When you choose to publish with PLOS, your research makes an impact. Make your work accessible to all, without restrictions, and accelerate scientific discovery with options like preprints and published peer review that make your work more Open.
- PLOS Biology
- PLOS Climate
- PLOS Complex Systems
- PLOS Computational Biology
- PLOS Digital Health
- PLOS Genetics
- PLOS Global Public Health
- PLOS Medicine
- PLOS Mental Health
- PLOS Neglected Tropical Diseases
- PLOS Pathogens
- PLOS Sustainability and Transformation
- PLOS Collections
- How to Write Discussions and Conclusions

The discussion section contains the results and outcomes of a study. An effective discussion informs readers what can be learned from your experiment and provides context for the results.
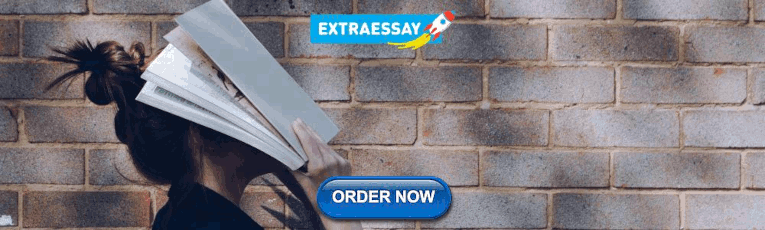
What makes an effective discussion?
When you’re ready to write your discussion, you’ve already introduced the purpose of your study and provided an in-depth description of the methodology. The discussion informs readers about the larger implications of your study based on the results. Highlighting these implications while not overstating the findings can be challenging, especially when you’re submitting to a journal that selects articles based on novelty or potential impact. Regardless of what journal you are submitting to, the discussion section always serves the same purpose: concluding what your study results actually mean.
A successful discussion section puts your findings in context. It should include:
- the results of your research,
- a discussion of related research, and
- a comparison between your results and initial hypothesis.
Tip: Not all journals share the same naming conventions.
You can apply the advice in this article to the conclusion, results or discussion sections of your manuscript.
Our Early Career Researcher community tells us that the conclusion is often considered the most difficult aspect of a manuscript to write. To help, this guide provides questions to ask yourself, a basic structure to model your discussion off of and examples from published manuscripts.

Questions to ask yourself:
- Was my hypothesis correct?
- If my hypothesis is partially correct or entirely different, what can be learned from the results?
- How do the conclusions reshape or add onto the existing knowledge in the field? What does previous research say about the topic?
- Why are the results important or relevant to your audience? Do they add further evidence to a scientific consensus or disprove prior studies?
- How can future research build on these observations? What are the key experiments that must be done?
- What is the “take-home” message you want your reader to leave with?
How to structure a discussion
Trying to fit a complete discussion into a single paragraph can add unnecessary stress to the writing process. If possible, you’ll want to give yourself two or three paragraphs to give the reader a comprehensive understanding of your study as a whole. Here’s one way to structure an effective discussion:
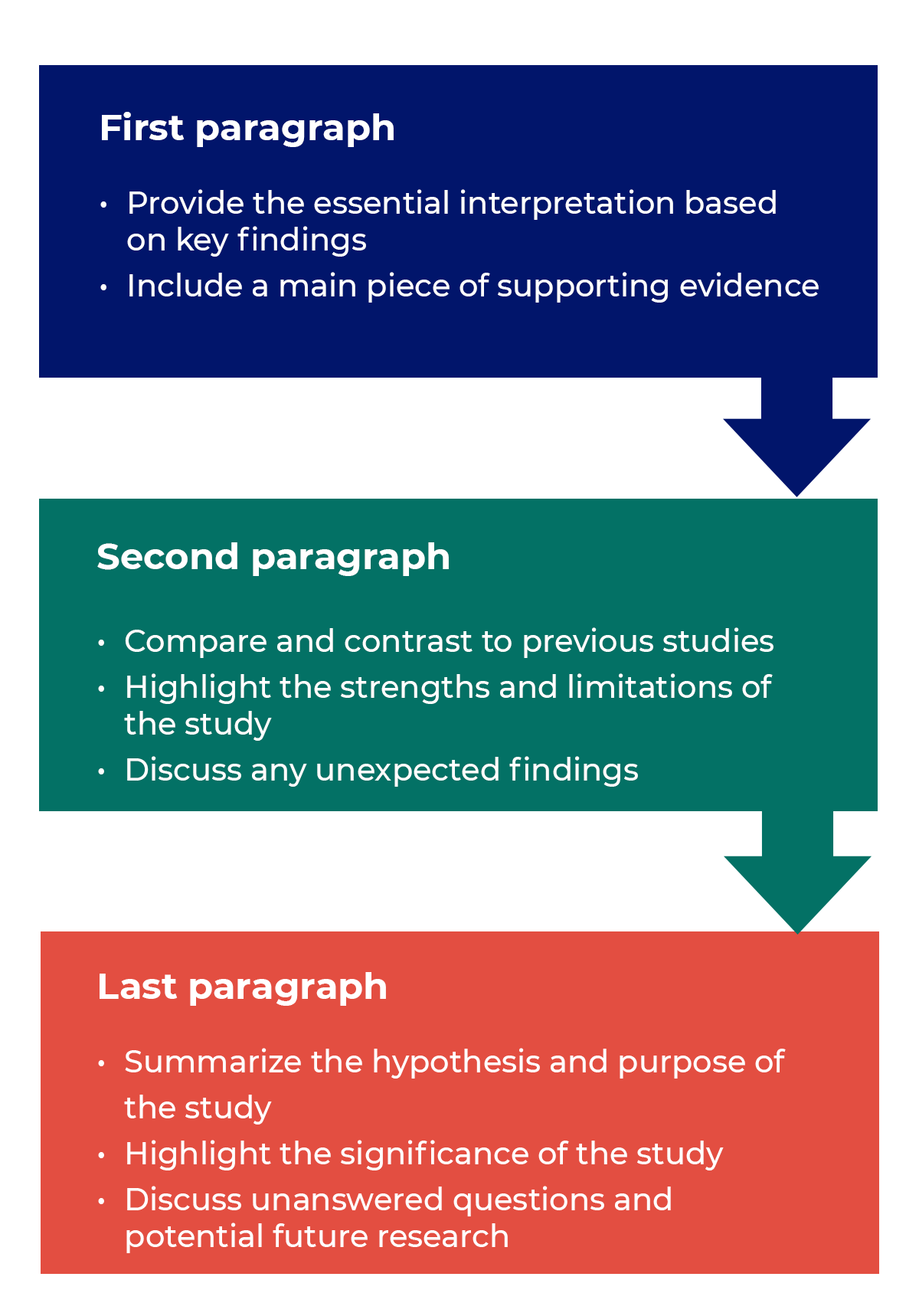
Writing Tips
While the above sections can help you brainstorm and structure your discussion, there are many common mistakes that writers revert to when having difficulties with their paper. Writing a discussion can be a delicate balance between summarizing your results, providing proper context for your research and avoiding introducing new information. Remember that your paper should be both confident and honest about the results!

- Read the journal’s guidelines on the discussion and conclusion sections. If possible, learn about the guidelines before writing the discussion to ensure you’re writing to meet their expectations.
- Begin with a clear statement of the principal findings. This will reinforce the main take-away for the reader and set up the rest of the discussion.
- Explain why the outcomes of your study are important to the reader. Discuss the implications of your findings realistically based on previous literature, highlighting both the strengths and limitations of the research.
- State whether the results prove or disprove your hypothesis. If your hypothesis was disproved, what might be the reasons?
- Introduce new or expanded ways to think about the research question. Indicate what next steps can be taken to further pursue any unresolved questions.
- If dealing with a contemporary or ongoing problem, such as climate change, discuss possible consequences if the problem is avoided.
- Be concise. Adding unnecessary detail can distract from the main findings.
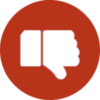
Don’t
- Rewrite your abstract. Statements with “we investigated” or “we studied” generally do not belong in the discussion.
- Include new arguments or evidence not previously discussed. Necessary information and evidence should be introduced in the main body of the paper.
- Apologize. Even if your research contains significant limitations, don’t undermine your authority by including statements that doubt your methodology or execution.
- Shy away from speaking on limitations or negative results. Including limitations and negative results will give readers a complete understanding of the presented research. Potential limitations include sources of potential bias, threats to internal or external validity, barriers to implementing an intervention and other issues inherent to the study design.
- Overstate the importance of your findings. Making grand statements about how a study will fully resolve large questions can lead readers to doubt the success of the research.
Snippets of Effective Discussions:
Consumer-based actions to reduce plastic pollution in rivers: A multi-criteria decision analysis approach
Identifying reliable indicators of fitness in polar bears
- How to Write a Great Title
- How to Write an Abstract
- How to Write Your Methods
- How to Report Statistics
- How to Edit Your Work
The contents of the Peer Review Center are also available as a live, interactive training session, complete with slides, talking points, and activities. …
The contents of the Writing Center are also available as a live, interactive training session, complete with slides, talking points, and activities. …
There’s a lot to consider when deciding where to submit your work. Learn how to choose a journal that will help your study reach its audience, while reflecting your values as a researcher…
- SpringerLink shop
Discussion and Conclusions
Your Discussion and Conclusions sections should answer the question: What do your results mean?
In other words, the majority of the Discussion and Conclusions sections should be an interpretation of your results. You should:
- Discuss your conclusions in order of most to least important.
- Compare your results with those from other studies: Are they consistent? If not, discuss possible reasons for the difference.
- Mention any inconclusive results and explain them as best you can. You may suggest additional experiments needed to clarify your results.
- Briefly describe the limitations of your study to show reviewers and readers that you have considered your experiment’s weaknesses. Many researchers are hesitant to do this as they feel it highlights the weaknesses in their research to the editor and reviewer. However doing this actually makes a positive impression of your paper as it makes it clear that you have an in depth understanding of your topic and can think objectively of your research.
- Discuss what your results may mean for researchers in the same field as you, researchers in other fields, and the general public. How could your findings be applied?
- State how your results extend the findings of previous studies.
- If your findings are preliminary, suggest future studies that need to be carried out.
- At the end of your Discussion and Conclusions sections, state your main conclusions once again .
Back │ Next
- USC Libraries
- Research Guides
Organizing Your Social Sciences Research Paper
- 9. The Conclusion
- Purpose of Guide
- Design Flaws to Avoid
- Independent and Dependent Variables
- Glossary of Research Terms
- Reading Research Effectively
- Narrowing a Topic Idea
- Broadening a Topic Idea
- Extending the Timeliness of a Topic Idea
- Academic Writing Style
- Applying Critical Thinking
- Choosing a Title
- Making an Outline
- Paragraph Development
- Research Process Video Series
- Executive Summary
- The C.A.R.S. Model
- Background Information
- The Research Problem/Question
- Theoretical Framework
- Citation Tracking
- Content Alert Services
- Evaluating Sources
- Primary Sources
- Secondary Sources
- Tiertiary Sources
- Scholarly vs. Popular Publications
- Qualitative Methods
- Quantitative Methods
- Insiderness
- Using Non-Textual Elements
- Limitations of the Study
- Common Grammar Mistakes
- Writing Concisely
- Avoiding Plagiarism
- Footnotes or Endnotes?
- Further Readings
- Generative AI and Writing
- USC Libraries Tutorials and Other Guides
- Bibliography
The conclusion is intended to help the reader understand why your research should matter to them after they have finished reading the paper. A conclusion is not merely a summary of the main topics covered or a re-statement of your research problem, but a synthesis of key points derived from the findings of your study and, if applicable, where you recommend new areas for future research. For most college-level research papers, two or three well-developed paragraphs is sufficient for a conclusion, although in some cases, more paragraphs may be required in describing the key findings and their significance.
Conclusions. The Writing Center. University of North Carolina; Conclusions. The Writing Lab and The OWL. Purdue University.
Importance of a Good Conclusion
A well-written conclusion provides you with important opportunities to demonstrate to the reader your understanding of the research problem. These include:
- Presenting the last word on the issues you raised in your paper . Just as the introduction gives a first impression to your reader, the conclusion offers a chance to leave a lasting impression. Do this, for example, by highlighting key findings in your analysis that advance new understanding about the research problem, that are unusual or unexpected, or that have important implications applied to practice.
- Summarizing your thoughts and conveying the larger significance of your study . The conclusion is an opportunity to succinctly re-emphasize your answer to the "So What?" question by placing the study within the context of how your research advances past research about the topic.
- Identifying how a gap in the literature has been addressed . The conclusion can be where you describe how a previously identified gap in the literature [first identified in your literature review section] has been addressed by your research and why this contribution is significant.
- Demonstrating the importance of your ideas . Don't be shy. The conclusion offers an opportunity to elaborate on the impact and significance of your findings. This is particularly important if your study approached examining the research problem from an unusual or innovative perspective.
- Introducing possible new or expanded ways of thinking about the research problem . This does not refer to introducing new information [which should be avoided], but to offer new insight and creative approaches for framing or contextualizing the research problem based on the results of your study.
Bunton, David. “The Structure of PhD Conclusion Chapters.” Journal of English for Academic Purposes 4 (July 2005): 207–224; Conclusions. The Writing Center. University of North Carolina; Kretchmer, Paul. Twelve Steps to Writing an Effective Conclusion. San Francisco Edit, 2003-2008; Conclusions. The Writing Lab and The OWL. Purdue University; Assan, Joseph. "Writing the Conclusion Chapter: The Good, the Bad and the Missing." Liverpool: Development Studies Association (2009): 1-8.
Structure and Writing Style
I. General Rules
The general function of your paper's conclusion is to restate the main argument . It reminds the reader of the strengths of your main argument(s) and reiterates the most important evidence supporting those argument(s). Do this by clearly summarizing the context, background, and necessity of pursuing the research problem you investigated in relation to an issue, controversy, or a gap found in the literature. However, make sure that your conclusion is not simply a repetitive summary of the findings. This reduces the impact of the argument(s) you have developed in your paper.
When writing the conclusion to your paper, follow these general rules:
- Present your conclusions in clear, concise language. Re-state the purpose of your study, then describe how your findings differ or support those of other studies and why [i.e., what were the unique, new, or crucial contributions your study made to the overall research about your topic?].
- Do not simply reiterate your findings or the discussion of your results. Provide a synthesis of arguments presented in the paper to show how these converge to address the research problem and the overall objectives of your study.
- Indicate opportunities for future research if you haven't already done so in the discussion section of your paper. Highlighting the need for further research provides the reader with evidence that you have an in-depth awareness of the research problem but that further investigations should take place beyond the scope of your investigation.
Consider the following points to help ensure your conclusion is presented well:
- If the argument or purpose of your paper is complex, you may need to summarize the argument for your reader.
- If, prior to your conclusion, you have not yet explained the significance of your findings or if you are proceeding inductively, use the end of your paper to describe your main points and explain their significance.
- Move from a detailed to a general level of consideration that returns the topic to the context provided by the introduction or within a new context that emerges from the data [this is opposite of the introduction, which begins with general discussion of the context and ends with a detailed description of the research problem].
The conclusion also provides a place for you to persuasively and succinctly restate the research problem, given that the reader has now been presented with all the information about the topic . Depending on the discipline you are writing in, the concluding paragraph may contain your reflections on the evidence presented. However, the nature of being introspective about the research you have conducted will depend on the topic and whether your professor wants you to express your observations in this way. If asked to think introspectively about the topics, do not delve into idle speculation. Being introspective means looking within yourself as an author to try and understand an issue more deeply, not to guess at possible outcomes or make up scenarios not supported by the evidence.
II. Developing a Compelling Conclusion
Although an effective conclusion needs to be clear and succinct, it does not need to be written passively or lack a compelling narrative. Strategies to help you move beyond merely summarizing the key points of your research paper may include any of the following:
- If your essay deals with a critical, contemporary problem, warn readers of the possible consequences of not attending to the problem proactively.
- Recommend a specific course or courses of action that, if adopted, could address a specific problem in practice or in the development of new knowledge leading to positive change.
- Cite a relevant quotation or expert opinion already noted in your paper in order to lend authority and support to the conclusion(s) you have reached [a good source would be from your literature review].
- Explain the consequences of your research in a way that elicits action or demonstrates urgency in seeking change.
- Restate a key statistic, fact, or visual image to emphasize the most important finding of your paper.
- If your discipline encourages personal reflection, illustrate your concluding point by drawing from your own life experiences.
- Return to an anecdote, an example, or a quotation that you presented in your introduction, but add further insight derived from the findings of your study; use your interpretation of results from your study to recast it in new or important ways.
- Provide a "take-home" message in the form of a succinct, declarative statement that you want the reader to remember about your study.
III. Problems to Avoid
Failure to be concise Your conclusion section should be concise and to the point. Conclusions that are too lengthy often have unnecessary information in them. The conclusion is not the place for details about your methodology or results. Although you should give a summary of what was learned from your research, this summary should be relatively brief, since the emphasis in the conclusion is on the implications, evaluations, insights, and other forms of analysis that you make. Strategies for writing concisely can be found here .
Failure to comment on larger, more significant issues In the introduction, your task was to move from the general [the field of study] to the specific [the research problem]. However, in the conclusion, your task is to move from a specific discussion [your research problem] back to a general discussion framed around the implications and significance of your findings [i.e., how your research contributes new understanding or fills an important gap in the literature]. In short, the conclusion is where you should place your research within a larger context [visualize your paper as an hourglass--start with a broad introduction and review of the literature, move to the specific analysis and discussion, conclude with a broad summary of the study's implications and significance].
Failure to reveal problems and negative results Negative aspects of the research process should never be ignored. These are problems, deficiencies, or challenges encountered during your study. They should be summarized as a way of qualifying your overall conclusions. If you encountered negative or unintended results [i.e., findings that are validated outside the research context in which they were generated], you must report them in the results section and discuss their implications in the discussion section of your paper. In the conclusion, use negative results as an opportunity to explain their possible significance and/or how they may form the basis for future research.
Failure to provide a clear summary of what was learned In order to be able to discuss how your research fits within your field of study [and possibly the world at large], you need to summarize briefly and succinctly how it contributes to new knowledge or a new understanding about the research problem. This element of your conclusion may be only a few sentences long.
Failure to match the objectives of your research Often research objectives in the social and behavioral sciences change while the research is being carried out. This is not a problem unless you forget to go back and refine the original objectives in your introduction. As these changes emerge they must be documented so that they accurately reflect what you were trying to accomplish in your research [not what you thought you might accomplish when you began].
Resist the urge to apologize If you've immersed yourself in studying the research problem, you presumably should know a good deal about it [perhaps even more than your professor!]. Nevertheless, by the time you have finished writing, you may be having some doubts about what you have produced. Repress those doubts! Don't undermine your authority as a researcher by saying something like, "This is just one approach to examining this problem; there may be other, much better approaches that...." The overall tone of your conclusion should convey confidence to the reader about the study's validity and realiability.
Assan, Joseph. "Writing the Conclusion Chapter: The Good, the Bad and the Missing." Liverpool: Development Studies Association (2009): 1-8; Concluding Paragraphs. College Writing Center at Meramec. St. Louis Community College; Conclusions. The Writing Center. University of North Carolina; Conclusions. The Writing Lab and The OWL. Purdue University; Freedman, Leora and Jerry Plotnick. Introductions and Conclusions. The Lab Report. University College Writing Centre. University of Toronto; Leibensperger, Summer. Draft Your Conclusion. Academic Center, the University of Houston-Victoria, 2003; Make Your Last Words Count. The Writer’s Handbook. Writing Center. University of Wisconsin Madison; Miquel, Fuster-Marquez and Carmen Gregori-Signes. “Chapter Six: ‘Last but Not Least:’ Writing the Conclusion of Your Paper.” In Writing an Applied Linguistics Thesis or Dissertation: A Guide to Presenting Empirical Research . John Bitchener, editor. (Basingstoke,UK: Palgrave Macmillan, 2010), pp. 93-105; Tips for Writing a Good Conclusion. Writing@CSU. Colorado State University; Kretchmer, Paul. Twelve Steps to Writing an Effective Conclusion. San Francisco Edit, 2003-2008; Writing Conclusions. Writing Tutorial Services, Center for Innovative Teaching and Learning. Indiana University; Writing: Considering Structure and Organization. Institute for Writing Rhetoric. Dartmouth College.
Writing Tip
Don't Belabor the Obvious!
Avoid phrases like "in conclusion...," "in summary...," or "in closing...." These phrases can be useful, even welcome, in oral presentations. But readers can see by the tell-tale section heading and number of pages remaining that they are reaching the end of your paper. You'll irritate your readers if you belabor the obvious.
Assan, Joseph. "Writing the Conclusion Chapter: The Good, the Bad and the Missing." Liverpool: Development Studies Association (2009): 1-8.
Another Writing Tip
New Insight, Not New Information!
Don't surprise the reader with new information in your conclusion that was never referenced anywhere else in the paper. This why the conclusion rarely has citations to sources. If you have new information to present, add it to the discussion or other appropriate section of the paper. Note that, although no new information is introduced, the conclusion, along with the discussion section, is where you offer your most "original" contributions in the paper; the conclusion is where you describe the value of your research, demonstrate that you understand the material that you’ve presented, and position your findings within the larger context of scholarship on the topic, including describing how your research contributes new insights to that scholarship.
Assan, Joseph. "Writing the Conclusion Chapter: The Good, the Bad and the Missing." Liverpool: Development Studies Association (2009): 1-8; Conclusions. The Writing Center. University of North Carolina.
- << Previous: Limitations of the Study
- Next: Appendices >>
- Last Updated: Apr 24, 2024 10:51 AM
- URL: https://libguides.usc.edu/writingguide

- Walden University
- Faculty Portal
General Research Paper Guidelines: Discussion
Discussion section.
The overall purpose of a research paper’s discussion section is to evaluate and interpret results, while explaining both the implications and limitations of your findings. Per APA (2020) guidelines, this section requires you to “examine, interpret, and qualify the results and draw inferences and conclusions from them” (p. 89). Discussion sections also require you to detail any new insights, think through areas for future research, highlight the work that still needs to be done to further your topic, and provide a clear conclusion to your research paper. In a good discussion section, you should do the following:
- Clearly connect the discussion of your results to your introduction, including your central argument, thesis, or problem statement.
- Provide readers with a critical thinking through of your results, answering the “so what?” question about each of your findings. In other words, why is this finding important?
- Detail how your research findings might address critical gaps or problems in your field
- Compare your results to similar studies’ findings
- Provide the possibility of alternative interpretations, as your goal as a researcher is to “discover” and “examine” and not to “prove” or “disprove.” Instead of trying to fit your results into your hypothesis, critically engage with alternative interpretations to your results.
For more specific details on your Discussion section, be sure to review Sections 3.8 (pp. 89-90) and 3.16 (pp. 103-104) of your 7 th edition APA manual
*Box content adapted from:
University of Southern California (n.d.). Organizing your social sciences research paper: 8 the discussion . https://libguides.usc.edu/writingguide/discussion
Limitations
Limitations of generalizability or utility of findings, often over which the researcher has no control, should be detailed in your Discussion section. Including limitations for your reader allows you to demonstrate you have thought critically about your given topic, understood relevant literature addressing your topic, and chosen the methodology most appropriate for your research. It also allows you an opportunity to suggest avenues for future research on your topic. An effective limitations section will include the following:
- Detail (a) sources of potential bias, (b) possible imprecision of measures, (c) other limitations or weaknesses of the study, including any methodological or researcher limitations.
- Sample size: In quantitative research, if a sample size is too small, it is more difficult to generalize results.
- Lack of available/reliable data : In some cases, data might not be available or reliable, which will ultimately affect the overall scope of your research. Use this as an opportunity to explain areas for future study.
- Lack of prior research on your study topic: In some cases, you might find that there is very little or no similar research on your study topic, which hinders the credibility and scope of your own research. If this is the case, use this limitation as an opportunity to call for future research. However, make sure you have done a thorough search of the available literature before making this claim.
- Flaws in measurement of data: Hindsight is 20/20, and you might realize after you have completed your research that the data tool you used actually limited the scope or results of your study in some way. Again, acknowledge the weakness and use it as an opportunity to highlight areas for future study.
- Limits of self-reported data: In your research, you are assuming that any participants will be honest and forthcoming with responses or information they provide to you. Simply acknowledging this assumption as a possible limitation is important in your research.
- Access: Most research requires that you have access to people, documents, organizations, etc.. However, for various reasons, access is sometimes limited or denied altogether. If this is the case, you will want to acknowledge access as a limitation to your research.
- Time: Choosing a research focus that is narrow enough in scope to finish in a given time period is important. If such limitations of time prevent you from certain forms of research, access, or study designs, acknowledging this time restraint is important. Acknowledging such limitations is important, as they can point other researchers to areas that require future study.
- Potential Bias: All researchers have some biases, so when reading and revising your draft, pay special attention to the possibilities for bias in your own work. Such bias could be in the form you organized people, places, participants, or events. They might also exist in the method you selected or the interpretation of your results. Acknowledging such bias is an important part of the research process.
- Language Fluency: On occasion, researchers or research participants might have language fluency issues, which could potentially hinder results or how effectively you interpret results. If this is an issue in your research, make sure to acknowledge it in your limitations section.
University of Southern California (n.d.). Organizing your social sciences research paper: Limitations of the study . https://libguides.usc.edu/writingguide/limitations
In many research papers, the conclusion, like the limitations section, is folded into the larger discussion section. If you are unsure whether to include the conclusion as part of your discussion or as a separate section, be sure to defer to the assignment instructions or ask your instructor.
The conclusion is important, as it is specifically designed to highlight your research’s larger importance outside of the specific results of your study. Your conclusion section allows you to reiterate the main findings of your study, highlight their importance, and point out areas for future research. Based on the scope of your paper, your conclusion could be anywhere from one to three paragraphs long. An effective conclusion section should include the following:
- Describe the possibilities for continued research on your topic, including what might be improved, adapted, or added to ensure useful and informed future research.
- Provide a detailed account of the importance of your findings
- Reiterate why your problem is important, detail how your interpretation of results impacts the subfield of study, and what larger issues both within and outside of your field might be affected from such results
University of Southern California (n.d.). Organizing your social sciences research paper: 9. the conclusion . https://libguides.usc.edu/writingguide/conclusion
- Previous Page: Results
- Next Page: References
- Office of Student Disability Services
Walden Resources
Departments.
- Academic Residencies
- Academic Skills
- Career Planning and Development
- Customer Care Team
- Field Experience
- Military Services
- Student Success Advising
- Writing Skills
Centers and Offices
- Center for Social Change
- Office of Academic Support and Instructional Services
- Office of Degree Acceleration
- Office of Research and Doctoral Services
- Office of Student Affairs
Student Resources
- Doctoral Writing Assessment
- Form & Style Review
- Quick Answers
- ScholarWorks
- SKIL Courses and Workshops
- Walden Bookstore
- Walden Catalog & Student Handbook
- Student Safety/Title IX
- Legal & Consumer Information
- Website Terms and Conditions
- Cookie Policy
- Accessibility
- Accreditation
- State Authorization
- Net Price Calculator
- Contact Walden
Walden University is a member of Adtalem Global Education, Inc. www.adtalem.com Walden University is certified to operate by SCHEV © 2024 Walden University LLC. All rights reserved.
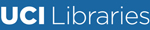
- Langson Library
- Science Library
- Grunigen Medical Library
- Law Library
- Connect From Off-Campus
- Accessibility
- Gateway Study Center
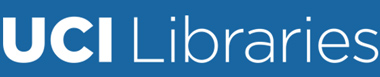
Email this link
Writing a scientific paper.
- Writing a lab report
- INTRODUCTION
Writing a "good" discussion section
"discussion and conclusions checklist" from: how to write a good scientific paper. chris a. mack. spie. 2018., peer review.
- LITERATURE CITED
- Bibliography of guides to scientific writing and presenting
- Presentations
- Lab Report Writing Guides on the Web
This is is usually the hardest section to write. You are trying to bring out the true meaning of your data without being too long. Do not use words to conceal your facts or reasoning. Also do not repeat your results, this is a discussion.
- Present principles, relationships and generalizations shown by the results
- Point out exceptions or lack of correlations. Define why you think this is so.
- Show how your results agree or disagree with previously published works
- Discuss the theoretical implications of your work as well as practical applications
- State your conclusions clearly. Summarize your evidence for each conclusion.
- Discuss the significance of the results
- Evidence does not explain itself; the results must be presented and then explained.
- Typical stages in the discussion: summarizing the results, discussing whether results are expected or unexpected, comparing these results to previous work, interpreting and explaining the results (often by comparison to a theory or model), and hypothesizing about their generality.
- Discuss any problems or shortcomings encountered during the course of the work.
- Discuss possible alternate explanations for the results.
- Avoid: presenting results that are never discussed; presenting discussion that does not relate to any of the results; presenting results and discussion in chronological order rather than logical order; ignoring results that do not support the conclusions; drawing conclusions from results without logical arguments to back them up.
CONCLUSIONS
- Provide a very brief summary of the Results and Discussion.
- Emphasize the implications of the findings, explaining how the work is significant and providing the key message(s) the author wishes to convey.
- Provide the most general claims that can be supported by the evidence.
- Provide a future perspective on the work.
- Avoid: repeating the abstract; repeating background information from the Introduction; introducing new evidence or new arguments not found in the Results and Discussion; repeating the arguments made in the Results and Discussion; failing to address all of the research questions set out in the Introduction.
WHAT HAPPENS AFTER I COMPLETE MY PAPER?
The peer review process is the quality control step in the publication of ideas. Papers that are submitted to a journal for publication are sent out to several scientists (peers) who look carefully at the paper to see if it is "good science". These reviewers then recommend to the editor of a journal whether or not a paper should be published. Most journals have publication guidelines. Ask for them and follow them exactly. Peer reviewers examine the soundness of the materials and methods section. Are the materials and methods used written clearly enough for another scientist to reproduce the experiment? Other areas they look at are: originality of research, significance of research question studied, soundness of the discussion and interpretation, correct spelling and use of technical terms, and length of the article.
- << Previous: RESULTS
- Next: LITERATURE CITED >>
- Last Updated: Aug 4, 2023 9:33 AM
- URL: https://guides.lib.uci.edu/scientificwriting
Off-campus? Please use the Software VPN and choose the group UCIFull to access licensed content. For more information, please Click here
Software VPN is not available for guests, so they may not have access to some content when connecting from off-campus.
How to Write the Discussion Section of a Research Paper
The discussion section of a research paper analyzes and interprets the findings, provides context, compares them with previous studies, identifies limitations, and suggests future research directions.
Updated on September 15, 2023

Structure your discussion section right, and you’ll be cited more often while doing a greater service to the scientific community. So, what actually goes into the discussion section? And how do you write it?
The discussion section of your research paper is where you let the reader know how your study is positioned in the literature, what to take away from your paper, and how your work helps them. It can also include your conclusions and suggestions for future studies.
First, we’ll define all the parts of your discussion paper, and then look into how to write a strong, effective discussion section for your paper or manuscript.
Discussion section: what is it, what it does
The discussion section comes later in your paper, following the introduction, methods, and results. The discussion sets up your study’s conclusions. Its main goals are to present, interpret, and provide a context for your results.
What is it?
The discussion section provides an analysis and interpretation of the findings, compares them with previous studies, identifies limitations, and suggests future directions for research.
This section combines information from the preceding parts of your paper into a coherent story. By this point, the reader already knows why you did your study (introduction), how you did it (methods), and what happened (results). In the discussion, you’ll help the reader connect the ideas from these sections.
Why is it necessary?
The discussion provides context and interpretations for the results. It also answers the questions posed in the introduction. While the results section describes your findings, the discussion explains what they say. This is also where you can describe the impact or implications of your research.
Adds context for your results
Most research studies aim to answer a question, replicate a finding, or address limitations in the literature. These goals are first described in the introduction. However, in the discussion section, the author can refer back to them to explain how the study's objective was achieved.
Shows what your results actually mean and real-world implications
The discussion can also describe the effect of your findings on research or practice. How are your results significant for readers, other researchers, or policymakers?
What to include in your discussion (in the correct order)
A complete and effective discussion section should at least touch on the points described below.
Summary of key findings
The discussion should begin with a brief factual summary of the results. Concisely overview the main results you obtained.
Begin with key findings with supporting evidence
Your results section described a list of findings, but what message do they send when you look at them all together?
Your findings were detailed in the results section, so there’s no need to repeat them here, but do provide at least a few highlights. This will help refresh the reader’s memory and help them focus on the big picture.
Read the first paragraph of the discussion section in this article (PDF) for an example of how to start this part of your paper. Notice how the authors break down their results and follow each description sentence with an explanation of why each finding is relevant.
State clearly and concisely
Following a clear and direct writing style is especially important in the discussion section. After all, this is where you will make some of the most impactful points in your paper. While the results section often contains technical vocabulary, such as statistical terms, the discussion section lets you describe your findings more clearly.
Interpretation of results
Once you’ve given your reader an overview of your results, you need to interpret those results. In other words, what do your results mean? Discuss the findings’ implications and significance in relation to your research question or hypothesis.
Analyze and interpret your findings
Look into your findings and explore what’s behind them or what may have caused them. If your introduction cited theories or studies that could explain your findings, use these sources as a basis to discuss your results.
For example, look at the second paragraph in the discussion section of this article on waggling honey bees. Here, the authors explore their results based on information from the literature.
Unexpected or contradictory results
Sometimes, your findings are not what you expect. Here’s where you describe this and try to find a reason for it. Could it be because of the method you used? Does it have something to do with the variables analyzed? Comparing your methods with those of other similar studies can help with this task.
Context and comparison with previous work
Refer to related studies to place your research in a larger context and the literature. Compare and contrast your findings with existing literature, highlighting similarities, differences, and/or contradictions.
How your work compares or contrasts with previous work
Studies with similar findings to yours can be cited to show the strength of your findings. Information from these studies can also be used to help explain your results. Differences between your findings and others in the literature can also be discussed here.
How to divide this section into subsections
If you have more than one objective in your study or many key findings, you can dedicate a separate section to each of these. Here’s an example of this approach. You can see that the discussion section is divided into topics and even has a separate heading for each of them.
Limitations
Many journals require you to include the limitations of your study in the discussion. Even if they don’t, there are good reasons to mention these in your paper.
Why limitations don’t have a negative connotation
A study’s limitations are points to be improved upon in future research. While some of these may be flaws in your method, many may be due to factors you couldn’t predict.
Examples include time constraints or small sample sizes. Pointing this out will help future researchers avoid or address these issues. This part of the discussion can also include any attempts you have made to reduce the impact of these limitations, as in this study .
How limitations add to a researcher's credibility
Pointing out the limitations of your study demonstrates transparency. It also shows that you know your methods well and can conduct a critical assessment of them.
Implications and significance
The final paragraph of the discussion section should contain the take-home messages for your study. It can also cite the “strong points” of your study, to contrast with the limitations section.
Restate your hypothesis
Remind the reader what your hypothesis was before you conducted the study.
How was it proven or disproven?
Identify your main findings and describe how they relate to your hypothesis.
How your results contribute to the literature
Were you able to answer your research question? Or address a gap in the literature?
Future implications of your research
Describe the impact that your results may have on the topic of study. Your results may show, for instance, that there are still limitations in the literature for future studies to address. There may be a need for studies that extend your findings in a specific way. You also may need additional research to corroborate your findings.
Sample discussion section
This fictitious example covers all the aspects discussed above. Your actual discussion section will probably be much longer, but you can read this to get an idea of everything your discussion should cover.
Our results showed that the presence of cats in a household is associated with higher levels of perceived happiness by its human occupants. These findings support our hypothesis and demonstrate the association between pet ownership and well-being.
The present findings align with those of Bao and Schreer (2016) and Hardie et al. (2023), who observed greater life satisfaction in pet owners relative to non-owners. Although the present study did not directly evaluate life satisfaction, this factor may explain the association between happiness and cat ownership observed in our sample.
Our findings must be interpreted in light of some limitations, such as the focus on cat ownership only rather than pets as a whole. This may limit the generalizability of our results.
Nevertheless, this study had several strengths. These include its strict exclusion criteria and use of a standardized assessment instrument to investigate the relationships between pets and owners. These attributes bolster the accuracy of our results and reduce the influence of confounding factors, increasing the strength of our conclusions. Future studies may examine the factors that mediate the association between pet ownership and happiness to better comprehend this phenomenon.
This brief discussion begins with a quick summary of the results and hypothesis. The next paragraph cites previous research and compares its findings to those of this study. Information from previous studies is also used to help interpret the findings. After discussing the results of the study, some limitations are pointed out. The paper also explains why these limitations may influence the interpretation of results. Then, final conclusions are drawn based on the study, and directions for future research are suggested.
How to make your discussion flow naturally
If you find writing in scientific English challenging, the discussion and conclusions are often the hardest parts of the paper to write. That’s because you’re not just listing up studies, methods, and outcomes. You’re actually expressing your thoughts and interpretations in words.
- How formal should it be?
- What words should you use, or not use?
- How do you meet strict word limits, or make it longer and more informative?
Always give it your best, but sometimes a helping hand can, well, help. Getting a professional edit can help clarify your work’s importance while improving the English used to explain it. When readers know the value of your work, they’ll cite it. We’ll assign your study to an expert editor knowledgeable in your area of research. Their work will clarify your discussion, helping it to tell your story. Find out more about AJE Editing.

Adam Goulston, PsyD, MS, MBA, MISD, ELS
Science Marketing Consultant
See our "Privacy Policy"
Ensure your structure and ideas are consistent and clearly communicated
Pair your Premium Editing with our add-on service Presubmission Review for an overall assessment of your manuscript.

Discussion Vs. Conclusion: Know the Difference Before Drafting Manuscripts
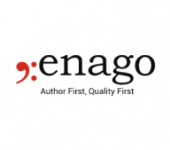
The discussion section of your manuscript can be one of the hardest to write as it requires you to think about the meaning of the research you have done. An effective discussion section tells the reader what your study means and why it is important. In this article, we will cover some pointers for writing clear/well-organized discussion and conclusion sections and discuss what should NOT be a part of these sections.
What Should be in the Discussion Section?
Your discussion is, in short, the answer to the question “what do my results mean?” The discussion section of the manuscript should come after the methods and results section and before the conclusion. It should relate back directly to the questions posed in your introduction, and contextualize your results within the literature you have covered in your literature review . In order to make your discussion section engaging, you should include the following information:
- The major findings of your study
- The meaning of those findings
- How these findings relate to what others have done
- Limitations of your findings
- An explanation for any surprising, unexpected, or inconclusive results
- Suggestions for further research
Your discussion should NOT include any of the following information:
- New results or data not presented previously in the paper
- Unwarranted speculation
- Tangential issues
- Conclusions not supported by your data
Related: Avoid outright rejection with a well-structured manuscript. Check out these resources and improve your manuscript now!
How to Make the Discussion Section Effective?
There are several ways to make the discussion section of your manuscript effective, interesting, and relevant. Hear from one of our experts on how to structure your discussion section and distinguish it from the results section:
Now that we have listened to how to approach writing a discussion section, let’s delve deeper into some essential tips with a few examples:
- Most writing guides recommend listing the findings of your study in decreasing order of their importance. You would not want your reader to lose sight of the key results that you found. Therefore, put the most important finding front and center. Example: Imagine that you conduct a study aimed at evaluating the effectiveness of stent placement in patients with partially blocked arteries. You find that despite this being a common first-line treatment, stents are not effective for patients with partially blocked arteries. The study also discovers that patients treated with a stent tend to develop asthma at slightly higher rates than those who receive no such treatment.
Which sentence would you choose to begin your discussion? Our findings suggest that patients who had partially blocked arteries and were treated with a stent as the first line of intervention had no better outcomes than patients who were not given any surgical treatments. Our findings noted that patients who received stents demonstrated slightly higher rates of asthma than those who did not. In addition, the placement of a stent did not impact their rates of cardiac events in a statistically significant way.
If you chose the first example, you are correct!
- If you are not sure which results are the most important, go back to your research question and start from there. The most important result is the one that answers your research question.
- It is also necessary to contextualize the meaning of your findings for the reader. What does previous literature say, and do your results agree? Do your results elaborate on previous findings, or differ significantly?
- In our stent example, if previous literature found that stents were an effective line of treatment for patients with partially blocked arteries, you should explore why your interpretation seems different in the discussion section. Did your methodology differ? Was your study broader in scope and larger in scale than the previous studies? Were there any limitations to previous studies that your study overcame? Alternatively, is it possible that your own study could be incorrect because of some difficulties you had in carrying it out? The discussion section should narrate a coherent story to the target audience.
- Finally, remember not to introduce new ideas/data, or speculate wildly on the possible future implications of your study in the discussion section. However, considering alternative explanations for your results is encouraged.
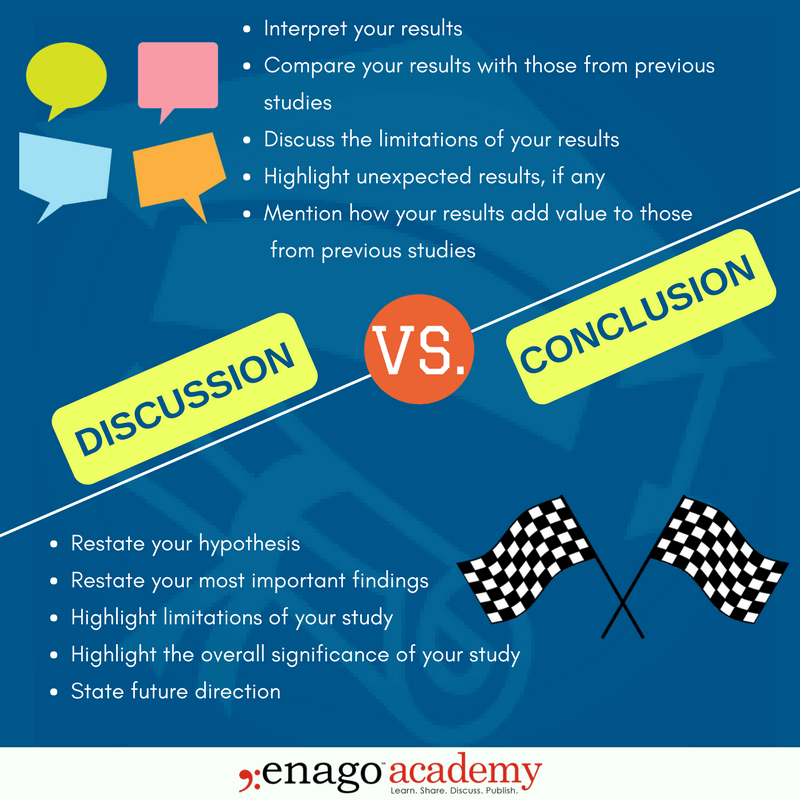
Avoiding Confusion in your Conclusion!
Many writers confuse the information they should include in their discussion with the information they should place in their conclusion. One easy way to avoid this confusion is to think of your conclusion as a summary of everything that you have said thus far. In the conclusion section, you remind the reader of what they have just read. Your conclusion should:
- Restate your hypothesis or research question
- Restate your major findings
- Tell the reader what contribution your study has made to the existing literature
- Highlight any limitations of your study
- State future directions for research/recommendations
Your conclusion should NOT:
- Introduce new arguments
- Introduce new data
- Fail to include your research question
- Fail to state your major results
An appropriate conclusion to our hypothetical stent study might read as follows:
In this study, we examined the effectiveness of stent placement. We compared the patients with partially blocked arteries to those with non-surgical interventions. After examining the five-year medical outcomes of 19,457 patients in the Greater Dallas area, our statistical analysis concluded that the placement of a stent resulted in outcomes that were no better than non-surgical interventions such as diet and exercise. Although previous findings indicated that stent placement improved patient outcomes, our study followed a greater number of patients than those in major studies conducted previously. It is possible that outcomes would vary if measured over a ten or fifteen year period. Future researchers should consider investigating the impact of stent placement in these patients over a longer period (five years or longer). Regardless, our results point to the need for medical practitioners to reconsider the placement of a stent as the first line of treatment as non-surgical interventions may have equally positive outcomes for patients.
Did you find the tips in this article relevant? What is the most challenging portion of a research paper for you to write? Let us know in the comments section below!

This is the most stunning and self-instructional site I have come across. Thank you so much for your updates! I will help me work on my dissertation.
Thank you so much!! It helps a lot!
very helpful, thank you
thanks a lot …
this is one of a kind! awesome, straight to the point and easy to understand! Thanks a lot
Thank you so much for this, I never comment on these types of sites but I just had too here as I’ve never seen an article that has answered everyone of the questions I wanted when I searched on Google. Certainly not to the extent and clear clarity that you have presented. Thanks so much for this it has put my mind to ease a bit with my terrible dissertation haha.
Have a nice day.
Helped massively with writing a good conclusion!
Extremely well explained all details in simple and applicable manner, Thank you very much for outstanding article. It really made life easy. Ravi, India.
Thanks a lot for such a nicely explained difference of discussion and conclusion. now got some basic idea to write what.
Thanks for clearing the great confusion. It gave real clarity to me!
Clarified my confusion. Thank you for this article
This website certainly has all of the information I wanted concerning this subject and didn’t know who to ask.
Rate this article Cancel Reply
Your email address will not be published.
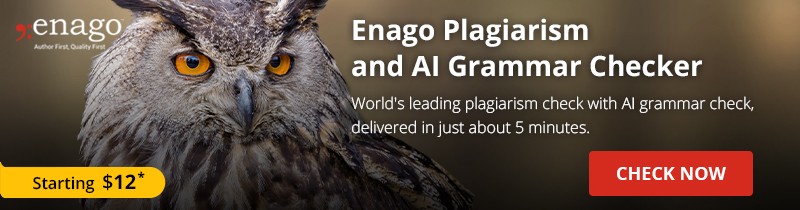
Enago Academy's Most Popular Articles
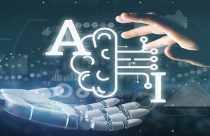
- AI in Academia
- Infographic
- Manuscripts & Grants
- Reporting Research
- Trending Now
Can AI Tools Prepare a Research Manuscript From Scratch? — A comprehensive guide
As technology continues to advance, the question of whether artificial intelligence (AI) tools can prepare…
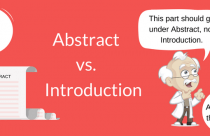
Abstract Vs. Introduction — Do you know the difference?
Ross wants to publish his research. Feeling positive about his research outcomes, he begins to…
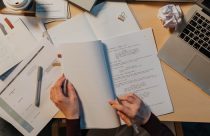
- Old Webinars
- Webinar Mobile App
Demystifying Research Methodology With Field Experts
Choosing research methodology Research design and methodology Evidence-based research approach How RAxter can assist researchers
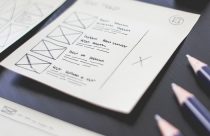
- Manuscript Preparation
- Publishing Research
How to Choose Best Research Methodology for Your Study
Successful research conduction requires proper planning and execution. While there are multiple reasons and aspects…
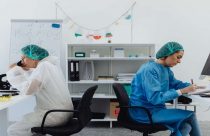
Top 5 Key Differences Between Methods and Methodology
While burning the midnight oil during literature review, most researchers do not realize that the…
How to Draft the Acknowledgment Section of a Manuscript
Annex Vs. Appendix: Do You Know the Difference?

Sign-up to read more
Subscribe for free to get unrestricted access to all our resources on research writing and academic publishing including:
- 2000+ blog articles
- 50+ Webinars
- 10+ Expert podcasts
- 50+ Infographics
- 10+ Checklists
- Research Guides
We hate spam too. We promise to protect your privacy and never spam you.
I am looking for Editing/ Proofreading services for my manuscript Tentative date of next journal submission:
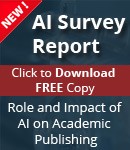
What should universities' stance be on AI tools in research and academic writing?
Get science-backed answers as you write with Paperpal's Research feature
How to Write a Conclusion for Research Papers (with Examples)
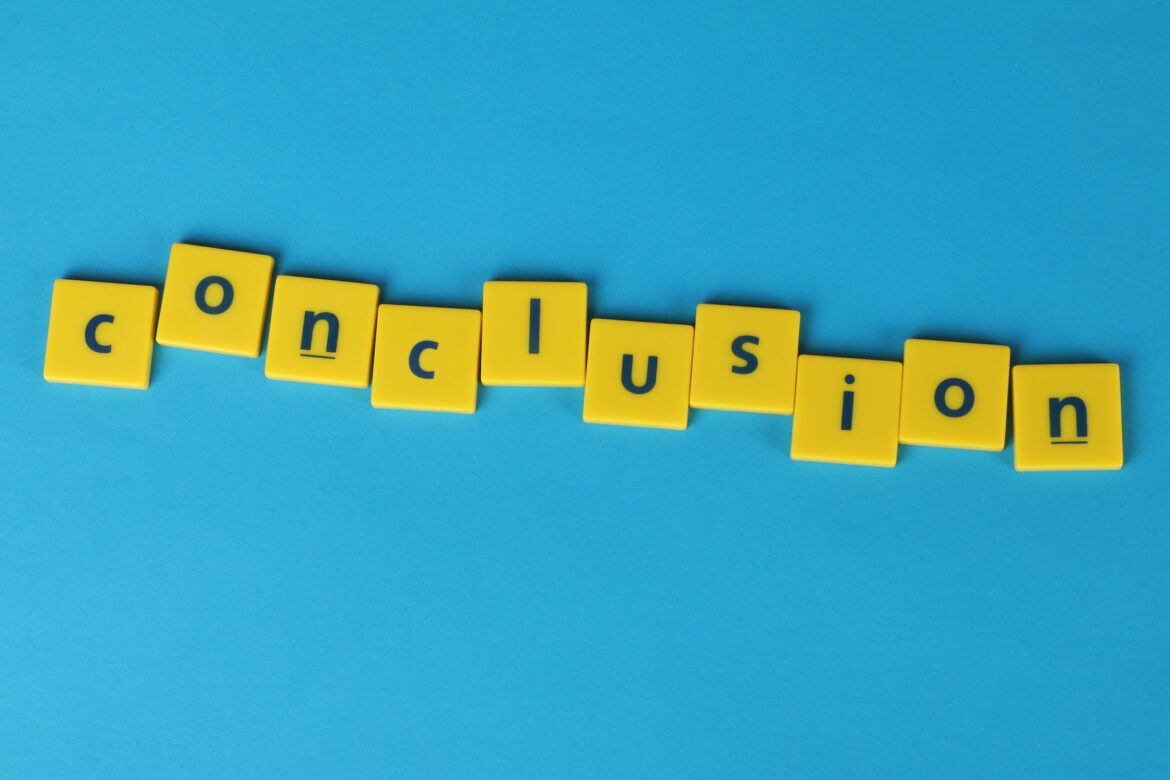
The conclusion of a research paper is a crucial section that plays a significant role in the overall impact and effectiveness of your research paper. However, this is also the section that typically receives less attention compared to the introduction and the body of the paper. The conclusion serves to provide a concise summary of the key findings, their significance, their implications, and a sense of closure to the study. Discussing how can the findings be applied in real-world scenarios or inform policy, practice, or decision-making is especially valuable to practitioners and policymakers. The research paper conclusion also provides researchers with clear insights and valuable information for their own work, which they can then build on and contribute to the advancement of knowledge in the field.
The research paper conclusion should explain the significance of your findings within the broader context of your field. It restates how your results contribute to the existing body of knowledge and whether they confirm or challenge existing theories or hypotheses. Also, by identifying unanswered questions or areas requiring further investigation, your awareness of the broader research landscape can be demonstrated.
Remember to tailor the research paper conclusion to the specific needs and interests of your intended audience, which may include researchers, practitioners, policymakers, or a combination of these.
Table of Contents
What is a conclusion in a research paper, summarizing conclusion, editorial conclusion, externalizing conclusion, importance of a good research paper conclusion, how to write a conclusion for your research paper, research paper conclusion examples.
- How to write a research paper conclusion with Paperpal?
Frequently Asked Questions
A conclusion in a research paper is the final section where you summarize and wrap up your research, presenting the key findings and insights derived from your study. The research paper conclusion is not the place to introduce new information or data that was not discussed in the main body of the paper. When working on how to conclude a research paper, remember to stick to summarizing and interpreting existing content. The research paper conclusion serves the following purposes: 1
- Warn readers of the possible consequences of not attending to the problem.
- Recommend specific course(s) of action.
- Restate key ideas to drive home the ultimate point of your research paper.
- Provide a “take-home” message that you want the readers to remember about your study.
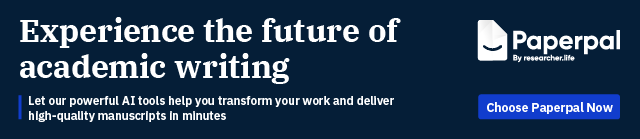
Types of conclusions for research papers
In research papers, the conclusion provides closure to the reader. The type of research paper conclusion you choose depends on the nature of your study, your goals, and your target audience. I provide you with three common types of conclusions:
A summarizing conclusion is the most common type of conclusion in research papers. It involves summarizing the main points, reiterating the research question, and restating the significance of the findings. This common type of research paper conclusion is used across different disciplines.
An editorial conclusion is less common but can be used in research papers that are focused on proposing or advocating for a particular viewpoint or policy. It involves presenting a strong editorial or opinion based on the research findings and offering recommendations or calls to action.
An externalizing conclusion is a type of conclusion that extends the research beyond the scope of the paper by suggesting potential future research directions or discussing the broader implications of the findings. This type of conclusion is often used in more theoretical or exploratory research papers.
Align your conclusion’s tone with the rest of your research paper. Start Writing with Paperpal Now!
The conclusion in a research paper serves several important purposes:
- Offers Implications and Recommendations : Your research paper conclusion is an excellent place to discuss the broader implications of your research and suggest potential areas for further study. It’s also an opportunity to offer practical recommendations based on your findings.
- Provides Closure : A good research paper conclusion provides a sense of closure to your paper. It should leave the reader with a feeling that they have reached the end of a well-structured and thought-provoking research project.
- Leaves a Lasting Impression : Writing a well-crafted research paper conclusion leaves a lasting impression on your readers. It’s your final opportunity to leave them with a new idea, a call to action, or a memorable quote.
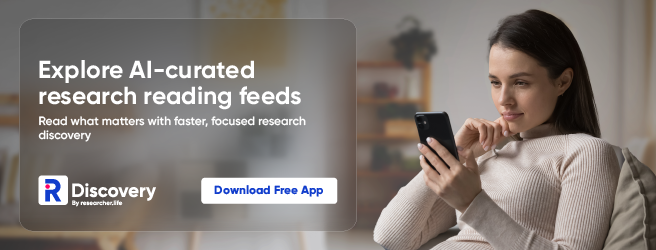
Writing a strong conclusion for your research paper is essential to leave a lasting impression on your readers. Here’s a step-by-step process to help you create and know what to put in the conclusion of a research paper: 2
- Research Statement : Begin your research paper conclusion by restating your research statement. This reminds the reader of the main point you’ve been trying to prove throughout your paper. Keep it concise and clear.
- Key Points : Summarize the main arguments and key points you’ve made in your paper. Avoid introducing new information in the research paper conclusion. Instead, provide a concise overview of what you’ve discussed in the body of your paper.
- Address the Research Questions : If your research paper is based on specific research questions or hypotheses, briefly address whether you’ve answered them or achieved your research goals. Discuss the significance of your findings in this context.
- Significance : Highlight the importance of your research and its relevance in the broader context. Explain why your findings matter and how they contribute to the existing knowledge in your field.
- Implications : Explore the practical or theoretical implications of your research. How might your findings impact future research, policy, or real-world applications? Consider the “so what?” question.
- Future Research : Offer suggestions for future research in your area. What questions or aspects remain unanswered or warrant further investigation? This shows that your work opens the door for future exploration.
- Closing Thought : Conclude your research paper conclusion with a thought-provoking or memorable statement. This can leave a lasting impression on your readers and wrap up your paper effectively. Avoid introducing new information or arguments here.
- Proofread and Revise : Carefully proofread your conclusion for grammar, spelling, and clarity. Ensure that your ideas flow smoothly and that your conclusion is coherent and well-structured.
Write your research paper conclusion 2x faster with Paperpal. Try it now!
Remember that a well-crafted research paper conclusion is a reflection of the strength of your research and your ability to communicate its significance effectively. It should leave a lasting impression on your readers and tie together all the threads of your paper. Now you know how to start the conclusion of a research paper and what elements to include to make it impactful, let’s look at a research paper conclusion sample.
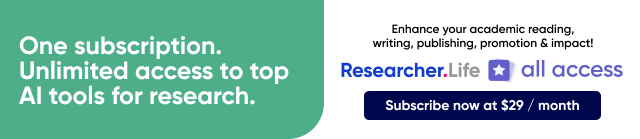
How to write a research paper conclusion with Paperpal?
A research paper conclusion is not just a summary of your study, but a synthesis of the key findings that ties the research together and places it in a broader context. A research paper conclusion should be concise, typically around one paragraph in length. However, some complex topics may require a longer conclusion to ensure the reader is left with a clear understanding of the study’s significance. Paperpal, an AI writing assistant trusted by over 800,000 academics globally, can help you write a well-structured conclusion for your research paper.
- Sign Up or Log In: Create a new Paperpal account or login with your details.
- Navigate to Features : Once logged in, head over to the features’ side navigation pane. Click on Templates and you’ll find a suite of generative AI features to help you write better, faster.
- Generate an outline: Under Templates, select ‘Outlines’. Choose ‘Research article’ as your document type.
- Select your section: Since you’re focusing on the conclusion, select this section when prompted.
- Choose your field of study: Identifying your field of study allows Paperpal to provide more targeted suggestions, ensuring the relevance of your conclusion to your specific area of research.
- Provide a brief description of your study: Enter details about your research topic and findings. This information helps Paperpal generate a tailored outline that aligns with your paper’s content.
- Generate the conclusion outline: After entering all necessary details, click on ‘generate’. Paperpal will then create a structured outline for your conclusion, to help you start writing and build upon the outline.
- Write your conclusion: Use the generated outline to build your conclusion. The outline serves as a guide, ensuring you cover all critical aspects of a strong conclusion, from summarizing key findings to highlighting the research’s implications.
- Refine and enhance: Paperpal’s ‘Make Academic’ feature can be particularly useful in the final stages. Select any paragraph of your conclusion and use this feature to elevate the academic tone, ensuring your writing is aligned to the academic journal standards.
By following these steps, Paperpal not only simplifies the process of writing a research paper conclusion but also ensures it is impactful, concise, and aligned with academic standards. Sign up with Paperpal today and write your research paper conclusion 2x faster .
The research paper conclusion is a crucial part of your paper as it provides the final opportunity to leave a strong impression on your readers. In the research paper conclusion, summarize the main points of your research paper by restating your research statement, highlighting the most important findings, addressing the research questions or objectives, explaining the broader context of the study, discussing the significance of your findings, providing recommendations if applicable, and emphasizing the takeaway message. The main purpose of the conclusion is to remind the reader of the main point or argument of your paper and to provide a clear and concise summary of the key findings and their implications. All these elements should feature on your list of what to put in the conclusion of a research paper to create a strong final statement for your work.
A strong conclusion is a critical component of a research paper, as it provides an opportunity to wrap up your arguments, reiterate your main points, and leave a lasting impression on your readers. Here are the key elements of a strong research paper conclusion: 1. Conciseness : A research paper conclusion should be concise and to the point. It should not introduce new information or ideas that were not discussed in the body of the paper. 2. Summarization : The research paper conclusion should be comprehensive enough to give the reader a clear understanding of the research’s main contributions. 3 . Relevance : Ensure that the information included in the research paper conclusion is directly relevant to the research paper’s main topic and objectives; avoid unnecessary details. 4 . Connection to the Introduction : A well-structured research paper conclusion often revisits the key points made in the introduction and shows how the research has addressed the initial questions or objectives. 5. Emphasis : Highlight the significance and implications of your research. Why is your study important? What are the broader implications or applications of your findings? 6 . Call to Action : Include a call to action or a recommendation for future research or action based on your findings.
The length of a research paper conclusion can vary depending on several factors, including the overall length of the paper, the complexity of the research, and the specific journal requirements. While there is no strict rule for the length of a conclusion, but it’s generally advisable to keep it relatively short. A typical research paper conclusion might be around 5-10% of the paper’s total length. For example, if your paper is 10 pages long, the conclusion might be roughly half a page to one page in length.
In general, you do not need to include citations in the research paper conclusion. Citations are typically reserved for the body of the paper to support your arguments and provide evidence for your claims. However, there may be some exceptions to this rule: 1. If you are drawing a direct quote or paraphrasing a specific source in your research paper conclusion, you should include a citation to give proper credit to the original author. 2. If your conclusion refers to or discusses specific research, data, or sources that are crucial to the overall argument, citations can be included to reinforce your conclusion’s validity.
The conclusion of a research paper serves several important purposes: 1. Summarize the Key Points 2. Reinforce the Main Argument 3. Provide Closure 4. Offer Insights or Implications 5. Engage the Reader. 6. Reflect on Limitations
Remember that the primary purpose of the research paper conclusion is to leave a lasting impression on the reader, reinforcing the key points and providing closure to your research. It’s often the last part of the paper that the reader will see, so it should be strong and well-crafted.
- Makar, G., Foltz, C., Lendner, M., & Vaccaro, A. R. (2018). How to write effective discussion and conclusion sections. Clinical spine surgery, 31(8), 345-346.
- Bunton, D. (2005). The structure of PhD conclusion chapters. Journal of English for academic purposes , 4 (3), 207-224.
Paperpal is a comprehensive AI writing toolkit that helps students and researchers achieve 2x the writing in half the time. It leverages 21+ years of STM experience and insights from millions of research articles to provide in-depth academic writing, language editing, and submission readiness support to help you write better, faster.
Get accurate academic translations, rewriting support, grammar checks, vocabulary suggestions, and generative AI assistance that delivers human precision at machine speed. Try for free or upgrade to Paperpal Prime starting at US$19 a month to access premium features, including consistency, plagiarism, and 30+ submission readiness checks to help you succeed.
Experience the future of academic writing – Sign up to Paperpal and start writing for free!
Related Reads:
- 5 Reasons for Rejection After Peer Review
- Ethical Research Practices For Research with Human Subjects
7 Ways to Improve Your Academic Writing Process
- Paraphrasing in Academic Writing: Answering Top Author Queries
Preflight For Editorial Desk: The Perfect Hybrid (AI + Human) Assistance Against Compromised Manuscripts
You may also like, measuring academic success: definition & strategies for excellence, phd qualifying exam: tips for success , ai in education: it’s time to change the..., is it ethical to use ai-generated abstracts without..., what are journal guidelines on using generative ai..., quillbot review: features, pricing, and free alternatives, what is an academic paper types and elements , should you use ai tools like chatgpt for..., 9 steps to publish a research paper, what are the different types of research papers.
Discussion and Conclusion
- First Online: 18 February 2020
Cite this chapter
- Coleen E. Toronto 3 &
- Ruth Remington 4
4228 Accesses
1 Citations
In the discussion section of the integrative review, the reviewer explains and evaluates the findings of the synthesis and how the findings relate to existing literature and the review question(s). Here, the reviewer interprets the meaning and relevance of the findings. Implications of the findings for research, practice, education, and/or policy are described. Writing the discussion requires reflection and critical thinking about the entire review process. This chapter can serve as a guide to writing a comprehensive discussion section.
This is a preview of subscription content, log in via an institution to check access.
Access this chapter
- Available as PDF
- Read on any device
- Instant download
- Own it forever
- Available as EPUB and PDF
- Compact, lightweight edition
- Dispatched in 3 to 5 business days
- Free shipping worldwide - see info
Tax calculation will be finalised at checkout
Purchases are for personal use only
Institutional subscriptions
Aveyard H (2019) Doing a literature review in health and social care: practical guide, 4th edn. Open University Press, Bethesda, MD, p 153
Google Scholar
Bettany-Saltikov J (2010) Learning how to undertake a systematic review: part 2. Nurs Stand 24:47–56
Article Google Scholar
Bowman KG (2007) A research synthesis overview. Nurs Sci Q 20:171–176. https://doi.org/10.1177/0894318407299575
Article PubMed Google Scholar
Brady S, Lee N, Gibbons K, Bogossian F (2019) Woman-centered care: an integrative review of empirical literature. Int J Nurs Stud 94:107–119. https://doi.org/10.1016/j.ijnurstu.2019.01.001
Cals JWL, Kotz D (2013) Effective writing and publishing scientific papers, part VI: Discussion. J Clin Epidemiol 66:1064. https://doi.org/10.1016/j.jclinepi.2013.04.017
Cartwright J, Atz T, Newman S, Mueller M, Demirci JR (2017) Integrative review of interventions to promote breastfeeding in the late preterm infant. JOGN Nurs 46:347–355. https://doi.org/10.1016/j.jogn.2017.01.006
Classen S, Winter SM, Brown C, Morgan-Daniel J, Medhizadah S, Agarwal N (2019) An Integrative Review on teen distracted driving for model program development. Front Public Health [Internet]. Frontiers Media SA 3:7. https://doi.org/10.3389/fpubh.2019.00111
Connelly LM (2009) The discussion section of a research report. Medsurg Nurs 18:300–301
PubMed Google Scholar
Cooper SA, Compton PA (2019) Nursing interventions for sexual dysfunction: an integrative review for the psychiatric nurse. Arch Psychiatr Nurs 33:389–399. https://doi.org/10.1016/j.apnu.2019.04.003
Coughlan M, Cronin P (2017) Doing a literature review in nursing, health and social care. Sage, London, UK, p 120
Coughlin MB, Sethares KA (2017) Chronic sorrow in parents of children with a chronic illness or disability: an integrative literature review. J Pediatr Nurs 37:108–116. https://doi.org/10.1016/j.pedn.2017.06.011
Dawidowicz P (2010) Literature reviews made easy: a quick guide to success. Information Age Publishing, Charlotte, NC, p 150
de Souza MT, da Silva MD, de Carvalho R (2010) Integrative review: what is it? How to do it? Einstein (São Paulo) 8:102–106. https://doi.org/10.1590/s1679-45082010rw1134
Flanagan J (2018) The integrative review. Int J Nurs Knowl. Wiley 29(2):81. https://doi.org/10.1111/2047-3095.12208
Foster M, Gilbert M, Hanson D, Whitcomb K, Graham C (2018) Use of simulation to develop teamwork skills in prelicensure nursing students: an integrative review. Nurse Educ 44:E7–E11. https://doi.org/10.1097/NNE.0000000000000616.
Ghazal LV, Ma C, Squires A (2019) Transition-to-U.S. practice experiences of internationally educated nurses: an integrative review. West J Nurs Res 4:193945919860855. https://doi.org/10.1177/0193945919860855
Gibbons SW, Ross A, Bevans M (2014) Liminality as a conceptual frame for understanding the family caregiving rite of passage: an integrative review. Res Nurs Health 37:423–436. https://doi.org/10.1002/nur.21622
Article PubMed PubMed Central Google Scholar
Hess DR (2004) How to write an effective discussion. Respir Care 49:1238–1241
Hooker SA, Grigsby ME, Riegel B, Bekelman DB (2015) The impact of relationship quality on health-related outcomes in heart failure patients and informal family caregivers: an integrative review. J Cardiovasc Nurs 30:552–563. https://doi.org/10.1097/JCN.000000000000270
Hopia H, Latvala E, Liimatainen L (2016) Reviewing the methodology of an integrative review. Scand J Caring Sci 30:662–669. https://doi.org/10.1111/scs.12327
Kearney MH (2017) The discussion section tells us where we are. Res Nurs Health 40:289–291. https://doi.org/10.1002/nur.21803
Lee SE, Scott LD, Dahinten VS, Vincent C, Dunn Lopez K, Park CG (2019) Safety culture, patient safety, and quality of care outcomes: a literature review. West J Nurs Res 4(2):279–304. https://doi.org/10.1177/019394591774716
Oermann MH, Hays JC (2019) Writing for publication in nursing, 4th edn. Springer, New York, NY, p 118
Radbron E, Wilson V, McCance T, Middleton R (2019) The use of data collected from mhealth apps to inform evidence-based quality improvement: an integrative review. Worldviews Evid Based Nurs 16:70–77
Robertson-Malt S (2014) Presenting and interpreting findings. Am J Nurs 114:49–54
Roush K (2019) A nurse’s step-by-step guide to writing a dissertation or scholarly project (2nd ed.). Sigma Theta Tau International (publisher). p 100
Rückholdt M, Toflera GH, Randall S, Buckley T (2019) Coping by family members of critically ill hospitalised patients: an integrative review. Int J Nurs Stud 97:40–54. https://doi.org/10.1016/j.ijnurstu.2019.04.016
Schulte B (2003) Scientific writing & the scientific method: parallel “Hourglass” structure in form & content. Am Biol Teach 65:591–594. https://doi.org/10.2307/4451568
Skelton JR, Edwards SJL (2000) The function of the discussion section in academic medical writing. Br Med J 320:1269–1270
Article CAS Google Scholar
Stamp KD, Machado MA, Allen NA (2014) Transitional care programs improve outcomes for heart failure patients. J Cardiovasc Nurs [Internet]. Ovid Technologies (Wolters Kluwer Health) 29(2):140–154. https://doi.org/10.1097/jcn.0b013e31827db560
Teunissen C, Burrell B, Maskill V (2019) Effective surgical teams: an integrative literature review. West J Nurs Res 41:1–35. https://doi.org/10.1177/0193945919834896
Toronto CE, LaRocco SA (2019) Family perception of and experience with family presence during cardiopulmonary resuscitation: an integrative review. J Clin Nurs 28:32–46. https://doi.org/10.1111/jocn.14649
Torraco RJ (2016) Writing integrative literature reviews: using the past and present to explore the future. Hum Resour Dev Rev 15:404–428. https://doi.org/10.1177/1534484316671606
Watson R (2018) The discussion section of a manuscript. Nurse, vol 28. Author, p 3
White LL, Cohen MZ, Berger AM, Kupzyk AZ, Bierman PJ (2019) Self-Efficacy for management of symptoms and symptom distress in adults with cancer: an integrative review. Oncol Nurs Forum 46:113–128
Download references
Author information
Authors and affiliations.
School of Nursing, Curry College, Milton, MA, USA
Coleen E. Toronto
Department of Nursing, Framingham State University, Framingham, MA, USA
Ruth Remington
You can also search for this author in PubMed Google Scholar
Corresponding author
Correspondence to Coleen E. Toronto .
Editor information
Editors and affiliations, rights and permissions.
Reprints and permissions
Copyright information
© 2020 Springer Nature Switzerland AG
About this chapter
Toronto, C.E., Remington, R. (2020). Discussion and Conclusion. In: Toronto, C., Remington, R. (eds) A Step-by-Step Guide to Conducting an Integrative Review. Springer, Cham. https://doi.org/10.1007/978-3-030-37504-1_6
Download citation
DOI : https://doi.org/10.1007/978-3-030-37504-1_6
Published : 18 February 2020
Publisher Name : Springer, Cham
Print ISBN : 978-3-030-37503-4
Online ISBN : 978-3-030-37504-1
eBook Packages : Medicine Medicine (R0)
Share this chapter
Anyone you share the following link with will be able to read this content:
Sorry, a shareable link is not currently available for this article.
Provided by the Springer Nature SharedIt content-sharing initiative
- Publish with us
Policies and ethics
- Find a journal
- Track your research
You are using an outdated browser. Please upgrade your browser to improve your experience.
- 321-251-6977
- Fiction Editing
- Nonfiction Editing
- Thesis & Dissertation Editing
- Instant Price Quotes
- Coupons and Discounts
- Free Editing Sample & Critique
- Editing eBook
- Your Editing Team
- CERTIFIED Story Editors
- PhD & Subject Matter Experts
- About First Editing
Editing Services BOOKS
- Authors Here >>
- Book Editing Services
- Compare Editing Services
- High-Impact Editing
- Executive Book Coaching
- Developmental Content Editing
- Advanced Line Editing
- Standard Copy Editing
- Basic Proofreading
- Private Consultations
Get Feedback
- Editorial Critique/Review
Academic English PAPERS
- Academics Here >>
- Dissertation
- Universities & Institutions
Journals and Scientific PUBLICATIONS
- Researchers Here >>
- Academic Editing
- Scientific Manuscript
- Subject Matter Experts
Marketing and Business DOCUMENTS
- Executives Here >>
- Business Editing
Editorial Support SERVICES
- Publishing Here >>
- Consultation
- Critiques & Reviews
- More…
Discussion vs Conclusion: Everything You Need to Know
- Academic Writing
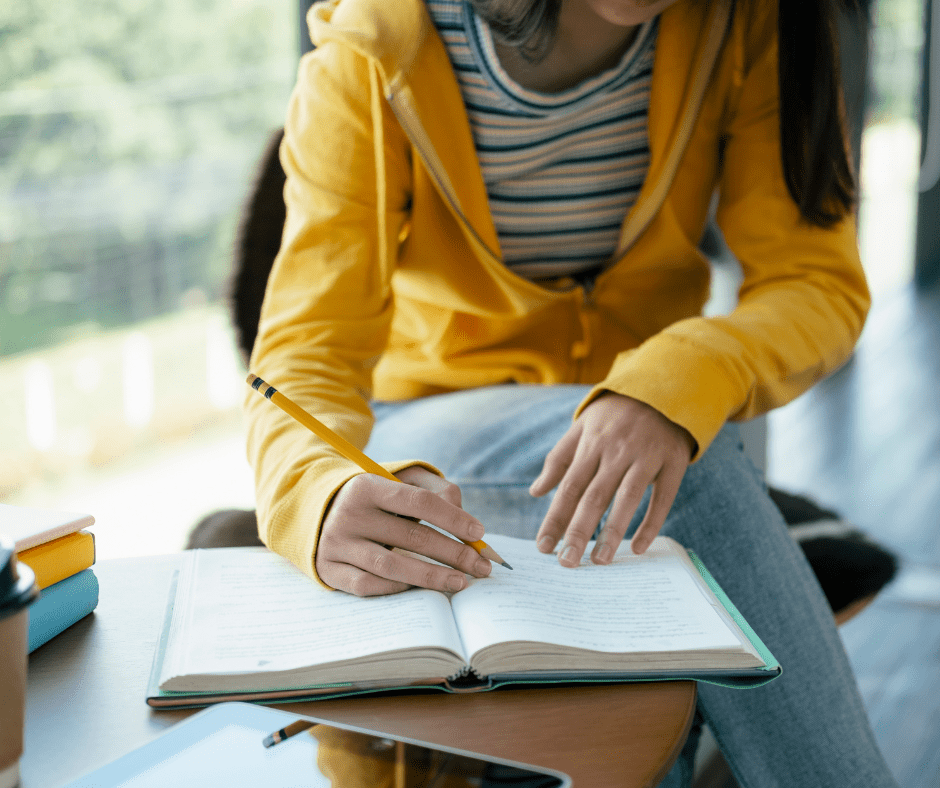
The discussion and conclusion sections are both essential parts of any academic thesis. In this short guide, I’ll define the content to include in each, as well as discuss the differences between the two sections, along with a few tips on what to include to ensure your thesis fulfills all the necessary criteria.
Discussion vs conclusion: what’s the difference?
When it comes to writing an academic thesis , the discussion and conclusion are two important sections to focus on. They serve different purposes and should be approached in their own ways to ensure a comprehensive and complete thesis.
The discussion section is where you’ll analyze and interpret your research findings in detail, providing a thorough explanation of your results. It’s not just a summary, but an opportunity to evaluate your work critically and answer any questions raised in the introduction. You should also mention any potential limitations and suggest any routes to explore in future research.
In contrast, the conclusion section summarizes your thesis, bringing together the key points from the introduction, literature review, methodology, and discussion. It’s your chance to restate your thesis statement and briefly summarize your findings in a concise and impactful way. Avoid introducing new information, as this section should focus solely on reinforcing the main points of your research and emphasizing its significance. The conclusion should leave the reader with a clear understanding of your research and its contribution to the field.
Here’s a simple table highlighting the major differences.
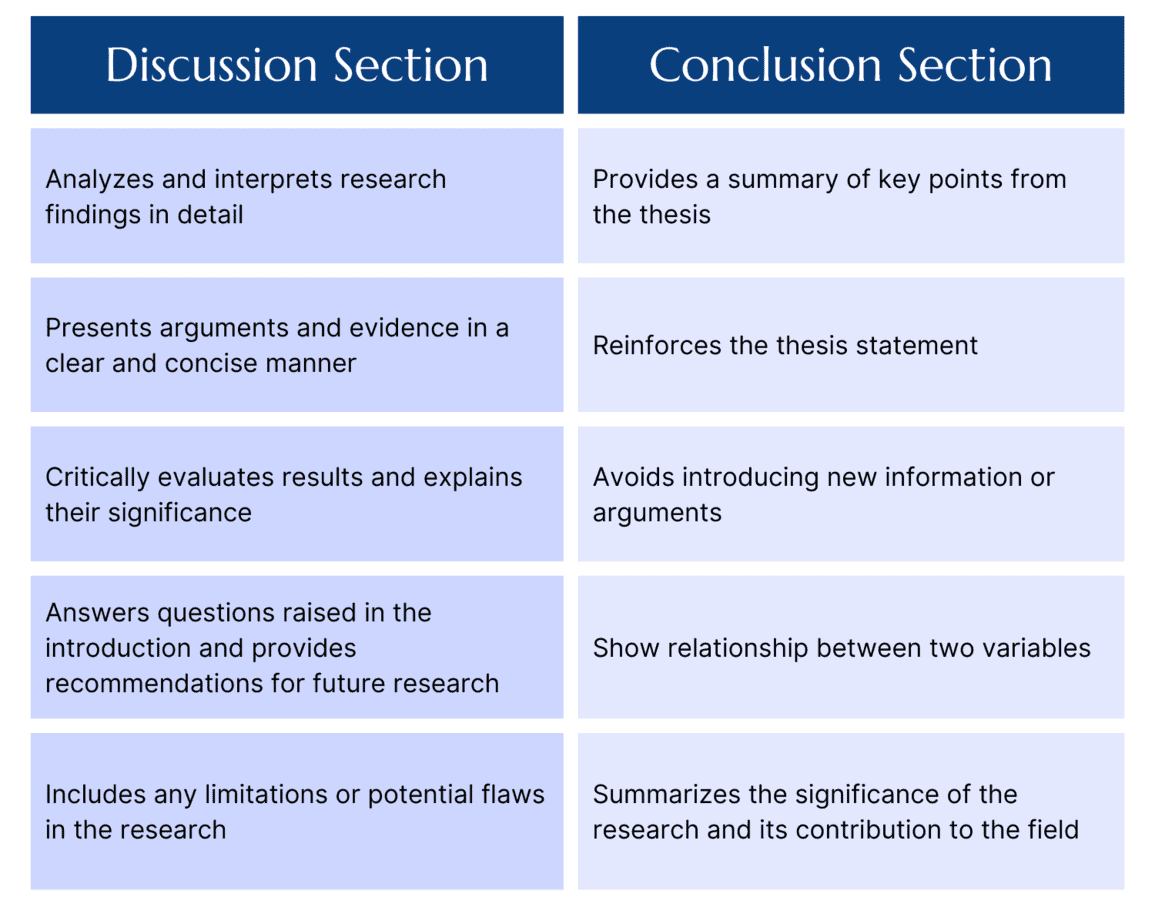
What should the discussion section include?
The discussion section can often prove to be one of the most challenging. Here, the author must condense their findings into a summary, along with a critical analysis and interpretation of the findings in relation to the existing literature. Essentially, it involves connecting the dots between one’s own work and the broader academic landscape.
In addition to writing a summary, a critical analysis, and an interpretation, the author must also consider the practical and theoretical implications of the research while clearly stating any potential limitations. Due to the high level of critical thinking researchers apply to the discussion section, it’s worth spending ample time on it.
The following are some common elements in a typical discussion section:
- Summary of findings: A brief summary of the main findings of the research.
- Interpretation of results: An explanation of what the findings mean, how they contribute to the existing literature, and how they relate to the research questions or hypotheses.
- Comparison with previous studies: A discussion of how the current study compares with previous research in the field.
- Limitations of the study: Here, the author describes any limitations of the research, such as sample size, methodology, or other factors that may have influenced the results.
- Implications of the findings: A discussion of the practical and theoretical implications of the research, and how it may impact future research or practice in the field.
- Recommendations for future research: Suggestions for future research that could build on the current study or address the limitations of the research.
- Conclusion: A summary of the main points discussed in the discussion section, and how they relate to the overall research aims and objectives.
Overall, the discussion section is an opportunity for the author to reflect on the significance and implications of their research, and to demonstrate their understanding of the field and its broader context.
As important components of the discussion section, the interpretations, limitations, and limitations help to demonstrate the author’s critical thinking and understanding of the research and are typically integrated into the discussion section, rather than included as independent sub-sections. Let’s take a look at the importance of each:
- Interpretations involve analyzing and interpreting the results of the research and explaining what they mean in the context of the existing literature. This includes addressing how the findings contribute to the field and what new insights they offer.
- Implications involve discussing the practical and theoretical implications of the research, such as how the findings could be applied in real-world situations, as well as any ethical or social implications of the research.
- Limitations involve acknowledging the limitations of the research, including any constraints on the research design or methodology, and suggesting ways that future research could address these limitations.
What makes an effective conclusion?
The conclusion of a thesis or dissertation should provide a summary of the key findings of the research and their implications, as well as offer suggestions for future research. Here are some specific elements to include:
- Restate the research questions or hypotheses and summarize the main findings of the study.
- Discuss the significance of the findings and their implications for the field, as well as any practical or theoretical implications.
- Provide a critical evaluation of the research and its limitations, and offer suggestions for future research that could build on the current study.
- Consider the broader implications of the research, including any ethical or social implications.
- End with a strong concluding statement that summarizes the main points of the conclusion and leaves a lasting impression on the reader.
Now, let’s summarize this information by comparing what should be included against what not to include:
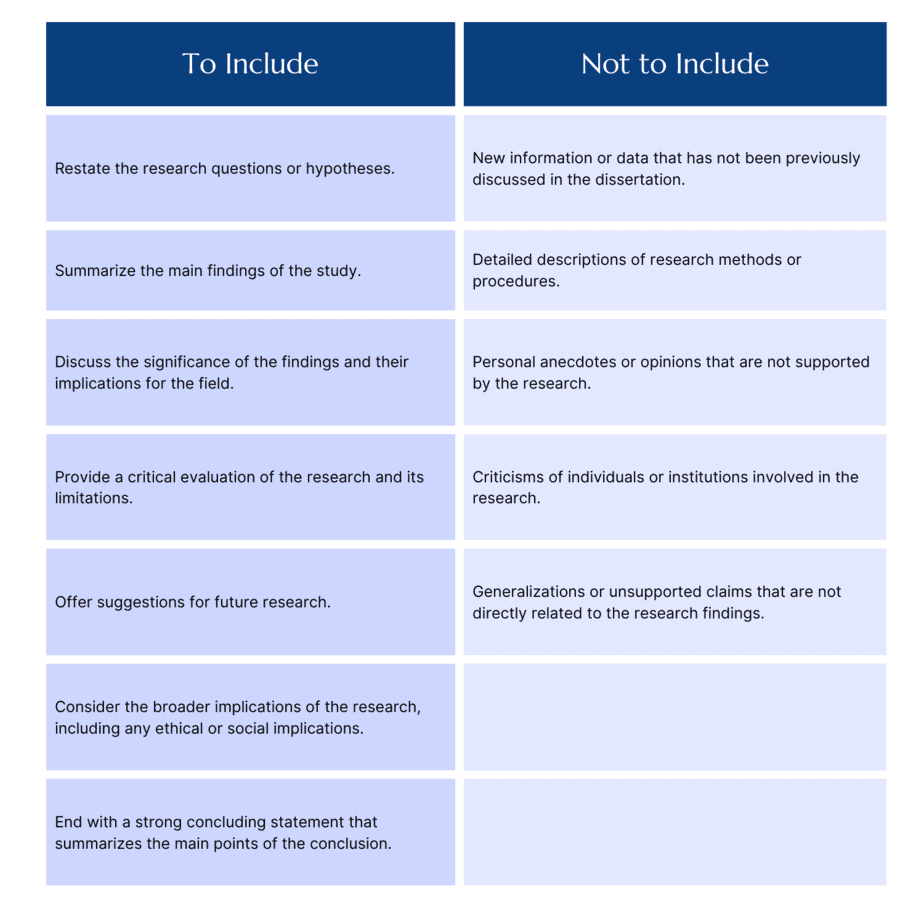
Overall, as the table above shows, it is clear that the conclusion should leave the reader with a clear understanding of the key contributions and limitations of the research, as well as the potential for future research in the field.
In conclusion, the discussion and conclusion sections are two essential components of a thesis that serve different purposes. Whereas the discussion section is where the author critically analyzes and interprets research findings in the context of existing literature, assesses the practical and theoretical implications of the research, and suggests directions for future research, in the conclusion section, they reinforce the thesis statement, summarize the key points, and highlight the significance of the research. To create an effective conclusion, the author should restate the research questions, summarize the main findings, discuss the significance and limitations of the findings, and provide suggestions for future research, without including new data or personal opinions.
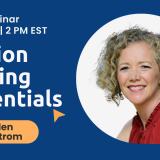

An official website of the United States government
The .gov means it's official. Federal government websites often end in .gov or .mil. Before sharing sensitive information, make sure you're on a federal government site.
The site is secure. The https:// ensures that you are connecting to the official website and that any information you provide is encrypted and transmitted securely.
- Publications
- Account settings
- Browse Titles
NCBI Bookshelf. A service of the National Library of Medicine, National Institutes of Health.
Trikalinos TA, Dahabreh IJ, Lee J, et al. Defining an Optimal Format for Presenting Research Needs [Internet]. Rockville (MD): Agency for Healthcare Research and Quality (US); 2011 Jun. (Methods Future Research Needs Reports, No. 3.)
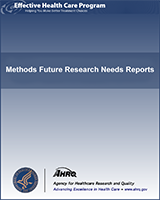
Defining an Optimal Format for Presenting Research Needs [Internet].
Discussion and conclusions.
Future research needs recommendations are valuable inputs for researchers, funders, and advocates making decisions about avenues for future scientific exploration. We performed an empirical evaluation of the published literature to appreciate the variability in the presentation of information on future research needs. We found that most systematic reviews, meta-analyses, or economic analyses do not focus on future research needs, and any referral to future research is most often cursory. Systematic reviews and meta-analyses often call for additional randomized trials, while economic analyses are more diverse in their suggestions.
In qualitative discussions with experts, we identified a number of general themes. The discussions were quickly saturated, and while additional one-to-one interviews could have generated more themes, it is unclear whether these would have been of major importance for defining the optimal format for presenting future research needs. An unanticipated theme from the qualitative interviews was that specificity in the recommendations for future research is not universally and unconditionally viewed as a desirable attribute. The rationale is that specific descriptions could be perceived as too prescriptive or restrictive, and in the extreme they may have unintended consequences, such as opposing the paradigm of investigator-initiated research. Experts disagreed on the importance of this point. Nevertheless it is probably prudent to take this point into account when reporting assessments of future research needs, and all experts agreed that proper framing of the future research needs documents could address this concern. A survey of a large and representative sample of potential users of future research needs assessments would clarify whether such reactions are prevalent. If yes, it would be also interesting to understand why some users are prone to seeing even moderately specific recommendations with skepticism. Ideology, cultural norms, or professional self-interests may be explanations.
Several limitations need to be considered when interpreting our results. First, we only recruited four experts for our qualitative interviews; hence, our results should be considered hypothesis forming and not representative of all stakeholders. However, the purpose of the qualitative interviews was to identify general themes with respect to the way different stakeholders would approach a document presenting and discussing future research needs, and not necessarily to get a representative quantification of different opinions. The optimal research design to quantify the frequency of different opinions is a survey using a representative sampling scheme, rather than qualitative interviews or even focus groups.
Regarding our empirical assessment of secondary research manuscripts, we focused on high impact general journals (for systematic reviews) or highly specialized technical journals (for cost-effectiveness/utility analyses). Thus our empirical assessment of future research presentation is not be generalizable to all journals. If anything, because even in these “top-tier” journals the presentation of implications for future research was fairly cursory and informal, the situation is unlikely to be better in other research outlets. Further, determining future research needs is not the primary focus of systematic reviews and cost-effectiveness/utility analyses. Therefore it is doubtful that including a broader set of journals would change the essence of our conclusions.
The concept of a stand-alone document on future research needs is probably new to most of the individuals approached, and additional discussion is likely needed regarding potential uses prior to making very specific recommendations on the optimal presentation of future research needs documents. We outline the following preliminary recommendations for presenting future research needs documents, based on the results of the empirical assessment and the qualitative interviews:
- Provide succinct yet adequate description of methods and results, following guidelines for reporting of health care research (for example, reporting guidelines for qualitative research 8–11 and modeling 12–15 ). Aim for a level of detail similar to that found in papers addressed to a general medical audience.
- Be cognizant of the importance of the face validity of the process. Justify the selection of the stakeholders who participated in the identification or prioritization of research needs, and be clear about their degree of engagement.
- Consider reporting the results of the future research needs assessment at two levels of detail. A suggestion would be to first present general areas that merit future research without specifying research designs or specific details on, for example, PICO elements. A second set of results could elaborate further on potential research designs, details on PICO elements, or other details as applicable.
- Frame the more specific set of results as “examples” rather than strict recommendations.
- Avoid explicit prioritization of research needs when there are no clear differences in the perceived strength of alternative recommendations. A general prioritization of future research recommendations as of “high,” “medium,” or “low” importance may be more appropriate than an explicit numerical ranking. Consider a grouped presentation of future research needs by thematic entities.
- Clearly define how the feasibility of future research was assessed. It may be instructive to perform power analyses for specific research designs for a range of assumptions, and to compare the results with the size and type of existing studies in the field. For example, trials are typically more resource-intensive than secondary analyses of existing data.
- Cite this Page Trikalinos TA, Dahabreh IJ, Lee J, et al. Defining an Optimal Format for Presenting Research Needs [Internet]. Rockville (MD): Agency for Healthcare Research and Quality (US); 2011 Jun. (Methods Future Research Needs Reports, No. 3.) Discussion and Conclusions.
- PDF version of this title (921K)
Other titles in this collection
- AHRQ Methods Future Research Needs Series
Recent Activity
- Discussion and Conclusions - Defining an Optimal Format for Presenting Research ... Discussion and Conclusions - Defining an Optimal Format for Presenting Research Needs
Your browsing activity is empty.
Activity recording is turned off.
Turn recording back on
Connect with NLM
National Library of Medicine 8600 Rockville Pike Bethesda, MD 20894
Web Policies FOIA HHS Vulnerability Disclosure
Help Accessibility Careers
- Privacy Policy

Home » Research Paper – Structure, Examples and Writing Guide
Research Paper – Structure, Examples and Writing Guide
Table of Contents

Research Paper
Definition:
Research Paper is a written document that presents the author’s original research, analysis, and interpretation of a specific topic or issue.
It is typically based on Empirical Evidence, and may involve qualitative or quantitative research methods, or a combination of both. The purpose of a research paper is to contribute new knowledge or insights to a particular field of study, and to demonstrate the author’s understanding of the existing literature and theories related to the topic.
Structure of Research Paper
The structure of a research paper typically follows a standard format, consisting of several sections that convey specific information about the research study. The following is a detailed explanation of the structure of a research paper:
The title page contains the title of the paper, the name(s) of the author(s), and the affiliation(s) of the author(s). It also includes the date of submission and possibly, the name of the journal or conference where the paper is to be published.
The abstract is a brief summary of the research paper, typically ranging from 100 to 250 words. It should include the research question, the methods used, the key findings, and the implications of the results. The abstract should be written in a concise and clear manner to allow readers to quickly grasp the essence of the research.
Introduction
The introduction section of a research paper provides background information about the research problem, the research question, and the research objectives. It also outlines the significance of the research, the research gap that it aims to fill, and the approach taken to address the research question. Finally, the introduction section ends with a clear statement of the research hypothesis or research question.
Literature Review
The literature review section of a research paper provides an overview of the existing literature on the topic of study. It includes a critical analysis and synthesis of the literature, highlighting the key concepts, themes, and debates. The literature review should also demonstrate the research gap and how the current study seeks to address it.
The methods section of a research paper describes the research design, the sample selection, the data collection and analysis procedures, and the statistical methods used to analyze the data. This section should provide sufficient detail for other researchers to replicate the study.
The results section presents the findings of the research, using tables, graphs, and figures to illustrate the data. The findings should be presented in a clear and concise manner, with reference to the research question and hypothesis.
The discussion section of a research paper interprets the findings and discusses their implications for the research question, the literature review, and the field of study. It should also address the limitations of the study and suggest future research directions.
The conclusion section summarizes the main findings of the study, restates the research question and hypothesis, and provides a final reflection on the significance of the research.
The references section provides a list of all the sources cited in the paper, following a specific citation style such as APA, MLA or Chicago.
How to Write Research Paper
You can write Research Paper by the following guide:
- Choose a Topic: The first step is to select a topic that interests you and is relevant to your field of study. Brainstorm ideas and narrow down to a research question that is specific and researchable.
- Conduct a Literature Review: The literature review helps you identify the gap in the existing research and provides a basis for your research question. It also helps you to develop a theoretical framework and research hypothesis.
- Develop a Thesis Statement : The thesis statement is the main argument of your research paper. It should be clear, concise and specific to your research question.
- Plan your Research: Develop a research plan that outlines the methods, data sources, and data analysis procedures. This will help you to collect and analyze data effectively.
- Collect and Analyze Data: Collect data using various methods such as surveys, interviews, observations, or experiments. Analyze data using statistical tools or other qualitative methods.
- Organize your Paper : Organize your paper into sections such as Introduction, Literature Review, Methods, Results, Discussion, and Conclusion. Ensure that each section is coherent and follows a logical flow.
- Write your Paper : Start by writing the introduction, followed by the literature review, methods, results, discussion, and conclusion. Ensure that your writing is clear, concise, and follows the required formatting and citation styles.
- Edit and Proofread your Paper: Review your paper for grammar and spelling errors, and ensure that it is well-structured and easy to read. Ask someone else to review your paper to get feedback and suggestions for improvement.
- Cite your Sources: Ensure that you properly cite all sources used in your research paper. This is essential for giving credit to the original authors and avoiding plagiarism.
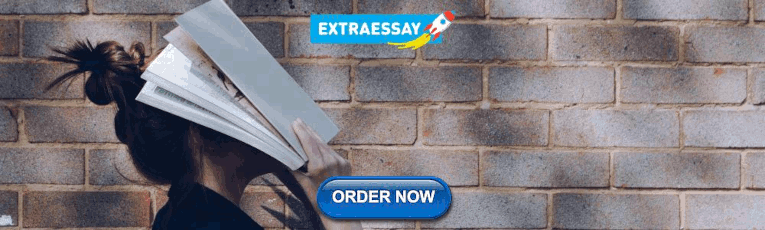
Research Paper Example
Note : The below example research paper is for illustrative purposes only and is not an actual research paper. Actual research papers may have different structures, contents, and formats depending on the field of study, research question, data collection and analysis methods, and other factors. Students should always consult with their professors or supervisors for specific guidelines and expectations for their research papers.
Research Paper Example sample for Students:
Title: The Impact of Social Media on Mental Health among Young Adults
Abstract: This study aims to investigate the impact of social media use on the mental health of young adults. A literature review was conducted to examine the existing research on the topic. A survey was then administered to 200 university students to collect data on their social media use, mental health status, and perceived impact of social media on their mental health. The results showed that social media use is positively associated with depression, anxiety, and stress. The study also found that social comparison, cyberbullying, and FOMO (Fear of Missing Out) are significant predictors of mental health problems among young adults.
Introduction: Social media has become an integral part of modern life, particularly among young adults. While social media has many benefits, including increased communication and social connectivity, it has also been associated with negative outcomes, such as addiction, cyberbullying, and mental health problems. This study aims to investigate the impact of social media use on the mental health of young adults.
Literature Review: The literature review highlights the existing research on the impact of social media use on mental health. The review shows that social media use is associated with depression, anxiety, stress, and other mental health problems. The review also identifies the factors that contribute to the negative impact of social media, including social comparison, cyberbullying, and FOMO.
Methods : A survey was administered to 200 university students to collect data on their social media use, mental health status, and perceived impact of social media on their mental health. The survey included questions on social media use, mental health status (measured using the DASS-21), and perceived impact of social media on their mental health. Data were analyzed using descriptive statistics and regression analysis.
Results : The results showed that social media use is positively associated with depression, anxiety, and stress. The study also found that social comparison, cyberbullying, and FOMO are significant predictors of mental health problems among young adults.
Discussion : The study’s findings suggest that social media use has a negative impact on the mental health of young adults. The study highlights the need for interventions that address the factors contributing to the negative impact of social media, such as social comparison, cyberbullying, and FOMO.
Conclusion : In conclusion, social media use has a significant impact on the mental health of young adults. The study’s findings underscore the need for interventions that promote healthy social media use and address the negative outcomes associated with social media use. Future research can explore the effectiveness of interventions aimed at reducing the negative impact of social media on mental health. Additionally, longitudinal studies can investigate the long-term effects of social media use on mental health.
Limitations : The study has some limitations, including the use of self-report measures and a cross-sectional design. The use of self-report measures may result in biased responses, and a cross-sectional design limits the ability to establish causality.
Implications: The study’s findings have implications for mental health professionals, educators, and policymakers. Mental health professionals can use the findings to develop interventions that address the negative impact of social media use on mental health. Educators can incorporate social media literacy into their curriculum to promote healthy social media use among young adults. Policymakers can use the findings to develop policies that protect young adults from the negative outcomes associated with social media use.
References :
- Twenge, J. M., & Campbell, W. K. (2019). Associations between screen time and lower psychological well-being among children and adolescents: Evidence from a population-based study. Preventive medicine reports, 15, 100918.
- Primack, B. A., Shensa, A., Escobar-Viera, C. G., Barrett, E. L., Sidani, J. E., Colditz, J. B., … & James, A. E. (2017). Use of multiple social media platforms and symptoms of depression and anxiety: A nationally-representative study among US young adults. Computers in Human Behavior, 69, 1-9.
- Van der Meer, T. G., & Verhoeven, J. W. (2017). Social media and its impact on academic performance of students. Journal of Information Technology Education: Research, 16, 383-398.
Appendix : The survey used in this study is provided below.
Social Media and Mental Health Survey
- How often do you use social media per day?
- Less than 30 minutes
- 30 minutes to 1 hour
- 1 to 2 hours
- 2 to 4 hours
- More than 4 hours
- Which social media platforms do you use?
- Others (Please specify)
- How often do you experience the following on social media?
- Social comparison (comparing yourself to others)
- Cyberbullying
- Fear of Missing Out (FOMO)
- Have you ever experienced any of the following mental health problems in the past month?
- Do you think social media use has a positive or negative impact on your mental health?
- Very positive
- Somewhat positive
- Somewhat negative
- Very negative
- In your opinion, which factors contribute to the negative impact of social media on mental health?
- Social comparison
- In your opinion, what interventions could be effective in reducing the negative impact of social media on mental health?
- Education on healthy social media use
- Counseling for mental health problems caused by social media
- Social media detox programs
- Regulation of social media use
Thank you for your participation!
Applications of Research Paper
Research papers have several applications in various fields, including:
- Advancing knowledge: Research papers contribute to the advancement of knowledge by generating new insights, theories, and findings that can inform future research and practice. They help to answer important questions, clarify existing knowledge, and identify areas that require further investigation.
- Informing policy: Research papers can inform policy decisions by providing evidence-based recommendations for policymakers. They can help to identify gaps in current policies, evaluate the effectiveness of interventions, and inform the development of new policies and regulations.
- Improving practice: Research papers can improve practice by providing evidence-based guidance for professionals in various fields, including medicine, education, business, and psychology. They can inform the development of best practices, guidelines, and standards of care that can improve outcomes for individuals and organizations.
- Educating students : Research papers are often used as teaching tools in universities and colleges to educate students about research methods, data analysis, and academic writing. They help students to develop critical thinking skills, research skills, and communication skills that are essential for success in many careers.
- Fostering collaboration: Research papers can foster collaboration among researchers, practitioners, and policymakers by providing a platform for sharing knowledge and ideas. They can facilitate interdisciplinary collaborations and partnerships that can lead to innovative solutions to complex problems.
When to Write Research Paper
Research papers are typically written when a person has completed a research project or when they have conducted a study and have obtained data or findings that they want to share with the academic or professional community. Research papers are usually written in academic settings, such as universities, but they can also be written in professional settings, such as research organizations, government agencies, or private companies.
Here are some common situations where a person might need to write a research paper:
- For academic purposes: Students in universities and colleges are often required to write research papers as part of their coursework, particularly in the social sciences, natural sciences, and humanities. Writing research papers helps students to develop research skills, critical thinking skills, and academic writing skills.
- For publication: Researchers often write research papers to publish their findings in academic journals or to present their work at academic conferences. Publishing research papers is an important way to disseminate research findings to the academic community and to establish oneself as an expert in a particular field.
- To inform policy or practice : Researchers may write research papers to inform policy decisions or to improve practice in various fields. Research findings can be used to inform the development of policies, guidelines, and best practices that can improve outcomes for individuals and organizations.
- To share new insights or ideas: Researchers may write research papers to share new insights or ideas with the academic or professional community. They may present new theories, propose new research methods, or challenge existing paradigms in their field.
Purpose of Research Paper
The purpose of a research paper is to present the results of a study or investigation in a clear, concise, and structured manner. Research papers are written to communicate new knowledge, ideas, or findings to a specific audience, such as researchers, scholars, practitioners, or policymakers. The primary purposes of a research paper are:
- To contribute to the body of knowledge : Research papers aim to add new knowledge or insights to a particular field or discipline. They do this by reporting the results of empirical studies, reviewing and synthesizing existing literature, proposing new theories, or providing new perspectives on a topic.
- To inform or persuade: Research papers are written to inform or persuade the reader about a particular issue, topic, or phenomenon. They present evidence and arguments to support their claims and seek to persuade the reader of the validity of their findings or recommendations.
- To advance the field: Research papers seek to advance the field or discipline by identifying gaps in knowledge, proposing new research questions or approaches, or challenging existing assumptions or paradigms. They aim to contribute to ongoing debates and discussions within a field and to stimulate further research and inquiry.
- To demonstrate research skills: Research papers demonstrate the author’s research skills, including their ability to design and conduct a study, collect and analyze data, and interpret and communicate findings. They also demonstrate the author’s ability to critically evaluate existing literature, synthesize information from multiple sources, and write in a clear and structured manner.
Characteristics of Research Paper
Research papers have several characteristics that distinguish them from other forms of academic or professional writing. Here are some common characteristics of research papers:
- Evidence-based: Research papers are based on empirical evidence, which is collected through rigorous research methods such as experiments, surveys, observations, or interviews. They rely on objective data and facts to support their claims and conclusions.
- Structured and organized: Research papers have a clear and logical structure, with sections such as introduction, literature review, methods, results, discussion, and conclusion. They are organized in a way that helps the reader to follow the argument and understand the findings.
- Formal and objective: Research papers are written in a formal and objective tone, with an emphasis on clarity, precision, and accuracy. They avoid subjective language or personal opinions and instead rely on objective data and analysis to support their arguments.
- Citations and references: Research papers include citations and references to acknowledge the sources of information and ideas used in the paper. They use a specific citation style, such as APA, MLA, or Chicago, to ensure consistency and accuracy.
- Peer-reviewed: Research papers are often peer-reviewed, which means they are evaluated by other experts in the field before they are published. Peer-review ensures that the research is of high quality, meets ethical standards, and contributes to the advancement of knowledge in the field.
- Objective and unbiased: Research papers strive to be objective and unbiased in their presentation of the findings. They avoid personal biases or preconceptions and instead rely on the data and analysis to draw conclusions.
Advantages of Research Paper
Research papers have many advantages, both for the individual researcher and for the broader academic and professional community. Here are some advantages of research papers:
- Contribution to knowledge: Research papers contribute to the body of knowledge in a particular field or discipline. They add new information, insights, and perspectives to existing literature and help advance the understanding of a particular phenomenon or issue.
- Opportunity for intellectual growth: Research papers provide an opportunity for intellectual growth for the researcher. They require critical thinking, problem-solving, and creativity, which can help develop the researcher’s skills and knowledge.
- Career advancement: Research papers can help advance the researcher’s career by demonstrating their expertise and contributions to the field. They can also lead to new research opportunities, collaborations, and funding.
- Academic recognition: Research papers can lead to academic recognition in the form of awards, grants, or invitations to speak at conferences or events. They can also contribute to the researcher’s reputation and standing in the field.
- Impact on policy and practice: Research papers can have a significant impact on policy and practice. They can inform policy decisions, guide practice, and lead to changes in laws, regulations, or procedures.
- Advancement of society: Research papers can contribute to the advancement of society by addressing important issues, identifying solutions to problems, and promoting social justice and equality.
Limitations of Research Paper
Research papers also have some limitations that should be considered when interpreting their findings or implications. Here are some common limitations of research papers:
- Limited generalizability: Research findings may not be generalizable to other populations, settings, or contexts. Studies often use specific samples or conditions that may not reflect the broader population or real-world situations.
- Potential for bias : Research papers may be biased due to factors such as sample selection, measurement errors, or researcher biases. It is important to evaluate the quality of the research design and methods used to ensure that the findings are valid and reliable.
- Ethical concerns: Research papers may raise ethical concerns, such as the use of vulnerable populations or invasive procedures. Researchers must adhere to ethical guidelines and obtain informed consent from participants to ensure that the research is conducted in a responsible and respectful manner.
- Limitations of methodology: Research papers may be limited by the methodology used to collect and analyze data. For example, certain research methods may not capture the complexity or nuance of a particular phenomenon, or may not be appropriate for certain research questions.
- Publication bias: Research papers may be subject to publication bias, where positive or significant findings are more likely to be published than negative or non-significant findings. This can skew the overall findings of a particular area of research.
- Time and resource constraints: Research papers may be limited by time and resource constraints, which can affect the quality and scope of the research. Researchers may not have access to certain data or resources, or may be unable to conduct long-term studies due to practical limitations.
About the author
Muhammad Hassan
Researcher, Academic Writer, Web developer
You may also like

How to Cite Research Paper – All Formats and...

Data Collection – Methods Types and Examples

Delimitations in Research – Types, Examples and...

Research Paper Format – Types, Examples and...

Research Process – Steps, Examples and Tips

Research Design – Types, Methods and Examples
- Methodology article
- Open access
- Published: 15 June 2022
Time of day as a critical variable in biology
- Randy J. Nelson ORCID: orcid.org/0000-0002-8194-4016 1 ,
- Jacob R. Bumgarner 1 ,
- Jennifer A. Liu 1 ,
- Jharnae A. Love 2 ,
- O. Hecmarie Meléndez-Fernández 1 ,
- Darius D. Becker-Krail 1 ,
- William H. Walker II 1 ,
- James C. Walton 1 ,
- A. Courtney DeVries 1 , 3 &
- Brian J. Prendergast 2
BMC Biology volume 20 , Article number: 142 ( 2022 ) Cite this article
4129 Accesses
15 Citations
63 Altmetric
Metrics details
Circadian rhythms are important for all aspects of biology; virtually every aspect of biological function varies according to time of day. Although this is well known, variation across the day is also often ignored in the design and reporting of research. For this review, we analyzed the top 50 cited papers across 10 major domains of the biological sciences in the calendar year 2015. We repeated this analysis for the year 2019, hypothesizing that the awarding of a Nobel Prize in 2017 for achievements in the field of circadian biology would highlight the importance of circadian rhythms for scientists across many disciplines, and improve time-of-day reporting.
Our analyses of these 1000 empirical papers, however, revealed that most failed to include sufficient temporal details when describing experimental methods and that few systematic differences in time-of-day reporting existed between 2015 and 2019. Overall, only 6.1% of reports included time-of-day information about experimental measures and manipulations sufficient to permit replication.
Conclusions
Circadian rhythms are a defining feature of biological systems, and knowing when in the circadian day these systems are evaluated is fundamentally important information. Failing to account for time of day hampers reproducibility across laboratories, complicates interpretation of results, and reduces the value of data based predominantly on nocturnal animals when extrapolating to diurnal humans.
Platt’s classic distillation of Francis Bacon’s inductive reasoning for the scientific enterprise has served as an essential guideline for generations of scientists [ 1 ]. In the model that he termed strong inference , Platt outlined four steps to be used for efficient scientific progress in any field: (1) formulate alternative hypotheses, (2) develop a decisive experiment (or a series of experiments) to rule out as many alternative hypotheses as possible, (3) conduct the experiment rigorously to obtain unambiguous results, and (4) recycle the process to test and refine remaining hypotheses [ 1 ]. As Platt noted, experiments must be conducted in a rigorous manner.
Experimental studies in biology require rigorous experimental design coupled with sufficiently detailed reporting of methods to allow other scientists to replicate and extend the results. Rigor and reproducibility have become a key initiative at the US National Institutes of Health (NIH) to improve the biomedical scientific enterprise (e.g., NIH guide notice NOT-OD-16–11) [ 2 , 3 ]. Training in rigor and transparency to increase reproducibility is now mandated for NIH-funded graduate and postdoctoral trainees [ 4 ]. Similarly, several scientific societies have revised their publishing guidelines to enhance rigor and reproducibility (e.g., [ 5 , 6 , 7 ]). Many of these have focused on providing details of statistical analyses and reagent identification and validation in response to new NIH guidelines. These guidelines require consideration of relevant biological variables, including sex, age, body mass, and underlying health conditions when seeking research funding through the NIH.
Some scientific society guidelines have rightfully called for more comprehensive details of experimental design and analysis in the method sections of published papers to enhance transparency, rigor, and reproducibility [ 7 ]. In a recent report, we examined the top 25 most cited papers in several domains of behavioral neuroscience in which previously documented significant time-of-day effects had been reported [ 8 ]; remarkably, many of the reviewed studies did not report the time of day when their data were collected (42%), and even when clearly reported, testing was almost as likely to have been performed during the light phase as during the dark phase. The vast majority of animal models used in behavioral neuroscience research are nocturnal rodents; thus, testing during the light phase (i.e., during the animals’ rest period) may confound results and introduce variability across studies [ 8 ]. Indeed, it has been reported that dramatic time-of-day effects on neuroprotection in animal models of stroke may contribute to the failures to translate these data to the treatment of stroke and central nervous system diseases in humans [ 9 ].
Virtually all physiological and behavioral processes display daily fluctuations driven by endogenous circadian clocks, and the neglect of time-of-day information in methods presents obvious challenges to experimental rigor and reproducibility [ 10 , 11 ]. In this report, we sought to determine how widespread the failure to report time-of-day information extends beyond behavioral neuroscience. We examined the top 50 cited papers across 10 major domains of the biological sciences in the calendar year 2015. We repeated this analysis for the year 2019, hypothesizing that the awarding of a Nobel Prize in 2017 for achievements in the field of circadian biology would highlight the importance of circadian rhythms for scientists across many disciplines, and improve time-of-day reporting. Time-of-day information was predicted to be more prevalent in the top 50 cited papers in 2019 compared to 2015.
Our analyses, however, revealed that most publications fail to include sufficient temporal details when describing their experimental methods and that there were no differences in time-of-day reporting between 2015 and 2019. Indeed, few studies that we examined included time-of-day factors when interpreting their data. We propose that failing to account for time-of-day as a key biological variable hampers reproducibility across biomedical laboratories, complicates interpretation of the results, and reduces the value of the data when extrapolating laboratory results based on (mainly nocturnal) animal studies to diurnal humans. Below, we review time-of-day reporting data from 10 major discipline areas of the biological sciences: (1) general biology, (2) immunology, (3) neuroscience, (4) physiology, (5) pharmacology and pharmacy, (6) reproductive biology, (7) endocrinology and metabolism, (8) behavioral sciences, (9) oncology, and (10) cardiac and cardiovascular systems. For each area, we (1) briefly highlight the relevance of daily rhythms to core tenets of the discipline and then (2) illustrate, using the 50 most highly cited publications in 2015 and 2019, the patterns of time-of-day reporting in each discipline. Our goal is to raise awareness of the importance of time-of-day as a biological variable that influences reproducibility, reliability, and validity across biological research.
As discussed above, nearly all biological processes display daily fluctuations driven by endogenous circadian clocks. Functional molecular circadian clocks and resultant circadian rhythms in biological processes are fundamental and thus have evolved independently multiple times across all domains of life on planet Earth [ 12 , 13 ]. Among eukaryotes, the Nobel Prize was awarded for identification of the molecular circadian clock in Drosophila [ 14 ]. In vertebrates, daily rhythmicity is controlled by a central circadian clock located in the suprachiasmatic nuclei (SCN) in the ventral hypothalamus, which is implicated in virtually all aspects of physiology and behavior (see below). Molecular clocks with similar organizational and functional characteristics, with different molecular mechanisms, have since been identified in all other domains of life. Circadian rhythms in fungi were first described in the genus Neurospora in 1959 [ 15 ], but the molecular mechanisms of the fungi circadian clock remained unidentified until 1989 [ 16 ]. In Neurospora, the molecular circadian clock controls physiology by directing nighttime growth and daytime catabolism [ 17 ], and disruption of the molecular clock can directly affect conidiation (reproduction) [ 18 ]. Among prokaryotes, circadian rhythms in cyanobacteria were first identified in 1986 [ 19 ], but the molecular mechanisms of these clocks remained unidentified for several more years [ 20 , 21 ]. In these cyanobacteria ( Synechococcus elongatus ) a functioning circadian clock is necessary for natural competence and allows for photoperiodic adaptation [ 22 ].
Despite the ubiquity of molecular clocks and circadian rhythms in most all living organisms, a review of the top 50 cited papers from general Biology journals in 2015 and 2019 revealed a consistent lack of time-of-day information provided in the methods. In 2015, time-of-day for dependent variables was not reported in 41 of the 50 top cited publications; similarly, 80% (40 of 50) of the top cited publications in 2019 failed to report time-of-day (Fig. 1 A) ( χ 2 = 1.82; p > 0.05) (Additional File 1 : Table 1.2). Among papers that did address time-of-day in their methods, this information was ambiguous in six papers in 2015 and in three papers during 2019. Some examples of ambiguity include reporting clock time without reporting time of lights on:off or environmental light schedules, as well as field studies not reporting dates or site location. Only three papers in 2015 and seven papers in 2019 unambiguously identified the time of day for the dependent variables. Likewise, environmental light-dark (LD) cycles were not reported in 35 of 50 of the top cited publications in 2015, nor were they reported in 26 of 50 top publications in 2019 (Fig. 1 B) ( χ 2 = 3.40; p > 0.05) (Additional File 1 : Table 1.2). However, some types of general biology studies reviewed here, such as papers describing protein structure or in vitro studies in cells or tissues with no circadian reporters, did not lend themselves for describing, or assuming, time-of-day for their dependent variables. Regardless, time-of-day and environmental photoperiod remain largely ignored as a biological variable, even in these publications that encompass studies across all kingdoms of life.
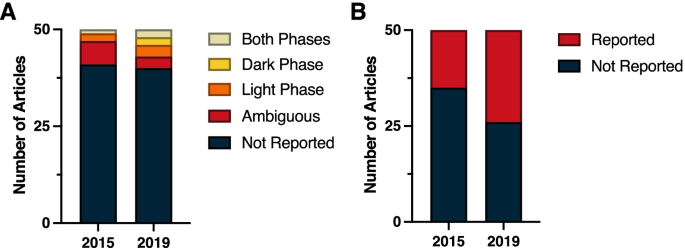
Stack plot indicating the incidence of reporting of ( A ) the time-of-day at which key experimental procedures were performed, and ( B ) experimental lighting cycles among the 50 highest-cited biology reports from 2015 and from 2019. See Methods (Measures and criteria) for time-of-day reporting criteria
Circadian rhythms in immune function are ubiquitous and arise from complex interactions among rhythmic features of the host and rhythmic processes intrinsic to immune cells themselves. Daily fluctuations in the numbers of circulating immune cells (e.g., circulating leukocytes or lymphocyte subpopulations) and in their trafficking into and out of the circulation are well-documented in laboratory animals [ 23 , 24 , 25 , 26 ] and in humans [ 27 , 28 ]. Innate immune responses to pathogens or pathogen-associated molecules likewise vary over the circadian cycle; in mice and other animal models, the severity of infection symptoms exhibits diurnal patterns [ 29 , 30 , 31 , 32 ], with more severe responses in animals challenged during the rest phase. Adaptive immune responses, [ 33 ] and T cell and B cell functions [ 34 ] change over the day, in a species- and trait-specific manner. Circadian clocks within cells of the immune system and circadian clocks distributed more broadly throughout the host interact to drive daily cycles in immune processes [ 35 ].
In light of the pervasive effects of circadian time on immune function, it is remarkable that not a single report from either 2015 or 2019 clearly indicated time-of-day information for experimental manipulations and measures (Fig. 2 A). In 2015, 12 papers were classified as ambiguous and 38 failed altogether to report time-of-day information; a similar breakdown was evident in 2019 (14 ambiguous, 36 not reported). Reports in the Immunology subdiscipline frequently contained manipulations that were performed in vitro, either in primary culture or on established, immortalized cell lines. Commonly occurring in vitro manipulations, such as temperature pulses and serum delivery, have the potential to induce transcription of circadian clock genes [ 36 , 37 , 38 , 39 ]. Analogous to information about light–dark cycles, it would be useful to indicate the time at which key experimental manipulations were performed relative to such events.
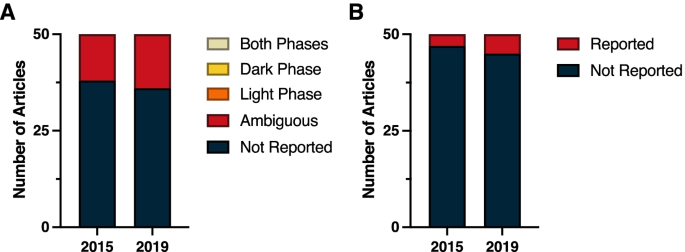
Reporting in immunology. A Time-of-day and B light cycle reporting
Finally, vivarium LD cycle information was seldom reported in the Immunology subdiscipline; this information was present in three reports from 2015 and in five reports from 2019 (Fig. 2 B) ( χ 2 = 0.54; p > 0.05) (Additional File 1 : Table 1.3).
- Neuroscience
The brain and virtually all aspects of neuroscience exhibit substantial fluctuations across the day. Circadian rhythms and time-of-day variations in physiological function have been well documented within all aspects of brain organization, which is important for synchronizing and adapting behavior to environmental conditions. For example, aspects of cellular and molecular neuroscience, including diurnal synaptic strength and protein markers [ 40 , 41 ], cortical and motor evoked response, and aspects of behavior including cognition [ 42 ], learning and memory [ 43 ], and sleep [ 44 ] are under clock control and display circadian rhythms. Furthermore, there have also been notable associations between disruptions to circadian rhythms and neurodegenerative diseases, including Alzheimer’s and other related dementias [ 45 ], mental health [ 46 ], and other neurological disorders [ 47 ], suggesting the relevance of time-of-day within translational and clinical neuroscience as well. The subfield of behavioral neuroscience is especially a critical area where the biological variable of time-of-day should be considered [ 48 ]. A lack of consistency is prevalent for reporting the timing of behavioral tests; publications fail to consider testing during the animal’s active period, suggesting that not accounting for this variable could affect behavioral outcomes and phenomena [ 8 ].
Even within this broad multidisciplinary field of Neuroscience, time-of-day as a crucial biological variable often remains unreported. A review of the top 50 cited papers in 2015 and 2019 revealed a substantial lack of time-of-day reporting in the methods. Within both years, time-of-day was not reported in 40 out of the 50 top publications (Fig. 3 A) ( χ 2 = 0.33; p > 0.05) (Additional File 1 : Table 1.2). Among papers that listed time of experiments, four papers in 2015 and two papers in 2019 were judged to be ambiguous because they failed to report time of lights on/off for the standard lighting conditions, and also did not report sufficient details to conclude what phase and time the experiments were conducted in relation to circadian time. Circadian time is a standardized 24-h notation of the phase within a circadian rhythm that represents an estimation of individuals’ subjective time. Vivarium light dark cycles were only reported in 29 of the studies in 2015, and 23 of the studies in 2019 (Fig. 3 B) ( χ 2 = 1.44; p > 0.05) (Additional File 1 : Table 1.3). However, in vitro studies did not describe or assume time-of-day as a dependent variable. Notably, four studies in 2019 reported circadian timing in the methods section, however, the report of time-of-day as a biological variable often remains neglected in the method sections of neuroscience papers.
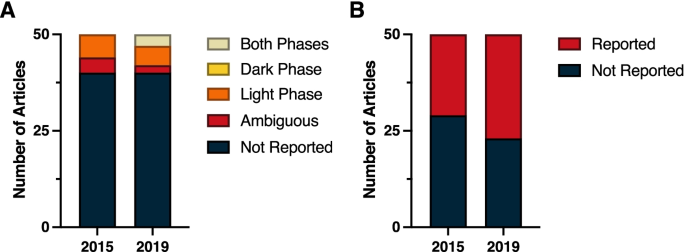
Reporting in neuroscience. A Time-of-day and B light cycle reporting
The Physiological Society defines physiology as a branch of biology that is distinguished from other physical sciences by an emphasis on the integration of molecular, cellular, systems, and whole organism function. Rusak and Zucker [ 49 ] published a seminal paper in the Journal of Physiology in 1979 that declared rhythms in temporal organization to be comparable to homeostasis in promoting organismal fitness. Michael Rosbash’s 2017 Nobel Lecture [ 50 ] emphasized that optimal circadian organization, including temporal coupling or separation of various physiological, metabolic, and behavioral processes, is closely tied to fitness. Given the longstanding emphasis on integration within the field of physiology and acknowledgment that many crucial physiological processes vary across the day, one might reasonably expect publications in the field to emphasize time-of-day information in the methods section.
The integrative approach to physiological research is evident from a perusal of highly cited papers from 2015 and 2019; the vast majority of these papers have both in vivo and in vitro/ex vivo experiments. Among the most common research outcomes of these select papers are inflammation, glucose regulation, blood pressure, generation of reactive oxygen species, and the microbiome. Each of these outcomes has well-characterized circadian rhythms. For example, daily oscillations in gene expression, surface marker expression, cytokine secretion, proliferation, trafficking, effector function, and responsiveness have been described for many immune cells [ 51 , 52 ]; a proposed adaptive explanation is that circadian regulation of immunity maximizes the benefits of immunity while minimizing the energetic costs and potential secondary damage to tissues (immunopathology) [ 53 ]. However, this organization creates a challenge in designing experiments because the peaks and nadirs of various immune cells occur at different points in the circadian cycle. Thus, immunological outcomes may vary significantly based on the time-of-day that the experiment is performed [ 54 ]. Similarly, both humans and rodents display daily rhythms in glucose tolerance, with a reduction in tolerance during the rest phase (dark phase in humans and light phase for most rodents) relative to the active phase (light phase in humans and dark phase for most rodents) [ 55 ]. Circadian rhythms in glucose availability likely reflect a combination of factors, including diurnal variation in food intake, insulin sensitivity, hepatic glucose production, and pancreatic beta-cell responsivity [ 55 ]. Thus, the specific timing of glucose samples, tolerance tests, and assays of processes related to glucose metabolism warrant mention in the “ Methods ” sections. There are also well-described cardiovascular rhythms in blood pressure and heart rate that tend to follow a 24-h rhythm in which there is a decline during the inactive phase and a rise in anticipation of the start of the active phase (see below and [ 56 ]). Not surprisingly, hundreds of genes related to metabolism, signal transduction, and transcription exhibit circadian oscillations in cardiomyocytes [ 57 ]. The risk of myocardial infarction follows a similar pattern to blood pressure in humans, with a three-fold increase at the 9:00 h peak compared to the 23:00 h trough [ 58 ]. In addition, myocardial infarctions that occur early in the active phase result in larger infarcts [ 59 ]. Likewise, in mice, myocardial infarction during the active phase produces larger infarcts and greater deficits in cardiac function than myocardial infarction during the inactive phase due to circadian differences in neutrophil trafficking and resulting inflammation in the myocardium [ 60 ]. Lastly, the gut microbiome oscillates in response to several factors including the circadian rhythm of food intake by the host [ 61 ], glucocorticoid concentrations, antimicrobial peptide concentrations, and intestinal mucus secretion [ 62 ]; there are documented circadian rhythms in microbiome biomass, production of microbially derived products, and gene expression in pathways associated with growth, energy metabolism, motility and detoxification [ 61 , 62 ]. Together, these studies emphasize the need for precisely timed experimental methods [ 62 ].
A common manipulation among the most highly cited physiology papers was exogenous melatonin administration. Melatonin is released from the pineal gland only during the dark phase, is suppressed upon exposure to light, and has potent chronobiotic properties; exogenous administration can phase shift the circadian clock and alter circadian rhythms in endogenous hormones, body temperature, and behavior [ 63 ]. Furthermore, time-of-day of administration may influence the physiological response to melatonin [ 64 ].
A review of the top 50 cited papers published in the Physiology category in 2015 and 2019 revealed a dearth of time-of-day information provided in the methods (Fig. 4 A) ( χ 2 = 1.01; p > 0.05) (Additional File 1 : Table 1.2). The most frequently reported time measure was the number of light versus dark hours in the LD cycle; in 2015, 23 of the 50 papers (46%) reported the LD cycle under which the animals were maintained, whereas in 2019 that number dropped to 15 of the 50 papers (30%; Fig. 4 B) ( χ 2 = 2.71; p > 0.05) (Additional File 1 : Table 1.3). Furthermore, 82% of the 2015 papers and 94% of the 2019 papers omitted the time-of-day that specific procedures were performed or samples were collected. Of the nine 2015 papers that provide at least one reference to time of day in the methods, eight were ambiguous (for example, stating that a procedure was performed in the afternoon or providing clock time without sufficient information to convert it to zeitgbeber time). Only one of these 2015 papers provided explicit time-of-day information for every procedure and sample collection in the methods section. Among the 2019 papers, three provided ambiguous reporting, with one reporting light phase sleep measurements and another reporting dark phase testing. But, none of these three 2019 papers provided explicit time-of-day information for every procedure and sample collection in the methods section. In sum, only one paper out of the 100 most highly cited papers in 2015 and 2019 within the Physiology category provided sufficient temporal information to allow replication with fidelity to time-of-day.
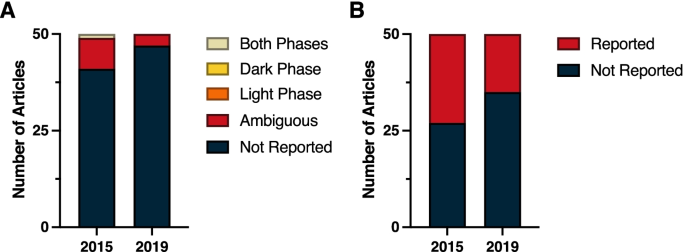
Reporting in physiology. A Time-of-day and B light cycle reporting
Pharmacology and pharmacy
In pharmacology, the efficacy and/or toxicity of a drug is heavily governed by dose, route, and timing of administration. In particular, drug timing has long been known to influence specific drug effects. One of the earliest experiments documenting this phenomenon demonstrated leukemic mice given the same dose of an anti-cancer drug had strikingly different outcomes depending on the time-of-day it was administered; i.e., the same dose yielded few adverse effects when given during the day or inactive phase, but proved lethal when given during the night or active phase [ 65 , 66 ]. Since then, this circadian variation in drug efficacy or toxicity has been seen in both clinical and preclinical settings, across many classes of compounds and therapies [ 67 ]. Accumulating evidence suggests this phenomenon is driven by circadian regulation of the physiology that governs a drug’s pharmacokinetics, now coined chronopharmacokinetics [ 68 ].
Chronopharmacokinetics describe a drug’s time-dependent variation across four main processes: absorption, distribution, metabolism, and excretion [ 69 ]. Circadian rhythms in gastrointestinal (GI) function directly impact the absorption of a drug, particularly with orally administered compounds (e.g., GI acid secretions, pH, motility, and blood flow all show circadian variation) [ 70 , 71 ]. Many of the important GI drug influx and efflux transporters show rhythms in expression [ 72 ], which may be directly regulated by BMAL1 and the molecular clock [ 73 ]. This is also true for many of the hepatic enzymes important for metabolism, including cytochrome P450 (CYP) phase I oxidation metabolism enzymes and multiple phase II conjugation metabolism enzymes [ 74 ]. Drug distribution is also heavily dependent on cardiac function, cardiac output, and blood flow, all of which are known to be under circadian regulation and show peaks during the active phase [ 75 , 76 , 77 ]. Finally, circadian rhythms in nearly all kidney functions have been documented and contribute to circadian variation in drug excretion [ 78 , 79 ], a critical last step in limiting a drug’s effects and/or toxicity.
Despite extensive literature underscoring the importance of the circadian system in regulating drug effects and/or toxicity, the field of Pharmacology and Pharmacy still largely lacks consistent reporting of time-of-day information. Upon reviewing the top 50 cited papers in 2015 and 2019, the overwhelming majority of papers lack time-of-day reporting. Strikingly, 44 out of 50 in 2015 and 46 out of 50 in 2019 did not report what time-of-day experiments were conducted (Fig. 5 A) ( χ 2 = 1.04; p > 0.05) (Additional File 1 : Table 1.2). In both years surveyed, each had three papers that reported time-of-day information, but they were ambiguous or did not report sufficient details to determine the time-of-day experiments were conducted. In 2015, one article reported testing during the light phase and two articles tested across both phases, whereas in 2019 just one article reported testing across both phases. Finally, upon reviewing light cycle reporting, only 21 articles in 2015 and 22 articles in 2019 reported light–dark cycle housing conditions (e.g., 12:12 light–dark cycle); however, none of the articles stated the time of light onset (Fig. 5 B) ( χ 2 = 0.04; p > 0.05) (Additional File 1 : Table 1.3).
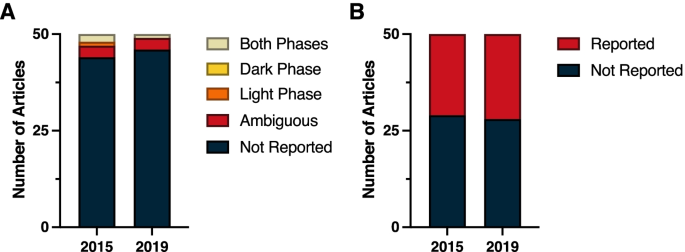
Reporting in pharmacology and pharmacy. A Time-of-day and B light cycle reporting
Reproductive biology
Reproductive function, from gametogenesis to reproductive behavior, varies across the day [ 80 ]. For example, the temporal functioning of ovaries is driven by circadian rhythms arising in the SCN. CLOCK, BMAL1, and CRY1 clock proteins display rhythmic expression in rat ovaries [ 81 ]. Clock genes are expressed throughout the ovarian granulosa, theca cells, and luteal cells of rats and other mammals [ 82 ]. BMAL1 is significantly elevated ~ 8–10 h after the peri-ovulatory luteinizing hormone (LH) surge at ZT18 on proestrus [ 81 ]; female BMAL1 gene knockout mice, however, are infertile likely due to a lack of phasic sensitivity to LH [ 83 ].
Importantly, ovulation displays strong time-of-day differences in mammals. For example, rodent ovaries display circadian rhythms of sensitivity to LH; rats injected with equine LH during the dark phase of either diestrus or proestrus ovulated more frequently and produced significantly more oocytes than did females injected during the middle of the light [ 84 ]. In contrast, diurnal cattle, gilts, and other female ungulates ovulate during the day [ 85 , 86 , 87 ].
Testicular function also displays daily fluctuations [ 80 ]. For instance, mice display increased spermatogenesis after the onset of dark compared to mice tested during the early daylight hours [ 88 ]. Meiosis of murine spermatids also displays daily rhythms [ 89 ]. Daily rhythms of core clock gene and protein expression have been reported in the testes of mice, rats, and hamsters, as well as bulls [ 90 , 91 ]. There have also been reports of clock gene and protein expression in the epididymis, vas deferens, seminal vesicles, and prostate (reviewed in [ 90 ]). In terms of mating behaviors, the vast majority of studies indicate that nocturnal animals mate during the dark (e.g., [ 92 ]), whereas diurnal animals such as livestock breed primarily during the day (e.g., [ 85 , 86 , 87 ]. Despite these strong circadian rhythms in reproductive biology, the vast majority of highly cited papers ignored time-of-day in their procedural descriptions.
A review of the top 50 cited papers in 2015 and 2019 revealed consistent lack of time-of-day information provided in the methods (Fig. 6 A) ( χ 2 = 1.04; p > 0.05) (Additional File 1 : Table 1.2). In many ways, the section on reproductive biology differs from other sections in that diurnal agricultural animals were primarily the topic of the studies, and it was presumed that tissue or other samples were collected during the day. Nonetheless, for many papers the time-of-day information was ambiguous (20 in 2015 and 22 in 2019), but again, inferred to be tested during the day. For example, in many of the highly cited papers in reproductive biology, ovaries or testes were obtained from cattle, swine, sheep, goats, or poultry from local abattoirs presumably during the day shift. In 2015, 29 papers did not report the time-of-day when experimental protocols were conducted, whereas in 2019, 25 papers failed to report time-of-day information (Fig. 6 A).
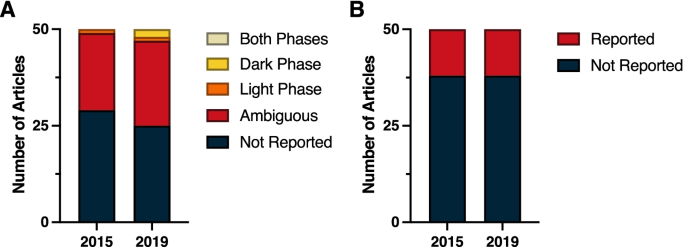
Reporting in reproductive biology. A Time-of-day and B light cycle reporting
Again, most of the studies in reproductive biology were conducted in livestock, and in 2015 the light cycle information (day length) was not provided in 38 cases. Light cycle information was reported in 12 instances (Fig. 6 B). One paper indicated that studies were conducted from February to December 2012 so photoperiod could be determined; two papers indicated that animals were in natural photoperiods, but did not report time of year. In 2019, 38 papers failed to provide light cycle information (Fig. 6 B) ( χ 2 = 0.0; p > 0.05) (Additional File 1 : Table 1.3). Twelve of the 50 most cited papers in 2019 reported light cycle information, including one study that collected data in Poland during December so photoperiod could be inferred, but was not explicitly reported.
- Endocrinology and metabolism
Metabolic and endocrine function in animals fluctuates across the day in response to shifts in energy requirements and environmental conditions. As time-of-day variation in food intake occurs, the metabolic systems of animals must adapt in response to varying needs for digestion or mobilization of energy stores [ 93 ]. For example, clock genes regulate lipogenesis [ 94 ] and glycogenesis [ 95 ] across the day in response to temporal variation in food intake. Moreover, the metabolic system also reciprocally interacts with circadian rhythms [ 96 , 97 ], highlighting the importance of considering time-of-day and circadian rhythms in metabolic research.
Circadian rhythms also regulate hormone production, release, and sensitivity [ 98 ]. Numerous hormones display circadian rhythms of secretion, including cortisol, melatonin, growth hormone, ghrelin, and insulin [ 99 ]. The rhythms of most hormones differ between nocturnal and diurnal animals, as they help to prepare the body for varying behavioral and physiological needs.
Over half of the top 50 cited papers from 2015 and 2019 in the field of endocrinology and metabolism did not report the time-of-day at which experiments were conducted; time-of-day reporting improved from 1 out of 50 in 2015 to 7 of 50 in 2019 (Fig. 7 A) ( χ 2 = 4.89; p < 0.05) (Additional File 1 : Table 1.2). Fourteen articles from 2015 and 9 articles from 2019 presented ambiguous time-of-day reporting, with a lack of details to sufficiently identify the timing of all experiments conducted. In 2015, only one article reported testing during the light phase, whereas in 2019 three articles reported light-phase testing and four articles conducted experiments across both phases. This improvement was significant based on a chi-squared analysis ( χ 2 = 4.89; p < 0.05) (Additional File 1 : Table 1.2).
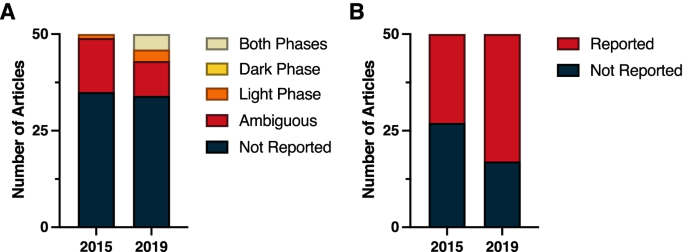
Reporting in endocrinology and metabolism. A Time-of-day and B light cycle reporting
In 2015, 27 of the 50 examined articles did not report light–dark cycle housing condition information (Fig. 7 B). In 2019, light–dark cycle reporting improved in this field ( χ 2 = 4.05; p < 0.05) (Additional File 1 : Table 1.3), as 33 of the 50 articles provided the hours of vivarium light–dark cycle conditions.
- Behavioral sciences
Daily rhythms in behavior are among the most well-established and well-studied rhythmic phenomena in complex, multicellular animals. Indeed, the discovery of the mammalian circadian pacemaker relied on the high-amplitude circadian rhythm in ingestive behavior of rats—and its elimination via lesions of the so-called master clock, the suprachiasmatic nuclei (SCN) [ 49 , 100 ]. So robust is the daily cycle of activity and rest that locomotor activity rhythms of mice are leveraged in high-throughput assays for genetic factors that impact the circadian clock [ 101 ]. The Behavioral Sciences subdiscipline contained a heterogeneous group of research reports, representing a diversity of species (13 different mammalian species, at least 8 different avian species, 6 fish, 2 lizards, 1 amphibian, 1 insect) and experimental venues (laboratory, field station, research farm, field study). As in many areas of biology, inbred laboratory mice were the modal research model (45 of 100 reports), but rats were commonly featured (24 reports); the linkage in this subdiscipline was an emphasis on behavioral manipulations and measures.
In contrast to many other subdisciplines surveyed here, LD cycle reporting was common in behavioral sciences (Fig. 8 B) ( χ 2 = 1.78; p > 0.05) (Additional File 1 : Table 1.3). In 2015, 43 of 50 (86%) papers clearly reported information on the photocycle exposure, and in 2019, 47 of 50 (94%) reported this information. Collapsing across both survey years, a total of 17 reports exposed animals to natural photoperiods, and 13 of these reports provided information sufficient to infer the photocycle.
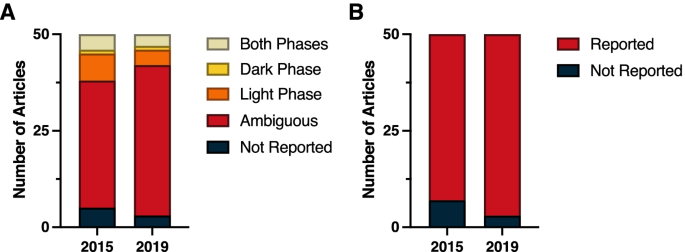
Reporting in behavioral sciences. A Time-of-day and B light cycle reporting
In common with other subdisciplines, very few reports indicated the phase of the circadian cycle when experimental manipulations were performed (Fig. 8 A) ( χ 2 = 1.00; p > 0.05) (Additional File 1 : Table 1.2). In 2015, time-of-day information was either not reported or ambiguous in over 75% (38 of 50) of the top 50 cited papers, and 2019 yielded a marginally lower ambiguous and non-reporting rate of 84% (42 of 50). A recurring feature across many papers reviewed in this subdiscipline was the use of standard laboratory-based behavioral tests of learning and memory, emotion, attention, and basic sensorimotor function; 55 of the 100 papers surveyed included one or more of these behavioral tests (range: 1–7 tests/report; mode: 3). Only 19 of these 55 (35%) reports indicated time-of-day information for the complete suite of behavioral tests performed: 14 performed testing in the light phase and 5 in the dark phase, although all 19 were conducted using nocturnal rats or mice. Ambiguous or absent reporting for other methodological steps in these 19 papers ultimately required classifying 12 as ambiguous.
Also commonplace among the top cited papers was the collection of blood, brain, feces, or other types of samples for analyses ex vivo. In total, 63 papers reported such collections, but in only 8 instances (13% of reports with collections) was the circadian phase of sample collection made clear. Ambiguous or absent reporting for other methodological steps in these 8 papers ultimately required classifying three as ambiguous.
Of all the described disciplines of animal biology, the field of oncology likely has one of the most integral, but least appreciated, relationship with circadian rhythms. There is a clear bidirectional relationships between core circadian clock genes and cell division [ 102 , 103 ]. In proliferating mammalian cells, the cell cycle and rhythmic circadian clock are phase-locked [ 104 ]. Altered circadian clock gene expression and by consequence altered circadian rhythms are considered a crucial factor for aberrant cell division (i.e., cancer). Indeed, numerous studies have demonstrated distorted clock gene expression in a broad spectrum of cancer types, and foundational sciences studies have demonstrated the functional consequence (i.e., increased cancer growth) of circadian rhythm disruption (reviewed [ 105 ]). Given the crucial relationship between circadian rhythms and cancer, it is not unexpected that multiple aspects of cancer biology display time-of-day effects. Indeed, recent studies have demonstrated time-of-day effects in circulating tumor cells [ 106 ]. Specifically, circulating tumor cells exhibited stochastic bursts throughout cancer progression with peaks at the onset of the active phase. Additionally, most pertinent to the current review, there is a time-of-day effect in tumor-take frequency following inoculation with cancer cells [ 107 ]. Subcutaneous injections of 2000–50,000 fibrosarcoma cells demonstrated a significantly reduced incidence of tumor-take at the sleep/wake transition relative to other times of day. Furthermore, iv injections of the B16 melanoma metastatic cell line exhibited similar time-of-day effects [ 107 ]. Time-of-day can also have indirect effects on cancer growth by affecting other systems in the body, for example, the immune system. The immune system displays clear time of day effects (see above), that can have dramatic consequences on cancer development and metastatic spread [ 108 ].
Despite the demonstrated relationship between oncology and circadian rhythms/time-of-day, none of the examined studies provided adequate details in describing the light cycle or timing of experimental manipulations. Of the 100 studies (50 from 2015 and 50 from 2019) examined in the field of oncology, only one study explicitly stated the light dark cycle (Fig. 9 B) ( χ 2 = 1.00; p > 0.05) (Additional File 1 : Table 1.3). This was not a consequence of a lack of detailed reporting as some studies mentioned the type of housing, temperature, and humidity, but failed to report the light dark cycle. Furthermore, no study in 2015 described the time-of-day of experimental manipulations, and only one study from 2019 provided an ambiguous description of the time of day of experimental manipulations (i.e., CHK1 inhibitor was injected subcutaneously at the nape of the neck every 12 h; Fig. 9 A). The vast majority of studies examined used xenograft or syngeneic tumor models that were then treated. One can assume that virtually all injections, implantations, and treatments likely occurred during the day. However, this is too ambiguous to allow meaningful replication and reproducibility.
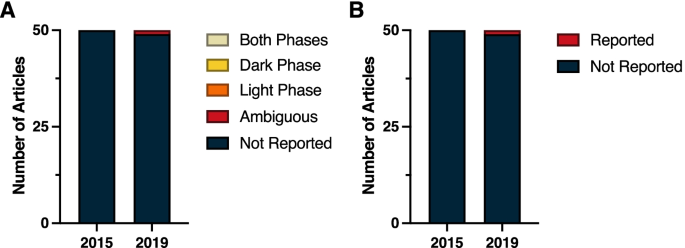
Reporting in oncology. A Time-of-day and B light cycle reporting
- Cardiac and cardiovascular systems
Cardiovascular function also displays daily variations [ 109 , 110 ] that are regulated by circadian rhythms; cardiovascular function is often linked with sleep–wake patterns [ 111 , 112 ] and the accompanying shift between sympathetic and parasympathetic innervation (reviewed in [ 113 ]). Several core aspects of cardiovascular physiology, such as heart rate and blood pressure variability are key indicators of proper vascular function and reflect predictable day-night fluctuations. Blunting of these circadian fluctuations often coincides with pathological cardiovascular events, such as myocardial infarction, ventricular tachycardia, and sudden cardiac death, peaking in the early morning [ 114 , 115 , 116 , 117 ]. Further, reduced daily variability of these parameters along with mistimed release of interdependent physiological factors such as endothelial [ 118 , 119 ], prothrombotic [ 120 ], clotting [ 121 ], and other core physiological biomarkers, in conjunction with altered immune vulnerability [ 122 ], interact to evoke a pathological response [ 56 ]. These data suggest circadian regulation over the cardiovascular system. Indeed, peripheral clocks and clock gene expression have been identified in these tissues [ 123 , 124 ], and differential responses to vasoactive drugs are well established [ 125 , 126 ]. Thus, time-of-day and circadian phase are important biological variables that need to be considered when choosing animal models to test drugs or other interventions, analyzing and interpreting data, and importantly, making conclusions for potential translatability.
Despite these well-characterized rhythmic fluctuations in cardiovascular function and physiology, few studies reported time-of-day, environmental light exposure, or circadian rhythm parameters in their research publications. Remarkably, in 2015, only 4 articles reported the animal housing light cycle (12:12) (Fig. 10 B) ( χ 2 = 2.99; p > 0.05)(Additional File 1 : Table 1.2), albeit, without a reference to the time of light onset (zeitgeber time, ZT), compared to 10 articles in 2019, with only one reporting ZT. ZT is a unit of time based on the period of a zeitgeber, such as a light–dark cycle of 12:12 h. In free-running animals housed in constant conditions, the onset of activity of diurnal animals is denoted as circadian time zero (CT0), whereas the onset of activity of nocturnal individuals is CT12. Moreover, none of the 100 articles analyzed for these two years sufficiently detailed the time-of-day (phase) during which experiments were conducted, although it is presumed that these occur during the daytime. Four articles in both 2015 and 2019 had ambiguous reporting, where only partial amounts of reporting were provided or hours of testing were reported without light cycle information.
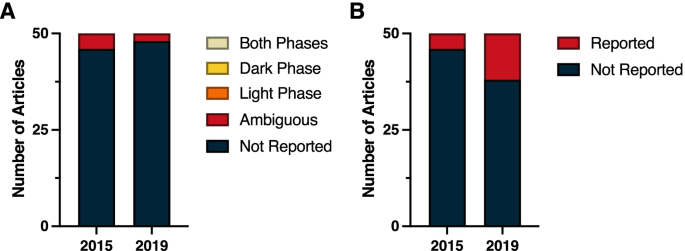
Reporting in cardiac and cardiovascular systems. A Time-of-day and B light cycle reporting
In this paper, we propose that time-of-day is a crucial biological variable across biological disciplines that should be cited in the methods sections of all published papers. It is well-known, and yet well-ignored, that temporal differences in physiology and behavior exist, and we assert that these time-of-day effects should be considered across all biological studies. For example, an anecdote was described how different results were obtained in the same lab examining a transcription factor. The ‘night owl’ postdoc was able to find this protein (albumin site D-binding protein (DBP)) abundant in hepatocytes, whereas a “morning lark” incoming graduate student could not detect DBP [ 127 ]. Similarly, in our lab, we initially reported that balance and motor coordination was unaffected in neuronal nitric oxide synthase (nNOS) knockout mice [ 128 ], which seemed inconsistent with reports that the cerebellum possesses the highest numbers of nNOS neurons in the brain. Our initial behavioral phenotyping study was conducted during the day (between 1400 and 1600; lights on at 0700). However, when locomotor behavior was examined during the dark phase, we observed striking differences in balance and motor coordination among the nNOS mice [ 129 ].
Our analyses in the present paper reveal that information regarding the time-of-day when studies are conducted is routinely omitted from the methods sections of research papers (Fig. 11 ). Our hypothesis that the 2017 Nobel Prize in Medicine and Physiology that highlighted the importance of circadian rhythms would improve appreciation and reporting of temporal variation in biological systems was not supported overall ( χ 2 = 0.86; p > 0.05)(Additional File 1 : Table 1.2). It is possible that 2 years was insufficient for the Nobel prize to have influenced reporting of time-of-day information in high-impact journal articles because such journals typically have longer editorial, review, and revision cycles. One positive note is that within the field of Endocrinology and Metabolism reporting of time-of-day improved from 2015 to 2019 ( χ 2 = 4.89; p < 0.05) (Additional File 1 : Table 1.2). Reporting of lighting conditions (i.e., light–dark cycles) also improved in this field from 2015 to 2019 ( χ 2 = 4.05; p < 0.05) (Additional File 1 : Table 1.3). Importantly, reporting of light–dark cycles also improved across all fields from 2015 to 2019 ( χ 2 = 4.16; p < 0.05) (Additional File 1 : Table 1.3).
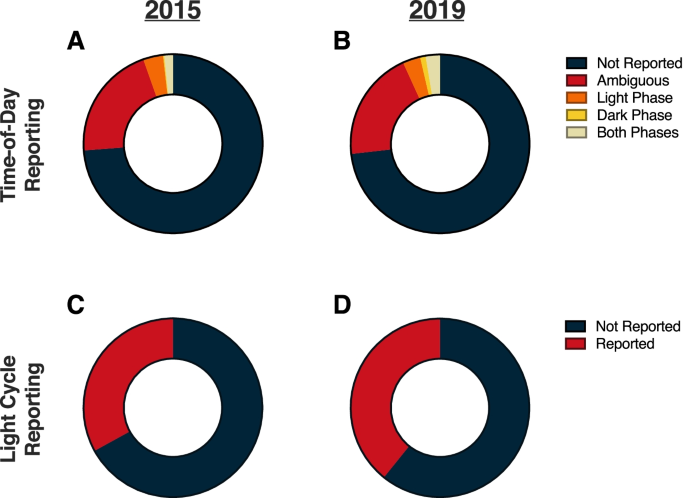
Time-of-day and light cycle reporting across 10 domains of biological sciences. A, B Time-of-day and C, D light cycle reporting in the top 50 papers across all 10 domains in 2015 and 2019. Each donut chart represents a total of 500 articles
We hope that the present analysis motivates improved reporting of time-of-day as an important biological variable in the future. We contend that this critical biological variable is necessary for reproducible, reliable, and transparent reporting of research.
In an effort to improve the reproducibility of biomedical research, journals are increasingly requiring submissions that involve animal research to adhere to minimum standards of methodological reporting. One such set of guidelines, the ARRIVE guidelines, includes information on sample sizes, randomization, blinding, statistical models, and results reporting, along with experimental procedures [ 130 ]. In this latter category, ARRIVE guidelines recommend information on what, how, where, why, and when procedures were performed, specifically that “Methods” sections: “Clearly report the frequency and timing of experimental procedures and measurements, including the light and dark cycle, circadian time cues, and experimental time sequence” [ 130 ]. It appears that very few journals require reporting of time-of-day information. This appears to be a joint failure of journals and reviewers to check that the ARRIVE guidelines are being enforced. We recommend that the ARRIVE guidelines should be enforced regarding time-of-day for experimental methods going forward to improve reliability and replicability.
Again, we assert that time-of-day is a controllable and critical biological factor that should be considered in the design, implementation, and analyses of experimental data. Importantly, time-of-day of animal testing, sample collection, as well as in vitro tests must be tightly controlled and described in detail. In some cases, it may be necessary to test during the light phase. For example, the use of some automated behavioral testing tools often requires animals to be tracked in the light. Nonetheless, to improve time-of-day reporting, details regarding time-of-day, photoperiod, time of testing (either clock time, circadian time, or zeitgeber time), and whether testing occurred during the dark or light should always be reported in every methods section. If testing occurs during the dark, then methods for protecting circadian rhythms such as using dim red lighting or night vision goggles must be described.
In sum, consideration of circadian rhythms across biological studies is critical to enhance experimental rigor and reproducibility and crucial for the interpretation of study results. Life on Earth is adapted to the 24-h solar day and adaptations to temporal niches have shaped virtually all aspects of biology during evolution to increase fitness. Ignoring these temporal influences during the conduct of animal studies influences the collected data, and muddles interpretation. Together, evidence-based decision-making in the timing of data collection, protection against exposure to extraneous light during dark phase testing, incorporation of temporal factors in data analysis and interpretation, and meticulous reporting of temporal factors in publications, have the potential to improve experimental rigor and reproducibility across all fields in biology.
Data sources
Using Web of Science, we examined the top cited 50 papers in several domains of biology for 2015 and 2019 to determine what time-of-day experiments were conducted or whether time of day was reported. These domains comprise: (1) general biology, (2) immunology, (3) neurosciences, (4) physiology, (5) pharmacology and pharmacy, (6) reproductive biology, (7) endocrinology and metabolism, (8) behavioral sciences, (9) oncology, and (10) cardiac and cardiovascular systems. Using Web of Science category domain searches with the results sorted by highest citation counts and examined studies that included living non-human animals in any aspect of the experimental design. Article duplications between the fields were also examined, and were found to be minimal (17 duplicated articles/1000 articles)(Additional File 2 : Fig. 1A-B). We excluded studies that exclusively examined humans, computational studies without animal data, or review papers from our analyses.
Measures and criteria
We recorded the species used in each study, the datapoints collected and reported, the experimental manipulations, and whether the study was in vivo, in vitro, or ex vivo. The principal data abstracted from each report were (1) indication of the circadian phase during which experimental manipulations were performed (i.e., light, dark, both, not reported) and (2) information about the vivarium photocycle under which experimental animals were tested.
Experimental manipulations were coded as being performed in the “light phase,” in the “dark phase,” or in “both phases,” if clear and consistent reporting was available for all procedures; we also noted whether circadian (zeitgeber) time was indicated for procedures. We categorized temporal information as “ambiguous” if clock time was stated without providing the light–dark cycle information or if the information could be inferred, but was not explicitly stated. We also categorized reports as “ambiguous” if circadian phase information was provided for some, but not all, key manipulations/procedures. Examples of “key” manipulations/procedures include behavioral tests, blood sampling, and tissue collection. The widespread practice of merely citing published methods in the description of an experimental manipulation was not regarded as adequate for the purposes of indicating vivarium LD cycle information or time-of-day information.
Vivarium photocycle information was coded as “reported” or “not reported” if such information was provided. If animals were exposed to natural photoperiods, then photocycle information was considered to be available if it could be determined from the latitude and time of year at which the experiment was performed.
Prevalent among the reports surveyed were common behavioral assays of learning and memory (Morris water maze, Barnes test, conditioned place preference, fear conditioning, spontaneous alternation, novel object recognition, avoidance tasks) emotion-like behavior (spontaneous locomotion, open field, elevated plus, tail suspension, marble burying, forced swim, tail suspension, sucrose preference, splash test, social motivation), motor function (limb strength, Rotarod) and attention (pre-pulse inhibition), many of which use bright light as a component of the motivational paradigm, but may also be performed under conditions of darkness. Because these tests may be performed in the light or dark phase of the circadian cycle, and consistent with prior analyses [ 8 ], we classified these tests as “ambiguous” if they failed to report time of testing, provided time of testing for some, but not all tests, or provided the time of testing without linkage to a specific phase of the LD cycle. In addition, many of the top cited papers performed collection of blood, brain, feces, and other types of samples for analyses ex vivo. Because such collections may be readily performed during the light or dark phase, unless information about the phase of collection was available, these procedures were coded as “not reported.”
Finally, although it may be reasonable to assume that many other manipulations and dependent measures were collected during the typical workday, which commonly overlaps with the light phase of the experimental animals, many of these same procedures can also be performed during the dark phase. Moreover, many laboratories house animals in reversed light–dark cycles. Thus, with few exceptions (discussed in the “Results” above), if time-of-day information was not explicitly available, such measures were coded as “not reported.”
Availability of data and materials
All data generated or analyzed during this study are included in this published article and its supplementary information files.
Platt JR. Strong Inference: Certain systematic methods of scientific thinking may produce much more rapid progress than others. Science. 1964;146:347–53.
Article CAS PubMed Google Scholar
Baker M. Reproducibility crisis. Nature. 2016;533:353–66.
CAS Google Scholar
Collins FS, Tabak LA. Policy: NIH plans to enhance reproducibility. Nature News. 2014;505:612.
Article Google Scholar
Lapane KL, Dube CE. Rigor and reproducibility training for first year medical students in research pathways. Clin Transl Sci. 2021;14:1026–36.
Article CAS PubMed PubMed Central Google Scholar
Landis SC, Amara SG, Asadullah K, Austin CP, Blumenstein R, Bradley EW, Crystal RG, Darnell RB, Ferrante RJ, Fillit H. A call for transparent reporting to optimize the predictive value of preclinical research. Nature. 2012;490:187–91.
Lindsey ML, Gray GA, Wood SK, Curran-Everett D. Statistical considerations in reporting cardiovascular research. Am J Physiol Heart Circ. 2018;315:H303–13.
Article CAS Google Scholar
Yosten GLC, Adams JC, Bennett CN, Bunnett NW, Scheman R, Sigmund CD, Yates BJ, Zucker IH, Samson WK. Revised guidelines to enhance the rigor and reproducibility of research published in American Physiological Society journals. Am J Physiol Regul Integr Comp Physiol. 2018;315:R1251–3.
Nelson RJ, Bumgarner JR, Walker WH, DeVries AC. Time-of-day as a critical biological variable. Neurosci Biobehav Rev. 2021;127:740–6.
Article PubMed Google Scholar
Esposito E, Li W, Mandeville ET, Park J-H, Şencan I, Guo S, Shi J, Lan J, Lee J, Hayakawa K. Potential circadian effects on translational failure for neuroprotection. Nature. 2020;582:395–8.
Burns ER. Biological time and in vivo research: a field guide to pitfalls. Anat Rec. 2000;261:141–52.
Dallmann R, Okyar A, Lévi F. Dosing-time makes the poison: circadian regulation and pharmacotherapy. Trends Mol Med. 2016;22:430–45.
Bell-Pedersen D, Cassone VM, Earnest DJ, Golden SS, Hardin PE, Thomas TL, Zoran MJ. Circadian rhythms from multiple oscillators: lessons from diverse organisms. Nat Rev Genet. 2005;6:544–56.
Saini R, Jaskolski M, Davis SJ. Circadian oscillator proteins across the kingdoms of life: structural aspects. BMC Biol. 2019;17:13.
Article PubMed PubMed Central Google Scholar
Huang R-C. The discoveries of molecular mechanisms for the circadian rhythm: The 2017 Nobel Prize in Physiology or Medicine. Biomed J. 2018;41:5–8.
Pittendrigh CS, Bruce VG, Rosensweig NS, Rubin ML. Growth patterns in Neurospora: a biological clock in Neurospora. Nature. 1959;184:169–70.
McClung CR, Fox BA, Dunlap JC. The Neurospora clock gene frequency shares a sequence element with the Drosophila clock gene period. Nature. 1989;339:558–62.
Hurley JM, Dasgupta A, Emerson JM, Zhou X, Ringelberg CS, Knabe N, Lipzen AM, Lindquist EA, Daum CG, Barry KW. Analysis of clock-regulated genes in Neurospora reveals widespread posttranscriptional control of metabolic potential. Proc Natl Acad Sci U S A. 2014;111:16995–7002.
Tan Y, Merrow M, Roenneberg T. Photoperiodism in Neurospora crassa. J Biol Rhythms. 2004;19:135–43.
Grobbelaar N, Huang TC, Lin HY, Chow TJ. Dinitrogen-fixing endogenous rhythm in Synechococcus RF-1. FEMS Microbiol Lett. 1986;37:173–7.
Ishiura M, Kutsuna S, Aoki S, Iwasaki H, Andersson CR, Tanabe A, Golden SS, Johnson CH, Kondo T. Expression of a gene cluster kaiABC as a circadian feedback process in cyanobacteria. Science. 1998;281:1519–23.
Kondo T, Strayer CA, Kulkarni RD, Taylor W, Ishiura M, Golden SS, Johnson CH. Circadian rhythms in prokaryotes: luciferase as a reporter of circadian gene expression in cyanobacteria. Proc Natl Acad Sci U S A. 1993;90:5672–6.
Taton A, Erikson C, Yang Y, Rubin BE, Rifkin SA, Golden JW, Golden SS. The circadian clock and darkness control natural competence in cyanobacteria. Nature Comm. 2020;11:1–11.
Méndez-Ferrer S, Lucas D, Battista M, Frenette PS. Haematopoietic stem cell release is regulated by circadian oscillations. Nature. 2008;452:442–7.
Article PubMed CAS Google Scholar
Scheiermann C, Kunisaki Y, Lucas D, Chow A, Jang J-E, Zhang D, Hashimoto D, Merad M, Frenette PS. Adrenergic nerves govern circadian leukocyte recruitment to tissues. Immunity. 2012;37:290–301.
Deprés-Brummer P, Bourin P, Pages N, Metzger G, Lévi F. Persistent T lymphocyte rhythms despite suppressed circadian clock outputs in rats. Am J Physiol Regul Integr Comp Physiol. 1997;273:R1891–9.
Dhabhar FS, Miller AH, Stein M, McEwen BS, Spencer RL. Diurnal and acute stress-induced changes in distribution of peripheral blood leukocyte subpopulations. Brain Behav Immun. 1994;8:66–79.
Born J, Lange T, Hansen K, Mölle M, Fehm H-L. Effects of sleep and circadian rhythm on human circulating immune cells. J Immunol. 1997;158:4454–64.
CAS PubMed Google Scholar
Dimitrov S, Benedict C, Heutling D, Westermann J, Born J, Lange T. Cortisol and epinephrine control opposing circadian rhythms in T cell subsets. Blood. 2009;113:5134–43.
Deng W, Zhu S, Zeng L, Liu J, Kang R, Yang M, Cao L, Wang H, Billiar TR, Jiang J. The circadian clock controls immune checkpoint pathway in sepsis. Cell Rep. 2018;24:366–78.
Feigin RD, Middelkamp JN, Reed C. Circadian rhythmicity in susceptibility of mice to sublethal Coxsackie B3 infection. Nature New Biol. 1972;240:57–8.
Scheiermann C, Gibbs J, Ince L, Loudon A. Clocking in to immunity. Nat Rev Immunol. 2018;18:423–37.
Sengupta S, Tang SY, Devine JC, Anderson ST, Nayak S, Zhang SL, Valenzuela A, Fisher DG, Grant GR, López CB. Circadian control of lung inflammation in influenza infection. Nature Comm. 2019;10:4107.
Pownall R, Kabler PA, Knapp MS. The time of day of antigen encounter influences the magnitude of the immune response. Clin Exp Immunol. 1979;36:347–54.
CAS PubMed PubMed Central Google Scholar
Fortier EE, Rooney J, Dardente H, Hardy M-P, Labrecque N, Cermakian N. Circadian variation of the response of T cells to antigen. J Immunol. 2011;187:6291–300.
Haspel JA, Anafi R, Brown MK, Cermakian N, Depner C, Desplats P, Gelman AE, Haack M, Jelic S, Kim BS. Perfect timing: circadian rhythms, sleep, and immunity—an NIH workshop summary. JCI insight. 2020;5: e131487.
Article PubMed Central Google Scholar
Balsalobre A, Damiola F, Schibler U. A serum shock induces circadian gene expression in mammalian tissue culture cells. Cell. 1998;93:929–37.
Garbarino-Pico E, Niu S, Rollag MD, Strayer CA, Besharse JC, Green CB. Immediate early response of the circadian polyA ribonuclease nocturnin to two extracellular stimuli. RNA. 2007;13:745–55.
Nagoshi E, Brown SA, Dibner C, Kornmann B, Schibler U. Circadian gene expression in cultured cells. Methods Enzymol. 2005;393:543–57.
Brown SA, Zumbrunn G, Fleury-Olela F, Preitner N, Schibler U. Rhythms of mammalian body temperature can sustain peripheral circadian clocks. Curr Biol. 2002;12:1574–83.
Gilestro GF, Tononi G, Cirelli C. Widespread changes in synaptic markers as a function of sleep and wakefulness in Drosophila. Science. 2009;324:109–12.
Liu ZW, Faraguna U, Cirelli C, Tononi G, Gao XB. Direct evidence for wake-related increases and sleep-related decreases in synaptic strength in rodent cortex. J Neurosci. 2010;30:8671–5.
Schmidt C, Collette F, Cajochen C, Peigneux P. A time to think: circadian rhythms in human cognition. Cogn Neuropsychol. 2007;24:755–89.
Smarr BL, Jennings KJ, Driscoll JR, Kriegsfeld LJ. A time to remember: the role of circadian clocks in learning and memory. Behav Neurosci. 2014;128:283–303.
Fisk AS, Tam SKE, Brown LA, Vyazovskiy VV, Bannerman DM, Peirson SN. Light and Cognition: Roles for Circadian Rhythms, Sleep, and Arousal. Front Neurol. 2018;9:56.
Leng Y, Musiek ES, Hu K, Cappuccio FP, Yaffe K. Association between circadian rhythms and neurodegenerative diseases. Lancet Neurol. 2019;18:307–18.
Walker WH, Walton JC, DeVries AC, Nelson RJ. Circadian rhythm disruption and mental health. Transl Psychiatry. 2020;10:28.
Videnovic A, Zee PC. Consequences of Circadian Disruption on Neurologic Health. Sleep Med Clin. 2015;10:469–80.
Gritton HJ, Kantorowski A, Sarter M, Lee TM. Bidirectional interactions between circadian entrainment and cognitive performance. Learn Mem. 2012;19:126–41.
Rusak B, Zucker I. Neural regulation of circadian rhythms. Physiol Rev. 1979;59:449–526.
Rosbash M. Circadian Rhythms and the Transcriptional Feedback Loop (Nobel Lecture). Angew Chem Int Ed. 2021;60:8650–66.
Keller M, Mazuch J, Abraham U, Eom GD, Herzog ED, Volk H-D, Kramer A, Maier B. A circadian clock in macrophages controls inflammatory immune responses. Proc Natl Acad Sci U S A. 2009;106:21407–12.
Scheiermann C, Kunisaki Y, Frenette PS. Circadian control of the immune system. Nat Rev Immunol. 2013;13:190–8.
Man K, Loudon A, Chawla A. Immunity around the clock. Science. 2016;354:999–1003.
Silver AC, Arjona A, Walker WE, Fikrig E. The circadian clock controls toll-like receptor 9-mediated innate and adaptive immunity. Immunity. 2012;36:251–61.
Qian J, Scheer FAJL. Circadian system and glucose metabolism: implications for physiology and disease. Trends Endocrinol Metab. 2016;27:282–93.
Rana S, Prabhu SD, Young ME. Chronobiological influence over cardiovascular function: the good, the bad, and the ugly. Circ Res. 2020;126:258–79.
Bray MS, Shaw CA, Moore MWS, Garcia RAP, Zanquetta MM, Durgan DJ, Jeong WJ, Tsai J-Y, Bugger H, Zhang D. Disruption of the circadian clock within the cardiomyocyte influences myocardial contractile function, metabolism, and gene expression. Am J Physiol Heart Circ. 2008;294:H1036–47.
Muller JE, Stone PH, Turi ZG, Rutherford JD, Czeisler CA, Parker C, Poole WK, Passamani E, Roberts R, Robertson T. Circadian variation in the frequency of onset of acute myocardial infarction. N Engl J Med. 1985;313:1315–22.
Suárez-Barrientos A, López-Romero P, Vivas D, Castro-Ferreira F, Núñez-Gil I, Franco E, Ruiz-Mateos B, García-Rubira JC, Fernández-Ortiz A, Macaya C. Circadian variations of infarct size in acute myocardial infarction. Heart. 2011;97:970–6.
Schloss MJ, Horckmans M, Nitz K, Duchene J, Drechsler M, Bidzhekov K, Scheiermann C, Weber C, Soehnlein O, Steffens S. The time-of-day of myocardial infarction onset affects healing through oscillations in cardiac neutrophil recruitment. EMBO Mol Med. 2016;8:937–48.
Thaiss CA, Zeevi D, Levy M, Zilberman-Schapira G, Suez J, Tengeler AC, Abramson L, Katz MN, Korem T, Zmora N. Transkingdom control of microbiota diurnal oscillations promotes metabolic homeostasis. Cell. 2014;159:514–29.
Frazier K, Chang EB. Intersection of the gut microbiome and circadian rhythms in metabolism. Trends Endocrinol Metab. 2020;31:25–36.
Arendt J, Skene DJ. Melatonin as a chronobiotic. Sleep Med Rev. 2005;9:25–39.
Tamarkin L, Westrom WK, Hamill AI, Goldman BD. Effect of melatonin on the reproductive systems of male and female Syrian hamsters: a diurnal rhythm in sensitivity to melatonin. Endocrinology. 1976;99:1534–41.
Cardoson SS, Scheving LE, Halberg F. Mortality of Mice as Influenced by Hour of Day of Drug (ARA-C) Administration. Pharmacologist. 1970;12:302.
Google Scholar
Haus E, Halberg F, Scheving LE, Pauly JE, Cardoso S, Kühl JFW, Sothern RB, Shiotsuka RN, Hwang DS. Increased tolerance of leukemic mice to arabinosyl cytosine with schedule adjusted to circadian system. Science. 1972;177:80–2.
Walton JC, Walker WH, Bumgarner JR, Meléndez-Fernández OH, Liu JA, Hughes HL, Kaper AL, Nelson RJ. Circadian variation in efficacy of medications. Clin Pharmacol Ther. 2021;109:1457–88.
Ayyar VS, Sukumaran S. Circadian rhythms: influence on physiology, pharacology, and therpeutic interventions. J Pharmacokinet Pharacodyn. 2021;48:321–8.
Dong D, Yang D, Lin L, Wang S, Wu B. Circadian rhythm in pharmacokinetics and its relevance to chronotherapy. Biochem Pharmacol. 2020;178:114045.
Bron R, Furness JB. Rhythm of digestion: keeping time in the gastrointestinal tract. Clin Exp Pharmacol P. 2009;36:1041–8.
Konturek PC, Brzozowski T, Konturek SJ. Gut clock: implication of circadian rhythms in the gastrointestinal tract. J Physiol Pharmacol. 2011;62:139–50.
Stearns AT, Balakrishnan A, Rhoads DB, Ashley SW, Tavakkolizadeh A. Diurnal rhythmicity in the transcription of jejunal drug transporters. J Pharmacol Sci. 2008;108:144–8.
Yu F, Zhang T, Zhou C, Xu H, Guo L, Chen M, Wu B. The circadian clock gene Bmal1 controls intestinal exporter MRP2 and drug disposition. Theranostics. 2019;9:2754.
Zhang Y-KJ, Yeager RL, Klaassen CD. Circadian expression profiles of drug-processing genes and transcription factors in mouse liver. Drug Metab Dispos. 2009;37:106–15.
Conroy DA, Spielman AJ, Scott RQ. Daily rhythm of cerebral blood flow velocity. J Circadian Rhythms. 2005;3:1–11.
Delp MD, Manning RO, Bruckner JV, Armstrong RB. Distribution of cardiac output during diurnal changes of activity in rats. Am J Physiol Heart Circ. 1991;261:H1487–93.
Lemmer B, Nold G. Circadian changes in estimated hepatic blood flow in healthy subjects. Br J Clin Pharmacol. 1991;32:627–9.
Firsov D, Bonny O. Circadian rhythms and the kidney. Nat Rev Nephrol. 2018;14:626–35.
Stow LR, Gumz ML. The circadian clock in the kidney. J Am Soc Nephrol. 2011;22:598–604.
Zhang WX, Chen SY, Liu C. Regulation of reproduction by the circadian rhythms. Sheng Li Xue Bao. 2016;68:799–808.
PubMed Google Scholar
Karman BN, Tischkau SA. Circadian clock gene expression in the ovary: effects of luteinizing hormone. Biol Reprod. 2006;75:624–32.
Fahrenkrug J, Georg B, Hannibal J, Hindersson P, Gräs S. Diurnal rhythmicity of the clock genes Per1 and Per2 in the rat ovary. Endocrinology. 2006;147:3769–76.
Alvarez JD, Hansen A, Ord T, Bebas P, Chappell PE, Giebultowicz JM, Williams C, Moss S, Sehgal A. The circadian clock protein BMAL1 is necessary for fertility and proper testosterone production in mice. J Biol Rhythms. 2008;23:26–36.
Sellix MT, Yoshikawa T, Menaker M. A circadian egg timer gates ovulation. Curr Biol. 2010;20(6):R266-7.
Garel M, Solberg EJ, Saether BE, Grøtan V, Tufto J, Heim M. Age, size, and spatiotemporal variation in ovulation patterns of a seasonal breeder, the Norwegian moose (Alces alces). Am Nat. 2009;173:89–104.
Brewster JE, Cole CL. The Time of Ovulation in Cattle. Int J Dairy Sci. 1941;24:111–5.
Sontakke SD. Monitoring and controlling ovarian activities in wild ungulates. Theriogenology. 2018;109:31–41.
Hacker-Klom UB. Diurnal changes in murine spermatogenesis. Z Naturforsch C. 1994;49(7–8):522–5.
Rienstein S, Dotan A, Avivi L, Ashkenazi I. Daily rhythms in male mice meiosis. Chronobiol Int. 1998;15:13–20.
Hatcher KM, Mahoney MM. Circadian Rhythms—Male. Encyclopedia of Reproduction. Oxford: Elsevier; 2018. p. 436–41.
Kumar S, Deb R, Singh U, Ganguly I, Mandal DK, Tyagi S, Kumar M, Sengar G, Sharma S, Singh R, Singh R. Bovine Circadian Locomotor Output Cycles Kaput (CLOCK) and Clusterin (CLU) mRNA Quantitation in Ejaculated Crossbred Bull Spermatozoa. Reprod Domest Anim. 2015;50:505–9.
Garey J, Kow LM, Huynh W, Ogawa S, Pfaff DW. Temporal and spatial quantitation of nesting and mating behaviors among mice housed in a semi-natural environment. Horm Behav. 2002;42(3):294–306.
Challet E. The circadian regulation of food intake. Nat Rev Endocrinol. 2019;15:393–405.
Sun Z, Feng D, Everett LJ, Bugge A, Lazar MA. Circadian epigenomic remodeling and hepatic lipogenesis: lessions from HDAC3. Cold Spring Harbor symposia on quantitative biology. Cold Spring Harbor Laboratory Press; 2011:76.
Doi R, Oishi K, Ishida N. CLOCK regulates circadian rhythms of hepatic glycogen synthesis through transcriptional activation of Gys2. J Biol Chem. 2010;285:22114–21.
Johnston JD, Ordovás JM, Scheer FA, Turek FW. Circadian rhythms, metabolism, and chrononutrition in rodents and humans. Adv Nutr. 2016;7:399–406.
Rutter J, Reick M, McKnight SL. Metabolism and the control of circadian rhythms. Annu Rev Biochem. 2002;71:307–31.
Gamble KL, Berry R, Frank SJ, Young ME. Circadian clock control of endocrine factors. Nat Rev Endocrinol. 2014;10:466–75.
Kriegsfeld LJ, LeSauter J, Hamada T, Pitts SM, Silver R. Circadian rhythms in the endocrine system. In: Pfaff DW, Arnold AP, Fahrbach SE, Etgen AM, RT R, editors. Hormones, Brain and Behavior. Cambridge: Elsevier; 2002. p. 33–91.
Chapter Google Scholar
Stephan FK, Zucker I. Circadian rhythms in drinking behavior and locomotor activity of rats are eliminated by hypothalamic lesions. Proc Natl Acad Sci U S A. 1972;69:1583–6.
Siepka SM, Yoo SH, Park J, Lee C, Takahashi JS. Genetics and neurobiology of circadian clocks in mammals. Cold Spring Harb Symp Quant Biol. 2007;72:251–9.
Farshadi E, van der Horst GTJ, Chaves I. Molecular Links between the Circadian Clock and the Cell Cycle. J Mol Biol. 2020;432:3515–24.
Yan J, Goldbeter A. Robust synchronization of the cell cycle and the circadian clock through bidirectional coupling. J R Soc Interface. 2019;16:20190376.
Feillet C, Krusche P, Tamanini F, Janssens RC, Downey MJ, Martin P, Teboul M, Saito S, Lévi FA, Bretschneider T, van der Horst GT, Delaunay F, Rand DA. Phase locking and multiple oscillating attractors for the coupled mammalian clock and cell cycle. Proc Natl Acad Sci U S A. 2014;111:9828–33.
Walker WH, Bumgarner JR, Walton JC, Liu JA, Meléndez-Fernández OH, Nelson RJ, DeVries AC. Light Pollution and Cancer. Int J Mol Sci. 2020;21:9360.
Article CAS PubMed Central Google Scholar
Zhu X, Suo Y, Fu Y, Zhang F, Ding N, Pang K, Xie C, Weng X, Tian M, He H, Wei X. In vivo flow cytometry reveals a circadian rhythm of circulating tumor cells. Light Sci Appl. 2021;10:110.
Hrushesky WJ, Lester B, Lannin D. Circadian coordination of cancer growth and metastatic spread. Int J Cancer. 1999;83:365–73.
Binnewies M, Roberts EW, Kersten K, Chan V, Fearon DF, Merad M, Coussens LM, Gabrilovich DI, Ostrand-Rosenberg S, Hedrick CC, Vonderheide RH, Pittet MJ, Jain RK, Zou W, Howcroft TK, Woodhouse EC, Weinberg RA, Krummel MF. Understanding the tumor immune microenvironment (TIME) for effective therapy. Nat Med. 2018;24:541–50.
Agarwal R. Regulation of circadian blood pressure: from mice to astronauts. Curr Opin Nephrol Hypertens. 2010;19:51–8.
Li P, Sur SH, Mistlberger RE, Morris M. Circadian blood pressure and heart rate rhythms in mice. Am J Physiol. 1999;276:R500–4.
Bastianini S, Silvani A, Berteotti C, Lo Martire V, Zoccoli G. Mice show circadian rhythms of blood pressure during each wake-sleep state. Chronobiol Int. 2012;29:82–6.
Smolensky MH, Hermida RC, Castriotta RJ, Portaluppi F. Role of sleep-wake cycle on blood pressure circadian rhythms and hypertension. Sleep Med. 2007;8:668–80.
Meléndez-Fernández OH, Walton JC, DeVries AC, Nelson RJ. Clocks, Rhythms, Sex, and Hearts: How Disrupted Circadian Rhythms, Time-of-Day, and Sex Influence Cardiovascular Health. Biomolecules. 2021;11:883.
Article PubMed PubMed Central CAS Google Scholar
Khan MS, Ahmad SI. Circadian variation-increased morning incidence of acute myocardial infarction in patients with coronary artery disease. J Pak Med Assoc. 2003;53(2):84–7.
Manfredini R, Boari B, Salmi R, Fabbian F, Pala M, Tiseo R, Portaluppi F. Twenty-four-hour patterns in occurrence and pathophysiology of acute cardiovascular events and ischemic heart disease. Chronobiol Int. 2013;30:6–16.
Muller JE, Ludmer PL, Willich SN, Tofler GH, Aylmer G, Klangos I, Stone PH. Circadian variation in the frequency of sudden cardiac death. Circulation. 1987;75:131–8.
Muller JE. Circadian variation and triggering of acute coronary events. Am Heart J. 1999;137:S1–8.
Bridges AB, McLaren M, Saniabadi A, Fisher TC, Belch JJ. Circadian variation of endothelial cell function, red blood cell deformability and dehydro-thromboxane B2 in healthy volunteers. Blood Coagul Fibrinolysis. 1991;2:447–52.
Etsuda H, Takase B, Uehata A, Kusano H, Hamabe A, Kuhara R, Akima T, Matsushima Y, Arakawa K, Satomura K, Kurita A, Ohsuzu F. Morning attenuation of endothelium-dependent, flow-mediated dilation in healthy young men: possible connection to morning peak of cardiac events. Clin Cardiol. 1999;22:417–21.
Takeda N, Maemura K, Horie S, Oishi K, Imai Y, Harada T, Saito T, Shiga T, Amiya E, Manabe I, Ishida N, Nagai R. Thrombomodulin is a clock-controlled gene in vascular endothelial cells. J Biol Chem. 2007;282:32561–7.
Dalby MC, Davidson SJ, Burman JF, Davies SW. Diurnal variation in platelet aggregation with the PFA-100 platelet function analyser. Platelets. 2000;11:320–4.
Curtis AM, Bellet MM, Sassone-Corsi P, O’Neill LA. Circadian clock proteins and immunity. Immunity. 2014;40:178–86.
Davidson AJ, London B, Block GD, Menaker M. Cardiovascular tissues contain independent circadian clocks. Clin Exp Hypertens. 2005;27:307–11.
Storch KF, Lipan O, Leykin I, Viswanathan N, Davis FC, Wong WH, Weitz CJ. Extensive and divergent circadian gene expression in liver and heart. Nature. 2002;417:78–83.
Keskil Z, Görgün CZ, Hodoğlugil U, Zengil H. Twenty-four-hour variations in the sensitivity of rat aorta to vasoactive agents. Chronobiol Int. 1996;13:465–75.
Tunçtan B, Weigl Y, Dotan A, Peleg L, Zengil H, Ashkenazi I, Abacioğlu N. Circadian variation of nitric oxide synthase activity in mouse tissue. Chronobiol Int. 2002;19:393–404.
Schibler U. The 2008 Pittendrigh/Aschoff lecture: peripheral phase coordination in the mammalian circadian timing system. J Biol Rhythms. 2009;24:3–15.
Nelson RJ, Demas GE, Huang PL, Fishman MC, Dawson VL, Dawson TM, Snyder SH. Behavioural abnormalities in male mice lacking neuronal nitric oxide synthase. Nature. 1995;378:383–6.
Kriegsfeld LJ, Eliasson MJL, Demas GE, Blackshaw S, Dawson TM, Nelson RJ, Snyder SH. Nocturnal motor coordination deficits in neuronal nitric oxide synthase knock-out mice. Neuroscience. 1999;89:311–5.
Percie du Sert N, Hurst V, Ahluwalia A, Alam S, Avey MT, Baker M, Browne WJ, Clark A, Cuthill IC, Dirnagl U, Emerson M, Garner P, Holgate ST, Howells DW, Karp NA, Lazic SE, Lidster K, MacCallum CJ, Macleod M, Pearl EJ, Petersen OH, Rawle F, Reynolds P, Rooney K, Sena ES, Silberberg SD, Steckler T, Würbel H. The ARRIVE guidelines 2.0: Updated guidelines for reporting animal research. J Cereb Blood Flow Metab. 2020;40:1769–77.
Download references
Acknowledgements
We thank Alexandra Richmond for assistance with references and Madelyn Brodie for guidance with figure production.
Preparation of this manuscript was supported by NIH Grants R01NS092388, R01CA194924, R21AT01128, and U54GM104942. O.H.M.F was supported by NIH NIGMS T32 GM132494. The content is solely the responsibility of the authors and does not necessarily represent the official views of the US National Institutes of Health.
Author information
Authors and affiliations.
Department of Neuroscience, Rockefeller Neuroscience Institute, West Virginia University, Morgantown, WV, 26505, USA
Randy J. Nelson, Jacob R. Bumgarner, Jennifer A. Liu, O. Hecmarie Meléndez-Fernández, Darius D. Becker-Krail, William H. Walker II, James C. Walton & A. Courtney DeVries
Department of Psychology, University of Chicago and Institute for Mind and Biology, IL, 60637, Chicago, USA
Jharnae A. Love & Brian J. Prendergast
Department of Medicine, Rockefeller Neuroscience Institute, West Virginia University, Morgantown, WV, 26505, USA
A. Courtney DeVries
You can also search for this author in PubMed Google Scholar
Contributions
RJN, JRB, and BJP conceived the study. All authors have contributed equally to the review and analysis of the various results sections within the review. JRB and DBK helped with graphics. All have corrected and modified the different versions of the manuscript as prepared by the corresponding and senior authors. All authors read and approved the final manuscript.
Corresponding author
Correspondence to Randy J. Nelson .
Ethics declarations
Ethics approval and consent to participate.
Not applicable.
Consent for publication
Competing interests.
The authors declare that they have no competing interests.
Additional information
Publisher’s note.
Springer Nature remains neutral with regard to jurisdictional claims in published maps and institutional affiliations.
Supplementary Information
Additional file 1., additional file 2., rights and permissions.
Open Access This article is licensed under a Creative Commons Attribution 4.0 International License, which permits use, sharing, adaptation, distribution and reproduction in any medium or format, as long as you give appropriate credit to the original author(s) and the source, provide a link to the Creative Commons licence, and indicate if changes were made. The images or other third party material in this article are included in the article's Creative Commons licence, unless indicated otherwise in a credit line to the material. If material is not included in the article's Creative Commons licence and your intended use is not permitted by statutory regulation or exceeds the permitted use, you will need to obtain permission directly from the copyright holder. To view a copy of this licence, visit http://creativecommons.org/licenses/by/4.0/ . The Creative Commons Public Domain Dedication waiver ( http://creativecommons.org/publicdomain/zero/1.0/ ) applies to the data made available in this article, unless otherwise stated in a credit line to the data.
Reprints and permissions
About this article
Cite this article.
Nelson, R.J., Bumgarner, J.R., Liu, J.A. et al. Time of day as a critical variable in biology. BMC Biol 20 , 142 (2022). https://doi.org/10.1186/s12915-022-01333-z
Download citation
Received : 21 December 2021
Accepted : 17 May 2022
Published : 15 June 2022
DOI : https://doi.org/10.1186/s12915-022-01333-z
Share this article
Anyone you share the following link with will be able to read this content:
Sorry, a shareable link is not currently available for this article.
Provided by the Springer Nature SharedIt content-sharing initiative
- Circadian rhythms
- Time of day
- Pharmacology
BMC Biology
ISSN: 1741-7007
- Submission enquiries: [email protected]
- General enquiries: [email protected]
- Open access
- Published: 17 April 2024
A data-driven combined prediction method for the demand for intensive care unit healthcare resources in public health emergencies
- Weiwei Zhang 1 &
- Xinchun Li 1
BMC Health Services Research volume 24 , Article number: 477 ( 2024 ) Cite this article
228 Accesses
1 Altmetric
Metrics details
Public health emergencies are characterized by uncertainty, rapid transmission, a large number of cases, a high rate of critical illness, and a high case fatality rate. The intensive care unit (ICU) is the “last line of defense” for saving lives. And ICU resources play a critical role in the treatment of critical illness and combating public health emergencies.
This study estimates the demand for ICU healthcare resources based on an accurate prediction of the surge in the number of critically ill patients in the short term. The aim is to provide hospitals with a basis for scientific decision-making, to improve rescue efficiency, and to avoid excessive costs due to overly large resource reserves.
A demand forecasting method for ICU healthcare resources is proposed based on the number of current confirmed cases. The number of current confirmed cases is estimated using a bilateral long-short-term memory and genetic algorithm support vector regression (BILSTM-GASVR) combined prediction model. Based on this, this paper constructs demand forecasting models for ICU healthcare workers and healthcare material resources to more accurately understand the patterns of changes in the demand for ICU healthcare resources and more precisely meet the treatment needs of critically ill patients.
Data on the number of COVID-19-infected cases in Shanghai between January 20, 2020, and September 24, 2022, is used to perform a numerical example analysis. Compared to individual prediction models (GASVR, LSTM, BILSTM and Informer), the combined prediction model BILSTM-GASVR produced results that are closer to the real values. The demand forecasting results for ICU healthcare resources showed that the first (ICU human resources) and third (medical equipment resources) categories did not require replenishment during the early stages but experienced a lag in replenishment when shortages occurred during the peak period. The second category (drug resources) is consumed rapidly in the early stages and required earlier replenishment, but replenishment is timelier compared to the first and third categories. However, replenishment is needed throughout the course of the epidemic.
The first category of resources (human resources) requires long-term planning and the deployment of emergency expansion measures. The second category of resources (drugs) is suitable for the combination of dynamic physical reserves in healthcare institutions with the production capacity reserves of corporations. The third category of resources (medical equipment) is more dependent on the physical reserves in healthcare institutions, but care must be taken to strike a balance between normalcy and emergencies.
Peer Review reports
Introduction
The outbreak of severe acute respiratory syndrome (SARS) in 2003 was the first global public health emergency of the 21st century. From SARS to the coronavirus disease (COVID-19) pandemic at the end of 2019, followed shortly by the monkeypox epidemic of 2022, the global community has witnessed eight major public health events within the span of only 20 years [ 1 ]. These events are all characterized by high infection and fatality rates. For example, the number of confirmed COVID-19 cases worldwide is over 700 million, and the number of deaths has exceeded 7 million [ 2 ]. Every major public health emergency typically consists of four stages: incubation, outbreak, peak, and decline. During the outbreak and transmission, surges in the number of infected individuals and the number of critically ill patients led to a corresponding increase in the urgent demand for intensive care unit (ICU) medical resources. ICU healthcare resources provide material security for rescue work during major public health events as they allow critically ill patients to be treated, which decreases the case fatality rate and facilitates the prevention and control of epidemics. Nevertheless, in actual cases of prevention and control, the surge in patients has often led to shortages of ICU healthcare resources and a short-term mismatch of supply and demand, which are problems that have occurred several times in different regions. These issues can drastically impact anti-epidemic frontline healthcare workers and the treatment outcomes of infected patients. According to COVID-19 data from recent years, many infected individuals take about two weeks to progress from mild to severe disease. As the peak of severe cases tends to lag behind that of infected cases, predicting the changes in the number of new infections can serve as a valuable reference for healthcare institutions in forecasting the demand for ICU healthcare resources. The accurate forecasting of the demand for ICU healthcare resources can facilitate the rational resource allocation of hospitals under changes in demand patterns, which is crucial for improving the provision of critical care and rescue efficiency. Therefore, in this study, we combined a support vector regression (SVR) prediction model optimized by a genetic algorithm (GA) with bidirectional long-short-term memory (BILSTM), with the aim of enhancing the dynamic and accurate prediction of the number of current confirmed cases. Based on this, we forecasted the demand for ICU healthcare resources, which in turn may enable more efficient resource deployment during severe epidemic outbreaks and improve the precise supply of ICU healthcare resources.
Research on the demand forecasting of emergency materials generally employs quantitative methods, and traditional approaches mainly include linear regression and GM (1,1). Linear regression involves the use of regression equations to make predictions based on data. Sui et al. proposed a method based on multiple regression that aimed to predict the demand for emergency supplies in the power grid system following natural disasters [ 3 ]. Historical data was used to obtain the impact coefficient of each factor on emergency resource forecasting, enabling the quick calculation of the demand for each emergency resource during a given type of disaster. However, to ensure prediction accuracy, regression analysis needs to be supported by data from a large sample size. Other researchers have carried out demand forecasting for emergency supplies from the perspective of grey prediction models. Li et al. calculated the development coefficient and grey action of the grey GM (1,1) model using the particle swarm optimization algorithm to minimize the relative errors between the real and predicted values [ 4 ]. Although these studies have improved the prediction accuracy of grey models, they mainly involve pre-processing the initial data series without considering the issue of the excessively fast increase in predicted values by traditional grey GM (1,1) models. In emergency situations, the excessively fast increase in predicted values compared to real values will result in the consumption of a large number of unnecessary resources, thereby decreasing efficiency and increasing costs. As traditional demand forecasting models for emergency supplies have relatively poor perfect order rates in demand analysis, which result in low prediction accuracy, they are not mainstream.
At present, dynamic models of infectious diseases and demand forecasting models based on machine learning are at the cutting edge of research. With regard to the dynamic models of infectious diseases, susceptible infected recovered model (SIR) is a classic mathematical model employed by researchers [ 5 , 6 , 7 ]. After many years of development, the SIR model has been expanded into various forms within the field of disease transmission, including susceptible exposed infected recovered model (SEIR) and susceptible exposed infected recovered dead model (SEIRD) [ 8 , 9 ]. Nevertheless, with the outbreak of COVID-19, dynamic models of infectious diseases have once again come under the spotlight, with researchers combining individual and group variables and accounting for different factors to improve the initial models and reflect the state of COVID-19 [ 10 , 11 , 12 , 13 ]. Based on the first round of epidemic data from Wuhan, Li et al. predicted the time-delay distributions, epidemic doubling time, and basic reproductive number [ 14 ]. Upon discovering the presence of asymptomatic COVID-19 infections, researchers began constructing different SEIR models that considered the infectivity of various viral incubation periods, yielding their respective predictions of the inflection point. Based on this, Anggriani et al. further considered the impact of the status of infected individuals and established a transmission model with seven compartments [ 15 ]. Efimov et al. set the model parameters for separating the recovered and the dead as uncertain and applied the improved SEIR model to analyze the transmission trend of the pandemic [ 16 ]. In addition to analyzing the transmission characteristics of normal COVID-19 infection to predict the status of the epidemic, many researchers have also used infectious disease models to evaluate the effects of various epidemic preventive measures. Lin et al. applied an SEIR model that considered individual behavioral responses, government restrictions on public gatherings, pet-related transmission, and short-term population movements [ 17 ]. Cao et al. considered the containment effect of isolation measures on the pandemic and solved the model using Euler’s numerical method [ 18 ]. Reiner et al. employed an improved SEIR model to study the impact of non-pharmaceutical interventions implemented by the government (e.g., restricting population movement, enhancing disease testing, and increasing mask use) on disease transmission and evaluated the effectiveness of social distancing and the closure of public spaces [ 19 ]. These studies have mainly focused on modeling the COVID-19 pandemic to perform dynamic forecasting and analyze the effectiveness of control measures during the epidemic. Infectious disease dynamics offer good predictions for the early transmission trends of epidemics. However, this approach is unable to accurately estimate the spread of the virus in open-flow environments. Furthermore, it is also impossible to set hypothetical parameters, such as disease transmissibility and the recovery probability constant, that are consistent with the conditions in reality. Hence, with the increase in COVID-19 data, this approach has become inadequate for the accurate long-term analysis of epidemic trends.
Machine learning has shown significant advantages in this regard [ 20 , 21 ]. Some researchers have adopted the classic case-based reasoning approach in machine learning to make predictions. However, it is not feasible to find historical cases that fully match the current emergency event, so this approach has limited operability. Other researchers have also employed neural network training in machine learning to make predictions. For example, Hamou et al. predicted the number of injuries and deaths, which in turn were used to forecast the demand for emergency supplies [ 22 ]. However, this approach requires a large initial dataset and a high number of training epochs, while uncertainty due to large changes in intelligence information can lead to significant errors in data prediction [ 23 , 24 , 25 ]. To address these problems, researchers have conducted investigations that account (to varying degrees) for data characterized by time-series and non-linearity and have employed time-series models with good non-linear fitting [ 26 , 27 , 28 ]. The use of LSTM to explore relationships within the data can improve the accuracy of predicting COVID-19 to some extent. However, there are two problems with this approach. First, LSTM neural networks require extremely large datasets, and each wave of the epidemic development cycle would be insufficient to support a dataset suitable for LSTM. Second, neural networks involve a large number of parameters and highly complex models and, hence, are susceptible to overfitting, which can prevent them from achieving their true and expected advantages in prediction.
Overall, Our study differs from other papers in the following three ways. First, the research object of this paper focuses on the specific point of ICU healthcare resource demand prediction, aiming to improve the rate of critical care patient treatment. However, past research on public health emergencies has focused more on resource prediction , such as N95 masks, vaccines, and generalized medical supplies during the epidemic , to mitigate the impact of rapid transmission and high morbidity rates. This has led to less attention being paid to the reality of the surge in critically ill patients due to their high rates of severe illness and mortality.
Second, the idea of this paper is to further forecast resource needs based on the projected number of people with confirmed diagnoses, which is more applicable to healthcare organizations than most other papers that only predict the number of people involved. However, in terms of the methodology for projecting the number of people, this paper adopts a combined prediction method that combines regression algorithms and recurrent neural networks to propose a BILSTM-GASVR prediction model for the number of confirmed diagnoses. It capitalizes on both the suitability of SVR for small samples and non-linear prediction as well as the learning and memory abilities of BILSTM in processing time-series data. On the basis of the prediction model for the number of infected cases, by considering the characteristics of ICU healthcare resources, we constructed a demand forecasting model of emergency healthcare supplies. Past public health emergencies are more likely to use infectious disease models or a single prediction model in deep learning. some of the articles, although using a combination of prediction, but also more for the same method domain combination, such as CNN-LSTM, GRU-LSTM, etc., which are all recurrent neural networks.
Third, in terms of specific categorization of resources to be forecasted, considering the specificity of ICU medical resources, we introduce human resource prediction on the basis of previous studies focusing on material security, and classified ICU medical resources into three categories: ICU human resources, drugs and medical equipment. The purpose of this classification is to match the real-life prediction scenarios of public health emergencies and improve the demand forecasting performance for local ICU healthcare resources. Thus, it is easy for healthcare institutions to grasp the overall development of events, optimizing decision-making, and reducing the risk of healthcare systems collapsing during the outbreak stage.
In this section, we accomplish the following two tasks. Firstly, we introduce the idea of predicting the number of infected cases and show the principle of the relevant models. Secondly, based on the number of infected cases, ICU healthcare resources are divided into two categories (healthcare workers and healthcare supplies), and their respective demand forecasting models are constructed.
Prediction model for the number of infected cases
Gasvr model.
Support vector machine (SVM) is a machine-learning language for classification developed by Vapnik [ 29 ]. Suppose there are two categories of samples: H1 and H2. If hyperplane H is able to correctly classify the samples into these two categories and maximize the margin between the two categories, it is known as the optimal separating hyperplane (OSH). The sample vectors closest to the OSH in H1 and H2 are known as the support vectors. To apply SVM to prediction, it is essential to perform regression fitting. By introducing the \(\varepsilon\) -insensitive loss function, SVM can be converted to a support vector regression machine, where the role of the OSH is to minimize the error of all samples from this plane. SVR has a theoretical basis in statistical learning and relatively high learning performance, making it suitable for performing predictions in small-sample, non-linear, and multi-dimensional fields [ 30 , 31 ].
Assume the training sample set containing \(l\) training samples is given by \(\{({x}_{i},{y}_{i}),i=\mathrm{1,2},...,l\}\) , where \({x}_{i}=[{x}_{i}^{1},{x}_{i}^{2},...,{x}_{i}^{d}{]}^{\rm T}\) and \({y}_{i}\in R\) are the corresponding output values.
Let the regression function be \(f(x)=w\Phi (x)+b\) , where \(\phi (x)\) is the non-linear mapping function. The linear \(\varepsilon\) -insensitive loss function is defined as shown in formula ( 1 ).
Among the rest, \(f(x)\) is the predicted value returned by the regression function, and \(y\) is the corresponding real value. If the error between \(f(x)\) and \(y\) is ≤ \(\varepsilon\) , the loss is 0; otherwise, the loss is \(\left|y-f(x)\right|-\varepsilon\) .
The slack variables \({\xi }_{i}\) and \({\xi }_{i}^{*}\) are introduced, and \(w\) , \(b\) are solved using the following equation as shown in formula ( 2 ).
Among the rest, \(C\) is the penalty factor, with larger values indicating a greater penalty for errors > \(\varepsilon\) ; \(\varepsilon\) is defined as the error requirement, with smaller values indicating a smaller error of the regression function.
The Lagrange function is introduced to solve the above function and transformed into the dual form to give the formula ( 3 ).
Among the rest, \(K({x}_{i},{x}_{j})=\Phi ({x}_{i})\Phi ({x}_{j})\) is the kernel function. The kernel function determines the structure of high-dimensional feature space and the complexity of the final solution. The Gaussian kernel is selected for this study with the function \(K({x}_{i},{x}_{j})=\mathit{exp}(-\frac{\Vert {x}_{i}-{x}_{j}\Vert }{2{\sigma }^{2}})\) .
Let the optimal solution be \(a=[{a}_{1},{a}_{2},...,{a}_{l}]\) and \({a}^{*}=[{a}_{1}^{*},{a}_{2}^{*},...,{a}_{l}]\) to give the formula ( 4 ) and formula ( 5 ).
Among the rest, \({N}_{nsv}\) is the number of support vectors.
In sum, the regression function is as shown in formula ( 6 ).
when some of the parameters are not 0, the corresponding samples are the support vectors in the problem. This is the principle of SVR. The values of the three unknown parameters (penalty factor C, ε -insensitive loss function, and kernel function coefficient \(\sigma )\) , can directly impact the model effect. The penalty factor C affects the degree of function fitting through the selection of outliers in the sample by the function. Thus, excessively large values lead to better fit but poorer generalization, and vice versa. The ε value in the ε-insensitive loss function determines the accuracy of the model by affecting the width of support vector selection. Thus, excessively large values lead to lower accuracy that does not meet the requirements and excessively small values are overly complex and increase the difficulty. The kernel function coefficient \(\sigma\) determines the distribution and range of the training sample by controlling the size of inner product scaling in high-dimensional space, which can affect overfitting.
Therefore, we introduce other algorithms for optimization of the three parameters in SVR. Currently the commonly used algorithms are 32and some heuristic algorithms. Although the grid search method is able to find the highest classification accuracy, which is the global optimal solution. However, sometimes it can be time-consuming to find the optimal parameters for larger scales. If a heuristic algorithm is used, we could find the global optimal solution without having to trace over all the parameter points in the grid. And GA is one of the most commonly used heuristic algorithms, compared to other heuristic algorithms, it has the advantages of strong global search, generalizability, and broader blending with other algorithms.
Given these factors, we employ a GA to encode and optimize the relevant parameters of the model. The inputs are the experimental training dataset, the Gaussian kernel function expression, the maximum number of generations taken by the GA, the accuracy range of the optimized parameters, the GA population size, the fitness function, the probability of crossover, and the probability of mutation. The outputs are the optimal penalty factor C, ε-insensitive loss function parameter \(\varepsilon ,\) and optimal Gaussian kernel parameter \(\sigma\) of SVR, thus achieving the optimization of SVR. The basic steps involved in GA optimization are described in detail below, and the model prediction process is shown in Fig. 1 .
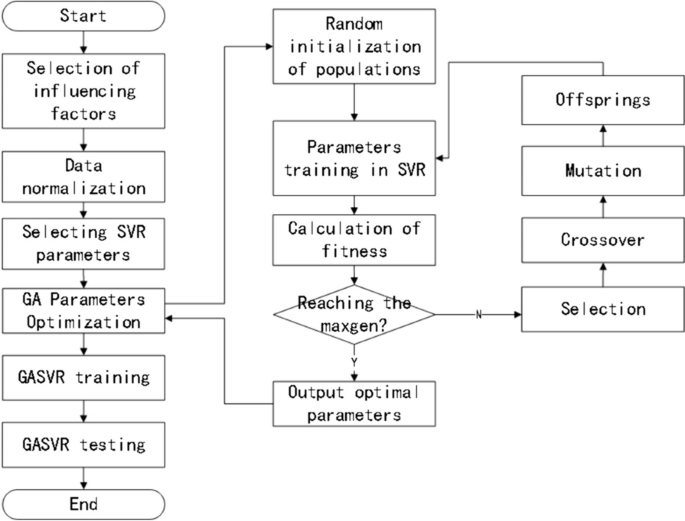
Prediction process of the GASVR model
Population initialization
The three parameters are encoded using binary arrays composed of 0–1 bit-strings. Each parameter consisted of six bits, and the initial population is randomly generated. The population size is set at 60, and the number of iterations is 200.
Fitness calculation
In the same dataset, the K-fold cross-validation technique is used to test each individual in the population, with K = 5. K-fold cross validation effectively avoids the occurrence of model over-learning and under-learning. For the judgment of the individual, this paper evaluates it in terms of fitness calculations. Therefore, combining the two enables the effective optimization of the model’s selected parameters and improves the accuracy of regression prediction.
Fitness is calculated using the mean error method, with smaller mean errors indicating better fitness. The fitness function is shown in formula ( 7 ) [ 32 ].
The individual’s genotype is decoded and mapped to the corresponding parameter value, which is substituted into the SVR model for training. The parameter optimization range is 0.01 ≤ C ≤ 100, 0.1 ≤ \(\sigma\) ≤ 20, and 0.001 ≤ ε ≤ 1.
Selection: The selection operator is performed using the roulette wheel method.
Crossover: The multi-point crossover operator, in which two chromosomes are selected and multiple crossover points are randomly chosen for swapping, is employed. The crossover probability is set at 0.9.
Mutation: The inversion mutation operator, in which two points are randomly selected and the gene values between them are reinserted to the original position in reverse order, is employed. The mutation probability is set at 0.09.
Decoding: The bit strings are converted to parameter sets.
The parameter settings of the GASVR model built in this paper are shown in Table 1 .
BILSTM model
The LSTM model is a special recurrent neural network algorithm that can remember the long-term dependencies of data series and has an excellent capacity for self-learning and non-linear fitting. LSTM automatically connects hidden layers across time points, such that the output of one time point can arbitrarily enter the output terminal or the hidden layer of the next time point. Therefore, it is suitable for the sample prediction of time-series data and can predict future data based on stored data. Details of the model are shown in Fig. 2 .
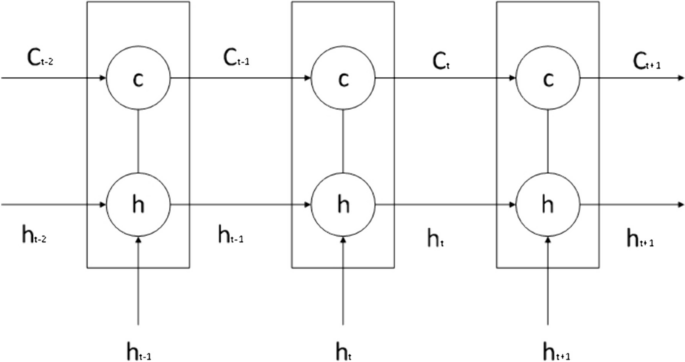
Schematic diagram of the LSTM model
LSTM consists of a forget gate, an input gate, and an output gate.
The forget gate combines the previous and current time steps to give the output of the sigmoid activation function. Its role is to screen the information from the previous state and identify useful information that truly impacts the subsequent time step. The equation for the forget gate is shown in formula ( 8 ).
Among the number, \(W_{f}\) is the weight of the forget gate, \({b}_{f}\) is the bias, \(\sigma\) is the sigmoid activation function, \({f}_{t}\) is the output of the sigmoid activation function, \(t-1\) is the previous time step, \(t\) is the current time step, and \({x}_{t}\) is the input time-series data at time step \(t\) .
The input gate is composed of the output of the sigmoid and tanh activation functions, and its role is to control the ratio of input information entering the information of a given time step. The equation for the input gate is shown in formula ( 9 ).
Among the number, \({W}_{i}\) is the output weight of the input gate, \({i}_{t}\) is the output of the sigmoid activation function, \({b}_{i}\) and \({b}_{C}\) are the biases of the input gate, and \({W}_{C}\) is the output of the tanh activation function.
The role of the output gate is to control the amount of information output at the current state, and its equation is shown in formula ( 10 ).
Among the number, \({W}_{o}\) is the weight of \({o}_{t}\) , and \({b}_{o}\) is the bias of the output gate.
The values of the above activation functions \(\sigma\) and tanh are generally shown in formulas ( 11 ) and ( 12 ).
\({C}_{t}\) is the data state of the current time step, and its value is determined by the input information of the current state and the information of the previous state. It is shown in formula ( 13 ).
Among the number, \(\widetilde{{C}_{t}}=\mathit{tan}h({W}_{c}[{h}_{t-1},{x}_{t}]+{b}_{c})\) .
\({h}_{t}\) is the state information of the hidden layer at the current time step, \({h}_{t}={o}_{t}\times \mathit{tan}h({c}_{t})\) .Each time step \({T}_{n}\) has a corresponding state \({C}_{t}\) . By undergoing the training process, the model can learn how to modify state \({C}_{t}\) through the forget, output, and input gates. Therefore, this state is consistently passed on, implying that important distant information will neither be forgotten nor significantly affected by unimportant information.
The above describes the principle of LSTM, which involves forward processing when applied. BILSTM consists of two LSTM networks, one of which processes the input sequence in the forward direction (i.e., the original order), while the other inputs the time series in the backward direction into the LSTM model. After processing both LSTM networks, the outputs are combined, which eventually gives the output results of the BILSTM model. Details of the model are presented in Fig. 3 .
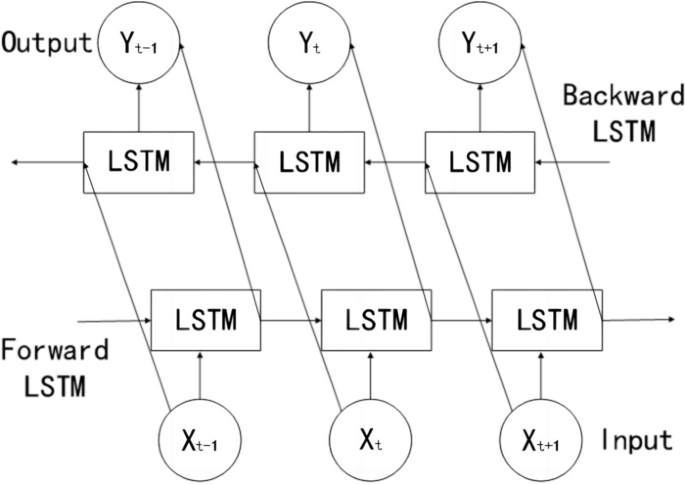
Schematic diagram of the BILSTM model
Compared to LSTM, BILSTM can achieve bidirectional information extraction of the time-series and connect the two LSTM layers onto the same output layer. Therefore, in theory, its predictive performance should be superior to that of LSTM. In BILSTM, the equations of the forward hidden layer( \(\overrightarrow{{h}_{t}}\) ) , backward hidden layer( \(\overleftarrow{{h}_{t}}\) ) , and output layer( \({o}_{t}\) ) are shown in formulas ( 14 ) , ( 15 ) and ( 16 ).
The parameter settings of the BILSTM model built in this paper are shown in Table 2 .
Informer model
The Informer model follows the compiler-interpreter architecture in the Transformer model, and based on this, structural optimizations have been made to reduce the computational time complexity of the algorithm and to optimize the output form of the interpreter. The two optimization methods are described in detail next.
With large amounts of input data, neural network models can have difficulty capturing long-term interdependencies in sequences, which can produce gradient explosions or gradient vanishing and affect the model's prediction accuracy. Informer model solves the existential gradient problem by using a ProbSparse Self-attention mechanism to make more efficient than conventional self-attention.
The value of Transformer self-attention is shown in formula ( 17 ).
Among them, \(Q\in {R}^{{L}_{Q}\times d}\) is the query matrix, \(K\in {R}^{{L}_{K}\times d}\) is the key matrix, and \(V\in {R}^{{L}_{V}\times d}\) is the value matrix, which are obtained by multiplying the input matrix X with the corresponding weight matrices \({W}^{Q}\) , \({W}^{K}\) , \({W}^{V}\) respectively, and d is the dimensionality of Q, K, and V. Let \({q}_{i}\) , \({k}_{i}\) , \(v_{i}\) represent the ith row in the Q, K, V matrices respectively, then the ith attention coefficient is shown in formula ( 18 ) as follows.
Therein, \(p({k}_{j}|{q}_{i})\) denotes the traditional Transformer's probability distribution formula, and \(k({q}_{i},{K}_{l})\) denotes the asymmetric exponential sum function. Firstly, q=1 is assumed, which implies that the value of each moment is equally important; secondly, the difference between the observed distribution and the assumed one is evaluated by the KL scatter, if the value of KL is bigger, the bigger the difference with the assumed distribution, which represents the more important this moment is. Then through inequality \(ln{L}_{k}\le M({q}_{i},K)\le {\mathit{max}}_{j}\left\{\frac{{q}_{i}{k}_{j}^{\rm T}}{\sqrt{d}}\right\}-\frac{1}{{L}_{k}}{\sum }_{j=1}^{{L}_{k}}\left\{\frac{{q}_{i}{k}_{j}^{\rm T}}{\sqrt{d}}\right\}+ln{L}_{k}\) , \(M({q}_{i},K)\) is transformed into \(\overline{M}({q}_{i},K)\) . According to the above steps, the ith sparsity evaluation formula is obtained as shown in formula ( 19 ) [ 33 ].
One of them, \(M({q}_{i},K)\) denotes the ith sparsity measure; \(\overline{M}({q}_{i},K)\) denotes the ith approximate sparsity measure; \({L}_{k}\) is the length of query vector. \(TOP-u\) quantities of \(\overline{M}\) are selected to form \(\overline{Q}\) , \(\overline{Q}\) is the first u sparse matrices, and the final sparse self-attention is shown in Formula ( 20 ). At this point, the time complexity is still \(O({n}^{2})\) , and to solve this problem, only l moments of M2 are computed to reduce the time complexity to \(O(L\cdot \mathit{ln}(L))\) .
Informer uses a generative decoder to obtain long sequence outputs.Informer uses the standard decoder architecture shown in Fig. 4 , in long time prediction, the input given to the decoder is shown in formula ( 21 ).
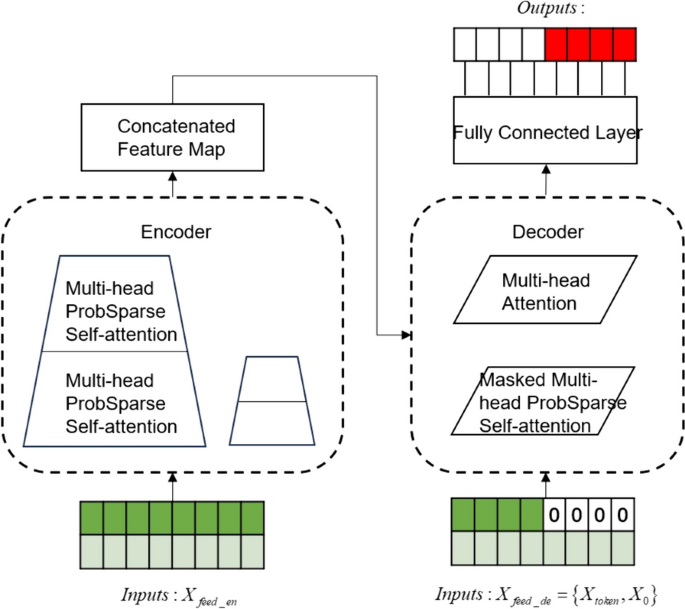
Informer uses a generative decoder to obtain long sequence outputs
Therein, \({X}_{de}^{t}\) denotes the input to the decoder; \({X}_{token}^{t}\in {R}^{({L}_{token}+{L}_{y})\times {d}_{\mathit{mod}el}}\) is the dimension of the encoder output, which is the starting token without using all the output dimensions; \({X}_{0}^{t}\in {R}^{({L}_{token}+{L}_{y})\times {d}_{\mathit{mod}el}}\) is the dimension of the target sequence, which is uniformly set to 0; and finally the splicing input is performed to the encoder for prediction.
The parameter settings of Informer model created in this paper are shown in Table 3 .
BILSTM-GASVR combined prediction model
SVR has demonstrated good performance in solving problems like finite samples and non-linearity. Compared to deep learning methods, it offers faster predictions and smaller empirical risks. BILSTM has the capacity for long-term memory, can effectively identify data periodicity and trends, and is suitable for the processing of time-series data. Hence, it can be used to identify the effect of time-series on the number of confirmed cases. Given the advantages of these two methods in different scenarios, we combined them to perform predictions using GASVR, followed by error repair using BILSTM. The basic steps for prediction based on the BILSTM-GASVR model are as follows:
Normalization is performed on the initial data.
The GASVR model is applied to perform training and parameter optimization of the data to obtain the predicted value \(\widehat{{y}_{i}}\) .
After outputting the predicted value of GASVR, the residual sequence between the predicted value and real data is extracted to obtain the error \({\gamma }_{i}\) (i.e., \({\gamma }_{i}={y}_{i}-\widehat{{y}_{i}}\) ).
The BILSTM model is applied to perform training of the error to improve prediction accuracy. The BILSTM model in this paper is a multiple input single output model. Its inputs are the true and predicted error values \({\gamma }_{i}\) and its output is the new error value \(\widehat{{\gamma }_{i}}\) predicted by BILSTM.
The final predicted value is the sum of the GASVR predicted value and the BILSTM residual predicted value (i.e., \({Y}_{i}=\widehat{{y}_{i}}+\widehat{{\gamma }_{i}}\) ).
The parameter settings of the BILSTM-GASVR model built in this paper are shown in Table 4 .
Model testing criteria
To test the effect of the model, the prediction results of the BILSTM-GASVR model are compared to those of GASVR, LSTM, BILSTM and Informer. The prediction error is mainly quantified using three indicators: mean squared error (MSE), root mean squared error (RMSE), and correlation coefficient ( \(R^{2}\) ). Their respective equations are shown in formulas ( 22 ), ( 23 ) and ( 24 ).
Demand forecasting model of ICU healthcare resources
ICU healthcare resources can be divided into human and material resources. Human resources refer specifically to the professional healthcare workers in the ICU. Material resources, which are combined with the actual consumption of medical supplies, can be divided into consumables and non-consumables. Consumables refer to the commonly used drugs in the ICU, which include drugs for treating cardiac insufficiency, vasodilators, anti-shock vasoactive drugs, analgesics, sedatives, muscle relaxants, anti-asthmatic drugs, and anticholinergics. Given that public health emergencies have a relatively high probability of affecting the respiratory system, we compiled a list of commonly used drugs for respiratory diseases in the ICU (Table 5 ).
Non-consumables refer to therapeutic medical equipment, including electrocardiogram machines, blood gas analyzers, electrolyte analyzers, bedside diagnostic ultrasound machines, central infusion workstations, non-invasive ventilators, invasive ventilators, airway clearance devices, defibrillators, monitoring devices, cardiopulmonary resuscitation devices, and bedside hemofiltration devices.
The demand forecasting model of ICU healthcare resources constructed in this study, as well as its relevant parameters and definitions, are described below. \({R}_{ij}^{n}\) is the forecasted demand for the \(i\) th category of resources on the \(n\) th day in region \(j\) . \({Y}_{j}^{n}\) is the predicted number of current confirmed cases on the \(n\) th day in region \(j\) . \({M}_{j}^{n}\) is the number of ICU healthcare workers on the \(n\) th day in region \(j\) , which is given by the following formula: number of healthcare workers the previous day + number of new recruits − reduction in number the previous day, where the reduction in number refers to the number of healthcare workers who are unable to work due to infection or overwork. In general, the number of ICU healthcare workers should not exceed 5% of the number of current confirmed cases (i.e., it takes the value range [0, \(Y_{j}^{n}\) ×5%]). \(U_{i}\) is the maximum working hours or duration of action of the \(i\) th resource category within one day. \({A}_{j}\) is the number of resources in the \(i\) th category allocated to patients (i.e., how many units of resources in the \(i\) th category is needed for a patient who need the \(i\) th unit of the given resource). \({\varphi }_{i}\) is the demand conversion coefficient (i.e., the proportion of the current number of confirmed cases who need to use the \(i\) th resource category). \({C}_{ij}^{n}\) is the available quantity of material resources of the \(i\) th category on the \(n\) th day in region \(j\) . At the start, this quantity is the initial reserve, and once the initial reserve is exhausted, it is the surplus from the previous day. The formula for this parameter is given as follows: available quantity from the previous day + replenishment on the previous day − quantity consumed on the previous day, where if \({C}_{ij}^{n}\) is a negative number, it indicates the amount of shortage for the given category of resources on the previous day.
In summary, the demand forecast for emergency medical supplies constructed in this study is shown in formula ( 25 ).
The number of confirmed cases based on data-driven prediction is introduced into the demand forecasting model for ICU resources to forecast the demand for the various categories of resources. In addition to the number of current confirmed cases, the main variables of the first demand forecasting model for human resources are the available quantity and maximum working hours. The main variable of the second demand forecasting model for consumable resources is the number of units consumed by the available quantity. The main variable of the third model for non-consumable resources is the allocated quantity. These three resource types can be predicted using the demand forecasting model constructed in this study.
Prediction of the number of current infected cases
The COVID-19 situation in Shanghai is selected for our experiment. A total of 978 entries of epidemic-related data in Shanghai between January 20, 2020, and September 24, 2022, are collected from the epidemic reporting platform. This dataset is distributed over a large range and belongs to a right-skewed leptokurtic distribution. The specific statistical description of data is shown in Table 6 . Part of the data is shown in Table 7 .
And we divided the data training set and test set in an approximate 8:2 ratio, namely, 798 days for training (January 20, 2020 to March 27, 2022) and 180 days for prediction (March 28, 2022 to September 24, 2022).
Due to the large difference in order of magnitude between the various input features, directly implementing training and model construction would lead to suboptimal model performance. Such effects are usually eliminated through normalization. In terms of interval selection, [0, 1] reflects the probability distribution of the sample, whereas [-1, 1] mostly reflects the state distribution or coordinate distribution of the sample. Therefore, [-1, 1] is selected for the normalization interval in this study, and the processing method is shown in formula ( 26 ).
Among the rest, \(X\) is the input sample, \({X}_{min}\) and \({X}_{max}\) are the minimum and maximum values of the input sample, and \({X}_{new}\) is the input feature after normalization.
In addition, we divide the data normalization into two parts, considering that the amount of data in the training set is much more than the test set in the real operating environment. In the first step, we normalize the training set data directly according to the above formula; in the second step, we normalize the test data set using the maximum and minimum values of the training data set.
The values of the preprocessed data are inserted into the GASVR, LSTM, Informer, BILSTM models and the BILSTM-GASVR model is constructed. Figures 5 , 6 , 7 , 8 and 9 show the prediction results. From Figs. 5 , 6 , and 7 , it can be seen that in terms of data accuracy, GASVR more closely matches the real number of infected people relative to BILSTM and LSTM. Especially in the most serious period of the epidemic in Shanghai (April 17, 2022 to April 30, 2022), the advantage of the accuracy of the predicted data of GASVR is even more obvious, which is due to the characteristics of GASVR for small samples and nonlinear prediction. However, in the overall trend of the epidemic, BILSTM and LSTM, which have the ability to learn and memorize to process time series data, are superior. It is clearly seen that in April 1, 2022-April 7, 2022 and May 10, 2022-May 15, 2022, there is a sudden and substantial increase in GASVR in these two time phases, and a sudden and substantial decrease in April 10, 2022-April 14, 2022. These errors also emphasize the stability of BILSTM and LSTM, which are more closely matched to the real epidemic development situation in the whole process of prediction, and the difference between BILSTM and LSTM prediction is that the former predicts data more accurately than the latter, which is focused on the early stage of prediction as well as the peak period of the epidemic. Informer is currently an advanced time series forecasting method. From Fig. 8 , it can be seen that the prediction data accuracy and the overall trend of the epidemic are better than the single prediction models of GASVR, LSTM and BILSTM. However, Informer is more suitable for long time series and more complex and large prediction problems, so the total sample size of less than one thousand cases is not in the comfort zone of Informer model. Figure 9 shows that the BILSTM-GASVR model constructed in this paper is more suitable for this smaller scale prediction problem, with the best prediction results, closest to the actual parameter (number of current confirmed cases), demonstrating small sample and time series advantages. In Short, the prediction effect of models is ranked as follows: BILSTM-GASVR> Informer> GASVR> BILSTM> LSTM.
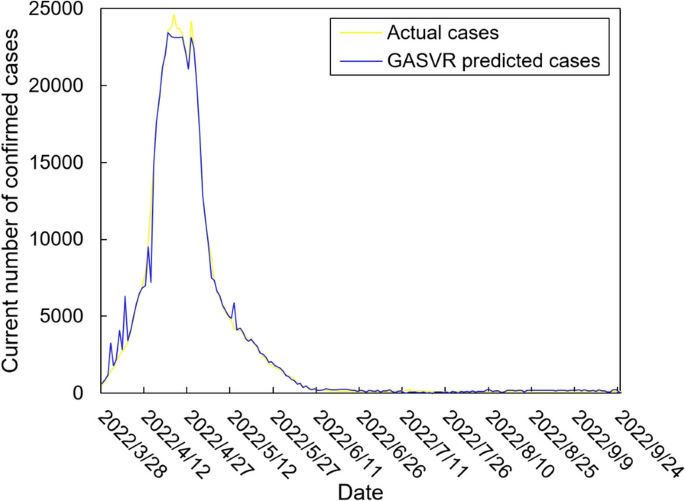
The prediction result of the GASVR model
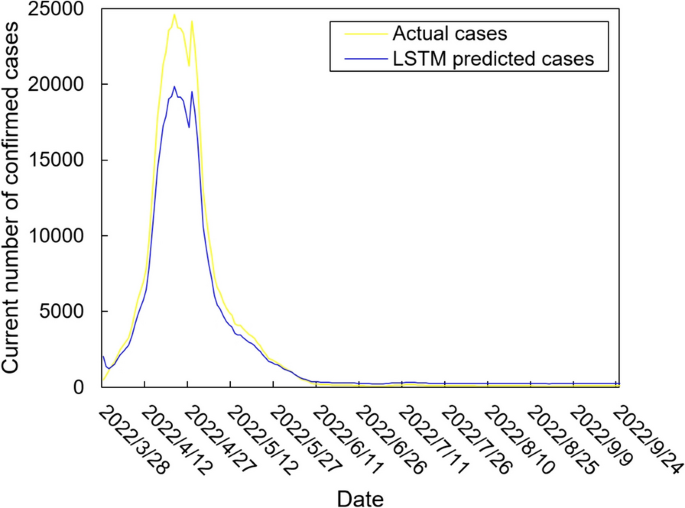
The prediction result of the LSTM model
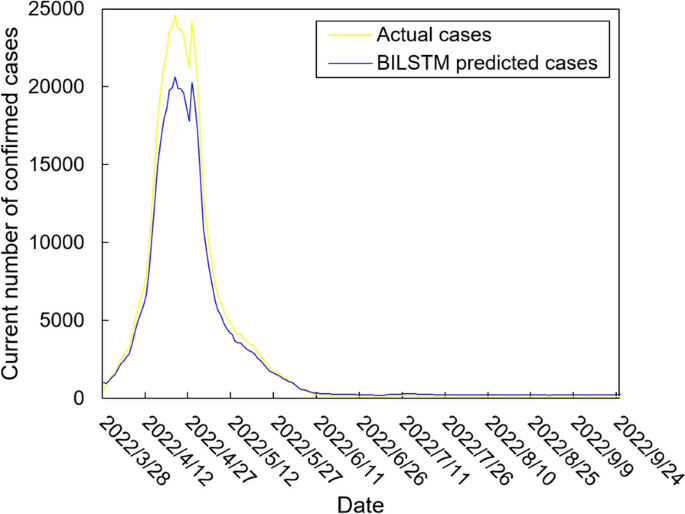
The prediction result of the BILSTM model
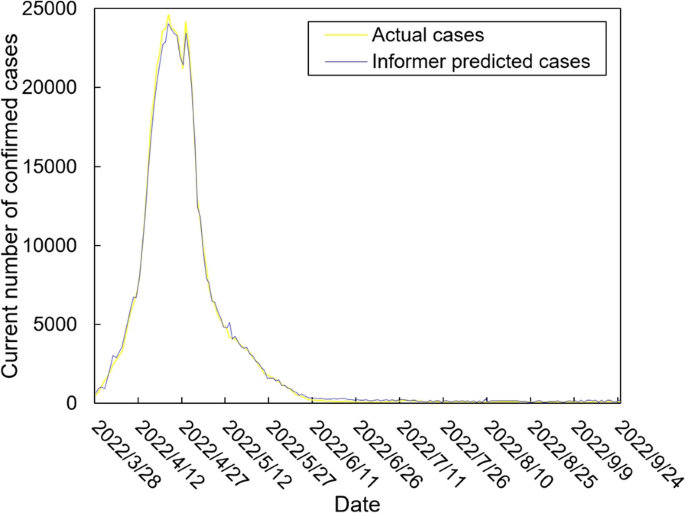
The prediction result of the Informer model
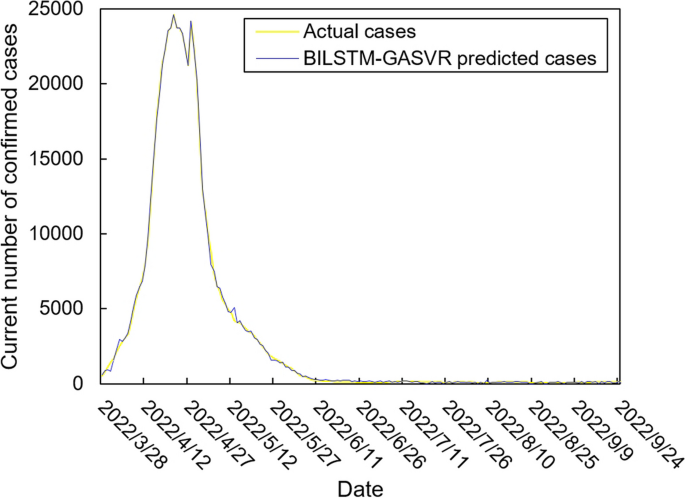
The prediction result of the BILSTM-GASVR model
The values of the three indicators (MSE, RMSE, and correlation coefficient \({R}^{2}\) ) for the five models are shown in Table 8 . MSE squares the error so that the larger the model error, the larger the value, which help capture the model's prediction error more sensitively. RMSE is MSE with a root sign added to it, which allows for a more intuitive representation of the order of magnitude difference from the true value. \({R}^{2}\) is a statistical indicator used to assess the overall goodness of fit of the model, which reflects the overall consistency of the predicted trend and does not specifically reflect the degree of data. The results in the Table 8 are consistent with the prediction results in the figure above, while the ranking of MSE, RMSE, and \({R}^{2}\) are also the same (i.e., BILSTM-GASVR> Informer> GASVR> BILSTM> LSTM).
In addition, we analyze the five model prediction data using significance tests as a way of demonstrating whether the model used is truly superior to the other baseline models. The test dataset with kurtosis higher than 4 does not belong to the approximate normal distribution, so parametric tests are not used in this paper. Given that the datasets predicted by each of the five models are continuous and independent datasets, this paper uses the Kruskal-Wallis test, which is a nonparametric test. The test steps are as follows.
Determine hypotheses (H0, H1) and significance level ( \(\alpha\) ).
For each data set, all its sample data are combined and ranked from smallest to largest. Then find the number of data items ( \({n}_{i}\) ), rank sum ( \({R}_{i}\) ) and mean rank of each group of data respectively.
Based on the rank sum, the test statistic (H) is calculated for each data set in the Kruskal-Wallis test. The specific calculation is shown in formula ( 27 ).
According to the test statistic and degrees of freedom, find the corresponding p-value in the Kruskal-Wallis distribution table. Based on the P-value, determine whether the original hypothesis is valid.
In the significance test, we set the significance setting original hypothesis (H0) as there is no significant difference between the five data sets obtained from the five predictive models. We set the alternative hypothesis (H1) as there is a significant difference between the five data sets obtained from the five predictive models. At the same time, we choose the most commonly used significance level taken in the significance test, namely 0.05. In this paper, multiple comparisons and two-by-two comparisons of the five data sets obtained from the five predictive models are performed through the SPSS software. The results of the test show that in the multiple comparison session, P=0.001<0.05, so H0 is rejected, which means that the difference between the five groups of data is significant. In the two-by-two comparison session, BILSTM-GASVR is less than 0.05 from the other four prediction models. The specific order of differences is Informer < GASVR < BILSTM < LSTM, which means that the BILSTM-GASVR prediction model does get a statistically significant difference between the dataset and the other models.
In summary, combined prediction using the BILSTM-GASVR model is superior to the other four single models in various aspects in the case study analysis of Shanghai epidemic with a sample size of 978.
Demand forecasting of ICU healthcare resources
Combined with the predicted number of current infected cases, representatives are selected from the three categories of resources for forecasting. The demand for nurses is selected as the representative for the first category of resources.
In view of the fact that there are currently no specific medications that are especially effective for this public health emergency, many ICU treatment measures involved helping patients survive as their own immune systems eliminated the virus. This involved, for example, administering antibiotics when patients developed a secondary bacterial infection. glucocorticoids are used to temporarily suppress the immune system when their immune system attacked and damaged lung tissues causing patients to have difficulty breathing. extracorporeal membrane oxygenation (ECMO) is used for performing cardiopulmonary resuscitation when patients are suffering from cardiac arrest. In this study, we take dexamethasone injection (5 mg), a typical glucocorticoid drug, as the second category of ICU resources (i.e., drugs); and invasive ventilators as the third category of ICU resources (i.e., medical equipment).
During the actual epidemic in Shanghai, the municipal government organized nine critical care teams, which are stationed in eight municipally designated hospitals and are dedicated to the treatment of critically ill patients. In this study, the ICU nurses, dexamethasone injections, and invasive ventilators in Shanghai are selected as the prediction targets and introduced into their respective demand forecasting models. Forecasting of ICU healthcare resources is then performed for the period from March 28, 2022, to April 28, 2022, as an example. Part of the parameter settings for the three types of resources are shown in Tables 9 , 10 , and 11 , respectively.
Table 12 shows the forecasting results of the demand for ICU nurses, dexamethasone injections, and invasive ventilators during the epidemic wave in Shanghai between March 28, 2022, and April 28, 2022.
For the first category (i.e., ICU nurses), human resource support is only needed near the peak period, but the supply could not be replenished immediately. In the early stages, Shanghai could only rely on the nurses’ perseverance, alleviating the shortage of human resources by reducing the number of shifts and increasing working hours. This situation persisted until about April 10 and is only resolved when nurses from other provinces and regions successively arrived in Shanghai.
The second category of ICU resources is drugs, which are rapidly consumed. The pre-event reserve of 30,000 dexamethasone injections could only be maintained for a short period and is fully consumed during the outbreak. Furthermore, daily replenishment is still needed, even when the epidemic has passed its peak and begun its decline.
The third category is invasive ventilators, which are non-consumables. Thus, the reserve lasted for a relatively long period of time in the early stages and did not require replenishment after its maximum usage during the peak period.
Demand forecasting models are constructed based on the classification of healthcare resources according to their respective features. We choose ICU nurses, dexamethasone injections, and invasive ventilators as examples, and then forecast demand for the epidemic wave in Shanghai between March 28, 2022, and April 28, 2022. The main conclusions are as follows:
A long period of time is needed to train ICU healthcare workers who can independently be on duty, taking at least one year from graduation to entering the hospital, in addition to their requiring continuous learning, regular theoretical training, and the accumulation of clinical experience during this process. Therefore, for the first category of ICU healthcare resources, in the long term, healthcare institutions should place a greater emphasis on their talent reserves. Using China as an example, according to the third ICU census, the ratio of the number of ICU physicians to the number of beds is 0.62:1 and the ratio of the number of nurses to the number of beds is 1.96:1, which are far lower than those stipulated by China itself and those of developed countries. Therefore, a fundamental solution is to undertake proactive and systematic planning and construction to ensure the more effective deployment of human resources in the event of a severe outbreak. In the short term, healthcare institutions should focus on the emergency expansion capacity of their human resources. In case there are healthcare worker shortages during emergencies, the situation can be alleviated by summoning retired workers back to work and asking senior medical students from various universities to help in the hospitals to prevent the passive scenario of severely compressing the rest time of existing staff or waiting for external aid. However, it is worth noting that to ensure the effectiveness of such a strategy of using retired healthcare workers or senior students of university medical faculties, it is necessary for healthcare organizations to provide them with regular training in the norm, such as organizing 2-3 drills a year, to ensure the professionalism and proficiency of healthcare workers who are temporarily and suddenly put on the job. At the same time, it is also necessary to fully mobilize the will of individuals. Medical institutions can provide certain subsidies to retired health-care workers and award them with honorable titles. For senior university medical students, volunteer certificates are issued and priority is given to their internships, so that health-care workers can be motivated to self-realization through spiritual and material rewards.
Regarding the second category of ICU resources (i.e., drugs), healthcare institutions perform the subdivision of drug types and carry out dynamic physical preparations based on 15–20% of the service recipient population for clinically essential drugs. This will enable a combination of good preparedness during normal times and emergency situations. In addition, in-depth collaboration with corporations is needed to fully capitalize on their production capacity reserves. This helps medical institutions to be able to scientifically and rationally optimize the structure and quantity of their drug stockpiles to prevent themselves from being over-stressed. Yet the lower demand for medicines at the end of the epidemic led to the problem of excess inventory of enterprises at a certain point in time must be taken into account. So, the medical institutions should sign a strategic agreement on stockpiling with enterprises, take the initiative to bear the guaranteed acquisition measures, and consider the production costs of the cooperative enterprises. These measures are used to truly safeguard the enthusiasm of the cooperative enterprises to invest in the production capacity.
Regarding the third category of ICU resources (i.e., medical equipment), large-scale medical equipment cannot be rapidly mass-produced due to limitations in the capacity for emergency production and conversion of materials. In addition, the bulk procurement of high-end medical equipment is also relatively difficult in the short term. Therefore, it is more feasible for healthcare institutions to have physical reserves of medical equipment, such as invasive ventilators. However, the investment costs of medical equipment are relatively high. Ventilators, for example, cost up to USD $50,000, and subsequent maintenance costs are also relatively high. After all, according to the depreciable life of specialized hospital equipment, the ventilator, as a surgical emergency equipment, is depreciated over five years. And its depreciation rate is calculated at 20% annually for the first five years, which means a monthly depreciation of $835. Thus, the excessively low utilization rate of such equipment will also impact the hospital. Healthcare institutions should, therefore, conduct further investigations on the number of beds and the reserves of ancillary large-scale medical equipment to find a balance between capital investment and patient needs.
The limitations of this paper are reflected in the following three points. Firstly, in the prediction of the number of infections, the specific research object in this paper is COVID-19, and other public health events such as SARS, H1N1, and Ebola are not comparatively analyzed. The main reason for this is the issue of data accessibility, and it is easier for us to analyze events that have occurred in recent years. In addition, using the Shanghai epidemic as a specific case may be more representative of the epidemic situation in an international metropolis with high population density and mobility. Hence, it has certain regional limitations, and subsequent studies should expand the scope of the case study to reflect the characteristics of epidemic transmission in different types of urban areas and enhance the generalizability.
Secondly, the main emphasis of this study is on forecasting the demand for ICU healthcare resources across the entire region of the epidemic, with a greater focus on patient demand during public emergencies. Our aims are to help all local healthcare institutions more accurately identify changes in ICU healthcare resource demand during this local epidemic wave, gain a more accurate understanding of the treatment demands of critically ill patients, and carry out comprehensive, scientifically based decision-making. Therefore, future studies can examine individual healthcare institutions instead and incorporate the actual conditions of individual units to construct multi-objective models. In this way, medical institutions can further grasp the relationship between different resource inputs and the recovery rate of critically ill patients, and achieve the balance between economic and social benefits.
Finally, for the BILSTM-GASVR prediction method, in addition to the number of confirmed diagnoses predicted for an outbreak in a given region, other potential applications beyond this type of medium-sized dataset still require further experimentation. For example, whether the method is suitable for procurement planning of a certain supply in production management, forecasting of goods sales volume in marketing management, and other long-period, large-scale and other situations.
Within the context of major public health events, the fluctuations and uncertainties in the demand for ICU resources can lead to large errors between the healthcare supply and actual demand. Therefore, this study focuses on the question of forecasting the demand for ICU healthcare resources. Based on the number of current confirmed cases, we construct the BILSTM-GASVR model for predicting the number of patients. By comparing the three indicators (MSE, MAPE, and correlation coefficient \(R^{2}\) ) and the results of the BILSTM, LSTM, and GASVR models, we demonstrate that our model have a higher accuracy. Our findings can improve the timeliness and accuracy of predicting ICU healthcare resources and enhance the dynamics of demand forecasting. Hence, this study may serve as a reference for the scientific deployment of ICU resources in healthcare institutions during major public events.
Given the difficulty in data acquisition, only the Shanghai epidemic dataset is selected in this paper, which is one of the limitations mentioned in Part 4. Although the current experimental cases of papers in the same field do not fully conform to this paper, the results of the study cannot be directly compared. However, after studying the relevant reviews and the results of the latest papers, we realize that there is consistency in the prediction ideas and prediction methods [ 34 , 35 ]. Therefore, we summarize the similarities and differences between the results of the study and other research papers in epidemic forecasting as shown below.
Similarities: on the one hand, we all characterize trends in the spread of the epidemic and predict the number of infections over 14 days. On the other hand, we all select the current mainstream predictive models as the basis and combine or improve them. Moreover, we all use the same evaluation method (comparison of metrics such as MSE and realistic values) to evaluate the improvements against other popular predictive models.
Differences: on the one hand, other papers focus more on predictions at the point of the number of patients, such as hospitalization rate, number of infections, etc. This paper extends the prediction from the number of patients to the specific healthcare resources. This paper extends the prediction from the number of patients to specific healthcare resources. We have divided the medical resources and summarized the demand regularities of the three types of information in the epidemic, which provides the basis for decision-making on epidemic prevention to the government or medical institutions. On the other hand, in addition to the two assessment methods mentioned in the same point, this paper assesses the performance of the prediction methods with the help of significance tests, which is a statistical approach to data. This can make the practicality of the forecasting methodology more convincing.
Availability of data and materials
The datasets used and/or analysed during the current study available from the corresponding author on reasonable request.
Yuan, R., Yang, Y., Wang, X., Duo, J. & Li, J. Study on the forecasting and allocation of emergency medical material needs in the event of a major infectious disease outbreak. J Saf Environ. https://doi.org/10.13637/j.issn.1009-6094.2023.2448 .
Total epidemic data (global). 2024. Retrieved March 30, 2024, from: https://www.sy72.com/world/ .
Sui, K., Wang, Y., Wang, S., Chen, C., & Sun, X. A multiple regression analysis-based method for forecasting the demand of emergency materials for power grids. Electron Technol Softw Eng. 2016; (23), 195-197.
Li K, Liu L, Zhai J, Khoshgoftaar TM, Li T. The improved grey model based on particle swarm optimization algorithm for time series prediction. Eng Appl Artif Intell. 2016;55:285–91. https://doi.org/10.1016/j.engappai.2016.07.005 .
Article Google Scholar
Fan R, Wang Y, Luo M. SEIR-based COVID-19 transmission model and inflection point prediction analysis. J Univ Electron Sci Technol China. 2020;49(3):369–74.
Google Scholar
Neves AGM, Guerrero G. Predicting the evolution of the COVID-19 epidemic with the A-SIR model: Lombardy, Italy and São Paulo state Brazil. Physica D. 2020;413:132693. https://doi.org/10.1016/j.physd.2020.132693 .
Article PubMed PubMed Central Google Scholar
Li R, Pei S, Chen B, Song Y, Zhang T, Wan Y, Shaman J. Substantial undocumented infection facilitates the rapid dissemination of novel coronavirus (SARS-CoV-2). Science. 2020;368(6490):489–93. https://doi.org/10.1126/science.abb3221 .
Article CAS PubMed PubMed Central Google Scholar
Kermack WO, McKendrick AG. A contribution to the mathematical theory of epidemics. Proc R Soc Lond. 1927;115(772):700–21. https://doi.org/10.1098/rspa.1927.0118 .
Hethcote HW. The mathematics of infectious diseases. Siam Rev. 2000;42(4):599–653. https://doi.org/10.1137/SIREAD000042000004000655000001 .
Estrada E. COVID-19 and SARS-CoV-2. Modeling the present, looking at the future. Phys Rep. 2020;869:1–51. https://doi.org/10.1016/j.physrep.2020.07.005 .
Mizumoto K, Chowell G. Transmission potential of the novel coronavirus (COVID-19) onboard the diamond Princess Cruises Ship, 2020. Infect Dis Model. 2020;5:264–70. https://doi.org/10.1016/j.idm.2020.02.003 .
Delamater PL, Street EJ, Leslie TF, Yang YT, Jacobsen KH. Complexity of the Basic Reproduction Number. Emerg Infect Dis. 2019;25(1):1–4. https://doi.org/10.3201/eid2501.171901 .
Annas S, Isbar Pratama M, Rifandi M, Sanusi W, Side S. Stability analysis and numerical simulation of SEIR model for pandemic COVID-19 spread in Indonesia. Chaos Solit Fract. 2020;139:110072. https://doi.org/10.1016/j.chaos.2020.110072 .
Li Q, Guan X, Wu P, Wang X, Zhou L, Tong Y, Ren R, Leung KSM. Early Transmission Dynamics in Wuhan, China, of Novel Coronavirus-Infected Pneumonia. N Engl J Med. 2020;382(13):1199–209. https://doi.org/10.1056/NEJMoa2001316 .
Anggriani N, Ndii MZ, Amelia R, Suryaningrat W, Pratama MAA. A mathematical COVID-19 model considering asymptomatic and symptomatic classes with waning immunity. Alexandria Eng J. 2022;61(1):113–24. https://doi.org/10.1016/j.aej.2021.04.104 .
Efimov D, Ushirobira R. On an interval prediction of COVID-19 development based on a SEIR epidemic model. Ann Rev Control. 2021;51:477–87. https://doi.org/10.1016/j.arcontrol.2021.01.006 .
Lin Q, Zhao S, Gao D, Lou Y, Yang S, Musa SS, Wang MH. A conceptual model for the coronavirus disease 2019 (COVID-19) outbreak in Wuhan, China with individual reaction and governmental action. Int J Infect Dis. 2020;93:211–16.
Chao L, Feng P, Shi P. Study on the epidemic development of COVID-19 in Hubei. J Zhejiang Univ (Med Sci). 2020;49(2):178–84.
Reiner RC, Barber RM, Collins JK, et al. Modeling COVID-19 scenarios for the United States. Nat Med. 2021;27(1):94–105. https://doi.org/10.1038/s41591-020-1132-9 .
Article CAS Google Scholar
Shahid F, Zameer A, Muneeb M. Predictions for COVID-19 with deep learning models of LSTM GRU and Bi-LSTM. Chaos Solit Fract. 2020;140:110212. https://doi.org/10.1016/j.chaos.2020.110212 .
Chimmula VKR, Zhang L. Time series forecasting of COVID-19 transmission in Canada using LSTM networks. Chaos Solit Fract. 2020;135:109864. https://doi.org/10.1016/j.chaos.2020.109864 .
Hamou AA, Azroul E, Hammouch Z, Alaoui AAL. A fractional multi-order model to predict the COVID-19 outbreak in Morocco. Appl Comput Math. 2021;20(1):177–203.
Zhou, L., Zhao, C., Liu, N., Yao, X., & Cheng, Z. Improved LSTM-based deep learning model for COVID-19 prediction using optimized approach. Eng Appl Artif Intell. 2023; 122. https://doi.org/10.1016/j.engappai.2023.106157 .
Huang C, Chen Y, Ma Y, Kuo P. Multiple-Input Deep Convolutional Neural Network Model for COVID-19 Forecasting in China. medRxiv. 2020;74822–34.
Gautam Y. Transfer Learning for COVID-19 cases and deaths forecast using LSTM network. Isa Trans. 2022;124:41–56. https://doi.org/10.1016/j.isatra.2020.12.057 .
Article PubMed Google Scholar
Ghany KKA, Zawbaa HM, Sabri HM. COVID-19 prediction using LSTM algorithm: GCC case study. Inform Med Unlock. 2021;23:100566. https://doi.org/10.1016/j.imu.2021.100566 .
Devaraj J, Madurai Elavarasan R, Pugazhendhi R, Shafiullah GM, Ganesan S, Jeysree AK, Khan IA, Hossain E. Forecasting of COVID-19 cases using deep learning models: Is it reliable and practically significant? Results Phys. 2021;21:103817. https://doi.org/10.1016/j.rinp.2021.103817 .
Arora P, Kumar H, Panigrahi BK. Prediction and analysis of COVID-19 positive cases using deep learning models: a descriptive case study of India. Chaos Solit Fract. 2020;139:110017. https://doi.org/10.1016/j.chaos.2020.110017 .
Liu Q, Fung DLX, Lac L, Hu P. A Novel Matrix Profile-Guided Attention LSTM Model for Forecasting COVID-19 Cases in USA. Front Public Health. 2021;9:741030. https://doi.org/10.3389/fpubh.2021.741030 .
Ribeiro MHDM, Da Silva RG, Mariani VC, Coelho LDS. Short-term forecasting COVID-19 cumulative confirmed cases: perspectives for Brazil. Chaos Solit Fract. 2020;135:109853. https://doi.org/10.1016/j.chaos.2020.109853 .
Shoko C, Sigauke C. Short-term forecasting of COVID-19 using support vector regression: an application using Zimbabwean data. Am J Infect Control. 2023. https://doi.org/10.1016/j.ajic.2023.03.010 .
Feng T, Peng Y, Wang J. ISGS: A Combinatorial Model for Hysteresis Effects. Acta Electronica Sinica. 2023;09:2504–9.
Zhou H, Zhang S, Peng J, Zhang S, Li J, Xiong H, Zhang W. Informer: Beyond Efficient Transformer for Long Sequence Time-Series Forecasting. AAAI Conference Artif Intell. 2020;35(12):11106–15.
Rahimi I, Chen F, Gandomi AH. A review on COVID-19 forecasting models. Neural Comput Applic. 2023;35:23671–81. https://doi.org/10.1007/s00521-020-05626-8 .
Chen J, Creamer GG, Ning Y, Ben-Zvi T. Healthcare Sustainability: Hospitalization Rate Forecasting with Transfer Learning and Location-Aware News Analysis. Sustainability. 2023;15(22):15840. https://doi.org/10.3390/su152215840 .
Download references
Acknowledgements
We would like to acknowledge the hard and dedicated work of all the staff that implemented the intervention and evaluation components of the study.
No external funding received to conduct this study.
Author information
Authors and affiliations.
School of Logistics, Beijing Wuzi University, No.321, Fuhe Street, Tongzhou District, Beijing, 101149, China
Weiwei Zhang & Xinchun Li
You can also search for this author in PubMed Google Scholar
Contributions
WWZ and XCL conceived the idea and conceptualised the study. XCL collected the data. WWZ analysed the data. WWZ and XCL drafted the manuscript, then WWZ and XCLreviewed the manuscript. WWZ and XCL read and approved the final draft.
Corresponding author
Correspondence to Xinchun Li .
Ethics declarations
Ethics approval and consent to participate.
Not applicable.
Consent for publication
Competing interests.
The authors declare no competing interests.
Additional information
Publisher’s note.
Springer Nature remains neutral with regard to jurisdictional claims in published maps and institutional affiliations.
Rights and permissions
Open Access This article is licensed under a Creative Commons Attribution 4.0 International License, which permits use, sharing, adaptation, distribution and reproduction in any medium or format, as long as you give appropriate credit to the original author(s) and the source, provide a link to the Creative Commons licence, and indicate if changes were made. The images or other third party material in this article are included in the article's Creative Commons licence, unless indicated otherwise in a credit line to the material. If material is not included in the article's Creative Commons licence and your intended use is not permitted by statutory regulation or exceeds the permitted use, you will need to obtain permission directly from the copyright holder. To view a copy of this licence, visit http://creativecommons.org/licenses/by/4.0/ . The Creative Commons Public Domain Dedication waiver ( http://creativecommons.org/publicdomain/zero/1.0/ ) applies to the data made available in this article, unless otherwise stated in a credit line to the data.
Reprints and permissions
About this article
Cite this article.
Zhang, W., Li, X. A data-driven combined prediction method for the demand for intensive care unit healthcare resources in public health emergencies. BMC Health Serv Res 24 , 477 (2024). https://doi.org/10.1186/s12913-024-10955-8
Download citation
Received : 21 September 2023
Accepted : 05 April 2024
Published : 17 April 2024
DOI : https://doi.org/10.1186/s12913-024-10955-8
Share this article
Anyone you share the following link with will be able to read this content:
Sorry, a shareable link is not currently available for this article.
Provided by the Springer Nature SharedIt content-sharing initiative
- Public health emergency
- ICU healthcare resource demand
- Machine learning
- Combined prediction
BMC Health Services Research
ISSN: 1472-6963
- General enquiries: [email protected]
This paper is in the following e-collection/theme issue:
Published on 29.4.2024 in Vol 26 (2024)
Effectiveness of a Smartphone App–Based Intervention With Bluetooth-Connected Monitoring Devices and a Feedback System in Heart Failure (SMART-HF Trial): Randomized Controlled Trial
Authors of this article:

Original Paper
- Minjae Yoon 1 , MD ;
- Seonhwa Lee 2 , MD ;
- Jah Yeon Choi 3 , MD, PhD ;
- Mi-Hyang Jung 4 , MD, PhD ;
- Jong-Chan Youn 4 , MD, PhD ;
- Chi Young Shim 5 , MD, PhD ;
- Jin-Oh Choi 6 , MD, PhD ;
- Eung Ju Kim 3 , MD, PhD ;
- Hyungseop Kim 2 , MD, PhD ;
- Byung-Su Yoo 7 , MD, PhD ;
- Yeon Joo Son 8 , BS ;
- Dong-Ju Choi 1 , MD, PhD
1 Division of Cardiology, Department of Internal Medicine, Seoul National University Bundang Hospital, Seoul National University College of Medicine, Seognam, Republic of Korea
2 Division of Cardiology, Department of Internal Medicine, Keimyung University Dongsan Hospital, Daegu, Republic of Korea
3 Cardiovascular Center, Korea University Guro Hospital, Korea University College of Medicine, Seoul, Republic of Korea
4 Division of Cardiology, Department of Internal Medicine, Seoul St. Mary's Hospital, Catholic Research Institute for Intractable Cardiovascular Disease, College of Medicine, The Catholic University of Korea, Seoul, Republic of Korea
5 Division of Cardiology, Department of Internal Medicine, Severance Hospital, Yonsei University College of Medicine, Seoul, Republic of Korea
6 Cardiac and Vascular Center, Samsung Medical Center, Sungkyunkwan University, Seoul, Republic of Korea
7 Department of Internal Medicine, Wonju Severance Christian Hospital, Yonsei University Wonju College of Medicine, Woonju, Republic of Korea
8 Healthcare Business Department, AI/DX Convergence Business Group, KT, Seoul, Republic of Korea
Corresponding Author:
Dong-Ju Choi, MD, PhD
Division of Cardiology, Department of Internal Medicine
Seoul National University Bundang Hospital, Seoul National University College of Medicine
82, Gumi-ro 173 Beon-gil, Bundang-gu
Seognam, 13620
Republic of Korea
Phone: 82 317877007
Fax:82 317877041
Email: [email protected]
Background: Current heart failure (HF) guidelines recommend a multidisciplinary approach, discharge education, and self-management for HF. However, the recommendations are challenging to implement in real-world clinical settings.
Objective: We developed a mobile health (mHealth) platform for HF self-care to evaluate whether a smartphone app–based intervention with Bluetooth-connected monitoring devices and a feedback system can help improve HF symptoms.
Methods: In this prospective, randomized, multicenter study, we enrolled patients 20 years of age and older, hospitalized for acute HF, and who could use a smartphone from 7 tertiary hospitals in South Korea. In the intervention group (n=39), the apps were automatically paired with Bluetooth-connected monitoring devices. The patients could enter information on vital signs, HF symptoms, diet, medications, and exercise regimen into the app daily and receive feedback or alerts on their input. In the control group (n=38), patients could only enter their blood pressure, heart rate, and weight using conventional, non-Bluetooth devices and could not receive any feedback or alerts from the app. The primary end point was the change in dyspnea symptom scores from baseline to 4 weeks, assessed using a questionnaire.
Results: At 4 weeks, the change in dyspnea symptom score from baseline was significantly greater in the intervention group than in the control group (mean –1.3, SD 2.1 vs mean –0.3, SD 2.3; P =.048). A significant reduction was found in body water composition from baseline to the final measurement in the intervention group (baseline level mean 7.4, SD 2.5 vs final level mean 6.6, SD 2.5; P =.003). App adherence, which was assessed based on log-in or the percentage of days when symptoms were first observed, was higher in the intervention group than in the control group. Composite end points, including death, rehospitalization, and urgent HF visits, were not significantly different between the 2 groups.
Conclusions: The mobile-based health platform with Bluetooth-connected monitoring devices and a feedback system demonstrated improvement in dyspnea symptoms in patients with HF. This study provides evidence and rationale for implementing mobile app–based self-care strategies and feedback for patients with HF.
Trial Registration: ClinicalTrials.gov NCT05668000; https://clinicaltrials.gov/study/NCT05668000
Introduction
Heart failure (HF) is a rapidly growing public health problem, with an estimated prevalence of 64 million people globally [ 1 - 5 ]. Although outcomes of HF have recently improved with the development of medications, a high rate of readmission remains after initial hospitalization for acute HF [ 6 - 10 ]. Recent studies have shown that 18%-23% of patients with acute HF were readmitted within 1 month, and these numbers have not decreased recently [ 6 , 11 , 12 ]. Therefore, the postdischarge management of patients with HF is important. In particular, multidisciplinary interventions, in addition to pharmacotherapy and discharge education, are known to improve quality of life and reduce hospitalizations [ 13 - 16 ]. A recent meta-analysis has shown that self-management interventions, including symptom and sign monitoring, education, and enhancement of drug adherence, improve outcomes of HF-related hospitalization and all-cause death, despite heterogeneity in the interventions [ 17 ]. Thus, current HF guidelines recommend multidisciplinary management, specific discharge education, and support to facilitate HF self-care [ 18 - 20 ].
Although encouraging self-care and providing feedback from health care providers are the most effective means, they are difficult to implement in a real-world clinical setting. These are also associated with higher costs and require more infrastructure and manpower. Smartphones are currently available to most of the general population at an affordable cost. The advent of mobile health (mHealth) technology and advances in artificial intelligence (AI) have enabled patient self-care, symptom, and sign monitoring, as well as mobile-based feedback via smartphone apps. Recent studies using smartphone apps for the self-management of cardiovascular disease demonstrated that this intervention led to better outcomes than the control groups [ 21 - 23 ]. Additionally, some recent studies and meta-analyses regarding mHealth apps have supported self-care among patients with HF [ 24 - 30 ]. However, these previous studies had a small number of participants and limited app functionality. Self-monitoring and feedback are particularly important, and the lack of these critical features limits the interpretation of the effectiveness of HF apps. Furthermore, functions provided in previous mobile-based interventions for HF were heterogeneous and varied between studies, which may lead to inconsistent results.
Given the uncertainty regarding the benefits of mobile apps for HF and their diverse functionality, we developed a mHealth platform to provide self-management interventions for patients with HF. This study aimed to evaluate whether this smartphone app–based intervention with Bluetooth-connected monitoring devices and a feedback system could improve the symptoms and clinical outcomes of HF.
Study Design and Population
The self-monitoring using a mobile app to improve symptoms and reduce rehospitalization and mortality in heart failure (SMART-HF) study is a prospective, multicenter, randomized, open-label trial to evaluate the efficacy of smartphone apps in improving the symptoms and outcomes of HF. A total of 7 tertiary university hospitals in South Korea participated in this study. The study design was registered at ClinicalTrials.gov (NCT05668000).
We enrolled patients aged older than 20 years who were hospitalized for acute HF with obvious symptoms or signs of HF at admission. Patients with N-terminal pro-B-type natriuretic peptide (NT-proBNP) levels ≥400 pg/mL or brain natriuretic peptide levels ≥100 pg/mL were enrolled. Since smartphone use was essential in this study, participants should be able to use Android smartphones well and be capable of following instructions on how to use apps. Patients with a baseline systolic blood pressure (BP) of <90 mm Hg or a resting heart rate (HR) of <50 beats per minute were excluded from this study. Additionally, patients were excluded if they had a cardiac [ 30 ] implantable electronic device that could interfere with body water analysis. Patients who were expected to have a prolonged hospital stay owing to medical problems other than HF were also excluded. The detailed inclusion and exclusion criteria are presented in Table S1 in Multimedia Appendix 1 .
Ethical Considerations
This clinical trial was approved by the institutional review boards of Seoul National University Bundang Hospital (B-2211-795-304) and other hospitals. The study was conducted in accordance with the Declaration of Helsinki, and all study data were deidentified. All patients provided written informed consent upon enrollment.
Patient Recruitment and Randomization
After a comprehensive interview, eligible participants were asked to provide written informed consent before being discharged from the hospital. At baseline (visit 1), all participants were assessed for sex, age, demographics, vital signs, comorbidities, laboratory data, and medication use. Eligible participants were randomized 1:1 to either the intervention group (app with Bluetooth-connected monitoring devices and feedback) or the control group (app only) using a web-based central randomization service ( Figure 1 A). Due to the nature of the intervention, the study participants and investigators interacting with the patients were not blinded to the group allocation.

Intervention and Follow-Up
We developed a mobile app and operating platform to improve the symptoms and outcomes of patients with HF. The app and platform comprised three parts: (1) a smartphone app for patients, (2) a dashboard system for physicians, and (3) a clinical decision support system (CDSS) on the platform ( Figure 1 B and Figures S1 and S2 in Multimedia Appendix 1 ).
The smartphone app comprised 4 menu screens ( Figure 2 ), and the detailed functions of the app are described in Table S2 in Multimedia Appendix 1 . In the “Today” menu, patients can enter their information, including body water, weight, BP, HR, symptom diary, medications, diet, and exercise. Patients can input their symptoms of HF, including dyspnea, fatigue, ankle edema, and palpitations, daily. Symptoms were scored as 0 (no symptoms), 1 (mild symptoms), 2 (moderate symptoms), and 3 (severe symptoms; Table S3 in Multimedia Appendix 1 ). Moreover, they could enter their daily diet or physical activity into the apps, and the amount of sodium in their diet was assessed through a camera image using AI (Figure S3 in Multimedia Appendix 1 ). Additionally, the BP, HR, weight, and body water could be entered into the app by the patients (intervention + control group) or automatically paired using Bluetooth-connected monitoring devices (intervention group only). In the “Records” menu, patients can access and review their historical data entered by date, as well as receive feedback regarding medication adherence, dietary habits, and exercise. Furthermore, the app can analyze and evaluate a patient’s symptom scores or measured vital signs and subsequently generate alerts based on a predefined algorithm (Tables S3 and S4 in Multimedia Appendix 1 ) using the CDSS. In the event of rapid changes in a patient’s symptoms or vital signs, the app prompts the patient to provide information regarding medication adherence, symptom severity, and any other concerns. Upon perceiving significant changes, it sends a message to the patients, advising them to contact their health care provider. In the “Contents” menu, the app can provide patients with information about HF, including symptoms, treatment, dietary guidelines, and exercise recommendations using chatbots.

The dashboard system was designed to present stored information to physicians (Figure S4 in Multimedia Appendix 1 ). This information included BP, HR, body weight, body water composition, HF symptom score, medications, diet, exercise, and any data entered by the patient. Physicians could easily observe the vital sign trends (daily, weekly, and monthly) of the participants. The CDSS analyzes the input data and generates recommendations for users using AI and algorithms. For example, it aggregates data and information from the patient and sends necessary messaging alerts to the patient’s app and, if needed, to the health care provider’s dashboard during office visits.
After randomization, both groups installed the study app on their Android smartphones, which the research nurse provided. Only the intervention group had full access to all functions of the app, and the apps were automatically paired with Bluetooth-connected monitoring devices, including a BP monitor, weight scale, and body water analyzer. The intervention group received feedback and alerts from the app. In the control group, patients could enter their BP, HR, and weight into the app using conventional non-Bluetooth devices only. Additionally, they received no feedback or alerts from the app. The “Contents” menu providing information about HF was available in both groups. The study aimed to evaluate the improvement in HF symptoms based on the functionality of the mobile app between the intervention and control groups, with a particular focus on Bluetooth-connected monitoring devices and the feedback function of the app.
We used a Bluetooth-enabled BP monitor BP170 (InBody Co). Bioimpedance analysis was performed using a portable multifrequency bioimpedance device (BWA ON; InBody Co) to analyze body water composition. Bioimpedance analysis has recently proven its efficacy in patients with acute HF with dyspnea [ 31 ], and the device can estimate body fluid status using extracellular water divided by total body water of the 4 limbs and trunk. The parameters of extracellular water divided by total body water <0.390, 0.390-0.400, and >0.400 were considered to be “normal,” “slightly over,” and “over,” respectively (Table S5 in Multimedia Appendix 1 ). The device classifies body water levels from levels 1 to 16.
Follow-up visits were scheduled at 2 (visit 2, optional) and 4 (visit 3) weeks after randomization. Both groups received standard care according to the current HF guidelines [ 20 , 32 ], and HF medication could be modified at the discretion of the treating physicians.
Study Outcomes
The primary end point of the study was the change in dyspnea symptom scores from baseline to 4 weeks, which was assessed using a questionnaire. We used a visual analog scale and a numerical rating scale for dyspnea ranging from 0 to 10 based on previous studies [ 33 , 34 ] (Figure S5 in Multimedia Appendix 1 ).
There were two secondary end points: (1) a composite outcome, including death, rehospitalization, and urgent visit for HF and (2) the change in body water composition from baseline to the last measurement in the intervention group. The exploratory clinical outcome was adherence to the app, which was calculated using either log-in access logs or the percentage of days when symptoms were entered into the app. App adherence was defined as follows:

We also compared app satisfaction scores at 4 weeks through a questionnaire, ranging from 0 to 10, between the intervention and control groups. If patients could not attend the scheduled study date, the outcome measures were assessed telephonically.
Sample Size and Statistical Analysis
Due to the absence of previous research and the pioneering nature of our intervention, our study adopts an exploratory approach. The primary objective was not to test a specific hypothesis regarding efficacy but rather to collect preliminary data and assess feasibility in preparation for a larger-scale study. Consequently, a sample size calculation was not conducted at this preliminary stage. Since this was a pilot study to explore the benefits of mobile apps for HF, we enrolled a total of 84 participants across 7 institutes, considering the duration of the study and the number of participating hospitals.
Categorical variables are reported as frequencies (percentages), and continuous variables are expressed as means (SD) or medians with IQR. Categorical variables were compared using the Pearson chi-square test or Fisher exact test, and continuous variables were compared using the Student t test or the Mann-Whitney U test. The change in body water composition from baseline to the last measurement after randomization was analyzed using the paired t test.
The intention-to-treat analysis included all randomized patients. The efficacy end points were primarily analyzed using the full analysis set, which included randomized participants who used the mobile app at least once. We also performed a per-protocol sensitivity analysis, including patients who completed the study protocol. For patients who dropped out before the end of the trial or had missing data, we used the latest available records for analysis, namely, the last observation carried forward method.
All tests were 2-tailed, and a P value <.05 was considered statistically significant. Statistical analyses were performed by using R (version 4.2.0; R Core Team).
Patient Enrollment and Clinical Characteristics
From October 2022 to January 2023, 132 patients from 7 centers were screened for eligibility, and 84 were randomly assigned to the intervention (n=43) or control (n=41) group ( Figure 3 and CONSORT [Consolidated Standards of Reporting Trials] checklist in Multimedia Appendix 2 ). After allocation, 7 patients (4 and 3 in the intervention and control groups, respectively) were excluded because they did not use the app at least once; thus, 77 patients (39 and 38 in the intervention and control groups, respectively) were included in the full analysis set.

The baseline characteristics of the groups are presented in Table 1 . The mean age of the total population was 62.1 (SD 14.7) years; 62% were male, 61% had de novo HF, and 30% had HF of an ischemic etiology. The median left ventricular ejection fraction was 38.7% (IQR 28%-58%) and the median NT-proBN P value was 2572 (IQR 1211-6651) pg/mL. The baseline characteristics, including age, sex, demographic data, laboratory values, and comorbidities, were well balanced between the 2 groups, except for diastolic BP. Additionally, the medication use at discharge was not significantly different between the 2 groups.
a HF: heart failure.
b NYHA: New York Heart Association.
c LVEF: left ventricular ejection fraction.
d eGFR: estimated glomerular filtration rate.
e NT-proBNP: N-terminal pro-B-type natriuretic peptide.
f RAS: renin-angiotensin system.
g SGLT2: sodium-glucose cotransporter 2.
Dyspnea Symptom Score
The dyspnea symptom scores assessed using the questionnaire are presented in Table 2 and Figure 4 . The baseline dyspnea symptom scores were not significantly different between the 2 groups (intervention vs control: mean 2.4, SD 2.8 vs mean 2.0, SD 2.0; P =.46). Regarding the primary end point, change in dyspnea symptom score from baseline to 4 weeks was significantly greater in the intervention group than in the control group (mean –1.3, SD 2.1 vs mean –0.3, SD 2.3; P =.048).

Secondary End Points
Secondary composite end points, including death, rehospitalization, and urgent visit for HF, were not significantly different between the 2 groups (3/39 vs 3/38; P >.99). A significant reduction was found in the level of body water composition from baseline to the last measurement in the intervention group (baseline level mean 7.4, SD 2.5 vs final level mean 6.6, SD 2.5; P =.003; Figure 5 ).

App Adherence and Patient Satisfaction
The distribution of app adherence among the treatment and control groups is presented in Figure 6 . App adherence, which was assessed based on log-in access logs was higher in the intervention group than in the control group (80.0%, IQR 45.0%-96.8% vs 39.3%, IQR 20.6%-77.4%; P =.003). Additionally, the app adherence evaluated based on the percentage of days when symptom was entered into the app was significantly higher in the intervention group than in the control group (58.6%, IQR 19.2%-79.5% vs 30.6%, IQR 9.1%-64.3%; P =.046).

We also analyzed differences in app adherence according to age. Patients aged 65 years and older showed lower app adherence, as indicated by log-in access logs than those aged younger than 65 years in the overall population (45.9%, IQR 8.9%-79.2% vs 74.9%, IQR 35.7%-95.5%; P =.02). The intervention group showed similar results (61.4%, IQR 13.5%-89.5% vs 92.9%, IQR 64.2%-98.5%; P =.04); however, in the control group, no significant difference was found in app adherence according to the age cutoff of 65 years (39.4%, IQR 5.7%-76.7% vs 39.3%, IQR 27.8%-74.6%; P =.40).
App satisfaction scores at 4 weeks tended to be higher in the intervention group than in the control group; however, the difference was not statistically significant (mean 7.1, SD 2.6 vs mean 6.2, SD 2.9; P =.15).
Sensitivity Analysis
Overall, 74 patients (37 in the intervention group and 37 in the control group) completed the trial over 4 weeks (per-protocol analysis; Figure 3 ). Table S6 and Figure S6 in Multimedia Appendix 1 show the results of sensitivity analyses of the per-protocol population. There was a trend toward a greater reduction in dyspnea symptom scores from baseline to 4 weeks in the intervention group than in the control group, similar to the primary analyses (mean –1.4, SD 2.1 vs mean –0.5, SD 2.1; P =.08). Regarding body water composition, the intervention group showed a greater reduction in the level of body water composition from baseline to last measurement, consistent with the main analysis (baseline level mean 7.4, SD 2.5 vs final level mean 6.6, SD 2.6; P =.006).
Principal Results
We developed a smartphone app and mHealth platform for patients with HF. The main findings of this study are (1) a mobile-based health platform with Bluetooth-connected monitoring devices and feedback improved dyspnea symptoms compared with the control group and (2) this app-based intervention led to an improvement in the level of body water composition and higher adherence to the app. We believe that the findings of this prospective, randomized study will help in formulating public health strategies to improve HF outcomes using smartphones.
Support for facilitating self-care is crucial for patients with HF [ 18 - 20 ]. However, proper patient self-care and delivery of health care provider feedback are challenging to implement in a real-world clinical setting because of the required time, financial, and human resources. It is challenging for patients to know how to respond to changes in their symptoms or vital signs. It is also impossible for medical staff to quickly check patient information or provide feedback 24 hours a day, 7 days a week. These disadvantages can be overcome by self-management interventions via smartphones, including self-care, symptom and sign monitoring, and mobile-based feedback, which are easily accessible anytime, anywhere. Considering these advantages of a smartphone app–based intervention, including cost-effective and accessible methods, our group has previously developed mobile app platforms for patients with atrial fibrillation [ 35 , 36 ] or hypertension [ 37 ]. Therefore, we planned to develop an app for patients with HF.
Our mobile app for HF provides diverse functions using the concept of mHealth platforms for personalized interventions. Vital signs and body water can be paired with Bluetooth-connected devices, and patients can enter their HF symptoms into the apps. Patients can also receive feedback or alerts from the app based on the information they have entered. Additionally, patients can obtain information about HF using chatbots. Although direct interaction between patients and health care providers may be an ideal situation, it may not be feasible owing to rising costs and a shortage of medical staff. Our app-based monitoring and feedback system had the advantage of being accessible and quick for patients to receive feedback promptly on their changes without high personnel or cost. Although patients in the control group had access to the smartphone app for HF self-care, the improvement in dyspnea symptoms was more pronounced in the intervention group than in the control group. Patients in the control group could only use the limited functionality of the app, were unable to use Bluetooth devices, and could not receive any feedback or alerts. Therefore, our study suggests that the comprehensive functionality of apps, especially in the monitoring and feedback system, is particularly important for their role.
Comparison With Prior Work
Some previous studies have focused on mHealth apps for HF [ 24 - 30 ]. However, these studies were limited by the small number of participants and limited app functionality. Additionally, previous mobile apps have heterogeneous functions and platforms [ 24 , 25 ], and this diversity might have influenced the benefits of mobile apps in HF. Considering the inconsistent benefits of mobile apps in HF [ 25 ], we developed a mobile app with multiple functions in collaboration with 1 of the largest high-tech information technology companies in Korea (KT). In our opinion, this app has a considerably wider range of features and is more efficiently organized than other existing apps [ 28 - 30 ]. Our app has the advantage of keeping patients engaged in their condition and self-management after leaving the hospital. Not only does this provide psychological reassurance, but it also serves the patient’s best interests by keeping a close eye on any changes in their clinical presentation or symptoms. Patients can assess their symptoms more frequently, make lifestyle changes, or contact health care providers earlier. The dashboard system also enables health care professionals to identify changes in a patient’s condition quickly. Furthermore, the app contains elements that may be of interest to patients, including sodium analysis in food using AI and various HF information. Overall, we assumed that the monitoring and feedback function and app adherence are especially important for the mobile app’s role in HF and believe that the benefits of our app could improve the symptoms of HF.
Increasing app adherence has been a common challenge in many studies. For example, a recent study showed that only approximately 30% of patients actively used the HF app, underscoring the importance of caution regarding the enrollment of critically ill, postacute, and older patients [ 30 ]. In our study, median adherence to apps based on log-in access logs was 80% and 39% in the intervention and control groups, respectively, which we believe should be further improved. In the intervention group, we believe that a more comprehensive functionality of apps, including Bluetooth-connected monitoring devices and a feedback system, may lead to higher adherence to apps than in the control group. Additionally, this disparity in app functionality may be associated with a higher trend in app satisfaction scores within the intervention group.
Particularly, as a large proportion of patients with HF tend to be older and app adherence appears to decline in those aged 65 years and older in our study, increasing adherence to apps for HF is crucial; this can be achieved by improving the platform to make it more user-friendly for older patients. Interestingly, at the cutoff age of 65 years, a difference was observed in app adherence according to age category in the intervention group but not in the control group, probably due to overall higher app adherence in the intervention group. In the subgroup analysis, no difference was found in the change of dyspnea symptom scores between the intervention and control groups according to an app adherence cutoff of 50%. This is probably due to the small sample size. Therefore, further research with larger study populations is warranted to confirm whether the frequency of app usage directly affects the outcomes.
Limitations and Strengths
This study had some limitations. First, our results are limited by the small sample size of the study. Second, we only enrolled patients who had smartphones and could use them; therefore, the results of our trial may not be applicable to patients who cannot use smartphones, such as very old adults. Since a large proportion of patients with HF are older and have difficulty using smartphones, excluding them from participation may lead to bias and study limitations. Third, the true engagement and use frequency of the apps and the real responses of participants to feedback could not be analyzed. There is a lack of clarity on exactly how the frequency of app use affects clinical outcomes. Additionally, there was a relatively large number of older patients, and adherence to the app declined toward the end of the study. These limitations are commonly observed in studies that use mobile apps. Fourth, we evaluated the dyspnea symptom score using a visual analog scale and a numerical rating scale ranging from 0 to 10. However, at baseline, the average of these scores was <3 points, suggesting that this study was conducted on patients who already had achieved significant improvement in their dyspnea, which limits surveying on a scale of 0-10 over 4 weeks. Fifth, the HF medications could be modified at the discretion of each physician, which could be a confounding factor. Sixth, baseline diastolic BP was higher in the intervention group than in the control group, while patients in the intervention group tended to be younger. This difference in baseline characteristics may limit the generalizability of our conclusions. Seventh, the follow-up period in our studies was only 4 weeks, which was shorter than that in a similar study [ 29 ]; therefore, the effect of mobile apps on patients’ symptoms for a long-term follow-up period was unknown. Furthermore, secondary clinical outcomes, including death, rehospitalization, and urgent visits for HF, were not significantly different between the 2 groups in this study, although a similar study using apps [ 28 ] for HF showed reduced hospitalization for HF by mobile app. Finally, we tested a single app, and our results may not be generalizable to other smartphone-based apps for patients with HF.
Despite these limitations, the major strength of this study is the use of a mobile app with various functions developed in collaboration with a cutting-edge technology company. We believe that the features of our app can be effective solutions for improving self-care in patients with HF.
Conclusions
The smartphone app–based intervention with Bluetooth-connected monitoring devices and feedback improved dyspnea symptoms among patients with HF compared with the control group. Considering the high cost of classical patient-provider interventions, self-care, and feedback through mobile apps are promising alternatives. Therefore, our study provides evidence and rationale for mobile app–based self-care and feedback for patients with HF.
Acknowledgments
The app used in this study was developed in collaboration between the Digital Health Council (DHC) from the Korean Society of Heart Failure and KT Corporation. This research was supported by KT’s Internalization of Digital Therapeutics Business project. This research was supported by a grant from the Korea Health Technology Research & Development Project through the Korea Health Industry Development Institute (KHIDI), funded by the Ministry of Health & Welfare, Republic of Korea (HI21C1074).
Data Availability
The data sets generated and analyzed during this study are available from the corresponding author upon reasonable request.
Conflicts of Interest
None declared.
App characteristics and functionalities, and participants’ clinical characteristics and outcomes.
CONSORT-EHEALTH checklist.
- Shahim B, Kapelios CJ, Savarese G, Lund LH. Global public health burden of heart failure: an updated review. Card Fail Rev. 2023;9:e11. [ FREE Full text ] [ CrossRef ] [ Medline ]
- GBD 2017 Disease and Injury Incidence and Prevalence Collaborators. Global, regional, and national incidence, prevalence, and years lived with disability for 354 diseases and injuries for 195 countries and territories, 1990-2017: a systematic analysis for the Global Burden of Disease Study 2017. Lancet. 2018;392(10159):1789-1858. [ FREE Full text ] [ CrossRef ] [ Medline ]
- Park JJ, Lee CJ, Park SJ, Choi JO, Choi S, Park SM, et al. Heart failure statistics in Korea, 2020: a report from the Korean Society of Heart Failure. Int J Heart Fail. 2021;3(4):224-236. [ FREE Full text ] [ CrossRef ] [ Medline ]
- Conrad N, Judge A, Tran J, Mohseni H, Hedgecott D, Crespillo AP, et al. Temporal trends and patterns in heart failure incidence: a population-based study of 4 million individuals. Lancet. 2018;391(10120):572-580. [ FREE Full text ] [ CrossRef ] [ Medline ]
- Cho JY, Cho DH, Youn JC, Kim D, Park SM, Jung MH, et al. Korean Society of Heart Failure guidelines for the management of heart failure: definition and diagnosis. Int J Heart Fail. 2023;5(2):51-65. [ FREE Full text ] [ CrossRef ] [ Medline ]
- Kimmoun A, Takagi K, Gall E, Ishihara S, Hammoum P, El Bèze N, et al. Temporal trends in mortality and readmission after acute heart failure: a systematic review and meta-regression in the past four decades. Eur J Heart Fail. 2021;23(3):420-431. [ FREE Full text ] [ CrossRef ] [ Medline ]
- Bueno H, Ross JS, Wang Y, Chen J, Vidán MT, Normand SLT, et al. Trends in length of stay and short-term outcomes among Medicare patients hospitalized for heart failure, 1993-2006. JAMA. 2010;303(21):2141-2147. [ FREE Full text ] [ CrossRef ] [ Medline ]
- Bozkurt B, Savarese G, Eryd SA, Bodegård J, Cleland JGF, Khordoc C, et al. Mortality, outcomes, costs, and use of medicines following a first heart failure hospitalization: EVOLUTION HF. JACC Heart Fail. 2023;11(10):1320-1332. [ FREE Full text ] [ CrossRef ] [ Medline ]
- Maggioni AP, Dahlström U, Filippatos G, Chioncel O, Leiro MC, Drozdz J, et al. EURObservational Research Programme: regional differences and 1-year follow-up results of the Heart Failure Pilot Survey (ESC-HF Pilot). Eur J Heart Fail. 2013;15(7):808-817. [ FREE Full text ] [ CrossRef ] [ Medline ]
- Lee SE, Lee HY, Cho HJ, Choe WS, Kim H, Choi JO, et al. Clinical characteristics and outcome of acute heart failure in Korea: results from the Korean Acute Heart Failure Registry (KorAHF). Korean Circ J. 2017;47(3):341-353. [ FREE Full text ] [ CrossRef ] [ Medline ]
- Khan MS, Sreenivasan J, Lateef N, Abougergi MS, Greene SJ, Ahmad T, et al. Trends in 30- and 90-day readmission rates for heart failure. Circ Heart Fail. 2021;14(4):e008335. [ FREE Full text ] [ CrossRef ] [ Medline ]
- Mozaffarian D, Benjamin EJ, Go AS, Arnett DK, Blaha MJ, Cushman M, et al. Heart disease and stroke statistics--2015 update: a report from the American Heart Association. Circulation. 2015;131(4):e29-322. [ FREE Full text ] [ CrossRef ] [ Medline ]
- Rich MW, Beckham V, Wittenberg C, Leven CL, Freedland KE, Carney RM. A multidisciplinary intervention to prevent the readmission of elderly patients with congestive heart failure. N Engl J Med. 1995;333(18):1190-1195. [ FREE Full text ] [ CrossRef ] [ Medline ]
- Koelling TM, Johnson ML, Cody RJ, Aaronson KD. Discharge education improves clinical outcomes in patients with chronic heart failure. Circulation. 2005;111(2):179-185. [ FREE Full text ] [ CrossRef ] [ Medline ]
- McAlister FA, Stewart S, Ferrua S, McMurray JJJV. Multidisciplinary strategies for the management of heart failure patients at high risk for admission: a systematic review of randomized trials. J Am Coll Cardiol. 2004;44(4):810-819. [ FREE Full text ] [ CrossRef ] [ Medline ]
- Bhatia A, Maddox TM. Remote patient monitoring in heart failure: factors for clinical efficacy. Int J Heart Fail. 2021;3(1):31-50. [ FREE Full text ] [ CrossRef ] [ Medline ]
- Jonkman NH, Westland H, Groenwold RHH, Ågren S, Atienza F, Blue L, et al. Do self-management interventions work in patients with heart failure? An individual patient data meta-analysis. Circulation. 2016;133(12):1189-1198. [ FREE Full text ] [ CrossRef ] [ Medline ]
- McDonagh TA, Metra M, Adamo M, Gardner RS, Baumbach A, Böhm M, et al. Corrigendum to: 2021 ESC Guidelines for the diagnosis and treatment of acute and chronic heart failure: developed by the Task Force for the diagnosis and treatment of acute and chronic heart failure of the European Society of Cardiology (ESC) with the special contribution of the Heart Failure Association (HFA) of the ESC. Eur Heart J. 2021;42(48):4901. [ FREE Full text ] [ CrossRef ] [ Medline ]
- Heidenreich PA, Bozkurt B, Aguilar D, Allen LA, Byun JJ, Colvin MM, et al. 2022 AHA/ACC/HFSA Guideline for the Management of Heart Failure: a report of the American College of Cardiology/American Heart Association joint committee on clinical practice guidelines. J Am Coll Cardiol. 2022;79(17):e263-e421. [ FREE Full text ] [ CrossRef ] [ Medline ]
- Youn JC, Kim D, Cho JY, Cho DH, Park SM, Jung MH, et al. Korean Society of Heart Failure guidelines for the management of heart failure: treatment. Int J Heart Fail. 2023;5(2):66-81. [ FREE Full text ] [ CrossRef ] [ Medline ]
- Cruz-Ramos NA, Alor-Hernández G, Colombo-Mendoza LO, Sánchez-Cervantes JL, Rodríguez-Mazahua L, Guarneros-Nolasco LR. mHealth apps for self-management of cardiovascular diseases: a scoping review. Healthcare (Basel). 2022;10(2):322. [ FREE Full text ] [ CrossRef ] [ Medline ]
- Kalagara R, Chennareddy S, Scaggiante J, Matsoukas S, Bhimani A, Smith C, et al. Blood pressure management through application-based telehealth platforms: a systematic review and meta-analysis. J Hypertens. 2022;40(7):1249-1256. [ CrossRef ] [ Medline ]
- Alessa T, Abdi S, Hawley MS, de Witte L. Mobile apps to support the self-management of hypertension: systematic review of effectiveness, usability, and user satisfaction. JMIR Mhealth Uhealth. 2018;6(7):e10723. [ FREE Full text ] [ CrossRef ] [ Medline ]
- Wali S, Demers C, Shah H, Wali H, Lim D, Naik N, et al. Evaluation of heart failure apps to promote self-care: systematic app search. JMIR Mhealth Uhealth. 2019;7(11):e13173. [ FREE Full text ] [ CrossRef ] [ Medline ]
- Athilingam P, Jenkins B. Mobile phone apps to support heart failure self-care management: integrative review. JMIR Cardio. 2018;2(1):e10057. [ FREE Full text ] [ CrossRef ] [ Medline ]
- Mortara A, Vaira L, Palmieri V, Iacoviello M, Battistoni I, Iacovoni A, et al. Would you prescribe mobile health apps for heart failure self-care? An integrated review of commercially available mobile technology for heart failure patients. Card Fail Rev. 2020;6:e13. [ FREE Full text ] [ CrossRef ] [ Medline ]
- Giordan LB, Tong HL, Atherton JJ, Ronto R, Chau J, Kaye D, et al. The use of mobile apps for heart failure self-management: systematic review of experimental and qualitative studies. JMIR Cardio. 2022;6(1):e33839. [ FREE Full text ] [ CrossRef ] [ Medline ]
- Sahlin D, Rezanezad B, Edvinsson ML, Bachus E, Melander O, Gerward S. Self-care management intervention in heart failure (SMART-HF): a multicenter randomized controlled trial. J Card Fail. 2022;28(1):3-12. [ FREE Full text ] [ CrossRef ] [ Medline ]
- Dorsch MP, Farris KB, Rowell BE, Hummel SL, Koelling TM. The effects of the ManageHF4Life mobile app on patients with chronic heart failure: randomized controlled trial. JMIR Mhealth Uhealth. 2021;9(12):e26185. [ FREE Full text ] [ CrossRef ] [ Medline ]
- Zisis G, Carrington MJ, Oldenburg B, Whitmore K, Lay M, Huynh Q, et al. An m-Health intervention to improve education, self-management, and outcomes in patients admitted for acute decompensated heart failure: barriers to effective implementation. Eur Heart J Digit Health. 2021;2(4):649-657. [ FREE Full text ] [ CrossRef ] [ Medline ]
- Park CS, Lee SE, Cho HJ, Kim YJ, Kang HJ, Oh BH, et al. Body fluid status assessment by bio-impedance analysis in patients presenting to the emergency department with dyspnea. Korean J Intern Med. 2018;33(5):911-921. [ FREE Full text ] [ CrossRef ] [ Medline ]
- Park SM, Lee SY, Jung MH, Youn JC, Kim D, Cho JY, et al. Korean Society of Heart Failure guidelines for the management of heart failure: management of the underlying etiologies and comorbidities of heart failure. Korean Circ J. 2023;53(7):425-451. [ FREE Full text ] [ CrossRef ] [ Medline ]
- Zhang X, Zhao C, Zhang H, Liu W, Zhang J, Chen Z, et al. Dyspnea measurement in acute heart failure: a systematic review and evidence map of randomized controlled trials. Front Med (Lausanne). 2021;8:728772. [ FREE Full text ] [ CrossRef ] [ Medline ]
- Placido R, Gigaud C, Gayat E, Ferry A, Cohen-Solal A, Plaisance P, et al. Assessment of dyspnoea in the emergency department by numeric and visual scales: a pilot study. Anaesth Crit Care Pain Med. 2015;34(2):95-99. [ CrossRef ] [ Medline ]
- Kim IC, Lee JH, Choi DJ, Park SJ, Lee JH, Park SM, et al. Rationale design and efficacy of a smartphone application for improving self-awareness of adherence to edoxaban treatment: study protocol for a randomised controlled trial (adhere app). BMJ Open. 2022;12(4):e048777. [ FREE Full text ] [ CrossRef ] [ Medline ]
- Yoon M, Park JJ, Hur T, Hua CH, Shim CY, Yoo BS, et al. The ReInforcement of adherence via self-monitoring app orchestrating biosignals and medication of RivaroXaban in patients with atrial fibrillation and co-morbidities: a study protocol for a randomized controlled trial (RIVOX-AF). Front Cardiovasc Med. 2023;10:1130216. [ FREE Full text ] [ CrossRef ] [ Medline ]
- Choi DJ, Park JJ, Yoon M, Park SJ, Jo SH, Kim EJ, et al. Self-monitoring of blood pressure and feed-back using APP in TReatment of UnconTrolled Hypertension (SMART-BP): a randomized clinical trial. Korean Circ J. 2022;52(10):785-794. [ FREE Full text ] [ CrossRef ] [ Medline ]
Abbreviations
Edited by A Mavragani; submitted 22.08.23; peer-reviewed by O El-Gayar, P Athilingam; comments to author 18.10.23; revised version received 07.11.23; accepted 12.03.24; published 29.04.24.
©Minjae Yoon, Seonhwa Lee, Jah Yeon Choi, Mi-Hyang Jung, Jong-Chan Youn, Chi Young Shim, Jin-Oh Choi, Eung Ju Kim, Hyungseop Kim, Byung-Su Yoo, Yeon Joo Son, Dong-Ju Choi. Originally published in the Journal of Medical Internet Research (https://www.jmir.org), 29.04.2024.
This is an open-access article distributed under the terms of the Creative Commons Attribution License (https://creativecommons.org/licenses/by/4.0/), which permits unrestricted use, distribution, and reproduction in any medium, provided the original work, first published in the Journal of Medical Internet Research, is properly cited. The complete bibliographic information, a link to the original publication on https://www.jmir.org/, as well as this copyright and license information must be included.
Zora Neale Hurston Research Paper
This essay about Zora Neale Hurston examines her profound impact on American literature. Highlighting her Southern heritage and influence from the Harlem Renaissance, it details her life’s story from her roots in Eatonville, Florida, to her academic and literary milestones. The essay reflects on her major works, including “Their Eyes Were Watching God,” emphasizing her exploration of Black womanhood and her resilient spirit despite personal hardships. Hurston is portrayed not just as a writer, but as a visionary who infused her work with the culture and experiences of African American life.
How it works
In the rich mosaic of American literature, few figures shine as brightly or contribute as distinctively as Zora Neale Hurston. Her writing, steeped in the essence of her Southern roots and the vibrant pulse of the Harlem Renaissance, represents a distinct blend of resilience, authenticity, and relentless determination.
Hurston was born in 1891 in Eatonville, Florida, a fully incorporated African American town where her father served as mayor. Surrounded by the evocative sights and sounds of this unique community, she absorbed the vibrant culture of African American life, which later vividly colored her narrative style.
Hurston’s literary career reflects a series of peaks and valleys, mirroring the turbulent times she lived through. From her educational beginnings at Howard University to her immersion in the dynamic scene of 1920s Harlem, she captured her experiences in a style rich with the texture of authenticity.
Her initial major work, “Jonah’s Gourd Vine” (1934), explores Southern life, weaving the region’s rich narratives into her storytelling. Through characters inspired by her own memories, Hurston crafted a world both enchanting and rooted in realism, showcasing the profound capability of stories to bridge time and place.
However, it was “Their Eyes Were Watching God” (1937) that elevated Hurston to literary prominence. Through the character of Janie Crawford, Hurston explored the depths of Black womanhood, creating a story that resonated with the universal themes of desire and resilience.
Despite her critical success, Hurston faced numerous personal and financial challenges. Yet, she continued to produce a wide range of literature, including novels, essays, and plays, demonstrating her unwavering creative spirit.
As we explore Hurston’s literary contributions today, we see her not just as an author, but as a creator of worlds, a dream weaver, and a keeper of her culture’s spirit. Her writings do more than recall the past; they serve as a perennial light, illuminating the complex journey of human experience.

Cite this page
Zora Neale Hurston Research Paper. (2024, Apr 29). Retrieved from https://papersowl.com/examples/zora-neale-hurston-research-paper/
"Zora Neale Hurston Research Paper." PapersOwl.com , 29 Apr 2024, https://papersowl.com/examples/zora-neale-hurston-research-paper/
PapersOwl.com. (2024). Zora Neale Hurston Research Paper . [Online]. Available at: https://papersowl.com/examples/zora-neale-hurston-research-paper/ [Accessed: 29 Apr. 2024]
"Zora Neale Hurston Research Paper." PapersOwl.com, Apr 29, 2024. Accessed April 29, 2024. https://papersowl.com/examples/zora-neale-hurston-research-paper/
"Zora Neale Hurston Research Paper," PapersOwl.com , 29-Apr-2024. [Online]. Available: https://papersowl.com/examples/zora-neale-hurston-research-paper/. [Accessed: 29-Apr-2024]
PapersOwl.com. (2024). Zora Neale Hurston Research Paper . [Online]. Available at: https://papersowl.com/examples/zora-neale-hurston-research-paper/ [Accessed: 29-Apr-2024]
Don't let plagiarism ruin your grade
Hire a writer to get a unique paper crafted to your needs.
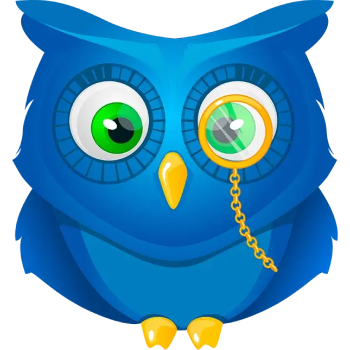
Our writers will help you fix any mistakes and get an A+!
Please check your inbox.
You can order an original essay written according to your instructions.
Trusted by over 1 million students worldwide
1. Tell Us Your Requirements
2. Pick your perfect writer
3. Get Your Paper and Pay
Hi! I'm Amy, your personal assistant!
Don't know where to start? Give me your paper requirements and I connect you to an academic expert.
short deadlines
100% Plagiarism-Free
Certified writers
Numbers, Facts and Trends Shaping Your World
Read our research on:
Full Topic List
Regions & Countries
- Publications
- Our Methods
- Short Reads
- Tools & Resources
Read Our Research On:
About 1 in 5 U.S. teens who’ve heard of ChatGPT have used it for schoolwork
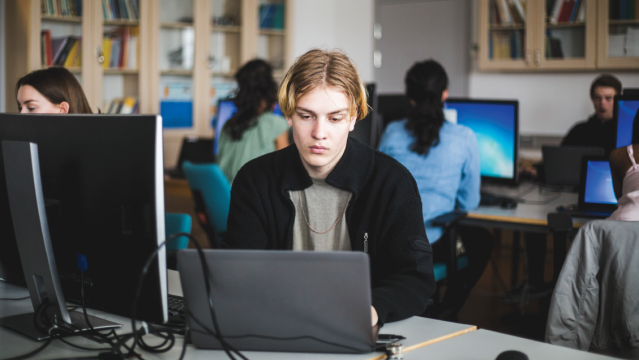
Roughly one-in-five teenagers who have heard of ChatGPT say they have used it to help them do their schoolwork, according to a new Pew Research Center survey of U.S. teens ages 13 to 17. With a majority of teens having heard of ChatGPT, that amounts to 13% of all U.S. teens who have used the generative artificial intelligence (AI) chatbot in their schoolwork.
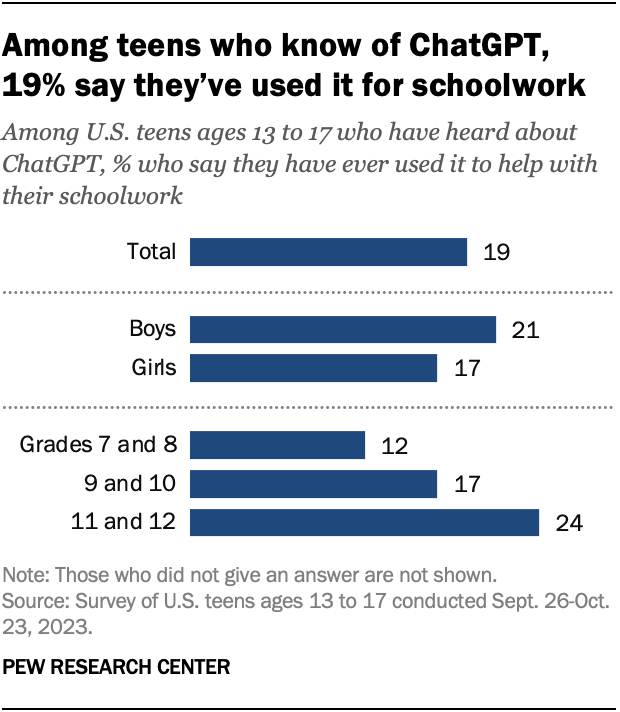
Teens in higher grade levels are particularly likely to have used the chatbot to help them with schoolwork. About one-quarter of 11th and 12th graders who have heard of ChatGPT say they have done this. This share drops to 17% among 9th and 10th graders and 12% among 7th and 8th graders.
There is no significant difference between teen boys and girls who have used ChatGPT in this way.
The introduction of ChatGPT last year has led to much discussion about its role in schools , especially whether schools should integrate the new technology into the classroom or ban it .
Pew Research Center conducted this analysis to understand American teens’ use and understanding of ChatGPT in the school setting.
The Center conducted an online survey of 1,453 U.S. teens from Sept. 26 to Oct. 23, 2023, via Ipsos. Ipsos recruited the teens via their parents, who were part of its KnowledgePanel . The KnowledgePanel is a probability-based web panel recruited primarily through national, random sampling of residential addresses. The survey was weighted to be representative of U.S. teens ages 13 to 17 who live with their parents by age, gender, race and ethnicity, household income, and other categories.
This research was reviewed and approved by an external institutional review board (IRB), Advarra, an independent committee of experts specializing in helping to protect the rights of research participants.
Here are the questions used for this analysis , along with responses, and its methodology .
Teens’ awareness of ChatGPT
Overall, two-thirds of U.S. teens say they have heard of ChatGPT, including 23% who have heard a lot about it. But awareness varies by race and ethnicity, as well as by household income:
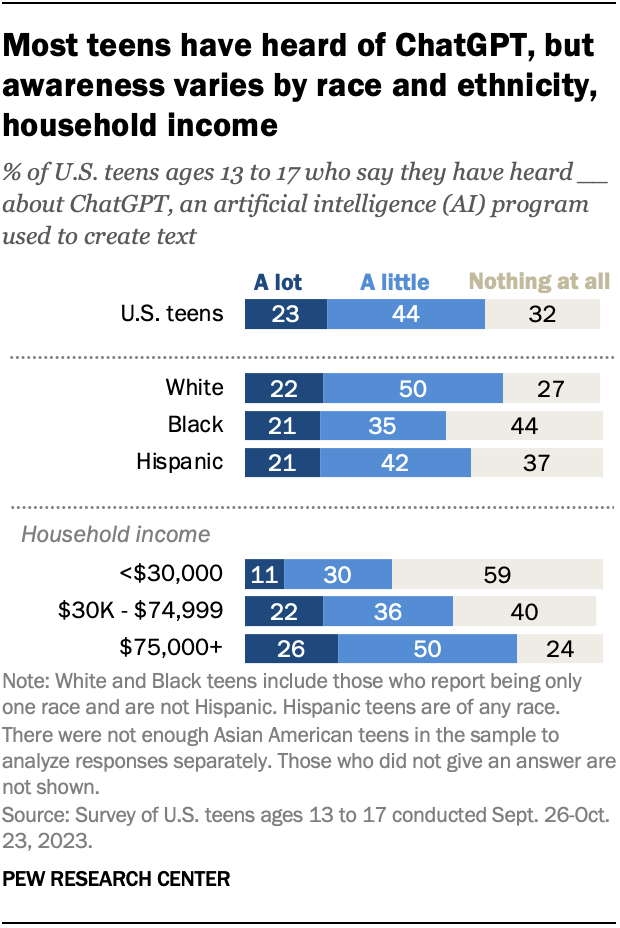
- 72% of White teens say they’ve heard at least a little about ChatGPT, compared with 63% of Hispanic teens and 56% of Black teens.
- 75% of teens living in households that make $75,000 or more annually have heard of ChatGPT. Much smaller shares in households with incomes between $30,000 and $74,999 (58%) and less than $30,000 (41%) say the same.
Teens who are more aware of ChatGPT are more likely to use it for schoolwork. Roughly a third of teens who have heard a lot about ChatGPT (36%) have used it for schoolwork, far higher than the 10% among those who have heard a little about it.
When do teens think it’s OK for students to use ChatGPT?
For teens, whether it is – or is not – acceptable for students to use ChatGPT depends on what it is being used for.
There is a fair amount of support for using the chatbot to explore a topic. Roughly seven-in-ten teens who have heard of ChatGPT say it’s acceptable to use when they are researching something new, while 13% say it is not acceptable.
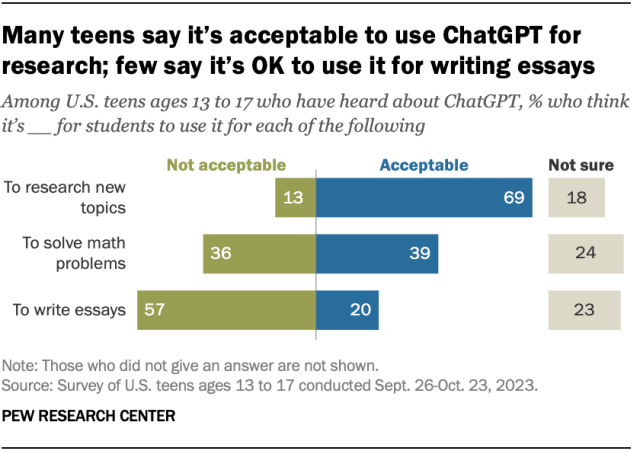
However, there is much less support for using ChatGPT to do the work itself. Just one-in-five teens who have heard of ChatGPT say it’s acceptable to use it to write essays, while 57% say it is not acceptable. And 39% say it’s acceptable to use ChatGPT to solve math problems, while a similar share of teens (36%) say it’s not acceptable.
Some teens are uncertain about whether it’s acceptable to use ChatGPT for these tasks. Between 18% and 24% say they aren’t sure whether these are acceptable use cases for ChatGPT.
Those who have heard a lot about ChatGPT are more likely than those who have only heard a little about it to say it’s acceptable to use the chatbot to research topics, solve math problems and write essays. For instance, 54% of teens who have heard a lot about ChatGPT say it’s acceptable to use it to solve math problems, compared with 32% among those who have heard a little about it.
Note: Here are the questions used for this analysis , along with responses, and its methodology .
- Artificial Intelligence
- Technology Adoption
- Teens & Tech
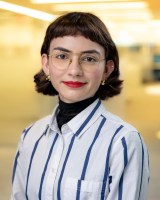
Olivia Sidoti is a research assistant focusing on internet and technology research at Pew Research Center
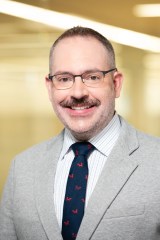
Jeffrey Gottfried is an associate director focusing on internet and technology research at Pew Research Center
Many Americans think generative AI programs should credit the sources they rely on
Americans’ use of chatgpt is ticking up, but few trust its election information, q&a: how we used large language models to identify guests on popular podcasts, striking findings from 2023, what the data says about americans’ views of artificial intelligence, most popular.
1615 L St. NW, Suite 800 Washington, DC 20036 USA (+1) 202-419-4300 | Main (+1) 202-857-8562 | Fax (+1) 202-419-4372 | Media Inquiries
Research Topics
- Age & Generations
- Coronavirus (COVID-19)
- Economy & Work
- Family & Relationships
- Gender & LGBTQ
- Immigration & Migration
- International Affairs
- Internet & Technology
- Methodological Research
- News Habits & Media
- Non-U.S. Governments
- Other Topics
- Politics & Policy
- Race & Ethnicity
- Email Newsletters
ABOUT PEW RESEARCH CENTER Pew Research Center is a nonpartisan fact tank that informs the public about the issues, attitudes and trends shaping the world. It conducts public opinion polling, demographic research, media content analysis and other empirical social science research. Pew Research Center does not take policy positions. It is a subsidiary of The Pew Charitable Trusts .
Copyright 2024 Pew Research Center
Terms & Conditions
Privacy Policy
Cookie Settings
Reprints, Permissions & Use Policy
- Share full article
Advertisement
Supported by
Guest Essay
Government Surveillance Keeps Us Safe

By Matthew Waxman and Adam Klein
Mr. Waxman served in senior national security roles in the George W. Bush administration. Mr. Klein served as the chairman of the Privacy and Civil Liberties Oversight Board from 2018 to 2021.
This is an extraordinarily dangerous time for the United States and our allies. Israel’s unpreparedness on Oct. 7 shows that even powerful nations can be surprised in catastrophic ways. Fortunately, Congress, in a rare bipartisan act, voted early Saturday to reauthorize a key intelligence power that provides critical information on hostile states and threats ranging from terrorism to fentanyl trafficking.
Civil libertarians argued that the surveillance bill erodes Americans’ privacy rights and pointed to examples when American citizens got entangled in investigations. Importantly, the latest version of the bill adds dozens of legal safeguards around the surveillance in question — the most expansive privacy reform to the legislation in its history. The result preserves critical intelligence powers while protecting Americans’ privacy rights in our complex digital age.
At the center of the debate is the Foreign Intelligence Surveillance Act. Originally passed in 1978, it demanded that investigators gain an order from a special court to surveil foreign agents inside the United States. Collecting the communications of foreigners abroad did not require court approval.
That line blurred in the digital age. Many foreign nationals rely on American providers such as Google and Meta, which route or store data in the United States, raising questions as to whether the rules apply to where the targets are or where their data is collected. In 2008, Congress addressed that conundrum with Section 702. Instead of requiring the government to seek court orders for each foreign target, that provision requires yearly judicial approval of the rules that govern the program as a whole. That way, the government can efficiently obtain from communication providers the calls and messages of large numbers of foreign targets — 246,073 in 2022 alone.
Since then, Section 702 has supplied extraordinary insight into foreign dangers, including military threats, theft of American trade secrets, terrorism, hacking and fentanyl trafficking. In 2022 intelligence from 702 helped the government find and kill the Qaeda leader Ayman al-Zawahri, one of the terrorists responsible for Sept. 11. Almost 60 percent of the articles in the president’s daily intelligence briefing include information from Section 702.
Although Section 702 can be used only to target foreigners abroad, it does include Americans when they interact with foreign targets. Not only is such incidental collection inevitable in today’s globalized world; it can be vital to U.S. security. If a terrorist or spy abroad is communicating with someone here, our government must find out why.
Some of what is found via Section 702 is therefore sent from the National Security Agency to the F.B.I. The F.B.I., which investigates threats to national security in the United States, can then check that database for Americans under investigation for national security reasons.
We agree that those queries raise legitimate privacy concerns. And those concerns are especially acute for public officials and journalists whose communications with foreign officials and other potential intelligence targets may be sensitive for political or professional reasons.
It is also true that the F.B.I. has broken the rules around these 702 database checks repeatedly in recent years. Agents ran improper queries related to elected officials and political protests. The wiretaps of Carter Page, a former Trump campaign adviser, also involved numerous violations of FISA rules. The Page wiretaps involved traditional FISA orders, not Section 702, but the bureau’s many errors there raised understandable doubts about whether it can be trusted to comply with other FISA rules.
Fortunately, there are ways to prevent abuses of Section 702 without compromising its critical national security value. The bill passed by Congress contains numerous reforms that will dramatically improve compliance. It sharply limits the number and ranks of F.B.I. agents who can run 702 queries, imposes strict penalties for misconduct and expands oversight by Congress and the courts.
Some of the bill’s critics argued that the F.B.I. should be required to obtain a warrant from a special FISA court before using the information collected under 702 when investigating Americans who may be involved in terrorism, espionage or other national security threats. But requiring such a warrant would have been unnecessary and unwise.
Getting a FISA court order is bureaucratically cumbersome and would slow down investigations — especially fast-moving cybercases, in which queries have proved especially useful. It would cause agents to miss important connections to national security threats. And because this information has already been lawfully collected and stored, its use in investigation doesn’t require a warrant under the Constitution.
Another problem is that the probable cause needed for a warrant is rarely available early in an investigation. But that’s precisely when these queries are most useful. Database checks allow an agent to quickly see whether there is a previously unnoticed connection to a foreign terrorist, spy or other adversary.
Balances struck between security and privacy need continual refinement. Recent years have shown Section 702’s great value for national security. But they have also revealed lax compliance at the F.B.I. The latest reauthorization boosts privacy without blinding our country to threats in today’s dangerous world.
Matthew Waxman is a Columbia University law professor who served in senior national security roles in the George W. Bush administration. Adam Klein is the director of the Strauss Center for International Security and Law at the University of Texas, Austin, and served as the chairman of the Privacy and Civil Liberties Oversight Board from 2018 to 2021.
The Times is committed to publishing a diversity of letters to the editor. We’d like to hear what you think about this or any of our articles. Here are some tips . And here’s our email: [email protected] .
Follow the New York Times Opinion section on Facebook , Instagram , TikTok , WhatsApp , X and Threads .
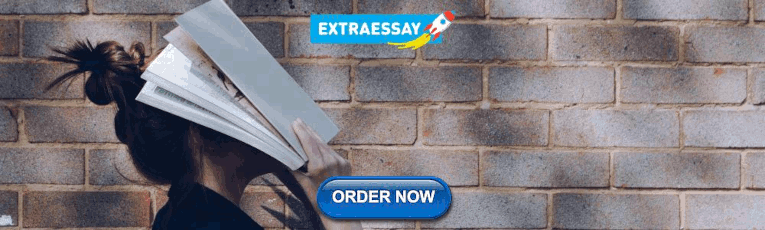
IMAGES
VIDEO
COMMENTS
Begin with a clear statement of the principal findings. This will reinforce the main take-away for the reader and set up the rest of the discussion. Explain why the outcomes of your study are important to the reader. Discuss the implications of your findings realistically based on previous literature, highlighting both the strengths and ...
You should: Discuss your conclusions in order of most to least important. Compare your results with those from other studies: Are they consistent? If not, discuss possible reasons for the difference. Mention any inconclusive results and explain them as best you can. You may suggest additional experiments needed to clarify your results.
The discussion section is where you delve into the meaning, importance, and relevance of your results.. It should focus on explaining and evaluating what you found, showing how it relates to your literature review and paper or dissertation topic, and making an argument in support of your overall conclusion.It should not be a second results section.. There are different ways to write this ...
The conclusion section of a research paper is where you summarize your research and its implications. Unlike the discussion section, the conclusion is not the place for a detailed analysis of your results. Instead, it's where you wrap up your argument and leave the reader with a clear understanding of your research and its significance.
Table of contents. Step 1: Restate the problem. Step 2: Sum up the paper. Step 3: Discuss the implications. Research paper conclusion examples. Frequently asked questions about research paper conclusions.
In a thesis or dissertation, the discussion is an in-depth exploration of the results, going into detail about the meaning of your findings and citing relevant sources to put them in context. The conclusion is more shorter and more general: it concisely answers your main research question and makes recommendations based on your overall findings.
The discussion section is often considered the most important part of your research paper because it: Most effectively demonstrates your ability as a researcher to think critically about an issue, to develop creative solutions to problems based upon a logical synthesis of the findings, and to formulate a deeper, more profound understanding of the research problem under investigation;
The conclusion is intended to help the reader understand why your research should matter to them after they have finished reading the paper. A conclusion is not merely a summary of the main topics covered or a re-statement of your research problem, but a synthesis of key points derived from the findings of your study and, if applicable, where you recommend new areas for future research.
Discussion Section. The overall purpose of a research paper's discussion section is to evaluate and interpret results, while explaining both the implications and limitations of your findings. Per APA (2020) guidelines, this section requires you to "examine, interpret, and qualify the results and draw inferences and conclusions from them ...
After a research article has presented the substantive background, the methods and the results, the discussion section assesses the validity of results and draws conclusions by interpreting them. The discussion puts the results into a broader context and reflects their implications for theoretical (e.g. etiological) and practical (e.g ...
Point out exceptions or lack of correlations. Define why you think this is so. State your conclusions clearly. Summarize your evidence for each conclusion. "Discussion and Conclusions Checklist" from: How to Write a Good Scientific Paper. Chris A. Mack. SPIE. 2018.
The discussion section is one of the final parts of a research paper, in which an author describes, analyzes, and interprets their findings. They explain the significance of those results and tie everything back to the research question(s). In this handout, you will find a description of what a discussion section does, explanations of how to ...
The discussion section of your research paper is where you let the reader know how your study is positioned in the literature, what to take away from your paper, and how your work helps them. It can also include your conclusions and suggestions for future studies. First, we'll define all the parts of your discussion paper, and then look into ...
Conclusion: Know the Difference Before Drafting Manuscripts. (average: 5 out of 5. Total: 2) The discussion section of your manuscript can be one of the hardest to write as it requires you to think about the meaning of the research you have done. An effective discussion section tells the reader what your study means and why it is important.
Papers usually end with a concluding section, often called the "Discussion.". The Discussion is your opportunity to evaluate and interpret the results of your study or paper, draw inferences and conclusions from it, and communicate its contributions to science and/or society. Use the present tense when writing the Discussion section.
The conclusion in a research paper is the final section, where you need to summarize your research, presenting the key findings and insights derived from your study. ... How to write effective discussion and conclusion sections. Clinical spine surgery, 31(8), 345-346. Bunton, D. (2005). The structure of PhD conclusion chapters. Journal of ...
The discussion section is the heart of any scientific paper. This is where new thoughts and directions are introduced and where reviewers provide context and meaning to their research findings (Hess 2004).Some suggest that the discussion is the most difficult part of a literature review to write (Aveyard 2019) and that it demands the most effort and critical thinking of reviewers (Kearney 2017).
In an empirical research paper, the purpose of the Discussion section is to interpret the results and discuss their implications, thereby establishing (and often qualifying) the practical and scholarly significance of the present study. It may be helpful to think of the Discussion section as the inverse of the introduction to an empirical ...
In academic writing, the discussion and conclusion sections of a thesis are important for presenting and summarizing research findings. This short guide explains the what to include, and what to avoid when writing each section. It also provides several tips for crafting an effective conclusion and discusses the significance of interpretations, implications, and limitations in the discussion ...
Future research needs recommendations are valuable inputs for researchers, funders, and advocates making decisions about avenues for future scientific exploration. We performed an empirical evaluation of the published literature to appreciate the variability in the presentation of information on future research needs. We found that most systematic reviews, meta-analyses, or economic analyses ...
Here are some steps you can follow to write an effective research paper conclusion: Restate the research problem or question: Begin by restating the research problem or question that you aimed to answer in your research. This will remind the reader of the purpose of your study. Summarize the main points: Summarize the key findings and results ...
How to Write an Effective Discussion in a Research Paper; a Guide to Writing the Discussion Section of a Research Article April 2022 DOI: 10.33552/OAJAP.2022.05.000609
The discussion section of a research paper interprets the findings and discusses their implications for the research question, the literature review, and the field of study. ... Write your Paper: Start by writing the introduction, followed by the literature review, methods, results, discussion, and conclusion. Ensure that your writing is clear ...
Background Circadian rhythms are important for all aspects of biology; virtually every aspect of biological function varies according to time of day. Although this is well known, variation across the day is also often ignored in the design and reporting of research. For this review, we analyzed the top 50 cited papers across 10 major domains of the biological sciences in the calendar year 2015 ...
However, after studying the relevant reviews and the results of the latest papers, we realize that there is consistency in the prediction ideas and prediction methods [34, 35]. Therefore, we summarize the similarities and differences between the results of the study and other research papers in epidemic forecasting as shown below.
Background: Current heart failure (HF) guidelines recommend a multidisciplinary approach, discharge education, and self-management for HF. However, the recommendations are challenging to implement in real-world clinical settings. Objective: We developed a mobile health (mHealth) platform for HF self-care to evaluate whether a smartphone app-based intervention with Bluetooth-connected ...
Essay Example: In the rich mosaic of American literature, few figures shine as brightly or contribute as distinctively as Zora Neale Hurston. Her writing, steeped in the essence of her Southern roots and the vibrant pulse of the Harlem Renaissance, represents a distinct blend of resilience,
Pew Research Center conducted this analysis to understand American teens' use and understanding of ChatGPT in the school setting. The Center conducted an online survey of 1,453 U.S. teens from Sept. 26 to Oct. 23, 2023, via Ipsos.
Since then, Section 702 has supplied extraordinary insight into foreign dangers, including military threats, theft of American trade secrets, terrorism, hacking and fentanyl trafficking. In 2022 ...