
- Search This Site All UCSD Sites Faculty/Staff Search Term
- Administration
- Climate Statement
- Research Cores & Facilities
- Course Offering
- NGP Course Catalog
- Winter Courses
- Advancement and Defense Exams
- Program Requirements
- Conferences & Courses
- Mentorship & Career Development
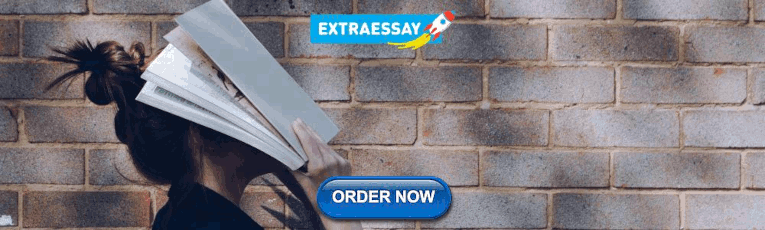
Computational Neuroscience
- Spring Courses
- Professional Development
- Degree Requirements
- Two-Column Template
- Fellowships
- Health & Wellness
- Reporting Harassment & Discrimination
- Student Directory
- Publications
- Seminar Series
- Events & Activities
- Apply: Admissions
- Funding, Housing & Benefits
Courses and Exams
The Computational Neuroscience specialization is a facet of the broader Neuroscience Graduate Program at UC San Diego . The goal of the specialization is to train the next generation of neuroscientists with the analytical and computational skills that are essential to understand the organization and function of neural systems. The specialization is open to all students and may be of particular interest to students with backgrounds in physics, computer science, engineering, and mathematics.
The specialization allows Neuroscience students to concentrate on a program of rigorous course work on fundamental aspects of computational neuroscience. Students are encouraged to pursue thesis projects that include both an experimental and a computational component, possibly arranged as a collaboration between two research groups. Upon achievement of degree requirements, students will receive a diploma indicating both their successful completion of the broader Neuroscience Program as well as their specialization in Computational Neuroscience.
The program is focused on these major themes relevant for computational neuroscience research:
- Cellular and Synaptic Dynamics - Anatomy, physiology, and electrical and chemical dynamics of individual neurons. Neuromorphic models.
- Biophysical Basis of Neuronal Computation - Collective properties and dynamics of neuronal systems, with emphasis on feedforward networks, associative networks, and networks of coupled oscillators.
- Algorithms for the Analysis of Neural Data - Characterization of spiking and continuous processes (ECoG, LFP, MEG, fMRI). Statistical aspects of genomics and neuroanatomy.
- Advanced Optical Tools in Quantitative Biology.
- Workshop in Electron Microscopy.
- Magnetic Resonance Imaging.
The program is currently led by David Kleinfeld (Physics and Neurobiology) and the primary teaching faculty also include Henry Abarbanel (Physics and Scripps Institution of Oceanography), Gert Cauwenberghs (Bioengineering), Eran Mukamel (Cognitive Science), Terrence Sejnowski (Salk Institute for Biological Studies and Neurobiology), Tatyana Sharpee (Salk Institute for Biological Studies and Physics) and Gabriel Silva (Ophthalmology and Bioengineering).
Application
All students admitted to the Neurosciences Graduate Program are eligible to pursue the CNS. Additionally, Ph.D. candidates in Physics and Bioengineering are also eligible to apply to the CNS. Upon completion of the CNS required coursework, a Neuroscience, Physics or Bioengineering student can apply for the specialization by completing the form below. After a preliminary review, our team will request a copy of their C.V., undergraduate transcripts, graduate transcripts, and a short description of their research interests. This application will be approved by the Computation Neuroscience Committee Chair, Dr. David Kleinfeld at [email protected] .
Upon achievement of degree requirements, students will receive a diploma indicating both their successful completion of their Ph.D. program as well as their specialization in Computational Neuroscience.
Thesis Research
All CNS students are expected to complete a Ph.D. dissertation connected with an issue in contemporary computational neuroscience. Either the student's primary advisor or close co-advisor (approved by the Computational Neuroscience Committee) must be a member of the Neuroscience Graduate Program faculty .
- Comp Neuro Resources
Emory University Laney Graduate School Neuroscience Graduate Program
Home » Overview » Computational Neuroscience
- Computational Neuroscience
Seeking a degree in Computational Neuroscience?
The graduate program in Neuroscience at Emory University is an interdisciplinary program, spanning many departments and priding itself on a collaborative atmosphere encouraging excellence. Our faculty and students have a broad scope of research interests within neuroscience, ranging from molecular to cellular to behavioral neuroscience.
Our program is one of eight Ph.D. programs that comprise the Emory Graduate Division of Biological and Biomedical Sciences (GDBBS). There are over 260 faculty members in the Division, and graduate students of any program in the Division face no departmental barriers. They can do laboratory rotations and research with any of the Division Faculty. The can also switch to one of the other programs, have an advisor from another program and take any course offered by the Division. This structure gives students tremendous flexibility in choosing coursework, advisors and research plans. There are currently 98 students enrolled in the program, and the average time to finish the degree is about 5.5 years.
Joint Degree Programs
- Neuroscience/Biomedical Engineering In 1997, Emory University and the Georgia Institute of Technology joined forces to create a joint Biomedical Engineering Department , which includes 15 Emory faculty members (4 from Neuroscience), and over 25 faculty members from Georgia Tech. The collaboration provides enormous opportunity and intellectual resources for students interested in neuroengineering, neuronal modeling, computational neuroscience and other cutting-edge challenges. Emory has eleven faculty that participate in this joint effort. The Chairman of the new department, Dr. Don Giddens, headed the Johns Hopkins College of Engineering before coming to Emory.
- MD/PhD Approximately 10-15% of Neuroscience graduate students are working towards an M.D. degree or already have one. MD/PhD students are admitted through the MD/PhD Program .
Financial Aid
In addition, all students must participate in weekly seminars for the first 2 years of the program. These seminars are informal venues where students present either relevant papers or their own research to their peers and a small group of faculty members. From this experience, students learn presentation and communication skills essential to a career in science.
Related Terms
- Behavioral Neuroscience
- Electrophysiology Research
- Molecular Neuroscience
- Neurodegeneration
- Neuropharmacology Research
- Neurophysiology PhD Graduate Programs
- Neuroscience Degree
- Neuroscience PhD
- Neuroscience PhD Graduate Programs
- Proteomics Research
- Systems Neuroscience
Information & Guides
- Prospective NS Students
- NS Students
Neuroscience Institute
Ph.d. in neural computation courses and activities.
The program consists of the following core activities:
- Coursework in computational neuroscience, quantitative methodologies and experimental neuroscience
- Exposure to experimental approaches through rotations or thesis research
- Training in teaching, scientific presentations and responsible conduct of research
- Successful defense of a Ph.D. Thesis
Additional satellite activities through the CNBC will also foster students’ professional and scientific development.
Course requirements
The coursework is designed to ensure that students are well trained in neuroscience and that they also receive in-depth training in a set of quantitative approaches relevant to the field of neural computation.
A typical student will take 2-3 courses per term in their first year and complete all coursework by the end of their third year in the program. Because of differences in background and educational goals, course requirements for each student in the program will be adapted to their individual needs.
CNBC Core Course Requirement
Students complete the four-course requirement of the CNBC graduate training program , with specific program related guidelines for the computational component. Students will gain graduate level training through coursework in the following three areas: (i) cell and molecular neuroscience/neurophysiology, (ii) systems neuroscience, and (iii) cognitive neuroscience. Courses fulfilling this requirement include
- (i) 03-762 Advanced Cellular Neuroscience (CMU) or NROSCI 2100/2101 Cellular and Molecular Neurobiology (Pitt)
- (ii) 03-763 Systems Neuroscience (CMU) or NROSCI 2102 Systems Neuroscience (Pitt), and
- (iii) 85-765 Cognitive Neuroscience.
Computational Neuroscience
Students are required to take at least three computational neuroscience courses, including mathematical, statistical and computational approaches.
To complete the computational requirement, students must take:
- 36-759 Statistical Models of the Brain (CMU)
- Two additional computational electives selected by the student.
Recommended courses fulfilling the computational elective requirement include:
- 10-733 Representation and Generation in Neuroscience and AI (CMU)
- 15-686 Neural Computation (CMU)
- 15-883 Computational Models of Neural Systems (CMU)
- 18-698/42-632 Neural Signals Processing (CMU)
- 85-719 Introduction to Parallel Distributed Processing (CMU)
- 86-631 Neural Data Analysis (CMU)
- 86-675 Computational Perception (CMU)
- BIOENG 2650 Mathematical Models of Biological Learning (Pitt)
- MATH 3370 Mathematical Neuroscience (Pitt)
Quantitative Methods
Students must take at least two graduate level courses in one quantitative subject (e.g. math, computer science or statistics) to ensure depth of knowledge in this area. Courses listed above under the Computational Neuroscience requirement are not eligible to fulfill this requirement. Under the quantitative methods requirement, we have identified two examples of focus areas:
Dynamical Systems focus
- MATH 2940 Applied Stochastic Methods (PITT)
- MATH 2950 Applied Math Methods (PITT)
Statistics and Machine Learning focus
- 10-701 or 10-715 Machine Learning (CMU)
- 36-705 Intermediate Statistics (CMU)
- 36-707 Regression Analysis (CMU)
Other foci, including “brain imaging and signal processing” have been discussed and may be added as recommended course sets, subject to approval by the program co-directors. Note that to be eligible to take certain of these course, students might first need to complete course pre-requisites. These pre-requisites would not count towards the two course depth requirement.
BACK TO TOP
Program Milestones
Progress in the program is tracked based in part on students’ successful completion of program milestones. A committee selected by the student and approved by the program director evaluates the performance on milestones.
First year research requirement
By the end of the first calendar year in the program, all students are required to complete a computational project. This project will be evaluated by a committee consisting of at least three faculty, of whom at least two are PNC training faculty. The project requires the student to identify a biological problem, understand the data collection process, articulate the goals of building a model or performing a particular kind of analysis and implement this computational approach. In some cases this project may be a precursor to the student’s eventual thesis project.
Second year research requirement
In the second year, students are expected to work on research about 1/3 of their time during the academic year and full time during the summer . By the end of the second full year in the program all students are required to complete a deeper computational project. The student’s work on the project should demonstrate that the student has 1) the ability to analyze and interpret experimental data in a particular area 2) the ability to develop and implement a computational approach incorporating the relevant level of biological detail and 3) the ability to organize, interpret and present the results of the computational work. This project should be a body of work suitable for publication .
Ph.D. Thesis proposal
Required coursework should be completed by the end of the third year. During the fourth year a Ph.D. candidate should present a thesis proposal to his or her thesis committee and the community. The thesis proposal should include: a succinct summary of the proposed research problem; the significance of the proposed research; a review of relevant literature relating to the problem; a review of the candidate’s work leading up to the thesis, including preliminary results; a clear statement of remaining research; and a tentative schedule for completing the work.
Ph.D. Thesis Defense
Normally, the dissertation is completed during the student’s fifth year. The thesis defense is your chance to present the culmination of all your work and research to the neuroscience community and field questions from your thesis committee.
Additional Requirements
- All students will complete Responsible Conduct of Research Training.
- In order to build skills in teaching, mentoring, communication and management, each student will be required to serve as a teaching assistant for two courses during their career as a graduate student in the program.
- Students will participate with CNBC graduate training students in the following co-curricular activities: CNBC colloquium series; Brain Bag research seminars; and CNBC retreat.
Neural Computation Home
COURSE REQUIREMENTS
PROGRAM MILESTONES
ADDITIONAL REQUIREMENTS
- Submit Media
- Report a Web Issue
Support Us
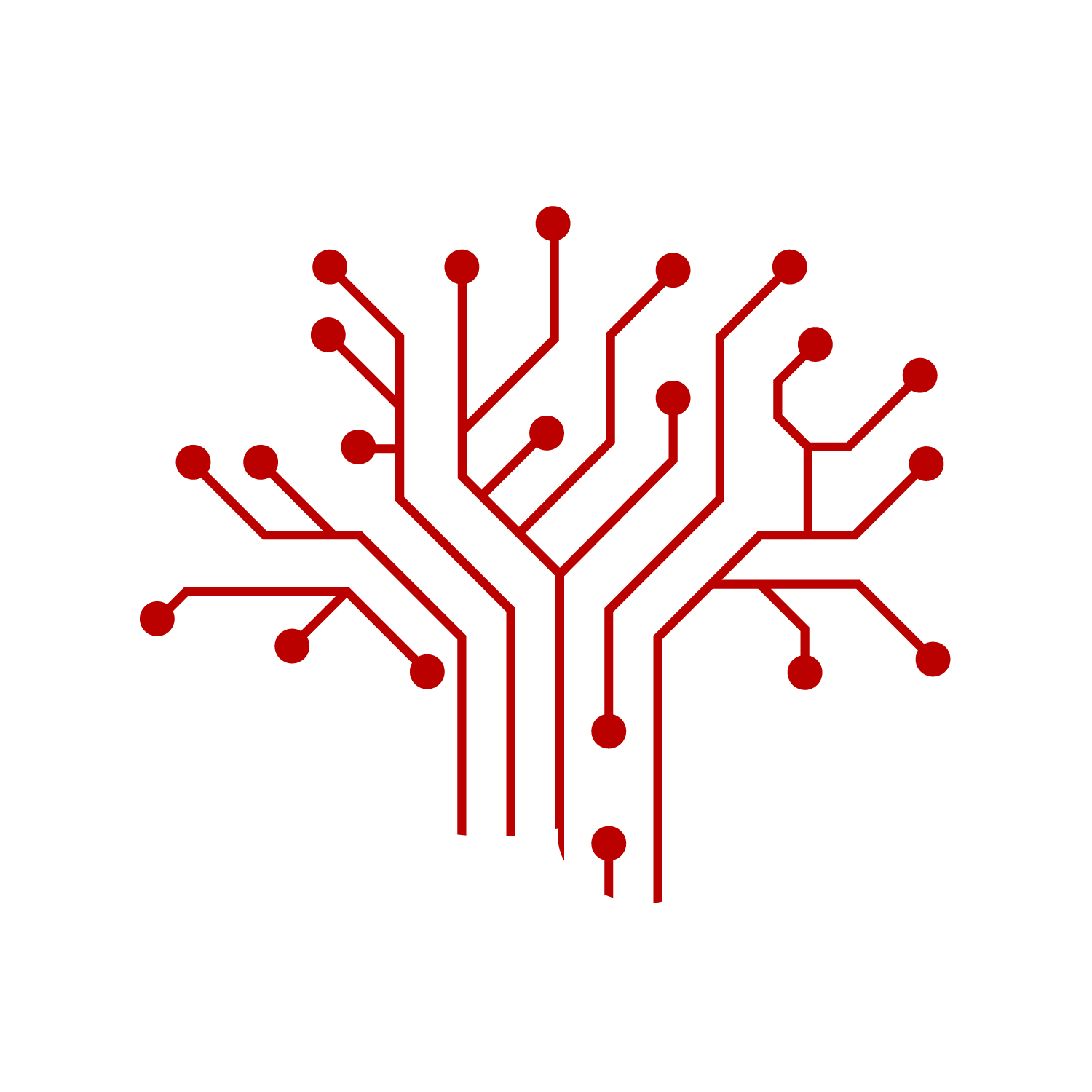
- Faculty of Arts and Sciences
- FAS Theses and Dissertations
- Communities & Collections
- By Issue Date
- FAS Department
- Quick submit
- Waiver Generator
- DASH Stories
- Accessibility
- COVID-related Research
Terms of Use
- Privacy Policy
- By Collections
- By Departments
The Computational Lens: from Quantum Physics to Neuroscience
Citable link to this page
Collections.
- FAS Theses and Dissertations [6136]
Contact administrator regarding this item (to report mistakes or request changes)
Thank you for visiting nature.com. You are using a browser version with limited support for CSS. To obtain the best experience, we recommend you use a more up to date browser (or turn off compatibility mode in Internet Explorer). In the meantime, to ensure continued support, we are displaying the site without styles and JavaScript.
- View all journals
- Explore content
- About the journal
- Publish with us
- Sign up for alerts
- Review Article
- Published: 20 August 2018
Cognitive computational neuroscience
- Nikolaus Kriegeskorte 1 &
- Pamela K. Douglas 2
Nature Neuroscience volume 21 , pages 1148–1160 ( 2018 ) Cite this article
38k Accesses
217 Citations
201 Altmetric
Metrics details
- Cognitive neuroscience
- Computational neuroscience
To learn how cognition is implemented in the brain, we must build computational models that can perform cognitive tasks, and test such models with brain and behavioral experiments. Cognitive science has developed computational models that decompose cognition into functional components. Computational neuroscience has modeled how interacting neurons can implement elementary components of cognition. It is time to assemble the pieces of the puzzle of brain computation and to better integrate these separate disciplines. Modern technologies enable us to measure and manipulate brain activity in unprecedentedly rich ways in animals and humans. However, experiments will yield theoretical insight only when employed to test brain-computational models. Here we review recent work in the intersection of cognitive science, computational neuroscience and artificial intelligence. Computational models that mimic brain information processing during perceptual, cognitive and control tasks are beginning to be developed and tested with brain and behavioral data.
This is a preview of subscription content, access via your institution
Access options
Access Nature and 54 other Nature Portfolio journals
Get Nature+, our best-value online-access subscription
24,99 € / 30 days
cancel any time
Subscribe to this journal
Receive 12 print issues and online access
195,33 € per year
only 16,28 € per issue
Buy this article
- Purchase on Springer Link
- Instant access to full article PDF
Prices may be subject to local taxes which are calculated during checkout
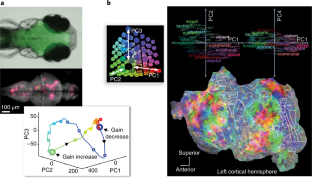
Similar content being viewed by others
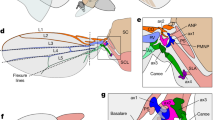
Machine learning reveals the control mechanics of an insect wing hinge
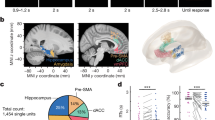
Control of working memory by phase–amplitude coupling of human hippocampal neurons
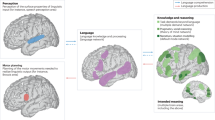
The language network as a natural kind within the broader landscape of the human brain
Newell, A. You can’t play 20 questions with nature and win: projective comments on the papers of this symposium. Technical Report, School of Computer Science, Carnegie Mellon University (1973).
Chapter Google Scholar
Lake, B. M., Ullman, T. D., Tenenbaum, J. B. & Gershman, S. J. Building machines that learn and think like people. Behav. Brain Sci. 40 , e253 (2017).
Article PubMed Google Scholar
Kriegeskorte, N. & Mok, R. M. Building machines that adapt and compute like brains. Behav. Brain Sci. 40 , e269 (2017).
Simon, H. A. & Newell, A. Human problem solving: the state of the theory in 1970. Am. Psychol. 26 , 145–159 (1971).
Article Google Scholar
Anderson, J. R. The Architecture of Cognition (Harvard Univ. Press, Cambridge, MA, USA, 1983).
McClelland, J. L. & Rumelhart, D. E. Parallel Distributed Processing (MIT Press, Cambridge, MA, USA, 1987).
Gazzaniga, M. S. ed. The Cognitive Neurosciences (MIT Press, Cambridge, MA, USA, 2004).
Fodor, J. A. Précis of The Modularity of Mind. Behav. Brain Sci. 8 , 1 (1985).
Chklovskii, D. B. & Koulakov, A. A. Maps in the brain: what can we learn from them? Annu. Rev. Neurosci. 27 , 369–392 (2004).
Article CAS PubMed Google Scholar
Szucs, D. & Ioannidis, J. P. A. Empirical assessment of published effect sizes and power in the recent cognitive neuroscience and psychology literature. PLoS Biol. 15 , e2000797 (2017).
Article PubMed PubMed Central CAS Google Scholar
Kriegeskorte, N., Simmons, W. K., Bellgowan, P. S. F. & Baker, C. I. Circular analysis in systems neuroscience: the dangers of double dipping. Nat. Neurosci. 12 , 535–540 (2009).
Article CAS PubMed PubMed Central Google Scholar
Kanwisher, N., McDermott, J. & Chun, M. M. The fusiform face area: a module in human extrastriate cortex specialized for face perception. J. Neurosci. 17 , 4302–4311 (1997).
Tsao, D. Y., Freiwald, W. A., Tootell, R. B. & Livingstone, M. S. A cortical region consisting entirely of face-selective cells. Science 311 , 670–674 (2006).
Freiwald, W. A. & Tsao, D. Y. Functional compartmentalization and viewpoint generalization within the macaque face-processing system. Science 330 , 845–851 (2010).
Grill-Spector, K., Weiner, K. S., Kay, K. & Gomez, J. The functional neuroanatomy of human face perception. Annu. Rev. Vis. Sci. 3 , 167–196 (2017).
Article PubMed PubMed Central Google Scholar
Yildirim, I. et al . Efficient and robust analysis-by-synthesis in vision: a computational framework, behavioral tests, and modeling neuronal representations. in Annual Conference of the Cognitive Science Society (eds. Noelle, D. C. et al.) (Cognitive Science Society, Austin, TX, USA, 2015).
Kriegeskorte, N., Formisano, E., Sorger, B. & Goebel, R. Individual faces elicit distinct response patterns in human anterior temporal cortex. Proc. Natl Acad. Sci. USA 104 , 20600–20605 (2007).
Anzellotti, S., Fairhall, S. L. & Caramazza, A. Decoding representations of face identity that are tolerant to rotation. Cereb. Cortex 24 , 1988–1995 (2014).
Chang, L. & Tsao, D. Y. The code for facial identity in the primate brain. Cell 169 , 1013–1028.e14 (2017).
Van Essen, D. C. et al. The Brain Analysis Library of Spatial maps and Atlases (BALSA) database. Neuroimage 144 (Pt. B), 270–274 (2017).
Griffiths, T. L., Chater, N., Kemp, C., Perfors, A. & Tenenbaum, J. B. Probabilistic models of cognition: exploring representations and inductive biases. Trends Cogn. Sci. 14 , 357–364 (2010).
Ernst, M. O. & Banks, M. S. Humans integrate visual and haptic information in a statistically optimal fashion. Nature 415 , 429–433 (2002).
Weiss, Y., Simoncelli, E. P. & Adelson, E. H. Motion illusions as optimal percepts. Nat. Neurosci. 5 , 598–604 (2002).
Körding, K. P. & Wolpert, D. M. Bayesian integration in sensorimotor learning. Nature 427 , 244–247 (2004).
Article PubMed CAS Google Scholar
MacKay, D. J. C. Information Theory, Inference, and Learning Algorithms . (Cambridge Univ. Press, Cambridge, 2003)
Murphy, K. P. Machine Learning: A Probabilistic Perspective (MIT Press, Cambridge, MA, USA, 2012).
Google Scholar
Dayan, P. & Abbott, L. F. Theoretical Neuroscience: Computational and Mathematical Modeling of Neural Systems (MIT Press, Cambridge, MA, USA, 2001).
Abbott, L. F. Theoretical neuroscience rising. Neuron 60 , 489–495 (2008).
Olshausen, B. A. & Field, D. J. Sparse coding of sensory inputs. Curr. Opin. Neurobiol. 14 , 481–487 (2004).
Simoncelli, E. P. & Olshausen, B. A. Natural image statistics and neural representation. Annu. Rev. Neurosci. 24 , 1193–1216 (2001).
Carandini, M. & Heeger, D. J. Normalization as a canonical neural computation. Nat. Rev. Neurosci. 13 , 51–62 (2011).
Chaudhuri, R. & Fiete, I. Computational principles of memory. Nat. Neurosci. 19 , 394–403 (2016).
Shadlen, M. N. & Kiani, R. Decision making as a window on cognition. Neuron 80 , 791–806 (2013).
Newsome, W. T., Britten, K. H. & Movshon, J. A. Neuronal correlates of a perceptual decision. Nature 341 , 52–54 (1989).
Wang, X.-J. Decision making in recurrent neuronal circuits. Neuron 60 , 215–234 (2008).
Diedrichsen, J., Shadmehr, R. & Ivry, R. B. The coordination of movement: optimal feedback control and beyond. Trends Cogn. Sci. 14 , 31–39 (2010).
Kriegeskorte, N. Deep neural networks: a new framework for modeling biological vision and brain information processing. Annu. Rev. Vis. Sci. 1 , 417–446 (2015).
Yamins, D. L. K. & DiCarlo, J. J. Using goal-driven deep learning models to understand sensory cortex. Nat. Neurosci. 19 , 356–365 (2016).
Krizhevsky, A., Sutskever, I. & Hinton, G. E. ImageNet classification with deep convolutional neural networks. in Advances in Neural Information Processing Systems 25 1097–1105 (Curran Associates, Red Hook, NY, USA, 2012).
Silver, D. et al. Mastering the game of Go with deep neural networks and tree search. Nature 529 , 484–489 (2016).
Mnih, V. et al. Human-level control through deep reinforcement learning. Nature 518 , 529–533 (2015).
LeCun, Y., Bengio, Y. & Hinton, G. Deep learning. Nature 521 , 436–444 (2015).
Cohen, J. D. et al. Computational approaches to fMRI analysis. Nat. Neurosci. 20 , 304–313 (2017).
Forstmann, B. U., Wagenmakers, E.-J., Eichele, T., Brown, S. & Serences, J. T. Reciprocal relations between cognitive neuroscience and formal cognitive models: opposites attract? Trends Cogn. Sci. 15 , 272–279 (2011).
Deco, G., Tononi, G., Boly, M. & Kringelbach, M. L. Rethinking segregation and integration: contributions of whole-brain modelling. Nat. Rev. Neurosci. 16 , 430–439 (2015).
Biswal, B., Yetkin, F. Z., Haughton, V. M. & Hyde, J. S. Functional connectivity in the motor cortex of resting human brain using echo-planar MRI. Magn. Reson. Med. 34 , 537–541 (1995).
Hyvarinen, A., Karhunen, J. & Oja, E. Independent Component Analysis (Wiley, Hoboken, NJ, USA, 2001).
Bullmore, E. T. & Bassett, D. S. Brain graphs: graphical models of the human brain connectome. Annu. Rev. Clin. Psychol. 7 , 113–140 (2011).
Deco, G., Jirsa, V. K. & McIntosh, A. R. Emerging concepts for the dynamical organization of resting-state activity in the brain. Nat. Rev. Neurosci. 12 , 43–56 (2011).
Friston, K. Dynamic causal modeling and Granger causality. Comments on: the identification of interacting networks in the brain using fMRI: model selection, causality and deconvolution. Neuroimage 58 , 303–305 (2011). author reply 310–311.
Dennett, D. C. The Intentional Stance (MIT Press, Cambridge, MA, USA, 1987).
Diedrichsen, J. & Kriegeskorte, N. Representational models: a common framework for understanding encoding, pattern-component, and representational-similarity analysis. PLoS Comput. Biol. 13 , e1005508 (2017).
Afraz, S.-R., Kiani, R. & Esteky, H. Microstimulation of inferotemporal cortex influences face categorization. Nature 442 , 692–695 (2006).
Parvizi, J. et al. Electrical stimulation of human fusiform face-selective regions distorts face perception. J. Neurosci. 32 , 14915–14920 (2012).
Norman, K. A., Polyn, S. M., Detre, G. J. & Haxby, J. V. Beyond mind-reading: multi-voxel pattern analysis of fMRI data. Trends Cogn. Sci. 10 , 424–430 (2006).
Tong, F. & Pratte, M. S. Decoding patterns of human brain activity. Annu. Rev. Psychol. 63 , 483–509 (2012).
Kriegeskorte, N. & Kievit, R. A. Representational geometry: integrating cognition, computation, and the brain. Trends Cogn. Sci. 17 , 401–412 (2013).
Haxby, J. V., Connolly, A. C. & Guntupalli, J. S. Decoding neural representational spaces using multivariate pattern analysis. Annu. Rev. Neurosci. 37 , 435–456 (2014).
Haynes, J.-D. A primer on pattern-based approaches to fMRI: principles, pitfalls, and perspectives. Neuron 87 , 257–270 (2015).
Jin, X. & Costa, R. M. Shaping action sequences in basal ganglia circuits. Curr. Opin. Neurobiol. 33 , 188–196 (2015).
DiCarlo, J. J. & Cox, D. D. Untangling invariant object recognition. Trends Cogn. Sci. 11 , 333–341 (2007).
Naselaris, T. & Kay, K. N. Resolving ambiguities of MVPA using explicit models of representation. Trends Cogn. Sci. 19 , 551–554 (2015).
Mitchell, T. M. et al. Predicting human brain activity associated with the meanings of nouns. Science 320 , 1191–1195 (2008).
Kay, K. N., Naselaris, T., Prenger, R. J. & Gallant, J. L. Identifying natural images from human brain activity. Nature 452 , 352–355 (2008).
Dumoulin, S. O. & Wandell, B. A. Population receptive field estimates in human visual cortex. Neuroimage 39 , 647–660 (2008).
Diedrichsen, J., Ridgway, G. R., Friston, K. J. & Wiestler, T. Comparing the similarity and spatial structure of neural representations: a pattern-component model. Neuroimage 55 , 1665–1678 (2011).
Kriegeskorte, N., Mur, M. & Bandettini, P. Representational similarity analysis - connecting the branches of systems neuroscience. Front. Syst. Neurosci. 2 , 4 (2008).
Nili, H. et al. A toolbox for representational similarity analysis. PLoS Comput. Biol. 10 , e1003553 (2014).
Devereux, B. J., Clarke, A., Marouchos, A. & Tyler, L. K. Representational similarity analysis reveals commonalities and differences in the semantic processing of words and objects. J. Neurosci. 33 , 18906–18916 (2013).
Huth, A. G., de Heer, W. A., Griffiths, T. L., Theunissen, F. E. & Gallant, J. L. Natural speech reveals the semantic maps that tile human cerebral cortex. Nature 532 , 453–458 (2016).
Markram, H. The Blue Brain Project. Nat. Rev. Neurosci. 7 , 153–160 (2006).
Eliasmith, C. & Trujillo, O. The use and abuse of large-scale brain models. Curr. Opin. Neurobiol. 25 , 1–6 (2014).
Eliasmith, C. et al. A large-scale model of the functioning brain. Science 338 , 1202–1205 (2012).
Hassabis, D., Kumaran, D., Summerfield, C. & Botvinick, M. Neuroscience-inspired artificial intelligence. Neuron 95 , 245–258 (2017).
Rumelhart, D. E., Hinton, G. E. & Williams, R. J. Learning representations by back-propagating errors. Nature 323 , 533–536 (1986).
Goodfellow, I., Bengio, Y. & Courville, A. Deep Learning (MIT Press, Cambridge, MA, USA, 2016).
Yamins, D. L. K. et al. Performance-optimized hierarchical models predict neural responses in higher visual cortex. Proc. Natl Acad. Sci. USA 111 , 8619–8624 (2014).
Khaligh-Razavi, S.-M. & Kriegeskorte, N. Deep supervised, but not unsupervised, models may explain IT cortical representation. PLoS Comput. Biol. 10 , e1003915 (2014).
Cadieu, C. F. et al. Deep neural networks rival the representation of primate IT cortex for core visual object recognition. PLoS Comput. Biol. 10 , e1003963 (2014).
Güçlü, U. & van Gerven, M. A. J. Deep neural networks reveal a gradient in the complexity of neural representations across the ventral stream. J. Neurosci. 35 , 10005–10014 (2015).
Article PubMed CAS PubMed Central Google Scholar
Eickenberg, M., Gramfort, A., Varoquaux, G. & Thirion, B. Seeing it all: convolutional network layers map the function of the human visual system. Neuroimage 152 , 184–194 (2017).
Cichy, R. M., Khosla, A., Pantazis, D., Torralba, A. & Oliva, A. Comparison of deep neural networks to spatio-temporal cortical dynamics of human visual object recognition reveals hierarchical correspondence. Sci. Rep. 6 , 27755 (2016).
Hong, H., Yamins, D. L. K., Majaj, N. J. & DiCarlo, J. J. Explicit information for category-orthogonal object properties increases along the ventral stream. Nat. Neurosci. 19 , 613–622 (2016).
Kubilius, J., Bracci, S. & Op de Beeck, H. P. Deep neural networks as a computational model for human shape sensitivity. PLoS Comput. Biol. 12 , e1004896 (2016).
Jozwik, K. M., Kriegeskorte, N., Storrs, K. R. & Mur, M. Deep convolutional neural networks outperform feature-based but not categorical models in explaining object similarity judgments. Front. Psychol. 8 , 1726 (2017).
Moore, C. & Mertens, S. The Nature of Computation . (Oxford Univ. Press, Oxford, 2011).
Book Google Scholar
Borst, J., Taatgen & Anderson, J. Using the ACT-R cognitive architecture in combination with fMRI data. in An Introduction to Model-Based Cognitive Neuroscience (eds. Forstmann, B. U. & Wagenmakers, E.-J.) (Springer, New York, 2014).
Sutton, R. & Barto, A. Reinforcement Learning: An Introduction Vol. 1 (MIT Press, Cambridge, MA, USA, 1998).
O’Doherty, J. P., Cockburn, J. & Pauli, W. M. Learning, reward, and decision making. Annu. Rev. Psychol. 68 , 73–100 (2017).
Daw, N. D. & Dayan, P. The algorithmic anatomy of model-based evaluation. Phil. Trans. R. Soc. Lond. B 369 , 20130478 (2014).
Lengyel, M. & Dayan, P. Hippocampal contributions to control: the third way in Advances in Neural Information Processing Systems 20 889–896 (MIT Press, Cambridge, MA, USA, 2008)..
Gershman, S. J. & Daw, N. D. Reinforcement learning and episodic memory in humans and animals: an integrative framework. Annu. Rev. Psychol. 68 , 101–128 (2017).
Schultz, W., Dayan, P. & Montague, P. R. A neural substrate of prediction and reward. Science 275 , 1593–1599 (1997).
Sutton, R. Integrated architectures for learning, planning, and reacting based on approximating dynamic programming. in Proceedings of the Seventh International Conference on Machine Learning 216–224 (Morgan Kaufmann, San Francisco, 1990).
Daw, N. D., Niv, Y. & Dayan, P. Uncertainty-based competition between prefrontal and dorsolateral striatal systems for behavioral control. Nat. Neurosci. 8 , 1704–1711 (2005).
Ma, W. J. Organizing probabilistic models of perception. Trends Cogn. Sci. 16 , 511–518 (2012).
Fiser, J., Berkes, P., Orbán, G. & Lengyel, M. Statistically optimal perception and learning: from behavior to neural representations. Trends Cogn. Sci. 14 , 119–130 (2010).
Tenenbaum, J. B., Kemp, C., Griffiths, T. L. & Goodman, N. D. How to grow a mind: statistics, structure, and abstraction. Science 331 , 1279–1285 (2011).
Tversky, A. & Kahneman, D. Judgment under uncertainty: heuristics and biases. in Utility, Probability, and Human Decision Making (eds. Wendt, D. & Vlek, C.) 141–162, https://doi.org/10.1007/978-94-010-1834-0_8 (Springer Netherlands, Dordrecht, the Netherlands, 1975).
Lake, B. M., Salakhutdinov, R. & Tenenbaum, J. B. Human-level concept learning through probabilistic program induction. Science 350 , 1332–1338 (2015).
Ullman, T. D., Spelke, E., Battaglia, P. & Tenenbaum, J. B. Mind games: game engines as an architecture for intuitive physics. Trends Cogn. Sci. 21 , 649–665 (2017).
Battaglia, P. W., Hamrick, J. B. & Tenenbaum, J. B. Simulation as an engine of physical scene understanding. Proc. Natl Acad. Sci. USA 110 , 18327–18332 (2013).
Kubricht, J. R., Holyoak, K. J. & Lu, H. Intuitive physics: current research and controversies. Trends Cogn. Sci. 21 , 749–759 (2017).
Pantelis, P. C. et al. Inferring the intentional states of autonomous virtual agents. Cognition 130 , 360–379 (2014).
Pouget, A., Beck, J. M., Ma, W. J. & Latham, P. E. Probabilistic brains: knowns and unknowns. Nat. Neurosci. 16 , 1170–1178 (2013).
Orhan, A. E. & Ma, W. J. Efficient probabilistic inference in generic neural networks trained with non-probabilistic feedback. Nat. Commun. 8 , 138 (2017).
Tervo, D. G. R., Tenenbaum, J. B. & Gershman, S. J. Toward the neural implementation of structure learning. Curr. Opin. Neurobiol. 37 , 99–105 (2016).
Buesing, L., Bill, J., Nessler, B. & Maass, W. Neural dynamics as sampling: a model for stochastic computation in recurrent networks of spiking neurons. PLoS Comput. Biol. 7 , e1002211 (2011).
Haefner, R. M., Berkes, P. & Fiser, J. Perceptual decision-making as probabilistic inference by neural sampling. Neuron 90 , 649–660 (2016).
Aitchison, L. & Lengyel, M. The Hamiltonian brain: efficient probabilistic inference with excitatory-inhibitory neural circuit dynamics. PLoS Comput. Biol. 12 , e1005186 (2016).
Sanborn, A. N. & Chater, N. Bayesian brains without probabilities. Trends Cogn. Sci. 20 , 883–893 (2016).
Dasgupta, I., Schulz, E., Goodman, N. & Gershman, S. Amortized hypothesis generation. Preprint at bioRxiv https://doi.org/10.1101/137190 (2017).
Krakauer, J. W., Ghazanfar, A. A., Gomez-Marin, A., MacIver, M. A. & Poeppel, D. Neuroscience needs behavior: correcting a reductionist bias. Neuron 93 , 480–490 (2017).
Gomez-Marin, A., Paton, J. J., Kampff, A. R., Costa, R. M. & Mainen, Z. F. Big behavioral data: psychology, ethology and the foundations of neuroscience. Nat. Neurosci. 17 , 1455–1462 (2014).
Marr, D. Vision: A Computational Investigation into the Human Representation and Processing of Visual Information (MIT Press, Cambridge, MA, USA, 2010).
Love, B. C. The algorithmic level is the bridge between computation and brain. Top. Cogn. Sci. 7 , 230–242 (2015).
Gal, Y. & Ghahramani, Z. Dropout as a Bayesian approximation: representing model uncertainty in deep learning. Preprint at https://arxiv.org/abs/1506.02142 (2016).
Rezende, D., Mohamed, S., Danihelka, I., Gregor, K. & Wierstra, D. One-shot generalization in deep generative models. Proc. Int. Conf. Mach. Learn. Appl. 48 , 1521–1529 (2016).
Kingma, D. & Welling, M. Auto-encoding variational Bayes. Preprint at https://arxiv.org/abs/1312.6114 (2013).
Naselaris, T. et al. Cognitive Computational Neuroscience: a new conference for an emerging discipline. Trends Cogn. Sci. 22 , 365–367 (2018).
Ahrens, M. B. et al. Brain-wide neuronal dynamics during motor adaptation in zebrafish. Nature 485 , 471–477 (2012).
Kietzmann, T., McClure, P. & Kriegeskorte, N. Deep neural networks in computational neuroscience. Preprint at bioRxiv https://doi.org/10.1101/133504 (2017).
Hornik, K. Approximation capabilities of multilayer feedforward networks. Neural Netw. 4 , 251–257 (1991).
Wyatte, D., Curran, T. & O’Reilly, R. The limits of feedforward vision: recurrent processing promotes robust object recognition when objects are degraded. J. Cogn. Neurosci. 24 , 2248–2261 (2012).
Spoerer, C. J., McClure, P. & Kriegeskorte, N. Recurrent convolutional neural networks: a better model of biological object recognition. Front. Psychol. 8 , 1551 (2017).
Hunt, L. T. & Hayden, B. Y. A distributed, hierarchical and recurrent framework for reward-based choice. Nat. Rev. Neurosci. 18 , 172–182 (2017).
Schäfer, A. M. & Zimmermann, H. G. Recurrent neural networks are universal approximators. Int. J. Neural Syst. 17 , 253–263 (2007).
O’Reilly, R. C., Hazy, T. E., Mollick, J., Mackie, P. & Herd, S. Goal-driven cognition in the brain: a computational framework. Preprint at http://arxiv.org/abs/1404.7591 (2014).
Whittington, J. C. R. & Bogacz, R. An approximation of the error backpropagation algorithm in a predictive coding network with local Hebbian synaptic plasticity. Neural Comput. 29 , 1229–1262 (2017).
Schiess, M., Urbanczik, R. & Senn, W. Somato-dendritic synaptic plasticity and error-backpropagation in active dendrites. PLoS Comput. Biol. 12 , e1004638 (2016).
Marblestone, A. H., Wayne, G. & Kording, K. P. Towards an integration of deep learning and neuroscience. Front. Comput. Neurosci. 10 , 94 (2016).
Shadlen, M. N. & Shohamy, D. Decision making and sequential sampling from memory. Neuron 90 , 927–939 (2016).
Roelfsema, P. R. & van Ooyen, A. Attention-gated reinforcement learning of internal representations for classification. Neural Comput. 17 , 2176–2214 (2005).
Goodfellow, I. et al. Generative adversarial nets. Preprint at https://arxiv.org/abs/1406.2661 (2014).
Kandel, E. R., Schwartz, J. H., Jessell, T. M., Siegelbaum, S. A. & Hudspeth, A. J. Principles of Neural Science (McGraw-Hill Professional, New York, 2013).
Bastos, A. M. et al. Canonical microcircuits for predictive coding. Neuron 76 , 695–711 (2012).
Larkum, M. A cellular mechanism for cortical associations: an organizing principle for the cerebral cortex. Trends Neurosci. 36 , 141–151 (2013).
Fries, P. A mechanism for cognitive dynamics: neuronal communication through neuronal coherence. Trends Cogn. Sci. 9 , 474–480 (2005).
Kumaran, D., Hassabis, D. & McClelland, J. L. What learning systems do intelligent agents need? complementary learning systems theory updated. Trends Cogn. Sci. 20 , 512–534 (2016).
Yuille, A. & Kersten, D. Vision as Bayesian inference: analysis by synthesis? Trends Cogn. Sci. 10 , 301–308 (2006).
Helmholtz, H. Handbuch der physiologischen Optik (Dover, New York, 1860).
Gershman, S. J., Horvitz, E. J. & Tenenbaum, J. B. Computational rationality: a converging paradigm for intelligence in brains, minds, and machines. Science 349 , 273–278 (2015).
Simon, H. A. Bounded rationality. in Utility and Probability (eds. Eatwell, J., Milgate, M. & Newman, P.) 15–18, https://doi.org/10.1007/978-1-349-20568-4_5 (Palgrave Macmillan, London, 1990).
Griffiths, T. L., Lieder, F. & Goodman, N. D. Rational use of cognitive resources: levels of analysis between the computational and the algorithmic. Top. Cogn. Sci. 7 , 217–229 (2015).
Srikumar, V., Kundu, G. & Roth, D. On amortizing inference cost for structured prediction Proceedings of the 2012 Joint Conference on Empirical Methods in Natural Language Processing and Computational Natural Language Learning 1114–1124 (Association for Computational Linguistics, Stroudsburg, PA, USA, 2012).
Bengio, Y., Scellier, B., Bilaniuk, O., Sacramento, J. & Senn, W. Feedforward initialization for fast inference of deep generative networks is biologically plausible. Preprint at https://arxiv.org/abs/1606.01651 (2016).
Ghahramani, Z. Bayesian non-parametrics and the probabilistic approach to modelling. Philos. Trans. A Math. Phys. Eng. Sci. 371 , 20110553 (2012).
Deng, J. et al. ImageNet: a large-scale hierarchical image database. in 2009 IEEE Conference on Computer Vision and Pattern Recognition 248–255, https://doi.org/10.1109/CVPR.2009.5206848 (IEEE, Piscataway, NJ, USA, 2009).
Beattie, C. et al. DeepMind Lab. Preprint at https://arxiv.org/abs/1612.03801 (2016).
Griffiths, T. L. Manifesto for a new (computational) cognitive revolution. Cognition 135 , 21–23 (2015).
Download references
Acknowledgements
This paper benefited from discussions in the context of the new conference Cognitive Computational Neuroscience, which had its inaugural meeting in New York City in September 2017 120 . We are grateful in particular to T. Naselaris, K. Kay, K. Kording, D. Shohamy, R. Poldrack, J. Diedrichsen, M. Bethge, R. Mok, T. Kietzmann, K. Storrs, M. Mur, T. Golan, M. Lengyel, M. Shadlen, D. Wolpert, A. Oliva, D. Yamins, J. Cohen, J. DiCarlo, T. Konkle, J. McDermott, N. Kanwisher, S. Gershman and J. Tenenbaum for inspiring discussions.
Author information
Authors and affiliations.
Department of Psychology, Department of Neuroscience, Department of Electrical Engineering, Zuckerman Mind Brain Behavior Institute, Columbia University, New York, NY, USA
Nikolaus Kriegeskorte
Center for Cognitive Neuroscience, University of California, Los Angeles, Los Angeles, CA, USA
Pamela K. Douglas
You can also search for this author in PubMed Google Scholar
Corresponding author
Correspondence to Nikolaus Kriegeskorte .
Ethics declarations
Competing interests.
The authors declare no competing interests.
Additional information
Publisher’s note: Springer Nature remains neutral with regard to jurisdictional claims in published maps and institutional affiliations.
Rights and permissions
Reprints and permissions
About this article
Cite this article.
Kriegeskorte, N., Douglas, P.K. Cognitive computational neuroscience. Nat Neurosci 21 , 1148–1160 (2018). https://doi.org/10.1038/s41593-018-0210-5
Download citation
Received : 07 November 2016
Revised : 09 June 2018
Accepted : 11 July 2018
Published : 20 August 2018
Issue Date : September 2018
DOI : https://doi.org/10.1038/s41593-018-0210-5
Share this article
Anyone you share the following link with will be able to read this content:
Sorry, a shareable link is not currently available for this article.
Provided by the Springer Nature SharedIt content-sharing initiative
This article is cited by
Understanding upper-limb movements via neurocomputational models of the sensorimotor system and neurorobotics: where we stand.
- Antonio Parziale
- Angelo Marcelli
Artificial Intelligence Review (2024)
Deep convolutional neural networks are not mechanistic explanations of object recognition
- Bojana Grujičić
Synthese (2024)
Wernicke’s functional neuroanatomy model of language turns 150: what became of its psychological reflex arcs?
- Ardi Roelofs
Brain Structure and Function (2024)
Local gradient analysis of human brain function using the Vogt-Bailey Index
- Christine Farrugia
- Paola Galdi
- Claude J. Bajada
Arithmetic value representation for hierarchical behavior composition
- Hiroshi Makino
Nature Neuroscience (2023)
Quick links
- Explore articles by subject
- Guide to authors
- Editorial policies
Sign up for the Nature Briefing newsletter — what matters in science, free to your inbox daily.

Johns Hopkins School of Medicine
The Solomon H. Snyder Department of Neuroscience
Welcome to the Graduate Program
Think of the Neuroscience Training Program at Johns Hopkins as an expedition, where you will search the frontiers of science for discoveries that explain the inner workings of the nervous system.
2023 Hopkins Neuroscience Graduate Program Virtual Open House Registration
November 2nd, 2023, 4-6 PM Please register below to receive the zoom link: https://forms.office.com/r/UdvVL6YtKq
Application Portal (JHU 2023-2024)
How to Apply (Neuroscience Training Program page)
Neuroscience Graduate Training Program Co-Directors: Chris Potter , Dan O'Connor Deputy Director: Hita Adwanikar Senior Academic Program Coordinator: Audrey Scriven Admissions Director: Hyungbae Kwon
Participation in extensive collaborations, access to cutting-edge resources, and exposure to world-class research, await students in our program.
The Neuroscience Training Program and the Neuroscience Department were among the first neuroscience-focused academic centers established in the United States, dating back to 1980. Our faculty have trained over 250 PhD and MD/PhD students and 500 postdoctoral fellows in just the past ten years, partnerships that have led to fundamental discoveries in the organization of the cerebral cortex, neurotransmitter signaling, neuronal and glial cell development, and circuit function.
Our students represent the brightest young scientific minds, and many have shown an early commitment to research. Because they enter our Program with different backgrounds, and the laboratories in which they choose to work are so diverse, our program is designed to be flexible. All doctoral candidates receive full tuition remission and a stipend for the duration of their studies. Currently, 177 doctoral candidates and 200 postdoctoral fellows work in the faculty laboratories, creating a diverse community that fosters development of novel approaches to answer complex questions.
The goal of the Program to ensure that our students obtain broad training in the neurosciences. Our curriculum spans the breadth of modern neuroscience, from molecular/cellular underpinnings to systems/cognitive integration, and offers a rich training experience that brings students to the forefront of research in their particular area of interest, in preparation for a rewarding, independent career in the sciences.
Core courses cover the basics of molecular and cellular neuroscience, neuroanatomy, and systems neuroscience. Electives and laboratory rotations provide students with specialized training, and the Department’s long-standing seminar series brings in weekly national and international luminaries, exposing students and fellows to the full spectrum of the world’s most exciting new discoveries in neuroscience.
Our 34 primary faculty , together with over 70 other faculty who have secondary appointments in the Department, offer graduate students and postdoctoral fellows an incomparable neuroscience training experience. Our students also have the opportunity perform laboratory rotations and conduct thesis research in the laboratory of scientists at Janelia Research Campus of the Howard Hughes Medical Institute, located near Leesburg Virginia. Faculty in the many departments associated with the Program share a commitment to training the next generation of scientists.
In recognition of this outstanding environment, our graduate program is consistently ranked among the best in the country, and our graduates have gone on to faculty positions at other leading institutions and senior research positions in pharmaceutical and biotech companies.
There has never been a more exciting time in the field of neuroscience. We hope you will join us in this journey of discovery.
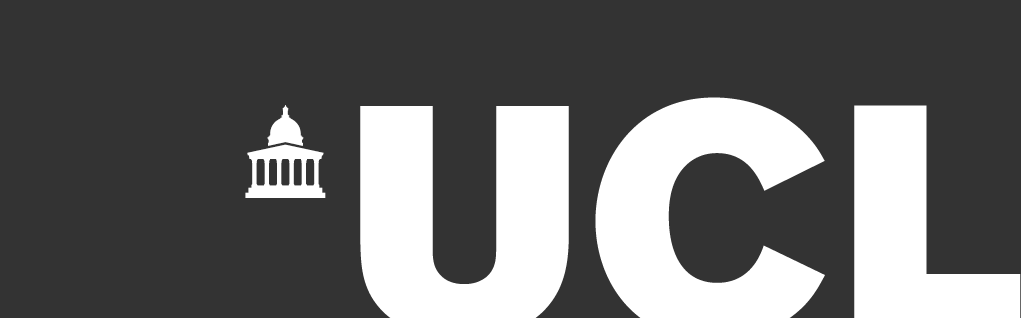
Gatsby Computational Neuroscience Unit MPhil/PhD
London, Bloomsbury
The Gatsby Unit PhD programme was the first to combine theoretical neuroscience and machine learning within the same programme. Our mathematical approach for developing novel algorithms and tools to understand learning, perception and action in brain and machines is unique. Applications to this programme must be submitted directly to the Gatsby Unit via its online portal.
UK tuition fees (2024/25)
Overseas tuition fees (2024/25), programme starts, applications accepted.
Applications closed
- Entry requirements
Applicants must have a strong analytical background, a keen interest in neuroscience or machine learning and a relevant first degree at a minimum of upper second-class UK Bachelor's level or an overseas equivalent, for example in Computer Science, Engineering, Mathematics, Neuroscience, Physics, Psychology or Statistics. Students seeking to combine work in neuroscience and machine learning are particularly encouraged to apply.
The English language level for this programme is: Level 3
UCL Pre-Master's and Pre-sessional English courses are for international students who are aiming to study for a postgraduate degree at UCL. The courses will develop your academic English and academic skills required to succeed at postgraduate level.
Further information can be found on our English language requirements page.
If you are intending to apply for a time-limited visa to complete your UCL studies (e.g., Student visa, Skilled worker visa, PBS dependant visa etc.) you may be required to obtain ATAS clearance . This will be confirmed to you if you obtain an offer of a place. Please note that ATAS processing times can take up to six months, so we recommend you consider these timelines when submitting your application to UCL.
Equivalent qualifications
Country-specific information, including details of when UCL representatives are visiting your part of the world, can be obtained from the International Students website .
International applicants can find out the equivalent qualification for their country by selecting from the list below. Please note that the equivalency will correspond to the broad UK degree classification stated on this page (e.g. upper second-class). Where a specific overall percentage is required in the UK qualification, the international equivalency will be higher than that stated below. Please contact Graduate Admissions should you require further advice.
About this degree
The Gatsby Unit is a world-class research centre for Computational and Theoretical Neuroscience and Machine Learning. Our research seeks to understand the principles of learning, perception and action in brains and machines by developing mathematical algorithms. We provide a unique opportunity for a critical mass of theoreticians to interact closely with each other and with other UCL research groups, in particular the Sainsbury Wellcome Centre for Neural Circuits and Behaviour (SWC) as well as the Centre for Computational Statistics and Machine Learning (CSML). Teaching is supplemented with regular research talks, journal clubs and reading groups, external seminar series and participation in relevant conferences and workshops. PhD students will be supported by all academic staff, not just their immediate supervisors.
Who this course is for
Applicants should have a strong analytical and mathematical background, a keen interest in neuroscience and/or machine learning, and a first degree in relevant fields such as Computer science, Engineering, Physics, Mathematics, Statistics, Neuroscience, or Cognitive Psychology. Many applicants to our programme will have a Master’s degree, but this is not essential. Each application is assessed on its individual merits. Candidates offered a place on our programme will be required to meet UCL's standard admissions requirements (including the English language proficiency requirements for international applicants).
What this course will give you
Students at the Gatsby Computational Neuroscience Unit study toward a PhD in Theoretical Neuroscience and Machine Learning. The Gatsby Unit is part of the Centre for Computational Statistics and Machine Learning, together with UCL Computer Science and Statistical Science. Approximately 90% of alumni have secured academic or industry positions. Many of the most successful leaders in the fields of computational neuroscience and machine learning have studied or worked in the unit, and the unit has a reputation for offering world-class training.
Our programme offers first-year teaching in Systems and Theoretical Neuroscience (jointly with SWC), Probabilistic and Unsupervised Learning, Approximate Inference and Learning in Probabilistic Models, Theoretical Neuroscience, and Advanced Methods in Machine Learning. In your second year, you will carry out a 2-month rotation project in a lab of your choice, either at or external to UCL. The rotation project should be on a research area not related to your PhD topic. Our course also offers a variety of career and skills development training.
We are based in a purpose-designed building in the heart of London and UCL. The building also houses the SWC, with which we interact closely. Our building offers two large seminar rooms, common areas for social interaction or quiet study, high-performance computing facilities, kitchen facilities, and a brasserie offering breakfast, lunch, coffee and snacks. There are also two large outdoor areas and an on-site bicycle rack. The surrounding area is vibrant with a variety of shops, restaurants, cafes and bars, and museums.
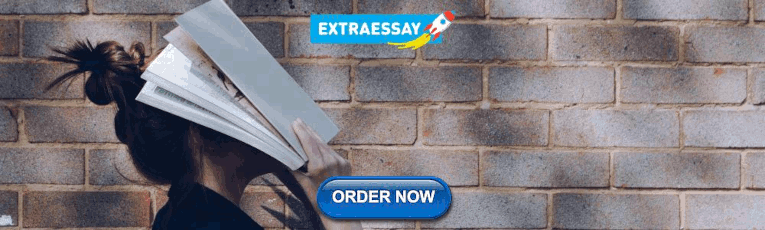
The foundation of your career
Students receive world-class training through our intense, rigorous and diverse research programme. As a result, the majority are highly employable. Students will develop interdisciplinarity and will be expected to collaborate widely. You will develop your communication skills by giving regular internal talks as well as writing scientific publications. Critical thinking is further developed through weekly journal clubs and lab meetings. You will be expected to teach during the second year of the programme and act as a mentor to junior PhDs. Participation in outreach and public engagement activities is also encouraged.
Employability
Most of our graduates have continued in the fields of computational neuroscience and/or machine learning. Alumni have secured academic positions in prestigious institutions such as Cambridge, Oxford, Edinburgh, Columbia, Princeton, Caltech, École Normale Supérieure, the Max Planck Institute for Intelligent Systems and Janelia Research Campus, or have gone on to work in companies such as Google DeepMind, Amazon, Facebook, Samsung and Babylon Health.
There are many networking opportunities, both within and external to the Gatsby Unit. Within the unit, there are daily tea hours where members take turns to present their research or a 10-minute talk on an interesting topic to the unit. Our external seminar series provides an opportunity to meet and interact with eminent speakers and external attendees.
Based in the same building as the SWC, students will have the opportunity to network extensively with experimentalists and to attend SWC seminars, lab meetings and joint social events. Through CSML you will network with peers in Computer Science and Statistics. All students are encouraged to attend relevant conferences and workshops.
Teaching and learning
The four-year PhD programme includes in its first year intensive courses that provide a comprehensive introduction to theoretical and systems neuroscience and machine learning:
- Systems Neuroscience and Theoretical Neuroscience
- Probabilistic and Unsupervised Learning (COMP0086)
- Approximate Inference and Learning in Probabilistic Models (COMP0085)
- Advanced Topics in Machine Learning (COMP0083)
- Reinforcement Learning (COMP0089)
Students will also be required to carry out a 2-month rotation project in a field different from the field of their PhD thesis.
We offer a supportive and interdisciplinary environment with close links to the SWC and the ELLIS Unit at UCL. For more details see https://www.ucl.ac.uk/gatsby/study-and-work/phd-programme/programme-structure.
Students are required to complete coursework for all first-year courses. There are three examinations in the first year of study.
Typical contact hours are 9am - 5pm.
Research areas and structure
- Analysis of neural data
- Neural dynamics
- Neural plasticity
- Perceptual processing of auditory and visual input
- Neural population coding
- Kernel methods
- Bayesian statistics
- Reinforcement learning
- Statistical machine learning
- Unsupervised learning
- Network and relational data
Research environment
The Gatsby Computational Neuroscience Unit was established by the Gatsby Charitable Foundation at UCL in 1998 to provide a unique opportunity for a critical mass of theoreticians to interact closely with each other and with other UCL research groups in neuroscience, machine learning and related areas. We are one of the first centres in the world to bring together the fields of theoretical neuroscience and machine learning, and our investigators have pioneered research into the mathematical underpinnings of learning, perception and action in natural and artificial systems.
In 2016 we moved from our original home in Queen Square to Fitzrovia to create a new collaborative partnership with the Sainsbury Wellcome Centre for Neural Circuits and Behaviour (SWC). The SWC and the Gatsby Unit work together closely, with joint research appointments, parallel PhD programmes, common day-to-day activities, and joint research projects that bring together theoretical and experimental neuroscience. We are also part of the cross-faculty Centre for Computational Statistics and Machine Learning (CSML) and work with other UCL research groups in neuroscience and machine learning .
There are many networking opportunities both within and external to the Gatsby Unit. Within the unit, there are regular talks where members take turns to give research talks to the rest of the unit. The unit also holds regular seminars to which eminent speakers are invited; these are open to other members of the university, and there is an opportunity to meet speakers and attendees after the talk. Students also run journal clubs and their own reading groups, and all students have the opportunity to attend relevant conferences and workshops.
The PhD programme lasts for four years, including first-year teaching in techniques and research in theoretical and systems neuroscience and machine learning. We only admit new students starting in September.
Courses in the first year, taught with colleagues from the SWC and CSML, provide a comprehensive introduction to theoretical and systems neuroscience and to machine learning; with multidisciplinary training in other areas of neuroscience also available. Students are encouraged to work and interact closely with peers and faculty in the SWC and CSML throughout their PhD to take advantage of this uniquely multidisciplinary research environment.
In Year 1, students take core courses in theoretical neuroscience (TN), systems neuroscience (taught with SWC) and machine learning (ML), after which they generally choose to concentrate on either TN or ML. Students sit exams for TN and ML during the second (third) term.
In Year 2, students are expected to work on their thesis project. Students also do a 2-month rotation in a field that is not related to their PhD thesis. At the end of the second year, students are expected to write an MPhil/PhD upgrade report on their progress and future plans and schedule to give a presentation on this report to the rest of the unit. Having passed all the required assessments, students then transfer from MPhil to PhD status and devote their remaining tenure to research.
Throughout the PhD programme, students are immersed in a highly stimulating educational environment, comprising regular talks, research reports, journal clubs and ad hoc reading groups; seminar series in the unit and other UCL departments (including SWC ; Institute of Cognitive Neuroscience ; Institute of Neurology , Department of Psychology ; Neuroscience, Physiology and Pharmacology ; the ELLIS Unit at UCL ; and Department of Statistical Science ), and participation in international conferences such as COSYNE, ICML and NeurIPS.
Accessibility
Details of the accessibility of UCL buildings can be obtained from AccessAble accessable.co.uk . Further information can also be obtained from the UCL Student Support and Wellbeing team .
Fees and funding
Fees for this course.
The tuition fees shown are for the year indicated above. Fees for subsequent years may increase or otherwise vary. Where the programme is offered on a flexible/modular basis, fees are charged pro-rata to the appropriate full-time Master's fee taken in an academic session. Further information on fee status, fee increases and the fee schedule can be viewed on the UCL Students website: ucl.ac.uk/students/fees .
Additional costs
There are no programme-specific additional costs.
For more information on additional costs for prospective students please go to our estimated cost of essential expenditure at Accommodation and living costs .
Funding your studies
A number of fully-funded studentships are available each year. Each studentship covers payment of full fees, provides a generous tax-free living stipend and a travel budget for conference/workshop attendance. Studentships are available to students of any nationality.
For a comprehensive list of the funding opportunities available at UCL, including funding relevant to your nationality, please visit the Scholarships and Funding website .
All applications must be made directly to the Gatsby Unit by the deadline stated on the Gatsby Unit website. Please refer to this web page on the Gatsby Unit website for how to apply to this programme. Late applications will be held until all applications received by the deadline have been assessed. It is very likely that all available positions will be filled by then.
Please note that you may submit applications for a maximum of two graduate programmes (or one application for the Law LLM) in any application cycle.
Got questions? Get in touch
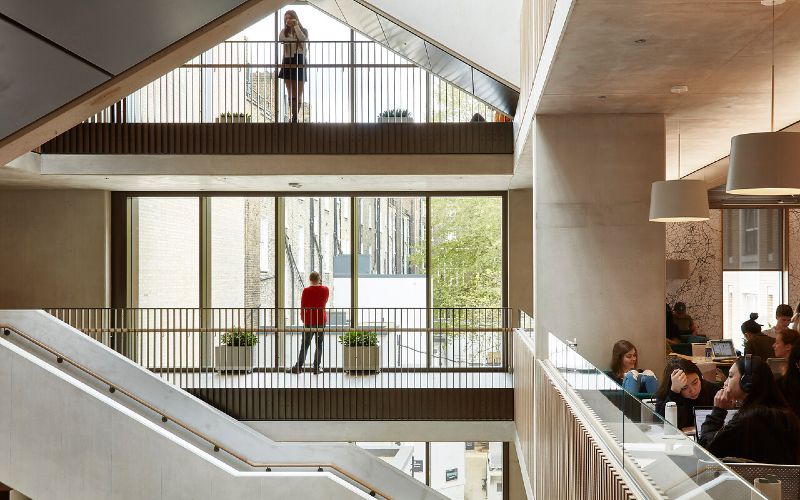
Gatsby Computational Neuroscience Unit
UCL is regulated by the Office for Students .
Prospective Students Graduate
- Graduate degrees
- Taught degrees
- Taught Degrees
- Applying for Graduate Taught Study at UCL
- Research degrees
- Research Degrees
- Funded Research Opportunities
- Doctoral School
- Funded Doctoral Training Programmes
- Applying for Graduate Research Study at UCL
- Teacher training
- Teacher Training
- Early Years PGCE programmes
- Primary PGCE programmes
- Secondary PGCE programmes
- Further Education PGCE programme
- How to apply
- The IOE approach
- Teacher training in the heart of London
- Why choose UCL?
- Entrepreneurship
- Inspiring facilities and resources
- Careers and employability
- Your global alumni community
- Your wellbeing
- Postgraduate Students' Association
- Your life in London
- Accommodation
- Funding your Master's
Boston University Academics
Boston University
- Campus Life
- Schools & Colleges
- Degree Programs
- Search Academics
PhD in Neuroscience (Program in Neuroscience)
The Graduate School of Arts & Sciences (GRS) no longer admits students to the PhD offered by the Program in Neuroscience (PIN). Students are now admitted into the Graduate Program for Neuroscience (GPN) , a University-wide effort administered by the Division of Graduate Medical Sciences (GMS). The following is provided as reference only for continuing GRS Neuroscience PhD students in PIN.
The goal for the majority of students will be to complete the requirements for elective study and the prescribed milestones for moving toward submission of the written thesis and the oral defense. This includes successful completion of the qualifying exam and biannual meetings of the dissertation advisory committee (DAC). Oversight of PIN students is now being conducted by the Graduate Education Committee of GPN. Please contact the GPN office for further information or guidelines.
For the post–bachelor’s PhD degree, a total of 64 course credits is required. Of these, at least 32 of the credits must come from lecture, methods, or seminar courses.
For the post–master’s PhD, 32 credits of coursework are required. The required courses are the same as for the post–bachelor’s PhD. However, depending on the student’s background, one or more of the required courses may be waived via petition to the BU Graduate Program for Neuroscience Graduate Education Committee (GEC).
Core Courses
An essential feature of the program is a set of core courses: these are taken by all students in GPN during their first year and are aimed at developing a community of thinkers who move through the training program together, building relationships that cross departmental and campus barriers, and foster cross-disciplinary collaborations.
Students complete 10 credits of core neuroscience coursework that provides a strong foundation in this diverse field of graduate study. There are two team-taught lecture/discussion courses that are given sequentially over the first year. Each course has two directors, each from a different campus. Courses are cross-listed to represent the cosponsorship of departments from both campuses (Charles River Campus and Medical Campus). GPN students register in the following:
- GRS BI 755 Cellular and Systems Neuroscience or GMS AN 810 Systems Neurobiology (4 cr)
- GRS BI 756/GRS PS 738 Systems and Behavioral Neuroscience or GMS AN 811 Cognitive Neuroscience (4 cr)
Additional core neuroscience requirements (2 cr) include: a 7-week intensive introductory course in data analysis and mathematical models for students who do not have a strong background in computation. This introductory course combines lectures and hands-on computer time to treat real laboratory data like case studies and motivates students to use the mathematical approach as a means to better understand their own research via statistical data analysis and modeling.
- GRS MA 665 Introduction to Mathematical Models and Data Analysis in Neuroscience (2 cr)
Students pursuing the PhD in Computational Neuroscience (or who have taken an undergraduate course in the area) can substitute a more advanced elective for this requirement. Likewise, students who have taken the required course and would like more exposure to the area can continue on in the class to take the next module that is offered sequentially (4 cr instead of 2 cr).
Additional Required Curriculum
In addition to the core curriculum, students take the following seminar coursework during their first year and enroll in laboratory rotations:
- GRS NE 500/501 Frontiers of Neuroscience (2 cr per semester)
All students attend a unique class on Friday afternoons that is managed by the Director or Associate Director of GPN. During the first semester, students are assigned key papers from a particular BU faculty member’s laboratory and supporting manuscripts in the field. During the session, student presenters review and critique experimental findings and approaches, building their skills in critical thinking and developing the basic tools for successful oral presentations. They also get to share their scientific ideas and interests with the leaders of neuroscience at Boston University, an activity that enriches the neuroscience community by building new relationships between faculty and students. Research from monthly GPN distinguished lecturers from across the world are integrated into the training experience to provide a balanced exposure for students to all areas of neuroscience and to give them firsthand interactions with exceptional individuals who are defining the field of the future. During the second semester, students focus on the development of professional skills, such as advanced techniques in oral presentations and the beginnings of scientific writing.
Laboratory Rotations
Providing an enriching set of laboratory research experiences directed by GPN faculty for students during their first year is a central feature of the graduate training program at Boston University. The multitude of highly talented mentors who have funded research projects provides the student with a large number of potential laboratories from which to choose their thesis research mentor that will complement their current interests, and through laboratory rotations, expand their horizons into different areas of investigation that they may grow toward in the future.
The majority of students pursuing the PhD in Neuroscience take three to four rotations, with at least one rotation in an area outside of their initial research interests; students pursuing the PhD in Computational Neuroscience take a minimum of two rotations, with at least one in an experimental laboratory. Students can also engage in additional rotations should they not find a mentor, or if they would like more exposure to other methodologies used in neuroscience. Credit for rotations is contingent upon receipt of a short (5-page) laboratory report for each experience that is reviewed by the rotation mentor.
Directed Study/Thesis
Additional program credits come from directed study during thesis research to make up the 64-credit PhD requirement. Students are also encouraged to take an additional course in probability and statistics that is appropriate to their area of thesis research and take required workshops in neuroscience ethics and responsible conduct of research. Students also attend the required bimonthly student seminar series, a graduate student forum where they can work on improving their oral communication skills throughout their graduate career in GPN. Finally, to be in good standing in the program, they must attend all GPN distinguished lectures and program events, workshops in professional development, and participate in at least one teaching or outreach activity. Optional paid teaching fellowships are available to students who want greater experience in the educational mission.
Students can also substitute additional coursework for directed study to make up the credit requirement for the degree, especially as needed based upon their choice of thesis research or to supplement a lack of certain background during undergraduate study.
Hands-On Laboratory Experience
During the summer before their first year of study, incoming students participate in the Tools of the Trade laboratory experience that is run by GPN faculty to provide students with the essential hands-on experience necessary to make their laboratory rotations in the fall meaningful for their graduate-level training. In Tools of the Trade, students learn some of the basic techniques necessary for conducting laboratory research in the field of neuroscience, independent of their current research interests.
Group activities might be organized around detection of an important neuronal RNA via real-time PCR, the identification of a single nucleotide polymorphism in a DNA sample from a patient with a neurodegenerative disease, identification of protein in brain slices using immunohistochemistry and fluorescence microscopy, electrophysiological measurements or calcium imaging of living neurons, interaction of transcription factors with DNA regulatory elements that control expression of neural-specific genes, neuroimaging of the brain to detect the activation of particular brain structures, and running of a behavioral task with animals to address questions of addiction or learning and memory. Projects vary with the expertise and interests of the participating GPN faculty. Tools of the Trade is coordinated with the university-wide Neuroscience Retreat.
Elective Study
The rest of the formal credits toward the PhD in Neuroscience come from a minimum of 12 credits of elective study that includes at least one course with clinical relevancy. For example, students with a background in molecular neuroscience (or equivalent biochemical/biological study) can take the following electives:
- GMS MS 783 Molecular Basis of Neurological Disease (2 cr)
- GMS PM 701 Molecular Neurobiology and Pharmacology (2 cr)
These advanced courses provide the focus for an understanding of how endogenous substances act in the brain, the challenges faced in the development of effective therapies that target the nervous system, and what molecules can tell us about disease etiology and the potential for future treatment.
Taking advantage of the translational research and history of clinical training at the Medical Campus, all students are required to participate in clinical rounds that are offered through the Boston VA hospital as well as the Alzheimer’s Disease Center. Options for clinically relevant courses specific to students with interests outside of molecular neuroscience cover topics ranging from neuropsychology to human imaging and neural engineering, as well as the rehabilitative sciences offered at BU’s Sargent College of Health & Rehabilitation Sciences.
Please see specific details for the PhD in Computational Neuroscience on the website as there is additional required coursework for this degree specialization.
Suggestions for elective study are provided by faculty curriculum committees in subdisciplines of neuroscience as a means to help guide students toward reaching their scholastic goals.
CURRENT OPTIONS FOR ELECTIVE STUDY
As a member of GPN, students will acquire their more advanced training from coursework offered in departments around the University in order to fulfill the credit requirements for the PhD degree. The following is a list of potential electives organized by topic area as a guide to help students choose their curriculum and to give them flexibility in the design of coursework that spans more than one area of interest. Suggestions on foundation coursework specific to a research area are updated by faculty curriculum committees and can be obtained by emailing [email protected] .
Relevant to Molecular, Cellular & Systems (see also Computational)
- CAS BI 520 Sensory Neurobiology (4 cr)
- CAS BI 545 Neurobiology of Motivated Behavior (4 cr)
- CAS BI 599 Neurobiology of Synapses (4 cr)
- CAS PS 530 Neural Models of Memory Function (4 cr)
- GMS AN 702 *Neurobiology of Learning and Memory (2 cr)
- GMS AN 709 *Neural Development and Plasticity (2 cr)
- GMS AN 804 *Methods in Neuroscience (4 cr)
- GMS AN 807 *Neurobiology of the Visual System (2 cr)
- GMS BN 798 *Functional Neuroanatomy in Neuropsychology (4 cr)
- GRS BI 644 Neuroethology (4 cr)
- GRS BI 645 Cellular and Molecular Neurophysiology (4 cr)
- GRS BI 655 Developmental Neurobiology (4 cr)
- GRS BI 681 Molecular Biology of the Neuron (4 cr)
- SAR HS 550 Neural Systems (4 cr)
- SAR HS 755 Readings in Neuroscience (4 cr)
Relevant to Biomedical & Translational
- CAS BI 554 Neuroendocrinology (4 cr)
- GMS AN 707 *Neurobiology of Aging (2 cr)
- GMS AN 713 *Autism: Clinical and Neuroscience Perspectives (2 cr)
- GMS AN 808 *Neuroanatomical Basis of Neurological Disorders (2 cr)
- GMS BN 782 *Forensic Neuropsychology (4 cr)
- GMS BN 793 *Adult Communication Disorders (4 cr)
- GMS BN 796 *Neuropsychological Assessment I (4 cr)
- GMS BN 797 *Neuropsychological Assessment II (4 cr)
- GMS BN 821 *Neuroimaging Seminar (2 cr)
- GMS BN 891 & 892 *Case Studies in Neuropsychology (three different clinical rounds, sections A1, B1, and C1) (2 credits each section)
- GMS BN 893 *Child Clinical Neuropsychology (4 cr)
- GMS IM 690 *Imaging of Neurologic Disease (2 cr)
- GMS PM 801 *Systems Pharmacology and Experimental Therapeutics I (2 cr)
- GMS PM 802 *Systems Pharmacology and Experimental Therapeutics II (2 cr)
- GMS PM 820 *Behavioral Pharmacology (2 cr)
- GMS PM 840 *Neuroendocrine Pharmacology (2 cr)
- GMS PM 843 *Pharmacologic Intervention in Inflammatory Responses (2 cr)
- GMS PM 850 *Biochemical Neuropharmacology (2 cr)
- GMS PM 881 *Drug Discovery and Development (2 cr)
Behavioral & Cognitive Neuroscience
- CAS PS 520 Research Methods in Perception and Cognition (4 cr)
- CAS PS 525 Cognitive Science (4 cr)
- CAS PS 528 Human Brain Mapping (4 cr)
- CAS PS 544 Developmental Neuropsychology (4 cr)
- CAS PS 737 Memory Systems of the Brain (4 cr)
- CAS PS 821 Learning (4 cr)
- ENG BE 715 Functional Neuroimaging (4 cr)
- GMS AN 716 *Developmental Cognitive Neuroscience (4 cr)
- GMS BN 795 *Neuropsychology of Perception and Memory (4 cr)
- GRS PS 721 General Experimental (4 cr)
- GRS PS 734 Psychopharmacology for the Behavioral Scientist (4 cr)
- GRS PS 738 Techniques in Systems & Behavioral Neuroscience (4 cr)
- GRS PS 822 Visual Perception (4 cr)
- GRS PS 824 Cognitive Psychology (4 cr)
- GRS PS 828 Seminar in Psycholinguistics (4 cr)
- GRS PS 829 Principles in Neuropsychology (4 cr)
- GRS PS 831 Seminar in Neuropsychology (4 cr)
- GRS PS 835 Attention (4 cr)
Theoretical & Computational Neuroscience
- CAS CN 500 Computational Methods in Cognitive and Neural Systems (4 cr)
- CAS CN 510 Principles and Methods of Cognitive and Neural Modeling I (4 cr)
- CAS CN 520 Principles and Methods of Cognitive and Neural Modeling II (4 cr)
- CAS CN 530 Neural and Computational Models of Vision (4 cr)
- CAS CN 540 Neural and Computational Models of Adaptive Movement and Planning Control (4 cr)
- CAS CN 550 Neural and Computational Models of Recognition, Memory, and Attention (4 cr)
- CAS CN 560 (colisted as BE 509) Neural and Computational Models of Speech and Hearing (4 cr)
- CAS CN 570 Neural and Computational Models of Conditioning, Reinforcement, Motivation, and Rhythm (4 cr)
- CAS CN 580 Introduction to Computational Neuroscience (4 cr)
- ENG BE 509 (colisted as CN 560) Quantitative Physiology of the Auditory System (4 cr)
- ENG BE 570 Introduction to Computational Vision (4 cr)
- ENG BE 701 Auditory Signal Processing: Peripheral (4 cr)
- ENG BE 702 Auditory Signal Processing: Central (4 cr)
- ENG BE 707 Quantitative Studies of Excitable Membranes (4 cr)
- ENG BE 710 Neural Plasticity and Perceptual Learning (4 cr)
- GRS CN 700 Computational and Mathematical Methods in Neural Modeling (4 cr)
- GRS CN 710 Advanced Topics in Neural Modeling: Comparative Analysis of Learning Systems (4 cr)
- GRS CN 720 Neural and Computational Models of Planning and Temporal Structure in Behavior (4 cr)
- GRS CN 730 Models of Visual Perception (4 cr)
- GRS CN 740 Topics in Sensory Motor Control (4 cr)
- GRS CN 760 Topics in Speech Perception and Recognition (4 cr)
- GRS CN 780 Topics in Computational Neuroscience (4 cr)
- GRS CS 640 Artificial Intelligence (4 cr)
Coursework in Related Disciplines
- CAS BI 551 Biology of Stem Cells (4 cr)
- CAS BI 552/553 Molecular Biology (4 cr, 4 cr)
- CAS BI 555 Techniques in Cell Biology (4 cr)
- CAS BI 556 Membrane Biochemistry and Cell Signaling (4 cr)
- CAS MA 565 Math Models in the Life Sciences (4 cr)
- CAS MA 573 Qualitative Theory of Ordinary Differential Equations (4 cr)
- CAS MA 581 Probability (4 cr)
- CAS MA 582 Mathematical Statistics (4 cr)
- CAS MA 583 Introduction to Stochastic Processes (4 cr)
- CAS MA 584 Multivariate Statistical Analysis (4 cr)
- CAS MA 585 Time Series and Forecasting (4 cr)
- CAS MA 684 Applied Multiple Regression and Multivariable Method (4 cr)
- ENG BE 515 Introduction to Medical Imaging (4 cr)
- ENG BE 540 Bioelectrical Signals: Analysis and Interpretation (4 cr)
- ENG BE 550 Bioelectromechanics (4 cr)
- ENG BE 560 Biomolecular Architecture (4 cr)
- ENG BE 561 DNA and Protein Sequence Analysis (4 cr)
- ENG BE 700 Advanced Topics in Biomedical Engineering (4 cr)
- ENG BE 740 Parameter Estimation and Systems Identification (4 cr)
- ENG BE 747 Advanced Signals and Systems Analysis for Biomedical Engineering (4 cr)
- GMS BI 782 *Molecular Biology (4 cr)
- GMS BI 789 *Physical Biochemistry (2 cr)
- GMS BI 776 *Gene Targeting in Transgenic Mice (2 cr)
- GMS BI 786 *Biochemical Mechanisms of Aging (2 cr)
- GMS BI 797 *Molecular Mechanisms of Growth and Development (2 cr)
- GMS BL 755/756 *Biochemistry (4 cr, 4 cr)
- GMS MI 713 *Comprehensive Immunology (4 cr)
- GMS MM 701 *Genetics and Epidemiology of Human Disease (2 cr)
- GMS MM 703 *Cancer Biology and Genetics (2 cr)
- GMS MM 710 *Molecules to Molecular Therapeutics: The Translation of Molecular Observations to Clinical Implementation (4 cr)
- GMS PM 832 *Pharmacogenomics (2 cr)
- GMS PM 880 *Gene Regulation and Pharmacology (2 cr)
- GRS BI 621/622 Biochemistry (4 cr, 4 cr)
- GRS BI 735 Advanced Cell Biology (4 cr)
- GRS MB 721 Graduate Biochemistry (4 cr)
- GRS MB 722 Advanced Biochemistry (4 cr)
- MET AD 893 Technology Commercialization: From Lab to Market (4 cr)
Qualifying Examination
Students must take their Qualifying Examination at the end of their second year of study to remain in good standing in the program. The Qualifying Examination tests the ability of the student to think experimentally by generating testable hypotheses based on a foundation of knowledge that can be communicated in a written document and defended orally in front of a committee of GPN members. It is recommended that at least one faculty member of the Qualifying Examination Committee be from the GPN faculty of a different campus than where the student is conducting their dissertation research. For instance, a student working in a laboratory at the Charles River Campus would also have a GPN faculty member on the examining committee from the Medical Campus. A minimum of three faculty members will be on the examining committee, with membership approved by the GEC (see above).
The exam will be structured around a written proposal that is in the form of an individual NIH training fellowship application within the student’s proposed area of thesis research as well as a written abstract and Specific Aims in an additional area to test the student’s ability to address topics outside of their comfort zone. Students will be given a small workshop run by faculty so that they first learn the basic structure of an NRSA-style fellowship application before beginning their writing exercise for the exam. During the oral exam, the examining committee evaluates the student’s core knowledge base in these two areas as well as basic neuroscience. Students must be able to propose and defend experimental and/or computational hypotheses with realistic approaches to answer key questions relevant to their Specific Aims. A successful completion of both the written and oral parts of the exam is necessary before advancing to doctoral candidacy and registering for the third year in the program.
Dissertation
Dissertation advisory committee (dac).
At the end of the second year, after successful completion of the Qualifying Exam, each student and the research mentor will put together a dissertation advisory committee (DAC) of five members that contains at least two faculty members from GPN and at least one member from outside of BU to serve as an outside reader. Composition of the DAC must be approved by the Program Director/Associate Director after review with the GEC. The thesis research mentor serves as the first reader. All students make a presentation to their DAC once a semester to get feedback on their progress, although not all members of the committee need to be present (a quorum of three is acceptable). Distant DAC members may participate by teleconference with the approval of the DAC chair. Evaluation of student progress by the DAC is recorded on a tracking sheet by the DAC chair (a GPN faculty member who is not the mentor) and is placed in the student’s file for future review.
Dissertation Prospectus and Progress Report Seminar
Students generate a written document of no more than 20 pages double-spaced (Dissertation Prospectus) that describes the Aims of their thesis research, its background and significance to the field, experimental design and methods, and a bibliography. They then make a formal oral presentation (Progress Report Seminar) to the neuroscience community that is followed by a meeting of the DAC, and approval to pursue the research direction is sent to the GEC for further administrative processing. This formal progress report occurs at least one year prior to the defense.
Predefense and Defense
A predefense meeting of the DAC occurs at least two to three weeks prior to the defense to make sure that the quality of the dissertation document is close to being acceptable for the degree and to review necessary paperwork. At this time, the committee reviews the abstract and title. The defense cannot be scheduled before the abstract is approved and copies sent to the GMS at least three weeks before the defense. At the time of their defense, students give a 50-minute oral presentation, followed by 10 minutes of questions, that is open to all members of the University. This public forum is followed by a closed session of the DAC, at which the student is asked to respond to questions put forth by the committee to test their ability to defend the work presented in the dissertation document.
Related Bulletin Pages
- Graduate School of Arts & Sciences Departments
- Graduate School of Arts & Sciences Courses
- Abbreviations and Symbols
Beyond the Bulletin
- Graduate School of Arts & Sciences
- Graduate Admissions
- Graduate Financial Aid
- BA/MA Program
- Master’s Degree Requirements
- PhD Degree Requirements
- African American Studies
- American & New England Studies
- Anthropology
- Archaeology
- Bioinformatics
- Biostatistics
- Classical Studies
- Cognitive & Neural Systems
- Computer Science
- Creative Writing
- Earth & Environment
- Editorial Studies
- History of Art & Architecture
- Latin American Studies
- Linguistics
- Literary Translation
- Mathematics & Statistics
- Molecular Biology, Cell Biology & Biochemistry
- MA in Neuroscience
- PhD in Neuroscience
- Pardee School of Global Studies
- Playwriting
- Political Science
- Preservation Studies
- Religious Studies
- Romance Studies
- Sociology & Social Work
- Statistical Practice
- African Studies Certificate
- Asian Studies Certificate
- Advanced Biogeoscience Certificate
- Holocaust, Genocide & Human Rights Studies Certificate
- Latin American Studies Certificate
- Linguistics Certificate
- Museum Studies Certificate
- Muslim Studies Certificate
- Teaching Language, Literature & Film Certificate
- Teaching Writing Certificate
- Women’s, Gender & Sexuality Studies Certificate
- Departments
- Research Centers & Institutes
Terms of Use
Note that this information may change at any time. Read the full terms of use .
related websites
Accreditation.
Boston University is accredited by the New England Commission of Higher Education (NECHE).
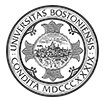
- © Copyright
- Mobile Version
- Graduate School
- Natural sciences
- Computer and information sciences
- Bioinformatics
Computational neurosciences
Parent category, graduate degree programs in computational neurosciences, doctor of philosophy in neuroscience (phd).
The Graduate Program in Neuroscience strives to educate and support graduate students as they expand the breadth and depth of their knowledge about the brain through enriching research experiences. The program embraces principles of equity, diversity and inclusion and recognizes and accommodates...
Master of Science in Neuroscience (MSc)
Academic units in computational neurosciences, neuroscience program, french name, french description, sign up for an information session to connect with students, advisors and faculty from across ubc and gain application advice and insight..
- Why Grad School at UBC?
- Graduate Degree Programs
- Application & Admission
- Info Sessions
- Research Supervisors
- Research Projects
- Indigenous Students
- International Students
- Tuition, Fees & Cost of Living
- Newly Admitted
- Student Status & Classification
- Student Responsibilities
- Supervision & Advising
- Managing your Program
- Health, Wellbeing and Safety
- Professional Development
- Dissertation & Thesis Preparation
- Final Doctoral Exam
- Final Dissertation & Thesis Submission
- Life in Vancouver
- Vancouver Campus
- Graduate Student Spaces
- Graduate Life Centre
- Life as a Grad Student
- Graduate Student Ambassadors
- Meet our Students
- Award Opportunities
- Award Guidelines
- Minimum Funding Policy for PhD Students
- Killam Awards & Fellowships
- Policies & Procedures
- Information for Supervisors
- Dean's Message
- Leadership Team
- Strategic Plan & Priorities
- Vision & Mission
- Equity, Diversity & Inclusion
- Initiatives, Plans & Reports
- Graduate Education Analysis & Research
- Media Enquiries
- Newsletters
- Giving to Graduate Studies
Strategic Priorities
- Strategic Plan 2019-2024
- Improving Student Funding
- Promoting Excellence in Graduate Programs
- Enhancing Graduate Supervision
- Advancing Indigenous Inclusion
- Supporting Student Development and Success
- Reimagining Graduate Education
- Enriching the Student Experience
Initiatives
- Public Scholars Initiative
- 3 Minute Thesis (3MT)
- PhD Career Outcomes
- Great Supervisor Week
Help | Advanced Search
Computer Science > Computational Complexity
Title: the computational lens: from quantum physics to neuroscience.
Abstract: Two transformative waves of computing have redefined the way we approach science. The first wave came with the birth of the digital computer, which enabled scientists to numerically simulate their models and analyze massive datasets. This technological breakthrough led to the emergence of many sub-disciplines bearing the prefix "computational" in their names. Currently, we are in the midst of the second wave, marked by the remarkable advancements in artificial intelligence. From predicting protein structures to classifying galaxies, the scope of its applications is vast, and there can only be more awaiting us on the horizon. While these two waves influence scientific methodology at the instrumental level, in this dissertation, I will present the computational lens in science, aiming at the conceptual level. Specifically, the central thesis posits that computation serves as a convenient and mechanistic language for understanding and analyzing information processing systems, offering the advantages of composability and modularity. This dissertation begins with an illustration of the blueprint of the computational lens, supported by a review of relevant previous work. Subsequently, I will present my own works in quantum physics and neuroscience as concrete examples. In the concluding chapter, I will contemplate the potential of applying the computational lens across various scientific fields, in a way that can provide significant domain insights, and discuss potential future directions.
Submission history
Access paper:.
- Other Formats

References & Citations
- INSPIRE HEP
- Google Scholar
- Semantic Scholar
BibTeX formatted citation

Bibliographic and Citation Tools
Code, data and media associated with this article, recommenders and search tools.
- Institution
arXivLabs: experimental projects with community collaborators
arXivLabs is a framework that allows collaborators to develop and share new arXiv features directly on our website.
Both individuals and organizations that work with arXivLabs have embraced and accepted our values of openness, community, excellence, and user data privacy. arXiv is committed to these values and only works with partners that adhere to them.
Have an idea for a project that will add value for arXiv's community? Learn more about arXivLabs .
Degree Programme Table: Informatics: ANC: Machine Learning, Computational Neuroscience, Computational Biology (PhD) (Full-time) (PRPHDINFMT1F)
Year 1 academic year: 2024/25, starting in: september.
There are no compulsory courses in this year of this programme.
PhD thesis in cognitive neuroscience (M/F)

Job Information
Offer description.
The successful candidate will join the Center de Recherche de Cerveau et Cognition (CerCo) in a dynamic research institute in Toulouse, a thriving city in the south of France. CerCo is a research center created by the National Center for Scientific Research (CNRS) and Paul Sabatier University, made up of more than 30 researchers and around 40 doctoral and post-doctoral students. The candidate will join the NeuroAI team, with a strong focus on computational neuroscience and artificial intelligence. The team has access to different experimental facilities, such as EEG, TMS and fMRI, as well as computing resources (clusters and local GPUs).
The project is part of an ERC starting grant investigating the role of oscillatory traveling waves in cognitive functions. Our primary goal is to study neural oscillations within and between cortical regions, as well as cortical-thalamic interactions. Our approach's novelty consists of framing the model in the light of Predictive Coding principles, using a computational approach based on spiking and deep neural networks. We'll compare the results of the simulations against experimental recordings of human participants (mostly EEG) to validate and assess the model's predictions.
Requirements
Additional information.
Please provide a transcript of the marks for the two years of the master's degree with details of the attended classes, a copy of the research dissertation carried out as part of the master's degree, a detailed CV, and a cover letter.
Work Location(s)
Where to apply.
MEMP PhD Thesis Defense (1:30pm): Jennifer Dawkins
Back to Events List
MIT E25-119/121 45 Carleton Street, Cambridge, MA 02142 and Zoom (See below for full information)
Computational prediction of health status from the human gut microbiome and metabolome
A healthy gut microbiome is crucial to overall human well-being. Gut microbiome dysfunction, or dysbiosis, has been implicated in a broad range of diseases, including inflammatory bowel diseases (IBDs), cardiovascular diseases, kidney diseases, metabolic diseases, and gastrointestinal infections like Clostridioides difficile infection (CDI). Often, microbiome-linked illnesses arise after the microbiome is disrupted, such as by antibiotic treatment. However, because the microbiome is so diverse and individual-specific, very little is known about the specific microbial changes that may lead to it human disease. Thus, it is extremely difficult to predict whether a given disruption to the microbiome will result in disease.
Of the diseases linked to gut microbial disfunction, dysbiosis is perhaps most prominently linked to CDI. As the most common health-care associate infection, CDI is thought to occur when an individual has had both exposure to the C. difficile pathogen and gut dysbiosis caused by a past perturbation, such as antibiotic treatment. Infection recurrence, with an estimated rate of 15.5%, is a particularly insidious problem, and there is currently no reliable method to predict which individuals will recur. There is a need for early prediction of CDI after a perturbation, as this can allow physicians to start or restart more effective treatments immediately and prevent further sickness and risk of death.
Current research into the microbiome and microbiome dysbiosis, including CDI, focuses heavily on identifying the microbial taxonomic composition using next generation sequencing. However, there is growing evidence that the gut metabolome may provide crucial information that cannot be gained from microbial composition alone, as metabolites provide the means by which host cells and microbe cells communicate with each-other. Predictive analysis is especially useful for uncovering links between metabolic or microbial composition features and host disease state as it models all input covariates simultaneously. However, current predictive methods often fall short when applied to the microbiome, as simpler methods lack the capabilities to model this complex system, whereas highly non-linear “black box” methods lack interpretability. When predicting from biological or medical data with the goals of clinical utility and advancement of scientific knowledge, a model that can explain its decisions is crucial for increasing physician trust and uncovering avenues for future investigation. There is a need for interpretable computational models that can learn non-linear relationships between host outcome and paired microbial composition and metabolomic profiles.
This thesis addresses these two challenges. First, we present the analysis of a novel longitudinal study of CDI recurrence in patients, including predictive analyses, which demonstrate that a small set of metabolites can accurately predict future recurrence. Our findings have clinical utility in the development of diagnostic tests and treatments that could ultimately short-circuit the cycle of CDI recurrence. Secondly, we present a novel predictive model developed specifically for making interpretable predictions on paired microbial composition and untargeted metabolic profiles. We demonstrate our model’s ability to predict a variety of host disease states accurately while providing clear and biologically compelling explanations of its decisions, thereby demonstrating high clinical and scientific utility.
Thesis Supervisor: Georg K. Gerber, MD, PhD Associate Professor of Pathology, HMS; Member of the Faculty, Harvard-MIT Program in Health Sciences and Technology
Thesis Committee Chair: Emery Brown, MD, PhD Warren M. Zapol Professor of Anesthesia, HMS, MGH; Edward Hood Taplin Professor of Medical Engineering and of Computational Neuroscience, MIT
Thesis Readers: Eric Alm, PhD Professor of Biological Engineering, MIT
Emily Balskus, PhD Thomas Dudley Cabot Professor of Chemistry and Chemical Biology, HU; Howard Hughes Medical Institute Investigator ------------------------------------------------------------------------------------------------------
Zoom invitation – Jennifer Dawkins is inviting you to a scheduled Zoom meeting.
Topic: Jennifer Dawkins MEMP PhD Thesis Defense Time: Tuesday, April 30, 2024, 1:30 PM Eastern Time (US and Canada)
Your participation is important to us: please notify hst [at] mit.edu (hst[at]mit[dot]edu) , at least 3 business days in advance, if you require accommodations in order to access this event.
Join Zoom Meeting https://mit.zoom.us/j/99749759912
Password: 216663
One tap mobile +16465588656,,99749759912# US (New York) +16699006833,,99749759912# US (San Jose)
Meeting ID: 997 4975 9912
US: +1 646 558 8656 or +1 669 900 6833
International Numbers: https://mit.zoom.us/u/aeD2jDIByj
Join by SIP 99749759912 [at] zoomcrc.com (99749759912[at]zoomcrc[dot]com)
Join by Skype for Business https://mit.zoom.us/skype/99749759912
Museum talks give UChicago graduate students’ research a new audience
April 25, 2024
By Benjamin Ransom/ UChicago News
When University of Chicago graduate student Mia Paletta recently delivered a gallery talk about her research at the Field Museum, she invited the audience to ponder: “What do birds dream about?”
A Ph.D. student studying computational neuroscience, Paletta is fascinated by the way that animal brains generate coordinated, complex movements like birdsong. Standing amid the Field’s eclectic collection of birds around the globe, Paletta explained that when songbirds are asleep, the neurons in their brains fire in the same patterns as those that are produced while the animal is awake and singing. She explained that songbirds may be mentally rehearsing and processing the complex motor actions required to sing their songs.
Paletta’s presentation was part of the UChicagoGRAD’s new Research Speaks event series, which provides unique opportunities for graduate students to share their research with the University community and wider public.
“As an early-career scientist that doesn’t get a lot of opportunities to talk about my research in front of a public audience, it was encouraging and fun to see that people were excited about what I was doing,” Paletta said. “I especially love talking to kids about science, and I want everyone to feel encouraged that they have a place in it if they want it.”
For the program’s inaugural event, 12 doctoral students in the humanities, social sciences, biological sciences, and engineering were invited to guide visitors through exhibits and specimens at the Field Museum—from ancient Egyptian scrolls to skeletal models of early human ancestors.
“Research Speaks is not only an exciting opportunity for graduate students’ professional development,” said Brooke Carrell, assistant provost and executive director of UChicagoGRAD Experience, “it’s a forum for them to engage with dynamic cultural institutions and practice sharing their ideas—maximizing the impact of their research with the broader public.”
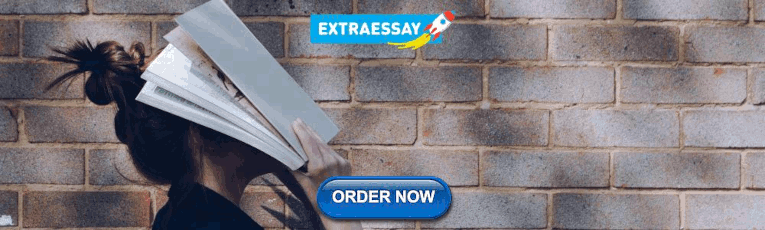
IMAGES
VIDEO
COMMENTS
The list of PhD theses defended so far in Alain Destexhe's lab is shown below together with links to PDF copies of the theses of summaries. The theses were defended successively in Laval University (Quebec, Canada) and at the UNIC (CNRS, Gif sur Yvette) and at ICN (CNRS, NeuroPSI, Paris-Saclay). Only PhD theses are listed here, by inverse ...
Computational neuroscience is an area of brain science that uses technology to develop and analyze large data sets that are used to understand the complexities of neurobiological systems. In recent years, these methods have become more and more vital to the field of neuroscience as a whole. ... Successful defense of a PhD Thesis;
The University of Chicago has a long tradition of innovative research in the neurosciences. K. C. Cole developed the voltage clamp here, Stephen Polyak and C. J. Herrick did pioneering work on the anatomy of the retina and brain, and Jack Cowan and Hugh Wilson were among the first to develop mathematical analyses of the dynamics of cortical ...
Thesis Research. All CNS students are expected to complete a Ph.D. dissertation connected with an issue in contemporary computational neuroscience. Either the student's primary advisor or close co-advisor (approved by the Computational Neuroscience Committee) must be a member of the Neuroscience Graduate Program faculty. Courses and Exams
MD/PhD Approximately 10-15% of Neuroscience graduate students are working towards an M.D. degree or already have one. MD/PhD students are admitted through the MD/PhD Program. Financial Aid All students receive a full tuition scholarship, complete health insurance coverage, and a stipend of $24,500 (for the academic year 2009-2010).
Students pursuing the PhD in Computational Neuroscience (or who have taken an undergraduate course in the area) can substitute a more advanced elective for this requirement. ... (GRS NE 901/902) during thesis research with the mentor, and attendance is required at neuroscience ethics and responsible conduct of research (RCR) workshops, at the ...
Most of the remaining time is devoted to thesis research, although students also take electives and can take training in teaching or other professional skills. The average time to Ph.D. is 5.7 years. Since its inception in 2001, the Program in Computational Neuroscience has awarded 47 Ph.D. degrees.
The Program in Neuroscience (PiN) is a full-time lab-based PhD program comprising a core curriculum that encompasses the interrelated disciplines of neuroscience, elective requirements in computational neuroscience and neuroanatomy, and training across multiple research areas and techniques through first-year lab rotations and dissertation research supported by a robust advising structure.
Students are required to take at least three computational neuroscience courses, including mathematical, statistical and computational approaches. To complete the computational requirement, students must take: 36-759 Statistical Models of the Brain (CMU) Two additional computational electives selected by the student.
Survey of Sytems Neuroscience: This lab-centered course teaches students the fundamental principles of vertebrate nervous system organization. Students learn the major structures and the basic circuitry of the brain, spinal cord, and peripheral nervous system. Somatic, visual, auditory, vestibular, and olfactory sensory systems are presented in ...
The work in this thesis was carried out at the Gatsby Computational Neuroscience Unit which is funded by the Gatsby Charitable Foundation. I am grateful to the Institute of Physics, the NIPS foundation, the UCL graduate school and Microsoft Research for generous travel grants. 3
The first year of the graduate program begins with the Neuro Boot Camp in August. All newly admitted Neuroscience graduate students are required to attend a 2-week course intended to ensure that new recruits have a basic understanding of molecular biology, as well as the core skills required to use mathematical and computational approaches to analyze neural systems and neural data.
This dissertation begins with an illustration of the blueprint of the computational lens, supported by a review of relevant previous work. Subsequently, I will present my own works in quantum physics and neuroscience as concrete examples. In the concluding chapter, I will contemplate the potential of applying the computational lens across ...
My laboratory focuses on translational research in primary and metastatic tumors of the central nervous system (CNS). Using genomic and transcriptomics... Read more. Lab Website. PubMed Link. 185 Cambridge St, CPZN3700/3800. Boston, MA 02114. [email protected]. p: 617-643-3431.
Nature Neuroscience (2023) To learn how cognition is implemented in the brain, we must build computational models that can perform cognitive tasks, and test such models with brain and behavioral ...
Neuroscience Graduate Training Program. Co-Directors: Chris Potter, Dan O'Connor. Deputy Director: Hita Adwanikar. Senior Academic Program Coordinator: Audrey Scriven. Admissions Director: Hyungbae Kwon. Participation in extensive collaborations, access to cutting-edge resources, and exposure to world-class research, await students in our program.
Year 1. Our PhD programme starts with a year of intensive courses in research and techniques in neuroscience and machine learning. New students are admitted at the start of each academic year in September. All students begin by attending Boot Camp, a three-week course covering the fundamentals of neuroscience and technology.
The Gatsby Unit PhD programme was the first to combine theoretical neuroscience and machine learning within the same programme. Our mathematical approach for developing novel algorithms and tools to understand learning, perception and action in brain and machines is unique. Applications to this programme must be submitted directly to the Gatsby Unit via its online portal.
University of Bristol School of Physiology, Pharmacology & Neuroscience. This project will be part of the Bristol-Macquarie Cotutelle Programme, funded by EPSRC. Read more. Supervisor: Prof J Hodge. 1 August 2024 PhD Research Project Competition Funded PhD Project (Students Worldwide) More Details.
For the post-master's PhD, 32 credits of coursework are required. The required courses are the same as for the post-bachelor's PhD. However, depending on the student's background, one or more of the required courses may be waived via petition to the BU Graduate Program for Neuroscience Graduate Education Committee (GEC).
Graduate Degree Programs in Computational neurosciences Doctor of Philosophy in Neuroscience (PhD) The Graduate Program in Neuroscience strives to educate and support graduate students as they expand the breadth and depth of their knowledge about the brain through enriching research experiences.
Graduate Programs: Computational Neuroscience, Neurobiology, UChicago Biosciences, PhD Program in Neurobiology Dense, Continuous Membrane Labeling and Expansion Microscopy Visualization of Ultrastructure in Tissues.
The Computational Lens: from Quantum Physics to Neuroscience. Chi-Ning Chou. Two transformative waves of computing have redefined the way we approach science. The first wave came with the birth of the digital computer, which enabled scientists to numerically simulate their models and analyze massive datasets. This technological breakthrough led ...
Degree Programme Table: Informatics: ANC: Machine Learning, Computational Neuroscience, Computational Biology (PhD) (Full-time) (PRPHDINFMT1F) Jump to: Year 1 Year 1 Academic year: 2024/25, Starting in: September Notes: PROGRAMME AIMS ... To be awarded a PhD you will submit a thesis and defend this thesis in an oral examination (assessed ...
CerCo is a research center created by the National Center for Scientific Research (CNRS) and Paul Sabatier University, made up of more than 30 researchers and around 40 doctoral and post-doctoral students. The candidate will join the NeuroAI team, with a strong focus on computational neuroscience and artificial intelligence.
Computational prediction of health status from the human gut microbiome and metabolomeA healthy gut microbiome is crucial to overall human well-being. Gut microbiome dysfunction, or dysbiosis, has been implicated in a broad range of diseases, including inflammatory bowel diseases (IBDs), cardiovascular diseases, kidney diseases, metabolic diseases, and gastrointestinal infections like ...
By Benjamin Ransom/ UChicago News. When University of Chicago graduate student Mia Paletta recently delivered a gallery talk about her research at the Field Museum, she invited the audience to ponder: "What do birds dream about?". A Ph.D. student studying computational neuroscience, Paletta is fascinated by the way that animal brains generate coordinated, complex movements like birdsong.