Thank you for visiting nature.com. You are using a browser version with limited support for CSS. To obtain the best experience, we recommend you use a more up to date browser (or turn off compatibility mode in Internet Explorer). In the meantime, to ensure continued support, we are displaying the site without styles and JavaScript.
- View all journals
- Explore content
- About the journal
- Publish with us
- Sign up for alerts
- Data Descriptor
- Open access
- Published: 24 June 2023
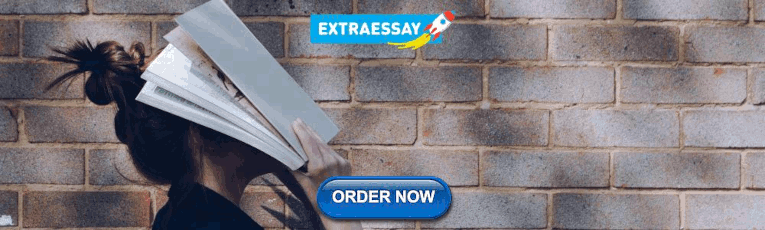
Comprehensive green growth indicators across countries and territories
- Samuel Asumadu Sarkodie ORCID: orcid.org/0000-0001-5035-5983 1 ,
- Phebe Asantewaa Owusu 1 &
- John Taden ORCID: orcid.org/0000-0002-3038-1831 2
Scientific Data volume 10 , Article number: 413 ( 2023 ) Cite this article
7837 Accesses
3 Altmetric
Metrics details
- Environmental economics
- Interdisciplinary studies
- Sustainability
A sustainable transition to green growth is crucial for climate change adaptation and mitigation. However, the lack of clear and consistent definitions and common measures for green growth implies a disagreement on its determinants which hampers the ability to proffer valuable guidance to policymakers. We contribute to the global debate on green economic development by constructing green growth measures from 1990 to 2021 across 203 countries. The pillars of green growth are anchored on five dimensions namely natural resource base, socio-economic outcomes, environmental productivity, environmental-related policy responses, and quality of life. Contrary to the aggregated methods used in constructing indices in the extant literature, we employ a novel summary index technique with generalized least squares attributed-standardized-weighted index that controls for highly correlated variables and missing values. The constructed indicators can be used for both country-specific and global data modeling on green economic development useful for policy formulation.
Similar content being viewed by others
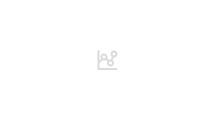
Green finance, renewable energy development, and climate change: evidence from regions of China
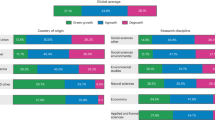
Shades of green growth scepticism among climate policy researchers
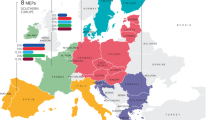
Perceptions of degrowth in the European Parliament
Background & summary.
Policies to promote green growth should be anchored on thorough knowledge and understanding of the concept. Likewise, tools to monitor green growth must have a reliable and comprehensive framework upon which progress can be recorded and compared across multiple entities. Yet, no two studies have a common measure or definition of green growth despite its widespread application in the scholarly and public policy discourse 1 . Not only does the lack of a common green growth measure stifle policymaking but also, hinders investments in its success 2 . The discourse on green growth is fairly new within international institutional development programs. As a multilateral agenda, green growth was first adopted in 2005 by 52 Asia-Pacific countries at Seoul’s 5 th Ministerial Conference on Environment and Development (MCED). The UN Economic and Social Commission for Asia and the Pacific (UNESCAP) then described the concept as a focus on sustained economic progress driven by environmental sustainability while improving low-carbon society and socially inclusive development (UNDESA, 2012, p. 35). The OECD in 2009 defined the term as achieving sustained economic development while reducing the negative environmental externalities including climate change, loss of biodiversity, and natural resource exploitation 3 . The organization also became the first to provide a cross-country ranking and comparative framework of green growth indicators for its industrialized economies in 2011 4 . The UN Environment Program (UNEP), under Towards Green Growth: Monitoring Progress program, also presented its first set of indicators in 2011. Per its definition, green economic growth improves well-being and social justice while reducing environmental risks and ecological footprint 5 . This infers that achieving green economic growth should prioritize green innovation, decarbonization, green trade, resource efficiency, and social inclusion 6 . Other international bodies such as the Global Green Growth Institute (GGGI), European Commission, and World Bank have all since proffered their definitions of the concept. In the wake of the discrepancies and unwieldy growth of the discourse surrounding green growth, the World Bank, UNIDO, OECD, and UNEP created the Green Growth Knowledge Platform (GGKP) in 2012. The GGKP is entrusted with the responsibility of collaboratively generating, managing, and sharing knowledge and data on green growth 7 . The GGGI produced the green growth index in 2019 aimed at preparing a measure that will enhance efforts to track the implementation of the Aichi Biodiversity Targets, Paris Accord, and SDGs 8 . At a country level, South Korea is widely recognized as the first to incorporate a comprehensive national strategy of green growth into its development plan in 2008 9 . Today, myriad countries across the globe have adopted various strategies to achieve green growth, even outside the frameworks of the SDGs and the Paris Climate Accord. Noticeably, developing countries such as Rwanda, Ethiopia, Vietnam, and Morocco have recently been commended for their track records on the incorporation of green growth into national development programs 10 .
In light of the growing adoption of green growth development into national development plans, researchers have recently focused on assessing performances and prospects of a green future across countries, but mostly relying on varied measures of the concept. For example, Houssini and Geng 10 assessed Morocco’s green growth performance between 2000 and 2018 using a self-derived green growth measure developed with a data envelopment (D.E) analysis technique. The assessment proceeded to score Morocco positively on several variables, commending the government on its conscious effort to promote green growth. Wang and Shao 11 developed the “Hybrid Global ML Index” to assess the effect of formal and informal national green growth performance among G20 economies. The study found that most countries in the G20 have achieved green growth benchmarks in the entire sample period, except from 2008 to 2009 11 . Several other studies used D.E analysis techniques but derived different green growth measures even when applied to the same units of analysis 12 , 13 , 14 , 15 , 16 . In related studies, Acosta, et al . 8 assessed and ranked 115 countries in 2019 after using composite (C.O) analysis to develop a green growth index that strayed significantly from what its member partners such as the OECD and the UNEP historically relied upon. Their measure particularly paid attention to the inclusivity of green growth by being one of the first and only known two [with Kararach et al . 17 ] to incorporate a gender dimension into the impacts of green growth policies. Though, using C.O analysis, other researchers 2 , however, arrived at measures that capture different sets of indicators for the same or different countries in their analyses.
Patently, the lack of clear and consistent definitions and common measures for green growth imperils attempts to compare findings across multiple studies 1 , discourages investments in its success 2 , and forces the “comparison of apples to oranges” when analyzing the extant literature 1 . Likewise, the lack of a common understanding of the meaning of green growth and the utilization of myriad varying sets of indicators implies a lack of agreement on its determinants 2 , which hampers the ability to proffer valuable guidance to policymakers. In this study, we propose a new set of green growth indicators that is founded on a thorough assessment of a wider range of factors contributing to green growth. Theoretically, we portend that the dynamic shift from brown to green growth entails strategic multifaceted actions that are informed by economic endowments, political choices, socio-economic capabilities, and environmental outcomes. Accordingly, we define green growth as a sustained economic development approach decoupled from negative environmental consequences but thrives on eco-technological efficiency, reduces poverty, and increases social inclusion. Empirically, we construct green growth measures whose pillars are anchored on the five dimensions of (i) natural asset base, (ii) policy responses, (iii) socio-economic outcomes, (iv) quality of life, and (v) environmental productivity (see Fig. 1 ). Contrary to the aggregated methods used in constructing indices, we employ a novel summary index technique with generalized least squares attributed-standardized-weighted index that control for highly correlated variables and missing values.
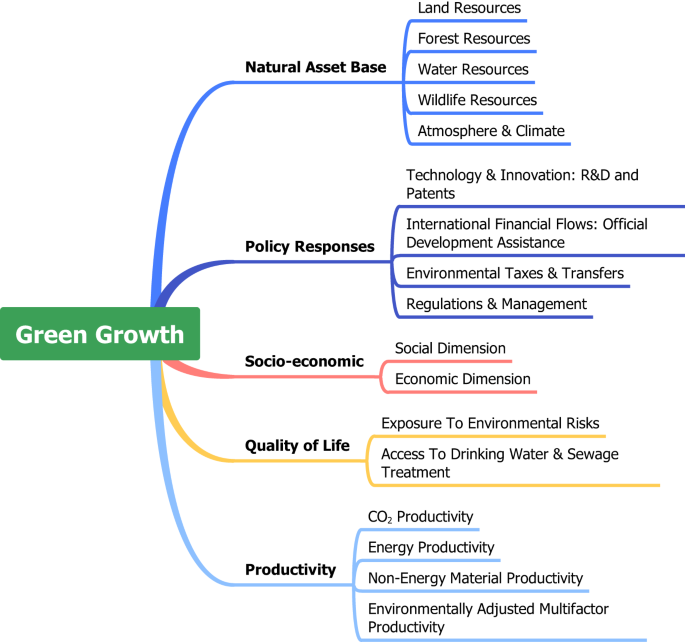
Dimensions of green growth. Data source: OECD ( https://buff.ly/43cKbKU ).
We analyze the frameworks and several indicators adopted by existing studies measuring green growth. For brevity, we analyze the indicators in light of our theoretical and empirical framework. Our empirical framework covers the dimensions of (i) natural asset base, (ii) policy responses, (iii) socio-economic outcomes, (iv) quality of life, and (v) environmental productivity (Fig. 1 ). To the best of our knowledge, these dimensions subsume the differing frameworks in the extant literature and, thus, allow us to review and analyze the individual indicators espoused in most studies. While not all studies in the extant literature are analyzed, Tables 1 , 2 describe all known studies, their measurement frameworks, and other applications.
Natural asset base
Natural resources are central to the purpose of all green growth or sustainable development initiatives under the Aichi Biodiversity Targets, Paris Climate Accord, and SDGs 8 . The Green Growth Performance Measurement (GGPM) Program, for instance, developed in 2019 utilizes data from 115 countries and relies on 36 sampled indicators categorized under four dimensions. The dimensions include sustainable and efficient resource utilization, economic opportunities, natural capital protection, and social inclusion. Within the dimension of natural capital protection, for example, the authors discussed 12 indicators that reflect components such as the proportion of forests, biodiversity cover, and marine protected areas. However, the authors also incorporated measures such as the share of non-carbon agricultural emissions, mean annual air pollution, and the level of recreation and tourism in marine areas. By this calibration, the authors developed factors such as ecosystem, environmental quality, GHG reductions, and the cultural value of resources useful in measuring green growth under natural capital protection.
To design a novel tool to measure the complete impacts of green growth policies, Kim et al . 4 synthesized a pool of 78 indicators in the extant literature down to what they describe as the 30 core and the 12 international indicators pertinent to assessing and comparing green growth across countries. Among the final 12 international indicators, two were used to measure the natural capital assets and environmental quality dimension which are: the inverse of domestic material consumption and the share of forest coverage per total land size. The authors’ omission of other non-forest resources in measuring natural capital is puzzling—as they offer no theoretical justification for the decision. However, the authors admit that by placing more weight on forest resources, the measure unfairly punishes countries with low forest cover such as Iceland. Baniya, et al . 18 utilized six indicators to analyze green growth in Bangladesh and Nepal from 1985 to 2016—and to project its 2030 prospects in both countries. Natural capital was measured using the share of land with forest coverage. The authors argued that the six indicators chosen for analyses are the most frequently used in the extant literature from the OECD (2017) framework. However, similar to Kim et al . 4 , the omission of indicators capturing non-forest resources poses a challenge to the generalizability of the final measure of green growth.
Kararach, et al . 17 developed the African Green Growth Index using data from 22 African countries. They featured 48 indicators representing five different dimensions including, socioeconomic context, productivity of resources and environment, natural asset base monitoring, gender, and governance. While natural assets include the usual measures of forests, land, agriculture, water, and aquatic resources, they also incorporated a disaster risk component. This measure tracks all disaster events from 1900 to 2014 and the population affected by disasters. Nonetheless, attempts to derive variables as far back as 1900 meant that the authors had to statistically impute a significant portion of the missing data, which admittedly leads to model uncertainties and affects the accuracy 17 . Inspired by the need to design a green growth index that accounts for the connection among society, economy, and nature, Li, et al . 19 employed factor analysis to design an inclusive green growth indicator covering four unique dimensions, viz. social inclusion, economic security, resource use, and sustainability. However, the design failed to provide a stand-alone measure for natural capital. Instead, the authors created a resource use dimension that is measured by a derivation of energy consumption and variables of natural capital including arable land holdings, forest cover, land yield efficiency, and freshwater resources.
Environmental productivity
The productivity dimension of green growth entails the efficient ways by which economic growth is decoupled from resource use. An existing study focused on both ecosystem services and the productive use of resources such as energy, water, and land 8 . The authors reinforced this dimension with eight indicators ranging from measures such as the share of primary energy provision to GDP to total material footprint per capita. Kim, et al . 4 dedicated two of the five dimensions of their framework to measuring productivity. The first dimension labeled as environmental efficiency of production is proxied by two indicators namely GHG emissions per GDP as well as the share of GDP from services. The second dimension labeled as environmental efficiency of consumption is proxied by three indicators including energy utilization per GDP, the share of renewable energy consumed, and withdrawal of both surface and groundwater out of total available water. The authors argued that their framework aligns with that of the OECD and purposely adopts the growth-accounting approach to reach a broader context of global decision-making. However, this also implies that unique country characteristics of certain measures are glossed over for the sake of generalizability. Similarly, four of the six indicators that constitute Baniya, et al .’s 18 framework have productivity connotations. These include the productivity of carbon, energy, materials, and the share of renewables. The authors, however, do not account for other crucial dimensions seen in other studies such as policy responses, quality of life, and social inclusion.
Socio-economic dimension
New and sustainable economic opportunities are crucial to the success of green growth strategies. Thus, some authors incorporated socio-economic variables of green growth into their frameworks. For instance, Acosta, et al . 8 employed a dimension termed green economic opportunities—that capture the share of economic opportunities that arise as investments shift from traditional activities to green sectors. They integrated this dimension with four indicators that capture environmental technology (i.e., number of patent publications), green employment, environmental goods exports, and net savings minus resource and pollution damages. On the other hand, Kararach, et al . 17 introduced an entire dimension for gender, into which seven different indicators are fed. They argued that green growth must be assessed by its impact on societal inequalities. However, it is unclear why such indicators (i.e., female HIV prevalence, female labor force, female literacy, parliamentary seats, and ministerial positions held by women) were used to measure the gender dimension. Li, et al . 19 argued that a green growth measure must quantitatively incorporate the themes of social equity, stability, and happiness. The authors provided an extensive set of indicators incorporated into the measure of social inclusion. However, unlike other studies that specifically addressed opportunities for women and minorities, Li, et al . 19 does not specify what constitutes fair opportunities and what specific group of people development must consciously cater for.
Quality of life
The quality-of-life dimension of green growth tracks individuals’ social well-being attributed to resources extracted for economic growth. The existing studies that incorporated aspects of quality of life conceptualize the measures with different but similar and overlapping terminologies. For example, Acosta, et al . 8 combined variables that track economic and social well-being into a single dimension described as social inclusion. They argued that inequality and poverty are directly affected by the availability of resources and crucial basic services. Conversely, people rely heavily on the environment such as forests, wildlife resources, and among other natural assets for livelihoods. They postulated that the social performance of green growth should be measured because sustained economic growth requires reduced inequality 8 . Consequently, they classified concepts such as “access to basic services and resources, gender balance, social equity, and social protection” under the social inclusion dimension. The authors supported this dimension with 12 indicators ranging from “access to safe drinking water and sanitation” to “proportion of urban population living in slums”. While theoretically cogent, this dimension does not distinguish traditional economic conditions such as income levels from social conditions or quality of life such as health. Kim et al . 4 measured the quality-of-life dimension with only one indicator, viz. the public transportation modal split. They justified the selection of this measure among other dimensions on a criterium of policy relevance, analytical soundness, and measurability.
Policy responses
The policy response dimension of green growth is represented in fewer studies. Although the OECD framework incorporates a policy response dimension, the Green Growth Index developed by Acosta, et al . 8 omits it. Among studies that justify the policy response dimension, Kim et al . 4 measured the response with four indicators: environmental expenditure, environmental patents, green ODA per GDP, and green R&D per government budget. While Kararach, et al . 17 does not incorporate a precise dimension for policy responses, they accentuated the distinct role of governance in their equation. Their “governance” dimension spells out four political indicators pertinent to measuring green growth. These indicators include “violence and/or terrorism, control of corruption, government effectiveness, political stability, rule of law, and regulatory quality”. Nonetheless, these variables primarily capture institutional quality and could have been complemented by other deliberate governmental efforts to promote green growth, such as eco-innovation R&D and environmental taxes.
Analysis of the literature
No two studies in the extant literature have the same or directly comparable measures of green growth 1 . From our review, no two studies have a common understanding of what set of indicators constitutes green growth or dimensions of green growth. In other scenarios, indicators used as proxies for one dimension in a study—for example, resource productivity—might be adopted as proxies for an entirely different dimension (such as economic opportunities) in another study. Consequently, their differential weightings in different dimensions imply they feature at different levels of importance in different studies. In such scenarios, comparing findings in the literature using these different measures might be tantamount to comparing apples to oranges 1 .
A few existing studies introduced distinct dimensions in their frameworks for various reasons. For instance, Li, et al . 19 utilized GDP as a measure of economic growth and termed as “economic prosperity”. They proceeded to introduce an economic prosperity dimension using indicators such as trade volumes, R&D expenditure, urbanization, and inflation in addition to GDP and GDP per capita. They argued that economic prosperity should be the primary criterion of green and inclusive growth, stating further that more focus must be placed on the growth, development, and stability potentials of the economy. Notably, these indicators were captured in different studies, even under different dimensions and for different conceptual purposes. Contrary to studies that employed C.O. analysis to measure green growth, a large share of the extant literature relies on D.E. analysis to derive the green growth index. Studies that used C.O. analysis adopted a range of diverse inputs. For instance, whereas Song, et al . 14 used labor and capital as inputs in their model, Cao, et al . 13 relied on capital, labor, technological innovation, and energy consumption. Tables 1 , 2 provide more information on the variability of datasets (used as inputs) and techniques in the existing studies.
Beyond studies utilizing C.O analysis and D.E techniques to measure green growth, a few other studies insist on simplifying the discourse further by adopting a single indicator out of the larger literature as a proxy for green growth. Accordingly, some researchers measure green growth using single indicators such as environmental and resource productivity 20 , carbon productivity 21 , and environmentally-adjusted-multifactor productivity 22 . In earnest, while these measures simplify analyses of green growth, they risk revealing only a portion of the picture. For instance, the use of carbon emissions as a sole indicator for green growth does not reveal any information beyond the pollution levels of the set of tools applied to achieving a certain level of growth 23 . Consequently, the contributions of other factors such as economic opportunities, policy responses, and resource protection to green growth are untenably omitted.
Index-making model
The schematic representation depicted in Fig. 2 shows the green growth index-making methodological structure used in this study. The steps include variable selection—used for categorization and classifying dimensions, income group & regional classifications, treatment of missing data, winsorization & normalization of data, adjusting the sign of variables where applicable, construction of weights, and construction of summary indices. While missing observations are often problematic in panel data, the estimation technique ignores missing outcomes (but also receives low weight) in creating new indices but utilizes all available data. Following the normalization procedure in developing the summary indicator, the technique further controls for missing data by fixing the values of the missing indicator to zero (0) — the mean of the reference group. This strategy further improves estimation efficiency by assigning lower weights to categories and dimensions with missing values 24 . The winsorizing technique entails generating new data by trimming 1 st and 99 th percentiles across countries and territories to treat extreme outliers 25 . Normalization \(\left[{\rm{norm}}=\left({y}_{i}-{y}_{min}\right)/\left({y}_{max}-{y}_{min}\right)\right]\) involves the generation of new data by normalization of scores ranging between 0 and 1 using the minimum ( y min ) and maximum ( y max ) observations while accounting for periodic data frequency across countries and territories. The summary index approach used to construct the green growth indicators can be expressed as 24 :
Where \({\bar{s}}_{i,j}\) is the constructed index (i.e., the outcomes can be categories, dimensions, and green growth indicators) with a weighted average W i,j . w j,k denotes the weight of the normalized output \([({y}_{i,j,k}-{\bar{y}}_{j,k})/{{\sigma }}_{j,k}^{y}]\) from inverse covariance matrix \({\widehat{\Sigma }}_{j}^{-1}\) , \({\widetilde{y}}_{i,j,k}\) is the inputs for individual i , indicators k , and country j . The normalized output is generated via effect sizes by dividing demeaned indicators \(({y}_{i,j,k}-{\bar{y}}_{j,k})\) by the standard deviation \({\sigma }_{j,k}^{y}\) of the reference group, whereas \({{\mathbb{K}}}_{i,j}\) represents non-missing outcomes for indicators k and country j . The summary index combines several variables into categories and several categories into dimensions and subsequently develops the green growth indicators. This test involves: (1) adjusting the sign of variables to indicate a better result, thus, a positive direction of the series always has positive consequences on the environment. (2) normalizing indicators into similar scales (3) constructing weights (4) constructing indices using weighted average and (5) normalizing indices. Thus, the winsorized and normalized dataset was classified into categories, dimensions, and green growth indicators. Contrary to the aggregated methods used in constructing indices 26 , the novel summary index technique with generalized least squares (GLS) attributed-standardized-weighted index used in this study controls for highly correlated variables and missing values.
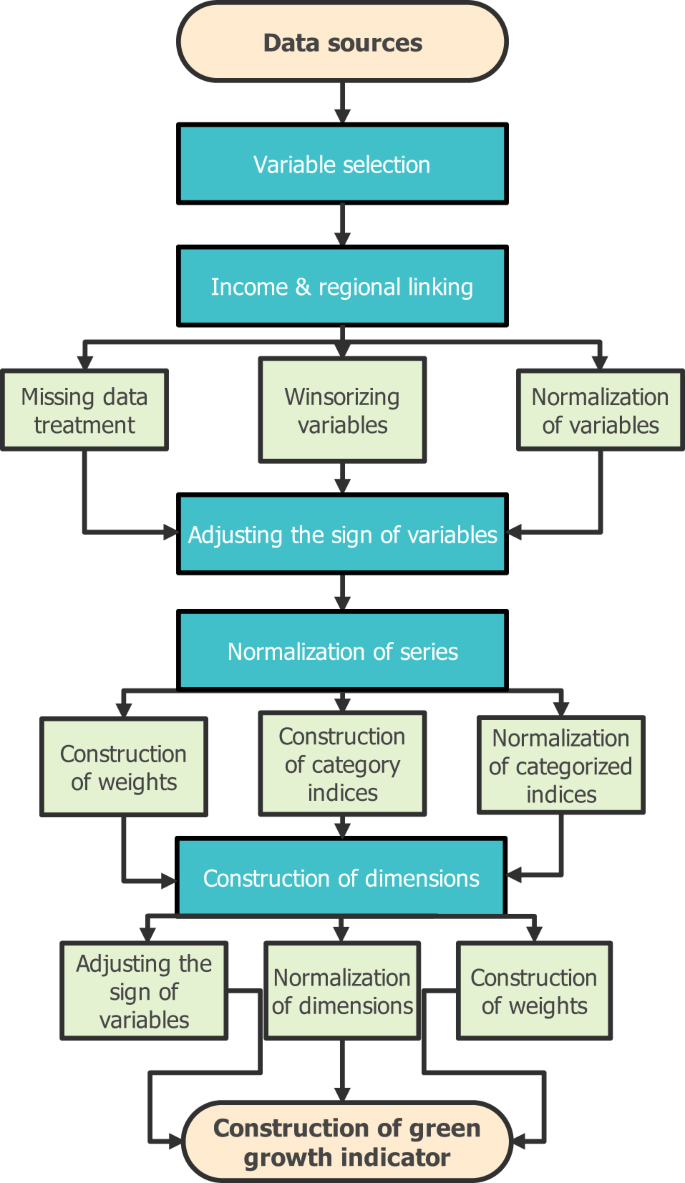
Green growth index-making methodological structure.
Category indices of green growth
Our empirical assessment began with a panel-based descriptive statistical analysis of 152 raw data series spanning over 30 years. The descriptive statistical metrics led to the identification of several missing and extreme distributions requiring winsorizing. The winsorized and normalized dataset was classified into 18 category indices with a composition of the estimated weight of 152 variables. As the rule of the GLS summary index algorithm presented in Schwab, et al . 27 , highly-correlated variables were apportioned offsetting or small constructed weights whereas less-correlated or unique variables were apportioned higher constructed weights (Tables 3 , 4 ). Positive weights signify a higher contribution to the summary index whereas negative weights signify factors that decrease the summary index. For example, demand-based CO 2 emissions (CO2_DBEM) contribute the highest weight (1.447) to the summary emission index whereas production-based CO 2 emissions (CO2_PBEM) show the highest weight (−0.865) that negatively enter the summary emission productivity index (Table 3 ). Energy consumption in other sectors (NRGC_OTH) and biomass (DMC_BIO) contribute the highest weights (0.350 and 0.278, respectively) to the energy productivity and non-energy material productivity indices whereas environmentally-adjusted multifactor productivity growth (EAMFP_EAMFPG) and relative advantage in environment-related technology (GPAT_DE_RTA) have the lowest weights (0.280 and 0.124, respectively) in the multifactor productivity and patents summary indices. Similarly, marine protected areas (PA_MARINE), allocable ODA to the environment sector (ODA_ENVSEC), and Petrol tax (FTAX_DIE_S) are assigned larger weights (0.520, 0.312, and 0.548, respectively) for environmental regulations, taxes & transfer, and official development assistance summary indices while purchasing power parity (PPP), environmentally-related R&D expenditure (ENVRD_GDP), and welfare costs of premature mortalities from exposure to PM 2.5 (PM_SC) are assigned offsetting or small weights (−0.011, 0.079, and −1.859, respectively) for the economic dimension, environmental risks, and R&D summary indices. Besides, the population connected to public sewerage (ASEW_POP), permanent surface water (SW_PERMWAT), threatened vascular plant species (WLIFE_PL), and net migration (POP_NETMIGR) show the lowest weights (−0.144, −0.044, −0.025, and 0.070, respectively) assigned for the summary indices of access to drinking water & sanitation, water resources, wildlife resources, and social dimension (Table 4 ).
Constructing green growth Indicators
We constructed 10 green growth indicators (Models 1–10) using all five dimensions in Fig. 1 but with varying input characteristics for users to choose from. In Model 2, the characteristics of all five dimensions (i.e., environmental productivity, environmental quality, natural asset base, policy response, and socioeconomics) show better environmental performance. In Model 1, the characteristics of policy response, and socioeconomics dimensions show better environmental impacts but environmental productivity, environmental quality, and natural asset base worsen the environment. In Model 3, the characteristics of environmental quality, natural asset base, policy response, and socioeconomics improve the environment but environmental productivity declines environmental sustainability. In Model 4, the characteristics of environmental productivity, natural asset base, policy response, and socioeconomics improve the environment but the environmental quality dimension spurs environmental degradation. In Model 5, the characteristics of environmental productivity, environmental quality, policy response, and socioeconomics promote sustainable environment whereas natural asset base is detrimental to the environment. In Model 6, the characteristics of natural asset base, policy response, and socioeconomics reduces environmental degradation whereas environmental productivity and environmental quality increase pollution. In Model 7, environmental quality, policy response, and socioeconomics improve sustainability while environmental productivity and natural asset base hamper clean environment. In Model 8, environmental productivity, policy response, and socioeconomics decline environmental threats whereas environmental quality and natural asset base escalate environmental consequences. In Model 9, the characteristics of environmental productivity, environmental quality, natural asset base, policy response, and socioeconomics deteriorate the environment and thwart sustainable transition. Model 10 incorporates all conditions in Models 1–9 except that the average weights of all indicators was used for its construction.
The negative characteristics of dimensions in Models 1–10 (used to construct the 10 green growth indicators) were determined using the flipping scenarios. The flipping scenarios used in calculating the ten green growth indicators are presented in Table 5 . The parenthesis (…%) denotes the percentage weight of dimensions (in Fig. 3 ) from the model used to construct green growth indicators. Model 1 covers the role of policy response (23.29%), natural asset base (21.97%), socioeconomics (20.97%), environmental productivity (17.69%), and environmental quality (16.07%) while altering the sign (i.e., flipping implies altering the sign to move in the opposite direction) of environmental productivity, environmental quality, and natural asset base. Model 2 captures socioeconomics (28.95%), environmental quality (19.74%), natural asset base (18.87%), policy response (16.25%), and environmental productivity (16.18%) with no flipping option. Model 3 comprises socioeconomics (26.62%), environmental quality (24.83%), environmental productivity (18.73%), natural asset base (15.92%), and policy response (13.89%) while altering the sign of environmental productivity. Model 4 encompasses environmental quality (22.04%), environmental productivity (21.38%), socioeconomics (19.85%), natural asset base (19.78%), and policy response (16.94%) while altering the sign of environmental quality. Model 5 includes socioeconomics (25.71%), natural asset base (21.83%), policy response (19.09%), environmental productivity (18.81%), and environmental quality (14.56%) while altering the sign of natural asset base. Model 6 captures socioeconomics (24.39%), natural asset base (22.55%), policy response (19.54%), environmental quality (18.14%), and environmental productivity (15.39%) while altering the sign of environmental productivity, and environmental quality. Model 7 entails natural asset base (25.23%), socioeconomics (22.26%), environmental productivity (19.13%), environmental quality (17.83%), and policy response (15.56%) while altering the sign of environmental productivity, and natural asset base. Model 8 covers natural asset base (22.24%), policy response (21.27%), environmental productivity (21.12%), socioeconomics (18.19%), and environmental quality (17.18%) while altering the sign of environmental quality, and natural asset base. Model 9 includes environmental productivity (28.95%), policy response (19.74%), natural asset base (18.87%), environmental quality (16.25%), and socioeconomics (16.18%) while altering the sign of socioeconomics, policy response, natural asset base, environmental quality, and environmental productivity. Finally, the weight of dimensions used to construct green growth indicators reveals an average weight contribution (Model 10) of 22.57%, 20.81%, 19.71%, 18.52%, and 18.40% in the order of dimensions: socioeconomics > natural asset base > environmental productivity > environmental quality > policy response (Fig. 3 ). Figure 4 presents the optimal green growth index across countries for the period 2021. Score 0 implies economies have low performance in transitioning toward green growth whereas Score 1 infers countries have high performance toward green growth. The comparisons between countries for other green growth indicators are presented in Supplementary Fig. 1 .
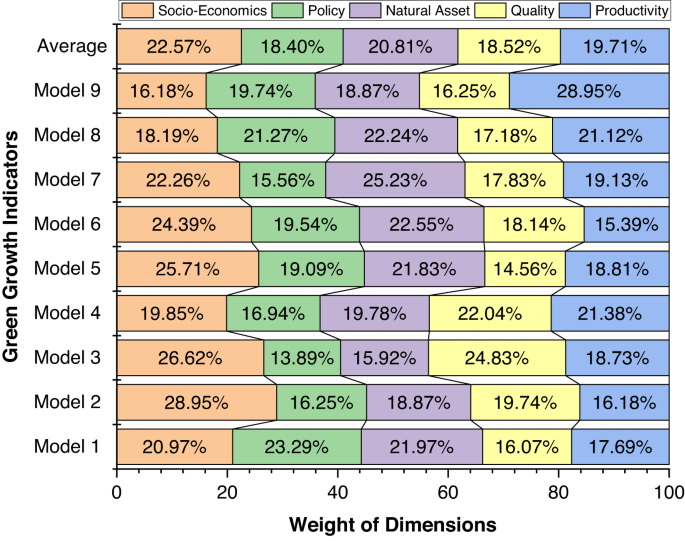
Weight of dimensions used to construct green growth indicators. Legend: Model 1- Comprise socioeconomics, policy response, natural asset base, environmental quality, and environmental productivity while altering the sign (i.e., flipping implies altering the sign to move in the opposite direction) of environmental productivity, environmental quality, and natural asset base. Model 2- Comprise socioeconomics, policy response, natural asset base, environmental quality, and environmental productivity with no flipping. Model 3- Comprise socioeconomics, policy response, natural asset base, environmental quality, and environmental productivity while altering the sign of environmental productivity. Model 4- Comprise socioeconomics, policy response, natural asset base, environmental quality, and environmental productivity while altering the sign of environmental quality. Model 5- Comprise socioeconomics, policy response, natural asset base, environmental quality, and environmental productivity while altering the sign of natural asset base. Model 6- Comprise socioeconomics, policy response, natural asset base, environmental quality, and environmental productivity while altering the sign of environmental productivity and quality. Model 7- Comprise socioeconomics, policy response, natural asset base, environmental quality, and environmental productivity while altering the sign of environmental productivity, and natural asset base. Model 8- Comprise socioeconomics, policy response, natural asset base, environmental quality, and environmental productivity while altering the sign of environmental quality, and natural asset base. Model 9- Comprise socioeconomics, policy response, natural asset base, environmental quality, and environmental productivity while altering the sign of socioeconomics, policy response, natural asset base, environmental quality, and environmental productivity. Model 10 (Average)- Comprise the average weight of socioeconomics, policy response, natural asset base, environmental quality, and environmental productivity in Models 1–9.
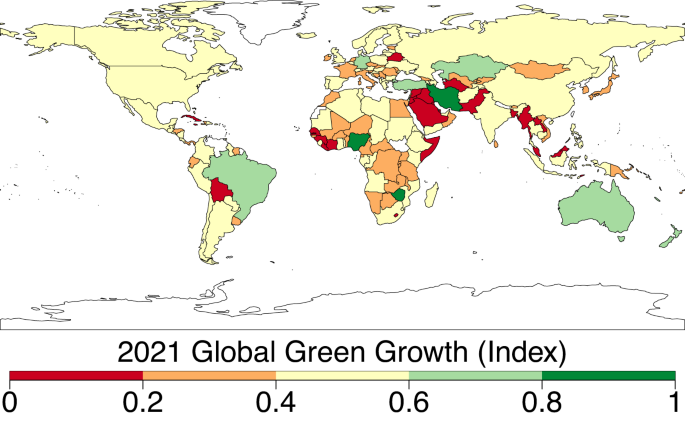
Global distribution of green growth (Index, Reference year: 2021, Model 2). Legend: Score 0 implies low green growth performance whereas Score 1 infers high green growth performance. The optimal green growth indicator (i.e., Model 2) captures 28.95% weight of socioeconomics dimension, 19.74% weight of environmental quality, 18.87% weight of natural asset base, 16.25% weight of policy response, and 16.18% weight of environmental productivity.
Data Records
We employed 152 variables (“OriginalData.xlsx” 28 ) for 203 economies (see sampled countries with ISO3 code in Supplementary Table 1 ) based on the theoretical and empirical framework presented in Tables 1 , 2 and Fig. 1 while integrating the sustainable development goals. We collected our global dataset spanning the period from 1990 to 2021 from OECD 29 with an initial 16,184 observations. The data were sorted into 17 categories (see Fig. 2 ) namely emissions (12 variables), energy (12 variables), non-energy (10 variables), multifactor productivity (3 variables), environmental risks (11 variables), access (5 variables), water (10 variables), land (17 variables), forest (5 variables), wildlife (4 variables), temperature (1 variable), patents (4 variables), research & development [R&D] (5 variables), official development assistance [ODA] (9 variables), taxes (27 variables), regulations (2 variables), economic (10 variables), and social (5 variables) [see details of the 152 variables in Supplementary Table 2 ]. The 17 categories are subsequently classified into 5 dimensions, viz. natural assets (5 categories—water, land, forest, wildlife, and temperature), policy responses (5 categories—patents, R&D, ODA, taxes, and regulations), socio-economic (2 categories—social, and economic), quality of life (2 categories—environmental risks, and access), and environmental productivity (4 categories—emissions, energy, non-energy, and multifactor productivity). Finally, we use permutation and combination strategies detailed in subsequent sub-sections to construct 10 global indicators of green growth labeled as Model 1 (GreenGrowth1), Model 2 (GreenGrowth2), …, and Model 10 (GreenGrowth10). Table 6 shows the data description of constructed categories, dimensions, and green growth indicators—which are publicly available in the Figshare repository 28 .
Technical Validation
To validate the quality of the dataset, we employed permutation and combination scenarios to construct 9 green growth indicators aside from the optimal indicator (labeled as Model 2). We further used statistical distribution 30 to examine variations across income groups. The Games-Howell test shows that the pairwise distribution in Figs. 5 – 14 (excluding Figs. 5 , 12 ) is not significantly different across groups, however, the mean of the optimal green growth (Fig. 6 ) indicator across income groups is in the descending order of high-income > upper-middle-income > lower-middle-income > low-income. This order is consistent with the environmental Kuznets curve (EKC) hypothesis 31 which underscores improved environmental performance with rising income. Changes in the order across income groups in Figs. 5 – 14 (excluding Fig. 6 ) can be explained by the scenarios presented in Fig. 3 . Yet, all constructed green growth indicators are heterogeneous across countries and territories (Fig. 15 ).
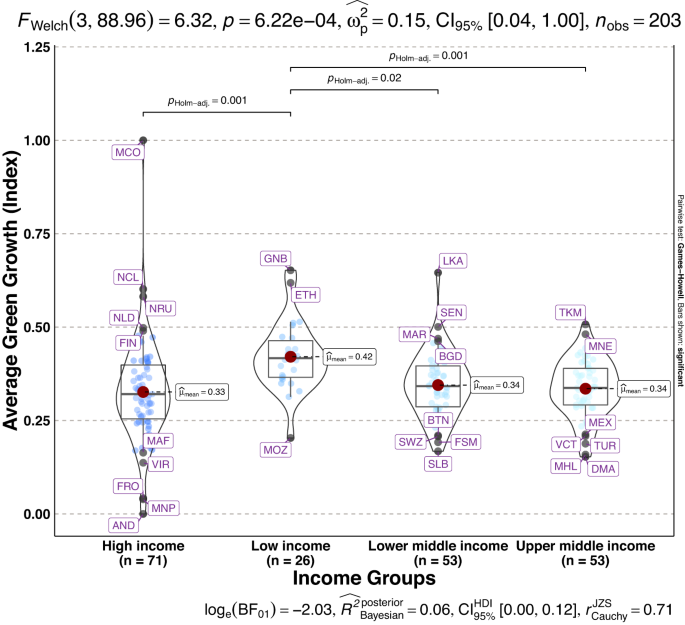
Statistical distribution of green growth indicator (Model 1) across income groups. Note: The country names with corresponding ISO3 codes are presented in Supplementary Table 1 .
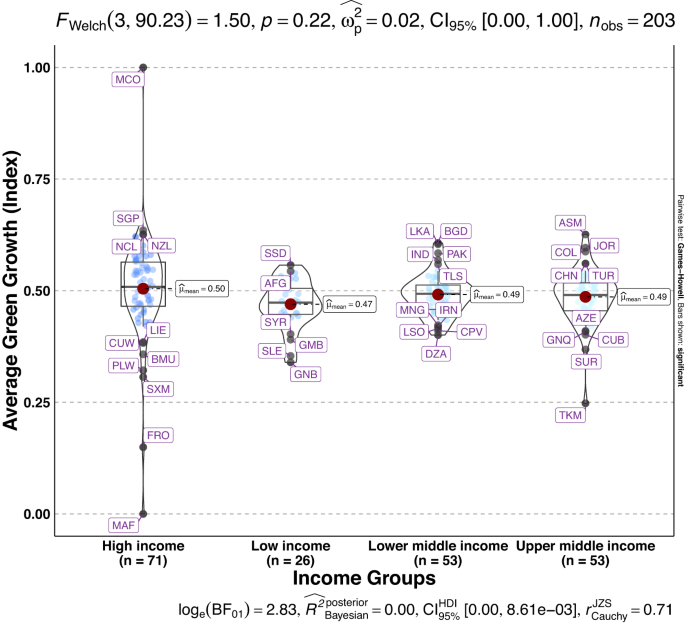
Statistical distribution of green growth indicator (Model 2) across income groups. Note: The country names with corresponding ISO3 codes are presented in Supplementary Table 1 .
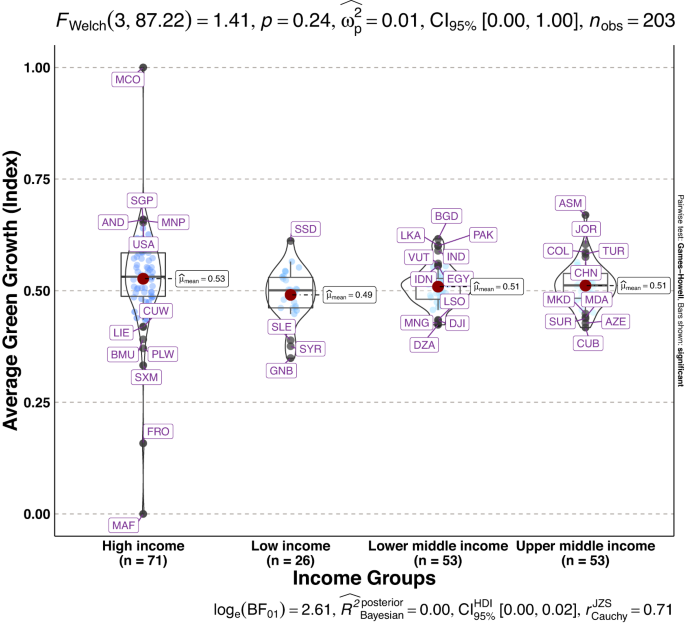
Statistical distribution of green growth indicator (Model 3) across income groups. Note: The country names with corresponding ISO3 codes are presented in Supplementary Table 1 .
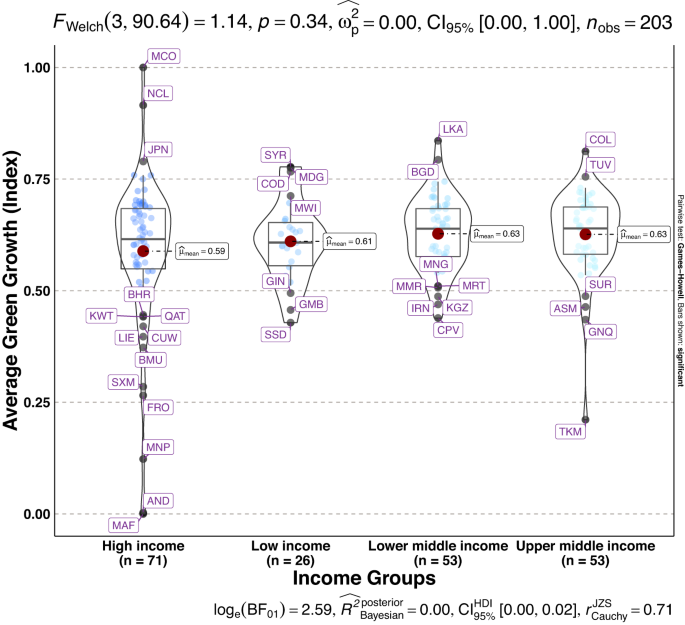
Statistical distribution of green growth indicator (Model 4) across income groups. Note: The country names with corresponding ISO3 codes are presented in Supplementary Table 1 .
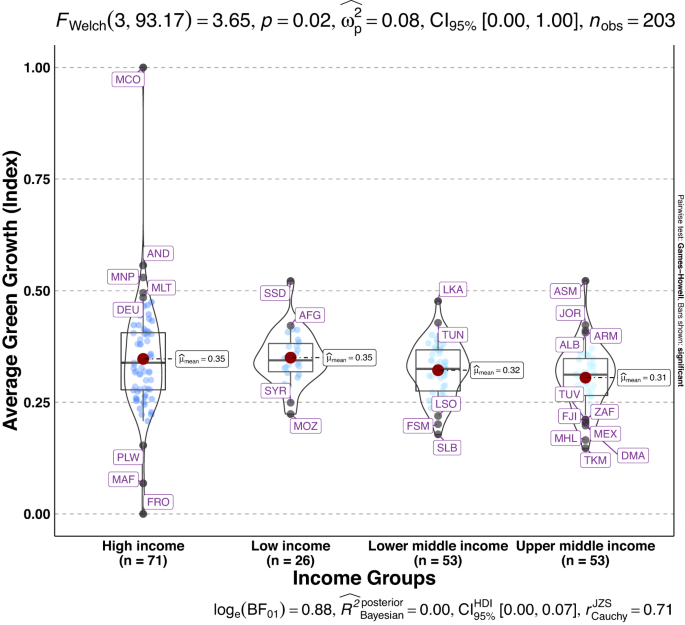
Statistical distribution of green growth indicator (Model 5) across income groups. Note: The country names with corresponding ISO3 codes are presented in Supplementary Table 1 .
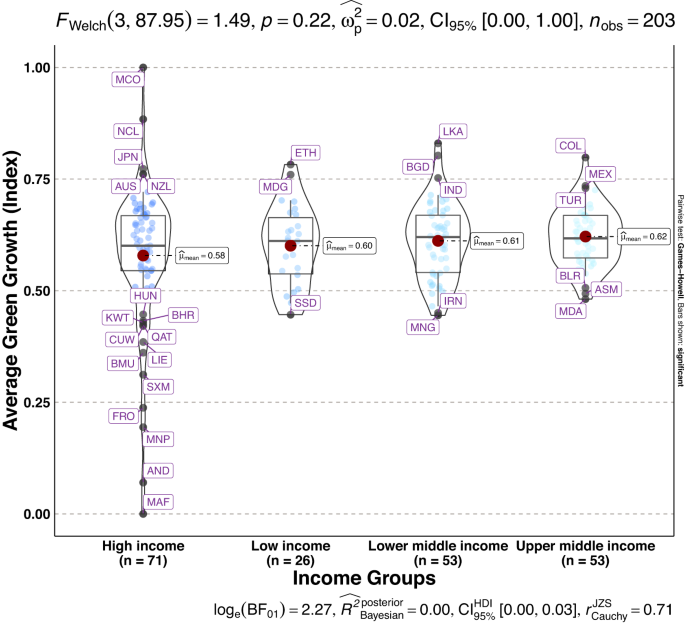
Statistical distribution of green growth indicator (Model 6) across income groups. Note: The country names with corresponding ISO3 codes are presented in Supplementary Table 1 .
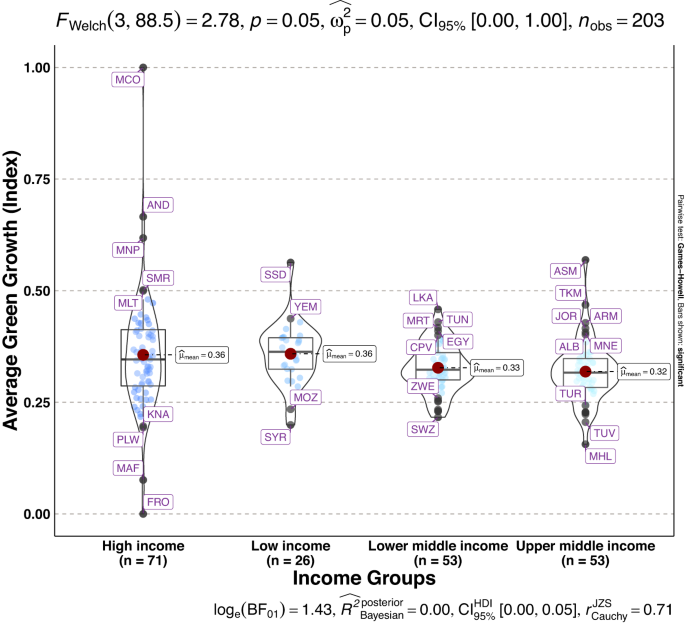
Statistical distribution of green growth indicator (Model 7) across income groups. Note: The country names with corresponding ISO3 codes are presented in Supplementary Table 1 .
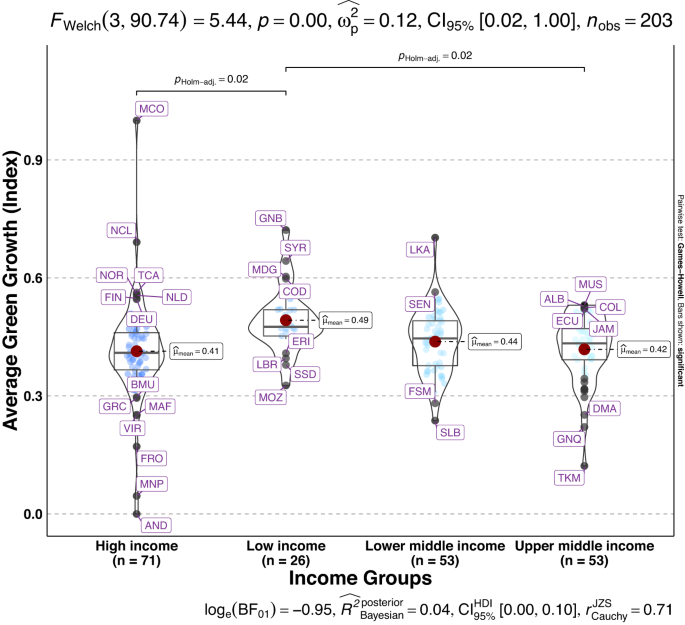
Statistical distribution of green growth indicator (Model 8) across income groups. Note: The country names with corresponding ISO3 codes are presented in Supplementary Table 1 .
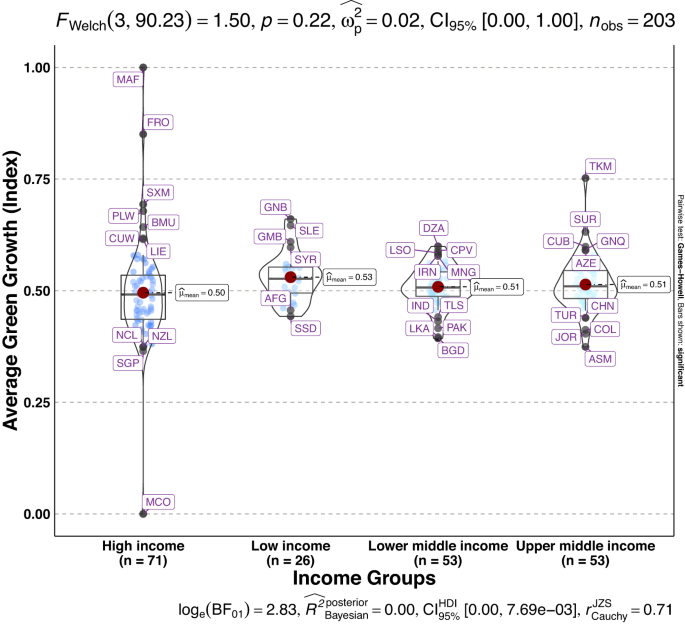
Statistical distribution of green growth indicator (Model 9) across income groups. Note: The country names with corresponding ISO3 codes are presented in Supplementary Table 1 .
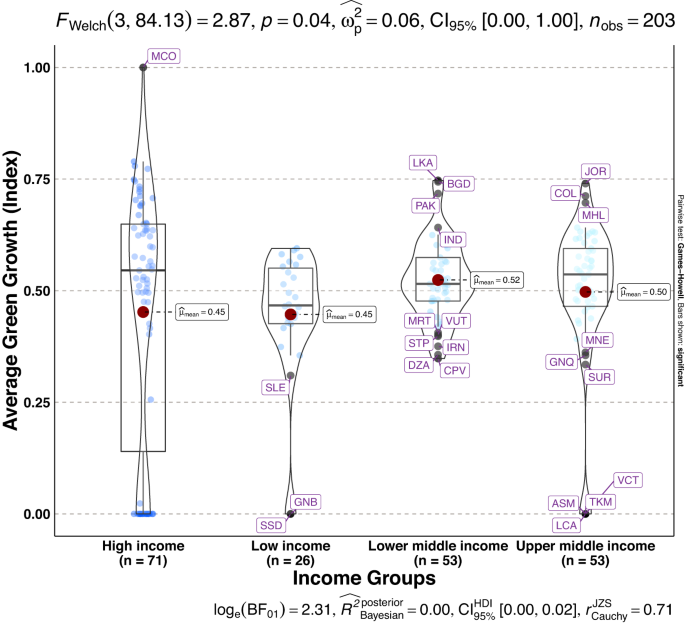
Statistical distribution of green growth indicator (Model 10) across income groups. Note: The country names with corresponding ISO3 codes are presented in Supplementary Table 1 .
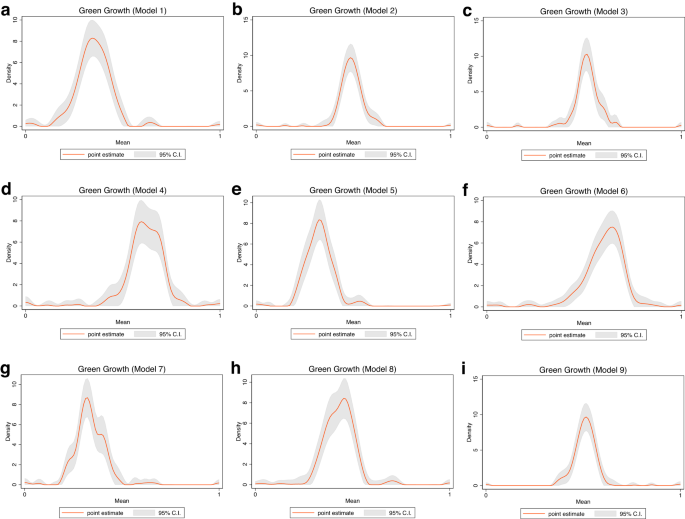
Panel heterogeneous effects of green growth indicators across economies ( a ) Model 1 ( b ) Model 2 ( c ) Model 3 ( d ) Model 4 ( e ) Model 5 ( f ) Model 6 ( g ) Model 7 ( h ) Model 8 ( i ) Model 9. Model 10 was ignored due to missing values with the function returning as an error.
Usage Notes
We developed 10 green growth indicators to examine the progress toward achieving a sustainable transition from a brown economy to a green economy. Each of the green growth indicators has underlying conditions and assumptions presented in Table 5 and Fig. 3 . However, Model 2 (GreenGrowth2) is the most optimal green growth indicator showing economies with high scores have better performance and sustainable transition to green economic development. Evidence from Fig. 15 shows that future research that employs our datasets for panel data modeling should control for heterogeneous effects across economies. The caveat: there may be an underestimation of the standard errors of constructed dimensions and indicators because the GLS-derived weights are inclusively estimated parameters.
Code availability
No custom code was used to generate or process the data described in the manuscript—however, we used the “swindex” package in Stata [Stata/SE 17.0 for Mac (Intel 64-bit)] software to execute the steps detailed in the Methods section.
Leth, N. E. The complications of measuring green growth: Current pitfalls, further developments, and impact on cross-country longitudinal analyses , Lund University (2022).
Ates, S. A. & Derinkuyu, K. Green growth and OECD countries: measurement of country performances through distance-based analysis (DBA). Environment, Development and Sustainability: A Multidisciplinary Approach to the Theory and Practice of Sustainable Development 23 , 15062–15073, https://doi.org/10.1007/s10668-021-01285-4 (2021).
Article Google Scholar
OECD. Declaration on Green Growth. (2009).
Kim, S. E., Kim, H. & Chae, Y. A new approach to measuring green growth: Application to the OECD and Korea. Futures Complete , 37–48, https://doi.org/10.1016/j.futures.2014.08.002 (2014).
UNEP. Towards a Green Economy Pathways to Sustainable Development and Poverty Eradication. UNEP - UN Environment Programme (2011).
UNEP. Green Economy and Trade: Trends, Challenges and Opportunities . (2013).
Green Growth Knowledge, P. About Us | Green Growth Knowledge Partnership. (2023).
Acosta, L. et al . Green growth index: Concepts, methods and applications. Report No. 5, (Global Green Growth Institute, Republic of Korea, 2019).
Kamal-Chaoui, L., Grazi, F., Joo, J. & Plouin, M. The Implementation of the Korean Green Growth Strategy in Urban Areas. (OECD, Paris, 2011).
Houssini, K. & Geng, Y. Measuring Morocco’s green growth performance. Environ. Sci. Pollut. Res. 29 , 1144–1154, https://doi.org/10.1007/s11356-021-15698-1 (2022).
Wang, X. & Shao, Q. Non-linear effects of heterogeneous environmental regulations on green growth in G20 countries: Evidence from panel threshold regression. Sci Total Environ 660 , 1346–1354, https://doi.org/10.1016/j.scitotenv.2019.01.094 (2019).
Article ADS CAS PubMed Google Scholar
Zhu, S. & Ye, A. Does foreign direct investment improve inclusive green growth? Empirical evidence from China. Economies 6 , 44 (2018).
Cao, Y., Liu, J., Yu, Y. & Wei, G. Impact of environmental regulation on green growth in China’s manufacturing industry-based on the Malmquist-Luenberger index and the system GMM model. Environ. Sci. Pollut. Res. 27 , 41928–41945 (2020).
Article CAS Google Scholar
Song, M., Zhu, S., Wang, J. & Zhao, J. Share green growth: Regional evaluation of green output performance in China. International Journal of Production Economics 219 , 152–163 (2020).
Sun, Y., Ding, W., Yang, Z., Yang, G. & Du, J. Measuring China’s regional inclusive green growth. Sci Total Environ 713 , 136367 (2020).
Qu, C., Shao, J. & Cheng, Z. Can embedding in global value chain drive green growth in China’s manufacturing industry? Journal of Cleaner Production 268 , 121962 (2020).
Kararach, G. et al . Reflections on the Green Growth Index for developing countries: A focus of selected African countries. Development Policy Review 36 , O432–O454, https://doi.org/10.1111/dpr.12265 (2018).
Baniya, B., Giurco, D. & Kelly, S. Green growth in Nepal and Bangladesh: Empirical analysis and future prospects. Energy Policy 149 , https://doi.org/10.1016/j.enpol.2020.112049 (2021).
Li, M., Zhang, Y., Fan, Z. & Chen, H. Evaluation and Research on the Level of Inclusive Green Growth in Asia-Pacific Region. Sustainability-Basel 13 , 7482, https://doi.org/10.3390/su13137482 (2021).
Fotros, M. H., Ferdosi, M. & Mehrpeyma, H. An examination of energy intensity and urbanization effect on environment degradation in Iran (a cointegration analysis). J. Environ. Stud. 37 , 13–22 (2012).
Google Scholar
Stoknes, P. E. & Rockström, J. Redefining green growth within planetary boundaries. Energy Research & Social Science 44 , 41–49, https://doi.org/10.1016/j.erss.2018.04.030 (2018).
Fernandes, C. I., Veiga, P. M., Ferreira, J. J. M. & Hughes, M. Green growth versus economic growth: Do sustainable technology transfer and innovations lead to an imperfect choice. Business Strategy and the Environment 30 , 2021–2037, https://doi.org/10.1002/bse.2730 (2021).
Sarkodie, S. A. The invisible hand and EKC hypothesis: what are the drivers of environmental degradation and pollution in Africa? Environ. Sci. Pollut. Res. 25 , 21993–22022, https://doi.org/10.1007/s11356-018-2347-x (2018).
Anderson, M. L. Multiple Inference and Gender Differences in the Effects of Early Intervention: A Reevaluation of the Abecedarian, Perry Preschool, and Early Training Projects. Journal of the American Statistical Association 103 , 1481–1495, https://doi.org/10.1198/016214508000000841 (2008).
Article MathSciNet CAS MATH Google Scholar
Barnett, V. & Lewis, T. Outliers in statistical data . Vol. 3 (Wiley New York, 1994).
Svirydzenka, K. Introducing a new broad-based index of financial development . (International Monetary Fund, 2016).
Schwab, B., Janzen, S., Magnan, N. P. & Thompson, W. M. Constructing a summary index using the standardized inverse-covariance weighted average of indicators. The Stata Journal 20 , 952–964 (2020).
Sarkodie, SA., Owusu, PA. & Taden, J. Comprehensive green growth indicators across countries and territories, figshare , https://doi.org/10.6084/m9.figshare.22291069.v2 (2023).
OECD. Green Growth Indicators https://stats.oecd.org/Index.aspx?DataSetCode=GREEN_GROWTH (2023).
Patil, I. Visualizations with statistical details: The’ggstatsplot’approach. Journal of Open Source Software 6 , 3167 (2021).
Article ADS Google Scholar
Sarkodie, S. A. & Strezov, V. A review on Environmental Kuznets Curve hypothesis using bibliometric and meta-analysis. Sci Total Environ 649 , 128–145, https://doi.org/10.1016/j.scitotenv.2018.08.276 (2019).
Huang, Y. & Quibria, M. G. Green growth: Theory and evidence. UNU-WIDER 2013/56 , 25 (2013).
Guo, L. L., Qu, Y. & Tseng, M.-L. The interaction effects of environmental regulation and technological innovation on regional green growth performance. Journal of Cleaner Production 162 , 894–902, https://doi.org/10.1016/j.jclepro.2017.05.210 (2017).
Jha, S., Sandhu, S. C. & Wachirapunyanont, R. Inclusive Green Growth Index: A New Benchmark for Quality of Growth . (Asian Development Bank, 2018).
Lee, C.-M. & Chou, H.-H. Green growth in taiwan — an application of the oecd green growth monitoring indicators. Singapore Econ. Rev. 63 , 249–274, https://doi.org/10.1142/S0217590817400100 (2018).
Šneiderienė, A., Viederytė, R. & Ābele, L. Green growth assessment discourse on evaluation indices in the European Union. Entrepreneurship and Sustainability Issues 8 , 360–369 (2020).
Gu, K., Dong, F., Sun, H. & Zhou, Y. How economic policy uncertainty processes impact on inclusive green growth in emerging industrialized countries: A case study of China. Journal of Cleaner Production 322 , 128963, https://doi.org/10.1016/j.jclepro.2021.128963 (2021).
Jadoon, I. A., Mumtaz, R., Sheikh, J., Ayub, U. & Tahir, M. The impact of green growth on financial stability. Journal of Financial Regulation and Compliance 29 , 533–560 (2021).
Liu, Z. et al . Inclusive Green Growth and Regional Disparities: Evidence from China. Sustainability-Basel 13 , 11651, https://doi.org/10.3390/su132111651 (2021).
Wu, Y. & Zhou, X. Research on the Efficiency of China’s Fiscal Expenditure Structure under the Goal of Inclusive Green Growth. Sustainability-Basel 13 , 9725, https://doi.org/10.3390/su13179725 (2021).
Zhang, X., Guo, W. & Bashir, M. B. Inclusive green growth and development of the high-quality tourism industry in China: The dependence on imports. Sustainable Production and Consumption 29 , 57–78, https://doi.org/10.1016/j.spc.2021.09.023 (2022).
Chen, G., Yang, Z. & Chen, S. Measurement and Convergence Analysis of Inclusive Green Growth in the Yangtze River Economic Belt Cities. Sustainability-Basel 12 , 2356, https://doi.org/10.3390/su12062356 (2020).
Download references
Acknowledgements
Open access funding provided by Nord University.
Author information
Authors and affiliations.
Nord University Business School (HHN), Post Box 1490, 8049, Bodø, Norway
Samuel Asumadu Sarkodie & Phebe Asantewaa Owusu
Pepperdine University, Malibu, California, USA
You can also search for this author in PubMed Google Scholar
Contributions
S.A.S. and P.O.A. designed the dataset; S.A.S. collated the data, S.A.S., P.O.A. and J.T. wrote the manuscript, and S.A.S. supervised the research.
Corresponding authors
Correspondence to Samuel Asumadu Sarkodie or Phebe Asantewaa Owusu .
Ethics declarations
Competing interests.
The authors declare no competing interests.
Additional information
Publisher’s note Springer Nature remains neutral with regard to jurisdictional claims in published maps and institutional affiliations.
Supplementary information
Supplementary information, rights and permissions.
Open Access This article is licensed under a Creative Commons Attribution 4.0 International License, which permits use, sharing, adaptation, distribution and reproduction in any medium or format, as long as you give appropriate credit to the original author(s) and the source, provide a link to the Creative Commons license, and indicate if changes were made. The images or other third party material in this article are included in the article’s Creative Commons license, unless indicated otherwise in a credit line to the material. If material is not included in the article’s Creative Commons license and your intended use is not permitted by statutory regulation or exceeds the permitted use, you will need to obtain permission directly from the copyright holder. To view a copy of this license, visit http://creativecommons.org/licenses/by/4.0/ .
Reprints and permissions
About this article
Cite this article.
Sarkodie, S.A., Owusu, P.A. & Taden, J. Comprehensive green growth indicators across countries and territories. Sci Data 10 , 413 (2023). https://doi.org/10.1038/s41597-023-02319-4
Download citation
Received : 28 March 2023
Accepted : 19 June 2023
Published : 24 June 2023
DOI : https://doi.org/10.1038/s41597-023-02319-4
Share this article
Anyone you share the following link with will be able to read this content:
Sorry, a shareable link is not currently available for this article.
Provided by the Springer Nature SharedIt content-sharing initiative
This article is cited by
A double machine learning model for measuring the impact of the made in china 2025 strategy on green economic growth.
- Shucheng Liu
Scientific Reports (2024)
The Dynamic Relationship Between Education and Green Growth in BRICS Countries
- Qasim Raza Syed
- Raja Fawad Zafar
Journal of the Knowledge Economy (2024)
Economic complexity and inclusive green growth: the moderating role of public expenditure on education
- Idrys Fransmel Okombi
- Niclaige Elion Lebomoyi
Journal of Environmental Studies and Sciences (2024)
New data and descriptor for crowdfunding and renewable energy
- Dario Salerno
- Andrea Gatto
- Simona Russo
Quality & Quantity (2024)
The impact of domestic materials and renewable energy consumption towards environmental sustainability: evidence from green growth policy across regional and global levels
- Jean Pierre Namahoro
- Wu Qiaosheng
Environment, Development and Sustainability (2024)
Quick links
- Explore articles by subject
- Guide to authors
- Editorial policies
Sign up for the Nature Briefing newsletter — what matters in science, free to your inbox daily.

Advertisement
Determinants of green growth in developed and developing countries
- Research Article
- Open access
- Published: 22 March 2021
- Volume 28 , pages 39227–39242, ( 2021 )
Cite this article
You have full access to this open access article
- Vincent Tawiah 1 ,
- Abdulrasheed Zakari 2 , 3 &
- Festus Fatai Adedoyin ORCID: orcid.org/0000-0002-3586-2570 4
15k Accesses
114 Citations
7 Altmetric
Explore all metrics
Considering the need for environmental sustainability while ensuring economic growth and development by 2030, this study uses data on 123 developed and developing countries to examine factors that influence green growth. The empirical results show that economic development positively influences green growth. However, trade openness is detrimental to green growth. Regarding energy-related factors, we find energy consumption negatively affecting green growth, but renewable energy consumption significantly improves green growth. In further analysis, we find that the influence of these factors differs between developed and developing countries. The result implies that countries at a different development level will require different strategies in achieving the Sustainable Development Goals in 2030. The results are robust to alternative identification strategies such as the System Generalised Method of Movement, which accounts for potential endogeneity.
Similar content being viewed by others
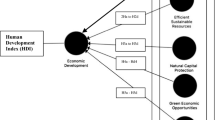
Diverging or converging to a green world? Impact of green growth measures on countries’ economic performance
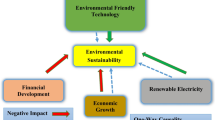
Green technology, green electricity, and environmental sustainability in Western European countries
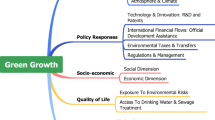
Comprehensive green growth indicators across countries and territories
Explore related subjects.
- Environmental Chemistry
Avoid common mistakes on your manuscript.
Introduction
The continuous rise in temperature and its concomitant effect on livelihood has put sustainable development as the top priority in international discourse (IPCC 2018 ) Footnote 1 . Many nations have also continuously look for avenues to address climate change. The Paris Agreement and 2030 Sustainable Development Agenda have renewed the actions towards a better environment (Organisation for Economic Co-operation and Development—OECD 2020 ). Indeed, OECD ( 2020 ) suggests that Agenda 2030 for sustainable development is the norm for all countries, been developed or developing country. Despite these commitments by almost all countries, there are significant variations at which countries are moving towards environmentally sustainable economic development. For example, among OECD members, Australia and Belgium are experiencing a significant increase in green growth, but Portugal and Turkey are stagnant or decreasing in green growth.
Reflecting on the multi-facet nature of environmental issues and the fact that one size fit all strategy cannot solve these issues in this age of increasing economic activities, we examine the different set of factors that drive green growth. According to OECD ( 2020 ), green growth indicates whether economic growth is becoming greener with more efficient use of natural capital. The green growth indicator monitors progress towards a sustainable and greener economy (OECD 2020 ). Attaining green growth simply implies the use of natural assets towards economic growth in a sustainable manner. The goal is to move towards an economy that leads to human well-being and reduce inequalities among people in the long run, and not exposing future generations to environmental risk (OECD 2018 ).
We employ a fixed effect estimation technique on a large panel data of 123 countries over 18 years. The results show that economic development is positive and significantly associated with green growth, while trade openness negatively impacts green growth. We find no significant relationship between institutional quality and green growth. Although we find energy consumption to impair green growth, renewable energy consumption significantly increases green growth. Our results remain robust to different model specifications, including country effect and accounting for CO 2 as an additional control variable. We also use the System Generalised Method of Movement to address any potential endogeneity issue. The results are also economically significant in explaining the variations of green growth across the world. For example, our results show that a 1% increase in trade openness, given its standard deviation, leads to 0.017 points decrease in green growth.
In further analyses, using sub-sampling techniques, we find interesting results between developed and developing countries. We find economic growth positive and significantly associated with green growth in developed countries but insignificant in developing countries. Next, while internationalization does not significantly impact green growth in developed countries, it is negatively and significantly associated with green growth in developing countries. Notwithstanding these contrasting findings, institutional quality and energy consumption remain similar for both developed and developing countries.
This study is timely as countries work towards achieving the Sustainable Development Goals, including high and progressive green growth by 2030. Our findings, which demonstrate the significant drivers of green growth, will enable policymakers to shape some of the country’s activities, leading to green growth. As evident in the results, countries at different development levels will require different strategies to achieve Sustainable Development Goals. For example, developing countries have to pay attention to foreign direct investment and trade they engage in to avoid harming the environment. Both developed and developing will also need to look closely at 'their institutional quality and regulations to boost green growth.
Our paper also makes an incremental contribution to the environmental literature by deviating from the traditional stream of carbon emission to a new area of improving its efficiency in using natural assets. More specifically, by focusing on green growth, we draw attention to factors that facilitate efficient and effective use of resources to achieve economic development and environmental sustainability.
The remainder of the paper is as follows. The next section presents a literature review on green energy determinants, capturing variables such as economic growth, foreign direct investment, foreign trade, and renewable energy, among others. Research methods, including data, description of variables, and econometric model, are presented in Section 3 , while results are discussed in Section 4 with implications of results. The study concludes in Section 5 with vital policy recommendations.
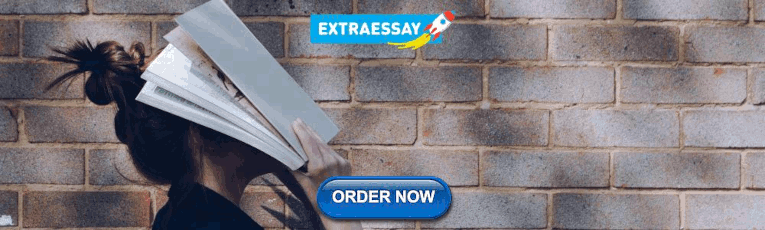
Review of prior studies
Economic factors and the environment.
Since the first Rio summit on environmental sustainability, the world falls short of numerous challenges, including the tense pressure to keep economic activities moving and the rising environmental degradation (The Washington Post 2017 ). Given these tense issues, green growth came to a place to bring these two issues together and address them (OECD 2011 ). In essence, green growth oversees economic growth and development while utilizing natural assets for the well-being of humanity. Despite this significant association between economic growth and green growth, there is scanty research on green growth. In light of the scarce literature on green growth, we present studies that may appear distinctively different but related to the environment and its consequence on green growth.
Alam and Kabir ( 2013 ) find that increase in economic growth sustains the environment by reducing carbon emissions. The author suggests joint pollution and eco-efficiency policies to enable environmental sustainability. Similarly, Rahman et al. ( 2020 ) posit the underline effect of dangerous emissions on economic growth to be approximately more than one digit, indicating that dangerous emissions alongside the population promote economic growth. However, the impact is insignificant because the trade openness causes a further decrease in economic growth. In another notion, investment in clean energy and advanced technologies is confirmed to effectively moderate pollution, which improves the economic growth of the host country (Muhammad and Khan 2019 ).
Furthermore, Mikayilov et al. ( 2018 ) confirm a long-run positive relationship between economic growth and a sustainable environment in Azerbaijan. These findings coined CO 2 mitigating policies such as implementing the carbon price mechanism, public enlighten on the cause and danger of carbon dioxide emissions, and the use of less pollution-intensive technologies. Equally, economic growth could influence environmental performance regardless of the level of the education system. What matters the most is the mediating effect of education on the nation’s cultures and demographic density, which could prompt the influence of environmental performance (Peng and Lin 2009 ). Chang and Hao ( 2017 ) also confirm that environmental performance positively interacts with economic growth in OECD and non-OECD countries.
However, when output and consumption increase, we are most likely to observe cost imposed on the environment, which, by implication, increased the consumption of non-renewable resources to increase pollution levels. Ardakani and Seyedaliakbar ( 2019 ) and Xie and Liu ( 2019 ) sustain this claim and argue that economic growth below the turning point could cause more carbon emissions, but economic growth crosses the turning point, and then environmental quality is achieved or improve. Although economic growth enables inhabitants to maintain a higher life expectancy and increase enrolment to school, this impact may not hold volume to contain the pollution (Cracolici et al. 2010 ). Wang et al. ( 2019 ) find that investment and economic growth jointly contribute to environmental quality. The authors suggest emission mitigation policies that encompass the efficient use of energy, clean technology investment, and promotion of labour standards will cut down the rising emissions.
Similarly, Shahbaz et al. ( 2013 ) confirm economic growth as the major contributor to CO 2 emission. The authors suggest a reduction at the cost of economic growth and financing the importation of environmentally friendly technologies. More so, the impact of economic growth may differ according to region. In China’s central and western region, Chen et al. ( 2019a , b ) suggest that economic growth increases CO2 emissions when it is below a threshold level but reduces CO 2 emission when the economic growth rises above a threshold. Adedoyin et al. ( 2020a , b ) demonstrate that the BRICS economic’ CO 2 emissions are aggravated by economic growth.
Internalization and the environment
In the quest to grow the economic base, nations are pushed to embrace foreign investment and trade. Through foreign direct investment, a country can improve technological progress as well as promote human capital. Trade helps achieve an efficiency of production by allocating resources in areas where a country has a comparative advantage. However, the effect of both foreign direct investment and trade on the environment has not always been straightforward. Existing studies provide two contrasting findings on the impact of internationalization on the environment. Following the pollution haven hypothesis (Walter and Ugelow 1979 ), critics of internationalization argue that foreign investment and trade serve as a channel for transferring pollution-intensive operations from one country to another. Hence, foreign investment and trade are associated with the poor environmental quality of the host country while lowering pollution (Beradovic 2009 ). Haug and Ucal ( 2019 ) and Salahuddin et al. ( 2018 ) validate this claim and report that the underlying effect of foreign direct investment on carbon emission is positive and implies that an increase in FDI leads to a deteriorating environment. However, the long-run effect shows no significant relationship between FDI and carbon emissions (Ayamba et al. 2020 ). Meanwhile, trade is found to be asymmetrically correlated with carbon emission; a rise in export causes an increase in carbon emission, while a rise in import reduces carbon emission in Turkey. The reduction in CO 2 emissions could have been caused by the transfer of highly emission-intensive production capacities from developed economies to low economies (Essandoh et al. 2020 ).
The pollution haven hypothesis is directly opposite to the pollution halo hypothesis, which states that internationalization facilitates the transfer of technology and acceptable practices from one country to another, particularly from developed to developing. Hence, foreign investment and trade improve the environmental quality of the host country (Birdsall and Wheeler 1993 ). There is a considerable amount of literature on which empirical evidence supports this hypothesis (Ayamba et al. 2019 ; Cole and Elliott 2003 ; Mihci et al. 2005 ; Pao and Tsai 2011 ; Romer 1993 , Zhu et al. 2016 ). Mihci et al. ( 2005 ) argue that international investment and trade help fill the technology gap among countries. Shahbaz et al. ( 2019 ) argue that allowing free trading in and out of the country can improve environmental quality, but an increase in foreign investment is detrimental to a greener environment. The author suggests investment in technological innovation, capital stock, and the implementation of stringent environmental policies that will re-direct FDI towards green investment.
Furthermore, trade openness and FDI has pushed for healthy economic development, but not without contributing to pollution. However, Muhammad et al. ( 2020 ) provide evidence of the positive impact of import and export on the environment. Bopkin ( 2017 ) suggests that FDI can positively impact the environment if there are quality institutions to monitor foreign investors.
Energy-related factors and the environment
High energy consumption is associated with low environmental quality (Bilgen 2014 ; Dincer 1998 ; Bailis et al. 2005 ; Fotis and Polemis 2018 ). Because energy consumption is inevitable in daily life, there is a strong argument for a different mix of energy sources of which renewable energy source has been considered the best alternative. Nonetheless, the empirical evidence on the impact of renewable energy on the environment remains very controversial, although it is perceived by many as a direct positive effect. Saidi and Omri ( 2020 ), for instance, claim that in order not to cause harm to the economy while promoting environmental quality, the private sector, such as industries, has to imbibe the use of nuclear and renewable energy during production. Equally, investment in human capital could cut-down emissions (Wang et al. 2020 ). Mendonca et al. ( 2020 ) confirm similar results in the 50 largest economies across the globe. According to Ikram et al. ( 2020 ), following the international standard’s issuance that specifies requirements for an effective environmental management system known as ISO 14001 has added a tally to factor helping to sustain the environment.
On the negative side, Jebli and Youssef ( 2017 ) argue that renewable energy consumption could raise CO 2 emissions because combustible and wastes renewable energy are highly pollution-intensive energy sources. Hence, carbon emissions are likely to increase. Furthermore, renewable energy alone as an indicator may not effectively reduce the CO 2 emissions until a fully renewable energy system is implemented, and then renewable energy will cut-down emissions (Pata 2018 ; Mitchell and Cleveland 1993 ). Kahouli ( 2018 ) reports that increasing renewable energy consumption could increase energy-intensive economic activities.
Institutional quality and the environment.
The institutional quality, which includes the quality of laws and the strength of enforcement agents can either promote or retard green growth. A well-established institutional quality promotes economic activities while reducing carbon emissions (Salman et al. 2019 ). Specifically, political-institutional quality provides all the social, governance, and economic readiness to cut down carbon emissions (Sarkodie and Adams 2018 ). High institutional quality is more beneficial in improving a sustainable environment through the effect of trade than in low institutional quality (Ibrahim and Law 2016 ). Quality institutions also ensure that firms are complying with environmental regulations.
However, a strong enforcement environment with ever-changing regulations will likely hamper the growth of firms, especially foreign business flow. There could be less innovation and creativity towards improving the environment (Nguyen et al. 2018 ). It should be noted that the evidence on the negative impact of institutional quality is fragile. Therefore, Abid ( 2017 ) suggests that some parts of institutional quality, such as government effectiveness and democracy, deteriorate environmental quality while regulatory quality and the rule of law increase sustainability. Democratic institution paves the way for foreign investment at the expense of the environment. The fact is that foreign investors tried to avoid environmental laws; thus, they invest in the economy where such policies are not in place and thereby raise the pollution (Kinda 2011 ).
Previous studies have been conducted linking economic growth, development, renewable energy, foreign direct investment, and trade on the environment. However, the previous failed to account for the green growth determinant. Therefore, we fill this gap by investigating the determinants of green growth across a panel of 123 countries.
Developed and developing countries
Given the difference between developed and developing countries regarding economic development and environmental degradation (De Angelis et al. 2019 ; Shahbaz et al. 2019 ), it is likely that their journey to green growth can be different. For example, whereas developing countries have a high pollution rate, developed countries are the largest contributors to CO 2 emission but at a decreasing rate (De Angelis et al. 2019 ). Shahbaz et al. ( 2019 ) argue that the developed countries such as the US maintained policies within “scale effect” to reduce carbon emissions. This is done through investment in technological innovation, addressing capital consumption, and providing informed knowledge about trade liberalization has helped reduce CO 2 emissions. Likewise, in Western Europe, CO 2 emissions are well managed because of the enforcement of policies targeted at improving economic growth and environmental protection simultaneously (Paramati et al. 2017 ), which is not the case of Eastern Europe, which is made of developing countries. In Eastern Europe, CO 2 emissions continue to surge beyond because the nation’s intent is directed towards tourism to grow its agenda for employment generation, income, and economic development without considering the environment (Paramati et al. 2017 ). This similar feature of most developing countries where there are weak institutions to enforce environmental laws.
In developing countries, Aye and Edoja ( 2017 ) found that economic growth has a negative impact on carbon emission in developing countries. However, Iwata et al. ( 2012 ) did not find any significant relationship between economic growth and carbon emission in developed countries. But the authors found a positive impact of energy consumption on carbon emission. Regarding the impact of foreign direct investment and trade, prior studies have found different results for developed and developing countries. Demena and Afesorgbor ( 2020 ) report that foreign direct investment reduces CO 2, but the result is stronger in developed countries than in developing countries. However, Khan et al. ( 2020 ) find FDI to negatively affect the environment in developing countries. Tang ( 2015 ) also reports a negative impact of FDI on the environment. Similarly, international trade has a negative impact on developing countries (Tawiah et al. 2021 ).
Data and methods
Data and sample selection.
The sample selection begins with all 134 countries listed in the OECD database. We drop six countries that have missing data for more than 3 years on green growth. Next, we drop five countries with missing data from World Development Indicators. Our final sample covers a large panel of data of 123 developed and developing countries over 18 years (2000–2017). We begin in 2000 and end in 2017 because the OCED data on green growth is limited to only this period.
Measurement of variables
- Green growth
According to the OECD Statistic ( 2020 ), green growth is the measure of efficient use of natural capital. Green growth indicates whether economic growth is becoming greener. It captures aspects of production which are rarely quantified in economic models and accounting frameworks. Green growth is measured by the environmental and resource productivity of a country. The higher the value, the more the country’s economic growth is becoming greener. Data is sourced from OECD statistics.
Economic factors
These factors include the general economic activities of a country. We use GDP per capita to measure the effect of economic development on green growth. Similarly, we use annualized GDP growth to measure the impact of economic growth . Both variables are collected from World Development Indicators.
Internationalization factors
These determinants include trade openness and foreign direct investment. These factors are used to examine how foreign activities drive green growth. Trade openness is measured by the sum of imports and export as a percentage of GDP. Foreign direct investment is measured by the net inflow of foreign direct investment as a percentage of GDP. Following the pollution haven and pollution halo hypothesis, we expect the relationship between internationalization factors and green growth to go either way. Data on import, export, and foreign direct investment are sourced from World Development Indicators.
Institutional quality factors
Although there are different measurements of the institutional quality of a country including, the legal origin, bureaucratic quality, the Worldwide Governance Indicators (WGI) by Kaufmann and Kraay ( 2018 ) is the most widely used proxy for institutional quality (Elamer et al. 2020 ; Konara and Shirodkar 2018 ; Tunyi et al. 2020 ). Therefore, we use the six WGI indicators to measure the institutional quality of a country. These six indicators are government effectiveness, political stability, absence of violence, regulatory quality, the rule of law, voice and accountability, and control of corruption. According to Kaufman and Kraay ( 2018 ), these indicators cover institutional quality and governance’s core areas. Although each indicator covers different aspects of institutional quality, including all the six indicators in a single model as individual variables will create multicollinearity due to the high correlation (seen Table 9 of Appendix 2). To capture all the six indicators without the problem of multicollinearity, we follow prior studies such as Elamer et al., (2020), Konara and Shirodkar ( 2018 ), and Tunyi et al. ( 2020 ) and use the principal component analysis (PCA) technique to construct a single composite index from all the six indicators. The PCA statistics presented in Table 10 of Appendix 2 show that the first principal component explains about 86.65% of the variance among the components, whereas the other five components altogether capture less than 2% of the variance. Consequently, we use only the first principal component to predict the sample countries’ institutional quality index. We find our constructed index to be positively and significantly correlated with the average of the six indicators.
Energy-related factors
This group of determinants is related to the type and level of energy consumption in the country. It includes energy consumption measured by energy use and the type of energy consumption measured by renewable energy . Data on energy-related variables are collected from World Development Indicators.
Control factors
We control for other factors that are likely to influence green growth. We use a log population and population growth to control the effect of growing human activities on green growth. A large population exerts an enormous influence on the environment (Aller et al. 2015 ); therefore, we expect a positive relationship between population and green growth. We also use the Forest area as a percentage of the total land area to control the effect of a green environment on green growth. Arguably, countries with large forest areas are most likely to have access to a large pool of greener resources and increase green growth. Natural resource extraction has been found to have negatively affected the environment (Bokpin 2017 ; López 1994 ); hence we use resource rent to control for the effect of continuous extraction of natural resources on green growth.
Pre-regression and econometric modelling
We perform different pre-regression tests to determine the appropriate estimate technique for the analysis. First, we test the correlation among the independent and control variables. Table 2 contains the result of the Pearson pairwise correlation matrix between the variables. The results show precursory evidence that selected variables are good for controlling for relevant factors that may influence domestic credit. However, none of the variables possesses any threat of multicollinearity, because all the coefficient is less than the standard threshold (Field 2000 ; Tabachnick and Fidell 2013 ). Next to minimize potential misspecification, we perform the Hausman test to choose between random effect and fixed effect estimation technique. The Hausman test results suggest that effects are not independent; hence, the use of the fixed effect is appropriate as opposed to the random effect.
Having established the data’s appropriateness for ordinary least square modelling, we fit our baseline as follows:
where Determinants take on economic factors , internationalization factors , institutional quality factor , or energy-related factor respective equations. it represent country and time respectively, and ε it is the associated error. All variables are defined in Tables 1 and 2 .
Results, discussion, and implications for policy
Descriptive statistics.
The descriptive statistics, including the mean, 25 th percentile, median 95 th percentile, and the standard deviation of all the variables, are presented in Table 3 . The mean of green growth is 5.977, with a large standard deviation of 4.058, indicating different stages of green growth among the sample size. The mean of economic growth of 3.930, which is lower than the mean of green growth, shows that environmental and resource productivity increases even when general economic growth is low. We observe large variations across almost all the independent variables, suggesting that these variables could explain the green growth variations. The number of observations (N) is not the same for all variables, resulting in unbalanced data in the estimations.
Main results
The fixed effect ordinary least square estimations are presented in Table 4 . To mitigate potential bias results, the country-fixed effect is included in all the models. The results of economic determinants are presented in column 1. The economic development proxy coefficient by GDP per capita is positive and significant at 1%, indicating a positive relationship between economic development and green growth. However, the coefficient of economic growth is not significant. Therefore, our results in column 1 imply that high economic development promotes sustainable environmental and economic growth. The results are consistent with the assumption that countries with high GDP per capita have the resources to provide incentives towards a greener environment.
In column 2, we regress the internationalization factors, namely, trade openness and foreign direct investment on green growth. The results show that trade openness has a negative and significant association with green growth, which is in line with the findings of Alola et al. ( 2019a , b ), whereas foreign direct investment also has a negative but insignificant relationship. These results suggest that countries that engage in more international business are more likely to experience a decline in green growth. The result is consistent with the pollution haven hypothesis, which states that the flow of international business deteriorates the environmental quality of the host country because of the transfer of pollution-intensive operations from one country to the other.
As presented in column 3 of Table 4 , the result of institutional quality is insignificant, suggesting that the level of institutional quality is not a significant determinant of green growth. This is probably because most countries still do not have specific regulations on the sustainable use of natural assets. This is because most regulations on environmental protection are viewed to be counterproductive to economic growth and development (De Angelis et al. 2019 ; Wolde-Rufael and Weldemeskel 2020 )
Column 4 contains the results of energy consumption as a determinant of green growth. As expected, high energy consumption is associated with a decrease in green growth. The coefficient of energy consumption (−0.000337***) is negative and highly significant, indicating an inverse relationship between the use of energy and green growth. However, the positive coefficient of renewable energy (0.117***) at a 1% significance level suggests that the type of energy matters in attaining green growth. More specifically, the results show that renewable energy consumption leads to an increase in green growth. Arguably, renewable energy sources make efficient and effective use of natural assets in general production and consumption than any other energy source.
Finally, in column 5, we combine all the factors, including the control variables, into a single equation. The results are not qualitatively different from the individual equations, confirming the results presented in columns 1–4. We find that both economic development and trade openness are larger in the combined equation than the individual equations.
Collectively, the results presented in Table 4 show that internationalization factors such as trade openness derive down green growth, while economic development is associated with increased green growth. Energy consumption has a negative impact on green growth; however, renewable energy consumption increases green growth.
The results of most control variables meet the standard assumption. For example, the population and extraction of natural resources are associated with a decrease in green growth. In contrast, countries with large forest areas experience green growth. The large and consistent R -squared across all the models signals how well the selected variables explain green growth variations.
To check the sanity of our results, we present the economic significance of the determinants that are statistically significant in Table 4 . The results in Table 5 show that all the statistically significant variables are also economically significant. For instance, the results in column 1 show that an increase in economic development leads to an increase in green growth by 0.0709. However, an increase in trade openness leads to a decrease in green growth by 0.017. Similar energy consumption decreases green growth by 0.172, but renewable energy consumption increases green growth by 0.538. The economic significance is calculated as (coefficient*standard deviation)/mean of green growth.
Given that our sample size is large, it is likely that our results could be driven by a particular set of countries, especially developed or developing countries. The level of environmental pollution differs between developed and developing. Whereas developing countries have a high pollution rate, developed countries are the largest contributors to CO 2 emission but at a decreasing rate (De Angelis et al. 2019 ). Furthermore, developed countries have high economic development but slow economic growth compared to the high economic growth but low economic development of developing countries. What is more, our large sample size contains 89 developing countries and 34 developed countries; hence, developing countries can drive our main results. Consequently, in this section, we use sub-sampling estimation techniques to check whether the results on green growth determinants differ between developed and developing countries.
The results which are presented in Table 6 reveal interesting and contrasting findings between developed countries and developing countries. First, on the economic determinants, economic development is positive and significant for both developed and developing countries, which is consistent with the full sample in Table 4 . However, economic growth is positive and significant for developed countries (Alola et al. 2019a , b ) but is insignificant for developing countries. This contrasting result suggests that developing countries that are growing fast are over-utilizing their natural assets, but developed countries experiencing fast economic growth are efficient in managing their natural assets towards green growth. This is consistent with Shahbaz et al. ( 2019 ) and Akadiri et al. ( 2019 ) findings that developed countries incorporate efficient technical process in their growth to reduce carbon emissions.
Second, on internationalization factors, the results show that both trade openness and foreign direct investment do not have a significant relationship with green growth in developed countries. In sharp contrast, the coefficient of both trade openness and foreign direct investment is negative and significant in column 7, indicating that internationalization is detrimental to green growth in developing countries. This negative result leans support to the pollution haven hypothesis that most international businesses in developing countries are pollution-intensive, as such foreign direct investment and trade reduce green growth in developing. These results are consistent with Khan et al. ( 2020 ) and Tawiah et al. ( 2021 ).
Third the results of institutional quality and energy-related factors are similar for both developed and developing countries and consistent with the main results as presented in Table 4 . There is an inverse relationship between energy consumption and green growth, but the consumption of renewable energy increases green growth in all countries regardless of whether it is classified as developed or developing.
In sum, the results in Table 6 highlight that the economic and internationalization determinants of green growth differ between developed and developing countries, but the relationship between green growth and energy consumption is similar for both sets of countries.
Accounting for the level of CO 2 emission
Although green growth may appear synonymous with CO 2 , they are quite different. CO 2 emission measures the environmental footprint, while green growth measures the action a country is taken to achieve growth and development, which is environmentally and economically sustainable. In other words, green growth involves reducing environmental footprint. Therefore, it is more likely that countries with high CO2 emissions have more incentive to engage in green growth due to the inherent obligation of solving the environmental problem of which they are the contributors. Arguably high CO 2 -emitting countries like Australia, China, the USA, and India have more incentives to increase green growth than countries like Portugal, Spain, Lao, and Angola, which have low emissions. Consequently, in this section, we test if the CO2 emission of the country drives our results.
The results are presented in Table 7 . We begin by establishing the relationship between CO2 emission and green growth without the determinants but including the control variables. The results presented in column 1 show that CO 2 emission has a negative effect on green growth. Next, we include all the determinants for the full sample in column 2, developed countries in column 3, and developing countries in column 4. The coefficient of CO 2 emission remains negative and significant for the full sample but insignificant for both developed and developing countries, suggesting that CO2 emission is less likely to be a significant determinant of green growth based on country classification. The other determinants remain qualitatively similar to the main results, which confirm our estimation’s robustness after accounting for the level of CO 2 emission.
Robustness check
In this section, we use the System Generalized Method of Movement (S-GMM) to test the robustness of the results to potential endogeneity problems. The S-GMM estimator can control for the presence of unobserved country-specific effects. The S-GMM estimation technique also has the advantage of controlling for or a simultaneity bias caused by the potential endogeneity of the explanatory variables (Arellano and Bond 1991 ).
The results of the S-GMM estimations are presented in Table 8 . The full sample results are presented in column 1, whereas those of developed and developing countries are presented in columns 2 and 3, respectively. The results are qualitatively similar to those in the main result of Tables 4 and 6 ; hence our results are not sensitive to potential endogeneity problems.
Conclusion and policy implications
This study investigates the determinants of green growth in 123 developing and developed countries listed in the OECD database between 2000 and 2017. Findings from the study show that high economic development promotes sustainable development and economic growth, which is in line with the assumption that countries with high GDP per capita possess adequate resources to support green growth incentives. This implies the need to adopt economic development policies, which leads to increased GDP since it is a determinant of achieving green growth goals and sustainability goals.
On the other hand, trade openness was found to exert a negative and significant relationship with green growth and foreign direct investments having a negative but insignificant relationship with green growth. This implies that increased international activities such as trade and foreign investments may deter or slow countries’ green goals. Therefore, it is important to ensure prompt restrictions and monitoring of foreign investments and movements to ensure the economic growth effect of FDI and trade is accompanied by environmental quality. The government and regulatory agencies of developed and particularly developing countries should be deliberate in their sustainability goals to ensure green growth. Meanwhile, the institutional quality is an insignificant determinant of green growth, i.e., considering the role of government and institutional policies in the success of economic goals such as green growth, the quality of environmental institutions in determining the green growth achievement is insignificant. Findings further show that high energy consumption leads to a decrease in green growth; however, renewable energy use improves green growth. This shows that despite energy consumption deterring green growth, renewable energy dominating the energy mix contributes positively to green growth and environmental sustainability.
In additional analyses, using the sub-sampling technique, we find that our results are sensitive to countries been classified as developed or developing countries. For instance, the economic development of green growth for both developed and developing countries is positive and significant. In contrast, economic growth is positive and significant for developed countries but insignificant for developing countries. On the other hand, the internationalization determinants of green growth, trade openness, and FDI relationship with green growth are insignificant for developed countries. The effect of trade openness and FDI on developing countries is negative and significant, causing FDI and trade openness to be detrimental to green growth. However, the effect of institutional quality in determining green growth increase is insignificant for both developed and developing countries.
Given our findings, we recommend maintaining a high level of GDP per capita to attain green growth through the purchase of resources tantamount to green growth. According to the IMF report (2019), trade and technology are famous for promoting GDP growth and GDP per capita. Hence, countries can gear up their policies towards the promotion of GDP per capita through technological innovations.
Second, government and policymakers should carefully choose international business policies because the foreign direct investment is often not targeted towards green growth. For instance, international business contract host countries often are at a disadvantage because of the transfer of pollution-intensive operations from one country to the other, thereby creating pollution. Against this background, we suggest the employment of minimal foreign investment with strict supervision in order not to give room for exploitation by foreign counterparts.
Third, renewable energy sources make efficient and effective use of natural assets in general production and consumption than any other energy source. As such, it is more likely to promote green growth than other sources of energy. For these reasons, we encourage governments and policymakers to promote renewable energy by offering grants and loans to investors in those areas. Also, a tax holiday could be another option to promote investment in renewable energy. With that, more users are likely to increase, thereby lowing the level of CO2 emissions and, consequently, achieving green growth.
Our results are not only statistically significant; they are also economically significant in explaining the variations in green growth across the world. Further, our results are robust to alternative identification, including System Generalised Method of Movements, which mitigate potential endogeneity.
Availability of data and materials
The data is accessible from the author upon request.
All over the world, many countries have recorded greater warming in their regions, with about half of the world populace experiencing warm temperatures of above 1.5°C in at least one period or the other (IPCC 2018 ).
Abid M (2017) Does economic, financial, and institutional developments matter for environmental quality? A comparative analysis of EU and MEA countries. J Environ Manag 188:183–194
Google Scholar
Adedoyin FF, Bekun FV, Alola AA (2020a) Growth impact of transition from non-renewable to renewable energy in the EU: the role of research and development expenditure. Renew, Energ
Adedoyin FF, Gumede MI, Bekun FV, Etokakpan MU, Balsalobre-lorente D (2020b) Modelling coal rent, economic growth, and CO2 emissions: does regulatory quality matter in BRICS economies? Sci Total Environ 710:136284
CAS Google Scholar
Akadiri SS, Alola AA, Akadiri AC, Alola UV (2019) Renewable energy consumption in EU-28 countries: policy toward pollution mitigation and economic sustainability. Energy Policy 132:803–810. https://doi.org/10.1016/j.enpol.2019.06.040
Article Google Scholar
Ardakani MK, Seyedaliakbar SM (2019) Impact of energy consumption and economic growth on CO2 emission using multivariate regression. Energ Strat Rev 26:100428
Alam MS, Kabir N (2013) Economic growth and environmental sustainability: empirical evidence from East and South-East Asia. Int J Econs Fin 5
Aller C, Ductor L, Herrerias MJ (2015) The world trade network and the environment. Energ Econs 52:55–68
Alola AA, Bekun FV, Sarkodie SA (2019a) Dynamic impact of trade policy, economic growth, fertility rate, renewable and non-renewable energy consumption on ecological footprint in Europe. Sci Total Environ 685:702–709. https://doi.org/10.1016/j.scitotenv.2019.05.139
Article CAS Google Scholar
Alola AA, Yalçiner K, Alola UV, Akadiri SS (2019b) The role of renewable energy, immigration and real income in environmental sustainability target. Evidence from Europe largest states. Sci Total Environ 674:307–315. https://doi.org/10.1016/j.scitotenv.2019.04.163
Arellano M, Bond S (1991) Some tests of specification for panel data: Monte Carlo evidence with an application for employment equation. Rev Econ Stud 58:277–297
Ayamba EC, Haibo C, Abdul-Rahaman A-R, Serwaa OE, Osei-Agyemang A (2020) The impact of foreign direct investment on sustainable development in China. Environ Sci Pollut Res 27(20):25625–25637
Ayamba EC, Haibo C, Ibn Musah A-A, Ruth A, Osei-Agyemang A (2019) An empirical model on the impact of foreign direct investment on China’s environmental pollution: analysis based on simultaneous equations. Environ Sci Pollut Res 26(16):16239–16248
Aye GC, Edoja PE (2017) Effect of economic growth on CO2 emission in developing countries: evidence from a dynamic panel threshold model. Cogent Econ Finance 5(1):1379239
Bailis R, Ezzati M, Kammen DM (2005) The role of technology management in the dynamics of greenhouse gas emissions from household energy use in Sub-Saharan Africa. J Environ Dev 14(1):149–174
Beradovic M (2009) The role of FDI and openness of trade in economic growth: a study of Africa and Asia. Unpublished Bachelor Thesis Submitted to JonkopinIntenational Business School, Jonkopin University
Bilgen S (2014) Structure and environmental impact of global energy consumption. Renew Sust Energ Rev 38:890–902
Birdsall N, Wheeler D (1993) Trade policy and industrial pollution in Latin America: where are the pollution havens? J Environ Dev 2:137–149
Bokpin GA (2017) Foreign direct investment and environmental sustainability in Africa: the role of institutions and governance. Res Int Bus Financ 39:239–247
Chang CP, Hao Y (2017) Environmental performance, corruption, and economic growth: global evidence using a new data set. Appl Econ 49:498–514
Chen Y, Zhao J, Lai Z, Wang Z, Xia H (2019a) Exploring the effects of economic growth, and renewable and non-renewable energy consumption on China's CO2 emissions: evidence from a regional panel analysis. Renew Energy 140:341–353
Chen Q, Löschel A, Pei J, Peters GP, Xue J, Zhao Z (2019b) Processing trade, foreign outsourcing, and carbon emissions in China. Struct Chang Econ Dyn 49:1–12
Cole MA, Elliott RJ (2003) Determining the trade–environment composition effect: the role of capital, labour, and environmental regulations. J Environ Econ Manag 46:363–383
Cracolici MF, Cuffaro M, Nijkamp P (2010) The measurement of economic, social, and environmental performance of countries: a novel approach. Soc Indic Res 95:339–356
De Angelis EM, Di Giacomo M, Vannoni D (2019) Climate change and economic growth: the role of environmental policy stringency. Sustainability 11:2273
Demena BA, Afesorgbor SK (2020) The effect of FDI on environmental emissions: evidence from a meta-analysis. Energy Policy 138:111192
Dincer I (1998) Energy and environmental impacts: present and future perspectives. Energy Sources 20:427–453
Elamer AA, Ntim CG, Abdou HA (2020) Islamic governance, national governance, and bank risk management and disclosure in MENA countries. Bus Soc 59:914–955
Essandoh OK, Islam M, Kakinaka M (2020) Linking international trade and foreign direct investment to CO2 emissions: any differences between developed and developing countries? SciTotal Environ 712:136437
Field AP (2000) Discovering statistics using SPSS for windows: advanced techniques for the beginner. SAGE.
Fotis P, Polemis M (2018) Sustainable development, environmental policy, and renewable energy use: a dynamic panel data approach. Sustain Dev 26(6):726–740
Khan A, Hussain J, Bano S, Chenggang Y (2020) The repercussions of foreign direct investment, renewable energy, and health expenditure on environmental decay? An econometric analysis of B&RI countries. J Environ Plan Manag 63:1965–1986
Haug AA, Ucal M (2019) The role of trade and FDI for CO2 emissions in Turkey: nonlinear relationships. Energ Econs 81:297–307
Ibrahim MH, Law SH (2016) Institutional quality and co2 emission–trade relations: evidence from sub-S Saharan Africa. South Afri J Econs 84:323–340
Ikram M, Zhang Q, Sroufe R, Shah SZA (2020) Towards a sustainable environment: the nexus between ISO 14001, renewable energy consumption, access to electricity, agriculture, and CO2 emissions in SAARC countries. Sust. Prod, Cons
IPCC (2018) Global warming of 1.5°C. An IPCC Special Report on the impacts of global warming of 1.5°C above pre-industrial levels and related global greenhouse gas emission pathways in the context of strengthening the global response to the threat of climate change. s.l.:In Press.
Iwata H, Okada K, Samreth S (2012) Empirical study on the determinants of CO2 emissions: evidence from OECD countries. Appl Econ 44(27):3513–3519
Jebli MB, Youssef SB (2017) The role of renewable energy and agriculture in reducing CO2 emissions: evidence for North Africa countries. Ecol Indic 74:295–301
Kahouli B (2018) The causality link between energy electricity consumption, CO2 emissions, R&D stocks, and economic growth in Mediterranean countries (MCs). Energy 145:388–399
Kaufmann D, Kraay A (2018) The worldwide governance indicators. The World Bank. https://info.worldbank.org/governance/wgi/
Kinda S (2011) Democratic institutions and environmental quality: effects and transmission channels. Available at SSRN 2714300
Konara P, Shirodkar V (2018) Regulatory institutional distance and MNCs’ subsidiary performance: climbing up vs. climbing down the institutional ladder. J Int Manag 24:333–347
López R (1994) The environment as a factor of production: the effects of economic growth and trade liberalization. J Environ Econ Manag 27:163–184
Mihci H, Cagatay S, Koska O (2005) The impact of environmental stringency on the foreign direct investments of the OECD countries. J Environ Assessment Policy Manage 7:679–704
Mikayilov JI, Galeotti M, Hasanov FJ (2018) The impact of economic growth on CO2 emissions in Azerbaijan. J Clean Prod 197:1558–1572. https://doi.org/10.1016/j.jclepro.2018.06.269
Mitchell C, Cleveland CJ (1993) Resource scarcity, energy use, and environmental impact: a case study of the New Bedford, Massachusetts, USA, fisheries. Environ Manag 17(3):305–317
Mendonça AK de S, de Andrade Conradi Barni G, Moro MF, Bornia AC, Kupek E, Fernandes L (2020) Hierarchical modeling of the 50 largest economies to verify the impact of GDP, population and renewable energy generation in CO2 emissions. Sustainable Production and Consumption 22:58–67. https://doi.org/10.1016/j.spc.2020.02.001
Muhammad B, Khan S (2019) Effect of bilateral FDI, energy consumption, CO2 emission, and capital on economic growth of Asia countries. Energy Rep 5:1305–1315
Muhammad S, Long X, Salman M, Dauda L (2020) Effect of urbanization and international trade on CO2 emissions across 65 belt and road initiative countries. Energy 196:117102. https://doi.org/10.1016/j.energy.2020.117102
Nguyen CP, Nguyen NA, Schinckus C, Su TD (2018) The ambivalent role of institutions in the CO2 emissions: the case of emerging countries. Int J Energ Econs Policy 8:7
OECD (2020) OECD work on green growth. https://issuu.com/oecd.publishing/docs/gg_brochure_2019_web
OECD (2011) Tackling climate change and growing the economy. Growth (Lakeland) 2050
OECD (2018) What is green growth, and how can it help deliver sustainable development?. http://www.oecd.org/greengrowth/whatisgreengrowthandhowcanithelpdeliversustainabledevelopment.htm
Pao HT, Tsai CM (2011) Multivariate Granger causality between CO2 emissions, energy consumption, FDI (foreign direct investment), and GDP (gross domestic product): evidence from a panel of BRIC (Brazil, Russian Federation, India, and China) countries. Energy 36:685–693
Paramati SR, Shahbaz M, Alam MS (2017) Does tourism degrade environmental quality? A comparative study of Eastern and Western European Union. Transp Res Part D: Transp Environ 50:1–13
Pata UK (2018) Renewable energy consumption, urbanization, financial development, income, and CO2 emissions in Turkey: testing EKC hypothesis with structural breaks. J Clean Prod 187:770–779
Peng YS, Lin SS (2009) National culture, economic development, population growth, and environmental performance: the mediating role of education. J Bus Ethics 90:203–219
Rahman MM, Saidi K, Mbarek MB (2020) Economic growth in South Asia: the role of CO2 emissions, population density, and trade openness. Heliyon 6:03903
Romer P (1993) Idea gaps and object gaps in economic development. J Mon Econs 32:543–573
Salahuddin M, Alam K, Ozturk I, Sohag K (2018) The effects of electricity consumption, economic growth, financial development, and foreign direct investment on CO2 emissions in Kuwait. Renew Sust Energ Rev 81:2002–2010
Salman M, Long X, Dauda L, Mensah CN (2019) The impact of institutional quality on economic growth and carbon emissions: evidence from Indonesia, South Korea, and Thailand. J Clean Prod 241:118331
Sarkodie SA, Adams S (2018) Renewable energy, nuclear energy, and environmental pollution: accounting for political, institutional quality in South Africa. Sci Total Environ 643:1590–1601
Saidi K, Omri A (2020) Reducing CO2 emissions in OECD countries: do renewable and nuclear energy matter? Prog Nucl Energy 126:103425
Shahbaz M, Gozgor G, Adom PK, Hammoudeh S (2019) The technical decomposition of carbon emissions and the concerns about FDI and trade openness effects in the United States. Int Econ 159:56–73
Shahbaz M, Ozturk I, Afza T, Ali A (2013) Revisiting the environmental Kuznets curve in a global economy. Renew Sust Energ Rev 25:494–502. https://doi.org/10.1016/j.rser.2013.05.021
Tabachnick BG, Fidell LS (2013) Using Multivariate Statistics. Pearson Education.
Tang J (2015) Testing the Pollution Haven Effect: Does the Type of FDI Matter? Environ Resour Econ 60(4):549–578. https://doi.org/10.1007/s10640-014-9779-7
Tawiah VK, Zakari A, Khan I (2021) The environmental footprint of China-Africa engagement: an analysis of the effect of China – Africa partnership on carbon emissions. Sci Total Environ 756:143603
The Washington Post (2017) Giant, 10-day-long UN conference on sustainable development gets underway in Rio de Janeiro. http://www.washingtonpost.com/world/the_americas/giant-10-day-long-un-conference-on-sustainable-development-gets-underway-in-rio-de-janeiro/2012/06/13/gJQAbPGVaV_story.html
Tunyi AA, Ehalaiye D, Gyapong E, Ntim CG (2020) The value of discretion in africa: evidence from acquired intangible assets under IFRS 3. Int J Account 2050008
Walter I, Ugelow JL (1979) Environmental policies in developing countries. Ambio:102–109
Wang R, Mirza N, Vasbieva DG, Abbas Q, Xiong D (2020) The nexus of carbon emissions, financial development, renewable energy consumption, and technological innovation: what should be the priorities in light of COP 21 Agreements? J Environ Manag 271:111027
Wang Q, Jiang XT, Ge S, Jiang R (2019) Is economic growth compatible with a reduction in CO2 emissions? Empirical analysis of the United States. Resour Conserv Recycl 151:104443
Wolde-Rufael Y, Weldemeskel EM (2020) Environmental policy stringency, renewable energy consumption, and CO2 emissions: panel cointegration analysis for BRIICTS countries. Int J Green Energ 17:568–582
Xie Q, Liu J (2019) Combined nonlinear effects of economic growth and urbanization on CO2 emissions in China: evidence from a panel data partially linear additive model. Energy 186:115868
Zhu H, Duan L, Guo Y, Yu K (2016) The effects of FDI, economic growth, and energy consumption on carbon emissions in ASEAN-5: evidence from panel quantile regression. Econ Model 58:237–248
Download references
Author information
Authors and affiliations.
DCU Business School, Dublin City University, Dublin, Ireland
Vincent Tawiah
Center for Energy and Environmental Policy Research, School of Management and Economics, Beijing Institute of Technology, Beijing, China
Abdulrasheed Zakari
Alma Mater Europaea ECM, Maribor, Slovenia
Department of Computing and Informatics, Bournemouth University, Poole, UK
Festus Fatai Adedoyin
You can also search for this author in PubMed Google Scholar
Contributions
Vincent Tawiah: idea conceptualization and data analysis. Abdulrasheed Zakari: literature review and conclusion. Festus Fatai Adedoyin: introduction and editing.
Corresponding author
Correspondence to Festus Fatai Adedoyin .
Ethics declarations
Ethics approval.
Not applicable
Consent to participate
Consent for publication.
The author has provided consent to publish this work is accepted.
Competing interests
The author declares no competing interests.
Additional information
Responsible editor: Nicholas Apergis
Publisher’s note
Springer Nature remains neutral with regard to jurisdictional claims in published maps and institutional affiliations.
Appendix 1. List of countries
Albania | Canada | Georgia | Kuwait | Nigeria | Spain |
---|---|---|---|---|---|
Algeria | Chile | Germany | Kyrgyz Republic | Norway | Sri Lanka |
Angola | China | Ghana | Latvia | Oman | Suriname |
Argentina | Colombia | Greece | Lebanon | Pakistan | Sweden |
Armenia | Congo, Dem. Rep. | Guatemala | Lithuania | Panama | Switzerland |
Australia | Congo, Rep. | Honduras | Luxembourg | Paraguay | Tanzania |
Austria | Costa Rica | Hungary | Malaysia | Peru | Thailand |
Azerbaijan | Cote d'Ivoire | Iceland | Malta | Philippines | Togo |
Bahrain | Croatia | India | Mauritius | Poland | Trinidad and Tobago |
Bangladesh | Cyprus | Indonesia | Mexico | Portugal | Tunisia |
Belarus | Czech Republic | Iran, Islamic Rep. | Moldova | Qatar | Ukraine |
Belgium | Denmark | Iraq | Mongolia | Romania | United Arab Emirates |
Benin | Dominica | Ireland | Morocco | Russian Federation | UK |
Bolivia | Ecuador | Israel | Mozambique | Saudi Arabia | USA |
Bosnia and Herzegovina | Egypt, Arab Rep. | Italy | Myanmar | Senegal | Uruguay |
Botswana | El Salvador | Jamaica | Namibia | Serbia | Uzbekistan |
Brazil | Estonia | Japan | Netherlands | Singapore | Vietnam |
Brunei Darussalam | Ethiopia | Jordan | New Zealand | Slovak Republic | Yemen, Rep. |
Bulgaria | Finland | Kazakhstan | Nicaragua | Slovenia | Zambia |
Cambodia | France | Kenya | Niger | South Africa | Zimbabwe |
Cameroon | Gabon | Korea, Rep. |
Appendix 2. PCA statistics
Rights and permissions.
Open Access This article is licensed under a Creative Commons Attribution 4.0 International License, which permits use, sharing, adaptation, distribution and reproduction in any medium or format, as long as you give appropriate credit to the original author(s) and the source, provide a link to the Creative Commons licence, and indicate if changes were made. The images or other third party material in this article are included in the article's Creative Commons licence, unless indicated otherwise in a credit line to the material. If material is not included in the article's Creative Commons licence and your intended use is not permitted by statutory regulation or exceeds the permitted use, you will need to obtain permission directly from the copyright holder. To view a copy of this licence, visit http://creativecommons.org/licenses/by/4.0/ .
Reprints and permissions
About this article
Tawiah, V., Zakari, A. & Adedoyin, F.F. Determinants of green growth in developed and developing countries. Environ Sci Pollut Res 28 , 39227–39242 (2021). https://doi.org/10.1007/s11356-021-13429-0
Download citation
Received : 09 February 2021
Accepted : 09 March 2021
Published : 22 March 2021
Issue Date : August 2021
DOI : https://doi.org/10.1007/s11356-021-13429-0
Share this article
Anyone you share the following link with will be able to read this content:
Sorry, a shareable link is not currently available for this article.
Provided by the Springer Nature SharedIt content-sharing initiative
- Energy consumption
- CO 2 emissions
- Environmental quality
- Find a journal
- Publish with us
- Track your research
Green growth -A synthesis of scientific findings
- September 2019
- Technological Forecasting and Social Change 146:390-402
- CC BY-NC-ND 4.0

- Nordic Institute for Studies in Innovation, Research and Education

- University of Copenhagen

- Eawag: Das Wasserforschungs-Institut des ETH-Bereichs

Abstract and Figures

Discover the world's research
- 25+ million members
- 160+ million publication pages
- 2.3+ billion citations
- POL J ENVIRON STUD
- Xuheng Wang

- Bui Huy Nhuong
- Ho Dinh Bao
- Le Thanh Ha
- NORSK GEOGR TIDSSKR

- Nghia Thi Minh Luu

- PAP REG SCI

- Alessandra Faggian

- Martina Fuchs

- Xue-Chao Wang

- Guoliang Zheng

- Stine Hach Juul Madsen

- AM J AGR ECON
- Kenneth E. Boulding
- Richard R. Nelson
- Sidney G. Winter
- Recruit researchers
- Join for free
- Login Email Tip: Most researchers use their institutional email address as their ResearchGate login Password Forgot password? Keep me logged in Log in or Continue with Google Welcome back! Please log in. Email · Hint Tip: Most researchers use their institutional email address as their ResearchGate login Password Forgot password? Keep me logged in Log in or Continue with Google No account? Sign up
Information
- Author Services
Initiatives
You are accessing a machine-readable page. In order to be human-readable, please install an RSS reader.
All articles published by MDPI are made immediately available worldwide under an open access license. No special permission is required to reuse all or part of the article published by MDPI, including figures and tables. For articles published under an open access Creative Common CC BY license, any part of the article may be reused without permission provided that the original article is clearly cited. For more information, please refer to https://www.mdpi.com/openaccess .
Feature papers represent the most advanced research with significant potential for high impact in the field. A Feature Paper should be a substantial original Article that involves several techniques or approaches, provides an outlook for future research directions and describes possible research applications.
Feature papers are submitted upon individual invitation or recommendation by the scientific editors and must receive positive feedback from the reviewers.
Editor’s Choice articles are based on recommendations by the scientific editors of MDPI journals from around the world. Editors select a small number of articles recently published in the journal that they believe will be particularly interesting to readers, or important in the respective research area. The aim is to provide a snapshot of some of the most exciting work published in the various research areas of the journal.
Original Submission Date Received: .
- Active Journals
- Find a Journal
- Journal Proposal
- Proceedings Series
- For Authors
- For Reviewers
- For Editors
- For Librarians
- For Publishers
- For Societies
- For Conference Organizers
- Open Access Policy
- Institutional Open Access Program
- Special Issues Guidelines
- Editorial Process
- Research and Publication Ethics
- Article Processing Charges
- Testimonials
- Preprints.org
- SciProfiles
- Encyclopedia
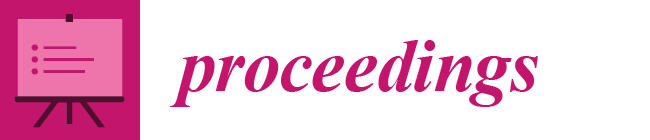
Article Menu
- Subscribe SciFeed
- Google Scholar
- on Google Scholar
- Table of Contents
Find support for a specific problem in the support section of our website.
Please let us know what you think of our products and services.
Visit our dedicated information section to learn more about MDPI.
JSmol Viewer
Green economy and green growth—opportunities for sustainable development †.
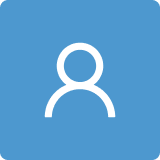
1. Introduction
2. the european framework of the green economy, 3. the national framework of the green economy: actualities and perspectives, 4. final conclusions.
- Kahle, L.R.; Gurel-Atay, E. Communicating Sustainability for the Green Economy , 1st ed.; Sharpe, M.E., Ed.; Routledge: New York, NY, USA, 2014; pp. 22–24. [ Google Scholar ]
- Grima, S. Eleftherios Thalassinos. In Contemporarry Issues in Business and Financial Management in Eastern Europe ; Contemporary Studies in Economic and Financial Analysis; Emerald Publishing House: Bingley, UK, 2018; Volume 100, pp. 1–141. [ Google Scholar ]
- Loiseau, E.; Saikku, L.; Antikainen, R.; Droste, N.; Hansjürgens, B.; Pitkänen, K.; Leskinen, P.; Kuikman, P.; Thomsen, M. Green Economy and Related Concepts: An Overview. J. Clean. Prod. 2016 , 139 , 361–363. [ Google Scholar ] [ CrossRef ]
- Barbier, E.; Markandya, A. A New Blueprint for a Green Economy ; Routledge: New York, NY, USA, 2013; pp. 141–145. [ Google Scholar ]
- Pollin, R.; Garrett-Peltier, H.; Heintz, J.; Hendricks, B. Green Growth: A U.S. Program for Controlling Climate Change and Expanding Job Opportunities, Center for American Progress ; Center for American Progress and Political Economy Research Institute, University of Massachusetts: Amherst, MA, USA, 2014; p. 2. [ Google Scholar ]
- Albekov, A.U.; Parkhomenko, T.V.; Polubotko, A.A. Green Economy: A Phenomenon of Progress and a Concept of Environmental Security ; Contemporary Studies in Economic and Financial Analysis; Emerald Publishing Limited: Bingley, UK, 2018; Volume 100, pp. 51–59. [ Google Scholar ]
- Scott, M. Green Growth ‘Tigers’ Can Help The Global Economy To Roar Once More ; Forbes Media LLC: New York, NY, USA, 2020; pp. 3–5. [ Google Scholar ]
- Galli, A.; Wackernagel, M.; Iha, K.; Lazarus, E. Ecological Footprint: Implications for Biodiversity ; Biological Conservation; Elsevier: Amsterdam, The Netherlands, 2014; Volume 173, pp. 121–132. [ Google Scholar ]
- Bina, O. The Green Economy and Sustainable Development: An Uneasy Balance? Environment and Planning C: Politics and Space. SAGE J. 2013 . [ Google Scholar ] [ CrossRef ] [ Green Version ]
- Vinuesa, R.; Azizpour, H.; Leite, I.; Balaam, M.; Dignum, V.; Domisch, S.; Felländer, A.; Langhans, S.D.; Tegmark, M.; Nerini, F.F. The role of artificial intelligence in achieving the Sustainable Development Goals. Nat. Commun. 2020 , 11 , 233. [ Google Scholar ] [ CrossRef ] [ PubMed ] [ Green Version ]
- Kajcsa, A. The Influence of Compliance with Environmental Requirements on Commercial Competition. Curentul Jurid. Jurid. Curr. Courant Jurid. 2011 , 44 , 143–150. [ Google Scholar ]
- Dalhammar, C. Promoting Energy and Resource Efficiency through the Eco-Design Directive ; Scandinavian Studies in Law: Stockholm, Sweden, 2014; pp. 162–176. [ Google Scholar ]
- Stahel, W.R. The Performance Economy , 2nd ed.; Palgrave MacMillan: London, UK, 2010; 350p. [ Google Scholar ]
- Howels, G.; Ramsay, I.; Wilhelmsson, T. Handbook of Research on International Consumer Law , 2nd ed.; Edward Elgar Publishing: Cheltenham, UK, 2016; pp. 7–12. [ Google Scholar ]
- Sharma, H.B.; Vanapalli, K.R.; Cheela, V.S.; Ranjan, V.P.; Jaglan, A.K.; Dubey, B.; Goel, S.; Bhattacharya, J. Challenges, Opportunities, And Innovations For Effective Solid Waste Management During And Post COVID-19 Pandemic ; PMC Elsevier Public Health Emergency Collection: Amsterdam, The Netherlands, 2020; Volume 162. [ Google Scholar ] [ CrossRef ]
- Diab, F.A.; Molinari, C. Interdisciplinarity: Practical approach to advancing education for sustainability and for the Sustainable Development Goals. Int. J. Educ. Manag. 2017 , 15 , 73–83. [ Google Scholar ] [ CrossRef ] [ Green Version ]
MDPI stays neutral with regard to jurisdictional claims in published maps and institutional affiliations. |
Share and Cite
Dogaru, L. Green Economy and Green Growth—Opportunities for Sustainable Development. Proceedings 2020 , 63 , 70. https://doi.org/10.3390/proceedings2020063070
Dogaru L. Green Economy and Green Growth—Opportunities for Sustainable Development. Proceedings . 2020; 63(1):70. https://doi.org/10.3390/proceedings2020063070
Dogaru, Lucreția. 2020. "Green Economy and Green Growth—Opportunities for Sustainable Development" Proceedings 63, no. 1: 70. https://doi.org/10.3390/proceedings2020063070
Article Metrics
Article access statistics, further information, mdpi initiatives, follow mdpi.
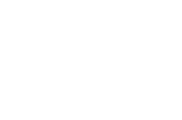
Subscribe to receive issue release notifications and newsletters from MDPI journals
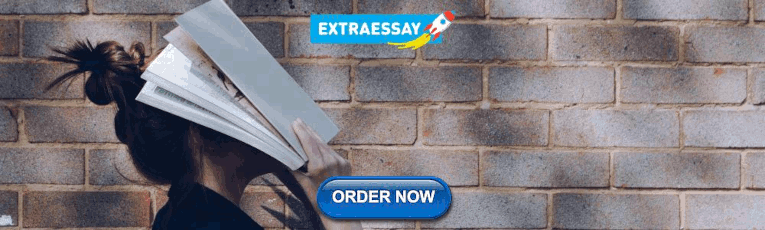
IMAGES
COMMENTS
This thesis aims at providing new policy-relevant understanding of how green growth climate mitigation policies can contribute towards rapid decarbonisation. The specific policies assessed in this thesis include green fiscal stimulus in the Republic of Korea, public financing for the development of low-carbon energy technologies in the Nordic
This paper aims at clarifying these concepts in an analytical framework and at proposing foundations for green growth, starting from and building on the long tradition of adapting growth theory to include the environment (e.g., Nordhaus, 1972; Dasgupta and Heal, 1974; Solow, 1974).
Based on our readings, the review highlights two aspects of green growth that any future research on the topic should not neglect: the different geographies involved in the triggering and diffusing green growth, and the intertwining of economic, social and political challenges which green transformative innovation entails.
In this study, we propose a new set of green growth indicators that is founded on a thorough assessment of a wider range of factors contributing to green growth.
The primary objective of this paper is to investigate the extent to which climate change adaptation and green technology diffusion serve as key drivers for green growth. Additionally, the study examines the influence of various economic, environmental, and social factors on green growth.
Considering the need for environmental sustainability while ensuring economic growth and development by 2030, this study uses data on 123 developed and developing countries to examine factors that influence green growth. The empirical results show that economic development positively influences green growth.
Governments in countries across the world increasingly adopt the “green growth” discourse to underline their ambition for the greening of their economies. The central tenet of this narrative ...
understand whether there is economic legitimacy to the notion of green growth, or whether it is an aspirational goal—something desirable from an environmental and ethical point of view, but unachievable given competing economic needs. So we examine green growth using the standard tools
Subsequently, we examine the main lines of research used by proponents of green growth to support their viewpoint, including theoretical arguments, exercises with integrated assessment models, and studies of the environmental Kuznets curve hypothesis.
In view of such issues, this paper seeks to provide some answers to the conceptual interrelationships between the green economy, green growth and sustainable development, through a harmonious blend with developments in the green economy at international, European and national levels.