
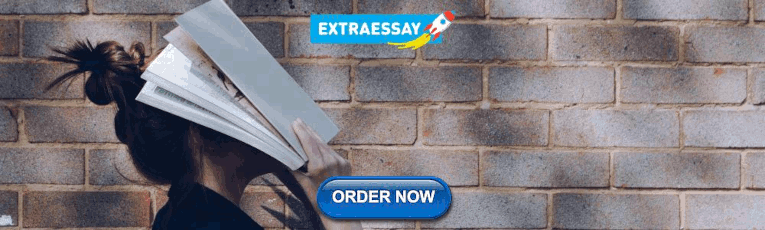
Research Design 101
Everything You Need To Get Started (With Examples)
By: Derek Jansen (MBA) | Reviewers: Eunice Rautenbach (DTech) & Kerryn Warren (PhD) | April 2023
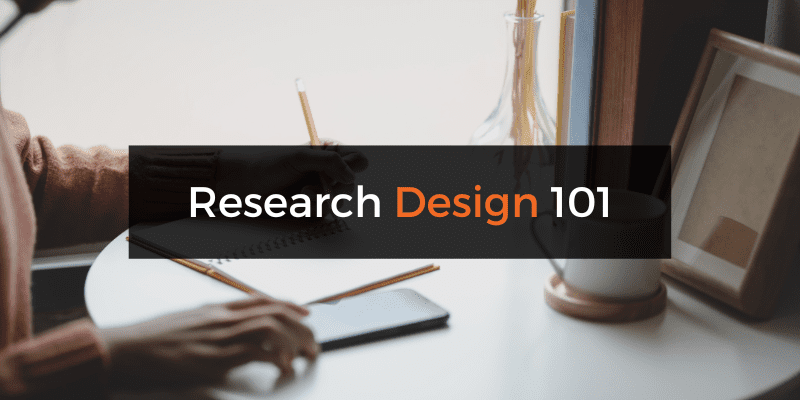
Navigating the world of research can be daunting, especially if you’re a first-time researcher. One concept you’re bound to run into fairly early in your research journey is that of “ research design ”. Here, we’ll guide you through the basics using practical examples , so that you can approach your research with confidence.
Overview: Research Design 101
What is research design.
- Research design types for quantitative studies
- Video explainer : quantitative research design
- Research design types for qualitative studies
- Video explainer : qualitative research design
- How to choose a research design
- Key takeaways
Research design refers to the overall plan, structure or strategy that guides a research project , from its conception to the final data analysis. A good research design serves as the blueprint for how you, as the researcher, will collect and analyse data while ensuring consistency, reliability and validity throughout your study.
Understanding different types of research designs is essential as helps ensure that your approach is suitable given your research aims, objectives and questions , as well as the resources you have available to you. Without a clear big-picture view of how you’ll design your research, you run the risk of potentially making misaligned choices in terms of your methodology – especially your sampling , data collection and data analysis decisions.
The problem with defining research design…
One of the reasons students struggle with a clear definition of research design is because the term is used very loosely across the internet, and even within academia.
Some sources claim that the three research design types are qualitative, quantitative and mixed methods , which isn’t quite accurate (these just refer to the type of data that you’ll collect and analyse). Other sources state that research design refers to the sum of all your design choices, suggesting it’s more like a research methodology . Others run off on other less common tangents. No wonder there’s confusion!
In this article, we’ll clear up the confusion. We’ll explain the most common research design types for both qualitative and quantitative research projects, whether that is for a full dissertation or thesis, or a smaller research paper or article.
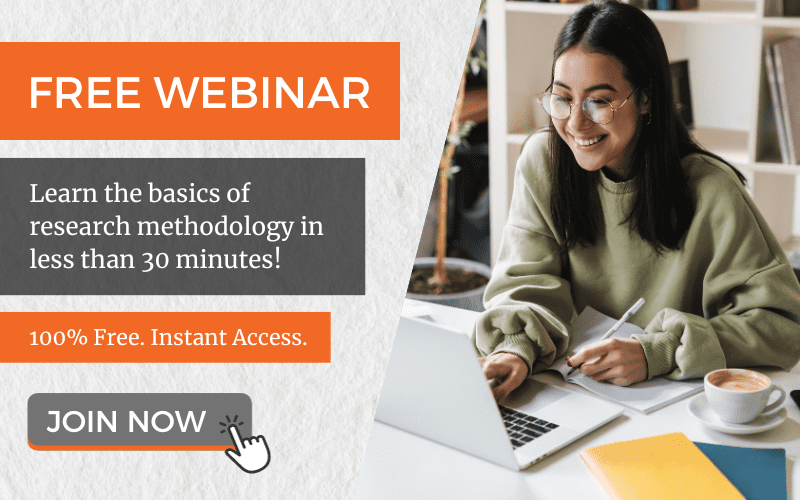
Research Design: Quantitative Studies
Quantitative research involves collecting and analysing data in a numerical form. Broadly speaking, there are four types of quantitative research designs: descriptive , correlational , experimental , and quasi-experimental .
Descriptive Research Design
As the name suggests, descriptive research design focuses on describing existing conditions, behaviours, or characteristics by systematically gathering information without manipulating any variables. In other words, there is no intervention on the researcher’s part – only data collection.
For example, if you’re studying smartphone addiction among adolescents in your community, you could deploy a survey to a sample of teens asking them to rate their agreement with certain statements that relate to smartphone addiction. The collected data would then provide insight regarding how widespread the issue may be – in other words, it would describe the situation.
The key defining attribute of this type of research design is that it purely describes the situation . In other words, descriptive research design does not explore potential relationships between different variables or the causes that may underlie those relationships. Therefore, descriptive research is useful for generating insight into a research problem by describing its characteristics . By doing so, it can provide valuable insights and is often used as a precursor to other research design types.
Correlational Research Design
Correlational design is a popular choice for researchers aiming to identify and measure the relationship between two or more variables without manipulating them . In other words, this type of research design is useful when you want to know whether a change in one thing tends to be accompanied by a change in another thing.
For example, if you wanted to explore the relationship between exercise frequency and overall health, you could use a correlational design to help you achieve this. In this case, you might gather data on participants’ exercise habits, as well as records of their health indicators like blood pressure, heart rate, or body mass index. Thereafter, you’d use a statistical test to assess whether there’s a relationship between the two variables (exercise frequency and health).
As you can see, correlational research design is useful when you want to explore potential relationships between variables that cannot be manipulated or controlled for ethical, practical, or logistical reasons. It is particularly helpful in terms of developing predictions , and given that it doesn’t involve the manipulation of variables, it can be implemented at a large scale more easily than experimental designs (which will look at next).
That said, it’s important to keep in mind that correlational research design has limitations – most notably that it cannot be used to establish causality . In other words, correlation does not equal causation . To establish causality, you’ll need to move into the realm of experimental design, coming up next…
Need a helping hand?
Experimental Research Design
Experimental research design is used to determine if there is a causal relationship between two or more variables . With this type of research design, you, as the researcher, manipulate one variable (the independent variable) while controlling others (dependent variables). Doing so allows you to observe the effect of the former on the latter and draw conclusions about potential causality.
For example, if you wanted to measure if/how different types of fertiliser affect plant growth, you could set up several groups of plants, with each group receiving a different type of fertiliser, as well as one with no fertiliser at all. You could then measure how much each plant group grew (on average) over time and compare the results from the different groups to see which fertiliser was most effective.
Overall, experimental research design provides researchers with a powerful way to identify and measure causal relationships (and the direction of causality) between variables. However, developing a rigorous experimental design can be challenging as it’s not always easy to control all the variables in a study. This often results in smaller sample sizes , which can reduce the statistical power and generalisability of the results.
Moreover, experimental research design requires random assignment . This means that the researcher needs to assign participants to different groups or conditions in a way that each participant has an equal chance of being assigned to any group (note that this is not the same as random sampling ). Doing so helps reduce the potential for bias and confounding variables . This need for random assignment can lead to ethics-related issues . For example, withholding a potentially beneficial medical treatment from a control group may be considered unethical in certain situations.
Quasi-Experimental Research Design
Quasi-experimental research design is used when the research aims involve identifying causal relations , but one cannot (or doesn’t want to) randomly assign participants to different groups (for practical or ethical reasons). Instead, with a quasi-experimental research design, the researcher relies on existing groups or pre-existing conditions to form groups for comparison.
For example, if you were studying the effects of a new teaching method on student achievement in a particular school district, you may be unable to randomly assign students to either group and instead have to choose classes or schools that already use different teaching methods. This way, you still achieve separate groups, without having to assign participants to specific groups yourself.
Naturally, quasi-experimental research designs have limitations when compared to experimental designs. Given that participant assignment is not random, it’s more difficult to confidently establish causality between variables, and, as a researcher, you have less control over other variables that may impact findings.
All that said, quasi-experimental designs can still be valuable in research contexts where random assignment is not possible and can often be undertaken on a much larger scale than experimental research, thus increasing the statistical power of the results. What’s important is that you, as the researcher, understand the limitations of the design and conduct your quasi-experiment as rigorously as possible, paying careful attention to any potential confounding variables .
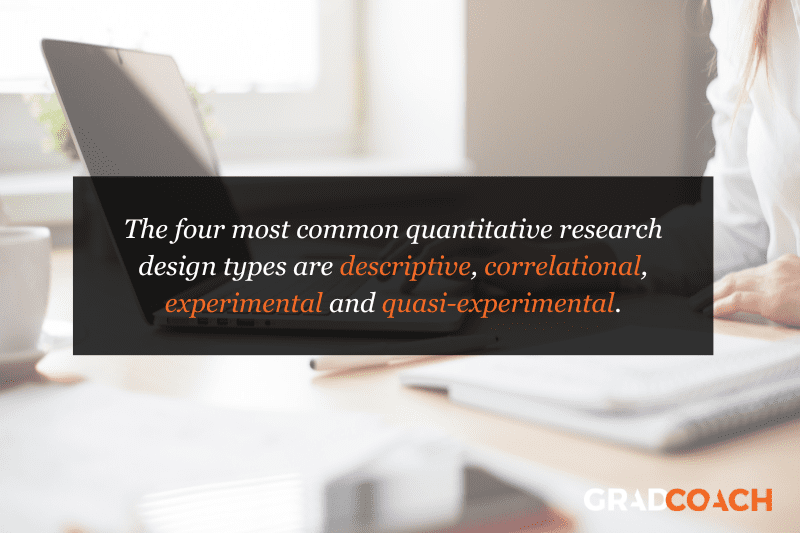
Research Design: Qualitative Studies
There are many different research design types when it comes to qualitative studies, but here we’ll narrow our focus to explore the “Big 4”. Specifically, we’ll look at phenomenological design, grounded theory design, ethnographic design, and case study design.
Phenomenological Research Design
Phenomenological design involves exploring the meaning of lived experiences and how they are perceived by individuals. This type of research design seeks to understand people’s perspectives , emotions, and behaviours in specific situations. Here, the aim for researchers is to uncover the essence of human experience without making any assumptions or imposing preconceived ideas on their subjects.
For example, you could adopt a phenomenological design to study why cancer survivors have such varied perceptions of their lives after overcoming their disease. This could be achieved by interviewing survivors and then analysing the data using a qualitative analysis method such as thematic analysis to identify commonalities and differences.
Phenomenological research design typically involves in-depth interviews or open-ended questionnaires to collect rich, detailed data about participants’ subjective experiences. This richness is one of the key strengths of phenomenological research design but, naturally, it also has limitations. These include potential biases in data collection and interpretation and the lack of generalisability of findings to broader populations.
Grounded Theory Research Design
Grounded theory (also referred to as “GT”) aims to develop theories by continuously and iteratively analysing and comparing data collected from a relatively large number of participants in a study. It takes an inductive (bottom-up) approach, with a focus on letting the data “speak for itself”, without being influenced by preexisting theories or the researcher’s preconceptions.
As an example, let’s assume your research aims involved understanding how people cope with chronic pain from a specific medical condition, with a view to developing a theory around this. In this case, grounded theory design would allow you to explore this concept thoroughly without preconceptions about what coping mechanisms might exist. You may find that some patients prefer cognitive-behavioural therapy (CBT) while others prefer to rely on herbal remedies. Based on multiple, iterative rounds of analysis, you could then develop a theory in this regard, derived directly from the data (as opposed to other preexisting theories and models).
Grounded theory typically involves collecting data through interviews or observations and then analysing it to identify patterns and themes that emerge from the data. These emerging ideas are then validated by collecting more data until a saturation point is reached (i.e., no new information can be squeezed from the data). From that base, a theory can then be developed .
As you can see, grounded theory is ideally suited to studies where the research aims involve theory generation , especially in under-researched areas. Keep in mind though that this type of research design can be quite time-intensive , given the need for multiple rounds of data collection and analysis.
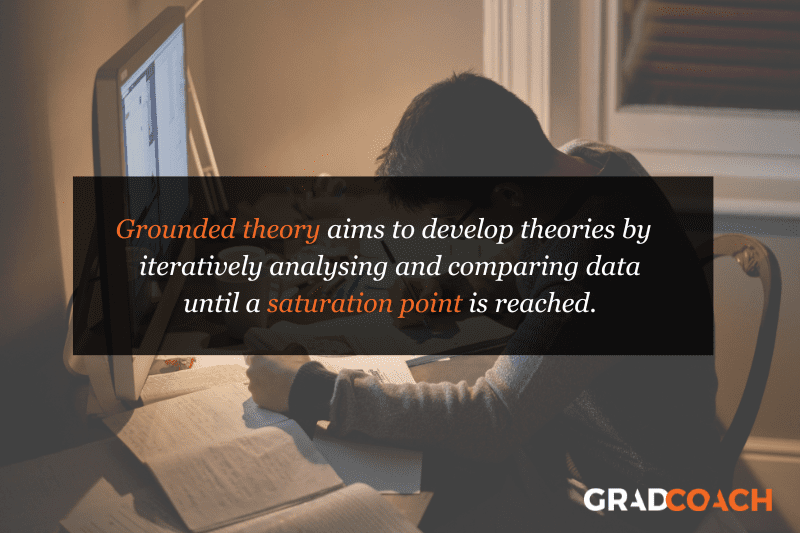
Ethnographic Research Design
Ethnographic design involves observing and studying a culture-sharing group of people in their natural setting to gain insight into their behaviours, beliefs, and values. The focus here is on observing participants in their natural environment (as opposed to a controlled environment). This typically involves the researcher spending an extended period of time with the participants in their environment, carefully observing and taking field notes .
All of this is not to say that ethnographic research design relies purely on observation. On the contrary, this design typically also involves in-depth interviews to explore participants’ views, beliefs, etc. However, unobtrusive observation is a core component of the ethnographic approach.
As an example, an ethnographer may study how different communities celebrate traditional festivals or how individuals from different generations interact with technology differently. This may involve a lengthy period of observation, combined with in-depth interviews to further explore specific areas of interest that emerge as a result of the observations that the researcher has made.
As you can probably imagine, ethnographic research design has the ability to provide rich, contextually embedded insights into the socio-cultural dynamics of human behaviour within a natural, uncontrived setting. Naturally, however, it does come with its own set of challenges, including researcher bias (since the researcher can become quite immersed in the group), participant confidentiality and, predictably, ethical complexities . All of these need to be carefully managed if you choose to adopt this type of research design.
Case Study Design
With case study research design, you, as the researcher, investigate a single individual (or a single group of individuals) to gain an in-depth understanding of their experiences, behaviours or outcomes. Unlike other research designs that are aimed at larger sample sizes, case studies offer a deep dive into the specific circumstances surrounding a person, group of people, event or phenomenon, generally within a bounded setting or context .
As an example, a case study design could be used to explore the factors influencing the success of a specific small business. This would involve diving deeply into the organisation to explore and understand what makes it tick – from marketing to HR to finance. In terms of data collection, this could include interviews with staff and management, review of policy documents and financial statements, surveying customers, etc.
While the above example is focused squarely on one organisation, it’s worth noting that case study research designs can have different variation s, including single-case, multiple-case and longitudinal designs. As you can see in the example, a single-case design involves intensely examining a single entity to understand its unique characteristics and complexities. Conversely, in a multiple-case design , multiple cases are compared and contrasted to identify patterns and commonalities. Lastly, in a longitudinal case design , a single case or multiple cases are studied over an extended period of time to understand how factors develop over time.
As you can see, a case study research design is particularly useful where a deep and contextualised understanding of a specific phenomenon or issue is desired. However, this strength is also its weakness. In other words, you can’t generalise the findings from a case study to the broader population. So, keep this in mind if you’re considering going the case study route.
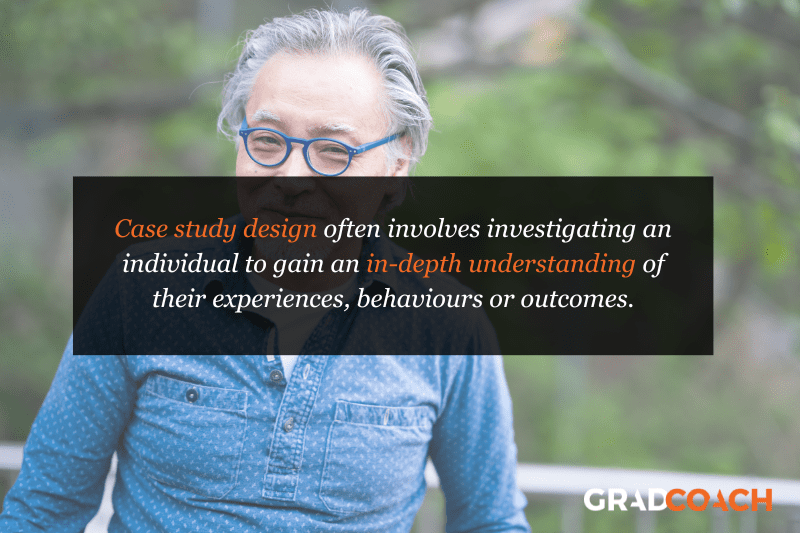
How To Choose A Research Design
Having worked through all of these potential research designs, you’d be forgiven for feeling a little overwhelmed and wondering, “ But how do I decide which research design to use? ”. While we could write an entire post covering that alone, here are a few factors to consider that will help you choose a suitable research design for your study.
Data type: The first determining factor is naturally the type of data you plan to be collecting – i.e., qualitative or quantitative. This may sound obvious, but we have to be clear about this – don’t try to use a quantitative research design on qualitative data (or vice versa)!
Research aim(s) and question(s): As with all methodological decisions, your research aim and research questions will heavily influence your research design. For example, if your research aims involve developing a theory from qualitative data, grounded theory would be a strong option. Similarly, if your research aims involve identifying and measuring relationships between variables, one of the experimental designs would likely be a better option.
Time: It’s essential that you consider any time constraints you have, as this will impact the type of research design you can choose. For example, if you’ve only got a month to complete your project, a lengthy design such as ethnography wouldn’t be a good fit.
Resources: Take into account the resources realistically available to you, as these need to factor into your research design choice. For example, if you require highly specialised lab equipment to execute an experimental design, you need to be sure that you’ll have access to that before you make a decision.
Keep in mind that when it comes to research, it’s important to manage your risks and play as conservatively as possible. If your entire project relies on you achieving a huge sample, having access to niche equipment or holding interviews with very difficult-to-reach participants, you’re creating risks that could kill your project. So, be sure to think through your choices carefully and make sure that you have backup plans for any existential risks. Remember that a relatively simple methodology executed well generally will typically earn better marks than a highly-complex methodology executed poorly.
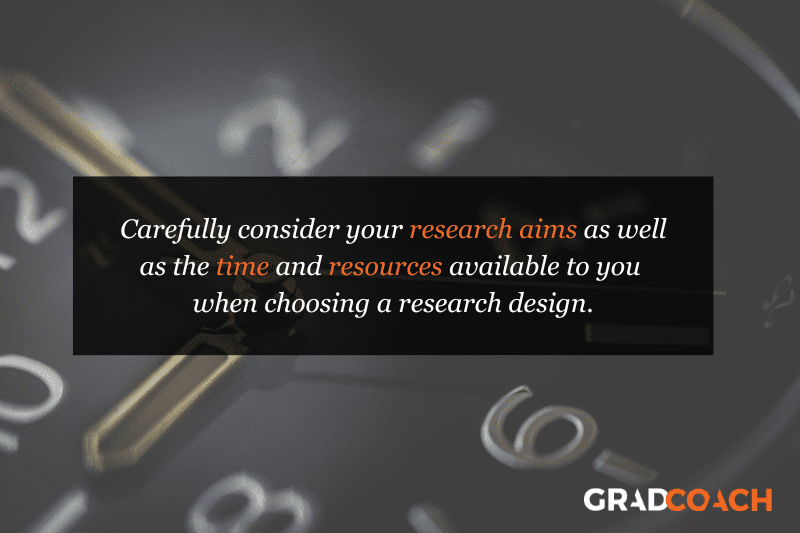
Recap: Key Takeaways
We’ve covered a lot of ground here. Let’s recap by looking at the key takeaways:
- Research design refers to the overall plan, structure or strategy that guides a research project, from its conception to the final analysis of data.
- Research designs for quantitative studies include descriptive , correlational , experimental and quasi-experimenta l designs.
- Research designs for qualitative studies include phenomenological , grounded theory , ethnographic and case study designs.
- When choosing a research design, you need to consider a variety of factors, including the type of data you’ll be working with, your research aims and questions, your time and the resources available to you.
If you need a helping hand with your research design (or any other aspect of your research), check out our private coaching services .
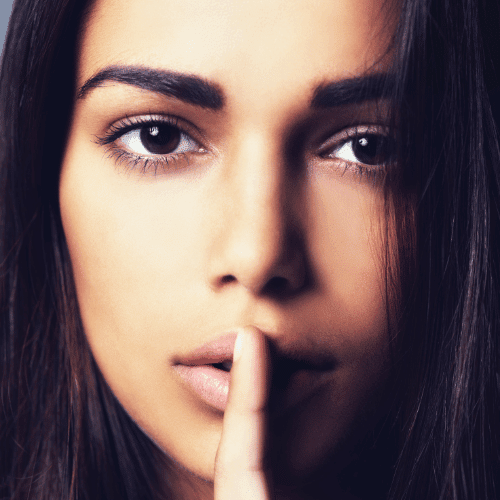
Psst... there’s more!
This post was based on one of our popular Research Bootcamps . If you're working on a research project, you'll definitely want to check this out ...
10 Comments
Is there any blog article explaining more on Case study research design? Is there a Case study write-up template? Thank you.
Thanks this was quite valuable to clarify such an important concept.
Thanks for this simplified explanations. it is quite very helpful.
This was really helpful. thanks
Thank you for your explanation. I think case study research design and the use of secondary data in researches needs to be talked about more in your videos and articles because there a lot of case studies research design tailored projects out there.
Please is there any template for a case study research design whose data type is a secondary data on your repository?
This post is very clear, comprehensive and has been very helpful to me. It has cleared the confusion I had in regard to research design and methodology.
This post is helpful, easy to understand, and deconstructs what a research design is. Thanks
how to cite this page
Thank you very much for the post. It is wonderful and has cleared many worries in my mind regarding research designs. I really appreciate .
how can I put this blog as my reference(APA style) in bibliography part?
Submit a Comment Cancel reply
Your email address will not be published. Required fields are marked *
Save my name, email, and website in this browser for the next time I comment.
- Print Friendly
- Privacy Policy

Home » Research Design – Types, Methods and Examples
Research Design – Types, Methods and Examples
Table of Contents

Research Design
Definition:
Research design refers to the overall strategy or plan for conducting a research study. It outlines the methods and procedures that will be used to collect and analyze data, as well as the goals and objectives of the study. Research design is important because it guides the entire research process and ensures that the study is conducted in a systematic and rigorous manner.
Types of Research Design
Types of Research Design are as follows:
Descriptive Research Design
This type of research design is used to describe a phenomenon or situation. It involves collecting data through surveys, questionnaires, interviews, and observations. The aim of descriptive research is to provide an accurate and detailed portrayal of a particular group, event, or situation. It can be useful in identifying patterns, trends, and relationships in the data.
Correlational Research Design
Correlational research design is used to determine if there is a relationship between two or more variables. This type of research design involves collecting data from participants and analyzing the relationship between the variables using statistical methods. The aim of correlational research is to identify the strength and direction of the relationship between the variables.
Experimental Research Design
Experimental research design is used to investigate cause-and-effect relationships between variables. This type of research design involves manipulating one variable and measuring the effect on another variable. It usually involves randomly assigning participants to groups and manipulating an independent variable to determine its effect on a dependent variable. The aim of experimental research is to establish causality.
Quasi-experimental Research Design
Quasi-experimental research design is similar to experimental research design, but it lacks one or more of the features of a true experiment. For example, there may not be random assignment to groups or a control group. This type of research design is used when it is not feasible or ethical to conduct a true experiment.
Case Study Research Design
Case study research design is used to investigate a single case or a small number of cases in depth. It involves collecting data through various methods, such as interviews, observations, and document analysis. The aim of case study research is to provide an in-depth understanding of a particular case or situation.
Longitudinal Research Design
Longitudinal research design is used to study changes in a particular phenomenon over time. It involves collecting data at multiple time points and analyzing the changes that occur. The aim of longitudinal research is to provide insights into the development, growth, or decline of a particular phenomenon over time.
Structure of Research Design
The format of a research design typically includes the following sections:
- Introduction : This section provides an overview of the research problem, the research questions, and the importance of the study. It also includes a brief literature review that summarizes previous research on the topic and identifies gaps in the existing knowledge.
- Research Questions or Hypotheses: This section identifies the specific research questions or hypotheses that the study will address. These questions should be clear, specific, and testable.
- Research Methods : This section describes the methods that will be used to collect and analyze data. It includes details about the study design, the sampling strategy, the data collection instruments, and the data analysis techniques.
- Data Collection: This section describes how the data will be collected, including the sample size, data collection procedures, and any ethical considerations.
- Data Analysis: This section describes how the data will be analyzed, including the statistical techniques that will be used to test the research questions or hypotheses.
- Results : This section presents the findings of the study, including descriptive statistics and statistical tests.
- Discussion and Conclusion : This section summarizes the key findings of the study, interprets the results, and discusses the implications of the findings. It also includes recommendations for future research.
- References : This section lists the sources cited in the research design.
Example of Research Design
An Example of Research Design could be:
Research question: Does the use of social media affect the academic performance of high school students?
Research design:
- Research approach : The research approach will be quantitative as it involves collecting numerical data to test the hypothesis.
- Research design : The research design will be a quasi-experimental design, with a pretest-posttest control group design.
- Sample : The sample will be 200 high school students from two schools, with 100 students in the experimental group and 100 students in the control group.
- Data collection : The data will be collected through surveys administered to the students at the beginning and end of the academic year. The surveys will include questions about their social media usage and academic performance.
- Data analysis : The data collected will be analyzed using statistical software. The mean scores of the experimental and control groups will be compared to determine whether there is a significant difference in academic performance between the two groups.
- Limitations : The limitations of the study will be acknowledged, including the fact that social media usage can vary greatly among individuals, and the study only focuses on two schools, which may not be representative of the entire population.
- Ethical considerations: Ethical considerations will be taken into account, such as obtaining informed consent from the participants and ensuring their anonymity and confidentiality.
How to Write Research Design
Writing a research design involves planning and outlining the methodology and approach that will be used to answer a research question or hypothesis. Here are some steps to help you write a research design:
- Define the research question or hypothesis : Before beginning your research design, you should clearly define your research question or hypothesis. This will guide your research design and help you select appropriate methods.
- Select a research design: There are many different research designs to choose from, including experimental, survey, case study, and qualitative designs. Choose a design that best fits your research question and objectives.
- Develop a sampling plan : If your research involves collecting data from a sample, you will need to develop a sampling plan. This should outline how you will select participants and how many participants you will include.
- Define variables: Clearly define the variables you will be measuring or manipulating in your study. This will help ensure that your results are meaningful and relevant to your research question.
- Choose data collection methods : Decide on the data collection methods you will use to gather information. This may include surveys, interviews, observations, experiments, or secondary data sources.
- Create a data analysis plan: Develop a plan for analyzing your data, including the statistical or qualitative techniques you will use.
- Consider ethical concerns : Finally, be sure to consider any ethical concerns related to your research, such as participant confidentiality or potential harm.
When to Write Research Design
Research design should be written before conducting any research study. It is an important planning phase that outlines the research methodology, data collection methods, and data analysis techniques that will be used to investigate a research question or problem. The research design helps to ensure that the research is conducted in a systematic and logical manner, and that the data collected is relevant and reliable.
Ideally, the research design should be developed as early as possible in the research process, before any data is collected. This allows the researcher to carefully consider the research question, identify the most appropriate research methodology, and plan the data collection and analysis procedures in advance. By doing so, the research can be conducted in a more efficient and effective manner, and the results are more likely to be valid and reliable.
Purpose of Research Design
The purpose of research design is to plan and structure a research study in a way that enables the researcher to achieve the desired research goals with accuracy, validity, and reliability. Research design is the blueprint or the framework for conducting a study that outlines the methods, procedures, techniques, and tools for data collection and analysis.
Some of the key purposes of research design include:
- Providing a clear and concise plan of action for the research study.
- Ensuring that the research is conducted ethically and with rigor.
- Maximizing the accuracy and reliability of the research findings.
- Minimizing the possibility of errors, biases, or confounding variables.
- Ensuring that the research is feasible, practical, and cost-effective.
- Determining the appropriate research methodology to answer the research question(s).
- Identifying the sample size, sampling method, and data collection techniques.
- Determining the data analysis method and statistical tests to be used.
- Facilitating the replication of the study by other researchers.
- Enhancing the validity and generalizability of the research findings.
Applications of Research Design
There are numerous applications of research design in various fields, some of which are:
- Social sciences: In fields such as psychology, sociology, and anthropology, research design is used to investigate human behavior and social phenomena. Researchers use various research designs, such as experimental, quasi-experimental, and correlational designs, to study different aspects of social behavior.
- Education : Research design is essential in the field of education to investigate the effectiveness of different teaching methods and learning strategies. Researchers use various designs such as experimental, quasi-experimental, and case study designs to understand how students learn and how to improve teaching practices.
- Health sciences : In the health sciences, research design is used to investigate the causes, prevention, and treatment of diseases. Researchers use various designs, such as randomized controlled trials, cohort studies, and case-control studies, to study different aspects of health and healthcare.
- Business : Research design is used in the field of business to investigate consumer behavior, marketing strategies, and the impact of different business practices. Researchers use various designs, such as survey research, experimental research, and case studies, to study different aspects of the business world.
- Engineering : In the field of engineering, research design is used to investigate the development and implementation of new technologies. Researchers use various designs, such as experimental research and case studies, to study the effectiveness of new technologies and to identify areas for improvement.
Advantages of Research Design
Here are some advantages of research design:
- Systematic and organized approach : A well-designed research plan ensures that the research is conducted in a systematic and organized manner, which makes it easier to manage and analyze the data.
- Clear objectives: The research design helps to clarify the objectives of the study, which makes it easier to identify the variables that need to be measured, and the methods that need to be used to collect and analyze data.
- Minimizes bias: A well-designed research plan minimizes the chances of bias, by ensuring that the data is collected and analyzed objectively, and that the results are not influenced by the researcher’s personal biases or preferences.
- Efficient use of resources: A well-designed research plan helps to ensure that the resources (time, money, and personnel) are used efficiently and effectively, by focusing on the most important variables and methods.
- Replicability: A well-designed research plan makes it easier for other researchers to replicate the study, which enhances the credibility and reliability of the findings.
- Validity: A well-designed research plan helps to ensure that the findings are valid, by ensuring that the methods used to collect and analyze data are appropriate for the research question.
- Generalizability : A well-designed research plan helps to ensure that the findings can be generalized to other populations, settings, or situations, which increases the external validity of the study.
Research Design Vs Research Methodology
Research Design | Research Methodology |
---|---|
The plan and structure for conducting research that outlines the procedures to be followed to collect and analyze data. | The set of principles, techniques, and tools used to carry out the research plan and achieve research objectives. |
Describes the overall approach and strategy used to conduct research, including the type of data to be collected, the sources of data, and the methods for collecting and analyzing data. | Refers to the techniques and methods used to gather, analyze and interpret data, including sampling techniques, data collection methods, and data analysis techniques. |
Helps to ensure that the research is conducted in a systematic, rigorous, and valid way, so that the results are reliable and can be used to make sound conclusions. | Includes a set of procedures and tools that enable researchers to collect and analyze data in a consistent and valid manner, regardless of the research design used. |
Common research designs include experimental, quasi-experimental, correlational, and descriptive studies. | Common research methodologies include qualitative, quantitative, and mixed-methods approaches. |
Determines the overall structure of the research project and sets the stage for the selection of appropriate research methodologies. | Guides the researcher in selecting the most appropriate research methods based on the research question, research design, and other contextual factors. |
Helps to ensure that the research project is feasible, relevant, and ethical. | Helps to ensure that the data collected is accurate, valid, and reliable, and that the research findings can be interpreted and generalized to the population of interest. |
About the author
Muhammad Hassan
Researcher, Academic Writer, Web developer
You may also like

Research Objectives – Types, Examples and...

Research Methodology – Types, Examples and...

Appendix in Research Paper – Examples and...

Research Summary – Structure, Examples and...

Research Topics – Ideas and Examples

Research Paper Outline – Types, Example, Template
Leave a comment x.
Save my name, email, and website in this browser for the next time I comment.
- En español – ExME
- Em português – EME
An introduction to different types of study design
Posted on 6th April 2021 by Hadi Abbas
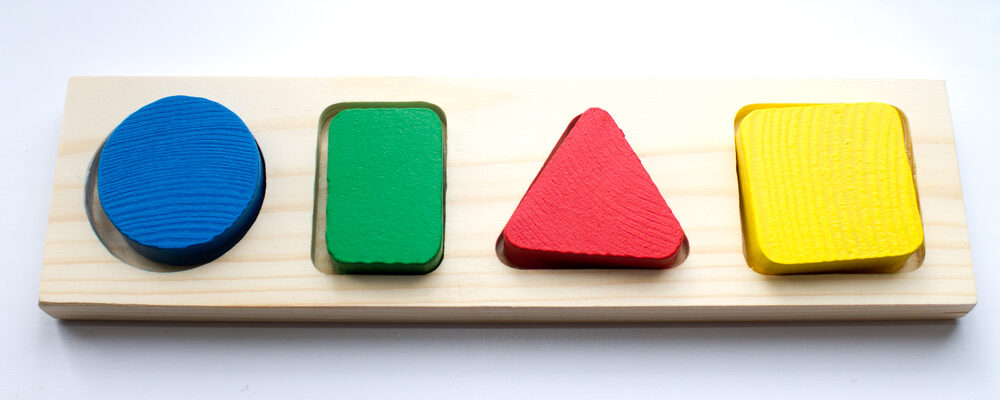
Study designs are the set of methods and procedures used to collect and analyze data in a study.
Broadly speaking, there are 2 types of study designs: descriptive studies and analytical studies.
Descriptive studies
- Describes specific characteristics in a population of interest
- The most common forms are case reports and case series
- In a case report, we discuss our experience with the patient’s symptoms, signs, diagnosis, and treatment
- In a case series, several patients with similar experiences are grouped.
Analytical Studies
Analytical studies are of 2 types: observational and experimental.
Observational studies are studies that we conduct without any intervention or experiment. In those studies, we purely observe the outcomes. On the other hand, in experimental studies, we conduct experiments and interventions.
Observational studies
Observational studies include many subtypes. Below, I will discuss the most common designs.
Cross-sectional study:
- This design is transverse where we take a specific sample at a specific time without any follow-up
- It allows us to calculate the frequency of disease ( p revalence ) or the frequency of a risk factor
- This design is easy to conduct
- For example – if we want to know the prevalence of migraine in a population, we can conduct a cross-sectional study whereby we take a sample from the population and calculate the number of patients with migraine headaches.
Cohort study:
- We conduct this study by comparing two samples from the population: one sample with a risk factor while the other lacks this risk factor
- It shows us the risk of developing the disease in individuals with the risk factor compared to those without the risk factor ( RR = relative risk )
- Prospective : we follow the individuals in the future to know who will develop the disease
- Retrospective : we look to the past to know who developed the disease (e.g. using medical records)
- This design is the strongest among the observational studies
- For example – to find out the relative risk of developing chronic obstructive pulmonary disease (COPD) among smokers, we take a sample including smokers and non-smokers. Then, we calculate the number of individuals with COPD among both.
Case-Control Study:
- We conduct this study by comparing 2 groups: one group with the disease (cases) and another group without the disease (controls)
- This design is always retrospective
- We aim to find out the odds of having a risk factor or an exposure if an individual has a specific disease (Odds ratio)
- Relatively easy to conduct
- For example – we want to study the odds of being a smoker among hypertensive patients compared to normotensive ones. To do so, we choose a group of patients diagnosed with hypertension and another group that serves as the control (normal blood pressure). Then we study their smoking history to find out if there is a correlation.
Experimental Studies
- Also known as interventional studies
- Can involve animals and humans
- Pre-clinical trials involve animals
- Clinical trials are experimental studies involving humans
- In clinical trials, we study the effect of an intervention compared to another intervention or placebo. As an example, I have listed the four phases of a drug trial:
I: We aim to assess the safety of the drug ( is it safe ? )
II: We aim to assess the efficacy of the drug ( does it work ? )
III: We want to know if this drug is better than the old treatment ( is it better ? )
IV: We follow-up to detect long-term side effects ( can it stay in the market ? )
- In randomized controlled trials, one group of participants receives the control, while the other receives the tested drug/intervention. Those studies are the best way to evaluate the efficacy of a treatment.
Finally, the figure below will help you with your understanding of different types of study designs.
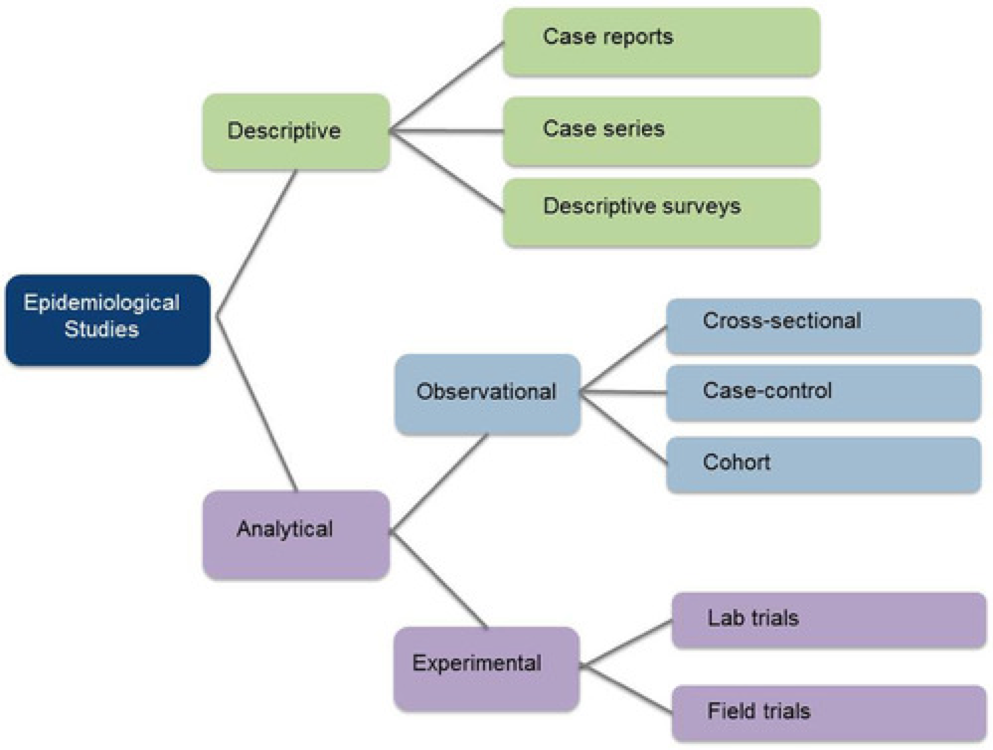
References (pdf)
You may also be interested in the following blogs for further reading:
An introduction to randomized controlled trials
Case-control and cohort studies: a brief overview
Cohort studies: prospective and retrospective designs
Prevalence vs Incidence: what is the difference?
Leave a Reply Cancel reply
Your email address will not be published. Required fields are marked *
Save my name, email, and website in this browser for the next time I comment.
No Comments on An introduction to different types of study design
you are amazing one!! if I get you I’m working with you! I’m student from Ethiopian higher education. health sciences student
Very informative and easy understandable
You are my kind of doctor. Do not lose sight of your objective.
Wow very erll explained and easy to understand
I’m Khamisu Habibu community health officer student from Abubakar Tafawa Balewa university teaching hospital Bauchi, Nigeria, I really appreciate your write up and you have make it clear for the learner. thank you
well understood,thank you so much
Well understood…thanks
Simply explained. Thank You.
Thanks a lot for this nice informative article which help me to understand different study designs that I felt difficult before
That’s lovely to hear, Mona, thank you for letting the author know how useful this was. If there are any other particular topics you think would be useful to you, and are not already on the website, please do let us know.
it is very informative and useful.
thank you statistician
Fabulous to hear, thank you John.
Thanks for this information
Thanks so much for this information….I have clearly known the types of study design Thanks
That’s so good to hear, Mirembe, thank you for letting the author know.
Very helpful article!! U have simplified everything for easy understanding
I’m a health science major currently taking statistics for health care workers…this is a challenging class…thanks for the simified feedback.
That’s good to hear this has helped you. Hopefully you will find some of the other blogs useful too. If you see any topics that are missing from the website, please do let us know!
Hello. I liked your presentation, the fact that you ranked them clearly is very helpful to understand for people like me who is a novelist researcher. However, I was expecting to read much more about the Experimental studies. So please direct me if you already have or will one day. Thank you
Dear Ay. My sincere apologies for not responding to your comment sooner. You may find it useful to filter the blogs by the topic of ‘Study design and research methods’ – here is a link to that filter: https://s4be.cochrane.org/blog/topic/study-design/ This will cover more detail about experimental studies. Or have a look on our library page for further resources there – you’ll find that on the ‘Resources’ drop down from the home page.
However, if there are specific things you feel you would like to learn about experimental studies, that are missing from the website, it would be great if you could let me know too. Thank you, and best of luck. Emma
Great job Mr Hadi. I advise you to prepare and study for the Australian Medical Board Exams as soon as you finish your undergrad study in Lebanon. Good luck and hope we can meet sometime in the future. Regards ;)
You have give a good explaination of what am looking for. However, references am not sure of where to get them from.
Subscribe to our newsletter
You will receive our monthly newsletter and free access to Trip Premium.
Related Articles
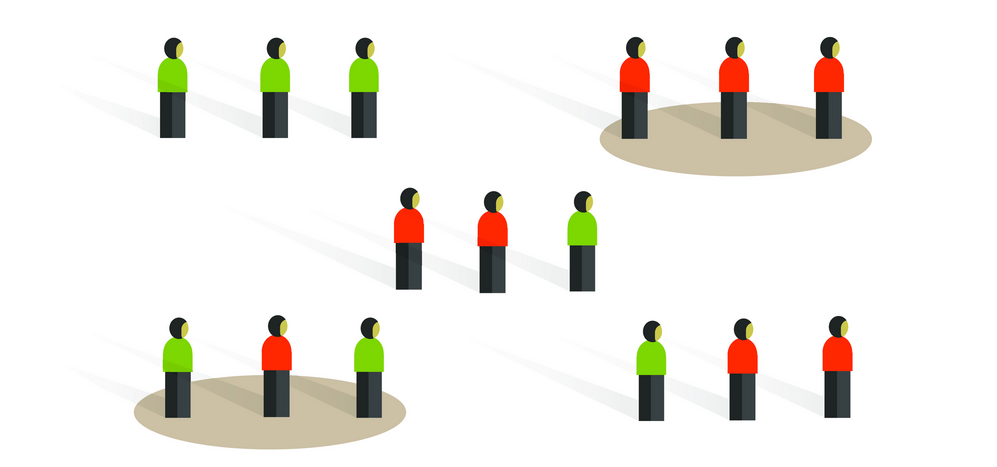
Cluster Randomized Trials: Concepts
This blog summarizes the concepts of cluster randomization, and the logistical and statistical considerations while designing a cluster randomized controlled trial.
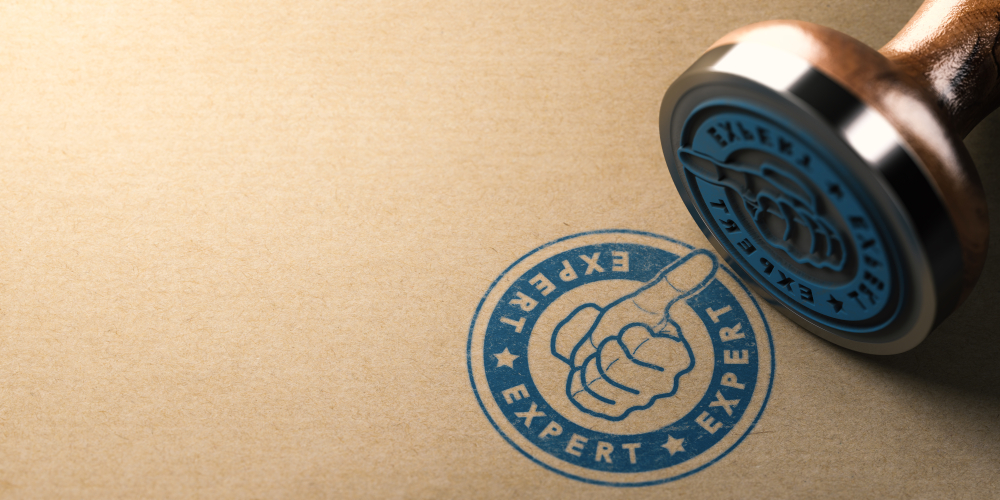
Expertise-based Randomized Controlled Trials
This blog summarizes the concepts of Expertise-based randomized controlled trials with a focus on the advantages and challenges associated with this type of study.
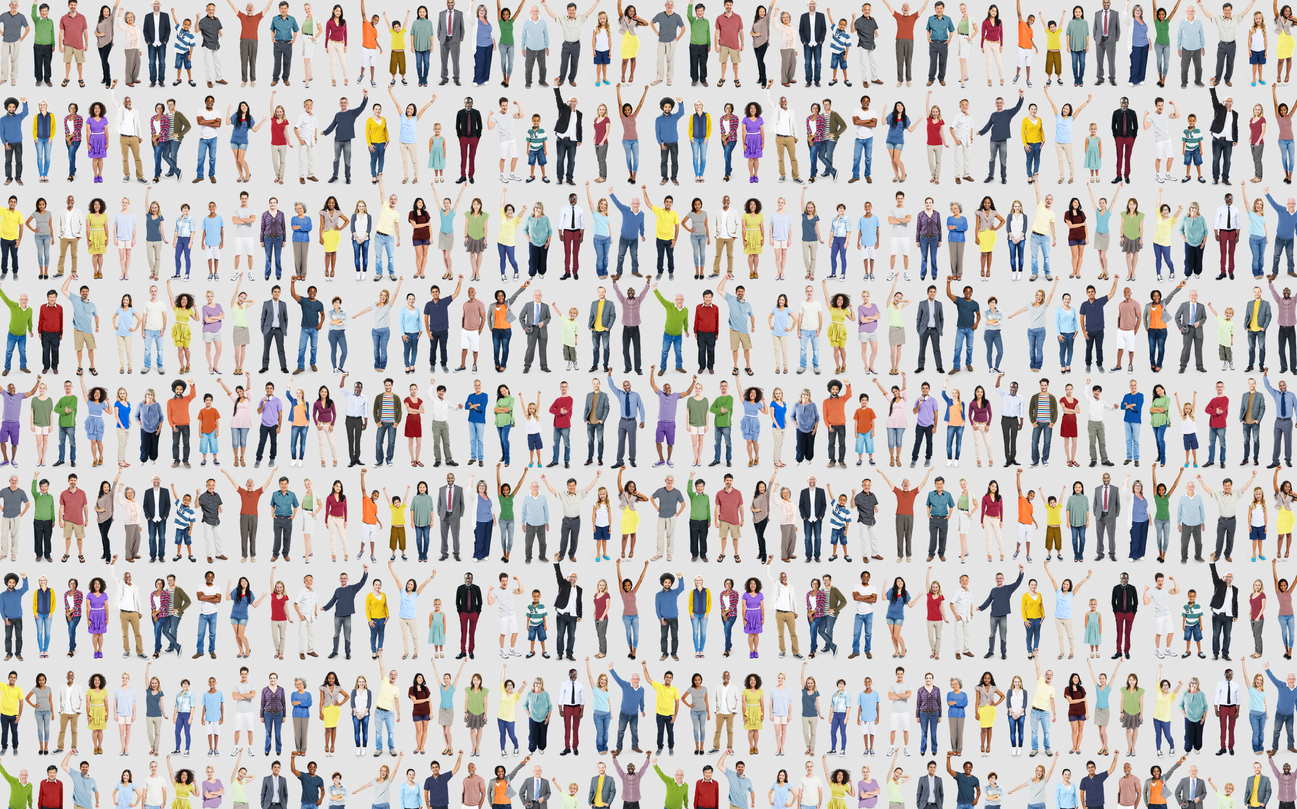
A well-designed cohort study can provide powerful results. This blog introduces prospective and retrospective cohort studies, discussing the advantages, disadvantages and use of these type of study designs.

An official website of the United States government
The .gov means it’s official. Federal government websites often end in .gov or .mil. Before sharing sensitive information, make sure you’re on a federal government site.
The site is secure. The https:// ensures that you are connecting to the official website and that any information you provide is encrypted and transmitted securely.
- Publications
- Account settings
Preview improvements coming to the PMC website in October 2024. Learn More or Try it out now .
- Advanced Search
- Journal List
- Indian J Crit Care Med
- v.23(Suppl 4); 2019 Dec
Understanding Research Study Designs
Priya ranganathan.
Department of Anesthesiology, Critical Care and Pain, Tata Memorial Hospital, Mumbai, Maharashtra, India
In this article, we will look at the important features of various types of research study designs used commonly in biomedical research.
How to cite this article
Ranganathan P. Understanding Research Study Designs. Indian J Crit Care Med 2019;23(Suppl 4):S305–S307.
We use a variety of research study designs in biomedical research. In this article, the main features of each of these designs are summarized.
TERMS USED IN RESEARCH DESIGNS
Exposure vs outcome.
Exposure refers to any factor that may be associated with the outcome of interest. It is also called the predictor variable or independent variable or risk factor. Outcome refers to the variable that is studied to assess the impact of the exposure on the population. It is also known as the predicted variable or the dependent variable. For example, in a study looking at nerve damage after organophosphate (OPC) poisoning, the exposure would be OPC and the outcome would be nerve damage.
Longitudinal vs Transversal Studies
In longitudinal studies, participants are followed over time to determine the association between exposure and outcome (or outcome and exposure). On the other hand, in transversal studies, observations about exposure and outcome are made at a single point in time.
Forward vs Backward Directed Studies
In forward-directed studies, the direction of enquiry moves from exposure to outcome. In backward-directed studies, the line of enquiry starts with outcome and then determines exposure.
Prospective vs Retrospective Studies
In prospective studies, the outcome has not occurred at the time of initiation of the study. The researcher determines exposure and follows participants into the future to assess outcomes. In retrospective studies, the outcome of interest has already occurred when the study commences.
CLASSIFICATION OF STUDY DESIGNS
Broadly, study designs can be classified as descriptive or analytical (inferential) studies.
Descriptive Studies
Descriptive studies describe the characteristics of interest in the study population (also referred to as sample, to differentiate it from the entire population in the universe). These studies do not have a comparison group. The simplest type of descriptive study is the case report. In a case report, the researcher describes his/her experience with symptoms, signs, diagnosis, or treatment of a patient. Sometimes, a group of patients having a similar experience may be grouped to form a case series.
Case reports and case series form the lowest level of evidence in biomedical research and, as such, are considered hypothesis-generating studies. However, they are easy to write and may be a good starting point for the budding researcher. The recognition of some important associations in the field of medicine—such as that of thalidomide with phocomelia and Kaposi's sarcoma with HIV infection—resulted from case reports and case series. The reader can look up several published case reports and case series related to complications after OPC poisoning. 1 , 2
Analytical (Inferential) Studies
Analytical or inferential studies try to prove a hypothesis and establish an association between an exposure and an outcome. These studies usually have a comparator group. Analytical studies are further classified as observational or interventional studies.
In observational studies, there is no intervention by the researcher. The researcher merely observes outcomes in different groups of participants who, for natural reasons, have or have not been exposed to a particular risk factor. Examples of observational studies include cross-sectional, case–control, and cohort studies.
Cross-sectional Studies
These are transversal studies where data are collected from the study population at a single point in time. Exposure and outcome are determined simultaneously. Cross-sectional studies are easy to conduct, involve no follow-up, and need limited resources. They offer useful information on prevalence of health conditions and possible associations between risk factors and outcomes. However, there are two major limitations of cross-sectional studies. First, it may not be possible to establish a clear cause–benefit relationship. For example, in a study of association between colon cancer and dietary fiber intake, it may be difficult to establish whether the low fiber intake preceded the symptoms of colon cancer or whether the symptoms of colon cancer resulted in a change in dietary fiber intake. Another important limitation of cross-sectional studies is survival bias. For example, in a study looking at alcohol intake vs mortality due to chronic liver disease, among the participants with the highest alcohol intake, several may have died of liver disease; this will not be picked up by the study and will give biased results. An example of a cross-sectional study is a survey on nurses’ knowledge and practices of initial management of acute poisoning. 3
Case–control Studies
Case–control studies are backward-directed studies. Here, the direction of enquiry begins with the outcome and then proceeds to exposure. Case–control studies are always retrospective, i.e., the outcome of interest has occurred when the study begins. The researcher identifies participants who have developed the outcome of interest (cases) and chooses matching participants who do not have the outcome (controls). Matching is done based on factors that are likely to influence the exposure or outcome (e.g., age, gender, socioeconomic status). The researcher then proceeds to determine exposure in cases and controls. If cases have a higher incidence of exposure than controls, it suggests an association between exposure and outcome. Case–control studies are relatively quick to conduct, need limited resources, and are useful when the outcome is rare. They also allow the researcher to study multiple exposures for a particular outcome. However, they have several limitations. First, matching of cases with controls may not be easy since many unknown confounders may affect exposure and outcome. Second, there may be biased in the way the history of exposure is determined in cases vs controls; one way to overcome this is to have a blinded assessor determining the exposure using a standard technique (e.g., a standardized questionnaire). However, despite this, it has been shown that cases are far more likely than controls to recall history of exposure—the “recall bias.” For example, mothers of babies born with congenital anomalies may provide a more detailed history of drugs ingested during their pregnancy than those with normal babies. Also, since case-control studies do not begin with a population at risk, it is not possible to determine the true risk of outcome. Instead, one can only calculate the odds of association between exposure and outcome.
Kendrick and colleagues designed a case–control study to look at the association between domestic poison prevention practices and medically attended poisoning in children. They identified children presenting with unintentional poisoning at home (cases with the outcome), matched them with community participants (controls without the outcome), and then elicited data from parents and caregivers on home safety practices (exposure). 4
Cohort Studies
Cohort studies resemble clinical trials except that the exposure is naturally determined instead of being decided by the investigator. Here, the direction of enquiry begins with the exposure and then proceeds to outcome. The researcher begins with a group of individuals who are free of outcome at baseline; of these, some have the exposure (study cohort) while others do not (control group). The groups are followed up over a period of time to determine occurrence of outcome. Cohort studies may be prospective (involving a period of follow-up after the start of the study) or retrospective (e.g., using medical records or registry data). Cohort studies are considered the strongest among the observational study designs. They provide proof of temporal relationship (exposure occurred before outcome), allow determination of risk, and permit multiple outcomes to be studied for a single exposure. However, they are expensive to conduct and time-consuming, there may be several losses to follow-up, and they are not suitable for studying rare outcomes. Also, there may be unknown confounders other than the exposure affecting the occurrence of the outcome.
Jayasinghe conducted a cohort study to look at the effect of acute organophosphorus poisoning on nerve function. They recruited 70 patients with OPC poisoning (exposed group) and 70 matched controls without history of pesticide exposure (unexposed controls). Participants were followed up or 6 weeks for neurophysiological assessments to determine nerve damage (outcome). Hung carried out a retrospective cohort study using a nationwide research database to look at the long-term effects of OPC poisoning on cardiovascular disease. From the database, he identified an OPC-exposed cohort and an unexposed control cohort (matched for gender and age) from several years back and then examined later records to look at the development of cardiovascular diseases in both groups. 5
Interventional Studies
In interventional studies (also known as experimental studies or clinical trials), the researcher deliberately allots participants to receive one of several interventions; of these, some may be experimental while others may be controls (either standard of care or placebo). Allotment of participants to a particular treatment arm is carried out through the process of randomization, which ensures that every participant has a similar chance of being in any of the arms, eliminating bias in selection. There are several other aspects crucial to the validity of the results of a clinical trial such as allocation concealment, blinding, choice of control, and statistical analysis plan. These will be discussed in a separate article.
The randomized controlled clinical trial is considered the gold standard for evaluating the efficacy of a treatment. Randomization leads to equal distribution of known and unknown confounders between treatment arms; therefore, we can be reasonably certain that any difference in outcome is a treatment effect and not due to other factors. The temporal sequence of cause and effect is established. It is possible to determine risk of the outcome in each treatment arm accurately. However, randomized controlled trials have their limitations and may not be possible in every situation. For example, it is unethical to randomize participants to an intervention that is likely to cause harm—e.g., smoking. In such cases, well-designed observational studies are the only option. Also, these trials are expensive to conduct and resource-intensive.
In a randomized controlled trial, Li et al. randomly allocated patients of paraquat poisoning to receive either conventional therapy (control group) or continuous veno-venous hemofiltration (intervention). Patients were followed up to look for mortality or other adverse events (outcome). 6
Researchers need to understand the features of different study designs, with their advantages and limitations so that the most appropriate design can be chosen for a particular research question. The Centre for Evidence Based Medicine offers an useful tool to determine the type of research design used in a particular study. 7
Source of support: Nil
Conflict of interest: None
Have a language expert improve your writing
Run a free plagiarism check in 10 minutes, automatically generate references for free.
- Knowledge Base
- Methodology
Types of Research Designs Compared | Examples
Published on 5 May 2022 by Shona McCombes . Revised on 10 October 2022.
When you start planning a research project, developing research questions and creating a research design , you will have to make various decisions about the type of research you want to do.
There are many ways to categorise different types of research. The words you use to describe your research depend on your discipline and field. In general, though, the form your research design takes will be shaped by:
- The type of knowledge you aim to produce
- The type of data you will collect and analyse
- The sampling methods , timescale, and location of the research
This article takes a look at some common distinctions made between different types of research and outlines the key differences between them.
Table of contents
Types of research aims, types of research data, types of sampling, timescale, and location.
The first thing to consider is what kind of knowledge your research aims to contribute.
Type of research | What’s the difference? | What to consider |
---|---|---|
Basic vs applied | Basic research aims to , while applied research aims to . | Do you want to expand scientific understanding or solve a practical problem? |
vs | Exploratory research aims to , while explanatory research aims to . | How much is already known about your research problem? Are you conducting initial research on a newly-identified issue, or seeking precise conclusions about an established issue? |
aims to , while aims to . | Is there already some theory on your research problem that you can use to develop , or do you want to propose new theories based on your findings? |
Prevent plagiarism, run a free check.
The next thing to consider is what type of data you will collect. Each kind of data is associated with a range of specific research methods and procedures.
Type of research | What’s the difference? | What to consider |
---|---|---|
Primary vs secondary | Primary data is (e.g., through interviews or experiments), while secondary data (e.g., in government surveys or scientific publications). | How much data is already available on your topic? Do you want to collect original data or analyse existing data (e.g., through a )? |
, while . | Is your research more concerned with measuring something or interpreting something? You can also create a research design that has elements of both. | |
vs | Descriptive research gathers data , while experimental research . | Do you want to identify characteristics, patterns, and or test causal relationships between ? |
Finally, you have to consider three closely related questions: How will you select the subjects or participants of the research? When and how often will you collect data from your subjects? And where will the research take place?
Type of research | What’s the difference? | What to consider |
---|---|---|
allows you to , while allows you to draw conclusions . | Do you want to produce knowledge that applies to many contexts or detailed knowledge about a specific context (e.g., in a )? | |
vs | Cross-sectional studies , while longitudinal studies . | Is your research question focused on understanding the current situation or tracking changes over time? |
Field vs laboratory | Field research takes place in , while laboratory research takes place in . | Do you want to find out how something occurs in the real world or draw firm conclusions about cause and effect? Laboratory experiments have higher but lower . |
Fixed vs flexible | In a fixed research design the subjects, timescale and location are begins, while in a flexible design these aspects may . | Do you want to test hypotheses and establish generalisable facts, or explore concepts and develop understanding? For measuring, testing, and making generalisations, a fixed research design has higher . |
Choosing among all these different research types is part of the process of creating your research design , which determines exactly how the research will be conducted. But the type of research is only the first step: next, you have to make more concrete decisions about your research methods and the details of the study.
Read more about creating a research design
Cite this Scribbr article
If you want to cite this source, you can copy and paste the citation or click the ‘Cite this Scribbr article’ button to automatically add the citation to our free Reference Generator.
McCombes, S. (2022, October 10). Types of Research Designs Compared | Examples. Scribbr. Retrieved 10 July 2024, from https://www.scribbr.co.uk/research-methods/types-of-research-designs/
Is this article helpful?
Shona McCombes
Other students also liked, sampling methods | types, techniques, & examples, qualitative vs quantitative research | examples & methods, between-subjects design | examples, pros & cons.
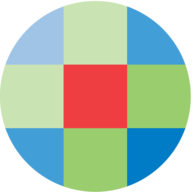
- Get new issue alerts Get alerts
- Submit a Manuscript
Secondary Logo
Journal logo.
Colleague's E-mail is Invalid
Your message has been successfully sent to your colleague.
Save my selection
Study designs
Part 1 – an overview and classification.
Ranganathan, Priya; Aggarwal, Rakesh 1
Department of Anaesthesiology, Tata Memorial Centre, Mumbai, Maharashtra, India
1 Department of Gastroenterology, Sanjay Gandhi Postgraduate Institute of Medical Sciences, Lucknow, Uttar Pradesh, India
Address for correspondence: Dr. Priya Ranganathan, Department of Anaesthesiology, Tata Memorial Centre, Ernest Borges Road, Parel, Mumbai - 400 012, Maharashtra, India. E-mail: [email protected]
This is an open access journal, and articles are distributed under the terms of the Creative Commons Attribution-NonCommercial-ShareAlike 4.0 License, which allows others to remix, tweak, and build upon the work non-commercially, as long as appropriate credit is given and the new creations are licensed under the identical terms.
There are several types of research study designs, each with its inherent strengths and flaws. The study design used to answer a particular research question depends on the nature of the question and the availability of resources. In this article, which is the first part of a series on “study designs,” we provide an overview of research study designs and their classification. The subsequent articles will focus on individual designs.
INTRODUCTION
Research study design is a framework, or the set of methods and procedures used to collect and analyze data on variables specified in a particular research problem.
Research study designs are of many types, each with its advantages and limitations. The type of study design used to answer a particular research question is determined by the nature of question, the goal of research, and the availability of resources. Since the design of a study can affect the validity of its results, it is important to understand the different types of study designs and their strengths and limitations.
There are some terms that are used frequently while classifying study designs which are described in the following sections.
A variable represents a measurable attribute that varies across study units, for example, individual participants in a study, or at times even when measured in an individual person over time. Some examples of variables include age, sex, weight, height, health status, alive/dead, diseased/healthy, annual income, smoking yes/no, and treated/untreated.
Exposure (or intervention) and outcome variables
A large proportion of research studies assess the relationship between two variables. Here, the question is whether one variable is associated with or responsible for change in the value of the other variable. Exposure (or intervention) refers to the risk factor whose effect is being studied. It is also referred to as the independent or the predictor variable. The outcome (or predicted or dependent) variable develops as a consequence of the exposure (or intervention). Typically, the term “exposure” is used when the “causative” variable is naturally determined (as in observational studies – examples include age, sex, smoking, and educational status), and the term “intervention” is preferred where the researcher assigns some or all participants to receive a particular treatment for the purpose of the study (experimental studies – e.g., administration of a drug). If a drug had been started in some individuals but not in the others, before the study started, this counts as exposure, and not as intervention – since the drug was not started specifically for the study.
Observational versus interventional (or experimental) studies
Observational studies are those where the researcher is documenting a naturally occurring relationship between the exposure and the outcome that he/she is studying. The researcher does not do any active intervention in any individual, and the exposure has already been decided naturally or by some other factor. For example, looking at the incidence of lung cancer in smokers versus nonsmokers, or comparing the antenatal dietary habits of mothers with normal and low-birth babies. In these studies, the investigator did not play any role in determining the smoking or dietary habit in individuals.
For an exposure to determine the outcome, it must precede the latter. Any variable that occurs simultaneously with or following the outcome cannot be causative, and hence is not considered as an “exposure.”
Observational studies can be either descriptive (nonanalytical) or analytical (inferential) – this is discussed later in this article.
Interventional studies are experiments where the researcher actively performs an intervention in some or all members of a group of participants. This intervention could take many forms – for example, administration of a drug or vaccine, performance of a diagnostic or therapeutic procedure, and introduction of an educational tool. For example, a study could randomly assign persons to receive aspirin or placebo for a specific duration and assess the effect on the risk of developing cerebrovascular events.
Descriptive versus analytical studies
Descriptive (or nonanalytical) studies, as the name suggests, merely try to describe the data on one or more characteristics of a group of individuals. These do not try to answer questions or establish relationships between variables. Examples of descriptive studies include case reports, case series, and cross-sectional surveys (please note that cross-sectional surveys may be analytical studies as well – this will be discussed in the next article in this series). Examples of descriptive studies include a survey of dietary habits among pregnant women or a case series of patients with an unusual reaction to a drug.
Analytical studies attempt to test a hypothesis and establish causal relationships between variables. In these studies, the researcher assesses the effect of an exposure (or intervention) on an outcome. As described earlier, analytical studies can be observational (if the exposure is naturally determined) or interventional (if the researcher actively administers the intervention).
Directionality of study designs
Based on the direction of inquiry, study designs may be classified as forward-direction or backward-direction. In forward-direction studies, the researcher starts with determining the exposure to a risk factor and then assesses whether the outcome occurs at a future time point. This design is known as a cohort study. For example, a researcher can follow a group of smokers and a group of nonsmokers to determine the incidence of lung cancer in each. In backward-direction studies, the researcher begins by determining whether the outcome is present (cases vs. noncases [also called controls]) and then traces the presence of prior exposure to a risk factor. These are known as case–control studies. For example, a researcher identifies a group of normal-weight babies and a group of low-birth weight babies and then asks the mothers about their dietary habits during the index pregnancy.
Prospective versus retrospective study designs
The terms “prospective” and “retrospective” refer to the timing of the research in relation to the development of the outcome. In retrospective studies, the outcome of interest has already occurred (or not occurred – e.g., in controls) in each individual by the time s/he is enrolled, and the data are collected either from records or by asking participants to recall exposures. There is no follow-up of participants. By contrast, in prospective studies, the outcome (and sometimes even the exposure or intervention) has not occurred when the study starts and participants are followed up over a period of time to determine the occurrence of outcomes. Typically, most cohort studies are prospective studies (though there may be retrospective cohorts), whereas case–control studies are retrospective studies. An interventional study has to be, by definition, a prospective study since the investigator determines the exposure for each study participant and then follows them to observe outcomes.
The terms “prospective” versus “retrospective” studies can be confusing. Let us think of an investigator who starts a case–control study. To him/her, the process of enrolling cases and controls over a period of several months appears prospective. Hence, the use of these terms is best avoided. Or, at the very least, one must be clear that the terms relate to work flow for each individual study participant, and not to the study as a whole.
Classification of study designs
Figure 1 depicts a simple classification of research study designs. The Centre for Evidence-based Medicine has put forward a useful three-point algorithm which can help determine the design of a research study from its methods section:[ 1 ]

- Does the study describe the characteristics of a sample or does it attempt to analyze (or draw inferences about) the relationship between two variables? – If no, then it is a descriptive study, and if yes, it is an analytical (inferential) study
- If analytical, did the investigator determine the exposure? – If no, it is an observational study, and if yes, it is an experimental study
- If observational, when was the outcome determined? – at the start of the study (case–control study), at the end of a period of follow-up (cohort study), or simultaneously (cross sectional).
In the next few pieces in the series, we will discuss various study designs in greater detail.
Financial support and sponsorship
Conflicts of interest.
There are no conflicts of interest.
Epidemiologic methods; research design; research methodology
- + Favorites
- View in Gallery
Readers Of this Article Also Read
Study designs: part 2 – descriptive studies, research studies on screening tests, study designs: part 3 - analytical observational studies, introduction to qualitative research methods – part i, investigator-initiated studies: challenges and solutions.
Educational resources and simple solutions for your research journey

What is Research Design? Understand Types of Research Design, with Examples
Have you been wondering “ what is research design ?” or “what are some research design examples ?” Are you unsure about the research design elements or which of the different types of research design best suit your study? Don’t worry! In this article, we’ve got you covered!
Table of Contents
What is research design?
Have you been wondering “ what is research design ?” or “what are some research design examples ?” Don’t worry! In this article, we’ve got you covered!
A research design is the plan or framework used to conduct a research study. It involves outlining the overall approach and methods that will be used to collect and analyze data in order to answer research questions or test hypotheses. A well-designed research study should have a clear and well-defined research question, a detailed plan for collecting data, and a method for analyzing and interpreting the results. A well-thought-out research design addresses all these features.
Research design elements
Research design elements include the following:
- Clear purpose: The research question or hypothesis must be clearly defined and focused.
- Sampling: This includes decisions about sample size, sampling method, and criteria for inclusion or exclusion. The approach varies for different research design types .
- Data collection: This research design element involves the process of gathering data or information from the study participants or sources. It includes decisions about what data to collect, how to collect it, and the tools or instruments that will be used.
- Data analysis: All research design types require analysis and interpretation of the data collected. This research design element includes decisions about the statistical tests or methods that will be used to analyze the data, as well as any potential confounding variables or biases that may need to be addressed.
- Type of research methodology: This includes decisions about the overall approach for the study.
- Time frame: An important research design element is the time frame, which includes decisions about the duration of the study, the timeline for data collection and analysis, and follow-up periods.
- Ethical considerations: The research design must include decisions about ethical considerations such as informed consent, confidentiality, and participant protection.
- Resources: A good research design takes into account decisions about the budget, staffing, and other resources needed to carry out the study.
The elements of research design should be carefully planned and executed to ensure the validity and reliability of the study findings. Let’s go deeper into the concepts of research design .

Characteristics of research design
Some basic characteristics of research design are common to different research design types . These characteristics of research design are as follows:
- Neutrality : Right from the study assumptions to setting up the study, a neutral stance must be maintained, free of pre-conceived notions. The researcher’s expectations or beliefs should not color the findings or interpretation of the findings. Accordingly, a good research design should address potential sources of bias and confounding factors to be able to yield unbiased and neutral results.
- Reliability : Reliability is one of the characteristics of research design that refers to consistency in measurement over repeated measures and fewer random errors. A reliable research design must allow for results to be consistent, with few errors due to chance.
- Validity : Validity refers to the minimization of nonrandom (systematic) errors. A good research design must employ measurement tools that ensure validity of the results.
- Generalizability: The outcome of the research design should be applicable to a larger population and not just a small sample . A generalized method means the study can be conducted on any part of a population with similar accuracy.
- Flexibility: A research design should allow for changes to be made to the research plan as needed, based on the data collected and the outcomes of the study
A well-planned research design is critical for conducting a scientifically rigorous study that will generate neutral, reliable, valid, and generalizable results. At the same time, it should allow some level of flexibility.
Different types of research design
A research design is essential to systematically investigate, understand, and interpret phenomena of interest. Let’s look at different types of research design and research design examples .
Broadly, research design types can be divided into qualitative and quantitative research.
Qualitative research is subjective and exploratory. It determines relationships between collected data and observations. It is usually carried out through interviews with open-ended questions, observations that are described in words, etc.
Quantitative research is objective and employs statistical approaches. It establishes the cause-and-effect relationship among variables using different statistical and computational methods. This type of research is usually done using surveys and experiments.
Qualitative research vs. Quantitative research
Deals with subjective aspects, e.g., experiences, beliefs, perspectives, and concepts. | Measures different types of variables and describes frequencies, averages, correlations, etc. |
Deals with non-numerical data, such as words, images, and observations. | Tests hypotheses about relationships between variables. Results are presented numerically and statistically. |
In qualitative research design, data are collected via direct observations, interviews, focus groups, and naturally occurring data. Methods for conducting qualitative research are grounded theory, thematic analysis, and discourse analysis.
| Quantitative research design is empirical. Data collection methods involved are experiments, surveys, and observations expressed in numbers. The research design categories under this are descriptive, experimental, correlational, diagnostic, and explanatory. |
Data analysis involves interpretation and narrative analysis. | Data analysis involves statistical analysis and hypothesis testing. |
The reasoning used to synthesize data is inductive.
| The reasoning used to synthesize data is deductive.
|
Typically used in fields such as sociology, linguistics, and anthropology. | Typically used in fields such as economics, ecology, statistics, and medicine. |
Example: Focus group discussions with women farmers about climate change perception.
| Example: Testing the effectiveness of a new treatment for insomnia. |
Qualitative research design types and qualitative research design examples
The following will familiarize you with the research design categories in qualitative research:
- Grounded theory: This design is used to investigate research questions that have not previously been studied in depth. Also referred to as exploratory design , it creates sequential guidelines, offers strategies for inquiry, and makes data collection and analysis more efficient in qualitative research.
Example: A researcher wants to study how people adopt a certain app. The researcher collects data through interviews and then analyzes the data to look for patterns. These patterns are used to develop a theory about how people adopt that app.
- Thematic analysis: This design is used to compare the data collected in past research to find similar themes in qualitative research.
Example: A researcher examines an interview transcript to identify common themes, say, topics or patterns emerging repeatedly.
- Discourse analysis : This research design deals with language or social contexts used in data gathering in qualitative research.
Example: Identifying ideological frameworks and viewpoints of writers of a series of policies.
Quantitative research design types and quantitative research design examples
Note the following research design categories in quantitative research:
- Descriptive research design : This quantitative research design is applied where the aim is to identify characteristics, frequencies, trends, and categories. It may not often begin with a hypothesis. The basis of this research type is a description of an identified variable. This research design type describes the “what,” “when,” “where,” or “how” of phenomena (but not the “why”).
Example: A study on the different income levels of people who use nutritional supplements regularly.
- Correlational research design : Correlation reflects the strength and/or direction of the relationship among variables. The direction of a correlation can be positive or negative. Correlational research design helps researchers establish a relationship between two variables without the researcher controlling any of them.
Example : An example of correlational research design could be studying the correlation between time spent watching crime shows and aggressive behavior in teenagers.
- Diagnostic research design : In diagnostic design, the researcher aims to understand the underlying cause of a specific topic or phenomenon (usually an area of improvement) and find the most effective solution. In simpler terms, a researcher seeks an accurate “diagnosis” of a problem and identifies a solution.
Example : A researcher analyzing customer feedback and reviews to identify areas where an app can be improved.
- Explanatory research design : In explanatory research design , a researcher uses their ideas and thoughts on a topic to explore their theories in more depth. This design is used to explore a phenomenon when limited information is available. It can help increase current understanding of unexplored aspects of a subject. It is thus a kind of “starting point” for future research.
Example : Formulating hypotheses to guide future studies on delaying school start times for better mental health in teenagers.
- Causal research design : This can be considered a type of explanatory research. Causal research design seeks to define a cause and effect in its data. The researcher does not use a randomly chosen control group but naturally or pre-existing groupings. Importantly, the researcher does not manipulate the independent variable.
Example : Comparing school dropout levels and possible bullying events.
- Experimental research design : This research design is used to study causal relationships . One or more independent variables are manipulated, and their effect on one or more dependent variables is measured.
Example: Determining the efficacy of a new vaccine plan for influenza.
Benefits of research design
T here are numerous benefits of research design . These are as follows:
- Clear direction: Among the benefits of research design , the main one is providing direction to the research and guiding the choice of clear objectives, which help the researcher to focus on the specific research questions or hypotheses they want to investigate.
- Control: Through a proper research design , researchers can control variables, identify potential confounding factors, and use randomization to minimize bias and increase the reliability of their findings.
- Replication: Research designs provide the opportunity for replication. This helps to confirm the findings of a study and ensures that the results are not due to chance or other factors. Thus, a well-chosen research design also eliminates bias and errors.
- Validity: A research design ensures the validity of the research, i.e., whether the results truly reflect the phenomenon being investigated.
- Reliability: Benefits of research design also include reducing inaccuracies and ensuring the reliability of the research (i.e., consistency of the research results over time, across different samples, and under different conditions).
- Efficiency: A strong research design helps increase the efficiency of the research process. Researchers can use a variety of designs to investigate their research questions, choose the most appropriate research design for their study, and use statistical analysis to make the most of their data. By effectively describing the data necessary for an adequate test of the hypotheses and explaining how such data will be obtained, research design saves a researcher’s time.
Overall, an appropriately chosen and executed research design helps researchers to conduct high-quality research, draw meaningful conclusions, and contribute to the advancement of knowledge in their field.
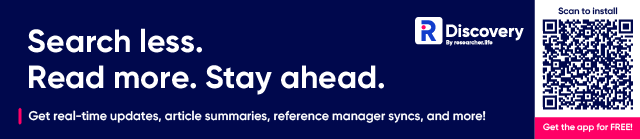
Frequently Asked Questions (FAQ) on Research Design
Q: What are th e main types of research design?
Broadly speaking there are two basic types of research design –
qualitative and quantitative research. Qualitative research is subjective and exploratory; it determines relationships between collected data and observations. It is usually carried out through interviews with open-ended questions, observations that are described in words, etc. Quantitative research , on the other hand, is more objective and employs statistical approaches. It establishes the cause-and-effect relationship among variables using different statistical and computational methods. This type of research design is usually done using surveys and experiments.
Q: How do I choose the appropriate research design for my study?
Choosing the appropriate research design for your study requires careful consideration of various factors. Start by clarifying your research objectives and the type of data you need to collect. Determine whether your study is exploratory, descriptive, or experimental in nature. Consider the availability of resources, time constraints, and the feasibility of implementing the different research designs. Review existing literature to identify similar studies and their research designs, which can serve as a guide. Ultimately, the chosen research design should align with your research questions, provide the necessary data to answer them, and be feasible given your own specific requirements/constraints.
Q: Can research design be modified during the course of a study?
Yes, research design can be modified during the course of a study based on emerging insights, practical constraints, or unforeseen circumstances. Research is an iterative process and, as new data is collected and analyzed, it may become necessary to adjust or refine the research design. However, any modifications should be made judiciously and with careful consideration of their impact on the study’s integrity and validity. It is advisable to document any changes made to the research design, along with a clear rationale for the modifications, in order to maintain transparency and allow for proper interpretation of the results.
Q: How can I ensure the validity and reliability of my research design?
Validity refers to the accuracy and meaningfulness of your study’s findings, while reliability relates to the consistency and stability of the measurements or observations. To enhance validity, carefully define your research variables, use established measurement scales or protocols, and collect data through appropriate methods. Consider conducting a pilot study to identify and address any potential issues before full implementation. To enhance reliability, use standardized procedures, conduct inter-rater or test-retest reliability checks, and employ appropriate statistical techniques for data analysis. It is also essential to document and report your methodology clearly, allowing for replication and scrutiny by other researchers.
Editage All Access is a subscription-based platform that unifies the best AI tools and services designed to speed up, simplify, and streamline every step of a researcher’s journey. The Editage All Access Pack is a one-of-a-kind subscription that unlocks full access to an AI writing assistant, literature recommender, journal finder, scientific illustration tool, and exclusive discounts on professional publication services from Editage.
Based on 22+ years of experience in academia, Editage All Access empowers researchers to put their best research forward and move closer to success. Explore our top AI Tools pack, AI Tools + Publication Services pack, or Build Your Own Plan. Find everything a researcher needs to succeed, all in one place – Get All Access now starting at just $14 a month !
Related Posts

How to Make a Graphical Abstract for Your Research Paper (with Examples)

Leveraging AI in Research: Kick-Start Your Academic Year with Editage All Access
- How it works
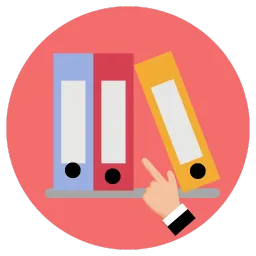
How to Write a Research Design – Guide with Examples
Published by Alaxendra Bets at August 14th, 2021 , Revised On June 24, 2024
A research design is a structure that combines different components of research. It involves the use of different data collection and data analysis techniques logically to answer the research questions .
It would be best to make some decisions about addressing the research questions adequately before starting the research process, which is achieved with the help of the research design.
Below are the key aspects of the decision-making process:
- Data type required for research
- Research resources
- Participants required for research
- Hypothesis based upon research question(s)
- Data analysis methodologies
- Variables (Independent, dependent, and confounding)
- The location and timescale for conducting the data
- The time period required for research
The research design provides the strategy of investigation for your project. Furthermore, it defines the parameters and criteria to compile the data to evaluate results and conclude.
Your project’s validity depends on the data collection and interpretation techniques. A strong research design reflects a strong dissertation , scientific paper, or research proposal .
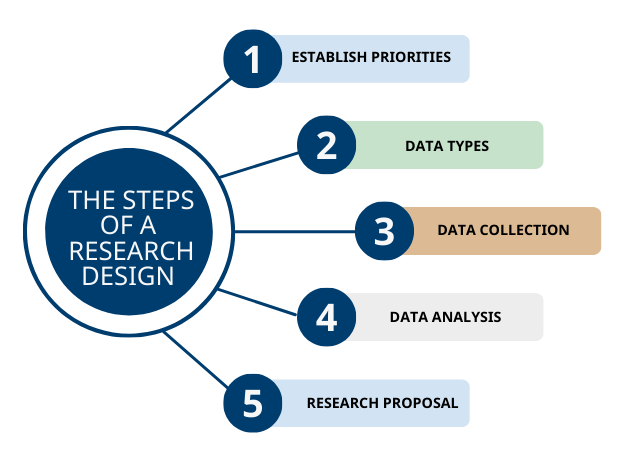
Step 1: Establish Priorities for Research Design
Before conducting any research study, you must address an important question: “how to create a research design.”
The research design depends on the researcher’s priorities and choices because every research has different priorities. For a complex research study involving multiple methods, you may choose to have more than one research design.
Multimethodology or multimethod research includes using more than one data collection method or research in a research study or set of related studies.
If one research design is weak in one area, then another research design can cover that weakness. For instance, a dissertation analyzing different situations or cases will have more than one research design.
For example:
- Experimental research involves experimental investigation and laboratory experience, but it does not accurately investigate the real world.
- Quantitative research is good for the statistical part of the project, but it may not provide an in-depth understanding of the topic .
- Also, correlational research will not provide experimental results because it is a technique that assesses the statistical relationship between two variables.
While scientific considerations are a fundamental aspect of the research design, It is equally important that the researcher think practically before deciding on its structure. Here are some questions that you should think of;
- Do you have enough time to gather data and complete the write-up?
- Will you be able to collect the necessary data by interviewing a specific person or visiting a specific location?
- Do you have in-depth knowledge about the different statistical analysis and data collection techniques to address the research questions or test the hypothesis ?
If you think that the chosen research design cannot answer the research questions properly, you can refine your research questions to gain better insight.
Step 2: Data Type you Need for Research
Decide on the type of data you need for your research. The type of data you need to collect depends on your research questions or research hypothesis. Two types of research data can be used to answer the research questions:
Primary Data Vs. Secondary Data
The researcher collects the primary data from first-hand sources with the help of different data collection methods such as interviews, experiments, surveys, etc. Primary research data is considered far more authentic and relevant, but it involves additional cost and time. | |
Research on academic references which themselves incorporate primary data will be regarded as secondary data. There is no need to do a survey or interview with a person directly, and it is time effective. The researcher should focus on the validity and reliability of the source. |
Qualitative Vs. Quantitative Data
This type of data encircles the researcher’s descriptive experience and shows the relationship between the observation and collected data. It involves interpretation and conceptual understanding of the research. There are many theories involved which can approve or disapprove the mathematical and statistical calculation. For instance, you are searching how to write a research design proposal. It means you require qualitative data about the mentioned topic. | |
If your research requires statistical and mathematical approaches for measuring the variable and testing your hypothesis, your objective is to compile quantitative data. Many businesses and researchers use this type of data with pre-determined data collection methods and variables for their research design. |
Also, see; Research methods, design, and analysis .
Need help with a thesis chapter?
- Hire an expert from ResearchProspect today!
- Statistical analysis, research methodology, discussion of the results or conclusion – our experts can help you no matter how complex the requirements are.
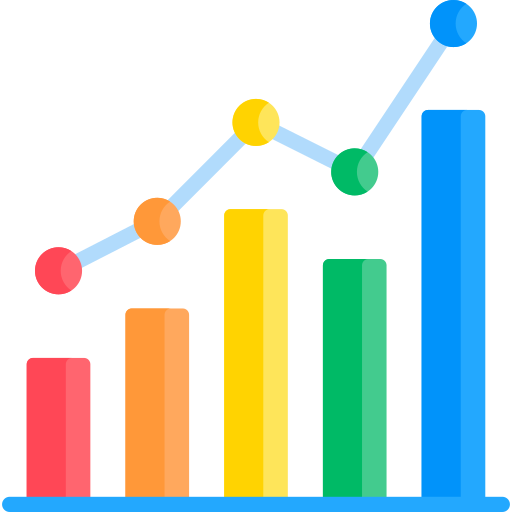
Step 3: Data Collection Techniques
Once you have selected the type of research to answer your research question, you need to decide where and how to collect the data.
It is time to determine your research method to address the research problem . Research methods involve procedures, techniques, materials, and tools used for the study.
For instance, a dissertation research design includes the different resources and data collection techniques and helps establish your dissertation’s structure .
The following table shows the characteristics of the most popularly employed research methods.
Research Methods
Methods | What to consider |
---|---|
Surveys | The survey planning requires; Selection of responses and how many responses are required for the research? Survey distribution techniques (online, by post, in person, etc.) Techniques to design the question |
Interviews | Criteria to select the interviewee. Time and location of the interview. Type of interviews; i.e., structured, semi-structured, or unstructured |
Experiments | Place of the experiment; laboratory or in the field. Measuring of the variables Design of the experiment |
Secondary Data | Criteria to select the references and source for the data. The reliability of the references. The technique used for compiling the data source. |
Step 4: Procedure of Data Analysis
Use of the correct data and statistical analysis technique is necessary for the validity of your research. Therefore, you need to be certain about the data type that would best address the research problem. Choosing an appropriate analysis method is the final step for the research design. It can be split into two main categories;
Quantitative Data Analysis
The quantitative data analysis technique involves analyzing the numerical data with the help of different applications such as; SPSS, STATA, Excel, origin lab, etc.
This data analysis strategy tests different variables such as spectrum, frequencies, averages, and more. The research question and the hypothesis must be established to identify the variables for testing.
Qualitative Data Analysis
Qualitative data analysis of figures, themes, and words allows for flexibility and the researcher’s subjective opinions. This means that the researcher’s primary focus will be interpreting patterns, tendencies, and accounts and understanding the implications and social framework.
You should be clear about your research objectives before starting to analyze the data. For example, you should ask yourself whether you need to explain respondents’ experiences and insights or do you also need to evaluate their responses with reference to a certain social framework.
Step 5: Write your Research Proposal
The research design is an important component of a research proposal because it plans the project’s execution. You can share it with the supervisor, who would evaluate the feasibility and capacity of the results and conclusion .
Read our guidelines to write a research proposal if you have already formulated your research design. The research proposal is written in the future tense because you are writing your proposal before conducting research.
The research methodology or research design, on the other hand, is generally written in the past tense.
How to Write a Research Design – Conclusion
A research design is the plan, structure, strategy of investigation conceived to answer the research question and test the hypothesis. The dissertation research design can be classified based on the type of data and the type of analysis.
Above mentioned five steps are the answer to how to write a research design. So, follow these steps to formulate the perfect research design for your dissertation .
ResearchProspect writers have years of experience creating research designs that align with the dissertation’s aim and objectives. If you are struggling with your dissertation methodology chapter, you might want to look at our dissertation part-writing service.
Our dissertation writers can also help you with the full dissertation paper . No matter how urgent or complex your need may be, ResearchProspect can help. We also offer PhD level research paper writing services.
Frequently Asked Questions
What is research design.
Research design is a systematic plan that guides the research process, outlining the methodology and procedures for collecting and analysing data. It determines the structure of the study, ensuring the research question is answered effectively, reliably, and validly. It serves as the blueprint for the entire research project.
How to write a research design?
To write a research design, define your research question, identify the research method (qualitative, quantitative, or mixed), choose data collection techniques (e.g., surveys, interviews), determine the sample size and sampling method, outline data analysis procedures, and highlight potential limitations and ethical considerations for the study.
How to write the design section of a research paper?
In the design section of a research paper, describe the research methodology chosen and justify its selection. Outline the data collection methods, participants or samples, instruments used, and procedures followed. Detail any experimental controls, if applicable. Ensure clarity and precision to enable replication of the study by other researchers.
How to write a research design in methodology?
To write a research design in methodology, clearly outline the research strategy (e.g., experimental, survey, case study). Describe the sampling technique, participants, and data collection methods. Detail the procedures for data collection and analysis. Justify choices by linking them to research objectives, addressing reliability and validity.
You May Also Like
Repository of ten perfect research question examples will provide you a better perspective about how to create research questions.
To help students organise their dissertation proposal paper correctly, we have put together detailed guidelines on how to structure a dissertation proposal.
Not sure how to approach a company for your primary research study? Don’t worry. Here we have some tips for you to successfully gather primary study.
USEFUL LINKS
LEARNING RESOURCES
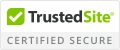
COMPANY DETAILS
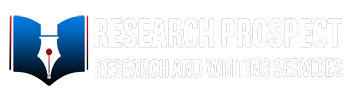
- How It Works

Types of Study Design
- 📖 Geeky Medics OSCE Book
- ⚡ Geeky Medics Bundles
- ✨ 1300+ OSCE Stations
- ✅ OSCE Checklist PDF Booklet
- 🧠 UKMLA AKT Question Bank
- 💊 PSA Question Bank
- 💉 Clinical Skills App
- 🗂️ Flashcard Collections | OSCE , Medicine , Surgery , Anatomy
- 💬 SCA Cases for MRCGP
To be the first to know about our latest videos subscribe to our YouTube channel 🙌
Table of Contents
Suggest an improvement
- Hidden Post Title
- Hidden Post URL
- Hidden Post ID
- Type of issue * N/A Fix spelling/grammar issue Add or fix a link Add or fix an image Add more detail Improve the quality of the writing Fix a factual error
- Please provide as much detail as possible * You don't need to tell us which article this feedback relates to, as we automatically capture that information for you.
- Your Email (optional) This allows us to get in touch for more details if required.
- Which organ is responsible for pumping blood around the body? * Enter a five letter word in lowercase
- Email This field is for validation purposes and should be left unchanged.
Introduction
Study designs are frameworks used in medical research to gather data and explore a specific research question .
Choosing an appropriate study design is one of many essential considerations before conducting research to minimise bias and yield valid results .
This guide provides a summary of study designs commonly used in medical research, their characteristics, advantages and disadvantages.
Case-report and case-series
A case report is a detailed description of a patient’s medical history, diagnosis, treatment, and outcome. A case report typically documents unusual or rare cases or reports new or unexpected clinical findings .
A case series is a similar study that involves a group of patients sharing a similar disease or condition. A case series involves a comprehensive review of medical records for each patient to identify common features or disease patterns. Case series help better understand a disease’s presentation, diagnosis, and treatment.
While a case report focuses on a single patient, a case series involves a group of patients to provide a broader perspective on a specific disease. Both case reports and case series are important tools for understanding rare or unusual diseases .
Advantages of case series and case reports include:
- Able to describe rare or poorly understood conditions or diseases
- Helpful in generating hypotheses and identifying patterns or trends in patient populations
- Can be conducted relatively quickly and at a lower cost compared to other research designs
Disadvantages
Disadvantages of case series and case reports include:
- Prone to selection bias , meaning that the patients included in the series may not be representative of the general population
- Lack a control group, which makes it difficult to conclude the effectiveness of different treatments or interventions
- They are descriptive and cannot establish causality or control for confounding factors
Cross-sectional study
A cross-sectional study aims to measure the prevalence or frequency of a disease in a population at a specific point in time. In other words, it provides a “ snapshot ” of the population at a single moment in time.
Cross-sectional studies are unique from other study designs in that they collect data on the exposure and the outcome of interest from a sample of individuals in the population. This type of data is used to investigate the distribution of health-related conditions and behaviours in different populations, which is especially useful for guiding the development of public health interventions .
Example of a cross-sectional study
A cross-sectional study might investigate the prevalence of hypertension (the outcome) in a sample of adults in a particular region. The researchers would measure blood pressure levels in each participant and gather information on other factors that could influence blood pressure, such as age, sex, weight, and lifestyle habits (exposure).
Advantages of cross-sectional studies include:
- Relatively quick and inexpensive to conduct compared to other study designs, such as cohort or case-control studies
- They can provide a snapshot of the prevalence and distribution of a particular health condition in a population
- They can help to identify patterns and associations between exposure and outcome variables, which can be used to generate hypotheses for further research
Disadvantages of cross-sectional studies include:
- They cannot establish causality , as they do not follow participants over time and cannot determine the temporal sequence between exposure and outcome
- Prone to selection bias , as the sample may not represent the entire population being studied
- They cannot account for confounding variables , which may affect the relationship between the exposure and outcome of interest
Case-control study
A case-control study compares people who have developed a disease of interest ( cases ) with people who have not developed the disease ( controls ) to identify potential risk factors associated with the disease.
Once cases and controls have been identified, researchers then collect information about related risk factors , such as age, sex, lifestyle factors, or environmental exposures, from individuals. By comparing the prevalence of risk factors between the cases and the controls, researchers can determine the association between the risk factors and the disease.
Example of a case-control study
A case-control study design might involve comparing a group of individuals with lung cancer (cases) to a group of individuals without lung cancer (controls) to assess the association between smoking (risk factor) and the development of lung cancer.
Advantages of case-control studies include:
- Useful for studying rare diseases , as they allow researchers to selectively recruit cases with the disease of interest
- Useful for investigating potential risk factors for a disease, as the researchers can collect data on many different factors from both cases and controls
- Can be helpful in situations where it is not ethical or practical to manipulate exposure levels or randomise study participants
Disadvantages of case-control studies include:
- Prone to selection bias , as the controls may not be representative of the general population or may have different underlying risk factors than the cases
- Cannot establish causality , as they can only identify associations between factors and disease
- May be limited by the availability of suitable controls , as finding appropriate controls who have similar characteristics to the cases can be challenging
Cohort study
A cohort study follows a group of individuals (a cohort) over time to investigate the relationship between an exposure or risk factor and a particular outcome or health condition. Cohort studies can be further classified into prospective or retrospective cohort studies.
Prospective cohort study
A prospective cohort study is a study in which the researchers select a group of individuals who do not have a particular disease or outcome of interest at the start of the study.
They then follow this cohort over time to track the number of patients who develop the outcome . Before the start of the study, information on exposure(s) of interest may also be collected.
Example of a prospective cohort study
A prospective cohort study might follow a group of individuals who have never smoked and measure their exposure to tobacco smoke over time to investigate the relationship between smoking and lung cancer .
Retrospective cohort study
In contrast, a retrospective cohort study is a study in which the researchers select a group of individuals who have already been exposed to something (e.g. smoking) and look back in time (for example, through patient charts) to see if they developed the outcome (e.g. lung cancer ).
The key difference in retrospective cohort studies is that data on exposure and outcome are collected after the outcome has occurred.
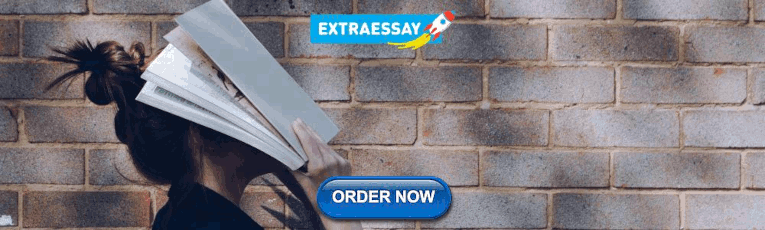
Example of a retrospective cohort study
A retrospective cohort study might look at the medical records of smokers and see if they developed a particular adverse event such as lung cancer.
Advantages of cohort studies include:
- Generally considered to be the most appropriate study design for investigating the temporal relationship between exposure and outcome
- Can provide estimates of incidence and relative risk , which are useful for quantifying the strength of the association between exposure and outcome
- Can be used to investigate multiple outcomes or endpoints associated with a particular exposure, which can help to identify unexpected effects or outcomes
Disadvantages of cohort studies include:
- Can be expensive and time-consuming to conduct, particularly for long-term follow-up
- May suffer from selection bias , as the sample may not be representative of the entire population being studied
- May suffer from attrition bias , as participants may drop out or be lost to follow-up over time
Meta-analysis
A meta-analysis is a type of study that involves extracting outcome data from all relevant studies in the literature and combining the results of multiple studies to produce an overall estimate of the effect size of an intervention or exposure.
Meta-analysis is often conducted alongside a systematic review and can be considered a study of studies . By doing this, researchers provide a more comprehensive and reliable estimate of the overall effect size and their confidence interval (a measure of precision).
Meta-analyses can be conducted for a wide range of research questions , including evaluating the effectiveness of medical interventions, identifying risk factors for disease, or assessing the accuracy of diagnostic tests. They are particularly useful when the results of individual studies are inconsistent or when the sample sizes of individual studies are small, as a meta-analysis can provide a more precise estimate of the true effect size.
When conducting a meta-analysis, researchers must carefully assess the risk of bias in each study to enhance the validity of the meta-analysis. Many aspects of research studies are prone to bias , such as the methodology and the reporting of results. Where studies exhibit a high risk of bias, authors may opt to exclude the study from the analysis or perform a subgroup or sensitivity analysis.
Advantages of a meta-analysis include:
- Combine the results of multiple studies, resulting in a larger sample size and increased statistical power, to provide a more comprehensive and precise estimate of the effect size of an intervention or outcome
- Can help to identify sources of heterogeneity or variability in the results of individual studies by exploring the influence of different study characteristics or subgroups
- Can help to resolve conflicting results or controversies in the literature by providing a more robust estimate of the effect size
Disadvantages of a meta-analysis include:
- Susceptible to publication bias , where studies with statistically significant or positive results are more likely to be published than studies with nonsignificant or negative results. This bias can lead to an overestimation of the treatment effect in a meta-analysis
- May not be appropriate if the studies included are too heterogeneous , as this can make it difficult to draw meaningful conclusions from the pooled results
- Depend on the quality and completeness of the data available from the individual studies and may be limited by the lack of data on certain outcomes or subgroups
Ecological study
An ecological study assesses the relationship between outcome and exposure at a population level or among groups of people rather than studying individuals directly.
The main goal of an ecological study is to observe and analyse patterns or trends at the population level and to identify potential associations or correlations between environmental factors or exposures and health outcomes.
Ecological studies focus on collecting data on population health outcomes , such as disease or mortality rates, and environmental factors or exposures, such as air pollution, temperature, or socioeconomic status.
Example of an ecological study
An ecological study might be used when comparing smoking rates and lung cancer incidence across different countries.
Advantages of an ecological study include:
- Provide insights into how social, economic, and environmental factors may impact health outcomes in real-world settings , which can inform public health policies and interventions
- Cost-effective and efficient, often using existing data or readily available data, such as data from national or regional databases
Disadvantages of an ecological study include:
- Ecological fallacy occurs when conclusions about individual-level associations are drawn from population-level differences
- Ecological studies rely on population-level (i.e. aggregate) rather than individual-level data; they cannot establish causal relationships between exposures and outcomes, as the studies do not account for differences or confounders at the individual level
Randomised controlled trial
A randomised controlled trial (RCT) is an important study design commonly used in medical research to determine the effectiveness of a treatment or intervention . It is considered the gold standard in research design because it allows researchers to draw cause-and-effect conclusions about the effects of an intervention.
In an RCT, participants are randomly assigned to two or more groups. One group receives the intervention being tested, such as a new drug or a specific medical procedure. In contrast, the other group is a control group and receives either no intervention or a placebo .
Randomisation ensures that each participant has an equal chance of being assigned to either group, thereby minimising selection bias . To reduce bias, an RCT often uses a technique called blinding , in which study participants, researchers, or analysts are kept unaware of participant assignment during the study. The participants are then followed over time, and outcome measures are collected and compared to determine if there is any statistical difference between the intervention and control groups.
Example of a randomised controlled trial
An RCT might be employed to evaluate the effectiveness of a new smoking cessation program in helping individuals quit smoking compared to the existing standard of care.
Advantages of an RCT include:
- Considered the most reliable study design for establishing causal relationships between interventions and outcomes and determining the effectiveness of interventions
- Randomisation of participants to intervention and control groups ensures that the groups are similar at the outset, reducing the risk of selection bias and enhancing internal validity
- Using a control group allows researchers to compare with the group that received the intervention while controlling for confounding factors
Disadvantages of an RCT include:
- Can raise ethical concerns ; for example, it may be considered unethical to withhold an intervention from a control group, especially if the intervention is known to be effective
- Can be expensive and time-consuming to conduct, requiring resources for participant recruitment, randomisation, data collection, and analysis
- Often have strict inclusion and exclusion criteria , which may limit the generalisability of the findings to broader populations
- May not always be feasible or practical for certain research questions, especially in rare diseases or when studying long-term outcomes
Dr Chris Jefferies
- Yuliya L, Qazi MA (eds.). Toronto Notes 2022. Toronto: Toronto Notes for Medical Students Inc; 2022.
- Le T, Bhushan V, Qui C, Chalise A, Kaparaliotis P, Coleman C, Kallianos K. First Aid for the USMLE Step 1 2023. New York: McGraw-Hill Education; 2023.
- Rothman KJ, Greenland S, Lash T. Modern Epidemiology. 3 rd ed. Philadelphia: Lippincott Williams & Wilkins; 2008.
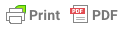
Other pages
- Product Bundles 🎉
- Join the Team 🙌
- Institutional Licence 📚
- OSCE Station Creator Tool 🩺
- Create and Share Flashcards 🗂️
- OSCE Group Chat 💬
- Newsletter 📰
- Advertise With Us
Join the community
Have a language expert improve your writing
Run a free plagiarism check in 10 minutes, generate accurate citations for free.
- Knowledge Base
Methodology
- Guide to Experimental Design | Overview, Steps, & Examples
Guide to Experimental Design | Overview, 5 steps & Examples
Published on December 3, 2019 by Rebecca Bevans . Revised on June 21, 2023.
Experiments are used to study causal relationships . You manipulate one or more independent variables and measure their effect on one or more dependent variables.
Experimental design create a set of procedures to systematically test a hypothesis . A good experimental design requires a strong understanding of the system you are studying.
There are five key steps in designing an experiment:
- Consider your variables and how they are related
- Write a specific, testable hypothesis
- Design experimental treatments to manipulate your independent variable
- Assign subjects to groups, either between-subjects or within-subjects
- Plan how you will measure your dependent variable
For valid conclusions, you also need to select a representative sample and control any extraneous variables that might influence your results. If random assignment of participants to control and treatment groups is impossible, unethical, or highly difficult, consider an observational study instead. This minimizes several types of research bias, particularly sampling bias , survivorship bias , and attrition bias as time passes.
Table of contents
Step 1: define your variables, step 2: write your hypothesis, step 3: design your experimental treatments, step 4: assign your subjects to treatment groups, step 5: measure your dependent variable, other interesting articles, frequently asked questions about experiments.
You should begin with a specific research question . We will work with two research question examples, one from health sciences and one from ecology:
To translate your research question into an experimental hypothesis, you need to define the main variables and make predictions about how they are related.
Start by simply listing the independent and dependent variables .
Research question | Independent variable | Dependent variable |
---|---|---|
Phone use and sleep | Minutes of phone use before sleep | Hours of sleep per night |
Temperature and soil respiration | Air temperature just above the soil surface | CO2 respired from soil |
Then you need to think about possible extraneous and confounding variables and consider how you might control them in your experiment.
Extraneous variable | How to control | |
---|---|---|
Phone use and sleep | in sleep patterns among individuals. | measure the average difference between sleep with phone use and sleep without phone use rather than the average amount of sleep per treatment group. |
Temperature and soil respiration | also affects respiration, and moisture can decrease with increasing temperature. | monitor soil moisture and add water to make sure that soil moisture is consistent across all treatment plots. |
Finally, you can put these variables together into a diagram. Use arrows to show the possible relationships between variables and include signs to show the expected direction of the relationships.
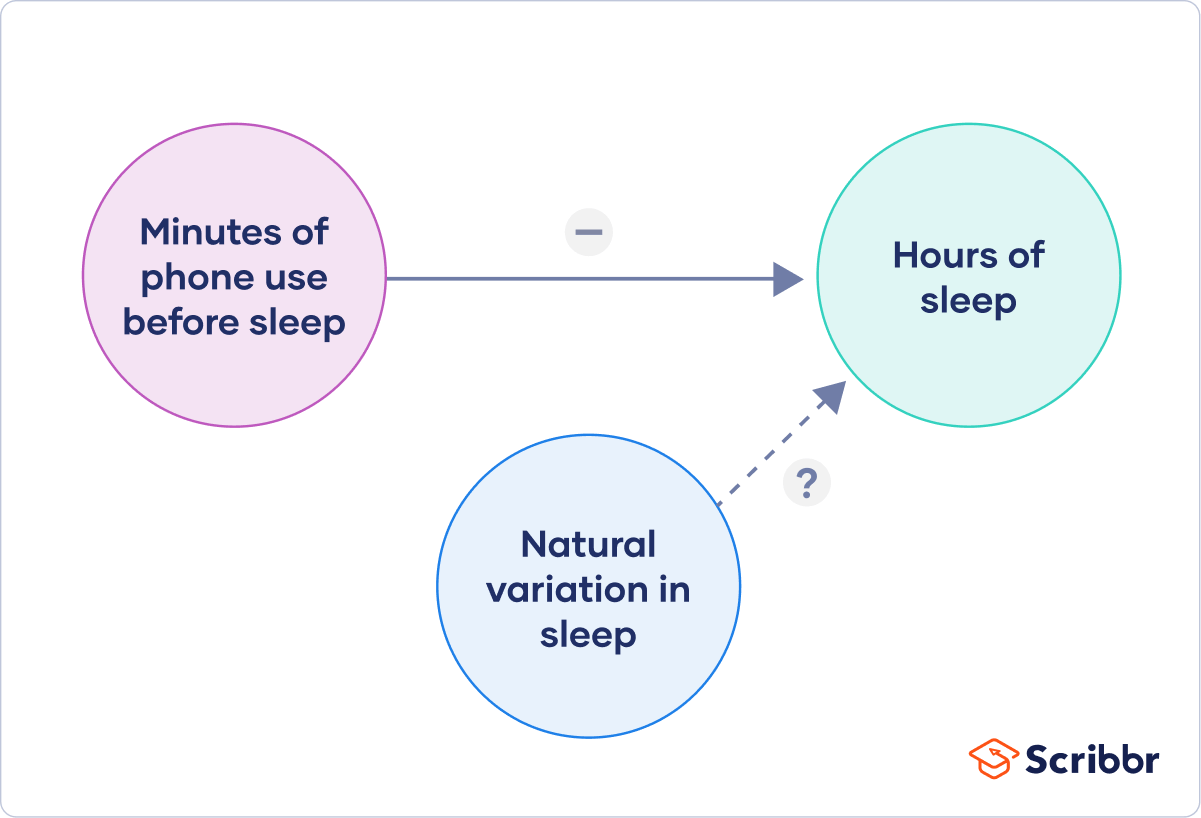
Here we predict that increasing temperature will increase soil respiration and decrease soil moisture, while decreasing soil moisture will lead to decreased soil respiration.
Prevent plagiarism. Run a free check.
Now that you have a strong conceptual understanding of the system you are studying, you should be able to write a specific, testable hypothesis that addresses your research question.
Null hypothesis (H ) | Alternate hypothesis (H ) | |
---|---|---|
Phone use and sleep | Phone use before sleep does not correlate with the amount of sleep a person gets. | Increasing phone use before sleep leads to a decrease in sleep. |
Temperature and soil respiration | Air temperature does not correlate with soil respiration. | Increased air temperature leads to increased soil respiration. |
The next steps will describe how to design a controlled experiment . In a controlled experiment, you must be able to:
- Systematically and precisely manipulate the independent variable(s).
- Precisely measure the dependent variable(s).
- Control any potential confounding variables.
If your study system doesn’t match these criteria, there are other types of research you can use to answer your research question.
How you manipulate the independent variable can affect the experiment’s external validity – that is, the extent to which the results can be generalized and applied to the broader world.
First, you may need to decide how widely to vary your independent variable.
- just slightly above the natural range for your study region.
- over a wider range of temperatures to mimic future warming.
- over an extreme range that is beyond any possible natural variation.
Second, you may need to choose how finely to vary your independent variable. Sometimes this choice is made for you by your experimental system, but often you will need to decide, and this will affect how much you can infer from your results.
- a categorical variable : either as binary (yes/no) or as levels of a factor (no phone use, low phone use, high phone use).
- a continuous variable (minutes of phone use measured every night).
How you apply your experimental treatments to your test subjects is crucial for obtaining valid and reliable results.
First, you need to consider the study size : how many individuals will be included in the experiment? In general, the more subjects you include, the greater your experiment’s statistical power , which determines how much confidence you can have in your results.
Then you need to randomly assign your subjects to treatment groups . Each group receives a different level of the treatment (e.g. no phone use, low phone use, high phone use).
You should also include a control group , which receives no treatment. The control group tells us what would have happened to your test subjects without any experimental intervention.
When assigning your subjects to groups, there are two main choices you need to make:
- A completely randomized design vs a randomized block design .
- A between-subjects design vs a within-subjects design .
Randomization
An experiment can be completely randomized or randomized within blocks (aka strata):
- In a completely randomized design , every subject is assigned to a treatment group at random.
- In a randomized block design (aka stratified random design), subjects are first grouped according to a characteristic they share, and then randomly assigned to treatments within those groups.
Completely randomized design | Randomized block design | |
---|---|---|
Phone use and sleep | Subjects are all randomly assigned a level of phone use using a random number generator. | Subjects are first grouped by age, and then phone use treatments are randomly assigned within these groups. |
Temperature and soil respiration | Warming treatments are assigned to soil plots at random by using a number generator to generate map coordinates within the study area. | Soils are first grouped by average rainfall, and then treatment plots are randomly assigned within these groups. |
Sometimes randomization isn’t practical or ethical , so researchers create partially-random or even non-random designs. An experimental design where treatments aren’t randomly assigned is called a quasi-experimental design .
Between-subjects vs. within-subjects
In a between-subjects design (also known as an independent measures design or classic ANOVA design), individuals receive only one of the possible levels of an experimental treatment.
In medical or social research, you might also use matched pairs within your between-subjects design to make sure that each treatment group contains the same variety of test subjects in the same proportions.
In a within-subjects design (also known as a repeated measures design), every individual receives each of the experimental treatments consecutively, and their responses to each treatment are measured.
Within-subjects or repeated measures can also refer to an experimental design where an effect emerges over time, and individual responses are measured over time in order to measure this effect as it emerges.
Counterbalancing (randomizing or reversing the order of treatments among subjects) is often used in within-subjects designs to ensure that the order of treatment application doesn’t influence the results of the experiment.
Between-subjects (independent measures) design | Within-subjects (repeated measures) design | |
---|---|---|
Phone use and sleep | Subjects are randomly assigned a level of phone use (none, low, or high) and follow that level of phone use throughout the experiment. | Subjects are assigned consecutively to zero, low, and high levels of phone use throughout the experiment, and the order in which they follow these treatments is randomized. |
Temperature and soil respiration | Warming treatments are assigned to soil plots at random and the soils are kept at this temperature throughout the experiment. | Every plot receives each warming treatment (1, 3, 5, 8, and 10C above ambient temperatures) consecutively over the course of the experiment, and the order in which they receive these treatments is randomized. |
Receive feedback on language, structure, and formatting
Professional editors proofread and edit your paper by focusing on:
- Academic style
- Vague sentences
- Style consistency
See an example
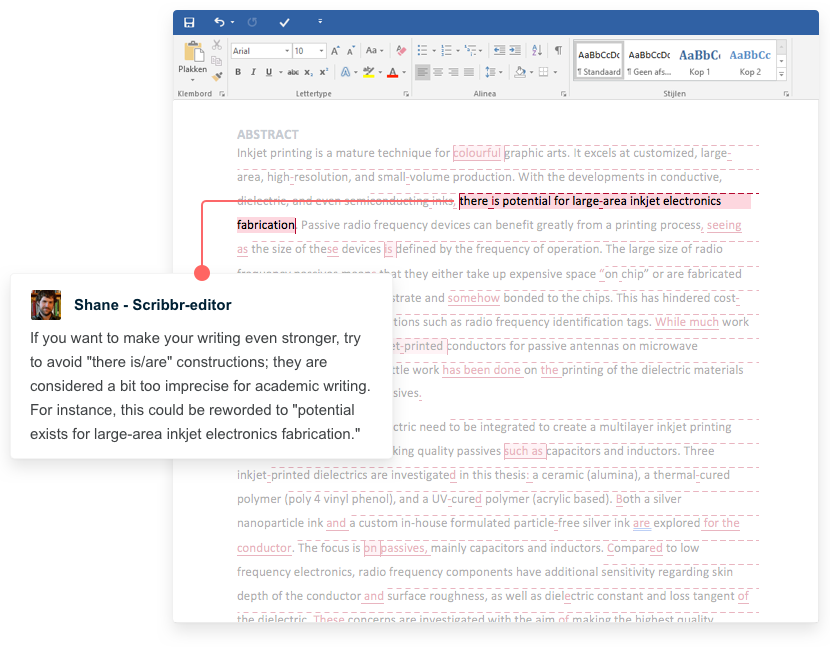
Finally, you need to decide how you’ll collect data on your dependent variable outcomes. You should aim for reliable and valid measurements that minimize research bias or error.
Some variables, like temperature, can be objectively measured with scientific instruments. Others may need to be operationalized to turn them into measurable observations.
- Ask participants to record what time they go to sleep and get up each day.
- Ask participants to wear a sleep tracker.
How precisely you measure your dependent variable also affects the kinds of statistical analysis you can use on your data.
Experiments are always context-dependent, and a good experimental design will take into account all of the unique considerations of your study system to produce information that is both valid and relevant to your research question.
If you want to know more about statistics , methodology , or research bias , make sure to check out some of our other articles with explanations and examples.
- Student’s t -distribution
- Normal distribution
- Null and Alternative Hypotheses
- Chi square tests
- Confidence interval
- Cluster sampling
- Stratified sampling
- Data cleansing
- Reproducibility vs Replicability
- Peer review
- Likert scale
Research bias
- Implicit bias
- Framing effect
- Cognitive bias
- Placebo effect
- Hawthorne effect
- Hindsight bias
- Affect heuristic
Experimental design means planning a set of procedures to investigate a relationship between variables . To design a controlled experiment, you need:
- A testable hypothesis
- At least one independent variable that can be precisely manipulated
- At least one dependent variable that can be precisely measured
When designing the experiment, you decide:
- How you will manipulate the variable(s)
- How you will control for any potential confounding variables
- How many subjects or samples will be included in the study
- How subjects will be assigned to treatment levels
Experimental design is essential to the internal and external validity of your experiment.
The key difference between observational studies and experimental designs is that a well-done observational study does not influence the responses of participants, while experiments do have some sort of treatment condition applied to at least some participants by random assignment .
A confounding variable , also called a confounder or confounding factor, is a third variable in a study examining a potential cause-and-effect relationship.
A confounding variable is related to both the supposed cause and the supposed effect of the study. It can be difficult to separate the true effect of the independent variable from the effect of the confounding variable.
In your research design , it’s important to identify potential confounding variables and plan how you will reduce their impact.
In a between-subjects design , every participant experiences only one condition, and researchers assess group differences between participants in various conditions.
In a within-subjects design , each participant experiences all conditions, and researchers test the same participants repeatedly for differences between conditions.
The word “between” means that you’re comparing different conditions between groups, while the word “within” means you’re comparing different conditions within the same group.
An experimental group, also known as a treatment group, receives the treatment whose effect researchers wish to study, whereas a control group does not. They should be identical in all other ways.
Cite this Scribbr article
If you want to cite this source, you can copy and paste the citation or click the “Cite this Scribbr article” button to automatically add the citation to our free Citation Generator.
Bevans, R. (2023, June 21). Guide to Experimental Design | Overview, 5 steps & Examples. Scribbr. Retrieved July 10, 2024, from https://www.scribbr.com/methodology/experimental-design/
Is this article helpful?
Rebecca Bevans
Other students also liked, random assignment in experiments | introduction & examples, quasi-experimental design | definition, types & examples, how to write a lab report, "i thought ai proofreading was useless but..".
I've been using Scribbr for years now and I know it's a service that won't disappoint. It does a good job spotting mistakes”
- Skip to main content
- Skip to primary sidebar
- Skip to footer
- QuestionPro

- Solutions Industries Gaming Automotive Sports and events Education Government Travel & Hospitality Financial Services Healthcare Cannabis Technology Use Case NPS+ Communities Audience Contactless surveys Mobile LivePolls Member Experience GDPR Positive People Science 360 Feedback Surveys
- Resources Blog eBooks Survey Templates Case Studies Training Help center

Home Market Research Research Tools and Apps
Research Design: What it is, Elements & Types
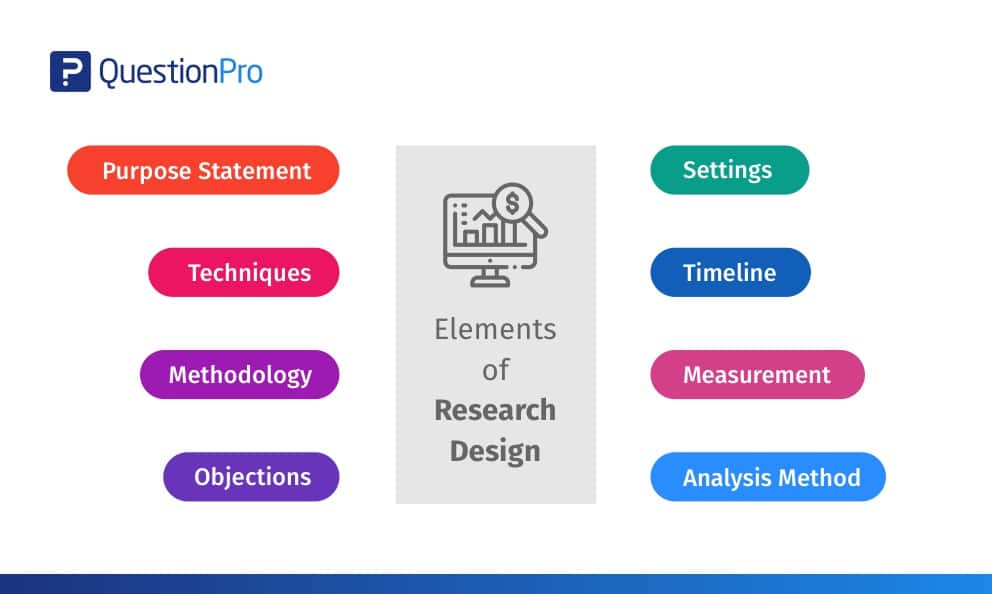
Can you imagine doing research without a plan? Probably not. When we discuss a strategy to collect, study, and evaluate data, we talk about research design. This design addresses problems and creates a consistent and logical model for data analysis. Let’s learn more about it.
What is Research Design?
Research design is the framework of research methods and techniques chosen by a researcher to conduct a study. The design allows researchers to sharpen the research methods suitable for the subject matter and set up their studies for success.
Creating a research topic explains the type of research (experimental, survey research , correlational , semi-experimental, review) and its sub-type (experimental design, research problem , descriptive case-study).
There are three main types of designs for research:
- Data collection
- Measurement
- Data Analysis
The research problem an organization faces will determine the design, not vice-versa. The design phase of a study determines which tools to use and how they are used.
The Process of Research Design
The research design process is a systematic and structured approach to conducting research. The process is essential to ensure that the study is valid, reliable, and produces meaningful results.
- Consider your aims and approaches: Determine the research questions and objectives, and identify the theoretical framework and methodology for the study.
- Choose a type of Research Design: Select the appropriate research design, such as experimental, correlational, survey, case study, or ethnographic, based on the research questions and objectives.
- Identify your population and sampling method: Determine the target population and sample size, and choose the sampling method, such as random , stratified random sampling , or convenience sampling.
- Choose your data collection methods: Decide on the data collection methods , such as surveys, interviews, observations, or experiments, and select the appropriate instruments or tools for collecting data.
- Plan your data collection procedures: Develop a plan for data collection, including the timeframe, location, and personnel involved, and ensure ethical considerations.
- Decide on your data analysis strategies: Select the appropriate data analysis techniques, such as statistical analysis , content analysis, or discourse analysis, and plan how to interpret the results.
The process of research design is a critical step in conducting research. By following the steps of research design, researchers can ensure that their study is well-planned, ethical, and rigorous.
Research Design Elements
Impactful research usually creates a minimum bias in data and increases trust in the accuracy of collected data. A design that produces the slightest margin of error in experimental research is generally considered the desired outcome. The essential elements are:
- Accurate purpose statement
- Techniques to be implemented for collecting and analyzing research
- The method applied for analyzing collected details
- Type of research methodology
- Probable objections to research
- Settings for the research study
- Measurement of analysis
Characteristics of Research Design
A proper design sets your study up for success. Successful research studies provide insights that are accurate and unbiased. You’ll need to create a survey that meets all of the main characteristics of a design. There are four key characteristics:
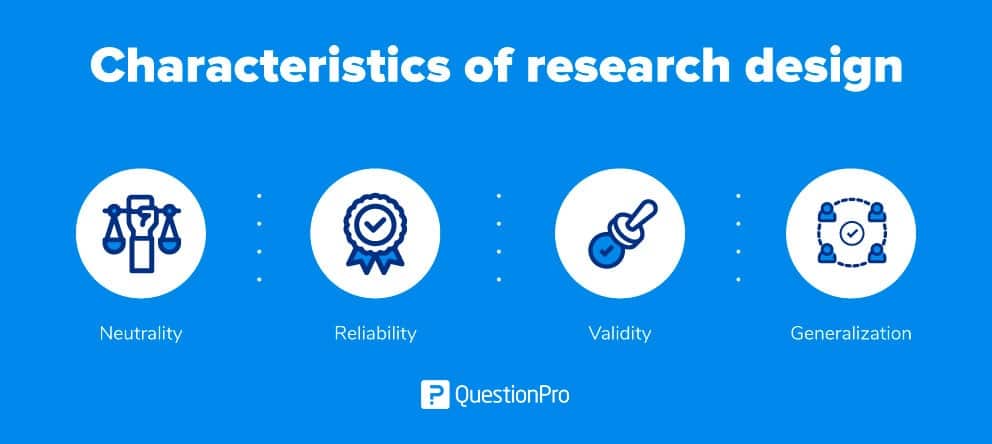
- Neutrality: When you set up your study, you may have to make assumptions about the data you expect to collect. The results projected in the research should be free from research bias and neutral. Understand opinions about the final evaluated scores and conclusions from multiple individuals and consider those who agree with the results.
- Reliability: With regularly conducted research, the researcher expects similar results every time. You’ll only be able to reach the desired results if your design is reliable. Your plan should indicate how to form research questions to ensure the standard of results.
- Validity: There are multiple measuring tools available. However, the only correct measuring tools are those which help a researcher in gauging results according to the objective of the research. The questionnaire developed from this design will then be valid.
- Generalization: The outcome of your design should apply to a population and not just a restricted sample . A generalized method implies that your survey can be conducted on any part of a population with similar accuracy.
The above factors affect how respondents answer the research questions, so they should balance all the above characteristics in a good design. If you want, you can also learn about Selection Bias through our blog.
Research Design Types
A researcher must clearly understand the various types to select which model to implement for a study. Like the research itself, the design of your analysis can be broadly classified into quantitative and qualitative.
Qualitative research
Qualitative research determines relationships between collected data and observations based on mathematical calculations. Statistical methods can prove or disprove theories related to a naturally existing phenomenon. Researchers rely on qualitative observation research methods that conclude “why” a particular theory exists and “what” respondents have to say about it.
Quantitative research
Quantitative research is for cases where statistical conclusions to collect actionable insights are essential. Numbers provide a better perspective for making critical business decisions. Quantitative research methods are necessary for the growth of any organization. Insights drawn from complex numerical data and analysis prove to be highly effective when making decisions about the business’s future.
Qualitative Research vs Quantitative Research
Here is a chart that highlights the major differences between qualitative and quantitative research:
Qualitative Research | Quantitative Research |
---|---|
Focus on explaining and understanding experiences and perspectives. | Focus on quantifying and measuring phenomena. |
Use of non-numerical data, such as words, images, and observations. | Use of numerical data, such as statistics and surveys. |
Usually uses small sample sizes. | Usually uses larger sample sizes. |
Typically emphasizes in-depth exploration and interpretation. | Typically emphasizes precision and objectivity. |
Data analysis involves interpretation and narrative analysis. | Data analysis involves statistical analysis and hypothesis testing. |
Results are presented descriptively. | Results are presented numerically and statistically. |
In summary or analysis , the step of qualitative research is more exploratory and focuses on understanding the subjective experiences of individuals, while quantitative research is more focused on objective data and statistical analysis.
You can further break down the types of research design into five categories:
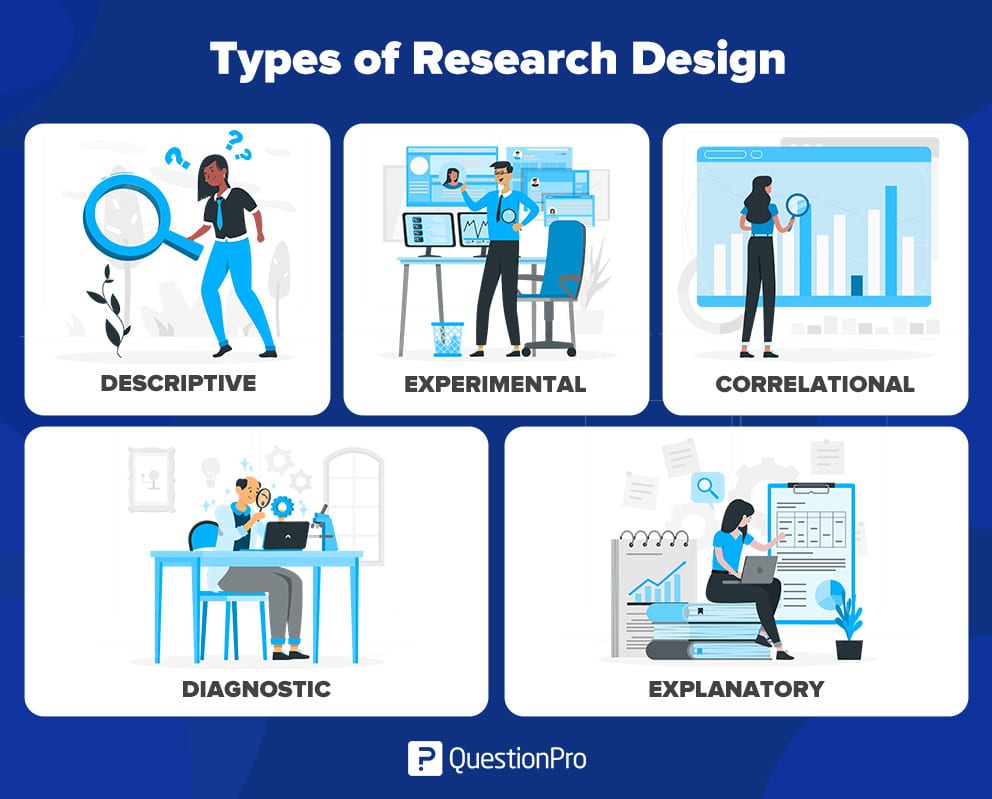
1. Descriptive: In a descriptive composition, a researcher is solely interested in describing the situation or case under their research study. It is a theory-based design method created by gathering, analyzing, and presenting collected data. This allows a researcher to provide insights into the why and how of research. Descriptive design helps others better understand the need for the research. If the problem statement is not clear, you can conduct exploratory research.
2. Experimental: Experimental research establishes a relationship between the cause and effect of a situation. It is a causal research design where one observes the impact caused by the independent variable on the dependent variable. For example, one monitors the influence of an independent variable such as a price on a dependent variable such as customer satisfaction or brand loyalty. It is an efficient research method as it contributes to solving a problem.
The independent variables are manipulated to monitor the change it has on the dependent variable. Social sciences often use it to observe human behavior by analyzing two groups. Researchers can have participants change their actions and study how the people around them react to understand social psychology better.
3. Correlational research: Correlational research is a non-experimental research technique. It helps researchers establish a relationship between two closely connected variables. There is no assumption while evaluating a relationship between two other variables, and statistical analysis techniques calculate the relationship between them. This type of research requires two different groups.
A correlation coefficient determines the correlation between two variables whose values range between -1 and +1. If the correlation coefficient is towards +1, it indicates a positive relationship between the variables, and -1 means a negative relationship between the two variables.
4. Diagnostic research: In diagnostic design, the researcher is looking to evaluate the underlying cause of a specific topic or phenomenon. This method helps one learn more about the factors that create troublesome situations.
This design has three parts of the research:
- Inception of the issue
- Diagnosis of the issue
- Solution for the issue
5. Explanatory research : Explanatory design uses a researcher’s ideas and thoughts on a subject to further explore their theories. The study explains unexplored aspects of a subject and details the research questions’ what, how, and why.
Benefits of Research Design
There are several benefits of having a well-designed research plan. Including:
- Clarity of research objectives: Research design provides a clear understanding of the research objectives and the desired outcomes.
- Increased validity and reliability: To ensure the validity and reliability of results, research design help to minimize the risk of bias and helps to control extraneous variables.
- Improved data collection: Research design helps to ensure that the proper data is collected and data is collected systematically and consistently.
- Better data analysis: Research design helps ensure that the collected data can be analyzed effectively, providing meaningful insights and conclusions.
- Improved communication: A well-designed research helps ensure the results are clean and influential within the research team and external stakeholders.
- Efficient use of resources: reducing the risk of waste and maximizing the impact of the research, research design helps to ensure that resources are used efficiently.
A well-designed research plan is essential for successful research, providing clear and meaningful insights and ensuring that resources are practical.
QuestionPro offers a comprehensive solution for researchers looking to conduct research. With its user-friendly interface, robust data collection and analysis tools, and the ability to integrate results from multiple sources, QuestionPro provides a versatile platform for designing and executing research projects.
Our robust suite of research tools provides you with all you need to derive research results. Our online survey platform includes custom point-and-click logic and advanced question types. Uncover the insights that matter the most.
FREE TRIAL RICHIEDI DEMO
MORE LIKE THIS
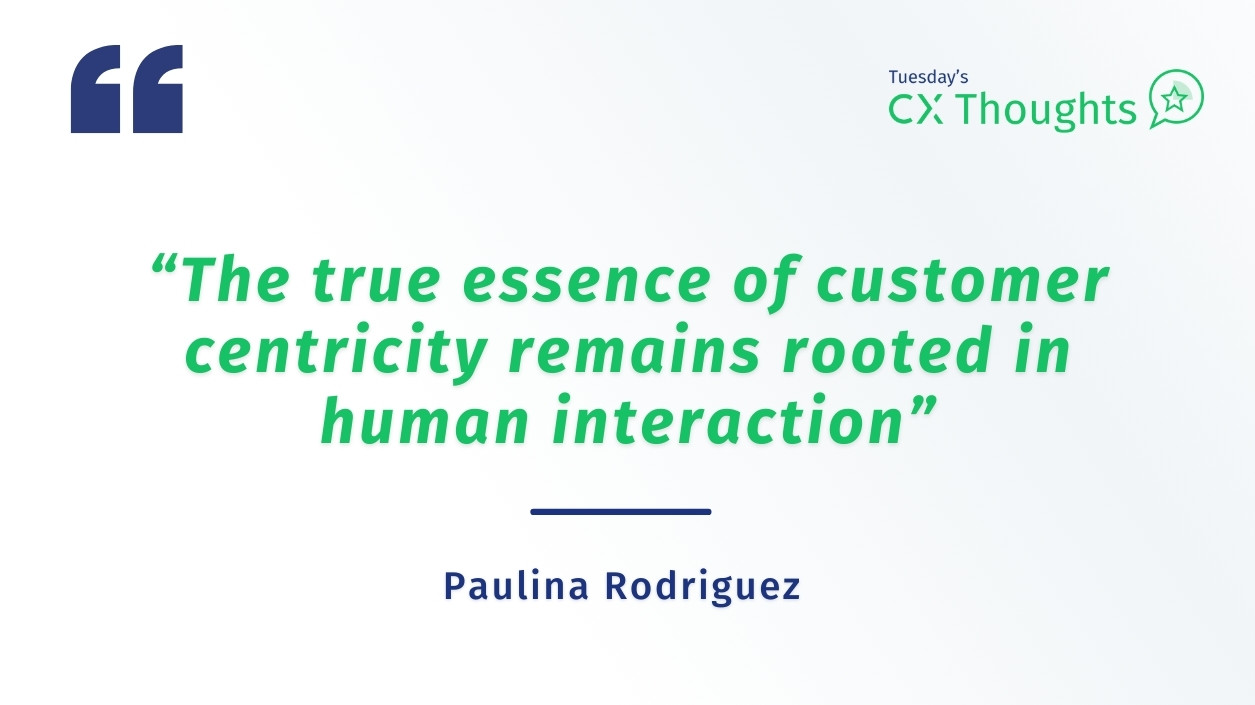
CX Shenanigans: Booth Duty and Beyond — Tuesday CX Thoughts
Jul 9, 2024
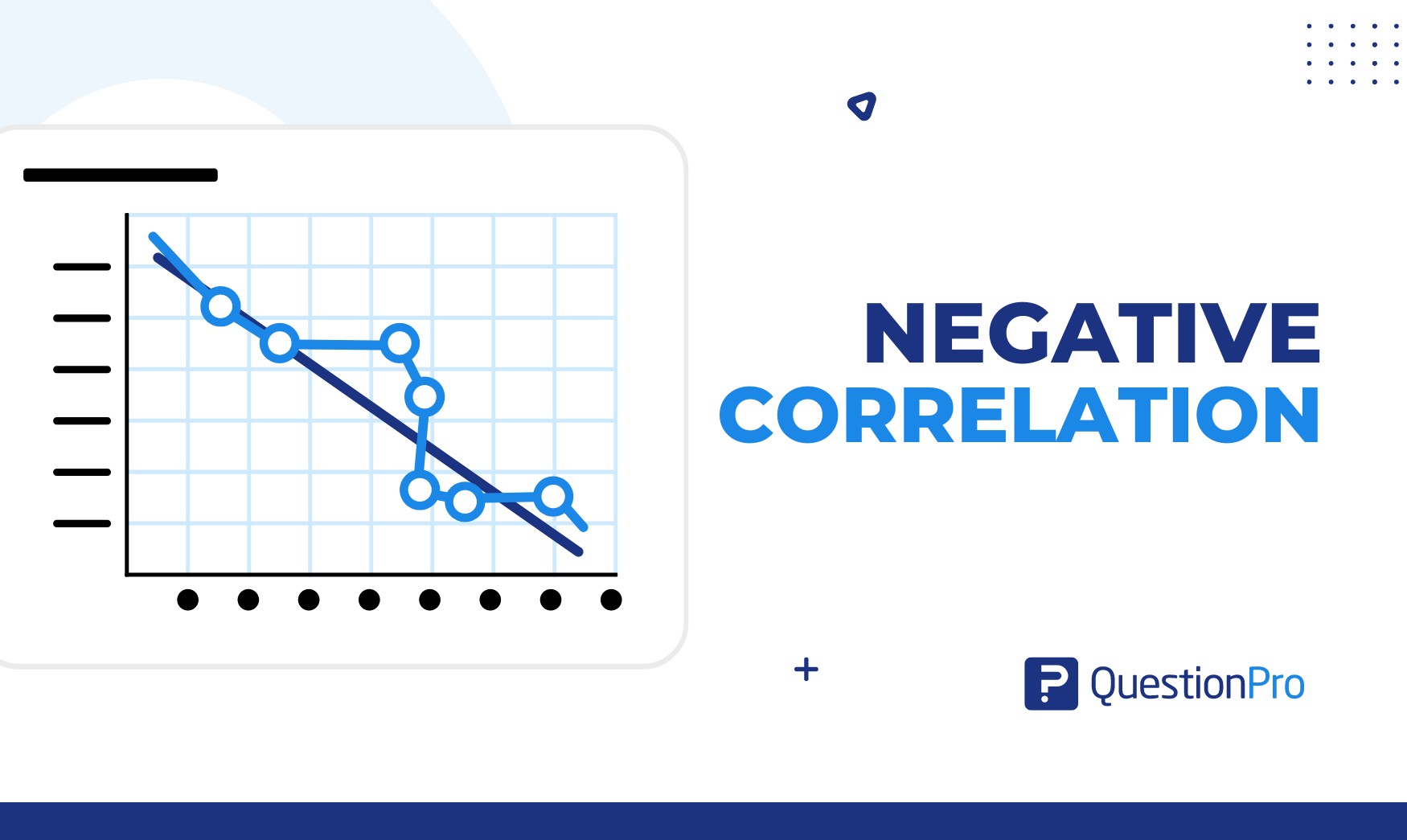
Negative Correlation: Definition, Examples + How to Find It?
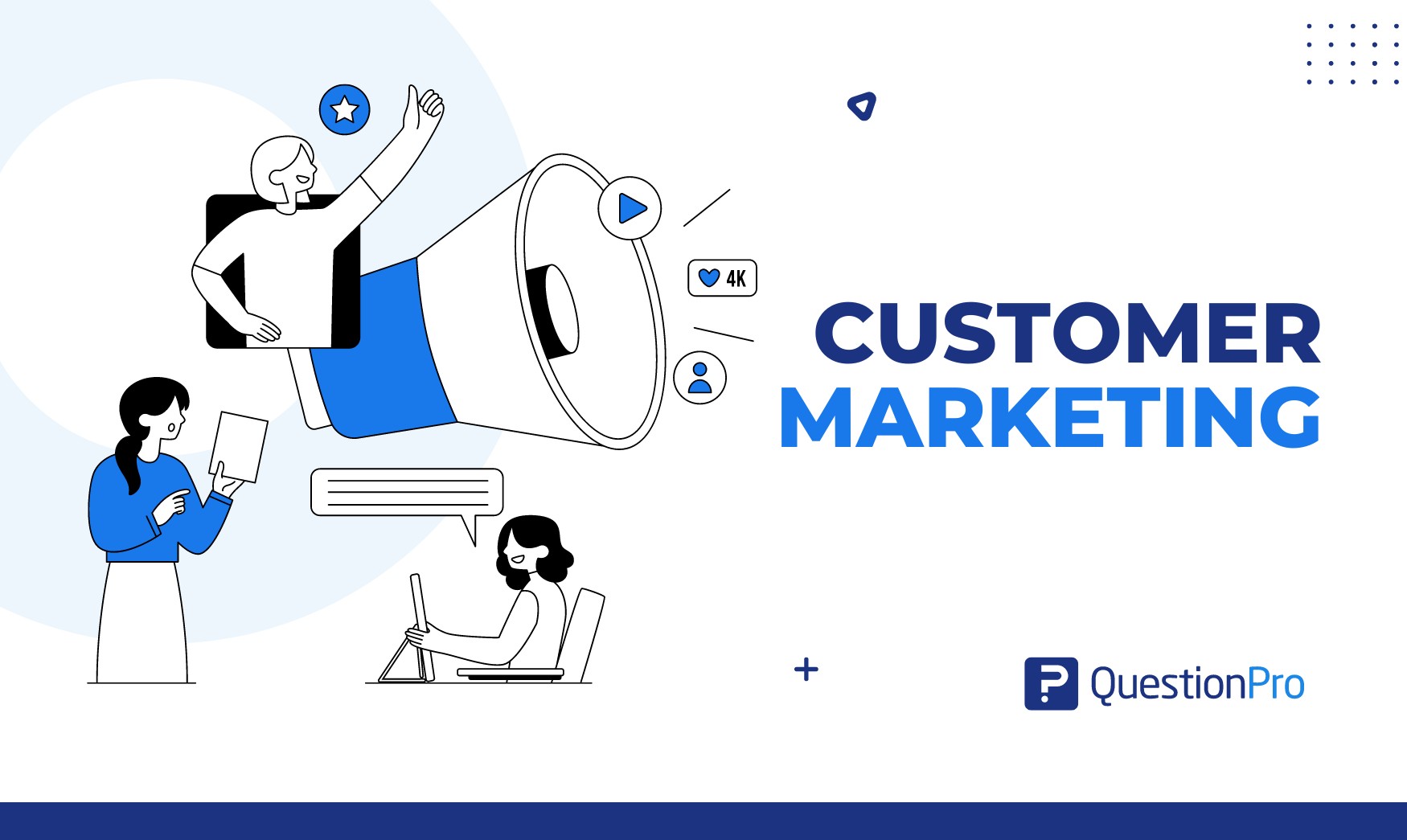
Customer Marketing: The Best Kept Secret of Big Brands
Jul 8, 2024
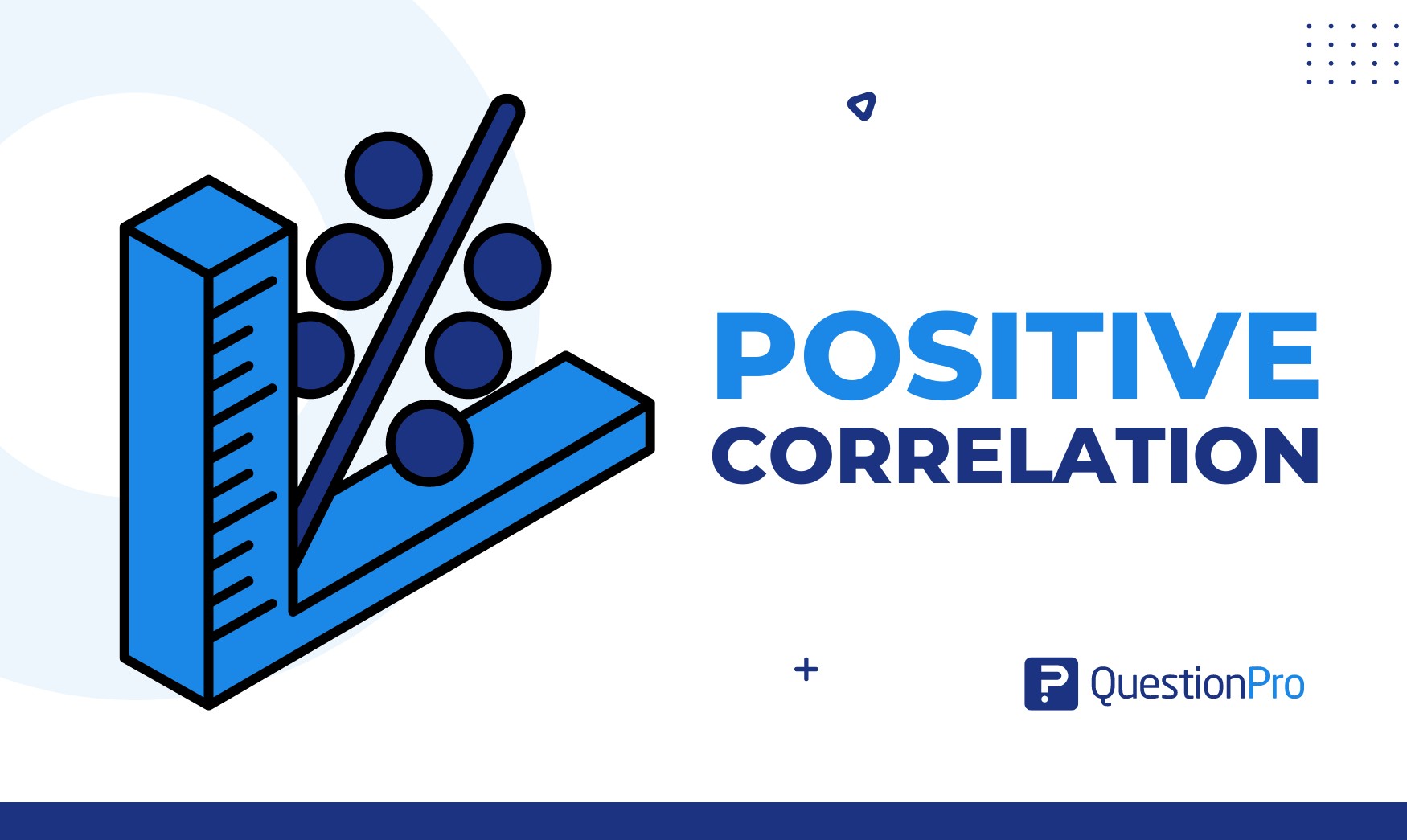
Positive Correlation: What It Is, Importance & How It Works
Jul 5, 2024
Other categories
- Academic Research
- Artificial Intelligence
- Assessments
- Brand Awareness
- Case Studies
- Communities
- Consumer Insights
- Customer effort score
- Customer Engagement
- Customer Experience
- Customer Loyalty
- Customer Research
- Customer Satisfaction
- Employee Benefits
- Employee Engagement
- Employee Retention
- Friday Five
- General Data Protection Regulation
- Insights Hub
- Life@QuestionPro
- Market Research
- Mobile diaries
- Mobile Surveys
- New Features
- Online Communities
- Question Types
- Questionnaire
- QuestionPro Products
- Release Notes
- Research Tools and Apps
- Revenue at Risk
- Survey Templates
- Training Tips
- Tuesday CX Thoughts (TCXT)
- Uncategorized
- What’s Coming Up
- Workforce Intelligence
Understanding Research Study Designs

Table of Contents
In order to find the best possible evidence, it helps to understand the basic designs of research studies. The following basic definitions and examples of clinical research designs follow the “ levels of evidence.”
Case Series and Case Reports
Case control studies, cohort studies, randomized controlled studies, double-blind method, meta-analyses, systematic reviews.
These consist either of collections of reports on the treatment of individual patients with the same condition, or of reports on a single patient.
- Case series/reports are used to illustrate an aspect of a condition, the treatment or the adverse reaction to treatment.
- Example : You have a patient that has a condition that you are unfamiliar with. You would search for case reports that could help you decide on a direction of treatment or to assist on a diagnosis.
- Case series/reports have no control group (one to compare outcomes), so they have no statistical validity.
- The benefits of case series/reports are that they are easy to understand and can be written up in a very short period of time.

Patients who already have a certain condition are compared with people who do not.
- Case control studies are generally designed to estimate the odds (using an odds ratio) of developing the studied condition/disease. They can determine if there is an associational relationship between condition and risk factor
- Example: A study in which colon cancer patients are asked what kinds of food they have eaten in the past and the answers are compared with a selected control group.
- Case control studies are less reliable than either randomized controlled trials or cohort studies.
- A major drawback to case control studies is that one cannot directly obtain absolute risk (i.e. incidence) of a bad outcome.
- The advantages of case control studies are they can be done quickly and are very efficient for conditions/diseases with rare outcomes.

Also called longitudinal studies, involve a case-defined population who presently have a certain exposure and/or receive a particular treatment that are followed over time and compared with another group who are not affected by the exposure under investigation.
- Cohort studies may be either prospective (i.e., exposure factors are identified at the beginning of a study and a defined population is followed into the future), or historical/retrospective (i.e., past medical records for the defined population are used to identify exposure factors).
- Cohort studies are used to establish causation of a disease or to evaluate the outcome/impact of treatment, when randomized controlled clinical trials are not possible.
- Example: One of the more well-know examples of a cohort study is the Framingham Heart Study, which followed generations of residents of Framingham, Massachusetts.
- Cohort studies are not as reliable as randomized controlled studies, since the two groups may differ in ways other than the variable under study.
- Other problems with cohort studies are that they require a large sample size, are inefficient for rare outcomes, and can take long periods of time.

This is a study in which 1) There are two groups, one treatment group and one control group. The treatment group receives the treatment under investigation, and the control group receives either no treatment (placebo) or standard treatment. 2) Patients are randomly assigned to all groups.
- Randomized controlled trials are considered the “gold standard” in medical research. They lend themselves best to answering questions about the effectiveness of different therapies or interventions.
- Randomization helps avoid the bias in choice of patients-to-treatment that a physician might be subject to. It also increases the probability that differences between the groups can be attributed to the treatment(s) under study.
- Having a control group allows for a comparison of treatments – e.g., treatment A produced favorable results 56% of the time versus treatment B in which only 25% of patients had favorable results.
- There are certain types of questions on which randomized controlled studies cannot be done for ethical reasons, for instance, if patients were asked to undertake harmful experiences (like smoking) or denied any treatment beyond a placebo when there are known effective treatments.

A type of randomized controlled clinical trial/study in which neither medical staff/physician nor the patient knows which of several possible treatments/therapies the patient is receiving.
- Example : Studies of treatments that consist essentially of taking pills are very easy to do double blind – the patient takes one of two pills of identical size, shape, and color, and neither the patient nor the physician needs to know which is which.
- A double blind study is the most rigorous clinical research design because, in addition to the randomization of subjects, which reduces the risk of bias, it can eliminate or minimize the placebo effect which is a further challenge to the validity of a study.

Meta-analysis is a systematic, objective way to combine data from many studies, usually from randomized controlled clinical trials, and arrive at a pooled estimate of treatment effectiveness and statistical significance.
- Meta-analysis can also combine data from case/control and cohort studies. The advantage to merging these data is that it increases sample size and allows for analyses that would not otherwise be possible.
- They should not be confused with reviews of the literature or systematic reviews.
- Two problems with meta-analysis are publication bias (studies showing no effect or little effect are often not published and just “filed” away) and the quality of the design of the studies from which data is pulled. This can lead to misleading results when all the data on the subject from “published” literature are summarized.

A systematic review is a comprehensive survey of a topic that takes great care to find all relevant studies of the highest level of evidence, published and unpublished, assess each study, synthesize the findings from individual studies in an unbiased, explicit and reproducible way and present a balanced and impartial summary of the findings with due consideration of any flaws in the evidence. In this way it can be used for the evaluation of either existing or new technologies and practices.
A systematic review is more rigorous than a traditional literature review and attempts to reduce the influence of bias. In order to do this, a systematic review follows a formal process:
- Clearly formulated research question
- Published & unpublished (conferences, company reports, “file drawer reports”, etc.) literature is carefully searched for relevant research
- Identified research is assessed according to an explicit methodology
- Results of the critical assessment of the individual studies are combined
- Final results are placed in context, addressing such issues are quality of the included studies, impact of bias and the applicability of the findings
- The difference between a systematic review and a meta-analysis is that a systematic review looks at the whole picture (qualitative view), while a meta-analysis looks for the specific statistical picture (quantitative view).

R esearch Process in the Health Sciences (35:37 min): Overview of the scientific research process in the health sciences. Follows the seven steps: defining the problem, reviewing the literature, formulating a hypothesis, choosing a research design, collecting data, analyzing the data and interpretation and report writing. Includes a set of additional readings and library resources.
Research Study Designs in the Health Sciences (29:36 min): An overview of research study designs used by health sciences researchers. Covers case reports/case series, case control studies, cohort studies, correlational studies, cross-sectional studies, experimental studies (including randomized control trials), systematic reviews and meta-analysis. Additional readings and library resources are also provided.
- University Libraries
- Research Guides
- Topic Guides
- Research Methods Guide
- Research Design & Method
Research Methods Guide: Research Design & Method
- Introduction
- Survey Research
- Interview Research
- Data Analysis
- Resources & Consultation
Tutorial Videos: Research Design & Method
Research Methods (sociology-focused)
Qualitative vs. Quantitative Methods (intro)
Qualitative vs. Quantitative Methods (advanced)
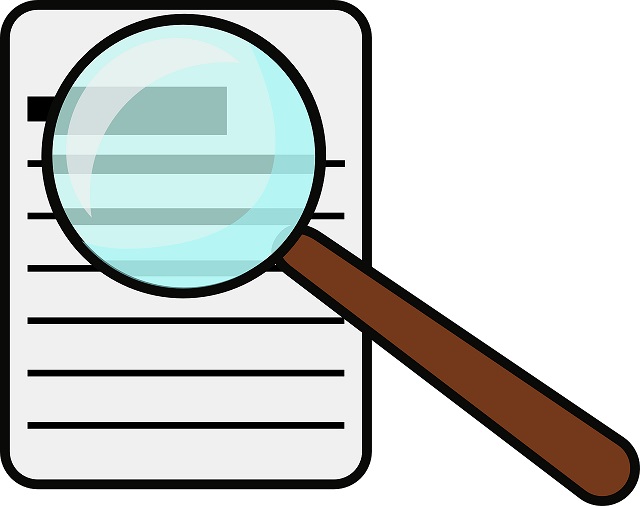
FAQ: Research Design & Method
What is the difference between Research Design and Research Method?
Research design is a plan to answer your research question. A research method is a strategy used to implement that plan. Research design and methods are different but closely related, because good research design ensures that the data you obtain will help you answer your research question more effectively.
Which research method should I choose ?
It depends on your research goal. It depends on what subjects (and who) you want to study. Let's say you are interested in studying what makes people happy, or why some students are more conscious about recycling on campus. To answer these questions, you need to make a decision about how to collect your data. Most frequently used methods include:
- Observation / Participant Observation
- Focus Groups
- Experiments
- Secondary Data Analysis / Archival Study
- Mixed Methods (combination of some of the above)
One particular method could be better suited to your research goal than others, because the data you collect from different methods will be different in quality and quantity. For instance, surveys are usually designed to produce relatively short answers, rather than the extensive responses expected in qualitative interviews.
What other factors should I consider when choosing one method over another?
Time for data collection and analysis is something you want to consider. An observation or interview method, so-called qualitative approach, helps you collect richer information, but it takes time. Using a survey helps you collect more data quickly, yet it may lack details. So, you will need to consider the time you have for research and the balance between strengths and weaknesses associated with each method (e.g., qualitative vs. quantitative).
- << Previous: Introduction
- Next: Survey Research >>
- Last Updated: Aug 21, 2023 10:42 AM
Organizing Your Social Sciences Research Paper: Types of Research Designs
- Purpose of Guide
- Writing a Research Proposal
- Design Flaws to Avoid
- Independent and Dependent Variables
- Narrowing a Topic Idea
- Broadening a Topic Idea
- The Research Problem/Question
- Academic Writing Style
- Choosing a Title
- Making an Outline
- Paragraph Development
- The C.A.R.S. Model
- Background Information
- Theoretical Framework
- Citation Tracking
- Evaluating Sources
- Reading Research Effectively
- Primary Sources
- Secondary Sources
- What Is Scholarly vs. Popular?
- Is it Peer-Reviewed?
- Qualitative Methods
- Quantitative Methods
- Common Grammar Mistakes
- Writing Concisely
- Avoiding Plagiarism [linked guide]
- Annotated Bibliography
- Grading Someone Else's Paper
Introduction
Before beginning your paper, you need to decide how you plan to design the study .
The research design refers to the overall strategy that you choose to integrate the different components of the study in a coherent and logical way, thereby, ensuring you will effectively address the research problem; it constitutes the blueprint for the collection, measurement, and analysis of data. Note that your research problem determines the type of design you should use, not the other way around!
De Vaus, D. A. Research Design in Social Research . London: SAGE, 2001; Trochim, William M.K. Research Methods Knowledge Base . 2006.
General Structure and Writing Style
The function of a research design is to ensure that the evidence obtained enables you to effectively address the research problem logically and as unambiguously as possible . In social sciences research, obtaining information relevant to the research problem generally entails specifying the type of evidence needed to test a theory, to evaluate a program, or to accurately describe and assess meaning related to an observable phenomenon.
With this in mind, a common mistake made by researchers is that they begin their investigations far too early, before they have thought critically about what information is required to address the research problem. Without attending to these design issues beforehand, the overall research problem will not be adequately addressed and any conclusions drawn will run the risk of being weak and unconvincing. As a consequence, the overall validity of the study will be undermined.
The length and complexity of describing research designs in your paper can vary considerably, but any well-developed design will achieve the following :
- Identify the research problem clearly and justify its selection, particularly in relation to any valid alternative designs that could have been used,
- Review and synthesize previously published literature associated with the research problem,
- Clearly and explicitly specify hypotheses [i.e., research questions] central to the problem,
- Effectively describe the data which will be necessary for an adequate testing of the hypotheses and explain how such data will be obtained, and
- Describe the methods of analysis to be applied to the data in determining whether or not the hypotheses are true or false.
The research design is usually incorporated into the introduction and varies in length depending on the type of design you are using. However, you can get a sense of what to do by reviewing the literature of studies that have utilized the same research design. This can provide an outline to follow for your own paper.
NOTE : Use the SAGE Research Methods Online and Cases and the SAGE Research Methods Videos databases to search for scholarly resources on how to apply specific research designs and methods . The Research Methods Online database contains links to more than 175,000 pages of SAGE publisher's book, journal, and reference content on quantitative, qualitative, and mixed research methodologies. Also included is a collection of case studies of social research projects that can be used to help you better understand abstract or complex methodological concepts. The Research Methods Videos database contains hours of tutorials, interviews, video case studies, and mini-documentaries covering the entire research process.
Creswell, John W. and J. David Creswell. Research Design: Qualitative, Quantitative, and Mixed Methods Approaches . 5th edition. Thousand Oaks, CA: Sage, 2018; De Vaus, D. A. Research Design in Social Research . London: SAGE, 2001; Gorard, Stephen. Research Design: Creating Robust Approaches for the Social Sciences . Thousand Oaks, CA: Sage, 2013; Leedy, Paul D. and Jeanne Ellis Ormrod. Practical Research: Planning and Design . Tenth edition. Boston, MA: Pearson, 2013; Vogt, W. Paul, Dianna C. Gardner, and Lynne M. Haeffele. When to Use What Research Design . New York: Guilford, 2012.

Videos in Business and Management , Criminology and Criminal Justice , Education , and Media, Communication and Cultural Studies specifically created for use in higher education.
A literature review tool that highlights the most influential works in Business & Management, Education, Politics & International Relations, Psychology and Sociology. Does not contain full text of the cited works. Dates vary.
Encyclopedias, handbooks, ebooks, and videos published by Sage and CQ Press. 2000 to present
Causal Design
Definition and Purpose
Causality studies may be thought of as understanding a phenomenon in terms of conditional statements in the form, “If X, then Y.” This type of research is used to measure what impact a specific change will have on existing norms and assumptions. Most social scientists seek causal explanations that reflect tests of hypotheses. Causal effect (nomothetic perspective) occurs when variation in one phenomenon, an independent variable, leads to or results, on average, in variation in another phenomenon, the dependent variable.
Conditions necessary for determining causality:
- Empirical association -- a valid conclusion is based on finding an association between the independent variable and the dependent variable.
- Appropriate time order -- to conclude that causation was involved, one must see that cases were exposed to variation in the independent variable before variation in the dependent variable.
- Nonspuriousness -- a relationship between two variables that is not due to variation in a third variable.
What do these studies tell you ?
- Causality research designs assist researchers in understanding why the world works the way it does through the process of proving a causal link between variables and by the process of eliminating other possibilities.
- Replication is possible.
- There is greater confidence the study has internal validity due to the systematic subject selection and equity of groups being compared.
What these studies don't tell you ?
- Not all relationships are casual! The possibility always exists that, by sheer coincidence, two unrelated events appear to be related [e.g., Punxatawney Phil could accurately predict the duration of Winter for five consecutive years but, the fact remains, he's just a big, furry rodent].
- Conclusions about causal relationships are difficult to determine due to a variety of extraneous and confounding variables that exist in a social environment. This means causality can only be inferred, never proven.
- If two variables are correlated, the cause must come before the effect. However, even though two variables might be causally related, it can sometimes be difficult to determine which variable comes first and, therefore, to establish which variable is the actual cause and which is the actual effect.
Beach, Derek and Rasmus Brun Pedersen. Causal Case Study Methods: Foundations and Guidelines for Comparing, Matching, and Tracing . Ann Arbor, MI: University of Michigan Press, 2016; Bachman, Ronet. The Practice of Research in Criminology and Criminal Justice . Chapter 5, Causation and Research Designs. 3rd ed. Thousand Oaks, CA: Pine Forge Press, 2007; Brewer, Ernest W. and Jennifer Kubn. “Causal-Comparative Design.” In Encyclopedia of Research Design . Neil J. Salkind, editor. (Thousand Oaks, CA: Sage, 2010), pp. 125-132; Causal Research Design: Experimentation. Anonymous SlideShare Presentation ; Gall, Meredith. Educational Research: An Introduction . Chapter 11, Nonexperimental Research: Correlational Designs. 8th ed. Boston, MA: Pearson/Allyn and Bacon, 2007; Trochim, William M.K. Research Methods Knowledge Base . 2006.
Cohort Design
Often used in the medical sciences, but also found in the applied social sciences, a cohort study generally refers to a study conducted over a period of time involving members of a population which the subject or representative member comes from, and who are united by some commonality or similarity. Using a quantitative framework, a cohort study makes note of statistical occurrence within a specialized subgroup, united by same or similar characteristics that are relevant to the research problem being investigated, r ather than studying statistical occurrence within the general population. Using a qualitative framework, cohort studies generally gather data using methods of observation. Cohorts can be either "open" or "closed."
- Open Cohort Studies [dynamic populations, such as the population of Los Angeles] involve a population that is defined just by the state of being a part of the study in question (and being monitored for the outcome). Date of entry and exit from the study is individually defined, therefore, the size of the study population is not constant. In open cohort studies, researchers can only calculate rate based data, such as, incidence rates and variants thereof.
- Closed Cohort Studies [static populations, such as patients entered into a clinical trial] involve participants who enter into the study at one defining point in time and where it is presumed that no new participants can enter the cohort. Given this, the number of study participants remains constant (or can only decrease).
- The use of cohorts is often mandatory because a randomized control study may be unethical. For example, you cannot deliberately expose people to asbestos, you can only study its effects on those who have already been exposed. Research that measures risk factors often relies upon cohort designs.
- Because cohort studies measure potential causes before the outcome has occurred, they can demonstrate that these “causes” preceded the outcome, thereby avoiding the debate as to which is the cause and which is the effect.
- Cohort analysis is highly flexible and can provide insight into effects over time and related to a variety of different types of changes [e.g., social, cultural, political, economic, etc.].
- Either original data or secondary data can be used in this design.
- In cases where a comparative analysis of two cohorts is made [e.g., studying the effects of one group exposed to asbestos and one that has not], a researcher cannot control for all other factors that might differ between the two groups. These factors are known as confounding variables.
- Cohort studies can end up taking a long time to complete if the researcher must wait for the conditions of interest to develop within the group. This also increases the chance that key variables change during the course of the study, potentially impacting the validity of the findings.
- Due to the lack of randominization in the cohort design, its external validity is lower than that of study designs where the researcher randomly assigns participants.
Healy P, Devane D. “Methodological Considerations in Cohort Study Designs.” Nurse Researcher 18 (2011): 32-36; Glenn, Norval D, editor. Cohort Analysis . 2nd edition. Thousand Oaks, CA: Sage, 2005; Levin, Kate Ann. Study Design IV: Cohort Studies. Evidence-Based Dentistry 7 (2003): 51–52; Payne, Geoff. “Cohort Study.” In The SAGE Dictionary of Social Research Methods . Victor Jupp, editor. (Thousand Oaks, CA: Sage, 2006), pp. 31-33; Study Design 101 . Himmelfarb Health Sciences Library. George Washington University, November 2011; Cohort Study . Wikipedia.
Cross-Sectional Design
Cross-sectional research designs have three distinctive features: no time dimension; a reliance on existing differences rather than change following intervention; and, groups are selected based on existing differences rather than random allocation. The cross-sectional design can only measure differences between or from among a variety of people, subjects, or phenomena rather than a process of change. As such, researchers using this design can only employ a relatively passive approach to making causal inferences based on findings.
- Cross-sectional studies provide a clear 'snapshot' of the outcome and the characteristics associated with it, at a specific point in time.
- Unlike an experimental design, where there is an active intervention by the researcher to produce and measure change or to create differences, cross-sectional designs focus on studying and drawing inferences from existing differences between people, subjects, or phenomena.
- Entails collecting data at and concerning one point in time. While longitudinal studies involve taking multiple measures over an extended period of time, cross-sectional research is focused on finding relationships between variables at one moment in time.
- Groups identified for study are purposely selected based upon existing differences in the sample rather than seeking random sampling.
- Cross-section studies are capable of using data from a large number of subjects and, unlike observational studies, is not geographically bound.
- Can estimate prevalence of an outcome of interest because the sample is usually taken from the whole population.
- Because cross-sectional designs generally use survey techniques to gather data, they are relatively inexpensive and take up little time to conduct.
- Finding people, subjects, or phenomena to study that are very similar except in one specific variable can be difficult.
- Results are static and time bound and, therefore, give no indication of a sequence of events or reveal historical or temporal contexts.
- Studies cannot be utilized to establish cause and effect relationships.
- This design only provides a snapshot of analysis so there is always the possibility that a study could have differing results if another time-frame had been chosen.
- There is no follow up to the findings.
Bethlehem, Jelke. "7: Cross-sectional Research." In Research Methodology in the Social, Behavioural and Life Sciences . Herman J Adèr and Gideon J Mellenbergh, editors. (London, England: Sage, 1999), pp. 110-43; Bourque, Linda B. “Cross-Sectional Design.” In The SAGE Encyclopedia of Social Science Research Methods . Michael S. Lewis-Beck, Alan Bryman, and Tim Futing Liao. (Thousand Oaks, CA: 2004), pp. 230-231; Hall, John. “Cross-Sectional Survey Design.” In Encyclopedia of Survey Research Methods . Paul J. Lavrakas, ed. (Thousand Oaks, CA: Sage, 2008), pp. 173-174; Helen Barratt, Maria Kirwan. Cross-Sectional Studies: Design, Application, Strengths and Weaknesses of Cross-Sectional Studies . Healthknowledge, 2009. Cross-Sectional Study . Wikipedia.
Descriptive Design
Descriptive research designs help provide answers to the questions of who, what, when, where, and how associated with a particular research problem; a descriptive study cannot conclusively ascertain answers to why. Descriptive research is used to obtain information concerning the current status of the phenomena and to describe "what exists" with respect to variables or conditions in a situation.
- The subject is being observed in a completely natural and unchanged natural environment. True experiments, whilst giving analyzable data, often adversely influence the normal behavior of the subject [a.k.a., the Heisenberg effect whereby measurements of certain systems cannot be made without affecting the systems].
- Descriptive research is often used as a pre-cursor to more quantitative research designs with the general overview giving some valuable pointers as to what variables are worth testing quantitatively.
- If the limitations are understood, they can be a useful tool in developing a more focused study.
- Descriptive studies can yield rich data that lead to important recommendations in practice.
- Appoach collects a large amount of data for detailed analysis.
- The results from a descriptive research cannot be used to discover a definitive answer or to disprove a hypothesis.
- Because descriptive designs often utilize observational methods [as opposed to quantitative methods], the results cannot be replicated.
- The descriptive function of research is heavily dependent on instrumentation for measurement and observation.
Anastas, Jeane W. Research Design for Social Work and the Human Services . Chapter 5, Flexible Methods: Descriptive Research. 2nd ed. New York: Columbia University Press, 1999; Given, Lisa M. "Descriptive Research." In Encyclopedia of Measurement and Statistics . Neil J. Salkind and Kristin Rasmussen, editors. (Thousand Oaks, CA: Sage, 2007), pp. 251-254; McNabb, Connie. Descriptive Research Methodologies . Powerpoint Presentation; Shuttleworth, Martyn. Descriptive Research Design , September 26, 2008. Explorable.com website.
Experimental Design
A blueprint of the procedure that enables the researcher to maintain control over all factors that may affect the result of an experiment. In doing this, the researcher attempts to determine or predict what may occur. Experimental research is often used where there is time priority in a causal relationship (cause precedes effect), there is consistency in a causal relationship (a cause will always lead to the same effect), and the magnitude of the correlation is great. The classic experimental design specifies an experimental group and a control group. The independent variable is administered to the experimental group and not to the control group, and both groups are measured on the same dependent variable. Subsequent experimental designs have used more groups and more measurements over longer periods. True experiments must have control, randomization, and manipulation.
- Experimental research allows the researcher to control the situation. In so doing, it allows researchers to answer the question, “What causes something to occur?”
- Permits the researcher to identify cause and effect relationships between variables and to distinguish placebo effects from treatment effects.
- Experimental research designs support the ability to limit alternative explanations and to infer direct causal relationships in the study.
- Approach provides the highest level of evidence for single studies.
- The design is artificial, and results may not generalize well to the real world.
- The artificial settings of experiments may alter the behaviors or responses of participants.
- Experimental designs can be costly if special equipment or facilities are needed.
- Some research problems cannot be studied using an experiment because of ethical or technical reasons.
- Difficult to apply ethnographic and other qualitative methods to experimentally designed studies.
Anastas, Jeane W. Research Design for Social Work and the Human Services . Chapter 7, Flexible Methods: Experimental Research. 2nd ed. New York: Columbia University Press, 1999; Chapter 2: Research Design, Experimental Designs . School of Psychology, University of New England, 2000; Chow, Siu L. "Experimental Design." In Encyclopedia of Research Design . Neil J. Salkind, editor. (Thousand Oaks, CA: Sage, 2010), pp. 448-453; "Experimental Design." In Social Research Methods . Nicholas Walliman, editor. (London, England: Sage, 2006), pp, 101-110; Experimental Research . Research Methods by Dummies. Department of Psychology. California State University, Fresno, 2006; Kirk, Roger E. Experimental Design: Procedures for the Behavioral Sciences . 4th edition. Thousand Oaks, CA: Sage, 2013; Trochim, William M.K. Experimental Design . Research Methods Knowledge Base. 2006; Rasool, Shafqat. Experimental Research . Slideshare presentation.
Exploratory Design
An exploratory design is conducted about a research problem when there are few or no earlier studies to refer to or rely upon to predict an outcome . The focus is on gaining insights and familiarity for later investigation or undertaken when research problems are in a preliminary stage of investigation. Exploratory designs are often used to establish an understanding of how best to proceed in studying an issue or what methodology would effectively apply to gathering information about the issue.
The goals of exploratory research are intended to produce the following possible insights:
- Familiarity with basic details, settings, and concerns.
- Well grounded picture of the situation being developed.
- Generation of new ideas and assumptions.
- Development of tentative theories or hypotheses.
- Determination about whether a study is feasible in the future.
- Issues get refined for more systematic investigation and formulation of new research questions.
- Direction for future research and techniques get developed.
- Design is a useful approach for gaining background information on a particular topic.
- Exploratory research is flexible and can address research questions of all types (what, why, how).
- Provides an opportunity to define new terms and clarify existing concepts.
- Exploratory research is often used to generate formal hypotheses and develop more precise research problems.
- In the policy arena or applied to practice, exploratory studies help establish research priorities and where resources should be allocated.
- Exploratory research generally utilizes small sample sizes and, thus, findings are typically not generalizable to the population at large.
- The exploratory nature of the research inhibits an ability to make definitive conclusions about the findings. They provide insight but not definitive conclusions.
- The research process underpinning exploratory studies is flexible but often unstructured, leading to only tentative results that have limited value to decision-makers.
- Design lacks rigorous standards applied to methods of data gathering and analysis because one of the areas for exploration could be to determine what method or methodologies could best fit the research problem.
Cuthill, Michael. “Exploratory Research: Citizen Participation, Local Government, and Sustainable Development in Australia.” Sustainable Development 10 (2002): 79-89; Streb, Christoph K. "Exploratory Case Study." In Encyclopedia of Case Study Research . Albert J. Mills, Gabrielle Durepos and Eiden Wiebe, editors. (Thousand Oaks, CA: Sage, 2010), pp. 372-374; Taylor, P. J., G. Catalano, and D.R.F. Walker. “Exploratory Analysis of the World City Network.” Urban Studies 39 (December 2002): 2377-2394; Exploratory Research . Wikipedia.
Historical Design
The purpose of a historical research design is to collect, verify, and synthesize evidence from the past to establish facts that defend or refute a hypothesis. It uses secondary sources and a variety of primary documentary evidence, such as, diaries, official records, reports, archives, and non-textual information [maps, pictures, audio and visual recordings]. The limitation is that the sources must be both authentic and valid.
- The historical research design is unobtrusive; the act of research does not affect the results of the study.
- The historical approach is well suited for trend analysis.
- Historical records can add important contextual background required to more fully understand and interpret a research problem.
- There is often no possibility of researcher-subject interaction that could affect the findings.
- Historical sources can be used over and over to study different research problems or to replicate a previous study.
- The ability to fulfill the aims of your research are directly related to the amount and quality of documentation available to understand the research problem.
- Since historical research relies on data from the past, there is no way to manipulate it to control for contemporary contexts.
- Interpreting historical sources can be very time consuming.
- The sources of historical materials must be archived consistently to ensure access. This may especially challenging for digital or online-only sources.
- Original authors bring their own perspectives and biases to the interpretation of past events and these biases are more difficult to ascertain in historical resources.
- Due to the lack of control over external variables, historical research is very weak with regard to the demands of internal validity.
- It is rare that the entirety of historical documentation needed to fully address a research problem is available for interpretation, therefore, gaps need to be acknowledged.
Howell, Martha C. and Walter Prevenier. From Reliable Sources: An Introduction to Historical Methods . Ithaca, NY: Cornell University Press, 2001; Lundy, Karen Saucier. "Historical Research." In The Sage Encyclopedia of Qualitative Research Methods . Lisa M. Given, editor. (Thousand Oaks, CA: Sage, 2008), pp. 396-400; Marius, Richard. and Melvin E. Page. A Short Guide to Writing about History . 9th edition. Boston, MA: Pearson, 2015; Savitt, Ronald. “Historical Research in Marketing.” Journal of Marketing 44 (Autumn, 1980): 52-58; Gall, Meredith. Educational Research: An Introduction . Chapter 16, Historical Research. 8th ed. Boston, MA: Pearson/Allyn and Bacon, 2007.
Longitudinal Design
A longitudinal study follows the same sample over time and makes repeated observations. For example, with longitudinal surveys, the same group of people is interviewed at regular intervals, enabling researchers to track changes over time and to relate them to variables that might explain why the changes occur. Longitudinal research designs describe patterns of change and help establish the direction and magnitude of causal relationships. Measurements are taken on each variable over two or more distinct time periods. This allows the researcher to measure change in variables over time. It is a type of observational study sometimes referred to as a panel study.
- Longitudinal data facilitate the analysis of the duration of a particular phenomenon.
- Enables survey researchers to get close to the kinds of causal explanations usually attainable only with experiments.
- The design permits the measurement of differences or change in a variable from one period to another [i.e., the description of patterns of change over time].
- Longitudinal studies facilitate the prediction of future outcomes based upon earlier factors.
- The data collection method may change over time.
- Maintaining the integrity of the original sample can be difficult over an extended period of time.
- It can be difficult to show more than one variable at a time.
- This design often needs qualitative research data to explain fluctuations in the results.
- A longitudinal research design assumes present trends will continue unchanged.
- It can take a long period of time to gather results.
- There is a need to have a large sample size and accurate sampling to reach representativness.
Anastas, Jeane W. Research Design for Social Work and the Human Services . Chapter 6, Flexible Methods: Relational and Longitudinal Research. 2nd ed. New York: Columbia University Press, 1999; Forgues, Bernard, and Isabelle Vandangeon-Derumez. "Longitudinal Analyses." In Doing Management Research . Raymond-Alain Thiétart and Samantha Wauchope, editors. (London, England: Sage, 2001), pp. 332-351; Kalaian, Sema A. and Rafa M. Kasim. "Longitudinal Studies." In Encyclopedia of Survey Research Methods . Paul J. Lavrakas, ed. (Thousand Oaks, CA: Sage, 2008), pp. 440-441; Menard, Scott, editor. Longitudinal Research . Thousand Oaks, CA: Sage, 2002; Ployhart, Robert E. and Robert J. Vandenberg. "Longitudinal Research: The Theory, Design, and Analysis of Change.” Journal of Management 36 (January 2010): 94-120; Longitudinal Study . Wikipedia.
Mixed-Method Design
- Narrative and non-textual information can add meaning to numeric data, while numeric data can add precision to narrative and non-textual information.
- Can utilize existing data while at the same time generating and testing a grounded theory approach to describe and explain the phenomenon under study.
- A broader, more complex research problem can be investigated because the researcher is not constrained by using only one method.
- The strengths of one method can be used to overcome the inherent weaknesses of another method.
- Can provide stronger, more robust evidence to support a conclusion or set of recommendations.
- May generate new knowledge new insights or uncover hidden insights, patterns, or relationships that a single methodological approach might not reveal.
- Produces more complete knowledge and understanding of the research problem that can be used to increase the generalizability of findings applied to theory or practice.
- A researcher must be proficient in understanding how to apply multiple methods to investigating a research problem as well as be proficient in optimizing how to design a study that coherently melds them together.
- Can increase the likelihood of conflicting results or ambiguous findings that inhibit drawing a valid conclusion or setting forth a recommended course of action [e.g., sample interview responses do not support existing statistical data].
- Because the research design can be very complex, reporting the findings requires a well-organized narrative, clear writing style, and precise word choice.
- Design invites collaboration among experts. However, merging different investigative approaches and writing styles requires more attention to the overall research process than studies conducted using only one methodological paradigm.
- Concurrent merging of quantitative and qualitative research requires greater attention to having adequate sample sizes, using comparable samples, and applying a consistent unit of analysis. For sequential designs where one phase of qualitative research builds on the quantitative phase or vice versa, decisions about what results from the first phase to use in the next phase, the choice of samples and estimating reasonable sample sizes for both phases, and the interpretation of results from both phases can be difficult.
- Due to multiple forms of data being collected and analyzed, this design requires extensive time and resources to carry out the multiple steps involved in data gathering and interpretation.
Burch, Patricia and Carolyn J. Heinrich. Mixed Methods for Policy Research and Program Evaluation . Thousand Oaks, CA: Sage, 2016; Creswell, John w. et al. Best Practices for Mixed Methods Research in the Health Sciences . Bethesda, MD: Office of Behavioral and Social Sciences Research, National Institutes of Health, 2010Creswell, John W. Research Design: Qualitative, Quantitative, and Mixed Methods Approaches . 4th edition. Thousand Oaks, CA: Sage Publications, 2014; Domínguez, Silvia, editor. Mixed Methods Social Networks Research . Cambridge, UK: Cambridge University Press, 2014; Hesse-Biber, Sharlene Nagy. Mixed Methods Research: Merging Theory with Practice . New York: Guilford Press, 2010; Niglas, Katrin. “How the Novice Researcher Can Make Sense of Mixed Methods Designs.” International Journal of Multiple Research Approaches 3 (2009): 34-46; Onwuegbuzie, Anthony J. and Nancy L. Leech. “Linking Research Questions to Mixed Methods Data Analysis Procedures.” The Qualitative Report 11 (September 2006): 474-498; Tashakorri, Abbas and John W. Creswell. “The New Era of Mixed Methods.” Journal of Mixed Methods Research 1 (January 2007): 3-7; Zhanga, Wanqing. “Mixed Methods Application in Health Intervention Research: A Multiple Case Study.” International Journal of Multiple Research Approaches 8 (2014): 24-35 .
Observational Design
This type of research design draws a conclusion by comparing subjects against a control group, in cases where the researcher has no control over the experiment. There are two general types of observational designs. In direct observations, people know that you are watching them. Unobtrusive measures involve any method for studying behavior where individuals do not know they are being observed. An observational study allows a useful insight into a phenomenon and avoids the ethical and practical difficulties of setting up a large and cumbersome research project.
- Observational studies are usually flexible and do not necessarily need to be structured around a hypothesis about what you expect to observe [data is emergent rather than pre-existing].
- The researcher is able to collect in-depth information about a particular behavior.
- Can reveal interrelationships among multifaceted dimensions of group interactions.
- You can generalize your results to real life situations.
- Observational research is useful for discovering what variables may be important before applying other methods like experiments.
- Observation research designs account for the complexity of group behaviors.
- Reliability of data is low because seeing behaviors occur over and over again may be a time consuming task and are difficult to replicate.
- In observational research, findings may only reflect a unique sample population and, thus, cannot be generalized to other groups.
- There can be problems with bias as the researcher may only "see what they want to see."
- There is no possibility to determine "cause and effect" relationships since nothing is manipulated.
- Sources or subjects may not all be equally credible.
- Any group that is knowingly studied is altered to some degree by the presence of the researcher, therefore, potentially skewing any data collected.
Atkinson, Paul and Martyn Hammersley. “Ethnography and Participant Observation.” In Handbook of Qualitative Research . Norman K. Denzin and Yvonna S. Lincoln, eds. (Thousand Oaks, CA: Sage, 1994), pp. 248-261; Observational Research . Research Methods by Dummies. Department of Psychology. California State University, Fresno, 2006; Patton Michael Quinn. Qualitiative Research and Evaluation Methods . Chapter 6, Fieldwork Strategies and Observational Methods. 3rd ed. Thousand Oaks, CA: Sage, 2002; Payne, Geoff and Judy Payne. "Observation." In Key Concepts in Social Research . The SAGE Key Concepts series. (London, England: Sage, 2004), pp. 158-162; Rosenbaum, Paul R. Design of Observational Studies . New York: Springer, 2010;Williams, J. Patrick. "Nonparticipant Observation." In The Sage Encyclopedia of Qualitative Research Methods . Lisa M. Given, editor.(Thousand Oaks, CA: Sage, 2008), pp. 562-563.
- << Previous: Writing a Research Proposal
- Next: Design Flaws to Avoid >>
- Last Updated: Sep 8, 2023 12:19 PM
- URL: https://guides.library.txstate.edu/socialscienceresearch

In order to continue enjoying our site, we ask that you confirm your identity as a human. Thank you very much for your cooperation.
- SSRS Opinion Panel
- Contacted by SSRS?
The Health of Health Panel Data
Comparing Survey Outcomes Between Probability Panelists and Address Based Sample Respondents

Research Objectives
- Compare the sample composition resulting from a fresh address-based sample (ABS) frame with the sample collected from a state health probability panel of randomly-recruited panel members in the 2023 Minnesota Health Access Survey (MNHA).
- Examine differences in responses to a range of healthcare and health outcome measures such as health insurance, health status, and chronic disease prevalence between the two samples.
- Assess the positive and negative aspects of using probability panels for state Health research.
Study Design
- The MNHA is a survey conducted every two years by The State Health Access Data Assistance Center (SHADAC) at the University of Minnesota and the Minnesota Department of Health (MDH) with SSRS as the data collection partner. The goal of this survey is to document health insurance coverage and access to health care for the non-institutionalized population of Minnesota.
- The 2023 MNHA utilized a combination of an ABS frame and, for the first time, a probability panel recruited sample, with 13,029 completes from the ABS frame and 2,191 from the panel. The general methodology is mail push-to-web.
- The survey uses a proxy methodology where the respondent answers on behalf of one randomly selected household member of any age, referred to as the “target”. Results represent data related to this target person.
- The Minnesota Voices on Health Panel is an emergent panel that was created for SHADAC and MDH. The panelists were recruited in two waves, once during a recontact survey consisting of respondents from the 2021 MNHA, and again during the 2023 MNHA.
- For the 2023 MNHA, the ABS recruitment protocol included three mailings: a letter with either a visible $1 or $2 bill included; a postcard; and a final letter. Sample in high-density Black/African American, low income, and Latino/a/Hispanic areas, as well as a residual stratum, received the third letter in a Fedex envelope. Respondents were also offered a phone number to call in to do the survey.
- The panel recruitment protocol involved an initial introduction letter and then subsequent email reminders and text messages where permitted. Panelists who do not use online technologies were called to complete the survey. All received a $5 post incentive for participation.
- The ABS portion of the survey had an AAPOR Response Rate 4 of 16.4%, and the panel portion had a Response Rate of 22.9%.
- Demographic and response data from the two sample frames are compared here to evaluate the similarities and differences between the two and to contribute to knowledge about how to use probability panels to increase efficiency of health data collection while maintaining data quality.
Principal Findings
Demographic differences between panel and abs sample.
There are distinct demographic differences between the panel sample and ABS respondents.
- The panel sample is older, less racially diverse, more educated, and has a slightly higher income.
- Correlations between these characteristics and health-related measures and outcomes contribute to differences in survey results between the sample types.

Health Insurance Status
Looking in aggregate, it appears that the panel sample overall represents a higher percentage of public insurance recipients. Uninsurance is significantly higher among the ABS members, which would be expected with the demographic profile described above.
Examining all panel members and the specific type of public insurance received, there is almost a 20% difference between the two sample sources in rates of Medicare, accounting for the higher public insurance finding for panelists. The higher SES of the panel respondents is reflected in the percent of Medicaid recipients found in the AB sample versus the panel sample, with the ABS having nearly twice as a high of a proportion of Medicaid respondents.

Reporting Of Health Status by Sample Type
Looking only at those younger than 65 to control for the age gap, panel respondents are significantly more likely to characterize their health as excellent or very good than members of the ABS sample, again reflecting expected characteristics of a more affluent, educated group of survey respondents. The basic relationship is the same when the older population is included, but the differences are smaller.

Reporting Of Chronic Condition by Sample Type
Surprisingly considering the SES differences noted and the pattern of health status response, panel respondents report higher rates of having one or more chronic conditions even when looking at only those younger than 65. Overall experience of these conditions is lower among the younger segment of the sample. Further research is needed to understand this finding.

Relevance To Policy Research
Conducting methodologically rigorous statewide health policy research is a challenging and expensive endeavor. One of the goals of such research is often to achieve a representative sample of the geographic and demographic diversity of a state with sufficient statistical power to meet analytic objectives. This requires large sample sizes. Often the populations most in need of effective health policy reform are the most traditionally underrepresented in surveys, requiring oversampling of these groups, generally at great cost. One avenue toward ameliorating these obstacles is creating a panel of randomly sampled survey respondents who agree to be interviewed for future surveys in exchange for small compensation.
As the demographics of our two sample groups demonstrate, the panel is not a panacea for providing access to underrepresented groups. However, completing interviews with more responsive groups through the panel can free up resources for enhanced outreach to populations of interest.
Weighting can correct for certain biases in the sample and combining cross-sectional samples with panel data can strike a balance between representativeness and efficiency. Understanding the relative benefits and skews of these samples will guide researchers and policy makers in determining tradeoffs and optimal balance between panel and new sample.

Susan Sherr, Ph.D.
Executive Vice President - Demographic and Policy Research
Susan is a long-time Pennsylvania resident with a New York soul who will never stop missing real bagels and pizza. She loves Broadway musicals and good books more than almost… Learn more

SSRS is an industry leader in health policy and health care research.
We are the preferred partner of many of the nation’s elite organizations working to improve public health and health policy, address health inequities, and increase citizens’ access to quality care.
Related Insights
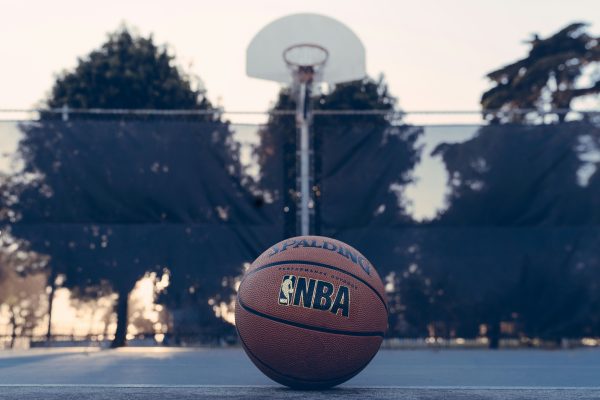
Who will be the next “face” of the NBA? Insights from 30 years of tracking NBA stars
Insight from Chad Menefee, Ph.D.
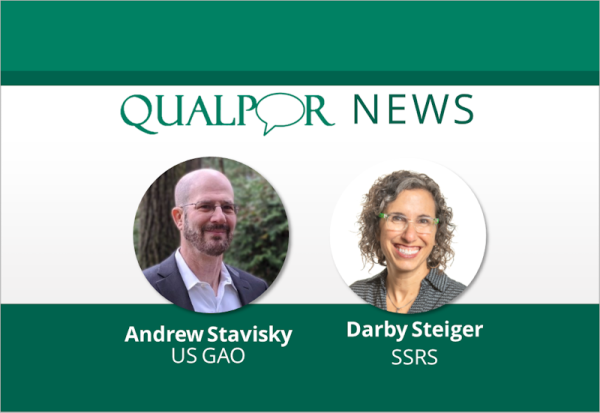
Perspectives on AI in Qualitative Research
Andrew Stavisky (US GAO*) and Darby Steiger (SSRS) have a conversation on AI in qualitative research.
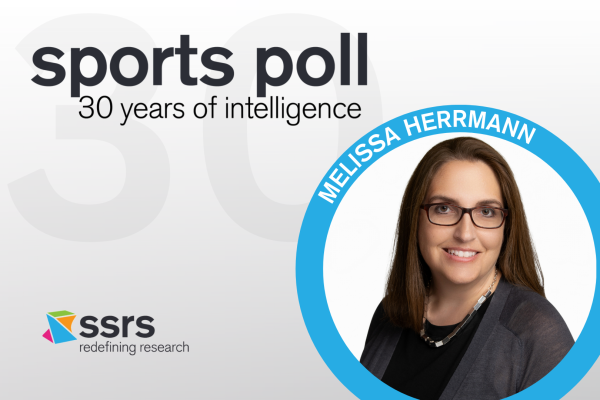
Baseball: A Universal Language
Melissa's Story
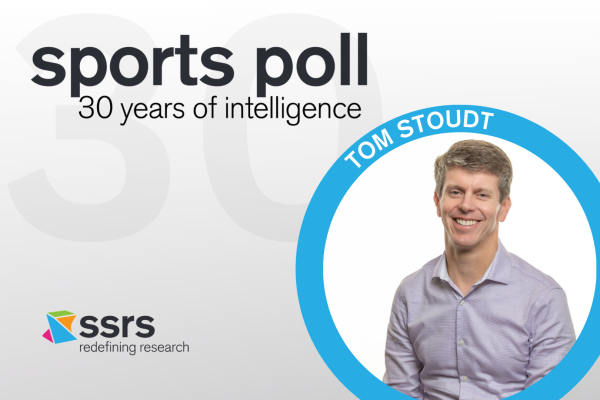
Athletes Make it Look Easy – We Know it’s Not
Tom's Story
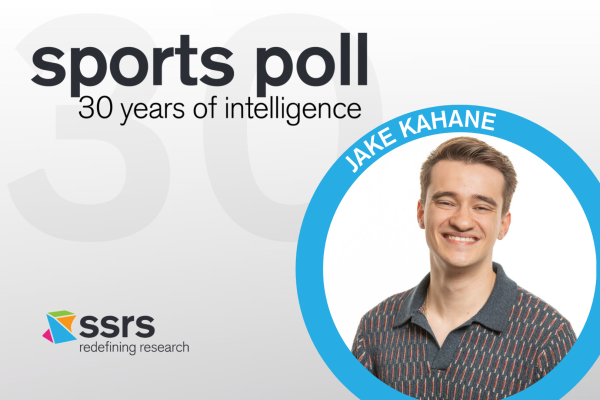
A Hockey Family Bond
Jake's Story
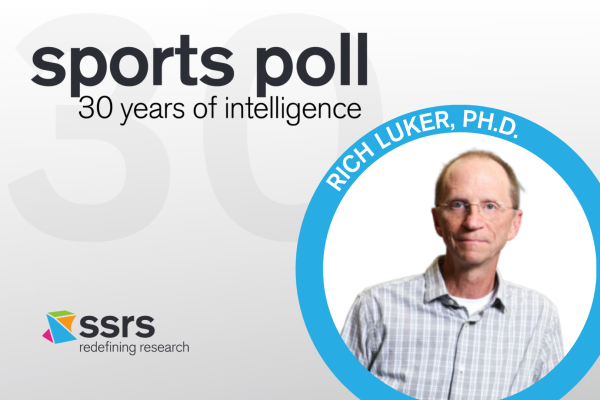
Lifelong Passion and Community in Sports
Rich's Story
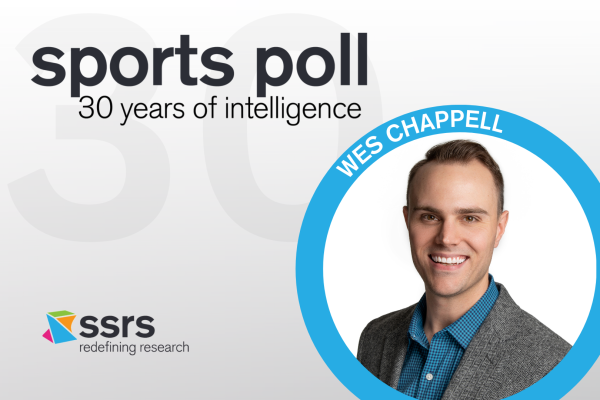
UVA Football: A Generational Legacy
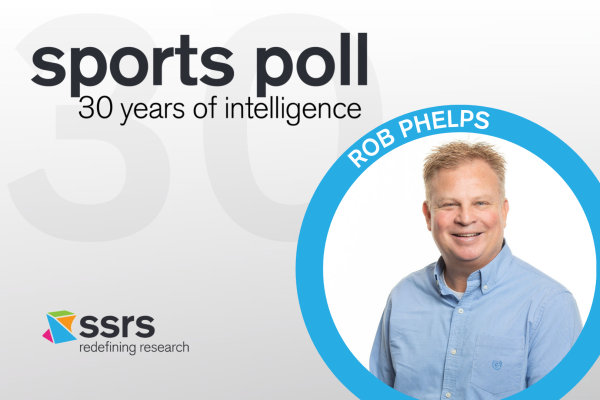
Sports as a Social Catalyst
Rob's Story
- Open access
- Published: 06 July 2024
Efficacy of relational agents for loneliness across age groups: a systematic review and meta-analysis
- Sia Sha ORCID: orcid.org/0009-0000-2027-1316 1 ,
- Kate Loveys 2 ,
- Pamela Qualter 3 ,
- Haoran Shi 1 ,
- Dario Krpan ORCID: orcid.org/0000-0002-3420-4672 1 &
- Matteo Galizzi 1
BMC Public Health volume 24 , Article number: 1802 ( 2024 ) Cite this article
241 Accesses
Metrics details
Loneliness is a serious public health concern. Although previous interventions have had some success in mitigating loneliness, the field is in search of novel, more effective, and more scalable solutions. Here, we focus on “relational agents”, a form of software agents that are increasingly powered by artificial intelligence and large language models (LLMs). We report on a systematic review and meta-analysis to investigate the impact of relational agents on loneliness across age groups.
In this systematic review and meta-analysis, we searched 11 databases including Ovid MEDLINE and Embase from inception to Sep 16, 2022. We included randomised controlled trials and non-randomised studies of interventions published in English across all age groups. These loneliness interventions, typically attempt to improve social skills, social support, social interaction, and maladaptive cognitions. Peer-reviewed journal articles, books, book chapters, Master’s and PhD theses, or conference papers were eligible for inclusion. Two reviewers independently screened studies, extracted data, and assessed risk of bias via the RoB 2 and ROBINS-I tools. We calculated pooled estimates of Hedge’s g in a random-effects meta-analysis and conducted sensitivity and sub-group analyses. We evaluated publication bias via funnel plots, Egger’s test, and a trim-and-fill algorithm.
Our search identified 3,935 records of which 14 met eligibility criteria and were included in our meta-analysis. Included studies comprised 286 participants with individual study sample sizes ranging from 4 to 42 participants ( x̄ = 20.43, s = 11.58, x̃ = 20). We used a Bonferroni correction with α Bonferroni = 0.05 / 4 = 0.0125 and applied Knapp-Hartung adjustments. Relational agents reduced loneliness significantly at an adjusted α Bonferroni ( g = -0.552; 95% Knapp-Hartung CI, -0.877 to -0.226; P = 0.003), which corresponds to a moderate reduction in loneliness.
Our results are currently the most comprehensive of their kind and provide promising evidence for the efficacy of relational agents. Relational agents are a promising technology that can alleviate loneliness in a scalable way and that can be a meaningful complement to other approaches. The advent of LLMs should boost their efficacy, and further research is needed to explore the optimal design and use of relational agents. Future research could also address shortcomings of current results, such as small sample sizes and high risk of bias. Particularly young audiences have been overlooked in past research.
Peer Review reports
Loneliness is a subjective experience that emerges when people feel that their social relationships are unsatisfactory [ 1 ]. For some people, loneliness is experienced when they want more people to interact with, but it is also often felt when one’s social relationships are not as fulfilling as one would like. Loneliness is not the same as social isolation (i.e., the objective lack of social interactions) but is often associated with it [ 2 ]. There is strong evidence of the risks associated with loneliness, including poorer physical health outcomes [ 3 ]. Loneliness also affects mental health and psychological wellbeing, with growing evidence that loneliness is associated with the onset of depression and other common mental health problems [ 4 ]. Crucially, poor health and wellbeing can, in turn, exacerbate loneliness, placing those who experience loneliness in a negative feedback loop [ 5 ]. Evidence for a wide range of health effects, therefore, has led scholars to propose that loneliness should be regarded as a public health priority. Governments have consequently looked to offer interventions for people reporting loneliness, and although evidence for intervention efficacy is increasing [ 6 ], the evidence base suffers from some gaps [ 7 ], and potentially effective interventions may lack scalability or fail to produce cost savings [ 8 ]. Governments therefore have developed an interest in digital interventions, such as mobile phone apps or virtual reality [ 9 ]. Yet despite their promise, the efficacy of digital interventions across recent systematic reviews and meta-analyses is mixed [ 10 ].
“Relational agents” are a technology that show promise for delivering loneliness interventions in a scalable and engaging manner. Relational agents are software agents that build relationships with users through their behaviours (e.g., personal conversation, play, empathy), and they may be embodied (e.g., take the shape of humans or animals) or lack embodiment (e.g., voice agents) [ 11 ]. Relational agents can be broadly separated into two types: social robotic agents (e.g., those that possess physical bodies made of carbon or steel), and app-based agents (e.g., those embedded in everyday hardware such as computers and smartphones). Relational agents increasingly employ artificial intelligence (AI) such as emotion recognition for enhanced interactions and large language models (LLMs) to generate highly tailored and relevant speech [ 12 ]. Relational agents may promote engagement with internet-based psychological interventions for loneliness because of the social engagement and presence that they provide [ 13 ]. Moreover, preliminary but promising evidence suggests that relational agents may reduce loneliness by directly providing companionship, and by serving as catalysts for social interaction [ 14 ]. Appendix E provides video links for relational agents.
There are three key reasons research and investment in relational agents are worthwhile. First, not everyone can socialise with other humans. Physical disability, for example, can impact mobility, which in turn can restrict opportunities for socialising, thus contributing to loneliness [ 15 ]. While interventions such as social visits can be effective to alleviate the loneliness of people with physical disabilities, these interventions are constrained: a person who is bedridden may wait for several days before his or her next visitation. Relational agents, on the other hand, can be an on-demand solution. Second, loneliness can be due to the feeling that one is not heard. This, for example, can occur when people do not feel comfortable sharing their secrets due to stigma, and there is indeed evidence that people prefer sharing some secrets with relational agents rather than humans [ 16 , 17 ]. Relational agents, then, are not just an intermediate solution: they are a separate class of intervention with a suitable audience. Here, one might raise the question of “understanding”: that is, whether AI can truly understand people’s self-disclosure. The answer is probably complex, but from a practical perspective it seems that the answer may not matter: people seem to benefit from relational agents as long as they feel they are understood and heard by them – irrespective of whether this is actually the case [ 18 ]. Third, both qualitative and quantitative metrics suggest that human–agent and human–human relationships may have some similar features at times [ 17 , 19 ]. For example, there is a vast literature on how people anthropomorphise machines, imbuing them with human-like traits, personalities, and motivations [ 20 , 21 , 22 ]. People often treat machines like other people, developing similar feelings for them such as pity and even love [ 23 ]. One participant said: “Yes, explicitly I will tell my Replika [relational agent] that I think he is wonderful, that he is fantastic and smart and helps me and makes me feel good about myself and that I enjoy our talks” [ 17 ].
Several scoping reviews have qualitatively summarised the efficacy of relational agents for loneliness [ 14 , 24 , 25 , 26 , 27 ]. Combined, these reviews concluded that some evidence for the efficacy of social robotic relational agents existed but that further work on app-based relational agents was needed. Additionally, one 2019 meta-analysis investigated a sub-set of social robotic relational agents (i.e., robotic pets), but failed to find significant results, most likely due to including only two studies [ 28 ]. Previous reviews, moreover, exclusively focused on elderly samples, and the literature is therefore in need of a comprehensive and up-to-date quantitative synthesis to evaluate the efficacy of relational agents to mitigate loneliness across all age groups.
We preregistered our methodology with PROSPERO: CRD42022359737. We have also made our full paper trail available on the Open Science Framework (OSF): https://osf.io/c6rdk/files/osfstorage . There, the reader can also find the full data set to reproduce the analyses.
Search strategy and selection criteria
In this systematic review and meta-analysis, we searched 11 databases from inception to Sep 16, 2022: Ovid MEDLINE, Ovid Embase, Ovid PsycINFO, Ovid Global Health, EBSCO CINAHL, Scopus, Web of Science, IEEE Xplore, ACM Digital Library, PROSPERO, and ProQuest Dissertations. We also manually searched the bibliographies of selected studies to identify additional papers. We searched titles and abstracts using a range of search terms such as lonel*, robot*, computer* agent*, and relation* agent*. Appendix A outlines the full search strategy.
We included randomised controlled trials (RCTs) and non-randomised studies of interventions (NRSIs). Factorial designs were eligible if they allowed us to collapse relevant intervention arms or drop irrelevant ones. Cluster-randomised trials were eligible if they included sufficient information (e.g., intra-cluster coefficient). Eligible studies had to be published in English and had to be peer-reviewed journal articles, books, book chapters, postgraduate theses, or conference papers. Government reports, company reports, newspaper articles, conference presentations, and similar were ineligible. There was no restriction on populations or settings. All eligible studies had to administer app-based or social robotic relational agents. Agents that did not use relational cues were ineligible. Any non-relational agent comparator made studies eligible (e.g., waiting lists). Finally, eligible studies had to report a quantitative, self-report loneliness outcome where follow-up was at least one week.
Coding of studies
SS, KL, and HS independently double-screened in Covidence the titles and abstracts of citations and then the full texts of remaining studies, using piloted and structured forms. We measured agreement between screeners via Cohen’s κ and resolved disagreements via discussion between screeners. SS, KL, and HS then extracted data in Covidence using a piloted and structured form, and we contacted primary study authors to obtain raw or missing data. Our data extraction forms are available on OSF, and we describe data imputations in Appendix B. Each study was coded for a range of variables such as sample size, research design, and loneliness scale used. Finally, SS, KL, HS, and DK independently double-assessed risk of bias in MS Excel, using the RoB 2 tool for RCTs and ROBINS-I tool for NRSIs.
Meta-analytic procedure
Our main outcome was loneliness for which we calculated a random-effects meta-analysis using the DerSimonian and Laird method because we expected the effects of relational agents to be heterogenous across populations, types of agents, etc. We used Hedge’s g to standardise results from diverse quantitative loneliness scales, and interpreted the magnitude of Hedge’s g according to the rules of thumb in the Cochrane Handbook for Systematic Reviews of Interventions . Hedge’s g itself was computed using standard formulas and relied on a range of data points such as group means and pooled standard deviations [ 29 ]. Our raw data on OSF show exactly how Hedge’s g was computed for each primary study.
We calculated four null hypothesis significance tests and applied a Bonferroni correction: α Bonferroni = 0.05 / 4 = 0.0125. We also applied Knapp-Hartung adjustments to our 95% confidence intervals. As measures of heterogeneity, we calculated Cochrane’s Q using a p value of 0.1, I 2 , τ 2 , and a prediction interval. We conducted an RCT-only sensitivity analysis and separate sub-group analyses for app-based and social robotic relational agents. We evaluated publication bias via funnel plots and Egger’s test, and we calculated an adjusted estimate of Hedge’s g using Duval and Tweedie’s trim-and-fill algorithm. We conducted all analyses in the Comprehensive Meta-Analysis Software package. The systematic review and meta-analysis followed PRISMA 2020 reporting guidelines [ 30 ].
Characteristics of studies
Our database searches identified 3,935 records and our manual searches 38 records, of which 1,910 were duplicates. We screened the titles and abstracts of 2,063 studies, deeming 1,908 irrelevant. We screened the full texts of 155 studies, with Fig. 1 detailing reasons for exclusions. In the end, we included 14 studies. When screening abstracts and titles, Cohen’s kappa ranged from κ = 0.46 to κ = 1 across reviewer pairs; when screening full texts, it ranged from κ = 0.71 to κ = 0.81 across pairs.
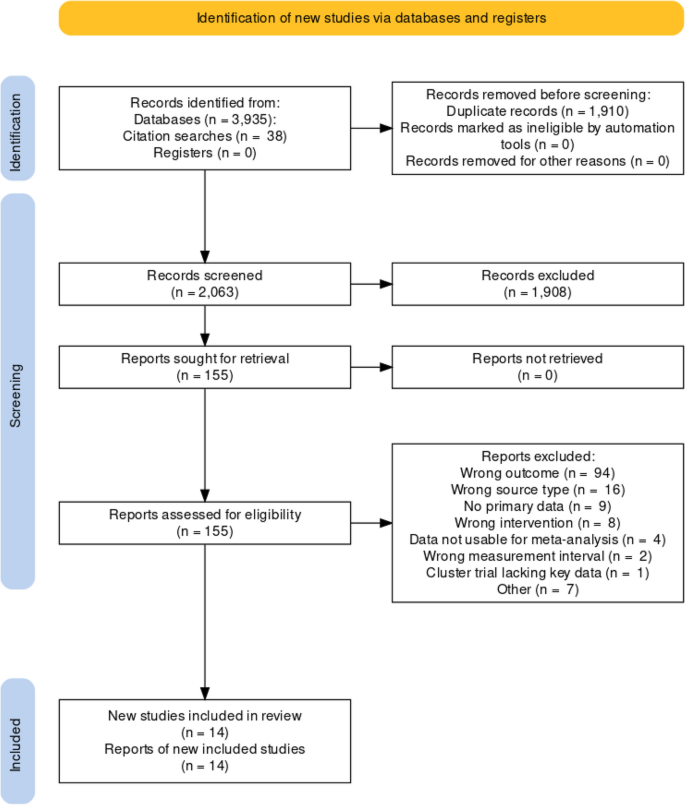
PRISMA flow diagram
Nine of the 14 included studies were NRSIs [ 31 , 32 , 33 , 34 , 35 , 36 , 37 , 38 , 39 ] and the rest RCTs [ 12 , 40 , 41 , 42 , 43 ]. All nine NRSIs were uncontrolled trials. Coding was generally straightforward, though some data points such as percentage of females in the sample were sometimes missing in manuscripts. Together, studies included 286 participants with individual study sample sizes ranging from 4 to 42 participants ( x̄ = 20.43, s = 11.58, x̃ = 20). Attrition rates ranged from 0 to 94% ( x̄ = 21.39%, s = 21.56%, x̃ = 16.50%). Based on guidance, we classified 86% of these studies as feasibility studies, since they included fewer than 25 participants in total, or fewer than 25 participants per group [ 44 ].
Figure A, Figure B, and Figure C provide a tabular summary of included studies, but we also provide below a prose summary. Participants’ age ranged from 19 to 100 years ( x̄ = 75.45, s = 12.89, x̃ = 77.55). Only two studies reported inclusion of participants younger than 50 years [ 12 , 32 ]. A third study is likely to have included them [ 36 ]. Nevertheless, none of the three studies focused on participants younger than 50 years exclusively, and hence studies only included young participants along with older ones. Remaining studies explicitly reported excluding those younger than 50 [ 31 , 33 , 35 , 37 , 38 , 40 , 41 , 43 ] or their sampling frames implied this [ 34 , 39 , 45 ]. Where reported, the percentages of both females and non-White participants were high in most studies.
Nine studies used social robotic relational agents [ 31 , 32 , 33 , 34 , 35 , 39 , 41 , 43 , 45 ] and five app-based relational agents [ 12 , 36 , 37 , 38 , 40 ]. The social robotic agents included Sony’s AIBO [ 32 , 34 , 41 ], PARO developed by ISRI [ 31 , 33 , 45 ], NAO developed by Aldebaran Robotics [ 35 ], Pepper developed by SoftBank Robotics [ 43 ], and either a robotic cat or dog developed by Joy for All [ 39 ]. The app-based agents included Laura developed by MIT [ 40 ], Elena + developed by ETH Zurich and the University of St. Gallen [ 36 ], Amazon’s Alexa [ 37 ], Bella by Soul Machines [ 46 ], and PACO developed by a consortium of Dutch organisations [ 38 ]. The relational behaviours of these agents varied. AIBO is a robotic puppy, PARO a robotic seal, and together with the robotic pets by Joy for All, these agents simulated live pet behaviour (e.g., the agents expressed emotions via facial cues and body language such as wagging of tails, played with users, learned their own names, and recognised users via their facial recognition capabilities) [ 32 ]. The agents responded to touch (e.g., petting) and adapted behaviour through reinforcement learning [ 33 ]. NAO and Pepper were humanoid robots that simulated human behaviours, customs, and speech. NAO, for example, would bow to users, extend its palm for a handshake, ask if participants would want to hear a poem, and only proceed once receiving a reply [ 35 ]. All app-based relational agents simulated humans. All were embodied, i.e., had a visual form, except for Amazon’s Alexa [ 37 ]. App-based agents primarily or to a significant degree used speech for relational behaviour. Laura, for example, expressed empathy (“I am sorry to hear that”), asked follow-up questions (“How tired are you feeling?”), and attempted to get to know users (“So, are you from the East Coast originally?”) [ 40 ]. Whilst in previous research several agents used “wizard-of-Oz methodologies”, i.e., agents controlled by humans pretending to be autonomous, all agents in this review were autonomous [ 47 ].
Most relational agents in our review acted as direct companions and did not seek to mitigate loneliness via other modalities [ 31 , 32 , 33 , 34 , 35 , 37 , 39 , 40 , 41 , 43 , 45 ], although exceptions existed. Elena + sought to remove cognitive biases and improve social skills [ 36 ]. PACO sought to create opportunities for socialising [ 38 ]. Bella sought to enhance social skills, increase social support, and increase opportunities for socialising [ 12 ].
Studies generally did not mention behavioural theories or behavioural change techniques (BCTs) that underpinned intervention design, although exceptions existed. One study based its intervention on Self-Determination Theory [ 38 ] and another study based its intervention on the COM-B model and the Theory of Planned Behaviour [ 36 ]. Nevertheless, these studies provided little detail on how exactly theories informed design. We classified BCTs according to the BCTTv1 by Michie et al., using below in quotation marks the labels of the original authors [ 48 ]. Only one study confirmed the full range of BCTs it used, and two other studies provided examples of BCTs. One study used six BCTs: “credible source”, “review behaviour goals”, “goal setting”, “instruction on how to perform a behaviour”, “social comparison”, and “social support” [ 38 ]. Another study mentioned seven BCTs: “information about emotional consequences”, “action planning”, “behavioural contract”, “instruction on how to perform a behaviour”, “review behaviour goals”, “reducing exposure to cues for the behaviour”, and “reduce negative emotions” [ 12 ]. A third study mentioned five BCTs: “information about emotional consequences”, “goal setting”, “instruction on how to perform a behaviour”, “reducing exposure to cues for the behaviour”, and “reduce negative emotions” [ 36 ].
All RCTs were at high risk of bias due to potential deviations from the intended interventions [ 40 , 41 , 43 , 45 ] except for one [ 12 ]. All NRSIs were at high risk due to confounds and potential biases in measurements [ 31 , 32 , 33 , 34 , 35 , 36 , 37 , 38 , 39 ]. Figures 2 and 3 illustrate these.
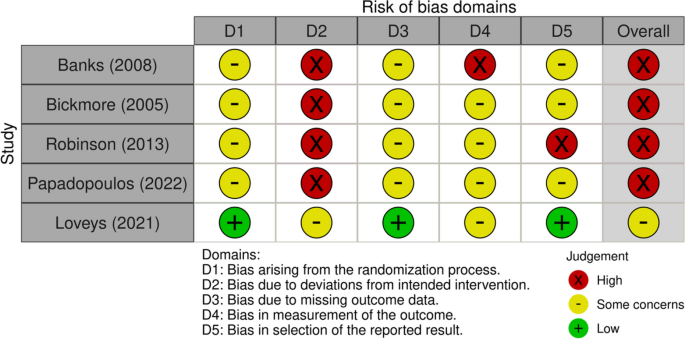
RCT risk of bias domains
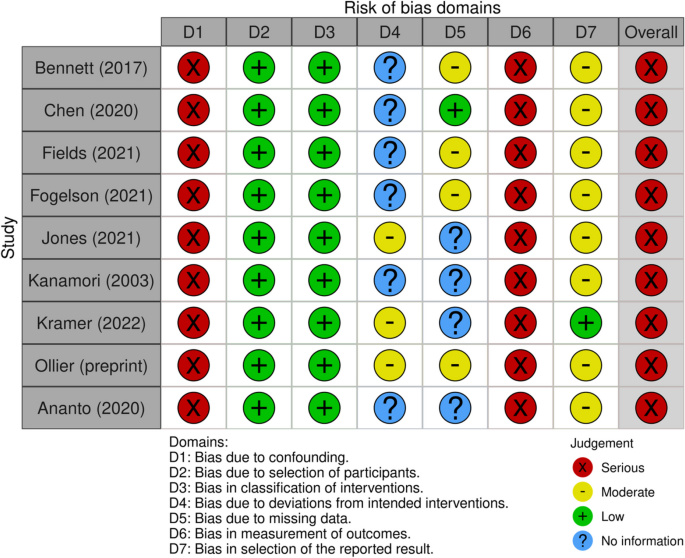
NRSI risk of bias domains
Meta-analysis
The pooled estimate of Hedge’s g was -0.552 ( Z = -3.833; 95% CI, -0.834 to -0.270; P < 0.001), indicating on average a moderate effect of relational agents on loneliness reduction. This is shown in Fig. 4 . Using a Bonferroni-corrected α Bonferroni = 0.0125, there was evidence to reject the null hypothesis. Using the Knapp-Hartung adjustment, there was also evidence to reject the null hypothesis ( t = -3.66; 95% Knapp-Hartung CI, -0.877 to -0.226; P = 0.003).
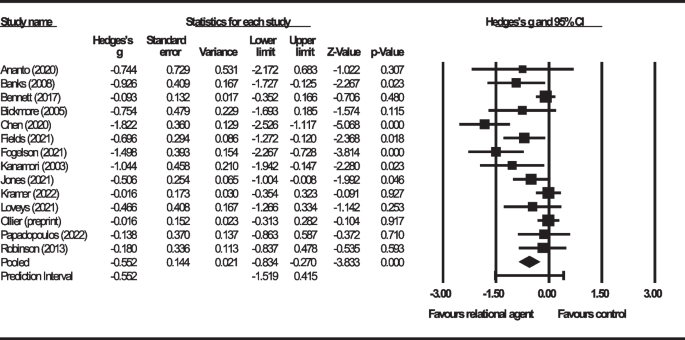
Main analysis forest plot
Heterogeneity measures indicated that, as anticipated, the true effect of relational agents varied ( Q = 45.073; I 2 = 71%; τ 2 = 0.176; τ = 0.420). Assuming a Gaussian distribution, the 95% prediction interval was estimated to range from -1.519 to 0.415, as seen in Fig. 5 .
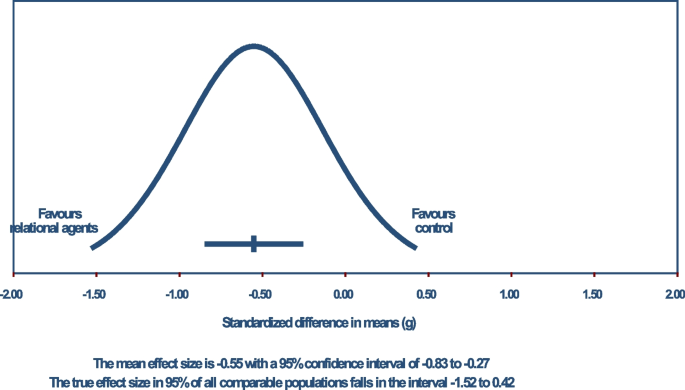
Main analysis prediction interval
Funnel plots as well as Egger’s test ( b = -2.81; t = 3.5; P = 0.004) suggested that a small study effect may exist. Figure 6 illustrates this. The small study effect could have been due to effect sizes being larger in smaller studies or due to publication bias. Assuming a severe publication bias, the trim-and-fill algorithm resulted in an adjusted estimate of g = -0.198 (95% CI, -0.505 to 0.109), which attenuated the original estimate by roughly 64%.
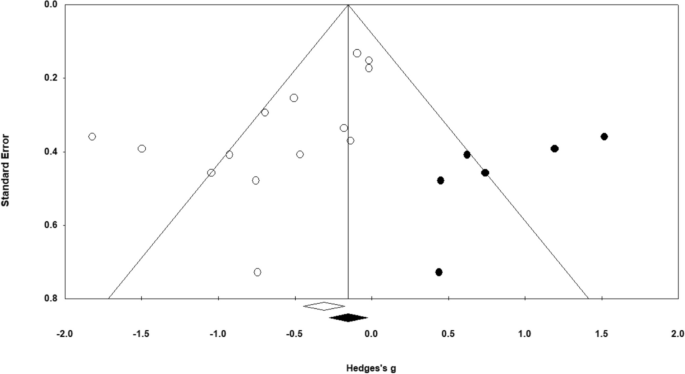
Funnel plot using standard error
Five studies were available for the RCT-only model. Hedge’s g was -0.437 ( Z = -2.495; 95% CI, -0.781 to -0.094; P = 0.013), which was 21% less than the estimate of the main model. The results were significant at a traditional α = 0.05 but not at the α Bonferroni . The Knapp-Hartung adjusted results were not significant ( t = -2.49; 95% Knapp-Hartung CI, -0.924 to 0.049).
Six studies were available for the app-based relational agent model. The pooled estimate of Hedge’s g was -0.286 ( Z = -1.611; 95% CI, -0.553 to -0.020; P = 0.035), which was significant at a traditional α but not α Bonferroni . The Knapp-Hartung adjustment resulted in non-significant results ( t = -2.11; 95% Knapp-Hartung CI, -0.636 to 0.063). Eight studies were available for the social robotic relational agent model. The pooled estimate of Hedge’s g was -0.774, which was significant at α Bonferroni ( Z = -2.909; 95% CI, -1.296 to -0.252; P = 0.004). Using a Knapp-Hartung adjustment, results were significant at a traditional α but not at α Bonferroni ( t = -2.91; 95% Knapp-Hartung CI, -1.403, -0.145, P = 0.023).
Our review is the first to provide quantitative evidence for the efficacy of relational agents to reduce loneliness in participants aged 19 to 100 years. Our results are promising, and although the effect size of g = -0.552 is likely somewhat inflated due to publication bias, it is probably less inflated than our trim-and-fill algorithm suggested. This is because the trim-and-fill algorithm assumed that several studies were suppressed in which relational agent interventions exacerbated loneliness. This, however, is unlikely. Failed loneliness interventions tend to have no effect on loneliness, not exacerbate it [ 10 ]. Our review could have used different algorithms to adjust for publication bias, and alternatives would probably have yielded different adjustments. Recently, for example, researchers have applied four different algorithms to a high-profile meta-analysis, resulting in a mix of significant and non-significant adjustments [ 49 , 50 , 51 ]. Nevertheless, no algorithm for publication bias would provide the “correct” effect size [ 52 ]. Instead, algorithms provide a sensitivity analysis assuming certain parameters, and sometimes these parameters lead to flawed results, e.g., the trim-and-fill algorithm overcorrects under heterogeneity, which was the very assumption of our analysis [ 53 ]. Ultimately, the most likely interpretation is that the true average effect size of relational agents was small to moderate. Table 1 provides a summary of our results.
We believe the above results have two important implications for the current loneliness literature. First, the literature is in search for novel and effective interventions that are scalable. The NHS is already facing resource constraints, these constraints are expected to exacerbate, and the NHS has consequently called for the increased adoption of AI to ease its burden [ 54 ]. Relational agents can be highly scalable, once some groundwork has been completed, and a possible follow-up from our results is a national or regional pilot. Such a pilot, of course, would entail the resolution of complex issues (e.g., digital literacy, access to technology, and privacy). Researchers, for example, will need to determine who will have access to user data and in what form, and such choices can fundamentally impact the success of a pilot.
The second implication of our results is that relational agents may act as a standalone intervention, but they are likely to be more useful in multi-component interventions that are tailored to individual needs. In the UK, the NHS’s current main strategy for loneliness is “social prescribing”, an outsourcing approach in which staff refer individuals to community schemes such as lifestyle interventions (e.g., physical exercise) or social activity interventions (e.g., volunteering) [ 7 , 55 ]. While there are alternative intervention approaches for loneliness, social prescribing is viewed by individuals and service providers as helpful [ 7 ] and cost-effective [ 56 , 57 ]. Social prescribing is, in essence, a sign-posting intervention, and it could sign-post, among other things, to relational agents. This could be valuable because there is currently a notion that interventions improve lives, but that people do not recover from loneliness [ 58 ]. Potentially, this may be because not all loneliness is the same. Two people may feel lonely for two different reasons, and these people may then require different sets of solutions [ 58 ]. Relational agents can extend the set of available solutions, and agents can complement existing human-centred interventions, rather than replace them.
Relational agents, thus, could help in the fight against loneliness. What is more, their full potential has not yet been realised. On the one hand, this is due to the absence of state-of-the-art knowledge integration. For example, the use of behavioural theories and BCTs can enhance intervention efficacy, yet studies in our sample generally did not discuss such theories and BCTs. Similarly, interventions can modify loneliness via multiple modalities. Studies in our review, however, generally used only one of these modalities, and the others—such as the debiasing of social cognition that has shown particular promise [ 56 ]—are yet to be integrated into relational agent design [ 14 ]. On the other hand, relational agents have not yet realised their full potential due to the nascency of AI. Increasingly, LLMs are powering relational agents. These models allow relational agents to produce open-ended, original, and highly tailored conversation, and although much of the conversation of relational agents has already become indistinguishable from human conversation [ 59 ], research on LLMs is burgeoning, and the race is on between organisations such as OpenAI and Google to develop the next generation of LLMs [ 60 ].
Limitations
Our review faced common limitations such as the exclusion of non-English sources and the quality of underlying primary studies, but a particular limitation of our review were the mixed results of the sensitivity and sub-group analyses. There are three potential explanations for this. First, sample sizes in these sub-group analyses were less than 10, and analyses with fewer than 10 studies tend to lack power [ 52 ]. At the same time, it is likely that underlying studies themselves lacked power due to small sample sizes [ 61 ]. Indeed, Appendix C demonstrates that power was likely well below the recommended level of 80% in our sub-group analyses, while Appendix D presents an additional sensitivity analysis indicating that further primary studies would have meaningfully reduced p levels [ 52 ]. Second, our review may have tested for results too conservatively. The Bonferroni correction, as applied in this review, results in Type 2 error rates of roughly 33%, which some have referred to as unacceptably high [ 62 ]. Finally, our review conducted two-tailed significance tests. This is usually anodyne—since interventions can both improve and exacerbate outcomes. Nevertheless, in cases where interventions are unlikely to exacerbate outcomes, one-tailed tests may be warranted [ 52 ]. This, as discussed, is likely to be the case with loneliness and relational agents. Had we conducted one-tailed tests, this would have entailed the halving of p values, which would have made some results statistically significant. Third, execution may have been a problem. Primary studies may not have sufficiently exposed participants to relational agents, or participants may not have interacted with relational agents, or relational agents may not have been correctly designed. Chen et al. [ 63 ], for example, found no significant difference between control and experimental groups at a four-week interval [ 63 ]. They did, however, find a significant difference at an eight-week interval. In our review, the mean time between pre-test and final post-test was 5.92 weeks.
Future research
We lack an understanding of relational agents in several areas, and we suggest that future research could focus on three. First, research on relational agents and loneliness in young people is scarce. Among some youth groups loneliness rates are higher than those of the elderly, and these rates of youth loneliness are increasing [ 64 ]. At the same time, smartphone ownership is high among the young [ 64 ]. Young people therefore are pertinent and amenable for the study of loneliness. Second, the efficacy of relational agents will depend on a variety of population and design factors. On the population side, we suspect that factors such as age, education, and digital literacy may impact efficacy. On the design side, we suspect that a hierarchy of features exists, e.g., certain design features will deliver more bang for your buck, although it is less clear which [ 65 ]. Third, although general attitudes towards relational agents may be favourable, some are concerned about the introduction of relational agents and similar technologies [ 66 ]. Future research could therefore explore how technology should be harnessed to increase its benefits and reduce unintended consequences. Finally, future research could address the shortcomings of current research. Almost all underlying studies in our review suffered from high risk of bias in one or several domains, sample sizes were small, and follow-up periods were brief. Particularly, there is a need for more high-quality RCTs.
The current study is the first meta-analysis to explore the effects of relational agents on loneliness across all age groups. It is also the first meta-analysis to provide statistically significant evidence for the efficacy of relational agents, which on average had a moderate effect on loneliness reduction. Loneliness has serious physical and mental health consequences for individuals, and the monetary costs to the state and employers are staggering [ 67 , 68 , 69 ]. Unfortunately, current interventions for loneliness can suffer from low engagement and scalability [ 58 ]. Relational agents, on the other hand, are an emerging technology that due to advances in AI and LLMs will increase in sophistication and realism. Although a multi-pronged approach is required, relational agents could play a significant role in alleviating a growing public health concern [ 64 ]. Future work is required that addresses weaknesses of current studies such as risk of bias, small study size, and brief follow-up periods.
Availability of data and materials
As a meta-analysis, this study used data reported in the literature. Appendices and derived data for meta-analytic calculations are available the Open Science Framework here https://osf.io/c6rdk/files/osfstorage . Reader can also write directly to the corresponding author.
Cacioppo JT. Loneliness: human nature and the need for social connection. New York, USA: W.W. Norton; 2010.
Google Scholar
Coyle CE, Dugan E. Social isolation, loneliness and health among older adults. J Aging Health. 2012;24:1346–63. https://doi.org/10.1177/0898264312460275 .
Article PubMed Google Scholar
Christiansen J, Lund R, Qualter P, Andersen CM, Pedersen SS, Lasgaard M. Loneliness, social isolation, and chronic disease outcomes. Ann Behav Med. 2021;55:203–15. https://doi.org/10.1093/abm/kaaa044 .
Beutel ME, Klein EM, Brähler E, Reiner I, Jünger C, Michal M, et al. Loneliness in the general population: prevalence, determinants and relations to mental health. BMC Psychiatry. 2017;17:97. https://doi.org/10.1186/s12888-017-1262-x .
Article PubMed PubMed Central Google Scholar
Qualter P, Vanhalst J, Harris R, Van Roekel E, Lodder G, Bangee M, et al. Loneliness across the life span. Perspect Psychol Sci. 2015;10:250–64. https://doi.org/10.1177/1745691615568999 .
Morrish N, Choudhury S, Medina-Lara A. What works in interventions targeting loneliness: a systematic review of intervention characteristics. BMC Public Health. 2023;23:2214. https://doi.org/10.1186/s12889-023-17097-2 .
Article PubMed PubMed Central CAS Google Scholar
Vidovic D, Reinhardt GY, Hammerton C. Can social prescribing foster individual and community well-being? A systematic review of the evidence. Int J Environ Res Public Health 2021;18. https://doi.org/10.3390/ijerph18105276 .
McDaid D, Park A-L. Modelling the economic impact of reducing loneliness in community dwelling older people in England. Int J Environ Res Public Health. 2021;18:1426. https://doi.org/10.3390/ijerph18041426 .
Kahlon MK, Aksan N, Aubrey R, Clark N, Cowley-Morillo M, Jacobs EA, et al. Effect of layperson-delivered, empathy-focused program of telephone calls on loneliness, depression, and anxiety among adults during the COVID-19 Pandemic: a randomized clinical trial. JAMA Psychiat. 2021;78:616–22. https://doi.org/10.1001/jamapsychiatry.2021.0113 .
Article Google Scholar
Shah SGS, Nogueras D, van Woerden HC, Kiparoglou V. Evaluation of the effectiveness of digital technology interventions to reduce loneliness in older adults: systematic review and meta-analysis. J Med Internet Res.2021;23:e24712. https://doi.org/10.2196/24712 .
Bickmore T. Relational agents: Effecting change through human-computer relationships. ProQuest Information & Learning. 2003.
Loveys K, Sagar M, Pickering I, Broadbent E. A digital human for delivering a remote loneliness and stress intervention to at-risk younger and older adults during the COVID-19 pandemic: randomized pilot trial. JMIR Ment Health. 2021;8:e31586. https://doi.org/10.2196/31586 .
Loveys K, Fricchione G, Kolappa K, Sagar M, Broadbent E. Reducing patient loneliness with artificial agents: design insights from evolutionary neuropsychiatry. J Med Internet Res. 2019;21:e13664. https://doi.org/10.2196/13664 .
Gasteiger N, Loveys K, Law M, Broadbent E. Friends from the future: a scoping review of research into robots and computer agents to combat loneliness in older people. Clin Interv Aging. 2021;16:941–71. https://doi.org/10.2147/CIA.S282709 .
Macdonald SJ, Deacon L, Nixon J, Akintola A, Gillingham A, Kent J, et al. ‘The invisible enemy’: disability, loneliness and isolation. Disabil Soc. 2018;33:1138–59. https://doi.org/10.1080/09687599.2018.1476224 .
Pickard MD, Roster CA, Chen Y. Revealing sensitive information in personal interviews: Is self-disclosure easier with humans or avatars and under what conditions? Comput Hum Behav. 2016;65:23–30. https://doi.org/10.1016/j.chb.2016.08.004 .
Skjuve M, Følstad A, Fostervold KI, Brandtzaeg PB. My chatbot companion - a study of human-chatbot relationships. Int J Hum-Comput Stud. 2021;149: 102601. https://doi.org/10.1016/j.ijhcs.2021.102601 .
Ho A, Hancock J, Miner AS. Psychological, relational, and emotional effects of self-disclosure after conversations with a chatbot. J Commun. 2018;68:712–33. https://doi.org/10.1093/joc/jqy026 .
Darcy A, Daniels J, Salinger D, Wicks P, Robinson A. Evidence of human-level bonds established with a digital conversational agent: cross-sectional. retrospective observational study. JMIR Form Res 2021;5:e27868. https://doi.org/10.2196/27868 .
Fussell SR, Kiesler S, Setlock LD, Yew V. How people anthropomorphize robots. Proc. 3rd ACMIEEE Int. Conf. Hum. Robot Interact., New York, NY, USA: Association for Computing Machinery; 2008, p. 145–52. https://doi.org/10.1145/1349822.1349842 .
Kühne R, Peter J. Anthropomorphism in human–robot interactions: a multidimensional conceptualization. Commun Theory. 2023;33:42–52. https://doi.org/10.1093/ct/qtac020 .
Dubois-Sage M, Jacquet B, Jamet F, Baratgin J. We Do Not Anthropomorphize a Robot Based Only on Its Cover: Context Matters too! Appl Sci. 2023;13:8743. https://doi.org/10.3390/app13158743 .
Article CAS Google Scholar
Riek LD, Rabinowitch T-C, Chakrabarti B, Robinson P, Empathizing with robots: Fellow feeling along the anthropomorphic spectrum. 3rd Int. Conf Affect Comput Intell Interact Workshop. 2009;2009:1–6. https://doi.org/10.1109/ACII.2009.5349423 .
Watford-Spence ATR. The effectiveness and usability of companion robots in combating loneliness in the elderly in mental healthcare : a scoping literature review 2022.
Bemelmans R, Gelderblom GJ, Jonker P, de Witte L. Socially assistive robots in elderly care: a systematic review into effects and effectiveness. J Am Med Dir Assoc. 2012;13:114-120.e1. https://doi.org/10.1016/j.jamda.2010.10.002 .
Pu L, Moyle W, Jones C, Todorovic M. The effectiveness of social robots for older adults: a systematic review and meta-analysis of randomized controlled studies. Gerontologist. 2019;59:e37-51. https://doi.org/10.1093/geront/gny046 .
Bousardt HM. Using embodied conversational agents to combat loneliness in older adults : a scoping review 2022.
Abbott R, Orr N, McGill P, Whear R, Bethel A, Garside R, et al. How do “robopets” impact the health and well-being of residents in care homes? A systematic review of qualitative and quantitative evidence. Int J Older People Nurs. 2019;14: e12239. https://doi.org/10.1111/opn.12239 .
Borenstein M, Hedges LV, Higgins JPT, Rothstein HR. Introduction to meta-analysis. Wiley: Hoboken; 2021.
Book Google Scholar
Page MJ, McKenzie JE, Bossuyt PM, Boutron I, Hoffmann TC, Mulrow CD, et al. The PRISMA 2020 statement: an updated guideline for reporting systematic reviews. BMJ. 2021;372: n71. https://doi.org/10.1136/bmj.n71 .
Chen S-C, Moyle W, Jones C, Petsky H. A social robot intervention on depression, loneliness, and quality of life for Taiwanese older adults in long-term care. Int Psychogeriatr. 2020;32:981–91. https://doi.org/10.1017/S1041610220000459 .
Rahatul A. Can Robots Help with Loneliness? University of Manitoba, 2020.
Bennett CC, Sabanovic S, Piatt JA, Nagata S, Eldridge L, Randall NA, Robot a Day Keeps the Blues Away. IEEE Int. Conf Healthc Inform ICHI. 2017;2017:536–40. https://doi.org/10.1109/ICHI.2017.43 .
Kanamori M, Suzuki M, Tanaka M. Maintenance and improvement of quality of life among elderly patients using a pet-type robot. Jpn J Geriatr. 2002;39:214–8. https://doi.org/10.3143/geriatrics.39.214 .
Fields N, Xu L, Greer J, Murphy E. Shall I compare thee...to a robot? An exploratory pilot study using participatory arts and social robotics to improve psychological well-being in later life. Aging Ment Health 2021;25:575–84. https://doi.org/10.1080/13607863.2019.1699016 .
Ollier J, Neff S, Dworschak C, Sejdiji A, Santhanam P, Keller R, et al. Elena+ Care for COVID-19, a Pandemic Lifestyle Care Intervention (preprint) Preprint.
Jones VK, Hanus M, Yan C, Shade MY, Blaskewicz Boron J, Maschieri BR. Reducing loneliness among aging adults: the roles of personal voice assistants and anthropomorphic interactions. Front Public Health. 2021;9:750736. https://doi.org/10.3389/fpubh.2021.750736 .
Kramer LL, van Velsen L, Clark JL, Mulder BC, de Vet E. Use and effect of embodied conversational agents for improving eating behavior and decreasing loneliness among community-dwelling older adults: randomized controlled trial. JMIR Form Res. 2022;6: e33974. https://doi.org/10.2196/33974 .
Fogelson DM, Rutledge C, Zimbro KS. The Impact of Robotic Companion Pets on Depression and Loneliness for Older Adults with Dementia During the COVID-19 Pandemic. J Holist Nurs Off J Am Holist Nurses Assoc 2021:8980101211064605. https://doi.org/10.1177/08980101211064605 .
Bickmore T, Caruso L, Clough-Gorr K, Heeren T. ‘It’s just like you talk to a friend’ relational agents for older adults. Interact Comput. 2005;17:711–35. https://doi.org/10.1016/j.intcom.2005.09.002 .
Banks MR, Willoughby LM, Banks WA. Animal-assisted therapy and loneliness in nursing homes: use of robotic versus living dogs. J Am Med Dir Assoc. 2008;9:173–7. https://doi.org/10.1016/j.jamda.2007.11.007 .
Robinson NL, Cottier TV, Kavanagh DJ. Psychosocial health interventions by social robots: systematic review of randomized controlled trials. J Med Internet Res. 2019;21: e13203. https://doi.org/10.2196/13203 .
Papadopoulos C, Castro N, Nigath A, Davidson R, Faulkes N, Menicatti R, et al. The CARESSES randomised controlled trial: exploring the health-related impact of culturally competent artificial intelligence embedded into socially assistive robots and tested in older adult care homes. Int J Soc Robot. 2022;14:245–56. https://doi.org/10.1007/s12369-021-00781-x .
Billingham SA, Whitehead AL, Julious SA. An audit of sample sizes for pilot and feasibility trials being undertaken in the United Kingdom registered in the United Kingdom Clinical Research Network database. BMC Med Res Methodol. 2013;13:104. https://doi.org/10.1186/1471-2288-13-104 .
Robinson H, Macdonald B, Kerse N, Broadbent E. The psychosocial effects of a companion robot: a randomized controlled trial. J Am Med Dir Assoc. 2013;14:661–7. https://doi.org/10.1016/j.jamda.2013.02.007 .
Loveys K. Developing engaging digital humans for psychotherapeutic applications. Thesis. ResearchSpace@Auckland, 2021.
Vardoulakis LP, Ring L, Barry B, Sidner CL, Bickmore T. Designing relational agents as long term social companions for older adults. In: Nakano Y, Neff M, Paiva A, Walker M, editors. Intell. Virtual Agents, Berlin, Heidelberg: Springer; 2012, p. 289–302. https://doi.org/10.1007/978-3-642-33197-8_30 .
Michie S, Richardson M, Johnston M, Abraham C, Francis J, Hardeman W, et al. The behavior change technique taxonomy (v1) of 93 hierarchically clustered techniques: building an international consensus for the reporting of behavior change interventions. Ann Behav Med. 2013;46:81–95. https://doi.org/10.1007/s12160-013-9486-6 .
Mertens S, Herberz M, Hahnel UJJ, Brosch T. The effectiveness of nudging: A meta-analysis of choice architecture interventions across behavioral domains. Cogn Sci n.d.:10.
Maier M, Bartoš F, Stanley TD, Shanks DR, Harris AJL, Wagenmakers E-J. No evidence for nudging after adjusting for publication bias. Proc Natl Acad Sci. 2022;119:e2200300119. https://doi.org/10.1073/pnas.2200300119 .
Szaszi B, Higney A, Charlton A, Gelman A, Ziano I, Aczel B, et al. No reason to expect large and consistent effects of nudge interventions. Proc Natl Acad Sci. 2022;119: e2200732119. https://doi.org/10.1073/pnas.2200732119 .
Borenstein M. Common mistakes in meta-analysis and how to avoid them. Englewood, NJ, USA: Biostat, Inc.; 2019.
Vevea JL, Woods CM. Publication bias in research synthesis: sensitivity analysis using a priori weight functions. Psychol Methods. 2005;10:428–43. https://doi.org/10.1037/1082-989X.10.4.428 .
NHS. The Topol Review: Preparing the healthcare workforce to deliver the digital future. London: Health Education England; 2019.
Husk K, Elston J, Gradinger F, Callaghan L, Asthana S. Social prescribing: where is the evidence? Br J Gen Pract. 2019;69:6–7. https://doi.org/10.3399/bjgp19X700325 .
Masi CM, Chen H-Y, Hawkley LC, Cacioppo JT. A Meta-Analysis of Interventions to Reduce Loneliness. Personal Soc Psychol Rev Off J Soc Personal Soc Psychol Inc 2011;15: https://doi.org/10.1177/1088868310377394 . https://doi.org/10.1177/1088868310377394 .
Kimberlee R, Bertotti M, Dayson C, Asthana S, Polley M, Burns L, et al. The economic impact of social prescribing 2022.
Akhter-Khan SC, Au R. Why Loneliness Interventions Are Unsuccessful: A Call for Precision Health. Adv Geriatr Med Res 2020;4. https://doi.org/10.20900/agmr20200016 .
Dale R. GPT-3: What’s it good for? Nat Lang Eng. 2021;27:113–8. https://doi.org/10.1017/S1351324920000601 .
The Economist. Is Google’s 20-year dominance of search in peril? The Economist 2023.
Quintana DS. A guide for calculating study-level statistical power for meta-analyses. Adv Methods Pract Psychol Sci. 2023;6:25152459221147260. https://doi.org/10.1177/25152459221147260 .
Nakagawa S. A farewell to Bonferroni: the problems of low statistical power and publication bias. Behav Ecol. 2004;15:1044–5. https://doi.org/10.1093/beheco/arh107 .
Chen S-C, Jones C, Moyle W. The Impact of Engagement with the PARO Therapeutic Robot on the Psychological Benefits of Older Adults with Dementia. Clin Gerontol 2022;0:1–13. https://doi.org/10.1080/07317115.2022.2117674 .
Buecker S, Mund M, Chwastek S, Sostmann M, Luhmann M. Is loneliness in emerging adults increasing over time? A preregistered cross-temporal meta-analysis and systematic review. Psychol Bull. 2021;147:787–805. https://doi.org/10.1037/bul0000332 .
ter Stal S, Kramer LL, Tabak M, op den Akker H, Hermens H. Design features of embodied conversational agents in ehealth: a literature review. Int J Hum-Comput Stud 2020;138:102409. https://doi.org/10.1016/j.ijhcs.2020.102409 .
Turkle S. Reclaiming Conversation: The Power of Talk in a Digital Age. New York: New York: Penguin Publishing Group; 2015.
Jeffrey K, Abdallah S, Michaelson J. The Cost of Loneliness to UK Employers. London: New Economics Foundations; 2017.
Mihalopoulos C, Le LK-D, Chatterton ML, Bucholc J, Holt-Lunstad J, Lim MH, et al. The economic costs of loneliness: a review of cost-of-illness and economic evaluation studies. Soc Psychiatry Psychiatr Epidemiol 2020;55:823–36. https://doi.org/10.1007/s00127-019-01733-7 .
Peytrignet S, Garforth-Bles S, Keohane K. Loneliness monetisation report. London: Simetrica; 2020.
Ollier J, Suryapalli P, Fleisch E, Wangenheim F von, Mair JL, Salamanca-Sanabria A, et al. Can digital health researchers make a difference during the pandemic? Results of the single-arm, chatbot-led Elena+: Care for COVID-19 interventional study. Front Public Health 2023;11. https://doi.org/10.3389/fpubh.2023.1185702 .
Download references
Acknowledgements
We would like to thank LSE staff for additional feedback, including Alina Velias, Andra Fry, Jessica Kong, and Georgia Nichols. We thank Nina Shahrizad for proofreading. We would also like to thank all primary authors who made this systematic review and meta-analysis possible. After our analysis was completed, a final version of one of our included studies [ 36 ] was published, which was a preprint. This final version is available here [ 70 ].
Not applicable.
Author information
Authors and affiliations.
Department of Psychological and Behavioural Science, LSE, London, UK
Sia Sha, Haoran Shi, Dario Krpan & Matteo Galizzi
Department of Psychological Medicine, The University of Auckland, Auckland, New Zealand
Kate Loveys
Manchester Institute of Education, University of Manchester, Manchester, UK
Pamela Qualter
You can also search for this author in PubMed Google Scholar
Contributions
SS drafted the first version of the protocol and search strategy. All other authors provided feedback and approved the final protocol. SS, KL, and HS screened the titles and abstracts and full texts of citations, and these authors also extracted data from included studies. SS, KL, HS, and DK conducted risk of bias assessment. SS completed the data analysis. SS, KL, and PQ co-wrote the first version of the final manuscript. All authors provided feedback and approved the final manuscript. All authors had access to the underlying data. SS, KL, and HS verified the data.
Corresponding author
Correspondence to Sia Sha .
Ethics declarations
Ethics approval and consent to participate.
Since the systematic review and meta-analysis synthesised publicly available data, ethics approval was not required.
Consent for publication
Competing interest.
The authors declare no competing interests.
Additional information
Publisher’s note.
Springer Nature remains neutral with regard to jurisdictional claims in published maps and institutional affiliations.
Supplementary Information
Supplementary material 1., supplementary material 2., rights and permissions.
Open Access This article is licensed under a Creative Commons Attribution 4.0 International License, which permits use, sharing, adaptation, distribution and reproduction in any medium or format, as long as you give appropriate credit to the original author(s) and the source, provide a link to the Creative Commons licence, and indicate if changes were made. The images or other third party material in this article are included in the article's Creative Commons licence, unless indicated otherwise in a credit line to the material. If material is not included in the article's Creative Commons licence and your intended use is not permitted by statutory regulation or exceeds the permitted use, you will need to obtain permission directly from the copyright holder. To view a copy of this licence, visit http://creativecommons.org/licenses/by/4.0/ . The Creative Commons Public Domain Dedication waiver ( http://creativecommons.org/publicdomain/zero/1.0/ ) applies to the data made available in this article, unless otherwise stated in a credit line to the data.
Reprints and permissions
About this article
Cite this article.
Sha, S., Loveys, K., Qualter, P. et al. Efficacy of relational agents for loneliness across age groups: a systematic review and meta-analysis. BMC Public Health 24 , 1802 (2024). https://doi.org/10.1186/s12889-024-19153-x
Download citation
Received : 11 October 2023
Accepted : 14 June 2024
Published : 06 July 2024
DOI : https://doi.org/10.1186/s12889-024-19153-x
Share this article
Anyone you share the following link with will be able to read this content:
Sorry, a shareable link is not currently available for this article.
Provided by the Springer Nature SharedIt content-sharing initiative
- Relational agents
- Social robots
BMC Public Health
ISSN: 1471-2458
- General enquiries: [email protected]
Automation has reached its tipping point for omnichannel warehouses
Never before has the competition for warehouse and fulfillment labor been so fierce, strongly driven by sustained growth in B2C channels. In the United States, for example, employment levels across distribution centers are at all-time highs and wages have risen to well above $18 an hour, yet attracting and retaining warehouse employees remains elusive. In the short term, strategies such as bonuses, accelerated pay raises, and tuition reimbursement are helping. But the long-term implications of a high reliance on labor are clear: automation in warehousing is no longer just nice to have but an imperative for sustainable growth.
About the authors
This article is a collaborative effort by John Barbee, Alan Davies, Raoul Dubeauclard, Tim Lange, and Christoph Lennartz, representing views from McKinsey’s Consumer & Retail Practice.
Fueled by venture capital over the past five years, the automation industry has seen increased availability of new warehouse-automation innovations, supply chain as a service (SCaaS) models, and technology that integrates multiple solutions to help retailers address some of these challenges.
For instance, the adoption of autonomous mobile robots (AMRs), technology that eliminates significant nonproductive walking time in warehouses, has progressed from early-stage pilots about four years ago to multiple at-scale deployments today. For example, DHL rolled out 1,000 Locus Robotics AMRs and will deploy up to 2,000 robots by 2022. 1 Steven Crowe, “Locus Robotics scaling AMR deployments with DHL supply chain,” The Robot Report , June 2, 2021, therobotreport.com. These technologies aren’t just for larger companies. German toy retailer Rofu Kinderland built a new warehouse that includes 57 robots retrieving 3,500 different products from more than 28,000 bins, increasing efficiency and delivery speeds. 2 Melanie Wack, “E-Commerce: AutoStore für Spiel-, Schreibwaren und Dekorationsartikel,” Logistik Heute , November 12, 2020, logistik-heute.de. Innovators are gaining momentum as well. For example, Alert Innovation developed a microfulfillment-center technology platform for Walmart to handle the demands of grocery e-commerce. The proof-of-concept pilot system is now in full operation.
Automation capabilities will play an influential role in the future size and scale of omnichannel networks. To successfully navigate the many choices for automation, retailers must have an informed perspective on where automation can create value, reduce risk, and improve reliability across an increasingly complex network of fulfillment nodes. Retailers should then use a three-step process—strategy, design, and implementation—to translate their vision into an optimal automated warehouse.
The changing face of the warehouse-automation industry
Investments from retailers in automation are poised to fuel significant industry growth: the warehouse-automation market is forecast to reach $51 billion by 2030, a CAGR of 23 percent. 3 David Edwards, “Revenues from robotics in warehouses to exceed $51 billion by 2030,” Robotics and Automation News , August 19, 2021, roboticsandautomationnews.com. The wave of innovation in warehousing has been fueled disproportionately by venture-capital funding for new start-ups, whose solutions increasingly influence the future of the omnichannel warehouse. In addition, private equity has provided a significant tailwind for key companies. For example, SoftBank invested $2.8 billion in AutoStore, an automation provider geared to the e-commerce and grocery industries. 4 Sam Shead, “SoftBank invests $2.8 billion in Norwegian robotics firm AutoStore,” CNBC, April 6, 2021, cnbc.com. Many of these technologies—for example, automated guided vehicles (AGVs) or the next level of automation AMRs as unmanned transport next to warehouse employees—have proved their effectiveness at scale in addressing the challenges of traditional e-commerce warehouses, such as labor shortages, SKU-complexity growth, and increasing service expectations.
Increased M&A and investment
Marketplaces and platform players have long recognized the importance of automation and have been rapidly acquiring robotics companies. Several at-scale investments have grabbed headlines. In 2019, the online marketplace Shopify spent $450 million to acquire automation provider 6 River Systems, with the goal of extending its AI-enabled fulfillment network. 5 Emil Protalinski, “Shopify acquires 6 River Systems for $450 million to expand its AI-powered fulfillment network,” VentureBeat, September 9, 2019, venturebeat.com. Amazon is developing proprietary automation solutions via Amazon Robotics to improve warehouse productivity and lessen the labor burden. Zalando has partnered with multiple automation partners to accelerate consumer-delivery times and improve operating efficiencies.
Several retailers have publicly committed significant capital toward their automation strategies. For instance, Walmart plans to allocate nearly $14 billion for warehouse automation and other business areas, 6 Tonya Garcia, “Walmart to invest nearly $14 billion in automation and other business areas in fiscal 2022,” MarketWatch, February 22, 2021, marketwatch.com. and ASOS announced $100 million in spending to expand the capacity and productivity of its warehouses. 7 “Retailer ASOS to spend $100M to automate Georgia warehouse,” U.S. News, July 12, 2021, usnews.com. These moves are indicative of an industry-wide focus on automation, now even further accelerated as a response to changing market conditions brought about by the COVID-19 pandemic. 8 “Warehouse automation investment up due to COVID-19,” Material Handling and Logistics, July 13, 2020, mhlnews.com; Mark Dunaway, “Why are so many companies investing in warehouse automation?,” Modern Materials Handling, January 10, 2020, mmh.com.
Larger players in the warehouse-automation industry have sought to create distinctive and integrated capabilities through acquisition. For instance, Toyota Material Handling has acquired integrators including Vanderlande and Bastian Solutions, Kion Group has acquired Dematic and software company Digital Applications International, and Honeywell has acquired Intelligrated and Transnorm. Acquirers are seeking to develop more end-to-end solution sets rather than point technologies as they seek to unlock greater value through integrated solutions. The automation market remains concentrated, with the top five automation and material-handling players still accounting for more than 50 percent of current market share. 9 “Warehouse automation market,” LogisticsIQ, 2021, thelogisticsiq.com. Beyond the top ten in each region, players mostly are specialty and niche automation providers.
Automation in action
Leading retailers are aiming to make warehouses responsive, resilient, and reliable to accommodate the ever-growing e-commerce market and incorporate lessons from the global pandemic. Along with improving existing warehouse capabilities and enabling new nodes of fulfillment (such as urban fulfillment centers), they view warehouse automation as an important part of the solution. In a recent McKinsey survey of 50 retailers across apparel, grocery, and other key sectors, more than 80 percent of respondents indicated they intend to increase automation investments over the next two to three years. 10 For more, see “ Retail speaks: Seven imperatives for the retail industry ” on McKinsey.com.
And it’s worth it: some retailers have cracked the code and have begun rolling out ambitious upgrades. As part of a €500 million initiative, Edeka invested €93 million to expand its existing warehouse in Berbersdorf, increasing the total number of SKUs from 2,900 to 12,700 while adding a 300,000-square-foot, partially automated picking-and-storage area. 11 Stefanie Schmitt, “EDEKA Nordbayern-Sachsen-Thüringen erweitert Logistikzentrum in Berbersdorf für rund 93 Mio. €,” EDEKA, March 12, 2021, verbund.edeka.
Leading retailers are aiming to make warehouses responsive, resilient, and reliable to accommodate the ever-growing e-commerce market.
Navigating automation choices
Multiple technological advancements have pushed the boundaries of what is possible in warehouse automation. As part of an overarching automation strategy, retailers that develop an end-to-end vision for the warehouse of the future have to identify the specific use cases and unlock value (Exhibit 1). Navigating the choices has become more complex, with new providers entering the market and larger conglomerates and venture-capital funds pursuing consolidation in an effort to build an integrated portfolio of solutions for clients. Acquisitions within the automation-provider landscape will continue, significantly increasing the pressure on automation companies to offer warehouses end-to-end solutions.
We envision three warehouse archetypes that will inform the design of automation systems: dedicated, shared, and integrated omnichannel (Exhibit 2). These archetypes can help retailers narrow down the set of use cases and solution sets and better understand the complex choices among automation providers, integrators, and start-ups.
Dedicated warehouses
This archetype consists of warehouses specifically designed for a given channel (such as e-commerce), product flow (for example, consolidation), or product type (apparel versus hard goods). Generally, dedicated warehouses solve for scale and cost efficiency in the network. Distribution formats can range from large-scale facilities that cover national distribution needs (more than one million square feet) to smaller, urban-based fulfillment centers (less than 20,000 square feet) that balance same-day and next-day speed with cost efficiency.
Given the specific focus of these distribution formats, integrated and specialized end-to-end automation concepts generally work best. These warehouses benefit from improved space efficiency, greater labor productivity, faster four-wall cycle time, 12 Cycle time from entering until exiting the warehouse. and downstream efficiencies (such as store-friendly pallets). Examples of dedicated warehouses include retail fulfillment (Amazon Go stores), national e-commerce fulfillment (Zara), store replenishment (such as Albertsons and Carrefour), delivery centers for small parcels (Post), and category-specific facilities (such as Reckitt Benckiser and Zalando).
Shared warehouses
Warehouses serving multiple channels or product segments, which may include wholesale and direct-to-consumer (DTC) channels or ambient and perishable-product segments, make up this archetype. While these warehouses exist under the same roof, the operations and inventories are independently managed by channel. This archetype offers greater flexibility than a dedicated warehouse in that multiple channels and categories may be served under one roof or in a campus setting. The multipurpose structure has several benefits: more efficient use of distribution space, cost savings from consolidated labor and overhead, and external advantages such as inbound consolidation. The systems technology and automation may need to support specific flows, handling requirements, and order profiles of each channel. As a result, individual warehouses still operate mostly independently. Automation solutions can still be integrated, but they may combine various fit-for-purpose technologies to address unique channel needs—for example, a retail-store warehouse could be on the side of the building (with automated pallet and case storage where store replenishment orders are prepared), while the e-commerce warehouse could be on mezzanines where individual units are picked with a multishuttle or autostore.
Integrated omnichannel warehouses
Omnichannel warehouses seamlessly serve all channels in the network and generally have the technology and systems to handle inventory across a mostly common stock pool (for example, the same picking locations or an automated storage system). These facilities offer the greatest flexibility in the network and reduce systemwide inventory-carrying costs, but retailers may have to make trade-offs on cycle time, dedicated capacity, and productivity. The set of automation solutions, which may be a hybrid of the capability or shared archetypes, could allow convergence in upstream warehouse processes such as inbound and storage. Distribution operations may have different requirements for fulfillment-execution processes to meet the needs of individual order profiles and channels. For example, online consumers might order small quantities and request a lead time of less than 24 hours, while stores might accept 48-hour or longer lead times with larger volumes being picked and shipped. Hence, the requirements in warehouse operations need to be matched along the steps across channels, balancing the trade-offs of solution benefits.
This archetype, which is best suited for stores that order in eaches 13 A unit of measure where each individual piece is tracked by the computer system. (an approach many apparel and electronics retailers take), can support a shared picking location between stores and online. It provides two benefits: First, it allows inventory pooling and the more efficient use of space. Second, it enables increased scale for automation and the better use of system capacity, with the ability to handle stores and online channels with different seasonality and peaks.
Because each type has its own advantages, identifying and implementing the optimal solution requires an informed decision-making process.
Selecting the right automation solutions
In response to a rapidly changing marketplace, many retailers are moving away from a single solution or turnkey provider and building a portfolio of solutions to fit their network. Traditionally, partnering with a turnkey provider offered advantages, such as integration across multiple solutions and pricing transparency. While this still holds true, the pace of innovation in solutions continues to accelerate, and innovations in technology and operating models provide compelling reasons to explore a multipartner strategy.
For instance, along with technological innovation, many new robotics and automation providers have innovated as-a-service models (XaaS), such as robotics as a service (RaaS) and fulfillment as a service (FaaS). These solutions alleviate the traditional hurdles of up-front capital risk (RaaS helps retailers overcome ROI hurdles such as a payback of two to three years) and offer retailers a variable cost structure better aligned to testing and learning across new technologies and concepts. Because of lower investment levels, retailers are now able to test and learn with selected partners, building up their automation capabilities. Companies can excel in innovation by replacing their tried-and-true approaches to warehouse automation with in-house capabilities to explore earlier-stage implementation. For example, companies can conduct a pilot with AMRs that assist picking operations alongside employees on site. The benefits of this experimentation can be significant—for example, progressing from proof of concept to large-scale implementations.
The range of design and implementation choices varies considerably depending on strategy. An AMR project may require six to eight weeks to pilot, whereas case multishuttles can take 12 months or more to accommodate infrastructure procurement and build-out. In our experience, a three-step process can help retailers determine the right approach to warehouse automation.
Traditionally, retailers might take a site-by-site view of their automation strategy. This exercise includes both establishing criteria for prioritizing automation opportunities and defining business cases to evaluate fit-for-purpose use cases and potential partners for a new or existing operation. We find the more innovative retailers are taking an end-to-end view of their network, developing scenarios for both productivity and short- and longer-term labor risks. A balanced approach to use cases may open up a variety of solutions, while the site-to-site approach focuses solely on payback for individual locations.
In this step—segmented into preconceptual, conceptual, and detailed design—retailers conduct a financial evaluation, create optimized warehouse designs, and select providers by stress testing simulations. The design workshops include retailers, joined by their chosen automation suppliers; beyond them, an objective, informed, third-party perspective—for example, via consultancy—is essential to reach the optimal design. The design process goes beyond the selection of automation to encompass warehouse analytics, strategic network effects, and much more.
Implementation
Where necessary, retailers can identify and select a warehouse-automation system integrator or can orchestrate across a set of partners to build the case-specific automated warehouse. Some companies may also select a logistics service provider to operate the new warehouses and orchestrate the warehouse launch, based on a case-by-case evaluation.
This process has repeatedly captured substantial value because even small decisions (for example, initial product-segment growth assumptions that, in the end, significantly influence automation-picking capacities) have a major impact on projects of this scale. By following this holistic approach, retailers can create a compelling business case for automation and gain buy-in for investments.
The rise of e-commerce in omnichannel has elevated the demand for warehouse automation across industries. Retailers that innovate in this space can keep pace with high consumer expectations for service and personalization. A structured approach helps to pinpoint their current status, identify available and suitable options, and implement warehouse automation and utilization—including harnessing analytics enabled by warehouse automation. With these insights, companies can select the optimal automation for their warehouses.
John Barbee is a partner in McKinsey’s Atlanta office, Alan Davies is a senior expert in the Miami office, Raoul Dubeauclard is a senior expert in the Lyon office, Tim Lange is a partner in the Cologne office, and Cristoph Lennartz is a consultant in the Düsseldorf office.
The authors wish to thank Lucas Clement, Katharina Hauck, and Michael Kalt for their contributions to this article.
Explore a career with us
Disease and Health Research - New Insights Vol. 1

Prevalence of Hypertension Disorders among Adolescent Students in Ghana
- Abena Sekyere
Disease and Health Research - New Insights Vol. 1 , 10 July 2024 , Page 1-10 https://doi.org/10.9734/bpi/dhrni/v1/909 Published: 2024-07-10
- View Article
Aims: The main purpose of this study is to investigate the prevalence of hypertension, associated risk factors, and its effect on physical activity in young people aged 14-19 years.
Study Design: This is a Cross-sectional study.
Place and Duration of Study: Sample: Department of Biochemistry, College of Science, Kwame Nkrumah University of Science and Technology between June 2009 and July 2023.
Methodology: A multistage sampling method was used to select 909 youth from three secondary schools (three towns in the Ashanti region of Ghana). The follow-up study was conducted on 142 people with high blood pressure. Anthropometric and blood pressure were measured with approved devices. Measurement of physical activity and dietary intake via survey. Blood pressure was measured using a validated automatic sphygmomanometer, with participants seated comfortably and resting for at least five minutes before measurement. Three readings were taken at one-minute intervals, and the average of the last two was recorded to reduce the impact of stress or short-term changes. Systolic and diastolic blood pressures were the main parameters recorded. Additionally, the study evaluated cardiovascular parameters (heart rate, echocardiography, ECG) and renal parameters (serum creatinine, GFR, urinalysis, BUN).
Results: Research shows that the prevalence of high blood pressure is 9.1% and the incidence of prehypertension is 24.8%. The prevalence of high blood pressure is significant by school choice. A majority of men have higher blood pressure than women, with 10.6% having high blood pressure, 46.6% having prehypertension, and 42.6% having high blood pressure. Data shows that 81.6% of participating parents are self-employed. The results show that there is little work, 72.73% of hypertensive patients are not working, while 40.32% and 59.97% of inactive and hypertensive patients are still sedentary. The results showed that MCV was negatively correlated with systolic blood pressure and diastolic blood pressure (r=-0.230, r=-0.183), while MCHC was negatively correlated with systolic blood pressure and diastolic blood pressure (r = 0.171). showed., r = 0.256). RDW-SD was found to be negatively associated with systolic and diastolic blood pressure. There was a negative correlation between P-LCR and systolic and diastolic blood pressure (r=-.185, r=-.167).
Conclusion: The research found that blood pressure distribution varied among students in different schools, with the highest levels in KASS (57% hypertensive) compared to KOSS (19%) and BONWIRE (7%) (X^2 = 32.993, df = 4, p < 0.001). Despite examining cultural and lifestyle factors, no significant determinants of hypertension were identified. Lipid analysis also showed no significant differences between blood pressure groups. These findings highlight the need for further research to explore other potential causes of high blood pressure and to develop effective prevention and management strategies.
- Hypertension
- organ function
- pre-hypertension
- normotensives
- blood pressure
- diastolic blood pressure
- hematological indice
- Review History
How to Cite
- Endnote/Zotero/Mendeley (RIS)
- Abstract Viewed : 0 times
- Review History Downloaded : 0 times
Download Statastics
Tablets and Apps for Promoting Nanoliteracy in Early Childhood Education: Results from an Experimental Study
- Published: 09 July 2024
Cite this article
- Pandora Dorouka ORCID: orcid.org/0000-0003-2267-7592 1 ,
- Michail Kalogiannakis ORCID: orcid.org/0000-0002-9124-2245 2 &
- Ron Blonder ORCID: orcid.org/0000-0003-4796-4678 3
Successful integration of digital technologies in the education of young children still needs to be solved. Despite a growing body of research focusing on learning through digital technologies in childhood, there are areas of knowledge where the impact of digital technologies has yet to be explored. A prominent example is nanoscience and nanotechnology (NST), a new interdisciplinary field that promises to solve long-standing global challenges. Considering that NST concerns elements that cannot be observed with the naked eye, their understanding by young children requires appropriate teaching methods. These distinctive aspects of NST align well with the capabilities of smart mobile devices, the critical feature of which is their ability to display interactive simulations and playful visualizations. This study investigates and compares the effect of using tablets and alternative experiential teaching on developing the ability to understand nanoscale elements. To implement the research, we conducted a week-long intervention, including experimental and control groups. Children in the experimental group participated in a nanoteaching session during the school curriculum, using educational software on tablets. The children in the control group participated in a precisely similar instruction but without using technology. To assess the children’s performance, the Nanoscale Elementary Knowledge Comprehension Test (TENANO) created for the needs of this study was used. The sample consisted of 101 s-grade primary school children in Greece. The results showed that teaching with tablets compared to alternative experiential teaching contributed significantly to developing young children’s nanoliteracy level. Moreover, gender and non-verbal cognitive ability did not seem to differentiate the development of children’s ability to understand nanoscale entities.
This is a preview of subscription content, log in via an institution to check access.
Access this article
Subscribe and save.
- Get 10 units per month
- Download Article/Chapter or Ebook
- 1 Unit = 1 Article or 1 Chapter
- Cancel anytime
Price includes VAT (Russian Federation)
Instant access to the full article PDF.
Rent this article via DeepDyve
Institutional subscriptions
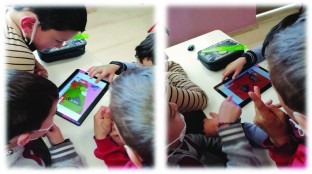
Availability of Data and Materials
The datasets used and/or analyzed during the current study are available from the corresponding author on reasonable request.
Akoumianakis, I., & Filippatos, T. (2020). The renin–angiotensin–aldosterone system as a link between obesity and coronavirus disease 2019 severity. Obesity Reviews, 21 (9), e13077.
Article Google Scholar
Aladé, F., Lauricella, A. R., Beaudoin-Ryan, L., & Wartella, E. (2016). Measuring with Murray: Touchscreen technology and preschoolers’ STEM learning. Computers in Human Behavior, 62 , 433–441.
Anthony, L., Brown, Q., Nias, J., Tate, B., & Mohan, S. (2012). Interaction and recognition challenges in interpreting children’s touch and gesture input on mobile devices. Proceedings of the 2012 ACM international conference on Interactive tabletops and surfaces (pp. 225–234). Association for Computing Machinery.
Chapter Google Scholar
Aunio, P., & Niemivirta, M. (2010). Predicting children’s mathematical performance in grade one by early numeracy. Learning and Individual Differences, 20 (5), 427–435.
Aunio, P., Aubrey, C., Godfrey, R., Pan, Y., & Liu, Y. (2008). Children’s early numeracy in England, Finland and People’s Republic of China. International Journal of Early Years Education, 16 (3), 203–221.
Aunola, K., Leskinen, E., Lerkkanen, M., & Nurmi, J. E. (2004). Developmental dynamics of math performance from preschool to grade 2. Journal of Educational Psychology, 96 , 699–713.
Beschorner, B., & Hutchison, A. (2013). iPads as a literacy teaching tool in early childhood. International Journal of Education in Mathematics, Science and Technology, 1 , 16–24.
Google Scholar
Blonder, R., & Rap, S. (2013). It’s a small world after all: A nanotechnology activity in a science festival. Journal of Nano Education, 4 , 47–56.
Blonder, R., & Sakhnini, S. (2012). Teaching two basic nanotechnology concepts in secondary school by using a variety of teaching methods. Chemistry Education Research and Practice, 13 , 500–516.
Blonder, R., & Yonai, E. (2020). Exposing school students to nanoscience: A review of published programs. In K. D. Sattler (Ed.), 21st century nanoscience – A handbook: Public policy, education, and global trends (1st ed., Vol. 10). Taylor & Francis (CRC Press).
Calder, N. (2015). Apps: Appropriate, applicable, and appealing? In T. Lowrie & R. Jorgensen (Zevenbergen) (Eds.), Digital games and mathematics learning, mathematics education in the digital era (Vol. 4, pp. 233–250). Netherlands: Springer.
Chen, G., Cheng, W., Chang, T. W., Zheng, X., & Huang, R. (2014). A comparison of reading comprehension across paper, computer screens, and tablets: Does tablet familiarity matter? Journal of Computers in Education, 1 (2–3), 213–225.
Chmiliar, L. (2017). Improving learning outcomes: The iPad and preschool children with disabilities. Frontiers in psychology, 8 , 660.
Ciampa, K., & Gallagher, T. L. (2013). Getting in touch: Use of mobile devices in the elementary classroom. Computers in the Schools, 30 (4), 309–328.
Clarke, L., & Abbott, L. (2016). Young pupils’, their teacher’s and classroom assistants’ experiences of iPads in a Northern Ireland school: “Four and five years old, who would have thought they could do that?” British Journal of Educational Technology, 47 (6), 1051–1064.
Clements, D. H. (1999). Playing math with young children. Curriculum Administrator, 35 (4), 25–28.
Clements, D. H., & Sarama, J. (2007). Effects of a preschool mathematics curriculum: Summative research on the Building Blocks project. Journal for Research in Mathematics Education, 38 , 136–163.
Clements, D. H., & Sarama, J. (2008). Mathematics and technology: Supporting learning for students and teachers. In O. N. Saracho & B. Spodek (Eds.), Contemporary perspectives on science and technology in early childhood education (pp. 127–147). Charlotte: Information Age.
Clements, D. H., & Sarama, J. (2013). Rethinking early mathematics: What is research based curriculum for young children? In L. D. English & J. T. Mulligan (Eds.), Reconceptualizing early mathematics learning (pp. 121–147). Springer.
Clements, D. H., Sarama, J., Spitler, M. E., Lange, A. A., & Wolfe, C. B. (2011). Mathematics learned by young children in an intervention based on learning trajectories: A large-scale cluster randomized trial. Journal for Research in Mathematics Education, 42 (2), 127–166.
Cohen, L., Manion, L., & Morrison, K. (2007). Research methods in education . Routledge.
Book Google Scholar
Cook, D. A., & Artino, A. R., Jr. (2016). Motivation to learn: An overview of contemporary theories. Medical Education, 50 (10), 997–1014.
Couse, L. J., & Chen, D. W. (2010). A Tablet computer for young children? Exploring its viability for early childhood education. Journal of Research on Technology in Education, 43 , 75–98.
Crescenzi, L., Jewitt, C., & Price, S. (2014). The role of touch in preschool children’s learning using iPad versus paper interaction. Australian Journal of Language and Literacy, 37 (2), 86–96.
Delgado, C., Stevens, S. Y., Shin, N., & Krajcik, J. (2015). A middle school instructional unit for size and scale contextualized in nanotechnology. Nanotechnology Reviews, 4 (1), 51–69.
Dorouka, P., & Kalogiannakis, M. (2023). Teaching nanotechnology concepts in early-primary education: an experimental study using digital games. International Journal of Science Education, 1–28.
Dorouka, P., Papadakis, S., & Kalogiannakis, M. (2020a). Tablets and apps for promoting robotics, mathematics, STEM education and literacy in early childhood education. International Journal of Mobile Learning and Organisation, 14 (2), 255–274.
Dorouka, P., Papadakis, St., & Kalogiannakis, M. (2020b). The influence of digital technology on young children’s “nano-literacy.” In K. Plakitsi, E. Kolokouri, & A.-C. Kornelaki (Eds.), ISCAR (International Society of Cultural-historical Activity Research) Regional Conference ‘Crisis in contexts’, e-proceedings (pp. 308–320). University of Ioannina. 19-24 March 2019.
Dorouka, P., Papadakis, St., & Kalogiannakis, M. (2021a). Nanotechnology and mobile learning: Perspectives and opportunities in young children’s education. International Journal of Technology Enhanced Learning, 13 (3), 237–252.
Dorouka, P., Papadakis, S., & Kalogiannakis, M. (2021b). The contribution of the health crisis to young children’s nano-literacy through STEAM education. Hellenic Journal of STEM Education, 2 (1), 1–7.
Dwyer, J. (2007). Computer-based learning in a primary school: Differences between the early and later years of primary schooling. Asia-Pacific Journal of Teacher Education, 35 (1), 89–103.
Goodwin, K. (2012). Use of tablet technology in the classroom (pp. 6–93). NSW Department of Education and Communities.
Guernsey, L. (2012). Can your preschooler learn anything from an iPad App? Retrieved on December 20, 2022, from http://goo.gl/en6Bme
Haßler, B., Major, L., & Hennessy, S. (2016). Tablet use in schools: A critical review of the evidence for learning outcomes. Journal of Computer Assisted Learning, 32 (2), 139–156.
Herodotou, C. (2017). Mobile games and science learning: A comparative study of 4 and 5 years old playing the game Angry Birds. British Journal of Educational Technology, 49 (1), 6–16.
Herodotou, C. (2018). Young children and tablets: A systematic review of effects on learning and development. Journal of Computer Assisted Learning, 34 (1), 1–9.
Hirsh-Pasek, K., Zosh, J. M., Golinkoff, R. M., Gray, J. H., Robb, M. B., & Kaufman, J. (2015). Putting education in Beducational^ apps: Lessons from the science of learning. Psychological Science in the Public Interest, 16 (1), 3–34.
Huber, B., Tarasuik, J., Antoniou, M. N., Garrett, C., Bowe, S. J., & Kaufman, J. (2016). Young children’s transfer of learning from a touchscreen device. Computers in Human Behavior, 56 , 56–64.
Hyde, J. S., & Mertz, J. E. (2009). Gender, culture, and mathematics performance. Proceedings of the National Academy of Sciences USA, 106 , 8801–8807.
İpek, Z., Atik, A. D., Tan, S., & Erkoç, F. (2020). Opinions of biology teachers about nanoscience and nanotechnology education in Turkey. International Journal of Progressive Education, 16 (1), 205–222.
Jayawardena, N. S., Ross, M., Quach, S., Behl, A., & Gupta, M. (2021). Effective online engagement strategies through gamification: A systematic literature review and a future research agenda. Journal of Global Information Management (JGIM), 30 (5), 1–25.
Jones, M. G., Blonder, R., & Kähkönen, A.-L. (2020). Challenges in nanoscience education. In K. D. Sattler (Ed.), 21st century nanoscience – A handbook: Public policy, education, and global trends. (Vol. 10). 10.1201/9780429351631: Taylor & Francis (CRC Press).
Jordan, N. C., Kaplan, D., Nabors Oláh, L., & Locuniak, M. N. (2006). Number sense growth in kindergarten: A longitudinal investigation of children at risk for mathematics difficulties. Child Development, 77 , 153–175.
Judge, S., Floyd, K., & Jeffs, T. (2015). Using mobile media devices and apps to promote young children’s learning. In K. Heider & M. Renck-Jalongo (Eds.), Young children and families in the information age. Educating the young child (pp. 117–131). Netherlands: Springer.
Kabali, H. K., Irigoyen, M. M., Nunez-Davis, R., Budacki, J. G., Mohanty, S. H., Leister, K. P., et al. (2015). Exposure and use of mobile media devices by young children. Pediatrics, 136 , 1044–1050.
Kähkönen, A. L., Laherto, A., Lindell, A., & Tala, S. (2016). Interdisciplinary nature of nanoscience: Implications for education. Global perspectives of nanoscience and engineering education (pp. 35–81). Springer.
Kalogiannakis, M., & Papadakis, S. (2020). The use of developmentally mobile applications for preparing pre-service teachers to promote STEM activities in preschool classrooms. In S. Papadakis & M. Kalogiannakis (Eds.), Mobile Learning applications in early childhood education (pp. 82–100). IGI Global.
Kalogiannakis, M., Papadakis, S., & Zourmpakis, A. I. (2021). Gamification in science education. A systematic review of the literature. Education Sciences, 11 (1), 22.
Kerckaert, St., Vanderlinde, R., & van Braak, J. (2015). The role of ICT in early childhood education: Scale development and research on ICT use and influencing factors. European Early Childhood Education Research Journal, 23 , 183–199.
Kolb, D. (1983). Experiential learning: Experience as the source of learning and development .
Kolb, D. A., Boyatzis, R. E., & Mainemelis, C. (2014). Experiential learning theory: Previous research and new directions. Perspectives on thinking, learning, and cognitive styles (pp. 227–247). Routledge.
Liu, N. S. H. (2013). iPad infuse creativity in solid geometry teaching. Turkish Online Journal of Education Technology, 12 , 177–192.
Lubienski, S. T., Robinson, J. P., Crane, C. C., & Ganley, C. M. (2013). Girls’ and boys’ mathematics achievement, affect, and experiences: Findings from ECLS-K. Journal for Research in Mathematics Education, 44 (4), 634–645.
Magana, A. J., Brophy, S. P., & Bryan, L. A. (2012). An integrated knowledge framework to characterize and scaffold size and scale cognition (FS2C). International Journal of Science Education, 34 (14), 2181–2203.
Mattoon, C., Bates, A., Shifflet, R., Latham, N., & Ennis, S. (2015). Examining computational skills in prekindergarteners: The effects of traditional and digital manipulatives in a prekindergarten classroom. Early Childhood Research and Practice, 17 (1), n1.
McManis, L. F. D., & Gunnewig, S. B. (2012). Finding the education in educational technology with early learners. YC Young Children, 67 , 14–24.
Mehdipour, Y., & Zerehkafi, H. (2013). Mobile learning for education: Benefits and challenges. International Journal of Computational Engineering Research, 3 (6), 93–101.
Miller, D., Robertson, D., Hudson, A., & Shimi, J. (2012). Signature pedagogy in early years education: A role for COTS game-based learning. Computers in the Schools, 29 (1–2), 227–247.
Milman, N. B., Carlson-Bancroft, A., & Boogart, A. V. (2014). Examining differentiation and utilization of iPads across content areas in an independent, preK–4th grade elementary school. Computers in the Schools, 31 (3), 119–133.
Mononen, R., & Aunio, P. (2013). Early mathematical performance in Finnish kindergarten and grade one. LUMAT, 1 (3), 245–261.
Murriello, S., Contier, D., & Knobel, M. (2006). Challenges of an exhibit on nanoscience and nanotechnology. Journal of Science Communication, 5 (4), A01.
Neumann, M. M. (2018). Using tablets and apps to enhance emergent literacy skills in young children. Early Childhood Research Quarterly, 42 , 239–246.
Neumann, M. M., & Neumann, D. L. (2014). Touch screen tablets and emergent literacy. Early Childhood Education Journal, 42 , 231–239.
NGSS Lead States. (2013). Next generation science standards: For states, by states . National Academies Press.
Nistor, G. C., & Iacob, A. (2018). The advantages of gamification and game-based learning and their benefits in the development of education. The International Scientific Conference eLearning and Software for Education (Vol. 1, pp. 308–312). “Carol I” National Defence University.
Nolan, J., & McBride, M. (2014). Beyond gamification: Reconceptualizing game-based learning in early childhood environments. Information, Communication & Society, 17 (5), 594–608.
Nunes, T., & Bryant, P. (1996). Children doing mathematics . Wiley-Blackwell.
Oakley, G., Wildy, H., & Berman, Y. E. (2018). Multimodal digital text creation using tablets and open-ended creative apps to improve the literacy learning of children in early childhood classrooms. Journal of Early Childhood Literacy . Online First Article.
Oliemat, E., Ihmeideh, F., & Alkhawaldeh, M. (2018). The use of touch-screen tablets in early childhood: Children’s knowledge, skills, and attitudes towards tablet technology. Children and Youth Services Review, 88 , 591–597.
Panagiotakopoulos, C. T., Sarris, M. E., & Koleza, E. G. (2013). Playing with numbers: Development issues and evaluation results of a computer game for primary school students. In T. Sobh & K. Elleithy (Eds.), Emerging trends in computing, informatics, systems sciences, and engineering (Vol. 151, pp. 263–275). Springer.
Papadakis, S., Kalogiannakis, M., & Zaranis, N. (2016). Comparing tablets and PCs in teaching mathematics: An attempt to improve mathematics competence in early 215 childhood education. Preschool and Primary Education, 4 (2), 241.
Papadakis, S., Kalogiannakis, M., & Zaranis, N. (2017). Improving mathematics teaching in kindergarten with realistic mathematical education. Early Childhood Education Journal, 45 , 369–378.
Papadakis, S., Kalogiannakis, M., & Zaranis, N. (2021). Teaching mathematics with mobile devices and the Realistic Mathematical Education (RME) approach in kindergarten. Advances in Mobile Learning Educational Research, 1 (1), 5–18.
Pasnik, S., & Llorente, C. (2013). Preschool teachers can use a PBS KIDS transmedia curriculum supplement to support young children’s mathematics learning: Results of a randomized controlled trial. Summative evaluation of the CPB-PBS ready to learn initiative . Waltham.
Pegrum, M., Oakley, G., & Faulkner, R. (2013). Schools going mobile: A study of the adoption of mobile handheld technologies in Western Australian independent schools. Australasian Journal of Educational Technology, 29 , 66–81.
Peikos, G., Spyrtou, A., Pnevmatikos, D., & Papadopoulou, P. (2020). Nanoscale science and technology education: Primary school students’ preconceptions of the lotus effect and the concept of size. Research in Science & Technological Education, 1–18.
Peikos, G., Spyrtou, A., Pnevmatikos, D., & Papadopoulou, P. (2022). A teaching learning sequence on nanoscience and nanotechnology content at primary school level: Evaluation of students’ learning. International Journal of Science Education, 44 (12), 1932–1957.
Peirce, N. (2013). Digital game-based learning for early childhood. A state of the art report . Learnovate Centre.
Penuel, W. R., Pasnik, S., Bates, L., Townsend, E., Gallagher, L. P., Llorente, C., & Hupert, N. (2009). Preschool teachers can use a mediarich curriculum to prepare lowincome children for school success: Results of a randomized controlled trial . Education Development Center Inc, and SRI International.
Plowman, L., Stevenson, O., Stephen, C., & McPake, J. (2012). Preschool children’s learning with technology at home. Computers & Education, 59 (1), 30–37.
Psycharis, S. (2016). The impact of computational experiment and formative assessment in inquiry-based teaching and learning approach in STEM education. Journal of Science Education and Technology, 25 (2), 316–326.
Reich, S. M., Yaw, J. C., & Warschauer, M. (2016). Tablet-based ebooks for young children: What does the research says? Journal of Developmental & Behavioral Pediatrics, 37 , 585–591.
Rideout, V. J. (2014). Learning at home: Families’ educational media use in America. A report of the families and media project . The Joan Ganz Cooney Center at Sesame Workshop.
Risconscente, M. (2012). Mobile learning games improves 5th graders’ fraction knowledge and attitudes . Los Angeles, CA: GameDesk Institute.
Roberts, T. A., Vadasy, P. F., & Sanders, E. A. (2018). Preschoolers’ alphabet learning: Letter name and sound instruction, cognitive processes, and English proficiency. Early Childhood Research Quarterly, 44 , 257–274.
Rogowsky, B. A., Terwilliger, C. C., Young, C. A., & Kribbs, E. E. (2018). Playful learning with technology: The effect of computer-assisted instruction on literacy and numeracy skills of preschoolers. International Journal of Play, 7 (1), 60–80.
Sakhnini, S., & Blonder, R. (2015). Essential concepts of nanoscale science and technology for high school students based on a Delphi study by the expert community. International Journal of Science Education, 37 (11), 1699–1738.
Schacter, J., & Jo, B. (2017). Improving preschoolers’ mathematics achievement with tablets: A randomized controlled trial. Mathematics Education Research Journal, 29 (3), 313–327.
Schroeder, E. L., & Kirkorian, H. L. (2016). When seeing is better than doing: Preschoolers’ transfer of STEM skills using touchscreen games. Frontiers in Psychology, 7 , 1–12.
Semmelmann, K., Nordt, M., Sommer, K., Röhnke, R., Mount, L., & Prüfer, H.,…& Weigelt, S. (2016). U can touch this: How tablets can be used to study cognitive development. Frontiers in Psychology, 7 , 1021.
Shamir, H., Feehan, K., & Yoder, E. (2017). Effects of personalized learning on kindergarten and first grade students’ early literacy skills. Proceedings of the 9th International Conference on Computer Supported Education (CSEDU 2017) (Vol. 2, pp. 273–279). Porto.
Sheehan, K. J., & Uttal, D. H. (2016). Children’s learning from touch screens: A dual representation perspective. Frontiers in Psychology, 7 , 1220.
Siraj-Blatchford, I., & Siraj-Blatchford, J. (2003). More than computers-information and communication technology in the early years . British Association for Early Childhood Education (Early Education).
Spelke, E. S. (2005). Sex differences in intrinsic attitude for mathematics and science. American Psychology, 60 , 950–958.
Spyrtou, A., Manou, L., Peikos, G., & Papadopoulou, P. (2018). Investigating the Secrets of the Nanoworld . Athens: Gutenberg - Dardanos. In Greek.
Starcic, A., & Bagon, S. (2014). ICT-supported learning for inclusion of people with special needs: Review of seven educational technology journals, 1970–2011. British Journal of Educational Technology, 45 (2), 202–230.
Stevens, S., Sutherland, L. M., & Krajcik, J. S. (2009). The big ideas of nanoscale science and engineering: A guidebook for secondary teachers . NSTA Press.
Tai, L., Zhu, G., Yang, M., Cao, L., Xing, X., Yin, G., & Zhu, Y. (2021). Nanometer-resolution in situ structure of the SARS-CoV-2 postfusion spike protein. Proceedings of the National Academy of Sciences, 118 (48), e2112703118.
Ting, Y. L. (2013). Using mobile technologies to create interwoven learning interactions: An intuitive design and its evaluation. Computers and Education, 60 , 1–13.
Tretter, T. R., Jones, M. G., Andre, T., Negishi, A., & Minogue, J. (2006a). Conceptual boundaries and distances: Students’ and experts’ concepts of the scale of scientific phenomena. Journal of Research in Science Teaching, 43 , 282–319.
Tretter, T. R., Jones, M. G., & Minogue, J. (2006b). Accuracy of scale conceptions in science: Mental maneuverings across many orders of spatial magnitude. Journal of Research in Science Teaching, 43 (10), 1061–1085.
Vavoula, G., & Karagiannidis, C. (2005). Designing mobile learning experiences. Panhellenic conference on informatics (pp. 534–544). Berlin, Heidelberg: Springer.
Verenikina, I., & Kervin, L. (2011). iPads, digital play and pre-schoolers. He Kupu, 2 , 4–19.
Wang, F., Xie, H., Wang, Y., Hao, Y., & An, J. (2016). Using touchscreen tablets to help young children learn to tell time. Frontiers in Psychology, 7 , 1800.
Watts, C. M., Moyer‐packenham, P. S., Tucker, S. I., Bullock, E. P., Shumway, J. F., Westenskow, A., & Jordan, K. (2016). An examination of children’s learning progression shifts while using touch screen virtual manipulative mathematics apps. Computers in Human Behavior, 64 , 814–828.
Wood, E., Petkovski, M., De Pasquale, D., Gottardo, A., Evans, M. A., & Savage, R. S. (2016). Parent scaffolding of young children when engaged with mobile technology. Frontiers in Psychology, 7 , 690.
Wu, W. H., Jim, Wu., & Y. C., Chen, C. Y., Kao, H. Y., Lin, C. H., & Huang, S. H. (2012). Review of trends from mobile learning studies: A meta-analysis. Computers in Education, 59 (2), 817–827.
Zaranis, N., Kalogiannakis, M., & Papadakis, S. (2013). Using mobile devices for teaching realistic mathematics in kindergarten education. Creative Education, 4 , 1–10.
Zevenbergen, R., & Logan, H. (2008). Computer use by preschool children: Rethinking practice as digital natives come to preschool. Australian Journal of Early Childhood, 33 , 37–44.
Zhou, S. (2022). Effect of mobile learning on the optimization of preschool education teaching mode under the epidemic. Wireless Communications and Mobile Computing, 2022 , 2194373.
Download references
The research work was supported by the Hellenic Foundation for Research and Innovation (HFRI) under the 3rd Call for HFRI PhD Fellowships (Fellowship Number 5503).
Author information
Authors and affiliations.
Department of Preschool Education, University of Crete, 74100, Rethymno, Greece
Pandora Dorouka
Department of Special Education, University of Thessaly, Volos, Greece
Michail Kalogiannakis
Department of Science Teaching, Weizmann Institute of Science, 76100, Rehovot, Israel
Ron Blonder
You can also search for this author in PubMed Google Scholar
Contributions
Conceptualization, investigation, drafting, and writing, PD; methodology, validation, review and editing, and visualization, PD; writing—review and editing, and supervision, PD; MK; RB. All authors have read and agreed to the published version of the manuscript.
Corresponding author
Correspondence to Pandora Dorouka .
Ethics declarations
Ethical approval.
Requirements regarding informed consent, confidentiality, and data use were carefully adhered to, both verbally and in writing, informing school staff, children, and guardians of the purpose of the study and their rights not to participate. In particular, before starting the first phase of the research, the research protocol was reviewed and approved by the Ethics Committee of the Department of Preschool Education of the University of Crete (1016/22-11-2021). The approval was approved by the Ethics and Research Ethics Committee of the University of Crete (REC-UOC) (no. 35/24.02.2022, https://www.ehde.uoc.gr/index.php/en ), as well as by the Hellenic Institute of Educational Policy (IEP) (no. 3578/14-–03-2022).
Informed Consent
Informed consent was obtained from all individual participants included in the study.
Consent to Participate
Consent to participate was obtained from all individual participants included in the study.
Consent for Publication
All authors have read and agreed to the published version of the manuscript.
Competing Interests
The authors declare no competing interests.
Statement Regarding Research Involving Human Participants and/or Animals
The research protocol was reviewed and approved by the Ethics Committee of the Department of Preschool Education of the University of Crete (1016/22–11-2021). The approval was approved by the Ethics and Research Ethics Committee of the University of Crete (REC-UOC) (no. 35/24.02.2022, https://www.ehde.uoc.gr/index.php/en ), as well as by the Hellenic Institute of Educational Policy (IEP) (no. 3578/14–03-2022).
Additional information
Publisher's note.
Springer Nature remains neutral with regard to jurisdictional claims in published maps and institutional affiliations.
Rights and permissions
Springer Nature or its licensor (e.g. a society or other partner) holds exclusive rights to this article under a publishing agreement with the author(s) or other rightsholder(s); author self-archiving of the accepted manuscript version of this article is solely governed by the terms of such publishing agreement and applicable law.
Reprints and permissions
About this article
Dorouka, P., Kalogiannakis, M. & Blonder, R. Tablets and Apps for Promoting Nanoliteracy in Early Childhood Education: Results from an Experimental Study. J Sci Educ Technol (2024). https://doi.org/10.1007/s10956-024-10132-w
Download citation
Accepted : 12 June 2024
Published : 09 July 2024
DOI : https://doi.org/10.1007/s10956-024-10132-w
Share this article
Anyone you share the following link with will be able to read this content:
Sorry, a shareable link is not currently available for this article.
Provided by the Springer Nature SharedIt content-sharing initiative
- Educational apps
- Early primary education
- Nanoscale elements
- Experimental design
- Find a journal
- Publish with us
- Track your research
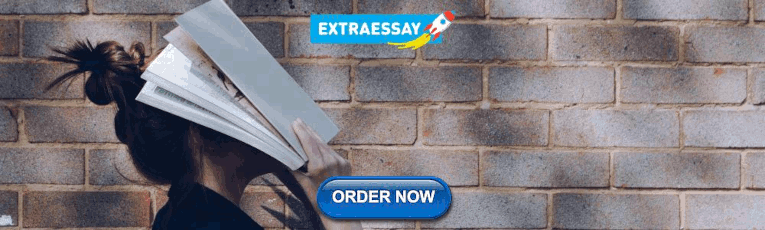
COMMENTS
A research design is a strategy for answering your research question using empirical data. Creating a research design means making decisions about: Your overall research objectives and approach. Whether you'll rely on primary research or secondary research. Your sampling methods or criteria for selecting subjects. Your data collection methods.
The study design used to answer a particular research question depends on the nature of the question and the availability of resources. In this article, which is the first part of a series on "study designs," we provide an overview of research study designs and their classification. The subsequent articles will focus on individual designs.
A research design is a strategy for answering your research question using empirical data and the right kind of analysis.
Research design refers to the overall plan, structure or strategy that guides a research project, from its conception to the final analysis of data. Research designs for quantitative studies include descriptive, correlational, experimental and quasi-experimenta l designs. Research designs for qualitative studies include phenomenological ...
In clinical research, our aim is to design a study which would be able to derive a valid and meaningful scientific conclusion using appropriate statistical methods. The conclusions derived from a research study can either improve health care or result in inadvertent harm to patients. Hence, this requires a well‐designed clinical research ...
This will guide your research design and help you select appropriate methods. Select a research design: There are many different research designs to choose from, including experimental, survey, case study, and qualitative designs. Choose a design that best fits your research question and objectives.
Study design is the key essential step in conducting successful research. There are many types of study designs in the biomedical field.
In this article, we will look at the important features of various types of research study designs used commonly in biomedical research.Ranganathan P. Understanding Research Study Designs. Indian J Crit Care Med 2019;23(Suppl 4):S305-S307.
Types of research can be categorized based on the research aims, the type of data, and the subjects, timescale, and location of the research.
Qualitative research methods focus on words and meanings, while quantitative research methods focus on numbers and statistics. Is your research more concerned with measuring something or interpreting something? You can also create a mixed methods research design that has elements of both. Descriptive vs experimental.
A research design is defined as the overall plan or structure that guides the process of conducting research. Learn more about research design types, methods and examples.
Research study design is a framework, or the set of methods and procedures used to collect and analyze data on variables specified in a particular research problem. Research study designs are of many types, each with its advantages and limitations. The type of study design used to answer a particular research question is determined by the ...
Have you ever wondered what is research design? Or wanted to know which type of research design is best for your study? We've got you covered! Read this article to understand what research design is, learn the different research design types and their characteristics, with examples.
Research design is a plan of investigation conceived to obtain an answer to research questions. Follow the steps for perfect research design.
A summary of study designs commonly used in medical research, including their characteristics, advantages and disadvantages.
Experimental design is the process of planning an experiment to test a hypothesis. The choices you make affect the validity of your results.
Research design is the framework of research methods and techniques chosen by a researcher to conduct a study. The design allows researchers to sharpen the research methods suitable for the subject matter and set up their studies for success. Creating a research topic explains the type of research (experimental,survey research,correlational ...
A research design typically outlines the theories and models underlying a project; the research question (s) of a project; a strategy for gathering data and information; and a strategy for producing answers from the data. [1] A strong research design yields valid answers to research questions while weak designs yield unreliable, imprecise or ...
Follows the seven steps: defining the problem, reviewing the literature, formulating a hypothesis, choosing a research design, collecting data, analyzing the data and interpretation and report writing. Includes a set of additional readings and library resources.
We believe novice qualitative researchers, students planning the design of a qualitative study or taking an introductory qualitative research course, and faculty teaching such courses can benefit from such a resource.
Research design is a plan to answer your research question. A research method is a strategy used to implement that plan. Research design and methods are different but closely related, because good research design ensures that the data you obtain will help you answer your research question more effectively.
The research design refers to the overall strategy that you choose to integrate the different components of the study in a coherent and logical way, thereby, ensuring you will effectively address the research problem; it constitutes the blueprint for the collection, measurement, and analysis of data. Note that your research problem determines the type of design you should use, not the other ...
Learn what research design is. Examine how to plan a research study, identify types of research design methods, and discover the purpose of...
Research Objectives Compare the sample composition resulting from a fresh address-based sample (ABS) frame with the sample collected from a state health probability panel of randomly-recruited panel members in the 2023 Minnesota Health Access Survey (MNHA). Examine differences in responses to a range of healthcare and health outcome measures such as health insurance, health status,...
The advent of LLMs should boost their efficacy, and further research is needed to explore the optimal design and use of relational agents. Future research could also address shortcomings of current results, such as small sample sizes and high risk of bias. Particularly young audiences have been overlooked in past research.
The design workshops include retailers, joined by their chosen automation suppliers; beyond them, an objective, informed, third-party perspective—for example, via consultancy—is essential to reach the optimal design.
Aims: The main purpose of this study is to investigate the prevalence of hypertension, associated risk factors, and its effect on physical activity in young people aged 14-19 years. Study Design: This is a Cross-sectional study. Place and Duration of Study: Sample: Department of Biochemistry, College of Science, Kwame Nkrumah University of Science and Technology between June 2009 and July 2023.
The novelty of this study lies in its integration of concepts pertaining to language acquisition, cooperative learning, and multimedia. This study addresses a significant research void by examining the multilingual experiences of both learners and instructors, offering valuable insights to enhance language education methodologies.
In particular, according to research (for example, Schacter & Jo, 2017), the educational use of new technologies offers new opportunities for early childhood children to engage effectively with mathematical concepts. ... 2014) since a between-subjects design was used. Future studies could turn their focus in this direction as well. Because ...