To read this content please select one of the options below:
Please note you do not have access to teaching notes, market segmentation, targeting and positioning.
Sustainability Marketing
ISBN : 978-1-80071-245-4 , eISBN : 978-1-80071-244-7
Publication date: 5 May 2021
Sharma, R.R. , Kaur, T. and Syan, A.S. (2021), "Market Segmentation, Targeting and Positioning", Sustainability Marketing , Emerald Publishing Limited, Leeds, pp. 119-132. https://doi.org/10.1108/978-1-80071-244-720211009
Emerald Publishing Limited
Copyright © 2021 by Emerald Publishing Limited
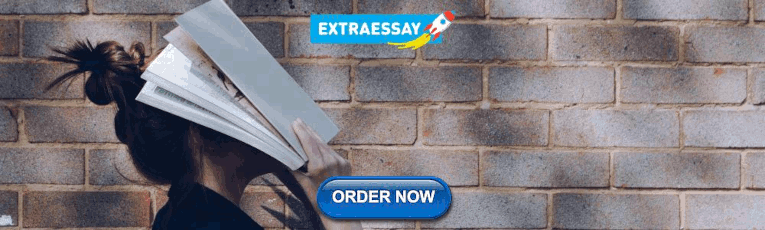
We’re listening — tell us what you think
Something didn’t work….
Report bugs here
All feedback is valuable
Please share your general feedback
Join us on our journey
Platform update page.
Visit emeraldpublishing.com/platformupdate to discover the latest news and updates
Questions & More Information
Answers to the most commonly asked questions here
Segmentation research: What is it and how to make it actionable
Segmentation research helps companies find target segments that can propel their growth and profitability. This article establishes what segmentation research is and how companies effectively implement it.
Segmentation in marketing research
Editor’s note: Michaela Mora is the CEO of Relevant Insights. This is an edited version of an article that originally appeared under the title “ How to Make Segmentation Research Actionable. ”
Segmentation research helps companies identify groups of current and potential customers or users with the highest profitability potential. This, along with product positioning and target marketing, is one of the pillars of strategic marketing and product development.
This is broadly called “market segmentation” in marketing and market research because we effectively study current and potential buyers and users of products and services. Buyer and user behaviors may differ depending on the roles they adopt at different points in the user experience journey. For example, in video game purchases for minors, in specific segments of the gaming population, the parents are more likely to play the role of buyers as they are the authority figures. At the same time, their kids are most likely to be product users, but they also influence the purchase decision, often by nagging their parents to grant permission or give them money. Depending on the business goals, we may focus on segmenting customers on their buying or user behaviors or a combination of both.
The division (or merging) of roles between buyers, decision-makers, decision influencers and users can be found in many product categories. It is a mistake to assume that only users play a particular role. The roles change based on many factors including demographics, psychographics, market trends and purchase and usage scenarios.
Addressing the myriad roles a customer can take requires different marketing tactics and product positioning to reach the intended market. On the product development side, it may require the development of features to satisfy different needs.
The key concepts
Markets are not homogeneous. They are comprised of individual consumers/users with unique needs and desires. This is why segmentation research is a powerful tool for creating better user experiences and leveraging competitive advantage. This applies to both B2C and B2B markets.
A market segment is a portion of a market whose needs differ from the larger market and potentially from other segments. When we do segmentation research, we need to consider the current and potential organizational capabilities. Capabilities include existing products and services, technologies, brand reputation, innovation pipeline, etc.
The first step is to identify the need sets the organization can meet. It is not pertinent to segment needs that lack the required skillset or resources.
We talk about need sets because most products satisfy more than one need. Customer needs are not restricted to those satisfied by product features or user interactions. Their needs also include those that happen at different points in the journey of becoming a customer connected to:
- Types and sources of information about the product.
- Channels where the product is available.
- Product price.
- Services associated with the product.
- Perceptions and image of the product or brand.
- Where and how the product is produced or developed.
- User’s life stages and lifestyle.
Identifying relevant need sets that the organization’s current and potential products may satisfy requires qualitative and quantitative research.
Product features vs. benefits
Customers buy need satisfaction, not product features or attributes. Behind a preference for a feature or attribute there is a need searching for satisfaction and driving behavior. A consumer may buy cosmetics to satisfy the need to feel beautiful or transformed. Another will buy a drill for a DIY project, giving them a sense of accomplishment. A product manager may buy software to save time and better manage her job’s daily tasks. Segmentation studies based on product features tend to be less actionable than those based on needs.
The role demographic variables play
Users’ needs don’t exist in a vacuum. They are often associated with demographic variables such as gender, age, ethnicity, marital status, family composition, education, social class, occupation and geographic location. A segmentation solution may start by grouping users with similar product need sets despite different demographics. However, it is essential to understand these demographic differences to design effective marketing programs to reach them through different channels and messages.
Demographic information provides insights into the context in which products are purchased and used, how they think about the products beyond its features and the language they use to describe their user experience. For example, while singles, young families with children and middle-aged couples may want the same features in a mobile app, website or car display, they likely differ in how they perceive different aspects of product design, messages, channels we use to communicate with each group and the points of friction in product interactions.
Excluding demographic information leads to a lack of diversity, unintended discrimination and missed product development opportunities. For specific product categories, demographic variables can be used as segmentation criteria if they identify segments with distinctive needs and behaviors. In other categories, demographic information may not be as discriminating but still can be used to profile the segments and understand the context in which products are purchased and used.
Key demographics that can affect product use
Research has shown that age shapes the products we buy, how we use them, where we shop, how we use technology and media and how we think and feel about marketing activities.
Many products are created with a gender in mind however sometimes gender-specific products can be based on obsolete ideas of what each gender may need or prefer. There are also product designs that intentionally or unintentionally ignore the needs of the other gender.
Race and ethnic origin are connected to ethnic subcultures in which members share unique behaviors based on a common racial, language or cultural background. It is important to remember that all subcultures are diverse and general descriptions don’t apply to every member. It is important to remain vigilant about unconscious biases that can lead to stereotypes.
Nonetheless, shared cultural traditions, values, language and behaviors within those subcultures are rooted in their histories that influence how some of their members see their needs represented in the products they buy and use. The cultures we identify with influence how we use language, how we interpret visual design elements and what mental models we have about how a product should work based on personal experiences connected to that culture.
Household cycle stages
As social species, we usually grow up in families and go through different stages in life, each with specific needs. As we age, we may get married, have children, become empty nesters or be caregivers of older parents. Our family may shrink or expand over time depending on the paths we take and the relationships we develop. The needs for products and services in each stage will change and influence what we buy and how we use products.
Income and education
Education often determines occupation and income and often influences our opportunities and purchasing abilities. Education also influences how we think, make decisions and relate to others.
Intersectionality
We don’t just identify with a gender, race or a particular age. We are all those things together all the time. This means research must consider the intersectionality of many of these variables at the segment level. The experiences of young Black American men in America are very different from that of young white American men. They will share preferences and use certain products in similar ways. Still, they could differ in perceived barriers to product use, depending on design elements and messaging about the product connected to their identity groups.
Firmographics
In B2B markets we use “firmographics” as equivalents to demographic information in B2C. Variables such as company size in terms of employees and revenue, industry, product category, structure, decision-making chain and processes and geographic location often correlate with the products and services they buy and how they are used internally.
These are just some of the demographics or firmographic variables that may be relevant for your product category. Qualitative and secondary research from internal data systems or prior primary research can shed light on which variables may influence product use in your case.
Segment profiling
The selection of a segmentation solution is often based on a combination of technical know-how and judgment calls that consider the consistency and viability of the segments. In exploratory segmentation research, in which we don’t know what the segments are a priori, we use multivariate statistical techniques to identify segments with similar needs sets, behaviors, attitudes, perceptions, demographics and other relevant variables. We also need to describe the segments across all the measured variables and check if their profiles make sense.
The smaller the segments, the more likely the product will meet the segment’s needs. Smaller segments tend to have dominant and specific needs and behaviors that separate them from the rest but servicing a small segment can be very costly. A segment must be large enough to be worth investing in to be viable.
Segment profiles, also called personas, should highlight the most prominent common traits within the segments that act as differentiators against other segments. These could be behaviors, use occasions, buyer and user roles, attitudes, barriers and pains, motivators, demographics, etc.
Segments are probabilistic constructs, which means they summarize needs sets, behaviors, attitudes, etc., that are more likely to be shared by a group of people (or companies in B2B). This doesn’t mean that each individual classified in a segment will perfectly fit the segment. We are all individuals with unique needs, yet we share commonalities with the different groups we belong to. Segment profiles help understand a group’s core needs and distinctive user behaviors so the company can develop products that satisfy those needs.
Making segmentation research actionable
Despite significant investment in segmentation studies, these may have little impact on organizational decision making unless some conditions are in place for insight implementation.
Define your desired business decisions and outcomes
The key to an actionable segmentation study is a precise translation of desired business actions and outcomes into the information needs the research should meet to support those actions and outcomes. In survey-based segmentation studies, this must go further to operationalizing those information needs into good question design grounded in how the team plans to use the results.
It’s not uncommon to see clients bring very indefinite descriptions of how they plan to use the research results. As someone who does market segmentation studies for clients, I often help them define the jobs they want done to support particular function(s) (marketing, product development, operations, etc.) and how they support business outcomes.
Failure to define the specific actions the team plans to make (create content for different media, identify keywords for SEO, etc.) to achieve business outcomes, can take the segmentation study in a direction that is likely to provide less than valuable insights.
Assign a C-suite research champion
Segmentation studies generate a lot of insights that are often difficult to socialize internally. The sheer amount of data can be overwhelming. Consequently, an action plan is needed to share the insights and help the organization to adopt them. Internal research teams are often responsible for this task but are rarely successful without a mandate from the top. Any strategic research effort needs a champion in the C-suite from its conception to its implementation.
With support from the executive team, researchers connected to marketing or product development need to educate internal stakeholders on the value of both the tactical and strategic implications of the segmentation research the company may have conducted. They need to understand the organization’s ability to adapt to the study’s findings and create an implementation plan to help manage internal clients’ expectations.
By connecting the tactical changes recommended by the findings to the overall strategic business goals, the research team can help internal teams, including the C-suite, to become educated on needed strategic changes.
Allow for a flexible organizational structure
Segmentation research provides insights with both tactical and strategic recommendations. Tactical recommendations may include changing a product configuration, adding new features, changing how is presented in advertising, etc. These changes can be implemented without significant organizational changes.
However, serving identified segments long-term may require a new structure to help manage them if the segmentation solution doesn’t align with the current organizational structure. In cases like these, the solution companies use is to create cross-functional teams, but depending on how rigid the structure is, these teams may get little accomplished.
To implement the strategic insights stemming from segmentation studies, the organization must be willing to change its structure to manage the market segments efficiently.
Create a balance between long- and short-term goals
In many organizations, there is often tension between marketing, sales and product development functions as they own channels and goals with different time horizons. A segmentation study may have recommendations that impact the design of channels these functions own. The marketing team may be receptive to changes the sales team resists because it may upset established client relationship patterns and short-term sales goals.
To balance short- and long-term goals, the management team must consider all research outcomes and decision possibilities of strategic value at the research design stage. If there is no commitment to implement strategic insights from the segmentation study, it is best to narrow its scope to find tactical solutions.
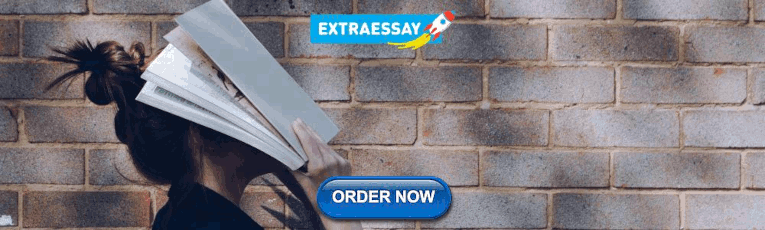
Prioritize certain market segments
A segmentation, by definition, implies discriminating among the segments in some respects. This means the marketing and product development will also discriminate certain segments if the segmentation solution is adopted. In practical terms, this will require prioritizing specific customer segments considering the risk of dedicating fewer resources to others.
If the company doesn’t want to take the risk of discriminating between segments and tries a middle-of-the-road strategy to reach all, it is likely to forfeit the competitive edge the segmentation insights may provide.
Have an experienced team
Understanding the value of the insights that can come from segmentation research and being willing to implement them requires prior experience with segmentation work. A marketing or product team not exposed to a well-designed segmentation study will have difficulty translating the insights into business implications.
If this is the case at your company, experienced internal researchers or external research suppliers should be called to help the teams think through the implications of decision-making based on different findings. Both internal researchers and external research suppliers should have experience in this methodology to help internal teams derive actionable insights.
Segmentation in the pharma industry: How to create resilient strategies Related Categories: Research Industry, Market Segmentation Studies, Segmentation Studies Research Industry, Market Segmentation Studies, Segmentation Studies, Health Care (Healthcare), Health Care (Healthcare) Research, Patients , Questionnaire Analysis, Sampling, Social Media Research, Survey Research
The use of synthetic data in marketing research Related Categories: Research Industry, Market Segmentation Studies, Segmentation Studies Research Industry, Market Segmentation Studies, Segmentation Studies, Artificial Intelligence / AI, Marketing Research-General, Predictive Markets, Software-Survey Design & Analysis, Survey Research
16 Top Consumer Research Companies Related Categories: Research Industry, Market Segmentation Studies, Segmentation Studies Research Industry, Market Segmentation Studies, Segmentation Studies, Consumer Research, Consumers, CX/UX-Customer/User Experience, Consumer Research Consultation, Consumer Services, Ethnographic Research, Focus Group-Online, Hybrid Research (Qual/Quant), Qualitative Research, Quantitative Research, Respondent Database/Recruiting System
Putting the next generation of segmentation tools to work Related Categories: Research Industry, Market Segmentation Studies, Segmentation Studies Research Industry, Market Segmentation Studies, Segmentation Studies, Automotive, CX/UX-Customer/User Experience, Consumer Research, Consumers, Data Analysis, Shopper Insights
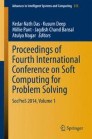
Proceedings of Fourth International Conference on Soft Computing for Problem Solving pp 87–98 Cite as
Data Mining in Market Segmentation: A Literature Review and Suggestions
- Saibal Dutta 7 ,
- Sujoy Bhattacharya 7 &
- Kalyan Kumar Guin 7
- Conference paper
- First Online: 25 December 2014
1725 Accesses
5 Citations
Part of the book series: Advances in Intelligent Systems and Computing ((AISC,volume 335))
The importance of data mining techniques for market segmentation is becoming indispensable in the field of marketing research. This is the first identified academic literature review of the available data mining techniques related to market segmentation. This research paper provides surveys of the available literature on data mining techniques in market segmentation. A categorization has been provided based on the available data mining techniques used in market segmentation. Eight online journal databases were used for searching, and finally, 103 articles were selected and categorized into 13 groups based on data mining techniques. The utility of data mining techniques and suggestions are also discussed. The findings of this study show that neural networks is the most used method, and kernel-based method is the most promising data mining techniques. Our research work provides a comprehensive understanding of past, present as well as future research trend on data mining techniques in market segmentation. We hope this paper provides reasonable insight and clear understating to both industry as well as academic researchers.
This is a preview of subscription content, log in via an institution .
Buying options
- Available as PDF
- Read on any device
- Instant download
- Own it forever
- Available as EPUB and PDF
- Compact, lightweight edition
- Dispatched in 3 to 5 business days
- Free shipping worldwide - see info
Tax calculation will be finalised at checkout
Purchases are for personal use only
Abdi, K., Fathian, M., Safari, E.: A novel algorithm based on hybridization of artificial immune system and simulated annealing for clustering problem. Int. J. Adv. Manuf. Technol. 60 (5–8), 723–732 (2012)
Article Google Scholar
Wildt, Albert R., McCann, John M.: Multicriterion market segmentation: a new model, implementation, and evaluation. J. Mark. Res. 17 (3), 335–340 (1980)
Babu, G.P., Murty, M.N.: A near-optimal initial seed value selection in K-means algorithm using a genetic algorithm. Pattern Recogn. Lett. 14 (10), 763–769 (1993)
Article MATH Google Scholar
Balakrishnan, P.V., Cooper, M.C., Jacob, V.S., Lewis, P.A.: Comparative performance of the FSCL neural net and K-means algorithm for market segmentation. Eur. J. Oper. Res. 93 , 346–357 (1996)
Bezdek, J.C., Pal, N.R.: Some new indexes of cluster validity. IEEE Trans. Syst. Man Cybern. Part B: Cybern. 28 (3), 301–315 (1998)
Blatt, M., Wiseman, S., Domany, E.: Clustering data through an analogy to the potts model. Adv. Neural Inf. Process. Syst. 8 , 416–422 (1996)
Google Scholar
Bloom, J.Z.: Tourist market segmentation with linear and non-linear techniques. Tour. Manage. 25 , 723–733 (2004)
Boone, D.S., Roehm, M.: Retail segmentation using artificial neural networks. Int. J. Res. Mark. 19 (3), 287–301 (2002)
Boone, D.S., Roehm, M.: Evaluating the appropriateness of market segmentation solutions using artificial neural networks and the membership clustering criterion. Market. Lett. 13 (4), 317–333 (2002)
Brida, J.G., Disegna, M., Osti, L.: Segmenting visitors of cultural events by motivation: A sequential non-linear clustering analysis of Italian Christmas market visitors. Expert Syst. Appl. 39 , 11349–11356 (2012)
Brida, J.G., Disegna, M., Scuderi, R.: Visitors of two types of museums: a segmentation study. Expert Syst. Appl. 40 (6), 2224–2232 (2012)
Bursco, M.J., Cradit, J.D., Stahl, S.: A simulated annealing heuristic for a bicriterion partitioning problem in marketing segmentation. J. Mark. Res. 39 (1), 99–109 (2002)
Bursco, M.J., Cradit, J.D., Stahl, S.: Multicriterion cluster wise regression for joint segmentation settings: an application to customer value. J. Mark. Res. 40 (2), 225–234 (2002)
Chen, C.H., Khoo, C.L., Yan, W.: A strategy for acquiring customer requirement patterns using laddering technique and ART2 neural network. Adv. Eng. Inform. 16 , 229–240 (2002)
Chen, M.C., Wu, H.P.: An association-based clustering approach to order batching considering customer demand patterns. Omega 33 (4), 333–343 (2005)
Cheng, C.H., Chen, Y.S.: Classifying the segmentation of customer value via RFM model and RS theory. Expert Syst. Appl. 36 , 4176–4184 (2009)
Chung, K.Y., Oh, S.Y., Kim, S.S., Han, S.Y.: Three representative market segmentation methodologies for hotel guest room customers. Tour. Manag. 25 (4), 429–441 (2004)
Croft, M.J.: Market Segmentation: A Step-by-Step Guide to Profitable New Business. Routledge, London, New York (1994)
Desarbo, W.S., Ramaswamy, V.: CRISP: customer response based iterative segmentation procedures for response modeling in direct marketing. J. Direct Market. 8 , 7–20 (1994)
Desarbo, W.S., Ramaswamy, V., Cohen, S.H.: market segmentation with choice-based conjoint analysis. Market. Lett. 6 (2), 137–147 (1995)
DeSarbo, W.S., Grisaffe, D.: Combinatorial optimization approaches to constrained market segmentation: an application to industrial market segmentation. Market. Lett. 9 , 115–134 (1998)
Desarbo, W.S., Grewal, R., Scott, C.J.: A cluster wise bilinear multidimensional scaling methodology for simultaneous segmentation and positioning analyses. J. Mark. Res. 45 , 280–292 (2008)
Frank, R.E., Massy, W.F., Wind, Y.: Market segmentation. Prentice Hall, Englewood Cliffs (1972)
Huang, J.H., Tzeng, G.H., Ong, C.S.: Marketing segmentation using support vector clustering. Expert Syst. Appl. 32 , 313–317 (2007)
Hammond, K., Ehrenberg, A.S.C., Goodhardt, G.J.: Market segmentation for competitive brands. Eur. J. Mark. 30 (12), 39–49 (1996)
Hruschka, H., Natter, M.: Comparing performance of feedforward neural nets and K-means for cluster-based market segmentation. Eur. J. Oper. Res. 114 , 346–353 (1999)
Hong, C.W.: Using the Taguchi method for effective market segmentation. Expert Syst. Appl. 39 , 5451–5459 (2012)
Kamakura, W.A., Russell, G.J.: A probabilistic choice model for market segmentation and elasticity structure. J. Market. Res. 26 , 379–390 (1989)
Kauko, T., Hooimeijer, P., Hakfoort, J.: Capturing housing market segmentation: an alternative approach based on neural network modeling. Hous. Stud. 17 (6), 875–894 (2002)
Kim, Y., Street, W.N.: An intelligent system for customer targeting: a data mining approach. Decis. Support Syst. 37 (2), 215–228 (2004)
Kotler, P., Gordon, M.: Principles of Market. Prentice Hall, Canada (1983)
Kuo, R.J., Ho, L.M., Hu, C.M.: Integration of self-organizing feature map and K-means algorithm for market segmentation. Comput. Oper. Res. 29 (11), 1475–1493 (2002)
Kuo, R.J., Chang, K., Chien, S.Y.: Integration of self-organizing feature maps and genetic algorithm- based clustering method for market segmentation. J. Organ. Comput. Electron. Commer. 14 (1), 43–60 (2004)
Li, Z., Wang, W., Yang, C., Ragland, D.R.: Bicycle commuting market analysis using attitudinal market segmentation approach. Transport. Res. Part A: Policy Pract. 47 , 56–68 (2013)
Maroosi, A., Amiri, B.: A new clustering algorithm based on hybrid global optimization based on a dynamical systems approach algorithm. Expert Syst. Appl. 37 (8), 5645–5652 (2010)
Maulik, U., Bandyopadhyay, S.: Genetic algorithm-based clustering technique. Pattern Recogn. 33 (9), 1455–1465 (2000)
Maulik, U., Bandyopadhyay, S.: Performance evaluation of some clustering algorithms and validity indices. IEEE Trans. Patt. Anal. Mach. Intell. 24 (12), 1650–1654 (2002)
Miguéis, V.L., Camanho, A.S., Falcão e Cunha, J.: Customer data mining for lifestyle segmentation. Expert Syst. Appl. 39 , 9359–9366 (2012)
Mostafa, M.M.: Clustering the ecological footprint of nations using Kohonen’s self-organizing maps. Expert Syst. Appl. 37 , 2747–2755 (2010)
Murthy, C.A., Chowdhury, N.: In search of optimal clusters using genetic algorithms. Pattern Recogn. Lett. 17 (8), 825–832 (1996)
Natter, M.: Conditional market segmentation by neural networks: a Monte-Carlo study. J. Retail. Consum. Serv. 6 (4), 237–248 (1999)
Ngai, E.W., Xiu, L., Chau, D.C.K.: Application of data mining techniques in customer relationship management: A literature review and classification. Expert Syst. Appl. 36 (2), 2592–2602 (2009)
Park, D.H., Kim, H.K., Choi, I.Y., Kim, J.K.: A literature review and classification of recommender systems research. Expert Syst. Appl. 39 (11), 10059–10072 (2012)
Punj, G., Stewart, D.W.: Cluster analysis in marketing research: review and suggestions for application. J. Market. Res. 20 , 134–148 (1983)
Smith, W.R.: Product differentiation and market segmentation as an alternative marketing strategy. J. Market. 21 , 3–8 (1956)
Vellido, A., Lisboa, P.J.G., Vaughan, J.: Neural networks in business: a survey of applications (1992–1998). Expert Syst. Appl. 17 (1), 51–70 (1999)
Vesanto, J., Alhoniemi, E.: Clustering of the self-organizing map. IEEE Trans. Neural Netw. 11 (3), 586–600 (2000)
Vishwanath, A., Chen, H.: Technology clusters: using multidimensional scaling to evaluate and structure technology clusters. J. Am. Soc. Inform. Sci. Technol. 57 (11), 1451–1460 (2006)
Vriens, M., Wedel, M., Wilms, T.: Metric conjoint segmentation methods: a Monte Carlo comparison. J. Mark. Res. 33 , 73–85 (1996)
Wang, C.H.: Apply robust segmentation to the service industry using kernel induced fuzzy clustering techniques. Expert Syst. Appl. 37 , 8395–8400 (2010)
Wedel, M., Kamakura, W.A.: Market segmentation: conceptual and methodological foundations. Kluwer Academic, Boston (2000)
Book Google Scholar
Wedel, M., Kistemaker, C.: Consumer benefit segmentation using cluster wise linear regression. Int. J. Res. Mark. 6 , 241–258 (1989)
Wei, J.T., Lin, S.Y., Weng, C.C., Wu, H.H.: A case study of applying LRFM model in market segmentation of a children’s dental clinic. Expert Syst. Appl. 39 (5), 5529–5533 (2012)
Wu, W.W.: Segmenting and mining the ERP users’ perceived benefits using the rough set approach. Expert Syst. Appl. 38 , 6940–6948 (2011)
Zhang, Y., Jiao, J., Ma, Y.: Market segmentation for product family positioning based on fuzzy clustering. J. Eng. Des. 18 (3), 227–241 (2007)
Download references
Author information
Authors and affiliations.
Indian Institute of Technology Kharagpur, Kharagpur, India
Saibal Dutta, Sujoy Bhattacharya & Kalyan Kumar Guin
You can also search for this author in PubMed Google Scholar
Corresponding author
Correspondence to Saibal Dutta .
Editor information
Editors and affiliations.
Mathematics, National Institute of Technology Silchar, Silchar, Assam, India
Kedar Nath Das
Department of Mathematics, Indian Institute of Technology Roorkee, Roorkee, India
Department of Paper Technology, Indian Institute of Technology Roorkee, Roorkee, India
Millie Pant
Department of Mathematics, South Asian University, New Delhi, India
Jagdish Chand Bansal
Department of Computer Science, Liverpool Hope University, Liverpool, United Kingdom
Atulya Nagar
Rights and permissions
Reprints and permissions
Copyright information
© 2015 Springer India
About this paper
Cite this paper.
Dutta, S., Bhattacharya, S., Guin, K.K. (2015). Data Mining in Market Segmentation: A Literature Review and Suggestions. In: Das, K., Deep, K., Pant, M., Bansal, J., Nagar, A. (eds) Proceedings of Fourth International Conference on Soft Computing for Problem Solving. Advances in Intelligent Systems and Computing, vol 335. Springer, New Delhi. https://doi.org/10.1007/978-81-322-2217-0_8
Download citation
DOI : https://doi.org/10.1007/978-81-322-2217-0_8
Published : 25 December 2014
Publisher Name : Springer, New Delhi
Print ISBN : 978-81-322-2216-3
Online ISBN : 978-81-322-2217-0
eBook Packages : Engineering Engineering (R0)
Share this paper
Anyone you share the following link with will be able to read this content:
Sorry, a shareable link is not currently available for this article.
Provided by the Springer Nature SharedIt content-sharing initiative
- Publish with us
Policies and ethics
- Find a journal
- Track your research
Monetary Policy, Segmentation, and the Term Structure
We develop a segmented markets model which rationalizes the effects of monetary policy on the term structure of interest rates. When arbitrageurs’ portfolio features positive duration, an unexpected rise in the short rate lowers their wealth and raises term premia. A calibration to the U.S. economy accounts for the transmission of monetary shocks to long rates. We discuss the additional implications of our framework for state-dependence in policy transmission, the volatility and slope of the yield curve, and trends in term premia accompanying trends in the natural rate.
An early version of this paper circulated under the title “Heterogeneity, Monetary Policy, and the Term Premium”. We thank Michael Bauer, Luigi Bocola, Anna Cieslak, James Costain, Vadim Elenev, Pierre-Olivier Gourinchas, Robin Greenwood, Sam Hanson, Ben Hebert, Christian Heyerdahl-Larsen, Sydney Ludvigson, Hanno Lustig, Anil Kashyap, Arvind Krishnamurthy, Matteo Maggiori, Stavros Panageas, Carolin Pflueger, Monika Piazzesi, Walker Ray, Alp Simsek, Eric Swanson, Dimitri Vayanos, Olivier Wang, Wei Xiong, and Motohiro Yogo for discussions. We thank Manav Chaudhary, Lipeng (Robin) Li, and Jihong Song for excellent research assistance. This research has been supported by the National Science Foundation grant SES-2117764. The views expressed herein are those of the authors and do not necessarily reflect the views of the National Bureau of Economic Research.
MARC RIS BibTeΧ
Download Citation Data
- data appendix
- Code to solve model
Conferences
More from nber.
In addition to working papers , the NBER disseminates affiliates’ latest findings through a range of free periodicals — the NBER Reporter , the NBER Digest , the Bulletin on Retirement and Disability , the Bulletin on Health , and the Bulletin on Entrepreneurship — as well as online conference reports , video lectures , and interviews .
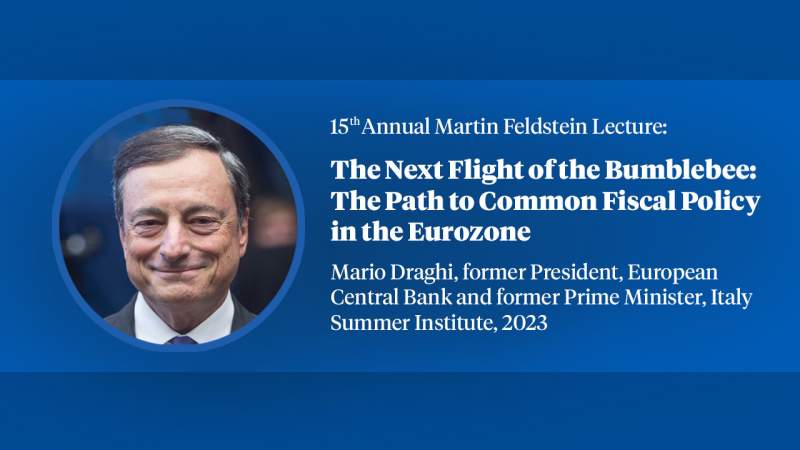
Help | Advanced Search
Computer Science > Computer Vision and Pattern Recognition
Title: visual prompting for generalized few-shot segmentation: a multi-scale approach.
Abstract: The emergence of attention-based transformer models has led to their extensive use in various tasks, due to their superior generalization and transfer properties. Recent research has demonstrated that such models, when prompted appropriately, are excellent for few-shot inference. However, such techniques are under-explored for dense prediction tasks like semantic segmentation. In this work, we examine the effectiveness of prompting a transformer-decoder with learned visual prompts for the generalized few-shot segmentation (GFSS) task. Our goal is to achieve strong performance not only on novel categories with limited examples, but also to retain performance on base categories. We propose an approach to learn visual prompts with limited examples. These learned visual prompts are used to prompt a multiscale transformer decoder to facilitate accurate dense predictions. Additionally, we introduce a unidirectional causal attention mechanism between the novel prompts, learned with limited examples, and the base prompts, learned with abundant data. This mechanism enriches the novel prompts without deteriorating the base class performance. Overall, this form of prompting helps us achieve state-of-the-art performance for GFSS on two different benchmark datasets: COCO-$20^i$ and Pascal-$5^i$, without the need for test-time optimization (or transduction). Furthermore, test-time optimization leveraging unlabelled test data can be used to improve the prompts, which we refer to as transductive prompt tuning.
Submission history
Access paper:.
- HTML (experimental)
- Other Formats

References & Citations
- Google Scholar
- Semantic Scholar
BibTeX formatted citation

Bibliographic and Citation Tools
Code, data and media associated with this article, recommenders and search tools.
- Institution
arXivLabs: experimental projects with community collaborators
arXivLabs is a framework that allows collaborators to develop and share new arXiv features directly on our website.
Both individuals and organizations that work with arXivLabs have embraced and accepted our values of openness, community, excellence, and user data privacy. arXiv is committed to these values and only works with partners that adhere to them.
Have an idea for a project that will add value for arXiv's community? Learn more about arXivLabs .
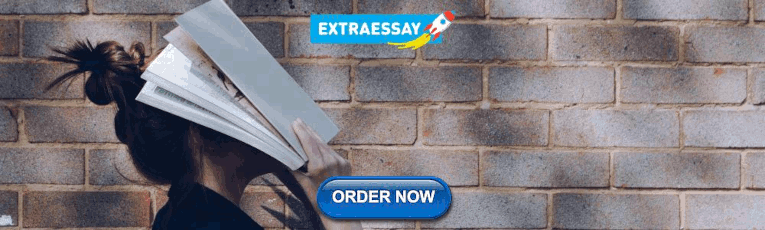
IMAGES
VIDEO
COMMENTS
The last major reviews of B2B market segmentation research were undertaken by Chéron and Kleinschmidt, ... In the reputable category, the journal with the most B2B market segmentation papers is EJM, with five. The most productive time period is 1997-2007, with 33 papers (37.5%), followed by 1986-96, with 30 papers (34.1%), and last, 2008 ...
In sum, this chapter explains the three stages of target marketing, including; market segmentation (ii) market targeting and (iii) market positioning. Discover the world's research 25+ million members
Recent research studies explore pricing dynamics and market segmentation, offering valuable insights into evolving patterns across different markets (Zhang & Chang, 2021;Zhao et al., 2020).
Market segmentation is defined as "breaking markets into slices" in a newsletter published by Grey Advertising Inc. and quoted in Haley (1985) as one of the simplest and clearest descriptions ...
Market segmentation is one of the ways in which such knowledge can be represented and make it new business opportunities." (Kim and Ahn 2004). Already in 2004, ... Yan E (2018) Web of science use in published research and review papers 1997-2017: a selective, dynamic, cross-domain, content based analysis. Scientometrics 115(1):1-20.
This paper performed market segmentation using self-organizing maps for business-to-business automation of markets in the United States. Their experimented results show the improved clustering performed and dimensionality reduction with SOM. ... Review of Marketing Research, A new spatial classification methodology for simultaneous segmentation ...
A systematic review is defined as "a research method and process for identifying and critically appraising relevant research as well as for collecting and analyzing data from said research" (Snyder, 2019, p. 334). Systematic reviews can be classified as (1) domain-based, (2) theory-based, or (3) method-based (Palmatier et al., 2018).
rather than simply describing market segmentation approaches, the emphasis will be on identifying key segmentation capabilities, which companies must develop in order to improve the outcomes they achieve from segmentation. Finally, the paper argues that market segmentation is comprised of five separate capabilities: segmentation research, segment
Despite the pervasive use of the terms "market segmentation" and "product differentiation," there has been and continues to be considerable misunderstanding about their meaning and use. ... SUBMIT PAPER. Journal of Marketing. Impact Factor: 12.9 / 5-Year ... and Jain Arun K. (1978), "An Approach to Normative Segmentation," Journal ...
Business success depends on understanding customers and their needs. A key method to achieve this is customer segmentation, i.e., dividing individual customers into groups based on their similarities and differences (Cooil et al. 2008).As postulated by Punj and Stewart (1983: 135), "All segmentation research, regardless of the method used, is designed to identify groups of entities (people ...
7) first introduced the concept of market segmentation in the marketing literature arguing that, in place of mass markets, goods would 'find their markets of maximu... Market Segmentation in Practice: Review of Empirical Studies, Methodological Assessment, and Agenda for Future Research: Journal of Strategic Marketing: Vol 16 , No 3 - Get Access
market segmentation research is part of corporate culture, providing discrete labels for groupings, which organize managerial thinking and facilitate communication by providing concrete characterizations of consumer wants within a market. In this paper, we examine the current state of market segmentation research and identify avenues for ...
Market Segmentation, Targeting and Positioning. Rishi Raj Sharma (Guru Nanak Dev University, India) Tanveer Kaur (Guru Nanak Dev University, India) Amanjot Singh Syan (Lovely Professional University, India) Sustainability Marketing. ISBN ...
It focuses on the definition, basis of market segmentation and issues related to market segmentation in detail. This research paper will provide information about the knowledge gap and will show a path for future research in the area of market segmentation, which is the heart of marketing now a day. Keywords: Market segmentation, basis of ...
Applied market segmentation: general observable bases - geo-demographics general unobservable bases - values and lifestyles - conjoint analysis conclusions and directions for future research. Expand 2,192
Rethinking Business Segmentation: A Conceptual Model and Strategic Insights. January 2018. Journal of Strategic Marketing 27 (2) DOI: 10.1080/0965254X.2017.1384750. Authors: Art Weinstein. Nova ...
A market segment is a portion of a market whose needs differ from the larger market and potentially from other segments. When we do segmentation research, we need to consider the current and potential organizational capabilities. Capabilities include existing products and services, technologies, brand reputation, innovation pipeline, etc.
The paper also studied a market segmentation policy for settings in which the firm is capable of detecting the consumer segment in advance. Under the segmentation policy, the products are presented to each consumer according to the quality ranking for their own class. ... The paper leaves some interesting questions for future research. The ...
March 08, 2020. Research Paper on Market Research and Market Segmentation for targeting the right Consumers . Market Segmentation and Targeting Consumers . Market segmentation is the research that ...
Abstract. The importance of data mining techniques for market segmentation is becoming indispensable in the field of marketing research. This is the first identified academic literature review of the available data mining techniques related to market segmentation. This research paper provides surveys of the available literature on data mining ...
Working Paper 32324. DOI 10.3386/w32324. Issue Date April 2024. We develop a segmented markets model which rationalizes the effects of monetary policy on the term structure of interest rates. When arbitrageurs' portfolio features positive duration, an unexpected rise in the short rate lowers their wealth and raises term premia. A calibration ...
Market segmentation can be defined as dividing a market into distinct groups of . ... (1989), and Wedel & Steenkamp (1 989, 1991) are seminal papers that extended . ... of a market research study .
The international digital twin market is a mix of well-established companies, possessing a deep market insight, and emerging start-ups aiming to carve out their niche in this intensely competitive arena. In 2022, dominant players held a substantial 71% of the market share, while start-ups made their mark by securing 29% of the industry.
The emergence of attention-based transformer models has led to their extensive use in various tasks, due to their superior generalization and transfer properties. Recent research has demonstrated that such models, when prompted appropriately, are excellent for few-shot inference. However, such techniques are under-explored for dense prediction tasks like semantic segmentation. In this work, we ...
Apple's smartphone sales tumbled a stunning 10% last quarter, according to market research firm IDC. The main cause: iPhone sales in China fell sharply. The company has lost momentum in China as ...
This paper explores contemporary market segm entation ne eds such as benefit segmentation and usage occasions to assess the attributes that customer's va lue, with a view to enhancing profitability.
Customer segmentation is defined as dividing company's customers. on the basis of demographic (age, gender, marital status) and behavioral (types of. products ordered, annual income) aspects ...