Click through the PLOS taxonomy to find articles in your field.
For more information about PLOS Subject Areas, click here .
Loading metrics
Open Access
Peer-reviewed
Research Article
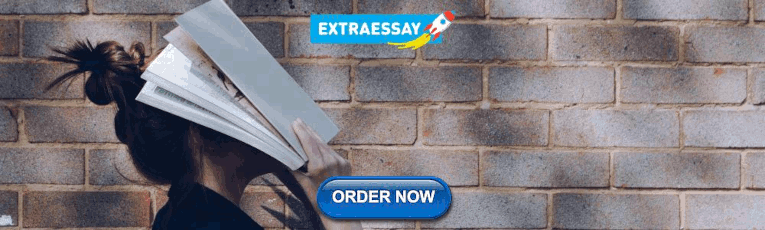
Personality types revisited–a literature-informed and data-driven approach to an integration of prototypical and dimensional constructs of personality description
Roles Conceptualization, Data curation, Formal analysis, Investigation, Methodology, Validation, Visualization, Writing – original draft
* E-mail: [email protected]
Affiliation Department of Psychology, Freie Universität Berlin, Berlin, Germany

Roles Conceptualization, Investigation, Methodology, Supervision, Validation, Writing – review & editing
Affiliation Department of Psychology, University of Duisburg-Essen, Duisburg Germany
Affiliation Personality Psychology and Psychological Assessment Unit, Helmut Schmidt University of the Federal Armed Forces Hamburg, Hamburg, Germany
- André Kerber,
- Marcus Roth,
- Philipp Yorck Herzberg
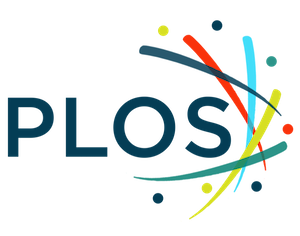
- Published: January 7, 2021
- https://doi.org/10.1371/journal.pone.0244849
- Peer Review
- Reader Comments
A new algorithmic approach to personality prototyping based on Big Five traits was applied to a large representative and longitudinal German dataset (N = 22,820) including behavior, personality and health correlates. We applied three different clustering techniques, latent profile analysis, the k-means method and spectral clustering algorithms. The resulting cluster centers, i.e. the personality prototypes, were evaluated using a large number of internal and external validity criteria including health, locus of control, self-esteem, impulsivity, risk-taking and wellbeing. The best-fitting prototypical personality profiles were labeled according to their Euclidean distances to averaged personality type profiles identified in a review of previous studies on personality types. This procedure yielded a five-cluster solution: resilient, overcontroller, undercontroller, reserved and vulnerable-resilient. Reliability and construct validity could be confirmed. We discuss wether personality types could comprise a bridge between personality and clinical psychology as well as between developmental psychology and resilience research.
Citation: Kerber A, Roth M, Herzberg PY (2021) Personality types revisited–a literature-informed and data-driven approach to an integration of prototypical and dimensional constructs of personality description. PLoS ONE 16(1): e0244849. https://doi.org/10.1371/journal.pone.0244849
Editor: Stephan Doering, Medical University of Vienna, AUSTRIA
Received: January 5, 2020; Accepted: December 17, 2020; Published: January 7, 2021
Copyright: © 2021 Kerber et al. This is an open access article distributed under the terms of the Creative Commons Attribution License , which permits unrestricted use, distribution, and reproduction in any medium, provided the original author and source are credited.
Data Availability: The data used in this article were made available by the German Socio-Economic Panel (SOEP, Data for years 1984-2015) at the German Institute for Economic Research, Berlin, Germany. To ensure the confidentiality of respondents’ information, the SOEP adheres to strict security standards in the provision of SOEP data. The data are reserved exclusively for research use, that is, they are provided only to the scientific community. To require full access to the data used in this study, it is required to sign a data distribution contract. All contact informations and the procedure to request the data can be obtained at: https://www.diw.de/en/diw_02.c.222829.en/access_and_ordering.html .
Funding: The author(s) received no specific funding for this work.
Competing interests: The authors have declared that no competing interests exist.
Introduction
Although documented theories about personality types reach back more than 2000 years (i.e. Hippocrates’ humoral pathology), and stereotypes for describing human personality are also widely used in everyday psychology, the descriptive and variable-oriented assessment of personality, i.e. the description of personality on five or six trait domains, has nowadays consolidated its position in modern personality psychology.
In recent years, however, the person-oriented approach, i.e. the description of an individual personality by its similarity to frequently occurring prototypical expressions, has amended the variable-oriented approach with the addition of valuable insights into the description of personality and the prediction of behavior. Focusing on the trait configurations, the person-oriented approach aims to identify personality types that share the same typical personality profile [ 1 ].
Nevertheless, the direct comparison of the utility of person-oriented vs. variable-oriented approaches to personality description yielded mixed results. For example Costa, Herbst, McCrae, Samuels and Ozer [ 2 ] found a higher amount of explained variance in predicting global functioning, geriatric depression or personality disorders for the variable-centered approach using Big Five personality dimensions. But these results also reflect a methodological caveat of this approach, as the categorical simplification of dimensionally assessed variables logically explains less variance. Despite this, the person-centered approach was found to heighten the predictability of a person’s behavior [ 3 , 4 ] or the development of adolescents in terms of internalizing and externalizing symptoms or academic success [ 5 , 6 ], problem behavior, delinquency and depression [ 7 ] or anxiety symptoms [ 8 ], as well as stress responses [ 9 ] and social attitudes [ 10 ]. It has also led to new insights into the function of personality in the context of other constructs such as adjustment [ 2 ], coping behavior [ 11 ], behavioral activation and inhibition [ 12 ], subjective and objective health [ 13 ] or political orientation [ 14 ], and has greater predictive power in explaining longitudinally measured individual differences in more temperamental outcomes such as aggressiveness [ 15 ].
However, there is an ongoing debate about the appropriate number and characteristics of personality prototypes and whether they perhaps constitute an methodological artifact [ 16 ].
With the present paper, we would like to make a substantial contribution to this debate. In the following, we first provide a short review of the personality type literature to identify personality types that were frequently replicated and calculate averaged prototypical profiles based on these previous findings. We then apply multiple clustering algorithms on a large German dataset and use those prototypical profiles generated in the first step to match the results of our cluster analysis to previously found personality types by their Euclidean distance in the 5-dimensional space defined by the Big Five traits. This procedure allows us to reliably link the personality prototypes found in our study to previous empirical evidence, an important analysis step lacking in most previous studies on this topic.
The empirical ground of personality types
The early studies applying modern psychological statistics to investigate personality types worked with the Q-sort procedure [ 1 , 15 , 17 ], and differed in the number of Q-factors. With the Q-Sort method, statements about a target person must be brought in an order depending on how characteristic they are for this person. Based on this Q-Sort data, prototypes can be generated using Q-Factor Analysis, also called inverse factor analysis. As inverse factor analysis is basically interchanging variables and persons in the data matrix, the resulting factors of a Q-factor analysis are prototypical personality profiles and not hypothetical or latent variable dimensions. On this basis, personality types (groups of people with similar personalities) can be formed in a second step by assigning each person to the prototype with whose profile his or her profile correlates most closely. All of these early studies determined at least three prototypes, which were labeled resilient, overcontroler and undercontroler grounded in Block`s theory of ego-control and ego-resiliency [ 18 ]. According to Jack and Jeanne Block’s decade long research, individuals high in ego-control (i.e. the overcontroler type) tend to appear constrained and inhibited in their actions and emotional expressivity. They may have difficulty making decisions and thus be non-impulsive or unnecessarily deny themselves pleasure or gratification. Children classified with this type in the studies by Block tend towards internalizing behavior. Individuals low in ego-control (i.e. the undercontroler type), on the other hand, are characterized by higher expressivity, a limited ability to delay gratification, being relatively unattached to social standards or customs, and having a higher propensity to risky behavior. Children classified with this type in the studies by Block tend towards externalizing behavior.
Individuals high in Ego-resiliency (i.e. the resilient type) are postulated to be able to resourcefully adapt to changing situations and circumstances, to tend to show a diverse repertoire of behavioral reactions and to be able to have a good and objective representation of the “goodness of fit” of their behavior to the situations/people they encounter. This good adjustment may result in high levels of self-confidence and a higher possibility to experience positive affect.
Another widely used approach to find prototypes within a dataset is cluster analysis. In the field of personality type research, one of the first studies based on this method was conducted by Caspi and Silva [ 19 ], who applied the SPSS Quick Cluster algorithm to behavioral ratings of 3-year-olds, yielding five prototypes: undercontrolled, inhibited, confident, reserved, and well-adjusted.
While the inhibited type was quite similar to Block`s overcontrolled type [ 18 ] and the well-adjusted type was very similar to the resilient type, two further prototypes were added: confident and reserved. The confident type was described as easy and responsive in social interaction, eager to do exercises and as having no or few problems to be separated from the parents. The reserved type showed shyness and discomfort in test situations but without decreased reaction speed compared to the inhibited type. In a follow-up measurement as part of the Dunedin Study in 2003 [ 20 ], the children who were classified into one of the five types at age 3 were administered the MPQ at age 26, including the assessment of their individual Big Five profile. Well-adjusteds and confidents had almost the same profiles (below-average neuroticism and above average on all other scales except for extraversion, which was higher for the confident type); undercontrollers had low levels of openness, conscientiousness and openness to experience; reserveds and inhibiteds had below-average extraversion and openness to experience, whereas inhibiteds additionally had high levels of conscientiousness and above-average neuroticism.
Following these studies, a series of studies based on cluster analysis, using the Ward’s followed by K-means algorithm, according to Blashfield & Aldenderfer [ 21 ], on Big Five data were published. The majority of the studies examining samples with N < 1000 [ 5 , 7 , 22 – 26 ] found that three-cluster solutions, namely resilients, overcontrollers and undercontrollers, fitted the data the best. Based on internal and external fit indices, Barbaranelli [ 27 ] found that a three-cluster and a four-cluster solution were equally suitable, while Gramzow [ 28 ] found a four-cluster solution with the addition of the reserved type already published by Caspi et al. [ 19 , 20 ]. Roth and Collani [ 10 ] found that a five-cluster solution fitted the data the best. Using the method of latent profile analysis, Merz and Roesch [ 29 ] found a 3-cluster, Favini et al. [ 6 ] found a 4-cluster solution and Kinnunen et al. [ 13 ] found a 5-cluster solution to be most appropriate.
Studies examining larger samples of N > 1000 reveal a different picture. Several favor a five-cluster solution [ 30 – 34 ] while others favor three clusters [ 8 , 35 ]. Specht et al. [ 36 ] examined large German and Australian samples and found a three-cluster solution to be suitable for the German sample and a four-cluster solution to be suitable for the Australian sample. Four cluster solutions were also found to be most suitable to Australian [ 37 ] and Chinese [ 38 ] samples. In a recent publication, the authors cluster-analysed very large datasets on Big Five personality comprising more than 1,5 million online participants using Gaussian mixture models [ 39 ]. Albeit their results “provide compelling evidence, both quantitatively and qualitatively, for at least four distinct personality types”, two of the four personality types in their study had trait profiles not found previously and all four types were given labels unrelated to previous findings and theory. Another recent publication [ 40 ] cluster-analysing data of over 270,000 participants on HEXACO personality “provided evidence that a five-profile solution was optimal”. Despite limitations concerning the comparability of HEXACO trait profiles with FFM personality type profiles, the authors again decided to label their personality types unrelated to previous findings instead using agency-communion and attachment theories.
We did not include studies in this literature review, which had fewer than 199 participants or those which restricted the number of types a priori and did not use any method to compare different clustering solutions. We have made these decisions because a too low sample size increases the probability of the clustering results being artefacts. Further, a priori limitation of the clustering results to a certain number of personality types is not well reasonable on the base of previous empirical evidence and again may produce artefacts, if the a priori assumed number of clusters does not fit the data well.
To gain a better overview, we extracted all available z-scores from all samples of the above-described studies. Fig 1 shows the averaged z-scores extracted from the results of FFM clustering solutions for all personality prototypes that occurred in more than one study. The error bars represent the standard deviation of the distribution of the z-scores of the respective trait within the same personality type throughout the different studies. Taken together the resilient type was replicated in all 19 of the mentioned studies, the overcontroler type in 16, the undercontroler personality type in 17 studies, the reserved personality type was replicated in 6 different studies, the confident personality type in 4 and the non-desirable type was replicated twice.
- PPT PowerPoint slide
- PNG larger image
- TIFF original image
Average Big Five z-scores of personality types based on clustering of FFM datasets with N ≥ 199 that were replicated at least once. Error bars indicate the standard deviation of the repective trait within the respective personality type found in the literature [ 5 , 6 , 10 , 22 – 25 , 27 – 31 , 33 – 36 , 38 , 39 , 41 ].
https://doi.org/10.1371/journal.pone.0244849.g001
Three implications can be drawn from this figure. First, although the results of 19 studies on 26 samples with a total N of 1,560,418 were aggregated, the Big Five profiles for all types can still be clearly distinguished. In other words, personality types seem to be a phenomenon that survives the aggregation of data from different sources. Second, there are more than three replicable personality types, as there are other replicated personality types that seem to have a distinct Big Five profile, at least regarding the reserved and confident personality types. Third and lastly, the non-desirable type seems to constitute the opposite of the resilient type. Looking at two-cluster solutions on Big Five data personality types in the above-mentioned literature yields the resilient opposed to the non-desirable type. This and the fact that it was only replicated twice in the above mentioned studies points to the notion that it seems not to be a distinct type but rather a combined cluster of the over- and undercontroller personality types. Further, both studies with this type in the results did not find either the undercontroller or the overcontroller cluster or both. Taken together, five distinct personality types were consistently replicated in the literature, namely resilient, overcontroller, undercontroller, reserved and confident. However, inferring from the partly large error margin for some traits within some prototypes, not all personality traits seem to contribute evenly to the occurrence of the different prototypes. While for the overcontroler type, above average neuroticism, below average extraversion and openness seem to be distinctive, only below average conscientiousness and agreeableness seemed to be most characteristic for the undercontroler type. The reserved prototype was mostly characterized by below average openness and neuroticism with above average conscientiousness. Above average extraversion, openness and agreeableness seemed to be most distinctive for the confident type. Only for the resilient type, distinct expressions of all Big Five traits seemed to be equally significant, more precisely below average neuroticism and above average extraversion, openness, agreeableness and conscientiousness.
Research gap and novelty of this study
The cluster methods used in most of the mentioned papers were the Ward’s followed by K-means method or latent profile analysis. With the exception of Herzberg and Roth [ 30 ], Herzberg [ 33 ], Barbaranelli [ 27 ] and Steca et. al. [ 25 ], none of the studies used internal or external validity indices other than those which their respective algorithm (in most cases the SPSS software package) had already included. Gerlach et al. [ 39 ] used Gaussian mixture models in combination with density measures and likelihood measures.
The bias towards a smaller amount of clusters resulting from the utilization of just one replication index, e.g. Cohen's Kappa calculated by split-half cross-validation, which was ascertained by Breckenridge [ 42 ] and Overall & Magee [ 43 ], is probably the reason why a three-cluster solution is preferred in most studies. Herzberg and Roth [ 30 ] pointed to the study by Milligan and Cooper [ 44 ], which proved the superiority of the Rand index over Cohen's Kappa and also suggested a variety of validity metrics for internal consistency to examine the construct validity of the cluster solutions.
Only a part of the cited studies had a large representative sample of N > 2000 and none of the studies used more than one clustering algorithm. Moreover, with the exception of Herzberg and Roth [ 30 ] and Herzberg [ 33 ], none of the studies used a large variety of metrics for assessing internal and external consistency other than those provided by the respective clustering program they used. This limitation further adds up to the above mentioned bias towards smaller amounts of clusters although the field of cluster analysis and algorithms has developed a vast amount of internal and external validity algorithms and criteria to tackle this issue. Further, most of the studies had few or no other assessments or constructs than the Big Five to assess construct validity of the resulting personality types. Herzberg and Roth [ 30 ] and Herzberg [ 33 ] as well, though using a diverse variety of validity criteria only used one clustering algorithm on a medium-sized dataset with N < 2000.
Most of these limitations also apply to the study by Specht et. al. [ 36 ], which investigated two measurement occasions of the Big Five traits in the SOEP data sample. They used only one clustering algorithm (latent profile analysis), no other algorithmic validity criteria than the Bayesian information criterion and did not utilize any of the external constructs also assessed in the SOEP sample, such as mental health, locus of control or risk propensity for construct validation.
The largest sample and most advanced clustering algorithm was used in the recent study by Gerlach et al. [ 39 ]. But they also used only one clustering algorithm, and had no other variables except Big Five trait data to assess construct validity of the resulting personality types.
The aim of the present study was therefore to combine different methodological approaches while rectifying the shortcomings in several of the studies mentioned above in order to answer the following exploratory research questions: Are there replicable personality types, and if so, how many types are appropriate and in which constellations are they more (or less) useful than simple Big Five dimensions in the prediction of related constructs?
Three conceptually different clustering algorithms were used on a large representative dataset. The different solutions of the different clustering algorithms were compared using methodologically different internal and external validity criteria, in addition to those already used by the respective clustering algorithm.
To further examine the construct validity of the resulting personality types, their predictive validity in relation to physical and mental health, wellbeing, locus of control, self-esteem, impulsivity, risk-taking and patience were assessed.
Mental health and wellbeing seem to be associated mostly with neuroticism on the variable-oriented level [ 45 ], but on a person-oriented level, there seem to be large differences between the resilient and the overcontrolled personality type concerning perceived health and well-being beyond mean differences in neuroticism [ 33 ]. This seems also to be the case for locus of control and self-esteem, which is associated with neuroticism [ 46 ] and significantly differs between resilient and overcontrolled personality type [ 33 ]. On the other hand, impulsivity and risk taking seem to be associated with all five personality traits [ 47 ] and e.g. risky driving or sexual behavior seem to occur more often in the undercontrolled personality type [ 33 , 48 ].
We chose these measures because of their empirically known differential associations to Big Five traits as well as to the above described personality types. So this both offers the opportunity to have an integrative comparison of the variable- and person-centered descriptions of personality and to assess construct validity of the personality types resulting from our analyses.
Materials and methods
The acquisition of the data this study bases on was carried out in accordance with the principles of the Basel Declaration and recommendations of the “Principles of Ethical Research and Procedures for Dealing with Scientific Misconduct at DIW Berlin”. The protocol was approved by the Deutsches Institut für Wirtschaftsforschung (DIW).
The data used in this study were provided by the German Socio-Economic Panel Study (SOEP) of the German institute for economic research [ 49 ]. Sample characteristics are shown in Table 1 . The overall sample size of the SOEP data used in this study, comprising all individuals who answered at least one of the Big-Five personality items in 2005 and 2009, was 25,821. Excluding all members with more than one missing answers on the Big Five assessment or intradimensional answer variance more than four times higher than the sample average resulted in a total Big Five sample of N = 22,820, which was used for the cluster analyses. 14,048 of these individuals completed, in addition to the Big Five, items relevant to further constructs examined in this study that were assessed in other years. The 2013 SOEP data Big Five assessment was used as a test sample to examine stability and consistency of the final cluster solution.
https://doi.org/10.1371/journal.pone.0244849.t001
The Big Five were assessed in 2005 2009 and 2013 using the short version of the Big Five inventory (BFI-S). It consists of 15 items, with internal consistencies (Cronbach’s alpha) of the scales ranging from .5 for openness to .73 for openness [ 50 ]. Further explorations showed strong robustness across different assessment methods [ 51 ].
To measure the predictive validity, several other measures assessed in the SOEP were included in the analyses. In detail, these were:
Patience was assessed in 2008 with one item: “Are you generally an impatient person, or someone who always shows great patience?”
Risk taking.
Risk-taking propensity was assessed in 2009 by six items asking about the willingness to take risks while driving, in financial matters, in leisure and sports, in one’s occupation (career), in trusting unknown people and the willingness to take health risks, using a scale from 0 (risk aversion) to 10 (fully prepared to take risks). Cronbach’s alpha was .82 for this scale in the current sample.
Impulsivity/Spontaneity.
Impulsivity/spontaneity was assessed in 2008 with one item: Do you generally think things over for a long time before acting–in other words, are you not impulsive at all? Or do you generally act without thinking things over for long time–in other words, are you very impulsive?
Affective and cognitive wellbeing.
Affect was assessed in 2008 by four items asking about the amount of anxiety, anger, happiness or sadness experienced in the last four weeks on a scale from 1 (very rare) to 5 (very often). Cronbach’s alpha for this scale was .66. The cognitive satisfaction with life was assessed by 10 items asking about satisfaction with work, health, sleep, income, leisure time, household income, household duties, family life, education and housing, with a Cronbach’s alpha of .67. The distinction between cognitive and affective wellbeing stems from sociological research based on constructs by Schimmack et al. [ 50 ].
Locus of control.
The individual attitude concerning the locus of control, the degree to which people believe in having control over the outcome of events in their lives opposed to being exposed to external forces beyond their control, was assessed in 2010 with 10 items, comprising four positively worded items such as “My life’s course depends on me” and six negatively worded items such as “Others make the crucial decisions in my life”. Items were rated on a 7-point scale ranging from “does not apply” to “does apply”. Cronbach’s alpha in the present sample for locus of control was .57.
Self-esteem.
Global self-esteem–a person’s overall evaluation or appraisal of his or her worth–was measured in 2010 with one item: “To what degree does the following statement apply to you personally?: I have a positive attitude toward myself”.
To assess subjective health, the 12-Item Short Form Health Survey (SF-12) was integrated into the SOEP questionnaire and assessed in 2002, 2004, 2006, 2008 and 2010. In the present study, we used the data from 2008 and 2010. The SF-12 is a short form of the SF-36, a self-report questionnaire to assess the non-disease-specific health status [ 52 ]. Within the SF-12, items can be grouped onto two subscales, namely the physical component summary scale, with items asking about physical health correlates such as how exhausting it is to climb stairs, and the mental component summary scale, with items asking about mental health correlates such as feeling sad and blue. The literature on health measures often distinguishes between subjective and objective health measures (e.g., BMI, blood pressure). From this perspective, the SF-12 would count as a subjective health measure. In the present sample, Cronbach’s alpha for the SF-12 items was .77.
Derivation of the prototypes
The first step was to administer three different clustering methods on the Big Five data of the SOEP sample: First, the conventional linear clustering method used by Asendorpf [ 15 , 35 , 53 ] and also Herzberg and Roth [ 30 ] combines the hierarchical clustering method of Ward [ 54 ] with the k-means algorithm [ 55 ]. This algorithm generates a first guess of personality types based on hierarchical clustering, and then uses this first guess as starting points for the k-means-method, which iteratively adjusts the personality profiles, i.e. the cluster means to minimize the error of allocation, i.e. participants with Big Five profiles that are allocated to two or more personality types. The second algorithm we used was latent profile analysis with Mclust in R [ 56 ], an algorithm based on probabilistic finite mixture modeling, which assumes that there are latent classes/profiles/mixture components underlying the manifest observed variables. This algorithm generates personality profiles and iteratively calculates the probability of every participant in the data to be allocated to one of the personality types and tries to minimize an error term using maximum likelihood method. The third algorithm was spectral clustering, an algorithm which initially computes eigenvectors of graph Laplacians of the similarity graph constructed on the input data to discover the number of connected components in the graph, and then uses the k-means algorithm on the eigenvectors transposed in a k-dimensional space to compute the desired k clusters [ 57 ]. As it is an approach similar to the kernel k-means algorithm [ 58 ], spectral clustering can discover non-linearly separable cluster formations. Thus, this algorithm is able, in contrast to the standard k-means procedure, to discover personality types having unequal or non-linear distributions within the Big-Five traits, e.g. having a small SD on neuroticism while having a larger SD on conscientiousness or a personality type having high extraversion and either high or low agreeableness.
Within the last 50 years, a large variety of clustering algorithms have been established, and several attempts have been made to group them. In their book about cluster analysis, Bacher et al. [ 59 ] group cluster algorithms into incomplete clustering algorithms, e.g. Q-Sort or multidimensional scaling, deterministic clustering, e.g. k-means or nearest-neighbor algorithms, and probabilistic clustering, e.g. latent class and latent profile analysis. According to Jain [ 60 ], cluster algorithms can be grouped by their objective function, probabilistic generative models and heuristics. In his overview of the current landscape of clustering, he begins with the group of density-based algorithms with linear similarity functions, e.g. DBSCAN, or probabilistic models of density functions, e.g. in the expectation-maximation (EM) algorithm. The EM algorithm itself also belongs to the large group of clustering algorithms with an information theoretic formulation. Another large group according to Jain is graph theoretic clustering, which includes several variants of spectral clustering. Despite the fact that it is now 50 years old, Jain states that k-means is still a good general-purpose algorithm that can provide reasonable clustering results.
The clustering algorithms chosen for the current study are therefore representatives of the deterministic vs. probabilistic grouping according to Bacher et. al. [ 59 ], as well as representatives of the density-based, information theoretic and graph theoretic grouping according to Jain [ 60 ].
Determining the number of clusters
There are two principle ways to determine cluster validity: external or relative criteria and internal validity indices.
External validity criteria.
External validity criteria measure the extent to which cluster labels match externally supplied class labels. If these external class labels originate from another clustering algorithm used on the same data sample, the resulting value of the external cluster validity index is relative. Another method, which is used in the majority of the cited papers in section 1, is to randomly split the data in two halves, apply a clustering algorithm on both halves, calculate the cluster means and allocate members of one half to the calculated clusters of the opposite half by choosing the cluster mean with the shortest Euclidean distance to the data member in charge. If the cluster algorithm allocation of one half is then compared with the shortest Euclidean distance allocation of the same half by means of an external cluster validity index, this results in a value for the reliability of the clustering method on the data sample.
As allocating data points/members by Euclidean distances always yields spherical and evenly shaped clusters, it will favor clustering methods that also yield spherical and evenly shaped clusters, as it is the case with standard k-means. The cluster solutions obtained with spectral clustering as well as latent profile analysis (LPA) are not (necessarily) spherical or evenly shaped; thus, allocating members of a dataset by their Euclidean distances to cluster means found by LPA or spectral clustering does not reliably represent the structure of the found cluster solution. This is apparent in Cohen’s kappa values <1 if one uses the Euclidean external cluster assignment method comparing a spectral cluster solution with itself. Though by definition, Cohen’s kappa should be 1 if the two ratings/assignments compared are identical, which is the case when comparing a cluster solution (assigning every data point to a cluster) with itself. This problem can be bypassed by allocating the members of the test dataset to the respective clusters by training a support vector machine classifier for each cluster. Support vector machines (SVM) are algorithms to construct non-linear “hyperplanes” to classify data given their class membership [ 61 ]. They can be used very well to categorize members of a dataset by an SVM-classifier trained on a different dataset. Following the rationale not to disadvantage LPA and spectral clustering in the calculation of the external validity, we used an SVM classifier to calculate the external validity criteria for all clustering algorithms in this study.
To account for the above mentioned bias to smaller numbers of clusters we applied three external validity criteria: Cohen’s kappa, the Rand index [ 62 ] and the Hubert-Arabie adjusted Rand index [ 63 ].
Internal validity criteria.
Again, to account for the bias to smaller numbers of clusters, we also applied multiple internal validity criteria selected in line with the the following reasoning: According to Lam and Yan [ 64 ], the internal validity criteria fall into three classes: Class one includes cost-function-based indices, e.g. AIC or BIC [ 65 ], whereas class two comprises cluster-density-based indices, e.g. the S_Dbw index [ 66 ]. Class three is grounded on geometric assumptions concerning the ratio of the distances within clusters compared to the distances between the clusters. This class has the most members, which differ in their underlying mathematics. One way of assessing geometric cluster properties is to calculate the within- and/or between-group scatter, which both rely on summing up distances of the data points to their barycenters (cluster means). As already explained in the section on external criteria, calculating distances to cluster means will always favor spherical and evenly shaped cluster solutions without noise, i.e. personality types with equal and linear distributions on the Big Five trait dimensions, which one will rarely encounter with natural data.
Another way not solely relying on distances to barycenters or cluster means is to calculate directly with the ratio of distances of the data points within-cluster and between-cluster. According to Desgraupes [ 67 ], this applies to the following indices: the C-index, the Baker & Hubert Gamma index, the G(+) index, Dunn and Generalized Dunn indices, the McClain-Rao index, the Point-Biserial index and the Silhouette index. As the Gamma and G(+) indices rely on the same mathematical construct, one can declare them as redundant. According to Bezdek [ 68 ], the Dunn index is very sensitive to noise, even if there are only very few outliers in the data. Instead, the authors propose several ways to compute a Generalized Dunn index, some of which also rely on the calculation of barycenters. The best-performing GDI algorithm outlined by Bezdek and Pal [ 68 ] which does not make use of cluster barycenters is a ratio of the mean distance of every point between clusters to the maximum distance between points within the cluster, henceforth called GDI31. According to Vendramin et al. [ 69 ], the Gamma, C-, and Silhouette indices are the best-performing (over 80% correct hit rate), while the worst-performing are the Point-Biserial and the McClain-Rao indices (73% and 51% correct hit rate, respectively).
Fig 2 shows a schematic overview of the procedure we used to determine the personality types Big Five profiles, i.e. the cluster centers. To determine the best fitting cluster solution, we adopted the two-step procedure proposed by Blashfield and Aldenfelder [ 21 ] and subsequently used by Asendorpf [ 15 , 35 , 53 ] Boehm [ 41 ], Schnabel [ 24 ], Gramzow [ 28 ], and Herzberg and Roth [ 30 ], with a few adjustments concerning the clustering algorithms and the validity criteria.
LPA = latent profile analysis, SVM = Support Vector Machine.
https://doi.org/10.1371/journal.pone.0244849.g002
First, we drew 20 random samples of the full sample comprising all individuals who answered the Big-Five personality items in 2005 and 2009 with N = 22,820 and split every sample randomly into two halves. Second, all three clustering algorithms described above were performed on each half, saving the 3-, 4-,…,9- and 10-cluster solution. Third, participants of each half were reclassified based on the clustering of the other half of the same sample, again for every clustering algorithm and for all cluster solutions from three to 10 clusters. In contrast to Asendorpf [ 35 ], this was implemented not by calculating Euclidean distances, but by training a support vector machine classifier for every cluster of a cluster solution of one half-sample and reclassifying the members of the other half of the same sample by the SVM classifier. The advantages of this method are explained in the section on external criteria. This resulted in 20 samples x 2 halves per sample x 8 cluster solutions x 3 clustering algorithms, equaling 960 clustering solutions to be compared.
The fourth step was to compute the external criteria comparing each Ward followed by k-means, spectral, or probabilistic clustering solution of each half-sample to the clustering by the SVM classifier trained on the opposite half of the same sample, respectively. The external calculated in this step were Cohen's kappa, Rand’s index [ 62 ] and the Hubert & Arabie adjusted Rand index [ 63 ]. The fifth step consisted of averaging: We first averaged the external criteria values per sample (one value for each half), and then averaged the 20x4 external criteria values for each of the 3-,4-…, 10-cluster solutions for each algorithm.
The sixth step was to temporarily average the external criteria values for the 3-,4-,… 10-cluster solution over the three clustering algorithms and discard the cluster solutions that had a total average kappa below 0.6.
As proposed by Herzberg and Roth [ 30 ], we then calculated several internal cluster validity indices for all remaining cluster solutions. The internal validity indices which we used were, in particular, the C-index [ 70 ], the Baker-Hubert Gamma index [ 71 ], the G + index [ 72 ], the Generalized Dunn index 31 [ 68 ], the Point-Biserial index [ 44 ], the Silhouette index [ 73 ], AIC and BIC [ 65 ] and the S_Dbw index [ 66 ]. Using all of these criteria, it is possible to determine the best clustering solution in a mathematical/algorithmic manner.
The resulting clusters where then assigned names by calculating Euclidean distances to the clusters/personality types found in the literature, taking the nearest type within the 5-dimensional space defined by the respective Big Five values.
To examine the stability and consistency of the final cluster solution, in a last step, we then used the 2013 SOEP data sample to calculate a cluster solution using the algorithm and parameters which generated the solution with the best validity criteria for the 2005 and 2009 SOEP data sample. The 2013 personality prototypes were allocated to the personality types of the solution from the previous steps by their profile similarity measure D. Stability then was assessed by calculation of Rand-index, adjusted Rand-index and Cohen’s Kappa for the complete solution and for every single personality type. To generate the cluster allocations between the different cluster solutions, again we used SVM classifier as described above.
To assess the predictive and the construct validity of the resulting personality types, the inversed Euclidean distance for every participant to every personality prototype (averaged Big Five profile in one cluster) in the 5-dimensional Big-Five space was calculated and correlated with further personality, behavior and health measures mentioned above. To ensure that longitudinal reliability was assessed in this step, Big Five data assessed in 2005 were used to predict measures which where assessed three, four or five years later. The selection of participants with available data in 2005 and 2008 or later reduced the sample size in this step to N = 14,048.
Internal and external cluster fit indices
Table 2 shows the mean Cohen’s kappa values, averaged over all clustering algorithms and all 20 bootstrapped data permutations.
https://doi.org/10.1371/journal.pone.0244849.t002
Whereas the LPA and spectral cluster solutions seem to have better kappa values for fewer clusters, the kappa values of the k-means clustering solutions have a peak at five clusters, which is even higher than the kappa values of the three-cluster solutions of the other two algorithms.
Considering that these values are averaged over 20 independent computations, there is very low possibility that this result is an artefact. As the solutions with more than five clusters had an average kappa below .60, they were discarded in the following calculations.
Table 3 shows the calculated external and internal validity indices for the three- to five-cluster solutions, ordered by the clustering algorithm. Comparing the validity criterion values within the clustering algorithms reveals a clear preference for the five-cluster solution in the spectral as well as the Ward followed by k-means algorithm.
https://doi.org/10.1371/journal.pone.0244849.t003
Looking solely at the cluster validity results of the latent profile models, they seem to favor the three-cluster model. Yet, in a global comparison, only the S_Dbw index continues to favor the three-cluster LPA model, whereas the results of all other 12 validity indices support five-cluster solutions. The best clustering solution in terms of the most cluster validity index votes is the five-cluster Ward followed by k-means solution, and second best is the five-cluster spectral solution. It is particularly noteworthy that the five-cluster K-means solution has higher values on all external validity criteria than all other solutions. As these values are averaged over 20 independent cluster computations on random data permutations, and still have better values than solutions with fewer clusters despite the fact that these indices have a bias towards solutions with fewer clusters [ 42 ], there seems to be a substantial, replicable five-component structure in the Big Five Data of the German SOEP sample.
Description of the prototypes
The mean z-scores on the Big Five factors of the five-cluster k-means as well as the spectral solution are depicted in Fig 2 . Also depicted is the five-cluster LPA solution, which is, despite having poor internal and external validity values compared to the other two solutions, more complicated to interpret. To find the appropriate label for the cluster partitions, the respective mean z-scores on the Big Five factors were compared with the mean z-scores found in the literature, both visually and by the Euclidean distance.
The spectral and the Ward followed by k-means solution overlap by 81.3%; the LPA solution only overlaps with the other two solutions by 21% and 23%, respectively. As the Ward followed by k-means solution has the best values both for external and internal validity criteria, we will focus on this solution in the following.
The first cluster has low neuroticism and high values on all other scales and includes on average 14.4% of the participants (53.2% female; mean age 53.3, SD = 17.3). Although the similarity to the often replicated resilient personality type is already very clear merely by looking at the z-scores, a very strong congruence is also revealed by computing the Euclidean distance (0.61). The second cluster is mainly characterized by high neuroticism, low extraversion and low openness and includes on average 17.3% of the participants (54.4% female; mean age 57.6, SD = 18.2). It clearly resembles the overcontroller type, to which it also has the shortest Euclidean distance (0.58). The fourth cluster shows below-average values on the factors neuroticism, extraversion and openness, as opposed to above-average values on openness and conscientiousness. It includes on average 22.5% of the participants (45% female; mean age 56.8, SD = 17.6). Its mean z-scores closely resemble the reserved personality type, to which it has the smallest Euclidean distance (0.36). The third cluster is mainly characterized by low conscientiousness and low openness, although in the spectral clustering solution, it also has above-average extraversion and openness values. Computing the Euclidean distance (0.86) yields the closest proximity to the undercontroller personality type. This cluster includes on average 24.6% of the participants (41.3% female; mean age 50.8, SD = 18.3). The fifth cluster exhibits high z-scores on every Big Five trait, including a high value for neuroticism. Computing the Euclidean distances to the previously found types summed up in Fig 1 reveals the closest resemblance with the confident type (Euclidean distance = 0.81). Considering the average scores of the Big Five traits, it resembles the confident type from Herzberg and Roth [ 30 ] and Collani and Roth [ 10 ] as well as the resilient type, with the exception of the high neuroticism score. Having above average values on the more adaptive traits while having also above average neuroticism values reminded a reviewer from a previous version of this paper of the vulnerable but invincible children of the Kauai-study [ 74 ]. Despite having been exposed to several risk factors in their childhood, they were well adapted in their adulthood except for low coping efficiency in specific stressful situations. Taken together with the lower percentage of participants in the resilient cluster in this study, compared to previous studies, we decided to name the 5 th cluster vulnerable-resilient. Consequently, only above or below average neuroticism values divided between resilient and vulnerable resilient. On average, 21.2% of the participants were allocated to this cluster (68.3% female; mean age 54.9, SD = 17.4).
Summarizing the descriptive statistics, undercontrollers were the “youngest” cluster whereas overcontrollers were the “oldest”. The mean age differed significantly between clusters ( F [4, 22820] = 116.485, p <0.001), although the effect size was small ( f = 0.14). The distribution of men and women between clusters differed significantly (c 2 [ 4 ] = 880.556, p <0.001). With regard to sex differences, it was particularly notable that the vulnerable-resilient cluster comprised only 31.7% men. This might be explained by general sex differences on the Big Five scales. According to Schmitt et al. [ 75 ], compared to men, European women show a general bias to higher neuroticism (d = 0.5), higher conscientiousness (d = 0.3) and higher extraversion and openness (d = 0.2). As the vulnerable-resilient personality type is mainly characterized by high neuroticism and above-average z-scores on the other scales, it is therefore more likely to include women. In turn, this implies that men are more likely to have a personality profile characterized mainly by low conscientiousness and low openness, which is also supported by our findings, as only 41.3% of the undercontrollers were female.
Concerning the prototypicality of the five-cluster solution compared to the mean values extracted from previous studies, it is apparent that the resilient, the reserved and the overcontroller type are merely exact replications. In contrast to previous findings, the undercontrollers differed from the previous findings cited above in terms of average neuroticism, whereas the vulnerable-resilient type differed from the previously found type (labeled confident) in terms of high neuroticism.
Stability and consistency
Inspecting the five cluster solution using the k-means algorithm on the Big Five data of the 2013 SOEP sample seemed to depict a replication of the above described personality types. This first impression was confirmed by the calculation of the profile similarity measure D between the 2005/2009 and 2013 SOEP sample cluster solutions, which yielded highest similarity for the undercontroler (D = 0.27) and reserved (D = 0.36) personality types, followed by the vulnerable-resilient (D = 0.37), overcontroler (D = 0.44) and resilient (D = 0.50) personality types. Substantial agreement was confirmed by the values of the Rand index (.84) and Cohen’ Kappa (.70) whereas the Hubert Arabie adjusted Rand Index (.58) indicated moderate agreement for the comparison between the kmeans cluster solution for the 2013 SOEP sample and the cluster allocation with an SVM classifier trained on the 2005 and 2009 kmeans cluster solution.
Predictive validity
In view of the aforementioned criticisms that (a) predicting dimensional variables will mathematically favor dimensional personality description models, and (b) using dichotomous predictors will necessarily provide less explanation of variance than a model using five continuous predictors, we used the profile similarity measure D [ 76 ] instead of dichotomous dummy variables accounting for the prototype membership. Correlations between the inversed Euclidean similarity measure D to the personality types and patience, risk-taking, spontaneity/impulsivity, locus of control, affective wellbeing, self-esteem and health are depicted in Table 4 .
https://doi.org/10.1371/journal.pone.0244849.t004
Patience had the highest association with the reserved personality type (r = .19, p < .001). The propensity to risky behavior, e.g. while driving (r = .17, p < .001), in financial matters (r = .17, p < .001) or in health decisions (r = .13, p < .001) was most highly correlated with the undercontroller personality type. This means that the more similar the Big-Five profile to the above-depicted undercontroller personality prototype, the higher the propensity for risky behavior. The average correlation across all three risk propensity scales with the undercontroller personality type is r = .21, with p < .001. This is in line with the postulations by Block and Block and subsequent replications by Caspi et al. [ 19 , 48 ], Robins et al. [ 1 ] and Herzberg [ 33 ] about the undercontroller personality type. Spontaneity/impulsivity showed the highest correlation with the overcontroller personality type (r = -.18, p<0.001). This is also in accordance with Block and Block, who described this type as being non-impulsive and appearing constrained and inhibited in actions and emotional expressivity.
Concerning locus of control, proximity to the resilient personality profile had the highest correlation with internal locus of control (r = .25, p < .001), and in contrast, the more similar the individual Big-Five profile was to the overcontroller personality type, the higher the propensity for external allocation of control (r = .22, p < .001). This is not only in line with Block and Block’s postulations that the resilient personality type has a good repertoire of coping behavior and therefore perceives most situations as “manageable” as well as with the findings by [ 33 ], but is also in accordance with findings regarding the construct and development of resilience [ 77 , 78 ].
Also in line with the predictions of Block and Block and replicating the findings of Herzberg [ 33 ], self-esteem was correlated the highest with the resilient personality profile similarity (r = .33, p < .001), second highest with the reserved personality profile proximity (r = .15, p < .001), and negatively correlated with the overcontroller personality type (r = -.27, p < .001).
This pattern also applies to affective and cognitive wellbeing as well as physical and mental health measured by the SF-12. Affective wellbeing was correlated the highest with similarity to the resilient personality type (r = .27, p < .001), and second highest with the reserved personality type (r = .23, p < .001). The overcontroller personality type, in contrast, showed a negative correlation with affective (r = -.16, p < .001) and cognitive (r = -21, p < .001) wellbeing. Concerning health, a remarkable finding is that lack of physical health impairment correlated the highest with the resilient personality profile similarity (p = -.23, p < .001) but lack of mental health impairment correlated the highest with the reserved personality type (r = -.15, p < .001). The highest correlation with mental health impairments (r = .11, p < .001), as well as physical health impairments (r = .16, p < .001) was with the overcontroller personality profile similarity. It is striking that although the undercontroller personality profile similarity was associated with risky health behavior, it had a negative association with health impairment measures, in contrast to the overcontroller personality type, which in turn had no association with risky health behavior. This result is in line with the link of internalizing and externalizing behavior with the overcontroller and undercontroller types [ 79 ], respectively. Moreover, it is also in accordance with the association of internalizing problems with somatic symptoms and/or symptoms of depressiveness and anxiety [ 80 ].
A further noteworthy finding is that these associations cannot be solely explained by the high neuroticism of the overcontroller personality type, as the vulnerable-resilient type showed a similar level of neuroticism but no correlation with self-esteem, the opposite correlation with impulsivity, and far lower correlations with health measures or locus of control. The vulnerable-resilient type showed also a remarkable distinction to the other types concerning the correlations to wellbeing. While for all other types, the direction and significance of the correlations to affective and cognitive measures of wellbeing were alike, the vulnerable-resilient type only had a significant negative correlation to affective wellbeing while having no significant correlation to measures of cognitive wellbeing.
To provide an overview of the particular associations of the Big Five values with all of the above-mentioned behavior and personality measures, Table 5 shows the bivariate correlations.
https://doi.org/10.1371/journal.pone.0244849.t005
Investigating the direction of the correlation and the relativity of each value to each other row-wise reveals, to some extent, a clear resemblance with the z-scores of the personality types shown in Fig 3 . Correlation profiles of risk taking, especially the facet risk-taking in health issues and locus of control, clearly resemble the undercontroller personality profile (negative correlations with openness and conscientiousness, positive but lower correlations with extraversion and openness). Patience had negative correlations with neuroticism and extraversion, and positive correlations with openness and conscientiousness, which in turn resembles the z-score profile of the reserved personality profile. Spontaneity/impulsivity had moderate to high positive correlations with extraversion and openness, and low negative correlations with openness and neuroticism, which resembles the inverse of the overcontroller personality profile. Self-esteem as well as affective and cognitive wellbeing correlations with the Big Five clearly resemble the resilient personality profile: negative correlations with neuroticism, and positive correlations with extraversion, openness, openness and conscientiousness. Inspecting the SF-12 health correlation, in terms of both physical and mental health, reveals a resemblance to the inversed resilient personality profile (high correlation with neuroticism, low correlation with extraversion, openness, openness and conscientiousness, as well as a resemblance with the overcontroller profile (positive correlation with neuroticism, negative correlation with extraversion).
https://doi.org/10.1371/journal.pone.0244849.g003
On the variable level, neuroticism had the highest associations with almost all of the predicted variables, with the exception of impulsivity, which was mainly correlated with extraversion and openness. It is also evident that all variables in question here are correlated with three or more Big Five traits. This can be seen as support for hypothesis that the concept of personality prototypes has greater utility than the variable-centered approach in understanding or predicting more complex psychological constructs that are linked to two or more Big Five traits.
The goal of this study was to combine different methodological approaches while overcoming the shortcomings of previous studies in order to answer the questions whether there are replicable personality types, how many of them there are, and how they relate to Big Five traits and other psychological and health-related constructs. The results revealed a robust five personality type model, which was able to significantly predict all of the psychological constructs in question longitudinally. Predictions from previous findings connecting the predicted variables to the particular Big Five dimensions underlying the personality type model were confirmed. Apparently, the person-centered approach to personality description has the most practical utility when predicting behavior or personality correlates that are connected to more than one or two of the Big Five traits such as self-esteem, locus of control and wellbeing.
This study fulfils all three criteria specified by von Eye & Bogat [ 81 ] regarding person-oriented research and considers the recommendations regarding sample size and composition by Herzberg and Roth [ 30 ]. The representative and large sample was analyzed under the assumption that it was drawn from more than one population (distinct personality types). Moreover, several external and internal cluster validity criteria were taken into account in order to validate the groupings generated by three different cluster algorithms, which were chosen to represent broad ranges of clustering techniques [ 60 , 82 ]. The Ward followed by K-means procedure covers hierarchical as well as divisive partitioning (crisp) clustering, the latent profile algorithm covers density-based clustering with probabilistic models and information theoretic validation (AIC, BIC), and spectral clustering represents graph theoretic as well as kernel-based non-linear clustering techniques. The results showed a clear superiority of the five-cluster solution. Interpreting this grouping based on theory revealed a strong concordance with personality types found in previous studies, which we could ascertain both in absolute mean values and in the Euclidean distances to mean cluster z-scores extracted from 19 previous studies. As no previous study on personality types used that many external and internal cluster validity indices and different clustering algorithms on a large data set of this size, the present study provides substantial support for the personality type theory postulating the existence of resilient, undercontroller, overcontroller, vulnerable-resilient and reserved personality types, which we will refer to with RUO-VR subsequently. Further, our findings concerning lower validity of the LPA cluster solutions compared to the k-means and spectral cluster solutions suggest that clustering techniques based on latent models are less suited for the BFI-S data of the SOEP sample than iterative and deterministic methods based on the k-means procedure or non-linear kernel or graph-based methods. Consequently, the substance of the clustering results by Specht et. al. [ 36 ], which applied latent profile analysis on the SOEP sample, may therefore be limited.
But the question, if the better validity values of the k-means and spectral clustering techniques compared to the LPA indicate a general superiority of these algorithms, a superiority in the field of personality trait clustering or only a superiority in clustering this specific personality trait assessment (BFI-S) in this specific sample (SOEP), remains subject to further studies on personality trait clustering.
When determining the longitudinal predictive validity, the objections raised by Asendorpf [ 53 ] concerning the direct comparison of person-oriented vs. variable-oriented personality descriptions were incorporated by using continuous personality type profile similarity based on Cronbach and Gleser [ 75 ] instead of dichotomous dummy variables as well as by predicting long-term instead of cross-sectionally assessed variables. Using continuous profile similarity variables also resolves the problem that potentially important information about members of the same class is lost in categorical personality descriptions [ 15 , 53 , 83 ]. Predictions regarding the association of the personality types with the assessed personality and behavior correlates, including risk propensity, impulsivity, self-esteem, locus of control, patience, cognitive and affective wellbeing as well as health measures, were confirmed.
Overcontrollers showed associations with lower spontaneity/impulsivity, with lower mental and physical health, and lower cognitive as well as affective wellbeing. Undercontrollers were mainly associated with higher risk propensity and higher impulsive behavior. These results can be explained through the connection of internalizing and externalizing behavior with the overcontroller and undercontroller types [ 5 – 7 , 78 ] and further with the connection of internalizing problems with somatic symptoms and/or symptoms of depressiveness and anxiety [ 79 ]. The dimensions or categories of internalizing and externalizing psychopathology have a long tradition in child psychopathology [ 84 , 85 ] and have been subsequently replicated in adult psychopathology [ 86 , 87 ] and are now basis of contemporary approaches to general psychopathology [ 88 ]. A central proceeding in this development is the integration of (maladaptive) personality traits into the taxonomy of general psychopathology. In the current approach, maladaptive personality traits are allocated to psychopathology spectra, such as the maladaptive trait domain negative affectivity to the spectrum of internalizing disorders. However, the findings of this study suggests that not specific personality traits are intertwined with the development or the occurrence of psychopathology but specific constellations of personality traits, in other words, personality profiles. This hypothesis is also supported by the findings of Meeus et al. [ 8 ], which investigated longitudinal transitions from one personality type to another with respect to symptoms of generalized anxiety disorder. Transitions from resilient to overcontroller personality profiles significantly predicted higher anxiety symptoms while the opposite was found for transitions from overcontroller to resilient personality profiles.
The resilient personality type had the strongest associations with external locus of control, higher patience, good health and positive wellbeing. This not only confirms the characteristics of the resilient type already described by Block & Block [ 18 ] and subsequently replicated, but also conveys the main characteristics of the construct of resilience itself. While the development of resiliency depends on the quality of attachment experiences in childhood and youth [ 89 ], resiliency in adulthood seems to be closely linked to internal locus of control, self-efficacy and self-esteem. In other words, the link between secure attachment experiences in childhood and resiliency in adulthood seems to be the development of a resilient personality trait profile. Seen the other way around, the link between traumatic attachment experiences or destructive environmental factors and low resiliency in adulthood may be, besides genetic risk factors, the development of personality disorders [ 90 ] or internalizing or externalizing psychopathology [ 91 ]. Following this thought, the p-factor [ 92 ], i.e. a general factor of psychopathology, may be an index of insufficient resilience. Although from the viewpoint of personality pathology, having a trait profile close to the resilient personality type may be an index of stable or good personality structure [ 93 ], i.e. personality functioning [ 94 ], which, though being consistently associated with general psychopathology and psychosocial functioning, should not be confused with it [ 95 ].
The reserved personality type had the strongest associations with higher patience as well as better mental health. The vulnerable-resilient personality type showed low positive correlations with spontaneity/impulsivity and low negative correlations with patience as well as health and affective wellbeing.
Analyzing the correlations of the dimensional Big Five values with the predicted variables revealed patterns similar to the mean z-scores of the personality types resilient, overcontrollers, undercontrollers and reserved. Most variables had a low to moderate correlation with just one personality profile similarity, while having at least two or three low to moderate correlations with the Big Five measures. This can be seen as support for the argument of Chapman [ 82 ] and Asendorpf [ 15 , 53 ] that personality types have more practical meaning in the prediction of more complex correlates of human behavior and personality such as mental and physical health, wellbeing, risk-taking, locus of control, self-esteem and impulsivity. Our findings further underline that the person-oritented approach may better be suited than variable-oriented personality descriptions to detect complex trait interactions [ 40 ]. E.g. the vulnerable-resilient and the overcontroller type did not differ in their high average neuroticism values, while differing in their correlations to mental and somatic health self-report measures. It seems that high neuroticism is far stronger associated to lower mental and physical health as well as wellbeing if it occurs together with low extraversion and low openness as seen in the overcontroller type. This differential association between the Big-Five traits also affects the correlation between neuroticism and self-esteem or locus of control. Not differing in their average neuroticism value, the overcontroller personality profile had moderate associations with low self-esteem and external locus of control while the vulnerable-resilient personality profile did only show very low or no association. Further remarkable is that the vulnerable-resilient profile similarity had no significant correlation with measures of cognitive wellbeing while being negatively correlated with affective wellbeing. This suggests that individuals with a Big-Five personality profile similar to the vulnerable-resilient prototype seem not to perceive impairments in their wellbeing, at least on a cognitive layer, although having high z-values in neuroticism. Another explanation for this discrepancy as well as for the lack of association of the vulnerable-resilient personality profile to low self-esteem and external locus of control though having high values in neuroticism could be found in the research on the construct of resilience. Personalities with high neuroticism values but stable self-esteem, internal locus of control and above average agreeableness and extraversion values may be the result of the interplay of multiple protective factors (e.g. close bond with primary caregiver, supportive teachers) with risk factors (e.g. parental mental illness, poverty). The development of a resilient personality profile with below average neuroticism values, on the other hand, may be facilitated if protective factors outweigh the risk factors by a higher ratio.
An interesting future research question therefore concerns to what extent personality types found in this study may be replicated using maladaptive trait assessments according to DSM-5, section III [ 96 ] or the ICD-11 personality disorder section [ 97 ] (for a comprehensive overview on that topic see e.g. [ 98 ]). As previous studies showed that both DSM-5 [ 99 ] and ICD-11 [ 100 ] maladaptive personality trait domains may be, to a large extent, conceptualized as maladaptive variants of Big Five traits, it is highly likely that also maladaptive personality trait domains align around personality prototypes and that the person-oriented approach may amend the research field of personality pathology [ 101 ].
Taken together, the findings of this study connect the variable centered approach of personality description, more precisely the Big Five traits, through the concept of personality types to constructs of developmental psychology (resiliency, internalizing and externalizing behavior and/or problems) as well as clinical psychology (mental health) and general health assessed by the SF-12. We could show that the distribution of Big Five personality profiles, at least in the large representative German sample of this study, aggregates around five prototypes, which in turn have distinct associations to other psychological constructs, most prominently resilience, internalizing and externalizing behavior, subjective health, patience and wellbeing.
Limitations
Several limitations of the present study need to be considered: One problem concerns the assessment of patience, self-esteem and impulsivity. From a methodological perspective, these are not suitable for the assessment of construct validity as they were assessed with only one item. A further weakness is the short Big Five inventory with just 15 items. Though showing acceptable reliability, 15 items are more prone to measurement errors than measures with more items and only allow a very broad assessment of the 5 trait domains, without information on individual facet expressions. A more big picture question is if the Big Five model is the best way to assess personality in the first place. A further limitation concerns the interpretation of the subjective health measures, as high neuroticism is known to bias subjective health ratings. But the fact that the vulnerable-resilient and the overcontroler type had similar average neuroticism values but different associations with the subjective health measures speaks against a solely neuroticism-based bias driven interpretation of the associations of the self-reported health measures with the found personality clusters. Another limitation is the correlation between the personality type similarities: As they are based on Euclidean distances and the cluster algorithms try to maximize the distances between the cluster centers, proximity to one personality type (that is the cluster mean) logically implies distance from the others. In the case of the vulnerable-resilient and the resilient type, the correlation of the profile similarities is positive, as they mainly differ on only one dimension (neuroticism). These high correlations between the profile similarities prevents or diminishes, due to the emerging high collinearity, the applicability of general linear models, i.e. regression to calculate the exact amount of variance explained by the profile similarities.
The latter issue could be bypassed by assessing types and dimensions with different questionnaires, i.e. as in Asendorpf [ 15 ] with the California Child Q-set to determine the personality type and the NEO-FFI for the Big Five dimensions. Another possibility is to design a new questionnaire based on the various psychological constructs that are distinctly associated with each personality type, which is probably a subject for future person-centered research.
Acknowledgments
The data used in this article were made available by the German Socio-Economic Panel (SOEP, Data for years 1984–2015) at the German Institute for Economic Research, Berlin, Germany. However, the findings and views reported in this article are those of the authors. To ensure the confidentiality of respondents’ information, the SOEP adheres to strict security standards in the provision of SOEP data. The data are reserved exclusively for research use, that is, they are provided only to the scientific community. All users, both within the EEA (and Switzerland) and outside these countries, are required to sign a data distribution contract.
- View Article
- PubMed/NCBI
- Google Scholar
- 21. Blashfield RK, Aldenderfer MS. The methods and problems of cluster analysis. Handbook of multivariate experimental psychology: Springer; 1988. p. 447–73. https://doi.org/10.1002/1097-4679(198801)44:1<47::aid-jclp2270440110>3.0.co;2-r pmid:3343363
- 48. Caspi A. Personality development across the life course (In Damon W.& Eisenberg N.(Eds.). Handbook of child psychology: Social, emotional, and personality development (Vol. 3, pp. 311–388). Hoboken. NJ: Wiley; 1998.
- 59. Bacher J, Pöge A, Wenzig K. Clusteranalyse: Anwendungsorientierte Einführung in Klassifikationsverfahren: Oldenbourg Verlag; 2010.
- 61. Cristianini N, Shawe-Taylor J. An introduction to support vector machines and other kernel-based learning methods: Cambridge university press; 2000.
- 66. Halkidi M, Vazirgiannis M, editors. Clustering validity assessment: Finding the optimal partitioning of a data set. Data Mining, 2001 ICDM 2001, Proceedings IEEE International Conference on; 2001: IEEE.
- 93. Kernberg OF. Borderline Personality Disorder and Borderline Personality Organization: Psychopathology and Psychotherapy. Handbook of Personality Disorders: Theory and Practice. Hoboken, NJ, US: John Wiley & Sons Inc; 2004. p. 92–119.
- 96. Diagnostic and statistical manual of mental disorders: DSM-5, (2013).
- 97. ICD-11 Clinical Descriptions and Diagnostic Guidelines for Mental and Behavioural Disorders. World Health Organisation, (2019).
METHODS article
Predicting personality and psychological distress using natural language processing: a study protocol.
- 1 School of Psychology, Korea University, Seoul, South Korea
- 2 Department of Software, Sejong University, Seoul, South Korea
- 3 KU Mind Health Institute, Korea University, Seoul, South Korea
Background: Self-report multiple choice questionnaires have been widely utilized to quantitatively measure one’s personality and psychological constructs. Despite several strengths (e.g., brevity and utility), self-report multiple choice questionnaires have considerable limitations in nature. With the rise of machine learning (ML) and Natural language processing (NLP), researchers in the field of psychology are widely adopting NLP to assess psychological construct to predict human behaviors. However, there is a lack of connections between the work being performed in computer science and that of psychology due to small data sets and unvalidated modeling practices.
Aims: The current article introduces the study method and procedure of phase II which includes the interview questions for the five-factor model (FFM) of personality developed in phase I. This study aims to develop the interview (semi-structured) and open-ended questions for the FFM-based personality assessments, specifically designed with experts in the field of clinical and personality psychology (phase 1), and to collect the personality-related text data using the interview questions and self-report measures on personality and psychological distress (phase 2). The purpose of the study includes examining the relationship between natural language data obtained from the interview questions, measuring the FFM personality constructs, and psychological distress to demonstrate the validity of the natural language-based personality prediction.
Methods: Phase I (pilot) study was conducted to fifty-nine native Korean adults to acquire the personality-related text data from the interview (semi-structured) and open-ended questions based on the FFM of personality. The interview questions were revised and finalized with the feedback from the external expert committee, consisting of personality and clinical psychologists. Based on the established interview questions, a total of 300 Korean adults will be recruited using a convenience sampling method via online survey. The text data collected from interviews will be analyzed using the natural language processing. The results of the online survey including demographic data, depression, anxiety, and personality inventories will be analyzed together in the model to predict individuals’ FFM of personality and the level of psychological distress (phase 2).
Introduction
Technological advances brought numerous changes in analyzing and predicting data in the field of psychology. In particular, the recent fourth industrial revolution and the development of computer technology made it possible to quickly and accurately analyze and predict human characteristics, with further innovations taking place. While many other fields (medicine, marketing, engineering, etc.) are rapidly integrating computer skills to develop technology that can be utilized in real life, the application of technology in the field of psychology remains limited and steady ( Ahmad et al., 2020 ; Le Glaz et al., 2021 ). Many attempts are being made to evaluate and identify the psychological state or characteristics of an individual. However, research and technological development face challenges due to numerous theories and immense structures of personality ( Zunic et al., 2020 ).
Traditional Personality Assessment
Understanding individuals’ personality gives substantial information about how people behave and adapt to the world. Personality psychology theories made attempts to explain human personality in a concrete and valid way, through accurately measuring individuals’ personality. In the field of clinical psychology and psychiatry, classifying personality disorders using personality measurements is a central objective. Disorders in personality have been categorically understood within the diagnostic system for a long time and assessing the presence or absence of the disorder has been an important topic. However, this method poses problems such as heterogeneity within the same category because the boundaries between disabilities are unclear, or having one disorder belonging to two or more categories at the same time ( Widiger and Trull, 2007 ; Livesley, 2010 ). In addition, the inter-rater reliability among experienced clinical psychologists and/or psychiatrists diagnosing personality disorders using the categorical approach did not reach a sufficient level ( Kraemer et al., 2012 ; Esbec and Echeburúa, 2015 ). For this reason, a dimensional model, which understands personality as a complex hierarchy of continuously distributed attributes rather than a categorical approach, is receiving attention in understanding personality and diagnosing disorders ( Costa and McCrae, 2017 ). Many empirical studies have proven its validity and usefulness ( Widiger, 2017 ). The Five-Factor Model (FFM), which explains personality with Neuroticism, Extraversion, Openness, Agreeableness, Conscientiousness, and their many facets, is a well-known dimensional model of personality ( McCrae and John, 1992 ).
Traditionally, a self-report multiple choice questionnaires have been widely utilized to quantitatively measure one’s personality and other psychological constructs. This measure has extreme practicality in that it simply requires the target person’s participation and can readily collect sufficient information in one sitting ( Paulhus and Vazire, 2007 ). Despite other definite strengths (e.g., brevity and utility), the self-report multiple choice questionnaires have several limitations in nature. First, it is possible for respondents to hide or distort their responses, especially in the context of forensic or evaluation settings for employment ( White et al., 2008 ; Fan et al., 2012 ). To prevent such manipulation, the L-scale was designed to detect and provide information on responses intentionally distorted or skewed toward socially desirable traits ( Furnham, 1986 ). Although L-scale can detect “faking” subjects, limitation remains in accurately discerning every faking subject from honest subjects ( Elliot et al., 1996 ). In addition, since item contents and anchors are pre-determined, test respondents cannot provide detailed information beyond test items ( Arntz et al., 2012 ). According to Paulhus and Vazire (2007) , this is especially evident in dichotomous response formats (e.g., Yes-No, True-False, and Agree-Disagree). Finally, test bias due to absolute or random responding also remains a critical issue in test administration ( Holden et al., 2012 ; Al-Mosaiwi and Johnstone, 2018 ).
Structured or semi-structured clinical interview methods are utilized in personality assessment and diagnosis since it allows more information than the self-reported multiple choice questionnaires, and participants are less likely to hide or distort their responses. These interview methods can also increase the reliability of personality disorder diagnosis in compliance to the diagnostic criteria ( Wood et al., 2002 ). Clinicians can identify discrepancies found in self-reported tests and obtain additional information on responses through follow-up questions, which is essential for diagnosing personality disorders ( Samuel et al., 2013 ). Especially, the Structured Interview for the FFM (SIFFM; Trull and Widiger, 1997 ) and the Structured Clinical Interview for the DSM-5 Alternative Model for Personality Disorder (SCID-5-AMPD) are representative interview instruments that evaluate personality traits in terms of dimensional aspect. These interviews may better describe behavioral symptoms and diagnostic criteria in a systematic and standardized manner because of their superior assessment of observable behavioral symptoms ( Hopwood et al., 2008 ). However, it is important to note that semi-structured interview requires a lot of time and manpower. Also, evaluation relying on clinician’s judgment may cause diagnosis bias or problems with reliability.
Machine Learning and Natural Language Processing in Psychology
With advancements in computer technology, new attempts have been made to analyze psychological traits through computer programming and to predict them quickly, efficiently, and accurately. Especially with the rise of Machine Learning (ML), Deep Learning (DL), and Natural Language Processing (NLP), researchers in the field of psychology are widely adopting NLP to assess psychological construct or to predict human behaviors. ML and DL mainly focus on developing algorithms to discover certain patterns and predict new data accumulated from prior experiences, learned by computer programs through previously performed similar tasks. ML and DL enable researchers to identify independent variables, which were previously under-recognized, and to handle tremendous data. Natural language processing (NLP), a branch of ML research and applications, incorporates computer programming that automatically understand and analyze natural language text. NLP researchers have developed appropriate tools and techniques to enable computer systems to understand and manipulate natural language to perform desired tasks ( Chowdhury, 2003 ). NLP also makes it possible to quantify and analyze qualitative text data.
According to previous studies, some researchers applied ML and NLP to measure and predict psychological traits such as personality and psychiatric disorders. For example, Al Hanai et al. (2018) attempted to predict depression by developing an automated depression-detection algorithm that learns from a sequence of questions and answers. Jayaratne and Jayatilleke (2020) sought to predict one’s personality as an indicator of job performance and satisfaction using the textual content of interview answers. Also, recent studies aim to identify psychotic symptoms and improve the efficient detection of individuals at risk for psychosis by applying NLP to language data ( Chandran et al., 2019 ; Corcoran and Cecchi, 2020 ; Irving et al., 2021 ). When Park et al. (2015) utilized ML and NLP to build the open-vocabulary language model with Facebook posts, the model appropriately predicted the participants’ personality based on the FFM.
Likewise, studies attempting to predict and diagnose individual psychological characteristics using ML and NLP techniques are gradually increasing in the field of psychology and mental health. This not only increases efficiency, but also reduces the influence of human bias of the existing measurements ( Oswald et al., 2020 ). However, it is notable that the prior studies still have limitations and many areas need to be supplemented. First, there is a lack of connections between the work being performed in computer science and that of psychology ( Stachl et al., 2020 ). Previous research works in computer science fall short in providing in-depth personality assessment and interpretation, only citing the psychological literature with respect to dependent (target) variables like personality inventories. The explanatory power of the results is questionable in that it overlooked the importance of personality theories. For instance, some studies not only applied non representative SNS profiles ( Amichai-Hamburger and Vinitzky, 2010 ; Lima and De Castro, 2014 ) or consumption data ( Gladstone et al., 2019 ) as independent (predictor) variables for personality prediction, but results of the MBTI (Myers-Briggs Type Indicators) test, which classify human characteristics into four domains, were also used as target variables despite lacking sufficient theoretical basis ( Cui and Qi, 2017 ; Gjurković and Šnajder, 2018 ; Sönmezöz et al., 2020 ). Using these variables makes it possible for convenient and quick big data collection and provides simple labeling for ML, but it does not give an adequate explanation for the predicted results. Second, studies published in the field of clinical and personality psychology often show potential overfitting problems caused by smaller datasets ( Yarkoni and Westfall, 2017 ) and raise concerns about questionable modeling practices ( Mønsted et al., 2018 ). Lastly, it should also be noted that compared to Western countries, studies on language-personality interconnectedness in the Eastern countries and cultures have been relatively less reported.
Traditional approaches using self-report multiple choice questionnaires and recent approaches using machine learning both have their strengths and limitations in personality assessment. Although ML allows faster mappings between data, the results are meaningful only when explanations for complex multidimensional human personality can be provided based on theory. The current study aims to examine the relationship between the FFM personality constructs, psychological distress, and natural language data, overcoming the lack of connection between the field of computer science and psychology. We developed the interview (semi-structured) and open-ended questions for the FFM-based personality assessments, specifically designed with experts in the field of clinical and personality psychology (phase 1). Developed interview questions that could extract linguistic data reflecting personality were formulated and will further be analyzed by NLP. This will help us acquire essential text data to increase the efficiency of ML analysis at the final research stage. We will collect the personality-related text data using the interview questions and extract linguistic features predicting the FFM of personality and psychological distress such as depression, anxiety, and risks for suicidality (phase 2). Finally, we will develop algorithm models that can predict personality with text data. We expect that the newly developed models will better predict personality compared to the traditional assessments. This will bring about important implications in that the research approach is not only exploratory but also theory driven, with sufficient amount of text data provided.
We hypothesize that the extracted linguistic feature (1) would identify individuals’ personality traits based on the FFM, having significant correlations with the self-reported FFM personality inventories and (2) would discern a linguistic marker of psychological distress. Also, (3) qualitative differences between the text data obtained from the video interview and the text data obtained from the online survey will be examined through an exploratory method.
Method and Analysis
Study design and procedure.
The current research consists of two phases to provide more explanatory power. In phase I, we conducted a pilot study to develop the semi-structured interview questions for the FFM of personality. In Phase II, the interview for the FFM of personality developed in phase I will be applied in conducting data collection to predict personality and psychological distress (study design and procedure are shown in Figure 1 ). All the courses of this study will be approved by Korea University’s Institutional Review Boards (IRB). Data will be collected by an online platform considering the COVID-19 pandemic. Finally, the current study will not include any intervention such as pharmacotherapy or psychotherapy.
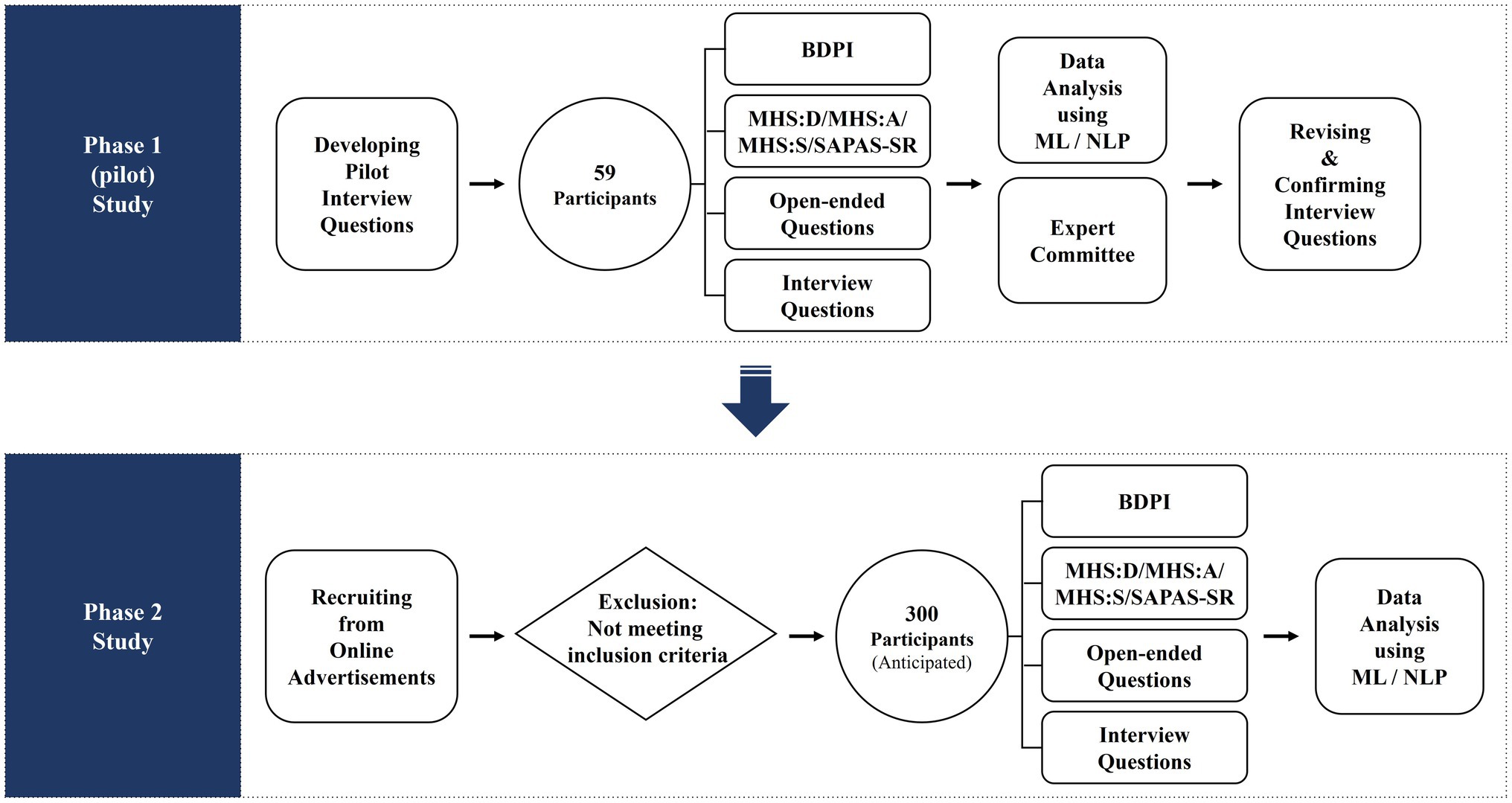
Figure 1 . Study design flow.
Phase 1 (Pilot) Study
A pilot study was conducted as a process for developing “semi-structured interview” questions based on the FFM of personality. The study was carried out for a year starting from September 2020. First, a preliminary question pool of 66 items was generated by licensed clinical psychologists, social and personality psychologists, psychometricians, and experts in human resource departments. All questions were designed to measure 10 domains and 33 sub-facets based on Costa and McCrae’s Five-Factor model ( McCrae and Costa, 1996 ). Then, we conducted a primary data collection on 59 participants using the 29 items selected through several additional review processes made by experts. All responses collected were once again empirically evaluated for adequacy from an external expert committee, consisting of psychologists with expertise in personality and psychopathology. In particular, differential validity, ambiguity in expressions, and the intention of the question were carefully considered. Furthermore, additional analysis based on ML and NLP for the text data was administered. At this stage, we applied information theory to identify the entropy of words that are highly correlated with specific personality traits, and Latent Dirichlet Allocation (LDA) was used to exploratorily analyze what kind of words related to which certain subjects are frequently used by people. Through these experts’ feedback and computing analysis process, the existing 29 questions were revised and finalized as a total of 18 questions.
Phase 2 Study
In phase 2 study, we will collect data using a semi-structured interview, developed in a pilot study and self-report inventories. Phase II started in November, 2021 and will be completed in August, 2023. Participants will first be asked to respond to the self-report questionnaire about depression, anxiety, suicide risk, and personality via online survey platform (Qualtrics). Then, they will participate in a semi-structured interview session with the researcher in an online meeting or chatting platform. All responses of the participants to the interview questions were stored and analyzed in the form of text. To extract text features, text data from the interview and the online survey will be preprocessed using morphological analysis and analyzed by applying the NLP and ML model. The results of the online survey including demographic data, depression, anxiety, suicidality, and personality inventories will be investigated together in the model to predict individuals’ personality based on the FFM and the level of psychological distress. The moderating role of qualitative differences of linguistic information, in terms of written text and transcribed speech, in the effects of personality on language patterns or expression needs to be further investigated. To explore this, the same questions will be asked to participants in both online survey (text submission) and online video interview (speech transcribed into text).
Participants
Participants will be recruited from online or local advertisements posted in university communities or job search websites. All participants will be provided with written informed consent before participating in the study. The inclusion criteria are (1) being over 18 years and (2) fluent in Korean language. The participants (1) who have a history of brain surgery or (2) intellectual disability will be excluded. A total of 59 participants were recruited in Phase 1, and in Phase 2, we will collect data from 300 (anticipated) Korean adults using a convenient sampling method. This number is considered appropriate in that several similar studies ( Hirsh and Peterson, 2009 ; Arntz et al., 2012 ; Al Hanai et al., 2018 ), which directly collected data (not based on big data) from about 100 to 400 people, reported appropriate results.
Online Survey
Participants will complete a battery of questionnaires designed to assess depression, anxiety, suicidality, personality disorders, personality characteristics, and data on demographic information. In addition, open-ended questions about individuals’ personality will be asked and collected.
Bright and Dark Personality Inventory
Bright and Dark Personality Inventory (BDPI; Kim et al., 2020 ) will be used to assess personality traits. The BDPI is a 173-item multidimensional personality inventory, which is a self-report measure with a 4-point Likert scale (1 = strongly disagree; 4 = strongly agree). BDPI was developed based on Five Factor Model of personality ( McCrae and Costa, 1996 ). BDPI measures personality in five-factor traits and maladaptive traits. Since BDPI measures both adaptive and maladaptive personality traits dimensionally, one’s personality can be described in more detail than traditional approaches which only measured adaptive or maladaptive traits of personality. This approach considering bipolarity of personality can be used to measure one’s personality in various settings from normal to clinical settings ( Lee et al., 2019 ; Kim et al., 2020 ). The five-factor adaptive personality traits include extraversion, agreeableness, conscientiousness, openness, and emotional stability, and the maladaptive personality traits include detachment, egocentrism, disinhibition, psychoticism, and negative affectivity. BDPI has total of 10 personality traits and each trait consists of three to four sub-facets. Each personality trait and facet will have its own score, which will be converted into T-score.
BDPI was psychometrically validated including Item Response Theory, reporting adequate reliability and validity ( Lee et al., 2019 ; Kim et al., 2020 ). Kim et al. (2020) reported that Cronbach’s alpha coefficient for adaptive personality scales was 0.924, ranging from 0.714 (conscientiousness) to 0.922 (extraversion) and maladaptive personality scales was 0.960, ranging from 0.848 (psychoticism) to 0.896 (egocentrism). Intraclass correlation coefficient was 0.731 and 0.707, respectively, for adaptive and maladaptive personality scales ( Kim et al., 2020 ).
Mental Health Screening Tool for Depressive Disorders
Mental Health Screening Tool for Depressive disorders (MHS:D; Yoon et al., 2018 ) will be used to assess depressive symptoms. The MHS:D is a 12-item self-report measure, with a 5-point Likert scale (0 = never, 4 = most of the time). Mental Health Screening Tools for depression, anxiety, and suicide risk were developed and validated for Korean adults. MHS:D was developed and validated using structured clinical interview and IRT ( Yoon et al., 2018 ). Weights calculated using IRT will be reflected on total score. In the previous study, Yoon et al. (2018) reported that Cronbach’s alpha coefficient was 0.99. Total score of MHS:D showed positive correlations of 0.74, 0.78, and 0.70 with those of CES-D, PHQ-9, and BDI-II. MHS:D showed 0.92 of sensitivity and 0.94 of specificity for depressive disorder diagnosed with M.I.N.I ( Lecrubier et al., 1997 ) at cut-off point of 13.
Mental Health Screening Tool for Anxiety Disorders
Mental Health Screening Tool for Anxiety Disorders (MHS:A; Kim et al., 2018 ) will be used to assess anxiety symptoms. The MHS:A is a 11-item self-report measure, with a 5-point Likert scale (0 = never, 4 = most of the time). MHS:A was developed and validated using IRT ( Kim et al., 2018 ). Weights calculated using IRT will be reflected on total score. In the previous study, Cronbach’s alpha coefficient was 0.92. Total score of MHS:A showed positive correlations of 0.821, 0.653, and 0.821 of total scores of GAD-7, PSWQ, and BAI, respectively ( Kim et al., 2018 ). MHS:A showed 0.795 of sensitivity and 0.937 of specificity for anxiety disorders at cut-off point of 25, when it showed 0.869 of sensitivity and 0.972 of specificity for GAD at cut-off point of 27.
Mental Health Screening Tool for Suicide Risk
Mental Health Screening Tool for Suicide Risk (MHS:S; Yoon et al., 2020 ) will be used to assess suicide risk. The MHS:S is a 4-item self-report measure, with a 5-point Likert scale (0 = never, 4 = always). MHS:S was developed and validated using IRT ( Yoon et al., 2020 ). Weights calculated using IRT will be reflected on total score. In the previous study, Cronbach’s alpha coefficient was 0.82 for both paper-based and online-based MHS:S. The optimal cut-off scores for risk positive and high risk group were total scores of 1 and 3, respectively ( Yoon et al., 2020 ).
Self-Report Standardized Assessment of Personality-Abbreviated Scale
Self-report Standardized Assessment of Personality-Abbreviated Scale (SAPAS-SR) is a self-report version of SAPAS, which is an interview for screening personality disorder ( Moran et al., 2003 ; Choi et al., 2015 ). It is an 8-item self-report measure, with a dichotomous scale (“Yes” or “No”). The scores on each scale are summed to obtain a total score. Cut-off score of Korean version of SAPAS-SR is 4 of 8, with 67.2% of patients with personality disorders were correctly classified with cut-off score of 4. In previous study, Cronbach’s alpha coefficient for SAPAS scales was 0.79 ( Choi et al., 2015 ).
Open-Ended Questions: Self-Description and Adjectives
Participants will be asked to describe their own personality in 300 characters in Korean. They will be also requested to write down six adjectives that describe themselves and another six adjectives which are important to them.
Interview Questions Developed for Personality Assessment
Interview questions will be administered to collect linguistic data for personality assessment. Interview questions designed to measure personality assessment consist of 18 questions about the adaptive personality traits (extraversion-introversion, agreeableness, conscientiousness, openness, and emotional stability) and the maladaptive personality traits (detachment, egocentrism, disinhibition, psychoticism, and negative affectivity) and 33 sub-facets based on the FFM (e.g., [Extraversion] “how do you want to spend your time for your routine daily hours?”). Final questionnaires were developed, revised, and confirmed in the pilot study (phase 1). Participants will be instructed to answer the questions reflecting their thoughts and ideas. There will be no time limit for the participants to answer. To exclude the impact of the interviewer on the responses, additional questions will be presented in a pre-determined manner.
Data Analysis
In this study, we will analyze textual data and explore its associations with personality traits through the following analysis plans: (1) Data preprocessing, (2) LDA (Latent Dirichlet Allocation), (3) Transformer-based Korean Language Model, and (4) Training, Cross-validation, and Testing. Python language and Gensim library are planning to be used in the analysis (see Figure 2 ).
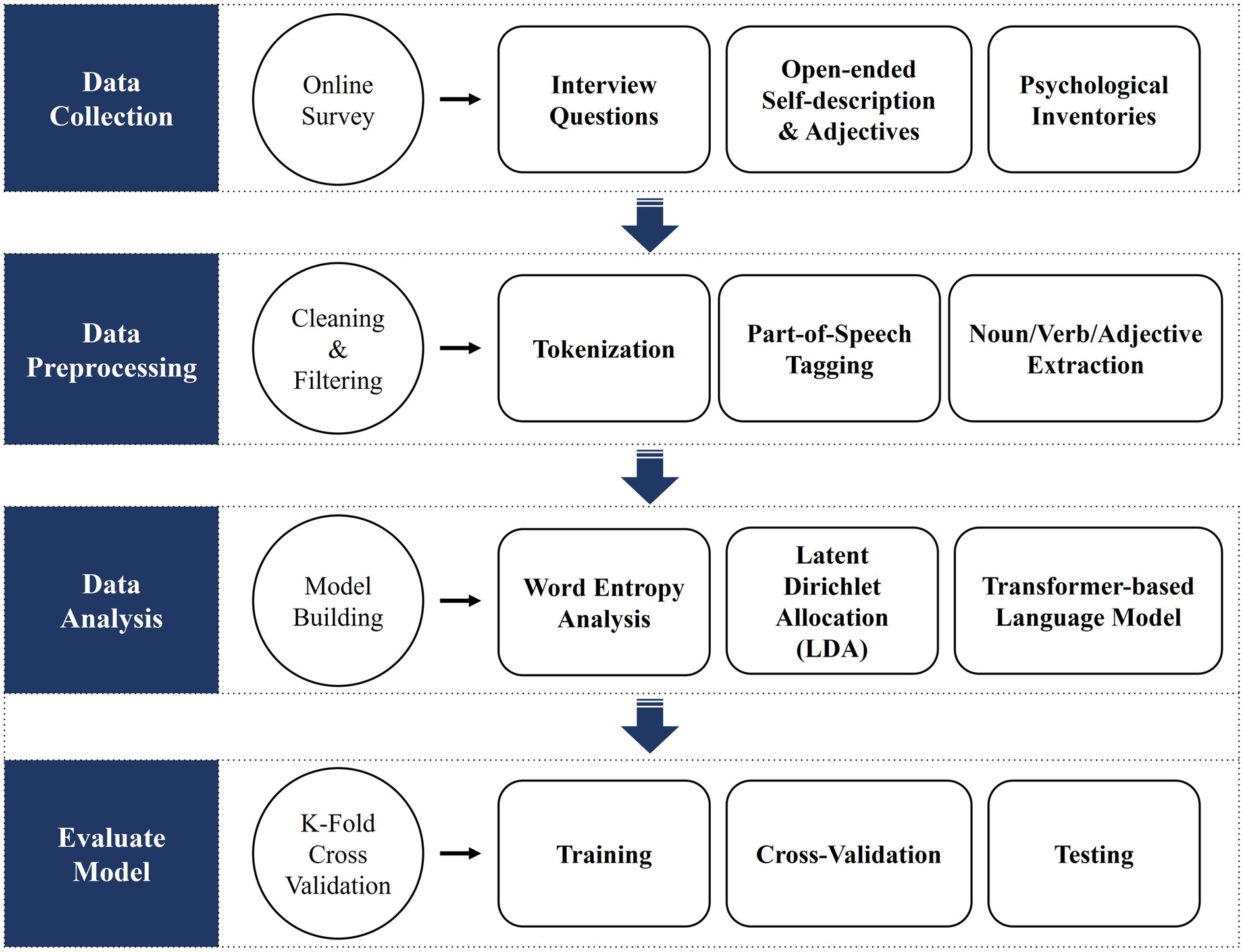
Figure 2 . Data analysis plan.
Data Preprocessing
After collecting the linguistic data for personality assessment, the data will be cleaned and filtered on the sentence units for analysis. In this step, we will use regular expressions (tokenization) to exclude other punctuation marks and symbols, and then perform part-of-speech tagging will be performed to extract only nouns, verbs, and adjectives which are used as input variables for the prediction model.
Latent Dirichlet Allocation
Latent Dirichlet Allocation is an unsupervised statistical language model which enables the discovery of latent topics in unlabeled data ( Andrzejewski and Zhu, 2009 ). By extracting the additional characteristics from the documents, it can be used to supplement the inputs to machine learning and clustering algorithms ( Campbell et al., 2015 ). This algorithm infers variables based on the words from the text data and generates topics for analyzing associations with personality traits. In other words, we will search for topics that can aggregate a large number of words contained in the data collected through LDA and select meaningful topics among them.
Transformer-Based Korean Language Model
Transformer-based pretrained language models have enabled neural network models to leverage raw textual data. After training the model with a large amount of unlabeled data in advance, transfer learning using the labeled data can be performed ( Devlin et al., 2018 ). The current study will utilize a transformer-based language model, additionally trained on Korean text. This will go through the process of learning, using text data obtained through the interview (unlabeled) and personality profile obtained through BDPI (labeled). Specifically, the embedding vectors of the sentences collected in the interview are extracted from the pretrained language model, and the scores of the measured personality characteristics are examined to explore words, sentences, and linguistic characteristics highly related to specific personality characteristics. As a result, the transformer model allows the discovery of sentence characteristics that can distinguish personality.
Training, Cross-Validation, and Testing
A 75% of the total data will be used for training and cross-validation, and the remaining 25% will be used to evaluate the performance of the trained model (the specific ratio may change depending on the final data size). The training dataset learns the process of finding answers through features, and the cross-validation dataset goes through assessing and comparing learning algorithms. Through the Testing process, we will identify the best fitting classifier and the best model.
Anticipated Results
Primary outcome.
Our primary objective is to identify specific linguistic features that correlate with individuals’ personality traits. In particular, we expect that the level of each factor that the FFM describes discovers and classifies linguistic variables that are highly relevant to high or low populations. In addition, we will extract text features that are helpful for predicting personality and apply them in machine learning algorithms to develop a Machine Learning Classification Model of the personality traits based on the FFM. We will examine predictive validity using data obtained from the interview questions as independent variables and individuals BDPI scores as dependent variables. This will add some evidence for the precision of algorithms using natural language processing to predict the ones from the traditional self-report personality questionnaire.
Secondary Outcome
We aim to detect linguistic markers of psychological distress including depressed symptoms and anxiety symptoms. In particular, words or language characteristics that highly reveal psychological distress in interview contents related to maladaptive facets or negative affectivity. This will enable distress to be quickly and accurately detected and diagnosed through an interview.
Exploratory Outcomes
Lastly, we hypothesized that there are qualitative differences between the text data obtained from the video interview and the text data obtained from the online survey. Responses to the same question obtained through video interview and online survey were compared and analyzed to see differences in the quality of information provided by face-to-face or non-contact method.
To the best of the author’s knowledge, this will be the first study to predict the FFM-based personality through machine learning technology, using both top-down method, based on personality theory and bottom-up approach, based on the data. Validity will be greater than previous studies in that interview questions are directly established on the FFM theory and that responses are analyzed through ML and NLP. Unlike this study, several studies in the past have used data lacking representativeness, such as Twitter ( Quercia et al., 2011 ) or Facebook ( Youyou et al., 2015 ), to evaluate personality. Correlation and predictive power can be reached by chance. However, it is very insufficient and error-prone to explain complex psychological characteristics such as personality without notable evidence. In other words, since such data are very limited, unexpected inferences can often be made from seemingly random data. But in the field of psychology, presenting a basis for the inference is essential. In this regard, this study will be able to provide evidence and explanation through the FFM.
In addition, the personality evaluation model and algorithm to be developed through this study may reveal better performance than the existing self-report multiple choice questionnaires or clinical interview measurements such as SCID-5-PD or SIFFM. The reason is that compared to the existing multiple choice type tests, questions are rich in information and are difficult to intentionally fake or deceive. Also, accurately predicting personality through statistical modeling and algorithms can reduce the inefficiency of one-on-one interviews while providing solutions to bias or reliability issues caused by relying on the clinician’s personal judgment. Moreover, this study can improve heterogeneity, comorbidity, misdiagnosis, stigma, or labelling problems since personality will be evaluated and diagnosed based on a dimensional approach of FFM, instead of the categorical approach used in the existing clinical field.
On the contrary, many existing studies were conducted on participants who spoke English as their mother tongue ( Wongkoblap et al., 2017 ; Shatte et al., 2019 ; Le Glaz et al., 2021 ), and Korean-based studies using the appropriate analysis methods were very limited. Specifically, Calvo et al. (2017) mentioned that studies applying NPL in mental health mostly consist of English-speaking participants, because predicting psychological characteristics by applying NLP to non-English languages is an unexplored area. This study can serve as a starting point for future studies that attempt to predict psychological characteristics by analyzing and learning Korean rather than English. However, problems arise in that psychological data are very sensitive and that it is difficult to obtain large amounts of information rapidly. Due to security issues, a lot of time and effort is needed in collecting large amounts of data unlike the other fields where pre-labeled information can be easily obtained through open source. Nevertheless, if data are collected and actively shared along with strict security management, sophisticated models and algorithms can be refined and the use of computer technology in the field of psychology can be further developed.
Unlike other fields that simply analyze large amounts of data, human psychology, mental characteristics, and personality characteristics require more explanations. We are confident that this will be a representative study meeting the criteria. We believe that this study will be of great interest to future studies seeking to improve the problems of the psychological evaluation methods through the advantages of using computers compared to humans, as well as combining advanced technologies such as psychology, machine learning, big data, and AI.
Data Availability Statement
The raw data supporting the conclusions of this article will be made available by the authors, without undue reservation.
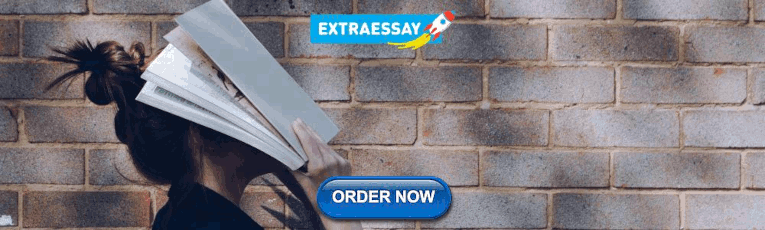
Ethics Statement
The studies involving human participants were reviewed and approved by the local Institutional Review Board (IRB) of Korea University. The patients/participants provided their written informed consent to participate in this study. The same ethical protocols will apply to ongoing research related to this study.
Author Contributions
JJ, SY, JC, and K-HC contributed to the conception and design of the study. K-HC administrated the overall study process. JC designed the overall analysis procedure and contributed to the revision of the semi-structured interview via pilot data analysis. JJ and SY contributed to acquisition of the phase 1 (pilot) data. JJ, SY, GS, and K-HC designed and revised the semi-structured interview. JJ, SY, and GS wrote the first draft of the manuscript. JJ, MK, JC, and K-HC contributed to editing the draft of the manuscript. All authors contributed to the article and approved the submitted version.
This work was supported by the National Research Foundation of Korea (NRF) grant funded by the Korea government (MSIT; no. NRF-2020R1A2C2099665).
Conflict of Interest
The authors declare that the research was conducted in the absence of any commercial or financial relationships that could be construed as a potential conflict of interest.
Publisher’s Note
All claims expressed in this article are solely those of the authors and do not necessarily represent those of their affiliated organizations, or those of the publisher, the editors and the reviewers. Any product that may be evaluated in this article, or claim that may be made by its manufacturer, is not guaranteed or endorsed by the publisher.
Acknowledgments
The authors would like to thank Heewon Seo, Minju Kim, Jueun Hwang, Seyeon Choi, Hyunjin Kim, Juhee Choi, and Euntaek Hong for assistance in subject recruitment, management, and overall data collection process. Also, Eunsil Cho and Yeoul Han contributed greatly to developing the interview questions in phase 1 (pilot) study.
Ahmad, H., Asghar, M. Z., Khan, A. S., and Habib, A. (2020). A systematic literature review of personality trait classification from textual content. Open Comput. Sci. 10, 175–193. doi: 10.1515/comp-2020-0188
CrossRef Full Text | Google Scholar
Al Hanai, T., Ghassemi, M. M., and Glass, J. R. (2018). “Detecting depression with audio/text sequence modeling of interviews.” in Proceedings of the Annual Conference of the International Speech Communication Association . INTERSPEECH; September 2018; 1716–1720.
Google Scholar
Al-Mosaiwi, M., and Johnstone, T. (2018). Linguistic markers of moderate and absolute natural language. Personal. Individ. Differ. 134, 119–124. doi: 10.1016/j.paid.2018.06.004
PubMed Abstract | CrossRef Full Text | Google Scholar
Amichai-Hamburger, Y., and Vinitzky, G. (2010). Social network use and personality. Comput. Hum. Behav. 26, 1289–1295. doi: 10.1016/j.chb.2010.03.018
Andrzejewski, D., and Zhu, X. (2009). “Latent dirichlet allocation with topic-in-set knowledge.” in Proceedings of the NAACL HLT 2009 Workshop on Semi-supervised Learning for Natural Language Processing . June 4, 2009; 43–48.
Arntz, A., Hawke, L. D., Bamelis, L., Spinhoven, P., and Molendijk, M. L. (2012). Changes in natural language use as an indicator of psychotherapeutic change in personality disorders. Behav. Res. Ther. 50, 191–202. doi: 10.1016/j.brat.2011.12.007
Calvo, R. A., Milne, D. N., Hussain, M. S., and Christensen, H. (2017). Natural language processing in mental health applications using non-clinical texts. Nat. Lang. Eng. 23, 649–685. doi: 10.1017/S1351324916000383
Campbell, J. C., Hindle, A., and Stroulia, E. (2015). “Latent dirichlet allocation: extracting topics from software engineering data,” in The Art and Science of Analyzing Software Data . eds. C. Bird, T. Menzies, and T. Zimmermann (United States: Morgan Kaufmann), 139–159.
Chandran, D., Robbins, D. A., Chang, C.-K., Shetty, H., Sanyal, J., Downs, J., et al. (2019). Use of natural language processing to identify obsessive compulsive symptoms in patients with schizophrenia, schizoaffective disorder or bipolar disorder. Sci. Rep. 9, 1–7. doi: 10.1038/s41598-019-49165-2
Choi, J., Hwang, S.-J., Pai, D., Hwang, S.-T., and Kim, Y.-R. (2015). Diagnostic efficiency of personality disorder screening tool; The Korean version of self-report standardized assessment of personality-abbreviated scale: preliminary validation study. J. Korean Neurol. Assoc. 54, 534–541. doi: 10.4306/jknpa.2015.54.4.534
Chowdhury, G. G. (2003). Natural language processing. Annu. Rev. Inf. Sci. Technol. 37, 51–89. doi: 10.1002/aris.1440370103
Corcoran, C. M., and Cecchi, G. A. (2020). Using language processing and speech analysis for the identification of psychosis and other disorders. Biol. Psych. Cogn. Neurosci. Neuro. 5, 770–779. doi: 10.1016/j.bpsc.2020.06.004
Costa, P. T. Jr., and McCrae, R. R. (2017). “The NEO inventories as instruments of psychological theory,” in The Oxford Handbook of the Five Factor Model . ed. T. A. Widiger (Oxford: Oxford University Press), 11–37.
Cui, B., and Qi, C. (2017). Survey analysis of machine learning methods for natural language processing for MBTI Personality Type Prediction.
Devlin, J., Chang, M. W., Lee, K., and Toutanova, K. (2018). Bert: pre-training of deep bidirectional transformers for language understanding. arXiv . [Epub ahead of print].
Elliot, S., Lawty-Jones, M., and Jackson, C. (1996). Effect of dissimulation on self-report and objective measures of personality. Personal. Individ. Differ. 21, 335–343. doi: 10.1016/0191-8869(96)00080-3
Esbec, E., and Echeburúa, E. (2015). The hybrid model for the classification of personality disorders in DSM-5: a critical analysis. Actas Esp. Psiquiatr. 43, 177–186.
Fan, J., Gao, D., Carroll, S. A., Lopez, F. J., Tian, T. S., and Meng, H. (2012). Testing the efficacy of a new procedure for reducing faking on personality tests within selection contexts. J. Appl. Psychol. 97, 866–880. doi: 10.1037/a0026655
Furnham, A. (1986). Response bias, social desirability and dissimulation. Personal. Individ. Differ. 7, 385–400. doi: 10.1016/0191-8869(86)90014-0
Gjurković, M., and Šnajder, J. (2018). “Reddit: A gold mine for personality prediction.” in Proceedings of the Second Workshop on Computational Modeling of PEOPLE’S Opinions, Personality, and Emotions in Social Media . June 6, 2018; New Orleans, SAD; 87-97.
Gladstone, J. J., Matz, S. C., and Lemaire, A. (2019). Can psychological traits be inferred from spending? Evidence from transaction data. Psychol. Sci. 30, 1087–1096. doi: 10.1177/0956797619849435
Hirsh, J. B., and Peterson, J. B. (2009). Personality and language use in self-narratives. J. Res. Pers. 43, 524–527. doi: 10.1016/j.jrp.2009.01.006
Holden, R. R., Wheeler, S., and Marjanovic, Z. (2012). When does random responding distort self-report personality assessment? An example with the NEO PI-R. Personal. Individ. Differ. 52, 15–20. doi: 10.1016/j.paid.2011.08.021
Hopwood, C. J., Morey, L. C., Edelen, M. O., Shea, M. T., Grilo, C. M., Sanislow, C. A., et al. (2008). A comparison of interview and self-report methods for the assessment of borderline personality disorder criteria. Psychol. Assess. 20, 81–85. doi: 10.1037/1040-3590.20.1.81
Irving, J., Patel, R., Oliver, D., Colling, C., Pritchard, M., Broadbent, M., et al. (2021). Using natural language processing on electronic health records to enhance detection and prediction of psychosis risk. Schizophr. Bull. 47, 405–414. doi: 10.1093/schbul/sbaa126
Jayaratne, M., and Jayatilleke, B. (2020). Predicting personality using answers to open-ended interview questions. IEEE Access 8, 115345–115355. doi: 10.1109/access.2020.3004002
Kim, M. G., Lee, S. G., Han, Y., Park, D. J., Choi, K. H., and Seo, D. G. (2020). Development and validation of bright and dark personality inventory (BDPI): integrating bright and dark sides of personality. Korean J. Psychology: General 39, 359–383. doi: 10.22257/kjp.2020.9.39.3.359
Kim, Y., Park, Y., Cho, G., Park, K., Kim, S.-H., Baik, S. Y., et al. (2018). Screening tool for anxiety disorders: development and validation of the Korean anxiety screening assessment. Psychiatry Investig. 15, 1053–1063. doi: 10.30773/pi.2018.09.27.2
Kraemer, H. C., Kupfer, D. J., Clarke, D. E., Narrow, W. E., and Regier, D. A. (2012). DSM-5: how reliable is reliable enough? Am. J. Psychiatr. 169, 13–15. doi: 10.1176/appi.ajp.2011.11010050
Lecrubier, Y., Sheehan, D. V., Weiller, E., Amorim, P., Bonora, I., Sheehan, K. H., et al. (1997). The MINI international neuropsychiatric interview (MINI). A short diagnostic structured interview: reliability and validity according to the CIDI. Eur. Psychiatry 12, 224–231. doi: 10.1016/S0924-9338(97)83296-8
Lee, S. J., Han, Y., Kim, H. W., Lee, H. J., Park, J. Y., Choi, K. H., et al. (2019). Development and validation of multi-dimensional personality inventory in preliminary study: integrating of bright and dark sides of personality. Korean J. Clin. Psychol. 38, 318–334. doi: 10.15842/kjcp.2019.38.3.005
Le Glaz, A., Haralambous, Y., Kim-Dufor, D. H., Lenca, P., Billot, R., Ryan, T. C., et al. (2021). Machine learning and natural language processing in mental health: systematic review. J. Med. Internet Res. 23:e15708. doi: 10.2196/15708
Lima, A. C. E., and De Castro, L. N. (2014). A multi-label, semi-supervised classification approach applied to personality prediction in social media. Neural Netw. 58, 122–130. doi: 10.1016/j.neunet.2014.05.020
Livesley, W. J. (2010). Confusion and incoherence in the classification of personality disorder: commentary on the preliminary proposals for DSM-5. Psychol. Injury Law 3, 304–313. doi: 10.1007/s12207-010-9094-8
McCrae, R. R., and Costa, P. T. (1996). “Toward a new generation of personality theories: theoretical contexts for the five-factor model,” in The Five-Factor Model of Personality. Theoretical Perspectives . ed. J. S. Wiggins (New York, NY: Guilford Press), 51–87.
McCrae, R. R., and John, O. P. (1992). An introduction to the five-factor model and its applications. J. Pers. 60, 175–215. doi: 10.1111/j.1467-6494.1992.tb00970.x
Mønsted, B., Mollgaard, A., and Mathiesen, J. (2018). Phone-based metric as a predictor for basic personality traits. J. Res. Pers. 74, 16–22. doi: 10.1016/j.jrp.2017.12.004
Moran, P., Leese, M., Lee, T., Walters, P., Thornicroft, G., and Mann, A. (2003). Standardised assessment of personality – abbreviated scale (SAPAS): preliminary validation of a brief screen for personality disorder. Br. J. Psychiatry 183, 228–232. doi: 10.1192/bjp.183.3.228
Oswald, F. L., Behrend, T. S., Putka, D. J., and Sinar, E. (2020). Big data in industrial-organizational psychology and human resource management: forward progress for organizational research and practice. Annu. Rev. Organ. Psych. Organ. Behav. 7, 505–533. doi: 10.1146/annurev-orgpsych-032117-104553
Park, G., Schwartz, H. A., Eichstaedt, J. C., Kern, M. L., Kosinski, M., Stillwell, D. J., et al. (2015). Automatic personality assessment through social media language. J. Pers. Soc. Psychol. 108, 934–952. doi: 10.1037/pspp0000020
Paulhus, D. L., and Vazire, S. (2007). “The self-report method,” in Handbook of Research Methods in Personality Psychology. Vol. 1. eds. R. W. Robins, R. C. Fraley, and R. F. Krueger (United states: Guilford Press), 224–239.
Quercia, D., Kosinski, M., Stillwell, D., and Crowcroft, J. (2011). “Our twitter profiles, Our selves: predicting personality with twitter.” in 2011 IEEE third International Conference on Privacy, Security, Risk and Trust and 2011 IEEE Third International Conference on Social Computing . IEEE. October 9-11, 2011; Boston, MA, USA; 180–185.
Samuel, D. B., Sanislow, C. A., Hopwood, C. J., Shea, M. T., Skodol, A. E., Morey, L., et al. (2013). Convergent and incremental predictive validity of clinician, self-report, and structured interview diagnoses for personality disorders over 5 years. J. Consult. Clin. Psychol. 81, 650–659. doi: 10.1037/a0032813
Shatte, A. B., Hutchinson, D. M., and Teague, S. J. (2019). Machine learning in mental health: a scoping review of methods and applications. Psychol. Med. 49, 1426–1448. doi: 10.1017/S0033291719000151
Sönmezöz, K., Uğur, Ö., and Diri, B. (2020). “MBTI personality prediction with machine learning,” in 2020 28th Signal Processing and Communications Applications Conference (SIU) IEEE. October 5-7, 2020; 1–4.
Stachl, C., Pargent, F., Hilbert, S., Harari, G. M., Schoedel, R., Vaid, S., et al. (2020). Personality research and assessment in the era of machine learning. Eur. J. Personal. 34, 613–631. doi: 10.1002/per.2257
Trull, T. J., and Widiger, T. A. (1997). Structured Interview for the Five-Factor Model of Personality . Odessa, FL: Psychological Assessment Resources.
White, L. A., Young, M. C., Hunter, A. E., and Rumsey, M. G. (2008). Lessons learned in transitioning personality measures from research to operational settings. Ind. Organ. Psychol. 1, 291–295. doi: 10.1111/j.1754-9434.2008.00049.x
Widiger, T. A. (ed.) (2017). “Introduction,” in The Oxford Handbook of the Five-Factor Model . New York: Oxford University Press.
Widiger, T. A., and Trull, T. J. (2007). Plate tectonics in the classification of personality disorder: shifting to a dimensional model. Am. Psychol. 62, 71–83. doi: 10.1037/0003-066X.62.2.71
Wongkoblap, A., Vadillo, M. A., and Curcin, V. (2017). Researching mental health disorders in the era of social media: systematic review. J. Med. Internet Res. 19:e228. doi: 10.2196/jmir.7215
Wood, J. M., Garb, H. N., Lilienfeld, S. O., and Nezworski, M. T. (2002). Clinical assessment. Annu. Rev. Psychol. 53, 519–543. doi: 10.1146/annurev.psych.53.100901.135136
Yarkoni, T., and Westfall, J. (2017). Choosing prediction over explanation in psychology: lessons from machine learning. Perspect. Psychol. Sci. 12, 1100–1122. doi: 10.1177/1745691617693393
Yoon, S., Lee, B., Park, K., Jung, S., Kim, S., Park, Y., et al. (2018). Development of Korean depression screening assessment: phase II preliminary validation study. Korean J. Clin. Psychol. 37, 254–264. doi: 10.15842/kjcp.2018.37.2.011
Yoon, S., Park, K., and Choi, K.-H. (2020). The ultra brief checklist for suicidality. J. Affect. Disord. 276, 279–286. doi: 10.1016/j.jad.2020.07.037
Youyou, W., Kosinski, M., and Stillwell, D. (2015). Computer-based personality judgments are more accurate than those made by humans. Proc. Natl. Acad. Sci. 112, 1036–1040. doi: 10.1073/pnas.1418680112
Zunic, A., Corcoran, P., and Spasic, I. (2020). Sentiment analysis in health and well-being: systematic review. JMIR Med. Inform. 8:e16023. doi: 10.2196/16023
Keywords: personality prediction, psychological distress prediction, natural language processing, machine learning, the five-factor model of personality
Citation: Jang J, Yoon S, Son G, Kang M, Choeh JY and Choi K-H (2022) Predicting Personality and Psychological Distress Using Natural Language Processing: A Study Protocol. Front. Psychol . 13:865541. doi: 10.3389/fpsyg.2022.865541
Received: 30 January 2022; Accepted: 22 March 2022; Published: 07 April 2022.
Reviewed by:
Copyright © 2022 Jang, Yoon, Son, Kang, Choeh and Choi. This is an open-access article distributed under the terms of the Creative Commons Attribution License (CC BY) . The use, distribution or reproduction in other forums is permitted, provided the original author(s) and the copyright owner(s) are credited and that the original publication in this journal is cited, in accordance with accepted academic practice. No use, distribution or reproduction is permitted which does not comply with these terms.
*Correspondence: Kee-Hong Choi, [email protected] ; Joon Yeon Choeh, [email protected]
Disclaimer: All claims expressed in this article are solely those of the authors and do not necessarily represent those of their affiliated organizations, or those of the publisher, the editors and the reviewers. Any product that may be evaluated in this article or claim that may be made by its manufacturer is not guaranteed or endorsed by the publisher.
Call +61 403 600 248
International Coach Academy
Coach Training School
Articles, Case Studies & Interviews
Research paper: psychometrics and the use of personality profile assessments as tools in the coaching process.

Introduction
The development and professionalization of the coaching industry have slowly expanded over the last 20+ years to become recognized, by corporations and individuals alike as a valid way to improve individual and organizational capacity to perform at higher levels of productivity.
As the coaching industry has professionalized it has segmented into a variety of niche areas. For example, leadership, life, performance, motivation, health, and a variety of other niche coaching areas. With the development and proliferation of coaching service providers and/or individual coaches, so the use of psychometric testing has expanded to enhance the offerings that coaches have, which provide data or results that be used as inputs for coaching sessions.
The list of potential psychometric tests available for coaches to use with clients is extensive. Below is a shortlist of example psychometric tests, that include MBTI, which is a personality profile test:
- Abstract Reasoning Test (DC3.1)
- Clerical Checking Test (CTB2)
- Decision-Making Test (MOST)
- Diagrammatic Reasoning Test (DIT6)
- Hogan Business Reasoning Inventory (HBRI)
- Hogan Development Survey (HDS)
- Hogan Personality Inventory (HPI)
- Interpreting Data Test (NC2.2)
- Manual Speed and Accuracy Test (EAS9)
- Motivation Questionnaire (MQ)
- Motives, Values & Preferences Inventory (MVPI)
- Myers Briggs Type Indicator (MBTI)
- NEO-FFI Assessment
- 15 Factor Questionnaire (15FQ)
NOTE: The list above is illustrative as there are potentially tens of different types of psychometric tests that can be used by coaches in consultation with their clients.
This research paper is interested in how, when, and where coaches can use psychometric tests that are designed to assist coaches and clients to understand a client’s personality and how that information might be used in individual and group coaching in the workplace.
Myers-Briggs – Foundation of Psychometric Testing for Personality Profiles
The Myers-Briggs Personality Type Indicator (MBTI) is a self-report inventory designed to identify a person’s personality type, strengths, and preferences. The questionnaire was developed by Isabel Myers and her mother Katherine Briggs based on their work with Carl Jung’s theory of personality types.
Based on the answers to the questions on the inventory, people are identified as having one of 16 personality types. The goal of the MBTI is to allow respondents to further explore and understand their own personalities including their likes, dislikes, strengths, weaknesses, possible career preferences, and compatibility with other people.
No one personality type is “best” or “better” than another. It isn’t a tool designed to look for dysfunction or abnormality. Instead, its goal is simply to help you learn more about yourself. The questionnaire itself is made up of four different scales as listed below.
- Extraversion (E) – Introversion (I)
- Sensing (S) – Intuition (N)
- Thinking (T) – Feeling (F)
- Judging (J) – Perceiving (P)
Today, the MBTI inventory is one of the most widely used psychological instruments in the world and consists of 16 different personality types listed in Table 1.0 below:
Table 1.0: MBTI 16 Personality Types
Personality profile assessments and understanding learning styles.
The use of personality profile assessments provides an opportunity for coach and client alike to learn about and/or classify cognitive learning and/or psychological types. There is a range of ways to classify learning styles drawing on a range of theories; for example, the VARK model [1] (Visual, Aural, Read/Write & Kinesthetic) that assists clients and coaches by suggesting appropriate learning styles for individuals, (Fleming & Mills, 1992) that can be utilized like the Myers Briggs (MBTI) psychological type theory based on Jung’s theory of psychological types.
MBTI instead of utilizing metrics like visual, aural, read/write, and kinesthetic (VARK) utilizes its own framework i.e. Extraversion (E) – Introversion (I), Sensing (S) – Intuition (N), Thinking (T) – Feeling (F); and, Judging (J) – Perceiving (P), which assists coaches and clients alike to understand individual interests, motivations and preferences as they relate to learning styles. For example, a theoretical client that is more introverted than extroverted may have a preferred learning style such as read/write, as opposed to an extrovert who may prefer to watch a video and/or receive some other external stimulation to promote their learning.
A coach needs to understand that personality type preferences may impact an individual’s motivation and interests as it relates to learning and studying, and the level of ease or difficulty that a particular person with a personality type may have when it comes to processing and/or recalling information. Thus, understanding personality type potentially has the potential to assist a coach and client in understanding the client’s learning preferences and how they help or hinder the individual’s and potentially group’s learning.
Application of Personality Profile Assessments
Personality assessments, sometimes known as personality questionnaires, inventories,s or profiles are part of the family of psychometric assessments available that coaches can use with clients and gives information about an individual’s personality preferences and qualities. Personality assessments can assist coaches and clients to better understand a variety of aspects of a person’s personality, preferences, and likely behavior.
Personality assessments can be used by coaches with clients in several ways as per Table 2.0 below:
Table 2.0: Uses for Personality Profiles

A Workplace Research Case Study – Personality Assessments for Workplace Performance Improvement
An example of how psychometric testing using personality profiles can be used and have an impact at multiple levels (individual and group) in the workplace is described below, based on the use of personality profiles working with three (3) local staff in my office in Yangon, Myanmar. In the activity described below the psychometric test used was https://www.16personalities.com/free-personality-test, which is based on Myers Briggs.
Methodology
The methodology used for the workplace research case study was as follows:
- A discussion was held with three (3) staff members to explain how personality tests can be used for individual and group professional development. With staff member agreement they were then invited to complete a free personality assessment ( https://www.16personalities.com/free-personality-test )
- The three (3) staff members shared the results of their personality tests with me.
- The three (3) staff members were encouraged to review their own personality test results.
- An individual 30-minute meeting was held with each staff member to discuss their personality profile results, what they might mean, how the staff member can use the results to become more aware of their own personality type, their preferences i.e. communication, strengths, weaknesses, etc.
- A group meeting was held for 60 minutes to discuss the results of staff member personality profiles. Each staff member shared their personality type and what they had learned about themselves (individual). A group discussion was then had to discuss what could be learned as a group from being aware of each other’s personality type and how these insights might be used to improve workplace performance (group).
Personality Profile Case Study
Soe, Aung, and Sung Sung [2] each completed their free personality profiles and received the following profile assessments:

Summary of Individual Team Member Learning
As a result of each team member completing their personality profiles and then discussing them with myself for 30 minutes (individually) [3] as their supervisor all three team members mentioned a range of learnings (new awareness) as follows:
- They become aware of personality profiles and key indicators of personality like ‘introversion and ‘extroversion’.
- They acknowledged that the results of their personal profile assessments confirmed how they had perceived themselves, building their awareness of who they are as individuals.
- They acknowledged that other aspects of their personality profiles, which detailed ‘strengths and weaknesses, ‘career-path and ‘work habits’ was useful to build their awareness of these aspects of their personalities. In particular, each of the team members indicated that they better understood how they can capitalize on their strengths while considering how understanding their weaknesses could empower them to mitigate weaknesses by addressing them as part of their own professional development plans.
- They acknowledged that it was just useful to better understand your own personality and how that matches with others in the workplace.
Summary of Team Group Learning
As a result of a group meeting for 60 minutes with the three (3) team members after completing individual interviews described above the three team members identified ways that individual awareness of each others personality might be utilized to enhance team performance and/or productivity as follows:
- They acknowledged that sharing their personality profile assessments with their fellow team members enabled them to better understand each other's personality types, preferences, strengths, and weaknesses, etc.
- They acknowledged that they had not considered how understanding another team member's personality profile, might be useful to strengthen internal communication and problem-solving. It was agreed that being aware of other's personality profiles and communication preferences could assist them to better work with each other (compromise) to ensure productive relationships are formed in the workplace through effective communication. Understanding other team member personality and communication preferences are therefore empowering to the team.
- They acknowledged and agreed that office performance could be further enhanced through additional facilitation focused on deepening understanding of individual and group dynamics i.e. results of personality profile assessments.
- They acknowledged that the use of tools like the personality assessments/profiles that they had completed, were very useful for building individual and group awareness for performance improvement. The personality profile assessments had enabled an ‘open’ and ‘transparent’ conversation to be facilitated at the ‘individual’ and ‘group’ levels. It was clear that team members participating in this exercise had become more informed about their individual personality profile and that of their team members. Team members wanted to deepen these conversations to further enhance individual and group performance.
- They acknowledged that they could see how the results of individual and group personality profile assessments could also be filtered into the drafting of annual individual and office performance plans. That is, where activities related to building individual or group performance can be further enhanced by holding additional meetings to discuss results, identify areas of improvement (individual/group) and execute activities that can bring about change in the workplace.
Research Paper Conclusion
That there are a large variety of psychometric tests available in the marketplace that can be utilized by coaches when working with clients to facilitate impactful coaching sessions. In particular, there are a wide variety of personality profile assessments available, of which many are based on the work of Myers Briggs, which identifies 16 basic personality types. The 16 personality types are comprised of eight (8) different dimensions that include, Extraversion (E) – Introversion (I), Sensing (S) – Intuition (N), Thinking (T) – Feeling (F); and, Judging (J) – Perceiving (P).
As a coaching tool, a personality assessment can be utilized by a coach in partnership with individual team members and/or teams to build awareness of individual and group preferences i.e. communication, strengths, weaknesses, and identification of areas of potential improvement that can assist individuals and teams alike to become more aware of who they are and how they can support and/or enhance performance improvement in the workplace.
References:
- An Overview of the Myers-Briggs Type Indicator (MBTI), https://www.verywellmind.com/the-myers-briggs-type-indicator-2795583
- 16 personalities free personality profile test, https://www.16personalities.com/free-personality-test
- Insights Discovery, https://www.insights.com/
- Learning Styles of the 16 Personality Types, http://www.humanmetrics.com/personality/learning-styles
- VARK (Visual, Aural, Read/Write and Kinesthetic), https://vark-learn.com/the-vark-questionnaire/
[1] VARK is another type of psychometric test focused on understanding a person’s learning style as it relates to the use of the VARK model.
[2] Real names changed to protect the identity of staff members.
[3] 30-minute interviews and group meetings all held December 2, 2020.
About Our School
Become a Coach Coaching Niches & Specialities Our Methodology Faculty Class Schedules Online Community
What is Coaching? Certification & Credentialing Guide Graduate Research Papers Graduate Yearbooks
Locations/Contact
Global: Clik Collective Asia: Duo Tower Postal: PO Box 3190 Mentone East, Melbourne AUSTRALIA, 3194
Email: [email protected] Questions: Ask here
Privacy Overview
Thank you for visiting nature.com. You are using a browser version with limited support for CSS. To obtain the best experience, we recommend you use a more up to date browser (or turn off compatibility mode in Internet Explorer). In the meantime, to ensure continued support, we are displaying the site without styles and JavaScript.
- View all journals
Personality articles from across Nature Portfolio
Personality refers to individual differences in characteristic patterns of thinking, feeling and behaving. It can also refer to how the patterns in different domains combine in each individual.
Latest Research and Reviews
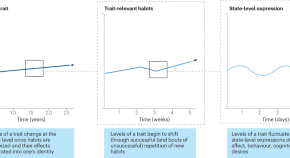
The process and mechanisms of personality change
Personality is relatively stable over long timescales but remains malleable to some degree. In this Review, Jackson and Wright examine the mechanisms responsible for intentional and naturally occurring change as well as mechanisms that promote stability, thereby limiting potential change.
- Joshua J. Jackson
- Amanda J. Wright
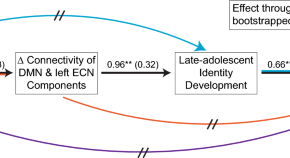
Diverse adolescents’ transcendent thinking predicts young adult psychosocial outcomes via brain network development
- Rebecca J. M. Gotlieb
- Xiao-Fei Yang
- Mary Helen Immordino-Yang
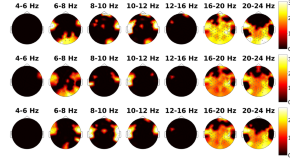
Detecting cognitive traits and occupational proficiency using EEG and statistical inference
- Ilya Mikheev
- Helen Steiner
- Olga Martynova
Digital media exposure and cognitive functioning in European children and adolescents of the I.Family study
- Christoph Buck
- Antje Hebestreit
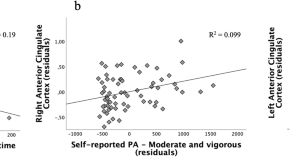
Relevance of the anterior cingulate cortex volume and personality in motivated physical activity behaviors
In a cohort of right-handed young adults, the volume of the right anterior cingulate cortex positively correlates with self-reported physical activity.
- Anna Miró-Padilla
- Jesús Adrián-Ventura
- César Ávila
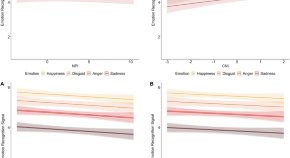
Narcissism predicts noise perception but not signal decoding in emotion
- Anna Z. Czarna
- Heidi Mauersberger
- Ursula Hess
News and Comment
Traits and states in mindfulness meditation.
- Yi-Yuan Tang
- Britta K. Hölzel
- Michael I. Posner
Placebo and personality
- Rachel Jones
Leadership theory: implications for developing dental surgeons in primary care?
- S. Willcocks
A risk marker for alcohol dependence on chromosome 2q35 is related to neuroticism in the general population
- M Rietschel
Variation in DISC1 is associated with anxiety, depression and emotional stability in elderly women
Amongst equals.
- Charvy Narain
Quick links
- Explore articles by subject
- Guide to authors
- Editorial policies

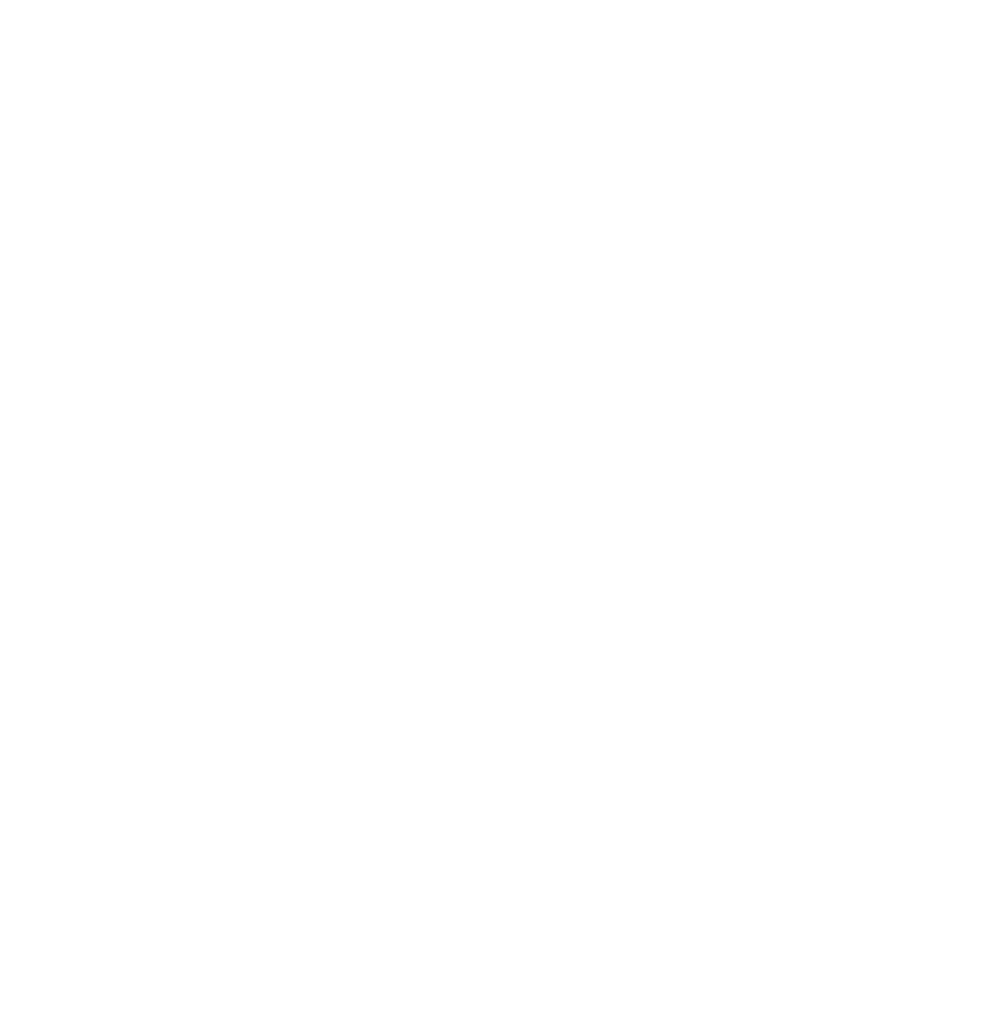
- Leadership Crisis
- Editor's Pick

‘Gender-Affirming Slay Fest’: Harvard College QSA Hosts Annual Queer Prom

‘Not Being Nerds’: Harvard Students Dance to Tinashe at Yardfest

Wrongful Death Trial Against CAMHS Employee Over 2015 Student Suicide To Begin Tuesday

Cornel West, Harvard Affiliates Call for University to Divest from ‘Israeli Apartheid’ at Rally

Harvard Launches Third Sexual Misconduct Climate Survey
Artist Profile: Kwesi James, Professional Trier, Is ‘Not Good at Anything’

The first thing Kwesi J. James did after agreeing to be interviewed for a profile was ask me — his interviewer — a question: “Who’s your favorite person to interview?”
Producer, internet personality, and world traveler, James has always steered away from the norm — of course when he was supposed to answer questions, he asked one.
“I'm not good at anything,” James said in an interview with The Harvard Crimson.
Regardless of whether that statement is true or not, James’s quest for knowledge and experience remains untempered. With an ambitious yet generous approach to life, he doesn’t take no for an answer and would give nearly anything in the world a try — particularly in his role as a frequent guest star on The Try Guys YouTube channel .
“Luckily, with The Try Guys, we’re trying things,” James said. “Throughout my life, I had to be comfortable with just getting a lot of things wrong. And that makes great comedy.”
The premise of much of James’s work is how open he is to trying and failing at anything — from cooking with a Michelin star chef to competing against a Scrabble World Champion .
Since “professional trier” isn’t exactly a job title, James tends to tell people that he’s a producer, especially when talking to his Carribean family and older generations who have limited exposure to internet media.
“Outside of Hollywood, no one knows what a producer does. But they know a producer does something,” James said, laughing. “I produce something, right? I produce laughs. I produce content.”
Clearly this definition is sufficient for James’s mom, who brags about James to her family to his evident delight.
“I was searching for that validation from my parents,” James said, with a grin. “I was the kid who felt like I was behind my whole life. I have dyslexia; I didn't know until I got into college. So for the majority of my life, it was like, ‘Kwesi needs extra time on everything.’”
His experience “working from behind” and “not being the fastest or smartest” translates perfectly to his current job of trying and failing at things professionally. This well-suited career path is no coincidence, though.
“I'm willing to try cool things that people who are smarter, faster, or better may not,” James said, acknowledging that more visibly-successful people are often more afraid of failure. Since he wasn’t the quickest along the traditional path, he grew to be resourceful and resilient instead.
“I had to learn how to go around doors,” James said. “How do I sneak through the window? To get in and get what I want, you know, and to live a cool, fulfilled life.”
A cool, fulfilled life isn’t easy to come by — and certainly not with the life circumstances that James was dealt. After living in Brooklyn and experiencing violence in his early life, he moved to Florida with his family and attended high school there, where he often skipped class and was ultimately expelled. Regardless, James still set his sights on film school.
“I went to this film school where if you have a student loan and you can open a door, you can get in,” James said. “I worked my ass off to try to learn as much as possible about this film industry.” Soon after, James made his way to L.A., eager to try his hand at the industry.
“It was the worst time to find a job in Los Angeles; it was 2008,” James said. He was living with his friends from film school at the time and knew, despite the tumultuous job market, that he owed it to himself to give the film world a “Kwesi James Try.” He put on his graduation suit — “the only suit that I had” — shipped his car from Florida to Los Angeles, and picked three L.A.-based production companies that had been ignoring his emails to visit in person and ask for a job.
“The first place I went to was a company called DNA,” James said. “I went in. I sat down. I named every director on their roster, and every single music video they shot — because I’d studied it. And she was like, ‘When do you want to start?’”
After DNA, James worked at BuzzFeed at the same time as other famous media personalities such as Quinta Brunson, Eugene Lee Yang, Zach Kornfeld, and Keith Habersberger. However, James was ultimately laid off. He eventually found his way back to the now-independent Try Guys, who he had worked with in his time at BuzzFeed. Currently James stars in many of their videos, while still working on other external projects.
Perhaps no one can sum up James’s approach to his life circumstances better than himself: “I'm not the fastest. I'm not the best. But man, I got some gumption in me.”
An exceptional thing about James — one that even he didn’t directly acknowledge in his interview — is how steadfast he is in self-direction. It’s hard not to marvel at his journey, at how confidently he depletes his energy to “try to accomplish dream[s] that I have,” and how he seems to know intuitively the next best move he should make to elevate his life.
“For me, it really feels like breathing. Like, do you think about breathing?” James said. “The only time when you feel like it's really hard to breathe is when something is suffocating you.” James paused, taking a breath himself. “I'm not the best at school. I don't think I'd be really that good if I worked in a cubicle. But performing with The Try Guys, creating videos, feels like breathing. It's that natural.”
James’s natural gift of creativity and his experience-honed tenacity to change his circumstances are exactly what pushed him from the well-trodden path of mediocrity towards one of true excellence — both as a producer and as a human being. In that way, he is more than just the “good at anything” label that he denies himself: James is ambitiously excellent.
—Staff writer Stella A. Gilbert can be reached at [email protected] .
Want to keep up with breaking news? Subscribe to our email newsletter.

An official website of the United States government
The .gov means it’s official. Federal government websites often end in .gov or .mil. Before sharing sensitive information, make sure you’re on a federal government site.
The site is secure. The https:// ensures that you are connecting to the official website and that any information you provide is encrypted and transmitted securely.
- Publications
- Account settings
Preview improvements coming to the PMC website in October 2024. Learn More or Try it out now .
- Advanced Search
- Journal List

Influence of Personality Traits on the Severity of Alcohol Use Disorders
Aida Álvarez.
1 Department of Psychiatry, Corporaciò Sanitária Parc Taulí, 08208 Sabadell, Spain; tac.iluat@oalapd
José J. Ávila
2 EUEF School, University of Salamanca, 37008 Salamanca, Spain; moc.liamg@onabircsealiva (J.J.Á.); se.lasu@ojetnoma (Á.L.M.)
Diego J. Palao
3 Centro de Investigación en Salud Mental (CIBERSAM), 28029 Madrid, Spain
Ángel L. Montejo
4 Institute of Biomedical Research of Salamanca (IBSAL), 37007 Salamanca, Spain
5 Departament of Psychiatry, University Clinical Hospital of Salamanca, 37007 Salamanca, Spain
Introduction: Alcohol Use Disorders (AUD) are the most prevalent psychiatric diagnosis in the general population. The study of personality characteristics, using Cloninger Personality Inventory (TCI-R), allows us to know the evolution of these patients at the beginning of treatment. Material and Method: We conducted a cross-sectional, observational and descriptive study for 3 years with a total of 304 patients. We studied the severity of their alcohol disorder by the Alcohol Dependency Intensity Scale (EIDA), Scale of Obsessive Consumption Compulsive (OCDS) and European version of the Addiction Severity Index (EUROPASI); we studied the relationship with the personality traits of TCI-R. Results and conclusions: The personality lines influence the evolution of alcohol use disorder (AUD). People with higher scores on Reward Dependency (RD), Persistence (P), Cooperation (CO) and Autotranscendence (ST) have a better prognosis while people with higher scores on Search for Novelty (SN) and Avoidance of Damage (AD) have a worst prognosis. Women present differences in consumption in relation to men, as a consequence of their personality. Women have lower scores in Persistence (P) y Self-Transcendence (ST) which are associated with the greater severity of their addiction.
1. Introduction
Alcohol use disorders (AUD) are the most prevalent psychiatric diagnosis in the general population. It is estimated that in Spain, around 7–10% of the adult population (3 million people) has problems related to alcohol consumption. This constitutes a high relevant public health problem [ 1 , 2 , 3 ].
Multiples studies have analyzed personality characteristics in patients with an AUD. The vast majority of studies conducted in patients with addiction problems, in order to know their personality traits, have been made through the Cloninger Temperament and Character Inventory (TCI), based on his psychobiological personality model (Cloninger, 1987) [ 4 ]. For Cloninger, the personality reflects differences in the responses of neuroadaptive systems related to learning mediating two concepts mainly: the concept of temperament and the concept of character. The temperament is defined as the individual differences in habits and abilities related to emotions, is inherited around 50%. Four features are described: Search for Novelty (BN), Avoidance of Damage (AD), Dependence on Reward (DR) and Persistence (P). The character of the personality is inherited to a lesser degree and is moderately influenced by social learning. There are three characteristic dimensions: self-direction (SD), cooperation (CO) and self-transcendence (ST) [ 1 , 2 , 3 ].
Differents studies found higher scores in people with AUD than in the general population, in terms of disinhibition, impulsivity, antisocial behaviour and aggressive personality. These features suggest low control capacity [ 5 , 6 ]. If we focus on the variables quantified by the Cloninger Personality Inventory (TCI-R), then the results are not as conclusive. Some authors report high scores in Avoidance of Damage (AD) [ 7 ], while others observe high scores in Search for Novelty (SN) and Avoidance of Damage with low scores in Self-Direction (SD) [ 8 ]. However, there are few studies that relate the personality traits of patients with the severity of their psychiatric pathology. The early detection of at-risk drinkers is a priority from a public health perspective because of the high prevalence of risk consumption and the effectiveness of treatment aimed at reducing consumption.
The main objective of this study doctoral thesis study was to investigate how—and to what extent—personality traits, at begining of the treatment, influence the severity of AUD and what differences exist according to gender [ 9 ].
2. Materials and Methods
This is a cross-sectional, observational and descriptive study carried out in the Alcoholism Treatment Unit of the Hospital Clínico Universitario de Salamanca with duration period of 3 years (from 2013 to 2016) including a sample of 304 patients patients (describir % hombres y mujeres y edad media con DS). The sample is composed of 304 patients affected by Alcohol Use Disorder (AUD), who attended the Alcohol Disorders Unit of Salamanca, of which 250 were men (82.2%), and 54 women (17.8%). The average age of men was 43.6 years (SD 9.7); and in the case of women of 43.8 (SD 9.8).
Regarding the diagnosis, 244 patients (80.3%) of the total sample fulfilled alcohol dependence criteria, whereas 60 (19.7%), suffered from an alcohol abuse disorder. 78.9% of men (197) had an alcohol dependence, while 21.2% (53 men) had an abuse disorder. In the case of women, 87.0% (47 of them), had a diagnosis of alcohol dependence, compared to 13.0% women (7), which presented a pattern of abuse, although these differences were not significant.
Patients had been diagnosed with an AUD and were between 18 and 70 years of age and of both sexes. They collaborated voluntarily and signed an Informed Consent. The study was approved by the Ethical Committee of the Clinical Hospital of Salamanca. Patients with concomitant consumption of other substances, with cognitive difficulties, illiteracy, under 18 years of age and with comorbidity with other serious mental disorders were excluded from the study.
Through the semi-structured interview, relevant data were collected, as follows: sociodemographic data, consumption pattern, consumption characteristics, type of alcoholic beverage and grams of alcohol consumed. In addition, the clinical course of the disorder, the diagnosis of AUD and comorbid entities were assessed. Finally the degree of motivation for the treatment and its type were also registered. The rest of the instrumental tests were then executed.
- EUROPASI scale (European version of the Addiction Severity Index) [ 10 , 11 ]: This basic instrument for clinical practice allows a multidimensional diagnosis of addiction problems. It assesses their severity and puts them in a bio-psycho and social context. This instrument evaluates different aspects of the lives of patients affected by an addictive pathology. Specifically, it explores the following six potentially problematic areas of life: Physical Health (16 items); Employment/Resources (26 items); Drugs/Alcohol (28 items); Legal situation (23 items); Family history (51 items); Family/Social Relations (26 items); Mental Health (22 items).
- EIDA Scale (Alcohol Dependency Intensity Scale) [ 12 ]: This is a self-applied measuring instrument that is composed of subscales: physical symptoms of abstinence, psychological symptoms of withdrawal, behaviours to relieve withdrawal symptoms, etc. It distinguishes between mild, moderate and severe dependence.
- Questionnaire on the obsessive-compulsive components of drinking (OCDS) [ 13 ]: This tool evaluates the intensity of the components obsessive (preoccupation with drinking) and compulsive nature of the drink. According to this model, both components have their common base in desire (“craving”). This questionnaire addresses alcoholism under the model of an obsessive-compulsive disorder.
The temperament of the personality is defined as the individual differences in habits and abilities related to emotions. It is inherited around 50%, moderately stable from childhood to adulthood, and it seems to be consistent in its structure in different cultures and ethnic groups. Four features or temperamental dimensions are described: search for novelty (BN), avoidance of harm (ED), dependence on reward (DR) and persistence (P).
- - Search for Novelty (SN): hereditary tendency towards the search for excitement and interest in novel stimuli. This trait is mediated by dopamine. These people would be impulsive, excitable, curious and enthusiastic.
- - Avoidance of Damage (AD): this is the hereditary tendency to respond intensely to signs of adverse stimuli stimulating the system of behavioral inhibition, probably through serotonin. Subjects with high values in this dimension develop conditioned avoidance responses to aversive stimuli, which makes them cautious, apprehensive and fearful.
- - Dependence of Reward (DR): corresponds to the tendency to respond intensely to reward stimuli or signals and to maintain the behavior previously associated with said reward. This response is mediated by norepinephrine in the maintenance system or behavioral persistence. It has to do with social reinforcement and sensitivity to social stimuli and discomfort for the separation of the group.
- - Persistence (P): which implies a neurobiological tendency to maintain behaviors under conditions of extinction. That is, the ability of the body to continue emitting behavior associated with reinforcement despite the disappearance of it. It would be associated with serotonergic transmission and orbitofrontal circuits.
The character or conceptual core of the personality is inherited to a lesser degree and is moderately influenced by social learning and cultural expectations about the social role in relation to age, occupation and other social circumstances (Cloninger, CR, Svrakic, DM; Przybeck, TR 1993) [ 13 ]. There are three characteristic dimensions: self-direction (AD), cooperation (CO) and self-transcendence (AT)
- - Self-direction: it is about the ability to regulate behavior and to commit oneself to the chosen goals
- - Cooperation: is the ability to identify and accept others. It refers to the prosocial behavior of the subject. It has to do with altruism, empathy and solidarity
- - Self-transcendence: represents the ability to identify as an integral part of the universe. It is related to creativity, fantasy and imagination (Bordalejo, D., Boullosa, O., Hadid, E., Puricell, M., Romero, E., Tannenhaus, L., Vieitez, A., Vázquez, G.; 2014 [ 15 ]).
The staircase consists of 240 elements that respond on a Likert scale of 5 options: 1 = False/2 = Almost all false/3 = True true than false/4 = Almost true/5 = True. Each of the dimensions of temperament and character is punctuated, according to a variable number of subscales, between 3 and 5.
For the TCI inventory, the revised version in Spanish (TCI-R) of Bayón (2004) was used and validated in our country by Gutiérrez-Zotes et al. (2004) In this version, the score of each trait is transformed into a “T” score (percentiles). Wong et al. (2010) define prominent features found in the upper third (67th percentile) or in the lower third (33th percentile) [ 15 ].
Statistical analysis used descriptive statistics, bivariate analyses and multivariate analysis.
Descriptive statistics: In all cases, the normality of the variables was verified with the Kolmogorov-Smirnov test. The quantitative variables were expressed as mean ± standard deviation and the qualitative variables were expressed as number and percentage.
Bivariate analyses: The Chi Square Test was used to analyze the association between quantitative variables. Using Student’s t -test or U Mann Whitney, according to the normality of the variables. To analyze the relationship between quantitative variables, the Pearson or Spearman correlation was used according to the normality of each variable.
Multivariate analysis: In the multivariate analysis, multiple linear regression analysis was used, using the multivariate linear general model (GLM), to analyze the relationship of personality with severity, intensity and the obsessive-compulsive component of consumption adjusted for age and sex.
In all cases, hypothesis testing used an alpha risk of 0.05 as the limit of statistical significance via IBM Statistical Package for the Social Sciences (SPSS) version 23.0 (IBM Corp., Armonk, NY, USA).
The sample consisted of 304 patients affected by Alcohol Use Disorder (AUD); 250 were male (82.2%) and 54 were female (17.8%). The average age of the men was 43.6 years (SD 9.7) and 43.8 (SD 9.8) for women. Most patients came from an urban environment (193 (63.5%)), while 111 patients (36.5%) came from a rural setting. Here, 163 (53.6%) had a partner, 86 (28.4%) were single and 54 (17.8%) were separated.
This cohort had high scores for Search for Novelty and Avoidance of Damage and a very low score in SD. According to Cloninger, this low score is associated with a personality disorder. Given that women represent only 17.8% of our study sample, we considered it relevant to analyse personality characteristics as independent samples according to sex. The women had significantly lower scores in Persistence ( p = 0.03) and Self-Autotranscendence ( p = 0.02) ( Figure 1 ).

Cloninger’s (TCI-R) personality scores as a function of gender (P* and ST* statistically significant).
The temperamental traits of Search for Novelty (SN) and Avoidance of Damage (AD) correspond to a higher degree of AUD severity. That is, people who are more impulsive and curious and/or more pessimistic and fearful of change present a greater severity of their AUD. They start consuming alcohol at a younger age and tend to do some in a pathological way. This consumption occurs with greater intensity and with serious dependence. In addition, this group of subjects has a greater compulsion and desire (“craving”) for the drink, which produces a greater medical severity ( Table 1 and Table 2 ).
Statistically significant correlations between Search for Novelty (TCI-R) and severity factors of the EIDA, OCDS and EuropAsi scales. (* p < 0.05; ** p < 0.001).
Statistically significant correlations between Avoidance of Damage (TCI-R) and severity factors of the EIDA, OCDS and EuropAsi scales. (* p < 0.05; ** p < 0.001).
However, the high scores in Reward Dependency (RD) and Persistence (P) are related to a lower severity of the disorder. In other words, people who were more sociable, ambitious and perfectionist had less intensity of dependence, less psychiatric severity and less craving for the substance. This translates into a less severe AUD ( Table 3 and Table 4 ).
Statistically significant correlations between Reward Dependency (TCI-R) and severity factors of the EIDA, OCDS and EuropAsi scales. (* p < 0.05; ** p < 0.001).
Statistically significant correlations between Persistence (TCI-R) and severity factors of the EIDA, OCDS and EuropAsi Scales. (* p < 0.05).
On the other hand, characteristic features, Self-Direction (SD), Cooperation (CO) and Self-Transcendence (ST) are unanimously related to a lower severity of the disorder by alcohol consumption. There is a lower intensity of dependency, less craving for drinking and less severe work and psychiatric severity ( Table 5 , Table 6 and Table 7 ).
Statistically significant correlations between SD (TCI-R) and severity factors of the EIDA, OCDS and EuropAsi scales. (* p < 0.05; ** p < 0.001).
Statistically significant correlations between Cooperation (TCI-R) and severity factors of the EIDA, OCDS and EuropAsi scales . (* p < 0.05; ** p < 0.001).
Statistically significant correlations between Self-Transcendence (TCI-R) and severity factors of the EIDA, OCDS and EuropAsi scales. (** p < 0.001).
Gender influences the severity of the alcohol consumption disorder via personality according to Cloninger. That is, three of the four temperamental variables (Search for Novelty, Avoidance of Damage, and Reward Dependency) are influenced by sex in their relationship with the severity of the AUD. This is seen through the duration of pathological consumption, medical severity, type of dependence, intensity of consumption, and objective family severity. On the other hand, SD and Self-Autotranscendence are influenced by sex in their relationship with the severity of the TCA through the obsession with drinking, the desire (“craving”) for it, and through psychiatric severity.
This means that the women in our study had significantly lower scores than men in Self-Autotranscendence and Persistence, i.e., they were less creative, spiritual, and ambitious than men—this translates into a greater severity of their addiction through a longer duration of pathological consumption, greater intensity of alcohol dependence, greater family and psychiatric severity, greater obsession and greater craving than drinking men ( Table 8 ).
Relationship of personality with the severity of the AUD accoing to Cloninger. This was influenced by gender in a statistically significant way.
4. Discussion
Through Through this study, we wanted to predict the severity of AUD by means of the assessment of their personality at the initial phase of treatment. This approach would allow us to know, at the initial stages of treatment, how many patients may probably have a more severe clinical course, how many patients would suffer from subsequent higher number of relapses and more alcohol consumption, greater craving and greater severity of dependence. The aim is to be able to optimize resources at the beginning of treatment. In our study we found that our AUD cohort had high scores SN, AD and SD. That is, AUD patients suffer from more impulsive personality, show a tendency to experience new emotions (SN) or are more fearful and apprehensive (AD). They may also have a lack of objectives or attribute their problems to others (SD) (Wong et al., 2010). These results are in line with some other authors who have studied the personality characteristics of these patients in similar settings [ 16 , 17 , 18 , 19 , 20 ].
Next we will expose the results we found between personality and severity of the AUD that we have found:
The scores described here are related to a higher severity of the AUD. The positive correlation of the Search for Novelty and Avoidance of Damage dimensions show a more severe dependence on alcohol (EIDA) and greater obsession and compulsion for drinking. These features indicate the relationship of these personality traits with a higher severity of AUD similar to other studies [ 18 , 19 ]. Nöel et al. (2011) concluded that alcohol-dependent subjects with high Search for Novelty scores have worse evolution and greater AUD severity and are more prone to relapse [ 8 ]. This may be due to the need of these people to experience new emotions even though this fact entails a risk to their physical and/or mental health.
The low score in Self-Direction observed here has been related to a greater severity of AUD through a more severe dependence on alcohol (EIDA) and more psychiatric pathology. On the other hand, high scores on this item are a protective factor—patients with a greater sense of responsibility and discipline have less severe AUD. Other studies have found similar results [ 20 ].
We conclude that people with high scores in Reward Dependency and Persistence as well as in Cooperation and Self-transcendence have less severe AUD. That is, people who are more social, hardworking, ambitious, empathetic and creative have a better prognosis in their AUD. Different authors corroborate our results and show that patients with higher scores in Persistence and Cooperation have a better evolution and less seriousness in their AUD [ 20 , 21 , 22 , 23 , 24 ].
The severity of alcohol dependence is determined by different variables, which show us statistically significant results if we analyze the sample according to gender. In relation to the severity of alcohol dependence by means of the EuropAsi scale, we find statistically significant gender differences. Women start drinking alcohol later compared to men (18 years vs. 15.6 years), this becomes problematic at older age (30.3 years vs. 22.9 years), the duration of this problematic consumption is lower (13.4 years vs. 20.3 years) and drink less alcohol (113 gr vs. 144), all of these differences being statistically significant ( p = 0.001). Although women drink for fewer years and consume less alcohol, we find that women’s addiction is more severe when atteding to some other variables:
- - The total score of the EIDA in our sample is 27.79 (SD 15.0), being 27.0 (SD 14.4), in the case of men; and of 32.5 (SD 16.7), in the case of women. Therefore, alcohol dependence is significantly more severe in women than in men ( p = 0.01).
- - In relation to the OCDS scale, the total score of the sample is 15.1 (SD 7.8). Men obtained a score of 14.6 (SD 7.2) and women 17.4 (SD 10.0), that is, women reported significantly more desire for alcohol than men. The average score on the obsession subscale is 6.3 (SD 4.1); in men the mean is 6.0 (SD 3.9) and in women 7.6 (SD 5.0), a very significant difference ( p = 0.008). On the other hand, the scores obtained in the compulsion subscale would be 8.87 for the total sample (SD 4.4); being in the case of men of 8.7 (SD 4.1) and in the case of women of 9.8 (SD 5.4). In conclusion, women have a greater obsession and compulsion for drinking than men in a significant way.
These scores are related to a worse evolution and a higher number of relapses, showing greater difficulty in maintaining abstinence (Pedrero et al., 2006) [ 25 ].
It is widely known that most of the addictive disorders are more frequent in men than in women. Particularly, in our study, 82.2% of the sample were men, whereas 17.8% were women. Despite this, women, who start drinking alcohol later and drink less alcohol than men, have a higher severity of their disorder, greater dependence on drinking and greater craving for alcohol, as exposed previously.
If we correlate these results with personality, we find that women show higher scores in Self-Transcendente and Persistence, which implies less ambition and/or greater difficulty in maintaining behaviors without positive reinforcement. They also present lower scores in Self-Transcendence, which is associated with less creative and intuitive people, these findings could be related to the fact that most of the women in our study do not perform paid work (25.9% vs. 22% of men), or are dedicated to the care sector (27.8% compared to 0.8% of men). In addition, there is a smaller number of women with both superior and elementary studies than men, which is justified in part, with the social reality in Spain to date, in which women have been relegated to a lower socio-economic status.
This study is limited in that it is an observational, naturalistic, epidemiological and usual clinical practice study; the sample was recruited in a non-experimental way. Enrolling more subjects would better approximate reality. Another limitation is that we used the TCI-R inventory that consists of 240 items. In some cases patients could not complete it due to lack of time or due to difficulties in compression. This could be solved via the abbreviated versions with 140 (Pedrero, 2006; Wedekind, 2013) [ 25 , 26 ] or 60 items (Pedrero, 2009) [ 27 ] although these are in the experimentation and validation phase. Finally, the gender differences do not follow a homogeneous distribution in our sample, following a naturalistic method in a real world setting.
By means of the present research work we were able to know the probable evolution of the AUD in the first visit of our patients, which allows us to carry out more specific interventions from the beginning oriented to avoid a bad evolution in the patients more serious and to optimize resources.
Future work will account for the information obtained about the personality profile of patients with greater severity of AUD. This information is only descriptive, and it is ideally suited for controlled designs that are different from this study. The effectiveness of a more targeted intervention could be assessed in those patients with a more serious profile.
5. Conclusions
The severity of AUD, in our study, is mainly given by the EUROPASI, EIDA, and OCDS scale. These are used to diagnose alcohol abuse and/or dependence. There were correlations between personality and the different variables. High Search for Novelty and Avoidance of Damage scores are related to a greater TCA severity, which is defined by an earlier age of onset in consumption, greater dependence on the substance (higher score of EIDA), and greater obsession and compulsion for drinking as well as a greater total score on the OCDS scale. High scores for Reward Dependency, Persistence, Self-Direction, Cooperation, and Self-Transcendence are associated with a lower severity of AUD and better prognosis. This is seen via a lower intensity of dependence (total EIDA), less obsession with the substance, and less psychiatric severity. The women in our study have a more severe AUD than men. They were less creative (Self-Transcendence) and ambitious (Persistence). This would imply that they had a greater dependence and “craving” for the drink than men do.
Author Contributions
A.Á., J.J.Á., D.J.P. and Á.L.M. conceived and designed the experiments; A.Á. and J.J.Á. performed the experiments and analyzed the data; D.J.P. and Á.L.M. contributed reagents/materials/analysis tools; A.Á. wrote the paper.
The present work has not received non-public and private funds.
Conflicts of Interest
Aida Alvarez, Jose J. Avila and D. J. Palao declare no conflict of interest. A. L. Montejo has been consultant or received research honoraria from Eli Lilly, Forum Pharmaceuti-cals, Rovi, Servier, Lundbeck, Otsuka, Janssen Cilag, Pfizery Roche, Instituto de Salud Carlos III and Junta de Castilla y León.
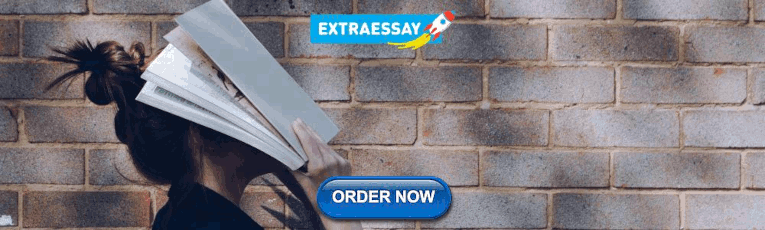
IMAGES
VIDEO
COMMENTS
The best-fitting prototypical personality profiles were labeled according to their Euclidean distances to averaged personality type profiles identified in a review of previous studies on personality types. This procedure yielded a five-cluster solution: resilient, overcontroller, undercontroller, reserved and vulnerable-resilient.
different aspects of personality traits assessment and cross cultural studies on personality traits. Keywords: Personality traits, current status, personality research, trait research 1.
A new algorithmic approach to personality prototyping based on Big Five traits was applied to a large representative and longitudinal German dataset (N = 22,820) including behavior, personality and health correlates. We applied three different clustering techniques, latent profile analysis, the k-means method and spectral clustering algorithms. The resulting cluster centers, i.e. the ...
Recent genomic, psychological, and developmental research shows that human personality is organized as a complex hierarchy that ascends from individual traits in many specific situations to multi ...
Personality types (profiles), on the other hand, can represent two- or k-way (k = 3 or more) interactions of certain trait levels, enabling all distinguished Big Five traits to simultaneously shape the interaction pattern (Asendorpf & Denissen, 2006). Most profile solutions (using the same sample and clustering method) vary from two to five ...
Using data from more than 370,000 individuals on the HEXACO-100 personality measure, Jose Espinoza and colleagues find that five profiles of the HEXACO-PI-R traits replicate robustly across ...
About the journal. Emphasizing experimental and descriptive research, the Journal of Research in Personality presents articles that examine important issues in the field of personality and in related fields basic to the understanding of personality. The subject matter includes treatments of genetic, physiological, …. View full aims & scope.
Another widely studied indicator is the facial width to height ratio (fWHR), which has been linked to various traits, such as achievement striving 10, deception 11, dominance 12, aggressiveness 13 ...
The personality's investigation has always been characterized as a central field of research for psychology, such that it was established in the 1920s as an autonomous scientific-disciplinary field. Personality can be defined as the set of characteristics of the person, capable of producing congruent patterns of thinking, feeling and behaving ...
Personality traits and personal values are important psychological characteristics, serving as important predictors of many outcomes. ... Sharpe J. P. (2000). Personality profiles of environmentally responsible groups. Psychological Reports, 87, 176-178. Crossref. PubMed. ISI. Google Scholar. Roberts B. W., Robins R. W. (2000). Broad ...
The personality questionnaire administered to the students is the Ten-Item Personality Inventory (TIPI) with the adaptation for Italian subjects by Chiorri et al. of the commonly adopted questionnaire of Gosling et al. . The TIPI is a short-form questionnaire based on a set of paired items, each one containing two descriptors that capture the ...
About this journal. Journal of Personality publishes scientific investigations in the field of personality. We focus particularly on personality and behavior dynamics, personality development, and individual differences in the cognitive, affective, and interpersonal domains. This social psychology journal reflects and stimulates interest in the ...
Personality is a mirror of what you do and say. Essentially, your personality defines who are you. Your behaviour reflects your personality and informs how different you are from others. A common ...
Personality is considered a quantitative variable, via Personality Inventory 16 PF. Sex is considered a qualitative variable with two dimensions, categorized M for men and F for women. For the quantitative academic performance variable, a measure was established according to the evaluation scale used by the university, i.e., one-five; being one ...
In addition, data were collected from essays on teachers personalities, evaluation scales, checklists, sociometry methods and personality and ability tests. ... of teachers (Harris, Vernon, Johnson, & Jang, Citation 2006), suggesting that teachers may have a distinctive personality profile as a professional group. Focusing specifically on ...
Abstract. In the current study, we set out to examine the viability of a novel approach to modeling human personality. Research in psychology suggests that people's personalities can be ...
1 School of Psychology, Korea University, Seoul, South Korea; 2 Department of Software, Sejong University, Seoul, South Korea; 3 KU Mind Health Institute, Korea University, Seoul, South Korea; Background: Self-report multiple choice questionnaires have been widely utilized to quantitatively measure one's personality and psychological constructs. Despite several strengths (e.g., brevity and ...
One hundred and forty nine (149) undergraduate students of a B - School were chosen. A comparative study of personality profiles for defined demographic variables were carried out. Dominant MBTI types found in this research are ESFJ (23.5%) and ESTJ (16.1%). However, ISTP (0.7%) and INTP (1.3%) are the least observed personality types.
Starting in the 1980s, personality psychology began a profound renaissance and has now become an extraordinarily diverse and intellectually stimulating field (Pervin & John, 1999).However, just because a field of inquiry is vibrant does not mean it is practical or useful—one would need to show that personality traits predict important life outcomes, such as health and longevity, marital ...
Research Paper By Robert Letchford (Leadership and Org Development, MYANMAR). Introduction. The development and professionalization of the coaching industry have slowly expanded over the last 20+ years to become recognized, by corporations and individuals alike as a valid way to improve individual and organizational capacity to perform at higher levels of productivity.
Personality is relatively stable over long timescales but remains malleable to some degree. In this Review, Jackson and Wright examine the mechanisms responsible for intentional and naturally ...
Producer, internet personality, and world traveler, James has always steered away from the norm — of course when he was supposed to answer questions, he asked one.
Discussion. The aim of the present study was to determine the relationship between self-care and the personality profile of patients with type 2 diabetes who were referred to the diabetes clinic of Imam Khomeini Medical Education Center in Ardabil city in 2021. The findings of the current research regarding the self-care status of the patients ...
Online internet personality group "Dude Perfect" has raised more than $100 million to expand its reach and create more content, the company announced Monday. Founded more than a decade ago ...
Future work will account for the information obtained about the personality profile of patients with greater severity of AUD. This information is only descriptive, and it is ideally suited for controlled designs that are different from this study. The effectiveness of a more targeted intervention could be assessed in those patients with a more ...