Random Assignment in Psychology: Definition & Examples
Julia Simkus
Editor at Simply Psychology
BA (Hons) Psychology, Princeton University
Julia Simkus is a graduate of Princeton University with a Bachelor of Arts in Psychology. She is currently studying for a Master's Degree in Counseling for Mental Health and Wellness in September 2023. Julia's research has been published in peer reviewed journals.
Learn about our Editorial Process
Saul Mcleod, PhD
Editor-in-Chief for Simply Psychology
BSc (Hons) Psychology, MRes, PhD, University of Manchester
Saul Mcleod, PhD., is a qualified psychology teacher with over 18 years of experience in further and higher education. He has been published in peer-reviewed journals, including the Journal of Clinical Psychology.
Olivia Guy-Evans, MSc
Associate Editor for Simply Psychology
BSc (Hons) Psychology, MSc Psychology of Education
Olivia Guy-Evans is a writer and associate editor for Simply Psychology. She has previously worked in healthcare and educational sectors.
In psychology, random assignment refers to the practice of allocating participants to different experimental groups in a study in a completely unbiased way, ensuring each participant has an equal chance of being assigned to any group.
In experimental research, random assignment, or random placement, organizes participants from your sample into different groups using randomization.
Random assignment uses chance procedures to ensure that each participant has an equal opportunity of being assigned to either a control or experimental group.
The control group does not receive the treatment in question, whereas the experimental group does receive the treatment.
When using random assignment, neither the researcher nor the participant can choose the group to which the participant is assigned. This ensures that any differences between and within the groups are not systematic at the onset of the study.
In a study to test the success of a weight-loss program, investigators randomly assigned a pool of participants to one of two groups.
Group A participants participated in the weight-loss program for 10 weeks and took a class where they learned about the benefits of healthy eating and exercise.
Group B participants read a 200-page book that explains the benefits of weight loss. The investigator randomly assigned participants to one of the two groups.
The researchers found that those who participated in the program and took the class were more likely to lose weight than those in the other group that received only the book.
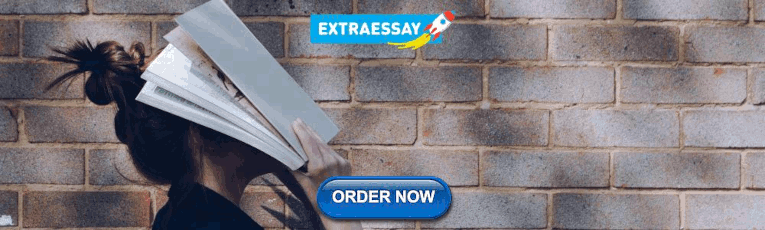
Importance
Random assignment ensures that each group in the experiment is identical before applying the independent variable.
In experiments , researchers will manipulate an independent variable to assess its effect on a dependent variable, while controlling for other variables. Random assignment increases the likelihood that the treatment groups are the same at the onset of a study.
Thus, any changes that result from the independent variable can be assumed to be a result of the treatment of interest. This is particularly important for eliminating sources of bias and strengthening the internal validity of an experiment.
Random assignment is the best method for inferring a causal relationship between a treatment and an outcome.
Random Selection vs. Random Assignment
Random selection (also called probability sampling or random sampling) is a way of randomly selecting members of a population to be included in your study.
On the other hand, random assignment is a way of sorting the sample participants into control and treatment groups.
Random selection ensures that everyone in the population has an equal chance of being selected for the study. Once the pool of participants has been chosen, experimenters use random assignment to assign participants into groups.
Random assignment is only used in between-subjects experimental designs, while random selection can be used in a variety of study designs.
Random Assignment vs Random Sampling
Random sampling refers to selecting participants from a population so that each individual has an equal chance of being chosen. This method enhances the representativeness of the sample.
Random assignment, on the other hand, is used in experimental designs once participants are selected. It involves allocating these participants to different experimental groups or conditions randomly.
This helps ensure that any differences in results across groups are due to manipulating the independent variable, not preexisting differences among participants.
When to Use Random Assignment
Random assignment is used in experiments with a between-groups or independent measures design.
In these research designs, researchers will manipulate an independent variable to assess its effect on a dependent variable, while controlling for other variables.
There is usually a control group and one or more experimental groups. Random assignment helps ensure that the groups are comparable at the onset of the study.
How to Use Random Assignment
There are a variety of ways to assign participants into study groups randomly. Here are a handful of popular methods:
- Random Number Generator : Give each member of the sample a unique number; use a computer program to randomly generate a number from the list for each group.
- Lottery : Give each member of the sample a unique number. Place all numbers in a hat or bucket and draw numbers at random for each group.
- Flipping a Coin : Flip a coin for each participant to decide if they will be in the control group or experimental group (this method can only be used when you have just two groups)
- Roll a Die : For each number on the list, roll a dice to decide which of the groups they will be in. For example, assume that rolling 1, 2, or 3 places them in a control group and rolling 3, 4, 5 lands them in an experimental group.
When is Random Assignment not used?
- When it is not ethically permissible: Randomization is only ethical if the researcher has no evidence that one treatment is superior to the other or that one treatment might have harmful side effects.
- When answering non-causal questions : If the researcher is just interested in predicting the probability of an event, the causal relationship between the variables is not important and observational designs would be more suitable than random assignment.
- When studying the effect of variables that cannot be manipulated: Some risk factors cannot be manipulated and so it would not make any sense to study them in a randomized trial. For example, we cannot randomly assign participants into categories based on age, gender, or genetic factors.
Drawbacks of Random Assignment
While randomization assures an unbiased assignment of participants to groups, it does not guarantee the equality of these groups. There could still be extraneous variables that differ between groups or group differences that arise from chance. Additionally, there is still an element of luck with random assignments.
Thus, researchers can not produce perfectly equal groups for each specific study. Differences between the treatment group and control group might still exist, and the results of a randomized trial may sometimes be wrong, but this is absolutely okay.
Scientific evidence is a long and continuous process, and the groups will tend to be equal in the long run when data is aggregated in a meta-analysis.
Additionally, external validity (i.e., the extent to which the researcher can use the results of the study to generalize to the larger population) is compromised with random assignment.
Random assignment is challenging to implement outside of controlled laboratory conditions and might not represent what would happen in the real world at the population level.
Random assignment can also be more costly than simple observational studies, where an investigator is just observing events without intervening with the population.
Randomization also can be time-consuming and challenging, especially when participants refuse to receive the assigned treatment or do not adhere to recommendations.
What is the difference between random sampling and random assignment?
Random sampling refers to randomly selecting a sample of participants from a population. Random assignment refers to randomly assigning participants to treatment groups from the selected sample.
Does random assignment increase internal validity?
Yes, random assignment ensures that there are no systematic differences between the participants in each group, enhancing the study’s internal validity .
Does random assignment reduce sampling error?
Yes, with random assignment, participants have an equal chance of being assigned to either a control group or an experimental group, resulting in a sample that is, in theory, representative of the population.
Random assignment does not completely eliminate sampling error because a sample only approximates the population from which it is drawn. However, random sampling is a way to minimize sampling errors.
When is random assignment not possible?
Random assignment is not possible when the experimenters cannot control the treatment or independent variable.
For example, if you want to compare how men and women perform on a test, you cannot randomly assign subjects to these groups.
Participants are not randomly assigned to different groups in this study, but instead assigned based on their characteristics.
Does random assignment eliminate confounding variables?
Yes, random assignment eliminates the influence of any confounding variables on the treatment because it distributes them at random among the study groups. Randomization invalidates any relationship between a confounding variable and the treatment.
Why is random assignment of participants to treatment conditions in an experiment used?
Random assignment is used to ensure that all groups are comparable at the start of a study. This allows researchers to conclude that the outcomes of the study can be attributed to the intervention at hand and to rule out alternative explanations for study results.
Further Reading
- Bogomolnaia, A., & Moulin, H. (2001). A new solution to the random assignment problem . Journal of Economic theory , 100 (2), 295-328.
- Krause, M. S., & Howard, K. I. (2003). What random assignment does and does not do . Journal of Clinical Psychology , 59 (7), 751-766.

If you're seeing this message, it means we're having trouble loading external resources on our website.
If you're behind a web filter, please make sure that the domains *.kastatic.org and *.kasandbox.org are unblocked.
To log in and use all the features of Khan Academy, please enable JavaScript in your browser.
AP®︎/College Statistics
Course: ap®︎/college statistics > unit 6.
- Statistical significance of experiment
Random sampling vs. random assignment (scope of inference)
- Conclusions in observational studies versus experiments
- Finding errors in study conclusions
- (Choice A) Just the residents involved in Hilary's study. A Just the residents involved in Hilary's study.
- (Choice B) All residents in Hilary's town. B All residents in Hilary's town.
- (Choice C) All residents in Hilary's country. C All residents in Hilary's country.
- (Choice A) Yes A Yes
- (Choice B) No B No
- (Choice A) Just the residents in Hilary's study. A Just the residents in Hilary's study.
Want to join the conversation?
- Upvote Button navigates to signup page
- Downvote Button navigates to signup page
- Flag Button navigates to signup page

- Bipolar Disorder
- Therapy Center
- When To See a Therapist
- Types of Therapy
- Best Online Therapy
- Best Couples Therapy
- Best Family Therapy
- Managing Stress
- Sleep and Dreaming
- Understanding Emotions
- Self-Improvement
- Healthy Relationships
- Student Resources
- Personality Types
- Guided Meditations
- Verywell Mind Insights
- 2023 Verywell Mind 25
- Mental Health in the Classroom
- Editorial Process
- Meet Our Review Board
- Crisis Support
The Definition of Random Assignment According to Psychology
Kendra Cherry, MS, is a psychosocial rehabilitation specialist, psychology educator, and author of the "Everything Psychology Book."
:max_bytes(150000):strip_icc():format(webp)/IMG_9791-89504ab694d54b66bbd72cb84ffb860e.jpg)
Emily is a board-certified science editor who has worked with top digital publishing brands like Voices for Biodiversity, Study.com, GoodTherapy, Vox, and Verywell.
:max_bytes(150000):strip_icc():format(webp)/Emily-Swaim-1000-0f3197de18f74329aeffb690a177160c.jpg)
Materio / Getty Images
Random assignment refers to the use of chance procedures in psychology experiments to ensure that each participant has the same opportunity to be assigned to any given group in a study to eliminate any potential bias in the experiment at the outset. Participants are randomly assigned to different groups, such as the treatment group versus the control group. In clinical research, randomized clinical trials are known as the gold standard for meaningful results.
Simple random assignment techniques might involve tactics such as flipping a coin, drawing names out of a hat, rolling dice, or assigning random numbers to a list of participants. It is important to note that random assignment differs from random selection .
While random selection refers to how participants are randomly chosen from a target population as representatives of that population, random assignment refers to how those chosen participants are then assigned to experimental groups.
Random Assignment In Research
To determine if changes in one variable will cause changes in another variable, psychologists must perform an experiment. Random assignment is a critical part of the experimental design that helps ensure the reliability of the study outcomes.
Researchers often begin by forming a testable hypothesis predicting that one variable of interest will have some predictable impact on another variable.
The variable that the experimenters will manipulate in the experiment is known as the independent variable , while the variable that they will then measure for different outcomes is known as the dependent variable. While there are different ways to look at relationships between variables, an experiment is the best way to get a clear idea if there is a cause-and-effect relationship between two or more variables.
Once researchers have formulated a hypothesis, conducted background research, and chosen an experimental design, it is time to find participants for their experiment. How exactly do researchers decide who will be part of an experiment? As mentioned previously, this is often accomplished through something known as random selection.
Random Selection
In order to generalize the results of an experiment to a larger group, it is important to choose a sample that is representative of the qualities found in that population. For example, if the total population is 60% female and 40% male, then the sample should reflect those same percentages.
Choosing a representative sample is often accomplished by randomly picking people from the population to be participants in a study. Random selection means that everyone in the group stands an equal chance of being chosen to minimize any bias. Once a pool of participants has been selected, it is time to assign them to groups.
By randomly assigning the participants into groups, the experimenters can be fairly sure that each group will have the same characteristics before the independent variable is applied.
Participants might be randomly assigned to the control group , which does not receive the treatment in question. The control group may receive a placebo or receive the standard treatment. Participants may also be randomly assigned to the experimental group , which receives the treatment of interest. In larger studies, there can be multiple treatment groups for comparison.
There are simple methods of random assignment, like rolling the die. However, there are more complex techniques that involve random number generators to remove any human error.
There can also be random assignment to groups with pre-established rules or parameters. For example, if you want to have an equal number of men and women in each of your study groups, you might separate your sample into two groups (by sex) before randomly assigning each of those groups into the treatment group and control group.
Random assignment is essential because it increases the likelihood that the groups are the same at the outset. With all characteristics being equal between groups, other than the application of the independent variable, any differences found between group outcomes can be more confidently attributed to the effect of the intervention.
Example of Random Assignment
Imagine that a researcher is interested in learning whether or not drinking caffeinated beverages prior to an exam will improve test performance. After randomly selecting a pool of participants, each person is randomly assigned to either the control group or the experimental group.
The participants in the control group consume a placebo drink prior to the exam that does not contain any caffeine. Those in the experimental group, on the other hand, consume a caffeinated beverage before taking the test.
Participants in both groups then take the test, and the researcher compares the results to determine if the caffeinated beverage had any impact on test performance.
A Word From Verywell
Random assignment plays an important role in the psychology research process. Not only does this process help eliminate possible sources of bias, but it also makes it easier to generalize the results of a tested sample of participants to a larger population.
Random assignment helps ensure that members of each group in the experiment are the same, which means that the groups are also likely more representative of what is present in the larger population of interest. Through the use of this technique, psychology researchers are able to study complex phenomena and contribute to our understanding of the human mind and behavior.
Lin Y, Zhu M, Su Z. The pursuit of balance: An overview of covariate-adaptive randomization techniques in clinical trials . Contemp Clin Trials. 2015;45(Pt A):21-25. doi:10.1016/j.cct.2015.07.011
Sullivan L. Random assignment versus random selection . In: The SAGE Glossary of the Social and Behavioral Sciences. SAGE Publications, Inc.; 2009. doi:10.4135/9781412972024.n2108
Alferes VR. Methods of Randomization in Experimental Design . SAGE Publications, Inc.; 2012. doi:10.4135/9781452270012
Nestor PG, Schutt RK. Research Methods in Psychology: Investigating Human Behavior. (2nd Ed.). SAGE Publications, Inc.; 2015.
By Kendra Cherry, MSEd Kendra Cherry, MS, is a psychosocial rehabilitation specialist, psychology educator, and author of the "Everything Psychology Book."
Browse Course Material
Course info.
- Prof. Scott Sheffield
Departments
- Mathematics
As Taught In
- Discrete Mathematics
- Probability and Statistics
Learning Resource Types
Probability and random variables, probability and random variables, problem set 1.
This resource contains information related to problem set 1.

You are leaving MIT OpenCourseWare
Have a language expert improve your writing
Run a free plagiarism check in 10 minutes, automatically generate references for free.
- Knowledge Base
- Methodology
- Random Assignment in Experiments | Introduction & Examples
Random Assignment in Experiments | Introduction & Examples
Published on 6 May 2022 by Pritha Bhandari . Revised on 13 February 2023.
In experimental research, random assignment is a way of placing participants from your sample into different treatment groups using randomisation.
With simple random assignment, every member of the sample has a known or equal chance of being placed in a control group or an experimental group. Studies that use simple random assignment are also called completely randomised designs .
Random assignment is a key part of experimental design . It helps you ensure that all groups are comparable at the start of a study: any differences between them are due to random factors.
Table of contents
Why does random assignment matter, random sampling vs random assignment, how do you use random assignment, when is random assignment not used, frequently asked questions about random assignment.
Random assignment is an important part of control in experimental research, because it helps strengthen the internal validity of an experiment.
In experiments, researchers manipulate an independent variable to assess its effect on a dependent variable, while controlling for other variables. To do so, they often use different levels of an independent variable for different groups of participants.
This is called a between-groups or independent measures design.
You use three groups of participants that are each given a different level of the independent variable:
- A control group that’s given a placebo (no dosage)
- An experimental group that’s given a low dosage
- A second experimental group that’s given a high dosage
Random assignment to helps you make sure that the treatment groups don’t differ in systematic or biased ways at the start of the experiment.
If you don’t use random assignment, you may not be able to rule out alternative explanations for your results.
- Participants recruited from pubs are placed in the control group
- Participants recruited from local community centres are placed in the low-dosage experimental group
- Participants recruited from gyms are placed in the high-dosage group
With this type of assignment, it’s hard to tell whether the participant characteristics are the same across all groups at the start of the study. Gym users may tend to engage in more healthy behaviours than people who frequent pubs or community centres, and this would introduce a healthy user bias in your study.
Although random assignment helps even out baseline differences between groups, it doesn’t always make them completely equivalent. There may still be extraneous variables that differ between groups, and there will always be some group differences that arise from chance.
Most of the time, the random variation between groups is low, and, therefore, it’s acceptable for further analysis. This is especially true when you have a large sample. In general, you should always use random assignment in experiments when it is ethically possible and makes sense for your study topic.
Prevent plagiarism, run a free check.
Random sampling and random assignment are both important concepts in research, but it’s important to understand the difference between them.
Random sampling (also called probability sampling or random selection) is a way of selecting members of a population to be included in your study. In contrast, random assignment is a way of sorting the sample participants into control and experimental groups.
While random sampling is used in many types of studies, random assignment is only used in between-subjects experimental designs.
Some studies use both random sampling and random assignment, while others use only one or the other.
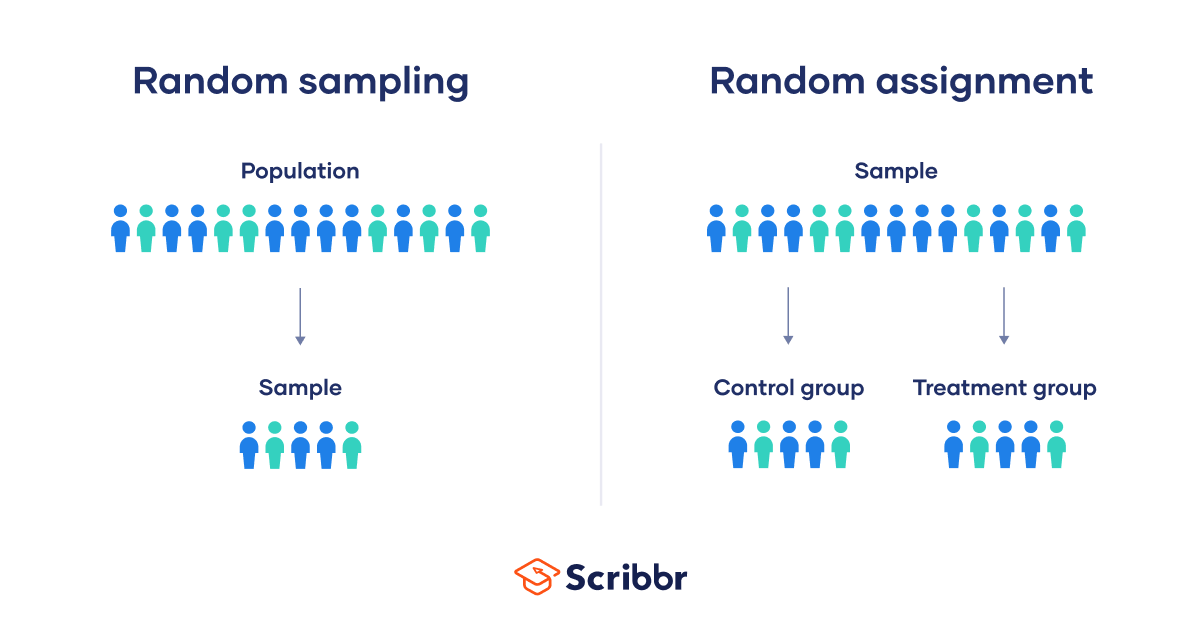
Random sampling enhances the external validity or generalisability of your results, because it helps to ensure that your sample is unbiased and representative of the whole population. This allows you to make stronger statistical inferences .
You use a simple random sample to collect data. Because you have access to the whole population (all employees), you can assign all 8,000 employees a number and use a random number generator to select 300 employees. These 300 employees are your full sample.
Random assignment enhances the internal validity of the study, because it ensures that there are no systematic differences between the participants in each group. This helps you conclude that the outcomes can be attributed to the independent variable .
- A control group that receives no intervention
- An experimental group that has a remote team-building intervention every week for a month
You use random assignment to place participants into the control or experimental group. To do so, you take your list of participants and assign each participant a number. Again, you use a random number generator to place each participant in one of the two groups.
To use simple random assignment, you start by giving every member of the sample a unique number. Then, you can use computer programs or manual methods to randomly assign each participant to a group.
- Random number generator: Use a computer program to generate random numbers from the list for each group.
- Lottery method: Place all numbers individually into a hat or a bucket, and draw numbers at random for each group.
- Flip a coin: When you only have two groups, for each number on the list, flip a coin to decide if they’ll be in the control or the experimental group.
- Use a dice: When you have three groups, for each number on the list, roll a die to decide which of the groups they will be in. For example, assume that rolling 1 or 2 lands them in a control group; 3 or 4 in an experimental group; and 5 or 6 in a second control or experimental group.
This type of random assignment is the most powerful method of placing participants in conditions, because each individual has an equal chance of being placed in any one of your treatment groups.
Random assignment in block designs
In more complicated experimental designs, random assignment is only used after participants are grouped into blocks based on some characteristic (e.g., test score or demographic variable). These groupings mean that you need a larger sample to achieve high statistical power .
For example, a randomised block design involves placing participants into blocks based on a shared characteristic (e.g., college students vs graduates), and then using random assignment within each block to assign participants to every treatment condition. This helps you assess whether the characteristic affects the outcomes of your treatment.
In an experimental matched design , you use blocking and then match up individual participants from each block based on specific characteristics. Within each matched pair or group, you randomly assign each participant to one of the conditions in the experiment and compare their outcomes.
Sometimes, it’s not relevant or ethical to use simple random assignment, so groups are assigned in a different way.
When comparing different groups
Sometimes, differences between participants are the main focus of a study, for example, when comparing children and adults or people with and without health conditions. Participants are not randomly assigned to different groups, but instead assigned based on their characteristics.
In this type of study, the characteristic of interest (e.g., gender) is an independent variable, and the groups differ based on the different levels (e.g., men, women). All participants are tested the same way, and then their group-level outcomes are compared.
When it’s not ethically permissible
When studying unhealthy or dangerous behaviours, it’s not possible to use random assignment. For example, if you’re studying heavy drinkers and social drinkers, it’s unethical to randomly assign participants to one of the two groups and ask them to drink large amounts of alcohol for your experiment.
When you can’t assign participants to groups, you can also conduct a quasi-experimental study . In a quasi-experiment, you study the outcomes of pre-existing groups who receive treatments that you may not have any control over (e.g., heavy drinkers and social drinkers).
These groups aren’t randomly assigned, but may be considered comparable when some other variables (e.g., age or socioeconomic status) are controlled for.
In experimental research, random assignment is a way of placing participants from your sample into different groups using randomisation. With this method, every member of the sample has a known or equal chance of being placed in a control group or an experimental group.
Random selection, or random sampling , is a way of selecting members of a population for your study’s sample.
In contrast, random assignment is a way of sorting the sample into control and experimental groups.
Random sampling enhances the external validity or generalisability of your results, while random assignment improves the internal validity of your study.
Random assignment is used in experiments with a between-groups or independent measures design. In this research design, there’s usually a control group and one or more experimental groups. Random assignment helps ensure that the groups are comparable.
In general, you should always use random assignment in this type of experimental design when it is ethically possible and makes sense for your study topic.
To implement random assignment , assign a unique number to every member of your study’s sample .
Then, you can use a random number generator or a lottery method to randomly assign each number to a control or experimental group. You can also do so manually, by flipping a coin or rolling a die to randomly assign participants to groups.
Cite this Scribbr article
If you want to cite this source, you can copy and paste the citation or click the ‘Cite this Scribbr article’ button to automatically add the citation to our free Reference Generator.
Bhandari, P. (2023, February 13). Random Assignment in Experiments | Introduction & Examples. Scribbr. Retrieved 15 April 2024, from https://www.scribbr.co.uk/research-methods/random-assignment-experiments/
Is this article helpful?
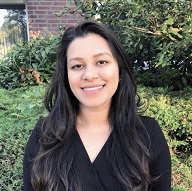
Pritha Bhandari
Other students also liked, a quick guide to experimental design | 5 steps & examples, controlled experiments | methods & examples of control, control groups and treatment groups | uses & examples.

An official website of the United States government
The .gov means it’s official. Federal government websites often end in .gov or .mil. Before sharing sensitive information, make sure you’re on a federal government site.
The site is secure. The https:// ensures that you are connecting to the official website and that any information you provide is encrypted and transmitted securely.
- Publications
- Account settings
Preview improvements coming to the PMC website in October 2024. Learn More or Try it out now .
- Advanced Search
- Journal List
- J Athl Train
- v.43(2); Mar-Apr 2008
Issues in Outcomes Research: An Overview of Randomization Techniques for Clinical Trials
Minsoo kang.
1 Middle Tennessee State University, Murfreesboro, TN
Brian G Ragan
2 University of Northern Iowa, Cedar Falls, IA
Jae-Hyeon Park
3 Korea National Sport University, Seoul, Korea
To review and describe randomization techniques used in clinical trials, including simple, block, stratified, and covariate adaptive techniques.
Background:
Clinical trials are required to establish treatment efficacy of many athletic training procedures. In the past, we have relied on evidence of questionable scientific merit to aid the determination of treatment choices. Interest in evidence-based practice is growing rapidly within the athletic training profession, placing greater emphasis on the importance of well-conducted clinical trials. One critical component of clinical trials that strengthens results is random assignment of participants to control and treatment groups. Although randomization appears to be a simple concept, issues of balancing sample sizes and controlling the influence of covariates a priori are important. Various techniques have been developed to account for these issues, including block, stratified randomization, and covariate adaptive techniques.
Advantages:
Athletic training researchers and scholarly clinicians can use the information presented in this article to better conduct and interpret the results of clinical trials. Implementing these techniques will increase the power and validity of findings of athletic medicine clinical trials, which will ultimately improve the quality of care provided.
Outcomes research is critical in the evidence-based health care environment because it addresses scientific questions concerning the efficacy of treatments. Clinical trials are considered the “gold standard” for outcomes in biomedical research. In athletic training, calls for more evidence-based medical research, specifically clinical trials, have been issued. 1 , 2
The strength of clinical trials is their superior ability to measure change over time from a treatment. Treatment differences identified from cross-sectional observational designs rather than experimental clinical trials have methodologic weaknesses, including confounding, cohort effects, and selection bias. 3 For example, using a nonrandomized trial to examine the effectiveness of prophylactic knee bracing to prevent medial collateral ligament injuries may suffer from confounders and jeopardize the results. One possible confounder is a history of knee injuries. Participants with a history of knee injuries may be more likely to wear braces than those with no such history. Participants with a history of injury are more likely to suffer additional knee injuries, unbalancing the groups and influencing the results of the study.
The primary goal of comparative clinical trials is to provide comparisons of treatments with maximum precision and validity. 4 One critical component of clinical trials is random assignment of participants into groups. Randomizing participants helps remove the effect of extraneous variables (eg, age, injury history) and minimizes bias associated with treatment assignment. Randomization is considered by most researchers to be the optimal approach for participant assignment in clinical trials because it strengthens the results and data interpretation. 4 – , 9
One potential problem with small clinical trials (n < 100) 7 is that conventional simple randomization methods, such as flipping a coin, may result in imbalanced sample size and baseline characteristics (ie, covariates) among treatment and control groups. 9 , 10 This imbalance of baseline characteristics can influence the comparison between treatment and control groups and introduce potential confounding factors. Many procedures have been proposed for random group assignment of participants in clinical trials. 11 Simple, block, stratified, and covariate adaptive randomizations are some examples. Each technique has advantages and disadvantages, which must be carefully considered before a method is selected. Our purpose is to introduce the concept and significance of randomization and to review several conventional and relatively new randomization techniques to aid in the design and implementation of valid clinical trials.
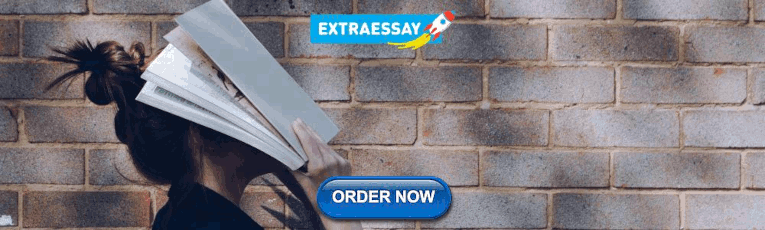
What Is Randomization?
Randomization is the process of assigning participants to treatment and control groups, assuming that each participant has an equal chance of being assigned to any group. 12 Randomization has evolved into a fundamental aspect of scientific research methodology. Demands have increased for more randomized clinical trials in many areas of biomedical research, such as athletic training. 2 , 13 In fact, in the last 2 decades, internationally recognized major medical journals, such as the Journal of the American Medical Association and the BMJ , have been increasingly interested in publishing studies reporting results from randomized controlled trials. 5
Since Fisher 14 first introduced the idea of randomization in a 1926 agricultural study, the academic community has deemed randomization an essential tool for unbiased comparisons of treatment groups. Five years after Fisher's introductory paper, the first randomized clinical trial involving tuberculosis was conducted. 15 A total of 24 participants were paired (ie, 12 comparable pairs), and by a flip of a coin, each participant within the pair was assigned to either the control or treatment group. By employing randomization, researchers offer each participant an equal chance of being assigned to groups, which makes the groups comparable on the dependent variable by eliminating potential bias. Indeed, randomization of treatments in clinical trials is the only means of avoiding systematic characteristic bias of participants assigned to different treatments. Although randomization may be accomplished with a simple coin toss, more appropriate and better methods are often needed, especially in small clinical trials. These other methods will be discussed in this review.
Why Randomize?
Researchers demand randomization for several reasons. First, participants in various groups should not differ in any systematic way. In a clinical trial, if treatment groups are systematically different, trial results will be biased. Suppose that participants are assigned to control and treatment groups in a study examining the efficacy of a walking intervention. If a greater proportion of older adults is assigned to the treatment group, then the outcome of the walking intervention may be influenced by this imbalance. The effects of the treatment would be indistinguishable from the influence of the imbalance of covariates, thereby requiring the researcher to control for the covariates in the analysis to obtain an unbiased result. 16
Second, proper randomization ensures no a priori knowledge of group assignment (ie, allocation concealment). That is, researchers, participants, and others should not know to which group the participant will be assigned. Knowledge of group assignment creates a layer of potential selection bias that may taint the data. Schulz and Grimes 17 stated that trials with inadequate or unclear randomization tended to overestimate treatment effects up to 40% compared with those that used proper randomization. The outcome of the trial can be negatively influenced by this inadequate randomization.
Statistical techniques such as analysis of covariance (ANCOVA), multivariate ANCOVA, or both, are often used to adjust for covariate imbalance in the analysis stage of the clinical trial. However, the interpretation of this postadjustment approach is often difficult because imbalance of covariates frequently leads to unanticipated interaction effects, such as unequal slopes among subgroups of covariates. 18 , 19 One of the critical assumptions in ANCOVA is that the slopes of regression lines are the same for each group of covariates (ie, homogeneity of regression slopes). The adjustment needed for each covariate group may vary, which is problematic because ANCOVA uses the average slope across the groups to adjust the outcome variable. Thus, the ideal way of balancing covariates among groups is to apply sound randomization in the design stage of a clinical trial (before the adjustment procedure) instead of after data collection. In such instances, random assignment is necessary and guarantees validity for statistical tests of significance that are used to compare treatments.
How To Randomize?
Many procedures have been proposed for the random assignment of participants to treatment groups in clinical trials. In this article, common randomization techniques, including simple randomization, block randomization, stratified randomization, and covariate adaptive randomization, are reviewed. Each method is described along with its advantages and disadvantages. It is very important to select a method that will produce interpretable, valid results for your study.
Simple Randomization
Randomization based on a single sequence of random assignments is known as simple randomization. 10 This technique maintains complete randomness of the assignment of a person to a particular group. The most common and basic method of simple randomization is flipping a coin. For example, with 2 treatment groups (control versus treatment), the side of the coin (ie, heads = control, tails = treatment) determines the assignment of each participant. Other methods include using a shuffled deck of cards (eg, even = control, odd = treatment) or throwing a die (eg, below and equal to 3 = control, over 3 = treatment). A random number table found in a statistics book or computer-generated random numbers can also be used for simple randomization of participants.
This randomization approach is simple and easy to implement in a clinical trial. In large trials (n > 200), simple randomization can be trusted to generate similar numbers of participants among groups. However, randomization results could be problematic in relatively small sample size clinical trials (n < 100), resulting in an unequal number of participants among groups. For example, using a coin toss with a small sample size (n = 10) may result in an imbalance such that 7 participants are assigned to the control group and 3 to the treatment group ( Figure 1 ).

Block Randomization
The block randomization method is designed to randomize participants into groups that result in equal sample sizes. This method is used to ensure a balance in sample size across groups over time. Blocks are small and balanced with predetermined group assignments, which keeps the numbers of participants in each group similar at all times. According to Altman and Bland, 10 the block size is determined by the researcher and should be a multiple of the number of groups (ie, with 2 treatment groups, block size of either 4 or 6). Blocks are best used in smaller increments as researchers can more easily control balance. 7 After block size has been determined, all possible balanced combinations of assignment within the block (ie, equal number for all groups within the block) must be calculated. Blocks are then randomly chosen to determine the participants' assignment into the groups.
For a clinical trial with control and treatment groups involving 40 participants, a randomized block procedure would be as follows: (1) a block size of 4 is chosen, (2) possible balanced combinations with 2 C (control) and 2 T (treatment) subjects are calculated as 6 (TTCC, TCTC, TCCT, CTTC, CTCT, CCTT), and (3) blocks are randomly chosen to determine the assignment of all 40 participants (eg, one random sequence would be [TTCC / TCCT / CTTC / CTTC / TCCT / CCTT / TTCC / TCTC / CTCT / TCTC]). This procedure results in 20 participants in both the control and treatment groups ( Figure 2 ).

Although balance in sample size may be achieved with this method, groups may be generated that are rarely comparable in terms of certain covariates. 6 For example, one group may have more participants with secondary diseases (eg, diabetes, multiple sclerosis, cancer) that could confound the data and may negatively influence the results of the clinical trial. Pocock and Simon 11 stressed the importance of controlling for these covariates because of serious consequences to the interpretation of the results. Such an imbalance could introduce bias in the statistical analysis and reduce the power of the study. 4 , 6 , 8 Hence, sample size and covariates must be balanced in small clinical trials.
Stratified Randomization
The stratified randomization method addresses the need to control and balance the influence of covariates. This method can be used to achieve balance among groups in terms of participants' baseline characteristics (covariates). Specific covariates must be identified by the researcher who understands the potential influence each covariate has on the dependent variable. Stratified randomization is achieved by generating a separate block for each combination of covariates, and participants are assigned to the appropriate block of covariates. After all participants have been identified and assigned into blocks, simple randomization occurs within each block to assign participants to one of the groups.
The stratified randomization method controls for the possible influence of covariates that would jeopardize the conclusions of the clinical trial. For example, a clinical trial of different rehabilitation techniques after a surgical procedure will have a number of covariates. It is well known that the age of the patient affects the rate of healing. Thus, age could be a confounding variable and influence the outcome of the clinical trial. Stratified randomization can balance the control and treatment groups for age or other identified covariates.
For example, with 2 groups involving 40 participants, the stratified randomization method might be used to control the covariates of sex (2 levels: male, female) and body mass index (3 levels: underweight, normal, overweight) between study arms. With these 2 covariates, possible block combinations total 6 (eg, male, underweight). A simple randomization procedure, such as flipping a coin, is used to assign the participants within each block to one of the treatment groups ( Figure 3 ).

Although stratified randomization is a relatively simple and useful technique, especially for smaller clinical trials, it becomes complicated to implement if many covariates must be controlled. 20 For example, too many block combinations may lead to imbalances in overall treatment allocations because a large number of blocks can generate small participant numbers within the block. Therneau 21 purported that a balance in covariates begins to fail when the number of blocks approaches half the sample size. If another 4-level covariate was added to the example, the number of block combinations would increase from 6 to 24 (2 × 3 × 4), for an average of fewer than 2 (40 / 24 = 1.7) participants per block, reducing the usefulness of the procedure to balance the covariates and jeopardizing the validity of the clinical trial. In small studies, it may not be feasible to stratify more than 1 or 2 covariates because the number of blocks can quickly approach the number of participants. 10
Stratified randomization has another limitation: it works only when all participants have been identified before group assignment. This method is rarely applicable, however, because clinical trial participants are often enrolled one at a time on a continuous basis. When baseline characteristics of all participants are not available before assignment, using stratified randomization is difficult. 7
Covariate Adaptive Randomization
Covariate adaptive randomization has been recommended by many researchers as a valid alternative randomization method for clinical trials. 9 , 22 In covariate adaptive randomization, a new participant is sequentially assigned to a particular treatment group by taking into account the specific covariates and previous assignments of participants. 9 , 12 , 18 , 23 , 24 Covariate adaptive randomization uses the method of minimization by assessing the imbalance of sample size among several covariates. This covariate adaptive approach was first described by Taves. 23
The Taves covariate adaptive randomization method allows for the examination of previous participant group assignments to make a case-by-case decision on group assignment for each individual who enrolls in the study. Consider again the example of 2 groups involving 40 participants, with sex (2 levels: male, female) and body mass index (3 levels: underweight, normal, overweight) as covariates. Assume the first 9 participants have already been randomly assigned to groups by flipping a coin. The 9 participants' group assignments are broken down by covariate level in Figure 4 . Now the 10th participant, who is male and underweight, needs to be assigned to a group (ie, control versus treatment). Based on the characteristics of the 10th participant, the Taves method adds marginal totals of the corresponding covariate categories for each group and compares the totals. The participant is assigned to the group with the lower covariate total to minimize imbalance. In this example, the appropriate categories are male and underweight, which results in the total of 3 (2 for male category + 1 for underweight category) for the control group and a total of 5 (3 for male category + 2 for underweight category) for the treatment group. Because the sum of marginal totals is lower for the control group (3 < 5), the 10th participant is assigned to the control group ( Figure 5 ).

The Pocock and Simon method 11 of covariate adaptive randomization is similar to the method Taves 23 described. The difference in this approach is the temporary assignment of participants to both groups. This method uses the absolute difference between groups to determine group assignment. To minimize imbalance, the participant is assigned to the group determined by the lowest sum of the absolute differences among the covariates between the groups. For example, using the previous situation in assigning the 10th participant to a group, the Pocock and Simon method would (1) assign the 10th participant temporarily to the control group, resulting in marginal totals of 3 for male category and 2 for underweight category; (2) calculate the absolute difference between control and treatment group (males: 3 control – 3 treatment = 0; underweight: 2 control – 2 treatment = 0) and sum (0 + 0 = 0); (3) temporarily assign the 10th participant to the treatment group, resulting in marginal totals of 4 for male category and 3 for underweight category; (4) calculate the absolute difference between control and treatment group (males: 2 control – 4 treatment = 2; underweight: 1 control – 3 treatment = 2) and sum (2 + 2 = 4); and (5) assign the 10th participant to the control group because of the lowest sum of absolute differences (0 < 4).
Pocock and Simon 11 also suggested using a variance approach. Instead of calculating absolute difference among groups, this approach calculates the variance among treatment groups. Although the variance method performs similarly to the absolute difference method, both approaches suffer from the limitation of handling only categorical covariates. 25
Frane 18 introduced a covariate adaptive randomization for both continuous and categorical types. Frane used P values to identify imbalance among treatment groups: a smaller P value represents more imbalance among treatment groups.
The Frane method for assigning participants to either the control or treatment group would include (1) temporarily assigning the participant to both the control and treatment groups; (2) calculating P values for each of the covariates using a t test and analysis of variance (ANOVA) for continuous variables and goodness-of-fit χ 2 test for categorical variables; (3) determining the minimum P value for each control or treatment group, which indicates more imbalance among treatment groups; and (4) assigning the participant to the group with the larger minimum P value (ie, try to avoid more imbalance in groups).
Going back to the previous example of assigning the 10th participant (male and underweight) to a group, the Frane method would result in the assignment to the control group. The steps used to make this decision were calculating P values for each of the covariates using the χ 2 goodness-of-fit test represented in the Table . The t tests and ANOVAs were not used because the covariates in this example were categorical. Based on the Table , the lowest minimum P values were 1.0 for the control group and 0.317 for the treatment group. The 10th participant was assigned to the control group because of the higher minimum P value, which indicates better balance in the control group (1.0 > 0.317).
Probabilities From χ 2 Goodness-of-Fit Tests for the Example Shown in Figure 5 (Frane 18 Method)

Covariate adaptive randomization produces less imbalance than other conventional randomization methods and can be used successfully to balance important covariates among control and treatment groups. 6 Although the balance of covariates among groups using the stratified randomization method begins to fail when the number of blocks approaches half the sample size, covariate adaptive randomization can better handle the problem of increasing numbers of covariates (ie, increased block combinations). 9
One concern of these covariate adaptive randomization methods is that treatment assignments sometimes become highly predictable. Investigators using covariate adaptive randomization sometimes come to believe that group assignment for the next participant can be readily predicted, going against the basic concept of randomization. 12 , 26 , 27 This predictability stems from the ongoing assignment of participants to groups wherein the current allocation of participants may suggest future participant group assignment. In their review, Scott et al 9 argued that this predictability is also true of other methods, including stratified randomization, and it should not be overly penalized. Zielhuis et al 28 and Frane 18 suggested a practical approach to prevent predictability: a small number of participants should be randomly assigned into the groups before the covariate adaptive randomization technique being applied.
The complicated computation process of covariate adaptive randomization increases the administrative burden, thereby limiting its use in practice. A user-friendly computer program for covariate adaptive randomization is available (free of charge) upon request from the authors (M.K., B.G.R., or J.H.P.). 29
Conclusions
Our purpose was to introduce randomization, including its concept and significance, and to review several randomization techniques to guide athletic training researchers and practitioners to better design their randomized clinical trials. Many factors can affect the results of clinical research, but randomization is considered the gold standard in most clinical trials. It eliminates selection bias, ensures balance of sample size and baseline characteristics, and is an important step in guaranteeing the validity of statistical tests of significance used to compare treatment groups.
Before choosing a randomization method, several factors need to be considered, including the size of the clinical trial; the need for balance in sample size, covariates, or both; and participant enrollment. 16 Figure 6 depicts a flowchart designed to help select an appropriate randomization technique. For example, a power analysis for a clinical trial of different rehabilitation techniques after a surgical procedure indicated a sample size of 80. A well-known covariate for this study is age, which must be balanced among groups. Because of the nature of the study with postsurgical patients, participant recruitment and enrollment will be continuous. Using the flowchart, the appropriate randomization technique is covariate adaptive randomization technique.

Simple randomization works well for a large trial (eg, n > 200) but not for a small trial (n < 100). 7 To achieve balance in sample size, block randomization is desirable. To achieve balance in baseline characteristics, stratified randomization is widely used. Covariate adaptive randomization, however, can achieve better balance than other randomization methods and can be successfully used for clinical trials in an effective manner.
Acknowledgments
This study was partially supported by a Faculty Grant (FRCAC) from the College of Graduate Studies, at Middle Tennessee State University, Murfreesboro, TN.
Minsoo Kang, PhD; Brian G. Ragan, PhD, ATC; and Jae-Hyeon Park, PhD, contributed to conception and design; acquisition and analysis and interpretation of the data; and drafting, critical revision, and final approval of the article.
Random Assignment Problems on 2 d Manifolds
- Open access
- Published: 12 May 2021
- Volume 183 , article number 34 , ( 2021 )
Cite this article
You have full access to this open access article
- D. Benedetto 1 ,
- E. Caglioti 1 ,
- S. Caracciolo 2 ,
- M. D’Achille ORCID: orcid.org/0000-0002-8750-1275 3 , 4 , 5 ,
- G. Sicuro ORCID: orcid.org/0000-0002-9258-2436 6 &
- A. Sportiello 7
1251 Accesses
4 Citations
3 Altmetric
Explore all metrics
We consider the assignment problem between two sets of N random points on a smooth, two-dimensional manifold \(\Omega \) of unit area. It is known that the average cost scales as \(E_{\Omega }(N)\sim {1}/{2\pi }\ln N\) with a correction that is at most of order \(\sqrt{\ln N\ln \ln N}\) . In this paper, we show that, within the linearization approximation of the field-theoretical formulation of the problem, the first \(\Omega \) -dependent correction is on the constant term, and can be exactly computed from the spectrum of the Laplace–Beltrami operator on \(\Omega \) . We perform the explicit calculation of this constant for various families of surfaces, and compare our predictions with extensive numerics.
Similar content being viewed by others
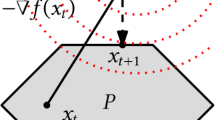
The Frank-Wolfe Algorithm: A Short Introduction
Sebastian Pokutta
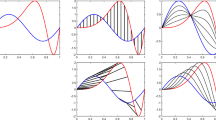
Shape-based functional data analysis
Yuexuan Wu, Chao Huang & Anuj Srivastava
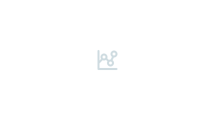
On the rate of convergence in Wasserstein distance of the empirical measure
Nicolas Fournier & Arnaud Guillin
Avoid common mistakes on your manuscript.
1 Introduction

It is a longstanding question to understand the asymptotic behaviour, for large N , of \(E_{\Omega }(N)\) , and, when \(n\ge 2\) , the results are very partial for any manifold \(\Omega \) , including the conceptually simplest ones (like the unit hypercube, or the unit hypertorus), and any value of p , including the special cases \(p=1\) and \(p=2\) . In particular, in the two-dimensional case for \(p=2\) (see [ 1 , 2 , 3 , 4 ]), it has been proved that, as long as \(\Omega \) has unit volume, the leading term is \(\Omega \) -independent:
The main goal of the present paper is to show that, at least in the case \(n=2\) , \(p=2\) , the leading behaviour in N of \(E_{\Omega }(N)\) which is \(\Omega \) -dependent is a constant, which can be calculated exactly . More precisely, we are not able to establish a full perturbative expansion for \(E_{\Omega }(N)\) , up to corrections o (1), for any \(\Omega \) . Nonetheless, for all pairs \((\Omega , \Omega ')\) , we predict that
where \(\{\lambda _i\}_{i \ge 1}\) is the set of eigenvalues of the Laplace–Beltrami operator on \(\Omega \) that are different from zero (if \(\Omega \) is a manifold with a boundary \(\partial \Omega \) of perimeter of order 1, it is the set of eigenvalues of the Laplace–Beltrami operator, with Neumann boundary conditions). That is, all terms in an asymptotic expansion of \(E_{\Omega }(N)\) which do not decrease with N must be ‘universal’.
One can notice that \(K_{\Omega }\) is a regularization of the trace of the Laplace–Beltrami operator. Another equivalent regularization is the so-called Robin mass \(R_\Omega ,\) see for instance [ 5 , 6 ]. In particular Eq. ( 2 ) can be equivalently written as
The definition of \(R_{\Omega }\) is postponed to Sect. 3 , while its relation with \(K_\Omega \) is described in Sect. 4 .
This fact is in striking contrast with the problem in Probability Theory, of understanding the asymptotic of the average cost, on various domains \(\Omega \) and statistical ensembles for the red and blue point processes. This random version of the problem has attracted much attention both in Mathematics and Physics. In the Physics community, the interest has come from the analogy with ‘spin glasses’ in Statistical Mechanics, and a seminal contribution was given in the eighties by Orland [ 10 ], Mézard and Parisi [ 11 ], that considered the problem “in infinite dimension”, by introducing the so-called “random-link” approximation. This version of the problem was addressed using (non-rigorous) statistical physics techniques such as the replica theory and the cavity method [ 12 ]. Their original results were later put on rigorous ground [ 13 , 14 , 15 ].
The extension to finite-dimension of the random-link results is, however, quite challenging. A first attempt was carried on by Mézard and Parisi [ 16 , 17 ] that showed how, for \(n>2\) , the random-link result can be used as a zero-order approximation for the finite-dimensional solution, adding perturbatively a series of corrections. In the same years, a remarkable result was obtained by Ajtai and coworkers [ 18 ] for \(n=2\) : they proved that, if the problem is considered on the unit square \(\Omega \equiv \mathcal {R}:=[0,1]^2\) , then \(E_{\Omega }(N)=\mathcal O(\ln N)\) . Footnote 2
Recently, the forementioned result has been refined. In particular, by means of non-rigorous arguments, in Refs. [ 1 , 2 ] it was claimed that, on the unit square \(\mathcal {R}\) ,
where \(c^\text {PP}_{\mathcal {R}}(N)=o(\ln N)\) (the factor 2 is for later convenience). This result has been later rigorously proved by Ambrosio and coworkers [ 3 ] and extended to any 2-dimensional compact closed manifold \(\Omega \) [ 4 ], where it is shown that
The latter paper also proves rigorous bounds for \(c^\text {PP}_\Omega \) , namely that \(c^\text {PP}_\Omega (N)=\mathcal {O}(\sqrt{\ln N\ln \ln N})\) . It has been recently conjectured that Eq. ( 6 ) holds also in the case of points generated from non-uniform densities [ 19 ].
In this paper we further investigate the problem of the estimation of \(c_\Omega (N)\) . Extending the arguments given in [ 2 ], we argue that, on a generic two-dimensional manifold of unit area, the correction \(c_\Omega \) in Eq. ( 6 ) can be written as
where \(c_*^\text {PP}(N)\) does not depend on \(\Omega \) . The index ‘PP’ is to denote that both the red and blue points are sampled with the ‘Poisson random process on \(\Omega \) ’ (that is, are i.i.d. and uniform). Numerical investigations are compatible with the possibility that \(c_*^\text {PP}(N)\) is indeed a constant, and, under this hypothesis, we can give the constant \(c_*^\text {PP}\) the approximate value
Analogous claims and results hold for other variants of the problem, most notably when one set of points is still sampled with the Poisson random process, and the other set is either a deterministic regular grid (we investigate here the cases of square (S), triangular (T) or “Fibonacci” (F) [ 20 , 21 ] grids), or, in the variant of the problem where T is the transportation between a discrete and a continuous measure, the uniform measure (U) on \(\Omega \) . In these three new cases, the factor 2 in Eq. ( 6 ) disappears, and we have the similar structure
Let us stress again that the functions \(c_*\) are ‘universal’, in the sense that they do not depend on the choice of manifold \(\Omega \) (but they do depend on the choice of local randomness, e.g. among P, S, T, F, U), while the geometric correction \(K_{\Omega }\) depends on the choice of manifold, but is ‘universal’ in a different sense, as it is independent of the choice of local randomness (provided that the extra factor 2 in the P case is taken into account). Just as well as Eq. ( 2 ), such a decomposition is not at all granted a priori , and is somewhat surprising.
We also give numerical estimates of the associated values of \(c_*\) , Footnote 3 under the hypothesis that they are indeed constant, namely
The paper is organized as follows. In Sect. 2 we define the random matching problems we are interested in. In Sect. 3 we present our functional approach for the derivation of the scaling of the optimal cost, including the finite-size corrections given in Eq. ( 7 ). For simplicity, we concentrate only on the Poisson–Poisson case. In Sect. 5 we apply our theory to different domains, giving an explicit computation of \(K_\Omega \) for all of them. In Sect. 6 we compare our predictions with numerical results obtained solving a large number of instances of the problem on the domains under investigation. Finally, in Sect. 7 we give our conclusions.
2 The Random Assignment Problem
Let us consider a connected, two-dimensional smooth Riemannian manifold \(\Omega \) having finite volume and, if with a non-empty boundary, finite perimeter, with metric g . Given a system of local coordinates \((x^1,x^2)\) around a point \(p\in \Omega \) , \(g=\sum _{ij}g_{ij}(p)\text {d}x^i\otimes \text {d}x^j\) , and given two elements v , w in the tangent bundle in p , we will denote by \(\langle v,w\rangle _p :=\sum _{ij}g_{ij}(p) v_iw_j\) . For the sake of generality, we will perform our analysis in the slightly more subtle case of \(\partial \Omega \ne \emptyset \) (the arguments below can be easily adapted to the case \(\partial \Omega =\emptyset \) ). We will denote \(\text {d}\sigma \) the Riemannian measure for \(\Omega \) , and we will assume the measure of \(\Omega \) to be equal to 1. Footnote 4 Moreover, we will denote by \(\delta _p(x)\text {d}\sigma (x)\) the unit measure concentrated in \(p\in \Omega \) , that is, given a test function \(\varphi (x)\) ,

and d ( x , y ) is the Riemann distance between the points x and y , i.e., the infimum of the lengths of the curves that join the two points.
Note that each feasible T corresponds to a permutation \(\pi \) of N elements, so that \(T(X_i)=Y_{\pi (i)}\) , and searching for the optimal map is equivalent to searching for the optimal permutation. If we introduce the two atomic measures
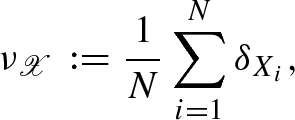
where the infimum is taken over the set \(\Gamma (\nu _1,\nu _2)\) of all the joint probability distributions J with first and second marginal given by \(\nu _1\) and \(\nu _2\) , respectively. It is well known (see for example [ 3 , 23 , 24 , 25 ]) that, in our setting, the set of the optimal joint probability distributions J is a convex polytope, called Birkhoff polytope, whose extreme points are all and only the permutations \(\pi \) which are optimal within the probability distributions of the form \(J(x,y)=\sum _{i} \delta _{X_i}(x) \delta _{Y_{\pi (i)}}(y)\) . Accordingly, the set of optimal maps \(T:\Omega \rightarrow \Omega \) pushing \(\nu _1\) to \(\nu _2\) , i.e., those realising the infimum in the expression

Together with the Poisson–Poisson, the most general and interesting case is the Uniform–Poisson case, in which the cost is the distance beetwen the Poisson random process and the uniform measure \(\text {d}\sigma \) :

As a discrete approximation of this case, we can introduce various grid–Poisson assignment problem (GP), interesting by themselves:
This is a subtle construction, adapted to the case of a sphere, see Fig. 1 b, and based on stereographic projection from a Fibonacci spiral on the plane (from which the name), described in [ 20 , 21 ]. The local aspect of this grid around one given point is somewhat intermediate between the one of a square and of a triangular lattice, with variations depending on the spherical coordinates of the point, and on the precise value of N . We will not enter in the detail of this construction, and the reader is referred to the forementioned papers.
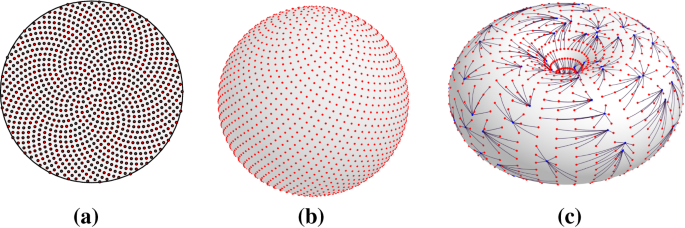
a Fibonacci lattice on the unit disc. b Fibonacci lattice on the unit sphere. c Transportation between a set of \(N=144\) random blue points and a square grid of 9 N red points on the flat torus (Color figure online)
In Appendix A we give more details about the relation between grid-Poisson assignment problems and the UP problem, with an estimation of the convergence rate of the optimal cost in the former to the optimal cost in the latter.
As anticipated, we are interested in the study of the asymptotic behaviour in N of the average optimal transportation cost, for which we will adopt the general notation

where the average \({\mathbb E\left[ {\cdot }\right] }\) is taken over the pertinent statistical ensemble for the point processes.
3 Main Conjecture
As we said above, the study of \(E_{\Omega }(N)\) in any dimension \(n\ne 1\) or \(\infty \) (i.e., in the random-link model) seems rather difficult. A possible approach to the study of the asymptotic behaviour for large N is based on the fact that, in this limit, T ( x ) is expected to be ‘close’ to the identity map (more precisely, from [ 18 ] we expect that \(d(X_i,Y_j) =\mathcal O(\sqrt{(\ln N)/ N})\) for pairs of points which are paired by an optimal matching), and an expansion in this small parameter, at the first non-trivial order, might still capture the relevant features of the solution of the problem. In [ 1 , 2 , 26 ] this approach has been applied to the study of the problem when \(\Omega \) is the square, or the torus with modular parameter \(\tau =i\) . The analysis leads to a result whose interpretation requires a regularization that takes into account the finite- N effects and avoid divergences, as we will see below.
In the approach in [ 1 , 2 , 26 ], a close analogy naturally emerges between the evaluation of the average optimal cost in the assignment problem and the evaluation of the electrostatic energy of 2 N particles, N of each charge sign, pinned in random positions on \(\Omega \) . This is a result a posteriori of the theory, as the obvious analogy just doesn’t hold as is (in the electrostatic problem, the energy is the sum of \(N(2N-1)\) pair contributions, not just N , which scale logarithmically with the distance of the pair, instead that quadratically). In a sense, the proposed linearization follows the opposite track of the suggestion by Born and Infeld [ 27 ] of a non-linear version of electrodynamics in order to solve the problem of divergencies. Similar ideas have been proposed recently by Brenier for fluid motion [ 28 , 29 ].
3.1 Linearization
Let us review the arguments of [ 2 ], in their natural generalisation to a Riemannian manifold. We start by introducing, for each map \(T:\Omega \rightarrow \Omega \) , the cost

The idea is now to write down a Lagrangian that combines the cost expression in Eq. ( 11 ) with the condition in Eq. ( 18 ) as

where \(\phi \) plays the role of a Lagrange multiplier. The optimal map \(T^*\) satisfies the Euler–Lagrange equations obtained from the Lagrangian above (which turn out to be nonlinear).
We shall now use the idea that, for \(N\rightarrow +\infty \) , we expect \(T(x)\rightarrow x\) for any \(x\in \Omega \) , due to the fact that the matched pairs become infinitesimally close under the scaling in which \(|\Omega |\) is kept fixed. Then, there exists a vector field \(\mu (x)\) on \(\Omega \) such that, at the leading order
The direction of the field is the one of the geodesic curve realising the distance of T ( x ) from x . Pictorially
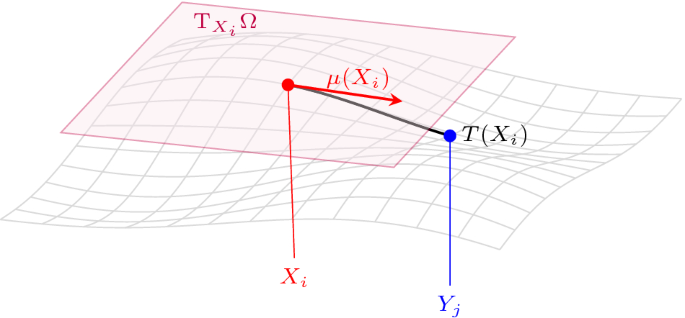
We shall now introduce
which is another perturbative parameter (when averaging over our statistical ensemble, monomials \(\mathbb {E}[\delta \nu (x_1) \delta \nu (x_2) \cdots \delta \nu (x_k)]\) have a definite scaling with N , and high powers are suppressed). The Lagrangian is approximated, in this limit, by its quadratic version,
which are the linearization of the original Euler–Lagrange equations in the fields \(\mu \) and \(\phi \) . In local coordinates:
where \(\partial _i\equiv \partial _{x^i}\) , the tensor \(g^{ij}\) is the inverse of g and \(|g|=\det g\) . The two equations imply the Poisson equation
to be solved with Neumann boundary conditions, since the flux of \(\mu (x)\) at the boundary is zero. Here \(-\Delta \) is the Laplace–Beltrami operator on \(\Omega \) , i.e., in local coordinates
3.2 The Divergence of the Cost and the Problem of Regularization
The functional approach above tells us that \(\mu =-\nabla \phi \) , where \(-\Delta \phi =\delta \nu \) . We can use the fact that Footnote 6
to write down an expression, valid for \(N\gg 1\) , for a quantity \(\epsilon (x)\) that we shall call the cost density
so that \(E_{\Omega }(N)=\int \limits _\Omega \epsilon (x)\text {d}\sigma (x)\) . Here we have introduced the Green function G ( x , y ) of \(-\Delta \) on the orthogonal complement of the locally constant functions. The Green function is a symmetric function that satisfies the equations
where \(\left. \partial _{n} G(x,y)\right| _{y\in \partial \Omega }\) is the normal derivative in x with respect to the boundary \(\partial \Omega \) of the domain. The equations above identify a unique Green function up to an additive constant: we will fix this constant adopting the convention

where \(\hat{\mu }(x)\) is such that \(\hat{\mu }(X_i)\) is a finite quantity (here we have used the diagonal expression for the Green function G , see below Eq. ( 33 )). Such an approximation could still be used through a delicate Cesàro limit, if we had to perform integrals in which \(\mu \) appears linearly. However our cost density is quadratic in these fields, and locally, at a formal level, for \(\delta \ll 1\) ,

that is, the appropriate result, which depends on the positions of the points and is finite at finite N , is shifted by a fixed but divergent quantity. Yet again, we observe a perfect analogy with 2-dimensional electrostatics, namely with the classical problem of the field self-energy for a distribution of point charges.
Analytically, we see this feature emerging from our result by observing that for \(d(x,y)\rightarrow 0\) , the Green function behaves as [ 5 , 6 ]
with a logarithmic divergence. We perform therefore a regularization of the logarithmic divergence, along the same lines of the classical treatment of electrostatics. Let us introduce \(\Omega _\delta (x)=\Omega \setminus B_\delta (x)\) , where \(B_\delta (x)=\{y\in \Omega :d(x,y)<\delta \}\) is the ball of radius \(0<\delta \ll 1\) centered in x . We can introduce a regularized expression
and a corresponding “regularized cost”
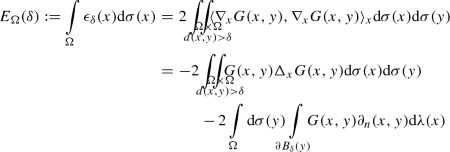
where the second integral runs over the border \(\partial B_{\delta }(y):=\{x\in \Omega :d(x,y)=\delta \}\) of \(B_{\delta }(y)\) , \(\text {d}\lambda (x)\) is the line element of \(\partial B_\delta (y)\) in x , and n is the outward normal to \(B_\delta \) . By Eq. ( 30 ) and Eq. ( 30c ) the first integral is infinitesimally small for \(\delta \rightarrow 0\) . Therefore

For \(0<\delta \ll 1\) , the inner integral can be estimated using the expression in Eq. ( 33 ), so that
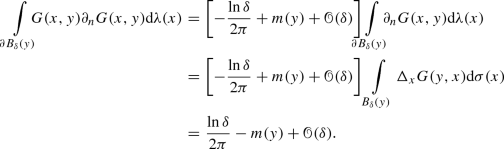
We finally get
The integral of m ( x )
is sometimes called Robin mass [ 5 , 6 ].
Now, we suppose that the regularization by the parameter \(\delta \) acts in the same way on all geometries. Under this assumption we can compare two different geometries, \(\Omega \) and \(\Omega '\) , obtaining the following conjecture.
Let \(\Omega \) , \(\Omega '\) be two regular two-dimensional manifolds, then
In other words, the differences of the average cost among different manifolds, in the large- N limit, are expected to be regularization-independent, and, in addition to this, can be expressed in terms of the Robin’s masses of the Laplace–Beltrami Green function on \(\Omega \) and \(\Omega '\) . The analytic evaluation of these differences will be the main object of our investigation, starting from Sect. 5 . The remaining of this section is instead devoted to a further justification of the assumption at the basis of Eq. ( 40 ).
One problem at this point is that our regularization parameter \(\delta \) does not have a clear relation with the perturbative parameter \(N^{-1}\) . In order to better understand what is the microscopic mechanism beyond the regularization, we observe that Eq. ( 38 ) can be formally written for \(\delta \rightarrow 0\) as
where the operator \(-\Delta ^{-1}\) is the inverse Laplace–Beltrami operator on \(\Omega \) (with Neumann boundary conditions, if the boundary exists)

The result of this analysis is that, if we decompose our fields in the basis of eigenfunctions of \(-\Delta \) , we can neglect the corrections coming from the further terms in the Taylor expansion, and the discreteness of the measure, only for those eigenfunctions with \(\lambda \lesssim N\) (up to possible factors \((\ln N)^{\gamma }\) in the scaling). Conversely, in the regime \(\lambda \gtrsim N\) some unknown mechanism comes into play, and we expect that its effect is to dump the sum \(\hbox {tr}\Delta ^{-1}\) appearing in ( 41 ), possibly at a scale \(\lambda \lesssim N\) . In [ 1 ], this unknown dumping mechanism is supposed to be encoded in a cut-off function \(F(\lambda /N)\) . Now, as this function is related to the local expansion of the fields \(\mu \) and \(\phi \) at high frequencies, and as the relation between eigenvalue \(\lambda \) and local wavelength is universal, the function \(F(\lambda /N)\) must have one of the two flavours of universality: it shall not depend on the manifold \(\Omega \) , while in general it must depend on the type of problem (among Poisson, various grids and uniform), that is, in a natural generalisation of the treatment of [ 1 ] to our setting, we should have some unknown functions \(F^{\bullet \text {P}} (\lambda /N)\) , with \(\bullet \) being one among P, S, T, F or U, and no dependence on \(\Omega \) . Going on with our analysis of the PP case (the reasoning can be repeated for all other cases similarly) and using \(F(\lambda /N)\) for \(F^\text {PP}(\lambda /N)\) for brevity, within the assumptions of [ 1 ] we should interpret the correspondence ( 41 ) above as

where \(\Lambda (\Omega )\) is the set of nonzero eigenvalues of the Laplace–Beltrami operator on \(\Omega \) . Following the analysis already performed in [ 1 ], this gives
for any domain of unit measure, for some constant \(c_\Omega \) depending on the cut-off, which cannot be determined if F is not known. We recall that, as anticipated in the introduction, the leading term in Eq. ( 46 ) is the correct asymptotic cost, as rigorously proved in Refs. [ 3 , 4 , 33 ], the presence of a logarithm being known since the eighties [ 18 ].
Note that there is no guarantee that the cut-off function scales exactly as \(F(\lambda /N)\) , as the mechanism beyond the dumping of the high-wavelength contributions, and the amount of this dumping, are not under control. It may well be, for example, that the function has the form \(F\big (\frac{\lambda }{N (\ln N)^\gamma }\big )\) , which would give a variant of ( 46 ) in which instead of the constant term it will appear an universal term \(O(\gamma \ln \ln N).\)
All these arguments lead us to reformulate our conjecture as follows.
(Alternative formulation) Let \(\Omega \) be a regular two-dimensional manifold, then
where \(c_*(N){=o(\ln N)}\) is an universal function not depending on \(\Omega \) .
Moreover, for \(\Omega \) , \(\Omega '\) different regular manifolds,
It is important to remark that, from the simulations made in Sect. 4 , we have evidence that the term \(c_*(N)\) can be chosen as a constant, i.e. that formula ( 46 ) is compatible with our numerical results. Obviously it is not possibile to deduce that \(c_*(N)=c_*\) from numerical simulation only. Anyway, in case, we would get ( 47 ) and \(c_{\Omega } = R_{\Omega }+c_*.\)
We notice that starting from Eq. ( 45 ) and comparing two manifolds, we get the interesting fact

The combination of the two integrals above can be rewritten as

4 Different Regularization Procedures
The expression ( 45 ) is, annoyingly, a diverging expression depending on \(\Omega .\) A way of studying this expression is by introducing a regularization parameter \(\epsilon \) for these contributions, and then deducing an evaluation of ( 51 ) from a singular expansion in \(\epsilon \) around zero.
One standard way to perform this programme is the so-called zeta regularization [ 34 ]. Let us introduce the generating function
which is known to be absolutely convergent for \(\mathfrak {R}(s) > 1\) , and in this case we recognise our scheme above under the identification \(s=1+\epsilon \) . Then \(-\hbox {tr}\Delta ^{-1}\) can be regularized by looking at \(Z_{\Omega }(s)\) near \(s=1\) [ 35 ]
and by removing the pole at \(s=1\) . That is, in equation ( 51 ),
For reasons that will appear clearer below, we will call Kronecker’s mass the constant \(K_\Omega \) . Despite the fact that there seems to be no reason a priori to believe that \(K_{\Omega }\) and \(R_{\Omega }\) are related, it has been proved by Morpurgo that \(R_{\Omega }-K_{\Omega }\) is a universal constant (that is, it does not depend on \(\Omega \) ), given by [ 5 , 36 , 37 , 38 ]
where \(\gamma _{\text {E}}=0.57721\dots \) is the Euler–Mascheroni constant. In particular, this universality result is crucial in checking a posteriori that our two predictions ( 40 ) and ( 54 ), obtained by two different analyses, are consistent, and also implies that our Conjecture is equivalent to the statement of E. ( 51 ). The computation of the Kronecker’s mass is often easier than the Robin’s mass, as we will show below. For a few manifolds \(\Omega \) , both computations, of \(R_{\Omega }\) and \(K_{\Omega }\) , can be performed with relatively small effort, and we will do this, for pedagogical reasons, in order to illustrate with an example the forementioned general result.
Another way of performing our programme is to consider the regularized sum
for \(p>0\) . This corresponds to a specific choice of function \(F(\lambda /N)\) , provided that \(\epsilon \) is identified with \(\gamma \big /N^p\) , for \(\gamma \) some constant. Also in this case we have, universally,
and this leads to the prediction
The analogue of the Morpurgo theorem reads in this case
as can be evinced by comparing the two regularizations for the diverging integral \(\frac{1}{4\pi }\int \nolimits _1^{\infty } \frac{\text {d}x}{x}\) (which, besides the fact that it is an integral rather than a sum, it has all the appropriate asymptotics properties implied by the Weyl law). Namely, for this choice we have
that is, \(K=0\) , and
that is, \(W^{(p)}=-{\gamma _E}/{4p\pi }\) .
Another regularization in the same spirit is through the regularized sums
with \(\theta (x)\) is the Heaviside step function, which, yet again, corresponds to a specific choice of function \(F(\lambda /N)\) , provided that \(\epsilon \) is identified with \(\gamma /N\) , for \(\gamma \) some constant. Also in this case we have a universal asymptotics
that is, \(W^\text {sharp}=0\) .
To verify our ansatz, we will compute the Kronecker’s mass and the Robin’s mass for different \(\Omega \) . We will compare our analytic results with numerical simulations in Sect. 6 . We will start considering flat manifolds having \(g(x)=\mathbb I\) , and consider manifolds with uniform curvature starting from Sect. 5.5 .
5.1 The Unit Rectangle
Let us start by considering the problem on the rectangle. We call \(\mathcal R(\rho )\) the rectangle \([0,\sqrt{\rho }] \times [0,1/\sqrt{\rho }]\) , and we consider the Laplace–Beltrami operator with Neumann boundary conditions. The eigenfunctions of \(-\Delta \) on \(\mathcal R(\rho )\) are given by
The corresponding eigenvalues are
We proceed computing the Kronecker mass using the regularized function
Here we have adopted \(\tau =i\rho \) , in compliance with standard notation for modular forms, and have introduced the lattice zeta function \(\zeta _\tau (s)\) defined in Appendix D. This calculation is readily performed thanks to a remarkable result due to Kronecker (and known as first limit formula of Kronecker ), reported in Appendix D, Eq. ( D.7 ), and that we repeat here:
where \(\eta (\tau )\) is the Dedekind \(\eta \) function. Kronecker’s formula allows us to immediately obtain Footnote 8
that for \(\rho =1\) (unit square) simplifies to
We will see in the following that the first limit formula of Kronecker will allow us to extract the Kronecker’s mass for many types of flat domains: this explains our choice of ‘Kronecker mass’ for denoting \(K_\Omega \) .
We can give a slightly more compact form to the function in ( 71 ), shifted by its minimal value above:
We shall make a remark on this expression. In the limit in which the rectangle is very elongated, we get Footnote 9
that is the average cost for the Poisson–Poisson one-dimensional assignment problem on the segment of unit length [ 39 ]. This is not by accident. Indeed, in any rectangular domain we can evaluate the average energy of the permutation in which the k -th red point counting from the left is matched to the k -th blue point counting from the left. This configuration is optimal w.h.p. in the limit \(\rho \rightarrow +\infty \) , and would be optimal, at any \(\rho \) , if the vertical coordinates of all the points were equal. On the other side, a worst case is when all the vertical coordinates of red points are zero, and all the vertical coordinates of blue points are \(1/\sqrt{\rho }\) , so that, calling \(E_{[0,1]}(N)\) the average energy for the 1-dimensional problem on the [0, 1] segment, we get
which, by substituting our scaling ansatz, gives
When we take a limit \(N \rightarrow \infty \) , \(\rho \rightarrow \infty \) on a direction \(\rho \gg \sqrt{N}\) , we thus get
which is consistent with ( 74 ). In the following we will encounter various other domains which allow a consistency check with a 1-dimensional limit. We will reach similar conclusions, without entering in the details of the estimates, as this is done by minor modifications of the reasonings presented here.
5.2 The Flat Torus
We shall now consider the problem on the flat torus \(\mathcal T(\tau )\) . To describe the corresponding manifold, let us first consider the lattice of points on \(\mathbb {R}^2\)
generated by the matrix

corresponding to the base vectors
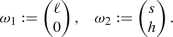
a Pictorial representation of an assignment on a torus generated by quotient of \(\mathbb {R}^2\) with a periodic lattice, with fundamental parallelogram and the corresponding base vectors. b Contour plot of \( \mathfrak {I}(\tau ) |\eta (\tau )|^4\) in the complex plane \(\tau \) . The triangoloid shape is the canonical fundamental region of the moduli space, given by \(|\tau | \le 1\) , \(|\tau \pm 1| \ge 1\) and \(\mathfrak {I}(\tau )>0\)
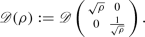
Given a lattice \(\Lambda \) generated by \(\underline{\omega }\) , it is possible to associate to it a dual lattice \(\Lambda ^*\) generated by \(\underline{\omega }^*\) , such that \(\underline{\omega }^*\ \underline{\omega }^T=\mathbb I\) , identity matrix, i.e.,
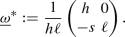
Each torus \(\mathcal T=\mathbb {R}^2/\Lambda \) is then naturally associated to a dual torus given by \(\mathcal T^*:=\mathbb {R}^2/\Lambda ^*\) .
In the following, we will restrict, without loss of generality, to the case in which the fundamental parallelograms have unit area, choosing

such that \(\rho \in \mathbb {R}^+\) and \(\sigma \in \mathbb {R}\) , and we will denote the corresponding torus by \(\mathcal T(\tau )\) , where \(\tau :=\sigma +i\rho \) is the half-period ratio.
The Kronecker’s mass. Due to the periodicity conditions, the eigenfunctions of \(-\Delta \) on \(\mathcal T(\tau )\) have the form
for all \(k^*=\underline{\omega }^*\ k\in \Lambda ^*\) , \(k=\left( {\begin{array}{c}n\\ -m\end{array}}\right) \in \mathbb {Z}^2\) . The corresponding eigenvalue is
We can compute now the Kronecker mass using the regularized function
and removing the pole in \(s\rightarrow 1\) , as discussed in Sect. 3 . This calculation is readily performed, again thanks to the first limit formula of Kronecker, Eq. ( D.7 ), which allows us to immediately obtain
In Fig. 2 b we present a contour-plot of the related expression \(\mathfrak {I}(\tau ) |\eta (\tau )|^4\) in the complex plane \(\tau \) , confined to the canonical fundamental region of the moduli space. In particular, this function diverges for \(\tau \rightarrow 0\) and has minimum at \(\tau =\exp (i \pi (\frac{1}{2} \pm \frac{1}{6}))\) . This implies that, among all unit tori equipped with the flat metric, the “hexagonal” one, that is the one for which \(\tau \) is a sixth root of unity, is the one in which the average cost of the Euclidean Random Assignment Problem is minimised. More strikingly, as deduced from results in [ 5 ] which are in turn based on the results in [ 35 ], the hexagonal torus is minimal also among unit surfaces with non-uniform metric.
Example: the rectangular and rhomboidal tori. We shall call “rectangular torus” a torus in which the fundamental parallelogram is a rectangle. This case corresponds to \(\tau =i\rho \) , with \(\rho >0\) real. Our formula specialises to
which is invariant under the map \(\rho \mapsto \rho ^{-1}\) , as it should. In the region \(\rho \in (0,1]\) the lowest value is achieved at \(\rho =1\) (see also Fig. 2 b), where
We can give a slightly more compact form to this function, shifted by its minimal value:
Similarly, we shall call “rhomboidal torus” a torus in which the fundamental parallelogram is a rhombus. This case corresponds to \(\tau =\hbox {e}^{i \theta }\) , with \(0< \theta \le \pi \big /2\) , and our formula specialises to
that is, again shifting by the value for the standard torus,
As was the case for the rectangle, the expression in Eq. ( 92 ), in the limit in which the torus is very “thin and long”, becomes
This happens to be the average cost for the Poisson–Poisson one-dimensional assignment problem on the circle of unit length [ 39 ], as was to be expected, by a reasoning analogous to the one presented for the case of the rectangle.
The Robin mass. Let us now evaluate, for the generic flat torus \(\mathcal T(\tau )\) , the Robin mass \(R_{\mathcal T}(\tau )\) . Calling \(z=z(x,y)= (x_1 -y_1) + i(x_2 - y_2)\) , the Green’s function on the torus is given in this case by [ 40 ]
where \(\theta _1(z; \tau )\) is an elliptic \(\theta \) function. The Robin mass is obtained from
It is immediately seen that, in agreement with the Morpurgo theorem, Eq. ( 55 ) is satisfied.
5.3 Other Boundary Conditions on the Unit Rectangle
5.3.1 the cylinder.
The corresponding eigenvalues are therefore
Repeating the same type of calculations performed for the rectangle (that is, expressing the regularised sum as a combination of \(\zeta _{\tau }(s)\) (for some \(\tau \) ’s) and \(\zeta (2s)\) ), we obtain
We also remark that
which is the cost density for the one-dimensional assignment problem with open boundary conditions (i.e., on the unit segment), while
which is the density of cost for the one-dimensional assignment problem with periodic boundary conditions (i.e., on the unit circle), again, as was to be expected. The nontrivial solution of Eq. \(K_{\mathcal C}(\rho )=K_{\mathcal C}\) is \(\rho =0.625352\dots \) , while the minimum value of the mass occurs for \(\rho =0.793439\dots \)
Remark that the constants above do not appear in the study of [ 35 ], because in our context, in presence of a boundary, we should impose Neumann boundary conditions (while the authors of [ 35 ] only analyse the case of Dirichlet boundary conditions).
5.3.2 The Möbius Strip
The parity constraint implements the twisted identification of the strip. The corresponding eigenvalues are
Repeating now the usual arguments we get
which is the average cost for the problem on the segment of length \(\frac{1}{2}\) (and the fact that the length is not 1 is related to the fact that the twisted boundary conditions are effectively ‘folding in two’ the segment), while
which is the cost for the problem on the unit circle. The non-trivial solution of Eq. \(K_{\mathcal M}(\rho ) = K_{\mathcal M}\) is found for \(\rho =4.1861\dots \) , whereas the minimum is achieved at \( \rho =2.30422\dots \)
5.3.3 The Klein Bottle
If we identify both pairs of opposite sides of the rectangle, one pair (say, the horizontal sides) in the ordinary way, and the other pair in the twisted way as in the Möbius strip, we obtain the Klein bottle \(\mathcal K(\rho )\) , see Fig. 4 c. The eigenfunctions of \(-\Delta \) are in this case
Proceeding as above, one can obtain
so that, in particular,
We also remark that both one-dimensional limits coincide with the corresponding constructions for the Möbius strip, and indeed the limits of the analytical expressions are the same, as
Here \(K_{\mathcal K}(\rho ) = K_{\mathcal K}\) for \(\rho = 1.09673\dots \) , whereas the minimum is obtained at \(\rho = 1.04689\dots \) .
5.3.4 The Boy Surface
The calculation proceeds as in the other cases, giving
which is symmetric for \(\rho \leftrightarrow \frac{1}{\rho }\) , as it must be. In particular
Now, both one-dimensional limits produce a domain corresponding to the segment of length \(\frac{1}{2}\) , and indeed
5.4 The Disc and the Cone
Up to now, we have mostly solved the problem using the zeta regularization of the Laplacian, and relying on Kronecker’s first limit formula. Only for the case of the torus, we have also performed the calculation of the Robin mass, and verified the prediction of the Morpurgo theorem. In this section we will give the results for a geometry \(\Omega \) in which the calculation of the Robin mass is done with relatively small effort, as the Green function can be guessed through the method of images, while the calculation of the Kronecker mass would require a sum over maxima and minima of Bessel functions. Footnote 10 Let us introduce the notation \(\mathcal D_p(r)\) for the circular sector of radius r and angle \(\frac{2\pi }{p}\) , see Fig. 6 a,
The unit area condition implies \(2\pi r^2=p\) . We considered the case \(p\in \mathbb {N}\) , and we choose periodic boundary conditions in the angular direction: this is equivalent to say that we identify the two radii of the sector, obtaining in this way a cone of height \(r\sqrt{1-p^{-2}}\) , see Fig. 6 b. This surface is interesting, as it is the first example in our list of a surface with singular curvature, the conical singularity being at the vertex of the cone. We have argued in the introduction that, because of the scaling \(\sim \sqrt{N^{-1}\ln N}\) of the field \(\mu \) , we expect that the same theory applies to the flat Euclidean space and to curved manifolds, as long as the curvature is non-singular . The case of surfaces with a finite number of conical singularities would require a different (although feasible) argument, and the verification of our theory on this family of surfaces (as well as the surfaces treated in Sect. 5.5 ) is an important validation of our predictions.
The Robin’s mass for this case is obtained in Appendix B, and it is equal to
where \(\phi (z)\) is the digamma function. In particular, for \(\alpha \rightarrow 2\pi \) we recover the case of the unit disc \(\mathcal D\equiv \mathcal D_{1}\) :
The Kronecker’s mass is readily obtained using Eq. ( 55 ).
5.5 The Unit Sphere and the Real Projective Sphere
An example of transportation problem on the surface of the sphere \(\mathcal S^2\) has been considered in [ 42 ], where the problem of transporting a uniform mass distribution into a set of random points on \(\mathcal S^2\) is analyzed. As an example of applications of our approach to non-flat manifolds, here we consider the problem in our usual setting, i.e., a transportation between two atomic measures of random points. As in the previous cases, the information on the finite-size corrections is related to the spectrum of the Laplace–Beltrami operator on the manifold. It is well known that the eigenfunctions of \(-\Delta \) on the surface of a sphere of radius r are the spherical harmonics \(Y_{l,m}(\theta ,\phi )\) with \(l\in \mathbb {N}\) and \(m\in \mathbb {Z}\) with \(-l\le m\le l\) . The corresponding eigenvalues are
that is, the eigenvalue \({l(l+1)}/{r^2}\) has multiplicity \(2l+1\) , for the range of integers \(-l \le m \le l\) .
We fix the surface area of the sphere to 1 (taking \(r=(4\pi )^{-1\big /2}\) ) and we proceed using the zeta regularization, computing
In this case, after some algebra, we are led to use ‘just’ the version of the zeta regularization for the Riemann zeta function (which is much simpler than the Kronecker formula)
and we obtain
The Kronecker mass for the unit sphere is therefore
Alternatively, we can use one of the regularizations illustrated in Sect. 4 . A convenient one is the evaluation of \(W^{(1\big /2)}\) , which gives
Recalling that in our case \(r^2=(4\pi )^{-1}\) , the final result is
that, in light of ( 59 ), allows to rederive equation ( 127 ).
The spherical lune. The calculation above can be extended to the spherical lune \(\mathcal S_k^2\) , a surface on a sphere of radius r , \(4\pi r^2=k\) , contained by two half great circles which meet at antipodal points with dihedral angle \(\frac{2\pi }{k}\) , see Fig. 7 . In Appendix C it is shown that the Kronecker’s mass corresponding to this manifold is
Choosing periodic boundary conditions on the two half great circles, the Kronecker’s mass takes an additional \(-\frac{1}{2\pi }\ln 2\) contribution, and we obtain
that reduces to ( 127 ) for \(k=1\) , as it should.
The projective sphere. A variation of the problem on the (unit) sphere is the problem on the (unit) real projective sphere \(\mathcal {PS}^2\) , that is, the sphere in which antipodal points are identified. The eigenfunctions of the Laplace–Beltrami operator are still the spherical harmonics \(Y_{l,m}(\theta ,\phi )\) with \(l\in \mathbb {N}\) and \(m\in \mathbb {Z}\) , \(-l\le m\le l\) , but we have to restrict ourselves to eigenfunctions that are invariant under the transformation \((\theta ,\pi ) \mapsto (\pi - \theta ,\phi + \pi )\) , i.e., to even values of l . Working on the unit-area manifold accounts to have \(2 \pi r^2=1\) . We get
so that the Kronecker’s mass is
Using a sharp cut-off instead
which, again by using ( 63 ) and ( 65 ), allows to rederive equation ( 133 ).
6 Numerical Results
We have numerically investigated all the cases described above to check our predictions. To solve the assignment problem we have used an implementation of the Jonker-Volgenant algorithm [ 8 ]. For each domain \(\Omega \) , we have computed the expected optimal cost averaging over at least \(10^4\) independent instances and different sizes N of the system, \(32\le N\le 1024\) . In each case, we have estimated \(c^{\bullet \text {P}}_\Omega (N)\) assuming that they are indeed constant at the leading order, i.e., \(c^{\bullet \text {P}}_\Omega (N)\equiv c^{\bullet \text {P}}_\Omega \) , via a least square regression. Here \(\bullet =\text {\{}P,S,T,F,U\}\) . Our results are given in Table 1 .
For each domain we have also computed \(c_*^{\bullet \text {P}}=c_\Omega ^{\bullet \text {P}}-K_\Omega \) that we expect to be domain-independent. In the PP case, our best estimation of \(c_*^\text {PP}\) , obtained for the PP problem on the torus (see Fig. 3 a) is
Numerically, however, we cannot rule out a weak N -dependence in \(c_*^\text {PP}\) . In a similar way we have obtained the results given in ( 9 ), that we repeat here,
To verify our results in Eqs. ( 8 ) we have also proceeded in this way. Given two unit-area domains \(\Omega \) , \(\bar{\Omega }\) , and a given type of the problem (PP, SP, etc), we have computed, for each N , \(\Delta E^{\bullet \text {P}}_{\Omega ,\bar{\Omega }}(N)=c^{\bullet \text {P}}_{\Omega }(N)-c^{\bullet \text {P}}_{\bar{\Omega }}(N)\) and then extrapolated to \(N\rightarrow +\infty \) . If the arguments above are correct, then
In Fig. 3 b we plot in particular (Fig. 4 )
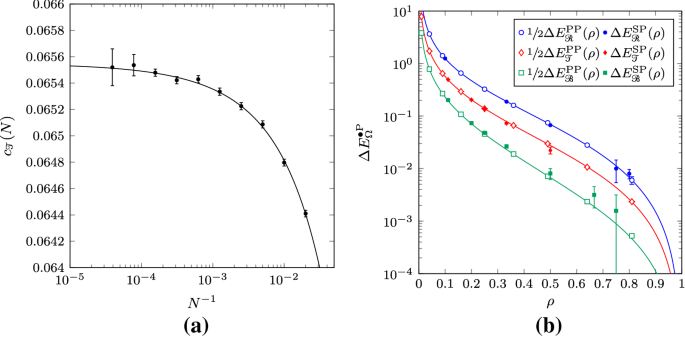
a Numerical estimation for \(c_{\mathcal T}(N)\) for different values of N . Each data point is obtained averaging the optimal cost over at least \(10^6\) instances and then removing the leading \(\frac{1}{2\pi }\ln N\) term. The fit is obtained using a quadratic function in \(1\big /\sqrt{N}\) . b Difference of average optimal costs for the assignment on the rectangle \(\mathcal R(\rho )\) , on the torus \(\mathcal T(i\rho )\) and on the Boy surface \(\mathcal B(\rho )\) with the corresponding costs for \(\rho =1\) . The numerical results, represented by the dots, are compared with the analytical prediction obtained from Kronecker’s masses
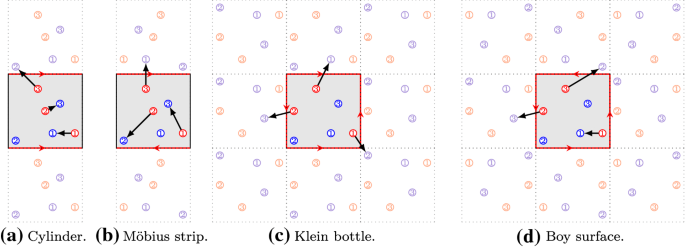
Pictorial representation of the different boundary conditions considered in Sect. 5.3 , with an example of assignment at \(N=3\) for each case. To obtain the corresponding surface, the red edges have to be considered joined in such a way that the directions of the arrows match (Color figure online)
as functions of \(\rho \) for \(\bullet =\text {\{}P,S\}\) . Similarly, in Fig. 5 we present our results for (the absolute value of)
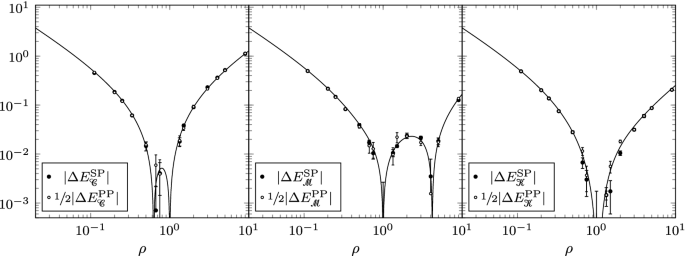
Absolute difference of average optimal costs for the assignment on the cylinder \(\mathcal C(\rho )\) , on the Möbius strip \(\mathcal M(\rho )\) and on the Klein bottle \(\mathcal K(\rho )\) with the corresponding costs for \(\rho =1\) . The numerical results, represented by the dots, are compared with the analytical prediction obtained from Kronecker’s masses
for \(\bullet =\text {\{}P,S\}\) . Footnote 11 In Fig. 6 c and in Fig. 7 b we have also considered the differences of average optimal costs in the case of the circular sector and of the spherical lune. In all investigated cases we found a perfect agreement with our predictions.
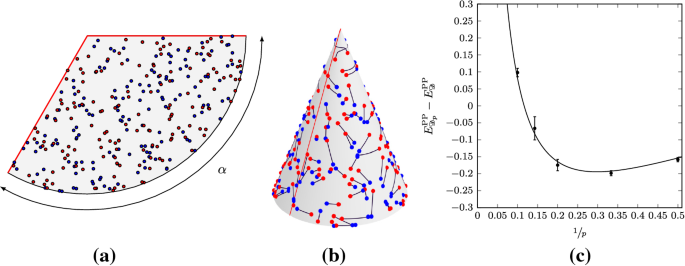
Random points on a circular sector ( a ) and optimal assignment on the corresponding cone ( b ) (in red, the part of the domain boundary where periodic boundary conditions are imposed). c Comparison between numerical results and our theoretical prediction for \(E^\text {PP}_{\mathcal D_p}-E^\text {PP}_{\mathcal D}\) (Color figure online)
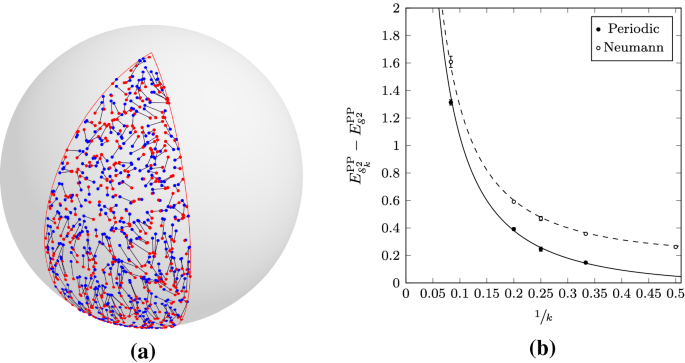
a Optimal assignment on a spherical lune. b Comparison between numerical results and our theoretical prediction for \(E^\text {PP}_{\mathcal S^2_k}-E^\text {PP}_{\mathcal S^2}\) with periodic (smooth line) and Neumann (dashed line) boundary conditions.
7 Conclusions and Perspectives
In this paper we have considered the assignment problem between two sets of random points on a generic two-dimensional smooth manifold of unit area. We have showed, by means of analytical arguments and numerical simulations, that the average optimal cost can be written as
if both sets of points are random (Poisson–Poisson case), and as
if one of the two sets is fixed on a grid (square, triangular, Fibonacci) or replaced with the uniform measure. In the equations above, \(K_\Omega \) is a precise quantity that can be obtained by a zeta-regularization of the trace of the inverse Laplace–Beltrami operator on \(\Omega \) . The contributions \(c^{\bullet \text {P}}_*\) are instead independent on \(\Omega \) and related to the ‘local details’ of the problem (i.e., if the assignment is between random points, or with a grid, or with the uniform measure). We have given an exact computation of \(K_\Omega \) for different domains, and using different procedures.
The quantity \(c_*^{\bullet \text {P}}(N)\) shows a weak dependence on N (if no dependence at all): it has been proven indeed that \(c_*^\text {UP}=\mathcal {O}(\sqrt{\ln N\ln \ln N})\) [ 4 ], a bound that, because of triangular inequality, holds for all the cases that we have considered. Assuming that \(c_*^{\bullet \text {P}}(N)\) are constants, we have verified, within our numerical precision, their independence on \(\Omega \) in all considered transportation cases (Poisson–Poisson, grid–Poisson, uniform–Poisson).
Our results reduce the computation of the (leading) finite-size correction to the optimal cost to the computation of the \(\Omega \) -independent contributions \(c_*^{\bullet \text {P}}(N)\) . These contributions are intrinsically dependent on the local nature of the problem (and therefore change if, for example, we fix on a grid one of the two sets of points) and are inherited by the regularization of the highest part of the spectrum of \(-\Delta \) , as discussed in Sect. 3 . What are the properties (and possibly the exact value, if they are constant) of \(c_*^{\bullet \text {P}}(N)\) remains an open question.
Finally, analogous results are expected to hold in higher dimension at the leading order. In particular, the analysis in [ 1 ] suggests that, for \(d > 2\) , the local properties of the problem affect the coefficient of the leading term, with a finite-size correction depending on the spectrum of the Laplacian only. This case may also be investigable with our tools, as indeed versions of the Weyl law, which we crucially use in Sect. 3.2 , exist in generic dimension. We leave the investigation of the higher dimensional problem for future works.
Of course, there may be in general more than one optimal map, however, in the random uniform ensemble, the optimal map is almost-surely unique.
More precisely Ajtai et al. studied the case \(p=1\) , but they also sketched how their analysis can be extended to p a positive integer, and predicted the scaling \(E_{\Omega }(N)=\mathcal O(N^{1-\frac{p}{2}} (\ln N)^{\frac{p}{2}})\) in this generality.
We do not give precise numerical estimates for the Fibonacci grid, as we suppose that, at the size we have investigated, the small variations in the realisation of the Fibonacci grid may affect this constant at an order of magnitude comparable to \(c_*^\text {SP}-c_*^\text {TP}\) , which is numerically rather small.
It is only at this point that the linearised theory for the Poisson–Poisson case differs from the theory for the grid–Poisson and the uniform–Poisson cases, as in these cases we would get 1/ N instead of 2/ N on the RHS of ( 28 ).
We recall here that \(\sqrt{\rho }\eta (i\rho )=\eta (i\big /\rho )\) .
The thumb rule in performing these limits is that, for \(\rho \rightarrow 0, +\infty \) ,
This is because we have to consider Neumann boundary conditions for \(\phi \) . The sum would run on the zeros of Bessel functions if we had Dirichlet boundary conditions [ 41 ].
Of course, all the signs just come out as predicted.
Caracciolo, S., Lucibello, C., Parisi, G., Sicuro, G.: Scaling Hypothesis for the Euclidean Bipartite Matching Problem. Phys. Rev. E 90 012118 (2014)
Caracciolo, S., Sicuro, G.: Quadratic Stochastic Euclidean Bipartite Matching Problem. Phys. Rev. Lett. 115 , 230601 (2015)
Ambrosio, L., Stra, F., Trevisan, D.: A PDE approach to a 2-dimensional matching problem. Prob. Theory Relat. Fields 173 , 433–477 (2019)
Article MathSciNet Google Scholar
Ambrosio, L., Glaudo, F.: Finer estimates on the 2-dimensional matching problem. J. Éc. Polytech. Math. 6 , 737–765 (2019)
Okikiolu, K.: A Negative Mass Theorem for the 2-Torus. Commun. Math. Phys. 284 , 775–802 (2008)
Article ADS MathSciNet Google Scholar
Okikiolu, K.: A negative mass theorem for surfaces of positive genus. Commun. Math. Phys. 290 , 1025–1031 (2009)
Kuhn, H.W.: The Hungarian method for the assignment problem. Nav. Res. Logist. 2 , 83–97 (1955)
Jonker, R., Volgenant, A.: A shortest augmenting path algorithm for dense and sparse linear assignment problems. Computing 38 , 325–340 (1987) ISSN 0010485X
Lovász, L., Plummer, M. D.: Matching Theory (AMS Chelsea Publishing Series vol 367) (North-Holland; Elsevier Science Publishers B.V.) (2009) ISBN 78-0-8218-4759-6
Orland, H.: Mean-field theory for optimization problems. Le J. Phys. (Paris) Lett. 46 , 770–773 (1985)
Google Scholar
Mézard, M., Parisi, G.: Replicas and optimization. J. Phys. (Paris) Lett. 46 , 771–778 (1985). ISSN 0302-072X
Mézard, M., Parisi, G.: Mean-field equations for the matching and the travelling salesman problems. Europhys. Lett. 2 , 913–918 (1986)
Article ADS Google Scholar
Aldous, D. J.: The \(\zeta \) (2) limit in the random assignment problem. Random Struct. Algorithms 2 , 381–418 (2001)
Nair, C., Prabhakar, B., Sharma, M.: Proofs of the Parisi and Coppersmith-Sorkin conjectures for the finite random assignment problem 44th Annual IEEE Symposium on Foundations of Computer Science, 2003. Proceedings. (IEEE Computer. Soc) pp 168–178 (2003). ISBN 0-7695-2040-5
Linusson, S., Wästlund, J.: A proof of Parisi’s conjecture on the random assignment problem. Prob. Theory Relat. Fields 128 , 419–440 (2004)
Mézard, M., Parisi, G.: The Euclidean matching problem. J. Phys. (Paris) 49 , 2019–2025 (1988)
Lucibello, C., Parisi, G., Sicuro, G.: One-loop diagrams in the random Euclidean matching problem. Phys. Rev. E 95 , 012302 (2017). ISSN 2470-0045 (Preprint 1609.09310)
Ajtai, M., Komlós, J., Tusnády, G.: On optimal Matchings. Combinatorica 4 , 259–264 (1984)
Benedetto, D., Caglioti, E.: Euclidean random matching in 2d for non-constant densities (Preprint 1911.10523) (2019)
Saff, E.B., Kuijlaars, A.B.J.: Distributing many points on a sphere. Math. Intell. 19 , 5–11 (1997)
Rakhmanov, E.A., Saff, E.B., Zhou, Y.M.: Minimal discrete energy on the sphere. Math. Res. Lett. 1 , 647–662 (1994)
Ambrosio, L.: Lecture notes on optimal transport problems (2003)
Ambrosio, L., Gigli, N., Savaré, G.: Gradient Flows: In Metric Spaces and in the Space of Probability Measures Lectures in Mathematics. ETH Zürich (Birkhäuser Basel) (2006). ISBN 9783764373092
Villani, C.: Optimal transport: old and new vol 338. Springer Science & Business Media (2008)
Fathi, A., Figalli, A.: Optimal transportation on non-compact manifolds. Isr. J. Math. 175 , 1–59 (2010)
Caracciolo, S., Sicuro, G.: Scaling hypothesis for the Euclidean bipartite matching problem. II. Correlation functions. Phys. Rev. E 91 , 062125 (2015)
Born, M., Infeld, L.: Foundations of the New Field. Proc. R. Soc. Lond. A 144 425–451 (1934)
Brenier, Y.: Derivation of the Euler Equations from a Caricature of Coulomb Interaction. Commun. Math. Phys. 212 , 93–104 (2000)
Brenier, Y.: A note on deformations of 2D fluid motions using 3D Born–Infeld equations. Monatsh. Math. 142 , 113–122 (2004)
Ivrii, V.: 100 years of Weyl’s law. Bull. Math. Sci. 6 , 379–452 (2016)
Duistermaat, J.J., Guillemin, V.W.: The spectrum of positive elliptic operators and periodic bicharacteristics. Invent. Math. 39–79 , (1975)
Strauss, W.A.: Partial Differential Equations. Wiley, New York (2008)
MATH Google Scholar
Ambrosio, L., Glaudo, F., Trevisan, D.: On the optimal map in the 2-dimensional random matching problem. Discrete Cont. Dyn. A 39 , 1078–0947 (2019)
Apostol, T.M.: Modular Functions and Dirichlet Series in Number Theory Graduate Texts in Mathematics. Springer, New York (2012)
Osgood, B., Phillips, R., Sarnak, P.: Extremals of determinants of Laplacians. J. Funct. Anal. 80 148 – 211 (1988). ISSN 0022-1236
Morpurgo, C.: Sharp inequalities for functional integrals and traces of conformally invariant operators. Duke Math. J. 114 , 477–553 (2002)
Steiner, J.: A geometrical mass and its extremal properties for metrics on \(S^2\) . Duke Math. J. 129 , 63–86 (2005)
Okikiolu, K.: Extremals for logarithmic Hardy–Littlewood–Sobelov inequalities on compact manifolds. Geom. Funct. Anal. 17 , 1655–1684 (2008)
Boniolo, E., Caracciolo, S., Sportiello, A.: Correlation function for the Grid-Poisson Euclidean matching on a line and on a circle. J. Stat. Mech. 11 P11023 (2014)
Lin, C.S., Wang, C.L.: Elliptic functions, Green functions and the mean field equations on tori. Ann. Math. 911–954 , (2010)
Elizalde, E., Leseduarte, S., Romeo, A.: Sum rules for zeros of Bessel functions and an application to spherical Aharonov–Bohm quantum bags. J. Phys. A Math. Gen. 26 , 2409–2419 (1993)
Holden, N., Peres, Y., Zhai, A.: Gravitational allocation on the sphere. Proc. Natl. Acad. Sci. USA 115 9666–9671 (2018). ISSN 0027-8424
Erdélyi, A., Magnus, W., Oberhettinger, F., Tricomi, F.G.: Higher Transcendental Functions (Krieger) (1981)
Siegel, C. L.: Lectures on Advanced Analytic Number Theory Lectures on mathematics and physics: Mathematics (Tata Institute of Fundamental Research) (1965)
Download references
Acknowledgements
E. Caglioti and G. Sicuro would like to thank Giorgio Parisi for putting them in contact. D. Benedetto and E. Caglioti thanks Gabriele Mondello and Riccardo Salvati Manni for clarifying discussions about the case of the torus. The authors are grateful to Jürg Fröhlich for his careful reading of the manuscript. A. Sportiello is partially supported by the Agence Nationale de la Recherche, Grant Number ANR-18-CE40-0033 (ANR DIMERS).
Author information
Authors and affiliations.
Dipartimento di Matematica, Sapienza Università di Roma, P.le Aldo Moro 5, 00185, Roma, Italy
D. Benedetto & E. Caglioti
Dipartimento di Fisica, Università di Milano, and INFN, sez. di Milano, Via Celoria 16, 20100, Milano, Italy
S. Caracciolo
Centre CEA de Saclay, Gif-sur-Yvette, France
M. D’Achille
CIRB Collège de France, 11 Place Marcelin Berthelot, 75231, Paris, France
LI-PaRAD Université de Versailles Saint-Quentin-en-Yvelines, Versailles and Université Paris Saclay, Gif-sur-Yvette, France
Department of Mathematics, King’s College London, Strand, London, WC2R 2LS, UK
LIPN, and CNRS, Université Paris 13, Sorbonne Paris Cité, 99 Av. J.-B. Clément, 93430, Villetaneuse, France
A. Sportiello
You can also search for this author in PubMed Google Scholar
Corresponding author
Correspondence to G. Sicuro .
Additional information
Communicated by Federico Ricci-Tersenghi.
Publisher's Note
Springer Nature remains neutral with regard to jurisdictional claims in published maps and institutional affiliations.
Comparison of GP Problem with UP Problem on the Flat Torus
As commented in the main text, the very same arguments presented for the PP case in Sect. 3 can be repeated for the GP case and the UP case, the only difference being that ( 28 ) has to be replaced by
The result is an overall \(\frac{1}{2}\) factor, as shown in the final formula ( 8b ). There is however no guarantee that \(c_\Omega ^\text {PP}(N)=c_\Omega ^{\bullet \text {P}}(N)\) for \(\bullet =\text {\{}S,T,F,U\}\) at fixed \(\Omega \) as one might naively expect from ( 8 ), because these quantities depends on the regularizing function \(F^{\bullet \text {P}}(\lambda /N)\) , that, even assuming that it exists, is expected to be in general different in each case. In [ 3 ] it is proved that
This equation, combined with ( 8 ), implies
As discussed in Sect. 3 , one way to numerically estimate \(c_*^\text {UP}(N)\) is to perform a transportation between two sets of points, supposing that one of them (e.g., the red ones) is fixed on a grid and not random. As intuitively expected, a grid approximation provides some information on \(c_*^\text {UP}\) .
where we denoted by a generic GP the correction in the discrete transportation problem (in particular, \(c^\text {GP}_{\mathcal T}(N)=c^\text {SP}_{\mathcal T}(N)\) for \(h=1\) ). By means of the GP problem, \(c^\text {UP}_{*}(N)\) can be estimated for each N in the limit \(h\rightarrow +\infty \) .
To prove Eq. ( A.4 ), first we notice that if a probability measure \(\nu _1\) is the convex combination of measures \(\nu _{\eta }\) , that is, if we can write
where \(\gamma (\eta )\) is a probability measure, then, for any measure \(\nu _2\)
Let us assume now that the following empirical measure is given on the torus \(\mathcal T\) ,
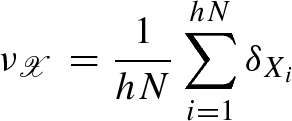
The points x joined with \(X_i\) by means of I ( x ) are the points in a square of side \((hN)^{-1\big /2}\) centered in \(X_i\) , therefore

so that Eq. ( A.11 ) becomes

i.e., \(E^\text {UP}_{\mathcal T}(N)\ge E^\text {GP}_{\mathcal T}(N)-\frac{1}{6h}\) and therefore
As expected, \(\lim _{h\rightarrow +\infty }c_{\mathcal T}^\text {GP}=c_{\mathcal T}^\text {UP}\) .
Robin’s Mass for the Circular Sector
In this appendix we compute the Robin mass of the Laplace–Beltrami Green function on the circular sector \(\mathcal D_p\) of angle \(\alpha =\frac{2\pi }{p}\) , with \(p\in \mathbb {N}\) , with periodic boundary conditions in the angular direction. We will work on the sector \(\mathcal D_p(r)\) defined in Eq. ( 120 ) and we will then restrict ourselves to the unit area case. Let us start considering the functions
where \(R_{\theta }\) is the rotation matrix of an angle \(\theta \) around the origin. The function \(A^{(p)}(x,y)\) is such that \(A^{(p)}(R_{\alpha }x,y)=A^{(p)}(x,y)\) . In the circular sector \(\mathcal D_p(r)\) we have
where \(\left. \partial _{n}A^{(p)}(x,y)\right| _{|y|=r}\) is the normal derivative in x with respect to the boundary \(|y|=r\) of the domain. The function
is therefore the Green function of the Laplacian on \(\mathcal D_p\) with Neumann boundary conditions on \(|y|=r\) . To impose ( 30c ) we compute
Observe that g ( x , y ) is periodic in y and therefore c ( y ) is periodic as well. The Green function is therefore
For \(y\rightarrow x\) we can write
with regular part \(\gamma (x,y)\) given by
To compute the Robin mass we have to estimate
where the four types of contributions that appear in the equation above, associated to the summands on the RHS of (B.7), are defined as

We will use the identities
Let us start from

Here the key observation is that \(|x|^{-1}r^2>r\) , i.e., \(x|x|^{-2}r^2\) always lies outside \(\mathcal D(r)\) , and therefore the integral in x is equal to the value of the integrand for \(x=0\) times the area of \(\mathcal D(r)\) ,
Similar arguments help us to evaluate \(I_2\) ,
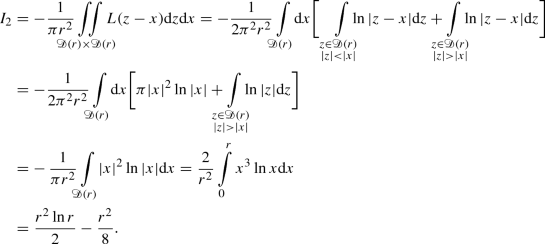
The integrals \(I_3(k)\) and \(I_4(k)\) can be computed introducing a complex representation of the integration variable, \(x= u\hbox {e}^{i\vartheta }\) , and then writing
After this change of variables, it is found that
Let us finally compute \(I_3(k)\) . Denoting by \(a=\cos (k\alpha )\) ,
Using now the fact that
Summing all contributions, we obtain
The last sum can be simplified as follows:
Applying the Gauss’s digamma theorem [ 43 ]
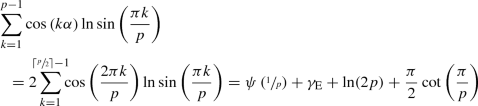
we can obtain the final expression
To restrict ourselves to the case of unit area, we impose \(\pi r^2 = p\) obtaining the searched Robin’s mass
For \(\alpha =2\pi \) , i.e., \(p=1\) , we obtain the Robin’s mass for the disc,
Kronecker’s Mass for the Spherical Lune
We consider the surface of the sphere but we wish to take only a portion \(\mathcal S^2_k\) around the z axis. Let us first observe that the eigenvectors of the Laplace–Beltrami operator on the sphere are the spherical harmonics,
The eigenvector \(Y^m_l(\theta ,\phi )\) has eigenvalue \(\frac{1}{r^2}l(l+1)\) . Here \(\theta \) is the colatitude and \(\phi \) the longitude on the sphere, whereas \(P_l^m(x)\) is an associated Legendre polynomial (we have omitted a normalization constant).
Let us now consider the lune \(\mathcal S^2_k\) with periodic boundary conditions. This means that we restrict ourselves to the span of eigenvectors having values m such that \(m\equiv 0\mod k\) . That is, the degeneracy in m of the eigenvalue \(l(l+1)r^{-2}\) of the Laplace–Beltrami operator, when \(l=nk+r\) with \(r=0,\dots ,k-1\) , is \(2n+1\) . The condition of unit area means \(4\pi r^2 = k\) , so that
The contribution obtained for \(n=0\) can be immediately summed,
The singular part for \(s=1\) comes from
where \(\psi (z):=\frac{\Gamma '(z)}{\Gamma (z)}\) is the digamma function and \(\psi (2)=1-\gamma _\text {E}\) . On the other hand
Collecting all the pieces we obtain
which reduces to the case of the surface of the sphere if we put \(k=1\) .
Similar arguments can be repeated if Neumann boundary conditions are chosen. In this case, the eigenfunctions of the Laplacian are
with corresponding eigenvalue
If \(k=2\kappa \) is even, then we have to compute
If \(k=2\kappa +1\) , then \(2m=0\mod k\) iff \(m=0\mod k\) : repeating the usual arguments, the same result is obtained, showing that the Kronecker’s mass in the case of Neumann conditions differs from the periodic boundary conditions case by an overall \(\frac{1}{2\pi }\ln 2\) constant.
Kronecker’s Limit Formulas
In this Appendix we will summarize some results obtained in the realm of analytic number theory. Let \(s\in \mathbb {C}\) . The Riemann \(\zeta \) -function \(\zeta (s)\) is defined in the half-plane \(\mathfrak {R}(s)>0\) by
The series converges absolutely for \(\mathfrak {R}(s)\ge 1 + \epsilon \) for every \(\epsilon > 0\) . Riemann proved that \(\zeta (s)\) has an analytic continuation in the whole s -plane which is regular except a simple pole at \(s=1\) with residue 1. At \(s=1\) , \(\zeta (s)\) has an expansion
As generalization of the Riemann \(\zeta \) -function, we consider a positive-definite binary quadratic form, in the real variables \(u,v \in \mathbb {R}\)
where \(a, b , c\in \mathbb {R}\) , \(a>0\) and \(d:=ac-b^2>0\) . Let us define
If \(d=1\) , \(\zeta _Q(s)\equiv \zeta _\tau (s)\) given in Eq. ( 70 ), associated to Q , is defined for \(\mathfrak {R}(s)>1\) can be analytically continued into a regular function for \(\mathfrak {R}(s)>1\big /2\) except for a simple pole at \(s=1\) with residue \(\pi \) , and the function \(\zeta _Q(s)\) , has an expansion (first limit formula of Kronecker)
is the Dedekind \(\eta \) -function, which satisfies the functional equations
Known particular values are
For a complete discussion of the results sketched here, see for example [ 44 ].
Rights and permissions
Open Access This article is licensed under a Creative Commons Attribution 4.0 International License, which permits use, sharing, adaptation, distribution and reproduction in any medium or format, as long as you give appropriate credit to the original author(s) and the source, provide a link to the Creative Commons licence, and indicate if changes were made. The images or other third party material in this article are included in the article’s Creative Commons licence, unless indicated otherwise in a credit line to the material. If material is not included in the article’s Creative Commons licence and your intended use is not permitted by statutory regulation or exceeds the permitted use, you will need to obtain permission directly from the copyright holder. To view a copy of this licence, visit http://creativecommons.org/licenses/by/4.0/ .
Reprints and permissions
About this article
Benedetto, D., Caglioti, E., Caracciolo, S. et al. Random Assignment Problems on 2 d Manifolds. J Stat Phys 183 , 34 (2021). https://doi.org/10.1007/s10955-021-02768-4
Download citation
Received : 20 February 2021
Accepted : 21 April 2021
Published : 12 May 2021
DOI : https://doi.org/10.1007/s10955-021-02768-4
Share this article
Anyone you share the following link with will be able to read this content:
Sorry, a shareable link is not currently available for this article.
Provided by the Springer Nature SharedIt content-sharing initiative
- random optimization problems
- optimal transportation
- finite-size corrections
- Find a journal
- Publish with us
- Track your research
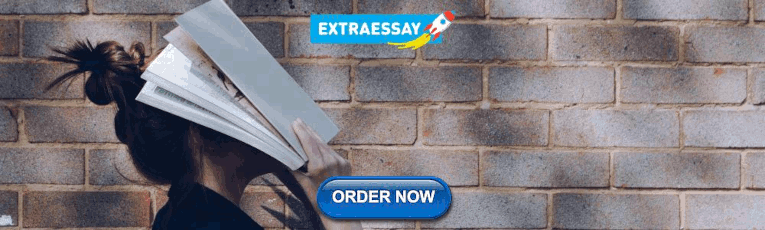
IMAGES
VIDEO
COMMENTS
other random assignment problems, on the probability of existence of a perfect matching in a graph selected uniformly from the class G.n;d/ of bipartite digraphs with n nodes in each class and outward degree d at each node. Namely, such a probability vanishes with n when d D 1, but for d 2 it approaches unity as
Random selection (also called probability sampling or random sampling) is a way of randomly selecting members of a population to be included in your study. On the other hand, random assignment is a way of sorting the sample participants into control and treatment groups. Random selection ensures that everyone in the population has an equal ...
Random Assignment in Experiments | Introduction & Examples. Published on March 8, 2021 by Pritha Bhandari.Revised on June 22, 2023. In experimental research, random assignment is a way of placing participants from your sample into different treatment groups using randomization. With simple random assignment, every member of the sample has a known or equal chance of being placed in a control ...
Random assignment is a simple, elegant solution to a complex problem. For any given study area, there can be a long list of confounding variables that you could worry about. However, using random assignment, you don't need to know what they are, how to detect them, or even measure them.
Random assignment or random placement is an experimental technique for assigning human participants or animal subjects to different groups in an experiment (e.g., a treatment group versus a control group) using randomization, such as by a chance procedure (e.g., flipping a coin) or a random number generator. This ensures that each participant or subject has an equal chance of being placed in ...
No random assignment: Can detect relationships in population, but cannot determine causality. This design is where many surveys and observational studies would fit. ... Problem A scenario 2 is absolutely ridiculous. Coincidence does not equal causality. Just because the ones taking vitamin D happened to have lower blood pressure absolutely does ...
The studies of random instances of assignment problems date back to as early as ( Donath, 1969 ), where limiting behavior of the linear assignment problem was investigated by solving small (by today's standards) randomly generated instances. In retrospect, probabilistic analysis of the linear assignment problem, whose deterministic instances ...
The Definition of Random Assignment According to Psychology. Random assignment refers to the use of chance procedures in psychology experiments to ensure that each participant has the same opportunity to be assigned to any given group in a study to eliminate any potential bias in the experiment at the outset. Participants are randomly assigned ...
A particular class of combinatorial optimization problems that have been studied extensively in the probabilistic context is represented by the assignment, or matching problems. In an assignment problem, one is looking to find an assignment, or matching, between the elements of two (or more) sets, such that the total cost of all matched pairs ...
The assignment problem is a fundamental combinatorial optimization problem. In its most general form, the problem is as follows: The problem instance has a number of agents and a number of tasks. Any agent can be assigned to perform any task, incurring some cost that may vary depending on the agent-task assignment.
A potential problem with random assignment is the temptation to ignore the random assignment procedures. For example, it may be tempting to assign an overweight participant to the treatment group that includes participation in a weight-loss program. Ignoring random assignment procedures in this study limits the ability to determine whether or ...
A random assignment is ordinally efficient if it is not stochastically dominated with respect to individual preferences over sure objects. Ordinal efficiency implies (is implied by) ex post (ex ante) efficiency. A simple algorithm characterizes ordinally efficient assignments: our solution, probabilistic serial (PS), is a central element within ...
a new mechanism (PS) for the random assignment problem. Their mechanism combines the attractive features of the random priority mechanism and the CEEI solution: it requires the agents to report their preferences over objects, not the complete utility functions, and yet computes a random assignment that is ordinally efficient and envy-free.
Probability and Random Variables, Problem Set 1. Description: This resource contains information related to problem set 1. Resource Type: Assignments. pdf. ... assignment Problem Sets. grading Exams with Solutions. notes Lecture Notes. menu_book Online Textbook. co_present Instructor Insights.
Random sampling (also called probability sampling or random selection) is a way of selecting members of a population to be included in your study. In contrast, random assignment is a way of sorting the sample participants into control and experimental groups. While random sampling is used in many types of studies, random assignment is only used ...
Random Assignment Problem By Anna Bogomolnaia, Herve Moulin Presented By Zach Jablons, Bharath Santosh. The Assignment Problem ... Random assignment Weighted sum of all Π ∈ D R is the set of all P > is all agents strict preference orders over A A is the domain of A.
Fair random assignment (also called probabilistic one-sided matching) is a kind of a fair division problem.. In an assignment problem (also called house-allocation problem or one-sided matching), there are m objects and they have to be allocated among n agents, such that each agent receives at most one object. Examples include the assignment of jobs to workers, rooms to housemates, dormitories ...
One potential problem with small clinical trials (n < 100) 7 is that conventional simple randomization ... design stage of a clinical trial (before the adjustment procedure) instead of after data collection. In such instances, random assignment is necessary and guarantees validity for statistical tests of significance that are used to compare ...
A random assignment is ordinally efficient if it is not stochastically dominated with respect to individual preferences over sure objects. Ordinal efficiency implies (is implied by) ex post (ex ante) efficiency. A simple algorithm characterizes ordinally efficient assignments: our solution, probabilistic serial (PS), is a central element within their set.
Summary. We show that, in the usual probabilistic model for the random assignment problem, the optimal cost tends to a limit constant in probability and in expectation. The method involves construction of an infinite limit structure, in terms of which the limit constant is defined. But we cannot improve on the known numerical bounds for the limit.
5.1 Misconception #1: Successful Random Assignment Guarantees Internal Validity. Although random assignment of participants (or other units) to treatment condition can greatly enhance the likelihood of internal validity, problems can still occur. Most widely recognized is that differential attrition may occur, with more (or different kinds of ...
We consider the assignment problem between two sets of N random points on a smooth, two-dimensional manifold \(\Omega \) of unit area. It is known that the average cost scales as \(E_{\Omega }(N)\sim {1}/{2\pi }\ln N\) with a correction that is at most of order \(\sqrt{\ln N\ln \ln N}\).In this paper, we show that, within the linearization approximation of the field-theoretical formulation of ...
Abstract. This paper proposes an uncertain random assignment problem in which random variables coexist with uncertain variables. Critical values of uncertain random variables are used to rank uncertain random variables. An uncertain random simulation algorithm is developed in order to obtain chance distributions and critical values of uncertain ...