
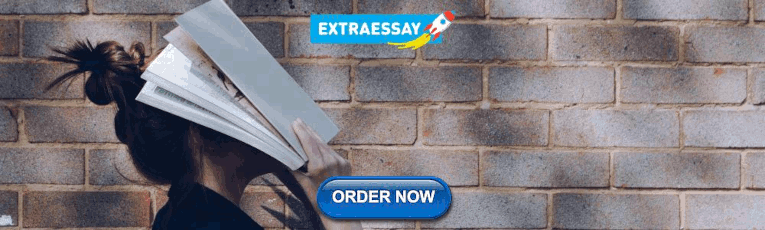
Academic Integrity at MIT
A handbook for students, search form, what is common knowledge.
You may have heard people say that you do not have to cite your source when the information you include is “common knowledge.” But what is common knowledge?
Broadly speaking, common knowledge refers to information that the average, educated reader would accept as reliable without having to look it up. This includes:
- Information that most people know , such as that water freezes at 32 degrees Fahrenheit or that Barack Obama was the first American of mixed race to be elected president.
- Information shared by a cultural or national group , such as the names of famous heroes or events in the nation’s history that are remembered and celebrated.
- Knowledge shared by members of a certain field , such as the fact that the necessary condition for diffraction of radiation of wavelength from a crystalline solid is given by Bragg’s law.
- However, what may be common knowledge in one culture, nation, academic discipline or peer group may not be common knowledge in another.
How do I determine if the information I am using is common knowledge?
To help you decide whether information can be considered common knowledge, ask yourself:
Who is my audience?
What can I assume they already know?
Will I be asked where I obtained my information?
Some examples:
A description of the symptoms of Asperger’s Syndrome would need to be cited for a composition in a general writing class but probably not need citation for an audience of graduate students in psychology.
A reference to the practice of fair value accounting would be understood by a group of economists, but would need citation to an audience of non-experts.
A statement reporting that 24% of children under the age of 18 live in households headed by single mothers would need to be cited. This is information that would not be known to the average reader, who would want to know where the figure was obtained.
The best advice is: When in doubt, cite your source.
Which of the following statements would be considered common knowledge which would need to be cited.
The Big Bang theory posits that the universe began billions of years ago with an enormous explosion.
The phrase “Big Bang” was coined by Sir Fred Hoyle, an English astronomer. Hoyle used the term to mock the theory, which he disagreed with.
According to the Big Bang model, the initial explosion was produced when an infinitely hot, dense center referred to as a singularity, began to expand, giving rise to the particles that eventually formed into our universe.
- Statement #1 is common knowledge – the Big Bang theory is widely accepted among scientists and the term is used regularly in everyday speech.
- Statement #2 needs citation; this information is very specific and may even be unknown to some physicists.
- Statement #3 would not need citation to an audience of physics students but would need citation in a paper for a non-expert audience.
What is not Common Knowledge?
Datasets generated by you or others.
Statistics obtained from sources such as the US Census Bureau and the Bureau of Labor Statistics.
References to studies done by others.
Reference to specific dates, numbers, or facts the reader would not know unless s/he had done the research.
Examples of statements that need citation - each refers to work done by others, statistics, or specific information that would not be known by the average reader:
Researchers have found that dispersants utilized to clean up oil spills can lead to lung damage when airborne particles of these dispersants combine with crude oil and are inhaled.
(Source: Wang, H., Shi, YL, Major, D. and Yang, HL (2012, August). Lung epithelial cell death induced by oil-dispersant mixtures. Toxicology in Vitro, 26, 5, 746-751. doi: 10.1016/j.tiv.2012.03.011)
A recent study done by scholars at the Brookings Institute found that the number of people living in poverty in America grew by 12.3 million between 2000 and 2010, so that by the end of 2010, 15% of the population was living under the poverty line.
(Source: Kneebone, E., C. Nadeau and Berube, A. (2011, November 3). The re-emergence of concentrated poverty: metropolitan trends in the 2000s. Brookings Metropolitan Opportunity Series. Retrieved from http://www.brookings.edu/research/papers/2011/11/03-poverty-kneebone-nadeau-berube .)
The energy of mixing per site for a binary polymer blend with differing degrees of polymerization can be described through the Flory-Huggins equation.
(Source: Flory, P.J. (1953). Principles of Polymer Chemistry. Ithaca, NY: Cornell University Press.)
Note: This equation is specific to the thermodynamics of macromolecular structures and would not be considered common knowledge by many scientists or engineers. For these reasons, they need to be cited.
Purdue Online Writing Lab Purdue OWL® College of Liberal Arts
Common Knowledge & Attribution
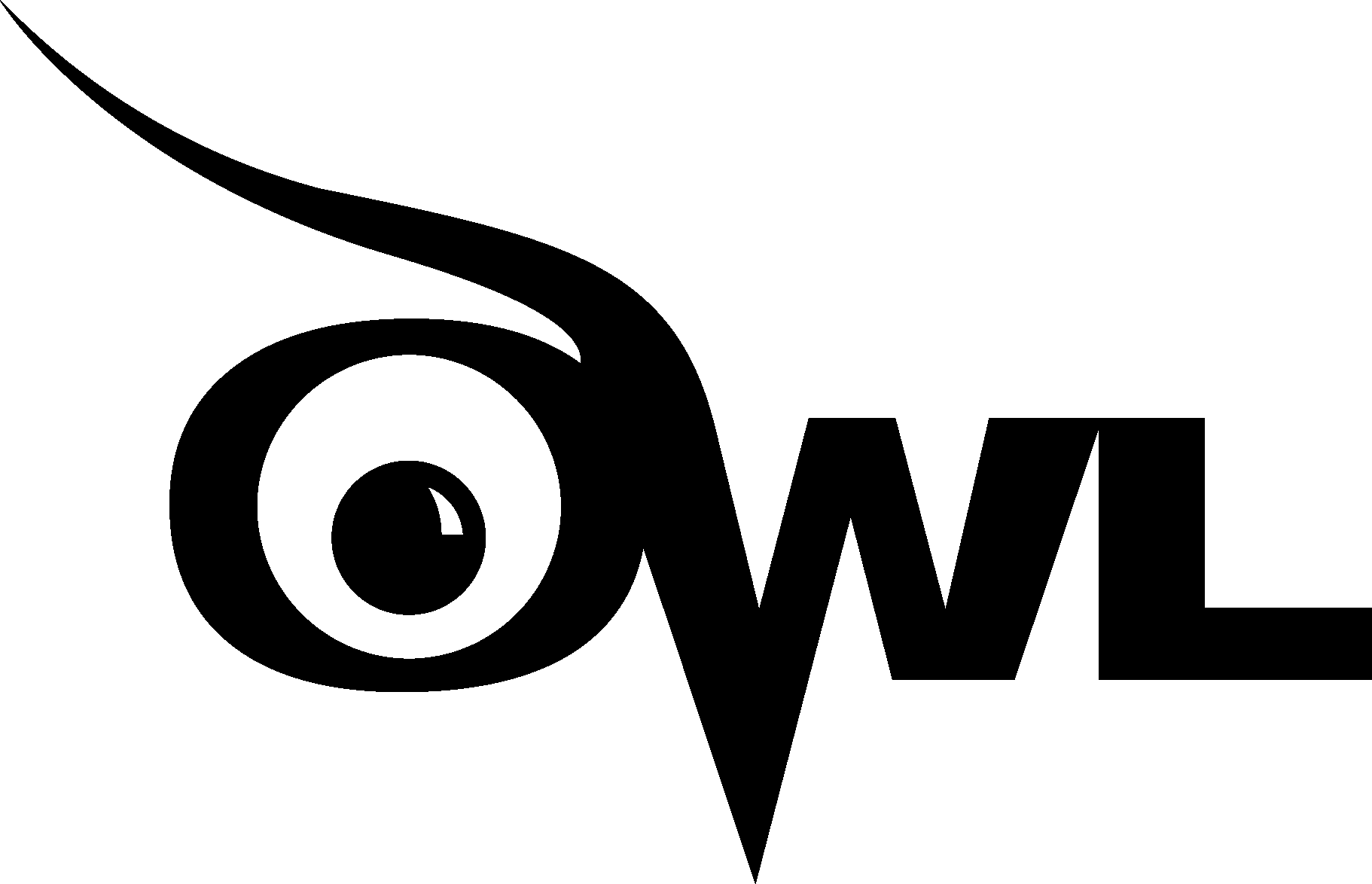
Welcome to the Purdue OWL
This page is brought to you by the OWL at Purdue University. When printing this page, you must include the entire legal notice.
Copyright ©1995-2018 by The Writing Lab & The OWL at Purdue and Purdue University. All rights reserved. This material may not be published, reproduced, broadcast, rewritten, or redistributed without permission. Use of this site constitutes acceptance of our terms and conditions of fair use.
What is ‘common knowledge’?
Generally, common knowledge is information that someone finds undocumented in at least five credible sources. For example, writing is difficult,” is considered common knowledge in the field of composition studies because at least five credible sources can back the claim up.
Remember the golden rule: When in doubt, just cite.
If the citation proves unnecessary or unnecessarily repetitive your instructor or supervisor will inform you.
How do es cit ation work ? What is attribution?
Many professional organizations, including the Modern Language Association (MLA) and the American Psychological Association (APA), have lengthy guidelines for citing sources. We have resources to help you use these guidelines (linked on the sidebar to the left, just underneath this page). But many students are so worried about how to cite a particular source that they never learn how the citation system works. This is often why students end up accidentally plagiarizing.
Different citation styles have different rules. You should look at the rules for your own (not just the examples that show you how to cite a book chapter). But generally, here is how citation works:
There are three elements: signal phrase (also called “signposting”), in-text citation (also called “parenthetical citation”) , bibliographic citation.
- A signal phrase is something like “According to Chen Li...”
- An in-text citation will look like (Li, 2020) or (Li 7)
- A bibliographic citation will look like: Li, C. (2020). Best practices to avoid plagiarism. Journal of Basic Writing, 42 (1), pp. 4-12.
The signal phrase and the in-text citation show up in the sentences of your paper. The bibliographic citation shows up in the references/works cited/bibliography at the end of your paper, or possibly footnotes at the bottom of each page. You need all three to effectively attribute ideas and words to their proper sources of origin.
The signal phrase and the in-text citation work together. The signal phrase shows up when you first start talking about a source. It says to the reader “hey! I am drawing on someone else’s idea.” You might use more than one signal phrase if you talk about a source for a few sentences or throughout a paragraph. Then, when you are done talking about that source, the in-text citation ends the sentence. It says to the reader “hey! This is the end of me talking about this source for now. Here is the information you will need to go to the bibliography and find the source I’m talking about.” The bibliographic citation then provides all the information the reader needs to go find the source you looked at. It also tells the reader a number of things before they even do that: the author, how current the source is, its title, and where it was published (like an academic journal, a news site, or a book with lots of chapters).
Here's an example of what all that looks like put together, using APA:
In this example, I opened with a signal phrase that included the author’s name and the year, to tell my reader who I am citing from. Then I summarized Li’s point in the rest of the first sentence. The second sentence includes paraphrase, where I restated her ideas in my own words, and a direct quote in quotation marks, where I used her words exactly. The in-text citation only includes the page number in this case, because I just gave the rest of the citational material a few words earlier. I could have also written (Li, 2020, pg. 7) and that would have been correct. Then I gave all the information about this (made-up) journal article in my references.
Look at some articles in a journal in your field — maybe class readings, or sources for your own research project. Pay attention to how the authors attribute sources. Look for signal phrases, in-text citations, and bibliographic citations, and try to see the patterns in how these authors use them. Each field is different — this is just one example.
See also our resource on quoting, paraphrasing, and summarizing. You can’t cite appropriately if you are not sure exactly how you are using source material.

- Walden University
- Faculty Portal
Using Evidence: Common Knowledge & Personal Experience
Common knowledge & personal experience.
Scholarly writing primarily relies on academic research as evidence. However, all writers bring previous knowledge to their writing, and Walden writers in particular might have years of experience in their field that they bring to their classroom writing. How to incorporate common knowledge and professional experience can cause confusion, since incorporating them inappropriately can create unintentional plagiarism .
- Writing About Common Knowledge & Personal Experience (video transcript)
Common Knowledge
Common knowledge is information or ideas that are widely known, accepted, and found in multiple places. Common knowledge is context dependent, meaning that something might be common knowledge to one audience but not another audience. If you are paraphrasing common knowledge , you do not need to cite that statement.
Let us look at a few statements and consider their context to see when they might be considered common knowledge:
Always consider your context and the audience you are writing for when determining whether a statement is common knowledge. Accidentally including a statement without a citation because you think it might be common knowledge can result in unintentional plagiarism . Ask your faculty if you are not sure, as your faculty can help guide you on what your audience is for an assignment and whether a statement is common knowledge for that audience.
Professional Experience
Many Walden students come with years of experience in their field, and you may find yourself writing about and researching topics you have engaged with in the past. The passion for and experience with the topics you are studying is one great advantage Walden students have.
Professional experience can cause a problem when students rely too heavily on their experience with a topic in their scholarly writing. Scholarly writing is meant to be informed by and supported by academic research, and so professional experience should not be the primary evidence you use for your ideas in your scholarly writing.
In fact, relying on professional experience too much or not clarifying when you are using professional experience in your scholarly writing can lead to questions about plagiarism. If you are writing a paper about handwashing practices for nurses, and throughout your paper you do not cite any sources, your faculty my interpret this lack of citations as passive plagiarism : Your faculty may think that you’re using evidence from sources but you just didn’t cite those sources. Although you know these ideas are based on your professional experience, your faculty may not, leading to confusion and possible misunderstandings.
We know that how and when to incorporate professional experience can be confusing if you are new to academic writing, and often students do not realize their approach could cause confusion. To avoid these issues and possible misunderstandings around plagiarism, we recommend three strategies:
- Use and Cite Evidence : Ensure you are adequately supporting your scholarly writing with academic evidence that is cited.
- Contextualize Professional Experience: If you do use professional experience to support your ideas, make it clear from context that you are doing so. Use phrasing like, “In my experience as a teacher…” or “I have found in my 10 years at my organization…” . These signal phrases help the reader know that the ideas that follow are based on your professional experience.
- Contact Your Faculty: Contact your faculty if you are not sure if professional experience is appropriate to use in your assignment or how to do so. Professional experience is more appropriate in some assignments more than others (e.g., a reflection paper versus a literature review). Your faculty can best guide you on how and when to include professional experience.
Related Webinar Resource

- Previous Page: Using Evidence
- Next Page: Summary
- Office of Student Disability Services
Walden Resources
Departments.
- Academic Residencies
- Academic Skills
- Career Planning and Development
- Customer Care Team
- Field Experience
- Military Services
- Student Success Advising
- Writing Skills
Centers and Offices
- Center for Social Change
- Office of Academic Support and Instructional Services
- Office of Degree Acceleration
- Office of Research and Doctoral Services
- Office of Student Affairs
Student Resources
- Doctoral Writing Assessment
- Form & Style Review
- Quick Answers
- ScholarWorks
- SKIL Courses and Workshops
- Walden Bookstore
- Walden Catalog & Student Handbook
- Student Safety/Title IX
- Legal & Consumer Information
- Website Terms and Conditions
- Cookie Policy
- Accessibility
- Accreditation
- State Authorization
- Net Price Calculator
- Contact Walden
Walden University is a member of Adtalem Global Education, Inc. www.adtalem.com Walden University is certified to operate by SCHEV © 2024 Walden University LLC. All rights reserved.
- Utility Menu

fa3d988da6f218669ec27d6b6019a0cd
A publication of the harvard college writing program.
Harvard Guide to Using Sources
- The Honor Code
The Exception: Common Knowledge
The only source material that you can use in an essay without attribution is material that is considered common knowledge and is therefore not attributable to one source. Common knowledge is information generally known to an educated reader, such as widely known facts and dates, and, more rarely, ideas or language. Facts, ideas, and language that are distinct and unique products of a particular individual's work do not count as common knowledge and must always be cited. Figuring out whether something is common knowledge can be tricky, and it's always better to cite a source if you're not sure whether the information or idea is common knowledge. If you err on the side of caution, the worst outcome would be that an instructor would tell you that you didn't need to cite; if you don't cite, you could end up with a larger problem.
If you have encountered the information in multiple sources but still think you should cite it, cite the source you used that you think is most reliable, or the one that has shaped your thinking the most.
Categories of Common Knowledge
Widely known facts
Widely known scientific and historical facts—such as the molecular structure of water (H2O), or that Andrew Jackson was the seventh president of the United States—generally count as common knowledge. You can include such facts in your writing without citation and without fear of committing plagiarism. Other facts that count as common knowledge—for instance, that Franz Boas, the distinguished American ethnologist, held the first academic appointment in anthropology in the United States—are widely known to some groups of people (professional anthropologists) but perhaps not to you. Nevertheless, you would not have to cite the fact about Boas, since it is common knowledge in the sense that no particular individual discovered this information (say, through archival research at Columbia University, where Boas taught). On the other hand, as soon as your discussion becomes more specific and puts forth assertions that would be the product of an individual's thought, research, or analysis, you do have to cite. For example, if you read Anthony Abraham Jack's The Privileged Poor and then wrote about the conclusions he drew from his study of economically disadvantaged students at elite colleges, you would need to cite his work.
Ideas or interpretations are usually not considered common knowledge, unless they are very widely held.
Ideas or interpretations generally do not count as common knowledge. If you read in R.A.C. Parker's history of World War II that British Prime Minister Neville Chamberlain did not have to agree to the 1938 Munich Pact with Hitler, and that he could have chosen an alternate path, you would need to acknowledge the source, since this judgment is Parker's theory rather than a widely agreed upon fact. (Historians disagree on the factors that led Chamberlain to agree to sign on to the Munich Pact.) Some interpretations or opinions (rather than facts) have entered the realm of common knowledge and need not be cited. If you were to introduce the claim that culture provides a means by which humans adapt to their environments, you would not need to cite a source for this claim, since it is almost universally held by anthropologists. But, if you were unsure that this was the consensus view among anthropologists, you would be best served simply to cite the source. On the whole, opinions or interpretations do not enter the realm of common knowledge as easily as historical or scientific facts.
Verbatim language drawn from a source is rarely common knowledge, unless the formulation is widely known.
You must always provide a citation for quotations you use in your writing. The only—and rare—exceptions to this rule concern well-known quotations that have entered the realm of common knowledge. For example, if you were writing a paper about President Obama's Inaugural Address, you would need to cite your source for any quotations you used from the speech. However, if in the course of that paper you compared one of President Obama's lines to this very well-known phrase from John F. Kennedy's Inaugural Address, "Ask not what your country can do for you—ask what you can do for your country," you would not need to provide a citation for that one phrase. However, if you were to analyze Kennedy's speech substantively and quote additional lines, then you would need to cite anything you quoted from his speech so that your readers could confirm the original language of the speech. If you are not sure whether a quotation is common knowledge, cite it.
Search form
- About Faculty Development and Support
- Programs and Funding Opportunities
- Consultations, Observations, and Services
- Strategic Resources & Digital Publications
- Canvas @ Yale Support
- Learning Environments @ Yale
- Teaching Workshops
- Teaching Consultations and Classroom Observations
- Teaching Programs
- Spring Teaching Forum
- Written and Oral Communication Workshops and Panels
- Writing Resources & Tutorials
- About the Graduate Writing Laboratory
- Writing and Public Speaking Consultations
- Writing Workshops and Panels
- Writing Peer-Review Groups
- Writing Retreats and All Writes
- Online Writing Resources for Graduate Students
- About Teaching Development for Graduate and Professional School Students
- Teaching Programs and Grants
- Teaching Forums
- Resources for Graduate Student Teachers
- About Undergraduate Writing and Tutoring
- Academic Strategies Program
- The Writing Center
- STEM Tutoring & Programs
- Humanities & Social Sciences
- Center for Language Study
- Online Course Catalog
- Antiracist Pedagogy
- NECQL 2019: NorthEast Consortium for Quantitative Literacy XXII Meeting
- STEMinar Series
- Teaching in Context: Troubling Times
- Helmsley Postdoctoral Teaching Scholars
- Pedagogical Partners
- Instructional Materials
- Evaluation & Research
- STEM Education Job Opportunities
- Yale Connect
- Online Education Legal Statements
You are here
Common knowledge.
If you are familiar with the notion of “common knowledge” from earlier writing experiences, you may have noticed that its definition is easy to state, but can be hard to apply in a particular case. The “common” way to talk about common knowledge is to say that it is knowledge that most educated people know or can find out easily in an encyclopedia or dictionary. Thus, you might not know the date of the most recent meeting of the Federal Reserve, but you can find it out quite easily. Further, the term “common knowledge” carries the sense of “communal” knowledge—it is community information that no particular individual can fairly claim to own. One sign that something is community knowledge is that it is stated in 5 or more sources. So, if it’s known to educated people, or can be easily looked up, or appears in many sources, it is likely to be “common knowledge” and so does not need to be cited.
But here is where things become tricky: As you write papers in college and move deeper into your field of study, what counts as common knowledge becomes much less clear. Within a given discipline, there is a body of common knowledge that an outsider (even an educated college student who doesn’t happen to be in your field) might not know. For example, within psychology, it is common knowledge that chimpanzees recognize themselves in a mirror; in literature, it is common knowledge that James Joyce is a major modernist author. In referring to the mirror test or calling James Joyce a modernist, you wouldn’t need to cite anyone. But as soon as you begin to say something, for instance, about what the results of the mirror test mean for a model of consciousness, you would need to cite a source. The point, then, is to think about your audience: What has been said in the class or repeated in textbooks and other sources often enough to suggest that it is common knowledge within the discipline?
Because the notion of “common knowledge” is ambiguous and depends on context, you should always check with a professor or TF if you have any doubts. Some reference books will say “if in doubt, cite it,” but you don’t want to over-cite, so check with your readers to try to fix the line between common and specialized knowledge.
Sometimes you become so conversant in a subject that you can explain complex theories, methodolologies, or historical timelines without reference to a source. You may notice this phenomenon as you research and write your senior essay. At this point, you’re becoming an expert in the field and things may start to seem obvious to you that are not obvious to an intelligent lay reader. You will want to check with your department about the level of expertise you’re expected to assume. You may also want to show your writing to a Residential College Writing Tutor, a Writing Partner, or a friend who’s a good reader. As a senior essay writer, you will probably need to cite less than you used to, but more than you may think.
This advice about “common knowledge” is true for all disciplines—think about your audience and the course attitude, recognize when you’re writing as an expert, and always check with professors if you’re in doubt. The sciences, however, have a somewhat different notion of “common knowledge,” coming partly out of research practice and partly out of more collaborative work methods. Ideas, findings, and methodologies that are new knowledge (and therefore specialized rather than common knowledge) become old knowledge more quickly in the sciences. The answer, again, is to consider the messages you’re getting from the course about what concepts are common or foundational, and to check in with professors or TFs.
YOU MAY BE INTERESTED IN
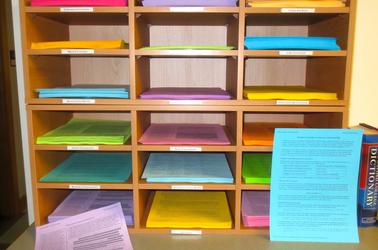
Writing Handouts
Principles, strategies, and models to deepen your understanding of what good writing looks like—and how to achieve it.
Drop-In Writing Partners
Writing Partners are Yale College or graduate school students selected for both their writing skills and their ability to talk about writing. They have a student’s-eye view of the challenges you’ll face in writing papers.

Reserve a Room
The Poorvu Center for Teaching and Learning partners with departments and groups on-campus throughout the year to share its space. Please review the reservation form and submit a request.
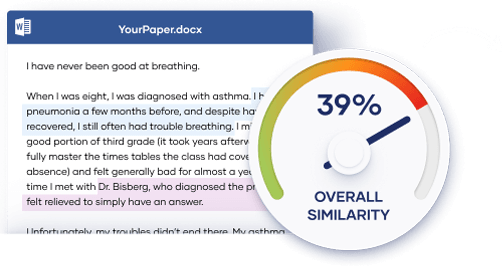
Prevent plagiarism, run a free plagiarism check.
- Knowledge Base
- What Is Common Knowledge? | Definition & Examples
What Is Common Knowledge? | Definition & Examples
Published on 18 July 2022 by Jack Caulfield and Shona McCombes.
In academic writing, ‘common knowledge’ refers to information that the average educated reader would accept without needing a source citation to back it up.
There are two main categories of common knowledge:
- Information that most people know
- Information shared by a specific group of people, such as a national or cultural group, or academics in a particular field of study
In academic writing, it’s normally essential to cite your sources , but statements that are considered common knowledge do not need a citation.
Table of contents
Examples of common knowledge, do i need a citation, common knowledge test, frequently asked questions about plagiarism.
Examples of common knowledge include:
- Information that appears across many sources without a clear origin, such as famous historical dates
- Long-established facts or theories that are foundational to a field, like fundamental equations in physics
It does not include:
- Data and statistics gathered in empirical studies. These should always be cited.
- Interpretations or arguments. If a statement could be reasonably disputed, provide a citation.
The examples below show how subtle the distinction can be:
- Statements about APA Style citation
- Statements about the Amazon rainforest
APA is a common citation style used by students. This is an undisputed and easily verified fact. It is common knowledge.
APA Style is mainly used in the social sciences, business, and nursing. For a reader familiar with research, this is likely to be accepted as common knowledge. For a more general readership, you might need to cite it.
APA Style is used in more than 90,000 papers per year. This is not an undisputed fact and should be attributed to one or more sources.
The Amazon is the world’s largest tropical rainforest. This is a widely known fact that you can assume any reader will accept. It’s common knowledge.
Due to deforestation, the Amazon has been rapidly decreasing in size since the 1960s. If you are writing an in-depth paper on the Amazon for an expert reader, this would likely be accepted as common knowledge. If you are writing a basic or introductory paper for a more general audience, you should provide a citation.
The cattle sector is responsible for 80% of deforestation in the Amazon. This is a statistic derived from specific research. It should be cited even if it is widely accepted to be true. You should always give the source of any figures or statistics.
Prevent plagiarism, run a free check.
Common knowledge does not need to be cited in your paper, because it is widely known, undisputed, easily verified, and generally not attributable to a specific author.
To avoid plagiarism , it can be tempting to include a citation for every sentence, but in some cases over-citation can weaken your academic writing. If you are unsure whether or not a statement counts as common knowledge, ask yourself the questions below.
Who is my reader, and what can I assume they know?
Are you writing for a general audience or for experts in the field?
In academic writing, it’s more likely that your reader will be an expert, and you can assume a certain level of shared knowledge. But if you include information from a different field, you should cite it.
Could my reader dispute this statement?
If your reader might be surprised by your statement, question its accuracy, or contest it with other data, it isn’t common knowledge, and you should provide a citation.
If it’s a foundational fact that everyone in the field agrees on, it’s probably common knowledge.
Can my reader easily verify this statement across multiple sources?
If you google it, can you easily find more than five scholarly sources that give the same information without citation? If so, it’s probably common knowledge.
If some of the search results contradict each other or you have to dig further to find the facts, you should provide a citation.
Common knowledge does not need to be cited. However, you should be extra careful when deciding what counts as common knowledge.
Common knowledge encompasses information that the average educated reader would accept as true without needing the extra validation of a source or citation.
Common knowledge should be widely known, undisputed, and easily verified. When in doubt, always cite your sources.
Accidental plagiarism is one of the most common examples of plagiarism . Perhaps you forgot to cite a source, or paraphrased something a bit too closely. Maybe you can’t remember where you got an idea from, and aren’t totally sure if it’s original or not.
These all count as plagiarism, even though you didn’t do it on purpose. When in doubt, make sure you’re citing your sources . Also consider running your work through a plagiarism checker tool prior to submission, which work by using advanced database software to scan for matches between your text and existing texts.
Scribbr’s Plagiarism Checker takes less than 10 minutes and can help you turn in your paper with confidence.
Plagiarism can be detected by your professor or readers if the tone, formatting, or style of your text is different in different parts of your paper, or if they’re familiar with the plagiarised source.
Many universities also use plagiarism detection software like Turnitin’s, which compares your text to a large database of other sources, flagging any similarities that come up.
It can be easier than you think to commit plagiarism by accident. Consider using a plagiarism checker prior to submitting your essay to ensure you haven’t missed any citations.
Cite this Scribbr article
If you want to cite this source, you can copy and paste the citation or click the ‘Cite this Scribbr article’ button to automatically add the citation to our free Reference Generator.
Caulfield, J. & McCombes, S. (2022, July 18). What Is Common Knowledge? | Definition & Examples. Scribbr. Retrieved 6 May 2024, from https://www.scribbr.co.uk/preventing-plagiarism/what-is-common-knowledge/
Is this article helpful?
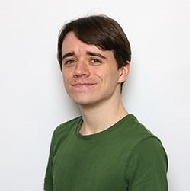
Jack Caulfield
Other students also liked, consequences of mild, moderate & severe plagiarism, how do plagiarism checkers work, the 5 types of plagiarism | explanations & examples.
Common Knowledge and Public Domain
The terms “common knowledge” and “public domain” often come up in discussions of plagiarism. In truth, neither term is really about plagiarism per se , but since they both relate to the proper use of outside sources, it’s important for writers to understand what they mean and how each term might apply to your work.
Common Knowledge
The term “common knowledge” refers to facts and information that are so well-known and clearly established that a writer can use them in their work without citing a specific source.
Like plagiarism, student writers tend to think of common knowledge as a universal distinction–either a given fact is common knowledge or it isn’t. Unfortunately, as with plagiarism, the rules for what qualifies as common knowledge actually vary from one situation to the next, and thus the responsibility falls on you as a writer to understand the general guidelines for common knowledge and apply them to any given assignment or piece that you write.
Generally speaking, something qualifies as common knowledge if it meets all of the criteria below:
1) The information is purely factual and easily known to your audience.
When you include facts or information in your writing that you can reasonably assume your readers already know, or that anyone in your audience could easily verify without doing any significant research, then it’s generally acceptable to present those facts as common knowledge.
For example, Carleton College, originally named Northfield College, was founded in 1866 by the General Conference of the Congregational Churches of Minnesota. This is a simple, objective set of facts that anyone familiar with Carleton might already know. Even if you didn’t know this, though, you can probably think of several places you could look to verify it ( the most obvious being the college’s website ). Since any reader could verify this fact in any number of ways, and any reasonably savvy reader could figure out on their own how to do this, you wouldn’t need to cite a specific source to use it in your writing.
By contrast, it is also an objective fact that in 1890, Carleton had three official political clubs for students: the Republican Club, the Democratic Club, and the Young Men’s Prohibition Club. However, even though this fact is probably available through multiple sources, none of them are particularly obvious or accessible to most readers (we discovered it by looking in the 1890 Algol Yearbook , which is only available through a secure login to the Carleton College Archives ). Since this information is obscure and difficult to verify, you would want to cite a specific source to prove that you’d done your research and to guide your readers, should they wish to check your facts or learn more.
2) The information is not disputed by your audience.
In order for something to qualify as common knowledge, you must be able to reasonably assume that your readers will accept it as true, without any significant qualification or skepticism. If there is likely to be any dispute or difference of opinion about a certain fact within your anticipated audience, then you would want to cite an informed and reliable source to verify that fact. This is where the definition of common knowledge begins to vary substantially from one audience to the next, because some audiences will accept certain facts uncritically that another audience might not.
For example, the international scientific community overwhelmingly agrees that the global climate is changing rapidly, and this change is primarily driven by human activity. Thus, if you were writing primarily to scientists, or even to readers who are reasonably familiar with climate science, you wouldn’t need to cite a source to assert that human induced global climate change is currently happening. The vast majority of your readers would already know and accept that fact, so it would qualify as common knowledge for that audience.
However, a portion of the American public does not accept that human induced global climate change is really happening. Thus, if you were writing to a general audience of Americans, it would be wise to cite a specific source for that fact, such as this report from the Intergovernmental Panel on Climate Change . This won’t necessarily persuade your audience that the information is true, but it will illustrate that other writers, with greater expertise in the subject than you, also believe that the information is true.
3) The information isn’t traceable to a single source.
When there is a single easily accessible origin point for a given piece of information, you should cite that source. Doing so allows you to acknowledge the intellectual work of whoever produced that information, and it demonstrates to your readers that you understand where the facts in your writing come from.
For example, in 2017 the Minneapolis/St. Paul area received a total of 32.36 inches of precipitation. There is no significant doubt or debate about this fact, and anyone who cares to look could easily find several reliable sources to verify it. However, every one of those sources would almost certainly get their information from the same place: the US National Weather Service , which is the official agency that collects and reports weather data in the United States. Since the NWS is generally considered the definitive source for US weather data, it would be wise to cite them directly, as opposed to citing a different source that uses the NWS’s data or not citing a source at all. This may mean that you need to do some additional research to find the original source of the data, but it’s work that’s worth doing. Citing the US-NWS directly recognizes their authority and signals to your readers that you know and used the best source for your information.
Beyond these guidelines, it’s important to understand that the concept of common knowledge only applies to situations in which it’s absolutely clear to readers that you, the author, are not the original source of the facts or information in question , and therefore there is no risk that you will accidentally take credit for intellectual work you didn’t do. If this isn’t clear from the context of your writing, or if you’re at all uncertain whether something qualifies as common knowledge, you should cite your source.
When in doubt, always cite your source!
Public Domain
Public domain is a legal term referring to documents, images, and other intellectual property that are not fully restricted by copyright. Intellectual property enters the public domain either when its copyright expires, when the copyright holder chooses to release it for public use, or in some rare cases where the law releases the work into the public domain automatically (as with much material produced by the U.S. Federal Government). Items in the public domain are “free” in the sense that you, as a writer, may access them and use them in your own work without paying fees or royalties.
However, this absolutely does not mean that you can use words, phrases, images, or ideas from the public domain in your academic writing without citing your sources or documenting exactly how you’ve used them.
The designation of public domain only applies to issues of copyright and intellectual property, and, as we noted on the “Rules and Principles” page , respecting intellectual property is only one reason that scholars document their sources. The more pressing reasons to document sources include honestly representing your intellectual work and acknowledging the ways you’ve benefited from the work of others. Thus, if you draw on an outside source and don’t clearly document how that source contributed to your writing, then you have committed plagiarism, even if the source is in the public domain.
Home / Guides / Citation Guides / Citation Basics / What is Considered Common Knowledge
What is Considered Common Knowledge
A cat is a common pet. Chocolate is a common candy. The fact that trees are plants is common knowledge… but what does ‘common knowledge’ mean exactly?
Whether your teacher requires MLA , APA, Chicago Manual of Style , or another citation format, you already know how important it is to cite the sources used in any academic papers you write. But you might not know about a little citation loophole that we call ‘common knowledge’. Interested?
What is common knowledge?
Common knowledge is, as the name suggests, information that could reasonably be known by the average person. For example:
- Grass is green
- Fish live in water
- China is a country in Asia
- An adverb is a part of speech
- Ice melts when exposed to heat
- Committing plagiarism is bad
Common knowledge could also be a fact that not everyone would know off the top of their heads, but that’s been generally verified and could be confirmed easily. For example:
- Missouri became a US state in 1821
- Water boils at 212 degrees Fahrenheit
- A subordinating conjunction joins independent and dependent clauses.
- Riga is the capital of Latvia
- Naira is the currency of Nigeria
- Depending on its use, “which” can be a pronoun or an adjective
When common knowledge not so common
When thinking about whether something is common knowledge or not for academic purposes, consider your audience and the context.
For example, if you’re a psychology major then you could reasonably assume that the person reading your paper (i.e., your psychology professor), will already have a good understanding of Pavlov’s classical conditioning theory and wouldn’t need you to cite a source about it. However, if you were referencing this theory as part of a history or English paper, it would be reasonable to cite your sources to allow your lecturer to look it up.
Ask yourself:
- Who is my audience?
- What can I reasonably assume they already know?
- Will they want to know where I got my information?
If in doubt, always cite
Using the above example, there are still many times when a psychology student would need to cite information when referencing Pavlov’s classical conditioning theory. It all hinges on the question: “What can I reasonably assume that they already know?”
For example, the following statement would need a source citation, as it’s very specific information that may not even be known to some psychologists:
The Nobel-prize winning physiologist Ivan Petrovich Pavlov was inspired by the progressive ideas of I.M. Sechenov, the father of Russian physiology .
You should also always follow with the question, “Will they want to know where I got my information?” Even if your subject professor knows something, they might still want to check where you found the information and which sources you used for your research.
If you need any help with formatting your citations correctly, you’ll find an MLA and APA citation generator at EasyBib.com (other citation styles are also available). You can also find information on how to set out a works cited page MLA , how to do an annotated bibliography MLA style, and much more.
How useful was this post?
Click on a star to rate it!
We are sorry that this post was not useful for you!
Let us improve this post!
Tell us how we can improve this post?
Citation Basics
Harvard Referencing
Plagiarism Basics
Plagiarism Checker
Upload a paper to check for plagiarism against billions of sources and get advanced writing suggestions for clarity and style.
Get Started

- Cambridge Libraries
Study Skills
Good academic practice and avoiding plagiarism.
- Introduction
- Taking good notes
- Managing your time
- Paraphrasing
- Summarising
Common knowledge
- Referencing styles
- Referencing tools
- Understanding plagiarism
- Final thoughts
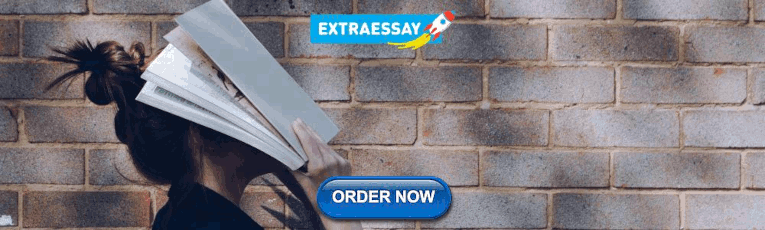
Creative Commons

Common Knowledge
The only source material you can legitimately use without quotation. Common knowledge is information that could be generally known to an educated reader. Examples of this might be facts such as Paris is the capital city of France or widely known historical or scientific facts, such as the molecular structure of water is H2O. However, ideas or interpretations would not normally be considered common knowledge. If you are in doubt as to whether something in your discipline is common knowledge or not it would be best to reference your source. When to Cite page provides more examples for common knowledge.
- << Previous: Summarising
- Next: Referencing styles >>
- Last Updated: Sep 12, 2023 8:59 AM
- URL: https://libguides.cam.ac.uk/plagiarism
© Cambridge University Libraries | Accessibility | Privacy policy | Log into LibApps

- Academic Integrity
- What Is Plagiarism?
- How to Avoid Plagiarism
- Paraphrasing
- Summarizing
- Using Direct Quotations
The Exception: Common Knowledge
- Code of Conduct
- Books at NIC
- How Do I...? This link opens in a new window
The only source material that you can use in an essay without attribution is material that is considered common knowledge and is therefore not attributable to one source. Common knowledge is information generally known to an educated reader, such as widely known facts and dates, and, more rarely, ideas or language. Figuring out whether something is common knowledge can be tricky, and it's always better to cite a source if you're not sure whether the information or idea is common knowledge.
Categories of Common Knowledge
Widely known facts
Widely known scientific and historical facts—such as the molecular structure of water (H2O), or that Ernest Manning was the eighth Premier of Alberta—generally count as common knowledge. You can include such facts in your writing without citation and without fear of committing plagiarism. Other facts that count as common knowledge—for instance, that Lap-Chee Tsui, a Canadian geneticist, led a team that identified the gene behind cystic fibrosis—are widely known to some groups of people (professional geneticists) but perhaps not to you. Nevertheless, you would not have to cite the fact about Tsui, since it is common knowledge in the sense that no particular individual discovered this information.
On the other hand, as soon as your discussion includes an individual's thought, research, or analysis, you do have to cite. For example, if you read Gregory Marchildon’s article on Ernest Manning and wrote a paper in which you repeated Marchildon's claim that Manning’s conservative ideals and religious impetus continue to have currency among those challenging universal healthcare, you would need to cite Marchildon as the source of this idea.
Ideas or interpretations are usually not considered common knowledge, unless they are very widely held.
Ideas or interpretations are usually not considered common knowledge, unless they are very widely held. If you read in R.A.C. Parker's history of World War II that British Prime Minister Neville Chamberlain did not have to agree to the 1938 Munich Pact with Hitler, and that he could have chosen an alternate path, you would need to acknowledge the source, since this judgment is Parker's theory rather than a widely agreed upon fact. (Historians disagree on the factors that led Chamberlain to agree to sign on to the Munich Pact.)
Some interpretations or opinions (rather than facts) have entered the realm of common knowledge and need not be cited. If you were to introduce the claim that culture provides a means by which humans adapt to their environments, you would not need to cite a source for this claim, since it is almost universally held by anthropologists. But, if you were unsure that this was the consensus view among anthropologists, you would be best served simply to cite the source. On the whole, opinions or interpretations do not enter the realm of common knowledge as easily as historical or scientific facts.
Verbatim language drawn from a source is rarely common knowledge, unless the formulation is widely known.
You must always provide a citation for quotations you use in your writing. The only—and rare—exceptions to this rule concern well-known quotations that have entered the realm of common knowledge. For example, if you were writing a paper about Justin Trudeau’s swearing-in address, you would need to cite your source for any quotations you used from the speech. However, if in the course of that paper you compared one of Prime Minister Trudeau’s lines to this very well-known phrase from John F. Kennedy's Inaugural Address, "Ask not what your country can do for you—ask what you can do for your country," you would not need to provide a citation for Kennedy’s phrase. However, if you were to analyze Kennedy's speech substantively and quote additional lines, then you would need to cite anything you quoted from his speech so that your readers could confirm the original language of the speech. If you are not sure whether a quotation is common knowledge, cite it.
Adapted from Harvard College Writing Program (2019) https://usingsources.fas.harvard.edu/exception-common-knowledge
- << Previous: Using Direct Quotations
- Next: Code of Conduct >>
- Last Updated: Feb 1, 2022 4:00 PM
- URL: https://library.nic.bc.ca/plagiarism
- Skip to Guides Search
- Skip to breadcrumb
- Skip to main content
- Skip to footer
- Skip to chat link
- Report accessibility issues and get help
- Go to Penn Libraries Home
- Go to Franklin catalog
Citation Practices and Avoiding Plagiarism: Examples of Common Knowledge
- Getting Started
- Understanding a Citation
- Penn's Plagiarism Policy
- Examples of Quotation
- Examples of Paraphrase
Examples of Common Knowledge
- Strategies for Avoiding Plagiarism
- Copyright This link opens in a new window
- Citation Management Tools This link opens in a new window
- Citation Styles
- Explore More and Practice
Not every fact in your paper needs to be documented. Facts that are widely known—common knowledge—can stand by themselves.
Examples of statements expressing common knowledge:
- George Washington was the first president.
- Plato spent most of his life in Athens.
- Maine has a lower yearly average temperature than Florida.
Any statement that originates from another source and contains information that is not common knowledge must be cited.
Examples of statements that don't express common knowledge:
- Civilizations that were aware of geese usually considered them intermediaries between heaven and earth (Toussaint-Samat 352).
- The Chinese fishing industry produces 800,000 tons of fish annually (Toussaint-Samat 328).
- The first international coffee syndicate was based in Germany (Toussaint-Samat 590).
These statements aren't surrounded by quotation marks because they aren't the original authors' words but must be cited because they express information that involves facts that are not commonly known.
- << Previous: Examples of Paraphrase
- Next: Strategies for Avoiding Plagiarism >>
- Last Updated: Jun 19, 2023 2:22 PM
- URL: https://guides.library.upenn.edu/citationpractices

- Table of Contents
- Random Entry
- Chronological
- Editorial Information
- About the SEP
- Editorial Board
- How to Cite the SEP
- Special Characters
- Advanced Tools
- Support the SEP
- PDFs for SEP Friends
- Make a Donation
- SEPIA for Libraries
- Entry Contents
Bibliography
Academic tools.
- Friends PDF Preview
- Author and Citation Info
- Back to Top
Common Knowledge
A proposition \(A\) is mutual knowledge among a set of agents if each agent knows that \(A\). Mutual knowledge by itself implies nothing about what, if any, knowledge anyone attributes to anyone else. Suppose each student arrives for a class meeting knowing that the instructor will be late. That the instructor will be late is mutual knowledge, but each student might think only she knows the instructor will be late. However, if one of the students says openly “Peter told me he will be late again,” then each student knows that each student knows that the instructor will be late, each student knows that each student knows that each student knows that the instructor will be late, and so on, ad infinitum. The announcement made the mutually known fact common knowledge among the students.
Common knowledge is a phenomenon which underwrites much of social life. In order to communicate or otherwise coordinate their behavior successfully, individuals typically require mutual or common understandings or background knowledge. Indeed, if a particular interaction results in “failure”, the usual explanation for this is that the agents involved did not have the common knowledge that would have resulted in success. If a married couple are separated in a department store, they stand a good chance of finding one another because their common knowledge of each others’ tastes and experiences leads them each to look for the other in a part of the store both know that both would tend to frequent. Since the spouses both love cappuccino, each expects the other to go to the coffee bar, and they find one another. But in a less happy case, if a pedestrian causes a minor traffic jam by crossing against a red light, she explains her mistake as the result of her not noticing, and therefore not knowing, the status of the traffic signal that all the motorists knew. The spouses coordinate successfully given their common knowledge, while the pedestrian and the motorists miscoordinate as the result of a breakdown in common knowledge.
Given the importance of common knowledge in social interactions, it is remarkable that only quite recently have philosophers and social scientists attempted to analyze the concept. David Hume (1740) was perhaps the first to make explicit reference to the role of mutual knowledge in coordination. In his account of convention in A Treatise of Human Nature , Hume argued that a necessary condition for coordinated activity was that agents all know what behavior to expect from one another. Without the requisite mutual knowledge, Hume maintained, mutually beneficial social conventions would disappear. Much later, J. E. Littlewood (1953) presented some examples of common-knowledge-type reasoning, and Thomas Schelling (1960) and John Harsanyi (1967–1968) argued that something like common knowledge is needed to explain certain inferences people make about each other. The philosopher Robert Nozick describes, but does not develop, the notion in his doctoral dissertation (Nozick 1963), while the first mathematical analysis and application of the notion of common knowledge is found in the technical report by Friedell (1967), then published as (Friedell 1969). [ 1 ] The first full-fledged philosophical analysis of common knowledge was offered by David Lewis (1969) in the monograph Convention. Stephen Schiffer (1972), Robert Aumann (1976), and Gilbert Harman (1977) independently gave alternate definitions of common knowledge. Jon Barwise (1988, 1989) gave a precise formulation of Harman’s intuitive account. Throughout the 1980s a number of epistemic logicians, both from philosophy and from computer science, studied the logical structure of common knowledge, and the interested reader should consult the relevant portions of the two important monographs (Fagin et al. 1995) and (Meyer and Van der Hoek 1995). Margaret Gilbert (1989) proposed a somewhat different account of common knowledge which she argues is preferable to the standard account. Others have developed accounts of mutual knowledge, approximate common knowledge , and common belief which require less stringent assumptions than the standard account, and which serve as more plausible models of what agents know in cases where strict common knowledge seems impossible (Brandenburger and Dekel 1987, Monderer and Samet 1989, Rubinstein 1992). The analysis and applications of common knowledge and related multi-agent knowledge concepts has become a lively field of research.
The purpose of this essay is to overview of some of the most important results stemming from this contemporary research. The topics reviewed in each section of this essay are as follows: Section 1 gives motivating examples which illustrate a variety of ways in which the actions of agents depend crucially upon their having, or lacking, certain common knowledge. Section 2 discusses alternative analyses of common knowledge. Section 3 reviews applications of multi-agent knowledge concepts, particularly to game theory (von Neumann and Morgenstern 1944), in which common knowledge assumptions have been found to have great importance in justifying solution concepts for mathematical games. Section 4 discusses skeptical doubts about the attainability of common knowledge. Finally, Section 5 discusses the common belief concept which result from weakening the assumptions of Lewis’ account of common knowledge.
- 1.1 The Clumsy Waiter
- 1.2 The Barbeque Problem
- 1.3 The Farmers’ Dilemma
1.4 The Centipede
1.5 the department store, 2.1 the hierarchical account, 2.2 lewis’ account, 2.3 aumann’s account, 2.4 barwise’s account, 2.5 gilbert’s account, 3.1 the “no disagreement” theorem, 3.2 convention, 3.3 strategic form games, 3.4 games of perfect information, 3.5 communication networks, 4. is common knowledge attainable.
- 5.1 The Email Coordination Example
5.2 Common \(p\)-Belief
Other internet resources, related entries, 1. motivating examples.
Most of the examples in this section are familiar in the common knowledge literature, although some of the details and interpretations presented here are new. Readers may want to ask themselves what, if any, distinctive aspects of mutual and common knowledge reasoning each example illustrates.
1.1. The Clumsy Waiter
A waiter serving dinner slips, and spills gravy on a guest’s white silk evening gown. The guest glares at the waiter, and the waiter declares “I’m sorry. It was my fault.” Why did the waiter say that he was at fault? He knew that he was at fault, and he knew from the guest’s angry expression that she knew he was at fault. However, the sorry waiter wanted assurance that the guest knew that he knew he was at fault. By saying openly that he was at fault, the waiter knew that the guest knew what he wanted her to know, namely, that he knew he was at fault. Note that the waiter’s declaration established at least three levels of nested knowledge. [ 2 ]
Certain assumptions are implicit in the preceding story. In particular, the waiter must know that the guest knows he has spoken the truth, and that she can draw the desired conclusion from what he says in this context. More fundamentally, the waiter must know that if he announces “It was my fault” to the guest, she will interpret his intended meaning correctly and will infer what his making this announcement ordinarily implies in this context. This in turn implies that the guest must know that if the waiter announces “It was my fault” in this context, then the waiter indeed knows he is at fault. Then on account of his announcement, the waiter knows that the guest knows that he knows he was at fault. The waiter’s announcement was meant to generate higher-order levels of knowledge of a fact each already knew.
Just a slight strengthening of the stated assumptions results in even higher levels of nested knowledge. Suppose the waiter and the guest each know that the other can infer what he infers from the waiter’s announcement. Can the guest now believe that the waiter does not know that she knows that he knows he is at fault? If the guest considers this question, she reasons that if the waiter falsely believes it is possible that she does not know that he knows he is at fault, then the waiter must believe it to be possible that she cannot infer that he knows he is at fault from his own declaration. Since she knows she can infer that the waiter knows he is at fault from his declaration, she knows that the waiter knows she can infer this, as well. Hence the waiter’s announcement establishes the fourth-order knowledge claim: The guest knows that the waiter knows that she knows that he knows he is at fault. By similar, albeit lengthier, arguments, the agents can verify that corresponding knowledge claims of even higher-order must also obtain under these assumptions.
1.2 The Barbecue Problem
This is a variation of an example first published by Littlewood (1953), although he notes that his version of the example was already well-known at the time. [ 3 ] \(N\) individuals enjoy a picnic supper together which includes barbecued spareribs. At the end of the meal, \(k \ge 1\) of these diners have barbecue sauce on their faces. Since no one can see her own face, none of the messy diners knows whether he or she is messy. Then the cook who served the spareribs returns with a carton of ice cream. Amused by what he sees, the cook rings the dinner bell and makes the following announcement: “At least one of you has barbecue sauce on her face. I will ring the dinner bell over and over, until anyone who is messy has wiped her face. Then I will serve dessert.” For the first \(k - 1\) rings, no one does anything. Then, at the \(k\) th ring, each of the messy individuals suddenly reaches for a napkin, and soon afterwards, the diners are all enjoying their ice cream.
How did the messy diners finally realize that their faces needed cleaning? The \(k = 1\) case is easy, since in this case, the lone messy individual will realize he is messy immediately, since he sees that everyone else is clean. Consider the \(k = 2\) case next. At the first ring, messy individual \(i_1\) knows that one other person, \(i_2\), is messy, but does not yet know about himself. At the second ring, \(i_1\) realizes that he must be messy, since had \(i_2\) been the only messy one, \(i_2\) would have known this after the first ring when the cook made his announcement, and would have cleaned her face then. By a symmetric argument, messy diner \(i_2\) also concludes that she is messy at the second ring, and both pick up a napkin at that time.
The general case follows by induction. Suppose that if \(k = j\), then each of the \(j\) messy diners can determine that he is messy after \(j\) rings. Then if \(k = j + 1\), then at the \(j + 1\) st ring, each of the \(j + 1\) individuals will realize that he is messy. For if he were not messy, then the other \(j\) messy ones would have all realized their messiness at the \(j\) th ring and cleaned themselves then. Since no one cleaned herself after the \(j\) th ring, at the \(j + 1\) st ring each messy person will conclude that someone besides the other \(j\) messy people must also be messy, namely, himself.
The “paradox” of this argument is that for \(k \gt 1\), like the case of the clumsy waiter of Example 1.1, the cook’s announcement told the diners something that each already knew. Yet apparently the cook’s announcement also gave the diners useful information. How could this be? By announcing a fact already known to every diner, the cook made this fact common knowledge among them, enabling each of them to eventually deduce the condition of his own face after sufficiently many rings of the bell. [ 4 ]
1.3 The Farmer’s Dilemma
Does meeting one’s obligations to others serve one’s self-interest? Plato and his successors recognized that in certain cases, the answer seems to be “No.” Hobbes (1651, pp. 101–102) considers the challenge of a “Foole”, who claims that it is irrational to honor an agreement made with another who has already fulfilled his part of the agreement. Noting that in this situation one has gained all the benefit of the other’s compliance, the Foole contends that it would now be best for him to break the agreement, thereby saving himself the costs of compliance. Of course, if the Foole’s analysis of the situation is correct, then would the other party to the agreement not anticipate the Foole’s response to agreements honored, and act accordingly?
Hume (1740, pp. 520–521) takes up this question, using an example: Two neighboring farmers each expect a bumper crop of corn. Each will require his neighbor’s help in harvesting his corn when it ripens, or else a substantial portion will rot in the field. Since their corn will ripen at different times, the two farmers can ensure full harvests for themselves by helping each other when their crops ripen, and both know this. Yet the farmers do not help each other. For the farmer whose corn ripens later reasons that if she were to help the other farmer, then when her corn ripens he would be in the position of Hobbes’ Foole, having already benefited from her help. He would no longer have anything to gain from her, so he would not help her, sparing himself the hard labor of a second harvest. Since she cannot expect the other farmer to return her aid when the time comes, she will not help when his corn ripens first, and of course the other farmer does not help her when her corn ripens later.
The structure of Hume’s Farmers’ Dilemma problem can be summarized using the following tree diagram:
Figure 1.1a
This tree is an example of a game in extensive form. At each stage \(i\), the agent who moves can either choose \(C^i\), which corresponds to helping or cooperating , or \(D^i\), which corresponds to not helping or defecting . The relative preferences of the two agents over the various outcomes are reflected by the ordered pairs of payoffs each receives at any particular outcome. If, for instance, Fiona chooses \(C^i\) and Alan chooses \(D^i\), then Fiona’s payoff is 0, her worst payoff, and Alan’s is 4, his best payoff. In a game such as the Figure 1.1.a game, agents are (Bayesian) rational if each chooses an act that maximizes her expected payoff, given what she knows.
In the Farmers’ Dilemma game, following the \(C^1,C^2\)-path is strictly better for both farmers than following the \(D^1,D^2\)-path. However, Fiona chooses \(D^1\), as the result of the following simple argument: “If I were to choose \(C^1\), then Alan, who is rational and who knows the payoff structure of the game, would choose \(D^2\). I am also rational and know the payoff structure of the game. So I should choose \(D^1\).” Since Fiona knows that Alan is rational and knows the game’s payoffs, she concludes that she need only analyze the reduced game in the following figure:
Figure 1.1b
In this reduced game, Fiona is certain to gain a strictly higher payoff by choosing \(D^1\) than if she chooses \(C^1\), so \(D^1\) is her unique best choice. Of course, when Fiona chooses \(D^1\), Alan, being rational, responds by choosing \(D^2\). If Fiona and Alan know: (i) that they are both rational, (ii) that they both know the payoff structure of the game, and (iii) that they both know (i) and (ii), then they both can predict what the other will do at every node of the Figure 1.1.a game, and conclude that they can rule out the \(D^1,C^2\)-branch of the Figure 1.1.b game and analyze just the reduced game of the following figure:
Figure 1.1c
On account of this mutual knowledge , both know that Fiona will choose \(D^1\), and that Alan will respond with \(D^2\). Hence, the \(D^1,D^2\)-outcome results if the Farmers’ Dilemma game is played by agents having this mutual knowledge, though it is suboptimal since both agents would fare better at the \(C^1,C^2\)-branch. [ 5 ] This argument, which in its essentials is Hume’s argument, is an example of a standard technique for solving sequential games known as backwards induction. [ 6 ] The basic idea behind backwards induction is that the agents engaged in a sequential game deduce how each will act throughout the entire game by ruling out the acts that are not payoff-maximizing for the agents who would move last, then ruling out the acts that are not payoff-maximizing for the agents who would move next-to-last, and so on. Clearly, backwards induction arguments rely crucially upon what, if any, mutual knowledge the agents have regarding their situation, and they typically require the agents to evaluate the truth values of certain subjunctive conditionals, such as “If I (Fiona) were to choose \(C^1\), then Alan would choose \(D^2\)”.
The mutual knowledge assumptions required to construct a backwards induction solution to a game become more complex as the number of stages in the game increases. To see this, consider the sequential Centipede game depicted in the following figure:
At each stage i\), the agent who moves can either choose \(R^i\), which in the first three stages gives the other agent an opportunity to move, or \(L^i\), which ends the game.
Like the Farmers’ Dilemma, this game is a commitment problem for the agents. If each agent could trust the other to choose \(R^i\) at each stage, then they would each expect to receive a payoff of 3. However, Alan chooses \(L^1\), leaving each with a payoff of only 1, as the result of the following backwards induction argument: “If node \(n_4\) were to be reached, then Fiona, (being rational) would choose \(L^4\). I, knowing this, would (being rational) choose \(L^3\) if node \(n_3\) were to be reached. Fiona, knowing this , would (being rational) choose \(L^2\) if node \(n_2\) were to be reached. Hence, I (being rational) should choose \(L^1\).” To carry out this backwards induction argument, Alan implicitly assumes that: (i) he knows that Fiona knows he is rational, and (ii) he knows that Fiona knows that he knows she is rational. Put another way, for Alan to carry out the backwards induction argument, at node \(n_1\) he must know what Fiona must know at node \(n_2\) to make \(L^2\) her best response should \(n_2\) be reached. While in the Farmer’s Dilemma Fiona needed only first-order knowledge of Alan’s rationality and second-order knowledge of Alan’s knowledge of the game to derive the backwards induction solution, in the Figure 1.2 game, for Alan to be able to derive the backwards induction solution, the agents must have third-order mutual knowledge of the game and second-order mutual knowledge of rationality, and Alan must have fourth-order knowledge of this mutual knowledge of the game and third-order knowledge of their mutual knowledge of rationality. This argument also involves several counterfactuals, since to construct it the agents must be able to evaluate conditionals of the form, “If node \(n_i\) were to be reached, Alan (Fiona) would choose \(L^i (R^i)\)”, which for \(i \gt 1\) are counterfactual, since third-order mutual knowledge of rationality implies that nodes \(n_2,\) \(n_3\), and \(n_4\) are never reached.
The method of backwards induction can be applied to any sequential game of perfect information , in which the agents can observe each others’ moves in turn and can recall the entire history of play. However, as the number of potential stages of play increases, the backwards induction argument evidently becomes harder to construct. This raises certain questions: (1) What precisely are the mutual or common knowledge assumptions that are required to justify the backwards induction argument for a particular sequential game? (2) As a sequential game increases in complexity, would we expect the mutual knowledge that is required for backwards induction to start to fail?
When a man loses his wife in a department store without any prior understanding on where to meet if they get separated, the chances are good that they will find each other. It is likely that each will think of some obvious place to meet, so obvious that each will be sure that it is “obvious” to both of them. One does not simply predict where the other will go, which is wherever the first predicts the second to predict the first to go, and so ad infinitum. Not “What would I do if I were she?” but “What would I do if I were she wondering what she would do if she were wondering what I would do if I were she … ?” —Thomas Schelling, The Strategy of Conflict
Schelling’s department store problem is an example of a pure coordination problem , that is, an interaction problem in which the interests of the agents coincide perfectly. Schelling (1960) and Lewis (1969), who were the first to make explicit the role common knowledge plays in social coordination, were also among the first to argue that coordination problems can be modeled using the analytic vocabulary of game theory. A very simple example of such a coordination problem is given in the next figure:
\(s_i =\) search on floor \(i\), \(1 \leq i \leq 4\)
The matrix of Figure 1.3 is an example of a game in strategic form. At each outcome of the game, which corresponds to a cell in the matrix, the row (column) agent receives as payoff the first (second) element of the ordered pair in the corresponding cell. However, in strategic form games, each agent chooses without first being able to observe the choices of any other agent, so that all must choose as if they were choosing simultaneously. The Figure 1.3 game is a game of pure coordination (Lewis 1969), that is, a game in which at each outcome, each agent receives exactly the same payoff. One interpretation of this game is that Schelling’s spouses, Liz and Robert, are searching for each other in the department store with four floors, and they find each other if they go to the same floor. Four outcomes at which the spouses coordinate correspond to the strategy profiles \((s_j, s_j), 1 \le j \le 4\), of the Figure 1.3 game. These four profiles are strict Nash equilibria (Nash 1950, 1951) of the game, that is, each agent has a decisive reason to follow her end of one of these strategy profiles provided that the other also follows this profile. [ 7 ]
The difficulty the agents face is trying to select an equilibrium to follow. For suppose that Robert hopes to coordinate with Liz on a particular equilibrium of the game, say \((s_2, s_2)\). Robert reasons as follows: “Since there are several strict equilibria we might follow, I should follow my end of \((s_2, s_2)\) if, and only if, I have sufficiently high expectations that Liz will follow her end of \((s_2, s_2)\). But I can only have sufficiently high expectations that Liz will follow \((s_2, s_2 )\) if she has sufficiently high expectations that I will follow \((s_2, s_2)\). For her to have such expectations, Liz must have sufficiently high (second-order) expectations that I have sufficiently high expectations that she will follow \((s_2, s_2)\), for if Liz doesn’t have these (second-order) expectations, then she will believe I don’t have sufficient reason to follow \((s_2, s_2)\) and may therefore deviate from \((s_2, s_2)\) herself. So I need to have sufficiently high (third-order) expectations that Liz has sufficiently high (second-order) expectations that I have sufficiently high expectations that she will follow \((s_2, s_2 )\), which involves her in fourth-order expectations regarding me, which involves me in fifth-order expectations regarding Liz, and so on.” What would suffice for Robert, and Liz, to have decisive reason to follow \((s_2, s_2)\) is that they each know that the other knows that … that the other will follow \((s_2, s_2)\) for any number of levels of knowledge, which is to say that between Liz and Robert it is common knowledge that they will follow \((s_2, s_2)\). If agents follow a strict equilibrium in a pure coordination game as a consequence of their having common knowledge of the game, their rationality and their intentions to follow this equilibrium, and no other, then the agents are said to be following a Lewis-convention (Lewis 1969).
Lewis’ theory of convention applies to a more general class of games than pure coordination games, but pure coordination games already model a variety of important social interactions. In particular, Lewis models conventions of language as equilibrium points of a pure coordination game. The role common knowledge plays in games of pure coordination sketched above of course raises further questions: (1) Can people ever attain the common knowledge which characterizes a Lewis-convention? (2) Would less stringent epistemic assumptions suffice to justify Nash equilibrium behavior in a coordination problem?
2. Alternative Accounts of Common Knowledge
Informally, a proposition \(A\) is mutually known among a set of agents if each agent knows that \(A\). Mutual knowledge by itself implies nothing about what, if any, knowledge anyone attributes to anyone else. Suppose each student arrives for a class meeting knowing that the instructor will be late. That the instructor will be late is mutual knowledge, but each student might think only she knows the instructor will be late. However, if one of the students says openly “Peter told me he will be late again,” then the mutually known fact is now commonly known. Each student now knows that the instructor will be late, and so on, ad infinitum. The agents have common knowledge in the sense articulated informally by Schelling (1960), and more precisely by Lewis (1969) and Schiffer (1972). Schiffer uses the formal vocabulary of epistemic logic (Hintikka 1962) to state his definition of common knowledge. Schiffer’s general approach was to augment a system of sentential logic with a set of knowledge operators corresponding to a set of agents, and then to define common knowledge as a hierarchy of propositions in the augmented system. Bacharach (1992) and Bicchieri (1993) adopt this approach, and develop logical theories of common knowledge which include soundness and completeness theorems in the style of (Fagin et al. 1995). One can also develop formal accounts of common knowledge in set-theoretic terms, as it was done in the early Friedell (1969) and in the economic literature after Aumann (1976). Such an approach, easily proven to be equivalent to the ones cast in epistemic logic, is taken also in this article. [ 8 ]
Monderer and Samet (1988) and Binmore and Brandenburger (1989) give a particularly elegant set-theoretic definition of common knowledge. I will review this definition here, and then show that it is logically equivalent to the ‘\(i\) knows that \(j\) knows that \(\ldots k\) knows that A’ hierarchy that Lewis (1969) and Schiffer (1972) argue characterizes common knowledge. [ 9 ]
Some preliminary notions must be stated first. Following C. I. Lewis (1943–1944) and Carnap (1947), propositions are formally subsets of a set \(\Omega\) of state descriptions or possible worlds. One can think of the elements of \(\Omega\) as representing Leibniz’s possible worlds or Wittgenstein’s possible states of affairs. Some results in the common knowledge literature presuppose that \(\Omega\) is of finite cardinality. If this admittedly unrealistic assumption is needed in any context, this will be explicitly stated in this essay, and otherwise one may assume that \(\Omega\) may be either a finite or an infinite set. A distinguished actual world \(\omega_{\alpha}\) is an element of \(\Omega\). A proposition \(A \subseteq \Omega\) obtains (or is true) if the actual world \(\omega_{\alpha} \in A\). In general, we say that \(A\) obtains at a world \(\omega \in \Omega\) if \(\omega \in A\). What an agent \(i\) knows about the possible worlds is stated formally in terms of a knowledge operator \(\mathbf{K}_i\). Given a proposition \(A \subseteq \Omega , \mathbf{K}_i (A)\) denotes a new proposition, corresponding to the set of possible worlds at which agent \(i\) knows that A obtains. \(\mathbf{K}_i (A)\) is read as ‘\(i\) knows (that) \(A\) (is the case)’. The knowledge operator \(\mathbf{K}_i\) satisfies certain axioms, including:
In words, K1 says that if \(i\) knows \(A\), then \(A\) must be the case. K2 says that \(i\) knows that some possible world in \(\Omega\) occurs no matter which possible world \(\omega\) occurs. K3 [ 10 ] says that \(i\) knows a conjunction if, and only if, \(i\) knows each conjunct. K4 is a reflection axiom , sometimes also presented as the axiom of transparency (or of positive introspection ), which says that if \(i\) knows \(A\), then \(i\) knows that she knows \(A\). Finally, K5 says that if the agent does not know an event, then she knows that she does not know. This axiom is presented as the axiom of negative introspection , or as the axiom of wisdom (since the agents possess Socratic wisdom, knowing that they do not know.) Note that by K3, if \(A \subseteq B\) then \(\mathbf{K}_i (A) \subseteq \mathbf{K}_i (B)\), by K1 and K2, \(\mathbf{K}_i (\Omega) = \Omega\), and by K1 and K4, \(\mathbf{K}_i (A) = \mathbf{K}_i \mathbf{K}_i (A)\). Any system of knowledge satisfying K1–K5 corresponds to the modal system S5, while any system satisfying K1–K4 corresponds to S4 (Kripke 1963). If one drops the K1 axiom and retains the others, the resulting system would give a formal account of what an agent believes , but does not necessarily know .
A useful notion in the formal analysis of knowledge is that of a possibility set. An agent i’s possibility set at a state of the world \(\Omega\) is the smallest set of possible worlds that \(i\) thinks could be the case if \(\omega\) is the actual world. More precisely,
Definition 2.1 Agent \(i\)’s possibility set \(\mathcal{H}_i (\omega)\) at \(\omega \in \Omega\) is defined as
The collection of sets
is \(i\)’s private information system .
Since in words, \(\mathcal{H}_i (\omega)\) is the intersection of all propositions which \(i\) knows at \(\omega , \mathcal{H}_i (\omega)\) is the smallest proposition in \(\Omega\) that \(i\) knows at \(\omega\). Put another way, \(\mathcal{H}_i (\omega)\) is the most specific information that \(i\) has about the possible world \(\omega\). The intuition behind assigning agents private information systems is that while an agent \(i\) may not be able to perceive or comprehend every last detail of the world in which \(i\) lives, \(i\) does know certain facts about that world. The elements of \(i\)’s information system represent what \(i\) knows immediately at a possible world. We also have the following:
Proposition 2.2 \(\mathbf{K}_i (A) = \{ \omega \mid \mathcal{H}_i (\omega) \subseteq A \}\)
In many formal analyses of knowledge in the literature, possibility sets are taken as primitive and Proposition 2.2 is given as the definition of knowledge. If one adopts this viewpoint, then the axioms K1–K5 follow as consequences of the definition of knowledge. In many applications, the agents’ possibility sets are assumed to partition [ 11 ] the set, in which case \(\mathcal{H}_i\) is called i’s private information partition . Notice that if axioms K1–K5 hold, then the possibility sets of each agent always partition the state set, and vice versa.
To illustrate the idea of possibility sets, let us return to the Barbecue Problem described in Example 1.2. Suppose there are three diners: Cathy, Jennifer and Mark. Then there are 8 relevant states of the world, summarized by Table 2.1:
Each diner knows the condition of the other diners’ faces, but not her own. Suppose the cook makes no announcement, after all. Then none of the diners knows the true state of the world whatever \(\omega \in \Omega\) the actual world turns out to be, but they do know a priori that certain propositions are true at various states of the world. For instance, Cathy’s information system before any announcement is made is depicted in Figure 2.1a:
Figure 2.1a
In this case, Cathy’s information system is a partition \(\mathcal{H}_1\) of \(\Omega\) defined by
Cathy knows immediately which cell \(\mathcal{H}_1 (\omega)\) in her partition is the case at any state of the world, but does not know which is the true state at any \(\omega \in \Omega\).
If we add in the assumption stated in Example 1.2 that if there is at least one messy diner, then the cook announces the fact, then Cathy’s information partition is depicted by Figure 2.1b:
Figure 2.1b
In this case, Cathy’s information partition is a refinement of the partition she has when there is no announcement, for in this case, then Cathy knows a priori that if \(\omega_1\) is the case there will be no announcement and will know immediately that she is clean, and Cathy knows a priori that if \(\omega_2\) is the case, then she will know immediately from the cook’s announcement that she is messy.
Similarly, if the cook makes an announcement only if he sees at least two messy diners, Cathy’s possibility set is the one represented in fig. 2.1c:
Figure 2.1c
Cathy’s information partition is now defined by
In this case, Cathy knows a priori that if \(\omega_3\) obtains there will be no announcement, and similarly for \(\omega_4\). Thus, she will be able to distinguish these states from \(\omega_5\) and \(\omega_6\), respectively.
As mentioned earlier in this subsection, the assumption that agents’ possibility sets partition the state space depends on the modeler’s choice of specific axioms for the knowledge operators. For example, if we drop axiom K5 (preserving the validity of K1–K4) the agent’s possibility sets need not partition the space set (follow the link for an example . For more details and applications, cf. Samet 1990.) It was conjectured (cf. Geanakoplos 1989) that lack of negative introspection (i.e. systems without K5) would allow to incorporate unforeseen contingencies in the epistemic model, by representing the agents’ unawareness of certain events (i.e. the case in which the agent does not know that an event occurs and also does not know that she does not know that.) It was later shown by Dekel et al. (1998) that standard models are not suitable to represent agents’ unawareness. An original non-standard model to represent unawareness is provided in Heifetz et al . (2006). For a comprehensive bibliography on modeling unawareness and applications of the notion, cf. the external links at the end on this entry.
We can now define mutual and common knowledge as follows:
Definition 2.3 Let a set \(\Omega\) of possible worlds together with a set of agents \(N\) be given.
1. The proposition that \(A\) is (first level or first order) mutual knowledge for the agents of N, \(\mathbf{K}^{1}_N (A)\), is the set defined by
2. The proposition that \(A\) is \(m\) th level (or \(m\) th order ) mutual knowledge among the agents of N , \(\mathbf{K}^m_N(A),\) is defined recursively as the set
3. The proposition that \(A\) is common knowledge among the agents of \(N, \mathbf{K}^{*}_N (A),\) is defined as the set [ 12 ]
Common knowledge of a proposition \(E\) implies common knowledge of all that \(E\) implies, as is shown in the following:
Proposition 2.4 If \(\omega \in \mathbf{K}^{*}_N (E)\) and \(E \subseteq F\), then \(\omega \in \mathbf{K}^{*}_N (F)\). Proof .
Note that \((\mathbf{K}^m_N(E))_{m\ge 1}\) is a decreasing sequence of events, in the sense that \(\mathbf{K}^{m+1}_N (E) \subseteq \mathbf{K}^m_N(E)\), for all \(m \ge 1\). It is also easy to check that if everyone knows \(E\), then \(E\) must be true, that is, \(\mathbf{K}^1_N (E) \subseteq E\). If \(\Omega\) is assumed to be finite, then if \(E\) is common knowledge at \(\omega\), this implies that there must be a finite \(m\) such that
The following result relates the set-theoretic definition of common knowledge to the hierarchy of ‘\(i\) knows that \(j\) knows that … knows \(A\)’ statements.
Proposition 2.5 \(\omega \in \mathbf{K}^m_N(A)\) iff
(1) For all agents \(i_1, i_2 , \ldots ,i_m \in N, \omega \in \mathbf{K}_{i_1}\mathbf{K}_{i_2} \ldots \mathbf{K}_{i_m}(A)\)
Hence, \(\omega \in \mathbf{K}^*_N (A)\) iff (1) is the case for each \(m \ge 1\). Proof .
The condition that \(\omega \in \mathbf{K}_{i_1}\mathbf{K}_{i_2} \ldots \mathbf{K}_{i_m}(A)\) for all \(m \ge 1\) and all \(i_1, i_2 , \ldots ,i_m \in N\) is Schiffer’s definition of common knowledge, and is often used as the definition of common knowledge in the literature.
Lewis is credited with the idea of characterizing common knowledge as a hierarchy of ‘\(i\) knows that \(j\) knows that … knows that \(A\)’ propositions. However, Lewis is aware of the difficulties that such an infinitary definition raises. A first problem is whether it is possible to reduce the infinity inherent in the hierarchical account into a workable finite definition. A second problem is the issue that finite agents cannot entertain the infinite amount of epistemic states which is necessary for common knowledge to obtain. Lewis tackles both problems, but his presentation is informal. Aumann is often credited with presenting the first finitary method of generating the common knowledge hierarchy (Aumann 1976), even though (Friedell 1969) in fact predates both Aumann’s and Lewis’s work. Recently, Cubitt and Sugden (2003) have argued that Aumann’s and Lewis’ accounts of common knowledge are radically different and irreconcilable.
Although Lewis introduced the technical term ‘common knowledge,’ his analysis is about belief, rather than knowledge. Indeed, Lewis offers his solution to the second problem mentioned above by introducing a distinction between actual belief and reason to believe . Reasons to believe are interpreted as potential beliefs of agents, so that the infinite hierarchy of epistemic states becomes harmless, consisting in an infinite number of states of potential belief. The solution to the first problem is given by providing a finite set of conditions that, if met, generate the infinite series of reasons to believe. Such conditions taken together represent Lewis’ official definition of common knowledge. Notice that it would be more appropriate to speak of ‘common reason to believe,’ or, at least, of ‘common belief.’ Lewis himself later acknowledges that “[t]hat term [common knowledge] was unfortunate, since there is no assurance that it will be knowledge, or even that it will be true.” Cf. (Lewis 1978, p. 44, n.13) Disregarding the distinction between reasons to believe and actual belief, we follow (Vanderschraaf 1998) to give the details of a formal account of Lewis’s definition here, and show that Lewis’ analysis does result in the common knowledge hierarchy following from a finite set of axioms. It is however debatable whether a possible worlds approach can properly render the subtleties of Lewis’ characterization. Cubitt and Sugden (2003), for example, abandon the possible worlds framework altogether and propose a different formal interpretation of Lewis in which, among other elements, the distinction between reasons to believe and actual belief is taken into account. An attempt to reconcile the two positions can be found in (Sillari 2005), where Lewis’ characterization is formalized in a richer possible worlds semantic framework where the distinction between reasons to believe and actual believe is represented.
Lewis presents his account of common knowledge on pp. 52–57 of Convention . Lewis does not specify what account of knowledge is needed for common knowledge. As it turns out, Lewis’ account is satisfactory for any formal account of knowledge in which the knowledge operators \(\mathbf{K}_i, i \in N\), satisfy K1, K2, and K3. A crucial assumption in Lewis’ analysis of common knowledge is that agents know they share the same “rationality, inductive standards and background information” (Lewis 1969, p. 53) with respect to a state of affairs \(A'\), that is, if an agent can draw any conclusion from \(A'\), she knows that all can do likewise. This idea is made precise in the following:
Definition 2.6 Given a set of agents \(N\) and a proposition \(A' \subseteq \Omega\), the agents of \(N\) are symmetric reasoners with respect to \(A' (or A'\) -symmetric reasoners ) iff, for each \(i, j \in N\) and for any proposition \(E \subseteq \Omega\), if \(\mathbf{K}_i (A') \subseteq \mathbf{K}_i (E)\) and \(\mathbf{K}_i (A') \subseteq \mathbf{K}_i\mathbf{K}_j(A')\), then \(\mathbf{K}_i (A') \subseteq \mathbf{K}_i\mathbf{K}_j(E)\). [ 13 ]
The definiens says that for each agent \(i\), if \(i\) can infer from \(A'\) that \(E\) is the case and that everyone knows that \(A'\) is the case, then \(i\) can also infer that everyone knows that \(E\) is the case.
\(A\)* is a basis for the agents’ common knowledge. \(\mathbf{L}*_N (E)\) denotes the proposition defined by L1–L3 for a set \(N\) of \(A\)*-symmetric reasoners, so we can say that \(E\) is Lewis-common knowledge for the agents of \(N\) iff \(\omega \in \mathbf{L}*_N (E)\).
In words, L1 says that \(i\) knows \(A\)* at \(\omega\). L2 says that if \(i\) knows that \(A\)* obtains, then \(i\) knows that everyone knows that \(A\)* obtains. This axiom is meant to capture the idea that common knowledge is based upon a proposition \(A\)* that is publicly known , as is the case when agents hear a public announcement. If the agents’ knowledge is represented by partitions, then a typical basis for the agents’ common knowledge would be an element \(\mathcal{M}(\omega)\) in the meet [ 14 ] of their partitions. L3 says that \(i\) can infer from \(A\)* that \(E\). Lewis’ definition implies the entire common knowledge hierarchy, as is shown in the following result.
Proposition 2.8 \(\mathbf{L}*_N (E) \subseteq \mathbf{K}*_N (E)\), that is, Lewis-common knowledge of \(E\) implies common knowledge of \(E\). Proof .
As mentioned above, it has recently come into question whether a formal rendition of Lewis’ definition as the one given above adequately represents all facets of Lewis’ approach. Cubitt and Sugden (2003) argue that it does not, their critique hinging on a feature of Lewis’ analysis that is lost in the possible worlds framework, namely the 3-place relation of indication used by Lewis. The definition of indication can be found at pp. 52–53 of Convention :
Definition 2.9 A state of affairs \(A\) indicates \(E\) to agent \(i\) \((A \indi E)\) if and only if, if \(i\) had reason to believe that \(A\) held, \(i\) would thereby have reason to believe that \(E\)
The wording of Lewis’ definition and the use he makes of the indication relation in the definitory clauses for common knowledge, suggest that Lewis is careful to distinguish indication and material implication. Cubitt and Sugden (2003) incorporate such distinction in their formal reconstruction. Paired with their interpretation of “\(i\) has reason to believe \(x\)” as “\(x\) is yielded by some logic of reasoning that \(i\) endorses,” we have that, if \(A \indi x,\) then \(i\)’s reason to believe \(A\) provides \(i\) with reason to believe \(x\) as well. Given that Lewis does want to endow agents with deductive reasoning, (Cubitt and Sugden 2003) list the following axioms, claiming that they capture the desired properties of indication. For all agents \(i, j,\) with \(\mathbf{R}_i A\) standing for “agent \(i\) has reason to believe A”, we have
The first axioms captures the intuition behind indication. It says that if an agent has reason to believe that \(A\) holds, then, if \(A\) indicates \(x\) to her, she has reason to believe \(x\) as well. CS2 says that indication extends material implication. CS3 says that if two propositions \(x\) and \(y\) are indicated to an agent by a proposition \(A\), then \(A\) indicates to her also the conjunction of \(x\) and \(y\). The next axiom states that indication is transitive. CS5 says that if a proposition \(A\) indicates to \(i\) that agent \(j\) has reason to believe \(B\), and \(i\) has reason to believe that \(B\) indicates \(x\) to \(j\), then \(A\) indicates to \(i\) also that \(j\) has reason to believe \(x\).
Armed with these axioms, it is possible to give the following definition.
Definition 2.10 In any given population \(P\) a proposition \(A\) is a reflexive common indicator that x if and only if, for all \(i, j \in P\) and all propositions \(x, y,\) the following four conditions hold:
Clauses RCI1–RCI3 above render L1–L3 of definition 2.7 above in the formal language that underlies axioms CS1–CS5; while RCI4 affirms (cf. definition 2.6 above) that agents are symmetric reasoners, i.e. that if a proposition indicates another proposition to a certain agent, then it does so to all agents in the population.
The following proposition shows that RCI1–RCI4 are sufficient conditions for ‘common reason to believe’ to arise:
Proposition 2.11 If \(A\) holds, and if \(A\) is a common reflexive indicator in the population \(P\) that \(x\), then there is common reason to believe in \(P\) that \(x\). Proof .
A group of (ideal) faultless reasoners who have common reason to believe that \(p\), will achieve common belief in \(p\).
Is it possible to take formally in account the insights of Lewis’ definition of common knowledge without abandoning the possible world framework? (Sillari 2005) puts forth an attempt to give a positive answer to that question by articulating in a possible world semantics the distinction between actual belief and reason to believe. As in (Cubitt and Sugden 2003), the basic epistemic operator represents reasons to believe. The idea is then to impose an awareness structure over possible worlds, adopting the framework first introduced by Fagin and Halpern (1988). Simply put, an awareness structure associates to each agent, for every possible world, a set of events of which the agent is said to be aware. An agent entertains an actual belief that a certain event occurs if and only if she has reason to believe that the event occurs and such event is in her awareness set at the world under consideration. A different avenue to the formalization of Lewis’s account of common knowledge is offered by Paternotte (2011), where the central notion is probabilistic common belief (see section 5.2 below).
Aumann (1976) gives a different characterization of common knowledge which gives another simple algorithm for determining what information is commonly known. Aumann’s original account assumes that the each agent’s possibility set forms a private information partition of the space \(\Omega\) of possible worlds. Aumann shows that a proposition C is common knowledge if, and only if, C contains a cell of the meet of the agents’ partitions. One way to compute the meet \(\mathcal{M}\) of the partitions \(\mathcal{H}_i, i \in N\) is to use the idea of “reachability”.
Definition 2.13 A state \(\omega ' \in \Omega\) is reachable from \(\omega \in \Omega\) iff there exists a sequence
such that for each \(k \in \{0,1, \ldots ,m-1\}\), there exists an agent \(i_k \in N\) such that \(\mathcal{H}_{i_{ k} }(\omega_k) = \mathcal{H}_{i_{ k} }(\omega_{k+1})\).
In words, \(\omega '\) is reachable from \(\omega\) if there exists a sequence or “chain” of states from \(\omega\) to \(\omega '\) such that two consecutive states are in the same cell of some agent’s information partition. To illustrate the idea of reachability, let us return to the modified Barbecue Problem in which Cathy, Jennifer and Mark receive no announcement. Their information partitions are all depicted in Figure 2.1d:
Figure 2.1d
One can understand the importance of the notion of reachability in the following way: If \(\omega '\) is reachable from \(\omega\), then if \(\omega\) obtains then some agent can reason that some other agent thinks that \(\omega '\) is possible. Looking at Figure 2.1d, if \(\omega = \omega_1\) occurs, then Cathy (who knows only that \(\{\omega_1, \omega_2\}\) has occurred) knows that Jennifer thinks that \(\omega_5\) might have occurred (even though Cathy knows that \(\omega_5\) did not occur). So Cathy cannot rule out the possibility that Jennifer thinks that Mark thinks that that \(\omega_8\) might have occurred. And Cathy cannot rule out the possibility that Jennifer thinks that Mark thinks that Cathy believes that \(\omega_7\) is possible. In this sense, \(\omega_7\) is reachable from \(\omega_1\). The chain of states which establishes this is \(\omega_1, \omega_2, \omega_5, \omega_8, \omega_7\), since \(\mathcal{H}_1 (\omega_1) = \mathcal{H}_1 (\omega_2),\) \(\mathcal{H}_2 (\omega_2) = \mathcal{H}_2 (\omega_5),\) \(\mathcal{H}_3 (\omega_5) = \mathcal{H}_3 (\omega_8),\) and \(\mathcal{H}_1 (\omega_8) = \mathcal{H}_1 (\omega_7)\). Note that one can show similarly that in this example any state is reachable from any other state. This example also illustrates the following immediate result:
Proposition 2.14 \(\omega'\) is reachable from \(\omega\) iff there is a sequence \(i_1, i_2 , \ldots ,i_m \in N\) such that
One can read (1) as: ‘At \(\omega , i_1\) thinks that \(i_2\) thinks that \(\ldots ,i_m\) thinks that \(\omega'\) is possible.’
We now have:
Lemma 2.15 \(\omega' \in \mathcal{M}(\omega)\) iff \(\omega '\) is reachable from \(\omega\). Proof .
Lemma 2.16 \(\mathcal{M}(\omega)\) is common knowledge for the agents of \(N\) at \(\omega\). Proof .
Proposition 2.17 (Aumann 1976) Let \(\mathcal{M}\) be the meet of the agents’ partitions \(\mathcal{H}_i\) for each \(i \in N\). A proposition \(E \subseteq \Omega\) is common knowledge for the agents of \(N\) at \(\omega\) iff \(\mathcal{M}(\omega) \subseteq E\). (In Aumann (1976), \(E\) is defined to be common knowledge at \(\omega\) iff \(\mathcal{M}(\omega) \subseteq E\).) Proof .
If \(E = \mathbf{K}^1_N (E)\), then \(E\) is a public event (Milgrom 1981) or a common truism (Binmore and Brandenburger 1989). Clearly, a common truism is common knowledge whenever it occurs, since in this case \(E = \mathbf{K}^1_N (E) = \mathbf{K}^2_N (E) =\ldots\) , so \(E = \mathbf{K}^*_N (E)\). The proof of Proposition 2.17 shows that the common truisms are precisely the elements of \(\mathcal{M}\) and unions of elements of \(\mathcal{M}\), so any commonly known event is the consequence of a common truism.
Barwise (1988) proposes another definition of common knowledge that avoids explicit reference to the hierarchy of ‘\(i\) knows that \(j\) knows that … knows that \(A\)’ propositions. Barwise’s analysis builds upon an informal proposal by Harman (1977). Consider the situation of the guest and clumsy waiter in Example 1 when he announces that he was at fault. They are now in a setting where they have heard the waiter’s announcement and know that they are in the setting. Harman adopts the circularity in this characterization of the setting as fundamental, and propses a definition of common knowledge in terms of this circularity. Barwise’s formal analysis gives a precise formulation of Harman’s intuitive analysis of common knowledge as a fixed point . Given a function \(f, A\) is a fixed point of \(f\) if \(f(A)=A.\) Now note that
So we have established that \(\mathbf{K}^{*}_N (E)\) is a fixed point of the function \(f_E\) defined by \(f_E (X) = \mathbf{K}^{1}_N (E \cap X). f_E\) has other fixed points. For instance, any contradiction \(B \cap B^c = \varnothing\) is a fixed point of \(f_E\). [ 15 ] Note also that if \(A \subseteq B\), then \(E \cap A \subseteq E \cap B\) and so
that is, \(f_E\) is monotone . (We saw that \(\mathbf{K}^1_N\) is also monotone in the proof of Proposition 2.4.) Barwise’s analysis of common knowledge can be developed using the following result from set theory:
Proposition A monotone function \(f\) has a unique fixed point \(C\) such that if \(B\) is a fixed point of \(f\), then \(B\subseteq C.\) \(C\) is the greatest fixed point of \(f.\)
This proposition establishes that \(f_E\) has a greatest fixed point, which characterizes common knowledge in Barwise’s account. As Barwise himself observes, the fixed point analysis of common knowledge is closely related to Aumann’s partition account. This is easy to see when one compares the fixed point analysis to the notion of common truisms that Aumann’s account generates. Some authors regard the fixed point analysis as an alternate formulation of Aumann’s analysis. Barwise’s fixed point analysis of common knowledge is favored by those who are especially interested in the applications of common knowledge to problems in logic, while the hierarchical and the partition accounts are favored by those who wish to apply common knowledge in social philosophy and social science. When knowledge operators satisfy the axioms (K1)-(K5), the Barwise account of common knowledge is equivalent to the hierarchical account.
Proposition 2.18 Let \(C^*_N\) be the greatest fixed point of \(f_E.\) Then \(C^{*}_N (E) = K^*_N (E).\) (In Barwise (1988, 1989), \(E\) is defined to be common knowledge at \(\omega\) iff \(\omega \in C^*_N(E).)\) Proof .
Barwise argues that in fact the fixed point analysis is more flexible and consequently more general than the hierarchical account. This may surprise readers in light of Proposition 2.18, which shows that Barwise’s fixed point definition is equivalent to the hierarchical account. Indeed, while Barwise (1988, 1989) proves a result showing that the fixed point account implies the hierarchical account and gives examples that satisfy the common knowledge hierarchy but fail to be fixed points, a number of authors who have written after Barwise have given various proofs of the equivalence of the two definitions, as was shown in Proposition 2.18. In fact, as (Heifetz 1999) shows, the hierarchical and fixed-point accounts are equivalent for all finite levels of iteration, while fixed-point common knowledge implies the conjunction of mutual knowledge up to any transfinite order, but it is never implied by any such conjunction.
Gilbert (1989, Chapter 3) presents an alternative account of common knowledge, which is meant to be more intuitively plausible than Lewis’ and Aumann’s accounts. Gilbert gives a highly detailed description of the circumstances under which agents have common knowledge.
Definition 2.19 A set of agents \(N\) are in a common knowledge situation \(\mathcal{S}(A)\) with respect to a proposition \(A\) if, and only if, \(\omega \in A\) and for each \(i \in N\),
Gilbert’s definition appears to contain some redundancy, since presumably an agent would not perceive A unless A is the case. Gilbert is evidently trying to give a more explicit account of single agent knowledge than Lewis and Aumann give. For Gilbert, agent \(i\) knows that a proposition \(E\) is the case if, and only if, \(\omega \in E\), that is, \(E\) is true, and either \(i\) perceives that the state of affairs \(E\) describes obtains or \(i\) can infer \(E\) as a consequence of other propositions \(i\) knows, given sufficient inferential capacity.
Like Lewis, Gilbert recognizes that human agents do not in fact have unlimited inferential capacity. To generate the infinite hierarchy of mutual knowledge, Gilbert introduces the device of an agent’s smooth-reasoner counterpart. The smooth-reasoner counterpart \(i'\) of an agent \(i\) is an agent that draws every logical conclusion from every fact that \(i\) knows. Gilbert stipulates that \(i'\) does not have any of the constraints on time, memory, or reasoning ability that \(i\) might have, so \(i'\) can literally think through the infinitely many levels of a common knowledge hierarchy.
Definition 2.20 If a set of agents \(N\) are in a common knowledge situation \(\mathcal{S}_N (A)\) with respect to \(A\), then the corresponding set \(N'\) of their smooth-reasoner counterparts is in a parallel situation \(\mathcal{S}'_{N'}(A)\) if, and only if, for each \(i' \in N\),
From this definition we get the following immediate consequence:
Proposition 2.21 If a set of smooth-reasoner counterparts to a set \(N\) of agents are in a situation \(\mathcal{S}'_{N'}(A)\) parallel to a common knowledge situation \(\mathcal{S}_N (A)\) of \(N\), then
for all \(m \in \mathbb{N}\) and for any \(i_1', \ldots ,i_m', \mathbf{K}_{i_1'}\mathbf{K}_{i_2'} \ldots \mathbf{K}_{i_m'}(A)\).
Consequently, \(\mathbf{K}^{m}_{N'}(A)\) for any \(m \in \mathbb{N}.\)
Gilbert argues that, given \(\mathcal{S}'_{N'}(A)\), the smooth-reasoner counterparts of the agents of \(N\) actually satisfy a much stronger condition, namely mutual knowledge \(\mathbf{K}^{\alpha}_{N'}(A)\) to the level of any ordinal number \(\alpha\), finite or infinite. When this stronger condition is satisfied, the proposition \(A\) is said to be open* to the agents of \(N\). With the concept of open*-ness, Gilbert gives her definition of common knowledge.
Definition 2.22 A proposition \(E \subseteq \Omega\) is Gilbert-common knowledge among the agents of a set \(N = \{1, \ldots,n\},\) if and only if,
\(\mathbf{G}_N^*(E)\) denotes the proposition defined by \(G_1^*\) and \(G_2^*\) for a set \(N\) of \(A^*\)-symmetric reasoners, so we can say that \(E\) is Lewis-common knowledge for the agents of \(N\) iff \(\omega \in \mathbf{G}_N^*(E)\).
One might think that an immediate corollary to Gilbert’s definition is that Gilbert-common knowledge implies the hierarchical common knowledge of Proposition 2.5. However, this claim follows only on the assumption that an agent knows all of the propositions that her smooth-reasoner counterpart reasons through. Gilbert does not explicitly endorse this position, although she correctly observes that Lewis and Aumann are committed to something like it. [ 17 ] Gilbert maintains that her account of common knowledge expresses our intuitions with respect to common knowledge better than Lewis’ and Aumann’s accounts, since the notion of open*-ness presumably makes explicit that when a proposition is common knowledge, it is “out in the open”, so to speak.
3. Applications of Mutual and Common Knowledge
Readers primarily interested in philosophical applications of common knowledge may want to focus on the No Disagreement Theorem and Convention subsections. Readers interested in applications of common knowledge in game theory may continue with the Strategic Form Games, and Games of Perfect Information subsections.
Aumann (1976) originally used his definition of common knowledge to prove a celebrated result that says that in a certain sense, agents cannot “agree to disagree” about their beliefs, formalized as probability distributions, if they start with common prior beliefs. Since agents in a community often hold different opinions and know they do so, one might attribute such differences to the agents’ having different private information. Aumann’s surprising result is that even if agents condition their beliefs on private information, mere common knowledge of their conditioned beliefs and a common prior probability distribution implies that their beliefs cannot be different, after all!
Proposition 3.1 Let \(\Omega\) be a finite set of states of the world. Suppose that
- Agents \(i\) and \(j\) have a common prior probability distribution \(\mu(\cdot)\) over the events of \(\Omega\) such that \(\mu(\omega) \gt 0\), for each \(\omega \in \Omega\), and
- It is common knowledge at \(\omega\) that \(i\)’s posterior probability of event \(E\) is \(q_i(E)\) and that \(j\)’s posterior probability of \(E\) is \(q_j(E)\).
Proof . [Note that in the proof of this proposition, and in the sequel, \(\mu(\cdot\mid B)\) denotes conditional probability; that is, given \(\mu(B)\gt 0, \mu(A\mid B) = \mu(A\cap B)/\mu(B)\).]
In a later article, Aumann (1987) argues that the assumptions that \(\Omega\) is finite and that \(\mu(\omega) \gt 0\) for each \(\omega \in \Omega\) reflect the idea that agents only regard as “really” possible a finite collection of salient worlds to which they assign positive probability, so that one can drop the states with probability 0 from the description of the state space. Aumann also notes that this result implicitly assumes that the agents have common knowledge of their partitions, since a description of each possible world includes a description of the agents’ possibility sets. And of course, this result depends crucially upon (i), which is known as the common prior assumption (CPA).
Aumann’s “no disagreement” theorem has been generalized in a number of ways in the literature. Cave 1983 generalizes the argument to 3 agents. Bacharach 1985 extends it to cases in which agents observe each other’s decisions rather than posteriors. Milgrom and Stokey, 1982 use it crucially for their no-trade theorem, applying no disagreement to show that speculative trade is impossible. Geanakoplos and Polemarchakis 1982 generalize the argument to a dynamic setting in which two agents communicate their posterior probabilities back and forth until they reach an agreement – this particular take on the agreement theorem has been characterized in terms of dynamic epistemic logic by Dégremont and Roy, 2009 and applied to cases of epistemic peer disagreement by Sillari 2019. McKelvey and Page 1986 further extend the results of Geanakoplos and Polemarchakis to the case of \(n\) individuals. (See also Monderer and Samet 1989 and, for a survey, Geanakoplos 1994.)
However, all of these “no disagreement” results raise the same philosophical puzzle raised by Aumann’s original result: How are we to explain differences in belief? Aumann’s result leaves us with two options: (1) admit that at some level, common knowledge of the agents’ beliefs or how they form their beliefs fails, or (2) deny the CPA. Thus, even if agents do assign precise posterior probabilities to an event, Aumann shows that if they have merely first-order mutual knowledge of the posteriors, they can “agree to disagree”. [ 18 ] Another way Aumann’s result might fail is if agents do not have common knowledge that they update their beliefs by Bayesian conditionalization. Then clearly, agents can explain divergent opinions as the result of others having modified their beliefs in the “wrong” way. However, there are cases in which neither explanation will seem convincing and denying the requisite common knowledge seems a rather ad hoc move. Why should one think that such failures of common knowledge provide a general explanation for divergent beliefs?
What of the second option, that is, denying the CPA? [ 19 ] The main argument put forward in favor of the CPA is that any differences in agents’ probabilities should be the result of their having different information only, that is, there is no reason to think that the different beliefs that agents have regarding the same event are the result of anything other than their having different information. However, one can reply that this argument amounts simply to a restatement of the Harsanyi Doctrine. [ 20 ]
Schelling’s Department Store problem of Example 1.5 is a very simple example in which the agents “solve” their coordination problem appropriately by establishing a convention. (see also the entry on convention in this encyclopedia.) Using the vocabulary of game theory, Lewis (1969) defines a convention as a strict coordination equilibrium of a game which agents follow on account of their common knowledge that they all prefer to follow this coordination equilibrium in a recurrent coordination problem. A coordination equilibrium of a game is a strategy combination such that no agent is better off if any agent unilaterally deviates from this combination. As with equilibria in general, a coordination equilibrium is strict if any agent who deviates unilaterally from the equilibrium is strictly worse off. The strategic form game of Figure 1.3 summarizes Liz’s and Robert’s situation. The Department Store game has four Nash equilibrium outcomes in pure strategies: \((s_1, s_1),\) \((s_2, s_2),\) \((s_3, s_3)\), and \((s_4, s_4)\). [ 21 ] These four equilibria are all strict coordination equilibria. If the agents follow either of these equilibria, then they coordinate successfully. For agents to be following a Lewis-convention in this situation, they must follow one of the game’s coordination equilibria. However, for Lewis to follow a coordination equilibrium is not a sufficient condition for agents to be following a convention. For suppose that Liz and Robert fail to analyze their predicament properly at all, but Liz chooses \(s_2\) and Robert chooses \(s_2\), so that they coordinate at \((s_2, s_2)\) by sheer luck. Lewis does not count accidental coordination of this sort as a convention.
Suppose next that both agents are Bayesian rational, and that part of what each agent knows is the payoff structure of the Intersection game. If the agents expect each other to follow \((s_2, s_2)\) and they consequently coordinate successfully, are they then following a convention? Not necessarily, contends Lewis in a subtle argument on p. 59 of Convention. For while each agent knows the game and that she is rational, still she might not attribute the same knowledge to the other agent. If each agent believes that the other agent will follow her end of the \((s_2, s_2)\) equilibrium mindlessly, then her best response is to follow her end of \((s_2, s_2)\). But in this case the agents coordinated as the result of their each falsely believing that the other acts like an automaton, and Lewis thinks that any proper account of convention must require that agents have correct beliefs about one another. In particular, Lewis requires that each agent involved in a convention must have mutual expectations that each is acting with the aim of coordinating with the other. The argument can be carried further on. What if both agents believe that they will follow \((s_2, s_2)\), and believe that each other will do so thinking that the other will choose \(s_2\) rationally and not mindlessly? Then, say, Liz would coordinate as the result of her false second-order belief that Robert believes that Liz acts mindlessly. Similarly for third-order beliefs and so on for any higher order of knowledge.
Lewis concludes that a necessary condition for agents to be following a convention is that their preferences to follow the corresponding coordination equilibrium be common knowledge (the issue whether conventions need to be common knowledge has been debated recently, cf. Cubitt and Sugden 2003, Binmore 2008, Sillari 2008, and, for an experimental approach, see Devetag et al. 2013, for a connection to the topic of rule-following, see Sillari 2013). So on Lewis’ account, a convention for a set of agents is a coordination equilibrium which the agents follow on account of their common knowledge of their rationality, the payoff structure of the relevant game and that each agent follows her part of the equilibrium.
A regularity \(R\) in the behavior of members of a population \(P\) when they are agents in a recurrent situation \(S\) is a convention if and only if it is true that, and it is common knowledge in \(P\) that, in any instance of \(S\) among the members of \(P\), everyone conforms to \(R\); everyone expects everyone else to conform to \(R\); everyone has approximately the same preferences regarding all possible combinations of actions; everyone prefers that everyone conform to \(R\), on condition that at least all but one conform to R; everyone would prefer that everyone conform to \(R'\), on condition that at least all but one conform to \(R'\), where \(R'\) is some possible regularity in the behavior of members of \(P\) in \(S\), such that no one in any instance of \(S\) among members of \(P\) could conform both to \(R'\) and to \(R\). (Lewis 1969, p. 76) [ 22 ]
Lewis includes the requirement that there be an alternate coordination equilibrium \(R'\) besides the equilibrium \(R\) that all follow in order to capture the fundamental intuition that how the agents who follow a convention behave depends crucially upon how they expect the others to behave.
Sugden (1986) and Vanderschraaf (1998) argue that it is not crucial to the notion of convention that the corresponding equilibrium be a coordination equilibrium. Lewis’ key insight is that a convention is a pattern of mutually beneficial behavior which depends on the agents’ common knowledge that all follow this pattern, and no other. Vanderschraaf gives a more general definition of convention as a strict equilibrium together with common knowledge that all follow this equilibrium and that all would have followed a different equilibrium had their beliefs about each other been different. An example of this more general kind of convention is given below in the discussion of the Figure 3.1 example.
Lewis formulated the notion of common knowledge as part of his general account of conventions. In the years following the publication of Convention , game theorists have recognized that any explanation of a particular pattern of play in a game depends crucially on mutual and common knowledge assumptions. More specifically, solution concepts in game theory are both motivated and justified in large part by the mutual or common knowledge the agents in the game have regarding their situation.
To establish the notation that will be used in the discussion that follows, the usual definitions of a game in strategic form, expected utility and agents’ distributions over their opponents’ strategies, are given here:
- A finite set \(N = \{1,2, \ldots ,n\}\), called the set of agents or players.
- For each agent \(k \in N\), there is a finite set \(S_k = \{s_{k1},s_{k2}, \ldots ,s_{kn_k}\}\), called the alternative pure strategies for agent \(k\). The Cartesian product \(S = S_1 \times \ldots \times S_n\) is called the pure strategy set for the game \(\Gamma\).
where \(I_k (\boldsymbol{x})\) projects \(\boldsymbol{x} \in \Re^n\) onto its \(k\) th component.
The subscript ‘\(-k\)’ indicates the result of removing the \(k\) th component of an \(n\)-tuple or an \(n\)-fold Cartesian product. For instance,
denotes the pure strategy combinations that agent \(k\)’s opponents may play.
Now let us formally introduce a system of the agents’ beliefs into this framework. \(\Delta_k (S_{-k})\) denotes the set of probability distributions over the measurable space \((S_{-k}, \mathfrak{F}_k)\), where \(\mathfrak{F}_k\) denotes the Boolean algebra generated by the strategy combinations \(S_{-k}\). Each agent \(k\) has a probability distribution \(\mu_k \in \Delta_k(S_{-k})\), and this distribution determines the ( Savage ) expected utilities for each of \(k\)’s possible acts:
If \(i\) is an opponent of \(k\), then \(i\)’s individual strategy \(s_{i j}\) may be characterized as a union of strategy combinations \(\bigcup\{\boldsymbol{s}_{-k}\mid s_{ij}\in \boldsymbol{s}_{-k}\} \in \mathfrak{F}_k\), and so \(k\)’s marginal probability for \(i\)’s strategy \(s_{i j}\) may be calculated as follows:
\(\mu_k(\cdot \mid A)\) denotes \(k\)’s conditional probability distribution given a set \(A\), and \(E(\cdot \mid A)\) denotes \(k\)’s conditional expectation given \(\mu_k(\cdot\mid A).\)
Suppose first that the agents have common knowledge of the full payoff structure of the game they are engaged in and that they are all rational, and that no other information is common knowledge. In other words, each agent knows that her opponents are expected utility maximizers, but does not in general know exactly which strategies they will choose or what their probabilities for her acts are. These common knowledge assumptions are the motivational basis for the solution concept for noncooperative games known as rationalizability , introduced independently by Bernheim (1984) and Pearce (1984). Roughly speaking, a rationalizable strategy is any strategy an agent may choose without violating common knowledge of Bayesian rationality. Bernheim and Pearce argue that when only the structure of the game and the agents’ Bayesian rationality are common knowledge, the game should be considered “solved” if every agent plays a rationalizable strategy. For instance, in the “Chicken” game with payoff structure defined by Figure 3.1,
if Joanna and Lizzi have common knowledge of all of the payoffs at every strategy combination, and they have common knowledge that both are Bayesian rational, then any of the four pure strategy profiles is rationalizable. For if their beliefs about each other are defined by the probabilities
so each agent maximizes her expected utility by playing \(s_1\) if \(\alpha_i + 2 \ge 4\alpha_i\) or \(\alpha_i \le 2/3\) and maximizes her expected utility by playing \(s_2\) if \(\alpha_i \ge 2/3\). If it so happens that \(\alpha_i \gt 2/3\) for both agents, then both conform with Bayesian rationality by playing their respective ends of the strategy combination \((s_2,s_2)\) given their beliefs , even though each would want to defect from this strategy combination were she to discover that the other is in fact going to play \(s_2\). Note that the game’s pure strategy Nash equilibria, \((s_1, s_2)\) and \((s_2, s_1)\), are rationalizable, since it is rational for Lizzi and Joanna to conform with either equilibrium given appropriate distributions. In general, the set of a game’s rationalizable strategy combinations contains the set of the game’s pure strategy Nash equilibria. [ 23 ]
Rationalizability can be defined formally in several ways. A variation of Bernheim’s original (1984) definition is given here.
is Bayes concordant if and only if,
and (3.i) is common knowledge. A pure strategy combination \(\boldsymbol{s} = (s_{1j_1}, \ldots ,s_{nj_n}) \in S\) is rationalizable if and only if the agents have a Bayes concordant system \(\mu\) of beliefs and, for each agent \(k \in N\),
The following result shows that the common knowledge restriction on the distributions in Definition 3.1 formalizes the assumption that the agents have common knowledge of Bayesian rationality.
Proposition 3.4 In a game \(\Gamma\), common knowledge of Bayesian rationality is satisfied if, and only if, (3.i) is common knowledge. Proof .
When agents have common knowledge of the game and their Bayesian rationality only, one can predict that they will follow a rationalizable strategy profile. However, rationalizability becomes an unstable solution concept if the agents come to know more about one another. For instance, in the Chicken example above with \(\alpha_i \gt 2/3, i = 1, 2\), if either agent were to discover the other agent’s beliefs about her, she would have good reason not to follow the \((s_2,s_2)\) profile and to revise her own beliefs regarding the other agent. If, in the other hand, it so happens that \(\alpha_1 = 1\) and \(\alpha_2 = 0\), so that the agents maximize expected payoff by following the \((s_2, s_1)\) profile, then should the agents discover their beliefs about each other, they will still follow \((s_2, s_1)\). Indeed, if their beliefs are common knowledge, then one can predict with certainty that they will follow \((s_2,s_1)\). The Nash equilibrium \((s_2,s_1)\) is characterized by the belief distributions defined by \(\alpha_1 = 1\) and \(\alpha_2 = 0\).
The Nash equilibrium is a special case of correlated equilibrium concepts , which are defined in terms of the belief distributions of the agents in a game. In general, a correlated equilibrium-in-beliefs is a system of agents’ probability distributions which remains stable given common knowledge of the game, rationality and the beliefs themselves . We will review two alternative correlated equilibrium concepts (Aumann 1974, 1987; Vanderschraaf 1995, 2001), and show how each generalizes the Nash equilibrium concept.
Definition 3.5 Given that each agent \(k \in N\) has a probability distribution \(\mu_k \in \Delta_k (s_{-k})\), the system of beliefs
is an endogenous correlated equilibrium if, and only if,
If \(\boldsymbol{\mu}^*\) is an endogenous correlated equilibrium a pure strategy combination \(\boldsymbol{s}^* = (s_1^*, \ldots ,s_n^*) \in S\) is an endogenous correlated equilibrium strategy combination given \(\boldsymbol{\mu}^*\) if, and only if, for each agent \(k \in N,\)
Hence, the endogenous correlated equilibrium \(\boldsymbol{\mu}^*\) restricts the set of strategies that the agents might follow, as do the Bayes concordant beliefs of rationalizability. However, the endogenous correlated equilibrium concept is a proper refinement of rationalizability, because the latter does not presuppose that condition (3.iii) holds with respect to the beliefs one’s opponents actually have. If exactly one pure strategy combination \(\boldsymbol{s}^*\) satisfies (3.iv) given \(\boldsymbol{\mu}^*\), then \(\boldsymbol{\mu}^*\) is a strict equilibrium , and in this case one can predict with certainty what the agents will do given common knowledge of the game, rationality and their beliefs. Note that Definition 3.5 says nothing about whether or not the agents regard their opponents’ strategy combinations as probabilistically independent. Also, this definition does not require that the agents’ probabilities are consistent , in the sense that agents’ probabilities for a mutual opponent’s acts agree. A simple refinement of the endogenous correlated equilibrium concept characterizes the Nash equilibrium concept.
- condition (3.iii) is satisfied,
- For each \(k \in N, \mu_k^*\) satisfies probabilistic independence, and
- For each \(s_{kj} \in s_k\), if \(i, l \ne k\) then \(\mu_i^*(s_{kj}) = \mu_l^*(s_{kj})\).
In other words, an endogenous correlated equilibrium is a Nash equilibrium-in-beliefs when each agent regards the moves of his opponents as probabilistically independent and the agents’ probabilities are consistent. Note that in the 2-agent case, conditions (b) and (c) of the Definition 3.6 are always satisfied, so for 2-agent games the endogenous correlated equilibrium concept reduces to the Nash equilibrium concept. Conditions (b) and (c) are traditionally assumed in game theory, but Skyrms (1991) and Vanderschraaf (1995, 2001) argue that there may be good reasons to relax these assumptions in games with 3 or more agents.
Brandenburger and Dekel (1988) show that in 2-agent games, if the beliefs of the agents are common knowledge, condition (3.iii) characterizes a Nash equilibrium-in-beliefs. As they note, condition (3.iii) characterizes a Nash equilibrium in beliefs for the \(n\)-agent case if the probability distributions are consistent and satisfy probabilistic independence. Proposition 3.7 extends Brandenburger and Dekel’s result to the endogenous correlated equilibrium concept by relaxing the consistency and probabilistic independence assumptions.
Proposition 3.7 Assume that the probabilities
are common knowledge. Then common knowledge of Bayesian rationality is satisfied if, and only if, \(\boldsymbol{\mu}\) is an endogenous correlated equilibrium. Proof .
In addition, we have:
Corollary 3.8 (Brandenburger and Dekel, 1988) Assume in a 2-agent game that the probabilities
are common knowledge. Then common knowledge of Bayesian rationality is satisfied if, and only if, \(\boldsymbol{\mu}\) is a Nash equilibrium.
Proof. The endogenous correlated equilibrium concept reduces to the Nash equilibrium concept in the 2-agent case, so the corollary follows by Proposition 3.7.
If \(\boldsymbol{\mu}^*\) is a strict equilibrium, then one can predict which pure strategy profile the agents in a game will follow given common knowledge of the game, rationality and \(\boldsymbol{\mu}^*.\) But if \(\boldsymbol{\mu}^*\) is such that several distinct pure strategy profiles satisfy (3.iv) with respect to \(\boldsymbol{\mu}^*\), then one can no longer predict with certainty what the agents will do. For instance, in the Chicken game of Figure 3.1, the belief distributions defined by \(\alpha_1 = \alpha_2 = 2/3\) together are a Nash equilibrium-in-beliefs. Given common knowledge of this equilibrium, either pure strategy is a best reply for each agent, in the sense that either pure strategy maximizes expected utility. Indeed, if agents can also adopt randomized or mixed strategies at which they follow one of several pure strategies according to the outcome of a chance experiment, then any of the infinitely mixed strategies an agent might adopt in Chicken is a best reply given \(\boldsymbol{\mu}^*\). [ 25 ] So the endogenous correlated equilibrium concept does not determine the exact outcome of a game in all cases, even if one assumes probabilistic consistency and independence so that the equilibrium is a Nash equilibrium.
Another correlated equilibrium concept formalized by Aumann (1974, 1987) does give a determinate prediction of what agents will do in a game given appropriate common knowledge. To illustrate Aumann’s correlated equilibrium concept, let us consider the Figure 3.1 game once more. If Joanna and Lizzi can tie their strategies to their knowledge of the possible worlds in a certain way, they can follow a system of correlated strategies which will yield a payoff vector they both prefer to that of the mixed Nash equilibrium and which is itself an equilibrium. One way they can achieve this is to have their friend Ron play a variation of the familiar shell game by hiding a pea under one of three walnut shells, numbered 1, 2 and 3. Joanna and Lizzi both think that each of the three relevant possible worlds corresponding to \(\omega_k = \{\)the pea lies under shell \(k\}\) is equally likely. Ron then gives Lizzi and Joanna each a private recommendation, based upon the outcome of the game, which defines a system of strategy combinations f as follows
\(f\) is a correlated strategy system because the agents tie their strategies, by following their recommendations, to the same set of states of the world \(\Omega\). \(f\) is also a strict Aumann correlated equilibrium , for if each agent knows how Ron makes his recommendations, but knows only the recommendation he gives her, either would do strictly worse were she to deviate from her recommendation. [ 26 ] Since there are several strict equilibria of Chicken, \(f\) corresponds to a convention as defined in Vanderschraaf (1998). The overall expected payoff vector of \(f\) is (3,3), which lies outside the convex hull of the payoffs for the game’s Nash equilibria and which Pareto-dominates the expected payoff vector (4/3, 4/3), of the mixed Nash equilibrium defined by \(\alpha_1 = 2/3\), \(i = 1, 2.\) [ 27 ] The correlated equilibrium f is characterized by the probability distribution of the agents’ play over the strategy profiles, given in Figure 3.3:
Aumann (1987) proves a result relating his correlated equilibrium concept to common knowledge. To review this result, we must give the formal definition of Aumann correlated equilibrium.
for each \(k \in N\) and for any function \(g_k\) that is a function of \(f_i\).
The agents are at Aumann correlated equilibrium if at each possible world \(\omega \in \Omega\), no agent will want to deviate from his recommended strategy, given that the others follow their recommended strategies. Hence, Aumann correlated equilibrium uniquely specifies the strategy of each agent, by explicitly introducing a space of possible worlds to which agents can correlate their acts. The deviations \(g_i\) are required to be functions of \(f_i\), that is, compositions of some other function with \(f_i\), because \(i\) is informed of \(f_i (\omega)\) only, and so can only distinguish between the possible worlds of \(\Omega\) that are distinguished by \(f_i\). As noted already, the primary difference between Aumann’s notion of correlated equilibrium and the endogenous correlated equilibrium is that in Aumann’s correlated equilibrium, the agents correlate their strategies to some event \(\omega \in \Omega\) that is external to the game. One way to view this difference is that agents who correlate their strategies exogenously can calculate their expected utilities conditional on their own strategies.
In Aumann’s model, a description of each possible world \(\omega\) includes descriptions of the following: the game \(\Gamma\), the agent’s private information partitions, and the actions chosen by each agent at \(\omega\), and each agent’s prior probability distribution \(\mu_k(\cdot)\) over \(\Omega\). The basic idea is that conditional on \(\omega\), everyone knows everything that can be the object of uncertainty on the part of any agent, but in general, no agent necessarily knows which world \(\omega\) is the actual world. The agents can use their priors to calculate the probabilities that the various act combinations \(\boldsymbol{s} \in S\) are played. If the agents’ priors are such that for all \(i, j \in N,\) \(\mu_i(\omega) = 0\) iff \(\mu_j (\omega) = 0,\) then the agents’ priors are mutually absolutely continuous . If the agents’ priors all agree, that is, \(\mu_1 (\omega) = \ldots = \mu_n (\omega) = \mu(\omega)\) for each \(\omega \in \Omega\), then it is said that the common prior assumption , or CPA, is satisfied. If agents are following an Aumann correlated equilibrium \(f\) and the CPA is satisfied, then \(f\) is an objective Aumann correlated equilibrium. An Aumann correlated equilibrium is a Nash equilibrium if the CPA is satisfied and the agents’ distributions satisfy probabilistic independence. [ 28 ]
Let \(s_i (\omega)\) denote the strategy chosen by agent \(i\) at possible world \(\omega\). Then \(s: \Omega \rightarrow S\) defined by \(s(\omega) = ( s_1 (\omega),\ldots ,s_n (\omega) )\) is a correlated \(n\)-tuple. Given that \(\mathcal{H}_i\) is a partition of \(\Omega\), [ 29 ] the function \(s_i : \Omega \rightarrow s_i\) defined by \(s\) is \(\mathcal{H}_i\)- measurable if for each \(\mathcal{H}_{ij} \in \mathcal{H}_{i}, s_i (\omega')\) is constant for each \(\omega' \in \mathcal{H}_{ij}.\) \(\mathcal{H}_i\)-measurability is a formal way of saying that \(i\) knows what she will do at each possible world, given her information.
Definition 3.10 Agent \(i\) is Bayes rational with respect to \(\omega \in \Omega\) (alternatively, \(\omega\) -Bayes rational ) iff \(s_i\) is \(\mathcal{H}_i\)-measurable and
for any \(\mathcal{H}_i\)-measurable function \(v_i : \Omega \rightarrow s_i\).
Note that Aumann’s definition of \(\omega\)-Bayesian rationality implies that \(\mu_i (\mathcal{H}_i (\omega)) \gt 0\), so that the conditional expectations are defined. Aumann’s main result, given next, implicitly assumes that \(\mu_i (\mathcal{H}_i (\omega)) \gt 0\) for every agent \(i \in N\) and every possible world \(\omega \in \Omega\). This poses no technical difficulties if the CPA is satisfied, or even if the priors are only mutually absolutely continuous, since if this is the case then one can simply drop any \(\omega\) with zero prior from consideration.
Proposition 3.11 (Aumann 1987) If each agent \(i \in N\) is \(\omega\)-Bayes rational at each possible world \(\omega \in \Omega\), then the agents are following an Aumann correlated equilibrium. If the CPA is satisfied, then the correlated equilibrium is objective. Proof .
Part of the uncertainty the agents might have about their situation is whether or not all agents are rational. But if it is assumed that all agents are \(\omega\)-Bayesian rational at each \(\omega \in \Omega\), then a description of this fact forms part of the description of each possible \(\omega\) and thus lies in the meet of the agents’ partitions. As noted already, descriptions of the agents’ priors, their partitions and the game also form part of the description of each possible world, so propositions corresponding to these facts also lie in the meet of the agents’ partitions. So another way of stating Aumann’s main result is as follows: Common knowledge of \(\omega\)- Bayesian rationality at each possible world implies that the agents follow an Aumann correlated equilibrium .
Propositions 3.7 and 3.11 are powerful results. They say that common knowledge of rationality and of agents beliefs about each other, quantified as their probability distributions over the strategy profiles they might follow, implies that the agents’ beliefs characterize an equilibrium of the game. Then if the agents’ beliefs are unconditional, Proposition 3.7 says that the agents are rational to follow a strategy profile consistent with the corresponding endogenous correlated equilibrium. If their beliefs are conditional on their private information partitions, then Proposition 3.11 says they are rational to follow the strategies the corresponding Aumann correlated equilibrium recommends. However, we must not overestimate the importance of these results, for they say nothing about the origins of the common knowledge of rationality and beliefs. For instance, in the Chicken game of Figure 3.1, we considered an example of a correlated equilibrium in which it was assumed that Lizzi and Joanna had common knowledge of the system of recommended strategies defined by \((\star).\) Given this common knowledge, Joanna and Lizzi indeed have decisive reason to follow the Aumann correlated equilibrium f. But where did this common knowledge come from? How, in general, do agents come to have the common knowledge which justifies their conforming to an equilibrium? Philosophers and social scientists have made only limited progress in addressing this question.
In extensive form games, the agents move in sequence. At each stage, the agent who is to move must base her decisions upon what she knows about the preceding moves. This part of the agent’s knowledge is characterized by an information set , which is the set of alternative moves that an agent knows her predecessor might have chosen. For instance, consider the extensive form game of Figure 3.4:
When Joanna moves she is at her information set \(I^{22} = \{C^1, D^1\},\) that is, she moves knowing that Lizzi might have chosen either \(C^1\) or \(D^1\), so this game is an extensive form representation of the Chicken game of Figure 3.1.
In a game of perfect information, each information set consists of a single node in the game tree, since by definition at each state the agent who is to move knows exactly how her predecessors have moved. In Example 1.4 it was noted that the method of backwards induction can be applied to any game of perfect information. [ 30 ] The backwards induction solution is the unique Nash equilibrium of a game of perfect information. The following result gives sufficient conditions to justify backwards induction play in a game of perfect information:
Proposition 3.12 (Bicchieri 1993) In an extensive form game of perfect information, the agents follow the backwards induction solution if the following conditions are satisfied for each agent \(i\) at each information set \(I^{ik}\):
- \(i\) is rational, \(i\) knows this and \(i\) knows the game, and
- At any information set \(I^{jk + 1}\) that immediately follows \(I^{ik}, i\) knows at \(I^{ik}\) what \(j\) knows at \(I^{jk + 1}\).
Proposition 3.12 says that far less than common knowledge of the game and of rationality suffices for the backwards induction solution to obtain in a game of perfect information. All that is needed is for each agent at each of her information sets to be rational, to know the game and to know what the next agent to move knows! For instance, in the Figure 1.2 game, if \(R_1 (R_2)\) stands for “Alan (Fiona) is rational” and \(\mathbf{K}_i (\Gamma)\) stands for “\(i\) knows the game \(\Gamma\)”, then the backwards induction solution is implied by the following:
- At \(I^{24}, R_2\) and \(\mathbf{K}_2 (\Gamma)\).
- At \(I^{13}, R_1, \mathbf{K}_1 (\Gamma), \mathbf{K}_1 (R_2)\), and \(\mathbf{K}_1\mathbf{K}_2 (\Gamma)\).
- At \(I^{22}, \mathbf{K}_2 (R_1), \mathbf{K}_2\mathbf{K}_1 (R_2)\), and \(\mathbf{K}_2\mathbf{K}_1\mathbf{K}_2 (\Gamma)\).
- At \(I ^{11}, \mathbf{K}_1\mathbf{K}_2 (R_1), \mathbf{K}_1\mathbf{K}_2\mathbf{K}_1 (R_2)\), and \(\mathbf{K}_1\mathbf{K}_2\mathbf{K}_1\mathbf{K}_2 (\Gamma)\). [ 31 ]
One might think that a corollary to Proposition 3.11 is that in a game of perfect information, common knowledge of the game and of rationality implies the backwards induction solution. This is the classical argument for the backwards induction solution. Many game theorists continue to accept the classical argument, but in recent years, the argument has come under strong challenge, led by the work of Reny (1988, 1992), Binmore (1987) and Bicchieri (1989, 1993). The basic idea underlying their criticisms of backwards induction can be illustrated with the Figure 1.2 game. According to the classical argument, if Alan and Fiona have common knowledge of rationality and the game, then each will predict that the other will follow her end of the backwards induction solution, to which his end of the backwards induction solution is his unique best response. However, what if Fiona reconsiders what to do if she finds herself at the information set \(I^{22}\)? If the information set \(I^{22}\) is reached, then Alan has of course not followed the backwards induction solution. If we assume that at \(I^{22}\), Fiona knows only what is stated in (iii), then she can explain her being at \(I^{22}\) as a failure of either \(\mathbf{K}_1\mathbf{K}_2\mathbf{K}_1 (R_2)\) or \(\mathbf{K}_1\mathbf{K}_2\mathbf{K}_1\mathbf{K}_2 (\Gamma)\) at \(I^{11}\). In this case, Fiona’s thinking that either \(\neg\mathbf{K}_1\mathbf{K}_2\mathbf{K}_1 (R_2)\) or \(\neg\mathbf{K}_1\mathbf{K}_2\mathbf{K}_1\mathbf{K}_2(\Gamma)\) at \(I^{11}\) is compatible with what Alan in fact does know at \(I^{11}\), so Fiona should not necessarily be surprised to find herself at \(I^{22}\), and given that what she knows there is characterized by (iii), following the backwards induction solution is her best strategy. But if rationality and the game are common knowledge, or even if Fiona and Alan both have just have mutual knowledge of the statements characterized by (iii) and (iv), then at \(I^{22}\), Fiona knows that \(\mathbf{K}_1\mathbf{K}_2\mathbf{K}_1 (R_2)\) or \(\mathbf{K}_1\mathbf{K}_2\mathbf{K}_1\mathbf{K}_2 (\Gamma)\) at \(I^{11}\). Hence given this much mutual knowledge, Fiona no longer can explain why Alan has deviated from the backwards induction solution, since this deviation contradicts part of what is their mutual knowledge. So if she finds herself at \(I^{22}\), Fiona does not necessarily have good reason to think that Alan will follow the backwards induction solution of the subgame beginning at \(I^{22}\), and hence she might not have good reason to follow the backwards induction solution, either. Bicchieri (1993), who along with Binmore (1987) and Reny (1988, 1992) extends this argument to games of perfect information with arbitrary length, draws a startling conclusion: If agents have strictly too few or strictly too many levels of mutual knowledge of rationality and the game relative to the number of potential moves, one cannot predict that they will follow the backwards induction solution. This would undermine the central role backwards induction has played in the analysis of extensive form games. For why should the number of levels of mutual knowledge the agents have depend upon the length of the game?
The classical argument for backwards induction implicitly assumes that at each stage of the game, the agents discount the preceding moves as strategically irrelevant. Defenders of the classical argument can argue that this assumption makes sense, since by definition at any agents’ decision node, the previous moves that led to this node are now fixed. Critics of the classical argument question this assumption, contending that when reasoning about how to move at any of his information sets, including those not on the backwards induction equilibrium path , part of what an agent must consider is what conditions might have led to his being at that information set. In other words, agents should incorporate reasoning about the reasoning of the previous movers, or forward induction reasoning, into their deliberations over how to move at a given information set. Binmore (1987) and Bicchieri (1993) contend that a backwards induction solution to a game should be consistent with the solution a corresponding forward induction argument recommends. As we have seen, given common knowledge of the game and of rationality, forward induction reasoning can lead the agents to an apparent contradiction: The classical argument for backwards induction is predicated on what agents predict they would do at nodes in the tree that are never reached. They make these predictions based upon their common knowledge of the game and of rationality. But forward induction reasoning seems to imply that if any off-equilibrium node had been reached, common knowledge of rationality and the game must have failed, so how could the agents have predicted what would happen at these nodes?
Situations in which a member of a population \(P\) is willing to engage in a certain course of action provided that a large enough portion of \(P\) engages in some appropriate behavior are typical problems of collective action . Consider the case of an agent who is debating whether to join a revolt. Her decision to join or not to join will depend on the number of other agents whom she expects to join the revolt. If such a number is too low, she will prefer not to revolt, while if the number is sufficiently large, she will prefer to revolt. Michael Chwe proposes a model where such a situation is modeled game-theoretically. Players’ knowledge about other players’ intentions depends on a social network in which players are located. The individual ‘thresholds’ for each player (the number of other agents that are needed for that specific player to revolt) are only known by the immediate neighbors in the network. Besides the intrinsic value of the results obtained by Chwe’s analysis regarding the subject of collective action, his model also provides insights about both the relation between social networks and common knowledge and about the role of common knowledge in collective action. For example, in some situations, first-order knowledge of other agents’ personal thresholds is not sufficient to motivate an agent to take action, whereas higher-order knowledge or, in the limit, common knowledge is.
We present Chwe’s model following (Chwe 1999) and (Chwe 2000). Suppose there is a group \(P\) of \(n\) people, and each agent has two strategies: \(r\) (revolt, that is participating in the collective action) and \(s\) (stay home and not participate). Each agent has her own individual threshold \(\theta \in \{1, 2,\ldots, n+1\}\) and she prefers \(r\) over \(s\) if and only if the total number of players who revolt is greater than or equal to her threshold. An agent with threshold 1 always revolts; an agent with threshold 2 revolts only if another agent does; an agent with threshold \(n\) revolts only if all agents do; an agent with threshold \(n+1\) never revolts, etc. The agents are located in a social network, represented by a binary relation \(\rightarrow\) over \(P\). The intended meaning of \(i \rightarrow j\) is that agent \(i\) ‘talks’ to agent \(j\), that is to say, agent \(i\) knows the threshold of agent \(j.\) If we define \(B(i)\) to be the set \(\{j \in P : j \rightarrow i\},\) we can interpret \(B(i)\) as \(i\)’s ‘neighborhood’ and say that, in general, \(i\) knows the thresholds of all agents in her neighborhood. A further assumption is that, for all \(j,k \in B(i),\) \(i\) knows whether \(j \rightarrow k\) or not, that is, every agent knows whether her neighbors are communicating with each other. The relation \(\rightarrow\) is taken to be reflexive (one knows her own threshold).
Players’ knowledge is represented as usual in a possible worlds framework. Consider for example the case in which there are two agents, both with one of thresholds 1, 2 or 3. There are nine possible worlds represented by ordered pairs of numbers, representing the first and second player’s individual thresholds respectively: 11, 12, 13,…, 32, 33. If the players do not communicate, each knows her own threshold only. Player 1’s information partition reflects her ignorance about player’s 2 threshold and it consists of the sets \(\{11, 12, 13\},\) \(\{21, 22, 23\},\) \(\{31, 32, 33\};\) whereas, similarly, player 2’s partition consists of the sets \(\{11, 21, 31\},\) \(\{12, 22, 32\},\) \(\{13, 23, 33\}.\) If player 1’s threshold is 1, she revolts no matter what player 2’s threshold is. Hence, player 1 revolts in \(\{11, 12, 13\}\). If player 1’s threshold is 3, she never revolts. Hence, she plays \(s\) in \(\{31, 32, 33\}\). If her threshold is 2, she revolts only if the other player revolts as well. Since in this example we are assuming that there is no communication between the agents, player 1 cannot be sure of player’s 2 action, and chooses the non-risky \(s\) in \(\{21, 22, 23\}\) as well. Similarly, player 2 plays \(r\) in \(\{11, 21, 31\}\) and \(s\) otherwise. Consider now the case in which \(1 \rightarrow 2\) and \(2 \rightarrow 1\). Both players have now the finest information partitions. Thresholds of 1 and 3 yield \(r\) and \(s\), respectively, for both players again. However, in player 1’s cells \(\{21\}\) and \(\{22\}\), she knows that player 2 will revolt, and, having threshold 2, she revolts as well. Similarly for player 2 in his cells \(\{12\}\) and \(\{22\}\). Note, that the case in which both players have threshold 2, yields both the equilibrium in which both players revolt and the equilibrium in which each player stays home. It is assumed that in the case of multiple equilibria, the one which results in the most revolt will obtain.
The analysis of the example above applies to general networks with \(n\) agents. Consider for example the three person network \(1 \rightarrow 2, 2 \rightarrow 1, 2 \rightarrow 3\), represented in figure 3.5a (notice that symmetric links are represented by a line without arrowheads) and assume that each player has threshold 2. The network between players 1 and 2 is the same as the one above, hence if they have threshold 2, they both revolt regardless of the threshold of player 3. Player 3, on the other hand, knows her own threshold and player 2’s. Hence, if they all have threshold 2, she cannot distinguish between the possibilities in the set \(\{122, 222, 322, 422\}\). At 422, in particular, neither player 1 nor player 2 revolt, hence player 3 cannot take the risk and does not revolt, even if , in fact, she has a neighbor who revolts. Adding the link \(1 \rightarrow 3\) to the network (cf. figure 3.5b) we provide player 3 with knowledge about player 1’s action, hence in this case, if they all have threshold 2, they all revolt. Notice that if we break the link between players 1 and 2 (so that the network is \(1 \rightarrow 3\) and \(2 \rightarrow 3)\), player 3 knows that 1 and 2 cannot communicate and hence do not revolt at 222, therefore she chooses \(s\) as well. Knowledge of what other players know about other players is crucial.
The next example reveals that in some cases not even first-order knowledge is sufficient to trigger action, and higher levels of knowledge are necessary. Consider four players, each with threshold 3, in the two different networks represented in figure 3.6 (‘square’, in figure 3.6a, and ‘kite’, in figure 3.6b.) In the square network, player 1 knows that both 2 and 4 have threshold 3. However, she does not know about player 3’s threshold. If player 3 has threshold 5, then player 2 will never revolt, since he does not know about player 4’s threshold and it is then possible for him that player 4 has threshold 5 as well. Player 1’s uncertainty about player 3 together with player 1’s knowledge of player 2’s uncertainty about player 4 force her not to revolt, although she has threshold 3 and two neighbors with threshold 3 as well. Similar reasoning applies to all other players, hence in the square no one revolts. Consider now the kite network. Player 4 ignores player 1’s and player 2’s thresholds, hence he does not revolt. However, player 1 knows that players 2 and 3 have threshold 3, that they know that they do, and that they know that player 1 knows that they do. This is enough to trigger action \(r\) for the three of them, and indeed if players 1, 2 and 3 all revolt in all states in \(\{3331, 3332, 3333, 3334, 3335\}\), this is an equilibrium since in all states at least three people revolt each with threshold three.
The difference between the square and the kite networks is that, although in the square enough agents are willing to revolt for a revolt to actually take place, and they all individually know this, no agent knows that others know it. In the kite, on the other hand, agents in the triangle not only know that there are three agents with threshold 3, but they also know that they all know it, know that they all know that they all know it, and so on. There is common knowledge of such fact among them. It is interesting to notice that in Chwe’s model, common knowledge obtains without there been a publicly known fact (cf. section 2.2). The proposition “players 1, 2 and 3 all have threshold 3” (semantically: the event \(\{3331, 3332, 3333, 3334, 3335\})\) is known by players 1, 2 and 3 because of the network structure, and becomes common knowledge because the network structure is known by the players. To be sure, the network structure is not just simply known, but it is actually commonly known by the players. Player 1, for example, does not only know that players 2 and 3 communicate with each other. She also knows that players 2 and 3 know that she knows that they communicate with each other, and so on.
In complete networks (networks in which all players communicate with everyone else, as within the triangle in the kite network) the information partitions of the players coincide, and they are the finest partitions of the set of possible worlds. Hence, if players have sufficiently low thresholds, such fact is commonly known and there is an equilibrium in which all players revolt.
Definition 3.13 We say that \(\rightarrow\) is a sufficient network if there is an equilibrium such that all players choose to revolt.
For a game in which all players have sufficiently low thresholds, the complete network is clearly sufficient. Is the complete network necessary to obtain an equilibrium in which all players revolt? It turns out that it is not. A crucial role is played by structures of the same kind as the ‘triangle’ group in the kite network, called cliques . In such structures, ‘local’ common knowledge (that is, limited to the players part of the structure) arises naturally. In a complete network (that is, a network in which there is sufficient but not superfluous communication for it to fully revolt) in which cliques cover the entire population, if one clique speaks to another then every member of that clique speaks to every member of the other clique. Moreover, for every two cliques such that one is talking to the other, there exists a ‘chain’ of cliques with a starting element. In other words, every pair of cliques in the relation are part of a chain (of length at least 2) with a starting element (a leading clique.) Revolt propagates in the network moving from ‘leading adopters’ to ‘followers’, according to the social role hierarchy defined by the cliques and their relation. Consider the following example, in which cliques are represented by circles and numbers represent the thresholds of individual players:
Here the threshold 3 clique is the leading clique, igniting revolt in the threshold 5 follower clique. In turn, the clique of a single threshold 3 element follows. Notice that although she does not need to know that the leading clique actually revolts to be willing to revolt, that information is needed to ensure that the threshold 5 clique does revolt, and hence that it is safe for her to join the revolt. While in each clique information about thresholds and hence willingness to revolt is common knowledge, in a chain of cliques information is ‘linear’; each clique knows about the clique of which it is a follower, but does not know about earlier cliques.
Analyzing Chwe’s models for collective action under the respect of weak versus strong links (cf. both Chwe 1999 and Chwe 2000) provides further insights about the interaction between communication networks and common knowledge. A strong link, roughly speaking, joins close friends, whereas a weak link joins acquaintances. Strong links tend to increase more slowly than weak ones, since people have common close friends more often than they share acquaintances. In terms of spreading information and connecting society, then, weak links do a better job than strong links, since they traverse society more quickly and have therefore larger reach. What role do strong and weak links play in collective action? In Chwe’s dynamic analysis, strong links fare better when thresholds are low, whereas weak links are better when players’ thresholds are higher. Intuitively, one sees that strong links tend to form small cliques right away (because of the symmetry intrinsic in them: my friends’ friends tend to be my friends as well); common knowledge arises quickly at the local level and, if thresholds are low, there is a better chance that a group tied by a strong link becomes a leading clique initiating revolt. If, on the other hand, thresholds are high, local common knowledge in small cliques is fruitless, and weak links, reaching further distances more quickly, speed up communication and building of the large cliques needed to sparkle collective action. Such considerations shed some light on the relation between social networks and common knowledge. While it is true that knowledge spreads faster in networks in which weak links predominate, higher-order knowledge (and, hence, common knowledge) tends to arise more slowly in this kind of networks. Networks with a larger number of strong links, on the other hand, facilitate the formation of common knowledge at the local level.
Lewis formulated an account of common knowledge which generates the hierarchy of ‘\(i\) knows that \(j\) knows that … \(k\) knows that \(A\)’ propositions in order to ensure that in his account of convention, agents have correct beliefs about each other. But since human agents obviously cannot reason their way through such an infinite hierarchy, it is natural to wonder whether any group of people can have full common knowledge of any proposition. More broadly, the analyses of common knowledge reviewed in §3 would be of little worth to social scientists and philosophers if this common knowledge lies beyond the reach of human agents.
Fortunately for Lewis’ program, there are strong arguments that common knowledge is indeed attainable. Lewis (1969) argues that the common knowledge hierarchy should be viewed as a chain of implications, and not as steps in anyone’s actual reasoning. He gives informal arguments that the common knowledge hierarchy is generated from a finite set of axioms. We saw in §2 that it is possible to formulate Lewis’ axioms precisely and to derive the common knowledge hierarchy from these axioms and a public event functioning as a basis for common knowledge. Again, the basic idea behind Lewis’ argument is that for a set of agents, if a proposition \(A\) is publicly known among them and each agent knows that everyone can draw the same conclusion \(p\) from \(A\) that she can, then \(p\) is common knowledge. These conditions are obviously context dependent, just as an individual’s knowing or not knowing a proposition is context dependent. Yet there are many cases where it is natural to assume that a public event generates common knowledge, because it is properly broadcast, agents in the group are in ideal conditions to perceive it, the inference from the public event to the object of common knowledge is immediate, etc. However, common knowledge could fail if some of the people failed to perceive the public event, or if some of them believed that some of the others could not understand the announcement, or hear it, or could not draw the necessary inferences, and so on.
In fact, skeptical doubt about the attainability of common knowledge is certainly possible. A strong skeptical argument has been recently put forth by Lederman (2018b). Lederman builds an argument meant to undermine the possibility of deriving the common knowledge hierarchy, as done in §2, on the basis of a public event or, as Lederman calls it, public information . The principle that Lederman targets is what he calls ideal common knowledge (or belief), that is: If \(p\) is public information in a group \(G\) then \(p\) is common knowledge in \(G\), provided the agents in \(G\) are ideal reasoners. The argument rests on the privacy and interpersonal incomparability of mental states among agents, and although it is offered in terms of perceptual knowledge, its scope goes beyond perception to question the possibility of common knowledge tout court.
Lederman (2018b) uses the following scenario: Two contestants, Alice and Bob, observe the height of the mast of a toy sailboat (100 cm) that is subsequently replaced with a randomly selected sailboat whose mast may be more or less tall than 100 cm. As a matter of fact, the mast of the selected boat is 300 cm tall. It is therefore public information that the mast is taller than 100 cm. The ideal common knowledge principle above, along with assumptions about Alice and Bob’s visual systems and their publicity, would entail that Alice and Bob have common knowledge that the mast is taller than 100 cm, and yet Lederman’s argument shows that they do not. The main idea is that there is some degree of approximation in how humans perceive, among other things, heights. Thus, for Alice it is epistemically compatible with the mast looking 300 cm tall to her, that the mast looks somewhat shorter than 300 cm to Bob, say 299 cm. Also, Alice knows that if the mast looks 299 cm tall to Bob, then it is epistemically compatible for him that the mast looks 298 cm tall to Alice. Also, Bob knows that Alice knows that if the mast looks 298 cm tall to Alice, then it is epistemically compatible for her that the mast looks 297 cm tall to Bob. The reasoning can be repeated until there it is epistemically compatible for Alice and Bob that the mast is not taller than 100 cm, against the intuition that they have common knowledge that the mast is over 100 cm tall!
Lederman (2018b) generalizes the argument to arbitrary cases and sources of public information, to conclude that people never achieve common knowledge or belief. In his view, the unattainability of common knowledge is not a concern in terms of a possible loss of explanatory power for social behavior. While common knowledge and public information from which it proceeds have long been considered crucial for coordinating behavior, Lederman claims that in fact coordination requires neither (see the discussion of Lederman 2018a in the next section.) Against Lederman, Immerman (2021) argues that the skeptical argument sketched here fails in a large set of circumstances, and hence fails to prove the unattainability of common knowledge. The key idea in Immerman’s attempt to refute Lederman’s argument is that there are many perceptual values that agents will not entertain to begin with, as if, in the original sailboat example, they knew that all masts within 100 and 300 cm tall had been stolen. According to Immerman, cases of such “knowledge of gaps” are not at all uncommon and their availability prevents Lederman’s argument to go through.
Even if one were to reject Lederman’s skeptical argument (be it by agreeing with Immerman’s argument above, or with the argument by Thomason (2021) addressed in the next section, or otherwise), care must be taken in ascribing common knowledge to a group of human agents. Common knowledge is a phenomenon highly sensitive to the agents’ circumstances. The following section gives an example that shows that in order for \(A\) to be a common truism for a set of agents, they ordinarily must perceive an event which implies \(A\) simultaneously and publicly.
5. Coordination and Common \(p\)-Belief
In certain contexts, agents might not be able to achieve common knowledge. The skeptical argument put forth by Lederman (2018b), indeed, rests on and generalizes related arguments about the attainability of common knowledge that were made in theoretical computer science in relation to the coordinated attack problem (see Lederman 2018a, Halpern and Moses, 1990 and Fagin et al. 1995, esp. chapters 6 and 11). In the context of distributed systems, using the formal systems of epistemic logic that, as mentioned above, are equivalent to the semantic approach privileged by economists, it can be proven formally that (i) common knowledge is necessary for coordination and that (ii) the attainability of common knowledge depends on assumptions made about the system. In particular, asynchronous systems do not allow for common knowledge of a communicated message to arise, making coordination impossible. Might the agents achieve something “close” to common knowledge? There are various weakenings of the notion of common knowledge that can be of use: \(\varepsilon\)-common knowledge (agents will achieve common knowledge within time \(\varepsilon\), hence they will coordinate within time \(\varepsilon)\), eventual common knowledge (agents will achieve common knowledge and therefore coordinate eventually), probabilistic common knowledge (agents will achieve probability \(p\) common belief, and hence with probability \(p\) successfully coordinate), etc. Such weakenings of the notion of common knowledge might prove useful depending on the intended application.
Another weakening of common knowledge to consider is of course \(m\) th level mutual knowledge. For a high value of \(m, \mathbf{K}^m_N(A)\) might seem a good approximation of \(\mathbf{K}^{*}_N(A)\). However, point (i) above maintains that no arbitrary high value of \(m\) will help for instance with the practical task of achieving coordination, so that the full force of common knowledge is needed. We illustrate the point through the following example, due to Rubinstein (1989, 1992), showing that simply truncating the common knowledge hierarchy at any finite level can lead agents to behave as if they had no mutual knowledge at all. [ 32 ]
5.1 The E-mail Coordination Example
Lizzi and Joanna are faced with the coordination problem summarized in the following figure:
Figure 5.1a \(\quad\omega_1, \mu(\omega_1)=0.51\)
Figure 5.1b \(\quad\omega_2, \mu(\omega_2)=0.49\)
In Figure 5.1, the payoffs are dependent upon a pair of possible worlds. World \(\omega_1\) occurs with probability \(\mu(\omega_1) = .51,\) while \(\omega_2\) occurs with probability \(\mu(\omega_2) = .49.\) Hence, they coordinate with complete success by both choosing \(A(B)\) only if the state of the world is \(\omega_1(\omega_2)\).
Suppose that Lizzi can observe the state of the world, but Joanna cannot. We can interpret this game as follows: Joanna and Lizzi would like to have a dinner together prepared by Aldo, their favorite chef. Aldo alternates between \(A\) and \(B\), the two branches of Sorriso, their favorite restaurant. State \(\omega_i\) is Aldo’s location that day. At state \(\omega_1 (\omega_2)\), Aldo is at \(A (B)\). Lizzi, who is on Sorriso’s special mailing list, receives notice of \(\omega_i\). Lizzi’s and Joanna’s best outcome occurs when they meet where Aldo is working, so they can have their planned dinner. If they meet but miss Aldo, they are disappointed and do not have dinner after all. If either goes to \(A\) and finds herself alone, then she is again disappointed and does not have dinner. But what each really wants to avoid is going to \(B\) if the other goes to \(A\). If either of them arrives at \(B\) alone, she not only misses dinner but must pay the exorbitant parking fee of the hotel which houses \(B\), since the headwaiter of \(B\) refuses to validate the parking ticket of anyone who asks for a table for two and then sits alone. This is what Harsanyi (1967) terms a game of incomplete information , since the game’s payoffs depend upon states which not all the agents know.
\(A\) is a “play-it-safe” strategy for both Joanna and Lizzi. [ 33 ] By choosing \(A\) whatever the state of the world happens to be, the agents run the risk that they will fail to get the positive payoff of meeting where Aldo is, but each is also sure to avoid the really bad consequence of choosing \(B\) if the other chooses \(A\). And since only Lizzi knows the state of the world, neither can use information regarding the state of the world to improve their prospects for coordination. For Joanna has no such information, and since Lizzi knows this, she knows that Joanna has to choose accordingly, so Lizzi must choose her best response to the move she anticipates Joanna to make regardless of the state of the world Lizzi observes. Apparently Lizzi and Joanna cannot achieve expected payoffs greater than 1.02 for each, their expected payoffs if they choose \((A, A)\) at either state of the world.
If the state \(\omega\) were common knowledge, then the conditional strategy profile \((A, A)\) if \(\omega = \omega_1\) and \((B, B)\), if \(\omega = \omega_2\) would be a strict Nash equilibrium at which each would achieve a payoff of 2. So the obvious remedy to their predicament would be for Lizzi to tell Joanna Aldo’s location in a face-to-face or telephone conversation and for them to agree to go where Aldo is, which would make the state \(\omega\) and their intentions to coordinate on the best outcome given \(\omega\) common knowledge between them. Suppose for some reason they cannot talk to each other, but they prearrange that Lizzi will send Joanna an e-mail message if, and only if, \(\omega_2\) occurs. Suppose further that Joanna’s and Lizzi’s e-mail systems are set up to send a reply message automatically to the sender of any message received and viewed, and that due to technical problems there is a small probability, \(\varepsilon \gt 0\), that any message can fail to arrive at its destination. Then if Lizzi sends Joanna a message, and receives an automatic confirmation, then Lizzi knows that Joanna knows that \(\omega_2\) has occurred. If Joanna receives an automatic confirmation of Lizzi’s automatic confirmation, then Joanna knows that Lizzi knows that Joanna knows that \(\omega_2\) occurred, and so on. That \(\omega_2\) has occurred would become common knowledge if each agent received infinitely many automatic confirmations, assuming that all the confirmations could be sent and received in a finite amount of time. [ 34 ] However, because of the probability \(\varepsilon\) of transmission failure at every stage of communication, the sequence of confirmations stops after finitely many stages with probability one. With probability one, therefore, the agents fail to achieve full common knowledge. But they do at least achieve something “close” to common knowledge. Does this imply that they have good prospects of settling upon \((B, B)\)?
Rubinstein shows by induction that if the number of automatically exchanged confirmation messages is finite, then \(A\) is the only choice that maximizes expected utility for each agent, given what she knows about what they both know.
Rubinstein’s Proof
So even if agents have “almost” common knowledge, in the sense that the number of levels of knowledge in “Joanna knows that Lizzi knows that … that Joanna knows that \(\omega_2\) occurred” is very large, their behavior is quite different from their behavior given common knowledge that \(\omega_2\) has occurred. Indeed, as Rubinstein points out, given merely “almost” common knowledge, the agents choose as if no communication had occurred at all! Rubinstein also notes that this result violates our intuitions about what we would expect the agents to do in this case. (See Rubinstein 1992, p. 324.) If \(T_i = 17\), wouldn’t we expect agent \(i\) to choose \(B\)? Indeed, in many actual situations we might think it plausible that the agents would each expect the other to choose \(B\) even if \(T_1 = T_2 = 2\), which is all that is needed for Lizzi to know that Joanna has received her original message and for Joanna to know that Lizzi knows this! Binmore and Samelson (2001) in fact show that if Joanna and Lizzi incur a cost when paying attention to the messages they exchange, or if sending a message is costly, then longer streams of messages are not paid attention to or do not occur, respectively.
Lederman (2018a) proposes a radical solution to the paradoxes. In the case of the coordinated attack, he argues that rational generals who commonly know that they are rational will attack if (and only if) they have common knowledge that they will attack; since common knowledge is not attainable by exchanging messages, they will not attack. However, admitting that the generals do not commonly believe that they are rational, a simple model can be built showing that such generals do attack without common knowledge that they will. Similarly, in the case of the e-mail game, he shows that if players can be of an irrational type (so that she chooses game \(B\) even if her expected payoff is lower than for choosing game \(A\),) and one player believes with sufficiently high probability that the other player is of the irrational type, then players can coordinate on game \(B\) after a finite number of messages have been exchanged. Thus, Lederman (2018a) argues that we should take common knowledge of rationality to be a simplifying assumption, useful to produce tractable mathematical models and yet generally false “in the wild,” where a commonsense notion of rationality does let generals and laymen easily coordinate after a small number of message exchanges. Thomason (2021) takes issue with Lederman’s use of the notion of commonsense rationality, and argues about the importance of considering instead the cognitive and deliberative processes that lead to the emergence of both individual and commonly held attitudes. Despite their disagreement, both Lederman (2018a, 2018b) and Thomason (2021) emphasize the importance of the relation between (commonly) held beliefs or knowledge and practical reasoning. An interesting application of practical issues pertaining to the attainability of common knowledge is offered in Halpern and Pass (2017), where a blockchain protocol (and consensus and hence coordination therein) is analyzed in terms of suitable weakenings of the notion of common knowledge. [ 35 ]
The example in Section 5.1 hints that mutual knowledge is not the only weakening of common knowledge that is relevant to coordination. Brandenburger and Dekel (1987) and Monderer and Samet (1989) explore another option, which is to weaken the properties of the \(\mathbf{K}^{*}_N\) operator. Monderer and Samet motivate this approach by noting that even if a mutual knowledge hierarchy stops at a certain level, agents might still have higher level mutual beliefs about the proposition in question. So they replace the knowledge operator \(\mathbf{K}_i\) with a belief operator \(\mathbf{B}^p_i\):
Definition 5.1 If \(\mu_i(\cdot)\) is agent \(i\)’s probability distribution over \(\Omega\), then
\(\mathbf{B}^p_i(A)\) is to be read ‘\(i\) believes \(A\) (given \(i\)’s private information) with probability at least \(p\) at \(\omega\)’, or ‘\(i\) \(p\)-believes \(A\)’. The belief operator \(\mathbf{B}^p_i\) satisfies axioms K2, K3, and K4 of the knowledge operator. \(\mathbf{B}^p_i\) does not satisfy K1, but does satisfy the weaker property
that is, if one believes \(A\) with probability at least \(p\), then the probability of \(A\) is indeed at least \(p\).
One can define mutual and common p-beliefs recursively in a manner similar to the definition of mutual and common knowledge:
(1) The proposition that \(A\) is (first level or first order) mutual p-belief for the agents of \(N, \mathbf{B}^p_{N^1}(A),\) is the set defined by
(2) The proposition that \(A\) is \(m\) th level (or \(m\) th order ) mutual \(p\)-belief among the agents of \(N, \mathbf{B}^p_{N^m}(A),\) is defined recursively as the set
(3) The proposition that \(A\) is common p-belief among the agents of \(N, \mathbf{B}^p_{N^*}(A),\) is defined as the set
If \(A\) is common (or \(m\) th level mutual) knowledge at world \(\omega\), then \(A\) is common \((m\) th level) \(p\)-belief at \(\omega\) for every value of \(p\). So mutual and common \(p\)-beliefs formally generalize the mutual and common knowledge concepts. However, note that \(\mathbf{B}^1_{N^*}(A)\) is not necessarily the same proposition as \(\mathbf{K}^{*}_N (A)\), that is, even if \(A\) is common 1-belief, \(A\) can fail to be common knowledge.
Common \(p\)-belief forms a hierarchy similar to a common knowledge hierarchy:
Proposition 5.3 \(\omega \in \mathbf{B}^{p}_{N^m}(A)\) iff
(∗) For all agents \(i_1, i_2 , \ldots ,i_m \in N,\) \(\omega \in \mathbf{B}^{p}_{i_1}\mathbf{B}^p_{i_2} \ldots \mathbf{B}^p_{i_m}(A)\)
Hence, \(\omega \in \mathbf{B}^{p}_{N^*}(A)\) iff (∗) is the case for each \(m \ge 1.\)
Proof . Similar to the Proof of Proposition 2.5 .
One can draw several morals from the e-mail game of Example 5.1. Rubinstein (1987) argues that his conclusion seems paradoxical for the same reason the backwards induction solution of Alan’s and Fiona’s perfect information game might seem paradoxical: Mathematical induction does not appear to be part of our “everyday” reasoning. This game also shows that in order for A to be a common truism for a set of agents, they ordinarily must perceive an event which implies A simultaneously in each others’ presence. A third moral is that in some cases, it may make sense for the agents to employ some solution concept weaker than Nash or correlated equilibrium. In their analysis of the e-mail game, Monderer and Samet (1989) introduce the notions of ex ante and ex post \(\varepsilon\)-equilibrium. An ex ante equilibrium \(h\) is a system of strategy profiles such that no agent \(i\) expects to gain more than \(\varepsilon\)-utiles if \(i\) deviates from \(h\). An ex post equilibrium \(h'\) is a system of strategy profiles such that no agent \(i\) expects to gain more than \(\varepsilon\)-utiles by deviating from \(h'\) given \(i\)’s private information. When \(\varepsilon = 0\), these concepts coincide, and \(h\) is a Nash equilibrium. Monderer and Samet show that, while the agents in the e-mail game can never achieve common knowledge of the world \(\omega\), if they have common \(p\)-belief of \(\omega\) for sufficiently high \(p\), then there is an ex ante equilibrium at which they follow \((A,A)\) if \(\omega = \omega_1\) and \((B,B)\), if \(\omega = \omega_2\). This equilibrium turns out not to be ex post. However, if the situation is changed so that there are no replies, then Lizzi and Joanna could have at most first order mutual knowledge that \(\omega = \omega_2\). Monderer and Samet show that in this situation, given sufficiently high common \(p\)-belief that \(\omega = \omega_2\), there is an ex post equilibrium at which Joanna and Lizzi choose \((B,B)\) if \(\omega = \omega_2\)! So another way one might view this third moral of the e-mail game is that agents’ prospects for coordination can sometimes improve dramatically if they rely on their common beliefs as well as their mutual knowledge. More recently, the notion of \(p\)-belief and \(p\)-common belief proved useful (Paternotte, 2011) to analyze and formalize Lewis’s account of common knowledge, while Paternotte (2017), establishing a link between “ordinary” common knowledge and \(p\)-common belief, uses the latter to show that only a limited number of exchanges in the e-mail game or coordinated attack paradox would be sufficient to determine coordination. The result, building on foundations provided by Leitgeb (2014), is used to show that our “ordinary” understanding of common knowledge is captured by probabilistic common belief, although at the price of decreased robustness relative to the number of individuals sharing common belief and their awareness.
Annotations
Lewis (1969) is the classic pioneering study of common knowledge and its potential applications to conventions and game theory. As Lewis acknowledges, parts of his work are foreshadowed in Hume (1740) and Schelling (1960).
Aumann (1976) gives the first mathematically rigorous formulation of common knowledge using set theory. Schiffer (1972) uses the formal vocabulary of epistemic logic (Hintikka 1962) to state his definition of common knowledge. Schiffer’s general approach is to augment a system of sentential logic with a set of knowledge operators corresponding to a set of agents, and then to define common knowledge as a hierarchy of propositions in the augmented system. Bacharach (1992), Bicchieri (1993) and Fagin, et al . (1995) adopt this approach, and develop logical theories of common knowledge which include soundness and completeness theorems. Fagin, et al. show that the syntactic and set-theoretic approaches to developing common knowledge are logically equivalent.
Aumann (1995) gives a recent defense of the classical view of backwards induction in games of imperfect information. For criticisms of the classical view, see Binmore (1987), Reny (1992), Bicchieri (1989) and especially Bicchieri (1993). Brandenburger (1992) surveys the known results connecting mutual and common knowledge to solution concepts in game theory. For more in-depth survey articles on common knowledge and its applications to game theory, see Binmore and Brandenburger (1989), Geanakoplos (1994) and Dekel and Gul (1997). For her alternate account of common knowledge along with an account of conventions which opposes Lewis’ account, see Gilbert (1989).
Monderer and Samet (1989) remains one of the best resources for the study of common p-belief.
- Alberucci, Luca and Jaeger, Gerhard, 2005, “About cut elimination for logics of common knowledge”, Annals of Pure and Applied Logic , 133(1–3): 73–99.
- Aumann, Robert, 1974, “Subjectivity and Correlation in Randomized Strategies”, Journal of Mathematical Economics , 1: 67–96.
- –––, 1976, “Agreeing to Disagree”, Annals of Statistics , 4: 1236–9.
- –––, 1987, “Correlated Equilibrium as an Expression of Bayesian Rationality”, Econometrica , 55: 1–18.
- –––, 1995, “Backward Induction and Common Knowledge of Rationality”, Games and Economic Behavior 8: 6–19.
- Bacharach, Michael, 1985 “Some Extensions of a Claim of Aumann in an Axiomatic Model of Knowledge”, Journal of Economic Theory , 37(1): 167–190.
- –––, 1992.“Backward Induction and Beliefs About Oneself”, Synthese , 91: 247–284.
- Barwise, Jon, 1988, “Three Views of Common Knowledge”, in Proceedings of the Second Conference on Theoretical Aspects of Reasoning About Knowledge , M.Y. Vardi (ed.), San Francisco: Morgan Kaufman, pp. 365–379.
- –––, 1989, The Situation in Logic , Stanford: Center for the Study of Language and Information.
- Bernheim, B. Douglas, 1984, “Rationalizable Strategic Behavior”, Econometrica , 52: 1007–1028.
- Bicchieri, Cristina, 1989, “Self Refuting Theories of Strategic Interaction: A Paradox of Common Knowledge”, Erkenntnis , 30: 69–85.
- –––, 1993, Rationality and Coordination , Cambridge: Cambridge University Press.
- –––, 2006, The Grammar of Society , Cambridge: Cambridge University Press.
- Binmore, Ken, 1987, “Modelling Rational Players I”, Economics and Philosophy , 3: 179–241.
- –––, 1992, Fun and Games , Lexington, MA: D. C. Heath.
- –––, 2008, “Do Conventions Need to be Common Knowledge?”, Topoi , 27: 17–27.
- Binmore, Ken and Brandenburger, Adam, 1988, “Common knowledge and Game theory” ST/ICERD Discussion Paper 88/167, London School of Economics.
- Binmore, Ken and Samuelson, Larry, 2001, “Coordinated Action in the Electronic Mail Game” Games and Economic Behavior , 35(1): 6–30.
- Bonanno, Giacomo and Battigalli, Pierpaolo, 1999, “Recent Results on Belief, Knowledge and the Epistemic Foundations of Game Theory”, Research in Economics , 53(2): 149–225.
- Bonnay, D. and Egré, Paul, 2009, “Inexact Knowledge with Introspection”, Journal of Philosophical Logic , 38: 179–227.
- Brandenburger, Adam, 1992, “Knowledge and Equilibrium in Games”, Journal of Economic Perspectives , 6: 83–101.
- Brandenburger, Adam, and Dekel, Eddie, 1987, “Common Knowledge with Probability 1”, Journal of Mathematical Economics , 16: 237–245.
- –––, 1988, “The Role of Common Knowledge Assumptions in Game Theory”, in The Economics of Missing Markets, Information and Games , Frank Hahn (ed.), Oxford: Clarendon Press, 46–61.
- Bruni, Riccardo and Giacomo Sillari, 2018, “A Rational Way of Playing: Revision Theory for Strategic Interaction”, Journal of Philosophical Logic , 47(3), 419–448.
- Carnap, Rudolf, 1947, Meaning and Necessity: A Study in Semantics and Modal Logic , Chicago, University of Chicago Press.
- Cave, Jonathan AK, 1983, “Learning to Agree”, Economics Letters , 12(2): 147–152.
- Chwe, Michael, 1999, “Structure and Strategy in Collective Action”, American Journal of Sociology 105: 128–56.
- –––, 2000, “Communcation and Coordination in Social Networks”, Review of Economic Studies , 67: 1–16.
- –––, 2001, Rational Ritual , Princeton, NJ: Princeton University Press
- Cubitt, Robin and Sugden, Robert, 2003, “Common Knowledge, Salience and Convention: A Reconstruction of David Lewis’ Game Theory”, Economics and Philosophy , 19: 175–210.
- Dégremont, Cédric, and Oliver Roy, 2012, “Agreement Theorems in Dynamic-Epistemic Logic”, Journal of Philosophical Logic , 41(4): 735-764.
- Dekel, Eddie and Gul, Faruk, 1997, “Rationality and Knowledge in Game Theory”, in Advances in Economic Theory: Seventh World Congress of the Econometric Society , D. Kreps and K. Wallace eds., Cambridge: Cambridge University Press.
- Dekel, Eddie, Lipman, Bart and Rustichini, Aldo, 1998, “Standard State-Space Models Preclude Unawareness,” Econometrica , 66: 159–173.
- Devetag, Giovanna, Hosni, Hykel and Sillari, Giacomo, 2013, “Play 7: Mutual Versus Common Knowledge of Advice in a Weak-Link Game,” Synthese , 190(8): 1351–1381
- Fagin, Ronald and Halpern, Joseph Y., 1988, “Awareness and Limited Reasoning,” Artificial Intelligence , 34: 39–76.
- Fagin, Ronald, Halpern, Joseph Y., Moses, Yoram and Vardi, Moshe Y., 1995, Reasoning About Knowledge , Cambridge, MA: MIT Press.
- Friedell, Morris, 1967, “On the Structure of Shared Awareness,” Working papers of the Center for Research on Social Organizations (Paper #27), Ann Arbor: University of Michigan.
- –––, 1969, “On the Structure of Shared Awareness,” Behavioral Science , 14(1): 28–39.
- Geanakoplos, John, 1989, “Games Theory without Partitions, and Applications to Speculation and Consensus,” Cowles Foundation Discussion Paper, No. 914.
- –––, 1994, “Common Knowledge”, in Handbook of Game Theory (Volume 2), Robert Aumann and Sergiu Hart (eds.), Amsterdam: Elsevier Science B.V., 1438–1496.
- Geanakoplos, John and Heraklis M. Polemarchakis, 1982, “We Can’t Disagree Forever” Journal of Economic theory 28(1): 192–200.
- Gilbert, Margaret, 1989, On Social Facts , Princeton: Princeton University Press.
- Halpern, Jospeh, 2001, “Alternative Semantics for Unawareness”, Games and Economic Behavior , 37(2): 321–339
- Halpern, J. Y., & Moses, Y. , 1990, “Knowledge and common Knowledge in a Distributed Environment”. Journal of the Association for Computing Machinery , 37(3): 549–587.
- Halpern, J. Y., & Pass, R., 2017, “A Knowledge-Based Analysis of the Blockchain Protocol”. arXiv preprint arXiv:1707.08751.
- Harman, Gilbert, 1977, “Review of Linguistic Behavior by Jonathan Bennett”, Language , 53: 417–424.
- Harsanyi, J., 1967, “Games with Incomplete Information Played by ”Bayesian“ Players, I: The basic model”, Management Science , 14: 159–82.
- –––, 1968a, “Games with Incomplete Information Played by ”Bayesian“ Players, II: Bayesian Equilibrium Points”, Management Science , 14: 320–324.
- –––, 1968b, “Games with Incomplete Information Played by ”Bayesian“ Players, III: The basic probability distribution of the game”, Management Science , 14: 486–502.
- Heifetz, Aviad, 1999, “Iterative and Fixed Point Common Belief”, Journal of Philosophical Logic , 28(1): 61–79.
- Heifetz, Aviad, Meier, Martin and Schipper, Burkhard, 2006, “Interactive Unawareness”, Journal of Economic Theory , 130: 78–94.
- Hintikka, Jaakko, 1962, Knowledge and Belief , Ithaca, NY: Cornell University Press.
- Hume, David, 1740 [1888, 1976], A Treatise of Human Nature , L. A. Selby-Bigge (ed.), rev. 2nd. edition P. H. Nidditch (ed.), Oxford: Clarendon Press.
- Immerman, D., 2021, “How Common Knowledge Is Possible”. Mind , first online 17 January 2021. doi:10.1093/mind/fzaa090
- Jäger, Gerhard and Michel Marti, 2016, “Intuitionistic Common Knowledge or Belief”, Journal of Applied Logic , 18: 150–163
- Lederman, Harvey, 2018a, “Two Paradoxes of Common Knowledge: Coordinated Attack and Electronic Mail”, Noûs , 52: 921–945.
- –––, 2018b, “Uncommon Knowledge” Mind 127, 1069–1105.
- Leitgeb, Hannes, 2014, “The Stability Theory of Belief”, The Philosophical Review , 123(2): 131–171.
- Lewis, C. I., 1943, “The Modes of Meaning”, Philosophy and Phenomenological Research , 4: 236–250.
- Lewis, David, 1969, Convention: A Philosophical Study , Cambridge, MA: Harvard University Press.
- –––, 1978, “Truth in Fiction”, American Philosophical Quarterly , 15: 37–46.
- Littlewood, J. E., 1953, A Mathematical Miscellany , London: Methuen; reprinted as Littlewood’s Miscellany , B. Bollobas (ed.), Cambridge: Cambridge University Press, 1986.
- McKelvey, Richard and Page, Talbot, 1986, “Common Knowledge, Consensus and Aggregate Information”, Econometrica , 54: 109–127.
- Meyer, J.-J.Ch. and van der Hoek, Wiebe, 1995, Epistemic Logic for Computer Science and Artificial Intelligence (Cambridge Tracts in Theoretical Computer Science 41), Cambridge: Cambridge University Press.
- Milgrom, Paul, 1981, “An Axiomatic Characterization of Common Knowledge”, Econometrica , 49: 219–222.
- Milgrom, Paul, and Nancy Stokey, 1982, “Information, Trade and Common Knowledge”, Journal of Economic Theory , 26(1): 17–27.
- Monderer, Dov and Samet, Dov, 1989, “Approximating Common Knowledge with Common Beliefs”, Games and Economic Behavior , 1: 170–190.
- Nash, John, 1950, “Equilibrium Points in N-person Games”. Proceedings of the National Academy of Sciences of the United States , 36: 48–49.
- –––, 1951, “Non-Cooperative Games”. Annals of Mathematics , 54: 286–295.
- Nozick, Robert, 1963, The Normative Theory of Individual Choice , Ph.D. dissertation, Princeton University
- Paternotte, Cédric, 2011, “Being Realistic about Common Knowledge: a Lewisian Approach”, Synthese , 183(2): 249–276.
- –––, 2017, “The Fragility of Common Knowledge”, Erkenntnis , 82(3): 451–472.
- Pearce, David, 1984, “Rationalizable Strategic Behavior and the Problem of Perfection”, Econometrica , 52: 1029–1050.
- Reny, Philip J, 1988, “Common Knowledge and Games with Perfect Information.” In PSA: Proceedings of the Biennial Meeting of the Philosophy of Science Association , vol. 1988, no. 2, pp. 363–369. East Lansing: Philosophy of Science Association.
- –––, 1992, “Rationality in Extensive Form Games”, Journal of Economic Perspectives , 6: 103–118.
- Rubinstein, Ariel, 1987, “A Game with ”Almost Common Knowledge“: An Example”, in Theoretical Economics , D. P. 87/165. London School of Economics.
- Samet, Dov, 1990, “Ignoring Ignorance and Agreeing to Disagree”, Journal of Economic Theory , 52: 190–207.
- Schelling, Thomas, 1960, The Strategy of Conflict , Cambridge, MA: Harvard University Press.
- Schiffer, Stephen, 1972, Meaning , Oxford: Oxford University Press.
- Sillari, Giacomo, 2005, “A Logical Framework for Convention”, Synthese , 147(2): 379–400.
- –––, 2008, “Common Knowledge and Convention”, Topoi , 27(1): 29–39.
- –––, 2013, “Rule-Following as Coordination: a Game-Theoretic Approach”, Synthese , 190(5): 871–890.
- –––, 2019, “Logics of Belief”, Rivista di Filosofia , 110(2): 243–262.
- Skyrms, Brian, 1984, Pragmatics and Empiricism , New Haven: Yale University Press.
- –––, 1990, The Dynamics of Rational Deliberation , Cambridge, MA: Harvard University Press
- –––, 1991, “Inductive Deliberation, Admissible Acts, and Perfect Equilibrium”, in Foundations of Decision Theory , Michael Bacharach and Susan Hurley eds., Cambridge, MA: Blackwell, pp. 220–241.
- –––, 1998, “The Shadow of the Future”, in Rational Commitment and Social Justice: Essays for Gregory Kavka , Jules Coleman and Christopher Morris eds., Cambridge: Cambridge University Press, pp. 12–22.
- Sugden, Robert, 1986, The Economics of Rights, Cooperation and Welfare , New York: Basil Blackwell.
- Thomason, R. H., 2021, “Common Knowledge, Common Attitudes and Social Reasoning”, Bulletin of the Section of Logic , 50(2): 229–247.
- Vanderschraaf, Peter, 1995, “Endogenous Correlated Equilibria in Noncooperative Games”, Theory and Decision , 38: 61–84.
- Vanderschraaf, Peter, 1998, “Knowledge, Equilibrium and Convention”, Erkenntnis , 49: 337–369.
- –––, 2001. A Study in Inductive Deliberation , New York: Routledge.
- von Neumann, John and Morgenstern, Oskar, 1944, Theory of Games and Economic Behavior , Princeton: Princeton University Press.
How to cite this entry . Preview the PDF version of this entry at the Friends of the SEP Society . Look up topics and thinkers related to this entry at the Internet Philosophy Ontology Project (InPhO). Enhanced bibliography for this entry at PhilPapers , with links to its database.
- Applications of Circumscription to Formalizing Common Sense Knowledge
- Burkhard C. Schipper’s Unawareness Bibliography
convention | game theory | logic: epistemic | prisoner’s dilemma | social norms
Copyright © 2022 by Peter Vanderschraaf Giacomo Sillari < gsillari @ luiss . it >
- Accessibility
Support SEP
Mirror sites.
View this site from another server:
- Info about mirror sites
The Stanford Encyclopedia of Philosophy is copyright © 2023 by The Metaphysics Research Lab , Department of Philosophy, Stanford University
Library of Congress Catalog Data: ISSN 1095-5054
Skip to Content
Massey University
- Search OWLL
- Handouts (Printable)
- Pre-reading Service
- StudyUp Recordings
- StudyUp Postgraduate
- Academic writing
- Intro to academic writing
- What is academic writing?
- Writing objectively
- Writing concisely
- 1st vs. 3rd person
- Inclusive language
- Te Reo Māori
- Assignment planning
- Assignment planning calculator
- Interpreting the assignment question
- Command words
- Organising points
- Researching
- Identifying academic sources
- Evaluating source quality
- Editing & proofreading
- Apostrophes
- Other punctuation
- Active voice
- American vs. British spelling
- Conditionals
- Prepositions
- Pronoun Reference
- Sentence fragments
- Sentence Structure
- Subject-verb agreement
- Formatting and layout
- Word limits and assignment length
- Commonly confused words
- How assignments are marked
- Marking guides
- Getting an A
- Levels of assessment
- Using feedback
- Professional emails
- Forum posts
- Forum netiquette guidelines
- Sharing personal information
- Writing about personal experiences
- Assignment types
- What is an essay?
- Essay planning and structure
- Introduction
- Thesis statement
- Body paragraphs
- Essay revision
- Essay writing resources
- What is a report?
- Report structure
- Analysing issues for a report
- Business report
- What is a business report?
- Business report structure
- Inductive vs. deductive reports
- Other kinds of business communication
- Business report format and layout
- What is a lab report?
- Lab report structure
- Science lab report writing resources
- Psychology lab report writing resources
- Lab report body paragraphs
- Literature review
- What is a literature review?
- Writing a literature review
- Literature review structure
- Literature review writing resources
- Research proposal
- Writing a research proposal
- Research proposal structure
- Other types
- Article critique
- Book review
- Annotated bibliography
- Reflective writing
- Oral presentation
- Thesis / dissertation
- Article / conference paper
- Shorter responses
- PhD confirmation report
- Computer skills
- Microsoft Word
- Basic formatting
- Images, tables, & figures
- Long documents
- Microsoft Excel
- Basic spreadsheets
- Navigating & printing spreadsheets
- Charts / graphs & formulas
- Microsoft PowerPoint
- Basic skills
- Advanced skills
- Distance study
- Getting started
- How to study
- Online study techniques
- Distance support
- Reading & writing
- Reading strategies
- Writing strategies
- Grammar resources
- Listening & speaking
- Listening strategies
- Speaking strategies
- Maths & statistics
- Trigonometry
- Finance formulas
- Postgraduate study
- Intro to postgrad study
- Planning postgrad study
- Postgrad resources
- Postgrad assignment types
- Referencing
- Intro to referencing
- What is referencing?
- Why reference?
Common knowledge
- Referencing styles
- What type of source is this?
- Reference list vs. bibliography
- Referencing software
- Quoting & paraphrasing
- Paraphrasing & summarising
- Paraphrasing techniques
- APA Interactive
- In-text citation
- Reference list
- Online material
- Other material
- Headings in APA
- Tables and Figures
- Referencing elements
- 5th vs. 6th edition
- 6th vs. 7th edition
- Chicago style
- Chicago Interactive
- About notes system
- Notes referencing elements
- Quoting and paraphrasing
- Author-date system
- MLA Interactive
- Abbreviations
- List of works cited
- Captions for images
- 8th vs 9th edition
- Oxford style
- Other styles
- Harvard style
- Vancouver style
- Legal citations
- Visual material
- Sample assignments
- Sample essay 1
- Sample essay 2
- Sample annotated bibliography
- Sample book review
- Study skills
- Time management
- Intro to time management
- Procrastination & perfectionism
- Goals & motivation
- Time management for internal students
- Time management for distance students
- Memory skills
- Principles of good memory
- Memory strategies
- Note-taking
- Note-taking methods
- Note-taking in lectures
- Note-taking while reading
- Digital note-taking
- Reading styles
- In-depth reading
- Reading comprehension
- Reading academic material
- Reading a journal article
- Reading an academic book
- Critical thinking
- What is critical thinking?
- Constructing an argument
- Critical reading
- Logical fallacies
- Tests & exams
- Exam & test study
- Planning exam study
- Gathering & sorting information
- Reviewing past exams
- Phases of revision
- Last-minute study strategies
- Question types
- Short answer
- Multi-choice
- Problem / computational
- Case-study / scenario
- Open book exam
- Open web exam or test
- Take home test
- In the exam
- Online exam
- Physical exam
Common knowledge refers to facts that are so widely known it is unnecessary to reference them. Common knowledge is general knowledge.
For example, the following points are common knowledge in New Zealand:
Beijing is the capital of China.
Wellington is the capital of New Zealand.
The Treaty of Waitangi was signed in 1840.
The Māori are the indigenous people of New Zealand.
These facts are so widely known that it is unnecessary to provide a source to support them.
Common knowledge may vary from country to country. In Argentina, for example, the signing date of the Treaty of Waitangi is not widely known.
Common knowledge may also vary from discipline to discipline. What is common knowledge in sociology, for example, may not be common knowledge in psychology. Some disciplines (such as in the sciences) incorporate facts into common knowledge faster than others. As you develop familiarity with a discipline you will become more aware of what is common knowledge and what is not.
Distinguishing common knowledge
Common knowledge can be identified by considering two important questions:
- Is the fact widely known?
- Is the fact disputed by anyone?
A widely known fact will appear in several different sources, particularly in general reference sources like encyclopædias or dictionaries. Common knowledge will also be widely known to your audience.
If there is academic disagreement about a fact it should not be treated as common knowledge. Likewise, interpretations of facts can be different between different sources, so the interpretation is not common knowledge.
Referencing common knowledge
Common knowledge does not need to be referenced. Most academic writing, however, does not focus on common knowledge. Instead, different interpretations and applications of facts and concepts are discussed, and these do need to be referenced appropriately.
Have a study or assignment writing question? Ask an expert at Academic Q+A forum
Get feedback on your draft assignment at Academic Writing and Learning Support
Need help with your assignment or referencing? Contact a consultant and receive online and/or face-to-face support
Page authorised by Director - Centre for Learner Success Last updated on 25 October, 2012
- Academic Q+A
Have a study or assignment writing question? Ask an expert at Academic Q+A
Live online workshops
- StudyUp (undergraduate)
- Campus workshops
- Albany (undergraduate)
- Albany (postgraduate)
- Albany (distance)
- Manawatu (undergraduate)
- Manawatu (postgraduate)
Upcoming events
- All upcoming events
- Academic writing and learning support
- 0800 MASSEY | (+64 6 350 5701)
- [email protected]
- Online form
Find and fix writing mistakes instantly
- Check for unintentional plagiarism
- Get instant grammar and style suggestions
Common knowledge is defined as factual information that you’d expect most people to know. For example, the capital of France is Paris. Or JK Rowling is the author of the Harry Potter books. You don’t need to cite a source when referencing common knowledge in your papers, as everyone knows it so it doesn’t usually need to be evidenced.
Imagine citing a world map to evidence that the capital of France is Paris — it would seem pretty silly and pointless, right? Plus, you’re also at risk of patronizing your reader!
So If I Know It, It’s Common Knowledge?
Not necessarily! We all have lots of information stored in the back of our mind that we’re not entirely sure how it got there. This could include anything from the fact that the Taj Mahal is a landmark in India to the ingredients in a good guacamole. But you knowing something doesn’t automatically mean that it’s common knowledge.
For example:
A big Harry Potter fan might know that the original book was named Harry Potter and the Philosopher’s Stone, with a release date of June 1997 in the UK. However, many readers in the US will know the book as Harry Potter and the Sorcerer’s Stone. And many more will have no idea of its release date in the US, let alone in the UK.
On the flip side, you not knowing something doesn’t necessarily mean that it’s not common knowledge.
Capital cities are normally taken as common knowledge, despite the fact that most of us would have to look up the capital city of Christmas Island (Flying Fish Cove!) or Luxembourg (Luxembourg!).
Likewise, many people wrongly assume that a certain city is a country’s capital — that Istanbul, and not the less well-known Ankara, is the capital of Turkey, for example. You still would not be expected to cite a source. However, you might wish to provide more clarification i.e. Istanbul (then known as Constantinople) was the capital of the former Ottoman Empire, but Ankara took over as capital city in 1923, when the Republic of Turkey was formed. In which case, you should cite the source of this info.
Know Your Audience
It’s a good idea to consider your intended audience when determining what constitutes common knowledge for the purpose of your paper or essay. For example, if you’re referencing Picasso for art history class, you might assume that certain facts are common knowledge — yet someone who’s never studied art might know nothing at all about the artist and his work.
Lies, Damned Lies, and Statistics
As the above popular quote alludes, statistics are often stated as fact but can actually be deceptive little things. There are many ways in which a statistic can be skewed — it might come from a sponsored study which leads to a bias result, or there could be a false causality or overgeneralization at play — therefore you should always cite a reputable source.
If you’re in doubt as to whether something that you’re referencing in your essay is common knowledge, the safest option is to add a citation. Citation Machine can help you to create and format citations correctly, using the popular MLA formatting , APA format , and Chicago formats, as well as other styles. Simply check with your teacher or tutors to check which style you should be using and Citation Machine will help you cite your work correctly.
How useful was this post?
Click on a star to rate it!
We are sorry that this post was not useful for you!
Let us improve this post!
Tell us how we can improve this post?
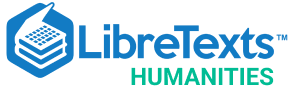
- school Campus Bookshelves
- menu_book Bookshelves
- perm_media Learning Objects
- login Login
- how_to_reg Request Instructor Account
- hub Instructor Commons
- Download Page (PDF)
- Download Full Book (PDF)
- Periodic Table
- Physics Constants
- Scientific Calculator
- Reference & Cite
- Tools expand_more
- Readability
selected template will load here
This action is not available.
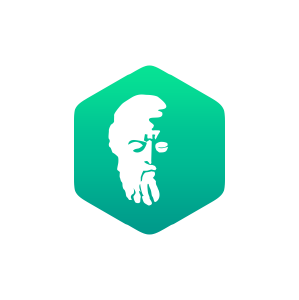
13: The Plot to Privatize Common Knowledge (Bollier)
- Last updated
- Save as PDF
- Page ID 21528
\( \newcommand{\vecs}[1]{\overset { \scriptstyle \rightharpoonup} {\mathbf{#1}} } \)
\( \newcommand{\vecd}[1]{\overset{-\!-\!\rightharpoonup}{\vphantom{a}\smash {#1}}} \)
\( \newcommand{\id}{\mathrm{id}}\) \( \newcommand{\Span}{\mathrm{span}}\)
( \newcommand{\kernel}{\mathrm{null}\,}\) \( \newcommand{\range}{\mathrm{range}\,}\)
\( \newcommand{\RealPart}{\mathrm{Re}}\) \( \newcommand{\ImaginaryPart}{\mathrm{Im}}\)
\( \newcommand{\Argument}{\mathrm{Arg}}\) \( \newcommand{\norm}[1]{\| #1 \|}\)
\( \newcommand{\inner}[2]{\langle #1, #2 \rangle}\)
\( \newcommand{\Span}{\mathrm{span}}\)
\( \newcommand{\id}{\mathrm{id}}\)
\( \newcommand{\kernel}{\mathrm{null}\,}\)
\( \newcommand{\range}{\mathrm{range}\,}\)
\( \newcommand{\RealPart}{\mathrm{Re}}\)
\( \newcommand{\ImaginaryPart}{\mathrm{Im}}\)
\( \newcommand{\Argument}{\mathrm{Arg}}\)
\( \newcommand{\norm}[1]{\| #1 \|}\)
\( \newcommand{\Span}{\mathrm{span}}\) \( \newcommand{\AA}{\unicode[.8,0]{x212B}}\)
\( \newcommand{\vectorA}[1]{\vec{#1}} % arrow\)
\( \newcommand{\vectorAt}[1]{\vec{\text{#1}}} % arrow\)
\( \newcommand{\vectorB}[1]{\overset { \scriptstyle \rightharpoonup} {\mathbf{#1}} } \)
\( \newcommand{\vectorC}[1]{\textbf{#1}} \)
\( \newcommand{\vectorD}[1]{\overrightarrow{#1}} \)
\( \newcommand{\vectorDt}[1]{\overrightarrow{\text{#1}}} \)
\( \newcommand{\vectE}[1]{\overset{-\!-\!\rightharpoonup}{\vphantom{a}\smash{\mathbf {#1}}}} \)
By David Bollier
#reportinginformation #descriptive #sharedvalues #currentevents #research #pathos #logos #kairos #cognitivebias #artsandculture #intellectualproperty
Over the past three decades, modern culture has become infatuated with the idea that knowledge should be owned like real estate or stock shares. The original idea, of course, is that copyrights, trademarks and patents reward people for their creative labors and thereby boosts the common good.
But this line of thinking has come to resemble a kind of Market Fundamentalism: copyrights, trademarks and patents are the only morally legitimate and practical method for managing creations of the mind. There is no middle ground. You either believe in intellectual property rights, or you support “theft” and “piracy.
This fundamentalist approach shuts down a broader discussion about how knowledge ought to circulate in our culture. To avoid any confusion, let me just say straight-up that I believe in copyrights and patents. In some cases, they provide significant and necessary incentives to invest in new works. But today, copyrights and patents are going far beyond their intended goals—such as the U.S. Constitution provision to “promote progress in science and the useful arts”-- to become ends in themselves. Instead of carefully balancing private interests and public needs, copyrights and patents are becoming crude, anti-social instruments of control and avarice.
This is the conclusion that I came to in my book Brand Name Bullies , which is filled with dozens of stories of copyright and trademark owners bullying citizens, artists, scholars and others with ridiculous legal threats.
Silent Campfires
One of my favorite stories about the alarming expansion of copyright law involves ASCAP, the American Society of Composers, Authors and Publishers, the organization that collects performance licensing fees from public establishments where recorded music is played.
ASCAP decided that their domain should be extended to summer camps. Why shouldn’t boys and girls singing around the campfire be considered a “public performance” that should pay royalties? A while back ASCAP approached the American Camping Association and said it wanted blanket performance licenses from hundreds of summer camps – something on the order of $300 to $1,400 per season per camp.
This caused quite a ruckus. When it was discovered that ASCAP wanted money for the Girl Scouts to sing “This Land Is Your Land” and “Puff, the Magic Dragon”, the press went nuts. There were stories about camps resorting to non-copyrighted songs like “The Bow-Legged Chicken.” An ASCAP official heartlessly told a reporter: “They [camps] buy paper, twine and glue for their crafts – they can pay for the music too.” Eventually, after a huge public outcry, ASCAP backed down. But its claim to legal authority in charging summer camps for their “public performances” of copyrighted songs remains intact.
Lawsuit Barbie
The issue in so many of these battles is: Who shall control the “public meaning” of familiar images? Mattel is legendary in trying to protect the cultural “meaning” of Barbie. It has gone after any unauthorized uses of Barbie. It went after a series of photographs by Mark Napier called Distorted Barbie, which dared to depict Barbie as fat or as having Down’s Syndrome. Even highly distorted images of Barbie that were essentially unrecognizable were deemed unacceptable by Mattel.
Mattel went after a magazine that caters to adult collectors of Barbie dolls. Mattel even pressured the Seattle publisher of a book, Adios, Barbie: Young Women Write About Body Image and Identity, to change the title. The book was reprinted as Body Outlaws. This extreme clampdown on free expression spurred culture-jammers, such as the self-styled Barbie Liberation Organization, which substituted voice boxes of GI Joe with those in Barbie, so that GI Joe would say, “Let’s plan our dream wedding,” and Barbie would yell, “Vengeance is mine!”
I am happy to report, a federal circuit court in the United States put a damper on Mattel’s bullying litigation. The case involved Utah photographer Tom Forsythe, who made a series of 78 photos of Barbie for his Food Chain Barbie exhibit. It featured Barbie in enchiladas, stuffed into a blender and in other kitchen poses. Only a few of Forsythe’s photos sold. He spent about $5,000 to mount the exhibit, and lost money.No matter; Mattel wanted to send a message that you can’t mess with Barbie. It spent years litigating the case, requiring Forsythe to find pro bono legal counsel, which spent nearly $2 million defending him. Forsythe prevailed in the circuit court, which delivered a stinging rebuke to Mattel for bringing a “groundless and unreasonable” trademark dilution claim.
Watch Your Words
The privatization of words—language is one of the most basic form of commons— is another disturbing trend. The Japanese corporation that owns the “Godzilla” trademark has a habit of threatening all sorts of people who use the phoneme “zilla,” including a website called “Davezilla” that featured a lizard-like cartoon character.
The corporate obsession with owning words is really quite extensive. McDonald’s claims to own 131 words and phrases. The San Diego-based McDonald’s actually claims to own the Irish prefix “Mc.” It has successfully prevented restaurant from naming their businesses McVegan, McSushi and McMunchies.
Ralph Lauren, the clothing line, went after Polo magazine, run by an equestrian organization, claiming it was a trademark infringement for the U.S. Polo Association to use the word “polo” on its line of clothing! MasterCard went after Ralph Nader for using “priceless” in his campaign ads when running for President in 2000. (Nader’s free speech rights ultimately prevailed.) But the gay athletes who wanted to host a series of athletic competitions in San Francisco could not use the phrase “Gay Olympics” because that phrase is owned by the U.S. Olympic Committee, who gets to decide who can use it. “Special Olympics” for disabled kids is OK, but not “Gay Olympics.”
The TV demagogue Bill O’Reilly reportedly went ballistic when he learned that the comedian (and now senator) Al Franken was using the words “fair and balanced” as a subtitle in his book that mocked various right-wing pundit, including him. The federal court laughed Fox News’ case out of court, and Franken won. But pity the people who can’t afford to hire Floyd Abrams, a prominent First Amendment attorney, to represent them. A woman from Los Angeles dared to name her neighborhood newspaper the Beechwood Voice. She was threatened with legal action by the Village Voice, which claimed that use of the word “voice” as a newspaper name diluted its trademark.
These stories illustrate just how far Market Fundamentalism is willing to go in order to enforce its vision of the world. It wants to commodify all of culture as private property, and require people to obtain permission (and to make royalties) before embarking on any modestly derivative new creativity. This approach, not coincidentally, favors the Disneys, Time Warners and Rupert Murdochs because it protects the market value of large inventories of copyrighted and trademarked works. It directly stifles expression that is local, amateur, small-scale or non-commercial in nature— the kind of expression that almost anyone outside a powerful corporation would engage in. This amounts to a wholesale privatization of our cultural commons.
Patents Privatize Taxpayer-Funded Research
The Market Fundamentalist worldview is even more infuriating, if that is possible, when applied to patents arising out of publicly funded research. Until 35 years ago, there had been a broad consensus that the intellectual property rights of federal research should stay in the public domain, or at least be licensed on a nonexclusive basis. That way, taxpayers could reap the full measure of value from their collective investments. In the late 1970s, however, large pharmaceutical, electronics and chemical companies mounted a bold lobbying campaign to reverse the public ownership of federal research. Since enactment of Bayh-Dole Act of 1980, authorizing universities to patent the fruits of federally funded research, we have seen a land rush to sell academic research that was once freely available to all.
Between 1980 and 2000, the number of patents secured by universities grew ten-fold, bringing in more than $1 billion in royalties and licensing fees – a windfall enjoyed mostly by a dozen top research universities. This, in reality, is a privatization of the public’s investments. Even though the public pays for the lion’s share of risky basic research for new drugs, the long-term equity returns tend to go to drug companies and a handful of top research universities. In the United States, we have seen this with the cancer drug Taxol; the antidepressant Prozac; the hypertension drug Capoten; and a number of HIV and AIDS therapies.
The upshot is that citizens often have to pay twice for pharmaceuticals and other medical treatments – first, as taxpayers who finance the research, and second, as consumers who pay monopoly prices for drugs. This is a pure giveaway because it’s not even clear that companies need exclusive patent rights as an incentive to commercialize new drug research.
Corporations Loot Indigenous People’s Knowledge
Multinational corporations are no longer content to simply claim ownership of commons knowledge at home. Now they scour the developing world-- in a practice known as biopiracy-- to claim patents on the botanical and ecological knowledge acquired by indigenous people through the centuries. They move into Madagascar, Brazil, Guatemala and other poor countries to find plants and microorganisms that might be used in making new medicines and genetically engineered crops. But as Seth Shulman writes in his book Owning the Future, “Who, if anyone, should be able to claim ownership rights to the globe’s genetic and cultural inheritance?”
Sir John Sulston answers this question eloquently in his book, The Common Thread , which chronicles the race to decode the human genome. A private startup company, Celera, was aggressively trying to put genomic sequences in one big privatized database. That way, it would have a monopoly over future use of the genomic data by licensing access to its database. Fortunately, a coalition of public-sector scientists published the data first, which is why the human genome is now in the public domain. Sulston answers, quite rightly, that the human genome must be treated as the “common heritage of humankind.”
Life Itself Can Now Be Owned
We dodged a bullet there when the publicly funded scientists won the race to decode human genome. Yet the threat of private ownership of essential knowledge for the sake of profits is not by any means over. Further attempts will by the logical culmination of a path first opened by the U.S. Supreme Court’s Diamond v. Chakrabarty ruling in 1980, which authorized the patenting of live, genetically altered microorganisms. The patenting of living organisms opened the way for an ecologically and ethically dubious future in which the life forms that are part of the sacred web of life can be owned and treated as commodities. Knowledge is treated as private property, not as a public good.
One inevitable result of all these new ownership claims is the rise of new barriers to open sharing, collaboration and discovery among researchers and scholars. Patents are increasingly being granted for “upstream” research, which means that basic knowledge that everyone else must use for the field to advance, is becoming proprietary. Harvard, MIT and the Whitehead Institute, for example, have a patent on all drugs that inhibit something known as NF-kB cell signaling. Since this physiological process is believed to have something to do with many diseases such as cancer and osteoporosis, the patent deters anyone else from pursuing their own scientific investigations in this area.
Things were not always this way concerning valuable knowledge. Contrast these stories with Jonas Salk, the inventor of the polio vaccine. When journalist Edward R. Murrow asked him, “Who owns the patent on this vaccine?” Salk replied, “Well, the people, I would say. There is no patent. Could you patent the sun?” This story helps us remember that current notions about ownership of knowledge are not inevitable and universal; they are the result of mounting market pressures to make our scientific and cultural commons into private property.
The privatization of knowledge has only intensified as the courts – in the United States, at least – have lowered the standards for obtaining patents while broadening the scope of what is patentable. It is now possible to own mathematical algorithms embedded in software programs. The very tools needed to conduct scientific research are now private property, available only for a steep fee.
Imagine what might have happened to biotechnology and computer science if contemporary patent rules had been in place in the 1950s and 1960s. Neither the biotech nor the computer revolution would have occurred in the first place. Too much fundamental knowledge would have been off limits due to patents.
Problem of the Anti-Commons
The over-patenting of knowledge sometimes results in what is called an “anti-commons” problem, in which property rights for a given field of research are so numerous and fragmented that it becomes very difficult to conduct research. The transaction costs for obtaining rights are simply too numerous and costly. For example, there are thirty-four “patent families” for a single malarial antigen, and those rights, applying to different pieces of the research agenda, are owned by different parties in many different countries. One reason that a malaria vaccine has been so elusive is because the patent rights are so complicated and expensive to secure.
It is worth noting that openness, sharing and the public domain do not harm the market. Quite the contrary. They invigorate it. In 2005, I co-hosted a conference called Ready to Share: Fashion and the Ownership of Creativity. It explored the power of openness in apparel design. Precisely because no one can own the creative design of clothes – they can only own the company name and logo, as trademarks – everyone can participate in the design commons. The result is a more robust, innovative and competitive marketplace. This is exactly the effect that Linux, the open-source computer operating system, had on the software sector. It has opened up new opportunities for value-added innovation and competition in a marketplace until then dominated by the Microsoft monopoly.
Yale Professor Yochai Benkler argues in his magisterial book, The Wealth of Networks , that a great deal of knowledge production is more effectively pursued through a commons than through markets. Questions of ethics aside, why doesn’t money succeed at simply “buying” the knowledge it needs? Because money tends to subvert the social dynamics that make the knowledge commons work. It can sabotage self-directed inquiry. It undermines the social trust, candor and ethics that are essential to creativity and good research.
____________________
David Bollier is an author, activist, blogger and consultant. He is the founding editor of On the Commons in which this essay first appeared. His 2014 book, Think Like a Commoner: A Short Introduction to the Commons describes his thinking about the commons.
The Plot to Privatize Common Knowledge by David Bollier is licensed under a Creative Commons Attribution-ShareAlike 4.0 International License .

31 Common Knowledge Examples (With Definition)
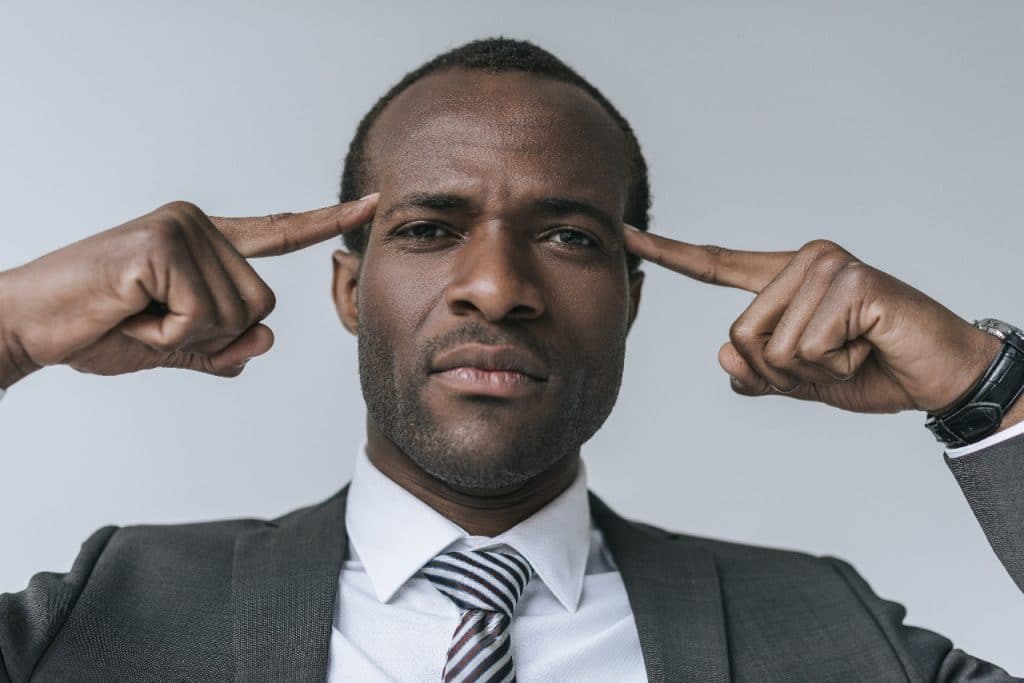
Common knowledge is information that is generally known by most people, and everyone knows that everyone else knows it.
This can include:
- Well-known facts
- Widely-accepted ideas
- Social norms, and
- Cultural concepts
But beware: common knowledge varies from society to society.
What’s common knowledge to you may not be common knowledge to someone on the other side of the world or even a different subculture in your city.
For example, it’s common knowledge among Christians that Judas betrayed Jesus. They were taught this lesson throughout their childhood.
But ask your Hindu friend down the street, and it may not be so commonly known to them!
As a result, “common knowledge” is a subjective and context-dependent term!
Definitions of Common Knowledge
One key feature of common knowledge is that it has to be known by a vast majority of people. But furthermore, people need to know that other people know that knowledge.
Here’s how one scholar says it:
“An event is common knowledge among a group of agents if each one knows it, if each one knows that the others know it, if each one knows that each one knows that the others know it, and so on.” (Geanakoplos, 1992).
This is an important detail if we take a case where ten people are in a room and they all know a fact, but they don’t realize that the people around them also know it! In this case, the thing is widely known, but not acknowledged to be common knowledge, because people don’t realize other people know it!
Common Knowledge Examples
Examples of common knowledge gained through education.
- The Earth is round.
- There are 365 days in the year.
- Water freezes at 32 degrees Fahrenheit.
- The French word for thankyou is “Merci”
- The capital of the United States is Washington, D.C.
- Humans have five senses – sight, smell, hearing, taste and touch.
- The colors of a rainbow are red, orange, yellow, green blue, indigo and violet (ROYGBIV).
- Oxygen is essential for human life.
- Gravity keeps us grounded on Earth.
- Objects in motion tend to stay in motion unless acted upon by an outside force.
Examples of Common Knowledge Gained through Experience
- If you touch something that’s hot, you will be burned.
- If someone finds out you lied to them, they will be offended.
- If you don’t wear sunblock, you will be sunburned.
- If you look at the sun, your eyes will hurt.
- Don’t touch wild animals.
- Books make you smarter.
Examples of Common Knowledge Gained through Social Norms
- It is polite to say “thank you”.
- Children aren’t allowed to drive.
- You need to respect the police.
- It’s polite to make eye contact with people.
- It’s polite to turn up to an appointment on time.
- If the teacher is talking, you need to be quiet and listen.
- Don’t talk in the library.
- Wait in line for your turn.
- It’s rude to tell people your income.
- Dresses are designed for women.
Sayings and quotes about Common Knowledge
- You catch more flies with honey (You’ll get your way more if you’re nice!)
- Let sleeping dogs lie (Don’t stir up trouble!)
- Don’t kick the hornet’s nest (Don’t stir up trouble!)
- If you don’t have anything nice to say, don’t say anything at all.
- The early bird gets the worm (Wake up early to have a good day)
Common Knowledge vs Common Sense
Common sense and common knowledge are terms that overlap, so examples will be similar .
Generally, common sense refers to things that are easy to deduce through logic.
For example, “don’t touch the hot plate” is common sense, but it’s also common knowledge.
Of course, we rightly assume that if something’s common sense then it’s also common knowledge.
But sometimes, people who lack street smarts might do things that aren’t common sense. One embarrassing example from my life was in science class, where we were given a science experiment involving acid. I touched the acid and said “wow, that tickles.” My friends looked at me like I was a fool.
The teacher thought this piece of common sense was such common knowledge that she didn’t bother telling the class not to touch the acid.
Here, we can return to the definition of common knowledge: everyone must know something, and everyone must know that everyone knows it.
By contrast, with common sense, you can know common sense because it makes sense and you would usually assume that others know it intuitively – unless they’re foolish, like me.
Migrants and Common Knowledge
Immigrants often find it very difficult to fit into a society because they’re often unaware of a culture’s common knowledge. This leads to culture shock .
For example, a migrant to my city of Vancouver might not realize that it’s common knowledge that you need to get a tap-on tap-off card to use public transit.
The migrant might get on a bus and try to give the bus driver cash. The bus driver will look at them like they’re ignorant.
They’re not ignorant, of course. They just lack common knowledge because they haven’t been in the society long enough and don’t have the social capital required to learn the things that are common knowledge in the society.
Common Knowledge and Cultural Capital
Common knowledge can be seen as a type of cultural capital . This is a term that refers to how well you know and fit-in within a cultural group.
In the example above of the migrant on the bus, they lack cultural capital in the form of common knowledge.
Turning to scholars like Pierre Bourdieu, we learn that cultural capital can be developed through time in the culture, education within the culture (see: cultural capital and education ), interaction with peer groups ( social capital ), and even by paying to learn about it ( economic capital ).
Common knowledge refers to information that is known by a group of people, and all people know that other people know it.
But as we’ve seen, common knowledge isn’t quite so common! If you move to another culture, you may find that you lack the common knowledge that all the people around you have!
Similarly, if you go back five years, new facts and even modern sayings and songs are not commonly known at all. So, what is considered “common knowledge” is always changing depending on the social and cultural context.
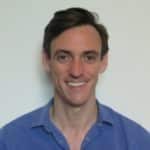
Chris Drew (PhD)
Dr. Chris Drew is the founder of the Helpful Professor. He holds a PhD in education and has published over 20 articles in scholarly journals. He is the former editor of the Journal of Learning Development in Higher Education. [Image Descriptor: Photo of Chris]
- Chris Drew (PhD) https://helpfulprofessor.com/author/chris-drew-phd/ Social-Emotional Learning (Definition, Examples, Pros & Cons)
- Chris Drew (PhD) https://helpfulprofessor.com/author/chris-drew-phd/ What is Educational Psychology?
- Chris Drew (PhD) https://helpfulprofessor.com/author/chris-drew-phd/ What is IQ? (Intelligence Quotient)
- Chris Drew (PhD) https://helpfulprofessor.com/author/chris-drew-phd/ 5 Top Tips for Succeeding at University
Leave a Comment Cancel Reply
Your email address will not be published. Required fields are marked *
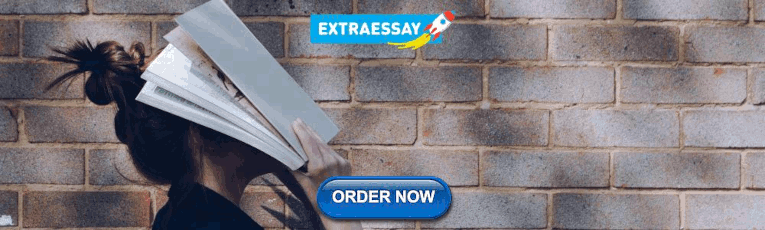
COMMENTS
In academic writing, "common knowledge" refers to information that the average educated reader would accept without needing a source citation to back it up. There are two main categories of common knowledge: Information that most people know. Information shared by a specific group of people, such as a national or cultural group, or ...
Broadly speaking, common knowledge refers to information that the average, educated reader would accept as reliable without having to look it up. This includes: Information that most people know, such as that water freezes at 32 degrees Fahrenheit or that Barack Obama was the first American of mixed race to be elected president.
Generally, common knowledge is information that someone finds undocumented in at least five credible sources. For example, writing is difficult," is considered common knowledge in the field of composition studies because at least five credible sources can back the claim up. Remember the golden rule: When in doubt, just cite.
Common Knowledge. Common knowledge is information or ideas that are widely known, accepted, and found in multiple places. Common knowledge is context dependent, meaning that something might be common knowledge to one audience but not another audience. If you are paraphrasing common knowledge, you do not need to cite that statement.
The Exception: Common Knowledge. The only source material that you can use in an essay without attribution is material that is considered common knowledge and is therefore not attributable to one source. Common knowledge is information generally known to an educated reader, such as widely known facts and dates, and, more rarely, ideas or language.
The "common" way to talk about common knowledge is to say that it is knowledge that most educated people know or can find out easily in an encyclopedia or dictionary. Thus, you might not know the date of the most recent meeting of the Federal Reserve, but you can find it out quite easily. Further, the term "common knowledge" carries the ...
Examples of common knowledge include: Information that appears across many sources without a clear origin, such as famous historical dates. Long-established facts or theories that are foundational to a field, like fundamental equations in physics. It does not include: Data and statistics gathered in empirical studies. These should always be cited.
Common Knowledge. The term "common knowledge" refers to facts and information that are so well-known and clearly established that a writer can use them in their work without citing a specific source. Like plagiarism, student writers tend to think of common knowledge as a universal distinction-either a given fact is common knowledge or it ...
When deciding whether or not to cite what might appear to be common knowledge, you can make use of these three general guidelines: 1. If in doubt, cite. 2. Always attribute arguments. 3. Seek advice from your instructors for further clarification. Look to style guides, published articles, as well as librarians, writing consultants, and ...
The announcement made the mutually known fact common knowledge among the students. ... The topics reviewed in each section of this essay are as follows: Section 1 gives motivating examples which illustrate a variety of ways in which the actions of agents depend crucially upon their having, or lacking, certain common knowledge. ...
Common knowledge is, as the name suggests, information that could reasonably be known by the average person. For example: Common knowledge could also be a fact that not everyone would know off the top of their heads, but that's been generally verified and could be confirmed easily. For example: A subordinating conjunction joins independent ...
Common Knowledge. The only source material you can legitimately use without quotation. Common knowledge is information that could be generally known to an educated reader. Examples of this might be facts such as Paris is the capital city of France or widely known historical or scientific facts, such as the molecular structure of water is H2O. ...
Common knowledge does not need to be cited. However, you should be extra careful when deciding what counts as common knowledge. Common knowledge encompasses information that the average educated reader would accept as true without needing the extra validation of a source or citation. Common knowledge should be widely known, undisputed and ...
Common knowledge is knowledge that is publicly known by everyone or nearly everyone, usually with reference to the community in which the knowledge is referenced. Common knowledge can be about a broad range of subjects, such as science, literature, history, or entertainment. Since individuals often have different knowledge bases, common knowledge can vary and it may sometimes take large-scale ...
not cited, but rather on how that common knowledge is rhetorically cued in the text. In her analysis of common knowledge in scienti‹c essays, Kout-santoni details the various markers of common knowledge expert writers employ and the effect those markers have on an audience. These markers generally fall into two categories: "evaluative ...
The Exception: Common Knowledge. The only source material that you can use in an essay without attribution is material that is considered common knowledge and is therefore not attributable to one source. Common knowledge is information generally known to an educated reader, such as widely known facts and dates, and, more rarely, ideas or language.
Examples of statements that don't express common knowledge: Civilizations that were aware of geese usually considered them intermediaries between heaven and earth (Toussaint-Samat 352). The Chinese fishing industry produces 800,000 tons of fish annually (Toussaint-Samat 328). The first international coffee syndicate was based in Germany ...
"Common knowledge" is a very hazy concept that is field-dependent, subfield-dependent, sub-sub-subfield dependent, and context-dependent as well (e.g. for a published paper, which journal the paper is published in can affect what is "common knowledge"). ... Anything else to either relegate to a bibliographical essay at the end or eliminate ...
Common Knowledge. First published Tue Aug 28, 2001; substantive revision Fri Aug 5, 2022. A proposition \ (A\) is mutual knowledge among a set of agents if each agent knows that \ (A\). Mutual knowledge by itself implies nothing about what, if any, knowledge anyone attributes to anyone else. Suppose each student arrives for a class meeting ...
Common knowledge. Common knowledge refers to facts that are so widely known it is unnecessary to reference them. Common knowledge is general knowledge. For example, the following points are common knowledge in New Zealand: Beijing is the capital of China. Wellington is the capital of New Zealand. The Treaty of Waitangi was signed in 1840.
4.3. ( 9) Common knowledge is defined as factual information that you'd expect most people to know. For example, the capital of France is Paris. Or JK Rowling is the author of the Harry Potter books. You don't need to cite a source when referencing common knowledge in your papers, as everyone knows it so it doesn't usually need to be ...
He is the founding editor of On the Commons in which this essay first appeared. His 2014 book, Think Like a Commoner: A Short Introduction to the Commons describes his thinking about the commons. The Plot to Privatize Common Knowledge by David Bollier is licensed under a Creative Commons Attribution-ShareAlike 4.0 International License.
Examples of Common Knowledge Gained through Social Norms. It is polite to say "thank you". Children aren't allowed to drive. You need to respect the police. It's polite to make eye contact with people. It's polite to turn up to an appointment on time. If the teacher is talking, you need to be quiet and listen.