Have a language expert improve your writing
Run a free plagiarism check in 10 minutes, generate accurate citations for free.
- Knowledge Base
Methodology
- Types of Research Designs Compared | Guide & Examples
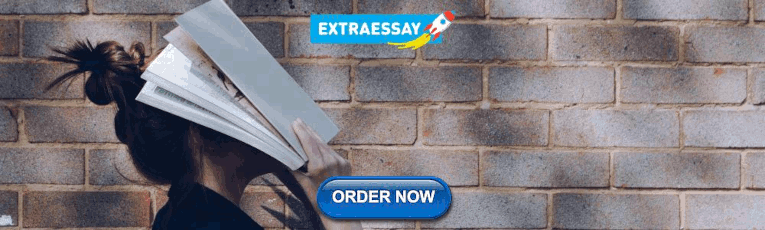
Types of Research Designs Compared | Guide & Examples
Published on June 20, 2019 by Shona McCombes . Revised on June 22, 2023.
When you start planning a research project, developing research questions and creating a research design , you will have to make various decisions about the type of research you want to do.
There are many ways to categorize different types of research. The words you use to describe your research depend on your discipline and field. In general, though, the form your research design takes will be shaped by:
- The type of knowledge you aim to produce
- The type of data you will collect and analyze
- The sampling methods , timescale and location of the research
This article takes a look at some common distinctions made between different types of research and outlines the key differences between them.
Table of contents
Types of research aims, types of research data, types of sampling, timescale, and location, other interesting articles.
The first thing to consider is what kind of knowledge your research aims to contribute.
Receive feedback on language, structure, and formatting
Professional editors proofread and edit your paper by focusing on:
- Academic style
- Vague sentences
- Style consistency
See an example
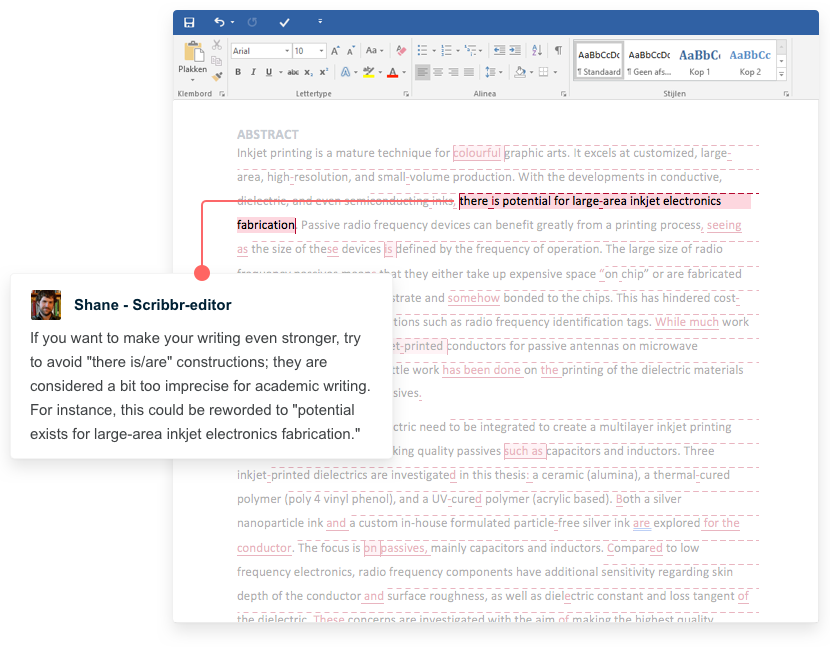
The next thing to consider is what type of data you will collect. Each kind of data is associated with a range of specific research methods and procedures.
Finally, you have to consider three closely related questions: how will you select the subjects or participants of the research? When and how often will you collect data from your subjects? And where will the research take place?
Keep in mind that the methods that you choose bring with them different risk factors and types of research bias . Biases aren’t completely avoidable, but can heavily impact the validity and reliability of your findings if left unchecked.
Choosing between all these different research types is part of the process of creating your research design , which determines exactly how your research will be conducted. But the type of research is only the first step: next, you have to make more concrete decisions about your research methods and the details of the study.
Read more about creating a research design
If you want to know more about statistics , methodology , or research bias , make sure to check out some of our other articles with explanations and examples.
- Normal distribution
- Degrees of freedom
- Null hypothesis
- Discourse analysis
- Control groups
- Mixed methods research
- Non-probability sampling
- Quantitative research
- Ecological validity
Research bias
- Rosenthal effect
- Implicit bias
- Cognitive bias
- Selection bias
- Negativity bias
- Status quo bias
Cite this Scribbr article
If you want to cite this source, you can copy and paste the citation or click the “Cite this Scribbr article” button to automatically add the citation to our free Citation Generator.
McCombes, S. (2023, June 22). Types of Research Designs Compared | Guide & Examples. Scribbr. Retrieved March 25, 2024, from https://www.scribbr.com/methodology/types-of-research/
Is this article helpful?
Shona McCombes
Other students also liked, what is a research design | types, guide & examples, qualitative vs. quantitative research | differences, examples & methods, what is a research methodology | steps & tips, unlimited academic ai-proofreading.
✔ Document error-free in 5minutes ✔ Unlimited document corrections ✔ Specialized in correcting academic texts
Have a language expert improve your writing
Run a free plagiarism check in 10 minutes, automatically generate references for free.
- Knowledge Base
- Methodology
Research Design | Step-by-Step Guide with Examples
Published on 5 May 2022 by Shona McCombes . Revised on 20 March 2023.
A research design is a strategy for answering your research question using empirical data. Creating a research design means making decisions about:
- Your overall aims and approach
- The type of research design you’ll use
- Your sampling methods or criteria for selecting subjects
- Your data collection methods
- The procedures you’ll follow to collect data
- Your data analysis methods
A well-planned research design helps ensure that your methods match your research aims and that you use the right kind of analysis for your data.
Table of contents
Step 1: consider your aims and approach, step 2: choose a type of research design, step 3: identify your population and sampling method, step 4: choose your data collection methods, step 5: plan your data collection procedures, step 6: decide on your data analysis strategies, frequently asked questions.
- Introduction
Before you can start designing your research, you should already have a clear idea of the research question you want to investigate.
There are many different ways you could go about answering this question. Your research design choices should be driven by your aims and priorities – start by thinking carefully about what you want to achieve.
The first choice you need to make is whether you’ll take a qualitative or quantitative approach.
Qualitative research designs tend to be more flexible and inductive , allowing you to adjust your approach based on what you find throughout the research process.
Quantitative research designs tend to be more fixed and deductive , with variables and hypotheses clearly defined in advance of data collection.
It’s also possible to use a mixed methods design that integrates aspects of both approaches. By combining qualitative and quantitative insights, you can gain a more complete picture of the problem you’re studying and strengthen the credibility of your conclusions.
Practical and ethical considerations when designing research
As well as scientific considerations, you need to think practically when designing your research. If your research involves people or animals, you also need to consider research ethics .
- How much time do you have to collect data and write up the research?
- Will you be able to gain access to the data you need (e.g., by travelling to a specific location or contacting specific people)?
- Do you have the necessary research skills (e.g., statistical analysis or interview techniques)?
- Will you need ethical approval ?
At each stage of the research design process, make sure that your choices are practically feasible.
Prevent plagiarism, run a free check.
Within both qualitative and quantitative approaches, there are several types of research design to choose from. Each type provides a framework for the overall shape of your research.
Types of quantitative research designs
Quantitative designs can be split into four main types. Experimental and quasi-experimental designs allow you to test cause-and-effect relationships, while descriptive and correlational designs allow you to measure variables and describe relationships between them.
With descriptive and correlational designs, you can get a clear picture of characteristics, trends, and relationships as they exist in the real world. However, you can’t draw conclusions about cause and effect (because correlation doesn’t imply causation ).
Experiments are the strongest way to test cause-and-effect relationships without the risk of other variables influencing the results. However, their controlled conditions may not always reflect how things work in the real world. They’re often also more difficult and expensive to implement.
Types of qualitative research designs
Qualitative designs are less strictly defined. This approach is about gaining a rich, detailed understanding of a specific context or phenomenon, and you can often be more creative and flexible in designing your research.
The table below shows some common types of qualitative design. They often have similar approaches in terms of data collection, but focus on different aspects when analysing the data.
Your research design should clearly define who or what your research will focus on, and how you’ll go about choosing your participants or subjects.
In research, a population is the entire group that you want to draw conclusions about, while a sample is the smaller group of individuals you’ll actually collect data from.
Defining the population
A population can be made up of anything you want to study – plants, animals, organisations, texts, countries, etc. In the social sciences, it most often refers to a group of people.
For example, will you focus on people from a specific demographic, region, or background? Are you interested in people with a certain job or medical condition, or users of a particular product?
The more precisely you define your population, the easier it will be to gather a representative sample.
Sampling methods
Even with a narrowly defined population, it’s rarely possible to collect data from every individual. Instead, you’ll collect data from a sample.
To select a sample, there are two main approaches: probability sampling and non-probability sampling . The sampling method you use affects how confidently you can generalise your results to the population as a whole.
Probability sampling is the most statistically valid option, but it’s often difficult to achieve unless you’re dealing with a very small and accessible population.
For practical reasons, many studies use non-probability sampling, but it’s important to be aware of the limitations and carefully consider potential biases. You should always make an effort to gather a sample that’s as representative as possible of the population.
Case selection in qualitative research
In some types of qualitative designs, sampling may not be relevant.
For example, in an ethnography or a case study, your aim is to deeply understand a specific context, not to generalise to a population. Instead of sampling, you may simply aim to collect as much data as possible about the context you are studying.
In these types of design, you still have to carefully consider your choice of case or community. You should have a clear rationale for why this particular case is suitable for answering your research question.
For example, you might choose a case study that reveals an unusual or neglected aspect of your research problem, or you might choose several very similar or very different cases in order to compare them.
Data collection methods are ways of directly measuring variables and gathering information. They allow you to gain first-hand knowledge and original insights into your research problem.
You can choose just one data collection method, or use several methods in the same study.
Survey methods
Surveys allow you to collect data about opinions, behaviours, experiences, and characteristics by asking people directly. There are two main survey methods to choose from: questionnaires and interviews.
Observation methods
Observations allow you to collect data unobtrusively, observing characteristics, behaviours, or social interactions without relying on self-reporting.
Observations may be conducted in real time, taking notes as you observe, or you might make audiovisual recordings for later analysis. They can be qualitative or quantitative.
Other methods of data collection
There are many other ways you might collect data depending on your field and topic.
If you’re not sure which methods will work best for your research design, try reading some papers in your field to see what data collection methods they used.
Secondary data
If you don’t have the time or resources to collect data from the population you’re interested in, you can also choose to use secondary data that other researchers already collected – for example, datasets from government surveys or previous studies on your topic.
With this raw data, you can do your own analysis to answer new research questions that weren’t addressed by the original study.
Using secondary data can expand the scope of your research, as you may be able to access much larger and more varied samples than you could collect yourself.
However, it also means you don’t have any control over which variables to measure or how to measure them, so the conclusions you can draw may be limited.
As well as deciding on your methods, you need to plan exactly how you’ll use these methods to collect data that’s consistent, accurate, and unbiased.
Planning systematic procedures is especially important in quantitative research, where you need to precisely define your variables and ensure your measurements are reliable and valid.
Operationalisation
Some variables, like height or age, are easily measured. But often you’ll be dealing with more abstract concepts, like satisfaction, anxiety, or competence. Operationalisation means turning these fuzzy ideas into measurable indicators.
If you’re using observations , which events or actions will you count?
If you’re using surveys , which questions will you ask and what range of responses will be offered?
You may also choose to use or adapt existing materials designed to measure the concept you’re interested in – for example, questionnaires or inventories whose reliability and validity has already been established.
Reliability and validity
Reliability means your results can be consistently reproduced , while validity means that you’re actually measuring the concept you’re interested in.
For valid and reliable results, your measurement materials should be thoroughly researched and carefully designed. Plan your procedures to make sure you carry out the same steps in the same way for each participant.
If you’re developing a new questionnaire or other instrument to measure a specific concept, running a pilot study allows you to check its validity and reliability in advance.
Sampling procedures
As well as choosing an appropriate sampling method, you need a concrete plan for how you’ll actually contact and recruit your selected sample.
That means making decisions about things like:
- How many participants do you need for an adequate sample size?
- What inclusion and exclusion criteria will you use to identify eligible participants?
- How will you contact your sample – by mail, online, by phone, or in person?
If you’re using a probability sampling method, it’s important that everyone who is randomly selected actually participates in the study. How will you ensure a high response rate?
If you’re using a non-probability method, how will you avoid bias and ensure a representative sample?
Data management
It’s also important to create a data management plan for organising and storing your data.
Will you need to transcribe interviews or perform data entry for observations? You should anonymise and safeguard any sensitive data, and make sure it’s backed up regularly.
Keeping your data well organised will save time when it comes to analysing them. It can also help other researchers validate and add to your findings.
On their own, raw data can’t answer your research question. The last step of designing your research is planning how you’ll analyse the data.
Quantitative data analysis
In quantitative research, you’ll most likely use some form of statistical analysis . With statistics, you can summarise your sample data, make estimates, and test hypotheses.
Using descriptive statistics , you can summarise your sample data in terms of:
- The distribution of the data (e.g., the frequency of each score on a test)
- The central tendency of the data (e.g., the mean to describe the average score)
- The variability of the data (e.g., the standard deviation to describe how spread out the scores are)
The specific calculations you can do depend on the level of measurement of your variables.
Using inferential statistics , you can:
- Make estimates about the population based on your sample data.
- Test hypotheses about a relationship between variables.
Regression and correlation tests look for associations between two or more variables, while comparison tests (such as t tests and ANOVAs ) look for differences in the outcomes of different groups.
Your choice of statistical test depends on various aspects of your research design, including the types of variables you’re dealing with and the distribution of your data.
Qualitative data analysis
In qualitative research, your data will usually be very dense with information and ideas. Instead of summing it up in numbers, you’ll need to comb through the data in detail, interpret its meanings, identify patterns, and extract the parts that are most relevant to your research question.
Two of the most common approaches to doing this are thematic analysis and discourse analysis .
There are many other ways of analysing qualitative data depending on the aims of your research. To get a sense of potential approaches, try reading some qualitative research papers in your field.
A sample is a subset of individuals from a larger population. Sampling means selecting the group that you will actually collect data from in your research.
For example, if you are researching the opinions of students in your university, you could survey a sample of 100 students.
Statistical sampling allows you to test a hypothesis about the characteristics of a population. There are various sampling methods you can use to ensure that your sample is representative of the population as a whole.
Operationalisation means turning abstract conceptual ideas into measurable observations.
For example, the concept of social anxiety isn’t directly observable, but it can be operationally defined in terms of self-rating scores, behavioural avoidance of crowded places, or physical anxiety symptoms in social situations.
Before collecting data , it’s important to consider how you will operationalise the variables that you want to measure.
The research methods you use depend on the type of data you need to answer your research question .
- If you want to measure something or test a hypothesis , use quantitative methods . If you want to explore ideas, thoughts, and meanings, use qualitative methods .
- If you want to analyse a large amount of readily available data, use secondary data. If you want data specific to your purposes with control over how they are generated, collect primary data.
- If you want to establish cause-and-effect relationships between variables , use experimental methods. If you want to understand the characteristics of a research subject, use descriptive methods.
Cite this Scribbr article
If you want to cite this source, you can copy and paste the citation or click the ‘Cite this Scribbr article’ button to automatically add the citation to our free Reference Generator.
McCombes, S. (2023, March 20). Research Design | Step-by-Step Guide with Examples. Scribbr. Retrieved 25 March 2024, from https://www.scribbr.co.uk/research-methods/research-design/
Is this article helpful?
Shona McCombes

Research Design 101
Everything You Need To Get Started (With Examples)
By: Derek Jansen (MBA) | Reviewers: Eunice Rautenbach (DTech) & Kerryn Warren (PhD) | April 2023
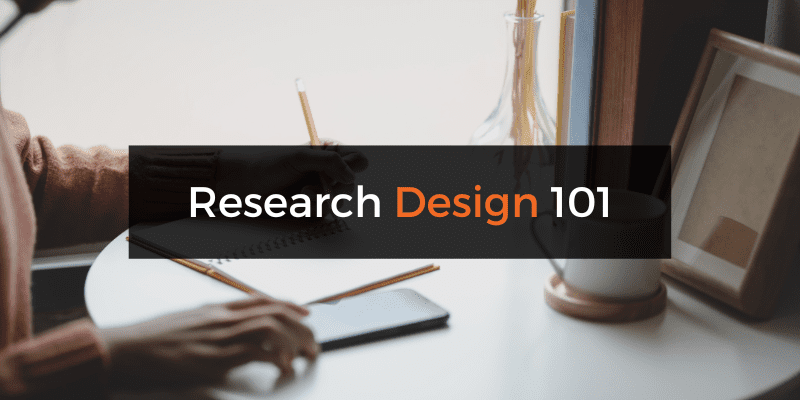
Navigating the world of research can be daunting, especially if you’re a first-time researcher. One concept you’re bound to run into fairly early in your research journey is that of “ research design ”. Here, we’ll guide you through the basics using practical examples , so that you can approach your research with confidence.
Overview: Research Design 101
What is research design.
- Research design types for quantitative studies
- Video explainer : quantitative research design
- Research design types for qualitative studies
- Video explainer : qualitative research design
- How to choose a research design
- Key takeaways
Research design refers to the overall plan, structure or strategy that guides a research project , from its conception to the final data analysis. A good research design serves as the blueprint for how you, as the researcher, will collect and analyse data while ensuring consistency, reliability and validity throughout your study.
Understanding different types of research designs is essential as helps ensure that your approach is suitable given your research aims, objectives and questions , as well as the resources you have available to you. Without a clear big-picture view of how you’ll design your research, you run the risk of potentially making misaligned choices in terms of your methodology – especially your sampling , data collection and data analysis decisions.
The problem with defining research design…
One of the reasons students struggle with a clear definition of research design is because the term is used very loosely across the internet, and even within academia.
Some sources claim that the three research design types are qualitative, quantitative and mixed methods , which isn’t quite accurate (these just refer to the type of data that you’ll collect and analyse). Other sources state that research design refers to the sum of all your design choices, suggesting it’s more like a research methodology . Others run off on other less common tangents. No wonder there’s confusion!
In this article, we’ll clear up the confusion. We’ll explain the most common research design types for both qualitative and quantitative research projects, whether that is for a full dissertation or thesis, or a smaller research paper or article.
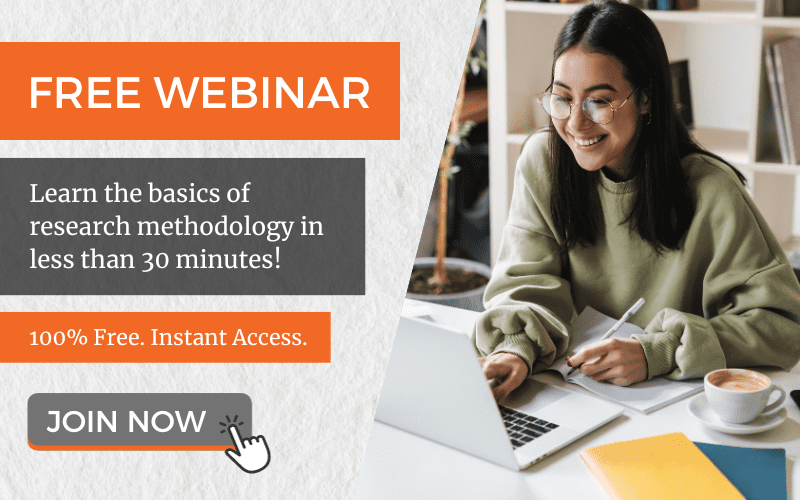
Research Design: Quantitative Studies
Quantitative research involves collecting and analysing data in a numerical form. Broadly speaking, there are four types of quantitative research designs: descriptive , correlational , experimental , and quasi-experimental .
Descriptive Research Design
As the name suggests, descriptive research design focuses on describing existing conditions, behaviours, or characteristics by systematically gathering information without manipulating any variables. In other words, there is no intervention on the researcher’s part – only data collection.
For example, if you’re studying smartphone addiction among adolescents in your community, you could deploy a survey to a sample of teens asking them to rate their agreement with certain statements that relate to smartphone addiction. The collected data would then provide insight regarding how widespread the issue may be – in other words, it would describe the situation.
The key defining attribute of this type of research design is that it purely describes the situation . In other words, descriptive research design does not explore potential relationships between different variables or the causes that may underlie those relationships. Therefore, descriptive research is useful for generating insight into a research problem by describing its characteristics . By doing so, it can provide valuable insights and is often used as a precursor to other research design types.
Correlational Research Design
Correlational design is a popular choice for researchers aiming to identify and measure the relationship between two or more variables without manipulating them . In other words, this type of research design is useful when you want to know whether a change in one thing tends to be accompanied by a change in another thing.
For example, if you wanted to explore the relationship between exercise frequency and overall health, you could use a correlational design to help you achieve this. In this case, you might gather data on participants’ exercise habits, as well as records of their health indicators like blood pressure, heart rate, or body mass index. Thereafter, you’d use a statistical test to assess whether there’s a relationship between the two variables (exercise frequency and health).
As you can see, correlational research design is useful when you want to explore potential relationships between variables that cannot be manipulated or controlled for ethical, practical, or logistical reasons. It is particularly helpful in terms of developing predictions , and given that it doesn’t involve the manipulation of variables, it can be implemented at a large scale more easily than experimental designs (which will look at next).
That said, it’s important to keep in mind that correlational research design has limitations – most notably that it cannot be used to establish causality . In other words, correlation does not equal causation . To establish causality, you’ll need to move into the realm of experimental design, coming up next…
Need a helping hand?
Experimental Research Design
Experimental research design is used to determine if there is a causal relationship between two or more variables . With this type of research design, you, as the researcher, manipulate one variable (the independent variable) while controlling others (dependent variables). Doing so allows you to observe the effect of the former on the latter and draw conclusions about potential causality.
For example, if you wanted to measure if/how different types of fertiliser affect plant growth, you could set up several groups of plants, with each group receiving a different type of fertiliser, as well as one with no fertiliser at all. You could then measure how much each plant group grew (on average) over time and compare the results from the different groups to see which fertiliser was most effective.
Overall, experimental research design provides researchers with a powerful way to identify and measure causal relationships (and the direction of causality) between variables. However, developing a rigorous experimental design can be challenging as it’s not always easy to control all the variables in a study. This often results in smaller sample sizes , which can reduce the statistical power and generalisability of the results.
Moreover, experimental research design requires random assignment . This means that the researcher needs to assign participants to different groups or conditions in a way that each participant has an equal chance of being assigned to any group (note that this is not the same as random sampling ). Doing so helps reduce the potential for bias and confounding variables . This need for random assignment can lead to ethics-related issues . For example, withholding a potentially beneficial medical treatment from a control group may be considered unethical in certain situations.
Quasi-Experimental Research Design
Quasi-experimental research design is used when the research aims involve identifying causal relations , but one cannot (or doesn’t want to) randomly assign participants to different groups (for practical or ethical reasons). Instead, with a quasi-experimental research design, the researcher relies on existing groups or pre-existing conditions to form groups for comparison.
For example, if you were studying the effects of a new teaching method on student achievement in a particular school district, you may be unable to randomly assign students to either group and instead have to choose classes or schools that already use different teaching methods. This way, you still achieve separate groups, without having to assign participants to specific groups yourself.
Naturally, quasi-experimental research designs have limitations when compared to experimental designs. Given that participant assignment is not random, it’s more difficult to confidently establish causality between variables, and, as a researcher, you have less control over other variables that may impact findings.
All that said, quasi-experimental designs can still be valuable in research contexts where random assignment is not possible and can often be undertaken on a much larger scale than experimental research, thus increasing the statistical power of the results. What’s important is that you, as the researcher, understand the limitations of the design and conduct your quasi-experiment as rigorously as possible, paying careful attention to any potential confounding variables .
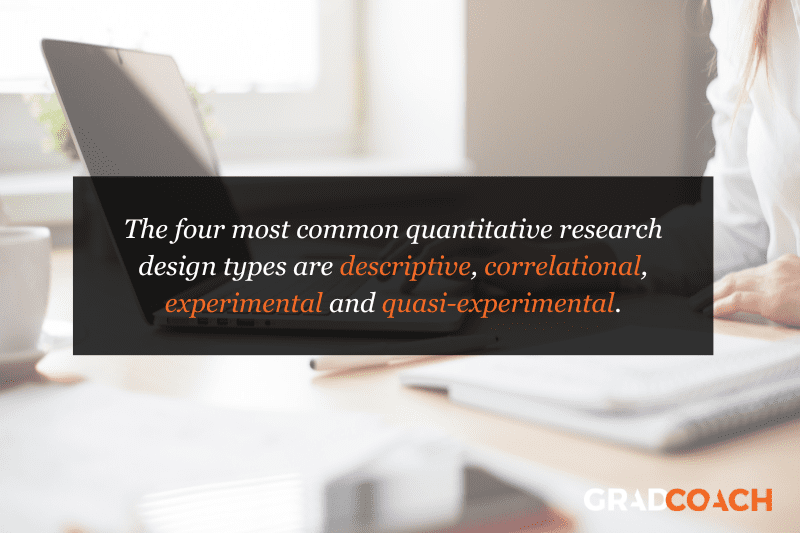
Research Design: Qualitative Studies
There are many different research design types when it comes to qualitative studies, but here we’ll narrow our focus to explore the “Big 4”. Specifically, we’ll look at phenomenological design, grounded theory design, ethnographic design, and case study design.
Phenomenological Research Design
Phenomenological design involves exploring the meaning of lived experiences and how they are perceived by individuals. This type of research design seeks to understand people’s perspectives , emotions, and behaviours in specific situations. Here, the aim for researchers is to uncover the essence of human experience without making any assumptions or imposing preconceived ideas on their subjects.
For example, you could adopt a phenomenological design to study why cancer survivors have such varied perceptions of their lives after overcoming their disease. This could be achieved by interviewing survivors and then analysing the data using a qualitative analysis method such as thematic analysis to identify commonalities and differences.
Phenomenological research design typically involves in-depth interviews or open-ended questionnaires to collect rich, detailed data about participants’ subjective experiences. This richness is one of the key strengths of phenomenological research design but, naturally, it also has limitations. These include potential biases in data collection and interpretation and the lack of generalisability of findings to broader populations.
Grounded Theory Research Design
Grounded theory (also referred to as “GT”) aims to develop theories by continuously and iteratively analysing and comparing data collected from a relatively large number of participants in a study. It takes an inductive (bottom-up) approach, with a focus on letting the data “speak for itself”, without being influenced by preexisting theories or the researcher’s preconceptions.
As an example, let’s assume your research aims involved understanding how people cope with chronic pain from a specific medical condition, with a view to developing a theory around this. In this case, grounded theory design would allow you to explore this concept thoroughly without preconceptions about what coping mechanisms might exist. You may find that some patients prefer cognitive-behavioural therapy (CBT) while others prefer to rely on herbal remedies. Based on multiple, iterative rounds of analysis, you could then develop a theory in this regard, derived directly from the data (as opposed to other preexisting theories and models).
Grounded theory typically involves collecting data through interviews or observations and then analysing it to identify patterns and themes that emerge from the data. These emerging ideas are then validated by collecting more data until a saturation point is reached (i.e., no new information can be squeezed from the data). From that base, a theory can then be developed .
As you can see, grounded theory is ideally suited to studies where the research aims involve theory generation , especially in under-researched areas. Keep in mind though that this type of research design can be quite time-intensive , given the need for multiple rounds of data collection and analysis.
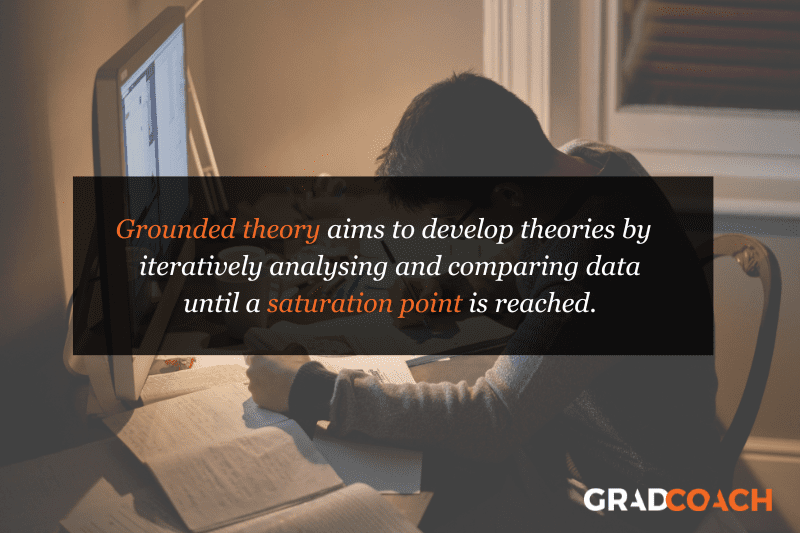
Ethnographic Research Design
Ethnographic design involves observing and studying a culture-sharing group of people in their natural setting to gain insight into their behaviours, beliefs, and values. The focus here is on observing participants in their natural environment (as opposed to a controlled environment). This typically involves the researcher spending an extended period of time with the participants in their environment, carefully observing and taking field notes .
All of this is not to say that ethnographic research design relies purely on observation. On the contrary, this design typically also involves in-depth interviews to explore participants’ views, beliefs, etc. However, unobtrusive observation is a core component of the ethnographic approach.
As an example, an ethnographer may study how different communities celebrate traditional festivals or how individuals from different generations interact with technology differently. This may involve a lengthy period of observation, combined with in-depth interviews to further explore specific areas of interest that emerge as a result of the observations that the researcher has made.
As you can probably imagine, ethnographic research design has the ability to provide rich, contextually embedded insights into the socio-cultural dynamics of human behaviour within a natural, uncontrived setting. Naturally, however, it does come with its own set of challenges, including researcher bias (since the researcher can become quite immersed in the group), participant confidentiality and, predictably, ethical complexities . All of these need to be carefully managed if you choose to adopt this type of research design.
Case Study Design
With case study research design, you, as the researcher, investigate a single individual (or a single group of individuals) to gain an in-depth understanding of their experiences, behaviours or outcomes. Unlike other research designs that are aimed at larger sample sizes, case studies offer a deep dive into the specific circumstances surrounding a person, group of people, event or phenomenon, generally within a bounded setting or context .
As an example, a case study design could be used to explore the factors influencing the success of a specific small business. This would involve diving deeply into the organisation to explore and understand what makes it tick – from marketing to HR to finance. In terms of data collection, this could include interviews with staff and management, review of policy documents and financial statements, surveying customers, etc.
While the above example is focused squarely on one organisation, it’s worth noting that case study research designs can have different variation s, including single-case, multiple-case and longitudinal designs. As you can see in the example, a single-case design involves intensely examining a single entity to understand its unique characteristics and complexities. Conversely, in a multiple-case design , multiple cases are compared and contrasted to identify patterns and commonalities. Lastly, in a longitudinal case design , a single case or multiple cases are studied over an extended period of time to understand how factors develop over time.
As you can see, a case study research design is particularly useful where a deep and contextualised understanding of a specific phenomenon or issue is desired. However, this strength is also its weakness. In other words, you can’t generalise the findings from a case study to the broader population. So, keep this in mind if you’re considering going the case study route.
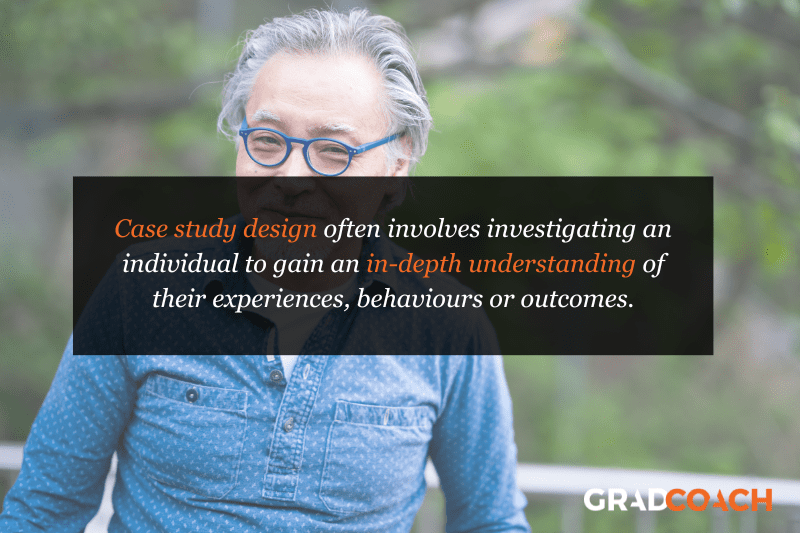
How To Choose A Research Design
Having worked through all of these potential research designs, you’d be forgiven for feeling a little overwhelmed and wondering, “ But how do I decide which research design to use? ”. While we could write an entire post covering that alone, here are a few factors to consider that will help you choose a suitable research design for your study.
Data type: The first determining factor is naturally the type of data you plan to be collecting – i.e., qualitative or quantitative. This may sound obvious, but we have to be clear about this – don’t try to use a quantitative research design on qualitative data (or vice versa)!
Research aim(s) and question(s): As with all methodological decisions, your research aim and research questions will heavily influence your research design. For example, if your research aims involve developing a theory from qualitative data, grounded theory would be a strong option. Similarly, if your research aims involve identifying and measuring relationships between variables, one of the experimental designs would likely be a better option.
Time: It’s essential that you consider any time constraints you have, as this will impact the type of research design you can choose. For example, if you’ve only got a month to complete your project, a lengthy design such as ethnography wouldn’t be a good fit.
Resources: Take into account the resources realistically available to you, as these need to factor into your research design choice. For example, if you require highly specialised lab equipment to execute an experimental design, you need to be sure that you’ll have access to that before you make a decision.
Keep in mind that when it comes to research, it’s important to manage your risks and play as conservatively as possible. If your entire project relies on you achieving a huge sample, having access to niche equipment or holding interviews with very difficult-to-reach participants, you’re creating risks that could kill your project. So, be sure to think through your choices carefully and make sure that you have backup plans for any existential risks. Remember that a relatively simple methodology executed well generally will typically earn better marks than a highly-complex methodology executed poorly.
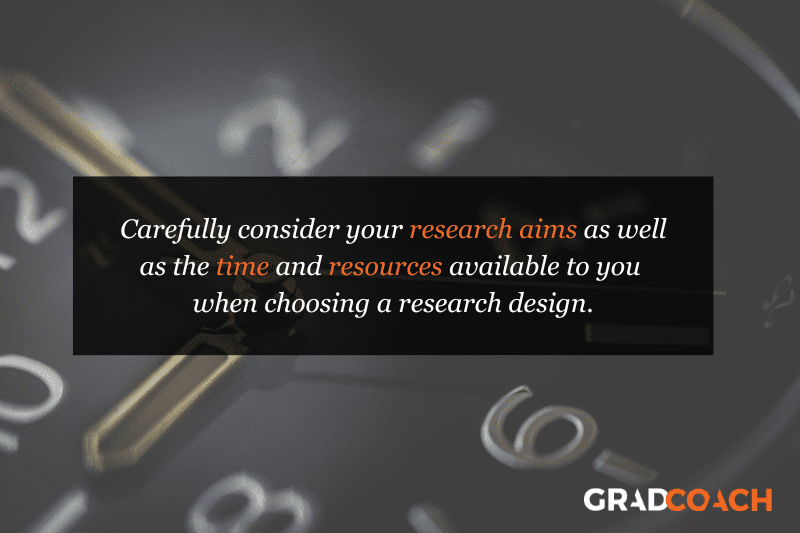
Recap: Key Takeaways
We’ve covered a lot of ground here. Let’s recap by looking at the key takeaways:
- Research design refers to the overall plan, structure or strategy that guides a research project, from its conception to the final analysis of data.
- Research designs for quantitative studies include descriptive , correlational , experimental and quasi-experimenta l designs.
- Research designs for qualitative studies include phenomenological , grounded theory , ethnographic and case study designs.
- When choosing a research design, you need to consider a variety of factors, including the type of data you’ll be working with, your research aims and questions, your time and the resources available to you.
If you need a helping hand with your research design (or any other aspect of your research), check out our private coaching services .
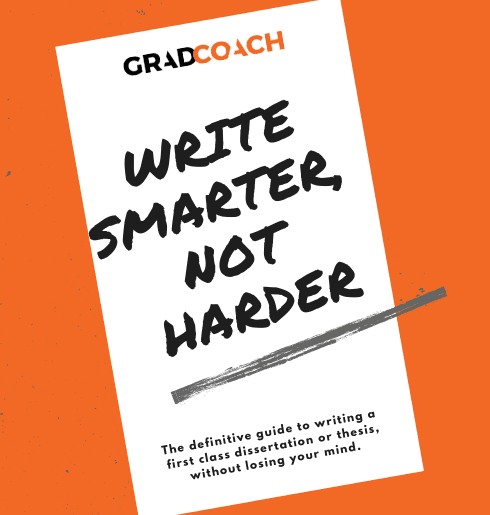
Psst… there’s more (for free)
This post is part of our dissertation mini-course, which covers everything you need to get started with your dissertation, thesis or research project.
You Might Also Like:
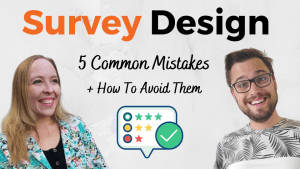
Is there any blog article explaining more on Case study research design? Is there a Case study write-up template? Thank you.
Thanks this was quite valuable to clarify such an important concept.
Thanks for this simplified explanations. it is quite very helpful.
This was really helpful. thanks
Thank you for your explanation. I think case study research design and the use of secondary data in researches needs to be talked about more in your videos and articles because there a lot of case studies research design tailored projects out there.
Please is there any template for a case study research design whose data type is a secondary data on your repository?
This post is very clear, comprehensive and has been very helpful to me. It has cleared the confusion I had in regard to research design and methodology.
This post is helpful, easy to understand, and deconstructs what a research design is. Thanks
how to cite this page
Thank you very much for the post. It is wonderful and has cleared many worries in my mind regarding research designs. I really appreciate .
Submit a Comment Cancel reply
Your email address will not be published. Required fields are marked *
Save my name, email, and website in this browser for the next time I comment.
- Print Friendly
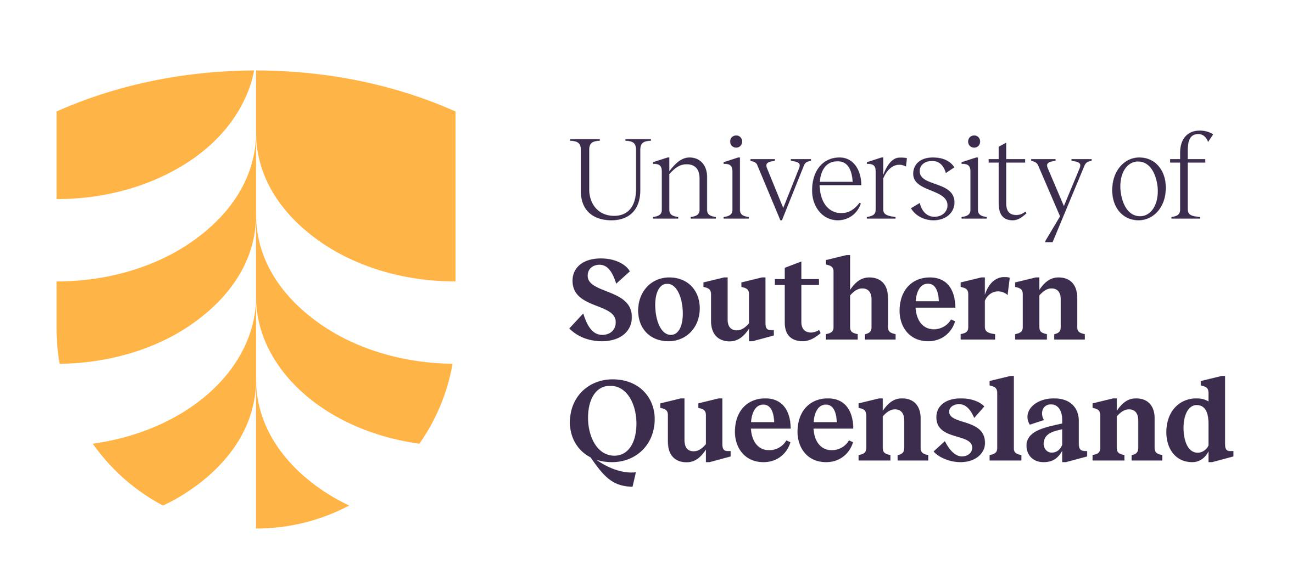
Want to create or adapt books like this? Learn more about how Pressbooks supports open publishing practices.
5 Research design
Research design is a comprehensive plan for data collection in an empirical research project. It is a ‘blueprint’ for empirical research aimed at answering specific research questions or testing specific hypotheses, and must specify at least three processes: the data collection process, the instrument development process, and the sampling process. The instrument development and sampling processes are described in the next two chapters, and the data collection process—which is often loosely called ‘research design’—is introduced in this chapter and is described in further detail in Chapters 9–12.
Broadly speaking, data collection methods can be grouped into two categories: positivist and interpretive. Positivist methods , such as laboratory experiments and survey research, are aimed at theory (or hypotheses) testing, while interpretive methods, such as action research and ethnography, are aimed at theory building. Positivist methods employ a deductive approach to research, starting with a theory and testing theoretical postulates using empirical data. In contrast, interpretive methods employ an inductive approach that starts with data and tries to derive a theory about the phenomenon of interest from the observed data. Often times, these methods are incorrectly equated with quantitative and qualitative research. Quantitative and qualitative methods refers to the type of data being collected—quantitative data involve numeric scores, metrics, and so on, while qualitative data includes interviews, observations, and so forth—and analysed (i.e., using quantitative techniques such as regression or qualitative techniques such as coding). Positivist research uses predominantly quantitative data, but can also use qualitative data. Interpretive research relies heavily on qualitative data, but can sometimes benefit from including quantitative data as well. Sometimes, joint use of qualitative and quantitative data may help generate unique insight into a complex social phenomenon that is not available from either type of data alone, and hence, mixed-mode designs that combine qualitative and quantitative data are often highly desirable.
Key attributes of a research design
The quality of research designs can be defined in terms of four key design attributes: internal validity, external validity, construct validity, and statistical conclusion validity.
Internal validity , also called causality, examines whether the observed change in a dependent variable is indeed caused by a corresponding change in a hypothesised independent variable, and not by variables extraneous to the research context. Causality requires three conditions: covariation of cause and effect (i.e., if cause happens, then effect also happens; if cause does not happen, effect does not happen), temporal precedence (cause must precede effect in time), and spurious correlation, or there is no plausible alternative explanation for the change. Certain research designs, such as laboratory experiments, are strong in internal validity by virtue of their ability to manipulate the independent variable (cause) via a treatment and observe the effect (dependent variable) of that treatment after a certain point in time, while controlling for the effects of extraneous variables. Other designs, such as field surveys, are poor in internal validity because of their inability to manipulate the independent variable (cause), and because cause and effect are measured at the same point in time which defeats temporal precedence making it equally likely that the expected effect might have influenced the expected cause rather than the reverse. Although higher in internal validity compared to other methods, laboratory experiments are by no means immune to threats of internal validity, and are susceptible to history, testing, instrumentation, regression, and other threats that are discussed later in the chapter on experimental designs. Nonetheless, different research designs vary considerably in their respective level of internal validity.
External validity or generalisability refers to whether the observed associations can be generalised from the sample to the population (population validity), or to other people, organisations, contexts, or time (ecological validity). For instance, can results drawn from a sample of financial firms in the United States be generalised to the population of financial firms (population validity) or to other firms within the United States (ecological validity)? Survey research, where data is sourced from a wide variety of individuals, firms, or other units of analysis, tends to have broader generalisability than laboratory experiments where treatments and extraneous variables are more controlled. The variation in internal and external validity for a wide range of research designs is shown in Figure 5.1.
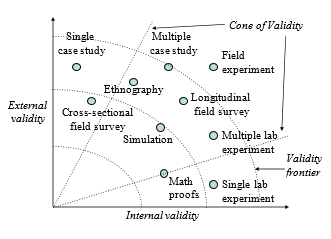
Some researchers claim that there is a trade-off between internal and external validity—higher external validity can come only at the cost of internal validity and vice versa. But this is not always the case. Research designs such as field experiments, longitudinal field surveys, and multiple case studies have higher degrees of both internal and external validities. Personally, I prefer research designs that have reasonable degrees of both internal and external validities, i.e., those that fall within the cone of validity shown in Figure 5.1. But this should not suggest that designs outside this cone are any less useful or valuable. Researchers’ choice of designs are ultimately a matter of their personal preference and competence, and the level of internal and external validity they desire.
Construct validity examines how well a given measurement scale is measuring the theoretical construct that it is expected to measure. Many constructs used in social science research such as empathy, resistance to change, and organisational learning are difficult to define, much less measure. For instance, construct validity must ensure that a measure of empathy is indeed measuring empathy and not compassion, which may be difficult since these constructs are somewhat similar in meaning. Construct validity is assessed in positivist research based on correlational or factor analysis of pilot test data, as described in the next chapter.
Statistical conclusion validity examines the extent to which conclusions derived using a statistical procedure are valid. For example, it examines whether the right statistical method was used for hypotheses testing, whether the variables used meet the assumptions of that statistical test (such as sample size or distributional requirements), and so forth. Because interpretive research designs do not employ statistical tests, statistical conclusion validity is not applicable for such analysis. The different kinds of validity and where they exist at the theoretical/empirical levels are illustrated in Figure 5.2.
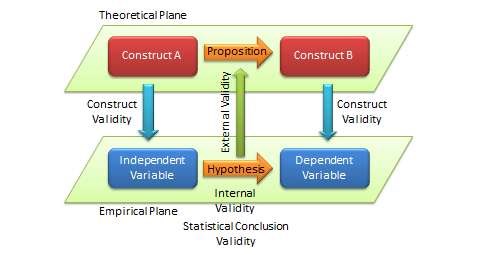
Improving internal and external validity
The best research designs are those that can ensure high levels of internal and external validity. Such designs would guard against spurious correlations, inspire greater faith in the hypotheses testing, and ensure that the results drawn from a small sample are generalisable to the population at large. Controls are required to ensure internal validity (causality) of research designs, and can be accomplished in five ways: manipulation, elimination, inclusion, and statistical control, and randomisation.
In manipulation , the researcher manipulates the independent variables in one or more levels (called ‘treatments’), and compares the effects of the treatments against a control group where subjects do not receive the treatment. Treatments may include a new drug or different dosage of drug (for treating a medical condition), a teaching style (for students), and so forth. This type of control is achieved in experimental or quasi-experimental designs, but not in non-experimental designs such as surveys. Note that if subjects cannot distinguish adequately between different levels of treatment manipulations, their responses across treatments may not be different, and manipulation would fail.
The elimination technique relies on eliminating extraneous variables by holding them constant across treatments, such as by restricting the study to a single gender or a single socioeconomic status. In the inclusion technique, the role of extraneous variables is considered by including them in the research design and separately estimating their effects on the dependent variable, such as via factorial designs where one factor is gender (male versus female). Such technique allows for greater generalisability, but also requires substantially larger samples. In statistical control , extraneous variables are measured and used as covariates during the statistical testing process.
Finally, the randomisation technique is aimed at cancelling out the effects of extraneous variables through a process of random sampling, if it can be assured that these effects are of a random (non-systematic) nature. Two types of randomisation are: random selection , where a sample is selected randomly from a population, and random assignment , where subjects selected in a non-random manner are randomly assigned to treatment groups.
Randomisation also ensures external validity, allowing inferences drawn from the sample to be generalised to the population from which the sample is drawn. Note that random assignment is mandatory when random selection is not possible because of resource or access constraints. However, generalisability across populations is harder to ascertain since populations may differ on multiple dimensions and you can only control for a few of those dimensions.
Popular research designs
As noted earlier, research designs can be classified into two categories—positivist and interpretive—depending on the goal of the research. Positivist designs are meant for theory testing, while interpretive designs are meant for theory building. Positivist designs seek generalised patterns based on an objective view of reality, while interpretive designs seek subjective interpretations of social phenomena from the perspectives of the subjects involved. Some popular examples of positivist designs include laboratory experiments, field experiments, field surveys, secondary data analysis, and case research, while examples of interpretive designs include case research, phenomenology, and ethnography. Note that case research can be used for theory building or theory testing, though not at the same time. Not all techniques are suited for all kinds of scientific research. Some techniques such as focus groups are best suited for exploratory research, others such as ethnography are best for descriptive research, and still others such as laboratory experiments are ideal for explanatory research. Following are brief descriptions of some of these designs. Additional details are provided in Chapters 9–12.
Experimental studies are those that are intended to test cause-effect relationships (hypotheses) in a tightly controlled setting by separating the cause from the effect in time, administering the cause to one group of subjects (the ‘treatment group’) but not to another group (‘control group’), and observing how the mean effects vary between subjects in these two groups. For instance, if we design a laboratory experiment to test the efficacy of a new drug in treating a certain ailment, we can get a random sample of people afflicted with that ailment, randomly assign them to one of two groups (treatment and control groups), administer the drug to subjects in the treatment group, but only give a placebo (e.g., a sugar pill with no medicinal value) to subjects in the control group. More complex designs may include multiple treatment groups, such as low versus high dosage of the drug or combining drug administration with dietary interventions. In a true experimental design , subjects must be randomly assigned to each group. If random assignment is not followed, then the design becomes quasi-experimental . Experiments can be conducted in an artificial or laboratory setting such as at a university (laboratory experiments) or in field settings such as in an organisation where the phenomenon of interest is actually occurring (field experiments). Laboratory experiments allow the researcher to isolate the variables of interest and control for extraneous variables, which may not be possible in field experiments. Hence, inferences drawn from laboratory experiments tend to be stronger in internal validity, but those from field experiments tend to be stronger in external validity. Experimental data is analysed using quantitative statistical techniques. The primary strength of the experimental design is its strong internal validity due to its ability to isolate, control, and intensively examine a small number of variables, while its primary weakness is limited external generalisability since real life is often more complex (i.e., involving more extraneous variables) than contrived lab settings. Furthermore, if the research does not identify ex ante relevant extraneous variables and control for such variables, such lack of controls may hurt internal validity and may lead to spurious correlations.
Field surveys are non-experimental designs that do not control for or manipulate independent variables or treatments, but measure these variables and test their effects using statistical methods. Field surveys capture snapshots of practices, beliefs, or situations from a random sample of subjects in field settings through a survey questionnaire or less frequently, through a structured interview. In cross-sectional field surveys , independent and dependent variables are measured at the same point in time (e.g., using a single questionnaire), while in longitudinal field surveys , dependent variables are measured at a later point in time than the independent variables. The strengths of field surveys are their external validity (since data is collected in field settings), their ability to capture and control for a large number of variables, and their ability to study a problem from multiple perspectives or using multiple theories. However, because of their non-temporal nature, internal validity (cause-effect relationships) are difficult to infer, and surveys may be subject to respondent biases (e.g., subjects may provide a ‘socially desirable’ response rather than their true response) which further hurts internal validity.
Secondary data analysis is an analysis of data that has previously been collected and tabulated by other sources. Such data may include data from government agencies such as employment statistics from the U.S. Bureau of Labor Services or development statistics by countries from the United Nations Development Program, data collected by other researchers (often used in meta-analytic studies), or publicly available third-party data, such as financial data from stock markets or real-time auction data from eBay. This is in contrast to most other research designs where collecting primary data for research is part of the researcher’s job. Secondary data analysis may be an effective means of research where primary data collection is too costly or infeasible, and secondary data is available at a level of analysis suitable for answering the researcher’s questions. The limitations of this design are that the data might not have been collected in a systematic or scientific manner and hence unsuitable for scientific research, since the data was collected for a presumably different purpose, they may not adequately address the research questions of interest to the researcher, and interval validity is problematic if the temporal precedence between cause and effect is unclear.
Case research is an in-depth investigation of a problem in one or more real-life settings (case sites) over an extended period of time. Data may be collected using a combination of interviews, personal observations, and internal or external documents. Case studies can be positivist in nature (for hypotheses testing) or interpretive (for theory building). The strength of this research method is its ability to discover a wide variety of social, cultural, and political factors potentially related to the phenomenon of interest that may not be known in advance. Analysis tends to be qualitative in nature, but heavily contextualised and nuanced. However, interpretation of findings may depend on the observational and integrative ability of the researcher, lack of control may make it difficult to establish causality, and findings from a single case site may not be readily generalised to other case sites. Generalisability can be improved by replicating and comparing the analysis in other case sites in a multiple case design .
Focus group research is a type of research that involves bringing in a small group of subjects (typically six to ten people) at one location, and having them discuss a phenomenon of interest for a period of one and a half to two hours. The discussion is moderated and led by a trained facilitator, who sets the agenda and poses an initial set of questions for participants, makes sure that the ideas and experiences of all participants are represented, and attempts to build a holistic understanding of the problem situation based on participants’ comments and experiences. Internal validity cannot be established due to lack of controls and the findings may not be generalised to other settings because of the small sample size. Hence, focus groups are not generally used for explanatory or descriptive research, but are more suited for exploratory research.
Action research assumes that complex social phenomena are best understood by introducing interventions or ‘actions’ into those phenomena and observing the effects of those actions. In this method, the researcher is embedded within a social context such as an organisation and initiates an action—such as new organisational procedures or new technologies—in response to a real problem such as declining profitability or operational bottlenecks. The researcher’s choice of actions must be based on theory, which should explain why and how such actions may cause the desired change. The researcher then observes the results of that action, modifying it as necessary, while simultaneously learning from the action and generating theoretical insights about the target problem and interventions. The initial theory is validated by the extent to which the chosen action successfully solves the target problem. Simultaneous problem solving and insight generation is the central feature that distinguishes action research from all other research methods, and hence, action research is an excellent method for bridging research and practice. This method is also suited for studying unique social problems that cannot be replicated outside that context, but it is also subject to researcher bias and subjectivity, and the generalisability of findings is often restricted to the context where the study was conducted.
Ethnography is an interpretive research design inspired by anthropology that emphasises that research phenomenon must be studied within the context of its culture. The researcher is deeply immersed in a certain culture over an extended period of time—eight months to two years—and during that period, engages, observes, and records the daily life of the studied culture, and theorises about the evolution and behaviours in that culture. Data is collected primarily via observational techniques, formal and informal interaction with participants in that culture, and personal field notes, while data analysis involves ‘sense-making’. The researcher must narrate her experience in great detail so that readers may experience that same culture without necessarily being there. The advantages of this approach are its sensitiveness to the context, the rich and nuanced understanding it generates, and minimal respondent bias. However, this is also an extremely time and resource-intensive approach, and findings are specific to a given culture and less generalisable to other cultures.
Selecting research designs
Given the above multitude of research designs, which design should researchers choose for their research? Generally speaking, researchers tend to select those research designs that they are most comfortable with and feel most competent to handle, but ideally, the choice should depend on the nature of the research phenomenon being studied. In the preliminary phases of research, when the research problem is unclear and the researcher wants to scope out the nature and extent of a certain research problem, a focus group (for an individual unit of analysis) or a case study (for an organisational unit of analysis) is an ideal strategy for exploratory research. As one delves further into the research domain, but finds that there are no good theories to explain the phenomenon of interest and wants to build a theory to fill in the unmet gap in that area, interpretive designs such as case research or ethnography may be useful designs. If competing theories exist and the researcher wishes to test these different theories or integrate them into a larger theory, positivist designs such as experimental design, survey research, or secondary data analysis are more appropriate.
Regardless of the specific research design chosen, the researcher should strive to collect quantitative and qualitative data using a combination of techniques such as questionnaires, interviews, observations, documents, or secondary data. For instance, even in a highly structured survey questionnaire, intended to collect quantitative data, the researcher may leave some room for a few open-ended questions to collect qualitative data that may generate unexpected insights not otherwise available from structured quantitative data alone. Likewise, while case research employ mostly face-to-face interviews to collect most qualitative data, the potential and value of collecting quantitative data should not be ignored. As an example, in a study of organisational decision-making processes, the case interviewer can record numeric quantities such as how many months it took to make certain organisational decisions, how many people were involved in that decision process, and how many decision alternatives were considered, which can provide valuable insights not otherwise available from interviewees’ narrative responses. Irrespective of the specific research design employed, the goal of the researcher should be to collect as much and as diverse data as possible that can help generate the best possible insights about the phenomenon of interest.
Social Science Research: Principles, Methods and Practices (Revised edition) Copyright © 2019 by Anol Bhattacherjee is licensed under a Creative Commons Attribution-NonCommercial-ShareAlike 4.0 International License , except where otherwise noted.
Share This Book
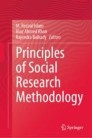
Principles of Social Research Methodology pp 59–71 Cite as
Inductive and/or Deductive Research Designs
- Md. Shahidul Haque 4
- First Online: 27 October 2022
2455 Accesses
1 Citations
This chapter aims to introduce the readers, especially the Bangladeshi undergraduate and postgraduate students to some fundamental considerations of inductive and deductive research designs. The deductive approach refers to testing a theory, where the researcher builds up a theory or hypotheses and plans a research stratagem to examine the formulated theory. On the contrary, the inductive approach intends to construct a theory, where the researcher begins by gathering data to establish a theory. In the beginning, a researcher must clarify which approach he/she will follow in his/her research work. The chapter discusses basic concepts, characteristics, steps and examples of inductive and deductive research designs. Here, also a comparison between inductive and deductive research designs is shown. It concludes with a look at how both inductive and deductive designs are used comprehensively to constitute a clearer image of research work.
- Deductive research design
- Inductive research design
- Research design
This is a preview of subscription content, log in via an institution .
Buying options
- Available as PDF
- Read on any device
- Instant download
- Own it forever
- Available as EPUB and PDF
- Compact, lightweight edition
- Dispatched in 3 to 5 business days
- Free shipping worldwide - see info
- Durable hardcover edition
Tax calculation will be finalised at checkout
Purchases are for personal use only
Beiske, B. (2007). Research methods: Uses and limitations of questionnaires, interviews and case studies . GRIN Verlag.
Google Scholar
Bhattacherjee, A. (2012). Social science research: Principles, methods, and practices (2nd ed.). Global Text Project.
Brewer, J., & Hunter, A. (1989). Multi method research: A synthesis of styles . Sage Publications Ltd.
Burns, N., & Grove, S. K. (2003). Understanding nursing research (3rd ed.). Saunders.
Cambridge Dictionary. (2016a). Hypothesis. In Dictionary.cambridge.org . Retrieved October, 15, 2016, from http://dictionary.cambridge.org/dictionary/english/hypothesis .
Corbin, J. M., & Strauss, A. (1990). Grounded theory research: Procedures, canons, and evaluative criteria. Qualitative Sociology, 13 , 3–21. https://doi.org/10.1007/BF00988593
Article Google Scholar
Creswell, J. W., & Plano Clark, V. L. (2017). Designing and conducting mixed methods research (3rd ed.). Sage Publications Inc.
Crowther, D., & Lancaster, G. (2009). Research methods: A concise introduction to research in management and business . Butterworth-Heinemann.
Easterby-Smith, M., Thorpe, R., & Lowe, A. (2002). Management research: An introduction . Sage Publications Ltd.
Engel, R. J., & Schutt, R. K. (2005). The practice of research in social work . Sage Publications Inc.
Gill, J., & Johnson, P. (2010). Research Methods for Managers (4th ed.). Sage Publications Ltd.
Goddard, W., & Melville, S. (2004). Research methodology: An introduction (2nd ed.). Blackwell Publishing.
Godfrey, J., Hodgson, A., Tarca, A., Hamilton, J., & Holmes, S. (2010). Accounting theory (7th ed). Wiley. ISBN: 978-0-470-81815-2.
Gray, D. E. (2004). Doing research in the real world . Sage Publications Ltd.
Hackley, C. (2003). Doing research projects in marketing, management and consumer research . Routledge.
Book Google Scholar
Lodico, M. G., Spaulding, D. T., & Voegtle, K. H. (2006). Methods in educational research: From theory to practice . John Wiley & Sons.
Merriam-Webster. (2016a). Inductive. In Merriam-Webster.com dictionary . Retrieved October 12, 2016a, from http://www.merriam-webster.com/dictionary/inductive .
Merriam-Webster. (2016b). Deductive. In Merriam-Webster.com dictionary . Retrieved October 12, 2016b, from http://www.merriam-webster.com/dictionary/deductive .
Morgan, D. L. (2014). Integrating Qualitative and Quantitative Methods: A Pragmatic Approach. SAGE Publications, Inc. https://dx.doi.org/10.4135/9781544304533
Neuman, W. L. (2003). Social research methods: Qualitative and quantitative approaches . Allyn and Bacon.
Oxford Dictionary. (2016a). Inductive. In Oxford online dictionary . Retrieved October 15, 2016a, from https://en.oxforddictionaries.com/definition/inductive .
Oxford Dictionary. (2016b). Deductive. In Oxford online dictionary . Retrieved October 15, 2016b, from https://en.oxforddictionaries.com/definition/deductive .
Oxford Dictionary. (2016c). Theory. In Oxford online dictionary . Retrieved October 15, 2016c, from https://en.oxforddictionaries.com/definition/theory .
Saunders, M., Lewis, P., & Thornhill, A. (2007). Research methods for business students (5th ed.). Prentice Hall.
Sherman, L. W., & Berk, R. A. (1984). The specific deterrent effects of arrest for domestic assault. American Sociological Review, 49 (2), 261–272.
Singh, K. (2006). Fundamental of research methodology and statistics. New Age International (P) Limited.
Snieder, R., & Larner, K. (2009). The art of being a scientist: A guide for graduate students and their mentors . Cambridge University Press.
Strauss, A., & Corbin, J. (1998). Basics of qualitative research (2nd ed.). Sage Publications Ltd.
Thomas, D. R. (2006). A general inductive approach for analyzing qualitative evaluation data. American Journal of Evaluation, 27 (2), 237–246. https://doi.org/10.1177/1098214005283748
Trochim, W. M. K. (2006). Research methods knowledge base. Retrieved on October 12, 2016, from http://www.socialresearchmethods.net .
Wilson, J. (2010). Essentials of business research: A guide to doing your research project . Sage Publishers Ltd.
Download references
Author information
Authors and affiliations.
Department of Social Work, Jagannath University, Dhaka, 1100, Bangladesh
Md. Shahidul Haque
You can also search for this author in PubMed Google Scholar
Corresponding author
Correspondence to Md. Shahidul Haque .
Editor information
Editors and affiliations.
Centre for Family and Child Studies, Research Institute of Humanities and Social Sciences, University of Sharjah, Sharjah, United Arab Emirates
M. Rezaul Islam
Department of Development Studies, University of Dhaka, Dhaka, Bangladesh
Niaz Ahmed Khan
Department of Social Work, School of Humanities, University of Johannesburg, Johannesburg, South Africa
Rajendra Baikady
Rights and permissions
Reprints and permissions
Copyright information
© 2022 The Author(s), under exclusive license to Springer Nature Singapore Pte Ltd.
About this chapter
Cite this chapter.
Haque, M.S. (2022). Inductive and/or Deductive Research Designs. In: Islam, M.R., Khan, N.A., Baikady, R. (eds) Principles of Social Research Methodology. Springer, Singapore. https://doi.org/10.1007/978-981-19-5441-2_5
Download citation
DOI : https://doi.org/10.1007/978-981-19-5441-2_5
Published : 27 October 2022
Publisher Name : Springer, Singapore
Print ISBN : 978-981-19-5219-7
Online ISBN : 978-981-19-5441-2
eBook Packages : Social Sciences
Share this chapter
Anyone you share the following link with will be able to read this content:
Sorry, a shareable link is not currently available for this article.
Provided by the Springer Nature SharedIt content-sharing initiative
- Publish with us
Policies and ethics
- Find a journal
- Track your research
Ohio State nav bar
The Ohio State University
- BuckeyeLink
- Find People
- Search Ohio State
Basic Research Design
What is research design.
- Definition of Research Design : A procedure for generating answers to questions, crucial in determining the reliability and relevance of research outcomes.
- Importance of Strong Designs : Strong designs lead to answers that are accurate and close to their targets, while weak designs may result in misleading or irrelevant outcomes.
- Criteria for Assessing Design Strength : Evaluating a design’s strength involves understanding the research question and how the design will yield reliable empirical information.
The Four Elements of Research Design (Blair et al., 2023)

- The MIDA Framework : Research designs consist of four interconnected elements – Model (M), Inquiry (I), Data strategy (D), and Answer strategy (A), collectively referred to as MIDA.
- Theoretical Side (M and I): This encompasses the researcher’s beliefs about the world (Model) and the target of inference or the primary question to be answered (Inquiry).
- Empirical Side (D and A): This includes the strategies for collecting (Data strategy) and analyzing or summarizing information (Answer strategy).
- Interplay between Theoretical and Empirical Sides : The theoretical side sets the research challenges, while the empirical side represents the researcher’s responses to these challenges.
- Relation among MIDA Components: The diagram above shows how the four elements of a design are interconnected and how they relate to both real-world and simulated quantities.
- Parallelism in Design Representation: The illustration highlights two key parallelisms in research design – between actual and simulated processes, and between the theoretical (M, I) and empirical (D, A) sides.
- Importance of Simulated Processes: The parallelism between actual and simulated processes is crucial for understanding and evaluating research designs.
- Balancing Theoretical and Empirical Aspects : Effective research design requires a balance between theoretical considerations (models and inquiries) and empirical methodologies (data and answer strategies).
Research Design Principles (Blair et al., 2023)
- Integration of Components: Designs are effective not merely due to their individual components but how these components work together.
- Focus on Entire Design: Assessing a design requires examining how each part, such as the question, estimator, and sampling method, fits into the overall design.
- Importance of Diagnosis: The evaluation of a design’s strength lies in diagnosing the whole design, not just its parts.
- Strong Design Characteristics: Designs with parallel theoretical and empirical aspects tend to be stronger.
- The M:I:D:A Analogy: Effective designs often align data strategies with models and answer strategies with inquiries.
- Flexibility in Models: Good designs should perform well even under varying world scenarios, not just under expected conditions.
- Broadening Model Scope: Designers should consider a wide range of models, assessing the design’s effectiveness across these.
- Robustness of Inquiries and Strategies: Inquiries should yield answers and strategies should be applicable regardless of variations in real-world events.
- Diagnosis Across Models: It’s important to understand for which models a design excels and for which it falters.
- Specificity of Purpose: A design is deemed good when it aligns with a specific purpose or goal.
- Balancing Multiple Criteria: Designs should balance scientific precision, logistical constraints, policy goals, and ethical considerations.
- Diverse Goals and Assessments: Different designs may be optimal for different goals; the purpose dictates the design evaluation.
- Early Planning Benefits: Designing early allows for learning and improving design properties before data collection.
- Avoiding Post-Hoc Regrets: Early design helps avoid regrets related to data collection or question formulation.
- Iterative Improvement: The process of declaration, diagnosis, and redesign improves designs, ideally done before data collection.
- Adaptability to Changes: Designs should be flexible to adapt to unforeseen circumstances or new information.
- Expanding or Contracting Feasibility: The scope of feasible designs may change due to various practical factors.
- Continual Redesign: The principle advocates for ongoing design modification, even post research completion, for robustness and response to criticism.
- Improvement Through Sharing: Sharing designs via a formalized declaration makes it easier for others to understand and critique.
- Enhancing Scientific Communication: Well-documented designs facilitate better communication and justification of research decisions.
- Building a Design Library: The idea is to contribute designs to a shared library, allowing others to learn from and build upon existing work.
The Basics of Social Science Research Designs (Panke, 2018)
Deductive and inductive research.

- Starting Point: Begins with empirical observations or exploratory studies.
- Development of Hypotheses: Hypotheses are formulated after initial empirical analysis.
- Case Study Analysis: Involves conducting explorative case studies and analyzing dynamics at play.
- Generalization of Findings: Insights are then generalized across multiple cases to verify their applicability.
- Application: Suitable for novel phenomena or where existing theories are not easily applicable.
- Example Cases: Exploring new events like Donald Trump’s 2016 nomination or Russia’s annexation of Crimea in 2014.
- Theory-Based: Starts with existing theories to develop scientific answers to research questions.
- Hypothesis Development: Hypotheses are specified and then empirically examined.
- Empirical Examination: Involves a thorough empirical analysis of hypotheses using sound methods.
- Theory Refinement: Results can refine existing theories or contribute to new theoretical insights.
- Application: Preferred when existing theories relate to the research question.
- Example Projects: Usually explanatory projects asking ‘why’ questions to uncover relationships.
Explanatory and Interpretative Research Designs

- Definition: Explanatory research aims to explain the relationships between variables, often addressing ‘why’ questions. It is primarily concerned with identifying cause-and-effect dynamics and is typically quantitative in nature. The goal is to test hypotheses derived from theories and to establish patterns that can predict future occurrences.
- Definition: Interpretative research focuses on understanding the deeper meaning or underlying context of social phenomena. It often addresses ‘how is this possible’ questions, seeking to comprehend how certain outcomes or behaviors are produced within specific contexts. This type of research is usually qualitative and prioritizes individual experiences and perceptions.
- Explanatory Research: Poses ‘why’ questions to explore causal relationships and understand what factors influence certain outcomes.
- Interpretative Research: Asks ‘how is this possible’ questions to delve into the processes and meanings behind social phenomena.
- Explanatory Research: Relies on established theories to form hypotheses about causal relationships between variables. These theories are then tested through empirical research.
- Interpretative Research: Uses theories to provide a framework for understanding the social context and meanings. The focus is on constitutive relationships rather than causal ones.
- Explanatory Research: Often involves studying multiple cases to allow for comparison and generalization. It seeks patterns across different scenarios.
- Interpretative Research: Typically concentrates on single case studies, providing an in-depth understanding of that particular case without necessarily aiming for generalization.
- Explanatory Research: Aims to produce findings that can be generalized to other similar cases or populations. It seeks universal or broad patterns.
- Interpretative Research: Offers detailed insights specific to a single case or context. These findings are not necessarily intended to be generalized but to provide a deep understanding of the particular case.
Qualitative, Quantitative, and Mixed-method Projects
- Definition: Qualitative research is exploratory and aims to understand human behavior, beliefs, feelings, and experiences. It involves collecting non-numerical data, often through interviews, focus groups, or textual analysis. This method is ideal for gaining in-depth insights into specific phenomena.
- Example in Education: A qualitative study might involve conducting in-depth interviews with teachers to explore their experiences and challenges with remote teaching during the pandemic. This research would aim to understand the nuances of their experiences, challenges, and adaptations in a detailed and descriptive manner.
- Definition: Quantitative research seeks to quantify data and generalize results from a sample to the population of interest. It involves measurable, numerical data and often uses statistical methods for analysis. This approach is suitable for testing hypotheses or examining relationships between variables.
- Example in Education: A quantitative study could involve surveying a large number of students to determine the correlation between the amount of time spent on homework and their academic achievement. This would involve collecting numerical data (hours of homework, grades) and applying statistical analysis to examine relationships or differences.
- Definition: Mixed-method research combines both qualitative and quantitative approaches, providing a more comprehensive understanding of the research problem. It allows for the exploration of complex research questions by integrating numerical data analysis with detailed narrative data.
- Example in Education: A mixed-method study might investigate the impact of a new teaching method. The research could start with quantitative methods, like administering standardized tests to measure learning outcomes, followed by qualitative methods, such as conducting focus groups with students and teachers to understand their perceptions and experiences with the new teaching method. This combination provides both statistical results and in-depth understanding.
- Research Questions: What kind of information is needed to answer the questions? Qualitative for “how” and “why”, quantitative for “how many” or “how much”, and mixed methods for a comprehensive understanding of both the breadth and depth of a phenomenon.
- Nature of the Study: Is the study aiming to explore a new area (qualitative), confirm hypotheses (quantitative), or achieve both (mixed-method)?
- Resources Available: Time, funding, and expertise available can influence the choice. Qualitative research can be more time-consuming, while quantitative research may require specific statistical skills.
- Data Sources: Availability and type of data also guide the methodology. Existing numerical data might lean towards quantitative, while studies requiring personal experiences or opinions might be qualitative.
References:
Blair, G., Coppock, A., & Humphreys, M. (2023). Research Design in the Social Sciences: Declaration, Diagnosis, and Redesign . Princeton University Press.
Panke, D. (2018). Research design & method selection: Making good choices in the social sciences. Research Design & Method Selection , 1-368.

Organizing Academic Research Papers: Types of Research Designs
- Purpose of Guide
- Design Flaws to Avoid
- Glossary of Research Terms
- Narrowing a Topic Idea
- Broadening a Topic Idea
- Extending the Timeliness of a Topic Idea
- Academic Writing Style
- Choosing a Title
- Making an Outline
- Paragraph Development
- Executive Summary
- Background Information
- The Research Problem/Question
- Theoretical Framework
- Citation Tracking
- Content Alert Services
- Evaluating Sources
- Primary Sources
- Secondary Sources
- Tertiary Sources
- What Is Scholarly vs. Popular?
- Qualitative Methods
- Quantitative Methods
- Using Non-Textual Elements
- Limitations of the Study
- Common Grammar Mistakes
- Avoiding Plagiarism
- Footnotes or Endnotes?
- Further Readings
- Annotated Bibliography
- Dealing with Nervousness
- Using Visual Aids
- Grading Someone Else's Paper
- How to Manage Group Projects
- Multiple Book Review Essay
- Reviewing Collected Essays
- About Informed Consent
- Writing Field Notes
- Writing a Policy Memo
- Writing a Research Proposal
- Acknowledgements
Introduction
Before beginning your paper, you need to decide how you plan to design the study .
The research design refers to the overall strategy that you choose to integrate the different components of the study in a coherent and logical way, thereby, ensuring you will effectively address the research problem; it constitutes the blueprint for the collection, measurement, and analysis of data. Note that your research problem determines the type of design you can use, not the other way around!
General Structure and Writing Style
Action research design, case study design, causal design, cohort design, cross-sectional design, descriptive design, experimental design, exploratory design, historical design, longitudinal design, observational design, philosophical design, sequential design.
Kirshenblatt-Gimblett, Barbara. Part 1, What Is Research Design? The Context of Design. Performance Studies Methods Course syllabus . New York University, Spring 2006; Trochim, William M.K. Research Methods Knowledge Base . 2006.
The function of a research design is to ensure that the evidence obtained enables you to effectively address the research problem as unambiguously as possible. In social sciences research, obtaining evidence relevant to the research problem generally entails specifying the type of evidence needed to test a theory, to evaluate a program, or to accurately describe a phenomenon. However, researchers can often begin their investigations far too early, before they have thought critically about about what information is required to answer the study's research questions. Without attending to these design issues beforehand, the conclusions drawn risk being weak and unconvincing and, consequently, will fail to adequate address the overall research problem.
Given this, the length and complexity of research designs can vary considerably, but any sound design will do the following things:
- Identify the research problem clearly and justify its selection,
- Review previously published literature associated with the problem area,
- Clearly and explicitly specify hypotheses [i.e., research questions] central to the problem selected,
- Effectively describe the data which will be necessary for an adequate test of the hypotheses and explain how such data will be obtained, and
- Describe the methods of analysis which will be applied to the data in determining whether or not the hypotheses are true or false.
Kirshenblatt-Gimblett, Barbara. Part 1, What Is Research Design? The Context of Design. Performance Studies Methods Course syllabus . New Yortk University, Spring 2006.
Definition and Purpose
The essentials of action research design follow a characteristic cycle whereby initially an exploratory stance is adopted, where an understanding of a problem is developed and plans are made for some form of interventionary strategy. Then the intervention is carried out (the action in Action Research) during which time, pertinent observations are collected in various forms. The new interventional strategies are carried out, and the cyclic process repeats, continuing until a sufficient understanding of (or implement able solution for) the problem is achieved. The protocol is iterative or cyclical in nature and is intended to foster deeper understanding of a given situation, starting with conceptualizing and particularizing the problem and moving through several interventions and evaluations.
What do these studies tell you?
- A collaborative and adaptive research design that lends itself to use in work or community situations.
- Design focuses on pragmatic and solution-driven research rather than testing theories.
- When practitioners use action research it has the potential to increase the amount they learn consciously from their experience. The action research cycle can also be regarded as a learning cycle.
- Action search studies often have direct and obvious relevance to practice.
- There are no hidden controls or preemption of direction by the researcher.
What these studies don't tell you?
- It is harder to do than conducting conventional studies because the researcher takes on responsibilities for encouraging change as well as for research.
- Action research is much harder to write up because you probably can’t use a standard format to report your findings effectively.
- Personal over-involvement of the researcher may bias research results.
- The cyclic nature of action research to achieve its twin outcomes of action (e.g. change) and research (e.g. understanding) is time-consuming and complex to conduct.
Gall, Meredith. Educational Research: An Introduction . Chapter 18, Action Research. 8th ed. Boston, MA: Pearson/Allyn and Bacon, 2007; Kemmis, Stephen and Robin McTaggart. “Participatory Action Research.” In Handbook of Qualitative Research . Norman Denzin and Yvonna S. Locoln, eds. 2nd ed. (Thousand Oaks, CA: SAGE, 2000), pp. 567-605.; Reason, Peter and Hilary Bradbury. Handbook of Action Research: Participative Inquiry and Practice . Thousand Oaks, CA: SAGE, 2001.
A case study is an in-depth study of a particular research problem rather than a sweeping statistical survey. It is often used to narrow down a very broad field of research into one or a few easily researchable examples. The case study research design is also useful for testing whether a specific theory and model actually applies to phenomena in the real world. It is a useful design when not much is known about a phenomenon.
- Approach excels at bringing us to an understanding of a complex issue through detailed contextual analysis of a limited number of events or conditions and their relationships.
- A researcher using a case study design can apply a vaiety of methodologies and rely on a variety of sources to investigate a research problem.
- Design can extend experience or add strength to what is already known through previous research.
- Social scientists, in particular, make wide use of this research design to examine contemporary real-life situations and provide the basis for the application of concepts and theories and extension of methods.
- The design can provide detailed descriptions of specific and rare cases.
- A single or small number of cases offers little basis for establishing reliability or to generalize the findings to a wider population of people, places, or things.
- The intense exposure to study of the case may bias a researcher's interpretation of the findings.
- Design does not facilitate assessment of cause and effect relationships.
- Vital information may be missing, making the case hard to interpret.
- The case may not be representative or typical of the larger problem being investigated.
- If the criteria for selecting a case is because it represents a very unusual or unique phenomenon or problem for study, then your intepretation of the findings can only apply to that particular case.
Anastas, Jeane W. Research Design for Social Work and the Human Services . Chapter 4, Flexible Methods: Case Study Design. 2nd ed. New York: Columbia University Press, 1999; Stake, Robert E. The Art of Case Study Research . Thousand Oaks, CA: SAGE, 1995; Yin, Robert K. Case Study Research: Design and Theory . Applied Social Research Methods Series, no. 5. 3rd ed. Thousand Oaks, CA: SAGE, 2003.
Causality studies may be thought of as understanding a phenomenon in terms of conditional statements in the form, “If X, then Y.” This type of research is used to measure what impact a specific change will have on existing norms and assumptions. Most social scientists seek causal explanations that reflect tests of hypotheses. Causal effect (nomothetic perspective) occurs when variation in one phenomenon, an independent variable, leads to or results, on average, in variation in another phenomenon, the dependent variable.
Conditions necessary for determining causality:
- Empirical association--a valid conclusion is based on finding an association between the independent variable and the dependent variable.
- Appropriate time order--to conclude that causation was involved, one must see that cases were exposed to variation in the independent variable before variation in the dependent variable.
- Nonspuriousness--a relationship between two variables that is not due to variation in a third variable.
- Causality research designs helps researchers understand why the world works the way it does through the process of proving a causal link between variables and eliminating other possibilities.
- Replication is possible.
- There is greater confidence the study has internal validity due to the systematic subject selection and equity of groups being compared.
- Not all relationships are casual! The possibility always exists that, by sheer coincidence, two unrelated events appear to be related [e.g., Punxatawney Phil could accurately predict the duration of Winter for five consecutive years but, the fact remains, he's just a big, furry rodent].
- Conclusions about causal relationships are difficult to determine due to a variety of extraneous and confounding variables that exist in a social environment. This means causality can only be inferred, never proven.
- If two variables are correlated, the cause must come before the effect. However, even though two variables might be causally related, it can sometimes be difficult to determine which variable comes first and therefore to establish which variable is the actual cause and which is the actual effect.
Bachman, Ronet. The Practice of Research in Criminology and Criminal Justice . Chapter 5, Causation and Research Designs. 3rd ed. Thousand Oaks, CA: Pine Forge Press, 2007; Causal Research Design: Experimentation. Anonymous SlideShare Presentation ; Gall, Meredith. Educational Research: An Introduction . Chapter 11, Nonexperimental Research: Correlational Designs. 8th ed. Boston, MA: Pearson/Allyn and Bacon, 2007; Trochim, William M.K. Research Methods Knowledge Base . 2006.
Often used in the medical sciences, but also found in the applied social sciences, a cohort study generally refers to a study conducted over a period of time involving members of a population which the subject or representative member comes from, and who are united by some commonality or similarity. Using a quantitative framework, a cohort study makes note of statistical occurrence within a specialized subgroup, united by same or similar characteristics that are relevant to the research problem being investigated, r ather than studying statistical occurrence within the general population. Using a qualitative framework, cohort studies generally gather data using methods of observation. Cohorts can be either "open" or "closed."
- Open Cohort Studies [dynamic populations, such as the population of Los Angeles] involve a population that is defined just by the state of being a part of the study in question (and being monitored for the outcome). Date of entry and exit from the study is individually defined, therefore, the size of the study population is not constant. In open cohort studies, researchers can only calculate rate based data, such as, incidence rates and variants thereof.
- Closed Cohort Studies [static populations, such as patients entered into a clinical trial] involve participants who enter into the study at one defining point in time and where it is presumed that no new participants can enter the cohort. Given this, the number of study participants remains constant (or can only decrease).
- The use of cohorts is often mandatory because a randomized control study may be unethical. For example, you cannot deliberately expose people to asbestos, you can only study its effects on those who have already been exposed. Research that measures risk factors often relies on cohort designs.
- Because cohort studies measure potential causes before the outcome has occurred, they can demonstrate that these “causes” preceded the outcome, thereby avoiding the debate as to which is the cause and which is the effect.
- Cohort analysis is highly flexible and can provide insight into effects over time and related to a variety of different types of changes [e.g., social, cultural, political, economic, etc.].
- Either original data or secondary data can be used in this design.
- In cases where a comparative analysis of two cohorts is made [e.g., studying the effects of one group exposed to asbestos and one that has not], a researcher cannot control for all other factors that might differ between the two groups. These factors are known as confounding variables.
- Cohort studies can end up taking a long time to complete if the researcher must wait for the conditions of interest to develop within the group. This also increases the chance that key variables change during the course of the study, potentially impacting the validity of the findings.
- Because of the lack of randominization in the cohort design, its external validity is lower than that of study designs where the researcher randomly assigns participants.
Healy P, Devane D. “Methodological Considerations in Cohort Study Designs.” Nurse Researcher 18 (2011): 32-36; Levin, Kate Ann. Study Design IV: Cohort Studies. Evidence-Based Dentistry 7 (2003): 51–52; Study Design 101 . Himmelfarb Health Sciences Library. George Washington University, November 2011; Cohort Study . Wikipedia.
Cross-sectional research designs have three distinctive features: no time dimension, a reliance on existing differences rather than change following intervention; and, groups are selected based on existing differences rather than random allocation. The cross-sectional design can only measure diffrerences between or from among a variety of people, subjects, or phenomena rather than change. As such, researchers using this design can only employ a relative passive approach to making causal inferences based on findings.
- Cross-sectional studies provide a 'snapshot' of the outcome and the characteristics associated with it, at a specific point in time.
- Unlike the experimental design where there is an active intervention by the researcher to produce and measure change or to create differences, cross-sectional designs focus on studying and drawing inferences from existing differences between people, subjects, or phenomena.
- Entails collecting data at and concerning one point in time. While longitudinal studies involve taking multiple measures over an extended period of time, cross-sectional research is focused on finding relationships between variables at one moment in time.
- Groups identified for study are purposely selected based upon existing differences in the sample rather than seeking random sampling.
- Cross-section studies are capable of using data from a large number of subjects and, unlike observational studies, is not geographically bound.
- Can estimate prevalence of an outcome of interest because the sample is usually taken from the whole population.
- Because cross-sectional designs generally use survey techniques to gather data, they are relatively inexpensive and take up little time to conduct.
- Finding people, subjects, or phenomena to study that are very similar except in one specific variable can be difficult.
- Results are static and time bound and, therefore, give no indication of a sequence of events or reveal historical contexts.
- Studies cannot be utilized to establish cause and effect relationships.
- Provide only a snapshot of analysis so there is always the possibility that a study could have differing results if another time-frame had been chosen.
- There is no follow up to the findings.
Hall, John. “Cross-Sectional Survey Design.” In Encyclopedia of Survey Research Methods. Paul J. Lavrakas, ed. (Thousand Oaks, CA: Sage, 2008), pp. 173-174; Helen Barratt, Maria Kirwan. Cross-Sectional Studies: Design, Application, Strengths and Weaknesses of Cross-Sectional Studies . Healthknowledge, 2009. Cross-Sectional Study . Wikipedia.
Descriptive research designs help provide answers to the questions of who, what, when, where, and how associated with a particular research problem; a descriptive study cannot conclusively ascertain answers to why. Descriptive research is used to obtain information concerning the current status of the phenomena and to describe "what exists" with respect to variables or conditions in a situation.
- The subject is being observed in a completely natural and unchanged natural environment. True experiments, whilst giving analyzable data, often adversely influence the normal behavior of the subject.
- Descriptive research is often used as a pre-cursor to more quantitatively research designs, the general overview giving some valuable pointers as to what variables are worth testing quantitatively.
- If the limitations are understood, they can be a useful tool in developing a more focused study.
- Descriptive studies can yield rich data that lead to important recommendations.
- Appoach collects a large amount of data for detailed analysis.
- The results from a descriptive research can not be used to discover a definitive answer or to disprove a hypothesis.
- Because descriptive designs often utilize observational methods [as opposed to quantitative methods], the results cannot be replicated.
- The descriptive function of research is heavily dependent on instrumentation for measurement and observation.
Anastas, Jeane W. Research Design for Social Work and the Human Services . Chapter 5, Flexible Methods: Descriptive Research. 2nd ed. New York: Columbia University Press, 1999; McNabb, Connie. Descriptive Research Methodologies . Powerpoint Presentation; Shuttleworth, Martyn. Descriptive Research Design , September 26, 2008. Explorable.com website.
A blueprint of the procedure that enables the researcher to maintain control over all factors that may affect the result of an experiment. In doing this, the researcher attempts to determine or predict what may occur. Experimental Research is often used where there is time priority in a causal relationship (cause precedes effect), there is consistency in a causal relationship (a cause will always lead to the same effect), and the magnitude of the correlation is great. The classic experimental design specifies an experimental group and a control group. The independent variable is administered to the experimental group and not to the control group, and both groups are measured on the same dependent variable. Subsequent experimental designs have used more groups and more measurements over longer periods. True experiments must have control, randomization, and manipulation.
- Experimental research allows the researcher to control the situation. In so doing, it allows researchers to answer the question, “what causes something to occur?”
- Permits the researcher to identify cause and effect relationships between variables and to distinguish placebo effects from treatment effects.
- Experimental research designs support the ability to limit alternative explanations and to infer direct causal relationships in the study.
- Approach provides the highest level of evidence for single studies.
- The design is artificial, and results may not generalize well to the real world.
- The artificial settings of experiments may alter subject behaviors or responses.
- Experimental designs can be costly if special equipment or facilities are needed.
- Some research problems cannot be studied using an experiment because of ethical or technical reasons.
- Difficult to apply ethnographic and other qualitative methods to experimental designed research studies.
Anastas, Jeane W. Research Design for Social Work and the Human Services . Chapter 7, Flexible Methods: Experimental Research. 2nd ed. New York: Columbia University Press, 1999; Chapter 2: Research Design, Experimental Designs . School of Psychology, University of New England, 2000; Experimental Research. Research Methods by Dummies. Department of Psychology. California State University, Fresno, 2006; Trochim, William M.K. Experimental Design . Research Methods Knowledge Base. 2006; Rasool, Shafqat. Experimental Research . Slideshare presentation.
An exploratory design is conducted about a research problem when there are few or no earlier studies to refer to. The focus is on gaining insights and familiarity for later investigation or undertaken when problems are in a preliminary stage of investigation.
The goals of exploratory research are intended to produce the following possible insights:
- Familiarity with basic details, settings and concerns.
- Well grounded picture of the situation being developed.
- Generation of new ideas and assumption, development of tentative theories or hypotheses.
- Determination about whether a study is feasible in the future.
- Issues get refined for more systematic investigation and formulation of new research questions.
- Direction for future research and techniques get developed.
- Design is a useful approach for gaining background information on a particular topic.
- Exploratory research is flexible and can address research questions of all types (what, why, how).
- Provides an opportunity to define new terms and clarify existing concepts.
- Exploratory research is often used to generate formal hypotheses and develop more precise research problems.
- Exploratory studies help establish research priorities.
- Exploratory research generally utilizes small sample sizes and, thus, findings are typically not generalizable to the population at large.
- The exploratory nature of the research inhibits an ability to make definitive conclusions about the findings.
- The research process underpinning exploratory studies is flexible but often unstructured, leading to only tentative results that have limited value in decision-making.
- Design lacks rigorous standards applied to methods of data gathering and analysis because one of the areas for exploration could be to determine what method or methodologies could best fit the research problem.
Cuthill, Michael. “Exploratory Research: Citizen Participation, Local Government, and Sustainable Development in Australia.” Sustainable Development 10 (2002): 79-89; Taylor, P. J., G. Catalano, and D.R.F. Walker. “Exploratory Analysis of the World City Network.” Urban Studies 39 (December 2002): 2377-2394; Exploratory Research . Wikipedia.
The purpose of a historical research design is to collect, verify, and synthesize evidence from the past to establish facts that defend or refute your hypothesis. It uses secondary sources and a variety of primary documentary evidence, such as, logs, diaries, official records, reports, archives, and non-textual information [maps, pictures, audio and visual recordings]. The limitation is that the sources must be both authentic and valid.
- The historical research design is unobtrusive; the act of research does not affect the results of the study.
- The historical approach is well suited for trend analysis.
- Historical records can add important contextual background required to more fully understand and interpret a research problem.
- There is no possibility of researcher-subject interaction that could affect the findings.
- Historical sources can be used over and over to study different research problems or to replicate a previous study.
- The ability to fulfill the aims of your research are directly related to the amount and quality of documentation available to understand the research problem.
- Since historical research relies on data from the past, there is no way to manipulate it to control for contemporary contexts.
- Interpreting historical sources can be very time consuming.
- The sources of historical materials must be archived consistentally to ensure access.
- Original authors bring their own perspectives and biases to the interpretation of past events and these biases are more difficult to ascertain in historical resources.
- Due to the lack of control over external variables, historical research is very weak with regard to the demands of internal validity.
- It rare that the entirety of historical documentation needed to fully address a research problem is available for interpretation, therefore, gaps need to be acknowledged.
Savitt, Ronald. “Historical Research in Marketing.” Journal of Marketing 44 (Autumn, 1980): 52-58; Gall, Meredith. Educational Research: An Introduction . Chapter 16, Historical Research. 8th ed. Boston, MA: Pearson/Allyn and Bacon, 2007.
A longitudinal study follows the same sample over time and makes repeated observations. With longitudinal surveys, for example, the same group of people is interviewed at regular intervals, enabling researchers to track changes over time and to relate them to variables that might explain why the changes occur. Longitudinal research designs describe patterns of change and help establish the direction and magnitude of causal relationships. Measurements are taken on each variable over two or more distinct time periods. This allows the researcher to measure change in variables over time. It is a type of observational study and is sometimes referred to as a panel study.
- Longitudinal data allow the analysis of duration of a particular phenomenon.
- Enables survey researchers to get close to the kinds of causal explanations usually attainable only with experiments.
- The design permits the measurement of differences or change in a variable from one period to another [i.e., the description of patterns of change over time].
- Longitudinal studies facilitate the prediction of future outcomes based upon earlier factors.
- The data collection method may change over time.
- Maintaining the integrity of the original sample can be difficult over an extended period of time.
- It can be difficult to show more than one variable at a time.
- This design often needs qualitative research to explain fluctuations in the data.
- A longitudinal research design assumes present trends will continue unchanged.
- It can take a long period of time to gather results.
- There is a need to have a large sample size and accurate sampling to reach representativness.
Anastas, Jeane W. Research Design for Social Work and the Human Services . Chapter 6, Flexible Methods: Relational and Longitudinal Research. 2nd ed. New York: Columbia University Press, 1999; Kalaian, Sema A. and Rafa M. Kasim. "Longitudinal Studies." In Encyclopedia of Survey Research Methods . Paul J. Lavrakas, ed. (Thousand Oaks, CA: Sage, 2008), pp. 440-441; Ployhart, Robert E. and Robert J. Vandenberg. "Longitudinal Research: The Theory, Design, and Analysis of Change.” Journal of Management 36 (January 2010): 94-120; Longitudinal Study . Wikipedia.
This type of research design draws a conclusion by comparing subjects against a control group, in cases where the researcher has no control over the experiment. There are two general types of observational designs. In direct observations, people know that you are watching them. Unobtrusive measures involve any method for studying behavior where individuals do not know they are being observed. An observational study allows a useful insight into a phenomenon and avoids the ethical and practical difficulties of setting up a large and cumbersome research project.
- Observational studies are usually flexible and do not necessarily need to be structured around a hypothesis about what you expect to observe (data is emergent rather than pre-existing).
- The researcher is able to collect a depth of information about a particular behavior.
- Can reveal interrelationships among multifaceted dimensions of group interactions.
- You can generalize your results to real life situations.
- Observational research is useful for discovering what variables may be important before applying other methods like experiments.
- Observation researchd esigns account for the complexity of group behaviors.
- Reliability of data is low because seeing behaviors occur over and over again may be a time consuming task and difficult to replicate.
- In observational research, findings may only reflect a unique sample population and, thus, cannot be generalized to other groups.
- There can be problems with bias as the researcher may only "see what they want to see."
- There is no possiblility to determine "cause and effect" relationships since nothing is manipulated.
- Sources or subjects may not all be equally credible.
- Any group that is studied is altered to some degree by the very presence of the researcher, therefore, skewing to some degree any data collected (the Heisenburg Uncertainty Principle).
Atkinson, Paul and Martyn Hammersley. “Ethnography and Participant Observation.” In Handbook of Qualitative Research . Norman K. Denzin and Yvonna S. Lincoln, eds. (Thousand Oaks, CA: Sage, 1994), pp. 248-261; Observational Research. Research Methods by Dummies. Department of Psychology. California State University, Fresno, 2006; Patton Michael Quinn. Qualitiative Research and Evaluation Methods . Chapter 6, Fieldwork Strategies and Observational Methods. 3rd ed. Thousand Oaks, CA: Sage, 2002; Rosenbaum, Paul R. Design of Observational Studies . New York: Springer, 2010.
Understood more as an broad approach to examining a research problem than a methodological design, philosophical analysis and argumentation is intended to challenge deeply embedded, often intractable, assumptions underpinning an area of study. This approach uses the tools of argumentation derived from philosophical traditions, concepts, models, and theories to critically explore and challenge, for example, the relevance of logic and evidence in academic debates, to analyze arguments about fundamental issues, or to discuss the root of existing discourse about a research problem. These overarching tools of analysis can be framed in three ways:
- Ontology -- the study that describes the nature of reality; for example, what is real and what is not, what is fundamental and what is derivative?
- Epistemology -- the study that explores the nature of knowledge; for example, on what does knowledge and understanding depend upon and how can we be certain of what we know?
- Axiology -- the study of values; for example, what values does an individual or group hold and why? How are values related to interest, desire, will, experience, and means-to-end? And, what is the difference between a matter of fact and a matter of value?
- Can provide a basis for applying ethical decision-making to practice.
- Functions as a means of gaining greater self-understanding and self-knowledge about the purposes of research.
- Brings clarity to general guiding practices and principles of an individual or group.
- Philosophy informs methodology.
- Refine concepts and theories that are invoked in relatively unreflective modes of thought and discourse.
- Beyond methodology, philosophy also informs critical thinking about epistemology and the structure of reality (metaphysics).
- Offers clarity and definition to the practical and theoretical uses of terms, concepts, and ideas.
- Limited application to specific research problems [answering the "So What?" question in social science research].
- Analysis can be abstract, argumentative, and limited in its practical application to real-life issues.
- While a philosophical analysis may render problematic that which was once simple or taken-for-granted, the writing can be dense and subject to unnecessary jargon, overstatement, and/or excessive quotation and documentation.
- There are limitations in the use of metaphor as a vehicle of philosophical analysis.
- There can be analytical difficulties in moving from philosophy to advocacy and between abstract thought and application to the phenomenal world.
Chapter 4, Research Methodology and Design . Unisa Institutional Repository (UnisaIR), University of South Africa; Labaree, Robert V. and Ross Scimeca. “The Philosophical Problem of Truth in Librarianship.” The Library Quarterly 78 (January 2008): 43-70; Maykut, Pamela S. Beginning Qualitative Research: A Philosophic and Practical Guide . Washington, D.C.: Falmer Press, 1994; Stanford Encyclopedia of Philosophy . Metaphysics Research Lab, CSLI, Stanford University, 2013.
- The researcher has a limitless option when it comes to sample size and the sampling schedule.
- Due to the repetitive nature of this research design, minor changes and adjustments can be done during the initial parts of the study to correct and hone the research method. Useful design for exploratory studies.
- There is very little effort on the part of the researcher when performing this technique. It is generally not expensive, time consuming, or workforce extensive.
- Because the study is conducted serially, the results of one sample are known before the next sample is taken and analyzed.
- The sampling method is not representative of the entire population. The only possibility of approaching representativeness is when the researcher chooses to use a very large sample size significant enough to represent a significant portion of the entire population. In this case, moving on to study a second or more sample can be difficult.
- Because the sampling technique is not randomized, the design cannot be used to create conclusions and interpretations that pertain to an entire population. Generalizability from findings is limited.
- Difficult to account for and interpret variation from one sample to another over time, particularly when using qualitative methods of data collection.
Rebecca Betensky, Harvard University, Course Lecture Note slides ; Cresswell, John W. Et al. “Advanced Mixed-Methods Research Designs.” In Handbook of Mixed Methods in Social and Behavioral Research . Abbas Tashakkori and Charles Teddle, eds. (Thousand Oaks, CA: Sage, 2003), pp. 209-240; Nataliya V. Ivankova. “Using Mixed-Methods Sequential Explanatory Design: From Theory to Practice.” Field Methods 18 (February 2006): 3-20; Bovaird, James A. and Kevin A. Kupzyk. “Sequential Design.” In Encyclopedia of Research Design . Neil J. Salkind, ed. Thousand Oaks, CA: Sage, 2010; Sequential Analysis . Wikipedia.
- << Previous: Purpose of Guide
- Next: Design Flaws to Avoid >>
- Last Updated: Jul 18, 2023 11:58 AM
- URL: https://library.sacredheart.edu/c.php?g=29803
- QuickSearch
- Library Catalog
- Databases A-Z
- Publication Finder
- Course Reserves
- Citation Linker
- Digital Commons
- Our Website
Research Support
- Ask a Librarian
- Appointments
- Interlibrary Loan (ILL)
- Research Guides
- Databases by Subject
- Citation Help
Using the Library
- Reserve a Group Study Room
- Renew Books
- Honors Study Rooms
- Off-Campus Access
- Library Policies
- Library Technology
User Information
- Grad Students
- Online Students
- COVID-19 Updates
- Staff Directory
- News & Announcements
- Library Newsletter
My Accounts
- Interlibrary Loan
- Staff Site Login

FIND US ON
- USC Libraries
- Research Guides
Organizing Your Social Sciences Research Paper
- Types of Research Designs
- Purpose of Guide
- Design Flaws to Avoid
- Independent and Dependent Variables
- Glossary of Research Terms
- Reading Research Effectively
- Narrowing a Topic Idea
- Broadening a Topic Idea
- Extending the Timeliness of a Topic Idea
- Academic Writing Style
- Choosing a Title
- Making an Outline
- Paragraph Development
- Research Process Video Series
- Executive Summary
- The C.A.R.S. Model
- Background Information
- The Research Problem/Question
- Theoretical Framework
- Citation Tracking
- Content Alert Services
- Evaluating Sources
- Primary Sources
- Secondary Sources
- Tiertiary Sources
- Scholarly vs. Popular Publications
- Qualitative Methods
- Quantitative Methods
- Insiderness
- Using Non-Textual Elements
- Limitations of the Study
- Common Grammar Mistakes
- Writing Concisely
- Avoiding Plagiarism
- Footnotes or Endnotes?
- Further Readings
- Generative AI and Writing
- USC Libraries Tutorials and Other Guides
- Bibliography
Introduction
Before beginning your paper, you need to decide how you plan to design the study .
The research design refers to the overall strategy and analytical approach that you have chosen in order to integrate, in a coherent and logical way, the different components of the study, thus ensuring that the research problem will be thoroughly investigated. It constitutes the blueprint for the collection, measurement, and interpretation of information and data. Note that the research problem determines the type of design you choose, not the other way around!
De Vaus, D. A. Research Design in Social Research . London: SAGE, 2001; Trochim, William M.K. Research Methods Knowledge Base. 2006.
General Structure and Writing Style
The function of a research design is to ensure that the evidence obtained enables you to effectively address the research problem logically and as unambiguously as possible . In social sciences research, obtaining information relevant to the research problem generally entails specifying the type of evidence needed to test the underlying assumptions of a theory, to evaluate a program, or to accurately describe and assess meaning related to an observable phenomenon.
With this in mind, a common mistake made by researchers is that they begin their investigations before they have thought critically about what information is required to address the research problem. Without attending to these design issues beforehand, the overall research problem will not be adequately addressed and any conclusions drawn will run the risk of being weak and unconvincing. As a consequence, the overall validity of the study will be undermined.
The length and complexity of describing the research design in your paper can vary considerably, but any well-developed description will achieve the following :
- Identify the research problem clearly and justify its selection, particularly in relation to any valid alternative designs that could have been used,
- Review and synthesize previously published literature associated with the research problem,
- Clearly and explicitly specify hypotheses [i.e., research questions] central to the problem,
- Effectively describe the information and/or data which will be necessary for an adequate testing of the hypotheses and explain how such information and/or data will be obtained, and
- Describe the methods of analysis to be applied to the data in determining whether or not the hypotheses are true or false.
The research design is usually incorporated into the introduction of your paper . You can obtain an overall sense of what to do by reviewing studies that have utilized the same research design [e.g., using a case study approach]. This can help you develop an outline to follow for your own paper.
NOTE : Use the SAGE Research Methods Online and Cases and the SAGE Research Methods Videos databases to search for scholarly resources on how to apply specific research designs and methods . The Research Methods Online database contains links to more than 175,000 pages of SAGE publisher's book, journal, and reference content on quantitative, qualitative, and mixed research methodologies. Also included is a collection of case studies of social research projects that can be used to help you better understand abstract or complex methodological concepts. The Research Methods Videos database contains hours of tutorials, interviews, video case studies, and mini-documentaries covering the entire research process.
Creswell, John W. and J. David Creswell. Research Design: Qualitative, Quantitative, and Mixed Methods Approaches . 5th edition. Thousand Oaks, CA: Sage, 2018; De Vaus, D. A. Research Design in Social Research . London: SAGE, 2001; Gorard, Stephen. Research Design: Creating Robust Approaches for the Social Sciences . Thousand Oaks, CA: Sage, 2013; Leedy, Paul D. and Jeanne Ellis Ormrod. Practical Research: Planning and Design . Tenth edition. Boston, MA: Pearson, 2013; Vogt, W. Paul, Dianna C. Gardner, and Lynne M. Haeffele. When to Use What Research Design . New York: Guilford, 2012.
Action Research Design
Definition and Purpose
The essentials of action research design follow a characteristic cycle whereby initially an exploratory stance is adopted, where an understanding of a problem is developed and plans are made for some form of interventionary strategy. Then the intervention is carried out [the "action" in action research] during which time, pertinent observations are collected in various forms. The new interventional strategies are carried out, and this cyclic process repeats, continuing until a sufficient understanding of [or a valid implementation solution for] the problem is achieved. The protocol is iterative or cyclical in nature and is intended to foster deeper understanding of a given situation, starting with conceptualizing and particularizing the problem and moving through several interventions and evaluations.
What do these studies tell you ?
- This is a collaborative and adaptive research design that lends itself to use in work or community situations.
- Design focuses on pragmatic and solution-driven research outcomes rather than testing theories.
- When practitioners use action research, it has the potential to increase the amount they learn consciously from their experience; the action research cycle can be regarded as a learning cycle.
- Action research studies often have direct and obvious relevance to improving practice and advocating for change.
- There are no hidden controls or preemption of direction by the researcher.
What these studies don't tell you ?
- It is harder to do than conducting conventional research because the researcher takes on responsibilities of advocating for change as well as for researching the topic.
- Action research is much harder to write up because it is less likely that you can use a standard format to report your findings effectively [i.e., data is often in the form of stories or observation].
- Personal over-involvement of the researcher may bias research results.
- The cyclic nature of action research to achieve its twin outcomes of action [e.g. change] and research [e.g. understanding] is time-consuming and complex to conduct.
- Advocating for change usually requires buy-in from study participants.
Coghlan, David and Mary Brydon-Miller. The Sage Encyclopedia of Action Research . Thousand Oaks, CA: Sage, 2014; Efron, Sara Efrat and Ruth Ravid. Action Research in Education: A Practical Guide . New York: Guilford, 2013; Gall, Meredith. Educational Research: An Introduction . Chapter 18, Action Research. 8th ed. Boston, MA: Pearson/Allyn and Bacon, 2007; Gorard, Stephen. Research Design: Creating Robust Approaches for the Social Sciences . Thousand Oaks, CA: Sage, 2013; Kemmis, Stephen and Robin McTaggart. “Participatory Action Research.” In Handbook of Qualitative Research . Norman Denzin and Yvonna S. Lincoln, eds. 2nd ed. (Thousand Oaks, CA: SAGE, 2000), pp. 567-605; McNiff, Jean. Writing and Doing Action Research . London: Sage, 2014; Reason, Peter and Hilary Bradbury. Handbook of Action Research: Participative Inquiry and Practice . Thousand Oaks, CA: SAGE, 2001.
Case Study Design
A case study is an in-depth study of a particular research problem rather than a sweeping statistical survey or comprehensive comparative inquiry. It is often used to narrow down a very broad field of research into one or a few easily researchable examples. The case study research design is also useful for testing whether a specific theory and model actually applies to phenomena in the real world. It is a useful design when not much is known about an issue or phenomenon.
- Approach excels at bringing us to an understanding of a complex issue through detailed contextual analysis of a limited number of events or conditions and their relationships.
- A researcher using a case study design can apply a variety of methodologies and rely on a variety of sources to investigate a research problem.
- Design can extend experience or add strength to what is already known through previous research.
- Social scientists, in particular, make wide use of this research design to examine contemporary real-life situations and provide the basis for the application of concepts and theories and the extension of methodologies.
- The design can provide detailed descriptions of specific and rare cases.
- A single or small number of cases offers little basis for establishing reliability or to generalize the findings to a wider population of people, places, or things.
- Intense exposure to the study of a case may bias a researcher's interpretation of the findings.
- Design does not facilitate assessment of cause and effect relationships.
- Vital information may be missing, making the case hard to interpret.
- The case may not be representative or typical of the larger problem being investigated.
- If the criteria for selecting a case is because it represents a very unusual or unique phenomenon or problem for study, then your interpretation of the findings can only apply to that particular case.
Case Studies. Writing@CSU. Colorado State University; Anastas, Jeane W. Research Design for Social Work and the Human Services . Chapter 4, Flexible Methods: Case Study Design. 2nd ed. New York: Columbia University Press, 1999; Gerring, John. “What Is a Case Study and What Is It Good for?” American Political Science Review 98 (May 2004): 341-354; Greenhalgh, Trisha, editor. Case Study Evaluation: Past, Present and Future Challenges . Bingley, UK: Emerald Group Publishing, 2015; Mills, Albert J. , Gabrielle Durepos, and Eiden Wiebe, editors. Encyclopedia of Case Study Research . Thousand Oaks, CA: SAGE Publications, 2010; Stake, Robert E. The Art of Case Study Research . Thousand Oaks, CA: SAGE, 1995; Yin, Robert K. Case Study Research: Design and Theory . Applied Social Research Methods Series, no. 5. 3rd ed. Thousand Oaks, CA: SAGE, 2003.
Causal Design
Causality studies may be thought of as understanding a phenomenon in terms of conditional statements in the form, “If X, then Y.” This type of research is used to measure what impact a specific change will have on existing norms and assumptions. Most social scientists seek causal explanations that reflect tests of hypotheses. Causal effect (nomothetic perspective) occurs when variation in one phenomenon, an independent variable, leads to or results, on average, in variation in another phenomenon, the dependent variable.
Conditions necessary for determining causality:
- Empirical association -- a valid conclusion is based on finding an association between the independent variable and the dependent variable.
- Appropriate time order -- to conclude that causation was involved, one must see that cases were exposed to variation in the independent variable before variation in the dependent variable.
- Nonspuriousness -- a relationship between two variables that is not due to variation in a third variable.
- Causality research designs assist researchers in understanding why the world works the way it does through the process of proving a causal link between variables and by the process of eliminating other possibilities.
- Replication is possible.
- There is greater confidence the study has internal validity due to the systematic subject selection and equity of groups being compared.
- Not all relationships are causal! The possibility always exists that, by sheer coincidence, two unrelated events appear to be related [e.g., Punxatawney Phil could accurately predict the duration of Winter for five consecutive years but, the fact remains, he's just a big, furry rodent].
- Conclusions about causal relationships are difficult to determine due to a variety of extraneous and confounding variables that exist in a social environment. This means causality can only be inferred, never proven.
- If two variables are correlated, the cause must come before the effect. However, even though two variables might be causally related, it can sometimes be difficult to determine which variable comes first and, therefore, to establish which variable is the actual cause and which is the actual effect.
Beach, Derek and Rasmus Brun Pedersen. Causal Case Study Methods: Foundations and Guidelines for Comparing, Matching, and Tracing . Ann Arbor, MI: University of Michigan Press, 2016; Bachman, Ronet. The Practice of Research in Criminology and Criminal Justice . Chapter 5, Causation and Research Designs. 3rd ed. Thousand Oaks, CA: Pine Forge Press, 2007; Brewer, Ernest W. and Jennifer Kubn. “Causal-Comparative Design.” In Encyclopedia of Research Design . Neil J. Salkind, editor. (Thousand Oaks, CA: Sage, 2010), pp. 125-132; Causal Research Design: Experimentation. Anonymous SlideShare Presentation; Gall, Meredith. Educational Research: An Introduction . Chapter 11, Nonexperimental Research: Correlational Designs. 8th ed. Boston, MA: Pearson/Allyn and Bacon, 2007; Trochim, William M.K. Research Methods Knowledge Base. 2006.
Cohort Design
Often used in the medical sciences, but also found in the applied social sciences, a cohort study generally refers to a study conducted over a period of time involving members of a population which the subject or representative member comes from, and who are united by some commonality or similarity. Using a quantitative framework, a cohort study makes note of statistical occurrence within a specialized subgroup, united by same or similar characteristics that are relevant to the research problem being investigated, rather than studying statistical occurrence within the general population. Using a qualitative framework, cohort studies generally gather data using methods of observation. Cohorts can be either "open" or "closed."
- Open Cohort Studies [dynamic populations, such as the population of Los Angeles] involve a population that is defined just by the state of being a part of the study in question (and being monitored for the outcome). Date of entry and exit from the study is individually defined, therefore, the size of the study population is not constant. In open cohort studies, researchers can only calculate rate based data, such as, incidence rates and variants thereof.
- Closed Cohort Studies [static populations, such as patients entered into a clinical trial] involve participants who enter into the study at one defining point in time and where it is presumed that no new participants can enter the cohort. Given this, the number of study participants remains constant (or can only decrease).
- The use of cohorts is often mandatory because a randomized control study may be unethical. For example, you cannot deliberately expose people to asbestos, you can only study its effects on those who have already been exposed. Research that measures risk factors often relies upon cohort designs.
- Because cohort studies measure potential causes before the outcome has occurred, they can demonstrate that these “causes” preceded the outcome, thereby avoiding the debate as to which is the cause and which is the effect.
- Cohort analysis is highly flexible and can provide insight into effects over time and related to a variety of different types of changes [e.g., social, cultural, political, economic, etc.].
- Either original data or secondary data can be used in this design.
- In cases where a comparative analysis of two cohorts is made [e.g., studying the effects of one group exposed to asbestos and one that has not], a researcher cannot control for all other factors that might differ between the two groups. These factors are known as confounding variables.
- Cohort studies can end up taking a long time to complete if the researcher must wait for the conditions of interest to develop within the group. This also increases the chance that key variables change during the course of the study, potentially impacting the validity of the findings.
- Due to the lack of randominization in the cohort design, its external validity is lower than that of study designs where the researcher randomly assigns participants.
Healy P, Devane D. “Methodological Considerations in Cohort Study Designs.” Nurse Researcher 18 (2011): 32-36; Glenn, Norval D, editor. Cohort Analysis . 2nd edition. Thousand Oaks, CA: Sage, 2005; Levin, Kate Ann. Study Design IV: Cohort Studies. Evidence-Based Dentistry 7 (2003): 51–52; Payne, Geoff. “Cohort Study.” In The SAGE Dictionary of Social Research Methods . Victor Jupp, editor. (Thousand Oaks, CA: Sage, 2006), pp. 31-33; Study Design 101. Himmelfarb Health Sciences Library. George Washington University, November 2011; Cohort Study. Wikipedia.
Cross-Sectional Design
Cross-sectional research designs have three distinctive features: no time dimension; a reliance on existing differences rather than change following intervention; and, groups are selected based on existing differences rather than random allocation. The cross-sectional design can only measure differences between or from among a variety of people, subjects, or phenomena rather than a process of change. As such, researchers using this design can only employ a relatively passive approach to making causal inferences based on findings.
- Cross-sectional studies provide a clear 'snapshot' of the outcome and the characteristics associated with it, at a specific point in time.
- Unlike an experimental design, where there is an active intervention by the researcher to produce and measure change or to create differences, cross-sectional designs focus on studying and drawing inferences from existing differences between people, subjects, or phenomena.
- Entails collecting data at and concerning one point in time. While longitudinal studies involve taking multiple measures over an extended period of time, cross-sectional research is focused on finding relationships between variables at one moment in time.
- Groups identified for study are purposely selected based upon existing differences in the sample rather than seeking random sampling.
- Cross-section studies are capable of using data from a large number of subjects and, unlike observational studies, is not geographically bound.
- Can estimate prevalence of an outcome of interest because the sample is usually taken from the whole population.
- Because cross-sectional designs generally use survey techniques to gather data, they are relatively inexpensive and take up little time to conduct.
- Finding people, subjects, or phenomena to study that are very similar except in one specific variable can be difficult.
- Results are static and time bound and, therefore, give no indication of a sequence of events or reveal historical or temporal contexts.
- Studies cannot be utilized to establish cause and effect relationships.
- This design only provides a snapshot of analysis so there is always the possibility that a study could have differing results if another time-frame had been chosen.
- There is no follow up to the findings.
Bethlehem, Jelke. "7: Cross-sectional Research." In Research Methodology in the Social, Behavioural and Life Sciences . Herman J Adèr and Gideon J Mellenbergh, editors. (London, England: Sage, 1999), pp. 110-43; Bourque, Linda B. “Cross-Sectional Design.” In The SAGE Encyclopedia of Social Science Research Methods . Michael S. Lewis-Beck, Alan Bryman, and Tim Futing Liao. (Thousand Oaks, CA: 2004), pp. 230-231; Hall, John. “Cross-Sectional Survey Design.” In Encyclopedia of Survey Research Methods . Paul J. Lavrakas, ed. (Thousand Oaks, CA: Sage, 2008), pp. 173-174; Helen Barratt, Maria Kirwan. Cross-Sectional Studies: Design Application, Strengths and Weaknesses of Cross-Sectional Studies. Healthknowledge, 2009. Cross-Sectional Study. Wikipedia.
Descriptive Design
Descriptive research designs help provide answers to the questions of who, what, when, where, and how associated with a particular research problem; a descriptive study cannot conclusively ascertain answers to why. Descriptive research is used to obtain information concerning the current status of the phenomena and to describe "what exists" with respect to variables or conditions in a situation.
- The subject is being observed in a completely natural and unchanged natural environment. True experiments, whilst giving analyzable data, often adversely influence the normal behavior of the subject [a.k.a., the Heisenberg effect whereby measurements of certain systems cannot be made without affecting the systems].
- Descriptive research is often used as a pre-cursor to more quantitative research designs with the general overview giving some valuable pointers as to what variables are worth testing quantitatively.
- If the limitations are understood, they can be a useful tool in developing a more focused study.
- Descriptive studies can yield rich data that lead to important recommendations in practice.
- Appoach collects a large amount of data for detailed analysis.
- The results from a descriptive research cannot be used to discover a definitive answer or to disprove a hypothesis.
- Because descriptive designs often utilize observational methods [as opposed to quantitative methods], the results cannot be replicated.
- The descriptive function of research is heavily dependent on instrumentation for measurement and observation.
Anastas, Jeane W. Research Design for Social Work and the Human Services . Chapter 5, Flexible Methods: Descriptive Research. 2nd ed. New York: Columbia University Press, 1999; Given, Lisa M. "Descriptive Research." In Encyclopedia of Measurement and Statistics . Neil J. Salkind and Kristin Rasmussen, editors. (Thousand Oaks, CA: Sage, 2007), pp. 251-254; McNabb, Connie. Descriptive Research Methodologies. Powerpoint Presentation; Shuttleworth, Martyn. Descriptive Research Design, September 26, 2008; Erickson, G. Scott. "Descriptive Research Design." In New Methods of Market Research and Analysis . (Northampton, MA: Edward Elgar Publishing, 2017), pp. 51-77; Sahin, Sagufta, and Jayanta Mete. "A Brief Study on Descriptive Research: Its Nature and Application in Social Science." International Journal of Research and Analysis in Humanities 1 (2021): 11; K. Swatzell and P. Jennings. “Descriptive Research: The Nuts and Bolts.” Journal of the American Academy of Physician Assistants 20 (2007), pp. 55-56; Kane, E. Doing Your Own Research: Basic Descriptive Research in the Social Sciences and Humanities . London: Marion Boyars, 1985.
Experimental Design
A blueprint of the procedure that enables the researcher to maintain control over all factors that may affect the result of an experiment. In doing this, the researcher attempts to determine or predict what may occur. Experimental research is often used where there is time priority in a causal relationship (cause precedes effect), there is consistency in a causal relationship (a cause will always lead to the same effect), and the magnitude of the correlation is great. The classic experimental design specifies an experimental group and a control group. The independent variable is administered to the experimental group and not to the control group, and both groups are measured on the same dependent variable. Subsequent experimental designs have used more groups and more measurements over longer periods. True experiments must have control, randomization, and manipulation.
- Experimental research allows the researcher to control the situation. In so doing, it allows researchers to answer the question, “What causes something to occur?”
- Permits the researcher to identify cause and effect relationships between variables and to distinguish placebo effects from treatment effects.
- Experimental research designs support the ability to limit alternative explanations and to infer direct causal relationships in the study.
- Approach provides the highest level of evidence for single studies.
- The design is artificial, and results may not generalize well to the real world.
- The artificial settings of experiments may alter the behaviors or responses of participants.
- Experimental designs can be costly if special equipment or facilities are needed.
- Some research problems cannot be studied using an experiment because of ethical or technical reasons.
- Difficult to apply ethnographic and other qualitative methods to experimentally designed studies.
Anastas, Jeane W. Research Design for Social Work and the Human Services . Chapter 7, Flexible Methods: Experimental Research. 2nd ed. New York: Columbia University Press, 1999; Chapter 2: Research Design, Experimental Designs. School of Psychology, University of New England, 2000; Chow, Siu L. "Experimental Design." In Encyclopedia of Research Design . Neil J. Salkind, editor. (Thousand Oaks, CA: Sage, 2010), pp. 448-453; "Experimental Design." In Social Research Methods . Nicholas Walliman, editor. (London, England: Sage, 2006), pp, 101-110; Experimental Research. Research Methods by Dummies. Department of Psychology. California State University, Fresno, 2006; Kirk, Roger E. Experimental Design: Procedures for the Behavioral Sciences . 4th edition. Thousand Oaks, CA: Sage, 2013; Trochim, William M.K. Experimental Design. Research Methods Knowledge Base. 2006; Rasool, Shafqat. Experimental Research. Slideshare presentation.
Exploratory Design
An exploratory design is conducted about a research problem when there are few or no earlier studies to refer to or rely upon to predict an outcome . The focus is on gaining insights and familiarity for later investigation or undertaken when research problems are in a preliminary stage of investigation. Exploratory designs are often used to establish an understanding of how best to proceed in studying an issue or what methodology would effectively apply to gathering information about the issue.
The goals of exploratory research are intended to produce the following possible insights:
- Familiarity with basic details, settings, and concerns.
- Well grounded picture of the situation being developed.
- Generation of new ideas and assumptions.
- Development of tentative theories or hypotheses.
- Determination about whether a study is feasible in the future.
- Issues get refined for more systematic investigation and formulation of new research questions.
- Direction for future research and techniques get developed.
- Design is a useful approach for gaining background information on a particular topic.
- Exploratory research is flexible and can address research questions of all types (what, why, how).
- Provides an opportunity to define new terms and clarify existing concepts.
- Exploratory research is often used to generate formal hypotheses and develop more precise research problems.
- In the policy arena or applied to practice, exploratory studies help establish research priorities and where resources should be allocated.
- Exploratory research generally utilizes small sample sizes and, thus, findings are typically not generalizable to the population at large.
- The exploratory nature of the research inhibits an ability to make definitive conclusions about the findings. They provide insight but not definitive conclusions.
- The research process underpinning exploratory studies is flexible but often unstructured, leading to only tentative results that have limited value to decision-makers.
- Design lacks rigorous standards applied to methods of data gathering and analysis because one of the areas for exploration could be to determine what method or methodologies could best fit the research problem.
Cuthill, Michael. “Exploratory Research: Citizen Participation, Local Government, and Sustainable Development in Australia.” Sustainable Development 10 (2002): 79-89; Streb, Christoph K. "Exploratory Case Study." In Encyclopedia of Case Study Research . Albert J. Mills, Gabrielle Durepos and Eiden Wiebe, editors. (Thousand Oaks, CA: Sage, 2010), pp. 372-374; Taylor, P. J., G. Catalano, and D.R.F. Walker. “Exploratory Analysis of the World City Network.” Urban Studies 39 (December 2002): 2377-2394; Exploratory Research. Wikipedia.
Field Research Design
Sometimes referred to as ethnography or participant observation, designs around field research encompass a variety of interpretative procedures [e.g., observation and interviews] rooted in qualitative approaches to studying people individually or in groups while inhabiting their natural environment as opposed to using survey instruments or other forms of impersonal methods of data gathering. Information acquired from observational research takes the form of “ field notes ” that involves documenting what the researcher actually sees and hears while in the field. Findings do not consist of conclusive statements derived from numbers and statistics because field research involves analysis of words and observations of behavior. Conclusions, therefore, are developed from an interpretation of findings that reveal overriding themes, concepts, and ideas. More information can be found HERE .
- Field research is often necessary to fill gaps in understanding the research problem applied to local conditions or to specific groups of people that cannot be ascertained from existing data.
- The research helps contextualize already known information about a research problem, thereby facilitating ways to assess the origins, scope, and scale of a problem and to gage the causes, consequences, and means to resolve an issue based on deliberate interaction with people in their natural inhabited spaces.
- Enables the researcher to corroborate or confirm data by gathering additional information that supports or refutes findings reported in prior studies of the topic.
- Because the researcher in embedded in the field, they are better able to make observations or ask questions that reflect the specific cultural context of the setting being investigated.
- Observing the local reality offers the opportunity to gain new perspectives or obtain unique data that challenges existing theoretical propositions or long-standing assumptions found in the literature.
What these studies don't tell you
- A field research study requires extensive time and resources to carry out the multiple steps involved with preparing for the gathering of information, including for example, examining background information about the study site, obtaining permission to access the study site, and building trust and rapport with subjects.
- Requires a commitment to staying engaged in the field to ensure that you can adequately document events and behaviors as they unfold.
- The unpredictable nature of fieldwork means that researchers can never fully control the process of data gathering. They must maintain a flexible approach to studying the setting because events and circumstances can change quickly or unexpectedly.
- Findings can be difficult to interpret and verify without access to documents and other source materials that help to enhance the credibility of information obtained from the field [i.e., the act of triangulating the data].
- Linking the research problem to the selection of study participants inhabiting their natural environment is critical. However, this specificity limits the ability to generalize findings to different situations or in other contexts or to infer courses of action applied to other settings or groups of people.
- The reporting of findings must take into account how the researcher themselves may have inadvertently affected respondents and their behaviors.
Historical Design
The purpose of a historical research design is to collect, verify, and synthesize evidence from the past to establish facts that defend or refute a hypothesis. It uses secondary sources and a variety of primary documentary evidence, such as, diaries, official records, reports, archives, and non-textual information [maps, pictures, audio and visual recordings]. The limitation is that the sources must be both authentic and valid.
- The historical research design is unobtrusive; the act of research does not affect the results of the study.
- The historical approach is well suited for trend analysis.
- Historical records can add important contextual background required to more fully understand and interpret a research problem.
- There is often no possibility of researcher-subject interaction that could affect the findings.
- Historical sources can be used over and over to study different research problems or to replicate a previous study.
- The ability to fulfill the aims of your research are directly related to the amount and quality of documentation available to understand the research problem.
- Since historical research relies on data from the past, there is no way to manipulate it to control for contemporary contexts.
- Interpreting historical sources can be very time consuming.
- The sources of historical materials must be archived consistently to ensure access. This may especially challenging for digital or online-only sources.
- Original authors bring their own perspectives and biases to the interpretation of past events and these biases are more difficult to ascertain in historical resources.
- Due to the lack of control over external variables, historical research is very weak with regard to the demands of internal validity.
- It is rare that the entirety of historical documentation needed to fully address a research problem is available for interpretation, therefore, gaps need to be acknowledged.
Howell, Martha C. and Walter Prevenier. From Reliable Sources: An Introduction to Historical Methods . Ithaca, NY: Cornell University Press, 2001; Lundy, Karen Saucier. "Historical Research." In The Sage Encyclopedia of Qualitative Research Methods . Lisa M. Given, editor. (Thousand Oaks, CA: Sage, 2008), pp. 396-400; Marius, Richard. and Melvin E. Page. A Short Guide to Writing about History . 9th edition. Boston, MA: Pearson, 2015; Savitt, Ronald. “Historical Research in Marketing.” Journal of Marketing 44 (Autumn, 1980): 52-58; Gall, Meredith. Educational Research: An Introduction . Chapter 16, Historical Research. 8th ed. Boston, MA: Pearson/Allyn and Bacon, 2007.
Longitudinal Design
A longitudinal study follows the same sample over time and makes repeated observations. For example, with longitudinal surveys, the same group of people is interviewed at regular intervals, enabling researchers to track changes over time and to relate them to variables that might explain why the changes occur. Longitudinal research designs describe patterns of change and help establish the direction and magnitude of causal relationships. Measurements are taken on each variable over two or more distinct time periods. This allows the researcher to measure change in variables over time. It is a type of observational study sometimes referred to as a panel study.
- Longitudinal data facilitate the analysis of the duration of a particular phenomenon.
- Enables survey researchers to get close to the kinds of causal explanations usually attainable only with experiments.
- The design permits the measurement of differences or change in a variable from one period to another [i.e., the description of patterns of change over time].
- Longitudinal studies facilitate the prediction of future outcomes based upon earlier factors.
- The data collection method may change over time.
- Maintaining the integrity of the original sample can be difficult over an extended period of time.
- It can be difficult to show more than one variable at a time.
- This design often needs qualitative research data to explain fluctuations in the results.
- A longitudinal research design assumes present trends will continue unchanged.
- It can take a long period of time to gather results.
- There is a need to have a large sample size and accurate sampling to reach representativness.
Anastas, Jeane W. Research Design for Social Work and the Human Services . Chapter 6, Flexible Methods: Relational and Longitudinal Research. 2nd ed. New York: Columbia University Press, 1999; Forgues, Bernard, and Isabelle Vandangeon-Derumez. "Longitudinal Analyses." In Doing Management Research . Raymond-Alain Thiétart and Samantha Wauchope, editors. (London, England: Sage, 2001), pp. 332-351; Kalaian, Sema A. and Rafa M. Kasim. "Longitudinal Studies." In Encyclopedia of Survey Research Methods . Paul J. Lavrakas, ed. (Thousand Oaks, CA: Sage, 2008), pp. 440-441; Menard, Scott, editor. Longitudinal Research . Thousand Oaks, CA: Sage, 2002; Ployhart, Robert E. and Robert J. Vandenberg. "Longitudinal Research: The Theory, Design, and Analysis of Change.” Journal of Management 36 (January 2010): 94-120; Longitudinal Study. Wikipedia.
Meta-Analysis Design
Meta-analysis is an analytical methodology designed to systematically evaluate and summarize the results from a number of individual studies, thereby, increasing the overall sample size and the ability of the researcher to study effects of interest. The purpose is to not simply summarize existing knowledge, but to develop a new understanding of a research problem using synoptic reasoning. The main objectives of meta-analysis include analyzing differences in the results among studies and increasing the precision by which effects are estimated. A well-designed meta-analysis depends upon strict adherence to the criteria used for selecting studies and the availability of information in each study to properly analyze their findings. Lack of information can severely limit the type of analyzes and conclusions that can be reached. In addition, the more dissimilarity there is in the results among individual studies [heterogeneity], the more difficult it is to justify interpretations that govern a valid synopsis of results. A meta-analysis needs to fulfill the following requirements to ensure the validity of your findings:
- Clearly defined description of objectives, including precise definitions of the variables and outcomes that are being evaluated;
- A well-reasoned and well-documented justification for identification and selection of the studies;
- Assessment and explicit acknowledgment of any researcher bias in the identification and selection of those studies;
- Description and evaluation of the degree of heterogeneity among the sample size of studies reviewed; and,
- Justification of the techniques used to evaluate the studies.
- Can be an effective strategy for determining gaps in the literature.
- Provides a means of reviewing research published about a particular topic over an extended period of time and from a variety of sources.
- Is useful in clarifying what policy or programmatic actions can be justified on the basis of analyzing research results from multiple studies.
- Provides a method for overcoming small sample sizes in individual studies that previously may have had little relationship to each other.
- Can be used to generate new hypotheses or highlight research problems for future studies.
- Small violations in defining the criteria used for content analysis can lead to difficult to interpret and/or meaningless findings.
- A large sample size can yield reliable, but not necessarily valid, results.
- A lack of uniformity regarding, for example, the type of literature reviewed, how methods are applied, and how findings are measured within the sample of studies you are analyzing, can make the process of synthesis difficult to perform.
- Depending on the sample size, the process of reviewing and synthesizing multiple studies can be very time consuming.
Beck, Lewis W. "The Synoptic Method." The Journal of Philosophy 36 (1939): 337-345; Cooper, Harris, Larry V. Hedges, and Jeffrey C. Valentine, eds. The Handbook of Research Synthesis and Meta-Analysis . 2nd edition. New York: Russell Sage Foundation, 2009; Guzzo, Richard A., Susan E. Jackson and Raymond A. Katzell. “Meta-Analysis Analysis.” In Research in Organizational Behavior , Volume 9. (Greenwich, CT: JAI Press, 1987), pp 407-442; Lipsey, Mark W. and David B. Wilson. Practical Meta-Analysis . Thousand Oaks, CA: Sage Publications, 2001; Study Design 101. Meta-Analysis. The Himmelfarb Health Sciences Library, George Washington University; Timulak, Ladislav. “Qualitative Meta-Analysis.” In The SAGE Handbook of Qualitative Data Analysis . Uwe Flick, editor. (Los Angeles, CA: Sage, 2013), pp. 481-495; Walker, Esteban, Adrian V. Hernandez, and Micheal W. Kattan. "Meta-Analysis: It's Strengths and Limitations." Cleveland Clinic Journal of Medicine 75 (June 2008): 431-439.
Mixed-Method Design
- Narrative and non-textual information can add meaning to numeric data, while numeric data can add precision to narrative and non-textual information.
- Can utilize existing data while at the same time generating and testing a grounded theory approach to describe and explain the phenomenon under study.
- A broader, more complex research problem can be investigated because the researcher is not constrained by using only one method.
- The strengths of one method can be used to overcome the inherent weaknesses of another method.
- Can provide stronger, more robust evidence to support a conclusion or set of recommendations.
- May generate new knowledge new insights or uncover hidden insights, patterns, or relationships that a single methodological approach might not reveal.
- Produces more complete knowledge and understanding of the research problem that can be used to increase the generalizability of findings applied to theory or practice.
- A researcher must be proficient in understanding how to apply multiple methods to investigating a research problem as well as be proficient in optimizing how to design a study that coherently melds them together.
- Can increase the likelihood of conflicting results or ambiguous findings that inhibit drawing a valid conclusion or setting forth a recommended course of action [e.g., sample interview responses do not support existing statistical data].
- Because the research design can be very complex, reporting the findings requires a well-organized narrative, clear writing style, and precise word choice.
- Design invites collaboration among experts. However, merging different investigative approaches and writing styles requires more attention to the overall research process than studies conducted using only one methodological paradigm.
- Concurrent merging of quantitative and qualitative research requires greater attention to having adequate sample sizes, using comparable samples, and applying a consistent unit of analysis. For sequential designs where one phase of qualitative research builds on the quantitative phase or vice versa, decisions about what results from the first phase to use in the next phase, the choice of samples and estimating reasonable sample sizes for both phases, and the interpretation of results from both phases can be difficult.
- Due to multiple forms of data being collected and analyzed, this design requires extensive time and resources to carry out the multiple steps involved in data gathering and interpretation.
Burch, Patricia and Carolyn J. Heinrich. Mixed Methods for Policy Research and Program Evaluation . Thousand Oaks, CA: Sage, 2016; Creswell, John w. et al. Best Practices for Mixed Methods Research in the Health Sciences . Bethesda, MD: Office of Behavioral and Social Sciences Research, National Institutes of Health, 2010Creswell, John W. Research Design: Qualitative, Quantitative, and Mixed Methods Approaches . 4th edition. Thousand Oaks, CA: Sage Publications, 2014; Domínguez, Silvia, editor. Mixed Methods Social Networks Research . Cambridge, UK: Cambridge University Press, 2014; Hesse-Biber, Sharlene Nagy. Mixed Methods Research: Merging Theory with Practice . New York: Guilford Press, 2010; Niglas, Katrin. “How the Novice Researcher Can Make Sense of Mixed Methods Designs.” International Journal of Multiple Research Approaches 3 (2009): 34-46; Onwuegbuzie, Anthony J. and Nancy L. Leech. “Linking Research Questions to Mixed Methods Data Analysis Procedures.” The Qualitative Report 11 (September 2006): 474-498; Tashakorri, Abbas and John W. Creswell. “The New Era of Mixed Methods.” Journal of Mixed Methods Research 1 (January 2007): 3-7; Zhanga, Wanqing. “Mixed Methods Application in Health Intervention Research: A Multiple Case Study.” International Journal of Multiple Research Approaches 8 (2014): 24-35 .
Observational Design
This type of research design draws a conclusion by comparing subjects against a control group, in cases where the researcher has no control over the experiment. There are two general types of observational designs. In direct observations, people know that you are watching them. Unobtrusive measures involve any method for studying behavior where individuals do not know they are being observed. An observational study allows a useful insight into a phenomenon and avoids the ethical and practical difficulties of setting up a large and cumbersome research project.
- Observational studies are usually flexible and do not necessarily need to be structured around a hypothesis about what you expect to observe [data is emergent rather than pre-existing].
- The researcher is able to collect in-depth information about a particular behavior.
- Can reveal interrelationships among multifaceted dimensions of group interactions.
- You can generalize your results to real life situations.
- Observational research is useful for discovering what variables may be important before applying other methods like experiments.
- Observation research designs account for the complexity of group behaviors.
- Reliability of data is low because seeing behaviors occur over and over again may be a time consuming task and are difficult to replicate.
- In observational research, findings may only reflect a unique sample population and, thus, cannot be generalized to other groups.
- There can be problems with bias as the researcher may only "see what they want to see."
- There is no possibility to determine "cause and effect" relationships since nothing is manipulated.
- Sources or subjects may not all be equally credible.
- Any group that is knowingly studied is altered to some degree by the presence of the researcher, therefore, potentially skewing any data collected.
Atkinson, Paul and Martyn Hammersley. “Ethnography and Participant Observation.” In Handbook of Qualitative Research . Norman K. Denzin and Yvonna S. Lincoln, eds. (Thousand Oaks, CA: Sage, 1994), pp. 248-261; Observational Research. Research Methods by Dummies. Department of Psychology. California State University, Fresno, 2006; Patton Michael Quinn. Qualitiative Research and Evaluation Methods . Chapter 6, Fieldwork Strategies and Observational Methods. 3rd ed. Thousand Oaks, CA: Sage, 2002; Payne, Geoff and Judy Payne. "Observation." In Key Concepts in Social Research . The SAGE Key Concepts series. (London, England: Sage, 2004), pp. 158-162; Rosenbaum, Paul R. Design of Observational Studies . New York: Springer, 2010;Williams, J. Patrick. "Nonparticipant Observation." In The Sage Encyclopedia of Qualitative Research Methods . Lisa M. Given, editor.(Thousand Oaks, CA: Sage, 2008), pp. 562-563.
Philosophical Design
Understood more as an broad approach to examining a research problem than a methodological design, philosophical analysis and argumentation is intended to challenge deeply embedded, often intractable, assumptions underpinning an area of study. This approach uses the tools of argumentation derived from philosophical traditions, concepts, models, and theories to critically explore and challenge, for example, the relevance of logic and evidence in academic debates, to analyze arguments about fundamental issues, or to discuss the root of existing discourse about a research problem. These overarching tools of analysis can be framed in three ways:
- Ontology -- the study that describes the nature of reality; for example, what is real and what is not, what is fundamental and what is derivative?
- Epistemology -- the study that explores the nature of knowledge; for example, by what means does knowledge and understanding depend upon and how can we be certain of what we know?
- Axiology -- the study of values; for example, what values does an individual or group hold and why? How are values related to interest, desire, will, experience, and means-to-end? And, what is the difference between a matter of fact and a matter of value?
- Can provide a basis for applying ethical decision-making to practice.
- Functions as a means of gaining greater self-understanding and self-knowledge about the purposes of research.
- Brings clarity to general guiding practices and principles of an individual or group.
- Philosophy informs methodology.
- Refine concepts and theories that are invoked in relatively unreflective modes of thought and discourse.
- Beyond methodology, philosophy also informs critical thinking about epistemology and the structure of reality (metaphysics).
- Offers clarity and definition to the practical and theoretical uses of terms, concepts, and ideas.
- Limited application to specific research problems [answering the "So What?" question in social science research].
- Analysis can be abstract, argumentative, and limited in its practical application to real-life issues.
- While a philosophical analysis may render problematic that which was once simple or taken-for-granted, the writing can be dense and subject to unnecessary jargon, overstatement, and/or excessive quotation and documentation.
- There are limitations in the use of metaphor as a vehicle of philosophical analysis.
- There can be analytical difficulties in moving from philosophy to advocacy and between abstract thought and application to the phenomenal world.
Burton, Dawn. "Part I, Philosophy of the Social Sciences." In Research Training for Social Scientists . (London, England: Sage, 2000), pp. 1-5; Chapter 4, Research Methodology and Design. Unisa Institutional Repository (UnisaIR), University of South Africa; Jarvie, Ian C., and Jesús Zamora-Bonilla, editors. The SAGE Handbook of the Philosophy of Social Sciences . London: Sage, 2011; Labaree, Robert V. and Ross Scimeca. “The Philosophical Problem of Truth in Librarianship.” The Library Quarterly 78 (January 2008): 43-70; Maykut, Pamela S. Beginning Qualitative Research: A Philosophic and Practical Guide . Washington, DC: Falmer Press, 1994; McLaughlin, Hugh. "The Philosophy of Social Research." In Understanding Social Work Research . 2nd edition. (London: SAGE Publications Ltd., 2012), pp. 24-47; Stanford Encyclopedia of Philosophy . Metaphysics Research Lab, CSLI, Stanford University, 2013.
Sequential Design
- The researcher has a limitless option when it comes to sample size and the sampling schedule.
- Due to the repetitive nature of this research design, minor changes and adjustments can be done during the initial parts of the study to correct and hone the research method.
- This is a useful design for exploratory studies.
- There is very little effort on the part of the researcher when performing this technique. It is generally not expensive, time consuming, or workforce intensive.
- Because the study is conducted serially, the results of one sample are known before the next sample is taken and analyzed. This provides opportunities for continuous improvement of sampling and methods of analysis.
- The sampling method is not representative of the entire population. The only possibility of approaching representativeness is when the researcher chooses to use a very large sample size significant enough to represent a significant portion of the entire population. In this case, moving on to study a second or more specific sample can be difficult.
- The design cannot be used to create conclusions and interpretations that pertain to an entire population because the sampling technique is not randomized. Generalizability from findings is, therefore, limited.
- Difficult to account for and interpret variation from one sample to another over time, particularly when using qualitative methods of data collection.
Betensky, Rebecca. Harvard University, Course Lecture Note slides; Bovaird, James A. and Kevin A. Kupzyk. "Sequential Design." In Encyclopedia of Research Design . Neil J. Salkind, editor. (Thousand Oaks, CA: Sage, 2010), pp. 1347-1352; Cresswell, John W. Et al. “Advanced Mixed-Methods Research Designs.” In Handbook of Mixed Methods in Social and Behavioral Research . Abbas Tashakkori and Charles Teddle, eds. (Thousand Oaks, CA: Sage, 2003), pp. 209-240; Henry, Gary T. "Sequential Sampling." In The SAGE Encyclopedia of Social Science Research Methods . Michael S. Lewis-Beck, Alan Bryman and Tim Futing Liao, editors. (Thousand Oaks, CA: Sage, 2004), pp. 1027-1028; Nataliya V. Ivankova. “Using Mixed-Methods Sequential Explanatory Design: From Theory to Practice.” Field Methods 18 (February 2006): 3-20; Bovaird, James A. and Kevin A. Kupzyk. “Sequential Design.” In Encyclopedia of Research Design . Neil J. Salkind, ed. Thousand Oaks, CA: Sage, 2010; Sequential Analysis. Wikipedia.
Systematic Review
- A systematic review synthesizes the findings of multiple studies related to each other by incorporating strategies of analysis and interpretation intended to reduce biases and random errors.
- The application of critical exploration, evaluation, and synthesis methods separates insignificant, unsound, or redundant research from the most salient and relevant studies worthy of reflection.
- They can be use to identify, justify, and refine hypotheses, recognize and avoid hidden problems in prior studies, and explain data inconsistencies and conflicts in data.
- Systematic reviews can be used to help policy makers formulate evidence-based guidelines and regulations.
- The use of strict, explicit, and pre-determined methods of synthesis, when applied appropriately, provide reliable estimates about the effects of interventions, evaluations, and effects related to the overarching research problem investigated by each study under review.
- Systematic reviews illuminate where knowledge or thorough understanding of a research problem is lacking and, therefore, can then be used to guide future research.
- The accepted inclusion of unpublished studies [i.e., grey literature] ensures the broadest possible way to analyze and interpret research on a topic.
- Results of the synthesis can be generalized and the findings extrapolated into the general population with more validity than most other types of studies .
- Systematic reviews do not create new knowledge per se; they are a method for synthesizing existing studies about a research problem in order to gain new insights and determine gaps in the literature.
- The way researchers have carried out their investigations [e.g., the period of time covered, number of participants, sources of data analyzed, etc.] can make it difficult to effectively synthesize studies.
- The inclusion of unpublished studies can introduce bias into the review because they may not have undergone a rigorous peer-review process prior to publication. Examples may include conference presentations or proceedings, publications from government agencies, white papers, working papers, and internal documents from organizations, and doctoral dissertations and Master's theses.
Denyer, David and David Tranfield. "Producing a Systematic Review." In The Sage Handbook of Organizational Research Methods . David A. Buchanan and Alan Bryman, editors. ( Thousand Oaks, CA: Sage Publications, 2009), pp. 671-689; Foster, Margaret J. and Sarah T. Jewell, editors. Assembling the Pieces of a Systematic Review: A Guide for Librarians . Lanham, MD: Rowman and Littlefield, 2017; Gough, David, Sandy Oliver, James Thomas, editors. Introduction to Systematic Reviews . 2nd edition. Los Angeles, CA: Sage Publications, 2017; Gopalakrishnan, S. and P. Ganeshkumar. “Systematic Reviews and Meta-analysis: Understanding the Best Evidence in Primary Healthcare.” Journal of Family Medicine and Primary Care 2 (2013): 9-14; Gough, David, James Thomas, and Sandy Oliver. "Clarifying Differences between Review Designs and Methods." Systematic Reviews 1 (2012): 1-9; Khan, Khalid S., Regina Kunz, Jos Kleijnen, and Gerd Antes. “Five Steps to Conducting a Systematic Review.” Journal of the Royal Society of Medicine 96 (2003): 118-121; Mulrow, C. D. “Systematic Reviews: Rationale for Systematic Reviews.” BMJ 309:597 (September 1994); O'Dwyer, Linda C., and Q. Eileen Wafford. "Addressing Challenges with Systematic Review Teams through Effective Communication: A Case Report." Journal of the Medical Library Association 109 (October 2021): 643-647; Okoli, Chitu, and Kira Schabram. "A Guide to Conducting a Systematic Literature Review of Information Systems Research." Sprouts: Working Papers on Information Systems 10 (2010); Siddaway, Andy P., Alex M. Wood, and Larry V. Hedges. "How to Do a Systematic Review: A Best Practice Guide for Conducting and Reporting Narrative Reviews, Meta-analyses, and Meta-syntheses." Annual Review of Psychology 70 (2019): 747-770; Torgerson, Carole J. “Publication Bias: The Achilles’ Heel of Systematic Reviews?” British Journal of Educational Studies 54 (March 2006): 89-102; Torgerson, Carole. Systematic Reviews . New York: Continuum, 2003.
- << Previous: Purpose of Guide
- Next: Design Flaws to Avoid >>
- Last Updated: Mar 26, 2024 10:40 AM
- URL: https://libguides.usc.edu/writingguide

An official website of the United States government
The .gov means it’s official. Federal government websites often end in .gov or .mil. Before sharing sensitive information, make sure you’re on a federal government site.
The site is secure. The https:// ensures that you are connecting to the official website and that any information you provide is encrypted and transmitted securely.
- Publications
- Account settings
Preview improvements coming to the PMC website in October 2024. Learn More or Try it out now .
- Advanced Search
- Journal List
- SAGE Open Med
Grounded theory research: A design framework for novice researchers
Ylona chun tie.
1 Nursing and Midwifery, College of Healthcare Sciences, James Cook University, Townsville, QLD, Australia
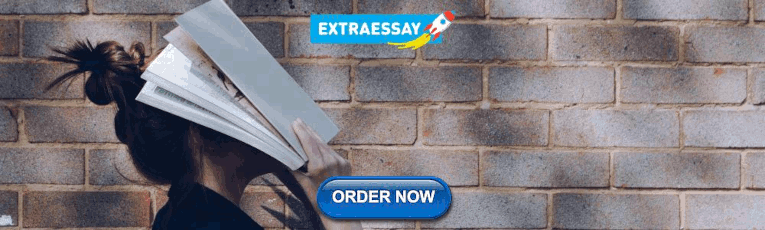
Melanie Birks
Karen francis.
2 College of Health and Medicine, University of Tasmania, Australia, Hobart, TAS, Australia
Background:
Grounded theory is a well-known methodology employed in many research studies. Qualitative and quantitative data generation techniques can be used in a grounded theory study. Grounded theory sets out to discover or construct theory from data, systematically obtained and analysed using comparative analysis. While grounded theory is inherently flexible, it is a complex methodology. Thus, novice researchers strive to understand the discourse and the practical application of grounded theory concepts and processes.
The aim of this article is to provide a contemporary research framework suitable to inform a grounded theory study.
This article provides an overview of grounded theory illustrated through a graphic representation of the processes and methods employed in conducting research using this methodology. The framework is presented as a diagrammatic representation of a research design and acts as a visual guide for the novice grounded theory researcher.
Discussion:
As grounded theory is not a linear process, the framework illustrates the interplay between the essential grounded theory methods and iterative and comparative actions involved. Each of the essential methods and processes that underpin grounded theory are defined in this article.
Conclusion:
Rather than an engagement in philosophical discussion or a debate of the different genres that can be used in grounded theory, this article illustrates how a framework for a research study design can be used to guide and inform the novice nurse researcher undertaking a study using grounded theory. Research findings and recommendations can contribute to policy or knowledge development, service provision and can reform thinking to initiate change in the substantive area of inquiry.
Introduction
The aim of all research is to advance, refine and expand a body of knowledge, establish facts and/or reach new conclusions using systematic inquiry and disciplined methods. 1 The research design is the plan or strategy researchers use to answer the research question, which is underpinned by philosophy, methodology and methods. 2 Birks 3 defines philosophy as ‘a view of the world encompassing the questions and mechanisms for finding answers that inform that view’ (p. 18). Researchers reflect their philosophical beliefs and interpretations of the world prior to commencing research. Methodology is the research design that shapes the selection of, and use of, particular data generation and analysis methods to answer the research question. 4 While a distinction between positivist research and interpretivist research occurs at the paradigm level, each methodology has explicit criteria for the collection, analysis and interpretation of data. 2 Grounded theory (GT) is a structured, yet flexible methodology. This methodology is appropriate when little is known about a phenomenon; the aim being to produce or construct an explanatory theory that uncovers a process inherent to the substantive area of inquiry. 5 – 7 One of the defining characteristics of GT is that it aims to generate theory that is grounded in the data. The following section provides an overview of GT – the history, main genres and essential methods and processes employed in the conduct of a GT study. This summary provides a foundation for a framework to demonstrate the interplay between the methods and processes inherent in a GT study as presented in the sections that follow.
Glaser and Strauss are recognised as the founders of grounded theory. Strauss was conversant in symbolic interactionism and Glaser in descriptive statistics. 8 – 10 Glaser and Strauss originally worked together in a study examining the experience of terminally ill patients who had differing knowledge of their health status. Some of these suspected they were dying and tried to confirm or disconfirm their suspicions. Others tried to understand by interpreting treatment by care providers and family members. Glaser and Strauss examined how the patients dealt with the knowledge they were dying and the reactions of healthcare staff caring for these patients. Throughout this collaboration, Glaser and Strauss questioned the appropriateness of using a scientific method of verification for this study. During this investigation, they developed the constant comparative method, a key element of grounded theory, while generating a theory of dying first described in Awareness of Dying (1965). The constant comparative method is deemed an original way of organising and analysing qualitative data.
Glaser and Strauss subsequently went on to write The Discovery of Grounded Theory: Strategies for Qualitative Research (1967). This seminal work explained how theory could be generated from data inductively. This process challenged the traditional method of testing or refining theory through deductive testing. Grounded theory provided an outlook that questioned the view of the time that quantitative methodology is the only valid, unbiased way to determine truths about the world. 11 Glaser and Strauss 5 challenged the belief that qualitative research lacked rigour and detailed the method of comparative analysis that enables the generation of theory. After publishing The Discovery of Grounded Theory , Strauss and Glaser went on to write independently, expressing divergent viewpoints in the application of grounded theory methods.
Glaser produced his book Theoretical Sensitivity (1978) and Strauss went on to publish Qualitative Analysis for Social Scientists (1987). Strauss and Corbin’s 12 publication Basics of Qualitative Research: Grounded Theory Procedures and Techniques resulted in a rebuttal by Glaser 13 over their application of grounded theory methods. However, philosophical perspectives have changed since Glaser’s positivist version and Strauss and Corbin’s post-positivism stance. 14 Grounded theory has since seen the emergence of additional philosophical perspectives that have influenced a change in methodological development over time. 15
Subsequent generations of grounded theorists have positioned themselves along a philosophical continuum, from Strauss and Corbin’s 12 theoretical perspective of symbolic interactionism, through to Charmaz’s 16 constructivist perspective. However, understanding how to position oneself philosophically can challenge novice researchers. Birks and Mills 6 provide a contemporary understanding of GT in their book Grounded theory: A Practical Guide. These Australian researchers have written in a way that appeals to the novice researcher. It is the contemporary writing, the way Birks and Mills present a non-partisan approach to GT that support the novice researcher to understand the philosophical and methodological concepts integral in conducting research. The development of GT is important to understand prior to selecting an approach that aligns with the researcher’s philosophical position and the purpose of the research study. As the research progresses, seminal texts are referred back to time and again as understanding of concepts increases, much like the iterative processes inherent in the conduct of a GT study.
Genres: traditional, evolved and constructivist grounded theory
Grounded theory has several distinct methodological genres: traditional GT associated with Glaser; evolved GT associated with Strauss, Corbin and Clarke; and constructivist GT associated with Charmaz. 6 , 17 Each variant is an extension and development of the original GT by Glaser and Strauss. The first of these genres is known as traditional or classic GT. Glaser 18 acknowledged that the goal of traditional GT is to generate a conceptual theory that accounts for a pattern of behaviour that is relevant and problematic for those involved. The second genre, evolved GT, is founded on symbolic interactionism and stems from work associated with Strauss, Corbin and Clarke. Symbolic interactionism is a sociological perspective that relies on the symbolic meaning people ascribe to the processes of social interaction. Symbolic interactionism addresses the subjective meaning people place on objects, behaviours or events based on what they believe is true. 19 , 20 Constructivist GT, the third genre developed and explicated by Charmaz, a symbolic interactionist, has its roots in constructivism. 8 , 16 Constructivist GT’s methodological underpinnings focus on how participants’ construct meaning in relation to the area of inquiry. 16 A constructivist co-constructs experience and meanings with participants. 21 While there are commonalities across all genres of GT, there are factors that distinguish differences between the approaches including the philosophical position of the researcher; the use of literature; and the approach to coding, analysis and theory development. Following on from Glaser and Strauss, several versions of GT have ensued.
Grounded theory represents both a method of inquiry and a resultant product of that inquiry. 7 , 22 Glaser and Holton 23 define GT as ‘a set of integrated conceptual hypotheses systematically generated to produce an inductive theory about a substantive area’ (p. 43). Strauss and Corbin 24 define GT as ‘theory that was derived from data, systematically gathered and analysed through the research process’ (p. 12). The researcher ‘begins with an area of study and allows the theory to emerge from the data’ (p. 12). Charmaz 16 defines GT as ‘a method of conducting qualitative research that focuses on creating conceptual frameworks or theories through building inductive analysis from the data’ (p. 187). However, Birks and Mills 6 refer to GT as a process by which theory is generated from the analysis of data. Theory is not discovered; rather, theory is constructed by the researcher who views the world through their own particular lens.
Research process
Before commencing any research study, the researcher must have a solid understanding of the research process. A well-developed outline of the study and an understanding of the important considerations in designing and undertaking a GT study are essential if the goals of the research are to be achieved. While it is important to have an understanding of how a methodology has developed, in order to move forward with research, a novice can align with a grounded theorist and follow an approach to GT. Using a framework to inform a research design can be a useful modus operandi.
The following section provides insight into the process of undertaking a GT research study. Figure 1 is a framework that summarises the interplay and movement between methods and processes that underpin the generation of a GT. As can be seen from this framework, and as detailed in the discussion that follows, the process of doing a GT research study is not linear, rather it is iterative and recursive.

Research design framework: summary of the interplay between the essential grounded theory methods and processes.
Grounded theory research involves the meticulous application of specific methods and processes. Methods are ‘systematic modes, procedures or tools used for collection and analysis of data’. 25 While GT studies can commence with a variety of sampling techniques, many commence with purposive sampling, followed by concurrent data generation and/or collection and data analysis, through various stages of coding, undertaken in conjunction with constant comparative analysis, theoretical sampling and memoing. Theoretical sampling is employed until theoretical saturation is reached. These methods and processes create an unfolding, iterative system of actions and interactions inherent in GT. 6 , 16 The methods interconnect and inform the recurrent elements in the research process as shown by the directional flow of the arrows and the encompassing brackets in Figure 1 . The framework denotes the process is both iterative and dynamic and is not one directional. Grounded theory methods are discussed in the following section.
Purposive sampling
As presented in Figure 1 , initial purposive sampling directs the collection and/or generation of data. Researchers purposively select participants and/or data sources that can answer the research question. 5 , 7 , 16 , 21 Concurrent data generation and/or data collection and analysis is fundamental to GT research design. 6 The researcher collects, codes and analyses this initial data before further data collection/generation is undertaken. Purposeful sampling provides the initial data that the researcher analyses. As will be discussed, theoretical sampling then commences from the codes and categories developed from the first data set. Theoretical sampling is used to identify and follow clues from the analysis, fill gaps, clarify uncertainties, check hunches and test interpretations as the study progresses.
Constant comparative analysis
Constant comparative analysis is an analytical process used in GT for coding and category development. This process commences with the first data generated or collected and pervades the research process as presented in Figure 1 . Incidents are identified in the data and coded. 6 The initial stage of analysis compares incident to incident in each code. Initial codes are then compared to other codes. Codes are then collapsed into categories. This process means the researcher will compare incidents in a category with previous incidents, in both the same and different categories. 5 Future codes are compared and categories are compared with other categories. New data is then compared with data obtained earlier during the analysis phases. This iterative process involves inductive and deductive thinking. 16 Inductive, deductive and abductive reasoning can also be used in data analysis. 26
Constant comparative analysis generates increasingly more abstract concepts and theories through inductive processes. 16 In addition, abduction, defined as ‘a form of reasoning that begins with an examination of the data and the formation of a number of hypotheses that are then proved or disproved during the process of analysis … aids inductive conceptualization’. 6 Theoretical sampling coupled with constant comparative analysis raises the conceptual levels of data analysis and directs ongoing data collection or generation. 6
The constant comparative technique is used to find consistencies and differences, with the aim of continually refining concepts and theoretically relevant categories. This continual comparative iterative process that encompasses GT research sets it apart from a purely descriptive analysis. 8
Memo writing is an analytic process considered essential ‘in ensuring quality in grounded theory’. 6 Stern 27 offers the analogy that if data are the building blocks of the developing theory, then memos are the ‘mortar’ (p. 119). Memos are the storehouse of ideas generated and documented through interacting with data. 28 Thus, memos are reflective interpretive pieces that build a historic audit trail to document ideas, events and the thought processes inherent in the research process and developing thinking of the analyst. 6 Memos provide detailed records of the researchers’ thoughts, feelings and intuitive contemplations. 6
Lempert 29 considers memo writing crucial as memos prompt researchers to analyse and code data and develop codes into categories early in the coding process. Memos detail why and how decisions made related to sampling, coding, collapsing of codes, making of new codes, separating codes, producing a category and identifying relationships abstracted to a higher level of analysis. 6 Thus, memos are informal analytic notes about the data and the theoretical connections between categories. 23 Memoing is an ongoing activity that builds intellectual assets, fosters analytic momentum and informs the GT findings. 6 , 10
Generating/collecting data
A hallmark of GT is concurrent data generation/collection and analysis. In GT, researchers may utilise both qualitative and quantitative data as espoused by Glaser’s dictum; ‘all is data’. 30 While interviews are a common method of generating data, data sources can include focus groups, questionnaires, surveys, transcripts, letters, government reports, documents, grey literature, music, artefacts, videos, blogs and memos. 9 Elicited data are produced by participants in response to, or directed by, the researcher whereas extant data includes data that is already available such as documents and published literature. 6 , 31 While this is one interpretation of how elicited data are generated, other approaches to grounded theory recognise the agency of participants in the co-construction of data with the researcher. The relationship the researcher has with the data, how it is generated and collected, will determine the value it contributes to the development of the final GT. 6 The significance of this relationship extends into data analysis conducted by the researcher through the various stages of coding.
Coding is an analytical process used to identify concepts, similarities and conceptual reoccurrences in data. Coding is the pivotal link between collecting or generating data and developing a theory that explains the data. Charmaz 10 posits,
codes rely on interaction between researchers and their data. Codes consist of short labels that we construct as we interact with the data. Something kinaesthetic occurs when we are coding; we are mentally and physically active in the process. (p. 5)
In GT, coding can be categorised into iterative phases. Traditional, evolved and constructivist GT genres use different terminology to explain each coding phase ( Table 1 ).
Comparison of coding terminology in traditional, evolved and constructivist grounded theory.
Adapted from Birks and Mills. 6
Coding terminology in evolved GT refers to open (a procedure for developing categories of information), axial (an advanced procedure for interconnecting the categories) and selective coding (procedure for building a storyline from core codes that connects the categories), producing a discursive set of theoretical propositions. 6 , 12 , 32 Constructivist grounded theorists refer to initial, focused and theoretical coding. 9 Birks and Mills 6 use the terms initial, intermediate and advanced coding that link to low, medium and high-level conceptual analysis and development. The coding terms devised by Birks and Mills 6 were used for Figure 1 ; however, these can be altered to reflect the coding terminology used in the respective GT genres selected by the researcher.
Initial coding
Initial coding of data is the preliminary step in GT data analysis. 6 , 9 The purpose of initial coding is to start the process of fracturing the data to compare incident to incident and to look for similarities and differences in beginning patterns in the data. In initial coding, the researcher inductively generates as many codes as possible from early data. 16 Important words or groups of words are identified and labelled. In GT, codes identify social and psychological processes and actions as opposed to themes. Charmaz 16 emphasises keeping codes as similar to the data as possible and advocates embedding actions in the codes in an iterative coding process. Saldaña 33 agrees that codes that denote action, which he calls process codes, can be used interchangeably with gerunds (verbs ending in ing ). In vivo codes are often verbatim quotes from the participants’ words and are often used as the labels to capture the participant’s words as representative of a broader concept or process in the data. 6 Table 1 reflects variation in the terminology of codes used by grounded theorists.
Initial coding categorises and assigns meaning to the data, comparing incident-to-incident, labelling beginning patterns and beginning to look for comparisons between the codes. During initial coding, it is important to ask ‘what is this data a study of’. 18 What does the data assume, ‘suggest’ or ‘pronounce’ and ‘from whose point of view’ does this data come, whom does it represent or whose thoughts are they?. 16 What collectively might it represent? The process of documenting reactions, emotions and related actions enables researchers to explore, challenge and intensify their sensitivity to the data. 34 Early coding assists the researcher to identify the direction for further data gathering. After initial analysis, theoretical sampling is employed to direct collection of additional data that will inform the ‘developing theory’. 9 Initial coding advances into intermediate coding once categories begin to develop.
Theoretical sampling
The purpose of theoretical sampling is to allow the researcher to follow leads in the data by sampling new participants or material that provides relevant information. As depicted in Figure 1 , theoretical sampling is central to GT design, aids the evolving theory 5 , 7 , 16 and ensures the final developed theory is grounded in the data. 9 Theoretical sampling in GT is for the development of a theoretical category, as opposed to sampling for population representation. 10 Novice researchers need to acknowledge this difference if they are to achieve congruence within the methodology. Birks and Mills 6 define theoretical sampling as ‘the process of identifying and pursuing clues that arise during analysis in a grounded theory study’ (p. 68). During this process, additional information is sought to saturate categories under development. The analysis identifies relationships, highlights gaps in the existing data set and may reveal insight into what is not yet known. The exemplars in Box 1 highlight how theoretical sampling led to the inclusion of further data.
Examples of theoretical sampling.
Thus, theoretical sampling is used to focus and generate data to feed the iterative process of continual comparative analysis of the data. 6
Intermediate coding
Intermediate coding, identifying a core category, theoretical data saturation, constant comparative analysis, theoretical sensitivity and memoing occur in the next phase of the GT process. 6 Intermediate coding builds on the initial coding phase. Where initial coding fractures the data, intermediate coding begins to transform basic data into more abstract concepts allowing the theory to emerge from the data. During this analytic stage, a process of reviewing categories and identifying which ones, if any, can be subsumed beneath other categories occurs and the properties or dimension of the developed categories are refined. Properties refer to the characteristics that are common to all the concepts in the category and dimensions are the variations of a property. 37
At this stage, a core category starts to become evident as developed categories form around a core concept; relationships are identified between categories and the analysis is refined. Birks and Mills 6 affirm that diagramming can aid analysis in the intermediate coding phase. Grounded theorists interact closely with the data during this phase, continually reassessing meaning to ascertain ‘what is really going on’ in the data. 30 Theoretical saturation ensues when new data analysis does not provide additional material to existing theoretical categories, and the categories are sufficiently explained. 6
Advanced coding
Birks and Mills 6 described advanced coding as the ‘techniques used to facilitate integration of the final grounded theory’ (p. 177). These authors promote storyline technique (described in the following section) and theoretical coding as strategies for advancing analysis and theoretical integration. Advanced coding is essential to produce a theory that is grounded in the data and has explanatory power. 6 During the advanced coding phase, concepts that reach the stage of categories will be abstract, representing stories of many, reduced into highly conceptual terms. The findings are presented as a set of interrelated concepts as opposed to presenting themes. 28 Explanatory statements detail the relationships between categories and the central core category. 28
Storyline is a tool that can be used for theoretical integration. Birks and Mills 6 define storyline as ‘a strategy for facilitating integration, construction, formulation, and presentation of research findings through the production of a coherent grounded theory’ (p. 180). Storyline technique is first proposed with limited attention in Basics of Qualitative Research by Strauss and Corbin 12 and further developed by Birks et al. 38 as a tool for theoretical integration. The storyline is the conceptualisation of the core category. 6 This procedure builds a story that connects the categories and produces a discursive set of theoretical propositions. 24 Birks and Mills 6 contend that storyline can be ‘used to produce a comprehensive rendering of your grounded theory’ (p. 118). Birks et al. 38 had earlier concluded, ‘storyline enhances the development, presentation and comprehension of the outcomes of grounded theory research’ (p. 405). Once the storyline is developed, the GT is finalised using theoretical codes that ‘provide a framework for enhancing the explanatory power of the storyline and its potential as theory’. 6 Thus, storyline is the explication of the theory.
Theoretical coding occurs as the final culminating stage towards achieving a GT. 39 , 40 The purpose of theoretical coding is to integrate the substantive theory. 41 Saldaña 40 states, ‘theoretical coding integrates and synthesises the categories derived from coding and analysis to now create a theory’ (p. 224). Initial coding fractures the data while theoretical codes ‘weave the fractured story back together again into an organized whole theory’. 18 Advanced coding that integrates extant theory adds further explanatory power to the findings. 6 The examples in Box 2 describe the use of storyline as a technique.
Writing the storyline.
Theoretical sensitivity
As presented in Figure 1 , theoretical sensitivity encompasses the entire research process. Glaser and Strauss 5 initially described the term theoretical sensitivity in The Discovery of Grounded Theory. Theoretical sensitivity is the ability to know when you identify a data segment that is important to your theory. While Strauss and Corbin 12 describe theoretical sensitivity as the insight into what is meaningful and of significance in the data for theory development, Birks and Mills 6 define theoretical sensitivity as ‘the ability to recognise and extract from the data elements that have relevance for the emerging theory’ (p. 181). Conducting GT research requires a balance between keeping an open mind and the ability to identify elements of theoretical significance during data generation and/or collection and data analysis. 6
Several analytic tools and techniques can be used to enhance theoretical sensitivity and increase the grounded theorist’s sensitivity to theoretical constructs in the data. 28 Birks and Mills 6 state, ‘as a grounded theorist becomes immersed in the data, their level of theoretical sensitivity to analytic possibilities will increase’ (p. 12). Developing sensitivity as a grounded theorist and the application of theoretical sensitivity throughout the research process allows the analytical focus to be directed towards theory development and ultimately result in an integrated and abstract GT. 6 The example in Box 3 highlights how analytic tools are employed to increase theoretical sensitivity.
Theoretical sensitivity.
The grounded theory
The meticulous application of essential GT methods refines the analysis resulting in the generation of an integrated, comprehensive GT that explains a process relating to a particular phenomenon. 6 The results of a GT study are communicated as a set of concepts, related to each other in an interrelated whole, and expressed in the production of a substantive theory. 5 , 7 , 16 A substantive theory is a theoretical interpretation or explanation of a studied phenomenon 6 , 17 Thus, the hallmark of grounded theory is the generation of theory ‘abstracted from, or grounded in, data generated and collected by the researcher’. 6 However, to ensure quality in research requires the application of rigour throughout the research process.
Quality and rigour
The quality of a grounded theory can be related to three distinct areas underpinned by (1) the researcher’s expertise, knowledge and research skills; (2) methodological congruence with the research question; and (3) procedural precision in the use of methods. 6 Methodological congruence is substantiated when the philosophical position of the researcher is congruent with the research question and the methodological approach selected. 6 Data collection or generation and analytical conceptualisation need to be rigorous throughout the research process to secure excellence in the final grounded theory. 44
Procedural precision requires careful attention to maintaining a detailed audit trail, data management strategies and demonstrable procedural logic recorded using memos. 6 Organisation and management of research data, memos and literature can be assisted using software programs such as NVivo. An audit trail of decision-making, changes in the direction of the research and the rationale for decisions made are essential to ensure rigour in the final grounded theory. 6
This article offers a framework to assist novice researchers visualise the iterative processes that underpin a GT study. The fundamental process and methods used to generate an integrated grounded theory have been described. Novice researchers can adapt the framework presented to inform and guide the design of a GT study. This framework provides a useful guide to visualise the interplay between the methods and processes inherent in conducting GT. Research conducted ethically and with meticulous attention to process will ensure quality research outcomes that have relevance at the practice level.
Declaration of conflicting interests: The author(s) declared no potential conflicts of interest with respect to the research, authorship, and/or publication of this article.
Funding: The author(s) received no financial support for the research, authorship, and/or publication of this article.

- Privacy Policy
Buy Me a Coffee

Home » Research Design – Types, Methods and Examples
Research Design – Types, Methods and Examples
Table of Contents

Research Design
Definition:
Research design refers to the overall strategy or plan for conducting a research study. It outlines the methods and procedures that will be used to collect and analyze data, as well as the goals and objectives of the study. Research design is important because it guides the entire research process and ensures that the study is conducted in a systematic and rigorous manner.
Types of Research Design
Types of Research Design are as follows:
Descriptive Research Design
This type of research design is used to describe a phenomenon or situation. It involves collecting data through surveys, questionnaires, interviews, and observations. The aim of descriptive research is to provide an accurate and detailed portrayal of a particular group, event, or situation. It can be useful in identifying patterns, trends, and relationships in the data.
Correlational Research Design
Correlational research design is used to determine if there is a relationship between two or more variables. This type of research design involves collecting data from participants and analyzing the relationship between the variables using statistical methods. The aim of correlational research is to identify the strength and direction of the relationship between the variables.
Experimental Research Design
Experimental research design is used to investigate cause-and-effect relationships between variables. This type of research design involves manipulating one variable and measuring the effect on another variable. It usually involves randomly assigning participants to groups and manipulating an independent variable to determine its effect on a dependent variable. The aim of experimental research is to establish causality.
Quasi-experimental Research Design
Quasi-experimental research design is similar to experimental research design, but it lacks one or more of the features of a true experiment. For example, there may not be random assignment to groups or a control group. This type of research design is used when it is not feasible or ethical to conduct a true experiment.
Case Study Research Design
Case study research design is used to investigate a single case or a small number of cases in depth. It involves collecting data through various methods, such as interviews, observations, and document analysis. The aim of case study research is to provide an in-depth understanding of a particular case or situation.
Longitudinal Research Design
Longitudinal research design is used to study changes in a particular phenomenon over time. It involves collecting data at multiple time points and analyzing the changes that occur. The aim of longitudinal research is to provide insights into the development, growth, or decline of a particular phenomenon over time.
Structure of Research Design
The format of a research design typically includes the following sections:
- Introduction : This section provides an overview of the research problem, the research questions, and the importance of the study. It also includes a brief literature review that summarizes previous research on the topic and identifies gaps in the existing knowledge.
- Research Questions or Hypotheses: This section identifies the specific research questions or hypotheses that the study will address. These questions should be clear, specific, and testable.
- Research Methods : This section describes the methods that will be used to collect and analyze data. It includes details about the study design, the sampling strategy, the data collection instruments, and the data analysis techniques.
- Data Collection: This section describes how the data will be collected, including the sample size, data collection procedures, and any ethical considerations.
- Data Analysis: This section describes how the data will be analyzed, including the statistical techniques that will be used to test the research questions or hypotheses.
- Results : This section presents the findings of the study, including descriptive statistics and statistical tests.
- Discussion and Conclusion : This section summarizes the key findings of the study, interprets the results, and discusses the implications of the findings. It also includes recommendations for future research.
- References : This section lists the sources cited in the research design.
Example of Research Design
An Example of Research Design could be:
Research question: Does the use of social media affect the academic performance of high school students?
Research design:
- Research approach : The research approach will be quantitative as it involves collecting numerical data to test the hypothesis.
- Research design : The research design will be a quasi-experimental design, with a pretest-posttest control group design.
- Sample : The sample will be 200 high school students from two schools, with 100 students in the experimental group and 100 students in the control group.
- Data collection : The data will be collected through surveys administered to the students at the beginning and end of the academic year. The surveys will include questions about their social media usage and academic performance.
- Data analysis : The data collected will be analyzed using statistical software. The mean scores of the experimental and control groups will be compared to determine whether there is a significant difference in academic performance between the two groups.
- Limitations : The limitations of the study will be acknowledged, including the fact that social media usage can vary greatly among individuals, and the study only focuses on two schools, which may not be representative of the entire population.
- Ethical considerations: Ethical considerations will be taken into account, such as obtaining informed consent from the participants and ensuring their anonymity and confidentiality.
How to Write Research Design
Writing a research design involves planning and outlining the methodology and approach that will be used to answer a research question or hypothesis. Here are some steps to help you write a research design:
- Define the research question or hypothesis : Before beginning your research design, you should clearly define your research question or hypothesis. This will guide your research design and help you select appropriate methods.
- Select a research design: There are many different research designs to choose from, including experimental, survey, case study, and qualitative designs. Choose a design that best fits your research question and objectives.
- Develop a sampling plan : If your research involves collecting data from a sample, you will need to develop a sampling plan. This should outline how you will select participants and how many participants you will include.
- Define variables: Clearly define the variables you will be measuring or manipulating in your study. This will help ensure that your results are meaningful and relevant to your research question.
- Choose data collection methods : Decide on the data collection methods you will use to gather information. This may include surveys, interviews, observations, experiments, or secondary data sources.
- Create a data analysis plan: Develop a plan for analyzing your data, including the statistical or qualitative techniques you will use.
- Consider ethical concerns : Finally, be sure to consider any ethical concerns related to your research, such as participant confidentiality or potential harm.
When to Write Research Design
Research design should be written before conducting any research study. It is an important planning phase that outlines the research methodology, data collection methods, and data analysis techniques that will be used to investigate a research question or problem. The research design helps to ensure that the research is conducted in a systematic and logical manner, and that the data collected is relevant and reliable.
Ideally, the research design should be developed as early as possible in the research process, before any data is collected. This allows the researcher to carefully consider the research question, identify the most appropriate research methodology, and plan the data collection and analysis procedures in advance. By doing so, the research can be conducted in a more efficient and effective manner, and the results are more likely to be valid and reliable.
Purpose of Research Design
The purpose of research design is to plan and structure a research study in a way that enables the researcher to achieve the desired research goals with accuracy, validity, and reliability. Research design is the blueprint or the framework for conducting a study that outlines the methods, procedures, techniques, and tools for data collection and analysis.
Some of the key purposes of research design include:
- Providing a clear and concise plan of action for the research study.
- Ensuring that the research is conducted ethically and with rigor.
- Maximizing the accuracy and reliability of the research findings.
- Minimizing the possibility of errors, biases, or confounding variables.
- Ensuring that the research is feasible, practical, and cost-effective.
- Determining the appropriate research methodology to answer the research question(s).
- Identifying the sample size, sampling method, and data collection techniques.
- Determining the data analysis method and statistical tests to be used.
- Facilitating the replication of the study by other researchers.
- Enhancing the validity and generalizability of the research findings.
Applications of Research Design
There are numerous applications of research design in various fields, some of which are:
- Social sciences: In fields such as psychology, sociology, and anthropology, research design is used to investigate human behavior and social phenomena. Researchers use various research designs, such as experimental, quasi-experimental, and correlational designs, to study different aspects of social behavior.
- Education : Research design is essential in the field of education to investigate the effectiveness of different teaching methods and learning strategies. Researchers use various designs such as experimental, quasi-experimental, and case study designs to understand how students learn and how to improve teaching practices.
- Health sciences : In the health sciences, research design is used to investigate the causes, prevention, and treatment of diseases. Researchers use various designs, such as randomized controlled trials, cohort studies, and case-control studies, to study different aspects of health and healthcare.
- Business : Research design is used in the field of business to investigate consumer behavior, marketing strategies, and the impact of different business practices. Researchers use various designs, such as survey research, experimental research, and case studies, to study different aspects of the business world.
- Engineering : In the field of engineering, research design is used to investigate the development and implementation of new technologies. Researchers use various designs, such as experimental research and case studies, to study the effectiveness of new technologies and to identify areas for improvement.
Advantages of Research Design
Here are some advantages of research design:
- Systematic and organized approach : A well-designed research plan ensures that the research is conducted in a systematic and organized manner, which makes it easier to manage and analyze the data.
- Clear objectives: The research design helps to clarify the objectives of the study, which makes it easier to identify the variables that need to be measured, and the methods that need to be used to collect and analyze data.
- Minimizes bias: A well-designed research plan minimizes the chances of bias, by ensuring that the data is collected and analyzed objectively, and that the results are not influenced by the researcher’s personal biases or preferences.
- Efficient use of resources: A well-designed research plan helps to ensure that the resources (time, money, and personnel) are used efficiently and effectively, by focusing on the most important variables and methods.
- Replicability: A well-designed research plan makes it easier for other researchers to replicate the study, which enhances the credibility and reliability of the findings.
- Validity: A well-designed research plan helps to ensure that the findings are valid, by ensuring that the methods used to collect and analyze data are appropriate for the research question.
- Generalizability : A well-designed research plan helps to ensure that the findings can be generalized to other populations, settings, or situations, which increases the external validity of the study.
Research Design Vs Research Methodology
About the author.
Muhammad Hassan
Researcher, Academic Writer, Web developer
You may also like

How to Cite Research Paper – All Formats and...

Data Collection – Methods Types and Examples

Delimitations in Research – Types, Examples and...

Research Paper Format – Types, Examples and...

Research Process – Steps, Examples and Tips

Institutional Review Board – Application Sample...
Leave a comment x.
Save my name, email, and website in this browser for the next time I comment.
- Our Mission
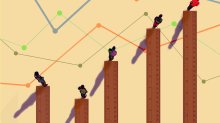
How to Design Better Tests, Based on the Research
A review of a dozen recent studies reveals that to design good tests, teachers need to consider bias, rigor, and mindset.
Claire Longmoor didn’t expect her math problem to go viral .
“An orchestra of 120 players takes 40 minutes to play Beethoven’s 9th symphony,” the question read. “How long would it take for 60 players to play the symphony?”
As with so many puzzles that find their way to the internet, the responses were radically split and mostly wrong: One group of people, who were perhaps reading too fast, confidently declared the answer to be 20 minutes. The second camp reasoned that half as many musicians would have to work twice as hard, so the answer must be 80 minutes.
Yet a third group was stupefied, questioning the teacher’s ability to write good questions. “Think the person who came up with that question really doesn’t know how an orchestra works!” the Wexford Sinfonia Orchestra tweeted.
It’s a trick question, Longmoor admitted, one designed to keep her students on their toes, echoing a common sentiment among test makers that such questions force students to read carefully, ensuring that they attend to substantive questions later on. But do trick questions actually work as intended?
Andrew Butler, a professor of psychological and brain sciences at Washington University, doesn’t think so. Trick questions are not “productive for learning” and can easily backfire, he says. The result: confused students, artificially reduced test performance, and a murkier picture of what students actually know.
Other research on test design suggests that all too often, we’re not just assessing what students know, but also getting a peek into the psychological and cognitive eddies that disrupt a student’s thinking—a high-stakes test that causes anxiety can become a barometer of a student’s poise, rather than their knowledge. A well-designed test is rigorous and keeps implicit bias in check, while being mindful of the role that confidence, mindset, and anxiety play in test taking. Here are eight tips to create effective tests, based on a review of more than a dozen recent studies.
1. HELP STUDENTS DEVELOP GOOD TEST PREP HABITS
Students often overestimate how prepared they are for an upcoming test, which can result in unexpected low performance, according to a 2017 study . Consider asking students to make and show you a study plan involving productive study strategies like self-quizzing, teaching the major concepts to peers, or spacing out their studying into multiple sessions instead of cramming the night before.
To help address test anxiety, researchers recommend setting aside a little time for simple writing or self-talk exercises before the test —they allow students to shore up their confidence, recall their test-taking strategies, and put the exam into perspective. In a 2019 study , for example, elementary students who spent a few minutes before a test and “silently spoke words of encouragement to themselves that were focused on effort” saw their math scores rise. And in a 2019 study of ninth graders, researchers found that a simple 10-minute expressive writing activity that reframed test anxiety as “a beneficial and energizing force” led to course failure rates being cut in half for vulnerable students.
2. FIND THE SWEET SPOT FOR RIGOR
Design tests so they’re at an appropriate level of difficulty for your students. Overly difficult tests not only sap students’ motivation but also increase the likelihood that students will remember the wrong answers, according to a 2018 study .
In the end, “tests that are extremely easy or difficult are essentially useless for both assessment and learning,” the study concludes. Students who study moderately should get roughly 70 to 80 percent of the questions correct.
3. BUT START WITH EASY QUESTIONS
Don’t start a test with challenging questions; let students ease into a test. Asking difficult questions to probe for deep knowledge is important, but remember that confidence and mindset can dramatically affect outcomes—and therefore muddy the waters of your assessment.
A 2021 study found that students were more likely to do worse on a test if difficult questions were at the beginning instead of nearer to the middle or the end of the test. “Students might be disheartened by seeing a hard question early in the test, as a signal of the general difficulty of the rest of the test,” the researchers explain.
4. BE AWARE OF IMPLICIT BIAS
Question format matters. In a 2018 study , researchers analyzed test scores for 8 million students and discovered that boys tend to outperform girls on multiple-choice questions, accounting for roughly 25 percent of the gender achievement gap. Girls performed significantly better than boys on open-ended questions. Consider the mix of your testing formats: Combine traditional testing formats—multiple choice, short answer, and essay questions—with creative, open-ended assessments that can elicit different strengths and interests.
Be mindful, as well, of how cultural or racial bias and background knowledge can infiltrate the language and framing of test questions. In an infamous example, an SAT analogy question required students to select “oarsman:regatta” in response to the word pair “runner:marathon,” an expectation that was fraught with classist, racial, and geographic overtones.
Other studies reveal that without a threshold of background knowledge, students fail to grasp the intent of their reading—an incorrect answer on a test may signify the failure to determine the meaning of the question, rather than measure the student’s understanding of the material. Keep test questions free of unnecessary jargon, revise tests to simplify questions, and consider allowing students to ask for clarification before you start the test.
5. AVOID TRICK QUESTIONS
While it may be tempting to include trick questions to make sure that students are paying attention, they can get stuck or confused, wasting precious time and compromising the rest of the test as a result, a 2018 study concludes.
Tests aren’t just tools to evaluate learning; they can also alter a student’s understanding of a topic. So if students try to recall information they’re unsure about, they may reconstruct it incorrectly, increasing the likelihood that they will retain false information. For example, if you asked, “What was George Washington’s goal with writing the Emancipation Proclamation?” some students may commit it to memory and connect the wrong president to the seminal historical document.
6. BREAK TESTS APART
Instead of a single high-stakes test, consider breaking it into smaller low-stakes tests that you can spread throughout the school year. That strategy alleviated test anxiety for 72 percent of middle and high school students, according to a 2014 study .
The likely reason? When students take high-stakes tests, their cortisol levels—a biological marker for stress—rise dramatically, impeding their ability to concentrate and artificially lowering test scores, a 2018 study found. Stress is a normal part of test-taking, but there are kinds of stress that should be avoided, such as whether the student thinks they’ll be able to finish.
7. TRY TO MINIMIZE THE EFFECTS OF TIME LIMITS
Time limits are unavoidable, but you can mitigate their pernicious effects on anxiety levels. “Evidence strongly suggests that timed tests cause the early onset of math anxiety for students across the achievement range,” explains Jo Boaler, mathematics professor at Stanford. This extends to other subjects as well, according to a 2020 study , which also found that timed tests disproportionately harm students with disabilities.
If a student aces most of the test but then gets the last few questions wrong or leaves them blank, it’s possible that they panicked as the time limit approached—or knew the information intimately but simply couldn’t finish the test. It may be helpful to time yourself taking the test and cut a few questions so that it’s clearly shorter than your class period.
8. PERIODICALLY, LET STUDENTS WRITE THEIR OWN TESTS
Sometimes, less design is better: The research suggests that one effective strategy, at least periodically, is to ask students to write their own test questions.
In a 2020 study , students who generated test questions scored 14 percentage points higher than students who simply reviewed the material. “Question generation promotes a deeper elaboration of the learning content,” explains psychology professor Mirjam Ebersbach. “One has to reflect what one has learned and how an appropriate knowledge question can be inferred from this knowledge.” Model question-asking for students —highlighting your own examples first—and then teach them how to ask good questions. They may start with simple factual questions, but with enough practice, they can propose questions that start with “Explain” or that dig deeper into a topic with how and why questions.
9. AFTER-TEST STRATEGIES
Beyond test design, there’s the important question of what happens after a test. All too often, students receive a test, glance at the grade, and move on. But that deprives them, and the teacher, of a valuable opportunity to address misconceptions and gaps in knowledge. Don’t think of tests as an endpoint to learning. Follow up with feedback , and consider strategies like “ exam wrappers ”— short metacognitive writing activities that ask students to review their performance on the test and think about ways they could improve in future testing scenarios.
You might also rethink your policy around test retakes. While students can certainly take unfair advantage of some test-retaking policies, there are innovative approaches that preserve the integrity of the initial test while allowing students to recover partial credit for materials they haven’t successfully learned. Set clear limits, pose a different set of questions—or allow partial credit for demonstrating deep knowledge of questions they missed on the test—or ask students to reflect on why they missed earlier questions and what they can do to improve in the future, teachers recommend .
This paper is in the following e-collection/theme issue:
Published on 29.3.2024 in Vol 26 (2024)
Developing and Testing the Usability of a Novel Child Abuse Clinical Decision Support System: Mixed Methods Study
Authors of this article:

Original Paper
- Amy Thomas 1 , MD ;
- Andrea Asnes 1 , MSW, MD ;
- Kyle Libby 2 , BA, MS ;
- Allen Hsiao 1 , MD, FAAP, FAMIA ;
- Gunjan Tiyyagura 1 , MHS, MD
1 Department of Pediatrics, Yale University School of Medicine, New Haven, CT, United States
2 3M | M*Modal, 3M Health Information Systems, 3M Company, Maplewood, MN, United States
Corresponding Author:
Gunjan Tiyyagura, MHS, MD
Department of Pediatrics
Yale University School of Medicine
Pediatric Emergency Medicine, PO Box 208064
New Haven, CT, 06520
United States
Phone: 1 203 464 6343
Fax:1 203 737 7447
Email: [email protected]
Background: Despite the impact of physical abuse on children, it is often underdiagnosed, especially among children evaluated in general emergency departments (EDs) and those belonging to racial or ethnic minority groups. Electronic clinical decision support (CDS) can improve the recognition of child physical abuse.
Objective: We aimed to develop and test the usability of a natural language processing–based child abuse CDS system, known as the Child Abuse Clinical Decision Support (CA-CDS), to alert ED clinicians about high-risk injuries suggestive of abuse in infants’ charts.
Methods: Informed by available evidence, a multidisciplinary team, including an expert in user design, developed the CA-CDS prototype that provided evidence-based recommendations for the evaluation and management of suspected child abuse when triggered by documentation of a high-risk injury. Content was customized for medical versus nursing providers and initial versus subsequent exposure to the alert. To assess the usability of and refine the CA-CDS, we interviewed 24 clinicians from 4 EDs about their interactions with the prototype. Interview transcripts were coded and analyzed using conventional content analysis.
Results: Overall, 5 main categories of themes emerged from the study. CA-CDS benefits included providing an extra layer of protection, providing evidence-based recommendations, and alerting the entire clinical ED team. The user-centered, workflow-compatible design included soft-stop alert configuration, editable and automatic documentation, and attention-grabbing formatting. Recommendations for improvement included consolidating content, clearer design elements, and adding a hyperlink with additional resources. Barriers to future implementation included alert fatigue, hesitancy to change, and concerns regarding documentation. Facilitators of future implementation included stakeholder buy-in, provider education, and sharing the test characteristics. On the basis of user feedback, iterative modifications were made to the prototype.
Conclusions: With its user-centered design and evidence-based content, the CA-CDS can aid providers in the real-time recognition and evaluation of infant physical abuse and has the potential to reduce the number of missed cases.
Introduction
Child physical abuse is commonly missed by emergency department (ED) providers, leading to escalating injuries and death [ 1 ]. More than 30% of children with serious injuries resulting from physical abuse have been previously evaluated for injuries that were not recognized as abusive [ 2 - 5 ]. This is amplified in general EDs, where most children receive emergency care and abuse is more frequently missed than in pediatric EDs [ 6 - 8 ]. Evaluation and reporting of child abuse are also impacted by provider biases [ 2 , 9 - 12 ]. Children belonging to racial or ethnic minority groups are more often evaluated for abusive head trauma than White or non-Hispanic children, and children with public insurance undergo increased testing and are reported more often to Child Protective Services (CPS) than privately insured children [ 9 , 12 ]. These findings highlight the need for systems that standardize care, improve clinical outcomes, and reduce bias.
Clinical decision support (CDS) integrated into the electronic health record (EHR) can present intelligently filtered, individualized, and timely information to enhance clinical decision-making [ 13 ]. Child abuse–specific CDS systems may improve outcomes and reduce bias in the evaluation and reporting of suspected abuse. Experts have shared consensus recommendations regarding developing, disseminating, and sustaining EHR-embedded child abuse CDS systems in the ED [ 14 ]. Key recommendations included universal, routine implementation of a child abuse CDS system in general and pediatric EDs for children aged <4 years; use of active alerts that share their reason for triggering; integration of a standardized system for reports to CPS; use of data warehouse reports to evaluate the CDS system’s efficacy; integration of a system that is feasible, sustainable, and easily disseminated; and personalized usability testing to ensure seamless integration of the system [ 14 ].
While reviewing the existing child abuse CDS systems [ 15 - 32 ], we found that a common limitation is their inability to be triggered by free text in an EHR encounter. To address this gap, our team previously developed and validated a natural language processing (NLP) algorithm that automatically and methodically examined the free text in the notes of nursing providers, medical providers, and social workers (SWs) to identify high-risk injuries associated with possible abuse in children [ 33 ]. The NLP algorithm would provide a positive alert when it identified preselected combinations of written terms associated with fractures, intracranial injuries, abdominal injuries, burns, bruising, or oral injuries. It was targeted to identify high-risk injuries in children aged <1 year (ie, infants) specifically given that infants are more than twice as likely to experience maltreatment and thrice as likely to experience fatality from maltreatment compared to older children of any age group [ 1 ]. Developing a novel child abuse CDS system triggered by this validated NLP algorithm may further increase the tool’s potential to reduce the number of missed cases and mitigate bias.
To change providers’ practice using CDS, it is crucial to understand the providers’ needs and priorities before development and implementation. Evaluating a system’s usability involves the assessment of its accommodation of users’ needs, ease of mastery, effects on workflow, and achievement of goals. Conducting evaluations during the design process is also important to identify shortcomings and incorporate user-centered modifications [ 34 , 35 ]. Usability testing can include direct observation, recording of user-system interactions, think-aloud sessions where users verbalize their thoughts while interacting with the system, near-live sessions where users test the system with simulated patient interactions, live testing, and quantitative measures [ 34 , 36 ]. However, to date, only 1 study has described the usability testing of child abuse CDS in local settings [ 31 ].
Therefore, in this study, we aimed to develop a novel child abuse CDS system—hereafter referred to as the Child Abuse Clinical Decision Support (CA-CDS)—which is triggered by a validated NLP algorithm and that both alerts ED providers to high-risk injuries in infants and provides evidence-based recommendations for evaluation and management. We also sought to test the usability of the CA-CDS and refine the system based on user feedback.
Study Design
The study consisted of 3 phases informed by the Guideline Implementation with Decision Support (GUIDES) checklist by Van de Velde et al [ 37 ], which describes factors relevant to the development of successful guideline-based CDS. The phases included the (1) development of a prototype, (2) mixed methods usability testing, and (3) iterative refinement of the CA-CDS based on stakeholder feedback. Participants were stakeholders from 4 EDs, including 1 (25%) academic pediatric ED (Yale New Haven Children’s Hospital) and 3 (75%) community pediatric and general EDs (Bridgeport Hospital, Lawrence + Memorial Hospital, and Saint Raphael Campus). All campuses use Epic (Epic Systems Corporation) as their EHR and can use the M*Modal Fluency Direct speech recognition technology and Natural Language Understanding platform (3M Company), which hosts the NLP algorithm and presents the CA-CDS via built-in computer-assisted physician documentation functionality.
Development of the Prototype
The initial CA-CDS was developed after literature review and discussions with local experts in child abuse, pediatric emergency medicine, and health informatics. The issues discussed included target users (medical and nursing providers in EDs), appropriate language, recommendations considering the local context (eg, using order sets vs consulting the local child protection team [CPT]), and degree of interruption (ie, hard-stop vs soft-stop alert in which the former requires alert completion to proceed with one’s workflow). The prototype, as depicted in Figures 1 - 3 , consisted of a card and protocol that appeared in the EHR once the NLP algorithm identified a high-risk injury within a note’s free text. A smaller card would first appear, stating that a high-risk injury was found, with the triggering language presented in a tooltip. The card would then allow providers to open a larger protocol that presented further information about the triggering language, suggested questions for evaluation, and suggested actions for management. Users could then select between 2 acknowledgment options regarding the likelihood of child abuse or neglect and select the actions taken, which would automatically be entered into an editable documentation field. Finally, they could click submit response to add the documentation to the bottom of their note or late r to minimize the CA-CDS such that it no longer blocked the provider’s view of the EHR but remained accessible via the Fluency Direct pop-up bar. The CA-CDS was designed as a soft-stop alert such that completion was not required and workflow was not permanently interrupted.
To tailor the CA-CDS to the needs of medical versus nursing providers, the content was customized for each provider type. For instance, for medical providers ( Figure 1 ), the suggested questions were based on the MORE (Mechanism, Others present, Review of development, and Examination details) mnemonic. The components of the mnemonic (“Mechanism: additional details about history and injury mechanism; Others present: witnesses to injury and history corroboration; Review of development: developmental ability; and Examination details: disrobed exam, specifically to examine for sentinel injuries, and additional details related to the physical examination”) aids providers in differentiating between accidental and abusive injuries [ 38 ]. For nurses ( Figure 2 ), the content was simplified and asked whether the history was consistent with the injury. The suggested actions included recommendations to contact the local CPT (known as the Detection, Assessment, Referral, and Treatment or DART team) and file a report with Connecticut’s CPS agency known as the Department of Children and Families (DCF) as appropriate. The nursing version also recommended discussing concerns with medical providers. A subsequent-provider version was also designed to be received after another provider had already submitted their response ( Figure 3 ). This version was equivalent to the first-provider version, except for text indicating that another provider had responded and its modified acknowledgment options, allowing the subsequent provider to disagree with the previous provider’s selection regarding the likelihood of abuse, agree without further action, or agree and take additional action.
A web-based prototype of the CA-CDS in a model EHR was designed using the InVision platform (InVisionApp Inc) for usability testing with the abovementioned features ( Figures 1 - 3 ). The model EHR showed a clinical vignette of an infant presenting for emergency care for whom a provider documented a high-risk injury that triggered the CA-CDS (Table S1 in Multimedia Appendix 1 ). For this study, the CA-CDS was tested solely in a model EHR before future live implementation.

Usability Testing
We tested the CA-CDS’s usability through a mixed methods approach. The research team, including a user design expert (KL) and researchers with qualitative research expertise (GT and AA), developed and iteratively refined an interview guide (Table S2 in Multimedia Appendix 1 ) with open-ended questions about topics including the CA-CDS’s design, strengths, deficits, and recommendations for improvement and future implementation. Purposive recruitment of stakeholders for interviews who represented the CA-CDS’s end users and local champions in child abuse care was conducted via email, in person, and through ED section meetings. Overall, 3 rounds of interviews were conducted by GT and AT, with audiovisual recording for documenting user-system interactions and transcript generation ( Figure 4 ). Interviews were conducted until thematic sufficiency was achieved [ 39 - 41 ].

The web-based prototype (accessed via hyperlink) and interview guide were pilot-tested through in-person, think-aloud interviews with 3 ED providers ( Figure 4 ). After further refinement of the interview guide, interviews were conducted via teleconferencing with 10 additional providers. Participants were instructed to think aloud while interacting with the prototype and then asked targeted questions using the interview guide. On the basis of the findings from the initial interviews, the CA-CDS was refined, and usability of the updated prototype was assessed with another round of interviews. Here, we sought to address topics that we felt needed more exploration such as preferred resources, documentation-related concerns, and target users for the subsequent-provider alert, and thus, we designed a more targeted interview guide (Table S2 in Multimedia Appendix 1 ). 11 additional ED providers were recruited in person in the ED to participate in a final round of interviews. The updated prototype was provided as a multipage PDF document on the interviewer’s tablet. The prototype was further refined based on these interviews.
Following each interview, participants were asked to complete a survey to capture demographic and quantitative usability data with adequate time and privacy for completion (Figure S1 in Multimedia Appendix 1 ). The survey requested the participants’ ID numbers to anonymously link their survey and interview transcript, profession and years in their role, employment site, and experience with suspected child abuse cases. To assess usability quantitatively, we used the System Usability Scale (SUS), which is a 5-point Likert scale with 10 questions exploring the different aspects of a tool’s usability and learnability. The SUS is a validated, frequently used scale that provides a quick, standardized, and easily interpretable measure for reporting and comparing a product’s usability [ 42 , 43 ].
The interview transcripts were anonymized and independently reviewed by a coding team consisting of 3 researchers, including 1 experienced in the analysis of qualitative data (GT) and 1 experienced in usability testing (KL), using conventional content analysis [ 44 ]. Team members applied codes to categorize data. Researchers then met to discuss the codes until consensus was reached, and a code list was subsequently generated and iteratively revised as new interviews were discussed [ 41 ]. The codes were then clustered into recurrent categories.
Regarding surveys, each participant’s SUS responses were scored, with the score ranging between 0 and 100 [ 45 ]. A cumulative SUS score for the CA-CDS was then obtained by calculating the median and IQR of the data set of participants’ scores. This cumulative score was compared against the curved grading scale developed by Lewis and Sauro [ 46 ] in which an SUS score of 68 corresponded to the 50th percentile of the range of scores included in their study and thus a “C” letter grade [ 46 , 47 ]. In their study and industry, an SUS score ≥80 indicated an above-average user experience.
Ethical Considerations
All participants provided verbal informed consent to be interviewed and recorded before starting the interviews. Participants received no compensation. This study was approved by the Yale Human Investigations Committee (2000029566).
Participant Characteristics
In total, 24 participants were interviewed in the study, and 23 participants completed the demographic survey. Most were physicians (13/23, 57%), from Yale New Haven Children’s Hospital (19/23, 83%), and held their current roles for >6 years (13/23, 57%; Table 1 ).
a In total, 24 participants were interviewed, but 1 (4%) participant was unable to complete the Qualtrics survey that requested demographic data due to conflicting clinical obligations.
Emerging Themes
Analysis of the interviews revealed 5 main categories of themes. These themes, along with sample subcategories and representative quotations, are presented in Table 2 .
a CA-CDS: Child Abuse Clinical Decision Support.
b DART: Detection, Assessment, Referral, and Treatment.
c ED: emergency department.
d DCF: Department of Children and Families.
CA-CDS Benefits
Participants discussed the challenges of recognizing abusive injuries, especially those that were subtle or “minor.” They expressed that the CA-CDS could provide an extra layer of protection against missing abuse by reminding providers in real time to consider abuse in their differential diagnosis. Users also appreciated the CA-CDS’s evidence-based recommendations for evaluation and management that included guidance about important historical information to be collected and about using the expertise of specialists. Specifically, they found the MORE mnemonic to be clear, memorable, and helpful to improve information gathering, decision-making, and documentation. Participants also valued the emphasis on consulting specialists to determine the appropriate workup as it enabled the CA-CDS to remain simple but adaptable despite case-specific variations. In addition, the users appreciated that the CA-CDS alerted both medical and nursing providers, allowing for open communication of concerns among the entire clinical team.
User-Centered, Workflow-Compatible Design
Participants discussed the elements of the CA-CDS that would optimize their workflow. First, they valued the CA-CDS’s customization based on provider type, which reflected workflow differences, and preferred that nurses and medical providers submit independent CA-CDS responses. For instance, nurses favored the recommendation to discuss concerns with the medical team rather than consulting the CPT directly as it reflected the typical nursing workflow. Second, users felt that the CA-CDS’s soft-stop alert configuration, which could be minimized and reaccessed on demand, would be more flexible around providers’ unpredictable workflow. Third, users expressed that the documentation component, which automatically populated the selected actions into the note while also remaining editable for providers to share their own decision-making, would avoid redundancy. Fourth, participants appreciated that the CA-CDS could be triggered by injuries in various providers’ notes. In particular, they expressed that by including nursing notes, which are often created before other clinicians’ notes, as a trigger source, the CA-CDS could allow for more timely evaluation. Fifth, users shared that having the alert explicitly identify the triggering documentation enabled all team members to quickly be on the same page regarding the specific injury causing concern for abuse and allowed providers to assess if their documentation was being construed as intended. Sixth, participants appreciated the CA-CDS’s attention-grabbing formatting. Features such as bold text, colorful symbols, and high-risk injury phrasing helped emphasize the alert’s significance. Finally, regarding the subsequent-provider CA-CDS version, participants valued that the protocol clearly displayed the actions selected by the provider who initially submitted a response. They found this helpful for handoffs, highlighting the salient concerns and workup for all team members.
Recommendations for Improvement
Users made several recommendations to improve the CA-CDS’s usability. Participants recommended consolidating the content to reduce information overload. For instance, they suggested removing the acknowledgment section to reduce redundant text, allow more flexibility for documentation, and circumvent the potential legal implications of documenting disagreement with previous providers. To improve clarity, users also suggested using obvious underlining and bold colors to highlight design elements such as hyperlinks and editable text fields. In addition, to improve providers’ case-specific and general knowledge about abuse, participants suggested adding a link to additional resources that providers could access for further support.
Next, users requested further information about the source of the triggering documentation, including its author and location, to better find and assess the triggering content. Finally, providers suggested modifications to improve their workflow and use of the CA-CDS. For example, nurses appreciated the card’s reminder to be in an appropriately private setting as they often charted near patients, whereas medical providers supported removing this component which was less relevant to their workflow. They also shared that the reappearance of the CA-CDS at discharge could serve as a reminder to those who had missed, ignored, or initially not felt ready to complete the alert and as an opportunity to add more information and reconsider abuse in their differential.
Barriers to Future Implementation
First, participants warned about the potential for alert fatigue, especially if there were several false positives or if excessive effort was required for completion. They discussed how alert fatigue may lead providers to ignore the alert or seek work-arounds such as documenting in a manner to avoid triggering the alert. Second, they shared that the CA-CDS, with its interruptive alert and recommendations to consult specialists, may be perceived by some providers as infringing on their autonomy. Third, users counseled that providers may be accustomed to a particular manner of providing care and hesitant to change.
Finally, users warned that providers may be wary of using the CA-CDS, especially its documentation component, given the potential consequences of documenting concerns about abuse in notes that are accessible to caregivers. These included liability, inadequate patient-sensitive language, caregivers learning about concerns before discussions with the medical team, and caregivers purposefully obstructing care or inflicting further harm. However, users also responded that they tried to document objectively, being mindful about how their documentation could be interpreted by caregivers. On the basis of these discussions, the following text was added to the protocol: “Consider ‘unsharing’ the note ‘to prevent substantive harm to patient or another person.’”
Facilitators of Future Implementation
Participants recommended several strategies to optimize the CA-CDS’s implementation. Users felt that stakeholder and leadership buy-in and support for the system would promote future use and sustainability. In addition, participants stated the importance of educating providers regarding how to use the system to appropriately manage the cases of potential abuse and how to approach caregivers based on evidence-based recommendations. Users also recommended communicating the accuracy of the CA-CDS and its triggering NLP algorithm by sharing the system’s validation data and instances where the system could have made a difference.
Prototype Revisions
On the basis of the interviews and feedback from our team of experts, multiple rounds of modifications were performed to create our final prototype ( Figures 5 and 6 ). All modifications are listed in Table S3 in Multimedia Appendix 1 .

Of the 24 interviewees, 23 (96%) completed the SUS. Scores ranged from 62.5 to 100, with a median of 80 (IQR 75-92.5). Compared with Lewis and Sauro’s [ 46 , 47 ] curved grading scale, our median corresponded to the 85th to 89th percentile and an A− letter grade.
Principal Findings
Usability testing of the CA-CDS revealed several key findings. Users valued the additional protection against missing abuse that is offered by the alert to the entire clinical team and the presence of evidence-based recommendations for the evaluation and management of suspected abuse. Users also appreciated the CA-CDS’s user-centered, workflow-compatible design elements that captured the user’s attention to provide timely, provider-specific information while minimizing interruptions and redundancy. However, they recommended improving the system’s clarity and brevity, highlighting critical features such as the triggering documentation’s source, and further supporting the users by offering additional resources and alert reappearance at discharge. User recommendations informed the iterative refinements of the CA-CDS prototype. Future studies will be directed toward the implementation and live testing of the revised, user-centric CA-CDS within our hospital system’s EHR.
Comparing the CA-CDS With Existing Systems
Researchers have described the development, implementation, and evaluation of a child abuse CDS system for pediatric and general EDs that identified high-risk injuries through a variety of alert triggers including specific screening results, orders, and discharge documentation [ 26 - 31 ]. Their CDS system notified providers about the concern for abuse and recommended direct connection to an age-appropriate, injury-specific order set or a CPS referral. While the CA-CDS similarly aimed to identify high-risk injuries and provide CDS regarding the evaluation of suspected abuse, there were numerous differences. The current CA-CDS was triggered via an NLP algorithm that examined all the free text in the notes of medical providers, nursing providers, and SWs, whereas previous CDS systems were triggered primarily by discrete fields, active screening such as those completed by nurses upon evaluation, or limited NLP function that could only examine the free text within the chief complaint and focused assessment fields [ 33 ]. An entirely NLP-triggered CDS system may allow for minimal interruptions to the workflow; be more acceptable to frontline providers; and allow the CA-CDS to be triggered as soon as there is any documentation, even as early as triage, without requiring actions outside the normal workflow.
While many existing CDS systems connect users to standardized order sets [ 26 - 31 ] and recent consensus guidelines also recommended the use of a physical abuse order set with consistent and evidence-based actions [ 14 ], most of our users (13/24, 54%) preferred simpler suggested actions with reminders to consult a SW or the CPT to aid in nuanced decision-making. Consultation with these specialists may facilitate appropriate decision-making around performing additional testing or reporting to CPS and reduce bias in evaluation and reporting of suspected child abuse [ 48 , 49 ]. However, to acknowledge the importance of autonomy for users, the CA-CDS was designed as a nonmandatory, soft-stop alert that included a free-text response option and a hyperlink to additional resources including local clinical pathway guidelines to provide either support or an avenue for independent decision-making depending on the provider’s needs. Next steps include comparing the outcomes of systems that recommend standardized order sets to those that recommend consulting clinicians.
Finally, the CA-CDS was hosted on external software from 3M Company and designed to be subsequently integrated into the EHR, rather than being directly built into the EHR. This design aligns with the Fast Healthcare Interoperability Resources (FHIR) data standard that standardizes how information is stored, used, and exchanged between computer systems and thereby streamlines software development to support health care needs [ 50 , 51 ]. EHRs with FHIR-enabled technology allow for the packaging of information from the EHR into discrete, standardized units that can be interpreted and acted upon by external applications including CDS systems. FHIR-based applications, such as those used in this study, allow the results of a CDS system to trigger the opening of order sets or to directly provide CDS within the EHR and may realistically solve the problem of 1 child abuse CDS system communicating with multiple EHRs [ 31 , 52 , 53 ]. With 84% of hospitals in the United States having adopted FHIR-enabled technology and 3M’s connection with hundreds of EHR systems [ 51 , 54 , 55 ], the CA-CDS’s FHIR-based application design may facilitate the system’s dissemination across numerous institutions and EHRs.
Examining the Rigor of the CA-CDS
Consistent with the recommendations for successful, guideline-based, computerized CDS as described by the GUIDES checklist and child abuse expert consensus recommendations [ 14 , 22 , 37 ], the CA-CDS was developed by a team of local experts to provide evidence-based guidelines for the evaluation and management of high-risk injuries, reflective of recent studies in the field. In addition, the CA-CDS was integrated with an objective and internally validated NLP algorithm that captured data widely in the notes of ED SWs, nursing providers, and medical providers [ 33 ]. Given that the system is triggered independent of the providers’ gestalt and background, the CA-CDS may improve the standardization of patient care and reduce the impact of providers’ implicit biases [ 49 , 56 ].
The CA-CDS met the recommended design standards in several ways. Users’ feedback demonstrated the system’s usability, with users finding the CA-CDS to be user friendly, concise, and clear. The CA-CDS was intentionally refined based on feedback to reflect the users’ preferences. Considerable effort was made to integrate the system into providers’ clinical and EHR workflow to minimize interruptions and redundancy. The CA-CDS also provided ample flexibility around decision-making through features such as editable and automatic documentation and soft-stop alert design. Interestingly, in contrast to experts’ recommendation to incorporate automated referrals, standardized CPS reporting, and a multidisciplinary audience [ 14 , 22 ], most users preferred to keep the CA-CDS simple without these features (19/24, 79%) and limit the alert’s recipients to the primary clinical team (7/13, 54%). Next steps include examining the real-time use of the CA-CDS by ED clinicians.
Similar to the consensus recommendations, participants discussed the importance of planning for future implementation [ 14 , 37 ]. They identified the facilitators of future implementation, such as stakeholder buy-in, education about the CA-CDS and the accuracy of the underlying trigger (ie, the NLP algorithm), and iterative refinement of the system based on user feedback. While participants discussed alert fatigue, or provider desensitization owing to excessive alerts [ 57 ], as a potential barrier to future implementation, the NLP algorithm’s relatively high specificity and the limited patient population may minimize this concern. However, continual improvement of any rule-based algorithm is critical to maintain its quality. While participants did not discuss the implications of receiving a CA-CDS alert after a patient’s discharge if a provider completes the documentation after a patient’s ED visit, encounters with injuries identified by the NLP algorithm undergo weekly routine case surveillance by the CPT [ 58 ]. This is especially important for cases in which documentation is completed after a patient’s discharge to assure that the identified injuries are not concerning for missed abuse. Such a monitoring system may facilitate the identification of cases that might have been missed in real time during the ED encounter [ 14 ].
Patient Access to Electronic Health Information
An important but underexplored aspect of child abuse CDS systems is the impact of the 21st Century Cures Act on providers’ EHR interactions. The Cures Act is a federal law that came into effect in April 2021, mandating the free, timely release of electronic health information to patients and their guardians unless the practice meets the condition of select exceptions, one of which is preventing harm in contexts such as child abuse [ 59 - 61 ]. Concurrently, adoption and use of the patient portal dramatically increased as a result of the COVID-19 pandemic, with much of patient care and communication shifting to electronic mediums. Given the increased ease of patient access to EHR content, it is especially important to understand how provider perspectives about documentation of suspected child abuse have been affected by the new law. This study was uniquely timed to explore these concerns following the Cures Act. Although users worried about the potential repercussions of using the CA-CDS to document suspicions about abuse in caregiver-accessible notes, their unease was alleviated by the clarity provided by the protocol regarding the destination of the automatic documentation into the note and the addition of a reminder to unshare the note if appropriate. Next steps include exploring caregivers’ perspectives about the documentation of suspected child abuse.
Limitations
This study had at least 3 limitations. First, although we tried to recruit representative participants, few community ED providers (4/23, 17%) versus pediatric ED providers (19/23, 83%) participated in the usability testing. As providers who work at sites that often see most of a community’s pediatric population and more often underdiagnose child abuse [ 6 - 8 ], feedback from community ED clinicians is uniquely valuable. Future system testing would benefit from having a more balanced or community-focused participant pool. Second, we modified the CA-CDS based on the majority’s preferences. As such, there may have been modifications desired by a notable percentage of our users that were not implemented, which may limit their interaction with the CA-CDS in the future. However, the high-risk injuries identified by the CA-CDS will be routinely reviewed by the CPT to assure that cases are not misdiagnosed. Third, while this system was developed by a local team of experts and through iterative usability testing with providers at different sites, the CA-CDS’s recommended management may be institution specific. Further usability testing may be required if the system is disseminated to other hospitals, especially those with limited resources.
Conclusions
In summary, with its user-centered design and evidence-based content, the CA-CDS offers a novel method to aid ED medical and nursing providers in the real-time recognition, evaluation, and management of infant physical abuse. Our system has the potential to reduce the number of missed cases and increase the provision of less biased and evidence-based care to all infants.
Acknowledgments
This study was supported in part by funds from the National Institute of Child Health and Human Development (grant K23HD107178 [GT]). The contents of this paper are solely the responsibility of the authors and do not necessarily represent the official views of the National Institutes of Health.
Data Availability
The data underlying this paper will be shared upon reasonable request to the corresponding author. The technical components used in this study were provided by 3M Company under license and will be shared upon request to the corresponding author with permission from 3M Company.
Authors' Contributions
AT, GT, and KL were involved in all steps of the study including conducting literature review, communicating with experts, developing the study material such as the interview guides and surveys, conducting interviews, analyzing the interview transcripts, and designing and refining the Child Abuse Clinical Decision Support (CA-CDS) prototypes. GT was also responsible for initial project conception, institutional review board submission, suggestion of candidates to participate in the study, and presentation of material at Yale New Haven Health stakeholder meetings. KL was also responsible for the technical components, designing and editing the prototype, and serving as our liaison with other 3M Company staff. AA and AH provided guidance and shared their clinical expertise in pediatric emergency medicine, child abuse, and health informatics as we developed and refined the CA-CDS. All authors collaborated to draft and review this paper.
Conflicts of Interest
KL serves as a consultant for 3M Health Information Systems Inc, 3M Company, and AH serves as a member of the Strategic Advisory Board for Johnson & Johnson. All other authors declare no other conflicts of interest.
Supplementary tables and figures including clinical vignettes, interview guide, demographic and System Usability Scale survey, and prototype modifications.
- Child maltreatment 2021. U.S. Department of Health & Human Services, Administration for Children and Families, Administration on Children, Youth and Families, Children’s Bureau. 2023. URL: https://www.acf.hhs.gov/sites/default/files/documents/cb/cm2021.pdf [accessed 2024-03-17]
- Jenny C, Hymel KP, Ritzen A, Reinert SE, Hay TC. Analysis of missed cases of abusive head trauma. JAMA. Feb 17, 1999;281(7):621-626. [ CrossRef ] [ Medline ]
- Letson MM, Cooper JN, Deans KJ, Scribano PV, Makoroff KL, Feldman KW, et al. Prior opportunities to identify abuse in children with abusive head trauma. Child Abuse Negl. Oct 2016;60:36-45. [ CrossRef ] [ Medline ]
- Thorpe EL, Zuckerbraun NS, Wolford JE, Berger RP. Missed opportunities to diagnose child physical abuse. Pediatr Emerg Care. Nov 2014;30(11):771-776. [ CrossRef ] [ Medline ]
- King WK, Kiesel EL, Simon HK. Child abuse fatalities: are we missing opportunities for intervention? Pediatr Emerg Care. Apr 2006;22(4):211-214. [ CrossRef ] [ Medline ]
- Ravichandiran N, Schuh S, Bejuk M, Al-Harthy N, Shouldice M, Au H, et al. Delayed identification of pediatric abuse-related fractures. Pediatrics. Jan 2010;125(1):60-66. [ CrossRef ] [ Medline ]
- Trokel M, Waddimba A, Griffith J, Sege R. Variation in the diagnosis of child abuse in severely injured infants. Pediatrics. Mar 2006;117(3):722-728. [ CrossRef ] [ Medline ]
- Ziegler DS, Sammut J, Piper AC. Assessment and follow-up of suspected child abuse in preschool children with fractures seen in a general hospital emergency department. J Paediatr Child Health. 2005;41(5-6):251-255. [ CrossRef ] [ Medline ]
- Hymel KP, Laskey AL, Crowell KR, Wang M, Armijo-Garcia V, Frazier TN, et al. Racial and ethnic disparities and bias in the evaluation and reporting of abusive head trauma. J Pediatr. Jul 2018;198:137-43.e1. [ FREE Full text ] [ CrossRef ] [ Medline ]
- Lane WG, Dubowitz H. What factors affect the identification and reporting of child abuse-related fractures? Clin Orthop Relat Res. Aug 2007;461:219-225. [ CrossRef ] [ Medline ]
- Wood JN, Christian CW, Adams CM, Rubin DM. Skeletal surveys in infants with isolated skull fractures. Pediatrics. Feb 2009;123(2):e247-e252. [ CrossRef ] [ Medline ]
- Wood JN, Hall M, Schilling S, Keren R, Mitra N, Rubin DM. Disparities in the evaluation and diagnosis of abuse among infants with traumatic brain injury. Pediatrics. Sep 2010;126(3):408-414. [ CrossRef ] [ Medline ]
- Clinical decision support. HealthIT.gov. Office of the National Coordinator for Health Information Technology URL: https://www.healthit.gov/topic/safety/clinical-decision-support [accessed 2021-12-23]
- Suresh S, Barata I, Feldstein D, Heineman E, Lindberg DM, Bimber T, et al. Clinical decision support for child abuse: recommendations from a consensus conference. J Pediatr. Jan 2023;252:213-8.e5. [ CrossRef ] [ Medline ]
- Low D. The Child Protection Information Sharing Project (CP-IS): electronic information sharing to improve the assessment of known vulnerable or at-risk children. Adopt Foster. 2016;40(3):293-296. [ CrossRef ]
- Child Protection - Information Sharing (CP-IS) service. National Health Service England. National Health Service England URL: https://digital.nhs.uk/services/child-protection-information-sharing-project [accessed 2021-12-24]
- Deutsch SA, Henry MK, Lin W, Valentine KJ, Valente C, Callahan JM, et al. Quality improvement initiative to improve abuse screening among infants with extremity fractures. Pediatr Emerg Care. Sep 2019;35(9):643-650. [ CrossRef ] [ Medline ]
- Erlanger AC, Heyman RE, Slep AM. Creating and testing the reliability of a family maltreatment severity classification system. J Interpers Violence. Apr 2022;37(7-8):NP5649-NP5668. [ CrossRef ] [ Medline ]
- Kelly P, Chan C, Reed P, Ritchie M. The national child protection alert system in New Zealand: a prospective multi-centre study of inter-rater agreement. Child Youth Serv Rev. Sep 2020;116:105174. [ CrossRef ]
- Thraen IM, Frasier L, Cochella C, Yaffe J, Goede P. The use of TeleCAM as a remote web-based application for child maltreatment assessment, peer review, and case documentation. Child Maltreat. Nov 2008;13(4):368-376. [ CrossRef ] [ Medline ]
- Child-at-risk electronic medical record alert. Agency for Clinical Innovation, New South Wales Government. New South Wales Government; Aug 25, 2017. URL: https://aci.health.nsw.gov.au/ie/projects/child-at-risk [accessed 2021-12-24]
- Gonzalez DO, Deans KJ. Hospital-based screening tools in the identification of non-accidental trauma. Semin Pediatr Surg. Feb 2017;26(1):43-46. [ CrossRef ] [ Medline ]
- Escobar MAJ, Pflugeisen BM, Duralde Y, Morris CJ, Haferbecker D, Amoroso PJ, et al. Development of a systematic protocol to identify victims of non-accidental trauma. Pediatr Surg Int. Apr 2016;32(4):377-386. [ CrossRef ] [ Medline ]
- Riney LC, Frey TM, Fain ET, Duma EM, Bennett BL, Murtagh Kurowski E. Standardizing the evaluation of nonaccidental trauma in a large pediatric emergency department. Pediatrics. Jan 2018;141(1):e20171994. [ CrossRef ] [ Medline ]
- Luo S, Botash AS. Designing and developing a mobile app for clinical decision support: an interprofessional collaboration. Comput Inform Nurs. Oct 2018;36(10):467-472. [ CrossRef ] [ Medline ]
- Berger RP, Saladino RA, Fromkin J, Heineman E, Suresh S, McGinn T. Development of an electronic medical record-based child physical abuse alert system. J Am Med Inform Assoc. Feb 01, 2018;25(2):142-149. [ FREE Full text ] [ CrossRef ] [ Medline ]
- Suresh S, Heineman E, Meyer L, Richichi R, Conger S, Young S, et al. Improved detection of child maltreatment with routine screening in a tertiary care pediatric hospital. J Pediatr. Apr 2022;243:181-7.e2. [ CrossRef ] [ Medline ]
- Suresh S, Saladino RA, Fromkin J, Heineman E, McGinn T, Richichi R, et al. Integration of physical abuse clinical decision support into the electronic health record at a Tertiary Care Children's Hospital. J Am Med Inform Assoc. Jul 01, 2018;25(7):833-840. [ FREE Full text ] [ CrossRef ] [ Medline ]
- Rumball-Smith J, Fromkin J, Rosenthal B, Shane D, Skrbin J, Bimber T, et al. Implementation of routine electronic health record-based child abuse screening in General Emergency Departments. Child Abuse Negl. Nov 2018;85:58-67. [ CrossRef ] [ Medline ]
- Rosenthal B, Skrbin J, Fromkin J, Heineman E, McGinn T, Richichi R, et al. Integration of physical abuse clinical decision support at 2 general emergency departments. J Am Med Inform Assoc. Oct 01, 2019;26(10):1020-1029. [ FREE Full text ] [ CrossRef ] [ Medline ]
- McGinn T, Feldstein DA, Barata I, Heineman E, Ross J, Kaplan D, et al. Dissemination of child abuse clinical decision support: moving beyond a single electronic health record. Int J Med Inform. Mar 2021;147:104349. [ FREE Full text ] [ CrossRef ] [ Medline ]
- Luo S, Botash AS. Testing a mobile app for child abuse treatment: a mixed methods study. Int J Nurs Sci. Jun 23, 2020;7(3):320-329. [ FREE Full text ] [ CrossRef ] [ Medline ]
- Tiyyagura G, Asnes AG, Leventhal JM, Shapiro ED, Auerbach M, Teng W, et al. Development and validation of a natural language processing tool to identify injuries in infants associated with abuse. Acad Pediatr. Aug 2022;22(6):981-988. [ FREE Full text ] [ CrossRef ] [ Medline ]
- Kushniruk AW, Patel VL. Cognitive and usability engineering methods for the evaluation of clinical information systems. J Biomed Inform. Feb 2004;37(1):56-76. [ FREE Full text ] [ CrossRef ] [ Medline ]
- Usability. Interaction Design Foundation. URL: https://www.interaction-design.org/literature/topics/usability [accessed 2021-12-26]
- Mann DM, Chokshi SK, Kushniruk A. Bridging the gap between academic research and pragmatic needs in usability: a hybrid approach to usability evaluation of health care information systems. JMIR Hum Factors. Nov 28, 2018;5(4):e10721. [ FREE Full text ] [ CrossRef ] [ Medline ]
- Van de Velde S, Kunnamo I, Roshanov P, Kortteisto T, Aertgeerts B, Vandvik PO, et al. The GUIDES checklist: development of a tool to improve the successful use of guideline-based computerised clinical decision support. Implement Sci. Jun 25, 2018;13(1):86. [ FREE Full text ] [ CrossRef ] [ Medline ]
- Shum M, Asnes A, Leventhal JM, Bechtel K, Gaither JR, Tiyyagura G. The use of experts to evaluate a child abuse guideline in community emergency departments. Acad Pediatr. Apr 2021;21(3):521-528. [ CrossRef ] [ Medline ]
- Varpio L, Ajjawi R, Monrouxe LV, O'Brien BC, Rees CE. Shedding the cobra effect: problematising thematic emergence, triangulation, saturation and member checking. Med Educ. Jan 2017;51(1):40-50. [ CrossRef ] [ Medline ]
- LaDonna KA, Artino ARJ, Balmer DF. Beyond the guise of saturation: rigor and qualitative interview data. J Grad Med Educ. Oct 2021;13(5):607-611. [ FREE Full text ] [ CrossRef ] [ Medline ]
- Hanson JL, Balmer DF, Giardino AP. Qualitative research methods for medical educators. Acad Pediatr. 2011;11(5):375-386. [ CrossRef ] [ Medline ]
- Johnson CM, Johnston D, Crowley PK, Culbertson H, Rippen HE, Damico DJ, et al. EHR usability toolkit: a background report on usability and electronic health records. Agency for Healthcare Research and Quality. U.S. Department of Health and Human Services; Aug 2011. URL: https://digital.ahrq.gov/sites/default/files/docs/citation/EHR_Usability_Toolkit_Background_Report.pdf [accessed 2024-03-11]
- Brooke J. SUS: a retrospective. J User Exp. 2013;8(2):29-40. [ FREE Full text ]
- Hsieh HF, Shannon SE. Three approaches to qualitative content analysis. Qual Health Res. Nov 2005;15(9):1277-1288. [ CrossRef ] [ Medline ]
- Brooke J. SUS: a 'quick and dirty' usability scale. In: Usability Evaluation In Industry. Boca Raton, FL. CRC Press; 1996.
- Lewis JR, Sauro J. Item benchmarks for the system usability scale. J Usab Stud. May 1, 2018;13(3):158-167. [ FREE Full text ]
- Sauro J. A Practical Guide to the System Usability Scale: Background, Benchmarks & Best Practices. Denver, CO. Measuring Usability LLC; 2011.
- Powers E, Tiyyagura G, Asnes AG, Leventhal JM, Moles R, Christison-Lagay E, et al. Early involvement of the child protection team in the care of injured infants in a pediatric emergency department. J Emerg Med. Jun 2019;56(6):592-600. [ CrossRef ] [ Medline ]
- Tiyyagura G, Emerson B, Gaither JR, Bechtel K, Leventhal JM, Becker H, et al. Child protection team consultation for injuries potentially due to child abuse in community emergency departments. Acad Emerg Med. Jan 2021;28(1):70-81. [ FREE Full text ] [ CrossRef ] [ Medline ]
- What is FHIR®? HealthIT.gov. The Office of the National Coordinator for Health Information Technology; 2019. URL: https://www.healthit.gov/sites/default/files/2019-08/ONCFHIRFSWhatIsFHIR.pdf [accessed 2024-03-11]
- Yan E. Catching FHIR: healthcare interoperability in 2023. Keysight Technologies. Jan 16, 2023. URL: https://www.keysight.com/blogs/en/tech/software-testing/2023/1/16/fhir-healthcare-interoperability-testing [accessed 2024-03-11]
- The FHIR® API. HealthIT.gov. The Office of the National Coordinator for Health Information Technology URL: https://www.healthit.gov/sites/default/files/page/2021-04/FHIR%20API%20Fact%20Sheet.pdf [accessed 2024-03-11]
- Vorisek CN, Lehne M, Klopfenstein SA, Mayer PJ, Bartschke A, Haese T, et al. Fast healthcare interoperability resources (FHIR) for interoperability in health research: systematic review. JMIR Med Inform. Jul 19, 2022;10(7):e35724. [ FREE Full text ] [ CrossRef ] [ Medline ]
- Barker W, Posnack S. The heat is on: US caught FHIR in 2019. Health IT Buzz. Office of the National Coordinator for Health Information Technology; Jul 29, 2021. URL: https://www.healthit.gov/buzz-blog/health-it/the-heat-is-on-us-caught-fhir-in-2019 [accessed 2024-03-11]
- 3M™ M*Modal fluency direct. 3M Company. URL: https://www.3m.com/3M/en_US/health-information-systems-us/create-time-to-care/clinician-solutions/speech-recognition/fluency-direct/ [accessed 2022-01-17]
- Rangel EL, Cook BS, Bennett BL, Shebesta K, Ying J, Falcone RA. Eliminating disparity in evaluation for abuse in infants with head injury: use of a screening guideline. J Pediatr Surg. Jun 2009;44(6):1229-1235. [ CrossRef ] [ Medline ]
- Alert fatigue. Patient Safety Network. Agency for Healthcare Research and Quality; Sep 07, 2019. URL: https://tinyurl.com/467dssaw [accessed 2024-03-10]
- Shum M, Hsiao A, Teng W, Asnes A, Amrhein J, Tiyyagura G. Natural language processing - a surveillance stepping stone to identify child abuse. Acad Pediatr. 2024;24(1):92-96. [ CrossRef ] [ Medline ]
- 21st century cures act. U.S. Food & Drug Administration. Jan 31, 2020. URL: https://www.fda.gov/regulatory-information/selected-amendments-fdc-act/21st-century-cures-act [accessed 2022-01-17]
- ONC's Cures Act Final Rule. HealthIT.gov. The Office of the National Coordinator for Health Information Technology URL: https://www.healthit.gov/topic/oncs-cures-act-final-rule [accessed 2022-01-17]
- Anthony ES. The cures act final rule: interoperability-focused policies that empower patients and support providers. Health IT Buzz. The Office of the National Coordinator for Health Information Technology; Mar 09, 2020. URL: https://www.healthit.gov/buzz-blog/21st-century-cures-act/the-cures-final-rule [accessed 2022-01-16]
Abbreviations
Edited by G Tsafnat; submitted 20.07.23; peer-reviewed by E Heiman, D Listman; comments to author 08.02.24; revised version received 23.02.24; accepted 23.02.24; published 29.03.24.
©Amy Thomas, Andrea Asnes, Kyle Libby, Allen Hsiao, Gunjan Tiyyagura. Originally published in the Journal of Medical Internet Research (https://www.jmir.org), 29.03.2024.
This is an open-access article distributed under the terms of the Creative Commons Attribution License (https://creativecommons.org/licenses/by/4.0/), which permits unrestricted use, distribution, and reproduction in any medium, provided the original work, first published in the Journal of Medical Internet Research, is properly cited. The complete bibliographic information, a link to the original publication on https://www.jmir.org/, as well as this copyright and license information must be included.

New Research First to Test 60-Year-Old Theory on Autism
By Emily Leclerc | Waisman Science Writer
*Note: The Travers lab has chosen to use identity first language in response to the growing preference for this type of language in the autism community. The language in this story reflects that choice.*
Autism is often associated with complex tasks like social processing and language and the later-developing brain regions that control them. But what if autism is more rooted in the earliest developing and most reflex-like part of the brain – the brainstem? This brainstem focused hypothesis about autism was put forth nearly 60 years ago by scientists but was left virtually untested due to the challenges of imaging the area in living individuals.

This new research by Brittany Travers, PhD , Waisman investigator and associate professor of kinesiology, is the first to officially test this hypothesis in children thanks to the advancements in brain imaging techniques. Her work reveals that the brainstem may indeed be central to core autism features. “It is a pivotal brain structure and deserves some attention, particularly in autism,” Travers says.
A stalk-like structure located at the base of the brain, the brainstem controls the body’s involuntary and automatic processes such as heart rate, blood pressure, breathing, digestion, and swallowing, among other functions. Even though we may not be aware of it, this autonomic nervous system – the name given to the specific bundle of nerves in the brainstem that regulate those processes – is in a constant state of responding to external and internal information.
The brainstem has reactions and sets of reactions to a variety of stimuli to try to keep our body in a state of balance, or homeostasis. Increase heart rate when this happens. Lower blood pressure when that happens. Disrupt digestion to reallocate energy when this happens. In turn, our behavior may be subtly or not so subtly affected by the underlying autonomic nervous system processing, even if we are not aware of it. So, what happens when this system is acting differently in a person?
“The brainstem is one of the earliest developing parts of the brain. So, it makes sense, in a neurodevelopmental condition like autism, that there would be differences that are happening in the brainstem that help explain the individual differences in autism,” Travers says.
Travers’ recent paper, “ Role of autonomic, nociceptive, and limbic brainstem nuclei in core autism features ” published in the journal Autism Research , shows that several core autism features, such as social communication differences and restrictive or repetitive behavior, may be directly related to the areas of the brainstem involved in autonomic functions, which may lead autistic individuals to experience or interpret the world’s stimuli in different ways.

Travers and her team utilized diffusion tensor imaging (DTI), a type of magnetic resonance imaging (MRI) that specifically measures how water diffuses through different tissue types, to look at the brainstem’s structure in autistic individuals and non-autistic individuals. Traditionally, the brainstem is a hard structure to image due to its location and the similarities in its tissue composition. DTI makes it possible to visualize the brainstem’s unique structure and tissue composition. Travers found differences in the autonomic nervous system’s structure between the two groups of participants that correlated with social communication differences and more restrictive or repetitive behavior.
DTI allowed Travers to focus specifically on the nuclei in the brainstem that are involved in our autonomic functions, pain systems, and the limbic system – which handles memory, emotion, and stress responses. “The autonomic nervous system is very much tied to the pain network and also to emotional structures. The original theories were also in line with these parts of the brainstem,” Travers says. “So, we chose to look at this particular grouping of brainstem structures.”
In particular, Travers found two nuclei in the brainstem that showed microstructural differences in autistic individuals and significant association with core autism features. The first nucleus, LPB, is involved in the pain processing system for internal organs and showed a significant relationship with an increase in repetitive behaviors. The second nucleus, PCRtA, is involved in digestion, swallowing, eating, and cardio-respiration and showed a meaningful relationship with more pronounced social communication challenges. Travers hypothesizes that structural changes in PCRtA could contribute to why it is fairly common for autistic individuals to have gastrointestinal discomfort and struggles eating or swallowing.
“This study was the first to be able to test this 60-year-old hypothesis in living children, and found that specific areas within the brainstem are linked to autism features,” Travers says. “This study directly tests and confirms this prior theory while also extending the literature to show that it is not all of the brainstem but some very specific nuclei that are involved in autonomic processing.”
Even the oldest and most rudimentary part of the brain still presents with great complexity. This work indicates that the brainstem likely plays an important role in the core features of autism but the mechanisms behind it are still a mystery. The study’s results did not reveal to Travers what exact changes to the brainstem are contributing to the core features. “We don’t know from our DTI if it is the myelination or the number of neurons or something else because we’re only looking at how water interacted with the tissues. This study helps us locate differences within the brainstem, but It brings up more questions than it solves,” Travers says. She hopes to answer those questions in future research.
“The brainstem is so important because it is this intersection between the brain and the rest of the body. So much information is transmitted through the brainstem and yet we’ve omitted it from most of our studies,” Travers says. “I’ve learned that the brainstem seems to be important in autism and it’s time that we really dug into this.”
- Facebook Logo
- Twitter Logo
- Linkedin Logo
- Request info
- Majors & Degrees
- Prospective Students
- Current Undergraduate Students
- Current Graduate Students
- Online Students
- Alumni and Friends
- Faculty and Staff
USM Honors College Students Present Thesis Research at Marketing Conference
Tue, 03/26/2024 - 09:14am | By: Van Arnold
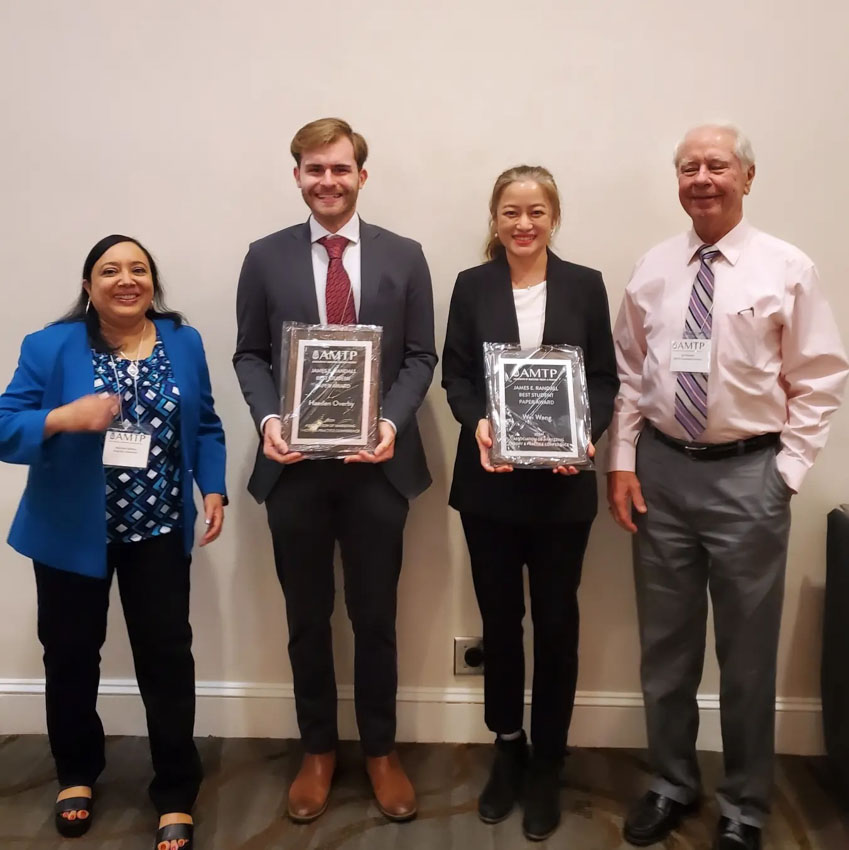
University of Southern Mississippi (USM) Honors College students Haeden Overby and Patrick Tyson presented their thesis research during the 2024 Association of Marketing Theory and Practice Conference (AMTP) held earlier this month in Hilton Head, S.C.
Overby, a hospitality and tourism management major, and Tyson, an anthropology, sociology, and Spanish major, presented individual research papers, as well as a collaborative project, at the international and interdisciplinary academic marketing conference which brings together academic theory and real-world marketing practices.
This year’s conference saw more than 100 submissions. Of the 33 student paper submissions across undergraduate, Master's, and Ph.D. levels, Overby’s thesis paper – “Service robots’ effect on branding and consumers' intentions through online reviews” - won the James E. Randall Best Student Paper Award.
“Haeden and Patrick are exceptional students in my class. Their passion for research and dedication to their thesis projects have impressed me,” said Dr. Wei Wang, Associate Professor, Hospitality and Tourism Management Program Coordinator who served as both students’ thesis advisor. “Moreover, their active involvement and leadership roles on campus exemplify their commitment to excellence beyond academics,”
Tyson presented a research paper titled, “Uncertainty avoidance moderation over film-motivated tourists' views on destination image, place attachment, and intentions.”
Collaboratively, they presented a paper titled, “Cookies and calamari: Squid Game’s “Dalgona” and cutting shapes from its impact on Korean product purchase and travel intentions.”
“My advisors, Dr. Wei Wang and Dr. Banu Bas, have been incredibly patient teaching me about the research process, and I would not have been able to succeed without their support,” said Overby. “Dr. Randall is retiring from the AMTP conference this year, and the award was named after him for the first time. I am pleased to be the first recipient of the award under his name and am incredibly thankful I had the chance to meet him when I was presented the award.”
Added Overby, “This accomplishment has become great motivation to continue my research in marketing and to cross the finish line of submitting my thesis. I am glad to bring home a new award for Southern Miss Business!"
More information about the conference can be found here .
To learn more about USM’s College of Business and Economic Development, call 601.266.4659.
Categories: Business and Economic Development Honors College
Recent News Articles
Six individuals to receive young professional scholarship at the 2024 national sports safety and security conference & exhibition, usm to host annual public health symposium april 3, usm names kennedy as associate vice president for research.
Find Info For
- Current Students
- Prospective Students
- Research and Partnerships
- Entrepreneurship and Commercialization
Quick Links
- Health and Life Sciences
- Info Security and AI
- Transformative Education
- Purdue Today
- Purdue Global
- Purdue in the News
March 27, 2024
Building the first highway segment in the U.S. that can charge electric vehicles big and small as they drive
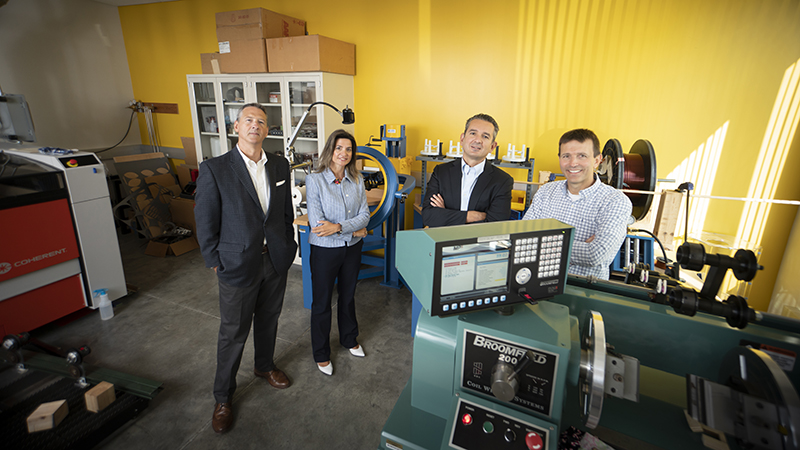
Purdue University engineers John Haddock (left), Nadia Gkritza, Dionysios Aliprantis and Steve Pekarek stand in the lab where they are testing technology they designed to enable all electric vehicle classes to receive power from the road. (Purdue University photo/Vincent Walter)
Construction to begin on test bed in Indiana to develop wireless charging for electric vehicles traveling at highway speeds
WEST LAFAYETTE, Ind. — At the “Crossroads of America,” Purdue University engineers and the Indiana Department of Transportation (INDOT) are working to make it possible for electric vehicles ranging from tractor-trailers to passenger cars to wirelessly charge while driving on highways.
Construction begins as soon as April 1 on a quarter-mile test bed on U.S. Highway 231/U.S. Highway 52 in West Lafayette that the team will use for testing how well a patent-pending system designed by Purdue engineers can provide power to a heavy-duty electric truck traveling at highway speeds.
“Thanks once again to some engineers and pioneers from Purdue, we’re developing the world’s first highway test bed for wireless charging,” said Indiana Gov. Eric Holcomb to attendees of COP27 , a United Nations environmental conference that took place in Egypt in 2022. “Please remember that one. Yes, we will be testing whether concrete can charge passing trucks — and don’t bet against a Purdue Boilermaker.”
The electric truck, provided by Indiana-based company Cummins Inc., will drive over the test bed as part of a pilot program tentatively planned to start next year. The hope is to electrify a section of an Indiana interstate in the next four to five years.
A few other states and countries have also begun testing roads that wirelessly charge EVs. But making this possible for highways — and heavy-duty trucks in particular — is a unique challenge. Because vehicles travel so much faster on highways than city roads, they need to be charged at higher power levels.
The Purdue-designed wireless charging system is intended to work at power levels much higher than what has been demonstrated in the U.S. so far. By accommodating the higher power needs for heavy-duty vehicles, the design is also able to support the lower power needs of other vehicle classes.
Why design electrified highways for trucks first?
An electrified highway in Indiana would serve much of the nation’s traffic. Eighty percent of the U.S. can be reached within a day’s drive from the state’s pass-through highways.
Building electrified highways with heavy-duty trucks in mind would maximize greenhouse gas reductions and the economic feasibility of developing infrastructure for EVs.
Heavy-duty trucks are one of the biggest sources of greenhouse gas emissions for the U.S. transportation sector because they make up a large portion of interstate traffic. Compared to passenger cars, these trucks also need a lot more fuel so that they can constantly transport everything from the packages we order to groceries.
“The so-called ‘middle mile’ of the supply chain, which refers to all the travel heavy-duty trucks have to do to carry goods from one major location to another, is the most challenging part of the transportation sector to decarbonize,” said Nadia Gkritza , a Purdue professor of civil engineering and agricultural and biological engineering .
But if electric heavy-duty trucks could charge or maintain their state-of-charge using highways, their batteries could be smaller in size and they could carry more cargo, significantly reducing the costs of using EVs for freight transportation. Since trucking contributes the most to U.S. gross domestic product compared to other modes of freight transportation, lowering costs for heavy-duty electric trucks could help attract more investment into electrifying highways that all vehicle classes would share.
“We’re developing a system that has the power to charge semitractor-trailers as they move 65 miles per hour down the road,” John Haddock , a professor in Purdue’s Lyles School of Civil Engineering, told U.S. News & World Report .
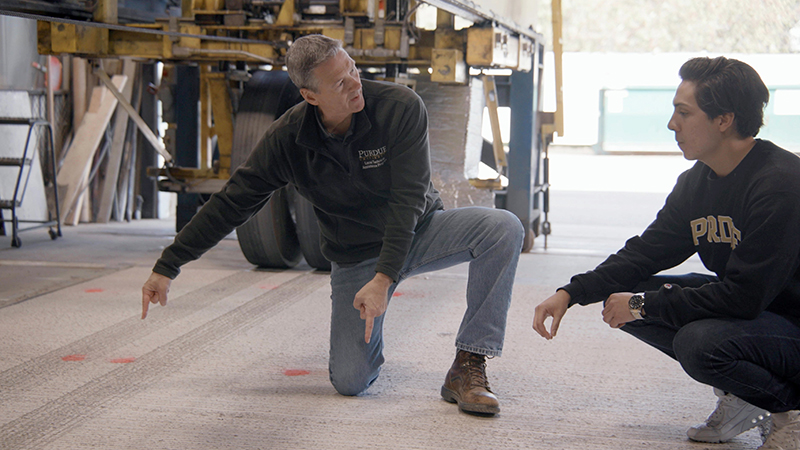
Highways that charge EVs like a smartphone
The technology Purdue is developing would enable highway pavement to provide power to EVs similarly to how newer smartphones use magnetic fields to wirelessly charge when placed on a pad.
“If you have a cellphone and you place it on a charger, there is what’s called magnetic fields that are coming up from the charger into that phone. We’re doing something similar. The only thing that’s different is the power levels are higher and you’re going out across a large distance from the roadway to the vehicle,” said Steve Pekarek , Purdue’s Edmund O. Schweitzer, III Professor of Electrical and Computer Engineering , in an episode of “American Innovators,” a Made in America series by Consensus Digital Media . “This is a simple solution. There are complicated parts of it, and that we leave to the vehicle manufacturers.”
In the wireless charging system that Purdue researchers have designed, transmitter coils would be installed in specially dedicated lanes underneath normal concrete pavement and send power to receiver coils attached to the underside of a vehicle.
Other wireless EV charging efforts are also using transmitter and receiver coils, but they haven’t been designed for the higher power levels that heavy-duty trucks need. The Purdue-designed coils accommodate a wider power range — larger vehicles wouldn’t need multiple low-power receiver coils on the trailer to charge from the road, which has been proposed to meet the high-power demands. Instead, in the Purdue design, a single receiver coil assembly is placed under the tractor, greatly simplifying the overall system.
Purdue researchers have also designed the transmitter coils to work within concrete pavement, which makes up 20% of the U.S. interstate system . Other coil designs have only been developed for use in asphalt pavement.
“The whole idea is if you can charge your car on the road while in motion, then you’re basically riding for free,” Aaron Brovont , a Purdue research assistant professor in Purdue’s Elmore Family School of Electrical and Computer Engineering, explained in a Scripps news segment .

The team has completed testing of how well 20-foot-long sections of concrete and asphalt could handle heavy loads with the transmitter coils embedded. The researchers imitated truck traffic by having a machine repeatedly drive a loaded one-half semi axle over the pavements.
Alongside the pavement mechanical tests, the team has also done lab tests verifying the electromagnetic performance of the bare transmitter coils and the receiver coils.
Laying the groundwork for highways that recharge EVs everywhere
As reported by The New York Times , CNBC , Scripps , Popular Mechanics and other news outlets, the research has the potential to define what EV charging looks like on highways.
The team’s partnerships are not just in Indiana, but also throughout the country. In addition to its funding from INDOT through the Joint Transportation Research Program at Purdue, the project is affiliated with a fourth-generation National Science Foundation Engineering Research Center called Advancing Sustainability through Powered Infrastructure for Roadway Electrification (ASPIRE) , dedicated to progressing the field of electrified transportation in all its forms.
Most real-world deployments of wireless pavement charging in the U.S. are led by members of ASPIRE. Purdue is a founding member of ASPIRE and Gkritza is the campus director of ASPIRE’s Purdue location.
Headquartered at Utah State University, ASPIRE integrates academia, scientific research, and real-world tests and deployments across more than 400 members from 10 partner universities: Purdue, the University of Colorado Boulder, the University of Texas at El Paso, the University of Auckland in New Zealand, Colorado State University, the University of Colorado Colorado Springs, Virginia Tech, Cornell University, and the University of Utah. These universities are joined by more than 60 industry, government and nonprofit members across all sections of the electric transportation ecosystem, as well as community partners and advisors.
ASPIRE’s members at Purdue and Cummins are also leading a project funded by the U.S. Department of Energy to develop an EV charging and hydrogen fueling plan for medium-duty and heavy-duty trucks on the Midwest’s Interstate 80 corridor. The corridor serves Indiana, Illinois and Ohio. The plan will examine the use of the wireless power transfer technology that Gkritza and her team are testing in West Lafayette.
“We don’t envision 100% of the roads being electrified,” Gkritza said in an episode of “Resources Radio,” a podcast by Washington, D.C., research institution Resources for the Future . “But we see the potential for dynamic wireless power pavement technology as complementary to an expanding network of EV charging stations that we will see very soon here in the U.S. We feel it would be useful in areas where charging stations are scarce in underserved communities, even supporting transit routes where initial charging at the depots and terminal stations might not be enough and there might need to be some charging in between the routes.”
The researchers anticipate that it may be 20 to 30 years before EVs can receive the full power they need while driving at highway speeds. It is up to EV manufacturers to decide whether to incorporate receiver coils into their vehicles.
“The technical obstacles that we need to overcome are not insurmountable. Those can be overcome with proper design,” Dionysios Aliprantis , a Purdue electrical and computer engineering professor, told The New York Times .
The team hopes that the results of their experiments could help convince the industry that electrified highways could work.
“We are Purdue University, where the difficult is done today and the impossible takes a bit longer,” Haddock said.
ASPIRE’s Purdue location is part of a new Purdue Engineering Initiative, Leading Energy-Transition Advances and Pathways to Sustainability (LEAPS) . The initiative’s mission is to spark and nurture innovations within Purdue to create scalable technologies for the energy transition, transform the nature of energy-focused learning, and accelerate the translation of these technologies through academic-industry synergies.
The researchers have disclosed their innovation to the Purdue Innovates Office of Technology Commercialization , which has applied for a patent on the intellectual property. Industry partners interested in developing or commercializing the work should contact Matt Halladay, senior business development manager and licensing manager, physical sciences, at [email protected] about track codes 2022-ALIP-69682, 2024-PEKA-70401 and 2024-PEKA-70402.
About Purdue University
Purdue University is a public research institution demonstrating excellence at scale. Ranked among top 10 public universities and with two colleges in the top four in the United States, Purdue discovers and disseminates knowledge with a quality and at a scale second to none. More than 105,000 students study at Purdue across modalities and locations, including nearly 50,000 in person on the West Lafayette campus. Committed to affordability and accessibility, Purdue’s main campus has frozen tuition 13 years in a row. See how Purdue never stops in the persistent pursuit of the next giant leap — including its first comprehensive urban campus in Indianapolis, the new Mitchell E. Daniels, Jr. School of Business, and Purdue Computes — at https://www.purdue.edu/president/strategic-initiatives .
Writer/Media contact: Kayla Albert, 765-494-2432, [email protected]
Nadia Gkritza, [email protected]
John Haddock, [email protected]
Dionysios Aliprantis, [email protected]
Steve Pekarek, [email protected]
Aaron Brovont, [email protected]
Note to journalists: Photos and video of the researchers and their experiments , in addition to b-roll of Purdue University’s campus , are available via Google Drive.
Research News
Communication.
- OneCampus Portal
- Brightspace
- BoilerConnect
- Faculty and Staff
- Human Resources
- Colleges and Schools
Info for Staff
- Purdue Moves
- Board of Trustees
- University Senate
- Center for Healthy Living
- Information Technology
- Ethics & Compliance
- Campus Disruptions
Purdue University, 610 Purdue Mall, West Lafayette, IN 47907, (765) 494-4600
© 2015-24 Purdue University | An equal access/equal opportunity university | Copyright Complaints | Maintained by Office of Strategic Communications
Trouble with this page? Disability-related accessibility issue? Please contact News Service at [email protected] .
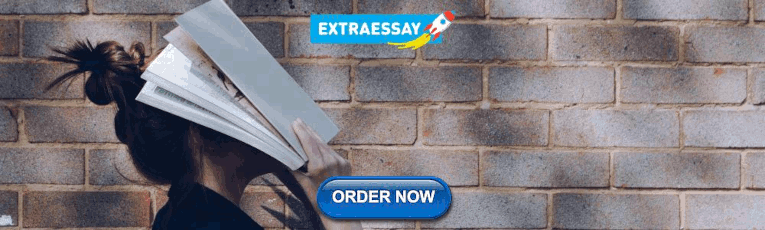
IMAGES
VIDEO
COMMENTS
A research design is a strategy for answering your research question using empirical data. Creating a research design means making decisions about: Your overall research objectives and approach. Whether you'll rely on primary research or secondary research. Your sampling methods or criteria for selecting subjects. Your data collection methods.
Types of Research Designs Compared | Guide & Examples. Published on June 20, 2019 by Shona McCombes.Revised on June 22, 2023. When you start planning a research project, developing research questions and creating a research design, you will have to make various decisions about the type of research you want to do.. There are many ways to categorize different types of research.
In this sense, building a research design for process-tracing is the same as in any other attempt at causal inference. There is, however, one important distinction. In process-tracing, we are concerned not only with our theory of interest; we also must juxtapose rival explanations that we intend to test (Hall Reference Hall 2013 ; Rohlfing ...
2. Research is the process of making claims and then refining or aban-doning some of them for other claims more strongly warranted. Most quantitative research, for example, starts with the test of a theory. 3. Data, evidence, and rational considerations shape knowledge. In prac-tice, the researcher collects information on instruments based on ...
Step 1: Consider your aims and approach. Step 2: Choose a type of research design. Step 3: Identify your population and sampling method. Step 4: Choose your data collection methods. Step 5: Plan your data collection procedures. Step 6: Decide on your data analysis strategies. Frequently asked questions.
Research design refers to the overall plan, structure or strategy that guides a research project, from its conception to the final analysis of data. Research designs for quantitative studies include descriptive, correlational, experimental and quasi-experimenta l designs. Research designs for qualitative studies include phenomenological ...
Research design is a comprehensive plan for data collection in an empirical research project. It is a 'blueprint' for empirical research aimed at answering specific research questions or testing specific hypotheses, and must specify at least three processes: the data collection process, the instrument development process, and the sampling process.
Purposive sampling is often used in qualitative research, with a goal of finding information-rich cases, not to generalize. 6. Be reflexive: Examine the ways in which your history, education, experiences, and worldviews have affected the research questions you have selected and your data collection methods, analyses, and writing. 13. Go to:
Research Design and Research Methods 49 your earlier observations and interviews. This approach calls for a flexible merger of data collection and analysis, since it is impossible to know when your observations will become analytic insights. The procedures associated with deduction are, necessarily, quite different. In particular, theory testing
The deductive approach involves formulating a hypothesis (or hypotheses) depending on the current theory and then devising a research procedure to test it (Wilson, 2010).According to Beiske (), the deductive research design investigates a familiar theory or phenomenon and examines whether it is true in a given situation.The deductive method is the one that most nearly follows the logical path.
Definition: Quantitative research seeks to quantify data and generalize results from a sample to the population of interest. It involves measurable, numerical data and often uses statistical methods for analysis. This approach is suitable for testing hypotheses or examining relationships between variables.
Effectively describe the data which will be necessary for an adequate test of the hypotheses and explain how such data will be obtained, and ... Sage, 2008), pp. 440-441; Ployhart, Robert E. and Robert J. Vandenberg. "Longitudinal Research: The Theory, Design, and Analysis of Change." Journal of Management 36 (January 2010): 94-120 ...
Before beginning your paper, you need to decide how you plan to design the study.. The research design refers to the overall strategy and analytical approach that you have chosen in order to integrate, in a coherent and logical way, the different components of the study, thus ensuring that the research problem will be thoroughly investigated. It constitutes the blueprint for the collection ...
Research design framework: summary of the interplay between the essential grounded theory methods and processes. Grounded theory research involves the meticulous application of specific methods and processes. Methods are 'systematic modes, procedures or tools used for collection and analysis of data'. 25 While GT studies can commence with a ...
There are at least three primary applications of theory in qualitative research: (1) theory of research paradigm and method (Glesne, 2011), (2) theory building as a result of data collection (Jaccard & Jacoby, 2010), and (3) theory as a framework to guide the study (Anfara & Mertz, 2015). Differentiation and clarification between these ...
The purpose of research design is to plan and structure a research study in a way that enables the researcher to achieve the desired research goals with accuracy, validity, and reliability. Research design is the blueprint or the framework for conducting a study that outlines the methods, procedures, techniques, and tools for data collection ...
Research Design A research design is the 'procedures for collecting, analyzing, interpreting and reporting data in research studies' (Creswell & Plano Clark 2007, p.58). ... use to test a theory ...
For instance, if the test scores of a class are an outcome of their efforts; efforts are an independent variable, and the score is a dependent variable. Hypothesis. ... Descriptive design is a theory-based research method describing the research's primary subject matter. This type of research design uses data collection techniques like ...
Köhler and Cortina (2021) described the types of research design and method issues associated with constructive replications including the use of more valid measures, more theoretically relevant control variables, more realistic experimental manipulations or tasks, a more representative sample, and stronger causal design. These have the virtue ...
The choice of the research design is influenced by the type of evidence needed to answer the research question (Akhtar, 2016), and it can be qualitative, quantitative, or a combination of both ...
In order to properly test Fischer and Verrecchia's (2000) theory, Ferri et al. necessarily make a host of auxiliary assumptions—many of which are implicit—to ensure that their research design "tests" (i.e., has the potential to falsify) Fischer and Verrecchia's theory. 14 It is important to acknowledge and justify such assumptions that ...
Research design is the plan, structure and strategy and investigation concaved so as to obtain search question and control variance" (Borwankar, 1995). Henry Manheim says that research design not ...
Here are eight tips to create effective tests, based on a review of more than a dozen recent studies. 1. HELP STUDENTS DEVELOP GOOD TEST PREP HABITS. Students often overestimate how prepared they are for an upcoming test, which can result in unexpected low performance, according to a 2017 study.
Background: Despite the impact of physical abuse on children, it is often underdiagnosed, especially among children evaluated in general emergency departments (EDs) and those belonging to racial or ethnic minority groups. Electronic clinical decision support (CDS) can improve the recognition of child physical abuse. Objective: We aimed to develop and test the usability of a natural language ...
This new research by Brittany Travers, PhD, Waisman investigator and associate professor of kinesiology, is the first to officially test this hypothesis in children thanks to the advancements in brain imaging techniques. Her work reveals that the brainstem may indeed be central to core autism features. "It is a pivotal brain structure and ...
Overby, a hospitality and tourism management major, and Tyson, an anthropology, sociology, and Spanish major, presented individual research papers, as well as a collaborative project, at the international and interdisciplinary academic marketing conference which brings together academic theory and real-world marketing practices.
Headquartered at Utah State University, ASPIRE integrates academia, scientific research, and real-world tests and deployments across more than 400 members from 10 partner universities: Purdue, the University of Colorado Boulder, the University of Texas at El Paso, the University of Auckland in New Zealand, Colorado State University, the ...