
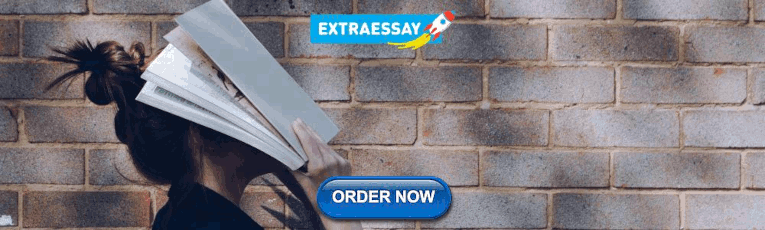
161+ Exciting Qualitative Research Topics For STEM Students

Are you doing Qualitative research? Looking for the best qualitative research topics for stem students? It is a most interesting and good field for research. Qualitative research allows STEM (Science, Technology, Engineering, and Mathematics) students to delve deeper into complex issues, explore human behavior, and understand the intricacies of the world around them.
In this article, we’ll provide you with an extensive list of 161+ qualitative research topics tailored to STEM students. We’ll also explore how to find and choose good qualitative research topics, and why these topics are particularly beneficial for students, including those in high school.
Also Like To Read: 171+ Brilliant Quantitative Research Topics For STEM Students
Table of Contents
What Are Qualitative Research Topics for STEM Students
Qualitative research topics for stem students are questions or issues that necessitate an in-depth exploration of people’s experiences, beliefs, and behaviors. STEM students can use this approach to investigate societal impacts, ethical dilemmas, and user experiences related to scientific advancements and innovations.
Unlike quantitative research, which focuses on numerical data and statistical analysis, qualitative research delves into the ‘whys’ and ‘hows’ of a particular phenomenon.
How to Find and Choose Good Qualitative Research Topics
Selecting qualitative research topics for stem students is a crucial step in the research process. Here are some tips to help you find and choose a suitable topic:

- Passion and Interest: Start by considering your personal interests and passions. What topics within STEM excite you? Research becomes more engaging when you’re genuinely interested in the subject.
- Relevance: Choose qualitative research topics for stem students. Look for gaps in the existing knowledge or unanswered questions.
- Literature Review: Conduct a thorough literature review to identify the latest trends and areas where qualitative research is lacking. This can guide you in selecting a topic that contributes to the field.
- Feasibility: Ensure that your chosen topic is feasible within the resources and time constraints available to you. Some research topics may require extensive resources and funding.
- Ethical Considerations: Be aware of ethical concerns related to your qualitative research topics for stem students, especially when dealing with human subjects or sensitive issues.
Here are the most exciting and very interesting Qualitative Research Topics For STEM Students, high school students, nursing students, college students, etc.
Biology Qualitative Research Topics
- Impact of Ecosystem Restoration on Biodiversity
- Ethical Considerations in Human Gene Editing
- Public Perceptions of Biotechnology in Agriculture
- Coping Mechanisms and Stress Responses in Marine Biologists
- Cultural Perspectives on Traditional Herbal Medicine
- Community Attitudes Toward Wildlife Conservation Efforts
- Ethical Issues in Animal Testing and Research
- Indigenous Knowledge and Ethnobotany
- Psychological Well-being of Conservation Biologists
- Attitudes Toward Endangered Species Protection
Chemistry Qualitative Research Topics For STEM Students
- Adoption of Green Chemistry Practices in the Pharmaceutical Industry
- Public Perception of Chemical Safety in Household Products
- Strategies for Improving Chemistry Education
- Art Conservation and Chemical Analysis
- Consumer Attitudes Toward Organic Chemistry in Everyday Life
- Ethical Considerations in Chemical Waste Disposal
- The Role of Chemistry in Sustainable Agriculture
- Perceptions of Nanomaterials and Their Applications
- Chemistry-Related Career Aspirations in High School Students
- Cultural Beliefs and Traditional Chemical Practices
Physics Qualitative Research Topics
- Gender Bias in Physics Education and Career Progression
- Philosophical Implications of Quantum Mechanics
- Public Understanding of Renewable Energy Technologies
- Influence of Science Fiction on Scientific Research
- Perceptions of Dark Matter and Dark Energy in the Universe
- Student Experiences in High School Physics Classes
- Physics Outreach Programs and Their Impact on Communities
- Cultural Variations in the Perception of Time and Space
- Role of Physics in Environmental Conservation
- Public Engagement with Science Through Astronomy Events
Engineering Qualitative Research Topics For STEM Students
- Ethics in Artificial Intelligence and Robotics
- Human-Centered Design in Engineering
- Innovation and Sustainability in Civil Engineering
- Public Perception of Self-Driving Cars
- Engineering Solutions for Climate Change Mitigation
- Experiences of Women in Male-Dominated Engineering Fields
- Role of Engineers in Disaster Response and Recovery
- Ethical Considerations in Technology Patents
- Perceptions of Engineering Education and Career Prospects
- Students Views on the Role of Engineers in Society
Computer Science Qualitative Research Topics
- Gender Diversity in Tech Companies
- Ethical Implications of AI-Powered Decision-Making
- User Experience and Interface Design
- Cybersecurity Awareness and Behaviors
- Digital Privacy Concerns and Practices
- Social Media Use and Mental Health in College Students
- Gaming Culture and its Impact on Social Interactions
- Student Attitudes Toward Coding and Programming
- Online Learning Platforms and Student Satisfaction
- Perceptions of Artificial Intelligence in Everyday Life
Mathematics Qualitative Research Topics For STEM Students
- Gender Stereotypes in Mathematics Education
- Cultural Variations in Problem-Solving Approaches
- Perception of Math in Everyday Life
- Math Anxiety and Coping Mechanisms
- Historical Development of Mathematical Concepts
- Attitudes Toward Mathematics Among Elementary School Students
- Role of Mathematics in Solving Real-World Problems
- Homeschooling Approaches to Teaching Mathematics
- Effectiveness of Math Tutoring Programs
- Math-Related Stereotypes in Society
Environmental Science Qualitative Research Topics
- Local Communities’ Responses to Climate Change
- Public Understanding of Conservation Practices
- Sustainable Agriculture and Farmer Perspectives
- Environmental Education and Behavior Change
- Indigenous Ecological Knowledge and Biodiversity Conservation
- Conservation Awareness and Behavior of Tourists
- Climate Change Perceptions Among Youth
- Perceptions of Water Scarcity and Resource Management
- Environmental Activism and Youth Engagement
- Community Responses to Environmental Disasters
Geology and Earth Sciences Qualitative Research Topics For STEM Students
- Geologists’ Risk Perception and Decision-Making
- Volcano Hazard Preparedness in At-Risk Communities
- Public Attitudes Toward Geological Hazards
- Environmental Consequences of Extractive Industries
- Perceptions of Geological Time and Deep Earth Processes
- Use of Geospatial Technology in Environmental Research
- Role of Geology in Disaster Preparedness and Response
- Geological Factors Influencing Urban Planning
- Community Engagement in Geoscience Education
- Climate Change Communication and Public Understanding
Astronomy and Space Science Qualitative Research Topics
- The Role of Science Communication in Astronomy Education
- Perceptions of Space Exploration and Colonization
- UFO and Extraterrestrial Life Beliefs
- Public Understanding of Black Holes and Neutron Stars
- Space Tourism and Future Space Travel
- Impact of Space Science Outreach Programs on Student Interest
- Cultural Beliefs and Rituals Related to Celestial Events
- Space Science in Indigenous Knowledge Systems
- Public Engagement with Astronomical Phenomena
- Space Exploration in Science Fiction and Popular Culture
Medicine and Health Sciences Qualitative Research Topics
- Patient-Physician Communication and Trust
- Ethical Considerations in Human Cloning and Genetic Modification
- Public Attitudes Toward Vaccination
- Coping Strategies for Healthcare Workers in Pandemics
- Cultural Beliefs and Health Practices
- Health Disparities Among Underserved Communities
- Medical Decision-Making and Informed Consent
- Mental Health Stigma and Help-Seeking Behavior
- Wellness Practices and Health-Related Beliefs
- Perceptions of Alternative and Complementary Medicine
Psychology Qualitative Research Topics
- Perceptions of Body Image in Different Cultures
- Workplace Stress and Coping Mechanisms
- LGBTQ+ Youth Experiences and Well-Being
- Cross-Cultural Differences in Parenting Styles and Outcomes
- Perceptions of Psychotherapy and Counseling
- Attitudes Toward Medication for Mental Health Conditions
- Psychological Well-being of Older Adults
- Role of Cultural and Social Factors in Psychological Well-being
- Technology Use and Its Impact on Mental Health
Social Sciences Qualitative Research Topics
- Political Polarization and Online Echo Chambers
- Immigration and Acculturation Experiences
- Educational Inequality and School Policy
- Youth Engagement in Environmental Activism
- Identity and Social Media in the Digital Age
- Social Media and Its Influence on Political Beliefs
- Family Dynamics and Conflict Resolution
- Social Support and Coping Strategies in College Students
- Perceptions of Cyberbullying Among Adolescents
- Impact of Social Movements on Societal Change
Interesting Sociology Qualitative Research Topics For STEM Students
- Perceptions of Racial Inequality and Discrimination
- Aging and Quality of Life in Elderly Populations
- Gender Roles and Expectations in Relationships
- Online Communities and Social Support
- Cultural Practices and Beliefs Related to Marriage
- Family Dynamics and Coping Mechanisms
- Perceptions of Community Safety and Policing
- Attitudes Toward Social Welfare Programs
- Influence of Media on Perceptions of Social Issues
- Youth Perspectives on Education and Career Aspirations
Anthropology Qualitative Research Topics
- Traditional Knowledge and Biodiversity Conservation
- Cultural Variation in Parenting Practices
- Indigenous Language Revitalization Efforts
- Social Impacts of Tourism on Indigenous Communities
- Rituals and Ceremonies in Different Cultural Contexts
- Food and Identity in Cultural Practices
- Traditional Healing and Healthcare Practices
- Indigenous Rights and Land Conservation
- Ethnographic Studies of Marginalized Communities
- Cultural Practices Surrounding Death and Mourning
Economics and Business Qualitative Research Topics
- Small Business Resilience in Times of Crisis
- Workplace Diversity and Inclusion
- Corporate Social Responsibility Perceptions
- International Trade and Cultural Perceptions
- Consumer Behavior and Decision-Making in E-Commerce
- Business Ethics and Ethical Decision-Making
- Innovation and Entrepreneurship in Startups
- Perceptions of Economic Inequality and Wealth Distribution
- Impact of Economic Policies on Communities
- Role of Economic Education in Financial Literacy
Good Education Qualitative Research Topics For STEM Students
- Homeschooling Experiences and Outcomes
- Teacher Burnout and Coping Strategies
- Inclusive Education and Special Needs Integration
- Student Perspectives on Online Learning
- High-Stakes Testing and Its Impact on Students
- Multilingual Education and Bilingualism
- Perceptions of Educational Technology in Classrooms
- School Climate and Student Well-being
- Teacher-Student Relationships and Their Effects on Learning
- Cultural Diversity in Education and Inclusion
Environmental Engineering Qualitative Research Topics
- Sustainable Transportation and Community Preferences
- Ethical Considerations in Waste Reduction and Recycling
- Public Attitudes Toward Renewable Energy Projects
- Environmental Impact Assessment and Community Engagement
- Sustainable Urban Planning and Neighborhood Perceptions
- Water Quality and Conservation Practices in Residential Areas
- Green Building Practices and User Experiences
- Community Resilience in the Face of Climate Change
- Role of Environmental Engineers in Disaster Preparedness
Why Qualitative Research Topics Are Good for STEM Students
- Deeper Understanding: Qualitative research encourages STEM students to explore complex issues from a human perspective. This deepens their understanding of the broader impact of scientific discoveries and technological advancements.
- Critical Thinking: Qualitative research fosters critical thinking skills by requiring students to analyze and interpret data, consider diverse viewpoints, and draw nuanced conclusions.
- Real-World Relevance: Many qualitative research topics have real-world applications. Students can address problems, inform policy, and contribute to society by investigating issues that matter.
- Interdisciplinary Learning: Qualitative research often transcends traditional STEM boundaries, allowing students to draw on insights from psychology, sociology, anthropology, and other fields.
- Preparation for Future Careers: Qualitative research skills are valuable in various STEM careers, as they enable students to communicate complex ideas and understand the human and social aspects of their work.
Qualitative Research Topics for High School STEM Students
High school STEM students can benefit from qualitative research by honing their critical thinking and problem-solving skills. Here are some qualitative research topics suitable for high school students:
- Perceptions of STEM Education: Investigate students’ and teachers’ perceptions of STEM education and its effectiveness.
- Environmental Awareness: Examine the factors influencing high school students’ environmental awareness and eco-friendly behaviors.
- Digital Learning in the Classroom: Explore the impact of technology on learning experiences and student engagement.
- STEM Gender Gap: Analyze the reasons behind the gender gap in STEM fields and potential strategies for closing it.
- Science Communication: Study how high school students perceive and engage with popular science communication channels, like YouTube and podcasts.
- Impact of Extracurricular STEM Activities: Investigate how participation in STEM clubs and competitions influences students’ interest and performance in science and technology.
In essence, these are the best qualitative research topics for STEM students in the Philippines and are usable for other countries students too. Qualitative research topics offer STEM students a unique opportunity to explore the multifaceted aspects of their fields, develop essential skills, and contribute to meaningful discoveries. With the right topic selection, a strong research design, and ethical considerations, STEM students can easily get the best knowledge on exciting qualitative research that benefits both their career growth. So, choose a topic that resonates with your interests and get best job in your interest field.
Leave a Reply Cancel reply
Your email address will not be published. Required fields are marked *
Save my name, email, and website in this browser for the next time I comment.

Best 151+ Quantitative Research Topics for STEM Students

In today’s rapidly evolving world, STEM (Science, Technology, Engineering, and Mathematics) fields have gained immense significance. For STEM students, engaging in quantitative research is a pivotal aspect of their academic journey. Quantitative research involves the systematic collection and interpretation of numerical data to address research questions or test hypotheses. Choosing the right research topic is essential to ensure a successful and meaningful research endeavor.
In this blog, we will explore 151+ quantitative research topics for STEM students. Whether you are an aspiring scientist, engineer, or mathematician, this comprehensive list will inspire your research journey. But we understand that the journey through STEM education and research can be challenging at times. That’s why we’re here to support you every step of the way with our Engineering Assignment Help service.
What is Quantitative Research in STEM?
Table of Contents
Quantitative research is a scientific approach that relies on numerical data and statistical analysis to draw conclusions and make predictions. In STEM fields, quantitative research encompasses a wide range of methodologies, including experiments, surveys, and data analysis. The key characteristics of quantitative research in STEM include:
- Data Collection: Systematic gathering of numerical data through experiments, observations, or surveys.
- Statistical Analysis: Application of statistical techniques to analyze data and draw meaningful conclusions.
- Hypothesis Testing: Testing hypotheses and theories using quantitative data.
- Replicability: The ability to replicate experiments and obtain consistent results.
- Generalizability: Drawing conclusions that can be applied to larger populations or phenomena.
Importance of Quantitative Research Topics for STEM Students
Quantitative research plays a pivotal role in STEM education and research for several reasons:
1. Empirical Evidence
It provides empirical evidence to support or refute scientific theories and hypotheses.
2. Data-Driven Decision-Making
STEM professionals use quantitative research to make informed decisions, from designing experiments to developing new technologies.
3. Innovation
It fuels innovation by providing data-driven insights that lead to the creation of new products, processes, and technologies.
4. Problem Solving
STEM students learn critical problem-solving skills through quantitative research, which are invaluable in their future careers.
5. Interdisciplinary Applications
Quantitative research transcends STEM disciplines, facilitating collaboration and the tackling of complex, real-world problems.
Also Read: Google Scholar Research Topics
Quantitative Research Topics for STEM Students
Now, let’s explore important quantitative research topics for STEM students:
Biology and Life Sciences
Here are some quantitative research topics in biology and life science:
1. The impact of climate change on biodiversity.
2. Analyzing the genetic basis of disease susceptibility.
3. Studying the effectiveness of vaccines in preventing infectious diseases.
4. Investigating the ecological consequences of invasive species.
5. Examining the role of genetics in aging.
6. Analyzing the effects of pollution on aquatic ecosystems.
7. Studying the evolution of antibiotic resistance.
8. Investigating the relationship between diet and lifespan.
9. Analyzing the impact of deforestation on wildlife.
10. Studying the genetics of cancer development.
11. Investigating the effectiveness of various plant fertilizers.
12. Analyzing the impact of microplastics on marine life.
13. Studying the genetics of human behavior.
14. Investigating the effects of pollution on plant growth.
15. Analyzing the microbiome’s role in human health.
16. Studying the impact of climate change on crop yields.
17. Investigating the genetics of rare diseases.
Let’s get started with some quantitative research topics for stem students in chemistry:
1. Studying the properties of superconductors at different temperatures.
2. Analyzing the efficiency of various catalysts in chemical reactions.
3. Investigating the synthesis of novel polymers with unique properties.
4. Studying the kinetics of chemical reactions.
5. Analyzing the environmental impact of chemical waste disposal.
6. Investigating the properties of nanomaterials for drug delivery.
7. Studying the behavior of nanoparticles in different solvents.
8. Analyzing the use of renewable energy sources in chemical processes.
9. Investigating the chemistry of atmospheric pollutants.
10. Studying the properties of graphene for electronic applications.
11. Analyzing the use of enzymes in industrial processes.
12. Investigating the chemistry of alternative fuels.
13. Studying the synthesis of pharmaceutical compounds.
14. Analyzing the properties of materials for battery technology.
15. Investigating the chemistry of natural products for drug discovery.
16. Analyzing the effects of chemical additives on food preservation.
17. Investigating the chemistry of carbon capture and utilization technologies.
Here are some quantitative research topics in physics for stem students:
1. Investigating the behavior of subatomic particles in high-energy collisions.
2. Analyzing the properties of dark matter and dark energy.
3. Studying the quantum properties of entangled particles.
4. Investigating the dynamics of black holes and their gravitational effects.
5. Analyzing the behavior of light in different mediums.
6. Studying the properties of superfluids at low temperatures.
7. Investigating the physics of renewable energy sources like solar cells.
8. Analyzing the properties of materials at extreme temperatures and pressures.
9. Studying the behavior of electromagnetic waves in various applications.
10. Investigating the physics of quantum computing.
11. Analyzing the properties of magnetic materials for data storage.
12. Studying the behavior of particles in plasma for fusion energy research.
13. Investigating the physics of nanoscale materials and devices.
14. Analyzing the properties of materials for use in semiconductors.
15. Studying the principles of thermodynamics in energy efficiency.
16. Investigating the physics of gravitational waves.
17. Analyzing the properties of materials for use in quantum technologies.
Engineering
Let’s explore some quantitative research topics for stem students in engineering:
1. Investigating the efficiency of renewable energy systems in urban environments.
2. Analyzing the impact of 3D printing on manufacturing processes.
3. Studying the structural integrity of materials in aerospace engineering.
4. Investigating the use of artificial intelligence in autonomous vehicles.
5. Analyzing the efficiency of water treatment processes in civil engineering.
6. Studying the impact of robotics in healthcare.
7. Investigating the optimization of supply chain logistics using quantitative methods.
8. Analyzing the energy efficiency of smart buildings.
9. Studying the effects of vibration on structural engineering.
10. Investigating the use of drones in agricultural practices.
11. Analyzing the impact of machine learning in predictive maintenance.
12. Studying the optimization of transportation networks.
13. Investigating the use of nanomaterials in electronic devices.
14. Analyzing the efficiency of renewable energy storage systems.
15. Studying the impact of AI-driven design in architecture.
16. Investigating the optimization of manufacturing processes using Industry 4.0 technologies.
17. Analyzing the use of robotics in underwater exploration.
Environmental Science
Here are some top quantitative research topics in environmental science for students:
1. Investigating the effects of air pollution on respiratory health.
2. Analyzing the impact of deforestation on climate change.
3. Studying the biodiversity of coral reefs and their conservation.
4. Investigating the use of remote sensing in monitoring deforestation.
5. Analyzing the effects of plastic pollution on marine ecosystems.
6. Studying the impact of climate change on glacier retreat.
7. Investigating the use of wetlands for water quality improvement.
8. Analyzing the effects of urbanization on local microclimates.
9. Studying the impact of oil spills on aquatic ecosystems.
10. Investigating the use of renewable energy in mitigating greenhouse gas emissions.
11. Analyzing the effects of soil erosion on agricultural productivity.
12. Studying the impact of invasive species on native ecosystems.
13. Investigating the use of bioremediation for soil cleanup.
14. Analyzing the effects of climate change on migratory bird patterns.
15. Studying the impact of land use changes on water resources.
16. Investigating the use of green infrastructure for urban stormwater management.
17. Analyzing the effects of noise pollution on wildlife behavior.
Computer Science
Let’s get started with some simple quantitative research topics for stem students:
1. Investigating the efficiency of machine learning algorithms for image recognition.
2. Analyzing the security of blockchain technology in financial transactions.
3. Studying the impact of quantum computing on cryptography.
4. Investigating the use of natural language processing in chatbots and virtual assistants.
5. Analyzing the effectiveness of cybersecurity measures in protecting sensitive data.
6. Studying the impact of algorithmic trading in financial markets.
7. Investigating the use of deep learning in autonomous robotics.
8. Analyzing the efficiency of data compression algorithms for large datasets.
9. Studying the impact of virtual reality in medical simulations.
10. Investigating the use of artificial intelligence in personalized medicine.
11. Analyzing the effectiveness of recommendation systems in e-commerce.
12. Studying the impact of cloud computing on data storage and processing.
13. Investigating the use of neural networks in predicting disease outbreaks.
14. Analyzing the efficiency of data mining techniques in customer behavior analysis.
15. Studying the impact of social media algorithms on user behavior.
16. Investigating the use of machine learning in natural language translation.
17. Analyzing the effectiveness of sentiment analysis in social media monitoring.
Mathematics
Let’s explore the quantitative research topics in mathematics for students:
1. Investigating the properties of prime numbers and their distribution.
2. Analyzing the behavior of chaotic systems using differential equations.
3. Studying the optimization of algorithms for solving complex mathematical problems.
4. Investigating the use of graph theory in network analysis.
5. Analyzing the properties of fractals in natural phenomena.
6. Studying the application of probability theory in risk assessment.
7. Investigating the use of numerical methods in solving partial differential equations.
8. Analyzing the properties of mathematical models for population dynamics.
9. Studying the optimization of algorithms for data compression.
10. Investigating the use of topology in data analysis.
11. Analyzing the behavior of mathematical models in financial markets.
12. Studying the application of game theory in strategic decision-making.
13. Investigating the use of mathematical modeling in epidemiology.
14. Analyzing the properties of algebraic structures in coding theory.
15. Studying the optimization of algorithms for image processing.
16. Investigating the use of number theory in cryptography.
17. Analyzing the behavior of mathematical models in climate prediction.
Earth Sciences
Here are some quantitative research topics for stem students in earth science:
1. Investigating the impact of volcanic eruptions on climate patterns.
2. Analyzing the behavior of earthquakes along tectonic plate boundaries.
3. Studying the geomorphology of river systems and erosion.
4. Investigating the use of remote sensing in monitoring wildfires.
5. Analyzing the effects of glacier melt on sea-level rise.
6. Studying the impact of ocean currents on weather patterns.
7. Investigating the use of geothermal energy in renewable power generation.
8. Analyzing the behavior of tsunamis and their destructive potential.
9. Studying the impact of soil erosion on agricultural productivity.
10. Investigating the use of geological data in mineral resource exploration.
11. Analyzing the effects of climate change on coastal erosion.
12. Studying the geomagnetic field and its role in navigation.
13. Investigating the use of radar technology in weather forecasting.
14. Analyzing the behavior of landslides and their triggers.
15. Studying the impact of groundwater depletion on aquifer systems.
16. Investigating the use of GIS (Geographic Information Systems) in land-use planning.
17. Analyzing the effects of urbanization on heat island formation.
Health Sciences and Medicine
Here are some quantitative research topics for stem students in health science and medicine:
1. Investigating the effectiveness of telemedicine in improving healthcare access.
2. Analyzing the impact of personalized medicine in cancer treatment.
3. Studying the epidemiology of infectious diseases and their spread.
4. Investigating the use of wearable devices in monitoring patient health.
5. Analyzing the effects of nutrition and exercise on metabolic health.
6. Studying the impact of genetics in predicting disease susceptibility.
7. Investigating the use of artificial intelligence in medical diagnosis.
8. Analyzing the behavior of pharmaceutical drugs in clinical trials.
9. Studying the effectiveness of mental health interventions in schools.
10. Investigating the use of gene editing technologies in treating genetic disorders.
11. Analyzing the properties of medical imaging techniques for early disease detection.
12. Studying the impact of vaccination campaigns on public health.
13. Investigating the use of regenerative medicine in tissue repair.
14. Analyzing the behavior of pathogens in antimicrobial resistance.
15. Studying the epidemiology of chronic diseases like diabetes and heart disease.
16. Investigating the use of bioinformatics in genomics research.
17. Analyzing the effects of environmental factors on health outcomes.
Quantitative research is the backbone of STEM fields, providing the tools and methodologies needed to explore, understand, and innovate in the world of science and technology . As STEM students, embracing quantitative research not only enhances your analytical skills but also equips you to address complex real-world challenges. With the extensive list of 155+ quantitative research topics for stem students provided in this blog, you have a starting point for your own STEM research journey. Whether you’re interested in biology, chemistry, physics, engineering, or any other STEM discipline, there’s a wealth of quantitative research topics waiting to be explored. So, roll up your sleeves, grab your lab coat or laptop, and embark on your quest for knowledge and discovery in the exciting world of STEM.
I hope you enjoyed this blog post about quantitative research topics for stem students.
Related Posts
8 easiest programming language to learn for beginners.
There are so many programming languages you can learn. But if you’re looking to start with something easier. We bring to you a list of…
10 Online Tutoring Help Benefits
Do you need a computer science assignment help? Get the best quality assignment help from computer science tutors at affordable prices. They always presented to help…

250+ Amazing Qualitative Research Topics for STEM Students
Students often focus on numbers and experiments in science, technology, engineering, and math (STEM). However, there’s one more side to explore that is similarly significant: subjective examination.
Qualitative research helps STEM students understand people’s thoughts, feelings, and experiences related to science and technology. Dissimilar to quantitative exploration, which manages numbers, subjective review examines the more profound implications behind things.
For STEM students, qualitative research has many benefits. It helps them think critically, understand others better, and see how science affects society. Through this blog series, we’ll investigate different research topics for STEM students, such as the moral side of biotechnology and the jobs of people in design.
Also Read : 270+ Best Physics Research Topics For High School Students.
In this day and age, where science and society meet, we want assorted points of view. By embracing qualitative research, STEM students can turn out to be better-adjusted specialists prepared to handle the difficulties. Go along with us as we find new bits of knowledge and extend our seeing together.
Table of Contents
What Are Qualitative Research Topics
Qualitative research in STEM (Science, Technology, Engineering, and Mathematics) examines many different areas. It helps us understand how people interact with science and technology. These research topics explore how people feel and think about different scientific fields. They also examine ethical issues, how society is affected, and how people are at the center of STEM subjects.
How Do I Choose The Right Qualitative Research Topics for STEM Students?

Choosing the right qualitative research topics for STEM students involves considering several key factors to ensure the research is meaningful, relevant, and feasible. Here are some tips to help you select appropriate topics:
1. Interest and Passion
Urge understudies to pick subjects that interest them and line up with their interests in STEM fields. Exploring a topic they find captivating will inspire them all through the exploration cycle.
2. Relevance to Current Issues
Select subjects addressing recent concerns, difficulties, or patterns in the selected STEM discipline. This guarantees that the exploration adds to existing information and resolves genuine issues.
3. Feasibility
Think about the assets, time, and aptitude expected to direct the examination. Guarantee that the picked subject is plausible inside the limitations of the understudy’s abilities, accessible assets, and period.
4. Scope and Manageability
Pick subjects that are sufficiently wide and thin in scope. The examination question should be focused on being sensible in the accessible time and assets yet expansive enough to consider significant investigation and investigation.
5. Originality and Innovation
Urge understudies to investigate exceptional or inventive examination points that still can’t be widely contemplated. Unique exploration propels information and permits understudies to affect their field significantly.
6. Interdisciplinary Connections
Consider interdisciplinary themes that span different STEM teaches or converge with fields like sociologies, humanities, or ecological examinations. Interdisciplinary exploration encourages joint effort and offers a comprehensive comprehension of intricate issues.
7. Ethical Considerations
Guarantee that the picked subject complies with moral rules and standards for the STEM discipline. Address expected moral worries about human subjects, creature government assistance, natural effects, or information protection.
List of Qualitative Research Topics for STEM Students
Here is a comprehensive list of qualitative research topics for STEM students.
Biology Research Topics for STEM Students
- The Impact of Climate Change on Wildlife in [Specific Region].
- Understanding How Nature Can Bounce Back in [Specific Habitat].
- What People Think About Changing Genes in Farming.
- Looking at What’s Right or Wrong in Testing Animals for Medicine.
- Learning About Tiny Creatures Living in Soil.
- How Our Genes Can Make Us More Likely to Get Sick: A Simple Look.
- What People Think is Okay About Editing Genes in Babies.
- Checking Out How Plants and Tiny Creatures Help Each Other on Farms.
- How People in Poor Countries See Science That Changes Living Things.
- Seeing What People Get About How Life Changes Over Time.
Chemistry Research Topics for STEM Students
- Looking at What People Think About Tiny Tech in Stuff We Use.
- Checking Out How Schools Teach Eco-Friendly Chemistry.
- Seeing What People Know About Keeping Workplaces Safe from Chemicals.
- Understanding How Chemistry Helps Us Make Energy That Won’t Run Out.
- Thinking About What’s Right and Wrong in Making Chemical Weapons.
- Studying Different Ways to Use Liquids in Chemistry Experiments.
- Seeing What People Think About Stuff Added to Food.
- Finding Out What People Think About Recycling Chemicals.
- Looking at How Chemicals Mess Up Cities.
- Understanding How Chemistry Helps Make New Medicines.
- Understanding What People Think About Nuclear Power as a Sustainable Energy Source.
- Looking at What Different Cultures Think About Exploring Space.
- Checking if Boys and Girls Get the Same Chance to Learn Physics.
- Looking at What People Think About Tiny Bits of Energy Science.
- Understanding How Physics Helps Us Make Renewable Energy.
- Thinking About What’s Right and Wrong in Studying Tiny Bits of Matter.
- Checking How Physics Helps Us See Inside Bodies.
- Looking at How Scientists Talk About Space Stuff.
- Understanding How Different People See Time.
- Seeing What People Think About Smart Computers in Physics.
Engineering
- Looking at Whether Boys and Girls Get the Same Chance to Learn Engineering.
- Understanding How Engineering Helps Us Build Things That Last.
- Thinking About What’s Right and Wrong in Cars That Drive Themselves.
- Studying How Engineers Make Things.
- Seeing What People Think About New Medical Tools Made by Engineers.
- Understanding How Different Cultures Deal with Water.
- Thinking About Tough Choices in Making Smart Computers.
- Looking at How Engineering Is Taught in Countryside Areas.
- Checking How Engineers Help During Disasters.
- Seeing What People Think About Making Stuff with 3D Printers.
Mathematics Qualitative Research Topics for STEM Students
- Looking at Whether Boys and Girls Get the Same Chance to Learn Math.
- Seeing How Different Cultures Solve Math Problems.
- Understanding What’s Right and Wrong in Making Computer Rules.
- Studying How Math is Taught in Poor Countries.
- Seeing What People Think About Secret Codes and Keeping Data Safe.
- Thinking About How Math Helps Make Art and Music.
- Understanding How Different Groups Do Math.
- Studying Why Some Students Worry About Math.
- Checking How Math Helps Study Diseases.
- Seeing How People in Different Cultures Do Math.
Interdisciplinary Topics
- Where Biology and Engineering Meet in Body Movements.
- Thinking About What’s Right and Wrong in Making Wearable Gadgets.
- Studying Programs That Teach Different Sciences Together.
- Seeing What People Think About Big Fixes for Climate Change.
- Looking at How Math Helps Make Computers Work.
- Understanding How Different Groups See Smart Computers and Robots.
- Studying How Computers Help with Health Care Using Biology.
- Checking How Learning Science Helps Fight Global Health Problems.
- Learning About Native Knowledge about Nature.
- Seeing What People Think About Talking to Computers.
Environmental Science Research Topics for STEM Students
- Seeing What People Think About Green Energy.
- Studying Projects That Protect Nature Made by Local Groups.
- Checking How Native Knowledge Helps Take Care of Nature.
- Understanding How Different Cultures Deal with Trash.
- Looking at How Climate Change Affects People.
- Studying How Schools Teach about Nature.
- Seeing What People Think About Saving Wild Places.
- Exploring How Regular People Help Watch Nature.
- Understanding What Different Cultures Think About City Parks.
- Looking at Movements That Fight for Fairness About Nature.
Computer Science
- Understanding How Different Cultures See Keeping Information Safe.
- Looking at Whether Computers Treat Men and Women Fairly.
- Studying How Computer Classes Are Taught in Poor Countries.
- Checking What People Think About Rules for Staying Safe Online.
- Thinking About What’s Right and Wrong in Making Computers That Think for Themselves.
- Understanding How Different Cultures Deal with Not Having Computers.
- Studying How People and Computers Work Together in Fake Worlds.
- Seeing What People Think About Keeping Computers Safe.
- Looking at How Computer Science Helps with Health Information.
- Understanding What People Think About Smart Computers Taking Jobs.
Technology Research Topics for STEM Students
- Whether Men and Women Get the Same Chance to Use and Like Tech.
- Seeing How Different Cultures Feel About New Technologies.
- Studying How Schools Use Tech.
- Checking What People Think About Making Cities Smart.
- Thinking About What’s Right and Wrong in Using Body Measurements for Tech.
- Understanding How Different Cultures Control the Internet.
- Studying Why Older People Like or Dislike Tech.
- Seeing What People Think About Rules for Flying Drones.
- Exploring Native Knowledge of Tech That Helps Nature.
- Understanding What Different Cultures Think About Health Gadgets You Wear.
Agriculture
- Studying How Men and Women Make Farming Choices.
- Looking at How Different Cultures Feel About Farming That Lasts.
- Studying Programs That Teach Farmers.
- Checking What People Think About Changing Food DNA.
- Learning About Native Farming Knowledge.
- Understanding How Different Cultures Feel About Bug Killers.
- Studying How Farmers Work in Small Farms with Nature.
- Checking What People Think About Farming Without Chemicals.
- Looking at How Farming Helps Deal with Climate Changes.
- Understanding What Different Cultures Think About Keeping Seeds and Different Plants.
Health Sciences Research Topics for STEM Students
- Looking at Whether Men and Women Get the Same Healthcare.
- Seeing How Different Cultures Feel About Treating the Mind.
- Studying How Doctors and Patients Talk.
- Checking What People Think About Vaccines.
- Learning About Native Ways to Heal and Traditional Medicine.
- Understanding How Different Cultures Deal with Dying.
- Studying How Doctors Work in Country Areas.
- Checking What People Think About Seeing a Doctor Online.
- Exploring What’s Right and Wrong in Studying People.
- Understanding How Different Cultures Feel About Services for Making Babies.
Social Sciences Research Topics for STEM Students
- Studying How Men and Women Choose Jobs in Science, Technology, Engineering, and Math.
- Looking at How Different Cultures Feel About Protecting Nature.
- Studying Programs That Help People Learn Science, Technology, Engineering, and Math.
- Checking What People Think About How Governments Make Science Rules.
- Learning About Native Ideas on How Machines Change Things.
- Understanding How Different Cultures Feel About Talking About Science.
- Studying How Much People Know About Science in Different Groups.
- Checking What People Think About Who Knows a Lot About Science.
- Exploring What’s Right and Wrong in Reporting Science News.
- Understanding How Different Cultures See Making Science Facts.
Neuroscience
- Studying How Boys’ and Girls’ Brains Grow and Work.
- Looking at How Different Cultures See Feeling Bad About Mental Health Problems.
- Studying How the Brain Can Change and Learn.
- Checking What People Think About Machines That Connect to the Brain.
- Seeing How the Brain Works in Addiction.
- Understanding How Different Cultures Feel About People’s Different Brain Types.
- Studying How Schools Teach Kids About Keeping Their Brains Healthy.
- Checking What People Think About Making Brains Work Better.
- Exploring What’s Right and Wrong in Studying Brains with Machines.
- Understanding How Different Cultures Feel Pain and Deal with It.
Biomedical Engineering Research Topics for STEM Students
- Studying Whether Men and Women Get Fair Treatment in Medical Engineering Research.
- Looking at How Different Cultures See Making Medical Tools.
- Studying Programs That Teach Medical Engineering.
- Checking What People Think About Printing Body Parts.
- Exploring How Medical Engineering Helps People Get Better.
- Understanding How Different Cultures Feel About Tools That Help People.
- Studying What’s Right and Wrong in Making People Better with Machines.
- Checking What People Think About Keeping Medical Implants Safe.
- Exploring Native Healing Tools.
- Understanding How Different Cultures Deal with Medical Trash.
Materials Science
- Studying Whether Men and Women Are Treated Fairly in Making New Materials.
- Looking at How Different Cultures See Making Things That Last.
- Studying Programs That Teach About Making Stuff.
- Checking What People Think About Keeping Tiny Stuff Safe.
- Exploring How Materials Help in Medicine.
- Understanding How Different Cultures Feel About Reusing Stuff.
- Studying What’s Right and Wrong in Studying Stuff.
- Checking What People Think About Materials That Change.
- Exploring Native Knowledge of How Stuff Works.
- Understanding How Different Cultures Make New Things in Art and Design.
Robotics Qualitative Research Topics for STEM Students
- Studying Whether Boys and Girls Get the Same Chance to Learn About Robots in School and Work.
- Looking at How Different Cultures See People and Machines Talking.
- Studying Programs That Teach About Robots.
- Checking What People Think About Robots Doing Stuff in Everyday Life.
- Exploring How Robots Help in Health Care.
- Understanding How Different Cultures Feel About What’s Right and Wrong for Robots to Do.
- Studying What’s Right and Wrong in Making Robots.
- Checking What People Think About Machines Doing Jobs.
- Exploring Native Ideas on Robots and Machines.
- Understanding How Different Cultures See Using Robots on Farms.
Renewable Energy Research Topics for STEM Students
- Studying Whether Men and Women Get Fair Jobs in Green Energy.
- Looking at How Different Cultures See Making Wind Power.
- Studying Rules for Making Green Energy Happen.
- Checking What People Think About Using Sun Power.
- Exploring How Green Energy Helps Light Up Country Areas.
- Understanding How Different Cultures Feel About Making Energy from Plants.
- Studying What’s Right and Wrong in Making Green Energy Projects.
- Checking What People Think About Making Power from Water.
- Exploring Native Knowledge of Green Power.
- Understanding How Different Cultures Feel About Digging for Heat Energy.
Best Quantitative Research Topics For STEM High School Students
Research topics for grade 11 stem students, research topics for grade 12 stem students, interesting research topics for senior high school stem students.
Also Read : 350+ Most Interesting Research Topics For ABM Students
Best Qualitative Research Topics for STEM Students PDF
10 major differences between qualitative and quantitative research .
Here are 10 major differences between qualitative and quantitative research:
Conclusion
In conclusion, picking the right research topic is important for science students because it lets them explore what interests them, develop their critical thinking skills, and contribute meaningfully to their studies.
The best research topics for science students are those that match their passions and curiosity and also give them chances to try things out, examine things closely, and generate new ideas.
Also, doing this kind of research helps students get better at talking with others, solving problems, and seeing how different fields connect, which helps them prepare for what comes next in school and their careers.
In the end, research like this helps science students keep learning, think hard, and be a positive force in science and technology.
FAQs- Qualitative Research Topics for STEM Students
What should stem students consider when choosing a research topic.
STEM students should consider their interests, career goals, available resources, and the potential impact of their research when choosing a topic. It’s also important to consider ethical considerations and the potential implications of the study.
Are there specific areas within STEM that offer promising research topics?
Yes, several areas within STEM offer promising research topics, including renewable energy, environmental sustainability, healthcare innovations, technology advancements, and interdisciplinary studies at the intersection of different STEM fields.
Can STEM students collaborate on research projects?
Yes, collaborating on research projects is common among STEM students. Collaboration allows students to leverage each other’s strengths, share resources, and foster interdisciplinary approaches to solving complex problems.
Leave a Comment Cancel Reply
Your email address will not be published. Required fields are marked *
Save my name, email, and website in this browser for the next time I comment.
Factors Influencing Student STEM Learning: Self-Efficacy and Outcome Expectancy, 21 st Century Skills, and Career Awareness
- Research Article
- Open access
- Published: 04 May 2021
- Volume 4 , pages 117–137, ( 2021 )
Cite this article
You have full access to this open access article
- Jung Han ORCID: orcid.org/0000-0003-0569-1552 1 ,
- Todd Kelley ORCID: orcid.org/0000-0001-6086-1927 1 &
- J. Geoff Knowles ORCID: orcid.org/0000-0001-6696-058X 2
41k Accesses
29 Citations
1 Altmetric
Explore all metrics
Social, motivational, and instructional factors impact students’ outcomes in STEM learning and their career paths. Based on prior research and expectancy-value theory, the study further explored how multiple factors affect students in the context of integrated STEM learning. High school STEM teachers participated in summer professional development and taught integrated STEM to students during the following school year, where scientific inquiry, biomimicry, 3D printing technology, and engineering design were integrated as instructional strategies. Surveys were conducted to measure teacher self-efficacy and outcome expectancy. Student STEM attitudes (self-efficacy and expectancy-value beliefs), 21st century skills, STEM career awareness, and STEM knowledge achievement were also measured using a survey and a custom-made knowledge test. Based on expectancy-value theory and literature, a path model was developed and tested to investigate causal relationships between these factors. The results revealed direct and indirect effects of teacher self-efficacy and outcome expectancy on students’ STEM knowledge achievements. Student STEM attitudes (self-efficacy and expectancy-value beliefs), 21st century skills, and STEM career awareness also significantly influenced STEM knowledge achievement directly or indirectly.
Similar content being viewed by others
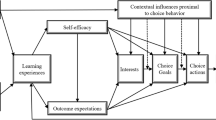
STEM stereotypes predict students’ STEM career interest via self-efficacy and outcome expectations
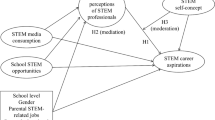
STEM learning opportunities and career aspirations: the interactive effect of students’ self-concept and perceptions of STEM professionals
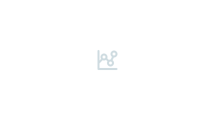
The role of parents’ beliefs in students’ motivation, achievement, and choices in the STEM domain: a review and directions for future research
Avoid common mistakes on your manuscript.
Introduction
The national efforts for advancing science, technology, engineering, and mathematics (STEM) education is becoming stronger as our society demands a global STEM workforce (Asunda, 2012 ; Keirl, 2006 ; Kelley & Knowles, 2016 ; Li et al., 2019 ). To help students enhance their achievements in STEM learning, teachers and educators should create appropriate instructional and social learning contexts and develop strategies that positively influence student learning. For this purpose, understanding factors that influence student STEM learning is imperative.
Social, motivational, and instructional factors greatly influence students’ achievements in STEM learning and their future careers. (Ketenci et al., 2020 ; Nugent et al., 2015 ; Wilson et al., 2015 ; Zeldin et al., 2008 ). Prior studies found that students’ academic achievements can be impacted by domain-specific self-efficacy, attitudes, and motivation (Pajares & Graham, 1999 ; Simon et al., 2014 ; Wiebe et al., 2018 ; Witt-Rose, 2003 ). However, although many studies have investigated the relationships between students’ self-efficacy, motivation, and learning outcomes, few studies were reported on the multiple factors influencing student learning in STEM. In addition, studies in this area typically have been conducted on a single STEM discipline, especially science and mathematics (Wiebe et al., 2018 ). Furthermore, research on how self-efficacy and outcome expectancy of both teachers and students collectively affect student learning outcomes is limited. Therefore, the current study examined multiple factors influencing student STEM learning, which include teacher self-efficacy and outcome expectancy, student STEM attitudes (self-efficacy and expectancy-value beliefs), 21st century skills, and STEM career awareness.
We used expectancy-value theory as a framework to hypothesize the path model. Findings will show the direct and indirect effects of multiple factors on student achievement in the integrated STEM teaching and learning context.
Theoretical framework
The current study is guided by expectancy-value theory (Eccles & Wigfield, 2002 ). Expectancy-value theory has been widely used to explain student performance (Berland & Steingut, 2016 ; Jackson et al., 2019 ; Jones et al., 2010 ). According to Eccles and Wigfield ( 2002 ), “expectancies refer to beliefs about how one will do on different tasks or activities, and values have to do with incentives or reasons for doing the activity” (p. 110). These expectancies and values are related to individual’s achievement, persistence, and choices in academic tasks (Atkinson, 1964 ; Eccles & Wigfield, 2002 ). Therefore, people who have strong beliefs about their competencies of success and efficacy tend to perform better and work on more challenging tasks (Bandura, 1994 ; Eccles & Wigfield, 2002 ).
Wiebe et al. ( 2018 ) stated that “expectancy-value theory helps frame both self-efficacy in terms of expectancies of success in a particular academic domain and outcome expectancy in terms of the value of this academic subject area to future goals” (p. 2). Bandura differentiated between efficacy expectation ( beliefs about what they can do ) and outcome expectation ( beliefs about the likely outcomes of performance ) and noted that both expectations are closely linked to academic outcomes (Trautwein et al., 2012 ). Previous research also revealed a significant relationship between expectancy-value beliefs and academic achievements (Bradley et al., 1999 ; Caraway et al., 2003 ; Nugent et al., 2015; Pajares & Miller, 1994 ; Yoon et al., 2012 ; Wood & Locke, 1987 ; Zimmerman et al., 1992 ). Moreover, many studies found “a dynamic, reciprocal nature of self-efficacy, expectancy outcomes, and academic career goals” (Wiebe et al., 2018 , p. 2). Specifically, Wiebe et al. ( 2018 ) examined the relationships between student attitudes (self-efficacy and expectancy-value beliefs) toward all core STEM subjects and their interests in future STEM careers using the S-STEM survey (Unfried et al., 2015) and found that student attitudes (expectancy-value beliefs) and their career interests are positively associated.
Teacher self-efficacy also has been emphasized as a strong predictor of student outcome and academic achievement (Nadelson et al., 2012 ; Ross, 1992 ; Tschannen-Moran & Barr, 2004 ; Yoon et al., 2012 ). Teachers' beliefs in their abilities to teach and motivate influence “the types of learning environments they create and the level of academic progress their students achieve” (Bandura, 1993 , p. 117), which in turn, significantly influence student STEM interests in future STEM careers (Autenrieth et al., 2018 ; Brophy et al., 2008 ; Kelley et al., 2020 ).
Based on expectancy-value theory and previous research findings, the present study created a hypothesized path model that displays the influence of teacher self-efficacy and outcome expectancy on student STEM attitudes, 21 st century skills, STEM career awareness, and STEM knowledge achievement. The results will show the relationship between these factors and the effects of teacher self-efficacy and outcome expectancy on student learning in integrated STEM.
Literature review
Self-efficacy and outcome expectancy.
Teachers’ self-efficacy can be defined as “teachers’ personal beliefs in their abilities to positively affect students for educational attainments” (Yoon et al., 2012 , p. 26). Prior studies provided empirical evidence that teachers’ beliefs in their teaching efficacy and successful outcome influence students’ self-efficacy, motivation, and performance (Cannon & Scharmann, 1996 ; Ross et al., 2001 ; Rutherford et al., 2017 ). Specifically, research on the relationship between teacher self-efficacy and student outcome in science learning (Bal-Taştan et al., 2018 ; Salgado et al., 2018 ) and mathematics learning (Borko & Whitcomb, 2008 ; Gulistan & Hussain, 2017 ; Perera & John, 2020 ) revealed that teachers’ self-efficacy and expectations significantly impact students’ academic achievement. According to researchers, teacher self-efficacy for successful teaching relates to content knowledge, quality pedagogy, and teaching strategies considerably (Knowles, 2017 , p. 25; Rutherford et al., 2017 ; Stohlmann et al., 2012 ; Yoon et al., 2012 ).
Students’ confidence in their abilities and perceptions of subjective values are also critical factors that influence their performances (Akey, 2006 ; Wigfield & Eccles, 2000 ). Many studies proved the positive association between student self-efficacy and academic success (Henson, 2001 ; Pajares, 1996 ; Reyes, 2010 ). Studies also found that student self-efficacy and expectancy-value beliefs significantly impact their career development and career choices (Ketenci et al., 2020 ; Lent et al., 2010 ; Zeldin et al., 2008 ). Unfried et al. ( 2015 ) used the term attitudes to indicate both self-efficacy and expectancy-value beliefs. They noted that students’ attitudes toward STEM content, as well as their interests in STEM careers and their 21 st century skills, can predict student participation in STEM-related careers. The present study also uses the term attitudes to indicate student self-efficacy in learning STEM content and their expectancy-value beliefs (Unfried et al., 2015 ; Wiebe et al., 2018 ).
21 st century skills
Increasing 21 st century skills through STEM education has been focused among educators (Bybee, 2010 ; Jang, 2016 ; Li et al., 2019 ). 21 st century skills, which include critical thinking, collaboration, creativity, and communication, are necessary skills in the future (International Technology and Engineering Educators Association [ITEEA], 2020 ; Partnership for 21st Century Skills [P21] n.d.). Li et al. ( 2019 ) posited that students can develop thinking skills in a new way in STEM education and that these new thinking skills are connected to 21 st century skills.
21 st century skills range from individual skills to workforce and social skills, which include skills in life and career, media and information, technology, and so on. (Kelley et al., 2019 ). Specifically, National Academy of Engineering (NAE and NRC, 2009 ) proposed engineering habits of mind as essential skills in the 21 st century, which include systems thinking, creativity, optimism, collaboration, communication, and attention to ethical considerations. Similarly, ITEEA ( 2020 ) proposed eight technology and engineering practices adopted from 21st century skills (Partnership for 21st Century Skills [P21], n.d. ) and engineering habits of mind, which include: 1) Systems thinking; 2) Creativity; 3) Making and Doing; 4) Critical thinking; 5) Optimism; 6) Collaboration; 7) Communication; 8) Attention to Ethics.
Contemporary educational standards indicate that students can enhance 21 st century skills and develop confidence through the integration of STEM subjects in project-based instruction. Accordingly, teachers are required to integrate science and engineering practices in their classrooms explicitly for the students to practice real-world problem-solving and increase 21 st century skills (Kelley et al., 2020 ; NGSS Lead States, 2013 ; NRC, 2012 ).
- STEM career awareness
The term career awareness implies “one's own talents and interests or understanding the opportunities and requirements of various career fields” (Braverman et al., 2002 , p. 55). There have been growing efforts to advance STEM education to increase students’ awareness of STEM careers as our society demands a competent STEM workforce (Kier et al., 2014 ; NGSS Lead States, 2013 ). Researchers claim that experiences of STEM practice through STEM education increase students’ interests in STEM-related careers and prepare them for future STEM job opportunities (Li et al., 2019 ; Zuo et al., 2020 ). Especially, as secondary school years are a critical period for students to decide their future careers, high school STEM teachers need to foster students’ STEM career awareness and job interest (Cohen et al., 2013 ).
STEM career-related instruction facilitates students’ interests in STEM learning and helps them be engaged in their learning activities (Salonen et al., 2018 ). To increase STEM career awareness, teachers are recommended to incorporate teaching strategies that students can research and solve real-world problems as scientists and engineers do. In doing so, students can enhance their understanding of the role of STEM in our society (Cohen et al., 2013 ; NGSS Lead States, 2013 ). Particularly, the Next Generation Science Standards (NGSS) present eight science and engineering practices, where students can experience what professional scientists and engineers do. The major practices of science and engineering suggested by the NGSS include: (1) Asking questions and defining problems; (2) Developing and using models; (3) Planning and carrying out investigations; (4) Analyzing and interpreting data; (5) Using mathematics and computational thinking; (6) Constructing explanations and designing solutions; (7) Engaging in argument from evidence; (8) Obtaining, evaluating, and communicating information (NGSS Lead States, 2013 ). By engaging in these science and engineering practices, students can acquire skills and knowledge needed for postsecondary careers, including the STEM field (NGSS Lead States, 2013 ).
The present study
The primary goal of the present study is to identify the factors influencing student STEM learning and determine if teacher self-efficacy and outcome expectancy beliefs affect student attitudes (self-efficacy and expectancy-value beliefs) and academic achievements in integrated STEM. A hypothesized path model was developed based on expectancy-value theory and previous research findings (see Fig. 1 ). The study was guided by two research questions:
Are teacher self-efficacy and outcome expectancy, student STEM attitudes, 21 st century skills, and STEM career awareness positively associated with student STEM knowledge achievement?
Are there any direct and indirect effects of teacher self-efficacy and outcome expectancy on students’ STEM attitudes, 21 st century skills, and STEM career awareness?
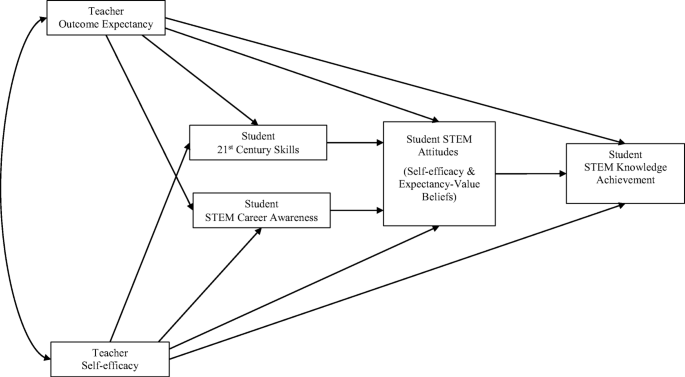
Conceptual model representing the influence of teacher self-efficacy and outcome-expectancy on students’ learning in STEM
Context of the study
The present study was conducted within an integrated STEM project named Teachers and Researchers Advancing Integrated Lessons in STEM (TRAILS) . TRAILS was a three-year-long project funded by the National Science Foundation (Award #DRL-1513248). Researchers, educators, and industry partners cooperated to develop an integrated STEM project and supported high school STEM teachers and their students through a community of practice during the 2016–2019 school years.
The TRAILS project consisted of three cohorts: Cohort 1 was the 2016–2017 school year, Cohort 2 was the 2017–2018 school year, and Cohort 3 was the 2018–2019 school year. Cohort 1–3 high school science teachers and engineering and technology education (ETE) teachers experienced the process of integrating science, technology, engineering, and mathematics into authentic contexts through TRAILS professional development. The participating teachers were selected among the applicants following the criteria: (1) The teachers are required to be high school biology or physics teachers or engineering and technology education (ETE) teachers; (2) The teachers are required to be able to participate in the summer professional development (PD).
A total of 30 STEM teachers (15 science teachers, 15 ETE teachers) were participated in the summer professional development (PD) for two weeks during summer vacation. During the PD, teachers were introduced to an exemplar lesson developed by the research team and learned the lesson from the student’s standpoint. The exemplar lesson, which was named Designing Bugs and Innovative Technology (D-BAIT) , employed biomimicry concepts for designing the fishing lure that mimics the functions of aquatic insects. The teachers also cogenerated their own integrated lessons as a science and engineering technology teacher pair. During the following year, the teachers taught both exemplar lesson D-BAIT and the custom lesson each teacher pair developed in their classrooms.
The D-BAIT unit consists of 10–12 sessions including: (1) entomology introductory lesson; (2) entomology field observation and collection of aquatic insects specimens; (3) analysis of the observed data using scientific inquiry and research on aquatic entomology taxonomy and food webs; (4) introduction to design and engineering design process; (5) introduction to CAD software and 3D printing; (6) design of a fishing lure using the biomimicry concept and mathematical modeling of a prototype (buoyancy concept); (7) testing and redesigning the prototype; and 8) evaluation of prototype lures (Han et al., 2020 , p. 27).
Data collection
The teachers completed the T-STEM survey, which consists of seven subscales including teaching self-efficacy toward educating STEM content and outcome expectancy , before and after the summer professional development. The survey scores of teachers increased after the summer PD (Kelley et al., 2020 ), and with the increased teaching efficacy and expectancy beliefs, they taught students during the following school year. Therefore, to see how teacher efficacy and expectancy affect student learning, we used the posttest scores (teacher scores at the point in time of teaching their students) from the T-STEM survey.
Student data were collected from high school science and ETE (engineering and technology education) students in the state of Indiana, who were enrolled in the 2016–2019 school years and experienced integrated STEM lessons from the TRAILS teachers. Students also took the S-STEM survey, which was developed to measure students’ attitudes toward STEM, 21 st century skills, and STEM career interest, and the D-BAIT STEM knowledge test two times respectively before and after they experienced integrated STEM lessons. As the S-STEM post-survey scores and the D-BAIT STEM knowledge posttest scores reflect student scores after they learned the D-BAIT lesson, these scores were used as student scores for the analysis.
All the surveys were done through the Qualtrics online survey system, and the Institutional Research Board (IRB) approval was obtained in advance.
Final data from the students, who submitted the IRB consent forms from both parents and themselves, are shown in Table 1 .
For the current study, a total of 507 data, which do not include missing data, were used for the analysis.
S-STEM survey
Friday Institute for Educational Innovation ( 2012b ) developed Student Attitudes toward STEM (S-STEM) survey for Elementary level and Middle/High School level. The present study used the S-STEM survey for Middle/High School Student level to measure high school students’ STEM attitudes. The S-STEM survey contains six survey sections. The first three sections ask the students about their attitudes toward math, science, engineering and technology, respectively. The fourth section measures students’ 21st century skills (21 st century learning confidence). The items in the next section ask students about their interests in STEM jobs and their attitudes toward 12 different STEM career areas. The survey items in the first four subscales ask respondents to report their levels of agreement on a five-point Likert-type scale ranging from “strongly disagree” to “strongly agree”. For the items in the fifth subscale, students are asked to rate on a four-point Likert-type scale with 1 being “Not at all interested,” 2 “Not so interested,” 3 “Interested,” and 4 being “Very interested”. While developing the S-STEM survey, Cronbach’s alpha was used to measure internal-consistency reliability for each of the subconstructs. The first four constructs (math attitudes, science attitudes, engineering and technology attitudes, and 21 st century skills) satisfied sufficient levels of reliability, 0.83–0.92, for both Elementary level and secondary level surveys (Unfried et al., 2015 ). Cronbach’s alpha for the fifth subscale, interests in STEM jobs, was not reported (Friday Institute for Educational Innovation, 2012b ). The items in the sixth survey section were not used for the present study. Table 2 summarizes the S-STEM survey.
STEM knowledge test
To measure the STEM knowledge of the students, the D-BAIT knowledge assessment was used. The D-BAIT knowledge test was developed by the TRAILS research team to evaluate students’ STEM knowledge before and after D-BAIT . The D-BAIT knowledge test consists of 20 items within three subject domains: engineering design, physics, and biology. The full score of the STEM knowledge test was 20.
The initial D-BAIT STEM knowledge test was drafted by a panel of six members including an entomology professor, a biology education professor, an engineering technology teacher educator, a two-year technical college faculty, an entomology major graduate student, and a technology major graduate student. The content and face validity of the instrument were checked by two high school biology and engineering technology teachers, who had more than 15 years of teaching experience. Then the instrument was pilot tested with 429 high school students from 18 STEM classrooms. With the results, item analysis was conducted, and the final version of the D-BAIT knowledge test with 20 items was obtained after four items were removed (see Appendix ). After removing four items, the overall Cronbach’s Alpha score of the final version of D-BAIT STEM knowledge test was over .70. The reliability score was also calculated using the adjusted Spearman-Brown prophecy formula (Brown, 1910 ; Spearman, 1910 ), and the score was 0.876.
T-STEM survey
For the measures of teacher self-efficacy and teaching outcome expectancy, the T-STEM Survey for technology (ETE) and science teachers was used (The Friday Institute for Educational Innovation, 2012a ). According to the survey developer, they adopted the existing survey, Science Teaching Efficacy Belief Instrument (STEBI) (Enochs & Riggs, 1990 ), for the Personal Teaching Efficacy and Beliefs (PTEB) construct and the Teaching Outcome Expectancy Beliefs (TOEB) construct. The T-STEM Survey consists of 7 subscales including: (1) teaching self-efficacy toward teaching STEM content (PTEB); (2) teacher’s expectancy on student learning outcome through effective teaching (TOEB); (3) technology use by students; (4) use of STEM instructional practices, (5) teacher attitudes toward 21 st century skills; (6) Teacher leadership attitudes; and (7) STEM career awareness (see Table 3 ).
For the construct reliability, developers calculated Cronbach’s alpha. For the science domain, Cronbach’s alpha for teaching efficacy and outcome expectancy were reported to be .908 and .814, respectively. However, the technology domain Cronbach alpha scores for both self-efficacy and outcome expectancy were not reported (Friday Institute for Educational Innovation T-STEM Survey, 2012a ). Therefore, we calculated Cronbach’s alpha for technology domains with our data, and the results were the following: technology teacher teaching efficacy = .915, technology teacher outcome expectancy = .800.
The survey items used a Likert-type scale with 1 being “Strongly Disagree,” 2 “Disagree,” 3 “Neither Agree Nor Disagree,” 4 “Disagree,” and 5 being “Strong Agree” (The Friday Institute for Educational Innovation, 2012a ). Table 3 demonstrates the summary of the T-STEM Survey.
Data analysis process
The first subscale, Teacher Self-efficacy , in the T-STEM survey consists of 11 Likert-style items, and the second subscale, Teaching Outcome Expectancy Beliefs , consists of 9 Likert-style items. All items ranged from 1 (Strongly Disagree) to 5 (Strongly Agree) points. The S-STEM survey for students consists of 49 Likert-style items with five subconstructs: math attitudes, science attitudes, engineering and technology attitudes, 21 st century skills, and STEM career awareness of their future. Each item’s score in the first four subconstructs ranged from 1(Strongly Disagree) to 5 (Strongly Agree). The items in the fifth subconstruct (career awareness) ranged from 1 (Not at all Interest) to 4 (Very Interest) points (The Friday Institute for Educational Innovation, 2012b ).
For each teacher’s T-STEM score and student’s S-STEM survey subscale scores, the researchers added the values across the questions for each respondent and treated the summed score as each individual’s score. As the context of the present study was integrated STEM, and the students experienced integrated STEM teaching and learning, score sums of math attitudes, science attitudes, and technology attitudes in the S-STEM survey were combined to be used as student STEM attitudes score. Each student’s ratings on the 21 st century skills items and career awareness items - subscales in the S-STEM survey- were summed to be used as student 21 st century skills score and STEM career awareness score, respectively. Each teacher’s ratings on the self-efficacy questionnaire, the first subconstruct in the T-STEM survey, and Teaching Outcome Expectancy Beliefs, the second subconstruct in the T-STEM survey, were also summed to be used as teacher scores (Teacher Self-efficacy & Outcome Expectancy).
Some scores (responses to the negative statements) were reversed in advance, and teacher scores were matched to their students. Table 4 shows all the variables and the full scores.
Data analysis method
Path analysis is known to be a useful method for identifying relationships among a set of variables as the structural model (path diagram) depicts a visual representation of relationships among variables. The procedure produces direct, indirect, and total effects represented by standardized coefficients. (Callaghan et al., 2018 ; Stage et al., 2004 ). We used the SPSS AMOS 26 software to test the hypothesized path model to investigate causal relationships between factors that could affect student learning in STEM. The path model was developed based on expectancy-value theory and previous research.
Table 5 shows the descriptive statistics of the data, and Table 6 displays correlations between the variables. Fig. 2 depicts the relationships among the factors that affect student knowledge achievement directly and indirectly in integrated STEM learning.
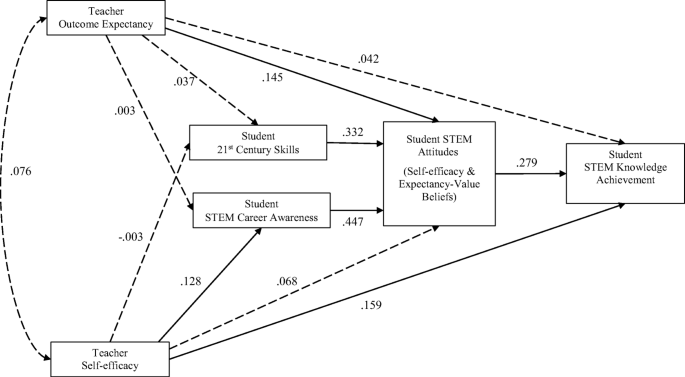
Path model of integrated STEM learning.
The test of the path model showed that the model was overall acceptable: χ2 (1) = 23.225, p < .001 ; Comparative Fit Index (CFI) = .940; Incremental Fit Index (IFI) = .942 ; Tucker-Lewis index (TLI) = .70; Root Mean Square Error of Approximation (RMSEA) = .115.
As Fig. 2 illustrates, teacher self-efficacy and outcome expectancy directly and indirectly affects student STEM knowledge achievement. The standardized direct effect of teacher self-efficacy on student STEM knowledge was .159 ( p < .001). The standardized indirect effect of teacher self-efficacy and teacher outcome expectancy on student STEM knowledge achievement was .035 ( p = .009) and .044 ( p = .002), respectively. Additionally, student STEM attitudes showed direct effects on student knowledge achievement ( B = .279, p < .001) while student 21 st century skills ( B = .093, p = .002) and STEM career awareness ( B = .125, p = .003) influenced STEM knowledge achievement indirectly when mediated by STEM attitudes. All significant direct and indirect effects were indicated in Table 7 .
Note: Standardized estimates. Solid line path coefficients are significant at p < .05 while the dotted line path coefficients are nonsignificant
The study investigated how multiple factors of both students and teachers influence students’ STEM learning with the two guiding questions as the following.
Are teacher self-efficacy and outcome expectancy, student STEM attitudes, 21st century skills, and STEM career awareness positively associated with student STEM knowledge achievement?
Are there any direct and indirect effects of teacher self-efficacy and outcome expectancy on students’ STEM attitudes, 21st century skills, and STEM career awareness?
For the first research question, the results reveal significant direct effects of teacher self-efficacy on students’ STEM knowledge achievement ( B = .159, p < .001). Student STEM attitudes also significantly influenced student STEM knowledge achievement ( B = .279, p < .001). Even though no significant direct effects of teacher outcome expectancy on student STEM knowledge achievement were found, it indirectly influenced student achievement by affecting their STEM attitudes. Additionally, indirect effects of 21st century skills (.093) and STEM career awareness (.125) on STEM knowledge achievement were found to be significant when mediated by STEM attitudes.
For the second research question, teacher outcome expectancy show significant direct effect on student STEM attitudes ( B = .145, p < .001). Teacher self-efficacy show significant direct effect on student STEM career awareness ( B = .128, p < .001) and indirect effect on STEM attitudes (.056). Both teacher self-efficacy and outcome expectancy did not show significant effects on student 21 st century skills.
The findings of this study indicate that self-efficacy and expectancy-value beliefs are critical for both teachers and students in teaching and learning integrated STEM. The standardized path diagram depicts the collective effects of teacher factors and student factors on student STEM attitudes and knowledge achievement (See Fig. 2 and Table 7 ).
Aiming to identity the factors that influence students’ learning in STEM, the current study investigated the relationships among teacher self-efficacy and outcome expectancy, student STEM attitudes (self-efficacy and expectancy-value beliefs), 21 st century skills, and STEM career awareness in an integrated STEM education context. According to the findings, the current study reinforces previous literature that teachers’ self-efficacy and expectancy beliefs are critical factors for enhancing students’ attitudes and performance, which sheds light on the importance of the teachers’ roles in student learning.
Integrating different subjects into one project is a relatively new way of teaching and learning. Consequently, the effect of teachers self-efficacy and student attitudes (self-efficacy and expectancy-value beliefs) on students’ achievement in an integrated STEM context was not researched as much as that of in general classrooms. The findings of the current study are consistent with the previous literature, which found that teachers’ beliefs in their teaching efficacy and success are strong predictors of students’ self-efficacy, motivation, and academic performance (Cannon & Scharmann, 1996 ; Muijs & Reynolds, 2015 ; Podell & Soodak, 1993 ; Ross, 1992 ; Ross et al., 2001 ; Rutherford et al., 2017 ; Shahzad & Naureen, 2017 ; Tschannen-Moran & Barr, 2004 ; Yoon et al., 2012 ). This result indicates the significance of educating teachers since teacher self-efficacy for successful teaching relates to content knowledge, quality pedagogy, and teaching strategies considerably (Knowles, 2017 , p. 25; Rutherford et al., 2017 ; Stohlmann et al., 2012 ; Yoon et al., 2012 ). Through professional development, teachers can construct a community of practice, where they could enhance knowledge, instructional skills, and pedagogical approaches (Kelley et al., 2020 ; Knowles et al., 2018 ).
Additionally, the present study draws attention to the importance of affective domains in STEM education (ITEEA, 2020 ; NGSS Lead States, 2013 ). Affective domain includes attitudes, interest, motivation, social skills, and so on, and researchers and instructional developers have been claimed to include affective domain in curriculum and instruction. However, the way of placing an affective domain within a curriculum can be different depending on the context, and many teachers lack attention to an affective domain (Hansen, 2009 ; Reigeluth, 1999 ). Therefore, teacher training programs that prepare teachers to teach students affective skills are recommended. For example, project-based instructions help students develop social and interpersonal skills (Hansen, 2009 ; Li et al., 2019 ). By learning how to incorporate project-based instruction in their teaching, teachers can enhance students’ attitudes, self-efficacy beliefs, and motivation to learn (Abdullah et al., 2010 ; Markham, 2011 ; Mataka & Kowalske, 2015 ),
To teach integrated STEM, further research is needed to develop instructional strategies which are “focusing teaching and learning across all three domains of learning: cognitive, affective, and psychomotor” (ITEEA, 2020 , p. 4; Griffith & Nguyen, 2006 ). Since integrated STEM education involves complex teaching strategies and requires insights into students’ educational and psychological needs, which are different from general education, further discussions based on more empirical research are required.
Implication
The current study provides some theoretical and practical implications. First, the present study contributes to the research in expectancy-value theory framework with empirical evidence. Consistent with previous studies, the current study demonstrates that self-efficacy and outcome expectancy of both teachers and students are significant predictors of student STEM knowledge achievement as a direct factor or a mediator (Bradley et al., 1999 ; Caraway et al., 2003 ; Nadelson et al., 2012 ; Nugentet et al., 2015; Ross, 1992 ; Tschannen-Moran & Barr, 2004 ; Pajares & Miller, 1994 ; Yoon et al., 2012 ; Wood & Locke, 1987 ; Zimmerman et al., 1992 ). As noted earlier, teachers’ self-efficacy and beliefs can influence the successful outcome of students’ performance (Bal-Taştan et al., 2018 ; Borko & Whitcomb, 2008 ; Gulistan & Hussain, 2017 ; Perera & John, 2020 ; Salgado et al., 2018 ), and students with strong competencies of success and efficacy beliefs tend to perform more challenging tasks and succeed more frequently (Bandura, 1994 ; Eccles & Wigfield, 2002 ). This finding confirms expectancy-value theory that expectations and task-value beliefs are linked to the achievement-related choice and performance of individuals (Eccles & Wigfield, 2002 ).
Second, this study indicates that students’ STEM knowledge achievement is influenced not only by a single factor but also by multiple factors of both teachers and students. Although many studies have investigated the relationships between students’ self-efficacy, motivation, and learning outcomes, few studies were reported on the multiple factors influencing student learning in STEM (Wiebe et al., 2018 ). Therefore, this study may provide implications by adding empirical evidence to the prior research. Specifically, as the path model illustrates, teacher self-efficacy and outcome expectancy are linked to student achievement directly or indirectly through student career interests and attitudes. Moreover, students’ 21 st century learning confidence and interests in future STEM careers significantly influenced their attitudes toward STEM, which in turn affected their academic achievement in STEM. Even though no significant direct effects of students’ STEM career awareness and 21 st century skills on their STEM knowledge achievement were found, indirect effects of STEM career awareness and 21 st century skills mediated by STEM attitudes (student self-efficacy and outcome expectancy) were detected. These results imply that multiple factors interplay and finally affect student STEM knowledge achievement collectively.
Finally, the present study focused on all core STEM disciplines: science, technology, engineering, and mathematics. As prior studies of the relationship between motivation and achievement have been conducted mostly on science or mathematics alone, the present study addresses the gap in this area by using the S-STEM survey focusing on student attitudes (expectancy-value beliefs) toward all STEM subjects (Wiebe et al., 2018 ).
This study has some limitations. Although construct validities of the instruments were confirmed, the respondents’ honesty, which is required for self-report surveys, cannot be verified. Additionally, as the current study investigated the relationships between the factors of both teachers and students, teacher career awareness and 21 st confidence may also need to be considered to draw conclusions that better discuss the findings. Finally, the variables of student STEM attitudes include both self-efficacy and expectancy-value beliefs while teacher variables include teacher self-efficacy and outcome expectancy separately. Following the instrument developers and previous studies, we used STEM attitudes , which indicate “a composite of both self-efficacy and expectancy-value beliefs” (Unfried et al., 2015 , p. 23; Wiebe et al., 2018). This may not fully explain the effect of each specific factor, self-efficacy and expectancy-value beliefs.
Abdullah, N. I., Tarmizi, R. A., & Abu, R. (2010). The effects of problem based learning on mathematics performance and affective attributes in learning statistics at form four secondary level. Procedia-Social and Behavioral Sciences, 8 , 370–376. https://doi.org/10.1016/j.sbspro.2010.12.052 .
Article Google Scholar
Akey, T. M. (2006). School context, student attitudes and behavior, and academic achievement: An exploratory analysis. MDRC.
Asunda, P. (2012). Standards for technological literacy and STEM education delivery through career and technical education programs. Journal of Technology Education, 23 ( 2 ), 44.
Atkinson, J.W. (1964). An introduction to motivation. Van Nostrand.
Autenrieth, R., Lewis, C., & Butler-Purry, K. (2018). Enrichment experiences in engineering (E3) summer teacher program: Analysis of student surveys regarding engineering awareness. Journal of STEM Education: Innovations and Research , 19 (4).
Bal-Taştan, S., Davoudi, S. M. M., Masalimova, A. R., Bersanov, A. S., Kurbanov, R. A., Boiarchuk, A. V., & Pavlushin, A. A. (2018). The impacts of teacher’s efficacy and motivation on student’s academic achievement in science education among secondary and high school students. EURASIA Journal of Mathematics, Science and Technology Education, 14 (6), 2353–2366. https://doi.org/10.29333/ejmste/89579 .
Bandura, A. (1993). Perceived self-efficacy in cognitive development and functioning. Educational Psychologist, 28 (2), 117–148. https://doi.org/10.1207/s15326985ep2802_3 .
Bandura, A. (1994). Self-efficacy. In Ramachaudran, V. S. (Ed.), Encyclopedia of human behavior (Vol. 4, pp. 71-81). New York: Academic Press. (Reprinted in H. Friedman [Ed.], Encyclopedia of mental health . San Diego: Academic Press, 1998).
Berland, L. K., & Steingut, R. (2016). Explaining variation in student efforts towards using math and science knowledge in engineering contexts. International Journal of Science Education, 38 (18), 2742–2761. https://doi.org/10.1080/09500.693.2016.12601.79 .
Borko, H., & Whitcomb, J. A. (2008). Teachers, teaching, and teacher education: Comments of the National Mathematics Advisory Panel's Report. Educational Researcher, 37 , 565–572. https://doi.org/10.3102/0013189X08328877 .
Bradley, J. C., Waliczek, T. M., & Zajicek, J. M. (1999). Relationship between environmental knowledge and environmental attitudes of high school students. The Journal of Environmental Education, 30 (3), 17–21.
Braverman, M. T., Young, J., King, N., Paterson, C. A., & Weisskirch, R. S. (2002). Career awareness and part-time work examined in lives of high school seniors. California Agriculture, 56 (2), 55–60. https://doi.org/10.3733/ca.v056n02p55 .
Brophy, S., Klein, S., Portsmore, M., & Rogers, C. (2008). Advancing engineering education in P-12 classrooms. Journal of Engineering Education, 97 (3), 369–387. https://doi.org/10.1002/j.2168-9830.2008.tb00985.x .
Brown, W. (1910). Some experimental results in the correlation of mental abilities. British Journal of Psychology, 3 , 296–322.
Google Scholar
Bybee, R. W. (2010). Advancing STEM education: A 2020 vision. Technology and engineering teacher, 70 (1), 30.
Callaghan, M. N., Long, J. J., Van Es, E. A., Reich, S. M., & Rutherford, T. (2018). How teachers integrate a math computer game: Professional development use, teaching practices, and student achievement. Journal of Computer Assisted Learning, 34 (1), 10–19. https://doi.org/10.1111/jcal.12209 .
Cannon, J. R., & Scharmann, L. C. (1996). Influence of a cooperative early field experience on preservice elementary teachers’ science self-efficacy. Science Education, 80 (4), 419–436. https://doi.org/10.1002/(SICI)1098-237X(199607)80:4<419::AID-SCE3>3.0.CO;2-G
Caraway, K., Tucker, C. M., Reinke, W. M., & Hall, C. (2003). Self-efficacy, goal orientation, and fear of failure as predictors of school engagement in high school students. Psychology in the Schools, 40 ( 4 ), 417–427.
Cohen, C., Patterson, D. G., Kovarik, D. N., & Chowning, J. T. (2013). Fostering STEM career awareness: emerging opportunities for teachers. WA State Kappan, 6 , 1–17.
Eccles, J. S., & Wigfield, A. (2002). Motivational beliefs, values, and goals. Annual Review of Psychology, 53 (1), 109–132. https://doi.org/10.1146/annurev.psych.53.100901.135153 .
Enochs, L. G., & Riggs, I. M. (1990). Further development of an elementary science teaching efficacy belief instrument: A preservice elementary scale . Proceedings of the National Association of Research in Science Teaching, Atlanta .
Friday Institute for Educational Innovation. (2012a). Teacher Efficacy and Attitudes toward STEM (T-STEM) survey: Development and psychometric properties . North Carolina State University Retrieved from: https://miso.fi.ncsu.edu/articles/t-stem-survey . Accessed 1 Apr 2021.
Friday Institute for Educational Innovation. (2012b). Student Attitudes toward STEM Survey – Middle and High School Students . North Carolina State University Retrieved from: https://miso.fi.ncsu.edu/articles/s-stem-survey . Accessed 7 Apr 2021.
Griffith, K. G., & Nguyen, A. D. (2006). Are educators prepared to affect the affective domain? National forum of teacher education journal, 16 (3), 1–4.
Gulistan, M., & Hussain, A. (2017). Relationship between mathematics teachers’ self efficacy and students’ academic achievement at secondary level. Bulletin of Education & Research, 39 (3), 171–182.
Han, J., Kelley, T. R., Bartholomew, S., & Knowles, J. G. (2020). Sharpening STEL with integrated STEM. Technology and Engineering Teacher, 80 (3), 24–29 Retrieved from https://search.proquest.com/scholarly-journals/sharpening-stel-with-integrated-stem/docview/2458779085/se-2?accountid=13360 . Accessed 7 Apr 2021.
Hansen, K. (2009). Strategies for developing effective teaching skills in the affective domain. Strategies, 23 (1), 14–19. https://doi.org/10.1080/08924562.2009.10590853 .
Henson, R. K. (2001). The effects of participation in teacher research on teacher efficacy. Teaching and Teacher Education, 17 , 819–836. https://doi.org/10.1016/S0742-051X(01)00033-6 .
International Technology and Engineering Educators Association [ITEEA]. (2020). Standards for technological and engineering literacy: Defining the role of technology and engineering in STEM education . Author.
Jackson, A., Mentzer, N., & Kramer-Bottiglio, R. (2019). Pilot analysis of the impacts of soft robotics design on high-school student engineering perceptions. International Journal of Technology and Design Education, 29 (5), 1083–1104. https://doi.org/10.1007/s10798-018-9478-8 .
Jang, H. (2016). Identifying 21st century STEM competencies using workplace data. Journal of Science Education and Technology, 25 (2), 284–301. https://doi.org/10.1007/s10956-015-9593-1 .
Jones, B. D., Paretti, M. C., Hein, S. F., & Knott, T. W. (2010). An analysis of motivation constructs with first-year engineering students: Relationships among expectancies, values, achievement, and career plans. Journal of Engineering Education, 99 , 319–336. https://doi.org/10.1002/j.2168-9830.2010.tb0106.x .
Keirl, S. (2006). Ethical technological literacy as democratic curriculum keystone. In In Defining Technological Literacy Towards an Epistemological Framework (pp. 81–102). Palgrave Macmillan.
Kelley, T. R., & Knowles, J. G. (2016). A conceptual framework for integrated STEM education. International Journal of STEM Education, 3 (1), 1–11. https://doi.org/10.1186/s40594-016-0046-z .
Kelley, T. R., Knowles, J. G., Han, J., & Sung, E. (2019). Creating a 21st century skills survey instrument for high school students. American Journal of Educational Research, 7 (8), 583–590 10.12691/education-7-8-7.
Kelley, T. R., Knowles, J. G., Holland, J. D., & Han, J. (2020). Increasing high school teachers self-efficacy for integrated STEM instruction through a collaborative community of practice. International Journal of STEM Education, 7 , 1–13. https://doi.org/10.1186/s40594-020-00211-w .
Ketenci, T., Leroux, A., & Renken, M. (2020). Beyond student factors: A study of the impact on STEM career attainment. Journal for STEM Education Research, 1-19. https://doi.org/10.1007/s41979-020-00037-9 .
Kier, M. W., Blanchard, M. R., Osborne, J. W., & Albert, J. L. (2014). The development of the STEM career interest survey (STEM-CIS). Research in Science Education, 44 (3), 461–481 https://doi.org/10.1007/s11165-013-9389-3 .
Knowles, J. G. (2017). Impacts of professional development in integrated STEM education on teacher self-efficacy, outcome expectancy, and STEM career awareness . Purdue University Doctoral Dissertation Retrieved from https://docs.lib.purdue.edu/techdissertations/4/ . Accessed 7 Apr 2021.
Knowles, J., Kelley, T., & Holland, J. (2018). Increasing teacher awareness of STEM careers. Journal of STEM Education, 19 (3), 47–55.
Lent, R. W., Sheu, H., Gloster, C. S., & Wilkins, G. (2010). Longitudinal test of the social cognitive model of choice in engineering students at historically Black universities. Journal of Vocational Behavior, 76 (3), 387–394.
Li, Y., Schoenfeld, A. H., diSessa, A. A., Graesser, A. C., Benson, L. C., English, L. D., & Duschl, L. D. (2019). On Thinking and STEM education. Journal for STEM Education Research, 2 , 1–13 https://doi.org/10.1007/s41979-019-00014-x .
Markham, T. (2011). Project based learning a bridge just far enough. Teacher Librarian, 39 (2), 38 https://search.proquest.com/magazines/project-based-learning-bridge-just-far-enough/docview/915254354/se-2?accountid=13360 . Accessed 7 Apr 2021.
Mataka, L. M., & Kowalske, M. G. (2015). The influence of PBL on students' self-efficacy beliefs in chemistry. Chemistry Education Research and Practice, 16 (4), 929–938. https://doi.org/10.1039/C5RP00099H .
Muijs, D., & Reynolds, D. (2015). Teachers’ beliefs and behaviors: What really matters? Journal of Classroom Interaction, 50 (1), 25–40.
Nadelson, L., Seifert, A., Moll, A., & Coats, B. (2012). i-STEM summer institute: An integrated approach to teacher professional development in STEM. Journal of STEM Education, 13 (2), 69–83.
National Academy of Engineering and National Research Council [NAE & NRC]. (2009). Engineering in K-12 education: Understanding the status and improving the prospects . National Academies Press.
National Research Council. (2012). A framework for K-12 science education: Practices, crosscutting concepts, and core ideas . National Academies Press.
NGSS Lead States. (2013). Next generation science standards . National Academies Press.
Nugent, G., Barker, B., Welch, G., Grandgenett, N., Wu, C., & Nelson, C. (2015). A model of factors contributing to STEM learning and career orientation. International Journal of Science Education, 37 (7), 1067–1088.
Pajares, F. (1996). Self-efficacy beliefs and mathematical problem-solving of gifted students. Contemporary Educational Psychology, 21 (4), 325–344. https://doi.org/10.1006/ceps.1996.0025 .
Pajares, F., & Graham, L. (1999). Self-efficacy, motivation constructs, and mathematics performance of entering middle school students. Contemporary Educational Psychology, 24 (2), 124–139. https://doi.org/10.1006/ceps.1998.0991 .
Pajares, F., & Miller, M. D. (1994). Role of self-efficacy and self-concept beliefs in mathematical problem solving: A pathanalysis. Journal of Educational Psychology, 86 , 193–203.
Partnership for 21st Century Skills. (n.d.) Retrieved from: http://www.p21.org/framework . Accessed 7 Apr 2021.
Perera, H. N., & John, J. E. (2020). Teachers’ self-efficacy beliefs for teaching math: Relations with teacher and student outcomes. Contemporary Educational Psychology, 61 , 101842. https://doi.org/10.1016/j.cedpsych.2020.101842 .
Podell, D. M., & Soodak, L. C. (1993). Teacher efficacy and bias in special education referrals. The Journal of Educational Research, 86 (4), 247–253. https://doi.org/10.1080/00220671.1993.9941836 .
Reigeluth, C. M. (1999). Affective education and the affective domain: Implications for instructional-design theories and models. In Instructional-design Theories and Models (pp. 497-522). Routledge.
Reyes, D. A. (2010). Academic performance and self efficacy of Filipino high school students on mathematics and english subjects. Retrieved from https://doi.org/10.2139/ssrn.2152791
Ross, J. A. (1992). Teacher efficacy and the effects of coaching on student achievement. Canadian Journal of Education/Revue canadienne de l'education, 51-65.
Ross, J. A., Hogaboam-Gray, A., & Hannay, L. (2001). Effects of teacher efficacy on computer skills and computer cognitions of Canadian students in grades K-3. The Elementary School Journal, 102 (2), 141–156.
Rutherford, T., Long, J. J., & Farkas, G. (2017). Teacher value for professional development, self-efficacy, and student outcomes within a digital mathematics intervention. Contemporary Educational Psychology, 51 , 22–36. https://doi.org/10.1016/j.cedpsych.2017.05.005 .
Salgado, R., Mundy, M. A., Kupczynski, L., & Challoo, L. (2018). Effects of teacher efficacy, certification route, content hours, experiences and class size on student achievement. Journal of Instructional Pedagogies, 21 .
Salonen, A., Kärkkäinen, S., & Keinonen, T. (2018). Career-related instruction promoting students’ career awareness and interest towards science learning. Chemistry Education Research and Practice, 19 (2), 474–483. https://doi.org/10.1039/C7RP00221A .
Shahzad, K., & Naureen, S. (2017). Impact of teacher self-efficacy on secondary school students’ academic achievement. Journal of Education and Educational Development, 4 (1), 48–72.
Simon, R. A., Aulls, M. W., Dedic, H., Hubbard, K., & Hall, N. C. (2014). Exploring student persistence in STEM programs: A motivational model. Canadian Journal of Education, 38 (1), 1–27.
Spearman, C. (1910). Correlation calculated from faulty data. British Journal of Psychology, 3 , 271–295.
Stage, F. K., Carter, H. C., & Nora, A. (2004). Path analysis: An introduction and analysis of a decade of research. The Journal of Educational Research, 98 (1), 5–13 https://doi-org.ezproxy.lib.purdue.edu/10.3200/JOER.98.1.5-13 .
Stohlmann, M., Moore, T. J., & Roehrig, G. H. (2012). Considerations for teaching integrated STEM education. Journal of Pre-College Engineering Education Research (J-PEER), 2 (1), 23–34. https://doi.org/10.5703/1288284314653 .
Trautwein, U., Marsh, H. W., Nagengast, B., Lüdtke, O., Nagy, G., & Jonkmann, K. (2012). Probing for the multiplicative term in modern expectancy-value theory: A latent interaction modeling study. Journal of Educational Psychology, 104 (3), 763–777. https://doi.org/10.1037/a0027470 .
Tschannen-Moran, M., & Barr, M. (2004). Fostering student learning: The relationship of collective teacher efficacy and student achievement. Leadership and Policy in Schools, 3 ( 3 ), 189–209.
Unfried, A., Faber, M., Stanhope, D. S., & Wiebe, E. (2015). The development and validation of a measure of student attitudes toward science, technology, engineering, and math (S-STEM). Journal of Psychoeducational Assessment, 33 (7), 622–639.
Wiebe, E., Unfried, A., & Faber, M. (2018). The relationship of STEM attitudes and career interest. EURASIA Journal of Mathematics, Science and Technology Education, 14 (10), em1580. https://doi.org/10.29333/ejmste/92286 .
Wigfield, A., & Eccles, J. S. (2000). Expectancy–value theory of achievement motivation. Contemporary Educational Psychology, 25 ( 1 ), 68–81.
Wilson, D., Jones, D., Bocell, F., Crawford, J., Kim, M. J., Veilleux, N., Floyd-Smith, T., Bates, R., & Plett, M. (2015). Belonging and academic engagement among undergraduate STEM students: A multi-institutional study. Research in Higher Education, 56 (7), 750–776.
Witt-Rose, D. (2003). Student self-efficacy in college science: An investigation of gender, age, and academic achievement . (Unpublished Master’s Thesis). University of Wisconsin-Stout.
Wood, R. E., & Locke, E. A. (1987). The relation of self-efficacy and grade goals to academic performance. Educational and Psychological Measurement, 47 , 1013–1024.
Yoon, S., Evans, M., & Strobel, J. (2012). Development of the teaching engineering self-efficacy scale (TESS) for K–12 teachers. In In Proceeding of the American Society of Engineering Education (ASEE) Annual Conference and Exposition . ASEE.
Zeldin, A. L., Britner, S. L., & Pajares, F. (2008). A comparative study of the self-efficacy beliefs of successful men and women in mathematics, science, and technology careers. Journal of Research in Science Teaching, 45 (9), 1036–1058 https://doi.org/10.1002/tea.20195 .
Zimmerman, B. J., Bandura, A., & Martinez-Pons, M. (1992). Self-motivation for academic attainment: The role of self-efficacy beliefs and personal goal setting. American Educational Research Journal, 29 (3), 663–676.
Zuo, H., Ferris, K. A., & LaForce, M. (2020). Reducing racial and gender gaps in mathematics attitudes: Investigating the use of instructional strategies in inclusive STEM high schools. Journal for STEM Educ Res, 3 , 125–146 https://doi.org/10.1007/s41979-019-00021-y .
Download references
Author information
Authors and affiliations.
Department of Technology Leadership Innovation, Purdue University, Lafayette, IN, USA
Jung Han & Todd Kelley
Ivy Tech Community College, Lafayette, IN, USA
J. Geoff Knowles
You can also search for this author in PubMed Google Scholar
Corresponding author
Correspondence to Todd Kelley .
Ethics declarations
Conflict of interest.
On behalf of all authors, the corresponding author states that there is no conflict of interest.
Additional information
Publisher’s note.
Springer Nature remains neutral with regard to jurisdictional claims in published maps and institutional affiliations.
Rights and permissions
Open Access This article is licensed under a Creative Commons Attribution 4.0 International License, which permits use, sharing, adaptation, distribution and reproduction in any medium or format, as long as you give appropriate credit to the original author(s) and the source, provide a link to the Creative Commons licence, and indicate if changes were made. The images or other third party material in this article are included in the article's Creative Commons licence, unless indicated otherwise in a credit line to the material. If material is not included in the article's Creative Commons licence and your intended use is not permitted by statutory regulation or exceeds the permitted use, you will need to obtain permission directly from the copyright holder. To view a copy of this licence, visit http://creativecommons.org/licenses/by/4.0/ .
Reprints and permissions
About this article
Han, J., Kelley, T. & Knowles, J.G. Factors Influencing Student STEM Learning: Self-Efficacy and Outcome Expectancy, 21 st Century Skills, and Career Awareness. Journal for STEM Educ Res 4 , 117–137 (2021). https://doi.org/10.1007/s41979-021-00053-3
Download citation
Accepted : 23 March 2021
Published : 04 May 2021
Issue Date : September 2021
DOI : https://doi.org/10.1007/s41979-021-00053-3
Share this article
Anyone you share the following link with will be able to read this content:
Sorry, a shareable link is not currently available for this article.
Provided by the Springer Nature SharedIt content-sharing initiative
- Integrated STEM education
- Self-efficacy
- STEM attitude
- 21 st century skill
- Find a journal
- Publish with us
- Track your research

60+ Best Quantitative Research Topics for STEM Students: Dive into Data
Embark on a captivating journey through the cosmos of knowledge with our curated guide on Quantitative Research Topics for STEM Students. Explore innovative ideas in science, technology, engineering, and mathematics, designed to ignite curiosity and shape the future.
Unleash the power of quantitative research and dive into uncharted territories that go beyond academics, fostering innovation and discovery.
Hey, you future scientists, tech wizards, engineering maestros, and math superheroes – gather ’round! We’re about to dive headfirst into the rad world of quantitative research topics, tailor-made for the rockstars of STEM.
In the crazy universe of science, technology, engineering, and math (STEM), quantitative research isn’t just a nerdy term—it’s your VIP pass to an interstellar adventure. Picture this: you’re strapping into a rocket ship, zooming through the cosmos, and decoding the universe’s coolest secrets, all while juggling numbers like a cosmic DJ.
But here’s the real scoop: finding the ultimate research topic is like picking the juiciest star in the galaxy. It’s about stumbling upon something so mind-blowing that you can’t resist plunging into the data. It’s about choosing questions that make your STEM-loving heart do the cha-cha.
In this guide, we’re not just your sidekicks; we’re your partners in crime through the vast jungle of quantitative research topics. Whether you’re a rookie gearing up for your first lab escapade or a seasoned explorer hunting for a new thrill, think of this article as your treasure map, guiding you to the coolest STEM discoveries.
From the teeny wonders of biology to the brain-bending puzzles of physics, the cutting-edge vibes of engineering, and the downright gorgeous dance of mathematics – we’ve got your back.
So, buckle up, fellow STEM enthusiasts! We’re setting sail on a cosmic adventure through the groovy galaxy of quantitative research topics. Get ready to unravel the secrets of science and tech, one sizzling digit at a time.
Stick around for a ride that’s part data, part disco, and all STEM swagger!
Table of Contents
Benefits of Choosing Quantitative Research
Embarking on the quantitative research journey is like stepping into a treasure trove of benefits across a spectrum of fields. Let’s dive into the exciting advantages that make choosing quantitative research a game-changer:
Numbers That Speak Louder
Quantitative research deals in cold, hard numbers. This means your data isn’t just informative; it’s objective, measurable, and has a voice of its own.
Statistical Swagger
Crunching numbers isn’t just for show. With quantitative research, statistical tools add a touch of pizzazz, boosting the validity of your findings and turning your study into a credible performance.
For the Masses
Quantitative research loves a crowd. Larger sample sizes mean your discoveries aren’t just for the lucky few – they’re for everyone. It’s the science of sharing the knowledge wealth.
Data Showdown
Ready for a duel between variables? Quantitative research sets the stage for epic battles, letting you compare, contrast, and uncover cause-and-effect relationships in the data arena.
Structured and Ready to Roll
Think of quantitative research like a well-organized party. It follows a structured plan, making replication a breeze. Because who doesn’t love a party that’s easy to recreate?
Data Efficiency Dance
Efficiency is the name of the game. Surveys, experiments, and structured observations make data collection a dance – choreographed, smooth, and oh-so-efficient.
Data Clarity FTW
No decoding needed here. Quantitative research delivers crystal-clear results. It’s like reading a good book without the need for interpretation – straightforward and to the point.
Spotting Trends Like a Pro
Ever wish you had a crystal ball for trends? Quantitative analysis is the next best thing. It’s like having a trend-spotting superpower, revealing patterns that might have otherwise stayed hidden.
Bias Be Gone
Quantitative research takes bias out of the equation. Systematic data collection and statistical wizardry reduce researcher bias, leaving you with results that are as unbiased as a judge at a talent show.
Key Components of a Quantitative Research Study
Launching into a quantitative research study is like embarking on a thrilling quest, and guess what? You’re the hero of this research adventure! Let’s unravel the exciting components that make your study a blockbuster:
Quest-Starter: Research Question or Hypothesis
It’s your “once upon a time.” Kick off your research journey with a bang by crafting a captivating research question or hypothesis. This is the spark that ignites your curiosity.
Backstory Bonanza: Literature Review
Think of it as your research Netflix binge. Dive into existing literature for the backstory. It’s not just research – it’s drama, plot twists, and the foundation for your epic tale.
Blueprint Brilliance: Research Design
Time to draw up the plans for your study castle. Choose your research design – is it a grand experiment or a cunning observational scheme? Your design is the architectural genius behind your research.
Casting Call: Population and Sample
Who’s in your star-studded lineup? Define your dream cast – your target population – and then handpick a sample that’s ready for the research red carpet.
Gear Up: Data Collection Methods
Choose your research tools wisely – surveys, experiments, or maybe a bit of detective work. Your methods are like the gadgets in a spy movie, helping you collect the data treasures.
The Numbers Game: Variables and Measures
What’s in the spotlight? Identify your main characters – independent and dependent variables. Then, sprinkle in some measures to add flair and precision to your study.
Magic Analysis Wand: Data Analysis Techniques
Enter the wizardry zone! Pick your magic wand – statistical methods, tests, or software – and watch as it unravels the mysteries hidden in your data.
Ethical Superhero Cape: Ethical Considerations
Every hero needs a moral compass. Clearly outline how you’ll be the ethical superhero of your study, protecting the well-being and secrets of your participants.
Grand Finale: Results and Findings
It’s showtime! Showcase your results like the grand finale of a fireworks display. Tables, charts, and statistical dazzle – let your findings steal the spotlight.
Wrap-Up Party: Conclusion and Implications
Bring out the confetti! Summarize your findings, discuss their VIP status in the research world, and hint at the afterparty – how your results shape the future.
Behind-the-Scenes Blooper Reel: Limitations and Future Research
No Hollywood film is perfect. Share the bloopers – the limitations of your study – and hint at the sequel with ideas for future research. It’s all part of the cinematic journey.
Roll Credits: References
Give a shout-out to the supporting cast! Cite your sources – it’s the credits that add credibility to your blockbuster.
Bonus Scene: Appendix
Think of it as the post-credits scene. Tuck in any extra goodies – surveys, questionnaires, or behind-the-scenes material – for those eager to dive deeper into your research universe.
By weaving these storylines together, your quantitative research study becomes a cinematic masterpiece, leaving a lasting impact on the grand stage of academia. Happy researching, hero!
Quantitative Research Topics for STEM Students
Check out the best quantitative research topics for STEM students:-
- Investigating the Effects of Different Soil pH Levels on Plant Growth.
- Analyzing the Impact of Pesticide Exposure on Bee Populations.
- Studying the Genetic Variability in Endangered Species.
- Quantifying the Relationship Between Temperature and Microbial Growth in Water.
- Analyzing the Effects of Ocean Acidification on Coral Reefs.
- Investigating the Correlation Between Pollinator Diversity and Crop Yield.
- Studying the Role of Gut Microbiota in Human Health and Disease.
- Quantifying the Impact of Antibiotics on Soil Microbial Communities.
- Analyzing the Effects of Light Pollution on Nocturnal Animal Behavior.
- Investigating the Relationship Between Altitude and Plant Adaptations in Mountain Ecosystems.
- Measuring the Speed of Light Using Interferometry Techniques.
- Investigating the Quantum Properties of Photons in Quantum Computing.
- Analyzing the Factors Affecting Magnetic Field Strength in Electromagnets.
- Studying the Behavior of Superfluids at Ultra-Low Temperatures.
- Quantifying the Efficiency of Energy Transfer in Photovoltaic Cells.
- Analyzing the Properties of Quantum Dots for Future Display Technologies.
- Investigating the Behavior of Particles in High-Energy Particle Accelerators.
- Studying the Effects of Gravitational Waves on Space-Time.
- Quantifying the Frictional Forces on Objects at Different Surfaces.
- Analyzing the Characteristics of Dark Matter and Dark Energy in the Universe.
Engineering
- Optimizing the Design of Wind Turbine Blades for Maximum Efficiency.
- Investigating the Use of Smart Materials in Structural Engineering.
- Analyzing the Impact of 3D Printing on Prototyping in Product Design.
- Studying the Behavior of Composite Materials Under Extreme Temperatures.
- Evaluating the Efficiency of Water Treatment Plants in Removing Contaminants.
- Investigating the Aerodynamics of Drones for Improved Flight Control.
- Quantifying the Effects of Traffic Flow on Roadway Maintenance.
- Analyzing the Impact of Vibration Damping in Building Structures.
- Studying the Mechanical Properties of Biodegradable Polymers in Medical Devices.
- Investigating the Use of Artificial Intelligence in Autonomous Robotic Systems.
Mathematics
- Exploring Chaos Theory and Its Applications in Nonlinear Systems.
- Modeling the Spread of Infectious Diseases in Population Dynamics.
- Analyzing Data Mining Techniques for Predictive Analytics in Business.
- Studying the Mathematics of Cryptography Algorithms for Data Security.
- Quantifying the Patterns in Stock Market Price Movements Using Time Series Analysis.
- Investigating the Applications of Fractal Geometry in Computer Graphics.
- Analyzing the Behavior of Differential Equations in Climate Modeling.
- Studying the Optimization of Supply Chain Networks Using Linear Programming.
- Investigating the Mathematical Concepts Behind Machine Learning Algorithms.
- Quantifying the Patterns of Prime Numbers in Number Theory.
- Investigating the Chemical Mechanisms Behind Enzyme Catalysis.
- Analyzing the Thermodynamic Properties of Chemical Reactions.
- Studying the Kinetics of Chemical Reactions in Different Solvents.
- Quantifying the Concentration of Pollutants in Urban Air Quality.
- Evaluating the Effectiveness of Antioxidants in Food Preservation.
- Investigating the Electrochemical Properties of Batteries for Energy Storage.
- Studying the Behavior of Nanomaterials in Drug Delivery Systems.
- Analyzing the Chemical Composition of Exoplanet Atmospheres Using Spectroscopy.
- Quantifying Heavy Metal Contamination in Soil and Water Sources.
- Investigating the Correlation Between Chemical Exposure and Human Health.
Computer Science
- Analyzing Machine Learning Algorithms for Natural Language Processing.
- Investigating Quantum Computing Algorithms for Cryptography Applications.
- Studying the Efficiency of Data Compression Methods for Big Data Storage.
- Quantifying Cybersecurity Threats and Vulnerabilities in IoT Devices.
- Evaluating the Impact of Cloud Computing on Distributed Systems.
- Investigating the Use of Artificial Intelligence in Autonomous Vehicles.
- Analyzing the Behavior of Neural Networks in Deep Learning Applications.
- Studying the Performance of Blockchain Technology in Supply Chain Management.
- Quantifying User Behavior in Social Media Analytics.
- Investigating Quantum Machine Learning for Enhanced Data Processing.
These additional project ideas provide a diverse range of opportunities for STEM students to engage in quantitative research and explore various aspects of their respective fields. Each project offers a unique avenue for discovery and contribution to the world of science and technology.
What is an example of a quantitative research?
Quantitative research is a powerful investigative approach, wielding numbers to shed light on intricate relationships and phenomena. Let’s dive into an example of quantitative research to understand its workings:
Research Question
What is the correlation between the time students devote to studying and their academic grades?
Students who invest more time in studying are likely to achieve higher grades.
Research Design
Imagine a researcher embarking on a journey within a high school. They distribute surveys to students, inquiring about their weekly study hours and their corresponding grades in core subjects.
Data Analysis
Equipped with statistical tools, our researcher scrutinizes the collected data. Lo and behold, a significant positive correlation emerges—students who dedicate more time to studying generally earn higher grades.
With data as their guide, the researcher concludes that indeed, a relationship exists between study time and academic grades. The more time students commit to their studies, the brighter their academic stars tend to shine.
This example merely scratches the surface of quantitative research’s potential. It can delve into an extensive array of subjects and investigate complex hypotheses. Here are a few more examples:
- Assessing a New Drug’s Effectiveness: Quantifying the impact of a novel medication in treating a specific illness.
- Socioeconomic Status and Crime Rates: Investigating the connection between economic conditions and criminal activity.
- Analyzing the Influence of an Advertising Campaign on Sales: Measuring the effectiveness of a marketing blitz on product purchases.
- Factors Shaping Customer Satisfaction: Using data to pinpoint the elements contributing to customer contentment.
- Government Policies and Employment Rates: Evaluating the repercussions of new governmental regulations on job opportunities.
Quantitative research serves as a potent beacon, illuminating the complexities of our world through data-driven inquiry. Researchers harness its might to collect, analyze, and draw valuable conclusions about a vast spectrum of phenomena. It’s a vital tool for unraveling the intricacies of our universe.
As we bid adieu to our whirlwind tour of quantitative research topics tailor-made for the STEM dreamers, it’s time to soak in the vast horizons that science, technology, engineering, and mathematics paint for us.
We’ve danced through the intricate tango of poverty and crime, peeked into the transformative realm of cutting-edge technologies, and unraveled the captivating puzzles of quantitative research. But these aren’t just topics; they’re open invitations to dive headfirst into the uncharted seas of knowledge.
To you, the STEM trailblazers, these research ideas aren’t mere academic pursuits. They’re portals to curiosity, engines of innovation, and blueprints for shaping the future of our world. They’re the sparks that illuminate the trail leading to discovery.
As you set sail on your research odyssey, remember that quantitative research isn’t just about unlocking answers—it’s about nurturing that profound sense of wonder, igniting innovation, and weaving your unique thread into the fabric of human understanding.
Whether you’re stargazing, decoding the intricate language of genes, engineering marvels, or tackling global challenges head-on, realize that your STEM and quantitative research journey is a perpetual adventure.
May your questions be audacious, your data razor-sharp, and your discoveries earth-shattering. Keep that innate curiosity alive, keep exploring, and let the spirit of STEM be your North Star, guiding you towards a future that’s not just brighter but brilliantly enlightened.
And with that, fellow adventurers, go forth, embrace the unknown, and let your journey in STEM be the epic tale that reshapes the narrative of tomorrow!
Frequently Asked Questions
How can i ensure the ethical conduct of my quantitative research project.
To ensure ethical conduct, obtain informed consent from participants, maintain data confidentiality, and adhere to ethical guidelines established by your institution and professional associations.
Are there any software tools recommended for data analysis in STEM research?
Yes, there are several widely used software tools for data analysis in STEM research, including R, Python, MATLAB, and SPSS. The choice of software depends on your specific research needs and familiarity with the tools.
Leave a Comment Cancel Reply
Your email address will not be published. Required fields are marked *
Save my name, email, and website in this browser for the next time I comment.

189+ Good Quantitative Research Topics For STEM Students
Quantitative research is an essential part of STEM (Science, Technology, Engineering, and Mathematics) fields. It involves collecting and analyzing numerical data to answer research questions and test hypotheses.
In 2023, STEM students have a wealth of exciting research opportunities in various disciplines. Whether you’re an undergraduate or graduate student, here are quantitative research topics to consider for your next project.
If you are looking for the best list of quantitative research topics for stem students, then you can check the given list in each field. It offers STEM students numerous opportunities to explore and contribute to their respective fields in 2023 and beyond.
Whether you’re interested in astrophysics, biology, engineering, mathematics, or any other STEM field.
Also Read: Most Exciting Qualitative Research Topics For Students
What Is Quantitative Research
Table of Contents
Quantitative research is a type of research that focuses on the organized collection, analysis, and evaluation of numerical data to answer research questions, test theories, and find trends or connections between factors. It is an organized, objective way to do study that uses measurable data and scientific methods to come to results.
Quantitative research is often used in many areas, such as the natural sciences, social sciences, economics, psychology, education, and market research. It gives useful information about patterns, trends, cause-and-effect relationships, and how often things happen. Quantitative tools are used by researchers to answer questions like “How many?” and “How often?” “Is there a significant difference?” or “What is the relationship between the variables?”
In comparison to quantitative research, qualitative research uses non-numerical data like conversations, notes, and open-ended surveys to understand and explore the ideas, experiences, and points of view of people or groups. Researchers often choose between quantitative and qualitative methods based on their research goals, questions, and the type of thing they are studying.
How To Choose Quantitative Research Topics For STEM
Here’s a step-by-step guide on how to choose quantitative research topics for STEM:
Step 1:- Identify Your Interests and Passions
Start by reflecting on your personal interests within STEM. What areas or subjects in STEM excite you the most? Choosing a topic you’re passionate about will keep you motivated throughout the research process.
Step 2:- Review Coursework and Textbooks
Look through your coursework, textbooks, and class notes. Identify concepts, theories, or areas that you found particularly intriguing or challenging. These can be a source of potential research topics.
Step 3:- Consult with Professors and Advisors
Discuss your research interests with professors, academic advisors, or mentors. They can provide valuable insights, suggest relevant topics, and guide you toward areas with research opportunities.
Step 4:- Read Recent Literature
Explore recent research articles, journals, and publications in STEM fields. This will help you identify current trends, gaps in knowledge, and areas where further research is needed.
Step 5:- Narrow Down Your Focus
Once you have a broad area of interest, narrow it down to a specific research focus. Consider questions like:
- What specific problem or phenomenon do you want to investigate?
- Are there unanswered questions or controversies in this area?
- What impact could your research have on the field or society?
Step 6:- Consider Resources and Access
Assess the resources available to you, including access to laboratories, equipment, databases, and funding. Ensure that your chosen topic aligns with the resources you have or can access.
Step 7:- Think About Practicality
Consider the feasibility of conducting research on your chosen topic. Are the data readily available, or will you need to collect data yourself? Can you complete the research within your available time frame?
Step 8:- Define Your Research Question
Formulate a clear and specific research question or hypothesis. Your research question should guide your entire study and provide a focus for your data collection and analysis.
Step 9:- Conduct a Literature Review
Dive deeper into the existing literature related to your chosen topic. This will help you understand the current state of research, identify gaps, and refine your research question.
Step 10:- Consider the Impact
Think about the potential impact of your research. How does your topic contribute to the advancement of knowledge in your field? Does it have practical applications or implications for society?
Step 11:- Brainstorm Research Methods
Determine the quantitative research methods and data collection techniques you plan to use. Consider whether you’ll conduct experiments, surveys, data analysis, simulations, or use existing datasets.
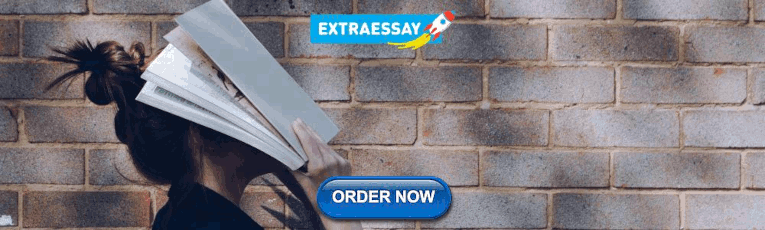
Step 12:- Seek Feedback
Share your research topic and ideas with peers, advisors, or mentors. They can provide valuable feedback and help you refine your research focus.
Step 13:- Assess Ethical Considerations
Consider ethical implications related to your research, especially if it involves human subjects, sensitive data, or potential environmental impacts. Ensure that your research adheres to ethical guidelines.
Step 14:- Finalize Your Research Topic
Once you’ve gone through these steps, finalize your research topic. Write a clear and concise research proposal that outlines your research question, objectives, methods, and expected outcomes.
Step 15:- Stay Open to Adjustments
Be open to adjusting your research topic as you progress. Sometimes, new insights or challenges may lead you to refine or adapt your research focus.
Following are the most interesting quantitative research topics for stem students. These are given below.
Quantitative Research Topics In Physics and Astronomy
- Quantum Computing Algorithms : Investigate new algorithms for quantum computers and their potential applications.
- Dark Matter Detection Methods : Explore innovative approaches to detect dark matter particles.
- Quantum Teleportation : Study the principles and applications of quantum teleportation.
- Exoplanet Characterization : Analyze data from telescopes to characterize exoplanets.
- Nuclear Fusion Modeling : Create mathematical models for nuclear fusion reactions.
- Superconductivity at High Temperatures : Research the properties and applications of high-temperature superconductors.
- Gravitational Wave Analysis : Analyze gravitational wave data to study astrophysical phenomena.
- Black Hole Thermodynamics : Investigate the thermodynamics of black holes and their entropy.
Quantitative Research Topics In Biology and Life Sciences
- Genome-Wide Association Studies (GWAS) : Conduct GWAS to identify genetic factors associated with diseases.
- Pharmacokinetics and Pharmacodynamics : Study drug interactions in the human body.
- Ecological Modeling : Model ecosystems to understand population dynamics.
- Protein Folding : Research the kinetics and thermodynamics of protein folding.
- Cancer Epidemiology : Analyze cancer incidence and risk factors in specific populations.
- Neuroimaging Analysis : Develop algorithms for analyzing brain imaging data.
- Evolutionary Genetics : Investigate evolutionary patterns using genetic data.
- Stem Cell Differentiation : Study the factors influencing stem cell differentiation.
Engineering and Technology Quantitative Research Topics
- Renewable Energy Efficiency : Optimize the efficiency of solar panels or wind turbines.
- Aerodynamics of Drones : Analyze the aerodynamics of drone designs.
- Autonomous Vehicle Safety : Evaluate safety measures for autonomous vehicles.
- Machine Learning in Robotics : Implement machine learning algorithms for robot control.
- Blockchain Scalability : Research methods to scale blockchain technology.
- Quantum Computing Hardware : Design and test quantum computing hardware components.
- IoT Security : Develop security protocols for the Internet of Things (IoT).
- 3D Printing Materials Analysis : Study the mechanical properties of 3D-printed materials.
Quantitative Research Topics In Mathematics and Statistics
Following are the best Quantitative Research Topics For STEM Students in mathematics and statistics.
- Prime Number Distribution : Investigate the distribution of prime numbers.
- Graph Theory Algorithms : Develop algorithms for solving graph theory problems.
- Statistical Analysis of Financial Markets : Analyze financial data and market trends.
- Number Theory Research : Explore unsolved problems in number theory.
- Bayesian Machine Learning : Apply Bayesian methods to machine learning models.
- Random Matrix Theory : Study the properties of random matrices in mathematics and physics.
- Topological Data Analysis : Use topology to analyze complex data sets.
- Quantum Algorithms for Optimization : Research quantum algorithms for optimization problems.
Experimental Quantitative Research Topics In Science and Earth Sciences
- Climate Change Modeling : Develop climate models to predict future trends.
- Biodiversity Conservation Analysis : Analyze data to support biodiversity conservation efforts.
- Geographic Information Systems (GIS) : Apply GIS techniques to solve environmental problems.
- Oceanography and Remote Sensing : Use satellite data for oceanographic research.
- Air Quality Monitoring : Develop sensors and models for air quality assessment.
- Hydrological Modeling : Study the movement and distribution of water resources.
- Volcanic Activity Prediction : Predict volcanic eruptions using quantitative methods.
- Seismology Data Analysis : Analyze seismic data to understand earthquake patterns.
Chemistry and Materials Science Quantitative Research Topics
- Nanomaterial Synthesis and Characterization : Research the synthesis and properties of nanomaterials.
- Chemoinformatics : Analyze chemical data for drug discovery and materials science.
- Quantum Chemistry Simulations : Perform quantum simulations of chemical reactions.
- Materials for Renewable Energy : Investigate materials for energy storage and conversion.
- Catalysis Kinetics : Study the kinetics of chemical reactions catalyzed by materials.
- Polymer Chemistry : Research the properties and applications of polymers.
- Analytical Chemistry Techniques : Develop new analytical techniques for chemical analysis.
- Sustainable Chemistry : Explore green chemistry approaches for sustainable materials.
Computer Science and Information Technology Topics
- Natural Language Processing (NLP) : Work on NLP algorithms for language understanding.
- Cybersecurity Analytics : Analyze cybersecurity threats and vulnerabilities.
- Big Data Analytics : Apply quantitative methods to analyze large data sets.
- Machine Learning Fairness : Investigate bias and fairness issues in machine learning models.
- Human-Computer Interaction (HCI) : Study user behavior and interaction patterns.
- Software Performance Optimization : Optimize software applications for performance.
- Distributed Systems Analysis : Analyze the performance of distributed computing systems.
- Bioinformatics Data Mining : Develop algorithms for mining biological data.
Good Quantitative Research Topics Students In Medicine and Healthcare
- Clinical Trial Data Analysis : Analyze clinical trial data to evaluate treatment effectiveness.
- Epidemiological Modeling : Model disease spread and intervention strategies.
- Healthcare Data Analytics : Analyze healthcare data for patient outcomes and cost reduction.
- Medical Imaging Algorithms : Develop algorithms for medical image analysis.
- Genomic Medicine : Apply genomics to personalized medicine approaches.
- Telemedicine Effectiveness : Study the effectiveness of telemedicine in healthcare delivery.
- Health Informatics : Analyze electronic health records for insights into patient care.
Agriculture and Food Sciences Topics
- Precision Agriculture : Use quantitative methods for optimizing crop production.
- Food Safety Analysis : Analyze food safety data and quality control.
- Aquaculture Sustainability : Research sustainable practices in aquaculture.
- Crop Disease Modeling : Model the spread of diseases in agricultural crops.
- Climate-Resilient Agriculture : Develop strategies for agriculture in changing climates.
- Food Supply Chain Optimization : Optimize food supply chain logistics.
- Soil Health Assessment : Analyze soil data for sustainable land management.
Social Sciences with Quantitative Approaches
- Educational Data Mining : Analyze educational data for improving learning outcomes.
- Sociodemographic Surveys : Study social trends and demographics using surveys.
- Psychometrics : Develop and validate psychological measurement instruments.
- Political Polling Analysis : Analyze political polling data and election trends.
- Economic Modeling : Develop economic models for policy analysis.
- Urban Planning Analytics : Analyze data for urban planning and infrastructure.
- Climate Policy Evaluation : Evaluate the impact of climate policies on society.
Environmental Engineering Quantitative Research Topics
- Water Quality Assessment : Analyze water quality data for environmental monitoring.
- Waste Management Optimization : Optimize waste collection and recycling programs.
- Environmental Impact Assessments : Evaluate the environmental impact of projects.
- Air Pollution Modeling : Model the dispersion of air pollutants in urban areas.
- Sustainable Building Design : Apply quantitative methods to sustainable architecture.
Quantitative Research Topics Robotics and Automation
- Robotic Swarm Behavior : Study the behavior of robot swarms in different tasks.
- Autonomous Drone Navigation : Develop algorithms for autonomous drone navigation.
- Humanoid Robot Control : Implement control algorithms for humanoid robots.
- Robotic Grasping and Manipulation : Study robotic manipulation techniques.
- Reinforcement Learning for Robotics : Apply reinforcement learning to robotic control.
Quantitative Research Topics Materials Engineering
- Additive Manufacturing Process Optimization : Optimize 3D printing processes.
- Smart Materials for Aerospace : Research smart materials for aerospace applications.
- Nanostructured Materials for Energy Storage : Investigate energy storage materials.
- Corrosion Prevention : Develop corrosion-resistant materials and coatings.
Nuclear Engineering Quantitative Research Topics
- Nuclear Reactor Safety Analysis : Study safety aspects of nuclear reactor designs.
- Nuclear Fuel Cycle Analysis : Analyze the nuclear fuel cycle for efficiency.
- Radiation Shielding Materials : Research materials for radiation protection.
Quantitative Research Topics In Biomedical Engineering
- Medical Device Design and Testing : Develop and test medical devices.
- Biomechanics Analysis : Analyze biomechanics in sports or rehabilitation.
- Biomaterials for Medical Implants : Investigate materials for medical implants.
Good Quantitative Research Topics Chemical Engineering
- Chemical Process Optimization : Optimize chemical manufacturing processes.
- Industrial Pollution Control : Develop strategies for pollution control in industries.
- Chemical Reaction Kinetics : Study the kinetics of chemical reactions in industries.
Best Quantitative Research Topics In Renewable Energy
- Energy Storage Systems : Research and optimize energy storage solutions.
- Solar Cell Efficiency : Improve the efficiency of photovoltaic cells.
- Wind Turbine Performance Analysis : Analyze and optimize wind turbine designs.
Brilliant Quantitative Research Topics In Astronomy and Space Sciences
- Astrophysical Simulations : Simulate astrophysical phenomena using numerical methods.
- Spacecraft Trajectory Optimization : Optimize spacecraft trajectories for missions.
- Exoplanet Detection Algorithms : Develop algorithms for exoplanet detection.
Quantitative Research Topics In Psychology and Cognitive Science
- Cognitive Psychology Experiments : Conduct quantitative experiments in cognitive psychology.
- Emotion Recognition Algorithms : Develop algorithms for emotion recognition in AI.
- Neuropsychological Assessments : Create quantitative assessments for brain function.
Geology and Geological Engineering Quantitative Research Topics
- Geological Data Analysis : Analyze geological data for mineral exploration.
- Geological Hazard Prediction : Predict geological hazards using quantitative models.
Top Quantitative Research Topics In Forensic Science
- Forensic Data Analysis : Analyze forensic evidence using quantitative methods.
- Crime Pattern Analysis : Study crime patterns and trends in urban areas.
Great Quantitative Research Topics In Cybersecurity
- Network Intrusion Detection : Develop quantitative methods for intrusion detection.
- Cryptocurrency Analysis : Analyze blockchain data and cryptocurrency trends.
Mathematical Biology Quantitative Research Topics
- Epidemiological Modeling : Model disease spread and control in populations.
- Population Genetics : Analyze genetic data to understand population dynamics.
Quantitative Research Topics In Chemical Analysis
- Analytical Chemistry Methods : Develop quantitative methods for chemical analysis.
- Spectroscopy Analysis : Analyze spectroscopic data for chemical identification.
Mathematics Education Quantitative Research Topics
- Mathematics Curriculum Analysis : Analyze curriculum effectiveness in mathematics education.
- Mathematics Assessment Development : Develop quantitative assessments for mathematics skills.
Quantitative Research Topics In Social Research
- Social Network Analysis : Analyze social network structures and dynamics.
- Survey Research : Conduct quantitative surveys on social issues and trends.
Quantitative Research Topics In Computational Neuroscience
- Neural Network Modeling : Model neural networks and brain functions computationally.
- Brain Connectivity Analysis : Analyze functional and structural brain connectivity.
Best Topics In Transportation Engineering
- Traffic Flow Modeling : Model and optimize traffic flow in urban areas.
- Public Transportation Efficiency : Analyze the efficiency of public transportation systems.
Good Quantitative Research Topics In Energy Economics
- Energy Policy Analysis : Evaluate the economic impact of energy policies.
- Renewable Energy Cost-Benefit Analysis : Assess the economic viability of renewable energy projects.
Quantum Information Science
- Quantum Cryptography Protocols : Develop and analyze quantum cryptography protocols.
- Quantum Key Distribution : Study the security of quantum key distribution systems.
Human Genetics
- Genome Editing Ethics : Investigate ethical issues in genome editing technologies.
- Population Genomics : Analyze genomic data for population genetics research.
Marine Biology
- Coral Reef Health Assessment : Quantitatively assess the health of coral reefs.
- Marine Ecosystem Modeling : Model marine ecosystems and biodiversity.
Data Science and Machine Learning
- Machine Learning Explainability : Develop methods for explaining machine learning models.
- Data Privacy in Machine Learning : Study privacy issues in machine learning applications.
- Deep Learning for Image Analysis : Develop deep learning models for image recognition.
Environmental Engineering
Robotics and automation, materials engineering, nuclear engineering, biomedical engineering, chemical engineering, renewable energy, astronomy and space sciences, psychology and cognitive science, geology and geological engineering, forensic science, cybersecurity, mathematical biology, chemical analysis, mathematics education, quantitative social research, computational neuroscience, quantitative research topics in transportation engineering, quantitative research topics in energy economics, topics in quantum information science, amazing quantitative research topics in human genetics, quantitative research topics in marine biology, what is a common goal of qualitative and quantitative research.
A common goal of both qualitative and quantitative research is to generate knowledge and gain a deeper understanding of a particular phenomenon or topic. However, they approach this goal in different ways:
1. Understanding a Phenomenon
Both types of research aim to understand and explain a specific phenomenon, whether it’s a social issue, a natural process, a human behavior, or a complex event.
2. Testing Hypotheses
Both qualitative and quantitative research can involve hypothesis testing. While qualitative research may not use statistical hypothesis tests in the same way as quantitative research, it often tests hypotheses or research questions by examining patterns and themes in the data.
3. Contributing to Knowledge
Researchers in both approaches seek to contribute to the body of knowledge in their respective fields. They aim to answer important questions, address gaps in existing knowledge, and provide insights that can inform theory, practice, or policy.
4. Informing Decision-Making
Research findings from both qualitative and quantitative studies can be used to inform decision-making in various domains, whether it’s in academia, government, industry, healthcare, or social services.
5. Enhancing Understanding
Both approaches strive to enhance our understanding of complex phenomena by systematically collecting and analyzing data. They aim to provide evidence-based explanations and insights.
6. Application
Research findings from both qualitative and quantitative studies can be applied to practical situations. For example, the results of a quantitative study on the effectiveness of a new drug can inform medical treatment decisions, while qualitative research on customer preferences can guide marketing strategies.
7. Contributing to Theory
In academia, both types of research contribute to the development and refinement of theories in various disciplines. Quantitative research may provide empirical evidence to support or challenge existing theories, while qualitative research may generate new theoretical frameworks or perspectives.
Conclusion – Quantitative Research Topics For STEM Students
So, selecting a quantitative research topic for STEM students is a pivotal decision that can shape the trajectory of your academic and professional journey. The process involves a thoughtful exploration of your interests, a thorough review of the existing literature, consideration of available resources, and the formulation of a clear and specific research question.
Your chosen topic should resonate with your passions, align with your academic or career goals, and offer the potential to contribute to the body of knowledge in your STEM field. Whether you’re delving into physics, biology, engineering, mathematics, or any other STEM discipline, the right research topic can spark curiosity, drive innovation, and lead to valuable insights.
Moreover, quantitative research in STEM not only expands the boundaries of human knowledge but also has the power to address real-world challenges, improve technology, and enhance our understanding of the natural world. It is a journey that demands dedication, intellectual rigor, and an unwavering commitment to scientific inquiry.
What is quantitative research in STEM?
Quantitative research in this context is designed to improve our understanding of the science system’s workings, structural dependencies and dynamics.
What are good examples of quantitative research?
Surveys and questionnaires serve as common examples of quantitative research. They involve collecting data from many respondents and analyzing the results to identify trends, patterns
What are the 4 C’s in STEM?
They became known as the “Four Cs” — critical thinking, communication, collaboration, and creativity.
Similar Articles

How To Do Homework Fast – 11 Tips To Do Homework Fast
Homework is one of the most important parts that have to be done by students. It has been around for…
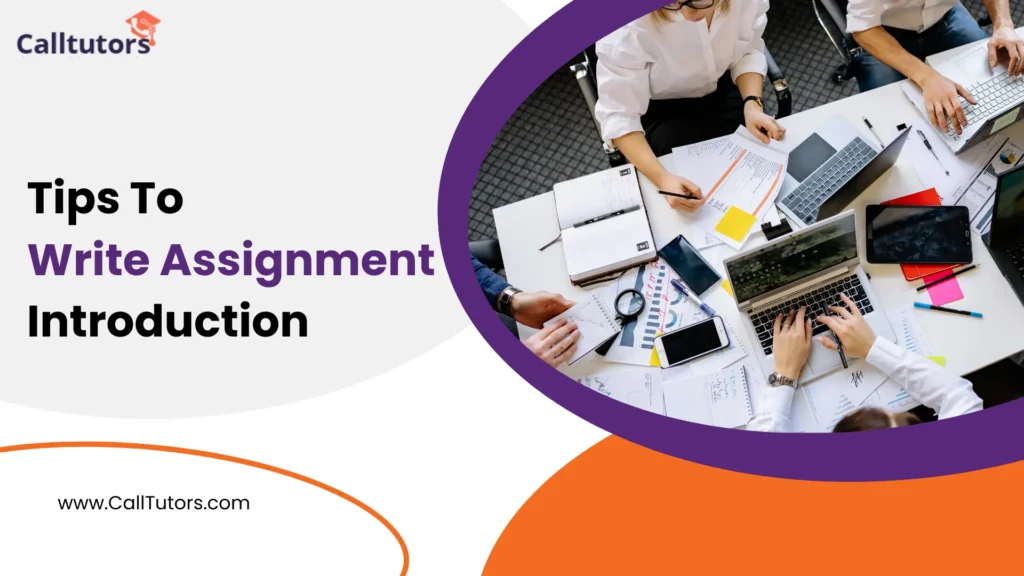
How to Write an Assignment Introduction – 6 Best Tips
In essence, the writing tasks in academic tenure students are an integral part of any curriculum. Whether in high school,…
Leave a Comment Cancel Reply
Your email address will not be published. Required fields are marked *
This site uses Akismet to reduce spam. Learn how your comment data is processed .
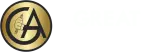
85 Unique Research Topics for STEM Students
Table of Contents
Are you a STEM (Science, Technology, Engineering, and Mathematics) student? If yes, then during your academic journey, you must do qualitative or quantitative research on your field of study. Generally, for doing research, an ideal topic is essential. Since STEM covers broad disciplines, it might be challenging for you to identify the right topic for your research. But, with our assistance, you can effectively handle your research topic selection process. Here, we have suggested 85 best research topics for STEM students on different subjects.
In addition to the list of STEM research topics, we have also shared the importance of STEM research and tips for choosing a perfect STEM research topic.
Explore this entire blog and get exclusive qualitative and quantitative STEM research ideas across a variety of fields.
What is STEM?
STEM refers to Science, Technology, Engineering, and Mathematics. It is a manner of discussing things like education, employment, and activities relating to these four fundamental areas.
Science is the study of the world around us. Technology is the use of tools and equipment to solve problems. Engineering is the design and construction of things. Mathematics is the study of numbers and their applications. STEM enables every student to research, discover, and build interesting things that make our world better and more enjoyable.
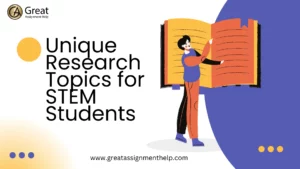
Importance of STEM Research
In recent times, our world has been facing tremendous growth in the science and technology fields. This advancement is a result of the continuous research in the STEM areas. Moreover, STEM research is also significant in several aspects as listed below.
- STEM research discovers new things and solves certain problems.
- It contributes to finding treatments for diseases.
- STEM research helps to develop new technology and makes human lives easier.
- Engineers create products that improve the quality of human life.
- Mathematics helps to comprehend and solve complicated problems.
STEM Research Type: Quantitative vs. Qualitative
STEM students can conduct either quantitative or qualitative research.
Quantitative research entails the methodical gathering and evaluation of numerical data to answer research questions, test hypotheses, identify trends, or find correlations between various factors. It is a systematic, objective approach to research that uses quantifiable data and scientific techniques to generate conclusions.
On the other hand, qualitative research is a methodical and exploratory method of research that focuses on comprehending and analyzing the challenges of human experiences, actions, and occurrences. Its goal is to provide deep insights into the “how” and “why” of various problems by studying them in their natural settings and surroundings.
When compared to quantitative research, qualitative research uses non-numerical data, such as discussions, notes, and open-ended surveys to investigate and comprehend the opinions, experiences, and ideas of individuals or groups.
STEM Researchers frequently select between quantitative and qualitative methods depending on their research objectives, questions, and the subject they are studying.
Know How to Choose a Good STEM Research Topic
As said earlier, for preparing a brilliant STEM research paper, an excellent topic is necessary. In case, you are unsure how to identify the right STEM research topic, follow the topic selection tips we have recommended below.
Determine Your Interests
Consider your interests and areas of excitement in science, technology, engineering, or math. It might be something you encountered in daily life, learned in school, or saw in the news. Simply, by selecting a topic that you are passionate about, you can enhance the pleasure of conducting research.
Examine Existing Subjects
Investigate several STEM research areas on the internet, in books, or at the library. Discover what subject specialists and scientists are researching. This can provide you with new ideas. Also, it can assist you in comprehending what is already known in your subject of choice.
Give Importance to Real-time Problems
Focus on the problems that exist around you. In specific, think about whether you can solve any issues in your community or world by using STEM concepts. Usually, selecting a study topic that fixes a real-world issue might bring more impact to your research.
Discuss with Teachers or Mentors
Talk to your teachers, mentors, or professors regarding what you are passionate about. They will offer assistance and propose STEM research topics that are relevant to your talents and goals. Furthermore, they may provide resources and help for your research.
Narrow Down the Topic
Once you’ve generated some ideas, limit them down to a specific study issue or project. Make sure the topic you select is not too wide or too narrow. Always pick a topic that you can thoroughly investigate within the boundaries of your STEM research paper.
Also Read: 200+ Excellent Research Paper Topics of 2023
List of the Best Research Topics for STEM Students
In case, you are confused about what STEM research topic to choose, then explore the list published below. In the list, you will get 85 outstanding STEM research topics on a wide range of subjects.
Quantitative Research Topics for STEM Students
- Measure the effect of different light wavelengths on plant growth.
- Examine the impact of pH levels on the rate of chemical reactions.
- Investigate the relation between the number of blades on a wind turbine and energy output
- Optimize algorithms for autonomous drone navigation in complex environments.
- Explore the use of artificial intelligence in predicting and preventing forest fires.
- Test the effectiveness of different insulating materials in conserving heat.
- Analyze the effect of different concentrations of a substance on bacterial growth.
- Investigate the effects of microplastic pollution on aquatic ecosystems.
- Analyze the efficiency of solar panels in converting sunlight into electricity under varying conditions.
- Study the behavior of magnets in different temperature conditions.
- Explore the ethical implications of gene editing in humans.
- Analyze the feasibility of harnessing geothermal energy from underwater volcanoes.
- Explain the use of machine learning and AI in predicting and mitigating the impact of natural disasters.
- Investigate the mechanisms of stem cell differentiation for regenerative medicine.
- Explore the science behind the formation of auroras and their cultural significance.
Qualitative Research Topics for STEM Students
- Share user experiences with augmented reality applications.
- Analyze the impact of social media on political activism.
- Present qualitative analysis of online gaming communities.
- Analyze the impact of educational apps on student engagement.
- Discuss ethical considerations in artificial intelligence development.
- Share the perceptions of online privacy and data security.
- Narratives of whistleblowers in scientific misconduct cases.
- Explain the experiences of individuals participating in virtual reality environments.
- Discuss the perceptions of artificial intelligence and automation among STEM Professionals.
- Qualitative exploration of team dynamics in engineering projects.
- Present the qualitative analysis of the digital divide in education.
- Analyze the role of ethics in emerging technology development.
- Discuss the perceptions of scientific responsibility in climate change.
- Explore the decision-making process in biomedical research.
- Qualitative analysis of the ethics of genetic engineering.
Science Research Topics for STEM Students
- Study the relationship between diet and lifespan.
- Analyze the synthesis of novel polymers with unique properties.
- Examine the properties of dark matter and dark energy.
- Study the effectiveness of various plant fertilizers.
- Explore the dynamics of black holes and their gravitational effects.
- Study the behavior of nanoparticles in different solvents.
- Analyze the impact of climate change on crop yields.
- Explore the physics of renewable energy sources like solar cells.
- Study the properties of superfluids at low temperatures.
- Investigate the chemistry of alternative fuels.
- Explore the quantum properties of entangled particles.
- Examine the physics of nanoscale materials and devices.
- Analyze the effects of chemical additives on food preservation.
- Investigate the chemistry of atmospheric pollutants.
- Examine the physics of gravitational waves.
Math Research Topics for STEM Students
- Analyze the properties of mathematical models for population dynamics.
- Investigate the use of mathematical modeling in epidemiology.
- Examine the use of numerical methods in solving partial differential equations.
- Analyze the properties of algebraic structures in coding theory.
- Explore the behavior of mathematical models in financial markets.
- Analyze the behavior of chaotic systems using differential equations.
- Examine the use of number theory in cryptography.
- Investigate the properties of prime numbers and their distribution.
- Analyze the behavior of mathematical models in climate prediction.
- Study the optimization of algorithms for solving complex mathematical problems.
Engineering Research Ideas for STEM Students
- Explore the efficiency of renewable energy storage systems.
- Examine the impact of machine learning in predictive maintenance.
- Study the impact of AI-driven design in architecture.
- Examine the optimization of supply chain logistics using quantitative methods.
- Analyze the effects of vibration on structural engineering.
- Discuss the efficiency of water treatment processes in civil engineering.
- Analyze the energy efficiency of smart buildings.
- Examine the impact of 3D printing on manufacturing processes.
- Explore the use of robotics in underwater exploration.
- Study the structural integrity of materials in aerospace engineering.
STEM Research Paper Ideas on Computer Science and Technology
- Analyze the effectiveness of recommendation systems in e-commerce.
- Study the impact of cloud computing on data storage and processing.
- Examine the use of neural networks in predicting disease outbreaks.
- Explore the efficiency of data mining techniques in customer behavior analysis.
- Examine the security of blockchain technology in financial transactions.
- Study the impact of quantum computing on cryptography.
- Analyze the effectiveness of sentiment analysis in social media monitoring.
- Analyze the effectiveness of cybersecurity measures in protecting sensitive data.
- Study the impact of algorithmic trading in financial markets.
- Analyze the efficiency of data compression algorithms for large datasets.
Also Read: 140 Captivating Public Health Topics for Academic Paper
STEM Research Paper Topics on Health and Medicine
- Analyze the impact of personalized medicine in cancer treatment.
- Examine the use of wearable devices in monitoring patient health.
- Study the epidemiology of chronic disease
- Analyze the behavior of pharmaceutical drugs in clinical trials.
- Investigate the use of bioinformatics in genomics research.
- Analyze the properties of medical imaging techniques for early disease detection.
- Study the impact of genetics in predicting disease susceptibility.
- Explore the use of regenerative medicine in tissue repair.
- Examine the use of artificial intelligence in medical diagnosis.
- Analyze the behavior of pathogens in antimicrobial resistance.
Wrapping Up
Out of the numerous ideas suggested above, choose any topic of your choice and compose a great STEM research paper . If it is more difficult for you to choose a good research topic, perform STEM research, and prepare a brilliant thesis, then call us immediately.
On our platform, we have plenty of well-qualified STEM assignment helpers. For your academic work on any topic related to STEM subjects, our professionals will provide the best assistance. Most importantly, by taking STEM assignment help online from our experts, you can finish your tasks accurately and on time at a nominal price.
Related Post
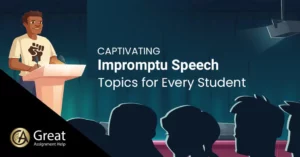
85 Engaging Impromptu Speech Topics and Prompts
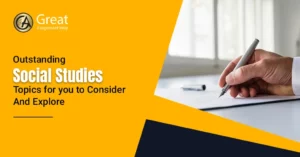
100 Impactful Social Studies Topics For Research Papers
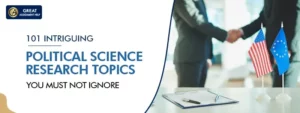
Top 100 Political Science Research Topics
About author.
Jacob Smith
Jacob Smith guides students with writing research paper topics and theses at greatassignmenthelp.com. Read about the author from this page
https://www.greatassignmenthelp.com/
Leave a Reply Cancel reply
Your email address will not be published. Required fields are marked *
- Featured Posts
200 Impressive Business Essay Topics
175 unique bioethics topics to consider for academic paper, apa vs. mla: know the major differences between the citation styles, top 155 java project ideas for beginners and experts, learn about the 12 popular applications of linear algebra, learn how to make money with chatgpt, 110 excellent romeo and juliet essay topics, a very old man with enormous wings summary, 215 unique economics essay topics and ideas, get help instantly.
Raise Your Grades with Great Assignment Help
- How To Find Articles with Databases
- How To Evaluate Articles
- How To Read A Scientific Paper
- How To Interpret Data
- How To Write A Lab Report
- How To Write A Scientific Paper
- Get More Help
- Reference: Encyclopedia, Handbooks & Dictionaries
- Research Tools: Databases, Protocols & Citation Locators
- E-Journal Lists by Subject
- Scholarly vs Popular
- Search Tips
- Open Resources
- E-Journal lists by subject
- Develop a Research Question
Developing a Research Question
From Laurier Library.
Selecting and Narrowing a Topic
When starting out on your research, it is important to choose a research topic that is not only of interest to you, but can also be covered effectively in the space that you have available. You may not know right away what your research question is - that's okay! Start out with a broad topic, then conduct some background research to explore possibilities and narrow your topic to something more manageable.
Choose an interesting general topic. If you’re interested in your topic, others probably will be too! And your research will be a lot more fun. Once you have a general topic of interest, you can begin to explore more focused areas within that broad topic.
Gather background information. Do a few quick searches in OneSearch@IU or in other relevant sources. See what other researchers have already written to help narrow your focus.
- What subtopics relate to the broader topic?
- What questions do these sources raise?
- What piques your interest? What might you like to say about the topic?
Consider your audience. Who would be interested in this issue? For whom are you writing?
Adapted from: George Mason University Writing Center. (2008). How to write a research question. Retrieved from http://writingcenter.gmu.edu/writing-resources/wc-quick-guides
From Topic to Research Question
Once you have done some background research and narrowed down your topic, you can begin to turn that topic into a research question that you will attempt to answer in the course of your research. Keep in mind that your question may change as you gather more information and as you write. However, having some sense of your direction can help you evaluate sources and identify relevant information throughout your research process.
Explore questions.
- Ask open-ended “how” and “why” questions about your general topic.
- Consider the “so what?” of your topic. Why does this topic matter to you? Why should it matter to others?
Evaluate your research question. Use the following to determine if any of the questions you generated would be appropriate and workable for your assignment.
- Is your question clear? Do you have a specific aspect of your general topic that you are going to explore further?
- Is your question focused? Will you be able to cover the topic adequately in the space available?
- Is your question sufficiently complex? (cannot be answered with a simple yes/no response, requires research and analysis)
Hypothesize. Once you have developed your research question, consider how you will attempt to answer or address it.
- If you are making an argument, what will you say?
- Why does your argument matter?
- What kinds of sources will you need in order to support your argument?
- How might others challenge your argument?
Adapted from: George Mason University Writing Center. (2008). How to write a research question. Retrieved from http://writingcenter.gmu.edu/writing-resources/wc-quick-guides
Sample Research Questions
A good research question is clear, focused, and has an appropriate level of complexity. Developing a strong question is a process, so you will likely refine your question as you continue to research and to develop your ideas.
Unclear : Why are social networking sites harmful?
Clear: How are online users experiencing or addressing privacy issues on such social networking sites as MySpace and Facebook?
Unfocused: What is the effect on the environment from global warming?
Focused: How is glacial melting affecting penguins in Antarctica?
Simple vs Complex
Too simple: How are doctors addressing diabetes in the U.S.?
Appropriately Complex: What are common traits of those suffering from diabetes in America, and how can these commonalities be used to aid the medical community in prevention of the disease?
Adapted from: George Mason University Writing Center. (2008). How to write a research question. Retrieved from http://writingcenter.gmu.edu/writing-resources/wc-quick-guides
General online reference sources.
Reference sources like dictionaries and encylopedias provide general information about various subjects. They also include definitions that may help you break down your topic and understand it better. Sources includes in these entries can be springboards for more in-depth research.
A note on citation: Reference sources are generally not cited since they usually consist of common knowledge (e.g. who was the first United States President). But if you're unsure whether to cite something it's best to do so. Specific pieces of information and direct quotes should always be cited.
Database of encyclopedias and specialized reference sources.
Encyclopedias and specialized reference resources in: Arts, Biography, History, Information and Publishing, Law, Literature, Medicine, Multicultural Studies, Nation and World, Religion, Science, Social Science
Why Use References Sources
Reference sources are a great place to begin your research. They can help you:
- gain an overview of a topic
- explore potential research areas
- identify key issues, publications, or authors in your research area
From here, you can narrow your search topic and look at more specialized sources.
- << Previous: Scholarly vs Popular
- Next: Search Tips >>
- Last Updated: Mar 8, 2024 2:26 PM
- URL: https://guides.libraries.indiana.edu/STEM
Social media
- Instagram for Herman B Wells Library
- Facebook for IU Libraries
Additional resources
Featured databases.
- Resource available to authorized IU Bloomington users (on or off campus) OneSearch@IU
- Resource available to authorized IU Bloomington users (on or off campus) Academic Search (EBSCO)
- Resource available to authorized IU Bloomington users (on or off campus) ERIC (EBSCO)
- Resource available to authorized IU Bloomington users (on or off campus) Nexis Uni
- Resource available without restriction HathiTrust Digital Library
- Databases A-Z
- Resource available to authorized IU Bloomington users (on or off campus) Google Scholar
- Resource available to authorized IU Bloomington users (on or off campus) JSTOR
- Resource available to authorized IU Bloomington users (on or off campus) Web of Science
- Resource available to authorized IU Bloomington users (on or off campus) Scopus
- Resource available to authorized IU Bloomington users (on or off campus) WorldCat
IU Libraries
- Diversity Resources
- About IU Libraries
- Alumni & Friends
- Departments & Staff
- Jobs & Libraries HR
- Intranet (Staff)
- IUL site admin

Explore 151 Amazing Topics For Survey Research (Updated 2024)
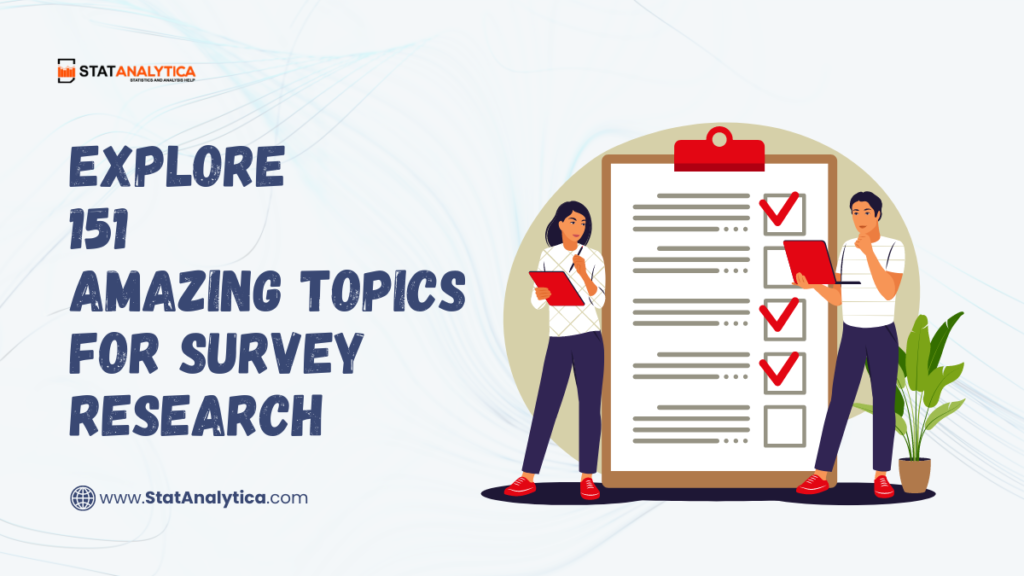
Hey everyone! Ready to dive into the world of surveys? We’ve got the ultimate guide “Explore 151 Amazing Topics for Survey Research (Updated 2024).” It’s like having a treasure map for cool and interesting stuff to ask people about. From what snacks folks love to the latest tech trends, we’ve got it all. No need for fancy words – just easy questions and tons of fun.
Ever wondered if more people like cats or dogs? Or maybe what games are ruling the playground? Well, get ready to reveal the answers. These topics aren’t just surveys; they’re your passport to understanding the world around you. So, grab a seat and let’s dive into the awesomeness of surveys – because every question is a key to unlocking something cool.
What Is Survey Research?
Table of Contents
Survey research is a method of gathering data from individuals to understand opinions, behaviors, or characteristics. Researchers use questionnaires, interviews, or other structured approaches to collect information.
This systematic approach allows for the analysis of trends, preferences, and attitudes within a targeted population, providing valuable information for various fields, including social sciences, market research, and public opinion studies.
151 Amazing Topics For survey Research
Top 10 topics for survey research on job satisfaction.
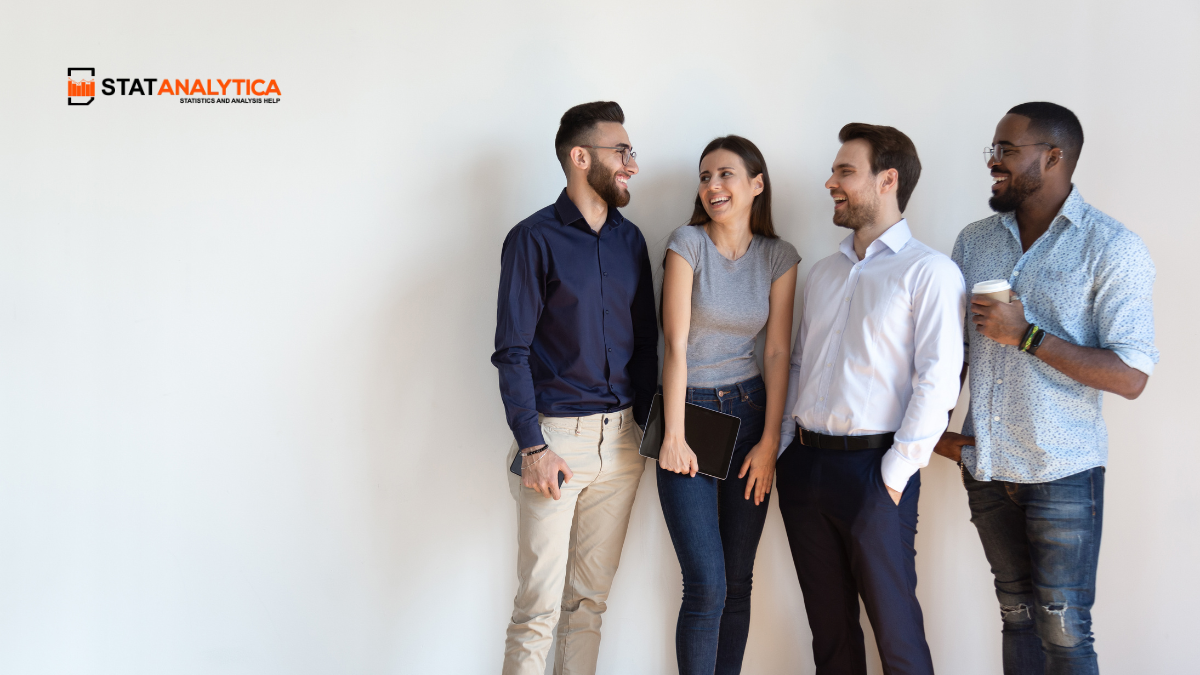
- Job Satisfaction Factors in Remote Work Environments
- The Impact of Leadership Styles on Employee Job Satisfaction
- Gender Disparities in Job Satisfaction Across Industries
- Job Satisfaction and Its Relation to Employee Productivity
- Influence of Organizational Culture on Job Satisfaction
- Job Satisfaction Among Healthcare Professionals
- Job Satisfaction Trends in the Gig Economy
- Job Satisfaction and Work-Life Balance in the Tech Industry
- Job Satisfaction Among Millennials in the Workplace
- The Role of Training and Development in Enhancing Job Satisfaction
Top 10 Survey Research Topics On Health and Wellness
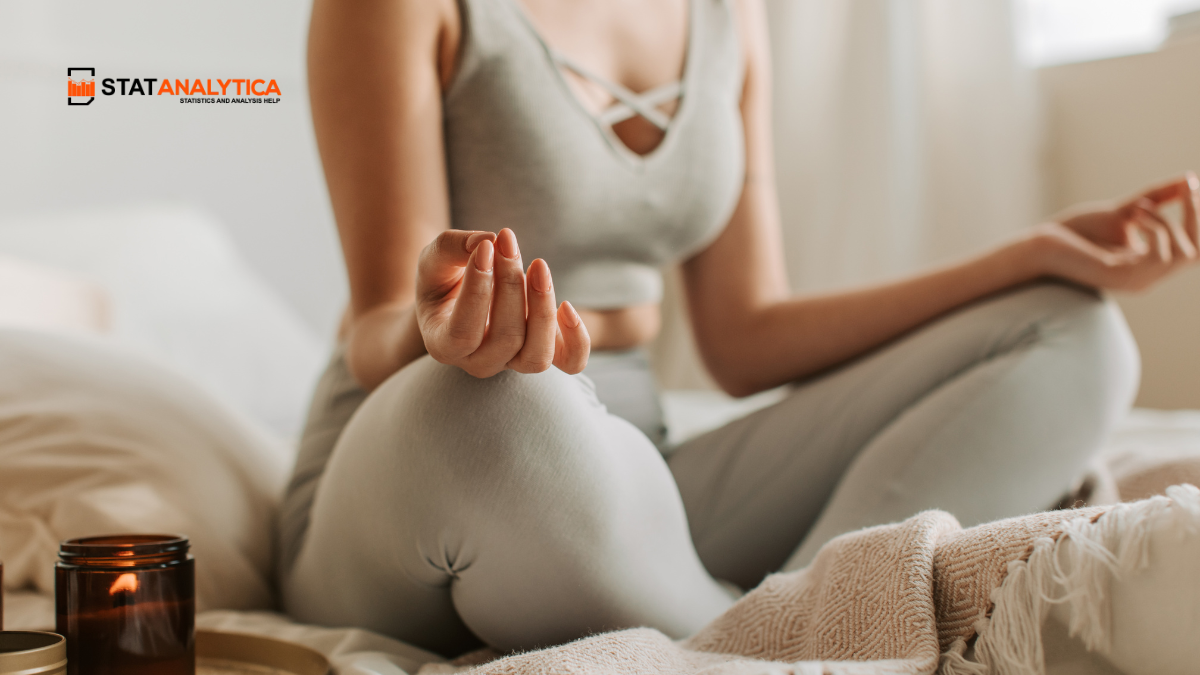
- Workplace Wellness Programs: Employee Perceptions and Participation
- Impact of Stress on Physical and Mental Health
- Effectiveness of Remote Work on Employee Wellbeing
- Health and Wellness Behaviors Among College Students
- Community Perspectives on Access to Healthcare Services
- Employee Engagement in Corporate Wellness Initiatives
- Mental Health Stigma: Public Attitudes and Awareness
- Impact of Physical Activity on Overall Wellbeing
- Dietary Habits and their Influence on Health
- Perceptions of Telehealth Services in Healthcare Accessibility
Top 10 Survey Research Topics On Education System Evaluation
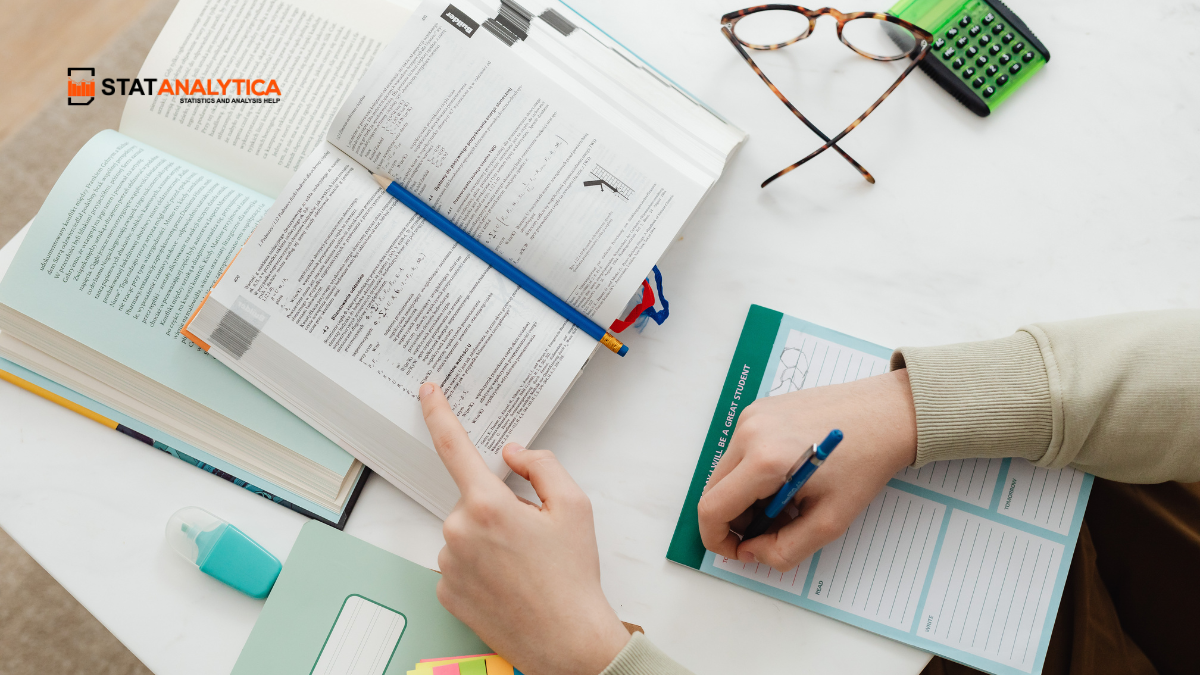
- Parental Satisfaction with Remote Learning Environments
- Student Perspectives on Online Education Effectiveness
- Teacher Feedback on the Implementation of Technology in the Classroom
- The Impact of Standardized Testing on Educational Quality
- Community Perceptions of School Safety Measures
- Teacher Training and Preparedness for Diverse Learning Needs
- Student Engagement in STEM (Science, Technology, Engineering, and Mathematics) Education
- Parental Involvement in School Decision-Making Processes
- The Effectiveness of Extracurricular Programs on Student Development
- Evaluation of Inclusivity and Diversity Initiatives in Educational Institutions
Top 10 Survey Research Topics On Consumer Preferences in Technology
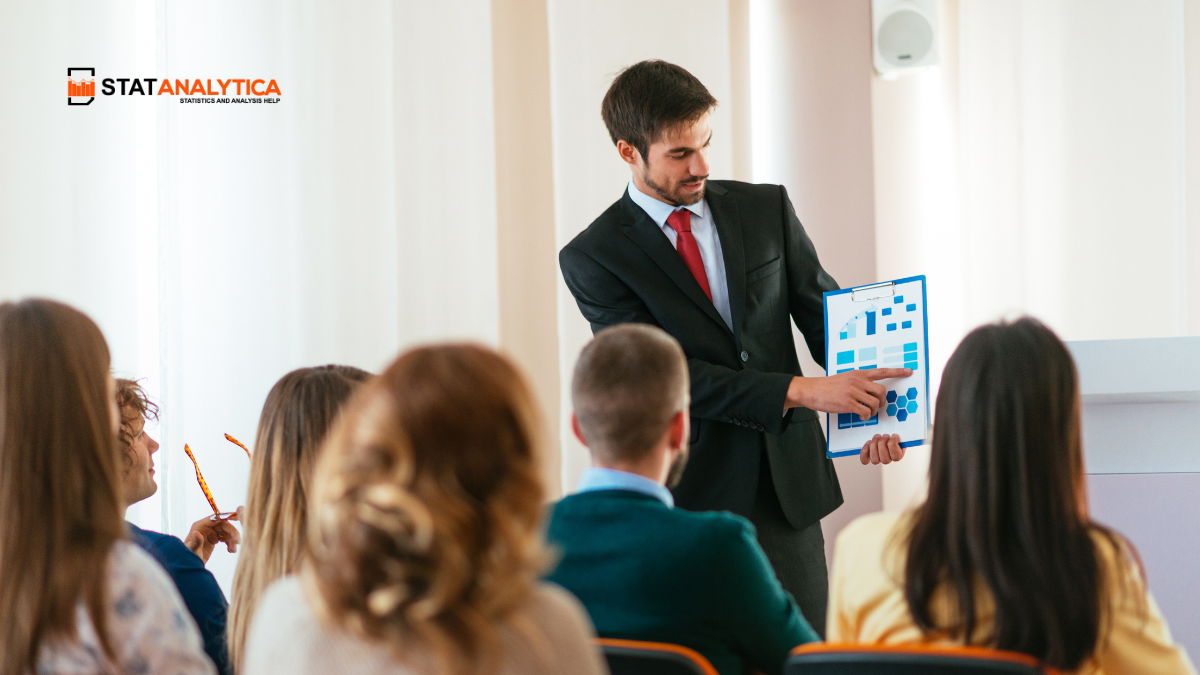
- Smartphone vs. Tablet Preferences: User Experiences
- Attitudes Towards Wearable Technology Adoption
- Consumer Perception of Artificial Intelligence in Everyday Devices
- Preferences in Operating Systems: iOS vs. Android
- Interest in Augmented Reality (AR) and Virtual Reality (VR) Experiences
- Security Concerns in Internet of Things (IoT) Devices
- Sustainable Technology: Consumer Awareness and Choices
- User Satisfaction with Smart Home Automation
- E-readers vs. Physical Books: Reading Preferences
- Preferences in Laptop vs. Desktop Computing for Various Tasks
Top 10 Survey Research Topics On Public Transportation Usage
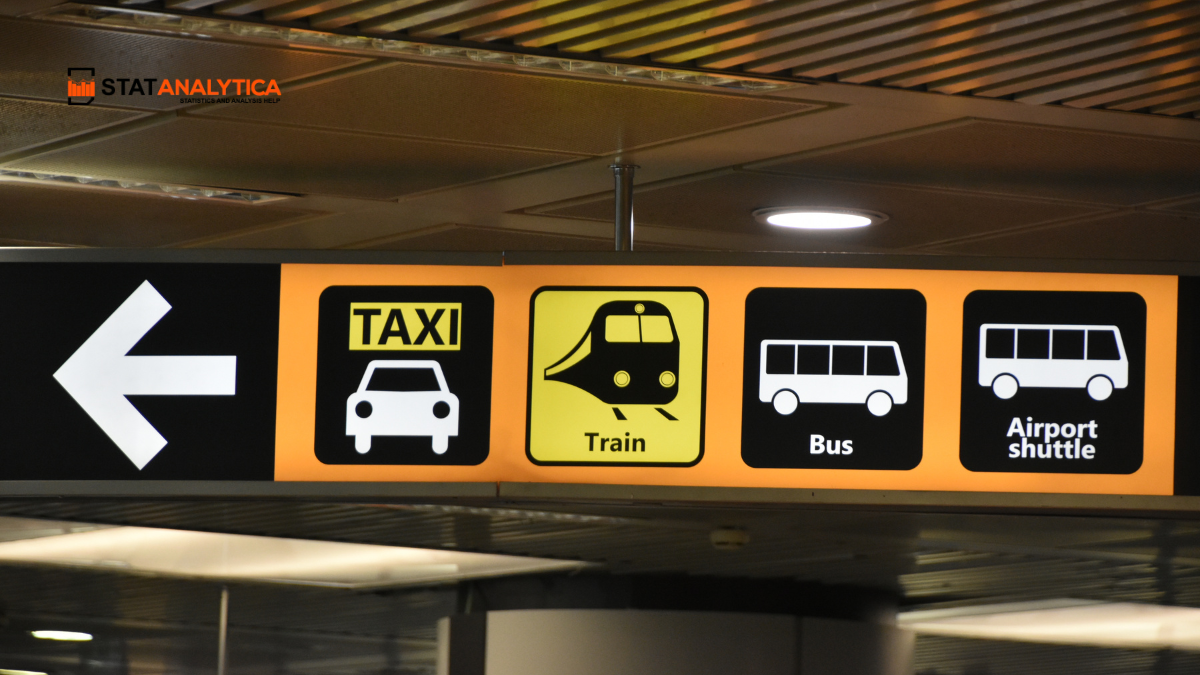
- Factors Influencing Public Transportation Commute Decisions
- User Satisfaction with Public Transit Services
- Impact of Commuting Time on Mode of Transportation
- Barriers to Increased Public Transportation Adoption
- Accessibility and Inclusivity in Public Transportation Systems
- Attitudes Towards Public Transportation Safety Measures
- Technology Integration in Public Transit: User Perspectives
- Public Transportation Usage Patterns During Peak vs. Off-Peak Hours
- The Role of Environmental Awareness in Transportation Choices
- Public Transportation’s Impact on Urban Planning and Development
Top 10 Topics For Survey Research On Environmental Awareness and Practices
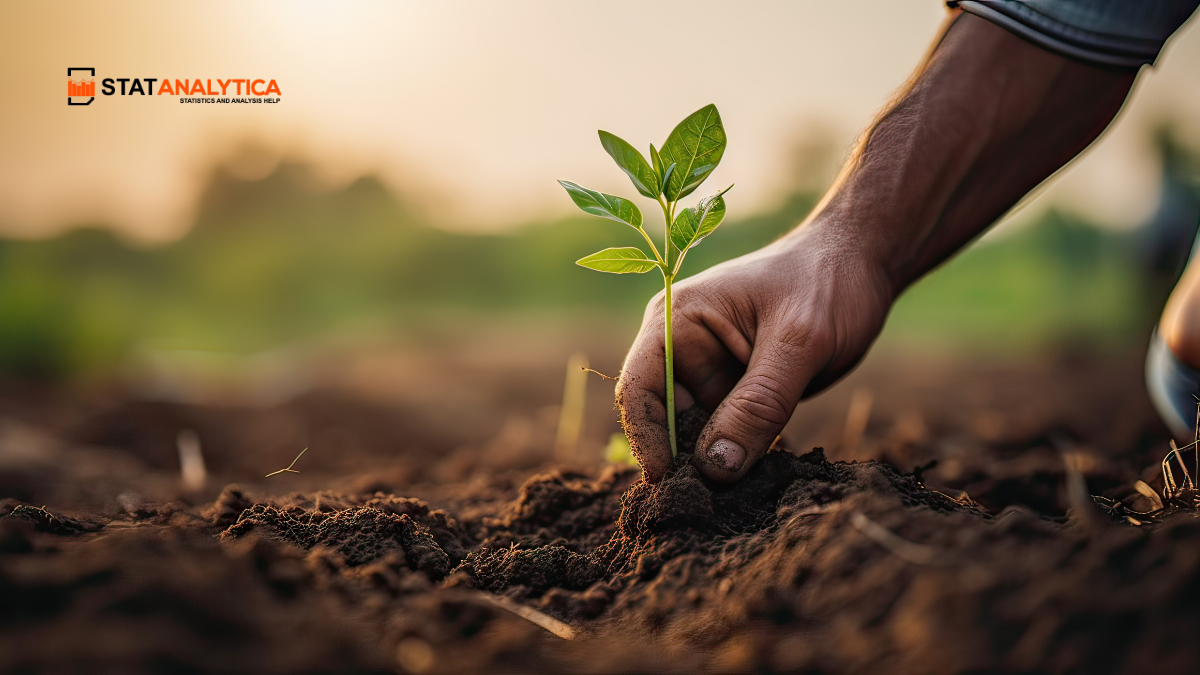
- Public Perceptions of Climate Change: Knowledge and Concerns
- Attitudes Towards Sustainable Energy Sources
- Consumer Preferences for Eco-friendly Products
- The Role of Environmental Education in Shaping Attitudes
- Waste Reduction and Recycling Behaviors
- Views on Government Policies Supporting Environmental Conservation
- Participation in Community Environmental Initiatives
- Water Conservation Practices in Urban and Rural Areas
- Urban Green Spaces and their Impact on Wellbeing
- The Influence of Corporate Sustainability Practices on Consumer Choices
Top 10 Survey Research Topics On Political Beliefs and Affiliations
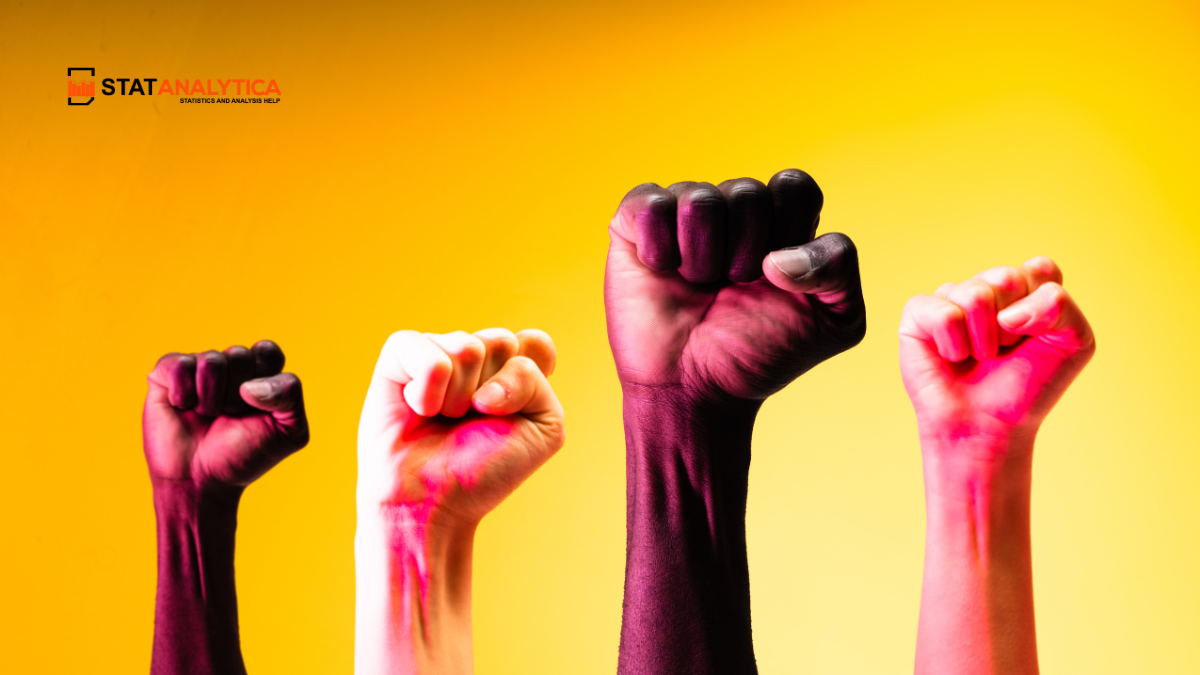
- Political Ideology Trends Among Different Age Groups
- Media Influence on Political Beliefs and Opinions
- Public Trust in Political Institutions and Leaders
- Social Media’s Role in Shaping Political Affiliations
- Factors Influencing Political Party Switching
- Attitudes Towards Political Activism and Protests
- The Impact of Economic Conditions on Political Preferences
- Civic Engagement: Voter Turnout and Political Participation
- Perspectives on Political Polarization and Unity
- Political Communication Channels and Effectiveness
Top 10 Survey Research Topics On Social Media Usage Patterns
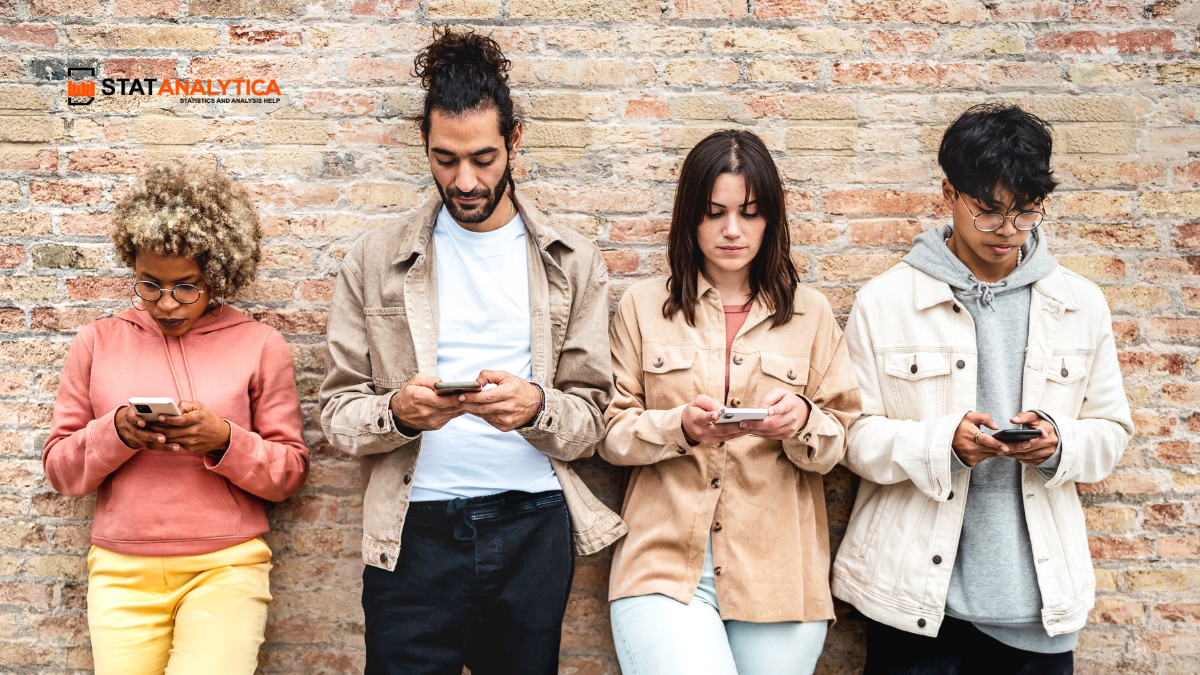
- Social Media Preferences Across Different Age Groups
- Influencers and their Impact on Social Media Choices
- Privacy Concerns and User Behavior on Social Platforms
- Trends in Social Media Engagement: Likes, Shares, and Comments
- The Role of Social Media in Shaping Personal Identity
- User Satisfaction with Social Media Algorithms
- Social Media’s Influence on News Consumption Habits
- Online Harassment and Cyberbullying on Social Platforms
- Attitudes Towards Social Media Advertising
- Impact of Social Media Detox Challenges on User Behavior
Top 10 Topics For Survey Research On Community Involvement
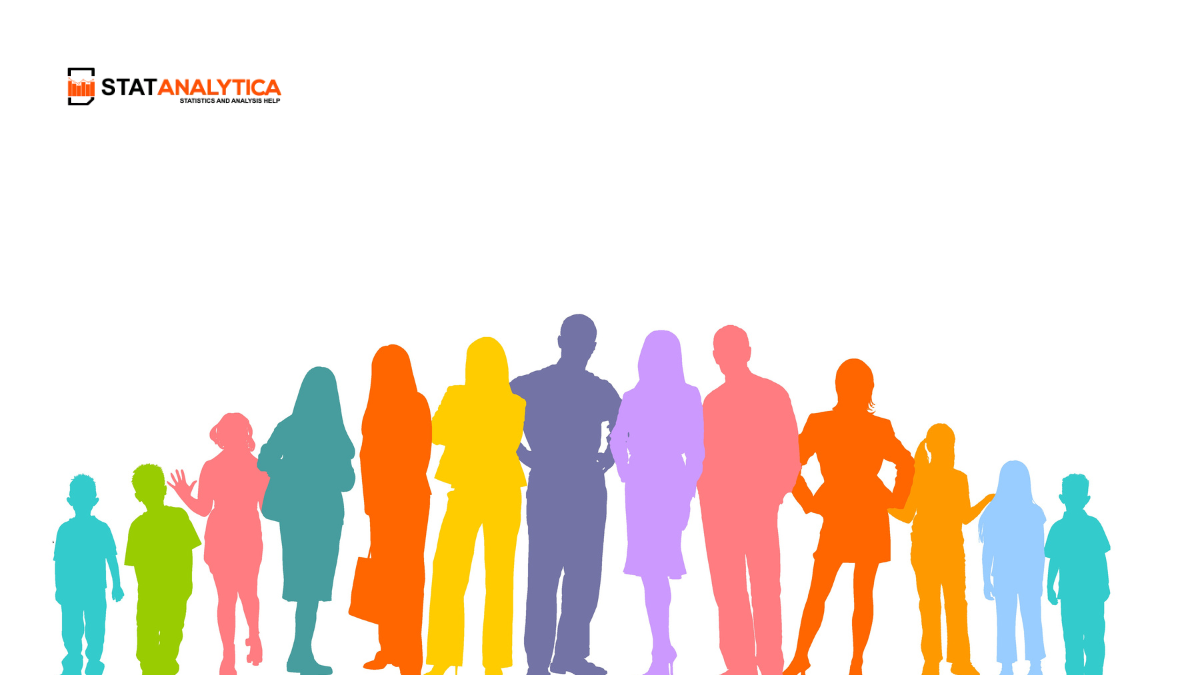
- Factors Influencing Community Volunteerism
- Public Awareness and Participation in Local Governance
- Community Perceptions of Civic Engagement Opportunities
- Attitudes Towards Community Improvement Initiatives
- Barriers to Active Community Participation
- Influence of Socioeconomic Factors on Community Involvement
- Perspectives on the Importance of Neighborhood Associations
- The Role of Social Media in Fostering Community Connections
- Civic Education and its Impact on Community Engagement
- Volunteer Motivations and Satisfaction in Community Service
Top 10 Survey Research Topics On Work-Life Balance
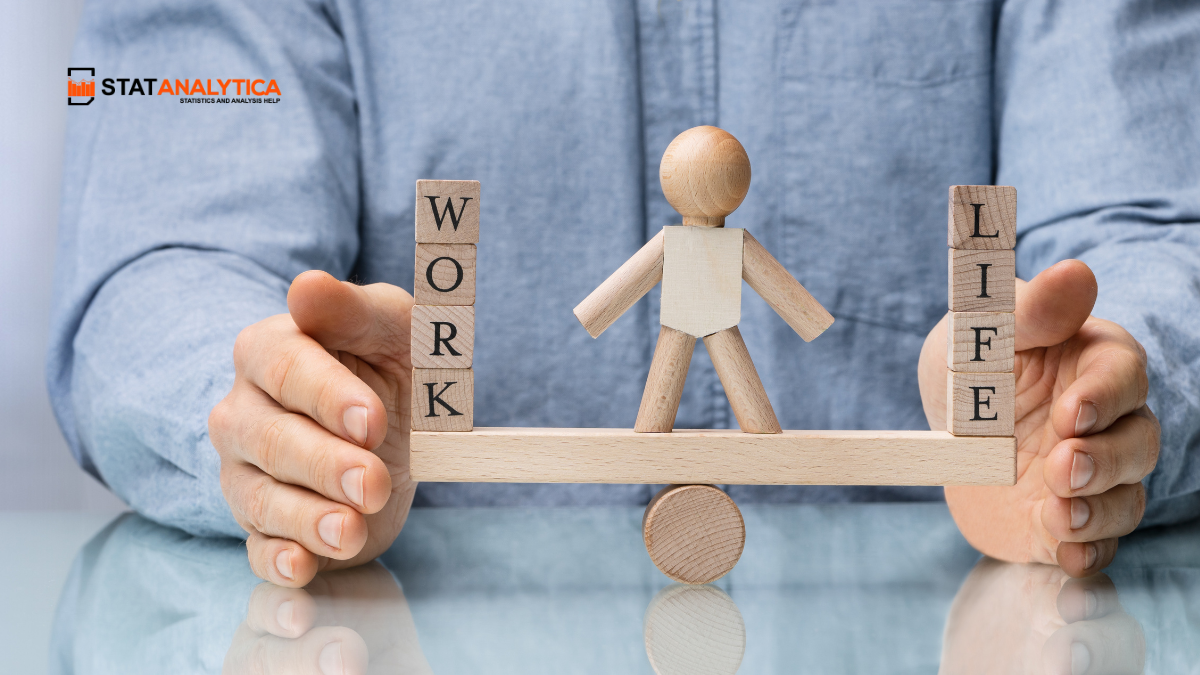
- Employee Perspectives on Work-Life Balance Policies
- The Impact of Flexible Work Arrangements on Wellbeing
- Burnout and Stress Levels in Different Professions
- Attitudes Towards Remote Work and its Effect on Work-Life Balance
- Parental Leave and its Influence on Work-Life Integration
- Job Satisfaction and its Relation to Work-Life Harmony
- Perceptions of Organizational Support for Work-Life Balance
- The Role of Technology in Balancing Work and Personal Life
- Gender Disparities in Work-Life Balance Experiences
- The Influence of Commute Length on Work-Life Equilibrium
Top 10 Topics For Survey Research On Financial Literacy
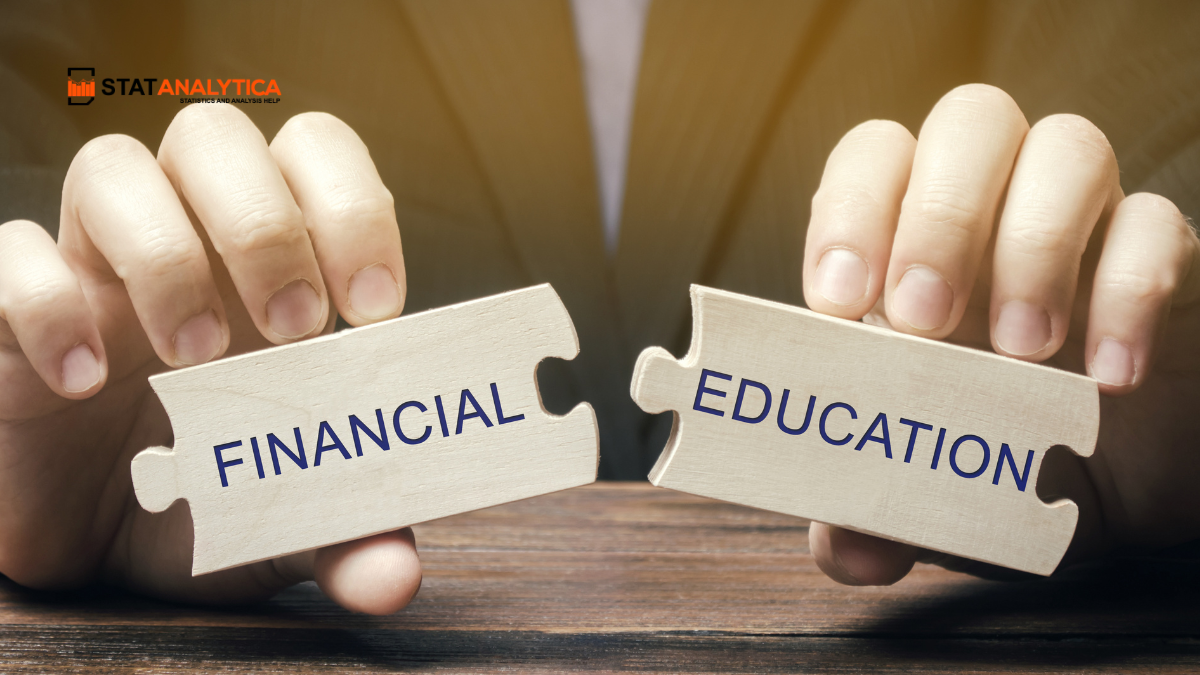
- Financial Literacy Among Different Age Groups
- Impact of Educational Background on Financial Knowledge
- Perspectives on Credit Card Usage and Debt Management
- Knowledge of Investment Strategies and Risk Tolerance
- Attitudes Towards Retirement Planning and Savings
- The Role of Financial Education Programs in Shaping Literacy
- Awareness and Utilization of Financial Planning Services
- Influence of Socioeconomic Factors on Financial Literacy
- Understanding of Taxation Systems and Financial Responsibilities
- Consumer Perspectives on Fintech and Digital Banking Literacy
Top 10 Survey Research Topics On E-commerce Shopping Habits
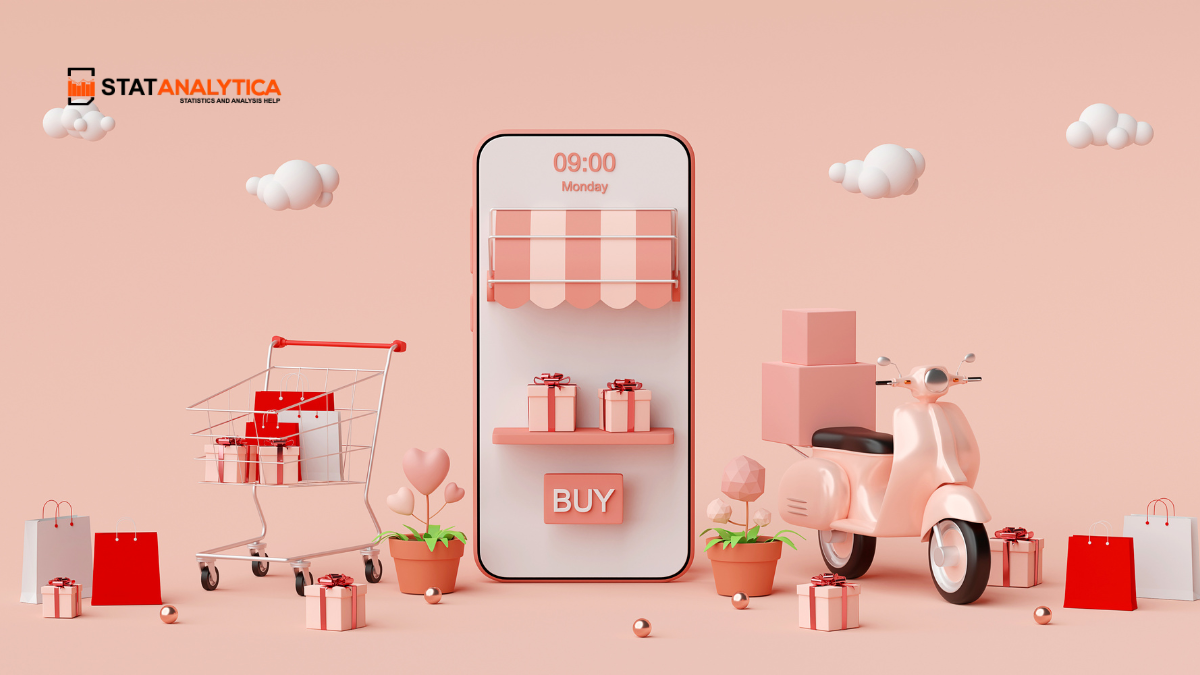
- Consumer Trust in Online Payment Security
- Preferences for Mobile vs. Desktop Shopping Experiences
- Factors Influencing Online Shopping Cart Abandonment
- Impact of Product Reviews on Purchasing Decisions
- The Role of Social Media in Influencing E-commerce Choices
- Customer Loyalty Programs: Effectiveness and Perception
- Cross-Border E-commerce Shopping Trends
- Sustainability and Ethical Considerations in Online Purchases
- Influencers and their Impact on E-commerce Sales
- Consumer Attitudes Towards Personalized Shopping Recommendations
Top 10 Topics For Survey Research On Entertainment Preferences
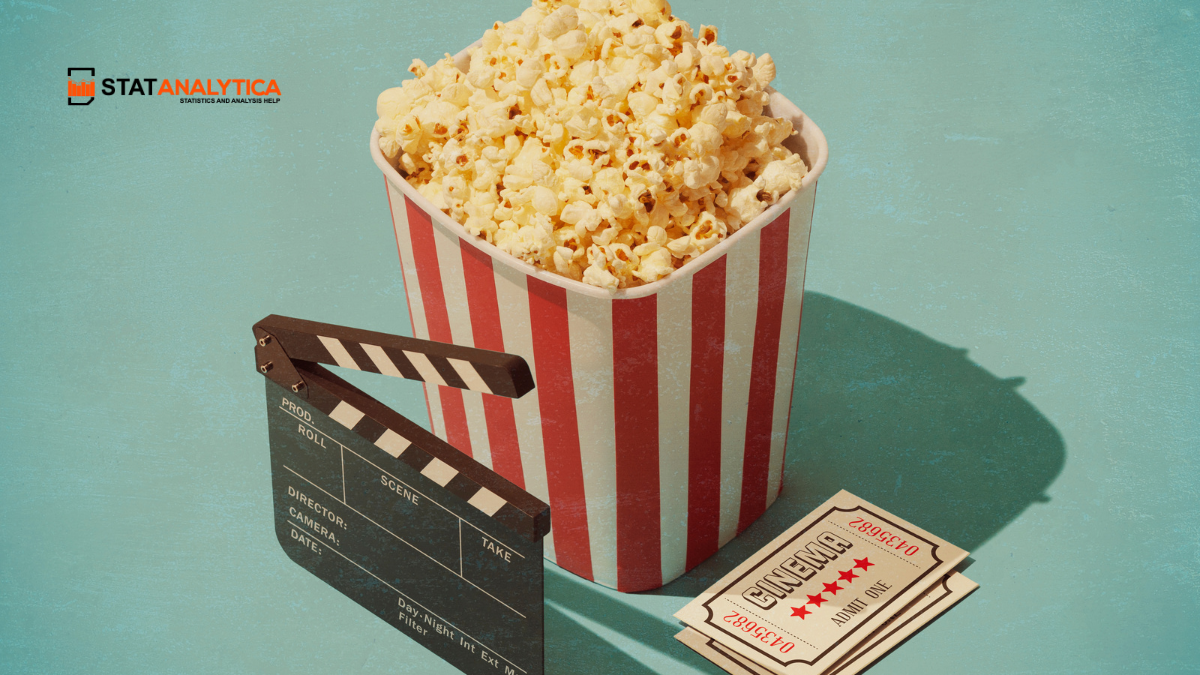
- Streaming Services vs. Traditional Cable: Consumer Preferences
- Influences on Music Genre Preferences Among Different Age Groups
- Viewer Satisfaction and Preferences in Online Video Content
- Impact of Social Media on Movie and TV Show Recommendations
- Trends in Live Events vs. Digital Entertainment Participation
- The Role of Podcasts in Consumer Entertainment Choices
- Gaming Preferences: Console vs. PC vs. Mobile
- User Engagement in Virtual and Augmented Reality Entertainment
- Attitudes Towards Celebrity Endorsements in Entertainment
- Impact of Cultural Background on Entertainment Choices
Top 10 Survey Research Topics On Housing and Living Conditions
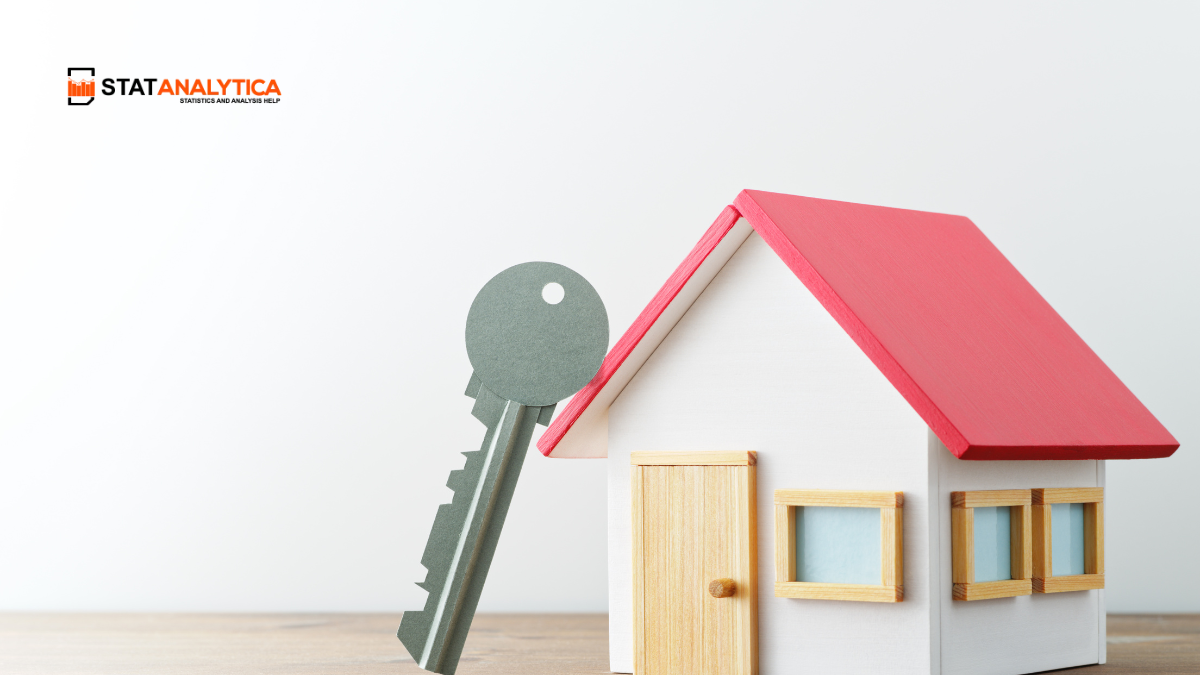
- Satisfaction with Affordable Housing Options
- Preferences for Urban vs. Suburban Living Environments
- Impact of Remote Work on Housing Choices and Locations
- Community Safety and its Influence on Housing Decisions
- The Role of Green Spaces in Residential Satisfaction
- Access to Public Transportation and Housing Selection
- Smart Home Technology Adoption and Preferences
- Perceptions of Gentrification in Local Neighborhoods
- Affordable Housing and its Impact on Local Communities
- Housing Accessibility and Satisfaction for Individuals with Disabilities
Top 10 Topics For Survey Research On Attitudes Towards Remote Work
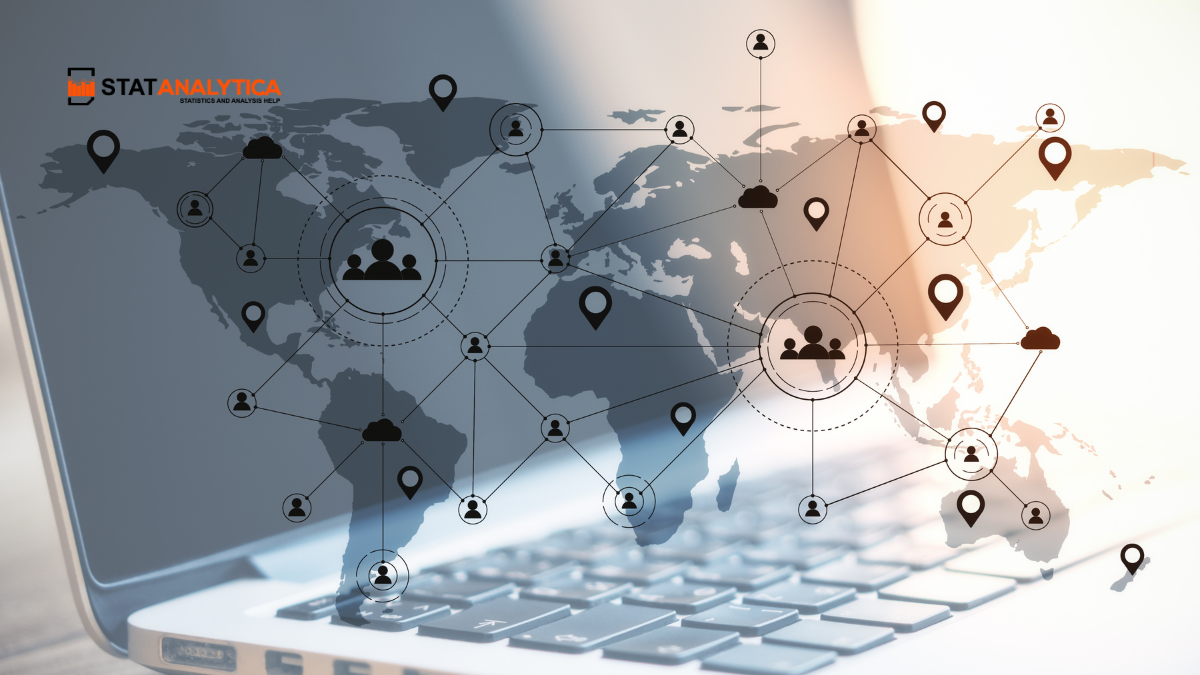
- Employee Satisfaction and Productivity in Remote Work Environments
- Technological Challenges in Adapting to Remote Work
- Employer Policies and Flexibility in Remote Work Arrangements
- Impact of Remote Work on Work-Life Balance
- Communication Effectiveness in Virtual Teams
- Employee Perspectives on Mental Health and Remote Work
- Remote Work’s Influence on Job Satisfaction and Career Advancement
- Employer and Employee Perceptions of Remote Work Sustainability
- Remote Work’s Effect on Organizational Culture
- Factors Influencing Employee Return-to-Office Preferences
30 Field Specific Topics on Survey Research
Top 10 survey research topics for marketing students.
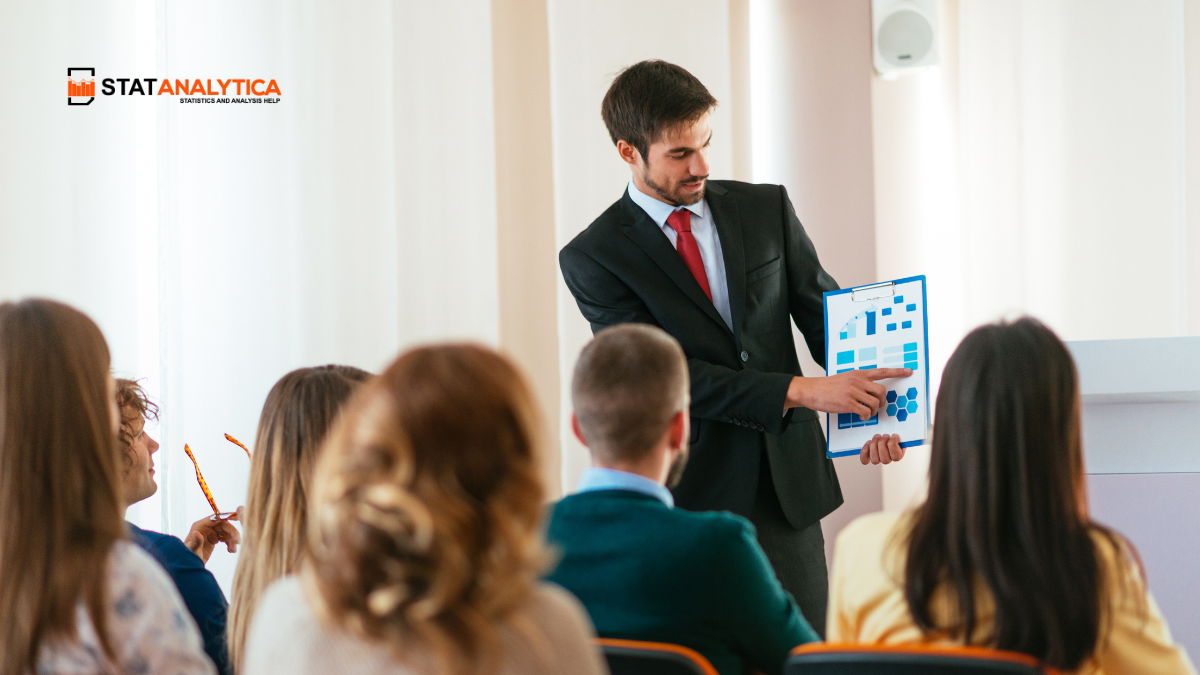
- Consumer Preferences in Online Shopping: A Comparative Analysis
- Effectiveness of Social Media Influencers in Product Endorsements
- Perceptions of Brand Loyalty Programs and Customer Retention
- Impact of E-commerce Trends on Traditional Retail Shopping Habits
- Evaluating Customer Satisfaction with Mobile App User Experiences
- Consumer Attitudes Towards Sustainable and Eco-Friendly Products
- Exploring the Influence of Celebrity Endorsements on Product Purchases
- Effectiveness of Personalized Marketing Strategies in Online Retail
- Customer Perception of Brand Authenticity in Marketing Campaigns
- Assessing the Impact of Online Reviews on Consumer Decision-Making
Top 10 Survey Research Topics For Medical Students
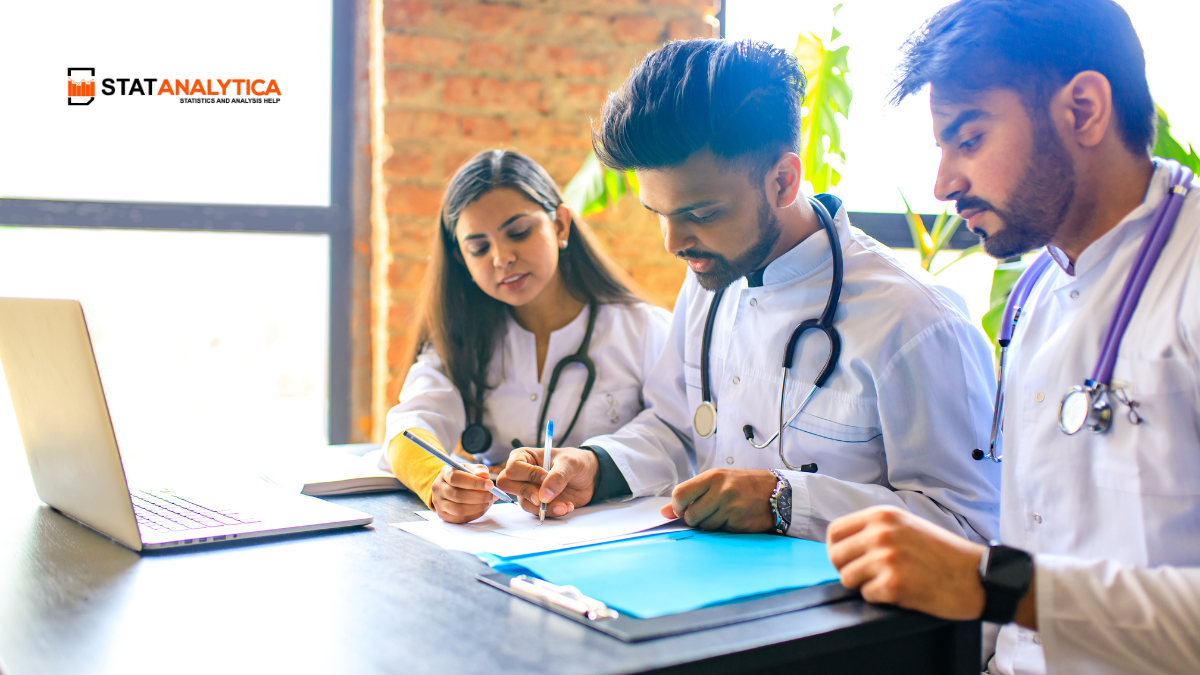
- Medical Students’ Perceptions of Virtual Learning Environments
- Evaluating the Impact of Clinical Rotations on Career Aspirations
- Assessment of Stress and Burnout Among Medical Students
- Effectiveness of Peer-Assisted Learning in Medical Education
- Attitudes Towards Integrating Technology in Medical Curriculum
- Exploring Medical Students’ Awareness of Mental Health Resources
- Medical Students’ Views on Cultural Competency Training
- Perceptions of the Clinical Clerkship Experience
- Understanding the Impact of Sleep Deprivation on Medical Students
- Medical Students’ Perspectives on Patient Interactions and Communication Skills Training
Top 10 Survey Research Topics For Psychology Students
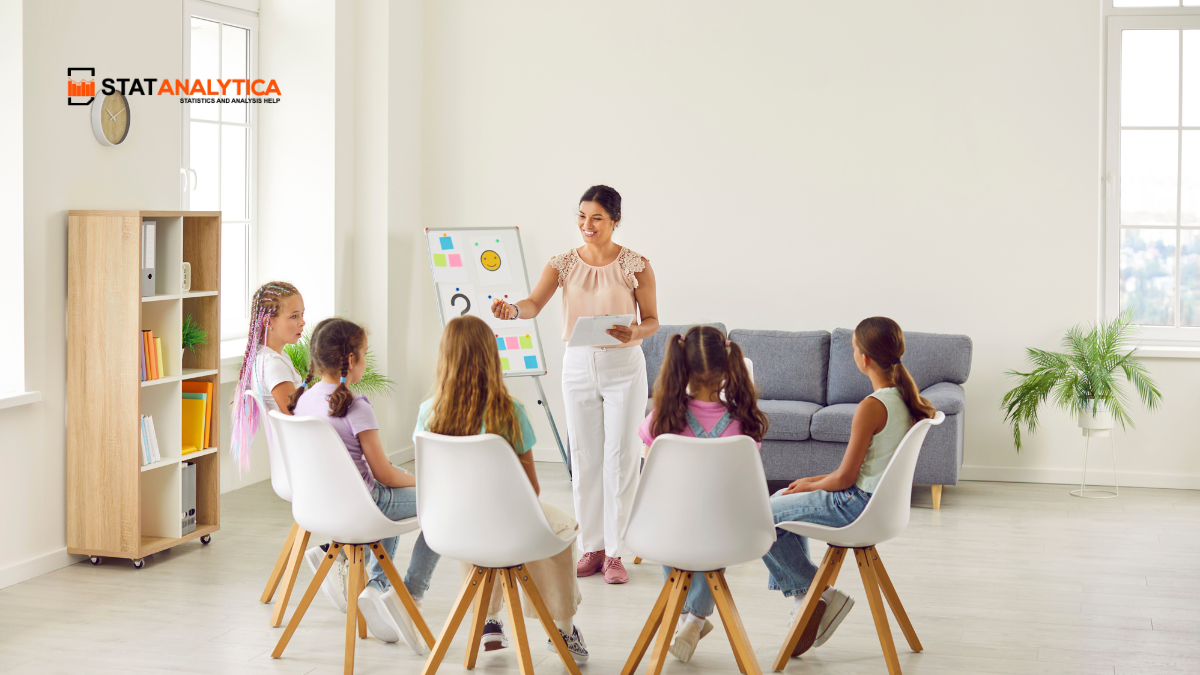
- The Impact of Social Media on Psychological Well being
- Perceptions of Therapy Effectiveness and Accessibility
- Factors Influencing Stress Levels Among College Students
- Understanding Attitudes Towards Mental Health in the Workplace
- Exploring Personality Traits and Coping Mechanisms
- Attitudes Towards Seeking Help for Mental Health Issues
- Impact of Childhood Experiences on Adult Mental Health
- Psychological Effects of Social Isolation and Loneliness
- Perceptions of Happiness and Life Satisfaction Across Age Groups
Top 15 Methods Of Survey Research
Survey research involves collecting data from a target audience to gain insights into opinions, behaviors, or characteristics. Several methods are employed in survey research, each with its strengths and limitations. Here are common methods:
- Questionnaires: Distributing written or online surveys with structured questions allows for standardized data collection.
- Interviews: Conducting one-on-one or group interviews enables in-depth exploration of responses and clarification of ambiguous answers.
- Telephone Surveys: Collecting data over the phone is a quick and cost-effective method for reaching a wide audience.
- Online Surveys: Utilizing web-based platforms for data collection offers convenience and access to a large and diverse participant pool.
- Face-to-Face Surveys: Interviewers administer surveys in person, fostering rapport and improving response rates.
- Mail Surveys: Sending questionnaires by mail is a traditional method, suitable for reaching specific demographic groups.
- Cross-Sectional Surveys: Collecting data from a diverse group at a single point in time helps capture a snapshot of opinions or behaviors.
- Longitudinal Surveys: Gathering data from the same participants over an extended period allows for the analysis of changes over time.
- Online Panel Surveys: Building a panel of participants who regularly respond to surveys facilitates repeated data collection.
- Randomized Controlled Trials (RCTs): Adding surveys within experimental designs helps assess causation and control for confounding variables.
- Mail Panels: Creating a group of individuals who regularly receive and respond to mail surveys allows for longitudinal data collection.
- Drop-Off/Pick-Up Surveys: Leaving surveys at a designated location for participants to complete and return later.
- Intercept Surveys: Approaching participants in public spaces, such as malls or events, for on the spot interviews.
- Ethnographic Surveys: Immersing researchers in the target environment to observe and understand behaviors and attitudes.
- Delphi Method: Repeating surveys with expert opinions to reach a consensus on complex topics.
Choosing the appropriate survey method depends on factors like research goals, budget, and the nature of the data needed. Researchers often use a combination of methods for a comprehensive understanding of their study area.
And that’s a wrap on our journey through 151 topics for survey research. Each question is a little window into people’s thoughts and preferences. We hope this updated list brings you not just data, but insights and a bit of fun too.
Surveys are like conversations with the world, and with these topics, you’re all set to start chatting. Whether it’s uncovering trends or settling debates, your survey adventure awaits. So, go ahead, dive into the questions, discover new perspectives, and enjoy the endless possibilities that survey research brings. Happy surveying, explorers.
Related Posts
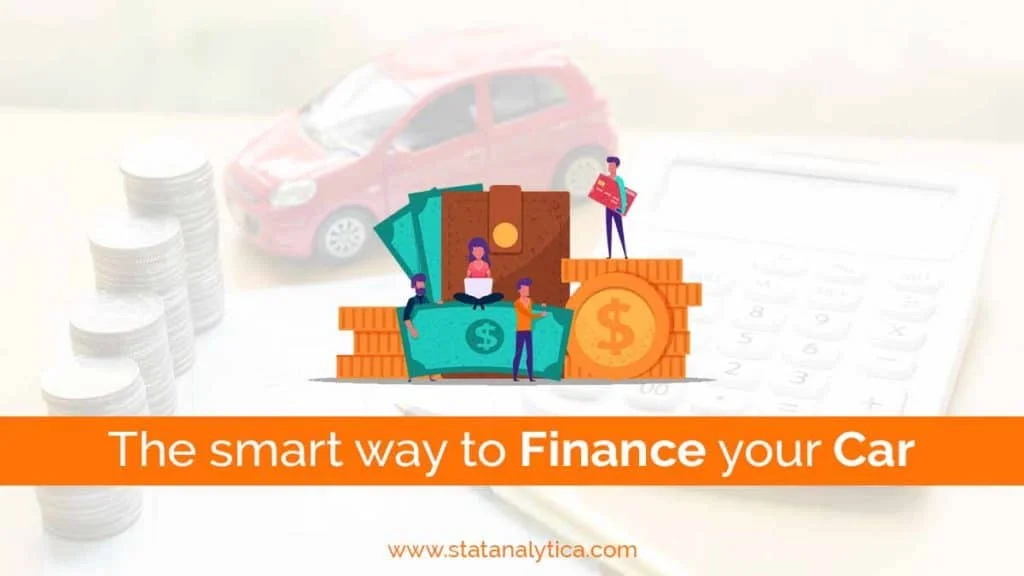
Step by Step Guide on The Best Way to Finance Car
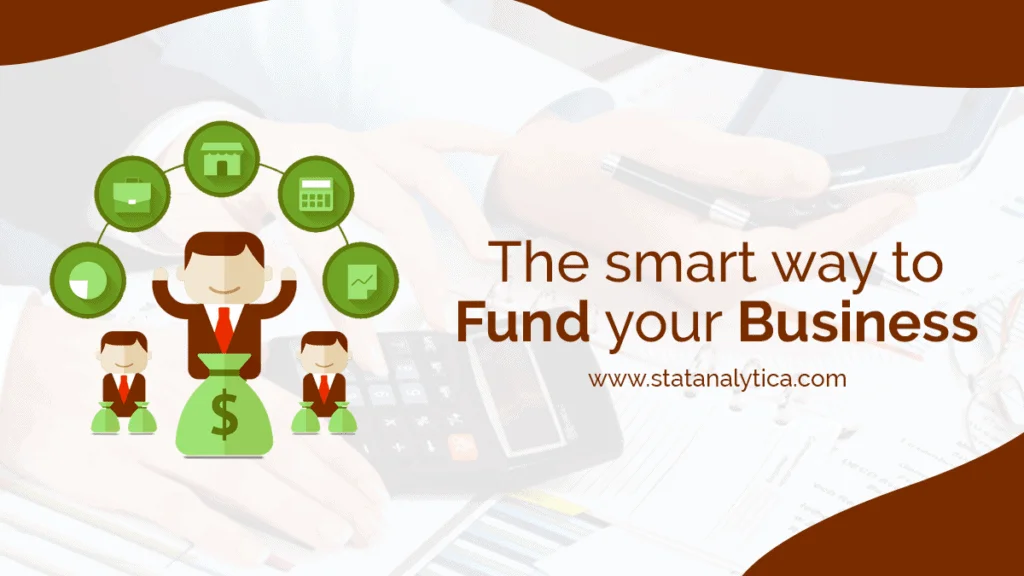
The Best Way on How to Get Fund For Business to Grow it Efficiently
- Open access
- Published: 12 August 2015
Refining questionnaire-based assessment of STEM students’ learning strategies
- Birgit Griese 1 ,
- Malte Lehmann 2 &
- Bettina Roesken-Winter 2
International Journal of STEM Education volume 2 , Article number: 12 ( 2015 ) Cite this article
17k Accesses
19 Citations
3 Altmetric
Metrics details
Learning strategies are considered a key aspect for academic performance not only for science, technology, engineering and mathematics (STEM) students. Refining their assessment thus constitutes a worthwhile research purpose. The aim of this study is to examine the 69-item LIST questionnaire (ZDDP 15:185-200, 1994) with the aim of shortening it while keeping its factor structure and thus its potential for describing learning behaviour and for identifying significant changes therein. This includes exploring if reduced scales remain internally reliable, both in terms of reliability measures and content, and to examine if they stay sensitive enough to capture developments in a pre-post design.
Our cohorts consist of STEM students ( N = 2374) from different engineering courses at Ruhr-Universität Bochum in Germany, typically predominantly males, some with insufficient background in mathematics or non-native speakers of German. The data was analysed using various statistic methods, e.g. reliability measurement and confirmatory factor analysis. Our findings show that about half of the original items (36 out of 69) are sufficient, reliability holds (Cronbach’s α > 0.7) and more variance is explained (56.17 % as compared to 45.65 %). Most content-related changes occurring when eliminating so many items survive critical scrutiny.
Conclusions
The study shows that it is possible to refine and considerably shorten the LIST questionnaire to the effect that all but one factor are kept intact. This will simplify future research enormously. Furthermore, the refined factor structure suggests reconsidering the postulate of metacognition as an easily accessed facet of learning behaviour—thus implying promising research perspectives.
In science, technology, engineering and mathematics (STEM) education, the passage from secondary to tertiary education is considered problematic, which is especially true regarding the challenges students encounter when being confronted with university mathematics (cf. Gueudet 2008 ; Henn et al. 2010 ). Students’ failure rates in STEM fields and particularly in engineering subjects are alarmingly high in many countries. In Germany, for instance, almost 48 % of engineering students fail in their first year of university studies (Heublein et al. 2012 ). In relation to other subjects, the gap between school and university mathematics seems to be extremely high and causes difficulties for students taking mathematics courses. The obstacles can be categorized in three categories, thus stressing the wide range of aspects: epistemological and cognitive difficulties, sociological and cultural difficulties and didactical difficulties (de Guzmán et al. 1998 ). Their dramatic character is depicted in the word “abstraction shock”, referring to the fact that university mathematics is adding a formal world to the mathematics encountered at school (e.g. Artigue et al. 2007 ; Tall 2004 ). The challenge of understanding (and influencing) how the learning of mathematics at university works is often addressed by the use of cognitive development theories, as mathematics is commonly regarded as a rational and cognitively demanding subject (cf. Dreyfus 1995 ). Studies therefore often elaborate on cognitive difficulties and conceptual obstacles experienced by students in how mathematics is communicated to them (Artigue et al. 2007 ), particularly referring to the formal level of university mathematics and the prevalent role of proofs (Selden and Selden 2005 ).
But mastering a university course containing mathematics requires more than a talent for abstract thought and formalities; it needs a combination of general skills and attitudes such as self-organisation, perseverance and frustration tolerance, as well as subject-specific abilities and meta-level capacities (cf. Pintrich et al. 1993 ; Weinstein and Palmer 2002 ; Wild and Schiefele 1994 ). It is interesting to detect in how far school leavers possess these different features, how these improve during the first year at the university and, importantly, what can be done to efficiently encourage their development. Students’ meta-level learning behaviour is crucial, taking account of the words of de Guzmán et al. ( 1998 ) who state:
Students’ success is linked to a great extent to their capacity of developing “meta-level” skills allowing them, for instance, to self-diagnose their difficulties and to overcome them, to ask proper questions to their tutors, to optimize their personal resources, to organize their knowledge, to learn to use it in a better way in various modes and not only at a technical level. (p. 760)
Thus, a promising perspective is provided by exploring general and meta-level skills in terms of learning strategies (e.g. Wild 2005 ; Rach and Heinze 2011 ), whose investigation allows for revealing both the cognitive dispositions as well as affective barriers and pathways—and finally the interrelations between them.
Numerous research and instruction projects that attend to these issues can be found in many countries (cf. Dunn et al. 2012 ). The causality between adequate learning strategies and successful learning seems well established (for example, cf. Erdem Keklik and Keklik 2013 ). Depending on the specific research interest, previous studies have different emphases, for example, affective aspects or problem-solving skills. Most of these studies include data collection via questionnaires. To meet the challenge of assessing learning strategies appropriately, in the past, questionnaires were developed aiming at capturing different facets of these skills. In this paper, we report on refining and adapting one commonly used German questionnaire for assessing students’ learning strategies. Our research is dedicated to gaining a reliable and valid instrument that simultaneously allows for making good economic sense. Often, research is not restricted to merely capturing students’ learning strategies but also includes interventions, leading to a pre-post design with repeated surveys, so one essential goal lies in keeping the load for students within reasonable limits.
All data was collected within the scope of the design-based research study MP 2 -Math/Plus/Practice, which focuses on supporting engineering students in mathematics (cf. Griese et al. 2013 ; Dehling et al. 2014 ). Among other issues, the project aims at supporting those with insufficient learning strategies and motivation by attempting to remedy these obstacles. To reveal the influence of the project interventions, the study draws on capturing students’ learning strategies and their development in the first semester at university.
- Learning strategies
Learning strategies as indications for learning behaviour
Recently, Blömeke et al. ( 2015 ) contributed to modelling competence “as a process, a continuum with many steps in between” (p. 7). In particular, they emphasized the following perspective:
Thus, we suggest that trait approaches recognize the necessity to measure behaviorally, and that behavioral approaches to competence recognize the role of cognitive, affective and conative resources. At this time, we encourage research on competence in higher education emanating from either perspective and paying attention particularly to the steps in between. (Blömeke et al. 2015 , p. 7)
Accordingly, in the paper at hand, learning strategies are understood as all kinds of planned and conscious learning behaviour and the attitudes behind it, involving observable actions (e.g. solving tasks, asking questions, taking notes) as well as thought processes (e.g. planning, reflecting) on the basis of both cognitive and affective-motivational dispositions. This extends to the lack of planning and conscious actions as it also presents a characteristic of an individual’s learning strategy.
Research on the significance of learning strategies in mathematics education has its roots in contributions highlighting the role of affect, motivation and beliefs (cf. McLeod 1992 , as a starting point), as all cognitive processes involve affective stances that moderate the tension between modes of intuitive and analytical thinking (e.g. Fischbein 1987 ; Stavy and Tirosh 2000 ). In particular, the theory of dual processes in cognitive psychology has been adapted to mathematics education, and the role of affective variables has been pointed out in this context (e.g. Evans 2007 ). These perspectives provide fresh views on learning processes and have done much to reach a deeper understanding of the obstacles involved. Findings reveal that students’ cognitive reflection, as a metacognitive variable, their beliefs about mathematics, and their self-efficacy, are all correlated positively and significantly with mathematical achievement (Gómez-Chacón et al. 2014 ). There is also evidence that metacognition impacts positively on learning strategies which in turn influences achievement (Griese et al. 2011 ).
In summary, it can be said, therefore, that this has led to a fortified interest in certain kinds of learning strategies. In the context of mathematics, overcoming motivational and affective barriers with the help of meta-skills, e.g. self-regulation, has become an important issue. What is more, mathematics demands the use of effective planning as well as organized and consistent work (cf. Rach and Heinze 2011 ). More than many other subjects, mathematics is cognitively challenging and needs motivational perseverance, thus representing an ideal research area for the influence of interventions addressing learning strategies, both on a general and a meta-level. However, the goals when assessing students’ learning behaviour in science are various: taking an inventory, describing the development, comparing or improving learning behaviour (cf. Lovelace and Brickman 2013 ), and so are the research interests, in mathematics, popularly performance prediction or the identification of at-risk students. Apart from more time-consuming methods, this has led to a great variety of questionnaires in many languages.
Questionnaires for assessing learning strategies
Questionnaires with different focal points (according to the background of the authors and their research interests) originate from this variety (cf. Pintrich et al. 1993 ; Weinstein and Palmer 2002 ). Schellings ( 2011 ) gives a comprehensive overview from an international and a Dutch perspective. Though her work is based on the text-heavy learning of history, the general categories of learning behaviour can be applied to other subjects as well. Differentiating between motivational and cognitive aspects when dealing with learning strategies is a widely accepted concept (cf. Nenniger 1999 ) and is in keeping with the understanding of affective aspects as a key issue.
In the following, approaches to capture learning strategies which have influenced subsequent research fundamentally will be outlined. The selection includes only those which reflect the importance of affective and motivational issues. Pintrich et al. ( 1993 ) developed a questionnaire “to measure college undergraduates’ motivation and self-regulated learning” (Artino 2005 , p. 3), the Motivated Strategies for Learning Questionnaire (MSLQ). The MSLQ measures motivation and self-regulated learning in general and for a particular course by means of six motivation and nine learning strategies subscales. Initially, Pintrich and De Groot ( 1990 ) started by postulating a five latent factor structure comprising expectancy, value, affect, learning strategies and self-regulation. The items that were developed for operationalizing these constructs later formed the basis for the 15 subscales mentioned above. MSLQ has been applied in many research studies (Duncan and McKeachie 2005 ), partly aiming at developing a new conceptualization with respect to the significance of the single sub-scales (Dunn et al. 2012 ; Hilpert et al. 2013 ). MSLQ’s reliability has proved “robust”, and its predictive validity to actual course performance is considered “reasonable” (Pintrich et al. 1993 , p. 801).
The Approaches to Studying Inventory (ASI) by Entwistle and Ramsden ( 1983 ) and its refinements (ASSIST by Tait et al. 1998 , ALSI by Entwistle and McCune 2004 ) feature the main distinction of categorizing learning behaviour as being of either strategic (deep) or of apathetic (surface) approach. The dichotomy forms the inventory’s two main factors which in turn contain up to 16 subscales, depending on the version of the questionnaire. Although the authors do not group their items into motivational and (meta-)cognitive scales, the object of research is nearly identical to that of MSLQ users. A specific feature of ASI and its variations is the idea to measure not only the desired learning behaviour ( strategic approach) but also what is hypothesized as less success-oriented ( apathetic approach). This produces a multifarious picture of learners’ behaviour.
Another well-known instrument to capture students learning strategies is the Learning and Study Strategies Inventory (LASSI) by Weinstein and Palmer ( 2002 ). LASSI covers thoughts, behaviours, attitudes and beliefs in relation to successful learning that can also be fostered by interventions. Its ten scales are classed into affective strategies, goal strategies and comprehension monitoring strategies, thus covering cognitive, metacognitive (particularly, self-regulative), affective and motivational aspects. LASSI is not only used for research purposes but is also recommended to students to use for themselves in order to get feedback on their strengths and weaknesses. LASSI’s reliability coefficients (Cronbach’s α ) for its different scales are reported to score between 0.86 and 0.68, the lowest often being considered insufficient (Weinstein et al. 1987 ). Its validity to academic performance depends on the specific scale, e.g. Cano ( 2006 ) found, using multiple regression, that two scales (namely Affective Strategies and Goal Strategies ) contributed to academic performance, whereas one ( Comprehension Monitoring Strategies ) did not.
All questionnaires described so far resort to self-assessment of student behaviour. It must be conceded that this entails the weakness that “the learner’s perceptions of his or her strategies are measured” (Schellings 2011 , p. 94), which need not coincide precisely with the strategies themselves. In this context, it is interesting to compare self-reported learning behaviour (especially concerning metacognition) to the results gained with other methods, e.g. Thinking Aloud (David 2013 ). When it comes to affective aspects, however, the learner’s subjective perspective is what counts. Other problems, like assessing the sufficiency or efficiency of study time or effort, might be harder to overcome. In our context, beginner students might initially judge their efforts as sufficient (in relation to what they have been used to at school), but later rate them as inadequate (when their frame of reference has shifted after some months at university). This can result in a seeming decrease, although objectively, they have actually increased their efforts. Particularly in pre-post designs, this must be taken into account when interpreting results. Some authors thus favour using a retrospective pretest data design to measure programme effectiveness (Nimon et al. 2011 ; Lam and Bengo 2003 ) which is easily applicable to inventories measuring learning strategies.
LIST inventory
For our research at Ruhr-Universität Bochum in Germany, the decision fell for the German LIST questionnaire ( Learning Strategies at University , Wild and Schiefele 1994 ), which is based on the same classification as MSLQ and takes up aspects from LASSI as well. LIST was invented for measuring learning strategies of medium generality, between learning styles and learning tactics (Wild 2000 ). The instrument distinguishes between cognitive, metacognitive and resource-related learning strategies and comprises dimensions of learning strategies grouped accordingly. This mirrors the acceptance of this taxonomy in the German-speaking community (Wild 2000 ). Just like its English predecessors, this approach originates from educational research and thus is not subject-specific. However, in STEM education, the instruments are frequently used to assess students’ learning behaviour on a general level while combining the results with subject-related measures (Lovelace and Brickman 2013 ). The LIST questionnaire for measuring learning strategies in academic studies was first compiled in the 1990s (Wild and Schiefele 1994 ) and has since been modified and tested several times. LIST has been applied in the context of many subjects, mathematics among them (cf. Liebendörfer et al. 2014 , for an overview), with overall satisfying results with regard to reliability (Wild and Schiefele 1994 found Cronbach’s α between 0.64 for Metacognition and 0.90 for Attention ) and validity (Wild 2000 , 2005 ). In the following, we explore in detail how the LIST takes up scales and items from MSLQ and LASSI, in order to understand its origins and to illustrate its structure.
Apart from Motivation , the scales from LIST are derived directly from MSLQ, although the number of items varies. Some items in LIST are translations of MSLQ items. In addition to LIST, MSLQ seems very differentiated in terms of motivation, it sports six Motivation scales ( Intrinsic Goal Orientation , Extrinsic Goal Orientation , Task Value , Control of Learning Beliefs , Self-Efficacy for Learning and Performance , Test Anxiety ) comprising 31 items. LIST does not have items with the label Motivation as such, but LIST’s six items on Attention (which are all reverse coded) and eight items on Effort more or less cover this aspect, for example, “I work late at night or at the weekends if necessary”. And other LIST scales, in particular, the resource-related ones, are meant to measure the degree of motivation a student possesses when preparing for an important exam, with items like “I fix the hours I spend daily on learning in a schedule”. The main difference between the two questionnaires is that MSLQ puts more emphasis on including different aspects of motivation as Goal Orientation or Control of Learning Beliefs . For LIST, on the other hand, the aim was to clearly keep apart cognitive and motivational aspects.
LASSI (Weinstein and Palmer 2002 ) also separates cognitive aspects but has much less communalities with LIST. LASSI scales partly cover the same contents though holding different names, e.g. Concentration and Attitude (LASSI) compared to Attention (LIST). The numbers of items in a scale are different, too: there are 3 to 8 in LIST (if Metacognitive Strategies are divided into three scales, 4 to 8 if not), 3 to 12 (3 to 8) in MSLQ, and a constant 8 items in all LASSI scales. This results in considerable differences in analogous scales between LIST and MSLQ: LIST has 31 in Cognitive Strategies whereas MSLQ has 19 in the respective scales. According to the inventors of LIST, scales were expanded in order to reach better reliability (Wild 2000 ). All three questionnaires use Likert scales, ranging from five points (LIST) over six (LASSI) to seven (MSLQ). An overview on how LIST is based on MSLQ and LASSI is provided in Table 1 .
As the comparison of sub-scales and items of the three instruments reveals, there are ample reasons for aiming at compactifying and balancing LIST, particularly when planning to use it with large samples. One side of the coin is receiving a reliable and valid instrument to access learning strategies. However, the next thing to consider is that the distribution, filling in and collection of the LIST questionnaire in a lecture hall holding several hundred students lasts almost 30 min, precious time that both lecturers and students would rather spend on mathematics. In addition, many studies aim at assessing learning strategies in a pre-post design at least twice, meaning that the drop-out rate is a serious issue to consider in any study design. That is, if there were a possibility to reduce the time to 15 min, participants’ cooperation would be easier to gain.
Study perspectives and research questions
The purpose of the project MP 2 -Math/Plus/Practice is to support engineering students in their first year at the university by enhancing their learning strategies and motivation. The length of MP 2 -Math/Plus/Practice, which has completed its fifth year, permits to change the project interventions according to the principles of educational design research (cf. McKenney and Reeves 2012 ). The fact that MP 2 -Math/Plus/Practice employs the LIST questionnaire in a pre-post design allows for conducting meta-analyses and prompted the study at hand which aims at exploring if the LIST questionnaire can be shortened while keeping the factor structure comparable to the original, and therefore its potential for describing learning strategies and significant changes therein:
(RQ 1): Do reduced LIST scales remain internally reliable both in terms of reliability measures and content?
(RQ 2): Are the reduced scales still sensitive enough to capture developments in a pre-post design?
The evaluations of the MP 2 -Math/Plus/Practice interventions, in which the results gained from LIST play an important role, are not the focus of this paper. They will address more content-related and less technical issues, e.g. in what respect students modify their learning strategies and to what extent this can be influenced by the project interventions (for more details, see Griese et al. 2011 ). We restrict ourselves to refer to MP 2 -Math/Plus/Practice only so far as information is needed to pursue the final goal of gaining a more workable version of LIST, making future research easier.
Participants of the study
LIST has been used for different groups of students from various backgrounds reading all sorts of subjects. Our research focuses on engineering students in their first semester at the university. Out of the students questioned, 77.70 % are males, 22.30 % females. The average age is M age = 20.34 years (SD age = 2.22 years). Almost one quarter (24.85 %) of the students have a first language different from German. Only 60.86 % of them attended an advanced course in mathematics at school, and no more than 57.55 % went to the preparation course in mathematics at the university prior to their engineering course. Moreover, 20.33 % of the students neither took part in an advanced mathematics course at school nor did they visit the preparation course at university. These last characteristics classify the academic qualifications of a substantial number of students for the chosen subject of engineering as insufficient.
Study design and instruments
In order to study the development of learning strategies, a pre-post design was decided upon, the post questionnaire consisting of identical items in retrospect, presented in the past tense. The questionnaires were distributed during the break or at the end of the mathematics lecture and collected on-site after completion. This lecture covers what is considered basic mathematics: linear algebra, differential and integral calculus. The approach is axiomatic, with an emphasis on calculation. On the whole, proofs are presented in the lectures but not tested in the examination. The pre-test took place around the second week of the regular lectures; the post-test around the second last week.
The modification of the original LIST used in the research at hand is minimal: the scale Critical Checks was eliminated as it did not seem appropriate for mathematics at the beginning of the university. The items referring to the use of reference works were reworded in order to relate to digital sources as well. A new starting item (I study for my courses) was introduced but not used for evaluation—and consequently not included in the following countings of items, which starts at 69 for the consequently named LIST69. Whereas MSLQ and LIST usually prefer five-point Likert scales, we opted for a four-point Likert scale (with poles very rarely and very often ) in order to get more concise results.
With respect to data collection, the number of filled-in questionnaires varied immensely, see Table 2 . In addition to that, some questionnaires were incomplete. Despite all this, well over 2000 data sets were collected. Before starting on shortening LIST69, it underwent exploratory and confirmatory factor analysis as well as tests for internal reliability. This was expected to set the limits for the refining process, as less input cannot carry more information, i.e. unreliable or inconclusive scales in LIST69 were not manipulated to become and well-cut and clear in shorter versions.
Process of data analysis
The objective of shortening LIST69 while keeping as much of the original scales as possible was conducted as follows, balancing consideration of content with statistical calculations: in a first step, the complete sample of data from 2011/2012 until 2013/2014 ( N = 2374) was randomly split in half. The first half ( N = 1187) was used to reduce the questionnaire by calculating Cronbach’s α values for each original scale, including how they would develop when single items were left out. Thus, a scale was shortened by eliminating the item whose deletion yielded the best alpha. This process was repeated as long as the alphas stayed good (>0.7), preferably until there were only half as many items as before, in order to produce a perceptible pruning. The shortened scales were tested on the second half of the sample ( N = 1187), meaning their Cronbach’s α values were calculated as well. This complete process was repeated three times for three different random splits of the sample, thus enabling an assessment for the stability of the process, in order to minimize the risk of coincidental results. Three rounds also mean that in case of incongruent results, a tendency might be detected.
As a second step, the shortened version of LIST69 (named LIST45 as it contained 45 items) then underwent tests on a second half of the sample, i.e. a principal component analysis (PCA) to explore its structure, followed by some minor changes in item deletion and further PCA as well as confirmatory factor analysis (CFA). The results were compared to the results of PCA and CFA of LIST69 from before, including model fit. Slight modifications of factor descriptions and changes in the loadings of single items onto factors were expected. As the pre and post scores would be explored separately, Cronbach’s α values for single questionnaires were calculated from the complete sample as well as a further test for scale reliability.
The aim of researching the development of learning strategies demands further investigation into whether significant developments can be identified with the help of the shortened questionnaire. For this purpose, the item scores of each scale were combined using the formula 100/3 · [1/ n · ( x 1 + … + x n ) − 1] = 100/3 · ( \( \overline{x} \) − 1), which renders values between 0 and 100 for a four-point Likert scale ( n = number of items in the scale; scale scores under 25 describing rare use, between 25 and under 50 infrequent use, between 50 and under 75 regular use, 75 or more continual use of the learning strategies). Using LIST69 and the reduced data, the differences of means of these combined scores were calculated in dependent t -tests (listwise exclusion of cases, level of significance 0.05).
The exploration of LIST69 identified the subscales of Metacognition ( Planning , Monitoring and Regulating ) as problematic, as all three had Cronbach’s α < 0.7 from the beginning. Thus, it was only possible to treat it as a complete scale where the eleven items rendered α = 0.728. PCA of LIST69 was conducted with orthogonal rotation (varix) and pairwise exclusion of cases on the complete sample. The chosen rotation takes into account that from the construction of LIST, the scales are expected to be unrelated. The Kaiser-Meyer-Olin (KMO) measures proved good, KMO LIST69 = 0.913, attesting the sample adequacy. All KMO values for individual items were greater than 0.76, which meets the accepted limit of 0.5. Bartlett’s test of sphericity χ 2 LIST69 (2346) = 37,916.098, p LIST69 = 0.000 indicated that correlations between items were sufficiently large for PCA. For LIST69, 13 components had eigenvalues over Kaiser’s criterion of 1 and in combination explained 50.59 % of variance. Traditionally, the LIST questionnaire is expected to render 10 scales (12 if Metacognition is regarded as having subscales Planning , Monitoring and Regulating ), whereas in our case, Kaiser’s criterion of extracting as many components as have eigenvalues >1 renders more. Extraction of ten factors in LIST69 resulted in explaining 45.65 % of variance. All this hints that the intended factor structure should be reconsidered.
The first step of reduction of the questionnaire was expected to lead to a distinct and reliable reduction of the questionnaire, due to the use of three different random splits. In effect, in seven scales, the reductions were identical in all three random splits. For three scales ( Elaborating , Metacognition and Time Management ), the reductions suggested that two out of the three random splits were chosen. As expected, when reducing the number of items in a scale, the α values suffer (see Table 3 ), due to the square of this number being a factor in their calculation. For Attention , however, the α profits from the reduced scale, an improvement which will have to pass the test with regard to content—as all scales must. On the whole, the shortened version of LIST69, LIST45, yields acceptable and often good α values, considering the nature of its items (self-reporting rather than testing) and the drastically reduced the number of items. They are all exactly in the range suggested by the analyses of the split sample, which once more hints at the stability of the procedure.
However, some items remained problematic in both versions of the questionnaire. The Metacognition items did not form a separate scale. This is in keeping with the results of other research (cf. Wild 2000 ), and the items were therefore candidates for deletion. The items from the Metacognition subscale, Planning , consistently loaded on the same component as Time Management , prompting a fresh inspection of this new scale. This lead to further deletion of items following the procedure described above, which quickly revealed all four Planning items as obsolete. Repeating items did not satisfy either; the items loaded on different scales. Not surprisingly, the Monitoring item that mentions fellow students (monitoring 4, see Additional file 1 ) loads on Peer Learning .
The PCA prompted the reduction to 36 items (keeping the Repeating items, but deleting the remaining 9 Metacognition items) in a new questionnaire correspondingly named LIST36, which was expected to yield nine factors that exactly match nine out of the ten scales from the original LIST69 (all but Metacognition ). A subsequent PCA of LIST36 (varimax rotation, pairwise exclusion of cases, number of factors set to be 9, KMO LIST36 = 0.821, all KMO values ≥ 0.617, χ 2 LIST36 (630) = 9340.628, p LIST36 = 0.000 resulted in Table 4 (for a second half of the randomly split sample) which illustrates how neatly the items load onto the remaining nine factors. Together, these factors explain 56.17 % of variance (whereas, extraction of ten factors in LIST69 only explained 45.65 % of variance, see above). The Cronbach’s α values are >0.7 without exception. This raises hopes for an imminent improvement of the instrument. The reduction to LIST36 also solves the problem of multicollinearity which exists in LIST69 (where the determinant of the correlation matrix is 1.151 × 10 −9 for the complete sample, cf. Field 2009 , p. 660), as it brings the determinant up to 8.765 × 10 −5 , meeting the criterion of exceeding 10 −5 .
All in all, the deletions show slight variations to our first attempt at refining the LIST questionnaire (cf. Griese et al. 2014 ), when we achieved a version with 32 items and slightly worse alphas, using data from earlier project years. As a side project, oblique rotation (direct oblimin) was also tried: the results stay the same, meaning that the same items load on the same factors as strongly as in orthogonal rotation, the same amount of variance is explained; and the structure matrix does not reveal any disturbing interconnections between the components.
To judge the development of the model fit, a comparative fit index (CFI) and two badness-of-fit indices (root mean square error of approximation (RMSEA) and standardized root mean residual (SRMR)) were calculated to balance for complexity of the model, sample size and robustness against violations of the underlying distribution (Table 5 ), revealing acceptable model fit in the shortened questionnaires (cf. Schreiber et al. 2006 ).
What is more, LIST36’s scales are quite stable in the single pre and post surveys conducted in the course of the study; out of the 54 Cronbach’s α values (nine scales in six surveys) only a few were <0.7, none were <0.6 (see Table 6 ).
The comparison of t -tests between the pre and post data of the project years was meant to reveal if the shortened questionnaire kept the significance of the originally used one, and also if there were scales that never produced significant differences. From the data available, it can be deduced that (a) the trends are always identical in the long and shortened questionnaires, (b) no significant developments are lost and (c) there were only two exceptions concerning significance of developments, i.e. Organizing in project year 2011/2012 where LIST36 changed the level of significance from 0.118 in LIST69 to under the customary level of 0.05 and similarly for Peer Learning in 2013/2014. Additional file 1 gives all LIST items in detail (translation from German by the first author), arranged by scale, with indications of deletion. Table 7 provides an overview of the evaluation of item deletions.
For the metacognitive scales Monitoring and Regulating , at a closer look, the aspects inquired for are mostly mirrored in Effort , though more superficially. One item in Monitoring (monitoring 4, see Additional file 1 ) can well be associated with Peer Learning , as it contains interacting with fellow students as much as it contains testing oneself. The metacognitive scale Planning , whose close connection to Time Management was obvious from the data analysis, nevertheless stresses substantial planning rather than simple timing. Its deletion means losing a more effective and more reflected learning strategy. It is doubtful, though, if techniques of this depth can be recorded well in a quick and self-reporting questionnaire. All in all it can be said that deleting the metacognitive scales must be regarded critically.
Investigations into an approved and validated questionnaire originally designed to explore learning strategies in general have led to a shorter version consisting of only 36 of the original 69 items, which will make it easier to ensure students’ cooperation for further research. LIST36 was developed using the data from research into STEM students’ learning strategies and mirrors nine out of the ten scales in LIST69 and has proven its reliability and its factors explain more variance. In addition, the shortened questionnaire appears as suitable for use in pre-post designs as its predecessor. If the differences in pre and post scores are a valid measurement for competence, development at all has to be viewed critically, though, as individual reference frames may have shifted. Furthermore, the validity in connection with academic performance will have to be further explored in our future research. Another point is that the generalizability of the new instrument will need to be tested on other populations than engineering students, as some of the content-related issues might be weighted differently for different groups of students.
Leaving aside the numerous calculations, it is nonetheless important to take a close look at which items have suffered deletion, if they contain any specific content that might get lost in the scale or if they involve a shift in content focus. For our purposes, we can keep in mind that we are dealing with first year engineering students and their learning of mathematics, which on the one hand makes some deletions acceptable but which, on the other hand, also represents a limitation. It still needs to be tested if the findings from our sample of first year engineering students can be generalized.
To avoid problems stemming from inter-language or inter-cultural differences, it seemed wise to use a German questionnaire in our research, although a transfer to another country and language can present a test for a questionnaire’s validity. International cooperation has been effected with colleagues from Spain (cf. Gómez-Chacón et al. 2015 , accepted for CERME9), showing that LIST keeps its qualities when being used in another country: After being translated into English and then into Spanish, the cognitive and metacognitive scales from LIST kept their reliability, an indication for the questionnaire’s universal applicability.
In addition to this, it has become apparent that some of our initial assumptions about the impact and measurability of metacognitive behaviour of STEM students might be incorrect, thus guiding further research towards other ways of examining this concept. This aspect represents a point that might interest researchers from other areas, as metacognition and its measurability often are popular research interests.
Depending on the purpose of the individual study, it might still be advisable to keep the Metacognition items in the questionnaire for further tests as the decision to consider deleting all of them was a hard one. Our research into the interventions of the project MP 2 -Math/Plus/Practice itself had been planned to keep a close eye on metacognitive learning strategies, hypothesizing that they make the difference when it comes to successful learning behaviour. However, the results of our analysis show that the characteristics formerly believed to be separate from more technical learning strategies actually load on already existing factors, and there they do not even contribute relevantly. One logical conclusion might be that metacognitive dispositions are latent factors which work in the background and become apparent only in other learning strategies—where they can then be measured accordingly. Another explanation could lie in the nature of the data acquisition used: metacognitive reflections might be inappropriate for being measured in self-assessing printed questionnaires. Other forms of procuring data, for example, from interviews, videographed peer discussions, classroom observations, flash interviews, reflective essays or learning diaries, might prove more adequate. Further research is required, and our data collected from interviews, learning diaries and other sources will be used for this purpose.
Artigue, M, Batanero, C, & Kent, P. (2007). Mathematics thinking and learning at post-secondary level. In F Lester (Ed.), Second handbook of research on mathematics teaching and learning (pp. 1011–1049). Greenwich, Connecticut: Information Age Publishing.
Google Scholar
Artino, AR (2005). A review of the motivated strategies for learning questionnaire. http://eric.ed.gov/?id=ED499083 . Accessed 1 December 2014.
Blömeke, S, Gustafsson, JE, & Shavelson, RJ. (2015). Beyond dichotomies. Competence viewed as a continuum. Zeitschrift für Psychologie, 223 (1), 3–13.
Article Google Scholar
Cano, F. (2006). An in-depth analysis of the learning and study strategies inventory (LASSI). Educational and Psychological Measurement, 66 (6), 1023–1038.
David, A (2013). Aufgabenspezifische Messung metakognitiver Aktivitäten im Rahmen von Lernaufgaben (PhD thesis). Technische Universität, Chemnitz. http://nbn-resolving.de/urn:nbn:de:bsz:ch1-qucosa-133054 . Accessed 7 April 2015.
de Guzmán, M, Hodgson, BR, Robert, A, & Villani, V. (1998). Difficulties in the passage from secondary to tertiary education. In G Fischer & U Rehmann (Eds.), Proceedings of the international congress of mathematicians—documenta mathematica extra volume III (pp. 747–762). Rosenheim: Geronimo GmbH.
Dehling, H, Glasmachers, E, Griese, B, Härterich, J, & Kallweit, M. (2014). MP 2 -Mathe/Plus/Praxis, strategien zur vorbeugung gegen studienabbruch. Zeitschrift für Hochschulentwicklung, 9 (4), 39–56.
Dreyfus, T (Guest Editor) (1995). Advanced mathematical thinking [special issue]. Educational Studies in Mathematics, 29(2).
Duncan, TG, & McKeachie, WJ. (2005). The making of the motivated strategies for learning questionnaire. Educational Psychologist, 40 , 117–128.
Dunn, KE, Lo, WJ, Mulvenon, SW, & Sutcliffe, R. (2012). Revisiting the motivated strategies for learning questionnaire: a theoretical and statistical reevaluation of the metacognitive self-regulation and effort regulation subscales. Educational and Psychological Measurement, 72 , 312–331.
Entwistle, N, & McCune, V. (2004). The conceptual bases of study strategies inventories. Educational Psychology Review, 16 (4), 325–346.
Entwistle, N, & Ramsden, P. (1983). Understanding student learning . London: Croom Helm.
Erdem Keklik, E, & Keklik, I. (2013). Motivation and learning strategies as predictors of high school students’ math achievement. Cukurova University Faculty of Education Journal, 42 (1), 96–109.
Evans, JSBT. (2007). Hypothetical thinking. Dual processes in reasoning and judgement . Hove: Psychology Press.
Field, A. (2009). Discovering statistics using SPSS (3rd ed.). Thousand Oaks: Sage Publications.
Fischbein, E. (1987). Intuition in science and mathematics. An educational approach . Dordrecht: Kluwer Academic Publishers.
Gómez-Chacón, IM, García-Madruga, JA, Vila, JO, Elosúa, MR, & Rodríguez, R. (2014). The dual processes hypothesis in mathematics performance: beliefs, cognitive reflection, reasoning and working memory. Learning and Individual Differences, 29 , 67–73.
Gómez-Chacón, IM, Griese, B, Rösken-Winter, B, & Gonzàlez-Guillén, C. (2015). Engineering students in Spain and Germany—varying and uniform learning strategies. In N Vondrova & K Krainer (Eds.), Proceedings of the 9th conference of european researchers in mathematics education . Prague, Czech Republic: Charles University. in press.
Griese, B, Glasmachers, E, Kallweit, M, & Roesken, B. (2011). Engineering students and their learning of mathematics. In B Roesken & M Casper (Eds.), Current state of research on mathematical beliefs XVII. Proceedings of the MAVI-17 conference (pp. 85–96) . Bochum: Professional School of Education, RUB.
Griese, B, Lehmann, M, & Roesken-Winter, B. (2014). Exploring university students’ learning strategies in mathematics: refining the LIST questionnaire. In S Oesterle, C Nicol, & P Liljedahl (Eds.), Proceedings of the joint meeting of PME 38 and PME-NA 36, Vol. 6 (p. 131). Vancouver, Canada: PME.
Griese, B, Roesken-Winter, B, Kallweit, M, & Glasmachers, E. (2013). Redesigning interventions for engineering students: learning from practice. In AM Lindmeier & A Heinze (Eds.), Proceedings of the 37th conference of the international group for the psychology of mathematics education vol. 5 (p. 65). Kiel, Germany: PME.
Gueudet, G. (2008). Investigating the secondary–tertiary transition. Educational Studies in Mathematics, 67 (3), 237–254.
Henn, HW, Bruder, R, Elschenbroich, J, Greefrath, G, Kramer, J, & Pinkernell, G. (2010). Schnittstelle schule - hochschule. In A Lindmeier & S Ufer (Eds.), Beiträge zum mathematikunterricht 2010 (pp. 75–82). Münster: WTM.
Heublein, U, Richter, J, Schmelzer, R, & Sommer, D. (2012). Die entwicklung der schwund- und studienabbruchquoten an den deutschen hochschulen: statistische berechnungen auf der basis des absolventenjahrgangs 2010. Forum hochschule: vol. 2012,3 . Hannover: HIS.
Hilpert, J, Stempien, J, van der Hoeven Kraft, KJ, & Husman, J. (2013). Evidence for the latent factor structure of the MSLQ: a new conceptualization of an established questionnaire. SAGE-Open, 3 , 1–10. doi: 10.1177/2158244013510305 .
Lam, TC, & Bengo, P. (2003). A comparison of three retrospective self-reporting methods of measuring change in instructional practice. American Journal of Evaluation, 223 (1), 3–13.
Liebendörfer, M, Hochmuth, R, Schreiber, S, Göller, R, Kolter, J, Biehler, R, Kortemeyer, J, & Ostsieker, L. (2014). Vorstellung eines fragebogens zur erfassung von lernstrategien in mathematikhaltigen studiengängen. In J Roth & J Ames (Eds.), Beiträge zum mathematikunterricht 2014 (pp. 739–742). Münster: WTM.
Lovelace, M, & Brickman, P. (2013). Best practices for measuring students’ attitudes toward learning science. CBE – Life Sciences Education, 12 , 606–617.
McKenney, SE, & Reeves, TC. (2012). Conducting educational design research . New York: Routledge.
McLeod, DB. (1992). Research on affect in mathematics education: a reconceptualization. In DA Grouws (Ed.), Handbook of research on mathematics teaching and learning (pp. 575–596). New York: Macmillan.
Nenniger, P. (1999). On the role of motivation in self-directed learning. The ‘two-shells-model of motivated self-directed learning’ as a structural explanatory concept. European Journal of Psychology of Education, 14 (1), 71–86.
Nimon, K, Zigarni, D, & Allen, J. (2011). Measures of program effectiveness based on retrospective pretest data: are all created equal? American Journal of Evaluation, 32 (1), 8–28.
Pintrich, P, & de Groot, E. (1990). Motivational and self-regulated learning components of classroom academic performance. Journal of Educational Psychology, 81 (1), 33–40.
Pintrich, P, Smith, D, Garcia, T, & McKeachie, W. (1993). Reliability and predictive validity of the motivated strategies for learning questionnaire (MSLQ). Educational and Psychological Measurement, 53 (3), 801–813.
Rach, S, & Heinze, A. (2011). Studying mathematics at the university: the influence of learning strategies. In B Ubuz (Ed.), Proceedings of the 35th conference of the international group for the psychology of mathematics education (Vol. 4, pp. 9–16). Ankara, Turkey: PME.
Schellings, C. (2011). Applying learning strategy questionnaires: problems and possibilities. Metacognition and Learning, 6 (2), 91–109.
Schreiber, JB, Nora, A, Stage, FK, Barlow, EA, & King, J. (2006). Reporting structural equation modeling and confirmatory factor analysis results: a review. The Journal of Educational Research, 99 (6), 323–337.
Selden, A, & Selden, J. (2005). Perspectives on advanced mathematical thinking. Mathematical Thinking and Learning, 7 (1), 1–13.
Stavy, R, & Tirosh, D. (2000). How students (mis-)understand science and mathematics: intuitive rules . New York: Teachers College Press.
Tait, H, Entwistle, N, & McCune, V. (1998). ASSIST: a reconceptualisation of the approaches to studying inventory. In C Rust (Ed.), Improving student learning: improving students as learners (pp. 262–271). Oxford: Oxford Centre for Staff and Learning Development.
Tall, DO. (2004). Building theories: the three worlds of mathematics. For the Learning of Mathematics, 24 (1), 29–33.
Weinstein, CE, & Palmer, DR. (2002). Learning and study strategies inventory (LASSI): user’s manual (2nd ed.). Clearwater: H & H Publishing.
Weinstein, CE, Schulte, AC, & Palmer, DR. (1987). Learning and study strategies inventory (LASSI) . Clearwater: H & H Publishing.
Wild, KP. (2000). Lernstrategien im studium: strukturen und bedingungen . Münster: Waxmann.
Wild, KP. (2005). Individuelle lernstrategien von studierenden. Konsequenzen für die hochschuldidaktik und die hochschullehre. Beiträge zur Lehrerbildung, 23 (2), 191–206.
Wild, KP, & Schiefele, U. (1994). Lernstrategien im studium. Ergebnisse zur Faktorenstruktur und Reliabilität eines neuen Fragebogens. Zeitschrift für Differentielle und Diagnostische Psychologie, 15 , 185–200.
Download references
Author information
Authors and affiliations.
Department of Mathematics, Ruhr-Universität Bochum, 44801, Bochum, Germany
Birgit Griese
Professional School of Education, Humboldt Universität zu Berlin, 10099, Berlin, Germany
Malte Lehmann & Bettina Roesken-Winter
You can also search for this author in PubMed Google Scholar
Corresponding author
Correspondence to Birgit Griese .
Additional information
Competing interests.
The authors declare that they have no competing interests.
Authors’ contributions
All data was collected in the context of the project MP²-Math/Plus at Ruhr-Universität Bochum in Germany. MP²-Math/Plus/Practice was initially (2010 – 2012) supported by Stifterverband für die Deutsche Wissenschaft in cooperation with Heinz-Nixdorf-Stiftung and is now an established part of the academic program. BG designed the study, collected the data, performed statistical analyses, drafted and revised the manuscript. ML performed some and reviewed all statistical analyses and reviewed the manuscript. BR-W participated in designing the study and drafting the manuscript, and supervised all work. All three authors read, re-read, discussed and approved the final manuscript.
Authors’ information
Bettina Rösken-Winter is a full professor for Mathematics education at the Humboldt-Universität zu Berlin (Germany). Her research interests include mathematical education in engineering studies, continuous teacher professional development, and design-based research. Birgit Griese is a mathematics teacher and a mathematics education researcher at Ruhr-Universität Bochum (Germany). She is currently working on her PhD thesis investigating engineering students’ learning strategies. Malte Lehmann is a mathematics education researcher at the Humboldt-Universität zu Berlin (Germany). He is interested in statistics and is currently working on his PhD project investigating students’ problem solving competences.
Additional file
Additional file 1:.
LIST questions (pre). Items (item names) deleted for LIST36.
Rights and permissions
Open Access This article is distributed under the terms of the Creative Commons Attribution 4.0 International License ( https://creativecommons.org/licenses/by/4.0 ), which permits use, duplication, adaptation, distribution, and reproduction in any medium or format, as long as you give appropriate credit to the original author(s) and the source, provide a link to the Creative Commons license, and indicate if changes were made.
Reprints and permissions
About this article
Cite this article.
Griese, B., Lehmann, M. & Roesken-Winter, B. Refining questionnaire-based assessment of STEM students’ learning strategies. IJ STEM Ed 2 , 12 (2015). https://doi.org/10.1186/s40594-015-0025-9
Download citation
Received : 14 January 2015
Accepted : 08 July 2015
Published : 12 August 2015
DOI : https://doi.org/10.1186/s40594-015-0025-9
Share this article
Anyone you share the following link with will be able to read this content:
Sorry, a shareable link is not currently available for this article.
Provided by the Springer Nature SharedIt content-sharing initiative
- Engineering education
- Mathematics courses
- LIST questionnaire
- Metacognition
Instruments
Stem semantics survey, description.
The STEM Semantics Survey measures student interest in science, technology, engineering and mathematics as well as interest in STEM careers. The survey is comprised of five scales each with five items measured on a 7-point scale. The STEM Semantic Survey is appropriate for administration to middle school students and teachers.
The link below provides access to the instrument as well as information about the instrument.
Authors provide instrument validity and/or reliability information.
All resources
STELAR is not the author of these materials and cannot provide information on validity or permission for use. Permissions must be requested through the publisher or authors listed below.
INSTRUMENT DETAILS
Document Instrument
Document Instrument Documentation
Dietrich College of Humanities and Social Sciences
Coding for animals key to engaging children in stem, an education pilot study bridges animal behavior research and computer coding to engage elementary school students in real-world, interdisciplinary science.
By Stacy Kish skish(through)andrew.cmu.edu
Teachers today are facing a bit of a conundrum. Their goal is to prepare their young students to enter a rapidly changing world. Even basic jobs require technical proficiency, which requires computational and analytical skills. To address this need, many educators are pushing to fold these important STEM skills into elementary curriculum.
Here’s the problem. Young students can lose interest and even develop an aversion to the tasks that build the skills associated with computational thinking. Past studies have pointed to historic low completion rates in STEM fields, with computer science among the lowest. A bridge is needed to engage students in the tasks to develop these important 21 st century skills.
Innovative Teaching Methods: Merging Interests for Effective Learning
“Sometimes students disengage from science, because they do not see the science that they are doing in the classroom as connected to the real world,” said Jessica Cantlon , the Ronald J. and Mary Ann Zdrojkowski Associate Professor of Developmental Neuroscience and Psychology at Carnegie Mellon University. “When young students engage in authentic science experiences, they can absorb facts more effectively.”
Unlike in the classroom, science does not fall into neat, separated boxes. Real-world science is interdisciplinary. Past attempts to build this bridge have focused on topics, such as robotics, gaming, or animations, but the niche nature of this subject often leaves many students uninterested.
Cantlon and her colleagues took a different approach. They merged a topic that younger children (grades 3 to 6) enjoy — animals — with one that most kids might look at like a plate of steaming Brussels sprouts — computer coding. The results of their pilot program are available in the April 2 issue of the journal STEM Education Research .
“The focus of this pilot study is whether, in principle, students can acquire skills in computational thinking during a relatively short, loose format, authentic science experience,” said Cantlon. “By learning these skills, the students also maintained or gained excitement throughout the project’s unique immersive experience in animal behavior.”
Integrating Coding and Animal Behavior
Cantlon and her colleagues developed an educational program in collaboration with the Primate Portal, an exhibit at the Seneca Park Zoo in Rochester, N.Y., in which the public can watch olive baboons solve problems presented as computerized tasks on a touchscreen computer
Through the program, students learned a basic coding language (Scratch) to develop a game that olive baboons at the zoo play to test their intelligence. While the students have the freedom to create their game, they are given different frameworks as a starting point, such as a matching game or a search game, like “Where’s Waldo.” At the end of the five-day programming course, the students took a field trip to the zoo to watch the primates play the games they programmed.
Insights and Challenges: Teacher and Student Perspectives
“The students definitely struggle with the complexity of the code as they had little to no experience with coding,” said Greg Booth, a teacher in the REACH program for gifted/talented students at QUEST Elementary in the Hilton Central School District who worked with the researchers on this project. “They were not given the opportunity to do [coding] in school prior to this, and they had a tremendous amount of intrinsic motivation to learn and develop their coding skills.”
In the first iteration of this pilot project, the team engaged 57 elementary-aged students from three elementary schools in Western New York, of which 36 completed pre- and post-surveys to evaluate the skills acquired during the class.
“It is rare for anyone to collect data from informal, teacher–scientist interventions,” said Cantlon, who is first author on the study. “[The study’s] effect size is large, because [the students] learned a lot of new computational thinking skills by completing the coding projects.”
Enhancing STEM Interest and Skills
According to Cantlon, the effect size of the study is large because students began the course with little to no knowledge about coding and developed definite coding skills that supported computational thinking. For example, the students learned to write conditional statements, a loop in code and interpret logical statements. In addition, the students experienced a significant increase in accuracy and problem-solving attempts. The project also showed that it is possible to integrate “learning and doing” into curriculum for elementary-aged students.
“I love seeing kids get interested in science, especially girls,” said Caroline DeLong , professor at Rochester Institute of Technology and co-author on the study. “This program is a fantastic way to utilize kids’ love of animals as a bridge to learning new computational skills and a way to show them how science works in real time.”
The students’ computational thinking scores improved by 17% from the beginning of the course to the end. There was no difference in the level of improvement between boys and girls who participated in the program. In addition, the students spoke highly of the program, citing their interest in creativity and independence during the learning process. According to Cantlon, the program shows that it is possible to enhance students’ interest in science and cultivate essential 21st-century skills.
“Yes it is possible to engage students early, in elementary school, and hook them into STEM interests in something that they might think is boring — coding,” said Cantlon. “It is important to engage students before they make up their mind that STEM isn’t for them and while they are still open to learning about STEM and hopefully come to a new conclusion that STEM is for them.”
Cantlon and her colleagues aim to expand this approach to engage a more diverse group of students in future studies.
Cantlon and DeLong were joined by Katherine Becker at the Rochester Institute of Technology on the project, titled “Computational thinking during a short, authentic, interdisciplinary STEM experience for elementary students.” The project received funding from the National Science Foundation and a fund associated with the Ronald and Mary Ann Zdrojkowski Chair in Developmental Neuroscience at CMU.
Student responses to a learning query from one of the post-course surveys
- “I learned that baboons are just like us.”
- “The coolest thing I learned was how to use the ‘if then else’ block.”
- “The thing I got to learn is that baboons can do math! (They are super intelligent.)”
- “I learned how to think mechanically.”

- CMU Directory
- Dietrich College Calendar
Numbers, Facts and Trends Shaping Your World
Read our research on:
Full Topic List
Regions & Countries
- Publications
- Our Methods
- Short Reads
- Tools & Resources
Read Our Research On:
- How Hispanic Americans Get Their News
Methodology
Table of contents.
- Where do Hispanic Americans get news?
- Major differences between U.S.-born Latinos and immigrants
- Bilingual Hispanics much more likely to get news in English than Spanish
- U.S.-born Hispanics less likely to engage with Hispanic news outlets
- Latinos with lower levels of income more likely to get news from Hispanic outlets and about origin countries
- Who gets news from Hispanic outlets and about origin countries?
- Acknowledgments
- The American Trends Panel survey methodology
- How family income tiers are calculated
The American Trends Panel (ATP), created by Pew Research Center, is a nationally representative panel of randomly selected U.S. adults. Panelists participate via self-administered web surveys. Panelists who do not have internet access at home are provided with a tablet and wireless internet connection. Interviews are conducted in both English and Spanish. The panel is being managed by Ipsos.
Most of the data in this report is drawn from ATP Wave 138, conducted from Nov. 6 to Nov. 19, 2023, among a sample of U.S. adults who identify as Hispanic. A total of 5,078 panelists responded out of 8,720 who were sampled, for a response rate of 61% (AAPOR RR3). This included 1,524 respondents from the ATP and an additional 3,554 from Ipsos’ KnowledgePanel (KP). The cumulative response rate accounting for nonresponse to the recruitment surveys and attrition is 1%. The break-off rate among panelists who logged on to the survey and completed at least one item is 3%. The margin of sampling error for the full sample of 5,078 respondents is plus or minus 2.0 percentage points.
The questions about how often people get news from various platforms, which platforms they prefer for getting news and which social media sites they get news from are from a different survey of 8,842 U.S. adults, including 1,193 Hispanic adults, conducted from Sept. 25 to Oct. 1, 2023, entirely on the ATP (Wave 135). Refer to the methodology for more details .
Pew Research Center is a subsidiary of The Pew Charitable Trusts, its primary funder. This is the latest report in Pew Research Center’s ongoing investigation of the state of news, information and journalism in the digital age, a research program funded by The Pew Charitable Trusts, with generous support from the John S. and James L. Knight Foundation.
Panel recruitment
The ATP was created in 2014, with the first cohort of panelists invited to join the panel at the end of a large, national, landline and cellphone random-digit-dial survey that was conducted in both English and Spanish. Two additional recruitments were conducted using the same method in 2015 and 2017, respectively. Across these three surveys, a total of 19,718 adults were invited to join the ATP, of whom 9,942 (50%) agreed to participate.
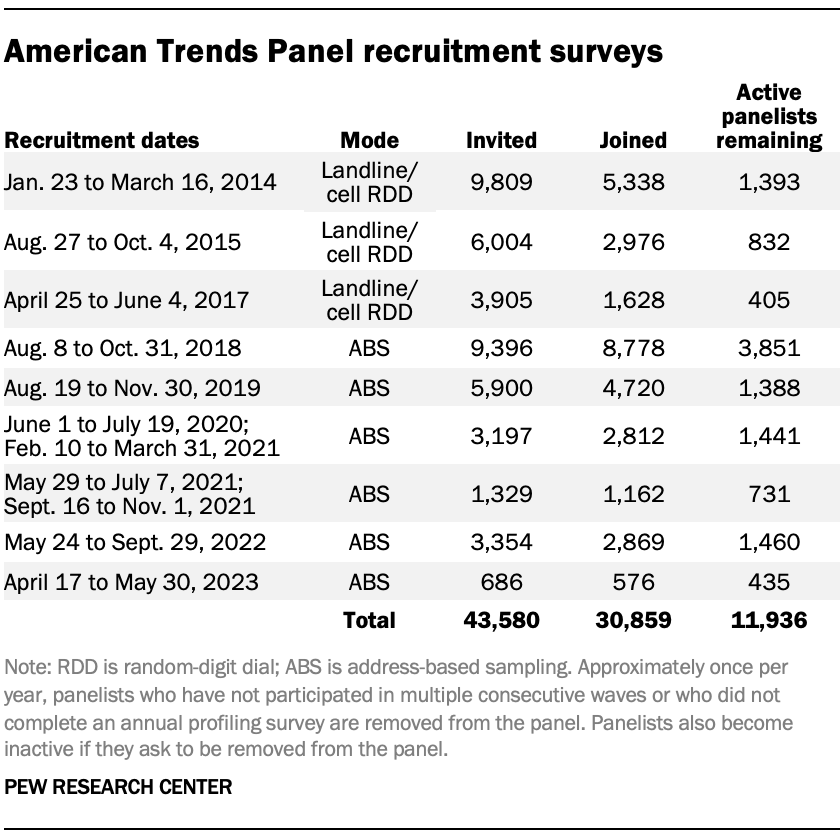
In August 2018, the ATP switched from telephone to address-based sampling (ABS) recruitment. A study cover letter and a pre-incentive are mailed to a stratified, random sample of households selected from the U.S. Postal Service’s Delivery Sequence File. This Postal Service file has been estimated to cover as much as 98% of the population, although some studies suggest that the coverage could be in the low 90% range. 1 Within each sampled household, the adult with the next birthday is asked to participate. Other details of the ABS recruitment protocol have changed over time but are available upon request. 2
We have recruited a national sample of U.S. adults to the ATP approximately once per year since 2014. In some years, the recruitment has included additional efforts (known as an “oversample”) to boost sample size with underrepresented groups. For example, Hispanic adults, Black adults and Asian adults were oversampled in 2019, 2022 and 2023, respectively.
Across the six address-based recruitments, a total of 23,862 adults were invited to join the ATP, of whom 20,917 agreed to join the panel and completed an initial profile survey. Of the 30,859 individuals who have ever joined the ATP, 11,936 remained active panelists and continued to receive survey invitations at the time this survey was conducted.
The American Trends Panel never uses breakout routers or chains that direct respondents to additional surveys.
Sample design
The overall target population for this survey was noninstitutionalized persons ages 18 and older living in the U.S., including Alaska and Hawaii who identify as Hispanic. All eligible members of the ATP and a random subsample of eligible members from Ipsos’ KnowledgePanel were invited to participate in this wave.
Questionnaire development and testing
The questionnaire was developed by Pew Research Center in consultation with Ipsos. The web program was rigorously tested on both PC and mobile devices by the Ipsos project management team and Pew Research Center researchers. The Ipsos project management team also populated test data that was analyzed in SPSS to ensure the logic and randomizations were working as intended before launching the survey.
All ATP respondents were offered a post-paid incentive for their participation. ATP respondents could choose to receive the post-paid incentive in the form of a check or a gift code to Amazon.com or could choose to decline the incentive. Incentive amounts ranged from $5 to $20 depending on whether the respondent belongs to a part of the population that is harder or easier to reach. Differential incentive amounts were designed to increase panel survey participation among groups that traditionally have low survey response propensities.
Ipsos operates an ongoing modest incentive program for KnowledgePanel to encourage participation and create member loyalty. The incentive program includes special raffles and sweepstakes with both cash rewards and other prizes to be won. Typically, panel members are assigned no more than one survey per week. On average, panel members complete two to three surveys per month with durations of 10 to 15 minutes per survey. An additional incentive is usually provided for longer surveys. For this survey, during the last few days of data collection, KnowledgePanel members who self-identified as Black were offered 10,000 points (equivalent to $10) in addition to the regular incentive program in an attempt to boost the number of responses from panel members who identified as Black American.
Data collection protocol
The data collection field period for this survey was Nov. 6-19, 2023. Postcard notifications were mailed to all ATP panelists with a known residential address on Nov. 6.
Invitations were sent out in two separate launches: soft launch and full launch. Sixty ATP panelists and 409 KP panelists were included in the soft launch, which began with an initial invitation sent on Nov 6. The ATP panelists chosen for the initial soft launch were known responders who had completed previous ATP surveys within one day of receiving their invitation. All remaining English- and Spanish-speaking sampled panelists were included in the full launch and were sent an invitation on Nov. 7.
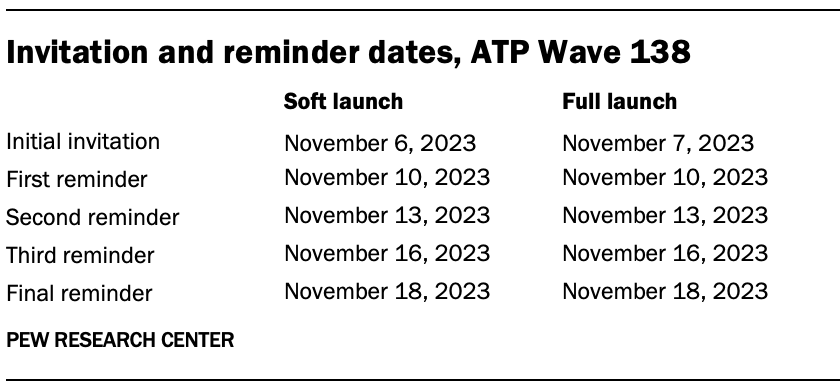
All panelists with an email address received an email invitation and up to four email reminders if they did not respond to the survey. All ATP panelists who consented to SMS messages received an SMS invitation and up to four SMS reminders.
Data quality checks
To ensure high-quality data, the Center’s researchers performed data quality checks to identify any respondents showing clear patterns of satisficing. This includes checking for whether respondents left questions blank at very high rates or always selected the first or last answer presented. As a result of this checking, six respondents were removed from the survey dataset prior to weighting and analysis. Another four respondents were removed from the survey dataset after providing feedback that they were not Hispanic, and therefore not eligible for the survey.
The ATP data is weighted in a multistep process that accounts for multiple stages of sampling and nonresponse that occur at different points in the survey process. First, each panelist begins with a base weight that reflects their probability of selection for their initial recruitment survey. These weights are then rescaled and adjusted to account for changes in the design of ATP recruitment surveys from year to year. Finally, the weights are calibrated to align with the population benchmarks in the accompanying table to correct for nonresponse to recruitment surveys and panel attrition. If only a subsample of panelists was invited to participate in the wave, this weight is adjusted to account for any differential probabilities of selection.
Among the panelists who completed the survey, this weight is then calibrated again to align with the population benchmarks identified in the accompanying table and trimmed at the 1st and 99th percentiles to reduce the loss in precision stemming from variance in the weights. Sampling errors and tests of statistical significance take into account the effect of weighting.
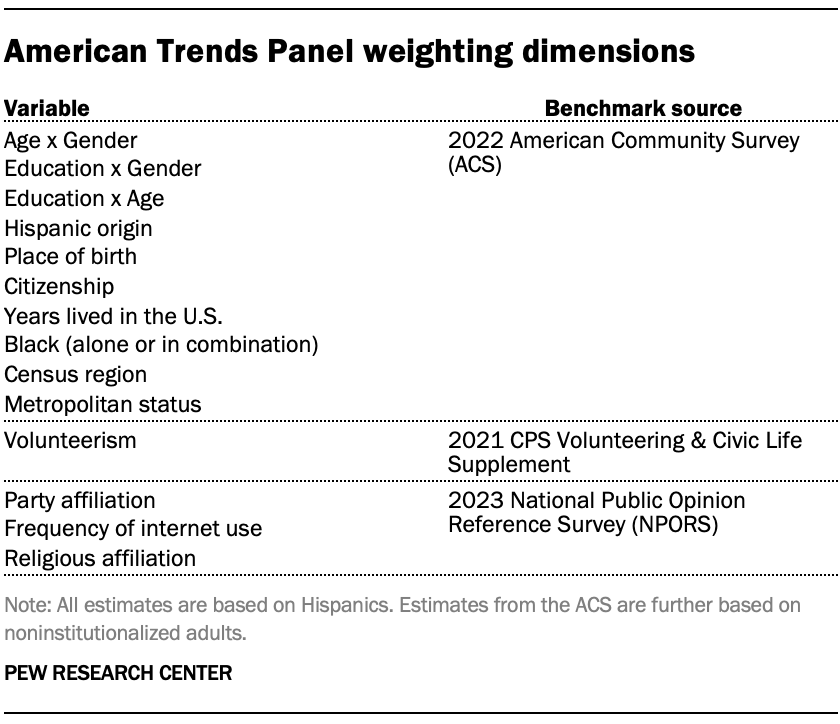
The following table shows the unweighted sample sizes and the error attributable to sampling that would be expected at the 95% level of confidence for different groups in the survey.
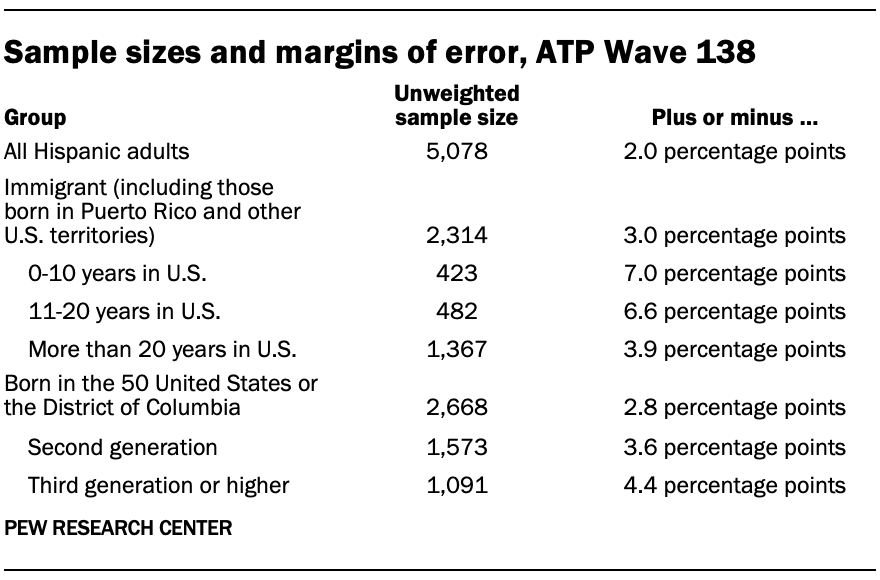
Sample sizes and sampling errors for other subgroups are available upon request. In addition to sampling error, one should bear in mind that question wording and practical difficulties in conducting surveys can introduce error or bias into the findings of opinion polls.
Dispositions and response rates
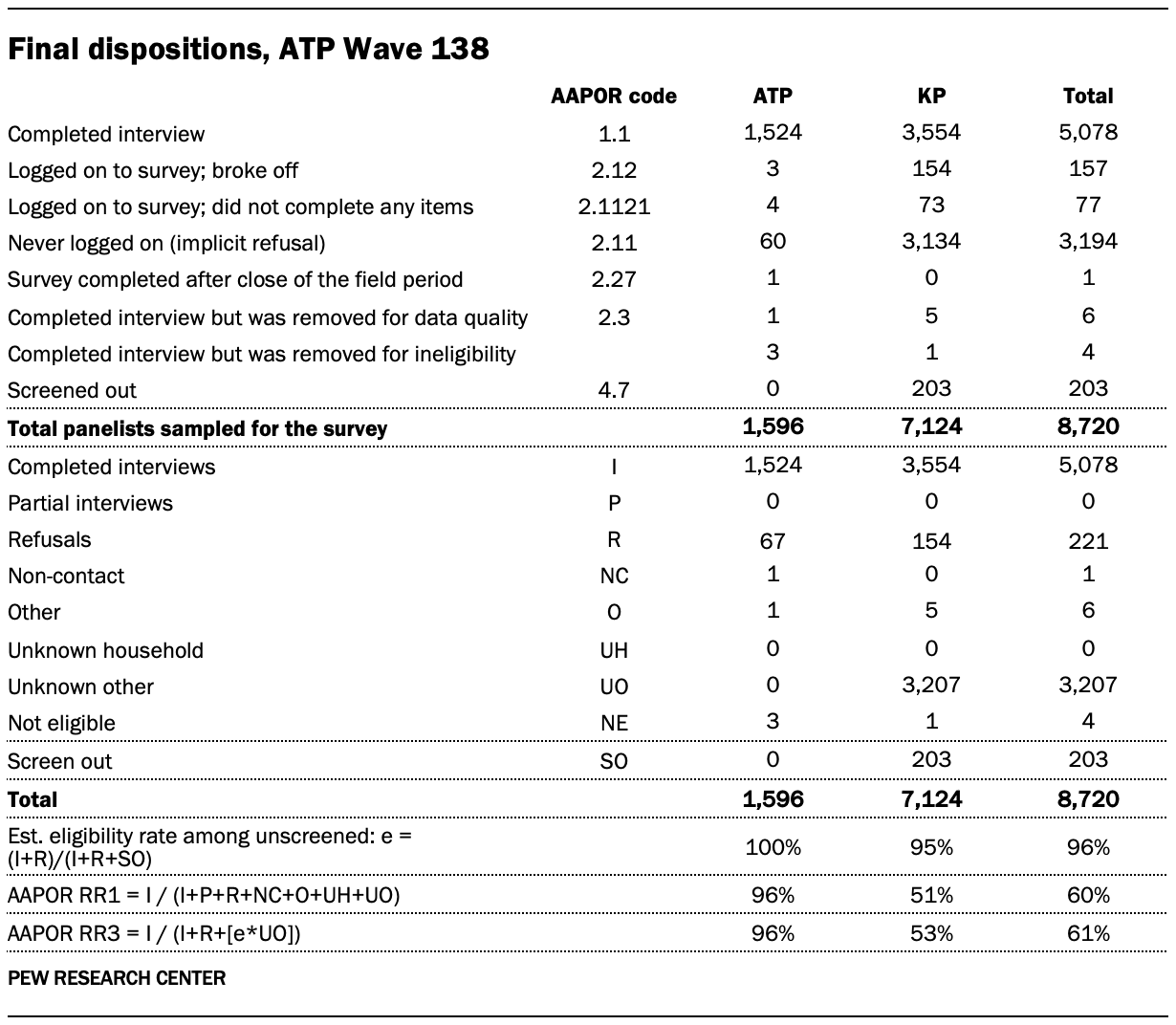
Family income data reported in this study is adjusted for household size and cost-of-living differences by geography. Panelists then are assigned to income tiers that are based on the median adjusted family income of all American Trends Panel members. The process uses the following steps:
- First, panelists are assigned to the midpoint of the income range they selected in a family income question that was measured on either the most recent annual profile survey or, for newly recruited panelists, their recruitment survey. This provides an approximate income value that can be used in calculations for the adjustment.
- Next, these income values are adjusted for the cost of living in the geographic area where the panelist lives. This is calculated using price indexes published by the U.S. Bureau of Economic Analysis. These indexes, known as Regional Price Parities (RPP), compare the prices of goods and services across all U.S. metropolitan statistical areas as well as non-metro areas with the national average prices for the same goods and services. The most recent available data at the time of the annual profile survey is from 2021. Those who fall outside of metropolitan statistical areas are assigned the overall RPP for their state’s non-metropolitan area.
- Family incomes are further adjusted for the number of people in a household using the methodology from Pew Research Center’s previous work on the American middle class . This is done because a four-person household with an income of say, $50,000, faces a tighter budget constraint than a two-person household with the same income.
- Panelists are then assigned an income tier. “Middle-income” adults are in families with adjusted family incomes that are between two-thirds and double the median adjusted family income for the full ATP at the time of the most recent annual profile survey. The median adjusted family income for the panel is roughly $71,800. Using this median income, the middle-income range is about $47,900 to $143,600. Lower-income families have adjusted incomes less than $47,900 and upper-income families have adjusted incomes greater than $143,600 (all figures expressed in 2022 dollars and scaled to a household size of three). If a panelist did not provide their income and/or their household size, they are assigned “no answer” in the income tier variable.
Two examples of how a given area’s cost-of-living adjustment was calculated are as follows: the Anniston-Oxford metropolitan area in Alabama is a relatively inexpensive area, with a price level that is 16.2% less than the national average. The San Francisco-Oakland-Berkeley metropolitan area in California is one of the most expensive areas, with a price level that is 19.8% higher than the national average. Income in the sample is adjusted to make up for this difference. As a result, a family with an income of $41,900 in the Anniston-Oxford area is as well off financially as a family of the same size with an income of $59,900 in San Francisco.
© Pew Research Center 2024
- AAPOR Task Force on Address-based Sampling. 2016. “AAPOR Report: Address-based Sampling.” ↩
- Email [email protected] . ↩
Sign up for our weekly newsletter
Fresh data delivery Saturday mornings
Sign up for The Briefing
Weekly updates on the world of news & information
- Digital News Landscape
- Hispanics/Latinos & Language
- Immigration & Language Adoption
- News Platforms & Sources
Key facts about U.S. Latinos for National Hispanic Heritage Month
Latinos’ views of and experiences with the spanish language, u.s. unauthorized immigrants are more proficient in english, more educated than a decade ago, most hispanic parents speak spanish to their children, but this is less the case in later immigrant generations, facts on foreign students in the u.s., most popular, report materials.
1615 L St. NW, Suite 800 Washington, DC 20036 USA (+1) 202-419-4300 | Main (+1) 202-857-8562 | Fax (+1) 202-419-4372 | Media Inquiries
Research Topics
- Age & Generations
- Coronavirus (COVID-19)
- Economy & Work
- Family & Relationships
- Gender & LGBTQ
- Immigration & Migration
- International Affairs
- Internet & Technology
- Methodological Research
- News Habits & Media
- Non-U.S. Governments
- Other Topics
- Politics & Policy
- Race & Ethnicity
- Email Newsletters
ABOUT PEW RESEARCH CENTER Pew Research Center is a nonpartisan fact tank that informs the public about the issues, attitudes and trends shaping the world. It conducts public opinion polling, demographic research, media content analysis and other empirical social science research. Pew Research Center does not take policy positions. It is a subsidiary of The Pew Charitable Trusts .
Copyright 2024 Pew Research Center
Terms & Conditions
Privacy Policy
Cookie Settings
Reprints, Permissions & Use Policy
- Make a Gift
- Directories
Search form
You are here.
- News & Events
- Advising News
JSIS 300 Climate Change Survey Request
Hello Political Science students,
We are students in JSIS 300 Claims and Evidence with Professor Porter and would appreciate your time in taking a quick and anonymous survey on Climate Change and Human Behavior. The data collected will contribute significantly to our research efforts and provide valuable insights for our study. The survey form will be open from Monday, May 6th to Saturday, May 11th and should take no longer than 5 minutes to complete.
Thank you for your help!
If you have any questions, please contact Jesse LaCross-Lambert at [email protected] .
Survey Link: https://form.jotform.com/241215580062144
- Newsletter
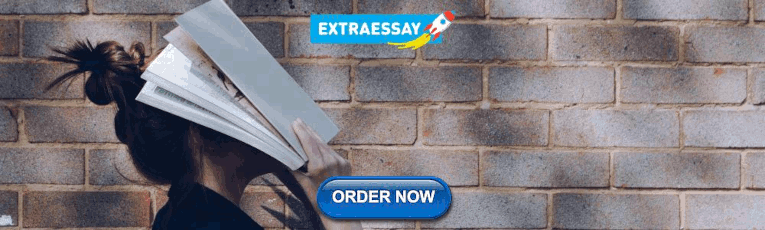
IMAGES
VIDEO
COMMENTS
Qualitative research topics for stem students are questions or issues that necessitate an in-depth exploration of people's experiences, beliefs, and behaviors. STEM students can use this approach to investigate societal impacts, ethical dilemmas, and user experiences related to scientific advancements and innovations. ...
Here are 10 practical research topics for STEM students: Developing an affordable and sustainable water purification system for rural communities. Designing a low-cost, energy-efficient home heating and cooling system. Investigating strategies for reducing food waste in the supply chain and households.
Qualitative Research Topics for STEM Students. 1. Studying how different types of dirt affect plant growth. 2. Checking how different plant foods impact how much crops grow. 3. Looking at how exercising affects your heart rate. 4. Testing which materials keep things warm the best.
Engineering. Let's explore some quantitative research topics for stem students in engineering: 1. Investigating the efficiency of renewable energy systems in urban environments. 2. Analyzing the impact of 3D printing on manufacturing processes. 3. Studying the structural integrity of materials in aerospace engineering.
Survey Research Topics For STEM Students; Interesting and Informative Research Topics For Senior High School STEM Students; List of Research Topics For STEM Students. Several research topics can be formulated in this field. They cut across STEM science, engineering, technology, and math. Here is a list of good research topics for STEM students.
Qualitative research in STEM (Science, Technology, Engineering, and Mathematics) examines many different areas. It helps us understand how people interact with science and technology. These research topics explore how people feel and think about different scientific fields. They also examine ethical issues, how society is affected, and how ...
Topic 1: Artificial Intelligence (AI) AI stands at the forefront of technological innovation. Students can engage in research on AI applications in various sectors and the ethical implications of AI. This field is suitable for students with interests in computer science, AI, data analytics, and related areas. Topic 2: Applied Math and AI.
Social, motivational, and instructional factors impact students' outcomes in STEM learning and their career paths. Based on prior research and expectancy-value theory, the study further explored how multiple factors affect students in the context of integrated STEM learning. High school STEM teachers participated in summer professional development and taught integrated STEM to students ...
Here's a list of over 200 qualitative research topics for STEM students: The Ethical Implications of CRISPR Technology in Genetic Engineering. Exploring the Societal Impact of Artificial Intelligence in Healthcare. User Experience and Human-Centered Design in Software Development.
Embark on a captivating journey through the cosmos of knowledge with our curated guide on Quantitative Research Topics for STEM Students. Explore innovative ideas in science, technology, engineering, and mathematics, designed to ignite curiosity and shape the future. Unleash the power of quantitative research and dive into uncharted territories ...
This systematic review provides an in-depth overview of international STEM researcher collaborations and trends in STEM education's most recent research topics. We examined 49 peer-reviewed articles selected from 244 articles published in three reputable international journals from January 2014 to December 2018.
Following are the best Quantitative Research Topics For STEM Students in mathematics and statistics. Prime Number Distribution: Investigate the distribution of prime numbers. Graph Theory Algorithms: Develop algorithms for solving graph theory problems. Statistical Analysis of Financial Markets: Analyze financial data and market trends.
Math Research Topics for STEM Students. Analyze the properties of mathematical models for population dynamics. Investigate the use of mathematical modeling in epidemiology. Examine the use of numerical methods in solving partial differential equations. Analyze the properties of algebraic structures in coding theory.
Environmental Science Experimental Research Topics for STEM Students. Studying the Impact of Deforestation on Local Climate Patterns. Investigating the Role of Ocean Acidification on Coral Reefs. Analyzing the Efficiency of Different Waste Management Strategies. Exploring the Effect of Air Pollution on Human Health.
Once you have done some background research and narrowed down your topic, you can begin to turn that topic into a research question that you will attempt to answer in the course of your research. Keep in mind that your question may change as you gather more information and as you write. However, having some sense of your direction can help you ...
UNDERGRADUA TE RESEARCH FOR STEM. STUDENTS, BENEFITS AND CHALLENGES. 1 Mohammed Mahmoud, 2 Mark Hoffmann. 1 [email protected], 2 [email protected]. 1 Department of Computer Science ...
Top 10 Topics For Survey Research On Entertainment Preferences. Streaming Services vs. Traditional Cable: Consumer Preferences. Influences on Music Genre Preferences Among Different Age Groups. Viewer Satisfaction and Preferences in Online Video Content. Impact of Social Media on Movie and TV Show Recommendations.
Rui could choose "distracted and aggressive driving" as an initial research topic. Tip #4: Think about something you've recently learned or read in a class. If a reading, assignment, or video from a class has stood out to you, explore that further. That topic or an aspect of it could serve as your initial research topic.
Learning strategies are considered a key aspect for academic performance not only for science, technology, engineering and mathematics (STEM) students. Refining their assessment thus constitutes a worthwhile research purpose. The aim of this study is to examine the 69-item LIST questionnaire (ZDDP 15:185-200, 1994) with the aim of shortening it while keeping its factor structure and thus its ...
These 5 steps are a useful process by which individuals/teams can organize efforts to learn about the impact of their program on participating students. (1) Develop Research Questions: determine important questions you want to know about program activities and impacts. (2) Identify Data: data that can help answer those questions, anything from ...
The STEM Semantics Survey measures student interest in science, technology, engineering and mathematics as well as interest in STEM careers. The survey is comprised of five scales each with five items measured on a 7-point scale. The STEM Semantic Survey is appropriate for administration to middle school students and teachers. The link below provides access to the instrument as well as ...
When Americans are asked why more students don't pursue a degree in science, technology, engineering or math (STEM), they are most likely to point to the difficulty of these subjects, according to a new Pew Research Center survey. About half of adults (52%) say the main reason young people don't pursue STEM degrees is they think these ...
Cantlon and her colleagues took a different approach. They merged a topic that younger children (grades 3 to 6) enjoy — animals — with one that most kids might look at like a plate of steaming Brussels sprouts — computer coding. The results of their pilot program are available in the April 2 issue of the journal STEM Education Research.
Is anyone here a STEM student, I'm currently doing a research study and have a survey that needs to be answered. ( Minimum of 2 mins)
George Mason University Systems Engineering and Operations Research students took first place in the Systems and Analytics Track of the Sage Memorial Design Competition and first place in the STEM Track of the Patriot Pitch Competition with their plan to deliver supplies to a hard-to-reach island in the Chesapeake Bay.
ABOUT PEW RESEARCH CENTER Pew Research Center is a nonpartisan fact tank that informs the public about the issues, attitudes and trends shaping the world. It conducts public opinion polling, demographic research, media content analysis and other empirical social science research. Pew Research Center does not take policy positions.
Hello Political Science students, We are students in JSIS 300 Claims and Evidence with Professor Porter and would appreciate your time in taking a quick and anonymous survey on Climate Change and Human Behavior. The data collected will contribute significantly to our research efforts and provide valuable insights for our study. The survey form will be open from Monday, May 6th to Saturday, May ...