
An official website of the United States government
The .gov means it’s official. Federal government websites often end in .gov or .mil. Before sharing sensitive information, make sure you’re on a federal government site.
The site is secure. The https:// ensures that you are connecting to the official website and that any information you provide is encrypted and transmitted securely.
- Publications
- Account settings
Preview improvements coming to the PMC website in October 2024. Learn More or Try it out now .
- Advanced Search
- Journal List
- HHS Author Manuscripts

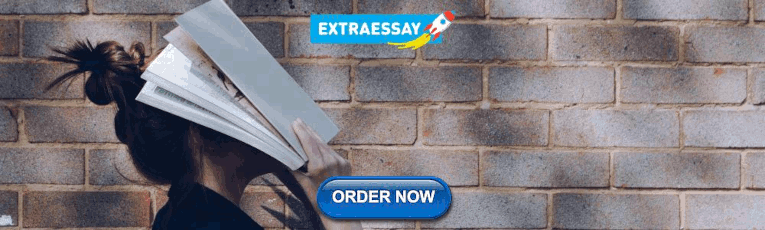
Trajectories of Big Five Personality Traits: A Coordinated Analysis of 16 Longitudinal Samples
Eileen k. graham.
1 Northwestern University, Chicago, IL USA
SARA J. WESTON
2 University of Oregon, Eugene, OR USA
DENIS GERSTORF
3 Humboldt University, Berlin, Germany
4 German Institute for Economic Research, Berlin, Germany
TOMIKO B. YONEDA
5 University of Victoria, Victoria, BC Canada
6 University of Edinburgh, Edinburgh, UK
CHRISTOPHER R. BEAM
7 University of Southern California, Los Angeles, CA USA
ANDREW J. PETKUS
Johanna drewelies, andrew n. hall, emily d. bastarache, ryne estabrook, mindy j. katz.
8 Albert Einstein College of Medicine, New York, NY USA
NICHOLAS A. TURIANO
9 University of West Virginia, Morgantown, WV USA
ULMAN LINDENBERGER
10 Max Planck Institute for Human Development, Berlin, Germany
JACQUI SMITH
11 University of Michigan, Ann Arbor, MI USA
GERT G. WAGNER
12 Berlin University of Technology, Berlin, Germany
NANCY L. PEDERSEN
13 Karolinska Institute, Solna, Sweden
MATHIAS ALLEMAND
14 University of Zurich, Zurich, Switzerland
AVRON SPIRO, III
15 VA Boston Healthcare System, Boston, MA USA
16 Boston University School of Public Health, Boston, MA USA
17 Boston University School of Medicine, Boston, MA USA
DORLY J.H. DEEG
18 VU University Medical Center, Amsterdam, The Netherlands
BOO JOHANSSON
19 University of Gothenburg, Gothenburg, Sweden
ANDREA M. PICCININ
Richard b. lipton, k. warner schaie.
20 Pennsylvania State University, State College, PA USA
SHERRY WILLIS
21 University of Washington, Seattle, WA USA
CHANDRA A. REYNOLDS
22 University of California, Riverside, Riverside, IL USA
IAN J. DEARY
Scott m. hofer, daniel k. mroczek, associated data.
This study assessed change in self-reported Big Five personality traits. We conducted a coordinated integrative data analysis using data from 16 longitudinal samples, comprising a total sample of over 60 000 participants. We coordinated models across multiple datasets and fit identical multi-level growth models to assess and compare the extent of trait change over time. Quadratic change was assessed in a subset of samples with four or more measurement occasions. Across studies, the linear trajectory models revealed declines in conscientiousness, extraversion, and openness. Non-linear models suggested late-life increases in neuroticism. Meta-analytic summaries indicated that the fixed effects of personality change are somewhat heterogeneous and that the variability in trait change is partially explained by sample age, country of origin, and personality measurement method. We also found mixed evidence for predictors of change, specifically for sex and baseline age. This study demonstrates the importance of coordinated conceptual replications for accelerating the accumulation of robust and reliable findings in the lifespan developmental psychological sciences.
INTRODUCTION
Questions remain regarding personality change in adulthood (especially in midlife and older adulthood), despite many studies over many decades. Recent work has embraced a more nuanced perspective than earlier approaches, which whipsawed from one extreme perspective (personality is unstable for nearly everyone) in the 1970s ( Mischel, 1969 , 1977 ), to its opposite (personality is stable for nearly everyone) in the 1980s and 1990s ( Costa & McCrae, 1980 , 1986 ). Over the last 10–15 years, a more integrative view has emerged, recognizing that personality does change, but likely in systematic ways, and that in any population or sample there will almost certainly be individual differences in change; that is, while some individuals may change, others may remain stable ( Roberts & Mroczek, 2008 ).
Recent work has also focused on antecedents to ( Allemand, Job, & Mroczek, 2019 ; Bleidorn et al., 2013 ; Mroczek & Spiro, 2003 ; Mühlig-Versen, Bowen, & Staudinger, 2012 ) or consequences of ( Graham & Lachman, 2012 ; Human et al., 2013 ; Mroczek & Spiro, 2007 ; Steiger, Allemand, Robins, & Fend, 2014 ; Turiano et al., 2011 ) personality change during middle and older adulthood. However, it is useful to step back from efforts to predict and explain personality change and carry out confirmatory work that circles back to fundamental questions of how personality changes. The current study aimed to address four basic research questions. First, what are the basic patterns of overall change for each of the Big Five traits, particularly in older adulthood? Second, are there individual differences in variations around those overall trajectories ( Nesselroade & Baltes, 1979 )? Third, if there are variations, what factors are associated with individual differences in personality change? Last, we posed a fourth question addressing issues of reproducibility, replicability, and generalizability ( Condon, Graham, & Mroczek, 2017 ). With the use of the language of multi-level (or mixed effects) modelling, the first question addressed the issue of fixed effects (average change), whereas the second two addressed random effects (individual variation around that average). Specifically, the second question concerned individual differences in rate of change, and the third question addressed predictors of these differences. We focused on sex and baseline age as variables that may be associated with individual differences in personality change, using 16 large longitudinal samples that were primarily comprised of midlife and older adults.
The fourth question is more meta-scientific in nature. Given that there is great heterogeneity of related findings in research on personality change, how can we enhance the reproducibility, replicability, and generalizability of our own findings? Using a methodological approach designed to enhance and inform replicability and drawing upon the data sharing network of the Integrative Analysis of Longitudinal Studies of Aging and Dementia (IALSA) project ( Hofer & Piccinin, 2009 ), we identified 16 longitudinal samples that included at least three occasions of personality assessment. Within each study, we independently estimated personality trait trajectories using the multi-level model (MLM) for change ( Singer & Willett, 2003 ), and then we summarized and synthesized the average trajectories applying meta-analytic techniques. This form of separate, but coordinated, modelling within each study is known as coordinated analysis and is a type of integrative data analysis ( Hofer & Piccinin, 2009 ). This technique is similar to individual participant data meta-analysis, which is being used increasingly in medicine and epidemiology to synthesize evidence across studies ( Riley, Lambert, & Abo-Zaid, 2010 ). This approach produces a group of replications (more accurately, a group of identical models fit to different samples) that otherwise would be very difficult to obtain owing to the extended data collection period of long-term longitudinal data. Additionally, a coordinated analysis preserves the separate findings across samples, permitting assessment of heterogeneity in effect sizes that may be due to sample differences (e.g. country of origin, age of sample, and measurement differences) or even to differences in true effect sizes across studies. This also permits an evaluation of the generalizability of the findings across varying samples, an issue we have discussed elsewhere in more detail ( Mroczek, Graham, Turiano, & Oro-Lambo, 2019 ; Mroczek, Weston, & Willroth, 2019 ). Thus, in addition to our substantive questions regarding the functional forms of the overall trajectories of Big Five traits, individual differences in trajectories, and predictors of change, our fourth question was more methodological, addressing whether we could identify between-sample variation in either overall patterns or individual differences in change.
Overall trajectories and current theoretical models of personality development
Overall or general patterns of personality change are nomothetic, or variable centred. These patterns capture how a given trait changes over time in a given sample, in contrast to how different persons change with time. They may be termed ‘meta-trajectories’ when they refer to population-level estimates of change ( Mroczek, Graham, et al., 2019 ). One of the more prominent theories of nomothetic or population-level trajectories is the neo-socioanalytic theory with its corollaries, the social investment principle, and the maturity principle ( Roberts & Mroczek, 2008 ; Roberts, Walton, & Viechtbauer, 2006 ; Roberts, Wood, & Caspi, 2008 ; Roberts, Wood, & Smith, 2005 ). These ideas posit that trait changes in adulthood tend to reflect an increase in socially acceptable characteristics (i.e. maturity) and a successful transition into adult roles that require additional responsibility (i.e. social investment: parenthood and work promotions) ( Caspi, Roberts, & Shiner, 2005 ; Roberts et al., 2006 , 2005 ; Roberts & Mroczek, 2008 ). Personality changes in young to mid-adulthood that are predicted by this theory include increasing conscientiousness, extraversion, openness, and agreeableness in young adulthood, along with declining neuroticism. Similarly, Denissen et al. (2013) proposed that norm-based reference values that operate via self-regulation strategies can bring about normative change such as that described by the maturity principle. This theory suggests that self-regulatory strategies are guided by social norms, as well as by personal values thus providing an explanation for both overall population-level trajectories (e.g. increasing conscientiousness) and variation around such overall trajectories (e.g. individual differences in rate of conscientiousness change). In essence, both the neo-socioanalytic ( Bogg, Voss, Wood, & Roberts, 2008 ) and self-regulation ( Denissen, van Aken, Penke, & Wood, 2013 ) theories argue that personality change is driven by the societal or cultural norms that govern adulthood roles, although Denissen et al. (2013) added personal values and expectations as a second set of influences.
However, the trait trends described above (decreasing neuroticism and increasing conscientiousness) are not consistent throughout older adulthood, suggesting that the above theories may be under-specified. One of the most theoretically compelling explanations for these personality trends in later life involves the concept of Baltes (1997) and Baltes and Baltes (1990) of selection, optimization, and compensation (SOC; ( Kandler, Kornadt, Hagemeyer, & Neyer, 2015 ; Mueller et al., 2019 ). The Baltes theory posits that development includes both gains and losses across most of the lifespan, whereby older adulthood is typically characterized by more losses. In order to optimize development in older adulthood, individuals must automatically or intentionally compensate for increasing losses and select by restricting domains and goals ( Baltes, 1987 ). The SOC theory of developmental regulation may help to explain trait trends that appear to decelerate or even reverse in later life ( Kandler et al., 2015 ; Mueller et al., 2016 ). For example, developing and maintaining a career and relevant skills may become less of a priority (or not a priority at all) in older adulthood and retirement. Thus, behaviours associated with accomplishing those goals are no longer needed and are ‘selected out’, thereby exerting downward pressure on traits such as conscientiousness that are associated with these behaviours. Similarly, the need to be vigilant against health risks in late life, alongside financial uncertainty when living on fixed or limited income, may promote selection and optimization processes that increase neuroticism. Perhaps the most obvious example involves extraversion, in which older adults select and pare down their social circles ( Carstensen, Isaacowitz, & Charles, 1999 ), allowing optimization of affect and behaviour. In turn, a smaller, but higher-quality, social network compensates for the loss of large (but lower quality) social networks typically seen in young adulthood and midlife.
Consistent with the broad array of theories described above, several studies that examined traits over different ages, or estimated overall trajectories (using either MLM or latent trajectory models), found that during the emerging adult and midlife years, agreeableness, conscientiousness, openness, and extraversion tend to increase and neuroticism tends to decrease ( Lucas & Donnellan, 2011 ; Marsh, Nagengast, & Morin, 2013 ; Roberts et al., 2006 , 2008 ). After midlife, these overall trajectories shift somewhat, with various studies showing decreases in most of the Big Five traits ( Berg & Johansson, 2013 ; Kandler et al., 2015 ; Lucas & Donnellan, 2011 ; Mõttus, Johnson, Starr, & Deary, 2012 ; Roberts & Mroczek, 2008 ). Some of these studies show that decreases occur mainly in older adulthood, particularly after age 65 or 70 ( Mõttus, Johnson, & Deary, 2012 ; Mroczek & Spiro, 2003 ). These findings also align with meta-analyses of mean-level change in traits ( Roberts et al., 2006 ), which indicate that certain traits (e.g. conscientiousness and emotional stability) increase in younger adulthood, whereas others (e.g. agreeableness) only change in older adulthood.
Interestingly, when the neo-socioanalytic (especially its maturity principle) and the SOC theories are combined, we would expect age-related average curvilinear personality change. Some traits may increase in the earlier part of adulthood, before decreasing in older adulthood. Several of the studies we analysed included four or more occasions, which allowed estimation of quadratic trajectories, and several studies had a wide age range. Thus, we were able to utilize several of these theoretical perspectives to guide our analysis plan to comprehensively test the different ways in which personality traits change in mid to late adulthood.
Individual differences in trajectories and lifespan developmental theory
Although overall patterns of change along with theories that explain them are important, individual differences that surround these overall trajectories may enable a more accurate and comprehensive description of personality change over time. Both SOC and Denissen’s self-regulation theory imply such individual variation. But can we empirically identify individual variation in trajectories around those population trajectory estimates? Even when overall patterns are well-replicated (e.g. decreasing neuroticism in adulthood), to what extent do individuals vary around that population-level trajectory, especially with respect to individual differences in rate of change, or slope? Large estimates of individual variation in rate of change tell us that the overall sample trajectory describes only a fraction of people, such that many individuals deviate from the average. Previously, many researchers had argued that personality is fixed and unlikely to change once adulthood is reached, commonly citing the popular William James quote that personality is set like plaster after age 30 ( Costa & McCrae, 1980 , 1986 ; McCrae & Costa, 1994 ). In general, a given collection of personality trajectories has historically been assumed to show very little variation around the population-level trajectory (meta-trajectory), thereby indicating stability. However, consideration and estimation of individual variation around such trajectories form a key tenet of lifespan developmental theory (LDT) ( Baltes, Reese, & Nesselroade, 1977 ), which posits that development (including personality development) is a lifelong process and that both general patterns and individual differences around these patterns are critical for understanding development of any particular variable over the lifespan ( Baltes, 1987 ; Baltes, Lindenberger, & Staudinger, 2006 ). As such, theories that focus on meta-trajectories, such as the maturity principle, are not mutually exclusive with LDT. The neo-socioanalytic theory, self-regulation theory, and SOC can explain the shape of the population-level trajectories, with self-regulation theory and LDT explaining individual differences around those overall patterns at the within-person level.
Existing literature examining adult personality change has reported estimates of individual variation that, in almost all cases, are consistent with the LDT principle of individual differences in person-level change ( Berg & Johansson, 2013 ; Bleidorn, Kandler, Riemann, Angleitner, & Spinath, 2009 ; Helson, Jones, & Kwan, 2002 ; Mroczek, Almeida, Spiro, & Pafford, 2006 ; Mroczek & Spiro, 2003 , 2007; Pedersen & Reynolds, 1998 ; Roberts et al., 2006 ; Terracciano, McCrae, Brant, & Costa, 2005 ; Vaidya, Gray, Haig, Mroczek, & Watson, 2008 ; Vecchione, Alessandri, Barbaranelli, & Caprara, 2012 ). However, systematic inquiry of consistency in individual differences in change is warranted, given the varying interpretations of the literature, and a coordinated analysis approach is aptly suited to investigate these cross-sample differences and similarities.
One goal of this project was to apply techniques designed to enhance replicability of results to longitudinal research on personality development across adulthood using existing data. Researchers across many fields are currently focused on enhancing replicability and reproducibility, and encouraging open science practices ( Condon et al., 2017 ; Nelson, Simmons, & Simonsohn, 2018 ; Vazire, 2018 ; Weston, Graham, & Piccinin, 2019 ). However, many of the shifts in research practices have focused on experimental sub-fields of psychology, leaving longitudinal researchers with more limited options regarding how to enhance the replicability of their findings. Given that longitudinal research often relies on large extant datasets, p-hacking and publication bias are concerns, and many published longitudinal studies may have capitalized on chance as a result.
With these issues in mind, we developed an analytic plan to replicate results within a set of analyses, chiefly through achieving model harmonization for optimal comparability across multiple distinct samples. This enabled us to approximate wide-scale replicability of results by applying the same analytic techniques to multiple samples in a controlled manner. The first step in this procedure was to identify the type of models we wanted to test. To examine stability and change of traits across time, we estimated MLMs for change in personality traits, using both linear slopes and (when appropriate, given the available data) quadratic slopes. To test predictors of personality change, we utilized a slopes-as-outcomes modelling technique to predict slopes from covariates of interest. Before fitting any models, we wrote analysis scripts in R, and we subsequently identified longitudinal studies that had the requisite data to fit those models (the minimum data required were three measurement occasions of at least one of the Big Five personality traits). Prior to analysis, we also identified sex and baseline age as predictors of change upon which to focus. See Table S1 for descriptive statistics, including reliability estimates (Cronbach’s alpha) for all traits.
We used the framework of the IALSA to identify participating longitudinal studies of aging that met study eligibility. IALSA is a platform for facilitating replication of analyses in multiple studies using longitudinal data ( Graham et al., 2017 ; Hofer & Piccinin, 2009 ). Longitudinal research requires years of data collection and substantial financial resources, thereby creating significant pragmatic barriers to replication. The IALSA network facilitates data access for researchers to test longitudinal models in a multi-study framework, allowing for conceptual replication. While direct replication is not feasible in this context, conceptual replication demonstrates the robustness and generalizability of effects across diverse methodologies and measures.
The current study used a coordinated analysis approach, which preserves measurement and sample heterogeneity, while allowing examination of the strengths and similarities in associations across studies ( Hofer & Piccinin, 2009 ). A coordinated analysis is different from a pooled analysis, which has strict harmonization requirements (usually identical measures) and merges each study’s data into a single dataset, thereby producing one set of models ( Curran et al., 2008 ). Pooled analysis assumes homogeneity across datasets and a single true effect size underlying all studies. This is often an untenable assumption ( Borenstein, Hedges, Higgins, & Rothstein, 2010 ); and coordinated analysis, by analysing each dataset separately and then synthesizing afterward, does not make this assumption. We identified 16 samples that achieved our data requirements, allowing analysis using multi-level growth models; see Table 1 for basic study-level descriptions.
Study descriptions
The Berlin Aging Study
The Berlin Aging Study (BASE) began in 1990 in Berlin, Germany. Neuroticism, extraversion, and openness were assessed using six items each, selected from the NEO-FFI ( Costa & McCrae, 1985a , 1985b) at baseline, with reassessment at four additional occasions (five total measurements) over the following 13 years ( Wagner, Ram, Smith, & Gerstorf, 2016 ). A total of 516 ( M age = 84.92, SD age = 8.66, 50% female) participants completed at least one measurement of personality. There were 132 individuals who completed three or more measurements and 83 who completed four or more measurements.
The Berlin Aging Study-II
The Berlin Aging Study-II (BASE-II) data used in the current report came from older adults (age range 60–84 at personality baseline) residing in the greater Berlin (East and West), Germany metropolitan area ( Bertram et al., 2013 ; Gerstorf et al., 2016 ; Mueller et al., 2016 ). Starting in 2009, a total of 1276 ( M age = 67.68, SD age = 3.73, 50% female) participants completed at least one measurement of personality. There were 957 individuals who completed three or more measurements and 671 participants who completed four or more measurements of the full Big Five personality traits using a short version of the Big Five Inventory (BFI-S; John & Srivastava, 1999 ; Lang, John, Lüdtke, Schupp, & Wagner, 2011 ).
The Einstein Aging Study
The Einstein Aging Study (EAS) is a sample of older, ethnically diverse, community-residing individuals from the Bronx. Data collection began in 1993, with rolling enrolment. At study entry, participant age ranged from 70 to 99 years, with follow-up occasions every 18 months ( Katz et al., 2012 ). Although some participants have as many as 16 measurement occasions of personality, most have 3 to 5. A total of 342 ( M age = 77.54, SD age = 4.99, 60% female) participants completed at least one measurement of personality, using adjectives from the International Personality Item Pool (IPIP) ( Goldberg, 1992 ). There were 214 individuals who completed three or more measurements and 179 participants who completed four or more measurements.
The Health and Retirement Study
The Health and Retirement Study (HRS) is a nationally representative longitudinal panel study of over 20 000 adults who were surveyed every 2 years starting in 1992 ( Juster & Suzman, 1995 ; Sonnega et al., 2014 ). Half of the sample completed a personality assessment in 2006, and the second half completed personality in 2008. The full Big Five was assessed using adjectives from the Midlife Development Inventory ( Lachman & Weaver, 1997 ). A total of 14 513 ( M age = 68.50, SD age = 10.50, 59% female) completed at least one measurement of personality. There were 8869 individuals who completed three or more measurements.
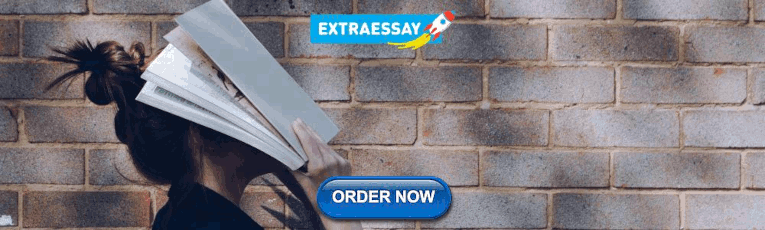
Interdisciplinary Longitudinal Study of Adult Development
The Interdisciplinary Longitudinal Study of Adult Development (ILSE and ILSE.Y) is a representative sample of 1390 individuals from two cohorts (1930–1932: older adults in their early 60s when ILSE was launched; 1950–1952: middle-aged adults in their early 40s at baseline) in Germany ( Sattler et al., 2015 ). For ILSE, three measurements of the full Big Five (NEO-FFI; Costa & McCrae, 1988 ) were collected in 1994, 1998, and 2006. A total of 485 ( M age = 62.50, SD age = 0.96, 48% female) completed at least one measurement of personality. There were 306 individuals who completed three or more measurements ( Allemand, Schaffhuser, & Martin, 2015 ; Allemand, Zimprich, & Martin, 2008 ). The younger cohort (ILSE.Y) was also included in this project, containing 496 ( M age = 43.78, SD age = 0.91, 48% female) who completed at least one measurement of personality and 328 individuals who completed three or more measurements.
Longitudinal Aging Study of Amsterdam
The Longitudinal Aging Study of Amsterdam (LASA) is a nationally representative cohort sample of 5132 older adults in the Netherlands. Of the Big Five traits, neuroticism was the sole trait collected in this sample, assessed using the neuroticism scale from the DPQ ( Luteijn, Starren, & van Dijk, 2000 ). Four measurements of neuroticism were taken in 1992, 1995, 1998, and 2001 ( Huisman et al., 2011 ). A total of 2111 ( M age = 69.75, SD age = 8.57, 52% female) participants completed at least one measurement of personality. There were 1662 individuals who completed three measurements and 1267 who completed four measurements.
Lothian Birth Cohort 1936
The Lothian Birth Cohort 1936 (LBC) consists of surviving participants of the 1947 Scottish Mental Health Survey. The 1936 cohort was recruited between 2004 and 2007 by identifying individuals from the original (1947) cohort who were residing in Edinburgh and the surrounding areas. In total, 1091 participants entered the study. Personality traits were measured using 50 items from the IPIP when participants were 67–71 years old in 2006 and then three times more in 2008, 2012, and 2016 for a total of four measurement occasions ( Deary, Gow, Pattie, & Starr, 2012 ). A total of 950 ( M age = 69.51, SD age = 0.84, 50% female) completed at least one measurement of personality. There were 680 individuals who completed three measurements and 528 individuals who completed four measurements.
Midlife in the United States
The Midlife in the United States (MIDUS) study is a national sample of 7108 adults, with a baseline age range of 28 to 74 years. The initial wave of measurement began in 1994–1995, and two additional waves of data collection took place in 2004–2005 ( N = 4963) and in 2013 ( N = 3294) ( Brim, Ryff, & Kessler, 2004 ). The Midlife Developmental Inventory (adjectives) was used to assess the full Big Five traits ( Lachman & Weaver, 1997 ). A total of 6265 ( M age = 46.80, SD age = 12.90, 53% female) completed at least one measurement of personality. There were 2717 individuals who completed three measurements.
VA Normative Aging Study
The VA Normative Aging Study (NAS) is a US Department of Veterans Affairs study, focused on the medical and psychosocial aspects of aging among men. The original sample consists of 2280 men living in Boston. At the first measurement occasion of personality, they had an age range of 30–78 years, with follow-up visits every 3–5 years. A total of 1645 ( M age = 51.40, SD age = 9.20, 0% female) completed at least one measurement of the Eysenck (EPI-Q, Floderus, 1974 ; based on Eysenck & Eysenck, 1968 , EPI) personality scale beginning in 1975. A total of 1423 individuals completed three measurements and 1255 who completed four measurements ( Bosse, Ekerdt, & Silbert, 1984 ).
The Origin of Variance in the Oldest-Old: Octogenerian Twins
The Origin of Variance in the Oldest-Old: Octogenerian Twins (Octo-Twin) study includes 351 Swedish twin pairs (702 individuals) aged 80 years and older (80–97). Baseline interviews occurred between 1991 and 1993 ( McClearn et al., 1997 ). Four additional waves of data were collected at 2-year intervals. Extraversion and neuroticism were assessed at the first four occasions using a shortened, 19-item, version of The Eysenck Personality Inventory (EPI-Q; Eysenck & Eysenck, 1968 ). A total of 469 ( M age = 83.17, SD age = 2.89, 64% female) completed at least one measurement of personality. There were 213 individuals who completed three measurements and 122 who completed four measurements.
Swedish Adoption/Twin Study of Aging
The Swedish Adoption/Twin Study of Aging (SATSA) began in 1984 on adults aged 26–93 years, with the objective of studying the genetic and environmental factors associated with aging ( Pedersen et al., 1991 ). Personality traits (neuroticism, extraversion, and openness) were assessed seven times between 1984 and 2010, using the NEO-PI ( Costa & McCrae, 1985a , 1985b ) inventory for openness and EPQ ( Eysenck, 1975 ) for neuroticism and extraversion. A total of 1925 ( M age = 59.80, SD age = 13.96, 58% female) participants completed at least one measurement of personality. There were 1438 individuals who completed three measurements and 1407 who completed four measurements.
Seattle Longitudinal Study
The Seattle Longitudinal Study (SLS) began in 1956 to study psychological development in adulthood ( Schaie, Willis, & Caskie, 2004 ). The full Big Five personality traits were assessed at four measurement occasions, beginning in 2001, using the NEO-PI-R Personality Inventory ( Costa & McCrae, 1992 ). A total of 1541 ( M age = 63.21, SD age = 15.61, 56% female) completed at least one measurement of personality. There were 785 individuals who completed three measurements and 639 who completed four measurements.
German Socio-Economic Panel
The German Socio-Economic Panel (SOEP) is an ongoing annual longitudinal study that began in 1984 with approximately 15 000 private households in Germany ( Goebel et al., 2018 ; Headey, Muffels, & Wagner, 2011 ; Wagner, Joachim, & Schupp, 2007 ). A total of 21 030 ( M age = 47.40, SD age = 17.58, 52% female) completed at least one measurement of personality, using a shortened version of the BFI personality inventory ( John & Srivastava, 1999 ; Lang et al., 2011 ). There were 19 076 individuals who completed three measurements and 13 229 who completed four measurements.
Wisconsin Longitudinal Study
The Wisconsin Longitudinal Study (WLSG/WLSS) contains two samples used in the current project. The first (WLSG) is a sample of Wisconsin residents who graduated from high school in 1957. Data collection was started in 1957, and participants were reassessed periodically over the following decades. Personality assessment was added to the study protocol in the early 1990s, providing personality data using the BFI personality inventory ( John, Donahue, & Kentle, 1991 ) in 1992, 2003, and 2011. Age at personality assessment baseline ranged from 51 to 56. A total of 6720 ( M age = 53.23, SD age = 0.64, 53% female) participants completed at least one measurement of personality and 5154 individuals completed three measurements. The second sample (WLSS) is composed of siblings of these graduates. Data collection for the sibling sample began in 1975, and personality data for these participants ( N = 4804) were collected in 1993, 2004, and 2011 ( Herd, Carr, & Roan, 2014 ; Sewell, Hauser, Springer, & Hauser, 2003 ). A total of 3987 ( M age = 53.27, SD age = 7.32, 53% female) completed at least one measurement of personality and 2853 individuals completed three measurements.
Personality
All studies contained at least a subset of the Big Five, assessed via different but reliable and validated measures of the Big Five. The IPIP ( Goldberg, 1992 ) was used to develop the measures for all five traits in the LBC1936 and EAS. Goldberg’s adjectives were also used to create the Midlife Development personality inventory, which was used in the MIDUS and HRS ( Lachman & Weaver, 1997 ). The Eysenck measures ( Eysenck, 1975 ; EPI-Q, EPQ; Eysenck & Eysenck, 1968 ) were used to measure neuroticism and extraversion in Octo-Twin, NAS, and SATSA. The NEO ( Costa Jr & McCrae, 1992 ) was used to assess the Big Five in ILSE, BASE (NEO-FFI), SLS (NEO-PI-R), and SATSA (NEO-PI; Openness only), while LASA measured neuroticism using the neuroticism scale from the DPQ ( Huisman et al., 2011 ). A BFI-S ( John et al., 1991 ; John & Srivastava, 1999 ; Lang et al., 2011 ) was used to assess the Big Five in the BASE-II, SOEP, and WLS samples. Each of the scales is measured in different units, with most on a 1–5 scale, but some used a 1–30 scale (sum scores). We transformed personality for comparability by converting each trait to a 1–10 scale. We then calculated the baseline mean and standard deviation of the transformed scale, standardized the transformed scores on the basis of these new baseline statistics, and finally, added back the baseline mean. For example, hypothetically, for a score of 3.5 on a Likert-type 1–5 scale, this would mean that this score would be converted to 6.25 [10 * (value – max/max – min)]. If this hypothetical sample had a transformed mean of 5 ( SD = 2), then this score would be standardized {[(value – mean)/ SD ] + mean} for a new score of 5.625. The units are interpreted in standard deviation units, and the mean is on a 1–10 scale. For the duration of this paper, ‘baseline’ refers to the initial assessment of personality for each study.
The time metric in our trajectory models was chronological age, centred at 60 and divided by 10 (to convert to change per decade). Centred age was squared for use in models estimating quadratic change. See Figure S1 for the distribution of age across all samples.
Sex and baseline age were used as predictors of personality change across studies. Each study used predictors from the same measurement occasion as the first personality assessment, to address the question of whether baseline predictor ‘status’ was prospectively associated with subsequent personality change. All predictors were added into separate models. Studies with less than 5% prevalence of a given predictor were dropped from those particular models. Models including health events, marital status, and retirement status as predictors of personality change are provided in the supplemental material ( https://osf.io/xqmfw/?view_only=126dbaafa46043038688e04bdcc23899 ). 1
All studies coded baseline sex in a binary manner such that 1 = female and 0 = male.
Baseline age
To explore whether personality change varied as a function of baseline age group, each study created a binary variable indicating whether a participant was over or under age 60 at study baseline, coded as 1 = 60 or over at baseline and 0 = under 60 at baseline. Individual studies had varying start years (1975–2009) with the majority being in the early 1990s, and a range of mean ages at baseline (average baseline age range = 44–85). Adding baseline age group as a level 2 predictor gave us a rough estimate of whether birth cohort in a given study start year (or more accurately, age group) could account for differences in personality trajectories within each study. The visualization of these trajectories across studies will give an illustration of age group differences in these trajectories, regardless of the year in which baseline age was assessed.
Data analysis
Individual study analyses.
We used MLMs for change, also known as individual growth or individual trajectory modelling ( Raudenbush & Bryk, 2002 ; Singer & Willett, 2003 ), to estimate trajectories of each personality trait in each sample. To provide a baseline for comparing subsequent models, we first tested intercept-only (unconditional means) models, expressed as Y ti = π 0 i + ε ti , where Y is personality trait at a given measurement occasion t for person i . This is a function of a person-level intercept (level of trait), plus the within-person residual. The variance, τ , is used in conjunction with the residual to calculate the intra-class correlation coefficient (ICC), or ρ . This is the ratio of between-person variance to total variation in each trait. We then fit a linear growth model (level 2 model) with the fixed-slope only, and next, we fit the linear growth model with both the fixed and random slopes. Likelihood ratio tests compared these two models so as to assess whether adding the random slopes improved model fit and thus whether there were individual differences in linear change. Next (for studies with 4+ measurement occasions) quadratic growth models were fit to assess non-linear change. We did this by squaring the time-metric (age) and entering this into the model. Lastly, using linear growth models, a series of slopes-as-outcomes models were fit, each with one of the above described predictors. Each predictor was added individually into separate models. The interaction of the binary predictor by the linear time metric (age) was calculated and added to the linear growth model.
All studies tested all models are based on data availability. Participants were included in the models if they had, at minimum, the initial assessment of personality. For a given trait, there were a total of six possible models (Intercept-Only, Fixed-Slope, Fixed+Random Slope, Quadratic Change, and two Slopes-As-Outcomes models). Because there were so many models estimated across the five traits and 16 studies, we included the most relevant model results in the main manuscript, and we put all other models in the supplemental material , available on OSF. All scripts and output files from this and prior drafts of this paper are available here ( http://osf.io/xqmfw/?view_only=126dbaafa46043038688e04bdcc23899 ). Analyses were completed using R ( R Core Team, 2014 ), including the packages lme4 ( Bates, Mächler, Bolker, & Walker, 2015 ), metafor ( Viechtbauer, 2019 ), and ggplot ( Wickham et al., 2019 ). Our analyses were not preregistered, as the planning stages of this project took place before pre-registration was common practice. In the results below, we report the plots for the linear and quadratic trajectories of each trait, as well as a selection of moderators. These plots include an average effect that is weighted by sample size. For interested readers, plots with the unweighted averages are available in the supplemental material ( https://osf.io/xqmfw/?view_only=126dbaafa46043038688e04bdcc23899 ).
Meta-analyses
We estimated a meta-analytic summary for linear, quadratic, and predictor models, to summarize the average effect across individual studies (e.g. linear slope estimate for neuroticism across samples; sex by extraversion slope estimate across samples). Each meta-analysis included an overall effect (weighted by sample size), with corresponding standard errors/confidence intervals, as well as estimates of heterogeneity ( I 2 , Q ) ( Borenstein, Higgins, Hedges, & Rothstein, 2017 ). We used the significance test of the Q statistic to guide our decision to report the study-level moderators of heterogeneity. For this project, three study-level moderators were considered: average baseline age (over or under 60), country that the study was based in, and personality scale used. We used random-effects models to meta-analyse each set of estimates.
Summaries for all individual study models, including tables, figures, and full meta-analytic summaries are included in the Supporting Information . For brevity, the main manuscript includes a table of the meta-analytic slope estimates for each model type (e.g. linear, quadratic, and predictors; Table 2 ) and key figures.
Meta analytic summary of slope estimates
The results reported below are organized by model. First, we describe the level 1 (intercept-only) models by reporting the between-study range of the ICC, which provides a proportion of between-person and within-person variability in repeated personality trait measurement, and related heterogeneity estimates for each trait. We then describe the trajectory models (linear and quadratic) by trait. When heterogeneity estimates for the growth trajectory models were substantial, predictor and subgroup analyses are warranted; we therefore added study-level moderators (age, country, and personality measurement) to the models to test if any of these predictors accounted for between-study variability. For each trait, we present a figure illustrating either the linear or non-linear trajectories, depending on which model was a better overall fit for a given trait. The trajectory figures not presented in the main manuscript can be found in the Supporting Information . Lastly, we discuss the slopes-as-outcomes models, and we describe the extent to which each predictor accounts for individual differences in change for each trait. Given the large number of analyses, we used a more conservative alpha ( α = .01) to evaluate statistical significance. For each of these models, there is a table, a figure, and a meta-analysis. All of these results can be found in the Supporting Information , organized by trait.
Intercept-only models
Neuroticism.
The ICC from the intercept-only model for neuroticism ranged across the 16 samples from 0.57 to 0.84 ( Table S2 ). The meta-analytic summary indicates that the average ICC across studies is B = 0.68 ( se = 0.02), with substantial heterogeneity ( I 2 = 99.09, Q = 2855.39, df = 15, p ≤ .001).
Extraversion
Of the 15 studies with extraversion data, the ICC ranged from 0.62 to 0.86 ( Table S18 ). The meta-analytic summary indicates that the average ICC across studies is B = 0.72 ( se = 0.02), with heterogeneity estimates of ( I 2 = 99.40, Q = 3782.33, df = 14, p ≤ .001).
Of the 13 samples with openness data, the ICC ranged from 0.59 to 0.88 ( Table S34 ). The meta-analytic summary indicates that the average ICC across studies is B = 0.70 ( se = 0.02), with heterogeneity estimates of ( I 2 = 99.54, Q = 4775.56, df = 12, p ≤ .001).
Conscientiousness
Of the 11 studies with conscientiousness data, the ICC ranged from 0.53 to 0.84 ( Table S50 ). The meta-analytic summary indicates that the average ICC across studies is B = 0.67 ( se = 0.03), with heterogeneity estimates of ( I 2 = 99.53, Q = 4013.80, df = 10, p < .001).
Agreeableness
Of the 11 studies with agreeableness data, the ICC ranged across these studies from 0.51 to 0.80 ( Table S66 ). The meta-analytic summary indicates that the average ICC across studies is B = 0.64 ( se = 0.02), with heterogeneity estimates of ( I 2 = 99.16, Q = 2121.47, df = 10, p < .001).
The ICCs indicate that across all studies and traits, half to over three-quarters of the total variation across persons and measurement occasions were between-person variation (individual differences in levels of trait). The remainder of variation is true trait change, plus variance due to measurement error. Variation in personality traits across individuals and occasions is not simply due to differences in level among persons but also includes variation over measurement occasions (across time) within persons, suggesting within-person change.
Average trajectories
Results from the linear growth models for neuroticism indicate that neuroticism changes over time. The likelihood ratio of the fixed ( Table S3 ) versus random ( Table S4 ) slope models indicate that, for most studies, there are individual differences in change. Across studies, there was a linear effect of age for all but five samples (ILSE.Y, LBC1936, Octo-Twin, SOEP, and LASA; Table S4 ). Most studies showed a decrease in neuroticism, with few showing an increase. The meta-analytic estimate for these models was not statistically significant ( p > .01) but suggests a pattern of decline over time ( Figures S2 and S3 ) ( B = – 0.05, 95% CI = [−0.09, – 0.01], p = .011). Because the meta-analysis also showed substantial heterogeneity ( I 2 = 98.67, Q = 1013.31, df = 15, p ≤ .001), moderators were added to account for this variability. Age and country did not account for the heterogeneity in neuroticism trajectories, but personality scale did, indicating that estimates of change in neuroticism were likely varying owing to measurement differences.
Ten studies had sufficient data to estimate quadratic growth models for neuroticism ( Table S5 ). Of these, three showed evidence for non-linear change. See Figure 1 for visualization of the quadratic trajectory. The thick black line indicates the overall average pattern, which suggests that neuroticism declines in early adulthood and increases again in older adulthood. See also Figures S4 and S5 for these figures in colour and with the unweighted average slopes. The meta-analysis suggests an overall quadratic effect ( B = – 0.10, 95% CI = [−0.15, – 0.04], p = .001), although there is also significant heterogeneity ( I 2 = 95.50, Q = 239.32, df =9, p ≤ .001). Neither country nor scale accounted for any of the heterogeneity, but age did. This result suggests that as average sample age increases, neuroticism slopes become less steep, indicating that the trajectory for neuroticism may be better described as a U-shaped trend.

Quadratic trajectories of neuroticism. The thick black line indicates the average trajectory weighted by N . At the individual study level, several studies showed evidence of a U-shaped curve, and the meta-analytic average was significant ( p < .001). [Colour figure can be viewed at wileyonlinelibrary.com ]
Results from the linear growth models for extraversion indicate that extraversion changes over time. The likelihood ratio of the fixed ( Table S19 ) versus random ( Table S20 ) slope models indicates that, for many studies, there are individual differences in change for all studies except with the exception of BASE, BASE-II, EAS, and Octo-Twin. Across studies, the linear effect of age was statistically significant ( p < .001) for over half of the studies, and these effects showed a consistent pattern of decline ( Table S20 ). This was confirmed in the meta-analytic summary, showing an overall decrease in extraversion ( B = – 0.09, 95% CI = [−0.13, – 0.04], p ≤ .001). See Figure 2 for a visualization of linear effects. The thick black line depicts the overall average trajectory, weighted by N , which shows an overall pattern of decline. The meta-analysis also showed significant heterogeneity ( I 2 = 99.07, Q = 431.15, df = 14, p < .001). Neither age, nor country, nor scale accounted for the variability in extraversion, suggesting that the detected heterogeneity is likely due to an untested moderator. The figure suggests that the older samples have somewhat steeper slopes than younger samples. See also Figures S30 and S31 for these figures in colour and with the unweighted average slopes.

Linear trajectories of extraversion. The thick black line indicates average trajectory weighted by N . At the individual study level, many showed evidence of decline, and the meta-analytic average was significant ( p < .001). [Colour figure can be viewed at wileyonlinelibrary.com ]
Nine of the studies had sufficient data to estimate quadratic growth models for extraversion. Three of the nine studies showed evidence for a non-linear trajectory of extraversion ( Table S21 ). The meta-analytic summary does not confirm this, showing a non-significant average effect ( B = 0.04, 95% CI = [−0.14,0.22], p = .645), which may be due to the smaller effects in the larger studies (e.g. SOEP). See Figures S32 and S33 . There was also high heterogeneity ( I 2 = 99.71, Q = 293.48, df =8, p ≤ .001) in the quadratic estimate. Neither country nor age accounted for any of the heterogeneity, but scale did. This result suggests that studies using the IPIP more frequently showed significant quadratic estimates (LBC, EAS). Overall, this indicates that the trajectory for extraversion over time may be better described as a linear trend.
Results from the linear growth models for openness indicate that openness changes over time. The likelihood ratio of the fixed ( Table S35 ) versus random ( Table S36 ) slope models indicate that, for most studies, there are individual differences in change, with the exception of BASE-II, EAS, ILSE, and SLS. There was a linear effect of age for most studies, and all trends showed a pattern of decline ( Table S36 ). This was supported in the meta-analytic summary, showing a decrease in openness ( B = – 0.09, 95% CI = [−0.13, – 0.05], p < .001). See Figure 3 for a visualization of linear effects. The thick black line depicts the overall average trajectory, weighted by N, which shows a pattern of decline. The meta-analysis also showed significant heterogeneity ( I 2 = 98.84, Q = 607.99, df = 12, p ≤ .001). The figure suggests that the older samples are less consistent in the pattern of decline than the younger samples. However, the tests of between-study moderators did not account for variation in these effects, suggesting the possibility that the effects estimated may be are due to some other untested moderator. See also Figures S58 and S59 for this figure in colour and with the unweighted average slopes.

Linear trajectories of openness. The thick black line indicates average trajectory weighted by N . At the individual study level, most showed evidence of decline, although the meta-analytic average was significant ( p = .001). [Colour figure can be viewed at wileyonlinelibrary.com ]
Seven of the studies had sufficient data to estimate quadratic growth models for openness ( Table S37 ). Of these, three showed evidence for non-linear change. The pattern depicted in the individual studies suggests that openness may be somewhat stable through middle adulthood and then decrease more sharply through older adulthood, although the older samples appear to have less consistency. The meta-analytic summary of the quadratic slopes was not significant ( B = – 0.07, 95% CI = [−0.14, – 0.01], p = .032). Based on the figure ( Figures S60 and S61 ), we tentatively conclude that the effects from the older samples are the estimates that deviate the most from the average trajectory.
The linear growth models for conscientiousness indicate that conscientiousness changes over time. The likelihood ratio of the fixed ( Table S51 ) versus random ( Table S52 ) slope models indicate that, for most studies, there are individual differences in change with the exception of BASE-II, EAS, and ILSE.Y. Results from the linear growth models for conscientiousness are more mixed than the other traits thus far. Several showed evidence of decline (WLSS, WLSG, HRS, EAS, and LBC1936), while others showed a weaker effect ( p > .01) but still in the negative direction (ILSE, BASE-II, and SLS). Only SOEP showed a significant increase in conscientiousness ( Table S52 ). See Figure 4 for a visualization, indicating an overall pattern of declining conscientiousness over time. The meta-analytic summary supports this interpretation, with an overall significant slope ( B = – 0.05, 95% CI = [−0.09, – 0.02], p = .004). This result was highly heterogeneous ( I 2 = 97.72, Q = 884.99, df = 10, p ≤ .001), and adding study age to the meta-analysis accounted for a significant amount of the variation in linear slope. See also Figures S86 and S87 for these figures in colour and with the unweighted average slopes.

Linear trajectories of conscientiousness. The thick black line indicates average trajectory weighted by N . At the individual study level, many showed evidence of decline, although the meta-analytic average was significant ( p = .004). [Colour figure can be viewed at wileyonlinelibrary.com ]
Five of the studies had sufficient data to estimate quadratic growth models for conscientiousness. Of these, two showed significant curvature (SLS and SOEP). The pattern depicted in the individual studies suggests that conscientiousness increases through younger and middle age and then decreases in older adulthood ( Table S53 , Figures S88 and S89 ). The meta-analytic summary of the quadratic estimate was not significant (B = – 0.02, 95% CI = [−0.07,0.03], p = .459).
The linear growth models for agreeableness were the least consistent of the five traits across individual studies. The likelihood ratios of the fixed ( Table S67 ) versus random ( Table S68 ) slope models indicate that, for approximately half of the studies, there are individual differences in change. Seven of the 11 studies showed evidence for significant ( p < .01) change in agreeableness; however, two of these studies showed decreasing agreeableness (HRS and BASE-II), while the other five showed increasing agreeableness (ILSE.Y, SLS, SOEP, WLSG, and WLSS). Two more (EAS and ILSE) showed an increase, but with p > .01. The last two (LBC1936 and MIDUS) showed an average decrease, but with p > .01 ( Table S68 ). The linear pattern depicted in Figure 5 shows this mix of findings, and the meta-analytic summary was not significant ( B = 0.02, 95% CI = [−0.02,0.07], p = .296). From these results, we tentatively suggest that agreeableness may increase over time, although not all samples replicate this finding. See Figures S114 and S115 .

Linear trajectories of agreeableness. The thick black line indicates the average trajectory weighted by N . At the individual study level, most showed evidence of decline, although some showed an increase. The meta-analytic average was not significant ( p = .296). [Colour figure can be viewed at wileyonlinelibrary.com ]
Five of the 11 studies had sufficient agreeableness data to estimate quadratic growth models for agreeableness ( Table S69 , Figures S116 and S117 ). Of these, two showed significant curvature (SLS and SOEP), but in opposite directions. The average pattern suggests that agreeableness may be relatively stable in younger adulthood and increase during older adulthood, although the individual studies do not show consistent patterns, and the meta-analytic summary was not significant ( B = 0.03, 95% CI = [−0.16,0.21], p = .777).
Predictors of change
Adding sex to the linear growth models for neuroticism yielded primarily null results with one exception (SOEP, Table S6 ). The overall pattern suggests that female participants may have slightly higher overall neuroticism levels and slightly steeper decreases, which was supported by the meta-analytic summary ( B = – 0.01, 95% CI = [−0.02,0.00], p = .002), and no observed heterogeneity ( I 2 = 0.00, Q = 18.35, df = 14, p = .191); see Figure 6 and Figures S6 and S7 .

Linear trajectories of neuroticism, moderated by sex. The thick black line indicates the average trajectory weighted by N . Female participants show slightly steeper decreases in neuroticism. [Colour figure can be viewed at wileyonlinelibrary.com ]
Adding sex to the linear growth models for all other traits yielded predominantly null results with a few exceptions. The overall trajectory pattern is very similar for male and female participants, and this is confirmed by the meta-analytic summary for extraversion ( B = 0.00, 95% CI = [−0.02,0.01], p = .517; Table S22 , Figure S34 and S35 ), openness ( B = 0.00, 95% CI = [−0.02,0.02], p = .851; see Table S38 , Figure S62 and S63 ), conscientiousness ( B = – 0.02, 95% CI = [−0.04,0.01], p = .169; see Table S54 , Figures S90 and S91 ), and agreeableness ( B = 0.00, 95% CI = [−0.02,0.02], p = .890; see Table S70 , Figure S118 and S119 ).
Adding baseline age to the linear neuroticism models yielded significant ( p < .01) effects at the individual study level for all but one study ( Table S17 ), and this is confirmed by the meta-analytic summary ( B = 0.11, 95% CI = [0.04,0.18], p = .001), although there is high heterogeneity across samples ( I 2 = 93.84, Q = 173.55, df = 7, p ≤ .001). These effects indicate that individuals who were under 60 at study baseline experienced overall decline in neuroticism, while those 60 and over tended to have flatter neuroticism slopes; see Figure 7 . The meta-analytic moderator results show that sample age accounts for some of the between-study variability.

Linear trajectories of neuroticism, moderated by baseline age. The thick black line indicates average trajectory weighted by N . At the individual study level, all showed evidence that being over 60 at baseline was associated with decreasing neuroticism, and the meta-analytic average was significant ( p < .001). [Colour figure can be viewed at wileyonlinelibrary.com ]
Adding baseline age to the linear extraversion models yielded significant effects for all but one study as well ( Table S33 , Figure S56 and S57 ), and this is confirmed by the meta-analytic summary ( B = – 0.09, 95% CI = [−0.12, – 0.06], p ≤ .001), and moderate heterogeneity ( I 2 = 60.10, Q = 12.84, df = 5, p = .025). These results suggest that while the overall pattern for both younger and older adults is that of decline, the decline is somewhat steeper for older adults; see Figure 8 .

Linear trajectories of extraversion, moderated by baseline age. The thick black line indicates average trajectory weighted by N . At the individual study level, all showed evidence that being over 60 at baseline was associated with decreasing extraversion, and the meta-analytic average was significant ( p < .01). [Colour figure can be viewed at wileyonlinelibrary.com ]
Adding baseline age to the linear openness models yielded significant effects for all but one study ( Table S49 , Figures S84 and S85 ), and this is confirmed by the meta-analytic summary ( B = – 0.12, 95% CI = [−0.15, – 0.08], p ≤ .001), and moderate heterogeneity ( I 2 = 67.10, Q = 11.68, df = 4, p = .020). These effects suggest that while there is an overall pattern of decline for both younger and older adults, this decline is steeper for older adults; see Figure 9 .

Linear trajectories of openness, moderated by baseline age. The thick black line indicates average trajectory weighted by N . At the individual study level, all showed evidence that being over 60 at baseline was associated with decreasing openness, and the meta-analytic average was significant ( p < .001). [Colour figure can be viewed at wileyonlinelibrary.com ]
Adding baseline age to the linear conscientiousness models yielded significant effects for all studies ( Table S65 , Figures S112 and S113 ), and this is confirmed by the meta-analytic summary ( B = – 0.18, 95% CI = [−0.21, – 0.16], p ≤ .001), and lack of observed heterogeneity ( I 2 = 0.00, Q = 2.02, df = 3, p = .568). The plot suggests that conscientiousness increases somewhat for individuals who were under 60 at baseline but declines for individuals who were older at baseline; see Figure 10 .

Linear trajectories of conscientiousness, moderated by baseline age. The thick black line indicates average trajectory weighted by N . At the individual study level, all showed evidence that being over 60 at baseline was associated with decreasing conscientiousness, and the meta-analytic average was significant ( p < .001). [Colour figure can be viewed at wileyonlinelibrary.com ]
Adding baseline age to the linear agreeableness models yielded effects for all but one study ( Table S81 , Figures S139 and S140 ), and this is confirmed by the meta-analytic summary ( B = – 0.10, 95% CI = [−0.14, – 0.05], p ≤ .001), and moderate heterogeneity ( I 2 = 68.02, Q = 8.38, df = 3, p = .039). These results indicate that agreeableness increased among individuals who were younger at baseline, and either stabilized or decreased among older individuals see Figure 11 .

Linear trajectories of agreeableness, moderated by baseline age. The thick black line indicates average trajectory weighted by N . At the individual study level, all showed evidence that being over 60 at baseline was associated with decreasing agreeableness, and the meta-analytic average was significant ( p < .001). [Colour figure can be viewed at wileyonlinelibrary.com ]
The current study had two major goals, one substantive and one methodological. The substantive aim was to examine the extent of change in the Big Five personality traits and whether sex and age at baseline were prospectively associated with these changes. The second was to demonstrate the utility of the coordinated analysis approach for enhancing replication efforts in long-term longitudinal research, an area where replication is typically quite difficult. This approach allows for conceptual replications of effects using longitudinal existing data and can help accelerate the accumulation of knowledge garnered from expensive long-term longitudinal datasets. Using the infrastructure of the IALSA and coordinated analysis ( Graham et al., 2017 ; Hofer & Piccinin, 2009 ), we identified 16 longitudinal samples in which Big Five personality traits were measured three or more times, providing an opportunity to examine up to 16 replications of personality trait trajectories. Using MLMs for change ( Singer & Willett, 2003 ), we identified patterns of overall change and of individual variation in change for the Big Five personality traits across a wide expanse of adulthood. In sum, we found evidence for personality change in midlife and older adulthood in ways that are consistent with theory. We also detected individual differences in change, which is also consistent with theory. However, clear and unambiguous evidence for predictors of change was not found, although relatively few individual difference factors were examined.
Synthesis of linear and quadratic models
Our consideration of overall trajectories (sample level and meta-trajectories) showed personality change over time for most of the Big Five Traits. Of the five traits, the models for extraversion and conscientiousness suggested that a linear pattern of decline best describes the data. Both of these traits show a steady (to a lesser degree for conscientiousness) decrease over time. For conscientiousness, while the quadratic model term was not significant, evidence from the baseline age models suggests that the decreasing pattern of conscientiousness is most evident among individuals who were over 60 at baseline. This is also consistent with several theories, including self-regulation theory, neo-socioanalytic theory (especially the maturity principle), and the SOC model ( Baltes & Baltes, 1990 ; Denissen et al., 2013 ; Marsh et al., 2013 ; Roberts et al., 2006 ), such that individuals are stable or increasing in conscientiousness through early and middle adulthood but may be unknowingly ‘selecting’ out of trait-typical behaviours (e.g. reducing the number of hours spent at their workplace) in older adulthood as the social demands on them begin to wane.
Neuroticism showed evidence for U-shaped change, and we can infer that neuroticism decreases through most of adulthood but may begin to increase again in older age. This is confirmed by both the quadratic models and linear models including baseline age as a predictor and is consistent with literature suggesting that increasing neuroticism in older adulthood may be a reflection of an increasing anxiety regarding onset of terminal diseases and approaching mortality ( Baltes, 1987 ; Mueller, Wagner, & Gerstorf, 2017 ). Openness remains somewhat stable through middle adulthood before decreasing in older age. Agreeableness is the only trait that did not yield a significant linear or quadratic effect. This could be due to study-level differences (including possible study differences in true effect size; e.g. Borenstein et al., 2010 ), or due to a true overall null effect: perhaps agreeableness is, in fact, relatively stable on average over the course of adulthood, although our analyses do reveal individual differences in agreeableness trajectories over time.
Our findings are consistent with much of the prior literature, suggesting that most or all traits decline over time ( Berg & Johansson, 2013 ; Kandler et al., 2015 ; Lucas & Donnellan, 2011 ; Mõttus, Johnson, Starr, & Deary, 2012 ; Roberts & Mroczek, 2008 ), and we found some evidence for non-linear change as well (see both the curvilinear results and baseline age predictor models). Our findings are inconsistent with recent work suggesting positive age differences in agreeableness and conscientiousness, and negative differences in neuroticism ( Bleidorn et al., 2009 ; Soto, John, Gosling, & Potter, 2011 ). However, the Soto et al. (2011) study was cross-sectional and did not test within-person change. The Bleidorn et al. (2009) report used a single small sample and a different modelling approach and should be interpreted within the context of other work (such as the current study). Additionally, several of the datasets included in the current study have published estimates of personality change, for example, the GSOEP ( Lucas & Donnellan, 2011 ), NAS ( Mroczek & Spiro, 2003 ), BASE-II ( Mueller et al., 2016 ), LASA ( Steunenberg, Twisk, Beekman, Deeg, & Kerkhof, 2005 ), and ILSE ( Allemand et al., 2015 ). Differences could be attributed to updated data (e.g. for NAS), or different modelling approaches (e.g. MLM vs. SEM), as well as different covariates and moderators.
Individual differences in personality change
There was evidence for individual differences in change in most traits across most samples. For the vast majority, there was support for the lifespan development principle of individual differences in intra-individual change ( Baltes, 1987 ) as well as self-regulation theory ( Denissen et al., 2013 ), both of which predict individual variation in trajectories. Not everyone changes at the same rate, nor in the same direction ( Mroczek & Spiro, 2003 ). This idea forms the basis of our efforts to identify predictors of personality change, and also indicates that the concept of individual differences applies to rate and direction of change, in addition to level or amount of a trait. Further, even if the models suggest only a small amount of intra-individual variation in slopes, we can still test predictors of variation.
Synthesis of predictor models
We tested a number of predictors of change to assess whether sex or age at baseline could reliably account for the within-study individual differences in change. We observed minimal evidence that sex accounted for individual differences. For most traits, the meta-analytic summary was null, with the exception of neuroticism. For neuroticism, it appears that female participants have slightly steeper declines in neuroticism than male participants. For the baseline age models, there was consistent evidence for an effect both at the individual study-level and in the meta-analytic summaries for all traits. This suggests that personality change may occur differently among younger and older adults. The differing patterns in linear change among younger and older adults, as evidenced by the baseline age predictor models, are consistent with the theories discussed above, such as neo-socioanalytic theory, self-regulation, and the SOC model ( Baltes & Baltes, 1990 ; Denissen et al., 2013 ; Marsh et al., 2013 ; Roberts et al., 2006 ). According to these theories, there is a clear benefit to having higher levels of conscientiousness, openness, agreeableness, and extraversion in younger and middle adulthood. As social demands begin to wane in older adulthood, so might these traits. Our findings support these theories. Similarly, the findings for neuroticism indicate that older individuals increase in neuroticism more than younger adults. This is consistent with the idea that, as a person ages, becomes more likely to develop serious conditions, and becomes more aware of their mortality, neuroticism increases as part of the heightened anxiety surrounding these new realities.
Variation in trajectories over samples
We observed statistical heterogeneity, as assessed by I 2 and Cochran’s Q , across samples, and we attempted to account for this variability using four study-level factors: sample age (average, at baseline), country, and personality scale. These analyses yielded mixed results. Even with a cross-study sample size of over 60 000, we were relatively low powered at the study level (maximum N = 16) to reliably account for variation in trajectories, and this became more apparent as the studies without a given variable, or measurement of a certain personality trait, were not included in the meta-analysis. A distribution of effects (in both magnitude and direction) is to be expected in any replication project, and the current study is no exception. Calculation of the average effect for each model helps to reduce this remaining uncertainty, and using identical variable transformations and harmonized models strengthens our confidence in these average estimates. However, heterogeneity is still widespread in our results and is not fully accounted for by our study-level moderators. The three most likely reasons for between-study heterogeneity are untested moderators, random variation due to measurement error, and variation in true effect sizes across studies due to different underlying populations. The latter reason is the basis of random-effects meta-analysis ( Borenstein et al., 2010 ), which does not assume that there is one true effect size in the population, and that different studies (because of variation on so many characteristics such as country of origin, age at entry, etc.) have different true effect sizes. Further, several personality scales were used to assess traits across our studies, and the measurement differences in these scales could be partially driving variation in a way that was not detected by our tests.
The value of coordinated analysis
These findings demonstrate the value of coordinated analysis as a technique for synthesizing long-term longitudinal findings over a shorter time frame than otherwise would be the case if each study published its own single-study analysis (some have called this technique coordinated replication, such as Duggan et al., 2019 ). Technically, coordinated analysis is not a replication study in the strict sense but rather a method that provides an initial set of multi-study findings that can be thought of as a set of replicates or as a synthesized group of findings centred around a common research question. It establishes a moderate-sized group of replications, along with synthesized products such as meta-trajectories, both of which enhance the robustness of future work.
Coordinated analysis has the additional advantage of preserving the heterogeneity in trajectories, as well as permitting analyses that identify factors that explain differences across samples. While we found limited evidence for between-study factors explaining variation in effects, future research should examine this further. Pooling of data is an alternative technique and can be quite valuable ( Jokela et al., 2014 ) but presents unique challenges with respect to data harmonization and therefore tends to use smaller subsets of studies (or downplays the issue of harmonization). Perhaps a bigger concern of pooled analysis is that it assumes a common true effect size underlying all of the included studies. By coordinating models across multiple datasets, we preserve the heterogeneity of the studies. By aligning with the assumptions of the random-effects model ( Borenstein et al., 2010 ), coordinated analysis allows the possibility of not one but many true effects. This touches on the issue of generalizability and the relationship between replicability and generalizability. Similarities in results across studies are an indication not only of replicability but also of generalizability. By contrast, single-study analysis has its own challenges. Any one of the samples used here could have comprised a publication on its own, with some claiming given trait increases, others claiming a decrease, and still others claiming no change at all. Add to this the inclusion of predictors, and the single-study publication possibilities from the current study could have yielded wildly different stories. This has the potential to sow great confusion and to send future researchers down ultimately fruitless pathways. The method used in this project thus demonstrates the utility and importance of coordinated analysis in enhancing the synthesis of findings across studies and in evaluating their replicability and generalizability.
Limitations and future directions
The samples used in the current study were primarily WEIRD (White, Educated, Industrialized, Rich, and Democratic) ( Henrich, Heine, & Norenzayan, 2010 ). As such, we cannot be confident that our results would translate to other cultures. An additional limitation of the current study is that we relied solely on self-reported measures of personality. This mono-method approach is limiting ( Costa, McCrae, & Lockenhoff, 2019 ), and future studies could execute a similar coordinated analysis of personality change using informant reports. It is also possible that the number of measurement occasions (i.e. test effects) could be partially responsible for differences in the trajectories. We added a measurement occasions variable to the meta-analysis as a study-level moderator, and we found that measurement occasions did not account for any of the observed heterogeneity. These additional analyses can be found in the Supporting Information . Also, the datasets used were somewhat asymmetrical; some were panel studies including individuals from across the entire adult lifespan, and others were focused solely on older adulthood. We used the datasets available as part of the IALSA network that had the requisite data for our analyses, and while IALSA is fairly comprehensive in its coverage of age-focused studies, it does not contain all available panel studies. The implications of this are that our selection of studies was unintentionally biased. We recommend that researchers with access to other panel data with longitudinal personality data to compare the trajectories reported in the current paper to those in their own data, to further enrich our understanding of personality change across the full adult lifespan.
Additionally, future research using ‘time-to-death’ as a temporal metric could yield theoretically interesting results ( Wagner et al., 2016 ). In most trajectory models, chronological time in the form of time-in-study or age is the preferred time metric. However, in studies where most participants are midlife or older a reverse time metric, time from death, is an alternative that has led to interesting results in the area of cognitive aging ( Gerstorf, Ram, Lindenberger, & Smith, 2013 ). Such alternative time metrics could be examined with respect to personality trajectories. Measurement differences are also a limitation. Some have found that different scales (e.g. BFI vs. NEO) measure certain traits (e.g. agreeableness) in categorically different ways and capture very different individual characteristics ( Miller, Gaughan, Maples, & Price, 2011 ). This being said, we acknowledge that the comparability of a given trait is limited by the scale used to measure it. However, in the context of a coordinated analysis, these measurement differences (as well as all study-level differences) are not strictly a liability: they can be considered an asset as well. Consistency in results in spite of study-level differences adds to the generalizability of the results ( Hofer & Piccinin, 2009 ). To limit our models to only a single measurement approach would constrain the external validity of our findings.
Across 16 diverse longitudinal samples of aging adults, we observed change in the Big Five personality traits. Linear models suggest that conscientiousness, extraversion, and openness decline on average over time, while agreeableness is relatively stable. Quadratic models pointed to potential late-life increases in neuroticism. Nearly all samples showed individual differences in change for all five traits. Age of samples, country, and scale account for some of the variations in sample trajectories.
This coordinated analysis makes three primary contributions to the field. The first contribution is empirical. We brought to bear a great deal of data to address continuing controversies regarding personality development over the lifespan. The second contribution is methodological and centres on replication and current concerns regarding reproducibility and robustness. We used a multi-study framework that by its very nature was designed to evaluate and promote replicability. This is particularly important at this point in the history of our science, given the need for greater credibility in many areas of psychology and other fields. Third, our analyses support current theory in lifespan personality development, as our findings are somewhat consistent with the Baltes theory of selection, optimization, and compensation (SOC), self-regulation theory ( Denissen et al., 2013 ), and neo-socioanalytic theory ( Roberts et al., 2008 ). Specifically, traits differed in patterns of change with age. However, people change differently on different traits, personality is not stable for everyone across the lifespan (but is for some people), and accounting for or explaining these changes is difficult. Overall, in addition to improving our understanding of typical personality development across the adult lifespan, as well as highlighting the prevalence of individual differences in personality trajectories, this research highlights the strengths and feasibility of a coordinated analysis approach.
Supplementary Material
Supplemental material, acknowledgement.
We would like to thank Martin Sliwinski for his contributions to this project as PI of the Einstein Aging Study.
1 We were not able to effectively account for anticipation effects or the timing of events in a coordinated manner across so many studies in a way that would be comparable and informative. As such, models including health events, marital status, and retirement status as predictors of personality change are not reported in the current manuscript, but interested readers may view the results from these models in the Supporting Information . See Tables S7 – S16 , S23 – 32 , S39 – S38 , S55 – S64 , and S71 – S80 and Figures S10 – S27 , S34 – 55 , S62 – S83 , S90 – S110 , and S118 – S138 .
SUPPORTING INFORMATION
Additional supporting information may be found online in the Supporting Information section at the end of the article.
Data S1. Supporting Information
- Allemand M, Job V, & Mroczek DK (2019). Self-control development in adolescence predicts love and work in adulthood . Journal of Personality and Social Psychology , 117 , 621–634. 10.1037/pspp0000229. [ PMC free article ] [ PubMed ] [ CrossRef ] [ Google Scholar ]
- Allemand M, Schaffhuser K, & Martin M (2015). Long-term correlated change between personality traits and perceived social support in middle adulthood . Personality and Social Psychology Bulletin , 41 , 420–432. 10.1177/0146167215569492. [ PubMed ] [ CrossRef ] [ Google Scholar ]
- Allemand M, Zimprich D, & Martin M (2008). Long-term correlated change in personality traits in old age . Psychology and Aging , 23 , 545–557. 10.1037/a0013239. [ PubMed ] [ CrossRef ] [ Google Scholar ]
- Baltes P, Reese H, & Nesselroade J (1977). Life-span developmental psychology: Introduction to research methods . Monterey, CA: Brooks. Cole Publishing Co. [ Google Scholar ]
- Baltes PB (1987). Theoretical propositions of life-span developmental psychology: On the dynamics between growth and decline . Developmental Psychology , 23 , 611–626. [ Google Scholar ]
- Baltes PB (1997). On the incomplete architecture of human ontogeny: Selection, optimization, and compensation as foundation of developmental theory . American Psychologist , 52 , 366–380. 10.1037//0003-066x.52.4.366. [ PubMed ] [ CrossRef ] [ Google Scholar ]
- Baltes PB, & Baltes MM (1990). Psychological perspectives on successful aging: The model of selective optimization with compensation . Successful Aging: Perspectives from the Behavioral Sciences , 1 , 1–34. [ Google Scholar ]
- Baltes PB, Lindenberger U, & Staudinger U (2006). Life span theory in developmental psychology In Damon W, & Lerner R (Eds.), Handbook of child psychology theoretical models of human development (− 569 , 595). New Jersey. [ Google Scholar ]
- Bates D, Mächler M, Bolker B, & Walker S (2015). Fitting linear mixed-effects models using lme4 . Journal of Statistical Software , 67 , 1–48. 10.18637/jss.v067.i01. [ CrossRef ] [ Google Scholar ]
- Berg AI, & Johansson B (2013). Personality change in the oldest-old: Is it a matter of compromised health and functioning? Journal of Personality , 82 , 25–31. 10.1111/jopy.12030. [ PubMed ] [ CrossRef ] [ Google Scholar ]
- Bertram L, Bockenhoff A, Demuth I, Duzel S, Eckardt R, Li S-C, ... Wagner GG (2013). Cohort profile: The Berlin Aging Study II (BASE-II) . International Journal of Epidemiology , 43 , 703–712. 10.1093/ije/dyt018. [ PubMed ] [ CrossRef ] [ Google Scholar ]
- Bleidorn W, Kandler C, Riemann R, Angleitner A, & Spinath FM (2009). Patterns and sources of adult personality development: Growth curve analyses of the NEO PI-R scales in a longitudinal twin study . Journal of Personality and Social Psychology , 97 , 142–155. 10.1037/a0015434. [ PubMed ] [ CrossRef ] [ Google Scholar ]
- Bleidorn W, Klimstra TA, Denissen JJA, Rentfrow PJ, Potter J, & Gosling SD (2013). Personality maturation around the world: A cross-cultural examination of social-investment theory . Psychological Science , 24 , 2530–2540. 10.1177/0956797613498396. [ PubMed ] [ CrossRef ] [ Google Scholar ]
- Bogg T, Voss MW, Wood D, & Roberts BW (2008). A hierarchical investigation of personality and behavior: Examining neo-socioanalytic models of health-related outcomes . Journal of Research in Personality , 42 , 183–207. https://doi.org/10.1016Zj.jrp.2007.05.003 . [ Google Scholar ]
- Borenstein M, Hedges LV, Higgins JP, & Rothstein HR (2010). A basic introduction to fixed-effect and random-effects models for meta-analysis . Research Synthesis Methods , 1 , 97–111. 10.1002/jrsm.12. [ PubMed ] [ CrossRef ] [ Google Scholar ]
- Borenstein M, Higgins JPT, Hedges LV, & Rothstein HR (2017). Basics of meta-analysis: I2 is not an absolute measure of heterogeneity . Research Synthesis Methods , 8 , 5–18. 10.1002/jrsm.1230. [ PubMed ] [ CrossRef ] [ Google Scholar ]
- Bosse R, Ekerdt D, & Silbert J (1984). The Veteran Administration Normative Aging Study In Mednick SA, Harway M, & Finello KM (Eds.), Handbook of longitudinal research vol. teen-age and adult cohorts (pp. 273–289). New York. [ Google Scholar ]
- Brim OG, Ryff CD, & Kessler RC (2004). How healthy are we? A national study of well-being at midlife . Chicago: University of Chicago Press. [ Google Scholar ]
- Carstensen LL, Isaacowitz DM, & Charles ST (1999). Taking time seriously: A theory of socioemotional selectivity . American Psychologist , 54 , 165–181. 10.1037//0003-066x.54.3.165. [ PubMed ] [ CrossRef ] [ Google Scholar ]
- Caspi A, Roberts BW, & Shiner RL (2005). Personality development: Stability and change . Annual Review of Psychology , 56 , 453–484. 10.1146/annurev.psych.55.090902.141913. [ PubMed ] [ CrossRef ] [ Google Scholar ]
- Condon DM, Graham EK, & Mroczek DK (2017). On replication research In The Wiley-Blackwell encyclopedia of personality and individual differences: Vol. II. Research methods and assessment techniques . Hoboken, NJ: John Wiley & Sons. [ Google Scholar ]
- Costa PT Jr, & McCrae RR (1980). Still stable after all these years: Personality as a key to some issues in adulthood and old age . Life-Span Development and Behavior . [ Google Scholar ]
- Costa PT Jr., & McCrae RR (1985a). The NEO Personality Inventory manual . Odessa, FL: Psychological Assessment Resources. [ Google Scholar ]
- Costa PT, & McCrae RR (1985b). The neo personality inventtory . FL: Psychological Assessment Resources Odessa. [ Google Scholar ]
- Costa PT Jr., & McCrae RR (1986). Personality stability and its implications for clinical psychology . Clinical Psychology Review , 6 , 407–423. [ Google Scholar ]
- Costa PT, & McCrae RR (1988). Personality in adulthood: A six-year longitudinal study of self-reports and spouse ratings on the neo personality inventory . Journal of Personality and Social Psychology , 54 , 853–863. [ PubMed ] [ Google Scholar ]
- Costa PT Jr., & McCrae RR (1992). Normal personality assessment in clinical practice: The NEO Personality Inventory . Psychological Assessment , 4 , 5–13. [ Google Scholar ]
- Costa PT Jr., McCrae RR, & Lockenhoff CE (2019). Personality across the life span . Annual Review of Psychology , 70 , 423–448. 10.1146/annurev-psych-010418-103244. [ PubMed ] [ CrossRef ] [ Google Scholar ]
- Curran PJ, Hussong AM, Cai L, Huang W, Chassin L, Sher KJ, & Zucker RA (2008). Pooling data from multiple longitudinal studies: The role of item response theory in integrative data analysis . Developmental Psychology , 44 , 365–380. 10.1037/0012-1649.44.2365. [ PMC free article ] [ PubMed ] [ CrossRef ] [ Google Scholar ]
- Deary IJ, Gow AJ, Pattie A, & Starr JM (2012). Cohort profile: The Lothian Birth Cohorts of 1921 and 1936 . International Journal of Epidemiology , 41 , 1576–1584. 10.1093/ije/dyr197. [ PubMed ] [ CrossRef ] [ Google Scholar ]
- Denissen JJ, van Aken MA, Penke L, & Wood D (2013). Self-regulation underlies temperament and personality: An integrative developmental framework . Child Development Perspectives , 7 , 255–260. 10.1111/cdep.12050. [ CrossRef ] [ Google Scholar ]
- Duggan EC, Piccinin AM, Clouston S, Koval AV, Robitaille A, Zammit AR, … Finkel D (2019). A multi-study coordinated meta-analysis of pulmonary function and cognition in aging . The Journals of Gerontology: Series A , 74 , 1793–1804. 10.1093/gerona/glz057. [ PMC free article ] [ PubMed ] [ CrossRef ] [ Google Scholar ]
- Eysenck HJ (1975). Manual of the Eysenck Personality Questionnaire (junior and adult) . London: Hodder; Stoughton. [ Google Scholar ]
- Eysenck HJ, & Eysenck SBG (1968). Manual for the Eysenck Personality Inventory . San Diego, CA: Education; Industrial Testing Service. [ Google Scholar ]
- Floderus B (1974). Psycho-social factors in relation to coronary heart disease and associated risk factors . Nordisk Hygienisk Tidskrift . [ Google Scholar ]
- Gerstorf D, Bertram L, Lindenberger U, Pawelec G, Demuth I, Steinhagen-Thiessen E, & Wagner GG (2016). The Berlin Aging Study II—An overview . Gerontology , 62 , 311–315. 10.1159/000441495. [ PubMed ] [ CrossRef ] [ Google Scholar ]
- Gerstorf D, Ram N, Lindenberger U, & Smith J (2013). Age and time-to-death trajectories of change in indicators of cognitive, sensory, physical, health, social, and self-related functions . Developmental Psychology , 49 , 1805–1821. 10.1037/a0031340. [ PubMed ] [ CrossRef ] [ Google Scholar ]
- Goebel J, Grabka MM, Liebig S, Kroh M, Richter D, Schroder C, & Schupp J (2018). The German Socio-Economic Panel (SOEP) . Jahrbücher Fur Nationalökonomie Und Statistik , 239 , 345–360. 10.1515/jbnst-2018-0022. [ CrossRef ] [ Google Scholar ]
- Goldberg LR (1992). The development of markers for the Big-Five factor structure . Psychological Assessment , 4 , 26–42. [ Google Scholar ]
- Graham EK, & Lachman ME (2012). Personality stability is associated with better cognitive performance in adulthood: Are the stable more able? Journal of Gerontology: Psychological Sciences , 67 , 545–554. 10.1093/geronb/gbr149. [ PMC free article ] [ PubMed ] [ CrossRef ] [ Google Scholar ]
- Graham EK, Rutsohn JP, Turiano NA, Bendayan R, Batterham PJ, Gerstorf D, … Mroczek DK (2017). Personality predicts mortality risk: An integrative data analysis of 15 international longitudinal studies . Journal of Research in Personality , 70 , 174–186. 10.1016/j.jrp.2017.07.005. [ PMC free article ] [ PubMed ] [ CrossRef ] [ Google Scholar ]
- Headey B, Muffels R, & Wagner G (2011). Choices which change life satisfaction: Replicating results for Australia, Britain and Germany . Social Indicators Research , 102 , 1–31. 10.1007/sl1205-012-0079-8. [ CrossRef ] [ Google Scholar ]
- Helson R, Jones C, & Kwan VSY (2002). Personality change over 40 years of adulthood: Hierarchical linear modeling analyses of two longitudinal samples . Journal of Personality and Social Psychology , 83 , 752–766. 10.1037//0022-3514.83.3.752. [ PubMed ] [ CrossRef ] [ Google Scholar ]
- Henrich J, Heine SJ, & Norenzayan A (2010). The weirdest people in the world? Behavioral and Brain Sciences , 33 , 61–83. 10.1017/S0140525X0999152X. [ PubMed ] [ CrossRef ] [ Google Scholar ]
- Herd P, Carr D, & Roan C (2014). Cohort profile: Wisconsin Longitudinal Study (WLS) . International Journal of Epidemiology , 43 , 34–41. 10.1093/ije/dys194. [ PMC free article ] [ PubMed ] [ CrossRef ] [ Google Scholar ]
- Hofer SM, & Piccinin AM (2009). Integrative data analysis through coordination of measurement and analysis protocol across independent longitudinal studies . Psychological Methods , 14 , 150–164. 10.1037/a0015566. [ PMC free article ] [ PubMed ] [ CrossRef ] [ Google Scholar ]
- Huisman M, Poppelaars J, van der Horst M, Beekman AT, Brug J, van Tilburg TG, & Deeg DJ (2011). Cohort profile: The longitudinal aging study Amsterdam . International Journal of Epidemiology , 40 , 868–876. 10.1093/ije/dyq219. [ PubMed ] [ CrossRef ] [ Google Scholar ]
- Human LJ, Biesanz JC, Miller GE, Chen E, Lachman ME, & Seeman TE (2013). Is change bad? Personality change is associated with poorer psychological health and greater metabolic syndrome in midlife . Journal of Personality , 81 , 249–260. 10.1111/jopy.12002. [ PMC free article ] [ PubMed ] [ CrossRef ] [ Google Scholar ]
- John OP, Donahue EM, & Kentle RL (1991). The Big Five Inventory—Versions 4a and 54 . Berkeley, CA: University of California, Berkeley, Institute of Personality. [ Google Scholar ]
- John OP, & Srivastava S (1999). The Big Five trait taxonomy: History, measurement, and theoretical perspectives . In Handbook of personality: Theory and research (pp. 102–138). [ Google Scholar ]
- Jokela M, Elovainio M, Nyberg ST, Tabak AG, Hintsa T, Batty GD, & Kivimaki M (2014). Personality and risk of diabetes in adults: Pooled analysis of 5 cohort studies . Health Psychology , 33 , 1618–1621. 10.1037/hea0000003. [ PubMed ] [ CrossRef ] [ Google Scholar ]
- Juster FT, & Suzman R (1995). An overview of the Health and Retirement Study . The Journal of Human Resources , 30 , S7–S56. [ Google Scholar ]
- Kandler C, Kornadt AE, Hagemeyer B, & Neyer FJ (2015). Patterns and sources of personality development in old age . Journal of Personality and Social Psychology , 109 , 175–191. 10.1037/pspp0000028. [ PubMed ] [ CrossRef ] [ Google Scholar ]
- Katz MJ, Lipton RB, Hall CB, Zimmerman ME, Sanders AE, Verghese J, … Derby CA (2012). Age-specific and sex-specific prevalence and incidence of mild cognitive impairment, dementia, and Alzheimer dementia in blacks and whites: A report from the Einstein Aging Study . Alzheimer Disease & Associated Disorders , 26 , 335–343. 10.1097/WAD.0b013e31823dbcfc. [ PMC free article ] [ PubMed ] [ CrossRef ] [ Google Scholar ]
- Lachman ME, & Weaver SL (1997). The Midlife Development Inventory (MIDI) Personality Scales: Scale construction and scoring (pp. 1–9). Waltham, MA: Brandeis University. [ Google Scholar ]
- Lang FR, John D, Lüdtke O, Schupp J, & Wagner GG (2011). Short assessment of the Big Five: Robust across survey methods except telephone interviewing . Behavior Research Methods , 43 , 548–567. 10.3758/s13428-011-0066-z. [ PMC free article ] [ PubMed ] [ CrossRef ] [ Google Scholar ]
- Lucas RE, & Donnellan MB (2011). Personality development across the life span: Longitudinal analyses with a national sample from Germany . Journal of Personality and Social Psychology , 101 , 847–861. 10.1037/a0024298. [ PubMed ] [ CrossRef ] [ Google Scholar ]
- Luteijn F, Starren JCMG, & van Dijk H (2000). Handleiding Nederlandse Persoonlijkheids Vragenlijst , NPV (herziene uitgave). [ Google Scholar ]
- Marsh HW, Nagengast B, & Morin AJS (2013). Measurement invariance of big-five factors over the life span: ESEM tests of gender, age, plasticity, maturity, and la dolce vita effects . Developmental Psychology , 49 , 1194–1218. 10.1037/a0026913. [ PubMed ] [ CrossRef ] [ Google Scholar ]
- McClearn GE, Johansson B, Berg S, Pedersen NL, Ahern E , Petrill SA, & Plomin R (1997). Substantial genetic influence on cognitive abilities in twins 80 or more years old . Science , 276 , 1560–1563. 10.1126/science.276.5318.1560. [ PubMed ] [ CrossRef ] [ Google Scholar ]
- McCrae RR, & Costa PT Jr. (1994). The stability of personality: Observations and evaluations . Current Directions in Psychological Science , 3 , 173–175. [ Google Scholar ]
- Miller JD, Gaughan ET, Maples J, & Price J (2011). A comparison of agreeableness scores from the Big Five Inventory and the NEO PI-R: Consequences for the study of narcissism and psychopathy . Assessment , 18 , 335–339. 10.1177/1073191111411671. [ PubMed ] [ CrossRef ] [ Google Scholar ]
- Mischel W (1969). Continuity and change in personality . American Psychologist , 24 , 1012–1018. 10.1037/h0028886. [ PubMed ] [ CrossRef ] [ Google Scholar ]
- Mischel W (1977). On the future of personality measurement . American Psychologist , 32 , 246–254. 10.1037/0003-066X.32.4.246. [ PubMed ] [ CrossRef ] [ Google Scholar ]
- Mõttus R, Johnson W, & Deary IJ (2012). Personality traits in old age: Measurement and rank-order stability and some mean-level change . Psychology and Aging , 27 , 243–249. 10.1037/a0023690. [ PubMed ] [ CrossRef ] [ Google Scholar ]
- Mõttus R, Johnson W, Starr JM, & Deary IJ (2012). Correlates of personality trait levels and their changes in very old age: The Lothian Birth Cohort 1921 . Journal of Research in Personality , 46 , 271–278. 10.1016/jjrp.2012.02.004. [ CrossRef ] [ Google Scholar ]
- Mroczek D, Weston SJ, & Willroth EC (2019). A lifespan perspective on the interconnections between personality, health, and optimal aging , 1–22. Doi: 10.31234/osf.io/sc74d [ CrossRef ] [ Google Scholar ]
- Mroczek DK, Almeida DM, Spiro A, & Pafford C (2006). Modeling intraindividual stability and change in personality In Mroczek DK, & Little TD (Eds.), Handbook of personality development (pp. 163–180). Mahwah: Lawrence Erlbaum Associates. [ Google Scholar ]
- Mroczek DK, Graham EK, Turiano NA, & Oro-Lambo MO (2019). Personality development in adulthood and later life In Handbook of personality: Theory and research (4th ed.). Guilford. [ Google Scholar ]
- Mroczek DK, & Spiro A (2003). Modeling intraindividual change in personality traits: Findings from the Normative Aging Study . The Journals of Gerontology Series B: Psychological Sciences and Social Sciences , 58 , P153–P165. 10.1093/geronb/58.3.P153. [ PubMed ] [ CrossRef ] [ Google Scholar ]
- Mroczek DK, & Spiro A (2007). Personality change influences mortality in older men . Psychological Science , 18 , 1–7. 10.1111/j.1467-9280.2007.01907.x. [ PMC free article ] [ PubMed ] [ CrossRef ] [ Google Scholar ]
- Mueller S, Ram N, Conroy DE, Pincus AL, Gerstorf D, & Wagner J (2019). Happy like a fish in water? The role of personality-situation fit for momentary happiness in social interactions across the adult lifespan . European Journal of Personality , 33 , 298–316. 10.1002/per.2198. [ CrossRef ] [ Google Scholar ]
- Mueller S, Wagner J, Drewelies J, Duezel S, Eibich P, Specht J, … Gerstorf D (2016). Personality development in old age relates to physical health and cognitive performance: Evidence from the Berlin Aging Study II . Journal of Research in Personality , 65 , 94–108. 10.1016/j.jrp.2016.08.007. [ CrossRef ] [ Google Scholar ]
- Mueller S, Wagner J, & Gerstorf D (2017). On the role of personality in late life In Personality development across the lifespan (pp. 69–84). Elsevier; doi: 10.1016/B978-0-12-804674-6.00006-5. [ CrossRef ] [ Google Scholar ]
- Mühlig-Versen A, Bowen CE, & Staudinger UM (2012). Personality plasticity in later adulthood: Contextual and personal resources are needed to increase openness to new experiences . Psychology and Aging , 27 , 855–866. 10.1037/a0029357. [ PubMed ] [ CrossRef ] [ Google Scholar ]
- Nelson LD, Simmons J, & Simonsohn U (2018). Psychology’s renaissance . Annual Review of Psychology , 69 , 511–534. 10.1146/annurev-psych-122216-011836. [ PubMed ] [ CrossRef ] [ Google Scholar ]
- Nesselroade JR, & Baltes PB (1979). Longitudinal research in the study of behavior and development . Academic Press. [ Google Scholar ]
- Pedersen NL, McClearn GE, Plomin R, Nesselroade JR, Berg S, & DeFaire U (1991). The Swedish Adoption Twin Study of Aging: An update . Acta Geneticae Medicae et Gemellologiae: Twin Research , 40 , 7–20. 10.1017/S0001566000006681. [ PubMed ] [ CrossRef ] [ Google Scholar ]
- Pedersen NL, & Reynolds CA (1998). Stability and change in adult personality: Genetic and environmental components . European Journal of Personality , 12 , 365–386. 10.1002/(SICI)1099-0984(1998090)12:5<365::AID-PER335>3.0.CO;2-N. [ CrossRef ] [ Google Scholar ]
- R Core Team (2014). R: A language and environment for statistical computing . Vienna, Austria: R Foundation for Statistical Com-puting; Retrieved from http://www.R-project.org/ . [ Google Scholar ]
- Raudenbush SW, & Bryk AS (2002). Hierarchical linear models: Applications and data analysis methods 1 . SAGE. [ Google Scholar ]
- Riley RD, Lambert PC, & Abo-Zaid G (2010). Meta-analysis of individual participant data: Rationale, conduct, and reporting . British Medical Journal , 340 , 521–525. 10.1136/bmj.c221. [ PubMed ] [ CrossRef ] [ Google Scholar ]
- Roberts BW, & Mroczek D (2008). Personality trait change in adulthood . Current Directions in Psychological Science , 17 , 31–35. 10.1111/j.1467-8721.2008.00543.x. [ PMC free article ] [ PubMed ] [ CrossRef ] [ Google Scholar ]
- Roberts BW, Walton KE, & Viechtbauer W (2006). Patterns of mean-level change in personality traits across the life course: A meta-analysis of longitudinal studies . Psychological Bulletin , 132 , 1–25. 10.1037/0033-2909.132.L1. [ PubMed ] [ CrossRef ] [ Google Scholar ]
- Roberts BW, Wood D, & Caspi A (2008). The development of personality traits in adulthood In John O, Robins R, & Pervin L (Eds.), Handbook of personality theory and research (pp. 375–398). [ Google Scholar ]
- Roberts BW, Wood D, & Smith JL (2005). Evaluating five factor theory and social investment perspectives on personality trait development . Journal of Research in Personality , 39 , 166–184. 10.1016/jjrp.2004.08.002. [ CrossRef ] [ Google Scholar ]
- Sattler C, Wahl H-W, Schroder J, Kruse A, Schonknecht P, Kunzmann U, & Zenthofer A (2015). Interdisciplinary Longitudinal Study on Adult Development and Aging (ILSE) In Pachana N (Ed.), Encyclopedia of geropsychology (pp. 1–10). [ Google Scholar ]
- Schaie KW, Willis SL, & Caskie GIL (2004). The Seattle Longitudinal Study: Relationship between personality and cognition . Aging, Neuropsychology, and Cognition , 11 , 304–324. 10.1080/13825580490511134. [ PMC free article ] [ PubMed ] [ CrossRef ] [ Google Scholar ]
- Sewell WH, Hauser RM, Springer KW, & Hauser TS (2003). As we age: A review of the Wisconsin Longitudinal Study, 1957-2001 . Research in Social Stratification and Mobility , 20 , 3–111. 10.1016/S0276-5624(03)20001-9. [ CrossRef ] [ Google Scholar ]
- Singer JD, & Willett JB (2003). Applied longitudinal data analysis . Oxford University Press. [ Google Scholar ]
- Sonnega A, Faul JD, Ofstedal MB, Langa KM, Phillips JW, & Weir DR (2014). Cohort profile: The Health and Retirement Study (HRS) . International Journal of Epidemiology , 43 , 576–585. 10.1093/ije/dyu067. [ PMC free article ] [ PubMed ] [ CrossRef ] [ Google Scholar ]
- Soto CJ, John OP, Gosling SD, & Potter J (2011). Age differences in personality traits from 10 to 65: Big five domains and facets in a large cross-sectional sample . Journal of Personality and Social Psychology , 100 , 330–348. 10.1037/a0021717. [ PubMed ] [ CrossRef ] [ Google Scholar ]
- Steiger AE, Allemand M, Robins RW, & Fend HA (2014). Low and decreasing self-esteem during adolescence predict adult depression two decades later . Journal of Personality and Social Psychology , 106 , 325–338. 10.1037/a0035133. [ PubMed ] [ CrossRef ] [ Google Scholar ]
- Steunenberg B, Twisk JW, Beekman AT, Deeg DJ, & Kerkhof AJ (2005). Stability and change of neuroticism in aging . The Journals of Gerontology Series B: Psychological Sciences and Social Sciences , 60 , P27–P33. 10.1093/geronb/60.1.P27. [ PubMed ] [ CrossRef ] [ Google Scholar ]
- Terracciano A, McCrae RR, Brant LJ, & Costa PT Jr. (2005). Hierarchical linear modeling analyses of the NEO-PI-R scales in the Baltimore Longitudinal Study of Aging . Psychology and Aging , 20 , 493–506. 10.1037/0882-7974.20.3.493. [ PMC free article ] [ PubMed ] [ CrossRef ] [ Google Scholar ]
- Turiano NA, Pitzer L, Armour C, Karlamangla A, Ryff CD, & Mroczek DK (2011). Personality trait level and change as predictors of health outcomes: Findings from a national study of Americans (MIDUS) . The Journals of Gerontology Series B: Psychological Sciences and Social Sciences , 67B , 4–12. 10.1093/geronb/gbr072. [ PMC free article ] [ PubMed ] [ CrossRef ] [ Google Scholar ]
- Vaidya JG, Gray EK, Haig JR, Mroczek DK, & Watson D (2008). Differential stability and individual growth trajectories of big five and affective traits during young adulthood . Journal of Personality , 76 , 267–304. 10.1111/j.1467-6494.2007.00486.x. [ PMC free article ] [ PubMed ] [ CrossRef ] [ Google Scholar ]
- Vazire S (2018). Implications of the credibility revolution for productivity, creativity, and progress . Perspectives on Psychological Science , 13 , 411–417. 10.1177/1745691617751884. [ PubMed ] [ CrossRef ] [ Google Scholar ]
- Vecchione M, Alessandri G, Barbaranelli C, & Caprara G (2012). Gender differences in the big five personality development: A longitudinal investigation from late adolescence to emerging adulthood . Personality and Individual Differences , 53 , 740–746. 10.1016/j.paid.2012.05.033. [ CrossRef ] [ Google Scholar ]
- Viechtbauer W (2019). Metafor: Meta-analysis package for r . Retrieved from https://CRAN.R-project.org/package=metafor [ Google Scholar ]
- Wagner GG, Joachim R, & Schupp J (2007). The German Socio-Economic Panel Study (SOEP)—Scope, evolution and enhancements . Schmollders Jahrbuch , 1 , 139–169. 10.2139/ssrn.1028709. [ CrossRef ] [ Google Scholar ]
- Wagner J, Ram N, Smith J, & Gerstorf D (2016). Personality trait development at the end of life: Antecedents and correlates of mean-level trajectories . Journal of Personality and Social Psychology , 111 , 411–429. 10.1037/pspp0000071. [ PubMed ] [ CrossRef ] [ Google Scholar ]
- Weston SJ, Graham EK, & Piccinin A (2019). Coordinated data analysis: A new method for the study of personality and health In Hill P, & Allemand M (Eds.), Personality and healthy aging in adulthood . Springer Nature; doi: 10.31234/osf.io/k9up8. [ CrossRef ] [ Google Scholar ]
- Wickham H, Chang W, Henry L, Pedersen TL, Takahashi K, Wilke C, & Woo K (2019). Ggplot2: Create elegant data visualisations using the grammar of graphics . Retrieved from https://CRAN.R-project.org/package=ggplot2 [ Google Scholar ]
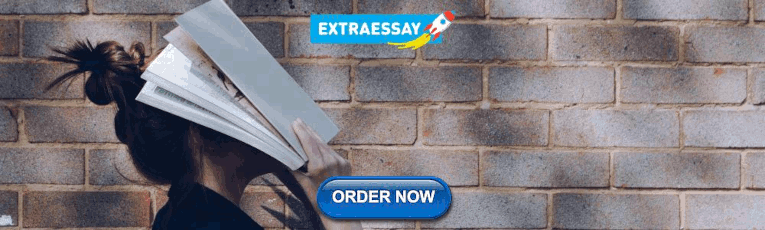
IMAGES
VIDEO
COMMENTS
Abstract. This study assessed change in self-reported Big Five personality traits. We conducted a coordinated integrative data analysis using data from 16 longitudinal samples, comprising a total sample of over 60 000 participants. We coordinated models across multiple datasets and fit identical multi-level growth models to assess and compare ...