your location and resolution will be provided based on the technician's evaluation report.
10 days Replacement only
This item is eligible for free replacement, within 10 days of delivery, in an unlikely event of damaged, defective or different/wrong item delivered to you. .
Please keep the item in its original condition, original packaging, with user manual, warranty cards, and original accessories in manufacturer packaging for a successful return pick-up.
If you report an issue with your Furniture,we may schedule a technician visit to your location. On the basis of the technician's evaluation report, we will provide resolution.
Large Appliances - Air Coolers, Air Conditioner, Refrigerator, Washing Machine, Dishwasher, Microwave
In certain cases, if you report an issue with your Air Conditioner, Refrigerator, Washing Machine or Microwave, we may schedule a technician visit to your location. On the basis of the technician's evaluation report, we'll provide a resolution.
Home and Kitchen
Grocery and gourmet, pet food, pet shampoos and conditioners, pest control and pet grooming aids, non-returnable, pet habitats and supplies, apparel and leashes, training and behavior aids, toys, aquarium supplies such as pumps, filters and lights, 7 days returnable.
All the toys item other than Vehicle and Outdoor Category are eligible for free replacement/refund, within 7 days of delivery, in an unlikely event of damaged, defective or different/wrong item delivered to you.
Vehicle and Outdoor category toys are eligible for free replacement, within 7 days of delivery, in an unlikely event of damaged, defective or different/wrong item delivered to you
Note: Please keep the item in its original condition, with outer box or case, user manual, warranty cards, and other accompaniments in manufacturer packaging for a successful return pick-up. We may contact you to ascertain the damage or defect in the product prior to issuing refund/replacement.
Sports, Fitness and Outdoors
Occupational health & safety products, personal care appliances, 7 days replacement only, health and personal care, clothing and accessories, 30 days returnable.
Lingerie, innerwear and apparel labeled as non-returnable on their product detail pages can't be returned.
Return the clothing in the original condition with the MRP and brand tag attached to the clothing to avoid pickup cancellation. We will not process a replacement or refund if the pickup is cancelled owing to missing MRP tag.
Precious Jewellery
Precious jewellery items need to be returned in the tamper free packaging that is provided in the delivery parcel. Returns in any other packaging will not be accepted.
Fashion or Imitation Jewellery, Eyewear and Watches
Return the watch in the original condition in brand box to avoid pickup cancellation. We will not process a replacement if the pickup is cancelled owing to missing/damaged contents.
Gold Coins / Gold Vedhanis / Gold Chips / Gold Bars
30 days; replacement/refund, 30 days, returnable, luggage and handbags.
Any luggage items with locks must be returned unlocked.
Car Parts and Accessories, Bike Parts and Accessories, Helmets and other Protective Gear, Vehicle Electronics
Items marked as non-returnable on detail page are not eligible for return.
Items that you no longer need must be returned in new and unopened condition with all the original packing, tags, inbox literature, warranty/ guarantee card, freebies and accessories including keys, straps and locks intact.
Fasteners, Food service equipment and supplies, Industrial Electrical, Lab and Scientific Products, Material Handling Products, Occupational Health and Safety Products, Packaging and Shipping Supplies, Professional Medical Supplies, Tapes, Adhesives and Sealants Test, Measure and Inspect items, Industrial Hardware, Industrial Power and Hand Tools.
Tyres (except car tyres), rims and oversized items (automobiles).
Car tyres are non-returnable and hence, not eligible for return.
Return pickup facility is not available for these items. You can self return these products using any courier/ postal service of your choice. Learn more about shipping cost refunds .
The return timelines for seller-fulfilled items sold on Amazon.in are equivalent to the return timelines mentioned above for items fulfilled by Amazon.
If you’ve received a seller-fulfilled product in a condition that is damaged, defective or different from its description on the product detail page on Amazon.in, returns are subject to the seller's approval of the return.
If you do not receive a response from the seller for your return request within two business days, you can submit an A-to-Z Guarantee claim. Learn more about returning seller fulfilled items.
Note : For seller fulfilled items from Books, Movies & TV Shows categories, the sellers need to be informed of the damage/ defect within 14 days of delivery.
For seller-fulfilled items from Fine Art category, the sellers need to be informed of the damage / defect within 10 days of delivery. These items are not eligible for self-return. The seller will arrange the return pick up for these items.
For seller-fulfilled items from Sports collectibles and Entertainment collectibles categories, the sellers need to be informed of the damage / defect within 10 days of delivery.
The General Return Policy is applicable for all Amazon Global Store Products (“Product”). If the Product is eligible for a refund on return, you can choose to return the Product either through courier Pickup or Self-Return**
Note: - Once the package is received at Amazon Export Sales LLC fulfillment center in the US, it takes 2 (two) business days for the refund to be processed and 2- 4 business days for the refund amount to reflect in your account. - If your return is due to an Amazon error you'll receive a full refund, else the shipping charges (onward & return) along with import fees will be deducted from your refund amount.
**For products worth more than INR 25000, we only offer Self-Return option.
2 Days, Refund
Refunds are applicable only if determined that the item was not damaged while in your possession, or is not different from what was shipped to you.
- ISBN-10 0201055945
- ISBN-13 978-0201055948
- Publisher Addison Wesley Longman Publishing Co
- Publication date 1 January 1984
- Language English
- Dimensions 17.15 x 1.91 x 24.13 cm
- Print length 399 pages
- See all details
Product details
- Publisher : Addison Wesley Longman Publishing Co (1 January 1984)
- Language : English
- Hardcover : 399 pages
- ISBN-10 : 0201055945
- ISBN-13 : 978-0201055948
- Item Weight : 680 g
- Dimensions : 17.15 x 1.91 x 24.13 cm
About the author
Judea pearl.
Discover more of the author’s books, see similar authors, read author blogs and more
Customer reviews
- Sort reviews by Top reviews Most recent Top reviews
Top reviews from India
Top reviews from other countries.

- Press Releases
- Amazon Science
- Sell on Amazon
- Sell under Amazon Accelerator
- Protect and Build Your Brand
- Amazon Global Selling
- Become an Affiliate
- Fulfilment by Amazon
- Advertise Your Products
- Amazon Pay on Merchants
- COVID-19 and Amazon
- Your Account
- Returns Centre
- 100% Purchase Protection
- Amazon App Download
- Conditions of Use & Sale
- Privacy Notice
- Interest-Based Ads
- Data Science
- Data Analysis
- Data Visualization
- Machine Learning
- Deep Learning
- Computer Vision
- Artificial Intelligence
- AI ML DS Interview Series
- AI ML DS Projects series
- Data Engineering
- Web Scrapping
- Difference between Best-First Search and A* Search?
- Difference Between Depth First Search, Breadth First Search and Depth Limit Search in AI
- Difference Between Search and Search All in COBOL
- Difference between Informed and Uninformed Search in AI
- Google's AI-Powered Search Makeover to Counter Microsoft's New Bing
- Breadth-first Search is a special case of Uniform-cost search
- Is Sentinel Linear Search better than normal Linear Search?
- Google Search AI-Powered Results Now Reaching More Users
- Which strategy can be used to solve branch and bound problem?
- Binary Search functions in C++ STL (binary_search, lower_bound and upper_bound)
- Best First Search (Informed Search)
- Episodic Memory and Deep Q-Networks
- Meta Binary Search | One-Sided Binary Search
- Allocating kernel memory (buddy system and slab system)
- GFact | Why s.lower_bound(x) is faster than lower_bound(s.begin(), s.end(), x) in C++ STL?
- Memory Organisation in Computer Architecture
- What is the difference between Binary Search and Jump Search?
- Linear Search vs Binary Search
- Is exponential search faster than binary search?
Memory-bounded search ( Memory Bounded Heuristic Search ) in AI
Search algorithms are fundamental techniques in the field of artificial intelligence (AI) that let agents or systems solve challenging issues. Memory-bounded search strategies are necessary because AI systems often encounter constrained memory resources in real-world circumstances. The notion of memory-bound search, often referred to as memory-bounded heuristic search, is examined in this article along with its importance in AI applications. We will review how AI effectively manages search jobs when memory resources are limited and provide a useful how-to manual for putting memory-bound search algorithms into practice.
Table of Content
Understanding Memory-Bound Search
Benefits of memory-bound search, implementing memory-bound search, pseudocode: memory-bounded a* algorithm, implemented of memory-bounded search strategy for the 8-puzzle problem, applying memory-bound search in ai, faqs on memory-bounded search (or memory bounded heuristic search).
When memory resources are restricted, AI uses a method called memory-bound search to solve issues and make judgments quickly. Conventional search methods, such as the A* or Dijkstra’s algorithms, sometimes require infinite memory, which may not be realistic in many circumstances.
Memory-bound search algorithms, on the other hand, are created with the limitation of finite memory in mind. The goal of these algorithms is to effectively use the memory that is available while finding optimum or nearly optimal solutions. They do this by deciding which information to keep and retrieve strategically, as well as by using heuristic functions to direct the search process.
Finding a balance between the quality of the answer produced and the quantity of memory consumed is the main notion underlying memory-bound search. Even with constrained resources, these algorithms may solve problems effectively by carefully allocating memory.
- Efficiency in Memory-Limited Situations: Memory-bound search algorithms perform well when memory is limited. They don’t need a lot of memory to hold the whole search space or exploration history to locate solutions.
- Real-world Applicability: Memory-bound search algorithms are useful for a variety of AI applications, particularly those integrated into hardware with constrained memory. IoT devices, robots, autonomous cars, and real-time systems fall under this category.
- Optimal or Near-Optimal Remedies: Memory-bound search looks for the optimal answer given the memory restrictions. These algorithms may often effectively provide optimum or almost ideal answers by using well-informed heuristics.
- Dynamic Memory Management: The memory allocation and deallocation techniques used by these algorithms are dynamic. They make decisions about what data to keep and when to remove or replace it, so memory is used effectively during the search process.
The method must be carefully designed to efficiently handle the memory resources available to implement memory-bound search. Let us go through a simple Pseudocode that illustrates the main ideas of creating memory-bound search code samples.
Explanation:
The MemoryBoundedSearch function in this pseudocode accepts an issue and a memory limit as input. The nodes to be investigated and the nodes that have already been explored are stored in the open and closed lists, respectively, which are initialized. The algorithm then goes into a loop where it keeps track of whether memory use goes above the predetermined threshold. If that’s the case, memory is freed up by pruning the open and closed lists.
Based on a heuristic assessment, the select_best_node function selects the most promising node from the open list. The answer is sent back if this node is in the desired state. If not, the node is transferred from the open list to the closed list, and if its heirs have previously been investigated or are already on the open list, they are created and added to the open list.
This is an example of a straightforward memory-bounded search strategy for the 8-puzzle problem implemented in Python:
code steps:
- Node Class : Defines a class representing nodes in the search tree, with attributes state , parent , and action .
- Heuristic Function : Calculates the Manhattan distance heuristic for a given state.
- Memory Usage Function : Computes the total memory usage based on the length of the open and closed lists.
- Prune Memory Function : Prunes the least promising nodes from the open list to reduce memory usage.
- Select Best Node Function : Selects the node with the lowest heuristic value from the open list.
- Goal State Check Function : Checks if a given node’s state matches the goal state.
- Generate Successors Function : Generates successor nodes by swapping the blank space with neighboring tiles.
- Redundancy Check Function : Determines if a successor node is redundant by checking if its state is already present in either the open or closed list.
- Memory-Bounded Search Function : Performs a memory-bounded search using A* algorithm, considering memory limit.
- Goal State Definition : Defines the goal state of the puzzle.
- Possible Moves Definition : Defines the possible moves for each position on the puzzle grid.
- Example Usage : Specifies an initial state of the puzzle and sets a memory limit for the search.
- Solution Found Check : Checks if a solution node is found within the memory limit.
- Print Solution Path : If a solution is found, prints the sequence of actions and states leading from the initial state to the goal state.
- Memory Limit Exceeded Check : Prints a message if the memory limit is exceeded without finding a solution.
Memory-bound search is useful in many AI disciplines, particularly for addressing complex problems or working in resource-constrained contexts. Here are few instances:
- Robotics Pathfinding: Robots that operate in complex surroundings often possess restricted memory and processing capabilities. They can effectively identify optimum or nearly optimal courses while avoiding obstacles and achieving their objectives thanks to memory-bound search techniques.
- Autonomous Vehicles: Due to limited onboard memory, autonomous vehicles must make judgments in real time. In order to provide effective and secure navigation, memory-bound search may help with route planning, obstacle avoidance, and traffic optimization.
- Agents who Play Games: Memory-bound search algorithms may assist AI agents in determining the optimal moves in games with enormous search spaces, like Go or Chess, while taking into account the limited memory available during gaming.
- Natural Language Processing: Memory-bound search may be used for jobs when the hardware’s memory limitations are present, such as machine translation or text summarization.
- Devices with Limited Resources: Embedded systems, mobile phones, and Internet of Things devices often have little memory. Memory-bound search may let these devices work within their memory limits to complete complicated tasks like data processing or picture recognition.
AI’s memory-bound search approach is essential for effectively solving problems and making decisions when memory is scarce. Memory consumption and solution quality are balanced by these algorithms via the use of heuristic functions and smart memory management. Memory-bound search guarantees that AI can function properly even with restricted memory availability, which has useful applications in robotics, autonomous systems, and resource-constrained devices. Memory-bound search has advantages in these kinds of situations, but it also has drawbacks, such as the possibility of less-than-ideal answers and a rise in computing complexity since effective memory management is required. For certain AI applications, memory-bound search algorithms must be carefully designed taking into account the trade-offs between memory utilization and result quality. All things considered, memory-bound search increases the capacity of AI systems, increasing their versatility and adaptability in a variety of real-world situations with few memory limits.
Q. What is the process by which memory-bound search manages to explore big search spaces?
A: To direct the search toward promising regions of the search space, memory-bound search algorithms make use of informed heuristics. Even with limited memory, these heuristics enable the algorithm to efficiently concentrate its research efforts by predicting the cost or distance to the target.
Q. How does memory-bound search balance the need for memory with the quality of the solutions it finds?
A: Memory management techniques and heuristic functions are used to handle the trade-off. While memory management strategies like dynamic allocation and eviction rules guarantee that the most relevant data is kept within the memory limit, informed heuristics direct the search towards promising regions.
Q. What data structures are often used in memory-bound search implementations?
A: Commonly utilized data structures in memory-bound search are priority queues and sets. While sets effectively record visited states or nodes, priority queues assist in ranking nodes according to their heuristic values. Furthermore, g-scores and f-scores are stored in dictionaries or hash tables for constant-time lookups and changes.
Q. What are some substitute methods for AI’s memory-bound search?
A: Alternate strategies include memory-aware algorithms, which dynamically modify their behavior depending on available memory, and anytime algorithms, which provide approximation answers that become better with time. Another method is incremental heuristic search, in which answers are improved little by little as additional memory becomes available.
Please Login to comment...
Similar reads.
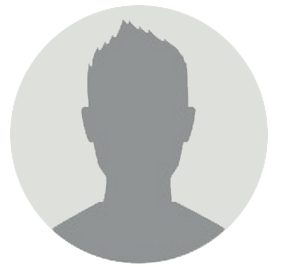
- Algorithms-Searching
- Data Science Blogathon 2024
Improve your Coding Skills with Practice
What kind of Experience do you want to share?
Enhancing cut selection through reinforcement learning
- AI Methods for Optimization Problems
- Published: 15 May 2024
Cite this article
- Shengchao Wang 1 ,
- Liang Chen 1 ,
- Lingfeng Niu 2 &
- Yu-Hong Dai 1
Explore all metrics
With the rapid development of artificial intelligence in recent years, applying various learning techniques to solve mixed-integer linear programming (MILP) problems has emerged as a burgeoning research domain. Apart from constructing end-to-end models directly, integrating learning approaches with some modules in the traditional methods for solving MILPs is also a promising direction. The cutting plane method is one of the fundamental algorithms used in modern MILP solvers, and the selection of appropriate cuts from the candidate cuts subset is crucial for enhancing efficiency. Due to the reliance on expert knowledge and problem-specific heuristics, classical cut selection methods are not always transferable and often limit the scalability and generalizability of the cutting plane method. To provide a more efficient and generalizable strategy, we propose a reinforcement learning (RL) framework to enhance cut selection in the solving process of MILPs. Firstly, we design feature vectors to incorporate the inherent properties of MILP and computational information from the solver and represent MILP instances as bipartite graphs. Secondly, we choose the weighted metrics to approximate the proximity of feasible solutions to the convex hull and utilize the learning method to determine the weights assigned to each metric. Thirdly, a graph convolutional neural network is adopted with a self-attention mechanism to predict the value of weighting factors. Finally, we transform the cut selection process into a Markov decision process and utilize RL method to train the model. Extensive experiments are conducted based on a leading open-source MILP solver SCIP. Results on both general and specific datasets validate the effectiveness and efficiency of our proposed approach.
This is a preview of subscription content, log in via an institution to check access.
Access this article
Price includes VAT (Russian Federation)
Instant access to the full article PDF.
Rent this article via DeepDyve
Institutional subscriptions
Similar content being viewed by others
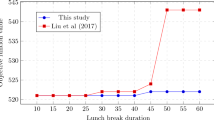
A novel MILP formulation and an efficient heuristic for the vehicle routing problem with lunch break
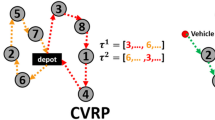
Multi-start team orienteering problem for UAS mission re-planning with data-efficient deep reinforcement learning
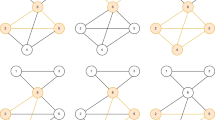
Solving maximum clique problem using chemical reaction optimization
Achterberg T. Constraint integer programming. PhD Thesis. Berlin: Technische Universität Berlin, 2007
Google Scholar
Achterberg T, Wunderling R. Mixed integer programming: Analyzing 12 years of progress. In: Jünger M, Reinelt G, eds. Facets of Combinatorial Optimization: Festschrift for Martin Grötschel. Berlin-Heidelberg: Springer, 2013, 449–481
Chapter Google Scholar
Alvarez A M, Louveaux Q, Wehenkel L. A machine learning-based approximation of strong branching. INFORMS J Comput, 2017, 29: 185–195
Article MathSciNet Google Scholar
Anderson L, Turner M, Koch T. Generative deep learning for decision making in gas networks. Math Methods Oper Res, 2022, 95: 503–532
Andreello G, Caprara A, Fischetti M. Embedding {0, 1/2}-cuts in a branch-and-cut framework: A computational study. INFORMS J Comput, 2007, 19: 229–238
Balcan M F, Dick T, Sandholm T, et al. Learning to branch. In: Proceedings of International Conference on Machine Learning. Ann Arbor: PMLR, 2018, 344–353
Balcan M F, Prasad S, Sandholm T, et al. Sample complexity of tree search configuration: Cutting planes and beyond. In: Proceedings of the 35th International Conference on Neural Information Processing Systems. San Francisco: Curran Associates, 2021, 4015–4027
Baltean-Lugojan R, Bonami P, Misener R, et al. Scoring positive semidefinite cutting planes for quadratic optimization via trained neural networks. https://optimization-online.org/2018/11/6943/ , 2019
Berthold T, Francobaldi M, Hendel G. Learning to use local cuts. arXiv:2206.11618, 2022
Bestuzheva K, Besançon M, Chen W K, et al. The SCIP Optimization Suite 8.0. Berlin: Zuse Institute Berlin, 2021, 21–41
Blieklú C, Bonami P, Lodi A. Solving mixed-integer quadratic programming problems with IBM-CPLEX: A progress report. In: Proceedings of the twenty-sixth RAMP Symposium. Montreal: ACM Press, 2014, 16–17
Bonami P, Cornuéjols G, Dash S, et al. Projected Chvatal-Gomory cuts for mixed integer linear programs. Math Program, 2008, 113: 241–257
Chen W K, Chen L, Dai Y H. Lifting for the integer knapsack cover polyhedron. J Global Optim, 2022: 1–45
Chvátal V. Edmonds polytopes and a hierarchy of combinatorial problems. Discrete Math, 1973, 4: 305–337
Dey S S, Molinaro M. Theoretical challenges towards cutting-plane selection. Math Program, 2018, 170: 237–266
Ding J Y, Zhang C, Shen L, et al. Accelerating primal solution findings for mixed integer programs based on solution prediction. AAAI J, 2020, 34: 1452–1459
Article Google Scholar
Gasse M, Chételat D, Ferroni N, et al. Exact combinatorial optimization with graph convolutional neural networks. In: Proceedings of the 32nd International Conference on Neural Information Processing Systems. San Francisco: Curran Associates, 2019, 15580–15592
Ge D, Huangfu Q, Wang Z, et al. Cardinal Optimizer (COPT) User Guide. arXiv:2208.14314, 2022
Gleixner A, Hendel G, Gamrath G, et al. MIPLIB 2017: Data-driven compilation of the 6th mixed-integer programming library. Math Program Comput, 2021, 13: 443–490
Gomory R. An algorithm for the mixed integer problem. RAND CORP SANTA MONICA CA, 1960
Gupta P, Gasse M, Khalil E, et al. Hybrid models for learning to branch. In: Proceedings of the 33rd International Conference on Neural Information Processing Systems. San Francisco: Curran Associates, 2020, 18087–18097
Gurobi Optimization L. Gurobi Optimizer Reference Manual. https://www.gurobi.com , 2023
Huang Z, Wang K, Liu F, et al. Learning to select cuts for efficient mixed-integer programming. Pattern Recogn, 2022, 123: 108353
Jia H, Shen S. Benders cut classification via support vector machines for solving two-stage stochastic programs. INFORMS J Optim, 2021, 3: 278–297
Kallrath J. Modeling Languages in Mathematical Optimization. New York: Springer, 2004
Book Google Scholar
Khalil E, Dilkina B, Nemhauser GL, et al. Learning to run heuristics in tree search. In: Proceedings of the Twenty-Sixth International Joint Conference on Artificial Intelligence. IJCAI, 2017, 659–666
Khalil E, Le Bodic P, Song L, et al. Learning to branch in mixed integer programming. AAAI J, 2016, 30: 724–731
Kingma DP, Ba J. Adam: A method for stochastic optimization. arXiv:1412.6980, 2014
Kool W, van Hoof H, Welling M. Attention, learn to solve routing problems! In: Proceedings of the International Conference on Learning Representations. ICLR, 2019, 3: 5057–5069
Land AH, Doig AG. An automatic method of solving discrete programming problems. Econometrica, 1960, 28: 497–520
Lodi A, Tramontani A. Performance variability in mixed-integer programming. In: Theory Driven by Influential Applications. Annapolis: INFORMS, 2013, 1–12
Marchand H, Martin A, Weismantel R, et al. Cutting planes in integer and mixed integer programming. Discrete Appl Math, 2002, 123: 397–446
Nair V, Bartunov S, Gimeno F, et al. Solving mixed integer programs using neural networks. arXiv:2012.13349, 2020
Nazari M, Oroojlooy A, Snyder L, et al. Reinforcement learning for solving the vehicle routing problem. In: Proceedings of the International Conference on Neural Information Processing Systems. San Francisco: Curran Associates, 2018, 94–99
Nickel S, Steinhardt C, Schlenker H, et al. IBM ILOG CPLEX Optimization Studio: Modellierung von Planungs-und Entscheidungsproblemen des Operations Research mit OPL, 2021, 9–23
Paszke A, Gross S, Massa F, et al. Pytorch: An imperative style, high-performance deep learning library. In: Proceedings of the 33rd International Conference on Neural Information Processing Systems. San Francisco: Curran Associates, 2019, 8026–8037
Paulus MB, Zarpellon G, Krause A, et al. Learning to cut by looking ahead: Cutting plane selection via imitation learning. In: Proceedings of International Conference on Machine Learning. Ann Arbor: PMLR, 2022, 17584–17600
Shen Y, Sun Y, Eberhard A, et al. Learning primal heuristics for mixed integer programs. In: Proceedings of the International Joint Conference on Neural Networks. San Francisco: IEEE, 2021, 1–8
Sutton R S, McAllester D, Singh S, et al. Policy gradient methods for reinforcement learning with function approximation. Adv Neural Inform Process Syst, 2000, 12: 1057–1063
Tang Y, Agrawal S, Faenza Y. Reinforcement learning for integer programming: Learning to cut. In: Proceedings of International Conference on Machine Learning. Ann Arbor: PMLR, 2020, 9367–9376
Turner M, Koch T, Serrano F, et al. Adaptive cut selection in mixed-integer linear programming. arXiv:2202.10962, 2022
Vasilyev I, Boccia M, Hanafi S. An implementation of exact knapsack separation. J Global Optim, 2016, 66: 127–150
Vinyals O, Fortunato M, Jaitly N. Pointer networks. In: Proceedings of the 28th International Conference on Neural Information Processing Systems. San Francisco: Curran Associates, 2015, 2692–2700
Wang Z, Li X, Wang J, et al. Learning cut selection for mixed-integer linear programming via hierarchical sequence model. arXiv:2302.00244, 2023
Wesselmann F, Stuhl U. Implementing cutting plane management and selection techniques. Technical Report. Paderborn: University of Paderborn, 2012
Wolsey LA. Integer Programming. New York: John Wiley & Sons, 2020
Yilmaz K, Yorke-Smith N. Learning efficient search approximation in mixed integer branch and bound. http://www.resolvertudelftnl/uuid:bce72457-108f-4215-b7e4-599866ba52aa , 2020
Download references
Acknowledgements
This work was supported by the National Key R&D Program of China (Grant No. 2022YFB2403400) and National Natural Science Foundation of China (Grant Nos. 11991021 and 12021001).
Author information
Authors and affiliations.
Academy of Mathematics and Systems Science, Chinese Academy of Sciences, Beijing, 100190, China
Shengchao Wang, Liang Chen & Yu-Hong Dai
Research Center on Fictitious Economy and Data Science, Chinese Academy of Sciences, Beijing, 100190, China
Lingfeng Niu
You can also search for this author in PubMed Google Scholar
Corresponding author
Correspondence to Yu-Hong Dai .
Rights and permissions
Reprints and permissions
About this article
Wang, S., Chen, L., Niu, L. et al. Enhancing cut selection through reinforcement learning. Sci. China Math. (2024). https://doi.org/10.1007/s11425-023-2294-3
Download citation
Received : 03 August 2023
Accepted : 18 April 2024
Published : 15 May 2024
DOI : https://doi.org/10.1007/s11425-023-2294-3
Share this article
Anyone you share the following link with will be able to read this content:
Sorry, a shareable link is not currently available for this article.
Provided by the Springer Nature SharedIt content-sharing initiative
- reinforcement learning
- mixed-integer linear programming
- cutting plane method
- cut selection
- Find a journal
- Publish with us
- Track your research
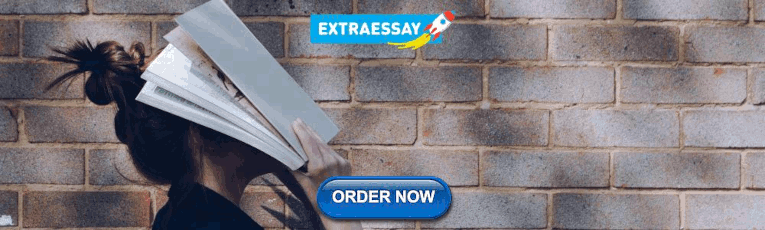
IMAGES
VIDEO
COMMENTS
Heuristics : intelligent search strategies for computer problem solving ... intelligent search strategies for computer problem solving by Pearl, Judea. ... 1985 Topics Artificial intelligence, Heuristic programming, Operations research, Problem solving Publisher Reading, Mass. : Collection inlibrary; printdisabled; internetarchivebooks ...
In particular, he defines these techniques as "strategies using readily accessible though loosely applicable information to control problem-solving processes in human beings and machine(s)1 (p. vii). As such, Pearl's heuristics that can be used in some cases but not in every case, where their utility in any particular case could be diminished ...
Pearl has gathered together, for the firs t time, almost all that is current in solving search problems in computer scienc e using heuristics. Pearl give some well known examples of the kinds of problems which with the book is concerned: the 8-Queens problem; the 8-Puzzle; the Traveling Salesman proble m; the Counterfeit Coin problem.
Heuristics : intelligent search strategies for computer problem solving. J. Pearl. Published 1 April 1984. Computer Science. TLDR. This book presents, characterizes and analyzes problem solving strategies that are guided by heuristic information and provides examples of how these strategies have changed over time. Expand.
OSTI ID: 5127296. Pearl, J. Heuristics stand for strategies using readily accessible information to control problem-solving processes in man and machine. This book presents an analysis of the nature and the power of typical heuristic methods, primarily those used in artificial intelligence and operations research, to solve problems in areas ...
This is a classic book essential for the study, research and teaching / learning of intelligent search strategies to solve problems guided by heuristic information. Read more. Edgar Altamirano. 5.0 out of 5 stars An essential book for the study and research of problem solving guided by heuristic information.
In this paper, a greedy heuristic and two local search algorithms, 1-opt local search and k-opt local search, are proposed for the unconstrained binary quadratic programming problem (BQP). These heuristics are well suited for the incorporation into meta-...
Click on the article title to read more.
Bibliographic information. Title. Heuristics: Intelligent Search Strategies for Computer Problem SolvingAddison-Wesley series in artificial intelligenceArtificial intelligence series, ISSN0272-1686. Author. Judea Pearl. Edition. illustrated, reprint. Publisher. Addison-Wesley Publishing Company, 1984.
Semantic Scholar extracted view of "Heuristics: Intelligent search strategies for computer problem solving: Judea PEARL Addison-Wesley, Reading, 1984" by H. Müller-Merbach
5.0 out of 5 stars An essential book for the study and research of problem solving guided by heuristic information. Reviewed in the United States on December 1, 2020 Verified Purchase
Problem-Solving Strategies and the Nature of Heuristic Information 1. Heuristics and Problem Representations 3 1.1 Typical Uses of Heuristics in Problem Solving 3 1.1.1 The 8-Queens Problem 4 / 1.1.2 The 8-Puzzle 6 / 1.1.3 The Road Map Problem 9 / 1.1.4 The Traveling Salesman Problem (TSP) 10 / 1.1.5 The Counterfeit Coin Problem 12
Buy Heuristics: Intelligent Search Strategies for Computer Problem Solving by Pearl, Judea (ISBN: 9780201055948) from Amazon's Book Store. Everyday low prices and free delivery on eligible orders. Heuristics: Intelligent Search Strategies for Computer Problem Solving: Amazon.co.uk: Pearl, Judea: 9780201055948: Books
As a book about search, it is thorough, at the state of the art, and contains expositions that will delight the expert with their clarity and depth. However, it is not, per se, a book about AI (nor was it intended to be) or about the history, philosophy, or cognitive aspects of heuristic knowledge.
Heuristics: Intelligent Search Strategies for Computer Problem Solving Hardcover - Import, 1 January 1984 by Judea Pearl (Author) 4.6 4.6 out of 5 stars 5 ratings
Judea Pearl. 4.07. 15 ratings1 review. Genres Artificial Intelligence Mathematics Computers Computer Science Technical. 399 pages, Hardcover. First published April 1, 1984. Book details & editions.
International Journal of Intelligent Systems. Volume 1, Issue 1 p. 69-70. Book Review. Heuristics—intelligent search strategies for computer problem solving, by Judea Pearl. (Reading, Ma: Addison-Wesley, 1984) ... Sabatier & I.N.P.T. Laboratoire Langages et Systèmes Informatiques 118, route de Narbonne, 31062 Toulouse Cedex, France. Search ...
Heuristics: Intelligent Search Strategies for Computer Problem Solving by Judea Pearl Addison-Wesley Publishing Company, Massachusetts, USA, 101985 (£43.95) - Volume 6 Issue 2
This document discusses the concept of an algorithm designed to locate the optimal solution to a problem in a (presumably) very large solution space by beginning a search at an arbitrary point in the solution space and then searching in the "local" area around the start point to find better solutions. Expand. 110. PDF.
The foregoing right shall not permit the posting of the article/paper in electronic or digital form on any computer network, except by the author or the author's employer, and then only on the author's or the employer's own web page or ftp site.
Intelligent Search Strategies f To fully appreciate Professor Pearl's book, begin with a careful reading of the title. It is a book about ". .Intelligent- ..Strategies. ." for the discovery and use of "Heuristics.. " to allow computers to solve " . . Search. . ' ' problems. Search
Heuristics: Intelligent Search Strategies for Computer Problem Solving : Pearl, Judea: Amazon.com.au: Books
Implemented of memory-bounded search strategy for the 8-puzzle problem . This is an example of a straightforward memory-bounded search strategy for the 8-puzzle problem implemented in Python: code steps: Node Class: Defines a class representing nodes in the search tree, with attributes state, parent, and action.
With the rapid development of artificial intelligence in recent years, applying various learning techniques to solve mixed-integer linear programming (MILP) problems has emerged as a burgeoning research domain. Apart from constructing end-to-end models directly, integrating learning approaches with some modules in the traditional methods for solving MILPs is also a promising direction. The ...