Jump to navigation

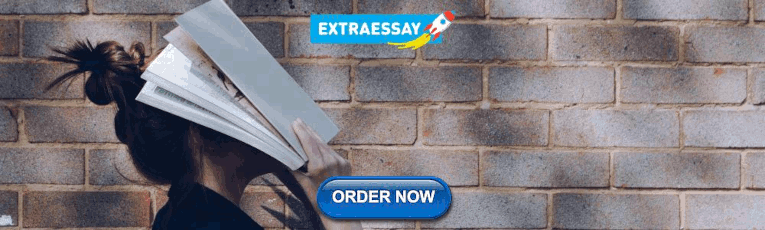
Cochrane Training
Chapter 5: collecting data.
Tianjing Li, Julian PT Higgins, Jonathan J Deeks
Key Points:
- Systematic reviews have studies, rather than reports, as the unit of interest, and so multiple reports of the same study need to be identified and linked together before or after data extraction.
- Because of the increasing availability of data sources (e.g. trials registers, regulatory documents, clinical study reports), review authors should decide on which sources may contain the most useful information for the review, and have a plan to resolve discrepancies if information is inconsistent across sources.
- Review authors are encouraged to develop outlines of tables and figures that will appear in the review to facilitate the design of data collection forms. The key to successful data collection is to construct easy-to-use forms and collect sufficient and unambiguous data that faithfully represent the source in a structured and organized manner.
- Effort should be made to identify data needed for meta-analyses, which often need to be calculated or converted from data reported in diverse formats.
- Data should be collected and archived in a form that allows future access and data sharing.
Cite this chapter as: Li T, Higgins JPT, Deeks JJ (editors). Chapter 5: Collecting data. In: Higgins JPT, Thomas J, Chandler J, Cumpston M, Li T, Page MJ, Welch VA (editors). Cochrane Handbook for Systematic Reviews of Interventions version 6.4 (updated August 2023). Cochrane, 2023. Available from www.training.cochrane.org/handbook .
5.1 Introduction
Systematic reviews aim to identify all studies that are relevant to their research questions and to synthesize data about the design, risk of bias, and results of those studies. Consequently, the findings of a systematic review depend critically on decisions relating to which data from these studies are presented and analysed. Data collected for systematic reviews should be accurate, complete, and accessible for future updates of the review and for data sharing. Methods used for these decisions must be transparent; they should be chosen to minimize biases and human error. Here we describe approaches that should be used in systematic reviews for collecting data, including extraction of data directly from journal articles and other reports of studies.
5.2 Sources of data
Studies are reported in a range of sources which are detailed later. As discussed in Section 5.2.1 , it is important to link together multiple reports of the same study. The relative strengths and weaknesses of each type of source are discussed in Section 5.2.2 . For guidance on searching for and selecting reports of studies, refer to Chapter 4 .
Journal articles are the source of the majority of data included in systematic reviews. Note that a study can be reported in multiple journal articles, each focusing on some aspect of the study (e.g. design, main results, and other results).
Conference abstracts are commonly available. However, the information presented in conference abstracts is highly variable in reliability, accuracy, and level of detail (Li et al 2017).
Errata and letters can be important sources of information about studies, including critical weaknesses and retractions, and review authors should examine these if they are identified (see MECIR Box 5.2.a ).
Trials registers (e.g. ClinicalTrials.gov) catalogue trials that have been planned or started, and have become an important data source for identifying trials, for comparing published outcomes and results with those planned, and for obtaining efficacy and safety data that are not available elsewhere (Ross et al 2009, Jones et al 2015, Baudard et al 2017).
Clinical study reports (CSRs) contain unabridged and comprehensive descriptions of the clinical problem, design, conduct and results of clinical trials, following a structure and content guidance prescribed by the International Conference on Harmonisation (ICH 1995). To obtain marketing approval of drugs and biologics for a specific indication, pharmaceutical companies submit CSRs and other required materials to regulatory authorities. Because CSRs also incorporate tables and figures, with appendices containing the protocol, statistical analysis plan, sample case report forms, and patient data listings (including narratives of all serious adverse events), they can be thousands of pages in length. CSRs often contain more data about trial methods and results than any other single data source (Mayo-Wilson et al 2018). CSRs are often difficult to access, and are usually not publicly available. Review authors could request CSRs from the European Medicines Agency (Davis and Miller 2017). The US Food and Drug and Administration had historically avoided releasing CSRs but launched a pilot programme in 2018 whereby selected portions of CSRs for new drug applications were posted on the agency’s website. Many CSRs are obtained through unsealed litigation documents, repositories (e.g. clinicalstudydatarequest.com ), and other open data and data-sharing channels (e.g. The Yale University Open Data Access Project) (Doshi et al 2013, Wieland et al 2014, Mayo-Wilson et al 2018)).
Regulatory reviews such as those available from the US Food and Drug Administration or European Medicines Agency provide useful information about trials of drugs, biologics, and medical devices submitted by manufacturers for marketing approval (Turner 2013). These documents are summaries of CSRs and related documents, prepared by agency staff as part of the process of approving the products for marketing, after reanalysing the original trial data. Regulatory reviews often are available only for the first approved use of an intervention and not for later applications (although review authors may request those documents, which are usually brief). Using regulatory reviews from the US Food and Drug Administration as an example, drug approval packages are available on the agency’s website for drugs approved since 1997 (Turner 2013); for drugs approved before 1997, information must be requested through a freedom of information request. The drug approval packages contain various documents: approval letter(s), medical review(s), chemistry review(s), clinical pharmacology review(s), and statistical reviews(s).
Individual participant data (IPD) are usually sought directly from the researchers responsible for the study, or may be identified from open data repositories (e.g. www.clinicalstudydatarequest.com ). These data typically include variables that represent the characteristics of each participant, intervention (or exposure) group, prognostic factors, and measurements of outcomes (Stewart et al 2015). Access to IPD has the advantage of allowing review authors to reanalyse the data flexibly, in accordance with the preferred analysis methods outlined in the protocol, and can reduce the variation in analysis methods across studies included in the review. IPD reviews are addressed in detail in Chapter 26 .
MECIR Box 5.2.a Relevant expectations for conduct of intervention reviews
5.2.1 Studies (not reports) as the unit of interest
In a systematic review, studies rather than reports of studies are the principal unit of interest. Since a study may have been reported in several sources, a comprehensive search for studies for the review may identify many reports from a potentially relevant study (Mayo-Wilson et al 2017a, Mayo-Wilson et al 2018). Conversely, a report may describe more than one study.
Multiple reports of the same study should be linked together (see MECIR Box 5.2.b ). Some authors prefer to link reports before they collect data, and collect data from across the reports onto a single form. Other authors prefer to collect data from each report and then link together the collected data across reports. Either strategy may be appropriate, depending on the nature of the reports at hand. It may not be clear that two reports relate to the same study until data collection has commenced. Although sometimes there is a single report for each study, it should never be assumed that this is the case.
MECIR Box 5.2.b Relevant expectations for conduct of intervention reviews
It can be difficult to link multiple reports from the same study, and review authors may need to do some ‘detective work’. Multiple sources about the same trial may not reference each other, do not share common authors (Gøtzsche 1989, Tramèr et al 1997), or report discrepant information about the study design, characteristics, outcomes, and results (von Elm et al 2004, Mayo-Wilson et al 2017a).
Some of the most useful criteria for linking reports are:
- trial registration numbers;
- authors’ names;
- sponsor for the study and sponsor identifiers (e.g. grant or contract numbers);
- location and setting (particularly if institutions, such as hospitals, are named);
- specific details of the interventions (e.g. dose, frequency);
- numbers of participants and baseline data; and
- date and duration of the study (which also can clarify whether different sample sizes are due to different periods of recruitment), length of follow-up, or subgroups selected to address secondary goals.
Review authors should use as many trial characteristics as possible to link multiple reports. When uncertainties remain after considering these and other factors, it may be necessary to correspond with the study authors or sponsors for confirmation.
5.2.2 Determining which sources might be most useful
A comprehensive search to identify all eligible studies from all possible sources is resource-intensive but necessary for a high-quality systematic review (see Chapter 4 ). Because some data sources are more useful than others (Mayo-Wilson et al 2018), review authors should consider which data sources may be available and which may contain the most useful information for the review. These considerations should be described in the protocol. Table 5.2.a summarizes the strengths and limitations of different data sources (Mayo-Wilson et al 2018). Gaining access to CSRs and IPD often takes a long time. Review authors should begin searching repositories and contact trial investigators and sponsors as early as possible to negotiate data usage agreements (Mayo-Wilson et al 2015, Mayo-Wilson et al 2018).
Table 5.2.a Strengths and limitations of different data sources for systematic reviews
5.2.3 Correspondence with investigators
Review authors often find that they are unable to obtain all the information they seek from available reports about the details of the study design, the full range of outcomes measured and the numerical results. In such circumstances, authors are strongly encouraged to contact the original investigators (see MECIR Box 5.2.c ). Contact details of study authors, when not available from the study reports, often can be obtained from more recent publications, from university or institutional staff listings, from membership directories of professional societies, or by a general search of the web. If the contact author named in the study report cannot be contacted or does not respond, it is worthwhile attempting to contact other authors.
Review authors should consider the nature of the information they require and make their request accordingly. For descriptive information about the conduct of the trial, it may be most appropriate to ask open-ended questions (e.g. how was the allocation process conducted, or how were missing data handled?). If specific numerical data are required, it may be more helpful to request them specifically, possibly providing a short data collection form (either uncompleted or partially completed). If IPD are required, they should be specifically requested (see also Chapter 26 ). In some cases, study investigators may find it more convenient to provide IPD rather than conduct additional analyses to obtain the specific statistics requested.
MECIR Box 5.2.c Relevant expectations for conduct of intervention reviews
5.3 What data to collect
5.3.1 what are data.
For the purposes of this chapter, we define ‘data’ to be any information about (or derived from) a study, including details of methods, participants, setting, context, interventions, outcomes, results, publications, and investigators. Review authors should plan in advance what data will be required for their systematic review, and develop a strategy for obtaining them (see MECIR Box 5.3.a ). The involvement of consumers and other stakeholders can be helpful in ensuring that the categories of data collected are sufficiently aligned with the needs of review users ( Chapter 1, Section 1.3 ). The data to be sought should be described in the protocol, with consideration wherever possible of the issues raised in the rest of this chapter.
The data collected for a review should adequately describe the included studies, support the construction of tables and figures, facilitate the risk of bias assessment, and enable syntheses and meta-analyses. Review authors should familiarize themselves with reporting guidelines for systematic reviews (see online Chapter III and the PRISMA statement; (Liberati et al 2009) to ensure that relevant elements and sections are incorporated. The following sections review the types of information that should be sought, and these are summarized in Table 5.3.a (Li et al 2015).
MECIR Box 5.3.a Relevant expectations for conduct of intervention reviews
Table 5.3.a Checklist of items to consider in data collection
*Full description required for assessments of risk of bias (see Chapter 8 , Chapter 23 and Chapter 25 ).
5.3.2 Study methods and potential sources of bias
Different research methods can influence study outcomes by introducing different biases into results. Important study design characteristics should be collected to allow the selection of appropriate methods for assessment and analysis, and to enable description of the design of each included study in a table of ‘Characteristics of included studies’, including whether the study is randomized, whether the study has a cluster or crossover design, and the duration of the study. If the review includes non-randomized studies, appropriate features of the studies should be described (see Chapter 24 ).
Detailed information should be collected to facilitate assessment of the risk of bias in each included study. Risk-of-bias assessment should be conducted using the tool most appropriate for the design of each study, and the information required to complete the assessment will depend on the tool. Randomized studies should be assessed using the tool described in Chapter 8 . The tool covers bias arising from the randomization process, due to deviations from intended interventions, due to missing outcome data, in measurement of the outcome, and in selection of the reported result. For each item in the tool, a description of what happened in the study is required, which may include verbatim quotes from study reports. Information for assessment of bias due to missing outcome data and selection of the reported result may be most conveniently collected alongside information on outcomes and results. Chapter 7 (Section 7.3.1) discusses some issues in the collection of information for assessments of risk of bias. For non-randomized studies, the most appropriate tool is described in Chapter 25 . A separate tool also covers bias due to missing results in meta-analysis (see Chapter 13 ).
A particularly important piece of information is the funding source of the study and potential conflicts of interest of the study authors.
Some review authors will wish to collect additional information on study characteristics that bear on the quality of the study’s conduct but that may not lead directly to risk of bias, such as whether ethical approval was obtained and whether a sample size calculation was performed a priori.
5.3.3 Participants and setting
Details of participants are collected to enable an understanding of the comparability of, and differences between, the participants within and between included studies, and to allow assessment of how directly or completely the participants in the included studies reflect the original review question.
Typically, aspects that should be collected are those that could (or are believed to) affect presence or magnitude of an intervention effect and those that could help review users assess applicability to populations beyond the review. For example, if the review authors suspect important differences in intervention effect between different socio-economic groups, this information should be collected. If intervention effects are thought constant over such groups, and if such information would not be useful to help apply results, it should not be collected. Participant characteristics that are often useful for assessing applicability include age and sex. Summary information about these should always be collected unless they are not obvious from the context. These characteristics are likely to be presented in different formats (e.g. ages as means or medians, with standard deviations or ranges; sex as percentages or counts for the whole study or for each intervention group separately). Review authors should seek consistent quantities where possible, and decide whether it is more relevant to summarize characteristics for the study as a whole or by intervention group. It may not be possible to select the most consistent statistics until data collection is complete across all or most included studies. Other characteristics that are sometimes important include ethnicity, socio-demographic details (e.g. education level) and the presence of comorbid conditions. Clinical characteristics relevant to the review question (e.g. glucose level for reviews on diabetes) also are important for understanding the severity or stage of the disease.
Diagnostic criteria that were used to define the condition of interest can be a particularly important source of diversity across studies and should be collected. For example, in a review of drug therapy for congestive heart failure, it is important to know how the definition and severity of heart failure was determined in each study (e.g. systolic or diastolic dysfunction, severe systolic dysfunction with ejection fractions below 20%). Similarly, in a review of antihypertensive therapy, it is important to describe baseline levels of blood pressure of participants.
If the settings of studies may influence intervention effects or applicability, then information on these should be collected. Typical settings of healthcare intervention studies include acute care hospitals, emergency facilities, general practice, and extended care facilities such as nursing homes, offices, schools, and communities. Sometimes studies are conducted in different geographical regions with important differences that could affect delivery of an intervention and its outcomes, such as cultural characteristics, economic context, or rural versus city settings. Timing of the study may be associated with important technology differences or trends over time. If such information is important for the interpretation of the review, it should be collected.
Important characteristics of the participants in each included study should be summarized for the reader in the table of ‘Characteristics of included studies’.
5.3.4 Interventions
Details of all experimental and comparator interventions of relevance to the review should be collected. Again, details are required for aspects that could affect the presence or magnitude of an effect or that could help review users assess applicability to their own circumstances. Where feasible, information should be sought (and presented in the review) that is sufficient for replication of the interventions under study. This includes any co-interventions administered as part of the study, and applies similarly to comparators such as ‘usual care’. Review authors may need to request missing information from study authors.
The Template for Intervention Description and Replication (TIDieR) provides a comprehensive framework for full description of interventions and has been proposed for use in systematic reviews as well as reports of primary studies (Hoffmann et al 2014). The checklist includes descriptions of:
- the rationale for the intervention and how it is expected to work;
- any documentation that instructs the recipient on the intervention;
- what the providers do to deliver the intervention (procedures and processes);
- who provides the intervention (including their skill level), how (e.g. face to face, web-based) and in what setting (e.g. home, school, or hospital);
- the timing and intensity;
- whether any variation is permitted or expected, and whether modifications were actually made; and
- any strategies used to ensure or assess fidelity or adherence to the intervention, and the extent to which the intervention was delivered as planned.
For clinical trials of pharmacological interventions, key information to collect will often include routes of delivery (e.g. oral or intravenous delivery), doses (e.g. amount or intensity of each treatment, frequency of delivery), timing (e.g. within 24 hours of diagnosis), and length of treatment. For other interventions, such as those that evaluate psychotherapy, behavioural and educational approaches, or healthcare delivery strategies, the amount of information required to characterize the intervention will typically be greater, including information about multiple elements of the intervention, who delivered it, and the format and timing of delivery. Chapter 17 provides further information on how to manage intervention complexity, and how the intervention Complexity Assessment Tool (iCAT) can facilitate data collection (Lewin et al 2017).
Important characteristics of the interventions in each included study should be summarized for the reader in the table of ‘Characteristics of included studies’. Additional tables or diagrams such as logic models ( Chapter 2, Section 2.5.1 ) can assist descriptions of multi-component interventions so that review users can better assess review applicability to their context.
5.3.4.1 Integrity of interventions
The degree to which specified procedures or components of the intervention are implemented as planned can have important consequences for the findings from a study. We describe this as intervention integrity ; related terms include adherence, compliance and fidelity (Carroll et al 2007). The verification of intervention integrity may be particularly important in reviews of non-pharmacological trials such as behavioural interventions and complex interventions, which are often implemented in conditions that present numerous obstacles to idealized delivery.
It is generally expected that reports of randomized trials provide detailed accounts of intervention implementation (Zwarenstein et al 2008, Moher et al 2010). In assessing whether interventions were implemented as planned, review authors should bear in mind that some interventions are standardized (with no deviations permitted in the intervention protocol), whereas others explicitly allow a degree of tailoring (Zwarenstein et al 2008). In addition, the growing field of implementation science has led to an increased awareness of the impact of setting and context on delivery of interventions (Damschroder et al 2009). (See Chapter 17, Section 17.1.2.1 for further information and discussion about how an intervention may be tailored to local conditions in order to preserve its integrity.)
Information about integrity can help determine whether unpromising results are due to a poorly conceptualized intervention or to an incomplete delivery of the prescribed components. It can also reveal important information about the feasibility of implementing a given intervention in real life settings. If it is difficult to achieve full implementation in practice, the intervention will have low feasibility (Dusenbury et al 2003).
Whether a lack of intervention integrity leads to a risk of bias in the estimate of its effect depends on whether review authors and users are interested in the effect of assignment to intervention or the effect of adhering to intervention, as discussed in more detail in Chapter 8, Section 8.2.2 . Assessment of deviations from intended interventions is important for assessing risk of bias in the latter, but not the former (see Chapter 8, Section 8.4 ), but both may be of interest to decision makers in different ways.
An example of a Cochrane Review evaluating intervention integrity is provided by a review of smoking cessation in pregnancy (Chamberlain et al 2017). The authors found that process evaluation of the intervention occurred in only some trials and that the implementation was less than ideal in others, including some of the largest trials. The review highlighted how the transfer of an intervention from one setting to another may reduce its effectiveness when elements are changed, or aspects of the materials are culturally inappropriate.
5.3.4.2 Process evaluations
Process evaluations seek to evaluate the process (and mechanisms) between the intervention’s intended implementation and the actual effect on the outcome (Moore et al 2015). Process evaluation studies are characterized by a flexible approach to data collection and the use of numerous methods to generate a range of different types of data, encompassing both quantitative and qualitative methods. Guidance for including process evaluations in systematic reviews is provided in Chapter 21 . When it is considered important, review authors should aim to collect information on whether the trial accounted for, or measured, key process factors and whether the trials that thoroughly addressed integrity showed a greater impact. Process evaluations can be a useful source of factors that potentially influence the effectiveness of an intervention.
5.3.5 Outcome s
An outcome is an event or a measurement value observed or recorded for a particular person or intervention unit in a study during or following an intervention, and that is used to assess the efficacy and safety of the studied intervention (Meinert 2012). Review authors should indicate in advance whether they plan to collect information about all outcomes measured in a study or only those outcomes of (pre-specified) interest in the review. Research has shown that trials addressing the same condition and intervention seldom agree on which outcomes are the most important, and consequently report on numerous different outcomes (Dwan et al 2014, Ismail et al 2014, Denniston et al 2015, Saldanha et al 2017a). The selection of outcomes across systematic reviews of the same condition is also inconsistent (Page et al 2014, Saldanha et al 2014, Saldanha et al 2016, Liu et al 2017). Outcomes used in trials and in systematic reviews of the same condition have limited overlap (Saldanha et al 2017a, Saldanha et al 2017b).
We recommend that only the outcomes defined in the protocol be described in detail. However, a complete list of the names of all outcomes measured may allow a more detailed assessment of the risk of bias due to missing outcome data (see Chapter 13 ).
Review authors should collect all five elements of an outcome (Zarin et al 2011, Saldanha et al 2014):
1. outcome domain or title (e.g. anxiety);
2. measurement tool or instrument (including definition of clinical outcomes or endpoints); for a scale, name of the scale (e.g. the Hamilton Anxiety Rating Scale), upper and lower limits, and whether a high or low score is favourable, definitions of any thresholds if appropriate;
3. specific metric used to characterize each participant’s results (e.g. post-intervention anxiety, or change in anxiety from baseline to a post-intervention time point, or post-intervention presence of anxiety (yes/no));
4. method of aggregation (e.g. mean and standard deviation of anxiety scores in each group, or proportion of people with anxiety);
5. timing of outcome measurements (e.g. assessments at end of eight-week intervention period, events occurring during eight-week intervention period).
Further considerations for economics outcomes are discussed in Chapter 20 , and for patient-reported outcomes in Chapter 18 .
5.3.5.1 Adverse effects
Collection of information about the harmful effects of an intervention can pose particular difficulties, discussed in detail in Chapter 19 . These outcomes may be described using multiple terms, including ‘adverse event’, ‘adverse effect’, ‘adverse drug reaction’, ‘side effect’ and ‘complication’. Many of these terminologies are used interchangeably in the literature, although some are technically different. Harms might additionally be interpreted to include undesirable changes in other outcomes measured during a study, such as a decrease in quality of life where an improvement may have been anticipated.
In clinical trials, adverse events can be collected either systematically or non-systematically. Systematic collection refers to collecting adverse events in the same manner for each participant using defined methods such as a questionnaire or a laboratory test. For systematically collected outcomes representing harm, data can be collected by review authors in the same way as efficacy outcomes (see Section 5.3.5 ).
Non-systematic collection refers to collection of information on adverse events using methods such as open-ended questions (e.g. ‘Have you noticed any symptoms since your last visit?’), or reported by participants spontaneously. In either case, adverse events may be selectively reported based on their severity, and whether the participant suspected that the effect may have been caused by the intervention, which could lead to bias in the available data. Unfortunately, most adverse events are collected non-systematically rather than systematically, creating a challenge for review authors. The following pieces of information are useful and worth collecting (Nicole Fusco, personal communication):
- any coding system or standard medical terminology used (e.g. COSTART, MedDRA), including version number;
- name of the adverse events (e.g. dizziness);
- reported intensity of the adverse event (e.g. mild, moderate, severe);
- whether the trial investigators categorized the adverse event as ‘serious’;
- whether the trial investigators identified the adverse event as being related to the intervention;
- time point (most commonly measured as a count over the duration of the study);
- any reported methods for how adverse events were selected for inclusion in the publication (e.g. ‘We reported all adverse events that occurred in at least 5% of participants’); and
- associated results.
Different collection methods lead to very different accounting of adverse events (Safer 2002, Bent et al 2006, Ioannidis et al 2006, Carvajal et al 2011, Allen et al 2013). Non-systematic collection methods tend to underestimate how frequently an adverse event occurs. It is particularly problematic when the adverse event of interest to the review is collected systematically in some studies but non-systematically in other studies. Different collection methods introduce an important source of heterogeneity. In addition, when non-systematic adverse events are reported based on quantitative selection criteria (e.g. only adverse events that occurred in at least 5% of participants were included in the publication), use of reported data alone may bias the results of meta-analyses. Review authors should be cautious of (or refrain from) synthesizing adverse events that are collected differently.
Regardless of the collection methods, precise definitions of adverse effect outcomes and their intensity should be recorded, since they may vary between studies. For example, in a review of aspirin and gastrointestinal haemorrhage, some trials simply reported gastrointestinal bleeds, while others reported specific categories of bleeding, such as haematemesis, melaena, and proctorrhagia (Derry and Loke 2000). The definition and reporting of severity of the haemorrhages (e.g. major, severe, requiring hospital admission) also varied considerably among the trials (Zanchetti and Hansson 1999). Moreover, a particular adverse effect may be described or measured in different ways among the studies. For example, the terms ‘tiredness’, ‘fatigue’ or ‘lethargy’ may all be used in reporting of adverse effects. Study authors also may use different thresholds for ‘abnormal’ results (e.g. hypokalaemia diagnosed at a serum potassium concentration of 3.0 mmol/L or 3.5 mmol/L).
No mention of adverse events in trial reports does not necessarily mean that no adverse events occurred. It is usually safest to assume that they were not reported. Quality of life measures are sometimes used as a measure of the participants’ experience during the study, but these are usually general measures that do not look specifically at particular adverse effects of the intervention. While quality of life measures are important and can be used to gauge overall participant well-being, they should not be regarded as substitutes for a detailed evaluation of safety and tolerability.
5.3.6 Results
Results data arise from the measurement or ascertainment of outcomes for individual participants in an intervention study. Results data may be available for each individual in a study (i.e. individual participant data; see Chapter 26 ), or summarized at arm level, or summarized at study level into an intervention effect by comparing two intervention arms. Results data should be collected only for the intervention groups and outcomes specified to be of interest in the protocol (see MECIR Box 5.3.b ). Results for other outcomes should not be collected unless the protocol is modified to add them. Any modification should be reported in the review. However, review authors should be alert to the possibility of important, unexpected findings, particularly serious adverse effects.
MECIR Box 5.3.b Relevant expectations for conduct of intervention reviews
Reports of studies often include several results for the same outcome. For example, different measurement scales might be used, results may be presented separately for different subgroups, and outcomes may have been measured at different follow-up time points. Variation in the results can be very large, depending on which data are selected (Gøtzsche et al 2007, Mayo-Wilson et al 2017a). Review protocols should be as specific as possible about which outcome domains, measurement tools, time points, and summary statistics (e.g. final values versus change from baseline) are to be collected (Mayo-Wilson et al 2017b). A framework should be pre-specified in the protocol to facilitate making choices between multiple eligible measures or results. For example, a hierarchy of preferred measures might be created, or plans articulated to select the result with the median effect size, or to average across all eligible results for a particular outcome domain (see also Chapter 9, Section 9.3.3 ). Any additional decisions or changes to this framework made once the data are collected should be reported in the review as changes to the protocol.
Section 5.6 describes the numbers that will be required to perform meta-analysis, if appropriate. The unit of analysis (e.g. participant, cluster, body part, treatment period) should be recorded for each result when it is not obvious (see Chapter 6, Section 6.2 ). The type of outcome data determines the nature of the numbers that will be sought for each outcome. For example, for a dichotomous (‘yes’ or ‘no’) outcome, the number of participants and the number who experienced the outcome will be sought for each group. It is important to collect the sample size relevant to each result, although this is not always obvious. A flow diagram as recommended in the CONSORT Statement (Moher et al 2001) can help to determine the flow of participants through a study. If one is not available in a published report, review authors can consider drawing one (available from www.consort-statement.org ).
The numbers required for meta-analysis are not always available. Often, other statistics can be collected and converted into the required format. For example, for a continuous outcome, it is usually most convenient to seek the number of participants, the mean and the standard deviation for each intervention group. These are often not available directly, especially the standard deviation. Alternative statistics enable calculation or estimation of the missing standard deviation (such as a standard error, a confidence interval, a test statistic (e.g. from a t-test or F-test) or a P value). These should be extracted if they provide potentially useful information (see MECIR Box 5.3.c ). Details of recalculation are provided in Section 5.6 . Further considerations for dealing with missing data are discussed in Chapter 10, Section 10.12 .
MECIR Box 5.3.c Relevant expectations for conduct of intervention reviews
5.3.7 Other information to collect
We recommend that review authors collect the key conclusions of the included study as reported by its authors. It is not necessary to report these conclusions in the review, but they should be used to verify the results of analyses undertaken by the review authors, particularly in relation to the direction of effect. Further comments by the study authors, for example any explanations they provide for unexpected findings, may be noted. References to other studies that are cited in the study report may be useful, although review authors should be aware of the possibility of citation bias (see Chapter 7, Section 7.2.3.2 ). Documentation of any correspondence with the study authors is important for review transparency.
5.4 Data collection tools
5.4.1 rationale for data collection forms.
Data collection for systematic reviews should be performed using structured data collection forms (see MECIR Box 5.4.a ). These can be paper forms, electronic forms (e.g. Google Form), or commercially or custom-built data systems (e.g. Covidence, EPPI-Reviewer, Systematic Review Data Repository (SRDR)) that allow online form building, data entry by several users, data sharing, and efficient data management (Li et al 2015). All different means of data collection require data collection forms.
MECIR Box 5.4.a Relevant expectations for conduct of intervention reviews
The data collection form is a bridge between what is reported by the original investigators (e.g. in journal articles, abstracts, personal correspondence) and what is ultimately reported by the review authors. The data collection form serves several important functions (Meade and Richardson 1997). First, the form is linked directly to the review question and criteria for assessing eligibility of studies, and provides a clear summary of these that can be used to identify and structure the data to be extracted from study reports. Second, the data collection form is the historical record of the provenance of the data used in the review, as well as the multitude of decisions (and changes to decisions) that occur throughout the review process. Third, the form is the source of data for inclusion in an analysis.
Given the important functions of data collection forms, ample time and thought should be invested in their design. Because each review is different, data collection forms will vary across reviews. However, there are many similarities in the types of information that are important. Thus, forms can be adapted from one review to the next. Although we use the term ‘data collection form’ in the singular, in practice it may be a series of forms used for different purposes: for example, a separate form could be used to assess the eligibility of studies for inclusion in the review to assist in the quick identification of studies to be excluded from or included in the review.
5.4.2 Considerations in selecting data collection tools
The choice of data collection tool is largely dependent on review authors’ preferences, the size of the review, and resources available to the author team. Potential advantages and considerations of selecting one data collection tool over another are outlined in Table 5.4.a (Li et al 2015). A significant advantage that data systems have is in data management ( Chapter 1, Section 1.6 ) and re-use. They make review updates more efficient, and also facilitate methodological research across reviews. Numerous ‘meta-epidemiological’ studies have been carried out using Cochrane Review data, resulting in methodological advances which would not have been possible if thousands of studies had not all been described using the same data structures in the same system.
Some data collection tools facilitate automatic imports of extracted data into RevMan (Cochrane’s authoring tool), such as CSV (Excel) and Covidence. Details available here https://documentation.cochrane.org/revman-kb/populate-study-data-260702462.html
Table 5.4.a Considerations in selecting data collection tools
5.4.3 Design of a data collection form
Regardless of whether data are collected using a paper or electronic form, or a data system, the key to successful data collection is to construct easy-to-use forms and collect sufficient and unambiguous data that faithfully represent the source in a structured and organized manner (Li et al 2015). In most cases, a document format should be developed for the form before building an electronic form or a data system. This can be distributed to others, including programmers and data analysts, and as a guide for creating an electronic form and any guidance or codebook to be used by data extractors. Review authors also should consider compatibility of any electronic form or data system with analytical software, as well as mechanisms for recording, assessing and correcting data entry errors.
Data described in multiple reports (or even within a single report) of a study may not be consistent. Review authors will need to describe how they work with multiple reports in the protocol, for example, by pre-specifying which report will be used when sources contain conflicting data that cannot be resolved by contacting the investigators. Likewise, when there is only one report identified for a study, review authors should specify the section within the report (e.g. abstract, methods, results, tables, and figures) for use in case of inconsistent information.
If review authors wish to automatically import their extracted data into RevMan, it is advised that their data collection forms match the data extraction templates available via the RevMan Knowledge Base. Details available here https://documentation.cochrane.org/revman-kb/data-extraction-templates-260702375.html.
A good data collection form should minimize the need to go back to the source documents. When designing a data collection form, review authors should involve all members of the team, that is, content area experts, authors with experience in systematic review methods and data collection form design, statisticians, and persons who will perform data extraction. Here are suggested steps and some tips for designing a data collection form, based on the informal collation of experiences from numerous review authors (Li et al 2015).
Step 1. Develop outlines of tables and figures expected to appear in the systematic review, considering the comparisons to be made between different interventions within the review, and the various outcomes to be measured. This step will help review authors decide the right amount of data to collect (not too much or too little). Collecting too much information can lead to forms that are longer than original study reports, and can be very wasteful of time. Collection of too little information, or omission of key data, can lead to the need to return to study reports later in the review process.
Step 2. Assemble and group data elements to facilitate form development. Review authors should consult Table 5.3.a , in which the data elements are grouped to facilitate form development and data collection. Note that it may be more efficient to group data elements in the order in which they are usually found in study reports (e.g. starting with reference information, followed by eligibility criteria, intervention description, statistical methods, baseline characteristics and results).
Step 3. Identify the optimal way of framing the data items. Much has been written about how to frame data items for developing robust data collection forms in primary research studies. We summarize a few key points and highlight issues that are pertinent to systematic reviews.
- Ask closed-ended questions (i.e. questions that define a list of permissible responses) as much as possible. Closed-ended questions do not require post hoc coding and provide better control over data quality than open-ended questions. When setting up a closed-ended question, one must anticipate and structure possible responses and include an ‘other, specify’ category because the anticipated list may not be exhaustive. Avoid asking data extractors to summarize data into uncoded text, no matter how short it is.
- Avoid asking a question in a way that the response may be left blank. Include ‘not applicable’, ‘not reported’ and ‘cannot tell’ options as needed. The ‘cannot tell’ option tags uncertain items that may promote review authors to contact study authors for clarification, especially on data items critical to reach conclusions.
- Remember that the form will focus on what is reported in the article rather what has been done in the study. The study report may not fully reflect how the study was actually conducted. For example, a question ‘Did the article report that the participants were masked to the intervention?’ is more appropriate than ‘Were participants masked to the intervention?’
- Where a judgement is required, record the raw data (i.e. quote directly from the source document) used to make the judgement. It is also important to record the source of information collected, including where it was found in a report or whether information was obtained from unpublished sources or personal communications. As much as possible, questions should be asked in a way that minimizes subjective interpretation and judgement to facilitate data comparison and adjudication.
- Incorporate flexibility to allow for variation in how data are reported. It is strongly recommended that outcome data be collected in the format in which they were reported and transformed in a subsequent step if required. Review authors also should consider the software they will use for analysis and for publishing the review (e.g. RevMan).
Step 4. Develop and pilot-test data collection forms, ensuring that they provide data in the right format and structure for subsequent analysis. In addition to data items described in Step 2, data collection forms should record the title of the review as well as the person who is completing the form and the date of completion. Forms occasionally need revision; forms should therefore include the version number and version date to reduce the chances of using an outdated form by mistake. Because a study may be associated with multiple reports, it is important to record the study ID as well as the report ID. Definitions and instructions helpful for answering a question should appear next to the question to improve quality and consistency across data extractors (Stock 1994). Provide space for notes, regardless of whether paper or electronic forms are used.
All data collection forms and data systems should be thoroughly pilot-tested before launch (see MECIR Box 5.4.a ). Testing should involve several people extracting data from at least a few articles. The initial testing focuses on the clarity and completeness of questions. Users of the form may provide feedback that certain coding instructions are confusing or incomplete (e.g. a list of options may not cover all situations). The testing may identify data that are missing from the form, or likely to be superfluous. After initial testing, accuracy of the extracted data should be checked against the source document or verified data to identify problematic areas. It is wise to draft entries for the table of ‘Characteristics of included studies’ and complete a risk of bias assessment ( Chapter 8 ) using these pilot reports to ensure all necessary information is collected. A consensus between review authors may be required before the form is modified to avoid any misunderstandings or later disagreements. It may be necessary to repeat the pilot testing on a new set of reports if major changes are needed after the first pilot test.
Problems with the data collection form may surface after pilot testing has been completed, and the form may need to be revised after data extraction has started. When changes are made to the form or coding instructions, it may be necessary to return to reports that have already undergone data extraction. In some situations, it may be necessary to clarify only coding instructions without modifying the actual data collection form.
5.5 Extracting data from reports
5.5.1 introduction.
In most systematic reviews, the primary source of information about each study is published reports of studies, usually in the form of journal articles. Despite recent developments in machine learning models to automate data extraction in systematic reviews (see Section 5.5.9 ), data extraction is still largely a manual process. Electronic searches for text can provide a useful aid to locating information within a report. Examples include using search facilities in PDF viewers, internet browsers and word processing software. However, text searching should not be considered a replacement for reading the report, since information may be presented using variable terminology and presented in multiple formats.
5.5.2 Who should extract data?
Data extractors should have at least a basic understanding of the topic, and have knowledge of study design, data analysis and statistics. They should pay attention to detail while following instructions on the forms. Because errors that occur at the data extraction stage are rarely detected by peer reviewers, editors, or users of systematic reviews, it is recommended that more than one person extract data from every report to minimize errors and reduce introduction of potential biases by review authors (see MECIR Box 5.5.a ). As a minimum, information that involves subjective interpretation and information that is critical to the interpretation of results (e.g. outcome data) should be extracted independently by at least two people (see MECIR Box 5.5.a ). In common with implementation of the selection process ( Chapter 4, Section 4.6 ), it is preferable that data extractors are from complementary disciplines, for example a methodologist and a topic area specialist. It is important that everyone involved in data extraction has practice using the form and, if the form was designed by someone else, receives appropriate training.
Evidence in support of duplicate data extraction comes from several indirect sources. One study observed that independent data extraction by two authors resulted in fewer errors than data extraction by a single author followed by verification by a second (Buscemi et al 2006). A high prevalence of data extraction errors (errors in 20 out of 34 reviews) has been observed (Jones et al 2005). A further study of data extraction to compute standardized mean differences found that a minimum of seven out of 27 reviews had substantial errors (Gøtzsche et al 2007).
MECIR Box 5.5.a Relevant expectations for conduct of intervention reviews
5.5.3 Training data extractors
Training of data extractors is intended to familiarize them with the review topic and methods, the data collection form or data system, and issues that may arise during data extraction. Results of the pilot testing of the form should prompt discussion among review authors and extractors of ambiguous questions or responses to establish consistency. Training should take place at the onset of the data extraction process and periodically over the course of the project (Li et al 2015). For example, when data related to a single item on the form are present in multiple locations within a report (e.g. abstract, main body of text, tables, and figures) or in several sources (e.g. publications, ClinicalTrials.gov, or CSRs), the development and documentation of instructions to follow an agreed algorithm are critical and should be reinforced during the training sessions.
Some have proposed that some information in a report, such as its authors, be blinded to the review author prior to data extraction and assessment of risk of bias (Jadad et al 1996). However, blinding of review authors to aspects of study reports generally is not recommended for Cochrane Reviews as there is little evidence that it alters the decisions made (Berlin 1997).
5.5.4 Extracting data from multiple reports of the same study
Studies frequently are reported in more than one publication or in more than one source (Tramèr et al 1997, von Elm et al 2004). A single source rarely provides complete information about a study; on the other hand, multiple sources may contain conflicting information about the same study (Mayo-Wilson et al 2017a, Mayo-Wilson et al 2017b, Mayo-Wilson et al 2018). Because the unit of interest in a systematic review is the study and not the report, information from multiple reports often needs to be collated and reconciled. It is not appropriate to discard any report of an included study without careful examination, since it may contain valuable information not included in the primary report. Review authors will need to decide between two strategies:
- Extract data from each report separately, then combine information across multiple data collection forms.
- Extract data from all reports directly into a single data collection form.
The choice of which strategy to use will depend on the nature of the reports and may vary across studies and across reports. For example, when a full journal article and multiple conference abstracts are available, it is likely that the majority of information will be obtained from the journal article; completing a new data collection form for each conference abstract may be a waste of time. Conversely, when there are two or more detailed journal articles, perhaps relating to different periods of follow-up, then it is likely to be easier to perform data extraction separately for these articles and collate information from the data collection forms afterwards. When data from all reports are extracted into a single data collection form, review authors should identify the ‘main’ data source for each study when sources include conflicting data and these differences cannot be resolved by contacting authors (Mayo-Wilson et al 2018). Flow diagrams such as those modified from the PRISMA statement can be particularly helpful when collating and documenting information from multiple reports (Mayo-Wilson et al 2018).
5.5.5 Reliability and reaching consensus
When more than one author extracts data from the same reports, there is potential for disagreement. After data have been extracted independently by two or more extractors, responses must be compared to assure agreement or to identify discrepancies. An explicit procedure or decision rule should be specified in the protocol for identifying and resolving disagreements. Most often, the source of the disagreement is an error by one of the extractors and is easily resolved. Thus, discussion among the authors is a sensible first step. More rarely, a disagreement may require arbitration by another person. Any disagreement that cannot be resolved should be addressed by contacting the study authors; if this is unsuccessful, the disagreement should be reported in the review.
The presence and resolution of disagreements should be carefully recorded. Maintaining a copy of the data ‘as extracted’ (in addition to the consensus data) allows assessment of reliability of coding. Examples of ways in which this can be achieved include the following:
- Use one author’s (paper) data collection form and record changes after consensus in a different ink colour.
- Enter consensus data onto an electronic form.
- Record original data extracted and consensus data in separate forms (some online tools do this automatically).
Agreement of coded items before reaching consensus can be quantified, for example using kappa statistics (Orwin 1994), although this is not routinely done in Cochrane Reviews. If agreement is assessed, this should be done only for the most important data (e.g. key risk of bias assessments, or availability of key outcomes).
Throughout the review process informal consideration should be given to the reliability of data extraction. For example, if after reaching consensus on the first few studies, the authors note a frequent disagreement for specific data, then coding instructions may need modification. Furthermore, an author’s coding strategy may change over time, as the coding rules are forgotten, indicating a need for retraining and, possibly, some recoding.
5.5.6 Extracting data from clinical study reports
Clinical study reports (CSRs) obtained for a systematic review are likely to be in PDF format. Although CSRs can be thousands of pages in length and very time-consuming to review, they typically follow the content and format required by the International Conference on Harmonisation (ICH 1995). Information in CSRs is usually presented in a structured and logical way. For example, numerical data pertaining to important demographic, efficacy, and safety variables are placed within the main text in tables and figures. Because of the clarity and completeness of information provided in CSRs, data extraction from CSRs may be clearer and conducted more confidently than from journal articles or other short reports.
To extract data from CSRs efficiently, review authors should familiarize themselves with the structure of the CSRs. In practice, review authors may want to browse or create ‘bookmarks’ within a PDF document that record section headers and subheaders and search key words related to the data extraction (e.g. randomization). In addition, it may be useful to utilize optical character recognition software to convert tables of data in the PDF to an analysable format when additional analyses are required, saving time and minimizing transcription errors.
CSRs may contain many outcomes and present many results for a single outcome (due to different analyses) (Mayo-Wilson et al 2017b). We recommend review authors extract results only for outcomes of interest to the review (Section 5.3.6 ). With regard to different methods of analysis, review authors should have a plan and pre-specify preferred metrics in their protocol for extracting results pertaining to different populations (e.g. ‘all randomized’, ‘all participants taking at least one dose of medication’), methods for handling missing data (e.g. ‘complete case analysis’, ‘multiple imputation’), and adjustment (e.g. unadjusted, adjusted for baseline covariates). It may be important to record the range of analysis options available, even if not all are extracted in detail. In some cases it may be preferable to use metrics that are comparable across multiple included studies, which may not be clear until data collection for all studies is complete.
CSRs are particularly useful for identifying outcomes assessed but not presented to the public. For efficacy outcomes and systematically collected adverse events, review authors can compare what is described in the CSRs with what is reported in published reports to assess the risk of bias due to missing outcome data ( Chapter 8, Section 8.5 ) and in selection of reported result ( Chapter 8, Section 8.7 ). Note that non-systematically collected adverse events are not amenable to such comparisons because these adverse events may not be known ahead of time and thus not pre-specified in the protocol.
5.5.7 Extracting data from regulatory reviews
Data most relevant to systematic reviews can be found in the medical and statistical review sections of a regulatory review. Both of these are substantially longer than journal articles (Turner 2013). A list of all trials on a drug usually can be found in the medical review. Because trials are referenced by a combination of numbers and letters, it may be difficult for the review authors to link the trial with other reports of the same trial (Section 5.2.1 ).
Many of the documents downloaded from the US Food and Drug Administration’s website for older drugs are scanned copies and are not searchable because of redaction of confidential information (Turner 2013). Optical character recognition software can convert most of the text. Reviews for newer drugs have been redacted electronically; documents remain searchable as a result.
Compared to CSRs, regulatory reviews contain less information about trial design, execution, and results. They provide limited information for assessing the risk of bias. In terms of extracting outcomes and results, review authors should follow the guidance provided for CSRs (Section 5.5.6 ).
5.5.8 Extracting data from figures with software
Sometimes numerical data needed for systematic reviews are only presented in figures. Review authors may request the data from the study investigators, or alternatively, extract the data from the figures either manually (e.g. with a ruler) or by using software. Numerous tools are available, many of which are free. Those available at the time of writing include tools called Plot Digitizer, WebPlotDigitizer, Engauge, Dexter, ycasd, GetData Graph Digitizer. The software works by taking an image of a figure and then digitizing the data points off the figure using the axes and scales set by the users. The numbers exported can be used for systematic reviews, although additional calculations may be needed to obtain the summary statistics, such as calculation of means and standard deviations from individual-level data points (or conversion of time-to-event data presented on Kaplan-Meier plots to hazard ratios; see Chapter 6, Section 6.8.2 ).
It has been demonstrated that software is more convenient and accurate than visual estimation or use of a ruler (Gross et al 2014, Jelicic Kadic et al 2016). Review authors should consider using software for extracting numerical data from figures when the data are not available elsewhere.
5.5.9 Automating data extraction in systematic reviews
Because data extraction is time-consuming and error-prone, automating or semi-automating this step may make the extraction process more efficient and accurate. The state of science relevant to automating data extraction is summarized here (Jonnalagadda et al 2015).
- At least 26 studies have tested various natural language processing and machine learning approaches for facilitating data extraction for systematic reviews.
· Each tool focuses on only a limited number of data elements (ranges from one to seven). Most of the existing tools focus on the PICO information (e.g. number of participants, their age, sex, country, recruiting centres, intervention groups, outcomes, and time points). A few are able to extract study design and results (e.g. objectives, study duration, participant flow), and two extract risk of bias information (Marshall et al 2016, Millard et al 2016). To date, well over half of the data elements needed for systematic reviews have not been explored for automated extraction.
- Most tools highlight the sentence(s) that may contain the data elements as opposed to directly recording these data elements into a data collection form or a data system.
- There is no gold standard or common dataset to evaluate the performance of these tools, limiting our ability to interpret the significance of the reported accuracy measures.
At the time of writing, we cannot recommend a specific tool for automating data extraction for routine systematic review production. There is a need for review authors to work with experts in informatics to refine these tools and evaluate them rigorously. Such investigations should address how the tool will fit into existing workflows. For example, the automated or semi-automated data extraction approaches may first act as checks for manual data extraction before they can replace it.
5.5.10 Suspicions of scientific misconduct
Systematic review authors can uncover suspected misconduct in the published literature. Misconduct includes fabrication or falsification of data or results, plagiarism, and research that does not adhere to ethical norms. Review authors need to be aware of scientific misconduct because the inclusion of fraudulent material could undermine the reliability of a review’s findings. Plagiarism of results data in the form of duplicated publication (either by the same or by different authors) may, if undetected, lead to study participants being double counted in a synthesis.
It is preferable to identify potential problems before, rather than after, publication of the systematic review, so that readers are not misled. However, empirical evidence indicates that the extent to which systematic review authors explore misconduct varies widely (Elia et al 2016). Text-matching software and systems such as CrossCheck may be helpful for detecting plagiarism, but they can detect only matching text, so data tables or figures need to be inspected by hand or using other systems (e.g. to detect image manipulation). Lists of data such as in a meta-analysis can be a useful means of detecting duplicated studies. Furthermore, examination of baseline data can lead to suspicions of misconduct for an individual randomized trial (Carlisle et al 2015). For example, Al-Marzouki and colleagues concluded that a trial report was fabricated or falsified on the basis of highly unlikely baseline differences between two randomized groups (Al-Marzouki et al 2005).
Cochrane Review authors are advised to consult with Cochrane editors if cases of suspected misconduct are identified. Searching for comments, letters or retractions may uncover additional information. Sensitivity analyses can be used to determine whether the studies arousing suspicion are influential in the conclusions of the review. Guidance for editors for addressing suspected misconduct will be available from Cochrane’s Editorial Publishing and Policy Resource (see community.cochrane.org ). Further information is available from the Committee on Publication Ethics (COPE; publicationethics.org ), including a series of flowcharts on how to proceed if various types of misconduct are suspected. Cases should be followed up, typically including an approach to the editors of the journals in which suspect reports were published. It may be useful to write first to the primary investigators to request clarification of apparent inconsistencies or unusual observations.
Because investigations may take time, and institutions may not always be responsive (Wager 2011), articles suspected of being fraudulent should be classified as ‘awaiting assessment’. If a misconduct investigation indicates that the publication is unreliable, or if a publication is retracted, it should not be included in the systematic review, and the reason should be noted in the ‘excluded studies’ section.
5.5.11 Key points in planning and reporting data extraction
In summary, the methods section of both the protocol and the review should detail:
- the data categories that are to be extracted;
- how extracted data from each report will be verified (e.g. extraction by two review authors, independently);
- whether data extraction is undertaken by content area experts, methodologists, or both;
- pilot testing, training and existence of coding instructions for the data collection form;
- how data are extracted from multiple reports from the same study; and
- how disagreements are handled when more than one author extracts data from each report.
5.6 Extracting study results and converting to the desired format
In most cases, it is desirable to collect summary data separately for each intervention group of interest and to enter these into software in which effect estimates can be calculated, such as RevMan. Sometimes the required data may be obtained only indirectly, and the relevant results may not be obvious. Chapter 6 provides many useful tips and techniques to deal with common situations. When summary data cannot be obtained from each intervention group, or where it is important to use results of adjusted analyses (for example to account for correlations in crossover or cluster-randomized trials) effect estimates may be available directly.
5.7 Managing and sharing data
When data have been collected for each individual study, it is helpful to organize them into a comprehensive electronic format, such as a database or spreadsheet, before entering data into a meta-analysis or other synthesis. When data are collated electronically, all or a subset of them can easily be exported for cleaning, consistency checks and analysis.
Tabulation of collected information about studies can facilitate classification of studies into appropriate comparisons and subgroups. It also allows identification of comparable outcome measures and statistics across studies. It will often be necessary to perform calculations to obtain the required statistics for presentation or synthesis. It is important through this process to retain clear information on the provenance of the data, with a clear distinction between data from a source document and data obtained through calculations. Statistical conversions, for example from standard errors to standard deviations, ideally should be undertaken with a computer rather than using a hand calculator to maintain a permanent record of the original and calculated numbers as well as the actual calculations used.
Ideally, data only need to be extracted once and should be stored in a secure and stable location for future updates of the review, regardless of whether the original review authors or a different group of authors update the review (Ip et al 2012). Standardizing and sharing data collection tools as well as data management systems among review authors working in similar topic areas can streamline systematic review production. Review authors have the opportunity to work with trialists, journal editors, funders, regulators, and other stakeholders to make study data (e.g. CSRs, IPD, and any other form of study data) publicly available, increasing the transparency of research. When legal and ethical to do so, we encourage review authors to share the data used in their systematic reviews to reduce waste and to allow verification and reanalysis because data will not have to be extracted again for future use (Mayo-Wilson et al 2018).
5.8 Chapter information
Editors: Tianjing Li, Julian PT Higgins, Jonathan J Deeks
Acknowledgements: This chapter builds on earlier versions of the Handbook . For details of previous authors and editors of the Handbook , see Preface. Andrew Herxheimer, Nicki Jackson, Yoon Loke, Deirdre Price and Helen Thomas contributed text. Stephanie Taylor and Sonja Hood contributed suggestions for designing data collection forms. We are grateful to Judith Anzures, Mike Clarke, Miranda Cumpston and Peter Gøtzsche for helpful comments.
Funding: JPTH is a member of the National Institute for Health Research (NIHR) Biomedical Research Centre at University Hospitals Bristol NHS Foundation Trust and the University of Bristol. JJD received support from the NIHR Birmingham Biomedical Research Centre at the University Hospitals Birmingham NHS Foundation Trust and the University of Birmingham. JPTH received funding from National Institute for Health Research Senior Investigator award NF-SI-0617-10145. The views expressed are those of the author(s) and not necessarily those of the NHS, the NIHR or the Department of Health.
5.9 References
Al-Marzouki S, Evans S, Marshall T, Roberts I. Are these data real? Statistical methods for the detection of data fabrication in clinical trials. BMJ 2005; 331 : 267-270.
Allen EN, Mushi AK, Massawe IS, Vestergaard LS, Lemnge M, Staedke SG, Mehta U, Barnes KI, Chandler CI. How experiences become data: the process of eliciting adverse event, medical history and concomitant medication reports in antimalarial and antiretroviral interaction trials. BMC Medical Research Methodology 2013; 13 : 140.
Baudard M, Yavchitz A, Ravaud P, Perrodeau E, Boutron I. Impact of searching clinical trial registries in systematic reviews of pharmaceutical treatments: methodological systematic review and reanalysis of meta-analyses. BMJ 2017; 356 : j448.
Bent S, Padula A, Avins AL. Better ways to question patients about adverse medical events: a randomized, controlled trial. Annals of Internal Medicine 2006; 144 : 257-261.
Berlin JA. Does blinding of readers affect the results of meta-analyses? University of Pennsylvania Meta-analysis Blinding Study Group. Lancet 1997; 350 : 185-186.
Buscemi N, Hartling L, Vandermeer B, Tjosvold L, Klassen TP. Single data extraction generated more errors than double data extraction in systematic reviews. Journal of Clinical Epidemiology 2006; 59 : 697-703.
Carlisle JB, Dexter F, Pandit JJ, Shafer SL, Yentis SM. Calculating the probability of random sampling for continuous variables in submitted or published randomised controlled trials. Anaesthesia 2015; 70 : 848-858.
Carroll C, Patterson M, Wood S, Booth A, Rick J, Balain S. A conceptual framework for implementation fidelity. Implementation Science 2007; 2 : 40.
Carvajal A, Ortega PG, Sainz M, Velasco V, Salado I, Arias LHM, Eiros JM, Rubio AP, Castrodeza J. Adverse events associated with pandemic influenza vaccines: Comparison of the results of a follow-up study with those coming from spontaneous reporting. Vaccine 2011; 29 : 519-522.
Chamberlain C, O'Mara-Eves A, Porter J, Coleman T, Perlen SM, Thomas J, McKenzie JE. Psychosocial interventions for supporting women to stop smoking in pregnancy. Cochrane Database of Systematic Reviews 2017; 2 : CD001055.
Damschroder LJ, Aron DC, Keith RE, Kirsh SR, Alexander JA, Lowery JC. Fostering implementation of health services research findings into practice: a consolidated framework for advancing implementation science. Implementation Science 2009; 4 : 50.
Davis AL, Miller JD. The European Medicines Agency and publication of clinical study reports: a challenge for the US FDA. JAMA 2017; 317 : 905-906.
Denniston AK, Holland GN, Kidess A, Nussenblatt RB, Okada AA, Rosenbaum JT, Dick AD. Heterogeneity of primary outcome measures used in clinical trials of treatments for intermediate, posterior, and panuveitis. Orphanet Journal of Rare Diseases 2015; 10 : 97.
Derry S, Loke YK. Risk of gastrointestinal haemorrhage with long term use of aspirin: meta-analysis. BMJ 2000; 321 : 1183-1187.
Doshi P, Dickersin K, Healy D, Vedula SS, Jefferson T. Restoring invisible and abandoned trials: a call for people to publish the findings. BMJ 2013; 346 : f2865.
Dusenbury L, Brannigan R, Falco M, Hansen WB. A review of research on fidelity of implementation: implications for drug abuse prevention in school settings. Health Education Research 2003; 18 : 237-256.
Dwan K, Altman DG, Clarke M, Gamble C, Higgins JPT, Sterne JAC, Williamson PR, Kirkham JJ. Evidence for the selective reporting of analyses and discrepancies in clinical trials: a systematic review of cohort studies of clinical trials. PLoS Medicine 2014; 11 : e1001666.
Elia N, von Elm E, Chatagner A, Popping DM, Tramèr MR. How do authors of systematic reviews deal with research malpractice and misconduct in original studies? A cross-sectional analysis of systematic reviews and survey of their authors. BMJ Open 2016; 6 : e010442.
Gøtzsche PC. Multiple publication of reports of drug trials. European Journal of Clinical Pharmacology 1989; 36 : 429-432.
Gøtzsche PC, Hróbjartsson A, Maric K, Tendal B. Data extraction errors in meta-analyses that use standardized mean differences. JAMA 2007; 298 : 430-437.
Gross A, Schirm S, Scholz M. Ycasd - a tool for capturing and scaling data from graphical representations. BMC Bioinformatics 2014; 15 : 219.
Hoffmann TC, Glasziou PP, Boutron I, Milne R, Perera R, Moher D, Altman DG, Barbour V, Macdonald H, Johnston M, Lamb SE, Dixon-Woods M, McCulloch P, Wyatt JC, Chan AW, Michie S. Better reporting of interventions: template for intervention description and replication (TIDieR) checklist and guide. BMJ 2014; 348 : g1687.
ICH. ICH Harmonised tripartite guideline: Struture and content of clinical study reports E31995. ICH1995. www.ich.org/fileadmin/Public_Web_Site/ICH_Products/Guidelines/Efficacy/E3/E3_Guideline.pdf .
Ioannidis JPA, Mulrow CD, Goodman SN. Adverse events: The more you search, the more you find. Annals of Internal Medicine 2006; 144 : 298-300.
Ip S, Hadar N, Keefe S, Parkin C, Iovin R, Balk EM, Lau J. A web-based archive of systematic review data. Systematic Reviews 2012; 1 : 15.
Ismail R, Azuara-Blanco A, Ramsay CR. Variation of clinical outcomes used in glaucoma randomised controlled trials: a systematic review. British Journal of Ophthalmology 2014; 98 : 464-468.
Jadad AR, Moore RA, Carroll D, Jenkinson C, Reynolds DJM, Gavaghan DJ, McQuay H. Assessing the quality of reports of randomized clinical trials: Is blinding necessary? Controlled Clinical Trials 1996; 17 : 1-12.
Jelicic Kadic A, Vucic K, Dosenovic S, Sapunar D, Puljak L. Extracting data from figures with software was faster, with higher interrater reliability than manual extraction. Journal of Clinical Epidemiology 2016; 74 : 119-123.
Jones AP, Remmington T, Williamson PR, Ashby D, Smyth RL. High prevalence but low impact of data extraction and reporting errors were found in Cochrane systematic reviews. Journal of Clinical Epidemiology 2005; 58 : 741-742.
Jones CW, Keil LG, Holland WC, Caughey MC, Platts-Mills TF. Comparison of registered and published outcomes in randomized controlled trials: a systematic review. BMC Medicine 2015; 13 : 282.
Jonnalagadda SR, Goyal P, Huffman MD. Automating data extraction in systematic reviews: a systematic review. Systematic Reviews 2015; 4 : 78.
Lewin S, Hendry M, Chandler J, Oxman AD, Michie S, Shepperd S, Reeves BC, Tugwell P, Hannes K, Rehfuess EA, Welch V, McKenzie JE, Burford B, Petkovic J, Anderson LM, Harris J, Noyes J. Assessing the complexity of interventions within systematic reviews: development, content and use of a new tool (iCAT_SR). BMC Medical Research Methodology 2017; 17 : 76.
Li G, Abbade LPF, Nwosu I, Jin Y, Leenus A, Maaz M, Wang M, Bhatt M, Zielinski L, Sanger N, Bantoto B, Luo C, Shams I, Shahid H, Chang Y, Sun G, Mbuagbaw L, Samaan Z, Levine MAH, Adachi JD, Thabane L. A scoping review of comparisons between abstracts and full reports in primary biomedical research. BMC Medical Research Methodology 2017; 17 : 181.
Li TJ, Vedula SS, Hadar N, Parkin C, Lau J, Dickersin K. Innovations in data collection, management, and archiving for systematic reviews. Annals of Internal Medicine 2015; 162 : 287-294.
Liberati A, Altman DG, Tetzlaff J, Mulrow C, Gøtzsche PC, Ioannidis JPA, Clarke M, Devereaux PJ, Kleijnen J, Moher D. The PRISMA statement for reporting systematic reviews and meta-analyses of studies that evaluate health care interventions: explanation and elaboration. PLoS Medicine 2009; 6 : e1000100.
Liu ZM, Saldanha IJ, Margolis D, Dumville JC, Cullum NA. Outcomes in Cochrane systematic reviews related to wound care: an investigation into prespecification. Wound Repair and Regeneration 2017; 25 : 292-308.
Marshall IJ, Kuiper J, Wallace BC. RobotReviewer: evaluation of a system for automatically assessing bias in clinical trials. Journal of the American Medical Informatics Association 2016; 23 : 193-201.
Mayo-Wilson E, Doshi P, Dickersin K. Are manufacturers sharing data as promised? BMJ 2015; 351 : h4169.
Mayo-Wilson E, Li TJ, Fusco N, Bertizzolo L, Canner JK, Cowley T, Doshi P, Ehmsen J, Gresham G, Guo N, Haythomthwaite JA, Heyward J, Hong H, Pham D, Payne JL, Rosman L, Stuart EA, Suarez-Cuervo C, Tolbert E, Twose C, Vedula S, Dickersin K. Cherry-picking by trialists and meta-analysts can drive conclusions about intervention efficacy. Journal of Clinical Epidemiology 2017a; 91 : 95-110.
Mayo-Wilson E, Fusco N, Li TJ, Hong H, Canner JK, Dickersin K, MUDS Investigators. Multiple outcomes and analyses in clinical trials create challenges for interpretation and research synthesis. Journal of Clinical Epidemiology 2017b; 86 : 39-50.
Mayo-Wilson E, Li T, Fusco N, Dickersin K. Practical guidance for using multiple data sources in systematic reviews and meta-analyses (with examples from the MUDS study). Research Synthesis Methods 2018; 9 : 2-12.
Meade MO, Richardson WS. Selecting and appraising studies for a systematic review. Annals of Internal Medicine 1997; 127 : 531-537.
Meinert CL. Clinical trials dictionary: Terminology and usage recommendations . Hoboken (NJ): Wiley; 2012.
Millard LAC, Flach PA, Higgins JPT. Machine learning to assist risk-of-bias assessments in systematic reviews. International Journal of Epidemiology 2016; 45 : 266-277.
Moher D, Schulz KF, Altman DG. The CONSORT Statement: revised recommendations for improving the quality of reports of parallel-group randomised trials. Lancet 2001; 357 : 1191-1194.
Moher D, Hopewell S, Schulz KF, Montori V, Gøtzsche PC, Devereaux PJ, Elbourne D, Egger M, Altman DG. CONSORT 2010 explanation and elaboration: updated guidelines for reporting parallel group randomised trials. BMJ 2010; 340 : c869.
Moore GF, Audrey S, Barker M, Bond L, Bonell C, Hardeman W, Moore L, O'Cathain A, Tinati T, Wight D, Baird J. Process evaluation of complex interventions: Medical Research Council guidance. BMJ 2015; 350 : h1258.
Orwin RG. Evaluating coding decisions. In: Cooper H, Hedges LV, editors. The Handbook of Research Synthesis . New York (NY): Russell Sage Foundation; 1994. p. 139-162.
Page MJ, McKenzie JE, Kirkham J, Dwan K, Kramer S, Green S, Forbes A. Bias due to selective inclusion and reporting of outcomes and analyses in systematic reviews of randomised trials of healthcare interventions. Cochrane Database of Systematic Reviews 2014; 10 : MR000035.
Ross JS, Mulvey GK, Hines EM, Nissen SE, Krumholz HM. Trial publication after registration in ClinicalTrials.Gov: a cross-sectional analysis. PLoS Medicine 2009; 6 .
Safer DJ. Design and reporting modifications in industry-sponsored comparative psychopharmacology trials. Journal of Nervous and Mental Disease 2002; 190 : 583-592.
Saldanha IJ, Dickersin K, Wang X, Li TJ. Outcomes in Cochrane systematic reviews addressing four common eye conditions: an evaluation of completeness and comparability. PloS One 2014; 9 : e109400.
Saldanha IJ, Li T, Yang C, Ugarte-Gil C, Rutherford GW, Dickersin K. Social network analysis identified central outcomes for core outcome sets using systematic reviews of HIV/AIDS. Journal of Clinical Epidemiology 2016; 70 : 164-175.
Saldanha IJ, Lindsley K, Do DV, Chuck RS, Meyerle C, Jones LS, Coleman AL, Jampel HD, Dickersin K, Virgili G. Comparison of clinical trial and systematic review outcomes for the 4 most prevalent eye diseases. JAMA Ophthalmology 2017a; 135 : 933-940.
Saldanha IJ, Li TJ, Yang C, Owczarzak J, Williamson PR, Dickersin K. Clinical trials and systematic reviews addressing similar interventions for the same condition do not consider similar outcomes to be important: a case study in HIV/AIDS. Journal of Clinical Epidemiology 2017b; 84 : 85-94.
Stewart LA, Clarke M, Rovers M, Riley RD, Simmonds M, Stewart G, Tierney JF, PRISMA-IPD Development Group. Preferred reporting items for a systematic review and meta-analysis of individual participant data: the PRISMA-IPD statement. JAMA 2015; 313 : 1657-1665.
Stock WA. Systematic coding for research synthesis. In: Cooper H, Hedges LV, editors. The Handbook of Research Synthesis . New York (NY): Russell Sage Foundation; 1994. p. 125-138.
Tramèr MR, Reynolds DJ, Moore RA, McQuay HJ. Impact of covert duplicate publication on meta-analysis: a case study. BMJ 1997; 315 : 635-640.
Turner EH. How to access and process FDA drug approval packages for use in research. BMJ 2013; 347 .
von Elm E, Poglia G, Walder B, Tramèr MR. Different patterns of duplicate publication: an analysis of articles used in systematic reviews. JAMA 2004; 291 : 974-980.
Wager E. Coping with scientific misconduct. BMJ 2011; 343 : d6586.
Wieland LS, Rutkow L, Vedula SS, Kaufmann CN, Rosman LM, Twose C, Mahendraratnam N, Dickersin K. Who has used internal company documents for biomedical and public health research and where did they find them? PloS One 2014; 9 .
Zanchetti A, Hansson L. Risk of major gastrointestinal bleeding with aspirin (Authors' reply). Lancet 1999; 353 : 149-150.
Zarin DA, Tse T, Williams RJ, Califf RM, Ide NC. The ClinicalTrials.gov results database: update and key issues. New England Journal of Medicine 2011; 364 : 852-860.
Zwarenstein M, Treweek S, Gagnier JJ, Altman DG, Tunis S, Haynes B, Oxman AD, Moher D. Improving the reporting of pragmatic trials: an extension of the CONSORT statement. BMJ 2008; 337 : a2390.
For permission to re-use material from the Handbook (either academic or commercial), please see here for full details.
- UNC Libraries
- HSL Academic Process
- Systematic Reviews
- Step 7: Extract Data from Included Studies
Systematic Reviews: Step 7: Extract Data from Included Studies
Created by health science librarians.

- Step 1: Complete Pre-Review Tasks
- Step 2: Develop a Protocol
- Step 3: Conduct Literature Searches
- Step 4: Manage Citations
- Step 5: Screen Citations
- Step 6: Assess Quality of Included Studies
About Step 7: Extract Data from Included Studies
About data extraction, select a data extraction tool, what should i extract, helpful tip- data extraction.
- Data extraction FAQs
- Step 8: Write the Review
Check our FAQ's
Email us
Call (919) 962-0800
Make an appointment with a librarian
Request a systematic or scoping review consultation
In Step 7, you will skim the full text of included articles to collect information about the studies in a table format (extract data), to summarize the studies and make them easier to compare. You will:
- Make sure you have collected the full text of any included articles.
- Choose the pieces of information you want to collect from each study.
- Choose a method for collecting the data.
- Create the data extraction table.
- Test the data collection table (optional).
- Collect (extract) the data.
- Review the data collected for any errors.
For accuracy, two or more people should extract data from each study. This process can be done by hand or by using a computer program.
Click an item below to see how it applies to Step 7: Extract Data from Included Studies.
Reporting your review with PRISMA
If you reach the data extraction step and choose to exclude articles for any reason, update the number of included and excluded studies in your PRISMA flow diagram.
Managing your review with Covidence
Covidence allows you to assemble a custom data extraction template, have two reviewers conduct extraction, then send their extractions for consensus.
How a librarian can help with Step 7
A librarian can advise you on data extraction for your systematic review, including:
- What the data extraction stage of the review entails
- Finding examples in the literature of similar reviews and their completed data tables
- How to choose what data to extract from your included articles
- How to create a randomized sample of citations for a pilot test
- Best practices for reporting your included studies and their important data in your review
In this step of the systematic review, you will develop your evidence tables, which give detailed information for each study (perhaps using a PICO framework as a guide), and summary tables, which give a high-level overview of the findings of your review. You can create evidence and summary tables to describe study characteristics, results, or both. These tables will help you determine which studies, if any, are eligible for quantitative synthesis.
Data extraction requires a lot of planning. We will review some of the tools you can use for data extraction, the types of information you will want to extract, and the options available in the systematic review software used here at UNC, Covidence .
How many people should extract data?
The Cochrane Handbook and other studies strongly suggest at least two reviewers and extractors to reduce the number of errors.
- Chapter 5: Collecting Data (Cochrane Handbook)
- A Practical Guide to Data Extraction for Intervention Systematic Reviews (Covidence)
Click on a type of data extraction tool below to see some more information about using that type of tool and what UNC has to offer.
Systematic Review Software (Covidence)
Most systematic review software tools have data extraction functionality that can save you time and effort. Here at UNC, we use a systematic review software called Covidence. You can see a more complete list of options in the Systematic Review Toolbox .
Covidence allows you to create and publish a data extraction template with text fields, single-choice items, section headings and section subheadings; perform dual and single reviewer data extraction ; review extractions for consensus ; and export data extraction and quality assessment to a CSV with each item in a column and each study in a row.
- Covidence@UNC Guide
- Covidence for Data Extraction (Covidence)
- A Practical Guide to Data Extraction for Intervention Systematic Reviews(Covidence)
Spreadsheet or Database Software (Excel, Google Sheets)
You can also use spreadsheet or database software to create custom extraction forms. Spreadsheet software (such as Microsoft Excel) has functions such as drop-down menus and range checks can speed up the process and help prevent data entry errors. Relational databases (such as Microsoft Access) can help you extract information from different categories like citation details, demographics, participant selection, intervention, outcomes, etc.
- Microsoft Products (UNC Information Technology Services)
Cochrane RevMan
RevMan offers collection forms for descriptive information on population, interventions, and outcomes, and quality assessments, as well as for data for analysis and forest plots. The form elements may not be changed, and data must be entered manually. RevMan is a free software download.
- Cochrane RevMan 5.0 Download
- RevMan for Non-Cochrane Reviews (Cochrane Training)
Survey or Form Software (Qualtrics, Poll Everywhere)
Survey or form tools can help you create custom forms with many different question types, such as multiple choice, drop downs, ranking, and more. Content from these tools can often be exported to spreadsheet or database software as well. Here at UNC we have access to the survey/form software Qualtrics & Poll Everywhere.
- Qualtrics (UNC Information Technology Services)
- Poll Everywhere (UNC Information Technology Services)
Electronic Documents or Paper & Pencil (Word, Google Docs)
In the past, people often used paper and pencil to record the data they extracted from articles. Handwritten extraction is less popular now due to widespread electronic tools. You can record extracted data in electronic tables or forms created in Microsoft Word or other word processing programs, but this process may take longer than many of our previously listed methods. If chosen, the electronic document or paper-and-pencil extraction methods should only be used for small reviews, as larger sets of articles may become unwieldy. These methods may also be more prone to errors in data entry than some of the more automated methods.
There are benefits and limitations to each method of data extraction. You will want to consider:
- The cost of the software / tool
- Shareability / versioning
- Existing versus custom data extraction forms
- The data entry process
- Interrater reliability
For example, in Covidence you may spend more time building your data extraction form, but save time later in the extraction process as Covidence can automatically highlight discrepancies for review and resolution between different extractors. Excel may require less time investment to create an extraction form, but it may take longer for you to match and compare data between extractors. More in-depth comparison of the benefits and limitations of each extraction tool can be found in the table below.

It may help to consult other similar systematic reviews to identify what data to collect or to think about your question in a framework such as PICO .
Helpful data for an intervention question may include:
- Information about the article (author(s), year of publication, title, DOI)
- Information about the study (study type, participant recruitment / selection / allocation, level of evidence, study quality)
- Patient demographics (age, sex, ethnicity, diseases / conditions, other characteristics related to the intervention / outcome)
- Intervention (quantity, dosage, route of administration, format, duration, time frame, setting)
- Outcomes (quantitative and / or qualitative)
If you plan to synthesize data, you will want to collect additional information such as sample sizes, effect sizes, dependent variables, reliability measures, pre-test data, post-test data, follow-up data, and statistical tests used.
Extraction templates and approaches should be determined by the needs of the specific review. For example, if you are extracting qualitative data, you will want to extract data such as theoretical framework, data collection method, or role of the researcher and their potential bias.
- Supplementary Guidance for Inclusion of Qualitative Research in Cochrane Systematic Reviews of Interventions (Cochrane Collaboration Qualitative Methods Group)
- Look for an existing extraction form or tool to help guide you. Use existing systematic reviews on your topic to identify what information to collect if you are not sure what to do.
- Train the review team on the extraction categories and what type of data would be expected. A manual or guide may help your team establish standards.
- Pilot the extraction / coding form to ensure data extractors are recording similar data. Revise the extraction form if needed.
- Discuss any discrepancies in coding throughout the process.
- Document any changes to the process or the form. Keep track of the decisions the team makes and the reasoning behind them.
- << Previous: Step 6: Assess Quality of Included Studies
- Next: Step 8: Write the Review >>
- Last Updated: May 10, 2024 5:39 PM
- URL: https://guides.lib.unc.edu/systematic-reviews
Search & Find
- E-Research by Discipline
- More Search & Find
Places & Spaces
- Places to Study
- Book a Study Room
- Printers, Scanners, & Computers
- More Places & Spaces
- Borrowing & Circulation
- Request a Title for Purchase
- Schedule Instruction Session
- More Services
Support & Guides
- Course Reserves
- Research Guides
- Citing & Writing
- More Support & Guides
- Mission Statement
- Diversity Statement
- Staff Directory
- Job Opportunities
- Give to the Libraries
- News & Exhibits
- Reckoning Initiative
- More About Us

- Search This Site
- Privacy Policy
- Accessibility
- Give Us Your Feedback
- 208 Raleigh Street CB #3916
- Chapel Hill, NC 27515-8890
- 919-962-1053
Research Methods
- Getting Started
- Literature Review Research
- Research Design
- Research Design By Discipline
- SAGE Research Methods
- Teaching with SAGE Research Methods
Literature Review
- What is a Literature Review?
- What is NOT a Literature Review?
- Purposes of a Literature Review
- Types of Literature Reviews
- Literature Reviews vs. Systematic Reviews
- Systematic vs. Meta-Analysis
Literature Review is a comprehensive survey of the works published in a particular field of study or line of research, usually over a specific period of time, in the form of an in-depth, critical bibliographic essay or annotated list in which attention is drawn to the most significant works.
Also, we can define a literature review as the collected body of scholarly works related to a topic:
- Summarizes and analyzes previous research relevant to a topic
- Includes scholarly books and articles published in academic journals
- Can be an specific scholarly paper or a section in a research paper
The objective of a Literature Review is to find previous published scholarly works relevant to an specific topic
- Help gather ideas or information
- Keep up to date in current trends and findings
- Help develop new questions
A literature review is important because it:
- Explains the background of research on a topic.
- Demonstrates why a topic is significant to a subject area.
- Helps focus your own research questions or problems
- Discovers relationships between research studies/ideas.
- Suggests unexplored ideas or populations
- Identifies major themes, concepts, and researchers on a topic.
- Tests assumptions; may help counter preconceived ideas and remove unconscious bias.
- Identifies critical gaps, points of disagreement, or potentially flawed methodology or theoretical approaches.
- Indicates potential directions for future research.
All content in this section is from Literature Review Research from Old Dominion University
Keep in mind the following, a literature review is NOT:
Not an essay
Not an annotated bibliography in which you summarize each article that you have reviewed. A literature review goes beyond basic summarizing to focus on the critical analysis of the reviewed works and their relationship to your research question.
Not a research paper where you select resources to support one side of an issue versus another. A lit review should explain and consider all sides of an argument in order to avoid bias, and areas of agreement and disagreement should be highlighted.
A literature review serves several purposes. For example, it
- provides thorough knowledge of previous studies; introduces seminal works.
- helps focus one’s own research topic.
- identifies a conceptual framework for one’s own research questions or problems; indicates potential directions for future research.
- suggests previously unused or underused methodologies, designs, quantitative and qualitative strategies.
- identifies gaps in previous studies; identifies flawed methodologies and/or theoretical approaches; avoids replication of mistakes.
- helps the researcher avoid repetition of earlier research.
- suggests unexplored populations.
- determines whether past studies agree or disagree; identifies controversy in the literature.
- tests assumptions; may help counter preconceived ideas and remove unconscious bias.
As Kennedy (2007) notes*, it is important to think of knowledge in a given field as consisting of three layers. First, there are the primary studies that researchers conduct and publish. Second are the reviews of those studies that summarize and offer new interpretations built from and often extending beyond the original studies. Third, there are the perceptions, conclusions, opinion, and interpretations that are shared informally that become part of the lore of field. In composing a literature review, it is important to note that it is often this third layer of knowledge that is cited as "true" even though it often has only a loose relationship to the primary studies and secondary literature reviews.
Given this, while literature reviews are designed to provide an overview and synthesis of pertinent sources you have explored, there are several approaches to how they can be done, depending upon the type of analysis underpinning your study. Listed below are definitions of types of literature reviews:
Argumentative Review This form examines literature selectively in order to support or refute an argument, deeply imbedded assumption, or philosophical problem already established in the literature. The purpose is to develop a body of literature that establishes a contrarian viewpoint. Given the value-laden nature of some social science research [e.g., educational reform; immigration control], argumentative approaches to analyzing the literature can be a legitimate and important form of discourse. However, note that they can also introduce problems of bias when they are used to to make summary claims of the sort found in systematic reviews.
Integrative Review Considered a form of research that reviews, critiques, and synthesizes representative literature on a topic in an integrated way such that new frameworks and perspectives on the topic are generated. The body of literature includes all studies that address related or identical hypotheses. A well-done integrative review meets the same standards as primary research in regard to clarity, rigor, and replication.
Historical Review Few things rest in isolation from historical precedent. Historical reviews are focused on examining research throughout a period of time, often starting with the first time an issue, concept, theory, phenomena emerged in the literature, then tracing its evolution within the scholarship of a discipline. The purpose is to place research in a historical context to show familiarity with state-of-the-art developments and to identify the likely directions for future research.
Methodological Review A review does not always focus on what someone said [content], but how they said it [method of analysis]. This approach provides a framework of understanding at different levels (i.e. those of theory, substantive fields, research approaches and data collection and analysis techniques), enables researchers to draw on a wide variety of knowledge ranging from the conceptual level to practical documents for use in fieldwork in the areas of ontological and epistemological consideration, quantitative and qualitative integration, sampling, interviewing, data collection and data analysis, and helps highlight many ethical issues which we should be aware of and consider as we go through our study.
Systematic Review This form consists of an overview of existing evidence pertinent to a clearly formulated research question, which uses pre-specified and standardized methods to identify and critically appraise relevant research, and to collect, report, and analyse data from the studies that are included in the review. Typically it focuses on a very specific empirical question, often posed in a cause-and-effect form, such as "To what extent does A contribute to B?"
Theoretical Review The purpose of this form is to concretely examine the corpus of theory that has accumulated in regard to an issue, concept, theory, phenomena. The theoretical literature review help establish what theories already exist, the relationships between them, to what degree the existing theories have been investigated, and to develop new hypotheses to be tested. Often this form is used to help establish a lack of appropriate theories or reveal that current theories are inadequate for explaining new or emerging research problems. The unit of analysis can focus on a theoretical concept or a whole theory or framework.
* Kennedy, Mary M. "Defining a Literature." Educational Researcher 36 (April 2007): 139-147.
All content in this section is from The Literature Review created by Dr. Robert Larabee USC
Robinson, P. and Lowe, J. (2015), Literature reviews vs systematic reviews. Australian and New Zealand Journal of Public Health, 39: 103-103. doi: 10.1111/1753-6405.12393
What's in the name? The difference between a Systematic Review and a Literature Review, and why it matters . By Lynn Kysh from University of Southern California
Systematic review or meta-analysis?
A systematic review answers a defined research question by collecting and summarizing all empirical evidence that fits pre-specified eligibility criteria.
A meta-analysis is the use of statistical methods to summarize the results of these studies.
Systematic reviews, just like other research articles, can be of varying quality. They are a significant piece of work (the Centre for Reviews and Dissemination at York estimates that a team will take 9-24 months), and to be useful to other researchers and practitioners they should have:
- clearly stated objectives with pre-defined eligibility criteria for studies
- explicit, reproducible methodology
- a systematic search that attempts to identify all studies
- assessment of the validity of the findings of the included studies (e.g. risk of bias)
- systematic presentation, and synthesis, of the characteristics and findings of the included studies
Not all systematic reviews contain meta-analysis.
Meta-analysis is the use of statistical methods to summarize the results of independent studies. By combining information from all relevant studies, meta-analysis can provide more precise estimates of the effects of health care than those derived from the individual studies included within a review. More information on meta-analyses can be found in Cochrane Handbook, Chapter 9 .
A meta-analysis goes beyond critique and integration and conducts secondary statistical analysis on the outcomes of similar studies. It is a systematic review that uses quantitative methods to synthesize and summarize the results.
An advantage of a meta-analysis is the ability to be completely objective in evaluating research findings. Not all topics, however, have sufficient research evidence to allow a meta-analysis to be conducted. In that case, an integrative review is an appropriate strategy.
Some of the content in this section is from Systematic reviews and meta-analyses: step by step guide created by Kate McAllister.
- << Previous: Getting Started
- Next: Research Design >>
- Last Updated: Aug 21, 2023 4:07 PM
- URL: https://guides.lib.udel.edu/researchmethods
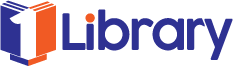
- No results found
Literature review
Chapter 3 research methodology, 3.5 data collection methods, 3.5.1 literature review.
A literature review is often undertaken prior to empirical research as it provides a synthesis of the extant knowledge on a given topic. The scope of a literature review can vary. The emphasis may be on a review of research methods to determine which approach to adopt or examination of current knowledge to inform policy decisions. An essay style review was criticised by Hakim (1992, pp.18-19) for its subjective approach and partial coverage. The preferred style is a meta-analysis which introduces more rigour into the process. Meta-analysis involves statistical analysis to highlight significance in reported study findings. It is a useful tool for reviews of quantitative studies but is not believed to be as appropriate for reviews of qualitative studies (Hakim 1992, pp.19-20). An alternative approach is to carry out a systematic review where explicit procedures are followed making bias less likely to occur (Bryman 2008, p.85). Systematic reviews involve a series of defined steps:
• purpose statement;
• criteria for selection of published works; • all in-scope works are included in the review;
• study features recorded against a defined protocol (location, sample size, data collection methods and key findings); and
• results summarised and synthesised, possibly presented in a table (Millar 2004, p.145).
One limitation of a systematic review is that differences between studies are not highlighted, resulting in a loss of important detail (Millar 2004, p.146).
A narrative or descriptive literature review is useful for gaining an insight into a topic which is further understood by empirical research. This form of review is more wide ranging, exploratory and not as clearly defined as other types of literature review (Bryman 2008, pp.92-93). Prior studies are compared for trends or patterns in their results (Millar 2004, p.142).
Literature reviews are advantageous because they can be conducted relatively quickly with little cost. They are, however, limited to published literature which may not adequately cover areas under investigation (Hakim 1992, p.24).
3.5.2 Questionnaires
The criteria for research questionnaires are that they should:
• collect information for analysis;
• comprise a set list of questions which is presented to all respondents; and • gather information directly from subjects (Denscombe 2007, pp.153-154)
They are ideal tools to use where the researcher wishes to gather information from a large number of individuals who are geographically dispersed, where standard data are required and respondents have the ability to understand the questions being asked. Questionnaires tend to gather information around ‘facts’ or ‘opinions’ and the researcher must have no ambiguities regarding the focus of their investigation (Denscombe 2007, pp.154-155).
The length and complexity of the questionnaire is a matter of judgement for the researcher. The decision needs to be made by taking into account the audience and time required to complete the questionnaire, however, a major deterrent to completion is its size. Therefore, key research issues should be addressed by the questionnaire (Denscombe 2007, pp.161-162). In addition, when compared with interviews, self-completion questionnaires need to be easy to follow, short to minimise the risk of survey fatigue, and have a limited number of open questions as closed questions are easier to answer in the absence of an interviewer to guide the process (Bryman 2008, p.217).
Prior to releasing a questionnaire to its intended audience it needs to be tested and refined. This pilot process ensures optimal wording and question ordering, tests letters of introduction and analysis of pilot data assists in developing a plan for final data analysis (Oppenheim 1992, pp.47-64).
One of the weaknesses of structured questionnaires is that they provide less depth of information than interviews (Hakim 1992, p.49). To be effective the researcher needs to ensure that questionnaire respondents mirror the wider target population. Failure to do so can introduce bias into the results. Responses also need to be an accurate measure of respondent characteristics (Fowler 2009, pp.12-14).
3.5.3 Interviews
Interviews are a useful source of preliminary information for the researcher and they can help to frame the research to follow (Blakeslee & Fleischer 2007, pp.30-31). In this respect they provide a mechanism for identifying issues and themes. They are also used to obtain in-depth data when “information based on insider experience, privileged insights and experiences” are required (Wisker 2001, p.165). Interviews can take a variety of formats from formal structured, through semi-structured to informal or opportunistic. Formal interviews follow a set structure and question list; for the researcher they are a way of gathering a standard set of data which is consistent across all
interviewees (Blakeslee & Fleischer 2007, p.133). Semi-structured interviews have a defined list of questions but provide scope for discussion (Wisker 2001, pp.168-169).
Interviews are conducted from the perspective of the interviewer; their views will have a bearing on the interview process and subsequent analysis of the transcript. It is therefore important to follow ethical practices, to avoid bias and to be open to the views of the interviewee (Wisker 2001, pp.142-143).
One of the drawbacks of adopting interviews as a research method is that they are time consuming (Gillham 2000, pp.65-66; Wisker 2001, 165). Thus, it is advisable to maintain a focus on the research topic (Blakeslee & Fleischer 2007, 138-139; Gillham 2000, pp.65-66).
3.5.4 Document analysis
Document analysis draws on written, visual and audio files from a range of sources. Written documents include Government publications, newspapers, meeting notes, letters, diaries or webpages. Particularly attractive sources of data for researchers are those which are freely available and accessible. Documents that are not freely available require the researcher to negotiate access or undertake undercover activities to source. Researchers need to assess the validity of the documents they examine; for a website this involves consideration of the authority of the source, trustworthiness of the website, whether information is up-to-date and the popularity of the website (Denscombe 2007, pp.227-234).
When conducting research based on documents the context within which these artefacts were created and the intended audience should be considered. Bryman (2008, p.527) offered the example of an organisation’s meeting minutes which may have been crafted to exclude certain discussions because they could be accessed by members of the public. Background information to meeting minutes might also be available internally, thus connecting them to wider internal events. Researchers may have to probe into the broader
3.6 Data analysis
In quantitative data analysis facts expressed in numerical form are used to test hypotheses (Neuman 2007, p.329). Raw data are processed by software and charts or graphs representing these data produced. Summaries of the data are explained and given meaning by the researcher (Merriam 1998, p.178; Neuman 2007, p.248). Qualitative data consists of words, photographs and other materials which require a different treatment for analysis. Researchers begin data analysis early in their research by looking for patterns and relationships in the data (Neuman 2007, p.329). Data analysis is achieved through a series of steps which involve preparing, coding, identifying themes and presentation (Creswell 2007, p.148). These activities are broken down into six stages: data managing, reading/memoing, describing, classifying, interpreting, and representing/visualising. The following activities are carried out during the process of collating and comparing these data:
• data managing: creating and organising files for the data;
• reading/memoing: reading, note taking in the margins and initial coding;
• describing, classifying and interpreting: describing the data and its context; analysing to identify themes and patterns; making sense of the data and bringing meaning to its interpretation; and
• representing/visualising: findings are presented by narration and visual representations (models, tables, figures or sketches) (Creswell 2007, pp.156-157).
Data analysis is designed to aid the understanding of an event; therefore, core elements of complex events are identified. Data are studied for themes, common issues, words or phrases. These are coded (tagged) into broad categories to develop an understanding of a phenomenon. Codes are not fixed; they change and develop as the research progresses. Thus, initial coding is descriptive and applied to broad chunks of text (open coding). Relationships between codes aids identification of key (axial) components and this leads on to a more focused effort on the core codes (selective coding) which are essential in explaining phenomena (Denscombe 2007, pp.97-98).
This approach is mirrored in the analysis of case study research data. Where data are interpreted and analysed for patterns in order to gain an understanding of the case and surrounding influences and conditions. The researcher questions the data, reading it over and again; taking the time to reflect on the data, their assumptions and analysis. In this way meaning and significance can be better understood and through coding and triangulation the process is enhanced (Stake 1995, pp.78-79).
Stake (1995, p.108) noted that “All researchers recognize the need not only for being accurate in measuring things but logical in interpreting the meaning of those measurements.” The protocol by which this validation is achieved is triangulation. There are four methods of triangulation:
1. data source triangulation: identifies whether a phenomenon occurs or carries the same meaning under different circumstances;
2. investigator triangulation: is achieved by having an independent observer of proceedings, or to present research observations and discuss appropriate interpretations with colleagues;
3. theory triangulation: data are compared by researchers with different theoretical perspectives and where agreement is reached triangulation is achieved. When different meanings are derived from the data, there is an opportunity to enhance understanding of the case; and
4. methodological triangulation: findings are confirmed by following a sequence of methods. In case study the most commonly used methods are observation, interview and document review. Adopting a range of methods can confirm events but it may also uncover an alternative perspective or reading of a situation (Stake 1995, pp112-115).
3.7 Research ethics
Research involving human subjects needs to be conducted in an ethical manner to ensure individuals are not adversely affected by the research (Fowler 2009. p.163). The standards for ethical research practice involve ensuring informed consent, data protection
and privacy (Pauwels 2007). Gaining informed consent from subjects willing to be involved in a research project necessitates that the following points are explained by the researcher and understood by the participant:
• research goals are clearly stated;
• side effects or potentially detrimental factors are transparent;
• gratuities do not act as an inducement to participate in the research; and • participants can withdraw at any time without prejudice (Pauwels 2007, p.20).
To this list Fowler (2009, p.164) added further guiding principles for research surveys involving general populations including:
• making participants aware of the name of the organisation under which the research is being conducted and providing the interviewer’s name;
• notifying subjects of any sponsoring body involved in the research; • stipulating terms of confidentiality; and
• ensuring there are no negative consequences for non-participation.
Data protection and privacy exist to ensure that data sharing does not infringe an individual’s right to privacy. Therefore, researchers are bound to protect identity by coding data during processing and anonymising it to ensure that the connection between an individual and data stored on them are not associated in any traceable way (Pauwels 2007, pp.27-28). Care should be taken when reporting data from small categories of respondents as they might be identifiable. In addition, completed responses should not be available to individuals beyond the project team. It is a researcher’s responsibility to ensure that the completed survey instrument is destroyed, or its continued storage is secure, once the research is completed (Fowler 2009, p.166).
Benefits to participating in research are usually altruistic and inducements should not be excessive so that the principle of voluntary participation is upheld. Researchers should not overstate any benefits and any promises made should be met (Fowler 2009, p.167).
- Website management and development
- Website metrics
- Conclusions
- Literature review (You are here)
- Research samples
- University policy guidance, website management and website template
- Library website governance and staffing
- Methods of website evaluation
- Use of data gathering methods
- Interview results
- Gaps in the survey data
Related documents
Eugene McDermott Library
Literature review.
- Collecting Resources for a Literature Review
- Organizing the Literature Review
- Writing the Literature Review
- Examples of Literature Reviews
Sources for Literature Review Items
Sources for a Literature Review will come from a variety of places, including:
•Books Use the Library Catalog to see what items McDermott Library has on your topic or if McDermott Library has a specific source you need. The WorldCat database allows you to search the catalogs on many, many libraries. WorldCat is a good place to find out what books exist on your topic.
•Reference Materials Reference Materials such as encyclopedias and dictionaries provide good overall views of topics and provide keyword hints for searching. Many will include lists of sources to consider for your literature review.
•Journals via Electronic Databases Journals are a major source of materials for a literature review. With the library's databases, you can search thousands of journals back a century or more.
•Conference Papers At conferences, professionals and scholars explore the latest trends, share new ideas, and present new research. Searching Conference papers allows you to see research before it is published and get a feel for what is going on in a particular organization or within a particular group.
Many electronic databases include conference proceedings, but with Conference Proceedings Citation Index database, you can search proceedings alone.
•Dissertations & Theses Here is a link to databases licensed by UTD McDermott library with full-text access to Dissertations and Theses
Some of these are specific to Texas or UTD produced studies. Choose the Global option to search more broadly.
•Internet The general internet can be a valuable resource for information. However, it is largely unregulated. Be sure to critically evaluate internet sources. Look at the Evaluating Websites LibGuide for suggestions on evaluating websites.
•Government Publications The U.S. government produces a wide variety of information sources, from consumer brochures to congressional reports to large amounts of data to longitudinal studies. For the United States, Usa.gov is a good place to start. Official state websites can be helpful for individual state statistics and information.
- << Previous: Home
- Next: Organizing the Literature Review >>
- Last Updated: Mar 22, 2024 12:28 PM
- URL: https://libguides.utdallas.edu/literature-review
- Open access
- Published: 17 August 2023
Data visualisation in scoping reviews and evidence maps on health topics: a cross-sectional analysis
- Emily South ORCID: orcid.org/0000-0003-2187-4762 1 &
- Mark Rodgers 1
Systematic Reviews volume 12 , Article number: 142 ( 2023 ) Cite this article
3626 Accesses
13 Altmetric
Metrics details
Scoping reviews and evidence maps are forms of evidence synthesis that aim to map the available literature on a topic and are well-suited to visual presentation of results. A range of data visualisation methods and interactive data visualisation tools exist that may make scoping reviews more useful to knowledge users. The aim of this study was to explore the use of data visualisation in a sample of recent scoping reviews and evidence maps on health topics, with a particular focus on interactive data visualisation.
Ovid MEDLINE ALL was searched for recent scoping reviews and evidence maps (June 2020-May 2021), and a sample of 300 papers that met basic selection criteria was taken. Data were extracted on the aim of each review and the use of data visualisation, including types of data visualisation used, variables presented and the use of interactivity. Descriptive data analysis was undertaken of the 238 reviews that aimed to map evidence.
Of the 238 scoping reviews or evidence maps in our analysis, around one-third (37.8%) included some form of data visualisation. Thirty-five different types of data visualisation were used across this sample, although most data visualisations identified were simple bar charts (standard, stacked or multi-set), pie charts or cross-tabulations (60.8%). Most data visualisations presented a single variable (64.4%) or two variables (26.1%). Almost a third of the reviews that used data visualisation did not use any colour (28.9%). Only two reviews presented interactive data visualisation, and few reported the software used to create visualisations.
Conclusions
Data visualisation is currently underused by scoping review authors. In particular, there is potential for much greater use of more innovative forms of data visualisation and interactive data visualisation. Where more innovative data visualisation is used, scoping reviews have made use of a wide range of different methods. Increased use of these more engaging visualisations may make scoping reviews more useful for a range of stakeholders.
Peer Review reports
Scoping reviews are “a type of evidence synthesis that aims to systematically identify and map the breadth of evidence available on a particular topic, field, concept, or issue” ([ 1 ], p. 950). While they include some of the same steps as a systematic review, such as systematic searches and the use of predetermined eligibility criteria, scoping reviews often address broader research questions and do not typically involve the quality appraisal of studies or synthesis of data [ 2 ]. Reasons for conducting a scoping review include the following: to map types of evidence available, to explore research design and conduct, to clarify concepts or definitions and to map characteristics or factors related to a concept [ 3 ]. Scoping reviews can also be undertaken to inform a future systematic review (e.g. to assure authors there will be adequate studies) or to identify knowledge gaps [ 3 ]. Other evidence synthesis approaches with similar aims have been described as evidence maps, mapping reviews or systematic maps [ 4 ]. While this terminology is used inconsistently, evidence maps can be used to identify evidence gaps and present them in a user-friendly (and often visual) way [ 5 ].
Scoping reviews are often targeted to an audience of healthcare professionals or policy-makers [ 6 ], suggesting that it is important to present results in a user-friendly and informative way. Until recently, there was little guidance on how to present the findings of scoping reviews. In recent literature, there has been some discussion of the importance of clearly presenting data for the intended audience of a scoping review, with creative and innovative use of visual methods if appropriate [ 7 , 8 , 9 ]. Lockwood et al. suggest that innovative visual presentation should be considered over dense sections of text or long tables in many cases [ 8 ]. Khalil et al. suggest that inspiration could be drawn from the field of data visualisation [ 7 ]. JBI guidance on scoping reviews recommends that reviewers carefully consider the best format for presenting data at the protocol development stage and provides a number of examples of possible methods [ 10 ].
Interactive resources are another option for presentation in scoping reviews [ 9 ]. Researchers without the relevant programming skills can now use several online platforms (such as Tableau [ 11 ] and Flourish [ 12 ]) to create interactive data visualisations. The benefits of using interactive visualisation in research include the ability to easily present more than two variables [ 13 ] and increased engagement of users [ 14 ]. Unlike static graphs, interactive visualisations can allow users to view hierarchical data at different levels, exploring both the “big picture” and looking in more detail ([ 15 ], p. 291). Interactive visualizations are often targeted at practitioners and decision-makers [ 13 ], and there is some evidence from qualitative research that they are valued by policy-makers [ 16 , 17 , 18 ].
Given their focus on mapping evidence, we believe that scoping reviews are particularly well-suited to visually presenting data and the use of interactive data visualisation tools. However, it is unknown how many recent scoping reviews visually map data or which types of data visualisation are used. The aim of this study was to explore the use of data visualisation methods in a large sample of recent scoping reviews and evidence maps on health topics. In particular, we were interested in the extent to which these forms of synthesis use any form of interactive data visualisation.
This study was a cross-sectional analysis of studies labelled as scoping reviews or evidence maps (or synonyms of these terms) in the title or abstract.
The search strategy was developed with help from an information specialist. Ovid MEDLINE® ALL was searched in June 2021 for studies added to the database in the previous 12 months. The search was limited to English language studies only.
The search strategy was as follows:
Ovid MEDLINE(R) ALL
(scoping review or evidence map or systematic map or mapping review or scoping study or scoping project or scoping exercise or literature mapping or evidence mapping or systematic mapping or literature scoping or evidence gap map).ab,ti.
limit 1 to english language
(202006* or 202007* or 202008* or 202009* or 202010* or 202011* or 202012* or 202101* or 202102* or 202103* or 202104* or 202105*).dt.
The search returned 3686 records. Records were de-duplicated in EndNote 20 software, leaving 3627 unique records.
A sample of these reviews was taken by screening the search results against basic selection criteria (Table 1 ). These criteria were piloted and refined after discussion between the two researchers. A single researcher (E.S.) screened the records in EPPI-Reviewer Web software using the machine-learning priority screening function. Where a second opinion was needed, decisions were checked by a second researcher (M.R.).
Our initial plan for sampling, informed by pilot searching, was to screen and data extract records in batches of 50 included reviews at a time. We planned to stop screening when a batch of 50 reviews had been extracted that included no new types of data visualisation or after screening time had reached 2 days. However, once data extraction was underway, we found the sample to be richer in terms of data visualisation than anticipated. After the inclusion of 300 reviews, we took the decision to end screening in order to ensure the study was manageable.
Data extraction
A data extraction form was developed in EPPI-Reviewer Web, piloted on 50 reviews and refined. Data were extracted by one researcher (E. S. or M. R.), with a second researcher (M. R. or E. S.) providing a second opinion when needed. The data items extracted were as follows: type of review (term used by authors), aim of review (mapping evidence vs. answering specific question vs. borderline), number of visualisations (if any), types of data visualisation used, variables/domains presented by each visualisation type, interactivity, use of colour and any software requirements.
When categorising review aims, we considered “mapping evidence” to incorporate all of the six purposes for conducting a scoping review proposed by Munn et al. [ 3 ]. Reviews were categorised as “answering a specific question” if they aimed to synthesise study findings to answer a particular question, for example on effectiveness of an intervention. We were inclusive with our definition of “mapping evidence” and included reviews with mixed aims in this category. However, some reviews were difficult to categorise (for example where aims were unclear or the stated aims did not match the actual focus of the paper) and were considered to be “borderline”. It became clear that a proportion of identified records that described themselves as “scoping” or “mapping” reviews were in fact pseudo-systematic reviews that failed to undertake key systematic review processes. Such reviews attempted to integrate the findings of included studies rather than map the evidence, and so reviews categorised as “answering a specific question” were excluded from the main analysis. Data visualisation methods for meta-analyses have been explored previously [ 19 ]. Figure 1 shows the flow of records from search results to final analysis sample.
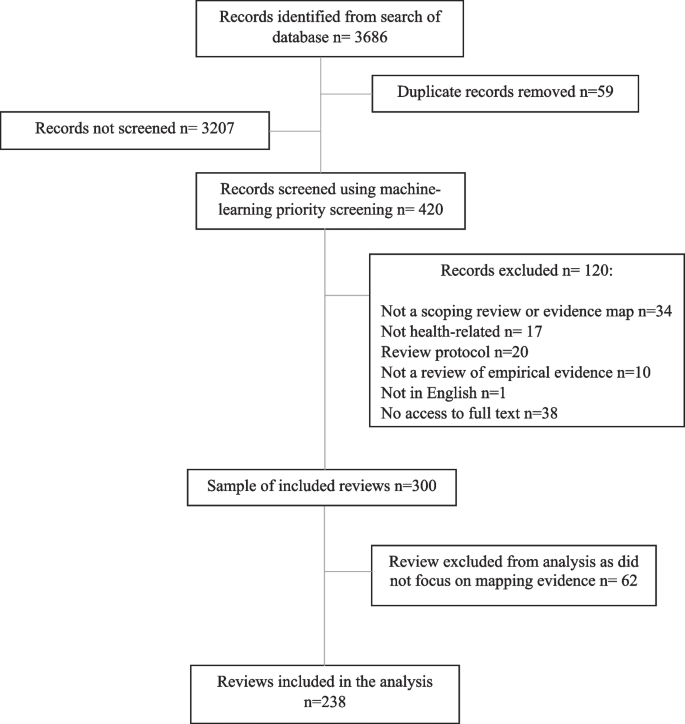
Flow diagram of the sampling process
Data visualisation was defined as any graph or diagram that presented results data, including tables with a visual mapping element, such as cross-tabulations and heat maps. However, tables which displayed data at a study level (e.g. tables summarising key characteristics of each included study) were not included, even if they used symbols, shading or colour. Flow diagrams showing the study selection process were also excluded. Data visualisations in appendices or supplementary information were included, as well as any in publicly available dissemination products (e.g. visualisations hosted online) if mentioned in papers.
The typology used to categorise data visualisation methods was based on an existing online catalogue [ 20 ]. Specific types of data visualisation were categorised in five broad categories: graphs, diagrams, tables, maps/geographical and other. If a data visualisation appeared in our sample that did not feature in the original catalogue, we checked a second online catalogue [ 21 ] for an appropriate term, followed by wider Internet searches. These additional visualisation methods were added to the appropriate section of the typology. The final typology can be found in Additional file 1 .
We conducted descriptive data analysis in Microsoft Excel 2019 and present frequencies and percentages. Where appropriate, data are presented using graphs or other data visualisations created using Flourish. We also link to interactive versions of some of these visualisations.
Almost all of the 300 reviews in the total sample were labelled by review authors as “scoping reviews” ( n = 293, 97.7%). There were also four “mapping reviews”, one “scoping study”, one “evidence mapping” and one that was described as a “scoping review and evidence map”. Included reviews were all published in 2020 or 2021, with the exception of one review published in 2018. Just over one-third of these reviews ( n = 105, 35.0%) included some form of data visualisation. However, we excluded 62 reviews that did not focus on mapping evidence from the following analysis (see “ Methods ” section). Of the 238 remaining reviews (that either clearly aimed to map evidence or were judged to be “borderline”), 90 reviews (37.8%) included at least one data visualisation. The references for these reviews can be found in Additional file 2 .
Number of visualisations
Thirty-six (40.0%) of these 90 reviews included just one example of data visualisation (Fig. 2 ). Less than a third ( n = 28, 31.1%) included three or more visualisations. The greatest number of data visualisations in one review was 17 (all bar or pie charts). In total, 222 individual data visualisations were identified across the sample of 238 reviews.
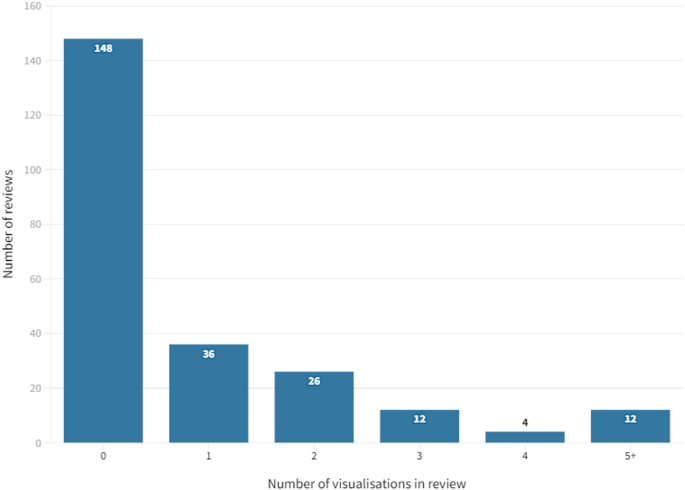
Number of data visualisations per review
Categories of data visualisation
Graphs were the most frequently used category of data visualisation in the sample. Over half of the reviews with data visualisation included at least one graph ( n = 59, 65.6%). The least frequently used category was maps, with 15.6% ( n = 14) of these reviews including a map.
Of the total number of 222 individual data visualisations, 102 were graphs (45.9%), 34 were tables (15.3%), 23 were diagrams (10.4%), 15 were maps (6.8%) and 48 were classified as “other” in the typology (21.6%).
Types of data visualisation
All of the types of data visualisation identified in our sample are reported in Table 2 . In total, 35 different types were used across the sample of reviews.
The most frequently used data visualisation type was a bar chart. Of 222 total data visualisations, 78 (35.1%) were a variation on a bar chart (either standard bar chart, stacked bar chart or multi-set bar chart). There were also 33 pie charts (14.9% of data visualisations) and 24 cross-tabulations (10.8% of data visualisations). In total, these five types of data visualisation accounted for 60.8% ( n = 135) of all data visualisations. Figure 3 shows the frequency of each data visualisation category and type; an interactive online version of this treemap is also available ( https://public.flourish.studio/visualisation/9396133/ ). Figure 4 shows how users can further explore the data using the interactive treemap.
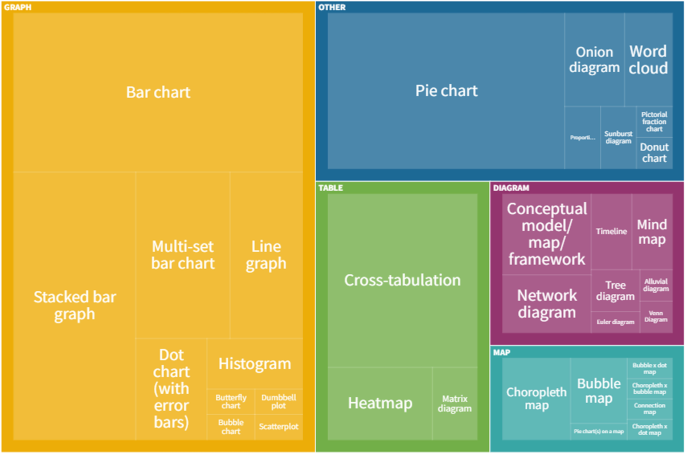
Data visualisation categories and types. An interactive version of this treemap is available online: https://public.flourish.studio/visualisation/9396133/ . Through the interactive version, users can further explore the data (see Fig. 4 ). The unit of this treemap is the individual data visualisation, so multiple data visualisations within the same scoping review are represented in this map. Created with flourish.studio ( https://flourish.studio )
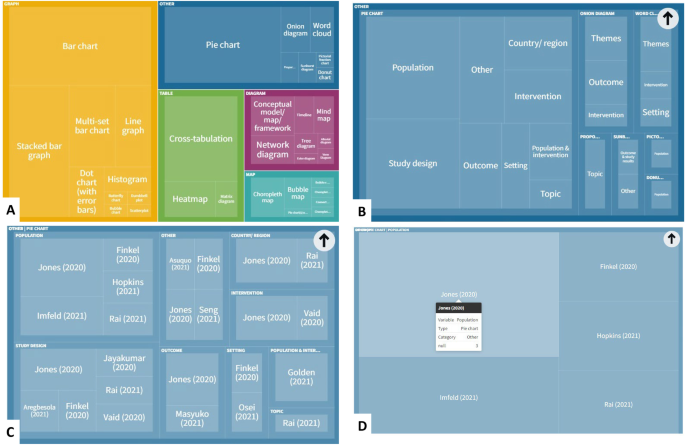
Screenshots showing how users of the interactive treemap can explore the data further. Users can explore each level of the hierarchical treemap ( A Visualisation category > B Visualisation subcategory > C Variables presented in visualisation > D Individual references reporting this category/subcategory/variable permutation). Created with flourish.studio ( https://flourish.studio )
Data presented
Around two-thirds of data visualisations in the sample presented a single variable ( n = 143, 64.4%). The most frequently presented single variables were themes ( n = 22, 9.9% of data visualisations), population ( n = 21, 9.5%), country or region ( n = 21, 9.5%) and year ( n = 20, 9.0%). There were 58 visualisations (26.1%) that presented two different variables. The remaining 21 data visualisations (9.5%) presented three or more variables. Figure 5 shows the variables presented by each different type of data visualisation (an interactive version of this figure is available online).
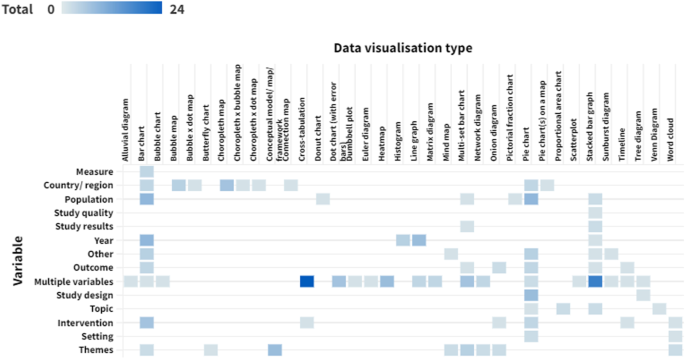
Variables presented by each data visualisation type. Darker cells indicate a larger number of reviews. An interactive version of this heat map is available online: https://public.flourish.studio/visualisation/10632665/ . Users can hover over each cell to see the number of data visualisations for that combination of data visualisation type and variable. The unit of this heat map is the individual data visualisation, so multiple data visualisations within a single scoping review are represented in this map. Created with flourish.studio ( https://flourish.studio )
Most reviews presented at least one data visualisation in colour ( n = 64, 71.1%). However, almost a third ( n = 26, 28.9%) used only black and white or greyscale.
Interactivity
Only two of the reviews included data visualisations with any level of interactivity. One scoping review on music and serious mental illness [ 22 ] linked to an interactive bubble chart hosted online on Tableau. Functionality included the ability to filter the studies displayed by various attributes.
The other review was an example of evidence mapping from the environmental health field [ 23 ]. All four of the data visualisations included in the paper were available in an interactive format hosted either by the review management software or on Tableau. The interactive versions linked to the relevant references so users could directly explore the evidence base. This was the only review that provided this feature.
Software requirements
Nine reviews clearly reported the software used to create data visualisations. Three reviews used Tableau (one of them also used review management software as discussed above) [ 22 , 23 , 24 ]. Two reviews generated maps using ArcGIS [ 25 ] or ArcMap [ 26 ]. One review used Leximancer for a lexical analysis [ 27 ]. One review undertook a bibliometric analysis using VOSviewer [ 28 ], and another explored citation patterns using CitNetExplorer [ 29 ]. Other reviews used Excel [ 30 ] or R [ 26 ].
To our knowledge, this is the first systematic and in-depth exploration of the use of data visualisation techniques in scoping reviews. Our findings suggest that the majority of scoping reviews do not use any data visualisation at all, and, in particular, more innovative examples of data visualisation are rare. Around 60% of data visualisations in our sample were simple bar charts, pie charts or cross-tabulations. There appears to be very limited use of interactive online visualisation, despite the potential this has for communicating results to a range of stakeholders. While it is not always appropriate to use data visualisation (or a simple bar chart may be the most user-friendly way of presenting the data), these findings suggest that data visualisation is being underused in scoping reviews. In a large minority of reviews, visualisations were not published in colour, potentially limiting how user-friendly and attractive papers are to decision-makers and other stakeholders. Also, very few reviews clearly reported the software used to create data visualisations. However, 35 different types of data visualisation were used across the sample, highlighting the wide range of methods that are potentially available to scoping review authors.
Our results build on the limited research that has previously been undertaken in this area. Two previous publications also found limited use of graphs in scoping reviews. Results were “mapped graphically” in 29% of scoping reviews in any field in one 2014 publication [ 31 ] and 17% of healthcare scoping reviews in a 2016 article [ 6 ]. Our results suggest that the use of data visualisation has increased somewhat since these reviews were conducted. Scoping review methods have also evolved in the last 10 years; formal guidance on scoping review conduct was published in 2014 [ 32 ], and an extension of the PRISMA checklist for scoping reviews was published in 2018 [ 33 ]. It is possible that an overall increase in use of data visualisation reflects increased quality of published scoping reviews. There is also some literature supporting our findings on the wide range of data visualisation methods that are used in evidence synthesis. An investigation of methods to identify, prioritise or display health research gaps (25/139 included studies were scoping reviews; 6/139 were evidence maps) identified 14 different methods used to display gaps or priorities, with half being “more advanced” (e.g. treemaps, radial bar plots) ([ 34 ], p. 107). A review of data visualisation methods used in papers reporting meta-analyses found over 200 different ways of displaying data [ 19 ].
Only two reviews in our sample used interactive data visualisation, and one of these was an example of systematic evidence mapping from the environmental health field rather than a scoping review (in environmental health, systematic evidence mapping explicitly involves producing a searchable database [ 35 ]). A scoping review of papers on the use of interactive data visualisation in population health or health services research found a range of examples but still limited use overall [ 13 ]. For example, the authors noted the currently underdeveloped potential for using interactive visualisation in research on health inequalities. It is possible that the use of interactive data visualisation in academic papers is restricted by academic publishing requirements; for example, it is currently difficult to incorporate an interactive figure into a journal article without linking to an external host or platform. However, we believe that there is a lot of potential to add value to future scoping reviews by using interactive data visualisation software. Few reviews in our sample presented three or more variables in a single visualisation, something which can easily be achieved using interactive data visualisation tools. We have previously used EPPI-Mapper [ 36 ] to present results of a scoping review of systematic reviews on behaviour change in disadvantaged groups, with links to the maps provided in the paper [ 37 ]. These interactive maps allowed policy-makers to explore the evidence on different behaviours and disadvantaged groups and access full publications of the included studies directly from the map.
We acknowledge there are barriers to use for some of the data visualisation software available. EPPI-Mapper and some of the software used by reviews in our sample incur a cost. Some software requires a certain level of knowledge and skill in its use. However numerous online free data visualisation tools and resources exist. We have used Flourish to present data for this review, a basic version of which is currently freely available and easy to use. Previous health research has been found to have used a range of different interactive data visualisation software, much of which does not required advanced knowledge or skills to use [ 13 ].
There are likely to be other barriers to the use of data visualisation in scoping reviews. Journal guidelines and policies may present barriers for using innovative data visualisation. For example, some journals charge a fee for publication of figures in colour. As previously mentioned, there are limited options for incorporating interactive data visualisation into journal articles. Authors may also be unaware of the data visualisation methods and tools that are available. Producing data visualisations can be time-consuming, particularly if authors lack experience and skills in this. It is possible that many authors prioritise speed of publication over spending time producing innovative data visualisations, particularly in a context where there is pressure to achieve publications.
Limitations
A limitation of this study was that we did not assess how appropriate the use of data visualisation was in our sample as this would have been highly subjective. Simple descriptive or tabular presentation of results may be the most appropriate approach for some scoping review objectives [ 7 , 8 , 10 ], and the scoping review literature cautions against “over-using” different visual presentation methods [ 7 , 8 ]. It cannot be assumed that all of the reviews that did not include data visualisation should have done so. Likewise, we do not know how many reviews used methods of data visualisation that were not well suited to their data.
We initially relied on authors’ own use of the term “scoping review” (or equivalent) to sample reviews but identified a relatively large number of papers labelled as scoping reviews that did not meet the basic definition, despite the availability of guidance and reporting guidelines [ 10 , 33 ]. It has previously been noted that scoping reviews may be undertaken inappropriately because they are seen as “easier” to conduct than a systematic review ([ 3 ], p.6), and that reviews are often labelled as “scoping reviews” while not appearing to follow any established framework or guidance [ 2 ]. We therefore took the decision to remove these reviews from our main analysis. However, decisions on how to classify review aims were subjective, and we did include some reviews that were of borderline relevance.
A further limitation is that this was a sample of published reviews, rather than a comprehensive systematic scoping review as have previously been undertaken [ 6 , 31 ]. The number of scoping reviews that are published has increased rapidly, and this would now be difficult to undertake. As this was a sample, not all relevant scoping reviews or evidence maps that would have met our criteria were included. We used machine learning to screen our search results for pragmatic reasons (to reduce screening time), but we do not see any reason that our sample would not be broadly reflective of the wider literature.
Data visualisation, and in particular more innovative examples of it, is currently underused in published scoping reviews on health topics. The examples that we have found highlight the wide range of methods that scoping review authors could draw upon to present their data in an engaging way. In particular, we believe that interactive data visualisation has significant potential for mapping the available literature on a topic. Appropriate use of data visualisation may increase the usefulness, and thus uptake, of scoping reviews as a way of identifying existing evidence or research gaps by decision-makers, researchers and commissioners of research. We recommend that scoping review authors explore the extensive free resources and online tools available for data visualisation. However, we also think that it would be useful for publishers to explore allowing easier integration of interactive tools into academic publishing, given the fact that papers are now predominantly accessed online. Future research may be helpful to explore which methods are particularly useful to scoping review users.
Availability of data and materials
The datasets used and/or analysed during the current study are available from the corresponding author on reasonable request.
Abbreviations
Organisation formerly known as Joanna Briggs Institute
Preferred Reporting Items for Systematic Reviews and Meta-Analyses
Munn Z, Pollock D, Khalil H, Alexander L, McLnerney P, Godfrey CM, Peters M, Tricco AC. What are scoping reviews? Providing a formal definition of scoping reviews as a type of evidence synthesis. JBI Evid Synth. 2022;20:950–952.
Peters MDJ, Marnie C, Colquhoun H, Garritty CM, Hempel S, Horsley T, Langlois EV, Lillie E, O’Brien KK, Tunçalp Ӧ, et al. Scoping reviews: reinforcing and advancing the methodology and application. Syst Rev. 2021;10:263.
Article PubMed PubMed Central Google Scholar
Munn Z, Peters MDJ, Stern C, Tufanaru C, McArthur A, Aromataris E. Systematic review or scoping review? Guidance for authors when choosing between a systematic or scoping review approach. BMC Med Res Methodol. 2018;18:143.
Sutton A, Clowes M, Preston L, Booth A. Meeting the review family: exploring review types and associated information retrieval requirements. Health Info Libr J. 2019;36:202–22.
Article PubMed Google Scholar
Miake-Lye IM, Hempel S, Shanman R, Shekelle PG. What is an evidence map? A systematic review of published evidence maps and their definitions, methods, and products. Syst Rev. 2016;5:28.
Tricco AC, Lillie E, Zarin W, O’Brien K, Colquhoun H, Kastner M, Levac D, Ng C, Sharpe JP, Wilson K, et al. A scoping review on the conduct and reporting of scoping reviews. BMC Med Res Methodol. 2016;16:15.
Khalil H, Peters MDJ, Tricco AC, Pollock D, Alexander L, McInerney P, Godfrey CM, Munn Z. Conducting high quality scoping reviews-challenges and solutions. J Clin Epidemiol. 2021;130:156–60.
Lockwood C, dos Santos KB, Pap R. Practical guidance for knowledge synthesis: scoping review methods. Asian Nurs Res. 2019;13:287–94.
Article Google Scholar
Pollock D, Peters MDJ, Khalil H, McInerney P, Alexander L, Tricco AC, Evans C, de Moraes ÉB, Godfrey CM, Pieper D, et al. Recommendations for the extraction, analysis, and presentation of results in scoping reviews. JBI Evidence Synthesis. 2022;10:11124.
Google Scholar
Peters MDJ GC, McInerney P, Munn Z, Tricco AC, Khalil, H. Chapter 11: Scoping reviews (2020 version). In: Aromataris E MZ, editor. JBI Manual for Evidence Synthesis. JBI; 2020. Available from https://synthesismanual.jbi.global . Accessed 1 Feb 2023.
Tableau Public. https://www.tableau.com/en-gb/products/public . Accessed 24 January 2023.
flourish.studio. https://flourish.studio/ . Accessed 24 January 2023.
Chishtie J, Bielska IA, Barrera A, Marchand J-S, Imran M, Tirmizi SFA, Turcotte LA, Munce S, Shepherd J, Senthinathan A, et al. Interactive visualization applications in population health and health services research: systematic scoping review. J Med Internet Res. 2022;24: e27534.
Isett KR, Hicks DM. Providing public servants what they need: revealing the “unseen” through data visualization. Public Adm Rev. 2018;78:479–85.
Carroll LN, Au AP, Detwiler LT, Fu T-c, Painter IS, Abernethy NF. Visualization and analytics tools for infectious disease epidemiology: a systematic review. J Biomed Inform. 2014;51:287–298.
Lundkvist A, El-Khatib Z, Kalra N, Pantoja T, Leach-Kemon K, Gapp C, Kuchenmüller T. Policy-makers’ views on translating burden of disease estimates in health policies: bridging the gap through data visualization. Arch Public Health. 2021;79:17.
Zakkar M, Sedig K. Interactive visualization of public health indicators to support policymaking: an exploratory study. Online J Public Health Inform. 2017;9:e190–e190.
Park S, Bekemeier B, Flaxman AD. Understanding data use and preference of data visualization for public health professionals: a qualitative study. Public Health Nurs. 2021;38:531–41.
Kossmeier M, Tran US, Voracek M. Charting the landscape of graphical displays for meta-analysis and systematic reviews: a comprehensive review, taxonomy, and feature analysis. BMC Med Res Methodol. 2020;20:26.
Ribecca, S. The Data Visualisation Catalogue. https://datavizcatalogue.com/index.html . Accessed 23 November 2021.
Ferdio. Data Viz Project. https://datavizproject.com/ . Accessed 23 November 2021.
Golden TL, Springs S, Kimmel HJ, Gupta S, Tiedemann A, Sandu CC, Magsamen S. The use of music in the treatment and management of serious mental illness: a global scoping review of the literature. Front Psychol. 2021;12: 649840.
Keshava C, Davis JA, Stanek J, Thayer KA, Galizia A, Keshava N, Gift J, Vulimiri SV, Woodall G, Gigot C, et al. Application of systematic evidence mapping to assess the impact of new research when updating health reference values: a case example using acrolein. Environ Int. 2020;143: 105956.
Article CAS PubMed PubMed Central Google Scholar
Jayakumar P, Lin E, Galea V, Mathew AJ, Panda N, Vetter I, Haynes AB. Digital phenotyping and patient-generated health data for outcome measurement in surgical care: a scoping review. J Pers Med. 2020;10:282.
Qu LG, Perera M, Lawrentschuk N, Umbas R, Klotz L. Scoping review: hotspots for COVID-19 urological research: what is being published and from where? World J Urol. 2021;39:3151–60.
Article CAS PubMed Google Scholar
Rossa-Roccor V, Acheson ES, Andrade-Rivas F, Coombe M, Ogura S, Super L, Hong A. Scoping review and bibliometric analysis of the term “planetary health” in the peer-reviewed literature. Front Public Health. 2020;8:343.
Hewitt L, Dahlen HG, Hartz DL, Dadich A. Leadership and management in midwifery-led continuity of care models: a thematic and lexical analysis of a scoping review. Midwifery. 2021;98: 102986.
Xia H, Tan S, Huang S, Gan P, Zhong C, Lu M, Peng Y, Zhou X, Tang X. Scoping review and bibliometric analysis of the most influential publications in achalasia research from 1995 to 2020. Biomed Res Int. 2021;2021:8836395.
Vigliotti V, Taggart T, Walker M, Kusmastuti S, Ransome Y. Religion, faith, and spirituality influences on HIV prevention activities: a scoping review. PLoS ONE. 2020;15: e0234720.
van Heemskerken P, Broekhuizen H, Gajewski J, Brugha R, Bijlmakers L. Barriers to surgery performed by non-physician clinicians in sub-Saharan Africa-a scoping review. Hum Resour Health. 2020;18:51.
Pham MT, Rajić A, Greig JD, Sargeant JM, Papadopoulos A, McEwen SA. A scoping review of scoping reviews: advancing the approach and enhancing the consistency. Res Synth Methods. 2014;5:371–85.
Peters MDJ, Marnie C, Tricco AC, Pollock D, Munn Z, Alexander L, McInerney P, Godfrey CM, Khalil H. Updated methodological guidance for the conduct of scoping reviews. JBI Evid Synth. 2020;18:2119–26.
Tricco AC, Lillie E, Zarin W, O’Brien KK, Colquhoun H, Levac D, Moher D, Peters MDJ, Horsley T, Weeks L, et al. PRISMA Extension for Scoping Reviews (PRISMA-ScR): checklist and explanation. Ann Intern Med. 2018;169:467–73.
Nyanchoka L, Tudur-Smith C, Thu VN, Iversen V, Tricco AC, Porcher R. A scoping review describes methods used to identify, prioritize and display gaps in health research. J Clin Epidemiol. 2019;109:99–110.
Wolffe TAM, Whaley P, Halsall C, Rooney AA, Walker VR. Systematic evidence maps as a novel tool to support evidence-based decision-making in chemicals policy and risk management. Environ Int. 2019;130:104871.
Digital Solution Foundry and EPPI-Centre. EPPI-Mapper, Version 2.0.1. EPPI-Centre, UCL Social Research Institute, University College London. 2020. https://eppi.ioe.ac.uk/cms/Default.aspx?tabid=3790 .
South E, Rodgers M, Wright K, Whitehead M, Sowden A. Reducing lifestyle risk behaviours in disadvantaged groups in high-income countries: a scoping review of systematic reviews. Prev Med. 2022;154: 106916.
Download references
Acknowledgements
We would like to thank Melissa Harden, Senior Information Specialist, Centre for Reviews and Dissemination, for advice on developing the search strategy.
This work received no external funding.
Author information
Authors and affiliations.
Centre for Reviews and Dissemination, University of York, York, YO10 5DD, UK
Emily South & Mark Rodgers
You can also search for this author in PubMed Google Scholar
Contributions
Both authors conceptualised and designed the study and contributed to screening, data extraction and the interpretation of results. ES undertook the literature searches, analysed data, produced the data visualisations and drafted the manuscript. MR contributed to revising the manuscript, and both authors read and approved the final version.
Corresponding author
Correspondence to Emily South .
Ethics declarations
Ethics approval and consent to participate.
Not applicable.
Consent for publication
Competing interests.
The authors declare that they have no competing interests.
Additional information
Publisher’s note.
Springer Nature remains neutral with regard to jurisdictional claims in published maps and institutional affiliations.
Supplementary Information
Additional file 1..
Typology of data visualisation methods.
Additional file 2.
References of scoping reviews included in main dataset.
Rights and permissions
Open Access This article is licensed under a Creative Commons Attribution 4.0 International License, which permits use, sharing, adaptation, distribution and reproduction in any medium or format, as long as you give appropriate credit to the original author(s) and the source, provide a link to the Creative Commons licence, and indicate if changes were made. The images or other third party material in this article are included in the article's Creative Commons licence, unless indicated otherwise in a credit line to the material. If material is not included in the article's Creative Commons licence and your intended use is not permitted by statutory regulation or exceeds the permitted use, you will need to obtain permission directly from the copyright holder. To view a copy of this licence, visit http://creativecommons.org/licenses/by/4.0/ . The Creative Commons Public Domain Dedication waiver ( http://creativecommons.org/publicdomain/zero/1.0/ ) applies to the data made available in this article, unless otherwise stated in a credit line to the data.
Reprints and permissions
About this article
Cite this article.
South, E., Rodgers, M. Data visualisation in scoping reviews and evidence maps on health topics: a cross-sectional analysis. Syst Rev 12 , 142 (2023). https://doi.org/10.1186/s13643-023-02309-y
Download citation
Received : 21 February 2023
Accepted : 07 August 2023
Published : 17 August 2023
DOI : https://doi.org/10.1186/s13643-023-02309-y
Share this article
Anyone you share the following link with will be able to read this content:
Sorry, a shareable link is not currently available for this article.
Provided by the Springer Nature SharedIt content-sharing initiative
- Scoping review
- Evidence map
- Data visualisation
Systematic Reviews
ISSN: 2046-4053
- Submission enquiries: Access here and click Contact Us
- General enquiries: [email protected]
Thank you for visiting nature.com. You are using a browser version with limited support for CSS. To obtain the best experience, we recommend you use a more up to date browser (or turn off compatibility mode in Internet Explorer). In the meantime, to ensure continued support, we are displaying the site without styles and JavaScript.
- View all journals
- My Account Login
- Explore content
- About the journal
- Publish with us
- Sign up for alerts
- Data Descriptor
- Open access
- Published: 03 May 2024
A dataset for measuring the impact of research data and their curation
- Libby Hemphill ORCID: orcid.org/0000-0002-3793-7281 1 , 2 ,
- Andrea Thomer 3 ,
- Sara Lafia 1 ,
- Lizhou Fan 2 ,
- David Bleckley ORCID: orcid.org/0000-0001-7715-4348 1 &
- Elizabeth Moss 1
Scientific Data volume 11 , Article number: 442 ( 2024 ) Cite this article
583 Accesses
8 Altmetric
Metrics details
- Research data
- Social sciences
Science funders, publishers, and data archives make decisions about how to responsibly allocate resources to maximize the reuse potential of research data. This paper introduces a dataset developed to measure the impact of archival and data curation decisions on data reuse. The dataset describes 10,605 social science research datasets, their curation histories, and reuse contexts in 94,755 publications that cover 59 years from 1963 to 2022. The dataset was constructed from study-level metadata, citing publications, and curation records available through the Inter-university Consortium for Political and Social Research (ICPSR) at the University of Michigan. The dataset includes information about study-level attributes (e.g., PIs, funders, subject terms); usage statistics (e.g., downloads, citations); archiving decisions (e.g., curation activities, data transformations); and bibliometric attributes (e.g., journals, authors) for citing publications. This dataset provides information on factors that contribute to long-term data reuse, which can inform the design of effective evidence-based recommendations to support high-impact research data curation decisions.
Similar content being viewed by others
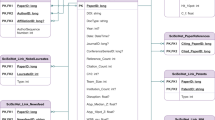
SciSciNet: A large-scale open data lake for the science of science research
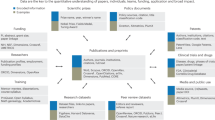
Data, measurement and empirical methods in the science of science
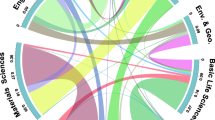
Interdisciplinarity revisited: evidence for research impact and dynamism
Background & summary.
Recent policy changes in funding agencies and academic journals have increased data sharing among researchers and between researchers and the public. Data sharing advances science and provides the transparency necessary for evaluating, replicating, and verifying results. However, many data-sharing policies do not explain what constitutes an appropriate dataset for archiving or how to determine the value of datasets to secondary users 1 , 2 , 3 . Questions about how to allocate data-sharing resources efficiently and responsibly have gone unanswered 4 , 5 , 6 . For instance, data-sharing policies recognize that not all data should be curated and preserved, but they do not articulate metrics or guidelines for determining what data are most worthy of investment.
Despite the potential for innovation and advancement that data sharing holds, the best strategies to prioritize datasets for preparation and archiving are often unclear. Some datasets are likely to have more downstream potential than others, and data curation policies and workflows should prioritize high-value data instead of being one-size-fits-all. Though prior research in library and information science has shown that the “analytic potential” of a dataset is key to its reuse value 7 , work is needed to implement conceptual data reuse frameworks 8 , 9 , 10 , 11 , 12 , 13 , 14 . In addition, publishers and data archives need guidance to develop metrics and evaluation strategies to assess the impact of datasets.
Several existing resources have been compiled to study the relationship between the reuse of scholarly products, such as datasets (Table 1 ); however, none of these resources include explicit information on how curation processes are applied to data to increase their value, maximize their accessibility, and ensure their long-term preservation. The CCex (Curation Costs Exchange) provides models of curation services along with cost-related datasets shared by contributors but does not make explicit connections between them or include reuse information 15 . Analyses on platforms such as DataCite 16 have focused on metadata completeness and record usage, but have not included related curation-level information. Analyses of GenBank 17 and FigShare 18 , 19 citation networks do not include curation information. Related studies of Github repository reuse 20 and Softcite software citation 21 reveal significant factors that impact the reuse of secondary research products but do not focus on research data. RD-Switchboard 22 and DSKG 23 are scholarly knowledge graphs linking research data to articles, patents, and grants, but largely omit social science research data and do not include curation-level factors. To our knowledge, other studies of curation work in organizations similar to ICPSR – such as GESIS 24 , Dataverse 25 , and DANS 26 – have not made their underlying data available for analysis.
This paper describes a dataset 27 compiled for the MICA project (Measuring the Impact of Curation Actions) led by investigators at ICPSR, a large social science data archive at the University of Michigan. The dataset was originally developed to study the impacts of data curation and archiving on data reuse. The MICA dataset has supported several previous publications investigating the intensity of data curation actions 28 , the relationship between data curation actions and data reuse 29 , and the structures of research communities in a data citation network 30 . Collectively, these studies help explain the return on various types of curatorial investments. The dataset that we introduce in this paper, which we refer to as the MICA dataset, has the potential to address research questions in the areas of science (e.g., knowledge production), library and information science (e.g., scholarly communication), and data archiving (e.g., reproducible workflows).
We constructed the MICA dataset 27 using records available at ICPSR, a large social science data archive at the University of Michigan. Data set creation involved: collecting and enriching metadata for articles indexed in the ICPSR Bibliography of Data-related Literature against the Dimensions AI bibliometric database; gathering usage statistics for studies from ICPSR’s administrative database; processing data curation work logs from ICPSR’s project tracking platform, Jira; and linking data in social science studies and series to citing analysis papers (Fig. 1 ).
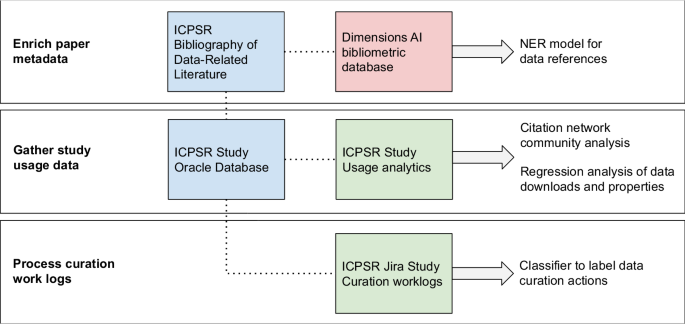
Steps to prepare MICA dataset for analysis - external sources are red, primary internal sources are blue, and internal linked sources are green.
Enrich paper metadata
The ICPSR Bibliography of Data-related Literature is a growing database of literature in which data from ICPSR studies have been used. Its creation was funded by the National Science Foundation (Award 9977984), and for the past 20 years it has been supported by ICPSR membership and multiple US federally-funded and foundation-funded topical archives at ICPSR. The Bibliography was originally launched in the year 2000 to aid in data discovery by providing a searchable database linking publications to the study data used in them. The Bibliography collects the universe of output based on the data shared in each study through, which is made available through each ICPSR study’s webpage. The Bibliography contains both peer-reviewed and grey literature, which provides evidence for measuring the impact of research data. For an item to be included in the ICPSR Bibliography, it must contain an analysis of data archived by ICPSR or contain a discussion or critique of the data collection process, study design, or methodology 31 . The Bibliography is manually curated by a team of librarians and information specialists at ICPSR who enter and validate entries. Some publications are supplied to the Bibliography by data depositors, and some citations are submitted to the Bibliography by authors who abide by ICPSR’s terms of use requiring them to submit citations to works in which they analyzed data retrieved from ICPSR. Most of the Bibliography is populated by Bibliography team members, who create custom queries for ICPSR studies performed across numerous sources, including Google Scholar, ProQuest, SSRN, and others. Each record in the Bibliography is one publication that has used one or more ICPSR studies. The version we used was captured on 2021-11-16 and included 94,755 publications.
To expand the coverage of the ICPSR Bibliography, we searched exhaustively for all ICPSR study names, unique numbers assigned to ICPSR studies, and DOIs 32 using a full-text index available through the Dimensions AI database 33 . We accessed Dimensions through a license agreement with the University of Michigan. ICPSR Bibliography librarians and information specialists manually reviewed and validated new entries that matched one or more search criteria. We then used Dimensions to gather enriched metadata and full-text links for items in the Bibliography with DOIs. We matched 43% of the items in the Bibliography to enriched Dimensions metadata including abstracts, field of research codes, concepts, and authors’ institutional information; we also obtained links to full text for 16% of Bibliography items. Based on licensing agreements, we included Dimensions identifiers and links to full text so that users with valid publisher and database access can construct an enriched publication dataset.
Gather study usage data
ICPSR maintains a relational administrative database, DBInfo, that organizes study-level metadata and information on data reuse across separate tables. Studies at ICPSR consist of one or more files collected at a single time or for a single purpose; studies in which the same variables are observed over time are grouped into series. Each study at ICPSR is assigned a DOI, and its metadata are stored in DBInfo. Study metadata follows the Data Documentation Initiative (DDI) Codebook 2.5 standard. DDI elements included in our dataset are title, ICPSR study identification number, DOI, authoring entities, description (abstract), funding agencies, subject terms assigned to the study during curation, and geographic coverage. We also created variables based on DDI elements: total variable count, the presence of survey question text in the metadata, the number of author entities, and whether an author entity was an institution. We gathered metadata for ICPSR’s 10,605 unrestricted public-use studies available as of 2021-11-16 ( https://www.icpsr.umich.edu/web/pages/membership/or/metadata/oai.html ).
To link study usage data with study-level metadata records, we joined study metadata from DBinfo on study usage information, which included total study downloads (data and documentation), individual data file downloads, and cumulative citations from the ICPSR Bibliography. We also gathered descriptive metadata for each study and its variables, which allowed us to summarize and append recoded fields onto the study-level metadata such as curation level, number and type of principle investigators, total variable count, and binary variables indicating whether the study data were made available for online analysis, whether survey question text was made searchable online, and whether the study variables were indexed for search. These characteristics describe aspects of the discoverability of the data to compare with other characteristics of the study. We used the study and series numbers included in the ICPSR Bibliography as unique identifiers to link papers to metadata and analyze the community structure of dataset co-citations in the ICPSR Bibliography 32 .
Process curation work logs
Researchers deposit data at ICPSR for curation and long-term preservation. Between 2016 and 2020, more than 3,000 research studies were deposited with ICPSR. Since 2017, ICPSR has organized curation work into a central unit that provides varied levels of curation that vary in the intensity and complexity of data enhancement that they provide. While the levels of curation are standardized as to effort (level one = less effort, level three = most effort), the specific curatorial actions undertaken for each dataset vary. The specific curation actions are captured in Jira, a work tracking program, which data curators at ICPSR use to collaborate and communicate their progress through tickets. We obtained access to a corpus of 669 completed Jira tickets corresponding to the curation of 566 unique studies between February 2017 and December 2019 28 .
To process the tickets, we focused only on their work log portions, which contained free text descriptions of work that data curators had performed on a deposited study, along with the curators’ identifiers, and timestamps. To protect the confidentiality of the data curators and the processing steps they performed, we collaborated with ICPSR’s curation unit to propose a classification scheme, which we used to train a Naive Bayes classifier and label curation actions in each work log sentence. The eight curation action labels we proposed 28 were: (1) initial review and planning, (2) data transformation, (3) metadata, (4) documentation, (5) quality checks, (6) communication, (7) other, and (8) non-curation work. We note that these categories of curation work are very specific to the curatorial processes and types of data stored at ICPSR, and may not match the curation activities at other repositories. After applying the classifier to the work log sentences, we obtained summary-level curation actions for a subset of all ICPSR studies (5%), along with the total number of hours spent on data curation for each study, and the proportion of time associated with each action during curation.
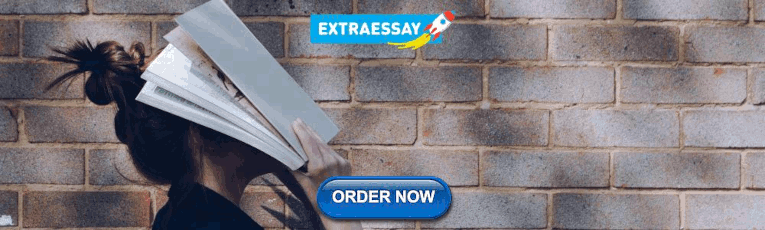
Data Records
The MICA dataset 27 connects records for each of ICPSR’s archived research studies to the research publications that use them and related curation activities available for a subset of studies (Fig. 2 ). Each of the three tables published in the dataset is available as a study archived at ICPSR. The data tables are distributed as statistical files available for use in SAS, SPSS, Stata, and R as well as delimited and ASCII text files. The dataset is organized around studies and papers as primary entities. The studies table lists ICPSR studies, their metadata attributes, and usage information; the papers table was constructed using the ICPSR Bibliography and Dimensions database; and the curation logs table summarizes the data curation steps performed on a subset of ICPSR studies.
Studies (“ICPSR_STUDIES”): 10,605 social science research datasets available through ICPSR up to 2021-11-16 with variables for ICPSR study number, digital object identifier, study name, series number, series title, authoring entities, full-text description, release date, funding agency, geographic coverage, subject terms, topical archive, curation level, single principal investigator (PI), institutional PI, the total number of PIs, total variables in data files, question text availability, study variable indexing, level of restriction, total unique users downloading study data files and codebooks, total unique users downloading data only, and total unique papers citing data through November 2021. Studies map to the papers and curation logs table through ICPSR study numbers as “STUDY”. However, not every study in this table will have records in the papers and curation logs tables.
Papers (“ICPSR_PAPERS”): 94,755 publications collected from 2000-08-11 to 2021-11-16 in the ICPSR Bibliography and enriched with metadata from the Dimensions database with variables for paper number, identifier, title, authors, publication venue, item type, publication date, input date, ICPSR series numbers used in the paper, ICPSR study numbers used in the paper, the Dimension identifier, and the Dimensions link to the publication’s full text. Papers map to the studies table through ICPSR study numbers in the “STUDY_NUMS” field. Each record represents a single publication, and because a researcher can use multiple datasets when creating a publication, each record may list multiple studies or series.
Curation logs (“ICPSR_CURATION_LOGS”): 649 curation logs for 563 ICPSR studies (although most studies in the subset had one curation log, some studies were associated with multiple logs, with a maximum of 10) curated between February 2017 and December 2019 with variables for study number, action labels assigned to work description sentences using a classifier trained on ICPSR curation logs, hours of work associated with a single log entry, and total hours of work logged for the curation ticket. Curation logs map to the study and paper tables through ICPSR study numbers as “STUDY”. Each record represents a single logged action, and future users may wish to aggregate actions to the study level before joining tables.
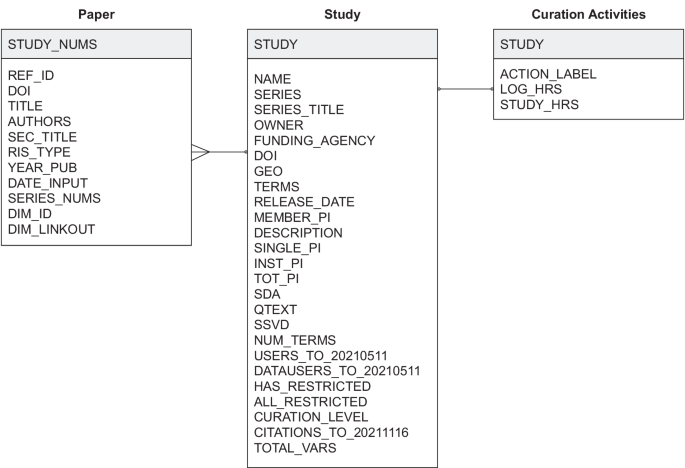
Entity-relation diagram.
Technical Validation
We report on the reliability of the dataset’s metadata in the following subsections. To support future reuse of the dataset, curation services provided through ICPSR improved data quality by checking for missing values, adding variable labels, and creating a codebook.
All 10,605 studies available through ICPSR have a DOI and a full-text description summarizing what the study is about, the purpose of the study, the main topics covered, and the questions the PIs attempted to answer when they conducted the study. Personal names (i.e., principal investigators) and organizational names (i.e., funding agencies) are standardized against an authority list maintained by ICPSR; geographic names and subject terms are also standardized and hierarchically indexed in the ICPSR Thesaurus 34 . Many of ICPSR’s studies (63%) are in a series and are distributed through the ICPSR General Archive (56%), a non-topical archive that accepts any social or behavioral science data. While study data have been available through ICPSR since 1962, the earliest digital release date recorded for a study was 1984-03-18, when ICPSR’s database was first employed, and the most recent date is 2021-10-28 when the dataset was collected.
Curation level information was recorded starting in 2017 and is available for 1,125 studies (11%); approximately 80% of studies with assigned curation levels received curation services, equally distributed between Levels 1 (least intensive), 2 (moderately intensive), and 3 (most intensive) (Fig. 3 ). Detailed descriptions of ICPSR’s curation levels are available online 35 . Additional metadata are available for a subset of 421 studies (4%), including information about whether the study has a single PI, an institutional PI, the total number of PIs involved, total variables recorded is available for online analysis, has searchable question text, has variables that are indexed for search, contains one or more restricted files, and whether the study is completely restricted. We provided additional metadata for this subset of ICPSR studies because they were released within the past five years and detailed curation and usage information were available for them. Usage statistics including total downloads and data file downloads are available for this subset of studies as well; citation statistics are available for 8,030 studies (76%). Most ICPSR studies have fewer than 500 users, as indicated by total downloads, or citations (Fig. 4 ).
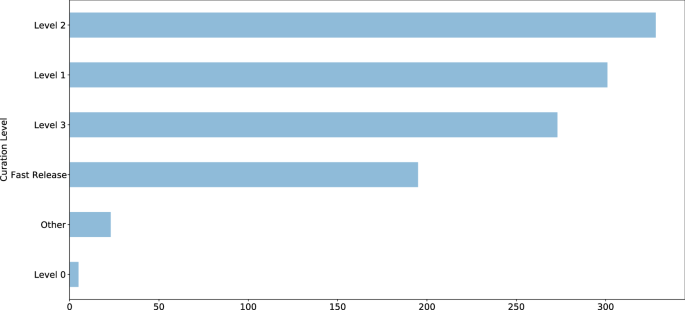
ICPSR study curation levels.
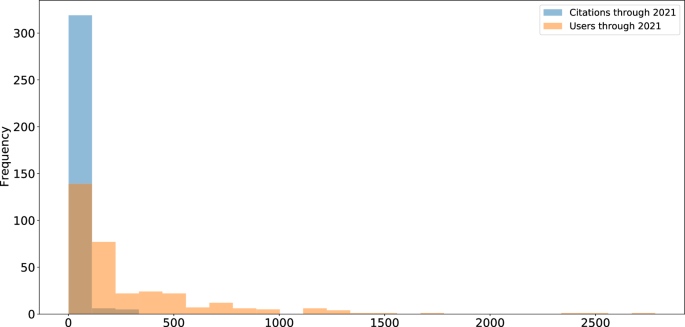
ICPSR study usage.
A subset of 43,102 publications (45%) available in the ICPSR Bibliography had a DOI. Author metadata were entered as free text, meaning that variations may exist and require additional normalization and pre-processing prior to analysis. While author information is standardized for each publication, individual names may appear in different sort orders (e.g., “Earls, Felton J.” and “Stephen W. Raudenbush”). Most of the items in the ICPSR Bibliography as of 2021-11-16 were journal articles (59%), reports (14%), conference presentations (9%), or theses (8%) (Fig. 5 ). The number of publications collected in the Bibliography has increased each decade since the inception of ICPSR in 1962 (Fig. 6 ). Most ICPSR studies (76%) have one or more citations in a publication.
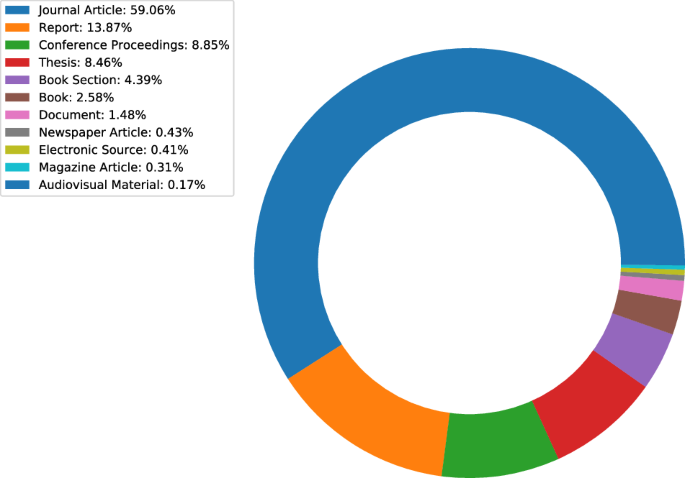
ICPSR Bibliography citation types.
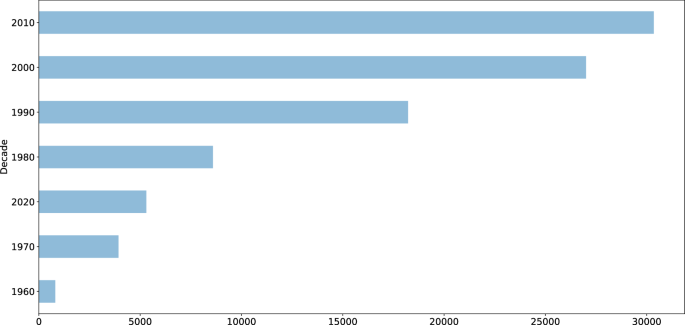
ICPSR citations by decade.
Usage Notes
The dataset consists of three tables that can be joined using the “STUDY” key as shown in Fig. 2 . The “ICPSR_PAPERS” table contains one row per paper with one or more cited studies in the “STUDY_NUMS” column. We manipulated and analyzed the tables as CSV files with the Pandas library 36 in Python and the Tidyverse packages 37 in R.
The present MICA dataset can be used independently to study the relationship between curation decisions and data reuse. Evidence of reuse for specific studies is available in several forms: usage information, including downloads and citation counts; and citation contexts within papers that cite data. Analysis may also be performed on the citation network formed between datasets and papers that use them. Finally, curation actions can be associated with properties of studies and usage histories.
This dataset has several limitations of which users should be aware. First, Jira tickets can only be used to represent the intensiveness of curation for activities undertaken since 2017, when ICPSR started using both Curation Levels and Jira. Studies published before 2017 were all curated, but documentation of the extent of that curation was not standardized and therefore could not be included in these analyses. Second, the measure of publications relies upon the authors’ clarity of data citation and the ICPSR Bibliography staff’s ability to discover citations with varying formality and clarity. Thus, there is always a chance that some secondary-data-citing publications have been left out of the bibliography. Finally, there may be some cases in which a paper in the ICSPSR bibliography did not actually obtain data from ICPSR. For example, PIs have often written about or even distributed their data prior to their archival in ICSPR. Therefore, those publications would not have cited ICPSR but they are still collected in the Bibliography as being directly related to the data that were eventually deposited at ICPSR.
In summary, the MICA dataset contains relationships between two main types of entities – papers and studies – which can be mined. The tables in the MICA dataset have supported network analysis (community structure and clique detection) 30 ; natural language processing (NER for dataset reference detection) 32 ; visualizing citation networks (to search for datasets) 38 ; and regression analysis (on curation decisions and data downloads) 29 . The data are currently being used to develop research metrics and recommendation systems for research data. Given that DOIs are provided for ICPSR studies and articles in the ICPSR Bibliography, the MICA dataset can also be used with other bibliometric databases, including DataCite, Crossref, OpenAlex, and related indexes. Subscription-based services, such as Dimensions AI, are also compatible with the MICA dataset. In some cases, these services provide abstracts or full text for papers from which data citation contexts can be extracted for semantic content analysis.
Code availability
The code 27 used to produce the MICA project dataset is available on GitHub at https://github.com/ICPSR/mica-data-descriptor and through Zenodo with the identifier https://doi.org/10.5281/zenodo.8432666 . Data manipulation and pre-processing were performed in Python. Data curation for distribution was performed in SPSS.
He, L. & Han, Z. Do usage counts of scientific data make sense? An investigation of the Dryad repository. Library Hi Tech 35 , 332–342 (2017).
Article Google Scholar
Brickley, D., Burgess, M. & Noy, N. Google dataset search: Building a search engine for datasets in an open web ecosystem. In The World Wide Web Conference - WWW ‘19 , 1365–1375 (ACM Press, San Francisco, CA, USA, 2019).
Buneman, P., Dosso, D., Lissandrini, M. & Silvello, G. Data citation and the citation graph. Quantitative Science Studies 2 , 1399–1422 (2022).
Chao, T. C. Disciplinary reach: Investigating the impact of dataset reuse in the earth sciences. Proceedings of the American Society for Information Science and Technology 48 , 1–8 (2011).
Article ADS Google Scholar
Parr, C. et al . A discussion of value metrics for data repositories in earth and environmental sciences. Data Science Journal 18 , 58 (2019).
Eschenfelder, K. R., Shankar, K. & Downey, G. The financial maintenance of social science data archives: Four case studies of long–term infrastructure work. J. Assoc. Inf. Sci. Technol. 73 , 1723–1740 (2022).
Palmer, C. L., Weber, N. M. & Cragin, M. H. The analytic potential of scientific data: Understanding re-use value. Proceedings of the American Society for Information Science and Technology 48 , 1–10 (2011).
Zimmerman, A. S. New knowledge from old data: The role of standards in the sharing and reuse of ecological data. Sci. Technol. Human Values 33 , 631–652 (2008).
Cragin, M. H., Palmer, C. L., Carlson, J. R. & Witt, M. Data sharing, small science and institutional repositories. Philosophical Transactions of the Royal Society A: Mathematical, Physical and Engineering Sciences 368 , 4023–4038 (2010).
Article ADS CAS Google Scholar
Fear, K. M. Measuring and Anticipating the Impact of Data Reuse . Ph.D. thesis, University of Michigan (2013).
Borgman, C. L., Van de Sompel, H., Scharnhorst, A., van den Berg, H. & Treloar, A. Who uses the digital data archive? An exploratory study of DANS. Proceedings of the Association for Information Science and Technology 52 , 1–4 (2015).
Pasquetto, I. V., Borgman, C. L. & Wofford, M. F. Uses and reuses of scientific data: The data creators’ advantage. Harvard Data Science Review 1 (2019).
Gregory, K., Groth, P., Scharnhorst, A. & Wyatt, S. Lost or found? Discovering data needed for research. Harvard Data Science Review (2020).
York, J. Seeking equilibrium in data reuse: A study of knowledge satisficing . Ph.D. thesis, University of Michigan (2022).
Kilbride, W. & Norris, S. Collaborating to clarify the cost of curation. New Review of Information Networking 19 , 44–48 (2014).
Robinson-Garcia, N., Mongeon, P., Jeng, W. & Costas, R. DataCite as a novel bibliometric source: Coverage, strengths and limitations. Journal of Informetrics 11 , 841–854 (2017).
Qin, J., Hemsley, J. & Bratt, S. E. The structural shift and collaboration capacity in GenBank networks: A longitudinal study. Quantitative Science Studies 3 , 174–193 (2022).
Article PubMed PubMed Central Google Scholar
Acuna, D. E., Yi, Z., Liang, L. & Zhuang, H. Predicting the usage of scientific datasets based on article, author, institution, and journal bibliometrics. In Smits, M. (ed.) Information for a Better World: Shaping the Global Future. iConference 2022 ., 42–52 (Springer International Publishing, Cham, 2022).
Zeng, T., Wu, L., Bratt, S. & Acuna, D. E. Assigning credit to scientific datasets using article citation networks. Journal of Informetrics 14 , 101013 (2020).
Koesten, L., Vougiouklis, P., Simperl, E. & Groth, P. Dataset reuse: Toward translating principles to practice. Patterns 1 , 100136 (2020).
Du, C., Cohoon, J., Lopez, P. & Howison, J. Softcite dataset: A dataset of software mentions in biomedical and economic research publications. J. Assoc. Inf. Sci. Technol. 72 , 870–884 (2021).
Aryani, A. et al . A research graph dataset for connecting research data repositories using RD-Switchboard. Sci Data 5 , 180099 (2018).
Färber, M. & Lamprecht, D. The data set knowledge graph: Creating a linked open data source for data sets. Quantitative Science Studies 2 , 1324–1355 (2021).
Perry, A. & Netscher, S. Measuring the time spent on data curation. Journal of Documentation 78 , 282–304 (2022).
Trisovic, A. et al . Advancing computational reproducibility in the Dataverse data repository platform. In Proceedings of the 3rd International Workshop on Practical Reproducible Evaluation of Computer Systems , P-RECS ‘20, 15–20, https://doi.org/10.1145/3391800.3398173 (Association for Computing Machinery, New York, NY, USA, 2020).
Borgman, C. L., Scharnhorst, A. & Golshan, M. S. Digital data archives as knowledge infrastructures: Mediating data sharing and reuse. Journal of the Association for Information Science and Technology 70 , 888–904, https://doi.org/10.1002/asi.24172 (2019).
Lafia, S. et al . MICA Data Descriptor. Zenodo https://doi.org/10.5281/zenodo.8432666 (2023).
Lafia, S., Thomer, A., Bleckley, D., Akmon, D. & Hemphill, L. Leveraging machine learning to detect data curation activities. In 2021 IEEE 17th International Conference on eScience (eScience) , 149–158, https://doi.org/10.1109/eScience51609.2021.00025 (2021).
Hemphill, L., Pienta, A., Lafia, S., Akmon, D. & Bleckley, D. How do properties of data, their curation, and their funding relate to reuse? J. Assoc. Inf. Sci. Technol. 73 , 1432–44, https://doi.org/10.1002/asi.24646 (2021).
Lafia, S., Fan, L., Thomer, A. & Hemphill, L. Subdivisions and crossroads: Identifying hidden community structures in a data archive’s citation network. Quantitative Science Studies 3 , 694–714, https://doi.org/10.1162/qss_a_00209 (2022).
ICPSR. ICPSR Bibliography of Data-related Literature: Collection Criteria. https://www.icpsr.umich.edu/web/pages/ICPSR/citations/collection-criteria.html (2023).
Lafia, S., Fan, L. & Hemphill, L. A natural language processing pipeline for detecting informal data references in academic literature. Proc. Assoc. Inf. Sci. Technol. 59 , 169–178, https://doi.org/10.1002/pra2.614 (2022).
Hook, D. W., Porter, S. J. & Herzog, C. Dimensions: Building context for search and evaluation. Frontiers in Research Metrics and Analytics 3 , 23, https://doi.org/10.3389/frma.2018.00023 (2018).
https://www.icpsr.umich.edu/web/ICPSR/thesaurus (2002). ICPSR. ICPSR Thesaurus.
https://www.icpsr.umich.edu/files/datamanagement/icpsr-curation-levels.pdf (2020). ICPSR. ICPSR Curation Levels.
McKinney, W. Data Structures for Statistical Computing in Python. In van der Walt, S. & Millman, J. (eds.) Proceedings of the 9th Python in Science Conference , 56–61 (2010).
Wickham, H. et al . Welcome to the Tidyverse. Journal of Open Source Software 4 , 1686 (2019).
Fan, L., Lafia, S., Li, L., Yang, F. & Hemphill, L. DataChat: Prototyping a conversational agent for dataset search and visualization. Proc. Assoc. Inf. Sci. Technol. 60 , 586–591 (2023).
Download references
Acknowledgements
We thank the ICPSR Bibliography staff, the ICPSR Data Curation Unit, and the ICPSR Data Stewardship Committee for their support of this research. This material is based upon work supported by the National Science Foundation under grant 1930645. This project was made possible in part by the Institute of Museum and Library Services LG-37-19-0134-19.
Author information
Authors and affiliations.
Inter-university Consortium for Political and Social Research, University of Michigan, Ann Arbor, MI, 48104, USA
Libby Hemphill, Sara Lafia, David Bleckley & Elizabeth Moss
School of Information, University of Michigan, Ann Arbor, MI, 48104, USA
Libby Hemphill & Lizhou Fan
School of Information, University of Arizona, Tucson, AZ, 85721, USA
Andrea Thomer
You can also search for this author in PubMed Google Scholar
Contributions
L.H. and A.T. conceptualized the study design, D.B., E.M., and S.L. prepared the data, S.L., L.F., and L.H. analyzed the data, and D.B. validated the data. All authors reviewed and edited the manuscript.
Corresponding author
Correspondence to Libby Hemphill .
Ethics declarations
Competing interests.
The authors declare no competing interests.
Additional information
Publisher’s note Springer Nature remains neutral with regard to jurisdictional claims in published maps and institutional affiliations.
Rights and permissions
Open Access This article is licensed under a Creative Commons Attribution 4.0 International License, which permits use, sharing, adaptation, distribution and reproduction in any medium or format, as long as you give appropriate credit to the original author(s) and the source, provide a link to the Creative Commons licence, and indicate if changes were made. The images or other third party material in this article are included in the article’s Creative Commons licence, unless indicated otherwise in a credit line to the material. If material is not included in the article’s Creative Commons licence and your intended use is not permitted by statutory regulation or exceeds the permitted use, you will need to obtain permission directly from the copyright holder. To view a copy of this licence, visit http://creativecommons.org/licenses/by/4.0/ .
Reprints and permissions
About this article
Cite this article.
Hemphill, L., Thomer, A., Lafia, S. et al. A dataset for measuring the impact of research data and their curation. Sci Data 11 , 442 (2024). https://doi.org/10.1038/s41597-024-03303-2
Download citation
Received : 16 November 2023
Accepted : 24 April 2024
Published : 03 May 2024
DOI : https://doi.org/10.1038/s41597-024-03303-2
Share this article
Anyone you share the following link with will be able to read this content:
Sorry, a shareable link is not currently available for this article.
Provided by the Springer Nature SharedIt content-sharing initiative
Quick links
- Explore articles by subject
- Guide to authors
- Editorial policies
Sign up for the Nature Briefing newsletter — what matters in science, free to your inbox daily.

- Open access
- Published: 06 May 2024
Scoping review of the recommendations and guidance for improving the quality of rare disease registries
- JE Tarride 1 , 2 , 3 ,
- A. Okoh 1 ,
- K. Aryal 1 ,
- C. Prada 1 ,
- Deborah Milinkovic 2 ,
- A. Keepanasseril 1 &
- A. Iorio 1
Orphanet Journal of Rare Diseases volume 19 , Article number: 187 ( 2024 ) Cite this article
197 Accesses
2 Altmetric
Metrics details
Rare disease registries (RDRs) are valuable tools for improving clinical care and advancing research. However, they often vary qualitatively, structurally, and operationally in ways that can determine their potential utility as a source of evidence to support decision-making regarding the approval and funding of new treatments for rare diseases.
The goal of this research project was to review the literature on rare disease registries and identify best practices to improve the quality of RDRs.
In this scoping review, we searched MEDLINE and EMBASE as well as the websites of regulatory bodies and health technology assessment agencies from 2010 to April 2023 for literature offering guidance or recommendations to ensure, improve, or maintain quality RDRs.
The search yielded 1,175 unique references, of which 64 met the inclusion criteria. The characteristics of RDRs deemed to be relevant to their quality align with three main domains and several sub-domains considered to be best practices for quality RDRs: (1) governance (registry purpose and description; governance structure; stakeholder engagement; sustainability; ethics/legal/privacy; data governance; documentation; and training and support); (2) data (standardized disease classification; common data elements; data dictionary; data collection; data quality and assurance; and data analysis and reporting); and (3) information technology (IT) infrastructure (physical and virtual infrastructure; and software infrastructure guided by FAIR principles (Findability; Accessibility; Interoperability; and Reusability).
Conclusions
Although RDRs face numerous challenges due to their small and dispersed populations, RDRs can generate quality data to support healthcare decision-making through the use of standards and principles on strong governance, quality data practices, and IT infrastructure.
Introduction
Randomized clinical trials (RCTs) for many years have been the main source of clinical evidence for regulatory and reimbursement decisions of healthcare technologies. However, as regulators and health technology assessment (HTA) agencies move towards a life cycle approach [ 1 , 2 ], there is an opportunity to broaden the evidence base and enhance decision-making through the integration of real-world evidence (RWE) into decision making. Based on real world data (RWD), RWE allows decision-makers to better understand how health technologies are being used, how they perform, and whether they are cost-effective in real-world healthcare settings. It is therefore not surprising that several frameworks have been developed over the past years to guide the use and reporting of RWD for decision making [ 3 , 4 , 5 , 6 , 7 , 8 , 9 ]. For common diseases, RWE is often provided by post-marketing phase IV clinical trials, administrative databases, or electronic medical records. In the case of rare diseases (RDs) characterized by small populations (e.g., fewer than one in 2,000 as per the Canadian or European definitions, or fewer than 200,000 in the US) [ 10 ], both traditional trials and common sources of RWD may be not providing sufficient evidence. For example, it may also not be feasible or ethical to conduct clinical trials for RDs [ 11 , 12 , 13 ]. Therefore, high-quality rare disease registries (RDRs) can play an important role in HTA, health policy, and clinical decision-making for RDs [ 14 , 15 ]. RDRs can improve our knowledge of RD conditions, support clinical research, improve patient care, and inform overall healthcare planning. However, RDRs are often diverse in nature, supported by different data governance and funding models, and may lack standardized data collection methods [ 16 ]. As such, HTA agencies may be reluctant to use RDR data to inform funding decisions on treatments for rare diseases [ 17 , 18 ].
To support acceptance of registry data by HTA bodies wishing to use registry data, the European Network for Health Technology Assessment (EUnetHTA) Joint Action 3 led the development of the “Registry Evaluation and Quality Standards Tool” (REQueST) [ 19 ] based on the Methodological Guidance on the Efficient and Rational Governance of Registries (PARENT) guidelines [ 20 ] and a series of HTA consultations [ 17 , 20 ]. Although not specific to RDRs, the REQueST tool includes 23 criteria to support the assessment of whether registries meet the needs of the regulatory and HTA bodies including eight criteria describing the methodology used (type of registry; use for registry-based studies and previous publications; geographical and organizational setting; duration; size; inclusion/exclusion criteria; follow-up and confounders), 11 criteria that are essential standards for good practices and data quality (registry aims and methodology; governance; informed consent; data dictionary; minimal data set; standard definitions; terminology and specifications; data collection; quality assurance; data cleaning; missing data; financing; protection; and security and safeguards) and three criteria that deal with information that may be required when evaluating a registry for a particular purpose (e.g., interoperability and readiness for data linkage; data sources; and ethics). The REQueST tool was piloted with two established European registries and results indicated that both registries performed well, with more than 70% of the domains rated satisfactory and none of the domains failed. However, results indicated that more information was required in terms of governance structure (e.g., the role of industry), data quality checks, and interoperability [ 17 ].
The REQueST tool was also used by the Canadian Agency for Drugs and Technologies in Health (CADTH) to describe 25 RDRs based on publicly available information reported by the RDRs [ 21 ]. Within the study limitations (e.g., an assessment with the REQueST tool should be completed by registry data holders and not based on public information), the results indicated that most Canadian RDRs scored well for the 8 methodological criteria, although no RDRs provided public information on methods used to measure and control confounding. While information on the RDR purpose, governance, and informed consent was publicly available for almost all RDRs, there was considerable variation in the amount of publicly available information on the other REQueST criteria for the 25 Canadian RDRs, thus prompting a call for the establishment of Canadian standards for RDRs [ 21 ]. Therefore, to support decision making around the approval or funding of treatments for RDs in Canada and elsewhere, the objective of this study was to identify best practices to improve the quality of RDRs.
A scoping review was conducted to meet the study objectives, as scoping review designs are particularly appropriate to answer broad research questions [ 22 ]. The scoping review included four steps: (1) developing the literature search strategy; (2) study selection; (3) data charting; and (4) summarizing and reporting the results.
Search strategy
The search strategy was developed by a librarian from CADTH. The search strategy (Appendix 1 ) included several search terms (e.g., rare disease, registry, recommendations, guidance, standards). Databases searched were MEDLINE and EMBASE and the search was restricted to articles published in English from 2010 to April 2023. The year 2010 was chosen as the cut-off point because 2010 corresponds to the guidance on RDRs published by the European Rare Disease Task Force initially published in 2009 and updated in 2011 [ 23 ]. Grey literature was searched from websites of regulatory bodies (e.g., European Medicines Agency, Food and Drug Administration, Health Canada) and HTA authorities (e.g., National Institute for Health and Care Excellence, CADTH).
Study selection
Screening for articles that met the inclusion was conducted using Rayyan [ 24 ]. Titles and abstracts were screened against the inclusion and exclusion criteria (Level I screening). Full texts of the publications that passed the Level I screening were retrieved before being screened for final inclusion and exclusion (Level II screening). The literature was screened by two pairs of independent reviewers (KA & CP; AK & AO) at each stage of the Level I and Level II screenings. Conflicts within each pair of reviewers were resolved through discussion. When consensus could not be reached an additional reviewer was consulted (JET). The same process was used for screening the grey literature.
Inclusion and exclusion criteria
Literature was included if it was reporting on standards, processes, guidance, or recommendations for improving the quality of RDRs. Exclusion criteria included: (1) non-English literature; (2) conference proceedings and letters; and (3) papers presenting clinical data based on an existing RDR without reporting on standards, guidance, or considerations relevant to RDR quality. The references cited in the included papers were also scanned to identify any relevant literature, including non-RDR guidance cited in the RDR literature.
Data charting
Based on the preliminary scoping of the literature, the following data were selected for abstraction: publication details and specific guidance related to RDRs’ governance; patient engagement and consent; diversity and equity issues; funding model and sustainability; ethical/legal/regulatory requirements; data quality and management; data elements; standardization; data linkage; data validity and audit; IT infrastructure; and barriers and facilitators for improving the quality of RDRs. Data was abstracted into a Microsoft Excel spreadsheet.
Data summary and synthesis
Once the data were abstracted, summaries were created by the team and the information was synthesized in terms of best practices for improving the quality of RDRs.
Results of the search strategy
Out of 1,135 unique citations identified by the search, 93 were assessed for eligibility based on a full-text review, and 47 studies were included for data abstraction. For the grey literature, 35 documents were identified, 18 were assessed for eligibility based on full-text review and 6 documents were included for data abstraction. In addition, 11 documents were identified by reviewing the references cited in the included papers, for a total of 64 documents included in our scoping review. Figure 1 presents the PRISMA diagram summarizing the screening process and key reasons for exclusion. Appendix 2 presents the list of the 64 documents identified through the literature review and used to develop the framework.
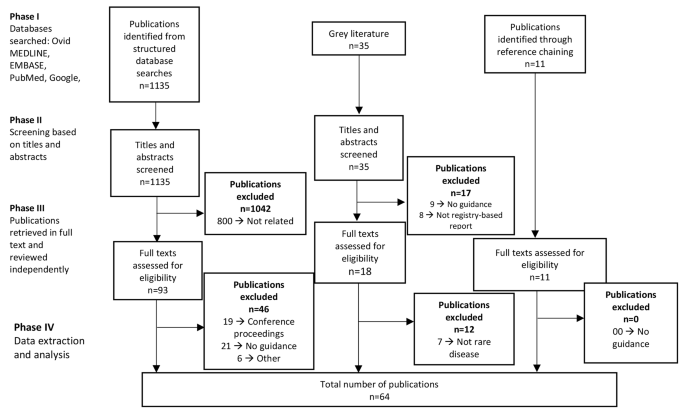
PRISMA diagram
Conceptual framework
Upon review of the evidence and authors’ discussion, the literature was synthesized according to three key quality domains (governance, data, and information technology) and several sub-domains: eight for governance (registry purpose and description; governance structure; stakeholder engagement; sustainability; ethics/legal/privacy; data governance; documentation and training and support), six for data (standardized disease classification; common data elements; data dictionary; data collection; data quality and assurance; and data analysis and reporting), and two for IT infrastructure (physical/virtual infrastructure; and software infrastructure).
Domain 1: RDR governance
Governance was the most discussed domain (48 of 64 sources), which was not surprising given that governance is foundational for quality and trust. Governance refers to the formalized structure that guides the RDR leadership and high-level decision-making required to achieve RDR’s objectives and long-term operational sustainability [ 13 , 16 , 25 ]. The following describes the guidance reported in the literature for each of the 8 governance sub-domains, while Table 1 summarizes the key guidance.
Sub-domain 1 — registry purpose and description
The critical first step in any registry description is to state its purpose and objectives since they establish the framework for all activities that follow (e.g., data collection, inclusion, and exclusion criteria). A comprehensive description of the registry, available through the registry website or publications, allows other stakeholders, including potential researchers or regulatory or HTA users, to understand and appraise the registry’s quality and potential usefulness. In addition to the RDR purpose and objectives, common attributes reported in the literature to describe a registry include registry design, timeframe, population characteristics, settings, geographical coverage area, type of data captured and data sources, data quality procedures, data access policies, ethics approvals and dissemination activities [ 17 , 20 , 23 , 25 , 26 , 27 , 28 , 29 , 30 , 31 , 32 , 33 , 34 , 35 , 36 , 37 , 38 , 39 , 40 , 41 , 42 ].
Sub-domain 2 — governance structure
The governance structure reflects the nature and extent of registry operations [ 13 ]. As for many organizations, the adoption of an organigram (a visual representation of the registry governance structure) helps clarify roles and responsibilities, reporting and decision-making flow, and how the different roles interconnect [ 29 , 43 ]. Examples of key roles and expertise reported in the RDR literature include: registry lead(s); project manager(s) and management team with financial and leadership experience; information technology experts; data entry personal; and team members with specific expertise (e.g., ethics, legal, statistics, population-based research) [ 25 , 31 , 37 , 38 , 42 , 44 ]. A central contact point for stakeholders is advisable [ 18 , 38 , 45 ].
Depending on the size and scope of the registry, a governing body, spanning from an independent Board of Directors to a Steering Committee comprised of various internal and external experts, has been recommended [ 35 , 36 ]. The role of the governing body is to direct daily operations and ensure compliance with applicable laws and regulations, directly or through small targeted work groups [ 42 , 45 , 46 ]. In addition, independent advisory boards can provide technical guidance and scientific independence [ 13 , 23 , 47 ]. Patient representation on governing bodies or committees facilitates patient centeredness and engagement in the decision-making process [ 17 , 20 , 25 ]. As for most public and private organizations, board and committee members should declare conflicts of interest to enhance transparency [ 17 , 35 , 48 ].
Sub-domain 3 — stakeholder engagement
Multi-stakeholder engagement (e.g., clinicians, patients and their families, patients organizations, provider organizations, regulators, payers, drug companies) is suggested to facilitate long-term sustainability of RDRs [ 23 , 25 , 37 , 38 , 41 , 46 , 49 , 50 , 51 , 52 , 53 ]. The integration of a broad group of stakeholders can also facilitate quality improvements [ 23 , 30 , 50 , 54 ]. Patient advocacy groups, for example, can enhance the accuracy and completeness of patient data [ 49 ]. However, the literature points out that decision-making may be challenging with a large number of stakeholders [ 25 ].
Sub-domain 4 — sustainability
Durable and long-term sustainability is dependent upon funding and is key to ensuring RDR quality [ 25 , 36 ]. Compared to non-RD registries that have large populations to draw upon, RDRs are constrained by small and dispersed patient populations and limited funding opportunities. These constraints can inhibit data accuracy, patient follow-up, standardization, and result in knowledge gaps [ 26 , 29 , 30 , 52 , 54 ]. Multiple funding sources (e.g., public or private organizations, public-private partnerships, non-profit foundations, patient groups, professional societies) may contribute to the long-term sustainability of RDRs [ 25 , 35 , 44 , 49 ], but for transparency, it is important that all funding sources are publicly disclosed [ 17 , 18 , 45 , 50 ]. Sustainability also necessitates a long-term comprehensive financial plan including future-oriented exit strategies and succession planning [ 39 , 47 , 50 ]. A registry’s utility, effectiveness, efficiency, and agility are also important to ensure its long-term sustainability [ 27 , 55 ].
Sub-domain 5 – ethics, legal, privacy
RDRs must comply with ethical, legal, and privacy regulations [ 25 , 32 , 33 , 35 , 36 , 39 , 42 ] for the collection, storage, and use and re-use of patient health data for RDR activities [ 23 , 27 , 28 , 29 , 30 , 34 , 38 , 45 ] or for regulatory purposes [ 12 , 45 ]. The collection of informed consent necessitates that participants understand the risks and benefits that might accrue to them specifically, who might have access to their data, how their data will be used and re-used including potential linkages to other registries or future research activities, and a participant’s right to withdraw their consent at any time [ 17 , 20 , 45 , 50 , 56 ]. Since the withdrawal of consent impacts both the current data holdings and past, present, and future research analyses, precise language in the consent about the withdrawal consent (e.g. what happens to the data of an individual withdrawing consent and how it impacts the analyses) will help mitigate potential misunderstanding and future conflicts [ 13 ]. Approaches used to encourage participation, if any (e.g., incentives) should be documented [ 26 ]. For international registries or RDRs intending to link to international registries, multiple statutes may apply (e.g., EU General Data Protection Regulation (GDPR), Canada’s Personal Information Protection and Electronic Documents Act (PIPEDA), various in the U.S.) [ 57 , 58 ]. For this reason, it is often recommended that RDRs strive to comply with international, national, and local ethical, legal, and privacy regulations as appropriate [ 23 , 25 , 36 , 49 , 50 ].
Sub-domain 6 - data governance
Data ownership and data custodianship are at the forefront of data governance [ 16 , 35 , 36 , 38 , 48 , 59 ]. Data ownership refers to the possession, responsibility, and control of data including the right to assign access privileges to others [ 60 ]. Patient participants may grant the registry authorization to access and use their data for research [ 16 , 29 , 38 ]. However, more than one entity (e.g., patients, clinicians, hospitals, funders) could have a claim to the aggregate data in the registry [ 16 , 18 , 38 , 46 ]; therefore, data ownership must be clearly defined. Data custodianship is the responsibility of the registry organization, which includes monitoring and managing registry use, data access policies, and data sharing agreements [ 18 , 45 , 46 ]. A protocol for third-party data requests, such as the administration of these requests through a data access committee, will ensure that requests are appropriately assessed and responded to in a timely manner [ 16 ]. Full disclosure of the registry’s fee structure (e.g., fees for ad-hoc requests versus subscription fee models) will mitigate potential miscommunication or misinterpretation of data access requirements [ 26 ].
Sub-domain 7 — documentation
Documentation is essential to maintaining a quality registry because it facilitates shared understanding and transparency around the registry activities. A Standard Operating Procedures (SOPs) manual that is updated regularly provides step-by-step guidance on the registry’s routine activities including performance targets [ 25 , 30 , 35 , 38 , 61 ]. Regular provision of activity reports (e.g., annual reports) and a repository of registry-based publications increase the transparency of the RDR processes and activities [ 17 , 25 , 48 , 62 ]. Similarly essential is the documentation of ethical and regulatory approvals for registry-based studies [ 12 , 32 , 33 , 47 ] and the adoption of standardized templates and forms (e.g., informed consent) that reflect the registry’s objective and use standardized language [ 33 , 40 , 61 ]. The adoption of an Investigator and User Declaration Form or similar document will affirm compliance with regulatory and operational processes [ 32 ]. The literature also recommends publishing study protocols and registering registry-based studies in a public register [ 18 , 45 ].
Sub-domain 8 – training and support
Training is essential for registry staff, data providers, and new users to ensure consistency and quality [ 25 , 38 , 45 , 63 ]. A training manual, “how-to videos”, and a comprehensive training plan that are updated regularly facilitate consistent training protocols [ 25 , 37 , 54 ]. A registry might also benefit from designated data entry personnel who can systematically monitor and evaluate data quality [ 38 ]. A Support Team or Help Desk is also beneficial to the operations of the registry [ 59 ].
Domain – data
Data was the second most discussed domain (45 of 64 sources). Data refers to the structures, policies, and processes required to ensure a RDR can maintain a high-quality database [ 13 ]. A high-quality database is characterized by completeness, accuracy, usefulness, and representativeness [ 13 , 25 , 64 ], which is paramount for meeting the needs of decision-makers.
Table 2 summarizes the guidance reported in the literature, which is described in more detail below in terms of 6 sub-domains (standardized disease classification; common data elements; data dictionary; data collection; data quality and assurance; data analysis and reporting).
Sub-domain 1 – standardized disease classifications
Standardized disease classifications such as the Orphanet Rare Disease Ontology (ORDO), Human Phenotype Ontology (HPO), ORPHA-codes or the International Classification of Disease ICD-9, ICD-10, or ICD-11 or some combination [ 31 , 32 , 50 , 65 , 66 ] have been proposed for data collection to ensure future interoperability and registry linkages. Being able to link to other registries facilitates knowledge creation, decision making, and improvements in clinical care that may not otherwise be possible for small RD patient populations [ 33 , 36 , 49 , 51 , 67 ]. When RDRs transfer or merge their data to or with other entities, the documentation of the process used to validate the data transfer ensures quality and consistency [ 68 ]. Although linking to international RDRs expands population reach, it poses some additional challenges (e.g., the regulatory environment) that need to be identified early in the registry design process [ 44 ]. The use of international standards and ontology codes apply in this context.
Sub-domain 2 – common data elements
Registries have to consider the informational needs of the registry against the needs of their other stakeholders and the available resources [ 25 ]. A minimum set of common data elements collected across the RDR sites (e.g., administrative data, socio-demographics, diagnosis, disease history, treatments, clinical and safety outcomes) that could be expanded upon to meet the specific needs of the registry is usually identified [ 37 , 41 , 50 , 53 , 56 , 69 ]. Ideally, these common data elements would be harmonized across all registries that represent the same rare disease when applicable [ 12 ]. However, the main challenge around common data elements is reaching a consensus regarding the choice, organization, and definition of the various elements [ 25 , 70 , 71 ]. Beyond simply determining the composition of the common data elements, other challenges include data coding standards (e.g., integer, float, string, date, derived data, and file names) [ 13 , 72 , 73 ], standardized data constructs, vocabulary and terminology [ 28 , 33 , 37 , 65 , 71 , 74 ], defined variable interpretation to avoid inconsistency (e.g., sex – genotypic sex or declared sex) [ 18 , 75 ] and ontology harmonization to facilitate convergence from different terms or languages [ 56 , 65 , 76 , 77 ]. The latter necessitates consistent agreed-upon disease classification standards [ 23 , 50 , 77 ].
Sub-domain 3 – data dictionary
A detailed data dictionary is an essential tool for quality data collection [ 17 , 20 , 25 ]. A data dictionary provides clear instructions for data entry and analysis by defining all data elements and their purpose as well as the coding values including permissible values, representation class, data type, and format [ 17 , 20 ]. Complete alignment between the variables described in the data dictionary and those captured by the registry’s interface is expected [ 55 ].
Sub-domain 4 – data collection
Procedures for documenting the entire data collection process including adverse event monitoring, baseline and follow-up data, causality assessment, and reporting timelines are recommended to improve data accuracy [ 18 , 51 ]. Standardized data collection forms (e.g., Clinical Data Interchange Standards Consortium Operational Data Model, Patient Records, and Outcome Management Information System) can facilitate the data collection process [ 35 ]. Training in data collection procedures is key to reducing information bias and data misclassification and to achieve consistency amongst users and high quality data collection [ 25 , 35 ]. Sustained investment in data collection and management is also critical as prospective data collection across the patient’s lifespan can be expensive and onerous [ 78 ]. The capacity for a registry to embed clinical studies into its own database can also help sustain the registry and reduce costs associated with duplicated data collection efforts when conducting additional studies [ 31 , 78 ].
Data collection tools such as computers, automation, smartphones, smartwatches, tablets, and medical devices (e.g., glucose monitors) can be valuable sources of electronic health data as well as increase registry participation, particularly from disparate geographic locations, which in turn can result in increased knowledge, improved patient outcomes, stronger patient advocacy, and enhanced equity through healthcare access [ 13 , 37 , 39 , 40 , 75 , 79 ]. However, internet-based data collection may impact equity for data providers with limited access to the internet. Relationship building with physicians and patient groups who serve often excluded groups can facilitate greater equity and inclusion through referrals and knowledge translation efforts that promote and encourage registry participation [ 32 , 40 ].
Sub-domain 5 – data quality and assurance
Data quality reflects various data attributes or dimensions that can be used to measure the calibre of the data [ 25 ] such as completeness (the extent that the stored data represents the potential data), uniqueness (no repeated or redundant data), timeliness (data is up to date at the time of release), validity (data conforms to the appropriate syntax [e.g., format, type, range]), accuracy (the data correctly reflects the object or event being described), consistency (there are no discrepancies when the data is compared across different databases or against its definition) [ 35 , 64 ], and usefulness (the extent to which the outputs provide value) [ 25 ].
Data quality and assurance plans which include data validation (e.g., medical, clinical, and record audit) [ 61 ] and a review of RDR-generated studies ensure compliance with RDR-based studies’ protocols and ethical and regulatory requirements [ 12 , 25 , 32 , 69 ]. Data quality and assurance processes necessitate routine data quality checks and data cleaning to ensure the enrolment of eligible patients, data completeness, validity, and coherence while mitigating record duplication and errors [ 26 , 31 , 35 , 45 , 55 ]. Data audits can be performed by internal registry staff or an external service provider, or some combination [ 37 ]. Regular feedback to data providers about these data quality activities and findings encourages prompt remedial action and learning, thus improving the quality of the RDR data [ 25 , 45 , 50 ].
Sub-domain 6 – data analysis and reporting
In addition to study protocols, RDR-based studies benefit from the development of statistical analysis plans (SAPs), whether for internal registry objectives or external research with third-party partners [ 12 , 13 , 25 , 45 ]. A SAP facilitates the production of trustworthy results that can be more easily interpreted and accepted by various stakeholders (e.g., registry participants, patient groups, researchers, decision-makers, or the general public) [ 25 ]. SAPs should provide a list of variables and confounders captured in the data and details on the statistical methods used to answer the study question(s) and to deal with missing or censored data [ 25 , 45 ]. Adoption of guidelines such as the Strengthening the Reporting of Observational Studies in Epidemiology (STROBE) Statement, or the Patient-Centered Outcomes Research Institute (PCORI) Methodology Report improves transparency and accuracy when reporting RDR findings [ 13 ]. Details about dissemination activities such as study reports and communication strategies are usually included in the study protocols [ 25 , 44 , 45 ].
Domain – information technology infrastructure
Information technology (IT) infrastructure was discussed in 29 of the 64 documents in terms of physical and virtual infrastructure, and software infrastructure. IT infrastructure refers to the critical infrastructure that is required to collect, share, link, and use patient and clinical data [ 16 , 32 , 37 ], and importantly, securely store, transmit, and manage this private data [ 32 , 33 , 63 ]. Table 3 summarizes the literature which is described below in more detail below.
Sub-domain 1 – physical and virtual infrastructure
High quality RDRs are characterized by procedures and processes that ensure the digitally stored private data is secure [ 29 , 32 , 35 , 62 , 80 ] by housing the data on dedicated servers with intrusion detection systems [ 35 ]. Critical decisions include where the server(s) are held (e.g., centralized database versus distributed); how these location(s) are secured, and how and who has access to the RDR data. Registries can safeguard their systems through several processes (e.g., analysis of threats and countermeasures [ 63 ]) and tools (e.g. data software and access policies [ 63 , 81 , 82 ]). An independent external security or threat risk assessment is recommended to document compliance with security and privacy standards [ 83 ].
Sub-domain 2 – software infrastructure
The adoption of FAIR principles at the data source can facilitate data connections and exchanges across multiple RDRs and bolster data quality that supports both clinical research and patient care [ 31 , 56 , 68 , 73 , 75 , 81 ]. FAIR data principles stand for Findability (easy to find for both humans and computers), Accessibility (easily retrievable and accessible by users), Interoperability (easily integrates with other data), and Reusability (well-described so it can be replicated or applied in another setting). Since FAIR principles enable the extensive and efficient use of registry data while mitigating duplication, recollection, and errors [ 25 , 56 ], it is recommended that the registry data infrastructure complies with FAIR principles [ 18 , 25 , 26 , 37 , 38 , 59 , 75 , 83 , 84 ].
The technology choices, software architecture design and software development practices have a dramatic impact on software sustainability, legacy software support, ease of software modification, enhancements and interoperability [ 81 ]. With this in mind, software solutions can either be “out-of-the-box” commercial software or “home-built”, custom-designed in-house software, the latter often being more powerful but more resource and time-intensive [ 25 ]. Either way, drop-down menus, pop-up explanatory notes, and tab-to-jump options will aid in rapid and user-friendly data entry [ 32 , 72 ]. A user-friendly web interface with the capacity to upload and download data can also facilitate data sharing [ 25 , 38 ]. Since registry data is often collected from several sources, machine-readable files could facilitate the interoperability of pseudonymized data subsets, reduce duplication, and make the data more findable [ 26 , 63 , 67 , 75 ]. However, data heterogeneity can prove a barrier to automation [ 79 ].
Regardless of the methods used to collect, store, and manage data sets, data encryption and firewalling of servers are standard [ 59 , 63 ]. The encryption of data while in transit is an added layer of data security [ 27 , 32 , 40 ]. As it might become necessary to delete data occasionally (e.g., participants who revoke their consent), standardized procedures for data deletion help maintain database integrity and mitigate errors [ 13 , 38 , 41 ]. Enhanced technological literacy and adoption of technology tools (e.g., electronic health records, automated data capture, internet and mobile devices) [ 44 , 54 ] and the integration of a broader group of stakeholders [ 23 , 30 , 54 ] can also facilitate quality improvements.
Because RDRs can be designed around different purposes (e.g., patient advocacy, enhanced clinical practice, epidemiological and research goals) [ 25 , 41 , 46 ], RDRs often vary in quality, and are structurally and operationally diverse [ 38 ]. As a result, their fitness for purpose as a source of data to support decision making around the approval or funding of treatments for rare diseases must be assessed on a case-by-case basis [ 17 , 18 ]. Fortunately, the RDR literature offers a range of quality standards that define the essential characteristics leading to the development and maintenance of a quality RDR. The guidance from the 64 sources captured by the scoping review is synthesized within three dominant domains: (1) governance, which represents the many operational features of governance such as the governance structure, stakeholder engagement, sustainability, ethic and regulatory oversight, and training; (2) data, which represents standardized ontology and common data elements and standardized processes for data entry, verification, and auditing and reporting; and (3) information technology, which represents physical and software infrastructure and security, guided by FAIR principles.
While many guidelines focused on certain dimensions of RDRs’ quality (e.g., governance, core data elements), only three papers provided overall guidance on an extensive set of elements required to set up and maintain high-quality RDRs. Among those, in 2018, Kodra et al. 2018 [ 25 ] reported on the set of 17 recommendations to improve the quality of RDRs developed by a select group of experts convened by the Italian National Center of Rare Diseases in collaboration with other European countries [ 25 ]. These recommendations touched on 11 topics (registry definition; registry classification; governance; data sources; data elements; case report form; IT infrastructure complying with FAIR principles; quality information; documentation; training; and data quality audit) [ 25 , 61 ]. Building on these recommendations and expert meetings, in 2020, Ali et al. [ 38 ] surveyed the RDR community to determine the consensus level regarding 17 criteria that could be considered essential when assessing the quality of a RDR in terms of registry governance (9 items), data quality (5 items), and IT infrastructure (6 items). The responses of 35 respondents representing 40 RDRs across the United States, Canada, the United Kingdom, and Europe indicated a high level of consensus among the RDRs with more than 90% of respondents agreeing with most of the 17 criteria. Of note, 30% of respondents did not feel that patient involvement in the registry governance was necessary, conceding that although patient involvement in RDR governance may be best practice, there may be a limited role for patients in some scenarios such as physician-driven registries. The 2021 European Medicine Agency (EMA) guidance [ 45 ] integrated guidelines from PARENT Joint Action Methodological Guidance [ 20 ], the EUnetHTA’s Registry Evaluation and Quality Standards Tool (REQueST) [ 19 ], the US Agency for Healthcare Research and Quality (AHRQ)’s Users’ Guide on registries [ 13 ], and the European Reference Network Patient Registry Platform [ 85 ]. The EMA guidance provides information regarding two main domains: Administrative Information (subdomains include governance, consent and data protection) and Methods (subdomains include objectives, data providers, population, data elements, infrastructure, quality requirements). Our review broadly aligns with all three of these sources in terms of content, but it is more closely aligned with Ali et al. 2021 in terms of organization and number of domains (registry governance, data quality, IT infrastructure). However, compared to these guidances based on consensus panels or surveys, the evidence leading to our framework was based on a scoping review of the literature and we synthesized a broader set of quality indicators deemed essential by the literature. In December 2023, the FDA released its finalized real-world data guidance regarding a registry’s fitness to support regulatory decision making, which is consistent with our framework (e.g., governance, data, information technology infrastructure) [ 86 ]. However, this guidance was published after the completion of the scoping review and as such was not included in this review.
Despite the literature on quality standards for RDRs, a review of 37 publications reporting on RDRs between 2001 and 2021 found that while most of these publications reported on collecting informed consent (81%) and provided information on data access, data sharing or data protection strategies (75%), fewer publications reported on quality management (51%) or maintenance (46%). Furthermore, fewer RDRs reported using core data elements (22%) or ontological coding systems (24%), which is key for interoperability and for linking registries [ 21 ]. It is however possible that RDRs had such policies in place but did not report on them. Initiatives such as those undertaken in Europe to develop guidance to improve the quality, reporting, and assessment of patient registries from both regulatory and HTA perspectives facilitate the integration of registry data into decision-making processes. For example, the REQueST tool has been used in Europe by HTA agencies to evaluate the quality of registries being used as a source of data to support decision-making [ 17 ]. However, the REQueST assessment of 25 Canadian RDRs based on publicly available information on these RDRs highlighted the importance of developing standards for Canadian RDRs [ 21 ]. In this context, the results of this scoping review could be used to help develop a Canadian consensus on the core standards defining high-quality RDRs from regulatory and HTA perspectives. Compliance with RWE guidance [ 87 , 88 ] and acceptance of evidence from other jurisdictions are other important considerations when using RDR data for decision-making, especially in countries with relatively small populations such as Canada.
While adhering to existing and future RDR and HTA guidance will certainly improve the quality of RDRs and their use in decision-making, it should be recognized that this may require significant investment in terms of human and financial resources which may not be easily available to all RDRs. However, at least for Canada, the recent announcement in March 2023 by Health Canada to invest $1.5 billion over three years in support of a National Strategy for Drugs for Rare Diseases [ 89 ] represents a unique opportunity to develop a national infrastructure of sustainable, standardized, and quality RDRs while aligning with the pan Canadian Health Data Strategy [ 90 ].
As a next step, for RDRs interested in the harmonization of their data collection with other registries, the European Platform on Rare Disease Registration (EU RD Platform) [ 91 ]serves as an example of how this might be achieved. With over 600 diverse and fragmented rare disease registries in Europe, the European Commission’s Joint Research Centre in collaboration with stakeholders took on the tremendous task of establishing standards for integration, training, and interoperability of RDR data across Europe. A core element of the EU RD Platform is the European Rare Disease Registry Infrastructure (ERDRI) [ 92 ], which consists of a directory of registries, a data repository, a pseudonymization tool and importantly the EU RD Platform comprising of a set of 16 common data elements [ 93 ] that capture the characteristics of rare disease patients such as demographic, and clinical, diagnostic, and genetic information [ 43 , 56 , 76 , 91 , 94 ].
Limitations
Before interpreting the results of this scoping review, several limitations should be considered. First, due to the unique characteristics of RDRs, we limited our scoping review to RDRs, and we did not search the literature to improve the quality of non-RD registries. However, we identified several non-RDR guidances [ 12 , 13 , 20 , 45 , 64 , 68 ] when checking the references of the RDR papers included in the scoping review. Second, although we took a systematic approach when selecting the papers to be included in our scoping review, it is always possible that we missed one or several studies, even though all included publications’ references were checked to identify relevant studies not included in our final list of documents. Third, while we summarized the guidance under three domains and 16 sub-domains, we did not develop recommendations. This is left for future research. Despite these limitations, the results of this scoping review of 64 documents published between 2010 and April 2023 add to the body of the literature offering suggestions to improve the quality of RDRs. The results of this scoping review provide the foundation to develop quality standards for RDRs in Canada or other countries lacking guidelines for quality RDRs. For example, these results could be used by a Delphi panel to develop standards and processes to enhance the quality of data in RDR registries.
This review has also identified a few areas which merit further consideration. First, from a Canadian standpoint, future work is needed to develop a database of Canadian RDRs along with information on their key characteristics (e.g., purpose, population, funding) and information regarding their governance, data and IT infrastructure. Second, although the literature agrees on the importance of being able to link with international registries, it is also important to be able to link RDRs with health administrative databases to provide HTA agencies and decision makers with information on short and long-term outcomes, healthcare resource utilization, and expenditures associated with RDs. Similarly, issues of equity and diversity were discussed by only a few papers in the context of data collection methods to encourage patient participation [ 27 , 40 , 80 ], and relationships with physicians and patient groups working with disadvantaged groups [ 32 , 40 ]. A broader RDR equity lens could be achieved by using equity tools such as the PROGRESS (Place of resident, Race, Occupation, Gender, Religion, Education, Socioeconomic status, Social capital) framework [ 95 ], which would facilitate a greater understanding of how equity-deserving populations are affected by RDs or represented in RDR registries. Finally, the integration of patients’ experiences and insights when designing and interpreting results is an important avenue of research to enhance the quality and acceptance of RDR studies by generating patient-centered RWE [ 96 ].
Data availability
The authors confirm that a complete list of the sources used for data analyzed during this study is available in Appendix 2 of this published article. Example: https://doi.org/10.3390/ijerph15081644 .
Canada’s Drug and Health Technology Agency (CADTH): Ahead of the Curve: Shaping Future-Ready Health System. (2022). https://strategicplan.cadth.ca/wp-content/uploads/2022/03/cadth_2022_2025_strategic_plan.pdf . Accessed March 15, 2023.
National Institute for Health and Care Excellence (NICE): NICE strategy 2021 to 2026 - Dynamic, Collaborative, Excellent. (2021). https://static.nice.org.uk/NICE%20strategy%202021%20to%202026%20-%20Dynamic,%20Collaborative,%20Excellent.pdf . Accessed March 15, 2023.
Wang SV, Pinheiro S, Hua W, Arlett P, Uyama Y, Berlin JA, et al. STaRT-RWE: structured template for planning and reporting on the implementation of real world evidence studies. BMJ. 2021;372:m4856. https://doi.org/10.1136/bmj.m4856 .
Article PubMed PubMed Central Google Scholar
ISPOR: New Real-World Evidence Registry Launches. (2021). https://www.ispor.org/heor-resources/news-top/news/view/2021/10/26/new-real-world-evidence-registry-launches . Accessed March 15, 2023.
Corrigan-Curay J, Sacks L, Woodcock J. JAMA. 2018;320(9):867–8. https://doi.org/10.1001/jama.2018.10136 . Real-World Evidence and Real-World Data for Evaluating Drug Safety and Effectiveness.
Finger RP, Daien V, Talks JS, Mitchell P, Wong TY, Sakamoto T, et al. A novel tool to assess the quality of RWE to guide the management of retinal disease. Acta Ophthalmol. 2021;99(6):604–10. https://doi.org/10.1111/aos.14698 .
Article PubMed Google Scholar
Schneeweiss S, Eichler HG, Garcia-Altes A, Chinn C, Eggimann AV, Garner S, et al. Real World Data in Adaptive Biomedical Innovation: a Framework for Generating evidence fit for decision-making. Clin Pharmacol Ther. 2016;100(6):633–46. https://doi.org/10.1002/cpt.512 .
Article CAS PubMed Google Scholar
Gliklich RE, Leavy MB. Ther Innov Regul Sci. 2020;54(2):303–7. https://doi.org/10.1007/s43441-019-00058-6 . Assessing Real-World Data Quality: The Application of Patient Registry Quality Criteria to Real-World Data and Real-World Evidence.
Reynolds MW, Bourke A, Dreyer NA. Considerations when evaluating real-world data quality in the context of fitness for purpose. Pharmacoepidemiol Drug Saf. 2020;29(10):1316–8. https://doi.org/10.1002/pds.5010 .
Government of Canada: Building a National Strategy for High-Cost Drugs for Rare Diseases: A Discussion Paper for Engaging Canadians: A discussion Paper for Engaging Canadians. (2021). https://www.canada.ca/content/dam/hc-sc/documents/services/health-related-consultation/National-Strategy-High-Cost-Drugs-eng.pdf . Accessed July 20, 2023.
The Canadian Forum for Rare Disease Innovators (RAREi). Unique approach needed: Addressing barriers to accessing rare disease treatments. Submission to House of Commons Standing Committee on Health (HESA). https://www.ourcommons.ca/Content/Committee/421/HESA/Brief/BR10189782/br-external/CanadianForumForRareDiseasesInnovators-e.pdf (2013). Accessed November 8, 2023.
European Medicines Agency: Discussion paper: Use of patient disease registries for regulatory purposes – methodological and operational considerations. (2018). https://view.officeapps.live.com/op/view.aspx?src=https%3A%2F%2Fwww.ema.europa.eu%2Fen%2Fdocuments%2Fother%2Fdiscussion-paper-use-patient-disease-registries-regulatory-purposes-methodological-operational_en.docx&wdOrigin=BROWSELINK . Accessed November 17, 2023.
Gliklich RE, Leavy MB, Dreyer NA. Registries for evaluating patient outcomes: a user’s guide (4th Eds.) (Prepared by L&M Policy Research, LLC under Contract No. 290-2014-00004-C with partners OM1 and IQVIA) (2020). https://effectivehealthcare.ahrq.gov/sites/default/files/pdf/registries-evaluating-patient-outcomes-4th-edition.pdf . Accessed March 7, 2023.
Canada’s Drug and Health Technology Agency (cadth). Optimizing the Integration of Real–World Evidence as Part of Decision-Making for Drugs for Rare Diseases. What We Learned https://www.cadth.ca/sites/default/files/RWE/pdf/optimizing_the_integration_of_real_world_evidence_as_part_of_decision-making_for_drugs_for_rare_diseases.pdf . Accessed November 8, 2023.
Canada’s Drug and Health Technology Agency (cadth): Report on a Best Brains Exchange. Optimizing the Use of Real-World Evidence as Part of Decision-Making for Drugs for Rare Diseases. (2022). https://www.cadth.ca/sites/default/files/RWE/pdf/MG0022_best_brains_exchange_optimizing_the_use_of_real_world_evidence_as_part_of_decision_making_for_drugs_for_rare_diseases.pdf . Accessed November 8, 2023.
Ali SR, Bryce J, Tan LE, Hiort O, Pereira AM, van den Akker ELT, et al. The EuRRECa Project as a model for Data Access and Governance policies for Rare Disease registries that collect clinical outcomes. Int J Environ Res Public Health. 2020;17(23). https://doi.org/10.3390/ijerph17238743 .
Allen A, Patrick H, Ruof J, Buchberger B, Varela-Lema L, Kirschner J, et al. Development and Pilot Test of the Registry evaluation and quality standards Tool: an Information Technology-based Tool to support and review registries. Value Health: J Int Soc Pharmacoeconomics Outcomes Res. 2022;25(8):1390–8. https://doi.org/10.1016/j.jval.2021.12.018 .
Article Google Scholar
Jonker CJ, de Vries ST, van den Berg HM, McGettigan P, Hoes AW, Mol PGM. Capturing data in Rare Disease registries to Support Regulatory decision making: a Survey Study among Industry and other stakeholders. Drug Saf. 2021;44(8):853–61. https://doi.org/10.1007/s40264-021-01081-z .
EUnetHTA. REQueST: Tool and its vision paper. https://eunethta.eu/request-tool-and-its-vision-paper/ Accessed February 14, 2024.
Zaletel M, Kralj M. Methodological guidelines and recommendations for efficient and rational governance of patient registries (2015). https://health.ec.europa.eu/system/files/2016-11/patient_registries_guidelines_en_0.pdf . Accessed September 14, 2023.
Boyle L, Gautam M, Gorospe M, Kleiman Y, Dan L, Lynn E et al. Assessing Canadian Rare Disease Patient Registries for Real-World Evidence Using REQueST (2022). https://www.cadth.ca/sites/default/files/pdf/es0369-cadth-poster-laurie-lambert-final.pdf . Accessed March 15, 2023.
Sucharew H, Macaluso M. Methods for Research evidence synthesis: the Scoping Review Approach. J Hosp Med. 2019;14:416–8. https://doi.org/10.12788/jhm.3248 .
Rare Disease Task Force: Patient registries in the field of rare diseases: overview of the issues surrounding the establishment, management, governance and financing of academic registries. (2011). https://www.orpha.net/actor/EuropaNews/2011/doc/RDTFReportRegistries2009Rev2011.pdf . Accessed March 7, 2023.
Ouzzani M, Hammady H, Fedorowicz Z, Elmagarmid A. Rayyan-a web and mobile app for systematic reviews. Syst Rev. 2016;5(1):210. https://doi.org/10.1186/s13643-016-0384-4 .
Kodra Y, Weinbach J, Posada-de-la-Paz M, Coi A, Lemonnier SL, van Enckevort D, et al. Recommendations for improving the quality of Rare Disease registries. Int J Environ Res Public Health. 2018;15(8). https://doi.org/10.3390/ijerph15081644 .
Gainotti S, Torreri P, Wang CM, Reihs R, Mueller H, Heslop E, et al. Eur J Hum genetics: EJHG. 2018;26(5):631–43. https://doi.org/10.1038/s41431-017-0085-z . The RD-Connect Registry & Biobank Finder: a tool for sharing aggregated data and metadata among rare disease researchers
Bellgard MI, Napier KR, Bittles AH, Szer J, Fletcher S, Zeps N, et al. Design of a framework for the deployment of collaborative independent rare disease-centric registries: Gaucher disease registry model. Blood Cells Mol Dis. 2018;68:232–8. https://doi.org/10.1016/j.bcmd.2017.01.013 .
Biedermann P, Ong R, Davydov A, Orlova A, Solovyev P, Sun H, et al. BMC Med Res Methodol. 2021;21(1):238. https://doi.org/10.1186/s12874-021-01434-3 . Standardizing registry data to the OMOP Common Data Model: experience from three pulmonary hypertension databases.
Boulanger V, Schlemmer M, Rossov S, Seebald A, Gavin P. Establishing patient registries for Rare diseases: Rationale and challenges. Pharm Med. 2020;34(3):185–90. https://doi.org/10.1007/s40290-020-00332-1 .
Busner J, Pandina G, Domingo SZ, Berger AK, Acosta MT, Fisseha N, et al. Clinician-and patient-reported endpoints in CNS orphan drug clinical trials: ISCTM position paper on best practices for Endpoint Selection, Validation, Training, and standardization. Innovations Clin Neurosci. 2021;18(10–12):15–22.
Google Scholar
Derayeh S, Kazemi A, Rabiei R, Hosseini A, Moghaddasi H. National information system for rare diseases with an approach to data architecture: a systematic review. Intractable rare Dis Res. 2018;7(3):156–63. https://doi.org/10.5582/irdr.2018.01065 .
Marques JP, Carvalho AL, Henriques J, Murta JN, Saraiva J, Silva R. Design, development and deployment of a web-based interoperable registry for inherited retinal dystrophies in Portugal: the IRD-PT. Orphanet J Rare Dis. 2020;15(1):304. https://doi.org/10.1186/s13023-020-01591-6 .
Rubinstein YR, Groft SC, Bartek R, Brown K, Christensen RA, Collier E, et al. Creating a global rare disease patient registry linked to a rare diseases biorepository database: Rare Disease-HUB (RD-HUB). Contemp Clin Trials. 2010;31(5):394–404. https://doi.org/10.1016/j.cct.2010.06.007 .
National Cancer Registry Ireland: Data confidentiality in the National Cancer Registry. (2007). https://www.ncri.ie/data.cgi/html/confidentialitypolicy.shtml . Accessed March 7, 2023.
Kourime M, Bryce J, Jiang J, Nixon R, Rodie M, Ahmed SF. An assessment of the quality of the I-DSD and the I-CAH registries - international registries for rare conditions affecting sex development. Orphanet J Rare Dis. 2017;12(1):56. https://doi.org/10.1186/s13023-017-0603-7 .
Article CAS PubMed PubMed Central Google Scholar
Coi A, Santoro M, Villaverde-Hueso A, Lipucci Di Paola M, Gainotti S, Taruscio D, et al. The quality of Rare Disease registries: evaluation and characterization. Public Health Genomics. 2016;19(2):108–15. https://doi.org/10.1159/000444476 .
Mordenti M, Boarini M, D’Alessandro F, Pedrini E, Locatelli M, Sangiorgi L. Remodeling an existing rare disease registry to be used in regulatory context: lessons learned and recommendations. Front Pharmacol. 2022;13(no pagination). https://doi.org/10.3389/fphar.2022.966081 .
Ali SR, Bryce J, Kodra Y, Taruscio D, Persani L, Ahmed SF. The Quality evaluation of Rare Disease Registries-An Assessment of the essential features of a Disease Registry. Int J Environ Res Public Health. 2021;18(22). https://doi.org/10.3390/ijerph182211968 .
Maruf N, Chanchu G. Planning a rare disease registry (2022). https://orphan-reach.com/planning-a-rare-disease-registry/ . Accessed September 29, 2023.
Hessl D, Rosselot H, Miller R, Espinal G, Famula J, Sherman SL, et al. The International Fragile X Premutation Registry: building a resource for research and clinical trial readiness. J Med Genet. 2022;59(12):1165–70. https://doi.org/10.1136/jmedgenet-2022-108568 .
Vitale A, Della Casa F, Lopalco G, Pereira RM, Ruscitti P, Giacomelli R, et al. Development and implementation of the AIDA International Registry for patients with still’s disease. Front Med. 2022;9:878797. https://doi.org/10.3389/fmed.2022.878797 .
Stanimirovic D, Murko E, Battelino T, Groselj U. Development of a pilot rare disease registry: a focus group study of initial steps towards the establishment of a rare disease ecosystem in Slovenia. Orphanet J Rare Dis. 2019;14(1):172. https://doi.org/10.1186/s13023-019-1146-x .
Kinsner-Ovaskainen A, Lanzoni M, Garne E, Loane M, Morris J, Neville A, et al. A sustainable solution for the activities of the European network for surveillance of congenital anomalies: EUROCAT as part of the EU platform on Rare diseases Registration. Eur J Med Genet. 2018;61(9):513–7. https://doi.org/10.1016/j.ejmg.2018.03.008 .
Pericleous M, Kelly C, Schilsky M, Dhawan A, Ala A. Defining and characterising a toolkit for the development of a successful European registry for rare liver diseases: a model for building a rare disease registry. Clin Med J Royal Coll Physicians Lond. 2022;22(4). https://doi.org/10.7861/CLINMED.2021-0725 .
European Medicines Agency: Guideline on registry-based studies. (2021). https://www.ema.europa.eu/en/documents/scientific-guideline/guideline-registry-based-studies_en-0.pdf . Accessed March 7, 2023.
Bellgard MI, Snelling T, McGree JM. RD-RAP: beyond rare disease patient registries, devising a comprehensive data and analytic framework. Orphanet J Rare Dis. 2019;14(1):176. https://doi.org/10.1186/s13023-019-1139-9 .
Isaacman D, Iliach O, Keefer J, Campion DM, Kelly B. Registries for rare diseases: a foundation for multi-arm, multi-company trials. (2019). https://www.iqvia.com/library/white-papers/registries-for-rare-diseases .
Chorostowska-Wynimko J, Wencker M, Horvath I. The importance of effective registries in pulmonary diseases and how to optimize their output. Chronic Resp Dis. 2019;16:1479973119881777. https://doi.org/10.1177/1479973119881777 .
Liu P, Gong M, Li J, Baynam G, Zhu W, Zhu Y, et al. Innovation in Informatics to Improve Clinical Care and Drug Accessibility for Rare diseases in China. Front Pharmacol. 2021;12:719415. https://doi.org/10.3389/fphar.2021.719415 .
EUCERD: EUCERD Core Recommendations on Rare Disease Patient Registration and Data Collection. (2013). http://www.rd-action.eu/eucerd/EUCERD_Recommendations/EUCERD_Recommendations_RDRegistryDataCollection_adopted.pdf . Accessed September 28, 2023.
Bellgard MI, Macgregor A, Janon F, Harvey A, O’Leary P, Hunter A, et al. A modular approach to disease registry design: successful adoption of an internet-based rare disease registry. Hum Mutat. 2012;33(10):E2356–66. https://doi.org/10.1002/humu.22154 .
Garcia M, Downs J, Russell A, Wang W. Impact of biobanks on research outcomes in rare diseases: a systematic review. Orphanet J Rare Dis. 2018;13(1):202. https://doi.org/10.1186/s13023-018-0942-z .
EURORDIS-NORD-CORD:, EURORDIS-NORD-CORD Joint Declaration of 10 Key Principles for Rare Disease Patient Registries. (2012). https://download2.eurordis.org/documents/pdf/EURORDIS_NORD_CORD_JointDec_Registries_FINAL.pdf . Accessed September 28, 2023.
Marques JP, Vaz-Pereira S, Costa J, Marta A, Henriques J, Silva R. Challenges, facilitators and barriers to the adoption and use of a web-based national IRD registry: lessons learned from the IRD-PT registry. Orphanet J Rare Dis. 2022;17(1):323. https://doi.org/10.1186/s13023-022-02489-1 .
Hageman IC, van der Steeg HJJ, Jenetzky E, Trajanovska M, King SK, de Blaauw I, et al. A Quality Assessment of the ARM-Net Registry Design and Data Collection. J Pediatr Surg. 2023;25:25. https://doi.org/10.1016/j.jpedsurg.2023.02.049 .
Kaliyaperumal R, Wilkinson MD, Moreno PA, Benis N, Cornet R, Dos Santos Vieira B, et al. Semantic modelling of common data elements for rare disease registries, and a prototype workflow for their deployment over registry data. J Biomedical Semant. 2022;13(1):9. https://doi.org/10.1186/s13326-022-00264-6 .
European Commission. Principles of the GDPR. https://commission.europa.eu/law/law-topic/data-protection/reform/rules-business-and-organisations/principles-gdpr_en .
Office of the Privacy Commissioner of Canada. PIPEDA in brief. https://www.priv.gc.ca/en/privacy-topics/privacy-laws-in-canada/the-personal-information-protection-and-electronic-documents-act-pipeda/pipeda_brief/ (2019).
Bettio C, Salsi V, Orsini M, Calanchi E, Magnotta L, Gagliardelli L, et al. The Italian National Registry for FSHD: an enhanced data integration and an analytics framework towards Smart Health Care and Precision Medicine for a rare disease. Orphanet J Rare Dis. 2021;16(1):470. https://doi.org/10.1186/s13023-021-02100-z .
U.S. Department of Health and Human Services. : Rare Disease Registry Program, Data Ownership https://registries.ncats.nih.gov/glossary/data-ownership/ .
Deserno TM, Haak D, Brandenburg V, Deserno V, Classen C, Specht P. Integrated image data and medical record management for rare disease registries. A general framework and its instantiation to theGerman Calciphylaxis Registry. J Digit Imaging. 2014;27(6):702–13. https://doi.org/10.1007/s10278-014-9698-8 .
Blumenthal S, The, NQRN Registry Maturational Framework: Evaluating the Capability and Use of Clinical Registries. EGEMS, Washington. DC). 2019;7(1):29. https://doi.org/10.5334/egems.278 .
Lautenschlager R, Kohlmayer F, Prasser F, Kuhn KA. A generic solution for web-based management of pseudonymized data. BMC Med Inf Decis Mak. 2015;15:100. https://doi.org/10.1186/s12911-015-0222-y .
DAMA U.K. Working Group: The Six Primary Dimensions For Data Quality Assessment, Defining Data Quality Dimensions. (2013). https://www.sbctc.edu/resources/documents/colleges-staff/commissions-councils/dgc/data-quality-deminsions.pdf . Accessed September 28, 2023.
McGlinn K, Rutherford MA, Gisslander K, Hederman L, Little MA, O’Sullivan D. FAIRVASC: a semantic web approach to rare disease registry integration. Comput Biol Med. 2022;145:105313. https://doi.org/10.1016/j.compbiomed.2022.105313 .
Song P, He J, Li F, Jin C. Innovative measures to combat rare diseases in China: the national rare diseases registry system, larger-scale clinical cohort studies, and studies in combination with precision medicine research. Intractable rare Dis Res. 2017;6(1):1–5. https://doi.org/10.5582/irdr.2017.01003 .
Sernadela P, Gonzalez-Castro L, Carta C, van der Horst E, Lopes P, Kaliyaperumal R, et al. Linked registries: connecting Rare diseases patient registries through a semantic web layer. Biomed Res Int. 2017;2017:8327980. https://doi.org/10.1155/2017/8327980 .
U.S. Food and Drug Administration. Real-World Data: Assessing Registries to Support Regulatory Decision-Making for Drug and Biological Products Guidance for Industry: Draft Guidance (2021). https://www.regulations.gov/document/FDA-2021-D-1146-0041 . Accessed August 15, 2023.
Santoro M, Coi A, Di Lipucci M, Bianucci AM, Gainotti S, Mollo E, et al. Rare disease registries classification and characterization: a data mining approach. Public Health Genomics. 2015;18(2):113–22. https://doi.org/10.1159/000369993 .
Daneshvari S, Youssof S, Kroth PJ. The NIH Office of Rare Diseases Research patient registry Standard: a report from the University of New Mexico’s Oculopharyngeal muscular dystrophy patient Registry. AMIA Annual Symp Proc AMIA Symp. 2013;2013:269–77.
Rubinstein YR, McInnes P, NIH/NCATS/GRDR. Contemp Clin Trials. 2015;42:78–80. https://doi.org/10.1016/j.cct.2015.03.003 . R Common Data Elements: A leading force for standardized data collection.
Bellgard MI, Render L, Radochonski M, Hunter A. Second generation registry framework. Source Code Biol Med. 2014;9:14. https://doi.org/10.1186/1751-0473-9-14 .
Choquet R, Maaroufi M, de Carrara A, Messiaen C, Luigi E, Landais P. J Am Med Inf Association: JAMIA. 2015;22(1):76–85. https://doi.org/10.1136/amiajnl-2014-002794 . A methodology for a minimum data set for rare diseases to support national centers of excellence for healthcare and research.
Mullin AP, Corey D, Turner EC, Liwski R, Olson D, Burton J, et al. Standardized data structures in Rare diseases: CDISC user Guides for Duchenne Muscular Dystrophy and Huntington’s Disease. Clin Transl Sci. 2021;14(1):214–21. https://doi.org/10.1111/cts.12845 .
Groenen KHJ, Jacobsen A, Kersloot MG, Dos Santos Vieira B, van Enckevort E, Kaliyaperumal R, et al. The de novo FAIRification process of a registry for vascular anomalies. Orphanet J Rare Dis. 2021;16(1):376. https://doi.org/10.1186/s13023-021-02004-y .
Taruscio D, Mollo E, Gainotti S, Posada de la Paz M, Bianchi F, Vittozzi L. Archives public health = Archives belges de sante publique. 2014;72(1):35. https://doi.org/10.1186/2049-3258-72-35 . The EPIRARE proposal of a set of indicators and common data elements for the European platform for rare disease registration.
Roos M, Lopez Martin E, Wilkinson MD. Adv Exp Med Biol. 2017;1031:165–79. https://doi.org/10.1007/978-3-319-67144-4_9 . Preparing Data at the Source to Foster Interoperability across Rare Disease Resources.
Ahern S, Sims G, Earnest A, Bell C. Optimism, opportunities, outcomes: the Australian cystic Fibrosis Data Registry. Intern Med J. 2018;48(6):721–3. https://doi.org/10.1111/imj.13807 .
Maaroufi M, Choquet R, Landais P, Jaulent M-C. Towards data integration automation for the French rare disease registry. AMIA Annual Symposium proceedings AMIA Symposium. 2015;2015:880-5.
Bellgard MI, Sleeman MW, Guerrero FD, Fletcher S, Baynam G, Goldblatt J, et al. Rare Disease Research Roadmap: navigating the bioinformatics and translational challenges for improved patient health outcomes. Health Policy Technol. 2014;3(4):325–35. https://doi.org/10.1016/j.hlpt.2014.08.007 .
Bellgard M, Beroud C, Parkinson K, Harris T, Ayme S, Baynam G, et al. Dispelling myths about rare disease registry system development. Source Code Biol Med. 2013;8(1):21. https://doi.org/10.1186/1751-0473-8-21 .
Vasseur J, Zieschank A, Gobel J, Schaaf J, Dahmer-Heath M, Konig J, et al. Development of an interactive dashboard for OSSE Rare Disease registries. Stud Health Technol Inform. 2022;293:187–8. https://doi.org/10.3233/SHTI220367 .
Amselem S, Gueguen S, Weinbach J, Clement A, Landais P. RaDiCo, the French national research program on rare disease cohorts. Orphanet J Rare Dis. 2021;16(1):454. https://doi.org/10.1186/s13023-021-02089-5 .
Hooshafza S, Mc Quaid L, Stephens G, Flynn R, O’Connor L. Development of a framework to assess the quality of data sources in healthcare settings. J Am Med Inf Assoc. 2022;29(5):944–52. https://doi.org/10.1093/jamia/ocac017 .
European Reference Network. ERN-RND Registry. https://www.ern-rnd.eu/ern-rnd-registry/#registry-objectives Accessed February 14, 2024.
U.S. Food and Drug Administration. Real-World Data: Assessing Registries To Support Regulatory Decision-Making for Drug and Biological Products. Final (2023). https://www.fda.gov/regulatory-information/search-fda-guidance-documents/real-world-data-assessing-registries-support-regulatory-decision-making-drug-and-biological-products . Accessed April 4, 2024.
Canada’s Drug and Health Technology Agency (cadth): Guidance for Reporting Real-World Evidence. (2023). https://www.cadth.ca/sites/default/files/RWE/MG0020/MG0020-RWE-Guidance-Report-Secured.pdf . Accessed November 16, 2023.
National Institute for Health and Care Excellence (NICE): NICE Real-World Evidence Framework. (2022). https://www.nice.org.uk/corporate/ecd9/resources/nice-realworld-evidence-framework-pdf-1124020816837 . Accessed November 16, 2023.
Government of Canada. Government of Canada improves access to affordable and effective drugs for rare diseases. https://www.canada.ca/en/health-canada/news/2023/03/government-of-canada-improves-access-to-affordable-and-effective-drugs-for-rare-diseases.html (2023).
Public Health Agency of Canada: Moving Forward on a Pan-Canadian Health Data Strategy. (2022). https://www.canada.ca/en/public-health/programs/pan-canadian-health-data-strategy.html . Accessed November 16, 2023.
European Commission: European Platform on Rare Disease Registration (EU RD Platform). https://eu-rd-platform.jrc.ec.europa.eu/_en Accessed February 9, 024.
European Commission. European Rare Disease Registry Infrastructure (ERDRI). https://eu-rd-platform.jrc.ec.europa.eu/erdri-description_en Accessed February 14, 2024.
European Commission. Set of Common Data Elements. https://eu- rd-platform.jrc.ec.europa.eu/set-of-common-data-elements_en Accessed February 14, 2024.
Abaza H, Kadioglu D, Martin S, Papadopoulou A, Dos Santos Vieira B, Schaefer F, et al. JMIR Med Inf. 2022;10(5):e32158. https://doi.org/10.2196/32158 . Domain-Specific Common Data Elements for Rare Disease Registration: Conceptual Approach of a European Joint Initiative Toward Semantic Interoperability in Rare Disease Research.
O’Neill J, Tabish H, Welch V, Petticrew M, Pottie K, Clarke M, et al. Applying an equity lens to interventions: using PROGRESS ensures consideration of socially stratifying factors to illuminate inequities in health. J Clin Epidemiol. 2014;67(1):56–64. https://doi.org/10.1016/j.jclinepi.2013.08.005 .
Oehrlein EM, Schoch S, Burcu M, McBeth JF, Bright J, Pashos CL, et al. Developing patient-centered real-world evidence: emerging methods recommendations from a Consensus process. Value Health. 2023;26(1):28–38. https://doi.org/10.1016/j.jval.2022.04.1738 .
Download references
Acknowledgements
The authors would like to acknowledge Farah Husein, Laurie Lambert, Patricia Caetano and Nicole Mittmann from CADTH for their support and suggestions on an earlier version of the manuscript.
Author’s Information (option) - Not applicable.
This work was supported with funding from the Canadian Agency for Drugs and Technologies in Health (CADTH).
Author information
Authors and affiliations.
Department of Health Research Methods, Evidence and Impact, Faculty of Health Sciences, McMaster University, Hamilton, Canada
JE Tarride, A. Okoh, K. Aryal, C. Prada, A. Keepanasseril & A. Iorio
Centre for Health Economics and Policy Analysis (CHEPA), McMaster University, Hamilton, Canada
JE Tarride & Deborah Milinkovic
Programs for the Assessment of Technologies in Health (PATH), The Research Institute of St. Joe’s Hamilton, St. Joseph’s Healthcare Hamilton, Hamilton, ON, Canada
You can also search for this author in PubMed Google Scholar
Contributions
JET and AI contributed to the concept of the study. JET, AO, KA, AK, CP contributed to the literature screening and data abstraction. JET, AO, DM contributed to the preparation of the draft manuscript. All authors contributed to the synthesis of the literature and the review/approval of the manuscript.
Corresponding author
Correspondence to Deborah Milinkovic .
Ethics declarations
Ethics approval and consent to participate.
not applicable.
Consent for publication
all authors give their consent to publish.
Competing interests
no conflict of interest.
Additional information
Publisher’s note.
Springer Nature remains neutral with regard to jurisdictional claims in published maps and institutional affiliations.
Electronic supplementary material
Below is the link to the electronic supplementary material.
Supplementary Material 1
Supplementary material 2, supplementary material 3, supplementary material 4, rights and permissions.
Open Access This article is licensed under a Creative Commons Attribution 4.0 International License, which permits use, sharing, adaptation, distribution and reproduction in any medium or format, as long as you give appropriate credit to the original author(s) and the source, provide a link to the Creative Commons licence, and indicate if changes were made. The images or other third party material in this article are included in the article’s Creative Commons licence, unless indicated otherwise in a credit line to the material. If material is not included in the article’s Creative Commons licence and your intended use is not permitted by statutory regulation or exceeds the permitted use, you will need to obtain permission directly from the copyright holder. To view a copy of this licence, visit http://creativecommons.org/licenses/by/4.0/ . The Creative Commons Public Domain Dedication waiver ( http://creativecommons.org/publicdomain/zero/1.0/ ) applies to the data made available in this article, unless otherwise stated in a credit line to the data.
Reprints and permissions
About this article
Cite this article.
Tarride, J., Okoh, A., Aryal, K. et al. Scoping review of the recommendations and guidance for improving the quality of rare disease registries. Orphanet J Rare Dis 19 , 187 (2024). https://doi.org/10.1186/s13023-024-03193-y
Download citation
Received : 21 November 2023
Accepted : 19 April 2024
Published : 06 May 2024
DOI : https://doi.org/10.1186/s13023-024-03193-y
Share this article
Anyone you share the following link with will be able to read this content:
Sorry, a shareable link is not currently available for this article.
Provided by the Springer Nature SharedIt content-sharing initiative
- Rare diseases
- Quality standards
Orphanet Journal of Rare Diseases
ISSN: 1750-1172
- Submission enquiries: Access here and click Contact Us
- General enquiries: [email protected]
Big data in transportation: a systematic literature analysis and topic classification
- Brief Report
- Open access
- Published: 08 May 2024
Cite this article
You have full access to this open access article
- Danai Tzika-Kostopoulou 1 ,
- Eftihia Nathanail 1 &
- Konstantinos Kokkinos 2
161 Accesses
Explore all metrics
This paper identifies trends in the application of big data in the transport sector and categorizes research work across scientific subfields. The systematic analysis considered literature published between 2012 and 2022. A total of 2671 studies were evaluated from a dataset of 3532 collected papers, and bibliometric techniques were applied to capture the evolution of research interest over the years and identify the most influential studies. The proposed unsupervised classification model defined categories and classified the relevant articles based on their particular scientific interest using representative keywords from the title, abstract, and keywords (referred to as top words). The model’s performance was verified with an accuracy of 91% using Naïve Bayesian and Convolutional Neural Networks approach. The analysis identified eight research topics, with urban transport planning and smart city applications being the dominant categories. This paper contributes to the literature by proposing a methodology for literature analysis, identifying emerging scientific areas, and highlighting potential directions for future research.
Avoid common mistakes on your manuscript.
1 Introduction
Urbanization, ongoing changes in mobility patterns, and rapid growth in freight transportation pose significant challenges to stakeholders and researchers within the transportation sector. New policies have focused on promoting sustainability and reducing emissions through smart and multimodal mobility [ 1 ]. To address these challenges, authorities are developing strategies that employ technological advances to gain a deeper understanding of travel behavior, produce more accurate travel demand estimates, and enhance transport system performance.
Undoubtedly, the development of Intelligent Transport Systems (ITS) and recent advances in Information and Communication Technology (ICT) have enabled the continuous generation, collection, and processing of data and the observation of mobility behavior with unprecedented precision [ 2 ]. Such data can be obtained from various sources, including ITS, cell phone call records, smart cards, geocoded social media, GPS, sensors, and video detectors.
Over the past decade, there has been increasing research interest in the application of big data in various transportation sectors, such as supply chain and logistics [ 3 ], traffic management [ 4 ], travel demand estimation [ 5 ], travel behavior [ 6 ], and real-time traffic operations [ 7 ]. Additionally, numerous studies in the field of transport planning and modeling have applied big data to extract vital attributes including trip identification [ 8 ] and activity inference [ 9 ]. Despite research efforts and existing applications of big data in transportation, many aspects remain unknown, and the prospects of big data to gain better insights into transport infrastructure and travel patterns have not yet been explored.
To maximize the benefits of big data in traffic operations, infrastructure maintenance, and predictive modeling, several challenges remain to be addressed. These include handling the high velocity and volume of streaming data for real-time applications, integrating multiple data sets with traditional information sources, ensuring that the data is representative of the entire population, and accessing big data without compromising privacy and ethical concerns. In terms of transport modeling and management, the research focuses on achieving short-term stability by incorporating more comprehensive data sets that cover hourly, daily, seasonal, or event-based variations, and on enhancing mobility on demand through real-time data processing and analysis. Additionally, it has become necessary to further investigate the methodology for processing large amounts of spatial and temporal information that has primarily been intended for non-transport purposes and to reconsider the existing analytical approaches to adapt to the changing data landscape.
There is an intention among policymakers, transport stakeholders, and researchers to better understand the relationship between big data and transport. The first step in this direction is to identify the key areas of big data utilization in the transport sector. Therefore, this study attempts to map big data applications within the transport domain. It provides a broad overview of big data applications in transport and contributes to the literature by introducing a methodology for identifying emerging areas where big data can be successfully applied and subfields that can be further developed.
The scope of the current study is twofold. First, a holistic literature analysis based on bibliometric techniques complemented by a topic classification model covering the complete domain of big data applications in the transportation sector was implemented. Despite numerous studies attempting to review the relevant literature, such as big data in public transport planning or transport management [ 10 , 11 ] to the best of our knowledge, no such investigation has produced a comprehensive and systematic cluster of multiple big data applications across the entire transportation domain based on a significant number of literature records. Therefore, the primary objective of this study is to classify the literature according to its particular interest and to pinpoint evolving scientific subfields and current research trends.
Second, as multiple studies have been conducted in this domain, the need to identify and assess them prior to running one’s own research through a thorough literature review is always necessary. However, the analysis and selection of appropriate studies can be challenging, particularly when the database is large. Therefore, this study aims to provide a comprehensive approach for evaluating and selecting appropriate literature that could be a methodological tool in any research field. Bibliometric methods have been widely applied in several scientific fields. Most of these studies use simple statistical methods to determine the evolution of the number of publications over the years, authors’ influence, or geographical distribution. There are also research works that attempt to categorize the literature mainly by manual content analysis [ 12 , 13 ] or by co-citation analysis, applying software for network and graphical analyses [ 14 , 15 ]. In this study, the review process also included an unsupervised topic model to classify literature into categories.
This paper presents a comprehensive evaluation of up-to-date published studies on big data and their applications in the transportation domain. A total of 2671 articles from Elsevier's Scopus database, published between 2012 and 2022 were analyzed. Bibliometric techniques were applied to capture the evolution of research over time and uncover emerging areas of interest. In addition, the focus of this study is to define categories and classify relevant papers based on their scientific interests. To achieve this, unsupervised classification was applied using the topic model proposed by Okafor [ 16 ] to identify clusters, extract the most representative topics, and group the documents accordingly.
The current study attempts to answer the following questions:
Which studies contribute the most to the field of big data in transportation?
What is the evolution of research over time in this field of interest?
What are the main research areas that have potential for further exploration?
What are the directions of future research?
This paper consists of six sections. Following the introduction, Sect. 2 provides a summary of previous research in this subject area. Section 3 outlines the methodology applied in this research. This includes the process of defining the eligible studies, bibliometric techniques utilized, and the topic model employed for paper classification. Section 4 presents the initial statistical results and the classification outcomes derived from the topic model. In Sect. 5 , the findings are summarized, and the results associated with the research questions are discussed. The final Section presents the general conclusions and research perspectives of the study.
2 Literature review
Due to the significant benefits of big data, several studies have been conducted in recent years to review and examine the existing applications of different big data sources in transportation. Most of these focus on a specific transport domain, such as transport planning, transport management and operations, logistics and supply chain and Intelligent Transportation Systems.
In the context of transport planning and modeling, Anda et al. [ 2 ] reviewed the current application of historical big data sources, derived from call data records, smart card data, and geocoded social media records, to understand travel behavior and to examine the methodologies applied to travel demand models. Iliashenko et al. [ 17 ] explored the potential of big data and Internet of Things technologies for transport planning and modeling needs, pointing out possible applications. Wang et al. [ 18 ] analyzed existing studies on travel behavior utilizing mobile phone data. They also identified the main opportunities in terms of data collection, travel pattern identification, modeling and simulation. Huang et al. [ 19 ] conducted a more specialized literature review focusing on the existing mode detection methods based on mobile phone network data. In the public transportation sector, Pelletier et al. [ 20 ] focused on the application of smart card data, showing that in addition to fare collection, these data can also be used for strategic purposes (long-term planning), tactical purposes (service adjustment and network development), and operational purposes (public transport performance indicators and payment management). Zannat et al. [ 10 ] provided an overview of big data applications focusing on public transport planning and categorized the reviewed literature into three categories: travel pattern analysis, public transport modeling, and public transport performance assessment.
In traffic forecasting, Lana et al. [ 21 ] conducted a survey to evaluate the challenges and technical advancements of traffic prediction models using big traffic data, whereas Miglani et al. [ 22 ] investigated different deep learning models for traffic flow prediction in autonomous vehicles. Regarding transport management, Pender et al. [ 23 ] examined social media use during transport network disruption events. Choi et al. [ 11 ] reviewed operational management studies associated with big data and identified key areas including forecasting, inventory management, revenue management, transportation management, supply chain management, and risk analysis.
There is also a range of surveys investigating the use of big data in other transport subfields. Ghofrani et al. [ 24 ] analyzed big data applications in railway engineering and transportation with a focus on three areas: operations, maintenance, and safety. In addition, Borgi et al. [ 25 ] reviewed big data in transport and logistics and highlighted the possibilities of enhancing operational efficiency, customer experience, and business models.
However, there is a lack of studies that have explored big data applications attempting to cover a wider range of transportation aspects. In this regard, Zhu et al. [ 26 ] examined the features of big data in intelligent transportation systems, the methods applied, and their applications in six subfields, namely road traffic accident analysis, road traffic flow prediction, public transportation service planning, personal travel route planning, rail transportation management and control, and asset management. Neilson et al. [ 27 ] conducted a review of big data usage obtained from traffic monitoring systems crowdsourcing, connected vehicles, and social media within the transportation domain and examined the storage, processing, and analytical techniques.
The study by Katrakazas et al. [ 28 ], conducted under the NOESIS project funded by the European Union's (EU) Horizon 2020 (H2020) program, is the only one we located that comprehensively covers the transportation field. Based on the reviewed literature, the study identified ten areas of focus that could further benefit from big data methods. The findings were validated by discussing with experts on big data in transportation. However, the disadvantage of this study lies in its dependence on a limited scope of the reviewed literature.
The majority of current review-based studies concentrate on one aspect of transportation, often analyzing a single big data source. Many of these studies rely on a limited literature dataset, and only a few have demonstrated a methodology for selecting the reviewed literature. Our review differs from existing surveys in the following ways: first, a methodology for defining the selected literature was developed, and the analysis was based on a large literature dataset. Second, this study is the only one to employ an unsupervised topic classification model to extract areas of interest and open challenges in the domain. Finally, it attempts to give an overview of the applications of big data across the entire field of transportation.
3 Research methodology
This study followed a three-stage literature analysis approach. The first stage includes defining the literature source and the papers' search procedures, as well as the “screening” to select the reviewed literature. The second stage involves statistics, which are widely employed in bibliometric analysis, to capture trends and primary insights. In the third stage, a topic classification model is applied to identify developing subfields and their applications. Eventually, the results are presented, and the findings are summarized.
3.1 Literature selection
The first step in this study was to define the reviewed literature. A bibliographic search was conducted using the Elsevier's Scopus database. Scopus and Web of Science (WOS) are the most extensive databases covering multiple scientific fields. However, Scopus offers wider overall coverage than WoS CC and provides a better representation of particular subject fields such as Computer Sciences [ 29 ] which is of interest in this study. Additionally, Scopus comprises 26591 peer-reviewed journals [ 30 ] including publications by Elsevier, Emerald, Informs, Taylor and Francis, Springer, and Interscience [ 15 ], covering the most representative journals in the transportation sector.
The relevant literature was identified and collected using the Scopus search API, which supports Boolean syntax. Four combinations of keywords were used in the “title, abstract, keywords” document search of the Scopus database including: “Big data” and “Transportation”, “Big data” and “Travel”, “Big data” and “Transport”, “Big data” and “Traffic”. The search was conducted in English as it offers a wider range of bibliographic sources. Only the last decade’s peer-reviewed research papers published in scientific journals and conference proceedings have been collected, written exclusively in English. Review papers and document types such as books and book chapters were excluded. As big data in transport is an interdisciplinary field addressed by different research areas, in order to cover the whole field of interest, the following subject areas were predefined in the Scopus search: computer sciences; engineering; social sciences; environmental sciences; energy; business, management and accounting. Fields considered irrelevant to the current research interest were filtered out.
The initial search resulted in a total of 5234 articles published between the period 2012–2022. The data was collected in December 2021 and last updated on the 5th of September 2023. The results were stored in a csv format, including all essential paper information such as paper title, authors’ names, source title, citations, abstracts, year of publication, and keywords. After removing duplications, a final dataset of 3532 papers remained.
The paper dataset went through a subject relevance review, at the first stage, by checking in the papers' title or keywords the presence of at least one combination of the search terms. If this condition was not met, a further review of the paper abstracts was conducted. From both stages, a filtered set of papers was selected, based on their relevance to the current study's areas of interest, associated with the search items. A total of 2671 selected papers formed the dataset which was further analyzed, evaluated, and categorized, based on clustering techniques.
3.2 Initial statistics
Once the dataset was defined, statistical analysis was performed to identify influential journals and articles within the study field. The first task was to understand the role of the different journals and conference proceedings. Those with the most publications were listed and further analyzed according to their publication rate and research area of interest. Second, the number of citations generated by the articles was analyzed as a measure of the quality of the published studies, and the content of the most cited articles was further discussed. The above provided essential insights into research trends and emerging topics.
3.3 Topic classification
A crucial step in our analysis was to extract the most representative sub-topics and classify the articles into categories by applying an unsupervised topic model [ 16 ]. Initially, the Excel file with the selected papers’ data (authors, year, title, abstract, and keywords) was imported into the model. Abstracts, titles, and keywords were analyzed and text-cleaning techniques were applied. This step includes normalizing text, removing punctuations, stop-words, and words of length less than three letters, as well as the lemmatization of the words. The most popular software tools/libraries used for text mining and cleaning, as well as natural language processing (NLP) in the topic model process, are implemented in Python programming and include NLTK ( https://www.nltk.org/ ), spaCy ( https://spacy.io/ ), Gensim ( https://radimrehurek.com/gensim/ ), scikit-learn ( https://scikit-learn.org/stable/ ), and Beautiful Soup ( https://www.crummy.com/software/BeautifulSoup/ ). NLTK is a powerful NLP library with various text preprocessing functions, while spaCy handles tokenization, stop word removal, stemming, lemmatization, and part-of-speech tagging. Gensim is a popular library for topic labeling and document similarity analysis. To process textual data, scikit-learn is a machine learning library with text preprocessing functions. Finally, Beautiful Soup is a web-based library for parsing HTML and XML documents. For the approach explained in the following sections, NLTK and Beautiful Soup were used to parse web metadata for the research papers. Moreover, bigrams and trigrams of two or three words, frequently occurring together in a document, were created.
The basic aim was to generate clusters (topics) using a topic model. The proposed model extracts representative words from the title, abstract, and keyword section of each paper, aiming to cluster research articles into neighborhoods of topics of interest without requiring any prior annotations or labeling of the documents. The method initially constructs a word graph model by counting the Term Frequency – Inverse Document Frequency (TF-IDF) [ 31 ].
The resulting topics are visualized through a diagram that shows the topics as circular areas. For the aforementioned mechanism, the Jensen-Shannon Divergence & Principal Components dimension reduction methodology was used [ 32 ]. The model is implemented in the LDAvis (Latent Dirichlet Allocation) Python library, resulting in two principal components (PC1 and PC2) that visualize the distance between the topics on a two-dimensional plane. The topic circles are created using a computationally greedy approach (the first in-order topic gets the largest area, and the rest of the topics get a proportional area according to their calculated significance). The method picks the circle centroids randomly for the first one (around the intersection of the two axes), and then the distance of the circle centroids is relevant to the overlapping of the top words according to the Jensen-Shannon Divergence model. The former model was applied for numerous numbers of topics, while its performance was experimentally verified using a combination of Naïve Bayesian Networks and a Convolutional Neural Network approach.
In the sequence of the two machine learning methodologies, supervised (Bayesian Neural Networks) and unsupervised (Deep Learning) research article classification were considered via sentiment analysis. For the supervised case, the relations among words were identified, offering interesting qualitative information from the outcome of the TF-IDF approach. The results show that the Bayesian Networks perform in accuracies near 90% of the corresponding statistical approach [ 33 ] and the same happens (somewhat inferior performance) for the unsupervised case [ 34 ]. The two methods validated the results of the TF-IDF, reaching accuracies within the acceptable limits of the aforementioned proven performances.
3.3.1 TF-IDF methodology
The TF-IDF (Term Frequency–Inverse Document Frequency) method is a weighted statistical approach primarily used for mining and textual analysis in large document collections. The method focuses on the statistical importance of a word value emanating by its existence in a document and the frequency of occurrences. In this context, the statistical significance of words grows in proportion to their frequency in the text, but also in inverse proportion to their frequency in the entire corpus of documents. Therefore, if a word or phrase appears with high frequency in an article (TF value is high), but simultaneously seldom appears in other documents (IDF value is low), it is considered a highly candidate word that may represent the article and can be used for classification [ 35 ]. In the calculation of TF-IDF, the TF word value is given as:
In Eq. 1 , \({n}_{ij}\) denotes the frequency of the word occurrence of the term \({t}_{i}\) in the document \({d}_{j}\) , and the denominator of the above fraction is the sum of the occurrence frequency of all terms in the document \({d}_{j}.\) At the same time, the calculation of the IDF value of a term \({t}_{i}\) , is found by dividing the total number of documents in the corpus by the number of documents containing the term \({t}_{i}\) , and then obtains the quotient logarithm:
In Eq . 2 , | D | denotes the total number of documents, and the denominator represents the number of documents j which contain the term \({t}_{i}\) in that specific document \({d}_{j}\) . In other words, we consider only the documents where \({n}_{ij}\ne 0\) in Eq. ( 1 ). In the case scenario in which the term \({t}_{i}\) does not appear in the corpus, it will cause the dividend to be zero in the above equation, causing the denominator to have the values of + 1. Using Eqs. ( 1 ) and ( 2 ), TF-IDF is given by:
From Eq. 3 , it can be assumed that high values of TF-IDF can be produced by high term frequencies in a document, while at the same time, low term frequencies occur in the entire document corpus. For this reason, TF-IDF succeeds in filtering out all high-frequency “common” words, while at the same time, retaining statistically significant words that can be the topic representatives [ 36 ]
3.3.2 Naïve bayes methodology
This methodology evaluates the performance of TF-IDF by following a purely “machine-learning” oriented approach, which is based on the Bayesian likelihood, i.e., reverse reasoning to discover arbitrary factor occurrences that impact a particular result. These arbitrary factors correspond to the corpus terms and their frequencies within each document and corpus. The model is a multinomial naive Bayes classifier that utilizes the Scikit-learn, which is a free programming Artificial Intelligence (AI) library for the Python programming language to support: a. training text, b. feature vectors, c. the predictive model, and d. the grouping mechanism.
The results of the TF-IDF method were imported into the Bayesian classifier. More specifically, the entire dataset was first prepared to be inserted by applying noise, stop-words, and punctuation removal. The text was then tokenized into words and phrases. For topic modeling, TF-IDF was used for feature extraction, creating the corresponding vectors as features for classification. In the next step, the Naive Bayes classifier is trained on the pre-processed and feature-extracted data. During training, it learns the likelihood of observing specific words or features given each topic and the prior probabilities of each topic occurring in the training data. Not all data was used for training. The model split the data into a 70% portion used for the unsupervised training, with the remaining 30% to be used for validation. This split ratio is a rule of thumb and not a strict requirement. However, this popular split ratio is to strike a balance between having enough data for training the machine learning model effectively and having enough data for validation or testing to evaluate the model's performance. The split was used within the k-fold cross-validation to assess the performance of the model while mitigating the risk of overfitting. While the dataset is divided into k roughly equal-sized "folds" or subsets, a fixed train-test split is used within each fold. The results from each fold were then averaged to obtain an overall estimate of model performance. This approach has the advantage of assessing the model's performance in a more granular way within each fold, similar to how it is assessed in a traditional train-test split, but at the same time, it provides additional information about how the model generalizes to different subsets of the data within each fold.
In the process of text examination, a cycle of weighting is dynamically updated for cases of term recurrences in the examined documents. The documents still contain only the title, abstract, and keywords for each article. For these cases, the Bayes theorem is used:
where \(P(c|x)\) is the posterior probability, \(P(x|c)\) is the likelihood, \(P(c)\) is the class prior probability, and \(P(x)\) is the predictor prior probability with \(P(c|x)\) resulting from the individual likelihoods of all documents \(P\left({x}_{i}|c\right),\) as depicted in Eq. ( 5 ):
This model was used as a validation method for the TF-IDF methodology, producing accuracy results reaching values of up to 91%. This value is widely acceptable for most cases of Bayesian classification and is expected to occur since prior classification has been applied [ 37 ].
3.3.3 Deep learning classification methodology
This is a secondary method of TF-IDF validation, which is based on the bibliometric coupling technique. However, this technique does not use the likelihood probability of the initial classification performed by TF-IDF but rather, this classifier deploys a character-based (i.e., the alphabetical letters composing the articles’ text) convolutional deep neural network. Using the letters as basic structural units, a Convolutional Neural Network [ 38 ] learns words and discovers features and word occurrences in various documents. The model has been primarily applied for computer vision and image machine learning techniques [ 39 ], but it is easily adapted for textual analysis.
All features previously used features (title, abstract, keywords) were kept and concatenated into a single string of text, which was itself truncated to a maximum length of 4000 characters. The size of the string can be dynamically adapted for each TensorFlow-model [ 40 ] according to the GPU performance of the graphics adapter of the hardware used, and basically represents the maximum allowable length for each feature in the analysis. The encoding involved all 26 English characters, 10 digits, all punctuation signs, and the space character. Other extraneous characters were eliminated. Furthermore, keyword information was considered primary in topic classification and encoded into a vector of the proportion of each subfield mentioned in the reference list of all documents/articles used in data. The system was rectified to behave as a linear unit, producing the activation function of the deep neural network between each layer. Only the last layer of the network utilized the SoftMax activation function for the final classification. The model was trained with a stochastic gradient descent as the optimizer and categorical cross-entropy as the loss function producing inferior results when compared with the corresponding Bayesian case as expected.
4.1 Source analysis
To understand the role of diverse academic sources, the leading eleven journals or conference proceedings were identified (Table 1 ), which have published a minimum of twenty papers between 2012 and 2022 in the field of interest. According to the preliminary data, 995 journals and conference proceedings have contributed to the publication of 2671 papers. Eleven sources have published 553 articles, representing the 2100% of all published papers.
Three sources in the field of computer science have published 239 articles. Five transportation journals and conference proceedings have published 208 papers, while there are journals on urban planning and policies (e.g., Cities) that have also significantly contributed to the research field (106 papers).
The research findings indicate the interdisciplinarity of the application of big data in transportation, encompassing not only computer science but also transport and urban planning journals, showing that transport specialists acknowledge the advantages of examining the applications of big data in the transport domain.
4.2 Citation analysis
Citation analysis classifies the papers by their citation frequency, aiming to point out their scientific research impact [ 14 ] and to identify influential articles within a research area. Table 2 demonstrates the top fifteen studies published between 2012 and 2022 (based on citation count on Scopus). Lv et al. [ 41 ] published the most influential paper in this period and received 2284 citations. This study applied a novel deep learning method to predict traffic flow. In this direction, four other articles focused on traffic flow prediction, real-time traffic operation, and transportation management [ 7 , 11 , 42 , 43 ].
Other important contributions highlighted the significance of big data generated in cities and analyzed challenges and possible applications in many aspects of the city, such as urban planning, transportation, and the environment [ 44 , 45 , 46 , 47 ]. Xu et al. [ 48 ] focused on the Internet of Vehicles and the generated big data. Finally, among the most influential works, there are papers that investigated big data usage in various subfields of spatial analysis, as well as urban transport planning and modeling, focusing on travel demand prediction, travel behavior analysis, and activity pattern identification [ 5 , 6 , 49 , 50 , 51 ].
4.3 Topic model
As previously mentioned, a topic model based on the TF-IDF methodology was used to categorize the papers under different topics. The basic goal was to identify subscientific areas of transportation where big data were implemented. A critical task was to define the appropriate parameters of the model, particularly the number of topics that could provide the most accurate and meaningful results, which would be further processed. To achieve this, a qualitative analysis was conducted taking into account the accuracy results obtained from the validation methodology. Therefore, to obtain a more precise view of the project, the model was implemented under various scenarios, increasing each time the number of topics by one, starting from four topics to fifteen.
The mapping of the tokenized terms and phrases to each other was used to understand the relationships between words in the context of topics and documents. The technique applied is the Multidimensional Scaling (MDS), which helps to visualize and analyze these relationships in a lower-dimensional space [ 52 , 53 ]. To highlight the relationships between terms, a matrix of tokens in the corpus was created based on their co-occurrence patterns. Each cell in the matrix represents how often two terms appear together in the same document. The MDS methodology provides the ability to view high-dimensional data in a lower-dimensional space while retaining as much of the pairwise differences or similarities between the terms as possible. More specifically, MDS translates the high-dimensional space of term co-occurrence relationships into a lower-dimensional space, where words that frequently co-occur are located closer to one another, and terms that infrequently co-occur are situated farther apart. When terms are displayed as points on a map or scatterplot via MDS, points that are close together in the scatterplot are used in the documents in a more connected manner.
As a consequence, the scatterplot that MDS produces can shed light on the structure of the vocabulary in relation to the topic model. It can assist in identifying groups of related terms that may indicate subjects or themes in the corpus. The pyLDAvis library of the Python programming language was used in conjunction with the sklearn library to incorporate the MDS into the clustering visualization process. This was done by superimposing the scatterplot with details about the subjects assigned to documents or the probability distributions over different topics.
Initially, the papers were divided into four topics. Figure 1 a displays four distinct clusters, each representing a scientific sub-area of interest. Clusters appear as circular areas, while the two principal components (PC1 and PC2) are used to visualize the distance between topics on a two-dimensional plane. Figure 1 b illustrates the most relevant terms for Topic 1 in abstracts, titles, and keywords. Table 3 contains the most essential words associated with each sub-area based on the TF-IDF methodology, representing the nature of the four topics. The results of the model, considering, also, the content of the most influential papers in each group, reveal that the biggest cluster is associated with “transport planning and travel behavior analysis”. In this topic, most papers have focused on long-term planning, utilizing big data mostly from smart cards, mobile phones, social media, and GPS trajectories to reveal travel and activity patterns or estimate crucial characteristics for transport planning, such as travel demand, number of trips, and trip purposes. The second topic refers to “smart cities and applications”, containing papers about how heterogeneous data generated in cities (streamed from sensors, devices, vehicles, or humans) can be used to improve the quality of human life, city operation systems, and the urban environment. Most studies have concentrated on short-term thinking about how innovative services and big data applications can support function and management of cities. In terms of transportation, several studies have managed to integrate Internet of Things (IoT) and big data analytics with intelligent transportation systems, including public transportation service plan, route optimization, parking, rail transportation, and engineering and supply chain management. Two smaller clusters were observed. One cluster is dedicated to “traffic forecasting and management” and includes papers related to traffic flow prediction or accident prediction, while many of them focus on real-time traffic management, city traffic monitoring, and control, mainly using real-time traffic data generated by sensors, cameras, and vehicles. The other is associated with “intelligent transportation systems and new technologies”, focusing on topics such as the connected vehicle-infrastructure environment and connected vehicle technologies as a promising direction to enhance the overall performance of the transportation system. Considerable attention has also been given to Internet of Vehicles (IoV) technology, autonomous vehicles, self-driving vehicle technology, and automatic traffic, while many contributions in this topic are related to green transportation and suggest solutions for optimizing emissions in urban areas with a focus on vehicle electrification. The four topics are represented as circular areas. The two principal components (PC1 and PC2) are used to visualize the distance between topics on a two-dimensional plane.
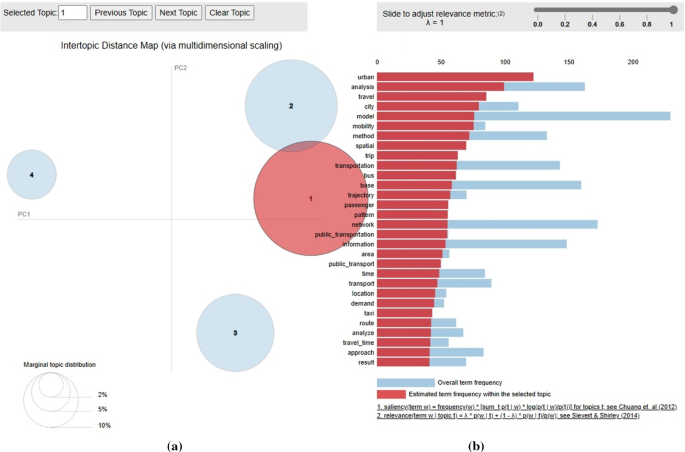
Four topics distance map, b Top 30 most relevant terms for Topic 1
By increasing the number of topics, various categories are becoming more specific, and it can be observed that there is a stronger co-relationship among clusters. The results of the eight topics’ categorization are presented in Fig. 2 a and b and Table 4 . The eight topics are represented as circular areas. The two principal components (PC1 and PC2) are used to visualize the distance between topics on a two-dimensional plane.
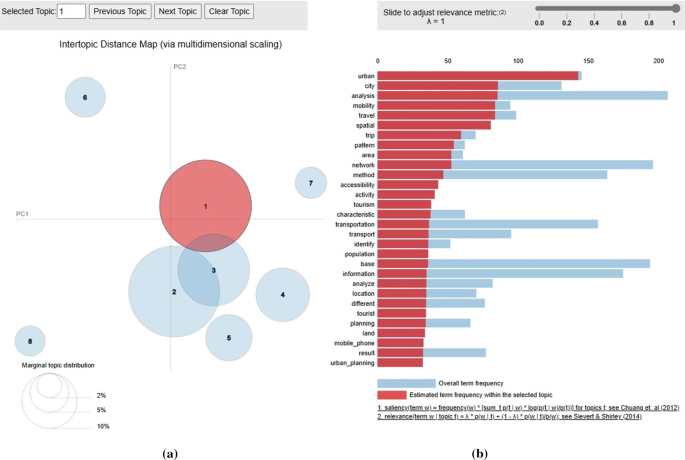
a Eight topics distance map, b Top 30 most relevant terms for Topic 1
According to the top words in each cluster and taking into consideration the content of top papers abstracts, the eight topics were specified as follows:
Topic 1 (742 papers), “Urban transport planning”: This topic concerns long-term transportation planning within cities, utilizing big and mainly historical data, such as call detail records from mobile phones and large-scale geo-location data from social media in conjunction with geospatial data, census records, and surveys. The emphasis is on analyzing patterns and trends in the urban environment. Most studies aim to investigate the spatial–temporal characteristics of urban movements, detect land use patterns, reveal urban activity patterns, and analyze travel behavior. Moreover, many papers focus on travel demand and origin–destination flow estimation or extract travel attributes, such as trip purpose and activity location.
Topic 2 (723 papers), “Smart cities and applications”: This topic remains largely consistent with the previous categorization. As above, the papers aim to take advantage of the various and diverse data generated in cities, analyze new challenges, and propose real-time applications to enhance the daily lives of individuals and city operation systems.
Topic 3 (438 papers), “Traffic flow forecasting and modeling”: This area of research involves the use of machine and deep learning techniques to analyze mainly historical data aiming to improve traffic prediction accuracy. The majority of these papers concentrate on short-term traffic flow forecasting, while a significant number of them address passenger flow and traffic accident prediction.
Topic 4 (231 papers), “Traffic management”: this topic concentrates on traffic management and real-time traffic control. City traffic monitoring, real-time video, and image processing techniques are gaining significant attention. Numerous studies utilize real-time data to evaluate traffic conditions by image-processing algorithms and provide real-time route selection guidance to users. Most of them manage to identify and resolve traffic congestion problems, as well as to detect anomalies or road traffic events, aiming to improve traffic operation and safety.
Topic 5 (194 papers), “Intelligent transportation systems and new technologies”: this topic remains nearly identical to the prior (4-clusters) classification, containing articles on emerging technologies implemented in an intelligent and eco-friendly transport system. Most studies focus on the connected vehicle-infrastructure environment and connected vehicle technologies as a promising direction for improving transportation system performance. Great attention is also given to Internet of Vehicles (IoV) technology and the efficient management of the generated and collected data. Autonomous and self-driving vehicle technologies are also crucial topics. Many papers, also, discuss green transportation and suggest ways to optimize emissions in urban areas, with a particular emphasis on vehicle electrification.
Topic 6 (144 papers), “Public transportation”: since public transportation gained special scientific interest in our database, a separate topic was created regarding public transport policy making, service, and management. Most publications focus on urban means of transport, such as buses, metro, and taxis, while a significant proportion refers to airlines. This topic covers studies related to public transportation network planning and optimization, performance evaluation, bus operation scheduling, analysis of passenger transit patterns, maximization of passenger satisfaction levels, and real-time transport applications. Moreover, smart cards and GPS data are extensively used to estimate origin–destination matrices.
Topic 7 (104 papers), “Railway”: this topic presents research papers that apply big data to railway transportation systems and engineering, encompassing three areas of interest: railway operations, maintenance, and safety. A significant proportion of studies focus on railway operations, including train delay prediction, timetabling improvement, and demand forecasting. Additionally, numerous researchers employ big data to support maintenance decisions and conduct risk analysis of railway networks, such as train derailments and failure prediction. These papers rely on diverse datasets, including GPS data, passenger travel information, as well as inspection, detectors, and failure data.
Topic 8 (95 papers), “GPS Trajectories”: This topic contains papers that take advantage of trajectory data primarily obtained from GPS devices installed in taxis. Most studies forecast the trip purpose of taxi passengers, trip destination, and travel time by analyzing historical GPS data. Additionally, a significant number of these studies focus on real-time analysis to provide passengers with useful applications and enhance the quality of taxi services. Finally, there is research interest in maritime routing and ship trajectory analysis to facilitate maritime traffic planning and service optimization.
In the eight-topic classification, the initial four clusters either remained almost unchanged or were divided into subcategories. For example, the previous cluster “transport planning and travel behavior analysis” is now divided into “urban transport planning” and “public transportation”, with “transport management” constituting a separate category. Moreover, several distinct smaller clusters have been identified (e.g., “railway” and “trajectories”). These, along with “public transportation”, are highly specialized categories with no correlation to the other clusters. Nevertheless, they constitute a significant proportion of the literature and merit separate analysis.
As the number of topics increased, so did the overlaps among the clusters. Thus, based on this observation and the accuracy results of the validation method, it was assumed that eight clusters were the most appropriate for further analysis.
Based on the results of eight-topic classification, Fig. 3 demonstrates the evolution of the number of published articles per topic and per year. As shown three topics have gained researchers’ interest: (1) urban transport planning, (2) smart cities and applications, and (3) traffic forecasting and modeling. Initially, the primary topic was “smart cities”, largely based on the computer science sector. Despite a slight decline in publications in 2019, there is an overall upward trend. “Urban transport planning” experienced a steady and notable increase until 2019. A sudden drop was recorded in 2022, but it is not clear whether this is a coincidence or a trend. However, it remains the dominant topic, with most publications over the years. The observed decrease could indicate further specialization and research evolution in the field, given it is also the topic with the most subcategories during the classification process.
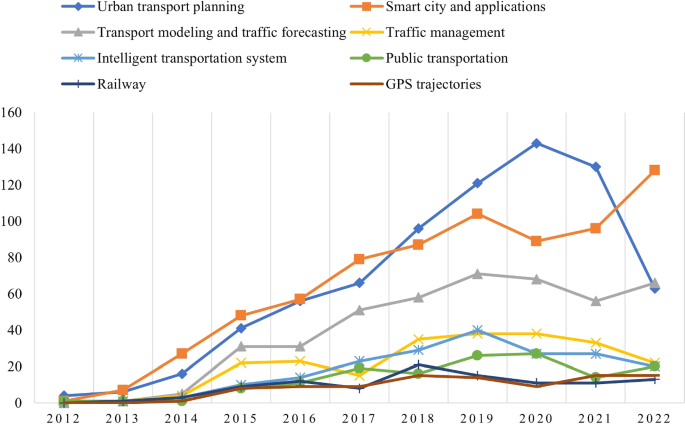
Number of papers per topic and year
5 Discussion
5.1 statistical analysis.
As shown in the analysis, there is an increasing research interest in big data usage in the transportation sector. It is remarkable that besides computer science journals and conferences, transportation journals have also published relevant articles representing a notable proportion of the research and indicating that transportation researchers acknowledge the significance of big data and its contribution to many aspects of transportation. According to the citation analysis, three research areas emerged among the most influential studies: (1) traffic flow prediction and management (2) new challenges of the cities (smart cities) and new technologies (3) urban transport planning and spatial analysis.
5.2 Topic classification
Following the topic model results, eight paper groups are proposed. Most articles (742) fall into the topic of “urban transport planning”. Several representative papers in this area attempted to estimate travel demand [ 5 ], analyze travel behavior [ 6 ], or investigate activity patterns [ 51 ] by utilizing big data sourced primarily from mobile phone records, social media, and smart card fare collection systems.
Big data also has significant impacts on “smart cities and applications”. Topic 2 is a substantial part of the dataset, which includes 723 papers. They mainly refer to new challenges arising from big data analytics to support various aspects of the city, such as transportation or energy [ 44 ] and investigate big data applications in intelligent transportation systems [ 26 ] or in the supply chain and logistics [ 54 ].
A total of 438 papers were categorized in Topic 3 labeled as “traffic flow forecasting and modeling”. The majority applied big data and machine learning techniques to predict traffic flow [ 41 , 42 , 43 ]. In risk assessment, Chen et al. [ 55 ] proposed a logit model to analyze hourly crash likelihood, considering temporal driving environmental data, whereas Yuan et al. [ 56 ] applied a Convolutional Long Short-Term Memory (ConvLSTM) neural network model to forecast traffic accidents.
Among the papers, a special focus is given to different aspects of “traffic management” (231 papers), largely utilizing real-time data. Shi and Abdel-Aty [ 7 ] employed random forest and Bayesian inference techniques in real-time crash prediction models to reduce traffic congestion and crash risk. Riswan et al. [ 57 ] developed a real-time traffic management system based on IoT devices and sensors to capture real-time traffic information. Meanwhile, He Z. et al. [ 58 ] suggested a low-frequency probe vehicle data (PVD)-based method to identify traffic congestion at intersections to solve traffic congestion problems.
Topic 5 includes 194 records on “intelligent transportation systems and new technologies”. It covers topics such as Internet of Vehicles [ 48 , 59 , 60 ], connected vehicle-infrastructure environment [ 61 ], electric vehicles [ 62 ], and the optimization of charging stations location [ 63 ], as well as autonomous vehicles (AV) and self-driving vehicle technology [ 64 ].
In recent years, three smaller and more specialized topics have gained interest. Within Topic 6, there are 144 papers discussing public transport. Tu et al. [ 65 ] examined the use of smart card data and GPS trajectories to explore multi-modal public ridership. Wang et al. [ 66 ] proposed a three-layer management system to support urban mobility with a focus on bus transportation. Tsai et al. [ 67 ] applied simulated annealing (SA) along with a deep neural network (DNN) to forecast the number of bus passengers. Liu and Yen [ 68 ] applied big data analytics to optimize customer complaint services and enhance management process in the public transportation system.
Topic 7 contains 104 papers on how big data is applied in “railway network”, focusing on three sectors of railway transportation and engineering. As mentioned in Ghofrani et al. [ 24 ], these sectors are maintenance [ 69 , 70 , 71 ], operation [ 72 , 73 ] and safety [ 74 ].
Topic 8 (95 papers) refers mainly to data deriving from “GPS trajectories”. Most researchers utilized GPS data from taxis to infer the trip purposes of taxi passengers [ 75 ], explore mobility patterns [ 76 ], estimate travel time [ 77 ], and provide real-time applications for taxi service improvement [ 78 , 79 ]. Additionally, there are papers included in this topic that investigate ship routes. Zhang et al. [ 80 ] utilized ship trajectory data to infer their behavior patterns and applied the Ant Colony Algorithm to deduce an optimal route to the destination, given a starting location, while Gan et al. [ 81 ] predicted ship travel trajectories using historical trajectory data and other factors, such as ship speed, with the Artificial Neural Network (ANN) model.
6 Conclusions
An extensive overview of the literature on big data and transportation from 2012 to 2022 was conducted using bibliometric techniques and topic model classification. This paper presents a comprehensive methodology for evaluating and selecting the appropriate literature. It identifies eight sub-areas of research and highlights current trends. The limitations of the study are as follows: (1) The dataset came up by using a single bibliographic database (Scopus). (2) Research sources, such as book chapters, were excluded. (3) Expanding the keyword combinations could result in a more comprehensive review. Despite these limitations, it is claimed that the reviewed dataset is representative, leading to accurate findings.
In the process of selecting the suitable literature, various criteria were defined in the Scopus database search, including the language, subject area, and document type. Subsequently, duplicate and non-scientific records were removed. However, the last screening of the titles and abstracts to determine the relevance of the studies to the paper’s research interests was conducted manually. This could not be possible for a larger dataset. Additionally, as previously stated, the dataset was divided into eight distinct topics due to multiple overlaps caused by an increase in the number of topics. Nevertheless, the topic of “smart cities and applications” remains broad, even with this division. This makes it challenging to gain in-depth insights into the field and identify specific applications, unlike in “transport planning”, where two additional topics were generated by the further classification. Applying the classification model to each topic separately could potentially overcome these constraints by revealing more precise applications and filtering out irrelevant studies.
Despite the above limitations and constraints, the current study provides an effective methodology for mapping the field of interest as a necessary step to define the areas of successful applications and identify open challenges and sub-problems that should be further investigated. It is worth mentioning that there is an intense demand from public authorities for a better understanding of the potential of big data applications in the transport domain towards more sustainable mobility [ 82 ]. In this direction, our methodology, along with the necessary literature review and discussion with relevant experts, can assist policymakers and transport stakeholders in identifying the specific domains in which big data can be applied effectively and planning future transport investments accordingly.
Having defined the critical areas of big data implementation within transportation, trends, and effective applications, the aim is to conduct a thorough literature review in a subarea of interest. This will focus on transport planning and modeling, and public transportation, which appears to be highly promising, based on our findings. A more extensive literature review and content analysis of key studies are crucial to further examine open challenges and subproblems as well as to investigate the applied methodologies for possible revision or further development.
The current study provides a broad overview of the applications of big data in transport areas, which is the initial step in understanding the characteristics and limitations of present challenges and opportunities for further research in the field.
European Commission (2021) Sustainable and Smart Mobility Strategy. https://ec.europa.eu/transport/sites/default/files/2021-mobility-strategy-and-action-plan.pdf . Accessed 10 Jul 2021
Anda C, Erath A, Fourie PJ (2017) Transport modelling in the age of big data. Int J Urban Sci 21:19–42. https://doi.org/10.1080/12265934.2017.1281150
Article Google Scholar
Zhong RY, Huang GQ, Lan S, Dai QY, Chen X, Zhang T (2015) A big data approach for logistics trajectory discovery from RFID-enabled production data. Int J Prod Econ 165:260–272. https://doi.org/10.1016/j.ijpe.2015.02.014
Nallaperuma D, Nawaratne R, Bandaragoda T, Adikari A, Nguyen S, Kempitiya T, de Silva D, Alahakoon D, Pothuhera D (2019) Online incremental machine learning platform for big data-driven smart traffic management. IEEE Trans Intell Transp Syst 20:4679–4690. https://doi.org/10.1109/TITS.2019.2924883
Toole JL, Colak S, Sturt B, Alexander LP, Evsukoff A, González MC (2015) The path most traveled: travel demand estimation using big data resources. Transp Res Part C Emerg Technol 58:162–177. https://doi.org/10.1016/j.trc.2015.04.022
Chen C, Ma J, Susilo Y, Liu Y, Wang M (2016) The promises of big data and small data for travel behavior (aka human mobility) analysis. Transp Res Part C Emerg Technol 68:285–299
Shi Q, Abdel-Aty M (2015) Big Data applications in real-time traffic operation and safety monitoring and improvement on urban expressways. Transp Res Part C Emerg Technol 58:380–394. https://doi.org/10.1016/j.trc.2015.02.022
Iqbal MS, Choudhury CF, Wang P, González MC (2014) Development of origin-destination matrices using mobile phone call data. Transp Res Part C Emerg Technol 40:63–74. https://doi.org/10.1016/j.trc.2014.01.002
Alexander L, Jiang S, Murga M, González MC (2015) Origin-destination trips by purpose and time of day inferred from mobile phone data. Transp Res Part C Emerg Technol 58:240–250. https://doi.org/10.1016/j.trc.2015.02.018
Zannat KE, Choudhury CF (2019) Emerging big data sources for public transport planning: a systematic review on current state of art and future research directions. J Indian Inst Sci 99:601–619
Choi TM, Wallace SW, Wang Y (2018) Big data analytics in operations management. Prod Oper Manag 27:1868–1883. https://doi.org/10.1111/poms.12838
Chalmeta R, Santos-deLeón NJ (2020) Sustainable supply chain in the era of industry 4.0 and big data: a systematic analysis of literature and research. Sustainability 12:4108. https://doi.org/10.3390/su12104108
De Bakker FGA, Groenewegen P, Den Hond F (2005) A bibliometric analysis of 30 years of research and theory on corporate social responsibility and corporate social performance. Bus Soc 44:283–317. https://doi.org/10.1177/0007650305278086
Mishra D, Gunasekaran A, Papadopoulos T, Childe SJ (2018) Big data and supply chain management: a review and bibliometric analysis. Ann Oper Res 270:313–336. https://doi.org/10.1007/s10479-016-2236-y
Fahimnia B, Sarkis J, Davarzani H (2015) Green supply chain management: a review and bibliometric analysis. Int J Prod Econ 162:101–114. https://doi.org/10.1016/j.ijpe.2015.01.003
Okafor O (2020) Automatic Topic classification of research papers using the NLP topic model NMF. https://obianuju-c-okafor.medium.com/automatic-topic-classification-of-research-papers-using-the-nlp-topic-model-nmf-d4365987ec82f . Accessed 10 Jul 2021
Iliashenko O, Iliashenko V, Lukyanchenko E (2021) Big data in transport modelling and planning. Transp Res Proced 54:900–908
Wang Z, He SY, Leung Y (2018) Applying mobile phone data to travel behaviour research: a literature review. Travel Behav Soc 11:141–155. https://doi.org/10.1016/j.tbs.2017.02.005
Huang H, Cheng Y, Weibel R (2019) Transport mode detection based on mobile phone network data: a systematic review. Transp Res Part C Emerg Technol 101:297–312
Pelletier MP, Trépanier M, Morency C (2011) Smart card data use in public transit: a literature review. Transp Res Part C Emerg Technol 19:557–568. https://doi.org/10.1016/j.trc.2010.12.003
Lana I, Del Ser J, Velez M, Vlahogianni EI (2018) Road traffic forecasting: recent advances and new challenges. IEEE Intell Transp Syst Mag 10:93–109
Miglani A, Kumar N (2019) Deep learning models for traffic flow prediction in autonomous vehicles: a review, solutions, and challenges. Veh Commun 20:100184. https://doi.org/10.1016/j.vehcom.2019.100184
Pender B, Currie G, Delbosc A, Shiwakoti N (2014) Social media use during unplanned transit network disruptions: a review of literature. Transp Rev 34:501–521. https://doi.org/10.1080/01441647.2014.915442
Ghofrani F, He Q, Goverde RMP, Liu X (2018) Recent applications of big data analytics in railway transportation systems: a survey. Transp Res Part C Emerg Technol 90:226–246. https://doi.org/10.1016/j.trc.2018.03.010
Borgi T, Zoghlami N, Abed M (2017). Big data for transport and logistics: a review. In: International conference on advanced systems and electric technologies (IC_ASET), pp 44–49
Zhu L, Yu FR, Wang Y, Ning B, Tang T (2019) Big data analytics in intelligent transportation systems: a survey. IEEE Trans Intell Transp Syst 20:383–398
Neilson A, Indratmo DB, Tjandra S (2019) Systematic review of the literature on big data in the transportation domain: concepts and applications. Big Data Res 17:35–44
Katrakazas C, Antoniou C, Sobrino N, Trochidis I, Arampatzis S (2019). Big data and emerging transportation challenges: findings from the NOESIS project. In: 6th IEEE International conference on models and technologies for intelligent transportation systems (MT-ITS), pp 1–9
Pranckutė R (2021) Web of science (WoS) and scopus: the titans of bibliographic information in today’s academic world. Publications 9(1):12. https://doi.org/10.3390/publications9010012
Elsevier Scopus (2023) Content coverage guide. https://www.elsevier.com/?a=69451 . Accessed 27 Sept 2023
Jiang Z, Gao B, He Y, Han Y, Doyle P, Zhu Q (2021) Text classification using novel term weighting scheme-based improved TF-IDF for internet media reports. Math Probl Eng. https://doi.org/10.1155/2021/6619088
Zhang X, Delpha C, Diallo D (2019) Performance of Jensen Shannon divergence in incipient fault detection and estimation. In: 2019 IEEE international conference on acoustics, speech and signal processing (ICASSP), pp 2742–2746
Ruz GA, Henríquez PA, Mascareño A (2020) Sentiment analysis of twitter data during critical events through Bayesian networks classifiers. Futur Gener Comput Syst 106:92–104. https://doi.org/10.1016/j.future.2020.01.005
Kumar A, Srinivasan K, Cheng WH, Zomaya AY (2020) Hybrid context enriched deep learning model for fine-grained sentiment analysis in textual and visual semiotic modality social data. Inf Process Manag 57:102–141. https://doi.org/10.1016/j.ipm.2019.102141
Pimpalkar AP, Retna Raj RJ (2020) Influence of pre-processing strategies on the performance of ML classifiers exploiting TF-IDF and BOW features. ADCAIJ Adv Distrib Comput Artif Intell J 9:49–68. https://doi.org/10.14201/adcaij2020924968
YueTing H, YiJia X, ZiHe C, Xin T (2019) Short text clustering algorithm based on synonyms and k-means. Computer knowledge and technology 15(1).
Bracewell DB, Yan J, Ren F, Kuroiwa S (2009) Category classification and topic discovery of japanese and english news articles. Electron Notes Theor Comput Sci 225:51–65. https://doi.org/10.1016/j.entcs.2008.12.066
Kalchbrenner N, Grefenstette E, Blunsom P (2014) A convolutional neural network for modelling sentences. In: 52nd Annual meeting of the association for computational linguistics, pp 655–665
Albawi S, Mohammed TA, Al-Zawi S (2017) Understanding of a convolutional neural network. In: International conference on engineering and technology (ICET), pp 1–6
Ertam F, Aydn G (2017) Data classification with deep learning using tensorflow. In: International conference on computer science and engineering (UBMK), pp 755–758
Lv Y, Duan Y, Kang W, Li Z, Wang FY (2015) Traffic flow prediction with big data: a deep learning approach. IEEE Trans Intell Transp Syst 16:865–873. https://doi.org/10.1109/TITS.2014.2345663
Wu Y, Tan H, Qin L, Ran B, Jiang Z (2018) A hybrid deep learning based traffic flow prediction method and its understanding. Transp Res Part C Emerg Technol 90:166–180. https://doi.org/10.1016/j.trc.2018.03.001
Tian Y, Pan L (2015) Predicting short-term traffic flow by long short-term memory recurrent neural network. In: IEEE International conference on smart city/socialcom/sustaincom (SmartCity). IEEE, pp 153–158
Al Nuaimi E, Al Neyadi H, Mohamed N, Al-Jaroodi J (2015) Applications of big data to smart cities. J Internet Serv Appl 6:1–15. https://doi.org/10.1186/s13174-015-0041-5
Batty M (2013) Big data, smart cities and city planning. Dialog Hum Geogr 3:274–279. https://doi.org/10.1177/2043820613513390
Zheng Y, Capra L, Wolfson O, Yang H (2014) Urban computing: concepts, methodologies, and applications. ACM Trans Intell Syst Technol 5(3):1–55. https://doi.org/10.1145/2629592
Mehmood Y, Ahmad F, Yaqoob I, Adnane A, Imran M, Guizani S (2017) Internet-of-things-based smart cities: recent advances and challenges. IEEE Commun Mag 55:16–24. https://doi.org/10.1109/MCOM.2017.1600514
Xu W, Zhou H, Cheng N, Lyu F, Shi W, Chen J, Shen X (2018) Internet of vehicles in big data era. IEEE/CAA J Autom Sin 5:19–35. https://doi.org/10.1109/JAS.2017.7510736
Zhong C, Arisona SM, Huang X, Batty M, Schmitt G (2014) Detecting the dynamics of urban structure through spatial network analysis. Int J Geogr Inf Sci 28:2178–2199. https://doi.org/10.1080/13658816.2014.914521
Yao H, Wu F, Ke J, Tang X, Jia Y, Lu S, Gong P, Ye J, Chuxing D, Li Z (2018) Deep multi-view spatial-temporal network for taxi demand prediction. In: AAAI Conference on artificial intelligence. pp 2588–2595
Hasan S, Ukkusuri SV (2014) Urban activity pattern classification using topic models from online geo-location data. Transp Res Part C Emerg Technol 44:363–381. https://doi.org/10.1016/j.trc.2014.04.003
Saeed N, Nam H, Haq MIU, Saqibm DBM (2018) A survey on multidimensional scaling. ACM Comput Surv (CSUR) 51:1–25
Hout MC, Papesh MH, Goldinger SD (2012) Multidimensional scaling. Wiley Interdiscip Rev Cogn Sci 4:93–103
Kaur H, Singh SP (2018) Heuristic modeling for sustainable procurement and logistics in a supply chain using big data. Comput Oper Res 98:301–321. https://doi.org/10.1016/j.cor.2017.05.008
Article MathSciNet Google Scholar
Chen F, Chen S, Ma X (2018) Analysis of hourly crash likelihood using unbalanced panel data mixed logit model and real-time driving environmental big data. J Saf Res 65:153–159. https://doi.org/10.1016/j.jsr.2018.02.010
Yuan Z, Zhou X, Yang T (2018) Hetero-ConvLSTM: a deep learning approach to traffic accident prediction on heterogeneous spatio-temporal data. In: Proceedings of the ACM SIGKDD international conference on knowledge discovery and data mining. Association for computing machinery, pp 984–992
Riswan P, Suresh K, Babu MR (2016) Real-time smart traffic management system for smart cities by using internet of things and big data. In: ICETT - 2016 : international conference on emerging technological trends in computing, communications and electrical engineering. IEEE, pp 1–7
He Z, Qi G, Lu L, Chen Y (2019) Network-wide identification of turn-level intersection congestion using only low-frequency probe vehicle data. Transp Res Part C Emerg Technol 108:320–339. https://doi.org/10.1016/j.trc.2019.10.001
Zhou Z, Gao C, Xu C, Zhang Y, Mumtaz S, Rodriguez J (2018) Social big-data-based content dissemination in internet of vehicles. IEEE Trans Ind Inf 14:768–777. https://doi.org/10.1109/TII.2017.2733001
Guo L, Dong M, Ota K, Li Q, Ye T, Wu J, Li J (2017) A secure mechanism for big data collection in large scale internet of vehicle. IEEE Internet Things J 4:601–610
Sumalee A, Ho HW (2018) Smarter and more connected: future intelligent transportation system. IATSS Res 42:67–71
Fetene GM, Kaplan S, Mabit SL, Jensen AF, Prato CG (2017) Harnessing big data for estimating the energy consumption and driving range of electric vehicles. Transp Res D Transp Environ 54:1–11. https://doi.org/10.1016/j.trd.2017.04.013
Tu W, Li Q, Fang Z, Shaw S, lung, Zhou B, Chang X, (2016) Optimizing the locations of electric taxi charging stations: a spatial–temporal demand coverage approach. Transp Res Part C Emerg Technol 65:172–189. https://doi.org/10.1016/j.trc.2015.10.004
Najada HA, Mahgoub I (2016) Autonomous vehicles safe-optimal trajectory selection based on big data analysis and predefined user preferences. In: EEE 7th annual ubiquitous computing, electronics mobile communication conference (UEMCON). IEEE, pp 1–6
Tu W, Cao R, Yue Y, Zhou B, Li Q, Li Q (2018) Spatial variations in urban public ridership derived from GPS trajectories and smart card data. J Transp Geogr 69:45–57. https://doi.org/10.1016/j.jtrangeo.2018.04.013
Wang Y, Ram S, Currim F, Dantas E, Sabóia L (2016) A big data approach for smart transportation management on bus network. In: IEEE international smart cities conference (ISC2), pp 1–6
Tsai CW, Hsia CH, Yang SJ, Liu SJ, Fang ZY (2020) Optimizing hyperparameters of deep learning in predicting bus passengers based on simulated annealing. Appl Soft Comput J. https://doi.org/10.1016/j.asoc.2020.106068
Liu WK, Yen CC (2016) Optimizing bus passenger complaint service through big data analysis: systematized analysis for improved public sector management. Sustainability 8:1319. https://doi.org/10.3390/su8121319
Li H, Parikh D, He Q, Qian B, Li Z, Fang D, Hampapur A (2014) Improving rail network velocity: a machine learning approach to predictive maintenance. Transp Res Part C Emerg Technol 45:17–26. https://doi.org/10.1016/j.trc.2014.04.013
Sharma S, Cui Y, He Q, Mohammadi R, Li Z (2018) Data-driven optimization of railway maintenance for track geometry. Transp Res Part C Emerg Technol 90:34–58. https://doi.org/10.1016/j.trc.2018.02.019
Jamshidi A, Hajizadeh S, Su Z, Naeimi M, Núñez A, Dollevoet R, de Schutter B, Li Z (2018) A decision support approach for condition-based maintenance of rails based on big data analysis. Transp Res Part C Emerg Technol 95:185–206. https://doi.org/10.1016/j.trc.2018.07.007
Thaduri A, Galar D, Kumar U (2015) Railway assets: a potential domain for big data analytics. Proced Comput Sci 53:457–467. https://doi.org/10.1016/j.procs.2015.07.323
Oneto L, Fumeo E, Clerico G, Canepa R, Papa F, Dambra C, Mazzino N, Anguita D (2017) Dynamic delay predictions for large-scale railway networks: deep and shallow extreme learning machines tuned via thresholdout. IEEE Trans Syst Man Cybern Syst 47:2754–2767. https://doi.org/10.1109/TSMC.2017.2693209
Sadler J, Griffin D, Gilchrist A, Austin J, Kit O, Heavisides J (2016) GeoSRM: online geospatial safety risk model for the GB rail network. IET Intell Transp Syst 10(1):17–24. https://doi.org/10.1049/iet-its.2015.0038
Gong L, Liu X, Wu L, Liu Y (2016) Inferring trip purposes and uncovering travel patterns from taxi trajectory data. Cartogr Geogr Inf Sci 43:103–114. https://doi.org/10.1080/15230406.2015.1014424
Xia F, Wang J, Kong X, Wang Z, Li J, Liu C (2018) Exploring human mobility patterns in urban scenarios: a trajectory data perspective. IEEE Commun Mag 56:142–149. https://doi.org/10.1109/MCOM.2018.1700242
Qiu J, Du L, Zhang D, Su S, Tian Z (2020) Nei-TTE: intelligent traffic time estimation based on fine-grained time derivation of road segments for smart city. IEEE Trans Ind Inf 16:2659–2666. https://doi.org/10.1109/TII.2019.2943906
Zhou Z, Dou W, Jia G, Hu C, Xu X, Wu X, Pan J (2016) A method for real-time trajectory monitoring to improve taxi service using GPS big data. Inf Manag 53:964–977. https://doi.org/10.1016/j.im.2016.04.004
Xu X, Zhou JY, Liu Y, Xu ZZ, Zha XW (2015) Taxi-RS: taxi-hunting recommendation system based on taxi GPS data. IEEE Trans Intell Transp Syst 16:1716–1727. https://doi.org/10.1109/TITS.2014.2371815
Zhang SK, Shi GY, Liu ZJ, Zhao ZW, Wu ZL (2018) Data-driven based automatic maritime routing from massive AIS trajectories in the face of disparity. Ocean Eng 155:240–250. https://doi.org/10.1016/j.oceaneng.2018.02.060
Gan S, Liang S, Li K, Deng J, Cheng T (2016) Ship trajectory prediction for intelligent traffic management using clustering and ANN. In: 2016 UKACC 11th international conference on control (CONTROL), pp 1–6
European Union (EU) Horizon 2020 (H2020) (2017) NOESIS: novel decision support tool for evaluating strategic big data investments in transport and intelligent mobility services. https://cordis.europa.eu/programme/id/H2020_MG-8-2-2017/en . Accessed 29 Sep 2023
Download references
Acknowledgements
This research is financed by the Research, Innovation and Excellence Program of the University of Thessaly.
Open access funding provided by HEAL-Link Greece. This research is supported by the Research, Innovation and Excellence Program of the University of Thessaly.
Author information
Authors and affiliations.
Department of Civil Engineering, University of Thessaly, Volos, Greece
Danai Tzika-Kostopoulou & Eftihia Nathanail
Department of Digital Systems, University of Thessaly, Larissa, Greece
Konstantinos Kokkinos
You can also search for this author in PubMed Google Scholar
Contributions
The authors confirm their contribution to the paper as follows: study conception and design done by DT and EN; DT helped in data collection; DT, EN, and KK done analysis and interpretation of results, draft manuscript preparation. All authors reviewed the results and approved the final version of the manuscript.
Corresponding author
Correspondence to Danai Tzika-Kostopoulou .
Ethics declarations
Conflict of interest.
The authors have no competing interests to declare that are relevant to the content of this article.
Additional information
Publisher's note.
Springer Nature remains neutral with regard to jurisdictional claims in published maps and institutional affiliations.
Rights and permissions
Open Access This article is licensed under a Creative Commons Attribution 4.0 International License, which permits use, sharing, adaptation, distribution and reproduction in any medium or format, as long as you give appropriate credit to the original author(s) and the source, provide a link to the Creative Commons licence, and indicate if changes were made. The images or other third party material in this article are included in the article's Creative Commons licence, unless indicated otherwise in a credit line to the material. If material is not included in the article's Creative Commons licence and your intended use is not permitted by statutory regulation or exceeds the permitted use, you will need to obtain permission directly from the copyright holder. To view a copy of this licence, visit http://creativecommons.org/licenses/by/4.0/ .
Reprints and permissions
About this article
Tzika-Kostopoulou, D., Nathanail, E. & Kokkinos, K. Big data in transportation: a systematic literature analysis and topic classification. Knowl Inf Syst (2024). https://doi.org/10.1007/s10115-024-02112-8
Download citation
Received : 06 August 2023
Revised : 26 January 2024
Accepted : 21 March 2024
Published : 08 May 2024
DOI : https://doi.org/10.1007/s10115-024-02112-8
Share this article
Anyone you share the following link with will be able to read this content:
Sorry, a shareable link is not currently available for this article.
Provided by the Springer Nature SharedIt content-sharing initiative
- Transportation
- Topic model
- Classification
- Term frequency–inverse document frequency method
- Find a journal
- Publish with us
- Track your research
Purdue Online Writing Lab Purdue OWL® College of Liberal Arts
Welcome to the Purdue Online Writing Lab
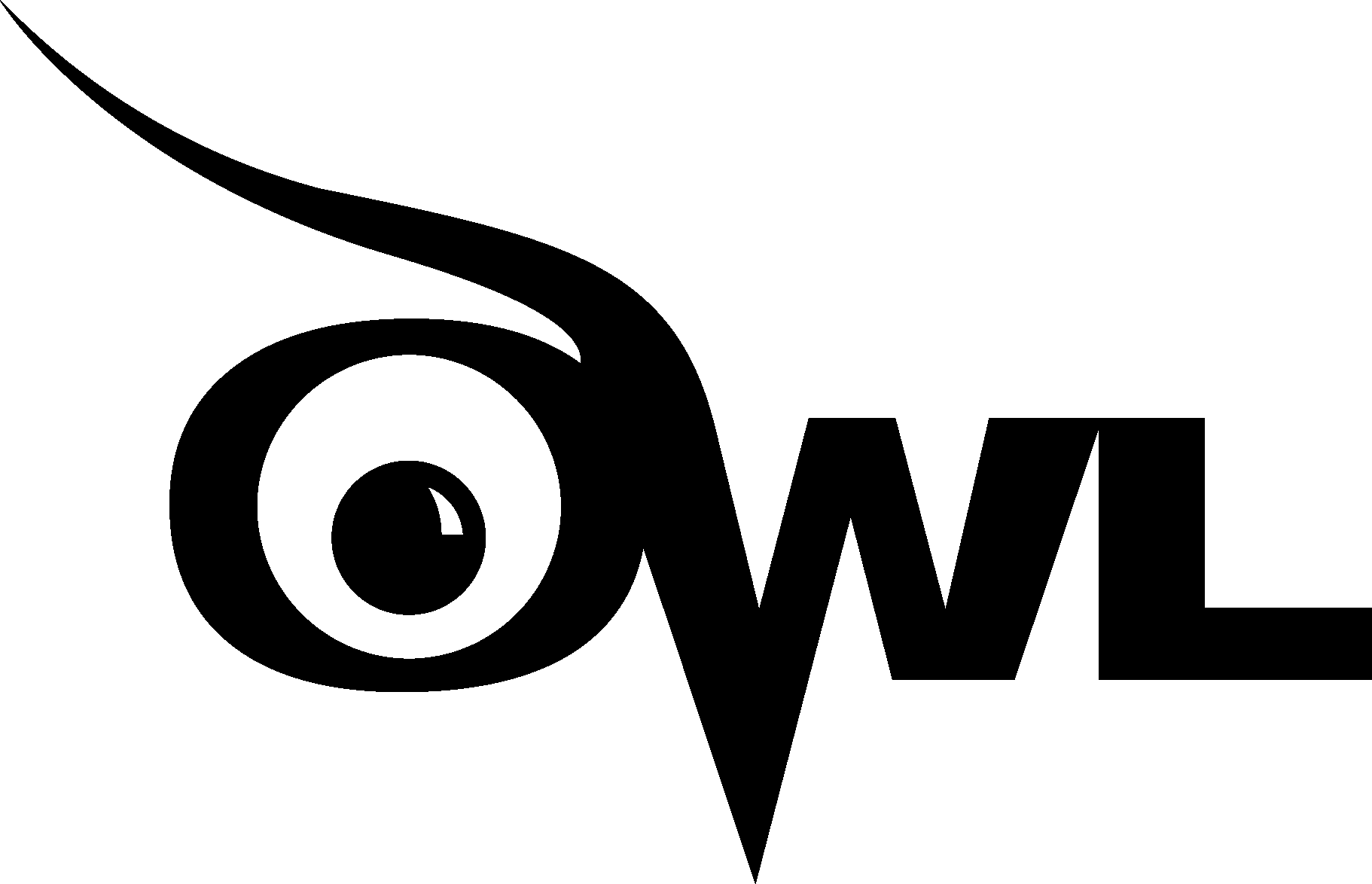
Welcome to the Purdue OWL
This page is brought to you by the OWL at Purdue University. When printing this page, you must include the entire legal notice.
Copyright ©1995-2018 by The Writing Lab & The OWL at Purdue and Purdue University. All rights reserved. This material may not be published, reproduced, broadcast, rewritten, or redistributed without permission. Use of this site constitutes acceptance of our terms and conditions of fair use.
The Online Writing Lab at Purdue University houses writing resources and instructional material, and we provide these as a free service of the Writing Lab at Purdue. Students, members of the community, and users worldwide will find information to assist with many writing projects. Teachers and trainers may use this material for in-class and out-of-class instruction.
The Purdue On-Campus Writing Lab and Purdue Online Writing Lab assist clients in their development as writers—no matter what their skill level—with on-campus consultations, online participation, and community engagement. The Purdue Writing Lab serves the Purdue, West Lafayette, campus and coordinates with local literacy initiatives. The Purdue OWL offers global support through online reference materials and services.
A Message From the Assistant Director of Content Development
The Purdue OWL® is committed to supporting students, instructors, and writers by offering a wide range of resources that are developed and revised with them in mind. To do this, the OWL team is always exploring possibilties for a better design, allowing accessibility and user experience to guide our process. As the OWL undergoes some changes, we welcome your feedback and suggestions by email at any time.
Please don't hesitate to contact us via our contact page if you have any questions or comments.
All the best,
Social Media
Facebook twitter.
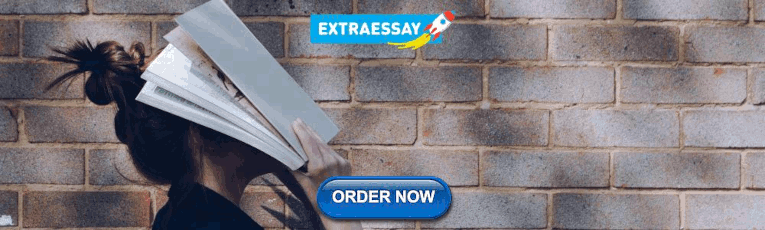
IMAGES
VIDEO
COMMENTS
This article is a practical guide to conducting data analysis in general literature reviews. The general literature review is a synthesis and analysis of published research on a relevant clinical issue, and is a common format for academic theses at the bachelor's and master's levels in nursing, physiotherapy, occupational therapy, public health and other related fields.
Review authors often have different backgrounds and level of systematic review experience. Using a data collection form ensures some consistency in the process of data extraction, and is necessary for comparing data extracted in duplicate. ... Systematic review authors can uncover suspected misconduct in the published literature. Misconduct ...
This is why the literature review as a research method is more relevant than ever. A literature review can broadly be described as a more or less systematic way of collecting and synthesizing previous research (Baumeister & Leary, 1997; Tranfield, ... In some cases, a research question requires a more creative collection of data, in these cases ...
reviewer collects to inform a literature review represents data. Thus, it stands to reason that the literature review process can be viewed as a data collection tool—that is, as a means of collecting a body of information per-tinent to a topic of interest. As a data collection tool, the literature review involves activities such as identi-
Literature reviews establish the foundation of academic inquires. However, in the planning field, we lack rigorous systematic reviews. In this article, through a systematic search on the methodology of literature review, we categorize a typology of literature reviews, discuss steps in conducting a systematic literature review, and provide suggestions on how to enhance rigor in literature ...
The most prevalent one is the "literature review" or "background" section within a journal paper or a chapter in a graduate thesis. ... research methods, data collection techniques, and direction or strength of research outcomes (e.g., positive, negative, or non-significant) in the form of frequency analysis to produce quantitative ...
A literature review should connect to the study question, guide the study methodology, and be central in the discussion by indicating how the analyzed data advances what is known in the field. A theoretical framework drives the question, guides the types of methods for data collection and analysis, informs the discussion of the findings, and ...
Those working on the literature review can access the data, add data such as appraisals of individual studies and revise them. Particularly, for protocol-driven literature reviews this is a necessity because normally at least two researchers are expected to appraise each study. ... The Cochrane Library is a collection of databases in medicine ...
Examples of literature reviews. Step 1 - Search for relevant literature. Step 2 - Evaluate and select sources. Step 3 - Identify themes, debates, and gaps. Step 4 - Outline your literature review's structure. Step 5 - Write your literature review.
A librarian can advise you on data extraction for your systematic review, including: What the data extraction stage of the review entails; Finding examples in the literature of similar reviews and their completed data tables; How to choose what data to extract from your included articles ; How to create a randomized sample of citations for a ...
Literature Review is a comprehensive survey of the works published in a particular field of study or line of research, usually over a specific period of time, in the form of an in-depth, ... research approaches and data collection and analysis techniques), enables researchers to draw on a wide variety of knowledge ranging from the conceptual ...
Contemporary Issue. A practical guide to data analysis in general. literature reviews. Rebecca P openoe, Ann Langius-Ekl €. of, Ewa Stenwall and. Anna Jervaeus. Abstract. Academic theses at the ...
Data Collection | Definition, Methods & Examples. Published on June 5, 2020 by Pritha Bhandari.Revised on June 21, 2023. Data collection is a systematic process of gathering observations or measurements. Whether you are performing research for business, governmental or academic purposes, data collection allows you to gain first-hand knowledge and original insights into your research problem.
This article is a practical guide to conducting data analysis in general literature reviews. The general literature review is a synthesis and analysis of published research on a rel-evant clinical issue, and is a common format for academic theses at the bachelor's and master's levels in nursing, physiotherapy, occupational therapy, public ...
Data Collection Methods and Tools for Research; A Step-by-Step Guide to Choose ... papers, the literature review section is based on secondary data sources. Thus, secondary data is an essential part of research that can help to get information from past studies as basis conduction for . Data Collection Methods and Tools for Research; A Step-by ...
THE COLLECTION OF STATISTICAL INFORMATION. 1. INTRODUCTION. The aim of this chapter is to discuss the research methods chosen for this study and the reasons for choosing them. These research methods include the literature review, interviews and the collection of statistical information. 2. RESEARCH METHODS. As explained in Section 6 of Chapter ...
Writing a literature review requires a range of skills to gather, sort, evaluate and summarise peer-reviewed published data into a relevant and informative unbiased narrative. ... Evaluation of the quality of studies and assessment of factors, such as study design, data collection, data analysis and interpretation and the conclusions drawn by ...
Thus, through an integrative literature review, the main objective of this work is to identify and evaluate digital health technology interventions designed to support the conduct of health research based on data quality. Methods. Study Design. ... Data Collection. First, 2 independent reviewers with expertise in information and data science ...
3.5 Data collection methods. 3.5.1 Literature review. A literature review is often undertaken prior to empirical research as it provides a synthesis of the extant knowledge on a given topic. The scope of a literature review can vary. The emphasis may be on a review of research methods to determine which approach to adopt or examination of ...
Sources for a Literature Review will come from a variety of places, including: •Books Use the Library Catalog to see what items McDermott Library has on your topic or if McDermott Library has a specific source you need. The WorldCat database allows you to search the catalogs on many, many libraries. WorldCat is a good place to find out what books exist on your topic.
Scoping reviews and evidence maps are forms of evidence synthesis that aim to map the available literature on a topic and are well-suited to visual presentation of results. A range of data visualisation methods and interactive data visualisation tools exist that may make scoping reviews more useful to knowledge users. The aim of this study was to explore the use of data visualisation in a ...
Data set creation involved: collecting and enriching metadata for articles indexed in the ICPSR Bibliography of Data-related Literature against the Dimensions AI bibliometric database; gathering ...
Methods and guidance to produce a reliable evidence synthesis. Several international consortiums of EBM experts and national health care organizations currently provide detailed guidance (Table (Table1). 1).They draw criteria from the reporting and methodological standards of currently recommended appraisal tools, and regularly review and update their methods to reflect new information and ...
We offer best-practice recommendations for journal reviewers, editors, and authors regarding data collection and preparation. Our recommendations are applicable to research adopting different epistemological and ontological perspectives—including both quantitative and qualitative approaches—as well as research addressing micro (i.e., individuals, teams) and macro (i.e., organizations ...
A scoping review was conducted to meet the study objectives, as scoping review designs are particularly appropriate to answer broad research questions [].The scoping review included four steps: (1) developing the literature search strategy; (2) study selection; (3) data charting; and (4) summarizing and reporting the results.
This paper identifies trends in the application of big data in the transport sector and categorizes research work across scientific subfields. The systematic analysis considered literature published between 2012 and 2022. A total of 2671 studies were evaluated from a dataset of 3532 collected papers, and bibliometric techniques were applied to capture the evolution of research interest over ...
The Online Writing Lab at Purdue University houses writing resources and instructional material, and we provide these as a free service of the Writing Lab at Purdue.
Literature reviews are essential in moving our evidence-base forward. "A literature review makes a significant contribution when the authors add to the body of knowledge through providing new insights" (Bearman, 2016, p. 383).Although there are many methods for conducting a literature review (e.g., systematic review, scoping review, qualitative synthesis), some commonalities in ...
The advent of the Internet of Things (IoT) has sparked the creation of numerous improved and new applications across numerous industries. Data collection from remote locations and remote object control are made possible by Internet of Things technology. The IoT has numerous applications in fields such as education, healthcare, agriculture, smart cities, and smart homes.