
- SUGGESTED TOPICS
- The Magazine
- Newsletters
- Managing Yourself
- Managing Teams
- Work-life Balance
- The Big Idea
- Data & Visuals
- Reading Lists
- Case Selections
- HBR Learning
- Topic Feeds
- Account Settings
- Email Preferences
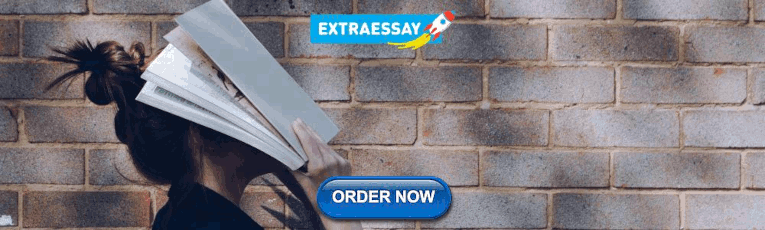
Visualizations That Really Work
- Scott Berinato

Not long ago, the ability to create smart data visualizations (or dataviz) was a nice-to-have skill for design- and data-minded managers. But now it’s a must-have skill for all managers, because it’s often the only way to make sense of the work they do. Decision making increasingly relies on data, which arrives with such overwhelming velocity, and in such volume, that some level of abstraction is crucial. Thanks to the internet and a growing number of affordable tools, visualization is accessible for everyone—but that convenience can lead to charts that are merely adequate or even ineffective.
By answering just two questions, Berinato writes, you can set yourself up to succeed: Is the information conceptual or data-driven? and Am I declaring something or exploring something? He leads readers through a simple process of identifying which of the four types of visualization they might use to achieve their goals most effectively: idea illustration, idea generation, visual discovery, or everyday dataviz.
This article is adapted from the author’s just-published book, Good Charts: The HBR Guide to Making Smarter, More Persuasive Data Visualizations.
Know what message you’re trying to communicate before you get down in the weeds.
Idea in Brief
Knowledge workers need greater visual literacy than they used to, because so much data—and so many ideas—are now presented graphically. But few of us have been taught data-visualization skills.
Tools Are Fine…
Inexpensive tools allow anyone to perform simple tasks such as importing spreadsheet data into a bar chart. But that means it’s easy to create terrible charts. Visualization can be so much more: It’s an agile, powerful way to explore ideas and communicate information.
…But Strategy Is Key
Don’t jump straight to execution. Instead, first think about what you’re representing—ideas or data? Then consider your purpose: Do you want to inform, persuade, or explore? The answers will suggest what tools and resources you need.
Not long ago, the ability to create smart data visualizations, or dataviz, was a nice-to-have skill. For the most part, it benefited design- and data-minded managers who made a deliberate decision to invest in acquiring it. That’s changed. Now visual communication is a must-have skill for all managers, because more and more often, it’s the only way to make sense of the work they do.
- Scott Berinato is a senior editor at Harvard Business Review and the author of Good Charts Workbook: Tips Tools, and Exercises for Making Better Data Visualizations and Good Charts: The HBR Guide to Making Smarter, More Persuasive Data Visualizations .

Partner Center
- Original Article
- Open access
- Published: 29 October 2021
Examining data visualization pitfalls in scientific publications
- Vinh T Nguyen 1 ,
- Kwanghee Jung ORCID: orcid.org/0000-0002-7459-7407 2 &
- Vibhuti Gupta 3
Visual Computing for Industry, Biomedicine, and Art volume 4 , Article number: 27 ( 2021 ) Cite this article
9652 Accesses
6 Citations
Metrics details
Data visualization blends art and science to convey stories from data via graphical representations. Considering different problems, applications, requirements, and design goals, it is challenging to combine these two components at their full force. While the art component involves creating visually appealing and easily interpreted graphics for users, the science component requires accurate representations of a large amount of input data. With a lack of the science component, visualization cannot serve its role of creating correct representations of the actual data, thus leading to wrong perception, interpretation, and decision. It might be even worse if incorrect visual representations were intentionally produced to deceive the viewers. To address common pitfalls in graphical representations, this paper focuses on identifying and understanding the root causes of misinformation in graphical representations. We reviewed the misleading data visualization examples in the scientific publications collected from indexing databases and then projected them onto the fundamental units of visual communication such as color, shape, size, and spatial orientation. Moreover, a text mining technique was applied to extract practical insights from common visualization pitfalls. Cochran’s Q test and McNemar’s test were conducted to examine if there is any difference in the proportions of common errors among color, shape, size, and spatial orientation. The findings showed that the pie chart is the most misused graphical representation, and size is the most critical issue. It was also observed that there were statistically significant differences in the proportion of errors among color, shape, size, and spatial orientation.
Introduction
With the advancement of the internet, data storage, and search methods, an increasing number of scientific publications and academic papers are being deposited online. This facilitates readers’ access to scientific knowledge and publications. To help in quick and efficient browsing, online resources allow full papers to be stored in several formats such as text, photographs, tables, and charts. This proprietary database helps the reader find it faster, while it raises some problems in understanding data. When tables and charts are positioned closest to their descriptions, the author’s intention will be delivered more clearly. However, it is not surprising that we see so many tables and charts that do not themselves contain information to convey. When a reader fails to read the description, the author loses the ability to communicate his or her information. As a result, the design, arrangement, and organization of data in tables and charts play an important role in information dissemination.
Visualization has long been used as an effective method for transcribing data into information and help people carry out data analysis tasks and make decisions. A number of visual encodings (such as color, shape, and size) can be used individually or together for representing various visualization tasks [ 1 , 2 , 3 ]. Misinformation in data visualization has become one of the main issues to convey knowledge more effectively [ 4 , 5 ]. As opposed to creating effective visualization, misinformation in data visualization receives less attention and becoming one of the major issues for conveying information [ 5 ]. Misinformation can be classified into two categories including intentional misinformation and unintentional misinformation [ 6 ]. Intentional misinformation [ 7 ] in data visualization refers to the use of charts, graphics to distort, hide, or fabricate data in an attempt to deceive users. On the other hand, unintentional misinformation [ 8 ] implies providing false and inaccurate information to the end-users because of human cognitive bias and carelessness in designing/selecting visual channels to encode the corresponded data. From the creator’s point of view, the former is controllable while the latter requires a lot of training. This work will be focusing more on the latter one as it is encouraged to build trust in visualization rather than lies [ 9 ].
A good visualization tool supports users to get insights (i.e., trends, patterns, and outliers) and extract meaningful information from data. Wilkinson [ 10 ] describes the building blocks for constructing a wide range of statistical graphics in his notable book “The Grammar of Graphics”. On the other hand, many articles on the web provide bad examples of visual designs leading to misinterpretation but they are usually viewed from different perspectives. In line with this research direction, Bresciani and Eppler [ 6 ] provided a comprehensive review on common errors in data visualization and classified these pitfalls into three categories: cognitive, emotional, and social. Their high-level abstract of the theoretically grounded classification can be used as a checklist for practitioners to examine the visualization for avoiding common drawbacks. However, it is challenging to go through the entire checklist due to the different human cognitive and one’s preference styles. As such, the authors suggested that a more rigorous study should be conducted to “rank the pitfalls according to how common or severe they are.” In response to these needs, in this paper, we try to compromise the most common errors into a structure based on the units of visualization. In another word, we address the following research questions: (R1) What are the most common effects resulting from visualization pitfalls? (R2) What are the most common errors in constructing a representation in terms of color, shape, size, and orientation? Or (R3) Is there any difference in the proportions of common errors among color, shape, size, and spatial orientation? We believe that going from these units would benefit practitioners, especially novice users when they are mostly working with these elements. We expect that the result of this study will be served as a reference for avoiding visualization pitfalls in general. Ultimately, in the long run, we will attempt to answer the questions such as what color [ 11 ] should we use to represent data? what visualization types [ 12 , 13 ] should be taking into account for analysis? what type of scale should we apply? As such, this is preliminary research work to accomplish the goals.
This study is a continuation of a previously published work at the symposium on visual information communication and interaction [ 14 ]. The new version was extended by expanding the existing database (Google Scholars) with Scopus, incorporating a text mining technique to extract practical insights from common visualization pitfalls, and examining if there is any difference in the proportions of common errors among color, shape, size, and spatial orientation with Cochran’s Q test and McNemar’s test.
The rest of this paper is structured as follows: First, a literature review relevant to our work was presented. Then, the instruments and processes to conduct the research were provided along with the analytics. After that, the results were presented. Finally, the findings were discussed and concluded.
Related work
Many recent works have addressed the issues of misinformation in data visualization. Huff [ 15 ] showed a variety of ways a designer can trick people, e.g., truncating certain portions of the graph. As such, the discrepancy is more obvious when seen as a whole. This goal can be accomplished by three common strategies [ 9 , 16 ]: (1) not revealing any data, (2) showing the data incorrectly, and (3) obfuscating the data.
Many authors attempted to provide designers with a boilerplate for creating a successful visualization, ranging from individual graph elements such as colors [ 17 , 18 , 19 ] and forms (or chart types) [ 20 ] to a detailed data visualization design [ 21 , 22 ]. However, bad visualization design persists in the presence of guidance, which leads to misinformation that can contribute to considerable problems, especially where individuals heavily rely on the data at hand when making business decisions. Study results raise concerns: what are the possible causes of disinformation visualization? This research is critical in developing a visualization platform that not only meets end-user needs but also mitigates the issues from misinformation and disinformation.
The pitfalls of visualization have been examined in a variety of fields such as information visualization [ 23 , 24 , 25 ] - psychological/aesthetic restrictions presented graphic format are highlighted, diagrammatic representations [ 13 , 26 , 27 , 28 ] using the diagram to understand concept or idea instead of algebraic means, human-computer interaction [ 21 , 29 ] - potential drawbacks of interactive visualizations, statistical graphic representations [ 22 , 30 ] - bad examples of representing data visually. The most comprehensive research was provided by Bresciani and Eppler [ 6 ], where the authors explored the potential drawbacks in graphic depictions. The authors compiled a list of 51 pitfalls of visualization drawbacks based on interviews with seven experts and divided them into three categories: cognitive, emotional, and social effects.
Unlike the previous research relying on high-level abstractions to avoid visualization errors, we focus on the basic elements that constitute visualization mapping. These elements are color, shape, size, and spatial orientation presented by Munzner [ 22 ]. According to Bresciani and Eppler’s research [ 6 ], there is a need to review a selection of images to assess the frequency and severity of the flaws. In this study, we aim to alleviate the problems of misinformation in data visualization.
Data sources
For the research, the preferred reporting items for systematic reviews and meta-analyses (PRISMA [ 31 ]) model served as a guideline. It is a bare-bones set of evidence-based features meant to help authors report on a wide range of systematic reviews and meta-analyses. Google Scholar and Scopus indexing databases were used to gather data for our study due to their popularity and credible results. The search terms were “misinformation visualization”, “misleading visualization”, “disinformation visualization”, “visualization pitfalls”, “bad visualization design”. Google Image search was used to collect representative images that illustrate visualization pitfalls to avoid direct criticism of previous studies. Table 1 provides the number of papers collected from the two indexing databases according to their keywords.
Data preprocessing
Search results from the database were consolidated into a single excel file format, and duplicated items were removed. The final list of items was filtered by the scientific articles’ abstracts and main contents. Here, we did not exclude items by their titles. Rather, we examined the details of abstracts and main contents to see where the keywords were positioned. It is noted that some of the collected papers contained keywords but were not relevant to our research goal, e.g., some papers did not contain figures to demonstrate the searching keywords. Such papers were excluded from our analysis. In the end, 178 papers were included in the study. Figure 1 depicts the flow of information through the different phases of the systematic review utilizing the PRISMA approach.
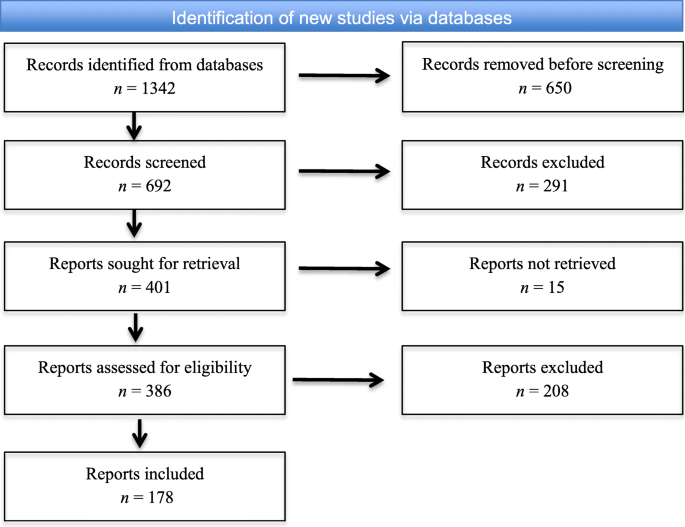
The PRISMA flow diagram
Data extraction
We extracted two types of data from the research papers: figures and their associated descriptions. Each figure is classified into one of four categories (i.e., color, shape, size, and spatial orientation) which indicates a type of violation in visualization. The relevant papers were screened through three phases: (1) checking the title, (2) reviewing the abstract, and (3) scrutinizing the full text of the paper.
Data analysis
For the first research question, we employed text mining techniques to gain practical insights from the collected data, including association rule mining to find out the relationships among the descriptions of figures. The inputs for this technique were taken from associated descriptions of each figure. We created a word cloud with the most often used words in related explanations, removing any stop words, non-ASCII characters, white space, and duplication. Word clouds are graphical representations of word frequency that highlight terms that appear regularly in a source document. The bigger the term in the visual, the more popular the word was in the text. This visualization method can help evaluators with exploratory textual research by highlighting terms that appear often in a series of interviews, records, or other languages. It may also be used to communicate the most important points or topics during the reporting stage. Association rule mining is a rule-based machine learning technique that seeks out interesting hidden relationships among variables in a dataset. Association rules have been used in a variety of applications, including opinion mining, intrusion prevention, continuous processing, and bioinformatics.
For the second research question, we grouped typical visualization pitfalls into four major categories denoted from M1 to M4 based on the basic elements (color, size, shape, and spatial orientation), as shown in Fig. 2 , where “red, blue, and green” represent the color, scaled squares represent the size, “circle, rectangle, and triangle” represent the shape, and scatter black dots represent the spatial orientation. As we move from left to right, misinformation for visual mapping can result from data itself, such as missing data or poor data, to cognitive perception, where color, shape, and scale are perfectly used but misinformation occurs due to cognitive mechanisms. Visual perception is the primary subject of this paper (for more information on data perception and cognitive perception, see Bresciani and Eppler’s study [ 6 ]).
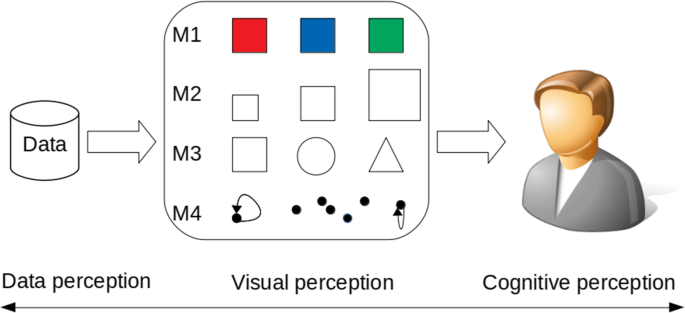
A visual approach for categorizing misinformation data visualization: M1 (color), M2 (size), M3 (shape), and M4 (spatial orientation)
For the third research question, we conducted Cochran’s Q test and McNemar’s test [ 32 ] to examine if there is any difference in the proportions of common errors among the fundamental units of color, shape, size, and spatial orientation. We examined each paper individually and extracted figures that were violated in terms of any of the four categories. It is noted that only figures generated from data by authors were included for the analysis. Figures taken from photos or other sources were excluded. We encoded “1” as being violated and “0” for not being violated. Thus, our data consists of four binary variables. Statistical analysis was performed with SPSS Version 25.
RQ1: What are the most common effects resulting from visualization pitfalls?
After removing special characters in the associated descriptions of figures, we had 363 items with 139 unique words. The items are the remaining words/keywords extracted from the descriptions after cleaning data. Figure 3 depicts the most frequently used terms for each input figure that encounter visualization pitfalls. Frequently used terms in Fig. 3 are shown in larger font sizes in the word cloud. In terms of dominant keywords, besides our proposed categories such as color (72), shape (36), size (132), a majority of misleading visualizations comes from pie chart (83) and bar chart (72), followed by using the wrong chart (63) that incur misinterpretation (21). In addition to the pie chart, donut (33) is another interesting finding that contributes to common errors, it seems that creators are interested in using circles to explain their messages. It is noticed that the terms “inform and axis” are also highlighted in our visual layout which indicates that creators and viewers have to take care of the axis while interpreting data to inform decision-making. We can see other terms that appear to be slightly different from others such as data, hard, and clutter, but they do not stand out apparently.
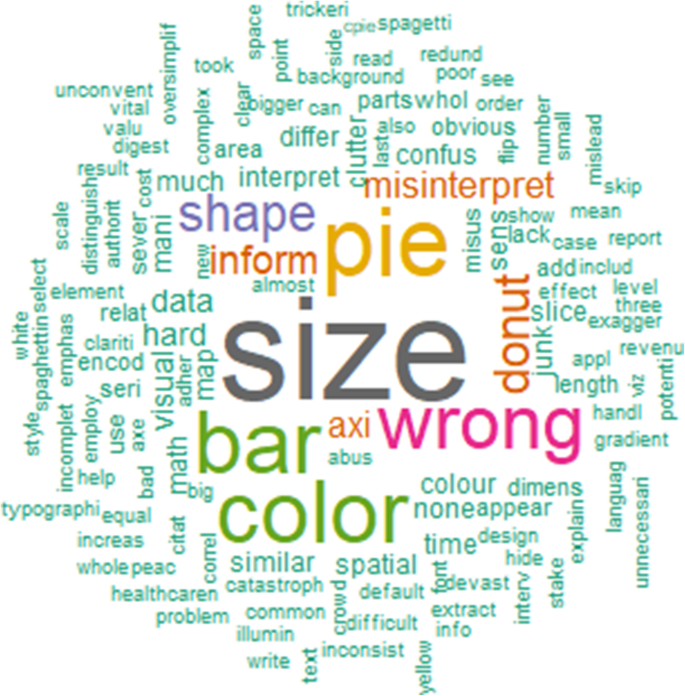
Word cloud constructed from associated descriptions about the figure that encounters visualization pitfalls
Figure 4 depicts the graphical layout of our transactions and items in the dataset, where black cells denote non-zero elements and empty cells represent zero elements. Rows (transactions) are associated description index of figures, while columns (items) are terms extracted from their corresponding descriptions. In total, we had 370 descriptions and 109 terms. The percentage of non-zero cells in this 370 × 109 matrix is shown by density. It is worth noting that a matrix is considered sparse if most of its elements are empty. On the other hand, if most of the elements are non-zero, the matrix is called dense. The sparsity of the matrix is defined as the number of zero-valued elements divided by the total number of elements. Our dataset showed a density of 0.0312 which means that on average, each description contains 3 to 4 keywords/terms. This is due to the data cleaning process, where all stop words were removed.
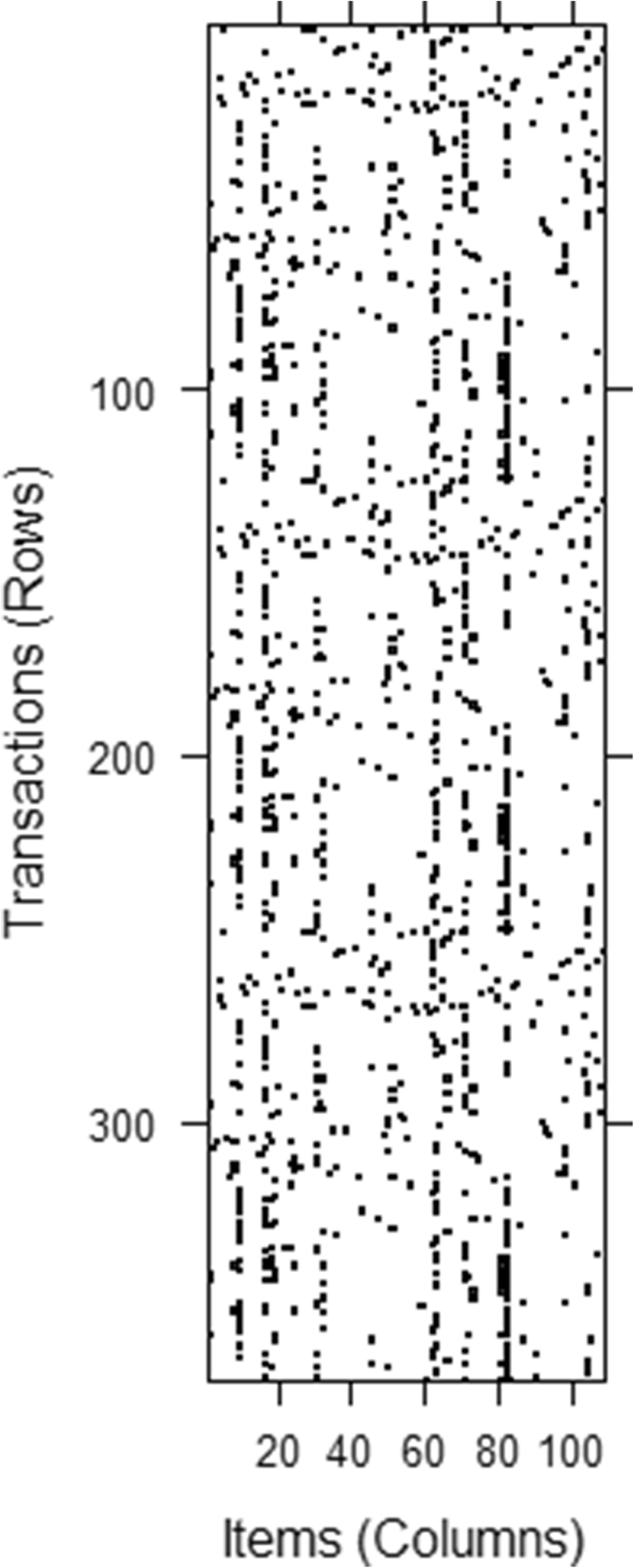
Transactions and items in a matrix
Table 2 showed a list of 14 rules generated from the A-Priori algorithm [ 33 ]. This algorithm necessitates both minimal support and a minimum confidence constraint. If no threshold value is defined, the function will consider the default values of support and confidence threshold as 0.1 and 0.8, respectively. Since the elements in the matrix are sparse, support and confidence are modified with lower values (support = 0.04 and confidence = 0.5) to mine the most potential rules rather than using default values. Support is the percentage of transactions (or figures) that contain both words in a rule and confidence is the strength of that rule. The A-Priori algorithm outputs the highest value of support (0.162), confidence (1), and lift (11.012) respectively. The lift value is an indicator of a rule’s significance. If the lift value is greater than 1, it indicates that the rule body (or Rhs) and the rule head (or Lhs) appear together more often than predicted, implying that the occurrence of the rule body has a positive impact on the occurrence of the rule head. On the other hand, if the lift value is less than 1, it implies that the occurrence of the Rhs has a negative effect on the occurrence of the Lhs. Table 2 shows that all lift values are greater than 1, indicating that items in the rules are positively correlated. It is noted that more than half of items (57.14 %) in the rule body involve ‘size’, which means that size is the most critical issue in visualization pitfalls. This statistical data is supported by the visual graph as depicted in Fig. 5 , where size is the center of the constructed network with a total of 10 connections from other rules. This network illustrates associations between items and rules. Bigger circles indicate higher support, while the red color implies higher lift. One interesting pattern revealed by the algorithm is when creators use size to express information in a bar chart, then misleading is likely to occur (rule 13, 14). In addition, incorrect usages of size can lead to confusion.
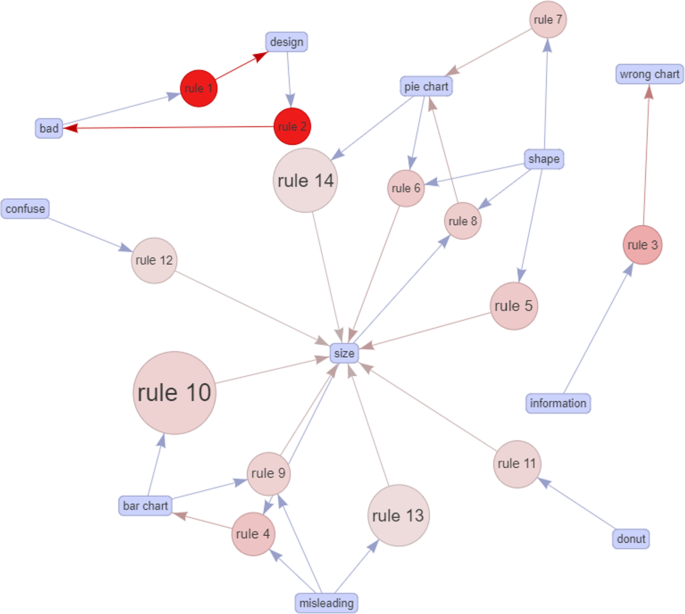
Graph-based visualization on the relationship between individual items in the ruleset
RQ2: What are the most common errors in constructing a representation in terms of color, shape, size, and orientation?
M1: misinformation due to color violation.
As shown in Fig. 6 , one of the most common visualization mistakes is the use of too many colors. Healey et al. [ 34 ] argued that the human eye can only process five to seven well-chosen colors pre-attentively, and qualitative color scales are thought to work best when three to five different colored categories are present. Choosing a color scheme is another critical issue when different categories are encoded by related color on a spectrum that makes it difficult to distinguish one from another as in Fig. 6 a - legend. In some cases, the use of continuous color even gets worse leading to misinterpretation of data [ 35 ], especially when using a non-monotonic scale or rainbow scale [ 11 ]. It can be seen from Fig. 6 d that the colors at the beginning and the end are almost equivalent (circulated color scales). Figure 6 b shows using the same color for different categorical data. Although each category can be annotated by a distinct shape, color is often misused to represent an additional dimension especially when the granularity of the new dimension is too low. It takes more time to locate the blue circle point of interest. Another common bad habit is using the color against the norm: it is often found in the practice that light color is usually for less density while darker color is for higher density or green for a healthy indicator vs. red for abnormal value. The example illustrated in Fig. 6 c misused red color business gains and green color for business losses. A first glance, this visualization misled us that the economy is flourishing.
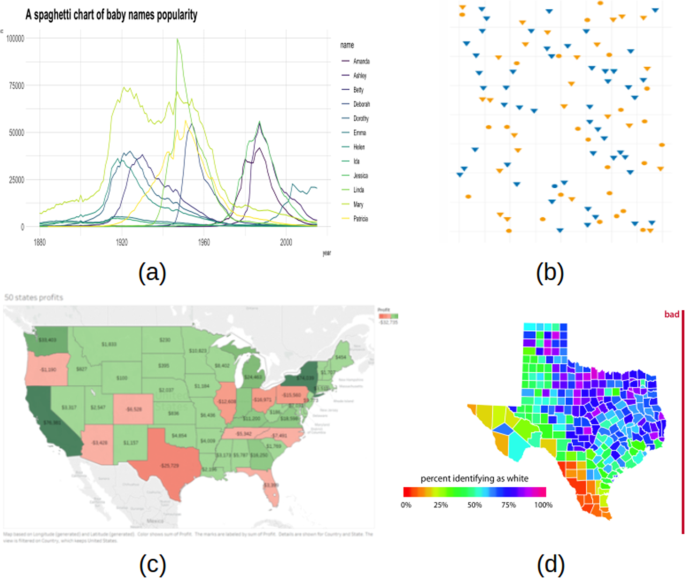
Misinformation due to color violation: a using too many colors [ 36 ], b unnecessary color usage [ 37 ], c color against the norm [ 38 ] and d rainbow color scale [ 39 ]
M2: Misinformation due to shape violation
The shape can be violated due to the use of inappropriate chart types. In fact, this is the most common misleading chart that we found in the literature. Particularly in the case of using a pie chart instead of a bar chart and vice versa. A pie chart is a type of graph in which a circle is broken down into segments (i.e., slices of pie) that each represent a proportion of the whole. Each slice of the pie chart is represented by a percentage value accumulating to 100%. Figure 7 a illustrates the improper use of the pie chart where each slice adds up to more than 100%. Even though the original intention was to compare values among different categories, this chart also violates color choices (so many colors, or similar colors for different categorical data). Common practice [ 37 ] suggested that the pie chart can be best used with less than seven categories (same magic number as in color) due to the difficulty for the eye to distinguish relativity of size between each segment. In this case, the bar chart should be a better choice. Figure 7 c shows an opposite case where the pie chart should be taken into consideration instead of using the bar chart for comparing proportional data as percentages add up to 100%.
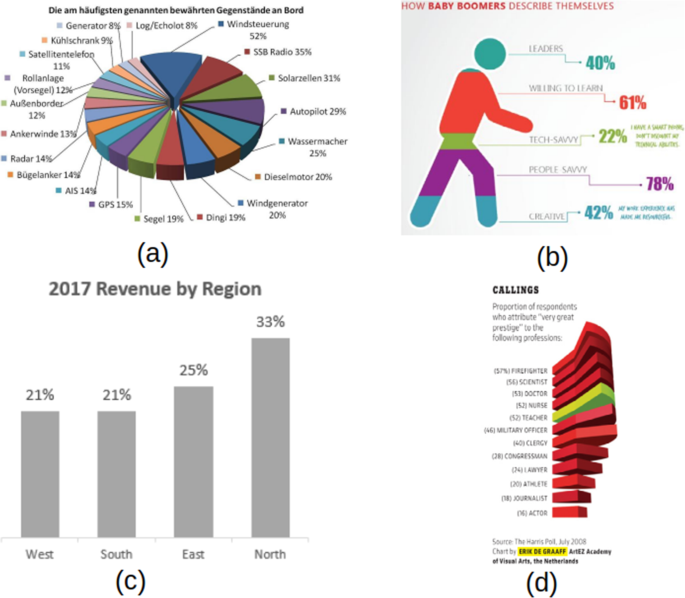
Misinformation due to shape violation: a using 3D pie chart for comparing proportion [ 40 ], b using wrong visualization chart type, c pie-chart should be used [ 41 ], and d bar chart should be used [ 42 ]
The second misleading information when performing visual mapping is to use a shape that does not reflect the information provided. It can be seen from Fig. 7 b that the body part misleads users from what it means. Like the case of the pie chart, proportionality does not add up to 100% or areas cannot be compared visually.
Another common misleading information is the use of a high-dimensional chart to represent lower-dimensional data. This is often due to the visual appeal of 3D charts or the priority of prioritizing design and technology over conveying information of the chart as it can be seen from Fig. 7 a and d where heights of each slice did not provide any additional information.
While doing visual mapping, the second deceptive information is to use a shape that does not represent the information presented. Figure 7 b demonstrates how the body part misleads people on what it means. Proportional, like the pie map, does not add up to 100%, or regions cannot be physically compared. Another example of deceptive evidence is the use of a high-dimensional map to display low-dimensional details. This is often attributed to the aesthetic appeal of 3D charts or the importance of prioritizing architecture and technology over conveying detail of the map, as seen in Fig. 7 a and d, where the heights of each slice provided no additional details.
M3: Misinformation due to size violation
Regarding size infringement, the most common problem is to use a single size dimension scale to express a two-dimensional value. For example, in Fig. 8 a, the diameter was used for the scale to display the proportion to GDP, but users often viewed the chart from the perspective of the region. In this case, the field expands exponentially (i.e., quadratic vs linear). Mathematically, 14.5 trillion is 2.56 times larger than 5.7 trillion, but the region seems to be 6.5 times larger.
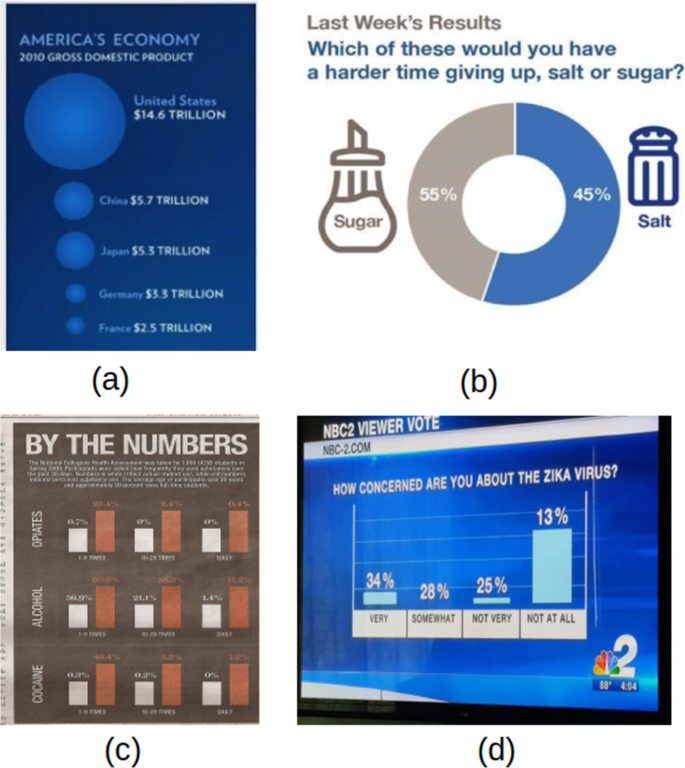
Misinformation due to size violation: a using wrong scale [ 43 ], b size is inverted [ 40 ], c all sizes are equal [ 44 ] and d size with no meaning [ 45 ]
Careless designers continue to apply incorrect labeling to visual mapping, especially where the chart is labeled manually without the assistance of automatic graphic software. Figure 8 b represented this scenario in which 55 % and 45 % could be swapped. Surprisingly, comparable findings in this form of pitfall are typically seen from news channel writers. Another method of softening the detrimental impact of a section is to use a light color, but we do not mention it in our study because it is considered an intention.
The use of equivalent scale shapes supplemented with numbers for contrast is the second common deceptive graph pitfall that belonged to this group, shown in Fig. 8 c. Similarly, to orange bars, all-white bars are the same height. Colors are often misused in three separate categories; however, the most serious flaw is the appearance of height where no meaning is present (0 % value of the white bars in the rightmost figure). These common pitfall patterns can also be seen in a pie chart of equal-sized parts.
Another pitfall is to use a graphic encoding that is unrelated to the values it contains, as seen in Fig. 8 d, where the height of each bar chart does not fit the value above it. Did the author use an inverted scale? Then 34 % should be the lowest, and where is the bar chart for the “SOMEWHAT” category? While this form of expression is uncommon in academia, social media has had many instances of it.
M4: Misinformation due to spatial orientation violation
In this study, we would use a subset of spatial features including spatial and temporal mapping which engages space and time, respectively.
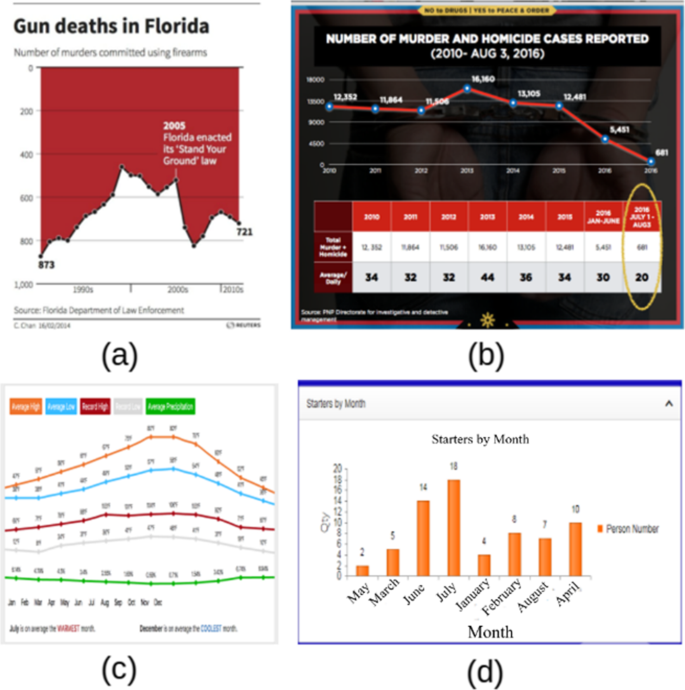
Misinformation due to spatial and temporal violation [ 46 ]: a inverted axis, b data split out, c missing axis to line up data, and d mixing time data
Inverting the vertical axis is a technique that allows viewers to see the flip side of the original data. In practice, the flip-side data is intentionally removed to convey other information. Figure 9 a is the most controversial chart being discussed whether it was created to deceive users [ 46 ]. For most viewers, this graph first gave the impression that gun deaths declined sharply after 2005 due to the illusion of the line chart, especially in the presence of black dots. Gun deaths increased. As indicated in ref. [ 46 ] “the artist does not appear to have intended the graph to be deceptive. Her view was that deaths are negative things and should be represented as such.” However, the focal point we want to address was that misleading information would make people feel that living in Florida get safer after the incident. A similar story can also be found in Fig. 9 b. Omitting data, truncating the axis (or non-zero-base axis), and over zooming to see the difference are popular techniques [ 9 , 16 ] to make a deceptive graph. From our point of view, in Fig. 9 b original data remains but misleading information still exists.
The most common problem with spatial orientation violation is reordering time series results. When creating a graph with a timestamp in one dimension, the order of data points at each time level is shown in Fig. 9 d, where months appear to be in a random position. The direction would be totally destroyed if the data are shuffled. Careless charting can also result in the lack of one dimension, shown in Fig. 9 c, where the author attempted to combine line graphs from different figures into one. We can see from the contradictory data unit and value location that does not match the upward trend. Misinformation due to data and visual interpretation is most often seen in the form of a table shown in Table 3 , where viewers must make at least two attempts to locate the absolute meaning (i.e., compare the length and compare the leftmost values). In this case, the order of magnitude in space is broken.
RQ3: Is there any difference in the proportions of common errors among color, shape, size, and spatial orientation?
Table 4 shows that the most frequently observed pitfalls were in size (69.4%). Color and shape showed a similar number of cases (39.7% and 37.2%, respectively). Spatial orientation had the least case (14.9%).
We conducted Cochran’s \(Q\) test and McNemar’s test [ 32 ] to examine if there is any difference in the proportions of common errors among color, shape, size, and spatial orientation. Cochran’s \(Q\) test determined that there was a statistically significant difference in the proportion of errors among the features of color, shape, size, and spatial orientation, \(Q\) (3) = 207.047, p < 0.001. This implies that at least two of the features had a different proportion of errors. Post hoc analysis with McNemar’s test was conducted. Table 5 shows the 2 × 2 classification tables for McNemar’s test. There were no significant differences between color and shape ( χ 2 = 0.547, p = 0.460). However, there was a statistically significant difference in proportions of errors between color and size ( χ 2 = 54.519, p < 0.001), color and spatial orientation ( χ 2 = 42.586, p < 0.001), shape and size ( χ 2 = 84.629, p < 0.001), and between size and spatial orientation ( χ 2 = 132.003, p < 0.001). The findings indicate that size is most frequent in the common pitfalls, which is statistically different in frequency from other features. Color and shape are the second most frequent common errors, which are statistically different from spatial orientation in terms of frequency. However, there is no significant difference between color and shape.
It is undeniable that visualization is becoming more common, especially in the age of the Internet of Things, where millions of data points are created every day. To succeed, we need to provide a big picture in a succinct graphic style to help customers digest details faster. When we screened the papers for the study, we discovered that the majority of the graphical layouts were created by hand or produced by a program using built-in features. Only a few studies used specialized software to present results. Part of the problem stems from developers’ lack of programming expertise, which forces them to rely on free software. In some cases, these tools have a fine, suggested interface (e.g., Google Form automatically generated charts based on survey data), but in others, the layout is much worse than if it were created manually (e.g., Google Form only generates one type of chart for numerical data without considering the number of categories). As a result, developers would use these preset visualizations without regard for visual interface clarity. Another risk that can trigger visualization pitfalls stems from the writing paper guidelines. Although there is a straightforward, concise description of how to write and section (e.g., abstract, introduction, methodology, and conclusion), there is no guidance on how to correctly present data in figures. As a result, authors place a greater emphasis on content rather than visual presentation. This problem is addressed in the area of data visualization, where instructions for presenting data can be found in the paper’s template. However, these recommendations are not systematic, making a boilerplate graphic suggestion for both inside and across fields challenging. We expect that artificial intelligence will be modified in the coming years to automatically screen and identify possible common errors based on research questions and the evidence presented. As a result, developers will be able to concentrate on mining the content rather than thinking about deceptive interfaces.
Conclusions
This paper dealt with typical visual representation pitfalls. We gathered data from two indexing databases, pooled it, and grouped it into four groups based on data representation units. The most popular visualization pitfalls from each segment were extracted, and data in each section was analyzed using both qualitative and quantitative methods. Word frequency from Word Cloud visualization shows that size is the most dominant keyword found in the descriptions of figures, followed by pie, bar charts, and color. Association rule mining reported that size is the center of the constructed network with a total of 10 connections from other rules and it plays a major concern in visualization pitfalls. Cochran’s Q test and McNemar’s test showed that there was a statistically significant difference in the proportion of errors among the fundamental units of color, shape, size, and spatial orientation. The size issue was most frequent in common pitfalls, followed by color and shape. Spatial orientation had the least case. This paper’s contribution can be thought of as a fine-grained (or subset) of general visualization pitfalls, with a focus on visual perception. We hoped that our findings would aid in the creation of a taxonomy of common errors at the information stage. User interface analysis on visual interpretation will be undertaken in the future with a more detailed test design, taking into account the user’s cognitive loads and cognitive style.
Availability of data and materials
The datasets used and/or analyzed during the current study are available from the corresponding author on reasonable request.
Abbreviations
Preferred reporting items for systematic reviews and meta-analyses
Ceneda D, Gschwandtner T, May T, Miksch S, Schulz HJ, Streit M et al (2016) Characterizing guidance in visual analytics. IEEE Trans Vis Comput Graph 23(1):111–120. https://doi.org/10.1109/TVCG.2016.2598468
Article Google Scholar
Nguyen VT, Namin AS, Dang T (2018) MalViz: an interactive visualization tool for tracing malware. In: Abstracts of the 27th ACM SIGSOFT international symposium on software testing and analysis, ACM, Amsterdam, 16–21 July 2018. https://doi.org/10.1145/3213846.3229501
Dang T, Nguyen VT (2018) ComModeler: topic modeling using community detection. In: Tominski C, von Landesberger T (eds) Eurovis workshop on visual analytics. The Eurographics Association, Brno, p 1–5
Google Scholar
Nguyen NVT, Nguyen VT, Dang T (2021) Color blind: can you sight? In: Abstracts of the 12th international conference on advances in information technology, Association for Computing Machinery, Bangkok, 29 June-1 July 2021. https://doi.org/10.1145/3468784.3471602
Bresciani S, Eppler MJ (2009) The risks of visualization: a classification of disadvantages associated with graphic representations of information. In: Schulz PJ, Hartung U, Keller S (eds) Identität und vielfalt der kommunikations-wissenschaft. UVK Verlagsgesellschaft mbH, Konstanz, p 165–178
Bresciani S, Eppler MJ (2015) The pitfalls of visual representations: a review and classification of common errors made while designing and interpreting visualizations. SAGE Open 5(4):2158244015611451. https://doi.org/10.1177/2158244015611451
Tufte ER (1983) The visual display of quantitative information. Graphics Press, Cheshire, p 200
Fishwick M (2004) Emotional design: why we love (or hate) everyday things. J Am Cult 27(2):234. https://doi.org/10.1111/j.1537-4726.2004.133_10.x
Cairo A (2015) Graphics lies, misleading visuals. In: Bihanic D (ed) New challenges for data design. Springer, London, p 103–116. https://doi.org/10.1007/978-1-4471-6596-5_5
Wilkinson L (2012) The grammar of graphics. In: Gentle JE, Härdle WK, Mori Y (eds) Handbook of computational statistics. Springer handbooks of computational statistics. Springer, Berlin, p 375–414. https://doi.org/10.1007/978-3-642-21551-3_13
Borland D, Taylor IIRM (2007) Rainbow color map (still) considered harmful. IEEE Comput Arch Lett 27(2):14–17. https://doi.org/10.1109/MCG.2007.323435
Acampora J (2018) When to use pie charts-best practices. https://www.excelcampus.com/charts/pie-charts-best-practices . Accessed 7 July 2019
Blackwell AF, Britton C, Cox A, Green TRG, Gurr C, Kadoda G et al (2001) Cognitive dimensions of notations: design tools for cognitive technology. In: Beynon M, Nehaniv CL, Dautenhahn K (eds) Cognitive technology: instruments of mind. 4th international conference, CT 2001, August 2001. Lecture notes in computer science, vol 2117. Springer, Berlin, Heidelberg, p 325–341. https://doi.org/10.1007/3-540-44617-6_31
Nguyen VT, Jung K, Dang T (2020) Revisiting common pitfalls in graphical representations utilizing a case-based learning approach. In: Abstracts of the 13th international symposium on visual information communication and interaction, ACM, Eindhoven, 8–10 December 2020. https://doi.org/10.1145/3430036.3430071
Huff D (1993) How to lie with statistics. W. W. Norton & Company, New York
Wainer H (2013) Visual revelations: graphical tales of fate and deception from napoleon bonaparte to ross perot. Psychology Press, Hove, p 56–61. https://doi.org/10.4324/9780203774793
Book Google Scholar
Silva S, Madeira J, Santos BS (2007) There is more to color scales than meets the eye: a review on the use of color in visualization. In: Abstracts of the 11th international conference information visualization, IEEE, Zurich, 4–6 July 2007. https://doi.org/10.1109/IV.2007.113
Wang LJ, Giesen J, McDonnell KT, Zolliker P, Mueller K (2008) Color design for illustrative visualization. IEEE Trans Vis Comput Graph 14(6):1739–1754. https://doi.org/10.1109/TVCG.2008.118
Stone M (2006) Choosing colors for data visualization. https://www.perceptualedge.com/articles/b-eye/choosing_colors.pdf . Accessed 22 July 2021.
Evergreen SDH (2019) Effective data visualization: the right chart for the right data, 2nd edn. SAGE Publications, Thousands Oaks, p 264
Nguyen HN, Nguyen VT, Dang T (2020) Interface design for HCI classroom: from learners’ perspective. In: Bebis G, Yin ZZ, Kim E, Bender J, Subr K, Kwon BC, et al (eds) Advances in visual computing. 15th international symposium, ISVC 2020, October 2020. Lecture notes in computer science, vol 12510. Springer, Cham, pp 545–557. https://doi.org/10.1007/978-3-030-64559-5_43 .
Munzner T (2014) Visualization analysis and design. CRC Press, Boca Ranton. https://doi.org/10.1201/b17511
Cawthon N (2007) Qualities of perceived aesthetic in data visualization. In: Abstracts of 2007 conference on designing for user eXperiences, ACM, Chicago, 5–7 November 2007. https://doi.org/10.1145/1389908.1389920
van Wijk JJ (2006) Views on visualization. IEEE Trans Vis Comput Graph 12(4):421–432. https://doi.org/10.1109/TVCG.2006.80
Kosslyn SM (2006) Graph design for the eye and mind. Oxford University Press, New York. https://doi.org/10.1093/acprof:oso/9780195311846.001.0001
Green TRG, Petre M (1996) Usability analysis of visual programming environments: a ‘cognitive dimensions’ framework. J Vis Lang Comput 7(2):131–174. https://doi.org/10.1006/jvlc.1996.0009
Crilly N, Blackwell AF, Clarkson PJ (2006) Graphic elicitation: using research diagrams as interview stimuli. Qual Res 6(3):341–366. https://doi.org/10.1177/1468794106065007
Nguyen QV, Zhang K, Simoff S (2015) Unlocking the complexity of port data with visualization. IEEE Trans Hum-Mach Syst 45(2):272–279. https://doi.org/10.1109/THMS.2014.2369375
Shneiderman B, Plaisant C, Cohen MS, Jacobs S, Elmqvist N, Diakopoulos N (2016) Designing the user interface: strategies for effective human-computer interaction. Pearson, Boston
Tufte ER (2006) Beautiful evidence. Graphics Press, Cheshire
Moher D, Liberati A, Tetzlaff J, Altman DG, PRISMA Group (2009) Preferred reporting items for systematic reviews and meta-analyses: the PRISMA statement. PLoS Med 6(7):e1000097. https://doi.org/10.1371/journal.pmed.1000097
Sheskin DJ (2007) Handbook of parametric and nonparametric statistical procedures. Chapman & Hall/CRC, Boca Raton
MATH Google Scholar
Agrawal R, Srikant R (1994) Fast algorithms for mining association rules in large databases. In: Abstracts of the 20th international conference on very large data bases, Morgan Kaufmann Publishers Inc., San Francisco, 12–15 September 1994
Healey CG, Booth KS, Enns JT (1995) Visualizing real-time multivariate data using preattentive processing. ACM Trans Model Comput Simul 5(3):190–221. https://doi.org/10.1145/217853.217855
Light A, Bartlein PJ (2004) The end of the rainbow? Color schemes for improved data graphics. Eos Trans Am Geophys Union 85(40):385–391. https://doi.org/10.1029/2004EO400002
Data toViz (2017) The spaghetti plot. https://www.data-to-viz.com/caveat/spaghetti.html . Accessed 22 July 2021
Healy K (2018) Data visualization: a practical introduction. Princeton University Press, Princeton
Shah S (2018) Fact or fiction: five common downfalls of data visualizations. https://www.business.com/articles/datavisualization-downfalls . Accessed 22 July 2021
Wilke CO (2019) Fundamentals of data visualization: a primer on making informative and compelling figures. O’Reilly Media, Sebastopol
Data toViz (2019) Calculation errors. https://www.data-to-viz.com/caveat/calculation_error.html . Accessed 22 July 2021.
Eckardt D (2019) Case study: how baby boomers describe themselves. https://vizfix.com/case-study-how-baby-boomers-describe-themselves/ . Accessed 22 July 2021.
Acampora J (2018) When to use pie charts - best practices. https://www.excelcampus.com/charts/pie-charts-best-practices/ . Accessed 22 July 2021
Meyer D (2011) [WCYDWT] obama botches SOTU infographic, stock market reels. https://blog.mrmeyer.com/2011/wcydwt-obama-botches-sotu-infographic-stock-market-reels . Accessed 22 July 2021
Hickey W (2013) The 27 worst charts of all time. https://www.businessinsider.com/the-27-worst-charts-of-all-time-2013-6 . Accessed 22 July 2021
Visualizations W (2016) How concerned are you about the Zika virus? https://viz.wtf/ . Accessed 22 July 2021
Visualizations W (2019) WTF visualizations. https://viz.wtf/ . Accessed 22 July 2021
Download references
Acknowledgements
Not applicable.
Author information
Authors and affiliations.
Department of Information Technology, TNU – University of Information and Communication Technology, Thai Nguyen, Vietnam
Vinh T Nguyen
Department of Educational Psychology, Leadership, and Counseling, Texas Tech University, Lubbock, TX, 79409, United States
Kwanghee Jung
Department of Computer Science and Data Science, Meharry Medical College, Nashville, TN, 37208, USA
Vibhuti Gupta
You can also search for this author in PubMed Google Scholar
Contributions
VTN and KJ provided conceptualization, formal analysis and investigation, methodology and visualization; KJ provided supervision; VTN, KJ and VG provided validation; VTN, KJ and VG wrote the paper. The authors read and approved the final manuscript.
Corresponding author
Correspondence to Kwanghee Jung .
Ethics declarations
Competing interests.
The authors declare that they have no competing interests.
Additional information
Publisher’s note.
Springer Nature remains neutral with regard to jurisdictional claims in published maps and institutional affiliations.
Rights and permissions
Open Access This article is licensed under a Creative Commons Attribution 4.0 International License, which permits use, sharing, adaptation, distribution and reproduction in any medium or format, as long as you give appropriate credit to the original author(s) and the source, provide a link to the Creative Commons licence, and indicate if changes were made. The images or other third party material in this article are included in the article's Creative Commons licence, unless indicated otherwise in a credit line to the material. If material is not included in the article's Creative Commons licence and your intended use is not permitted by statutory regulation or exceeds the permitted use, you will need to obtain permission directly from the copyright holder. To view a copy of this licence, visit http://creativecommons.org/licenses/by/4.0/ .
Reprints and permissions
About this article
Cite this article.
Nguyen, V.T., Jung, K. & Gupta, V. Examining data visualization pitfalls in scientific publications. Vis. Comput. Ind. Biomed. Art 4 , 27 (2021). https://doi.org/10.1186/s42492-021-00092-y
Download citation
Received : 03 May 2021
Accepted : 19 September 2021
Published : 29 October 2021
DOI : https://doi.org/10.1186/s42492-021-00092-y
Share this article
Anyone you share the following link with will be able to read this content:
Sorry, a shareable link is not currently available for this article.
Provided by the Springer Nature SharedIt content-sharing initiative
- Data visualization
- Graphical representations
- Misinformation
- Visual encodings
- Association rule mining
- Cochran’s Q test
- McNemar’s test
Advertisement
The Impact of Visual Displays on Learning Across the Disciplines: A Systematic Review
- Review Article
- Published: 09 March 2020
- Volume 32 , pages 627–656, ( 2020 )
Cite this article
- Daibao Guo ORCID: orcid.org/0000-0001-7624-6718 1 ,
- Erin M. McTigue 2 ,
- Sharon D. Matthews 3 &
- Wendi Zimmer 3
2995 Accesses
15 Citations
9 Altmetric
Explore all metrics
The current systematic review aimed to investigate in what ways the incorporation of visual display tasks benefits K-12 students’ content-area learning . After screening 1693 articles at abstract level and a systematic evaluation of methodological quality, we synthesized 44 articles for this review. The qualitative synthesis of the studies is organized by categories of interaction with visual displays (ViDis), instructional support, and types of knowledge and learning. Overall findings indicate the simple inclusion of visual displays does not guarantee a positive learning effect. More detailed findings distinguish three categories of ViDis: author-provided, student-filled-in, and student-created visual displays. Furthermore, we discuss each category’s effectiveness for students’ learning. Additionally, findings on retention and information comprehension are mixed when students are either provided with ViDis or complete ViDis themselves. However, the integration of ViDis in K-12 classrooms indicate highly promising results for enhancing students’ higher-level learning (i.e., analyzing, evaluating, applying, and creating). Finally, we provided practical implications for K-12 teachers and recommendations for future research.
This is a preview of subscription content, log in via an institution to check access.
Access this article
Price includes VAT (Russian Federation)
Instant access to the full article PDF.
Rent this article via DeepDyve
Institutional subscriptions
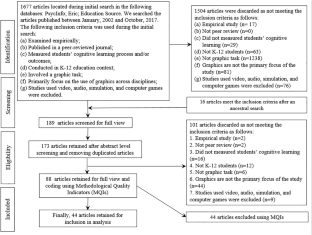
Similar content being viewed by others
Conditions for the effectiveness of multiple visual representations in enhancing stem learning.
Martina A. Rau
Studying Visual Displays: How to Instructionally Support Learning
Alexander Renkl & Katharina Scheiter
Representing Visually: What Teachers Know and What They Prefer
*samples included in the present study.
*Åberg-Bengtsson, L. (2006). “Then you can take half… almost”—elementary students learning bar graphs and pie charts in a computer-based context. The Journal of Mathematical Behavior, 25 , 116–135. https://doi.org/10.1016/j.jmathb.2006.02.007 .
Article Google Scholar
*Acha, J. (2009). The effectiveness of multimedia programmes in children’s vocabulary learning. British Journal of Educational Technology, 40 , 23–31. https://doi.org/10.1111/j.1467-8535.2007.00800.x .
Anderson, L. W., Krathwohl, D. R., & Bloom, B. S. (2001). A taxonomy for learning, teaching, and assessing: a revision of Bloom’s taxonomy of educational objectives . New York: Longman.
Google Scholar
*Arya, P., & Feathers, K. M. (2012). Reconsidering children’s readings: insights into the reading process. Reading Psychology, 33 , 301–322. https://doi.org/10.1080/02702711.2010.518881 .
*Bergey, B. W., Cromley, J. G., Kirchgessner, M. L., & Newcombe, N. S. (2015). Using diagrams versus text for spaced restudy: effects on learning in 10th grade biology classes. British Journal of Educational Psychology, 85 (1), 59–74. https://doi.org/10.1111/bjep.12062 .
Bergey, B. W., Cromley, J. G., & Newcombe, N. S. (2015b). Teaching high school biology students to coordinate text and diagrams: Relations with transfer, effort, and spatial skill. International Journal of Science Education, 37 (15), 2476–2502.
*Berthold, K., & Renkl, A. (2009). Instructional aids to support a conceptual understanding of multiple representations. Journal of Educational Psychology, 101 , 70–87. https://doi.org/10.1037/a0013247 .
Bertin, J. (1983). Semiology of graphics: diagrams, networks, maps (2nd ed.). Madison: University of Wisconsin Press.
Bloom, B.S. (Ed.). Engelhart, M.D., Furst, E.J., Hill, W.H., Krathwohl, D.R. (1956). Taxonomy of educational objectives . Handbook I: the cognitive domain . New York: David McKay Co Inc.
*Boulineau, T., Fore Iii, C., Hagan-Burke, S., & Burke, M. D. (2004). Use of story-mapping to increase the story-grammar text comprehension of elementary students with learning disabilities. Learning Disability Quarterly, 27 , 105–121. https://doi.org/10.2307/1593645 .
Britt, M. A., & Gabrys, G. L. (2001). Teaching advanced literacy skills for the world wide web. In C. Wolfe (Ed.), Learning and teaching on the world wide web (pp. 73–90). Cambridge: Academic Press.
Chapter Google Scholar
Brookshire, J., Scharff, L. F., & Moses, L. E. (2002). The influence of illustrations on children’s book preferences and comprehension. Reading Psychology, 23 , 323–339. https://doi.org/10.1080/713775287 .
Carney, R. N., & Levin, J. R. (2002). Pictorial illustrations still improve students’ learning from text. Educational Psychology Review, 14 , 5–26. https://doi.org/10.1023/A:1013176309260 .
Chi, M. (2000). Self-explaining expository texts: The dual processes of generating inferences and repairing mental models. Advances in instructional psychology, 5 , 161–238.
*Cho, Y. H., & Jonassen, D. H. (2012). Learning by self-explaining causal diagrams in high-school biology. Asia Pacific Education Review, 13 (1), 171–184. https://doi.org/10.1007/s12564-011-9187-4 .
*Ciullo, S., Falcomata, T. S., Pfannenstiel, K., & Billingsley, G. (2015a). Improving learning with science and social studies text using computer-based concept maps for students with disabilities. Behavior Modification, 39 , 117–135. https://doi.org/10.1177/0145445514552890 .
*Ciullo, S., Falcomata, T., & Vaughn, S. (2015b). Teaching social studies to upper elementary students with learning disabilities: graphic organizers and explicit instruction. Learning Disability Quarterly, 38 , 15–26. https://doi.org/10.1177/0731948713516767 .
Clark, J. M., & Paivio, A. (1991). Dual coding theory and education. Educational Psychology Review, 3 , 149–210. https://doi.org/10.1007/BF01320076 .
*Cohen, M. T., & Johnson, H. L. (2011). Improving the acquisition of novel vocabulary through the use of imagery interventions. Early Childhood Education Journal, 38 (5), 357–366. https://doi.org/10.1007/s10643-010-0408-y .
*Cohen, M. T., & Johnson, H. L. (2012). Improving the acquisition and retention of science material by fifth grade students through the use of imagery interventions. Instructional Science, 40 , 925–955. https://doi.org/10.1007/s11251-011-9197-y .
Coleman, J. M., McTigue, E. M., & Dantzler, J. A. (2018). What makes a diagram easy or hard? The impact of diagram design on fourth-grade students’ comprehension of science texts. The elementary school journal, 119 (1), 122–151.
Common Core State Standards. (2009). English language arts—literacy Retrieved from http://www.corestandards.org/ELA-Literacy/RI/4/ .
Cooper, H. M. (1982). Scientific guidelines for conducting integrative research reviews. Review of Educational Research, 52 , 291–302.
*Cromley, J. G., Bergey, B. W., Fitzhugh, S. L., Newcombe, N., Wills, T. W., Shipley, T. F., & Tanaka, J. C. (2013a). Effectiveness of student-constructed diagrams and self-explanation instruction. Learning and Instruction, 26 , 45–58.
*Cromley, J. G., Perez, T. C., Fitzhugh, S. L., Newcombe, N. S., Wills, T. W., & Tanaka, J. C. (2013b). Improving students’ diagram comprehension with classroom instruction. The Journal of Experimental Education, 81 , 511–537. https://doi.org/10.1080/00220973.2012.745465 .
*Cromley, J. G., Weisberg, S. M., Dai, T., Newcombe, N. S., Schunn, C. D., Massey, C., & Merlino, F. J. (2016). Improving middle school science learning using diagrammatic reasoning. Science Education, 100 , 1184–1213. https://doi.org/10.1002/sce.21241 .
*DiCecco, V. M., & Gleason, M. M. (2002). Using graphic organizers to attain relational knowledge from expository text. Journal of Learning Disabilities, 35 (4), 306–320.
Duke, N. K., & Bennett-Armistead, V. S. (2003). Reading and writing informational text in the primary grades: research based practices . New York: Scholastic.
*Elia, I., Gagatsis, A., & Demetriou, A. (2007). The effects of different modes of representation on the solution of one-step additive problems. Learning and Instruction, 17 , 658–672. https://doi.org/10.1016/j.learninstruc.2007.09.011 .
*Enyedy, N. (2005). Inventing mapping: creating cultural forms to solve collective problems. Cognition and Instruction, 23 , 427–466. https://doi.org/10.1207/s1532690xci2304_1 .
Fingeret, L. (2012). Visuals in children’s informational texts: a content analysis. (Unpublished doctoral dissertation). Michigan State University, East Lansing, MI.
Gatto, J. A., Porter, A. W., & Selleck, J. (2011). Exploring visual design: the elements and principles (4th ed.). Worcester: Davis Publications.
*Gerstner, S., & Bogner, F. X. (2009). Concept map structure, gender and teaching methods: an investigation of students’ science learning. Educational Research, 51 , 425–438. https://doi.org/10.1080/00131880903354758
Guo, D., Wright, K. L., & McTigue, E. M. (2018). A content analysis of visuals in elementary school textbooks. The elementary school journal, 119 (2), 244–269.
Guo, D., Zimmer, W., Matthews, S. D., & McTigue, E. M. (2019). Critical analysis of research on the impact of visual literacy for learning: strengths, weaknesses and recommendations for improvement. Journal of Visual Literacy, 38 (3), 181–198.
Guo, D., Zhang, S., Wright, K. L., & McTigue, E. M. (2020). Do You Get the Picture? A Meta-Analysis of the Effect of Graphics on Reading Comprehension. AERA Open, 6(1), 2332858420901696.
Hannus, M., & Hyönä, J. (1999). Utilization of illustrations during learning of science textbook passages among low-and high-ability children. Contemporary Educational Psychology, 24 (2), 95–123.
Hunter, B., Crismore, A., & Pearson, P. D. (1997). Visual displays in basal readers and social studies textbooks. In H. A. Houghton & D. M. Willows (Eds.), The psychology of illustration (pp. 116–135). Harrisonburg: R. R. Donnelly & Sons.
*Jian, Y. C. (2017). Eye-movement patterns and reader characteristics of students with good and poor performance when reading scientific text with diagrams. Reading and Writing, 30 (7), 1447–1472. https://doi.org/10.1007/s11145-017-9732-6 .
Kress, G., & Van Leeuwen, T. (1996). Reading images: the grammar of visual design . London: Routledge.
Kulhavy, R. W., Stock, W. A., Woodard, K. A., & Haygood, R. C. (1993). Comparing elaboration and dual coding theories: the case of maps and text. Contemporary Educational Psychology, 106 , 483–498.
Kulhavy, R. W., Stock, W. A., & Caterino, L. C. (1994). Reference maps as a framework for remembering text. In W. Schnotz & R. W. Kulhavy (Eds.), Comprehension of graphics (pp. 153–162). New York: Elsevier Science.
*Kwon, S. Y., & Cifuentes, L. (2009). The comparative effect of individually-constructed vs. collaboratively-constructed computer-based concept maps. Computers & Education, 52 , 365–375.
*Lenzner, A., Schnotz, W., & Müller, A. (2013). The role of decorative pictures in learning. Instructional Science, 41 (5), 811–831. https://doi.org/10.1007/s11251-012-9256-z .
*Leopold, C., Doerner, M., Leutner, D., & Dutke, S. (2015). Effects of strategy instructions on learning from text and pictures. Instructional Science, 43 (3), 345–364. https://doi.org/10.1007/s11251-014-9336-3 .
Levie, W. H., & Lentz, R. (1982). Effects of text illustrations: a review of research. Educational Communication and Technology, 30 , 195–232.
*Marley, S. C., & Szabo, Z. (2010). Improving children’s listening comprehension with a manipulation strategy. The Journal of Educational Research, 103 , 227–238. https://doi.org/10.1080/00220670903383036 .
*Mason, L., Pluchino, P., Tornatora, M. C., & Ariasi, N. (2013a). An eye-tracking study of learning from science text with concrete and abstract illustrations. The Journal of Experimental Education, 81 , 356–384. https://doi.org/10.1080/00220973.2012.727885 .
*Mason, L., Tornatora, M. C., & Pluchino, P. (2013b). Do fourth graders integrate text and picture in processing and learning from an illustrated science text? Evidence from eye-movement patterns. Computers & Education, 60 , 95–109. https://doi.org/10.1016/j.compedu.2012.07.011 .
*Mason, L., Baldi, R., Di Ronco, S., Scrimin, S., Danielson, R. W., & Sinatra, G. M. (2017). Textual and graphical refutations: effects on conceptual change learning. Contemporary Educational Psychology, 49 , 275–288. https://doi.org/10.1016/j.cedpsych.2017.03.007 .
Mayer, R. E. (1989). Systematic thinking fostered by illustrations in scientific text. Journal of educational psychology, 81 (2), 240–246.
Mayer, R. (2001). Multi-media learning . Cambridge, UK: Cambridge University Press.
Book Google Scholar
Mayer, R. E., & Gallini, J. K. (1990). When is an illustration worth ten thousand words? Journal of Educational Psychology, 82 , 715–726.
Mayer, R. E. (2006). Ten research-based principles of multimedia learning. Web-based learning: Theory, research, and practice, 371–390.
Mayer, R. E., & Johnson, C. I. (2008). Revising the redundancy principle in multimedia learning. Journal of Educational Psychology, 100, 380.
*McTigue, E. M. (2009). Does multimedia learning theory extend to middle-school students? Contemporary Educational Psychology, 34 , 143–153. https://doi.org/10.1016/j.cedpsych.2008.12.003 .
McTigue, E. M., & Flowers, A. C. (2011). Science visual literacy: Learners’ perceptions and knowledge of diagrams. The Reading Teacher, 64 (8), 578–589.
Metros, S. E. (2008). The educator’s role in preparing visually literate learners. Theory into Practice, 47 . https://doi.org/10.1080/00405840801992264 .
*Miller, B. W., Cromley, J. G., & Newcombe, N. S. (2016). Improving diagrammatic reasoning in middle school science using conventions of diagrams instruction. Journal of Computer Assisted Learning, 32 , 374–390. https://doi.org/10.1111/jcal.12143 .
*Moreno, R., Ozogul, G., & Reisslein, M. (2011). Teaching with concrete and abstract visual representations: effects on students’ problem solving, problem representations, and learning perceptions. Journal of Educational Psychology, 103 , 32. https://doi.org/10.1037/a0021995 .
*Muthersbaugh, D., Kern, A. L., & Charvoz, R. (2014). Impact through images: exploring student understanding of environmental science through integrated place-based lessons in the elementary classroom. Journal of Research in Childhood Education, 28 , 313–326.
*Norman, R. R. (2012). Reading the graphics: what is the relationship between graphical reading processes and student comprehension? Reading and Writing, 25 , 739–774.
Paivio, A. (1971). Imagery and verbal processes . New York: Holt, Rinehart & Winston.
Paivio, A. (1986). Mental representations: a dual coding approach . New York: Oxford University Press.
Peeck, J. (1993). Increasing picture effects in learning from illustrated text. Learning and Instruction, 3 , 227–238.
Polkinghorne, D. E. (1995). Narrative configuration in qualitative analysis. International Journal of Qualitative Studies in Education, 8 , 5–23.
*Pyke, C. L. (2003). The use of symbols, words, and diagrams as indicators of mathematical cognition: a causal model. Journal for Research in Mathematics Education, 34 , 406–432. https://doi.org/10.2307/30034794 .
Renkl, A., & Scheiter, K. (2017). Studying visual displays: how to instructionally support learning. Educational Psychology Review, 29 (3), 599–621. https://doi.org/10.1007/s10648-015-9340-4 .
Rimrodt, S., Lightman, A., Roberts, L., Denckla, M. B., & Cutting, L. E. (2005). Are all tests of reading comprehension the same? Paper presented at the 33rd Annual International Neuropsychological Society Meeting.
Risko, V. J., Roller, C. M., Cummins, C., Bean, R. M., Block, C. C., Anders, P. L., & Flood, J. (2008). A critical analysis of research on reading teacher education. Reading Research Quarterly, 43 , 252–288. https://doi.org/10.1598/RRQ.43.3.3 .
Roberts, K. L., & Brugar, K. A. (2017). The view from here: emergence of graphical literacy. Reading Psychology, 38 , 733–777. https://doi.org/10.1080/02702711.2017.1336661 .
*Roberts, K. L., Norman, R. R., & Cocco, J. (2015). Relationship between graphical device comprehension and overall text comprehension for third-grade children. Reading Psychology, 36 , 389–420. https://doi.org/10.1080/02702711.2013.865693 .
Robinson, R. S. (1984). Learning to see: developing visual literacy through film. Top of the News, 40 , 267–275.
Sadoski, M., Goetz, E. T., Stricker, A. G., & Burdenski, T. K. (2003). New findings for concreteness and imagery effects in written composition. Reading and Writing, 16 , 443–453.
*Schmeck, A., Mayer, R. E., Opfermann, M., Pfeiffer, V., & Leutner, D. (2014). Drawing pictures during learning from scientific text: testing the generative drawing effect and the prognostic drawing effect. Contemporary Educational Psychology, 39 , 275–286. https://doi.org/10.1016/j.cedpsych.2014.07.003 .
Scott, C. E., McTigue, E. M., Miller, D. M., & Washburn, E. K. (2018). The what, when, and how of preservice teachers and literacy across the disciplines: a systematic literature review of nearly 50 years of research. Teaching and Teacher Education, 73 , 1–13.
*Segers, E., Verhoeven, L., & Hulstijn-Hendrikse, N. (2008). Cognitive processes in children’s multimedia text learning. Applied Cognitive Psychology, 22 , 375–387.
Shanahan, T., & Shanahan, C. (2008). Teaching disciplinary literacy to adolescents: rethinking content-area literacy. Harvard Educational Review, 78 , 40–59.
Slough, S. W., & McTigue, E. M. (2010). Introduction to the integration of verbal and visual information in science texts. Reading Psychology, 31 (3), 206–212.
*Sun, J. C. Y., & Chen, A. Y. Z. (2016). Effects of integrating dynamic concept maps with Interactive Response System on elementary school students’ motivation and learning outcome: the case of anti-phishing education. Computers & Education, 102 , 117–127. https://doi.org/10.1016/j.compedu.2016.08.002 .
*Sun, J. C. Y., & Lee, K. H. (2016). Which teaching strategy is better for enhancing anti-phishing learning motivation and achievement? The concept maps on tablet PCs or worksheets? Journal of Educational Technology & Society, 19 , 87.
*Swanson, H. L. (2015). Cognitive strategy interventions improve word problem solving and working memory in children with math disabilities. Frontiers in Psychology, 6 , 1099. https://doi.org/10.3389/fpsyg.2015.01099 .
*Swanson, H. L., Lussier, C., & Orosco, M. (2013). Effects of cognitive strategy interventions and cognitive moderators on word problem solving in children at risk for problem solving difficulties. Learning Disabilities Research & Practice, 28 , 170–183. https://doi.org/10.1111/ldrp.12019 .
*Terwel, J., van Oers, B., van Dijk, I., & van den Eeden, P. (2009). Are representations to be provided or generated in primary mathematics education? Effects on transfer. Educational Research and Evaluation, 15 , 25–44. https://doi.org/10.1080/13803610802481265 .
Texas Education Agency. (2017). Texas Essential Knowledge and Skills for Science. Retrieved from http://ritter.tea.state.tx.us/rules/tac/chapter112/
Vacca, R. T., Vacca, J. A. L., & Mraz, M. E. (2005). Content area reading: literacy and learning across the curriculum . England: Pearson.
*Van Garderen, D. (2006). Spatial visualization, visual imagery, and mathematical problem solving of students with varying abilities. Journal of Learning Disabilities, 39 (6), 496–506. https://doi.org/10.1177/00222194060390060201 .
*Van Garderen, D. (2007). Teaching students with LD to use diagrams to solve mathematical word problems. Journal of Learning Disabilities, 40 (6), 540–553.
Vekiri, I. (2002). What is the value of graphical displays in learning? Educational Psychology Review, 14 , 261–312.
Vinter, A., & Perruchet, P. (2000). Implicit learning in children is not related to age: evidence from drawing behavior. Child Development, 71 (5), 1223–1240.
Waller, R. (1981). Understanding network diagrams. Paper presented at the Annual Meeting of the American Educational Research Association , Los Angeles, April 1981.
*Wang, H. Y., Huang, I., & Hwang, G. J. (2016). Effects of a question prompt-based concept mapping approach on students’ learning achievements, attitudes and 5C competences in project-based computer course activities. Journal of Educational Technology & Society, 19 , 351.
Watkins, N. M., & Lindahl, K. M. (2010). Targeting content area literacy instruction to meet the needs of adolescent English language learners. Middle School Journal, 4 , 23–33.
Wileman, R. E. (1993). Visual communicating . Englewood Cliffs: Educational Technology Publications.
*Zheng, R. Z., Yang, W., Garcia, D., & McCadden, E. P. (2008). Effects of multimedia and schema induced analogical reasoning on science learning. Journal of Computer Assisted Learning, 24 , 474–482. https://doi.org/10.1111/j.1365-2729.2008.00282.x .
Download references
Author information
Authors and affiliations.
Department of Literacy, Language, & Culture, Boise State University, Boise, ID, USA
Norwegian National Centre for Reading and Reading Research, University of Stavanger, Stavanger, Norway
Erin M. McTigue
Department of Teaching, Learning, & Culture, Texas A&M University, College Station, TX, USA
Sharon D. Matthews & Wendi Zimmer
You can also search for this author in PubMed Google Scholar
Corresponding author
Correspondence to Daibao Guo .
Additional information
Publisher’s note.
Springer Nature remains neutral with regard to jurisdictional claims in published maps and institutional affiliations.
Electronic supplementary material
(DOCX 36 kb)
(DOCX 25 kb)
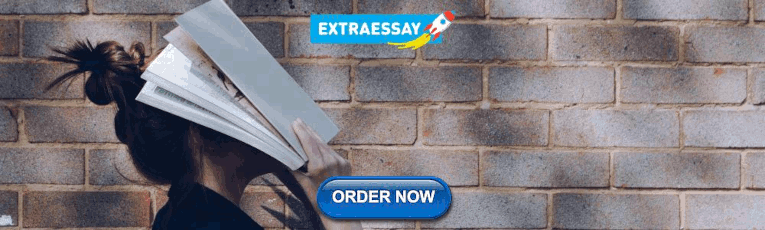
Rights and permissions
Reprints and permissions
About this article
Guo, D., McTigue, E.M., Matthews, S.D. et al. The Impact of Visual Displays on Learning Across the Disciplines: A Systematic Review. Educ Psychol Rev 32 , 627–656 (2020). https://doi.org/10.1007/s10648-020-09523-3
Download citation
Published : 09 March 2020
Issue Date : September 2020
DOI : https://doi.org/10.1007/s10648-020-09523-3
Share this article
Anyone you share the following link with will be able to read this content:
Sorry, a shareable link is not currently available for this article.
Provided by the Springer Nature SharedIt content-sharing initiative
- Visual displays
- Systematic review
- Find a journal
- Publish with us
- Track your research
- Abbot’s Blog
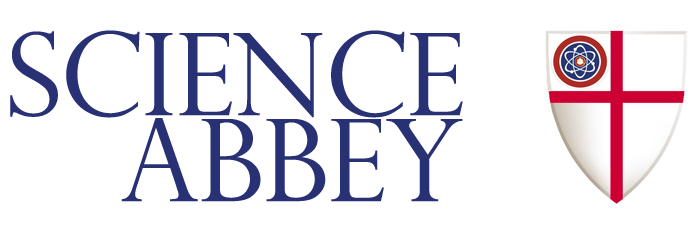
Power of the Mind 1: The Science of Visualization

CONTENTS PART 1: Creative Visualization | Guided Meditation | Relaxation | Health and Immunity | Healing | Athletics | Life Goals | Holistic Imagery for Success
Contents part 2: studying and professional skill building | mnemonics | the memory palace | artistic creativity | enlightenment | the universal person | archetypes | inner alchemy | spirituality | meditation, mental health and enlightenment | mindfulness meditation, contents part 3: meditation, the nervous system and immunity | anatomical basics | neuroendocrinology | psychoneuroendocrinoimmunology | the sam | the hpa | endorphins: the body’s natural pain-killers | how visualization works | how meditation works | how enlightenment works.
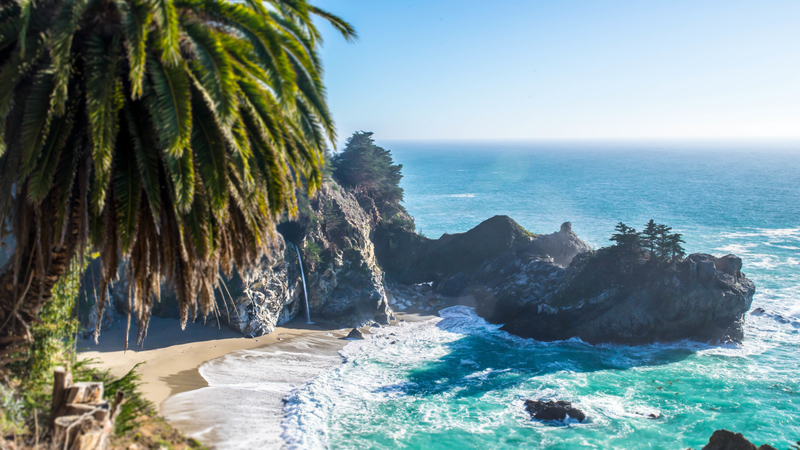
Creative Visualization
When I was a gymnast as a child, my teammates and I were taught to visualize ourselves doing each routine while we were waiting our turn to practice the event. This technique helps gymnasts prepare for difficult exercises that are judged to the tenth of a point on form and execution. Toes must be pointed, knees straight, body in alignment, and advanced skills must be performed with speed and precision. Creative visualization plays an essential part in such serious training because in large part, where the mind goes, the body will follow.
Creative visualization has many forms and uses. Its benefits are manifold and really quite astonishing in depth and scope. Scientific studies have shown the positive effects of creative visualization on health, such as immunity, stress, healing and pain management. Science also shows that it improves many facets of life, from athletic ability to cognitive performance, to self esteem, to goal achievement.
Treatment of anger, stress, anxiety, depression and other emotional issues, along with letting go of bad habits, can have a major impact on self esteem and personal relationships. [1] Visualization techniques may also aid in the attainment of higher states of consciousness. Whatever you need, whatever you do, whatever your abilities, visualization will help.
Mental imagery is a reproduction of perceived aspects of the physical world within a subject’s mind. [2] Neuroscience has demonstrated that the brain can reproduce mental imagery from all of the senses, including sights, sounds, tastes, smells, pressures, textures, temperatures and movements. [3] Mental imagery may be associated with positive or negative emotions.
Experimental psychology has developed therapeutic methods to replace a patient’s imagery that causes pain, depression, anxiety or stress with imagery that is calming, relaxing, comforting, and conducive to cognitive clarity and emotional stability. Visualization acts directly on the brain, altering brainwave activity and biochemistry, and its effects branch out into every aspect of life. There is a lot of research that shows how mental practice boosts confidence, enhances mood, helps the healing process and has a positive effect on performance of cognitive and physical tasks . [4] [5] [6] [7] [8]
As a general introduction to the subject of creative visualization, this article will not go into a lot of detail, but citations are given so the interested reader can pursue further information. Future articles will delve deeper into topics like traditional religious visualizations; relaxation exercises; healing with intent; guided meditation; goal-setting; the biological sciences that examine visualization; and the relationship between science and spirituality.
[1] Susan Heitler, PhD, “Advanced Visualization Techniques for Individual and Couples Therapy,” 29 Sept., 2017, https://www.goodtherapy.org/advanced-visualization-techniques-therapy-web-conference.html
[2] “mental image,” wikipedia, accessed 24 oct., 2018, https://en.wikipedia.org/wiki/mental_image, [3] “creative visualization,” wikipedia, accessed 24 oct., 2018, https://en.wikipedia.org/wiki/creative_visualization#visual_and_non-visual_mental_imagery, [4] naparstek, “guided imagery 101,” excerpted from “staying well with guided imagery,” naparstek, 1994 and “invisible heroes” naparstek, 2005, https://www.healthjourneys.com/whatisguidedimagery ., [5] tracy c. ekeocha, b.s., “the effects of visualization & guided imagery in sports performance,” may 2015, https://digital.library.txstate.edu/bitstream/handle/10877/5548/ekeocha-thesis-2015.pdfsequence=1, [6] “research on meditation,” wikipedia, accessed 24 oct., 2018, https://en.wikipedia.org/wiki/research_on_meditation, [7] alvin powell, “when science meets mindfulness,” the harvard gazette , 9 april, 2018, https://news.harvard.edu/gazette/story/2018/04/harvard-researchers-study-how-mindfulness-may-change-the-brain-in-depressed-patients/, [8] “meditation: in depth,” the national institutes of health (nih), accessed 24 oct., 2018, https://nccih.nih.gov/health/meditation/overview.htm.
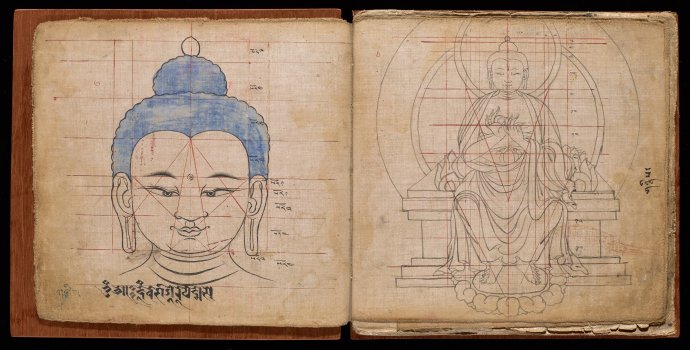
Guided Meditation
Guided meditation is meditation led by a trained expert or teacher who verbally guides an audience through a meditation in person or via audio or video recording. The technical term “guided meditation” is used in academic research, clinical practice of contemporary medicine, and scientific experimentation. Guided meditation may involve relaxation techniques, hypnosis, mindfulness meditation and journaling. Sometimes music or sounds are used to elicit certain moods or emotions as a complement to the verbal instruction and guided imagery.
Guided meditation can be purely instructional, but usually it involves visualization. Guided imagery is the primary element of visualization-centered guided meditation. Guided imagery is a form of therapy used by health professionals to treat disorders by generating, maintaining, inspecting and transforming mental images recreated from sights, sounds, scents, tastes, or feelings.
According to S. M. Kosslyn’s [1] computational theory of visualization, mental imagery may be held in the mind to different degrees, expressed in four stages. Beginning with the simple generation of images, the mind proceeds to maintenance of imagery and then inspection of the object(s) of the image. Finally, there may be the transformation of imagery from negative and painful images to positive and healthy images. [2]
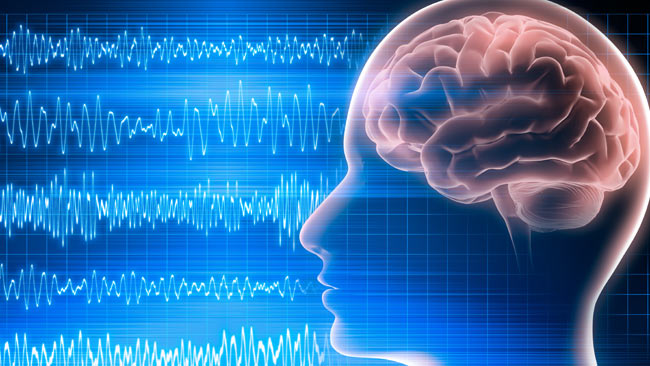
Guided Meditations from UCLA
Sense objects are often used in guided imagery meditation, especially sounds, usually music or recordings of nature. Worship music, chanting, and other kinds of song can be experienced as guided visualizations. [3] [4] Classical music or relaxing music is often used, but studies show that various genres are effective, especially when lyrics contain what are perceived to be meaningful affirmations about success. [5] Visual aids like mandalas and visual symbols are found in religious ceremonies and in modern psychotherapy. Ritual, itself, is a form of guided imagery that relies heavily on sensory stimulation.
Not all sense objects used in meditation are related to guided imagery; the senses can be used in several ways to aid meditation. The color and tidiness of a room can have significant effects on the mind. Light can be dimmed for a calming atmosphere. Read material, such as poetry or instruction, may be part of meditation without any visualization involved. Music, chanting, bells, nature, water or other sounds may be simply pleasurable and relaxing.
Scents may be used to induce a specific state of mind, such as the calming effect of lavender, often in the form of essential oils as aromatherapy, or incense. Massage therapy is a touch-based healing modality that may use pressure, joint movements and heat to help induce a meditative state of mind.
Often the initial phase of guided meditation is relaxation. Self-hypnosis or hypnosis by a professional therapist utilizes visualization and deep relaxation to alter brainwaves from Beta (12 to 30 Hz), the normal state, to Alpha (8 to 12 Hz) or Theta (4 to 8 Hz), suggestible states wherein the subconscious can be explored and conditioned. Repeatedly visualizing achieving one’s goals can change patterns of failure to establish a successful mindset that overcomes psychological obstructions and produces positive results. [6]
[1] Stephen M. Kosslyn: https://psychology.fas.harvard.edu/people/stephen-m-kosslyn
[2] “creative visualization,” wikipedia, accessed 24 oct., 2018, https://en.wikipedia.org/wiki/creative_visualization#stages, [3] e. pickett, “introduction,” guided imagery and music (bruscia ke & grocke de eds), barcelona publishers, gilsum, nh, 2002, pp. 15– 25., [4] m. lin, m. hsu, h. chang, y. hsu, m. chou, & p. crawford,”pivotal moments and changes in the bonny method of guided imagery and music for patients with depression.” journal of clinical nursing , 19, 2010, pp. 1139–1148., [5] f. goldberg, “the bonny method of guided imagery and music,” the art & science of music therapy: a handbook, 1995, (t. a. wigram, b. saperston, & r. west, eds.) sydney, australia, harwood academic publishers, pp.112-128., [6] emilie pelletier, “4 scientific reasons why visualization will increase your chances to succeed,” 24 jan., 2018, https://entrepreneurs.maqtoob.com/4-scientific-reasons-why-visualization-will-increase-your-chances-to-succeed-5515ef2dbdb7.
Visualization is one of several meditation methods that can induce relaxation and relieve stress for improved health. [1] [2] Imagery may be focused upon a remembered location or imaginary environment, such as a beach, an island, a jungle, or any other peaceful, enjoyable setting that makes one comfortable. This kind of meditation may be supplemented with music or sounds related to the images within the visualization, such as sounds of the ocean, birds or wind through the trees. [3] Visualization can be self-directed or it may be assisted by a guided meditation. It can be joined with other types of relaxation meditations and modalities.
Mental relaxation techniques include the body scan meditation, progressive muscle relaxation, deep breathing, movement meditation like yoga or taijiquan (Tai Chi), guided imagery and mindfulness meditation. [4] The Relaxation Response is a term coined by Harvard University’s Dr. Herbert Benson to indicate meditation techniques that elicit deep relaxation. [5]
It is important to note that different meditations achieve varying positive results. Whereas mindfulness meditation has been shown to affect the sensory awareness and perception regions of the brain, the Relaxation Response exercises the deliberate control neural mechanisms. [6] It follows that a routine that utilized complementary modalities would be ideal for a comprehensive meditation program.
It should be noted that while relaxation techniques are certainly helpful in reducing stress, other psychological modalities, such as cognitive-behavioral therapy, psychotherapy or medication, may be even more helpful. It is essential to one’s health that one seek the advice of a qualified professional medical practitioner for any health-related complaints. That said, visualization techniques have proven to be a significant part of a basic health regimen.
[1] Julie Corliss, “Six relaxation techniques to reduce stress,” Sept., 2016, https://www.health.harvard.edu/mind-and-mood/six-relaxation-techniques-to-reduce-stress
[2] katherine ellen foley, “science finally proves that meditation helps make your body markedly less stressed,” 20, feb., 2016, https://qz.com/620839/science-finally-proves-that-meditation-makes-your-body-markedly-less-stressed/, [3] “relaxation techniques for stress relief,” pdf, united nations high commissioner for refugees, retrieved 24 oct., 2018, https://cms.emergency.unhcr.org/documents/11982/34619/relaxation+techniques+for+stress+relief/3b3ae17a-2dbf-4cc4-b2b8-569c805bd379, [4] lawrence robinson, robert segal, m.a., jeanne segal, ph.d., and melinda smith, m.a., “relaxation techniques: using the relaxation response to relieve stress,” sept., 2018, https://www.helpguide.org/articles/stress/relaxation-techniques-for-stress-relief.htm, [5] marilyn mitchell m.d., “dr. herbert benson’s relaxation response,” psychology today , 29 march, 2013, https://www.psychologytoday.com/us/blog/heart-and-soul-healing/201303/dr-herbert-benson-s-relaxation-response, [6] mgh public affairs, “mindfulness meditation and relaxation response affect brain differently,” the harvard gazette , 20 june, 2018, https://news.harvard.edu/gazette/story/2018/06/mindfulness-meditation-and-relaxation-response-affect-brain-differently/.
Health and Immunity
Psychoneuroendocrinoimmunology shows how the nervous, endocrine and immune systems are intertwined and how disorders can arise from stress. The article, “Visualization and Stress Relief” goes into more detail on the anatomy and physiology of this process. Stress in the form of loneliness, fear, anger, anxiety, or depression can raise heart rate and blood pressure while lowering immunity. Chronic stress of this kind often undermines homeostasis and health.
Visualization is a uniquely attractive form of medicine. It is an effective preventative therapy as well as a benign remedy for stress and many of its associated disorders. Pharmaceuticals such as antidepressants and psychedelics may be used for similar purposes, but they may come with negative side-effects, which visualization does not. Furthermore, stress relief and managing the many side effects of stress are not the only ways visualization and other forms of meditation can help maintain and improve health.
Visualization may be effective in helping to treat addiction. In one study, seventy-six outpatients with a chemical dependency treatment program were given six hour-long relaxation and visualization exercises over a period of three weeks, replacing the usual treatment of psychoeducation (problem-solving skills). Standard tests revealed that visualization and psychoeducation produced equally positive results. [1] The authors of the study recommend that further research be undertaken to understand the benefits of visualization as a health and healing modality.
Visualization is also used in therapeutic guided imagery. Learning techniques to control one’s mental imagery can be vital to healing from serious psychological afflictions. Mental imagery can exacerbate certain disorders such as PTSD (post-traumatic stress disorder), eating disorders, multiple sclerosis, bipolar disorder, schizophrenia, phobias, anxiety and depression. [2]
[1] Kathryn D. Kominars, PhD, “A study of visualization and addiction treatment,” PDF, Journal of Substance Abuse Treatment, Volume 14, Issue 3, May-June, 1997, Pages 213–223, https://www.journalofsubstanceabusetreatment.com/article/S0740-5472(96)00068-2/pdf
[2] “guided imagery,” wikipedia, accessed 24 oct., 2018, https://en.wikipedia.org/wiki/guided_imagery#mental_imagery_and_ill_health.
Painful memories or imagined scenarios about the future can cause distress, whether anxiety, depression, or both. Spontaneous vivid mental images called flashbacks can cause anxiety and stress. A flashback is an involuntary onslaught of vivid imagery from past situations that caused intense fear, discomfort, pain or suffering. Similarly, negative imagery can arise that causes or perpetuates depression. Anxiety and depression can exist simultaneously or cycle through the mind.
Just as illness can cause pain through mental imagery, so can creative visualization be a source of healing. Guided imagery can increase relaxation and reduce stress. Cancer patients trained in relaxation techniques and/or guided imagery experience less pain, anxiety and depression. [1] [2] Guided imagery has been shown scientifically to be an effective treatment for both physical and psychological pain, and is an important part of a holistic therapeutic plan. [3]
Guided imagery is used in therapy to teach the patient how to replace painful images, like distressing memories, negative thoughts or worries about the future, with images that calm, comfort, encourage and empower. One can go to a therapist to receive guided meditation face-to-face, or find the appropriate verbal guided meditations recorded on media.
Therapeutic guided meditation may involve several types of guided imagery. Soothing words may be used to calm the mind and relax the body. Instruction may focus on breath control or progressive relaxation techniques. There may be multi-sensory visualizations of real or imaginary peaceful locations, either natural or architectural. A patient may be led to change attitudes, perspectives, ways of thinking, or behaviors, through mental rehearsals of healthier patterns of volitional action and reaction to the environment.
Medical science has made it clear that guided imagery is useful for relaxation, health and healing. Visualization has also been shown to be a key element of physical fitness.
[1] Leo, Pizarro, Gich, Barthe, Rovirosa, Farru, Casas, Arcusa, “A randomized trial of the effect of training in relaxation and guided imagery techniques in improving psychological and quality-of-life indices for gynecologic and breast brachytherapy patients.” PsychoOncology , 16, 2007, pp. 971-979.
[2] walker, walker, ogston, heys, ah-see, miller, eremin, “psychological, clinical and pathological effects of relaxation training and guided imagery during primary chemotherapy.” british journal of cancer , 80(1-2), 1999, pp. 262-268, https://www.nature.com/articles/6690349, [3] shapiro, pisetsky, crenshaw, spainhour, hamer, dymek, valentine, and bulik, “exploratory study to decrease postprandial anxiety: just relax” international journal of eating disorders , 41(8), 2008, pp. 728-733, https://onlinelibrary.wiley.com/doi/10.1002/eat.20552..
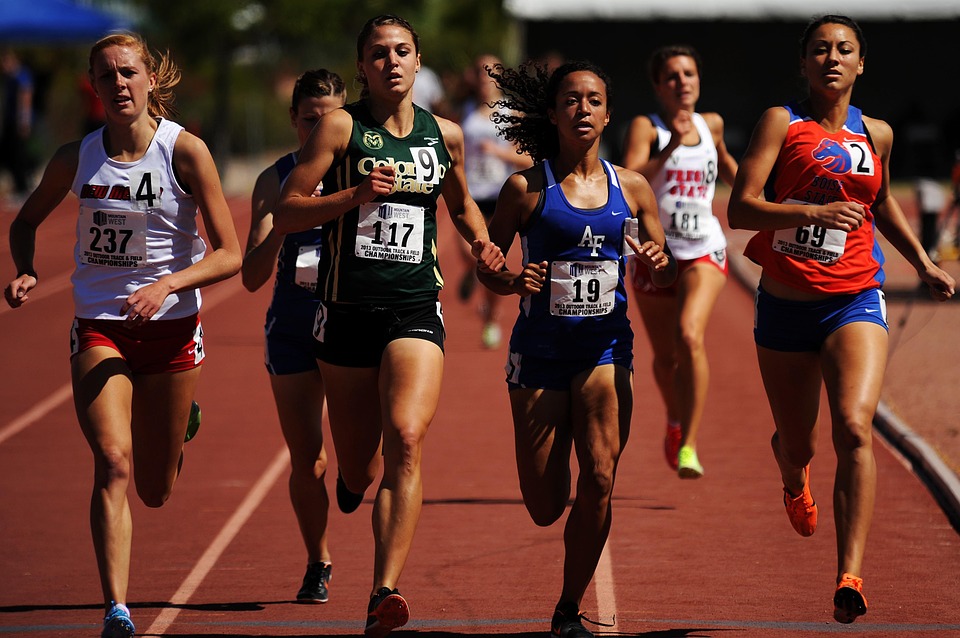
Guided imagery has been shown to improve motivation, pain management and endurance in sports. [1] Visualizing athletic skills during downtime improves performance. [2] Many scientific studies have proven that mental training with visualization is an effective strategy for many forms of athletics, from throwing darts and basketball, [3] [4] to sprinting and weight training, [5] to Olympic competitions. [6] [7] [8] [9] Olympic athletes from various disciplines, including gymnastics, diving, judo and fencing, use visualization to prepare for competition. [10]
There is a study supposed to have occured in Russia in 1980, described by the authors of Peak Performance: Mental Training Techniques of the World’s Greatest Athletes , published in 1985. As already stated, many later studies have verified the benefits of visualization on athletic performance. However, I have not personally confirmed the authenticity of these Russian experiments, as I cannot find them recorded by an original source. Until the original source is discovered or the experiment reproduced, and the study reviewed by scientists in related fields, one ought not consider the study to be verified as factual.
The claim in Peak Performance is that Soviet Olympic athletes were divided into four groups to train for hours several times each week. Each group had a different percentage of physical versus mental training.
Group 1 – 100% physical training, 0% mental training Group 2 – 75% physical training, 25% mental training Group 3 – 50% physical training, 50% mental training Group 4 – 25% physical training, 75% mental training
When compared for performance, Group 4 had the best outcomes of all four groups, and the less mental training a group practiced, the worse were their outcomes. [11] I am not sure I believe the claims of this legendary experiment, but I would like to believe them. I will suspend judgment until such time as the results can be verified and I can make a proper citation.
In 2012 Thomas Newmark made the claim that Russian research on Olympic athletes indicated that visualization techniques improved athletic performance in the 1984 Olympics. [12] Due to evidence from many other later studies it is clear that the more mental training one adds to one’s physical regimen (considering its quality, i.e., vividness, accuracy and relevance), the better performance one will achieve.
Mental practice can be a time-saving and cost-efficient method of simulation for training in certain occupations beyond sports, like dance, marksmanship or combat. [14] [15] [16] Scientific studies have shown that visualization has many of the same effects on the brain as physical activity. Studies have shown that while physical practice improves performance more than anything else, mental practice can enhance performance. [17]
Visualization practices help wire the brain for perception, motor control and memory, incidentally boosting confidence. Research on golf performance suggests that it is important to use visualization away from events that require present awareness, for example, between practices. Optimum performance is achieved when one uses an uninhibited state of awareness at the time of execution. [18] [19] Focusing the attention in the present moment during performance, a form of mindfulness meditation, is proven to increase chances of success. [20] [21] [22] [23]
Performance is enhanced by visualization through purely physical benefits as well as mental benefits. Scientific studies are showing that visualization by itself actually helps build muscle strength. [24] [25] [26] One study revealed a 13.5 percent improvement in strength in subjects who merely imagined maximum flexing of biceps. [27] [28] Of course, more muscle strength can be achieved with isometric training, where the muscle is contracted but without moving the joint, and the most expedient method of growing strength is by contracting muscles against resistance, such as weights. [29] [30]
Creative visualization can improve fitness in many ways. It can also affect your diet. Targeted mental imagery has been scientifically shown to improve success in achieving dietary goals. Periodically visualizing the steps of eating a piece of fruit seems to encourage eating more fruit, according to a study carried out in 2011 by Bärbel Knäuper, PhD. at McGill University. [31] Diet, like exercise, affects health and many other aspects of life, like self-esteem, motivation, cognitive ability and relationships. [32] Visualization, too, can have an impact on these and other realms of life. It is not just athletes who can use visualization to achieve their life goals.
ARTICLE CONTINUES BELOW NOTES
[1] R. C. Thelwell and I. A. Greenless, “Developing competitive endurance performance using mental skills training,” The Sport Psychologist , 17, 2003, pp. 318–337.
[2] k. a. t. eddy and s. d. mellalieu, “mental imagery in athletes with visual impairments,” adapted physical activity quarterly , 20(4), 2003, pp. 347–368., [3] m. l. epstein, “the relationship of mental imagery and mental rehearsal to performance of a motor task,” journal of sport psychology , 2, 1980, pp. 211-220., [4] e. g. hall and e. s. erffmeyer, “the effect of visuo-motor behavior rehearsal with videotaped modeling on free throw accuracy of intercollegiate female basketball players,” journal of sport psychology , 5, 1983, pp. 343-346., [5] richter, jeremy ma, cscs; gilbert, jenelle n. phd; baldis, mark phd, cscs, “maximizing strength training performance using mental imagery,” strength and conditioning journal , october, 2012, volume 34 – issue 5, pp. 65–69, https://journals.lww.com/nsca-scj/fulltext/2012/10000/maximizing_strength_training_performance_using.10.aspx, [6] d. druckman and j. a. swets (eds.), “enhancing human performance,” national academy press , 1988., [7] j. b. oxendine, “effect of mental and physical practice on the learning of three motor skills,” research quarterly , 40(4), 1969, pp. 755-763., [8] a. paivio, “cognitive and motivational functions of imagery in human performance,” canadian journal of applied sport sciences , 10(4), 1985, pp. 225-285., [9] g. h. van gyn, h. a. wenger, and c. a. gaul, “imagery as a method of enhancing transfer from training to performance,” journal of sport and exercise psychology , 12, 1990, pp. 366-375., [10] sarah schmalbruch, “here’s the trick olympic athletes use to achieve their goals,” business insider , 28 jan., 2015, https://www.businessinsider.com/olympic-athletes-and-power-of-visualization-2015-1, [11] logan christopher, “the history, science, and how-to of visualization,” accessed 24 oct., 2018, https://breakingmuscle.com/fitness/the-history-science-and-how-to-of-visualization, [12] t. newmark, md., “cases in visualization for improved athletic performance,” healio psychiatric annals , 42(10), 22 oct., 2012, https://www.healio.com/psychiatry/journals/psycann/2012-10-42-10/%7bb39a71aa-2e68-49c7-9367-dd3eed3f4f52%7d/cases-in-visualization-for-improved-athletic-performance, [14] thomas s. whetstone, “enhancing psychomotor skill development through the use of mental practice,” 1995, https://scholar.lib.vt.edu/ejournals/jite/v32n4/whetstone.html#hird, [15] alyssa robinson, “a dancer’s introduction to visualization,” 6 may, 2018, http://www.danceadvantage.net/visualization-for-dancers/, [16] kevin cull, “visualization for martial arts: get better without training,” retrieved 24 oct., 2018, http://www.doboksquawk.com/visualization-for-martial-arts/, [17] l. v. clark, “effect of mental practice on the development of a certain motor skill,” research quarterly of the american association for health, physical education, & recreation , 31, 1960, pp. 560-569, http://psycnet.apa.org/record/1962-00248-001, [18] michael hebron, “visualization hurting golf performance” golf science lab , retrieved 24 oct., 2018, http://golfsciencelab.com/visualization-hurting-golf-performance/, [19] david mackenzie, “visualization: the most powerful thing in golf,” 10 jan., 2018, https://golfstateofmind.com/powerful-visualization-golf/, [20] jim sliwa, “new mindfulness method helps coaches, athletes score,” american psychological association, 4 aug., 2017, http://www.apa.org/news/press/releases/2017/08/mindfulness-method.aspx, [21] crystal chariton, “mindfulness: improving sports performance & reducing sport anxiety,” retrieved 24 oct., 2018, https://believeperform.com/performance/mindfulness-improving-sports-performance-reducing-sport-anxiety/, [22] christopher heckman, “the effect of mindfulness and meditation in sports performance,” pdf, kinesiology, sport studies, and physical education synthesis projects 47, 18 may, 2018, https://digitalcommons.brockport.edu/cgi/viewcontent.cgiarticle=1049&context=pes_synthesis, [23] a. j. adams, mapp, “seeing is believing: the power of visualization,” psychology today , 3 dec., 2009, https://www.psychologytoday.com/us/blog/flourish/200912/seeing-is-believing-the-power-visualization, [24] v. k. ranganathan, v. siemionow, j. z. liu, v. sahgal, g. h. yue, “from mental power to muscle power–gaining strength by using the mind,” neuropsychologia, 2004, 42(7), pp. 944-56, https://www.ncbi.nlm.nih.gov/pubmed/14998709 , or pdf, https://lecerveau.mcgill.ca/flash/capsules/articles_pdf/gaining_strength.pdf, [25] clayton mosher, “how to grow stronger without lifting weights,” scientific american , 23 dec., 2014, https://www.scientificamerican.com/article/how-to-grow-stronger-without-lifting-weights/, [26] brian c. clark, niladri k. mahato, masato nakazawa, timothy d. law, and james s. thomas, “the power of the mind: the cortex as a critical determinant of muscle strength/weakness,” journal of neurophysiology , 15 dec., 2014, https://www.physiology.org/doi/abs/10.1152/jn.00386.2014, [27] v. k. ranganathan, v. siemionow, j. z. liu, v. sahgal, g. h. yue, “from mental power to muscle power–gaining strength by using the mind,” neuropsychologia, 42(7), 2004, pp. 944-56, https://www.ncbi.nlm.nih.gov/pubmed/14998709 , or pdf, https://lecerveau.mcgill.ca/flash/capsules/articles_pdf/gaining_strength.pdf, [28] v. k. ranganathan, v. siemionow, j. z. liu, v. sahgal, g. h. yue, “from mental power to muscle power–gaining strength by using the mind,” neuropsychologia , 42(7), 2004, pp. 944-56, https://www.sciencedirect.com/science/article/pii/s0028393203003257via%3dihub, [29] edward r. laskowski, m.d., “are isometric exercises a good way to build strength” retrieved 24 oct., 2018, https://www.mayoclinic.org/healthy-lifestyle/fitness/expert-answers/isometric-exercises/faq-20058186, [30] benjamin wedro, md, facep, faaem and richard weil, med, cde, “anatomy and physiology of skeletal muscles,” 12 dec., 2016, https://www.medicinenet.com/how_muscles_work_and_respond_to_resistance_exercise/views.htm, [31] b. knäuper, a. mccollam, a. rosen-brown, j. lacaille, e. kelso, m. roseman, “fruitful plans: adding targeted mental imagery to implementation intentions increases fruit consumption,” epub , 18 feb., 2011, https://www.ncbi.nlm.nih.gov/pubmed/21337259, [32] tiffany l. carson, phd, bertha hidalgo, phd, jamy d. ard, md, and olivia affuso, phd, rd, “dietary interventions and quality of life: a systematic review of the literature,” 30 oct., 2013, https://www.ncbi.nlm.nih.gov/pmc/articles/pmc3982833/.
Everyone can achieve a higher quality of life by using visualization to help accomplish life goals. A study on golfers in 1995 observed how negative visualizations of a golf putt affected the performance of golfers. The study found that visualizations of negative outcomes resulted in a decline of accuracy . [1] This study, along with the storehouse of information on the beneficial effects of positive visualization, makes a convincing assertion that the way we visualize outcomes has a direct and significant impact on the results of our efforts in life.
Visualizations and accomplishments can be based on short-term actions or cultivated with long-term goals. Hollywood actors are taught special methods to breathe, visualize and act. [2] This allows them to better understand human behavior and control what they express to an audience. This kind of meditative visualization of one’s own character in the theatre of life can give anyone a safe place and time for self-reflection and planning. It can help form goals and offer inspiration how to achieve one’s goals.
A few simple methods define goal-achievement meditation. Choose one realistic and important short-term goal that you could accomplish within a couple of days. [3] Make sure the goal is significant to your life, rather than random or irrelevant to your goals in life, and make it something you can reasonably achieve.
Choose from any number of goals, for example, to get to bed on time, to study, to exercise, to get rid of your superfluous possessions, to stop eating beef by finding and making new recipes, to vote in upcoming elections for public office, to save $5000, to invest in some mutual funds, or to start a family. These goals can turn into long-term goals, but they begin as short-term, one day at a time.
Goals obviously possess different levels of difficulty, from easy tasks like cleaning a room, to starting a new career, to overcoming addiction. Visualizing the steps that lead to your goal being reached will make any undertaking easier. Begin by writing a list of the steps necessary to reaching your goal. In business this is called an action plan. For example, a blueprint for various goals might include:
- Write the action plan
- Visualization
- Make an appointment or schedule
- Dress appropriately
- Organize your tools
- Second Step
- Goal completion
Once these steps are written down in a list, determine a time to practice these visualization techniques, such as early morning or in the evening before you sleep. Sit in a meditative posture, close your eyes and state your intent to achieve your goal. This is an affirmation of what you will to happen and your confidence in achieving your goal. Visualize each step being carried out, one at a time.
[1] Woolfolk, Murphy, Gottesfeld and Aitken, “Effects of mental rehearsal of task motor activity and mental depiction of task outcome on motor skill performance,” Journal of Sport Psychology , 7, 1985, pp. 191-197.
[2] filmnutlive, acting coach larry moss, youtube video, 55:29, 14 april, 2010, https://www.youtube.com/watchv=y1t0icpgmu8, [3] “goal visualization,” greater good in action, uc berkeley, retrieved 24 oct. 2018, https://ggia.berkeley.edu/practice/goal_visualization#.
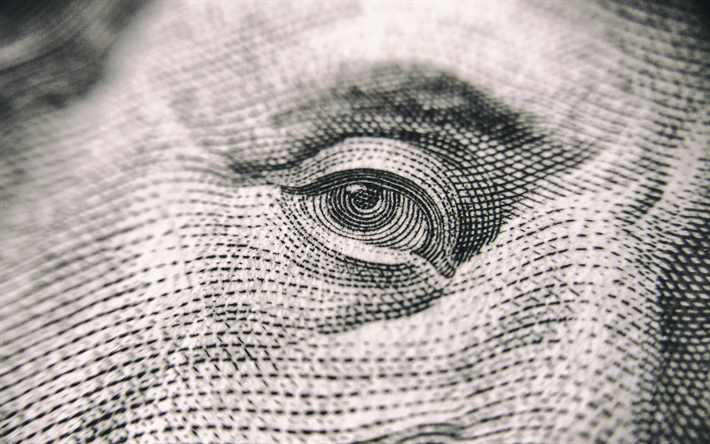
Holistic Imagery for Success
The most effective visualization uses a multi-sensory approach known as holistic imagery. Imagery is usually centered on visualization, that is, a visual picture within the mind’s eye. However, the more the senses are engaged, the more powerful the meditation becomes. [1] [2] [3] Imagery should include sights, sounds, smells, tactile feelings and kinesthetics. [4] More vivid imagery has a more profound effect on the mind. [5]
Imagine the environment at each step along the way toward your goal. Feel the emotions you will feel. Conjure the scents and the sounds as if you were there. Finally, create within the mind’s eye a detailed future scene of that goal being just accomplished. Imagine you are there in the moment. Evoke a strong positive emotional state to leave a powerful and productive impression. [6] Take a minute to return to the present moment and reaffirm your intention of fulfilling your goal.
Techniques for visualization will not work if you just read about them and think about them: these techniques must be actually carried out in reality. It does not take a lot of time and it costs nothing. There is evidence that vision and vision communication in business can result in venture growth. [7] Although we can discount claims of universal forces working on us via laws of visualization and success as pure pseudoscience , the consequences of vision and visualization in various business settings certainly deserves more research.
[Edit 21 Sept. 2019: the original article included the sentence: “When visualization techniques are taken seriously and applied to business, they can result in amazing financial gains, concrete success, as in tens of millions of dollars in revenue.” This was deemed hyperbolic and included a link to an article with an anecdotal account of success linked to visualization that did not consider that correlation does not equal causation.]
Visualization has been shown to play a unique part in physical and material accomplishments. Meditation with imagery is also useful in mental and spiritual endeavors. There are imagery techniques proven to be excellent solutions for studying and memorization. Visualization can be helpful in professional artistic creativity and science communication. Other methods have been used to induce spiritual experiences for enlightenment and the betterment of the personality.
[1] Andre and Means, “Rate of imagery in mental practice: An experimental investigation,” Journal of Sport Psychology , 8, 1986, pp. 124-128.
[2] paivio, “cognitive and motivational functions of imagery in human performance,” canadian journal of applied sport sciences , 10(4), 1985, pp. 225-285., [3] m. denis, “visual imagery and the use of mental practice in the development of motor skills,” canadian journal of applied sport sciences , 10(4), 1985, pp. 45-165., [4] farah, hammond, levine, and calvanio, “visual and spatial mental imagery: dissociable systems of representation,” cognitive psychology , 20, 1988, pp. 439-462., [5] e. d. ryan and j. simons, “efficacy of mental imagery in enhancing mental rehearsal of motor skills.” journal of sport psychology , 4, 1982, pp. 41-51., [6] a. j. adams, mapp, “seeing is believing: the power of visualization,” psychology today, 3 dec., 2009, https://www.psychologytoday.com/us/blog/flourish/200912/seeing-is-believing-the-power-visualization, [7] robert j. baum, edwin a. locke, shelley a. kirkpatrick, “a longitudinal study of the relation of vision and vision communication to venture growth in entrepreneurial firms,” journal of applied psychology , 1998, 83(1), pp. 43-54..
Medically Reviewed by Dr. Andreas Laurencius, MD .
Power of the Mind: The Science of Visualization Page 1
Read page 2: visualization and the mind.
- Terms & Conditions
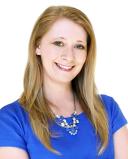
Seeing Is Believing: The Power of Visualization
Your best life, from the comfort of your armchair..
Posted December 3, 2009 | Reviewed by Jessica Schrader
Despite the great case for getting off our duffs, there are some amazingly cool and effective practices we can do from the comfort of our own recliners—without even budging a finger. For instance, you could practice your golf swing, work out your muscles, prepare to climb Mount Kilimanjaro, hone your chess skills, practice for tomorrow’s surgery, and you can even prepare for your best life!
Mental practice can get you closer to where you want to be in life, and it can prepare you for success! For instance, Natan Sharansky, a computer specialist who spent nine years in prison in the USSR after being accused of spying for U.S., has a lot of experience with mental practices. While in solitary confinement, he played himself in mental chess, saying: “I might as well use the opportunity to become the world champion!” Remarkably, in 1996, Sharansky beat world champion chess player Garry Kasparov!
A study looking at brain patterns in weightlifters found that the patterns activated when a weightlifter lifted hundreds of pounds were similarly activated when they only imagined lifting. In some cases, research has revealed that mental practices are almost effective as true physical practice . For instance, in his study on everyday people, Guang Yue , an exercise psychologist from Cleveland Clinic Foundation in Ohio, compared results of those who did physical exercises to the results of those who carried out virtual workouts in their heads. In the physical exercise group, finger abduction strength increased by 53%. In the group that did "mental contractions", their finger abduction strength increased by 35%. However, "the greatest gain (40%) was not achieved until 4 weeks after the training had ended" (Ranganathan et al., 2004). This demonstrates the mind's incredible power over the body and its muscles.
Noted as one form of mental rehearsal, visualization has been popular since the Soviets started using it back in the 1970s to compete in sports. Now, many athletes employ this technique, including Tiger Woods who has been using it since his pre- teen years. Seasoned athletes use vivid, highly detailed internal images and run-throughs of the entire performance, engaging all their senses in their mental rehearsal, and they combine their knowledge of the sports venue with mental rehearsal. World Champion Golfer, Jack Nicklaus has said: “I never hit a shot, not even in practice, without having a very sharp in-focus picture of it in my head." Even heavyweight champion Muhammad Ali used different mental practices to enhance his performance in the ring such as: “affirmation; visualization; mental rehearsal; self-confirmation; and perhaps the most powerful epigram of personal worth ever uttered: 'I am the greatest.'"
Brain studies now reveal that thoughts produce the same mental instructions as actions. Mental imagery impacts many cognitive processes in the brain: motor control, attention , perception, planning, and memory . So the brain is getting trained for actual performance during visualization. It’s been found that mental practices can enhance motivation , increase confidence and self-efficacy , improve motor performance, prime your brain for success, and increase states of flow—all relevant to achieving your best life!
For someone like Matthew Nagle who is paralyzed in all four limbs, mental practices have transformed his entire way of life . Matthew had a silicone chip implanted in brain. Astonishingly, after just four days of mental practice, he could move a computer cursor on a screen, open email, play a computer game, and control a robotic arm. While our circumstances may be less stringent than those that Matthew endures, it’s quite obvious that every person can benefit from mental practices.
So, if athletes and chess players use this technique to enhance performance, how can it enhance the lives of the "average Joe"? First, study results highlight the strength of the mind-body connection, or in other words the link between thoughts and behaviors—a very important connection for achieving your best life. While your future may not include achieving a great physique or becoming the heavyweight champ or winning the Masters Tournament, mental practice has a lot to offer you. Try it here!
Begin by establishing a highly specific goal. Imagine the future; you have already achieved your goal. Hold a mental "picture" of it as if it were occurring to you right at that moment. Imagine the scene in as much detail as possible. Engage as many of the five senses as you can in your visualization. Who are you with? Which emotions are you feeling right now? What are you wearing? Is there a smell in the air? What do you hear? What is your environment? Sit with a straight spine when you do this. Practice at night or in the morning (just before/after sleep). Eliminate any doubts, if they come to you. Repeat this practice often. Combine with meditation or an affirmation (e.g. “I am courageous; I am strong,” or to borrow from Ali, “I am the greatest!”).
Angie LeVan, MAPP , is a resilience coach, speaker, trainer, and writer, dedicated to helping individuals and organizations/businesses thrive! For more information, visit ajlevan.com .
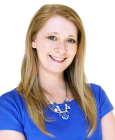
A.J. Adams, MAPP , specializes in workplace well-being, resilience, and burnout prevention.
- Find a Therapist
- Find a Treatment Center
- Find a Psychiatrist
- Find a Support Group
- Find Teletherapy
- United States
- Brooklyn, NY
- Chicago, IL
- Houston, TX
- Los Angeles, CA
- New York, NY
- Portland, OR
- San Diego, CA
- San Francisco, CA
- Seattle, WA
- Washington, DC
- Asperger's
- Bipolar Disorder
- Chronic Pain
- Eating Disorders
- Passive Aggression
- Personality
- Goal Setting
- Positive Psychology
- Stopping Smoking
- Low Sexual Desire
- Relationships
- Child Development
- Therapy Center NEW
- Diagnosis Dictionary
- Types of Therapy
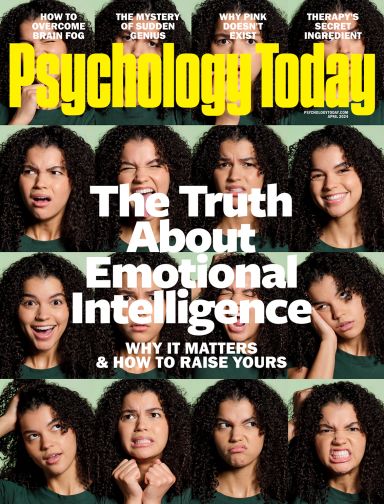
Understanding what emotional intelligence looks like and the steps needed to improve it could light a path to a more emotionally adept world.
- Coronavirus Disease 2019
- Affective Forecasting
- Neuroscience

An official website of the United States government
The .gov means it’s official. Federal government websites often end in .gov or .mil. Before sharing sensitive information, make sure you’re on a federal government site.
The site is secure. The https:// ensures that you are connecting to the official website and that any information you provide is encrypted and transmitted securely.
- Publications
- Account settings
Preview improvements coming to the PMC website in October 2024. Learn More or Try it out now .
- Advanced Search
- Journal List
- Vis Comput Ind Biomed Art
- v.4; 2021 Dec

Examining data visualization pitfalls in scientific publications
Vinh t nguyen.
1 Department of Information Technology, TNU – University of Information and Communication Technology, Thai Nguyen, Vietnam
Kwanghee Jung
2 Department of Educational Psychology, Leadership, and Counseling, Texas Tech University, Lubbock, TX 79409 United States
Vibhuti Gupta
3 Department of Computer Science and Data Science, Meharry Medical College, Nashville, TN 37208 USA
Associated Data
The datasets used and/or analyzed during the current study are available from the corresponding author on reasonable request.
Data visualization blends art and science to convey stories from data via graphical representations. Considering different problems, applications, requirements, and design goals, it is challenging to combine these two components at their full force. While the art component involves creating visually appealing and easily interpreted graphics for users, the science component requires accurate representations of a large amount of input data. With a lack of the science component, visualization cannot serve its role of creating correct representations of the actual data, thus leading to wrong perception, interpretation, and decision. It might be even worse if incorrect visual representations were intentionally produced to deceive the viewers. To address common pitfalls in graphical representations, this paper focuses on identifying and understanding the root causes of misinformation in graphical representations. We reviewed the misleading data visualization examples in the scientific publications collected from indexing databases and then projected them onto the fundamental units of visual communication such as color, shape, size, and spatial orientation. Moreover, a text mining technique was applied to extract practical insights from common visualization pitfalls. Cochran’s Q test and McNemar’s test were conducted to examine if there is any difference in the proportions of common errors among color, shape, size, and spatial orientation. The findings showed that the pie chart is the most misused graphical representation, and size is the most critical issue. It was also observed that there were statistically significant differences in the proportion of errors among color, shape, size, and spatial orientation.
Introduction
With the advancement of the internet, data storage, and search methods, an increasing number of scientific publications and academic papers are being deposited online. This facilitates readers’ access to scientific knowledge and publications. To help in quick and efficient browsing, online resources allow full papers to be stored in several formats such as text, photographs, tables, and charts. This proprietary database helps the reader find it faster, while it raises some problems in understanding data. When tables and charts are positioned closest to their descriptions, the author’s intention will be delivered more clearly. However, it is not surprising that we see so many tables and charts that do not themselves contain information to convey. When a reader fails to read the description, the author loses the ability to communicate his or her information. As a result, the design, arrangement, and organization of data in tables and charts play an important role in information dissemination.
Visualization has long been used as an effective method for transcribing data into information and help people carry out data analysis tasks and make decisions. A number of visual encodings (such as color, shape, and size) can be used individually or together for representing various visualization tasks [ 1 – 3 ]. Misinformation in data visualization has become one of the main issues to convey knowledge more effectively [ 4 , 5 ]. As opposed to creating effective visualization, misinformation in data visualization receives less attention and becoming one of the major issues for conveying information [ 5 ]. Misinformation can be classified into two categories including intentional misinformation and unintentional misinformation [ 6 ]. Intentional misinformation [ 7 ] in data visualization refers to the use of charts, graphics to distort, hide, or fabricate data in an attempt to deceive users. On the other hand, unintentional misinformation [ 8 ] implies providing false and inaccurate information to the end-users because of human cognitive bias and carelessness in designing/selecting visual channels to encode the corresponded data. From the creator’s point of view, the former is controllable while the latter requires a lot of training. This work will be focusing more on the latter one as it is encouraged to build trust in visualization rather than lies [ 9 ].
A good visualization tool supports users to get insights (i.e., trends, patterns, and outliers) and extract meaningful information from data. Wilkinson [ 10 ] describes the building blocks for constructing a wide range of statistical graphics in his notable book “The Grammar of Graphics”. On the other hand, many articles on the web provide bad examples of visual designs leading to misinterpretation but they are usually viewed from different perspectives. In line with this research direction, Bresciani and Eppler [ 6 ] provided a comprehensive review on common errors in data visualization and classified these pitfalls into three categories: cognitive, emotional, and social. Their high-level abstract of the theoretically grounded classification can be used as a checklist for practitioners to examine the visualization for avoiding common drawbacks. However, it is challenging to go through the entire checklist due to the different human cognitive and one’s preference styles. As such, the authors suggested that a more rigorous study should be conducted to “rank the pitfalls according to how common or severe they are.” In response to these needs, in this paper, we try to compromise the most common errors into a structure based on the units of visualization. In another word, we address the following research questions: (R1) What are the most common effects resulting from visualization pitfalls? (R2) What are the most common errors in constructing a representation in terms of color, shape, size, and orientation? Or (R3) Is there any difference in the proportions of common errors among color, shape, size, and spatial orientation? We believe that going from these units would benefit practitioners, especially novice users when they are mostly working with these elements. We expect that the result of this study will be served as a reference for avoiding visualization pitfalls in general. Ultimately, in the long run, we will attempt to answer the questions such as what color [ 11 ] should we use to represent data? what visualization types [ 12 , 13 ] should be taking into account for analysis? what type of scale should we apply? As such, this is preliminary research work to accomplish the goals.
This study is a continuation of a previously published work at the symposium on visual information communication and interaction [ 14 ]. The new version was extended by expanding the existing database (Google Scholars) with Scopus, incorporating a text mining technique to extract practical insights from common visualization pitfalls, and examining if there is any difference in the proportions of common errors among color, shape, size, and spatial orientation with Cochran’s Q test and McNemar’s test.
The rest of this paper is structured as follows: First, a literature review relevant to our work was presented. Then, the instruments and processes to conduct the research were provided along with the analytics. After that, the results were presented. Finally, the findings were discussed and concluded.
Related work
Many recent works have addressed the issues of misinformation in data visualization. Huff [ 15 ] showed a variety of ways a designer can trick people, e.g., truncating certain portions of the graph. As such, the discrepancy is more obvious when seen as a whole. This goal can be accomplished by three common strategies [ 9 , 16 ]: (1) not revealing any data, (2) showing the data incorrectly, and (3) obfuscating the data.
Many authors attempted to provide designers with a boilerplate for creating a successful visualization, ranging from individual graph elements such as colors [ 17 – 19 ] and forms (or chart types) [ 20 ] to a detailed data visualization design [ 21 , 22 ]. However, bad visualization design persists in the presence of guidance, which leads to misinformation that can contribute to considerable problems, especially where individuals heavily rely on the data at hand when making business decisions. Study results raise concerns: what are the possible causes of disinformation visualization? This research is critical in developing a visualization platform that not only meets end-user needs but also mitigates the issues from misinformation and disinformation.
The pitfalls of visualization have been examined in a variety of fields such as information visualization [ 23 – 25 ] - psychological/aesthetic restrictions presented graphic format are highlighted, diagrammatic representations [ 13 , 26 – 28 ] using the diagram to understand concept or idea instead of algebraic means, human-computer interaction [ 21 , 29 ] - potential drawbacks of interactive visualizations, statistical graphic representations [ 22 , 30 ] - bad examples of representing data visually. The most comprehensive research was provided by Bresciani and Eppler [ 6 ], where the authors explored the potential drawbacks in graphic depictions. The authors compiled a list of 51 pitfalls of visualization drawbacks based on interviews with seven experts and divided them into three categories: cognitive, emotional, and social effects.
Unlike the previous research relying on high-level abstractions to avoid visualization errors, we focus on the basic elements that constitute visualization mapping. These elements are color, shape, size, and spatial orientation presented by Munzner [ 22 ]. According to Bresciani and Eppler’s research [ 6 ], there is a need to review a selection of images to assess the frequency and severity of the flaws. In this study, we aim to alleviate the problems of misinformation in data visualization.
Data sources
For the research, the preferred reporting items for systematic reviews and meta-analyses (PRISMA [ 31 ]) model served as a guideline. It is a bare-bones set of evidence-based features meant to help authors report on a wide range of systematic reviews and meta-analyses. Google Scholar and Scopus indexing databases were used to gather data for our study due to their popularity and credible results. The search terms were “misinformation visualization”, “misleading visualization”, “disinformation visualization”, “visualization pitfalls”, “bad visualization design”. Google Image search was used to collect representative images that illustrate visualization pitfalls to avoid direct criticism of previous studies. Table 1 provides the number of papers collected from the two indexing databases according to their keywords.
Data collection results from Google Scholar and Scopus indexing databases
Data preprocessing
Search results from the database were consolidated into a single excel file format, and duplicated items were removed. The final list of items was filtered by the scientific articles’ abstracts and main contents. Here, we did not exclude items by their titles. Rather, we examined the details of abstracts and main contents to see where the keywords were positioned. It is noted that some of the collected papers contained keywords but were not relevant to our research goal, e.g., some papers did not contain figures to demonstrate the searching keywords. Such papers were excluded from our analysis. In the end, 178 papers were included in the study. Figure 1 depicts the flow of information through the different phases of the systematic review utilizing the PRISMA approach.

The PRISMA flow diagram
Data extraction
We extracted two types of data from the research papers: figures and their associated descriptions. Each figure is classified into one of four categories (i.e., color, shape, size, and spatial orientation) which indicates a type of violation in visualization. The relevant papers were screened through three phases: (1) checking the title, (2) reviewing the abstract, and (3) scrutinizing the full text of the paper.
Data analysis
For the first research question, we employed text mining techniques to gain practical insights from the collected data, including association rule mining to find out the relationships among the descriptions of figures. The inputs for this technique were taken from associated descriptions of each figure. We created a word cloud with the most often used words in related explanations, removing any stop words, non-ASCII characters, white space, and duplication. Word clouds are graphical representations of word frequency that highlight terms that appear regularly in a source document. The bigger the term in the visual, the more popular the word was in the text. This visualization method can help evaluators with exploratory textual research by highlighting terms that appear often in a series of interviews, records, or other languages. It may also be used to communicate the most important points or topics during the reporting stage. Association rule mining is a rule-based machine learning technique that seeks out interesting hidden relationships among variables in a dataset. Association rules have been used in a variety of applications, including opinion mining, intrusion prevention, continuous processing, and bioinformatics.
For the second research question, we grouped typical visualization pitfalls into four major categories denoted from M1 to M4 based on the basic elements (color, size, shape, and spatial orientation), as shown in Fig. 2 , where “red, blue, and green” represent the color, scaled squares represent the size, “circle, rectangle, and triangle” represent the shape, and scatter black dots represent the spatial orientation. As we move from left to right, misinformation for visual mapping can result from data itself, such as missing data or poor data, to cognitive perception, where color, shape, and scale are perfectly used but misinformation occurs due to cognitive mechanisms. Visual perception is the primary subject of this paper (for more information on data perception and cognitive perception, see Bresciani and Eppler’s study [ 6 ]).

A visual approach for categorizing misinformation data visualization: M1 (color), M2 (size), M3 (shape), and M4 (spatial orientation)
For the third research question, we conducted Cochran’s Q test and McNemar’s test [ 32 ] to examine if there is any difference in the proportions of common errors among the fundamental units of color, shape, size, and spatial orientation. We examined each paper individually and extracted figures that were violated in terms of any of the four categories. It is noted that only figures generated from data by authors were included for the analysis. Figures taken from photos or other sources were excluded. We encoded “1” as being violated and “0” for not being violated. Thus, our data consists of four binary variables. Statistical analysis was performed with SPSS Version 25.
RQ1: What are the most common effects resulting from visualization pitfalls?
After removing special characters in the associated descriptions of figures, we had 363 items with 139 unique words. The items are the remaining words/keywords extracted from the descriptions after cleaning data. Figure 3 depicts the most frequently used terms for each input figure that encounter visualization pitfalls. Frequently used terms in Fig. 3 are shown in larger font sizes in the word cloud. In terms of dominant keywords, besides our proposed categories such as color (72), shape (36), size (132), a majority of misleading visualizations comes from pie chart (83) and bar chart (72), followed by using the wrong chart (63) that incur misinterpretation (21). In addition to the pie chart, donut (33) is another interesting finding that contributes to common errors, it seems that creators are interested in using circles to explain their messages. It is noticed that the terms “inform and axis” are also highlighted in our visual layout which indicates that creators and viewers have to take care of the axis while interpreting data to inform decision-making. We can see other terms that appear to be slightly different from others such as data, hard, and clutter, but they do not stand out apparently.

Word cloud constructed from associated descriptions about the figure that encounters visualization pitfalls
Figure 4 depicts the graphical layout of our transactions and items in the dataset, where black cells denote non-zero elements and empty cells represent zero elements. Rows (transactions) are associated description index of figures, while columns (items) are terms extracted from their corresponding descriptions. In total, we had 370 descriptions and 109 terms. The percentage of non-zero cells in this 370 × 109 matrix is shown by density. It is worth noting that a matrix is considered sparse if most of its elements are empty. On the other hand, if most of the elements are non-zero, the matrix is called dense. The sparsity of the matrix is defined as the number of zero-valued elements divided by the total number of elements. Our dataset showed a density of 0.0312 which means that on average, each description contains 3 to 4 keywords/terms. This is due to the data cleaning process, where all stop words were removed.

Transactions and items in a matrix
Table 2 showed a list of 14 rules generated from the A-Priori algorithm [ 33 ]. This algorithm necessitates both minimal support and a minimum confidence constraint. If no threshold value is defined, the function will consider the default values of support and confidence threshold as 0.1 and 0.8, respectively. Since the elements in the matrix are sparse, support and confidence are modified with lower values (support = 0.04 and confidence = 0.5) to mine the most potential rules rather than using default values. Support is the percentage of transactions (or figures) that contain both words in a rule and confidence is the strength of that rule. The A-Priori algorithm outputs the highest value of support (0.162), confidence (1), and lift (11.012) respectively. The lift value is an indicator of a rule’s significance. If the lift value is greater than 1, it indicates that the rule body (or Rhs) and the rule head (or Lhs) appear together more often than predicted, implying that the occurrence of the rule body has a positive impact on the occurrence of the rule head. On the other hand, if the lift value is less than 1, it implies that the occurrence of the Rhs has a negative effect on the occurrence of the Lhs. Table 2 shows that all lift values are greater than 1, indicating that items in the rules are positively correlated. It is noted that more than half of items (57.14 %) in the rule body involve ‘size’, which means that size is the most critical issue in visualization pitfalls. This statistical data is supported by the visual graph as depicted in Fig. 5 , where size is the center of the constructed network with a total of 10 connections from other rules. This network illustrates associations between items and rules. Bigger circles indicate higher support, while the red color implies higher lift. One interesting pattern revealed by the algorithm is when creators use size to express information in a bar chart, then misleading is likely to occur (rule 13, 14). In addition, incorrect usages of size can lead to confusion.
Association rules mining in the figures’ descriptions
A-Priori Algorithm was used with a support threshold of 0.04 and a confidence threshold of 0.5

Graph-based visualization on the relationship between individual items in the ruleset
RQ2: What are the most common errors in constructing a representation in terms of color, shape, size, and orientation?
M1: misinformation due to color violation.
As shown in Fig. 6 , one of the most common visualization mistakes is the use of too many colors. Healey et al. [ 34 ] argued that the human eye can only process five to seven well-chosen colors pre-attentively, and qualitative color scales are thought to work best when three to five different colored categories are present. Choosing a color scheme is another critical issue when different categories are encoded by related color on a spectrum that makes it difficult to distinguish one from another as in Fig. 6 a - legend. In some cases, the use of continuous color even gets worse leading to misinterpretation of data [ 35 ], especially when using a non-monotonic scale or rainbow scale [ 11 ]. It can be seen from Fig. 6 d that the colors at the beginning and the end are almost equivalent (circulated color scales). Figure 6 b shows using the same color for different categorical data. Although each category can be annotated by a distinct shape, color is often misused to represent an additional dimension especially when the granularity of the new dimension is too low. It takes more time to locate the blue circle point of interest. Another common bad habit is using the color against the norm: it is often found in the practice that light color is usually for less density while darker color is for higher density or green for a healthy indicator vs. red for abnormal value. The example illustrated in Fig. 6 c misused red color business gains and green color for business losses. A first glance, this visualization misled us that the economy is flourishing.

Misinformation due to color violation: a using too many colors [ 36 ], b unnecessary color usage [ 37 ], c color against the norm [ 38 ] and d rainbow color scale [ 39 ]
M2: Misinformation due to shape violation
The shape can be violated due to the use of inappropriate chart types. In fact, this is the most common misleading chart that we found in the literature. Particularly in the case of using a pie chart instead of a bar chart and vice versa. A pie chart is a type of graph in which a circle is broken down into segments (i.e., slices of pie) that each represent a proportion of the whole. Each slice of the pie chart is represented by a percentage value accumulating to 100%. Figure 7 a illustrates the improper use of the pie chart where each slice adds up to more than 100%. Even though the original intention was to compare values among different categories, this chart also violates color choices (so many colors, or similar colors for different categorical data). Common practice [ 37 ] suggested that the pie chart can be best used with less than seven categories (same magic number as in color) due to the difficulty for the eye to distinguish relativity of size between each segment. In this case, the bar chart should be a better choice. Figure 7 c shows an opposite case where the pie chart should be taken into consideration instead of using the bar chart for comparing proportional data as percentages add up to 100%.

Misinformation due to shape violation: a using 3D pie chart for comparing proportion [ 40 ], b using wrong visualization chart type, c pie-chart should be used [ 41 ], and d bar chart should be used [ 42 ]
The second misleading information when performing visual mapping is to use a shape that does not reflect the information provided. It can be seen from Fig. 7 b that the body part misleads users from what it means. Like the case of the pie chart, proportionality does not add up to 100% or areas cannot be compared visually.
Another common misleading information is the use of a high-dimensional chart to represent lower-dimensional data. This is often due to the visual appeal of 3D charts or the priority of prioritizing design and technology over conveying information of the chart as it can be seen from Fig. 7 a and d where heights of each slice did not provide any additional information.
While doing visual mapping, the second deceptive information is to use a shape that does not represent the information presented. Figure 7 b demonstrates how the body part misleads people on what it means. Proportional, like the pie map, does not add up to 100%, or regions cannot be physically compared. Another example of deceptive evidence is the use of a high-dimensional map to display low-dimensional details. This is often attributed to the aesthetic appeal of 3D charts or the importance of prioritizing architecture and technology over conveying detail of the map, as seen in Fig. 7 a and d, where the heights of each slice provided no additional details.
M3: Misinformation due to size violation
Regarding size infringement, the most common problem is to use a single size dimension scale to express a two-dimensional value. For example, in Fig. 8 a, the diameter was used for the scale to display the proportion to GDP, but users often viewed the chart from the perspective of the region. In this case, the field expands exponentially (i.e., quadratic vs linear). Mathematically, 14.5 trillion is 2.56 times larger than 5.7 trillion, but the region seems to be 6.5 times larger.

Misinformation due to size violation: a using wrong scale [ 43 ], b size is inverted [ 40 ], c all sizes are equal [ 44 ] and d size with no meaning [ 45 ]
Careless designers continue to apply incorrect labeling to visual mapping, especially where the chart is labeled manually without the assistance of automatic graphic software. Figure 8 b represented this scenario in which 55 % and 45 % could be swapped. Surprisingly, comparable findings in this form of pitfall are typically seen from news channel writers. Another method of softening the detrimental impact of a section is to use a light color, but we do not mention it in our study because it is considered an intention.
The use of equivalent scale shapes supplemented with numbers for contrast is the second common deceptive graph pitfall that belonged to this group, shown in Fig. 8 c. Similarly, to orange bars, all-white bars are the same height. Colors are often misused in three separate categories; however, the most serious flaw is the appearance of height where no meaning is present (0 % value of the white bars in the rightmost figure). These common pitfall patterns can also be seen in a pie chart of equal-sized parts.
Another pitfall is to use a graphic encoding that is unrelated to the values it contains, as seen in Fig. 8 d, where the height of each bar chart does not fit the value above it. Did the author use an inverted scale? Then 34 % should be the lowest, and where is the bar chart for the “SOMEWHAT” category? While this form of expression is uncommon in academia, social media has had many instances of it.
M4: Misinformation due to spatial orientation violation
In this study, we would use a subset of spatial features including spatial and temporal mapping which engages space and time, respectively.
Inverting the vertical axis is a technique that allows viewers to see the flip side of the original data. In practice, the flip-side data is intentionally removed to convey other information. Figure 9 a is the most controversial chart being discussed whether it was created to deceive users [ 46 ]. For most viewers, this graph first gave the impression that gun deaths declined sharply after 2005 due to the illusion of the line chart, especially in the presence of black dots. Gun deaths increased. As indicated in ref. [ 46 ] “the artist does not appear to have intended the graph to be deceptive. Her view was that deaths are negative things and should be represented as such.” However, the focal point we want to address was that misleading information would make people feel that living in Florida get safer after the incident. A similar story can also be found in Fig. 9 b. Omitting data, truncating the axis (or non-zero-base axis), and over zooming to see the difference are popular techniques [ 9 , 16 ] to make a deceptive graph. From our point of view, in Fig. 9 b original data remains but misleading information still exists.

Misinformation due to spatial and temporal violation [ 46 ]: a inverted axis, b data split out, c missing axis to line up data, and d mixing time data
The most common problem with spatial orientation violation is reordering time series results. When creating a graph with a timestamp in one dimension, the order of data points at each time level is shown in Fig. 9 d, where months appear to be in a random position. The direction would be totally destroyed if the data are shuffled. Careless charting can also result in the lack of one dimension, shown in Fig. 9 c, where the author attempted to combine line graphs from different figures into one. We can see from the contradictory data unit and value location that does not match the upward trend. Misinformation due to data and visual interpretation is most often seen in the form of a table shown in Table Table3, 3 , where viewers must make at least two attempts to locate the absolute meaning (i.e., compare the length and compare the leftmost values). In this case, the order of magnitude in space is broken.
Misinformation due to data and cognitive perception
RQ3: Is there any difference in the proportions of common errors among color, shape, size, and spatial orientation?
Table 4 shows that the most frequently observed pitfalls were in size (69.4%). Color and shape showed a similar number of cases (39.7% and 37.2%, respectively). Spatial orientation had the least case (14.9%).
Frequency table of color, shape, size, and spatial orientation in visualization pitfalls
We conducted Cochran’s Q test and McNemar’s test [ 32 ] to examine if there is any difference in the proportions of common errors among color, shape, size, and spatial orientation. Cochran’s Q test determined that there was a statistically significant difference in the proportion of errors among the features of color, shape, size, and spatial orientation, Q (3) = 207.047, p < 0.001. This implies that at least two of the features had a different proportion of errors. Post hoc analysis with McNemar’s test was conducted. Table 5 shows the 2 × 2 classification tables for McNemar’s test. There were no significant differences between color and shape ( χ 2 = 0.547, p = 0.460). However, there was a statistically significant difference in proportions of errors between color and size ( χ 2 = 54.519, p < 0.001), color and spatial orientation ( χ 2 = 42.586, p < 0.001), shape and size ( χ 2 = 84.629, p < 0.001), and between size and spatial orientation ( χ 2 = 132.003, p < 0.001). The findings indicate that size is most frequent in the common pitfalls, which is statistically different in frequency from other features. Color and shape are the second most frequent common errors, which are statistically different from spatial orientation in terms of frequency. However, there is no significant difference between color and shape.
2 × 2 classification tables for McNemar analysis
It is undeniable that visualization is becoming more common, especially in the age of the Internet of Things, where millions of data points are created every day. To succeed, we need to provide a big picture in a succinct graphic style to help customers digest details faster. When we screened the papers for the study, we discovered that the majority of the graphical layouts were created by hand or produced by a program using built-in features. Only a few studies used specialized software to present results. Part of the problem stems from developers’ lack of programming expertise, which forces them to rely on free software. In some cases, these tools have a fine, suggested interface (e.g., Google Form automatically generated charts based on survey data), but in others, the layout is much worse than if it were created manually (e.g., Google Form only generates one type of chart for numerical data without considering the number of categories). As a result, developers would use these preset visualizations without regard for visual interface clarity. Another risk that can trigger visualization pitfalls stems from the writing paper guidelines. Although there is a straightforward, concise description of how to write and section (e.g., abstract, introduction, methodology, and conclusion), there is no guidance on how to correctly present data in figures. As a result, authors place a greater emphasis on content rather than visual presentation. This problem is addressed in the area of data visualization, where instructions for presenting data can be found in the paper’s template. However, these recommendations are not systematic, making a boilerplate graphic suggestion for both inside and across fields challenging. We expect that artificial intelligence will be modified in the coming years to automatically screen and identify possible common errors based on research questions and the evidence presented. As a result, developers will be able to concentrate on mining the content rather than thinking about deceptive interfaces.
Conclusions
This paper dealt with typical visual representation pitfalls. We gathered data from two indexing databases, pooled it, and grouped it into four groups based on data representation units. The most popular visualization pitfalls from each segment were extracted, and data in each section was analyzed using both qualitative and quantitative methods. Word frequency from Word Cloud visualization shows that size is the most dominant keyword found in the descriptions of figures, followed by pie, bar charts, and color. Association rule mining reported that size is the center of the constructed network with a total of 10 connections from other rules and it plays a major concern in visualization pitfalls. Cochran’s Q test and McNemar’s test showed that there was a statistically significant difference in the proportion of errors among the fundamental units of color, shape, size, and spatial orientation. The size issue was most frequent in common pitfalls, followed by color and shape. Spatial orientation had the least case. This paper’s contribution can be thought of as a fine-grained (or subset) of general visualization pitfalls, with a focus on visual perception. We hoped that our findings would aid in the creation of a taxonomy of common errors at the information stage. User interface analysis on visual interpretation will be undertaken in the future with a more detailed test design, taking into account the user’s cognitive loads and cognitive style.
Acknowledgements
Not applicable.
Abbreviations
Authors’ contributions.
VTN and KJ provided conceptualization, formal analysis and investigation, methodology and visualization; KJ provided supervision; VTN, KJ and VG provided validation; VTN, KJ and VG wrote the paper. The authors read and approved the final manuscript.
Availability of data and materials
Declarations.
The authors declare that they have no competing interests.
Publisher’s Note
Springer Nature remains neutral with regard to jurisdictional claims in published maps and institutional affiliations.
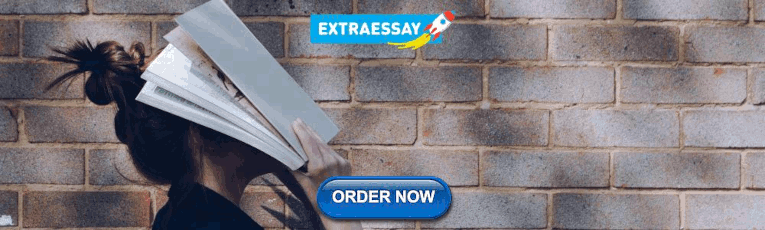
IMAGES
VIDEO
COMMENTS
A data visualization based around the median is the box plot, pioneered by Spear (1952) and enhanced into its current form by Tukey (1977). For a dated visualization, the box plot remains extremely effective in conveying a large amount of information about the underlying data. Yet modern improvements have been made.
Data visualization is the same, with the added benefit that most software is readily available, inexpensive, or free, and many come with large online help resources. This article does not promote any specific software, and readers are encouraged to reference other work 14 for an overview of software resources.
The visualization of information is a widely used tool to improve comprehension and, ultimately, decision-making in strategic management decisions as well as in a diverse array of other domains. Across social science research, many findings have supported this rationale. However, empirical results vary significantly in terms of the variables and mechanisms studied as well as their resulting ...
Summary. Not long ago, the ability to create smart data visualizations (or dataviz) was a nice-to-have skill for design- and data-minded managers. But now it's a must-have skill for all managers ...
Research article. First published online December 15, 2021. The Science of Visual Data Communication: What Works ... information visualization, data-based reasoning, uncertainty representation, and health risk communication. These research communities study similar questions and use complementary expertise and styles of inquiry, yet they too ...
As a preview, we will illustrate Type 1 and 2 processing in decision making with visualizations using four cross-domain findings that we observed in the literature review. Our focus here is on demonstrating how dual-processing can be a useful framework for examining visualization decision-making research.
This statement emphasizes that data visualization as scientific research relies on computing technology and its utilization for the process of information. For this research, it is necessary to initially define the concept of data visualization in order to explore and identify the key forms and characteristics for designing a theoretical ...
New visualization endeavors built on imaging data, such as the Allen Cell Explorer (Fig 1), will allow cell biologists to delve deeper into the architecture, composition, and dynamics of normal and diseased cells, potentially informing research and diagnosis. Visualization bridges the scales from the atomic and molecular levels, to that of ...
In this section, we illustrate the detailed processes of visualizing qualitative data through two research projects from prior empirical work, focusing on technology-enhanced STEM learning (Huang & Parker, 2022; Jiang et al., 2019).Through these examples, we discuss the use of visualization processing flow by employing rich qualitative data in practice and thereby highlight possibilities for ...
Data visualization blends art and science to convey stories from data via graphical representations. Considering different problems, applications, requirements, and design goals, it is challenging to combine these two components at their full force. While the art component involves creating visually appealing and easily interpreted graphics for users, the science component requires accurate ...
Diana Garbinsky. Although the use of tables, graphs, and figures to summarize information has long existed, the advent of the big data era and improved computing power has brought renewed attention to the field of data visualization. As such, it is crucial that introductory statistics courses train students to become critical authors and ...
The research on data visualization has gradually increased, and the total number of articles has increased from 138 in 2012 to 306 in 2021. Over the 10 years, data visualization has become the focus of research. However, the research on information visualization has fluctuated and become stable. The total number of articles is 299.
We catalog collections of visualization resources (as opposed to individual items), e.g. a survey of surveys, and state-of-the-art reports rather than an individual survey, or a survey of books rather than an individual book. There are too many visualization research papers (estimated in the thousands) to list individually.
The current systematic review aimed to investigate in what ways the incorporation of visual display tasks benefits K-12 students' content-area learning. After screening 1693 articles at abstract level and a systematic evaluation of methodological quality, we synthesized 44 articles for this review. The qualitative synthesis of the studies is organized by categories of interaction with visual ...
In summative research articles, illustrations primarily serve to assist the reader in visualizing interpretation of data after they have been collated and statistically analysed. This second form of visualization generally requires different strategies from those for visualizing primary data sets.
Data visualization is the same, with the added benefit that most software is readily available, inexpensive, or free, and many come with large online help resources. This article does not promote any specific software, and readers are encouraged to reference other work 14 for an overview of software resources.
Visualization acts directly on the brain, altering brainwave activity and biochemistry, and its effects branch out into every aspect of life. There is a lot of research that shows how mental practice boosts confidence, enhances mood, helps the healing process and has a positive effect on performance of cognitive and physical tasks. [4] [5] [6 ...
Mental imagery impacts many cognitive processes in the brain: motor control, attention, perception, planning, and memory. So the brain is getting trained for actual performance during ...
Pedagogical Research 2022, 7(2), em0119 e-ISSN: 2468-4929 https://www.pedagogicalresearch.com Research Article OPEN ACCESS Visualization and Problem-based earning Approaches and Students' Attitude toward earning athematics Abera Abate 1* , Mulugeta Atnafu 1, Kassa Michael 1 1 Addis Ababa University, ETHIOPIA
The review criteria focus on incorporating the available studies in the review context and identifying recent research on visualization techniques in healthcare. The selected articles were obtained from various databases (SLD portal, PubMed, and Google Scholar). ... This review fully relied on published articles of the research that other ...
According to the Cambridge Dictionary, visualization is "the act of visualizing something or someone" or "forming a picture of it in your mind". It's almost like looking through a ...
Data visualization blends art and science to convey stories from data via graphical representations. Considering different problems, applications, requirements, and design goals, it is challenging to combine these two components at their full force. While the art component involves creating visually appealing and easily interpreted graphics for ...