Popular searches
- How to Get Participants For Your Study
- How to Do Segmentation?
- Conjoint Preference Share Simulator
- MaxDiff Analysis
- Likert Scales
- Reliability & Validity
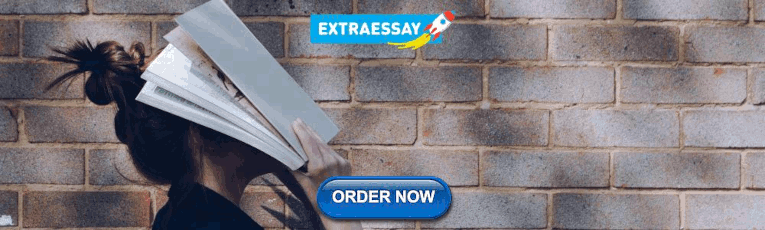
Request consultation
Do you need support in running a pricing or product study? We can help you with agile consumer research and conjoint analysis.
Looking for an online survey platform?
Conjointly offers a great survey tool with multiple question types, randomisation blocks, and multilingual support. The Basic tier is always free.
Research Methods Knowledge Base
- Navigating the Knowledge Base
- Foundations
- Measurement
- Research Design
- Threats to Conclusion Validity
- Improving Conclusion Validity
- Statistical Power
- Data Preparation
- Descriptive Statistics
- Inferential Statistics
- Table of Contents
Fully-functional online survey tool with various question types, logic, randomisation, and reporting for unlimited number of surveys.
Completely free for academics and students .
Conclusion Validity
Of the four types of validity (see also internal validity , construct validity and external validity ) conclusion validity is undoubtedly the least considered and most misunderstood. That’s probably due to the fact that it was originally labeled ‘statistical’ conclusion validity and you know how even the mere mention of the word statistics will scare off most of the human race!
In many ways, conclusion validity is the most important of the four validity types because it is relevant whenever we are trying to decide if there is a relationship in our observations (and that’s one of the most basic aspects of any analysis). Perhaps we should start with an attempt at a definition:
Conclusion validity is the degree to which conclusions we reach about relationships in our data are reasonable.
For instance, if we’re doing a study that looks at the relationship between socioeconomic status (SES) and attitudes about capital punishment, we eventually want to reach some conclusion. Based on our data, we may conclude that there is a positive relationship, that persons with higher SES tend to have a more positive view of capital punishment while those with lower SES tend to be more opposed. Conclusion validity is the degree to which the conclusion we reach is credible or believable.
Although conclusion validity was originally thought to be a statistical inference issue, it has become more apparent that it is also relevant in qualitative research. For example, in an observational field study of homeless adolescents the researcher might, on the basis of field notes, see a pattern that suggests that teenagers on the street who use drugs are more likely to be involved in more complex social networks and to interact with a more varied group of people. Although this conclusion or inference may be based entirely on impressionistic data, we can ask whether it has conclusion validity, that is, whether it is a reasonable conclusion about a relationship in our observations.
Whenever you investigate a relationship, you essentially have two possible conclusions — either there is a relationship in your data or there isn’t. In either case, however, you could be wrong in your conclusion. You might conclude that there is a relationship when in fact there is not, or you might infer that there isn’t a relationship when in fact there is (but you didn’t detect it!). So, we have to consider all of these possibilities when we talk about conclusion validity.
It’s important to realize that conclusion validity is an issue whenever you conclude there is a relationship, even when the relationship is between some program (or treatment) and some outcome. In other words, conclusion validity also pertains to causal relationships. How do we distinguish it from internal validity which is also involved with causal relationships? Conclusion validity is only concerned with whether there is a relationship. For instance, in a program evaluation, we might conclude that there is a positive relationship between our educational program and achievement test scores — students in the program get higher scores and students not in the program get lower ones. Conclusion validity is essentially whether that relationship is a reasonable one or not, given the data. But it is possible that we will conclude that, while there is a relationship between the program and outcome, the program didn’t cause the outcome. Perhaps some other factor, and not our program, was responsible for the outcome in this study. For instance, the observed differences in the outcome could be due to the fact that the program group was smarter than the comparison group to begin with. Our observed posttest differences between these groups could be due to this initial difference and not be the result of our program. This issue — the possibility that some other factor than our program caused the outcome — is what internal validity is all about. So, it is possible that in a study we can conclude that our program and outcome are related (conclusion validity) and also conclude that the outcome was caused by some factor other than the program (i.e. we don’t have internal validity).
We’ll begin this discussion by considering the major threats to conclusion validity , the different reasons you might be wrong in concluding that there is or isn’t a relationship. You’ll see that there are several key reasons why reaching conclusions about relationships is so difficult. One major problem is that it is often hard to see a relationship because our measures or observations have low reliability — they are too weak relative to all of the ’noise’ in the environment. Another issue is that the relationship we are looking for may be a weak one and seeing it is a bit like looking for a needle in the haystack. Sometimes the problem is that we just didn’t collect enough information to see the relationship even if it is there. All of these problems are related to the idea of statistical power and so we’ll spend some time trying to understand what ‘power’ is in this context. Finally, we need to recognize that we have some control over our ability to detect relationships, and we’ll conclude with some suggestions for improving conclusion validity .
Cookie Consent
Conjointly uses essential cookies to make our site work. We also use additional cookies in order to understand the usage of the site, gather audience analytics, and for remarketing purposes.
For more information on Conjointly's use of cookies, please read our Cookie Policy .
Which one are you?
I am new to conjointly, i am already using conjointly.
Validity In Psychology Research: Types & Examples
Saul Mcleod, PhD
Editor-in-Chief for Simply Psychology
BSc (Hons) Psychology, MRes, PhD, University of Manchester
Saul Mcleod, PhD., is a qualified psychology teacher with over 18 years of experience in further and higher education. He has been published in peer-reviewed journals, including the Journal of Clinical Psychology.
Learn about our Editorial Process
Olivia Guy-Evans, MSc
Associate Editor for Simply Psychology
BSc (Hons) Psychology, MSc Psychology of Education
Olivia Guy-Evans is a writer and associate editor for Simply Psychology. She has previously worked in healthcare and educational sectors.
In psychology research, validity refers to the extent to which a test or measurement tool accurately measures what it’s intended to measure. It ensures that the research findings are genuine and not due to extraneous factors.
Validity can be categorized into different types based on internal and external validity .
The concept of validity was formulated by Kelly (1927, p. 14), who stated that a test is valid if it measures what it claims to measure. For example, a test of intelligence should measure intelligence and not something else (such as memory).
Internal and External Validity In Research
Internal validity refers to whether the effects observed in a study are due to the manipulation of the independent variable and not some other confounding factor.
In other words, there is a causal relationship between the independent and dependent variables .
Internal validity can be improved by controlling extraneous variables, using standardized instructions, counterbalancing, and eliminating demand characteristics and investigator effects.
External validity refers to the extent to which the results of a study can be generalized to other settings (ecological validity), other people (population validity), and over time (historical validity).
External validity can be improved by setting experiments more naturally and using random sampling to select participants.
Types of Validity In Psychology
Two main categories of validity are used to assess the validity of the test (i.e., questionnaire, interview, IQ test, etc.): Content and criterion.
- Content validity refers to the extent to which a test or measurement represents all aspects of the intended content domain. It assesses whether the test items adequately cover the topic or concept.
- Criterion validity assesses the performance of a test based on its correlation with a known external criterion or outcome. It can be further divided into concurrent (measured at the same time) and predictive (measuring future performance) validity.
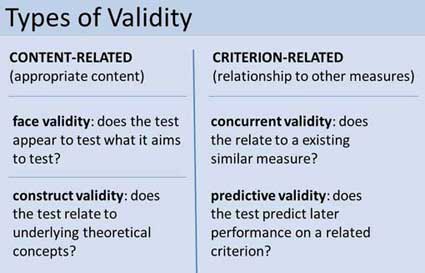
Face Validity
Face validity is simply whether the test appears (at face value) to measure what it claims to. This is the least sophisticated measure of content-related validity, and is a superficial and subjective assessment based on appearance.
Tests wherein the purpose is clear, even to naïve respondents, are said to have high face validity. Accordingly, tests wherein the purpose is unclear have low face validity (Nevo, 1985).
A direct measurement of face validity is obtained by asking people to rate the validity of a test as it appears to them. This rater could use a Likert scale to assess face validity.
For example:
- The test is extremely suitable for a given purpose
- The test is very suitable for that purpose;
- The test is adequate
- The test is inadequate
- The test is irrelevant and, therefore, unsuitable
It is important to select suitable people to rate a test (e.g., questionnaire, interview, IQ test, etc.). For example, individuals who actually take the test would be well placed to judge its face validity.
Also, people who work with the test could offer their opinion (e.g., employers, university administrators, employers). Finally, the researcher could use members of the general public with an interest in the test (e.g., parents of testees, politicians, teachers, etc.).
The face validity of a test can be considered a robust construct only if a reasonable level of agreement exists among raters.
It should be noted that the term face validity should be avoided when the rating is done by an “expert,” as content validity is more appropriate.
Having face validity does not mean that a test really measures what the researcher intends to measure, but only in the judgment of raters that it appears to do so. Consequently, it is a crude and basic measure of validity.
A test item such as “ I have recently thought of killing myself ” has obvious face validity as an item measuring suicidal cognitions and may be useful when measuring symptoms of depression.
However, the implication of items on tests with clear face validity is that they are more vulnerable to social desirability bias. Individuals may manipulate their responses to deny or hide problems or exaggerate behaviors to present a positive image of themselves.
It is possible for a test item to lack face validity but still have general validity and measure what it claims to measure. This is good because it reduces demand characteristics and makes it harder for respondents to manipulate their answers.
For example, the test item “ I believe in the second coming of Christ ” would lack face validity as a measure of depression (as the purpose of the item is unclear).
This item appeared on the first version of The Minnesota Multiphasic Personality Inventory (MMPI) and loaded on the depression scale.
Because most of the original normative sample of the MMPI were good Christians, only a depressed Christian would think Christ is not coming back. Thus, for this particular religious sample, the item does have general validity but not face validity.
Construct Validity
Construct validity assesses how well a test or measure represents and captures an abstract theoretical concept, known as a construct. It indicates the degree to which the test accurately reflects the construct it intends to measure, often evaluated through relationships with other variables and measures theoretically connected to the construct.
Construct validity was invented by Cronbach and Meehl (1955). This type of content-related validity refers to the extent to which a test captures a specific theoretical construct or trait, and it overlaps with some of the other aspects of validity
Construct validity does not concern the simple, factual question of whether a test measures an attribute.
Instead, it is about the complex question of whether test score interpretations are consistent with a nomological network involving theoretical and observational terms (Cronbach & Meehl, 1955).
To test for construct validity, it must be demonstrated that the phenomenon being measured actually exists. So, the construct validity of a test for intelligence, for example, depends on a model or theory of intelligence .
Construct validity entails demonstrating the power of such a construct to explain a network of research findings and to predict further relationships.
The more evidence a researcher can demonstrate for a test’s construct validity, the better. However, there is no single method of determining the construct validity of a test.
Instead, different methods and approaches are combined to present the overall construct validity of a test. For example, factor analysis and correlational methods can be used.
Convergent validity
Convergent validity is a subtype of construct validity. It assesses the degree to which two measures that theoretically should be related are related.
It demonstrates that measures of similar constructs are highly correlated. It helps confirm that a test accurately measures the intended construct by showing its alignment with other tests designed to measure the same or similar constructs.
For example, suppose there are two different scales used to measure self-esteem:
Scale A and Scale B. If both scales effectively measure self-esteem, then individuals who score high on Scale A should also score high on Scale B, and those who score low on Scale A should score similarly low on Scale B.
If the scores from these two scales show a strong positive correlation, then this provides evidence for convergent validity because it indicates that both scales seem to measure the same underlying construct of self-esteem.
Concurrent Validity (i.e., occurring at the same time)
Concurrent validity evaluates how well a test’s results correlate with the results of a previously established and accepted measure, when both are administered at the same time.
It helps in determining whether a new measure is a good reflection of an established one without waiting to observe outcomes in the future.
If the new test is validated by comparison with a currently existing criterion, we have concurrent validity.
Very often, a new IQ or personality test might be compared with an older but similar test known to have good validity already.
Predictive Validity
Predictive validity assesses how well a test predicts a criterion that will occur in the future. It measures the test’s ability to foresee the performance of an individual on a related criterion measured at a later point in time. It gauges the test’s effectiveness in predicting subsequent real-world outcomes or results.
For example, a prediction may be made on the basis of a new intelligence test that high scorers at age 12 will be more likely to obtain university degrees several years later. If the prediction is born out, then the test has predictive validity.
Cronbach, L. J., and Meehl, P. E. (1955) Construct validity in psychological tests. Psychological Bulletin , 52, 281-302.
Hathaway, S. R., & McKinley, J. C. (1943). Manual for the Minnesota Multiphasic Personality Inventory . New York: Psychological Corporation.
Kelley, T. L. (1927). Interpretation of educational measurements. New York : Macmillan.
Nevo, B. (1985). Face validity revisited . Journal of Educational Measurement , 22(4), 287-293.

Related Articles
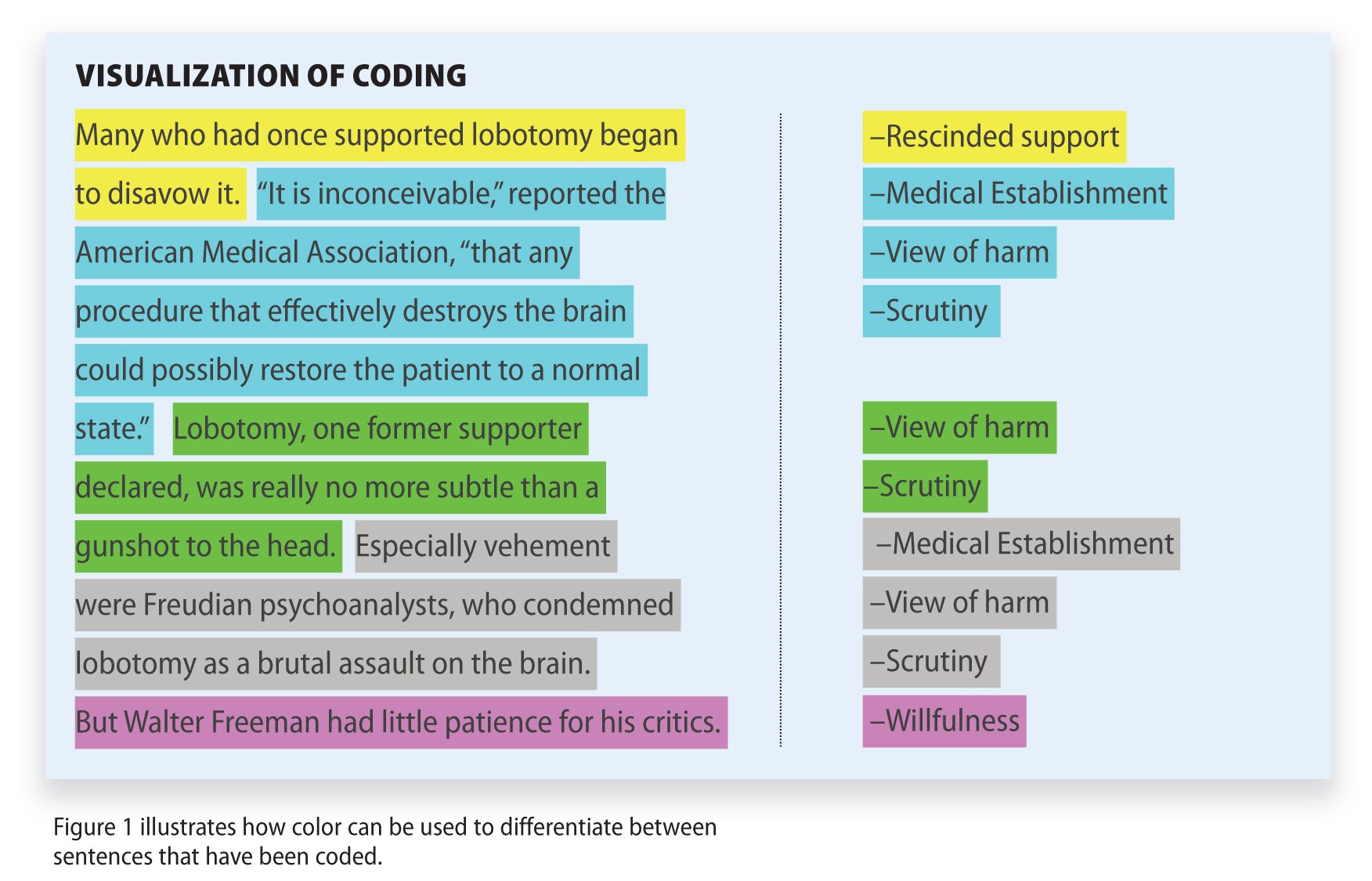
Research Methodology
Qualitative Data Coding
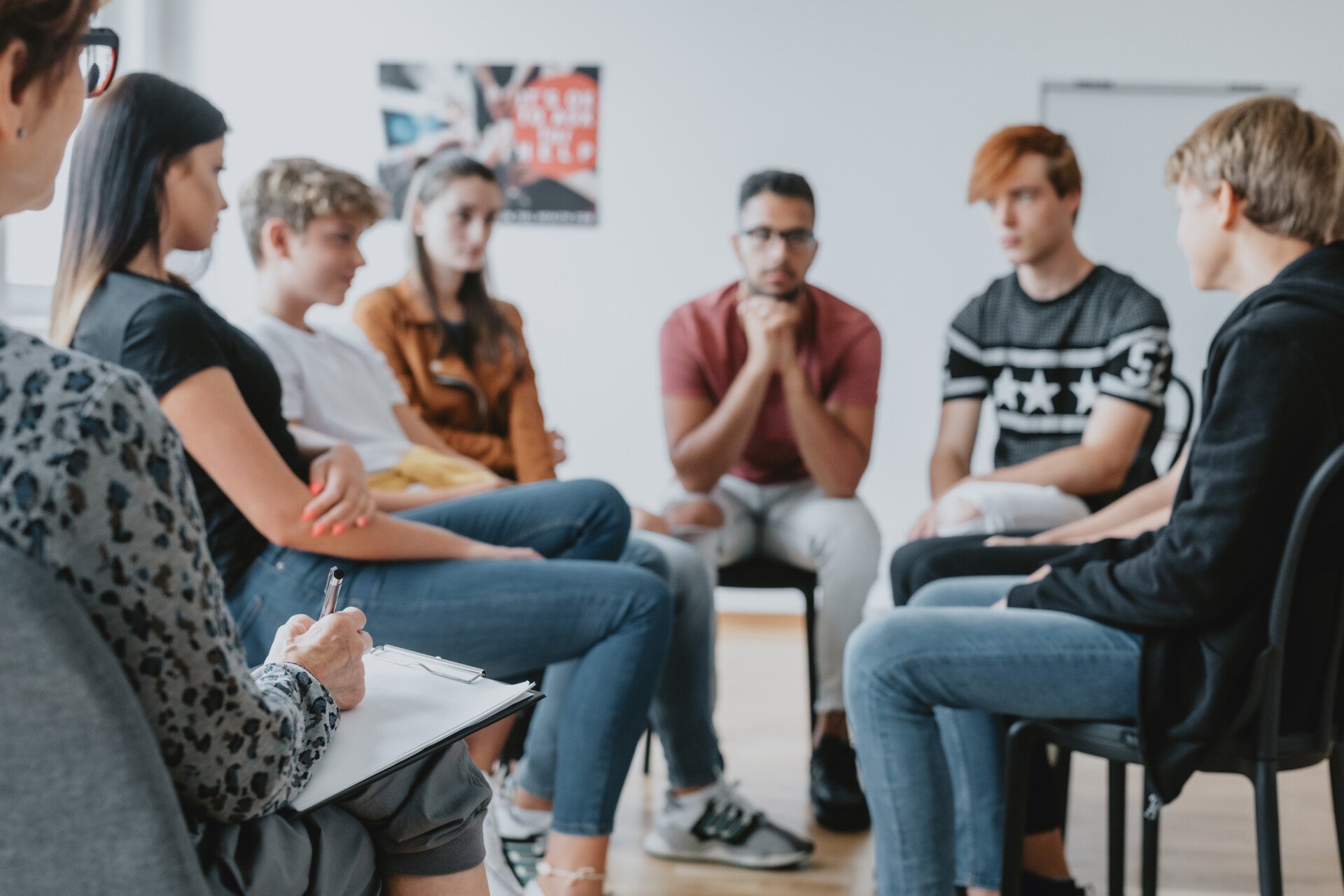
What Is a Focus Group?
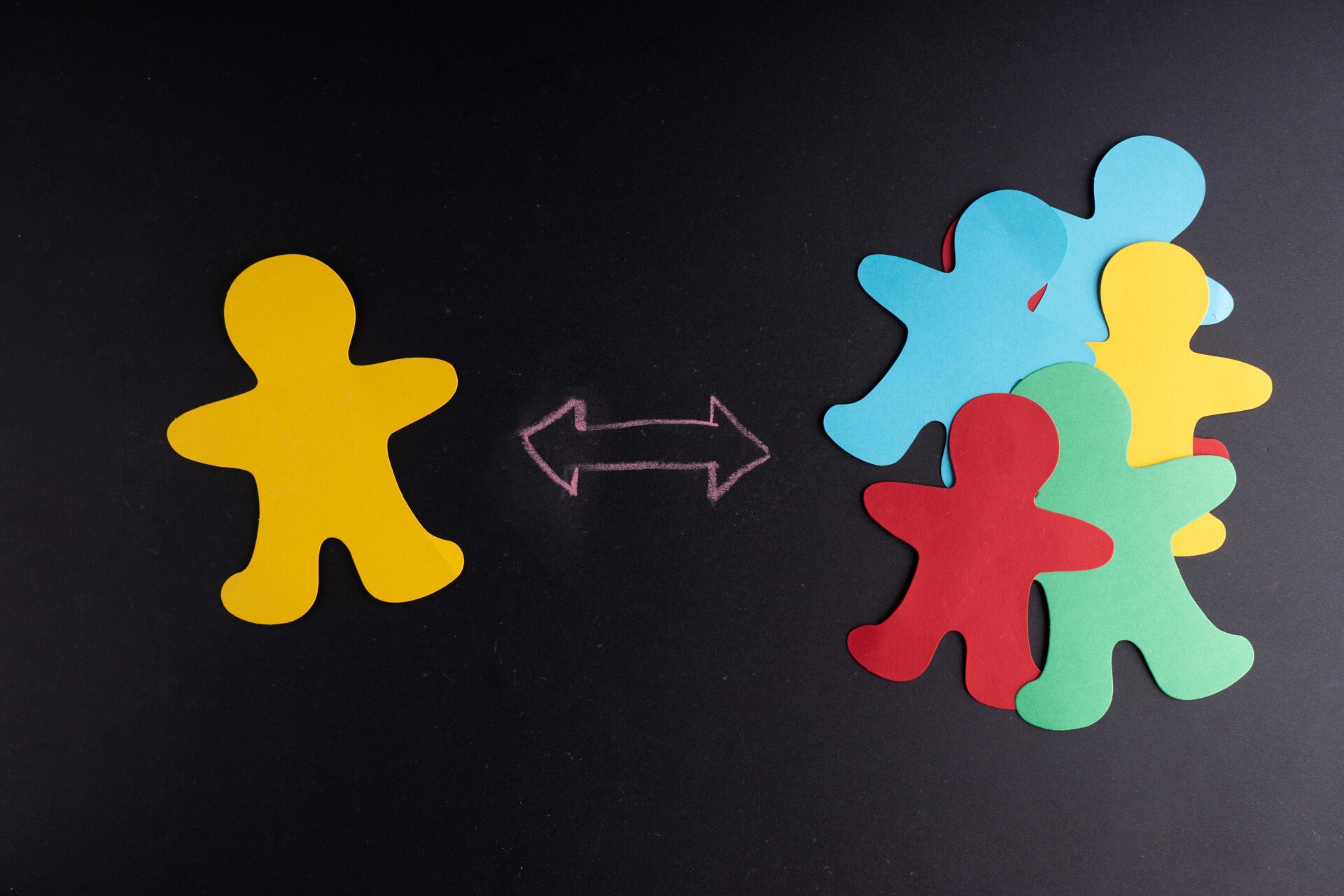
Cross-Cultural Research Methodology In Psychology
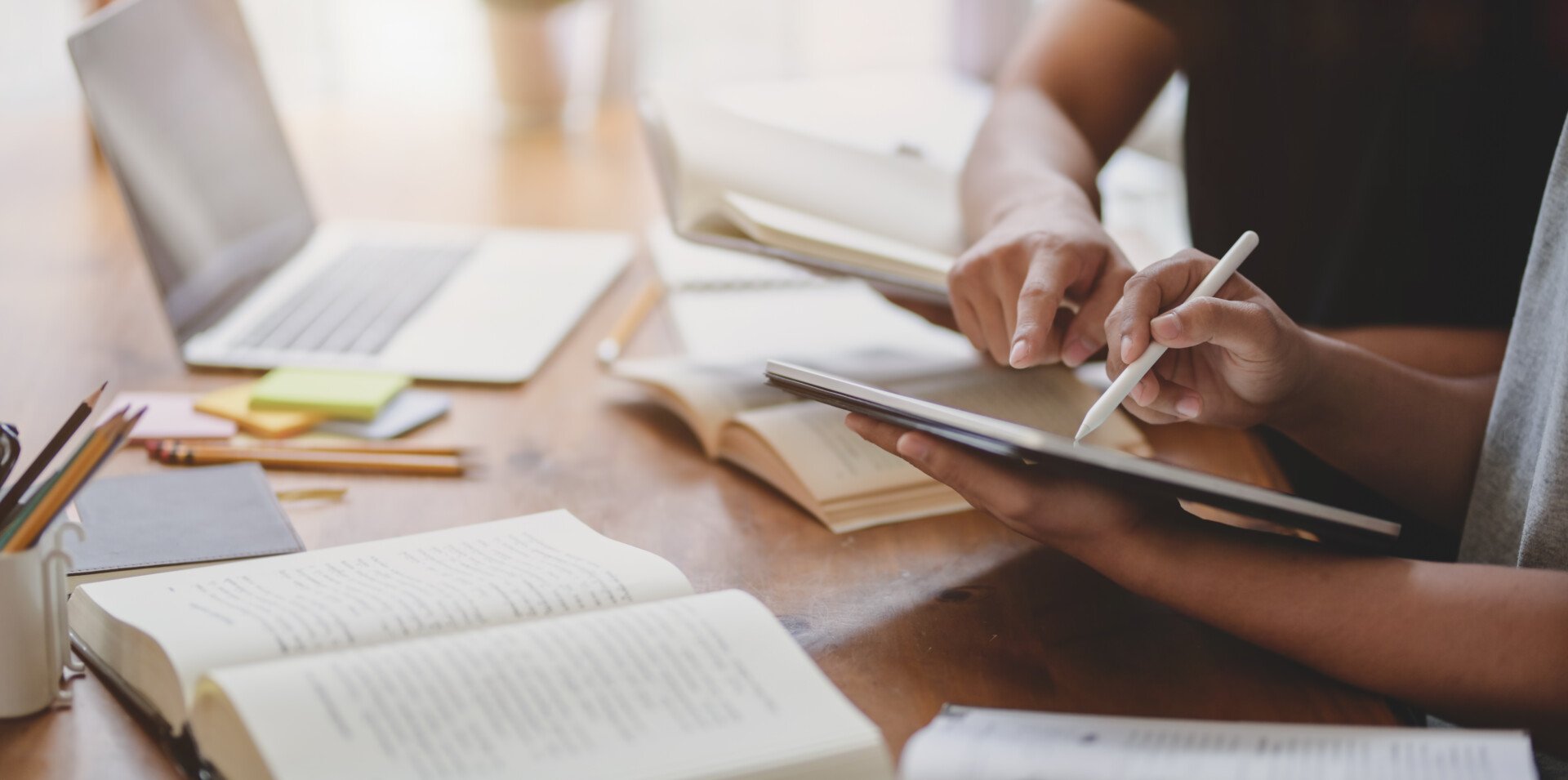
What Is Internal Validity In Research?
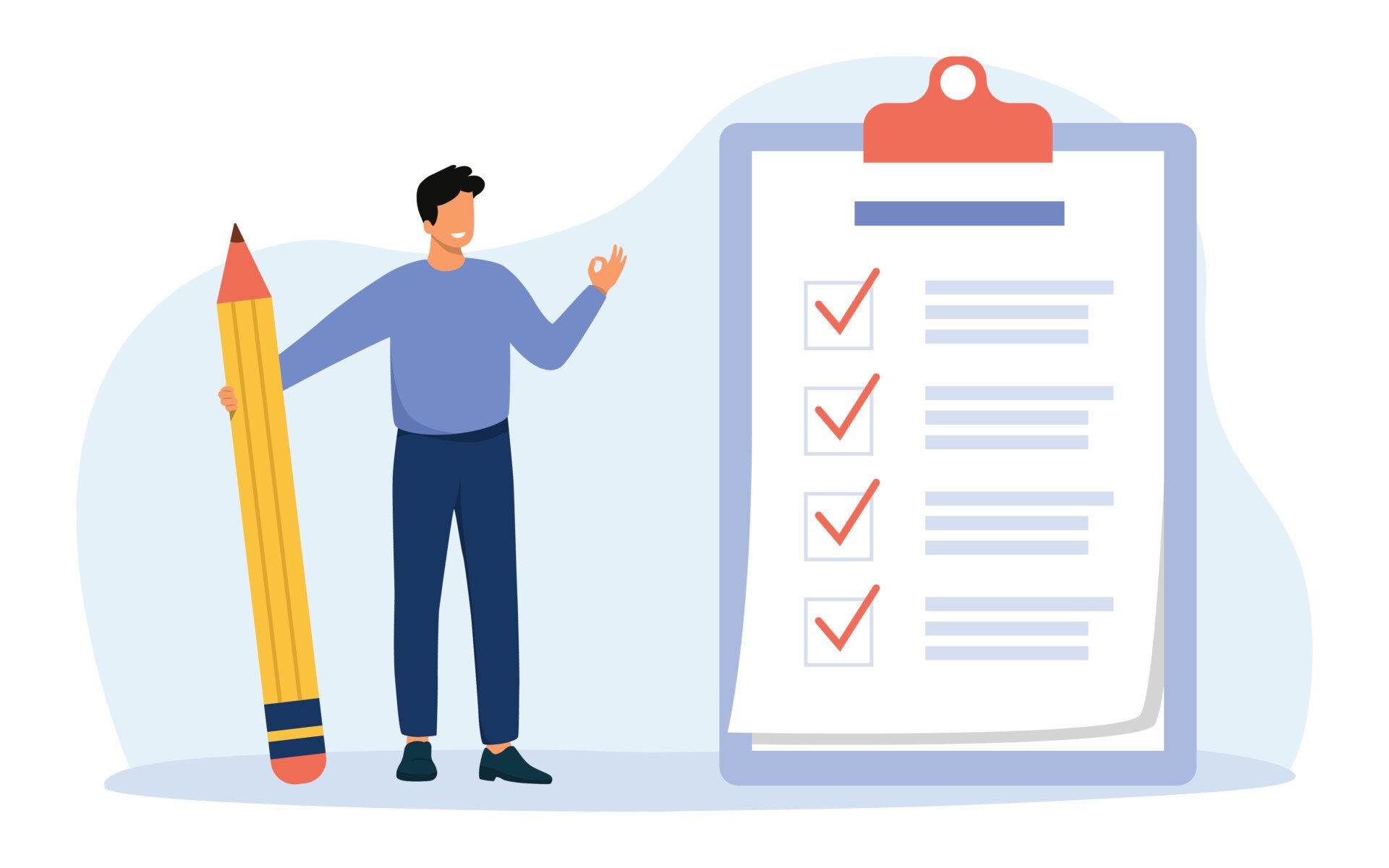
Research Methodology , Statistics
What Is Face Validity In Research? Importance & How To Measure
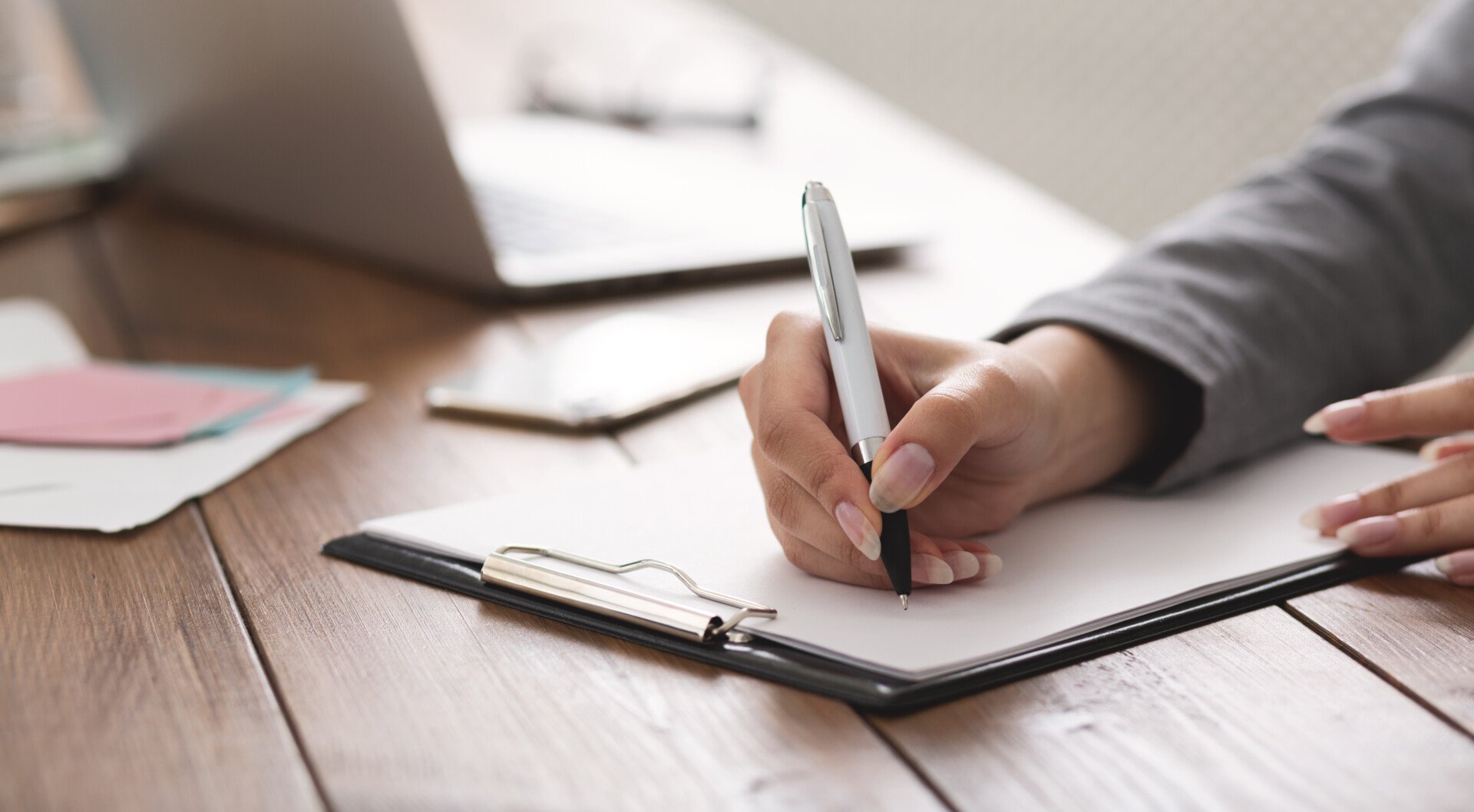
Criterion Validity: Definition & Examples
Have a language expert improve your writing
Run a free plagiarism check in 10 minutes, automatically generate references for free.
- Knowledge Base
- Methodology
- Reliability vs Validity in Research | Differences, Types & Examples
Reliability vs Validity in Research | Differences, Types & Examples
Published on 3 May 2022 by Fiona Middleton . Revised on 10 October 2022.
Reliability and validity are concepts used to evaluate the quality of research. They indicate how well a method , technique, or test measures something. Reliability is about the consistency of a measure, and validity is about the accuracy of a measure.
It’s important to consider reliability and validity when you are creating your research design , planning your methods, and writing up your results, especially in quantitative research .
Table of contents
Understanding reliability vs validity, how are reliability and validity assessed, how to ensure validity and reliability in your research, where to write about reliability and validity in a thesis.
Reliability and validity are closely related, but they mean different things. A measurement can be reliable without being valid. However, if a measurement is valid, it is usually also reliable.
What is reliability?
Reliability refers to how consistently a method measures something. If the same result can be consistently achieved by using the same methods under the same circumstances, the measurement is considered reliable.
What is validity?
Validity refers to how accurately a method measures what it is intended to measure. If research has high validity, that means it produces results that correspond to real properties, characteristics, and variations in the physical or social world.
High reliability is one indicator that a measurement is valid. If a method is not reliable, it probably isn’t valid.
However, reliability on its own is not enough to ensure validity. Even if a test is reliable, it may not accurately reflect the real situation.
Validity is harder to assess than reliability, but it is even more important. To obtain useful results, the methods you use to collect your data must be valid: the research must be measuring what it claims to measure. This ensures that your discussion of the data and the conclusions you draw are also valid.
Prevent plagiarism, run a free check.
Reliability can be estimated by comparing different versions of the same measurement. Validity is harder to assess, but it can be estimated by comparing the results to other relevant data or theory. Methods of estimating reliability and validity are usually split up into different types.
Types of reliability
Different types of reliability can be estimated through various statistical methods.
Types of validity
The validity of a measurement can be estimated based on three main types of evidence. Each type can be evaluated through expert judgement or statistical methods.
To assess the validity of a cause-and-effect relationship, you also need to consider internal validity (the design of the experiment ) and external validity (the generalisability of the results).
The reliability and validity of your results depends on creating a strong research design , choosing appropriate methods and samples, and conducting the research carefully and consistently.
Ensuring validity
If you use scores or ratings to measure variations in something (such as psychological traits, levels of ability, or physical properties), it’s important that your results reflect the real variations as accurately as possible. Validity should be considered in the very earliest stages of your research, when you decide how you will collect your data .
- Choose appropriate methods of measurement
Ensure that your method and measurement technique are of high quality and targeted to measure exactly what you want to know. They should be thoroughly researched and based on existing knowledge.
For example, to collect data on a personality trait, you could use a standardised questionnaire that is considered reliable and valid. If you develop your own questionnaire, it should be based on established theory or the findings of previous studies, and the questions should be carefully and precisely worded.
- Use appropriate sampling methods to select your subjects
To produce valid generalisable results, clearly define the population you are researching (e.g., people from a specific age range, geographical location, or profession). Ensure that you have enough participants and that they are representative of the population.
Ensuring reliability
Reliability should be considered throughout the data collection process. When you use a tool or technique to collect data, it’s important that the results are precise, stable, and reproducible.
- Apply your methods consistently
Plan your method carefully to make sure you carry out the same steps in the same way for each measurement. This is especially important if multiple researchers are involved.
For example, if you are conducting interviews or observations, clearly define how specific behaviours or responses will be counted, and make sure questions are phrased the same way each time.
- Standardise the conditions of your research
When you collect your data, keep the circumstances as consistent as possible to reduce the influence of external factors that might create variation in the results.
For example, in an experimental setup, make sure all participants are given the same information and tested under the same conditions.
It’s appropriate to discuss reliability and validity in various sections of your thesis or dissertation or research paper. Showing that you have taken them into account in planning your research and interpreting the results makes your work more credible and trustworthy.
Cite this Scribbr article
If you want to cite this source, you can copy and paste the citation or click the ‘Cite this Scribbr article’ button to automatically add the citation to our free Reference Generator.
Middleton, F. (2022, October 10). Reliability vs Validity in Research | Differences, Types & Examples. Scribbr. Retrieved 21 May 2024, from https://www.scribbr.co.uk/research-methods/reliability-or-validity/
Is this article helpful?
Fiona Middleton
Other students also liked, the 4 types of validity | types, definitions & examples, a quick guide to experimental design | 5 steps & examples, sampling methods | types, techniques, & examples.
- Search Menu
- Browse content in Arts and Humanities
- Browse content in Archaeology
- Anglo-Saxon and Medieval Archaeology
- Archaeological Methodology and Techniques
- Archaeology by Region
- Archaeology of Religion
- Archaeology of Trade and Exchange
- Biblical Archaeology
- Contemporary and Public Archaeology
- Environmental Archaeology
- Historical Archaeology
- History and Theory of Archaeology
- Industrial Archaeology
- Landscape Archaeology
- Mortuary Archaeology
- Prehistoric Archaeology
- Underwater Archaeology
- Urban Archaeology
- Zooarchaeology
- Browse content in Architecture
- Architectural Structure and Design
- History of Architecture
- Residential and Domestic Buildings
- Theory of Architecture
- Browse content in Art
- Art Subjects and Themes
- History of Art
- Industrial and Commercial Art
- Theory of Art
- Biographical Studies
- Byzantine Studies
- Browse content in Classical Studies
- Classical History
- Classical Philosophy
- Classical Mythology
- Classical Literature
- Classical Reception
- Classical Art and Architecture
- Classical Oratory and Rhetoric
- Greek and Roman Papyrology
- Greek and Roman Epigraphy
- Greek and Roman Law
- Greek and Roman Archaeology
- Late Antiquity
- Religion in the Ancient World
- Digital Humanities
- Browse content in History
- Colonialism and Imperialism
- Diplomatic History
- Environmental History
- Genealogy, Heraldry, Names, and Honours
- Genocide and Ethnic Cleansing
- Historical Geography
- History by Period
- History of Emotions
- History of Agriculture
- History of Education
- History of Gender and Sexuality
- Industrial History
- Intellectual History
- International History
- Labour History
- Legal and Constitutional History
- Local and Family History
- Maritime History
- Military History
- National Liberation and Post-Colonialism
- Oral History
- Political History
- Public History
- Regional and National History
- Revolutions and Rebellions
- Slavery and Abolition of Slavery
- Social and Cultural History
- Theory, Methods, and Historiography
- Urban History
- World History
- Browse content in Language Teaching and Learning
- Language Learning (Specific Skills)
- Language Teaching Theory and Methods
- Browse content in Linguistics
- Applied Linguistics
- Cognitive Linguistics
- Computational Linguistics
- Forensic Linguistics
- Grammar, Syntax and Morphology
- Historical and Diachronic Linguistics
- History of English
- Language Evolution
- Language Reference
- Language Acquisition
- Language Variation
- Language Families
- Lexicography
- Linguistic Anthropology
- Linguistic Theories
- Linguistic Typology
- Phonetics and Phonology
- Psycholinguistics
- Sociolinguistics
- Translation and Interpretation
- Writing Systems
- Browse content in Literature
- Bibliography
- Children's Literature Studies
- Literary Studies (Romanticism)
- Literary Studies (American)
- Literary Studies (Asian)
- Literary Studies (European)
- Literary Studies (Eco-criticism)
- Literary Studies (Modernism)
- Literary Studies - World
- Literary Studies (1500 to 1800)
- Literary Studies (19th Century)
- Literary Studies (20th Century onwards)
- Literary Studies (African American Literature)
- Literary Studies (British and Irish)
- Literary Studies (Early and Medieval)
- Literary Studies (Fiction, Novelists, and Prose Writers)
- Literary Studies (Gender Studies)
- Literary Studies (Graphic Novels)
- Literary Studies (History of the Book)
- Literary Studies (Plays and Playwrights)
- Literary Studies (Poetry and Poets)
- Literary Studies (Postcolonial Literature)
- Literary Studies (Queer Studies)
- Literary Studies (Science Fiction)
- Literary Studies (Travel Literature)
- Literary Studies (War Literature)
- Literary Studies (Women's Writing)
- Literary Theory and Cultural Studies
- Mythology and Folklore
- Shakespeare Studies and Criticism
- Browse content in Media Studies
- Browse content in Music
- Applied Music
- Dance and Music
- Ethics in Music
- Ethnomusicology
- Gender and Sexuality in Music
- Medicine and Music
- Music Cultures
- Music and Media
- Music and Religion
- Music and Culture
- Music Education and Pedagogy
- Music Theory and Analysis
- Musical Scores, Lyrics, and Libretti
- Musical Structures, Styles, and Techniques
- Musicology and Music History
- Performance Practice and Studies
- Race and Ethnicity in Music
- Sound Studies
- Browse content in Performing Arts
- Browse content in Philosophy
- Aesthetics and Philosophy of Art
- Epistemology
- Feminist Philosophy
- History of Western Philosophy
- Metaphysics
- Moral Philosophy
- Non-Western Philosophy
- Philosophy of Language
- Philosophy of Mind
- Philosophy of Perception
- Philosophy of Science
- Philosophy of Action
- Philosophy of Law
- Philosophy of Religion
- Philosophy of Mathematics and Logic
- Practical Ethics
- Social and Political Philosophy
- Browse content in Religion
- Biblical Studies
- Christianity
- East Asian Religions
- History of Religion
- Judaism and Jewish Studies
- Qumran Studies
- Religion and Education
- Religion and Health
- Religion and Politics
- Religion and Science
- Religion and Law
- Religion and Art, Literature, and Music
- Religious Studies
- Browse content in Society and Culture
- Cookery, Food, and Drink
- Cultural Studies
- Customs and Traditions
- Ethical Issues and Debates
- Hobbies, Games, Arts and Crafts
- Lifestyle, Home, and Garden
- Natural world, Country Life, and Pets
- Popular Beliefs and Controversial Knowledge
- Sports and Outdoor Recreation
- Technology and Society
- Travel and Holiday
- Visual Culture
- Browse content in Law
- Arbitration
- Browse content in Company and Commercial Law
- Commercial Law
- Company Law
- Browse content in Comparative Law
- Systems of Law
- Competition Law
- Browse content in Constitutional and Administrative Law
- Government Powers
- Judicial Review
- Local Government Law
- Military and Defence Law
- Parliamentary and Legislative Practice
- Construction Law
- Contract Law
- Browse content in Criminal Law
- Criminal Procedure
- Criminal Evidence Law
- Sentencing and Punishment
- Employment and Labour Law
- Environment and Energy Law
- Browse content in Financial Law
- Banking Law
- Insolvency Law
- History of Law
- Human Rights and Immigration
- Intellectual Property Law
- Browse content in International Law
- Private International Law and Conflict of Laws
- Public International Law
- IT and Communications Law
- Jurisprudence and Philosophy of Law
- Law and Politics
- Law and Society
- Browse content in Legal System and Practice
- Courts and Procedure
- Legal Skills and Practice
- Primary Sources of Law
- Regulation of Legal Profession
- Medical and Healthcare Law
- Browse content in Policing
- Criminal Investigation and Detection
- Police and Security Services
- Police Procedure and Law
- Police Regional Planning
- Browse content in Property Law
- Personal Property Law
- Study and Revision
- Terrorism and National Security Law
- Browse content in Trusts Law
- Wills and Probate or Succession
- Browse content in Medicine and Health
- Browse content in Allied Health Professions
- Arts Therapies
- Clinical Science
- Dietetics and Nutrition
- Occupational Therapy
- Operating Department Practice
- Physiotherapy
- Radiography
- Speech and Language Therapy
- Browse content in Anaesthetics
- General Anaesthesia
- Neuroanaesthesia
- Clinical Neuroscience
- Browse content in Clinical Medicine
- Acute Medicine
- Cardiovascular Medicine
- Clinical Genetics
- Clinical Pharmacology and Therapeutics
- Dermatology
- Endocrinology and Diabetes
- Gastroenterology
- Genito-urinary Medicine
- Geriatric Medicine
- Infectious Diseases
- Medical Toxicology
- Medical Oncology
- Pain Medicine
- Palliative Medicine
- Rehabilitation Medicine
- Respiratory Medicine and Pulmonology
- Rheumatology
- Sleep Medicine
- Sports and Exercise Medicine
- Community Medical Services
- Critical Care
- Emergency Medicine
- Forensic Medicine
- Haematology
- History of Medicine
- Browse content in Medical Skills
- Clinical Skills
- Communication Skills
- Nursing Skills
- Surgical Skills
- Browse content in Medical Dentistry
- Oral and Maxillofacial Surgery
- Paediatric Dentistry
- Restorative Dentistry and Orthodontics
- Surgical Dentistry
- Medical Ethics
- Medical Statistics and Methodology
- Browse content in Neurology
- Clinical Neurophysiology
- Neuropathology
- Nursing Studies
- Browse content in Obstetrics and Gynaecology
- Gynaecology
- Occupational Medicine
- Ophthalmology
- Otolaryngology (ENT)
- Browse content in Paediatrics
- Neonatology
- Browse content in Pathology
- Chemical Pathology
- Clinical Cytogenetics and Molecular Genetics
- Histopathology
- Medical Microbiology and Virology
- Patient Education and Information
- Browse content in Pharmacology
- Psychopharmacology
- Browse content in Popular Health
- Caring for Others
- Complementary and Alternative Medicine
- Self-help and Personal Development
- Browse content in Preclinical Medicine
- Cell Biology
- Molecular Biology and Genetics
- Reproduction, Growth and Development
- Primary Care
- Professional Development in Medicine
- Browse content in Psychiatry
- Addiction Medicine
- Child and Adolescent Psychiatry
- Forensic Psychiatry
- Learning Disabilities
- Old Age Psychiatry
- Psychotherapy
- Browse content in Public Health and Epidemiology
- Epidemiology
- Public Health
- Browse content in Radiology
- Clinical Radiology
- Interventional Radiology
- Nuclear Medicine
- Radiation Oncology
- Reproductive Medicine
- Browse content in Surgery
- Cardiothoracic Surgery
- Gastro-intestinal and Colorectal Surgery
- General Surgery
- Neurosurgery
- Paediatric Surgery
- Peri-operative Care
- Plastic and Reconstructive Surgery
- Surgical Oncology
- Transplant Surgery
- Trauma and Orthopaedic Surgery
- Vascular Surgery
- Browse content in Science and Mathematics
- Browse content in Biological Sciences
- Aquatic Biology
- Biochemistry
- Bioinformatics and Computational Biology
- Developmental Biology
- Ecology and Conservation
- Evolutionary Biology
- Genetics and Genomics
- Microbiology
- Molecular and Cell Biology
- Natural History
- Plant Sciences and Forestry
- Research Methods in Life Sciences
- Structural Biology
- Systems Biology
- Zoology and Animal Sciences
- Browse content in Chemistry
- Analytical Chemistry
- Computational Chemistry
- Crystallography
- Environmental Chemistry
- Industrial Chemistry
- Inorganic Chemistry
- Materials Chemistry
- Medicinal Chemistry
- Mineralogy and Gems
- Organic Chemistry
- Physical Chemistry
- Polymer Chemistry
- Study and Communication Skills in Chemistry
- Theoretical Chemistry
- Browse content in Computer Science
- Artificial Intelligence
- Computer Architecture and Logic Design
- Game Studies
- Human-Computer Interaction
- Mathematical Theory of Computation
- Programming Languages
- Software Engineering
- Systems Analysis and Design
- Virtual Reality
- Browse content in Computing
- Business Applications
- Computer Security
- Computer Games
- Computer Networking and Communications
- Digital Lifestyle
- Graphical and Digital Media Applications
- Operating Systems
- Browse content in Earth Sciences and Geography
- Atmospheric Sciences
- Environmental Geography
- Geology and the Lithosphere
- Maps and Map-making
- Meteorology and Climatology
- Oceanography and Hydrology
- Palaeontology
- Physical Geography and Topography
- Regional Geography
- Soil Science
- Urban Geography
- Browse content in Engineering and Technology
- Agriculture and Farming
- Biological Engineering
- Civil Engineering, Surveying, and Building
- Electronics and Communications Engineering
- Energy Technology
- Engineering (General)
- Environmental Science, Engineering, and Technology
- History of Engineering and Technology
- Mechanical Engineering and Materials
- Technology of Industrial Chemistry
- Transport Technology and Trades
- Browse content in Environmental Science
- Applied Ecology (Environmental Science)
- Conservation of the Environment (Environmental Science)
- Environmental Sustainability
- Environmentalist Thought and Ideology (Environmental Science)
- Management of Land and Natural Resources (Environmental Science)
- Natural Disasters (Environmental Science)
- Nuclear Issues (Environmental Science)
- Pollution and Threats to the Environment (Environmental Science)
- Social Impact of Environmental Issues (Environmental Science)
- History of Science and Technology
- Browse content in Materials Science
- Ceramics and Glasses
- Composite Materials
- Metals, Alloying, and Corrosion
- Nanotechnology
- Browse content in Mathematics
- Applied Mathematics
- Biomathematics and Statistics
- History of Mathematics
- Mathematical Education
- Mathematical Finance
- Mathematical Analysis
- Numerical and Computational Mathematics
- Probability and Statistics
- Pure Mathematics
- Browse content in Neuroscience
- Cognition and Behavioural Neuroscience
- Development of the Nervous System
- Disorders of the Nervous System
- History of Neuroscience
- Invertebrate Neurobiology
- Molecular and Cellular Systems
- Neuroendocrinology and Autonomic Nervous System
- Neuroscientific Techniques
- Sensory and Motor Systems
- Browse content in Physics
- Astronomy and Astrophysics
- Atomic, Molecular, and Optical Physics
- Biological and Medical Physics
- Classical Mechanics
- Computational Physics
- Condensed Matter Physics
- Electromagnetism, Optics, and Acoustics
- History of Physics
- Mathematical and Statistical Physics
- Measurement Science
- Nuclear Physics
- Particles and Fields
- Plasma Physics
- Quantum Physics
- Relativity and Gravitation
- Semiconductor and Mesoscopic Physics
- Browse content in Psychology
- Affective Sciences
- Clinical Psychology
- Cognitive Psychology
- Cognitive Neuroscience
- Criminal and Forensic Psychology
- Developmental Psychology
- Educational Psychology
- Evolutionary Psychology
- Health Psychology
- History and Systems in Psychology
- Music Psychology
- Neuropsychology
- Organizational Psychology
- Psychological Assessment and Testing
- Psychology of Human-Technology Interaction
- Psychology Professional Development and Training
- Research Methods in Psychology
- Social Psychology
- Browse content in Social Sciences
- Browse content in Anthropology
- Anthropology of Religion
- Human Evolution
- Medical Anthropology
- Physical Anthropology
- Regional Anthropology
- Social and Cultural Anthropology
- Theory and Practice of Anthropology
- Browse content in Business and Management
- Business Ethics
- Business Strategy
- Business History
- Business and Technology
- Business and Government
- Business and the Environment
- Comparative Management
- Corporate Governance
- Corporate Social Responsibility
- Entrepreneurship
- Health Management
- Human Resource Management
- Industrial and Employment Relations
- Industry Studies
- Information and Communication Technologies
- International Business
- Knowledge Management
- Management and Management Techniques
- Operations Management
- Organizational Theory and Behaviour
- Pensions and Pension Management
- Public and Nonprofit Management
- Strategic Management
- Supply Chain Management
- Browse content in Criminology and Criminal Justice
- Criminal Justice
- Criminology
- Forms of Crime
- International and Comparative Criminology
- Youth Violence and Juvenile Justice
- Development Studies
- Browse content in Economics
- Agricultural, Environmental, and Natural Resource Economics
- Asian Economics
- Behavioural Finance
- Behavioural Economics and Neuroeconomics
- Econometrics and Mathematical Economics
- Economic History
- Economic Systems
- Economic Methodology
- Economic Development and Growth
- Financial Markets
- Financial Institutions and Services
- General Economics and Teaching
- Health, Education, and Welfare
- History of Economic Thought
- International Economics
- Labour and Demographic Economics
- Law and Economics
- Macroeconomics and Monetary Economics
- Microeconomics
- Public Economics
- Urban, Rural, and Regional Economics
- Welfare Economics
- Browse content in Education
- Adult Education and Continuous Learning
- Care and Counselling of Students
- Early Childhood and Elementary Education
- Educational Equipment and Technology
- Educational Strategies and Policy
- Higher and Further Education
- Organization and Management of Education
- Philosophy and Theory of Education
- Schools Studies
- Secondary Education
- Teaching of a Specific Subject
- Teaching of Specific Groups and Special Educational Needs
- Teaching Skills and Techniques
- Browse content in Environment
- Applied Ecology (Social Science)
- Climate Change
- Conservation of the Environment (Social Science)
- Environmentalist Thought and Ideology (Social Science)
- Natural Disasters (Environment)
- Social Impact of Environmental Issues (Social Science)
- Browse content in Human Geography
- Cultural Geography
- Economic Geography
- Political Geography
- Browse content in Interdisciplinary Studies
- Communication Studies
- Museums, Libraries, and Information Sciences
- Browse content in Politics
- African Politics
- Asian Politics
- Chinese Politics
- Comparative Politics
- Conflict Politics
- Elections and Electoral Studies
- Environmental Politics
- European Union
- Foreign Policy
- Gender and Politics
- Human Rights and Politics
- Indian Politics
- International Relations
- International Organization (Politics)
- International Political Economy
- Irish Politics
- Latin American Politics
- Middle Eastern Politics
- Political Behaviour
- Political Economy
- Political Institutions
- Political Methodology
- Political Communication
- Political Philosophy
- Political Sociology
- Political Theory
- Politics and Law
- Politics of Development
- Public Policy
- Public Administration
- Quantitative Political Methodology
- Regional Political Studies
- Russian Politics
- Security Studies
- State and Local Government
- UK Politics
- US Politics
- Browse content in Regional and Area Studies
- African Studies
- Asian Studies
- East Asian Studies
- Japanese Studies
- Latin American Studies
- Middle Eastern Studies
- Native American Studies
- Scottish Studies
- Browse content in Research and Information
- Research Methods
- Browse content in Social Work
- Addictions and Substance Misuse
- Adoption and Fostering
- Care of the Elderly
- Child and Adolescent Social Work
- Couple and Family Social Work
- Developmental and Physical Disabilities Social Work
- Direct Practice and Clinical Social Work
- Emergency Services
- Human Behaviour and the Social Environment
- International and Global Issues in Social Work
- Mental and Behavioural Health
- Social Justice and Human Rights
- Social Policy and Advocacy
- Social Work and Crime and Justice
- Social Work Macro Practice
- Social Work Practice Settings
- Social Work Research and Evidence-based Practice
- Welfare and Benefit Systems
- Browse content in Sociology
- Childhood Studies
- Community Development
- Comparative and Historical Sociology
- Economic Sociology
- Gender and Sexuality
- Gerontology and Ageing
- Health, Illness, and Medicine
- Marriage and the Family
- Migration Studies
- Occupations, Professions, and Work
- Organizations
- Population and Demography
- Race and Ethnicity
- Social Theory
- Social Movements and Social Change
- Social Research and Statistics
- Social Stratification, Inequality, and Mobility
- Sociology of Religion
- Sociology of Education
- Sport and Leisure
- Urban and Rural Studies
- Browse content in Warfare and Defence
- Defence Strategy, Planning, and Research
- Land Forces and Warfare
- Military Administration
- Military Life and Institutions
- Naval Forces and Warfare
- Other Warfare and Defence Issues
- Peace Studies and Conflict Resolution
- Weapons and Equipment

- < Previous chapter
- Next chapter >
6 Statistical Conclusion Validity
- Published: May 2017
- Cite Icon Cite
- Permissions Icon Permissions
Chapter 6 addresses the sub-category of internal validity defined by Shadish et al., as statistical conclusion validity, or “validity of inferences about the correlation (covariance) between treatment and outcome.” The common threats to statistical conclusion validity can arise, or become plausible through either model misspecification or through hypothesis testing. The risk of a serious model misspecification is inversely proportional to the length of the time series, for example, and so is the risk of mistating the Type I and Type II error rates. Threats to statistical conclusion validity arise from the classical and modern hybrid significance testing structures, the serious threats that weigh heavily in p-value tests are shown to be undefined in Beyesian tests. While the particularly vexing threats raised by modern null hypothesis testing are resolved through the elimination of the modern null hypothesis test, threats to statistical conclusion validity would inevitably persist and new threats would arise.
Signed in as
Institutional accounts.
- GoogleCrawler [DO NOT DELETE]
- Google Scholar Indexing
Personal account
- Sign in with email/username & password
- Get email alerts
- Save searches
- Purchase content
- Activate your purchase/trial code
- Add your ORCID iD
Institutional access
Sign in with a library card.
- Sign in with username/password
- Recommend to your librarian
- Institutional account management
- Get help with access
Access to content on Oxford Academic is often provided through institutional subscriptions and purchases. If you are a member of an institution with an active account, you may be able to access content in one of the following ways:
IP based access
Typically, access is provided across an institutional network to a range of IP addresses. This authentication occurs automatically, and it is not possible to sign out of an IP authenticated account.
Sign in through your institution
Choose this option to get remote access when outside your institution. Shibboleth/Open Athens technology is used to provide single sign-on between your institution’s website and Oxford Academic.
- Click Sign in through your institution.
- Select your institution from the list provided, which will take you to your institution's website to sign in.
- When on the institution site, please use the credentials provided by your institution. Do not use an Oxford Academic personal account.
- Following successful sign in, you will be returned to Oxford Academic.
If your institution is not listed or you cannot sign in to your institution’s website, please contact your librarian or administrator.
Enter your library card number to sign in. If you cannot sign in, please contact your librarian.
Society Members
Society member access to a journal is achieved in one of the following ways:
Sign in through society site
Many societies offer single sign-on between the society website and Oxford Academic. If you see ‘Sign in through society site’ in the sign in pane within a journal:
- Click Sign in through society site.
- When on the society site, please use the credentials provided by that society. Do not use an Oxford Academic personal account.
If you do not have a society account or have forgotten your username or password, please contact your society.
Sign in using a personal account
Some societies use Oxford Academic personal accounts to provide access to their members. See below.
A personal account can be used to get email alerts, save searches, purchase content, and activate subscriptions.
Some societies use Oxford Academic personal accounts to provide access to their members.
Viewing your signed in accounts
Click the account icon in the top right to:
- View your signed in personal account and access account management features.
- View the institutional accounts that are providing access.
Signed in but can't access content
Oxford Academic is home to a wide variety of products. The institutional subscription may not cover the content that you are trying to access. If you believe you should have access to that content, please contact your librarian.
For librarians and administrators, your personal account also provides access to institutional account management. Here you will find options to view and activate subscriptions, manage institutional settings and access options, access usage statistics, and more.
Our books are available by subscription or purchase to libraries and institutions.
- About Oxford Academic
- Publish journals with us
- University press partners
- What we publish
- New features
- Open access
- Rights and permissions
- Accessibility
- Advertising
- Media enquiries
- Oxford University Press
- Oxford Languages
- University of Oxford
Oxford University Press is a department of the University of Oxford. It furthers the University's objective of excellence in research, scholarship, and education by publishing worldwide
- Copyright © 2024 Oxford University Press
- Cookie settings
- Cookie policy
- Privacy policy
- Legal notice
This Feature Is Available To Subscribers Only
Sign In or Create an Account
This PDF is available to Subscribers Only
For full access to this pdf, sign in to an existing account, or purchase an annual subscription.
Statistical conclusion validity: some common threats and simple remedies
Affiliation.
- 1 Facultad de Psicología, Departamento de Metodología, Universidad Complutense Madrid, Spain.
- PMID: 22952465
- PMCID: PMC3429930
- DOI: 10.3389/fpsyg.2012.00325
The ultimate goal of research is to produce dependable knowledge or to provide the evidence that may guide practical decisions. Statistical conclusion validity (SCV) holds when the conclusions of a research study are founded on an adequate analysis of the data, generally meaning that adequate statistical methods are used whose small-sample behavior is accurate, besides being logically capable of providing an answer to the research question. Compared to the three other traditional aspects of research validity (external validity, internal validity, and construct validity), interest in SCV has recently grown on evidence that inadequate data analyses are sometimes carried out which yield conclusions that a proper analysis of the data would not have supported. This paper discusses evidence of three common threats to SCV that arise from widespread recommendations or practices in data analysis, namely, the use of repeated testing and optional stopping without control of Type-I error rates, the recommendation to check the assumptions of statistical tests, and the use of regression whenever a bivariate relation or the equivalence between two variables is studied. For each of these threats, examples are presented and alternative practices that safeguard SCV are discussed. Educational and editorial changes that may improve the SCV of published research are also discussed.
Keywords: data analysis; preliminary tests; regression; stopping rules; validity of research.

An official website of the United States government
The .gov means it’s official. Federal government websites often end in .gov or .mil. Before sharing sensitive information, make sure you’re on a federal government site.
The site is secure. The https:// ensures that you are connecting to the official website and that any information you provide is encrypted and transmitted securely.
- Publications
- Account settings
Preview improvements coming to the PMC website in October 2024. Learn More or Try it out now .
- Advanced Search
- Journal List
- J Family Med Prim Care
- v.4(3); Jul-Sep 2015
Validity, reliability, and generalizability in qualitative research
Lawrence leung.
1 Department of Family Medicine, Queen's University, Kingston, Ontario, Canada
2 Centre of Studies in Primary Care, Queen's University, Kingston, Ontario, Canada
In general practice, qualitative research contributes as significantly as quantitative research, in particular regarding psycho-social aspects of patient-care, health services provision, policy setting, and health administrations. In contrast to quantitative research, qualitative research as a whole has been constantly critiqued, if not disparaged, by the lack of consensus for assessing its quality and robustness. This article illustrates with five published studies how qualitative research can impact and reshape the discipline of primary care, spiraling out from clinic-based health screening to community-based disease monitoring, evaluation of out-of-hours triage services to provincial psychiatric care pathways model and finally, national legislation of core measures for children's healthcare insurance. Fundamental concepts of validity, reliability, and generalizability as applicable to qualitative research are then addressed with an update on the current views and controversies.
Nature of Qualitative Research versus Quantitative Research
The essence of qualitative research is to make sense of and recognize patterns among words in order to build up a meaningful picture without compromising its richness and dimensionality. Like quantitative research, the qualitative research aims to seek answers for questions of “how, where, when who and why” with a perspective to build a theory or refute an existing theory. Unlike quantitative research which deals primarily with numerical data and their statistical interpretations under a reductionist, logical and strictly objective paradigm, qualitative research handles nonnumerical information and their phenomenological interpretation, which inextricably tie in with human senses and subjectivity. While human emotions and perspectives from both subjects and researchers are considered undesirable biases confounding results in quantitative research, the same elements are considered essential and inevitable, if not treasurable, in qualitative research as they invariable add extra dimensions and colors to enrich the corpus of findings. However, the issue of subjectivity and contextual ramifications has fueled incessant controversies regarding yardsticks for quality and trustworthiness of qualitative research results for healthcare.
Impact of Qualitative Research upon Primary Care
In many ways, qualitative research contributes significantly, if not more so than quantitative research, to the field of primary care at various levels. Five qualitative studies are chosen to illustrate how various methodologies of qualitative research helped in advancing primary healthcare, from novel monitoring of chronic obstructive pulmonary disease (COPD) via mobile-health technology,[ 1 ] informed decision for colorectal cancer screening,[ 2 ] triaging out-of-hours GP services,[ 3 ] evaluating care pathways for community psychiatry[ 4 ] and finally prioritization of healthcare initiatives for legislation purposes at national levels.[ 5 ] With the recent advances of information technology and mobile connecting device, self-monitoring and management of chronic diseases via tele-health technology may seem beneficial to both the patient and healthcare provider. Recruiting COPD patients who were given tele-health devices that monitored lung functions, Williams et al. [ 1 ] conducted phone interviews and analyzed their transcripts via a grounded theory approach, identified themes which enabled them to conclude that such mobile-health setup and application helped to engage patients with better adherence to treatment and overall improvement in mood. Such positive findings were in contrast to previous studies, which opined that elderly patients were often challenged by operating computer tablets,[ 6 ] or, conversing with the tele-health software.[ 7 ] To explore the content of recommendations for colorectal cancer screening given out by family physicians, Wackerbarth, et al. [ 2 ] conducted semi-structure interviews with subsequent content analysis and found that most physicians delivered information to enrich patient knowledge with little regard to patients’ true understanding, ideas, and preferences in the matter. These findings suggested room for improvement for family physicians to better engage their patients in recommending preventative care. Faced with various models of out-of-hours triage services for GP consultations, Egbunike et al. [ 3 ] conducted thematic analysis on semi-structured telephone interviews with patients and doctors in various urban, rural and mixed settings. They found that the efficiency of triage services remained a prime concern from both users and providers, among issues of access to doctors and unfulfilled/mismatched expectations from users, which could arouse dissatisfaction and legal implications. In UK, a care pathways model for community psychiatry had been introduced but its benefits were unclear. Khandaker et al. [ 4 ] hence conducted a qualitative study using semi-structure interviews with medical staff and other stakeholders; adopting a grounded-theory approach, major themes emerged which included improved equality of access, more focused logistics, increased work throughput and better accountability for community psychiatry provided under the care pathway model. Finally, at the US national level, Mangione-Smith et al. [ 5 ] employed a modified Delphi method to gather consensus from a panel of nominators which were recognized experts and stakeholders in their disciplines, and identified a core set of quality measures for children's healthcare under the Medicaid and Children's Health Insurance Program. These core measures were made transparent for public opinion and later passed on for full legislation, hence illustrating the impact of qualitative research upon social welfare and policy improvement.
Overall Criteria for Quality in Qualitative Research
Given the diverse genera and forms of qualitative research, there is no consensus for assessing any piece of qualitative research work. Various approaches have been suggested, the two leading schools of thoughts being the school of Dixon-Woods et al. [ 8 ] which emphasizes on methodology, and that of Lincoln et al. [ 9 ] which stresses the rigor of interpretation of results. By identifying commonalities of qualitative research, Dixon-Woods produced a checklist of questions for assessing clarity and appropriateness of the research question; the description and appropriateness for sampling, data collection and data analysis; levels of support and evidence for claims; coherence between data, interpretation and conclusions, and finally level of contribution of the paper. These criteria foster the 10 questions for the Critical Appraisal Skills Program checklist for qualitative studies.[ 10 ] However, these methodology-weighted criteria may not do justice to qualitative studies that differ in epistemological and philosophical paradigms,[ 11 , 12 ] one classic example will be positivistic versus interpretivistic.[ 13 ] Equally, without a robust methodological layout, rigorous interpretation of results advocated by Lincoln et al. [ 9 ] will not be good either. Meyrick[ 14 ] argued from a different angle and proposed fulfillment of the dual core criteria of “transparency” and “systematicity” for good quality qualitative research. In brief, every step of the research logistics (from theory formation, design of study, sampling, data acquisition and analysis to results and conclusions) has to be validated if it is transparent or systematic enough. In this manner, both the research process and results can be assured of high rigor and robustness.[ 14 ] Finally, Kitto et al. [ 15 ] epitomized six criteria for assessing overall quality of qualitative research: (i) Clarification and justification, (ii) procedural rigor, (iii) sample representativeness, (iv) interpretative rigor, (v) reflexive and evaluative rigor and (vi) transferability/generalizability, which also double as evaluative landmarks for manuscript review to the Medical Journal of Australia. Same for quantitative research, quality for qualitative research can be assessed in terms of validity, reliability, and generalizability.
Validity in qualitative research means “appropriateness” of the tools, processes, and data. Whether the research question is valid for the desired outcome, the choice of methodology is appropriate for answering the research question, the design is valid for the methodology, the sampling and data analysis is appropriate, and finally the results and conclusions are valid for the sample and context. In assessing validity of qualitative research, the challenge can start from the ontology and epistemology of the issue being studied, e.g. the concept of “individual” is seen differently between humanistic and positive psychologists due to differing philosophical perspectives:[ 16 ] Where humanistic psychologists believe “individual” is a product of existential awareness and social interaction, positive psychologists think the “individual” exists side-by-side with formation of any human being. Set off in different pathways, qualitative research regarding the individual's wellbeing will be concluded with varying validity. Choice of methodology must enable detection of findings/phenomena in the appropriate context for it to be valid, with due regard to culturally and contextually variable. For sampling, procedures and methods must be appropriate for the research paradigm and be distinctive between systematic,[ 17 ] purposeful[ 18 ] or theoretical (adaptive) sampling[ 19 , 20 ] where the systematic sampling has no a priori theory, purposeful sampling often has a certain aim or framework and theoretical sampling is molded by the ongoing process of data collection and theory in evolution. For data extraction and analysis, several methods were adopted to enhance validity, including 1 st tier triangulation (of researchers) and 2 nd tier triangulation (of resources and theories),[ 17 , 21 ] well-documented audit trail of materials and processes,[ 22 , 23 , 24 ] multidimensional analysis as concept- or case-orientated[ 25 , 26 ] and respondent verification.[ 21 , 27 ]
Reliability
In quantitative research, reliability refers to exact replicability of the processes and the results. In qualitative research with diverse paradigms, such definition of reliability is challenging and epistemologically counter-intuitive. Hence, the essence of reliability for qualitative research lies with consistency.[ 24 , 28 ] A margin of variability for results is tolerated in qualitative research provided the methodology and epistemological logistics consistently yield data that are ontologically similar but may differ in richness and ambience within similar dimensions. Silverman[ 29 ] proposed five approaches in enhancing the reliability of process and results: Refutational analysis, constant data comparison, comprehensive data use, inclusive of the deviant case and use of tables. As data were extracted from the original sources, researchers must verify their accuracy in terms of form and context with constant comparison,[ 27 ] either alone or with peers (a form of triangulation).[ 30 ] The scope and analysis of data included should be as comprehensive and inclusive with reference to quantitative aspects if possible.[ 30 ] Adopting the Popperian dictum of falsifiability as essence of truth and science, attempted to refute the qualitative data and analytes should be performed to assess reliability.[ 31 ]
Generalizability
Most qualitative research studies, if not all, are meant to study a specific issue or phenomenon in a certain population or ethnic group, of a focused locality in a particular context, hence generalizability of qualitative research findings is usually not an expected attribute. However, with rising trend of knowledge synthesis from qualitative research via meta-synthesis, meta-narrative or meta-ethnography, evaluation of generalizability becomes pertinent. A pragmatic approach to assessing generalizability for qualitative studies is to adopt same criteria for validity: That is, use of systematic sampling, triangulation and constant comparison, proper audit and documentation, and multi-dimensional theory.[ 17 ] However, some researchers espouse the approach of analytical generalization[ 32 ] where one judges the extent to which the findings in one study can be generalized to another under similar theoretical, and the proximal similarity model, where generalizability of one study to another is judged by similarities between the time, place, people and other social contexts.[ 33 ] Thus said, Zimmer[ 34 ] questioned the suitability of meta-synthesis in view of the basic tenets of grounded theory,[ 35 ] phenomenology[ 36 ] and ethnography.[ 37 ] He concluded that any valid meta-synthesis must retain the other two goals of theory development and higher-level abstraction while in search of generalizability, and must be executed as a third level interpretation using Gadamer's concepts of the hermeneutic circle,[ 38 , 39 ] dialogic process[ 38 ] and fusion of horizons.[ 39 ] Finally, Toye et al. [ 40 ] reported the practicality of using “conceptual clarity” and “interpretative rigor” as intuitive criteria for assessing quality in meta-ethnography, which somehow echoed Rolfe's controversial aesthetic theory of research reports.[ 41 ]
Food for Thought
Despite various measures to enhance or ensure quality of qualitative studies, some researchers opined from a purist ontological and epistemological angle that qualitative research is not a unified, but ipso facto diverse field,[ 8 ] hence any attempt to synthesize or appraise different studies under one system is impossible and conceptually wrong. Barbour argued from a philosophical angle that these special measures or “technical fixes” (like purposive sampling, multiple-coding, triangulation, and respondent validation) can never confer the rigor as conceived.[ 11 ] In extremis, Rolfe et al. opined from the field of nursing research, that any set of formal criteria used to judge the quality of qualitative research are futile and without validity, and suggested that any qualitative report should be judged by the form it is written (aesthetic) and not by the contents (epistemic).[ 41 ] Rolfe's novel view is rebutted by Porter,[ 42 ] who argued via logical premises that two of Rolfe's fundamental statements were flawed: (i) “The content of research report is determined by their forms” may not be a fact, and (ii) that research appraisal being “subject to individual judgment based on insight and experience” will mean those without sufficient experience of performing research will be unable to judge adequately – hence an elitist's principle. From a realism standpoint, Porter then proposes multiple and open approaches for validity in qualitative research that incorporate parallel perspectives[ 43 , 44 ] and diversification of meanings.[ 44 ] Any work of qualitative research, when read by the readers, is always a two-way interactive process, such that validity and quality has to be judged by the receiving end too and not by the researcher end alone.
In summary, the three gold criteria of validity, reliability and generalizability apply in principle to assess quality for both quantitative and qualitative research, what differs will be the nature and type of processes that ontologically and epistemologically distinguish between the two.
Source of Support: Nil.
Conflict of Interest: None declared.
Understanding External Validity and its Role in Research Design
This essay discusses external validity in scientific research and its significance in ensuring that study findings are applicable beyond specific experimental conditions. It examines how external validity helps researchers determine whether results can be generalized to different populations, settings, and contexts. The essay highlights challenges in achieving high external validity due to the specificity of research environments, participant characteristics, and timing. It also explains strategies like replication studies and field experiments that help increase the generalizability of results. The essay emphasizes the importance of balancing internal and external validity, acknowledging that while highly controlled studies can ensure internal accuracy, they might not reflect real-world conditions. Ultimately, external validity is crucial for producing research that informs effective policies, clinical guidelines, and societal changes.
How it works
Within the domain of scientific inquiry, the concept of external validity assumes paramount importance, serving as a linchpin for ensuring the relevance and broad applicability of research findings beyond the confines of a singular study. Put succinctly, external validity scrutinizes the extent to which the outcomes of a given investigation can be extrapolated to diverse populations, environments, temporal epochs, or contextual milieus. This conceptual framework assumes significance by bridging the chasm between meticulously controlled research settings and the kaleidoscopic intricacies of real-world scenarios, thereby furnishing researchers with the assurance that their conclusions possess trans-situational validity.
External validity assumes a pivotal role in buttressing the overall veracity of scientific inquiry, furnishing scientists with the means to discern whether their revelations hold substantive import and utility for wider swathes of humanity. Nonetheless, attaining elevated levels of external validity often presents an arduous undertaking owing to the idiosyncratic nature of experimental methodologies. Numerous investigations are conducted within controlled environs or with highly circumscribed samples to mitigate the influence of confounding variables, thereby complicating the process of extrapolating findings to disparate cohorts. For instance, a psychological study confined to the precincts of university campuses might furnish insights germane solely to that demographic stratum, precluding facile generalization to alternative age cohorts or cultural constellations.
Pivotal determinants impinging upon external validity encompass the demographic attributes of study participants, the ambient ambiance of experimental locales, and the temporal dynamics of data accrual. Researchers routinely resort to random sampling methodologies to obviate selection biases and assemble a heterogeneous cohort reflective of the broader populace. The ambient setting assumes salience as outcomes gleaned within sterile laboratory settings might not seamlessly transmute into real-world vicinities. Furthermore, external validity can be influenced by the temporal dimension of investigations, particularly when grappling with phenomena susceptible to temporal vicissitudes induced by cultural, economic, or technological flux.
Researchers endeavor to fortify external validity through recourse to replication studies, which seek to reproduce experimental paradigms under varying conditions to corroborate the robustness of findings. Engaging in multi-site investigations spanning disparate geographical realms or demographic constituencies also serves to validate the generalizability of findings across multifarious locales and participant profiles. Augmenting the verisimilitude of study designs by approximating real-world conditions more faithfully or deploying field experiments conducted within natural habitats constitutes an additional stratagem employed to enhance external validity.
Nonetheless, a judicious equilibrium between internal and external validity is indispensable. While internal validity safeguards against spurious attributions of causality by delineating the genuine effects attributable to independent variables as opposed to extraneous factors, an undue emphasis on this facet may inadvertently compromise external validity. For instance, a rigorously controlled laboratory investigation might succeed in eradicating most confounding variables but might concurrently engender an artificial milieu divorced from real-world veracity.
On occasion, external validity is consciously sacrificed to scrutinize a phenomenon within a specific subgroup or contextual milieu. Clinical trials, for instance, might pivot towards patients exhibiting highly circumscribed medical afflictions or demographic profiles. While such findings might lack broad generalizability, they furnish invaluable insights germane to the target demographic.
In summation, the salience of external validity cannot be overstated. Research endeavors endeavoring to inform policy formulation, clinical dictums, or societal transformations necessitate a degree of generalizability to obviate the formulation of recommendations that are ineffectual or even deleterious. Researchers ought to meticulously orchestrate their investigations, cognizant of the demographic cohorts and environmental terrains that their findings aspire to impact. By acknowledging and redressing the vicissitudes of external validity, scientists can engender more resilient and universally applicable research findings that withstand the rigors of real-world complexity.

Cite this page
Understanding External Validity and Its Role in Research Design. (2024, May 21). Retrieved from https://papersowl.com/examples/understanding-external-validity-and-its-role-in-research-design/
"Understanding External Validity and Its Role in Research Design." PapersOwl.com , 21 May 2024, https://papersowl.com/examples/understanding-external-validity-and-its-role-in-research-design/
PapersOwl.com. (2024). Understanding External Validity and Its Role in Research Design . [Online]. Available at: https://papersowl.com/examples/understanding-external-validity-and-its-role-in-research-design/ [Accessed: 22 May. 2024]
"Understanding External Validity and Its Role in Research Design." PapersOwl.com, May 21, 2024. Accessed May 22, 2024. https://papersowl.com/examples/understanding-external-validity-and-its-role-in-research-design/
"Understanding External Validity and Its Role in Research Design," PapersOwl.com , 21-May-2024. [Online]. Available: https://papersowl.com/examples/understanding-external-validity-and-its-role-in-research-design/. [Accessed: 22-May-2024]
PapersOwl.com. (2024). Understanding External Validity and Its Role in Research Design . [Online]. Available at: https://papersowl.com/examples/understanding-external-validity-and-its-role-in-research-design/ [Accessed: 22-May-2024]
Don't let plagiarism ruin your grade
Hire a writer to get a unique paper crafted to your needs.
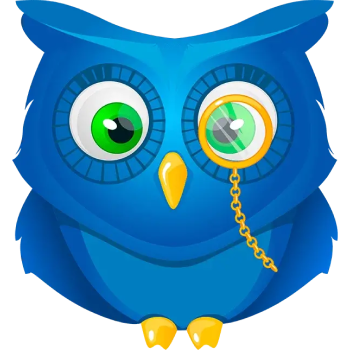
Our writers will help you fix any mistakes and get an A+!
Please check your inbox.
You can order an original essay written according to your instructions.
Trusted by over 1 million students worldwide
1. Tell Us Your Requirements
2. Pick your perfect writer
3. Get Your Paper and Pay
Hi! I'm Amy, your personal assistant!
Don't know where to start? Give me your paper requirements and I connect you to an academic expert.
short deadlines
100% Plagiarism-Free
Certified writers
- Open access
- Published: 18 May 2024
Psychometric properties and criterion related validity of the Norwegian version of hospital survey on patient safety culture 2.0
- Espen Olsen 1 ,
- Seth Ayisi Junior Addo 1 ,
- Susanne Sørensen Hernes 2 , 3 ,
- Marit Halonen Christiansen 4 ,
- Arvid Steinar Haugen 5 , 6 &
- Ann-Chatrin Linqvist Leonardsen 7 , 8
BMC Health Services Research volume 24 , Article number: 642 ( 2024 ) Cite this article
97 Accesses
1 Altmetric
Metrics details
Several studies have been conducted with the 1.0 version of the Hospital Survey on Patient Safety Culture (HSOPSC) in Norway and globally. The 2.0 version has not been translated and tested in Norwegian hospital settings. This study aims to 1) assess the psychometrics of the Norwegian version (N-HSOPSC 2.0), and 2) assess the criterion validity of the N-HSOPSC 2.0, adding two more outcomes, namely ‘pleasure of work’ and ‘turnover intention’.
The HSOPSC 2.0 was translated using a sequential translation process. A convenience sample was used, inviting hospital staff from two hospitals ( N = 1002) to participate in a cross-sectional questionnaire study. Data were analyzed using Mplus. The construct validity was tested with confirmatory factor analysis (CFA). Convergent validity was tested using Average Variance Explained (AVE), and internal consistency was tested with composite reliability (CR) and Cronbach’s alpha. Criterion related validity was tested with multiple linear regression.
The overall statistical results using the N-HSOPSC 2.0 indicate that the model fit based on CFA was acceptable. Five of the N-HSOPSC 2.0 dimensions had AVE scores below the 0.5 criterium. The CR criterium was meet on all dimensions except Teamwork (0.61). However, Teamwork was one of the most important and significant predictors of the outcomes. Regression models explained most variance related to patient safety rating (adjusted R 2 = 0.38), followed by ‘turnover intention’ (adjusted R 2 = 0.22), ‘pleasure at work’ (adjusted R 2 = 0.14), and lastly, ‘number of reported events’ (adjusted R 2= 0.06).
The N-HSOPSC 2.0 had acceptable construct validity and internal consistency when translated to Norwegian and tested among Norwegian staff in two hospitals. Hence, the instrument is appropriate for use in Norwegian hospital settings. The ten dimensions predicted most variance related to ‘overall patient safety’, and less related to ‘number of reported events’. In addition, the safety culture dimensions predicted ‘pleasure at work’ and ‘turnover intention’, which is not part of the original instrument.
Peer Review reports
Patient harm due to unsafe care is a large and persistent global public health challenge and one of the leading causes of death and disability worldwide [ 1 ]. Improving safety in healthcare is central in governmental policies, though progress in delivering this has been modest [ 2 ]. Patient safety culture surveys have been the most frequently used approach to measure and monitor perception of safety culture [ 3 ]. Safety culture is defined as “the product of individual and group values, attitudes, perceptions, competencies and patterns of behavior that determine the commitment to, and the style and proficiency of, an organization’s health and safety management” [ 4 ]. Moreover, safety culture refers to the perceptions, beliefs, values, attitudes, and competencies within an organization pertaining to safety and prevention of harm [ 5 ]. The importance of measuring patient safety culture was underlined by the results in a 2023 scoping review, where 76 percent of the included studies observed associations between improved safety culture and reduction of adverse events [ 6 ].
To assess patient safety culture in hospitals the US Agency for Healthcare Research and Quality (AHRQ) launched the Hospital Survey on Patient Safety Culture (HSOPSC) version 1.0 in 2004 [ 7 , 8 ]. Since then, HSOPSC 1.0 has become one of the most used tools to evaluate patient safety culture in hospitals, administered to approximately hundred countries and translated into 43 languages as of September 2022 [ 9 ]. HSOPSC 1.0 has generally been considered to be one of the most robust instrument measuring patient safety culture, and it has adequate psychometric properties [ 10 ]. In Norway, the first studies using N-HSOPSC 1.0 concluded that the psychometric properties of the instrument were satisfactory for use in Norwegian hospital settings [ 11 , 12 , 13 ]. A recent review of literature revealed 20 research articles using the N-HSOPSC 1.0 [ 14 ].
Studies of safety culture perceptions in hospitals require valid and psychometric sound instruments [ 12 , 13 , 15 ]. First, an accurate questionnaire structure should demonstrate a match between the theorized content structure and the actual content structure [ 16 , 17 ]. Second, psychometric properties of instruments developed in one context is required to demonstrate appropriateness in other cultures and settings [ 16 , 17 ]. Further, psychometric concepts need to demonstrate relationships with other related and valid criteria. For example, data on criterion validity can be compared with criteria data collected at the same time (concurrent validity) or with similar data from a later time point (predictive validity) [ 12 , 16 , 17 ]. Finally, researchers need to demonstrate a match between the content theorized to be related to the actual content in empirical data [ 15 ]. If these psychometric areas are not taken seriously, this may lead to many pitfalls both for researchers and practitioners [ 14 ]. Pitfalls might be imprecise diagnostics of the patient safety level and failure to evaluate effect of improvement initiative. Moreover, researchers can easily erroneously confirm or reject research hypothesis when applying invalid and inaccurate measurement tools.
Patient safety cannot be understood as an isolated phenomenon, but is influenced by general job characteristics and the well-being of the individual health care workers. Karsh et al. [ 18 ] found that positive staff perceptions of their work environment and low work pressure were significantly related to greater job satisfaction and work commitment. A direct association has also been reported between turnover and work strain, burnout and stress [ 19 ] Zarei et al. [ 20 ] showed a significant relationship between patient safety (safety climate) and unit type, job satisfaction, job interest, and stress in hospitals. This study also illustrated a strong relationship between lack of personal accomplishment, job satisfaction, job interest and stress. Also, there was a negative correlation between occupational burnout and safety climate, where a decrease in the latter was associated with an increase in the former. Hence, patient safety researchers should look at healthcare job characteristics in combination with patient safety culture.
Recently, the AHRQ revised the HSOPSC 1.0 to a 2.0 version, to improve the quality and relevance of the instrument. HSOPSC 2.0 is shorter, with 25 items removed or with changes made for response options and ten additional items added. HSOPSC 2.0 was validated during the revision process [ 21 ], but the psychometric qualities across cultures, countries and in different settings need further investigation. Consequently, the overall aim of this study was to investigate the psychometric properties of the HSOPSC 2.0 [ 21 ] (see supplement 1) in a Norwegian hospital setting. Specifically, the aims were to 1) assess the psychometrics of the Norwegian version (N-HSOPSC 2.0), and 2) assess the criterion validity of the N-HSOPSC 2.0, adding two more outcomes, namely’ pleasure of work’ and ‘turnover intention’.
This study had cross‐sectional design, using a web-based survey solution called “Nettskjema” to distribute questionnaires in two Norwegian hospitals. The study adheres to The Strengthening the Reporting of Observational Studies in Epidemiology (STROBE)Statement guidelines for reporting observational studies [ 22 ].
Translation of the HSOPSC 2.0
We conducted a «forward and backward» translation in-line with recommendations from Brislin [ 23 ]. First, the questionnaires were translated from English to Norwegian by a bilingual researcher. The Norwegian version was then translated back to English by another bilingual researcher. Thereafter, the semantic, idiopathic and conceptual equivalence between the two versions were compared by the research group, consisting of experienced researchers. The face value of the N-HSOPSC 2.0-version was considered to be adequate and the items lend themselves well to the corresponding latent concepts.
The N-HSOPSC 2.0 was pilot-tested with focus on content and face validity. Six randomly selected healthcare personnel were asked to assess whether the questionnaire was adequate, appropriate, and understandable regarding language, instructions, and scores. In addition, an expert group consisting of senior researchers ( n = 4) and healthcare personnel ( n = 6), with competence in patient safety culture was asked to assess the same.
The questionnaire
The HSOSPS 2.0 (supplement 1) consists of 32 items using 5-point Likert-like scales of agreement (from 1 = strongly disagree to 5 = strongly agree) or frequency (from 1 = never to 5 = always), as well as an option for “does not apply/do now know”. The 32 items are distributed over ten dimensions. Additionally, 2-single item patient safety culture outcome measures, and 6-item background information measures are included. The patient safety culture single item outcome measures evaluate the overall ‘patient safety rating’ for the work area, and ‘reporting patient safety events’.
In addition to the N-HSOPSC 2.0, participants were asked to respond to three questions about their ‘pleasure at work’ (measure if staff enjoy their work, and are pleased with their work, scored from 1 = never, to 4 = always) [ 24 ], two questions about their ‘intention to quit’ (measure is staff are considering to quit their job, scored on a 5-point likert scale where 1 = strongly agree to 5 = strongly disagree) [ 25 ], as well as demographic variables (gender, age, professional background, primary work area, years of work experience).
Participants and procedure
The data collection was conducted in two phases: the first phase (Nov-Dec 2021) at Hospital A and the second phase at Hospital B (Feb-March 2022)). We used a purposive sampling strategy: At Hospital A (two locations), all employees were invited to participate ( N = 6648). This included clinical staff, administrators, managers, and technical staff. At Hospital B (three locations) all employees from the anesthesiology, intensive care and operation wards were invited to participate ( N = 655).
The questionnaire was distributed by e-mail, including a link to a digital survey solution delivered by the University of Oslo, and gathered and stored on a safe research platform: TSD (services for sensitive data). This is a service with two-factor authentication, allowing data-sharing between the collaborating institutions without having to transfer data between them. The system allows for storage of indirectly identifying data, such as gender, age, profession and years of experience, as well as hospital. Reminders were sent out twice.
Statistical analyses
Data were analyzed using Mplus. Normality was assessed for each item using skewness and kurtosis, where values between + 2 and -2 are deemed acceptable for normal distribution [ 26 ]. Missing value analysis was conducted using frequencies, to check the percentage of missing responses for each item. Correlations were assessed using Spearman’s correlation analysis, reported as Cronbach’s alpha.
Confirmatory factor analysis (CFA) was conducted to test the ten-dimension structure of the N-HSOPSC 2.0 using Mplus and Mplus Microsoft Excel Macros. The structure was then tested for fitness using Comparative Fit Index (CFI), Tucker-Lewis Index (TLI), Root Mean Square Error of Approximation (RMSEA) and Standardized Root Mean Square Residual (SRMR) [ 27 ]. Table 1 shows the fitness indices and acceptable thresholds.
Reliability of the 10 predicting dimensions were also assessed using composite reliability (CR) values, where 0.7 or above is deemed acceptable for ascertaining internal consistency [ 25 ].
Convergent validity was assessed using the Average Variance Explained (AVE), where a value of at least 0.5 is deemed acceptable [ 28 ], indicating that at least 50 percent of the variance is explained by the items in a dimension. Criterion-related validity was tested using linear regression, adding ‘turnover intention’ and ‘pleasure at work’ to the two single item outcomes of the N-HSOPSC 2.0.
Internal consistency and reliability were assessed using Cronbach’s alpha, where values > 0.9 is assumed excellent, > 0.8 = good, > 0.7 = acceptable, > 0.6 = questionable, > 0.5 = poor and < 0.5 = unacceptable [ 29 ].
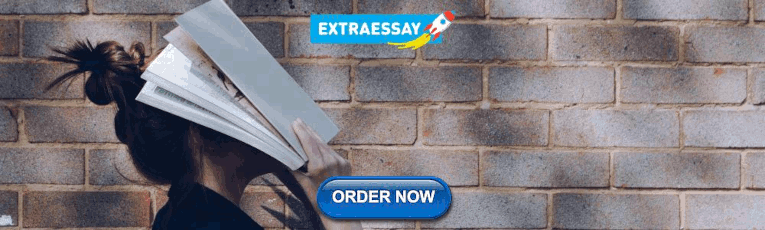
Ethical considerations
The study was conducted in-line with principles for ethical research in the Declaration of Helsinki, and informed consent was obtained from all the participants [ 30 ]. Completed and submitted questionnaires were assumed as consent to participate. Data privacy protection was reviewed by the respective hospitals’ data privacy authority, and assessed by the Norwegian Center for Research Data (NSD, project number 322965).
In total, 1002 participants responded to the questionnaire, representing a response rate of 12.6 percent. As seen in Table 2 , 83.7% of the respondents worked in Hospital A and the remaining 16.3% in Hospital B. The majority of respondents (75.7%) were female, and 75.9 percent of respondents worked directly with patients.
The skewness and kurtosis were between + 2 and -2, indicating that the data were normally distributed. All items had less than two percent of missing values, hence no methods for calculating missing values were used.
Correlations
Correlations and Cronbach’s alpha are displayed in Table 3 .
The following dimensions had the highest correlations; ‘teamwork’, ‘staffing and work pace’, ‘organizational learning-continuous improvement’, ‘response to error’, ‘supervisor support for patient safety’, ‘communication about error’ and ‘communication openness’. Only one dimension, ‘teamwork’ (0.58), had a Cronbach’s alpha below 0.7 (acceptable). Hence, most of the dimensions indicated adequate reliability. Higher levels of the 10 safety dimensions correlate positively with patient safety ratings.
Confirmatory Factor Analysis (CFA)
Table 4 shows the results from the CFA. CFA ( N = 1002) showed acceptable fitness values [CFI = 0.92, TLI = 0.90, RMSEA = 0.045, SRMR = 0.053] and factor loadings ranged from 0.51–0.89 (see Table 1 ). CR was above the 0.70 criterium on all dimensions except on ‘teamwork’ (0.61). AVE was above the 0.50 criterium except on ‘teamwork’ (0.35), ‘staffing and work pace’ (0.44), ‘organizational learning-continuous improvement’ (0.47), ‘response to error’ (0.47), and communication openness.
Criterion validity
Independent dimensions of HSOPSC 2.0 were employed to predict four different criteria: 1) ‘number of reported events’, 2) ‘patient safety rating’, 3) ‘pleasure at work’, and 4) ‘turnover intentions’. The composite measures explained variance of all the outcome variables significantly thereby ascertaining criterion-related validity (Table 5 ). Regression models explained most variance related to ‘patient safety rating’ (adjusted R 2 = 0.38), followed by ‘turnover intention’ (adjusted R 2 = 0.22), ‘pleasure at work’ (adjusted R 2 = 0.14), and lastly, number of reported events (adjusted R 2 = 0.06).
In this study we have investigated the psychometric properties of the N-HSOPSC 2.0. We found the face and content validity of the questionnaire satisfactory. Moreover, the overall statistical results indicate that the model fit based on CFA was acceptable. Five of the N-HSOPSC 2.0 dimensions had AVE scores below the 0.5 criterium, but we consider this to be the strictest criterium employed in the evaluations of the psychometric properties. The CR criterium was met on all dimensions except ‘teamwork’ (0.61). However, ‘teamwork’ was one of the most important and significant predictors of the outcomes. One the positive side, the CFA results supports the dimensional structure of N-HSOPSC 2.0, and the regression results indicate a satisfactory explanation of the outcomes. On the more critical side, particularly AVE scores reflect threshold below 0.5 on five dimensions, indicating items have certain levels of measurement error as well.
In our study, regression models explained most variance related to ‘patient safety rating’ (R 2 = 0.38), followed by ‘turnover intention’ (R 2 = 0. 22), ‘pleasure at work’ (R 2 = 0.14), and lastly, number of reported events (R 2 = 0.06). This supports the criterion validity of the independent dimensions of N-HSOSPC 2.0, also when adding ‘turnover intention’ and ‘pleasure at work’. These results confirm previous research on the original N-HSOPSC 1.0 [ 12 , 13 ]. The current study also found that ‘number of reported events’ was negatively related to safety culture dimensions, which is also similar to the N-HSOPSC 1.0 findings [ 12 , 13 ].
The current study did more psychometric assessments compared to the first Norwegian studies using HSOPSC 1.0 [ 11 , 12 , 13 ]. However, results from the current study still support that the overall reliability and validity of N-HSOPSC 2.0 when comparing the results with the first studies using N-HSOPSC 1.0 [ 11 , 12 , 13 ]. Also, based on theory and expectations, the dimensions predicted ‘pleasure at work’ and ‘overall safety rating’ positively, and ‘turnover intentions’ and ‘number of reported events’ negatively. The directions of the relations thereby support the overall criterion validity. Some of the dimensions do not predict the outcome variables significantly, nonetheless, each criterion related significantly to at least two dimensions on the HSOPSC 2.0. It is also worth noticing that ‘teamwork’ was generally one of the most important predictors even thought this dimension had the lowest convergent validity (AVE) in the previous findings [ 11 , 12 , 13 ], even if the strict AVE criterium was not satisfactory on the teamwork dimension and CR was also below 0.7. Since the explanatory power of teamwork was satisfactory, this illustrate that the AVE and CR criteria are maybe too strict.
The sample in the current study consisted of 1009 employees at two different hospital trusts in Norway and across different professions. The gender and ages are representative for Norwegian health care workers. In total 760 workers had direct patient contact, 167 had not, and 74 had patient contact sometimes. We think this mix is interesting, since a system perspective is key to establishing patient safety [ 31 ]. The other background variables (work experience, age, primary work area, and gender) indicate a satisfactory spread and mix of personnel in the sample, which is an advantage since then the sample to a large extend represent typical healthcare settings in Norway.
In the current study, N-HSOPSC 2.0 had higher levels of Cronbach’s alpha than in the first N-HSOPSC 1.0 studies [ 11 , 13 ], but more in-line with results from a longitudinal Norwegian study using the N-HSOPSC 1.0 in 2009, 2010 and 2017 respectively [ 23 ]. Moreover, the estimates in the current study reveal a higher level of factor loading on the N-HSOPSC 2.0, ranging from 0.51 to 0.89. This is positive since CFA is a key method when assessing the construct validity [ 16 , 17 , 32 ].
AVE and CR were not estimated in the first Norwegian HSOPSC 1.0 studies [ 11 , 13 ]. The results in this study indicate some issues regarding particularly AVE (convergent validity) since five of the concepts were below the recommended 0.50 threshold [ 32 ]. It is also worth noticing that all measures in the N-HSOPSC 2.0, except ‘teamwork’ (CR = 61), had CR values above 0.70, which is satisfactory. AVE is considered a strict and more conservative measure than CR. The validity of a construct may be adequate even though more than 50% of the variance is due to error [ 33 ]. Hence, some AVE values below 0.50 is not considered critical since the overall results are generally satisfactory.
The first estimate of the criterion related validity of the N-HSOPSC 2.0 using multiple regression indicated that two dimensions where significantly related to ‘number of reported events’, while six dimensions were significantly related to ‘patient safety rating’. The coefficients were negatively related with number of reported events, and positively related with patient safety rating, as expected. In the first Norwegian study in Norway on the N-HSOPSC 1.0 [ 13 ], five dimensions were significantly related to ‘number of reported events’, and seven dimensions were significantly related to ‘patient safety ratings’. The relations with ‘numbers of events reported’ were then both positive and negative, which is not optimal when assessing criterion validity. Hence, since all significant estimates are in the expected directions, the criterion validity of N-HSOPSC 2.0 has generally improved compared to the previous version.
In the current study we added ‘pleasure at work’ and ‘turnover intention’ to extend the assessment of criterion related validity. The first assessment indicated that ‘teamwork’ had a very substantial and positive influence on ‘pleasure at work’. Moreover, ‘staffing and work pace’ also had a positive influence on ‘pleasure at work’, but none of the other concepts were significant predictors. Hence, the teamwork dimension is key in driving ‘pleasure at work’, then followed by ‘staffing and working pace’. ‘Turnover intentions’ was significantly and negatively related to ‘teamwork’, ‘staffing and working pace’, ‘response to error’ and ‘hospital management support’. Hence, the results indicate these dimensions are key drivers in avoiding turnover intentions among staff in hospitals. A direct association has been reported between turnover and work strain, burnout and stress [ 19 ]. Zarei et al. [ 20 ] showed a significant relationship between patient safety (safety climate) and unit type, job satisfaction, job interest, and stress in hospitals. This study also illustrated a strong relationship between lack of personal accomplishment, job satisfaction, job interest and stress. Furthermore, a negative correlation between occupational burnout and safety climate was discovered, where a decrease in the latter is associated with an increase in the former [ 20 ]. Hence, patient safety researchers should look at health care job characteristics in combination with patient safety culture.
Assessment of psychometrics must consider other issues beyond statistical assessments such as theoretical consideration and face validity [ 16 , 17 ]; we believe one of the strengths of the HSOPSC 1.0 is that the instrument was operationalized based on theoretical concepts. This has been a strength, as opposed to other instruments built on EFA and a random selection of items included in the development process. We believe this is also the case in relation to HSOPSC 2.0; the instrument is theoretically based, easy to understand, and most importantly, can function as a tool to improve patient safety in hospitals. Moreover, when assessing the items that belongs to the different latent constructs, item-dimension relationships indicate a high face validity.
Forthcoming studies should consider predicting other outcomes, such as for instance mortality, morbidity, length of stay and readmissions, with the use of N-HSOPSC 2.0.
Limitations
This study is conducted in two Norwegian public hospital trusts, indicating some limitations about generalizability. The response rate within hospitals was low and therefore we could not benchmark subgroups. However, this was not part of the study objectives. The response rate may be hampered by the pandemic workload, and high workload in the hospitals. However, based on the diversity of the sample, we find the study results robust and adequate to explore the psychometric properties of N-HSOPSC 2.0. For the current study, we did not perform sample size calculations. With over 1000 respondents, we consider the sample size adequate to assess psychometric properties. Moreover, the low level of missing responses indicate N-HSOPSC 2.0 was relevant for the staff included in the study.
There are many alternative ways of exploring psychometric capabilities of instruments. For example, we did not investigate alternative factorial structures, e.g. including hierarchical factorial models or try to reduce the factorial structure which has been done with N-HSOPSC 1.0 short [ 34 ]. Lastly, we did not try to predict patient safety indicators over time using a longitudinal design and other objective patient safety indicators.
The results from this study generally support the validity and reliability of the N-HSOPSC 2.0. Hence, we recommend that the N-HSOPSC 2.0 can be applied without any further adjustments. However, future studies should potentially develop structural models to strengthen the knowledge and relationship between the factors included in the N-HSOPSC 2.0/ HSOPSC 2.0. Both improvement initiatives and future research projects can consider including the ‘pleasure at work’ and ‘turnover intentions’ indicators, since N-HSOPSC 2.0 explain a substantial level of variance relating to these criteria. This result also indicates an overlap between general pleasure at work and patient safety culture which is important when trying to improve patient safety.
Availability of data and materials
Datasets generated and/or analyzed during the current study are not publicly available due to local ownership of data, but aggregated data are available from the corresponding author on reasonable request.
World Health Organization. Global patient safety action plan 2021–2030: towards eliminating avoidable harm in health care. 2021. https://www.who.int/teams/integrated-health-services/patient-safety/policy/global-patient-safety-action-plan .
Rafter N, Hickey A, Conroy RM, Condell S, O’Connor P, Vaughan D, Walsh G, Williams DJ. The Irish National Adverse Events Study (INAES): the frequency and nature of adverse events in Irish hospitals—a retrospective record review study. BMJ Qual Saf. 2017;26(2):111–9.
Article PubMed Google Scholar
O’Connor P, O’Malley R, Kaud Y, Pierre ES, Dunne R, Byrne D, Lydon S. A scoping review of patient safety research carried out in the Republic of Ireland. Irish J Med. 2022;192:1–9.
Google Scholar
Halligan M, Zecevic A. Safety culture in healthcare: a review of concepts, dimensions, measures and progress. BMJ Qual Saf. 2011;20(4):338–43.
Weaver SJ, Lubomksi LH, Wilson RF, Pfoh ER, Martinez KA, Dy SM. Promoting a culture of safety as a patient safety strategy: a systematic review. Ann Intern Med. 2013;158(5):369–74.
Article PubMed PubMed Central Google Scholar
Vikan M, Haugen AS, Bjørnnes AK, Valeberg BT, Deilkås ECT, Danielsen SO. The association between patient safety culture and adverse events – a scoping review. BMC Health Serv Res 2023;300. https://doi.org/10.1186/s12913-023-09332-8 .
Sorra J, Nieva V. Hospital survey on patient safety culture. AHRQ publication no. 04–0041. Rockville: Agency for Healthcare Research and Quality; 2004.
Nieva VF, Sorra J. Safety culture assessment: a tool for improving patient safety in healthcare organizations. Qual Saf Health Car. 2003;12:II17–23.
Agency for Healthcare Research and Quality (AHQR). International use of SOPS. https://www.ahrq.gov/sops/international/index.html .
Flin R, Burns C, Mearns K, Yule S, Robertson E. Measuring safety climate in health care. Qual Saf Health Care. 2006;15(2):109–15.
Article CAS PubMed PubMed Central Google Scholar
Olsen E, Aase K. The challenge of improving safety culture in hospitals: a longitudinal study using hospital survey on patient safety culture. International Probabilistic Safety Assessment and Management Conference and the Annual European Safety and Reliability Conference. 2012;2012:25–9.
Olsen E. Safety climate and safety culture in health care and the petroleum industry: psychometric quality, longitudinal change, and structural models. PhD thesis number 74. University of Stavanger; 2009.
Olsen E. Reliability and validity of the Hospital Survey on Patient Safety Culture at a Norwegian hospital. Quality and safety improvement research: methods and research practice from the International Quality Improvement Research Network (QIRN) 2008:173–186.
Olsen E, Leonardsen ACL. Use of the Hospital Survey of Patient Safety Culture in Norwegian Hospitals: A Systematic Review. Int J Environment Res Public Health. 2021;18(12):6518.
Article Google Scholar
Hughes DJ. Psychometric validity: Establishing the accuracy and appropriateness of psychometric measures. The Wiley handbook of psychometric testing: A multidisciplinary reference on survey, scale and test development; 2018:751–779.
DeVillis RF. Scale development: Theory and application. Thousands Oaks: Sage Publications; 2003.
Netemeyer RG, Bearden WO, Sharma S. Scaling procedures: Issues and application. London: SAGE Publications Ltd; 2003.
Book Google Scholar
Karsh B, Booske BC, Sainfort F. Job and organizational determinants of nursing home employee commitment, job satisfaction and intent to turnover. Ergonomics. 2005;48:1260–81. https://doi.org/10.1080/00140130500197195 .
Article CAS PubMed Google Scholar
Hayes L, O’Brien-Pallas L, Duffield C, Shamian J, Buchan J, Hughes F, Spence Laschinger H, North N, Stone P. Nurse turnover: a literature review. Int J Nurs Stud. 2006;43:237–63.
Zarei E, Najafi M, Rajaee R, Shamseddini A. Determinants of job motivation among frontline employees at hospitals in Teheran. Electronic Physician. 2016;8:2249–54.
Agency for Healthcare Research and Quality (AHQR). Hospital Survey on Patient Safety Culture. https://www.ahrq.gov/sops/surveys/hospital/index.html .
von Elm E, Altman DG, Egger M, Pocock SJ, Gotzsche PC, Vandenbroucke JP. The Strengthening the Reporting of Observational Studies in Epidemiology (STROBE) Statement: guidelines for reporting observational studies. BMJ. 2007;335(7624):806–8.
Brislin R. Back translation for cross-sectional research. J Cross-Cultural Psychol. 1970;1(3):185–216.
Notelaers G, De Witte H, Van Veldhoven M, Vermunt JK. Construction and validation of the short inventory to monitor psychosocial hazards. Médecine du Travail et Ergonomie. 2007;44(1/4):11.
Bentein K, Vandenberghe C, Vandenberg R, Stinglhamber F. The role of change in the relationship between commitment and turnover: a latent growth modeling approach. J Appl Psychol. 2005;90(3):468.
Tabachnick B, Fidell L. Using multivariate statistics. 6th ed. Boston: Pearson; 2013.
Hu L, Bentler P. Cutoff criteria for fit indexes in covariance structure analysis: Conventional criteria versus new alternatives. Structural Equation Modelling. 1999;6(1):1–55.
Hair J, Sarstedt M, Hopkins L, Kuppelwieser V. Partial least squares structural equation modeling (PLS-SEM): An emerging tool in business research. Eur Business Rev. 2014;26:106–21.
George D, Mallery P. SPSS for Windows step by step: A simple guide and reference. 11.0 update. Boston: Allyn & Bacon; 2003.
World Medical Association. Declaration of Helsinki- Ethical Principles for Medical Research Involving Human Subjects. 2018. http://www.wma.net/en/30publications/10policies/b3 .
Farup PG. Are measurements of patient safety culture and adverse events valid and reliable? Results from a cross sectional study. BMC Health Serv Res. 2015;15(1):1–7.
Hair JF, Black WC, Babin BJ, Anderson RE. Applications of SEM. Multivariate data analysis. Upper Saddle River: Pearson; 2010.
Malhotra NK, Dash S. Marketing research an applied orientation (paperback). London: Pearson Publishing; 2011.
Olsen E, Aase K. A comparative study of safety climate differences in healthcare and the petroleum industry. Qual Saf Health Care. 2010;19(3):i75–9.
Download references
Acknowledgements
Master student Linda Eikemo is acknowledged for participating in the data collection in Hospital A, and Nina Føreland in Hospital B.
Not applicable.
Author information
Authors and affiliations.
UiS Business School, Department of Innovation, Management and Marketing, University of Stavanger, Stavanger, Norway
Espen Olsen & Seth Ayisi Junior Addo
Hospital of Southern Norway, Flekkefjord, Norway
Susanne Sørensen Hernes
Department of Clinical Sciences, University of Bergen, Bergen, Norway
Department of Obstetrics and Gynecology, Stavanger University Hospital, Stavanger, Norway
Marit Halonen Christiansen
Faculty of Health Sciences Department of Nursing and Health Promotion Acute and Critical Illness, OsloMet - Oslo Metropolitan University, Oslo, Norway
Arvid Steinar Haugen
Department of Anaesthesia and Intensive Care, Haukeland University Hospital, Bergen, Norway
Faculty of Health, Welfare and Organization, Østfold University College, Fredrikstad, Norway
Ann-Chatrin Linqvist Leonardsen
Department of anesthesia, Østfold Hospital Trust, Grålum, Norway
You can also search for this author in PubMed Google Scholar
Contributions
EO, ASH and ACLL initiated the study. All authors (EO, SA, SSH, MHC, ASH, ACLL) participated in the translation process. SSH and ACLL were responsible for data collection. EO and SA performed the statistical analysis, which was reviewed by ASH and ACLL. EO, SA and ACLL wrote the initial draft of the manuscript, and all authors (EO, SA, SSH, MHC, ASH, ACLL) critically reviewed the manuscript. All authors(EO, SA, SSH, MHC, ASH, ACLL) have read and approved the final version of the manuscript.
Corresponding author
Correspondence to Ann-Chatrin Linqvist Leonardsen .
Ethics declarations
Ethics approval and consent to participate.
The study was conducted in-line with principles for ethical research in the Declaration of Helsinki, and informed consent was obtained from all the participants [ 30 ]. Eligible healthcare personnel were informed of the study through hospital e-mails and by text messages. Completed and submitted questionnaires were assumed as consent to participate. According to the Norwegian Health Research Act §4, no ethics approval is needed when including healthcare personnel in research.
Consent for publication
Competing interests.
The authors declare no competing interests.
Additional information
Publisher’s note.
Springer Nature remains neutral with regard to jurisdictional claims in published maps and institutional affiliations.
Supplementary Information
Supplementary material 1., rights and permissions.
Open Access This article is licensed under a Creative Commons Attribution 4.0 International License, which permits use, sharing, adaptation, distribution and reproduction in any medium or format, as long as you give appropriate credit to the original author(s) and the source, provide a link to the Creative Commons licence, and indicate if changes were made. The images or other third party material in this article are included in the article's Creative Commons licence, unless indicated otherwise in a credit line to the material. If material is not included in the article's Creative Commons licence and your intended use is not permitted by statutory regulation or exceeds the permitted use, you will need to obtain permission directly from the copyright holder. To view a copy of this licence, visit http://creativecommons.org/licenses/by/4.0/ . The Creative Commons Public Domain Dedication waiver ( http://creativecommons.org/publicdomain/zero/1.0/ ) applies to the data made available in this article, unless otherwise stated in a credit line to the data.
Reprints and permissions
About this article
Cite this article.
Olsen, E., Addo, S.A.J., Hernes, S.S. et al. Psychometric properties and criterion related validity of the Norwegian version of hospital survey on patient safety culture 2.0. BMC Health Serv Res 24 , 642 (2024). https://doi.org/10.1186/s12913-024-11097-7
Download citation
Received : 03 April 2023
Accepted : 09 May 2024
Published : 18 May 2024
DOI : https://doi.org/10.1186/s12913-024-11097-7
Share this article
Anyone you share the following link with will be able to read this content:
Sorry, a shareable link is not currently available for this article.
Provided by the Springer Nature SharedIt content-sharing initiative
- Hospital survey on patient safety culture
- Patient safety culture
- Psychometric testing
BMC Health Services Research
ISSN: 1472-6963
- General enquiries: [email protected]
The short Thai version of functional outcomes of sleep questionnaire (FOSQ-10T): reliability and validity in patients with sleep-disordered breathing
- Sleep Breathing Physiology and Disorders • Original Article
- Open access
- Published: 15 May 2024
Cite this article
You have full access to this open access article
- Kawisara Chaiyaporntanarat 1 ,
- Wish Banhiran ORCID: orcid.org/0000-0002-4029-6657 1 , 2 ,
- Phawin Keskool 1 ,
- Sarin Rungmanee 2 ,
- Chawanont Pimolsri 2 ,
- Wattanachai Chotinaiwattarakul 2 , 3 &
- Auamporn Kodchalai 2 , 4
326 Accesses
Explore all metrics
The study is to evaluate reliability and validity of the short Thai version of Functional Outcome of Sleep Questionnaire (FOSQ-10T), in patients with sleep disordered breathing (SDB).
Inclusion criteria were Thai patients with SDB age ≥ 18 years old who had polysomnography results available. Exclusion criteria were patients unable to complete questionnaire for any reason, patients with a history of continuous antidepressant or alcohol use, and underlying disorders including unstable cardiovascular, pulmonary, or neurological conditions. All participants were asked to complete the FOSQ-10 T and Epworth sleepiness scales (ESS). Of these, 38 patients were required to retake FOSQ-10 T at 2–4 weeks later to assess test–retest reliability, and 19 OSA patients treated with CPAP were asked to do so at 4 weeks following therapy to assess questionnaire’s responsiveness to treatment.
There were 42 participants (24 men, 18 women), with a mean age of 48.3 years. The internal consistency of the FOSQ-10T was good, as indicated by Cronbach’s alpha coefficient of 0.85. The test–retest reliability was good, as indicated by intraclass correlation coefficient of 0.77. The correlation between the FOSQ-10T and ESS scores (concurrent validity) was moderate ( r = − 0.41). The scores of FOSQ-10T significantly increased after receiving adequate CPAP therapy, showing an excellent responsiveness to treatment. However, there was no significant association between FOSQ-10T scores and OSA severity measured by apnea–hypopnea index.
Conclusions
The FOSQ-10T has good reliability and validity to use as a tool to assess QOL in Thai patients with SDB. It is convenient and potentially useful in both clinical and research settings.
Similar content being viewed by others
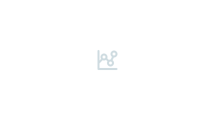
The DS-14 questionnaire: psychometric characteristics and utility in patients with obstructive sleep apnea
Psychometric properties of the turkish version of modified freedman questionnaire for sleep quality.
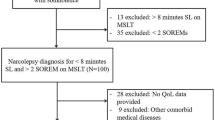
The influential factor of narcolepsy on quality of life: compared to obstructive sleep apnea with somnolence or insomnia
Avoid common mistakes on your manuscript.
Introduction
The term “sleep-disordered breathing” (SDB) refers to a category of high prevalent sleep disorders that are distinguished by abnormal breathing patterns when the patient is asleep. Its negative consequences, especially obstructive sleep apnea (OSA), include excessive daytime sleepiness, high blood pressure, poor quality of life (QOL), cardiometabolic diseases, and sensorineural hearing loss [ 1 , 2 , 3 , 4 , 5 , 6 ]. In addition to lowering these possible morbidities and deaths, improving the patients’ QOL is a key goal of appropriately treating SDB [ 7 ].
Currently available instruments to assess health-related QOL in individuals with sleep disorders include general and disease-specific questionnaires [ 5 , 8 , 9 ]. The Functional Outcomes of Sleep Questionnaire (FOSQ-30), however, is perhaps one of the most widely utilized [ 10 ]. The questionnaire is a standardized self-report form consisting of 30 items that cover various domains including sexual relationships, general productivity, activity level, vigilance, and social consequence. Each of the FOSQ-30 items is given a score between 0 and 4, with a higher score representing a higher quality of life. However, one of the FOSQ-30’s drawbacks is the somewhat lengthy time required to respond to all questions (a total of 20–25 min). The original authors subsequently developed a shortened version (FOSQ-10) to make the QOL assessment easier and more efficient while still maintaining all crucial components [ 11 ]. Unfortunately, there is currently no validated version of this tool available for Thai patients.
The FOSQ-10 has been used to study the effects of therapeutic interventions such as functional septorhinoplasty [ 12 ], continuous positive airway pressure (CPAP) treatment [ 13 , 14 ], oral appliance [ 15 , 16 ], and the effects of gastroesophageal reflux disease [ 17 ]. Previous research also showed that the FOSQ-10 was validated across a number of languages and ethnics [ 18 , 19 , 20 ]. In Iranians, a study found that the FOSQ-10 was comparable in meaning to the original version [ 18 ]. In Peruvians, the Spanish version of FOSQ-10 showed good internal consistency, construct validity, and sensitivity to change in patients with OSA who received treatment [ 19 ]. In Chinese, a study reported that the FOSQ-10 was a valid and reliable instrument for identifying the effects of sleep-related impairment in women during pregnancy [ 20 ]. Yet, no study has looked into its use in Thai people.
The primary objective of this study was to evaluate reliability and validity of the short Thai version of Functional Outcomes of Sleep Questionnaire (FOSQ-10T). The secondary objectives were to evaluate (1) QOL of OSA patients pre- and post-CPAP treatment, (2) QOL of SDB patients across different AHI severity, and (3) the correlation between scores of the FOSQ-10T and Epworth sleepiness scale (ESS).
Material and methods
This observational, prospective research was approved by the Siriraj Institutional Review (SIRB), COA Si 258/2021. The study was conducted between November 2021 and February 2022. All participants gave their informed consent.
Subjects and allocation
The inclusion criteria were Thai patients with SDB who were at least 18 years old and had polysomnography (PSG) results available. The exclusion criteria were patients who were unable to complete questionnaire for any reason, those with a history of long-term sedatives, antidepressants, or alcohol use, and those with underlying medical conditions that would significantly impair QOL.
All participants were asked to complete the FOSQ-10T and ESS. Of these, 38 patients were asked to retake the FOSQ-10T between two and four weeks later in order to evaluate test–retest reliability, and 19 patients with OSA who were receiving CPAP were asked to retake the questionnaire 4 weeks later in order to evaluate the questionnaire’s responsiveness to treatment, as presented in the flow chart (Fig. 1 ).
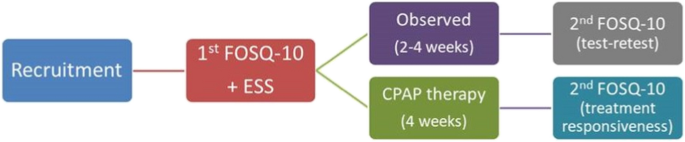
The flow chart of the study; FOSQ-10, the short form of the Functional Outcomes of Sleep Questionnaire; ESS, Epworth Sleepiness scale; CPAP, continuous positive airway pressure
The short Thai version of Functional Outcomes of Sleep Questionnaire (FOSQ-10T)
The FOSQ-10T is a 10-item self-reported questionnaire that measures the impact of sleep disturbances on daily functioning. Though it is a condensed form of the FOSQ-30, it still includes all of the important domains: activity level (three items), vigilance (three items), sexual relationships (one item), general productivity (two items), and social outcome (one item). Every item is assigned a number between 0 and 4, where a higher number corresponds to a higher QOL. With permission of Professor Terri Weaver, the original developers [ 10 ] graciously provided us with the FOSQ-10T (see Supplementary information the appendix ) for use in this study. Standard processes were used to translate it both forward and backward from English to Thai.
Epworth sleepiness scales (ESS)
The ESS is a self-administered questionnaire used to assess an individual's subjective level of sleepiness. It comprises of eight questions that ask the respondent to rate their likelihood of dozing off or falling asleep in a range of everyday scenarios, such as sitting and conversing with someone, watching television, or riding as a passenger in a car. The scores range from 0 to 3, where a higher number indicates greater sleepiness. In this study, we use a validated Thai version of ESS with permission [ 21 ] (see Supplementary information the appendix ).
Statistical analysis
The categorical data were presented as numbers and percentages, whereas the continuous data were presented as mean ± standard deviation (SD). The Cronbach’s alpha coefficient was used to evaluate the internal consistency for reliability and the intraclass coefficient (ICC) was used to evaluate test–retest reliability. The discriminant validity of the FOSQ-10T among each SDB severity was evaluated using a Kruskal–Wallis one-way analysis of variance (ANOVA), and the concurrent validity of the FOSQ-10T and ESS was evaluated using a scatter plot and a Pearson correlation coefficient. A significance level of p < 0.05 was employed to denote statistical significance. The Statistical Package for the Social Science (SPSS) version 22, International Business Machines Corporation, Armonk, NY, USA, was utilized to conduct the statistical analyses.
For this study, 42 participants (24 men and 18 women) with a mean age of 48.3 ± 15.3 years and a mean BMI of 27.9 ± 5.4 kg/m 2 were recruited. The apnea–hypopnea index (AHI) and mean ESS scores for the group were 38.6 ± 29.8 events/h and 7 ± 3.8, respectively. Among the participants, 16 (38.1%) had hypertension, 11 (26.2%) had dyslipidemia, and 7 (16.7%) had underlying diabetes mellitus.
Reliability
Cronbach’s alpha coefficient of the FOSQ-10T ranged from 0.82 to 0.85 for all 10 items, and the removal of any items did not significantly alter the result (Table 1 ). This suggested a high level of internal consistency. With an ICC of 0.77 and a 95% confidence interval (CI) of 0.60–0.88, the FOSQ-10T demonstrated good test–retest reliability.
The concurrent validity between FOSQ-10T and ESS scores was shown by a scatter plot (Fig. 1 ) and Pearson correlation coefficient of − 0.41 ( p = 0.01) which indicated that there was a moderate correlation. However, the results of the discriminant validity analysis using the Kruskal–Wallis one-way ANOVA indicated that there was no significant correlation between the OSA severity as determined by the AHI and the FOSQ-10T scores (Table 2 ).
Responsiveness
The mean FOSQ-10 T scores across all domains and the overall scores considerably improved after receiving adequate CPAP therapy (Table 3 ). This proved that the questionnaire had an excellent responsiveness to treatment (Fig. 2 ).
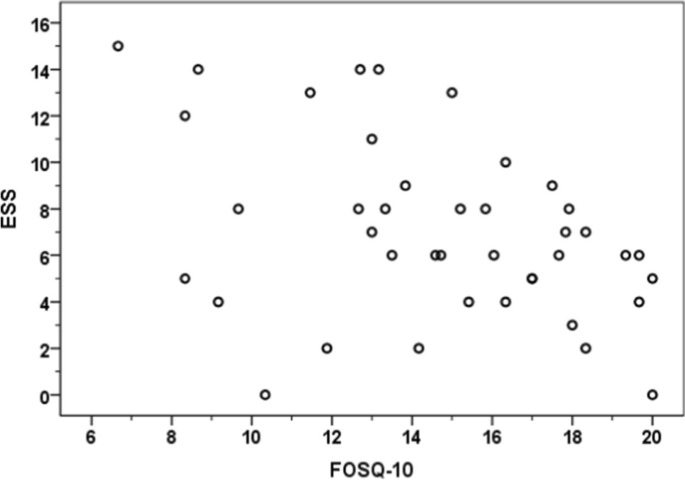
The scatter plot of ESS score and FOSQ-10; FOSQ-10, the short Form of the Functional Outcomes of Sleep Questionnaire; ESS, Epworth sleepiness scale; CPAP, continuous positive airway pressure
When treating patients with SDB, the QOL of the patients is an important consideration that cannot be overlooked. While there are other instruments to assess this issue, the FOSQ-30 is likely one of the most widely utilized disease-specific questionnaires. However, its disadvantage is that answering every question takes a substantial amount of time. As a result, the original authors eventually developed a shortened version (FOSQ-10) to streamline and improve the effectiveness of the QOL evaluation while maintaining all crucial elements [ 11 ]. Comparable to the original, this updated version has demonstrated good validity and reliability [ 10 ]. It has been used to evaluate the effects of various therapeutic interventions [ 12 , 14 , 17 ] and has been validated in several other languages, including Spanish, Persian, and Chinese [ 18 , 19 , 20 ].
This study is most likely the first to report on the validity and reliability of the FOSQ-10T in Thai patients with SDB. The results of our study showed that the Cronbach’s alpha coefficient, which measures internal consistency, was 0.85, indicating good reliability. This closely resembles the original version [ 10 ] and studies conducted in Chinese, Spanish, and Persian [ 18 , 19 , 20 ] that found a Cronbach’s alpha of 0.84–0.87.
The results of the present study showed that the FOSQ-10T has good test–retest reliability with an ICC of 0.77. This could suggest that the tool is reliable when applied repeatedly to the same Thai population. It should be mentioned that the ICC of this study was comparable to that of the Chinese study (ICC of 0.73) and another Thai version of the FOSQ-30 (ICC of 0.70) [ 5 ], but it was lower than that of the Iranian study (ICC of 0.92). A straight comparison of ICC values across studies, however, would not always be appropriate because different study designs, populations, and measuring techniques can all have an impact on the values.
According to the study, there was a moderately negative correlation between the FOSQ-10T and the ESS ( r = − 0.41). This association is similar to that of the Iranian version [ 18 ] and another Thai version of FOSQ-30 [ 5 ]. Our finding, however, diverged from that of the Spanish [ 19 ] and Chinese studies [ 20 ], which did not discover any significant relationships between the FOSQ-10 T and ESS scores.
Among the OSA patients with different AHI severities in this study, there were no statistically significant differences in the FOSQ-10T scores. Mild OSA had the lowest scores, whereas moderate OSA had the highest. These, however, differ from the original English [ 11 ], Iranian [ 18 ], and Spanish [ 18 , 19 ] versions which showed moderate degrees of discriminating validity.
Not surprisingly, after therapy, the FOSQ-10T scores of participants in this study who used CPAP appropriately improved significantly. These findings were in line with a number of other studies; thus, it may indicate that the questionnaire had a high degree of treatment responsiveness.
This study may have some limitations. First, because the FOSQ-10T scores were subjectively evaluated by individuals, bias cannot be avoided. Second, the FOSQ-10T and the original FOSQ-30 were not directly compared, so there is a chance that both of them will produce different results when applied. Furthermore, only relatively healthy SDB patients were assessed in this study. For this reason, our findings could not be directly applied to patients suffering from critical illnesses such as heart failure, stroke, or chronic renal diseases, or to patients with other sleep disorders including insomnia or hypersomnolence due to central origin. It is recommended that further study be done in populations with varying characteristics or manifestations.
The results of this study indicate that the FOSQ-10T is a valid and reliable tool for evaluating QOL in Thai patients with SDB. In clinical practice, physicians may utilize the questionnaire to monitor therapy results and customize interventions to fit specific patient needs. In research, the FOSQ-10T may be used to evaluate the effectiveness of various therapeutic or diagnostic approaches.
Data availability
To comply with general data protection regulation and to protect people’s privacy, the raw data for this dataset is not publicly accessible.
Kasemsuk N, Chayopasakul V, Banhiran W, Prakairungthong S, Rungmanee S, Suvarnsit K et al (2023) Obstructive sleep apnea and sensorineural hearing loss: a systematic review and meta-analysis. Otolaryngol Head Neck Surg 169:201–209
Article PubMed Google Scholar
Sangchan T, Banhiran W, Chotinaiwattarakul W, Keskool P, Rungmanee S, Pimolsri C (2023) Association between REM-related mild obstructive sleep apnea and common cardiometabolic diseases. Sleep Breath 27:2265–2271
Uataya M, Banhiran W, Chotinaiwattarakul W, Keskool P, Rungmanee S, Pimolsri C (2023) Association between hypoxic burden and common cardiometabolic diseases in patients with severe obstructive sleep apnea. Sleep Breath 27:2423–2428
Baldwin CM, Griffith KA, Nieto FJ, O’Connor GT, Walsleben JA, Redline S (2001) The association of sleep-disordered breathing and sleep symptoms with quality of life in the Sleep Heart Health Study. Sleep 24:96–105
Article CAS PubMed Google Scholar
Banhiran W, Assanasen P, Metheetrairut C, Nopmaneejumruslers C, Chotinaiwattarakul W, Kerdnoppakhun J (2012) Functional outcomes of sleep in Thai patients with obstructive sleep-disordered breathing. Sleep Breath 16:663–675
Lal C, Weaver TE, Bae CJ, Strohl KP (2021) Excessive daytime sleepiness in obstructive sleep apnea. mechanisms and clinical management. Ann Am Thorac Soc 18:757–768
Article PubMed PubMed Central Google Scholar
Cai Y, Tripuraneni P, Gulati A, Stephens EM, Nguyen DK, Durr ML et al (2022) Patient-defined goals for obstructive sleep apnea treatment. Otolaryngol Head Neck Surg 167:791–798
Banhiran W, Assanasen P, Metheetrairut C, Chotinaiwattarakul W (2013) Health-related quality of life in Thai patients with obstructive sleep disordered breathing. J Med Assoc Thai 96:209–216
PubMed Google Scholar
Rahavi-Ezabadi S, Amali A, Sadeghniiat-Haghighi K, Montazeri A, Nedjat S (2016) Translation, cultural adaptation, and validation of the Sleep Apnea Quality of Life Index (SAQLI) in Persian-speaking patients with obstructive sleep apnea. Sleep Breath 20:523–528
Weaver TE, Laizner AM, Evans LK, Maislin G, Chugh DK, Lyon K et al (1997) An instrument to measure functional status outcomes for disorders of excessive sleepiness. Sleep 20:835–843
CAS PubMed Google Scholar
Chasens ER, Ratcliffe SJ, Weaver TE (2009) Development of the FOSQ-10: a short version of the Functional Outcomes of Sleep Questionnaire. Sleep 32:915–919
Hismi A, Yu P, Locascio J, Levesque PA, Lindsay RW (2020) The impact of nasal obstruction and functional septorhinoplasty on sleep quality. Facial Plast Surg Aesthet Med 22:412–419
Lam AS, Collop NA, Bliwise DL, Dedhia RC (2017) Validated measures of insomnia, function, sleepiness, and nasal obstruction in a CPAP alternatives clinic population. J Clin Sleep Med 13:949–957
Boyer L, Philippe C, Covali-Noroc A, Dalloz MA, Rouvel-Tallec A, Maillard D et al (2019) OSA treatment with CPAP: randomized crossover study comparing tolerance and efficacy with and without humidification by ThermoSmart. Clin Respir J 13:384–390
Banhiran W, Assanasen P, Nopmaneejumrudlers C, Nujchanart N, Srechareon W, Chongkolwatana C et al (2018) Adjustable thermoplastic oral appliance versus positive airway pressure for obstructive sleep apnea. Laryngoscope 128:516–522
Banhiran W, Durongphan A, Keskool P, Chongkolwatana C, Metheetrairut C (2020) Randomized crossover study of tongue-retaining device and positive airway pressure for obstructive sleep apnea. Sleep Breath 24:1011–1018
Laohasiriwong S, Johnston N, Woodson BT (2013) Extra-esophageal reflux, NOSE score, and sleep quality in an adult clinic population. Laryngoscope 123:3233–3238
Rahavi-Ezabadi S, Amali A, Sadeghniiat-Haghighi K, Montazeri A (2016) Adaptation of the 10-item Functional Outcomes of Sleep Questionnaire to Iranian patients with obstructive sleep apnea. Qual Life Res 25:337–341
Rey de Castro J, Rosales-Mayor E, Weaver TE (2018) Reliability and validity of the Functional Outcomes of Sleep Questionnaire - Spanish Short Version (FOSQ-10SV) in Peruvian patients with obstructive sleep apnea. J Clin Sleep Med 14:615–621
Tsai SY, Shun SC, Lee PL, Lee CN, Weaver TE (2016) Validation of the Chinese version of the Functional Outcomes of Sleep Questionnaire-10 in pregnant women. Res Nurs Health 39:463–471
Banhiran W, Assanasen P, Nopmaneejumruslers C, Metheetrairut C (2011) Epworth sleepiness scale in obstructive sleep disordered breathing: the reliability and validity of the Thai version. Sleep Breath 15:571–577
Download references
Acknowledgements
The authors express their gratitude to Jeerapa Kerdnoppakhun for all of her research assistance including paperwork and working process procedures, as well as to Chulaluk Komoltri for her statistical analysis and sample size computation. Along with all of the patients who participated in this study, the authors also acknowledge the kind cooperation of the medical staffs at the Siriraj Sleep Center and the Department of Otorhinolaryngology.
Open access funding provided by Mahidol University This research project was supported by Siriraj Research Fund, grant number (IO) R016531056, Faculty of Medicine Siriraj Hospital, Mahidol University, Thailand.
Author information
Authors and affiliations.
Department of Otorhinolaryngology, Faculty of Medicine Siriraj Hospital, Mahidol University, Bangkok, Thailand
Kawisara Chaiyaporntanarat, Wish Banhiran & Phawin Keskool
Siriraj Sleep Center, Faculty of Medicine Siriraj Hospital, Mahidol University, Bangkok, Thailand
Wish Banhiran, Sarin Rungmanee, Chawanont Pimolsri, Wattanachai Chotinaiwattarakul & Auamporn Kodchalai
Neurology Division, Department of Medicine, Faculty of Medicine Siriraj Hospital, Mahidol University, Bangkok, Thailand
Wattanachai Chotinaiwattarakul
American Board of Sleep Medicine, Department of Otorhinolaryngology, Faculty of Medicine Siriraj Hospital, Certified International Sleep Specialist, Mahidol University, 2 Wanglang Road, Bangkok Noi, Bangkok, 10700, Thailand
Auamporn Kodchalai
You can also search for this author in PubMed Google Scholar
Contributions
Kawisara Chaiyaporntanarat: conception and design, data acquisition, collection, analysis, interpretation, drafting the article, final approval; Wish Banhiran: conception and design, data acquisition, interpretation, critical revisions, drafting the article, final approval, and being the corresponding author; Wattanachai Chotinaiwattarakul, Phawin Keskool, Sarin Rungmanee, Chawanont Pimolsri, Auamporn Kodchalai: data acquisition, interpretation, critical revisions, final approval.
Corresponding author
Correspondence to Wish Banhiran .
Ethics declarations
Ethical approval.
This study was approved by the Siriraj Institutional Review Board (SIRB).
Competing interests
The authors declare no competing interests.
Additional information
Publisher's note.
Springer Nature remains neutral with regard to jurisdictional claims in published maps and institutional affiliations.
Supplementary Information
Below is the link to the electronic supplementary material.
Supplementary file1 (PDF 163 KB)
Rights and permissions.
Open Access This article is licensed under a Creative Commons Attribution 4.0 International License, which permits use, sharing, adaptation, distribution and reproduction in any medium or format, as long as you give appropriate credit to the original author(s) and the source, provide a link to the Creative Commons licence, and indicate if changes were made. The images or other third party material in this article are included in the article's Creative Commons licence, unless indicated otherwise in a credit line to the material. If material is not included in the article's Creative Commons licence and your intended use is not permitted by statutory regulation or exceeds the permitted use, you will need to obtain permission directly from the copyright holder. To view a copy of this licence, visit http://creativecommons.org/licenses/by/4.0/ .
Reprints and permissions
About this article
Chaiyaporntanarat, K., Banhiran, W., Keskool, P. et al. The short Thai version of functional outcomes of sleep questionnaire (FOSQ-10T): reliability and validity in patients with sleep-disordered breathing. Sleep Breath (2024). https://doi.org/10.1007/s11325-024-03024-1
Download citation
Received : 09 January 2024
Revised : 28 February 2024
Accepted : 15 March 2024
Published : 15 May 2024
DOI : https://doi.org/10.1007/s11325-024-03024-1
Share this article
Anyone you share the following link with will be able to read this content:
Sorry, a shareable link is not currently available for this article.
Provided by the Springer Nature SharedIt content-sharing initiative
- Functional Outcome of Sleep Questionnaire
- Obstructive sleep apnea
- Sleep-disordered breathing
- Find a journal
- Publish with us
- Track your research
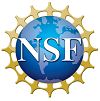
SCIENCE & ENGINEERING INDICATORS
Research and development: u.s. trends and international comparisons.
- Report PDF (808 KB)
- Report - All Formats .ZIP (5.2 MB)
- Supplemental Materials - All Formats .ZIP (513 KB)
- MORE DOWNLOADS OPTIONS
- Share on X/Twitter
- Share on Facebook
- Share on LinkedIn
- Send as Email
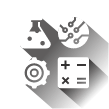
U.S. GERD grew at a faster rate than GDP over 2010–21 on a compound annual growth rate basis. And while the United States remains the top R&D performer globally, other countries show continued growth in GERD and R&D intensity (R&D-to-GDP ratio). In 2021, the U.S. R&D intensity was 3.5%, based on internationally comparable OECD statistics. Other economies with R&D intensities above 3.0% include Israel and South Korea (both with intensities above 4.0%). Eight economies had intensities between 3.0% and 4.0%, including Taiwan, the United States, Japan, and Germany. Countries with intensities above 2.0% included the United Kingdom and China.
For the United States, the business sector continued to be the leading performer and funder of R&D. Manufacturing industries accounted for the largest proportion of R&D for companies with 10 or more employees, whereas the professional, scientific, and R&D services industry accounted for the largest proportion of R&D by microbusinesses. And U.S.-located companies continue to invest in software, AI, biotechnology, and nanotechnology R&D.
Consistent federal government support for R&D is a key feature of the U.S. R&D enterprise. The CHIPS and Science Act of 2022 appropriated $52.7 billion to revitalize the U.S. semiconductor industry along the supply chain, including $13.7 billion supporting R&D, workforce development, and related programs. More broadly, federal R&D funding constitutes the second-largest overall funding source and the largest source for U.S. basic research performance. The higher education sector was the largest performer of basic research and the largest recipient of federal R&D funding; in 2022, however, total R&D performance by the higher education sector did not increase after adjusting for inflation.
Related Content
- Skip to main content
- Skip to FDA Search
- Skip to in this section menu
- Skip to footer links

The .gov means it’s official. Federal government websites often end in .gov or .mil. Before sharing sensitive information, make sure you're on a federal government site.
The site is secure. The https:// ensures that you are connecting to the official website and that any information you provide is encrypted and transmitted securely.
U.S. Food and Drug Administration
- Search
- Menu
- Science & Research
- About Science & Research at FDA
- The FDA Science Forum
A protocol to differentiate drug unbinding characteristics from cardiac sodium channel for proarrhythmia risk assessment
2023 FDA Science Forum
Background:
Class I antiarrhythmic drugs (AADs) are sodium channel blockers that have been used to treat cardiac arrhythmias that arise through either abnormal automaticity or reentry. Class I AADs can be further classified into Class IA, IB and IC subgroups. Class IC AADs (flecainide or encainide) are associated with increased mortality in patients with structural heart diseases. Thus, identifying drug-sodium channel interaction characteristics to distinguish subgroups of Class I AAD is important for proarrhythmic risk assessment. Indeed, FDA recently issued notifications of Post-marketing Requirements for several antiepileptic drugs that are sodium channel blockers to request sponsors to characterize drug - sodium channel interaction characteristics for comparison with class I AADs.
There is no standardized protocol to characterize blocking kinetics of drug on cardiac sodium channels (NaV1.5). This creates an uncertainty and burden for regulatory reviews of such data, because electrophysiology results are dependent on protocols used. To enable a more efficient review process, this study aimed to test the utility of one protocol to characterize unbinding kinetics from cardiac NaV1.5 channel by classic IA (quinidine), IB (mexiletine) and IC (flecainide) AADs. Methodology: Whole cell recordings of NaV1.5 currents were made using overexpression cells. Channel properties, block potencies, and block kinetics of the aforementioned drugs were assessed at room temperature and near physiological temperature.
NaV1.5 peak current exhibited large magnitude, extremely fast activation and inactivation, and required a high degree of series resistance compensation to maintain adequate voltage control. Mexiletine, quinidine and flecainide showed fast, intermediate, and slow dissociation rates, respectively, at room temperature and near physiological temperature. Both association and dissociation rates of the three drugs increased by 3~5-times at physiological temperature compared with at room temperature. However, the potencies of the three drugs on NaV1.5 current were not impacted by recording temperature.
Conclusion:
The dissociation time constants of quinidine, mexiletine and flecainide as determined using this protocol are consistent with their classification in the Class IA, IB, and IC subgroups. Adoption of this protocol for proarrhythmia risk assessment based on drug-cardiac sodium channel interactions should facilitate the review process of drugs with sodium channel blockade.

Download the Poster (PDF; 0.61 MB)
ORIGINAL RESEARCH article
Statistical conclusion validity: some common threats and simple remedies.

- Facultad de Psicología, Departamento de Metodología, Universidad Complutense, Madrid, Spain
The ultimate goal of research is to produce dependable knowledge or to provide the evidence that may guide practical decisions. Statistical conclusion validity (SCV) holds when the conclusions of a research study are founded on an adequate analysis of the data, generally meaning that adequate statistical methods are used whose small-sample behavior is accurate, besides being logically capable of providing an answer to the research question. Compared to the three other traditional aspects of research validity (external validity, internal validity, and construct validity), interest in SCV has recently grown on evidence that inadequate data analyses are sometimes carried out which yield conclusions that a proper analysis of the data would not have supported. This paper discusses evidence of three common threats to SCV that arise from widespread recommendations or practices in data analysis, namely, the use of repeated testing and optional stopping without control of Type-I error rates, the recommendation to check the assumptions of statistical tests, and the use of regression whenever a bivariate relation or the equivalence between two variables is studied. For each of these threats, examples are presented and alternative practices that safeguard SCV are discussed. Educational and editorial changes that may improve the SCV of published research are also discussed.
Psychologists are well aware of the traditional aspects of research validity introduced by Campbell and Stanley (1966) and further subdivided and discussed by Cook and Campbell (1979) . Despite initial criticisms of the practically oriented and somewhat fuzzy distinctions among the various aspects (see Cook and Campbell, 1979 , pp. 85–91; see also Shadish et al., 2002 , pp. 462–484), the four facets of research validity have gained recognition and they are currently covered in many textbooks on research methods in psychology (e.g., Beins, 2009 ; Goodwin, 2010 ; Girden and Kabacoff, 2011 ). Methods and strategies aimed at securing research validity are also discussed in these and other sources. To simplify the description, construct validity is sought by using well-established definitions and measurement procedures for variables, internal validity is sought by ensuring that extraneous variables have been controlled and confounds have been eliminated, and external validity is sought by observing and measuring dependent variables under natural conditions or under an appropriate representation of them. The fourth aspect of research validity, which Cook and Campbell called statistical conclusion validity (SCV), is the subject of this paper.
Cook and Campbell, 1979 , pp. 39–50) discussed that SCV pertains to the extent to which data from a research study can reasonably be regarded as revealing a link (or lack thereof) between independent and dependent variables as far as statistical issues are concerned . This particular facet was separated from other factors acting in the same direction (the three other facets of validity) and includes three aspects: (1) whether the study has enough statistical power to detect an effect if it exists, (2) whether there is a risk that the study will “reveal” an effect that does not actually exist, and (3) how can the magnitude of the effect be confidently estimated. They nevertheless considered the latter aspect as a mere step ahead once the first two aspects had been satisfactorily solved, and they summarized their position by stating that SCV “refers to inferences about whether it is reasonable to presume covariation given a specified α level and the obtained variances” ( Cook and Campbell, 1979 , p. 41). Given that mentioning “the obtained variances” was an indirect reference to statistical power and mentioning α was a direct reference to statistical significance, their position about SCV may have seemed to only entail consideration that the statistical decision can be incorrect as a result of Type-I and Type-II errors. Perhaps as a consequence of this literal interpretation, review papers studying SCV in published research have focused on power and significance (e.g., Ottenbacher, 1989 ; Ottenbacher and Maas, 1999 ), strategies aimed at increasing SCV have only considered these issues (e.g., Howard et al., 1983 ), and tutorials on the topic only or almost only mention these issues along with effect sizes (e.g., Orme, 1991 ; Austin et al., 1998 ; Rankupalli and Tandon, 2010 ). This emphasis on issues of significance and power may also be the reason that some sources refer to threats to SCV as “any factor that leads to a Type-I or a Type-II error” (e.g., Girden and Kabacoff, 2011 , p. 6; see also Rankupalli and Tandon, 2010 , Section 1.2), as if these errors had identifiable causes that could be prevented. It should be noted that SCV has also occasionally been purported to reflect the extent to which pre-experimental designs provide evidence for causation ( Lee, 1985 ) or the extent to which meta-analyses are based on representative results that make the conclusion generalizable ( Elvik, 1998 ).
But Cook and Campbell’s (1979 , p. 80) aim was undoubtedly broader, as they stressed that SCV “is concerned with sources of random error and with the appropriate use of statistics and statistical tests ” (italics added). Moreover, Type-I and Type-II errors are an essential and inescapable consequence of the statistical decision theory underlying significance testing and, as such, the potential occurrence of one or the other of these errors cannot be prevented. The actual occurrence of them for the data on hand cannot be assessed either. Type-I and Type-II errors will always be with us and, hence, SCV is only trivially linked to the fact that research will never unequivocally prove or reject any statistical null hypothesis or its originating research hypothesis. Cook and Campbell seemed to be well aware of this issue when they stressed that SCV refers to reasonable inferences given a specified significance level and a given power. In addition, Stevens (1950 , p. 121) forcefully emphasized that “ it is a statistician’s duty to be wrong the stated number of times,” implying that a researcher should accept the assumed risks of Type-I and Type-II errors, use statistical methods that guarantee the assumed error rates, and consider these as an essential part of the research process. From this position, these errors do not affect SCV unless their probability differs meaningfully from that which was assumed. And this is where an alternative perspective on SCV enters the stage, namely, whether the data were analyzed properly so as to extract conclusions that faithfully reflect what the data have to say about the research question. A negative answer raises concerns about SCV beyond the triviality of Type-I or Type-II errors. There are actually two types of threat to SCV from this perspective. One is when the data are subjected to thoroughly inadequate statistical analyses that do not match the characteristics of the design used to collect the data or that cannot logically give an answer to the research question. The other is when a proper statistical test is used but it is applied under conditions that alter the stated risk probabilities. In the former case, the conclusion will be wrong except by accident; in the latter, the conclusion will fail to be incorrect with the declared probabilities of Type-I and Type-II errors.
The position elaborated in the foregoing paragraph is well summarized in Milligan and McFillen’s (1984 , p. 439) statement that “under normal conditions (…) the researcher will not know when a null effect has been declared significant or when a valid effect has gone undetected (…) Unfortunately, the statistical conclusion validity, and the ultimate value of the research, rests on the explicit control of (Type-I and Type-II) error rates.” This perspective on SCV is explicitly discussed in some textbooks on research methods (e.g., Beins, 2009 , pp. 139–140; Goodwin, 2010 , pp. 184–185) and some literature reviews have been published that reveal a sound failure of SCV in these respects.
For instance, Milligan and McFillen’s (1984 , p. 438) reviewed evidence that “the business research community has succeeded in publishing a great deal of incorrect and statistically inadequate research” and they dissected and discussed in detail four additional cases (among many others that reportedly could have been chosen) in which a breach of SCV resulted from gross mismatches between the research design and the statistical analysis. Similarly, García-Pérez (2005) reviewed alternative methods to compute confidence intervals for proportions and discussed three papers (among many others that reportedly could have been chosen) in which inadequate confidence intervals had been computed. More recently, Bakker and Wicherts (2011) conducted a thorough analysis of psychological papers and estimated that roughly 50% of published papers contain reporting errors, although they only checked whether the reported p value was correct and not whether the statistical test used was appropriate. A similar analysis carried out by Nieuwenhuis et al. (2011) revealed that 50% of the papers reporting the results of a comparison of two experimental effects in top neuroscience journals had used an incorrect statistical procedure. And Bland and Altman (2011) reported further data on the prevalence of incorrect statistical analyses of a similar nature.
An additional indicator of the use of inadequate statistical procedures arises from consideration of published papers whose title explicitly refers to a re-analysis of data reported in some other paper. A literature search for papers including in their title the terms “a re-analysis,” “a reanalysis,” “re-analyses,” “reanalyses,” or “alternative analysis” was conducted on May 3, 2012 in the Web of Science (WoS; http://thomsonreuters.com ), which rendered 99 such papers with subject area “Psychology” published in 1990 or later. Although some of these were false positives, a sizeable number of them actually discussed the inadequacy of analyses carried out by the original authors and reported the results of proper alternative analyses that typically reversed the original conclusion. This type of outcome upon re-analyses of data are more frequent than the results of this quick and simple search suggest, because the information for identification is not always included in the title of the paper or is included in some other form: For a simple example, the search for the clause “a closer look” in the title rendered 131 papers, many of which also presented re-analyses of data that reversed the conclusion of the original study.
Poor design or poor sample size planning may, unbeknownst to the researcher, lead to unacceptable Type-II error rates, which will certainly affect SCV (as long as the null is not rejected; if it is, the probability of a Type-II error is irrelevant). Although insufficient power due to lack of proper planning has consequences on statistical tests, the thread of this paper de-emphasizes this aspect of SCV (which should perhaps more reasonably fit within an alternative category labeled design validity ) and emphasizes the idea that SCV holds when statistical conclusions are incorrect with the stated probabilities of Type-I and Type-II errors (whether the latter was planned or simply computed). Whether or not the actual significance level used in the research or the power that it had is judged acceptable is another issue, which does not affect SCV: The statistical conclusion is valid within the stated (or computed) error probabilities. A breach of SCV occurs, then, when the data are not subjected to adequate statistical analyses or when control of Type-I or Type-II errors is lost.
It should be noted that a further component was included into consideration of SCV in Shadish et al.’s (2002) sequel to Cook and Campbell’s (1979 ) book, namely, effect size. Effect size relates to what has been called a Type-III error ( Crawford et al., 1998 ), that is, a statistically significant result that has no meaningful practical implication and that only arises from the use of a huge sample. This issue is left aside in the present paper because adequate consideration and reporting of effect sizes precludes Type-III errors, although the recommendations of Wilkinson and The Task Force on Statistical Inference (1999) in this respect are not always followed. Consider, e.g., Lippa’s (2007) study of the relation between sex drive and sexual attraction. Correlations generally lower than 0.3 in absolute value were declared strong as a result of p values below 0.001. With sample sizes sometimes nearing 50,000 paired observations, even correlations valued at 0.04 turned out significant in this study. More attention to effect sizes is certainly needed, both by researchers and by journal editors and reviewers.
The remainder of this paper analyzes three common practices that result in SCV breaches, also discussing simple replacements for them.
Stopping Rules for Data Collection without Control of Type-I Error Rates
The asymptotic theory that provides justification for null hypothesis significance testing (NHST) assumes what is known as fixed sampling , which means that the size n of the sample is not itself a random variable or, in other words, that the size of the sample has been decided in advance and the statistical test is performed once the entire sample of data has been collected. Numerous procedures have been devised to determine the size that a sample must have according to planned power ( Ahn et al., 2001 ; Faul et al., 2007 ; Nisen and Schwertman, 2008 ; Jan and Shieh, 2011 ), the size of the effect sought to be detected ( Morse, 1999 ), or the width of the confidence intervals of interest ( Graybill, 1958 ; Boos and Hughes-Oliver, 2000 ; Shieh and Jan, 2012 ). For reviews, see Dell et al. (2002) and Maxwell et al. (2008) . In many cases, a researcher simply strives to gather as large a sample as possible. Asymptotic theory supports NHST under fixed sampling assumptions, whether or not the size of the sample was planned.
In contrast to fixed sampling, sequential sampling implies that the number of observations is not fixed in advance but depends by some rule on the observations already collected ( Wald, 1947 ; Anscombe, 1953 ; Wetherill, 1966 ). In practice, data are analyzed as they come in and data collection stops when the observations collected thus far satisfy some criterion. The use of sequential sampling faces two problems ( Anscombe, 1953 , p. 6): (i) devising a suitable stopping rule and (ii) finding a suitable test statistic and determining its sampling distribution. The mere statement of the second problem evidences that the sampling distribution of conventional test statistics for fixed sampling no longer holds under sequential sampling. These sampling distributions are relatively easy to derive in some cases, particularly in those involving negative binomial parameters ( Anscombe, 1953 ; García-Pérez and Núñez-Antón, 2009 ). The choice between fixed and sequential sampling (sometimes portrayed as the “experimenter’s intention”; see Wagenmakers, 2007 ) has important ramifications for NHST because the probability that the observed data are compatible (by any criterion) with a true null hypothesis generally differs greatly across sampling methods. This issue is usually bypassed by those who look at the data as a “sure fact” once collected, as if the sampling method used to collect the data did not make any difference or should not affect how the data are interpreted.
There are good reasons for using sequential sampling in psychological research. For instance, in clinical studies in which patients are recruited on the go, the experimenter may want to analyze data as they come in to be able to prevent the administration of a seemingly ineffective or even hurtful treatment to new patients. In studies involving a waiting-list control group, individuals in this group are generally transferred to an experimental group midway along the experiment. In studies with laboratory animals, the experimenter may want to stop testing animals before the planned number has been reached so that animals are not wasted when an effect (or the lack thereof) seems established. In these and analogous cases, the decision as to whether data will continue to be collected results from an analysis of the data collected thus far, typically using a statistical test that was devised for use in conditions of fixed sampling. In other cases, experimenters test their statistical hypothesis each time a new observation or block of observations is collected, and continue the experiment until they feel the data are conclusive one way or the other. Software has been developed that allows experimenters to find out how many more observations will be needed for a marginally non-significant result to become significant on the assumption that sample statistics will remain invariant when the extra data are collected ( Morse, 1998 ).
The practice of repeated testing and optional stopping has been shown to affect in unpredictable ways the empirical Type-I error rate of statistical tests designed for use under fixed sampling ( Anscombe, 1954 ; Armitage et al., 1969 ; McCarroll et al., 1992 ; Strube, 2006 ; Fitts, 2011a ). The same holds when a decision is made to collect further data on evidence of a marginally (non) significant result ( Shun et al., 2001 ; Chen et al., 2004 ). The inaccuracy of statistical tests in these conditions represents a breach of SCV, because the statistical conclusion thus fails to be incorrect with the assumed (and explicitly stated) probabilities of Type-I and Type-II errors. But there is an easy way around the inflation of Type-I error rates from within NHST, which solves the threat to SCV that repeated testing and optional stopping entail.
In what appears to be the first development of a sequential procedure with control of Type-I error rates in psychology, Frick (1998) proposed that repeated statistical testing be conducted under the so-called COAST (composite open adaptive sequential test) rule: If the test yields p < 0.01, stop collecting data and reject the null; if it yields p > 0.36, stop also and do not reject the null; otherwise, collect more data and re-test. The low criterion at 0.01 and the high criterion at 0.36 were selected through simulations so as to ensure a final Type-I error rate of 0.05 for paired-samples t tests. Use of the same low and high criteria rendered similar control of Type-I error rates for tests of the product-moment correlation, but they yielded slightly conservative tests of the interaction in 2 × 2 between-subjects ANOVAs. Frick also acknowledged that adjusting the low and high criteria might be needed in other cases, although he did not address them. This has nevertheless been done by others who have modified and extended Frick’s approach (e.g., Botella et al., 2006 ; Ximenez and Revuelta, 2007 ; Fitts, 2010a , b , 2011b ). The result is sequential procedures with stopping rules that guarantee accurate control of final Type-I error rates for the statistical tests that are more widely used in psychological research.
Yet, these methods do not seem to have ever been used in actual research, or at least their use has not been acknowledged. For instance, of the nine citations to Frick’s (1998) paper listed in WoS as of May 3, 2012, only one is from a paper (published in 2011) in which the COAST rule was reportedly used, although unintendedly. And not a single citation is to be found in WoS from papers reporting the use of the extensions and modifications of Botella et al. (2006) or Ximenez and Revuelta (2007) . Perhaps researchers in psychology invariably use fixed sampling, but it is hard to believe that “data peeking” or “data monitoring” was never used, or that the results of such interim analyses never led researchers to collect some more data. Wagenmakers (2007 , p. 785) regretted that “it is not clear what percentage of p values reported in experimental psychology have been contaminated by some form of optional stopping. There is simply no information in Results sections that allows one to assess the extent to which optional stopping has occurred.” This incertitude was quickly resolved by John et al. (2012) . They surveyed over 2000 psychologists with highly revealing results: Respondents affirmatively admitted to the practices of data peeking, data monitoring, or conditional stopping in rates that varied between 20 and 60%.
Besides John et al.’s (2012) proposal that authors disclose these details in full and Simmons et al.’s (2011) proposed list of requirements for authors and guidelines for reviewers, the solution to the problem is simple: Use strategies that control Type-I error rates upon repeated testing and optional stopping. These strategies have been widely used in biomedical research for decades ( Bauer and Köhne, 1994 ; Mehta and Pocock, 2011 ). There is no reason that psychological research should ignore them and give up efficient research with control of Type-I error rates, particularly when these strategies have also been adapted and further developed for use under the most common designs in psychological research ( Frick, 1998 ; Botella et al., 2006 ; Ximenez and Revuelta, 2007 ; Fitts, 2010a , b ).
It should also be stressed that not all instances of repeated testing or optional stopping without control of Type-I error rates threaten SCV. A breach of SCV occurs only when the conclusion regarding the research question is based on the use of these practices. For an acceptable use, consider the study of Xu et al. (2011) . They investigated order preferences in primates to find out whether primates preferred to receive the best item first rather than last. Their procedure involved several experiments and they declared that “three significant sessions (two-tailed binomial tests per session, p < 0.05) or 10 consecutive non-significant sessions were required from each monkey before moving to the next experiment. The three significant sessions were not necessarily consecutive (…) Ten consecutive non-significant sessions were taken to mean there was no preference by the monkey” (p. 2304). In this case, the use of repeated testing with optional stopping at a nominal 95% significance level for each individual test is part of the operational definition of an outcome variable used as a criterion to proceed to the next experiment. And, in any event, the overall probability of misclassifying a monkey according to this criterion is certainly fixed at a known value that can easily be worked out from the significance level declared for each individual binomial test. One may object to the value of the resultant risk of misclassification, but this does not raise concerns about SCV.
In sum, the use of repeated testing with optional stopping threatens SCV for lack of control of Type-I and Type-II error rates. A simple way around this is to refrain from these practices and adhere to the fixed sampling assumptions of statistical tests; otherwise, use the statistical methods that have been developed for use with repeated testing and optional stopping.
Preliminary Tests of Assumptions
To derive the sampling distribution of test statistics used in parametric NHST, some assumptions must be made about the probability distribution of the observations or about the parameters of these distributions. The assumptions of normality of distributions (in all tests), homogeneity of variances (in Student’s two-sample t test for means or in ANOVAs involving between-subjects factors), sphericity (in repeated-measures ANOVAs), homoscedasticity (in regression analyses), or homogeneity of regression slopes (in ANCOVAs) are well known cases. The data on hand may or may not meet these assumptions and some parametric tests have been devised under alternative assumptions (e.g., Welch’s test for two-sample means, or correction factors for the degrees of freedom of F statistics from ANOVAs). Most introductory statistics textbooks emphasize that the assumptions underlying statistical tests must be formally tested to guide the choice of a suitable test statistic for the null hypothesis of interest. Although this recommendation seems reasonable, serious consequences on SCV arise from following it.
Numerous studies conducted over the past decades have shown that the two-stage approach of testing assumptions first and subsequently testing the null hypothesis of interest has severe effects on Type-I and Type-II error rates. It may seem at first sight that this is simply the result of cascaded binary decisions each of which has its own Type-I and Type-II error probabilities; yet, this is the result of more complex interactions of Type-I and Type-II error rates that do not have fixed (empirical) probabilities across the cases that end up treated one way or the other according to the outcomes of the preliminary test: The resultant Type-I and Type-II error rates of the conditional test cannot be predicted from those of the preliminary and conditioned tests. A thorough analysis of what factors affect the Type-I and Type-II error rates of two-stage approaches is beyond the scope of this paper but readers should be aware that nothing suggests in principle that a two-stage approach might be adequate. The situations that have been more thoroughly studied include preliminary goodness-of-fit tests for normality before conducting a one-sample t test ( Easterling and Anderson, 1978 ; Schucany and Ng, 2006 ; Rochon and Kieser, 2011 ), preliminary tests of equality of variances before conducting a two-sample t test for means ( Gans, 1981 ; Moser and Stevens, 1992 ; Zimmerman, 1996 , 2004 ; Hayes and Cai, 2007 ), preliminary tests of both equality of variances and normality preceding two-sample t tests for means ( Rasch et al., 2011 ), or preliminary tests of homoscedasticity before regression analyses ( Caudill, 1988 ; Ng and Wilcox, 2011 ). These and other studies provide evidence that strongly advises against conducting preliminary tests of assumptions. Almost all of these authors explicitly recommended against these practices and hoped for the misleading and misguided advice given in introductory textbooks to be removed. Wells and Hintze(2007 , p. 501) concluded that “checking the assumptions using the same data that are to be analyzed, although attractive due to its empirical nature, is a fruitless endeavor because of its negative ramifications on the actual test of interest.” The ramifications consist of substantial but unknown alterations of Type-I and Type-II error rates and, hence, a breach of SCV.
Some authors suggest that the problem can be solved by replacing the formal test of assumptions with a decision based on a suitable graphical display of the data that helps researchers judge by eye whether the assumption is tenable. It should be emphasized that the problem still remains, because the decision on how to analyze the data is conditioned on the results of a preliminary analysis. The problem is not brought about by a formal preliminary test, but by the conditional approach to data analysis. The use of a non-formal preliminary test only prevents a precise investigation of the consequences on Type-I and Type-II error rates. But the “out of sight, out of mind” philosophy does not eliminate the problem.
It thus seems that a researcher must make a choice between two evils: either not testing assumptions (and, thus, threatening SCV as a result of the uncontrolled Type-I and Type-II error rates that arise from a potentially undue application of the statistical test) or testing them (and, then, also losing control of Type-I and Type-II error rates owing to the two-stage approach). Both approaches are inadequate, as applying non-robust statistical tests to data that do not satisfy the assumptions has generally as severe implications on SCV as testing preliminary assumptions in a two-stage approach. One of the solutions to the dilemma consists of switching to statistical procedures that have been designed for use under the two-stage approach. For instance, Albers et al. (2000) used second-order asymptotics to derive the size and power of a two-stage test for independent means preceded by a test of equality of variances. Unfortunately, derivations of this type are hard to carry out and, hence, they are not available for most of the cases of interest. A second solution consists of using classical test statistics that have been shown to be robust to violation of their assumptions. Indeed, dependable unconditional tests for means or for regression parameters have been identified (see Sullivan and D’Agostino, 1992 ; Lumley et al., 2002 ; Zimmerman, 2004 , 2011 ; Hayes and Cai, 2007 ; Ng and Wilcox, 2011 ). And a third solution is switching to modern robust methods (see, e.g., Wilcox and Keselman, 2003 ; Keselman et al., 2004 ; Wilcox, 2006 ; Erceg-Hurn and Mirosevich, 2008 ; Fried and Dehling, 2011 ).
Avoidance of the two-stage approach in either of these ways will restore SCV while observing the important requirement that statistical methods should be used whose assumptions are not violated by the characteristics of the data.
Regression as a Means to Investigate Bivariate Relations of all Types
Correlational methods define one of the branches of scientific psychology ( Cronbach, 1957 ) and they are still widely used these days in some areas of psychology. Whether in regression analyses or in latent variable analyses ( Bollen, 2002 ), vast amounts of data are subjected to these methods. Regression analyses rely on an assumption that is often overlooked in psychology, namely, that the predictor variables have fixed values and are measured without error. This assumption, whose validity can obviously be assessed without recourse to any preliminary statistical test, is listed in all statistics textbooks.
In some areas of psychology, predictors actually have this characteristic because they are physical variables defining the magnitude of stimuli, and any error with which these magnitudes are measured (or with which stimuli with the selected magnitudes are created) is negligible in practice. Among others, this is the case in psychophysical studies aimed at estimating psychophysical functions describing the form of the relation between physical magnitude and perceived magnitude (e.g., Green, 1982 ) or psychometric functions describing the form of the relation between physical magnitude and performance in a detection, discrimination, or identification task ( Armstrong and Marks, 1997 ; Saberi and Petrosyan, 2004 ; García-Pérez et al., 2011 ). Regression or analogous methods are typically used to estimate the parameters of these relations, with stimulus magnitude as the independent variable and perceived magnitude (or performance) as the dependent variable. The use of regression in these cases is appropriate because the independent variable has fixed values measured without error (or with a negligible error). Another area in which the use of regression is permissible is in simulation studies on parameter recovery ( García-Pérez et al., 2010 ), where the true parameters generating the data are free of measurement error by definition.
But very few other predictor variables used in psychology meet this requirement, as they are often test scores or performance measures that are typically affected by non-negligible and sometimes large measurement error. This is the case of the proportion of hits and the proportion of false alarms in psychophysical tasks, whose theoretical relation is linear under some signal detection models ( DeCarlo, 1998 ) and, thus, suggests the use of simple linear regression to estimate its parameters. Simple linear regression is also sometimes used as a complement to statistical tests of equality of means in studies in which equivalence or agreement is assessed (e.g., Maylor and Rabbitt, 1993 ; Baddeley and Wilson, 2002 ), and in these cases equivalence implies that the slope should not differ significantly from unity and that the intercept should not differ significantly from zero. The use of simple linear regression is also widespread in priming studies after Greenwald et al. (1995 ; see also Draine and Greenwald, 1998 ), where the intercept (and sometimes the slope) of the linear regression of priming effect on detectability of the prime are routinely subjected to NHST.
In all the cases just discussed and in many others where the X variable in the regression of Y on X is measured with error, a study of the relation between X and Y through regression is inadequate and has serious consequences on SCV. The least of these problems is that there is no basis for assigning the roles of independent and dependent variable in the regression equation (as a non-directional relation exists between the variables, often without even a temporal precedence relation), but regression parameters will differ according to how these roles are assigned. In influential papers of which most researchers in psychology seem to be unaware, Wald (1940) and Mandansky (1959) distinguished regression relations from structural relations, the latter reflecting the case in which both variables are measured with error. Both authors illustrated the consequences of fitting a regression line when a structural relation is involved and derived suitable estimators and significance tests for the slope and intercept parameters of a structural relation. This topic was brought to the attention of psychologists by Isaac (1970) in a criticism of Treisman and Watts’ (1966) use of simple linear regression to assess the equivalence of two alternative estimates of psychophysical sensitivity ( d ′ measures from signal detection theory analyses). The difference between regression and structural relations is briefly mentioned in passing in many elementary books on regression, the issue of fitting structural relations (sometimes referred to as Deming’s regression or the errors-in-variables regression model ) is addressed in detail in most intermediate and advance books on regression (e.g., Fuller, 1987 ; Draper and Smith, 1998 ) and hands-on tutorials have been published (e.g., Cheng and Van Ness, 1994 ; Dunn and Roberts, 1999 ; Dunn, 2007 ). But this type of analysis is not in the toolbox of the average researcher in psychology 1 . In contrast, recourse to this type analysis is quite common in the biomedical sciences.
Use of this commendable method may generalize when researchers realize that estimates of the slope β and the intercept α of a structural relation can be easily computed through
where X ̄ , Ȳ , S x 2 , S y 2 , and S x y are the sample means, variances, and covariance of X and Y , and λ = σ ε y 2 ∕ σ ε x 2 is the ratio of the variances of measurement errors in Y and in X . When X and Y are the same variable measured at different times or under different conditions (as in Maylor and Rabbitt, 1993 ; Baddeley and Wilson, 2002 ), λ = 1 can safely be assumed (for an actual application, see Smith et al., 2004 ). In other cases, a rough estimate can be used, as the estimates of α and β have been shown to be robust except under extreme departures of the guesstimated λ from its true value ( Ketellapper, 1983 ).
For illustration, consider Yeshurun et al. (2008) comparison of signal detection theory estimates of d ′ in each of the intervals of a two alternative forced-choice task, which they pronounced different as revealed by a regression analysis through the origin. Note that this is the context in which Isaac (1970) had illustrated the inappropriateness of regression. The data are shown in Figure 1 , and Yeshurun et al. rejected equality of d 1 ′ and d 2 ′ because the regression slope through the origin (red line, whose slope is 0.908) differed significantly from unity: The 95% confidence interval for the slope ranged between 0.844 and 0.973. Using Eqs 1 and 2, the estimated structural relation is instead given by the blue line in Figure 1 . The difference seems minor by eye, but the slope of the structural relation is 0.963, which is not significantly different from unity ( p = 0.738, two-tailed; see Isaac, 1970 , p. 215). This outcome, which reverses a conclusion raised upon inadequate data analyses, is representative of other cases in which the null hypothesis H 0 : β = 1 was rejected. The reason is dual: (1) the slope of a structural relation is estimated with severe bias through regression ( Riggs et al., 1978 ; Kalantar et al., 1995 ; Hawkins, 2002 ) and (2) regression-based statistical tests of H 0 : β = 1 render empirical Type-I error rates that are much higher than the nominal rate when both variables are measured with error ( García-Pérez and Alcalá-Quintana, 2011 ).
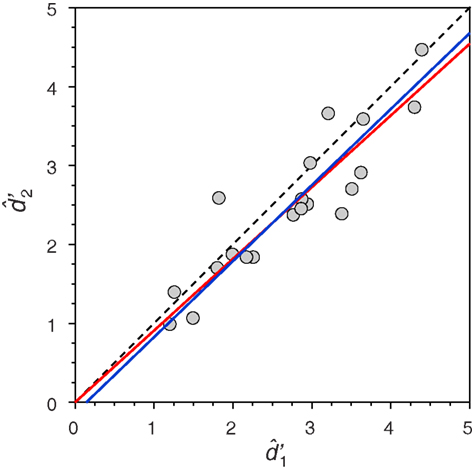
Figure 1. Replot of data from Yeshurun et al. (2008 , their Figure 8) with their fitted regression line through the origin (red line) and a fitted structural relation (blue line) . The identity line is shown with dashed trace for comparison. For additional analyses bearing on the SCV of the original study, see García-Pérez and Alcalá-Quintana ( 2011 ).
In sum, SCV will improve if structural relations instead of regression equations were fitted when both variables are measured with error.
Type-I and Type-II errors are essential components of the statistical decision theory underlying NHST and, therefore, data can never be expected to answer a research question unequivocally. This paper has promoted a view of SCV that de-emphasizes consideration of these unavoidable errors and considers instead two alternative issues: (1) whether statistical tests are used that match the research design, goals of the study, and formal characteristics of the data and (2) whether they are applied in conditions under which the resultant Type-I and Type-II error rates match those that are declared as limiting the validity of the conclusion. Some examples of common threats to SCV in these respects have been discussed and simple and feasible solutions have been proposed. For reasons of space, another threat to SCV has not been covered in this paper, namely, the problems arising from multiple testing (i.e., in concurrent tests of more than one hypothesis). Multiple testing is commonplace in brain mapping studies and some implications on SCV have been discussed, e.g., by Bennett et al. (2009) , Vul et al. (2009a , b ), and Vecchiato et al. (2010) .
All the discussion in this paper has assumed the frequentist approach to data analysis. In closing, and before commenting on how SCV could be improved, a few words are worth about how Bayesian approaches fare on SCV.
The Bayesian Approach
Advocates of Bayesian approaches to data analysis, hypothesis testing, and model selection (e.g., Jennison and Turnbull, 1990 ; Wagenmakers, 2007 ; Matthews, 2011 ) overemphasize the problems of the frequentist approach and praise the solutions offered by the Bayesian approach: Bayes factors (BFs) for hypothesis testing, credible intervals for interval estimation, Bayesian posterior probabilities, Bayesian information criterion (BIC) as a tool for model selection and, above all else, strict reliance on observed data and independence of the sampling plan (i.e., fixed vs. sequential sampling). There is unquestionable merit in these alternatives and a fair comparison with their frequentist counterparts requires a detailed analysis that is beyond the scope of this paper. Yet, I cannot resist the temptation of commenting on the presumed problems of the frequentist approach and also on the standing of the Bayesian approach with respect to SCV.
One of the preferred objections to p values is that they relate to data that were never collected and which, thus, should not affect the decision of what hypothesis the observed data support or fail to support. Intuitively appealing as it may seem, the argument is flawed because the referent for a p value is not other data sets that could have been observed in undone replications of the same experiment. Instead, the referent is the properties of the test statistic itself, which is guaranteed to have the declared sampling distribution when data are collected as assumed in the derivation of such distribution. Statistical tests are calibrated procedures with known properties, and this calibration is what makes their results interpretable. As is the case for any other calibrated procedure or measuring instrument, the validity of the outcome only rests on adherence to the usage specifications. And, of course, the test statistic and the resultant p value on application cannot be blamed for the consequences of a failure to collect data properly or to apply the appropriate statistical test.
Consider a two-sample t test for means. Those who need a referent may want to notice that the p value for the data from a given experiment relates to the uncountable times that such test has been applied to data from any experiment in any discipline. Calibration of the t test ensures that a proper use with a significance level of, say, 5% will reject a true null hypothesis on 5% of the occasions, no matter what the experimental hypothesis is, what the variables are, what the data are, what the experiment is about, who carries it out, or in what research field. What a p value indicates is how tenable it is that the t statistic will attain the observed value if the null were correct, with only a trivial link to the data observed in the experiment of concern. And this only places in a precise quantitative framework the logic that the man on the street uses to judge, for instance, that getting struck by lightning four times over the past 10 years is not something that could identically have happened to anybody else, or that the source of a politician’s huge and untraceable earnings is not the result of allegedly winning top lottery prizes numerous times over the past couple of years. In any case, the advantage of the frequentist approach as regards SCV is that the probability of a Type-I or a Type-II error can be clearly and unequivocally stated, which is not to be mistaken for a statement that a p value is the probability of a Type-I error in the current case, or that it is a measure of the strength of evidence against the null that the current data provide. The most prevalent problems of p values are their potential for misuse and their widespread misinterpretation ( Nickerson, 2000 ). But misuse or misinterpretation do not make NHST and p values uninterpretable or worthless.
Bayesian approaches are claimed to be free of these presumed problems, yielding a conclusion that is exclusively grounded on the data. In a naive account of Bayesian hypothesis testing, Malakoff (1999) attributes to biostatistician Steven Goodman the assertion that the Bayesian approach “says there is an X% probability that your hypothesis is true–not that there is some convoluted chance that if you assume the null hypothesis is true, you will get a similar or more extreme result if you repeated your experiment thousands of times.” Besides being misleading and reflecting a poor understanding of the logic of calibrated NHST methods, what goes unmentioned in this and other accounts is that the Bayesian potential to find out the probability that the hypothesis is true will not materialize without two crucial extra pieces of information. One is the a priori probability of each of the competing hypotheses, which certainly does not come from the data. The other is the probability of the observed data under each of the competing hypothesis, which has the same origin as the frequentist p value and whose computation requires distributional assumptions that must necessarily take the sampling method into consideration.
In practice, Bayesian hypothesis testing generally computes BFs and the result might be stated as “the alternative hypothesis is x times more likely than the null,” although the probability that this type of statement is wrong is essentially unknown. The researcher may be content with a conclusion of this type, but how much of these odds comes from the data and how much comes from the extra assumptions needed to compute a BF is undecipherable. In many cases research aims at gathering and analyzing data to make informed decisions such as whether application of a treatment should be discontinued, whether changes should be introduced in an educational program, whether daytime headlights should be enforced, or whether in-car use of cell phones should be forbidden. Like frequentist analyses, Bayesian approaches do not guarantee that the decisions will be correct. One may argue that stating how much more likely is one hypothesis over another bypasses the decision to reject or not reject any of them and, then, that Bayesian approaches to hypothesis testing are free of Type-I and Type-II errors. Although this is technically correct, the problem remains from the perspective of SCV: Statistics is only a small part of a research process whose ultimate goal is to reach a conclusion and make a decision, and researchers are in a better position to defend their claims if they can supplement them with a statement of the probability with which those claims are wrong.
Interestingly, analyses of decisions based on Bayesian approaches have revealed that they are no better than frequentist decisions as regards Type-I and Type-II errors and that parametric assumptions (i.e., the choice of prior and the assumed distribution of the observations) crucially determine the performance of Bayesian methods. For instance, Bayesian estimation is also subject to potentially large bias and lack of precision ( Alcalá-Quintana and García-Pérez, 2004 ; García-Pérez and Alcalá-Quintana, 2007 ), the coverage probability of Bayesian credible intervals can be worse than that of frequentist confidence intervals ( Agresti and Min, 2005 ; Alcalá-Quintana and García-Pérez, 2005 ), and the Bayesian posterior probability in hypothesis testing can be arbitrarily large or small ( Zaslavsky, 2010 ). On another front, use of BIC for model selection may discard a true model as often as 20% of the times, while a concurrent 0.05-size chi-square test rejects the true model between 3 and 7% of times, closely approximating its stated performance (García-Pérez and Alcalá-Quintana, 2012 ). In any case, the probabilities of Type-I and Type-II errors in practical decisions made from the results of Bayesian analyses will always be unknown and beyond control.
Improving the SCV of Research
Most breaches of SCV arise from a poor understanding of statistical procedures and the resultant inadequate usage. These problems can be easily corrected, as illustrated in this paper, but the problems will not have arisen if researchers had had a better statistical training in the first place. There was a time in which one simply could not run statistical tests without a moderate understanding of NHST. But these days the application of statistical tests is only a mouse-click away and all that students regard as necessary is learning the rule by which p values pouring out of statistical software tell them whether the hypothesis is to be accepted or rejected, as the study of Hoekstra et al. (2012) seems to reveal.
One way to eradicate the problem is by improving statistical education at undergraduate and graduate levels, perhaps not just focusing on giving formal training on a number of methods but by providing students with the necessary foundations that will subsequently allow them to understand and apply methods for which they received no explicit formal training. In their analysis of statistical errors in published papers, Milligan and McFillen(1984 , p. 461) concluded that “in doing projects, it is not unusual for applied researchers or students to use or apply a statistical procedure for which they have received no formal training. This is as inappropriate as a person conducting research in a given content area before reading the existing background literature on the topic. The individual simply is not prepared to conduct quality research. The attitude that statistical technology is secondary or less important to a person’s formal training is shortsighted. Researchers are unlikely to master additional statistical concepts and techniques after leaving school. Thus, the statistical training in many programs must be strengthened. A single course in experimental design and a single course in multivariate analysis is probably insufficient for the typical student to master the course material. Someone who is trained only in theory and content will be ill-prepared to contribute to the advancement of the field or to critically evaluate the research of others.” But statistical education does not seem to have changed much over the subsequent 25 years, as revealed by survey studies conducted by Aiken et al. (1990) , Friedrich et al. (2000) , Aiken et al. (2008) , and Henson et al. (2010) . Certainly some work remains to be done in this arena, and I can only second the proposals made in the papers just cited. But there is also the problem of the unhealthy over-reliance on narrow-breadth, clickable software for data analysis, which practically obliterates any efforts that are made to teach and promote alternatives (see the list of “Pragmatic Factors” discussed by Borsboom, 2006 , pp. 431–434).
The last trench in the battle against breaches of SCV is occupied by journal editors and reviewers. Ideally, they also watch for problems in these respects. There is no known in-depth analysis of the review process in psychology journals (but see Nickerson, 2005 ) and some evidence reveals that the focus of the review process is not always on the quality or validity of the research ( Sternberg, 2002 ; Nickerson, 2005 ). Simmons et al. (2011) and Wicherts et al. (2012) have discussed empirical evidence of inadequate research and review practices (some of which threaten SCV) and they have proposed detailed schemes through which feasible changes in editorial policies may help eradicate not only common threats to SCV but also other threats to research validity in general. I can only second proposals of this type. Reviewers and editors have the responsibility of filtering out (or requesting amendments to) research that does not meet the journal’s standards, including SCV. The analyses of Milligan and McFillen (1984) and Nieuwenhuis et al. (2011) reveal a sizeable number of published papers with statistical errors. This indicates that some remains to be done in this arena too, and some journals have indeed started to take action (see Aickin, 2011 ).
Conflict of Interest Statement
The author declares that the research was conducted in the absence of any commercial or financial relationships that could be construed as a potential conflict of interest.
Acknowledgments
This research was supported by grant PSI2009-08800 (Ministerio de Ciencia e Innovación, Spain).
- ^ SPSS includes a regression procedure called “two-stage least squares” which only implements the method described by Mandansky (1959) as “use of instrumental variables” to estimate the slope of the relation between X and Y . Use of this method requires extra variables with specific characteristics (variables which may simply not be available for the problem at hand) and differs meaningfully from the simpler and more generally applicable method to be discussed next
Agresti, A., and Min, Y. (2005). Frequentist performance of Bayesian confidence intervals for comparing proportions in 2 × 2 contingency tables. Biometrics 61, 515–523.
Pubmed Abstract | Pubmed Full Text | CrossRef Full Text
Ahn, C., Overall, J. E., and Tonidandel, S. (2001). Sample size and power calculations in repeated measurement analysis. Comput. Methods Programs Biomed. 64, 121–124.
Aickin, M. (2011). Test ban: policy of the Journal of Alternative and Complementary Medicine with regard to an increasingly common statistical error. J. Altern. Complement. Med. 17, 1093–1094.
Aiken, L. S., West, S. G., and Millsap, R. E. (2008). Doctoral training in statistics, measurement, and methodology in psychology: replication and extension of Aiken, West, Sechrest, and Reno’s (1990) survey of PhD programs in North America. Am. Psychol. 63, 32–50.
Aiken, L. S., West, S. G., Sechrest, L., and Reno, R. R. (1990). Graduate training in statistics, methodology, and measurement in psychology: a survey of PhD programs in North America. Am. Psychol. 45, 721–734.
CrossRef Full Text
Albers, W., Boon, P. C., and Kallenberg, W. C. M. (2000). The asymptotic behavior of tests for normal means based on a variance pre-test. J. Stat. Plan. Inference 88, 47–57.
Alcalá-Quintana, R., and García-Pérez, M. A. (2004). The role of parametric assumptions in adaptive Bayesian estimation. Psychol. Methods 9, 250–271.
Alcalá-Quintana, R., and García-Pérez, M. A. (2005). Stopping rules in Bayesian adaptive threshold estimation. Spat. Vis. 18, 347–374.
Anscombe, F. J. (1953). Sequential estimation. J. R. Stat. Soc. Series B 15, 1–29.
Anscombe, F. J. (1954). Fixed-sample-size analysis of sequential observations. Biometrics 10, 89–100.
Armitage, P., McPherson, C. K., and Rowe, B. C. (1969). Repeated significance tests on accumulating data. J. R. Stat. Soc. Ser. A 132, 235–244.
Armstrong, L., and Marks, L. E. (1997). Differential effect of stimulus context on perceived length: implications for the horizontal–vertical illusion. Percept. Psychophys. 59, 1200–1213.
Austin, J. T., Boyle, K. A., and Lualhati, J. C. (1998). Statistical conclusion validity for organizational science researchers: a review. Organ. Res. Methods 1, 164–208.
Baddeley, A., and Wilson, B. A. (2002). Prose recall and amnesia: implications for the structure of working memory. Neuropsychologia 40, 1737–1743.
Bakker, M., and Wicherts, J. M. (2011). The (mis) reporting of statistical results in psychology journals. Behav. Res. Methods 43, 666–678.
Bauer, P., and Köhne, K. (1994). Evaluation of experiments with adaptive interim analyses. Biometrics 50, 1029–1041.
Beins, B. C. (2009). Research Methods. A Tool for Life , 2nd Edn. Boston, MA: Pearson Education.
Bennett, C. M., Wolford, G. L., and Miller, M. B. (2009). The principled control of false positives in neuroimaging. Soc. Cogn. Affect. Neurosci. 4, 417–422.
Bland, J. M., and Altman, D. G. (2011). Comparisons against baseline within randomised groups are often used and can be highly misleading. Trials 12, 264.
Bollen, K. A. (2002). Latent variables in psychology and the social sciences. Annu. Rev. Psychol. 53, 605–634.
Boos, D. D., and Hughes-Oliver, J. M. (2000). How large does n have to be for Z and t intervals? Am. Stat. 54, 121–128.
Borsboom, D. (2006). The attack of the psychometricians. Psychometrika 71, 425–440.
Botella, J., Ximenez, C., Revuelta, J., and Suero, M. (2006). Optimization of sample size in controlled experiments: the CLAST rule. Behav. Res. Methods Instrum. Comput. 38, 65–76.
Campbell, D. T., and Stanley, J. C. (1966). Experimental and Quasi-Experimental Designs for Research . Chicago, IL: Rand McNally.
Caudill, S. B. (1988). Type I errors after preliminary tests for heteroscedasticity. Statistician 37, 65–68.
Chen, Y. H. J., DeMets, D. L., and Lang, K. K. G. (2004). Increasing sample size when the unblinded interim result is promising. Stat. Med. 23, 1023–1038.
Cheng, C. L., and Van Ness, J. W. (1994). On estimating linear relationships when both variables are subject to errors. J. R. Stat. Soc. Series B 56, 167–183.
Cook, T. D., and Campbell, D. T. (1979). Quasi-Experimentation: Design and Analysis Issues for Field Settings . Boston, MA: Houghton Mifflin.
Crawford, E. D., Blumenstein, B., and Thompson, I. (1998). Type III statistical error. Urology 51, 675.
Cronbach, L. J. (1957). The two disciplines of scientific psychology. Am. Psychol. 12, 671–684.
DeCarlo, L. T. (1998). Signal detection theory and generalized linear models. Psychol. Methods 3, 186–205.
Dell, R. B., Holleran, S., and Ramakrishnan, R. (2002). Sample size determination. ILAR J. 43, 207–213.
Pubmed Abstract | Pubmed Full Text
Draine, S. C., and Greenwald, A. G. (1998). Replicable unconscious semantic priming. J. Exp. Psychol. Gen. 127, 286–303.
Draper, N. R., and Smith, H. (1998). Applied Regression Analysis , 3rd Edn. New York: Wiley.
Dunn, G. (2007). Regression models for method comparison data. J. Biopharm. Stat. 17, 739–756.
Dunn, G., and Roberts, C. (1999). Modelling method comparison data. Stat. Methods Med. Res. 8, 161–179.
Easterling, R. G., and Anderson, H. E. (1978). The effect of preliminary normality goodness of fit tests on subsequent inference. J. Stat. Comput. Simul. 8, 1–11.
Elvik, R. (1998). Evaluating the statistical conclusion validity of weighted mean results in meta-analysis by analysing funnel graph diagrams. Accid. Anal. Prev. 30, 255–266.
Erceg-Hurn, C. M., and Mirosevich, V. M. (2008). Modern robust statistical methods: an easy way to maximize the accuracy and power of your research. Am. Psychol. 63, 591–601.
Faul, F., Erdfelder, E., Lang, A.-G., and Buchner, A. (2007). G*Power 3: a flexible statistical power analysis program for the social, behavioral, and biomedical sciences. Behav. Res. Methods 39, 175–191.
Fitts, D. A. (2010a). Improved stopping rules for the design of efficient small-sample experiments in biomedical and biobehavioral research. Behav. Res. Methods 42, 3–22.
Fitts, D. A. (2010b). The variable-criteria sequential stopping rule: generality to unequal sample sizes, unequal variances, or to large ANOVAs. Behav. Res. Methods 42, 918–929.
Fitts, D. A. (2011a). Ethics and animal numbers: Informal analyses, uncertain sample sizes, inefficient replications, and Type I errors. J. Am. Assoc. Lab. Anim. Sci. 50, 445–453.
Fitts, D. A. (2011b). Minimizing animal numbers: the variable-criteria sequential stopping rule. Comp. Med. 61, 206–218.
Frick, R. W. (1998). A better stopping rule for conventional statistical tests. Behav. Res. Methods Instrum. Comput. 30, 690–697.
Fried, R., and Dehling, H. (2011). Robust nonparametric tests for the two-sample location problem. Stat. Methods Appl. 20, 409–422.
Friedrich, J., Buday, E., and Kerr, D. (2000). Statistical training in psychology: a national survey and commentary on undergraduate programs. Teach. Psychol. 27, 248–257.
Fuller, W. A. (1987). Measurement Error Models . New York: Wiley.
Gans, D. J. (1981). Use of a preliminary test in comparing two sample means. Commun. Stat. Simul. Comput. 10, 163–174.
García-Pérez, M. A. (2005). On the confidence interval for the binomial parameter. Qual. Quant. 39, 467–481.
García-Pérez, M. A., and Alcalá-Quintana, R. (2007). Bayesian adaptive estimation of arbitrary points on a psychometric function. Br. J. Math. Stat. Psychol. 60, 147–174.
García-Pérez, M. A., and Alcalá-Quintana, R. (2011). Testing equivalence with repeated measures: tests of the difference model of two-alternative forced-choice performance. Span. J. Psychol. 14, 1023–1049.
García-Pérez, M. A., and Alcalá-Quintana, R. (2012). On the discrepant results in synchrony judgment and temporal-order judgment tasks: a quantitative model. Psychon. Bull. Rev. (in press). doi:10.3758/s13423-012-0278-y
García-Pérez, M. A., Alcalá-Quintana, R., and García-Cueto, M. A. (2010). A comparison of anchor-item designs for the concurrent calibration of large banks of Likert-type items. Appl. Psychol. Meas. 34, 580–599.
García-Pérez, M. A., Alcalá-Quintana, R., Woods, R. L., and Peli, E. (2011). Psychometric functions for detection and discrimination with and without flankers. Atten. Percept. Psychophys. 73, 829–853.
García-Pérez, M. A., and Núñez-Antón, V. (2009). Statistical inference involving binomial and negative binomial parameters. Span. J. Psychol. 12, 288–307.
Girden, E. R., and Kabacoff, R. I. (2011). Evaluating Research Articles. From Start to Finish , 3rd Edn. Thousand Oaks, CA: Sage.
Goodwin, C. J. (2010). Research in Psychology. Methods and Design , 6th Edn. Hoboken, NJ: Wiley.
Graybill, F. A. (1958). Determining sample size for a specified width confidence interval. Ann. Math. Stat. 29, 282–287.
Green, B. G. (1982). The perception of distance and location for dual tactile figures. Percept. Psychophys. 31, 315–323.
Greenwald, A. G., Klinger, M. R., and Schuh, E. S. (1995). Activation by marginally perceptible (“subliminal”) stimuli: dissociation of unconscious from conscious cognition. J. Exp. Psychol. Gen. 124, 22–42.
Hawkins, D. M. (2002). Diagnostics for conformity of paired quantitative measurements. Stat. Med. 21, 1913–1935.
Hayes, A. F., and Cai, L. (2007). Further evaluating the conditional decision rule for comparing two independent means. Br. J. Math. Stat. Psychol. 60, 217–244.
Henson, R. K., Hull, D. M., and Williams, C. S. (2010). Methodology in our education research culture: toward a stronger collective quantitative proficiency. Educ. Res. 39, 229–240.
Hoekstra, R., Kiers, H., and Johnson, A. (2012). Are assumptions of well-known statistical techniques checked, and why (not)? Front. Psychol. 3:137. doi:10.3389/fpsyg.2012.00137
Howard, G. S., Obledo, F. H., Cole, D. A., and Maxwell, S. E. (1983). Linked raters’ judgments: combating problems of statistical conclusion validity. Appl. Psychol. Meas. 7, 57–62.
Isaac, P. D. (1970). Linear regression, structural relations, and measurement error. Psychol. Bull. 74, 213–218.
Jan, S.-L., and Shieh, G. (2011). Optimal sample sizes for Welch’s test under various allocation and cost considerations. Behav. Res. Methods 43, 1014–1022.
Jennison, C., and Turnbull, B. W. (1990). Statistical approaches to interim monitoring of clinical trials: a review and commentary. Stat. Sci. 5, 299–317.
John, L. K., Loewenstein, G., and Prelec, D. (2012). Measuring the prevalence of questionable research practices with incentives for truth telling. Psychol. Sci. 23, 524–532.
Kalantar, A. H., Gelb, R. I., and Alper, J. S. (1995). Biases in summary statistics of slopes and intercepts in linear regression with errors in both variables. Talanta 42, 597–603.
Keselman, H. J., Othman, A. R., Wilcox, R. R., and Fradette, K. (2004). The new and improved two-sample t test. Psychol. Sci. 15, 47–51.
Ketellapper, R. H. (1983). On estimating parameters in a simple linear errors-in-variables model. Technometrics 25, 43–47.
Lee, B. (1985). Statistical conclusion validity in ex post facto designs: practicality in evaluation. Educ. Eval. Policy Anal. 7, 35–45.
Lippa, R. A. (2007). The relation between sex drive and sexual attraction to men and women: a cross-national study of heterosexual, bisexual, and homosexual men and women. Arch. Sex. Behav. 36, 209–222.
Lumley, T., Diehr, P., Emerson, S., and Chen, L. (2002). The importance of the normality assumption in large public health data sets. Annu. Rev. Public Health 23, 151–169.
Malakoff, D. (1999). Bayes offers a “new” way to make sense of numbers. Science 286, 1460–1464.
Mandansky, A. (1959). The fitting of straight lines when both variables are subject to error. J. Am. Stat. Assoc. 54, 173–205.
Matthews, W. J. (2011). What might judgment and decision making research be like if we took a Bayesian approach to hypothesis testing? Judgm. Decis. Mak. 6, 843–856.
Maxwell, S. E., Kelley, K., and Rausch, J. R. (2008). Sample size planning for statistical power and accuracy in parameter estimation. Annu. Rev. Psychol. 59, 537–563.
Maylor, E. A., and Rabbitt, P. M. A. (1993). Alcohol, reaction time and memory: a meta-analysis. Br. J. Psychol. 84, 301–317.
McCarroll, D., Crays, N., and Dunlap, W. P. (1992). Sequential ANOVAs and type I error rates. Educ. Psychol. Meas. 52, 387–393.
Mehta, C. R., and Pocock, S. J. (2011). Adaptive increase in sample size when interim results are promising: a practical guide with examples. Stat. Med. 30, 3267–3284.
Milligan, G. W., and McFillen, J. M. (1984). Statistical conclusion validity in experimental designs used in business research. J. Bus. Res. 12, 437–462.
Morse, D. T. (1998). MINSIZE: a computer program for obtaining minimum sample size as an indicator of effect size. Educ. Psychol. Meas. 58, 142–153.
Morse, D. T. (1999). MINSIZE2: a computer program for determining effect size and minimum sample size for statistical significance for univariate, multivariate, and nonparametric tests. Educ. Psychol. Meas. 59, 518–531.
Moser, B. K., and Stevens, G. R. (1992). Homogeneity of variance in the two-sample means test. Am. Stat. 46, 19–21.
Ng, M., and Wilcox, R. R. (2011). A comparison of two-stage procedures for testing least-squares coefficients under heteroscedasticity. Br. J. Math. Stat. Psychol. 64, 244–258.
Nickerson, R. S. (2000). Null hypothesis significance testing: a review of an old and continuing controversy. Psychol. Methods 5, 241–301.
Nickerson, R. S. (2005). What authors want from journal reviewers and editors. Am. Psychol. 60, 661–662.
Nieuwenhuis, S., Forstmann, B. U., and Wagenmakers, E.-J. (2011). Erroneous analyses of interactions in neuroscience: a problem of significance. Nat. Neurosci. 14, 1105–1107.
Nisen, J. A., and Schwertman, N. C. (2008). A simple method of computing the sample size for chi-square test for the equality of multinomial distributions. Comput. Stat. Data Anal. 52, 4903–4908.
Orme, J. G. (1991). Statistical conclusion validity for single-system designs. Soc. Serv. Rev. 65, 468–491.
Ottenbacher, K. J. (1989). Statistical conclusion validity of early intervention research with handicapped children. Except. Child. 55, 534–540.
Ottenbacher, K. J., and Maas, F. (1999). How to detect effects: statistical power and evidence-based practice in occupational therapy research. Am. J. Occup. Ther. 53, 181–188.
Rankupalli, B., and Tandon, R. (2010). Practicing evidence-based psychiatry: 1. Applying a study’s findings: the threats to validity approach. Asian J. Psychiatr. 3, 35–40.
Rasch, D., Kubinger, K. D., and Moder, K. (2011). The two-sample t test: pre-testing its assumptions does not pay off. Stat. Pap. 52, 219–231.
Riggs, D. S., Guarnieri, J. A., and Addelman, S. (1978). Fitting straight lines when both variables are subject to error. Life Sci. 22, 1305–1360.
Rochon, J., and Kieser, M. (2011). A closer look at the effect of preliminary goodness-of-fit testing for normality for the one-sample t-test. Br. J. Math. Stat. Psychol. 64, 410–426.
Saberi, K., and Petrosyan, A. (2004). A detection-theoretic model of echo inhibition. Psychol. Rev. 111, 52–66.
Schucany, W. R., and Ng, H. K. T. (2006). Preliminary goodness-of-fit tests for normality do not validate the one-sample Student t. Commun. Stat. Theory Methods 35, 2275–2286.
Shadish, W. R., Cook, T. D., and Campbell, D. T. (2002). Experimental and Quasi-Experimental Designs for Generalized Causal Inference . Boston, MA: Houghton Mifflin.
Shieh, G., and Jan, S.-L. (2012). Optimal sample sizes for precise interval estimation of Welch’s procedure under various allocation and cost considerations. Behav. Res. Methods 44, 202–212.
Shun, Z. M., Yuan, W., Brady, W. E., and Hsu, H. (2001). Type I error in sample size re-estimations based on observed treatment difference. Stat. Med. 20, 497–513.
Simmons, J. P., Nelson, L. D., and Simoshohn, U. (2011). False-positive psychology: undisclosed flexibility in data collection and analysis allows presenting anything as significant. Psychol. Sci. 22, 1359–1366.
Smith, P. L., Wolfgang, B. F., and Sinclair, A. J. (2004). Mask-dependent attentional cuing effects in visual signal detection: the psychometric function for contrast. Percept. Psychophys. 66, 1056–1075.
Sternberg, R. J. (2002). On civility in reviewing. APS Obs. 15, 34.
Stevens, W. L. (1950). Fiducial limits of the parameter of a discontinuous distribution. Biometrika 37, 117–129.
Strube, M. J. (2006). SNOOP: a program for demonstrating the consequences of premature and repeated null hypothesis testing. Behav. Res. Methods 38, 24–27.
Sullivan, L. M., and D’Agostino, R. B. (1992). Robustness of the t test applied to data distorted from normality by floor effects. J. Dent. Res. 71, 1938–1943.
Treisman, M., and Watts, T. R. (1966). Relation between signal detectability theory and the traditional procedures for measuring sensory thresholds: estimating d’ from results given by the method of constant stimuli. Psychol. Bull. 66, 438–454.
Vecchiato, G., Fallani, F. V., Astolfi, L., Toppi, J., Cincotti, F., Mattia, D., Salinari, S., and Babiloni, F. (2010). The issue of multiple univariate comparisons in the context of neuroelectric brain mapping: an application in a neuromarketing experiment. J. Neurosci. Methods 191, 283–289.
Vul, E., Harris, C., Winkielman, P., and Pashler, H. (2009a). Puzzlingly high correlations in fMRI studies of emotion, personality, and social cognition. Perspect. Psychol. Sci. 4, 274–290.
Vul, E., Harris, C., Winkielman, P., and Pashler, H. (2009b). Reply to comments on “Puzzlingly high correlations in fMRI studies of emotion, personality, and social cognition.” Perspect. Psychol. Sci. 4, 319–324.
Wagenmakers, E.-J. (2007). A practical solution to the pervasive problems of p values. Psychon. Bull. Rev. 14, 779–804.
Wald, A. (1940). The fitting of straight lines if both variables are subject to error. Ann. Math. Stat. 11, 284–300.
Wald, A. (1947). Sequential Analysis . New York: Wiley.
Wells, C. S., and Hintze, J. M. (2007). Dealing with assumptions underlying statistical tests. Psychol. Sch. 44, 495–502.
Wetherill, G. B. (1966). Sequential Methods in Statistics . London: Chapman and Hall.
Wicherts, J. M., Kievit, R. A., Bakker, M., and Borsboom, D. (2012). Letting the daylight in: reviewing the reviewers and other ways to maximize transparency in science. Front. Comput. Psychol. 6:20. doi:10.3389/fncom.2012.00020
Wilcox, R. R. (2006). New methods for comparing groups: strategies for increasing the probability of detecting true differences. Curr. Dir. Psychol. Sci. 14, 272–275.
Wilcox, R. R., and Keselman, H. J. (2003). Modern robust data analysis methods: measures of central tendency. Psychol. Methods 8, 254–274.
Wilkinson, L.The Task Force on Statistical Inference. (1999). Statistical methods in psychology journals: guidelines and explanations. Am. Psychol. 54, 594–604.
Ximenez, C., and Revuelta, J. (2007). Extending the CLAST sequential rule to one-way ANOVA under group sampling. Behav. Res. Methods Instrum. Comput. 39, 86–100.
Xu, E. R., Knight, E. J., and Kralik, J. D. (2011). Rhesus monkeys lack a consistent peak-end effect. Q. J. Exp. Psychol. 64, 2301–2315.
Yeshurun, Y., Carrasco, M., and Maloney, L. T. (2008). Bias and sensitivity in two-interval forced choice procedures: tests of the difference model. Vision Res. 48, 1837–1851.
Zaslavsky, B. G. (2010). Bayesian versus frequentist hypotheses testing in clinical trials with dichotomous and countable outcomes. J. Biopharm. Stat. 20, 985–997.
Zimmerman, D. W. (1996). Some properties of preliminary tests of equality of variances in the two-sample location problem. J. Gen. Psychol. 123, 217–231.
Zimmerman, D. W. (2004). A note on preliminary tests of equality of variances. Br. J. Math. Stat. Psychol. 57, 173–181.
Zimmerman, D. W. (2011). A simple and effective decision rule for choosing a significance test to protect against non-normality. Br. J. Math. Stat. Psychol. 64, 388–409.
Keywords: data analysis, validity of research, regression, stopping rules, preliminary tests
Citation: García-Pérez MA (2012) Statistical conclusion validity: some common threats and simple remedies. Front. Psychology 3 :325. doi: 10.3389/fpsyg.2012.00325
Received: 10 May 2012; Paper pending published: 29 May 2012; Accepted: 14 August 2012; Published online: 29 August 2012.
Reviewed by:
Copyright: © 2012 García-Pérez. This is an open-access article distributed under the terms of the Creative Commons Attribution License , which permits use, distribution and reproduction in other forums, provided the original authors and source are credited and subject to any copyright notices concerning any third-party graphics etc.
*Correspondence: Miguel A. García-Pérez, Facultad de Psicología, Departamento de Metodología, Campus de Somosaguas, Universidad Complutense, 28223 Madrid, Spain. e-mail: miguel@psi.ucm.es
Disclaimer: All claims expressed in this article are solely those of the authors and do not necessarily represent those of their affiliated organizations, or those of the publisher, the editors and the reviewers. Any product that may be evaluated in this article or claim that may be made by its manufacturer is not guaranteed or endorsed by the publisher.
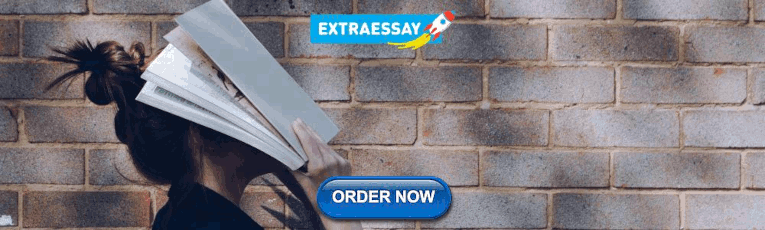
COMMENTS
Conclusion validity is the degree to which the conclusion we reach is credible or believable. Although conclusion validity was originally thought to be a statistical inference issue, it has become more apparent that it is also relevant in qualitative research. For example, in an observational field study of homeless adolescents the researcher ...
It is a fundamental concept in research and assessment that assesses the soundness and appropriateness of the conclusions, inferences, or interpretations made based on the data or evidence collected. Research Validity. Research validity refers to the degree to which a study accurately measures or reflects what it claims to measure.
Face validity. Face validity considers how suitable the content of a test seems to be on the surface. It's similar to content validity, but face validity is a more informal and subjective assessment. Example. You create a survey to measure the regularity of people's dietary habits.
The fourth aspect of research validity, which Cook and Campbell called statistical conclusion validity (SCV), is the subject of this paper. Cook and Campbell, 1979 , pp. 39-50) discussed that SCV pertains to the extent to which data from a research study can reasonably be regarded as revealing a link (or lack thereof) between independent and ...
Reliability is about the consistency of a measure, and validity is about the accuracy of a measure.opt. It's important to consider reliability and validity when you are creating your research design, planning your methods, and writing up your results, especially in quantitative research. Failing to do so can lead to several types of research ...
Table 6.1 Validity questions in analysis, interpretation, and conclusion. Generally, the validity at this stage has to do with coherence or consistence in the story that an evaluation is trying to tell (Peck et al., 2012 ). The consistency of an evaluation's story definitely affects the persuasiveness of its argument.
Statistical Conclusion Validity (SCV), or just Conclusion Validity is a measure of how reasonable a research or experimental conclusion is. For example, let's say you ran some research to find out if two years of preschool is more effective than one. Based on the data, you conclude that there's a positive relationship between how well a ...
Statistical conclusion validity is the degree to which conclusions about the relationship among variables based on the data are correct or "reasonable". This began as being solely about whether the statistical conclusion about the relationship of the variables was correct, but now there is a movement towards moving to "reasonable" conclusions that use: quantitative, statistical, and ...
Table 6.1 Validity questions in analysis, interpretation, and conclusion. Full size table. Generally, the validity at this stage is related to coherence or consistence in the story that an evaluation is trying to tell (Peck et al. 2012 ). The consistency of an evaluation's story definitely affects the persuasiveness of its argument.
The ultimate goal of research is to produce dependable knowledge or to provide the evidence that may guide practical decisions. Statistical conclusion validity (SCV) holds when the conclusions of a research study are founded on an adequate analysis of the data, generally meaning that adequate statistical methods are used whose small-sample behavior is accurate, besides being logically capable ...
In psychology research, validity refers to the extent to which a test or measurement tool accurately measures what it's intended to measure. It ensures that the research findings are genuine and not due to extraneous factors. Validity can be categorized into different types, including construct validity (measuring the intended abstract trait), internal validity (ensuring causal conclusions ...
Revised on 10 October 2022. Reliability and validity are concepts used to evaluate the quality of research. They indicate how well a method, technique, or test measures something. Reliability is about the consistency of a measure, and validity is about the accuracy of a measure. It's important to consider reliability and validity when you are ...
38) define statistical conclusion validity as the "validity of inferences about the correlation (covariance) between treatment and outcome.". In principle, all nine of the common threats to statistical conclusion validity identified by Shadish et al. (Table 6.1) apply to time series designs.
Statistical Conclusion Validity. Shadish et al. define statistical conclusion validity as the validity of the conclusion that the dependent variable covaries with the independent variable, as well as that of any conclusions regarding the degree of their covariation. In other words, it concerns the question of whether an effect was observed in ...
Validity is the main extent to which a concept, conclusion, or measurement is well-founded and likely corresponds accurately to the real world. The word "valid" is derived from the Latin validus, meaning strong. The validity of a measurement tool (for example, a test in education) is the degree to which the tool measures what it claims to measure. Validity is based on the strength of a ...
Statistical conclusion validity (SCV) holds when the conclusions of a research study are founded on an adequate analysis of the data, generally meaning that adequate statistical methods are used whose small-sample behavior is accurate, besides being logically capable of providing an answer to the research question. Compared to the three other ...
Whether the research question is valid for the desired outcome, the choice of methodology is appropriate for answering the research question, the design is valid for the methodology, the sampling and data analysis is appropriate, and finally the results and conclusions are valid for the sample and context.
Internal validity makes the conclusions of a causal relationship credible and trustworthy. Without high internal validity, an experiment cannot demonstrate a causal link between two variables. Research example. You want to test the hypothesis that drinking a cup of coffee improves memory. You schedule an equal number of college-aged ...
3.5.2. Validity assessment. In this research, validity assessment, as recommended by scholars like Kothari (Citation 2004), encompassed three key methods: content validity, construct validity, and criterion validity. Content validity was ensured through an extensive literature review and consultation with experts to guarantee that all observed ...
This essay discusses external validity in scientific research and its significance in ensuring that study findings are applicable beyond specific experimental conditions. It examines how external validity helps researchers determine whether results can be generalized to different populations, settings, and contexts.
Background Several studies have been conducted with the 1.0 version of the Hospital Survey on Patient Safety Culture (HSOPSC) in Norway and globally. The 2.0 version has not been translated and tested in Norwegian hospital settings. This study aims to 1) assess the psychometrics of the Norwegian version (N-HSOPSC 2.0), and 2) assess the criterion validity of the N-HSOPSC 2.0, adding two more ...
Purpose The study is to evaluate reliability and validity of the short Thai version of Functional Outcome of Sleep Questionnaire (FOSQ-10T), in patients with sleep disordered breathing (SDB). Methods Inclusion criteria were Thai patients with SDB age ≥ 18 years old who had polysomnography results available. Exclusion criteria were patients unable to complete questionnaire for any reason ...
Investment in research and development (R&D) is essential for a country's success in the global economy and for its ability to address challenges and opportunities. R&D contributes to innovation and competitiveness. In 2021, the business sector was the leading performer and funder of U.S. R&D. The federal government was the second-largest overall funding source and the largest funding source ...
Conclusion: The dissociation time constants of quinidine, mexiletine and flecainide as determined using this protocol are consistent with their classification in the Class IA, IB, and IC subgroups.
Table of contents. Step 1: Restate the problem. Step 2: Sum up the paper. Step 3: Discuss the implications. Research paper conclusion examples. Frequently asked questions about research paper conclusions.
There is much to take away from these case studies and readers are encouraged to reflect on how the case narratives relate to and inform their own scholarship and practice. As a way to frame these recommendations, this feature conclusion applies the idea of collective action formations in discussing the Feature articles.
The fourth aspect of research validity, which Cook and Campbell called statistical conclusion validity (SCV), is the subject of this paper. Cook and Campbell, 1979 , pp. 39-50) discussed that SCV pertains to the extent to which data from a research study can reasonably be regarded as revealing a link (or lack thereof) between independent and ...