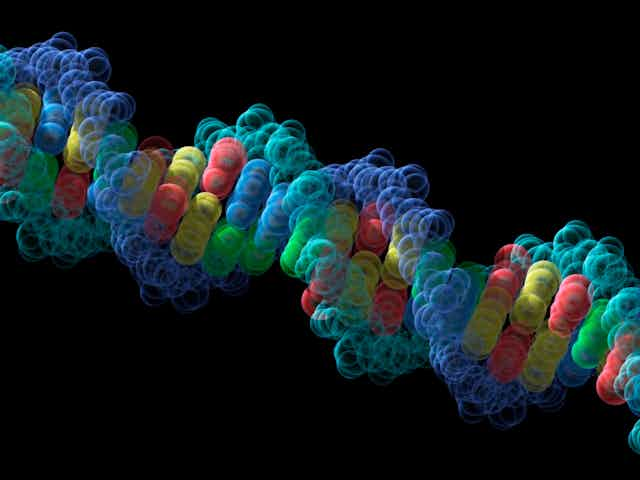
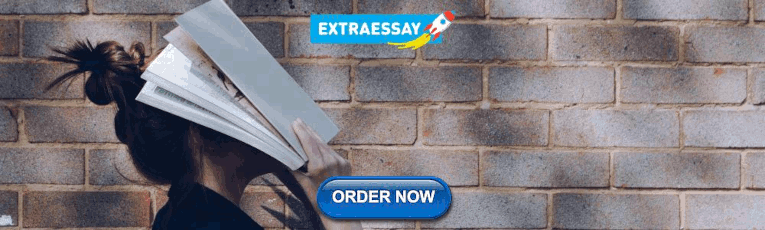
Quantum physics proposes a new way to study biology – and the results could revolutionize our understanding of how life works
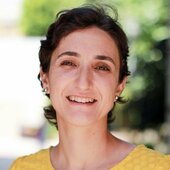
Quantum Biology Tech (QuBiT) Lab, Assistant Professor of Electrical and Computer Engineering, University of California, Los Angeles
Disclosure statement
Clarice D. Aiello receives funding from NSF, ONR, IDOR Foundation, Faggin Foundation, Templeton Foundation.
University of California, Los Angeles provides funding as a member of The Conversation US.
View all partners
Imagine using your cellphone to control the activity of your own cells to treat injuries and disease. It sounds like something from the imagination of an overly optimistic science fiction writer. But this may one day be a possibility through the emerging field of quantum biology.
Over the past few decades, scientists have made incredible progress in understanding and manipulating biological systems at increasingly small scales, from protein folding to genetic engineering . And yet, the extent to which quantum effects influence living systems remains barely understood.
Quantum effects are phenomena that occur between atoms and molecules that can’t be explained by classical physics. It has been known for more than a century that the rules of classical mechanics, like Newton’s laws of motion, break down at atomic scales . Instead, tiny objects behave according to a different set of laws known as quantum mechanics .
For humans, who can only perceive the macroscopic world, or what’s visible to the naked eye, quantum mechanics can seem counterintuitive and somewhat magical. Things you might not expect happen in the quantum world, like electrons “tunneling” through tiny energy barriers and appearing on the other side unscathed, or being in two different places at the same time in a phenomenon called superposition .
I am trained as a quantum engineer . Research in quantum mechanics is usually geared toward technology. However, and somewhat surprisingly, there is increasing evidence that nature – an engineer with billions of years of practice – has learned how to use quantum mechanics to function optimally . If this is indeed true, it means that our understanding of biology is radically incomplete. It also means that we could possibly control physiological processes by using the quantum properties of biological matter.
Quantumness in biology is probably real
Researchers can manipulate quantum phenomena to build better technology. In fact, you already live in a quantum-powered world : from laser pointers to GPS, magnetic resonance imaging and the transistors in your computer – all these technologies rely on quantum effects.
In general, quantum effects only manifest at very small length and mass scales, or when temperatures approach absolute zero. This is because quantum objects like atoms and molecules lose their “quantumness” when they uncontrollably interact with each other and their environment. In other words, a macroscopic collection of quantum objects is better described by the laws of classical mechanics. Everything that starts quantum dies classical. For example, an electron can be manipulated to be in two places at the same time, but it will end up in only one place after a short while – exactly what would be expected classically.
In a complicated, noisy biological system, it is thus expected that most quantum effects will rapidly disappear, washed out in what the physicist Erwin Schrödinger called the “ warm, wet environment of the cell .” To most physicists, the fact that the living world operates at elevated temperatures and in complex environments implies that biology can be adequately and fully described by classical physics: no funky barrier crossing, no being in multiple locations simultaneously.
Chemists, however, have for a long time begged to differ. Research on basic chemical reactions at room temperature unambiguously shows that processes occurring within biomolecules like proteins and genetic material are the result of quantum effects. Importantly, such nanoscopic, short-lived quantum effects are consistent with driving some macroscopic physiological processes that biologists have measured in living cells and organisms. Research suggests that quantum effects influence biological functions, including regulating enzyme activity , sensing magnetic fields , cell metabolism and electron transport in biomolecules .
How to study quantum biology
The tantalizing possibility that subtle quantum effects can tweak biological processes presents both an exciting frontier and a challenge to scientists. Studying quantum mechanical effects in biology requires tools that can measure the short time scales, small length scales and subtle differences in quantum states that give rise to physiological changes – all integrated within a traditional wet lab environment.
In my work , I build instruments to study and control the quantum properties of small things like electrons. In the same way that electrons have mass and charge, they also have a quantum property called spin . Spin defines how the electrons interact with a magnetic field, in the same way that charge defines how electrons interact with an electric field. The quantum experiments I have been building since graduate school , and now in my own lab, aim to apply tailored magnetic fields to change the spins of particular electrons.
Research has demonstrated that many physiological processes are influenced by weak magnetic fields. These processes include stem cell development and maturation , cell proliferation rates , genetic material repair and countless others . These physiological responses to magnetic fields are consistent with chemical reactions that depend on the spin of particular electrons within molecules. Applying a weak magnetic field to change electron spins can thus effectively control a chemical reaction’s final products, with important physiological consequences.
Currently, a lack of understanding of how such processes work at the nanoscale level prevents researchers from determining exactly what strength and frequency of magnetic fields cause specific chemical reactions in cells. Current cellphone, wearable and miniaturization technologies are already sufficient to produce tailored, weak magnetic fields that change physiology , both for good and for bad. The missing piece of the puzzle is, hence, a “deterministic codebook” of how to map quantum causes to physiological outcomes.
In the future, fine-tuning nature’s quantum properties could enable researchers to develop therapeutic devices that are noninvasive, remotely controlled and accessible with a mobile phone. Electromagnetic treatments could potentially be used to prevent and treat disease, such as brain tumors , as well as in biomanufacturing, such as increasing lab-grown meat production .
A whole new way of doing science
Quantum biology is one of the most interdisciplinary fields to ever emerge. How do you build community and train scientists to work in this area?
Since the pandemic, my lab at the University of California, Los Angeles and the University of Surrey’s Quantum Biology Doctoral Training Centre have organized Big Quantum Biology meetings to provide an informal weekly forum for researchers to meet and share their expertise in fields like mainstream quantum physics, biophysics, medicine, chemistry and biology.
Research with potentially transformative implications for biology, medicine and the physical sciences will require working within an equally transformative model of collaboration. Working in one unified lab would allow scientists from disciplines that take very different approaches to research to conduct experiments that meet the breadth of quantum biology from the quantum to the molecular, the cellular and the organismal.
The existence of quantum biology as a discipline implies that traditional understanding of life processes is incomplete. Further research will lead to new insights into the age-old question of what life is, how it can be controlled and how to learn with nature to build better quantum technologies.
- Quantum mechanics
- Quantum physics
- Electromagnetic radiation
- Magnetic fields
- atomic scale
- Quantum technologies
- Subatomic particles
- Interdisciplinary research
- Quantum science
- Quantum superposition
- Electromagnetic energy
- Quantum technology
- Magnetic field
- Science collaboration
- Interdisciplinary science

Sydney Horizon Educators (Identified)

Senior Disability Services Advisor

Deputy Social Media Producer

Associate Professor, Occupational Therapy

GRAINS RESEARCH AND DEVELOPMENT CORPORATION CHAIRPERSON
- Frontiers in Photonics
- Biophotonics
- Research Topics
Quantum Biology
Total Downloads
Total Views and Downloads
About this Research Topic
Quantum biology is an interdisciplinary field that explores the application of quantum mechanics principles and phenomena to biological systems. It seeks to understand how quantum processes and interactions influence the behavior, functioning, and dynamics of biological systems at various scales, from molecules and cells to organisms and ecosystems. By investigating the potential role of quantum effects in biological processes, quantum biology aims to uncover new insights and mechanisms that may govern biological phenomena. This Research Topic aims to showcase recent advancements, discoveries, and applications at the intersection of Quantum Physics and Biology and correlated matters. It will provide a platform for leading scientists and researchers to share their groundbreaking work, foster collaborations, and contribute to the growing field of Quantum Biology. Research in quantum biology encompasses a wide range of topics: - Photobiology and Photomedicine: Investigating the quantum processes involved in light absorption, energy transfer, and charge separation within chromophores complexes. - Magnetic Navigation: Exploring the mechanisms behind the navigation abilities of certain animals, such as birds and insects. - Quantum Biology in Brain Function: Investigating quantum processes in neural systems and exploring potential quantum effects in cognition, information processing, and consciousness. - Quantum Sensing in Biological Systems: Exploring the use of quantum phenomena in biological sensing (In humans and other animals). - Enzymatic Reactions: Studying quantum tunneling, coherence, and entanglement effects in enzymatic reactions. - Electron Transport in Proteins: Investigating how proteins can transport electrons efficiently over long distances. - Quantum Effects in Biological Systems: Studying how quantum effects (at atomic and molecular levels) influence cell processes. Quantum biology is a rapidly evolving field and ongoing research continues to uncover new aspects and applications. Therefore, quantum biology is broader than the listed topics above. Submissions to this Research Topic are by invitation only. We acknowledge the funding of the manuscripts published in this Research Topic by IDOR. We hereby state publicly that IDOR has had no editorial input in articles included in this Research Topic, thus ensuring that all aspects of this Research Topic are evaluated objectively, unbiased by any specific policy or opinion of IDOR.
Keywords : Photobiomedicine, Enzymatic Reactions, Magnetic Navigation, Electron Transport in Proteins, Quantum Sensing, Effects in Biological Systems
Important Note : All contributions to this Research Topic must be within the scope of the section and journal to which they are submitted, as defined in their mission statements. Frontiers reserves the right to guide an out-of-scope manuscript to a more suitable section or journal at any stage of peer review.
Topic Editors
Topic coordinators, submission deadlines, participating journals.
Manuscripts can be submitted to this Research Topic via the following journals:
total views
- Demographics
No records found
total views article views downloads topic views
Top countries
Top referring sites, about frontiers research topics.
With their unique mixes of varied contributions from Original Research to Review Articles, Research Topics unify the most influential researchers, the latest key findings and historical advances in a hot research area! Find out more on how to host your own Frontiers Research Topic or contribute to one as an author.
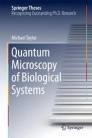
Quantum Microscopy of Biological Systems
- © 2015
- Michael Taylor 0
School of Mathematics and Physics, The University of Queensland, Brisbane, Australia
You can also search for this author in PubMed Google Scholar
- Nominated as an outstanding Ph.D. thesis by the University of Queensland, Australia
- Describes the design and construction of the first quantum-enhanced microscope
- Reports on the first biophysics experiments to break the quantum shot-noise limit
- Provides insight into the significance and potential of quantum measurements in biology
- Includes supplementary material: sn.pub/extras
Part of the book series: Springer Theses (Springer Theses)
10k Accesses
13 Citations
This is a preview of subscription content, log in via an institution to check access.
Access this book
- Available as EPUB and PDF
- Read on any device
- Instant download
- Own it forever
- Compact, lightweight edition
- Dispatched in 3 to 5 business days
- Free shipping worldwide - see info
- Durable hardcover edition
Tax calculation will be finalised at checkout
Other ways to access
Licence this eBook for your library
Institutional subscriptions
Table of contents (14 chapters)
Front matter, introduction.
Michael Taylor
The Quantum Limit to Particle Tracking Sensitivity
The total information carried by the light, the quantum noise limit for a specific measurement, characterizing quadrant detection, classically optimizing sensitivity, interferometer enhanced particle tracking, homodyne based particle tracking, lock-in particle tracking, selective measurement by optimized dark-field illumination angle, technical constraints on sensitivity, quantum enhanced optical tweezers, surpassing the quantum limit, biological measurement beyond the quantum limit, subdiffraction-limited quantum imaging of a living cell, further extensions, summary and conclusion, back matter.
- Optical Tweezers
- Optomechanics and Quantum Imaging
- Particle Tracking
- Quantum Enhanced Microscope
- Quantum Imaging in Biology
- Quantum Metrology
- Quantum Microscope
- Quantum Shot Noise Limit
- Squeezed Light
About this book
This thesis reports on the development of the first quantum enhanced microscope and on its applications in biological microscopy. The first quantum particle-tracking microscope, described in detail here, represents a pioneering advance in quantum microscopy, which is shown to be a powerful and relevant technique for future applications in science and medicine.
The microscope is used to perform the first quantum-enhanced biological measurements -- a central and long-standing goal in the field of quantum measurement. Sub diffraction-limited quantum imaging is achieved, also for the first time, with a scanning probe imaging configuration allowing 10-nanometer resolution.
Authors and Affiliations
Bibliographic information.
Book Title : Quantum Microscopy of Biological Systems
Authors : Michael Taylor
Series Title : Springer Theses
DOI : https://doi.org/10.1007/978-3-319-18938-3
Publisher : Springer Cham
eBook Packages : Physics and Astronomy , Physics and Astronomy (R0)
Copyright Information : Springer International Publishing Switzerland 2015
Hardcover ISBN : 978-3-319-18937-6 Published: 09 June 2015
Softcover ISBN : 978-3-319-36948-8 Published: 09 October 2016
eBook ISBN : 978-3-319-18938-3 Published: 26 May 2015
Series ISSN : 2190-5053
Series E-ISSN : 2190-5061
Edition Number : 1
Number of Pages : XIX, 195
Number of Illustrations : 39 b/w illustrations, 27 illustrations in colour
Topics : Spectroscopy and Microscopy , Quantum Optics , Biological and Medical Physics, Biophysics
- Publish with us
Policies and ethics
- Find a journal
- Track your research

An official website of the United States government
The .gov means it’s official. Federal government websites often end in .gov or .mil. Before sharing sensitive information, make sure you’re on a federal government site.
The site is secure. The https:// ensures that you are connecting to the official website and that any information you provide is encrypted and transmitted securely.
- Publications
- Account settings
Preview improvements coming to the PMC website in October 2024. Learn More or Try it out now .
- Advanced Search
- Journal List
- Front Mol Neurosci
Revisiting the Quantum Brain Hypothesis: Toward Quantum (Neuro)biology?
The nervous system is a non-linear dynamical complex system with many feedback loops. A conventional wisdom is that in the brain the quantum fluctuations are self-averaging and thus functionally negligible. However, this intuition might be misleading in the case of non-linear complex systems. Because of an extreme sensitivity to initial conditions, in complex systems the microscopic fluctuations may be amplified and thereby affect the system’s behavior. In this way quantum dynamics might influence neuronal computations. Accumulating evidence in non-neuronal systems indicates that biological evolution is able to exploit quantum stochasticity. The recent rise of quantum biology as an emerging field at the border between quantum physics and the life sciences suggests that quantum events could play a non-trivial role also in neuronal cells. Direct experimental evidence for this is still missing but future research should address the possibility that quantum events contribute to an extremely high complexity, variability and computational power of neuronal dynamics.
Introduction
The view of the nervous system as a linear, computer-like machine performing classical, deterministic input-output or stimulus-response computations is still very popular in neuroscience. However, this view is challenged by experimental findings and theoretical analyses indicating that the nervous system is a non-linear dynamical complex system ( Deco et al., 2008 ; Singer, 2009 ; Tognoli and Scott Kelso, 2014 ; Wolf et al., 2014 ) exhibiting highly stochastic activity ( Deco et al., 2009 ). There is a need for a “paradigm shift from behaviorist stimulus-response concepts toward notions of predictive coding in self-organizing recurrent networks with high dimensional dynamics” ( Singer, 2015 ). Neural networks consist of nerve cells that are linked by many reciprocal connections ( Markov and Kennedy, 2013 ; Singer, 2013 ) and are capable of non-linear computations ( London and Häusser, 2005 ). Neuronal networks with non-linear neurons and densely connected feedback loops can generate dynamics that is more complex, variable and rich than expected ( Deco and Jirsa, 2012 ; Singer, 2013 ; Singer and Lazar, 2016 ).
The complexity of neuronal dynamics and its capability of fast parallel processing of information is thought to arise from powerful but purely classical computing strategies. The high diversity of neural network dynamics is considered to emerge primarily from non-linearities in the behavior of network nodes and from high variability in the strength and conduction delays of network connections. A conventional wisdom is that in macroscopic objects such as our brain the quantum fluctuations are self-averaging and thus cannot contribute to its rich dynamics. Indeed, it is very likely that the nervous system cannot display macroscopic quantum (classically impossible) behaviors such as quantum entanglement, superposition or tunneling ( Koch and Hepp, 2006 ). Therefore, the prevailing view has been that quantum processes are irrelevant for the brain function. However, in contrast to quantum brain proposals based on implausible quantum mechanisms, there is an alternative, more realistic and subtle way in which quantum events might influence the brain activity and increase its computational power and information coding abilities. Part of this article has been published as a part of a book chapter and is adapted here with permission ( Jedlicka, 2014 ).
Two Arguments Against the Quantum Brain Hypothesis
There are two main arguments, which are usually raised against the quantum brain hypothesis:
(1) Neuronal signaling molecules, neurons and neural networks are too large for quantum phenomena to play a significant role in their functioning. The conventional wisdom is that all quantum events are averaging out , so that fluctuations among quantum particles are not important. As expressed by Daniel Dennett:
“Most biologists think that quantum effects all just cancel out in the brain, that there’s no reason to think they’re harnessed in any way. Of course they’re there; quantum effects are there in your car, your watch, and your computer. But most things — most macroscopic objects — are, as it were, oblivious to quantum effects. They don’t amplify them; they don’t hinge on them” ( Penrose and Dennett, 1995 ).
Christoph Koch and Klaus Hepp, too, identify the large size of neuronal objects and the huge number of particles involved in neuronal signaling as one of the critical weak points of quantum brain hypothesis:
“Although brains obey quantum mechanics, they do not seem to exploit any of its special features. Molecular machines, such as the light-amplifying components of photoreceptors, pre- and post-synaptic receptors and the voltage- and ligand-gated channel proteins that span cellular membranes and underpin neuronal excitability, are so large that they can be treated as classical objects. … Two key biophysical operations underlie information processing in the brain: chemical transmission across the synaptic cleft, and the generation of action potentials. These both involve 1000s of ions and neurotransmitter molecules, coupled by diffusion or by the membrane potential that extends across 10s of micrometers. Both processes will destroy any coherent quantum states. Thus, spiking neurons can only receive and send classical, rather than quantum, information. It follows that a neuron either spikes at a particular point in time or it does not, but is not in a superposition of spike and non-spike states” ( Koch and Hepp, 2006 ).
(2) The second important criticism is that the interaction of neuronal molecules, neurons, or neuronal networks with their noisy, wet and warm environment will destroy any non-trivial quantum states such as superpositions or entanglements. If this were true, only trivial quantum effects could be present in the nervous system. But what is the difference between trivial and non-trivial quantum effects? Trivial quantum effects provide the basis for the structure and chemical properties of molecules and they are ubiquitous (also in cars and watches). Hence, trivial quantum effects are crucial for the basic biochemistry of neuronal molecules, but when considering the neuronal function, these trivial quantum features can be ignored and the molecules important for neuronal signaling can, allegedly, be treated as essentially classical. All interesting coherent quantum states which are necessary for any non-trivial quantum computation ( Davies, 2004 ) can, allegedly, exist only in well-isolated quantum states and are rapidly destroyed by the environment. Since the brain is “a 300-degrees Kelvin tissue strongly coupled to its environment” ( Koch and Hepp, 2006 ), decoherence will prevail and no neuronal quantum computation will be possible. Because of the extremely high speed of environment-induced decoherence, the brain “should be thought of as a classical rather than quantum system” ( Tegmark, 2000 ).
Two Possibilities of the Impact by Quantum Events
The two arguments against the hypothesis that quantum dynamics play a non-trivial role in the nervous system seem convincing and are accepted by most scientists. However, a growing body of empirical evidence indicates that the second argument is false and that the first argument is also very likely false. Exciting recent research shows that non-trivial quantum effects are present in biological systems – and not just in spite of, but sometimes because of, the interaction with the noisy and warm environment. Furthermore, because the brain is a complex non-linear system with high sensitivity to small fluctuations, it is likely that it can amplify microscopic quantum effects . Specifically, there are two alternative but interrelated ways in which quantum events may influence the activity of the brain ( Satinover, 2001 , see also Jedlicka, 2005 , 2009 ): (1) Non-trivial quantum effects can speed up the computational processes in living organisms at the microscopic level. (2) Non-linear chaotic dynamics can amplify lowest-level quantum fluctuations upward, modulating even larger-scale mesoscopic and maybe also macroscopic neuronal activity.
What is the experimental evidence for these two claims?
(1) Contrary to expectations, non-trivial quantum processes have been observed in living systems ( Arndt et al., 2009 ; Brookes, 2017 ). Experiments provide evidence for unexpectedly long-lasting quantum coherence in the electron transfer which is involved in photosynthesis ( Engel et al., 2007 ; Lee et al., 2007 ; Collini et al., 2010 ; Panitchayangkoon et al., 2010 , 2011 ; Sarovar et al., 2010 ). This quantum-mechanical process is thought to improve the efficiency of energy transfer in photopigment molecules ( Panitchayangkoon et al., 2010 ). The pigment molecules seem to implement an efficient quantum algorithm to find the fastest route for the light-induced excitation of electrons ( Sension, 2007 ). Quantum coherence ( Chenu and Scholes, 2015 ) has been found in photosynthetic bacteria as well as in marine algae. This suggests that evolution has been able to select and exploit quantum-mechanical features for fast and efficient computation in two evolutionary distinct organisms (but see also Duan et al., 2017 ). Another example of quantum dynamics in living systems has been found in photoreceptors, which are important for vision. Photoreceptor cells of the retina contain a protein called rhodopsin. Experiments using high-resolution spectroscopic and nuclear-magnetic resonance techniques revealed coherent quantum waves in the rhodopsin molecule ( Wang et al., 1994 ; Loewenstein, 2013b ). As summarized by Werner Loewenstein: “Quantum mechanics, not classical mechanics, rules the roost at this sensory outpost of the brain ” ( Loewenstein, 2013b ).
Quantum effects have been described also in the olfactory system. Electron tunneling has been suggested to play an important role in the detection of odorants by olfactory receptors ( Huelga and Plenio, 2013 ). Avian magnetoreception is yet another example of potentially beneficial quantum effects in biology. Long-lived quantum entanglements in the cryptochromes of the retina seem to support the sensitivity of a bird’s eye to magnetic fields ( Arndt et al., 2009 ; Ball, 2011 ; Huelga and Plenio, 2013 ). Recent simulations show that quantum mechanical coherences in cryptochrome models can account for the precision of the avian magnetic compass ( Hiscock et al., 2016 ). In addition, quantum tunneling has been observed in other biomolecules, such as enzymes ( Klinman and Kohen, 2013 ) or motor proteins ( Hunter, 2006 ). Most importantly, contrary to the long-held view, under some conditions, the strong coupling to the noisy and warm environment is able to promote rather than hinder long-lasting quantum coherence in biological systems ( Plenio and Huelga, 2008 ; Huelga and Plenio, 2013 ). Because of the accumulating evidence that quantum phenomena need to be considered explicitly and in detail when studying living organisms, quantum biology has recently emerged as a new field at the border between quantum physics and the life sciences ( Ball, 2011 ; Tarlac, 2011 ; Lambert et al., 2012 ; Al-Khalili and McFadden, 2014 ; Al-Khalili, 2017 ).
“Physicists thought the bustle of living cells would blot out quantum phenomena. Now they find that cells can nurture these phenomena—and exploit them” ( Vedral, 2011 ).
So far, we have focused on non-trivial quantum processes in sensory cells. But are non-trivial quantum effects also present elsewhere in the nervous system? It is very likely, but direct experimental evidence is still missing. Where should we look for further instances of neuronal quantum effects? There are many stochastic neuronal mechanisms, which may be driven by quantum events. Although it is true that “the main sources of neural noise are forces that can be characterized as thermal and chaotic rather than quantum in nature” ( Sompolinsky, 2005 ), quantum physics is expected to shape at least some stochastic events in the brain, such as the opening of ion channels ( Vaziri and Plenio, 2010 ). In this way microscopic quantum events might affect electrical signals in neurons, as proposed by Paul Glimcher:
“[T]hese data suggest that membrane voltage is the product of interactions at the atomic level, many of which are governed by quantum physics and thus are truly indeterminate events. Because of the tiny scale at which these processes operate, interactions between action potentials and transmitter release as well as interactions between transmitter molecules and post-synaptic receptors may be, and indeed seem likely to be, fundamentally indeterminate” ( Glimcher, 2005 ).
Johnjoe McFadden has made a similar suggestion:
“If neurons poised on the dynamics of individual membrane proteins are critical to the initiation of a particular course of motor action or cognitive process, then the consequent action or cognitive processes will be subject to non- deterministic quantum dynamics” ( McFadden, 2002 ).
It is in itself important that quantum coherence in living organisms has been experimentally demonstrated at the microscopic level. But what are the spatial and temporal limits of these quantum effects? Can we discover quantum coherence in more than just a few molecules? How long can it persist? Some quantum brain proposals, focusing on explaining consciousness ( Hameroff and Penrose, 2014 ), would require coherent quantum waves on much larger and longer scales than those found so far. The honest reaction to the questions just posed is that we do not know the answers to them and that only future research can provide those answers. The ‘orchestrated objective reduction’ (Orch-OR) hypothesis based on putative quantum computations in microtubules has been criticized for various reasons ( Seife, 2000 ; Tegmark, 2000 ; McKemmish et al., 2009 ), e.g., for not being able to distinguish between neural mechanisms of conscious and unconscious information processing ( Baars and Edelman, 2012 ). Although the authors of the Orch-OR model have tried to address most of the criticisms ( Craddock et al., 2014 ; Hameroff and Penrose, 2014 ; Hameroff et al., 2014 ), their and other quantum consciousness hypotheses remain controversial and difficult to test experimentally. Interestingly, a new quantum cognition model, based on entangled calcium phosphate molecules in neurons, has recently been suggested and seems better testable than previous proposals ( Fisher, 2015 ; Weingarten et al., 2016 ). One might speculate that future research on quantum cognition and consciousness might benefit from developing a quantum version of the integrated information theory ( Tononi et al., 2016 ). Nevertheless, it is very important to emphasize that the main idea of this article, namely that biological evolution is able to take advantage of non-trivial quantum events at the microscopic level (see above) and even at larger-scale levels [by non-linear amplification, see point (2) below], is not dependent on the plausibility or validity of quantum consciousness proposals.
(2) If it turned out that quantum effects cannot be observed in living systems at the macroscopic level, would that mean that living systems can be fully described by classical physics? Or is there another plausible way in which small-scale quantum effects – there is evidence for their occurrence [see (1)] – might influence large-scale neuronal activity and behavior? Yes, there is. The common view that minuscule fluctuations, including quantum events, cancel out in larger systems need not be true in highly non-linear systems like our brain. The nervous system can be seen as a nested hierarchy of non-linear complex networks of molecules, cells, microcircuits, and brain regions. In iterative hierarchies with non-linear dynamics (at the edge of chaos), small (even infinitesimal) fluctuations are not averaged out, but can be amplified. Quantum fluctuations on the lowest level of scale may influence the initial state of the next level of scale, while the higher levels shape the boundary conditions of the lower ones. This hierarchy of nested networks with many feedback loops exploits rather than cancels out the quantum effects as proposed by Jeffrey Satinover:
“[Q]quantum dynamics alters the final outcomes of computation at all levels – not by producing classically impossible solutions but by having a profound effect on which of many possible solutions are actually selected” ( Satinover, 2001 ).
In his essay on free will and neuroscience, Haim Sompolinsky has also mentioned this possibility:
“Chaos within the brain may amplify enormously the small quantum fluctuations … to a degree that will affect the timing of spikes in neurons” ( Sompolinsky, 2005 ).
Similarly, even Christof Koch, one of the major critics of quantum brain ideas, had to admit:
“What cannot be ruled out is that tiny quantum fluctuations deep in the brain are amplified by deterministic chaos and will ultimately lead to behavioral choices” ( Koch, 2009 ).
The quantum amplification mechanism has been adopted also by Scott Aaronson whose recent “freebit” theory of free will “postulates that chaotic dynamics in the brain can have the effect of amplifying freebits to macroscopic scale” ( Aaronson, 2016 , but see also criticism of this quantum ampflification idea in Clarke, 2014 ). A similar theory has already been proposed by Jordan (1938) .
What is the evidence for the proposal that the brain is a complex non-linear system, capable of chaotic dynamics ( van Vreeswijk and Sompolinsky, 1996 ; Harish and Hansel, 2015 )? Beggs and Plenz (2003 , 2004) provided experimental evidence that neuronal networks can produce complex patterns of collective activity, which are called neuronal avalanches . These avalanches have a characteristic distribution: each avalanche engages a variable number of neurons, but, on average, many more small avalanches are observed than large ones. This indicates that neuronal networks are poised near criticality (near phase transition, Beggs and Timme, 2012 ) and are prone to displaying emergent complex activity ( Chialvo, 2010 ). Similar results supporting criticality in the brain have been obtained on a larger spatial scale from fMRI data ( Deco and Jirsa, 2012 ) and from local field potential and spike recordings ( Hahn et al., 2010 , 2017 and references therein; but see also Touboul and Destexhe, 2010 ; Priesemann et al., 2014 ; Nonnenmacher et al., 2017 ). In general, we can observe three types of dynamics in the brain: (1) ordered/subcritical dynamics consisting of oscillatory synchronous activity with the characteristic features of high coordination and low variability, (2) random/supracritical dynamics consisting of asynchronous irregular activity with low coordination and high variability, and (3) complex/critical dynamics with high coordination and high variability. Brain states exhibiting complex/critical dynamics are the most interesting ones because they support the most efficient information processing ( Beggs and Timme, 2012 ). At the critical point between order and disorder (i.e., at the edge of chaos and instability), neurons can communicate best, since at that point they are coordinated but not stuck in a certain state for a long time and can establish long-range dynamical correlations. Furthermore, neuronal networks in near critical states display, because of largest fluctuations, the largest repertoire of network activity. Finally, at the critical point, the highest sensitivity to small fluctuations ( Fujisawa et al., 2006 ; London et al., 2010 ) is observed: even a single neuron perturbation has a small but non-zero chance to trigger an avalanche. As pointed out by Dante Chialvo, there are convincing Darwinian reasons for supposing that (parts of) our brains operate near the critical point ( Chialvo, 2010 ): in a subcritical world, everything would always be uniform, there would be nothing new to learn and hence no critical and plastic brain would be needed; memories might as well be unchanging. In a supracritical world, everything would always be changing with no regularities to be learnt. No long-term plasticity and memory would be of any help. In our critical (complex) world, surprising events do occur, but regularities, too, are present so that the brain needs to register but also to update the stored memories.
“[B]rains seem “balanced on a knife-edge” between order and chaos: were they as orderly as a pendulum, they couldn’t support interesting behavior; were they as chaotic as the weather, they couldn’t support rationality” ( Aaronson, 2016 ).
Thus, it is plausible, that small quantum fluctuations can be amplified, since brain activity can develop to the critical point: the point of complex neuronal dynamics. Interestingly, recent calculations suggest that quantum coherence can become long-lived in complex systems which are in a critical state between chaos and regularity – at the edge of quantum chaos ( Vattay et al., 2014 ).
In summary, the intricate interplay between quantum effects and non-linear complex dynamics might be able (a) to generate new persistent quantum-chaotic patterns at a microscopic scale, (b) to amplify quantum effects to a macroscopic scale ( Satinover, 2001 ). How exactly the indeterminacy of complex quantum dynamics of the brain is embedded in classical neuronal mechanisms of decision making ( Rolls, 2012 ) remains to be determined.
Toward Quantum Neurobiology?
How could the two scales (the microscopic and the macroscopic one) be interconnected? Is there a biologically plausible way in which small-scale quantum effects could influence large-scale neuronal activity and behavior? In their book, Al-Khalili and McFadden (2014) have made a specific proposal of such interconnection. According to their hypothesis, quantum events, e.g., at the level of ion channels, might affect and/or be affected by extracellular electric fields, which are generated at the level of neurons and their networks. This proposal is based on recent evidence that extracellular fields are able to influence membrane potential of neurons and their spiking activity by so called ephaptic coupling ( Fröhlich and McCormick, 2010 ; Anastassiou et al., 2011 ; Anastassiou and Koch, 2015 ). There is evidence for a causal loop between brain’s endogenous electrical fields and neuronal firing mediated by voltage-gated ion channels. Electrical fields may guide and synchronize firing of many neurons and thus affect cognition and behavior. Thus it is possible that, by modulating (and being modulated by) ion channel activity, electrical fields are coupled to the level of quantum coherent events in many neurons, potentially affecting behaviorally relevant synchrony of neural firing ( Al-Khalili and McFadden, 2014 ).
Interestingly, recent research in psychology has provided another hint that the brain displays quantum-like behavior ( Bruza et al., 2015 ). This lead to the emergence of a new field of quantum cognition ( Busemeyer and Wang, 2014 ). Several studies have shown that certain aspects of decision-making behavior are better described by quantum probability framework than classical probability framework ( Mogiliansky et al., 2009 ; Pothos and Busemeyer, 2009 ; Yukalov and Sornette, 2011 ; Wang et al., 2014 ). However, the quantum cognition theory and its results do not necessarily imply that the brain employs quantum events to implement quantum cognition ( Busemeyer et al., 2017 ). Whether quantum physics is related to the emergence of cognition from neural activity is still an open question ( Bruza et al., 2015 ). Therefore, it will be exciting to see whether future experiments will find or disprove a link between ion channel coherence, field potentials and the above mentioned quantum-like decision behavior. Computational neuroscience may help design the appropriate experimental tests. Large-scale and highly detailed but still purely classical models of neurons and neural circuits are currently being developed (e.g., Markram et al., 2015 ). Quantum brain hypothesis predicts that these models will fail to account for some observed cognitive phenomena (such as quantum-like decision-making). It predicts that new mechanisms from quantum physics will have to be incorporated in these models. A more specific prediction of Al-Khalili and McFadden’s theory (see above) would be that successful models will have to include quantum coherence in ion channels as well as ephaptic coupling between endogenous electrical field potentials and cellular membrane potentials. Even if details of such quantum brain proposals will turn out to be incorrect, a thorough study of the possible contribution of quantum events to the computational aspects of the neural networks is worthwhile because it may lead to the discovery of novel principles of information processing in neuroscience as well as in artificial intelligence and neurorobotics.
Learning from Non-Neuronal Biological Systems?
Long-lasting quantum coherence phenomena have been first observed in non-neuronal systems. Therefore, non-neuronal organisms such as bacteria, algae or land plants can be useful model systems to study the relationship between biological information processing and quantum events. Many molecular mechanisms, which support complex information processing, including neurotransmitter molecules, ion channels, gap junctions, evolved before the emergence of the nervous system during evolution ( Baluska and Levin, 2016 ). Molecular and functional similarities between plants and neurons provide a hint that the notion of cognition defined as complex signal processing and signal integration, including perception, memory and decision-making, should be extended from neural to aneural systems ( Baluska and Mancuso, 2009a ; Baluska and Levin, 2016 ). Even though the concept of plant neurobiology ( Brenner et al., 2006 ; Baluska and Mancuso, 2009b ) has been controversially debated ( Alpi et al., 2007 ; Brenner et al., 2007 ; Trewavas, 2007 ) and the essential requirements for conscious as compared to unconscious cognition remain the topic of investigation and discussion ( Jedlicka, 2007 ; Trewavas and Baluska, 2011 ; Boly et al., 2017 ; Odegaard et al., 2017 ), an exploration of common principles underlying biological information processing in plants and animals leads to new insights and ideas. For instance, it has recently been suggested that both animals and plants are capable of active inference and anticipatory behavior ( Calvo et al., 2016 ; Calvo and Friston, 2017 ). It is tempting to speculate that to achieve optimal accumulation of information ( Jedlicka, 2007 ; Tkaćik and Bialek, 2014 ) and predictive coding, evolution would have developed strategies for exploiting the benefits of both classical as well as quantum information processing. Maybe our brain is not only a classical ( Knill and Pouget, 2004 ; Friston, 2010 ) but, at least to some extent, also a quantum Bayesian inference machine ( Haven and Khrennikov, 2016 ). Using simple organisms to better understand complex functions of the brain such as learning and memory has a great tradition in neuroscience ( Kandel, 2001 ). Thus, studying bacteria or plants in the context of quantum biology ( Lambert et al., 2012 ) may bring new insights also on quantum aspects of computations in the brain.
Although new ideas and concepts are emerging ( Van Regenmortel, 2004 ; Noble, 2010 ), reductionism and determinism are still the major paradigms in current biology, including neurobiology. “[Physicists] invented the deterministic-reductionistic philosophy and taught it to the biologists, only to walk from it themselves” ( Loewenstein, 2013a ). The dominant belief is that “anything can be reduced to simple, obvious mechanical interactions. The cell is a machine. The animal is a machine. Man is a machine” ( Monod, 1974 ). Direct experimental evidence in favor of the quantum brain hypothesis would challenge the mainstream reductionistic-deterministic view of the human brain as a sophisticated machine performing classical computations.
Author Contributions
The author confirms being the sole contributor of this work and approved it for publication.
Conflict of Interest Statement
The author declares that the research was conducted in the absence of any commercial or financial relationships that could be construed as a potential conflict of interest.
- Aaronson S. (2016). “ The ghost in the quantum Turing machine ,” in The Once and Future Turing: Computing the World , eds Cooper S. B., Hodges A. (Cambridge: Cambridge University Press; ), 193–294. 10.1017/CBO9780511863196.018 [ CrossRef ] [ Google Scholar ]
- Al-Khalili J. (2017). Overview of the quantum biology session at the 19th IUPAB congress and 11th EBSA congress. Biophys. Rev. 9 293–294. 10.1007/s12551-017-0283-5 [ PMC free article ] [ PubMed ] [ CrossRef ] [ Google Scholar ]
- Al-Khalili J., McFadden J. (2014). Life on the Edge: The Coming of Age of Quantum Biology. London: Bantam Press. [ Google Scholar ]
- Alpi A., Amrhein N., Bertl A., Michael R., Blumwald E., Cervone F., et al. (2007). Plant neurobiology: No brain, no Gain? Trends Plant Sci. 12 135–136. 10.1016/j.tplants.2007.03.002 [ PubMed ] [ CrossRef ] [ Google Scholar ]
- Anastassiou C. A., Koch C. (2015). Ephaptic coupling to endogenous electric field activity: Why bother? Curr. Opin. Neurobiol. 31 95–103. 10.1016/j.conb.2014.09.002 [ PubMed ] [ CrossRef ] [ Google Scholar ]
- Anastassiou C. A., Perin R., Markram H., Koch C. (2011). Ephaptic coupling of cortical neurons. Nat. Neurosci. 14 217–223. 10.1038/nn.2727 [ PubMed ] [ CrossRef ] [ Google Scholar ]
- Arndt M., Juffmann T., Vedral V. (2009). Quantum physics meets biology. HFSP J. 3 386–400. 10.2976/1.3244985 [ PMC free article ] [ PubMed ] [ CrossRef ] [ Google Scholar ]
- Baars B. J., Edelman D. B. (2012). Consciousness, biology and quantum hypotheses. Phys. Life Rev. 9 285–294. 10.1016/j.plrev.2012.07.001 [ PubMed ] [ CrossRef ] [ Google Scholar ]
- Ball P. (2011). Physics of life: the dawn of quantum biology. Nature 474 272–274. 10.1038/474272a [ PubMed ] [ CrossRef ] [ Google Scholar ]
- Baluska F., Levin M. (2016). On having no head: cognition throughout biological systems. Front. Psychol. 7 : 902 . 10.3389/fpsyg.2016.00902 [ PMC free article ] [ PubMed ] [ CrossRef ] [ Google Scholar ]
- Baluska F., Mancuso S. (2009a). Deep evolutionary origins of neurobiology: turning the essence of ‘neural’ upside-down. Commun. Integr. Biol. 2 60–65. [ PMC free article ] [ PubMed ] [ Google Scholar ]
- Baluska F., Mancuso S. (2009b). Plant neurobiology: from stimulus perception to adaptive behavior of plants, via integrated chemical and electrical signaling. Plant Signal. Behav. 4 475–476. 10.4161/psb.4.6.8870 [ PMC free article ] [ PubMed ] [ CrossRef ] [ Google Scholar ]
- Beggs J. M., Plenz D. (2003). Neuronal avalanches in neocortical circuits. J. Neurosci. 23 11167–11177. [ PMC free article ] [ PubMed ] [ Google Scholar ]
- Beggs J. M., Plenz D. (2004). Neuronal avalanches are diverse and precise activity patterns that are stable for many hours in cortical slice cultures. J. Neurosci. 24 5216–5229. 10.1523/JNEUROSCI.0540-04.2004 [ PMC free article ] [ PubMed ] [ CrossRef ] [ Google Scholar ]
- Beggs J. M., Timme N. (2012). Being critical of criticality in the brain. Front. Physiol. 3 : 163 10.3389/fphys.2012.00163 [ PMC free article ] [ PubMed ] [ CrossRef ] [ Google Scholar ]
- Boly M., Massimini M., Tsuchiya N., Postle B. R., Koch C., Tononi G. (2017). Are the neural correlates of consciousness in the front or in the back of the cerebral cortex? Clinical and neuroimaging evidence. J. Neurosci. 37 9603–9613. 10.1523/JNEUROSCI.3218-16.2017 [ PMC free article ] [ PubMed ] [ CrossRef ] [ Google Scholar ]
- Brenner E. D., Stahlberg R., Mancuso S., Baluška F., Van Volkenburgh E. (2007). Response to Alpi et al.,: plant neurobiology: the gain is more than the name. Trends Plant Sci. 12 285–286. 10.1016/j.tplants.2007.06.005 [ PubMed ] [ CrossRef ] [ Google Scholar ]
- Brenner E. D., Stahlberg R., Mancuso S., Vivanco J., Baluška F., Van Volkenburgh E. (2006). Plant neurobiology: an integrated view of plant signaling. Trends Plant Sci. 11 413–419. 10.1016/j.tplants.2006.06.009 [ PubMed ] [ CrossRef ] [ Google Scholar ]
- Brookes J. C. (2017). Quantum effects in biology: golden rule in enzymes, olfaction, photosynthesis and magnetodetection. Proc. R. Soc. A Math. Phys. Eng. Sci. 473 : 20160822 . 10.1098/rspa.2016.0822 [ PMC free article ] [ PubMed ] [ CrossRef ] [ Google Scholar ]
- Bruza P. D., Wang Z., Busemeyer J. R. (2015). Quantum cognition: a new theoretical approach to psychology. Trends Cogn. Sci. 19 383–393. 10.1016/j.tics.2015.05.001 [ PubMed ] [ CrossRef ] [ Google Scholar ]
- Busemeyer J. R., Fakhari P., Kvam P. (2017). Neural implementation of operations used in quantum cognition. Prog. Biophys. Mol. Biol. 130 53–60. 10.1016/j.pbiomolbio.2017.04.007 [ PubMed ] [ CrossRef ] [ Google Scholar ]
- Busemeyer J. R., Wang Z. (2014). Quantum cognition: key issues and discussion. Top. Cogn. Sci. 6 43–46. 10.1111/tops.12074 [ PubMed ] [ CrossRef ] [ Google Scholar ]
- Calvo P., Baluska F., Sims A. (2016). “Feature detection” vs. “predictive coding”’ models of plant behavior. Front. Psychol. 7 : 1505 . 10.3389/fpsyg.2016.01505 [ PMC free article ] [ PubMed ] [ CrossRef ] [ Google Scholar ]
- Calvo P., Friston K. (2017). Predicting green: really radical (plant) predictive processing. J. R. Soc. Interface 14 : 20170096 . 10.1098/rsif.2017.0096 [ PMC free article ] [ PubMed ] [ CrossRef ] [ Google Scholar ]
- Chenu A., Scholes G. D. (2015). Coherence in energy transfer and photosynthesis. Annu. Rev. Phys. Chem. 66 69–96. 10.1146/annurev-physchem-040214-121713 [ PubMed ] [ CrossRef ] [ Google Scholar ]
- Chialvo D. R. (2010). Emergent complex neural dynamics. Nat. Phys. 6 744–750. 10.1038/nphys1803 [ CrossRef ] [ Google Scholar ]
- Clarke P. G. (2014). Neuroscience, quantum indeterminism and the cartesian soul. Brain Cogn. 84 109–117. 10.1016/j.bandc.2013.11.008 [ PubMed ] [ CrossRef ] [ Google Scholar ]
- Collini E., Wong C., Wilk K., Curmi P., Brume P., Scholes G. D. (2010). Coherently wired light-harvesting in photosynthetic marine algae at ambient temperature. Nature 463 644–647. 10.1038/nature08811 [ PubMed ] [ CrossRef ] [ Google Scholar ]
- Craddock T. J., Friesen D., Mane J., Hameroff S., Tuszynski J. A. (2014). The feasibility of coherent energy transfer in microtubules. J. R. Soc. Interface 11 : 20140677 . 10.1098/rsif.2014.0677 [ PMC free article ] [ PubMed ] [ CrossRef ] [ Google Scholar ]
- Davies P. C. W. (2004). Does quantum mechanics play a non-trivial role in life? Biosystems 78 69–79. 10.1016/j.biosystems.2004.07.001 [ PubMed ] [ CrossRef ] [ Google Scholar ]
- Deco G., Jirsa V. K. (2012). Ongoing cortical activity at rest: criticality, multistability, and ghost attractors. J. Neurosci. 32 3366–3375. 10.1523/JNEUROSCI.2523-11.2012 [ PMC free article ] [ PubMed ] [ CrossRef ] [ Google Scholar ]
- Deco G., Jirsa V. K., Robinson P. A., Breakspear M., Friston K. (2008). The dynamic brain: from spiking neurons to neural masses and cortical fields. PLOS Comput. Biol. 4 : e1000092 . 10.1371/journal.pcbi.1000092 [ PMC free article ] [ PubMed ] [ CrossRef ] [ Google Scholar ]
- Deco G., Rolls E. T., Romo R. (2009). Stochastic dynamics as a principle of brain function. Prog. Neurobiol. 88 1–16. 10.1016/j.pneurobio.2009.01.006 [ PubMed ] [ CrossRef ] [ Google Scholar ]
- Duan H.-G., Prokhorenko V. I., Cogdell R. J., Ashraf K., Stevens A. L., Thorwart M., et al. (2017). Nature does not rely on long-lived electronic quantum coherence for photosynthetic energy transfer. Proc. Natl. Acad. Sci. 114 8493–8498. 10.1073/pnas.1702261114 [ PMC free article ] [ PubMed ] [ CrossRef ] [ Google Scholar ]
- Engel G. S., Calhoun T. R., Read E. L., Ahn T.-K., Manćal T., Cheng Y.-C., et al. (2007). Evidence for wavelike energy transfer through quantum coherence in photosynthetic systems. Nature 446 782–786. 10.1038/nature05678 [ PubMed ] [ CrossRef ] [ Google Scholar ]
- Fisher M. P. A. (2015). Quantum cognition: the possibility of processing with nuclear spins in the brain. Ann. Phys. 362 593–602. 10.1016/j.aop.2015.08.020 [ CrossRef ] [ Google Scholar ]
- Friston K. (2010). The free-energy principle: a unified brain theory? Nat. Rev. Neurosci. 11 127–138. 10.1038/nrn2787 [ PubMed ] [ CrossRef ] [ Google Scholar ]
- Fröhlich F., McCormick D. A. (2010). Endogenous electric fields may guide neocortical network activity. Neuron 67 129–143. 10.1016/j.neuron.2010.06.005 [ PMC free article ] [ PubMed ] [ CrossRef ] [ Google Scholar ]
- Fujisawa S., Matsuki N., Ikegaya Y. (2006). Single neurons can induce phase transitions of cortical recurrent networks with multiple internal states. Cereb. Cortex 16 639–654. 10.1093/cercor/bhj010 [ PubMed ] [ CrossRef ] [ Google Scholar ]
- Glimcher P. W. (2005). Indeterminacy in brain and behavior. Annu. Rev. Psychol. 56 25–56. 10.1146/annurev.psych.55.090902.141429 [ PubMed ] [ CrossRef ] [ Google Scholar ]
- Hahn G., Petermann T., Havenith M. N., Yu S., Singer W., Plenz D., et al. (2010). Neuronal avalanches in spontaneous activity in vivo. J. Neurophysiol. 104 3312–3322. 10.1152/jn.00953.2009 [ PMC free article ] [ PubMed ] [ CrossRef ] [ Google Scholar ]
- Hahn G., Ponce-Alvarez A., Monier C., Benvenuti G., Kumar A., Chavane F., et al. (2017). Spontaneous cortical activity is transiently poised close to criticality. PLOS Comput. Biol. 13 : e1005543 . 10.1371/journal.pcbi.1005543 [ PMC free article ] [ PubMed ] [ CrossRef ] [ Google Scholar ]
- Hameroff S., Penrose R. (2014). Consciousness in the Universe: a review of the ‘Orch OR’ theory. Phys. Life Rev. 11 39–78. 10.1016/j.plrev.2013.08.002 [ PubMed ] [ CrossRef ] [ Google Scholar ]
- Hameroff S. R., Craddock T. J., Tuszynski J. A. (2014). Quantum effects in the understanding of consciousness. J. Integr. Neurosci. 13 229–252. 10.1142/S0219635214400093 [ PubMed ] [ CrossRef ] [ Google Scholar ]
- Harish O., Hansel D. (2015). Asynchronous rate chaos in spiking neuronal circuits. PLOS Comput. Biol. 11 : e1004266 . 10.1371/journal.pcbi.1004266 [ PMC free article ] [ PubMed ] [ CrossRef ] [ Google Scholar ]
- Haven E., Khrennikov A. (2016). Statistical and subjective interpretations of probability in quantum-like models of cognition and decision making. J. Math. Psychol. 74 82–91. 10.1016/J.JMP.2016.02.005 [ CrossRef ] [ Google Scholar ]
- Hiscock H. G., Worster S., Kattnig D. R., Steers C., Jin Y., Manolopoulos D. E., et al. (2016). The quantum needle of the avian magnetic compass. Proc. Natl. Acad. Sci. U.S.A. 113 4634–4639. 10.1073/pnas.1600341113 [ PMC free article ] [ PubMed ] [ CrossRef ] [ Google Scholar ]
- Huelga S. F., Plenio M. B. (2013). Vibrations, quanta and biology. Contemp. Phys. 54 181–207. 10.1080/00405000.2013.829687 [ CrossRef ] [ Google Scholar ]
- Hunter P. (2006). A quantum leap in biology. One inscrutable field helps another, as quantum physics unravels consciousness. EMBO Rep. 7 971–974. 10.1038/sj.embor.7400802 [ PMC free article ] [ PubMed ] [ CrossRef ] [ Google Scholar ]
- Jedlicka P. (2005). Neuroethics, reductionism and dualism. Trends Cogn. Sci. 9 172 ; author reply 173. 10.1016/j.tics.2005.02.010 [ PubMed ] [ CrossRef ] [ Google Scholar ]
- Jedlicka P. (2007). “ Physical complexity and cognitive evolution ,” in Worldviews, Science and Us: Philosophy and Complexity , eds Gershenson C., Aerts D., Edmonds B. (Singapore: World Scientific; ), 221–231. 10.1142/9789812707420_0014 [ CrossRef ] [ Google Scholar ]
- Jedlicka P. (2009). Quantum Stochasticity and Neuronal Computations. Available at: http://dx.doi.org/10.1038/npre.2009.3702.1 [ Google Scholar ]
- Jedlicka P. (2014). “ Quantum stochasticity and (the end of) neurodeterminism ,” in Quantum Physics Meets the Philosophy of Mind New Essays on the Mind-Body Relation in Quantum-Theoretical Perspective , eds Corradini A., Meixner U. (Berlin: De Gruyer; ), 183–198. [ Google Scholar ]
- Jordan P. (1938). Die verstärkertheorie der organismen in ihrem gegenwärtigen stand. Naturwissenschaften 26 537–545. 10.1007/BF01675497 [ CrossRef ] [ Google Scholar ]
- Kandel E. R. (2001). The molecular biology of memory storage: a dialogue between genes and synapses. Science 294 1030–1038. 10.1126/science.1067020 [ PubMed ] [ CrossRef ] [ Google Scholar ]
- Klinman J. P., Kohen A. (2013). Hydrogen tunneling links protein dynamics to enzyme catalysis. Annu. Rev. Biochem. 82 471–496. 10.1146/annurev-biochem-051710-133623 [ PMC free article ] [ PubMed ] [ CrossRef ] [ Google Scholar ]
- Knill D. C., Pouget A. (2004). The Bayesian brain: the role of uncertainty in neural coding and computation. Trends Neurosci. 27 712–719. 10.1016/j.tins.2004.10.007 [ PubMed ] [ CrossRef ] [ Google Scholar ]
- Koch C. (2009). “ Free will, physics, biology, and the brain ,” in Downward Causation and the Neurobiology of Free Will , eds Murphy N., Ellis G. F. R., O T.’Connor (Berlin: Springer Verlag), 31–52. 10.1007/978-3-642-03205-9_2 [ CrossRef ] [ Google Scholar ]
- Koch C., Hepp K. (2006). Quantum mechanics in the brain. Nature 440 611–612. 10.1038/440611a [ PubMed ] [ CrossRef ] [ Google Scholar ]
- Lambert N., Chen Y.-N., Cheng Y.-C., Li C.-M., Chen G.-Y., Nori F. (2012). Quantum biology. Nat. Phys. 9 10–18. 10.1038/nphys2474 [ CrossRef ] [ Google Scholar ]
- Lee H., Cheng Y.-C., Fleming G. R. (2007). Coherence dynamics in photosynthesis: protein protection of excitonic coherence. Science 316 1462–1465. 10.1126/science.1142188 [ PubMed ] [ CrossRef ] [ Google Scholar ]
- Loewenstein W. R. (ed.) (2013a). “ Consciousness and quantum information ,” in Physics in Mind: A Quantum View of the Brain , Chap. 17 (New York, NY: Basic Books; ). [ Google Scholar ]
- Loewenstein W. R. (ed.) (2013b). “ Quantum Sensing ,” in Physics in Mind: A Quantum View of the Brain , Chap. 5 (New York, NY: Basic Books; ). [ Google Scholar ]
- London M., Häusser M. (2005). Dendritic computation. Annu. Rev. Neurosci. 28 503–532. 10.1146/annurev.neuro.28.061604.135703 [ PubMed ] [ CrossRef ] [ Google Scholar ]
- London M., Roth A., Beeren L., Häusser M., Latham P. E. (2010). Sensitivity to perturbations in vivo implies high noise and suggests rate coding in cortex. Nature 466 123–127. 10.1038/nature09086 [ PMC free article ] [ PubMed ] [ CrossRef ] [ Google Scholar ]
- Markov N. T., Kennedy H. (2013). The importance of being hierarchical. Curr. Opin. Neurobiol. 23 187–194. 10.1016/j.conb.2012.12.008 [ PubMed ] [ CrossRef ] [ Google Scholar ]
- Markram H., Muller E., Ramaswamy S., Reimann M. W., Abdellah M., Sanchez C. A., et al. (2015). Reconstruction and simulation of neocortical microcircuitry. Cell 163 456–492. 10.1016/j.cell.2015.09.029 [ PubMed ] [ CrossRef ] [ Google Scholar ]
- McFadden J. (2002). The conscious electromagnetic information (cemi) field theory: the hard problem made easy? J. Conscious. Stud. 9 45–60. [ Google Scholar ]
- McKemmish L., Reimers J., McKenzie R., Mark A., Hush N. (2009). Penrose-Hameroff orchestrated objective-reduction proposal for human consciousness is not biologically feasible. Phys. Rev. E 80 : 021912 . 10.1103/PhysRevE.80.021912 [ PubMed ] [ CrossRef ] [ Google Scholar ]
- Mogiliansky A. L., Zamir S., Zwirn H. (2009). Type indeterminacy: a model of the KT(Kahneman–Tversky)-man. J. Math. Psychol. 53 349–361. 10.1016/j.jmp.2009.01.001 [ CrossRef ] [ Google Scholar ]
- Monod J. (1974). “ BBC lecture ,” in Beyond Chance and Necessity , ed. Lewis J. (London: Garnstone Press; ). [ Google Scholar ]
- Noble D. (2010). Biophysics and systems biology. Philos. Trans. A Math. Phys. Eng. Sci. 368 1125–1139. 10.1098/rsta.2009.0245 [ PMC free article ] [ PubMed ] [ CrossRef ] [ Google Scholar ]
- Nonnenmacher M., Behrens C., Berens P., Bethge M., Macke J. H. (2017). Signatures of criticality arise from random subsampling in simple population models. PLOS Comput. Biol. 13 : e1005718 . 10.1371/journal.pcbi.1005718 [ PMC free article ] [ PubMed ] [ CrossRef ] [ Google Scholar ]
- Odegaard B., Knight R. T., Lau H. (2017). Should a few null findings falsify prefrontal theories of conscious perception? J. Neurosci. 37 9593–9602. 10.1523/JNEUROSCI.3217-16.2017 [ PMC free article ] [ PubMed ] [ CrossRef ] [ Google Scholar ]
- Panitchayangkoon G., Hayes D., Fransted K. A., Caram J. R., Harel E., Wen J., et al. (2010). Long-lived quantum coherence in photosynthetic complexes at physiological temperature. Proc. Natl. Acad. Sci. U.S.A. 107 12766–12770. 10.1073/pnas.1005484107 [ PMC free article ] [ PubMed ] [ CrossRef ] [ Google Scholar ]
- Panitchayangkoon G., Voronine D. V., Abramavicius D., Caram J. R., Lewis N. H. C., Mukamel S., et al. (2011). Direct evidence of quantum transport in photosynthetic light-harvesting complexes. Proc. Natl. Acad. Sci. U.S.A. 108 20908–20912. 10.1073/pnas.1105234108 [ PMC free article ] [ PubMed ] [ CrossRef ] [ Google Scholar ]
- Penrose R., Dennett D. C. (1995). “ Consciousness involves noncomputable ingredients ,” in The Third Culture: Beyond the Scientific Revolution , ed. Brockman J. (New York City, NY: Simon & Schuster; ). [ Google Scholar ]
- Plenio M. B., Huelga S. F. (2008). Dephasing-assisted transport: quantum networks and biomolecules. New J. Phys. 10 : 113019 10.1088/1367-2630/10/11/113019 [ CrossRef ] [ Google Scholar ]
- Pothos E. M., Busemeyer J. R. (2009). A quantum probability explanation for violations of ‘rational’ decision theory. Proc. Biol. Sci. 276 2171–2178. 10.1098/rspb.2009.0121 [ PMC free article ] [ PubMed ] [ CrossRef ] [ Google Scholar ]
- Priesemann V., Wibral M., Valderrama M., Pröpper R., Le Van Quyen M., Geisel T., et al. (2014). Spike avalanches in vivo suggest a driven, slightly subcritical brain state. Front. Syst. Neurosci. 8 : 108 . 10.3389/fnsys.2014.00108 [ PMC free article ] [ PubMed ] [ CrossRef ] [ Google Scholar ]
- Rolls E. T. (2012). Willed action, free will, and the stochastic neurodynamics of decision-making. Front. Integr. Neurosci. 6 : 68 . 10.3389/fnint.2012.00068 [ PMC free article ] [ PubMed ] [ CrossRef ] [ Google Scholar ]
- Sarovar M., Ishizaki A., Fleming G. R., Birgitta Whaley K. (2010). Quantum entanglement in photosynthetic light-harvesting complexes. Nat. Phys. 6 462–467. 10.1038/nphys1652 [ CrossRef ] [ Google Scholar ]
- Satinover J. (2001). The Quantum Brain: The Search for Freedom and the next Generation of Man. New York, NY: John Wiley & Sons, Inc. [ Google Scholar ]
- Seife C. (2000). Neuroscience. Cold numbers unmake the quantum mind. Science 287 791 . 10.1126/science.287.5454.791 [ PubMed ] [ CrossRef ] [ Google Scholar ]
- Sension R. J. (2007). Biophysics: quantum path to photosynthesis. Nature 446 740–741. 10.1038/446740a [ PubMed ] [ CrossRef ] [ Google Scholar ]
- Singer W. (2009). The brain, a complex self-organizing system. Eur. Rev. 17 321–329. 10.1017/S1062798709000751 [ CrossRef ] [ Google Scholar ]
- Singer W. (2013). Cortical dynamics revisited. Trends Cogn. Sci. 17 616–626. 10.1016/j.tics.2013.09.006 [ PubMed ] [ CrossRef ] [ Google Scholar ]
- Singer W. (2015). “ Complexity as substrate for neuronal computations ,” in Complexity and Analogy in Science: Theoretical, Methodological and Epistemological Aspects , eds Arber W., Mittelstra J.ß, Sánchez Sorondo M. (Vatican City: Pontifical Academy of Sciences; ), 209–218. [ Google Scholar ]
- Singer W., Lazar A. (2016). Does the cerebral cortex exploit high-dimensional, non-linear dynamics for information processing? Front. Comput. Neurosci. 10 : 99 . 10.3389/fncom.2016.00099 [ PMC free article ] [ PubMed ] [ CrossRef ] [ Google Scholar ]
- Sompolinsky H. (2005). “ Scientific perspective on human choice ,” in Judaism, Science, and Moral Responsibility , eds Shatz D., Berger Y. (New York, NY: Rowman & Littlefield; ), 13–44. [ Google Scholar ]
- Tarlac S. (2011). Quantum physics in living matter: from quantum biology to quantum neurobiology. Neuroquantology 9 692–701. 10.1016/j.ijpsycho.2015.02.016 [ PubMed ] [ CrossRef ] [ Google Scholar ]
- Tegmark M. (2000). Importance of quantum decoherence in brain processes. Phys. Rev. E Stat. Phys. Plasmas Fluids Relat. Interdiscip. Topics 61(4 Pt B), 4194–4206. 10.1103/PhysRevE.61.4194 [ PubMed ] [ CrossRef ] [ Google Scholar ]
- Tkaćik G., Bialek W. (2014). Information Processing in Living Systems. Available at: http://arxiv.org/abs/1412.8752 [ Google Scholar ]
- Tognoli E., Scott Kelso J. A. (2014). The metastable brain. Neuron 81 35–48. 10.1016/j.neuron.2013.12.022 [ PMC free article ] [ PubMed ] [ CrossRef ] [ Google Scholar ]
- Tononi G., Boly M., Massimini M., Koch C. (2016). Integrated information theory: from consciousness to its physical substrate. Nat. Rev. Neurosci. 17 450–461. 10.1038/nrn.2016.44 [ PubMed ] [ CrossRef ] [ Google Scholar ]
- Touboul J., Destexhe A. (2010). Can power-law scaling and neuronal avalanches arise from stochastic dynamics? PLOS ONE 5 : e8982 . 10.1371/journal.pone.0008982 [ PMC free article ] [ PubMed ] [ CrossRef ] [ Google Scholar ]
- Trewavas A. (2007). Response to Alpi et al.,: plant neurobiology – all metaphors have value. Trends Plant Sci. 12 231–233. 10.1016/j.tplants.2007.04.006 [ PubMed ] [ CrossRef ] [ Google Scholar ]
- Trewavas A., Baluska F. (2011). The ubiquity of consciousness. EMBO Rep. 12 1221–1225. 10.1038/embor.2011.218 [ PMC free article ] [ PubMed ] [ CrossRef ] [ Google Scholar ]
- Van Regenmortel M. H. V. (2004). Reductionism and complexity in molecular biology. EMBO Rep. 5 1016–1020. 10.1038/sj.embor.7400284 [ PMC free article ] [ PubMed ] [ CrossRef ] [ Google Scholar ]
- van Vreeswijk C., Sompolinsky H. (1996). Chaos in neuronal networks with balanced excitatory and inhibitory activity. Science 274 1724–1726. 10.1126/science.274.5293.1724 [ PubMed ] [ CrossRef ] [ Google Scholar ]
- Vattay G., Kauffman S., Niiranen S. (2014). Quantum biology on the edge of quantum chaos. PLOS ONE 9 : e89017 . 10.1371/journal.pone.0089017 [ PMC free article ] [ PubMed ] [ CrossRef ] [ Google Scholar ]
- Vaziri A., Plenio M. (2010). Quantum coherence in ion channels: resonances, transport and verification. New J. Phys. 12 : 85001 10.1088/1367-2630/12/8/085001 [ CrossRef ] [ Google Scholar ]
- Vedral V. (2011). Living in a quantum world. Sci. Am. 304 38–43. 10.1038/scientificamerican0611-38 [ PubMed ] [ CrossRef ] [ Google Scholar ]
- Wang Q., Schoenlein R. W., Peteanu L. A., Mathies R. A., Shank C. V. (1994). Vibrationally coherent photochemistry in the femtosecond primary event of vision. Science 266 422–424. 10.1126/science.7939680 [ PubMed ] [ CrossRef ] [ Google Scholar ]
- Wang Z., Solloway T., Shiffrin R. M., Busemeyer J. R. (2014). Context effects produced by question orders reveal quantum nature of human judgments. Proc. Natl. Acad. Sci. U.S.A. 111 9431–9436. 10.1073/pnas.1407756111 [ PMC free article ] [ PubMed ] [ CrossRef ] [ Google Scholar ]
- Weingarten C. P., Murali Doraiswamy P., Fisher M. P. A. (2016). A new spin on neural processing: quantum cognition. Front. Hum. Neurosci. 10 : 541 . 10.3389/fnhum.2016.00541 [ PMC free article ] [ PubMed ] [ CrossRef ] [ Google Scholar ]
- Wolf F., Engelken R., Puelma-Touzel M., Weidinger J. D., Neef A. (2014). Dynamical models of cortical circuits. Curr. Opin. Neurobiol. 25 228–236. 10.1016/j.conb.2014.01.017 [ PubMed ] [ CrossRef ] [ Google Scholar ]
- Yukalov V. I., Sornette D. (2011). Decision theory with prospect interference and entanglement. Theory Decis. 70 283–328. 10.1007/s11238-010-9202-y [ CrossRef ] [ Google Scholar ]
Raptor Research Center
- Share on Facebook
- Share on Twitter
- Share on LinkedIn
- Share through Email
- Student & Alumni Updates
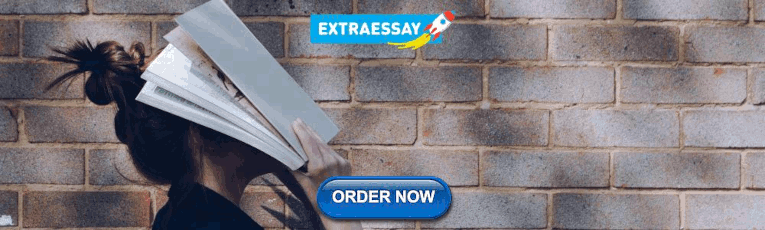
Ashley Santiago Thesis Defense
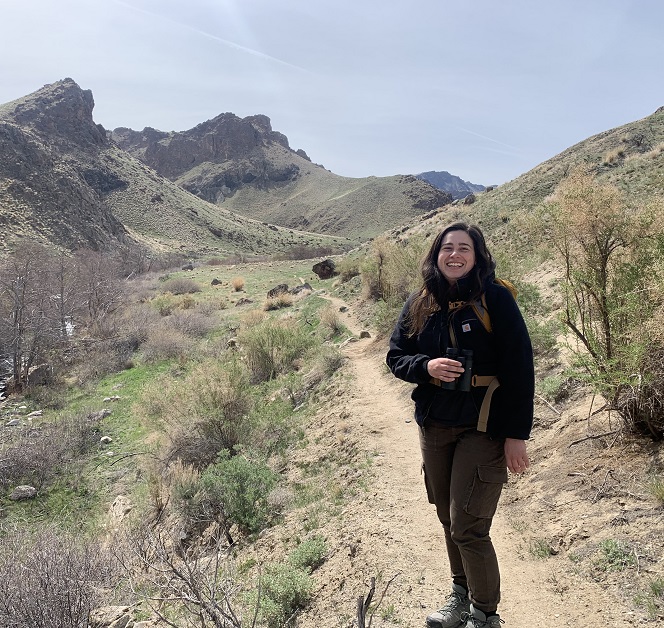
Boise State University MS Raptor Biology student Ashley Santiago defended her thesis, “Associations Between Landscape Changes And Declines In Golden Eagle Territory Occupancy In The Morley Nelson Snake River Birds Of Prey National Conservation Area ” on the morning of April 19, 2024. Santiago’s main advisor is Julie Heath (MS, Raptor Biology, ’96; Boise State University Raptor Research Center , Biological Sciences ) , and her committee includes Jen Cruz ( Boise State University Raptor Research Center , Biological Sciences) and Donna Delparte (Boise State University Biological Sciences).
Rapid loss of native shrubs and expansion of invasive annuals like cheatgrass (Bromus tectorum) have extensively altered shrub-steppe systems. Vegetation changes can have bottom-up effects on trophic interactions and ecosystem dynamics. Predators, like Golden Eagles (Aquila chrysaetos) that forage on shrub-reliant prey, may increase their territory size to cope with degraded habitat or abandon historically occupied territories sites. We used a multi-season occupancy model that accounted for imperfect detection to investigate the associations between landscape cover (i.e., shrub and annual herbaceous), fire history, neighbor density, and eagle territory occupancy of 36 Golden Eagle nesting territories in the Morley Nelson Snake River Birds of Prey National Conservation Area (NCA), Idaho, USA from 1986–2022.
The probability of Golden Eagle occupancy decreased 23% from 0.82 in 1986 to 0.59 in 2022. Further, once territories became vacant, they tended to remain vacant, suggesting that the territories were no longer suitable for eagles or no new eagles were recruiting into the population. Territory occupancy was negatively associated with annual herbaceous cover (β: -0.86, 95% CI: -1.53 to -0.24) and tended to be positively associated with shrub cover (β: 0.63, 95% CI: -0.11 to 1.39) and time since last burn (β: 0.25, 95% CI: -0.28 to 0.83). Territories that were farther from other occupied territories had a higher probability of occupancy than territories with closer neighbors (β: -3.56, 95% CI: -4.22 to -2.92), likely because as habitat degraded, eagles compensated by expanding their territories into nearby vacant territories. The probability of detection was high at 0.90 but improved in surveys conducted earlier in the day and later in the breeding season.
Together, these results show that landscape change and habitat degradation have bottom-up effects on Golden Eagle behavior and territory occupancy, resulting in an overall decrease in the local carrying capacity for eagles. Understanding associations between environmental change and territory occupancy has important implications for predicting population persistence and eagle adaptability to changing landscapes.
Thank you for visiting nature.com. You are using a browser version with limited support for CSS. To obtain the best experience, we recommend you use a more up to date browser (or turn off compatibility mode in Internet Explorer). In the meantime, to ensure continued support, we are displaying the site without styles and JavaScript.
- View all journals
- My Account Login
- Explore content
- About the journal
- Publish with us
- Sign up for alerts
- Open access
- Published: 22 October 2018
Efficient quantum simulation of photosynthetic light harvesting
- Bi-Xue Wang 1 na1 ,
- Ming-Jie Tao 1 , 2 na1 ,
- Qing Ai ORCID: orcid.org/0000-0002-0116-7844 2 , 3 na1 ,
- Tao Xin 1 ,
- Neill Lambert 3 ,
- Dong Ruan 1 ,
- Yuan-Chung Cheng 4 ,
- Franco Nori ORCID: orcid.org/0000-0003-3682-7432 3 , 5 ,
- Fu-Guo Deng 2 , 6 &
- Gui-Lu Long 1 , 7 , 8 , 9 , 10
npj Quantum Information volume 4 , Article number: 52 ( 2018 ) Cite this article
8133 Accesses
93 Citations
83 Altmetric
Metrics details
- Quantum information
- Quantum optics
Near-unity energy transfer efficiency has been widely observed in natural photosynthetic complexes. This phenomenon has attracted broad interest from different fields, such as physics, biology, chemistry, and material science, as it may offer valuable insights into efficient solar-energy harvesting. Recently, quantum coherent effects have been discovered in photosynthetic light harvesting, and their potential role on energy transfer has seen the heated debate. Here, we perform an experimental quantum simulation of photosynthetic energy transfer using nuclear magnetic resonance (NMR). We show that an N -chromophore photosynthetic complex, with arbitrary structure and bath spectral density, can be effectively simulated by a system with log 2 N qubits. The computational cost of simulating such a system with a theoretical tool, like the hierarchical equation of motion, which is exponential in N , can be potentially reduced to requiring a just polynomial number of qubits N using NMR quantum simulation. The benefits of performing such quantum simulation in NMR are even greater when the spectral density is complex, as in natural photosynthetic complexes. These findings may shed light on quantum coherence in energy transfer and help to provide design principles for efficient artificial light harvesting.
Similar content being viewed by others
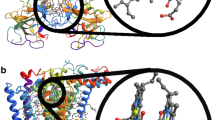
Exact simulation of pigment-protein complexes unveils vibronic renormalization of electronic parameters in ultrafast spectroscopy
F. Caycedo-Soler, A. Mattioni, … M. B. Plenio
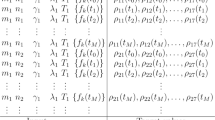
Predicting the future of excitation energy transfer in light-harvesting complex with artificial intelligence-based quantum dynamics
Arif Ullah & Pavlo O. Dral
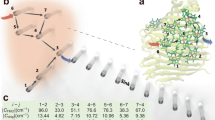
Simulating photosynthetic energy transport on a photonic network
Hao Tang, Xiao-Wen Shang, … Xian-Min Jin
Introduction
Efficient exciton energy transfer (EET) is crucial in photosynthesis and solar cells, 1 , 2 especially when the systems are large, 3 e.g., as in Photosystem I (PSI) and Photosystem II (PSII), which have hundreds of chromophores. A comprehensive knowledge of the quantum dynamics of such systems would be of potential importance to the study on EET. 4 Much effort has been made to reveal the effects of quantum coherence on efficient energy transfer. 4 , 5 , 6 , 7 , 8 , 9 , 10 , 11 In order to try to mimic EET, a Frenkel-exciton Hamiltonian is required and this can be studied with quantum chemistry approaches, 1 e.g., fitting experimental spectra, or calculations by density functional theory. Because photosynthetic pigment–protein complexes are intrinsically open quantum systems, with system-bath couplings comparable to the intra-system couplings, it is difficult to faithfully mimic the exact quantum dynamics of EET. Among the methods for describing EET, 1 , 11 , 12 , 13 the hierarchical equation of motion (HEOM) yields a numerically exact solution at the cost of considerable computing time. 11 , 14 For the widely-studied Fenna–Matthews–Olson (FMO) complex, 5 , 6 , 11 , 13 , 15 , 16 a reliable result could be produced by 16,170 coupled differential equations at low temperatures, based on the HEOM 11 , 14 with 4 layers. Moreover, the HEOM encounters difficulties when the system has non-trivial spectral density, making it often difficult to verify the results. On the other hand, due to heterogeneity, e.g., static disorder and conformational change, it is difficult to experimentally verify the theoretical predictions in natural photosynthetic systems. Because quantum simulations with N qubits can powerfully mimic the quantum dynamics of 2 N states by virtue of quantum mechanics, 17 , 18 , 19 the quantum simulation of photosynthetic energy transfer with an arbitrary Hamiltonian by nuclear magnetic resonance (NMR) provides an intriguing approach to verify theoretical predictions. Recently, a newly-developed technique which could simulate the effect of a bath with an arbitrary spectral density by a set of classical pulses has been successfully realized with ion traps 20 and NMR. 21 Therefore, photosynthetic light-harvesting with an arbitrary Hamiltonian and spectral density, describing a structured environment, can be experimentally simulated by NMR.
NMR is an excellent platform for quantum simulation since it is easy to operate and it can have long coherence times. 22 , 23 In this paper, NMR is utilized to simulate the quantum coherent dynamics in photosynthetic light harvesting. As a prototype, a tetramer including four chlorophylls, 13 as schematically illustrated in Fig. 1a , is employed in the NMR quantum simulation. In a previous investigation, 13 the tetramer model was exploited to study the clustered geometry utilizing exciton delocalization and energy matching to accelerate the energy transfer. The EET in photosynthesis is described by the Frenkel-exciton Hamiltonian
where \(\left| i \right\rangle\) ( i = 1, 2, 3, 4) is the state with a single excitation at site i and other sites at the ground state, ε i is the site energy of \(\left| i \right\rangle\) , J ij is the excitonic interaction between sites i and j . In our quantum simulations, we adopt the site energies 13 ε 1 = 13,000 cm −1 , ε 2 = 12,900 cm −1 , ε 3 = 12,300 cm −1 , and ε 4 = 12,200 cm −1 , and the couplings J 12 = J 34 = 126 cm −1 , J 13 = J 24 = 16 cm −1 , J 23 = 132 cm −1 , and J 14 = 5 cm −1 , which are typical parameters in photosynthetic systems.
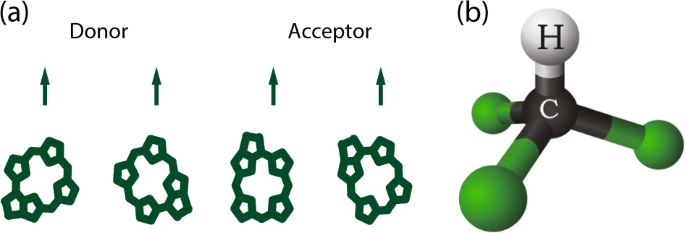
Photosynthetic chromophore arrangement and physical system for NMR simulation. a Linear geometry with four chromophores for photosynthetic energy transfer. b Chemical structure for a 13 C-labeled chloroform molecule, where the H and C nuclear spins are chosen as the two qubits
For a photosynthetic complex with N chlorophylls, there are only N single-excitation states involved in the energy transfer. Therefore, only log 2 N qubits are required to realize the quantum simulation. To mimic the energy transfer described by the above Hamiltonian ( 1 ), two qubits are necessary for the quantum simulation. In this case, the photosynthetic single-excitation state \(\left| i \right\rangle\) is encoded as a two-qubit product state, i.e., \(\left| {00} \right\rangle\) , \(\left| {01} \right\rangle\) , \(\left| {10} \right\rangle\) , and \(\left| {11} \right\rangle\) . By a straightforward calculation, the Frenkel-exciton Hamiltonian can be mapped to the NMR Hamiltonian as
where \(\sigma _j^u\) ( j = 1, 2, u = x , y , z ) is the Pauli operator for qubit j , numerically \(\varepsilon _j^\prime = \pi \varepsilon _j{\mathrm{/}}10\) and \(J_{ij}^\prime = \pi J_{ij}{\mathrm{/}}10\) , but the dimension cm −1 should be replaced by kHz. In other words, all realistic parameters have been scaled down in energy by a factor of 1 cm −1 /( π /10 kHz) = 3 × 10 8 / π .
The excitonic coupling J ij between sites i and j makes the exciton energy hop in both directions, i.e., \(\left| i \right\rangle\) ⇆ \(\left| j \right\rangle\) . Apart from this, the system-bath couplings will facilitate the energy flow towards an energy trap, where the captured photon energy is converted into chemical energy. 2 , 3 The interaction between the system and bath in photosynthetic complexes can be described by
where \(a_{ik}^\dagger\) is the creation operator for the k th phonon mode of chlorophyll i with coupling strength g ik . H SB will induce pure-dephasing on the i -th chromophore when it is in the excited state. Generally, the system-bath couplings are given by the spectral density
which we assume identical for all chromophores. For typical photosynthetic complexes, the system-bath couplings are of the same order as the intra-system couplings. In order to mimic the effects of noise, we utilize the bath-engineering technique which has been successfully implemented in ion traps and NMR. The implementation of a pure-dephasing Hamiltonian
relies on generating stochastic errors by performing phase modulations on a constant-amplitude carrier, i.e.,
where Ω 0 is the constant amplitude of a magnetic field with driving frequency ω μ , ψ j is a random number, ω j = jω 0 with ω 0 and ω J = Jω 0 being base and cutoff frequencies, respectively, and α is a global scaling factor. The power-spectral density \(S_z(\omega ) \equiv {\int} {\kern 1pt} d\tau \left\langle {\dot \phi _N(t + \tau )\dot \phi _N(t)} \right\rangle e^{i\omega \tau }\) is the Fourier transform of the autocorrelation function of \(\dot \phi _N(t)\) ,
By appropriately choosing F ( j ) and the cutoff J , an arbitrary power-spectral density of the bath can be realized, e.g., white, Ohmic, or Drude–Lorentz spectral densities. For the details, please refer to the Supplementary Information .
As illustrated in Fig. 1b , the nuclear spins of the carbon atom and hydrogen atom in a chloroform molecule are chosen to encode the two qubits with the Hamiltonian written as
where ω 1 = 3206.5 Hz, ω 2 = 7787.9 Hz are the chemical shifts of the two spins, and J = 215.1 Hz (ref. 24 ). Therefore, in order to simulate the quantum dynamics of energy transfer, the entire evolution is decomposed into repetitive identical cycles. After each cycle, there is a small difference between the exact evolution and the simulated one. However, because there are tens of thousands of cycles before completing the energy transfer, the accumulated error might be significant so that the simulation becomes unreliable. To avoid this problem, we utilize the gradient ascent pulse engineering (GRAPE) algorithm, 25 which has been successfully applied to a number of quantum simulations in NMR, 23 , 26 , 27 to mimic the quantum dynamics of light harvesting.
Before studying the population dynamics, we shall explore the characteristics of the artificially injected noise. Generally, the noise is characterized by Ramsey interference measurements, as shown in Fig. 2 . When there is no noise applied to the system, we would expect coherent evolution without damping. However, as the artificial noise is applied, the oscillations become damped. The envelope of the damped oscillations is fully determined by the noise strength. Interestingly, for the parameters employed in this work for a broad-frequency background bath, the NMR experimental results exactly fit the theoretical prediction by HEOM. This suggests that our technique for injecting artificial noise can, in this limit, faithfully reproduce the effect of the protein environment on natural photosynthetic complexes.
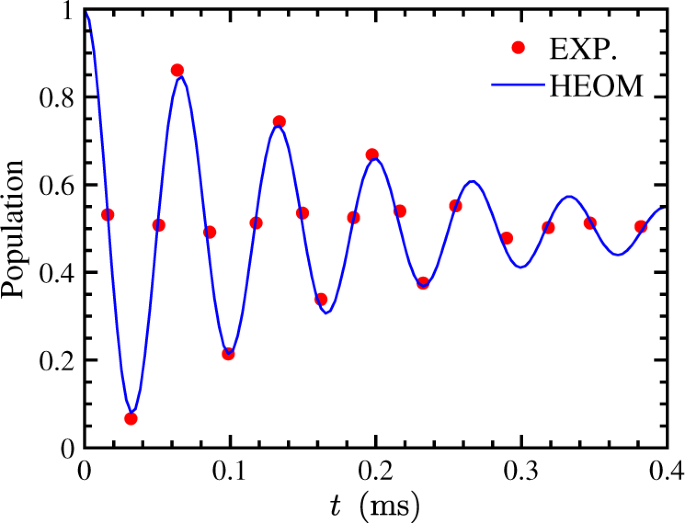
The decay of the population of the state \(\left| 0 \right\rangle\) . The theoretical simulation adopting HEOM is shown by the blue curve, and the experimental data by the red dots. In our experiment, the parameters are ε D − ε A = 2 π × 15 kHz, dt = 20 μs, and M = 50. The parameters of the Drude–Lorentz noise are γ NMR = 2 π × 45 kHz, λ NMR = 2 π × 0.01 kHz, T EET = 300 K, ω 0 = 2 π × 5 Hz, and ω J = 2 π × 25 kHz
In Fig. 3a , the quantum coherent energy transfer for the above Hamiltonian H NMR + H PDN , using an initial condition where an excitation is localized on the first chromophore, is simulated in NMR. In the short-time regime, cf. Fig. 3b , there are Rabi-like oscillations of coherent energy transfer between the two levels with the highest energies, because there is a strong coupling between these two levels. Furthermore, the oscillation quickly damps as the energy transfer is irreversible due to the pure-dephasing noise. After an exponential decay process, the populations on all levels reach thermal equilibrium. Noticeably, there are small oscillations for the two lowest-energy levels as a result of their strong coupling. For each point in the quantum simulation, the data is averaged over M random realizations. For a given realization, the system undergoes a coherent evolution by applying a time-dependent magnetic field with fluctuating phases, as shown in Eqs. ( 6 ) and ( 7 ). However, for an ensemble of random realizations, since each realization experiences a different phase at a given time, the ensemble average manifests itself as a single realization undergoing a pure-dephasing noise. In this regard, the deviation of the NMR simulation from that predicted by the HEOM decreases as the number of realizations in the ensemble increases. In Fig. 4 , we show the effect on the simulation error of the number of realizations in each ensemble. Both results, by NMR and by HEOM, converge as M increases, such that the difference between them can hardly be noticed when M ~ 500. This effect would be more remarkable if M were increased further, as confirmed by our theoretical simulations in the Supplementary Information . In conclusion, the dephasing Hamiltonian ( 3 ) in photosynthesis is effectively mimicked by a classical time-fluctuating magnetic field (( 6 ) and ( 7 )).
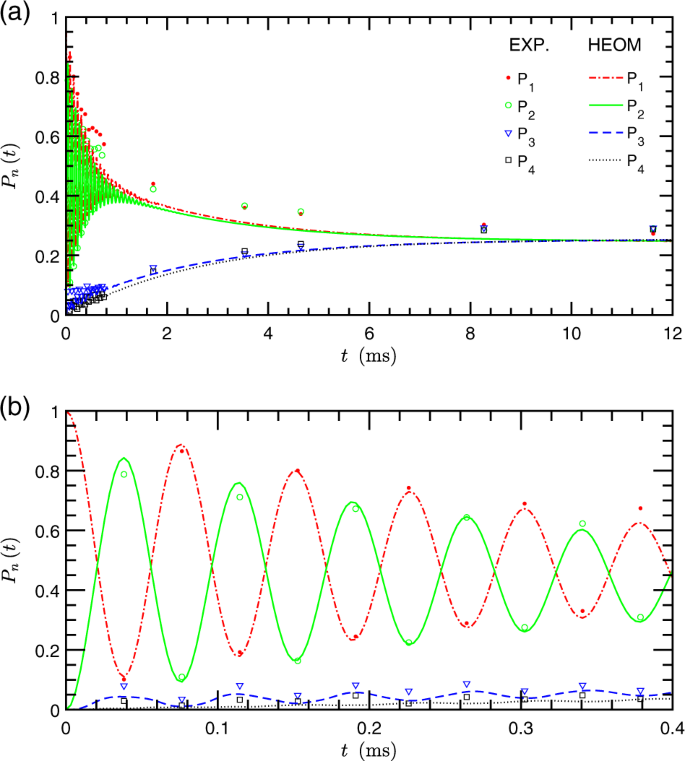
Simulation of the energy transfer governed by H NMR + H PDN for M = 150 random realizations. a Long-time quantum dynamics; b short-time quantum dynamics. The symbols show the experimental data, and the curves are obtained from the numerical simulation using the HEOM. In all figures, the horizontal coordinates of the curves for EET dynamics have been magnified by 3 × 10 8 / π times
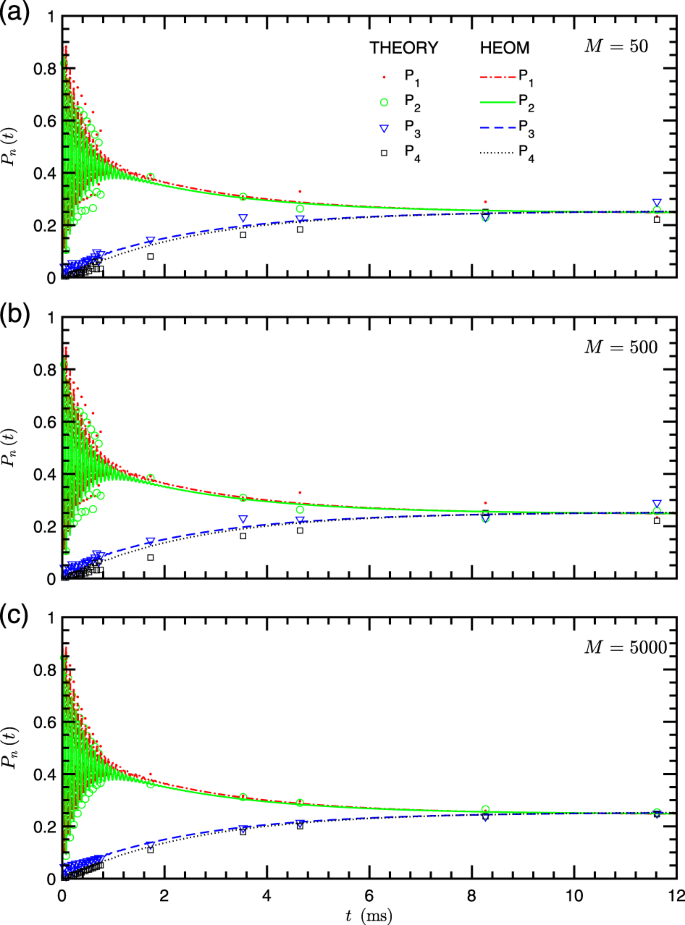
Effect of the ensemble size on the simulations. The symbols show the theoretical results and the curves show the HEOM results. The number of realizations in each ensemble are M = 50 a , 500 b , and 5000 c
It has been suggested in the literature 6 , 28 , 29 that the long-lasting electronic coherence observed in experiments might be attributed to strong coupling to vibrational modes. Therefore, it is interesting to consider whether it is possible to simulate some features of underdamped vibrational modes in the NMR platform. In photosynthesis, the coupling to a vibrational mode can be described by \(\mathop {\sum}\nolimits_i {\kern 1pt} g_{iv}\left| i \right\rangle \left\langle i \right|\left( {a_{iv}^\dagger + a_{iv}} \right)\) . In order to simulate the underdamped vibrational mode in our protocol, we can supplement an additional term, i.e., αF ( v ) sin( ω v t ) into Eq. ( 7 ). In Fig. 5 , we show the numerical simulation for the above 4-level system including such a “semi-classical” vibrational mode. Because we set the vibrational mode to be closely-resonant with the two lower exciton states, there is significantly prolonged coherence in the two lower energy states in the short-time regime, as compared to Fig. 3 . Since there is no random phase associated with this modulation, and quantum properties of the vibrational mode are neglected, each realization will evolve uniformly in Fig. 5 . Therefore, the long-lasting coherence is driven by the nearly-resonant driving. Going beyond a semi-classical approach, and accurately capturing the quantum features of this mode, requires the use of ancillary degrees of freedom, as discussed in the conclusions.
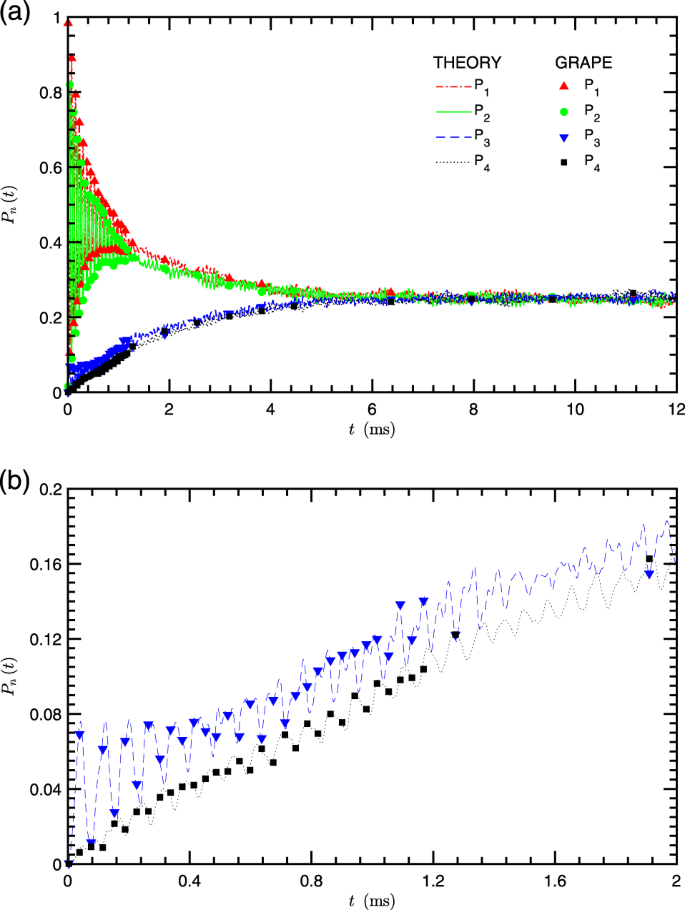
The long-lasting electronic coherence due to coupling to a vibrational mode. a Long-time quantum dynamics; b short-time quantum dynamics to show the prolonged coherence of the two lower levels. The symbols show the GRAPE results and the curves show theoretical simulations. The number of realizations in each ensemble is M = 500
Note that the proof-of-concept NMR experiment presented in this work goes beyond reproducing the HEOM results. To faithfully simulate the EET dynamics on a large photosynthetic system, using the HEOM would be computationally unaffordable. In addition, the HEOM 11 , 14 is known to encounter difficulties when the spectral density is not in a simple Drude–Lorentz form. As shown in the Supplementary Information , the computational cost of HEOM scales exponentially with the system size and the number of exponentials in the bath correlation function. 11 , 14 Our NMR simulations do not have such shortcomings, as it scales polynomially with respect to the system size. 30 , 31 Moreover, the quantum simulation algorithm also scales more favorably in terms of the number of exponentials in the bath correlation function. Notice that our simulation proposal is designed for a Gaussian bath. Interestingly, a recently-developed stochastic Liouville equation approach 32 can effectively handle open-system dynamics for spin and fermion bath. In the future it might be beneficial to generalize our approach to handle non-Gaussian baths. With our current approach, in principle, a photosynthetic system with ~100 sites (e.g., the PSI complex) requires a 7-qubit quantum simulator. This means that the coherent EET dynamics of a full functional biological unit for photosynthesis (e.g., the PSII supercomplex that contains ~300 sites) can be faithfully simulated by a 9-qubit NMR quantum computer. Recently, the quantum control on 12 qubits has been successfully demonstrated on an NMR system, 31 where the shortest \(T_2^ \ast\) is 150 ms. In natural photosynthetic complexes, the energy transfer is completed within 50 ps. For the scaling factor in our proposal, it corresponds to 7.5 ms, which is smaller than \(T_2^ \ast\) by one order of magnitude. Therefore, it is reasonable to simulate the coherent energy transfer within the intrinsic decoherence time of NMR systems. Thus, we believe that NMR quantum simulation has the potential to help clarify the mysteries of light harvesting in natural photosynthesis. In this regard, other approaches, which do not take advantage of this scaling provided by encoding multiple sites into a single qubit, 33 currently lack the required size to simulate such large photosynthetic systems, and thus may also benefit from the approach we develop here.
On the other hand, in natural photosynthetic complexes, the energy is transferred from the outer antenna to the reaction center. In principle, the excitation depletion at the reaction center could be simulated in the NMR platform by coupling auxiliary spins to the energy sink site to remove the population, which is similar to introducing an amplitude damping error in the simulations. 34 In order to prevent the back transfer of energy, there is a large energy gap between the lowest eigenstate of the outer antenna and the reaction center. Thus, the transfer rate from the lowest-energy state to the reaction center is much smaller than the transfer rate within the outer antenna. It is reasonable to simulate the energy transfer without the reaction center. 35 In our theoretical exploration, 13 we investigate the geometrical effects on the energy transfer. In another exploration 36 , it was shown that tuning the phases of the inter-molecular couplings can further optimize the energy-transfer efficiency. In future work, it might be interesting to investigate the effect of the couplings’ phases on the NMR quantum simulation.
In this paper, the photosynthetic energy transfer is experimentally simulated using NMR. As a prototype, a two-qubit NMR system is utilized to demonstrate both: coherent oscillations in the short-time regime and steady-state thermalization for long times. By using the GRAPE technique, an arbitrary photosynthetic system can be faithfully mapped to the NMR system. Moreover, the effect of the environment on EET can be effectively mimicked by a set of well-designed pulses, which act as a classical pure-dephasing noise. The quantum simulation of photosynthetic energy transfer in NMR would facilitate the investigation of quantum coherent effects on the EET and provide clearer design principles for artificial light-harvesting devices together with structured baths. 13 , 37
It was experimentally verified in circuit QED 33 that a structured bath can optimize the energy transfer in EET. 37 Vibrationally assisted energy transfer was demonstrated in a trapped-ion quantum simulator. 38 In both cases, the physical setups therein showed their potential to verify the design principles in optimal photosynthetic light harvesting. However, here we showed that we can use NMR and bath-engineering techniques to efficiently mimic exactly photosynthetic light harvesting in large systems with arbitrary system structure. On the other hand, with our current approach, the simulation may not capture quantum features of the system–environment interaction that arises at stronger couplings and lower temperatures. Capturing such correlations by encoding them in ancillary qubits 39 is a potentially rich avenue for future explorations.
Physical parameters
The Hamiltonian H NMR implemented in our NMR simulations is the same as the photosynthetic Hamiltonian H EET , but with the unit cm −1 divided by 3 × 10 4 / π . The diagonal terms are
The inter-level couplings are: the nearest neighboring couplings
the next-nearest-neighboring couplings
and the coupling between the two ends
The temperature of the photosynthetic energy transfer and NMR experiment are respectively T EET = 3 × 10 4 K and T NMR = 5 × 10 −5 K. The reorganization energy of the bath is λ EET = 0.2 cm −1 . The cutoff frequency of the bath is γ EET = 900 cm −1 .
Both the Hamiltonian and bath parameters for NMR experiment are those for the photosynthetic energy transfer which are scaled down by a factor of 3 × 10 8 / π .
Initialization
Starting from the thermal-equilibrium state, we prepare a pseudo-pure state 40 , 41 using the spatial-average technique. 41
Measurement
The goal is to acquire probability distributions of four states \(\left| {00} \right\rangle\) , \(\left| {01} \right\rangle\) , \(\left| {10} \right\rangle\) , and \(\left| {11} \right\rangle\) after the pulse sequences that we designed are implemented on the two-qubit NMR system, namely, four diagonal values of the final density matrix. The density matrices of the output states are reconstructed completely via quantum state tomography. 42 Therefore, the density matrix of the system can be estimated from ensemble averages of a set of observables. For the one-qubit system, the observable set is { σ i } ( i = 0, 1, 2, 3). Here, σ 0 = I , σ 1 = σ x , σ 2 = σ y , σ 3 = σ z . For the two-qubit system, the observable set is { σ i ⊗ σ j } ( i , j = 0, 1, 2, 3). In our experiments, the complete density matrix tomography is not necessary. All we need is to perform two experiments in which the reading-out pulses exp(− iπσ y /4) ⊗ I and I ⊗ exp(− iπσ y /4) are respectively implemented on the final states of 1 H and 13 C. The symbols in Fig. 3 are obtained by averaging over M = 150 random realizations.
Ramsey spectroscopy
In our experiments, we perform Ramsey spectroscopy for a single qubit subject to Drude–Lorentz noise. In particular, the experiment is divided into three steps:
Preparing the state \(\left| 0 \right\rangle\) and rotating it to the xy plane by a π /2 pulse along the x -axis.
Free evolution while adding the Drude–Lorentz noise.
Applying a reverse π /2 pulse and measuring the population of the state \(\left| 0 \right\rangle\) .
We observe that the decay time constant of the population of the state \(\left| 0 \right\rangle\) is significantly reduced in the presence of the Drude–Lorentz noise, as shown in Fig. 2 . Ramsey fringes are a fit to a cosine with a simple exponential decay envelope. The result by the HEOM theory (blue curve in Fig. 2 ) is consistent with that in experiments (red dots in Fig. 2 ). In other words, we demonstrate that χ ( t ) = Re[ g ( t )].
Vibrational mode
In photosynthetic energy transfer, the coupling to a vibrational mode is described by \(\mathop {\sum}\nolimits_i {\kern 1pt} g_{iv}\left| i \right\rangle \left\langle i \right|\left( {a_{iv}^\dagger + a_{iv}} \right)\) . In order to simulate the underdamped vibrational mode, we can supplement an additional term into Eq. ( 7 ), i.e., αF ( v ) sin( \(\omega _{{\mathrm{NMR}}}^vt\) ). Here we choose \(\omega _{{\mathrm{NMR}}}^v\) = 2 π × 12.5 kHz and \(\alpha F(v) = \sqrt {0.1} \times \omega _{{\mathrm{NMR}}}^v\) . Since there is no random phase associated with this modulation, i.e., ψ v = 0, each realization will be driven uniformly. Because we set the vibrational mode to be closely-resonant with the two lower exciton states, i.e.,
and the Huang–Rhys factor ( g iv / \(\omega _{{\mathrm{EET}}}^v\) ) 2 = 0.1, the long-lived coherence observed in experiments can be effectively simulated, as shown in Fig. 5 .
Data availability
The data that support the findings of this study are available from the corresponding author upon reasonable request.
Cheng, Y.-C. & Fleming, G. R. Dynamics of light harvesting in photosynthesis. Annu. Rev. Phys. Chem. 60 , 241–262 (2009).
Article ADS Google Scholar
Romero, E., Novoderezhkin, V. I. & van Grondelle, R. Quantum design of photosynthesis for bio-inspired solar-energy conversion. Nature 543 , 647–656 (2017).
Article Google Scholar
Scholes, G. D. et al. Using coherence to enhance function in chemical and biophysical systems. Nature 543 , 647–656 (2017).
Lambert, N., Chen, Y.-N., Cheng, Y.-C., Chen, G.-Y. & Nori, F. Quantum biology. Nat. Phys. 9 , 10–18 (2013).
Engel, G. S. et al. Evidence for wavelike energy transfer through quantum coherence in photosynthetic systems. Nature 4 , 782–786 (2007).
Chin, A. W. et al. The role of non-equilibrium vibrational structures in electronic coherence and recoherence in pigment–protein complexes. Nat. Phys. 9 , 1–6 (2013).
Collini, E. et al. Coherently wired light-harvesting in photosynthetic marine algae at ambient temperature. Nature 463 , 644–647 (2010).
Hildner, R., Brinks, D., Nieder, J. B., Cogdell, R. J. & van Hulst, N. F. Quantum coherent energy transfer over varying pathways in single light-harvesting complexes. Science 340 , 1448–1451 (2013).
Dong, H., Xu, D. Z., Huang, J.-F. & Sun, C.-P. Coherent excitation transfer via the dark-state channel in a bionic system. Light Sci. Appl. 1 , e2 (2012).
Xu, L., Gong, Z. R., Tao, M. J. & Ai, Q. Artificial light harvesting by dimerized Möbius ring. Phys. Rev. E 97 , 042124 (2018).
Article ADS MathSciNet Google Scholar
Ishizaki, A. & Fleming, G. R. Theoretical examination of quantum coherence in a photosynthetic system at physiological temperature. Proc. Natl. Acad. Sci. U.S.A. 106 , 17255–17260 (2009).
Ai, Q., Fan, Y.-J., Jin, B.-Y. & Cheng, Y.-C. An efficient quantum jump method for coherent energy transfer dynamics in photosynthetic systems under the influence of laser fields. New J. Phys. 16 , 053033 (2014).
Ai, Q., Yen, T.-C., Jin, B.-Y. & Cheng, Y.-C. Clustered geometries exploiting quantum coherence effects for efficient energy transfer in light harvesting. J. Phys. Chem. Lett. 4 , 2577–2584 (2013).
Tanimura, Y. Stochastic Liouville, Langevin, Fokker–Planck, and master equation approaches to quantum dissipative systems. J. Phys. Soc. Jpn. 75 , 082001 (2006).
Li, C. M., Lambert, N., Chen, Y. N., Chen, G. Y. & Nori, F. Witnessing quantum coherence: from solid-state to biological systems. Sci. Rep. 2 , 885 (2012).
Tao, M.-J., Ai, Q., Deng, F.-G. & Cheng, Y.-C. Proposal for probing energy transfer pathway by single molecule pump–dump experiment. Sci. Rep. 6 , 27535 (2016).
Buluta, I. & Nori, F. Quantum simulators. Science 326 , 108–111 (2009).
Georgescu, I., Ashhab, S. & Nori, F. Quantum simulation. Rev. Mod. Phys. 86 , 153–185 (2014).
Buluta, I., Ashhab, S. & Nori, F. Natural and artificial atoms for quantum computation. Rep. Prog. Phys. 74 , 104401 (2011).
Soare, A. et al. Experimental noise filtering by quantum control. Nat. Phys. 10 , 825–829 (2014).
Zhen, X.-L., Zhang, F.-H., Feng, G. R., Li, H. & Long, G.-L. Optimal experimental dynamical decoupling of both longitudinal and transverse relaxations. Phys. Rev. A 93 , 022304 (2016).
Luo, Z. H. et al. Experimentally probing topological order and its breakdown through modular matrices. Nat. Phys. 14 , 160–165 (2018).
Feng, G. R., Xu, G. F. & Long, G. L. Experimental realization of nonadiabatic holonomic quantum computation. Phys. Rev. Lett. 110 , 190501 (2013).
Chuang, I. L., Vandersypen, L. M. K., Zhou, X. L., Leung, D. W. & Lloyd, S. Experimental realization of a quantum algorithm. Nature 393 , 143–146 (1998).
Khaneja, N., Reiss, T., Kehlet, C. & Schulte-Herbrüggen, T. Optimal control of coupled spin dynamics: design of NMR pulse sequences by gradient ascent algorithms. J. Magn. Reson. 172 , 296–305 (2005).
Zhang, J. F., Wei, T.-C. & Laflamme, R. Experimental quantum simulation of entanglement in many-body systems. Phys. Rev. Lett. 107 , 010501 (2011).
Peng, X. H. et al. Experimental implementation of adiabatic passage between different topological orders. Phys. Rev. Lett. 113 , 080404 (2014).
Santamore, D. H., Lambert, N. & Nori, F. Vibrationally mediated transport in molecular transistors. Phys. Rev. B 87 , 075422 (2013).
Wertnik, M., Chin, A. W., Nori, F. & Lambert, N. Optimizing co-operative multi-environment dynamics in a dark-state-enhanced photosynthetic heat engine. J. Chem. Phys. 149 , 084112 (2018).
Li, J., Yang, X. D., Peng, X. H. & Sun, C.-P. Hybrid quantum-classical approach to quantum optimal control. Phys. Rev. Lett. 118 , 150503 (2017).
Lu, D. W. et al. Enhancing quantum control by bootstrapping a quantum processor of 12 qubits. npj Quantum Inf. 3 , 45 (2017).
Hsieh, C.-Y. & Cao, J. S. A unified stochastic formulation of dissipative quantum dynamics. I. Generalized hierarchical equations. J. Chem. Phys. 148 , 014103 (2018).
Potǒnik, A. et al. Studying light-harvesting models with superconducting circuits. Nat. Commun. 9 , 904 (2018).
Nielsen, M. & Chuang, I. Quantum Computation and Quantum Information . (University Press, Cambridge, 2000).
MATH Google Scholar
Moix, J. M., Zhao, Y. & Cao, J. S. Equilibrium-reduced density matrix formulation: Influence of noise, disorder, and temperature on localization in excitonic systems. Phys. Rev. B 85 , 115412 (2012).
Cao, J. S. & Silbey, R. J. Optimization of exciton trapping in energy transfer processes. J. Phys. Chem. A 113 , 13825–13838 (2009).
del Rey, M., Chin, A. W., Huelga, S. F. & Plenio, M. B. Exploiting structured environments for efficient energy transfer: the phonon antenna mechanism. J. Phys. Chem. Lett. 4 , 903–907 (2013).
Gorman, D. J. et al. Engineering vibrationally assisted energy transfer in a trapped-ion quantum simulator. Phys. Rev. X 8 , 011038 (2018).
Google Scholar
Chen, H.-B., Gneiting, C., Lo, P.-Y., Chen, Y.-N. & Nori, F. Simulating open quantum systems with Hamiltonian ensembles and the nonclassicality of the dynamics. Phys. Rev. Lett. 120 , 030403 (2018).
Gershenfeld, N. A. & Chuang, I. L. Bulk spin-resonance quantum computation. Science 275 , 350–356 (1997).
Article MathSciNet Google Scholar
Cory, D. G., Fahmy, A. F. & Havel, T. F. Ensemble quantum computing by NMR spectroscopy. Proc. Natl. Acad. Sci. U.S.A. 94 , 1634–1639 (1997).
Xin, T. et al. Quantum state tomography via reduced density matrices. Phys. Rev. Lett. 118 , 020401 (2017).
Download references
Acknowledgements
We thank Xing-Long Zhen and Ke-Ren Li for discussions. G.L.L. was supported by National Natural Science Foundation of China under Grant Nos. 11175094 and 91221205, and the National Basic Research Program of China under Grant No. 2015CB921001. F.G.D. was supported by the National Natural Science Foundation of China under Grant Nos. 11474026 and 11674033. F.N. was supported in part by the MURI Center for Dynamic Magneto-Optics via the Air Force Office of Scientific Research (AFOSR) (FA9550-14-1-0040), Army Research Office (ARO) (Grant No. 73315PH), Asian Office of Aerospace Research and Development (AOARD) (Grant No. FA2386-18-1-4045), Japan Science and Technology Agency (JST) (the ImPACT program and CREST Grant No. JPMJCR1676), Japan Society for the Promotion of Science (JSPS) (JSPS-RFBR Grant No. 17-52-50023), RIKEN-AIST Challenge Research Fund, and the John Templeton Foundation. Q.A. was supported by the National Natural Science Foundation of China under Grant No. 11505007. This work was supported in part by the Beijing Advanced Innovation Center for Future Chip (ICFC).
Author information
These authors contributed equally: Bi-Xue Wang, Ming-Jie Tao, Qing Ai.
Authors and Affiliations
State Key Lab for Low-dimensional Quantum Physics, Department of Physics, Tsinghua University, Beijing, 100084, China
Bi-Xue Wang, Ming-Jie Tao, Tao Xin, Dong Ruan & Gui-Lu Long
Department of Physics, Applied Optics Beijing Area Major Laboratory, Beijing Normal University, Beijing, 100875, China
Ming-Jie Tao, Qing Ai & Fu-Guo Deng
Theoretical Quantum Physics Laboratory, RIKEN Cluster for Pioneering Research, Wako-shi, Saitama, 351-0198, Japan
Qing Ai, Neill Lambert & Franco Nori
Department of Chemistry, National Taiwan University, Taipei City, 106, Taiwan
Yuan-Chung Cheng
Physics Department, The University of Michigan, Ann Arbor, MI, 48109-1040, USA
Franco Nori
NAAM-Research Group, Department of Mathematics, Faculty of Science, King Abdulaziz University, Jeddah, 21589, Saudi Arabia
Fu-Guo Deng
Beijing National Research Center on Information Science and Technology, Beijing, 100084, China
Gui-Lu Long
School of Information Science and Technology, Tsinghua University, Beijing, 100084, China
Innovation Center of Quantum Matter, Beijing, 100084, China
Beijing Academy of Quantum Information, Beijing, 100085, China
You can also search for this author in PubMed Google Scholar
Contributions
All work was carried out under the supervision of G.L.L., F.G.D., Y.C.C., and F.N. B.X.W. and T.X. performed the experiments. Y.C.C., B.X.W., Q.A., M.J.T., and N.L. analyzed the experimental data. M.J.T., Q.A., and N.L. wrote the HEOM simulation code and performed the numerical simulation. Y.C.C. and Q.A. contributed the theory. All authors contributed to writing the manuscript.
Corresponding authors
Correspondence to Fu-Guo Deng or Gui-Lu Long .
Ethics declarations
Competing interests.
The authors declare no competing interests.
Additional information
Publisher's note: Springer Nature remains neutral with regard to jurisdictional claims in published maps and institutional affiliations.
Electronic supplementary material
Supplementary information, rights and permissions.
Open Access This article is licensed under a Creative Commons Attribution 4.0 International License, which permits use, sharing, adaptation, distribution and reproduction in any medium or format, as long as you give appropriate credit to the original author(s) and the source, provide a link to the Creative Commons license, and indicate if changes were made. The images or other third party material in this article are included in the article’s Creative Commons license, unless indicated otherwise in a credit line to the material. If material is not included in the article’s Creative Commons license and your intended use is not permitted by statutory regulation or exceeds the permitted use, you will need to obtain permission directly from the copyright holder. To view a copy of this license, visit http://creativecommons.org/licenses/by/4.0/ .
Reprints and permissions
About this article
Cite this article.
Wang, BX., Tao, MJ., Ai, Q. et al. Efficient quantum simulation of photosynthetic light harvesting. npj Quantum Inf 4 , 52 (2018). https://doi.org/10.1038/s41534-018-0102-2
Download citation
Received : 15 March 2018
Revised : 10 August 2018
Accepted : 24 September 2018
Published : 22 October 2018
DOI : https://doi.org/10.1038/s41534-018-0102-2
Share this article
Anyone you share the following link with will be able to read this content:
Sorry, a shareable link is not currently available for this article.
Provided by the Springer Nature SharedIt content-sharing initiative
This article is cited by
- Xiao-Wen Shang
- Xian-Min Jin
npj Quantum Information (2024)
Entanglement concentration of W state using linear optics with a higher success probability
- Fang-Fang Du
Quantum Information Processing (2024)
Euclidean time method in generalized eigenvalue equation
- Mi-Ra Hwang
- DaeKil Park
Criticality-based quantum metrology in the presence of decoherence
- Wan-Ting He
- Cong-Wei Lu
Frontiers of Physics (2023)
Implementations of heralded quantum Toffoli and Fredkin gates assisted by waveguide-mediated photon scattering
Quantum Information Processing (2023)
Quick links
- Explore articles by subject
- Guide to authors
- Editorial policies
Sign up for the Nature Briefing newsletter — what matters in science, free to your inbox daily.

Department of Biology
Graduate Studies
The application deadline for the 2024-2025 cohort is February 1, 2024. There is no application fee.
The Portland State Biology graduate program offers M.S.T, M.A., M.S, and Ph.D degree options. Admission into our program is highly competitive. Our multi-disciplinary faculty offer admitted graduate students the opportunity to conduct cutting edge research, gain professional teaching experience, and pursue an academically rigorous program which fosters professional research development. Our students graduate with the skills, knowledge, and experience necessary to be successful contributors to the scientific community.
Our campus is located in the heart of Downtown Portland, and our program draws top graduate students from around the world. Our research faculty expertise spans a broad range of disciplines within the field of Biology. Research is conducted in state-of-the-art laboratories and in field locations, from the glaciers of Antarctica, to the jungles of Central and South America, to the bamboo forests of central China. Regional collaborators include public, state and federal agencies as well as other regional institutions including, but not limited to, the Oregon Zoo, Oregon Museum of Science & Industry (OMSI), Oregon Health & Science University, and Oregon State University.
Degree Requirements
- Biology M.A./M.S.
- Biology M.A.T./M.S.T.
- Biology Ph.D.
Graduate Thesis or Dissertation
All Biology graduate students must defend a thesis or dissertation to complete the degree program. The first part of the graduate defense is a one-hour seminar that is open to the public followed by a closed-door review of the student's research with the student and the student's graduate committee.
PDXScholar maintains a complete list of all theses and dissertations submitted to the University.
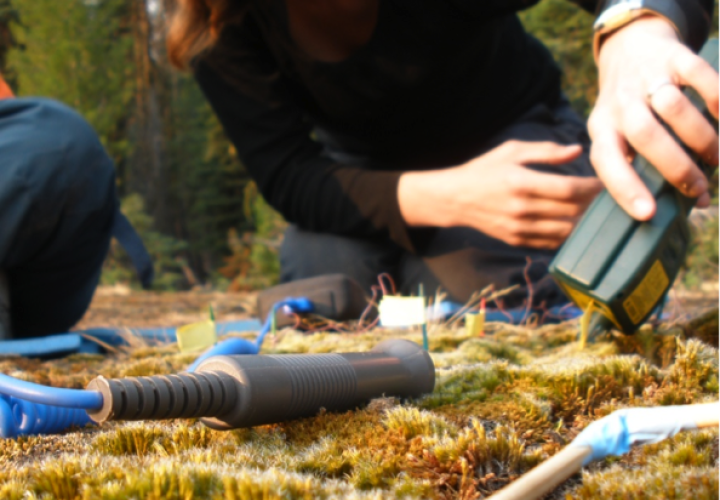
How to Apply
Applications to the Biology Graduate Program must be made through the Graduate School ; please do not send any application materials directly to the Biology Department.
Applications are reviewed only for admission for fall term. There is no application fee.
- Graduate School
- Thesis & Dissertation Info
- Grad Dates & Deadlines
- Graduate School Forms
- Joint Campus Registration
- Biology Grad Handbook
- Graduate Learning Objectives
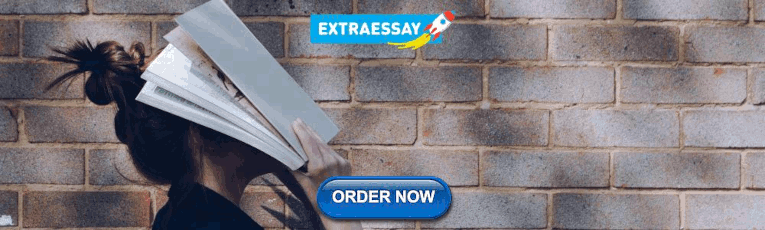
COMMENTS
Quantum biology is the study of such processes, and here we provide an outline of the current state of the field, as well as insights into future directions. Keywords: artificial photosynthesis, light harvesting, charge transfer, enzyme catalysis, sensing, quantum technology. Go to: 1. Introduction.
Quantum biology is usually considered to be a new discipline, arising from recent research that suggests that biological phenomena such as photosynthesis, enzyme catalysis, avian navigation or olfaction may not only operate within the bounds of classical physics but also make use of a number of the non-trivial features of quantum mechanics, such as coherence, tunnelling and, perhaps, entanglement.
Over the past decade, the field of quantum biology has seen an enormous increase in activity, with detailed studies of phenomena ranging from the primary processes in vision and photosynthesis to avian navigation (1, 2).In principle, the study of quantum effects in complex biological systems has a history stretching back to the early years of quantum mechanics (); however, only recently has it ...
Quantum biology is the study of such quantum aspects of living systems. In this review, we summarise the latest progress in quantum biology, including the areas of enzyme-catalysed reactions, photosynthesis, spin-dependent reactions, DNA, fluorescent proteins, and ion channels. Many of these results are expected to be fundamental building ...
Tunnelling of light-mass particles is a quintessential quantum effect well studied even at the dawn of quantum physics. In biology, it is evident that long-range electron tunnelling 81, 82, 83 and ...
Quantum biology has emerged as a space for true scientific discourse, where cross-disciplinary research has built and is continuing to improve upon a scientific foundation that promises to drive the scientific revolutions of the past century into a new era of discovery. To capitalize on the successes of modern interdisciplinary fields like ...
Biology has a knack for using what works, says Seth Lloyd, a physicist at the Massachusetts Institute of Technology in Cambridge. And if that means "quantum hanky-panky", he says, "then quantum ...
Quantum computing devices have the potential to solve a variety of problems arising in computational biology (Table 1).Being limited in their present abilities, quantum devices of various types ...
In this contribution, the thesis that quantum mechanics is a powerful tool for explaining the characteristics of biological systems will be defended and some (speculative, at the moment) lines of research will be suggested. From a certain point of view, the use of quantum mechanics in biology might seem logical since it is the fundamental theory
The existence of quantum biology as a discipline implies that traditional understanding of life processes is incomplete. Further research will lead to new insights into the age-old question of ...
Quantum biology is an interdisciplinary field that explores the application of quantum mechanics principles and phenomena to biological systems. It seeks to understand how quantum processes and interactions influence the behavior, functioning, and dynamics of biological systems at various scales, from molecules and cells to organisms and ecosystems. By investigating the potential role of ...
Quantum biology is usually considered to be a new discipline, arising from recent research that suggests that biological phenomena such as photosynthesis, enzyme catalysis, avian navigation or olfaction may not only operate within the bounds of classical physics but also make use of a number of the non-trivial features of quantum mechanics, such as coherence, tunnelling and, perhaps, entanglement.
The review article presents the recent progress in quantum computing and simulation within the field of biological sciences. The article is designed mainly into two portions: quantum computing and quantum simulation. In the first part, significant aspects of quantum computing was illustrated, such as quantum hardware, quantum RAM and big data, modern quantum processors, qubit, superposition ...
Thesis advisor Author Al an Aspuru-Guzik Sangwoo Shim Quantum Dynamics in Biological Systems Abstract In the rst part of this dissertation, recent e orts to understand quantum mechanical e ects in biological systems are discussed. Especially, long-lived quantum coherences observed during the electronic energy transfer process in the Fenna ...
INTRODUCTION. Over the past decade, the field of quantum biology has seen an enormous increase in activity, with detailed studies of phenomena ranging from the primary processes in vision and photosynthesis to avian navigation (1, 2).In principle, the study of quantum effects in complex biological systems has a history stretching back to the early years of quantum mechanics (); however, only ...
This thesis reports on the development of the first quantum enhanced microscope and on its applications in biological microscopy. The first quantum particle-tracking microscope, described in detail here, represents a pioneering advance in quantum microscopy, which is shown to be a powerful and relevant technique for future applications in science and medicine.
QUANTUM BIOLOGY ALESSANDRO SERGI a ABSTRACT.A critical assessment of the recent developments of molecular biology is pre- ... In this contribution, the thesis that quantum mechanics is a powerful tool for explain-ing the characteristics of biological systems will be defended and some (speculative, at ...
Quantum biology. Quantum biology is the study of applications of quantum mechanics and theoretical chemistry to aspects of biology that cannot be accurately described by the classical laws of physics. [1] An understanding of fundamental quantum interactions is important because they determine the properties of the next level of organization in ...
This Review overviews the application of single photons in quantum metrology, quantum biology and the foundations of quantum physics, discussing specific needs and requirements, achieved ...
The recent rise of quantum biology as an emerging field at the border between quantum physics and the life sciences suggests that quantum events could play a non-trivial role also in neuronal cells. ... (the end of) neurodeterminism," in Quantum Physics Meets the Philosophy of Mind New Essays on the Mind-Body Relation in Quantum-Theoretical ...
Thesis advisor: Professor T. Senthil Liujun Zou An odyssey in modern quantum many-body physics Abstract This thesis is a collection of research work in quantum many-body physics that I have done during the past few years. The topics include: 1. a theory of anomalous magnetotransport from
Quantum biology is the study of such processes, and here we provide an outline of the current state of the field, as well as insights into future directions. 1. Introduction. Quantum mechanics is the fundamental theory that describes the properties of subatomic particles, atoms, molecules, molecular assemblies and possibly beyond.
Boise State University MS Raptor Biology student Ashley Santiago defended her thesis, "Associations Between Landscape Changes And Declines In Golden Eagle Territory Occupancy In The Morley Nelson Snake River Birds Of Prey National Conservation Area" on the morning of April 19, 2024. Santiago's main advisor is Julie Heath (MS, Raptor Biology, '96; Boise State University Raptor Research ...
Quantum Information Processing (2023) Near-unity energy transfer efficiency has been widely observed in natural photosynthetic complexes. This phenomenon has attracted broad interest from ...
Biology Ph.D. Graduate Thesis or Dissertation. All Biology graduate students must defend a thesis or dissertation to complete the degree program. The first part of the graduate defense is a one-hour seminar that is open to the public followed by a closed-door review of the student's research with the student and the student's graduate committee.