Representation Words
Words related to representation.
Below is a massive list of representation words - that is, words related to representation. The top 4 are: image , model , diversity and inclusion . You can get the definition(s) of a word in the list below by tapping the question-mark icon next to it. The words at the top of the list are the ones most associated with representation, and as you go down the relatedness becomes more slight. By default, the words are sorted by relevance/relatedness, but you can also get the most common representation terms by using the menu below, and there's also the option to sort the words alphabetically so you can get representation words starting with a particular letter. You can also filter the word list so it only shows words that are also related to another word of your choosing. So for example, you could enter "image" and click "filter", and it'd give you words that are related to representation and image.
You can highlight the terms by the frequency with which they occur in the written English language using the menu below. The frequency data is extracted from the English Wikipedia corpus, and updated regularly. If you just care about the words' direct semantic similarity to representation, then there's probably no need for this.
There are already a bunch of websites on the net that help you find synonyms for various words, but only a handful that help you find related , or even loosely associated words. So although you might see some synonyms of representation in the list below, many of the words below will have other relationships with representation - you could see a word with the exact opposite meaning in the word list, for example. So it's the sort of list that would be useful for helping you build a representation vocabulary list, or just a general representation word list for whatever purpose, but it's not necessarily going to be useful if you're looking for words that mean the same thing as representation (though it still might be handy for that).
If you're looking for names related to representation (e.g. business names, or pet names), this page might help you come up with ideas. The results below obviously aren't all going to be applicable for the actual name of your pet/blog/startup/etc., but hopefully they get your mind working and help you see the links between various concepts. If your pet/blog/etc. has something to do with representation, then it's obviously a good idea to use concepts or words to do with representation.
If you don't find what you're looking for in the list below, or if there's some sort of bug and it's not displaying representation related words, please send me feedback using this page. Thanks for using the site - I hope it is useful to you! 🐏

- representative
- participation
- proportional representation
- recognition
- illustration
- typification
- adumbration
- interpretation
- instantiation
- pictorial representation
- jurisdiction
- representation
- unrepresented
- underrepresented
- mental representation
- manifestation
- internal representation
- representatives
- representational
- equitable distribution
- disenfranchised
- proportionally
- participatory democracy
- inclusivity
- marginalized
- ethnic minorities
- affiliations
- distinction
- constituencies
- description
- understanding
- unrepresentative
- geographic diversity
- proportionate
- constitutionally
- treated unfairly
- decisionmaking
- discriminated against
- representations
- presentation
- proportional
- performance
- normalization
- advertising
- representing
- represented
- histrionics
- incarnation
- appreciation
- personification
- appropriation
- dramatization
- dramatisation
- empowerment
- explanation
- cooperation
- cosmography
- objectification
- affiliation
- consultation
- convergence
- intersection
- phantasmagoria
- abstractionism
- psychosexuality
- schematization
- diagramming
- schematisation
- visualization
- theatrical performance
- iconography
- corresponding
- geographical
- furthermore
- delineative
- constituted
- determining
- portraiture
- consideration
- independent
- corresponds
- fundamental
- constitutional
- appropriate
- orientation
- characteristics
- prototypical
- resemblance
- hyperrealism
- delineation
- apportionment
- legal representation
- cutaway model
- free agency
- mental image
- concrete representation
- public presentation
- station of the cross
- cutaway drawing
- perceptual experience
- mental object
- picturesque
- cognitive content
- portraitist
- videography
- photomontage
- decision-making
- shadowgraph
- approximation
- photographer
- illustrator
- miniaturist
- backgrounds
- commissions
- nonrepresentational
- printmaking
- discrimination
- transpressionism
- disenfranchisement
- reapportionment
- remonstrate
- photography
- gerrymandering
- photographic
- consultative
- astrophotography
- articulation
- homogeneity
- misrepresent
- accountability
- pseudophotograph
- cartography
- victimization
- transparency
- marginalization
- inclusiveness
- photomicrograph
- composition
- photoengraving
- autoradiograph
- visual information source
- emancipation
- wordshaping
- photomechanical
- telephotograph
- enfranchisement
- disfranchisement
- definiteness
- disproportion
- particularity
- elaboration
- involvement
- distinctness
- pictorialism
- stereomonoscope
- telephotography
- instantiate
- unrepresentable
- scenography
- abstractionist
- artsploitation
- underexpose
- magnetic resonance image
- positron emission tomography
- districting
- answerability
- reputability
- deconcentration
- objectiveness
- bicameralism
- corroboration
- consociational
- fashion model
- photo realism
- technical draw
- screen capture
- mathematical model
- conecept design
- block diagram
- station of cross
- scale model
- pictorial convention
- hang on wall
- graphic art
- logic diagram
- paint on canvas
- gallery open
- partiya karkeran kurdistan
- pro bono publico
That's about all the representation related words we've got! I hope this list of representation terms was useful to you in some way or another. The words down here at the bottom of the list will be in some way associated with representation, but perhaps tenuously (if you've currenly got it sorted by relevance, that is). If you have any feedback for the site, please share it here , but please note this is only a hobby project, so I may not be able to make regular updates to the site. Have a nice day! 👽

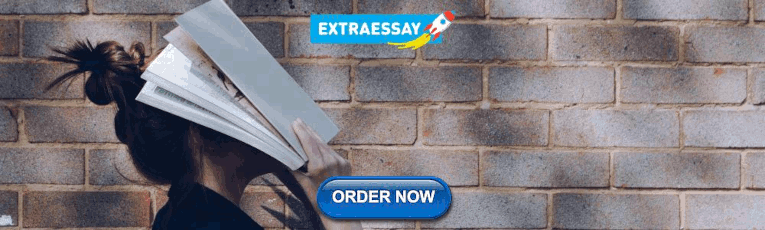
Synonyms of 'representation' in American English
- representation
Synonyms of 'representation' in British English
Additional synonyms, video: pronunciation of representation.
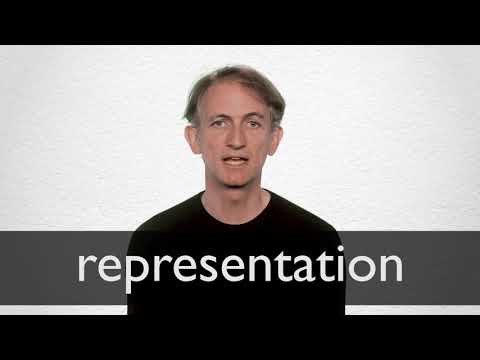
Browse alphabetically representation
- represent someone as something or someone
- represent yourself as something or someone
- representative
- All ENGLISH synonyms that begin with 'R'
Quick word challenge
Quiz Review
Score: 0 / 5

Wordle Helper

Scrabble Tools
- TheFreeDictionary
- Word / Article
- Starts with
- Free toolbar & extensions
- Word of the Day
- Free content
- representation
Synonyms for representation
- body of representatives
- illustration
- resemblance
- description
- delineation
- explanation
- remonstrance
- expostulation
the act or process of describing in lifelike imagery
A presentation to the mind in the form of an idea or image.
- internal representation
- mental representation
Related Words
- convergence
- intersection
- cognitive content
- mental object
- instantiation
- mental image
- interpretation
- phantasmagoria
- psychosexuality
- perceptual experience
- abstractionism
- concrete representation
a creation that is a visual or tangible rendering of someone or something
- adumbration
- cosmography
- cutaway drawing
- cutaway model
- presentation
- objectification
- Station of the Cross
the act of representing
- cooperation
- proportional representation
the state of serving as an official and authorized delegate or agent
- free agency
- legal representation
a body of legislators that serve in behalf of some constituency
A factual statement made by one party in order to induce another party to enter into a contract, a performance of a play.
- histrionics
- theatrical performance
- performance
- public presentation
a statement of facts and reasons made in appealing or protesting
The right of being represented by delegates who have a voice in some legislative body, an activity that stands as an equivalent of something or results in an equivalent.
- dramatisation
- dramatization
- diagramming
- schematisation
- schematization
- pictorial representation
- typification
- reporting weight
- repositioning
- repossession
- reprehensibility
- reprehensible
- reprehensibly
- reprehension
- reprehensively
- representable
- representational
- representational process
- representative
- representative sample
- representative sampling
- represented
- repressor gene
- reproachful
- reproachfully
- represent to
- represent to (someone or something)
- Represent to Witness
- represent us as
- represent us in
- represent us to
- represent you as
- represent you in
- represent you to
- represent yourself as
- represent yourself in
- represent yourself to
- Represent/Represented
- representability
- Representable functor
- representablely
- Representaciones Riquelme y Cerramientos Murcia
- Representamen
- Representance
- Representant
- Representante Especial del Secretario General
- Representatieve Vakorganisaties
- Representation (arts)
- Representation (disambiguation)
- Representation (psychology)
- Representation Agreement Resource Centre
- Representation and Maintenance of Process Knowledge
- Representation and Transportation Allowances
- Representation and Welfare Unit
- representation condition
- Représentation des Institutions Françaises de Sécurité Sociale
- Representation Fund Custodian
- Representation Language Language
- Représentation Militaire Française
- Representation of People's Act
- Representation of persons; a fiction of the law
- Representation of Stimuli as Neural Activity Project
- Representation of the People Act
- Representation of the People Acts
- Representation of the People Order
- Representation oligonucleotide microarray analysis
- Representation Oligonucleotide Microarray Analysis (ROMA)
- Representation Quality
- Representation theory
- Facebook Share
Related Words and Phrases
Bottom_desktop desktop:[300x250].
- More from M-W
- To save this word, you'll need to log in. Log In
representation
Definition of representation
Examples of representation in a sentence.
These examples are programmatically compiled from various online sources to illustrate current usage of the word 'representation.' Any opinions expressed in the examples do not represent those of Merriam-Webster or its editors. Send us feedback about these examples.
Word History
15th century, in the meaning defined at sense 1
Phrases Containing representation
- proportional representation
- self - representation
Dictionary Entries Near representation
representant
representationalism
Cite this Entry
“Representation.” Merriam-Webster.com Dictionary , Merriam-Webster, https://www.merriam-webster.com/dictionary/representation. Accessed 14 Apr. 2024.
Kids Definition
Kids definition of representation, legal definition, legal definition of representation, more from merriam-webster on representation.
Thesaurus: All synonyms and antonyms for representation
Nglish: Translation of representation for Spanish Speakers
Britannica English: Translation of representation for Arabic Speakers
Britannica.com: Encyclopedia article about representation
Subscribe to America's largest dictionary and get thousands more definitions and advanced search—ad free!
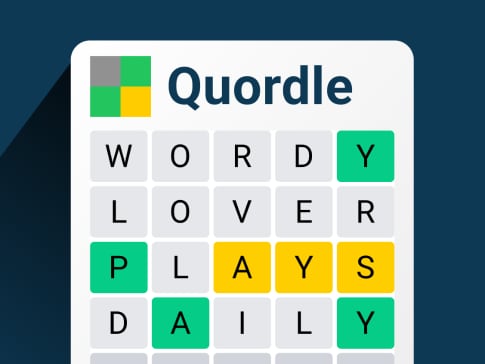
Can you solve 4 words at once?
Word of the day.
See Definitions and Examples »
Get Word of the Day daily email!
Popular in Grammar & Usage
Your vs. you're: how to use them correctly, every letter is silent, sometimes: a-z list of examples, more commonly mispronounced words, how to use em dashes (—), en dashes (–) , and hyphens (-), absent letters that are heard anyway, popular in wordplay, the words of the week - apr. 12, 10 scrabble words without any vowels, 12 more bird names that sound like insults (and sometimes are), 8 uncommon words related to love, 9 superb owl words, games & quizzes.
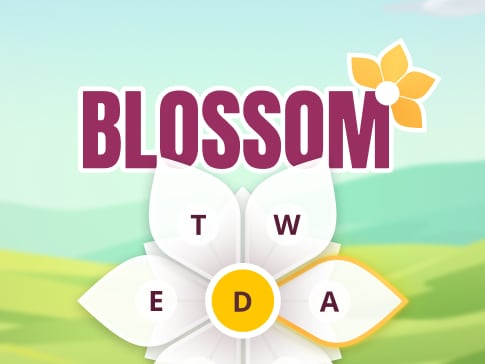

- Cambridge Dictionary +Plus
Meaning of representation in English
Your browser doesn't support HTML5 audio
representation noun ( ACTING FOR )
- Defendants have a right to legal representation and must be informed of that right when they are arrested .
- The farmers demanded greater representation in parliament .
- The main opposing parties have nearly equal representation in the legislature .
- The scheme is intended to increase representation of minority groups .
- The members are chosen by a system of proportional representation.
- admissibility
- extinguishment
- extrajudicial
- extrajudicially
- out-of-court
- pay damages
- plea bargain
- walk free idiom
representation noun ( DESCRIPTION )
- anti-realism
- anti-realist
- complementary
- confederate
- naturalistically
- non-figurative
- non-representational
- poetic license
- symbolization
representation noun ( INCLUDING ALL )
- all manner of something idiom
- alphabet soup
- it takes all sorts (to make a world) idiom
- non-segregated
- odds and ends
- of every stripe/of all stripes idiom
- this and that idiom
- variety is the spice of life idiom
- wide choice
representation | Business English
Examples of representation, collocations with representation.
- representation
These are words often used in combination with representation .
Click on a collocation to see more examples of it.
Translations of representation
Get a quick, free translation!
Word of the Day
pitch-perfect
singing each musical note perfectly, at exactly the right pitch (= level)

Alike and analogous (Talking about similarities, Part 1)

Learn more with +Plus
- Recent and Recommended {{#preferredDictionaries}} {{name}} {{/preferredDictionaries}}
- Definitions Clear explanations of natural written and spoken English English Learner’s Dictionary Essential British English Essential American English
- Grammar and thesaurus Usage explanations of natural written and spoken English Grammar Thesaurus
- Pronunciation British and American pronunciations with audio English Pronunciation
- English–Chinese (Simplified) Chinese (Simplified)–English
- English–Chinese (Traditional) Chinese (Traditional)–English
- English–Dutch Dutch–English
- English–French French–English
- English–German German–English
- English–Indonesian Indonesian–English
- English–Italian Italian–English
- English–Japanese Japanese–English
- English–Norwegian Norwegian–English
- English–Polish Polish–English
- English–Portuguese Portuguese–English
- English–Spanish Spanish–English
- English–Swedish Swedish–English
- Dictionary +Plus Word Lists
- representation (ACTING FOR)
- representation (DESCRIPTION)
- representation (INCLUDING ALL)
- make representations to sb
- Collocations
- Translations
- All translations
Add representation to one of your lists below, or create a new one.
{{message}}
Something went wrong.
There was a problem sending your report.
- Dictionaries home
- American English
- Collocations
- German-English
- Grammar home
- Practical English Usage
- Learn & Practise Grammar (Beta)
- Word Lists home
- My Word Lists
- Recent additions
- Resources home
- Text Checker
Definition of representation noun from the Oxford Advanced American Dictionary
representation
Join our community to access the latest language learning and assessment tips from Oxford University Press!
- 3 representations [ plural ] ( formal ) formal statements made to someone in authority, especially in order to make your opinions known or to protest We have made representations to the mayor but without success.
Other results
Nearby words.
- Daily Crossword
- Word Puzzle
- Word Finder
- Word of the Day
Synonym of the Day
- Word of the Year
- Language stories
- All featured
- Gender and sexuality
- All pop culture
- Grammar Coach ™
- Writing hub
- Grammar essentials
- Commonly confused
- All writing tips
- Pop culture
- Writing tips
Advertisement
- representational
adjective as in graphic
Weak matches
- blocked-out
- descriptive
- diagrammatic
- iconographic
- illustrated
- illustrational
- illustrative
- photographic
adjective as in lifelike
Strongest match
- representative
- true to life
adjective as in pictographic
- hieroglyphic
adjective as in pictorial
Strongest matches
- pictographic
- picturesque
adjective as in realistic
adjective as in schematic
Strong match
- delineative
Discover More
Related words.
Words related to representational are not direct synonyms, but are associated with the word representational . Browse related words to learn more about word associations.
adjective as in pictorial, visible
adjective as in genuine
Viewing 5 / 6 related words
Example Sentences
Of the two teams that produced representational paintings, only one explicitly depicts the newlyweds.
Despite representational gestures, there’s not structural change or a redistribution of money.
Imbalances like this threaten core values of representational democracy like fairness, inclusion and equality.
The cyanotypes and photograms are the closest things to representational works in the show, and they’re deliberately cryptic and detached.
The selection includes abstract works, but most of those seem less urgent than the representational ones.
This is jaw-droppingly strange since perfumes, like paintings and sculpture, are often hyper-representational.
The idea of placing atop this perfect thing a big granite planith surmounted by a representational bronze ... ugh.
The very fact of depicting at one-to-one carries special representational weight.
In fact, when used correctly (i.e., by the Democrats), the filibuster can help right this representational wrong.
Voit documents a perceptual anomaly and allows it to trick us—or not—without any representational manipulation.
To justify our presence there the only thing demanded of us is that we shall have felt the representational impulse.
Strictly representational it may not be, but there are none of your whorls and cylinders and angles and what nots.
On the functional theory of ideas, their value does not rest at all upon their representational nature.
His books are neither documentary nor representational; his characters are symbols of human desires and motives.
He wondered what on earth "anti-representational" could mean.
Start each day with the Synonym of the Day in your inbox!
By clicking "Sign Up", you are accepting Dictionary.com Terms & Conditions and Privacy Policies.
On this page you'll find 115 synonyms, antonyms, and words related to representational, such as: blocked-out, delineated, depicted, descriptive, diagrammatic, and drawn.
From Roget's 21st Century Thesaurus, Third Edition Copyright © 2013 by the Philip Lief Group.
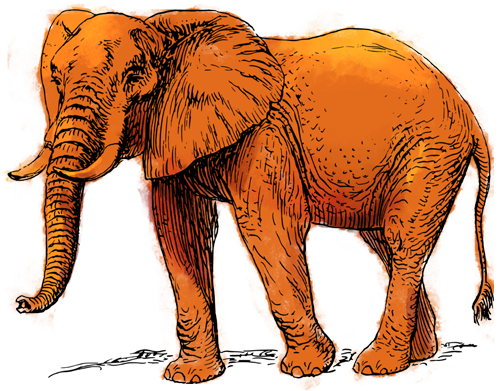
Related Words
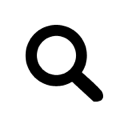
This tool helps you find words that are related to a specific word or phrase. Also check out ReverseDictionary.org and DescribingWords.io . Here are some words that are associated with representation : . You can get the definitions of these representation related words by clicking on them. Also check out describing words for representation and find more words related to representation using ReverseDictionary.org
Click words for definitions
Our algorithm is scanning multiple databases for related words. Please be patient! :)
Words Related to representation
Below is a list of words related to representation . You can click words for definitions. Sorry if there's a few unusual suggestions! The algorithm isn't perfect, but it does a pretty good job for common-ish words. Here's the list of words that are related to representation :
- illustration
- typification
- adumbration
- instantiation
- pictorial representation
- interpretation
- internal representation
- mental representation
- proportional representation
- distinction
- representative
- recognition
- participation
- jurisdiction
- constituencies
- histrionics
- dramatisation
- dramatization
- performance
- cooperation
- cosmography
- presentation
- objectification
- convergence
- intersection
- phantasmagoria
- abstractionism
- psychosexuality
- diagramming
- schematization
- schematisation
- visualization
Popular Searches
As you've probably noticed, words related to " representation " are listed above. Hopefully the generated list of term related words above suit your needs.
P.S. There are some problems that I'm aware of, but can't currently fix (because they are out of the scope of this project). The main one is that individual words can have many different senses (meanings), so when you search for a word like mean , the engine doesn't know which definition you're referring to ("bullies are mean " vs. "what do you mean ?", etc.), so consider that your search query for words like term may be a bit ambiguous to the engine in that sense, and the related terms that are returned may reflect this. You might also be wondering: What type of word is ~term~ ?
Also check out representation words on relatedwords.io for another source of associations.
Related Words runs on several different algorithms which compete to get their results higher in the list. One such algorithm uses word embedding to convert words into many dimensional vectors which represent their meanings. The vectors of the words in your query are compared to a huge database of of pre-computed vectors to find similar words. Another algorithm crawls through Concept Net to find words which have some meaningful relationship with your query. These algorithms, and several more, are what allows Related Words to give you... related words - rather than just direct synonyms.
As well as finding words related to other words, you can enter phrases and it should give you related words and phrases, so long as the phrase/sentence you entered isn't too long. You will probably get some weird results every now and then - that's just the nature of the engine in its current state.
Special thanks to the contributors of the open-source code that was used to bring you this list of representation themed words: @Planeshifter , @HubSpot , Concept Net , WordNet , and @mongodb .
There is still lots of work to be done to get this to give consistently good results, but I think it's at the stage where it could be useful to people, which is why I released it.
Please note that Related Words uses third party scripts (such as Google Analytics and advertisements) which use cookies. To learn more, see the privacy policy .
Recent Queries

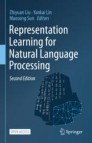
Representation Learning for Natural Language Processing pp 29–68 Cite as
Word Representation Learning
- Shengding Hu 4 ,
- Zhiyuan Liu 4 ,
- Yankai Lin 5 &
- Maosong Sun 4
- Open Access
- First Online: 24 August 2023
1964 Accesses
Words are the building blocks of phrases, sentences, and documents. Word representation is thus critical for natural language processing (NLP). In this chapter, we introduce the approaches for word representation learning to show the paradigm shift from symbolic representation to distributed representation. We also describe the valuable efforts in making word representations more informative and interpretable. Finally, we present applications of word representation learning to NLP and interdisciplinary fields, including psychology, social sciences, history, and linguistics.
You have full access to this open access chapter, Download chapter PDF
2.1 Introduction
The nineteenth-century philosopher Wilhelm von Humboldt described language as the infinite use of finite means , which is frequently quoted by many linguists such as Noam Chomsky, the father of modern linguistics. Apparently, the vocabulary in human language is a finite set of words that can be regarded as a kind of finite means . Words can be infinitely used as building blocks of phrases, sentences, and documents. As human beings start learning languages from words, machines need to understand each word first so as to master the sophisticated meanings of human languages. Hence, effective word representations are essential for natural language processing (NLP), and it is also a good start for introducing representation learning in NLP.
We can consider word representations as the knowledge of the semantic meanings of words. As discussed in Chap. 1 , we can investigate word representations from two aspects, how knowledge is organized and where knowledge is from, i.e., the form and source of word representations.
The form of word representation can be divided into the symbolic representation (Sect. 2.2 ) and the distributed representation (Sect. 2.3 ), which respectively correspond to symbolism and connectionism mentioned in Chap. 1 . Both forms represent words into vectors to facilitate computer processing. The essential difference between these two approaches lies in the meaning of each dimension. In symbolic word representation, each dimension has clear meanings, corresponding to concrete concepts such as words and topics. The symbolic representation form is straightforward to human understanding and has been adopted by linguists and old-fashioned AI (OFAI). However, it’s not optimal for computers due to high dimensionality and sparsity issues: computers need large storage for these high-dimensional representations, and computation is less meaningful because most entries of the representations are zeros. Fortunately, the distributed word representation overcomes these problems by representing words as low-dimensional and real-valued dense vectors. In distributed word representation, each dimension in isolation is meaningless because semantics is distributed over all dimensions of the vector. Distributed representations can be obtained by factorizing the matrices of symbolic representations or learned by gradient descent optimization from data. In addition to overcoming the aforementioned problems of symbolic representation, it handles emerging words easily and accurately.
The effectiveness of word representation is also determined by the source of word semantics. A word in most alphabetic languages, such as English, is usually a sequence of characters. The internal structure usually reflects its speech or sound but helps little in understanding word semantics, except for some informative prefixes and suffixes. By taking human languages as a typical and complicated symbolic system as structuralism suggests (Chap. 1 ), words obtain their semantics from their relationship to other words . Given a word, we can find its hypernyms, synonyms, hyponyms, and antonyms from a human-organized linguistic knowledge base (KB) like WordNet [ 52 ] to represent word semantics. By extending structuralism to the distributional hypothesis , i.e., you shall know a word by the company it keeps [ 24 ], we can build word representations from their rich context in large-scale text corpora . Since most linguistic knowledge graphs are usually annotated by linguists, they are convenient to be used by humans but difficult to comprehensively and immediately reflect the dynamics of human languages. Meanwhile, word representations obtained from large-scale text corpora can capture up-to-date semantics of words in the real world with few subjective biases.
We can summarize existing methods of word representation as a mix of the above two perspectives. In the era of statistical NLP, word representation follows the symbolic form, obtained either from a linguistic knowledge graph (Fig. 2.1 a) or from large-scale text corpora (Fig. 2.1 b), which will be introduced in Sect. 2.2 .
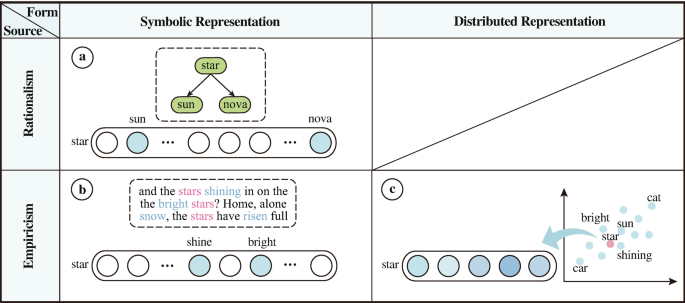
The word representations can be divided according to their form of representation and source of the semantics: ( a ) shows the symbolic representations that use the knowledge base as the source, which is adopted by conventional linguistics; ( b ) shows the symbolic representations that adopt the distributional hypothesis as the foundation of the semantic source; ( c ) shows the distributed representation learned from large-scale corpora based on the distributional hypothesis, which is the mainstream of nowadays word representation learning
In the era of deep learning, distributed word representation follows the spirits of connectionism and empiricism . It learns powerful low-dimensional word vectors from large-scale text corpora and achieves ground-breaking performance on numerous tasks (Fig. 2.1 c). In Sect. 2.3 , we will present representative works of distributed word representation such as word2vec [ 48 ] and GloVe [ 57 ]. These methods typically assign a fixed vector for each word and learn from text corpora. To address those words with multiple meanings under different contexts, researchers further propose contextualized word representation to capture sophisticated word semantics dynamically. The idea also inspires subsequent pre-trained models, which will be introduced in Chap. 5 .
Many efforts have been devoted to constructing more informative word representations by encoding more information, such as multilingual data, internal character information, morphology information, syntax information, document-level information, and linguistic knowledge, as introduced in Sect. 2.4 . Moreover, it would be a bonus if some degree of interpretability is added to word representation, and we will also briefly describe improvements in interpretable word representation.
Word representation learning has been widely used in many applications in NLP and other areas. In NLP, word representations can be applied to word-level tasks such as word similarity and analogy and simple downstream tasks such as sentiment analysis. We note that, with the advancement of deep learning and pre-trained models, word representations are less used in isolation in NLP but more as building blocks of neural language models, as shown in Chaps. 3 , 4 , and 5 . Meanwhile, word representations play indispensable roles in interdisciplinary fields such as computational social sciences for studying social bias and historical change.
2.2 Symbolic Word Representation
Since the ancient days of knotted strings, human ancestors have used symbols to record and share information. As time progressed, isolated symbols gradually merged to form a symbol system. This system is human language. In fact, human language is probably the most complex and systematic symbol system that humans have ever built. In human language, each word is a discrete symbol that contains a wealth of semantic meaning. Therefore, ancient linguists also regard each word as a discrete symbol.
This common practice can also apply to NLP in modern computer science. In this section, we introduce three traditional symbolic approaches to word representations, i.e., one-hot word representation, linguistic KB-based word representation, and corpus-based word representation.
2.2.1 One-Hot Word Representation
One-hot representation is the simplest way for symbol-based word representation, which can be formalized as follows. Given a finite set of word vocabulary V = { w (1) , w (2) , …, w (| V |) }, where | V | is the vocabulary size, one-hot representation represents an i -th word w ( i ) with a | V |-dimensional vector w ( i ) , where only the i -th dimension has a value 1 while all other dimensions are 0. That is, each dimension \({\mathbf {w}}^{(i)}_j\) is defined as:
In essence, the one-hot word representation maps each word to an index of the vocabulary. However, it can only distinguish between different words and does not contain any syntactic or semantic information. For any two words, their one-hot vectors are orthogonal to each other. That is, the cosine similarity between cat and dog is the same as the similarity between cat and sun , which are both zeros.
Although we do not have much to talk about one-hot word representation itself, it is the foundation of bag-of-words models for document representations, which are widely used in information retrieval and text classification. Readers can refer to document representation learning methods in Chap. 4 .
As mentioned, there is no internal semantic structure in the one-hot representation. To incorporate semantics in the representation, we will present two methods with different sources of semantics: linguistic KB and natural corpus.
2.2.2 Linguistic KB-based Word Representation
As we introduced in Chap. 1 , rationalism regards the introspective reasoning process as the source of knowledge. Therefore, the researchers construct a complex word-to-word network by reflecting on the relationship between words. For example, human linguists manually annotate the synonyms and hypernyms Footnote 1 of each word. In the well-known linguistic knowledge base WordNet [ 52 ], the hypernyms and hyponyms of dog are annotated as Fig. 2.2 . To represent a word, we can use the vector forms just like one-hot representation as follows:
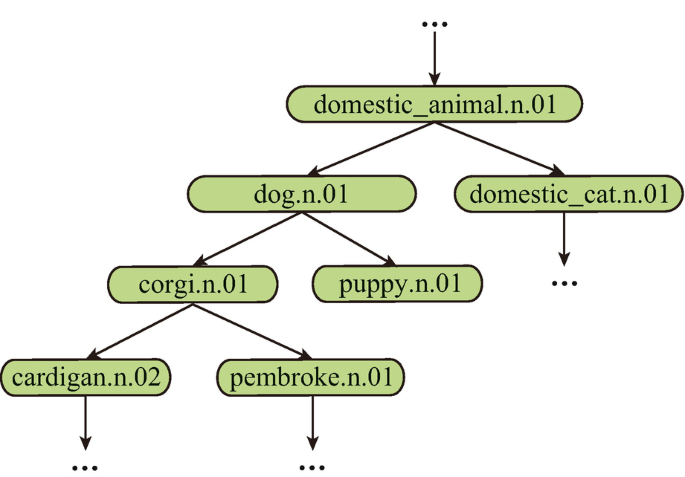
Hypernyms and hyponyms of dog in WordNet [ 52 ]. The dog.n.01 denotes the first synset of dog used as a noun
But it is clear that this representation has limited expressive power, where the similarity of two words without common hypernyms and hyponyms is 0. It would be better to directly adopt the original graph form, where the similarity between the two words can be derived using metrics on the graph. For synonym networks, we can calculate the distance between two words on the network as their semantic similarity (i.e., the shortest path length between the two words). Hierarchical information can be utilized to better measure the similarity for hypernym-hyponym networks. For example, the information content (IC) approach [ 61 ] is proposed to calculate the similarity based on the assumption that the lower the frequency of the closest hypernym of two words is, the closer the two words are.
Formally, we define the similarity \(\operatorname {s}\) as follows:
where C ( w 1 , w 2 ) is the common hypernym set of w 1 and w 2 and P ( w ) is the probability of word w ’s appearance in the corpus. Footnote 2 Intuitively, P ( w ) is the generality of the word w . It indicates that if all common hypernyms of w 1 and w 2 are very general, then \(\operatorname {s}(w_1, w_2)\) will be very small. But if some hypernyms of w 1 and w 2 are specific, \(\operatorname {s}(w_1, w_2)\) will have a higher score, which indicates that these two words are closely related to each other. A vivid example is shown in Fig. 2.3 .
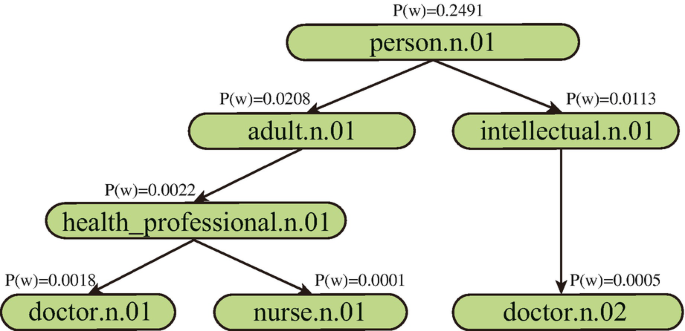
doctor.n.01 and nurse.n.01 share a rare ancestor health_professional.n.01 , their similarity is large. But the closest common ancestor of doctor.n.02 and nurse.n.01 is people.n.01 , which is common. Therefore the similarity between them is small
2.2.3 Corpus-based Word Representation
The process of constructing a linguistic KB is labor-intensive. In contrast, it is much easier to collect a corpus. This motivation is also supported by empiricism , which emphasizes knowledge from naturally produced data.
The correctness of automatically derived representations from a corpus relies on the linguistic hypothesis behind them. We start with the bag-of-words hypothesis . To illustrate this hypothesis, we temporarily shift our attention to document representation. This hypothesis states that we can ignore the order of words in a document and simply treat the document as a bag (i.e., a multiset Footnote 3 ) of words. Then the frequencies of the words in the bag can reflect the content of the document [ 66 ]. In this way, a document is represented by a row vector in which each element indicates the presence or frequency of a word in the document. For example, the value of the entry corresponding to word cat being 3 means that cat occurs three times in the document, and an entry corresponding to a word being 0 means that the word is not in the document [ 67 ]. In this way, we have automatically constructed a representation of the document.
How does this inspire us to construct the word representations of greater interest in this chapter? In fact, as we stack the row vectors of each document to form a document (row)-word (column) matrix, we can shift our attention from rows to columns [ 17 ]. Each column now represents the occurrence of a word in a stack of documents. Intuitively, if the words rat and cat tend to occur in the same documents, their statistics in the columns will be similar.
In the above approach, a document can be considered as the context of a word. Actually, more flexibility can be added in defining the context of a word to obtain other kinds of representations. For example, we can define a fixed-size window centered on a word and use the words inside the window as the context of that word. This corresponds to the well-known distributional hypothesis that the meaning of a word is described by its companions [ 24 ]. Then we count the words that appear in a word’s neighborhood and use a dictionary as a word representation, where each key is a context word whose value is the frequency of the occurrence of that context word within a certain distance.
To further extend the context of a word, several works propose to include dependency links [ 56 ] or links induced by argument positions [ 21 ]. Interested readers can refer to a summary of various contexts used for corpus-based distributional representations [ 65 ].
In summary, in symbolic representations, each entry of the representation has a clear and interpretable meaning. The clear interpretable meaning can correspond to a specific word, synset, or term, and that is why we call it “symbolic representation.”
2.3 Distributed Word Representation
Although simple and interpretable, symbolic representations are not the best choice for computation. For example, the very sparse nature of the symbolic representation makes it difficult to compute word-to-word similarities. Methods like information content [ 61 ] cannot naturally generalize to other symbolic representations.
The difficulty of symbolic representation is solved by the distributed representation. Footnote 4 Distributed representation represents a subject (here is a word) as a fixed-length real-valued vector, where no clear meaning is assigned to every single dimension of the vector. More specifically, semantics is scattered over all (or a large portion) of the dimensions of the representation, and one dimension contributes to the semantics of all (or a large proportion) of the words.
We must emphasize that the “ distributed representation ” is completely different from and orthogonal to the “ distributional representation ” (induced by “ distributional hypothesis ”). Distributed representation describes the form of a representation, while distributional hypothesis (representation) describes the source of semantics.
2.3.1 Preliminary: Interpreting the Representation
Although each dimension is uninterpretable in distributed representation, we still want ways to interpret the meaning conveyed by the representation approximately. We introduce two basic computational methods to understand distributed word representation: similarity and dimension reduction.
Suppose the representations of two words are u = [ u 1 , …, u d ] and v = [ v 1 , …, v d ], Footnote 5 we can calculate the similarity or perform dimension reduction as follows.
The Euclidean distance is the L 2-norm of the difference vector of u and v .
Then the Euclidean similarity can be defined as the inverse of distance, i.e.,
Cosine similarity is also common. It measures the similarity by the angle between the two vectors:
Dimension Reduction
Distributed representations, though being lower dimensional than symbolic representations, still exist in manifolds higher than three dimensions. To visualize them, we need to reduce the dimension of the vector to 2 or 3. Many methods have been proposed for this purpose. We will briefly introduce principal component analysis (PCA).
PCA transforms the vectors into a set of new coordinates using an orthogonal linear transformation. In the new coordinate system, an axis is pointed in the direction which explains the data’s most variance while being orthogonal to all other axes. Under this construction, the later constructed axes explain less variance and therefore are less important to fit the data. Then we can use only the first two to three axes as the principal components and omit the later axes. A case of PCA on two-dimensional data is in Fig. 2.4 . Formally, denoting the new axes by a set of unit row vectors { d j | j = 1, …, k }, where k is the number of unit row vectors. An original vector u of the sample u can be represented in the new coordinates by
where a j is the weight of the vector d j for representing u , and a = [ a 1 , …, a r ] forms the new vector representation of u . In practice, we only set r = 2 or 3 for visualization.
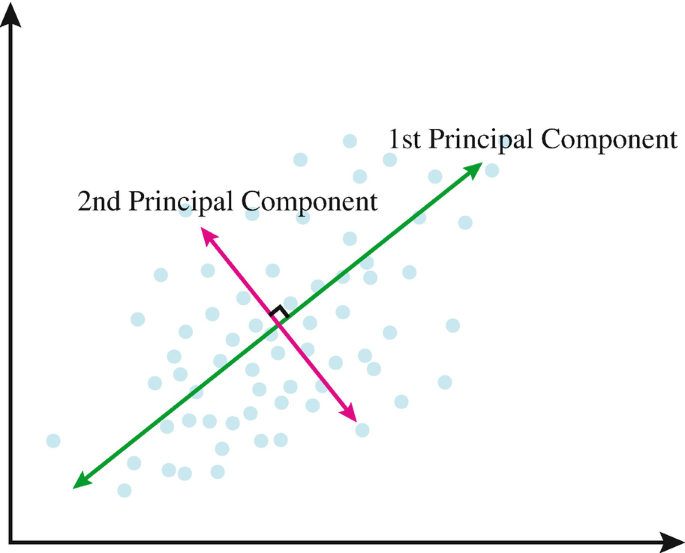
The PCA specifies new axis directions (called principal components), and the axes are ordered from largest to smallest variance in their directions so that keeping the coordinates on the first few axes retains most of the distribution information
The set of new coordinates { d j | j = 1, …, k } can be computed by eigendecomposition of the covariance matrix or using singular value decomposition (SVD) . Then we introduce SVD-based PCA and present its resemblance to latent semantic analysis (LSA) in the next subsection. In SVD, a real-valued matrix can be decomposed into
such that Σ is a diagonal matrix of positive real numbers, i.e., the singular values, and W and D are singular matrices formed by orthogonal vectors. For a data sample (e.g., i -th row in U ):
Thus in Eq. ( 2.7 ), a j = σ j W i , j and d j = D j ,: .
Although widely adopted in high-dimensional data visualization, PCA is unable to visualize representations that form nonlinear manifolds. Other dimensionality reduction methods, such as t-SNE [ 73 ], can solve this problem.
2.3.2 Matrix Factorization-based Word Representation
Distributed representations can be transformed from symbolic representations by matrix factorization or neural networks. In this subsection, we introduce the matrix factorization-based methods. We introduce latent semantic analysis (LSA), its probabilistic version PLSA, and latent Dirichlet allocation (LDA) as the representative approaches. Readers who are only interested in neural networks can jump to the next section to continue reading.
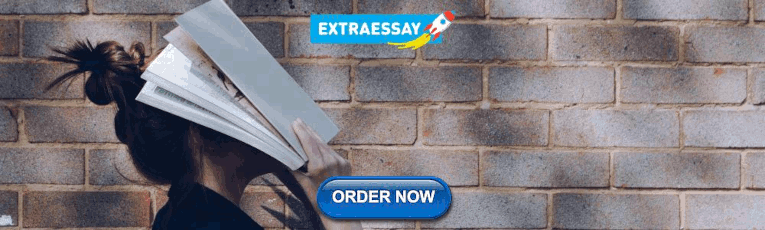
Latent Semantic Analysis (LSA)
LSA [ 17 ] utilizes singular value decomposition (SVD) to perform the transformation from matrices of symbolic representations. Suppose we have a word-document matrix \(\mathbf {M}\in \mathbb {R}^{n\times d}\) , where n is the number of words and d is the number of documents. By linear algebra, it can be uniquely Footnote 6 decomposed into the multiplication of three matrices \(\mathbf {W}\in \mathbb {R}^{n\times n}\) , \(\varSigma \in \mathbb {R}^{n\times d}\) , and \(\mathbf {D}\in \mathbb {R}^{d\times d}\) :
such that Σ is a diagonal matrix of positive real numbers, i.e., the singular values, and columns of W , D are left-singular vectors and right-singular vectors, respectively. Footnote 7
Now let’s try to interpret the two orthogonal matrices. The i -th row of the matrix M that represents the i -th word’s symbolic representation (denoted by M i ,: ) is decomposed into:
From Eq. ( 2.11 ), we can see only the i -th row of W contributes to the i -th word’s symbolic representation. More importantly, since D is an orthogonal matrix, the similarity between words w i and w j is given by:
Thus we can take W i ,: Σ as the distributed representation for word w i . Note that taking W i ,: Σ or W i ,: as the distributed representation is both ok because W i ,: Σ is W i ,: stretched along each axis j with ratio Σ j , j , and the relative positions of points in the two spaces are similar.
Suppose we arrange the eigenvalues in descending order. In that case, the largest K singular values (and their singular vectors) contribute the most to matrix M . Thus we only use them to approximate M . Now Eq. ( 2.11 ) becomes:
where ⊙ is the element-wise multiplication and σ i is the i -th diagonal element of Σ .
To sum up, in LSA, we perform SVD to the counting statistics to get the distributed representation W i ,: K . Usually, with a much smaller K , the approximation can be sufficiently good, which means the semantics in the high-dimensional symbolic representation are now compressed and distributed into a much lower-dimensional real-valued vector. LSA has been widely used to improve the recall of query-based document ranking in information retrieval since it supports ambiguous semantic matching.
One challenge of LSA comes from the computational cost. A full SVD on an n × d matrix requires \(\mathcal {O}(\min \{n^2d, nd^2\})\) time, and the parallelization of SVD is not trivial. A solution is random indexing [ 36 , 64 ] that overcomes the computational difficulties of SVD-based LSA and avoids expensive preprocessing of a huge word-document matrix. In random indexing, each document is assigned a randomly-generated high-dimensional sparse ternary vector (named as index vector ). Note that random vectors in high-dimensional space should be (nearly) orthogonal, analogous to the orthogonal matrix D in SVD-based LSA. For each word in a document, we add the document’s index vector to the word’s vector. After passing the whole text corpora, we can get accumulated word vectors. Random indexing is simple to parallelize and implement, and its performance is comparable to the SVD-based LSA [ 64 ].
Probabilistic LSA (PLSA)
LSA further evolves into PLSA [ 34 ]. To understand PLSA based on LSA, we can treat the W i ,: K as a distribution over latent factors { z k | k = 1, …, K }, where
We can understand these factors as “topics” as we will assign meanings to them later. Similarly, D i ,: K can also be regarded as a distribution, where
And the { σ k | k = 1, …, K } are the prior probabilities of factors z k , i.e., P ( z k ) = σ k . Thus, the word-document matrix becomes a joint probability of word w j and document d j :
With the help of Bayes’ theorem and Eq. ( 2.16 ), we can compute the conditional probability of w i given a document d j is
Now we can see a generative process is defined from Eq. ( 2.17 ). To generate a word in the document d j , we first sample a latent factor z k from P ( z k | d j ) and then sample a word w i from the conditional probability P ( w i | z k ). The process is represented in Fig. 2.5 .
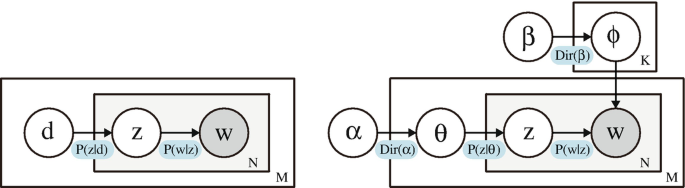
The generative process of words in documents in the PLSA model (left) and LDA model (right). N is the number of words in a document, M is the number of documents, and K is the number of topics. w is the only observed variable, and we need to estimate the other white variables based on the observation. The figure is redrawn according to the Wikipedia entry “Latent Dirichlet allocation”
Note that to make Eq. ( 2.14 )∼Eq. ( 2.17 ) rigorous, the elements of W , Σ , D have to be nonnegative. To do optimization under such probabilistic constraints, a different loss from SVD is used [ 34 ]:
The following works prove that optimizing the above objective is the same as nonnegative matrix factorization [ 20 , 38 ]. We omit the mathematical details here.
Latent Dirichlet Allocation (LDA)
PLSA is further developed into latent Dirichlet allocation (LDA) [ 10 ], a popular topic model that is widely used in document retrieval. LDA adds hierarchical Bayesian priors to the generative process defined by Eq. ( 2.14 ). The generative process for words in document j becomes:
Choose \(\boldsymbol {\theta }_j \in \mathbb {R}^{K}\sim \operatorname {Dir}(\boldsymbol {\alpha })\) , where \(\operatorname {Dir}(\boldsymbol {\alpha })\) is a Dirichlet distribution (typically each dimension of α <1), where K is the number of topics. This is the probability distribution of topics in the document d j .
Choose \(\boldsymbol {\phi }_z\in \mathbb {R}^{|V|}\sim \operatorname {Dir}(\boldsymbol {\beta })\) for each topic z , where | V | is the size of the vocabulary. Typically each dimension of β is less than 1. This is the probability distribution of words produced by topic z .
For each word w i in the document d j :
Choose a topic \(z_{i,j}\sim \operatorname {Multinomial}(\boldsymbol {\theta }_j)\) .
Choose a word from \(w_{i,j} = P(w_j|d_j) \sim \operatorname {Multinomial}(\boldsymbol {\phi }_{z_{i,j}})\) .
The generative process in LDA is in Fig. 2.5 (right). We will not dive into the mathematical details of LDA.
We would like to emphasize two points about LDA: (1) the hyper-parameter α , β in Dirichlet prior is typically set to be less than 1, resulting in a “sparse prior”, i.e., most dimensions of the sampled θ and ϕ are close to zero, and the mass of the distribution will be concentrated in a few values. This is consistent with our common sense that a document will always have only a few topics and that a topic will only produce a small number of words. Moreover, the total number of topics K is pre-defined as a relatively small integer. The sparsity and interpretability make LDA essentially a kind of symbolic representation , and LDA can be seen as a bridge between distributed representations and symbolic representations . (2) Although PLSA and LDA are more often used in document retrieval, the distribution of a word over different topics (latent factors) P ( w | z i ) can be used as an effective word representation, i.e., w = [ P ( w | z 1 ), …, P ( w | z K )].
However, the information source, i.e., counting matrix M , of matrix factorization-based methods is still based on the bag-of-words hypothesis. These methods lose the word order information in the documents, so their expressiveness capability remains limited. Therefore, these classical methods are less often used when neural network-based methods that can model word order information emerge.
2.3.3 Word2vec and GloVe
The neural networks, revived in the 2010s, are similar to the neurons of the human brain, where the neurons inside a neural network perform distributed computation. One neuron is responsible for the computation of multiple pieces of information, and one input activates multiple neurons at the same time. This property coincides with distributed representation. Hence, distributed representation plays a dominant role in the era of neural networks. Moreover, neural models are optimized on large-scale data. The data dependency makes the distributional hypothesis particularly important in optimizing such distributed representations. In the following section, we first present word2vec [ 48 ], a milestone work of distributional distributed word representation using neural approaches. After that, we introduce GloVe [ 57 ] that improves word2vec with a global word-occurrence matrix.
Word2vec adopts the distributional hypothesis but does not take a count-based approach. It directly uses gradient descent to optimize the representation of a word toward its neighbors’ representations. Word2vec has two specifications, namely, continuous bag-of-words (CBOW) and skip-gram . The difference is that CBOW predicts a center word based on multiple context words, while skip-gram predicts multiple context words based on the center word.
CBOW predicts the center word given a window of context. Figure 2.6 shows the idea of CBOW with a window of five words.
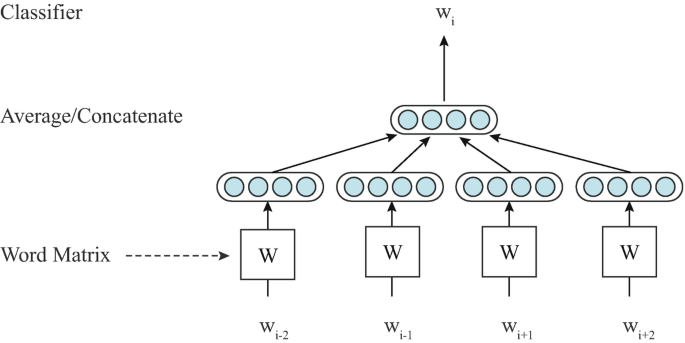
The architecture of the CBOW model. (The figure is redrawn according to Fig. 1 from Mikolov et al. [ 49 ])
Formally, CBOW predicts w i according to its contexts as:
where P ( w i | w i − l , …, w i −1 , w i +1 , …, w i + l ) is the probability of word w i given its contexts, 2 l + 1 is the size of training contexts, w j is the word vector of word w j , W is the weight matrix in \(\mathbb {R}^{|{V}|\times m}\) , V indicates the vocabulary, and m is the dimension of the word vector.
The CBOW model is optimized by minimizing the sum of the negative log probabilities:
Here, the window size l is a hyper-parameter to be tuned. A larger window size may lead to higher accuracy as well as longer training time.
Contrary to CBOW, skip-gram predicts the context given the center word. Figure 2.7 shows the model.
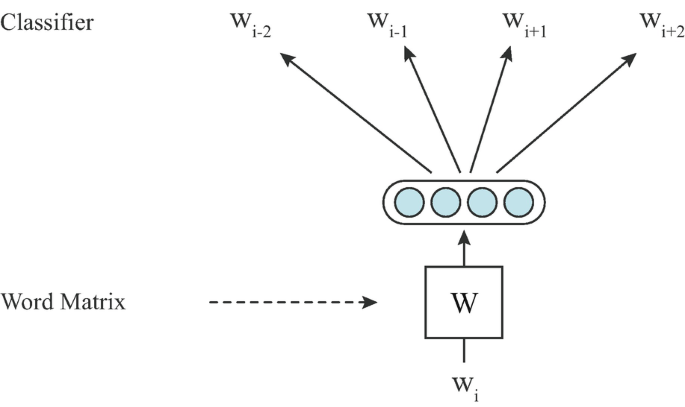
The architecture of the skip-gram model. (The figure is redrawn according to Fig. 1 from Mikolov et al. [ 49 ])
Formally, given a word w i , skip-gram predicts its context as:
where P ( w j | w i ) is the probability of context word w j given w i and W is the weight matrix. The loss function is similar to CBOW but needs to sum over multiple context words:
In the early stages of the deep learning renaissance, computational resources are still limited, and it is time-consuming to optimize the above objectives directly. The most time-consuming part is the softmax layer since the softmax layer uses the scores of predicting all words in the vocabulary V in the denominator:
An intuitive idea to improve efficiency is obtaining a reasonable but faster approximation of the softmax score. Here, we present two typical approximation methods, including hierarchical softmax and negative sampling . We explain these two methods using CBOW as an example.
The idea of hierarchical softmax is to build hierarchical classes for all words and to estimate the probability of a word by estimating the conditional probability of its corresponding hierarchical classes. Figure 2.8 gives an example. Each internal node of the tree indicates a hierarchical class and has a feature vector, while each leaf node of the tree indicates a word. The conditional probabilities, e.g., p 0 and p 1 in Fig. 2.8 , of two child nodes are computed by the feature vector of each node and the context vector. For example,
where w c is the context vector, w 0 and w 1 are the feature vectors.
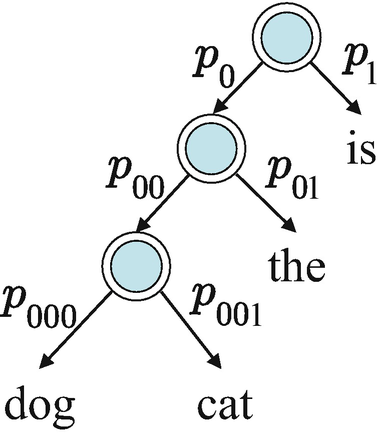
An illustration of hierarchical softmax
Then, the probability of a word can be obtained by multiplying the probabilities of all nodes on the path from the root node to the corresponding leaf node. For example, the probability of the word the is p 0 × p 01 , while the probability of cat is p 0 × p 00 × p 001 .
The tree of hierarchical classes is generated according to the word frequencies, which is called the Huffman tree. Through this approximation, the computational complexity of the probability of each word is \(\mathcal {O}(\log |V|)\) .
Negative sampling is a more straightforward technique. It directly samples k words as negative samples according to the word frequency. Then, it computes a softmax over the k + 1 words (1 for the positive sample, i.e., the target word) to approximate the conditional probability of the target word.
The word2vec and matrix factorization-based methods have complementary advantages and disadvantages. In terms of learning efficiency and scalability, word2vec is superior because word2vec uses an online learning (or batch learning paradigm in deep learning) approach and is able to learn over large corpora. However, considering the preciseness of distribution modeling, the matrix factorization-based methods can exploit global co-occurrence information by building a global co-occurrence matrix. In comparison, word2vec is a local window-based method that cannot see the frequency of word pairs in a global corpus in a single optimization step. Therefore, GloVe [ 57 ] is proposed to combine the advantages of word2vec and matrix factorization-based methods.
To learn from global count statistics, GloVe firstly builds a co-occurrence matrix M over the entire corpus but does not directly factorize it. Instead, it takes each entry in the co-occurrence matrix M ij and optimizes the following target:
The d ( w i , w j , M ij ) is a metric that compares the distributed representations of word w i and w j with the ground-truth statistics M ij . f ( M ij ) is a weight term measuring the importance of the word pair w i , w j . Specifically, GloVe adopts the following form as the metric d :
where b i and b j are bias terms for word w i and w j . Interested readers can read the original paper [ 57 ] for the derivation details.
For the weight term f ( M ij ), most previous approaches set the weight of all word pairs to 1. However, common word pairs may have too weak semantics, so we should lower their weights and increase the weights of rare word pairs slightly. Thus, GloVe observes that it should satisfy three constraints:
f (0) = 0 and \(\operatorname {lim}_{x\rightarrow 0}f(x)\log ^2x\) is finite.
A nondecreasing function.
Truncated for large values of x to avoid overfitting to common words (stop words).
A possible choice is the following, where α is taken as \(\frac {3}{4}\) in the original GloVe paper:
In summary, GloVe uses weighted squared loss to optimize the representation of words based on the elements in the global co-occurrence matrix. Compared with word2vec, it captures global statistics. Compared with matrix factorization, it (1) reasonably reduces the weights of the most frequent words at the level of matrix entries, (2) reduces the noise caused by non-discriminative word pairs by implicitly optimizing the ratio of co-occurrence frequencies, and (3) enables fitting on large corpus by iterative optimization. Since the number of nonzero elements of the co-occurrence matrix is much smaller than | V | 2 , the efficiency of GloVe is ensured in practice.
Word2vec as Implicit Matrix Factorization
It seems so far that the neural network-based methods like word2vec and matrix factorization-based methods are two distinct paradigms for deriving distributed representation. But in fact, they have close theoretical connections. Omer et al. [ 41 ] prove that word2vec is factorizing pointwise mutual information matrix ( \(\operatorname {PMI}\) ), where
Omer et al. [ 41 ] then compare the performance of factoring the PMI matrix using SVD and skip-gram with the negative sampling (SGNS) model. SVD achieves a significantly better objective value when the embedding size is smaller than 500 dimensions and the number of negative samples is 1. With more negative samples and higher embedding dimensions, SGNS gets a better objective value. For downstream tasks, under several conditions, SVD achieves slightly better performance on word analogy and word similarity. In contrast, skip-gram with negative sampling achieves better performance by 2% on syntactical analogy.
2.3.4 Contextualized Word Representation
In natural language, the semantic meaning of an individual word can usually be different with respect to its context in a sentence. For example, in the two sentences: “ willows lined the bank of the stream. ”, “ a bank account. ”, Footnote 8 although the word bank is always the same, their meanings are different. This phenomenon is prevalent in any language. However, most of the traditional word embeddings (CBOW, skip-gram, GloVe, etc.) cannot well understand the different nuances of the meanings of words in the different surrounding texts. The reason is that these models only learn a unique and specific representation for each word. Therefore these models cannot capture how the meanings of words change based on their surrounding contexts.
Matthew et al. [ 58 ] propose ELMo to address this issue, whose word representation is a function of the whole input. More specifically, rather than having a look-up table of word embedding matrix, ELMo converts words into low-dimensional vectors on-the-fly by feeding the word and its context into a deep neural network. ELMo utilizes a bidirectional language model to conduct word representation. Formally, given a sequence of N words ( w 1 , …, w N ), a forward language model (LM) Footnote 9 models the probability of the sequence by predicting the probability of each word w k according to the historical context:
The forward LM in ELMo is a multilayer long short-term memory (LSTM) network [ 33 ], which is a kind of widely used neural network for modeling sequential data, and the j -th layer of the LSTM-based forward LM will generate the context-dependent word representation \(\overrightarrow {\mathbf {h}}^{\text{LM}}_{k,j}\) for the word w k . The backward LM is similar to the forward LM. The only difference is that it reverses the input word sequence to ( w N , w N −1 , …, w 1 ) and predicts each word according to the future context:
Similar to the forward LM, the j -th backward LM layer generates the representations \(\overleftarrow {\mathbf {h}}^{\text{LM}}_{k,j}\) for the word w k .
When used in a downstream task, ELMo combines all layer representations of the bidirectional LM into a single vector as the contextualized word representation . The way to do the combination is flexible. For example, the final representation can be the weighting of all bidirectional LM layer’s hidden representation, and the weights are task-specific:
where \({\mathbf {s}}^{\text{task}} = [{s}^{\text{task}}_1, \ldots , {s}^{\text{task}}_L]\) are softmax-normalized weights and α task allows the task to scale the whole representation, and \({\mathbf {h}}^{\text{LM}}_{k,j} = \operatorname {concat}(\overrightarrow {\mathbf {h}}^{\text{LM}}_{k,j}; \overleftarrow {\mathbf {h}}^{\text{LM}}_{k,j})\) .
Due to the superiority of contextualized representations, a group of works led by ELMo [ 58 ], BERT [ 19 ], and GPT [ 59 ] has started to emerge since 2017, eventually leading to a unified paradigm of pre-training-fine-tuning across NLP. Please refer to Chap. 5 for further reading.
2.4 Advanced Topics
In the previous section, we introduced the basic models of word representation learning. These studies promoted more work on pursuing better word representations. In this section, we introduce the improvement from different aspects. Before we dive into the specific methods, let’s first discuss the essential features of a good word representation.
Informative Word Representation
A key point where representation learning differs from traditional prediction tasks is that when we construct representations, we do not know what information is needed for downstream tasks. Therefore, we should compress as much information as possible into the representation to facilitate various downstream tasks. From the development of one-hot representations to distributional and contextualized representations, the information in the representations is indeed increasing. And we still expect to incorporate more information into the representations.
Interpretable Word Representation
For distributed representations, a single dimension is not responsible for explaining the factors of semantic change, and the semantics is entangled in multiple dimensions. As a result, distributed representations are difficult to interpret. As Bengio et al. [ 9 ] pointed out, a good distributed representation should “ disentangle the factors of variation .” There is always a desire for an interpretable distributed representation. Although PLSA and LDA have already increased interpretability, we would like to see more developments in this direction.
In this section, we will introduce the efforts that enhance the distributed word representations in terms of the above criteria.
2.4.1 Informative Word Representation
To make the representations informative, we can learn word representations from universal training data, including multilingual corpus. Another key direction for being informative is incorporating as much additional information into the representation as possible. From small to large information granularity, we can utilize character, morphological, syntactic, document, and knowledge base information. We will describe the related work in detail.
Multilingual Word Representation
There are thousands of languages in the world. Making the vector space applicable for multiple languages not only improves the performance of word representation in low-resource languages but also can absorb information from the corpora of multiple languages. The bilingual word embedding model [ 78 ] proposes to make use of the word alignment pairs available in machine translation. It maps the embeddings of the source language to the embeddings of the target language and vice versa.
Specifically, a set of source words’ representations that are trained on monolingual source language corpus is used to initialize the words in the target language:
where w s is the trained embeddings of the source word and w t-init is the initial embedding of the target word, respectively. N ts is the number of times that the target word t is aligned with the source word s . N t is the total times of word t in the target corpus. The add-on terms + 1 and + S are the Laplace smoothing. S is the number of source words. Intuitively, the initialization of target word embedding is the weighted average of the aligned words in the source corpus, which ensure the two sets of embeddings are in the same space initially.
Then the source and target representation is optimized on their unlabeled corpora with target \(\mathcal {L}_{s}\) and \(\mathcal {L}_{t}\) , respectively. To improve the alignment during training, alignment matrices \({\mathbf {N}}_{t\rightarrow s}\) and \({\mathbf {N}}_{s\rightarrow t}\) are used, where each element N ij denotes the count of a word w i is aligned with source word w j normalized across all source words. Then a translation equivalence objective is used:
Thus the unified objective becomes \(\mathcal {L}_s + \lambda _1 \mathcal {L}_{t\rightarrow s} \mathcal {L}_t + \lambda _2 \mathcal {L}_{s\rightarrow t}\) for source words and target words, respectively, where λ 1 and λ 2 are the coefficients to weight the different sub-objectives.
However, this model performs poorly when the seed lexicon is small. Some works introduce virtual alignment between languages to tackle this limitation. Let’s take Zhang et al. [ 77 ] as an example. In addition to monolingual word embedding learning and bilingual word embedding alignment based on seed lexicon, this work proposes an integer latent variable vector \(\mathbf {m}\in \mathbb {N}^{V^T}\) ( V T is the size of target vocabulary, and \(\mathbb {N}\) is the set of natural numbers) representing which source word is linked by a target word w t . So m t ∈{0, 1, …, V S }. m is randomly initialized and then optimized through an expectation-maximization algorithm [ 18 ] together with the word representations. In the E-step, the algorithm fixes the current word representation and finds the best matching m that can align the source and target representations. And in the M-step, it treats the mapping as fixed and known, just like Zou et al. [ 78 ], and optimizes the source and target word representations.
Character-Enhanced Word Representation
Many languages, such as Chinese and Japanese, have thousands of characters compared to other languages containing only dozens of characters. And the words in Chinese and Japanese are composed of several characters. Characters in these languages have richer semantic information. Hence, the meaning of a word can be learned not only from its context but also from the composition of characters. This intuitive idea drives Chen et al. [ 14 ] to propose a joint learning model for character and word embeddings (CWE). In CWE, a word representation w is a composition of the original word embedding w 0 trained on corpus and its character embeddings c i . Formally,
where | w | is the number of characters in the word. Note that this model can be integrated with various models such as skip-gram, CBOW, and GloVe.
Further, position-based and cluster-based methods are proposed to address the issue that characters are highly ambiguous. In the position-based approach, each character is assigned three vectors that appear in begin , middle , and end of a word, respectively. Since the meaning of a character varies when it appears in the different positions of a word, this method can significantly resolve the ambiguity problem. However, characters that appear in the same position may also have different meanings. In the cluster-based method, a character is assigned K different vectors for its different meanings, in which a word’s context is used to determine which vector to be used for the characters in this word.
Introducing character embeddings can significantly improve the representation of low-frequency words. Besides, this method can deal with new words while other methods fail. Experiments show that the joint learning method can perform better on both word similarity and analogy tasks.
Morphology-Enhanced Word Representation
Many languages, such as English, have rich morphological information and plenty of rare words. However, most word representation models ignore the rich morphology information. This is a limitation because a word’s affixes can help infer a word’s meaning. Moreover, in traditional models, word representation is independent of each other. So when facing rare words without enough context to learn the representations, the representations tend to be inaccurate.
Fortunately, in morphology-enhanced word representation, morphologies’ representation can enrich word embeddings and are shared among words to assist the representation of rare words. Piotr et al. [ 11 ] propose to represent a word as a bag of morphology n -grams. This model substitutes word vectors in skip-gram with the sum of morphology n -gram vectors. When creating the dictionary of morphology n -grams, they select all morphology n -grams with a length greater or equal to 3 and smaller or equal to 6. To distinguish prefixes and suffixes from other affixes, they also add special characters to indicate the beginning and the end of a word. This model is efficient and straightforward, which achieves good performance on word similarity and word analogy tasks, especially when the training set is small. Ling et al. [ 44 ] further use a bidirectional LSTM to generate word representation by composing morphologies. This model significantly reduces the number of parameters since only the morphology representations and the weights of LSTM need to be stored.
Syntax-Enhanced Word Representation
Continuous word embeddings should combine the semantic and syntactic information of words. However, existing word representation models depend solely on linear contexts and have more semantic information than syntactic information. To inject the embeddings with more syntactic information, the dependency-based word embedding [ 40 ] uses the dependency-based context. Dependency-based representation contains less topical information than the original skip-gram representation and shows more functional similarity. It considers the dependency parsing tree’s information when learning word representations. The contexts of a target word w are the modifiers m i of this word, i.e., ( m 1 , r 1 ), …, ( m k , r k ), where r i is the type of dependency relation between the target node and the modifier. During training, the model optimizes the probability of dependency-based contexts rather than neighboring contexts. This model gains some improvements on word similarity benchmarks compared with skip-gram. Experiments also show that words with syntactic similarity are more similar in the vector space.
Document-Enhanced Word Representation
Word embedding methods like skip-gram simply consider the context information within a window to learn word representation. However, the information in the whole document, e.g., the topics of the document, could also help word representation learning. Topical word embedding (TWE) [ 45 ] introduces topic information generated by latent Dirichlet allocation (LDA) to help distinguish different meanings of a word. The model is defined to minimize the following objective:
where w i is the word embedding and z i is the topic embedding of w i . Each word w i is assigned a unique topic, and each topic has a topic embedding. The topical word embedding model shows the advantage of contextual word similarity and document classification tasks.
TopicVec [ 42 ] further improves the TWE model. TWE simply combines the LDA with word embeddings and lacks statistical foundations. Moreover, the LDA topic model needs numerous documents to learn semantically coherent topics. TopicVec encodes words and topics in the same semantic space. It can learn coherent topics when only one document is presented.
Knowledge-Enhanced Word Representation
People have also annotated many knowledge bases that can be used in word representation learning as additional information. Yu et al. [ 76 ] introduce relational objectives into the CBOW model. With the objective, the embeddings can predict their contexts and words with relations. The objective is to minimize the sum of the negative log probability of all relations as:
where \({R}_{w_i}\) indicates a set of words that have a relation with w i . The external information helps train a better word representation, showing significant improvements in word similarity benchmarks.
Moreover, retrofitting [ 22 ] introduces a post-processing step that can introduce knowledge bases into word representation learning. It is more modular than other approaches which consider knowledge base during training. Let the word embeddings learned by existing word representation approaches be \(\hat {\mathbf {W}}\) . Retrofitting attempts to find a knowledgeable embedding space W , which is close to \(\hat {\mathbf {W}}\) but considers the relations in the knowledge base. It optimizes W toward \(\hat {\mathbf {W}}\) and simultaneously shrinks the distance between knowledgeable representations of words w i , w j with relations. Formally,
where α and β are hyper-parameters indicating the strength of the associations, and R is a set of relations in the knowledge base. With knowledge bases such as the paraphrase database [ 28 ], WordNet [ 52 ], and FrameNet [ 3 ], this model can achieve consistent improvement on word similarity tasks. But it may also reduce the performance of the analogy of syntactic relations if it emphasizes semantic knowledge. Since it is a post-processing approach, it is compatible with various distributed representation models.
In addition to the aforementioned synonym-based knowledge bases, there are also sememe-based knowledge bases, in which the sememe is defined as the minimum semantic unit of word meanings. Due to the importance of sememe in computational linguistics, we introduce it in detail in Chap. 10 .
2.4.2 Interpretable Word Representation
Although distributed word representation achieves ground-breaking performance on numerous tasks, it is less interpretable than conventional symbolic word representations. It would be a bonus if the distributed representations also enjoy some degree of interpretability. We can improve the interpretability from three directions. The first is to increase the interpretability of the vector representation among its neighbors. Since a word has multiple meanings, especially those polysemy words, the vectors of different meanings should locate in different neighborhoods. Therefore, we introduce work on disambiguated word representations. Another direction is to increase the interpretability of each dimension of the representation. A group of nonnegative and sparse word representations is shown to be well interpretable in each dimension. The third direction is to increase the interpretability of the embedding space by introducing more spatial properties in addition to the translational semantics in word2vec. In this section, we illustrate related work in these three directions.
Disambiguated Word Representation
Using only one single vector to represent a word is problematic due to the ambiguity of words. A single vector that implies multiple meanings is naturally difficult to interpret, and distinguishing different meanings can lead to a more accurate representation.
In the multi-prototype vector space model, Banerjee et al. [ 5 ] use clustering algorithms to cluster different word meanings. Formally, it assigns a different word representation w i ( w 1 ) to the same word w 1 in each different cluster i . When the multi-prototype embedding is used, the similarity between two words w 1 , w 2 is computed by comparing each pair of prototypes, i.e.,
where K is a hyper-parameter indicating the number of clusters and s (⋅) is a similarity function of two vectors, such as cosine similarity. When contexts are available, the similarity can be computed more precisely as
where \(s_{c, {w_1}, i}=s(\mathbf {w}(c),{\mathbf {w}}_i({w_1}))\) is the likelihood of context c belonging to cluster i , w ( c ) is the context representation, and \(\hat {\mathbf {w}}({w_1})={\mathbf {w}}_{\arg \max _{1\leq i \leq K}s_{c,{w_1},i}}({w_1})\) is the maximum likelihood cluster for w 1 in context c . With multi-prototype embeddings, the accuracy of the word similarity task is significantly improved, but the performance is still sensitive to the number of clusters.
The multi-prototype embedding method can effectively cluster different meanings of a word via its contexts. However, the clustering is offline, and the number of clusters is fixed and needs to be pre-defined. It is difficult for a model to select an appropriate amount of meanings for different words; to adapt to new senses, new words, or new data; and to align the senses with prototypes. A unified model is proposed for both word representation and word sense disambiguation [ 13 ]. It uses available knowledge bases such as WordNet [ 52 ] to provide the list of possible senses of a word, perform the disambiguation based on the original word vectors, and update the word vectors and sense vectors. More specifically, as shown in Fig. 2.9 , it first initalizes the word vectors to be the skip-gram vectors learned from large-scale corpora. And then, it aggregates the words in the definition of the sense (provided by knowledge bases) to form the sense initialization, where only the words in the definition whose similarity with the original word is larger than a threshold are considered. After initialization, it uses the sense vector to update the context vectors. For example, in the sentence “ He sat on the bank of the lake ,” the “ bank ” which means “ the land alongside the lake ” is closer to the context vectors formed by words “ sat, bank, lake ,” and then the representation of bank 1 is utilized to update the context vectors. The process is repeated for all words with multiple senses. After the disambiguation, a joint objective is used to optimize the senses and word vectors together
where M ( w j ) is the disambiguated sense of word w j and 2 l + 1 is the window size. With the joint learning framework, not only performance on word representation learning tasks are enhanced, but the representations of concrete senses are more interpretable than the representations of polysemy words.
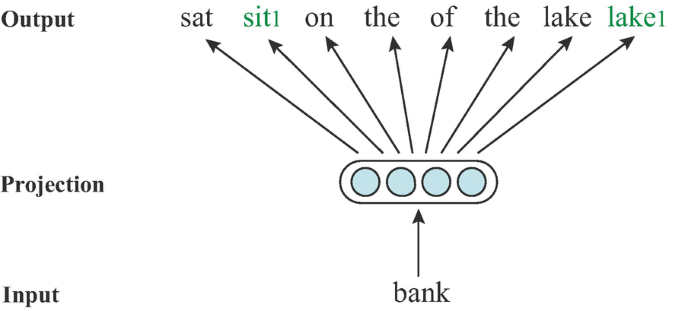
The framework of Chen et al. [ 13 ]. We use the center word to predict both the context word and the context word’s sense. The figure is redrawn according to Fig. 1 from Chen et al. [ 13 ]
Nonnegative and Sparse Word Representation
Another aspect of interpretability comes from the interpretability of each dimension of distributed word representations. Murphy et al. [ 53 ] introduce nonnegative and sparse embeddings (NNSE), where each dimension indicates a unique concept. This method factorizes the corpus statistics matrix \(\mathbf {M}\in \mathbb {R}^{|V|\times |D|}\) into a word embedding matrix \(\mathbf {W}\in \mathbb {R}^{|V|\times m}\) and a document statistics matrix \(\mathbf {D}\in \mathbb {R}^{m\times |D|}\) , where | V |, | D | and m are the vocabulary size, the number of documents, and the dimension of the distributed representation, respectively. Its training objective is
The sparsity is ensured by λ ∥ W i ,: ∥ 1 , and non-negativity is guaranteed by W i , j ≥ 0. By iteratively optimizing W and D via gradient descent, this model can learn nonnegative and sparse embeddings for words. Since embeddings are nonnegative, words with the highest scores on each dimension show high similarity to more words. Therefore, they can be regarded as superordinate concepts of more specific words. Again, since embeddings are sparse and only a few words correspond to each dimension, each dimension can be interpreted as the concept (word) with the highest value in that dimension.
A word intrusion task is designed to assess the interpretability of the word representation. For each dimension, we pick the N words with the largest value for that dimension as the positive words. Then we select the noisy words with the value of that dimension in the small half. Finally, we let human annotators pick out these noise words. The performance of the human annotators in all dimensions is the interpretability score of the model.
Fyshe et al. [ 27 ] further improve NNSE by enforcing the compositionality of the interpretable dimensions. For a phrase p composed of words w i and w j , the following constraint can be applied:
Therefore, the objective becomes
where λ 1 and λ 2 are the coefficients to weigh different sub-objectives.
The f has many choices. The authors define it to be a weighted addition between W i ,: and W j ,: , i.e.,
The resulting word representations are more interpretable since the multiple dimensions can form compositional meanings.
The above method applies to the matrix factorization paradigm, which encounters difficulty when the corpus and global co-occurrence is large. Can we apply the same nonnegative regularization for neural word representations such as word2vec? Luo et al. [ 47 ] present a nonnegative skip-gram model OIWE (online interpretable word embeddings), which adds constraints to the gradient descent process. Specifically, the update rule of the parameter is
where w is the word representation that needs to be updated, k is its k -th dimension, and γ is the learning rate.
However, directly using this update rule leads to unstable optimization because the update deviates from the true update too much. What we need is to make fewer dimensions of w k + γ ∇ f ( w k ) less than 0. To achieve this goal, we use a dynamic learning rate. The learning rate is chosen to make the following violation ratio small:
where K is the number of dimensions.
The resulting word representation exceeds NNSE in both word similarity and word intrusion detection tasks.
Non-Euclidean Word Representation
Interpretability also comes from an embedding space with comprehensible spatial properties. For example, the translation property of word2vec makes the difference between male and female interpretable (i.e., relation gender ). Therefore, we are looking for more interpretable spatial properties. We introduce two special embedding spaces, i.e., Gaussian distribution space and hyperbolic space. Both of them enjoy hierarchical spatial properties that are understandable by humans. Vilnis et al. [ 74 ] propose to encode words into Gaussian distribution N ( x ; μ , Σ ), where the mean μ of the Gaussian distribution is similar to traditional word embedding and the variance Σ becomes the uncertainty of the word meaning. The similarity between two representations can be defined either using asymmetric similarity (e.g., the KL divergence) or symmetric similarity (e.g., the continuous inner product between two Gaussian distributions):
where n is the dimension of vectors.
Note that the focus of Vilnis et al. [ 74 ] is on the uncertainty estimation of word meanings, which increases the interpretability of word meanings in terms of uncertainty estimation. But on the other hand, it is very easy to define entailment relations between two Gaussian embeddings of different sizes (variances), thus natural to encode the hierarchy into the representation, which increases the interpretability of the embedding in terms of ontology. This line of work further develops into representations based on the Gaussian mixture model [ 2 ] and elliptical word embedding [ 54 ].
Another line of work focuses on hyperbolic embeddings. Hyperbolic spaces \(\mathbb {H}^n\) are spaces with constant negative curvature. The volume of the circle in hyperbolic space grows exponentially with radius. This property makes it suitable for encoding the tree structure, where the number of nodes grows exponentially with depth. Hence, it is a suitable space for encoding hierarchical structures. For example, Nickel et al. [ 55 ] use a special hyperbolic space, namely, Poincaré Ball, as the embedding space. They propose to encode word relations such as those explicitly given by the hierarchy in WordNet using supervised learning. A subsequent work [ 72 ] successfully applies Poincaré embeddings in a completely unsupervised manner. Specifically, they propose Poincaré GloVe, a modified target of GloVe, for encoding hyperbolic geometry. Considering the GloVe target in Eq. ( 2.26 ), it can be generalized into more general metrics as follows:
where M ij is the global co-occurrence matrix and h Euclidean = (⋅) 2 is the metric for accessing the similarity of the two embeddings. Now it can be substituted with \(h_{\text{hyperbolic}} = \operatorname {cosh}^2(\cdot )\) . The embedding is optimized using Riemannian optimization [ 8 ]. The use of this word vector for inference (e.g., word analogy tasks) requires using the corresponding hyperbolic space operators, which we omit the details.
In summary, encoding more information and improving interpretability have been pursued by researchers. With such efforts, word representations have become the basis of modern NLP and are widely used in many practical tasks.
2.5 Applications
Word representation, as a milestone breakthrough in NLP, has not only spawned subsequent work in NLP itself but has also been widely applied in other disciplines, catalyzing many highly influential interdisciplinary works. Therefore, in this section, we first introduce the applications of NLP itself, and then we introduce interdisciplinary works such as in psychology, history, and social science.
In the early stages of the introduction of neural networks into NLP, research on the application of word representations was very vigorous. For example, word representations are helpful in word-level tasks such as word similarity, word analogy, and ontology construction. They can also be applied to simple higher-level downstream tasks such as sentiment analysis. The performance of word representations on these tasks can measure the quality of word representations, so they can also be considered as evaluation tasks of word representations . Next, we introduce word similarity, word analogy, ontology construction, and sentence-level tasks.
Word Similarity and Relatedness
Word similarity and relatedness both measure how close a word is to another word. Similarity means that the two words express similar meanings. And relatedness refers to a close linguistic relationship between the two words. Words that are not semantically similar could still be related in many ways, such as meronymy ( car and wheel ) or antonymy ( hot and cold ).
To measure word similarity and relatedness, researchers collect a set of word pairs and compute the correlation between human judgment and predictions made by word representations. So far, many datasets have been collected and made public. Some datasets focus on word similarity, such as RG-65 [ 62 ] and SimLex-999 [ 32 ]. Other datasets concern word relatedness, such as MTurk [ 60 ]. WordSim-353 [ 23 ] is a very popular dataset for word representation evaluation, but its annotation guideline does not differentiate similarity and relatedness. Agirre et al. [ 1 ] conduct another round of annotation based on WordSim-353 and generate two subsets, one for similarity and the other for relatedness. We summarize some information about these datasets in Table 2.1 .
After collecting the datasets, quantitative evaluations of the word representations for the datasets are needed. Researchers usually select cosine similarity as the metric. After that, Spearman’s correlation coefficient ρ is then used to evaluate the coherence between human annotators and the word representation. A higher Spearman’s correlation coefficient indicates they are more similar.
Given the datasets and evaluation metrics, different kinds of word representations can be compared. Agirre et al. [ 1 ] address different word representations with different advantages. They point out that linguistic KB-based methods perform better on similarity than on relatedness, while distributed word representation shows similar performance on both. With the development of distributed representations, a study [ 68 ] in 2015 compares a series of word representations on a wide variety of datasets and gives the conclusion that distributed representations achieve state of the art in both similarity and relatedness.
Besides evaluation with deliberately collected datasets, the word similarity measurement can come in an alternative format, the TOEFL synonyms test. In this test, a cue word is given, and the test is required to choose one from four words that are the synonym of the cue word. The exciting part of this task is that the performance of a system could be compared with human beings. Landauer et al. [ 39 ] evaluate the system with the TOEFL synonyms test to address the knowledge inquiring and representing of LSA. The reported score is 64.4%, which is very close to the average rating of the human test-takers. On this test set with 80 queries, Sahlgren et al. [ 63 ] report a score of 72.0%. Freitag et al. [ 25 ] extend the original dataset with the help of WordNet and generate a new dataset (named as WordNet-based synonymy test) containing thousands of queries.
Word Analogy
Besides word similarity, the word analogy task is an interesting task to infer words and serves as an alternative way to measure the quality of word representations. This task gives three words w 1 , w 2 , and w 3 , and then it requires the system to predict a word w 4 such that the relation between w 1 and w 2 is the same as that between w 3 and w 4 . This task has been used since the proposal of word2vec [ 49 , 51 ] to exploit the structural relations among words. Here, word relations can be divided into two categories, including semantic relations and syntactic relations. Word analogy quickly becomes a standard evaluation metric once the dataset is released. Unlike the TOEFL synonyms test, the prediction is chosen from the whole vocabulary instead of the provided options. This test favors distributed word representations because it emphasizes the structure of word space. The comparison between different models on the word analogy task measured by accuracy could be found in [ 7 , 68 , 70 , 75 ].
Ontology Construction
Another usage of word representation is to construct the ontology knowledge bases. Section 2.4.1 talked about injecting knowledge base information into word representations. But conversely, learned word embeddings also help build the knowledge base. Since word representation is better at common words than rare words, word representations are more suitable for building ontology graphs Footnote 10 than building factual knowledge graphs.
In ontologies, perhaps the most important relation is the is_a relation. Traditional word2vec models are good at expressing analogous relations, such as man - woman ≈ king - queen but not good at hierarchical relations, such as mammal - cat ≉ celestial body - sun . To model such relationships, Fu et al. [ 26 ] propose to use a linear projection rather than a simple embedding offset to represent the relationship. The model optimizes the projection as
where w i and w j are hypernym and hyponym embeddings and W is the transformation matrix.
The non-Euclidean word representations introduced in Sect. 2.4.2 also help build the ontology network. Another knowledge base that word embedding can help is the sememe knowledge introduced in Chap. 10 .
Sentence-Level Tasks
Besides word-level tasks, word representations can also be used alone in some simple sentence-level tasks. However, word representations trained under purely co-occurrence objectives may not be optimal for a given task, and we can include task-relevant objectives in training. Take sentiment analysis as an example. Most word representation methods capture syntactic and semantic information while ignoring the sentiment of the text. This is questionable because words with similar syntactic polarity but opposite sentiment polarity obtain close word vectors. Tang et al. [ 71 ] propose to learn sentiment-specific word embeddings (SSWE). An intuitive idea is to jointly optimize the sentiment classification model using word embeddings as its feature, and SSWE minimizes the cross entropy loss to achieve this goal. To better combine the unsupervised word embedding method and the supervised discriminative model, they further use the words in a window rather than a whole sentence to classify sentiment polarity. To get massive training data, they use distant supervision to generate sentiment labels for a document. On sentiment classification tasks, sentiment embeddings outperform other strong baselines, including SVM [ 15 ] and other word embedding methods. SSWE also shows strong polarity consistency, where the closest words of a word are more likely to have the same sentiment polarity compared with existing word representation models. This sentiment-specific word embedding method provides us with a general way to learn task-specific word embeddings, which is to design a joint loss function and generate massive labeled data automatically.
Interestingly, as subsequent research in NLP progressed, including the development of sentence representations (Chap. 4 ) and the introduction of pre-trained models (Chap. 5 ), simple word vectors gradually ceased to be used alone. We point out the following reasons for this:
High-level (e.g., sentence level) semantic units require combinations between words, and simple arithmetic operations between word representations are not sufficient to model high-level semantic models.
Most word representation models do not consider word order and cannot model utterance probabilities, much less generate language.
We recommend that readers continue reading subsequent chapters to become familiar with more advanced methods.
2.5.2 Cognitive Psychology
In cognitive psychology, a famous behavioral test examines the correlation in the subconscious mind, named the implicit association test (IAT) [ 30 ]. This test is widely used to detect biases, such as gender biases, religion biases, occupation biases, etc.
IAT is based on a hypothesis. The hypothesis says that people’s reaction time decreases when faced with similar concepts and increases when faced with conflicting concepts. For example, given target words ( woman , man ) and attribute words ( beautiful , strong ), we want to test a subject’s perspective: which attribute is associated more closely with each target. If a person believes that woman and beautiful are close and man and strong are close, then, when faced with category A ( woman , beautiful ) and category B ( man , strong ), he/she will quickly categorize the word charming into the former group. Whereas if he/she is faced with two categories A ( woman , strong ) and B ( man , beautiful ), then faced with the word charming , he/she will hesitate between the two pairs of words, thus increasing the reaction time substantially, although the correct answer is clear that charming should be grouped into B since it’s an attribute word, and thus should be classified according to beautiful or strong . A part of an IAT is shown in Fig. 2.10 .
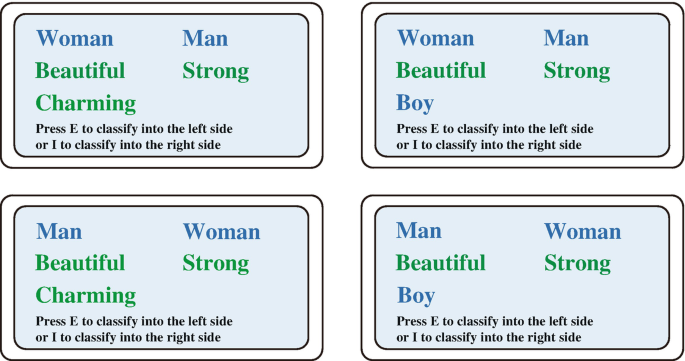
Parts of the IAT. The box in the second row is considered to be harder than the first row for people who have an implicit association between women and beautiful ( man and strong )
IAT can detect implicit thoughts and biases in the human mind. Considering that bias is so prevalent in people’s perceptions, it will likely be reflected in the texts written by humans. A pioneering article [ 12 ] in Science magazine proposes WEAT (word-embedding association test) to detect bias in texts. Given two sets X , Y as the target words and A , B as two sets of attribute words, WEAT defines a difference between the target sets to the two sets of attribute words as follows:
where x , y , a , b are the vector representation of word x , y , a , b . \(\operatorname {cos} (\mathbf {x},\mathbf {a})\) that can be treated as the response time in the IAT. And \(\operatorname {mean}(\cdot )\) is the average function. Thus, s ( x ; A , B ) measures the closeness of x to two sets of attributes. And s ( X , Y ; A , B ) measures the bias difference between X and Y to A and B . If X , Y are not biased differently for A and B , then they should not exceed at least the bias difference of ( X i , Y i ), where X ∩ Y is randomly divided into two sets X i , Y i of equal size. Hence, we test whether the following metric is small:
After some statistical derivations, we are able to calculate the above probabilities. In their experiments, WEAT is capable of capturing the occupational gender bias, where the occupational gender association calculated from the word representation is highly correlated with the publicly available proportion of female workers in each industry. For the name gender association, WEAT can find a similar pattern.
2.5.3 History and Social Science
It is possible to detect human thoughts without conducting live experiments using tests built on texts. This makes it very helpful to study the thoughts of ancient people. That is, we can explore the thoughts of the ancients through the texts they wrote, and this is precisely the important role of word representations in history and social sciences. In this section, we talk about how to use word representations to study the changes in history and society across time.
In order to track the chronological changes in word meanings, we first need to have a corpus of different chronologies. Google NGram Book Corpus [ 43 ] is a relatively early chronological corpus. This dataset counts the words/phrases’ frequency used during the last five centuries and includes 6% of all published books, which is a very large dataset. Another COHA dataset [ 16 ] has 400 million words, documenting the development of American English between 1810 and 2009, contains a wide variety of genres, and is relatively balanced across genres.
The work on tracking word sense changes [ 31 , 37 ] divides these datasets into bins of equal size by time. They then train word representation models on the text within each period. For example, in the work [ 31 ], the SVD decomposition of the PPMI (positive pointwise mutual information) matrix and SGNS (skip-gram with negative sampling) are used as two base models. As mentioned earlier, the dimensions are not aligned for different groups of distributed representations, even if they are derived from the same counting matrix. Therefore, the authors propose to optimize a mapping matrix that maps the word vectors of the previous period to the word vector space of the latter period.
Aligning the word vectors after training them usually does not yield satisfying results because simple transformations cannot always align the two vector spaces, and complex transformations carry the risk of overfitting. Time-sensitive word representation is developed to address these issues. Bamler et al. [ 4 ] propose a dynamic skip-gram model which connects several Bayesian skip-gram models [ 6 ] using Kalman filters [ 35 ]. In this model, the embeddings of words in different periods could affect each other. For example, a word that appears in the 1990s document can affect the embeddings of that word in the 1980s and 2000s. Moreover, this model puts all the embeddings into the same semantic space, significantly improving against other methods and making word embeddings in different periods comparable. Experimental results show that the cosine distance between two words changes much more smoothly in this model than in those that simply divide the corpus into bins.
We can arrive at interesting societal observations using word representations from different periods. Hamilton et al. [ 31 ] perform two analyses, the first of which computes the time series formed by the cosine value of a word pair over time. They use Spearman correlation coefficients of the time series against time to estimate whether this change is an upward or downward trend and how significant the trend is. For the second analysis, the authors track the degree of change in the word vector of the same word over time to see the semantic drifts of a word across periods. They have come to some interesting conclusions. (1) Some established word sense shifts can be confirmed from the corpus. For example, the shift of gay change from happy to homosexual is observed from the word representation. (2) The authors also find the ten words that changed the most from 1900 to 1990. (3) Combining some experimental observations, the authors found two statistical rules of semantic variation. The first is that common words change their meanings more slowly, and rare words change their meanings more quickly. The second is that words with multiple meanings change their meanings more quickly.
The follow-up work [ 29 ] is based on the same diachronic word vectors as Hamilton et al. [ 31 ] but makes some observations with more depth in social science. Specifically, it compares trends in gender and race stereotypes over 100 years. To get the stereotype information from word representations, this article computes the difference in the association scores of an attribute word (e.g., intelligent ) to two groups of words (e.g., woman , female versus man , male ). The association score can be calculated by either cosine similarity or Euclidean distance. This is similar to the WEAT mentioned in Sect. 2.5.2 . The work further compares the association score with publicly available data about the gender per occupation statistics over the year. They find the two trends match almost exactly. When studying the association of adjectives to genders, a clear phase shift is found. The similarity of adjectives’ association scores to genders is similar within the 1910s∼1960s and the 1960s∼1990s, respectively, but differs substantially between the two time periods. The phase shift in the 1960s corresponds to the US women’s movement in history.
2.6 Summary and Further Readings
In this chapter, we focus on words, which are the basic semantic units. We introduce the representative methods in symbolic representation and distributed representation. Some well-known models are introduced, such as one-hot representation, LSA, PLSA, LDA, word2vec, GloVe, and ELMo. We also present the methods to make the representations more informative and interpretable. At last, the applications of word representations are introduced, where interdisciplinary applications are emphasized. For further readings, firstly, we encourage the readers to read Chaps. 3 and 4 for higher-level representations as they are more widely used in practical tasks. We also encourage the readers to review historical research, such as the review paper on representation learning by Yoshua Bengio [ 9 ], and the review paper on pre-trained distributed word representations by Tomas Mikolov, the author of word2vec [ 50 ].
Hypernyms are words whose meaning includes a group of other words, which are instances of the former. Word u is a hyponym of word v if and only if word v is a hypernym of word u .
Estimated by dividing the frequency of a word by the total number of words in the corpus.
A multiset is a set where duplicated elements are allowed, e.g., (1,2,2,3) and (2,2,3,1) are the same multiset.
Models of distributed representations are also called vector space models (VSMs).
In the following sections, we use the row vector for distributed word representation.
Up to permutations of rows, columns, and signs.
In fact, the mathematical backbone of LSA is the same as PCA. We repeat it here for the convenience of those readers who skipped the previous section.
Examples are taken from the Oxford Dictionary of English [ 69 ].
The details of the language model are in Chap. 5 .
Ontology graph connects different abstract concepts in a graph according to their semantic relationships.
Eneko Agirre, Enrique Alfonseca, Keith Hall, Jana Kravalova, Marius Paşca, and Aitor Soroa. A study on similarity and relatedness using distributional and WordNet-based approaches. In Proceedings of NAACL-HLT , 2009.
Google Scholar
Ben Athiwaratkun and Andrew Wilson. Multimodal word distributions. In Proceedings of ACL , 2017.
Collin F Baker, Charles J Fillmore, and John B Lowe. The berkeley framenet project. In Proceedings of ACL , 1998.
Robert Bamler and Stephan Mandt. Dynamic word embeddings via skip-gram filtering. arXiv preprint arXiv:1702.08359 , 2017.
Arindam Banerjee, Inderjit S Dhillon, Joydeep Ghosh, and Suvrit Sra. Clustering on the unit hypersphere using von Mises-Fisher distributions. Journal of Machine Learning Research , 2005.
Oren Barkan. Bayesian neural word embedding. In Proceedings of AAAI , 2017.
Marco Baroni, Georgiana Dinu, and Germán Kruszewski. Don’t count, predict! a systematic comparison of context-counting vs. context-predicting semantic vectors. In Proceedings of ACL , 2014.
Gary Bécigneul and Octavian-Eugen Ganea. Riemannian adaptive optimization methods. In Proceedings of ICLR , 2019.
Yoshua Bengio, Aaron Courville, and Pascal Vincent. Representation learning: A review and new perspectives. IEEE Transactions on Pattern Analysis and Machine Intelligence , 2013.
David M. Blei, Andrew Y. Ng, and Michael I. Jordan. Latent Dirichlet Allocation. In Proceedings of NeurIPS , 2001.
Piotr Bojanowski, Edouard Grave, Armand Joulin, and Tomas Mikolov. Enriching word vectors with subword information. Transactions of the Association for Computational Linguistics , 2017.
Aylin Caliskan, Joanna J Bryson, and Arvind Narayanan. Semantics derived automatically from language corpora contain human-like biases. Science , 2017.
Xinxiong Chen, Zhiyuan Liu, and Maosong Sun. A unified model for word sense representation and disambiguation. In Proceedings of EMNLP , 2014.
Xinxiong Chen, Lei Xu, Zhiyuan Liu, Maosong Sun, and Huanbo Luan. Joint learning of character and word embeddings. In Proceedings of IJCAI , 2015.
Corinna Cortes and Vladimir Vapnik. Support-vector networks. Machine Learning , 1995.
Mark Davies. The corpus of historical American english: 400 million words, (1810-2009), 2010.
Scott Deerwester, Susan T Dumais, George W Furnas, Thomas K Landauer, and Richard Harshman. Indexing by latent semantic analysis. Journal of the American Society for Information Science , 1990.
Arthur P Dempster, Nan M Laird, and Donald B Rubin. Maximum likelihood from incomplete data via the EM algorithm. Journal of the Royal Statistical Society: Series B (Methodological) , 1977.
Jacob Devlin, Ming-Wei Chang, Kenton Lee, and Kristina Toutanova. BERT: pre-training of deep bidirectional transformers for language understanding. In Proceedings of NAACL-HLT , 2019.
Chris Ding, Tao Li, and Wei Peng. On the equivalence between non-negative matrix factorization and probabilistic latent semantic indexing. Computational Statistics & Data Analysis , 2008.
Katrin Erk and Sebastian Padó. A structured vector space model for word meaning in context. In Proceedings of EMNLP , 2008.
Manaal Faruqui, Jesse Dodge, Sujay Kumar Jauhar, Chris Dyer, Eduard Hovy, and Noah A Smith. Retrofitting word vectors to semantic lexicons. In Proceedings of NAACL-HLT , 2015.
Lev Finkelstein, Evgeniy Gabrilovich, Yossi Matias, Ehud Rivlin, Zach Solan, Gadi Wolfman, and Eytan Ruppin. Placing search in context: The concept revisited. In Proceedings of WWW , 2001.
John R Firth. A synopsis of linguistic theory, 1930-1955. Studies in Linguistic Analysis , 1957.
Dayne Freitag, Matthias Blume, John Byrnes, Edmond Chow, Sadik Kapadia, Richard Rohwer, and Zhiqiang Wang. New experiments in distributional representations of synonymy. In Proceedings of CoNLL , 2005.
Ruiji Fu, Jiang Guo, Bing Qin, Wanxiang Che, Haifeng Wang, and Ting Liu. Learning semantic hierarchies via word embeddings. In Proceedings of ACL , 2014.
Alona Fyshe, Leila Wehbe, Partha Pratim Talukdar, Brian Murphy, and Tom M Mitchell. A compositional and interpretable semantic space. In Proceedings of NAACL-HLT , 2015.
Juri Ganitkevitch, Benjamin Van Durme, and Chris Callison-Burch. PPDB: The paraphrase database. In Proceedings of NAACL-HLT , 2013.
Nikhil Garg, Londa Schiebinger, Dan Jurafsky, and James Zou. Word embeddings quantify 100 years of gender and ethnic stereotypes. Proceedings of the National Academy of Sciences , 2018.
Anthony G Greenwald and Shelly D Farnham. Using the implicit association test to measure self-esteem and self-concept. Journal of Personality and Social Psychology , 2000.
William L. Hamilton, Jure Leskovec, and Dan Jurafsky. Diachronic word embeddings reveal statistical laws of semantic change. In Proceedings of ACL , 2016.
Felix Hill, Roi Reichart, and Anna Korhonen. Simlex-999: Evaluating semantic models with (genuine) similarity estimation. Computational Linguistics , 2015.
Sepp Hochreiter and Jürgen Schmidhuber. Long short-term memory. Neural Computation , 1997.
Thomas Hofmann. Probabilistic latent semantic indexing. In Proceedings of SIGIR , 1999.
Rudolph Emil Kalman et al. A new approach to linear filtering and prediction problems. Journal of Basic Engineering , 1960.
Pentti Kanerva, Jan Kristofersson, and Anders Holst. Random indexing of text samples for latent semantic analysis. In Proceedings of CogSci , 2000.
Yoon Kim, Yi-I Chiu, Kentaro Hanaki, Darshan Hegde, and Slav Petrov. Temporal analysis of language through neural language models. In Proceedings of ACL Workshop , 2014.
Da Kuang, Jaegul Choo, and Haesun Park. Nonnegative matrix factorization for interactive topic modeling and document clustering. Partitional Clustering Algorithms , page 215, 2014.
Thomas K Landauer and Susan T Dumais. A solution to Plato’s problem: The latent semantic analysis theory of acquisition, induction, and representation of knowledge. Psychological Review , 1997.
Omer Levy and Yoav Goldberg. Dependency-based word embeddings. In Proceedings of ACL , 2014.
Omer Levy and Yoav Goldberg. Neural word embedding as implicit matrix factorization. In Proceedings of NeurIPS , 2014.
Shaohua Li, Tat-Seng Chua, Jun Zhu, and Chunyan Miao. Generative topic embedding: a continuous representation of documents. In Proceedings of ACL , 2016.
Yuri Lin, Jean-Baptiste Michel, Erez Aiden Lieberman, Jon Orwant, Will Brockman, and Slav Petrov. Syntactic annotations for the Google Books NGram corpus. In Proceedings of the ACL , 2012.
Wang Ling, Chris Dyer, Alan W Black, Isabel Trancoso, Ramón Fermandez, Silvio Amir, Luis Marujo, and Tiago Luís. Finding function in form: Compositional character models for open vocabulary word representation. In Proceedings of EMNLP , 2015.
Yang Liu, Zhiyuan Liu, Tat-Seng Chua, and Maosong Sun. Topical word embeddings. In Proceedings of AAAI , 2015.
Zhiyuan Liu, Yankai Lin, and Maosong Sun. Representation Learning for Natural Language Processing . Springer, 2020.
Book Google Scholar
Hongyin Luo, Zhiyuan Liu, Huanbo Luan, and Maosong Sun. Online learning of interpretable word embeddings. In Proceedings of EMNLP , 2015.
T Mikolov and J Dean. Distributed representations of words and phrases and their compositionality. In Proceedings of NeurIPS , 2013.
Tomas Mikolov, Kai Chen, Greg Corrado, and Jeffrey Dean. Efficient estimation of word representations in vector space. In Proceedings of ICLR , 2013.
Tomas Mikolov, Edouard Grave, Piotr Bojanowski, Christian Puhrsch, and Armand Joulin. Advances in pre-training distributed word representations. In Proceedings of LREC , 2018.
Tomas Mikolov, Wen-tau Yih, and Geoffrey Zweig. Linguistic regularities in continuous space word representations. In Proceedings of NAACL-HLT , 2013.
George A Miller. WordNet: a lexical database for English. Communications of the ACM , 1995.
Brian Murphy, Partha Talukdar, and Tom Mitchell. Learning effective and interpretable semantic models using non-negative sparse embedding. In Proceedings of COLING , 2012.
Boris Muzellec and Marco Cuturi. Generalizing point embeddings using the Wasserstein space of elliptical distributions. In Proceedings of NeurIPS , 2018.
Maximillian Nickel and Douwe Kiela. Poincare embeddings for learning hierarchical representations. In Proceedings of NeurIPS , 2017.
Sebastian Padó and Mirella Lapata. Dependency-based construction of semantic space models. Computational Linguistics , 2007.
Jeffrey Pennington, Richard Socher, and Christopher Manning. GloVe: Global vectors for word representation. In Proceedings of EMNLP , 2014.
Matthew Peters, Mark Neumann, Mohit Iyyer, Matt Gardner, Christopher Clark, Kenton Lee, and Luke Zettlemoyer. Deep contextualized word representations. In Proceedings of NAACL-HLT , 2018.
Alec Radford, Karthik Narasimhan, Tim Salimans, and Ilya Sutskever. Improving language understanding with unsupervised learning. 2018.
Kira Radinsky, Eugene Agichtein, Evgeniy Gabrilovich, and Shaul Markovitch. A word at a time: computing word relatedness using temporal semantic analysis. In Proceedings of WWW , 2011.
Philip Resnik. Semantic similarity in a taxonomy: An information-based measure and its application to problems of ambiguity in natural language. Journal of artificial intelligence research , 1999.
Herbert Rubenstein and John B Goodenough. Contextual correlates of synonymy. Communications of the ACM , 1965.
Magnus Sahlgren. Vector-based semantic analysis: Representing word meanings based on random labels. In Proceedings of SKAC Workshop , 2001.
Magnus Sahlgren. An introduction to random indexing. In Proceedings of TKE , 2005.
Magnus Sahlgren. The Word-Space Model: Using Distributional Analysis to represent syntagmatic and paradigmatic relations between words in high-dimensional vector spaces . PhD thesis, Institutionen för lingvistik, 2006.
Gerard Salton. The SMART retrieval system—experiments in automatic document processing . Prentice-Hall, Inc., 1971.
Gerard Salton, Anita Wong, and Chung-Shu Yang. A vector space model for automatic indexing. Communications of the ACM , 1975.
Tobias Schnabel, Igor Labutov, David Mimno, and Thorsten Joachims. Evaluation methods for unsupervised word embeddings. In Proceedings of EMNLP , 2015.
Angus Stevenson. Oxford dictionary of English . 2010.
Fei Sun, Jiafeng Guo, Yanyan Lan, Jun Xu, and Xueqi Cheng. Learning word representations by jointly modeling syntagmatic and paradigmatic relations. In Proceedings of ACL , 2015.
Duyu Tang, Furu Wei, Nan Yang, Ming Zhou, Ting Liu, and Bing Qin. Learning sentiment-specific word embedding for Twitter sentiment classification. In Proceedings of ACL , 2014.
Alexandru Tifrea, Gary Bécigneul, and Octavian-Eugen Ganea. Poincaré glove: Hyperbolic word embeddings. arXiv preprint arXiv:1810.06546 , 2018.
Laurens Van der Maaten and Geoffrey Hinton. Visualizing data using t-SNE. Journal of Machine Learning Research , 2008.
Luke Vilnis and Andrew McCallum. Word representations via Gaussian embedding. In Proceedings of ICLR , 2015.
Dani Yogatama, Manaal Faruqui, Chris Dyer, and Noah A Smith. Learning word representations with hierarchical sparse coding. In Proceedings of ICML , 2015.
Mo Yu and Mark Dredze. Improving lexical embeddings with semantic knowledge. In Proceedings of ACL , 2014.
Meng Zhang, Haoruo Peng, Yang Liu, Huan-Bo Luan, and Maosong Sun. Bilingual lexicon induction from non-parallel data with minimal supervision. In Proceedings of AAAI , 2017.
Will Y Zou, Richard Socher, Daniel M Cer, and Christopher D Manning. Bilingual word embeddings for phrase-based machine translation. In Proceedings of EMNLP , 2013.
Download references
Acknowledgements
Zhiyuan Liu, Yankai Lin, and Maosong Sun designed the overall architecture of this chapter; Shengding Hu drafted this chapter. Zhiyuan Liu and Yankai Lin proofread and revised this chapter.
We also thank Ning Ding, Yujia Qin, Si Sun, Yusheng Su, Zhitong Wang, Xingyu Shen, Zheni Zeng, and Ganqu Cui for proofreading the chapter and Lei Xu for preparing the initial draft materials for the first edition.
This is the word representation learning chapter about of the second edition of the book Representation Learning for Natural Language Processing , with its first edition published in 2020 [ 46 ]. Compared to the first edition of this chapter, the main changes include the following: (1) we rewrote the sections before Sect. 2.4 by systematically restructuring the works, (2) we restructured and summarized the advanced topics into two directions and polish the writing of advanced topics, and (3) we added a new section to introduce word representation’s applications.
Author information
Authors and affiliations.
Department of Computer Science and Technology, Tsinghua University, Beijing, China
Shengding Hu, Zhiyuan Liu & Maosong Sun
Gaoling School of Artificial Intelligence, Renmin University of China, Beijing, China
You can also search for this author in PubMed Google Scholar
Corresponding author
Correspondence to Zhiyuan Liu .
Editor information
Editors and affiliations.
Zhiyuan Liu
Maosong Sun
Rights and permissions
Open Access This chapter is licensed under the terms of the Creative Commons Attribution 4.0 International License ( http://creativecommons.org/licenses/by/4.0/ ), which permits use, sharing, adaptation, distribution and reproduction in any medium or format, as long as you give appropriate credit to the original author(s) and the source, provide a link to the Creative Commons license and indicate if changes were made.
The images or other third party material in this chapter are included in the chapter's Creative Commons license, unless indicated otherwise in a credit line to the material. If material is not included in the chapter's Creative Commons license and your intended use is not permitted by statutory regulation or exceeds the permitted use, you will need to obtain permission directly from the copyright holder.
Reprints and permissions
Copyright information
© 2023 The Author(s)
About this chapter
Cite this chapter.
Hu, S., Liu, Z., Lin, Y., Sun, M. (2023). Word Representation Learning. In: Liu, Z., Lin, Y., Sun, M. (eds) Representation Learning for Natural Language Processing. Springer, Singapore. https://doi.org/10.1007/978-981-99-1600-9_2
Download citation
DOI : https://doi.org/10.1007/978-981-99-1600-9_2
Published : 24 August 2023
Publisher Name : Springer, Singapore
Print ISBN : 978-981-99-1599-6
Online ISBN : 978-981-99-1600-9
eBook Packages : Computer Science Computer Science (R0)
Share this chapter
Anyone you share the following link with will be able to read this content:
Sorry, a shareable link is not currently available for this article.
Provided by the Springer Nature SharedIt content-sharing initiative
- Publish with us
Policies and ethics
- Find a journal
- Track your research
Help | Advanced Search
Computer Science > Computation and Language
Title: reft: representation finetuning for language models.
Abstract: Parameter-efficient fine-tuning (PEFT) methods seek to adapt large models via updates to a small number of weights. However, much prior interpretability work has shown that representations encode rich semantic information, suggesting that editing representations might be a more powerful alternative. Here, we pursue this hypothesis by developing a family of $\textbf{Representation Finetuning (ReFT)}$ methods. ReFT methods operate on a frozen base model and learn task-specific interventions on hidden representations. We define a strong instance of the ReFT family, Low-rank Linear Subspace ReFT (LoReFT). LoReFT is a drop-in replacement for existing PEFTs and learns interventions that are 10x-50x more parameter-efficient than prior state-of-the-art PEFTs. We showcase LoReFT on eight commonsense reasoning tasks, four arithmetic reasoning tasks, Alpaca-Eval v1.0, and GLUE. In all these evaluations, LoReFT delivers the best balance of efficiency and performance, and almost always outperforms state-of-the-art PEFTs. We release a generic ReFT training library publicly at this https URL .
Submission history
Access paper:.
- Other Formats

References & Citations
- Google Scholar
- Semantic Scholar
BibTeX formatted citation

Bibliographic and Citation Tools
Code, data and media associated with this article, recommenders and search tools.
- Institution
arXivLabs: experimental projects with community collaborators
arXivLabs is a framework that allows collaborators to develop and share new arXiv features directly on our website.
Both individuals and organizations that work with arXivLabs have embraced and accepted our values of openness, community, excellence, and user data privacy. arXiv is committed to these values and only works with partners that adhere to them.
Have an idea for a project that will add value for arXiv's community? Learn more about arXivLabs .
Dev Patel on ‘Monkey Man’ Sequel Possibilities and Trans Representation: ‘This Is an Anthem for the Underdogs, the Voiceless and the Marginalized’
By Marc Malkin
Marc Malkin
Senior Editor, Culture and Events
- Nicole Richie on the 20th Anniversary of ‘The Simple Life,’ Starring in ‘Don’t Tell Mom the Babysitter’s Dead’ Remake and Writing a Horror-Comedy 5 days ago
- ‘Femme’ Star George MacKay Spent Eight Weeks Bulking Up to Become a Violent Street Thug in the Queer Revenge Thriller: ‘It’s an Animal Thing’ 6 days ago
- ‘We Were the Lucky Ones’ Actor Amit Rahav Honors His Grandmother, a Holocaust Survivor, With Hulu’s WWII Drama 1 week ago

Dev Patel refused to let the slew of injuries he suffered while filming his elaborate fight scenes in “ Monkey Man ” get in the way of him completing the movie.
Patel not only directed and co-wrote the action movie, but he also stars as Kid, a young man who seeks to avenge his mother’s death at the hands of India’s corrupt law enforcement officials, political figures and spiritual leaders. He earns money by competing in a brutally violent underground fight club.
Eye infections?
“I was crawling on a bathroom floor and it’s flooding. We were shooting this scene for three days,” Patel explained. “All the crew were coming in with their dirty shoes and I’m drinking this water literally. It was grimy stuff.”
Sikandar Kher, whose villainous Rana spars with Patel, revealed that the director-star immediately returned to filming after undergoing surgery for his broken hand. “I was like, ‘Man, this is too much because you don’t want to hurt the guy anymore. It’s enough,’” Kher said. “But he mustered through it.”
The movie also includes a group of trans and gender-nonconforming characters who join Kid in his fight against India’s elite. “For me, this is an anthem for the underdogs, the voiceless and the marginalized,” Patel said. “Together they wage this war for the good and the just, and for me, I really wanted to include the hijra community, the third gender in India.”
He added, “We should be fighting for each other, not against each other.”
Vipin Sharma, who plays trans woman Alpha, recently attended a screening of the movie for the trans community. “I was almost in tears when they said they loved it, they loved the representation and they were very happy about it,” he said. “That just touched my heart.”
“Monkey Man” is in theaters April 5.

More From Our Brands
Drake responds to rick ross’ nose job claim, warns rapper after diss, ‘don’t worry we’ll handle it’, how cartier’s tiniest new tank made big waves at watches & wonders, timberwolves can clinch west title, but ownership remains in flux, be tough on dirt but gentle on your body with the best soaps for sensitive skin, the masters 2024: how to watch the final round online, verify it's you, please log in.
Gary Brown: Paying our fair share is not fun

"I just filled out my income tax," comedian Milton Berle once quipped. "Who says you can't get killed by a blank."
Many of us consider the day that is the deadline for filing state and federal income taxes – April 15, or tomorrow if you've been trying to forget – as a dark and looming moment to be postponed as long as possible. I've been in my share of vehicle lines in front of the post office late on the night of tax day, handing to a postal worker the envelopes that mail my returns to the federal and state money grabbers ... I mean taxation office workers.
Few of us are really happy about paying taxes. As an unidentified observer once said, "People who complain about taxes can be divided into two classes: men and women." Undoubtedly, animals would whine, too, if they ever got W-2 or 1099 forms for their farm work.
Still, paying taxes is as old as the country and can be traced to colonial times. We started the country, for heaven's sake, on the protest of "No taxation without representation."
As humorist Gerald Barzan noted, "Taxation with representation ain't so hot either."
Filling out our forms
Now, with only a day to go, the most tardy among us finally must sit down and tackle the difficult and time-consuming task of filling out our tax forms.
The job isn't simple even for the smartest among us.
"This is too difficult for a mathematician," Albert Einstein is once supposed to have claimed about filing taxes. "It takes a philosopher."
Forms are varied and can be confusing. Instructions can seem vague, which is convenient to some taxpayers. It enhances our powers of deduction.
"The income tax," humorist Will Rogers, "has made more liars out of the American people than golf."
Our will to delay the inevitable is strong. People some states even seem to have used the calendar to occasionally get more time before taxing ourselves. Patriots Day is Monday April 15, 2024, in six states – Massachusetts, Maine, Florida, Wisconsin, Connecticut and North Dakota – pushing the need to file and pay up income taxes forward this year. Since the next day, Tuesday April 16, is Emancipation Day in Washington, D.C., the deadline for taxpayers in those states is delayed until Wednesday April 17.
And, yes, it's probably too late to move to any of those states.
A few patriotic words
Still, in a country where people long to enjoy a higher standard of living than much of the world, why are we so disenchanted with paying taxes to achieve it? I mean beyond what President Calvin Coolidge said, which was "Collecting more taxes than is absolutely necessary is legalized robbery." And, well, don't most of us believe that our personal taxes are more than necessary?
With all due patriotism, author, columnist and policy analyst Holly Sklar once explained the reason for taxes.
"Taxes are how we pool our money for public health and safety, infrastructure, research, and services – from the development of vaccines and the Internet to public schools and universities, transportation, courts, police, parks, and safe drinking water."
I like all those things.
"The point to remember is that what the government gives," said John S. Coleman, described as "a savvy business executive" during the Eisenhower administration, "it first must take away."
The "taking away" part is what becomes is so unpalatable for us this time of year.
It puts many of us in the camp of radio and television personality Arthur Godfrey, who before his passing in the 1980s boasted that "I am proud to be paying taxes in the United States."
"The only thing is I could be just as proud for half the money."
Reach Gary at [email protected] . On Twitter: @gbrownREP
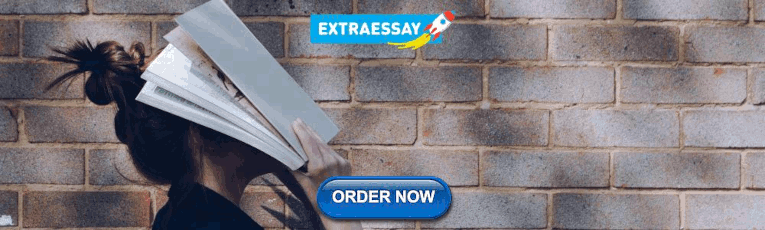
IMAGES
VIDEO
COMMENTS
Find 40 different ways to say REPRESENTATION, along with antonyms, related words, and example sentences at Thesaurus.com.
Below is a massive list of representation words - that is, words related to representation. The top 4 are: image, model, diversity and inclusion. You can get the definition(s) of a word in the list below by tapping the question-mark icon next to it. The words at the top of the list are the ones most associated with representation, and as you go ...
Words related to representation play a crucial role in shaping our understanding of various concepts, ideas, and experiences. Through language, we are able to express our perspectives, advocate for marginalized communities, and challenge existing power structures.
REPRESENTATION - Synonyms, related words and examples | Cambridge English Thesaurus
Synonyms for REPRESENTATION: depiction, image, portrait, drawing, picture, illustration, photograph, view, resemblance, delineation
Most related words/phrases with sentence examples define Representation meaning and usage. Thesaurus for Representation. Related terms for representation- synonyms, antonyms and sentences with representation. Lists. synonyms. antonyms. definitions. sentences. thesaurus. Parts of speech. nouns. verbs. adjectives. Synonyms
Words Related to Representation Related words are words that are directly connected to each other through their meaning, even if they are not synonyms or antonyms. This connection may be general or specific, or the words may appear frequently together. Related: show; interpretation; representational;
Another way to say Representation? Synonyms for Representation (other words and phrases for Representation). Synonyms for Representation. 1 852 other terms for representation- words and phrases with similar meaning. Lists. synonyms. antonyms. definitions. sentences. thesaurus. words. phrases. idioms. Parts of speech. nouns. verbs. adjectives ...
REPRESENT - Synonyms, related words and examples | Cambridge English Thesaurus
The representation of something through art or imagery. The description or portrayal of someone or something in a particular way. A theatrical performance. (politics) The role of a representative in government. ( law) The lawyers and staff who argue on behalf of another in court. ( representations) Formal statements made to an authority.
Synonyms and related words for representation from OneLook Thesaurus, a powerful English thesaurus and brainstorming tool that lets you describe what you're looking for in plain terms. Search Advanced filters. Enter a word, phrase, description, or pattern above to find synonyms, related words, and more. ...
Synonyms for REPRESENTATION: portrayal, account, depiction, description, illustration, image, likeness, model, picture, portrait, …
Synonyms for REPRESENTATIONS: pictures, depictions, portraits, drawings, images, photographs, illustrations, resemblances, likenesses, views
Most related words/phrases with sentence examples define Represent meaning and usage. Thesaurus for Represent Related terms for represent - synonyms, antonyms and sentences with represent
Synonyms for representation in Free Thesaurus. Antonyms for representation. 40 synonyms for representation: body of representatives, committee, embassy, delegates ...
Another way to say Representations? Synonyms for Representations (other words and phrases for Representations).
Plural for the description or portrayal of someone or something in a particular way. (politics) Plural for the role of a representative in government. ( law) Plural for the lawyers and staff who argue on behalf of another in court. ( representations) Plural for formal statements made to an authority. Plural for a visual graph or map, especially ...
representation: [noun] one that represents: such as. an artistic likeness or image. a statement or account made to influence opinion or action. an incidental or collateral statement of fact on the faith of which a contract is entered into. a dramatic production or performance. a usually formal statement made against something or to effect a ...
REPRESENTATION meaning: 1. a person or organization that speaks, acts, or is present officially for someone else: 2. the…. Learn more.
Definition of representation noun in Oxford Advanced American Dictionary. Meaning, pronunciation, picture, example sentences, grammar, usage notes, synonyms and more.
Find 109 different ways to say REPRESENTATIONAL, along with antonyms, related words, and example sentences at Thesaurus.com.
Words Related to representation. Below is a list of words related to representation. You can click words for definitions. Sorry if there's a few unusual suggestions! The algorithm isn't perfect, but it does a pretty good job for common-ish words. Here's the list of words that are related to representation: image;
The word representations can be divided according to their form of representation and source of the semantics: (a) shows the symbolic representations that use the knowledge base as the source, which is adopted by conventional linguistics; (b) shows the symbolic representations that adopt the distributional hypothesis as the foundation of the semantic source; (c) shows the distributed ...
ReFT methods operate on a frozen base model and learn task-specific interventions on hidden representations. We define a strong instance of the ReFT family, Low-rank Linear Subspace ReFT (LoReFT). LoReFT is a drop-in replacement for existing PEFTs and learns interventions that are 10x-50x more parameter-efficient than prior state-of-the-art PEFTs.
Dev Patel on 'Monkey Man' Sequel Possibilities and Trans Representation: 'This Is an Anthem for the Underdogs, the Voiceless and the Marginalized'. Dev Patel refused to let the slew of ...
Gary Brown: Paying our fair share is not fun. "I just filled out my income tax," comedian Milton Berle once quipped. "Who says you can't get killed by a blank." Many of us consider the day that is ...