Confirmation bias studies: towards a scientific theory in the humanities
- Original Paper
- Open access
- Published: 22 July 2023
- Volume 3 , article number 123 , ( 2023 )
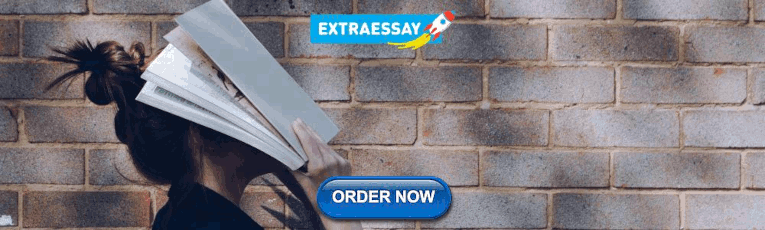
Cite this article
You have full access to this open access article
- Thomas Rist ORCID: orcid.org/0000-0002-2651-5163 1
1051 Accesses
Explore all metrics
This article argues that a global crisis of interpretation can and should be confronted by humanities programmes in UK and similar universities. It contends that raising the standards of proof for theoretical models of interpretation in the humanities will help reverse erosions of trust undermining democratic institutions and expertise. To this end, it considers how financial challenges facing UK universities mould the teaching of theory in the humanities and the knowledge this teaching gives rise to. The article considers how standards of proof from the social sciences can interrogate theory in these conditions, developing it and increasing its assurance. The essay illustrates this claim through a series of sample theories and literary works: Roland Barthes’ ‘Death of the Author’, the Orientalism derived of Edward Said, Chinua Achebe’s novel Things Fall Apart and Jung Chang’s Wild Swans: Three Daughters of China . From these examples the essay draws larger conclusions, the biggest of which is a new subfield of study for the humanities: Confirmation Bias Studies. The article is structured as follows. In ‘Part 1: Introduction’, the article considers the openness of literary theory to confirmation bias, which is considered historically, in cognitive processes, especially those processes in the age of the internet, and in educational processes in the humanities. In ‘Part 2: Procedure’, the article explores the challenges of applying a confirmation bias approach to literary theory as a means of interpretation. In ‘Part 3: Conclusion’, the article summarises the key strategies for overcoming confirmation bias in theoretical approaches to the humanities discovered in the article.
Similar content being viewed by others
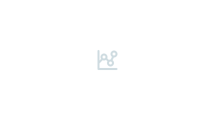
What Is the Function of Confirmation Bias?
Social Constructionism
C. wright mills’ the sociological imagination and the construction of talcott parsons as a conservative grand theorist.
Avoid common mistakes on your manuscript.
Introduction
Confirmation bias studies does not yet exist in the humanities, but in theory it should. Theory, especially literary theory, is an evolving discipline, defined by its willingness to take on new thoughts, and so, paradoxically, by its lack of definition. To earlier theories like structuralism, poststructuralism and feminism, more recently have been added postmodernism, postcolonialism, gender studies, trauma theory and queer theory among other theoretical models. More theory—a lot more of it—seems likely to follow. In one definition, literary theory is ‘increasingly hybrid’, with an ‘endlessly fecundating terminology’. Footnote 1 A consequence is the ‘resurgence of scepticism about the possibility of verifiable knowledge’, to which considerations of confirmation bias provide a response. Footnote 2
There is a place, therefore, for study of confirmation bias in the theoretical humanities. Yet presently this study exists on no humanities curriculum, and it is only here, in this article, that the study receives a name. Nevertheless, ‘Confirmation Bias Studies’ has been stirring for aeons. It begins with the ancients, with the contention of Plato, Aristotle and others that a world of fact exists that is independent of our ideas and judgements, a contention implying some ideas are correct while others are not. Footnote 3 For some, the debate reached its zenith in the ‘fake news’ crisis of the Trump presidency, in which we learnt of ‘alternative facts’—a crisis to which, in his inauguration speech, President Biden replied directly: ‘There is truth, and there are lies—lies told for power, and for profit … And each of us has a duty and a responsibility as citizens … to defend the truth, to defeat the lies’. Footnote 4 Yet one would be an optimist to think a contention that began before Plato will end with Biden. In the internet’s global village, Plato’s old enemy, opinion, is alive and kicking. If you ‘like’ an idea, and then others like it, before long it’s around the world, apparently as good as true.
An aspect of ‘likes’ of the kind is that they please. One ‘likes’ it because one likes it, not because one considers it to have a basis in fact or to be more than an opinion. Footnote 5 The criteria on which public discourses of this kind are judged is desire. I desire to believe this . Or, I desire to please this friend . Or, simply, I desire to have a say in the public discourse . With a public discourse based on these criteria, anything and everything becomes say-able; and if one searches far enough, on the net, one finds this everything and anything. It behoves the academy to do better.
This is where confirmation bias theory, from departments of Psychology, comes in. Defined as ‘the seeking or interpreting of evidence in ways that are partial to existing beliefs, expectations, or a hypothesis in hand’, confirmation bias has hyperbolically been called ‘the single problematic aspect of human reasoning that deserves attention above all others’. Footnote 6 Confirmation bias has been identified in fields as diverse as strategy formation, enhanced commitment to unprofitable courses of action, risk assessment, software testing, criminal investigations, medical diagnoses, judicial decisions, bullet comparisons, skull sexing, animal behaviour research, psychiatric confinement examinations and visual perception. Footnote 7 Closer to home, it is found in political debate, academic discussion and, most importantly, literary criticism and interpretation. Footnote 8 This focus of confirmation bias theory on interpretation gives it an important place in literary theory and in the humanities. Like these disciplines, confirmation bias study is concerned with interpretation, but it approaches the topic from the point of view of the prevalence of misinterpretation. At the heart of the problem is a human tendency to seek and endorse viewpoints and information confirming one’s existing, subjective beliefs. Footnote 9
The far-reaching implications for literary and wider cultural scholarship are only beginning to be understood. In 2014 Maurice Lee observed there is ‘no sustained work’ in literary studies on evidentiary superabundance: a crisis in literary interpretation caused not by a lack of evidence, but by its inexhaustible profusion. Footnote 10 As in the wider world, the internet plays a significant role in the crisis: ‘targeted searches of immense databases combined with evidentiary inclusiveness and confirmation bias allow literary critics to discover support for an amazing range of claims’. Footnote 11 Lee compared this to playing tennis with the net down, which invites the question: why play the game at all? Yet this fundamental challenge to the value of literary (and wider cultural) studies is as nothing when placed beside the wider crisis of truth at large. Since superabundance characterises academic and non-academic search engines alike, the problems of modern literary interpretation are the problems of our age. Footnote 12
Two answers to this problem one encounters in cultural debates are censorship and peer-review. Footnote 13 Peer-review, it is argued, guarantees quality interpretation because the reviewers are experts. Censorship, it is also argued, guarantees truth by weeding out falsehoods. Yet both approaches suffer from the same weakness and are open to the same challenge. Both depend on a higher-order interpreter, or ‘specialised class’, who may or may not be reliable. Footnote 14 One hopes, of course, that an academic reviewer will be better informed and motivated than the censor for a totalitarian state, but it is not guaranteed. Academics can be partisan and—as importantly—everyone knows it. Footnote 15 Especially in the humanities, too, some academics reject the existence of objective truths, reasonably raising the question of why they should be trusted to review objectively. The question arises even when these academics review honestly. Footnote 16
Despite President Biden’s resolve to restore it in public life, trust alone is not the answer. There must be good reason to trust. Footnote 17 For literary study in the age of superabundance, that reason comes from the application of confirmation bias theory. Reading literary theories for their capacity to misinterpret, rather than to interpret, provides unusual evidence of their value. The argument that a theory proves its worth by the interpretations it enables is circular. Footnote 18 If the theory is wrong, the interpretation arising from it will likely contain errors. For this reason, it is argued, all literary interpretation requires faith. Footnote 19 Yet testing a literary theory for confirmation bias before putting it to interpretative use helps validate the theory, allowing us to apply it with greater faith thereafter. Just as one wouldn’t expect to take a covid vaccine without the assurance of validating procedures beforehand, so one shouldn’t expect to apply a theoretical model to interpretation without a proper initial test. Footnote 20
It’s worth emphasising here how our age of superabundance creates the need for a new standard of proof. Scientific standards of proof lean towards two critical but adverse models, those of T.S. Kuhn and Karl Popper. Footnote 21 Kuhn noted confirmation-seeking is fundamental in scientific practice, but Popper observed the method is also likely to produce pseudoscience. Footnote 22 Finding examples which confirm your hypothesis can produce vaccinations against Covid-19 and adherents to Daesh or Q-Anon. It is not enough, therefore, to seek new examples substantiating your hypothesis. For a theory to be valid, one needs to know not only how true it is, but also how false. Footnote 23
This is old hat in the social sciences, but despite their interdisciplinary turns, it is mostly absent in the theoretical humanities; and, therefore, in the bright young things it sends out to the wider world. One reason for this is the traditional aim of the humanities to explain primary sources. Footnote 24 Explaining a source means interpreting it, so theoretical models are valued for their capacity to interpret rather than for their capacity not to interpret. Footnote 25 The bias operates at several levels. If it’s at all concerned with a theory, the one-hour undergraduate lecture must show students the theory’s value. In practice, this means first explaining the theory and then applying it in interpretation, so that the undergraduate audience can see its usefulness. Footnote 26 That leaves little or no time to address its deficiencies; and being as interested as their teachers in interpreting texts, or artworks or historical documents, students are likely to resist as obfuscation or irrelevance critiques of theories they are barely accustomed to. Footnote 27
At the postgraduate level, the problem is different. Footnote 28 Postgraduates tend to be undergraduates who rose to the top, so some capacity for critical thinking inheres. Yet as in the undergraduate scenario, where the single hour of the lecture (or tutorial) limits a theoretical enquiry, the pressure of income-streams on universities means doctoral students often lack time for their deepest research. Footnote 29 In UK universities graduate students (especially those paying higher fees, from abroad) are a main source of revenue. Humanities especially tend to be cash-strapped, meaning they are under-staffed and want as many doctoral students as possible. Footnote 30 Churning out contributions to knowledge (the definition of the PhD) becomes a desideratum, and the easiest model for it goes like this: here’s a theory, here’s a text (or artwork or document), here’s a new interpretation. Footnote 31 Operating under financial and temporal constraints, what this process aimed at interpretation does not do is produce many theses on misinterpretation. And since new theories encourage fresh interpretations, there is the additional pressure to side-line older theories in favour of newer ones that are less tried. Footnote 32 Sniffing out misinterpretation is a part of most doctoral projects, but rarely is it the main part, not least because misinterpretation in the Humanities has come widely to be seen as ‘a misnomer for an opinion delinquent enough to differ from our own’. Footnote 33 Moreover, in the humanities only the mainly theoretical or philosophical doctorate might attend to large-scale misinterpretation; yet as we have seen, there’s no such thing as yet as Confirmation Bias Studies in the humanities.
How, though, might such study work? In 2014, Lee noted literary critics weren’t yet under a mandate to meet scientific standards of proof, but he saw the moment coming and as he observed, sampling is the answer. Footnote 34 The trick in this process is to find the universal claim in a hypothesis and then to test it for shortcomings; if counter-examples to the hypothesis can be found, then the hypothesis needs to be modified. As a simple example, consider the so-called death of the author. Clearly some authors (including the present one) are alive, ergo the theoretical hypothesis needs at least some qualification and modification. If authors aren’t all dead in the same way, then in what sense are they dead? What does it mean to be dead in this sense and (by extension) what might it mean for an author to be alive? These and deeper questions proliferate. If, more substantively, authors are just products of their societies, then Hitler would seem reducible to the 1918 Armistice—to which many will reply: really? Footnote 35 It was part of the magisterial genius of Roland Barthes that his essay proclaiming the death of the author gave only sweeping readings of texts and authors, most of which look questionable today. Footnote 36 Yet to question Barthes on these terms is only to do the everyday work of literary criticism: challenging interpretations. The Confirmation Bias Studies approach to ‘The Death of the Author’, by contrast, challenges the fundamental hypothesis: since, biologically, some authors are alive (and since Hitler seems irreducible to the Armistice) the hypothesis of the death of the author needs modification. Footnote 37 The Death of Some Authors is not as poetic a title as Barthes provided, but considered for confirmation bias, it probably has more mileage.
Tilting at Barthes is fun and in view of my previous comments about truth (and Hitler) it also has social use. Yet for all its influence, ‘The Death of the Author’ is a small essay based on smaller evidence: barely worth considering from the confirmation bias viewpoint. A much bigger example (but one just as influential today) might be Edward Said’s Orientalism . Here we have a full-scale study devoted to a defined hypothesis: western texts fundamentally misrepresent the east as a strategy of domination. Unlike Barthes, whose attention to texts in ‘The Death of the Author’ is cursory, Said supported his claim with detailed analyses of central western texts and many others have followed suit. Orientalism as first hypothesised by Said, therefore, can be said to have attained the level of proof required by T.S. Kuhn, since repeated confirmations of the hypothesis have been found. This is the case even if some of these confirmations are more persuasive than others. Yet confirmation bias remains a problem and to be fully validated the theory must also pass the criteria for proof of Popper; or, if it cannot be so validated, it needs to be rendered more precise through modification. As with the challenge of biological and undead authors to Barthes, what’s needed is counter examples.
I stress at this point that it is not this essay’s aim to disprove Orientalism. Far more substantial critiques of Orientalism already exist than this essay could aspire to and (depending on the author in question) more and less inverted versions of Orientalism already exist in Occidentalism. Footnote 38 The aim of the essay here is to propose a process for Confirmation Bias Studies. Like Barthes’ ‘Death of the Author’, Orientalism serves in the process as an illustrative theory. In so far as it shows shortcomings, what follows not so much disproves the theory as reveals areas to be developed.
Objectors might want to claim at this point that there are no counter examples to Said’s thesis, arguing that is why it is so powerful. They might add, too, that any cultural interpretation found counter to Said can itself be revealed to be a misinterpretation, leaving his thesis intact. This may be true. Yet the claim that something can be done is not the same as that it ought to be done; and unless it passes the falsifiability test, the thesis will remain open to doubt: one of the many theories circulating in the world of alternative facts, as likely to inflame opposition as to be found persuasive. Footnote 39 For scholarship, too, failing the falsifiability test is serious. A theory found to be unfalsifiable cannot be improved, even in the limited, Popperian sense of improvement ‘criticising our guesses’. Footnote 40 Scholarship following such unfalsified theory is therefore doomed to repetition.
This essay can only sketch out what a Confirmation Bias Studies response to this problem might look like. Only a consideration of every possible counter example to a prevailing thesis would finally reveal what qualifications the thesis would need to obtain Popper’s criteria of truth; even though for Popper (dealing in inductive science) a single counter example was enough. In this sense, one needs not individual case studies, but a continual testing of theory (established, emerging, or new) for confirmation bias. If that sounds like a lot of work, take comfort. If getting the full picture is going to keep us busy, we can make consistent, if incremental, gains along the way. Knowledge can develop. The humanities can address the need for truth in the public sphere with new commitment and energy. Yet methodology needs emphasis. Since counter examples are necessary in falsifiability tests, to begin work one needs a clear example of the theory under consideration. Clarifications, therefore, are likely to be an initial part of the procedure. In the case of Orientalism, this requires a little work.
Orientalism depends on the view that (in Said’s words) ‘European culture was able to manage—and even produce—the Orient politically, sociologically, militarily, scientifically and imaginatively during the post-Enlightenment period’. Footnote 41 There would seem to be slippage here between ‘manage’ and ‘produce’, which points to stronger and weaker versions of the thesis. The claim that Europe ‘managed’ large parts of the Orient is uncontentious, but that it ‘produced’ that world—a much stronger claim—is remarkable and has stood out. This slippage in Said’s thesis, I think, continued throughout his work, but it is the stronger claim that is interesting, and which has been widely taken as representative. Interpreting Said here, for example, Shehla Burney writes: ‘In other words, Said argues that Orientalism is a built in system or method by which the West not only socially constructed and actually produced the Orient, but controlled and managed it through the tropes, images, and representations of literature, art, visual media, film and travel writing, among other aspects of cultural and political appropriation’. Footnote 42 This is both the more interesting and stronger of Said’s claims and—while I do not think Said ever clearly distinguished the two Footnote 43 —it is this remarkable claim that has had most purchase and merits attention.
Having clarified the thesis to be tested, the next step in a confirmation bias study will be to find counter examples. In the case of Orientalism, that means finding examples of oriental culture isolated from western influence. For a thesis like Said’s, a counter example requires isolation from western influence to qualify. That puts a premium on oriental works predating western influence, a premium on scholars who can read these works in their original language, and an onus on western scholars unable to read them to listen to those who can. Footnote 44 Evidence from these sources and scholars are precisely relevant to the claim that western influence ‘produced’ the Orient. If the pre-western sources reveal similar behaviours and attitudes in oriental countries to those found after the arrival of the west, the sources confirm a bias in the Orientalist thesis. Modifications to the thesis will therefore need to be made, for example regarding indigenous and local structures of power and morality; and these modifications will themselves eventually need testing for confirmation bias, since scholars today, including non-westerners, are rarely if ever entirely isolated from western influence. Clearly, there is a lot of work from scholars who can read pre-western, oriental languages for us to attend to.
Counter examples to Said’s thesis (in its stronger form) might also be found in other kinds of oriental isolation from the west. Since isolation is key, many works post-dating east–west engagement will likely not qualify for a confirmation bias study. With western assumptions, for example about moral behaviour, they do not provide the truly counter example.
Yet greater and lesser degrees of isolation can exist and eastern artefacts post-dating western colonisation can be more and less isolated from the experience: more and less valuable, therefore, as relevant evidence in confirmation bias studies. Footnote 45 Though long post-dating African westernisation, for example, Chinua Achebe’s novel Things Fall Apart purports to tell an African history before and after westernisation. Though scholars might argue the novel’s presentation of pre-colonised Africa is damaged by western influences on Achebe, few would argue these so entirely harm his presentation of pre-colonial Africa that it has no merit. Footnote 46 For confirmation bias studies, the lesson is that partial counter examples too have a place in the testing procedure, albeit in these cases conclusions may be more provisional.
In such examples, the case for their isolation—as perhaps for any sample—needs to be made, which means assessing their eastern components against their western ones. Only through this procedure can distinctions necessary for conclusions about confirmation bias be drawn between their eastern and western elements. In the case of Achebe, for example, the validity of his portrait of the pre-colonial Igbo depends on his intimate, first-person and inherited knowledge of the Igbo through membership. Footnote 47 Yet his membership is also of the Igbo after colonisation, taking in experiences, for instance, like his study of English literature at University College in Nigeria. Footnote 48 Evaluating post-colonial portraits of pre-colonial cultures means surrounding analysis of the sample texts with a ledger explaining the strengths and weaknesses of the evidence. Its provisional nature will then be clear, but that does not amount to ‘the impossibility of objectivity and impartiality in the human sciences’. Footnote 49 Rather, it ensures the evidence is understood in its proper context: neither over-estimated nor underestimated.
Studying isolated examples of this kind may also bring insights that are otherwise unobtainable. As a narrative about China by a native Chinese, for instance, Jung Chang’s Wild Swans: Three Daughters of China makes a claim to stand largely outside an Orientalist framework. Isolating Europe and America from its main narrative, stories of personal and local experience dominate in the work. The driving, though not exclusive, motive is the experience of the three daughters, to appropriate which would invite orientalist charges. Footnote 50 As with the veracity of Achebe’s portrait of the pre-western Igbo, the strength of Chang’s portrait in Wild Swans is its seeming reliance on first-person experience and the oral and cultural evidence gathered from forbears. From the point of view of Confirmation Bias Studies, therefore, Wild Swans provides the prima facie counter example required to test the Orientalist thesis.
Much as a reading of Things Fall Apart observes the evidence of gradual Igbo disruption caused by the west, a full-scale confirmation bias study of Wild Swans will detail the forms of unfolding disruption. In Chang’s story, though, the failings catalogued, largely emerging in isolation from western influence, signal oriental failings firstly, rather than western ones. Each example of Chinese abuse or atrocity in Wild Swans stands as a counter example to the thesis that Europe (or America) produced oriental China; and the hundreds of examples in the work (or in sampled works like it) each imply a need to modify Said’s stronger thesis. This is despite the role of colonisation and Marx in nineteenth and twentieth century Chinese history. Footnote 51 Chinese agency is paramount in Wild Swans . Although its author is of China, the work in this aligns with wider ‘China-centred’ histories, which through research into Chinese archives, have brought to attention Chinese agency in historical processes of change. Footnote 52
As the present plight of the Uighurs illustrates, the proposed, literary work of cataloguing and detailing is important, yet there are wider points to observe here too. According to the Global Slavery Index, in 2016 there were 3.8 million people in China living in conditions of modern slavery. Footnote 53 From its opening sentence, older and newer forms of slavery are the abiding topic of Wild Swans :
At the age of fifteen my grandmother became the concubine of a warlord general, the police chief of a tenuous national government of China. The year was 1924 and China was in chaos. Much of it, including Manchuria, where my grandmother lived, was ruled by warlords. The liaison was arranged by her father, a police chief official in the provincial town of Yixian in southwest Manchuria, about a hundred miles north of the Great wall and 250 miles northeast of Peking. Like most towns in China, Yixian was built like a fortress. It was encircled by walls thirty feet high and twelve feet thick dating from the Tang dynasty (AD 618-907), surmounted by battlements, dotted with sixteen forts at regular intervals, and wide enough to ride a horse quite easily along the top. There were four gates into the city, one at each point of the compass, with outer protecting gates, and the fortifications were surrounded by a deep moat. Footnote 54
The slavery of concubinage, which is soon revealed as a commonplace deal made for advantage by the girl’s father, stands out here in a series of images of Chinese and Manchurian isolation dating back to the Tang dynasty. The implication is that this slavery is natively Chinese, though the originally western name ‘Peking’ and the dating anno domini offer subtle modifications.
Whether this view is entirely correct is the task of historical criticism to discern and here the process of confirmation bias studies stands out. Isolating and so distinguishing the properly Chinese elements of the girl’s concubinage from features externally derived allows the girl’s representative and personal concubinage to be understood. Said, who claimed Orientalism is an ally of women’s studies, in principle approves this procedure. Footnote 55 In the case of Manchuria, non-Chinese elements include the differently oriental influences of Russia and Japan: by no means isolated from the west (especially Russia), yet modulating its influence with their own oriental features. The slavery and misery of the 15-year-old girl sold into concubinage, Chang’s grandmother, opens oriental histories in which the west plays a distant third fiddle. This is the origin of older and newer forms of enslavement in Chang’s narrative, as Chang’s two following daughters of China—her mother and then Chang herself—grind out lives defined either by, or by reaction to, concubinage as the original sin.
Other forms of enslavement loom along the way. The puppet state of Manchukuo which followed the Japanese invasion of 1931 stands out both as an external influence on Manchuria’s isolation and for its brutality. The Chinese Kuomintang (backed by America) is also brutal, inviting both condemnation and critical procedure. The torture of Chang’s mother by the Kuomintang, for example, entails putting her through a mock execution by firing squad—not an especially Chinese means of intimidation—but also one in which Chinese agents and agency stand out. In a passage like this ( Wild Swans , p. 137) the isolating procedure of confirmation bias studies gives a basis for the apportioning of responsibility, providing some affirmation of the strong Orientalist thesis and, also, correctives to it.
Sampled scenes of this kind provide a very large terrain for literary study along confirmation bias lines, but they also invite theoretical considerations important for procedure. There is a case for western disruption of Chinese lifestyles in the Kuomintang passages of Wild Swans , but transparently the passages are part of a very much bigger story. In its evaluative procedure, therefore, Confirmation Bias Studies will want to indicate the frequency of the kinds of passages it samples vis a vis passages and themes of other kinds.
The relations of parts to the whole raise bigger questions too. The forgoing analysis holds oriental (Chinese, and more briefly, Japanese) culture largely responsible for enslavements and abuse in Wild Swans and America somewhat so. A larger analysis of Wild Swans would need, too, fully to consider the relation of Marxism and Enlightenment ideas of progress to the Cultural Revolution, addressing the agency and responsibility of each. Footnote 56 Yet at stake here are also literary issues of time and representation. It is perfectly possible to imagine a version of Wild Swans focusing exclusively on the Kuomintang era to bring out its (and America’s) abuses, just as it is possible to imagine a Wild Swans that does not open with an isolated Chinese history dating back to the Tang dynasty; and just as it would be possible to write a Wild Swans with the Enlightenment and Marx to the fore. Literature constructs sequences of cause and effect that need recognition, especially if a confirmation bias approach to it is to be persuasive. Footnote 57 Chang writes: ‘Manchuria was the key battleground in the civil war, and what happened in Jinzhou was becoming more and more critical to the outcome of the whole struggle for China’. Footnote 58 This is, in effect, the historical justification for opening the work in the earlier Manchuria of the warlords and in the province’s Tang origins. Reading works for confirmation bias in theories will mean recognising the way they set events within temporal parameters of cause and effect. It will also mean testing those parameters against other historical accounts. In a case like Wild Swans , these accounts will be somewhat self-selecting. Since Wild Swans is banned in China, official or state sponsored accounts of Chinese history disqualify themselves as historical tests of the work since they do not recognise it.
No account of the ledger of evidence needed for assessing works like Wild Swans or Things Fall Apart is complete without comment on English, the medium where east meets west. Footnote 59 Despite Achebe’s defence of English in colonial representation, the confirmation bias approach (like any truth-seeking approach) should here too stress the provisional nature of its findings. Footnote 60 To be stressed too is that cultural translation need not work against the country described in English, especially if the describer is from that country. Critiques of Wild Swans tend not to fault its broad picture of Chinese (and from 1931, Japanese) atrocities performed on Chinese people. Rather, they criticise a tale of the Cultural Revolution too sharply dividing the evils perpetrated by an elite cadre (the so-called Gang of Four) from the actions of the wider populace. Wild Swans implies Chang and most students avoided violence during the revolution, even dividing Red Guards into a peaceful majority and a small minority ‘actually involved in cruelty or violence’. Footnote 61 It is here, claims Shuyu Kong, that Chang’s English readership most exerts its influence on the narrative of China:
the account is plausible and seductive precisely because we can imagine ourselves adopting a similarly detached, sceptical attitude in episodes of mass hysteria. We like to think that we, too, are sensitive individuals, would avoid violence and would be among the first to challenge it, or at least would avoid participating wherever possible. This is, after all, how rational people behave. Footnote 62
The rational ‘we’, here, are the English speakers who are Chang’s target readership. For many, Wild Swans is a bridge to a country largely unknown, raising questions of the relation of geography and distance to responsibility. Yet Kong’s point is that the meeting of the oriental author and the western readership produces a happier picture of the Chinese than is due. Since the author is a native of China, this is neither the idealisation nor demonization of the east by western authors posited by Said. It is, rather, a third thing, which we might call ‘angelification’, in which identification by the eastern author with the west enhances the moral presentation of the east. Considering English works on the orient by its natives, Confirmation Bias Studies will need to keep the phenomenon in mind.
What bearing have these academic points on our global crisis of interpretation? As consequences of a Confirmation Bias procedure, each point reveals an aspect of Orientalism needing development. They reveal the efficacy of the confirmation bias method and so the capacity of the humanities to grapple with hypothetical and theoretical truths. In the age of superabundance, they reveal the academy setting a systematic example of how to tell fake theoretical news from true; and they suggest a procedure by which the humanities can prepare students for superabundance, as a model of truth in the public sphere.
Just as there is no bias without a category of truth, the fore-mentioned terms (idealisation, demonization, angelification) base standards of judgement on the putative existence of facts; what Said called, ‘the world of reality’. Footnote 63 Confirmation Bias Studies seeks this putative world through patient testing of the theories about it. As an academic field, it is largely untrodden in the humanities and so ripe for development. Yet it is also the means by which the academe can set an interpretative example, presenting itself to the world of superabundance as a model for telling theoretical wheat from chaff. Were the next generation of humanities graduates to be trained in confirmation bias theory, they would be more habituated to making critical distinctions and less susceptible to fake news. Public discourse and our democratic institutions would benefit.
Conclusion: nine features of confirmation bias study for the humanities of the future
To summarise, the confirmation bias study proposed here for the humanities has eight distinct features. Its principal address is to theories and to theory. Its focus is on misinterpretation, rather than interpretation. Its aim is to develop theory through modification. Its means is the identification and analysis of counter examples. Its conclusions will explain how the examples modify the theory. The conclusions will be provisional. It is a contribution from the humanities to public discourse in the age of superabundance. It maintains a category of truth.
Since it answers human tendencies to seek and interpret evidence in ways partial to existing beliefs, a ninth and last feature will be reflection on oneself as the reasoning analyser. In this essay, I have argued for a crisis of truth in the public sphere that is reflected in the academy and to which the humanities can respond. I have illustrated a procedure for this response through consideration of the ‘Death of the Author’ and Orientalism as sample theories, giving main attention to Said’s stronger theory of the relations of east and west, which has garnered attention in both spheres. I have done so as a middle-aged man who spent his first 9 years in Canada, who has lived in the UK, Europe and Israel, travelled in Egypt and Turkey, and resides in Scotland. From around the eighteenth century, the Rist family were farmers in Suffolk, but the name Rist is much more common in Germany and Estonia than in England, so an earlier immigrant history seems likely. On my mother’s side, my grandmother was English but with family origins in France, while my Jewish grandfather’s family had come from Poland. How far these identities shed light on this essay is for me unclear. As a middle-class academic born into an academic family, I engage actively with academic (including political) topics, especially in the humanities. As someone who would have been executed under Nazi race laws, and whose Jewish forbears died by those laws, I am alive to antisemitism, racism, dictatorships and the distortions of truth on which they depend. No doubt this last has a bearing on this essay, for it inculcates the view that truth, in culture, is a matter of life and death; and that in the age of superabundance, maintaining truth requires of us a coherent process of reason.
Data availability
Not applicable.
See Wolfreys et al. ( 2014 , p. x).
See McSweeney, ‘Part 2’ ( 2021b , p. 842).
On this history, see Rorty ( 2009 ); for an influential modern defence of this philosophical position, see Nagel ( 1997 ).
On ‘fake news’ and the ‘“post-truth”/“post factual” politics characterised by the diminishing importance of anchoring utterances in verifiable facts’, see McSweeney, ‘Part 1’ ( 2021a , p. 1065).
See Dhir et al. ( 2019 , pp. 544–545), Lesley et al. ( 2017 , p. 153).
See Nickerson ( 1998 , p. 175).
McSweeney, ‘Part 1’ ( 2021a , p. 1065).
See Lee ( 2012 , pp. 87–94).
Lee ( 2012 , p. 163).
On social media creating confirmation bias today, see Lokot and Diakopolous ( 2016 , pp. 682–699), Dubois and Blank ( 2018 , pp. 729–745), McSweeney, ‘Part 1’ ( 2021a , p. 1065).
See Brady ( 2010 , pp. 10–11), Unerman ( 2020 , pp. 1–26), King et al. ( 2018 , pp. 843–855), Nickerson ( 2020 , pp. 224–225).
See Herman ( 2000 , p. 101).
Unerman ( 2020 , pp. 1–26), King et al. ( 2018 , pp. 843–855), McSweeney, ‘Part 1’ ( 2021a , p. 1064).
McSweeney, ‘Part 2’ ( 2021b , p. 842).
On distinctions between good and bad reasoning, see Mercier ( 2012 , pp. 244–245).
See Simpson ( 2003 , pp. 215, 221).
Simpson ( 2003 , p. 229).
On the importance of scientific tests, in this respect, see Zu et al. ( 2022 , p. 1).
For introduction, see Noturno ( 1984 , pp. 273–289).
See Rosende ( 2009 , pp. 135–154), Robergs ( 2017 , pp. 1–11)
Robergs, 4. On good and bad theory in Popper, see also Boyer ( 2009 , p. 247).
See Jay ( 2014 , p. 142).
See Jay ( 2014 , pp. 114–142).
See Byrne ( 2011 , pp. 117–118).
Byrne ( 2011 , p. 118).
See Mattison ( 2012 , pp. 5–10).
See Levin ( 2005 , pp. 17–28).
See Collini ( 2012 , p. 31).
My characterisation of the theoretical doctorate develops Carter’s description of humanities doctorates as ‘creating and occupying a gap in existing knowledge, making an original contribution that is accepted by the community … transforming its author from novice to licenced practitioner’. See Carter ( 2011 , p. 730).
On the ‘capitalist presentism’ driving this tendency, see McGlazer ( 2020 , p. 23).
Lang ( 2005 , p. 159).
Lee ( 2014 , p. 163).
Lang ( 2005 , p. xii and pp. 15–16).
See Ryan ( 2022 , pp. 83–84). Notably, Barthes’ essay was ‘ never [ sic. ] meant to be a traditional literary or scholarly essay’. See Logie ( 2013 , p. 494).
For a sample of the variety, see Salhi ( 2019 ), and Chen ( 2002 ).
For a recent discussion of Hume’s Law (‘you cannot derive an “ought” from an “is”’) see Radcliffe ( 2022 , p. 44).
Boyer ( 2009 , p. 246).
Said ( 1978 , p. 11).
Burney ( 2012 , p. 23).
Vacillation between the stronger and weaker theses is implicit, for example, in Said’s later claim that Orientalism concerns ‘overlapping domains’. See Said ( 1985 , p. 90).
For example, Warscheid ( 2018 , pp. 1–10).
Hence the recent call for ‘African language independence’ from ‘the hegemony of European languages in African Literature’. See Cantalupo ( 2016 , p. 1).
See Morrison ( 2014 , pp. 177–192), Tiffin ( 1995 , pp. 97–98).
AbdelRahman ( 2005 , p. 179).
AbdelRahman ( 2005 , pp. 178–180).
AbdelRahman ( 2005 , p. 189).
On orientalist charges, see Spivak ( 1995 , p. 24).
For discussion, see Huters ( 2005 ).
See, notably, Cohen ( 1984 , 2003 ).
See ‘Global Slavery Index’, at China | Global Slavery Index (accessed, 31/10/22).
Chang ( 1993 , p. 27).
Said ( 1985 , p. 106).
Chen comments relevantly here: ‘it would not be accurate to say that Chinese political and intellectual culture is nothing more than an outpost of mindlessly replicated Western thought. However Western these ‘Chinese’ ideas may be in their origins, it is undeniable that their mere utterance in a non-Western context inevitably creates a modification of their form and content’. See Chen ( 2002 , p. 2).
See de Guevara and Kostic ( 2017 , p. 13), Fernandez ( 2018 , p. 80). More generally, see, for example, Andrews ( 2007 ); on cause and effect in Wild Swans , see Li and Li ( 2021 , pp. 54–77), though the comparative (rather than historical) approach limits the article’s conclusions.
Chang ( 1993 , p. 128).
On language creating worldviews, see Underhill ( 2011 ).
On Achebe’s defence of English and questions arising, see Lynn ( 2017 , pp. 77–95).
Kong (quoting Wild Swans ): ( 1999 , p. 245).
Kong ( 1999 , p. 249).
Said ( 1985 , p. 100). See, more recently, Fischer and Klazar ( 2020 , p. 6), Brown ( 2016 , pp. 1–2), Ryan ( 2022 , pp. 84–87).
AbdelRahman F (2005) Said and Achebe: writers at the crossroads of culture. In: Journal of Comparative Poetics. Edward Said and critical decolonisation, vol 25. pp 177–192
Andrews M (2007) Shaping history: narratives of political change. Cambridge University Press, Cambridge
Book Google Scholar
Boyer A (2009) Open rationality: making guesses about nature, society and justice. In: Parusnikova Z, Cohen R (eds) Rethinking Popper. Boston studies in the philosophy of science, vol 272. Springer, Dordrecht, pp 245–55
Google Scholar
Brady A-M (2010) Propaganda and thought work in contemporary China. Rowman & Littlefield Publishers, Lantham
Brown T (2016) Evidence, expertise, and facts in a “post-truth” society. Br Med J. https://doi.org/10.1136/bmj.i6467
Article Google Scholar
Burney S (2012) Orientalism: the making of the other. In: Counterpoints: studies in criticality. Pedagogy of the other: Edward Said, postcolonial theory and strategies for critique, vol 417. Peter Lang, New York, pp 23–39
Byrne K (2011) From theory to practice: literary theory in the classroom. In: Bradford R (ed) Teaching theory. Palgrave Macmillan, New York
Cantalupo C (2016) Africa antitranslation. Res Afr Lit 47(3):1–14
Carter S (2011) Doctorate as genre: supporting thesis writing across campus. High Educ Res Dev 30(6):725–736
Chakravorty Spivak G (1995) Can the subaltern speak? In: Ashcroft B, Griffiths G, Tiffin H (eds) The post-colonial studies reader. Routledge, London, pp 24–8
Chang J (1991) Wild swans: three daughters of China. Harper Collins, London. Reprint 1993
Chen X (2002) Occidentalism: a theory of counter-discourse in post-Mao China, 2nd edn. Rowan & Littlefield, Lanham
Cohen P (1984) Discovering history in China: American historical writing on the recent Chinese past. Colombia University Press, New York
Cohen P (2003) China unbound: evolving perspectives on the Chinese past. Routledge, London
Collini S (2012) What are universities for? Penguin, London
De Guevara BB, Kostic R (2017) Knowledge production in/about conflict and intervention: finding “facts”, telling “truth.” J Interv State Build 11(2):1–20
Dhir A, Khalil A, Kaur P, Rajala R (2019) Rationale for “liking” on social networking cites. Soc Sci Comput Rev 37(4):529–550
Dubois E, Blank G (2018) The echo chamber is overstated: the moderating effects of political interest and diverse media. Inf Commun Soc 21:729–745
Fernandez J (2018) Story makes history, theory makes story: developing Rusen’s Historik in logical and semiotic directions. Hist Theory 57(1):75–103
Fischer R, Klazar E (2020) Facts, truth and post-truth: access to cognitively and socially just information. Int J Inf Divers Incl 4(3/4):5–19
Herman ES (2000) The propaganda model: a retrospective. Journal Stud 1(1):101–112
Huters T (2005) Bringing the world home: appropriating the west in late Qing and early Republican China. University of Hawai’i Press, Honolulu
Jay P (2014) The humanities ‘crisis’ and the future of literary studies. Palgrave Macmillan, New York
King E et al (2018) Systematic subjectivity: how subtle biases infect the scholarship review process. J Manag 44(3):843–855
Kong S (1999) Swan and spider eater in problematic memoires of cultural revolution. Positions East Asia Cult Crit 7(1):239–52
Lang B (2005) Post-holocaust: interpretation, misinterpretation and the claims of history. Indiana University Press, Bloomington
Lee M (2012) Evidence, coincidence and superabundant information. Vic Stud 54(1):87–94
Lee M (2014) Falsifiability, confirmation bias, and textual promiscuity. J Ninet Century Am 2(1):162–71
Lesley LK, Emrich O, Gupta S, Norton MI (2017) ‘Does “liking” lead to loving?: the impact of joining a brand’s social network on marketting outcomes. Am Mark Assoc 54(1):144–55
Levin J (2005) The business culture of the community college: students as consumers, students as commodities. New Dir High Educ 129:11–26
Li L, Li X (2021) Who “let all this happen”?: shifts of responsibilities in representing the cultural revolution in Jung Chang’s Wild Swans . Lang Lit 30(1):54–77
Logie J (2013) 1967: The birth of “The Death of the Author.” Coll Engl 75(5):493–512
Lokot T, Diakopolous N (2016) New bots; automating news and information dissemination on twitter. Digit Journal 4:682–699
Lynn T (2017) Chinua Achebe and the politics of narration: envisioning language, African histories and modernities. Palgrave MacMillan, Cham
Mattison J (2012) Literary theory in the postgraduate classroom: it’s role and challenges. In: Karlsson L (ed) Lärarlärdom: högskolepedagogisk konferens. Kristianstad University Press, Kristianstad
McGlazer R (2020) Old schools: modernism, education and the critique of progress. Fordham University Press, New York
McSweeney B (2021a) Fooling ourselves and others: confirmation bias and the trustworthiness of qualitative research, part 1. J Organ Change Manag 34(5):1063–1075
McSweeney B (2021b) Fooling ourselves and others: confirmation bias and the trustworthiness of qualitative research, part 2. J Organ Change Manag 34(5):848–859
Mercier H (2012) Reason is for arguing: understanding the success and failures of deliberation. Polit Psychol 33(2):243–258
Morrisson J (2014) Chinua Achebe. Manchester University Press, Manchester
Nagel T (1997) The last word. Oxford University Press, Oxford
Nickerson R (1998) Confirmation bias: a ubiquitous phenomenon in many guises. Rev Gen Psychol 2(2):175–222
Nickerson R (2020) Argumentation: the art of persuasion. Cambridge University Press, Cambridge
Noturno MA (1984) The Popper/Kuhn debate: truth and the two faces of relativism. Psychol Med 14:273–289
Radcliffe E (2022) Hume on the nature of morality. Cambridge University Press, Cambridge
Robergs R (2017) Lessons from Popper for science, pardigm shifts, scientific revoltuions and exercise psychology. BMJ Open Sports and Exercise Medicine 3/1:1–11
Rorty R (1979) Philosophy and the mirror of nature, 13th edn. Princeton University Press, Princeton. Reprint 2009
Rosende D (2009) Popper on refutability: some philosophical and historical questions. In: Parusnikova Z, Cohen R (eds) Rethinking popper. Boston studies in the philosophy of science, vol 272. Springer, Dordrecht, pp 135–54
Ryan M-L (2022) Media, genres, facts and truths: revisiting basic categories of narrative diversification. Neohelicon 49:75–88
Said E (1978) Orientalism. Routledge and Kegan Paul, London
Said E (1985) Orientalism reconsidered. Cult Crit 1:89–10
Salhi ZS (2019) Occidentalism: literary representations of the Maghrebi experience of the east–west encounter, Edinburgh studies in modern Arabic literature. Edinburgh University Press, Edinburgh
Simpson J (2003) Faith and hermeneutics: pragmatism vs pragmatism. J Mediev Early Mod Stud 33(2):215–239
Spivak GC (1995) Can the subaltern speak? In: Ashcroft B, Griffiths G, Tiffin H (eds) The post-colonial studies reader. Routledge, London, pp 24–28
Svensson G, Wood G (2007) Are university students really customers? When illusion may lead to delusion for all! Int J Educ Manag 21(1):17–28
Tiffin H (1995) Post-colonial literatures and counter-discourse. In: Ashcroft B, Griffiths G, Tiffin H (eds) The post-colonial studies reader. Routledge, London, pp 95–98
Underhill J (2011) Creating worldviews: metaphor, ideology and language. Edinburgh University Press, Edinburgh
Unerman J (2020) Risks from self-referential peer-review echo chambers developing in research fields; 2018 keynote address presented at the British accounting review 50th anniversary celebrations, British accounting and finance association annual conference, London. Br Account Rev 52(5):1–26
Warscheid I (2018) The Islamic literature of the precolonial Sahara: sources and approaches. Hist Compass 16(5):1–10
Wolfreys J, Womack K, Robbins R (2014) Key concepts in literary theory, 3rd edn. Edinburgh University Press, Edinburgh
Zu S et al (2022) Exposure effects or confirmation bias? Examining reciprocal dynamics of misinformation, misperceptions, and attitudes towards Covid-19 vaccines. Health Commun. https://doi.org/10.1080/10410236.2022.2059802
Download references
Acknowledgements
No funding was received by the author in relation to this article.
Author information
Authors and affiliations.
Department of English, King’s College, University of Aberdeen, Aberdeen, UK
Thomas Rist
You can also search for this author in PubMed Google Scholar
Contributions
I, Thomas Rist, am the sole author of this article.
Corresponding author
Correspondence to Thomas Rist .
Ethics declarations
Conflicts of interest.
There are no conflict of interest.
Ethical approval
No committee was involved in this research or therefore approved it. All research was performed in accordance with relevant guidelines and regulations.
Informed consent
This article does not contain any studies with human participants performed by any of the authors.
Rights and permissions
Open Access This article is licensed under a Creative Commons Attribution 4.0 International License, which permits use, sharing, adaptation, distribution and reproduction in any medium or format, as long as you give appropriate credit to the original author(s) and the source, provide a link to the Creative Commons licence, and indicate if changes were made. The images or other third party material in this article are included in the article's Creative Commons licence, unless indicated otherwise in a credit line to the material. If material is not included in the article's Creative Commons licence and your intended use is not permitted by statutory regulation or exceeds the permitted use, you will need to obtain permission directly from the copyright holder. To view a copy of this licence, visit http://creativecommons.org/licenses/by/4.0/ .
Reprints and permissions
About this article
Rist, T. Confirmation bias studies: towards a scientific theory in the humanities. SN Soc Sci 3 , 123 (2023). https://doi.org/10.1007/s43545-023-00689-5
Download citation
Received : 18 October 2021
Accepted : 01 June 2023
Published : 22 July 2023
DOI : https://doi.org/10.1007/s43545-023-00689-5
Share this article
Anyone you share the following link with will be able to read this content:
Sorry, a shareable link is not currently available for this article.
Provided by the Springer Nature SharedIt content-sharing initiative
- Confirmation Bias
- Public Discourse
- Find a journal
- Publish with us
- Track your research

An official website of the United States government
The .gov means it’s official. Federal government websites often end in .gov or .mil. Before sharing sensitive information, make sure you’re on a federal government site.
The site is secure. The https:// ensures that you are connecting to the official website and that any information you provide is encrypted and transmitted securely.
- Publications
- Account settings
Preview improvements coming to the PMC website in October 2024. Learn More or Try it out now .
- Advanced Search
- Journal List
- Front Psychol
Characterizing the Influence of Confirmation Bias on Web Search Behavior
Associated data.
The raw data supporting the conclusions of this article will be made available by the authors, without undue reservation.
In this study, we analyzed the relationship between confirmation bias, which causes people to preferentially view information that supports their opinions and beliefs, and web search behavior. In an online user study, we controlled confirmation bias by presenting prior information to participants that manipulated their impressions of health search topics and analyzed their behavioral logs during web search tasks. We found that web search users with poor health literacy and negative prior beliefs about the health search topic did not spend time examining the list of web search results, and these users demonstrated bias in webpage selection. In contrast, web search users with high health literacy and negative prior beliefs about the search topic spent more time examining the list of web search results. In addition, these users attempted to browse webpages that present different opinions. No significant difference in web search behavior was observed between users with positive prior beliefs about the search topic and those with neutral belief.
1. Introduction
The credibility of web information has become a serious social issue. For example, Sillence et al. reported that more than half of the health information available on the web has not been verified by experts (Sillence et al., 2004 ). Therefore, if web search users may believe misinformation, they cannot distinguish correct and incorrect web information.
In addition, problems with web information credibility are amplified due to the personalization of information delivery, e.g., web search engines and recommendation systems. The “filter bubble,” which is phenomenon where users only access information they are interested in due to the optimization of information access, is becoming a social problem because it deprives users of the opportunity to examine information from broader perspectives to facilitate careful and effective decision making (Le et al., 2019 ; Yamamoto and Yamamoto, 2020 ).
People can believe incorrect or low-quality information due to “confirmation bias,” which is a concept defined in cognitive psychology. In cognitive psychology, confirmation bias, i.e., the tendency to preferentially view information that is consistent with one's opinions or hypotheses, has a significant impact on decision making (Nickerson, 1998 ; Kahneman, 2011 ). Confirmation bias occurs frequently in web searches. For example, assume that user X, who is health conscious, learns on TV that food Y, which uses genetic modification, is harmful to health and distrusts food Y. When user X performs a web search to obtain information about food Y's safety, they unconsciously seek to support the idea that food Y is harmful to their health; therefore, user X will preferentially browse negative information about food Y, even if that information is incorrect or low-quality. Thus, confirmation bias can be a significant problem in web search behavior because confirmation bias that occurs when users search the web for information about food, clothing, housing, and politics can significantly impact society.
There are several studies on the relationship between confirmation bias and web search behaviors (White, 2013 ; Schweiger et al., 2014 ; Pothirattanachaikul et al., 2019 ). For instance, White investigated the impact of prior beliefs on web search behaviors and demonstrated that the prior beliefs of web search users are likely to be strengthened by web search when their prior beliefs about the search topics are not strong (White, 2013 ). White also found that web search users are more susceptible to positive search results. Pothirattanachaikul et al. studied how opinion polarity and document credibility affect the search behavior and prior belief of web search users (Pothirattanachaikul et al., 2019 ). They found that web search users spent more time on search tasks when they viewed webpages with opinions that are inconsistent with their existing beliefs. Schweiger et. al. focused on treatment for manic depression and studied the relationship between confirmation bias toward psychotherapy and searchers' belief change on the treatment after reading web pages (Schweiger et al., 2014 ). Their study suggested that showing experts' evaluation on treatment could reduce confirmation bias and change the prior belief. Like the above studies, many have focused on investigating how confirmation bias influences searcher belief on topics via web searches. However, few studies have characterized the influence of confirmation bias on behaviors on search engine results pages (SERPs) and webpages as well as belief change via web searches, based on log-based analysis (e.g., number of clicks, dwell time on webpages, and click depth). Moreover, few studies have examined the relationship between confirmation bias, web search behaviors, and critical information-seeking skills, i.e., information literacy.
In the fields of information retrieval and human-computer interaction, several studies have investigated how to present information to enhance critical information seeking on the web (Liao and Fu, 2014a ; Liao et al., 2015 ; Yamamoto and Yamamoto, 2018 , 2020 ). For instance, Liao et al. revealed that indication of the opinion stance and expertise of the information sender can mitigate the confirmation bias (Liao and Fu, 2014a ). Yamamoto et al. proposed the Q uery P riming system, which facilitates careful information retrieval by showing keywords that evoke critical thinking on web search systems (Yamamoto and Yamamoto, 2018 ). Q uery P riming employs keyword auto-completion and keyword suggestion to present search terms that stimulate critical thinking and encourages careful information seeking and decision making. In addition, Yamamoto et al. proposed the P ersonalization F inder , web browser extension to reveal the effects of web search personalization and promote careful web search practices (Yamamoto and Yamamoto, 2020 ). The P ersonalization F inder exposes search results personalized/hidden by web search engines so that searchers can get aware that web search engines provide them with a biased list of web pages according to the searchers' preference. However, these methods were designed for situations where useful meta-information can be obtained to mitigate confirmation bias, e.g., information provider's expertise/perspective, typical search queries used by careful web searchers, and user preference models. If the typical behaviors of web search users with confirmation bias can be identified and compared to those of users with critical information search skills, we believe it will be possible to design web search systems that consider and reduce confirmation bias.
Previously, we conducted a pilot-study to investigate the relationship between confirmation bias and web search behaviors (Suzuki and Yamamoto, 2020 ). Although the results of that study suggested that people with confirmation bias can perform web search differently to people without the bias, the study design was not sufficiently rigorous to validate the findings because it was difficult to clearly distinguish participants with confirmation bias from those without the bias. Thus, in this study, we quantitatively analyzed the relationship between confirmation bias, information literacy, and web search behavior on health topics by generating pseudo-confirmation bias in participants. We had participants conduct online search tasks by manipulating prior information about health topics to control confirmation. We then analyzed the differences in the web search behaviors of users with and without confirmation bias. We believe it is essential to design information access systems such as web search engines ans web browsers that considers confirmation bias to encourage users to avoid incorrect information for critical health information seeking on the web.
Ennis defined critical thinking as logical and reflective thinking to determine what to believe or do (Ennis, 1987 ). Ennis also claimed that ideal critical thinkers are disposed to: seek reasons, consider the total situation, look for alternatives, and use logical thinking, e.g., deductive reasoning. Kusumi et al. stated that accurate evaluations of information require searchers to possess critical thinking attitudes and critical thinking skills, e.g., language and reasoning skills (Kusumi et al., 2017 ). In addition, using the elaboration likelihood model (ELM), Petty et al. indicated that possessing motivation to scrutinize information is a prerequisite for people to utilize critical thinking skills (Petty and Cacioppo, 1986 ). Confirmation bias can influence people's attitudes about evaluating information. We expect that, if search users have no confirmation bias and do web searches as critical thinkers, to obtain correct and information from the web during web search processes, they will behave in the same manner which the information literacy researchers or librarians think is important. According to Meola ( 2004 ) and Yamamoto et al. ( 2018 ), the following actions are necessary to obtain correct information on the web: (1) spending more time searching, (2) browsing more webpages for comparison, (3) browse web pages in lower-ranked web search results as well as higher-ranked ones, and (4) checking evidence to support webpage content, such as the expertise of webpage authors, existence of valid references, and the freshness of webpages. Therefore, we set the following hypotheses H1 and H2 for our online user study.
- H1 Web searchers with confirmation bias preferentially browse information that is consistent with their beliefs and do not carefully examine which information they should view. Thus, they spend less time browsing the search results list and preferentially browse higher-ranked pages in the results.
- H2 Web searchers with confirmation bias only view information that is consistent with their beliefs and do not browse information carefully. Thus, they spend less time browsing webpages and view fewer webpages.
As mentioned above, the ELM theory indicates that if people are more willing to understand information about a topic, they often make more efforts to scrutinize its quality and modify their prior belief if necessary (Petty and Cacioppo, 1986 ). On the other hand, White found that web search users often strengthen their own beliefs through search (White, 2013 ). Based on these two studies, we also set the following hypothesis H3 for the user study.
- H3 Web searchers with confirmation bias do not change their beliefs significantly when they search the web, compared to users without confirmation bias.
Lopes et al. analyzed the relationship between health literacy and web search behavior using eye-tracking analysis (Teixeira Lopes and Ramos, 2020 ). They found that web search users with higher health literacy visited more webpages and spent more time reading webpages. Furthremore, Yamamoto et al. revealed that the higher health information literacy web searchers have, the more tolerant they are for cognitive biases in web searches (Yamamoto et al., 2018 ). Therefore, we set the following hypothesis H4 .
- H4 The degrees of H1 , H2 , and H3 are influenced by the web search user's degree of information literacy.
2. Materials and Methods
This section describes the methodology employed to analyze the impact of confirmation bias and information literacy on web search behavior. The details of the experiment are described in the following. Note that we refer to the group with negative beliefs about the search topic as the biased(−) group, we refer to the group with positive beliefs as the biased(+) group, and we refer to the group with no bias as the neutral group.
2.1. Procedures
We conducted an online user study in Japanese according to the following procedure: (1) user registration; (2) prior belief questionnaire; (3) presentation of prior information about the search topic; (4) search task; and (5) post-task questionnaire.
First, the participants visited the experimental site prepared by our laboratory after they registered as users at Lancers.jp, which is a Japanese crowdsourcing service 1 . Then, the participants answered a questionnaire on their prior beliefs about a given search topic. In the prior belief questionnaire, we asked the participants to answer the following question on a five-point Likert scale: “How do you feel about the safety of eating GM (genetically modified) foods?” (“1. Danger;” “2. Somewhat danger;”, “3. Neither danger nor safe;” “4. Somewhat safe;” to “5. Safe”).
We then assigned participants to specific experimental conditions based on their answers regarding their prior beliefs about the search topic.
- - biased(−) group: Participants who answered “Dangerous” or “Somewhat dangerous.”
- - biased(+) group: Participants who answered “Safe” or “Somewhat safe.”
- - neutral group: Participants who answered “Neither danger nor safe.”
Next, we presented prior information to strengthen the participants' prior beliefs to introduce confirmation bias during the search task. Here, the presented information comprised a section 1 that described the search task and a section about GM foods. Note that we used the same description for all participants; however, we presented different descriptions about GM foods depending on the participants' prior beliefs.
The introduction for the search task is as follows.
You pick up a bottle of rapeseed oil that was on sale, and you notice a label that states that “it may contain GM rapeseed.” You have always been a little curious about GM foods. Then, you asked your friend to give you some advice about GM foods .
In addition, we presented different information to strengthen the participants' prior beliefs depending on the experimental group. The information presented to each group is described as follows.
- - biased(−) group: This group was shown a 200-word negative description of GM foods (e.g., “Europe has strict regulations against GM foods.”) and a 2-min video 2 against GM foods.
- - biased(+) group: This group was shown a 200-word positive description of GM foods (e.g., “Japan's Ministry of Health, Labor and Welfare (MHLW) carries out strict screening, and many Japanese people eat GM foods.”) and a 2-min video 3 supporting GM foods.
- - neutral group: This group was shown the negative and positive information presented to the biased(−) and (+) groups so that the participants in this group could understand there is controversy about whether or not GM foods are safe to eat.
To ensure all participants viewed the preliminary information, we asked them to summarize the content in approximately 100 words after viewing the video.
The participants performed the search task after viewing the preliminary information. The following instructions were presented to the participants when they began the search task.
Follow the steps below to complete the task of investigating whether or not it is safe to eat GM foods. Click on the “Start the search” button below and browse a list of search results and their links. When you have reached a satisfactory conclusion about “whether it is safe to eat GM foods,” please stop searching the web and report your final opinion and the reasons for it in the form .
After participants clicked the “Start the search” button, they browsed a search engine results page (SERP) and the documents linked from the SERP to collect information about the safety of eating GM foods.
When the participants were satisfied with the obtained information, they completed the search and reported their responses to the search task (posterior beliefs). Here, the participants were asked to answer a questionnaire about whether it is safe to eat GM foods using the same five-point Likert scale used in the prior belief questionnaire. Note that we did not set a time limit in this search task because the goal was to analyze how participants searched and browsed at their discretion.
After completing the search task, the participants answered the post-task questionnaire about health literacy and demographic characteristics. We used the eHealth Literacy Scale (eHEALS) to survey information literacy on health topics, i.e., the ability to search for reliable health information on the web (health literacy) (Norman and Skinner, 2006 ). The participants answered the eight questions on a five-point Likert scale (“1: I never agree” to “5: Completely agree”). Here, we used the total eHEALS score as an indicator of the degree of each participant's health literacy. In addition, in the demographic characteristics questionnaire, we investigated the participants' gender, age, and educational background.
2.2. Search Task and Search Results List
We set a search task for a search topic that increases the polarity's variance and degree of prior beliefs. In this experiment, we selected “GM foods,” which is a controversial topic in Japan, as the search topic.
In the search task, we presented the participants with a list of search results that imitated those returned by common web search engines, e.g., Google 4 and Yahoo! 5 The search result list included 30 search results prepared in advance for the given search topic. Figure 1 shows the search result list used in the search task.

SERP presented to participants in the user experiment.
Before starting the task, we performed a Google search using the queries “GM foods safe” and “GM foods dangerous” to obtain 15 search results containing the words “safe” and “dangerous” in the title or summary (referred to as a snippet). We defined the search results collected by the former query as search results containing positive information about prior beliefs and search results collected by the latter query as search results containing negative information about prior beliefs . We then created a list of search results by alternately displaying the results of the two queries from the top ( Figure 2 ). We displayed the positive and negative results alternately to present both types of information as equally as possible to the participants. Although the search results imitate the results screen of a general web search, the system was configured such that participants could not modify the search queries.

Allocation of search results on SERP. Red and blue search results contain the terms “safe” and “dangerous” in their title or summary, respectively.
When the participants clicked each search result, an archived version of the corresponding webpage was displayed. Here, we embedded JavaScript code in the archived webpages to measure the browsing time on each webpage. In addition, we disabled hyperlinks in the documents; thus, the participants could not view documents other than those displayed in the search results list. As a result, we measured the page browsing time for only the webpages in the search result list.
2.3. Participants
We recruited 300 Japanese participants using Lancers.jp. We excluded data for participants who failed to complete the task or worked on the task multiple times for some reasons. After selecting the data to exclude, we used the data from a total of 275 participants in our analysis.
We then assigned the participants to specific groups according to their prior beliefs. In the biased(−) group, 148 participants completed the task, and 96 and 31 participants completed the task in the neutral group and biased(+) group, respectively. Note that we paid 100 Japanese yen to each participant who completed the task.
2.4. Monitored Data
We collected data on the following items during the search task to analyze the relationship between confirmation bias and web search behavior.
- - Dwell time on search engine results page (SERP)
- - Dwell time on webpages
- - Search session time
- - Clickthrough of search results.
The dwell time on SERP is the total time the participants browsed the SERP, and the dwell time on webpages is the time the participants spent browsing the webpages linked from the SERP. The search session time is the total time the participants browsed the webpages and SERP, and the clickthrough of search results is the information in the search results the participants clicked on the SERP. The clickthrough information includes the title, summary text, URL, search result rank, and belief polarity (i.e., whether the search result contains “safe” or “dangerous” in the title or summary text). We set up these indicators in reference to the paper by White et al., which analyzed web search behavior logs (White and Morris, 2007 ).
2.5. Analyses
We employed the generalized linear mixed model (GLMM) (Barr et al., 2013 ) to analyze the users' behavioral logs. The GLMM can separate the main effect of the intervention from the random effect, which is the effect of individual differences among the participants and tasks. Note that the GLMM can analyze small-scale data more accurately than methods that employ frequentist statistics (Kay et al., 2016 ). The GLMM is becoming an increasingly established method to model user behavior in the information retrieval and human-computer interaction fields (Kim et al., 2017 ). In this study, we modeled the behavioral data using the GLMM extended by the Bayesian statistical model.
Here, we assumed that search session time and dwell time on SERP follow a Weibull distribution (Liu et al., 2010 ). We also assumed that the number of page views and maximum click depth follow a Poisson distribution, and that the amount of belief change follows a normal distribution.
In the GLMM, we set the two factors, i.e., confirmation bias (condition) and health literacy score (eHEALS), as the main effects and the participant as a random effect. Following the literature (Barr et al., 2013 ), we modeled the behavioral indicator measured in the user experiment as follows 6 :
where Y is the target variable, Cond is a binary value indicating the presence or absence of confirmation bias for each participant, and eHEALS is the health literacy score. Here, (x|y) means that y is a random effect of x.
We used the highest density interval (HDI) as a measure to investigate the effect of the condition and eHEALS factors. The HDI represents the possible range of the parameter, where the parameter is considered effective if the HDI does not contain zero. Note that this is equivalent to rejecting the null hypothesis in frequentist statistics. Following Kruschke's point, we set the HDI for the parameter to be effective at 90% (Kruschke, 2014 ).
We used a non-parametric test to analyze the results of the post-task questionnaire.
From the user experiment, we collected behavioral and questionnaire data from the 275 participants. Here, we describe the results of the analyses of the behavioral data, the pre-task questionnaire, and post-task questionnaires.
We analyzed the effects of two factors, i.e., the presence of condition and eHEALS, on search/browsing behavior and information scrutiny perspectives. Here, we set three levels for the condition: (1) with negative confirmation bias ( biased(−) group), (2) without confirmation bias ( neutral group), and (3) with positive confirmation bias ( biased(+) group). We then analyzed the differences between the biased(−) and biased(+) groups compared to the neutral group.
Table 1 shows the mean values and standard deviations of the various behavioral indices for each condition.
Mean and standard deviation of condition in each behavioral index.
3.1. Search Session Time
To analyze how carefully participants performed their search and browsing behavior, we compared the search session time for each group of participants. Table 2 shows that the 90% HDI of the coefficient of the condition did not contain zero in the analysis comparing the biased(−) and neutral groups. Note that this is equivalent to rejecting the null hypothesis in frequentist statistics.
GLMM results compared to neutral group.
Numbers represent the median and interval of 90% HDI. Bold numbers do not contain zero in the 90% HDI .
These results demonstrate that the biased(−) group tended to have shorter search session time than that of the neutral group. However, the 90% HDI of the coefficients of the eHEALS and interaction contained zeros, which is equivalent to not rejecting the null hypothesis in frequentist statistics. In addition, we observed that eHEALS and interaction had no effect on the search session time.
The 90% HDI for condition, eHEALS, and interaction coefficients contained zero in the analysis comparing the biased(+) and neutral groups. Therefore, the presence or absence of positive confirmation bias had no effect on the search session time.
3.2. Dwell Time on SERP
We compared the SERP browsing time to analyze how carefully the participants browsed the list of search results while collecting information. We found that the 90% HDI of the coefficient of the condition and interaction did not contain zero in the analysis comparing the biased(−) and neutral groups.
The interaction was confirmed; thus, we conducted a simple main effect analysis, and the results are shown in Figure 3 . As can be seen, when the participant's eHEALS was low, the biased(−) group tended to spend less time browsing SERP compared to the neutral group. However, when the eHEALS was high, the biased(−) group tended to spend more time browsing the SERP compared to the neutral group.

Estimated effect of condition and eHEALS on SERP dwell time. The red line represents the neutral group, and the blue line represents the biased(−) group. The background color indicates the confidence interval.
As shown in Table 2 , the 90% HDI of the coefficients of condition and interaction contained zero in the analysis comparing the biased(−) and neutral groups. Therefore, the presence or absence of positive confirmation bias had no effect on SERP dwell time.
3.3. Maximum Dwell Time on Webpage
To analyze how carefully the participants browsed the webpages in the SERP, we compared the participants' maximum webpage browsing time during the search task. Compared to the neutral group, the 90% HDI of the condition, eHEALS, and interaction coefficients contained zero for the biased(−) and biased(+) groups ( Table 2 ), which indicates that the presence or absence of confirmation bias had no effect on maximum dwell time.
3.4. Number of Page Views
We also evaluated the number of webpages viewed by the participants during the search task to analyze how intensively the participants attempted to collect evidence when they assessed the truth of the given search topic. Compared to the neutral group, the 90% HDI of the condition, eHEALS, and interaction coefficients contained zero for both the biased(−) and biased(+) groups ( Table 2 ), which indicates that the presence or absence of confirmation bias had no effect on the number of page views.
We also analyzed the extent to which participants viewed webpages containing information that was consistent with their prior beliefs. Here, the number of clicks on a webpage that included the word “dangerous” in the title or summary of the search result was defined as the number of pageviews(−) . In addition, we defined the number of clicks on a webpage that included the word “safe” as the number of pageviews(+) .
For the number of pageviews(−) , the 90% HDI of the condition, eHEALS, and interaction coefficients contained zero for both the biased(−) and biased(+) groups ( Table 2 ), which indicates that the number of pageviews(−) was not affected by the presence or absence of confirmation bias.
For the number of pageviews(+) , the 90% HDI of the condition and interaction coefficients did not contain zero in the analysis comparing the biased(−) and neutral groups ( Table 2 ). Here, as we observed the interaction, we conducted a simple main effect analysis, and the results are shown in Figure 4 . As can be seen, when the participant's eHEALS was low, the biased(−) group tended to have fewer pageviews(+) than the neutral group. However, when the participant's eHEALS was high, the biased(−) group tended to have more pageviews(+) than the neutral group.

Estimated effect of condition and eHEALS on number of page views(+). The red line represents the neutral group and the blue line represents the biased(−) group. The background color indicates the confidence interval.
For the number of pageviews(+) , the 90% HDI of the condition and interaction coefficients did not contain zero in the analysis comparing the biased(+) and neutral groups ( Table 2 ). This indicates that the presence or absence of positive confirmation bias had no effect on the number of pageviews(+) .
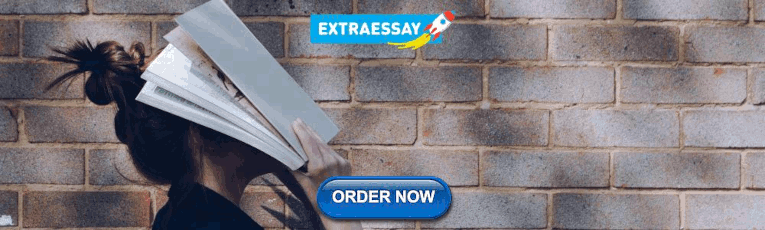
3.5. Maximum Click Depth
To analyze how deeply the participants scanned the search result list, we investigated the order of the search results the participants clicked on to analyze the maximum search result rank, i.e., the maximum click depth. Table 2 shows that the 90% HDI of the condition and interaction coefficients did not contain zero in the analysis comparing the biased(−) and neutral groups. Here, we conducted a simple main effect analysis because we observed the interaction, and the results are shown in Figure 5 . As can be seen, when the participant's eHEALS was low, the biased(−) group tended to click on higher search results than the neutral group. However, when the participant's eHEALS was high, the biased(−) group tended to click on lower search results than the neutral group.

Estimated effect of condition and eHEALS on maximum click depth. The red line represents the neutral group, and the blue line represents the biased(−) group. The background color indicates the confidence interval.
As shown in Table 2 , the 90% HDI of the condition, eHEALS, and interaction coefficients contained zero in the analysis comparing the biased(+) and neutral groups, which indicates that the presence or absence of positive confirmation bias had no effect on the maximum click depth.
3.6. Belief Change
We evaluated the difference between the posterior and prior beliefs to analyze the extent to which the participants' prior beliefs changed as a result of the search task. Table 2 shows that the 90% HDI of the condition, eHEALS, and interaction coefficients included zero for both the biased(−) and biased(−) groups compared to the neutral group. These results indicate that participants did not change their prior beliefs much over the course of the search task regardless of the presence of positive or negative confirmation bias.
4. Discussion
4.1. hypothesis verification.
In this study, we analyzed the SERP browsing time and maximum click depth to verify H1 regarding the webpage selection behavior. The results demonstrated that when the participant's eHEALS score was low, the biased(−) group spent less time browsing the SERPs than the neutral group, tended to click on the higher (shallower)-ranked search results, and viewed pages that were inconsistent with their prior belief less frequently. When the participants' eHEALS score was high, the biased(−) group spent more time browsing the SERPs than the neutral group, tended to click on lower (deeper)-rank search results, and viewed pages that were inconsistent with their prior belief more often. In contrast, no difference was observed in SERP browsing time and maximum click depth for the biased(+) and neutral groups.
The eHEALS score is a scale that reflects the information literacy required to obtain and view health information on the web carefully (health literacy). Therefore, even if participants with high health literacy had negative confirmation bias for the search topic, they could reduce the negative confirmation bias and carefully select webpages to view. In contrast, when participants with low health literacy had negative confirmation bias about the search topic, they could not reduce the negative confirmation bias and spent much attention and time selecting the webpages to view from the search result list. Thus, we believe that hypotheses H1 and H4 regarding webpage selection are supported only when web search users have negative confirmation bias for the given search topic.
We also analyzed the maximum page browsing time and number of page views to verify H2 regarding webpage browsing behavior. Here, we did not find that maximum page browsing time was affected by confirmation bias. For the number of page views, the biased(−) group with low eHEALS score tended to view fewer webpages containing the word “safe” in the title or summary text compared to the neutral group. In contrast, the biased(−) group with a high eHEALS score tended to view more webpages with the word “safe” in the title or summary text compared to the neutral group. Similar to the results of the analyses of dwell time on SERP and maximum click depth, these results suggest that the participants with low health literacy could not control the effects of confirmation bias when they had negative confirmation bias for the given search topic. In addition, the results indicate that the participants did not actively browse webpages that were inconsistent with their belief (i.e., webpages that refers to GM foods as safe). In contrast, participants with high health literacy were able to reduce the impact of negative confirmation bias and actively browsed webpages that were inconsistent with their prior belief in the search results. Therefore, we believe that H2 and H4 were supported only when the participants had a negative confirmation bias about the given search topic.
We analyzed the difference in belief before and after performing the web search task to verify H3 regarding belief change after web searches. The results demonstrate that no significant difference was observed in terms of the amount of belief change in the biased(−) and biased(+) groups compared to the neutral group. Thus, we consider that H3 was not supported. The results for H1 and H2 indicate that even if web search users with high health literacy had negative confirmation bias for the given search topic, they viewed pages with different positions actively. Thus, the results for H3 suggest that it is difficult for users with high health literacy to change their beliefs in a significant way, even if they are able to reduce the negative effects of confirmation bias and perform careful search browsing behavior.
Finally, we discuss the differences in the various behavioral indexes only for the biased(−) group. Rozin et al. found that humans are more influenced by negative information than positive information (Rozin and Royzman, 2001 ); therefore, we expected that the negative confirmation bias for search topics would impact search browsing behavior more than positive confirmation bias. We found that the biased(−) group was more affected by confirmation bias than the biased(+) group, and the values of the various behavioral indexes decreased significantly compared to those of the neutral group.
In summary, our study revealed that when web searchers with poor health literacy have negative prior beliefs about health topics, they could not examine web search results and preferentially view web pages supporting their beliefs. On the other hand, if web searchers with high health literacy have negative prior beliefs about health topics, they could spend more time examining web search results and browsing web pages that present different opinions. However, the study results indicate that their prior belief could not change so much even if they browse various opinions. In the case where web searchers have positive prior beliefs about health search topics, we did not observe the relationship between health literacy and web search behaviors.
The study results imply several points to design classes and information access systems for critical information seeking on the web. Firstly, we might need to develop educational classes related to information literacy so that people can reflect and improve their web search behaviors toward critical information seeking. It might be good to collaborate with computer scientists to develop a function on web search/browsing systems that general web searchers can use to reflect their search behaviors. As our study revealed, web searchers with poor health literacy did not often examine web search results and compare them with various web pages. Consequently, they lost opportunities to check if their prior belief could be wrong or disputed. Bateman et al. proposed a search user interface that summarizes web search histories of users and revealed that the interface could help users modify their search behavior to improve search performance (Bateman et al., 2012 ). For supporting web searchers with low health literacy, one possible application is a web browser extension to visualize user behavior tendencies in order to encourage people to improve deficiencies relative to behaviors of web searchers with high health literacy.
The second point is prediction of the extent of health literacy. Our study revealed that if web searchers with poor health literacy have negative prior beliefs about health search topics, they often make less effort to examine web search results than those with high health literacy. For supporting web searchers with poor health literacy efficiently, we need a method to find such searchers. We observed specific web search behaviors to distinguish web searchers with poor health literacy and those with high literacy (e.g., dwell time on SERP, number of page views, and maximum click depth) through the online study. In the field of computer science, machine learning is a popular technique to make predictions with data. We plan to apply machine learning techniques to web search behavior data to build a predictor for the health literacy of web searchers.
The third point is mitigation of confirmation bias in web searches. Although our study suggests that it is difficult for web searchers to change their prior beliefs, we need to support web searchers mitigating their confirmation bias or doing web searches objectively. One possible application is interactive chat-bot systems that ask web searchers which evidence supports the belief and show contradictory opinions while searching for web information. If computer scientists collaborate with experts from the field of health psychology, we believe that they can develop such systems and contribute to reducing confirmation bias.
4.2. Limitations
To realize more accurate analyses, at least two issues must be considered and improved in this user experiment. The first is the generalizability of the results of the online study. In this study, we considered “GM foods” as a search topic in the health field. To confirm whether this study's findings can be generalized to other topics, we must conduct search task experiments in other fields and examine the effects of confirmation bias in each field.
The second issue is the quality of the webpages in the list of search results in the given search task. In our user experiment, we used the results of a Google search with a query pair of the words “safe” or “dangerous” and “GM foods” to create the list of search results. However, when we investigated the domains of the collected webpages, we found that many of the webpages containing the word “safe” were authorized by public organizations, which are generally considered reliable. The “GM foods” chosen as the search topic in this user experiment represents foods that have been confirmed as safe by the Ministry of Health, Labor, and Welfare in Japan (MHLW). Therefore, the list of results including the word “safe” collected by the Google search also contained a significant amount of information from national public organizations, e.g., the MHLW. According to Liao et al., even if information is inconsistent with one's beliefs, users are more likely to view the information if the information provider is identified as having a high level of expertise (Liao and Fu, 2014b ). In other words, users with negative confirmation bias may be more likely to click on positive information if it contains reliable information regardless of the polarity of their beliefs. Therefore, it is difficult to precisely analyze why participants with negative confirmation bias viewed the search results containing the word “safe” actively in the current experimental design. Thus, we must conduct user experiments by creating search results for both negative and positive information with the same level of reliability.
5. Conclusion
In this paper, we have described an online experiment using crowdsourcing that was conducted to identify web search behaviors in consideration of confirmation bias. To divide users into groups with and without confirmation bias, we provided the participants with prior information to manipulate their impressions of the given search topic. We then analyzed the logs of their search and browsing.
We found that participants with negative beliefs about the given search topic often spent less time browsing the search result list page, clicked on higher-ranked search results, and did not browse search results about positive opinions when they had low health literacy. In contrast, participants with high health literacy, even if they had negative beliefs about the given search topic, often spent more time browsing the search results page, scanned lower ranked search results, and browsed more actively for search results containing positive opinions. However, the results also suggest that it was difficult for participants with high health literacy to remove the negative effects of confirmation bias and change their beliefs, even if they were able to perform careful search browsing behavior. We conclude from these results that web searchers with confirmation bias are unlikely to change their prior beliefs even if they spend a lot of effort searching for information. Therefore, we consider that the most important issue is to design a function on web access systems that supports web searchers to mitigate confirmation bias. Moreover, we need to develop a function of the systems to detect web searchers with poor health literacy and improve their health literacy and web search behaviors toward critical information seeking on the web.
In the future, we plan to challenge the following several issues based on our study results. First, we must conduct additional user experiments with different search topics and search result lists to obtain a deeper understanding of user web search behaviors in consideration of confirmation bias and generalize our findings to other fields. Secondly, we need to develop a function on web search/browsing systems that general web searchers can use to reflect their search behaviors toward critical information seeking. Furthermore, we need to build a system that predicts the health literacy of web searchers and encourages the searchers with poor health literacy to make more efforts for critical web searches. Finally, we need to support web searchers mitigating their confirmation bias by showing contradictory opinions in web searches.
Data Availability Statement
Ethics statement.
Ethical review and approval was not required for the study on human participants in accordance with the local legislation and institutional requirements. Written informed consent for participation was not required for this study in accordance with the national legislation and the institutional requirements.
Author Contributions
MS and YY contributed to conception and design of the study. MS developed an experimental system and wrote the first draft of the manuscript. YY performed the statistical analysis. Both authors contributed to manuscript revision, read, and approved the submitted version.
This work was supported by JSPS KAKENHI Grant Numbers JP18H03244, JP21H03554, and JP21H03775.
Conflict of Interest
The authors declare that the research was conducted in the absence of any commercial or financial relationships that could be construed as a potential conflict of interest.
Publisher's Note
All claims expressed in this article are solely those of the authors and do not necessarily represent those of their affiliated organizations, or those of the publisher, the editors and the reviewers. Any product that may be evaluated in this article, or claim that may be made by its manufacturer, is not guaranteed or endorsed by the publisher.
1 https://www.lancers.jp/ .
2 https://www.youtube.com/watch?v=umXN64zIH-8 (in Japanese).
3 https://www.youtube.com/watch?v=zMnX3qS6Dj4 (in Japanese).
4 https://www.google.co.jp/ .
5 https://www.yahoo.co.jp/ .
6 The brms package in R was used for modeling.
- Barr D., Levy R., Scheepers C., Tily H. (2013). Random effects structure for confirmatory hypothesis testing: keep it maximal . J. Memory Lang. 68 , 255–278. 10.1016/j.jml.2012.11.001 [ PMC free article ] [ PubMed ] [ CrossRef ] [ Google Scholar ]
- Bateman S., Teevan J., White R. W. (2012). The search dashboard: how reflection and comparison impact search behavior, in Proceedings of the 30th ACM International Conference on Human Factors in Computing Systems (CHI 2012) (New York, NY: ACM; ), 1785–1794. [ Google Scholar ]
- Ennis R. H.. (1987). A taxonomy of critical thinking dispositions and abilities, in Teaching Thinking Skills: Theory and Practice , eds Baron J. B., Sternberg R. J. (New York, NY: WH Freeman/Times Books/ Henry Holt & Co.), 9–26. [ Google Scholar ]
- Kahneman D.. (2011). Thinking, Fast and Slow . London: Macmillan. [ Google Scholar ]
- Kay M., Nelson G. L., Hekler E. B. (2016). Researcher-centered design of statistics: why Bayesian statistics better fit the culture and incentives of HCI, in Proceedings of the 2016 CHI Conference on Human Factors in Computing Systems (New York, NY: ), 4521–4532. [ Google Scholar ]
- Kim J., Thomas P., Sankaranarayana R., Gedeon T., Yoon H. (2017). What snippet size is needed in mobile web search? in Proc. of the 2017 Conference on Conference Human Information Interaction and Retrieval (CHIIR 2017) (New York, NY: ), 97–106. [ Google Scholar ]
- Kruschke J.. (2014). Doing Bayesian Data Analysis: A Tutorial with R, JAGS, and Stan . Cambridge, MA: Academic Press. [ Google Scholar ]
- Kusumi T., Hirayama R., Kashima Y. (2017). Risk perception and risk talk: the case of the Fukushima Daiichi nuclear radiation risk . Risk Anal. 37 , 2305–2320. 10.1111/risa.12784 [ PubMed ] [ CrossRef ] [ Google Scholar ]
- Le H., Maragh R., Ekdale B., High A., Havens T., Shafiq Z. (2019). Measuring political personalization of google news search,” in The World Wide Web Conference (WebConf 2019) (New York, NY: ), 2957–2963. [ Google Scholar ]
- Liao Q. V., Fu W.-T. (2014a). Can you hear me now?: mitigating the echo chamber effect by source position indicators, in Proceedings of the 17th ACM Conference on Computer supported Cooperative Work & Social Computing (CSCW 2014) (New York, NY: ), 184–196. [ Google Scholar ]
- Liao Q. V., Fu W.-T. (2014b). Expert voices in echo chambers: effects of source expertise indicators on exposure to diverse opinions, in Proceedings of the SIGCHI Conference on Human Factors in Computing Systems (CHI 2014) (New York, NY: ), 2745–2754. [ Google Scholar ]
- Liao Q. V., Fu W.-T., Mamidi S. S. (2015). It is all about perspective: an exploration of mitigating selective exposure with aspect indicators, in Proceedings of the 33rd Annual ACM Conference on Human Factors in Computing Systems (CHI 2015) (New York, NY: ), 1439–1448. [ Google Scholar ]
- Liu C., White R. W., Dumais S. (2010). Understanding web browsing behaviors through weibull analysis of dwell time, in Proceedings of the 33rd International ACM SIGIR Conference on Research and Development in Information Retrieval (New York, NY: ), 379–386. [ Google Scholar ]
- Meola M.. (2004). Chucking the checklist: a contextual approach to teaching undergraduates web-site evaluation . Port. Lib. Acad. 4 , 331–344. 10.1353/pla.2004.0055 [ CrossRef ] [ Google Scholar ]
- Nickerson R. S.. (1998). Confirmation bias: a ubiquitous phenomenon in many guises . Rev. Gen. Psychol. 2 , 175–220. 10.1037/1089-2680.2.2.175 [ CrossRef ] [ Google Scholar ]
- Norman C. D., Skinner H. A. (2006). eheals: the ehealth literacy scale . J. Med. Internet Res. 8 :e27. 10.2196/jmir.8.4.e27 [ PMC free article ] [ PubMed ] [ CrossRef ] [ Google Scholar ]
- Petty R., Cacioppo J. (1986). The elaboration likelihood model of persuasion . Adv. Exp. Soc. Psychol. 19 , 123–205. 10.1016/S0065-2601(08)60214-2 [ CrossRef ] [ Google Scholar ]
- Pothirattanachaikul S., Yamamoto T., Yamamoto Y., Yoshikawa M. (2019). Analyzing the effects of document's opinion and credibility on search behaviors and belief dynamics, in Proceedings of the 28th ACM International Conference on Information and Knowledge Management (CIKM 2019) (New York, NY: ), 1653–1662. [ Google Scholar ]
- Rozin P., Royzman E. B. (2001). Negativity bias, negativity dominance, and contagion . Pers. Soc. Psychol. Rev. (Thousand Oaks, California: ), 5 , 296–320. 10.1207/S15327957PSPR0504_2 [ CrossRef ] [ Google Scholar ]
- Schweiger S., Oeberst A., Cress U. (2014). Confirmation bias in web-based search: a randomized online study on the effects of expert information and social tags on information search and evaluation . J. Med. Internet Res. 16 :e94. 10.2196/jmir.3044 [ PMC free article ] [ PubMed ] [ CrossRef ] [ Google Scholar ]
- Sillence E., Briggs P., Fishwick L., Harris P. (2004). Trust and mistrust of online health sites, in Proceedings of the SIGCHI Conference on Human Factors in Computing Systems (CHI 2004) (New York, NY: ), 663–670. [ Google Scholar ]
- Suzuki M., Yamamoto Y. (2020). Analysis of relationship between confirmation bias and web search behavior, in Proceedings of the 22nd International Conference on Information Integration and Web-Based Applications & Services (iiWAS 2020) (New York, NY: ), 184–191. [ Google Scholar ]
- Teixeira Lopes C., Ramos E. (2020). Studying how health literacy influences attention during online information seeking, in Proceedings of the 2020 Conference on Human Information Interaction and Retrieval (CHIIR 2020) (New York, NY: ), 283–291. [ Google Scholar ]
- White R.. (2013). Beliefs and biases in web search, in Proceedings of the 36th International ACM SIGIR Conference on Research and Development in Information Retrieval (SIGIR 2013) (New York, NY: ), 3–12. [ Google Scholar ]
- White R. W., Morris D. (2007). Investigating the querying and browsing behavior of advanced search engine users, in Proceedings of the 30th Annual International ACM SIGIR Conference on Research and Development in Information Retrieval (SIGIR 2007) (New York, NY: ), 255–262. [ Google Scholar ]
- Yamamoto Y., Yamamoto T. (2018). Query priming for promoting critical thinking in web search, in Proceedings of the 2018 Conference on Human Information Interaction & Retrieval (CHIIR 2018) (New York, NY: ), 12–21. [ Google Scholar ]
- Yamamoto Y., Yamamoto T. (2020). Personalization finder: a search interface for identifying and self-controlling web search personalization, in Proceedings of the ACM/IEEE Joint Conference on Digital Libraries in 2020 (JCDL 2020) (New York, NY: ), 37–46. [ Google Scholar ]
- Yamamoto Y., Yamamoto T., Ohshima H., Kawakami H. (2018). Web access literacy scale to evaluate how critically users can browse and search for web information, in Proceedings of the 10th ACM Conference on Web Science (WebSci 2018) (New York, NY: ACM; ), 97–106. [ Google Scholar ]
Loading metrics
Open Access
Peer-reviewed
Research Article
A confirmation bias in perceptual decision-making due to hierarchical approximate inference
Roles Conceptualization, Data curation, Formal analysis, Investigation, Methodology, Software, Validation, Visualization, Writing – original draft, Writing – review & editing
* E-mail: [email protected] (RDL); [email protected] (RMH)
Current address: Department of Neurobiology, University of Pennsylvania, Philadelphia, Pennsylvania, United States of America
Affiliations Brain and Cognitive Sciences, University of Rochester, Rochester, New York, United States of America, Computer Science, University of Rochester, Rochester, New York, United States of America
Roles Conceptualization, Data curation, Formal analysis, Investigation, Methodology, Software, Validation, Visualization, Writing – review & editing
Affiliation Brain and Cognitive Sciences, University of Rochester, Rochester, New York, United States of America

Roles Formal analysis, Writing – review & editing
Affiliation Department of Neurobiology, Duke University, Durham, North Carolina, United States of America
Roles Methodology, Resources, Software, Writing – review & editing
Current address: Department of Biology, University of Maryland, College Park, Maryland, United States of America
Roles Conceptualization, Formal analysis, Funding acquisition, Methodology, Resources, Supervision, Writing – original draft, Writing – review & editing
- Richard D. Lange,
- Ankani Chattoraj,
- Jeffrey M. Beck,
- Jacob L. Yates,
- Ralf M. Haefner
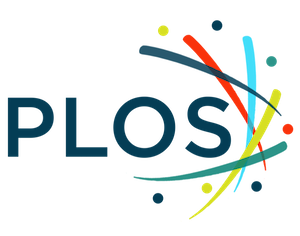
- Published: November 29, 2021
- https://doi.org/10.1371/journal.pcbi.1009517
- Peer Review
- Reader Comments
Making good decisions requires updating beliefs according to new evidence. This is a dynamical process that is prone to biases: in some cases, beliefs become entrenched and resistant to new evidence (leading to primacy effects), while in other cases, beliefs fade over time and rely primarily on later evidence (leading to recency effects). How and why either type of bias dominates in a given context is an important open question. Here, we study this question in classic perceptual decision-making tasks, where, puzzlingly, previous empirical studies differ in the kinds of biases they observe, ranging from primacy to recency, despite seemingly equivalent tasks. We present a new model, based on hierarchical approximate inference and derived from normative principles, that not only explains both primacy and recency effects in existing studies, but also predicts how the type of bias should depend on the statistics of stimuli in a given task. We verify this prediction in a novel visual discrimination task with human observers, finding that each observer’s temporal bias changed as the result of changing the key stimulus statistics identified by our model. The key dynamic that leads to a primacy bias in our model is an overweighting of new sensory information that agrees with the observer’s existing belief—a type of ‘confirmation bias’. By fitting an extended drift-diffusion model to our data we rule out an alternative explanation for primacy effects due to bounded integration. Taken together, our results resolve a major discrepancy among existing perceptual decision-making studies, and suggest that a key source of bias in human decision-making is approximate hierarchical inference.
Author summary
When humans and animals accumulate evidence over time, they are often biased. Identifying the mechanisms underlying these biases can lead to new insights into principles of neural computation. The confirmation bias, in which new evidence is given more weight when it agrees with existing beliefs, is a ubiquitous yet poorly understood example of such biases. Here we report that a confirmation bias arises even during perceptual decision-making, and propose an approximate hierarchical inference model as the underlying mechanism. Our model correctly predicts for what stimuli and tasks this bias will be strong, and when it will be weak, a critical prediction that we confirm using old and new data. A quantitative model comparison clearly favors our model over a key alternative: integration to bound. The key dynamic driving the confirmation bias in our model is an interaction between inferences on different timescales, a common scenario in decision-making more generally.
Citation: Lange RD, Chattoraj A, Beck JM, Yates JL, Haefner RM (2021) A confirmation bias in perceptual decision-making due to hierarchical approximate inference. PLoS Comput Biol 17(11): e1009517. https://doi.org/10.1371/journal.pcbi.1009517
Editor: Megan A. K. Peters, UC Irvine: University of California Irvine, UNITED STATES
Received: June 12, 2021; Accepted: October 1, 2021; Published: November 29, 2021
Copyright: © 2021 Lange et al. This is an open access article distributed under the terms of the Creative Commons Attribution License , which permits unrestricted use, distribution, and reproduction in any medium, provided the original author and source are credited.
Data Availability: Location of data and code: https://osf.io/mxw5v/ .
Funding: This work was supported by National Eye Institute/NIH awards R01 EY028811 (RMH) and T32 EY007125 (RDL,JLY), as well as an National Science Foundation/NRT graduate training grant NSF-1449828 (RDL). The funders had no role in study design, data collection and analysis, decision to publish, or preparation of the manuscript.
Competing interests: The authors have declared that no competing interests exist.
Introduction
Human decisions are known to be systematically biased, from high-level planning and reasoning to low-level perceptual decisions [ 1 , 2 ]. Decisions are especially difficult when they require synthesizing multiple pieces of noisy or ambiguous evidence for or against multiple alternatives [ 3 – 6 ]. Perceptual decision-making studies across multiple species and sensory modalities have exposed systematic biases that differ in ways that are not well understood. Here, we focus on temporal biases, which range from over-weighting early evidence (a primacy effect) to over-weighting late evidence (a recency effect) ( Fig 1A ) even in situations when an equal weighting of evidence would be optimal. Despite seemingly comparable tasks, existing studies are surprisingly heterogeneous in the biases they find: some report primacy effects [ 7 – 9 ], some find that information is weighted equally over time [ 10 – 12 ], and some find recency effects [ 13 ] without a clear pattern emerging from the data.
- PPT PowerPoint slide
- PNG larger image
- TIFF original image
a) A observer’s “temporal weighting strategy” is an estimate of how their choice is based on a weighted sum of each frame of evidence e f (more precisely, a weighted sum of the log odds at each frame). Three commonly observed motifs are decreasing weights (primacy), constant weights (optimal), or increasing weights (recency). b) Information in the stimulus about the category may be decomposed into information in each frame about a sensory variable (“sensory information”) and information about the category given the sensory variable (“category information”). c) Category information and sensory information may be manipulated independently, creating a two-dimensional space of possible tasks. Any level of task performance can be the result of different combinations of sensory and category information. A qualitative placement of previous work into this space separates those that find primacy effects in the upper-left (low sensory/high category information or LSHC regime) from those that find recency effects or optimal weights in the lower right (high sensory/low category information or HSLC regime). Numbered references are: [ 1 ] Kiani et al (2008), [ 2 ] Nienborg and Cumming (2009), [ 3 ] Brunton et al (2013), [ 4 ] Wyart et al (2012), [ 5 ] Raposo et al (2014), [ 6 ] Drugowitsch et al (2016). See S1 Text for justifications of placements.
https://doi.org/10.1371/journal.pcbi.1009517.g001
Existing models propose mechanisms for either primacy [ 7 ] or recency [ 14 ] effects alone, or are flexible enough to account for either type of bias [ 3 , 4 , 11 , 13 , 15 – 18 ], but none identifies or predicts factors that cause one bias or the other to appear in a given experimental context. All of these models are based on a variant of the classic drift-diffusion model [ 5 ]. For example, Kiani et al (2008) proposed that evidence integration stops when an internal bound is reached, even during fixed stimulus duration tasks. Averaged over many trials in which the bound is reached at different times, this leads to a primacy effect. Alternatively, a primacy effect is also expected if evidence integration is implemented by an attractor network [ 16 , 17 , 19 ], or mutual inhibition of competing accumulators [ 15 , 18 , 20 ]. However, neither of these mechanisms can account for recency effects. On the other hand, including a “forgetting” or “leak” term in the updating of the decision-variable leads to a recency effect [ 3 , 4 , 14 , 15 , 17 , 18 , 20 ]. The analysis by Glaze et al (2015) shows that a recency bias is optimal in a volatile environment, but such mechanisms cannot explain primacy effects [ 14 ]. Deneve (2012)’s normative analysis predicts that primacy and recency should depend on trial-by-trial changes in difficulty [ 21 ], while Prat-Ortega et al (2021) find that primacy and recency can change as a function of the variability of the input to a attractor-based decision-circuit [ 22 ]. However, neither account alone, or in combination, can explain the differences found across experiments. It is thus an open question whether the disparate biases observed empirically are due to differences in species, sensory modalities, training, experimental design, or individual observers.
Here, we propose a new model that not only accounts for the existing findings in the literature, but also predicts which key aspect of the stimulus determines the specific temporal bias shown by an observer. Our model extends classic ideal observer models to the hierarchical case by explicitly including the intermediate sensory representation. This reveals that task difficulty is modulated by two distinct types of information: the information between the stimulus and sensory representation (“sensory information”), and the information between sensory representation and category (“category information”) ( Fig 1B ). We show that approximate inference in such a model predicts characteristic temporal biases in a way that can explain prior empirical findings. Furthermore, our model makes a critical prediction: that the temporal bias of an individual observer should change from primacy to recency as the balance in the types of information is changed. We verify this critical prediction of our model using newly collected data from a novel pair of visual discrimination tasks. Finally, we perform a quantitative model comparison demonstrating that inference dynamics, not a finite integration bound, explain our observers’ biases, consistent with our theory.
“Sensory information” vs “Category information”
In the brain, however, a decision-making area cannot base its decision on the externally presented stimulus, e f , directly, but must rely on intermediate sensory features, which we call x f ( Fig 1B ). Accounting for the intervening sensory representation implies that LLO f cannot be computed directly, but only in stages. The information between the stimulus and category ( e f to C ) is therefore partitioned into two stages: the information between the stimulus and the sensory features ( e f to x f ), and the information between sensory features and category ( x f to C ). We call these “sensory information” and “category information,” respectively ( Fig 1B ). These two kinds of information define a two-dimensional space in which a given task is located as a single point ( Fig 1C ). For example, in a visual task, each e f would be the image on the screen while x f could be the instantaneous orientation or motion direction.
An evidence integration task may be challenging either because each frame is perceptually unclear (low “sensory information”), or because the relationship between sensory features and category is ambiguous in each frame (low “category information”). Consider the classic dot motion task [ 24 ] and the Poisson clicks task [ 11 ], which occupy opposite locations in the space. In the classic low-coherence dot motion task, observers view a cloud of moving dots, a small percentage of which move “coherently” in one direction. Here, sensory information is low since the percept of net motion is weak on each frame. Category information, on the other hand, is high, since knowing the true net motion on a single frame would be highly predictive of the correct choice (and of motion on subsequent frames). In the Poisson clicks task, on the other hand, observers hear a random sequence of clicks in each ear and must report the side with the higher rate. Here, sensory information is high since each click is well above sensory thresholds. Category information, however, is low, since knowing the side on which a single click was presented provides only little information about the correct choice for the trial as a whole (and the side of the other clicks). When frames are sequential, another way to think about category information is as “temporal coherence” of the stimulus: the more each frame of evidence is predictive of the correct choice, the more the frames must be predictive of each other, whether a frame consists of visual dots or of auditory clicks. Note that our distinction between sensory and category information is different from the well-studied distinction between internal and external noise; in general, both internal and external noise will reduce the amount of sensory and category information.
In general, sensory and category information depends on the nature of the sensory features x relative to e and C , and those relationships depend on the sensory system under consideration. For instance, a high spatial frequency grating may contain high sensory information to a primate, but low sensory information to a species with lower acuity. Similarly, when “frames” are presented quickly, they may be temporally integrated, with the effect of both reducing sensory information and increasing category information.
Qualitatively placing prior studies in the space spanned by these two kinds of information results in two clusters: the studies that report primacy effects are located in the upper left quadrant (low-sensory/high-category or LSHC) and studies with flat weighting or recency effects are in the lower right quadrant (high-sensory/low-category or HSLC) ( Fig 1C ; see S1 Text for justifications of placements). This provides initial empirical evidence that the trade-off between sensory information and category information may underlie differences in temporal weighting seen in previous studies. Unfortunately, since our placement of prior studies is only qualitative this observation only constitutes weak evidence in favor of this hypothesis. However, this hypothesis makes the strong prediction that a simple change in the stimulus statistics corresponding to sensory and category information, while holding everything else constant, should change the temporal weighting found in these previous studies (predictions provided in Table A in S1 Text ). Below we will present new data from an experiment in which we did exactly that and found that biases indeed shifted from primacy to optimal/recency as predicted.
Approximate hierarchical inference explains transition from primacy to recency
If stimuli were processed by the brain in a purely feedforward fashion, then a decision-making area could simply integrate the evidence in sensory features ( x f ) directly. This is consistent with some theories of inference in the brain in which sensory areas represent a likelihood function over stimuli [ 25 – 28 ]. However, activity in sensory areas does not rigidly track the stimulus, but is known to be influenced by past stimuli [ 29 , 30 ], as well as by feedback from the rest of the brain [ 31 , 32 ]. In fact, the intermediate sensory representation is itself often assumed to be the result of an inference process over latent variables in an internal model of the world [ 33 – 35 ]. This process is naturally formalized as hierarchical inference ( Fig 2A ) in which feedforward connections communicate the likelihood and feedback communicates the prior or other contextual expectations, and sensory areas combine these to represent a posterior distribution [ 27 , 36 – 39 ].
a) Generative model that we assume the brain has learned for a discrimination task, which specifies how sensory observations e f depend on the category for the trial, C , in two stages: each sensory observation e f is assumed to be a noisy realization of underlying sensory features, x f , and each frame of sensory features is itself assumed to be selected according to the trial’s category. b-c) Integrating evidence about C requires updating the current belief about C with new information derived from the sensory representation (left-right “integration” and bottom-up “update” arrows). The posterior distribution over x combines top-down expectations (diagonal “prior” arrows) with new evidence from the stimulus, e f (bottom-up “likelihood” arrows). Width of arrows indicates average amount of information communicated; red and blue arrows indicate changes in information flow between conditions. Note that when inference is exact , the prior is subtracted from the information in the update during the integration to prevent double-counting early evidence. While the generative model in (a) operates with discrete frames, f , inference in the brain happens in continuous time, t . b) LSHC: Low sensory information means little information in the likelihood about sensory features x f . High category information means that most of this information is also informative about C . It also means high information in the prior that is fed back to the sensory representation. c) HSLC: High sensory information means high information in the likelihood about sensory features x f . Low category information means that this information is only weakly predictive of C . It also means little information in the prior that is being fed back to the sensory representation.
https://doi.org/10.1371/journal.pcbi.1009517.g002
We hypothesize that feedback of “decision-related” information to sensory areas [ 40 , 41 ] implements a prior that reflects current beliefs about the stimulus category [ 39 , 42 , 43 ]. While such a prior is optimal from the perspective of estimating the sensory features, x f , this complicates evidence accumulation ( Methods ). When x f is influenced by prior beliefs about the stimulus category, the calculation of the “update” (log likelihood odds or LLO f ) cannot simply replace p( e f | C ) by p( x f | C ); instead, the decision-making area would need to account for or “divide out” the influence of the top-down prior on the sensory representation to avoid a double-counting of the prior ( Fig 2B and 2C ). For an ideal observer performing exact inference, this process would not entail any suboptimalities or biases. However, inference in the brain is necessarily approximate, with the potential to induce a bias.
Under -correcting for this prior would lead to earlier frames entering into the update multiple times, giving them a larger weight in the final decision. Over multiple frames, the effect is a positive feedback loop between estimates of sensory features x f and the belief in C . This mechanism constitutes a “perceptual confirmation bias,” since belief updates are biased towards confirmatory evidence [ 2 , 44 ], and leads to primacy effects. Over -correcting for the prior, on the other hand, would lead to information from earlier frames decaying away, giving earlier frames less influence on the final decision and manifesting as recency effects. In either case, the strength of any bias is directly related to the strength of the prior.
Importantly, the strength of the prior only depends on the amount of category information, and not the amount of sensory information (unlike task performance which depends on both). For instance, in a task with high category information such as the classic dot motion task [ 24 ], high certainty in the trial category, based on stimulus frames seen so far within a trial, directly translates into high certainty about the net motion direction in subsequent frames of that trial ( Fig 2B ). In a low category information task such as the Poisson clicks task [ 11 ], on the other hand, certainty in the trial category is only weakly predictive whether any one click is on the left or on the right ( Fig 2C ). In the motion dots task, the relevant sensory feature, x , is the net motion of all dots, not the motion of any one dot. The net motion in later frames is highly predictable from the net motion in earlier frames (i.e. high category-information) even if the motion of any one dot is not, and it is known that the sensory neurons involved in the task represent the net motion by averaging over the motion of many neurons within their receptive field [ 5 ].
We implemented two canonical models, corresponding to each of the two major classes of approximate inference schemes known from statistics and machine learning: sampling-based and variational inference [ 45 , 46 ], and both of which have previously been proposed models for inference in the brain [ 27 , 36 , 37 , 47 ]. In both cases, we assumed that sensory areas of the brain approximate the posterior, incorporating both the current sensory input and expectations based on past frames. Interestingly, both sampling-based and variational inference models behaved similarly in terms of performance and biases, and so here only show the results from the sampling-based model, and provide the corresponding variational results in the SI. The performance of our approximate models ( Fig 3B ) largely matched that of an exact inference model ( Fig 3A ), with accuracy somewhat reduced for high category information. We computed the temporal biases of the approximate inference models for each combination of sensory information and category information, and found that both models showed a primacy effect whose magnitude decreased with the amount of category information ( Fig 3B, 3C and 3D ). Both hierarchical inference models under -corrected for the influence of prior expectations on the sensory representation. Over the course of a trial, this lead to a positive feedback loop between the evidence-integration part of the model and the sensory representation, with the strength of this loop being strong in the LSHC and weak in the HSLC condition ( Fig 2B and 2C ). Importantly, this bias is a direct consequence of the approximate nature of the representation of the posterior distribution; for instance, in the sampling model, the bias disappears as the number of samples gets large ( Methods ).
a) Performance of an ideal observer reporting C given ten frames of evidence. White line shows threshold performance, defined as 70% correct. The ideal observer’s temporal weights are always flat (not shown). b) Performance of our sampling-based approximate inference model with no leak ( Methods ). Colored dots correspond to lines in the next panel. c) Temporal weights in the model transition from flat to a strong primacy effect, all at threshold performance, as the stimulus transitions from the HSLC to the LSHC conditions. d) Visualization of how temporal biases change across the entire task space. Red corresponds to primacy, and blue to recency. White contour as in (b). Black lines are iso-contours for slopes corresponding to highlighted points in (b). e-g) Same as (b-d) but with leaky integration, which lessens primacy effects and produces recency effects when category information is low.
https://doi.org/10.1371/journal.pcbi.1009517.g003
Results for the variational and sampling-based inference models are qualitatively the same (Fig D in S1 Text ), as are results from simulating a larger neurally-inspired sampling model (Fig H in S1 Text ) [ 42 ]. This indicates that the observed pattern of biases is not tied to a particular representation scheme—sampling or parametric—but to the approximate and hierarchical nature of inference.
Previous studies further suggest that evidence integration in the brain may be “leaky” or “forgetful,” which can be motivated either mechanistically [ 3 , 4 , 13 , 17 ], or as an adaptation to non-stationary environments in normative models [ 14 ]. Including leaky integration, our final approximate inference models contain two competing mechanisms: first, they exhibit a confirmation bias as a consequence of approximate hierarchical inference, which is strongest when category information is high, leading to a primacy effect. Second, they contain leaky integration dynamics, which dampens the primacy effect and results in recency effects when category information is low and confirmation bias dynamics are weak ( Fig 3E, 3F and 3G ). While the exact magnitude of the leak is a free parameter in our model, to be constrained by data, the change in bias with changes in category information is a strong prediction, i.e. changing from strong primacy to no bias, or changing from weak primacy to recency, depending on the magnitude of the leak.
We performed additional simulations to explore the interaction between leaky integration and hierarchical inference. First, we observed that leaky integration can, surprisingly, improve performance, since it counteracts the confirmation bias when category information is high (Fig E in S1 Text ). We further observed that optimizing the magnitude of the leak for maximum performance, separately for each combination of sensory information and category information, always resulted in flat temporal weights (Fig F in S1 Text ).
Our models make a critical prediction that is not shared by any other model: that the temporal bias of the very same observer should change from primacy to flat or recency in a task in which nothing changes apart from the balance between category and sensory information.
Changing category information in a visual discrimination task
To test this prediction, we designed an orientation discrimination task with two stimulus conditions that correspond to the two opposite sides of this task space (LSHC and HSLC), while keeping all other aspects of the design the same ( Fig 4A and 4B ). Importantly, in this experiment, within-observer comparisons between the two task conditions isolate relative changes in sensory information and category information. This overcomes the difficulties in directly quantifying sensory information and category information as “high” or “low” in an isolated task, which requires additional assumptions, as discussed above.
a) Each trial consisted of a 200ms start cue, followed by 10 stimulus frames presented for 83ms each, followed by a single mask frame of zero-coherence noise. After a 750ms delay, left or right targets appeared and participants pressed a button to categorize the stimulus as “left” or “right.” Stimulus contrast is amplified and spatial frequency reduced in this illustration. b) Category information is determined by the expected ratio of frames in which the orientation matches the correct category, and sensory information is determined by a parameter κ determining the degree of spatial orientation coherence ( Methods ). At the start of each block, we reset the staircase to the same point, with category information at 9: 1 and κ at 0.8. We then ran a 2-to-1 staircase either on κ or on category information. The Low-Sensory-High-Category (LSHC) and High-Category-Low-Sensory (HSLC) ovals indicate sub-threshold trials; only these trials were used in the regression to infer observers’ temporal weights. c) Visualization of a noisy stimulus in the LSHC condition. All frames are oriented to the left. d) Psychometric curves for all observers (thin lines) and averaged (thick line) over the κ staircase. Shaded gray area indicates the median threshold level across all observers. e) Example frames in the HSLC condition. The orientation of each frame is clear, but orientations change from frame to frame. f) Psychometric curves over frame ratios, plotted as in (d).
https://doi.org/10.1371/journal.pcbi.1009517.g004
The stimulus in our task consisted of a sequence of ten visual frames ( Fig 4A ). Each frame consisted of band-pass-filtered white noise with excess orientation power either in the −45° or the +45° orientation [ 48 ] ( Fig 4B and 4D ). On each trial, there was a single true orientation category, but individual frames might differ in their orientation. At the end of each trial, observers reported whether the stimulus was oriented predominantly in the −45° or the +45° orientation ( Methods ).
Sensory information in our task is determined by how well each image determines the orientation of that frame (i.e. the amount of “noise” in each frame), and category information is determined by the probability that any given frame’s orientation matches the trial’s category. We used signal detection theory to quantify both sensory information and category information as the area under the receiver-operating-characteristic curve for e f and x f (sensory information), and for x f and C (category information). Hence for a ratio of 5 : 5 frames of each orientation, a frame’s orientation does not predict the correct choice and category information is 0.5. For a ratio of 10 : 0, knowledge of the orientation of a single frame is sufficient to determine the correct choice and category information is 1. Quantifying sensory information depends on individual observer’s sensory noise, but likewise ranges from 0.5 to 1 (see S1 Text ).
For each observer, we compared two conditions intended to probe the difference between the LSHC and HSLC regimes. Starting with a stimulus containing both high sensory and high category information, we either ran a 2:1 staircase lowering the sensory information while keeping category information high, or we ran a 2:1 staircase lowering category information while keeping sensory information high ( Fig 4B ). Sub-threshold trials in each condition define the LSHC and HSLC regimes, respectively ( Fig 4C and 4E ). For each condition and each observer, we used logistic regression to infer the influence of each frame onto their choice. observers’ overall performance was matched in the two conditions by setting a performance threshold below which trials were included in the analysis ( Methods ).
In agreement with our hypothesis, we find predominantly flat or decreasing temporal weights in the LSHC condition ( Fig 5A ), and when the information is partitioned differently—in the HSLC condition—we find flat or increasing weights ( Fig 5B ). To quantify this change, we first used cross-validation to select a method for quantifying temporal slopes, and found that constraining weights to be either a linear or exponential function of time worked equally well, and both outperformed logistic regression with a smoothness prior (Fig B in S1 Text ; Methods ). A within-observer comparison revealed that the change in slope between the two conditions was as predicted for all observers ( Fig 5H ) ( p < 0.05 for 9 of 12 observers, bootstrap). The effect was also highly significant on a population level ( p < 0.01, sign test on median slope parameters for each observer). This demonstrates that the trade-off between sensory and category information in a task robustly changes observers’ temporal weighting strategy as we predicted.
a-b) Temporal weights from logistic regression of choices from sub-threshold frames for individual observers. Weights are regularized by a cross-validated smoothness term, and are normalized to have a mean of 1. c-d) To summarize temporal biases, we constrained weights to be an exponential function of time and re-fit them to observers’ choices. Exponential weights had higher cross-validated performance than regularized logistic regression, supporting their use to summarize temporal biases (Fig B in S1 Text ; Methods ). e) The change in the temporal bias, quantified as the exponential slope parameter ( β ), between the two task contexts for each observer is consistently positive (combined, p < 0.01, sign test on median slope from bootstrapping). This result is individually significant in 9 of 12 observers by bootstrapping ( p < 0.05, p < 0.01, and p < 0.001 indicated by *, **, and *** respectively; non-significant observers plotted with dashed lines). Points are median slope values after bootstrap-resampling each observer’s sub-threshold trials. A slope parameter β > 0 corresponds to a recency bias and β < 0 to a primacy bias. We found similar results using linear rather than exponential weight functions (Fig C in S1 Text ).
https://doi.org/10.1371/journal.pcbi.1009517.g005
Confirmation bias, not bounded integration, explains primacy effects
The primary alternative explanation for primacy effects in fixed-duration integration tasks proposes that observers integrate evidence to an internal bound , at which point they cease paying attention to the stimulus [ 7 ]. In this scenario, early evidence always enters the decision-making process while evidence late in trial is often ignored. Averaged over many trials, this results in early evidence having a larger effect on the final decision than late evidence, and hence decreasing regression weights (and psychophysical kernels) just as we found in the LSHC condition. Both models reflect very different underlying mechanism: in our approximate hierarchical inference models, a confirmation bias ensures that early evidence has a larger effect on the final decision than late evidence for every single trial. In the integration-to-bound (ITB) model, in a single trial, all evidence is weighed exactly the same before the bound is reached, and not at all afterwards.
a) Illustration of Extended ITB model. As in classic drift-diffusion models with an absorbing bound, evidence is integrated to an internal bound, after which new evidence is ignored. Compared to perfect integration ( α = 0), a positive leak ( α > 0) decays information away and results in a recency bias, and a negative leak ( α < 0) amplifies already integrated information, resulting in a primacy bias. Since α < 0 may also result in more bound crossings, both leak and bound together determine the shape of the temporal weights. b) Inferred values of the bound and leak parameters in each condition, shown as median±68% credible intervals. The classic ITB explanation of primacy effects corresponds to a non-negative leak and a small bound—illustrated here as a shaded green area. Note that the three observers near the ITB regime are points from the HSLC task—two still exhibit mild recency effects and one exhibits a mild primacy effect as predicted by ITB. c) Across both conditions, the temporal slopes ( β ) implied by the full model fits closely match the slopes in the data. β < 0 corresponds to primacy, and β > 0 to recency. Error bars indicate 68% confidence intervals from bootstrapping trials on β data and from posterior samples on β fit . d) Median temporal biases implied by the full model (middle) and by the model with either zero leak (left) or infinite bound (right). Each line corresponds to a single observer. (LSHC condition only—HSLC condition in Fig L in S1 Text ). d) Across the population, the negative leak (confirmation bias) accounted for 99% (68%CI = [93%, 106%]), and bounded integration accounted for 18% (68%CI = [13%, 23%]) of the primacy bias captured by the model. (Additional analyses in Fig L in S1 Text ).
https://doi.org/10.1371/journal.pcbi.1009517.g006
The Extended ITB model produces three distinct patterns in the data (colored text in Fig 6B ). First, when α is positive and the bound is large, it produces recency biases. Second, when the bound is small, it produces primacy biases [ 7 ], as long as α is also small so that it does not prevent the bound from being crossed. Third, when the bound is large and α is negative , it also produces primacy biases but now due to confirmation-bias-like dynamics rather than due to bounded integration. Crucially, this single model can account for both primacy due to a bound and primacy due to a confirmation bias by different parameter values (recovery of ground-truth mechanisms shown in Figs J and K in S1 Text ). Examining the parameters of this model fit to data therefore allows us to determine the relative contributions of bounded integration and confirmation bias dynamics in cases where observers show primacy effects.
We fit the Extended ITB model to individual choices on sub-threshold trials, separately for the LSHC and the HSLC conditions. Fig 6B shows the posterior mean and 68% credible interval for the dynamics parameter, α , and bound parameter inferred for each observer. The model consistently inferred a negative α in the LSHC condition and a positive α in the HSLC condition for all observers, suggesting that confirmation-bias dynamics are crucial to explain observer’s primacy biases in the LSHC condition, as well as the change in bias from LSHC to HSLC conditions. Note that the leak term in the Extended ITB model reflects a combination of both the confirmation bias dynamic and a mechanistic “forgetting” term in the accumulation of the decision variable. Those two effects are opposite in nature. As a result, the acceleration inferred by our function model fit to data is likely a lower bound on the actual strength of the confirmation bias dynamics. However, while the inferred bound for every single observer is so high as not to contribute at all if the leak was zero , it is possible that bounded integration still contributes to primacy effects, given that a stronger confirmation bias ( α < 0) will hit a bound more often.
We therefore performed an ablation analysis to quantify the relative contribution of the leak and bound parameters to the primacy effect in the LSHC condition ( Methods ). We first asked whether the inferred model parameters reproduced the observed biases. Indeed, Fig 6C shows near-perfect agreement between the temporal biases implied by simulating choices from the fitted models and the biases inferred directly from observers’ choices. Given this, if setting the bound to ∞ leaves temporal biases unchanged, then we can conclude that biases were driven by the leak, and conversely, a temporal bias that remains after setting α to zero must be due to the bound. Fig 6D shows that primacy effects largely disappear when α is ablated, but not when the bound is ablated. To summarize ablation effects across the population, we used a hierarchical regression model to compute a population-level “ablation index” for each parameter, which is 0 if removing the parameter has no effect on temporal slopes, β , and is 1 if removing it destroys all temporal biases ( Methods ). The ablation index can therefore be interpreted as the fraction of the observers’ primacy or recency biases that are attributable to each parameter (but they do not necessarily sum to 1 because the slope is a nonlinear combination of both parameters). In the LSHC condition, the ablation index for the leak term was 0.99 (68% CI = [0.93, 1.06]), and for the bound term it was 0.18 (68% CI = [0.13, 0.23]) ( Fig 6E ). This indicates that although both mechanisms are present, primacy effects in our data are dominated by the self-reinforcing dynamics of a negative leak. Results for the HSLC condition are shown in Fig L in S1 Text .
Interestingly, one observer exhibited a slight primacy effect in the HSLC condition, and our analyses suggest this was primarily due to bounded integration dynamics as proposed by Kiani et al (2008). This outlier observer is marked with a diamond symbol throughout Fig 6 , and is further highlighted in Fig L in S1 Text . However, even this observer’s primacy effect in the LSHC condition was driven by a confirmation bias (negative α ), and their change in slope between LSHC and HSLC conditions was in the same direction as the other observers. Importantly, finding a primacy effect due to an internal bound confirms that our model fitting procedure is able to detect such effects when they are, in fact, present. Two additional observers appear to have low bounds in the HSLC condition ( Fig 6C ), but are dominated by leaky integration ( α > 0), resulting in an overall recency bias.
Our work makes three main contributions. First, we extended ideal observer models of evidence integration tasks by explicitly accounting for the intermediate sensory representation. We showed that this partitions the information in the stimulus about the category into two parts—“sensory information” and “category information”—defining a novel two-dimensional space of possible tasks. Second, we found that two classes of biologically-plausible approximate inference algorithms entailed a confirmation bias whose strength strongly varied across this task space. Interestingly, the location of tasks in existing studies qualitatively predicted the bias they found across species, sensory modalities and task designs. Third, we collected new data and confirmed a critical prediction of our theory, namely that individual observers’ temporal biases should change depending on the balance of sensory information and category information in the stimulus. Finally, by fitting an extended integration to bound (Extended ITB) model to individual observer choices, we confirmed that these changes in biases are due to a change in integration dynamics rather than bounded integration.
The “confirmation bias” emerges in our hierarchical inference models as the result of three key assumptions. Our first assumption is that inference in evidence integration tasks is in fact hierarchical, and that the brain approximates the posterior distribution over the intermediate sensory variables, x . This is in line with converging evidence that populations of sensory neurons encode posterior distributions of corresponding sensory variables [ 34 , 35 , 50 , 51 ] incorporating dynamic prior beliefs via feedback connections [ 34 , 35 , 39 , 42 , 43 , 51 – 53 ]. This is in contrast to other probabilistic theories in which only the likelihood is represented in sensory areas [ 25 , 26 , 28 , 54 ], which would not predict primacy effects due to confirmation bias dynamics.
Our second key assumption is that evidence is accumulated online. In our models, the belief over C is updated based only on the posterior from the previous step and the current posterior over x . This can be thought of as an assumption that the brain does not have a mechanism to store and retrieve earlier frames of evidence directly, and is consistent with drift-diffusion models of decision-making [ 5 ]. As mentioned in the main text, the assumptions until now—hierarchical inference with online updates—do not entail any temporal biases for an ideal observer. Further, the use of discrete time in our experiment and models is only for mathematical convenience—analogous dynamics emerge in continuous-time, and in fact we implemented our models at a finer time scale than at which evidence frames are presented.
Third, we assumed that inference in the brain is approximate—a safe assumption due to the intractable nature of exact inference in large models. In the sampling model, we assumed that the brain can draw a limited number of independent samples of x per update to C , and found that for a finite number of samples the model is inherently unable to account for all of the prior bias of C on x in its updates to C . Existing neural models of sampling typically assume that samples are distributed temporally [ 36 , 42 , 53 , 55 , 56 ], but it has also been proposed that the brain could process multiple sampling “chains” distributed spatially [ 57 ]. The relevant quantity for our model is the number of independent samples that can be tallied per update: the more samples, the smaller the bias. The variational model’s representational capacity was limited by enforcing that the posterior over x is unimodal, and that there is no explicit representation of dependencies between x and C . Importantly, this does not imply that x and C do not influence each other. Rather, the Variational Bayes algorithm expresses these dependencies in the dynamics between the two areas: each update that makes C = +1 more likely pushes the distribution over x further towards +1, and vice versa. Because the number of dependencies between variables grows exponentially, such approximates are necessary in variational inference with many variables [ 36 ]. The Mean Field Variational Bayes algorithm that we use here has been previously proposed as a candidate algorithm for neural inference [ 58 ].
The assumptions up to now predict a primacy effect but cannot account for the observed recency effects. When we incorporate a forgetting term in our models, they reproduce the observed range of biases from primacy to recency. The existence of such a forgetting term is supported by previous literature [ 4 , 15 ]. Further, it is normative in our framework in the sense that reducing the bias in the above models improves performance (Fig D in S1 Text through Fig F in S1 Text ). The optimal amount of bias correction depends on the task statistics: for high category information where the confirmation bias is strongest, a stronger forgetting term is needed to correct for it. While it is conceivable that the brain would optimize this term to the task [ 11 , 59 , 60 ], our data suggest it is stable across our LSHC and HSLC conditions, or only adapts slowly.
It has been proposed that post-decision feedback biases subsequent perceptual estimations [ 61 – 65 ]. While in spirit similar to our confirmation bias model, there are two conceptual differences between these models and our own: First, the feedback from decision area to sensory area in our model is both continuous and online, rather than conditioned on a single choice after a decision is made. Second, our models are derived from an ideal observer and only incur bias due to algorithmic approximations, while previously proposed “self-consistency” biases are not normative and require separate justification. However, these previous findings on the effect of commitment to a choice on weighing subsequent evidence can easily be accommodated in our framework by plausibly proposing that the act of committing to a choice increases one’s subjective certainty about that choice being correct. In our model, such an increase in certainty would directly translate into an increase in feedback from decision to sensory areas, and hence increased confirmation bias.
Our analysis decisively shows that accelerating dynamics, rather than reaching a bound before the end of the trial, explains the primacy effect in our data. Prior work has suggested that such accelerating dynamics may arise from mutual inhibition of two accumulators [ 15 , 18 , 20 ], or two attractor states corresponding to the two choices [ 16 , 19 , 66 – 68 ]—all within a decision-area, and that the nature of the temporal bias depends on the volatility of the integrated signal [ 22 ]. Importantly, decision-dynamics alone cannot explain our results, since the input to these models usually reflects the total information in each frame about the choice, i.e. combining both sensory and category information. In other words, these models usually integrate log odds , which we kept approximately constant between LSHC and HSLC conditions. The same argument applies to other models that do no distinguish between sensory and category information, whether based on mixing trials of different difficulty [ 21 ] or differential accumulation of consistent and inconsistent evidence [ 63 , 64 , 69 , 70 ].
In contrast, in our explanation based on approximate hierarchical inference, attractor dynamics arise across sensory and decision areas, as the result of cortical inter-area feedback whose strength is monotonically related to category information. Holding the task difficulty (and hence the magnitude of the log odds of each frame) constant, our model nonetheless predicts stronger inter-area attractor dynamics when category information is high. Given recent evidence that noise correlations contain a task-dependent feedback component [ 71 ], we therefore predict a reduction of task-dependent noise correlations in comparable tasks with lower category information. The confirmation bias mechanism may also account for the recent finding that stronger attractor dynamics are seen in a categorization task than in a comparable estimation task [ 38 ].
Rollwage et al (2020) recently presented evidence for a relationship between decision confidence and confirmation bias: when observers are more confident about a decision, they will be more biased in how they interpret subsequent information [ 65 ]. Interestingly, our model makes a closely related prediction: that positive feedback loop between decision and sensory area also increases confidence beyond that of an ideal observer. In particular, it predicts that confidence judgements in the LSHC condition when the primacy effect is strong will be higher than in the HSLC condition when the primacy effect is weak—a prediction that we found to be confirmed in follow-up work [ 72 ].
It has also been proposed that primacy effects could be the result of near-perfect integration of an adapting sensory population [ 29 , 68 ]. For this mechanism to explain our full results, however, the sensory population would need to become less adapted over the course of a trial in our HSLC condition, while at the same time more adapted in the LSHC condition. We are unaware of such an adaptation mechanism in the literature. Further, such stimulus-dependent circuit dynamics would not predict top-down neural effects such as the task-dependence of the dynamics of sensory populations [ 38 ] nor the origin and prevalence of differential correlations [ 71 ], both of which are consistent with hierarchical inference [ 39 , 42 ].
While our focus is on the perceptual domain in which observers integrate evidence over a timescale on the order of tens or hundreds of milliseconds, analogous computational principles hold in the cognitive domain over longer timescales. The crucial computational motif underlying our model of the confirmation bias is approximate hierarchical inference over multiple timescales. An agent in such a setting must simultaneously make accurate judgments of current data (based on the current posterior) and track long-term trends (based on all likelihoods). For instance, Zylberberg et al. (2018) identified an analogous challenge when observers must simultaneously make categorical decisions each trial (their “fast” timescale) while tracking the stationary statistics of a block of trials (their “slow” timescale), with trial-by-trial statistics analogous to the frame-by-frame statistics in our LSHC condition. As the authors describe, if observers base model updates on posteriors rather than likelihoods, they will further entrench existing beliefs [ 73 ]. However, the authors did not investigate order effects; our proposed confirmation bias models would predict that observers’ estimates of block statistics is biased towards earlier trials in the block (primacy). Schustek et al. (2018) likewise asked observers to track information across trials in a cognitive task more analogous to our HSLC condition, and report close to flat weighting of evidence across trials [ 74 ] in agreement with our model.
The strength of the perceptual confirmation bias is directly related to the integration of internal “top-down” beliefs and external “bottom-up” evidence previously implicated in clinical dysfunctions of perception [ 75 ]. Therefore, the differential effect of sensory and category information may be useful in diagnosing clinical conditions that have been hypothesized to be related to abnormal integration of sensory information with internal expectations [ 76 ].
Hierarchical (approximate) inference on multiple timescales is a common motif across perception, cognition, and machine learning. We suspect that all of these areas will benefit from the insights on the causes of the confirmation bias mechanism that we have described here and how they depend on the statistics of the inputs in a task.
Ethics statement
This study was approved by the Institutional Research Review Board of the University of Rochester (RSRB #55456).
Visual discrimination task
We recruited twelve students at the University of Rochester as observers in our study. All non-author participants were compensated for their time. We found no difference between naive observers and authors, so all main-text analyses are combined, with data points belonging to authors and naive observers indicated in Fig 5D .
Our stimulus consisted of ten frames of band-pass filtered noise [ 48 , 77 ] masked by a soft-edged annulus, leaving a “hole” in the center for a small cross on which observers fixated. The stimulus subtended 2.6 degrees of visual angle around fixation. Stimuli were presented using Matlab and Psychtoolbox on a 1920x1080px 120 Hz monitor with gamma-corrected luminance [ 78 ]. Observers kept a constant viewing distance of 36 inches using a chin-rest. Each trial began with a 200ms “start” cue consisting of a black ring around the location of the upcoming stimulus. Each frame lasted 83.3ms (12 frames per second). The last frame was followed by a single double-contrast noise mask with no orientation energy. Observers then had a maximum of 1s to respond, or the trial was discarded ( Fig 4A ). The stimulus was designed to minimize the effects of small fixational eye movements: (i) small eye movements do not provide more information about either orientation, and (ii) each 83ms frame was too fast for observers to make multiple fixations on a single frame.
The stimulus was constructed from white noise that was then masked by a kernel in the Fourier domain to include energy at a range of orientations and spatial frequencies but random phases [ 48 , 71 , 77 ] (a complete description and parameters can be found in Table B in S1 Text ). We manipulated sensory information by broadening or narrowing the distribution of orientations present in each frame, centered on either +45° or −45° depending on the chosen orientation of each frame. We manipulated category information by changing the proportion of frames that matched the orientation chosen for that trial. The range of spatial frequencies was kept constant for all observers and in all conditions.
Trials were presented in blocks of 100, with typically 8 blocks per session (about 1 hour). Each session consisted of blocks of only HSLC or only LSHC trials ( Fig 4 ). Observers completed between 1500 and 4400 trials in the LSHC condition, and between 1500 and 3200 trials in the HSLC condition. After each block, observers were given an optional break and the staircase was reset to κ = 0.8 and p match = 0.9. p match is defined as the probability that a single frame matched the category for a given trial. In each condition, psychometric curves were fit to the concatenation of all trials from all sessions using the Psignifit Matlab package [ 79 ], and temporal weights were fit to all trials below each observer’s threshold.
Low sensory-, high category-information (LSHC) condition.
In the LSHC condition, a continuous 2-to-1 staircase on κ was used to keep observers near threshold ( κ was incremented after each incorrect response, and decremented after two correct responses in a row). p match was fixed to 0.9. On average, observers had a threshold (defined as 70% correct) of κ = 0.17 ± 0.07 (1 standard deviation). Regression of temporal weights was done on all sub-threshold trials, defined per-observer.
High sensory-, low category-information (HSLC) condition.
In the HSLC condition, the staircase acted on p match while keeping κ fixed at 0.8. Although p match is a continuous parameter, observers always saw 10 discrete frames, hence the true ratio of frames ranged from 5:5 to 10:0 on any given trial. Observers were on average 69.5% ± 4.7% (1 standard deviation) correct when the ratio of frame types was 6:4, after adjusting for individual biases in the 5:5 case. Regression of temporal weights was done on all 6:4 and 5:5 ratio trials for all observers, regardless of the underlying p match parameter.
Logistic regression of temporal weights
We constructed a matrix of per-frame signal strengths S on sub-threshold trials by measuring the empirical signal level in each frame. This was done by taking the dot product of the Fourier-domain energy of each frame as it was displayed on the screen (that is, including the annulus mask applied in pixel space) with a difference of Fourier-domain kernels at +45° and −45° with κ = 0.16. This gives a scalar value per frame that is positive when the stimulus contained more +45° energy and negative when it contained more −45° energy. Signals were z-scored before performing logistic regression, and weights were normalized to have a mean of 1 after fitting.
Temporal weights were first fit using (regularized) logistic regression with different types of regularization. The first regularization method consisted of an AR0 (ridge) prior, and an AR2 (curvature penalty) prior. We did not use an AR1 prior to avoid any bias in the slopes, which is central to our analysis.
To visualize regularized weights in Fig 5 , the ridge and AR2 hyperparameters were chosen using 10-fold cross-validation for each observer, then averaging the optimal hyperparameters across observers for each task condition. This cross validation procedure was used only for display purposes for individual observers in Fig 5A and 5B of the main text, while the linear and exponential fits (described below) were used for Fig 5C and 5D and statistical comparisons. Fig A in S1 Text shows individual observers’ weights for all regression models.
Fig 5E shows the median exponential shape parameter ( β ) after bootstrapped resampling of trials 500 times for each observer. Both the exponential and linear weights give comparable results (Fig C in S1 Text ).
Because we are not explicitly interested in the magnitude of w but rather its shape over stimulus frames, we always plot a “normalized” weight, w /mean( w ), both for our experimental results ( Fig 5A–5D ) and for the model ( Fig 3C and 3F ).
Approximate inference models
We model evidence integration as Bayesian inference in a three-variable generative model ( Fig 2A ) that distills the key features of online evidence integration in a hierarchical model [ 42 ]. The variables in the model are mapped onto the sensory periphery ( e ), sensory cortex ( x ), and a decision-making area ( C ) in the brain. For simulations, the same model was used both to generate data ( C → x f → e f ), and, in the reverse direction, as a model of inference dynamics ( e f → p( x f | …) ↔ p( C | …)).
Simulations of both models were done with 10000 trials per task type and 10 frames per trial. To quantify the evidence-weighting of each model, we used the same logistic regression procedure that was used to analyze human observers’ behavior. In particular, temporal weights in the model are best described by the exponential weights ( Eq (3) ), so we use β to characterize the model’s biases.
Sampling model.
The full sampling model is given in Algorithm A in S1 Text . Simulations in the main text were done with S = 5, n U = 5, normalized importance weights, and γ = 0 or γ = 0.1.
Variational model.
The following variational model produces qualitatively similar patterns of temporal biases to the IS model (Fig D in S1 Text ).
By restricting the updates to be online (one frame at a time, in order), this model can be seen as an instance of “Streaming Variational Bayes” [ 82 ]. That is, the model computes a sequence of approximate posteriors over C using the same update rule for each frame. We thus only need to derive the update rules for a single frame and a given prior over C ; this is extended to multiple frames by re-using the posterior from frame f − 1 as the prior on frame f .
As in the sampling model, this model is unable to completely discount the added prior over x . Intuitively, since the mean-field assumption removes explicit correlations between x and C , the model is forced to commit to a marginal posterior in favor of C = +1 or C = −1 and x > 0 or x < 0 after each update, which then biases subsequent judgments of each.
Analogously to the sampling model we assume a number of updates n U reflecting the speed of relevant computations in the brain relative to how quickly stimulus frames are presented. Unlike for the sampling model, naively amortizing the updates implied by Eq (21) n U times results in a stronger primacy effect than observed in the data, since the Variational Bayes algorithm naturally has attractor dynamics built in. Allowing for an additional parameter η scaling this update (corresponding to the step size in Stochastic Variational Inference [ 83 ]) seems biologically plausible because it simply corresponds to a coupling strength in the feed-forward direction. Decreasing η both reduces the primacy effect and improves the model’s performance. Here we used η = 0.05 in all simulations based on a qualitative match with the data. The full variational model is given in Algorithm B in S1 Text .
Fitting the extended ITB model to data.
Per observer per condition, we used Metropolis Hastings (MH) to infer the joint posterior over seven parameters: the category prior ( p C ), lapse rate (λ), decision temperature ( T ), integration noise ( ϵ ), bound ( B ), leak ( α ), and evidence scale ( s ). One challenge for fitting models is that the mapping from signal in the images ( S ) to “log odds” to be integrated (LLO) depends on category information, sensory information, and on unknown properties of each observer’s visual system. The evidence scale parameter, s , was introduced because although we can estimate the ground truth category information in each task (0.6 for HSLC and 0.9 for LSHC), the effective sensory information depends on each observer’s visual system and will differ between the two tasks. Using logistic regression, we explored plausible nonlinear monotonic mappings between signals S and log-odds, but found that none performed better than linear scaling when applied to sub-threshold trials. We therefore used LLO ≈ g ( S / s ), where g is a sigmoidal function that accounts for category information being less than 1, and inferred s jointly along with other parameters of the model. The scale s was fixed to 1 when fitting the ground-truth models, as the mapping between evidence and log odds is completely known in those cases.
The priors over each parameter were set as follows. p( p C ) was set to Beta(2, 2). p(λ) was set to Beta(1, 10). p( α ) was uniform in [−1, 1]. p( s ) was set to an exponential distribution with mean 20. p( ϵ ) was set to an exponential distribution with mean 0.25. p( T ) was set to an exponential distribution with mean 4. p( B ) was set to a Gamma distribution with (shape,scale) parameters (2, 3) (mean 6). MH proposal distributions were chosen to minimize the autocorrelation time when sampling each parameter in isolation.
Estimating temporal slopes and ablation indices implied by model samples.
To estimate the the shape of temporal weights implied by the model fits, we simulated choices from the model once for each posterior sample after thinning to 500 samples per chain for a total of 6k samples per observer and condition. We then fit the slope of the exponential weight function, β , to these simulated choices using logistic regression constrained to be an exponential function of time as described earlier ( Eq (3) ). This is the β fit plotted on the y-axis of Fig 6C . For the ablation analyses, we again fit β to choices simulated once per posterior sample of model parameters, but setting α = 0 in one case or ( B = ∞, ϵ = 0) in the other.
Ground-truth models.
Based on observations of the temporal weighting profile alone, the transition between primacy and recency could be explained by bounded integration with a changing leak amount in the LSHC condition and high bound in the HSLC condition (Fig G in S1 Text ). To verify that all of the above fitting and ablation procedures could distinguish a confirmation bias from bounded integration, we tested them on two ground-truth models: one where choices were simulated from a hierarchical inference (IS) model, and one where choices were simulated from an ITB model. All ground-truth parameter values are given in Table C in S1 Text , which were chosen to meet two criteria: first, constant performance at 70% in both LSHC and HSLC regimes, and second, matched temporal slopes (a primacy effect with shape β ≈ −0.1 in the LSHC condition and a recency effect with shape β ≈ 0.1 in the HSLC condition for both models). This analysis confirmed that bounded integration is indeed distinguishable from a confirmation bias ( α < 0), in terms of the quality of the fit (Fig I in S1 Text ), different inferred parameter values (Fig J in S1 Text ), and the ablation tests (Fig K in S1 Text ).
Supporting information
Combined Supplemental Text, Figs A-L, Tables A-C, and Algorithms A-B. Table A : Sensory Information, Category Information, and biases in previous studies . Justification of placement of example prior studies in Fig 1C and description of stimulus manipulations that will move it to the opposite side of the category–sensory–information space. Each manipulation corresponds to a prediction about how temporal weighting of evidence should change from primacy (red) to flat/recency (blue), or vice versa, as a result. Table B : Stimulus parameters . Table C : Parameters of ground-truth models used to test model-fitting . SI = sensory information. CI = category information. γ / α = leak. S = samples per batch (IS model only). B = bound (ITB model only). ϵ = integration noise. T = decision temperature. λ = lapse rate. Fig A : Temporal kernels for each condition (LSHC and HSLC), and their difference between conditions, for each of four regularization techniques . In all panels, weights are normalized to have a mean of 1, individual observers are shown as faint thin lines, and the average across observers as a darker bold line. First row (“Logisitic Regression”) is the result of ridge regression for predicting choices from per-frame signal levels with no further regularization. Second row (“Smooth Logistic Regression”) includes a second-order autoregressive penalty, resulting in smoother kernels. Third row (“Linear Kernels”) is a three-parameter model that constrains weights to be a linear function of time. The three parameters control the slope and intercept of the kernel, and the choice bias ( Methods ). Fourth row (“Exponential Kernels”) is a similar three-parameter model that instead constrains weights to be an exponential function of time. Fig B : Cross-validation selects linear or exponential shapes for temporal weights, compared to both unregularized and smoothness-regularized logistic regression . Panels show 20-fold cross-validation performance of four regression methods to predict choices from sub-threshold trials, separated by task type and by observer. All values are relative to the log-likelihood, per fold, of the unregularized model. Error bars show standard error of the mean difference in performance across folds of shuffled data. “Unregularized LR” refers to standard ridge regression with no regularization of the temporal shape. “Regularized LR” refers to the AR2-penalized logistic regression objective, where the hyperparameters were chosen to maximize cross-validated fitting performance separately for each observer. “Exponential” is the 3-parameter model where weights are an exponential function of time ( Eq (3) plus a bias term). Similarly, the “Linear” model constrains the weights to be a linear function of time as in Eq (4) , plus a bias term. Fig C : Comparing exponential and linear regression weights . Left panel is the same as Fig 5E in the main text, comparing slope of temporal weights by constraining weights to be an exponential function of time. The right panel shows the same analysis with weights constrained to be a linear function of time. In both cases, 9 of 12 observers individually have a significant increase in slope ( p < 0.05, bootstrap). A one-sided sign test on the medians for each observer reveals a significant population effect with p = .0032 (**)for the exponential method and p = 0.00024 (***) for the linear method. Fig D : Effect of leak ( γ ) parameter in hierarchical inference models . In both models, larger γ increases the prevalence of recency effects across the entire task space. Panels are as in Fig 3 in the main text. a-c sampling model with γ = 0. d-f sampling model with γ = 0.1. g-i sampling model with γ = 0.2. j-l variational model with γ = 0. m-o variational model with γ = 0.1. p-r variational model with γ = 0.2. Fig E : Performance of hierarchical inference model using optimal leak ( γ ) . Optimizing performance with respect to γ (see also Fig F in S1 Text). a) Sampling model performance across task space with S = 5 and γ = 0.5 (compare with Fig 3C in which γ = 0.1). b) Difference in performance for γ = 0.5 versus γ = 0.1. Higher γ improves performance in the upper part of the space where the confirmation bias is strongest. c) Optimizing for performance, the optimal γ * depends on the task. Where the confirmation bias had been strongest, optimal performance is achieved with a stronger leak term. d) Model performance when the optimal γ * from (c) is used in each task. e) Comparing the ideal observer to (d), the ideal observer still outperforms the model but only in the upper part of the space. f) Temporal weight slopes when using the optimal γ * are flat everywhere. The models reproduce the change in slopes seen in the data only when γ is fixed across tasks (compare Fig D in S1 Text). Fig F : Further investigation of optimal leak ( γ ) . Simulation results for optimal leak ( γ ) for two further model variations, panels as in Fig E in S1 Text. a-f Variational model results. As in the sampling model, we see that the optimal value of γ * increases with category information, or with the strength of the confirmation bias. h-l Sampling model results with S = 1 (in the main text and Fig E in S1 Text we used S = 5). Since the sampling model without a leak term approaches the ideal observer in the limit of S → ∞, the optimal γ * was close to 0 for much of the space in the main text figure. Here, by comparison, γ * > 0 is more common because the S = 1 model is more biased. Fig G : Simulation of bounded integration (ITB) model . a) Performance of an ITB model is not differentially modulated by sensory and category information. b) ITB consistently produces primacy effects, as in [ 7 ]. c) The primacy effect becomes more extreme in regions where evidence is stronger, since the bound is hit earlier in the trial. d-f) As in (a-c), but with an additional leak term, resulting in less extreme primacy effects and a transition to recency for difficult tasks, but no transition from primacy to recency along the iso-performance contour. (Also note the departure from monotonic exponential-like weight profiles). g-i) We now vary the leak term, α , as a function of category information. This reproduces the qualitative transition from primacy in LSHC to recency in HSLC. As measured by an exponential fit ( β ), slopes are matched to those in the confirmation bias models ( Fig 3D and 3G ). Fig H : Simulation results on the larger model of Haefner et al (2016) [ 42 ]. a) Performance as a function of sensory information (grating contrast) and category information (probability that each frame matches the trial category). White line is iso-performance contour at 70%, and dots correspond to LSHC and HSLC parameter regimes plotted in (b). Simulation details in S1 Text. b) Temporal weights from LSHC and HSLC simulations corresponding to colored points in (a), normalized in each condition so the weights have mean 1. As in the reduced models in the main text, we see a transition from primacy to recency. Fig I : Results of direct model comparison between IS model and ITB model(s) fit to ground-truth data . Lower AIC indicates better fit. An ideal integrator (gold) and ground-truth (gray) values serve as upper- and lower-bounds, respectively, on plausible AIC values. In all cases, the best fitting model recovered parameters that are as good as the ground truth. The standard ITB model (with positive leak enforced) is distinguishable from the IS model in the LSHC simulation (top row). However, an Extended ITB model that allows for negative leak (purple), fits all data in all conditions as well as the ground-truth. For this reason, we state in the main text that a negative leak is functionally indistinguishable from the true IS model. We pursued parameter comparison within this Extended ITB model class, rather than model comparison between IS and ITB, in the main text. Fig J : Box and whisker plots of inferred parameter values . Showing inferred parameter values in the extended ITB model for each of 12 observers as well as the ground truth models (IS and ITB—see Table C in S1 Text). Each parameter and observer has two fits, one for the LSHC condition (lower/red) and one for the HSLC condition (upper/blue). Thin lines are 95% posterior interval, thick lines are 50% interval, and points are posterior median. Parameter names are as in the main paper, restated here: p C = prior over categories, λ = symmetric lapse rate, T = decision temperature, s = signal scale (fixed to 1 for ground truth models), α = leak, B = bound, ϵ = noise. Fig K : Parameter ablation analysis on ground-truth models . Recall that the ITB model has a primacy effect in the LSHC condition driven by bounded integration. The key signature of bounded integration dynamics is that when the bound is ablated, the leak takes over and it flips to no bias or a recency bias. The key signature of a hierarchical inference model (here, Importance Sampling or IS), on the other hand, is that the primacy bias is unaffected by ablating the bound, but disappears when the leak term is ablated, since a negative leak acts as a confirmation bias. In the HSLC condition (right panel), both models’ recency effects are driven by leaky integration. The ITB model’s bound competes with the leak, however, so ablating the bound results in exaggerated recency effects, and ablating the leak results in primacy effects. The key signature of a hierarchical inference model, on the other hand, is a recency effect that is unaffected by ablating the bound and that disappears when the leak is ablated. Fig L : Additional information on fits of the Extended ITB model to empirical data and ablation analyses . a) Copy of Fig 6D . Comparing with Fig K in S1 Text suggests that primacy effects are largely driven by confirmation-bias dynamics rather than by bounded integration. b) Temporal bias of the full Extended ITB model (x-axis) versus the ablated model (y-axis) for each observer and each ablated parameter in the LSHC condition (each observer has two points at the same x coordinate, offset for visualization). We regressed a single slope for each ablated parameter to summarize the fraction of bias in the population explained by the leak parameter (green) or the bound parameter (purple). c) Copy of Fig 6E from the main text. The fact that the leak parameter explains 99.4% of the population primacy effects corresponds to the green regression line being nearly horizontal in (b). d-f) Same as (a-c) but for the HSLC condition. As in Fig 6 , outlier observer in—who had a primacy bias in the HSLC condition—is shown as a diamond symbol in panels (a), (d), and (f). Algorithm A : Hierarchical inference using Importance Sampling . Algorithm B : Hierarchical inference using Variational Bayes .
https://doi.org/10.1371/journal.pcbi.1009517.s001
Acknowledgments
We thank Matthew Hochberg for help with initial implementations of the experiment software, and Chris Summerfield for early comments on our experiment design. We also thank Richard Born, Hendrikje Nienborg, Martina Poletti, Duje Tadin, and Ariel Zylberberg for feedback on the manuscript.
- View Article
- PubMed/NCBI
- Google Scholar
- 37. Gershman SJ, Beck JM. Complex Probabilistic Inference: From Cognition to Neural Computation. In: Moustafa A, editor. Computational Models of Brain and Behavior. Wiley-Blackwell; 2016. p. 1–17.
- 45. Bishop CM. Pattern Recognition and Machine Learning. Information science and statistics. Springer (New York); 2006.
- 46. Murphy KP. Machine Learning: A Probabilistic Perspective. The MIT Press; 2012.
- 72. Chattoraj A, Snarskis M, Haefner RM. Relating confidence judgements to temporal biases in perceptual decision-making. Proceedings of the 36th Annual Conference of the Cognitive Science Society. 2021.
- 80. Owen AB. Importance Sampling. In: Monte Carlo theory, methods and examples. 2013
- 81. Cremer C, Morris Q, Duvenaud D. Reinterpreting Importance-Weighted Autoencoders. arXiv. 2017; p. 1–6.
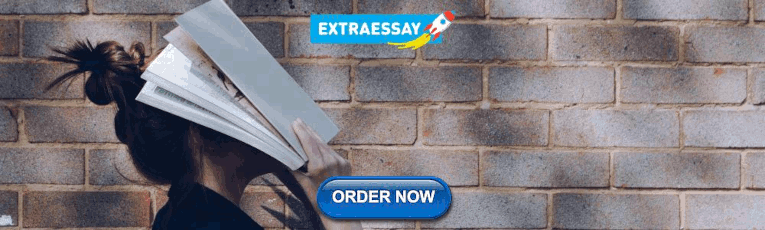
IMAGES
VIDEO
COMMENTS
This confirmation bias has significant impact on domains ranging from politics to science and education. Little is known about the mechanisms underlying this fundamental characteristic of belief ...
This confirmation bias has significant impact on domains ranging from politics to science and education. Little is known about the mechanisms underlying this fundamental characteristic of belief ...
1. Introduction. Polarization between opposing viewpoints is increasingly prevalent in discussions surrounding political and societal issues [].An important cognitive driver of this polarization is the human tendency to discount evidence against one's current position [2-5], a phenomenon known as confirmation bias [].Confirmation bias has been reported in a variety of settings [], including ...
Confirmation bias is one of the most widely discussed epistemically problematic cognitions, challenging reliable belief formation and the correction of inaccurate views. Given its problematic nature, it remains unclear why the bias evolved and is still with us today. To offer an explanation, several philosophers and scientists have argued that the bias is in fact adaptive. I critically discuss ...
Finally, the third hypothesis-"confirmation bias"—was that valence would affect factual and counterfactual learning in opposing directions, such that negative unchosen prediction errors would be more likely to be taken into account than positive unchosen prediction errors. In this scenario, factual and counterfactual learning biases ...
The term ' confirmation bias ' usually refers to the tendency to search for evidence. supporting an already stated hypothesis, or to the tendency to interpret evidence in a favoring. manner to ...
a hypothesis, a confirmation bias would be a bias to confirm the individual's own belief, namely that the hypothesis in question is false. A Long-Recognized Phenomenon Motivated confirmation bias has long been believed by philosophers to be an important determinant of thought and behavior. Francis Bacon (1620/1939) had this to say about it, for ...
Nature Communications 11, Article number: 2634 ( 2020 ) Cite this article. A prominent source of polarised and entrenched beliefs is confirmation bias, where evidence against one's position is ...
Abstract. Confirmation bias is one of the most widely discussed epistemically problematic cognitions, challenging reliable belief formation and the correction of inaccurate views. Given its problematic nature, it remains unclear why the bias evolved and is still with us today.
As hypothesized, we found that after high con fidence (vs. low con fidence) decisions, accumulation of neural evidence was facilitated if it was con rmatory, but largely fi abolished if it was ...
Abstract. Confirmation bias, as the term is typically used in the psychological literature, connotes the seeking or interpreting of evidence in ways that are partial to existing beliefs, expectations, or a hypothesis in hand. The author reviews evidence of such a bias in a variety of guises and gives examples of its operation in several ...
The confirmation bias, in which new evidence is given more weight when it agrees with existing beliefs, is a ubiquitous yet poorly understood example of such biases. Here we report that a confirmation bias arises even during perceptual decision-making, and propose an approximate hierarchical inference model as the underlying mechanism. ...
Confirmation bias, also called my-side bias, is the tendency to search for, interpret, favor, and recall information in a way that confirms one's beliefs or hypotheses, while giving disproportionately less attention to information that contradicts it.
Conclusion: nine features of confirmation bias study for the humanities of the future. To summarise, the confirmation bias study proposed here for the humanities has eight distinct features. Its principal address is to theories and to theory. Its focus is on misinterpretation, rather than interpretation.
information, confirmation bias causes the learner to interpret ambiguous new information as a reinforcement of prior beliefs. Thus in contrast to learning models that allow people to give higher weight to new signals from specific sources (Camacho et al. 2011), learning under confirmation bias not only leads to different weighting
Confirmation bias is the tendency to seek out and prefer information that supports our preexisting beliefs. As a result, we tend to ignore any information that contradicts those beliefs. Confirmation bias is often unintentional but can still lead to poor decision-making in (psychology) research and in legal or real-life contexts.
In cognitive psychology, confirmation bias, i.e., the tendency to preferentially view information that is consistent with one's opinions or hypotheses, has a significant impact on decision making (Nickerson, 1998; Kahneman, 2011 ). Confirmation bias occurs frequently in web searches.
history of confirmation cannot be attempted because of time constraints, but we will give a general overview of the development of confirmation through the various periods of church history, allowing the voices of the past to speak for themselves whenever possible. 6 Arthur C. Repp, Confirmation in the Lutheran Church. (St.
The confirmation bias, in which new evidence is given more weight when it agrees with existing beliefs, is a ubiquitous yet poorly understood example of such biases. Here we report that a confirmation bias arises even during perceptual decision-making, and propose an approximate hierarchical inference model as the underlying mechanism. ...
Abstract. Confirmation bias is a common problem for customers, brands, and decision-makers across society. And collectively, it can positively or negatively impact the accuracy and quality of ...
Part of aiding in this process is the requirement of a Confirmation Saint paper. Explanation: For Confirmation, each student candidate must choose a Confirmation name. Candidates may use their baptismal name or can choose the name of a canonized saint. The saint should hold personal significance for the student.
This exploratory study investigates one aspect of automation, outsourcing the bank. confirmation process to an outside service bureau. Electronically confirming cash and debt. balances with banks ...
According to the text, confirmation bias is defined as the tendency to seek out information that reaffirms past choices and to discount information that contradicts past judgements. In other words, individuals favor information that confirms previously existing beliefs or biases, despite attaining information that challenges the assumption (s).