
- Walden University
- Faculty Portal
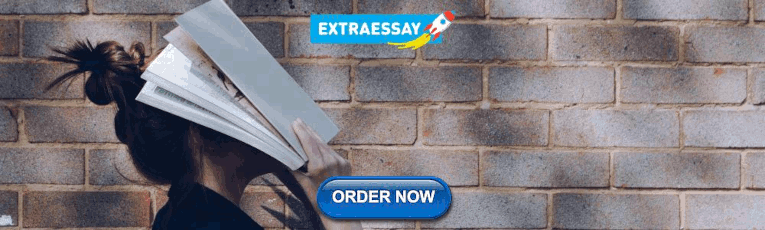
Writing a Paper: Thesis Statements
Basics of thesis statements.
The thesis statement is the brief articulation of your paper's central argument and purpose. You might hear it referred to as simply a "thesis." Every scholarly paper should have a thesis statement, and strong thesis statements are concise, specific, and arguable. Concise means the thesis is short: perhaps one or two sentences for a shorter paper. Specific means the thesis deals with a narrow and focused topic, appropriate to the paper's length. Arguable means that a scholar in your field could disagree (or perhaps already has!).
Strong thesis statements address specific intellectual questions, have clear positions, and use a structure that reflects the overall structure of the paper. Read on to learn more about constructing a strong thesis statement.
Being Specific
This thesis statement has no specific argument:
Needs Improvement: In this essay, I will examine two scholarly articles to find similarities and differences.
This statement is concise, but it is neither specific nor arguable—a reader might wonder, "Which scholarly articles? What is the topic of this paper? What field is the author writing in?" Additionally, the purpose of the paper—to "examine…to find similarities and differences" is not of a scholarly level. Identifying similarities and differences is a good first step, but strong academic argument goes further, analyzing what those similarities and differences might mean or imply.
Better: In this essay, I will argue that Bowler's (2003) autocratic management style, when coupled with Smith's (2007) theory of social cognition, can reduce the expenses associated with employee turnover.
The new revision here is still concise, as well as specific and arguable. We can see that it is specific because the writer is mentioning (a) concrete ideas and (b) exact authors. We can also gather the field (business) and the topic (management and employee turnover). The statement is arguable because the student goes beyond merely comparing; he or she draws conclusions from that comparison ("can reduce the expenses associated with employee turnover").
Making a Unique Argument
This thesis draft repeats the language of the writing prompt without making a unique argument:
Needs Improvement: The purpose of this essay is to monitor, assess, and evaluate an educational program for its strengths and weaknesses. Then, I will provide suggestions for improvement.
You can see here that the student has simply stated the paper's assignment, without articulating specifically how he or she will address it. The student can correct this error simply by phrasing the thesis statement as a specific answer to the assignment prompt.
Better: Through a series of student interviews, I found that Kennedy High School's antibullying program was ineffective. In order to address issues of conflict between students, I argue that Kennedy High School should embrace policies outlined by the California Department of Education (2010).
Words like "ineffective" and "argue" show here that the student has clearly thought through the assignment and analyzed the material; he or she is putting forth a specific and debatable position. The concrete information ("student interviews," "antibullying") further prepares the reader for the body of the paper and demonstrates how the student has addressed the assignment prompt without just restating that language.
Creating a Debate
This thesis statement includes only obvious fact or plot summary instead of argument:
Needs Improvement: Leadership is an important quality in nurse educators.
A good strategy to determine if your thesis statement is too broad (and therefore, not arguable) is to ask yourself, "Would a scholar in my field disagree with this point?" Here, we can see easily that no scholar is likely to argue that leadership is an unimportant quality in nurse educators. The student needs to come up with a more arguable claim, and probably a narrower one; remember that a short paper needs a more focused topic than a dissertation.
Better: Roderick's (2009) theory of participatory leadership is particularly appropriate to nurse educators working within the emergency medicine field, where students benefit most from collegial and kinesthetic learning.
Here, the student has identified a particular type of leadership ("participatory leadership"), narrowing the topic, and has made an arguable claim (this type of leadership is "appropriate" to a specific type of nurse educator). Conceivably, a scholar in the nursing field might disagree with this approach. The student's paper can now proceed, providing specific pieces of evidence to support the arguable central claim.
Choosing the Right Words
This thesis statement uses large or scholarly-sounding words that have no real substance:
Needs Improvement: Scholars should work to seize metacognitive outcomes by harnessing discipline-based networks to empower collaborative infrastructures.
There are many words in this sentence that may be buzzwords in the student's field or key terms taken from other texts, but together they do not communicate a clear, specific meaning. Sometimes students think scholarly writing means constructing complex sentences using special language, but actually it's usually a stronger choice to write clear, simple sentences. When in doubt, remember that your ideas should be complex, not your sentence structure.
Better: Ecologists should work to educate the U.S. public on conservation methods by making use of local and national green organizations to create a widespread communication plan.
Notice in the revision that the field is now clear (ecology), and the language has been made much more field-specific ("conservation methods," "green organizations"), so the reader is able to see concretely the ideas the student is communicating.
Leaving Room for Discussion
This thesis statement is not capable of development or advancement in the paper:
Needs Improvement: There are always alternatives to illegal drug use.
This sample thesis statement makes a claim, but it is not a claim that will sustain extended discussion. This claim is the type of claim that might be appropriate for the conclusion of a paper, but in the beginning of the paper, the student is left with nowhere to go. What further points can be made? If there are "always alternatives" to the problem the student is identifying, then why bother developing a paper around that claim? Ideally, a thesis statement should be complex enough to explore over the length of the entire paper.
Better: The most effective treatment plan for methamphetamine addiction may be a combination of pharmacological and cognitive therapy, as argued by Baker (2008), Smith (2009), and Xavier (2011).
In the revised thesis, you can see the student make a specific, debatable claim that has the potential to generate several pages' worth of discussion. When drafting a thesis statement, think about the questions your thesis statement will generate: What follow-up inquiries might a reader have? In the first example, there are almost no additional questions implied, but the revised example allows for a good deal more exploration.
Thesis Mad Libs
If you are having trouble getting started, try using the models below to generate a rough model of a thesis statement! These models are intended for drafting purposes only and should not appear in your final work.
- In this essay, I argue ____, using ______ to assert _____.
- While scholars have often argued ______, I argue______, because_______.
- Through an analysis of ______, I argue ______, which is important because_______.
Words to Avoid and to Embrace
When drafting your thesis statement, avoid words like explore, investigate, learn, compile, summarize , and explain to describe the main purpose of your paper. These words imply a paper that summarizes or "reports," rather than synthesizing and analyzing.
Instead of the terms above, try words like argue, critique, question , and interrogate . These more analytical words may help you begin strongly, by articulating a specific, critical, scholarly position.
Read Kayla's blog post for tips on taking a stand in a well-crafted thesis statement.
Related Resources

Didn't find what you need? Email us at [email protected] .
- Previous Page: Introductions
- Next Page: Conclusions
- Office of Student Disability Services
Walden Resources
Departments.
- Academic Residencies
- Academic Skills
- Career Planning and Development
- Customer Care Team
- Field Experience
- Military Services
- Student Success Advising
- Writing Skills
Centers and Offices
- Center for Social Change
- Office of Academic Support and Instructional Services
- Office of Degree Acceleration
- Office of Research and Doctoral Services
- Office of Student Affairs
Student Resources
- Doctoral Writing Assessment
- Form & Style Review
- Quick Answers
- ScholarWorks
- SKIL Courses and Workshops
- Walden Bookstore
- Walden Catalog & Student Handbook
- Student Safety/Title IX
- Legal & Consumer Information
- Website Terms and Conditions
- Cookie Policy
- Accessibility
- Accreditation
- State Authorization
- Net Price Calculator
- Contact Walden
Walden University is a member of Adtalem Global Education, Inc. www.adtalem.com Walden University is certified to operate by SCHEV © 2024 Walden University LLC. All rights reserved.
Thank you for visiting nature.com. You are using a browser version with limited support for CSS. To obtain the best experience, we recommend you use a more up to date browser (or turn off compatibility mode in Internet Explorer). In the meantime, to ensure continued support, we are displaying the site without styles and JavaScript.
- View all journals
- My Account Login
- Explore content
- About the journal
- Publish with us
- Sign up for alerts
- Review Article
- Open access
- Published: 10 August 2016
Learning strategies: a synthesis and conceptual model
- John A C Hattie 1 &
- Gregory M Donoghue 1
npj Science of Learning volume 1 , Article number: 16013 ( 2016 ) Cite this article
173k Accesses
185 Citations
680 Altmetric
Metrics details
- Social sciences
The purpose of this article is to explore a model of learning that proposes that various learning strategies are powerful at certain stages in the learning cycle. The model describes three inputs and outcomes (skill, will and thrill), success criteria, three phases of learning (surface, deep and transfer) and an acquiring and consolidation phase within each of the surface and deep phases. A synthesis of 228 meta-analyses led to the identification of the most effective strategies. The results indicate that there is a subset of strategies that are effective, but this effectiveness depends on the phase of the model in which they are implemented. Further, it is best not to run separate sessions on learning strategies but to embed the various strategies within the content of the subject, to be clearer about developing both surface and deep learning, and promoting their associated optimal strategies and to teach the skills of transfer of learning. The article concludes with a discussion of questions raised by the model that need further research.
Similar content being viewed by others
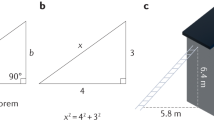
The science of effective learning with spacing and retrieval practice
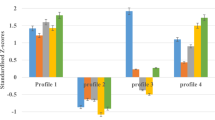
Profiles of learners based on their cognitive and metacognitive learning strategy use: occurrence and relations with gender, intrinsic motivation, and perceived autonomy support
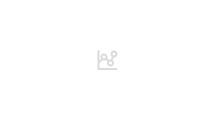
Looking back to move forward: comparison of instructors’ and undergraduates’ retrospection on the effectiveness of online learning using the nine-outcome influencing factors
There has been a long debate about the purpose of schooling. These debates include claims that schooling is about passing on core notions of humanity and civilisation (or at least one’s own society’s view of these matters). They include claims that schooling should prepare students to live pragmatically and immediately in their current environment, should prepare students for the work force, should equip students to live independently, to participate in the life of their community, to learn to ‘give back’, to develop personal growth. 1
In the past 30 years, however, the emphasis in many western systems of education has been more on enhancing academic achievement—in domains such as reading, mathematics, and science—as the primary purpose of schooling. 2 Such an emphasis has led to curricula being increasingly based on achievement in a few privileged domains, and ‘great’ students are deemed those who attain high levels of proficiency in these narrow domains.
This has led to many countries aiming to be in the top echelon of worldwide achievement measures in a narrow range of subjects; for example, achievement measures such as PISA (tests of 15-year olds in mathematics, reading and science, across 65 countries in 2012) or PIRLS (Year-5 tests of mathematics, reading and science, across 57 countries in 2011). Indeed, within most school systems there is a plethora of achievement tests; many countries have introduced accountability pressures based on high levels of testing of achievement; and communities typically value high achievement or levels of knowledge. 3 The mantra underpinning these claims has been cast in terms of what students know and are able to do; the curriculum is compartmentalised into various disciplines of achievement; and students, teachers, parents and policy makers talk in terms of success in these achievement domains.
Despite the recent emphasis on achievement, the day-to-day focus of schools has always been on learning—how to know, how to know more efficiently and how to know more effectively. The underlying philosophy is more about what students are now ready to learn, how their learning can be enabled, and increasing the ‘how to learn’ proficiencies of students. In this scenario, the purpose of schooling is to equip students with learning strategies, or the skills of learning how to learn. Of course, learning and achievement are not dichotomous; they are related. 4 Through growth in learning in specific domains comes achievement and from achievement there can be much learning. The question in this article relates to identifying the most effective strategies for learning.
In our search, we identified >400 learning strategies: that is, those processes which learners use to enhance their own learning. Many were relabelled versions of others, some were minor modifications of others, but there remained many contenders purported to be powerful learning strategies. Such strategies help the learner structure his or her thinking so as to plan, set goals and monitor progress, make adjustments, and evaluate the process of learning and the outcomes. These strategies can be categorised in many ways according to various taxonomies and classifications (e.g., references 5 , 6 , 7 ). Boekaerts, 8 for example, argued for three types of learning strategies: (1) cognitive strategies such as elaboration, to deepen the understanding of the domain studied; (2) metacognitive strategies such as planning, to regulate the learning process; and (3) motivational strategies such as self-efficacy, to motivate oneself to engage in learning. Given the advent of newer ways to access information (e.g., the internet) and the mountain of information now at students’ fingertips, it is appropriate that Dignath, Buettner and Langfeldt 9 added a fourth category—management strategies such as finding, navigating, and evaluating resources.
But merely investigating these 400-plus strategies as if they were independent is not defensible. Thus, we begin with the development of a model of learning to provide a basis for interpreting the evidence from our meta-synthesis. The argument is that learning strategies can most effectively enhance performance when they are matched to the requirements of tasks (cf. 10 ).
A model of learning
The model comprises the following components: three inputs and three outcomes; student knowledge of the success criteria for the task; three phases of the learning process (surface, deep and transfer), with surface and deep learning each comprising an acquisition phase and a consolidation phase; and an environment for the learning ( Figure 1 ). We are proposing that various learning strategies are differentially effective depending on the degree to which the students are aware of the criteria of success, on the phases of learning process in which the strategies are used, and on whether the student is acquiring or consolidating their understanding. The following provides an overview of the components of the model (see reference 11 for a more detailed explanation of the model).
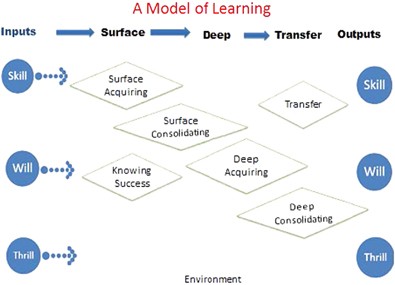
A model of learning.
Input and outcomes
The model starts with three major sources of inputs: the skill, the will and the thrill. The ‘skill’ is the student’s prior or subsequent achievement, the ‘will’ relates to the student’s various dispositions towards learning, and the ‘thrill’ refers to the motivations held by the student. In our model, these inputs are also the major outcomes of learning. That is, developing outcomes in achievement (skill) is as valuable as enhancing the dispositions towards learning (will) and as valuable as inviting students to reinvest more into their mastery of learning (thrill or motivations).
The first component describes the prior achievement the student brings to the task. As Ausubel 12 claimed ‘if I had to reduce all of educational psychology to just one principle, I would say this ‘The most important single factor influencing learning is what the leaner already knows. Ascertain this and teach him accordingly. Other influences related to the skills students bring to learning include their working memory, beliefs, encouragement and expectations from the student’s cultural background and home.
Dispositions are more habits of mind or tendencies to respond to situations in certain ways. Claxton 13 claimed that the mind frame of a ‘powerful learner’ is based on the four major dispositions: resilience or emotional strength, resourcefulness or cognitive capabilities, reflection or strategic awareness, and relating or social sophistication. These dispositions involve the proficiency to edit, select, adapt and respond to the environment in a recurrent, characteristic manner. 14 But dispositions alone are not enough. Perkins et al. 15 outlined a model with three psychological components which must be present in order to spark dispositional behaviour: sensitivity—the perception of the appropriateness of a particular behaviour; inclination—the felt impetus toward a behaviour; and ability—the basic capacity and confidence to follow through with the behaviour.
There can be a thrill in learning but for many students, learning in some domains can be dull, uninviting and boring. There is a huge literature on various motivational aspects of learning, and a smaller literature on how the more effective motivational aspects can be taught. A typical demarcation is between mastery and performance orientations. Mastery goals are seen as being associated with intellectual development, the acquisition of knowledge and new skills, investment of greater effort, and higher-order cognitive strategies and learning outcomes. 16 Performance goals, on the other hand, have a focus on outperforming others or completing tasks to please others. A further distinction has been made between approach and avoidance performance goals. 17 – 19 The correlations of mastery and performance goals with achievement, however, are not as high as many have claimed. A recent meta-analysis found 48 studies relating goals to achievement (based on 12,466 students), and the overall correlation was 0.12 for mastery and 0.05 for performance goals on outcomes. 20 Similarly, Hulleman et al. 21 reviewed 249 studies ( N =91,087) and found an overall correlation between mastery goal and outcomes of 0.05 and performance goals and outcomes of 0.14. These are small effects and show the relatively low importance of these motivational attributes in relation to academic achievement.
An alternative model of motivation is based on Biggs 22 learning processes model, which combines motivation (why the student wants to study the task) and their related strategies (how the student approaches the task). He outlined three common approaches to learning: deep, surface and achieving. When students are taking a deep strategy, they aim to develop understanding and make sense of what they are learning, and create meaning and make ideas their own. This means they focus on the meaning of what they are learning, aim to develop their own understanding, relate ideas together and make connections with previous experiences, ask themselves questions about what they are learning, discuss their ideas with others and compare different perspectives. When students are taking a surface strategy, they aim to reproduce information and learn the facts and ideas—with little recourse to seeing relations or connections between ideas. When students are using an achieving strategy, they use a ‘minimax’ notion—minimum amount of effort for maximum return in terms of passing tests, complying with instructions, and operating strategically to meet a desired grade. It is the achieving strategy that seems most related to school outcomes.
Success criteria
The model includes a prelearning phase relating to whether the students are aware of the criteria of success in the learning task. This phase is less about whether the student desires to attain the target of the learning (which is more about motivation), but whether he or she understands what it means to be successful at the task at hand. When a student is aware of what it means to be successful before undertaking the task, this awareness leads to more goal-directed behaviours. Students who can articulate or are taught these success criteria are more likely to be strategic in their choice of learning strategies, more likely to enjoy the thrill of success in learning, and more likely to reinvest in attaining even more success criteria.
Success criteria can be taught. 23 , 24 Teachers can help students understand the criteria used for judging the students’ work, and thus teachers need to be clear about the criteria used to determine whether the learning intentions have been successfully achieved. Too often students may know the learning intention, but do not how the teacher is going to judge their performance, or how the teacher knows when or whether students have been successful. 25 The success criteria need to be as clear and specific as possible (at surface, deep, or transfer level) as this enables the teacher (and learner) to monitor progress throughout the lesson to make sure students understand and, as far as possible, attain the intended notions of success. Learning strategies that help students get an overview of what success looks like include planning and prediction, having intentions to implement goals, setting standards for judgement success, advance organisers, high levels of commitment to achieve success, and knowing about worked examples of what success looks like. 23
Environment
Underlying all components in the model is the environment in which the student is studying. Many books and internet sites on study skills claim that it is important to attend to various features of the environment such as a quiet room, no music or television, high levels of social support, giving students control over their learning, allowing students to study at preferred times of the day and ensuring sufficient sleep and exercise.
The three phases of learning: surface, deep and transfer
The model highlights the importance of both surface and deep learning and does not privilege one over the other, but rather insists that both are critical. Although the model does seem to imply an order, it must be noted that these are fuzzy distinctions (surface and deep learning can be accomplished simultaneously), but it is useful to separate them to identify the most effective learning strategies. More often than not, a student must have sufficient surface knowledge before moving to deep learning and then to the transfer of these understandings. As Entwistle 26 noted, ‘The verb ‘to learn’ takes the accusative’; that is, it only makes sense to analyse learning in relation to the subject or content area and the particular piece of work towards which the learning is directed, and also the context within which the learning takes place. The key debate, therefore, is whether the learning is directed content that is meaningful to the student, as this will directly affect student dispositions, in particular a student’s motivation to learn and willingness to reinvest in their learning.
A most powerful model to illustrate this distinction between surface and deep is the structure of observed learning outcomes, or SOLO, 27 , 28 as discussed above. The model has four levels: unistructural, multistructural, relational and extended abstract. A unistructural intervention is based on teaching or learning one idea, such as coaching one algorithm, training in underlining, using a mnemonic or anxiety reduction. The essential feature is that this idea alone is the focus, independent of the context or its adaption to or modification by content. A multistructural intervention involves a range of independent strategies or procedures, but without integrating or orchestration as to the individual differences or demands of content or context (such as teaching time management, note taking and setting goals with no attention to any strategic or higher-order understandings of these many techniques). Relational interventions involve bringing together these various multistructural ideas, and seeing patterns; it can involve the strategies of self-monitoring and self-regulation. Extended abstract interventions aim at far transfer (transfer between contexts that, initally, appear remote to one another) such that they produce structural changes in an individual’s cognitive functioning to the point where autonomous or independent learning can occur. The first two levels (one then many ideas) refer to developing surface knowing and the latter two levels (relate and extend) refer to developing deeper knowing. The parallel in learning strategies is that surface learning refers to studying without much reflecting on either purpose or strategy, learning many ideas without necessarily relating them and memorising facts and procedures routinely. Deep learning refers to seeking meaning, relating and extending ideas, looking for patterns and underlying principles, checking evidence and relating it to conclusions, examining arguments cautiously and critically, and becoming actively interested in course content (see reference 29 ).
Our model also makes a distinction between first acquiring knowledge and then consolidating it. During the acquisition phase, information from a teacher or instructional materials is attended to by the student and this is taken into short-term memory. During the consolidation phase, a learner then needs to actively process and rehearse the material as this increases the likelihood of moving that knowledge to longer-term memory. At both phases there can be a retrieval process, which involves transferring the knowing and understanding from long-term memory back into short-term working memory. 30 , 31
Acquiring surface learning
In their meta-analysis of various interventions, Hattie et al. 32 found that many learning strategies were highly effective in enhancing reproductive performances (surface learning) for virtually all students. Surface learning includes subject matter vocabulary, the content of the lesson and knowing much more. Strategies include record keeping, summarisation, underlining and highlighting, note taking, mnemonics, outlining and transforming, organising notes, training working memory, and imagery.
Consolidating surface learning
Once a student has begun to develop surface knowing it is then important to encode it in a manner such that it can retrieved at later appropriate moments. This encoding involves two groups of learning strategies: the first develops storage strength (the degree to which a memory is durably established or ‘well learned’) and the second develops strategies that develop retrieval strength (the degree to which a memory is accessible at a given point in time). 33 ‘Encoding’ strategies are aimed to develop both, but with a particular emphasis on developing retrieval strength. 34 Both groups of strategies invoke an investment in learning, and this involves ‘the tendency to seek out, engage in, enjoy and continuously pursue opportunities for effortful cognitive activity. 35 Although some may not ‘enjoy’ this phase, it does involve a willingness to practice, to be curious and to explore again, and a willingness to tolerate ambiguity and uncertainty during this investment phase. In turn, this requires sufficient metacognition and a calibrated sense of progress towards the desired learning outcomes. Strategies include practice testing, spaced versus mass practice, teaching test taking, interleaved practice, rehearsal, maximising effort, help seeking, time on task, reviewing records, learning how to receive feedback and deliberate practice (i.e., practice with help of an expert, or receiving feedback during practice).
Acquiring deep learning
Students who have high levels of awareness, control or strategic choice of multiple strategies are often referred to as ‘self-regulated’ or having high levels of metacognition. In Visible Learning , Hattie 36 described these self-regulated students as ‘becoming like teachers’, as they had a repertoire of strategies to apply when their current strategy was not working, and they had clear conceptions of what success on the task looked like. 37 More technically, Pintrich et al. 38 described self-regulation as ‘an active, constructive process whereby learners set goals for their learning and then attempt to monitor, regulate and control their cognition, motivation and behaviour, guided and constrained by their goals and the contextual features in the environment’. These students know the what, where, who, when and why of learning, and the how, when and why to use which learning strategies. 39 They know what to do when they do not know what to do. Self-regulation strategies include elaboration and organisation, strategy monitoring, concept mapping, metacognitive strategies, self-regulation and elaborative interrogation.
Consolidating deep learning
Once a student has acquired surface and deep learning to the extent that it becomes part of their repertoire of skills and strategies, we may claim that they have ‘automatised’ such learning—and in many senses this automatisation becomes an ‘idea’, and so the cycle continues from surface idea to deeper knowing that then becomes a surface idea, and so on. 40 There is a series of learning strategies that develop the learner’s proficiency to consolidate deeper thinking and to be more strategic about learning. These include self-verbalisation, self-questioning, self-monitoring, self-explanation, self-verbalising the steps in a problem, seeking help from peers and peer tutoring, collaborative learning, evaluation and reflection, problem solving and critical thinking techniques.
There are skills involved in transferring knowledge and understanding from one situation to a new situation. Indeed, some have considered that successful transfer could be thought as synonymous with learning. 41 , 42 There are many distinctions relating to transfer: near and far transfer, 43 low and high transfer, 44 transfer to new situations and problem solving transfer, 5 and positive and negative transfer. 45 Transfer is a dynamic, not static, process that requires learners to actively choose and evaluate strategies, consider resources and surface information, and, when available, to receive or seek feedback to enhance these adaptive skills. Reciprocal teaching is one program specifically aiming to teach these skills; for example, Bereiter and Scardamalia 46 have developed programs in the teaching of transfer in writing, where students are taught to identify goals, improve and elaborate existing ideas, strive for idea cohesion, present their ideas to groups and think aloud about how they might proceed. Similarly, Schoenfeld 47 outlined a problem-solving approach to mathematics that involves the transfer of skills and knowledge from one situation to another. Marton 48 argued that transfer occurs when the learner learns strategies that apply in a certain situation such that they are enabled to do the same thing in another situation when they realise that the second situation resembles (or is perceived to resemble) the first situation. He claimed that not only sameness, similarity, or identity might connect situations to each other, but also small differences might connect them as well. Learning how to detect such differences is critical for the transfer of learning. As Heraclitus claimed, no two experiences are identical; you do not step into the same river twice.
Overall messages from the model
There are four main messages to be taken from the model. First, if the success criteria is the retention of accurate detail (surface learning) then lower-level learning strategies will be more effective than higher-level strategies. However, if the intention is to help students understand context (deeper learning) with a view to applying it in a new context (transfer), then higher level strategies are also needed. An explicit assumption is that higher level thinking requires a sufficient corpus of lower level surface knowledge to be effective—one cannot move straight to higher level thinking (e.g., problem solving and creative thought) without sufficient level of content knowledge. Second, the model proposes that when students are made aware of the nature of success for the task, they are more likely to be more involved in investing in the strategies to attain this target. Third, transfer is a major outcome of learning and is more likely to occur if students are taught how to detect similarities and differences between one situation and a new situation before they try to transfer their learning to the new situation. Hence, not one strategy may necessarily be best for all purposes. Fourth, the model also suggests that students can be advantaged when strategy training is taught with an understanding of the conditions under which the strategy best works—when and under what circumstance it is most appropriate.
The current study
The current study synthesises the many studies that have related various learning strategies to outcomes. This study only pertains to achievement outcomes (skill, on the model of learning); further work is needed to identify the strategies that optimise the dispositions (will) and the motivation (thrill) outcomes. The studies synthesised here are from four sources. First, there are the meta-analyses among the 1,200 meta-analyses in Visible Learning that relate to strategies for learning. 36 , 49 , 50 Second, there is the meta-analysis conducted by Lavery 51 on 223 effect-sizes derived from 31 studies relating to self-regulated learning interventions. The third source is two major meta-analyses by a Dutch team of various learning strategies, especially self-regulation. And the fourth is a meta-analysis conducted by Donoghue et al. 52 based on a previous analysis by Dunlosky et al. 53
The data in Visible Learning is based on 800 meta-analyses relating influences from the home, school, teacher, curriculum and teaching methods to academic achievement. Since its publication in 2009, the number of meta-analyses now exceeds 1,200, and those influences specific to learning strategies are retained in the present study. Lavery 51 identified 14 different learning strategies and the overall effect was 0.46—with greater effects for organising and transforming (i.e., deliberate rearrangement of instructional materials to improve learning, d =0.85) and self-consequences (i.e., student expectation of rewards or punishment for success or failure, d =0.70). The lowest effects were for imagery (i.e., creating or recalling vivid mental images to assist learning, d =0.44) and environmental restructuring (i.e., efforts to select or arrange the physical setting to make learning easier, d =0.22). She concluded that the higher effects involved ‘teaching techniques’ and related to more ‘deep learning strategies’, such as organising and transforming, self-consequences, self-instruction, self-evaluation, help-seeking, keeping records, rehearsing/memorising, reviewing and goal-setting. The lower ranked strategies were more ‘surface learning strategies’, such as time management and environmental restructuring.
Of the two meta-analyses conducted by the Dutch team, the first study, by Dignath et al. 9 analysed 357 effects from 74 studies ( N =8,619). They found an overall effect of 0.73 from teaching methods of self-regulation. The effects were large for achievement (elementary school, 0.68; high school, 0.71), mathematics (0.96, 1.21), reading and writing (0.44, 0.55), strategy use (0.72, 0.79) and motivation (0.75, 0.92). In the second study, Donker et al. 54 reviewed 180 effects from 58 studies relating to self-regulation training, reporting an overall effect of 0.73 in science, 0.66 in mathematics and 0.36 in reading comprehension. The most effective strategies were cognitive strategies (rehearsal 1.39, organisation 0.81 and elaboration 0.75), metacognitive strategies (planning 0.80, monitoring 0.71 and evaluation 0.75) and management strategies (effort 0.77, peer tutoring 0.83, environment 0.59 and metacognitive knowledge 0.97). Performance was almost always improved by a combination of strategies, as was metacognitive knowledge. This led to their conclusion that students should not only be taught which strategies to use and how to apply them (declarative knowledge or factual knowledge) but also when (procedural or how to use the strategies) and why to use them (conditional knowledge or knowing when to use a strategy).
Donoghue et al. 52 conducted a meta-analysis based on the articles referenced in Dunlosky et al. 53 They reviewed 10 learning strategies and a feature of their review is a careful analysis of possible moderators to the conclusions about the effectiveness of these learning strategies, such as learning conditions (e.g., study alone or in groups), student characteristics (e.g., age, ability), materials (e.g., simple concepts to problem-based analyses) and criterion tasks (different outcome measures).
In the current study, we independently assigned all strategies to the various parts of the model—this was a straightforward process, and the few minor disagreements were resolved by mutual agreement. All results are presented in Appendix 1.
Results: the meta-synthesis of learning strategies
There are 302 effects derived from the 228 meta-analyses from the above four sources that have related some form of learning strategy to an achievement outcome. Most are experimental–control studies or pre–post studies, whereas some are correlations ( N =37). There are 18,956 studies (although some may overlap across meta-analyses). Only 125 meta-analyses reported the sample size ( N =11,006,839), but if the average (excluding the outlier 7 million from one meta-analysis) is used for the missing sample sizes, the best estimate of sample size is between 13 and 20 million students.
The average effect is 0.53 but there is considerable variance ( Figure 2 ), and the overall number of meta-analyses, studies, number of people (where provided), effects and average effect-sizes for the various phases of the model are provided in Table 1 . The effects are lowest for management of the environment and ‘thrill’ (motivation), and highest for developing success criteria across the learning phases. The variance is sufficiently large, however, that it is important to look at specific strategies within each phase of the model.
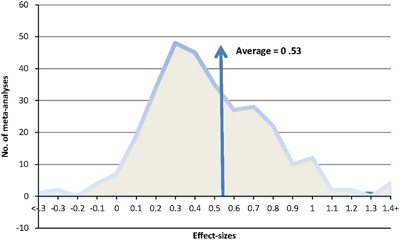
The average and the distribution of all effect sizes.
Synthesis of the input phases of the model
The inputs: skills.
There are nine meta-analyses that have investigated the relation between prior achievement and subsequent achievement, and not surprisingly these relations are high ( Table 2 ). The average effect-size is 0.77 (s.e.=0.10), which translates to a correlation of 0.36—substantial for any single variable. The effects of prior achievement are lowest in the early years, and highest from high school to university. One of the purposes of school, however, is to identify those students who are underperforming relative to their abilities and thus to not merely accept prior achievement as destiny. The other important skill is working memory—which relates to the amount of information that can be retained in short-term working memory when engaged in processing, learning, comprehension, problem solving or goal-directed thinking. 55 Working memory is strongly related to a person’s ability to reason with novel information (i.e., general fluid intelligence. 56
The inputs: will
There are 28 meta-analyses related to the dispositions of learning from 1,304 studies and the average effect-size is 0.48 (s.e.=0.09; Table 3 ). The effect of self-efficacy is highest ( d =0.90), followed by increasing the perceived value of the task ( d = 0.46), reducing anxiety ( d =0.45) and enhancing the attitude to the content ( d =0.35). Teachers could profitably increase students’ levels of confidence and efficacy to tackle difficult problems; not only does this increase the probability of subsequent learning but it can also help reduce students’ levels of anxiety. It is worth noting the major movement in the anxiety and stress literature in the 1980s moved from a preoccupation on understanding levels of stress to providing coping strategies—and these strategies were powerful mediators in whether people coped or not. 57 Similarly in learning, it is less the levels of anxiety and stress but the development of coping strategies to deal with anxiety and stress. These strategies include being taught to effectively regulate negative emotions; 58 increasing self-efficacy, which relates to developing the students conviction in their own competence to attain desired outcomes; 59 focusing on the positive skills already developed; increasing social support and help seeking; reducing self-blame; and learning to cope with error and making mistakes. 60 Increasing coping strategies to deal with anxiety and promoting confidence to tackle difficult and challenging learning tasks frees up essential cognitive resources required for the academic work.
There has been much discussion about students having growth—or incremental—mindsets (human attributes are malleable not fixed) rather than fixed mindsets (attributes are fixed and invariant). 61 However, the evidence in Table 3 ( d =0.19) shows how difficult it is to change to growth mindsets, which should not be surprising as many students work in a world of schools dominated by fixed notions—high achievement, ability groups, and peer comparison.
The inputs: thrill
The thrill relates to the motivation for learning: what is the purpose or approach to learning that the student adopts? Having a surface or performance approach motivation (learning to merely pass tests or for short-term gains) or mastery goals is not conducive to maximising learning, whereas having a deep or achieving approach or motivation is helpful ( Table 4 ). A possible reason why mastery goals are not successful is that too often the outcomes of tasks and assessments are at the surface level and having mastery goals with no strategic sense of when to maximise them can be counter-productive. 62 Having goals, per se , is worthwhile—and this relates back to the general principle of having notions of what success looks like before investing in the learning. The first step is to teach students to have goals relating to their upcoming work, preferably the appropriate mix of achieving and deep goals, ensure the goals are appropriately challenging and then encourage students to have specific intentions to achieve these goals. Teaching students that success can then be attributed to their effort and investment can help cement this power of goal setting, alongside deliberate teaching.
The environment
Despite the inordinate attention, particularly by parents, on structuring the environment as a precondition for effective study, such effects are generally relatively small ( Table 5 ). It seems to make no differences if there is background music, a sense of control over learning, the time of day to study, the degree of social support or the use of exercise. Given that most students receive sufficient sleep and exercise, it is perhaps not surprising that these are low effects; of course, extreme sleep or food deprivation may have marked effects.
Knowing the success criteria
A prediction from the model of learning is that when students learn how to gain an overall picture of what is to be learnt, have an understanding of the success criteria for the lessons to come and are somewhat clear at the outset about what it means to master the lessons, then their subsequent learning is maximised. The overall effect across the 31 meta-analyses is 0.54, with the greatest effects relating to providing students with success criteria, planning and prediction, having intentions to implement goals, setting standards for self-judgements and the difficulty of goals ( Table 6 ). All these learning strategies allow students to see the ‘whole’ or the gestalt of what is targeted to learn before starting the series of lessons. It thus provides a ‘coat hanger’ on which surface-level knowledge can be organised. When a teacher provides students with a concept map, for example, the effect on student learning is very low; but in contrast, when teachers work together with students to develop a concept map, the effect is much higher. It is the working with students to develop the main ideas, and to show the relations between these ideas to allow students to see higher-order notions, that influences learning. Thus, when students begin learning of the ideas, they can begin to know how these ideas relate to each other, how the ideas are meant to form higher order notions, and how they can begin to have some control or self-regulation on the relation between the ideas.
Synthesis of the learning phases of the model
There are many strategies, such as organising, summarising, underlining, note taking and mnemonics that can help students master the surface knowledge ( Table 7 ). These strategies can be deliberately taught, and indeed may be the only set of strategies that can be taught irrespective of the content. However, it may be that for some of these strategies, the impact is likely to be higher if they are taught within each content domain, as some of the skills (such as highlighting, note taking and summarising) may require specific ideas germane to the content being studied.
While it appears that training working memory can have reasonable effects ( d =0.53) there is less evidence that training working memory transfers into substantial gains in academic attainment. 63 There are many emerging and popular computer games that aim to increase working memory. For example, CogMed is a computer set of adaptive routines that is intended to be used 30–40 min a day for 25 days. A recent meta-analysis (by the commercial owners 64 ) found average effect-sizes (across 43 studies) exceed 0.70, but in a separate meta-analysis of 21 studies on the longer term effects of CogMed, there was zero evidence of transfer to subjects such as mathematics or reading 65 . Although there were large effects in the short term, they found that these gains were not maintained at follow up (about 9 months later) and no evidence to support the claim that working memory training produces generalised gains to the other skills that have been investigated (verbal ability, word decoding or arithmetic) even when assessment takes place immediately after training. For the most robust studies, the effect of transfer is zero. It may be better to reduce working memory demands in the classroom. 66
The investment of effort and deliberate practice is critical at this consolidation phase, as are the abilities to listen, seek and interpret the feedback that is provided ( Table 8 ). At this consolidation phase, the task is to review and practice (or overlearn) the material. Such investment is more valuable if it is spaced over time rather than massed. Rehearsal and memorisation is valuable—but note that memorisation is not so worthwhile at the acquisition phase. The difficult task is to make this investment in learning worthwhile, to make adjustments to the rehearsal as it progresses in light of high levels of feedback, and not engage in drill and practice. These strategies relating to consolidating learning are heavily dependent on the student’s proficiency to invest time on task wisely, 67 to practice and learn from this practice and to overlearn such that the learning is more readily available in working memory for the deeper understanding.
Acquiring deeper learning
Nearly all the strategies at this phase are powerful in enhancing learning ( Table 9 ). The ability to elaborate and organise, monitor the uses of the learning strategies, and have a variety of metacognitive strategies are the critical determinants of success at this phase of learning. A major purpose is for the student to deliberately activate prior knowledge and then make relations and extensions beyond what they have learned at the surface phase.
At this phase, the power of working with others is most apparent ( Table 10 ). This involves skills in seeking help from others, listening to others in discussion and developing strategies to ‘speak’ the language of learning. It is through such listening and speaking about their learning that students and teachers realise what they do deeply know, what they do not know and where they are struggling to find relations and extensions. An important strategy is when students become teachers of others and learn from peers, as this involves high levels of regulation, monitoring, anticipation and listening to their impact on the learner.
There has been much research confirming that teaching help-seeking strategies is successful, but how this strategy then works in classrooms is more complex. Teachers have to welcome students seeking help, and there needs to be knowledgeable others (e.g., peers) from whom to seek the help—too often students left in unsupported environments can seek and gain incorrect help and not know the help is incorrect. 68 Ryan and Shin 69 also distinguished between adaptive help seeking (seeking help from others, such as an explanation, a hint, or an example, that would further learning and promote independent problem solving in the future) and expedient help seeking (seeking help that expedites task completion, such as help that provides the answer and is not focused on learning). They showed that adaptive help seeking from peers declines and expedient help seeking increases during early adolescence. Further, increases in expedient help seeking were associated with declines in achievement but changes in adaptive help seeking were unrelated to achievement. The key is for teachers to teach adaptive help seeking, to ensure the help is dependable and correct and to see this more of a student than a teacher skill. Help seeking needs to be welcomed before it can have an effect.
The transfer model promoted by Marton 48 seems to be supported in that a key in teaching for transfer involves understanding the patterns, similarities and differences in the transfer before applying the strategies to new task ( Table 11 ). Marton argued that transfer occurs when students learn strategies that apply in a certain situation such that they are enabled to do the same thing in another situation to the degree that they realise how the second situation does (or does not) resemble the first situation. It is learning to detect differences and similarities that is the key that leads to transfer of learning.
Discussion and Conclusions
There is much debate about the optimal strategies of learning, and indeed we identified >400 terms used to describe these strategies. Our initial aim was to rank the various strategies in terms of their effectiveness but this soon was abandoned. There was too much variability in the effectiveness of most strategies depending on when they were used during the learning process, and thus we developed the model of learning presented in this article. Like all models, it is a conjecture, it aims to say much and it is falsifiable. The efficacy of any model can be seen as an expression of its capacity to generate a scalable solution to a problem or need in ways that resolve more issues than prevailing theories or approaches. 70 The model posits that learning must be embedded in some content (something worth knowing) and thus the current claims about developing 21st century skills sui generis are most misleading. These skills often are promoted as content free and are able to be developed in separate courses (e.g., critical thinking, resilience). Our model, however, suggests that such skills are likely to be best developed relative to some content. There is no need to develop learning strategy courses, or teach the various strategies outside the context of the content. Instead, the strategies should be an integral part of the teaching and learning process, and can be taught within this process.
The model includes three major inputs and outcomes. These relate to what the students bring to the learning encounter (skill), their dispositions about learning (will) and their motivations towards the task (thrill). The first set of strategies relate to teaching students the standards for what is to be learned (the success criteria). We propose that effective learning strategies will be different depending on the phase of the learning—the strategies will be different when a student is first acquiring the matters to be learnt compared with when the student is embedding or consolidating this learning. That is, the strategies are differentially effective depending on whether the learning intention is surface learning (the content), deep learning (the relations between content) or the transfer of the skills to new situations or tasks. In many ways this demarcation is arbitrary (but not capricious) and more experimental research is needed to explore these conjectures. Further, the model is presented as linear whereas there is often much overlap in the various phases. For example, to learn subject matter (surface) deeply (i.e., to encode in memory) is helped by exploring and understanding its meaning; success criteria can have a mix of surface and deep and even demonstrate the transfer to other (real world) situations; and often deep learning necessitates returning to acquire specific surface level vocabulary and understanding. In some cases, there can be multiple overlapping processes. A reviewer provided a clear example: in learning that the internal angles of a quadrilateral add up to 360°, this might involve surface learning, which then requires rehearsal to consolidate, some self-questioning to apply, some detection of similarities to then work out what the internal angles of a hexagon might be, and spotting similarities to the triangle rule. There may be no easy way to know the right moment, or no easy demarcation of the various phases. The proposal in this paper is but a ‘model’ to help clarify the various phases of learning, and in many real world situations there can be considerable overlap.
We have derived six sets of propositions from our conceptual model of learning and the results of our meta-synthesis of research on learning strategies. The first set relates to the differential role played by what students bring to and take from the learning encounter—the inputs and outcomes. Second, there are some strategies that are more effective than others—but their relative effectiveness depends on the phase in the model of learning in which they take place. Third is the distinction between surface learning, deep learning and the transfer of learning. The fourth set relates to the skills of transfer, the fifth to how the model of learning can be used to resolve some unexpected findings about the effectiveness of some strategies, and the sixth set discusses the question ‘what is learning?’.
The intertwining role of skill, will, and thrill
Our first set of claims relates to the differential role of what students bring to and take from the learning encounter. Rather than arguing that many factors contribute to achievement (an important but sometimes the only privileged outcome of learning), we are promoting the notion that the skill, will and thrill can intertwine during learning and that these three inputs are also important outcomes of learning—the aim is to enhance the will (e.g., the willingness to reinvest in more and deeper learning), the thrill (e.g., the emotions associated with successful learning, the curiosity and the willingness to explore what one does not know) and the skills (e.g., the content and the deeper understanding). The relation between the thrill, will and skill can vary depending on the student and the requirements of the task. Certainly, negative emotions, such as those induced by fear, anxiety, and stress can directly and negatively affect learning and memory. Such negative emotions block learning: ‘If the student is faced with sources of stress in an educational context which go beyond the positive challenge threshold—for instance, aggressive teachers, bullying students or incomprehensible learning materials whether books or computers—it triggers fear and cognitive function is negatively affected. 71 Our argument is that learning can lead to enhanced skills, dispositions, motivations and excitements that can be reinvested in learning, and can lead to students setting higher standards for their success criteria. When skill, will, and thrill overlap, this should be considered a bonus; developing each is a worthwhile outcome of schooling in its own right.
It is all in the timing
Our second set of claims is that while it is possible to nominate the top 10 learning strategies the more critical conclusion is that the optimal strategies depend on where in the learning cycle the student is located. This strategic skill in using the strategies at the right moment is akin to the message in the Kenny Rogers song—you need to ‘know when to hold ‘em, know when to fold ‘em’. For example, when starting a teaching sequence, it is most important to be concerned that students have confidence they can understand the lessons, see value in the lessons and are not overly anxious about their skills to be mastered. Providing them early on with an overview of what successful learning in the lessons will look like (knowing the success criteria) will help them reduce their anxiety, increase their motivation, and build both surface and deeper understandings.
To acquire surface learning, it is worthwhile knowing how to summarise, outline and relate the learning to prior achievement; and then to consolidate this learning by engaging in deliberate practice, rehearsing over time and learning how to seek and receive feedback to modify this effort. To acquire deep understanding requires the strategies of planning and evaluation and learning to monitor the use of one’s learning strategies; and then to consolidate deep understanding calls on the strategy of self-talk, self-evaluation and self-questioning and seeking help from peers. Such consolidation requires the learner to think aloud, learn the ‘language of thinking’, 72 know how to seek help, self-question and work through the consequences of the next steps in learning. To transfer learning to new situations involves knowing how to detect similarities and differences between the old and the new problem or situations.
We recommend that these strategies are developed by embedding them into the cycle of teaching rather than by running separate sessions, such as ‘how to learn’ or study skills courses. There is a disappointing history of educational programs aimed at teaching students how to learn. 30 , 73 , 74 Wiliam 75 made this case for why teaching these learning strategies (e.g., critical thinking) out of context is unlikely to develop a generic skill applicable to many subjects. He noted that in a ‘mathematics proof, critical thinking might involve ensuring that each step follows from the previous one (e.g., by checking that there has not been a division by zero). In reading a historical account, critical thinking might involve considering the author of the account, the potential biases and limitations that the author may be bringing to the account, and what other knowledge the reader has about the events being described. The important point here is that although there is some commonality between the processes in mathematics and history, they are not the same. Developing a capacity for critical thinking in history does not make one better at critical thinking in mathematics. For all of the apparent similarities, critical thinking in history and critical thinking in mathematics are different, and they are developed in different ways’. Many others have noted that metacognition is not knowledge-free but needs to be taught in the context of the individual subject areas. 76 , 77 Perkins 78 also noted that there is a certain art to infusing the teaching of thinking into content learning. Sometimes, ‘teachers think it is enough simply to establish a generally thoughtful atmosphere in a classroom, with regular expectations for thinking critically and creatively...teaching for know-how about learning to learn is a much more time-consuming enterprise than teaching for just learning the ideas... Building active know-how requires much more attention’.
Another aspect to consider is the difference, identified in the model, between being first exposed to learning and the consolidation of this learning. This distinction is far from novel. Shuell, 79 for example, distinguished between initial, intermediate, and final phases of learning. In the initial phase, the students can encounter a ‘large array of facts and pieces of information that are more-or-less isolated conceptually... there appears to be little more than a wasteland with few landmarks to guide the traveller on his or her journey towards understanding and mastery’. Students can use existing schema to make sense of this new information, or can be guided to have more appropriate schema (and thus experience early stages of concept learning and relation between ideas) otherwise the information may remain as isolated facts, or be linked erroneously to previous understandings. At the intermediate phase, the learner begins to see similarities and relationships among these seemingly conceptually isolated pieces of information. ‘The fog continues to lift but still has not burnt off completely’. During the final phase, the knowledge structure becomes well integrated and functions more autonomously, and the emphasis is more on performance or exhibiting the outcome of learning.
Horses for courses: matching strategies with phases
The third set of claims relates to the distinction between surface, deep, and transfer of learning. Although not a hard and fast set of demarcations, surface learning refers more to the content and underlying skills; deep learning to the relationships between, and extensions of, ideas; and transfer to the proficiency to apply learning to new problems and situations. During the surface learning phase, an aim is to assist students to overlearn certain ideas and thus reduce the needs of their working memory to work with these new facts when moving into the deeper understanding phase. Note, for example, that Marton et al. 80 made an important distinction between memorising without understanding first and called this rote memorisation (which has long term effect), and memorisation when you have understood and called this meaningful memorisation (which can be powerful). The evidence in the current study supports this distinction.
It is when students have much information, or many seemingly unrelated ideas, that the learning strategies for the deep phase are optimally invoked. This is when they should be asked to integrate ideas with previous schema or modify their previous schema to integrate new ideas and ways of thinking. The key to this process is first gaining ideas—a fact often missed by those advocating deeper thinking strategies when they try to teach these skills prior to developing sufficient knowledge within the content domain. The students need to first have ideas before they can relate them. The model does not propose discarding the teaching or learning skills that have been developed to learn surface knowing, but advocates the benefits of a more appropriate balance of surface and deeper strategies and skills that then lead to transfer. The correct balance of surface to deep learning depends on the demands of the task. It is likely that more emphasis on surface strategies is probably needed as students learn new ideas, moving to an emphasis on deeper strategies as they become more proficient.
Pause and reflect: detecting similarities and differences
The fourth set of claims relate to the skills of transfer, and how important it is to teach students to pause and detect the similarities and differences between previous tasks and the new one, before attempting to answer a new problem. Such transfer can be positive, such as when a learner accurately remembers a learning outcome reached in a certain situation and appropriately applies it in a new and similar situation, or negative, such as when a learner applies a strategy used successfully in one situation in a new situation where this strategy is not appropriate. Too many (particularly struggling) students over-rehearse a few learning strategies (e.g., copying and highlighting) and apply them in situations regardless of the demands of new tasks. Certainly, the fundamental skill for positive transfer is stopping before addressing the problem and asking about the differences and similarities of the new to any older task situation. This skill can be taught.
This ability to notice similarities and differences over content is quite different for novices and experts 81 , 82 and we do not simply learn from experience but we also learn to experience. 83 Preparation for future learning involves opportunities to try our hunches in different contexts, receive feedback, engage in productive failure and learn to revise our knowing based on feedback. The aim is to solve problems more efficiently, and also to ‘let go’ of previously acquired knowledge in light of more sophisticated understandings—and this can have emotional consequences: ‘Failure to change strategies in new situations has been described as the tyranny of success’. 84 It is not always productive for students to try the same thing that worked last time. Hence there may need to be an emphasis on knowledge-building rather than knowledge-telling, 85 and systematic inquiry based on theory-building and disconfirmation rather than simply following processes for how to find some result.
Why some strategies do not work
The fifth set of claims relate to how the model can be used to resolve some of the unexpected findings about the impact of various teaching methods. In Visible Learning , 36 it was noted that many programs that seem to lead to developing deeper processing have very low effect sizes (e.g., inquiry based methods, d =0.31; problem-based learning, d =0.15). For example, there have been 11 meta-analyses relating to problem-based learning based on 509 studies, leading to an average small effect ( d =0.15). It hardly seems necessary to run another problem-based program (particularly in first-year medicine, where four of the meta-analyses were completed) to know that the effects of problem-based learning on outcomes are small. The reason for this low effect seems to be related to using problem-based methods before attaining sufficient surface knowledge. When problem-based learning is used in later medical years, the effects seem to increase. Albanese and Mitchell 86 claimed that increased years of exposure to medical education increases the effect of problem-based learning. They argued that lack of experience (and lack of essential surface knowledge) leads the student to make more errors in their knowledge base, add irrelevant material to their explanations and engage in backward reasoning (from the unknown to the givens), whereas experts engaged in forward reasoning (also see references 87 , 88 ). Walker et al. 89 also noted that novice problem-based learning students tended to engage in far more backward-driven reasoning, which results in more errors during problem solving and may persist even after the educational intervention is complete. It is likely that problem-based learning works more successfully when students engage in forward reasoning and this depends on having sufficient content knowledge to make connections.
Deep understanding in problem-based learning requires a differentiated knowledge structure, 90 and this may need to be explicitly taught—as there is no assumption that students will see similarities and differences in contexts by themselves. There is a limit to what we can reasonably expect students to discover, and it may require teaching students to make predictions based on features that were told to them and that they may not notice on their own. Deliberate teaching of these surface features can offer a higher level of explanation that would be difficult or time consuming to discover. A higher level explanation is important because it provides a generative framework that can extend one understanding beyond the specific cases that have been analysed and experienced. On the other hand, the problems need not be too overly structured, as then students do not gain experience of searching out conceptual tools or homing in on particular cases of application. 78
Another example of the different requirements of surface and deep learning is the effect of asking students to explore errors and misconceptions during their learning. Using meta-analysis, Keith and Frese 91 found that the average effect of using these strategies when the outcome was surface learning was −0.15 and when the outcome was deep learning and far transfer to new problems, it was 0.80.
So: what is learning?
The sixth set of claims relate to the notion of ‘what is learning?’. The argument in this article is that learning is the outcome of the processes of moving from surface to deep to transfer. Only then will students be able to go beyond the information given to ‘figure things out’, which is one of the few untarnishable joys of life. 92 One of the greatest triumphs of learning is what Perkins 78 calls ‘knowing one’s way around’ a particular topic or ‘playing the whole game’ of history, mathematics, science or whatever. This is a function of knowing much and then using this knowledge in the exploration of relations and to make extensions to other ideas, and being able to know what to do when one does not know what to do (the act of transfer).
Concluding comments
Like all models, the one proposed in this article invites as many conjectures and directions for further research as it provide a basis for interpreting the evidence from the meta-synthesis. It helps make sense of much of the current literature but it is speculative in that it also makes some untested predictions. There is much solace in Popper's 93 claim that ‘Bold ideas, unjustified anticipations, and speculative thought, are our only means for interpreting nature: our only organon, our only instrument, for grasping her. And we must hazard them to win our prize. Those among us who are unwilling to expose their ideas to the hazard of refutation do not take part in the scientific game.’ Further research is needed, for example, to better understand the optimal order through the various phases; there may be circumstances where it may be beneficial to learn the deeper notions before developing the surface knowledge. It is highly likely that as one develops many ideas and even relates and extends them, these become ‘ideas’ and the cycle continues. 94 We know much, but we need to know much more, and in particular we need to know how these many learning strategies might be better presented in another competing model. Such testing of a bold model and making predictions from models is, according to Popper, how science progresses.
Further research is needed that asks whether the distinction between the acquisition and the consolidation of learning is a distinctive difference, a melding from one to the other or whether both can occur simultaneously. If there is a difference, then more research on ascertaining the best time to move from acquisition to consolidation would be informative. Similarly, there is no hard rule in the model of a sequence from surface to deep to transfer. In some ways, teaching the strategies of knowing what success looks like upfront implies an exposure to both surface and deep learning. Also, the many arguments (but surprisingly there is a lack of evidence) for the popular notions of flipped classrooms could be supported with more evidence of introducing the success criteria upfront to students. A typical flipped lesson starts with students accessing online video lectures or resources prior to in-class sessions so that students are prepared to participate in more interactive and higher-order activities such as problem solving, discussions and debates. 95 The most needed research concerns transfer—the variation theory of Marton, 48 the claims by Perkins 78 and others need more focused attention and the usual (and often unsubstantiated) claims that doing x will assist learning y should come back as a focus of learning sciences.
We are proposing that it is worthwhile to develop the skill, will and thrill of learning, and that there are many powerful strategies for learning. Students can be taught these strategies (declarative knowledge), how to use them (procedural knowledge), under what conditions it may be more or less useful to apply them (conditional knowledge) and how to evaluate them. It may be necessary to teach when best to use these strategies according the nature of the outcomes (surface and deep), according to the timing of learning (first acquiring and then consolidating learning) and to teach the skill of transferring learning to new situations. We need to think in terms of ‘surface to deep’ and not one alone; we need to think in terms of developing dispositions, motivations and achievement, and not one alone. This invites considering multiple outcomes from our schools. Singapore, 96 for example, is now committed to developing an educational system which will produce young people who have the moral courage to stand up for what is right; pursue a healthy lifestyle and have an appreciation of aesthetics; are proud to be Singaporeans; are resilient in the face of difficulty, innovative and enterprising; are purposeful in the pursuit of excellence; are able to collaborate across cultures; and can think critically and communicate persuasively. Academic achievement is but one desirable learning outcomes of many.
Another important message is that developing a few learning strategies may not be optimal. The failure to change strategies in new situations has been described as the tyranny of success; 84 and the current meta-synthesis suggests that choosing different strategies as one progresses through the learning cycle (from first exposure to embedding, from surface to deep to transfer) demands cognitive flexibility. It may not be the best option for students to use the same strategies that worked last time, as when the context is changed the old strategies may no longer work.
Widdowson, D. A., Dixon, R. S., Peterson, E. R., Rubie-Davies, C. M. & Irving, S. E. Why go to school? Student, parent and teacher beliefs about the purposes of schooling. Asia Pac. J. Educ. 35 , 1–14 (2014).
Google Scholar
Biesta, G. J. Good Education in an Age of Measurement: Ethics, Politics, democracy , (Routledge, 2015).
Tröhler, D., Meyer, H. D., Labaree, D. F. & Hutt, E. L. Accountability: antecedents, power, and processes. Teach. Coll. Rec. 116 , 1–12 (2014).
Soderstrom, N. C. & Bjork, R. A. Learning versus performance: an integrative review. Perspect. Psychol. Sci. 10 , 176–199 (2015).
Article Google Scholar
Mayer, R. E. Applying the science of learning: Evidence-based principles for the design of multimedia instruction. Am. Psychol. 63 , 760–769 (2008).
Pressley, M. Comprehension Instruction: Research-Based Best Practices 11–27 (Guilford Press, 2002).
Weinstein, C. E. & Mayer, R. E. in Handbook of Research on Teaching: a Project of the American Educational Research Association (ed. Wittrock, M. C.) 3rd edn., 315–327 (Macmillan, 1986).
Boekaerts, M. Self-regulated learning: a new concept embraced by researchers, policy makers, educators, teachers, and students. Learn. Instruct. 7 , 161–186 (1997).
Dignath, C., Buettner, G. & Langfeldt, H. P. How can primary school students learn self-regulated learning strategies most effectively? A meta-analysis on self-regulation training programmes. Educ. Res. Rev. 3 , 101–129 (2008).
Pressley, M., Goodchild, F., Fleet, J., Zajchowski, R. & Evans, E. D. The challenges of classroom strategy instruction. The Elementary School Journal 89 , 301–342 (1989).
Hattie, J. A. C. The 34th Vernon-Wall Lecture (British Psychological Society, 2014).
Ausubel, D. P. Educational Psychology: a Cognitive View (Holt, Rinehart, & Winston, 1968).
Claxton, G. What's the Point of School? Rediscovering the Heart of Education (Oneworld Publications, 2013).
Carr, M. & Claxton, G. Tracking the development of learning dispositions. Assessment in Education: Principles, Policy & Practice 9 , 9–37 (2002).
Perkins, D. N., Jay, E. & Tishman, S. Beyond abilities: A dispositional theory of thinking. Merrill-Palmer Quarterly 39 , 1–21 (1993).
Anderman, E. M. & Patrick, H. in Handbook of Research on Student Engagement , 173–191 (Springer, USA, 2012).
Elliot, A. J. & Harackiewicz, J. M. Approach and avoidance achievement goals and intrinsic motivation: A mediational analysis. J. Personal. Soc. Psychol. 70 , 461–475 (1996).
Middleton, M. J. & Midgley, C. Avoiding the demonstration of lack of ability: An underexplored aspect of goal theory. J. Educ. Psychol. 89 , 710 (1997).
Skaalvik, E. M. in Advances in Motivation and Achievement (eds Maehr, M. L. & Pintrich, P. R.) 51–97 (JAI Press, 1997).
Carpenter, S. L. A Comparison of the Relationships of Students' Self-Efficacy, Goal Orientation, and Achievement Across Grade Levels: a Meta-Analysis (Unpublished doctoral dissertation Faculty of Education, Simon Fraser Univ., 2007).
Hulleman, C. S., Schrager, S. M., Bodmann, S. M. & Harackiewicz, J. M. A meta-analytic review of achievement goal measures: Different labels for the same constructs or different constructs with similar labels? Psychol. Bull. 136 , 422 (2010).
Biggs, J. B. What do inventories of students’ learning processes really measure? A theoretical review and clarification. British J. Educ. Psychol. 63 , 3–19 (1993).
Clarke, S. Formative assessment in the secondary classroom (Hodder Murray, 2005).
Heritage, M. Formative assessment: What do teachers need to know and do? Phi Delta Kappan 89 , 140–145 (2007).
Boekaerts, M. Self-regulated learning: Where we are today. Int. J. Educ. Res. 31 , 445–457 (1999).
Entwistle, N. J. The verb ‘to learn’takes the accusative. Br. J. Educ. Psychol. 46 , 1–3 (1976).
Biggs, J. B. & Collis, K. F. Evaluating the Quality of Learning: the SOLO Taxonomy (Structure of the Observed Learning Outcome) (Academic Press, 1982).
Hattie, J. A. C. & Brown, G. T. L. Cognitive processes in asTTle: the SOLO taxonomy. asTTle technical report (No. 43) (University of Auckland & Ministry of Education, 2004).
Enwistle, N. J. Approaches to studying and levels of understanding: the influences of teaching and assessment . High. Educ. 15 , 156–218. (2000).
Mayer, R. E. The Cambridge Handbook of Multimedia Learning , 2nd edn, 43–71 (Cambridge Univ. Press, 2014).
Robertson, E. M., Pascual-Leone, A. & Miall, R. C. Current concepts in procedural consolidation. Nat. Rev. Neurosci. 5 , 576–582 (2004).
Article CAS Google Scholar
Hattie, J. A. C., Biggs, J. & Purdie, N. Effects of learning skills interventions on student learning: A meta-analysis. Rev. Educ. Res. 66 , 99–136 (1996).
Bjork, R. A. & Bjork, E. L. in Learning Processes to Cognitive Processes: essays in Honor of William K. Estes (eds Healy, A. et al. ) Vol. 2, 35–67 (Erlbaum, 1992).
Bjork, E. L., de Winstanley, P. A. & Storm, B. C. Learning how to learn: can experiencing the outcome of different encoding strategies enhance subsequent encoding? Psychon. Bull. Rev. 14 , 207–211 (2007).
von Stumm, S., Chamorro-Premuzic, T. & Ackerman, P. L. in Wiley-Blackwell Handbook of Individual Differences (eds Chamorr-Premuzic, T. et al. ) 217–241 (Wiley-Blackwell, 2011).
Hattie, J. A. C. Visible Learning: a Synthesis of Over 800 Meta-Analyses Relating to Achievement (Routledge, 2009).
Purdie, N. & Hattie, J. A. C. Assessing students’ conceptions of learning. Austr. J. Dev. Educ. Psychol. 2 , 17–32 (2002).
Pintrich, P. R. Multiple goals, multiple pathways: the role of goal orientation in learning and achievement. J. Educ. Psychol. 92 , 544–55 (2000).
Schraw, G. & Dennison, R. S. Assessing metacognitive awareness. Contemp. Educ. Psychol. 19 , 460–475 (1994).
Pegg, J. & Tall, D. in Theories of Mathematics Education 173–192 (Springer, 2010).
Perkins, D. N. & Salomon, G. Knowledge to go: a motivational and dispositional view of transfer. Educ. Psychol. 47 , 248–258 (2012).
Smedslund, J. The problem of “what is learned?”. Psychol. Rev. 60 , 157–158 (1953).
Barnett, S. M. & Ceci, S. J. When and where do we apply what we learn? A taxonomy for far transfer. Psychol. Bull. 128 , 612–637 (2002).
Salomon, G. & Perkins, D. N. Rocky roads to transfer: rethinking mechanism of a neglected phenomenon. Educ. Psychol. 24 , 113–142 (1989).
Osgood, C. E. The similarity paradox in Hum. Learn.: A resolution. Psychol. Rev. 56 , 132–143 (1949).
Bereiter, C. & Scardamalia, M. in Knowledge Creation in Education (eds Tan, S. C. et al. ) 35–52 (Springer, 2014).
Schoenfeld, A. H. in Handbook of Research on Mathematics Learning and Teaching (ed. Grouws, D. A.) 334–370 (Macmillan, 1992).
Marton, F. Sameness and difference in transfer. J. Learn. Sci. 15 , 499–535 (2006).
Hattie, J. A. C. Visible Learning for Teachers (Routledge, 2012).
Book Google Scholar
Hattie, J. A. C. The applicability of Visible Learning to higher education. Scholarship Teach. Learn. Psychol. 1 , 79–91 (2015).
Lavery, L. Self-regulated Learning For Academic Success: an Evaluation Of Instructional Techniques (Unpublished doctoral thesis Univ. Auckland, 2008).
Donoghue, G. & Hattie, J. A. C. A Meta-Analysis of Learning Strategies based on Dunlosky et al. (Unpublished paper Science of Learning Research Centre, 2015).
Dunlosky, J., Rawson, K. A., Marsh, E. J., Nathan, M. J. & Willingham, D. T. Improving students’ learning with effective learning techniques promising directions from cognitive and educational psychology. Psychol. Sci. Public Interest 14 , 4–58 (2013).
Donker, A. S., de Boer, H., Kostons, D., van Ewijk, C. D. & van der Werf, M. P. C. Effectiveness of learning strategy instruction on academic performance: A meta-analysis. Educ. Res. Rev. 11 , 1–26 (2013).
Cowan, N. in Neuroscience in Education: the Good, the Bad and the Ugly (eds Della Sala, S. & Anderson, M.) 111–127 (Oxford Univ. Press, 2012).
Ackerman, P. L., Beier, M. E. & Boyle, M. O. Working memory and intelligence: The same or different constructs? Psychol. Bull. 131 , 30–60 (2005).
Frydenberg, E. & Lewis, R. Coping Scale for Adults (ACER, 2015).
Galla, B. M. & Wood, J. J. Emotional self-efficacy moderates anxiety-related impairments in math performance in elementary school-age youth. Pers. Indiv. Differ. 52 , 118–122 (2012).
Bandura, A. Self-efficacy: the Exercise of Control (Macmillan, 1997).
Frydenberg, E. Think positively!: a Course For Developing Coping Skills In Adolescents (A&C Black, 2010).
Dweck, C. Mindset: How You Can Fulfil Your Potential (Hachette, 2012).
Watkins, D. & Hattie, J. A. A longitudinal study of the approaches to learning of Australian Tertiary students. Hum. Learn. 4 , 127–141 (1985).
Turley-Ames, K. J. & Whitfield, M. M. Strategy training and working memory task performance. J. Mem. Lang. 49 , 446–468 (2003).
Owen, A. M. et al. Putting brain training to the test. Nature 465 , 775–778 (2010).
Melby-Lervåg, M. & Hulme, C. Is working memory training effective? A meta-analytic review. Dev. Psychol. 49 , 270–291 (2013).
Alloway, T. P. How does working memory work in the classroom? Educ. Res. Rev. 1 , 134–139 (2006).
Claessens, B. J., Van Eerde, W., Rutte, C. G. & Roe, R. A. Things to do today: a daily diary study on task completion at work. Appl. Psychol. 59 , 273–295 (2010).
Nuthall, G. A. The Hidden Lives of Learners (New Zealand Council for Educational Research, 2007).
Ryan, A. M. & Shin, H. Help-seeking tendencies during early adolescence: an examination of motivational correlates and consequences for achievement. Learn. Instruct. 21 , 247–256 (2011).
Kuhn, T. S. The structure of scientific revolutions (The University of Chicago Press, 1962).
CERI. 40th Anniversary International Conference on Learning in the 21st century , May 2008. (2008).
Zohar, A. in Metacognition in Science Education 197–223 (Springer, 2012).
Lockhead J. & Clement J. (eds) Research on Teaching Thinking Skills (Franklin Institute Press, 1979).
Mayer, R. E. Multimedia learning: Are we asking the right questions? Educ. Psychol. 32 , 1–19 (1997).
Wiliam, D. Presentation to the Salzburg Global Seminar. Available at www.dylanwiliam.org/Dylan_Wiliams.../Salzburg%20Seminar%20talk (2014).
Donovan M. S. & Bransford J. D. (eds). How Students Learn: History in the Classroom (National Academies Press, 2005).
Vye, N., Schwartz, D. L., Bransford, J. D., Barron, B. J. & Zech, L. in Metacognition in Educational Theory And Practice (eds Dunlosky, H. & Graesser, A.) 305–347 (Lawrence Erlbaum, 1998).
Perkins, D. Future Wise: Educating our Children for a Changing World (John Wiley & Sons, 2014).
Shuell, T. J. Teaching and learning as problem solving. Theor. Pract. 29 , 102–108 (1990).
Marton, F., Wen, Q. & Wong, K. C. ‘Read a hundred times and the meaning will appear’. Changes in Chinese university students’ views of the temporal structure of learning. High. Educ. 49 , 291–318 (2005).
Chase, W. G. & Simon, H. A. Perception in chess. Cogn. Psychol. 4 , 55–81 (1973).
Berliner, D. C. Learning about and learning from expert teachers. Int. J. Educ. Res. 35 , 463–482 (2001).
Bransford, J. D., Brown, A. L. & Cocking, R. R. How People Learn: Brain, Mind, Experience, and School (National Academy Press, 1999).
Robinson, A. G., Stern, S. & Stern, S. Corporate creativity: How Innovation and Improvement Actually Happen (Berrett-Koehler Publishers, 1997).
Bereiter, C. & Scardamalia, M. An Inquiry Into the Nature and Implications of Expertise , (Open Court, 1993).
Albanese, M. A. & Mitchell, S. Problem-based learning: A review of literature on its outcomes and implementation issues. Acad. Med. 68 , 52–81 (1993).
Gijbels, D., Dochy, F., Van den Bossche, P. & Segers, M. Effects of problem-based learning: A meta-analysis from the angle of assessment. Rev. Educ. Res. 75 , 27–61 (2005).
Gilhooly, K. J. Cognitive psychology and medical diagnosis. Appl. Cogn. Psychol. 4 , 261–272 (1990).
Walker, A. & Leary, H. M. A problem based learning meta-analysis: differences across problem types, implementation types, disciplines, and assessment levels. Interdiscipl. J. Probl. Based Learn. 3 , 12–43 (2009).
Schwartz, D. L. & Bransford, J. D. A time for telling. Cogn. Instruct. 16 , 475–522 (1998).
Keith, N. & Frese, M. Effectiveness of error management training: A meta-analysis. J. Appl. Psychol. 93 , 59 (2008).
Bruner, J. S. The Culture of Education (Harvard Univ. Press, 1996).
Popper, K. R. The Logic of Scientific Discovery , 3rd edn (Hutchinson, 1968).
Pegg, J. in Encyclopedia of Mathematics Education , 570–572 (Springer, 2014).
DeWitt, P. M. Flipping Leadership Doesn’t Mean Reinventing the Wheel (Corwin Press, 2014).
Tan, C. Y. & Dimmock, C. How a ‘top performing’ Asian school system formulates and implements policy: The case of Singapore. Educ. Manage. Admin. Leadership 42 , 743–763 (2014).
Download references
Acknowledgements
The Science of Learning Research Centre is a Special Research Initiative of the Australian Research Council. Project Number SR120300015. We thank the following for critiquing earlier drafts of this article: Dan Willingham, Jason Lodge, Debra Masters, Rob Hester, Jared Horvath and Luke Rowe.
Author information
Authors and affiliations.
Science of Learning Research Centre, Graduate School of Education, University of Melbourne, Carlton, VIC, Australia
John A C Hattie & Gregory M Donoghue
You can also search for this author in PubMed Google Scholar
Contributions
The authors contributed equally to the project and writing of this paper.
Corresponding author
Correspondence to John A C Hattie .
Ethics declarations
Competing interests.
The authors declare no conflict of interest.
Supplementary information
Supplementary appendix (doc 899 kb), rights and permissions.
This work is licensed under a Creative Commons Attribution 4.0 International License. The images or other third party material in this article are included in the article’s Creative Commons license, unless indicated otherwise in the credit line; if the material is not included under the Creative Commons license, users will need to obtain permission from the license holder to reproduce the material. To view a copy of this license, visit http://creativecommons.org/licenses/by/4.0/
Reprints and permissions
About this article
Cite this article.
Hattie, J., Donoghue, G. Learning strategies: a synthesis and conceptual model. npj Science Learn 1 , 16013 (2016). https://doi.org/10.1038/npjscilearn.2016.13
Download citation
Received : 30 December 2015
Revised : 12 April 2016
Accepted : 23 May 2016
Published : 10 August 2016
DOI : https://doi.org/10.1038/npjscilearn.2016.13
Share this article
Anyone you share the following link with will be able to read this content:
Sorry, a shareable link is not currently available for this article.
Provided by the Springer Nature SharedIt content-sharing initiative
This article is cited by
Effect of instruction and experience on students’ learning strategies.
- Ezgi Melisa Yüksel
- C. Shawn Green
- Haley A. Vlach
Metacognition and Learning (2024)
Key antecedents of maximal levels of aspiration and minimal boundary goals: a structural equation modeling analysis
- Flaviu A. Hodis
- Georgeta M. Hodis
Asia Pacific Education Review (2024)
Where is Ethics in the Evolution of AIED’s Future?
- Arthur C. Graesser
- Colin M. Carmon
International Journal of Artificial Intelligence in Education (2024)
Variable- and Person-centred meta-re-analyses of university students' learning strategies from a cross-cultural perspective
- Luke K. Fryer
Higher Education (2024)
Analyzing the associations between motivation and academic performance via the mediator variables of specific mathematic cognitive learning strategies in different subject domains of higher education
- Steffen Wild
- Christoph Neef
International Journal of STEM Education (2023)
Quick links
- Explore articles by subject
- Guide to authors
- Editorial policies
Sign up for the Nature Briefing newsletter — what matters in science, free to your inbox daily.


Thesis Statements
What this handout is about.
This handout describes what a thesis statement is, how thesis statements work in your writing, and how you can craft or refine one for your draft.
Introduction
Writing in college often takes the form of persuasion—convincing others that you have an interesting, logical point of view on the subject you are studying. Persuasion is a skill you practice regularly in your daily life. You persuade your roommate to clean up, your parents to let you borrow the car, your friend to vote for your favorite candidate or policy. In college, course assignments often ask you to make a persuasive case in writing. You are asked to convince your reader of your point of view. This form of persuasion, often called academic argument, follows a predictable pattern in writing. After a brief introduction of your topic, you state your point of view on the topic directly and often in one sentence. This sentence is the thesis statement, and it serves as a summary of the argument you’ll make in the rest of your paper.
What is a thesis statement?
A thesis statement:
- tells the reader how you will interpret the significance of the subject matter under discussion.
- is a road map for the paper; in other words, it tells the reader what to expect from the rest of the paper.
- directly answers the question asked of you. A thesis is an interpretation of a question or subject, not the subject itself. The subject, or topic, of an essay might be World War II or Moby Dick; a thesis must then offer a way to understand the war or the novel.
- makes a claim that others might dispute.
- is usually a single sentence near the beginning of your paper (most often, at the end of the first paragraph) that presents your argument to the reader. The rest of the paper, the body of the essay, gathers and organizes evidence that will persuade the reader of the logic of your interpretation.
If your assignment asks you to take a position or develop a claim about a subject, you may need to convey that position or claim in a thesis statement near the beginning of your draft. The assignment may not explicitly state that you need a thesis statement because your instructor may assume you will include one. When in doubt, ask your instructor if the assignment requires a thesis statement. When an assignment asks you to analyze, to interpret, to compare and contrast, to demonstrate cause and effect, or to take a stand on an issue, it is likely that you are being asked to develop a thesis and to support it persuasively. (Check out our handout on understanding assignments for more information.)
How do I create a thesis?
A thesis is the result of a lengthy thinking process. Formulating a thesis is not the first thing you do after reading an essay assignment. Before you develop an argument on any topic, you have to collect and organize evidence, look for possible relationships between known facts (such as surprising contrasts or similarities), and think about the significance of these relationships. Once you do this thinking, you will probably have a “working thesis” that presents a basic or main idea and an argument that you think you can support with evidence. Both the argument and your thesis are likely to need adjustment along the way.
Writers use all kinds of techniques to stimulate their thinking and to help them clarify relationships or comprehend the broader significance of a topic and arrive at a thesis statement. For more ideas on how to get started, see our handout on brainstorming .
How do I know if my thesis is strong?
If there’s time, run it by your instructor or make an appointment at the Writing Center to get some feedback. Even if you do not have time to get advice elsewhere, you can do some thesis evaluation of your own. When reviewing your first draft and its working thesis, ask yourself the following :
- Do I answer the question? Re-reading the question prompt after constructing a working thesis can help you fix an argument that misses the focus of the question. If the prompt isn’t phrased as a question, try to rephrase it. For example, “Discuss the effect of X on Y” can be rephrased as “What is the effect of X on Y?”
- Have I taken a position that others might challenge or oppose? If your thesis simply states facts that no one would, or even could, disagree with, it’s possible that you are simply providing a summary, rather than making an argument.
- Is my thesis statement specific enough? Thesis statements that are too vague often do not have a strong argument. If your thesis contains words like “good” or “successful,” see if you could be more specific: why is something “good”; what specifically makes something “successful”?
- Does my thesis pass the “So what?” test? If a reader’s first response is likely to be “So what?” then you need to clarify, to forge a relationship, or to connect to a larger issue.
- Does my essay support my thesis specifically and without wandering? If your thesis and the body of your essay do not seem to go together, one of them has to change. It’s okay to change your working thesis to reflect things you have figured out in the course of writing your paper. Remember, always reassess and revise your writing as necessary.
- Does my thesis pass the “how and why?” test? If a reader’s first response is “how?” or “why?” your thesis may be too open-ended and lack guidance for the reader. See what you can add to give the reader a better take on your position right from the beginning.
Suppose you are taking a course on contemporary communication, and the instructor hands out the following essay assignment: “Discuss the impact of social media on public awareness.” Looking back at your notes, you might start with this working thesis:
Social media impacts public awareness in both positive and negative ways.
You can use the questions above to help you revise this general statement into a stronger thesis.
- Do I answer the question? You can analyze this if you rephrase “discuss the impact” as “what is the impact?” This way, you can see that you’ve answered the question only very generally with the vague “positive and negative ways.”
- Have I taken a position that others might challenge or oppose? Not likely. Only people who maintain that social media has a solely positive or solely negative impact could disagree.
- Is my thesis statement specific enough? No. What are the positive effects? What are the negative effects?
- Does my thesis pass the “how and why?” test? No. Why are they positive? How are they positive? What are their causes? Why are they negative? How are they negative? What are their causes?
- Does my thesis pass the “So what?” test? No. Why should anyone care about the positive and/or negative impact of social media?
After thinking about your answers to these questions, you decide to focus on the one impact you feel strongly about and have strong evidence for:
Because not every voice on social media is reliable, people have become much more critical consumers of information, and thus, more informed voters.
This version is a much stronger thesis! It answers the question, takes a specific position that others can challenge, and it gives a sense of why it matters.
Let’s try another. Suppose your literature professor hands out the following assignment in a class on the American novel: Write an analysis of some aspect of Mark Twain’s novel Huckleberry Finn. “This will be easy,” you think. “I loved Huckleberry Finn!” You grab a pad of paper and write:
Mark Twain’s Huckleberry Finn is a great American novel.
You begin to analyze your thesis:
- Do I answer the question? No. The prompt asks you to analyze some aspect of the novel. Your working thesis is a statement of general appreciation for the entire novel.
Think about aspects of the novel that are important to its structure or meaning—for example, the role of storytelling, the contrasting scenes between the shore and the river, or the relationships between adults and children. Now you write:
In Huckleberry Finn, Mark Twain develops a contrast between life on the river and life on the shore.
- Do I answer the question? Yes!
- Have I taken a position that others might challenge or oppose? Not really. This contrast is well-known and accepted.
- Is my thesis statement specific enough? It’s getting there–you have highlighted an important aspect of the novel for investigation. However, it’s still not clear what your analysis will reveal.
- Does my thesis pass the “how and why?” test? Not yet. Compare scenes from the book and see what you discover. Free write, make lists, jot down Huck’s actions and reactions and anything else that seems interesting.
- Does my thesis pass the “So what?” test? What’s the point of this contrast? What does it signify?”
After examining the evidence and considering your own insights, you write:
Through its contrasting river and shore scenes, Twain’s Huckleberry Finn suggests that to find the true expression of American democratic ideals, one must leave “civilized” society and go back to nature.
This final thesis statement presents an interpretation of a literary work based on an analysis of its content. Of course, for the essay itself to be successful, you must now present evidence from the novel that will convince the reader of your interpretation.
Works consulted
We consulted these works while writing this handout. This is not a comprehensive list of resources on the handout’s topic, and we encourage you to do your own research to find additional publications. Please do not use this list as a model for the format of your own reference list, as it may not match the citation style you are using. For guidance on formatting citations, please see the UNC Libraries citation tutorial . We revise these tips periodically and welcome feedback.
Anson, Chris M., and Robert A. Schwegler. 2010. The Longman Handbook for Writers and Readers , 6th ed. New York: Longman.
Lunsford, Andrea A. 2015. The St. Martin’s Handbook , 8th ed. Boston: Bedford/St Martin’s.
Ramage, John D., John C. Bean, and June Johnson. 2018. The Allyn & Bacon Guide to Writing , 8th ed. New York: Pearson.
Ruszkiewicz, John J., Christy Friend, Daniel Seward, and Maxine Hairston. 2010. The Scott, Foresman Handbook for Writers , 9th ed. Boston: Pearson Education.
You may reproduce it for non-commercial use if you use the entire handout and attribute the source: The Writing Center, University of North Carolina at Chapel Hill
Make a Gift
While Sandel argues that pursuing perfection through genetic engineering would decrease our sense of humility, he claims that the sense of solidarity we would lose is also important.
This thesis summarizes several points in Sandel’s argument, but it does not make a claim about how we should understand his argument. A reader who read Sandel’s argument would not also need to read an essay based on this descriptive thesis.
Broad thesis (arguable, but difficult to support with evidence)
Michael Sandel’s arguments about genetic engineering do not take into consideration all the relevant issues.
This is an arguable claim because it would be possible to argue against it by saying that Michael Sandel’s arguments do take all of the relevant issues into consideration. But the claim is too broad. Because the thesis does not specify which “issues” it is focused on—or why it matters if they are considered—readers won’t know what the rest of the essay will argue, and the writer won’t know what to focus on. If there is a particular issue that Sandel does not address, then a more specific version of the thesis would include that issue—hand an explanation of why it is important.
Arguable thesis with analytical claim
While Sandel argues persuasively that our instinct to “remake” (54) ourselves into something ever more perfect is a problem, his belief that we can always draw a line between what is medically necessary and what makes us simply “better than well” (51) is less convincing.
This is an arguable analytical claim. To argue for this claim, the essay writer will need to show how evidence from the article itself points to this interpretation. It’s also a reasonable scope for a thesis because it can be supported with evidence available in the text and is neither too broad nor too narrow.
Arguable thesis with normative claim
Given Sandel’s argument against genetic enhancement, we should not allow parents to decide on using Human Growth Hormone for their children.
This thesis tells us what we should do about a particular issue discussed in Sandel’s article, but it does not tell us how we should understand Sandel’s argument.
Questions to ask about your thesis
- Is the thesis truly arguable? Does it speak to a genuine dilemma in the source, or would most readers automatically agree with it?
- Is the thesis too obvious? Again, would most or all readers agree with it without needing to see your argument?
- Is the thesis complex enough to require a whole essay's worth of argument?
- Is the thesis supportable with evidence from the text rather than with generalizations or outside research?
- Would anyone want to read a paper in which this thesis was developed? That is, can you explain what this paper is adding to our understanding of a problem, question, or topic?
- picture_as_pdf Thesis
Create Your Course
The 7 main types of learning styles (and how to teach to them), share this article.
Understanding the 7 main types of learning styles and how to teach them will help both your students and your courses be more successful.
When it comes to learning something new, we all absorb information at different rates and understand it differently too. Some students get new concepts right away; others need to sit and ponder for some time before they can arrive at similar conclusions.
Why? The answer lies in the type of learning styles different students feel more comfortable with. In other words, we respond to information in different ways depending on how it is presented to us.
Clearly, different types of learning styles exist, and there are lots of debates in pedagogy about what they are and how to adapt to them.
For practical purposes, it’s recommended to ensure that your course or presentation covers the 7 main types of learning.
In this article, we’ll break down the 7 types of learning styles, and give practical tips for how you can improve your own teaching styles , whether it’s in higher education or an online course you plan to create on the side.
Skip ahead:
What are the 7 types of learning styles?
How to accommodate different types of learning styles online.
- How to help students understand their different types of learning styles
How to create an online course for all
In the academic literature, the most common model for the types of learning you can find is referred to as VARK.
VARK is an acronym that stands for Visual, Auditory, Reading & Writing, and Kinesthetic. While these learning methods are the most recognized, there are people that do not fit into these boxes and prefer to learn differently. So we’re adding three more learning types to our list, including Logical, Social, and Solitary.
Visual learners
Visual learners are individuals that learn more through images, diagrams, charts, graphs, presentations, and anything that illustrates ideas. These people often doodle and make all kinds of visual notes of their own as it helps them retain information better.
When teaching visual learners, the goal isn’t just to incorporate images and infographics into your lesson. It’s about helping them visualize the relationships between different pieces of data or information as they learn.
Gamified lessons are a great way to teach visual learners as they’re interactive and aesthetically appealing. You should also give handouts, create presentations, and search for useful infographics to support your lessons.
Since visual information can be pretty dense, give your students enough time to absorb all the new knowledge and make their own connections between visual clues.
Auditory/aural learners
The auditory style of learning is quite the opposite of the visual one. Auditory learners are people that absorb information better when it is presented in audio format (i.e. the lessons are spoken). This type of learner prefers to learn by listening and might not take any notes at all. They also ask questions often or repeat what they have just heard aloud to remember it better.
Aural learners are often not afraid of speaking up and are great at explaining themselves. When teaching auditory learners, keep in mind that they shouldn’t stay quiet for long periods of time. So plan a few activities where you can exchange ideas or ask questions. Watching videos or listening to audio during class will also help with retaining new information.
Reading and writing (or verbal) learners
Reading & Writing learners absorb information best when they use words, whether they’re reading or writing them. To verbal learners, written words are more powerful and granular than images or spoken words, so they’re excellent at writing essays, articles, books, etc.
To support the way reading-writing students learn best, ensure they have time to take ample notes and allocate extra time for reading. This type of learner also does really well at remote learning, on their own schedule. Including reading materials and writing assignments in their homework should also yield good results.
Kinesthetic/tactile learners
Kinesthetic learners use different senses to absorb information. They prefer to learn by doing or experiencing what they’re being taught. These types of learners are tactile and need to live through experiences to truly understand something new. This makes it a bit challenging to prepare for them in a regular class setting.
As you try to teach tactile learners, note that they can’t sit still for long and need more frequent breaks than others. You need to get them moving and come up with activities that reinforce the information that was just covered in class. Acting out different roles is great; games are excellent; even collaborative writing on a whiteboard should work fine. If applicable, you can also organize hands-on laboratory sessions, immersions, and workshops.
In general, try to bring every abstract idea into the real world to help kinesthetic learners succeed.
Logical/analytical learners
As the name implies, logical learners rely on logic to process information and understand a particular subject. They search for causes and patterns to create a connection between different kinds of information. Many times, these connections are not obvious to people to learn differently, but they make perfect sense to logical learners.
Logical learners generally do well with facts, statistics, sequential lists, and problem-solving tasks to mention a few.
As a teacher, you can engage logical learners by asking open-ended or obscure questions that require them to apply their own interpretation. You should also use teaching material that helps them hone their problem-solving skills and encourages them to form conclusions based on facts and critical thinking.
Social/interpersonal learners
Social or interpersonal learners love socializing with others and working in groups so they learn best during lessons that require them to interact with their peers . Think study groups, peer discussions, and class quizzes.
To effectively teach interpersonal learners, you’ll need to make teamwork a core part of your lessons. Encourage student interaction by asking questions and sharing stories. You can also incorporate group activities and role-playing into your lessons, and divide the students into study groups.
Solitary/intrapersonal learners
Solitary learning is the opposite of social learning. Solitary, or solo, learners prefer to study alone without interacting with other people. These learners are quite good at motivating themselves and doing individual work. In contrast, they generally don’t do well with teamwork or group discussions.
To help students like this, you should encourage activities that require individual work, such as journaling, which allows them to reflect on themselves and improve their skills. You should also acknowledge your students’ individual accomplishments and help them refine their problem-solving skills.
Are there any unique intelligence types commonly shared by your students? Adapting to these different types of intelligence can help you can design a course best suited to help your students succeed.
Launch your online learning product for free
Use Thinkific to create, market, and sell online courses, communities, and memberships — all from a single platform.
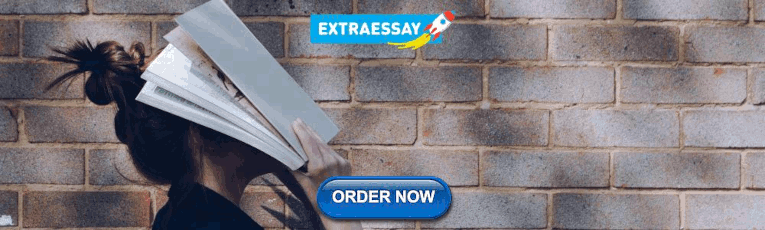
How to help students understand their different types of learning styles
Unless you’re teaching preschoolers, most students probably already realize the type of learning style that fits them best. But some students do get it wrong.
The key here is to observe every student carefully and plan your content for different learning styles right from the start.
Another idea is to implement as much individual learning as you can and then customize that learning for each student. So you can have visual auditory activities, riddles for logical learners, games for kinesthetic learners, reading activities, writing tasks, drawing challenges, and more.
When you’re creating your first course online, it’s important to dedicate enough time to planning out its structure. Don’t just think that a successful course consists of five uploaded videos.
Think about how you present the new knowledge. Where it makes sense to pause and give students the time to reflect. Where to include activities to review the new material. Adapting to the different learning types that people exhibit can help you design an online course best suited to help your students succeed.
That being said, here are some tips to help you tailor your course to each learning style, or at least create enough balance.
Visual learners
Since visual learners like to see or observe images, diagrams, demonstrations, etc., to understand a topic, here’s how you can create a course for them:
- Include graphics, cartoons, or illustrations of concepts
- Use flashcards to review course material
- Use flow charts or maps to organize materials
- Highlight and color code notes to organize materials
- Use color-coded tables to compare and contrast elements
- Use a whiteboard to explain important information
- Have students play around with different font styles and sizes to improve readability
Auditory learners prefer to absorb information by listening to spoken words, so they do well when teachers give spoken instructions and lessons. Here’s how to cater to this learning type through your online course:
- Converse with your students about the subject or topic
- Ask your students questions after each lesson and have them answer you (through the spoken word)
- Have them record lectures and review them with you
- Have articles, essays, and comprehension passages out to them
- As you teach, explain your methods, questions, and answers
- Ask for oral summaries of the course material
- If you teach math or any other math-related course, use a talking calculator
- Create an audio file that your students can listen to
- Create a video of you teaching your lesson to your student
- Include a YouTube video or podcast episode for your students to listen to
- Organize a live Q & A session where students can talk to you and other learners to help them better understand the subject
Reading and writing (or verbal) learners
This one is pretty straightforward. Verbal learners learn best when they read or write (or both), so here are some practical ways to include that in your online course:
- Have your students write summaries about the lesson
- If you teach language or literature, assign them stories and essays that they’d have to read out loud to understand
- If your course is video-based, add transcripts to aid your students’ learning process
- Make lists of important parts of your lesson to help your students memorize them
- Provide downloadable notes and checklists that your students can review after they’ve finished each chapter of your course
- Encourage extra reading by including links to a post on your blog or another website in the course
- Use some type of body movement or rhythm, such as snapping your fingers, mouthing, or pacing, while reciting the material your students should learn
Since kinesthetic learners like to experience hands-on what they learn with their senses — holding, touching, hearing, and doing. So instead of churning out instructions and expecting to follow, do these instead:
- Encourage them to experiment with textured paper, and different sizes of pencils, pens, and crayons to jot down information
- If you teach diction or language, give them words that they should incorporate into their daily conversations with other people
- Encourage students to dramatize or act out lesson concepts to understand them better
Logical learners are great at recognizing patterns, analyzing information, and solving problems. So in your online course, you need to structure your lessons to help them hone these abilities. Here are some things you can do:
- Come up with tasks that require them to solve problems. This is easy if you teach math or a math-related course
- Create charts and graphs that your students need to interpret to fully grasp the lesson
- Ask open-ended questions that require critical thinking
- Create a mystery for your students to solve with clues that require logical thinking or math
- Pose an issue/topic to your students and ask them to address it from multiple perspectives
Since social learners prefer to discuss or interact with others, you should set up your course to include group activities. Here’s how you can do that:
- Encourage them to discuss the course concept with their classmates
- Get your students involved in forum discussions
- Create a platform (via Slack, Discord, etc.) for group discussions
- Pair two or more social students to teach each other the course material
- If you’re offering a cohort-based course , you can encourage students to make their own presentations and explain them to the rest of the class
Solitary learners prefer to learn alone. So when designing your course, you need to take that into consideration and provide these learners a means to work by themselves. Here are some things you can try:
- Encourage them to do assignments by themselves
- Break down big projects into smaller ones to help them manage time efficiently
- Give them activities that require them to do research on their own
- When they’re faced with problems regarding the topic, let them try to work around it on their own. But let them know that they are welcome to ask you for help if they need to
- Encourage them to speak up when you ask them questions as it builds their communication skills
- Explore blended learning , if possible, by combining teacher-led classes with self-guided assignments and extra ideas that students can explore on their own.
Now that you’re ready to teach something to everyone, you might be wondering what you actually need to do to create your online courses. Well, start with a platform.
Thinkific is an intuitive and easy-to-use platform any instructor can use to create online courses that would resonate with all types of learning styles. Include videos, audio, presentations, quizzes, and assignments in your curriculum. Guide courses in real-time or pre-record information in advance. It’s your choice.
In addition, creating a course on Thinkific doesn’t require you to know any programming. You can use a professionally designed template and customize it with a drag-and-drop editor to get exactly the course you want in just a few hours. Try it yourself to see how easy it can be.
This blog was originally published in August 2017, it has since been updated in March 2023.
Althea Storm is a B2B SaaS writer who specializes in creating data-driven content that drives traffic and increases conversions for businesses. She has worked with top companies like AdEspresso, HubSpot, Aura, and Thinkific. When she's not writing web content, she's curled up in a chair reading a crime thriller or solving a Rubik's cube.
- The 5 Most Effective Teaching Styles (Pros & Cons of Each)
- 7 Top Challenges with Online Learning For Students (and Solutions)
- 6 Reasons Why Creators Fail To Sell Their Online Courses
- The Advantages and Disadvantages of Learning in Online Classes in 2023
- 10 Steps To Creating A Wildly Successful Online Course
Related Articles
Building a b2b online learning experience to grow your business.
Read this blog post to learn how to successfully build, pitch, and sell your online courses to clients in the B2B space.
Say Thanks to Your Online Course Students with Handwritten Cards
Thanks to Thankster, sending handwritten thank you cards to your online course students has never been easier.
How to Create a Membership Site for your Thinkific Course
Want to create a membership site for your online course? No problem! Thanks to Thinkific's subscription plans, setting up your membership site is easy.
Try Thinkific for yourself!
Accomplish your course creation and student success goals faster with thinkific..
Download this guide and start building your online program!
It is on its way to your inbox
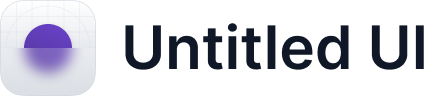
10 Types of Learning and How to Teach Them: A Complete Guide to Learning Styles
If you have been a part of the education sector, you probably already know how different each child is. Every student has their own set of strengths and weaknesses which make them unique. Similarly, every student has a preferred way of learning and retaining the material. These preferences are called “learning styles”.
Each learning style describes how a learner best receives information, interprets it, organizes it, and stores it. A majority of the learners today even have multiple or overlapping learning styles, also called multimodal learning . As an educator, it is extremely important to know the different types of learning – since this will help your students build on their strengths and retain information better. The original model of VARK only describes four of these - visual, auditory, reading/writing, and kinesthetic. However, in this article we explore 10 types of learning and how to teach them.
Another factor that contributes to learning and retaining information is the subject of the information. It is a well known fact that some people excel better in creative fields like design, fine arts, photography, while others might excel in practical or calculative fields like mathematics, engineering, science, and so on.
Thus, different people have different interests and these interests contribute to different learning areas or subjects as well.
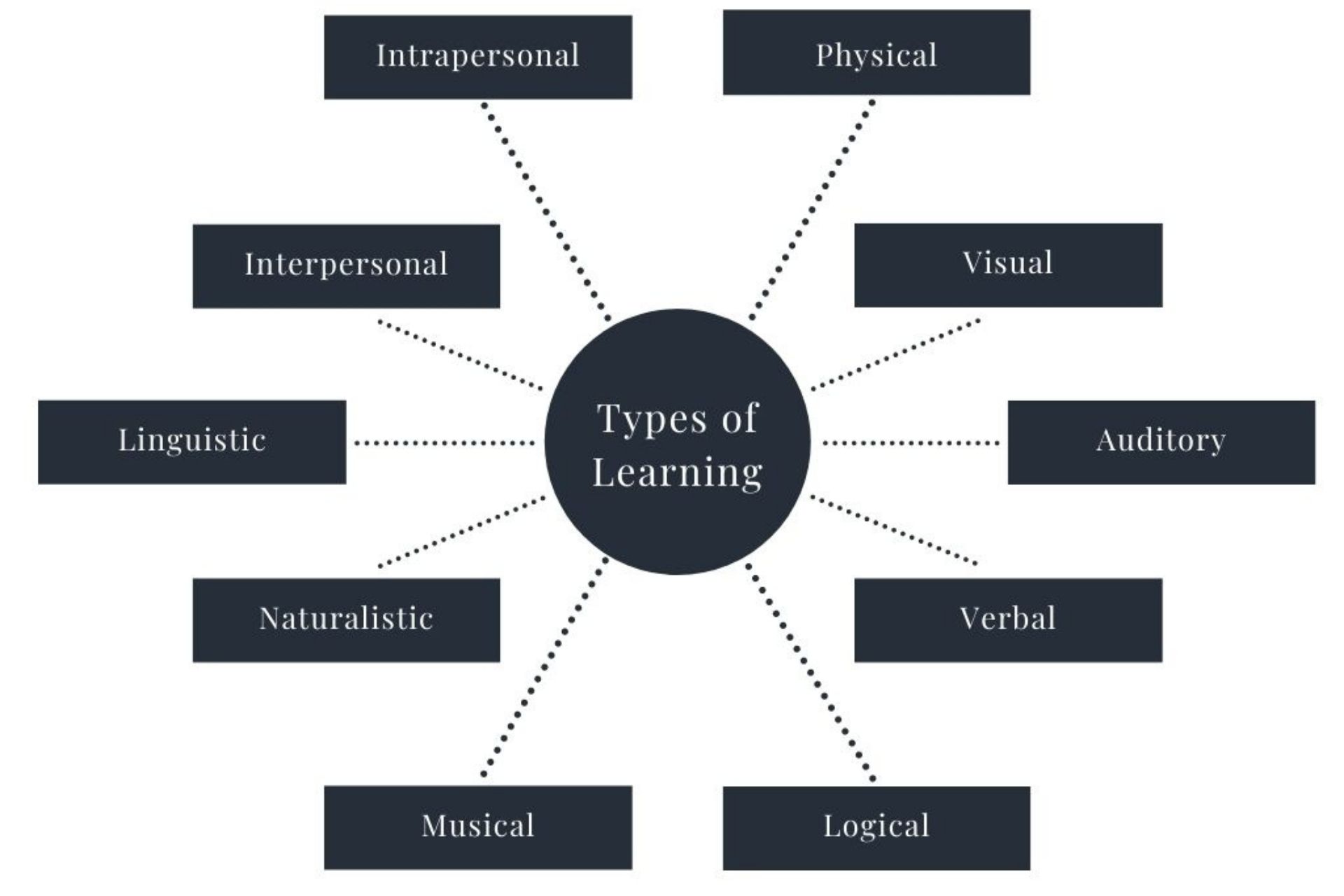
1. Physical (Kinesthetic) Learning
Physical or kinesthetic learners prefer a hands-on experience rather than listening to lectures or sitting in a class. They like interacting physically with things that are tangible in nature. These learners could see the idea of studying for hours as a daunting experience but are better with actually doing things themselves. They possess qualities like being restless, preferring to get their hands “dirty”, outgoing and energetic.
Ways to engage physical learners:
- Encourage movement within lessons. Example: role play
- Give them well-spaced breaks between lessons to move around
- Use props and interactive models
- Declutter desks to promote better focus
2. Visual (Spatial) Learning
Visual or spatial learners learn best with the help of visual cues like charts, images, diagrams, graphs, etc. These learners respond best to colours and mind maps . They use their visual memory to retain information for longer periods of time. Many visual learners possess characteristics like frequent planning and doodling, they have a good attention span and are extremely observant, and they prefer visual directions.
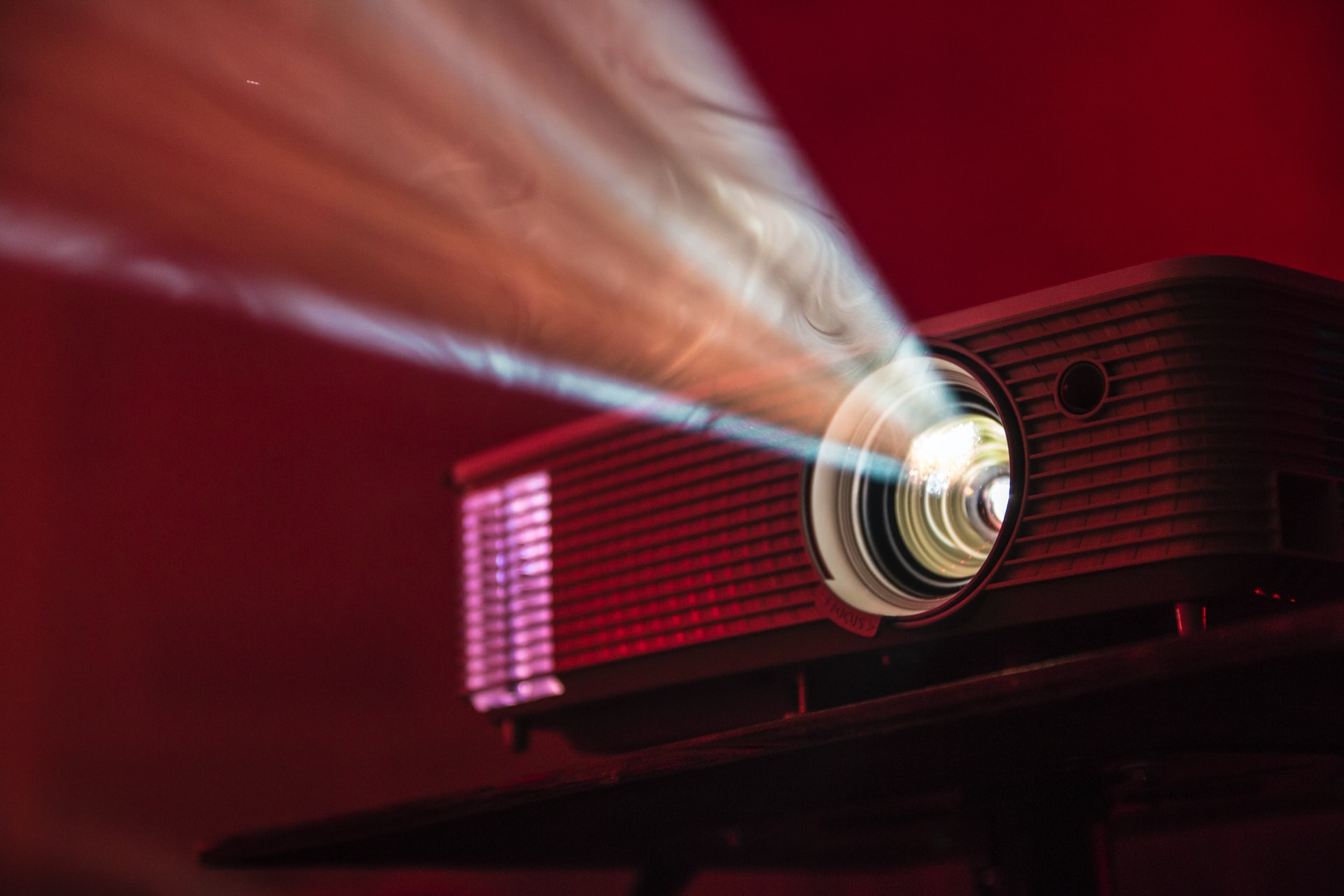
Ways to engage visual learners:
- Use maps, diagrams, imagery
- Include technology like projectors
- Use colour coding techniques
- Encourage mind maps and flowcharts
3. Auditory Learning
People who tend to understand and retain information by hearing it or saying it out loud (oral) are called auditory learners. These types of learners can quickly notice the change in someone’s pitch, tone, and other voice qualities. They usually prefer discussing topics, participating in debates, and conversing about things to remember them. Most auditory learners are easy to distract and might even hum, sing, or talk to self frequently.
Ways to engage auditory learners:
- Try using different pitches and tones while reading the material
- Record voice lessons
- Encourage class presentations, group discussions, debates
- Ask them to teach others verbally
4. Verbal (Read/Write) Learning
These types of learners prefer traditional methods like using multiple written resources for learning. Verbal learners learn best through written material or by writing the material themselves. They usually possess a broad vocabulary and might even like using tools like acronyms, rhymes, tongue twisters, among others. Verbal learners are known to be bookworms.
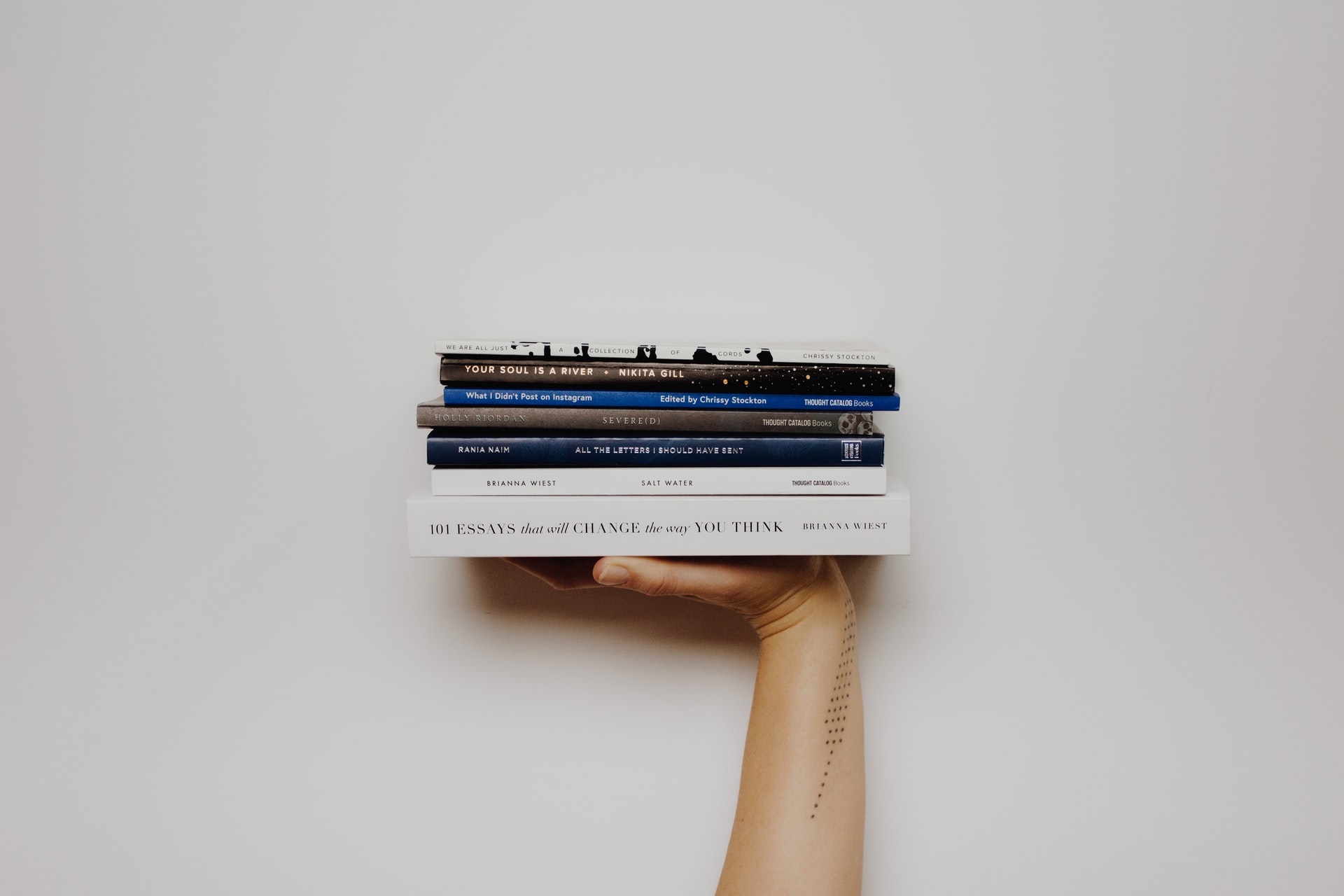
Ways to engage verbal learners:
- Make use of mnemonics while teaching (song, rhyme, acronym, phrase)
- Inculcate scripts
- Encourage students to jot down and voice their ideas
- Include word games like crossword
5. Logical (Mathematical) Learning
Logical or mathematical learners tend to categorize information into groups to learn them better. They have a knack for quickly recognizing patterns and sequences; and understand equations, numbers, and relationships easily. These learners love structure and logic to things. Naturally, mathematics comes easy to them.
Ways to engage logical learners:
- Create an easy to navigate system to your lessons
- Try and inculcate statistics to subjects other than mathematics
- Classify concepts into groups or categories
- Generate cause-effect relationships between variables throughout all subject areas
6. Musical Learning
Where music or background noise is a distraction to most of us, musical learners prefer them. They tend to learn better with music, beats, and rhythm. Like logical learners, they too find patterns and relationships, but between different sounds. Some sources say they even think in sounds and rhythms instead of words and pictures.
Clearly, these learners often grow up to be musicians or instrumentalists. More often than never, some people are a combination of auditory and musical learners. This is why strategies to engage these two kinds aren’t too different.
Ways to engage musical learners:
- Encourage listening to soft background music
- Promote podcasts
7. Naturalist Learners
Naturalist learners learn best through experimentation and practical experiences. They like making observations of the world around them. Just like the name suggests, naturalistic learners are also said to be one with nature. They retain information best when they are outdoors, around plants, animals, among others.
These types can also be somewhat related to kinesthetic learners since they appreciate tactile sensations. All-in-all, they apply scientific reasoning to the world around them and are highly interested in nature, as well as the things created by man.
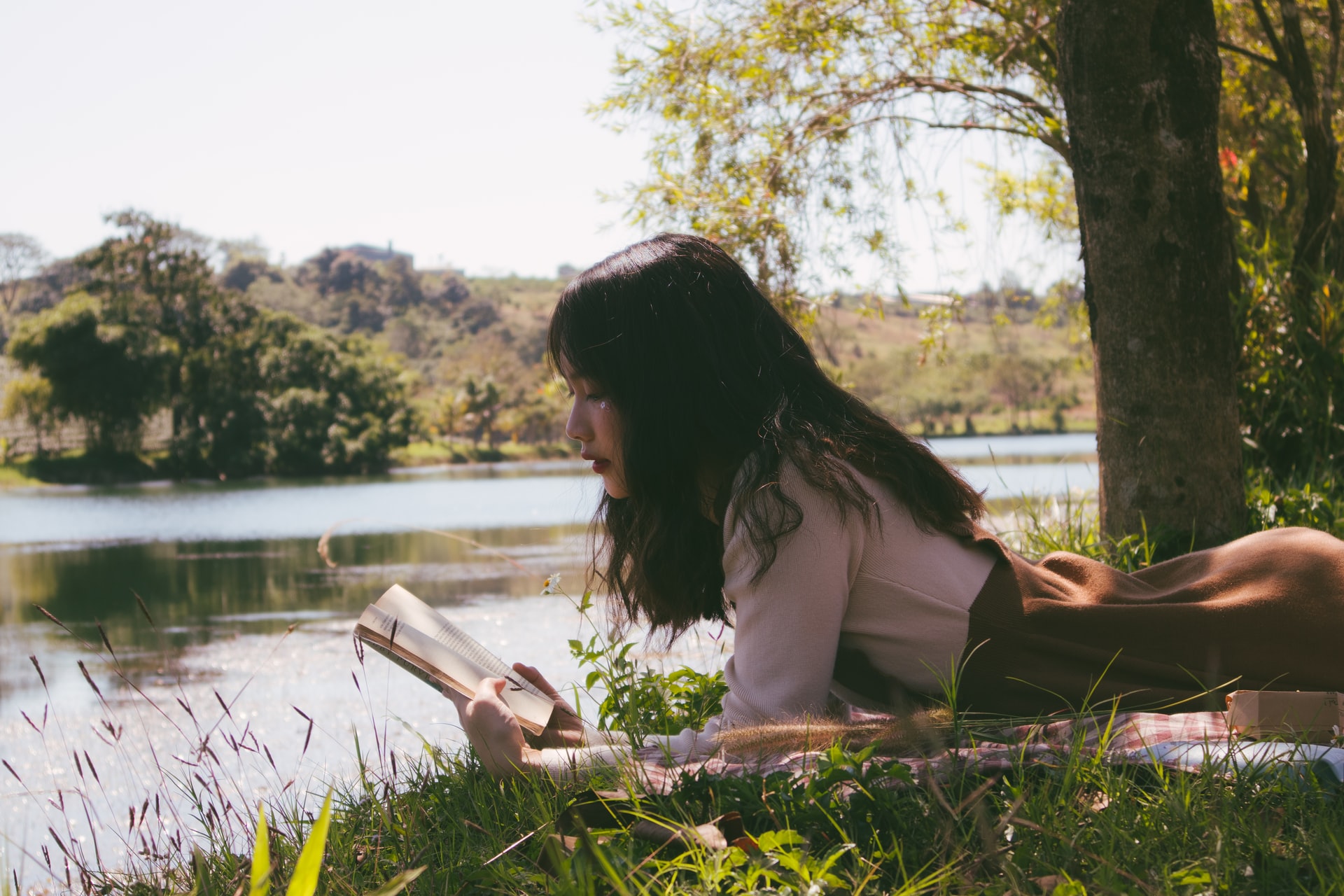
Ways to engage naturalistic learners:
- Take students out for a field trip
- Give lessons in outdoor spaces
- Promote journaling, drawing, sketching, photographing or natural phenomena
- Encourage work that involves getting into nature (especially in subjects like biology)
8. Linguistic Learners
Linguistic learners are the combination of auditory and verbal learners. They absorb knowledge best by writing, reading, and sounding the material out. These learners can use the traditional methods of learning just like verbal learners and also prefer listening to the information. Linguistic learners also make their own notes while studying.
Ways to engage linguistic learners:
- Read out to them and have them read it back to you
- Include written projects and assignments
- Avoid using too many diagrams; use verbal methods of engaging them
- Avoid using a monotonous voice; use different pitches, voices, and characters
9. Interpersonal (Social) Learners
Social or interpersonal learners learn best while working in groups or with other people. They often make good leaders and others even come for advice to them. Social learners learn by relating their ideas and thoughts to the lives of other people. These learners are usually empaths and possess qualities like sensitivity to others, excellent communication, leadership skills, and problem-solving skills. This type of learning can fall adjacent to one or more types mentioned above.
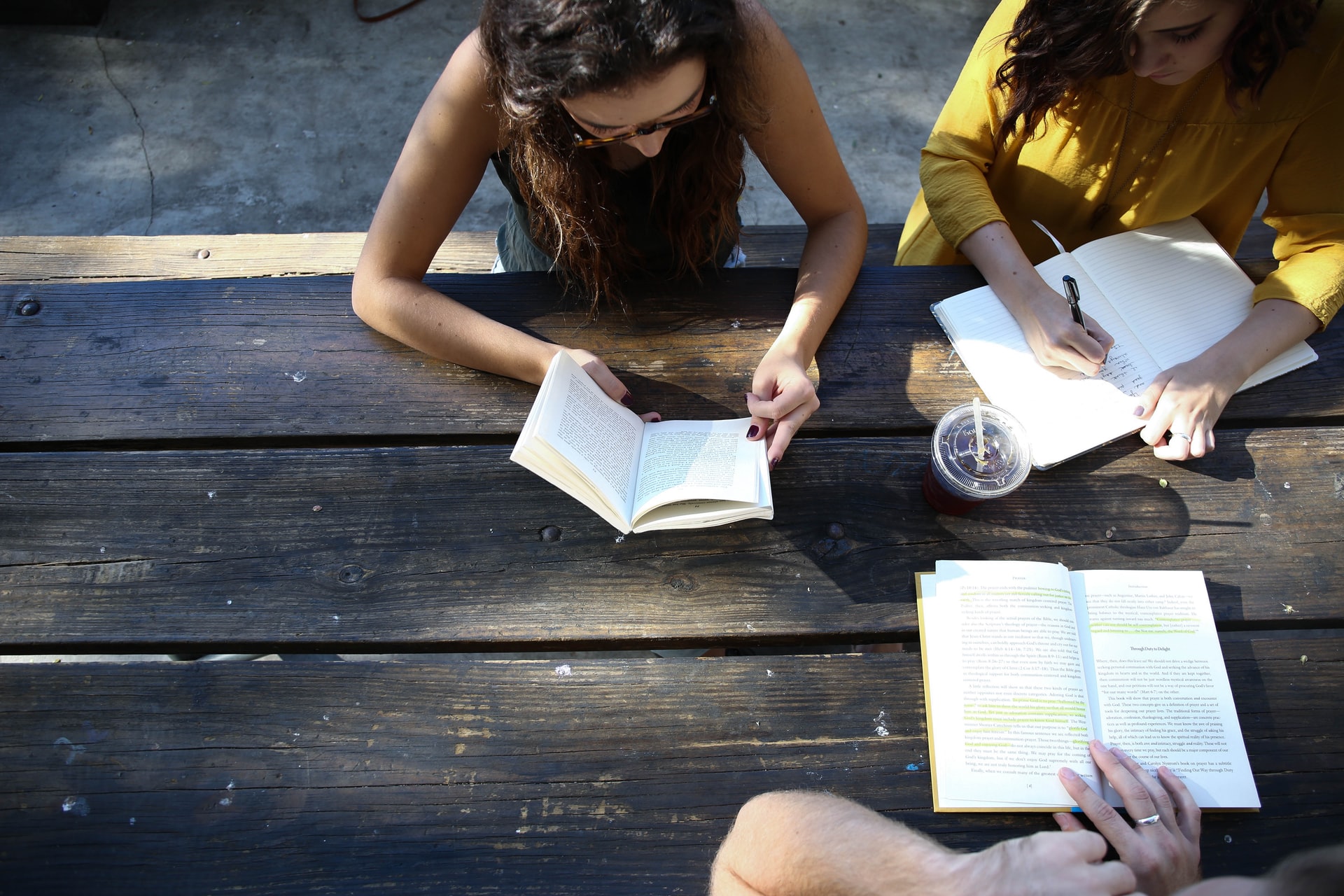
Ways to engage social learners:
- Figure out their adjacent learning style and inculcate those strategies
- Encourage role-playing
- Assign group activities and projects
10. Intrapersonal (Solitary) Learners
In a complete contrast to interpersonal or social learners, intrapersonal or solitary learners prefer solitude while studying. They are more independent and introspective by nature and prefer to be with their own thoughts and ideas without too much external interference. Usually, you can find these types sitting at the back of the class or you might refer to them as the “quiet kid” but they may end up acing the exam. Solitary learning too can fall adjacent with other learning styles.
Ways to engage solitary learners:
- Designate a quiet area
- Check in with them every once in a while
- Define a specific time for collaboration so they feel prepared enough
To summarize, remember to not put your students in a box. It is not necessary and probably unlikely that a student is only one type of learner. There can exist multiple variations and combinations between their learning styles. This is also called a multimodal approach. According to statistics, more than 60% of people are multimodal learners.
Acknowledge that each child is different and you might have the need to find varying ways to approach their education. Again, these differences can also exist with regards to the subject area. Certain people are simply better suited and more interested in some, while others might have completely opposing interests. These learning styles only provide you with a framework to follow.
Organize and sell classes online
Creator Educators - Redefining Education in the Digital Age
Somersault to success: effective gymnastics school management and growth, start, grow, and thrive: essential tips for running a teaching and learning business.
Click through the PLOS taxonomy to find articles in your field.
For more information about PLOS Subject Areas, click here .
Loading metrics
Open Access
Peer-reviewed
Research Article
Differentiating the learning styles of college students in different disciplines in a college English blended learning setting
Roles Conceptualization, Formal analysis, Funding acquisition, Methodology, Supervision, Writing – original draft, Writing – review & editing
* E-mail: [email protected]
Affiliations Department of Linguistics, School of International Studies, Zhejiang University, Hangzhou City, Zhejiang Province, China, Center for College Foreign Language Teaching, Zhejiang University, Hangzhou City, Zhejiang Province, China, Institute of Asian Civilizations, Zhejiang University, Hangzhou City, Zhejiang Province, China

Roles Formal analysis, Project administration, Writing – review & editing
Affiliation Department of Linguistics, School of International Studies, Zhejiang University, Hangzhou City, Zhejiang Province, China
Roles Formal analysis, Writing – original draft
Roles Writing – review & editing
- Jie Hu,
- Yi Peng,
- Xueliang Chen,
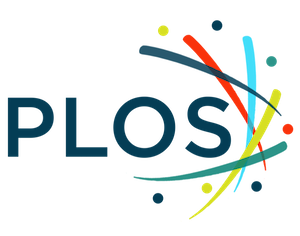
- Published: May 20, 2021
- https://doi.org/10.1371/journal.pone.0251545
- Peer Review
- Reader Comments
Learning styles are critical to educational psychology, especially when investigating various contextual factors that interact with individual learning styles. Drawing upon Biglan’s taxonomy of academic tribes, this study systematically analyzed the learning styles of 790 sophomores in a blended learning course with 46 specializations using a novel machine learning algorithm called the support vector machine (SVM). Moreover, an SVM-based recursive feature elimination (SVM-RFE) technique was integrated to identify the differential features among distinct disciplines. The findings of this study shed light on the optimal feature sets that collectively determined students’ discipline-specific learning styles in a college blended learning setting.
Citation: Hu J, Peng Y, Chen X, Yu H (2021) Differentiating the learning styles of college students in different disciplines in a college English blended learning setting. PLoS ONE 16(5): e0251545. https://doi.org/10.1371/journal.pone.0251545
Editor: Haoran Xie, Lingnan University, HONG KONG
Received: May 15, 2020; Accepted: April 29, 2021; Published: May 20, 2021
Copyright: © 2021 Hu et al. This is an open access article distributed under the terms of the Creative Commons Attribution License , which permits unrestricted use, distribution, and reproduction in any medium, provided the original author and source are credited.
Data Availability: All relevant data are within the paper and its Supporting Information files.
Funding: This research was supported by the Philosophical and Social Sciences Planning Project of Zhejiang Province in 2020 [grant number 20NDJC01Z] with the recipient Jie Hu, Second Batch of 2019 Industry-University Collaborative Education Project of Chinese Ministry of Education [grant number 201902016038] with the recipient Jie Hu, SUPERB College English Action Plan with the recipient Jie Hu, and the Fundamental Research Funds for the Central Universities of Zhejiang University with the recipient Jie Hu.
Competing interests: The authors have declared that no competing interests exist.
Introduction
Research background.
Learning style, as an integral and vital part of a student’s learning process, has been constantly discussed in the field of education and pedagogy. Originally developed from the field of psychology, psychological classification, and cognitive research several decades ago [ 1 ], the term “learning style” is generally defined as the learner’s innate and individualized preference for ways of participation in learning practice [ 2 ]. Theoretically, learning style provides a window into students’ learning processes [ 3 , 4 ], predicts students’ learning outcomes [ 5 , 6 ], and plays a critical role in designing individualized instruction [ 7 ]. Knowing a student’s learning style and personalizing instruction to students’ learning style could enhance their satisfaction [ 8 ], improve their academic performance [ 9 ], and even reduce the time necessary to learn [ 10 ].
Researchers in recent years have explored students’ learning styles from various perspectives [ 11 – 13 ]. However, knowledge of the learning styles of students from different disciplines in blended learning environments is limited. In an effort to address this gap, this study aims to achieve two major objectives. First, it investigates how disciplinary background impacts students’ learning styles in a blended learning environment based on data collected in a compulsory college English course. Students across 46 disciplines were enrolled in this course, providing numerous disciplinary factor resources for investigating learning styles. Second, it introduces a novel machine learning method named the SVM to the field of education to identify an optimal set of factors that can simultaneously differentiate students of different academic disciplines. Based on data for students from 46 disciplines, this research delves into the effects of a massive quantity of variables related to students’ learning styles with the help of a powerful machine learning algorithm. Considering the convergence of a wide range of academic disciplines and the detection of latent interactions between a large number of variables, this study aims to provide a clear picture of the relationship between disciplinary factors and students’ learning styles in a blended learning setting.
Literature review
Theories of learning styles..
Learning style is broadly defined as the inherent preferences of individuals as to how they engage in the learning process [ 2 ], and the “cognitive, affective and physiological traits” of students have received special attention [ 14 ]. To date, there has been a proliferation of learning style definitions proposed to explain people’s learning preferences, each focusing on different aspects. Efforts to dissect learning style have been contested, with some highlighting the dynamic process of the learner’s interaction with the learning environment [ 14 ] and others underlining the individualized ways of information processing [ 15 ]. One vivid explication involved the metaphor of an onion, pointing out the multilayer nature of learning styles. It was proposed that the outermost layer of the learning style could change in accordance with the external environment, while the inner layer is relatively stable [ 16 , 17 ]. In addition, a strong concern in this field during the last three decades has led to a proliferation of models that are germane to learning styles, including the Kolb model [ 18 ], the Myers-Briggs Type Indicator model [ 19 ] and the Felder-Silverman learning style model (FSLSM) [ 20 ]. These learning style models have provided useful analytical lenses for analyzing students’ learning styles. The Kolb model focuses on learners’ thinking processes and identifies four types of learning, namely, diverging, assimilating, converging, and accommodating [ 18 ]. The Myers-Briggs Type Indicator model classifies learners into extraversion and introversion types, with the former preferring to learn from interpersonal communication and the latter inclining to benefit from personal experience [ 19 ]. As the most popular available model, the FSLSM identifies eight categories of learners according to the four dimensions of perception, input, processing and understanding [ 20 ]. In contrast to other learning style models that divided students into only a few groups, the FSLSM describes students’ learning styles in a more detailed manner. The four paired dimensions delicately distinguish students’ engagement in the learning process, providing a solid basis for a steady and reliable learning style analysis [ 21 ]. In addition, it has been argued that the FSLSM is the most appropriate model for a technology-enhanced learning environment because it involves important theories of cognitive learning behaviors [ 22 , 23 ]. Therefore, a large number of scholars have based their investigations of students’ learning styles in the e-learning/computer-aided learning environment on FSLSM [ 24 – 28 ].
Learning styles and FSLSM.
Different students receive, process, and respond to information with different learning styles. A theoretical model of learning style can be used to categorize people according to their idiosyncratic learning styles. In this study, the FSLSM was adopted as a theoretical framework to address the collective impacts of differences in students’ learning styles across different disciplines (see Fig 1 ).
- PPT PowerPoint slide
- PNG larger image
- TIFF original image
This model specifies the four dimensions of the construct of learning style: visual/verbal, sensing/intuitive, active/reflective, and sequential/global. These four dimensions correspond to four psychological processes: input, perception, processing, and understanding.
https://doi.org/10.1371/journal.pone.0251545.g001
The FSLSM includes learning styles scattered among four dimensions.
Visual learners process information best when it is presented as graphs, pictures, etc., while verbal learners prefer spoken cues and remember best what they hear. Sensory learners like working with facts, data, and experimentation, while intuitive learners prefer abstract principles and theories. Active learners like to try things and learn through experimentation, while reflective learners prefer to think things through before taking action. Sequential learners absorb knowledge in a linear fashion and make progress step by step, while global learners tend to grasp the big picture before filling in all the details.
Learning styles and academic disciplines.
Learning styles vary depending on a series of factors, including but not limited to age [ 29 ], gender [ 30 ], personality [ 2 , 31 ], learning environment [ 32 ] and learning experience [ 33 ]. In the higher education context, the academic discipline seems to be an important variable that influences students’ distinctive learning styles, which echoes a multitude of investigations [ 29 , 34 – 41 ]. One notable study explored the learning styles of students from 4 clusters of disciplines in an academic English language course and proposed that the academic discipline is a significant predictor of students’ learning styles, with students from the soft-pure, soft-applied, hard-pure and hard-applied disciplines each favoring different learning modes [ 42 ]. In particular, researchers used the Inventory of Learning Styles (ILS) questionnaire and found prominent disparities in learning styles between students from four different disciplinary backgrounds in the special educational field of vocational training [ 43 ]. These studies have found significant differences between the learning styles of students from different academic disciplines, thus supporting the concept that learning style could be domain dependent.
Learning styles in an online/blended learning environment.
Individuals’ learning styles reflect their adaptive orientation to learning and are not fixed personality traits. Consequently, learning styles can vary among diverse contexts, and related research in different contexts is vital to understanding learning styles in greater depth. Web-based technologies eliminate barriers of space and time and have become integrated in individuals’ daily lives and learning habits. Online and blended learning have begun to pervade virtually every aspect of the education landscape [ 40 ], and this warrants close attention. In addition to a series of studies that reflected upon the application of information and communication technology in the learning process [ 44 , 45 ], recent studies have found a mixed picture of whether students in a web-based/blended learning environment have a typical preference for learning.
Online learning makes it possible for students to set their goals and develop an individualized study plan, equipping them with more learning autonomy [ 46 ]. Generally, students with a more independent learning style, greater self-regulating behavior and stronger self-efficacy are found to be more successful in an online environment [ 47 ]. For now, researchers have made substantial contributions to the identification and prediction of learning styles in an online learning environment [ 27 , 48 – 51 ]. For instance, an inspiring study focused on the manifestation of college students’ learning styles in a purely computer-based learning environment to evaluate the different learning styles of web-learners in the online courses, indicating that students’ learning styles were significantly related to online participation [ 49 ]. Students’ learning styles in interactive E-learning have also been meticulously investigated, from which online tutorials have been found to be contributive to students’ academic performance regardless of their learning styles [ 51 ].
As a flexible learning method, blended courses have combined the advantages of both online learning and traditional teaching methods [ 52 ]. Researchers have investigated students’ learning styles within this context and have identified a series of prominent factors, including perceived satisfaction and technology acceptance [ 53 ], the dynamics of the online/face-to-face environment [ 54 ], and curriculum design [ 55 ]. Based on the Visual, Aural, Reading or Write and Kinesthetic model, a comprehensive study scrutinized the learning styles of K12 students in a blended learning environment, elucidating the effect of the relationship between personality, learning style and satisfaction on educational outcomes [ 56 ]. A recent study underscored the negative effects of kinesthetic learning style, whereas the positive effects of visual or auditory learning styles on students’ academic performance, were also marked in the context of blended learning [ 57 ].
Considering that academic disciplines and learning environment are generally regarded as essential predictors of students’ learning styles, some studies have also concentrated on the effects of academic discipline in a blended learning environment. Focusing on college students’ learning styles in a computer-based learning environment, an inspiring study evaluated the different learning styles of web learners, namely, visual, sensing, global and sequential learners, in online courses. According to the analysis, compared with students from other colleges, liberal arts students, are more susceptible to the uneasiness that may result from remote teaching because of their learning styles [ 11 ]. A similar effort was made with the help of the CMS tool usage logs and course evaluations to explore the learning styles of disciplinary quadrants in the online learning environment. The results indicated that there were noticeable differences in tool preferences between students from different domains [ 12 ]. In comparison, within the context of blended learning, a comprehensive study employed chi-square statistics on the basis of the Community of Inquiry (CoI) presences framework, arguing that soft-applied discipline learners in the blended learning environment prefer the kinesthetic learning style, while no correlations between the learning style of soft-pure and hard-pure discipline students and the CoI presences were identified. However, it is noted that students’ blended learning experience depends heavily on academic discipline, especially for students in hard-pure disciplines [ 13 ].
Research gaps and research questions
Overall, the research seems to be gaining traction, and new perspectives are continually introduced. The recent literature on learning styles mostly focuses on the exploration of the disciplinary effects on the variation in learning styles, and some of these studies were conducted within the blended environment. However, most of the studies focused only on several discrete disciplines or included only a small group of student samples [ 34 – 41 ]. Data in these studies were gathered through specialized courses such as academic English language [ 42 ] rather than the compulsory courses available to students from all disciplines. Even though certain investigations indeed boasted a large number of samples [ 49 ], the role of teaching was emphasized rather than students’ learning style. In addition, what is often overlooked is that a large number of variables related to learning styles could distinguish students from different academic disciplines in a blended learning environment, whereas a more comprehensive analysis that takes into consideration the effects of a great quantity of variables related to learning styles has remained absent. Therefore, one goal of the present study is to fill this gap and shed light on this topic.
Another issue addressed in this study is the selection of an optimal measurement that can effectively identify and differentiate individual learning styles [ 58 ]. The effective identification and differentiation of individual learning styles can not only help students develop greater awareness of their learning but also provide teachers with the necessary input to design tailor-made instructions in pedagogical practice. Currently, there are two general approaches to identify learning styles: a literature-based approach and a data-driven approach. The literature-based approach tends to borrow established rules from the existing literature, while the data-driven approach tends to construct statistical models using algorithms from fields such as machine learning, artificial intelligence, and data mining [ 59 ]. Research related to learning styles has been performed using predominantly traditional instruments, such as descriptive statistics, Spearman’s rank correlation, coefficient R [ 39 ], multivariate analysis of variance [ 56 ] and analysis of variance (ANOVA) [ 38 , 43 , 49 , 57 ]. Admittedly, these instruments have been applied and validated in numerous studies, in different disciplines, and across multiple timescales. Nevertheless, some of the studies using these statistical tools did not identify significant results [ 36 , 53 , 54 ] or reached only loose conclusions [ 60 ]; this might be because of the inability of these methods to probe into the synergistic effects of variables. However, the limited functions of comparison, correlation, prediction, etc. are being complemented by a new generation of technological innovations that promise more varied approaches to addressing social and scientific issues. Machine learning is one such approach that has received much attention both in academia and beyond. As a subset of artificial intelligence, machine learning deals with algorithms and statistical models on computer systems, performing tasks based on patterns and inference instead of explicit instruction. As such, it can deal with high volumes of data at the same time, perform tasks automatically and independently, and continuously improve its performance based on past experience [ 54 ]. Similar machine learning approaches have been proposed and tested by different scholars to identify students’ learning styles, with varying results regarding the classification of learning styles. For instance, a study that examined the precision levels of four computational intelligence approaches, i.e., artificial neural network, genetic algorithm, ant colony system and particle swarm optimization, found that the average precision of learning style differentiation ranged between 66% and 77% [ 61 ]. Another study that classified learning styles through SVM reported accuracy levels ranging from 53% to 84% [ 62 ]. A comparison of the prediction performance of SVM and artificial neural networks found that SVM has higher prediction accuracy than the latter [ 63 ]. This was further supported by another study, which yielded a similar result between SVM and the particle swarm optimization algorithm [ 64 ]. Moreover, when complemented by a genetic algorithm [ 65 ] and ant colony system [ 66 ], SVM has also shown improved results. These findings across different fields point to the reliability of SVM as an effective statistical tool for identification and differentiation analysis.
Therefore, a comprehensive investigation across the four general disciplines in Biglan’s taxonomy using a strong machine learning approach is needed. Given the existence of the research gaps discussed above, this exploratory study seeks to address the following questions:
- Can students’ learning styles be applied to differentiate various academic disciplines in the blended learning setting? If so, what are the differentiability levels among different academic disciplines based on students’ learning styles?
- What are the key features that can be selected to determine the collective impact on differentiation by a machine learning algorithm?
- What are the collective impacts of optimal feature sets?
Materials and methods
This study adopted a quantitative approach for the analysis. First, a modified and translated version of the original ILS questionnaire was administered to collect scores for students’ learning styles. Then, two alternate data analyses were performed separately. One analysis involved a traditional ANOVA, which tested the main effect of discipline on students’ learning styles in each ILS dimension. The other analysis involved the support vector machine (SVM) technique to test its performance in classifying students’ learning styles in the blended learning course among 46 specializations. Then, SVM-based recursive feature elimination (SVM-RFE) was employed to specify the impact of students’ disciplinary backgrounds on their learning styles in blended learning. By referencing the 44 questions (operationalized as features in this study) in the ILS questionnaire, SVM-RFE could rank these features based on their relative importance in differentiating different disciplines and identify the key features that collectively differentiate the students’ learning style. These steps are intended to not only identify students’ learning style differences but also explain such differences in relation to their academic disciplinary backgrounds.
Participants
The participants included 790 sophomores taking the blended English language course from 46 majors at Z University. Sophomore students were selected for this study for two reasons. First, sophomores are one of the only two groups of students (the other group being college freshmen) who take a compulsory English language course, namely, the College English language course. Second, of these two groups of students, sophomores have received academic discipline-related education, while their freshmen counterparts have not had disciplinary training during the first year of college. In the College English language course, online activities, representing 55% of the whole course, include e-course teaching designed by qualified course teachers or professors, courseware usage for online tutorials, forum discussion and essay writing, and two online quizzes. Offline activities, which represent 45% of the whole course, include role-playing, ice-breaker activities, group presentations, an oral examination, and a final examination. Therefore, the effects of the academic discipline on sophomores’ learning styles might be sufficiently salient to warrant a comparison in a blended learning setting [ 67 ]. Among the participants, 420 were male, and 370 were female. Most participants were aged 18 to 19 years and had taken English language courses for at least 6 years. Based on Biglan’s typology of disciplinary fields, the students’ specializations were classified into the four broad disciplines of hard-applied (HA, 289/37.00%), hard-pure (HP, 150/19.00%), soft-applied (SA, 162/20.00%), and soft-pure (SP, 189/24.00%).
Biglan’s classification scheme of academic disciplines (hard (H) vs. soft (S) disciplines and pure (P) vs. applied (A) disciplines) has been credited as the most cited organizational system of academic disciplines in tertiary education [ 68 – 70 ]. Many studies have also provided evidence supporting the validity of this classification [ 69 ]. Over the years, research has indicated that Biglan’s typology is correlated with differences in many other properties and serves as an appropriate mechanism to organize discipline-specific knowledge or epistemologies [ 38 ] and design and deliver courses for students with different learning style preferences [ 41 ]. Therefore, this classification provides a convenient framework to explore differences across disciplinary boundaries. In general, HA disciplines include engineering, HP disciplines include the so-called natural sciences, SA disciplines include the social sciences, and SP disciplines include the humanities [ 41 , 68 , 71 ].
In learning style research, it is difficult to select an instrument to measure the subjects’ learning styles [ 72 ]. The criteria used for the selection of a learning style instrument in this study include the following: 1) successful use of the instrument in previous studies, 2) demonstrated validity and reliability, 3) a match between the purpose of the instrument and the aim of this study and 4) open access to the questionnaire.
The Felder and Soloman’s ILS questionnaire, which was built based on the FSLSM, was adopted in the present study to investigate students’ learning styles across different disciplines. First, the FSLSM is recognized as the most commonly used model for measuring individual learning styles on a general scale [ 73 ] in higher education [ 74 ] and has remained popular for many years across different disciplines in university settings and beyond. In the age of personalized instruction, this model has breathed new life into areas such as blended learning [ 75 ], online distance learning [ 76 ], courseware design [ 56 ], and intelligent tutoring systems [ 77 , 78 ]. Second, the FSLSM is based on previous learning style models; the FSLSM integrates all their advantages and is, thus, more comprehensive in delineating students’ learning styles [ 79 , 80 ]. Third, the FSLSM has a good predictive ability with independent testing sets (i.e., unknown learning style objects) [ 17 ], which has been repeatedly proven to be a more accurate, reliable, and valid model than most other models for predicting students’ learning performance [ 10 , 80 ]. Fourth, the ILS is a free instrument that can be openly accessed online (URL: https://www.webtools.ncsu.edu/learningstyles/ ) and has been widely used in the research context [ 81 , 82 ].
The modified and translated version of the original ILS questionnaire includes 44 questions in total, and 11 questions correspond to each dimension of the Felder-Silverman model as follows: questions 1–11 correspond to dimension 1 (active vs. reflective), questions 12–22 correspond to dimension 2 (sensing vs. intuitive), questions 23–33 correspond to dimension 3 (visual vs. verbal), and questions correspond 34–44 to dimension 4 (sequential vs. global). Each question is followed by five choices on a five-point Likert scale ranging from “strongly agree with A (1)”, “agree with A (2)”, “neutral (3)”, “agree with B (4)” and “strongly agree with B (5)”. Option A and option B represent the two choices offered in the original ILS questionnaire.
Ethics statements
The free questionnaires were administered in a single session by specialized staff who collaborated on the investigation. The participants completed all questionnaires individually. The study procedures were in accordance with the ethical standards of the Helsinki Declaration and were approved by the Ethics Committee of the School of International Studies, Zhejiang University. All participants signed written informed consent to authorize their participation in this research. After completion of the informed consent form, each participant was provided a gift (a pen) in gratitude for their contribution and participation.
Data collection procedure
Before the questionnaires were distributed, the researchers involved in this study contacted faculty members from various departments and requested their help. After permission was given, the printed questionnaires were administered to students under the supervision of their teachers at the end of their English language course. The students were informed of the purpose and importance of the study and asked to carefully complete the questionnaires. The students were also assured that their personal information would be used for research purposes only. All students provided written informed consent (see S2 File ). After the questionnaires were completed and returned, they were thoroughly examined by the researchers such that problematic questionnaires could be identified and excluded from further analysis. All questionnaires eligible for the data analysis had to meet the following two standards: first, all questions must be answered, and second, the answered questions must reflect a reasonable logic. Regarding the few missing values, the median number of a given individual’s responses on 11 questions per dimension included in the ILS questionnaire was used to fill the void in each case. In statistics, using the median number to impute missing values is common and acceptable because missing values represent only a small minority of the entire dataset and are assumed to not have a large impact on the final results [ 83 , 84 ].
In total, 850 questionnaires were administered to the students, and 823 of these questionnaires were retrieved. Of the retrieved questionnaires, the remaining 790 questionnaires were identified as appropriate for further use. After data screening, these questionnaires were organized, and their respective results were translated into an Excel format.
Data analysis method
During the data analysis, as a library of the SVM, the free package LIBSVM ( https://www.csie.ntu.edu.tw/~cjlin/libsvm/ ) was first applied as an alternative method of data analysis. Then, a traditional ANOVA was performed to examine whether there was a main effect of academic discipline on Chinese students’ learning styles. ANOVA could be performed using SPSS, a strong data analysis software that supports a series of statistical analyses. In regard to the examination of the effect of a single or few independent variables, SPSS ANOVA can produce satisfactory results. However, SVM, a classic data mining algorithm, outperforms ANOVA for dataset in which a large number of variables with multidimensions are intertwined and their combined/collective effects influence the classification results. In this study, the research objective was to efficiently differentiate and detect the key features among the 44 factors. Alone, a single factor or few factors might not be significant enough to discriminate the learning styles among the different disciplines. Selected by the SVM, the effects of multiple features may collectively enhance the classification performance. Therefore, the reason for selecting SVM over ANOVA is that in the latter case, the responses on all questions in a single dimension are summed instead of treated as individual scores; thus, the by-item variation is concealed. In addition, the SVM is especially suitable for statistical analysis with high-dimensional factors (usually > 10; 44-dimensional factors were included in this study) and can detect the effects collectively imposed by a feature set [ 85 ].
Originally proposed in 1992 [ 86 ], the SVM is a supervised learning model related to machine learning algorithms that can be used for classification, data analysis, pattern recognition, and regression analysis. The SVM is an efficient classification model that optimally divides data into two categories and is ranked among the top methods in statistical theory due to its originality and practicality [ 85 ]. Due to its robustness, accurate classification, and prediction performance [ 87 – 89 ], the SVM has high reproducibility [ 90 , 91 ]. Due to the lack of visualization of the computing process of the SVM, the SVM has been described as a “black box” method [ 92 ]; however, future studies in the emerging field of explainable artificial intelligence can help solve this problem and convert this approach to a “glass box” method [ 67 ]. This algorithm has proven to have a solid theoretical foundation and excellent empirical application in the social sciences, including education [ 93 ] and natural language processing [ 94 ]. The mechanism underlying the SVM is also presented in Fig 2 .
Hyperplanes 1 and 2 are two regression lines that divide the data into two groups. Hyperplane 1 is considered the best fitting line because it maximizes the distance between the two groups.
https://doi.org/10.1371/journal.pone.0251545.g002
The SVM contains the following two modules: one module is a general-purpose machine learning method, and the other module is a domain-specific kernel function. The SVM training algorithm is used to build a training model that is then used to predict the category to which a new sample instance belongs [ 95 ]. When a set of training samples is given, each sample is given the label of one of two categories. To evaluate the performance of SVM models, a confusion matrix, which is a table describing the performance of a classifier on a set of test data for which the true values are known, is used (see Table 1 ).
https://doi.org/10.1371/journal.pone.0251545.t001
ACC represents the proportion of true results, including both positive and negative results, in the selected population;
SPE represents the proportion of actual negatives that are correctly identified as such;
SEN represents the proportion of actual positives that are correctly identified as such;
AUC is a ranking-based measure of classification performance that can distinguish a randomly chosen positive example from a randomly chosen negative example; and
F-measure is the harmonic mean of precision (another performance indicator) and recall.
The ACC is a good metric frequently applied to indicate the measurement of classification performance, but the combination of the SPE, SEN, AUC, F-measure and ACC may be a measure of enhanced performance assessment and was frequently applied in current studies [ 96 ]. In particular, the AUC is a good metric frequently applied to validate the measurement of the general performance of models [ 97 ]. The advantage of this measure is that it is invariant to relative class distributions and class-specific error costs [ 98 , 99 ]. Moreover, to some extent, the AUC is statistically consistent and more discriminating than the ACC with balanced and imbalanced real-world data sets [ 100 ], which is especially suitable for unequal samples, such as the HA-HP model in this study. After all data preparations were completed, the data used for the comparisons were extracted separately. First, the processed data of the training set were run by using optimized parameters. Second, the constructed model was used to predict the test set, and the five indicators of the fivefold cross-validation and fivefold average were obtained. Cross-validation is a general validation procedure used to assess how well the results of a statistical analysis generalize to an independent data set, which is used to evaluate the stability of the statistical model. K-fold cross-validation is commonly used to search for the best hyperparameters of SVM to achieve the highest accuracy performance [ 101 ]. In particular, fivefold, tenfold, and leave-one-out cross-validation are typically used versions of k-fold cross-validation [ 102 , 103 ]. Fivefold cross-validation was selected because fivefold validation can generally achieve a good prediction performance [ 103 , 104 ] and has been commonly used as a popular rule of thumb supported by empirical evidence [ 105 ]. In this study, five folds (groups) of subsets were randomly divided from the entire set by the SVM, and four folds (training sample) of these subsets were randomly selected to develop a prediction model, while the remaining one fold (test sample) was used for validation. The above functions were all implemented with Python Programming Language version 3.7.0 (URL: https://www.python.org/ ).
Then, SVM-RFE, which is an embedded feature selection strategy that was first applied to identify differentially expressed genes between patients and healthy individuals [ 106 ], was adopted. SVM-RFE has proven to be more robust to data overfitting than other feature selection techniques and has shown its power in many fields [ 107 ]. This approach works by removing one feature each time with the smallest weight iteratively to a feature rank until a group of highly weighted features were selected. After this feature selection procedure, several SVM models were again constructed based on these selected features. The performance of the new models is compared to that of the original models with all features included. The experimental process is provided in Fig 3 for the ease of reference.
https://doi.org/10.1371/journal.pone.0251545.g003
The classification results produced by SVM and the ranking of the top 20 features produced by SVM-RFE were listed in Table 2 . Twenty variables have been selected in this study for two reasons: a data-based reason and a literature-based reason. First, it is clear that models composed of 20 features generally have a better performance than the original models. The performance of models with more than 20 is negatively influenced. Second, SVM-based studies in the social sciences have identified 20 to 30 features as a good number for an optimal feature set [ 108 ], and 20 features were selected for inclusion in the optimal feature set [ 95 ]. Therefore, in this study, the top 20 features were selected for subsequent analysis, as proposed in previous analyses that yielded accepted measurement rates. These 20 features retained most of the useful information from all 44 factors but with fewer feature numbers, which showed satisfactory representation [ 96 ].
https://doi.org/10.1371/journal.pone.0251545.t002
Results of RQ (1) What are the differentiability levels among different academic disciplines based on students’ learning styles?
To further measure the performance of the differentiability among students’ disciplines, the collected data were examined with the SVM algorithm. As shown in Table 2 , the five performance indicators, namely, the ACC, SPE, SEN, AUC and F-measure, were utilized to measure the SVM models. Regarding the two general performance indicators, i.e., the ACC value and AUC value, the HA-HP, HA-SA, and HA-SP-based models yielded a classification capacity of approximately 70.00%, indicating that the students in these disciplines showed a relatively large difference. In contrast, the models based on the H-S, A-P, HP-SA, HP-SP, and SA-SP disciplines only showed a moderate classification capacity (above 55.00%). This finding suggests that these five SVM models were not as effective as the other three models in differentiating students among these disciplines based on their learning styles. The highest ACC and AUC values were obtained in the model based on the HA-HP disciplines, while the lowest values were obtained in the model based on the HP-SA disciplines. As shown in Table 2 , the AUCs of the different models ranged from 57.76% (HP-SA) to 73.97% (HA-HP).
To compare the results of the SVM model with another statistical analysis, an ANOVA was applied. Prior to the main analysis, the students’ responses in each ILS dimension were summed to obtain a composite score. All assumptions of ANOVA were checked, and no serious violations were observed. Then, an ANOVA was performed with academic discipline as the independent variable and the students’ learning styles as the dependent variable. The results of the ANOVA showed that there was no statistically significant difference in the group means of the students’ learning styles in Dimension 1, F(3, 786) = 2.56, p = .054, Dimension 2, F(3, 786) = 0.422, p = .74, or Dimension 3, F(3, 786) = 0.90, p = .443. However, in Dimension 4, a statistically significant difference was found in the group means of the students’ learning styles, F (3, 786) = 0.90, p = .005. As the samples in the four groups were unbalanced, post hoc comparisons using Scheffé’s method were performed, demonstrating that the means of the students’ learning styles significantly differed only between the HA (M = 31.04, SD = 4.986) and SP (M = 29.55, SD = 5.492) disciplines, 95.00% CI for MD [0.19, 2.78], p = .016, whereas the other disciplinary models showed no significant differences. When compared with the results obtained from the SVM models, the three models (HA-HP, HA-SA, and HA-SP models) presented satisfactory differentiability capability of approximately 70.00% based on the five indicators.
In the case of a significant result, it was difficult to determine which questions were representative of the significant difference. With a nonsignificant result, it was possible that certain questions might be relevant in differentiating the participants. However, this problem was circumvented in the SVM, where each individual question was treated as a variable and a value was assigned to indicate its relative importance in the questionnaire. Using SVM also circumvented the inherent problems with traditional significance testing, especially the reliance on p-values, which might become biased in the case of multiple comparisons [ 109 ].
Results of RQ (2) What are the key features that can be selected to determine the collective impact on differentiation by a machine learning algorithm?
To examine whether the model performance improved as a result of this feature selection procedure, the 20 selected features were submitted to another round of SVM analysis. The same five performance indicators were used to measure the model performance (see Table 2 ). By comparing the performance of the SVM model and that of the SVM-RFE model presented in Table 2 , except for the HA-SP model, all other models presented a similar or improved performance after the feature selection process. In particular, the improvement in the HA-HP and HP-SA models was quite remarkable. For instance, in the HA-HP model, the ACC value increased from 69.32% in the SVM model to 82.59% in the SVM-RFE model, and the AUC score substantially increased from 73.97% in the SVM model to 89.13% in the SVM-RFE model. This finding suggests that the feature selection process refined the model’s classification accuracy and that the 20 features selected, out of all 44 factors, carry substantive information that might be informative for exploring disciplinary differences. Although results for the indicators of the 20 selected features were not very high, all five indicators above 65.00% showed that the model was still representative because only 20 of 44 factors could present the classification capability. Considering that there was a significant reduction in the number of questions used for the model construction in SVM-RFE (compared with those used for the SVM model), the newly identified top 20 features by SVM-RFE were effective enough to preserve the differential ability of all 44 questions. Thus, these newly identified top 20 factors could be recognized as key differential features for distinguishing two distinct disciplines.
To identify these top 20 features in eight models (see Table 2 ), SVM-RFE was applied to rank order all 44 features contained in the ILS questionnaire. To facilitate a detailed understanding of what these features represent, the questions related to the top 20 features in the HA-HP model are listed in Table 3 for ease of reference.
https://doi.org/10.1371/journal.pone.0251545.t003
Results of RQ (3) What are the collective impacts of optimal feature sets?
The collective impacts of optimal feature sets could be interpreted from four aspects, namely, the complexities of students’ learning styles, the appropriate choice of SVM, the ranking of SVM-RFE and multiple detailed comparisons between students from different disciplines. First, the FSLSM considers the fact that students’ learning styles are shaped by a series of factors during the growth process, which intertwine and interact with each other. Considering the complex dynamics of the learning style, selecting an approach that could detect the combined effects of a group of variables is needed. Second, recent years have witnessed the emergence of data mining approaches to explore students learning styles [ 28 , 48 – 50 , 110 ]. Specifically, as one of the top machine learning algorithms, the SVM excels in identifying the combined effects of high-order factors [ 87 ]. In this study, the SVM has proven to perform well in classifying students’ learning styles across different disciplines, with every indicator being acceptable. Third, the combination of SVM with RFE could enable the simultaneous discovery of multiple features that collectively determine classification. Notably, although SVM-FRE could rank the importance of the features, they should be regarded as an entire optimal feature set. In other words, the combination of these 20 features, rather than a single factor, could differentiate students’ learning styles across different academic disciplines. Last but not least, the multiple comparisons between different SVM models of discipline provide the most effective learning style factors, giving researchers clues to the nuanced differences between students’ learning styles. It can be seen that students from different academic disciplines understand, see and reflect things from individualized perspectives. The 20 most effective factors for all models scattered within 1 to 44, verifying students’ different learning styles in 4 dimensions. Therefore, the FSLSM provides a useful and effective tool for evaluating students’ learning styles from a rather comprehensive point of view.
The following discussions address the three research questions explored in the current study.
Levels of differentiability among various academic disciplines based on students’ learning styles with SVM
The results suggest that SVM is an effective approach for classification in the blended learning context in which students with diverse disciplinary backgrounds can be distinguished from each other according to their learning styles. All performance indicators presented in Tables 2 and 3 remain above the baseline of 50.00%, suggesting that between each two disciplines, students’ learning style differences can be identified. To some extent, these differences can be identified with a relatively satisfactory classification capability (e.g., 69.32% of the ACC and 73.97% of the AUC in the HA-HP model shown in Table 2 ). Further support for the SVM algorithm is obtained from the SVM-RFE constructed to assess the rank of the factors’ classification capacity, and all values also remained above the baseline value, while some values reached a relatively high classification capability (e.g., 82.59% of the ACC and 89.13% of the AUC in the HA-HP model shown in Table 2 ). While the results obtained mostly show a moderate ACC and AUC, they still provide some validity evidence supporting the role of SVM as an effective binary classifier in the educational context. However, while these differences are noteworthy, the similarities among students in different disciplines also deserve attention. The results reported above indicate that in some disciplines, the classification capacity is not relatively high; this was the case for the model based on the SA-SP disciplines.
Regarding low differentiability, one explanation might be the indistinct classification of some emerging “soft disciplines.” It was noted that psychology, for example, could be identified as “a discipline that can be considered predominantly ‘soft’ and slightly ‘purer’ than ‘applied’ in nature” [ 111 ] (p. 43–53), which could have blurred the line between the SA and SP disciplines. As there is now no impassable gulf separating the SA and SP disciplines, their disciplinary differences may have diminished in the common practice of lecturing in classrooms. Another reason comes from the different cultivation models of “soft disciplines” and “hard disciplines” for sample students. In their high school, sample students are generally divided into liberal art students and science students and are then trained in different environments of knowledge impartation. The two-year unrelenting and intensive training makes it possible for liberal art students to develop a similar thinking and cognitive pattern that is persistent. After the college entrance examination, most liberal art students select SA or SP majors. However, a year or more of study in university does not exert strong effects on their learning styles, which explains why a multitude of researchers have traditionally investigated the SA and SP disciplines together, calling them simply “social science” or “soft disciplines” compared with “natural science” or “hard disciplines”. There have been numerous contributions pointing out similarities in the learning styles of students from “soft disciplines” [ 37 , 112 – 114 ]. However, students majoring in natural science exhibit considerable differences in learning styles, demonstrating that the talent cultivation model of “hard disciplines” in universities is to some extent more influential on students’ learning styles than that of the “soft disciplines”. Further compelling interpretations of this phenomenon await only the development of a sufficient level of accumulated knowledge among scholars in this area.
In general, these results are consistent with those reported in many previous studies based on the Felder-Silverman model. These studies tested the precision of different computational approaches in identifying and differentiating the learning styles of students. For example, by means of a Bayesian network (BN), an investigation obtained an overall precision of 58.00% in the active/reflective dimension, 77.00% in the sensing/intuitive dimension and 63.00% in the sequential/global dimension (the visual/verbal dimension was not considered) [ 81 ]. With the help of the keyword attributes of learning objects selected by students, a precision of 70.00% in the active/reflective dimension, 73.30% in the sensing/intuitive dimension, 73.30% in the sequential/global dimension and 53.30% in the visual/verbal dimension was obtained [ 115 ].
These results add to a growing body of evidence expanding the scope of the application of the SVM algorithm. Currently, the applications of the SVM algorithm still reside largely in engineering or other hard disciplines despite some tentative trials in the humanities and social sciences [ 26 ]. In addition, as cross-disciplines increase in current higher education, it is essential to match the tailored learning styles of students and researchers studying interdisciplinary subjects, such as the HA, HP, SA and SP disciplines. Therefore, the current study is the first to incorporate such a machine learning algorithm into interdisciplinary blended learning and has broader relevance to further learning style-related theoretical or empirical investigations.
Verification of the features included in the optimal feature sets
Features included in the optimal feature sets provided mixed findings compared with previous studies. Some of the 20 identified features are verified and consistent with previous studies. A close examination of the individual questions included in the feature sets can offer some useful insights into the underlying psychological processes. For example, in six of the eight models constructed, Question 1 (“I understand something better after I try it out/think it through”) appears as the feature with the number 1 ranking, highlighting the great importance attached to this question. This question mainly reflects the dichotomy between experimentation and introspection. A possible revelation is that students across disciplines dramatically differ in how they process tasks, with the possible exception of the SA-SP disciplines. This difference has been supported by many previous studies. For example, it was found that technical students tended to be more tactile than those in the social sciences [ 116 ], and engineering students (known as HA in this study) were more inclined toward concrete and pragmatic learning styles [ 117 ]. Similarly, it was explored that engineering students prefer “a logical learning style over visual, verbal, aural, physical or solitary learning styles” [ 37 ] (p. 122), while social sciences (known as SA in this study) students prefer a social learning style to a logical learning style. Although these studies differ in their focus to a certain degree, they provide an approximate idea of the potential differences among students in their relative disciplines. In general, students in the applied disciplines show a tendency to experiment with tasks, while those in the pure disciplines are more inclined towards introspective practices, such as an obsession with theories. For instance, in Biglan’s taxonomy of academic disciplines, students in HP disciplines prefer abstract rules and theories, while students in SA disciplines favor application [ 67 ]. Additionally, Question 10 (“I find it easier to learn facts/to learn concepts”) is similar to Question 1, as both questions indicate a certain level of abstraction or concreteness. The difference between facts and concepts is closely related to the classification difference between declarative knowledge and procedural knowledge in cognitive psychology [ 35 , 38 ]. Declarative knowledge is static and similar to facts, while procedural knowledge is more dynamic and primarily concerned with operational steps. Students’ preferences for facts or concepts closely correspond to this psychological distinction.
In addition, Questions 2, 4, 7, and 9 also occur frequently in the 20 features selected for the different models. Question 2 (“I would rather be considered realistic/innovative”) concerns taking chances. This question reflects a difference in perspective, i.e., whether the focus should be on obtaining pragmatic results or seeking original solutions. This difference cannot be easily connected to the disciplinary factor. Instead, there are numerous factors, e.g., genetic, social and psychological factors, that may play a strong role in defining this trait. The academic discipline only serves to strengthen or diminish this difference. For instance, decades of research in psychology have shown that males are more inclined towards risk taking than females [ 118 – 121 ]. A careful examination of the current academic landscape reveals a gender difference; more females choose soft disciplines than males, and more males choose hard disciplines than females. This situation builds a disciplinary wall classifying students into specific categories, potentially strengthening the disciplinary effect. For example, Question 9 (“In a study group working on difficult material, I am more likely to jump in and contribute ideas/sit back and listen”) emphasizes the distinction between active participation and introspective thinking, reflecting an underlying psychological propensity in blended learning. Within this context, the significance of this question could also be explained by the psychological evaluation of “loss and gain”, as students’ different learning styles are associated with expected reward values and their internal motivational drives, which are determined by their personality traits [ 122 ]. When faced with the risk of “losing face”, whether students will express their ideas in front of a group of people depends largely on their risk and stress management capabilities and the presence of an appropriate motivation system.
The other two questions also convey similar messages regarding personality differences. Question 4 concerns how individuals perceive the world, while Question 7 concerns the preferred modality of information processing. Evidence of disciplinary differences in these respects was also reported [ 35 , 123 – 125 ]. The other questions, such as Questions 21, 27, and 39, show different aspects of potential personality differences and are mostly consistent with the previous discussion. This might also be a vivid reflection of the multi-faceted effects of blended learning, which may differ in their consonance with the features of each discipline. First, teachers from different domains use technology in different ways, and student from different disciplines may view blended learning differently. For instance, the characteristics of soft-applied fields entail specialized customization in blended courses, further broadening the gulf between different subjects [ 126 ]. Second, although blended learning is generally recognized as a stimulus to students’ innovation [ 127 ], some students who are used to an instructivist approach in which the educator acts as a ‘sage on the stage’ will find it difficult to adapt to a social constructivist approach in which the educator serves as a ‘guide on the side’ [ 128 ]. This difficulty might not only negatively affect students’ academic performance but also latently magnify the effects of different academic disciplines.
Interpretation of the collective impact of optimal feature sets
In each SVM model based on a two-discipline model, the 20 key features (collectively known as an optimal feature set) selected exert a concerted effect on students’ learning styles across different disciplines (see Table 2 ). A broad examination of the distribution of collective impact of each feature set with 20 features in the eight discipline models suggests that it is especially imperative considering the emerging cross-disciplines in academia. Current higher education often involves courses with crossed disciplines and students with diverse disciplinary backgrounds. In addition, with the rise of technology-enhanced learning, the design of personalized tutoring systems requires more nuanced information related to student attributes to provide greater adaptability [ 59 ]. By identifying these optimal feature sets, such information becomes accessible. Therefore, understanding such interdisciplinary factors and designing tailor-made instructions are essential for promoting learning success [ 9 ]. For example, in an English language classroom in which the students are a blend of HP and SP disciplines, instructors might consider integrating a guiding framework at the beginning of the course and stepwise guidelines during the process such that the needs of both groups are met. With the knowledge that visual style is dominant across disciplines, instructors might include more graphic presentations (e.g., Question 11) in language classrooms rather than continue to use slides or boards filled with words. Furthermore, to achieve effective communication with students and deliver effective teaching, instructors may target these students’ combined learning styles. While some methods are already practiced in real life, this study acts as a further reminder of the rationale underlying these practices and thus increases the confidence of both learners and teachers regarding these practices. Therefore, the practical implications of this study mainly concern classroom teachers and educational researchers, who may draw some inspiration for interdisciplinary curriculum design and the tailored application of learning styles to the instructional process.
Conclusions
This study investigated learning style differences among students with diverse disciplinary backgrounds in a blended English language course based on the Felder-Silverman model. By introducing a novel machine learning algorithm, namely, SVM, for the data analysis, the following conclusions can be reached. First, the multiple performance indicators used in this study confirm that it is feasible to apply learning styles to differentiate various disciplines in students’ blended learning processes. These disciplinary differences impact how students engage in their blended learning activities and affect students’ ultimate blended learning success. Second, some questions in the ILS questionnaire carry more substantive information about students’ learning styles than other questions, and certain underlying psychological processes can be derived. These psychological processes reflect students’ discipline-specific epistemologies and represent the possible interaction between the disciplinary background and learning style. In addition, the introduction of SVM in this study can provide inspiration for future studies of a similar type along with the theoretical significance of the above findings.
Despite the notable findings of this study, it is subject to some limitations that may be perfected in further research. First, the current analysis examined the learning styles without allowing for the effects of other personal or contextual factors. The educational productivity model proposed by Walberg underlines the significance of the collected influence of contextual factors on individuals’ learning [ 129 ]. For example, teachers from different backgrounds and academic disciplines are inclined to select various teaching methods and to create divergent learning environments [ 130 ], which should also be investigated thoroughly. The next step is therefore to take into account the effects of educational background, experience, personality and learning experience to gain a more comprehensive understanding of students’ learning process in the blended setting.
In conclusion, the findings of this research validate previous findings and offer new perspectives on students’ learning styles in a blended learning environment, which provides future implications for educational researchers, policy makers and educational practitioners (i.e., teachers and students). For educational researchers, this study not only highlights the merits of using machine learning algorithms to explore students’ learning styles but also provides valuable information on the delicate interactions between blended learning, academic disciplines and learning styles. For policy makers, this analysis provides evidence for a more inclusive but personalized educational policy. For instance, in addition to learning styles, the linkage among students’ education in different phases should be considered. For educational practitioners, this study plays a positive role in promoting student-centered and tailor-made teaching. The findings of this study can help learners of different disciplines develop a more profound understanding of their blended learning tendencies and assist teachers in determining how to bring students’ learning styles into full play pedagogically, especially in interdisciplinary courses [ 131 – 134 ].
Supporting information
https://doi.org/10.1371/journal.pone.0251545.s001
S2 File. Informed consent for participants.
https://doi.org/10.1371/journal.pone.0251545.s002
S1 Dataset.
https://doi.org/10.1371/journal.pone.0251545.s003
Acknowledgments
The authors would like to thank the anonymous reviewers for their constructive comments on this paper and Miss Ying Zhou for her suggestions during the revision on this paper.
- View Article
- Google Scholar
- 15. Dunn R, Dunn K, Perrin J. Teaching young children through their individual learning styles. Boston, MA: Allyn & Bacon, Inc;1994.
- 17. Curry L. Integrating concepts of cognitive or learning style: A review with attention to psychometric standards. Ottawa, ON: Canadian College of Health Service Executives;1987.
- 18. Kolb DA. Experiential learning: experience as the source of learning and development. Englewood Cliffs, NJ: Prentice-Hall;1984.
- 21. Anitha D, Deisy C, Lakshmi SB, Meenakshi MK. Proposing a Classification Methodology to Reduce Learning Style Combinations for Better Teaching and Learning. 2014 IEEE Sixth International Conference on Technology for Education, Amritapuri, India, 2014; 208–211. https://doi.org/10.1109/T4E.2014.5
- 23. Kuljis J, Liu F. A comparison of learning style theories on the suitability for e-learning. Web Technologies, Applications, and Services, 2005; 191–197. Retrieved from: https://www.mendeley.com/catalogue/da014340-bfb5-32d1-b144-73545a86d440/
- 30. Richardson JTE. Researching student learning. Buckingham: SRHE and Open University Press; 2000.
- 33. Marton F, Säljö R. Approaches to learning. Edinburgh: Scottish Academic Press; 1984.
- PubMed/NCBI
- 52. Thorne K. Blended learning: How to integrate online and traditional learning, London: Kogan Page; 2003.
- 59. Graf S. Adaptivity in learning management systems focusing on learning styles. Vienna, Austria: Vienna University of Technology;2007.
- 79. Crockett K, Latham A, Mclean D, Bandar Z, O’Shea J. On predicting learning styles in conversational intelligent tutoring systems using fuzzy classification trees. IEEE International Conference on Fuzzy Systems. 2011; 2481–2488. https://doi.org/10.1109/FUZZY.2011.6007514
- 82. Coffield, Ecclestone K, Moseley, Hall E. Learning styles and pedagogy in post 16 education: A critical and systematic review. London, UK: Learning and Skills Research Centre;2004.
- 86. Acuna E, Rodriguez C. The Treatment of Missing Values and its Effect on Classifier Accuracy. In Banks D, House L, McMorris FR, Arabie P, Gaul W, editors. Classification, Clustering, and Data Mining Applications. Springer Berlin Heidelberg;2004. p. 639–647.
- 88. Boser BE, Guyon IM, Vapnik VN. A training algorithm for optimal margin classifiers. A training algorithm for optimal margin classifiers. Proceedings of The Fifth Annual Workshop on Computational Learning Theory. New York: ACM Press, 1992: 144–152. https://doi.org/10.1145/130385.130401
- 100. Maloof MA. Learning when data sets are imbalanced and when costs are unequal and unknown. Proceedings of the 20th International Conference on Machine Learning (ICML-2003). 2003. Retrieved from: http://www.site.uottawa.ca/~nat/Workshop2003/maloof-icml03-wids.pdf
- 101. Yan L, Rodier R, Mozer M, Wolniewicz R. Optimizing classifier performance via the Wilcoxon-Mann-Withney statistics. Proceedings of the 20th International Conference on Machine Learning (ICML-2003). 2003. Retrieved from: http://citeseerx.ist.psu.edu/viewdoc/download?doi=10.1.1.324.1091&rep=rep1&type=pdf
- 105. James G, Witten D, Hastie T, Tibshirani R. An introduction to statistical learning: with applications in R (Springer Texts in Statistics). 1st ed. New York, NY: Springer Verlag;2013.
- 118. Anderson JR. Language, memory, and thought. Mahwah, NJ: Lawrence Erlbaum Associates;1976.
- 119. Anderson JR. The architecture of cognition. Cambridge, Massachusetts: Harvard University Press;1983.
Purdue Online Writing Lab Purdue OWL® College of Liberal Arts
Welcome to the Purdue Online Writing Lab
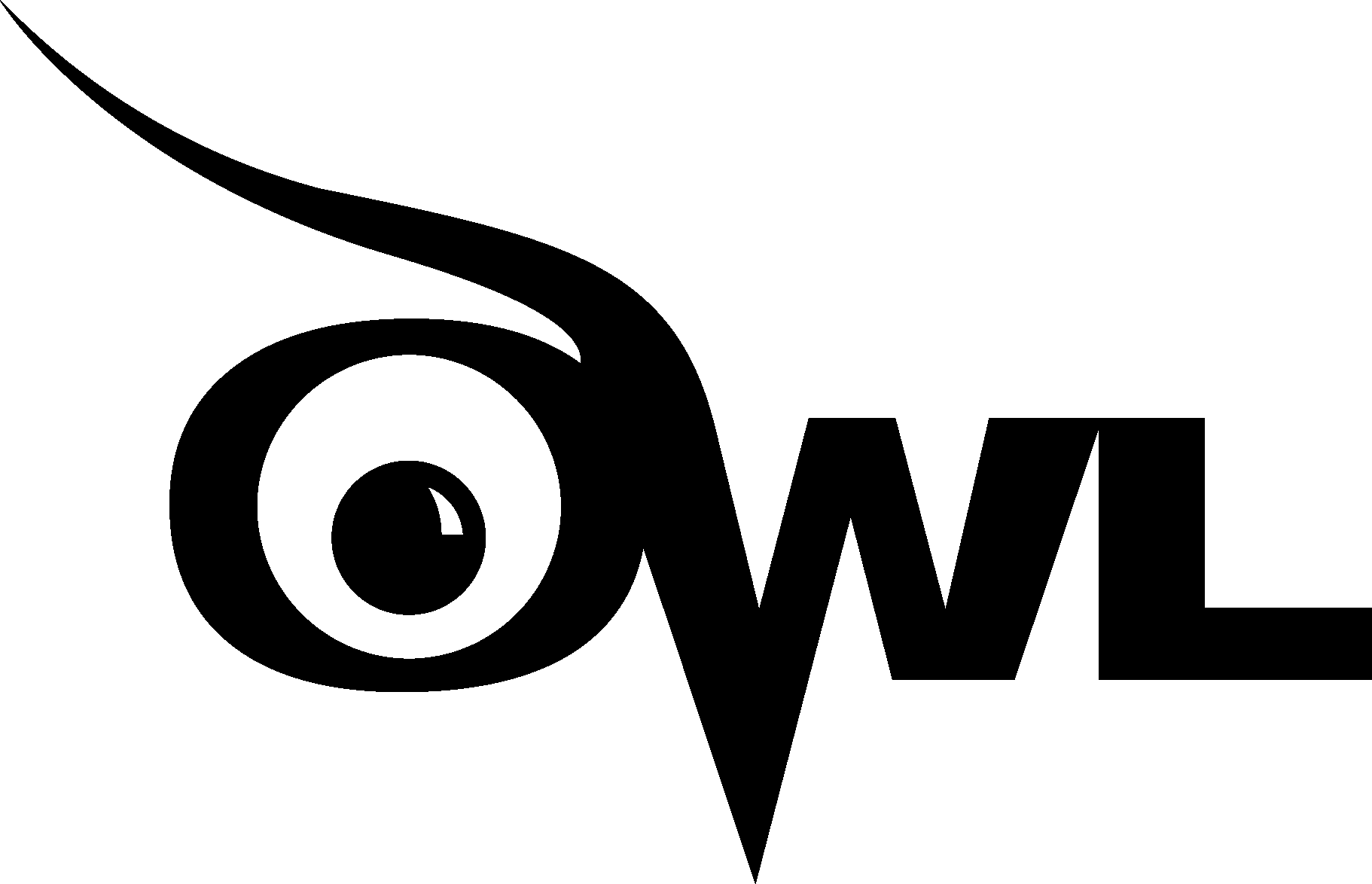
Welcome to the Purdue OWL
This page is brought to you by the OWL at Purdue University. When printing this page, you must include the entire legal notice.
Copyright ©1995-2018 by The Writing Lab & The OWL at Purdue and Purdue University. All rights reserved. This material may not be published, reproduced, broadcast, rewritten, or redistributed without permission. Use of this site constitutes acceptance of our terms and conditions of fair use.
The Online Writing Lab at Purdue University houses writing resources and instructional material, and we provide these as a free service of the Writing Lab at Purdue. Students, members of the community, and users worldwide will find information to assist with many writing projects. Teachers and trainers may use this material for in-class and out-of-class instruction.
The Purdue On-Campus Writing Lab and Purdue Online Writing Lab assist clients in their development as writers—no matter what their skill level—with on-campus consultations, online participation, and community engagement. The Purdue Writing Lab serves the Purdue, West Lafayette, campus and coordinates with local literacy initiatives. The Purdue OWL offers global support through online reference materials and services.
A Message From the Assistant Director of Content Development
The Purdue OWL® is committed to supporting students, instructors, and writers by offering a wide range of resources that are developed and revised with them in mind. To do this, the OWL team is always exploring possibilties for a better design, allowing accessibility and user experience to guide our process. As the OWL undergoes some changes, we welcome your feedback and suggestions by email at any time.
Please don't hesitate to contact us via our contact page if you have any questions or comments.
All the best,
Social Media
Facebook twitter.

What Are The Different Types Of Learning Styles?
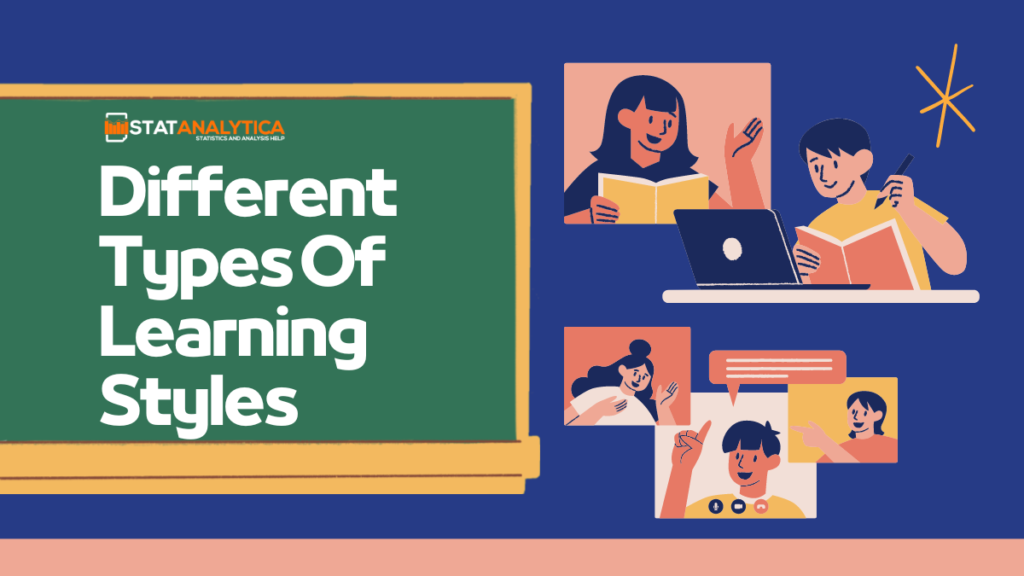
Learning is a complex process, and each person has their own unique way of absorbing information. Understanding different types of learning styles can significantly enhance the effectiveness of education and training. In this blog, we’ll explore the various types of learning styles, how they impact learning, and why it’s essential to recognize and accommodate them.
What Is The Theory Of Learning Styles?
Table of Contents
The theory of learning styles suggests that individuals have preferred ways of learning, and understanding these preferences can enhance educational outcomes.
For example, someone with a visual learning style may learn best through diagrams, charts, and other visual aids, while an auditory learner may prefer listening to lectures or discussions.
By recognizing and accommodating these preferences, educators can tailor their teaching strategies to better suit the needs of diverse learners, ultimately leading to improved comprehension and retention.
How To Identify Different Learning Styles?
Identifying different learning styles involves observing how individuals prefer to absorb and process information. Here are some methods to help identify learning styles:
- Self-assessment: Encourage people to reflect on their own learning preferences by considering how they best understand and retain information. This can involve thinking about whether they prefer visual aids, auditory explanations, hands-on activities, or reading/writing tasks.
- Learning style inventories: Utilize standardized assessments or quizzes designed to identify learning styles. These tools often present scenarios or questions related to learning preferences and provide insights into an individual’s dominant learning style(s).
- Observation: Observe how individuals engage with learning materials and activities in various settings. Pay attention to their reactions, preferences, and behaviors, such as whether they gravitate towards visual aids, actively participate in discussions, or prefer hands-on activities.
- Feedback: Seek feedback from learners themselves, as well as from peers, teachers, or trainers who have interacted with them in learning environments. They may provide valuable insights into the individual’s strengths and preferences in learning.
- Trial and error: Try out different ways of teaching and using different things to see which ones the child likes the most. By observing their responses and performance across various learning activities, you can gather clues about their preferred learning style(s).
- Multiple modalities: Keep in mind that individuals may exhibit characteristics of multiple learning styles or may prefer different styles in different contexts. Therefore, it’s essential to offer a variety of learning opportunities that cater to different modalities to accommodate diverse learning preferences effectively.
Visual Learning Style
Characteristics.
- Visual learners like to understand things by looking at pictures, drawings, graphs, or any other things they can see.
Preferred learning methods
- Watching videos or demonstrations
- Studying infographics, diagrams, or maps
- Using flashcards or visual organizers
- Understanding a concept better by viewing a graph or chart that illustrates the information.
- Remembering details of a story by visualizing scenes in their mind.
- Learning vocabulary words by associating them with corresponding images.
Auditory Learning Style
- Auditory learners like to learn by listening and talking.
- Participating in discussions or group activities
- Listening to lectures, podcasts, or audiobooks
- Using verbal repetition or mnemonics
- Remembering information from a lecture or podcast discussion.
- Understanding musical concepts by listening to examples and analyzing their sound.
- Memorizing information by reciting it out loud or creating rhymes to remember key points.
Kinesthetic Learning Style
- Kinesthetic learners prefer hands-on experiences and physical activities.
- Engaging in experiments, simulations, or role-plays
- Using manipulatives or interactive materials
- Incorporating movement or gestures into learning activities
- Understanding physics concepts by conducting experiments or building models.
- Learning a new dance routine by physically practicing the steps.
- Memorizing multiplication tables by using finger counting or physical movements.
Reading/Writing Learning Style
- Reading/writing learners prefer to learn through written materials and text-based activities.
- Reading textbooks, articles, or written instructions
- Taking notes, writing summaries, or creating outlines
- Engaging in writing exercises, essays, or journaling
- Understanding historical events by reading primary sources and analyzing written accounts.
- Learning grammar rules by reading explanations and practicing writing sentences.
- Retaining information by writing down key points or summarizing text in their own words.
Multimodal Learning Style
- Multimodal learners incorporate elements of two or more learning styles.
- Engaging with a variety of learning materials and activities that cater to different modalities
- Combining visual, auditory, kinesthetic, and reading/writing approaches to learning
- Watching a video lecture (visual) while taking notes (reading/writing).
- Participating in a group discussion (auditory) while using visual aids (visual).
- Engaging in a hands-on experiment (kinesthetic) while verbally explaining the process (auditory).
What Is The Most Common Learning Style?
The way people learn the best can change from person to person. It depends on what they like, their culture, and what they’ve learned before. However, research suggests that the visual learning style is often considered one of the most prevalent among learners.
Lots of folks understand and remember stuff better when they see it with their eyes, like pictures, drawings, charts, or videos. Visual learning is particularly common in educational settings where visual aids are frequently used to convey information and enhance understanding.
However, it’s important to note that learning styles can be diverse, and individuals may exhibit characteristics of multiple styles or prefer different styles in different contexts.
How Do You Identify A Child’s Learning Style?
Identifying a child’s learning style involves observing their behaviors, preferences, and responses to various learning activities. Here are some strategies to help identify a child’s learning style:
- Observe their preferences: Pay attention to how the child naturally engages with learning materials and activities. Notice if they show a preference for certain types of activities, such as drawing, listening to stories, or participating in hands-on experiments.
- Ask questions: Encourage the child to express their preferences and experiences with different learning activities. Ask them about their favorite subjects, how they like to study or learn new things, and what activities they find most enjoyable or challenging.
- Provide variety: Offer a variety of learning materials and activities that cater to different learning styles, including visual, auditory, kinesthetic, and reading/writing approaches. Observe which types of activities the child gravitates towards and performs well in.
- Use learning style inventories: Utilize standardized assessments or quizzes designed to identify learning styles in children. These tools often present scenarios or questions related to learning preferences and provide insights into the child’s dominant learning style (s).
- Encourage self-reflection: Help the child become more aware of their own learning preferences by encouraging them to reflect on how they best understand and retain information. Prompt them to think about whether they prefer looking at pictures, listening to explanations, or engaging in hands-on activities.
- Seek feedback: Talk to parents, teachers, or other caregivers who interact with the child regularly. They may provide valuable insights into the child’s strengths, weaknesses, and learning preferences based on their observations and interactions.
In conclusion, understanding different types of learning styles is essential for educators, trainers, and learners alike. By recognizing and accommodating individual differences in learning preferences, we can create more inclusive and effective learning environments.
Whether you’re a visual learner, auditory learner, kinesthetic learner, reading/writing learner, or a combination of these, embracing your unique learning style can empower you to achieve your full potential.
So, next time you embark on a learning journey, remember to consider your learning style and explore diverse teaching methods that cater to your needs. Happy learning!
Related Posts
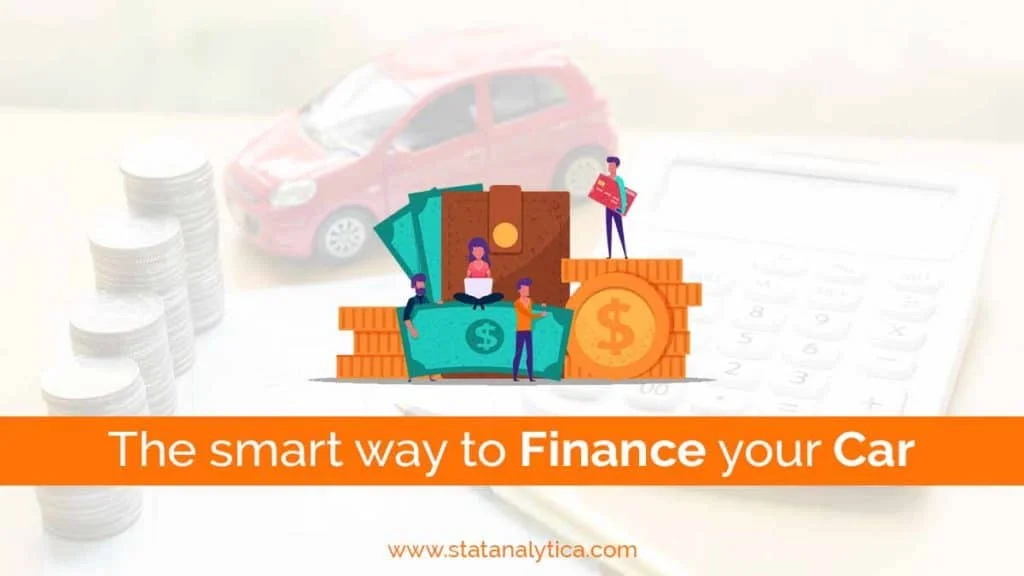
Step by Step Guide on The Best Way to Finance Car
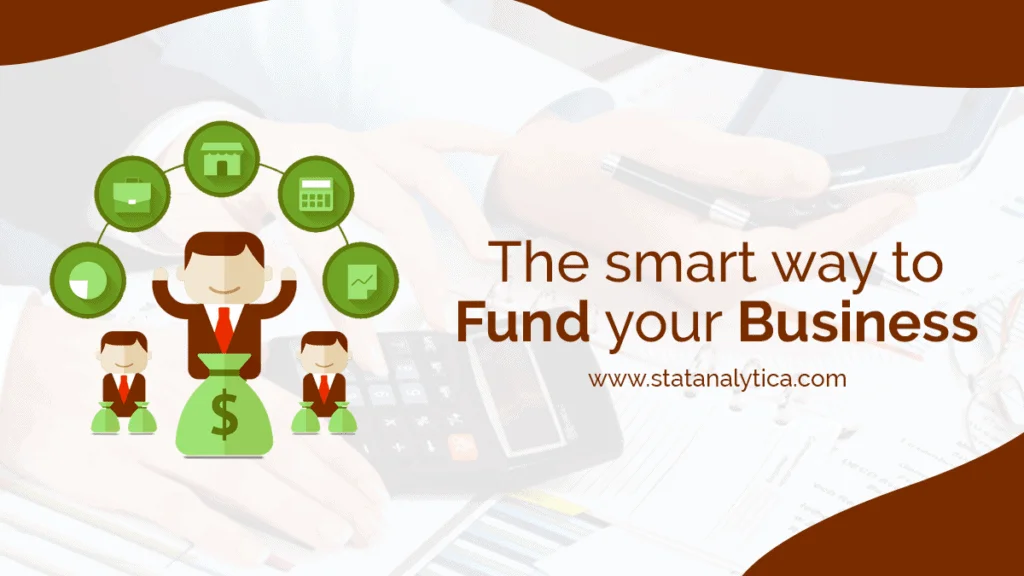
The Best Way on How to Get Fund For Business to Grow it Efficiently
Leave a comment cancel reply.
Your email address will not be published. Required fields are marked *
2024 Theses Doctoral
Deep Learning Strategies for Pandemic Preparedness and Post-Infection Management
Lee, Sang Won
The global transmission of Severe Acute Respiratory Syndrome Coronavirus-2 (SARS-CoV-2) has resulted in over 677 million infections and 6.88 million tragic deaths worldwide as of March 10th, 2023. During the pandemic, the ability to effectively combat SARS-CoV-2 had been hindered by the lack of rapid, reliable, and cost-effective testing platforms for readily screening patients, discerning incubation stages, and accounting for variants. The limited knowledge of the viral pathogenesis further hindered rapid diagnosis and long-term clinical management of this complex disease. While effective in the short term, measures such as social distancing and lockdowns have resulted in devastating economic loss, in addition to material and psychological hardships. Therefore, successfully reopening society during a pandemic depends on frequent, reliable testing, which can result in the timely isolation of highly infectious cases before they spread or become contagious. Viral loads, and consequently an individual's infectiousness, change throughout the progression of the illness. These dynamics necessitate frequent testing to identify when an infected individual can safely interact with non-infected individuals. Thus, scalable, accurate, and rapid serial testing is a cornerstone of an effective pandemic response, a prerequisite for safely reopening society, and invaluable for early containment of epidemics. Given the significant challenges posed by the pandemic, the power of artificial intelligence (AI) can be harnessed to create new diagnostic methods and be used in conjunction with serial tests. With increasing utilization of at-home lateral flow immunoassay (LFIA) tests, the National Institutes of Health (NIH) and Centers for Disease Control and Prevention (CDC) have consistently raised concerns about a potential underreporting of actual SARS-CoV-2-positive cases. When AI is paired with serial tests, it could instantly notify, automatically quantify, aid in real-time contact tracing, and assist in isolating infected individuals. Moreover, the computer vision-assisted methodology can help objectively diagnose conditions, especially in cases where subjective LFIA tests are employed. Recent advances in the interdisciplinary scientific fields of machine learning and biomedical engineering support a unique opportunity to design AI-based strategies for pandemic preparation and response. Deep learning algorithms are transforming the interpretation and analysis of image data when used in conjunction with biomedical imaging modalities such as MRI, Xray, CT scans, confocal microscopes, etc. These advances have enabled researchers to carry out real-time viral infection diagnostics that were previously thought to be impossible. The objective of this thesis is to use SARS-CoV-2 as a model virus and investigate the potential of applying multi-class instance segmentation deep learning and other machine learning strategies to build pandemic preparedness for rapid, in-depth, and longitudinal diagnostic platforms. This thesis encompasses three research tasks: 1) computer vision-assisted rapid serial testing, 2) infected cell phenotyping, and 3) diagnosing the long-term consequences of infection (i.e., long-term COVID). The objective of Task 1 is to leverage the power of AI, in conjunction with smartphones, to rapidly and simultaneously diagnose COVID-19 infections for millions of people across the globe. AI not only makes it possible for rapid and simultaneous screenings of millions but can also aid in the identification and contact tracing of individuals who may be carriers of the virus. The technology could be used, for example, in university settings to manage the entry of students into university buildings, ensuring that only students who test negative for the virus are allowed within campus premises, while students who test positive are placed in quarantine until they recover. The technology could also be used in settings where strict adherence to COVID-19 prevention protocols is compromised, for example, in an Emergency Room. This technology could also help with CDC’s concern on growing incidences of underreporting positive COVID-19 cases with growing utilization of at-home LFIA tests. AI can address issues that arise from relying solely on the visual interpretation of LFIA tests to make accurate diagnoses. One problem is that LFIA test results may be subjective or ambiguous, especially when the test line of the LFIA displays faint color, indicating a low analyte abundance. Therefore, reaching a decisive conclusion regarding the patient's diagnosis becomes challenging. Additionally, the inclusion of a secondary source for verifying the test results could potentially increase the test's cost, as it may require the purchase of complementary electronic gadgets. To address these issues, our innovation would be accurately calibrated with appropriate sensitivity markers, ensuring increased accuracy of the diagnostic test and rapid acquisition of test results from the simultaneous classification of millions of LFIA tests as either positive or negative. Furthermore, the designed network architecture can be utilized to detect other LFIA-based tests, such as early pregnancy detection, HIV LFIA detection, and LFIA-based detection of other viruses. Such minute advances in machine learning and artificial intelligence can be leveraged on many different scales and at various levels to revolutionize the health sector. The motivating purpose of Task 2 is to design a highly accurate instance segmentation network architecture not only for the analysis of SARS-CoV-2 infected cells but also one that yields the highest possible segmentation accuracy for all applications in biomedical sciences. For example, the designed network architecture can be utilized to analyze macrophages, stem cells, and other types of cells. Task 3 focuses on conducting studies that were previously considered computationally impossible. The invention will assist medical researchers and dentists in automatically calculating alveolar crest height (ACH) in teeth using over 500 dental Xrays. This will help determine if patients diagnosed with COVID-19 by a positive PCR test exhibited more alveolar bone loss and had greater bone loss in the two years preceding their COVID-positive test when compared to a control group without a positive COVID-19 test. The contraction of periodontal disease results in higher levels of transmembrane serine protease 2 (TMPRSS2) within the buccal cavity, which is instrumental in enabling the entry of SARS-CoV-2. Gum inflammation, a symptom of periodontal disease, can lead to alterations in the ACH of teeth within the oral mucosa. Through this innovation, we can calculate ACHs of various teeth and, therefore, determine the correlation between ACH and the risk of contracting SARS-CoV-2 infection. Without the invention, extensive manpower and time would be required to make such calculations and gather data for further research into the effects of SARS-CoV-2 infection, as well as other related biological phenomena within the human body. Furthermore, the novel network framework can be modified and used to calculate dental caries and other periodontal diseases of interest.
- Biomedical engineering
- Deep learning (Machine learning)
- Artificial intelligence
- Imaging systems in biology
- COVID-19 Pandemic (2020-)
- COVID-19 (Disease)--Testing
- Macrophages
- Centers for Disease Control and Prevention (U.S.)
- National Institutes of Health (U.S.)
This item is currently under embargo. It will be available starting 2026-04-29.
More About This Work
- DOI Copy DOI to clipboard
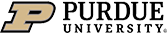
File(s) under embargo
until file(s) become available
A Study on the Use of Unsupervised, Supervised, and Semi-supervised Modeling for Jamming Detection and Classification in Unmanned Aerial Vehicles
In this work, first, unsupervised machine learning is proposed as a study for detecting and classifying jamming attacks targeting unmanned aerial vehicles (UAV) operating at a 2.4 GHz band. Three scenarios are developed with a dataset of samples extracted from meticulous experimental routines using various unsupervised learning algorithms, namely K-means, density-based spatial clustering of applications with noise (DBSCAN), agglomerative clustering (AGG) and Gaussian mixture model (GMM). These routines characterize attack scenarios entailing barrage (BA), single- tone (ST), successive-pulse (SP), and protocol-aware (PA) jamming in three different settings. In the first setting, all extracted features from the original dataset are used (i.e., nine in total). In the second setting, Spearman correlation is implemented to reduce the number of these features. In the third setting, principal component analysis (PCA) is utilized to reduce the dimensionality of the dataset to minimize complexity. The metrics used to compare the algorithms are homogeneity, completeness, v-measure, adjusted mutual information (AMI) and adjusted rank index (ARI). The optimum model scored 1.00, 0.949, 0.791, 0.722, and 0.791, respectively, allowing the detection and classification of these four jamming types with an acceptable degree of confidence.
Second, following a different study, supervised learning (i.e., random forest modeling) is developed to achieve a binary classification to ensure accurate clustering of samples into two distinct classes: clean and jamming. Following this supervised-based classification, two-class and three-class unsupervised learning is implemented considering three of the four jamming types: BA, ST, and SP. In this initial step, the four aforementioned algorithms are used. This newly developed study is intended to facilitate the visualization of the performance of each algorithm, for example, AGG performs a homogeneity of 1.0, a completeness of 0.950, a V-measure of 0.713, an ARI of 0.557 and an AMI of 0.713, and GMM generates 1, 0.771, 0.645, 0.536 and 0.644, respectively. Lastly, to improve the classification of this study, semi-supervised learning is adopted instead of unsupervised learning considering the same algorithms and dataset. In this case, GMM achieves results of 1, 0.688, 0.688, 0.786 and 0.688 whereas DBSCAN achieves 0, 0.036, 0.028, 0.018, 0.028 for homogeneity, completeness, V-measure, ARI and AMI respectively. Overall, this unsupervised learning is approached as a method for jamming classification, addressing the challenge of identifying newly introduced samples.
Collaborative Research: SaTC: CORE: Small: UAV-NetSAFE.COM: UAV Network Security Assessment and Fidelity Enhancement through Cyber-Attack-Ready Optimized Machine-Learning Platforms
Directorate for Computer & Information Science & Engineering
Degree Type
- Master of Science
- Electrical and Computer Engineering
Campus location
Advisor/supervisor/committee chair, additional committee member 2, additional committee member 3, usage metrics.
- Other engineering not elsewhere classified
- Machine learning not elsewhere classified

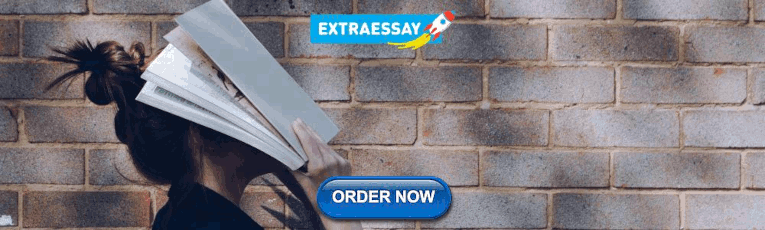
IMAGES
VIDEO
COMMENTS
Argumentative essays test your ability to research and present your own position on a topic. This is the most common type of essay at college level—most papers you write will involve some kind of argumentation. The essay is divided into an introduction, body, and conclusion: The introduction provides your topic and thesis statement
Overview of Learning Theories Although there are many different approaches to learning, there are three basic types of learning theory: behaviorist, cognitive constructivist, and social constructivist. This section provides a brief introduction to each type of learning theory. The theories are
Placement of the thesis statement. Step 1: Start with a question. Step 2: Write your initial answer. Step 3: Develop your answer. Step 4: Refine your thesis statement. Types of thesis statements. Other interesting articles. Frequently asked questions about thesis statements.
A good thesis has two parts. It should tell what you plan to argue, and it should "telegraph" how you plan to argue—that is, what particular support for your claim is going where in your essay. Steps in Constructing a Thesis. First, analyze your primary sources. Look for tension, interest, ambiguity, controversy, and/or complication.
When drafting your thesis statement, avoid words like explore, investigate, learn, compile, summarize, and explain to describe the main purpose of your paper. These words imply a paper that summarizes or "reports," rather than synthesizing and analyzing. Instead of the terms above, try words like argue, critique, question, and interrogate.
Boekaerts, 8 for example, argued for three types of learning strategies: (1) ... an Evaluation Of Instructional Techniques (Unpublished doctoral thesis Univ. Auckland, 2008).
A thesis statement: tells the reader how you will interpret the significance of the subject matter under discussion. is a road map for the paper; in other words, it tells the reader what to expect from the rest of the paper. directly answers the question asked of you. A thesis is an interpretation of a question or subject, not the subject itself.
Thesis. Your thesis is the central claim in your essay—your main insight or idea about your source or topic. Your thesis should appear early in an academic essay, followed by a logically constructed argument that supports this central claim. A strong thesis is arguable, which means a thoughtful reader could disagree with it and therefore ...
The authors of the present review were charged with determining whether these practices are supported by scientific evidence. We concluded that any credible validation of learning-styles-based instruction requires robust documentation of a very particular type of experimental finding with several necessary criteria. First, students must be divided into groups on the basis of their learning ...
LEARNING STYLES. A benchmark definition of "learning styles" is "characteristic cognitive, effective, and psychosocial behaviors that serve as relatively stable indicators of how learners perceive, interact with, and respond to the learning environment. 10 Learning styles are considered by many to be one factor of success in higher education. . Confounding research and, in many instances ...
What is a Thesis Statement? A thesis statement identifies the main point or central idea of your paper. Where should I put it? In most, but not all, academic papers, your thesis will be explicitly stated in one or two sentences near the beginning of your paper. What are the most common kinds of thesis statements? 1.
The way classes are taught in school may only use one or two learning styles and if you don't have one of those learning styles, it can be a struggle. ... If you write amazing essays, know how to review books, and don't mind speaking in public, you're probably a verbal or linguistic learner. Verbal learners rarely struggle at school as they ...
Reading and writing (or verbal) learners. Reading & Writing learners absorb information best when they use words, whether they're reading or writing them. To verbal learners, written words are more powerful and granular than images or spoken words, so they're excellent at writing essays, articles, books, etc.
"The Journey to Learning: Through the Learning Styles of the Senior High School Academic Strand Students A.Y. 2019-2020" March 2020 DOI: 10.13140/RG.2.2.10443.62240
of major learning strategies used by the successful language learner. Meanwhile, the data obtained through the interview was analyzed by transcribing the voice recording during the interview. Then, it was analyzed to categorize them appropriately between learning styles and learning strategies. Some relevant quotations were chosen and put in the
THE DESIGN OF ONLINE LEARNING ENVIRONMENTS. A Thesis submitted to the Faculty of the Graduate School of Arts and Sciences of Georgetown University in partial fulfillment of the requirements for the degree of Master of Arts in Communication, Culture, and Technology. By. Daniel John Davis, B.A.
Declutter desks to promote better focus. 2. Visual (Spatial) Learning. Visual or spatial learners learn best with the help of visual cues like charts, images, diagrams, graphs, etc. These learners respond best to colours and mind maps. They use their visual memory to retain information for longer periods of time.
forms of e-learning (e.g. computer assisted learning, blended learning, massive online open courses (MOOCs), etc.) has become reality at most universities. The principal objective of the research presented in the study was to find out whether learning styles of students and the form of teaching generate interaction effects on
Learning styles are critical to educational psychology, especially when investigating various contextual factors that interact with individual learning styles. Drawing upon Biglan's taxonomy of academic tribes, this study systematically analyzed the learning styles of 790 sophomores in a blended learning course with 46 specializations using a novel machine learning algorithm called the ...
More specifically, the study aims to identify the most preferred learning styles of students learning the 1119 English paper according to gender and academic streams (Pure Science and Account).
Universities strive to ensure quality education focused on the diversity of the student body. According to experiential learning theory, students display different learning preferences. This study has a three-fold objective: to compare learning styles based on personal and educational variables, to analyze the association between learning styles, the level of academic performance, and ...
The seven types of learning. New Zealand educator Neil Fleming developed the VARK model in 1987. It's one of the most common methods to identify learning styles. Fleming proposed four primary learning preferences—visual, auditory, reading/writing, and kinesthetic. The first letter of each spells out the acronym (VARK).
The Online Writing Lab at Purdue University houses writing resources and instructional material, and we provide these as a free service of the Writing Lab at Purdue.
In conclusion, understanding different types of learning styles is essential for educators, trainers, and learners alike. By recognizing and accommodating individual differences in learning preferences, we can create more inclusive and effective learning environments.
The objective of this thesis is to use SARS-CoV-2 as a model virus and investigate the potential of applying multi-class instance segmentation deep learning and other machine learning strategies to build pandemic preparedness for rapid, in-depth, and longitudinal diagnostic platforms.
In this work, first, unsupervised machine learning is proposed as a study for detecting and classifying jamming attacks targeting unmanned aerial vehicles (UAV) operating at a 2.4 GHz band. Three scenarios are developed with a dataset of samples extracted from meticulous experimental routines using various unsupervised learning algorithms, namely K-means, density-based spatial clustering of ...