Hypothesis Testing Calculator
Related: confidence interval calculator, type ii error.
The first step in hypothesis testing is to calculate the test statistic. The formula for the test statistic depends on whether the population standard deviation (σ) is known or unknown. If σ is known, our hypothesis test is known as a z test and we use the z distribution. If σ is unknown, our hypothesis test is known as a t test and we use the t distribution. Use of the t distribution relies on the degrees of freedom, which is equal to the sample size minus one. Furthermore, if the population standard deviation σ is unknown, the sample standard deviation s is used instead. To switch from σ known to σ unknown, click on $\boxed{\sigma}$ and select $\boxed{s}$ in the Hypothesis Testing Calculator.
Next, the test statistic is used to conduct the test using either the p-value approach or critical value approach. The particular steps taken in each approach largely depend on the form of the hypothesis test: lower tail, upper tail or two-tailed. The form can easily be identified by looking at the alternative hypothesis (H a ). If there is a less than sign in the alternative hypothesis then it is a lower tail test, greater than sign is an upper tail test and inequality is a two-tailed test. To switch from a lower tail test to an upper tail or two-tailed test, click on $\boxed{\geq}$ and select $\boxed{\leq}$ or $\boxed{=}$, respectively.
In the p-value approach, the test statistic is used to calculate a p-value. If the test is a lower tail test, the p-value is the probability of getting a value for the test statistic at least as small as the value from the sample. If the test is an upper tail test, the p-value is the probability of getting a value for the test statistic at least as large as the value from the sample. In a two-tailed test, the p-value is the probability of getting a value for the test statistic at least as unlikely as the value from the sample.
To test the hypothesis in the p-value approach, compare the p-value to the level of significance. If the p-value is less than or equal to the level of signifance, reject the null hypothesis. If the p-value is greater than the level of significance, do not reject the null hypothesis. This method remains unchanged regardless of whether it's a lower tail, upper tail or two-tailed test. To change the level of significance, click on $\boxed{.05}$. Note that if the test statistic is given, you can calculate the p-value from the test statistic by clicking on the switch symbol twice.
In the critical value approach, the level of significance ($\alpha$) is used to calculate the critical value. In a lower tail test, the critical value is the value of the test statistic providing an area of $\alpha$ in the lower tail of the sampling distribution of the test statistic. In an upper tail test, the critical value is the value of the test statistic providing an area of $\alpha$ in the upper tail of the sampling distribution of the test statistic. In a two-tailed test, the critical values are the values of the test statistic providing areas of $\alpha / 2$ in the lower and upper tail of the sampling distribution of the test statistic.
To test the hypothesis in the critical value approach, compare the critical value to the test statistic. Unlike the p-value approach, the method we use to decide whether to reject the null hypothesis depends on the form of the hypothesis test. In a lower tail test, if the test statistic is less than or equal to the critical value, reject the null hypothesis. In an upper tail test, if the test statistic is greater than or equal to the critical value, reject the null hypothesis. In a two-tailed test, if the test statistic is less than or equal the lower critical value or greater than or equal to the upper critical value, reject the null hypothesis.
When conducting a hypothesis test, there is always a chance that you come to the wrong conclusion. There are two types of errors you can make: Type I Error and Type II Error. A Type I Error is committed if you reject the null hypothesis when the null hypothesis is true. Ideally, we'd like to accept the null hypothesis when the null hypothesis is true. A Type II Error is committed if you accept the null hypothesis when the alternative hypothesis is true. Ideally, we'd like to reject the null hypothesis when the alternative hypothesis is true.
Hypothesis testing is closely related to the statistical area of confidence intervals. If the hypothesized value of the population mean is outside of the confidence interval, we can reject the null hypothesis. Confidence intervals can be found using the Confidence Interval Calculator . The calculator on this page does hypothesis tests for one population mean. Sometimes we're interest in hypothesis tests about two population means. These can be solved using the Two Population Calculator . The probability of a Type II Error can be calculated by clicking on the link at the bottom of the page.
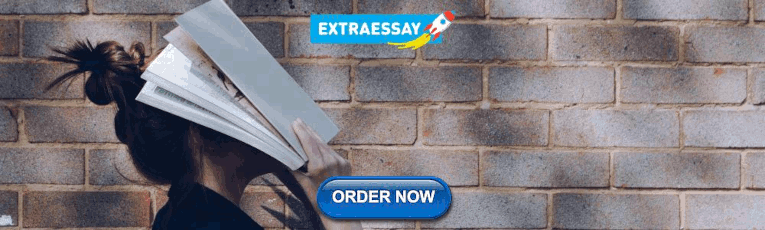
Have a language expert improve your writing
Run a free plagiarism check in 10 minutes, generate accurate citations for free.
- Knowledge Base
Hypothesis Testing | A Step-by-Step Guide with Easy Examples
Published on November 8, 2019 by Rebecca Bevans . Revised on June 22, 2023.
Hypothesis testing is a formal procedure for investigating our ideas about the world using statistics . It is most often used by scientists to test specific predictions, called hypotheses, that arise from theories.
There are 5 main steps in hypothesis testing:
- State your research hypothesis as a null hypothesis and alternate hypothesis (H o ) and (H a or H 1 ).
- Collect data in a way designed to test the hypothesis.
- Perform an appropriate statistical test .
- Decide whether to reject or fail to reject your null hypothesis.
- Present the findings in your results and discussion section.
Though the specific details might vary, the procedure you will use when testing a hypothesis will always follow some version of these steps.
Table of contents
Step 1: state your null and alternate hypothesis, step 2: collect data, step 3: perform a statistical test, step 4: decide whether to reject or fail to reject your null hypothesis, step 5: present your findings, other interesting articles, frequently asked questions about hypothesis testing.
After developing your initial research hypothesis (the prediction that you want to investigate), it is important to restate it as a null (H o ) and alternate (H a ) hypothesis so that you can test it mathematically.
The alternate hypothesis is usually your initial hypothesis that predicts a relationship between variables. The null hypothesis is a prediction of no relationship between the variables you are interested in.
- H 0 : Men are, on average, not taller than women. H a : Men are, on average, taller than women.
Prevent plagiarism. Run a free check.
For a statistical test to be valid , it is important to perform sampling and collect data in a way that is designed to test your hypothesis. If your data are not representative, then you cannot make statistical inferences about the population you are interested in.
There are a variety of statistical tests available, but they are all based on the comparison of within-group variance (how spread out the data is within a category) versus between-group variance (how different the categories are from one another).
If the between-group variance is large enough that there is little or no overlap between groups, then your statistical test will reflect that by showing a low p -value . This means it is unlikely that the differences between these groups came about by chance.
Alternatively, if there is high within-group variance and low between-group variance, then your statistical test will reflect that with a high p -value. This means it is likely that any difference you measure between groups is due to chance.
Your choice of statistical test will be based on the type of variables and the level of measurement of your collected data .
- an estimate of the difference in average height between the two groups.
- a p -value showing how likely you are to see this difference if the null hypothesis of no difference is true.
Based on the outcome of your statistical test, you will have to decide whether to reject or fail to reject your null hypothesis.
In most cases you will use the p -value generated by your statistical test to guide your decision. And in most cases, your predetermined level of significance for rejecting the null hypothesis will be 0.05 – that is, when there is a less than 5% chance that you would see these results if the null hypothesis were true.
In some cases, researchers choose a more conservative level of significance, such as 0.01 (1%). This minimizes the risk of incorrectly rejecting the null hypothesis ( Type I error ).
The results of hypothesis testing will be presented in the results and discussion sections of your research paper , dissertation or thesis .
In the results section you should give a brief summary of the data and a summary of the results of your statistical test (for example, the estimated difference between group means and associated p -value). In the discussion , you can discuss whether your initial hypothesis was supported by your results or not.
In the formal language of hypothesis testing, we talk about rejecting or failing to reject the null hypothesis. You will probably be asked to do this in your statistics assignments.
However, when presenting research results in academic papers we rarely talk this way. Instead, we go back to our alternate hypothesis (in this case, the hypothesis that men are on average taller than women) and state whether the result of our test did or did not support the alternate hypothesis.
If your null hypothesis was rejected, this result is interpreted as “supported the alternate hypothesis.”
These are superficial differences; you can see that they mean the same thing.
You might notice that we don’t say that we reject or fail to reject the alternate hypothesis . This is because hypothesis testing is not designed to prove or disprove anything. It is only designed to test whether a pattern we measure could have arisen spuriously, or by chance.
If we reject the null hypothesis based on our research (i.e., we find that it is unlikely that the pattern arose by chance), then we can say our test lends support to our hypothesis . But if the pattern does not pass our decision rule, meaning that it could have arisen by chance, then we say the test is inconsistent with our hypothesis .
If you want to know more about statistics , methodology , or research bias , make sure to check out some of our other articles with explanations and examples.
- Normal distribution
- Descriptive statistics
- Measures of central tendency
- Correlation coefficient
Methodology
- Cluster sampling
- Stratified sampling
- Types of interviews
- Cohort study
- Thematic analysis
Research bias
- Implicit bias
- Cognitive bias
- Survivorship bias
- Availability heuristic
- Nonresponse bias
- Regression to the mean
Hypothesis testing is a formal procedure for investigating our ideas about the world using statistics. It is used by scientists to test specific predictions, called hypotheses , by calculating how likely it is that a pattern or relationship between variables could have arisen by chance.
A hypothesis states your predictions about what your research will find. It is a tentative answer to your research question that has not yet been tested. For some research projects, you might have to write several hypotheses that address different aspects of your research question.
A hypothesis is not just a guess — it should be based on existing theories and knowledge. It also has to be testable, which means you can support or refute it through scientific research methods (such as experiments, observations and statistical analysis of data).
Null and alternative hypotheses are used in statistical hypothesis testing . The null hypothesis of a test always predicts no effect or no relationship between variables, while the alternative hypothesis states your research prediction of an effect or relationship.
Cite this Scribbr article
If you want to cite this source, you can copy and paste the citation or click the “Cite this Scribbr article” button to automatically add the citation to our free Citation Generator.
Bevans, R. (2023, June 22). Hypothesis Testing | A Step-by-Step Guide with Easy Examples. Scribbr. Retrieved April 15, 2024, from https://www.scribbr.com/statistics/hypothesis-testing/
Is this article helpful?
Rebecca Bevans
Other students also liked, choosing the right statistical test | types & examples, understanding p values | definition and examples, what is your plagiarism score.
“extremely user friendly”
“truly amazing!”
“so easy to use!”
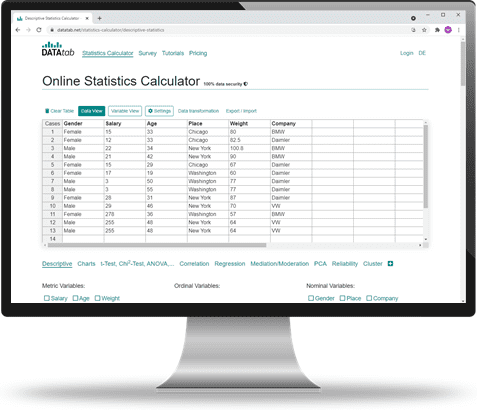
Statistics Calculator
You want to analyze your data effortlessly? DATAtab makes it easy and online.
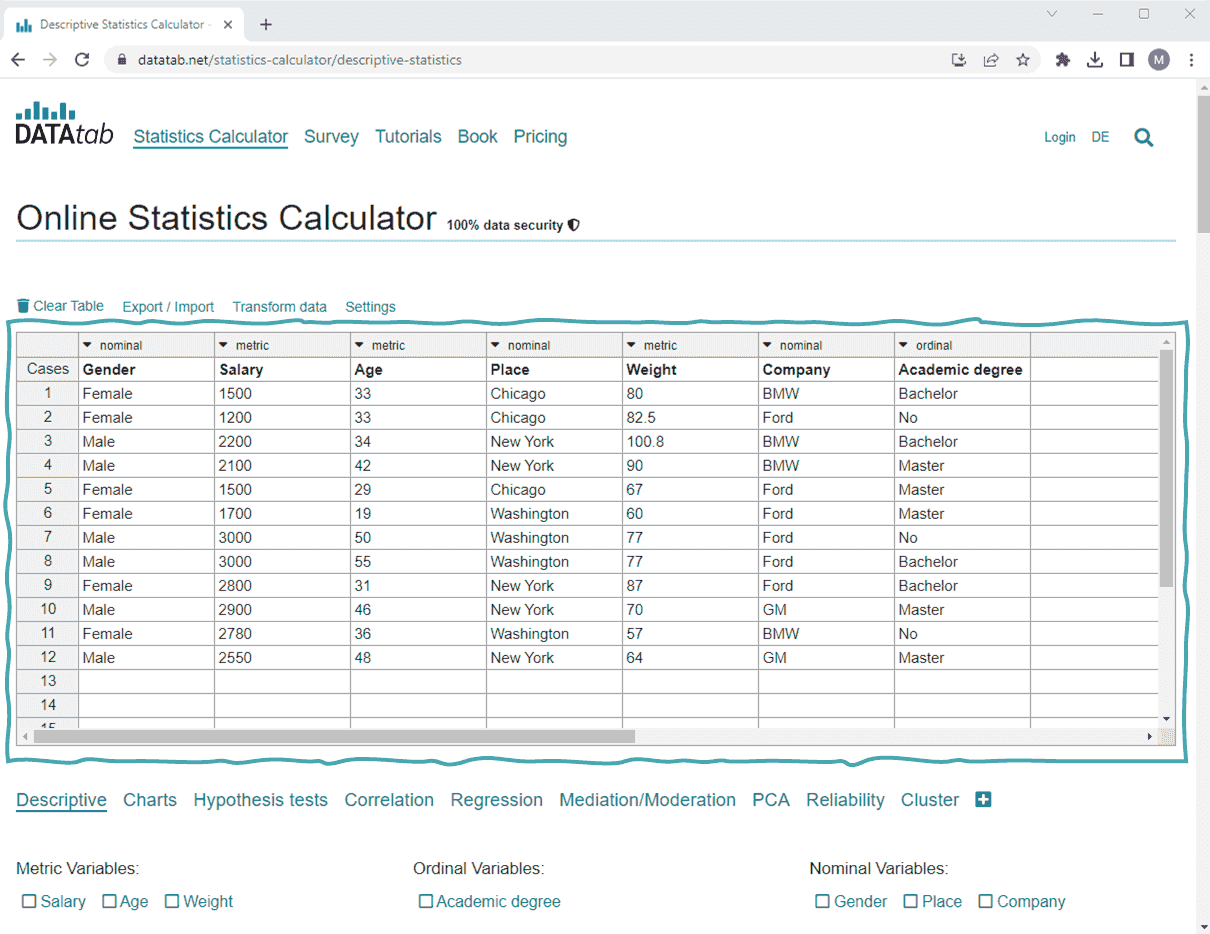
Online Statistics Calculator
What do you want to calculate online? The online statistics calculator is simple and uncomplicated! Here you can find a list of all implemented methods!
Create charts online with DATAtab
Create your charts for your data directly online and uncomplicated. To do this, insert your data into the table under Charts and select which chart you want.
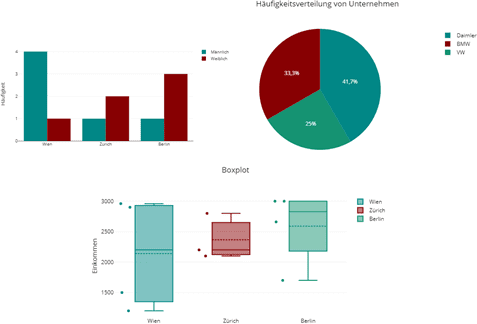
The advantages of DATAtab
Statistics, as simple as never before..
DATAtab is a modern statistics software, with unique user-friendliness. Statistical analyses are done with just a few clicks, so DATAtab is perfect for statistics beginners and for professionals who want more flow in the user experience.
Directly in the browser, fully flexible.
Directly in the browser, fully flexible. DATAtab works directly in your web browser. You have no installation and maintenance effort whatsoever. Wherever and whenever you want to use DATAtab, just go to the website and get started.
All the statistical methods you need.
DATAtab offers you a wide range of statistical methods. We have selected the most central and best known statistical methods for you and do not overwhelm you with special cases.
Data security is a top priority.
All data that you insert and evaluate on DATAtab always remain on your end device. The data is not sent to any server or stored by us (not even temporarily). Furthermore, we do not pass on your data to third parties in order to analyze your user behavior.
Many tutorials with simple examples.
In order to facilitate the introduction, DATAtab offers a large number of free tutorials with focused explanations in simple language. We explain the statistical background of the methods and give step-by-step explanations for performing the analyses in the statistics calculator.
Practical Auto-Assistant.
DATAtab takes you by the hand in the world of statistics. When making statistical decisions, such as the choice of scale or measurement level or the selection of suitable methods, Auto-Assistants ensure that you get correct results quickly.
Charts, simple and clear.
With DATAtab data visualization is fun! Here you can easily create meaningful charts that optimally illustrate your results.
New in the world of statistics?
DATAtab was primarily designed for people for whom statistics is new territory. Beginners are not overwhelmed with a lot of complicated options and checkboxes, but are encouraged to perform their analyses step by step.
Online survey very simple.
DATAtab offers you the possibility to easily create an online survey, which you can then evaluate immediately with DATAtab.
Our references
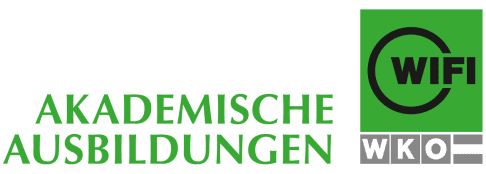
Alternative to statistical software like SPSS and STATA
DATAtab was designed for ease of use and is a compelling alternative to statistical programs such as SPSS and STATA. On datatab.net, data can be statistically evaluated directly online and very easily (e.g. t-test, regression, correlation etc.). DATAtab's goal is to make the world of statistical data analysis as simple as possible, no installation and easy to use. Of course, we would also be pleased if you take a look at our second project Statisty .
Extensive tutorials
Descriptive statistics.
Here you can find out everything about location parameters and dispersion parameters and how you can describe and clearly present your data using characteristic values.
Hypothesis Test
Here you will find everything about hypothesis testing: One sample t-test , Unpaired t-test , Paired t-test and Chi-square test . You will also find tutorials for non-parametric statistical procedures such as the Mann-Whitney u-Test and Wilcoxon-Test . mann-whitney-u-test and the Wilcoxon test
The regression provides information about the influence of one or more independent variables on the dependent variable. Here are simple explanations of linear regression and logistic regression .
Correlation
Correlation analyses allow you to analyze the linear association between variables. Learn when to use Pearson correlation or Spearman rank correlation . With partial correlation , you can calculate the correlation between two variables to the exclusion of a third variable.
Partial Correlation
The partial correlation shows you the correlation between two variables to the exclusion of a third variable.
Levene Test
The Levene Test checks your data for variance equality. Thus, the levene test is used as a prerequisite test for many hypothesis tests .
The p-value is needed for every hypothesis test to be able to make a statement whether the null hypothesis is accepted or rejected.
Distributions
DATAtab provides you with tables with distributions and helpful explanations of the distribution functions. These include the Table of t-distribution and the Table of chi-squared distribution
Contingency table
With a contingency table you can get an overview of two categorical variables in the statistics.
Equivalence and non-inferiority
In an equivalence trial, the statistical test aims at showing that two treatments are not too different in characteristics and a non-inferiority trial wants to show that an experimental treatment is not worse than an established treatment.
If there is a clear cause-effect relationship between two variables, then we can speak of causality. Learn more about causality in our tutorial.
Multicollinearity
Multicollinearity is when two or more independent variables have a high correlation.
Effect size for independent t-test
Learn how to calculate the effect size for the t-test for independent samples.
Reliability analysis calculator
On DATAtab, Cohen's Kappa can be easily calculated online in the Cohen’s Kappa Calculator . there is also the Fleiss Kappa Calculator . Of course, the Cronbach's alpha can also be calculated in the Cronbach's Alpha Calculator .
Analysis of variance with repeated measurement
Repeated measures ANOVA tests whether there are statistically significant differences in three or more dependent samples.
Cite DATAtab: DATAtab Team (2024). DATAtab: Online Statistics Calculator. DATAtab e.U. Graz, Austria. URL https://datatab.net
- Comprehensive Learning Paths
- 150+ Hours of Videos
- Complete Access to Jupyter notebooks, Datasets, References.

Hypothesis Testing – A Deep Dive into Hypothesis Testing, The Backbone of Statistical Inference
- September 21, 2023
Explore the intricacies of hypothesis testing, a cornerstone of statistical analysis. Dive into methods, interpretations, and applications for making data-driven decisions.

In this Blog post we will learn:
- What is Hypothesis Testing?
- Steps in Hypothesis Testing 2.1. Set up Hypotheses: Null and Alternative 2.2. Choose a Significance Level (α) 2.3. Calculate a test statistic and P-Value 2.4. Make a Decision
- Example : Testing a new drug.
- Example in python
1. What is Hypothesis Testing?
In simple terms, hypothesis testing is a method used to make decisions or inferences about population parameters based on sample data. Imagine being handed a dice and asked if it’s biased. By rolling it a few times and analyzing the outcomes, you’d be engaging in the essence of hypothesis testing.
Think of hypothesis testing as the scientific method of the statistics world. Suppose you hear claims like “This new drug works wonders!” or “Our new website design boosts sales.” How do you know if these statements hold water? Enter hypothesis testing.
2. Steps in Hypothesis Testing
- Set up Hypotheses : Begin with a null hypothesis (H0) and an alternative hypothesis (Ha).
- Choose a Significance Level (α) : Typically 0.05, this is the probability of rejecting the null hypothesis when it’s actually true. Think of it as the chance of accusing an innocent person.
- Calculate Test statistic and P-Value : Gather evidence (data) and calculate a test statistic.
- p-value : This is the probability of observing the data, given that the null hypothesis is true. A small p-value (typically ≤ 0.05) suggests the data is inconsistent with the null hypothesis.
- Decision Rule : If the p-value is less than or equal to α, you reject the null hypothesis in favor of the alternative.
2.1. Set up Hypotheses: Null and Alternative
Before diving into testing, we must formulate hypotheses. The null hypothesis (H0) represents the default assumption, while the alternative hypothesis (H1) challenges it.
For instance, in drug testing, H0 : “The new drug is no better than the existing one,” H1 : “The new drug is superior .”
2.2. Choose a Significance Level (α)
When You collect and analyze data to test H0 and H1 hypotheses. Based on your analysis, you decide whether to reject the null hypothesis in favor of the alternative, or fail to reject / Accept the null hypothesis.
The significance level, often denoted by $α$, represents the probability of rejecting the null hypothesis when it is actually true.
In other words, it’s the risk you’re willing to take of making a Type I error (false positive).
Type I Error (False Positive) :
- Symbolized by the Greek letter alpha (α).
- Occurs when you incorrectly reject a true null hypothesis . In other words, you conclude that there is an effect or difference when, in reality, there isn’t.
- The probability of making a Type I error is denoted by the significance level of a test. Commonly, tests are conducted at the 0.05 significance level , which means there’s a 5% chance of making a Type I error .
- Commonly used significance levels are 0.01, 0.05, and 0.10, but the choice depends on the context of the study and the level of risk one is willing to accept.
Example : If a drug is not effective (truth), but a clinical trial incorrectly concludes that it is effective (based on the sample data), then a Type I error has occurred.
Type II Error (False Negative) :
- Symbolized by the Greek letter beta (β).
- Occurs when you accept a false null hypothesis . This means you conclude there is no effect or difference when, in reality, there is.
- The probability of making a Type II error is denoted by β. The power of a test (1 – β) represents the probability of correctly rejecting a false null hypothesis.
Example : If a drug is effective (truth), but a clinical trial incorrectly concludes that it is not effective (based on the sample data), then a Type II error has occurred.
Balancing the Errors :
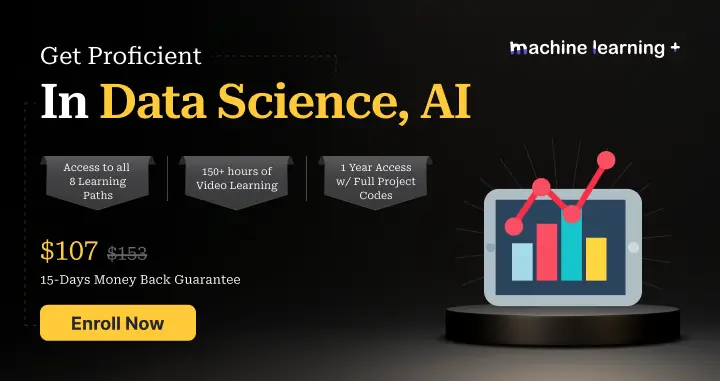
In practice, there’s a trade-off between Type I and Type II errors. Reducing the risk of one typically increases the risk of the other. For example, if you want to decrease the probability of a Type I error (by setting a lower significance level), you might increase the probability of a Type II error unless you compensate by collecting more data or making other adjustments.
It’s essential to understand the consequences of both types of errors in any given context. In some situations, a Type I error might be more severe, while in others, a Type II error might be of greater concern. This understanding guides researchers in designing their experiments and choosing appropriate significance levels.
2.3. Calculate a test statistic and P-Value
Test statistic : A test statistic is a single number that helps us understand how far our sample data is from what we’d expect under a null hypothesis (a basic assumption we’re trying to test against). Generally, the larger the test statistic, the more evidence we have against our null hypothesis. It helps us decide whether the differences we observe in our data are due to random chance or if there’s an actual effect.
P-value : The P-value tells us how likely we would get our observed results (or something more extreme) if the null hypothesis were true. It’s a value between 0 and 1. – A smaller P-value (typically below 0.05) means that the observation is rare under the null hypothesis, so we might reject the null hypothesis. – A larger P-value suggests that what we observed could easily happen by random chance, so we might not reject the null hypothesis.
2.4. Make a Decision
Relationship between $α$ and P-Value
When conducting a hypothesis test:
We then calculate the p-value from our sample data and the test statistic.
Finally, we compare the p-value to our chosen $α$:
- If $p−value≤α$: We reject the null hypothesis in favor of the alternative hypothesis. The result is said to be statistically significant.
- If $p−value>α$: We fail to reject the null hypothesis. There isn’t enough statistical evidence to support the alternative hypothesis.
3. Example : Testing a new drug.
Imagine we are investigating whether a new drug is effective at treating headaches faster than drug B.
Setting Up the Experiment : You gather 100 people who suffer from headaches. Half of them (50 people) are given the new drug (let’s call this the ‘Drug Group’), and the other half are given a sugar pill, which doesn’t contain any medication.
- Set up Hypotheses : Before starting, you make a prediction:
- Null Hypothesis (H0): The new drug has no effect. Any difference in healing time between the two groups is just due to random chance.
- Alternative Hypothesis (H1): The new drug does have an effect. The difference in healing time between the two groups is significant and not just by chance.
Calculate Test statistic and P-Value : After the experiment, you analyze the data. The “test statistic” is a number that helps you understand the difference between the two groups in terms of standard units.
For instance, let’s say:
- The average healing time in the Drug Group is 2 hours.
- The average healing time in the Placebo Group is 3 hours.
The test statistic helps you understand how significant this 1-hour difference is. If the groups are large and the spread of healing times in each group is small, then this difference might be significant. But if there’s a huge variation in healing times, the 1-hour difference might not be so special.
Imagine the P-value as answering this question: “If the new drug had NO real effect, what’s the probability that I’d see a difference as extreme (or more extreme) as the one I found, just by random chance?”
For instance:
- P-value of 0.01 means there’s a 1% chance that the observed difference (or a more extreme difference) would occur if the drug had no effect. That’s pretty rare, so we might consider the drug effective.
- P-value of 0.5 means there’s a 50% chance you’d see this difference just by chance. That’s pretty high, so we might not be convinced the drug is doing much.
- If the P-value is less than ($α$) 0.05: the results are “statistically significant,” and they might reject the null hypothesis , believing the new drug has an effect.
- If the P-value is greater than ($α$) 0.05: the results are not statistically significant, and they don’t reject the null hypothesis , remaining unsure if the drug has a genuine effect.
4. Example in python
For simplicity, let’s say we’re using a t-test (common for comparing means). Let’s dive into Python:
Making a Decision : “The results are statistically significant! p-value < 0.05 , The drug seems to have an effect!” If not, we’d say, “Looks like the drug isn’t as miraculous as we thought.”
5. Conclusion
Hypothesis testing is an indispensable tool in data science, allowing us to make data-driven decisions with confidence. By understanding its principles, conducting tests properly, and considering real-world applications, you can harness the power of hypothesis testing to unlock valuable insights from your data.
More Articles
Correlation – connecting the dots, the role of correlation in data analysis, sampling and sampling distributions – a comprehensive guide on sampling and sampling distributions, law of large numbers – a deep dive into the world of statistics, central limit theorem – a deep dive into central limit theorem and its significance in statistics, skewness and kurtosis – peaks and tails, understanding data through skewness and kurtosis”, similar articles, complete introduction to linear regression in r, how to implement common statistical significance tests and find the p value, logistic regression – a complete tutorial with examples in r.
Subscribe to Machine Learning Plus for high value data science content
© Machinelearningplus. All rights reserved.

Machine Learning A-Z™: Hands-On Python & R In Data Science
Free sample videos:.

- Business Essentials
- Leadership & Management
- Credential of Leadership, Impact, and Management in Business (CLIMB)
- Entrepreneurship & Innovation
- *New* Digital Transformation
- Finance & Accounting
- Business in Society
- For Organizations
- Support Portal
- Media Coverage
- Founding Donors
- Leadership Team
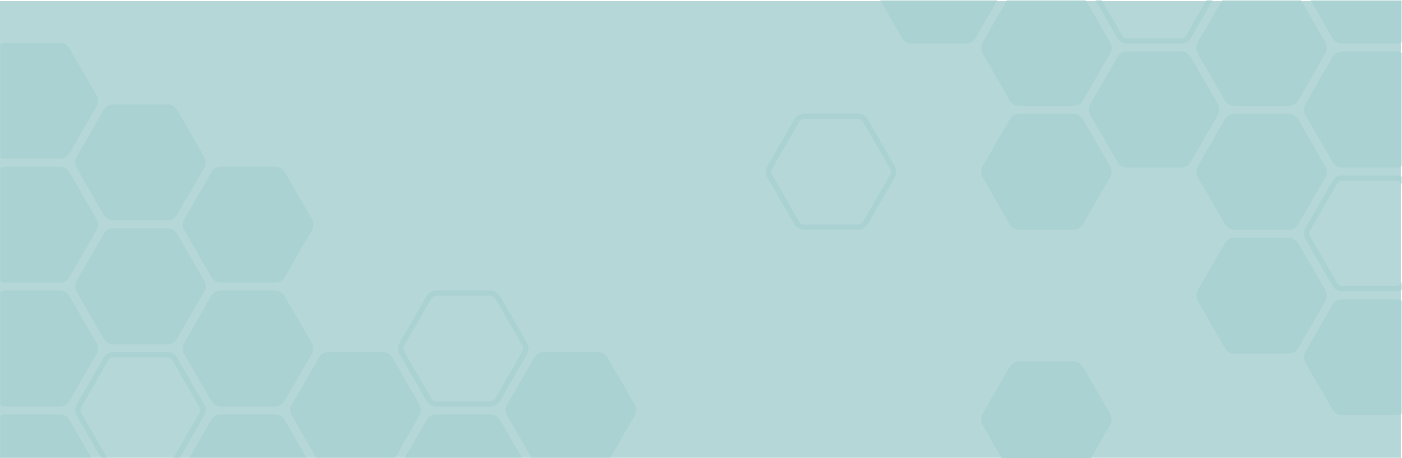
- Harvard Business School →
- HBS Online →
- Business Insights →
Business Insights
Harvard Business School Online's Business Insights Blog provides the career insights you need to achieve your goals and gain confidence in your business skills.
- Career Development
- Communication
- Decision-Making
- Earning Your MBA
- Negotiation
- News & Events
- Productivity
- Staff Spotlight
- Student Profiles
- Work-Life Balance
- Alternative Investments
- Business Analytics
- Business Strategy
- Business and Climate Change
- Design Thinking and Innovation
- Digital Marketing Strategy
- Disruptive Strategy
- Economics for Managers
- Entrepreneurship Essentials
- Financial Accounting
- Global Business
- Launching Tech Ventures
- Leadership Principles
- Leadership, Ethics, and Corporate Accountability
- Leading with Finance
- Management Essentials
- Negotiation Mastery
- Organizational Leadership
- Power and Influence for Positive Impact
- Strategy Execution
- Sustainable Business Strategy
- Sustainable Investing
- Winning with Digital Platforms
A Beginner’s Guide to Hypothesis Testing in Business
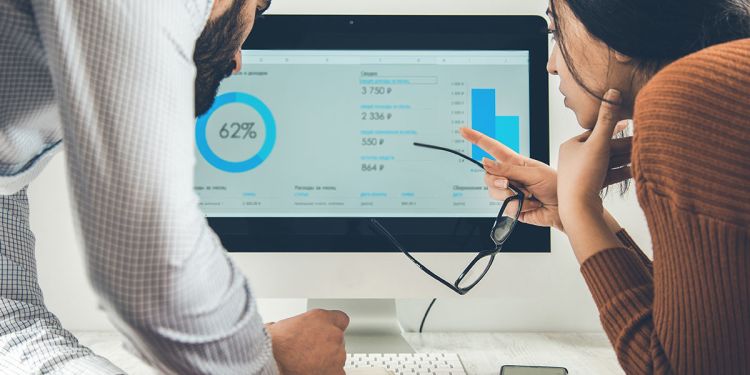
- 30 Mar 2021
Becoming a more data-driven decision-maker can bring several benefits to your organization, enabling you to identify new opportunities to pursue and threats to abate. Rather than allowing subjective thinking to guide your business strategy, backing your decisions with data can empower your company to become more innovative and, ultimately, profitable.
If you’re new to data-driven decision-making, you might be wondering how data translates into business strategy. The answer lies in generating a hypothesis and verifying or rejecting it based on what various forms of data tell you.
Below is a look at hypothesis testing and the role it plays in helping businesses become more data-driven.
Access your free e-book today.
What Is Hypothesis Testing?
To understand what hypothesis testing is, it’s important first to understand what a hypothesis is.
A hypothesis or hypothesis statement seeks to explain why something has happened, or what might happen, under certain conditions. It can also be used to understand how different variables relate to each other. Hypotheses are often written as if-then statements; for example, “If this happens, then this will happen.”
Hypothesis testing , then, is a statistical means of testing an assumption stated in a hypothesis. While the specific methodology leveraged depends on the nature of the hypothesis and data available, hypothesis testing typically uses sample data to extrapolate insights about a larger population.
Hypothesis Testing in Business
When it comes to data-driven decision-making, there’s a certain amount of risk that can mislead a professional. This could be due to flawed thinking or observations, incomplete or inaccurate data , or the presence of unknown variables. The danger in this is that, if major strategic decisions are made based on flawed insights, it can lead to wasted resources, missed opportunities, and catastrophic outcomes.
The real value of hypothesis testing in business is that it allows professionals to test their theories and assumptions before putting them into action. This essentially allows an organization to verify its analysis is correct before committing resources to implement a broader strategy.
As one example, consider a company that wishes to launch a new marketing campaign to revitalize sales during a slow period. Doing so could be an incredibly expensive endeavor, depending on the campaign’s size and complexity. The company, therefore, may wish to test the campaign on a smaller scale to understand how it will perform.
In this example, the hypothesis that’s being tested would fall along the lines of: “If the company launches a new marketing campaign, then it will translate into an increase in sales.” It may even be possible to quantify how much of a lift in sales the company expects to see from the effort. Pending the results of the pilot campaign, the business would then know whether it makes sense to roll it out more broadly.
Related: 9 Fundamental Data Science Skills for Business Professionals
Key Considerations for Hypothesis Testing
1. alternative hypothesis and null hypothesis.
In hypothesis testing, the hypothesis that’s being tested is known as the alternative hypothesis . Often, it’s expressed as a correlation or statistical relationship between variables. The null hypothesis , on the other hand, is a statement that’s meant to show there’s no statistical relationship between the variables being tested. It’s typically the exact opposite of whatever is stated in the alternative hypothesis.
For example, consider a company’s leadership team that historically and reliably sees $12 million in monthly revenue. They want to understand if reducing the price of their services will attract more customers and, in turn, increase revenue.
In this case, the alternative hypothesis may take the form of a statement such as: “If we reduce the price of our flagship service by five percent, then we’ll see an increase in sales and realize revenues greater than $12 million in the next month.”
The null hypothesis, on the other hand, would indicate that revenues wouldn’t increase from the base of $12 million, or might even decrease.
Check out the video below about the difference between an alternative and a null hypothesis, and subscribe to our YouTube channel for more explainer content.
2. Significance Level and P-Value
Statistically speaking, if you were to run the same scenario 100 times, you’d likely receive somewhat different results each time. If you were to plot these results in a distribution plot, you’d see the most likely outcome is at the tallest point in the graph, with less likely outcomes falling to the right and left of that point.

With this in mind, imagine you’ve completed your hypothesis test and have your results, which indicate there may be a correlation between the variables you were testing. To understand your results' significance, you’ll need to identify a p-value for the test, which helps note how confident you are in the test results.
In statistics, the p-value depicts the probability that, assuming the null hypothesis is correct, you might still observe results that are at least as extreme as the results of your hypothesis test. The smaller the p-value, the more likely the alternative hypothesis is correct, and the greater the significance of your results.
3. One-Sided vs. Two-Sided Testing
When it’s time to test your hypothesis, it’s important to leverage the correct testing method. The two most common hypothesis testing methods are one-sided and two-sided tests , or one-tailed and two-tailed tests, respectively.
Typically, you’d leverage a one-sided test when you have a strong conviction about the direction of change you expect to see due to your hypothesis test. You’d leverage a two-sided test when you’re less confident in the direction of change.

4. Sampling
To perform hypothesis testing in the first place, you need to collect a sample of data to be analyzed. Depending on the question you’re seeking to answer or investigate, you might collect samples through surveys, observational studies, or experiments.
A survey involves asking a series of questions to a random population sample and recording self-reported responses.
Observational studies involve a researcher observing a sample population and collecting data as it occurs naturally, without intervention.
Finally, an experiment involves dividing a sample into multiple groups, one of which acts as the control group. For each non-control group, the variable being studied is manipulated to determine how the data collected differs from that of the control group.

Learn How to Perform Hypothesis Testing
Hypothesis testing is a complex process involving different moving pieces that can allow an organization to effectively leverage its data and inform strategic decisions.
If you’re interested in better understanding hypothesis testing and the role it can play within your organization, one option is to complete a course that focuses on the process. Doing so can lay the statistical and analytical foundation you need to succeed.
Do you want to learn more about hypothesis testing? Explore Business Analytics —one of our online business essentials courses —and download our Beginner’s Guide to Data & Analytics .

About the Author
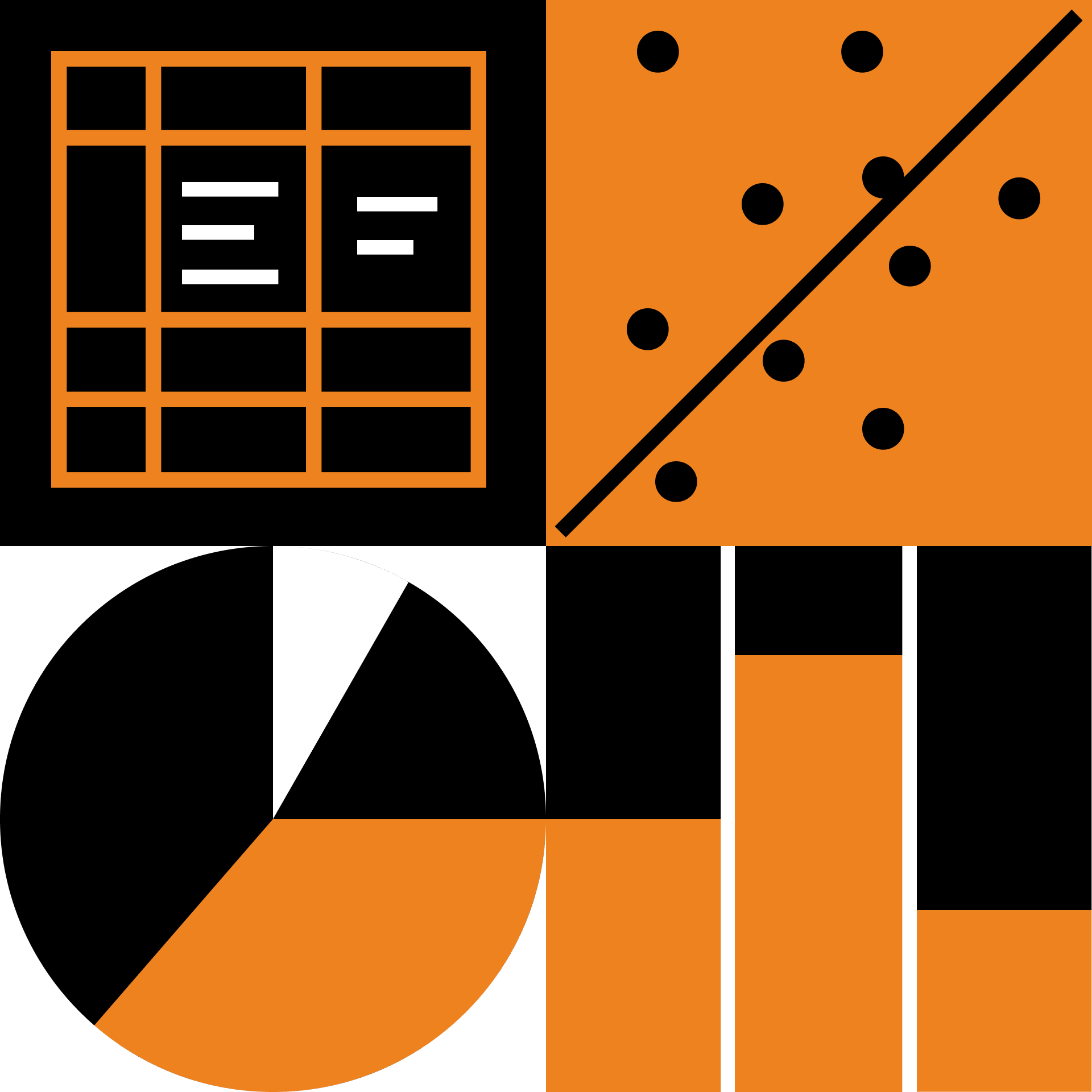
Hypothesis Testing
Expand your statistics toolkit, learning how to make good decisions with limited data.
Journey into the core ideas of statistics.
The Z-statistic
Measure the "extremeness" of your data.
Apply probability concepts to test a hypothesis.
End of Unit 1
Complete all lessons above to reach this milestone.
0 of 3 lessons complete
Understanding p-values
Explore the essential piece of many popular hypothesis tests.
Discover how p-values can be misused.
Learn to measure the quality of your experiment.
Practice: Power & Error
Put your skills to the test with a real-world scenario.
Confidence Intervals
Gain confidence in estimating a population's mean.
End of Unit 2
0 of 5 lessons complete
The Chi-Square Statistic I
Venture into the world of hypothesis testing with chi-square statistics.
The Chi-Square Statistic II
Apply the chi-square statistic to a goodness of fit test.
Chi-Square Random Variables
Gain insight into chi-square statistics and their distributions.
End of Unit 3
Degrees of Freedom I
Deduce properties of dice from a distance.
Point Estimates
Learn to estimate population parameters with data.
Degrees of Freedom II
Find the right distribution by counting degrees of freedom.
End of Unit 4
Homogeneity Tests
Determine if two samples share the same distribution.
Independence Tests
Rule out relationships with chi-square.
End of Unit 5
0 of 2 lessons complete
A Tale of Two Cities' Proportions
Use data to compare the means of two binomially distributed populations.
Intro to t-variables
Find out how to handle small samples and unknown variances.
Pooled Variance
Test for changes in population mean over time.
Unpooled Variance
Compare the means of two normally distributed populations.
End of Unit 6
0 of 4 lessons complete
Take the first steps towards a test for comparing multiple means.
Linear Regression: The Simplest Model
Explore the concepts at the heart of linear regression.
Best-Fit Lines
Learn how to find the best possible linear fit to your data.
The Linear Regression F-statistic
Construct the go-to statistic for linear regression tests.
Linear Regression ANOVA Tables
Summarize a linear regression analysis like a professional.
ANOVA and Mean Comparisons
Compare many means with the F-test.
End of Unit 7
0 of 6 lessons complete
Course description
Have you ever wanted to use data to test a hypothesis, prove a point, or even just make meaning of the world? Statistics is essential for achieving all of those goals, and this course will teach you the methods you need to make the most of your data. You'll gain hands-on experience designing experiments and framing questions for statistical analysis. You'll also expand your statistics toolkit to include a suite of powerful hypothesis tests.
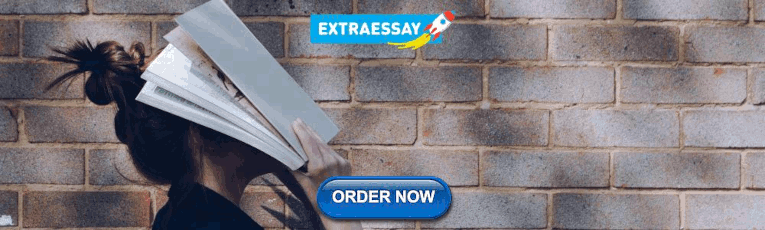
Topics covered
- Chi-square test
- Contingency tables
- Goodness of fit
Prerequisites and next steps
The basics of statistics covered in a first semester stats course is crucial. Familiarity with some basic concepts from probability, such as distribution functions, mean, variance, and the central limit theorem, is necessary. Some calculus knowledge will be helpful, but not essential.
Two sample t test
Kruskal wallis
Pearson product moment
Two sample f test
One way anova
Bartlett test
Chi square goodness of fit
Fligner killeen
Hypothesis Test Calculator
Upload your data set below to get started
Or input your data as csv
Sharing helps us build more free tools

Hypothesis Testing Calculator
Understanding Hypothesis Testing: A Guide to the Hypothesis Testing Calculator
Hypothesis testing is a crucial statistical method used to make informed decisions about data and draw conclusions. Whether you’re a student, researcher, or professional, a Hypothesis Testing Calculator can be an invaluable tool in your statistical toolkit. Let’s explore what hypothesis testing is and how this calculator can assist you:
Hypothesis Testing Basics:
- Null Hypothesis (H0): This is the default assumption or claim that there is no significant difference or effect. It’s often denoted as H0.
- Alternative Hypothesis (Ha): This is the statement that contradicts the null hypothesis. It suggests that there is a significant difference or effect. It’s denoted as Ha.
- Significance Level (α): This is the predetermined threshold (e.g., 0.05 or 5%) used to determine statistical significance. If the calculated p-value is less than α, you reject the null hypothesis.
- p-value: This is the probability of observing the results (or more extreme results) if the null hypothesis is true. A small p-value suggests that the results are unlikely under the null hypothesis.
Key Features of the Hypothesis Testing Calculator:
- Input Parameters: The calculator typically requires you to input sample data, choose the type of test (e.g., t-test, chi-square test), specify the null and alternative hypotheses, and set the significance level.
- Calculations: Once you input the data and parameters, the calculator performs the necessary statistical tests and calculations. It generates results such as the test statistic, degrees of freedom, and the p-value.
- Interpretation: Based on the results, the calculator helps you determine whether to reject or fail to reject the null hypothesis. It provides an interpretation of the findings, which is crucial for drawing conclusions.
- Visual Representation: Some calculators may offer visual aids like graphs or charts to help you better understand the data distribution and test results.
Significance of the Hypothesis Testing Calculator:
- Scientific Research: Researchers across various fields use hypothesis testing to validate their hypotheses and draw meaningful conclusions from data.
- Quality Control: Industries use hypothesis testing to ensure the quality and consistency of products and processes.
- Medical Studies: In medical research, hypothesis testing helps assess the effectiveness of treatments or interventions.
- Academics: Students and educators use hypothesis testing to teach and learn statistical concepts and conduct experiments.
- Data-Driven Decisions: Businesses use hypothesis testing to make data-driven decisions, such as whether to launch a new product based on market research.
Conclusion:
The Hypothesis Testing Calculator is a powerful tool that simplifies complex statistical analysis and enables data-driven decision-making. Whether you’re conducting experiments, analyzing survey data, or performing quality control, understanding hypothesis testing and using this calculator can help you make informed choices and contribute to evidence-based research and decision-making.

Statistics Made Easy
Introduction to Hypothesis Testing
A statistical hypothesis is an assumption about a population parameter .
For example, we may assume that the mean height of a male in the U.S. is 70 inches.
The assumption about the height is the statistical hypothesis and the true mean height of a male in the U.S. is the population parameter .
A hypothesis test is a formal statistical test we use to reject or fail to reject a statistical hypothesis.
The Two Types of Statistical Hypotheses
To test whether a statistical hypothesis about a population parameter is true, we obtain a random sample from the population and perform a hypothesis test on the sample data.
There are two types of statistical hypotheses:
The null hypothesis , denoted as H 0 , is the hypothesis that the sample data occurs purely from chance.
The alternative hypothesis , denoted as H 1 or H a , is the hypothesis that the sample data is influenced by some non-random cause.
Hypothesis Tests
A hypothesis test consists of five steps:
1. State the hypotheses.
State the null and alternative hypotheses. These two hypotheses need to be mutually exclusive, so if one is true then the other must be false.
2. Determine a significance level to use for the hypothesis.
Decide on a significance level. Common choices are .01, .05, and .1.
3. Find the test statistic.
Find the test statistic and the corresponding p-value. Often we are analyzing a population mean or proportion and the general formula to find the test statistic is: (sample statistic – population parameter) / (standard deviation of statistic)
4. Reject or fail to reject the null hypothesis.
Using the test statistic or the p-value, determine if you can reject or fail to reject the null hypothesis based on the significance level.
The p-value tells us the strength of evidence in support of a null hypothesis. If the p-value is less than the significance level, we reject the null hypothesis.
5. Interpret the results.
Interpret the results of the hypothesis test in the context of the question being asked.
The Two Types of Decision Errors
There are two types of decision errors that one can make when doing a hypothesis test:
Type I error: You reject the null hypothesis when it is actually true. The probability of committing a Type I error is equal to the significance level, often called alpha , and denoted as α.
Type II error: You fail to reject the null hypothesis when it is actually false. The probability of committing a Type II error is called the Power of the test or Beta , denoted as β.
One-Tailed and Two-Tailed Tests
A statistical hypothesis can be one-tailed or two-tailed.
A one-tailed hypothesis involves making a “greater than” or “less than ” statement.
For example, suppose we assume the mean height of a male in the U.S. is greater than or equal to 70 inches. The null hypothesis would be H0: µ ≥ 70 inches and the alternative hypothesis would be Ha: µ < 70 inches.
A two-tailed hypothesis involves making an “equal to” or “not equal to” statement.
For example, suppose we assume the mean height of a male in the U.S. is equal to 70 inches. The null hypothesis would be H0: µ = 70 inches and the alternative hypothesis would be Ha: µ ≠ 70 inches.
Note: The “equal” sign is always included in the null hypothesis, whether it is =, ≥, or ≤.
Related: What is a Directional Hypothesis?
Types of Hypothesis Tests
There are many different types of hypothesis tests you can perform depending on the type of data you’re working with and the goal of your analysis.
The following tutorials provide an explanation of the most common types of hypothesis tests:
Introduction to the One Sample t-test Introduction to the Two Sample t-test Introduction to the Paired Samples t-test Introduction to the One Proportion Z-Test Introduction to the Two Proportion Z-Test

Published by Zach
Leave a reply cancel reply.
Your email address will not be published. Required fields are marked *
Descriptive Statistics
Hypothesis test, online statistics calculator.
On Statisty you can statistically analyse your data online. Simply copy your own data into the table above and select the variables you want to analyse.
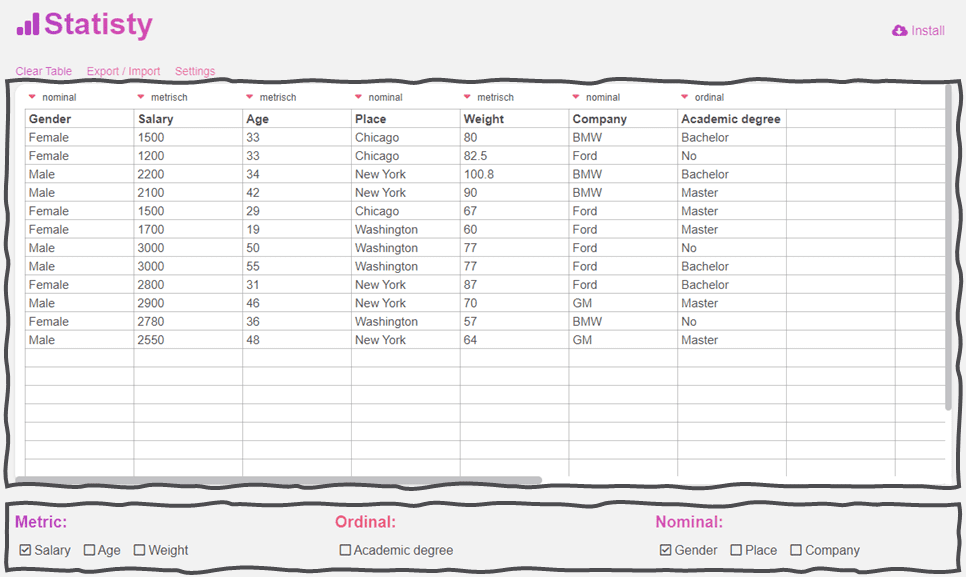
Statisty thus is a free statistical software that makes your calculations directly online. In contrast to SPSS, JASP or Excel, nothing needs to be installed in order to statistically evaluate your data.
Depending on how many variables you click on and what scale level they have, the appropriate tests are calculated.
- One sample t-Test
- Independent t-test
- Paired t-Test
- Binomial Test
- Chi-Square Test
- One-way ANOVA
- Two-way ANOVA
- Repeated measures ANOVA
- Two-way ANOVA with repeated measures
- Mann-Whitney U-test
- Wilcoxon Signed-Rank test
- Kruskal-Wallis Test
- Friedman-Test
- Correlation analysis
- Pearson correlation
- Spearman correlation
- Simple Lineare Regression
- Multiple Lineare Regression
- Logistische Regression
Statistics App
The results are then displayed clearly. First you get the descriptive statistics and then the appropriate hypothesis test. Of course, you can also calculate a linear regression or a logistic regression .
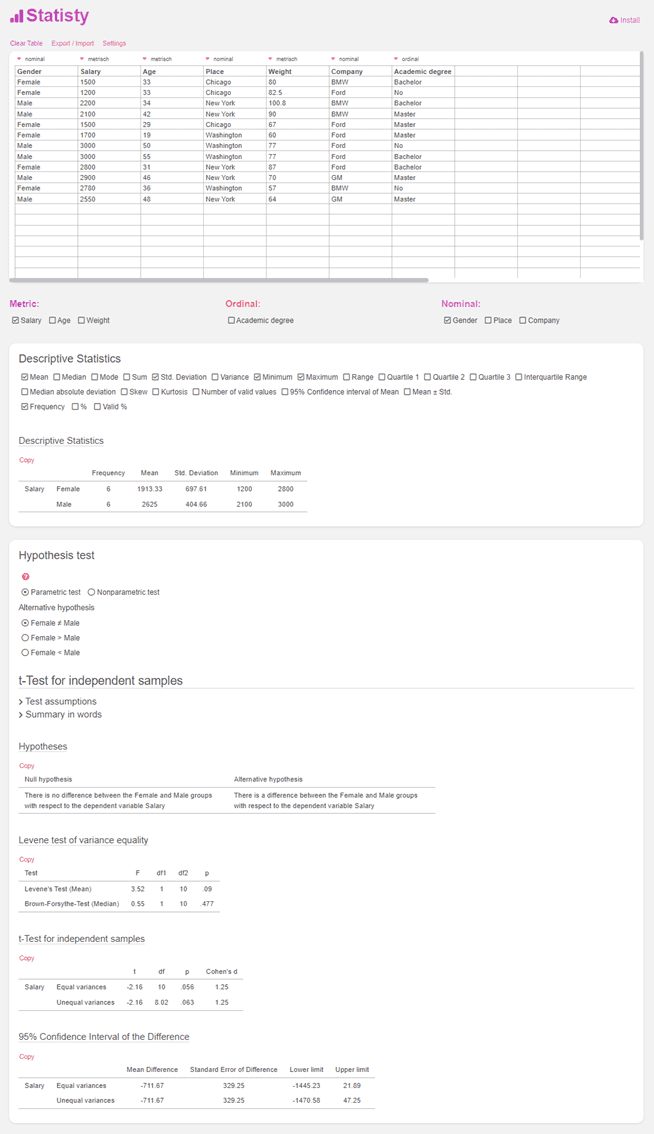
If you like also have a look at the Online Statistics Calculator at DATAtab
Welcome Back!
It looks like you already have created an account in GreatLearning with email . Would you like to link your Google account?
1000+ Courses for Free
Forgot password.
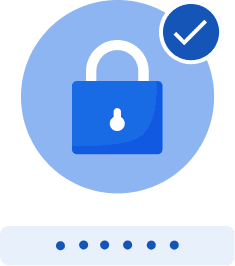
If an account with this email id exists, you will receive instructions to reset your password.
Get free access to
1000+ courses with certificates
Live sessions from industry experts
Industry salary insights and benchmarks
Have an account?
By signing up/logging in, you agree to our Terms and condition • Privacy Policy
We've sent an OTP to CHANGE
Setting up your account...
- Great Learning
- Free Courses
- Machine Learning
Hypothesis Testing

Get familiar with the significant concept of Hypothesis Testing through this free online course. Learn types of errors, Z-test, T-test, sample, and population. Enroll and learn Hypothesis Testing in detail with the hands-on demo.
Certificate of completion
Presented to
Ajith Singh
For successfully completing a free online course
Provided by
Great Learning Academy
(on JAN XXXX)
What you learn in Hypothesis Testing ?
About this free certificate course.
This free Hypothesis Testing course will help you comprehend the major concepts that help to carry it out successfully. You will learn about Type 1 & 2 errors and understand the Z-test in detail. You will learn about the Student’s T-test where you will go through types of T-tests, and their uses, and solve real-world examples to understand them better. You will further understand random sample and population size and their role in Hypothesis Testing. Lastly, you will learn independent T-test two sample and T-test outcome and paired T-test for student’s score. You will solve real-world examples along with a hands-on demo through which you will also gain the required practical knowledge. Complete the modules and a quiz to earn a free certificate of Hypothesis Testing course completion certificate.
Check out Great Learning’s Best Data Science Courses to gain in-depth knowledge in this field and earn the certificate that helps you grab better job opportunities.
- Course Outline
This module discusses the important concept of hypothesis testing: type 1 and type 2 errors. You will go through them in detail and understand the various assumptions concerned along with solving a Z test result-related problem.
In this module, you will learn about the T-test from scratch. You will understand its uses, types, and solve an example to gain practical knowledge.
This module introduces you to the important terms in hypothesis testing called sample and population. You will understand how random sample and population size plays role in hypothesis testing.
This module in detail explains to you about independent T-test two sample and T-test outcome. You will go through a hands-on demo to understand how code works in each case.
In this module, you will go through an example where you will apply paired T-test for student scores and understand the implementation of the test and carry out the hypothesis testing.
Share your certificate & get noticed
Showcase your skills
Gain a competitive edge
Stand out to recruiters
Land your dream job
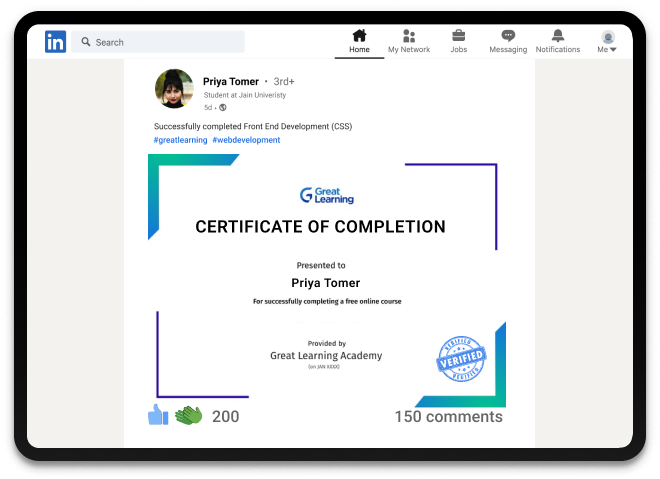
What our learners say about the course
Find out how our platform helped our learners to upskill in their career.
With this course, you get
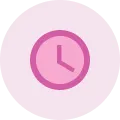
Free lifetime access
Learn anytime, anywhere
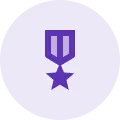
Completion Certificate
Stand out to your professional network
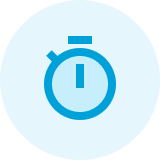
of self-paced video lectures

Share with friends
Frequently Asked Questions
What are the prerequisites required to learn this free Hypothesis Testing course?
This Hypothesis Testing course is suitable for beginners, so there are no prerequisites for enrollment.
How long does it take to complete this free Hypothesis Testing course?
There are two hours of self-paced video content in this course that learners can learn at their own pace and comfort.
Will I have lifetime access to the free course?
Yes, you can access any of the Great Learning Academy’s free courses at your preferred period and resume learning.
What are my next learning options after this Hypothesis Testing course?
You can go through Great Learning’s PG Data Science and Machine Learning Course by MIT.
Is it worth learning about Hypothesis Testing?
Yes, hypothesis testing is a useful tool for determining whether a claim is supported by evidence. It can help you to decide whether to accept or reject a claim and can also be used to compare different claims.
What is Hypothesis Testing used for?
Hypothesis testing is used to determine whether there is sufficient evidence to support a claim.
Why is Hypothesis Testing so popular?
Hypothesis testing is a powerful concept that allows researchers to test ideas and make inferences about a population based on a sample. Hypothesis testing is popular because it is relatively simple to understand and use, and it can be applied to a wide range of research questions.
What jobs demand that you learn Hypothesis Testing?
Many jobs demand that you learn Hypothesis Testing, such as:
- Statistician
Data Analyst
- Research Analyst
- Market Research Analyst
- Business Analyst
- Financial Analyst
Will I get a certificate after completing this Hypothesis Testing course?
Yes. You can proudly display your newly acquired skills through the free Hypothesis Testing certificate after completing all the modules and a quiz at the end of the course.
What knowledge and skills will I gain upon completing this Insurance in Analytics course?
You will learn about type 1&2 errors, Z-test, T-test, sample, population, independent T-test two sample and T-test outcome and paired T-test for student’s score in-depth.
How much does this Hypothesis Testing course cost?
This is a free Hypothesis Testing course offered by Great Learning and any learner can enroll for free and start learning.
Is there a limit on how many times I can take this Hypothesis Testing course?
No, there is no limit on the number of times you can attain this free Hypothesis Testing course.
Can I sign up for multiple courses from Great Learning Academy at the same time?
Yes, you can anytime register for multiple courses that will help you establish a successful profession.
Success stories
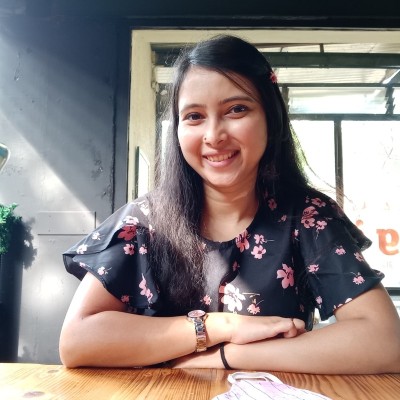
SecOps Engineer

Bandhan Bank
Team Trainer
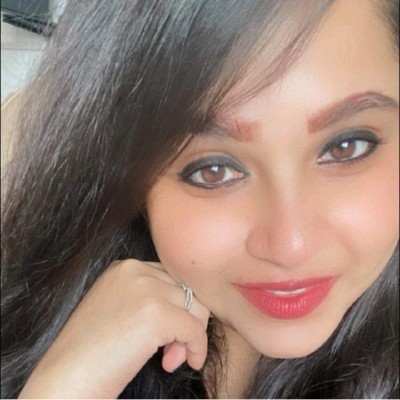
Altem Technology
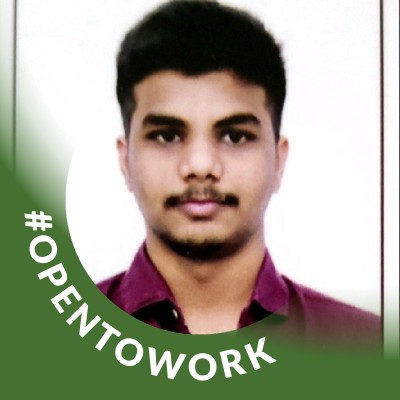
Soft Talk India
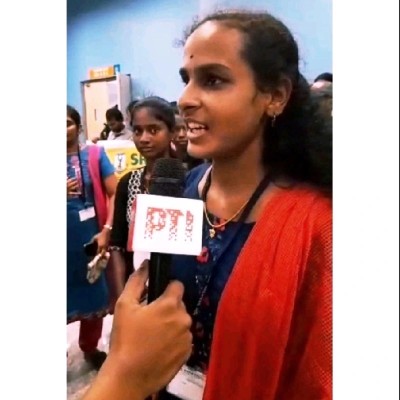
And thousands more such success stories..
Top Free Machine Learning Courses >
Related data science courses.
Placement assistance
Personalized mentorship
Detailed curriculum
Learn from world-class faculties
Scholarships upto ₹25K
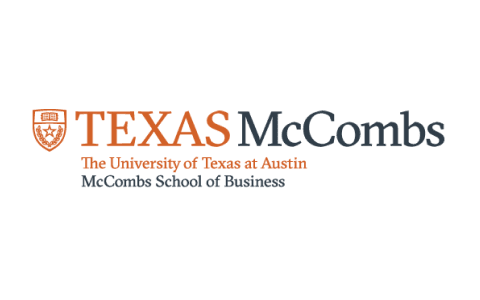
University of Texas - McCombs
PG Program in Artificial Intelligence & Machine Learning
#1 Ranked AI Program

Artificial Intelligence PG Program for Leaders
No Programming Exp Required
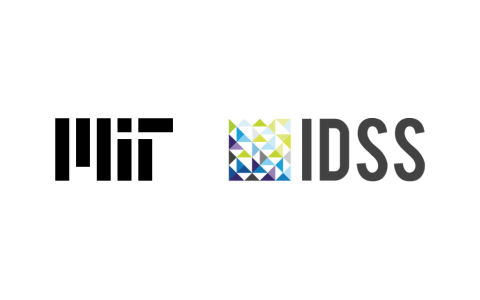
MIT Data Science and Machine Learning Program
Learn from MIT Faculty
Popular Upskilling Programs
PGP in Data Science and Business Analytics
Dedicated Career Support
Scholarships upto ₹50K
PGP in Data Science and Engineering (Bootcamp)
Dedicated Placement Assistance
Post Graduate Diploma in Management (Online)
DEDICATED CAREER SUPPORT
Scholarships upto ₹15K
PG Program in Cloud Computing
360° Cloud Learning
Generative AI for Business with Microsoft Azure OpenAI Program
MS in Data Science Programme
Live Sessions
Design Thinking: From Insights to Viability
Live Faculty Interaction
Explore new and trending free online courses

Getting Started with Bard
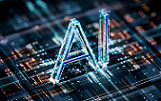
Generative AI for beginners
ChatGPT for HR
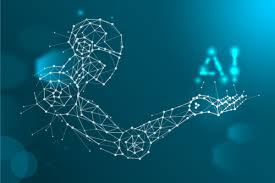
Artificial Intelligence with Python
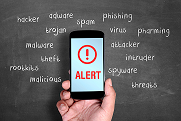
Introduction to Cyber Attacks
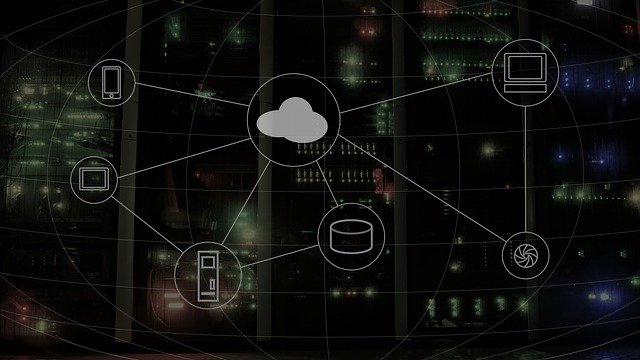
Microsoft Azure Application
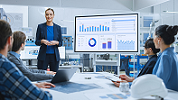
Business Intelligence using Excel
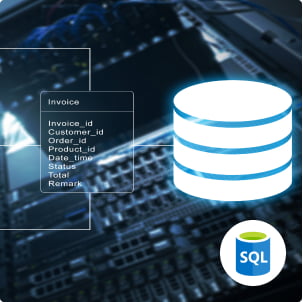
SQL for Data Science
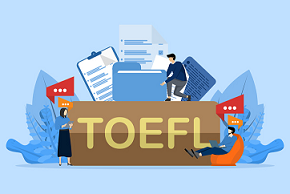
Complete TOEFL Prep Course
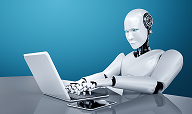
Interview Preparation using Bard
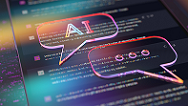
Introduction to Claude
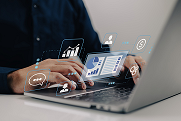
Customer Service Essentials
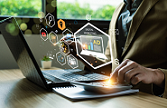
Introduction to Google Ads Campaign
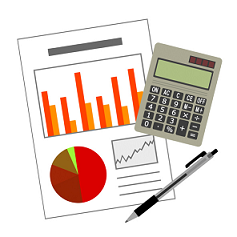
TallyPrime Tutorial
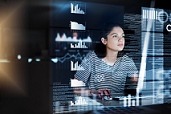
Google Bard for Coders
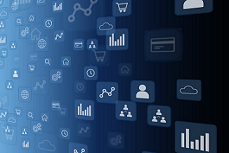
Basics of Data Visualization for Data Science
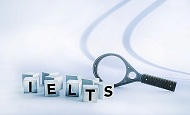
Complete IELTS Prep Course
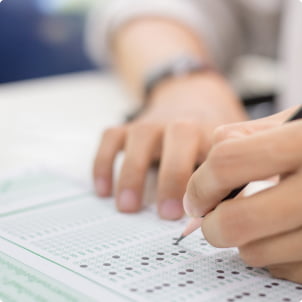
GRE Prep Course
Popular topics to explore.
- Data Science SoapUI
- Data Analytics ChatGPT
- AI Microsoft Excel
- Python Machine Learning
Relevant Career Paths >
Data Scientist
Data Engineer
Other Data Science tutorials for you
- Data Science
Hypothesis Testing
What is hypothesis-testing?
Hypothesis testing is when an assumption is made and evidence is collected in favor of the assumption. In Statistics, hypothesis-testing is performed using sample data to draw inferences regarding a given population parameter or population probability distribution. An analyst decides which hypothesis-testing methodology to be catered to on the basis of the nature of data used and the analysis reason.
The sample data used while testing a hypothesis might come from a large population or a process of data generation. The term ‘population’ is used to refer to this sample data irrespective of its source.
The stats library of Python allows you to perform hypothesis-testing. There is a Python package, named ht, exclusively available for carrying out hypothesis tests in Machine Learning.
Hypothesis-Testing Terminology
There are some commonly used terms in hypothesis testing that one must know before conducting a hypothesis test. Those commonly used terms are:
Null Hypothesis: An assertion concerned with a population that you want to test. It is called the ‘null hypothesis in the sense that it assumes the contrast of our belief. For instance, if we are testing the presence of a specific ingredient in hair oil, our null hypothesis would state the absence of that ingredient in the oil. It is denoted by H0.
Alternative Hypothesis: The opposite of a null hypothesis is the alternative hypothesis in a test. Evidence for approval of this hypothesis is to be found through a hypothesis test. For the example mentioned above of hair oil, the alternative hypothesis would state the ingredient’s presence in the oil. It is denoted by HA.
Null and alternative hypotheses are always mutually exclusive of each other.
Test Statistic: A random variable computed from a random population sample is known as a test statistic. It is used in a hypothesis test to decide the rejection of the null hypothesis. For example, the test statistic for a t-test is t-statistic.
P-value: The probability of getting a value of the test static at least as extreme as the computed value in the null hypothesis of the hypothesis test is known as p-value. The smaller the p-value, the stronger is the evidence in support of the alternative hypothesis.
Significance Level: The significance level represents the probability of rejecting the null hypothesis when it is true. It is denoted by alpha (α). For example, a significance level of 0.01 marks a 1% risk of drawing an inference even though there is no actual difference.
Steps of Hypothesis-Testing
The entire process of testing a hypothesis is carried out in the following order of events:
State the Null and Alternative Hypotheses: The very first step that an analyst takes is to specify the null and alternative hypothesis for the corresponding hypothesis test.
Formulate an Analysis Plan: The second step is to map out an analysis plan depending on the nature of the data and the reason behind the analysis.
Analyze the Sample Data: In the next step, the analyst carries out the formulated plan to analyze the sample data based on the determined hypothesis.
Determine plausibility of Null Hypothesis: The last step that comes is to decide whether to reject the null hypothesis or state the plausibility of the null hypothesis for the given sample data. The decision made is determined by the p-value and significance value of the hypothesis test.
Need of Hypothesis-Testing in Machine Learning
All the discoveries made based on hypotheses need to pass the hypothesis test. The result of a hypothesis test is used to assert the statistical significance of a finding. As mentioned in the hypothesis-testing introduction, the result of a hypothesis test tells us whether the statement of the null hypothesis or the statement of the alternative hypothesis best reflects the sample data of the population parameter.
In Machine Learning, when we train an ML model, we must be confident about the population itself first. Thus, to analyze the nature and trend of a population, we need to conduct a hypothesis test as it tells whether a speculated hypothesis about the population is true.
Basis of Hypothesis
The entire hypothesis revolves around the basics of normalization and standard normalization. A variable is normally distributed when its curve is a normal bell-shaped curve representing the equal mean, median, and mode. On the other hand, we say that a variable has standard normal distribution when its mean 0 and the standard deviation is 1.
Types of Hypothesis Test
Four types of hypothesis tests can be performed. These types are:
- Z-test: This test concludes whether two population means are different for known variances and a large volume of sample data. The test static, in this case, is z-static and the formula used is:
Z= X- µₒs
Here, Z = Z-test
X = sample average
µₒ = mean
s = standard deviation
T-test (Student t-test): Another hypothesis-testing tool differentiates between the means of two groups when their variances are not given. The test static, in this case, is t-static. It is further categorized into three kinds which are as follows:
One sample t-test
Independent two-sample t-test,
Paired sample t-test
t-tests have common applications in the field of Data Science, CS Research, and ML.
ANOVA test: ANOVA stands for Analysis of Variance. This test reports a statistically significant result when one of the groups differs significantly from the overall mean of the groups. It can be one-way, two-way, or n-way. The test static here is F-static.
Chi-square test: This hypothesis test is used to test the independence of two variables. It is sensitive to the size of the test sample. A chi-square test holds a comparison between expectations and model results. The test static in this type of test is chi-squared static.
Alpha Risk & Beta Risk
In statistical hypothesis-testing, there might be a risk of rejecting the null hypothesis when it is true. This risk is referred to as alpha risk. The other term for alpha risks is Type-I error and false positive. The amount of alpha risk is mainly governed by the size of the sample used. A large sample size corresponds to low alpha risk.
In contrast to alpha risk, there is a beta risk, commonly known as Type-II error. It is the likelihood of approving the null hypothesis when it is actually false, i.e., it is a false negative.
Real-World Examples of Hypothesis Tests
The real-world examples in various fields that involve hypothesis-testing are given below:
Example-1: Hypothesis tests are done in different clinical trials to check the effectiveness of a new drug for curing a certain symptom.
Example-2: To test the presence of a specific ingredient in any cosmetic item, food item, etc.
Example-3: A hypothesis test can be used to determine whether a new type of manure will increase soil fertility.
Example-4: Hypothesis tests are useful to find evidence in favor of a certain marketing strategy by analyzing the business growth.
Example-5: A hypothesis test can be run to determine whether an ML model fulfills the requirement in concern.
Likewise, endless real-world examples can be cited to show the implementation of hypothesis tests.
About the Course – Hypothesis Testing
The planned curriculum of this course will highly benefit the enthusiastic learners seeking opportunities in the field of Machine Learning. The hypothesis-testing course of Great Learning will help the learners to build a strong base in hypothesis-testing. Taking up this course enables you to get an insight into of Machine Learning Analytics job. Another advantage of this online course is that it is going to cost you nothing except for your time and dedication.
The content of this online course is well structured to give a boost to beginners. It starts with a descriptive hypothesis-testing introduction and ends with a quiz to test your skills. On qualifying for the quiz, you can claim your free course completion certificate. Hence, along with learning new skills, you get a certificate to upgrade your CV and LinkedIn profile. This course contains two hours of video content presenting lectures on the basics of hypothesis-testing, including a description of student t-test and paired tests, for example.
Refer & Win >
Premium course worth ₹15,000/-
Oops!! Something went wrong, Please try again.
*Students less than 14 years of age are not eligible
By submitting the form, you agree to our Terms and Conditions and our Privacy Policy .
Form submitted successfully
We are allocating a suitable domain expert to help you out with your queries. Expect to receive a call in the next 4 hours.
Hypothesis Maker Online
Looking for a hypothesis maker? This online tool for students will help you formulate a beautiful hypothesis quickly, efficiently, and for free.
Are you looking for an effective hypothesis maker online? Worry no more; try our online tool for students and formulate your hypothesis within no time.
- 🔎 How to Use the Tool?
- ⚗️ What Is a Hypothesis in Science?
👍 What Does a Good Hypothesis Mean?
- 🧭 Steps to Making a Good Hypothesis
🔗 References
📄 hypothesis maker: how to use it.
Our hypothesis maker is a simple and efficient tool you can access online for free.
If you want to create a research hypothesis quickly, you should fill out the research details in the given fields on the hypothesis generator.
Below are the fields you should complete to generate your hypothesis:
- Who or what is your research based on? For instance, the subject can be research group 1.
- What does the subject (research group 1) do?
- What does the subject affect? - This shows the predicted outcome, which is the object.
- Who or what will be compared with research group 1? (research group 2).
Once you fill the in the fields, you can click the ‘Make a hypothesis’ tab and get your results.
⚗️ What Is a Hypothesis in the Scientific Method?
A hypothesis is a statement describing an expectation or prediction of your research through observation.
It is similar to academic speculation and reasoning that discloses the outcome of your scientific test . An effective hypothesis, therefore, should be crafted carefully and with precision.
A good hypothesis should have dependent and independent variables . These variables are the elements you will test in your research method – it can be a concept, an event, or an object as long as it is observable.
You can observe the dependent variables while the independent variables keep changing during the experiment.
In a nutshell, a hypothesis directs and organizes the research methods you will use, forming a large section of research paper writing.
Hypothesis vs. Theory
A hypothesis is a realistic expectation that researchers make before any investigation. It is formulated and tested to prove whether the statement is true. A theory, on the other hand, is a factual principle supported by evidence. Thus, a theory is more fact-backed compared to a hypothesis.
Another difference is that a hypothesis is presented as a single statement , while a theory can be an assortment of things . Hypotheses are based on future possibilities toward a specific projection, but the results are uncertain. Theories are verified with undisputable results because of proper substantiation.
When it comes to data, a hypothesis relies on limited information , while a theory is established on an extensive data set tested on various conditions.
You should observe the stated assumption to prove its accuracy.
Since hypotheses have observable variables, their outcome is usually based on a specific occurrence. Conversely, theories are grounded on a general principle involving multiple experiments and research tests.
This general principle can apply to many specific cases.
The primary purpose of formulating a hypothesis is to present a tentative prediction for researchers to explore further through tests and observations. Theories, in their turn, aim to explain plausible occurrences in the form of a scientific study.
It would help to rely on several criteria to establish a good hypothesis. Below are the parameters you should use to analyze the quality of your hypothesis.
🧭 6 Steps to Making a Good Hypothesis
Writing a hypothesis becomes way simpler if you follow a tried-and-tested algorithm. Let’s explore how you can formulate a good hypothesis in a few steps:
Step #1: Ask Questions
The first step in hypothesis creation is asking real questions about the surrounding reality.
Why do things happen as they do? What are the causes of some occurrences?
Your curiosity will trigger great questions that you can use to formulate a stellar hypothesis. So, ensure you pick a research topic of interest to scrutinize the world’s phenomena, processes, and events.
Step #2: Do Initial Research
Carry out preliminary research and gather essential background information about your topic of choice.
The extent of the information you collect will depend on what you want to prove.
Your initial research can be complete with a few academic books or a simple Internet search for quick answers with relevant statistics.
Still, keep in mind that in this phase, it is too early to prove or disapprove of your hypothesis.
Step #3: Identify Your Variables
Now that you have a basic understanding of the topic, choose the dependent and independent variables.
Take note that independent variables are the ones you can’t control, so understand the limitations of your test before settling on a final hypothesis.
Step #4: Formulate Your Hypothesis
You can write your hypothesis as an ‘if – then’ expression . Presenting any hypothesis in this format is reliable since it describes the cause-and-effect you want to test.
For instance: If I study every day, then I will get good grades.
Step #5: Gather Relevant Data
Once you have identified your variables and formulated the hypothesis, you can start the experiment. Remember, the conclusion you make will be a proof or rebuttal of your initial assumption.
So, gather relevant information, whether for a simple or statistical hypothesis, because you need to back your statement.
Step #6: Record Your Findings
Finally, write down your conclusions in a research paper .
Outline in detail whether the test has proved or disproved your hypothesis.
Edit and proofread your work, using a plagiarism checker to ensure the authenticity of your text.
We hope that the above tips will be useful for you. Note that if you need to conduct business analysis, you can use the free templates we’ve prepared: SWOT , PESTLE , VRIO , SOAR , and Porter’s 5 Forces .
❓ Hypothesis Formulator FAQ
Updated: Oct 25th, 2023
- How to Write a Hypothesis in 6 Steps - Grammarly
- Forming a Good Hypothesis for Scientific Research
- The Hypothesis in Science Writing
- Scientific Method: Step 3: HYPOTHESIS - Subject Guides
- Hypothesis Template & Examples - Video & Lesson Transcript
- Free Essays
- Writing Tools
- Lit. Guides
- Donate a Paper
- Referencing Guides
- Free Textbooks
- Tongue Twisters
- Job Openings
- Expert Application
- Video Contest
- Writing Scholarship
- Discount Codes
- IvyPanda Shop
- Terms and Conditions
- Privacy Policy
- Cookies Policy
- Copyright Principles
- DMCA Request
- Service Notice
Use our hypothesis maker whenever you need to formulate a hypothesis for your study. We offer a very simple tool where you just need to provide basic info about your variables, subjects, and predicted outcomes. The rest is on us. Get a perfect hypothesis in no time!
User Preferences
Content preview.
Arcu felis bibendum ut tristique et egestas quis:
- Ut enim ad minim veniam, quis nostrud exercitation ullamco laboris
- Duis aute irure dolor in reprehenderit in voluptate
- Excepteur sint occaecat cupidatat non proident
Keyboard Shortcuts
10: hypothesis testing, lesson overview section .
In Lesson 2 we saw the value of random assignment in designed experiments. Random assignment alleviates the bias that might cause a systematic difference between groups unrelated to the treatment itself. Precautions like blinding that ensure that the subjects are treated the same during the experiment then leave us with just two possibilities for the cause of differences seen between groups. Either:
- the treatment was effective in producing the changes (the research hypothesis), or
- differences were just the result of the luck of the draw (the null hypothesis).
This shows the importance of addressing the concept of statistical significance. If it is very unlikely that the results of a randomized experiment are just the result of random chance, then we are left with the treatment itself as the probable cause of any relationship seen. Even in an observational study, being able to show that random chance is a poor explanation of the data is still good evidence for a true association in the population (even though it is poor evidence of causality).
This lesson focuses on Statistical hypothesis testing. In a significance test, you carry out a probability calculation assuming the null hypothesis is true to see if random chance is a plausible explanation for the data. Let's illustrate the process with an example.
Example 10.1 Section
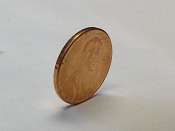
Physical theory suggests that when a coin is spun on a table (rather than flipped in the air) the probability it lands heads up is less than 0.5. We are hesitant to believe this without proof.
To test the theory we carry out an experiment and independently spin a penny 100 times getting 37 heads and 63 tails. Thus, the observed proportion of heads is 37 / 100 = 0.37
We have two possible explanations for the data:
Null Hypothesis : The data is merely a reflection of chance variation. The probability of heads when a penny is spun is really p = 0.5
Alternative Hypothesis : The probability of heads when a penny is spun is really < 0.5.
A statistical hypothesis test is designed to answer the question: "Does the Null Hypothesis provide a reasonable explanation of the data?”
To answer this question we carry out a probability calculation. First, we can calculate a
Test Statistic = a measure of the difference between the data and what is expected when the null hypothesis is true.
In our example, the null hypothesis says the number of heads in 100 spins would closely follow the normal distribution with p = 0.5. So, if the null hypothesis is true, we expect half (0.5) heads give or take a standard deviation of
\[\sqrt{\frac{0.5(1-0.5)}{100}}=0.05\]
Further, we can see how unusual our data is if the null hypothesis is true by finding the standard score z for the test statistic and using the normal curve:
\[z = (0.37-0.5)/0.05 = -2.6\]
How unusual is the value we got, assuming the null hypothesis (i.e., the real proportion is 0.5) is true? We know that standard scores of -2.6 or lower only happen about 0.5% of the time. So the null hypothesis provides a poor explanation for our data. This would seem to provide strong evidence that spinning a coin has less than a 50% chance of landing heads.
- Formulate appropriate null and alternative hypotheses.
- Identify the type 1 and the type 2 error in the context of the problem.
- Use the four basic steps to carry out a significance test in some basic situations.
- Interpret a p -value in terms of the problem.
- State an appropriate conclusion for a hypothesis test.
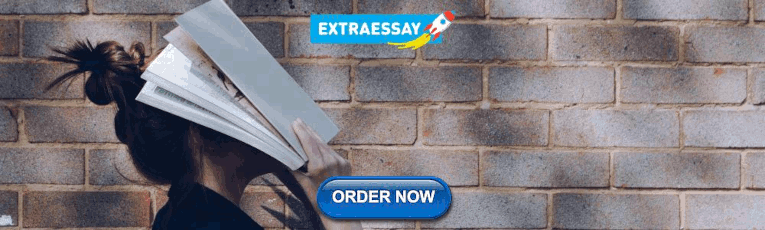
IMAGES
VIDEO
COMMENTS
Hypothesis Testing Calculator. The first step in hypothesis testing is to calculate the test statistic. The formula for the test statistic depends on whether the population standard deviation (σ) is known or unknown. If σ is known, our hypothesis test is known as a z test and we use the z distribution. If σ is unknown, our hypothesis test is ...
This section answers some common questions about . Use this Hypothesis Test Calculator for quick results in Python and R. Learn the step-by-step hypothesis test process and why hypothesis testing is important.
Present the findings in your results and discussion section. Though the specific details might vary, the procedure you will use when testing a hypothesis will always follow some version of these steps. Table of contents. Step 1: State your null and alternate hypothesis. Step 2: Collect data. Step 3: Perform a statistical test.
Hypothesis Test. Here you will find everything about hypothesis testing: One sample t-test, Unpaired t-test, Paired t-test and Chi-square test. You will also find tutorials for non-parametric statistical procedures such as the Mann-Whitney u-Test and Wilcoxon-Test. mann-whitney-u-test and the Wilcoxon test
S.3 Hypothesis Testing. In reviewing hypothesis tests, we start first with the general idea. Then, we keep returning to the basic procedures of hypothesis testing, each time adding a little more detail. The general idea of hypothesis testing involves: Making an initial assumption. Collecting evidence (data).
With online courses on Coursera, you also have the opportunity to learn the essential building blocks of hypothesis testing, which include choosing the right hypothesis testing tool and performing hypothesis tests using chi-square tests, correlation, t-tests, simple regression, logistic regression, and analysis of variance (ANOVA).
Hypothesis testing is an indispensable tool in data science, allowing us to make data-driven decisions with confidence. By understanding its principles, conducting tests properly, and considering real-world applications, you can harness the power of hypothesis testing to unlock valuable insights from your data.
Unit test. Significance tests give us a formal process for using sample data to evaluate the likelihood of some claim about a population value. Learn how to conduct significance tests and calculate p-values to see how likely a sample result is to occur by random chance. You'll also see how we use p-values to make conclusions about hypotheses.
Basic approach to hypothesis testing. State a model describing the relationship between the explanatory variables and the outcome variable (s) in the population and the nature of the variability. State all of your assumptions. Specify the null and alternative hypotheses in terms of the parameters of the model.
If the biologist set her significance level \(\alpha\) at 0.05 and used the critical value approach to conduct her hypothesis test, she would reject the null hypothesis if her test statistic t* were less than -1.6939 (determined using statistical software or a t-table):s-3-3. Since the biologist's test statistic, t* = -4.60, is less than -1.6939, the biologist rejects the null hypothesis.
3. One-Sided vs. Two-Sided Testing. When it's time to test your hypothesis, it's important to leverage the correct testing method. The two most common hypothesis testing methods are one-sided and two-sided tests, or one-tailed and two-tailed tests, respectively. Typically, you'd leverage a one-sided test when you have a strong conviction ...
Course description. Have you ever wanted to use data to test a hypothesis, prove a point, or even just make meaning of the world? Statistics is essential for achieving all of those goals, and this course will teach you the methods you need to make the most of your data. You'll gain hands-on experience designing experiments and framing questions ...
Hypothesis Test Calculator. Upload your data set below to get started. Upload File. Or input your data as csv. column_one,column_two,column_three 1,2,3 4,5,6 7,8,9. Submit CSV. Sharing helps us build more free tools.
The Hypothesis Testing Calculator is a powerful tool that simplifies complex statistical analysis and enables data-driven decision-making. Whether you're conducting experiments, analyzing survey data, or performing quality control, understanding hypothesis testing and using this calculator can help you make informed choices and contribute to ...
Simple hypothesis testing. Niels has a Magic 8 -Ball, which is a toy used for fortune-telling or seeking advice. To consult the ball, you ask the ball a question and shake it. One of 5 different possible answers then appears at random in the ball. Niels sensed that the ball answers " Ask again later " too frequently.
The P -value is, therefore, the area under a tn - 1 = t14 curve to the left of -2.5 and to the right of 2.5. It can be shown using statistical software that the P -value is 0.0127 + 0.0127, or 0.0254. The graph depicts this visually. Note that the P -value for a two-tailed test is always two times the P -value for either of the one-tailed tests.
Step 2: State the Alternate Hypothesis. The claim is that the students have above average IQ scores, so: H 1: μ > 100. The fact that we are looking for scores "greater than" a certain point means that this is a one-tailed test. Step 3: Draw a picture to help you visualize the problem. Step 4: State the alpha level.
A hypothesis test consists of five steps: 1. State the hypotheses. State the null and alternative hypotheses. These two hypotheses need to be mutually exclusive, so if one is true then the other must be false. 2. Determine a significance level to use for the hypothesis. Decide on a significance level.
First you get the descriptive statistics and then the appropriate hypothesis test. Of course, you can also calculate a linear regression or a logistic regression. If you like also have a look at the Online Statistics Calculator at DATAtab Free Statistics App: t-test, chi-square, correlation, ANOVA, Regression ...
This free Hypothesis Testing course will help you comprehend the major concepts that help to carry it out successfully. You will learn about Type 1 & 2 errors and understand the Z-test in detail. You will learn about the Student's T-test where you will go through types of T-tests, and their uses, and solve real-world examples to understand ...
The critical value for conducting the left-tailed test H0 : μ = 3 versus HA : μ < 3 is the t -value, denoted -t( α, n - 1), such that the probability to the left of it is α. It can be shown using either statistical software or a t -table that the critical value -t0.05,14 is -1.7613. That is, we would reject the null hypothesis H0 : μ = 3 ...
Our hypothesis maker is a simple and efficient tool you can access online for free. If you want to create a research hypothesis quickly, you should fill out the research details in the given fields on the hypothesis generator. Below are the fields you should complete to generate your hypothesis:
Test Statistic = a measure of the difference between the data and what is expected when the null hypothesis is true. In our example, the null hypothesis says the number of heads in 100 spins would closely follow the normal distribution with p = 0.5. So, if the null hypothesis is true, we expect half (0.5) heads give or take a standard deviation of.