
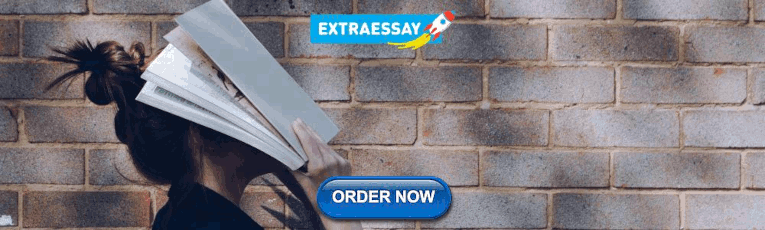
Global Dimensions of Intellectual Property Rights in Science and Technology (1993)
Chapter: 12 a case study on computer programs, 12 a case study on computer programs.
PAMELA SAMUELSON
HISTORICAL OVERVIEW
Phase 1: the 1950s and early 1960s.
When computer programs were first being developed, proprietary rights issues were not of much concern. Software was often developed in academic or other research settings. Much progress in the programming field occurred as a result of informal exchanges of software among academics and other researchers. In the course of such exchanges, a program developed by one person might be extended or improved by a number of colleagues who would send back (or on to others) their revised versions of the software. Computer manufacturers in this period often provided software to customers of their machines to make their major product (i.e., computers) more commercially attractive (which caused the software to be characterized as "bundled" with the hardware).
To the extent that computer programs were distributed in this period by firms for whom proprietary rights in software were important, programs tended to be developed and distributed through restrictive trade secret licensing agreements. In general, these were individually negotiated with customers. The licensing tradition of the early days of the software industry has framed some of the industry expectations about proprietary rights issues, with implications for issues still being litigated today.
In the mid-1960s, as programs began to become more diverse and complex, as more firms began to invest in the development of programs, and as
some began to envision a wider market for software products, a public dialogue began to develop about what kinds of proprietary rights were or should be available for computer programs. The industry had trade secrecy and licensing protection, but some thought more legal protection might be needed.
Phase 2: Mid-1960s and 1970s
Copyright law was one existing intellectual property system into which some in the mid-1960s thought computer programs might potentially fit. Copyright had a number of potential advantages for software: it could provide a relatively long term of protection against unauthorized copying based on a minimal showing of creativity and a simple, inexpensive registration process. 1 Copyright would protect the work's ''expression," but not the "ideas" it contained. Others would be free to use the same ideas in other software, or to develop independently the same or a similar work. All that would be forbidden was the copying of expression from the first author's work.
In 1964, the U.S. Copyright Office considered whether to begin accepting registration of computer programs as copyrightable writings. It decided to do so, but only under its "rule of doubt" and then only on condition that a full text of the program be deposited with the office, which would be available for public review. 2
The Copyright Office's doubt about the copyrightability of programs
arose from a 1908 Supreme Court decision that had held that a piano roll was not an infringing "copy" of copyrighted music, but rather part of a mechanical device. 3 Mechanical devices (and processes) have traditionally been excluded from the copyright domain. 4 Although the office was aware that in machine-readable form, computer programs had a mechanical character, they also had a textual character, which was why the Copyright Office decided to accept them for registration.
The requirement that the full text of the source code of a program be deposited in order for a copyright in the program to be registered was consistent with a long-standing practice of the Copyright Office, 5 as well as with what has long been perceived to be the constitutional purpose of copyright, namely, promoting the creation and dissemination of knowledge. 6
Relatively few programs, however, were registered with the Copyright Office under this policy during the 1960s and 1970s. 7 Several factors may have contributed to this. Some firms may have been deterred by the requirement that the full text of the source code be deposited with the office and made available for public inspection, because this would have dispelled its trade secret status. Some may have thought a registration certificate issued under the rule of doubt might not be worth much. However, the main reason for the low number of copyright registrations was probably that a mass market in software still lay in the future. Copyright is useful mainly to protect mass-marketed products, and trade secrecy is quite adequate for programs with a small number of distributed copies.
Shortly after the Copyright Office issued its policy on the registrability of computer programs, the U.S. Patent Office issued a policy statement concerning its views on the patentability of computer programs. It rejected the idea that computer programs, or the intellectual processes that might be embodied in them, were patentable subject matter. 8 Only if a program was
claimed as part of a traditionally patentable industrial process (i.e., those involving the transformation of matter from one physical state to another) did the Patent Office intend to issue patents for program-related innovations. 9
Patents are typically available for inventive advances in machine designs or other technological products or processes on completion of a rigorous examination procedure conducted by a government agency, based on a detailed specification of what the claimed invention is, how it differs from the prior art, and how the invention can be made. Although patent rights are considerably shorter in duration than copyrights, patent rights are considered stronger because no one may make, use, or sell the claimed invention without the patent owner's permission during the life of the patent. (Patents give rights not just against someone who copies the protected innovation, but even against those who develop it independently.) Also, much of what copyright law would consider to be unprotectable functional content ("ideas") if described in a book can be protected by patent law.
The Patent Office's policy denying the patentability of program innovations was consistent with the recommendations of a presidential commission convened to make suggestions about how the office could more effectively cope with an "age of exploding technology." The commission also recommended that patent protection not be available for computer program innovations. 10
Although there were some appellate decisions in the late 1960s and
early 1970s overturning Patent Office rejections of computer program-related applications, few software developers looked to the patent system for protection after two U.S. Supreme Court decisions in the 1970s ruled that patent protection was not available for algorithms. 11 These decisions were generally regarded as calling into question the patentability of all software innovations, although some continued to pursue patents for their software innovations notwithstanding these decisions. 12
As the 1970s drew to a close, despite the seeming availability of copyright protection for computer programs, the software industry was still relying principally on trade secrecy and licensing agreements. Patents seemed largely, if not totally, unavailable for program innovations. Occasional suggestions were made that a new form of legal protection for computer programs should be devised, but the practice of the day was trade secrecy and licensing, and the discourse about additional protection was focused overwhelmingly on copyright.
During the 1960s and 1970s the computer science research community grew substantially in size. Although more software was being distributed under restrictive licensing agreements, much software, as well as innovative ideas about how to develop software, continued to be exchanged among researchers in this field. The results of much of this research were published and discussed openly at research conferences. Toward the end of this period, a number of important research ideas began to make their way into commercial projects, but this was not seen as an impediment to research by computer scientists because the commercial ventures tended to arise after the research had been published. Researchers during this period did not, for the most part, seek proprietary rights in their software or software ideas, although other rewards (such as tenure or recognition in the field) were available to those whose innovative research was published.
Phase 3: The 1980s
Four significant developments in the 1980s changed the landscape of the software industry and the intellectual property rights concerns of those who developed software. Two were developments in the computing field; two were legal developments.
The first significant computing development was the introduction to the market of the personal computer (PC), a machine made possible by improvements in the design of semiconductor chips, both as memory storage
devices and as processing units. A second was the visible commercial success of some early PC applications software—most notably, Visicalc, and then Lotus 1-2-3—which significantly contributed to the demand for PCs as well as making other software developers aware that fortunes could be made by selling software. With these developments, the base for a large mass market in software was finally in place.
During this period, computer manufacturers began to realize that it was to their advantage to encourage others to develop application programs that could be executed on their brand of computers. One form of encouragement involved making available to software developers whatever interface information would be necessary for development of application programs that could interact with the operating system software provided with the vendor's computers (information that might otherwise have been maintained as a trade secret). Another form of encouragement was pioneered by Apple Computer, which recognized the potential value to consumers (and ultimately to Apple) of having a relatively consistent "look and feel" to the applications programs developed to run on Apple computers. Apple developed detailed guidelines for applications developers to aid in the construction of this consistent look and feel.
The first important legal development—one which was in place when the first successful mass-marketed software applications were introduced into the market—was passage of amendments to the copyright statute in 1980 to resolve the lingering doubt about whether copyright protection was available for computer programs. 13 These amendments were adopted on the recommendation of the National Commission on New Technological Uses of Copyrighted Works (CONTU), which Congress had established to study a number of "new technology" issues affecting copyrighted works. The CONTU report emphasized the written nature of program texts, which made them seem so much like written texts that had long been protected by copyright law. The CONTU report noted the successful expansion of the boundaries of copyright over the years to take in other new technology products, such as photographs, motion pictures, and sound recordings. It predicted that computer programs could also be accommodated in the copyright regime. 14
Copyright law was perceived by CONTU as the best alternative for protection of computer programs under existing intellectual property regimes. Trade secrecy, CONTU noted, was inherently unsuited for mass-marketed products because the first sale of the product on the open market would dispel the secret. CONTU observed that Supreme Court rulings had cast
doubts on the availability of patent protection for software. CONTU's confidence in copyright protection for computer programs was also partly based on an economic study it had commissioned. This economic study regarded copyright as suitable for protecting software against unauthorized copying after sale of the first copy of it in the marketplace, while fostering the development of independently created programs. The CONTU majority expressed confidence that judges would be able to draw lines between protected expression and unprotected ideas embodied in computer programs, just as they did routinely with other kinds of copyrighted works.
A strong dissenting view was expressed by the novelist John Hersey, one of the members of the CONTU commission, who regarded programs as too mechanical to be protected by copyright law. Hersey warned that the software industry had no intention to cease the use of trade secrecy for software. Dual assertion of trade secrecy and copyright seemed to him incompatible with copyright's historical function of promoting the dissemination of knowledge.
Another development during this period was that the Copyright Office dropped its earlier requirement that the full text of source code be deposited with it. Now only the first and last 25 pages of source code had to be deposited to register a program. The office also decided it had no objection if the copyright owner blacked out some portions of the deposited source code so as not to reveal trade secrets. This new policy was said to be consistent with the new copyright statute that protected both published and unpublished works alike, in contrast to the prior statutes that had protected mainly published works. 15
With the enactment of the software copyright amendments, software developers had a legal remedy in the event that someone began to mass-market exact or near-exact copies of the developers' programs in competition with the owner of the copyright in the program. Unsurprisingly, the first software copyright cases involved exact copying of the whole or substantial portions of program code, and in them, the courts found copyright infringement. Copyright litigation in the mid- and late 1980s began to grapple with questions about what, besides program code, copyright protects about computer programs. Because the "second-generation" litigation affects the current legal framework for the protection of computer programs, the issues raised by these cases will be dealt with in the next section.
As CONTU Commissioner Hersey anticipated, software developers did not give up their claims to the valuable trade secrets embodied in their programs after enactment of the 1980 amendments to the copyright statute.
To protect those secrets, developers began distributing their products in machine-readable form, often relying on "shrink-wrap" licensing agreements to limit consumer rights in the software. 16 Serious questions exist about the enforceability of shrink-wrap licenses, some because of their dubious contractual character 17 and some because of provisions that aim to deprive consumers of rights conferred by the copyright statute. 18 That has not led, however, to their disuse.
One common trade secret-related provision of shrink-wrap licenses, as well as of many negotiated licenses, is a prohibition against decompilation or disassembly of the program code. Such provisions are relied on as the basis of software developer assertions that notwithstanding the mass distribution of a program, the program should be treated as unpublished copyrighted works as to which virtually no fair use defenses can be raised. 19
Those who seek to prevent decompilation of programs tend to assert that since decompilation involves making an unauthorized copy of the program, it constitutes an improper means of obtaining trade secrets in the program. Under this theory, decompilation of program code results in three unlawful acts: copyright infringement (because of the unauthorized copy made during the decompilation process), trade secret misappropriation (because the secret has been obtained by improper means, i.e., by copyright
infringement), and a breach of the licensing agreement (which prohibits decompilation).
Under this theory, copyright law would become the legal instrument by which trade secrecy could be maintained in a mass-marketed product, rather than a law that promotes the dissemination of knowledge. Others regard decompilation as a fair use of a mass-marketed program and, shrink-wrap restrictions to the contrary, as unenforceable. This issue has been litigated in the United States, but has not yet been resolved definitively. 20 The issue remains controversial both within the United States and abroad.
A second important legal development in the early 1980s—although one that took some time to become apparent—was a substantial shift in the U.S. Patent and Trademark Office (PTO) policy concerning the patentability of computer program-related inventions. This change occurred after the 1981 decision by the U.S. Supreme Court in Diamond v. Diehr, which ruled that a rubber curing process, one element of which was a computer program, was a patentable process. On its face, the Diehr decision seemed consistent with the 1966 Patent Office policy and seemed, therefore, not likely to lead to a significant change in patent policy regarding software innovations. 21 By the mid-1980s, however, the PTO had come to construe the Court's ruling broadly and started issuing a wide variety of computer program-related patents. Only "mathematical algorithms in the abstract" were now thought unpatentable. Word of the PTO's new receptivity to software patent applications spread within the patent bar and gradually to software developers.
During the early and mid-1980s, both the computer science field and the software industry grew very significantly. Innovative ideas in computer science and related research fields were widely published and disseminated. Software was still exchanged by researchers, but a new sensitivity to intellectual property rights began to arise, with general recognition that unauthorized copying of software might infringe copyrights, especially if done with a commercial purpose. This was not perceived as presenting a serious obstacle to research, for it was generally understood that a reimplementation of the program (writing one's own code) would be
noninfringing. 22 Also, much of the software (and ideas about software) exchanged by researchers during the early and mid-1980s occurred outside the commercial marketplace. Increasingly, the exchanges took place with the aid of government-subsidized networks of computers.
Software firms often benefited from the plentiful availability of research about software, as well as from the availability of highly trained researchers who could be recruited as employees. Software developers began investing more heavily in research and development work. Some of the results of this research was published and/or exchanged at technical conferences, but much was kept as a trade secret and incorporated in new products.
By the late 1980s, concerns began arising in the computer science and related fields, as well as in the software industry and the legal community, about the degree of intellectual property protection needed to promote a continuation of the high level of innovation in the software industry. 23 Although most software development firms, researchers, and manufacturers of computers designed to be compatible with the leading firms' machines seemed to think that copyright (complemented by trade secrecy) was adequate to their needs, the changing self-perception of several major computer manufacturers led them to push for more and "stronger" protection. (This concern has been shared by some successful software firms whose most popular programs were being "cloned" by competitors.) Having come to realize that software was where the principal money of the future would be made, these computer firms began reconceiving themselves as software developers. As they did so, their perspective on software protection issues changed as well. If they were going to invest in software development, they wanted "strong'' protection for it. They have, as a consequence, become among the most vocal advocates of strong copyright, as well as of patent protection for computer programs. 24
CURRENT LEGAL APPROACHES IN THE UNITED STATES
Software developers in the United States are currently protecting software products through one or more of the following legal protection mechanisms: copyright, trade secret, and/or patent law. Licensing agreements often supplement these forms of protection. Some software licensing agreements are negotiated with individual customers; others are printed forms found under the plastic shrink-wrap of a mass-marketed package. 25 Few developers rely on only one form of legal protection. Developers seem to differ somewhat on the mix of legal protection mechanisms they employ as well as on the degree of protection they expect from each legal device.
Although the availability of intellectual property protection has unquestionably contributed to the growth and prosperity of the U.S. software industry, some in the industry and in the research community are concerned that innovation and competition in this industry will be impeded rather than enhanced if existing intellectual property rights are construed very broadly. 26 Others, however, worry that courts may not construe intellectual property rights broadly enough to protect what is most valuable about software, and if too little protection is available, there may be insufficient incentives to invest in software development; hence innovation and competition may be retarded through underprotection. 27 Still others (mainly lawyers) are confident that the software industry will continue to prosper and grow under the existing intellectual property regimes as the courts "fill out" the details of software protection on a case-by-case basis as they have been doing for the past several years. 28
What's Not Controversial
Although the main purpose of the discussion of current approaches is to give an overview of the principal intellectual property issues about which there is controversy in the technical and legal communities, it may be wise to begin with a recognition of a number of intellectual property issues as to which there is today no significant controversy. Describing only the aspects of the legal environment as to which controversies exist would risk creating a misimpression about the satisfaction many software developers and lawyers have with some aspects of intellectual property rights they now use to protect their and their clients' products.
One uncontroversial aspect of the current legal environment is the use of copyright to protect against exact or near-exact copying of program code. Another is the use of copyright to protect certain aspects of user interfaces, such as videogame graphics, that are easily identifiable as "expressive" in a traditional copyright sense. Also relatively uncontroversial is the use of copyright protection for low-level structural details of programs, such as the instruction-by-instruction sequence of the code. 29
The use of trade secret protection for the source code of programs and other internally held documents concerning program design and the like is similarly uncontroversial. So too is the use of licensing agreements negotiated with individual customers under which trade secret software is made available to licensees when the number of licensees is relatively small and when there is a reasonable prospect of ensuring that licensees will take adequate measures to protect the secrecy of the software. Patent protection for industrial processes that have computer program elements, such as the rubber curing process in the Diehr case, is also uncontroversial.
Substantial controversies exist, however, about the application of copyright law to protect other aspects of software, about patent protection for other kinds of software innovations, about the enforceability of shrink-wrap licensing agreements, and about the manner in which the various forms of legal protection seemingly available to software developers interrelate in the protection of program elements (e.g., the extent to which copyright and trade secret protection can coexist in mass-marketed software).
Controversies Arising From Whelan v. Jaslow
Because quite a number of the most contentious copyright issues arise from the Whelan v. Jaslow decision, this subsection focuses on that case. In the summer of 1986, the Third Circuit Court of Appeals affirmed a trial court decision in favor of Whelan Associates in its software copyright lawsuit against Jaslow Dental Laboratories. 30 Jaslow's program for managing dental lab business functions used some of the same data and file structures as Whelan's program (to which Jaslow had access), and five subroutines of Jaslow's program functioned very similarly to Whelan's. The trial court inferred that there were substantial similarities in the underlying structure of the two programs based largely on a comparison of similarities in the user interfaces of the two programs, even though user interface similarities were not the basis for the infringement claim. Jaslow's principal defense was that Whelan's copyright protected only against exact copying of program code, and since there were no literal similarities between the programs, no copyright infringement had occurred.
In its opinion on this appeal, the Third Circuit stated that copyright protection was available for the "structure, sequence, and organization" (sso) of a program, not just the program code. (The court did not distinguish between high- and low-level structural features of a program.) The court analogized copyright protection for program sso to the copyright protection available for such things as detailed plot sequences in novels. The court also emphasized that the coding of a program was a minor part of the cost of development of a program. The court expressed fear that if copyright protection was not accorded to sso, there would be insufficient incentives to invest in the development of software.
The Third Circuit's Whelan decision also quoted with approval from that part of the trial court opinion stating that similarities in the manner in which programs functioned could serve as a basis for a finding of copyright infringement. Although recognizing that user interface similarities did not necessarily mean that two programs had similar underlying structures (thereby correcting an error the trial judge had made), the appellate court thought that user interface similarities might still be some evidence of underlying structural similarities. In conjunction with other evidence in the case, the Third Circuit decided that infringement had properly been found.
Although a number of controversies have arisen out of the Whelan opinion, the aspect of the opinion that has received the greatest attention is the test the court used for determining copyright infringement in computer
program cases. The " Whelan test" regards the general purpose or function of a program as its unprotectable "idea." All else about the program is, under the Whelan test, protectable "expression'' unless there is only one or a very small number of ways to achieve the function (in which case idea and expression are said to be "merged," and what would otherwise be expression is treated as an idea). The sole defense this test contemplates for one who has copied anything more detailed than the general function of another program is that copying that detail was "necessary" to perform that program function. If there is in the marketplace another program that does the function differently, courts applying the Whelan test have generally been persuaded that the copying was unjustified and that what was taken must have been "expressive."
Although the Whelan test has been used in a number of subsequent cases, including the well-publicized Lotus v. Paperback case, 31 some judges have rejected it as inconsistent with copyright law and tradition, or have found ways to distinguish the Whelan case when employing its test would have resulted in a finding of infringement. 32
Many commentators assert that the Whelan test interprets copyright
protection too expansively. 33 Although the court in Whelan did not seem to realize it, the Whelan test would give much broader copyright protection to computer programs than has traditionally been given to novels and plays, which are among the artistic and fanciful works generally accorded a broader scope of protection than functional kinds of writings (of which programs would seem to be an example). 34 The Whelan test would forbid reuse of many things people in the field tend to regard as ideas. 35 Some commentators have suggested that because innovation in software tends to be of a more incremental character than in some other fields, and especially given the long duration of copyright protection, the Whelan interpretation of the scope of copyright is likely to substantially overprotect software. 36
One lawyer-economist, Professor Peter Menell, has observed that the model of innovation used by the economists who did the study of software for CONTU is now considered to be an outmoded approach. 37 Those econo-
mists focused on a model that considered what incentives would be needed for development of individual programs in isolation. Today, economists would consider what protection would be needed to foster innovation of a more cumulative and incremental kind, such as has largely typified the software field. In addition, the economists on whose work CONTU relied did not anticipate the networking potential of software and consequently did not study what provisions the law should make in response to this phenomenon. Menell has suggested that with the aid of their now more refined model of innovation, economists today might make somewhat different recommendations on software protection than they did in the late 1970s for CONTU. 38
As a matter of copyright law, the principal problem with the Whelan test is its incompatibility with the copyright statute, the case law properly interpreting it, and traditional principles of copyright law. The copyright statute provides that not only ideas, but also processes, procedures, systems, and methods of operation, are unprotectable elements of copyrighted works. 39 This provision codifies some long-standing principles derived from U.S. copyright case law, such as the Supreme Court's century-old Baker v. Selden decision that ruled that a second author did not infringe a first author's copyright when he put into his own book substantially similar ledger sheets to those in the first author's book. The reason the Court gave for its ruling was that Selden's copyright did not give him exclusive rights to the bookkeeping system, but only to his explanation or description of it. 40 The ordering and arrangement of columns and headings on the ledger sheets were part of the system; to get exclusive rights in this, the Court said that Selden would have to get a patent.
The statutory exclusion from copyright protection for methods, processes, and the like was added to the copyright statute in part to ensure that the scope of copyright in computer programs would not be construed too broadly. Yet, in cases in which the Whelan test has been employed, the courts have tended to find the presence of protectable "expression" when they perceive there to be more than a couple of ways to perform some function, seeming not to realize that there may be more than one "method" or "system" or "process" for doing something, none of which is properly protected by copyright law. The Whelan test does not attempt to exclude
methods or processes from the scope of copyright protection, and its recognition of functionality as a limitation on the scope of copyright is triggered only when there are no alternative ways to perform program functions.
Whelan has been invoked by plaintiffs not only in cases involving similarities in the internal structural design features of programs, but also in many other kinds of cases. sso can be construed to include internal interface specifications of a program, the layout of elements in a user interface, and the sequence of screen displays when program functions are executed, among other things. Even the manner in which a program functions can be said to be protectable by copyright law under Whelan . The case law on these issues and other software issues is in conflict, and resolution of these controversies cannot be expected very soon.
Traditionalist Versus Strong Protectionist View of What Copyright Law Does and Does Not Protect in Computer Programs
Traditional principles of copyright law, when applied to computer programs, would tend to yield only a "thin" scope of protection for them. Unquestionably, copyright protection would exist for the code of the program and the kinds of expressive displays generated when program instructions are executed, such as explanatory text and fanciful graphics, which are readily perceptible as traditional subject matters of copyright law. A traditionalist would regard copyright protection as not extending to functional elements of a program, whether at a high or low level of abstraction, or to the functional behavior that programs exhibit. Nor would copyright protection be available for the applied know-how embodied in programs, including program logic. 41 Copyright protection would also not be available for algorithms or other structural abstractions in software that are constituent elements of a process, method, or system embodied in a program.
Efficient ways of implementing a function would also not be protectable by copyright law under the traditionalist view, nor would aspects of software design that make the software easier to use (because this bears on program functionality). The traditionalist would also not regard making a limited number of copies of a program to study it and extract interface information or other ideas from the program as infringing conduct, because computer programs are a kind of work for which it is necessary to make a copy to "read" the text of the work. 42 Developing a program that incorporates interface information derived from decompilation would also, in the traditionalist view, be noninfringing conduct.
If decompilation and the use of interface information derived from the study of decompiled code were to be infringing acts, the traditionalist would regard copyright as having been turned inside out, for instead of promoting the dissemination of knowledge as has been its traditional purpose, copyright law would become the principal means by which trade secrets would be maintained in widely distributed copyrighted works. Instead of protecting only expressive elements of programs, copyright would become like a patent: a means by which to get exclusive rights to the configuration of a machine—without meeting stringent patent standards or following the strict procedures required to obtain patent protection. This too would seem to turn copyright inside out.
Because interfaces, algorithms, logic, and functionalities of programs are aspects of programs that make them valuable, it is understandable that some of those who seek to maximize their financial returns on software investments have argued that "strong" copyright protection is or should be available for all valuable features of programs, either as part of program sso or under the Whelan "there's-another-way-to-do-it" test. 43 Congress seems to have intended for copyright law to be interpreted as to programs on a case-by-case basis, and if courts determine that valuable features should be considered "expressive," the strong protectionists would applaud this common law evolution. If traditional concepts of copyright law and its purposes do not provide an adequate degree of protection for software innovation, they see it as natural that copyright should grow to provide it. Strong protectionists tend to regard traditionalists as sentimental Luddites who do not appreciate that what matters is for software to get the degree of protection it needs from the law so that the industry will thrive.
Although some cases, most notably the Whelan and Lotus decisions, have adopted the strong protectionist view, traditionalists will tend to regard these decisions as flawed and unlikely to be affirmed in the long run because they are inconsistent with the expressed legislative intent to have traditional principles of copyright law applied to software. Some copyright traditionalists favor patent protection for software innovations on the ground that the valuable functional elements of programs do need protection to create proper incentives for investing in software innovations, but that this protection should come from patent law, not from copyright law.
Controversy Over "Software Patents"
Although some perceive patents as a way to protect valuable aspects of programs that cannot be protected by copyright law, those who argue for patents for software innovations do not rely on the "gap-filling" concern alone. As a legal matter, proponents of software patents point out that the patent statute makes new, nonobvious, and useful "processes" patentable. Programs themselves are processes; they also embody processes. 44 Computer hardware is clearly patentable, and it is a commonplace in the computing field that any tasks for which a program can be written can also be implemented in hardware. This too would seem to support the patentability of software.
Proponents also argue that protecting program innovations by patent law is consistent with the constitutional purpose of patent law, which is to promote progress in the "useful arts." Computer program innovations are technological in nature, which is said to make them part of the useful arts to which the Constitution refers. Proponents insist that patent law has the same potential for promoting progress in the software field as it has had for promoting progress in other technological fields. They regard attacks on patents for software innovations as reflective of the passing of the frontier in the software industry, a painful transition period for some, but one necessary if the industry is to have sufficient incentives to invest in software development.
Some within the software industry and the technical community, however, oppose patents for software innovations. 45 Opponents tend to make two kinds of arguments against software patents, often without distinguishing between them. One set of arguments questions the ability of the PTO to deal well with software patent applications. Another set raises more fundamental questions about software patents. Even assuming that the PTO could begin to do a good job at issuing software patents, some question whether
innovation in the software field will be properly promoted if patents become widely available for software innovations. The main points of both sets of arguments are developed below.
Much of the discussion in the technical community has focused on "bad" software patents that have been issued by the PTO. Some patents are considered bad because the innovation was, unbeknownst to the PTO, already in the state of the art prior to the date of invention claimed in the patent. Others are considered bad because critics assert that the innovations they embody are too obvious to be deserving of patent protection. Still others are said to be bad because they are tantamount to a claim for performing a particular function by computer or to a claim for a law of nature, neither of which is regarded as patentable subject matter. Complaints abound that the PTO, after decades of not keeping up with developments in this field, is so far out of touch with what has been and is happening in the field as to be unable to make appropriate judgments on novelty and nonobviousness issues. Other complaints relate to the office's inadequate classification scheme for software and lack of examiners with suitable education and experience in computer science and related fields to make appropriate judgments on software patent issues. 46
A somewhat different point is made by those who assert that the software industry has grown to its current size and prosperity without the aid of patents, which causes them to question the need for patents to promote innovation in this industry. 47 The highly exclusionary nature of patents (any use of the innovation without the patentee's permission is infringing) contrasts sharply with the tradition of independent reinvention in this field. The high expense associated with obtaining and enforcing patents raises concerns about the increased barriers to entry that may be created by the patenting of software innovations. Since much of the innovation in this industry has come from small firms, policies that inhibit entry by small firms may not promote innovation in this field in the long run. Similar questions arise as to whether patents will promote a proper degree of innovation in an incremental industry such as the software industry. It would be possible to undertake an economic study of conditions that have promoted and are promoting progress in the software industry to serve as a basis for a policy decision on software patents, but this has not been done to date.
Some computer scientists and mathematicians are also concerned about patents that have been issuing for algorithms, 48 which they regard as dis-
coveries of fundamental truths that should not be owned by anyone. Because any use of a patented algorithm within the scope of the claims—whether by an academic or a commercial programmer, whether one knew of the patent or not—may be an infringement, some worry that research on algorithms will be slowed down by the issuance of algorithm patents. One mathematical society has recently issued a report opposing the patenting of algorithms. 49 Others, including Richard Stallman, have formed a League for Programming Freedom.
There is substantial case law to support the software patent opponent position, notwithstanding the PTO change in policy. 50 Three U.S. Supreme Court decisions have stated that computer program algorithms are unpatentable subject matter. Other case law affirms the unpatentability of processes that involve the manipulation of information rather than the transformation of matter from one physical state to another.
One other concern worth mentioning if both patents and copyrights are used to protect computer program innovations is whether a meaningful boundary line can be drawn between the patent and copyright domains as regards software. 51 A joint report of the U.S. PTO and the Copyright Office optimistically concludes that no significant problems will arise from the coexistence of these two forms of protection for software because copyright law will only protect program "expression" whereas patent law will only protect program "processes." 52
Notwithstanding this report, I continue to be concerned with the patent/ copyright interface because of the expansive interpretations some cases, particularly Whelan, have given to the scope of copyright protection for programs. This prefigures a significant overlap of copyright and patent law as to software innovations. This overlap would undermine important economic and public policy goals of the patent system, which generally leaves in the public domain those innovations not novel or nonobvious enough to be patented. Mere "originality" in a copyright sense is not enough to make an innovation in the useful arts protectable under U.S. law. 53
A concrete example may help illustrate this concern. Some patent lawyers report getting patents on data structures for computer programs.
The Whelan decision relied in part on similarities in data structures to prove copyright infringement. Are data structures "expressive" or "useful"? When one wants to protect a data structure of a program by copyright, does one merely call it part of the sso of the program, whereas if one wants to patent it, one calls it a method (i.e., a process) of organizing data for accomplishing certain results? What if anything does copyright's exclusion from protection of processes embodied in copyrighted works mean as applied to data structures? No clear answer to these questions emerges from the case law.
Nature of Computer Programs and Exploration of a Modified Copyright Approach
It may be that the deeper problem is that computer programs, by their very nature, challenge or contradict some fundamental assumptions of the existing intellectual property regimes. Underlying the existing regimes of copyright and patent law are some deeply embedded assumptions about the very different nature of two kinds of innovations that are thought to need very different kinds of protection owing to some important differences in the economic consequences of their protection. 54
In the United States, these assumptions derive largely from the U.S. Constitution, which specifically empowers Congress "to promote the progress of science [i.e., knowledge] and useful arts [i.e., technology], by securing for limited times to authors and inventors the exclusive right to their respective writings and discoveries." 55 This clause has historically been parsed as two separate clauses packaged together for convenience: one giving Congress power to enact laws aimed at promoting the progress of knowledge by giving authors exclusive rights in their writings, and the other giving Congress power to promote technological progress by giving inventors exclusive rights in their technological discoveries. Copyright law implements the first power, and patent law the second.
Owing partly to the distinctions between writings and machines, which the constitutional clause itself set up, copyright law has excluded machines
and other technological subject matters from its domain. 56 Even when described in a copyrighted book, an innovation in the useful arts was considered beyond the scope of copyright protection. The Supreme Court's Baker v. Selden decision reflects this view of the constitutional allocation. Similarly, patent law has historically excluded printed matter (i.e., the contents of writings) from its domain, notwithstanding the fact that printed matter may be a product of a manufacturing process. 57 Also excluded from the patent domain have been methods of organizing, displaying, and manipulating information (i.e., processes that might be embodied in writings, for example mathematical formulas), notwithstanding the fact that "processes" are named in the statute as patentable subject matter. They were not, however, perceived to be "in the useful arts" within the meaning of the constitutional clause.
The constitutional clause has been understood as both a grant of power and a limitation on power. Congress cannot, for example, grant perpetual patent rights to inventors, for that would violate the "limited times" provision of the Constitution. Courts have also sometimes ruled that Congress cannot, under this clause, grant exclusive rights to anyone but authors and inventors. In the late nineteenth century, the Supreme Court struck down the first federal trademark statute on the ground that Congress did not have power to grant rights under this clause to owners of trademarks who were neither "authors" nor "inventors." 58 A similar view was expressed in last year's Feist Publications v. Rural Telephone Services decision by the Supreme Court, which repeatedly stated that Congress could not constitutionally protect the white pages of telephone books through copyright law because to be an "author" within the meaning of the Constitution required some creativity in expression that white pages lacked. 59
Still other Supreme Court decisions have suggested that Congress could not constitutionally grant exclusive rights to innovators in the useful arts who were not true "inventors." 60 Certain economic assumptions are connected with this view, including the assumption that more modest innovations in the useful arts (the work of a mere mechanic) will be forthcoming without the grant of the exclusive rights of a patent, but that the incentives of patent rights are necessary to make people invest in making significant technological advances and share the results of their work with the public instead of keeping them secret.
One reason the United States does not have a copyright-like form of protection for industrial designs, as do many other countries, is because of lingering questions about the constitutionality of such legislation. In addition, concerns exist that the economic consequences of protecting uninventive technological advances will be harmful. So powerful are the prevailing patent and copyright paradigms that when Congress was in the process of considering the adoption of a copyright-like form of intellectual property protection for semiconductor chip designs, there was considerable debate about whether Congress had constitutional power to enact such a law. It finally decided it did have such power under the commerce clause, but even then was not certain.
As this discussion reveals, the U.S. intellectual property law has long assumed that something is either a writing (in which case it is protectable, if at all, by copyright law) or a machine (in which case it is protectable, if at all, by patent law), but cannot be both at the same time. However, as Professor Randall Davis has so concisely said, software is "a machine whose medium of construction happens to be text." 61 Davis regards the act of creating computer programs as inevitably one of both authorship and invention. There may be little or nothing about a computer program that is not, at base, functional in nature, and nothing about it that does not have roots in the text. Because of this, it will inevitably be difficult to draw meaningful boundaries for patents and copyrights as applied to computer programs.
Another aspect of computer programs that challenges the assumptions of existing intellectual property systems is reflected in another of Professor Davis's observations, namely, that "programs are not only texts; they also behave." 62 Much of the dynamic behavior of computer programs is highly functional in nature. If one followed traditional copyright principles, this functional behavior—no matter how valuable it might be—would be considered outside the scope of copyright law. 63 Although the functionality of program behavior might seem at first glance to mean that patent protection would be the obvious form of legal protection for it, as a practical matter, drafting patent claims that would adequately capture program behavior as an invention is infeasible. There are at least two reasons for this: it is partly because programs are able to exhibit such a large number and variety of states that claims could not reasonably cover them, and partly because of
the ''gestalt"-like character of program behavior, something that makes a more copyright-like approach desirable.
Some legal scholars have argued that because of their hybrid character as both writings and machines, computer programs need a somewhat different legal treatment than either traditional patent or copyright law would provide. 64 They have warned of distortions in the existing legal systems likely to occur if one attempts to integrate such a hybrid into the traditional systems as if it were no different from the traditional subject matters of these systems. 65 Even if the copyright and patent laws could be made to perform their tasks with greater predictability than is currently the case, these authors warn that such regimes may not provide the kind of protection that software innovators really need, for most computer programs will be legally obvious for patent purposes, and programs are, over time, likely to be assimilated within copyright in a manner similar to that given to "factual" and "functional" literary works that have only "thin" protection against piracy. 66
Professor Reichman has reported on the recurrent oscillations between states of under- and overprotection when legal systems have tried to cope with another kind of legal hybrid, namely, industrial designs (sometimes referred to as "industrial art"). Much the same pattern seems to be emerging in regard to computer programs, which are, in effect, "industrial literature." 67
The larger problems these hybrids present is that of protecting valuable forms of applied know-how embodied in incremental innovation that cannot successfully be maintained as trade secrets:
[M]uch of today's most advanced technology enjoys a less favorable competitive position than that of conventional machinery because the unpatentable, intangible know-how responsible for its commercial value becomes embodied in products that are distributed on the open market. A product of the new technologies, such as a computer program, an integrated circuit
design, or even a biogenetically altered organism may thus bear its know-how on its face, a condition that renders it as vulnerable to rapid appropriation by second-comers as any published literary or artistic work.
From this perspective, a major problem with the kinds of innovative know-how underlying important new technologies is that they do not lend themselves to secrecy even when they represent the fruit of enormous investment in research and development. Because third parties can rapidly duplicate the embodied information and offer virtually the same products at lower prices than those of the originators, there is no secure interval of lead time in which to recuperate the originators' initial investment or their losses from unsuccessful essays, not to mention the goal of turning a profit. 68
From a behavioral standpoint, investors in applied scientific know-how find the copyright paradigm attractive because of its inherent disposition to supply artificial lead time to all comers without regard to innovative merit and without requiring originators to preselect the products that are most worthy of protection. 69
Full copyright protection, however, with its broad notion of equivalents geared to derivative expressions of an author's personality is likely to disrupt the workings of the competitive market for industrial products. For this and other reasons, Professor Reichman argues that a modified copyright approach to the protection of computer programs (and other legal hybrids) would be a preferable framework for protecting the applied know-how they embody than either the patent or the copyright regime would presently provide. Similar arguments can be made for a modified form of copyright protection for the dynamic behavior of programs. A modified copyright approach might involve a short duration of protection for original valuable functional components of programs. It could be framed to supplement full copyright protection for program code and traditionally expressive elements of text and graphics displayed when programs execute, features of software that do not present the same dangers of competitive disruption from full copyright protection.
The United States is, in large measure, already undergoing the development of a sui generis law for protection of computer software through case-by-case decisions in copyright lawsuits. Devising a modified copyright approach to protecting certain valuable components that are not suitably protected under the current copyright regime would have the advantage of allowing a conception of the software protection problem as a whole, rather than on a piecemeal basis as occurs in case-by-case litigation in which the
skills of certain attorneys and certain facts may end up causing the law to develop in a skewed manner. 70
There are, however, a number of reasons said to weigh against sui generis legislation for software, among them the international consensus that has developed on the use of copyright law to protect software and the trend toward broader use of patents for software innovations. Some also question whether Congress would be able to devise a more appropriate sui generis system for protecting software than that currently provided by copyright. Some are also opposed to sui generis legislation for new technology products such as semiconductor chips and software on the ground that new intellectual property regimes will make intellectual property law more complicated, confusing, and uncertain.
Although there are many today who ardently oppose sui generis legislation for computer programs, these same people may well become among the most ardent proponents of such legislation if the U.S. Supreme Court, for example, construes the scope of copyright protection for programs to be quite thin, and reiterates its rulings in Benson, Flook, and Diehr that patent protection is unavailable for algorithms and other information processes embodied in software.
INTERNATIONAL PERSPECTIVES
After adopting copyright as a form of legal protection for computer programs, the United States campaigned vigorously around the world to persuade other nations to protect computer programs by copyright law as well. These efforts have been largely successful. Although copyright is now an international norm for the protection of computer software, the fine details of what copyright protection for software means, apart from protection against exact copying of program code, remain somewhat unclear in other nations, just as in the United States.
Other industrialized nations have also tended to follow the U.S. lead concerning the protection of computer program-related inventions by patent
law. 71 Some countries that in the early 1960s were receptive to the patenting of software innovations became less receptive after the Gottschalk v. Benson decision by the U.S. Supreme Court. Some even adopted legislation excluding computer programs from patent protection. More recently, these countries are beginning to issue more program-related patents, once again paralleling U.S. experience, although as in the United States, the standards for patentability of program-related inventions are somewhat unclear. 72 If the United States and Japan continue to issue a large number of computer program-related patents, it seems quite likely other nations will follow suit.
There has been strong pressure in recent years to include relatively specific provisions about intellectual property issues (including those affecting computer programs) as part of the international trade issues within the framework of the General Agreement on Tariffs and Trade (GATT). 73 For a time, the United States was a strong supporter of this approach to resolution of disharmonies among nations on intellectual property issues affecting software. The impetus for this seems to have slackened, however, after U.S. negotiators became aware of a lesser degree of consensus among U.S. software developers on certain key issues than they had thought was the case. Since the adoption of its directive on software copyright law, the European Community (EC) has begun pressing for international adoption of its position on a number of important software issues, including its copyright rule on decompilation of program code.
There is a clear need, given the international nature of the market for software, for a substantial international consensus on software protection issues. However, because there are so many hotly contested issues concerning the extent of copyright and the availability of patent protection for computer programs yet to be resolved, it may be premature to include very specific rules on these subjects in the GATT framework.
Prior to the adoption of the 1991 European Directive on the Protection of Computer Programs, there was general acceptance in Europe of copyright as a form of legal protection for computer programs. A number of nations had interpreted existing copyright statutes as covering programs. Others took legislative action to extend copyright protection to software. There was, however, some divergence in approach among the member nations of the EC in the interpretation of copyright law to computer software. 74
France, for example, although protecting programs under its copyright law, put software in the same category as industrial art, a category of work that is generally protected in Europe for 25 years instead of the life plus 50-year term that is the norm for literary and other artistic works. German courts concluded that to satisfy the "originality" standard of its copyright law, the author of a program needed to demonstrate that the program was the result of more than an average programmer's skill, a seemingly patentlike standard. In 'addition, Switzerland (a non-EC member but European nonetheless) nearly adopted an approach that treated both semiconductor chip designs and computer programs under a new copyright-like law.
Because of these differences and because it was apparent that computer programs would become an increasingly important item of commerce in the European Community, the EC undertook in the late 1980s to develop a policy concerning intellectual property protection for computer programs to which member nations should harmonize their laws. There was some support within the EC for creating a new law for the protection of software, but the directorate favoring a copyright approach won this internal struggle over what form of protection was appropriate for software.
In December 1988 the EC issued a draft directive on copyright protection for computer programs. This directive was intended to spell out in considerable detail in what respects member states should have uniform rules on copyright protection for programs. (The European civil law tradition generally prefers specificity in statutory formulations, in contrast with the U.S. common law tradition, which often prefers case-by-case adjudication of disputes as a way to fill in the details of a legal protection scheme.)
The draft directive on computer programs was the subject of intense debate within the European Community, as well as the object of some intense lobbying by major U.S. firms who were concerned about a number of issues, but particularly about what rule would be adopted concerning decompilation of program code and protection of the internal interfaces of
programs. Some U.S. firms, among them IBM Corp., strongly opposed any provision that would allow decompilation of program code and sought to have interfaces protected; other U.S. firms, such as Sun Microsystems, sought a rule that would permit decompilation and would deny protection to internal interfaces. 75
The final EC directive published in 1991 endorses the view that computer programs should be protected under member states' copyright laws as literary works and given at least 50 years of protection against unauthorized copying. 76 It permits decompilation of program code only if and to the extent necessary to obtain information to create an interoperable program. The inclusion in another program of information necessary to achieve interoperability seems, under the final directive, to be lawful.
The final EC directive states that "ideas" and "principles" embodied in programs are not protectable by copyright, but does not provide examples of what these terms might mean. The directive contains no exclusion from protection of such things as processes, procedures, methods of operation, and systems, as the U.S. statute provides. Nor does it clearly exclude protection of algorithms, interfaces, and program logic, as an earlier draft would have done. Rather, the final directive indicates that to the extent algorithms, logic, and interfaces are ideas, they are unprotectable by copyright law. In this regard, the directive seems, quite uncharacteristically for its civil law tradition, to leave much detail about how copyright law will be applied to programs to be resolved by litigation.
Having just finished the process of debating the EC directive about copyright protection of computer programs, intellectual property specialists in the EC have no interest in debating the merits of any sui generis approach to software protection, even though the only issue the EC directive really resolved may have been that of interoperability. Member states will likely have to address another controversial issue—whether or to what extent user interests in standardization of user interfaces should limit the scope of copyright
protection for programs—as they act on yet another EC directive, one that aims to standardize user interfaces of computer programs. Some U.S. firms may perceive this latter directive as an effort to appropriate valuable U.S. product features.
Japan was the first major industrialized nation to consider adoption of a sui generis approach to the protection of computer programs. 77 Its Ministry of International Trade and Industry (MITI) published a proposal that would have given 15 years of protection against unauthorized copying to computer programs that could meet a copyright-like originality standard under a copyright-like registration regime. MITI attempted to justify its proposed different treatment for computer programs as one appropriate to the different character of programs, compared with traditional copyrighted works. 78 The new legal framework was said to respond and be tailored to the special character of programs. American firms, however, viewed the MITI proposal, particularly its compulsory license provisions, as an effort by the Japanese to appropriate the valuable products of the U.S. software industry. Partly as a result of U.S. pressure, the MITI proposal was rejected by the Japanese government, and the alternative copyright proposal made by the ministry with jurisdiction over copyright law was adopted.
Notwithstanding their inclusion in copyright law, computer programs are a special category of protected work under Japanese law. Limiting the scope of copyright protection for programs is a provision indicating that program languages, rules, and algorithms are not protected by copyright law. 79 Japanese case law under this copyright statute has proceeded along lines similar to U.S. case law, with regard to exact and near-exact copying of program code and graphical aspects of videogame programs, 80 but there have been some Japanese court decisions interpreting the exclusion from protection provisions in a manner seemingly at odds with some U.S. Decisions.
The Tokyo High Court, for example, has opined that the processing flow of a program (an aspect of a program said to be protectable by U.S. law in the Whelan case) is an algorithm within the meaning of the copyright limitation provision. 81 Another seems to bear out Professor Karjala's prediction that Japanese courts would interpret the programming language limitation to permit firms to make compatible software. 82 There is one Japanese decision that can be read to prohibit reverse engineering of program code, but because this case involved not only disassembly of program code but also distribution of a clearly infringing program, the legality of intermediate copying to discern such things as interface information is unclear in Japan. 83
Other Nations
The United States has been pressing a number of nations to give "proper respect" to U.S. intellectual property products, including computer programs. In some cases, as in its dealings with the People's Republic of China, the United States has been pressing for new legislation to protect software under copyright law. In some cases, as in its dealings with Thailand, the United States has been pressing for more vigorous enforcement of intellectual property laws as they affect U.S. intellectual property products. In other cases, as in its dealings with Brazil, the United States pressed for repeal of sui generis legislation that disadvantaged U.S. software producers, compared with Brazilian developers. The United States has achieved some success in these efforts. Despite these successes, piracy of U.S.-produced software and other intellectual property products remains a substantial source of concern.
FUTURE CHALLENGES
Many of the challenges posed by use of existing intellectual property laws to protect computer programs have been discussed in previous sections. This may, however, only map the landscape of legal issues of widespread concern today. Below are some suggestions about issues as to which computer programs may present legal difficulties in the future.
Advanced Software Systems
It has thus far been exceedingly difficult for the legal system to resolve even relatively simple disputes about software intellectual property rights, such as those involved in the Lotus v. Paperback Software case. This does not bode well for how the courts are likely to deal with more complex problems presented by more complex software in future cases. The difficulties arise partly from the lack of familiarity of judges with the technical nature of computers and software, and partly from the lack of close analogies within the body of copyright precedents from which resolutions of software issues might be drawn. The more complex the software, the greater is the likelihood that specially trained judges will be needed to resolve intellectual property disputes about the software. Some advanced software systems are also likely to be sufficiently different from traditional kinds of copyrighted works that the analogical distance between the precedents and a software innovation may make it difficult to predict how copyright law should be applied to it. What copyright protection should be available, for example, to a user interface that responds to verbal commands, gestures, or movements of eyeballs?
Digital Media
The digital medium itself may require adaptation of the models underlying existing intellectual property systems. 84 Copyright law is built largely on the assumption that authors and publishers can control the manufacture and distribution of copies of protected works emanating from a central source. The ease with which digital works can be copied, redistributed, and used by multiple users, as well as the compactness and relative invisibility of works in digital form, have already created substantial incentives for developers of digital media products to focus their commercialization efforts on controlling the uses of digital works, rather than on the distribution of copies, as has more commonly been the rule in copyright industries.
Rules designed for controlling the production and distribution of copies may be difficult to adapt to a system in which uses need to be controlled. Some digital library and hypertext publishing systems seem to be designed to bypass copyright law (and its public policy safeguards, such as the fair use rule) and establish norms of use through restrictive access licensing
agreements. 85 Whether the law will eventually be used to regulate conditions imposed on access to these systems, as it has regulated access to such communication media as broadcasting, remains to be seen. However, the increasing convergence of intellectual property policy, broadcast and telecommunications policy, and other aspects of information policy seems inevitable.
There are already millions of people connected to networks of computers, who are thereby enabled to communicate with one another with relative ease, speed, and reliability. Plans are afoot to add millions more and to allow a wide variety of information services to those connected to the networks, some of which are commercial and some of which are noncommercial in nature. Because networks of this type and scope are a new phenomenon, it would seem quite likely that some new intellectual property issues will arise as the use of computer networks expands. The more commercial the uses of the networks, the more likely intellectual property disputes are to occur.
More of the content distributed over computer networks is copyrighted than its distributors seem to realize, but even as to content that has been recognized as copyrighted, there is a widespread belief among those who communicate over the net that at least noncommercial distributions of content—no matter the number of recipients—are "fair uses" of the content. Some lawyers would agree with this; others would not. Those responsible for the maintenance of the network may need to be concerned about potential liability until this issue is resolved.
A different set of problems may arise when commercial uses are made of content distributed over the net. Here the most likely disputes are those concerning how broad a scope of derivative work rights copyright owners should have. Some owners of copyrights can be expected to resist allowing anyone but themselves (or those licensed by them) to derive any financial benefit from creating a product or service that is built upon the value of their underlying work. Yet value-added services may be highly desirable to consumers, and the ability of outsiders to offer these products and services may spur beneficial competition. At the moment, the case law generally regards a copyright owner's derivative work right as infringed only if a recognizable block of expression is incorporated into another work. 86 How-
ever, the ability of software developers to provide value-added products and services that derive value from the underlying work without copying expression from it may lead some copyright owners to seek to extend the scope of derivative work rights.
Patents and Information Infrastructure of the Future
If patents are issued for all manner of software innovations, they are likely to play an important role in the development of the information infrastructure of the future. Patents have already been issued for hypertext navigation systems, for such things as latent semantic indexing algorithms, and for other software innovations that might be used in the construction of a new information infrastructure. Although it is easy to develop a list of the possible pros and cons of patent protection in this domain, as in the more general debate about software patents, it is worth noting that patents have not played a significant role in the information infrastructure of the past or of the present. How patents would affect the development of the new information infrastructure has not been given the study this subject may deserve.
Conflicts Between Information Haves and Have-Nots on an International Scale
When the United States was a developing nation and a net importer of intellectual property products, it did not respect copyright interests of any authors but its own. Charles Dickens may have made some money from the U.S. tours at which he spoke at public meetings, but he never made a dime from the publication of his works in the United States. Now that the United States is a developed nation and a net exporter of intellectual property products, its perspective on the rights of developing nations to determine for themselves what intellectual property rights to accord to the products of firms of the United States and other developed nations has changed. Given the greater importance nowadays of intellectual property products, both to the United States and to the world economy, it is foreseeable that there will be many occasions on which developed and developing nations will have disagreements on intellectual property issues.
The United States will face a considerable challenge in persuading other nations to subscribe to the same detailed rules that it has for dealing with intellectual property issues affecting computer programs. It may be easier for the United States to deter outright ''piracy" (unauthorized copying of the whole or substantially the whole of copyrighted works) of U.S. intellectual property products than to convince other nations that they must adopt the same rules as the United States has for protecting software.
It is also well for U.S. policymakers and U.S. firms to contemplate the possibility that U.S. firms may not always have the leading position in the world market for software products that they enjoy today. When pushing for very "strong" intellectual property protection for software today in the expectation that this will help to preserve the U.S. advantage in the world market, U.S. policymakers should be careful not to push for adoption of rules today that may substantially disadvantage them in the world market of the future if, for reasons not foreseen today, the United States loses the lead it currently enjoys in the software market.
As technological developments multiply around the globe—even as the patenting of human genes comes under serious discussion—nations, companies, and researchers find themselves in conflict over intellectual property rights (IPRs). Now, an international group of experts presents the first multidisciplinary look at IPRs in an age of explosive growth in science and technology.
This thought-provoking volume offers an update on current international IPR negotiations and includes case studies on software, computer chips, optoelectronics, and biotechnology—areas characterized by high development cost and easy reproducibility. The volume covers these and other issues:
- Modern economic theory as a basis for approaching international IPRs.
- U.S. intellectual property practices versus those in Japan, India, the European Community, and the developing and newly industrializing countries.
- Trends in science and technology and how they affect IPRs.
- Pros and cons of a uniform international IPRs regime versus a system reflecting national differences.
READ FREE ONLINE
Welcome to OpenBook!
You're looking at OpenBook, NAP.edu's online reading room since 1999. Based on feedback from you, our users, we've made some improvements that make it easier than ever to read thousands of publications on our website.
Do you want to take a quick tour of the OpenBook's features?
Show this book's table of contents , where you can jump to any chapter by name.
...or use these buttons to go back to the previous chapter or skip to the next one.
Jump up to the previous page or down to the next one. Also, you can type in a page number and press Enter to go directly to that page in the book.
Switch between the Original Pages , where you can read the report as it appeared in print, and Text Pages for the web version, where you can highlight and search the text.
To search the entire text of this book, type in your search term here and press Enter .
Share a link to this book page on your preferred social network or via email.
View our suggested citation for this chapter.
Ready to take your reading offline? Click here to buy this book in print or download it as a free PDF, if available.
Get Email Updates
Do you enjoy reading reports from the Academies online for free ? Sign up for email notifications and we'll let you know about new publications in your areas of interest when they're released.
University Case Studies
Mills college, stanford university, carnegie mellon university.
- U.C. Berkeley: Reentering the Pipeline
- Women Enrollment Committee Report, MIT Dept. of Electrical Engineering and Computer Science
The Women's Science and Engineering Network (WSEN) pairs up female undergraduate and graduate students in computer science in mentor relationships. The Stanford Undergraduate Engineering Handbook states, "networking with a mentor promotes self-confidence and assertiveness; helps students clarify their career goals; teaches them how to interact better with faculty and peers; and offers them the support needed for dealing with the pressures of being in male-dominated fields." The Stanford WSEN brings undergraduates and graduate students and faculty through receptions, workshops, panel discussion, quarterly programs, field trips, as well as a monthly newsletter. Such relationships not only encourage female undergraduate students by having graduate students act as role models, but also graduate students feel more respected and accomplished (Pearl, et al. 52).
Incoming Freshmen in CS at CMU: Percentage of Women Students
U.c. berkely: reentering the pipeline.
Over 170 women have participated in the program since the program's inceptions in 1984. Women whose undergraduate degrees were in fields like biology, drama, classics, and mathematics have gone on to attain Ph.D.'s in computer science. Unfortunately, the passage of Proposition 209 in California has imposed legal constraints on the Computer Science Reentry Program. The program is currently under review. (Humphreys.)
Advertisement
Teaching Mathematics to Computer Scientists: Reflections and a Case Study
- Review Article
- Open access
- Published: 05 February 2021
- Volume 2 , article number 75 , ( 2021 )
Cite this article
You have full access to this open access article
- Ferrante Neri ORCID: orcid.org/0000-0002-6100-6532 1
2 Citations
1 Altmetric
Explore all metrics
Mathematics, despite being the foundation of computer science, is nowadays often considered a totally separate subject. The fact that many jobs in computer science do not explicitly require any specific mathematical knowledge posed questions about the importance of mathematics within computer science undergraduate curricula. In many educational systems, a prior high school knowledge of mathematics is often not a mandatory requirement to be enrolled into a degree of computer science. On the other hand, several studies report that mathematics is important to computer scientists since it provides essential analytical and critical skills and since many professional and research tasks in computer science require an in-depth understanding of mathematical concepts. From this assumption, this article proposes an analysis of the cohort of computer science’ students, with a specific reference to British Universities, and identifies some challenges that lecturers of mathematical subjects normally face. On the basis of this analysis this article proposes two teaching techniques to promote effective learning. The proposed techniques aim at addressing the diversity of cohorts in terms of mathematical background and skepticism from part of the cohort of students to consider mathematics as an essential element of their education. Numerical results indicate the validity and effectiveness of the proposed teaching techniques.
Similar content being viewed by others
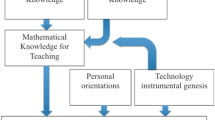
Teaching with digital technology
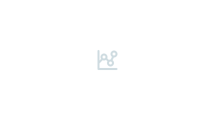
Research on early childhood mathematics teaching and learning
Science Education in the Philippines
Avoid common mistakes on your manuscript.
Introduction
In the 1830s, Charles Babbage developed the idea of an automatic calculator and in the 1840s Ada Lovelace conceptualised computer programming. These scientific contributions are allegedly the first visionary foundations of computer science [ 24 ]. However, the beginning of modern computer science is usually dated about one century later, when Alan Turing and Alonzo Church introduced the concepts of algorithm and model of computation , see [ 12 , 13 , 49 ]. An important stepping stone from theoretical model to hardware implementation is that the computer architecture formalised by John von Neumann in the 1940s [ 32 ].
These pioneers of computer science have something in common: they were all mathematicians. Hence, the research published at the time was presented and perceived as part of mathematics. Thus, we may observe that computer science originated as a branch of mathematics that over the second half of the twentieth century became a discipline separate and independent from it.
On the other hand, when we analyse computer science today, it appears like a broad and complex subject composed of heterogeneous parts and whose specialists possess diverse and heterogeneous skills. For example, among the plethora of its sub-fields, computer science (and its taught curricula) includes subjects very close to mathematics like theory of computation and algorithmics [ 46 ], programming subjects whose focus is in the computer implementation and hardware exploitation [ 36 ], subjects that focus on the human user, their psychology and aesthetic preferences to build efficient front-end interfaces [ 9 ].
By analysing the job market in computer science, many of the jobs most in demand, like Applications developer , Game designer/developer , Information systems manager , IT consultant do not require any specific mathematical training. Hence, by echoing the (rhetorical) question posed by Anthony Ralston in [ 38 ]:
The answer to this question is not straightforward and is controversial, see [ 37 , 39 ]. Ralston acknowledges the importance of mathematics in computer science degrees and points out that it is important “to insure that mathematics does play a proper role in CS/SE programs and, in particular, to do so by breaking the stranglehold of calculus on first and second year college mathematics”. By paraphrasing this statement, mathematics should harmonically sit within a computer science degree taking into account the learners, the job market, and the nature of the subject.
The role of mathematics within computer science education has been recently discussed by Lincoln Sedlacek in [ 45 ] where it is stated that mathematics is an essential subject of computer science education and the following four reasons are given
Mathematics teaches understanding and communication through an abstract language. This general argument, also mentioned in [ 38 ], means that mathematics “rewires the brain” of the learner and enables a general broader understanding, see in the context of school education [ 3 ]. The abstract nature of programming and other areas of computer science would greatly benefit from this skill.
Mathematics teaches how to work with algorithms. Algorithms are a fundamental part of computer science and appear explicitly or implicitly in most computer related tasks. The skill of conceptualising algorithms as mathematical entity helps to better understand and solve these tasks, [ 3 , 19 , 26 ].
Mathematics teaches computer scientists how to analyse their work. The analytical skills provided by the study and understanding of mathematics enable students to strengthen their critical skills. These skills are useful to programmers, designers, and developers to assess their own work and that made by others to identify mistakes and areas for improvement, see [ 15 , 47 ].
A lot of computer science still involves mathematics. Many computer-related tasks require knowledge and understanding of mathematics. For example, the programming of 3D graphics and animation in games requires the implementation of mathematical equations [ 17 , 27 ]. There is a degree of presence of mathematics in various computer science tasks such as cybersecurity [ 5 , 40 ], artificial intelligence [ 18 , 43 ] and data science [ 11 ].
While assuming, on the basis of considerations above, that some degree of mathematics provision is crucially important in computer science education, the present paper offers reflections about how mathematics can be effectively and efficiently taught to computer science undergraduates. In other words, this paper addresses the following research question:
This research question makes an implicit assumption: there is a specific way to efficiently teach mathematics in a computer science degree (which would differ from the way mathematics is taught to mathematics students). More generally, this article puts the learners at the centre of the attention of the lecturer who adapts their teaching on the basis of the inclinations (what they easily understand) needs (what can be useful in their professional life) of the cohort. This is in line with the study reported in [ 10 ] where some tangible tools are proposed to enhance the understanding of mathematics among engineering students.
To address this question, this paper proposes an analysis of the features of a computer science undergraduate cohort and two teaching techniques that, on the basis of the experience of the author, promote a large-scale engagement, understanding of mathematics, and improved exam results.
To further clarify the main purpose and significance of this study, mathematics, albeit very impactful on the careers of computer scientists, is often overlooked in computer science’ curricula and its importance in teaching practice often not enough recognised.
In the literature, numerous studies are devoted to the teaching of mathematics with several journals focussed solely on mathematics’ education. The link between mathematics and computer science/engineering has also been intensively studied. However, the most popular approaches revolve around the use of computer technologies to enhance the learning of mathematics, see, e.g. [ 21 , 34 , 44 ]. Furthermore, several books of mathematics refer to a computer science audience, e.g. [ 22 , 50 ], thus implicitly proposing examples of teaching practice. The present paper proposes the first study, to the knowledge of the author, that conceptualises some educational techniques specific to the teaching of mathematics to computer science’ cohorts.
The remainder of this paper is organised in the following way. The next section provides some observations about cohorts of undergraduate students of computer science and their attitude towards modules of mathematics. The subsequent section outlines the developed teaching techniques.
Computer Science Cohorts
As a premise of this work, the observations reported in this section are the result of a decade of teaching experience of mathematics in Schools of Computer Science across two British institutions, De Montfort University and the university of Nottingham. During this time, the author published a textbook entitled “Linear Algebra for Computational Sciences and Engineering” [ 28 ] which then has been substantially re-written in a second edition by taking into account the feedback of multiple cohorts of students, see [ 29 ].
With respect to the learning of mathematics, the following challenges associated with the (often large) cohorts of students have been noted:
Since in many universities there are no specific mathematical pre-requisites, the cohorts can be very diverse in terms of mathematics’ background . Some students may have encountered advanced mathematical studies in high school (A levels in further maths), some others may have studied basic mathematics in high school and others may have not studied mathematics at school in the two years immediately preceding university education. Furthermore, international students may have a strong mathematical background and have not necessarily met the same content as local students in their high schools, see [ 7 , 25 ].
Thus, the preparation of a lecture of mathematics that is suitable for the entire cohort is a challenging task. The lecture is likely to be either excessively demanding for some students or not stimulating enough for others. The search for the correct balance can easily lead to ineffective learning since it would not target large portions of the cohorts.
In continuity with the Ralston’s observations [ 38 ], part of the cohort is likely to not fully appreciate the importance of mathematics within their curriculum. To the experience of the author, many computer science students, especially in the early undergraduate years, do not see the benefits of mathematics to their future career . Mathematics is sometimes perceived as an abstract subject that has no relation at all with the work of a professional computer scientist.
Another challenge for the lecturer is to motivate the entire cohort and overcome the initial resistance of many students to learn mathematics. This attitude may also link to individual psychological issues such as maths anxiety, see, e.g. [ 23 , 48 ] in case of students who have not studied any mathematics in the two years preceding the university studies.
On the other hand, these challenges can be mitigated by an important feature of the cohort: since there are normally pre-requisites in computer science discipline, the entire cohort is guaranteed to have a minimum understanding of programming, Information Technology, and computing disciplines. In the opinion of the author, this feature can be exploited by the lecturer of mathematics designing a module that is interesting and engaging for all the students and contains new learning material and approaches for the entire cohort of students.
Teaching Mathematics to Computer Scientist: Two Proposed Techniques
This section describes at the conceptual level and by means of a concrete example two proposed teaching techniques used to address the two challenges outlined in Sect. “ Computer Science Cohorts ”.
Addressing the Diversity in Mathematical Background
The research question above is broken into two question to address the challenges outlined in Sect. “ Computer Science Cohorts ”. With reference to the first challenge and with the purpose of proposing a technique addressing it, let us formulate the first research sub-question.
How to design a lecture (entire module) that is interesting for a cohort with a diverse mathematical background and promote the learning for all the students regardless of their starting point?
The first underpinning principle embraced by the author in his teaching and in his textbook [ 29 ] (as explicitly declared on the back cover), is that no compromises should be made on the content nor on the mathematical rigour of the lectures. To enable that computer science students benefit in their career from modules of mathematics, it is fundamental that the four points outlined by Lincoln Sedlacek [ 45 ] are covered. This means that a number of mathematical topics relevant to computer science are presented and assessed. Furthermore, rigorous mathematical reasoning must be used throughout the mathematical modules and be part of the assessment. This is done to allow students to develop analytical and critical skills that will then be transferred to their professional life.
On the other hand, in the opinion of the author, the way mathematics is taught to students of computer science should take into great consideration the composition and features of the audience/cohort. To address the diversity in mathematical background, the author proposes to introduce and explain each mathematical topic in three different ways and from different perspectives. More specifically, each topic is presented
By formal mathematics . This presentation immediately targets that part of the cohort with prior mathematical studies and is available to the other students after they achieved an intuitive understanding of the concept.
By abstraction of an example . This presentation allows an initial understanding to the students without solid prior mathematical bases. These students have an opportunity to quickly achieve some degree of understanding of the explained mathematical concept and remain engaged throughout the lecture. Then, following an initial understanding of the subject, these students can revise the formal presentation of the concept and understand it more in depth and at a more general level. In the meantime, students with a solid mathematical background have the opportunity to check and consolidate their understanding of the formal presentation by seeing this second presentation as its numerical example.
By “algorithmification” of formal mathematics . Mathematical concepts and proofs can be interpreted and presented as procedures/algorithms that achieve a numerical result or a logical goal. Since the entire cohort is already familiar with programming, and procedural description of instructions, the author exploits the common background of the cohort to offer an alternative (and original) view of the subject that is easily accessible to everybody. It must be remarked, that this algorithmification, albeit a powerful teaching tool, always allows a procedural understanding of mathematics, i.e. what needs to be done to achieve a goal, but not always allows an in-depth understanding of the concept for which a revision of the formal presentation may be necessary. On the other hand, the algorithmification of mathematics enables the development of a common language, understandable by all students and offers a further support to better learn and understand rigorous mathematics.
Figure 1 displays in a schematic way the proposed teaching technique and displays the three ways the mathematical concept is explained, categorising the learners on the basis of their mathematical background. Two learning phases are included, a first approach where the students are introduced to the topic and revision where the students study the topic again after having familiarised with the multiple explanations. As shown, in the first phase, students with a mathematical background are expected to prefer a formal approach whereas students without a mathematical background are likely to prefer an intuitive explanation. During the revision, the background becomes less relevant since the students had the opportunity to study the concept and reflect about it. In revision phase, students are expected to choose the approach they prefer on the basis of their personal inclinations and are expected to refer to both formal and intuitive approach to study the concept from complementary perspectives. The explanation by algorithmification is expected to be easily accessible for the entire cohort and be a further form of support to enable another level of understanding of the subject. The proposed approach is in agreement with the inclusive education theories [ 2 ] and in particular with the cognitivism-based inclusive education practices and constructivism-based inclusive education practices . The former focuses on the mental information processing of the learners, see [ 1 ] while the latter makes use of real-life experiences as learning tools, see [ 14 ].
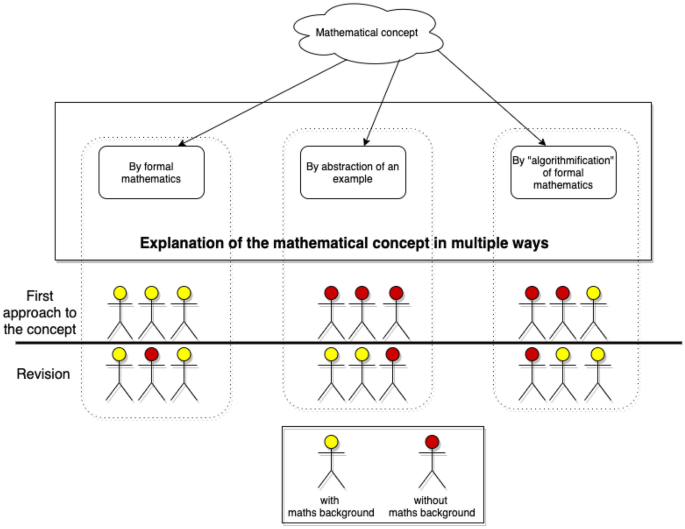
Scheme of the teaching technique to address the diversity in mathematical background
To better demonstrate the proposed teaching technique, in the following example a mathematical concept is explained in the three different ways outlined above.
Example: \({\mathbf {LU}}\) Factorisation Explained to a Computer Science Cohort
Let us consider a popular topic in mathematics which is fundamental in the career of a computer scientist, that is the solution a large system of linear questions. Let us assume that the problem has been presented as
that is a matrix equation of the type \({\mathbf {A}}{\mathbf {x}}={\mathbf {b}}\) . In the following, the solution of this problem by a direct method called \(\mathbf {LU}\) factorisation is presented, see [ 29 ]. At first, a general premise is made and then the concept is explained by means of the three different ways explained above.
Premise . The LU factorization is a direct method that transforms a matrix \({\mathbf {A}}\) into a matrix product \({\mathbf {L}}{\mathbf {U}}\) where \({\mathbf {L}}\) is a lower triangular matrix having the diagonal elements all equal to 1 and \({\mathbf {U}}\) is an upper triangular matrix. Thus, if we aim at solving a system of linear equations \({\mathbf {A}}{\mathbf {x}}={\mathbf {b}}\) , we obtain
If we pose \({\mathbf {U}} {\mathbf {x}}={\mathbf {y}}\) , we solve at first the triangular system \({\mathbf {L}}{\mathbf {y}}={\mathbf {b}}\) and then extract \({\mathbf {x}}\) from the triangular system \({\mathbf {U}} {\mathbf {x}}={\mathbf {y}}\) . Thus, instead of solving a computationally complex system of linear equations \({\mathbf {L}}{\mathbf {U}}\) factorisation transforms \({\mathbf {A}}\) into the product \(\mathbf {LU}\) and then poses two extremely straightforward systems (triangular systems are immediate to solve by substitution).
Explanation by formal mathematics .
Let \({\mathbf {A}}\in {\mathbb {R}}_{n,n}\) be a non-singular matrix. Let us indicate with \(\mathbf {A}_{\mathbf{k}}\) the submatrix having order k composed of the first k rows and k columns of \({\mathbf {A}}\) . If \(\det \mathbf {A}_{\mathbf{k}} \ne 0\) for \(k=1,2,\ldots ,n\) then \(\exists !\) lower triangular matrix \({\mathbf {L}}\) having all the diagonal elements equal to 1 and \(\exists !\) upper triangular matrix \({\mathbf {U}}\) such that \({\mathbf {A}}={\mathbf {L}}{\mathbf {U}}\) .
Let us now derive the general transformation formulas. Let \({\mathbf {A}}\) be
while \({\mathbf {L}}\) and \({\mathbf {U}}\) are, respectively,
If we impose \({\mathbf {A}}={\mathbf {L}}{\mathbf {U}}\) , we obtain
for \(i,j = 1,2,\ldots ,n\) .
In the case \(i \le j\) , i.e. in the case of the triangular upper part of the matrix, we have
This equation is equivalent to
that is the formula to determine the elements of \({\mathbf {U}}\) .
Let us consider the case \(j<i\) , i.e. the lower triangular part of the matrix
that is the formula to determine the elements of \({\mathbf {L}}\) .
Explanation by abstraction of an example . If we consider the following system of linear equations
and the corresponding incomplete matrix \({\mathbf {A}}\)
we can impose the factorization \({\mathbf {A}}={\mathbf {L}}{\mathbf {U}}\) . This means
If we perform the multiplication of the two matrices we obtain the following system of 9 equations in 12 variables.
Since this system has infinite solutions we can impose some extra equations. Let us impose that \(l_{1,1}=l_{2,2}=l_{3,3}=1\) . By substitution, we find that
The \({\mathbf {A}}={\mathbf {L}}{\mathbf {U}}\) factorization is then
Explanation by “algorithmification” of formal mathematics . The \(\mathbf {LU}\) factorisation can be expressed by the equation
where \(\forall i,j\) , \(a_{i,j}\) are known while \(l_{i,j}\) and \(u_{i,j}\) must be found. We may consider the matrices \({\mathbf {L}}\) and \({\mathbf {U}}\) as data structures that can be viewed as vectors of row vectors \(\mathbf {l_i}\) and column vector \(\mathbf {u^j}\) , respectively
Let us indicate with \({\mathbf {l}}_{\mathbf{i}}{} \mathbf{u}^{\mathbf{j}}\) the scalar product of the vector \({\mathbf {l}}_{\mathbf{i}}\) by \({\mathbf {u}}^{\mathbf{j}}\) that is \(a_{i,j}\) :
If the equations are performed in a certain order, from each scalar product an element \(l_{i,j}\) or \(u_{i,j}\) can be calculated. Then we may think about an empty data structure \({\mathbf {B}}\) that will store the representation of the result of the \(\mathbf {LU}\) factorisation. The algorithm initialises the first row of the matrix \({\mathbf {B}}\) as the first row of \({\mathbf {A}}\) . The following rows of the matrix \({\mathbf {B}}\) are filled by solving the equations \(a_{i,j}={\mathbf {l}}_{\mathbf{i}}{} \mathbf{u}^{\mathbf{j}}\) with the data previously calculated and allocated in \({\mathbf {B}}\) . More specifically, each of these equations is a simple linear equation with only one unknown. The value of this unknown is allocated in \(b_{i,j}\) . At the end of this procedure the matrix \({\mathbf {B}}\) contains the data of the factorisation:
Algorithm 1 displays the pseudocode of the \(\mathbf {LU}\) factorisation.
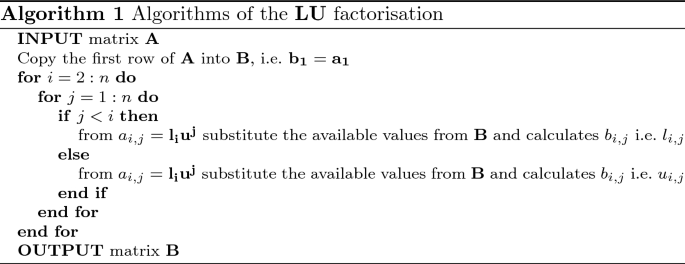
Addressing the Resisting Attitude to Mathematics
With reference to the second challenge, let us formulate the corresponding research sub-question.
How to keep the full computer science cohort engaged and interested in learning mathematics?
On the basis of trials and errors and observations of the behaviour in the classroom as well as the results at the exam, the author argues that a good strategy is to explicitly highlight the impact of mathematics on the career of a computer scientist. When a mathematical topic is introduced, some context about the practical use of mathematics in computer science should be provided. Two types of contextualisation have been identified.
Report the links between mathematics and computer science professions . As mentioned above, computer science jobs can be of various type. Students are likely to have heard of some types of profession and may even have the ambition of undertaking one of them (or one among some of them). The author observed that references to the links between mathematical theory and computer science professions greatly help to keep the audience engaged and willing to learn.
Share personal experience of mathematics in research/profession . As a computer scientist who actively (and enthusiastically) uses mathematics in his research and profession, the author can share his personal experience. This approach may genuinely interest and enthuse part of the student cohort who may decide to continue their studies in a final year project (thesis) and can be considered part of Research Informed Teaching (RIT). With reference to the theory reported in [ 6 ] that classifies different types of RIT, the proposed approach is a combination of research-led , research-oriented and research-tutored learning. The first refers to the illustration of research concept during the teaching, the second refers to the research methodologies, the third refers to critical discussions about research. Furthermore, even when the students do not share the same scientific interest of the lecturer, they may appreciate and participate the passion for the subject that naturally the lecturer would share when talking about their research experience and achievements, see [ 35 , 41 ]. One of the purposes of sharing the personal professional experience is to be inspirational and promote, among students, reflections about their own skills, passions, and ambitions, see [ 42 ].
The proposed approach is in line with the relevance aspect of the Attention Relevance Confidence Satisfaction (ARCS) instructional design model designed by Keller, see [ 20 ]. In this model Relevance refers to the usefulness of the information to motivate the learners. Following this principle, the author suggests that the integration of examples related to the prospective careers to the students supports the student to remain motivated and catalyses effective learning sessions.
The following example shows how one of the most abstract and difficult concept of undergraduate mathematics, eigenvalues and eigenvectors, can be linked to computer science profession and research.
Example: The Importance of Eigenvectors in Computer Science Profession
Before entering into the details, let us informally introduce the context of the topic. When a multivariate linear mapping is considered, its eigenvector is a special direction along which the function behaves like a multiplier of a scalar by a vector [ 29 ]. A function of n variables has n eigenvectors. These eigenvectors can be seen a new reference system, a new set of variables that can replace the original one. In this new reference system, the original function (and thus the mathematical model approximating the reality), is very easy to handle since its variables are independent on each other. This transformation is called diagonalisation .
Link between eigenvectors and a computer science profession . One popular profession in computer science is the data scientist. When a large number of data are handled, it is fundamental to extract the most useful piece of information so that the data set can be interpreted correctly. Data can be viewed as multivariate distributions (distributions of vectors) characterised by a mean vector and a covariance matrix. A covariance matrix can be interpreted as a linear mapping and its diagonalisation allows the detection of the direction that best fits the data. This method, commonly known as Principal Component Analysis (PCA) [ 16 ], enables the detection of the most represented variables in the dataset that are the most important ones.
Link between eigenvectors and personal experience . Eigenvectors can play a very important role also in the specific research field of the author that is optimisation. When the optimum of a multivariate function is searched, a set of candidate solutions can be interpreted as a multivariate distribution, see [ 4 , 8 ]. If only a distribution of points whose objective function value is below a threshold (in a minimisation problem) are saved in the data set, then this distribution describes the geometry of the optimisation problem, see [ 31 ]. Like for the case of the PCA, the diagonalisation of the associated covariance matrix, that is the detection of its eigenvectors provides the optimisation algorithm with a set of preferential search directions to perform the search for the the optimum, see [ 30 ]. However, unlike the case of the PCA, the most important direction (variable) is the least represented one as it would correspond to the direction with maximum directional gradient.
To provide a graphical representation of the research idea, let us consider a problem in two variables and let us assume we generated a set of points whose objective function value is below a certain threshold. Figure 2 shows this distribution as blue points with a simple geometry, that is a line. The dashed lines indicate the directions of the eigenvectors. Then Fig. 2 displays the trajectory of a classical algorithm named Pattern Search (PS) using the standard set of variables (line with yellow markers) and the eigenvectors of the covariance matrix of the distribution. The latter algorithm, namely Covariance Pattern Search (CPS, line with red markers) is identical to PS except it used a different set of variables (it works in a different reference system). We may observe that the version that exploits the mathematics of eigenvectors achieves a result that is seventeen orders of magnitude better than its vanilla version.
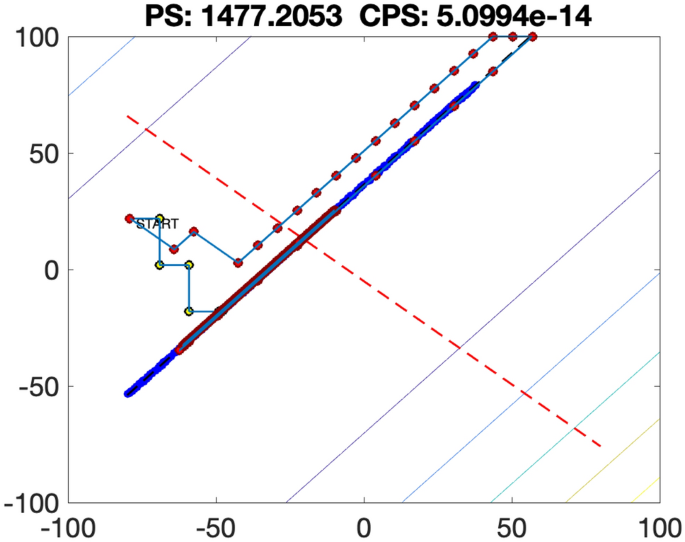
Functioning and performance of standard Pattern Search (PS) and its enhanced version that exploits the mathematical knowledge about eigenvectors (CPS)
The outlined teaching techniques have been tested in the classrooms over the years 2014–2019 in the School of Computer Science and Informatics at De Montfort University. More specifically the author designed and taught two modules of 30 Credits each (one fourth of the year credits) to undergraduate students in Years 1 and 2, respectively. Data have been collected for four cohorts of students corresponding to
Year 1 - Academic Years from 2014/2015 to 2017/2018
Year 2 - Academic Years from 2015/2016 to 2018/2019
The assessment of each module, in each year, was composed of two classroom tests and one exam. Each piece of assessment had the same structure: \(50\%\) of the marks we assigned to numerical exercises while \(50\%\) were theoretical questions (which imposed the use of formal mathematics). The minimum average mark to pass each module is 40/100. While the structure of the assessment remained unaltered throughout the 2014–2019 period, the student feedback coming from explicit comments and through their performance affected the way the modules were delivered by progressively adopting the teaching techniques above. This iterative process affected the classroom teaching as well as the study material, see [ 29 ], which eventually presented, for almost each topic, an explanation in multiple ways as in Fig. 1 and its applicability in the context of the computer science job market.
For each cohort, the performance of a group of students has been monitored. Each group has been selected to represent the diversity in terms of mathematical prior competence, since some of the students had a limited mathematical background (in the British education systems no A levels in Mathematics or less than C in A levels), some students had a moderate mathematical background (passed A levels in Mathematics with at least C), some students had an advanced mathematical background (passed A levels in Further Mathematics with at least C). For each group and each module (1) pass rate (percentage of students achieving at least 40/100); (2) first rate (percentage of students achieving at least 70/100); (3) average mark of the group ± standard deviation \(\sigma \) have been recorded at each June exam board. Table 1 displays these data.
Figure 3 displays the trend over the years of the student average with the respective error bars.
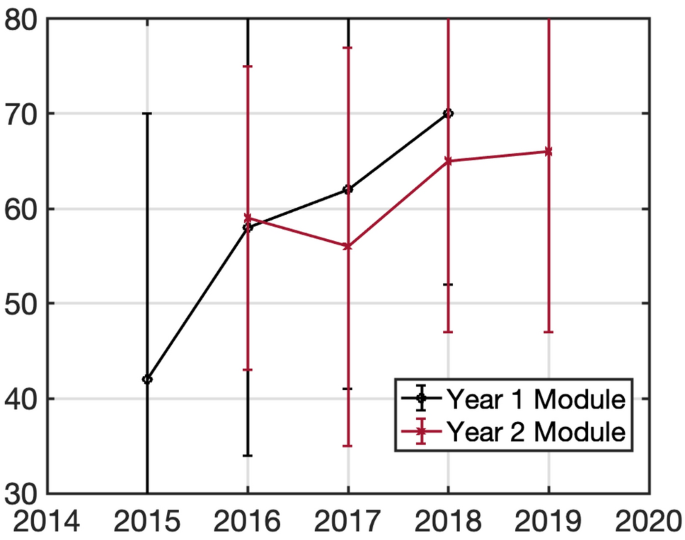
Trend of the average mark of the students over the years
The results on the cohorts show that year after year the students achieve better results. Although many factor may have contributed to this outcome, the consistent updates in the material and teaching style indicate the effectiveness of the proposed teaching techniques.
To provide further (qualitative) evidence of the effectiveness of the proposed teaching techniques, some of the comments given by the students in the questionnaire of the module are given in the following. In these comments, the students refer to the approach of explaining topics from different perspectives and to the book [ 29 ] as a study manual.
“Theory part always was clearly shown in practice part of module, we had support of book to complete our knowledge, and answer any queries.” (Year 1 student 2018)
“Really enjoyed this module and the teaching of the module is clear and well explained and examples are good to help with the learning of the theory” (Year 2 student 2018)
The results of the Year 1 Module are especially interesting since students performed way better from 2016 onward. From the academic year 2015/2016, many topics have been re-written and the algorithmified explanation has been added to the formal mathematical description and the abstraction by example. Furthermore, from the academic year 2015/2016, a lot of attention had been paid to link the topics of the module to realistic scenarios of the job market. It must be remarked that the composition of mathematical background of each group was broadly constant. While in the Academic Year 2014/2015, various students without a high-school mathematical background failed the module (at the first sit), in the following years the lack of prior mathematical knowledge no longer appeared to be a clear disadvantage when the proposed teaching techniques were applied. Figure 4 shows that prior mathematical knowledge may be an advantage to achieve a better performance. However, this is not always true: students with no prior mathematics successfully passed the module and students with a modest mathematical background achieved marks greater than 70. This tendency mitigates over the academic years. In the group of 2018, the performance of students in the Year 1 Module did not appear to be related to their high-school education in mathematics. This fact seems to demonstrate the effectiveness of the proposed teaching.
The results of the Year 2 Module also show an overall improvement of pass rate and average mark, thus indicating that the suggested teaching techniques may have a successful impact on the cohorts. However, since these students previously progressed to from Year 1 to Year 2, the dependency between their pre-university education and results in the module do not appear to be correlated. To exemplify this fact, Fig. 5 shows the scatter plot of the marks of the Year 2 Module in year 2018 against the prior mathematical background. It can be observed that very high marks have been achieved by students in Year 2 regardless of their mathematical knowledge achieved in high school.
To study the correlation between marks in the module and prior mathematical knowledge, a score has been assigned to quantify the mathematical background of each student: 0 has been assigned in case of no A levels, 20, 40, 60, 80, 100 have been assigned students achieving E, D, C, B, A in A levels in Mathematics and Further mathematics. The the score has been normalised to the highest value and expressed within the range [0, 100].
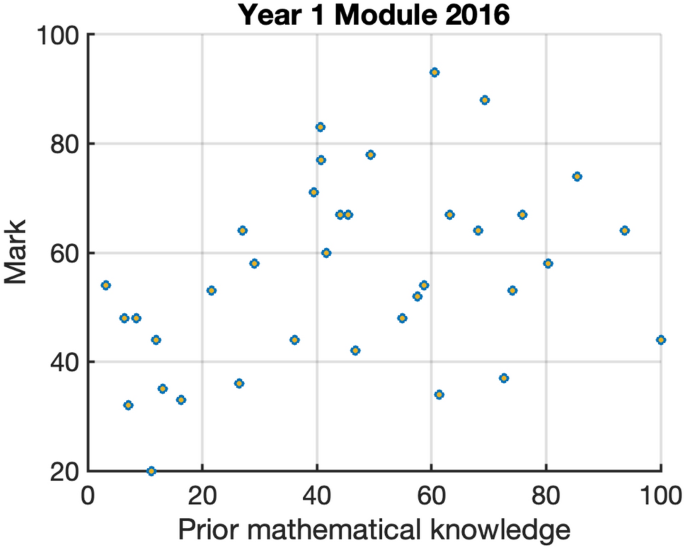
Scatter plot of the marks against the prior mathematical background for the group of 2016 and Year 1 Module. Although students with prior mathematical knowledge appear to achieve better marks, also students with a limited prior knowledge in mathematics may achieve high marks
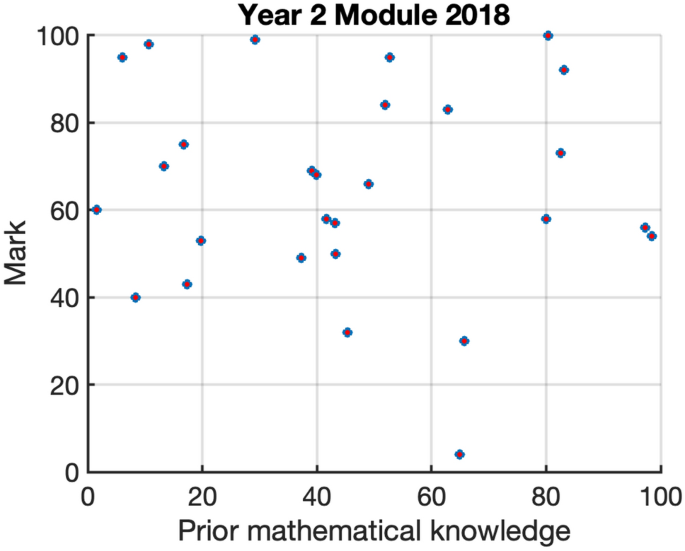
Scatter plot of the marks against the prior mathematical background for the group of 2018 and Year 2 Module. Most of the students appear to perform well regardless of their prior background
The correlation displayed in Figs. 4 and 5 has been quantitatively studied by calculating the Pearson’s coefficient r [ 33 ]. Table 2 lists the Pearson’s coefficients for the cohorts studied in this paper. We may observe that Pearson’s coefficients are thirty to forty times higher for the Year 1 data than the coefficients for Year 2 data. Thus, the quantitative analysis confirms that whilst there is a correlation between prior mathematical knowledge and performance in Year 1, the correlation is negligible in Year 2 ( r is close to zero).
This paper investigates the teaching of mathematics in schools of computer science with specific reference to British Universities. After embracing the assumption that teaching mathematics is beneficial to computer science students and to the professional career of computer scientists, this article provides some suggestions to engage the students and teach effectively.
Two specific challenges have been identified and discussed: (1) computer science students typically have a diverse mathematical background; (2) often mathematics is not perceived as a subject that relates directly to computer science jobs. Two teaching techniques are proposed on the basis of the experience of teaching and writing a textbook in these specific circumstances. The first technique, in line with the literature proposes the explanation of each mathematical topic in different ways including the explanation of mathematics as an algorithm (algorithmification of mathematics). The second technique blends research informed teaching with a provision of references how mathematics impacts the every day job of a computer scientists. Specific classroom tested examples are provided to enrich and clarify the proposed techniques. In any case, it is advocated that no compromises are made on the taught content or on the mathematical rigour of the teaching.
A case study based on multiple years teaching indicates that the proposed techniques can be effective to enhance the performance of the students. Furthermore, some observations based on the correlation between prior mathematical knowledge and performance show that in Year 1 prior mathematical education may have a bias on the student performance which appears to be mitigated by the proposed techniques (students without prior mathematics can perform equally well as their colleagues with prior advanced mathematical studies). The performance of students in Year 2 does not appear to be correlated anymore with their high school history.
This study implicates that teaching of mathematics should be targeted to the specific cohorts/degrees where the teaching occurs. Since mathematics plays a fundamental role in various programmes of applied sciences and engineering, the proposed study indicates that an adaptation of context-related teaching techniques can enhance the engagement of the students and the efficacy of the learning experience. Hence, the proposed teaching techniques can be interpreted as a template expandable to a broader context such as physics and engineering degrees.
Al-Shammari Z. Using evidence-based cognitive teaching strategies with effect size in inclusion classrooms in kuwait. Saudi J Spec Educ. 2019;10
Al-Shammari Z, Faulkner PE, Forlin C. Theories-based inclusive education practices. Educ Q Rev. 2019;2:408–14.
Google Scholar
Benton L, Hoyles C, Kalas I, Noss R. Bridging primary programming and mathematics: some findings of design research in england. Digit Exp Math Educ. 2017;3:115–38.
Article Google Scholar
Blum C, Chiong R, Clerc M, Jong KD, Michalewicz Z, Neri F, Weise T. Evolutionary optimization. In: R. Chiong, T. Weise, Z. Michalewicz (eds.) Variants of Evolutionary Algorithms for Real-World Applications. Berlin, Heidelberg
Boavida F, Praitano A, Lioudakis GV. Topical issue on privacy, data protection, and digital identity. SN Comput. Sci. 2020;1(5):250.
Burgum S, Stoakes G. What does research informed teaching look like? HEA booklet 2016; URL https://www.heacademy.ac.uk/blog/what-does-research-informed-teaching-look
Byram M. Internationalisation in higher education—an internationalist perspective. On the Horizon. 2018;26(2):148–56.
Caraffini F, Neri F, Cheng J, Zhang G, Picinali L, Iacca G, Mininno E. Super-fit multicriteria adaptive differential evolution. In: 2013 IEEE congress on evolutionary computation, 2013; 1678–1685
Card SK, Newell A, Moran TP. The Psychology of Human-Computer Interaction. USA: L. Erlbaum Associates Inc.; 1983.
Ceragioli F, Spreafico M. Tangible tools in mathematics for engineering students: experimental activity at politecnico di torino. Digit Exp Math Educ. 2020;6:244–56.
Ceri S, Pinoli P. Data science for genomic data management: challenges, resources, experiences. SN Comput Sci. 2020;1(1):5:1–7.
Church A. On carnap’s analysis of statements of assertion and belief. Analysis. 1950;10(5):97–9.
Church A. Introduction to Mathematical Logic. Annals of Mathematics Studies. Princeton University Press 1996; URL https://books.google.co.uk/books?id=JDLQOMKbdScC
Ertmer PA, Newby TJ. Behaviorism, cognitivism, constructivism: comparing critical features from an instructional design perspective. Performance Improvement Quarterly. 2013;26:43–71.
Firdaus F, Kailani I, Bakar M, Bakry B. Developing critical thinking skills of students in mathematics learning. J Educ Learn (EduLearn). 2015;9:226.
F.R.S., K.P.: Liii. on lines and planes of closest fit to systems of points in space. The London, Edinburgh, and Dublin Philosophical Magazine and Journal of Science 2 (11), 559–572 (1901)
Ghorashi A, Ghorashi M. Theoretical and computational analysis of the falling ladder problem. SN Comput Sci. 2020;1(1):20:1–11.
Islam MM, Haque MR, Iqbal H, Hasan MM, Hasan M, Kabir MN. Breast cancer prediction: A comparative study using machine learning techniques. SN Comput Sci. 2020;1(5):290.
Jonsson B, Norqvist M, Liljekvist Y, Lithner J. Learning mathematics through algorithmic and creative reasoning. J Math Behav. 2014;36:20–32.
Keller JM. Motivational design of instruction. In: C.M. Reigeluth (ed.) Instructional-design theories and models: an overview of their current status, pp. 386–434. Lawrence Erlbaum Associates 1983.
Kumar A, Kumaresan S. Use of mathematical software for teaching and learning mathematics. In: Proceedings of the 11th international congress on mathematical education, 2008;373–388
Lehman E, Thomson Leighton F, Meyer AR. Mathematics for Computer Science Hardcover. Cambridge: MIT Press; 2017.
Luttenberger S, Wimmer S, Paechter M. Spotlight on math anxiety. Psychol Res Behav Manag. 2018;11:311–22.
Menabrea L, Babbage C, Lovelace, A., L, A. Sketch of the Analytical Engine invented by Charles Babbage with notes by the translator. Extracted from the ’Scientific Memoirs. R. & J. E. Taylor 1843;
Mittelmeier J, Rienties B, Tempelaar D, Whitelock D. Overcoming cross-cultural group work tensions: mixed student perspectives on the role of social relationships. High Educ. 2018;75:149–66.
Morley A. Teaching and learning algorithms. Learning Math. 1981;2(2):50–1.
Musse SR, Thalmann D. Hierarchical model for real time simulation of virtual human crowds. IEEE Trans Vis Comput Graph. 2001;7(2):152–64.
Neri F. Linear algebra computational sciences and engineering. Berlin: Springer; 2016.
Book Google Scholar
Neri F. Linear algebra for computational sciences and engineering. 2nd ed. Berlin: Springer; 2019.
Neri F, Rostami S. A local search for numerical optimisation based on covariance matrix diagonalisation. In: P.A. Castillo, J.L.J. Laredo, F.F. de Vega (eds.) Applications of evolutionary computation—23rd European conference, EvoApplications 2020, Held as Part of EvoStar 2020, Seville, Spain, April 15-17, 2020, Proceedings, Lecture Notes in Computer Science , vol. 12104, pp. 3–19. Springer 2020.
Neri F, Zhou Y. Covariance local search for memetic frameworks: A fitness landscape analysis approach. In: 2020 IEEE congress on evolutionary computation (CEC), 2020;1–8
von Neumann J. First draft of a report on the edvac. Tech. rep. 1945.
NIST/SEMATECH: e-Handbook of Statistical Methods 2003; http://www.itl.nist.gov/div898/handbook/
Ochkov VF, Bogomolova EP. Teaching mathematics with mathematical software. J Humanistic Math. 2015;5:265–85.
Palmer BL. Teacher passion as a teaching tool. In: Electronic Theses and Dissertations, 2017;3269
Press W, Teukolsky S, Vetterling W, Flannery B. Numerical recipes in C. 2nd ed. Cambridge: Cambridge University Press; 1992.
MATH Google Scholar
Ralston A. The first course in computer science needs a mathematics corequisite. Commun ACM. 1984;27(10):1002–5.
Ralston A. Do we need ANY mathematics in computer science curricula? ACM SIGCSE Bull. 2005;37(2):6–9.
Ralston A, Shaw M. Curriculum ’78—is computer science really that unmathematical? Commun. ACM. 1980;23(2):67–70.
Reddy NCS, Madhuravani B, Sneha DP. An approach for efficient and secure data encryption scheme for spatial data. SN Compu Sci. 2020;1(3):117.
Revell A, Wainwright E. What makes lectures ‘unmissable’? insights into teaching excellence and active learning. J Geography Higher Educ. 2009;33(2):209–23.
Robinson K, Aronica L. Finding Your Element: How to Discover Your Talents and Passions and Transform Your Life. penguin books 2014.
Rostami S, Neri F, Epitropakis MG. Progressive preference articulation for decision making in multi-objective optimisation problems. Integr Comput Aided Eng. 2017;24(4):315–35.
Rønning F. Influence of computer-aided assessment on ways of working with mathematics. Teaching Math Appl. 2017;36(2):94–107.
Sedlacek L. Math education: The roots of computer science. https://www.edutopia.org/blog/math-education-roots-computer-science-lincoln-sedlacek 2016.
Sipser M. Introduction to the Theory of Computation. 1st ed. : International Thomson Publishing; 1996.
Su H, Ricci FA, Mnatsakanian M. Mathematical teaching strategies: pathways to critical thinking and metacognition. J Res Educ Sci (IJRES). 2016;1:190–200.
Tobias S, Weissbrod C. Anxiety and mathematics: an update. Harvard Educ Rev. 1980;50(1):63–70.
Turing A. On computable numbers, with an application to the entscheidungsproblem. Proc Lond Math Soc. 1936;42(1):230–65. https://doi.org/10.2307/2268810 .
Article MathSciNet MATH Google Scholar
Vince J. Foundation mathematics for computer science: a visual approach. Berlin: Springer; 2015.
Download references
This study was funded by the institutions indicated in the list of affiliations.
Author information
Authors and affiliations.
COL Laboratory, School of Computer Science, University of Nottingham, Nottingham, NG8 1BB, UK
Ferrante Neri
You can also search for this author in PubMed Google Scholar
Corresponding author
Correspondence to Ferrante Neri .
Ethics declarations
Conflict of interest.
The authors declare that they have no conflict of interest.
Additional information
Publisher's note.
Springer Nature remains neutral with regard to jurisdictional claims in published maps and institutional affiliations.
Rights and permissions
Open Access This article is licensed under a Creative Commons Attribution 4.0 International License, which permits use, sharing, adaptation, distribution and reproduction in any medium or format, as long as you give appropriate credit to the original author(s) and the source, provide a link to the Creative Commons licence, and indicate if changes were made. The images or other third party material in this article are included in the article's Creative Commons licence, unless indicated otherwise in a credit line to the material. If material is not included in the article's Creative Commons licence and your intended use is not permitted by statutory regulation or exceeds the permitted use, you will need to obtain permission directly from the copyright holder. To view a copy of this licence, visit http://creativecommons.org/licenses/by/4.0/ .
Reprints and permissions
About this article
Neri, F. Teaching Mathematics to Computer Scientists: Reflections and a Case Study. SN COMPUT. SCI. 2 , 75 (2021). https://doi.org/10.1007/s42979-021-00461-7
Download citation
Received : 30 September 2020
Accepted : 08 January 2021
Published : 05 February 2021
DOI : https://doi.org/10.1007/s42979-021-00461-7
Share this article
Anyone you share the following link with will be able to read this content:
Sorry, a shareable link is not currently available for this article.
Provided by the Springer Nature SharedIt content-sharing initiative
- Mathematics for computer science
- British educational system
- Research informed teaching
- Find a journal
- Publish with us
- Track your research
Gamification for Engaging Computer Science Students in Learning Activities: A Case Study
Ieee account.
- Change Username/Password
- Update Address
Purchase Details
- Payment Options
- Order History
- View Purchased Documents
Profile Information
- Communications Preferences
- Profession and Education
- Technical Interests
- US & Canada: +1 800 678 4333
- Worldwide: +1 732 981 0060
- Contact & Support
- About IEEE Xplore
- Accessibility
- Terms of Use
- Nondiscrimination Policy
- Privacy & Opting Out of Cookies
A not-for-profit organization, IEEE is the world's largest technical professional organization dedicated to advancing technology for the benefit of humanity. © Copyright 2024 IEEE - All rights reserved. Use of this web site signifies your agreement to the terms and conditions.
- The University Library
Case studies: Embedding IDL as part of a programme level approach
Case study of the BSc Computer Science (COMU01)
Overview and outcomes of the BSc Computer Science project to embed IDL.
The Library's support for Computer Science undergraduate students has traditionally been provided through induction sessions at the beginning of each academic year.
The aim of this project was to explore where information and digital literacy (IDL) support could be embedded within the curriculum to provide the right support, in the right format, at the most appropriate time.
The Library Student Associate and Liaison Librarian explored the IDL resources available, assessed the relevance of IDL resources to their own studies, and identified gaps in the IDL offer from the perspective of a Computer Science student.
Recommendations from the project:
Developments will be made to the Library's online resources to address the identified need for guidance on referencing code in assignments and finding and using images in website creation.
The Library will co-investigate with the department guidance on the ethical reuse and referencing of code with a view to update the Library's Referencing Guides.
The Discovering images tutorial has been identified as a resource which can be modified to include specific information about using images in creating websites.
The Library Student Associates identified the need to promote the Library's IDL offer and that awareness of the online tutorials could be raised with academic staff with a view to embedding these into Blackboard modules. Modules identified were:
COM1001, COM1008, COM3420 Images: Discover, understand, reference Creating and using images
COM2009 Questioning and evaluating information
- The relevant referencing guides should be embedded in all applicable modules and assessment.
The Library will continue to collaborate with the department to embed the IDL support that is available and to promote this to students.
Reflections
Sofia bodurova, library student associate.
From the very beginning until the end I have felt great being able to participate in the programme development, doing research on how things can be done. This participation guided me in many ways about all of the resources and support that are provided by the Library.
Many students from the University, including me, have had a hard time finding information about plagiarism. However, during that time the Library has supported us.
After this project I would definitely start looking a bit more into the Library, many topics not covered by lectures are provided as workshops by the Library. The key idea of ours was to identify the gaps in the department which could be filled by the Library.
There are two key concepts that are used but not explained in many modules; they are 'Images: Discover, understand, reference' and 'Preventing plagiarism and reference management'.
What can be better in our case is to reduce the time it takes for a student to find everything on their own and have information provided on Blackboard. This can also save time for many lecturers who have to deal with enquiries in these areas.
Emily Herron, Liaison Librarian
It has been interesting to discover more about the assignments that students are working on and to learn from Sofia how the Library's resources could provide support with these.
One of the main messages we heard from all the Library Student Associates was how hidden the Library's online tutorials are. They need to be embedded within Blackboard modules at the point of student need and made easier to find.
The PLA lead and the department have been very supportive of the work that Sofia and the Library have undertaken. The next steps will be to work on the outcomes identified within the project.
This will be done in collaboration with the department with a particular emphasis on the need for guidance on referencing code in assignments and finding and using images in website creation.
Related information
Ask a question.
Email: [email protected]
Phone: +44 114 222 7200
Sheffield is a research university with a global reputation for excellence. We're a member of the Russell Group: one of the 24 leading UK universities for research and teaching.
Automated Content Analysis: A Case Study of Computer Science Student Summaries
Yanjun Gao , Patricia M. Davies , Rebecca J. Passonneau
Export citation
- Preformatted
Markdown (Informal)
[Automated Content Analysis: A Case Study of Computer Science Student Summaries](https://aclanthology.org/W18-0531) (Gao et al., BEA 2018)
- Automated Content Analysis: A Case Study of Computer Science Student Summaries (Gao et al., BEA 2018)
- Yanjun Gao, Patricia M. Davies, and Rebecca J. Passonneau. 2018. Automated Content Analysis: A Case Study of Computer Science Student Summaries . In Proceedings of the Thirteenth Workshop on Innovative Use of NLP for Building Educational Applications , pages 264–272, New Orleans, Louisiana. Association for Computational Linguistics.
- Dean’s Office
- External Advisory Council
- Computing Council
- Extended Computing Council
- Undergraduate Advisory Group
- Break Through Tech AI
- Building 45 Event Space
- Infinite Mile Awards: Past Winners
- Frequently Asked Questions
- Undergraduate Programs
- Graduate Programs
- Educating Computing Bilinguals
- Online Learning
- Industry Programs
- AI Policy Briefs
- Envisioning the Future of Computing Prize
- SERC Symposium 2023
- SERC Case Studies
- SERC Scholars Program
- SERC Postdocs
- Common Ground Subjects
- For First-Year Students and Advisors
- For Instructors: About Common Ground Subjects
- Common Ground Award for Excellence in Teaching
- New & Incoming Faculty
- Faculty Resources
- Faculty Openings
- Search for: Search
- MIT Homepage
Case Studies in Social and Ethical Responsibilities of Computing
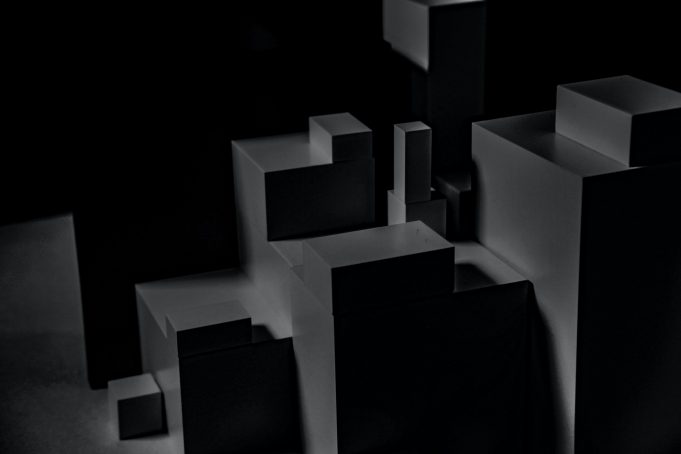
The MIT Case Studies in Social and Ethical Responsibilities of Computing (SERC) aims to advance new efforts within and beyond the Schwarzman College of Computing. The specially commissioned and peer-reviewed cases are brief and intended to be effective for undergraduate instruction across a range of classes and fields of study, and may also be of interest for computing professionals, policy specialists, and general readers. The series editors interpret “social and ethical responsibilities of computing” broadly. Some cases focus closely on particular technologies, others on trends across technological platforms. Others examine social, historical, philosophical, legal, and cultural facets that are essential for thinking critically about present-day efforts in computing activities. Special efforts are made to solicit cases on topics ranging beyond the United States and that highlight perspectives of people who are affected by various technologies in addition to perspectives of designers and engineers. New sets of case studies, produced with support from the MIT Press’ Open Publishing Services program, will be published twice a year and made available via the Knowledge Futures Group’s PubPub platform. The SERC case studies are made available for free on an open-access basis , under Creative Commons licensing terms. Authors retain copyright, enabling them to re-use and re-publish their work in more specialized scholarly publications. If you have suggestions for a new case study or comments on a published case, the series editors would like to hear from you! Please reach out to [email protected] .
Winter 2024
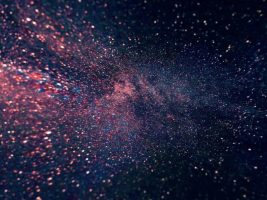
Integrals and Integrity: Generative AI Tries to Learn Cosmology
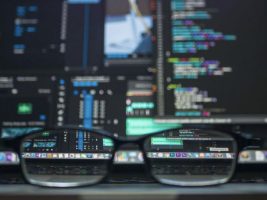
How Interpretable Is “Interpretable” Machine Learning?
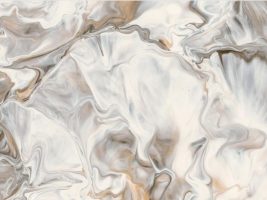
AI’s Regimes of Representation: A Community-Centered Study of Text-to-Image Models in South Asia
Past issues, summer 2023.
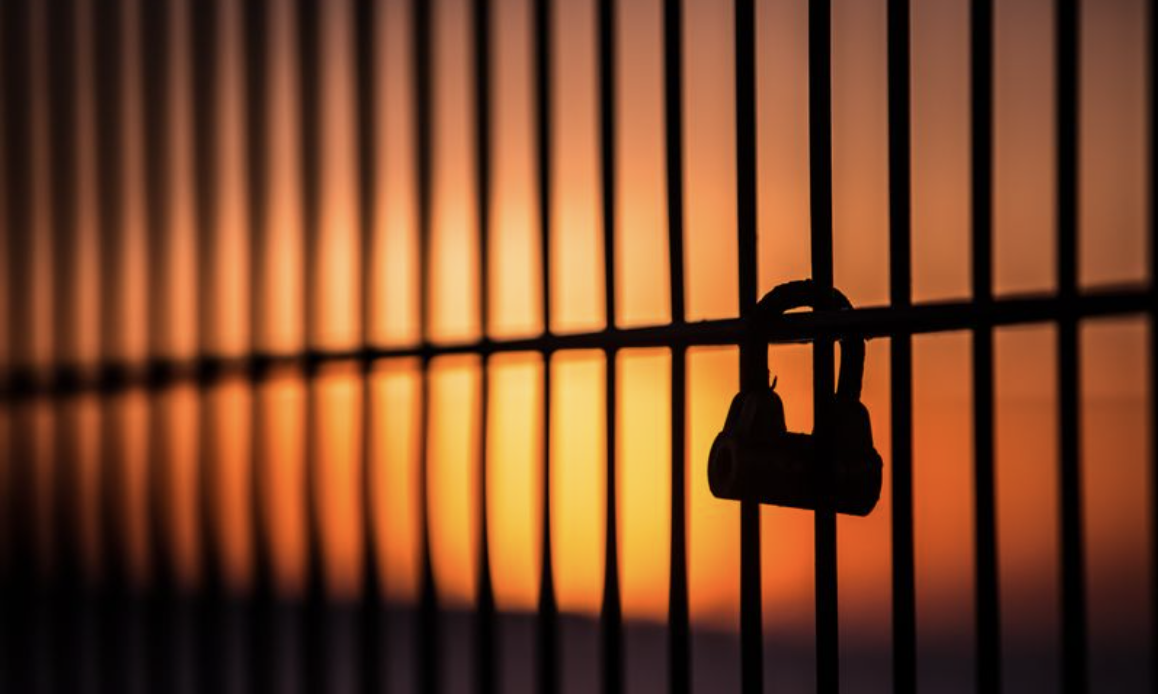
Pretrial Risk Assessment on the Ground: Algorithms, Judgments, Meaning, and Policy by Cristopher Moore, Elise Ferguson, and Paul Guerin
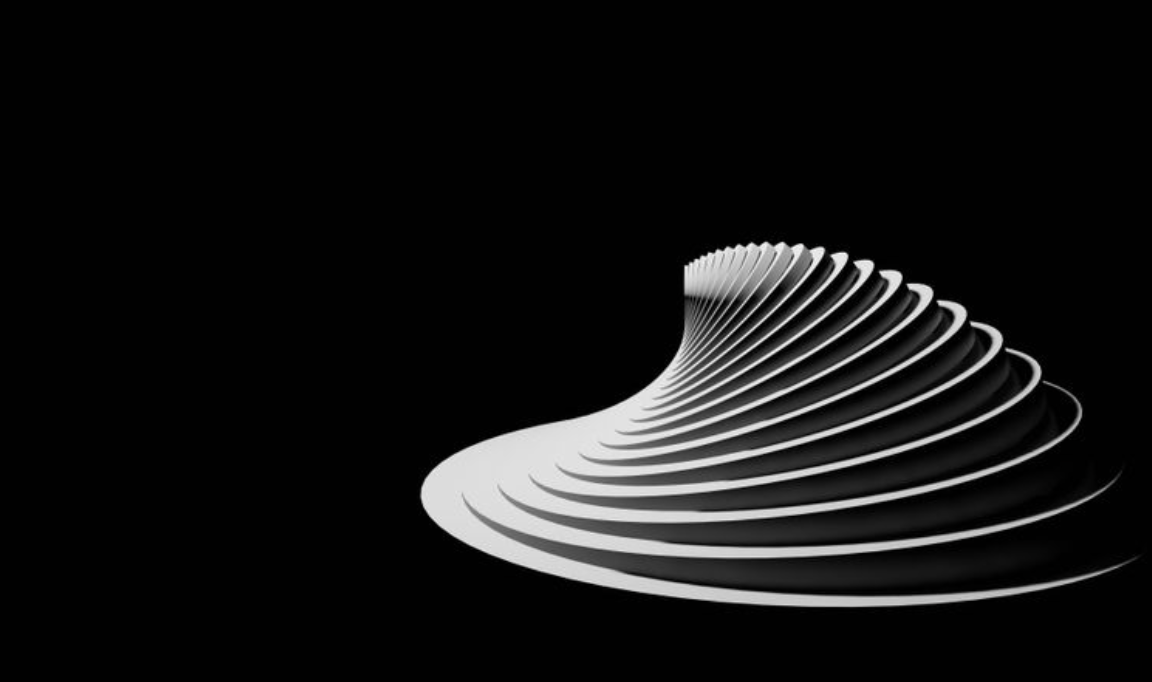
To Search and Protect? Content Moderation and Platform Governance of Explicit Image Material by Mitali Thakor, Sumaiya Sabnam, Ransho Ueno, and Ella Zaslow
Winter 2023
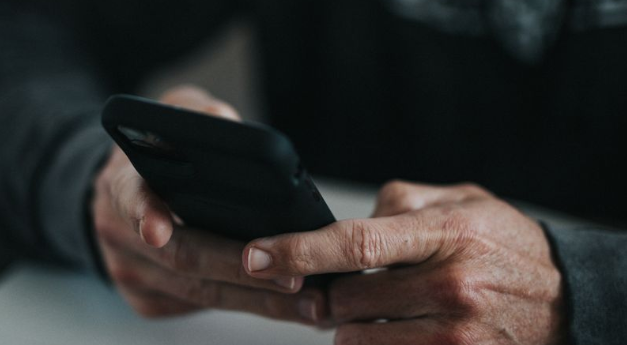
Emotional Attachment to AI Companions and European Law by Claire Boine
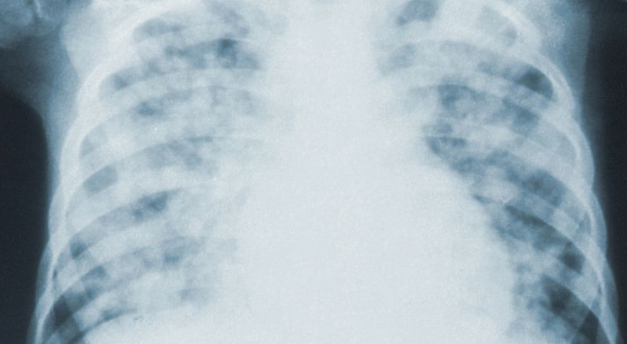
Algorithmic Fairness in Chest X-ray Diagnosis: A Case Study by Haoran Zhang, Thomas Hartvigsen, and Marzyeh Ghassemi
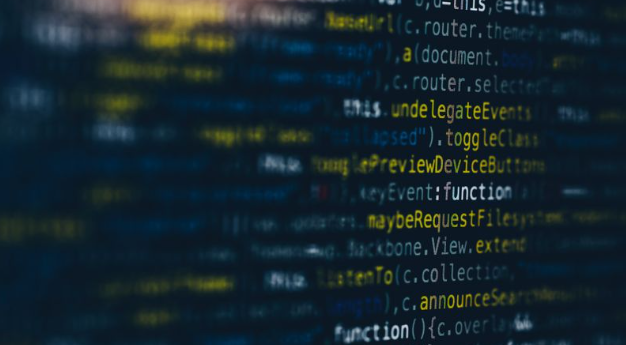
The Right to Be an Exception to a Data-Driven Rule by Sarah H. Cen and Manish Raghavan
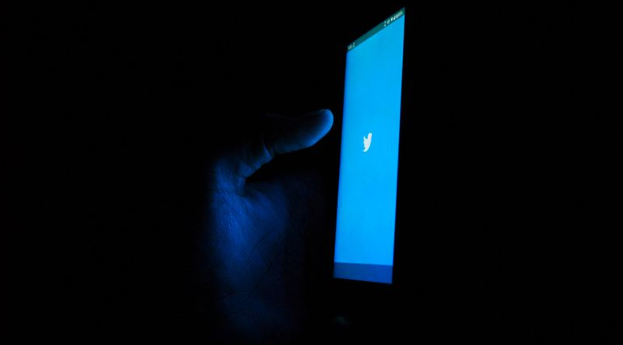
Twitter Gamifies the Conversation by C. Thi Nguyen, Meica Magnani, and Susan Kennedy
Summer 2022
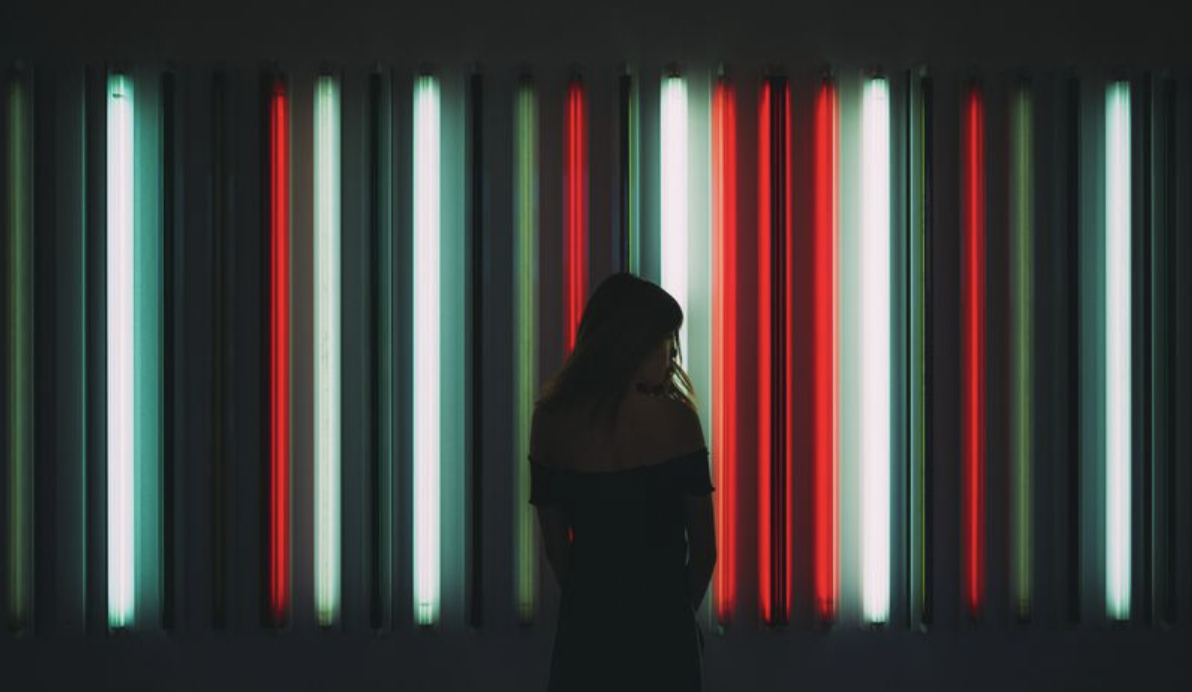
“Porsche Girl”: When a Dead Body Becomes a Meme by Nadia de Vries
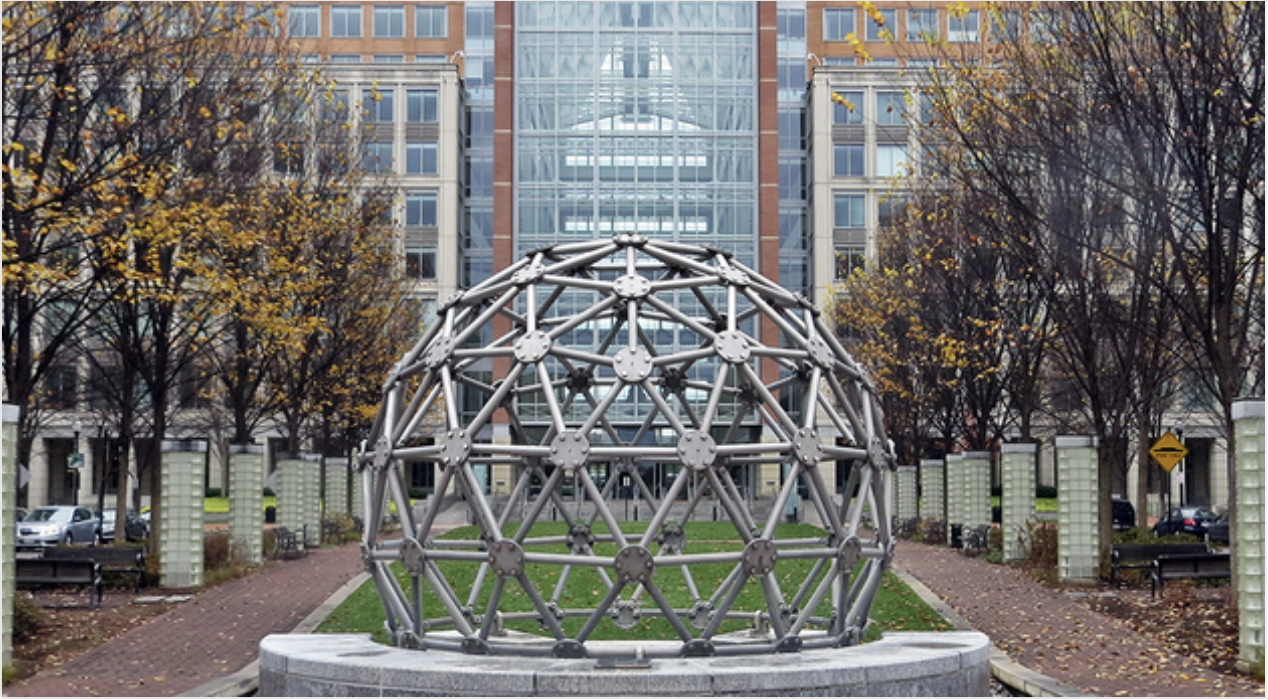
Patenting Bias: Algorithmic Race and Ethnicity Classifications, Proprietary Rights, and Public Data by Tiffany Nichols
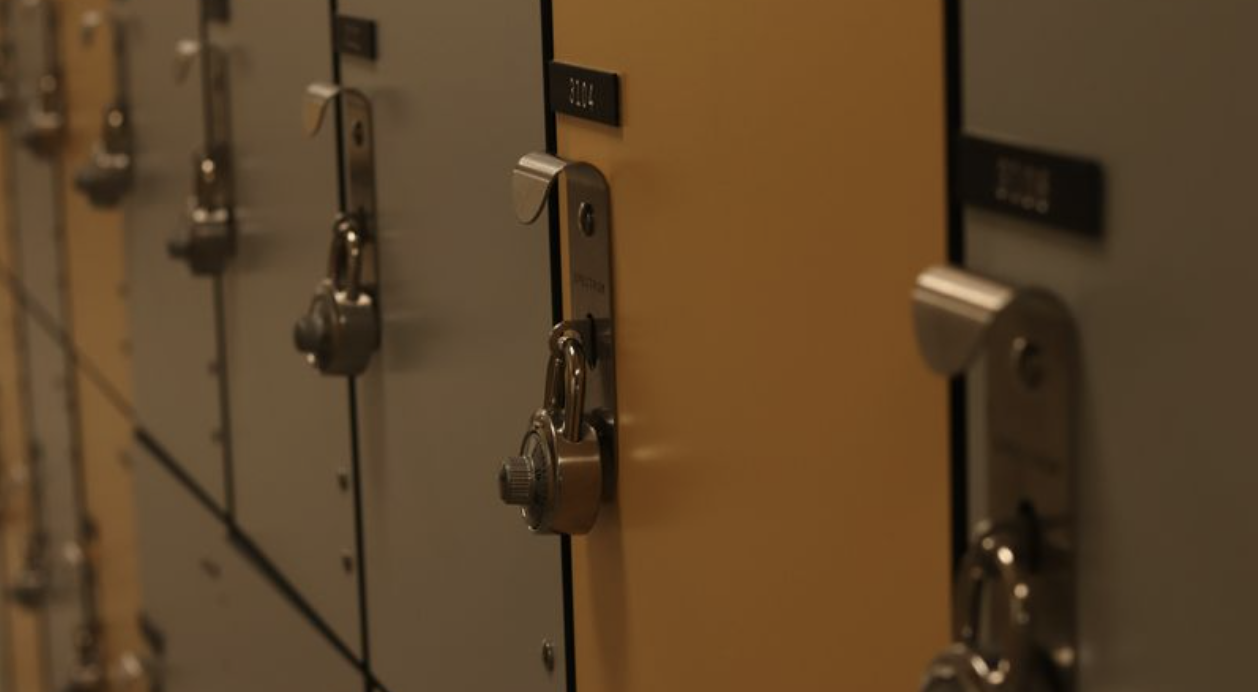
Privacy and Paternalism: The Ethics of Student Data Collection by Kathleen Creel and Tara Dixit
Winter 2022
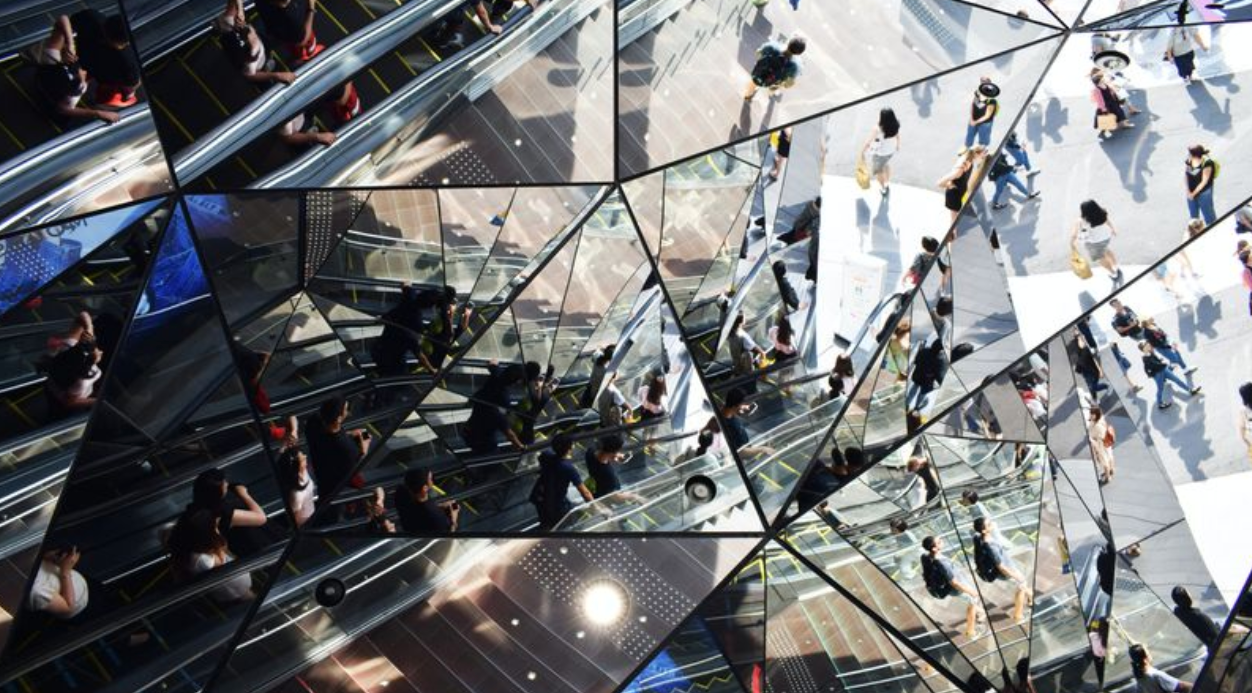
Differential Privacy and the 2020 US Census by Simson Garfinkel
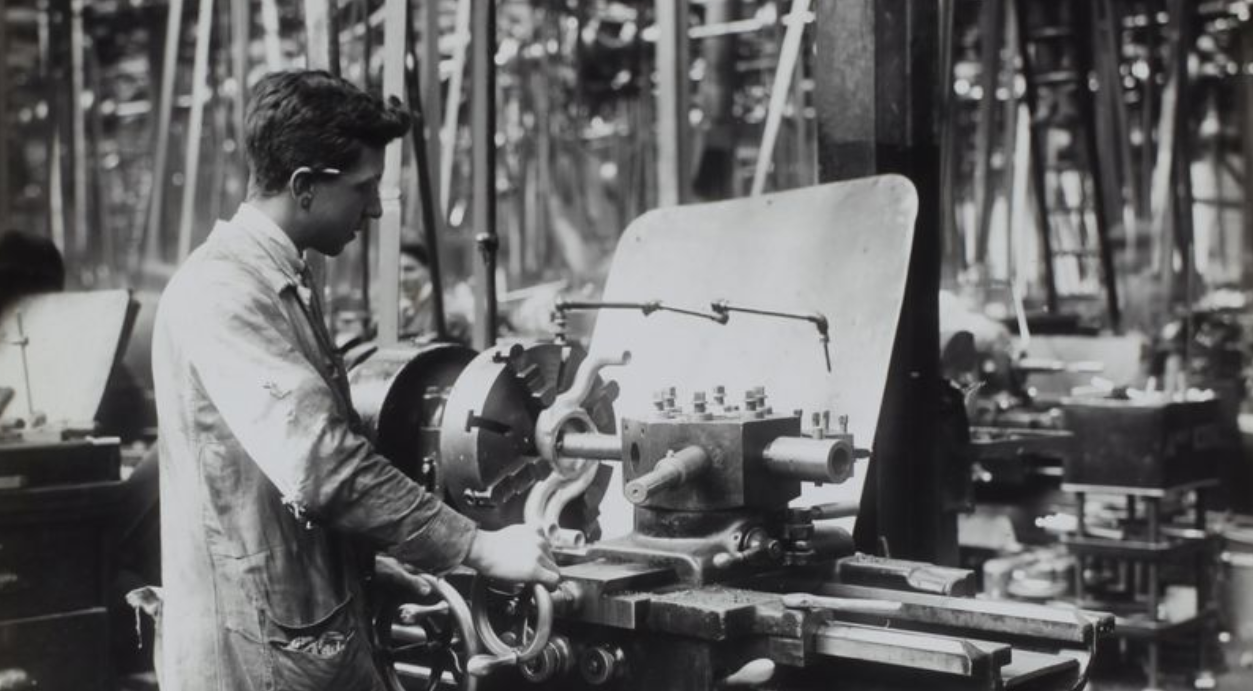
The Puzzle of the Missing Robots by Suzanne Berger and Benjamin Armstrong
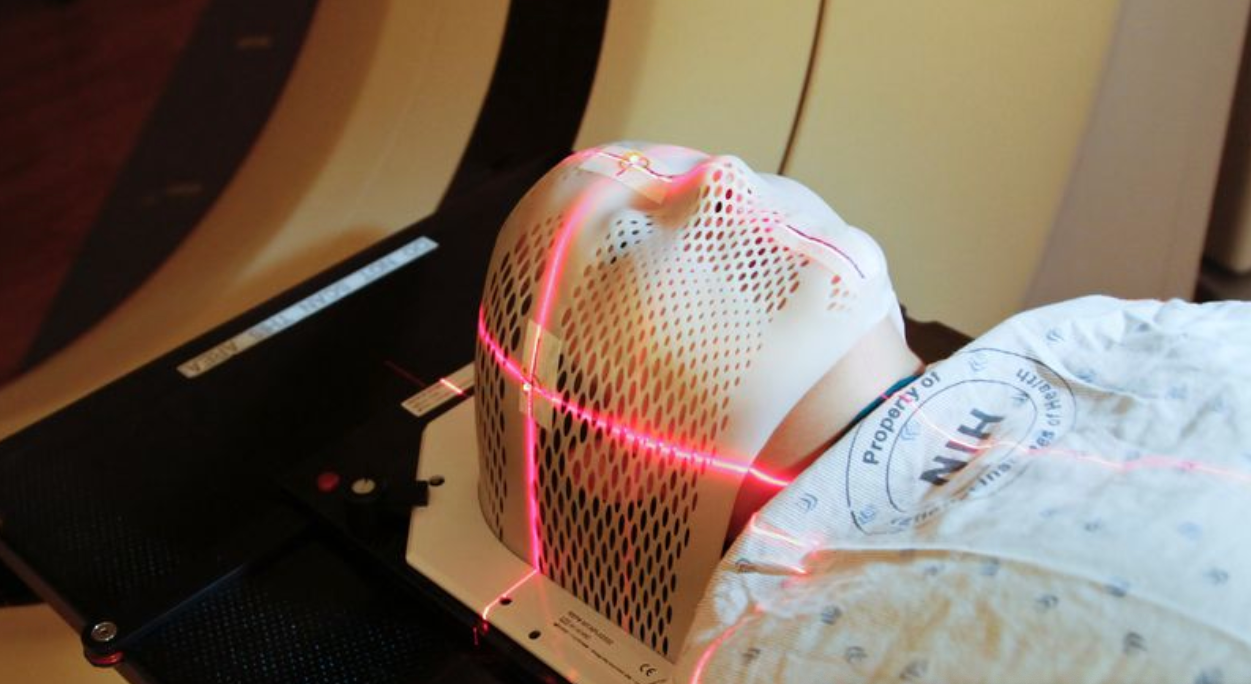
Protections for Human Subjects in Research: Old Models, New Needs? by Laura Stark
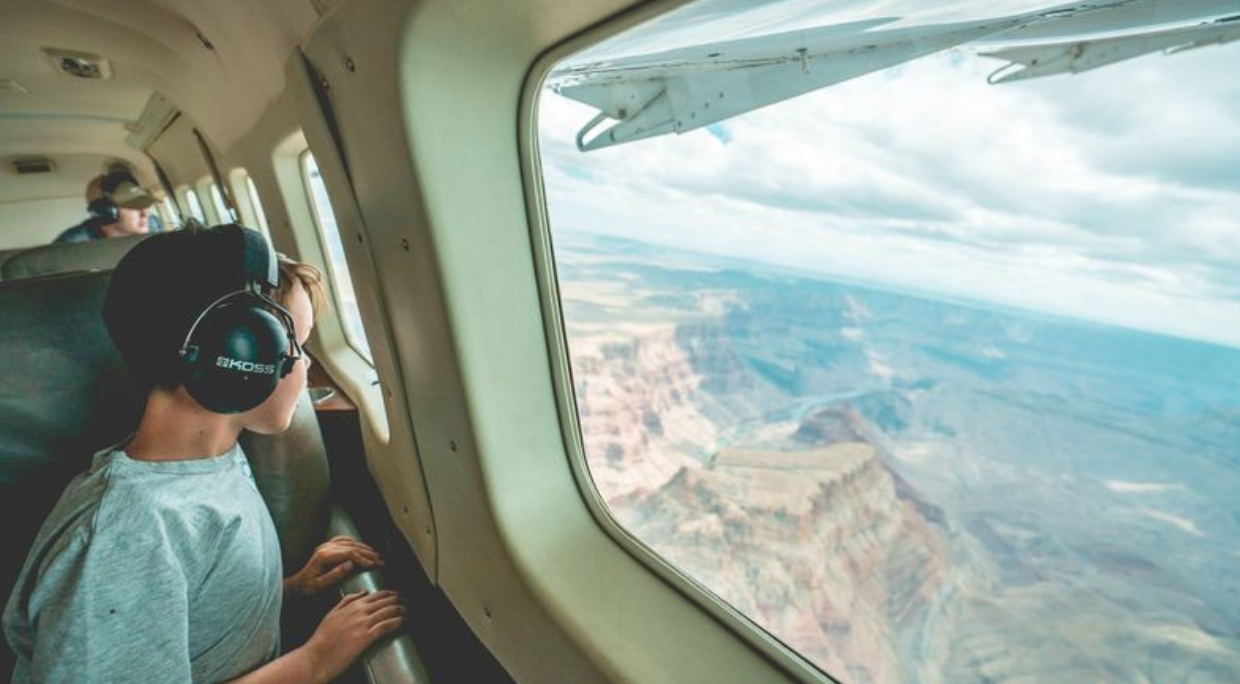
The Cloud Is Material: On the Environmental Impacts of Computation and Data Storage by Steven Gonzalez Monserrate
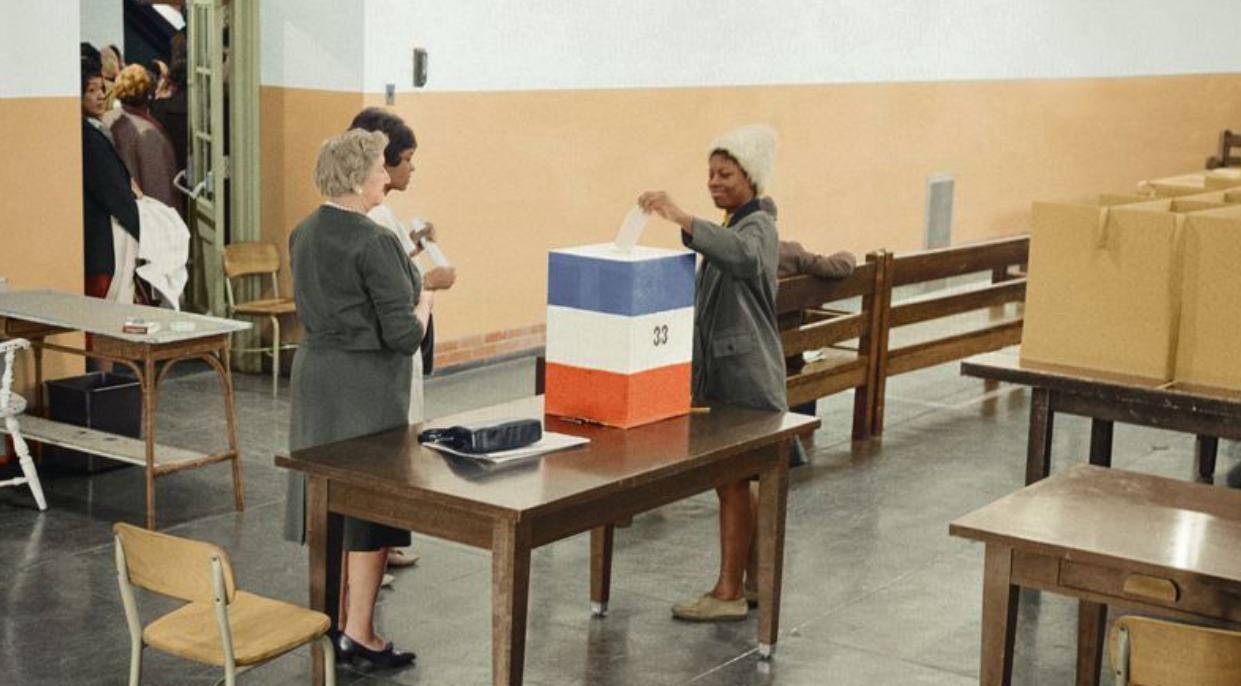
Algorithmic Redistricting and Black Representation in US Elections by Zachary Schutzman
Summer 2021
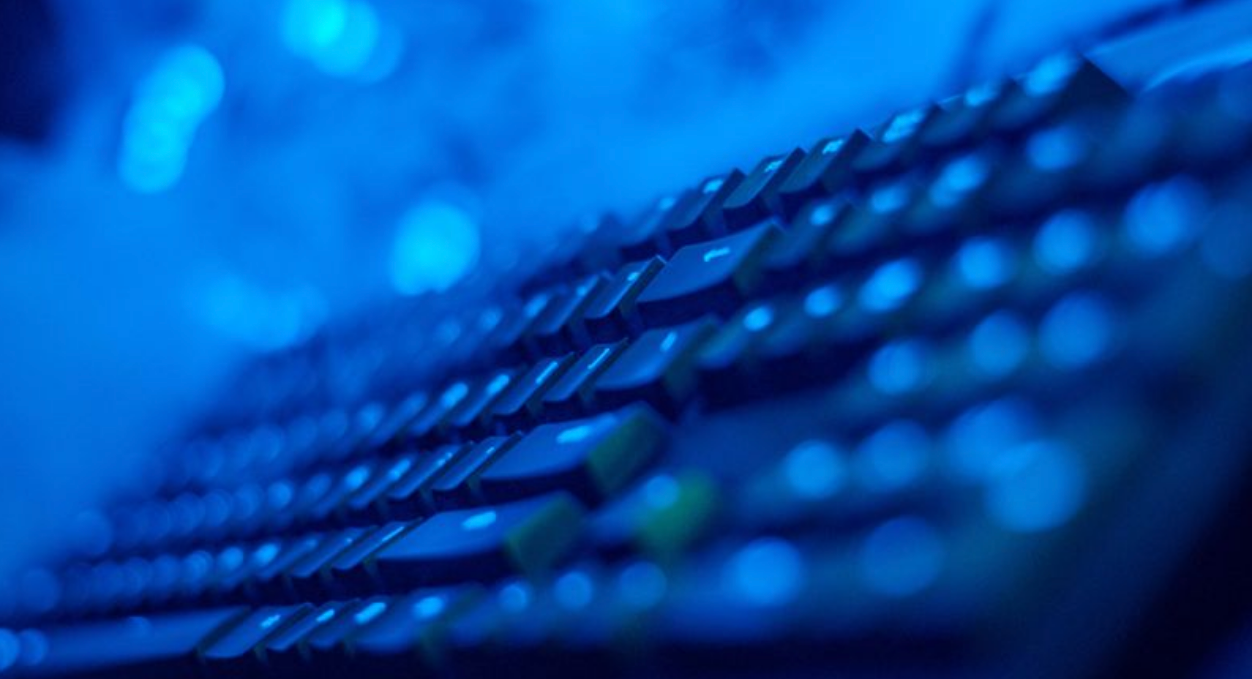
Hacking Technology, Hacking Communities: Codes of Conduct and Community Standards in Open Source by Christina Dunbar-Hester
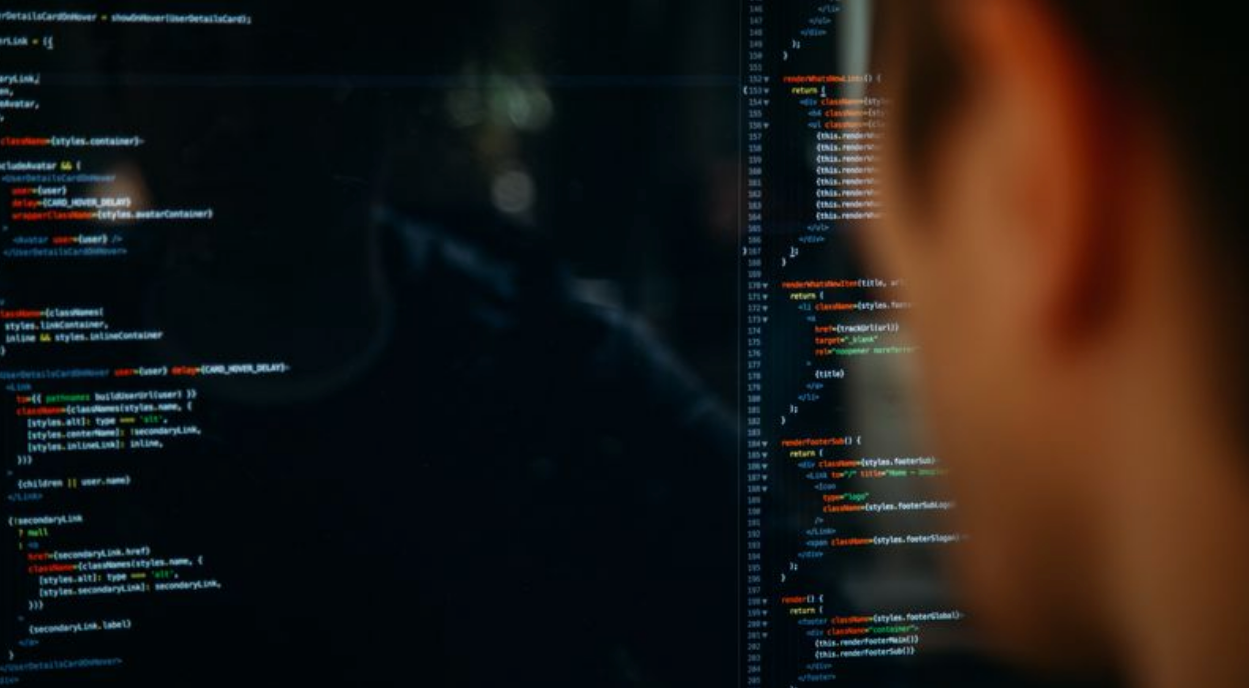
Understanding Potential Sources of Harm throughout the Machine Learning Life Cycle by Harini Suresh and John Guttag
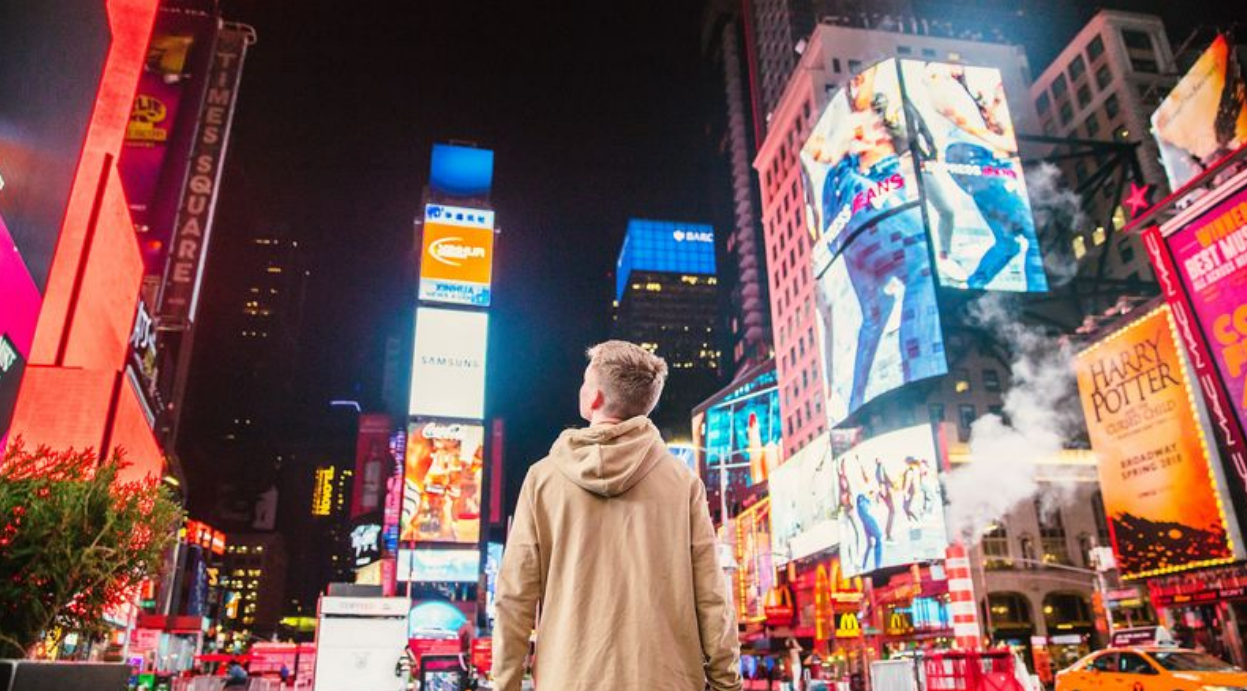
Identity, Advertising, and Algorithmic Targeting: Or How (Not) to Target Your “Ideal User” by Tanya Kant
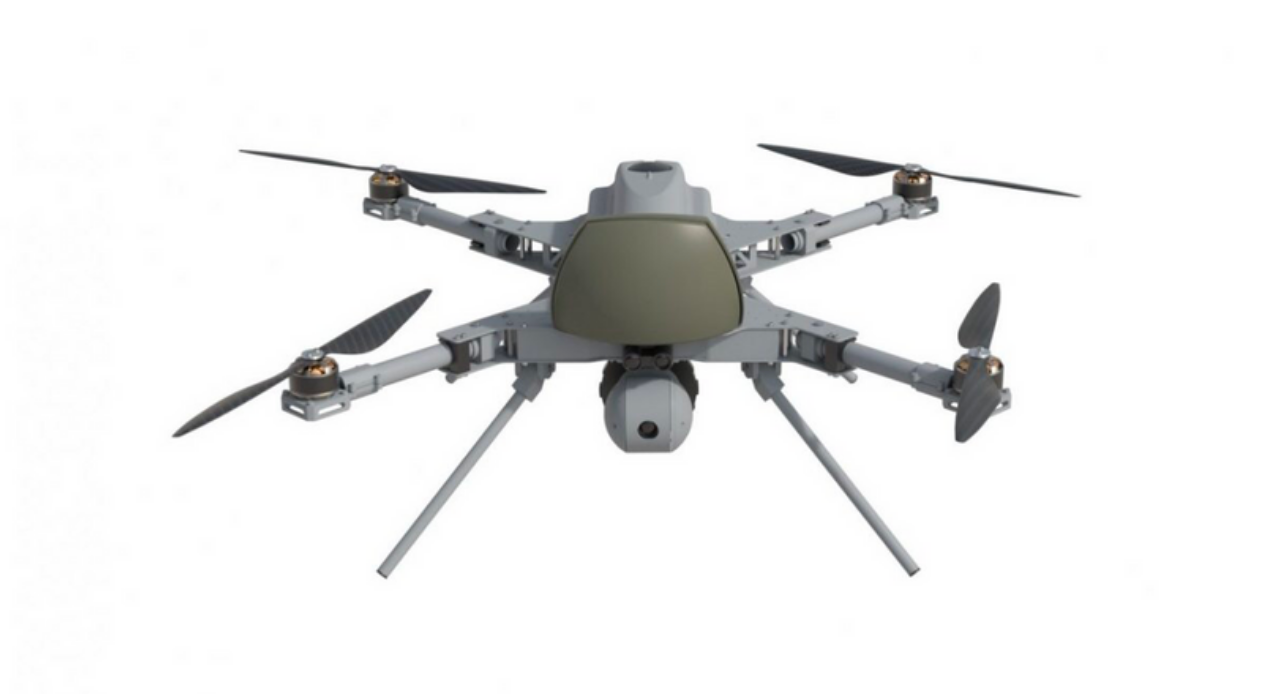
Wrestling with Killer Robots: The Benefits and Challenges of Artificial Intelligence for National Security by Erik Lin-Greenberg
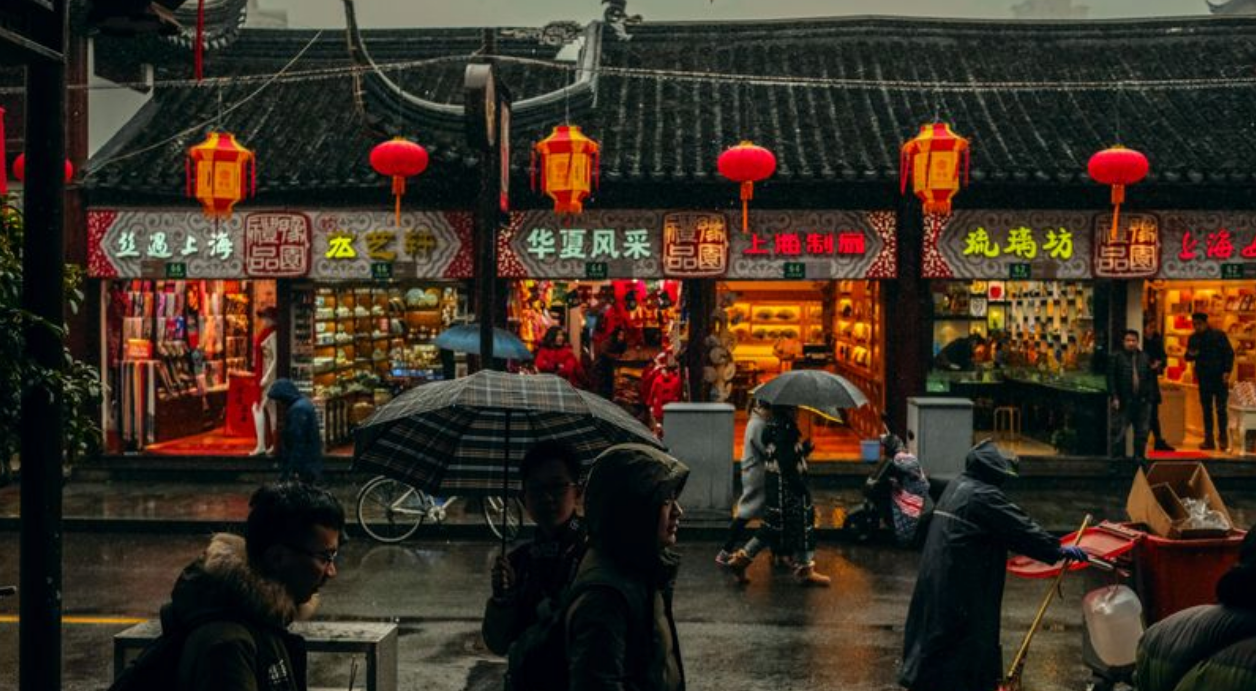
Public Debate on Facial Recognition Technologies in China by Tristan G. Brown, Alexander Statman, and Celine Sui
Winter 2021
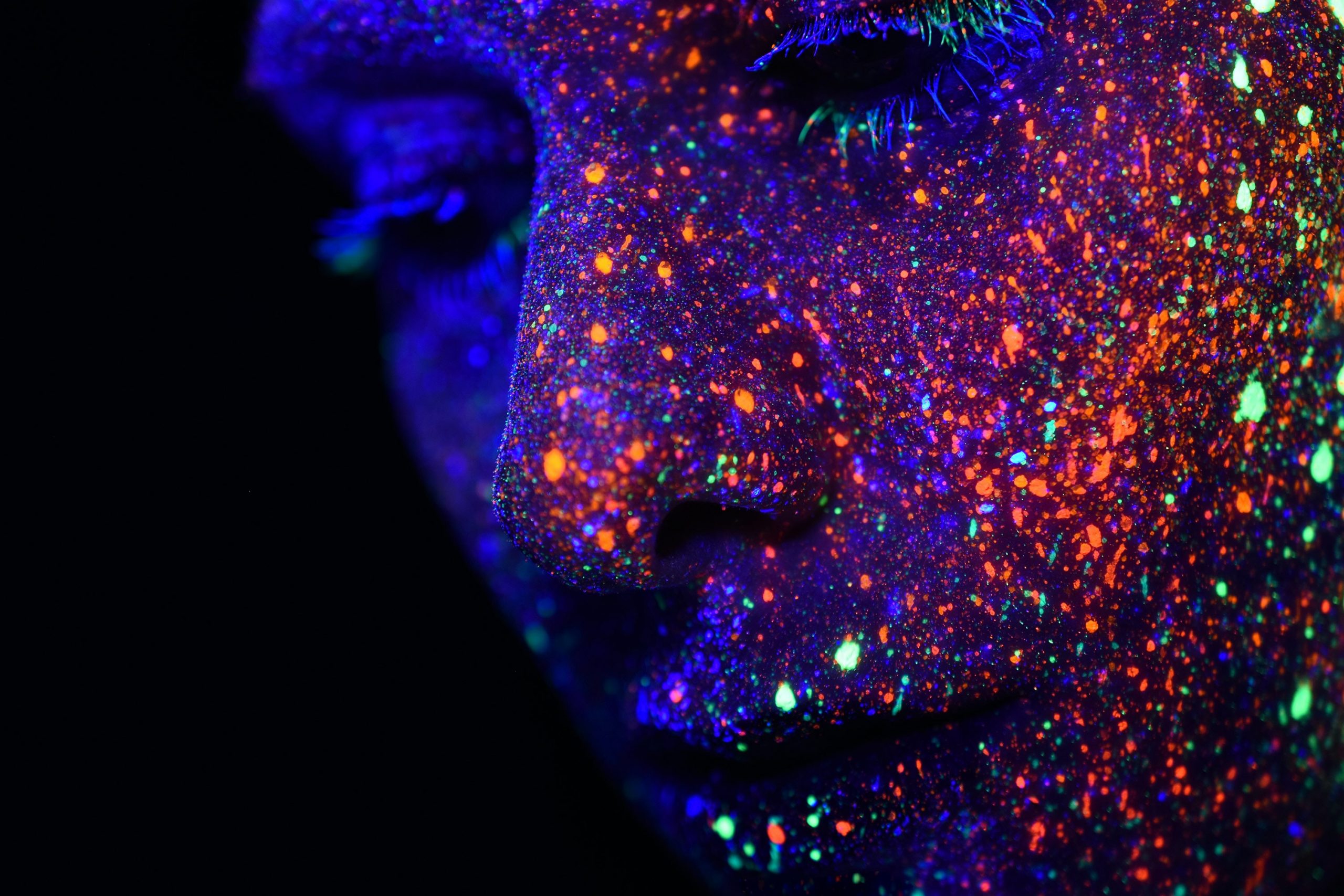
The Case of the Nosy Neighbors by Johanna Gunawan and Woodrow Hartzog
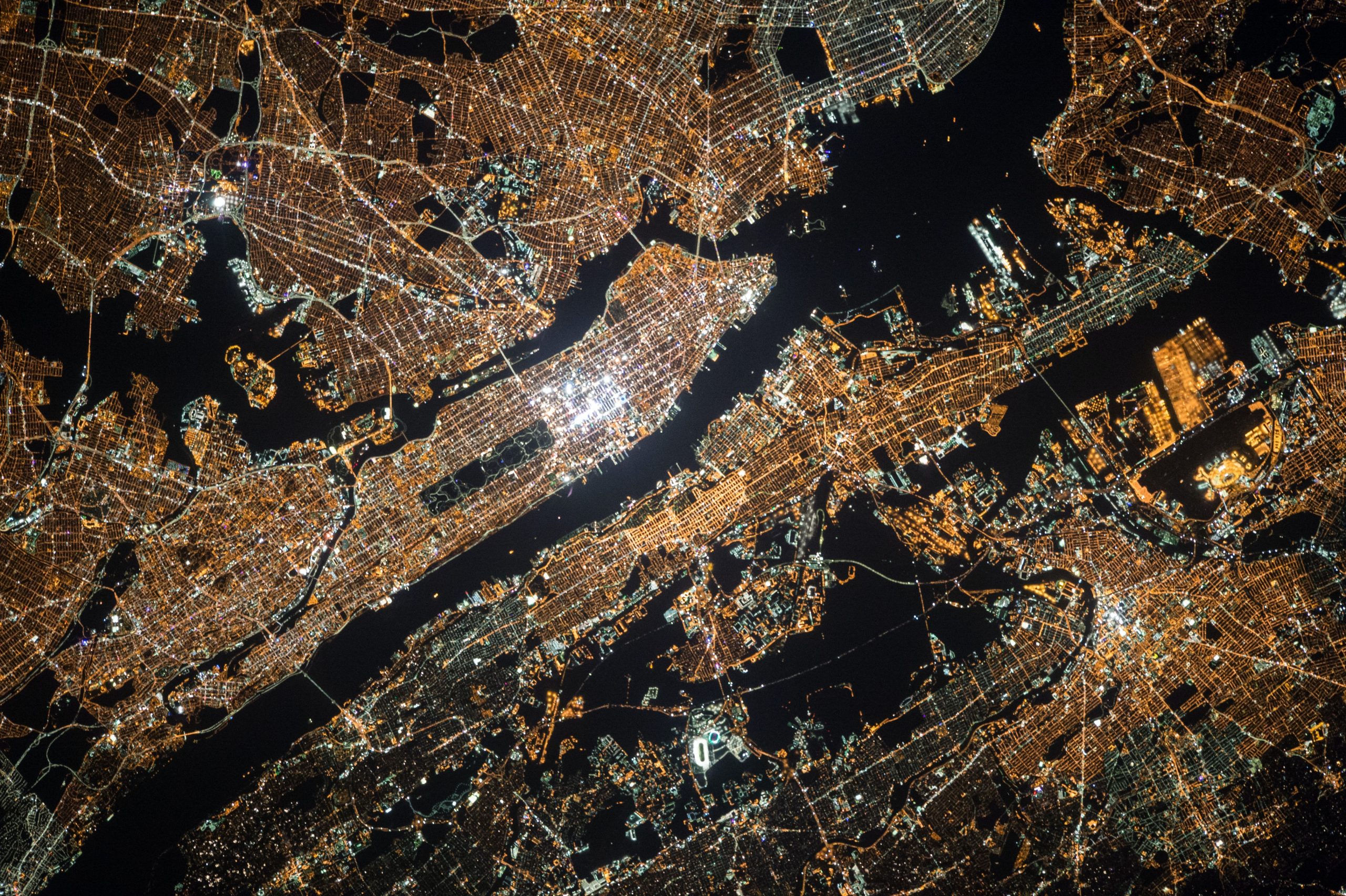
Who Collects the Data? A Tale of Three Maps by Catherine D’Ignazio and Lauren Klein
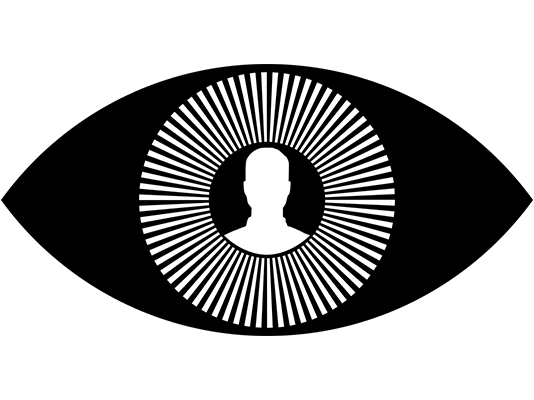
The Bias in the Machine: Facial Recognition Technology and Racial Disparities by Sidney Perkowitz
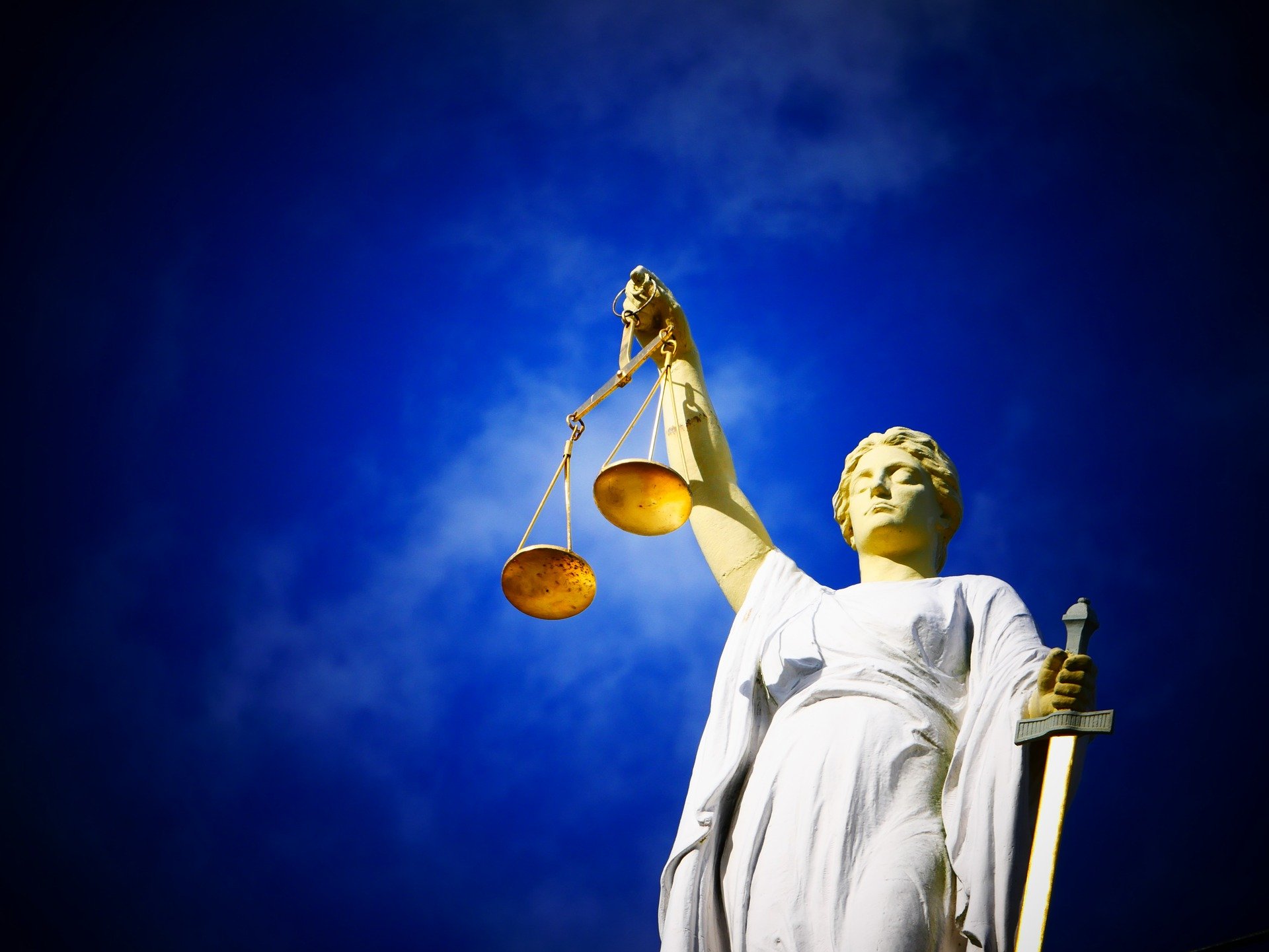
The Dangers of Risk Prediction in the Criminal Justice System by Julia Dressel and Hany Farid
- Skip to Content
- Bulletin Home
2023-24 General Bulletin

Computer Science, BS
Degree: Bachelor of Science (BS) Major: Computer Science
Program Overview
The Bachelor of Science degree program in computer science is designed to give a student a strong background in the fundamentals of mathematics and computer science. The curriculum is designed according to the latest ACM/IEEE computer science curriculum guidelines. A graduate of this program should be able to use these fundamentals to analyze and evaluate software systems and the underlying abstractions upon which they are based. A graduate should also be able to design and implement software systems that are state-of-the-art solutions to a variety of computing problems; this includes problems that are sufficiently complex to require the evaluation of design alternatives and engineering trade-offs. In addition to these program-specific objectives, students can use their technical and open electives to pursue interests in software engineering, algorithms, artificial intelligence, databases, data mining, bioinformatics, security, computer systems, and computer networks, and all students in the Case School of Engineering are exposed to societal issues, professionalism, and are provided opportunities to develop leadership skills.
The Bachelor of Science degree program in computer science is accredited by the Computing Accreditation Commission of ABET .
The mission of the Bachelor of Science degree program in computer science is to graduate students who have fundamental technical knowledge of their profession and the requisite technical breadth and communications skills to become leaders in creating the new techniques and technologies which will advance the field of computer science and its application to other disciplines.
Program Educational Objectives
To educate and train students in the fundamentals of computer science and mathematics
To educate students with an understanding of real-world computing needs
To train students to work effectively, professionally and ethically in computing-related professions
Learning Outcomes
As preparation for achieving the above educational objectives, the Bachelor of Science degree program in computer science is designed so that students attain the ability to:
- Analyze a complex computing problem and to apply principles of computing and other relevant disciplines to identify solutions.
- Design, implement, and evaluate a computing-based solution to meet a given set of computing requirements in the context of the program’s discipline.
- Communicate effectively in a variety of professional contexts.
- Recognize professional responsibilities and make informed judgments in computing practice based on legal and ethical principles.
- Function effectively as a member or leader of a team engaged in activities appropriate to the program’s discipline.
- Apply computer science theory and software development fundamentals to produce computing-based solutions.
Co-op and Internship Programs
Opportunities are available for students to alternate studies with work in industry or government as a co-op student, which involves paid full-time employment over seven months (one semester and one summer). Students may work in one or two co-ops, beginning in the third year of study. Co-ops provide students the opportunity to gain valuable hands-on experience in their field by completing a significant engineering project while receiving professional mentoring. During a co-op placement, students do not pay tuition but maintain their full-time student status while earning a salary. Alternatively or additionally, students may obtain employment as summer interns.
Undergraduate Policies
For undergraduate policies and procedures, please review the Undergraduate Academics section of the General Bulletin.
Accelerated Master's Programs
Undergraduate students may participate in accelerated programs toward graduate or professional degrees. For more information and details of the policies and procedures related to accelerated studies, please visit the Undergraduate Academics section of the General Bulletin.
Program Requirements
Students seeking to complete this major and degree program must meet the general requirements for bachelor's degrees and the Unified General Education Requirements . Students completing this program as a secondary major while completing another undergraduate degree program do not need to satisfy the school-specific requirements associated with this major.
Each student is required to complete at least 20 computer science and computer science related courses totaling at least 63 credit hours plus additional courses in mathematics, science, engineering and statistics.
The 20 computer science and computer science related courses must include:
- all six core courses;
- at least two courses from each of the four breadth areas;
- and a course from the secure computing requirement list.
The remaining courses needed to fulfill the 20 course and 63 credit hour requirement may come from the list of approved technical electives with at most two Group 2 courses.
Other computer science related courses not listed here may be used with prior permission from the student’s academic advisor.
Computer Science Core Requirement
Computer science breadth requirement.
BS students are required to complete at least two courses from each of the four breadth areas for a total of eight computer science breadth courses.
Computer Science Secure Computing Requirement
Students pursuing the BS degree must demonstrate competence in the principles and practices of secure computing by completing one of the following courses as part of their 20 computer science or computer science related courses. This course may be double counted as a computer science breadth course or technical elective course, as appropriate.
Mathematics, Science and Engineering Requirement
Statistics requirement, list of approved technical electives.
This list of approved technical electives is divided into groups according to how closely a course is related to the core knowledge areas as defined in the ACM/IEEE computer science curriculum guidelines. Computer Science BS students may use up to two courses from Group 2 as technical electives toward the computer science degree. Computer science related courses not listed below may be used as a technical elective but require prior permission from the student’s academic advisor.
Sample Plan of Study
Unified General Education Requirement .
Print Options
Print this page.
The PDF will include all information unique to this page.
- Research article
- Open access
- Published: 31 January 2023
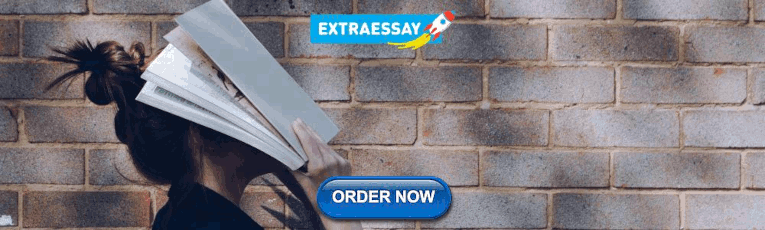
Student-centered case-based teaching and online–offline case discussion in postgraduate courses of computer science
- Xinhong Zhang 1 ,
- Boyan Zhang 1 &
- Fan Zhang ORCID: orcid.org/0000-0003-2176-3835 2
International Journal of Educational Technology in Higher Education volume 20 , Article number: 6 ( 2023 ) Cite this article
6702 Accesses
10 Citations
3 Altmetric
Metrics details
This study explores a student-centered teaching method in postgraduate courses. Teacher-centered classroom teaching cannot fully stimulate learning initiative and enthusiasm of students. Student-centered means that students actively learn and construct knowledge by participating in teaching activities. This study presents a student-centered online–offline hybrid teaching method, which adopts student-centered case-based teaching and online–offline case discussion in the postgraduate courses of computer science. The latest engineering cases are integrated into teaching and a case library is constructed. Taking the digital image processing course as an example, student-centered teaching allows students to choose what to learn and how to learn. Case-based teaching makes students better understand the application of theory of knowledge. It can introduce multiple perspectives, promote understanding and reflection on problems, and help students develop higher-level thinking, analysis, and synthesis skills. This study explores online–offline case discussion method in the student-centered teaching and proposes the principles of case design of postgraduate courses. Revised Bloom’s taxonomy is used for teaching assessment. The actual teaching effect shows that student-centered case-based teaching and online–offline case discussion have achieved better teaching effect.
Introduction
Teacher-centered classroom teaching is the main mode adopted in current postgraduate courses of computer science. However, this traditional teaching mode cannot fully stimulate the learning initiative and enthusiasm of students, and is also not conducive to cultivating their innovative thinking ability. We find it difficult to achieve the teaching goal by completely adopting teacher-centered classroom teaching for science and engineering graduate students, because their studies focus more on engineering and practical applications. So, it is necessary to explore a new teaching mode. Case-based teaching (CBT) or case-based learning (CBL) provides a solution to solve the problems above (Sangam et al., 2021 ).
In case-based teaching, a case is defined as a description based on a real event or situation in which sufficient detail is provided to assist students in the analysis and solution of problems (Prada et al., 2020 ; Tan et al., 2014 ). The development of information technology has created a variety of possibilities for the design of cases. Therefore, cases are also defined as the typical teaching events using multimedia formats, such as video, audio, pictures, animation, and web pages. The case-based teaching method guides students to carry out a series of learning activities, including analysis, discussion, problem-solving, evaluation, reflection etc., which is helpful for students to develop higher-level thinking, analytical and integrative skills (Tawfik et al., 2017 ; Strobel et al., 2013 ). Some studies have shown that case-based teaching makes up for the deficiency of passive acceptance of learning, and has a significant impact on promoting knowledge transfer and knowledge application.
This paper presents a student-centered online–offline hybrid teaching method for the postgraduate courses of computer science, which adopts case-based teaching and online–offline case discussion. The latest engineering cases are integrated into teaching and a case library is constructed. Taking the digital image processing course as an example, student-centered teaching allows students to choose what to learn and how to learn. Case-based teaching makes students better understand the application of theory. It can introduce multiple perspectives, promote understanding and reflection on problems, and help students develop higher-level thinking, analysis, and synthesis skills. Revised Bloom’s taxonomy is used for teaching assessment.
The main contributions of this study are as follows:
Exploring student-centered teaching in postgraduate courses.
Using cases as the main contents of teaching.
Adopting the case-based teaching method.
Exploring the online–offline case discussions in the student-centered teaching.
Proposing the principles of case design of postgraduate courses.
This paper is organized as follows: " Introduction " section deals with the introduction. " Literature review " section reviews the relevant literature. " Methods " section describes the method of case library construction and the method of student-centered case-based teaching. " Result " section provides the implementation results of our teaching method. " Discussion " section discusses this study. Finally, " Conclusion " section draws conclusion.
Literature review
Case-based teaching
The design and implementation of case-based teaching activities create opportunities for an exploratory new learning mode (Goeze et al., 2014 ). By participating in a series of activities in case-based teaching, students actively develop skills of knowledge application and problem-solving, and conduct abilities of higher-level thinking, analysis and synthesis (Newton et al., 2015b ). Several studies have highlighted how case-based teaching enhances students’ comprehension and critical thinking skills (Leon et al., 2015 ). Students’ reflective and critical thinking skills are promoted as they work on cases that challenge them to deal with issues of multiple layers and complex dimensions.
With the development of information technology, the cases used for teaching have been transformed from the textual narration to the multimedia-based presentation. Multimedia cases are gradually applied to the online learning environment (Luo et al., 2018 ). Multimedia case teaching has its unique advantages. It can better simulate the complexity of real-world problems (Rico & Ertmer, 2015 ). For example, the cases presented by interactive multimedia can attract and motivate students, and can effectively promote knowledge transfer. Hewitt et al. use video cases as the carrier of case-based teaching. They encourage students to think, discuss, solve, and reflect the problem through pause and interaction of the video case at each key point. The final results prove that video case teaching promotes the learning interest and motivation of students (Hewitt et al., 2003 ). In the exploration of learning effects, Choi et al. use the interaction and feedback functions of multimedia cases to provide feedbacks of experts at each decision point of case problems. During the case learning process, students can view expert opinions to gain an in-depth understanding of the problem. The results of the learning effect evaluation demonstrate the effectiveness of multimedia case teaching (Choi & Lee, 2008 ). Research results show that multimedia case teaching improves learning motivation of students, helps students better master knowledge, and improves their problem-solving ability. Yoon et al. use learning analytics to gain useful insights into student learning in a video-based online learning environment (Yoon et al., 2021 ). Based on the observed patterns of log behavior, students can be divided into two categories: active learners and passive learners. Aactive learners have higher academic performance than passive learners.
When constructing teaching cases, teachers should start by identifying goals, identifying skills, and deciding which concepts students should learn. Through this process, teachers carefully consider the learning outcomes that students should achieve (Jevne et al., 2021 ). Newton et al. argue that case production can be either open-ended or guided by challenges or problems, depending on the teaching purpose and student population. The case should enhance students’ interest by using stories they can relate to (Newton et al., 2015a ).
Case discussion
Case discussion is an important part of case-based teaching. It is regarded as the key to the success of case-based teaching. Teachers guide students to express their personal opinions on the case, and realize the sharing process of problem exploration and knowledge construction (Ertmer & Koehler, 2014 ). Some studies have shown that case discussions can introduce a variety of viewpoints, promote students’ understanding and reflection on problems, and help students transfer and apply knowledge. In general, case discussion has a good role in promoting case-based teaching (Ertmer & Koehler, 2015 ). Yew et al. believe that students’ participation in the interactive case activities can help students to actively construct knowledge, improve learning interest and learning engagement, and enhance learning performance (Yew & Yong, 2014 ). The targeted guidance of teachers also improves the learning experience and learning effect (Long & Koehler, 2021 ; Kim, 2022 ; Roels et al., 2021 ; Zhang et al., 2019 , 2022a ). Lock et al. provide expert understandings of online discussions. These understandings address real-world issues related to diverse and digital classrooms (Lock & Redmond, 2021 ). Zhang et al. use epistemic network analysis (ENA) to explore the collaborative problem-solving processes of students and teachers in different online collaborative learning tasks (Zhang et al., 2022b ). By investigating the academic performance of collaborative problem-solving patterns, they reveal in detail the relationship between cooperative problem-solving and students’ academic performance.
The online learning environment presents both opportunities and challenges for case discussions (Mcpartlan et al., 2021 ). Broadbent et al. evaluate whether self-regulated learning (SRL) impacts with students’ academic performance in both online and offline learning environments (Broadbent et al., 2021 ). Among students who study online, those who benefit the most are those who are confident, able to manage their time and discipline their efforts. Turk et al. believe that online course instructors should provide self-supporting goals, choices, guidance, and feedbacks. They should also ensure their effective interactions with students. The interactive learning environment for students to interact with their peers should be socially and emotionally trusting (Turk et al., 2022 ).
As an important activity of the case-based teaching method, online discussions create conditions for online teaching or online–offline hybrid teaching. online–offline hybrid teaching is a kind of teaching that combines online teaching with traditional teaching (Zhao et al., 2022 ; Yi, 2022 ; Peng & Wei, 2021 ). online–offline hybrid case discussion has special advantages. (1) online–offline hybrid case discussion breaks the limitation of time and space. It realizes a more flexible and free way of asynchronous discussion. Online case discussion prolongs the timeliness of classroom discussion and provides students with a more personalized learning pace, more flexible problem-solving and reflection space. (2) online–offline hybrid case discussion creates more favorable conditions for the participation of teachers and invited experts. Flexible online and offline interaction helps teachers to provide more accurate guidance and feedback, which makes it possible for highly interactive case teaching. However, the asynchronous discussion makes the problem discussion lose the characteristics of timely feedback, and the online discussion weakens the guiding role of teachers to a certain extent (Wu, 2022 ; Li et al., 2021 ).
- Student-centered teaching
The student-centered teaching concept reflects the principles of constructivism theory. Student-centered means that students actively learn and construct knowledge by participating in teaching activities (Zhienbayeva & Abdigapbarova, 2021 ; Mamnpoba, 2021 ). In student-centered teaching, the teaching method changes from teaching to guiding; The teaching subject changes from teacher to student; The teaching content changes from textbook to practice; The assessment method changes from traditional examinations to diversified procedural examinations. Student-centered teaching is closely related to students’ learning enthusiasm. Specifically, student-centered teaching can help students actively participate in learning and achieve better grades. When students’ needs are more comprehensively met, student attendance will increase, and the possibility of dropping out will be reduced. They will focus more on their studies and be better prepared for graduation. Constantinou et al. point out that student-centered teaching involves not only academic learning, but also other skills, such as active participation in society or community, professionalism, mental health, etc. Therefore, student-centered teaching requires a holistic view of the learning and development of students (Constantinou, 2020 ). The corona virus 2019 (COVID-19) global pandemic has forced higher education to transform to the online learning mode. This provides an opportunity to adopt student-centered teaching. Active learning can improve students’ performance and close the achievement gaps for underachievers (Sandrone et al., 2021 ). Angel et al. adopt the method of flipped classroom to carry out student-centered teaching (Mingorance Estrada et al., 2019 ). Compared with the traditional teaching, this method significantly improves student performance, increases student interaction, and improves classroom attendance and engagement. Teachers’ feedback and teacher-student interaction will effectively mobilize students’ learning enthusiasm. Moges et al. believe that in order to improve the teaching effect, teachers should innovate and diversify teaching methods to attract students to participate. In addition, teachers and students need to be properly trained. Both of them need to understand the impact of student-centered education so that they have a clear understanding of their roles and responsibilities (Moges, 2019 ).
Both the teacher-centered teaching method and the student-centered teaching method are useful. The best teaching method is comprehensive. Different teaching methods can learn from each other and complement each other. Several studies have revealed the value of combining traditional teaching with student-centered teaching. A way of combined approach is for students to try to solve problems on their own. The teacher then teaches the correct problem-solving steps and compares the student’s solution to a standard problem-solving solution. This model can be called learning before teaching. Exploratory learning is a teaching method of learning before teaching. Exploratory learning refers to the exploration of new problems by students before they are taught related concepts and solutions (Chung & Ho, 2021 ). The purpose of exploratory learning is to give students the opportunity to explore new topics for themselves before accepting traditional teaching (Weaver et al., 2018 ; Schalk et al., 2017 ). Another way of combining application is to teach the relevant knowledge and correct solution directly, and then ask the students to do problem-solving exercises using the method taught by the teacher. This model can be called teaching before learning. The most typical example of teaching before learning is problem-based learning (PBL). PBL is a teaching method that students are presented with a real or realistic problem, such as a case, and use inductive reasoning to learn both information about the topic and how to think critically about it. Through PBL, students can acquire both knowledge and skills of collaboration, communication, and reflection (Kapur, 2016 ; Armstrong et al., 2021 ).
Self-regulated learning and Bloom’s taxonomy
Self-regulated learning (SRL) refers to the process by which students activate and maintain their own thoughts, feelings and behaviors, and systematically achieve learning goals (Song et al., 2021 ; Tran et al., 2022 ). Learning goal, efficacy and learning strategy are three important components of self-regulated learning (Granberg et al., 2021 ). The most striking feature of self-regulated learning is that students have actual control over their own learning. They can cognitive and control the processes directly to achieve their learning goals (Callan et al., 2021 ; Guo et al., 2021a ; Tuti et al., 2021 ). Rovers et al. compare the validity of several different methods of self-regulated learning (Rovers et al., 2019 ). The self-reported questionnaire can reflect the overall level of students’ self-regulated learning. In contrast, behavioral measures provide more accurate explanations when students are asked to report specific self-regulated learning strategies. Many studies have shown that the external feedback from teachers could promote students’ self-regulated learning (Yunus et al., 2021 ; Aguilar et al., 2021 ). Teacher’s feedback and evaluation could increase the intrinsic motivation of students. For example, encouraging students to participate in more challenging tasks can improve the self-regulated learning level of students. Students typically exhibit more academic help-seeking behavior and make more efforts in response to teachers’ support (Guo et al., 2021b ).
Bloom’s taxonomy is a hierarchical model that divides learning into levels of complexity. The revised Bloom’s taxonomy divides the cognitive process dimensions in six levels (Krathwohl, 2002 ). The six levels from low to high are: Remember, Understand, Apply, Analyze, Evaluate, and Create. Figure 1 shows the revised Bloom’s taxonomy. Bloom’s taxonomy is a hierarchical model designating learning into levels of complexity and is often used to structure course experiences such as learning objectives, assessments, and pedagogical choices (Killion et al., 2022 ). Bloom’s taxonomy of educational goals reflects the relationship between knowledge learning and ability development through the structure of knowledge dimension and cognitive process dimension. It is also a tool for the evaluation of teaching objectives and the assessment of examinations (Vieyra & Gonzlez, 2020 ). Desha et al. propose a new model to assess the development of problem-solving skills based on Bloom’s taxonomy (Desha et al., 2021 ). They wonder how the design might have stimulated or dampened student appreciation of complexity, and how these findings aligned with desired expectations. To explore this, the learning materials are evaluated through Bloom’s taxonomy. The goal is to understand the extent to which the course content exposed students to the spectrum of problem-solving contexts. Dolan et al. propose some recommendations for the use of virtual simulations in the current learning environment by studying learning theories, learning styles, and Bloom’s revised taxonomy (Dolan et al., 2021 ). Synchronous debriefing with students, faculty, preceptors, and peers provides the opportunity for scaffolding to support students’ learning needs and foster reflection.
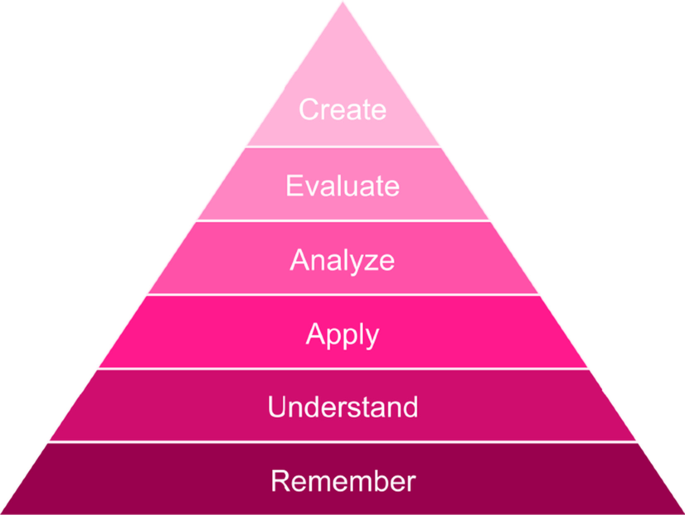
The revised Bloom’s taxonomy
Construction of a case library for the digital image processing course
Digital image processing is a course for computer science graduate students. This course is both theoretical and practical. At present, the cases in the teaching materials are relatively outdated and cannot reflect the latest research progress. In addition, because there is no experiment arranged, students’ sense of participation is not strong. This paper explores the method of student-centered case-based teaching and online–offline case discussion during digital image processing.
The construction objectives of the case library (or case base) of digital image processing course are as follows. The design of case library is student-centered. The information and data in the case should fully consider correctness and reliability. Case library requires constant maintenance and updating. Cases should meet the requirements of typicality, objectivity, advancement, and innovation. Students can acquire the knowledge of image processing efficiently and quickly from cases. Through case-based teaching, students should be able to broaden their horizons, stimulate their learning interest and improve their practical abilities.
Principles of case design
We propose the following principles of case design for the engineering courses:
Comprehensiveness. Multiple knowledge points are run through case-based teaching. Knowledge points are presented to students in the form of interconnected case applications. Students can discover and master knowledge in the practice process of problem-solving. Therefore, it is necessary to ensure comprehensive requirements in case design, so that the designed cases can be seamlessly connected with the knowledge points of the textbooks.
Advanced. The latest research results are collated into teaching cases to replace the outdated cases of the textbooks. The teaching case should be advanced and innovative. For example, choosing teaching cases using artificial intelligence (AI) and other new technologies can make up for the insufficient introduction of new knowledge and new technologies in the textbooks.
Engineering. The practical engineering problems are transformed into teaching cases to reflect the practicability of the digital image processing course. For example, we invite engineering experts from partner companies to write cases together. These cases place more emphasis on the combination of theory and practice.
Case design and selection
The design and selection of cases should give students a solid understanding of the application and implementation of theories, methods, and models. Well-designed cases can guide students to discover, analyze, and solve problems. Cases should involve all the knowledge points and their applications of each chapter of the digital image processing course.
Digital image processing has been widely used in many fields. The applications of digital image processing are interconnected with many disciplines, such as mathematics, physics, biology, medicine, and computer science. At the same time, it is supported by many new theories, new tools, and new technologies. Artificial intelligence (AI) is the main application field of digital image processing. The digital image processing course intersects with many courses, such as pattern recognition, machine vision, computer graphics and other courses. Therefore, the design and selection of cases should avoid being limited to the knowledge points of a digital image processing course, but should try to reflect interdisciplinary characteristics and interdisciplinary integration. Figure 2 shows the relationship between a digital image processing course and other disciplines or courses.
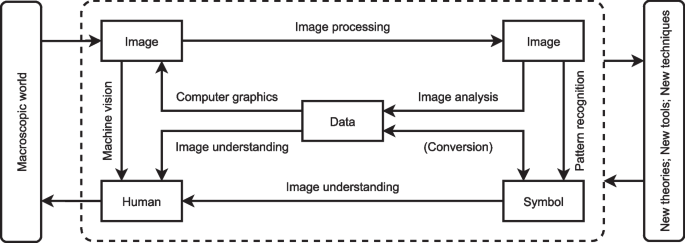
The relationship between a digital image processing course and other disciplines or courses
According to the case design principles mentioned above, we designed and selected some teaching cases for the digital image processing course. In order to facilitate students’ extracurricular study and online–offline discussions, we provide the case study documents for each case. The contents of case study documents include preparatory knowledge, theoretical knowledge, technical points, implementation process, results, and demonstration programs. Source codes are also provided in most of the cases. Case study documents are distributed online for students to study and practice after class. Some of the case study documents of the digital image processing course are shown in Table 1 .
Arrangements of student-centered case-based teaching
Our student-centered case-based teaching process adopts an online–offline hybrid approach. The teaching arrangement includes theoretical knowledge lectures (offline), case introduction (offline or online), extracurricular literature reading, extracurricular experiments, and group discussions (online or offline).
Lectures on theoretical knowledge
Classroom teaching is used to describe the knowledge background and the application fields of cases. Problems are elicited through cases, and theoretical concepts and knowledge points related to cases are explained. In the teaching of theoretical knowledge, we only teach selected contents of the textbook to save the limited classroom teaching time. The rest of the contents are left to students for self-study and discussion.
Introduction of cases
When and how to introduce cases is also considered. According to the teaching plan, we determine which cases are introduced in which chapters, how each case is presented, and how long it takes to explain or demonstrate the case. The introduction of cases not only enables students to better understand the practical application of theoretical knowledge, but also enables these cases to effectively support the relevant knowledge points in the textbook.
Extracurricular literature reading and extracurricular experiments
We arrange for students to consult the literature on the content of theoretical knowledge of the introduced cases. Students should run the source codes provided by case after class and improve it, or design new codes according to the requirements of the case and the theoretical knowledge they have learned. They need to implement the codes themselves to achieve the required functions of the case.
online–offline discussion
online–offline group discussions are conducted on the theoretical knowledge lectures, introduced cases, and experimental results. We encourage students to ask questions and encourage their sense of innovation. When necessary, we also arrange for oral presentations by group representatives.
Implementations of student-centered case-based teaching
The case-based teaching process is designed as student-centered. The main teaching content is cases and textbooks. The implementations of student-centered case-based teaching for digital image processing course is shown in Fig. 3 .
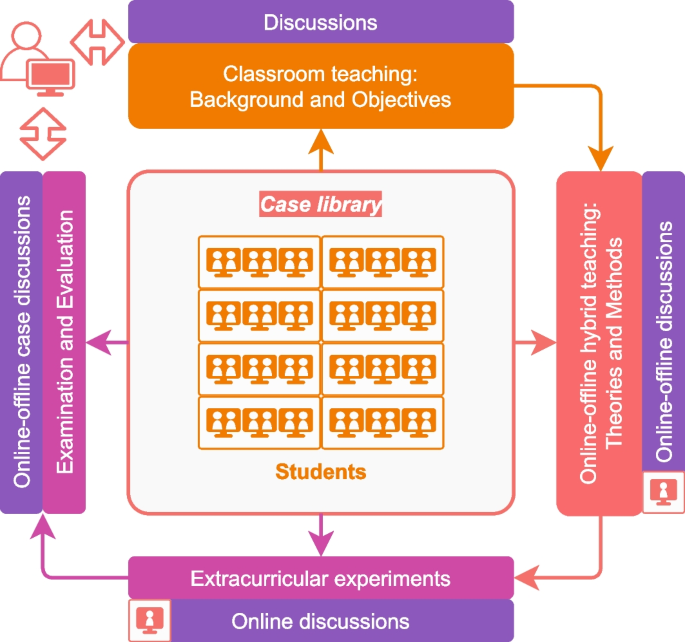
The implementations of student-centered case-based teaching for the digital image processing course
Providing case documents online and arranging students to preview before class.
Introducing the background and objectives of the case in traditional classroom teaching mode, and teaching relevant knowledge and theories. Classroom group discussions are arranged during this process.
Guiding students to explore cases in online and offline hybrid teaching mode. Students learn relevant theories and methods through case studies. Students are encouraged to come up with their own solutions based on the theories and methods they have learned. This is a learning-imitation-exploration-innovation process (innovation is optional). Online and offline discussions are arranged during this process.
Students implement extracurricular experiments based on the source codes provided by the case or design new codes by themselves. The analysis of the experimental results also needs to be done themselves. They can communicate about the problems they encountered, seek help, or discuss solutions of problems and experimental results through online or offline discussions.
Introducing, sharing, and demonstrating the learning results of the case in online or classroom teaching mode. Finally, we organize exams and evaluate the exam results.
Assessment methods
The revised Bloom’s taxonomy is used for teaching objective evaluation and examination assessment. After the introduction of the case-based teaching mode, the assessment method also needs to be adjusted accordingly. We no longer only use the static indicators, such as exam scores, but introduce the dynamic indicators for the assessments, such as case study reports, experiment reports, literature reading reports, oral reports, and records of participation in online–offline group discussions. These assessments consider the characteristics of case-based teaching and realize individualized evaluation.
Research object
This research is based on the digital image processing course for graduate students of computer science. Full-time postgraduate students of three consecutive years participated in this research. Since the number of graduate students varies from year to year, we randomly selected 100 students from each year as one group. All the students in the three groups are to study the digital image processing course for the first time, and they had never learned any cases used in this research before. The three groups of students are roughly equivalent in gender ratio, age distribution, and course-related prior knowledge. In addition, when the questionnaire of learning interest and learning motivation were scored, the feedbacks of the three groups of students are not significantly different. Which indicates that they have similar learning interests and learning motivations.
Different teaching methods, teaching contents, and assessment methods were adopted to the three groups of students. The differences between them are shown in Table 2 .
Student-centered case-based learning
The case-based teaching process is designed as student-centered. After the traditional classroom teaching for the introduction of the cases background and objectives, and the online–offline hybrid teaching for the study of relevant theories and methods, students are encouraged to propose their own solutions based on the theories and methods they have learned. Students can design and implement personalized solutions, and in the process, further learn and understand the theories and methods they want to use.
Example of a case: Text recognition of images.
Some X-ray images of welding seam inspection are provided. The goal of this case is to identify all the text on the X-ray images. This is a case with practical engineering needs.
According to the procedure of non-destructive testing (NDT), operators (welding workers) place some leaden markers beside the welding seam. The leaden markers are photographed together with the welding seam. The leaden markers include image quality indicator, positioning markers (center markers, overlap markers) and other identification markers. These identification markers can display the project number, pipe number, welding seam number, welding worker’s ID, welding date, etc.
The welding seam films will be scanned as high-quality digital images by using an industrial X-ray film digitizer. A scanned example image is shown in Fig. 4 . The resolution of scanned image is \(4242\times 882\times 3\) , and the image format is TIFF.
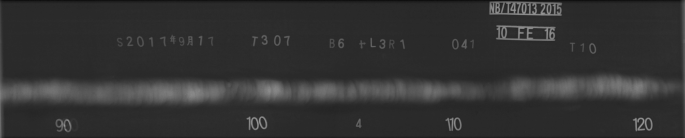
The scanned X-ray imaging film
Usually, the solution of this case includes three main parts: image preprocessing, image segmentation, and text recognition. Each part can be implemented in many different methods or a combination of several methods. For example, the methods of image preprocessing include: contrast enhancement, binarization, histogram equalization, geometric transformation, gray level interpolation, noise removal and so on. The methods of image segmentation include: threshold-based segmentation methods (such as Otsu’s method), region-based segmentation methods, and edge-based segmentation methods. In addition, image segmentation may also involve other related technologies, such as Radon transform. The methods of text recognition include: the traditional machine learning methods, such as artificial neural network (ANN), support vector machine (SVM), etc., and the deep learning methods, such as deep convolutional neural network (DCNN), recurrent neural network (RNN), etc. Here, deep learning is the learning technology in the sense of artificial intelligence (AI) rather than the learning method in the sense of education.
In each part, students can choose one or more methods they want to learn and use according to their own learning ability and learning interest. After making their choice, they need to conduct an in-depth study of these methods. They can download the source codes or write their own codes to implement these methods. Finally, the three parts of the codes are combined to generate their own personalized solution. Because the methods that students choose to learn and use are not the same, the combination of these methods results in a variety of personalized solutions. These solutions need to be tested and evaluated experimentally. Students can communicate any issues they encounter and share their learning experiences through online and offline case discussions. In this process, we encourage students to innovate their own methods or adopt novel ways of combining methods. For this case, the student-centered case-based teaching process is shown in Fig. 5 .
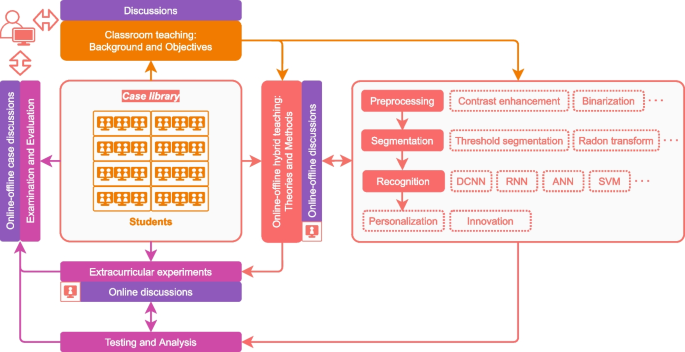
An example (text recognition of images) of the student-centered case-based teaching process
Learning behavior comparison
We compared the learning behaviors of Group B and Group C (Group A was the traditional teaching model without providing new cases). Group B adopted extracurricular self-regulated case learning mode. Group C adopted the student-centered case-based teaching and online–offline case discussion mode. According to the statistics, the times of online–offline discussions, the time of discussion, the times of asking questions, and the times of answering questions of Group C students was much larger than that of Group C students. The number of completed cases and the implement quality of cases of Group C students was better than that of Group C students. A radar chart of learning behavior comparison is shown as Fig. 6 . The comparison of the two groups of students’ learning behavior shows that the student-centered case-based teaching and online–offline case discussion teaching mode could indeed improve the students’ learning interest and initiative.
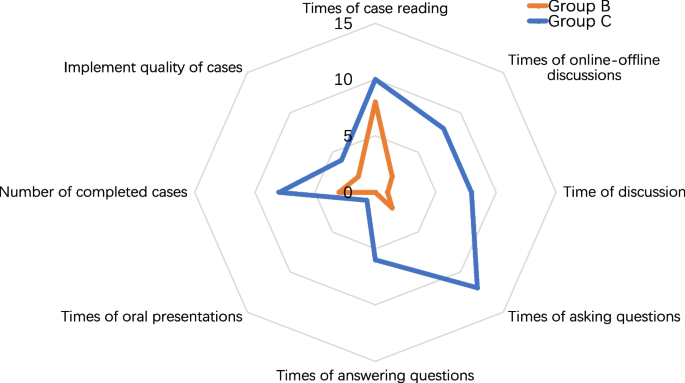
Radar chart of learning behavior comparison
Assessments
In the teaching of Group A, Group B and Group C, Groups A and B adopted the traditional teaching mode. The teaching of Group A did not involve new cases. Students of Group B were provided with case study documents and were arranged for extracurricular self-regulated case learning. Group C adopted the student-centered case-based teaching mode. The assessment methods of three groups were also different. Both Group A and Group B used the traditional examination method. Although students of Group B were arranged to study the case by themselves, the examination contents of Group B did not involve these cases. The assessment methods of Group C included traditional examination, case study reports, experiment reports, etc. In Group B, because the cases are self-regulated learned and the exam did not involve cases, many students did not put a lot of effort into the case study. The examination scores of Group B only improved slightly compared to Group A. The student-centered case-based teaching mode adopted by Group C greatly stimulated students’ learning interest, and their examination scores improved significantly. The comparison results show that the examination scores of Group C are significantly better than those of Group A and Group B. The comparison of the examination scores of the three groups is shown in Fig. 6 .
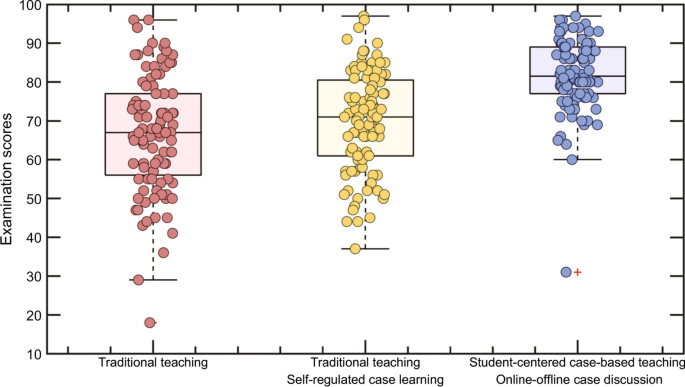
Comparison of examination scores of the three groups
Bloom’s taxonomy can be used as a tool for objective evaluation and examination assessment. It reflects the relationship between knowledge learning and ability development. In the assessments of Group C, we assigned six weights for each examination question according to the revised Bloom’s taxonomy in six aspects: Remember, Understand, Apply, Analyze, Evaluate, and Create. Table 3 is an example of student-centered case-based teaching and online–offline case discussion applying the revised Bloom’s taxonomy. Each cognitive skill corresponds to a specific teaching objective, and these teaching objectives are reflected in the specific test questions.
We conducted a comparison of the examination performance of students in Group A and Group C according to the revised Bloom’s taxonomy. The test data of the two groups approximately obey the normal distribution and meet the requirement of parameter test. The joint hypotheses test ( F -test) is used to analyze whether there are significant differences in the knowledge and ability levels of the two groups of students. The F -test results are shown in Table 4 , where MS represents mean squares, df represents degrees of freedom. Degrees of freedom refers to the number of variables that can be evaluated without restriction when calculating a uniform measure. F -value (or F -statistic) is the test statistic. P -value is the observed significance level. F crit represents the F -critical value, which is a specific value that F -value is compared with. It can be seen from Table 4 that, for Remember, Analyze, and Evaluate, their F -values are less than F crit and P -values are higher than 0.05. This indicates that there is no significant difference between the two groups in these three aspects. For Understand, Apply, and Create, their F -values are greater than F crit and P -value are less than 0.01. This indicates that the two groups of data have very significant differences in these three aspects. The F -test results show that, in three aspects of Understand, Apply, and Create, our teaching method has a very significant improvement over the traditional teaching method.
These results can be interpreted as follows. Traditional classroom teaching methods emphasize memorization of basic theories and concepts, based on which students can use these insights to solve problems and pass exams. Therefore, students are fully trained in the three cognitive skills of Remember, Analyze, and Evaluate. In these three aspects, its learning effect is no less than the student-centered case teaching. However, due to the lack of specific application training, traditional classroom teaching methods do not allow students to understand the basic concepts more deeply. Students’ ability of association and innovation has not been fully trained. This is reflected in students’ difficulty in applying what they have learned to solve complex engineering problems.
Student-centered case-based teaching and online–offline case discussion provide students with an environment and opportunity to carry out specific application training, which help students actively explore and understand basic concepts, and apply the knowledge learned to practical engineering problems. Compared with the traditional classroom teaching mode, student-centered case-based teaching mode can improve students’ enthusiasm and initiative in learning, and improve their ability to solve complex engineering problems. This is reflected in the improvement of three cognitive skills: Understand, Apply, and Create.
Digital image processing is a highly theoretical and practical course. When using the case-based teaching method, the physical concepts and meanings behind mathematical formulas should be emphasized in classroom teaching, and the methods and principles should be explained thoroughly. We try to let students truly grasp the theoretical principles and understand engineering applications through the introduction of engineering cases. The main characteristics of our student-centered case-based teaching are as follows.
Using cases as the main content of teaching
At present, the digital image processing teaching materials used in this course cannot fully meet the needs of postgraduate teaching, and we do not find a better alternative textbook. Therefore, changing the main teaching content from textbook to cases is a solution. In our student-centered case-based teaching, cases are the main content of teaching. This method stimulates learning interest of students. Students can deepen their understanding of knowledge in the process of solving engineering problems.
Adopting a case-based teaching method
Knowledge points of the textbook are guided by the needs of engineering applications. The knowledge points are presented to students in the way of interconnected applications, so that students can discover and master knowledge in the practice process of solving-problems.
Student-centered teaching design
Through case-based teaching, the student-centered teaching design is truly realized. The student-centered learning approach not only allows students to choose what to learn, but also to choose how to learn.
Online and offline case discussions
Case discussion promotes knowledge construction through the process of shared exploration. Case discussion is the key to the success of case-based teaching. In the teaching method we designed, the case discussions can be transferred to online, and the real-time synchronous discussions can be transferred to the online asynchronous discussions.
Compared with the traditional teaching mode, the student-centered case-based teaching and online–offline case discussions proposed in this paper have achieved the following improvements in the teaching of postgraduate courses of computer science.
Students have a deeper and better understanding of the digital image processing course. The case-based learning model enables personalized learning by using offline-online hybrid approaches, supported by expanded learning options and multiple case resources (Jevne et al., 2021 ; Yoon et al., 2021 ). Through case-based teaching, they have fully realized the importance and practicability of this course.
Through the student-centered case-based teaching method, the boring knowledge teaching is replaced by the flexible and diverse case teaching, which arouses learning enthusiasm and interest of students. The study found that students can organize their learning process, and students’ time management flexibility and flexibility content are quite high, which were stated in the literature (Endedijk et al., 2016 ; Turan et al., 2022 ).
Teaching effect is improved. Students’ theoretical level, practical level, ability of analyze and solve problems, innovative thinking mode and literature reading level are improved to a certain extent. This result can be confirmed by the comparison of examination scores of the three groups (Fig. 7 ) and the learning behavior comparison of the two groups (Fig. 6 ). Empirical studies on how students learn, including brain development, motivation, creativity, perseverance, self-regulation, knowledge application, etc., also confirm the effectiveness of student-centered learning approaches (Goodell & Thai, 2020 ).
Students’ horizons are broadened. Students can understand the knowledge structure and problem-solving methods of different disciplines and courses, and fully realize the advantages of interdisciplinary learning. In case-based learning, there is a need to relate prior knowledge within and between disciplines to external lived experiences. In the process, students are trained in critical thinking, creative thinking, and problem-solving skills and strategies (Jung, 2013 ).
Teaching level of teachers is improved. After the introduction of the student-centered case-based teaching method, the teaching process is no longer completely based on textbooks. Teachers need to think more about the selection and design of cases. This process deepens teachers’ understanding of the curriculum and improves teachers’ knowledge structure. Student-centered case-based teaching can help teachers update teaching concepts, improve teaching methods, and continuously improve teaching levels in subsequent teaching. In student-centered teaching, teachers need to solve problems in communication between students and teachers so that students can receive correct feedback when they need it. Therefore, the teaching management ability of teachers has also been improved (Yan et al., 2021 ).
Graduate students of science and engineering usually focus on engineering applications and practices. It is difficult to achieve teaching goals by completely adopting teacher-centered classroom teaching. Case-based teaching can greatly improve the teaching effect. By constructing a case library and integrating the latest engineering cases into teaching, students can better understand the practical application of theoretical knowledge, and generate strong interest in learning and research. We practice the student-centered case-based teaching and online–offline case discussion in a digital image processing course for graduate students in computer science, and propose an actionable case-based teaching scheme. Case-based teaching is a systematic project. In addition to the construction of a case library and the introduction of cases in teaching, it also involves a series of problems, such as the adjustment of teaching plans and the changes of assessment methods. There are still many aspects of case-based teaching that need to be explored and perfected. Through the verification of actual teaching, the student-centered case-based teaching can stimulate learning enthusiasm and interest of students, and help them to cultivate innovative thinking modes and practical abilities. The joint hypotheses test is used to analyze whether there are significant differences in the knowledge and ability levels of students in different learning modes. The F -test results show that, in three aspects (Understand, Apply, and Create), our teaching method shows a very significant improvement over the traditional teaching method.
Availability of data and materials
The data of this study is not open to the public due to participant privacy.
Abbreviations
- Case-based learning
Epistemic network analysis
Self-regulated learning
Coronavirus disease 2019
Pproblem based learning
Artificial intelligence
Non-destructive testing
Aartificial neural network
Support vector machine
Deep convolutional neural network
Recurrent neural network
Aguilar, S. J., Karabenick, S. A., Teasley, S. D., & Baek, C. (2021). Associations between learning analytics dashboard exposure and motivation and self-regulated learning. Computers & Education, 162 , 104085.
Article Google Scholar
Armstrong, D., Murck, B., & Poe, J. C. (2021). Service learning opportunity for undergraduate science students: Integrating problem-based learning in the high school science curriculum. Journal of Chemical Education, 98 (4), 1275–1282.
Broadbent, J., Sharman, S., Panadero, E., & Fuller-Tyszkiewicz, M. (2021). How does self-regulated learning influence formative assessment and summative grade? Comparing online and blended learners. The Internet and Higher Education, 50 (4), 100805.
Callan, G. L., Rubenstein, D. V., Ridgley, L. M., Neumeister, K. S., Finch, M. H., & Longhurst, D. (2021). Measuring and predicting divergent thinking with a self-report questionnaire, teacher rating scale, and self-regulated learning microanalysis. Journal of Psychoeducational Assessment, 39 (5), 549–562.
Choi, I., & Lee, K. (2008). Designing and implementing a case-based learning environment for enhancing ill-structured problem solving: Classroom management problems for prospective teachers. Educational Technology Research and Development, 57 , 99–129.
Chung, H., & Ho, M. (2021). International competitive strategies, organizational learning and export performance: A match and mismatch conceptualization. European Journal of Marketing, 55, 2794–2822.
Constantinou, C. (2020). A reflexive goal framework for achieving student-centered learning in European higher education: From class learning to community engagement. Societies, 10, 1–11.
Desha, C., Caldera, S., & Hutchinson, D. (2021). Exploring the development of context appreciation in coursework that targets problem-solving for sustainable development. International Journal of Sustainability in Higher Education, 22 (5), 1186–1224.
Dolan, H., Amidon, B., & Gephart, S. (2021). Evidentiary and theoretical foundations for virtual simulation in nursing education. Journal of Professional Nursing, 37 , 810–815.
Endedijk, M., Brekelmans, M., Sleegers, P., & Vermunt, J. D. (2016). Measuring students’ self-regulated learning in professional education: Bridging the gap between event and aptitude measurements. Quality & Quantity, 50, 2141–2164.
Ertmer, P., & Koehler, A. (2014). Online case-based discussions: Examining coverage of the afforded problem space. Educational Technology Research and Development, 62, 617–636.
Ertmer, P., & Koehler, A. (2015). Facilitated versus non-facilitated online case discussions: Comparing differences in problem space coverage. Journal of Computing in Higher Education, 27, 69–93.
Goeze, A., Zottmann, J., Vogel, F., Fischer, F., & Schrader, J. (2014). Getting immersed in teacher and student perspectives? Facilitating analytical competence using video cases in teacher education. Instructional Science, 42, 91–114.
Goodell, J., & Thai, K. (2020). A learning engineering model for learner-centered adaptive systems. vol 12425, pp 557–573. https://doi.org/10.1007/978-3-030-60128-7_41 .
Granberg, C., Palm, T., & Palmberg, B. (2021). A case study of a formative assessment practice and the effects on students’ self-regulated learning. Studies in Educational Evaluation, 68 (3), 100955.
Guo, H., Tong, F., Wang, Z., Tang, S., Yoon, M., Ying, M., & Yu, X. (2021a). Examining self-regulated learning strategy model: A measurement invariance analysis of mslq-cal among college students in china. Sustainability, 13 , 10133.
Guo, W., Bai, B., & Song, H. (2021b). Influences of process-based instruction on students’ use of self-regulated learning strategies in efl writing. System, 101 , 102578.
Hewitt, J., Pedretti, E., Bencze, L., Vaillancourt, B., & Yoon, S. (2003). New applications for multimedia cases: Promoting reflective practice in preservice teacher education. Journal of Technology and Teacher Education, 11 , 483–500.
Google Scholar
Jevne, K., Ulleberg, I., & Oien, I. (2021). Why and how? Case-based teaching in interprofessional and interdisciplinary education. Nordisk tidsskrift for utdanning og praksis, 15, 51–68.
Jung, S. (2013). Experiences in developing an experimental robotics course program for undergraduate education. IEEE Transactions on Education, 56 (1), 129–136.
Kapur, M. (2016). Examining productive failure, productive success, unproductive failure, and unproductive success in learning. Educational Psychologist, 51 , 1–11.
Killion, A. K., Michel, J. O., & Hawes, J. K. (2022). Toward identifying sustainability leadership competencies: Insights from mapping a graduate sustainability education curriculum. Sustainability, 14 , 1–18.
Kim, J. (2022). A case study on an online Korean discussion class with foreign undergraduate students. The Korean Association of General Education, 16, 167–178.
Krathwohl, D. (2002). A revision of bloom’s taxonomy: An overview. Theory into Practice, 41 (4), 212–218.
Leon, J., Winskell, K., Mcfarland, D., & Del Rio, C. (2015). A case-based, problem-based learning approach to prepare master of public health candidates for the complexities of global health. American Journal of Public Health, 105, S92–S96.
Li, J., Yao, Y., & Guo, Q. (2021). Online and offline hybrid teaching in the luminescent materials and applications course. Journal of Contemporary Educational Research, 5 , 51–56.
Lock, J., & Redmond, P. (2021). Embedded experts in online collaborative learning: A case study. The Internet and Higher Education, 48 , 100773.
Long, Y., & Koehler, A. (2021). Student participation and interaction in online case-based discussions: Comparing expert and novice facilitation. Online Learning, 25 , 220–246.
Luo, H., Koszalka, T., Arnone, M., & Choi, I. (2018). Applying case-based method in designing self-directed online instruction: A formative research study. Educational Technology Research and Development, 66, 515–544.
Mamnpoba, K. (2021). Principles and methods of student-centered learning (scl). Scientific Work, 69 , 16–19.
Mcpartlan, P., Rutherford, T., Rodriguez, F., Schaffer, J. F., & Holton, A. (2021). Modality motivation: Selection effects and motivational differences in students who choose to take courses online. The Internet and Higher Education, 49 (5), 100793.
Mingorance Estrada, A., Vera, G., Ruiz, R., & Arrebola, I. (2019). Flipped classroom to improve university student centered learning and academic performance. Social Sciences, 8 , 315.
Moges, B. (2019). Contemporary teaching-learning practices: Implementation and challenges of student-centered learning approach in higher education of Ethiopia. Journal of Education, Society and Behavioural Science, 32 (4), 1–16. https://doi.org/10.9734/jesbs/2019/v32i430181
Newton, C., Bainbridge, L., Ball, V., Baum, K. D., Bontje, P., Boyce, R. A., Moran, M., Richardson, B., Tamura, Y., Uden, D., Wagner, S. J., & Wood, V. (2015a). The health care team challenge: Developing an international interprofessional education research collaboration. Nurse Education Today, 35 (1), 4–8.
Newton, C., Bainbridge, L., Ball, V., Baum, K. D., & Wood, V. (2015b). The health care team challenge: Developing an international interprofessional education research collaboration. Nurse Education Today, 35 (1), 4–8.
Peng, X., & Wei, L. (2021). Exploration of online and offline hybrid teaching of pathophysiology. Open Journal of Social Sciences, 09 , 433–438.
Prada, M., Dominguez, M., Lopez Vicario, J., Alves, P., Barbu, M., Podpora, M., Spagnolini, U., Pereira, M., & Vilanova, R. (2020). Educational data mining for tutoring support in higher education: A web-based tool case study in engineering degrees. IEEE Access, 8 , 212818–212836.
Rico, R., & Ertmer, P. (2015). Examining the role of the instructor in problem-centered instruction. TechTrends, 59 , 96–103.
Roels, N., Ghidinelli, M., Cunningham, M., & Bilici, M. (2021). What are learner and instructor preferences for group size and composition for a series of synchronous online case discussions for upper extremity trauma surgeons? Journal of European CME, 10 , 1–10.
Rovers, S., Clarebout, G., Savelberg, H., de Bruin, A., & Van Merrienboer, J. J. G. (2019). Granularity matters: Comparing different ways of measuring self-regulated learning. Metacognition and Learning, 14, 1–19.
Sandrone, S., Scott, G., Anderson, W., & Musunuru, K. (2021). Active learning-based stem education for in-person and online learning. Cell, 184 , 1409–1414.
Sangam, M., Kalatharan, P., Bokan, G. V., Deka, R., & Kaur, A. (2021). Efficacy of case-based learning in anatomy. Cureus, 13 , e20472.
Schalk, L., Schumacher, R., Barth, A., & Stern, E. (2017). When problem-solving followed by instruction is superior to the traditional tell-and-practice sequence. Journal of Educational Psychology, 110 (4), 596–610. https://doi.org/10.1037/edu0000234
Song, D., Hong, H., & Oh, E. Y. (2021). Applying computational analysis of novice learners’ computer programming patterns to reveal self-regulated learning, computational thinking, and learning performance. Computers in Human Behavior, 120 (6), 106746.
Strobel, J., Wang, J., Weber, N., & Dyehouse, M. (2013). The role of authenticity in design-based learning environments: The case of engineering education. Computers & Education, 64 , 143–152.
Tan, J., Guo, X., Zheng, W., & Zhong, M. (2014). Case-based teaching using the laboratory animal system for learning c/c++ programming. Computers & Education, 77 (Aug), 39–49.
Tawfik, A., Giabbanelli, P., Hogan, M., Msilu, F., Gill, A., & York, C. (2017). Effects of success v failure cases on learner-learner interaction. Computers & Education, 118 , 120–132.
Tran, H., Capps, D., & Hodges, G. (2022). Preservice science teachers’ perspectives on and practices related to self-regulated learning after a brief learning opportunity. Sustainability, 14 , 5923.
Turan, Z., Kucuk, S., & Karabey, S. C. (2022). The university students’ self-regulated effort, flexibility and satisfaction in distance education. International Journal of Educational Technology in Higher Education, 19 (35), 1–19. https://doi.org/10.1186/s41239-022-00342-w
Turk, M., Heddy, B. C., & Danielson, R. W. (2022). Teaching and social presences supporting basic needs satisfaction in online learning environments: How can presences and basic needs happily meet online? Computers & Education, 180 , 104432.
Tuti, T., Paton, C., & Winters, N. (2021). The counterintuitive self-regulated learning behaviours of healthcare providers from low-income settings. Computers & Education, 166 (1), 104136.
Vieyra, G. Q., & Gonzlez, L. (2020). Learning objects in online education: A systemic approach. European Journal of Education, 3 , 1186–1224.
Weaver, J., Chastain, R., DeCaro, D., & DeCaro, M. (2018). Reverse the routine: Problem solving before instruction improves conceptual knowledge in undergraduate physics. Contemporary Educational Psychology, 52 , 36–47.
Wu, X. (2022). Cloud-based collaborative English online and offline hybrid teaching. Wireless Communications and Mobile Computing, 2022, 1–11.
Yan, L., Whitelock-Wainwright, A., Guan, Q., Wen, G., Gasevic, D., & Chen, G. (2021). Students’ experience of online learning during the COVID-19 pandemic: A province-wide survey study. British Journal of Educational Technology, 52 (5), 2038–2057.
Yew, E., & Yong, J. (2014). Student perceptions of facilitators’ social congruence, use of expertise and cognitive congruence in problem-based learning. Instructional Science, 42 , 795–815.
Yi, M. (2022). Study on the effectiveness of online and offline hybrid teaching. International Journal of Computing and Information Technology, 1 , 50–53.
Yoon, M., Lee, J., & Jo, I. H. (2021). Video learning analytics: Investigating behavioral patterns and learner clusters in video-based online learning. The Internet and Higher Education, 50 (3), 100806.
Yunus, F. W., Bissett, M., Penkala, S., Kadar, M., & Liu, K. (2021). Self-regulated learning versus activity-based intervention to reduce challenging behaviors and enhance school-related function for children with autism spectrum disorders: A randomized controlled trial. Research in Developmental Disabilities, 114 , 103986.
Zhang, F., Li, Z., Zhang, B., Du, H., Wang, B., & Zhang, X. (2019). Multi-modal deep learning model for auxiliary diagnosis of Alzheimer’s disease. Neurocomputing, 361, 185–195.
Zhang, F., Zhang, Y., Zhu, X., Chen, X., Du, H., & Zhang, X. (2022a). Preggan: A prognosis prediction model for breast cancer based on conditional generative adversarial networks. Computer Methods and Programs in Biomedicine, 224 , 107026.
Zhang, S., Gao, Q., Sun, M., Cai, Z., Li, H., Tang, Y., & Liu, Q. (2022b). Understanding student teachers’ collaborative problem solving: Insights from an epistemic network analysis (ena). Computers & Education, 183 , 104485.
Zhao, W., Tan, F., Huang, H., & Liang, S. (2022). The design and implementation of a medical parasitology teaching case database based on the online and offline hybrid teaching model. Department of Parasitology, 6 (3), 49–53.
Zhienbayeva, N., & Abdigapbarova, U. (2021). The mechanism of transformation of student-centered learning. Pedagogy and Psychology, 47 , 120–128.
Download references
Acknowledgements
Not applicable.
This research was supported by the Key scientific and technological project (222102310090) and the Postgraduate Education Reform and Quality Improvement Project (YJS2022AL098 and YJS2022JD26).
Author information
Authors and affiliations.
School of Software, Henan University, Kaifeng, 475004, China
Xinhong Zhang & Boyan Zhang
Henan Key Laboratory of Big Data Analysis and Processing, Henan University, Kaifeng, 475004, China
You can also search for this author in PubMed Google Scholar
Contributions
XZ: Conceptualization, Methodology, Formal analysis, Resources, Writing-original draft, Writing-original draft. BZ: Investigation, Data collection and analysis, Writing-original draft preparation. FZ: Conceptualization, Methodology, Writing-review and editing, Supervision. All authors read and approved the final manuscript.
Corresponding author
Correspondence to Fan Zhang .
Ethics declarations
Competing interests.
The authors declare no competing interests.
Additional information
Publisher’s note.
Springer Nature remains neutral with regard to jurisdictional claims in published maps and institutional affiliations.
Rights and permissions
Open Access This article is licensed under a Creative Commons Attribution 4.0 International License, which permits use, sharing, adaptation, distribution and reproduction in any medium or format, as long as you give appropriate credit to the original author(s) and the source, provide a link to the Creative Commons licence, and indicate if changes were made. The images or other third party material in this article are included in the article's Creative Commons licence, unless indicated otherwise in a credit line to the material. If material is not included in the article's Creative Commons licence and your intended use is not permitted by statutory regulation or exceeds the permitted use, you will need to obtain permission directly from the copyright holder. To view a copy of this licence, visit http://creativecommons.org/licenses/by/4.0/ .
Reprints and permissions
About this article
Cite this article.
Zhang, X., Zhang, B. & Zhang, F. Student-centered case-based teaching and online–offline case discussion in postgraduate courses of computer science. Int J Educ Technol High Educ 20 , 6 (2023). https://doi.org/10.1186/s41239-022-00374-2
Download citation
Received : 05 September 2022
Accepted : 21 November 2022
Published : 31 January 2023
DOI : https://doi.org/10.1186/s41239-022-00374-2
Share this article
Anyone you share the following link with will be able to read this content:
Sorry, a shareable link is not currently available for this article.
Provided by the Springer Nature SharedIt content-sharing initiative
- online–offline case discussion
- Postgraduate teaching
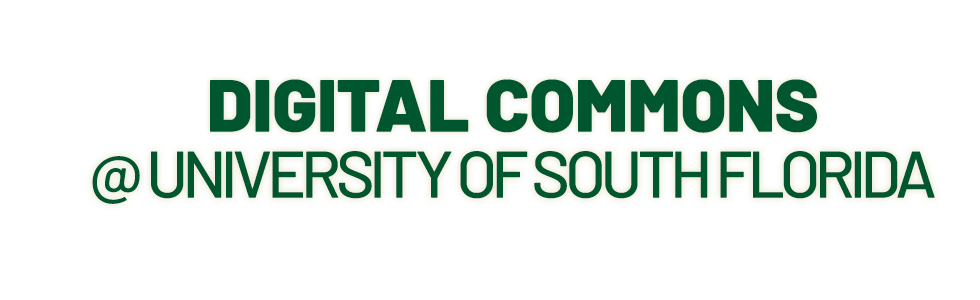
- USF Research
- USF Libraries
Digital Commons @ USF > College of Engineering > Computer Science and Engineering > Theses and Dissertations
Computer Science and Engineering Theses and Dissertations
Theses/dissertations from 2023 2023.
Refining the Machine Learning Pipeline for US-based Public Transit Systems , Jennifer Adorno
Insect Classification and Explainability from Image Data via Deep Learning Techniques , Tanvir Hossain Bhuiyan
Brain-Inspired Spatio-Temporal Learning with Application to Robotics , Thiago André Ferreira Medeiros
Evaluating Methods for Improving DNN Robustness Against Adversarial Attacks , Laureano Griffin
Analyzing Multi-Robot Leader-Follower Formations in Obstacle-Laden Environments , Zachary J. Hinnen
Secure Lightweight Cryptographic Hardware Constructions for Deeply Embedded Systems , Jasmin Kaur
A Psychometric Analysis of Natural Language Inference Using Transformer Language Models , Antonio Laverghetta Jr.
Graph Analysis on Social Networks , Shen Lu
Deep Learning-based Automatic Stereology for High- and Low-magnification Images , Hunter Morera
Deciphering Trends and Tactics: Data-driven Techniques for Forecasting Information Spread and Detecting Coordinated Campaigns in Social Media , Kin Wai Ng Lugo
Deciphering Trends and Tactics: Data-driven Techniques for Forecasting Information Spread and Detecting Coordinated Campaigns in Social Media , Kin Wai NG Lugo
Automated Approaches to Enable Innovative Civic Applications from Citizen Generated Imagery , Hye Seon Yi
Theses/Dissertations from 2022 2022
Towards High Performing and Reliable Deep Convolutional Neural Network Models for Typically Limited Medical Imaging Datasets , Kaoutar Ben Ahmed
Task Progress Assessment and Monitoring Using Self-Supervised Learning , Sainath Reddy Bobbala
Towards More Task-Generalized and Explainable AI Through Psychometrics , Alec Braynen
A Multiple Input Multiple Output Framework for the Automatic Optical Fractionator-based Cell Counting in Z-Stacks Using Deep Learning , Palak Dave
On the Reliability of Wearable Sensors for Assessing Movement Disorder-Related Gait Quality and Imbalance: A Case Study of Multiple Sclerosis , Steven Díaz Hernández
Securing Critical Cyber Infrastructures and Functionalities via Machine Learning Empowered Strategies , Tao Hou
Social Media Time Series Forecasting and User-Level Activity Prediction with Gradient Boosting, Deep Learning, and Data Augmentation , Fred Mubang
A Study of Deep Learning Silhouette Extractors for Gait Recognition , Sneha Oladhri
Analyzing Decision-making in Robot Soccer for Attacking Behaviors , Justin Rodney
Generative Spatio-Temporal and Multimodal Analysis of Neonatal Pain , Md Sirajus Salekin
Secure Hardware Constructions for Fault Detection of Lattice-based Post-quantum Cryptosystems , Ausmita Sarker
Adaptive Multi-scale Place Cell Representations and Replay for Spatial Navigation and Learning in Autonomous Robots , Pablo Scleidorovich
Predicting the Number of Objects in a Robotic Grasp , Utkarsh Tamrakar
Humanoid Robot Motion Control for Ramps and Stairs , Tommy Truong
Preventing Variadic Function Attacks Through Argument Width Counting , Brennan Ward
Theses/Dissertations from 2021 2021
Knowledge Extraction and Inference Based on Visual Understanding of Cooking Contents , Ahmad Babaeian Babaeian Jelodar
Efficient Post-Quantum and Compact Cryptographic Constructions for the Internet of Things , Rouzbeh Behnia
Efficient Hardware Constructions for Error Detection of Post-Quantum Cryptographic Schemes , Alvaro Cintas Canto
Using Hyper-Dimensional Spanning Trees to Improve Structure Preservation During Dimensionality Reduction , Curtis Thomas Davis
Design, Deployment, and Validation of Computer Vision Techniques for Societal Scale Applications , Arup Kanti Dey
AffectiveTDA: Using Topological Data Analysis to Improve Analysis and Explainability in Affective Computing , Hamza Elhamdadi
Automatic Detection of Vehicles in Satellite Images for Economic Monitoring , Cole Hill
Analysis of Contextual Emotions Using Multimodal Data , Saurabh Hinduja
Data-driven Studies on Social Networks: Privacy and Simulation , Yasanka Sameera Horawalavithana
Automated Identification of Stages in Gonotrophic Cycle of Mosquitoes Using Computer Vision Techniques , Sherzod Kariev
Exploring the Use of Neural Transformers for Psycholinguistics , Antonio Laverghetta Jr.
Secure VLSI Hardware Design Against Intellectual Property (IP) Theft and Cryptographic Vulnerabilities , Matthew Dean Lewandowski
Turkic Interlingua: A Case Study of Machine Translation in Low-resource Languages , Jamshidbek Mirzakhalov
Automated Wound Segmentation and Dimension Measurement Using RGB-D Image , Chih-Yun Pai
Constructing Frameworks for Task-Optimized Visualizations , Ghulam Jilani Abdul Rahim Quadri
Trilateration-Based Localization in Known Environments with Object Detection , Valeria M. Salas Pacheco
Recognizing Patterns from Vital Signs Using Spectrograms , Sidharth Srivatsav Sribhashyam
Recognizing Emotion in the Wild Using Multimodal Data , Shivam Srivastava
A Modular Framework for Multi-Rotor Unmanned Aerial Vehicles for Military Operations , Dante Tezza
Human-centered Cybersecurity Research — Anthropological Findings from Two Longitudinal Studies , Anwesh Tuladhar
Learning State-Dependent Sensor Measurement Models To Improve Robot Localization Accuracy , Troi André Williams
Human-centric Cybersecurity Research: From Trapping the Bad Guys to Helping the Good Ones , Armin Ziaie Tabari
Theses/Dissertations from 2020 2020
Classifying Emotions with EEG and Peripheral Physiological Data Using 1D Convolutional Long Short-Term Memory Neural Network , Rupal Agarwal
Keyless Anti-Jamming Communication via Randomized DSSS , Ahmad Alagil
Active Deep Learning Method to Automate Unbiased Stereology Cell Counting , Saeed Alahmari
Composition of Atomic-Obligation Security Policies , Yan Cao Albright
Action Recognition Using the Motion Taxonomy , Maxat Alibayev
Sentiment Analysis in Peer Review , Zachariah J. Beasley
Spatial Heterogeneity Utilization in CT Images for Lung Nodule Classication , Dmitrii Cherezov
Feature Selection Via Random Subsets Of Uncorrelated Features , Long Kim Dang
Unifying Security Policy Enforcement: Theory and Practice , Shamaria Engram
PsiDB: A Framework for Batched Query Processing and Optimization , Mehrad Eslami
Composition of Atomic-Obligation Security Policies , Danielle Ferguson
Algorithms To Profile Driver Behavior From Zero-permission Embedded Sensors , Bharti Goel
The Efficiency and Accuracy of YOLO for Neonate Face Detection in the Clinical Setting , Jacqueline Hausmann
Beyond the Hype: Challenges of Neural Networks as Applied to Social Networks , Anthony Hernandez
Privacy-Preserving and Functional Information Systems , Thang Hoang
Managing Off-Grid Power Use for Solar Fueled Residences with Smart Appliances, Prices-to-Devices and IoT , Donnelle L. January
Novel Bit-Sliced In-Memory Computing Based VLSI Architecture for Fast Sobel Edge Detection in IoT Edge Devices , Rajeev Joshi
Edge Computing for Deep Learning-Based Distributed Real-time Object Detection on IoT Constrained Platforms at Low Frame Rate , Lakshmikavya Kalyanam
Establishing Topological Data Analysis: A Comparison of Visualization Techniques , Tanmay J. Kotha
Machine Learning for the Internet of Things: Applications, Implementation, and Security , Vishalini Laguduva Ramnath
System Support of Concurrent Database Query Processing on a GPU , Hao Li
Deep Learning Predictive Modeling with Data Challenges (Small, Big, or Imbalanced) , Renhao Liu
Countermeasures Against Various Network Attacks Using Machine Learning Methods , Yi Li
Towards Safe Power Oversubscription and Energy Efficiency of Data Centers , Sulav Malla
Design of Support Measures for Counting Frequent Patterns in Graphs , Jinghan Meng
Automating the Classification of Mosquito Specimens Using Image Processing Techniques , Mona Minakshi
Models of Secure Software Enforcement and Development , Hernan M. Palombo
Functional Object-Oriented Network: A Knowledge Representation for Service Robotics , David Andrés Paulius Ramos
Lung Nodule Malignancy Prediction from Computed Tomography Images Using Deep Learning , Rahul Paul
Algorithms and Framework for Computing 2-body Statistics on Graphics Processing Units , Napath Pitaksirianan
Efficient Viewshed Computation Algorithms On GPUs and CPUs , Faisal F. Qarah
Relational Joins on GPUs for In-Memory Database Query Processing , Ran Rui
Micro-architectural Countermeasures for Control Flow and Misspeculation Based Software Attacks , Love Kumar Sah
Efficient Forward-Secure and Compact Signatures for the Internet of Things (IoT) , Efe Ulas Akay Seyitoglu
Detecting Symptoms of Chronic Obstructive Pulmonary Disease and Congestive Heart Failure via Cough and Wheezing Sounds Using Smart-Phones and Machine Learning , Anthony Windmon
Toward Culturally Relevant Emotion Detection Using Physiological Signals , Khadija Zanna
Theses/Dissertations from 2019 2019
Beyond Labels and Captions: Contextualizing Grounded Semantics for Explainable Visual Interpretation , Sathyanarayanan Narasimhan Aakur
Empirical Analysis of a Cybersecurity Scoring System , Jaleel Ahmed
Phenomena of Social Dynamics in Online Games , Essa Alhazmi
A Machine Learning Approach to Predicting Community Engagement on Social Media During Disasters , Adel Alshehri
Interactive Fitness Domains in Competitive Coevolutionary Algorithm , ATM Golam Bari
Measuring Influence Across Social Media Platforms: Empirical Analysis Using Symbolic Transfer Entropy , Abhishek Bhattacharjee
A Communication-Centric Framework for Post-Silicon System-on-chip Integration Debug , Yuting Cao
Authentication and SQL-Injection Prevention Techniques in Web Applications , Cagri Cetin
Multimodal Emotion Recognition Using 3D Facial Landmarks, Action Units, and Physiological Data , Diego Fabiano
Robotic Motion Generation by Using Spatial-Temporal Patterns from Human Demonstrations , Yongqiang Huang
Advanced Search
- Email Notifications and RSS
- All Collections
- USF Faculty Publications
- Open Access Journals
- Conferences and Events
- Theses and Dissertations
- Textbooks Collection
Useful Links
- Rights Information
- SelectedWorks
- Submit Research
Home | About | Help | My Account | Accessibility Statement | Language and Diversity Statements
Privacy Copyright
- Biomedical Engineering
- Chemical & Biomolecular Engineering
- Civil and Environmental Engineering
- Computer & Data Sciences
- Electrical, Computer and Systems Engineering
- Macromolecular Science & Engineering
- Materials Science & Engineering
- Mechanical & Aerospace Engineering
- Intranet Login
Search form
Case school of engineering.
- Global Opportunities
- Outside The Classroom
Computer and Data Sciences
Helpful Links
Financial Aid
Register for Classes
Visit Campus
Academic Calendar
Student Resources
Housing and Dining
International Student Resources
Graduation Application
Enrollment Data
Looking to expand your options with a minor in Computer Science, or AI? Explore options and minor requirements in the university's General Bulletin.
Student Opportunities
From research to student groups to career development, explore all the resources available to you.
View Student Opportunities >>
Gain real experience with a full-time, paid co-op
BS in Computer Science
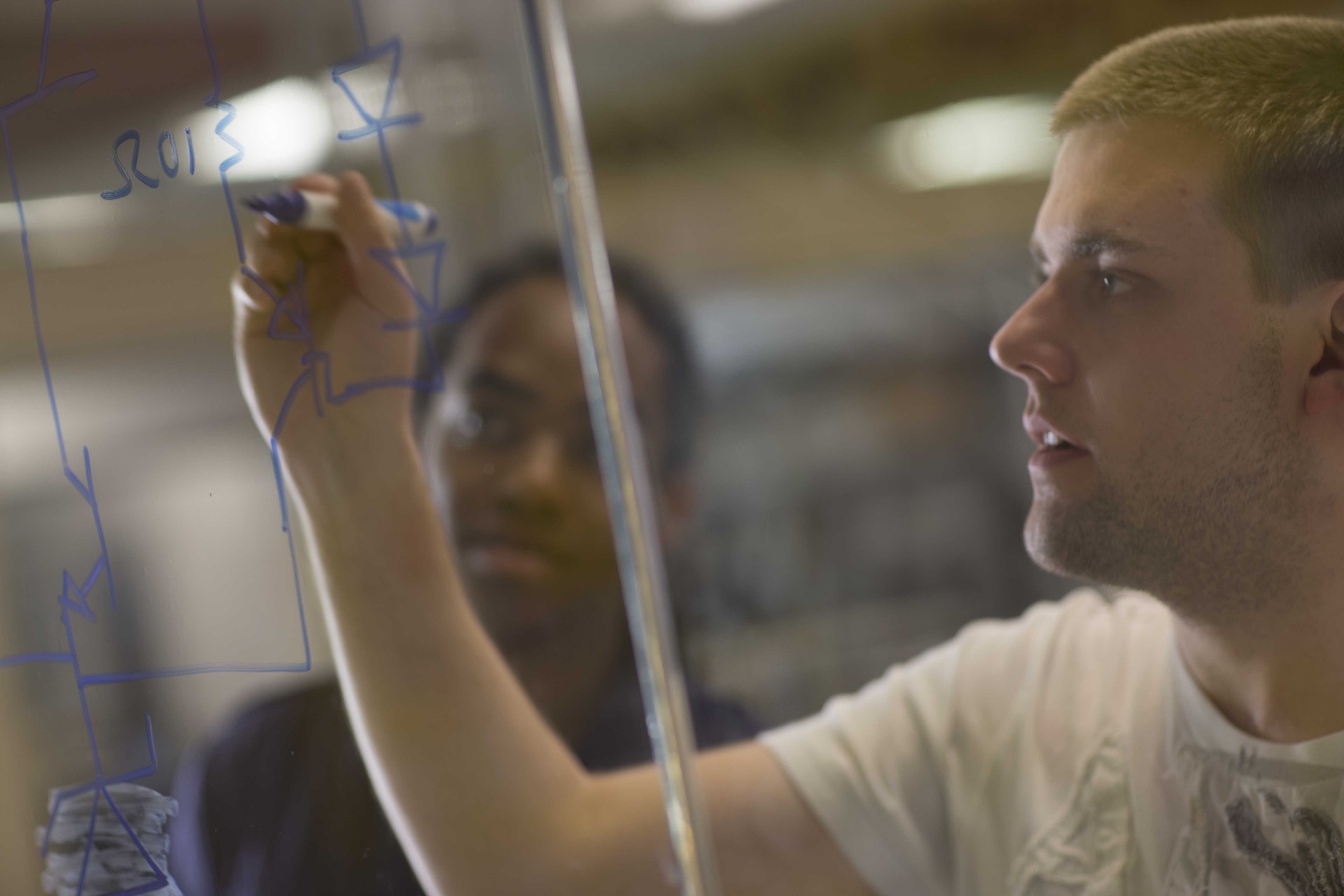
In our undergraduate program in Computer Science, we’re looking for problem-solvers who relish the challenge of using computers to find solutions to difficult issues.
No prior experience with programming? No problem!
Our program welcomes all, and you will not be alone. A large number of our majors come to Case Western Reserve having never written a computer program.
We have the educational expertise to get you up to speed, quickly.
Our Bachelor of Science in Computer Science is an ABET accredited degree program designed for students who want a deep dive into computer science. In this program, you will master the fundamentals of computer science, explore the breadth of computing, and choose one of six areas of specialization for further in-depth study:
- Algorithms and Theory
- Artificial Intelligence
- Bioinformatics
- Computer Systems, Networks and Security
- Databases and Data Mining
- Software Engineering
Not sure which computer science degree is right for you?
The Bachelor of Science and the Bachelor of Arts programs provide the same foundational courses in computer science. The difference is in the elective courses you choose.
Students pursuing the Bachelor of Science degree take a majority of their courses in computer science, mathematics, engineering and the natural sciences. Students pursuing the Bachelor of Arts degree have fewer required computer science courses and at the same time, many open electives, allowing students to easily explore other interests in fields outside of computer science or engineering.
Still not sure? At Case Western Reserve University, you can change your program at any time.
Both our degree programs provide a lot of flexibility so wherever you are on the spectrum between wanting to focus on computer science versus wanting a broad liberal arts education, our faculty advisors will help you craft the education that fits you.
Computer Undergrad Apply
Learn more and apply now.
Ready to start engineering your future at Case Western Reserve? Learn more about how to apply.
Computer Undergrad Contact
Find your geographic-specific admissions counselor here , or contact [email protected] .
Computer Undergrad Faculty
Meet faculty.
Meet the faculty members who will be your teachers and mentors.
Being a part of the Case School of Engineering means challenging your limits inside and outside of the classroom, and getting leading-edge education and experience. For example: Our “Introduction to Connected Devices” course, jointly offered to Case Western Reserve and Cleveland State University students as part of the partnership between the two universities via the IoT Collaborative , gives students the chance to cover the full spectrum of work of a multidisciplinary team at a real-world software firm.
Looking to have your work published? You can easily team up with other departments and industry professionals in our research facilities to create work that can be published, showcased or presented at conferences.
Have an idea you aren’t sure how to execute—or don’t have a clue where to begin? That’s where we thrive.
You’ll study and learn from peers and professors who can guide you toward solutions—and support you no matter what.
Get Hands-On Experience
Want to make the most of your time as a CWRU student? Be proactive and take part in the programs, volunteer opportunities, and competitions Case Western Reserve has to offer.
Our Cooperative Education Program allows you to pursue a unique paid experience relevant to your course of study. Most of our students participate in summer internships and/or research experiences.
You can also get involved in Hackathon , or teach local middle school and high school girls programming through the Girls Who Code .
The Bachelor of Science degree program in Computer Science is accredited by the Computing Accreditation Commission of ABET .
Explore degree requirements, courses and more in the university’s General Bulletin
Degree FAQs
Visit the Office of Undergraduate Admissions to apply and learn more about admissions requirements.
When you’re ready, the Office of Undergraduate Studies will guide you through the process .
Visit the university's General Bulletin for specific course requirements.
Sphero makes remarkably cool, programmable robots and STEAM-based educational tools that transform the way kids learn, create and invent through coding, science, music, and the arts.
- Lessons & Resources
- Schedule a Virtual Demo
- Professional Development
- Standards Alignment
- Funding & Grants
- Case Studies & Whitepapers
- App Downloads
- Sphero BOLT
- Sphero indi
- Sphero RVR+
- Sphero littleBits
- Sphero Blueprint
- Sphero Mini
- Computer Science Foundations (Sphero BOLT)
- Early Elementary
- Upper Elementary
- Middle School
- Past Year's Challenges
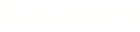
Enter your e-mail and password:
New customer? Create your account
Lost password? Recover password
Recover password
Enter your email:
Remembered your password? Back to login
Create my account
Please fill in the information below:
Already have an account? Login here
Case Studies & Whitepapers
Our library of insightful case studies and whitepapers is designed with educators in mind. We regularly publish original content to inform and educate on the importance and power of STEM education. Download the topics that interest you most and be the first to learn when new articles are published by signing up for our newsletter!

Sphero & littleBits K-12 STEAM & Computer Science Case Studies

Harlem Children's Zone Case Study

Improved Student Ambition, Resilience and Engagement

Making littleBits a Part of the Curriculum
Sphero & littlebits steam & computer science whitepapers.
Download our whitepapers to learn more about timely education topics, like why professional development is so critical for teachers or how to get STEM funding for your school or district.
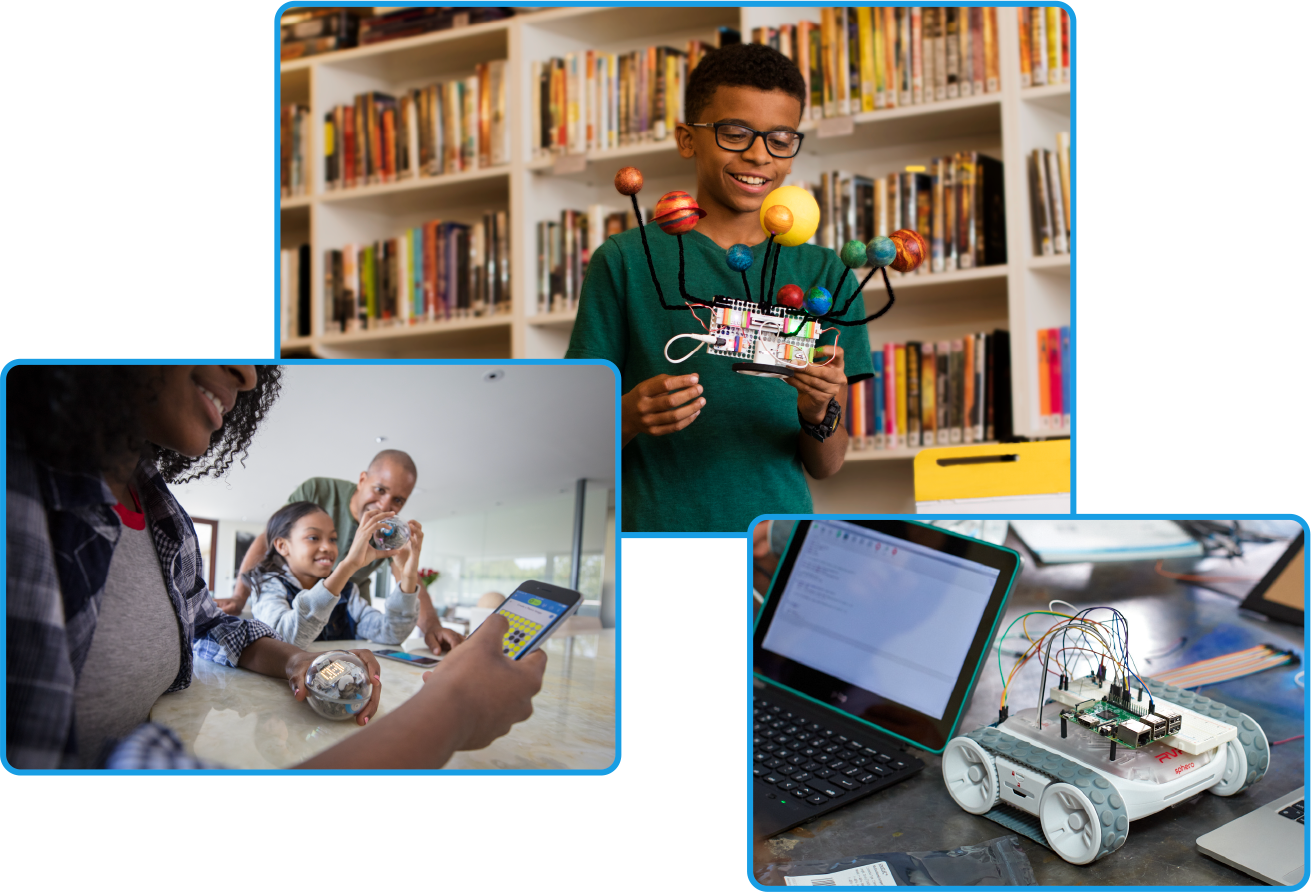
Integrating Play-based Learning in a Hybrid Classroom to Accelerate STEM Education
While we’re all adjusting to working and learning in new ways this year, as an educator you may wonder why it's important to integrate play-based learning in a hybrid classroom or blended classroom. This whitepaper will dive deeper into what play-based learning is, the main benefits of play-based learning, and feature examples of PBL activities and lessons that yield the best outcome for students no matter where they are learning right now.
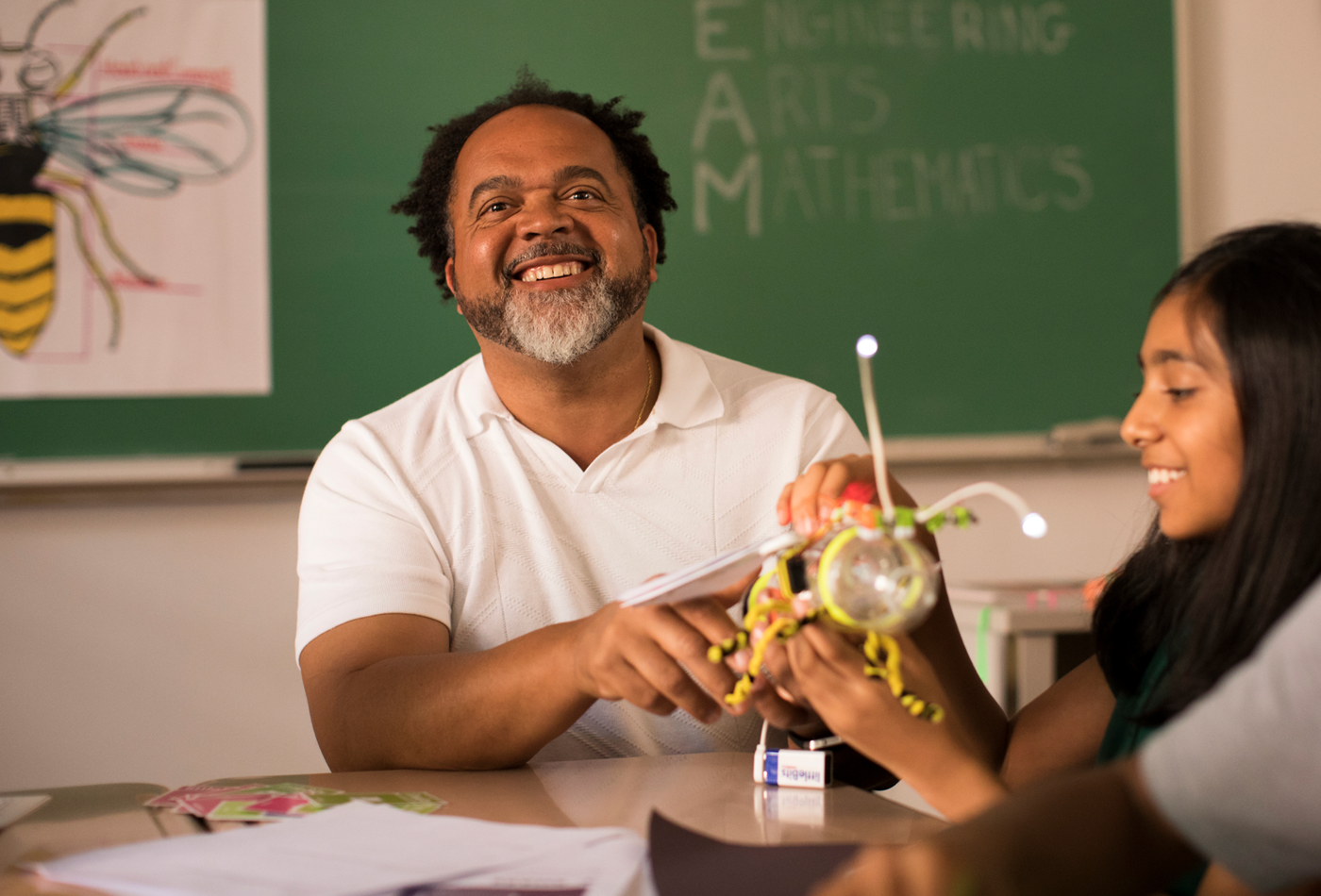
11 Reasons Why Professional Development is Critical for Teachers
In this whitepaper, we’ll look at professional development for teachers, why it matters, how it benefits not only teachers but their students, and how to discern you’re getting the best educational opportunities for your efforts.
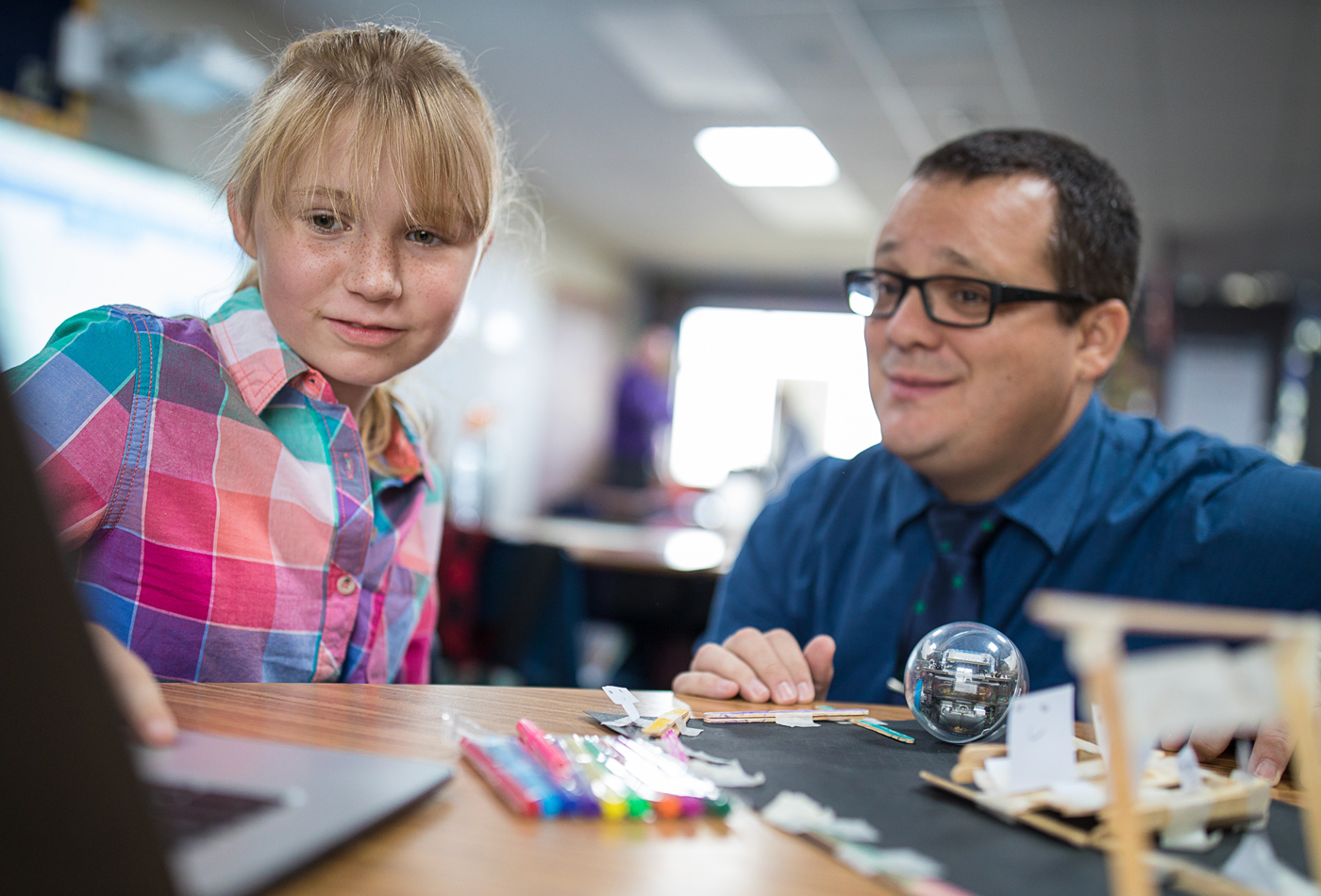
7 Misconceptions About Introducing Computer Science to your Classroom
In this whitepaper, we'll dispel some of the most common myths. The goal is to give you a better understanding of why, perhaps now more than ever before, it's not only a good idea, but critical to teach your students the basics of computer science in and beyond in the classroom. (Hint: Their futures may depend on it.)
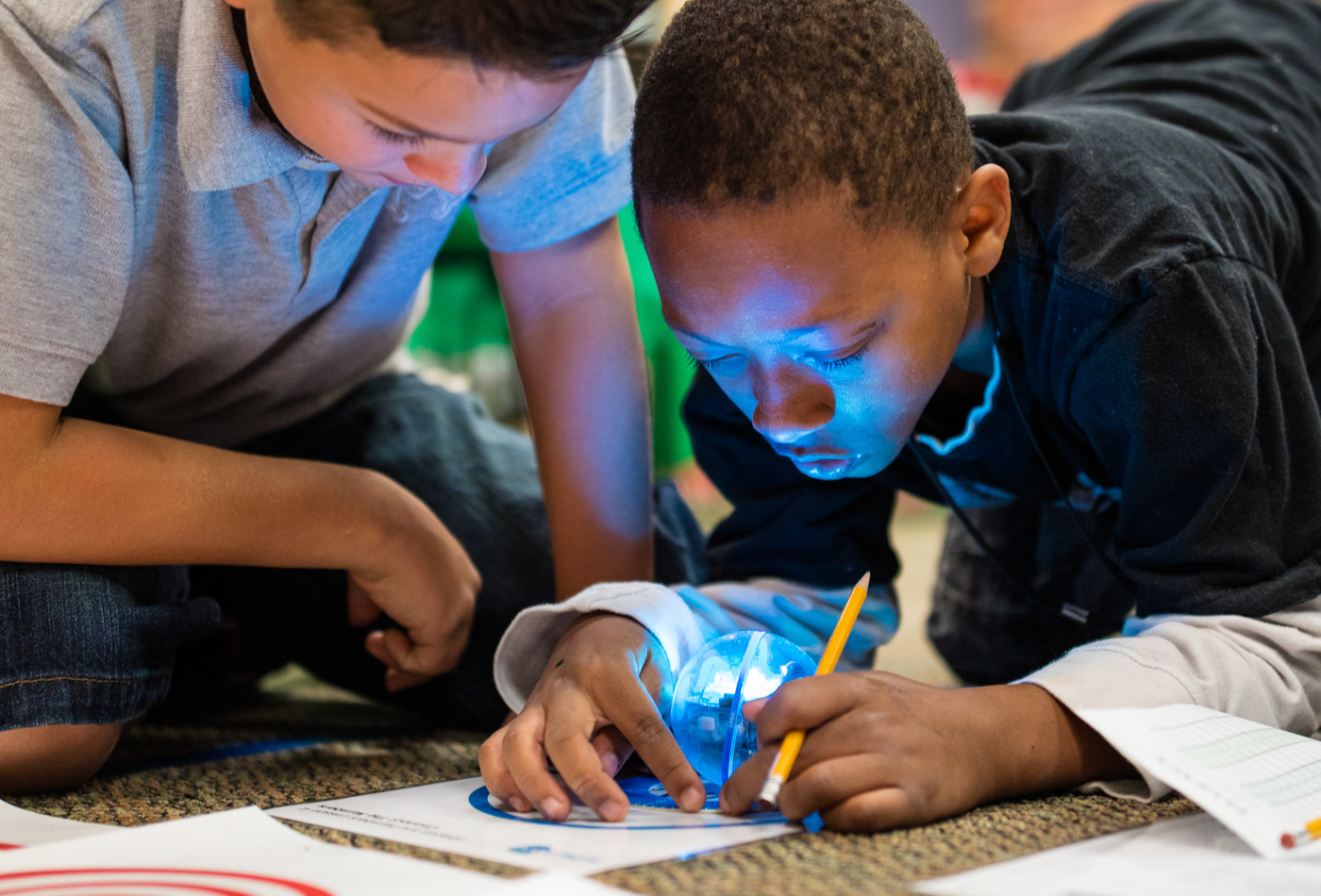
Guide to STEM Funding
When it comes to incorporating STEAM into the classroom, support can come from a surprising number of sources -- and sometimes, all you have to do is ask. That’s why we’ve compiled a list of resources to help drive innovative tech adoption.
Full STEAM Ahead!
Ready to get started with Sphero, littleBits or teaching STEAM and computer science in general? Our team of Education Experts is happy to answer any questions you may have.
- Publications
- Conferences & Events
- Professional Learning
- Science Standards
- Awards & Competitions
- Instructional Materials
- Free Resources
- American Rescue Plan
- For Preservice Teachers
NCCSTS Case Collection
- Science and STEM Education Jobs
- Interactive eBooks+
- Digital Catalog
- Regional Product Representatives
- e-Newsletters
- Bestselling Books
- Latest Books
- Popular Book Series
- Prospective Authors
- Web Seminars
- Exhibits & Sponsorship
- Conference Reviewers
- National Conference • Denver 24
- Leaders Institute 2024
- National Conference • New Orleans 24
- Submit a Proposal
- Latest Resources
- Professional Learning Units & Courses
- For Districts
- Online Course Providers
- Schools & Districts
- College Professors & Students
- The Standards
- Teachers and Admin
- eCYBERMISSION
- Toshiba/NSTA ExploraVision
- Junior Science & Humanities Symposium
- Teaching Awards
- Climate Change
- Earth & Space Science
- New Science Teachers
- Early Childhood
- Middle School
- High School
- Postsecondary
- Informal Education
- Journal Articles
- Lesson Plans
- e-newsletters
- Science & Children
- Science Scope
- The Science Teacher
- Journal of College Sci. Teaching
- Connected Science Learning
- NSTA Reports
- Next-Gen Navigator
- Science Update
- Teacher Tip Tuesday
- Trans. Sci. Learning
MyNSTA Community
- My Collections
Case Study Listserv
Permissions & Guidelines
Submit a Case Study
Resources & Publications
Enrich your students’ educational experience with case-based teaching
The NCCSTS Case Collection, created and curated by the National Center for Case Study Teaching in Science, on behalf of the University at Buffalo, contains over a thousand peer-reviewed case studies on a variety of topics in all areas of science.
Cases (only) are freely accessible; subscription is required for access to teaching notes and answer keys.
Subscribe Today
Browse Case Studies
Latest Case Studies

Development of the NCCSTS Case Collection was originally funded by major grants to the University at Buffalo from the National Science Foundation , The Pew Charitable Trusts , and the U.S. Department of Education .
- MyU : For Students, Faculty, and Staff
ML/AI Self Study Links
If you are aware of good online resources that you think we should include, please email us .
The following websites are useful for people interested in machine learning with Python.
Minnesota Supercomputing Institute
The Minnesota Supercomputing Institute has a YouTube channel with a number of tutorials on it. In addition, they regularly offer tutorials and other events. Check out their webpage for current offerings.
Tutorials available on their YouTube channel include:
- Python for Scientific Computing
- Advanced Python for Scientific Computing
- Getting Started with PyTorch: Setup
- Getting Started with PyTorch: Example
- Programming with Python
- Programming with Python: Fundamentals and Working with Data
- Programming with Python: Making Choices and Creating Functions
- Programming with Python: Loops, Lists, and Automating Analysis
Below we provide a selection of resources for Python, a general purpose programming language that has become popular for use in data science. One reason for that popularity is the existence of a number of packages for Python that implement various mathematical, statistical, and machine learning-related functions. Another attractive feature of Python is the widespread use of Jupyter notebooks that allow for interactive programming in Python (as well as other languages). Jupyter notebooks are also an easy way to share code and create tutorials for various programming tasks. Of course, Python programs can also be created using standard editors used for programming languages. There will be more on this below.
General Python background
- Python website
- Python Wikipedia Entr y
Getting Started with Python
The first task in getting started with Python is to get access. You can do this by installing it on a machine you have access to, such as your personal computer, or you can get access through a computing facility in your organization.
Access Python without installing it on your personal machine
Option 1: Google Colab This puts you directly into a Python notebook, which has a link to an introductory video, and to a new Jupyter notebook. Other links on the side of this notebook have links to examples, etc. If you just want to see the webpage, you can hit the cancel button at the bottom of the notebook. Colab allows you to get started with coding in Python right away. Access to Python's packages and GPUs is provided in this environment, but it is not intended for running compute-intensive programs.
Option 2: MSI Jupyter Notebook Server Those who have an account at the Minnesota Supercomputing Institute can go directly to the Jupyter notebook server. You must have an MSI account to use MSI resources. You must also connect either from a machine on a UMN network or via VPN. See the MSI website for additional documentation . You can perform moderate-size tasks using this option, but for programs that use more resources, you should create and submit jobs via the batch system at MSI.
Option 3: Code in the Cloud from Anaconda Anaconda also provides an environment that you can use to install and use Python and its packages. If you just want to have access to a Jupyter notebook and play around with learning and light use of Python, this could be another possibility. You will have to create an account with Anaconda.
Install Python on your personal machine
We recommend installing Anaconda as it will install Python and also install other regularly used packages for scientific computing and data science. It will also help make sure all your packages remain compatible with one another. Much of the functionality of Python, like R, Matlab, and other languages, comes from the packages.
The Anaconda website also provides a webpage for Getting started with Anaconda .
Anaconda takes a fair amount of space on the disk and time to install, so if you have an older computer with limited disk space or a limited internet connection, you should consider lighter-weight options such as Miniconda .
Alternatively, you can download Python from python.org . In that case, you will probably want to use a package manager such as pip , which comes with Python if you install it from python.org. This gives you more control but requires more understanding of Python, its packages, and how they are managed.
Learning to program in Python
For those unfamiliar with Python 3 and Jupyter notebooks, this is a relatively easy environment to learn. Learning Python 3 will help you advance your knowledge of data analytics, as most big data platforms and data mining/machine learning projects require a working knowledge of Python. The following resources can help you learn Python and get started in using Python for machine learning.
- Learn Python (book wesbite)
- Google's Python Class (website and videos)
- Python for Data Analysis, 3E (book website)
- W3Schools Python Tutorials (a collection of short Python tutorials on various aspects of the Python language)
- Data Analysis with Python and Pandas (videos) Pandas is a Python package for handling and analyzing data.
- Learn to Program with Python (videos)
Running Jupyter Notebooks
If you have installed Anaconda, you can run Anaconda Navigator and then click on the Jupyter notebook icon to start the Jupyter notebook. (Once you become familiar with a Jupyter notebook, you may want to run JupyterLab, a more advanced Notebook Interface.)
You can also log onto the MSI Jupyter notebook server.
- How to Use Jupyter Notebook: A Beginner's Tutorial (webpage)
- Running the Notebook (webpage) For those not using Anaconda
General Machine Learning
- An introduction to machine learning with the Python library, scikit-learn
- Machine Learning for Absolute Beginners , 3e by Oliver Theobald. 2nd Edition, 2017.
- Stanford machine learning playlist on YouTube by Andrew Ng
- Introduction to Statistical Learning , by James, Witten, Hastie, Tibshirani, and Taylor. (Available to download with R or Python.)
- Introduction to Data Mining , 2nd edition by Tan, Steinbach, Karpatne, and Kumar, which has three chapters available online, two of which provide a basic introduction to Classification and Clustering.
- Mathematics for Machine Learning . Deisenroth, Faisal, and Ong.
- Understanding Machine Learning: From Theory to Algorithms , Shalev-Schwartz and Sai Ben-David. Choose the download option on the bottom left.
- The website, KDD Nuggets , has many resources, including additional links to Free Books for Machine Learning .
Deep Learning with Python
- Deep Learning , by Goodfellow, Bengio, and Courville An online book that gives a good, although demanding, introduction to deep learning.
- Dive into Deep Learning , by by Zhang, Lipton, Li, and Smola. This interactive online book includes concepts, exercises, and code.
- Practical Deep Learning for Coders . This interactive online book also includes concepts, exercises, and code. It now has a part 2, From Deep Learning Foundations to Stable Diffusion .
- Deep Learning . This website features instructors Yann LeCun and Alfredo Canziani teaching a course on deep learning in Spring 2020 at the NYU Center for Data Science. It includes YouTube videos, slides, and Jupyter notebooks. The course concerns the latest techniques in deep learning and representation learning.
- Welcome to the UVA Deep Learning Tutorials! The University of Amsterdam has a set of Python notebook tutorials for deep learning. These tutorial notebooks are fairly self-contained but are accompanied by videos available on YouTube.
- Future undergraduate students
- Future transfer students
- Future graduate students
- Future international students
- Diversity and Inclusion Opportunities
- Learn abroad
- Living Learning Communities
- Mentor programs
- Programs for women
- Student groups
- Visit, Apply & Next Steps
- Information for current students
- Departments and majors overview
- Departments
- Undergraduate majors
- Graduate programs
- Integrated Degree Programs
- Additional degree-granting programs
- Online learning
- Academic Advising overview
- Academic Advising FAQ
- Academic Advising Blog
- Appointments and drop-ins
- Academic support
- Commencement
- Four-year plans
- Honors advising
- Policies, procedures, and forms
- Career Services overview
- Resumes and cover letters
- Jobs and internships
- Interviews and job offers
- CSE Career Fair
- Major and career exploration
- Graduate school
- Collegiate Life overview
- Scholarships
- Diversity & Inclusivity Alliance
- Anderson Student Innovation Labs
- Information for alumni
- Get engaged with CSE
- Upcoming events
- CSE Alumni Society Board
- Alumni volunteer interest form
- Golden Medallion Society Reunion
- 50-Year Reunion
- Alumni honors and awards
- Outstanding Achievement
- Alumni Service
- Distinguished Leadership
- Honorary Doctorate Degrees
- Nobel Laureates
- Alumni resources
- Alumni career resources
- Alumni news outlets
- CSE branded clothing
- International alumni resources
- Inventing Tomorrow magazine
- Update your info
- CSE giving overview
- Why give to CSE?
- College priorities
- Give online now
- External relations
- Giving priorities
- CSE Dean's Club
- Donor stories
- Impact of giving
- Ways to give to CSE
- Matching gifts
- CSE directories
- Invest in your company and the future
- Recruit our students
- Connect with researchers
- K-12 initiatives
- Diversity initiatives
- Research news
- Give to CSE
- CSE priorities
- Corporate relations
- Information for faculty and staff
- Administrative offices overview
- Office of the Dean
- Academic affairs
- Finance and Operations
- Communications
- Human resources
- Undergraduate programs and student services
- CSE Committees
- CSE policies overview
- Academic policies
- Faculty hiring and tenure policies
- Finance policies and information
- Graduate education policies
- Human resources policies
- Research policies
- Research overview
- Research centers and facilities
- Research proposal submission process
- Research safety
- Award-winning CSE faculty
- National academies
- University awards
- Honorary professorships
- Collegiate awards
- Other CSE honors and awards
- Staff awards
- Performance Management Process
- Work. With Flexibility in CSE
- K-12 outreach overview
- Summer camps
- Outreach events
- Enrichment programs
- Field trips and tours
- CSE K-12 Virtual Classroom Resources
- Educator development
- Sponsor an event
Computer Science & Engineering

CSE 190 - TOPICS IN COMPUTER SCIENCE AND ENGINEERING (2024-2025)
Updated: May 23rd, 2024
CSE 190 - Topics in Computer Science and Engineering
CSE 190 is a topics of special interest in Computer Science and Engineering course. Topics may vary from quarter to quarter.
Prerequisites also vary per course/per instructor. Department approval required.
CSE 190 is typically offered every quarter as staffing allows.
CSE 190 may be repeated for credit a maximum of 3 times (maximum of 12 units; assuming courses taken for a different topic)
A maximum of one CSE 190 may enrolled/waitlisted per quarter
Note: For the Fall 2023 Computer Science (CS26) curriculum, all CSE 190 courses will be labeled with a corresponding "Tag(s)" (Systems, Theory/Abstraction, and/or Applications of Computing). CSE 190 offerings before Fall 2023 are untagged but may be used as an Open CSE Elective for Computer Science majors who have changed to the FA23 CS26 curriculum.
____________________________________________________________________________
CSE 190 A00: Human-Centered AI with Kristen Vaccaro
Prerequisite: No course prerequisites.
CS Curriculum Tag(s): Applications of Computing
To enroll: Submit course clearance request via Enrollment Authorization System (EASy)
Description: This course provides an introduction to harnessing the power of AI so that it benefits people and communities. Topics will include: agency and initiative, fairness and bias, transparency and explainability, confidence and errors, and privacy, ethics, and trust. Students will build a number of interactive technologies powered by AI, gain practical experience with what makes them more or less usable, and learn to evaluate their impact on individuals and communities. Students will learn to think critically (but also optimistically) about what AI systems can do and how they should be integrated into society.
___________________
CSE 190 B00: Programmers are People Too with Michael Coblenz
Prerequisite: CSE 110 or CSE 130. Class is unofficially co-scheduled with CSE 291 Section B00. Students will not receive credit for both classes.
Description: Programmers of all kinds express their ideas using programming languages. Unfortunately, languages can be hard to use correctly, resulting in lengthy development times and buggy software. How can these languages be designed to make programmers as effective as possible?
In this course, we will learn research methods for analyzing and improving the usability of programming languages. Students will apply these techniques to languages of current and historical interest, and in the process, expand their knowledge of different ways to design languages. This course is intended as preparation for conducting independent research on the usability of programming languages. The first part of the course will emphasize research methods from human-computer interaction research, with examples drawn from programming languages and development environments. The second part of the course will focus on reading research papers that describe key results from the field. The course will include homework assignments as well as a group research project.
Video: Why Kenyan Student Regrets Pursuing Computer Science
- A young lady pursuing a computer science at the university wondered why she chose the course
- The student posted a video, seemingly perplexed by the course, just two weeks after studies commence
- Kenyans reacted by recounting dropping the course, while others encouraged her to keep going
A Kenyan student is the talk on social media after revealing that she was overwhelmed by her computer science course.
Is Computer Science hard?
Candidly opening up just two weeks after studies commenced, the student, Christine Keni, said the course was proving difficult.
Keni expressed regret, telling her TikTok followers that she could not identify precisely what convinced her to take the course.
"Guys, I don't know what convinced me to take computer science, but wueh! Wueh," said Keni.
The post drew interest from current and former computer science students, who urged her to reconsider withdrawing from the course.
"Don't worry; you will make it through."
“You have not started doing data structures and algorithms, object-oriented programming, etc., but don't drop out. We are making money here, even without a corporate job."
"I think computer science is a good course. My bro did that course and has a KSh 100k job while still in school.”
"I saw this immediately after applying for this course. Mayoooo."
Rose Wanjiru:
“I dropped it in my second year and did nursing. No regrets."
"I almost dropped out due to the artificial intelligence and machine learning unit in 4th year. Five years later I'm grateful."
Mutuanicholas7:
"I just heard 2 'wuehs' so you still have to top up 998 more to catch up with the 'wueh' speed."
Why did student kneel for lecturer?
Mid this month, a engineering student warmed hearts after kneeling before her lecturer to express gratitude for encouraging her not to drop out.
A video immortalising the moment showed the young woman thanking the lecturer for convincing her to stay in school when she was on the brink of giving up.
The lecturer encouraged her to make the most of her Engineering degree, highlighting the many opportunities it would bring.
Unlock the best of TUKO.co.ke on Pinterest! Subscribe now and get your daily inspiration!
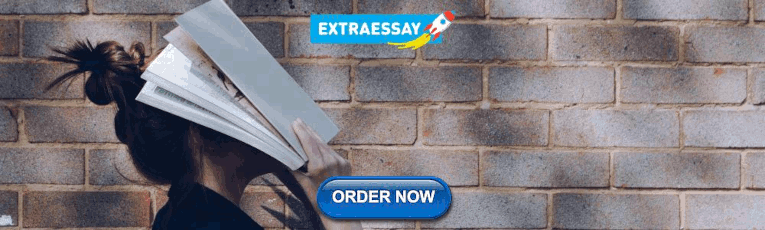
IMAGES
VIDEO
COMMENTS
the subject of this case study. Genetic algorithms Genetic algorithms mimic the process of natural selection in an attempt to evolve solutions to otherwise computationally intractable problems. Implementation details vary considerably, but a standard genetic algorithm includes the following steps: Initialize While true Evaluate
1. A Real-W orld Case S tudy in Information T echnology for. Undergraduate S tudents. Klaas Sikkel (1), Ton A.M. Spil (2), Rob L. W. van de Weg (1) (1) Faculty of Computer Science. (2) Faculty of ...
Computer Science Education in North-Rhine Westphalia, Germany—A Case Study Special Issue II on Computer Science Education in K-12 Schools In North-Rhine Westphalia, the most populated state in Germany, Computer Science (CS) has been taught in secondary schools since the early 1970s.
Women are in short supply in the computer science classroom. In the UK in 2017, women or girls made up 21% of secondary school pupils (aged 11- 16), 14.5% of college level students (aged 16-18), and 19% of university undergraduates studying computer science (Higher Education Statistics Agency, 2018; Joint Council for Qualifications, 2018).The trend continues beyond education and into the ...
Case Study. Is p53 a Smoking Gun? By Michèle I. Shuster, Joann Mudge, Meghan Hill, Katelynn James, Gabriella A. DeFrancesco, Maria P. Chadiarakou, Anitha Sundararajan
Suggested Citation:"12 A Case Study on Computer Programs."National Research Council. 1993. Global Dimensions of Intellectual Property Rights in Science and Technology.Washington, DC: The National Academies Press. doi: 10.17226/2054.
This small program offers support and personal attention while students study complex computer science concepts. It also provides substantial opportunity for hands-on experience with computer programming on a variety of computer systems. Each student's course of study is tailored to individual needs and includes independent study options.
From this assumption, this article proposes an analysis of the cohort of computer science' students, with a specific reference to British Universities, and identifies some challenges that lecturers of mathematical subjects normally face. ... A case study based on multiple years teaching indicates that the proposed techniques can be effective ...
2Department of Computer Science, University of Wolverhampton. [email protected] Abstract. Technology is transforming Higher Education learning and teaching. This paper reports on a project to examine how and why automated content analysis could be used to assess precis´ writing by university students.
A Case Study of Computer Science Students Abstract: This paper presents the development of a new learning platform in Virtual Reality to create a more immersive and intuitive learning experience for introduction of programming courses at an intermediate level. This platform is designed to create a central hub for interactive courseware and ...
Gamification is the use of game design elements in non-game settings to engage participants and encourage desired behaviors. It has been identified as a promising technique to improve students' engagement which could have a positive impact on learning. This study evaluated the learning effectiveness and engagement appeal of a gamified learning activity targeted at the learning of C-programming ...
This case study uses the PBL approach in an introductory course of computer architecture to overcome the main drawbacks of more traditional teaching schemes. Moreover, through PBL, students ...
Overview. The Library's support for Computer Science undergraduate students has traditionally been provided through induction sessions at the beginning of each academic year. The aim of this project was to explore where information and digital literacy (IDL) support could be embedded within the curriculum to provide the right support, in the ...
This paper reports on a project to examine how and why automated content analysis could be used to assess precis writing by university students. We examine the case of one hundred and twenty-two summaries written by computer science freshmen. The texts, which had been hand scored using a teacher-designed rubric, were autoscored using the ...
The MIT Case Studies in Social and Ethical Responsibilities of Computing (SERC) aims to advance new efforts within and beyond the Schwarzman College of Computing. The specially commissioned and peer-reviewed cases are brief and intended to be effective for undergraduate instruction across a range of classes and fields of study, and may also be ...
The Bachelor of Science degree program in computer science is designed to give a student a strong background in the fundamentals of mathematics and computer science. The curriculum is designed according to the latest ACM/IEEE computer science curriculum guidelines. A graduate of this program should be able to use these fundamentals to analyze ...
Student-centered means that students actively learn and construct knowledge by participating in teaching activities. This study presents a student-centered online-offline hybrid teaching method, which adopts student-centered case-based teaching and online-offline case discussion in the postgraduate courses of computer science.
Turkic Interlingua: A Case Study of Machine Translation in Low-resource Languages, Jamshidbek Mirzakhalov. PDF. Automated Wound Segmentation and Dimension Measurement Using RGB-D Image, Chih-Yun Pai. PDF. Constructing Frameworks for Task-Optimized Visualizations, Ghulam Jilani Abdul Rahim Quadri. PDF
1. Introduction. To learn computer programming is part of many study programmes in higher education. A multitude of reports of high failure and dropout rates (Ben-Ari Citation 1998; McCracken et al. Citation 2001; Robins, Rountree, and Rountree Citation 2003; Lister et al. Citation 2004; Kinnunen and Malmi Citation 2006; Kinnunen Citation 2009; Sorva Citation 2013) indicate that students ...
13 computer science student study tips. You can use the 13 tips below to help you succeed as a computer science student: 1. Pursue knowledge outside of courses. While a fair amount of the knowledge you learn comes from classroom instruction, you can also pursue topics in your own time. For example, if you discover you enjoy a topic that only ...
Our Bachelor of Science in Computer Science is an ABET accredited degree program designed for students who want a deep dive into computer science. In this program, you will master the fundamentals of computer science, explore the breadth of computing, and choose one of six areas of specialization for further in-depth study: Algorithms and Theory.
STEM skills grant students the 21st-century abilities needed to perform a wide range of both essential and highly-skilled jobs. Risk-taking, leaping, trying again, and failing are part of our genetic material. ... Sphero & littleBits K-12 STEAM & Computer Science Case Studies. Explore first-hand accounts of Sphero and littleBits success with ...
Enrich your students' educational experience with case-based teaching. The NCCSTS Case Collection, created and curated by the National Center for Case Study Teaching in Science, on behalf of the University at Buffalo, contains over a thousand peer-reviewed case studies on a variety of topics in all areas of science.
12 Computer Science case study question 2. Rohit, a student of class 12th, is learning CSV File Module in Python. During examination, he has been assigned an incomplete python code (shown below) to create a CSV File 'Student.csv' (content shown below). Help him in completing the code which creates the desired CSV File. CSV File.
If you are aware of good online resources that you think we should include, please email us.The following websites are useful for people interested in machine learning with Python.Minnesota Supercomputing InstituteThe Minnesota Supercomputing Institute has a YouTube channel with a number of tutorials on it. In addition, they regularly offer tutorials and other events. Check out their webpage ...
Note: For the Fall 2023 Computer Science (CS26) curriculum, all CSE 190 courses will be labeled with a corresponding "Tag(s)" (Systems, Theory/Abstraction, and/or Applications of Computing). CSE 190 offerings before Fall 2023 are untagged but may be used as an Open CSE Elective for Computer Science majors who have changed to the FA23 CS26 ...
A young lady pursuing a computer science at the university wondered why she chose the course; The student posted a video, seemingly perplexed by the course, just two weeks after studies commence