- Skip to Content
- Catalog Home
- Institution Home
- The University of Chicago
- Academic Calendar
- General Information
- Interdivisional Programs
- The Division of the Biological Sciences and the Pritzker School of Medicine
- The Pritzker School of Medicine
- The Division of the Humanities
- Master of Science Program in Applied Data Science
- Master of Science Program in Applied Data Science-Online
- Master of Science Program in Computer Science
- Master of Science Program in Financial Mathematics
- Department of Astronomy and Astrophysics
- Graduate Program in Biophysical Sciences
- Department of Chemistry
- Committee on Computational and Applied Mathematics
- Department of Computer Science
- Department of the Geophysical Sciences
- Department of Mathematics
- Department of Physics
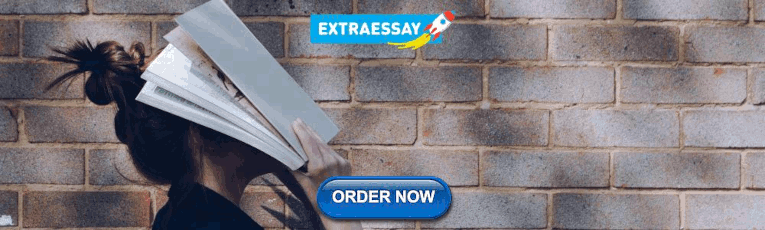
Department of Statistics
- The Division of the Social Sciences
- The William B. and Catherine V. Graham School of Continuing Liberal and Professional Studies
- The University of Chicago Booth School of Business
- The Divinity School
- The Law School
- The Pritzker School of Molecular Engineering
- The Irving B. Harris Graduate School of Public Policy Studies
- The Crown Family School of Social Work, Policy, and Practice
- Matthew Stephens, Statistics and Human Genetics
- Yali Amit, Statistics
- Mihai Anitescu, Statistics and Argonne National Laboratory
- Guillaume Bal, Statistics and Mathematics
- Rina Foygel Barber
- Brent Doiron, Neurobiology and Statistics
- Lars Peter Hansen, Economics and Statistics
- Gregory F. Lawler, Mathematics and Statistics
- Lek-Heng Lim
- Mary Sara McPeek, Statistics and Human Genetics
- Per Mykland, Statistics and the Stevanovich Center
- Dan Liviu Nicolae, Statistics and Medicine
- John Reinitz, Statistics, Ecology and Evolution, and Molecular Genetics and Cell Biology
- Mary Silber
- Rebecca Willett, Statistics and Computer Science
- Kirk M. Wolter
- Wei Biao Wu
Associate Professors
- Imre Risi Kondor, Computer Science and Statistics
Assistant Professors
- Claire Donnat
- Jeremy Hoskins
- Yuehaw Khoo
- Alisa Knizel
- Daniel Sanz-Alonso
- Victor Veitch
- Jingshu Wang
Senior Instructional Professors
- David Biron
- Kendra Burbank
Instructional Professors
Instructors
- Daniel Massatt
- Bradley J. Nelson
- Benjamin Palacios
- Alexander Strang
- Fatma Terzioglu
- Zhongjian Wang
- Pierre Yves Gaudreau Lamarre
The Department of Statistics offers an exciting and revamped graduate program that prepares students for cutting-edge interdisciplinary research in a wide variety of fields. The field of statistics has become a core component of research in the biological, physical, and social sciences, as well as in traditional computer science domains such as artificial intelligence. In light of this, the Department of Statistics is currently undergoing a major expansion of approximately ten new faculty into fields of Computational and Applied Mathematics. The massive increase in the data acquired, through scientific measurement on one hand and through web-based collection on the other, makes the development of statistical analysis and prediction methodologies more relevant than ever. Our graduate program aims to prepare students to address these issues through rigorous training in theory, methodology, and applications of statistics; rigorous training in scientific computation; and research projects in core methodology of statistics and computation as well as in a wide variety of interdisciplinary fields.
The Department of Statistics offers two tracks of graduate study, one leading to the Master of Science (M.S.) degree, the other to the Doctorate of Philosophy (Ph.D.). The M.S. degree is a professional degree. Students who receive this degree are prepared for nonacademic careers in which the use of advanced statistical and computational methods is of central importance. The program also prepares students for possible further graduate study.
During the first year of the Ph.D. program, students are given a thorough grounding in material that forms the foundations of modern statistics and scientific computation, including data analysis, mathematical statistics, probability theory, applied probability and modeling, and computational methods. Throughout the entire program, students attend a weekly consulting seminar where researchers from across the University come to get advice on modeling, statistical analysis, and computation. This seminar is often the source of interesting and ongoing research projects.
In the second year, students have a wide range of choices of topics they can pursue further, based on their interests, through advanced courses and reading courses with faculty. During the second year, students will typically identify their subfield of interest, take some advanced courses in the subject, and interact with the relevant faculty members. The Department maintains very strong connections to numerous other units on campus, either through joint appointments of the faculty or through ongoing collaborations. Students have easy access to faculty in other departments, which allows them to expand their interactions and develop new interdisciplinary research projects. Examples include joint projects with Human Genetics, Ecology and Evolution, Neurobiology, Chemistry, Economics, Health Studies, and Astronomy.
Programs and Requirements for the Ph.D.
The program offers four core sequences:
- Probability (STAT 30400, 38100, 38300)
- Mathematical statistics (STAT 30400, 30100, 30210)
- Applied statistics (STAT 34300, 34700, 34800)
- Computational mathematics and machine learning (STAT 30900, 31015/31020, 37710)
All students must take the applied statistics sequence and one of the two theoretical sequences: mathematical statistics or probability. At the start of their second year, the students take preliminary examinations covering the two sequences they have taken. In addition, it is highly recommended that students take a third core sequence based on their interests and in consultation with the Department Graduate Adviser (DGA). Incoming first-year students may request the DGA to take one or both of their preliminary exams. If approved, and if the student passes one or more of these, the student will then be excused from the requirement of taking the first-year courses in that subject. Incoming students are advised by the DGA until they find a faculty adviser for their Ph.D. thesis work.
In their second year, Ph.D. students typically take a number of advanced topics: courses in statistics, probability, computation, and applications. These should be selected with the dual objective of (i) acquiring a broad overview of current research areas, and (ii) settling on a particular research topic and dissertation supervisor. It is recommended that the students take at least one regular class based course each quarter. In addition, students can ask to take reading courses with faculty to learn more in depth about their fields of research. Students have considerable latitude in selecting their second-year courses, but their programs must be approved by the Department Graduate Adviser. Students are expected to find a dissertation adviser by the end of the second year. The detailed process is listed here .
The Ph.D.: Training in Teaching, Presentation, and Consulting
Part of every statistician's job is to evaluate the work of others and to communicate knowledge, experience, and insights. Every statistician is, to some extent, an educator, and the department provides graduate students with training for this aspect of their professional lives. The department expects all doctoral students, regardless of their professional objectives and sources of financial support, to take part in a graduated program of participation in some or all phases of instruction, from grading, course assisting, and conducting discussion sections, to being a lecturer with responsibility for an entire course.
Students also receive training in how to present research in short seminars in the first and second years of study. Later, students present their own work in a dissertation proposal and, eventually, in a thesis defense. The student seminars are listed here .
Ph.D. students should also participate in the department's consulting program , which is led by faculty members and exposes the students to empirical projects inside the university. Projects are carried out by groups of students under the guidance of a faculty member. The client is a researcher in an applied area, usually associated with the university. An informal seminar meets regularly over lunch to provide a forum for presenting and discussing problems, solutions, and topics in statistical consultation. Students present interesting or difficult consulting problems to the seminar as a way of stimulating wider consideration of the problem and as a means of developing familiarity with the kinds of problems and lines of attack involved. Often the client will participate in the presentation and discussion.
Programs and Requirements for the M.S. degree
The main requirements of the M.S. program are a sequence of at least nine approved courses plus a Master's paper. Students may take up to two years of courses. A detailed set of regulations can be found here . A substantial fraction of available courses are the same as for the Ph.D. degree.
Almost all departmental activities–classes, seminars , computation , and student and faculty offices –are located in Jones Laboratory . Each student is assigned a desk in one of several offices. The major computing facilities of the department are based upon a network of PCs running mainly Linux. One computer room currently houses many of these PCs; these rooms are directly and primarily for graduate students in the Statistics Department. In addition, all student offices have limited computer facilities. For further information, consult the department’s computing policies .
Statistics Throughout the University
In addition to the courses, seminars, and programs in the Department of Statistics, courses and workshops of direct interest to statisticians occur throughout the University, most notably in the programs in statistics and econometrics in the Booth School of Business and in the research programs in Public Health Sciences , Human Genetics , Financial Mathematics and Econometrics , Computer Science , Economics , and NORC (formerly the National Opinion Research Center). The large number of statistics related seminars is perhaps the best indication of the vibrancy of the statistics research community here at the University of Chicago.
Statistics Courses
STAT 30030. Statistical Theory and Methods Ia. 100 Units.
This course is the first quarter of a two-quarter sequence providing a principled development of statistical methods, including practical considerations in applying these methods to the analysis of data. The course begins with a brief review of probability and some elementary stochastic processes, such as Poisson processes, that are relevant to statistical applications. The bulk of the quarter covers principles of statistical inference from both frequentist and Bayesian points of view. Specific topics include maximum likelihood estimation, posterior distributions, confidence and credible intervals, principles of hypothesis testing, likelihood ratio tests, multinomial distributions, and chi-square tests. Additional topics may include diagnostic plots, bootstrapping, a critical comparison of Bayesian and frequentist inference, and the role of conditioning in statistical inference. Examples are drawn from the social, physical, and biological sciences. The statistical software package R will be used to analyze datasets from these fields and instruction in the use of R is part of the course.
Instructor(s): Staff Terms Offered: Autumn Prerequisite(s): STAT 25100 or STAT 25150 or MATH 23500. This course is only open to graduate students in Statistics, Applied Mathematics, and Financial Mathematics, and to undergraduate Statistics majors, or by consent of instructor. Note(s): Some previous experience with statistics helpful but not required. Concurrent or prior linear algebra (MATH 18600 or 19620 or 20250 or 20700 or STAT 24300 or equivalent) is recommended for students continuing to STAT 24510. Students may count either STAT 24400 or STAT 24410, but not both, toward the forty-two credits required for graduation. Equivalent Course(s): STAT 24410
STAT 30040. Statistical Theory and Methods IIa. 100 Units.
This course is a continuation of STAT 24410. The focus is on theory and practice of linear models, including the analysis of variance, regression, correlation, and some multivariate analysis. Additional topics may include bootstrapping for regression models, nonparametric regression, and regression models with correlated errors.
Terms Offered: Winter Prerequisite(s): STAT 24410 and linear algebra (MATH 18600 or 19620 or 20250 or 20700 or STAT 24300 or equivalent). This course is only open to graduate students in Statistics, Applied Mathematics, and Financial Mathematics, and to undergraduate Statistics majors, or by consent of instructor. Note(s): Students may count either STAT 24500 or STAT 24510, but not both, toward the forty-two credits required for graduation. Equivalent Course(s): STAT 24510
STAT 30100. Mathematical Statistics-1. 100 Units.
This course is part of a two-quarter sequence on the theory of statistics. Topics will include exponential, curved exponential, and location-scale families; mixtures, hierarchical, and conditional modeling including compatibility of conditional distributions; principles of estimation; identifiability, sufficiency, minimal sufficiency, ancillarity, completeness; properties of the likelihood function and likelihood-based inference, both univariate and multivariate, including examples in which the usual regularity conditions do not hold; elements of Bayesian inference and comparison with frequentist methods; and multivariate information inequality. Part of the course will be devoted to elementary asymptotic methods that are useful in the practice of statistics, including methods to derive asymptotic distributions of various estimators and test statistics, such as Pearson's chi-square, standard and nonstandard asymptotics of maximum likelihood estimators and Bayesian estimators, asymptotics of order statistics and extreme order statistics, Cramer's theorem including situations in which the second-order term is needed, and asymptotic efficiency. Other topics (e.g., methods for dependent observations) may be covered if time permits.
Instructor(s): Staff Terms Offered: Winter Prerequisite(s): STAT 30400 or consent of instructor
STAT 30200. Mathematical Statistics-2. 100 Units.
This course continues the development of Mathematical Statistics, with an emphasis on hypothesis testing. Topics include comparison of Bayesian and frequentist hypothesis testing; admissibility of Bayes' rules; confidence and credible sets; likelihood ratio tests and their asymptotics; Bayes factors; methods for assessing predictions for normal means; shrinkage and thresholding methods; sparsity; shrinkage as an example of empirical Bayes; multiple testing and false discovery rates; Bayesian approach to multiple testing; sparse linear regressions (subset selection and LASSO, proof of estimation errors for LASSO, Bayesian perspective of sparse regressions); and Bayesian model averaging.
Instructor(s): Staff Terms Offered: Spring Prerequisite(s): STAT 24500 or STAT 30100
STAT 30400. Distribution Theory. 100 Units.
This course is a systematic introduction to random variables and probability distributions. Topics include standard distributions (i.e. uniform, normal, beta, gamma, F, t, Cauchy, Poisson, binomial, and hypergeometric); properties of the multivariate normal distribution and joint distributions of quadratic forms of multivariate normal; moments and cumulants; characteristic functions; exponential families; modes of convergence; central limit theorem; and other asymptotic approximations.
Instructor(s): Staff Terms Offered: Autumn Prerequisite(s): STAT 24500 or STAT 24510 and MATH 20500 or MATH 20510, or consent of instructor.
STAT 30600. Adv. Statistical Inference 1. 100 Units.
Topics covered in this course will include: Gaussian distributions; conditional distributions; maximum likelihood and REML; Laplace approximation and associated expansion; combinatorics and the partition lattice; Mobius inversion; moments, cumulants symmetric functions, and $k$-statistics; cluster expansions; Bartlett identities and Bartlett adjustment; random partitions, partition processes, and CRP process; Gauss-Ewens cluster process; classification models; trees rooted and unrooted; exchangeable random trees; and Cox processes used for classification.
Terms Offered: To be determined; may not offered in 2020-2021. Prerequisite(s): Consent of instructor
STAT 30800. Advanced Statistical Inference II. 100 Units.
This course will discuss the following topics in high-dimensional statistical inference: random matrix theory and asymptotics of its eigen-decompositions, estimation and inference of high-dimensional covariance matrices, large dimensional factor models, multiple testing and false discovery control and high-dimensional semiparametrics. On the methodological side, probability inequalities, including exponential, Nagaev, and Rosenthal-type inequalities will be introduced.
Terms Offered: To be determined; may not be offered in 2020-2021. Prerequisite(s): STAT 30400, STAT 30100, and STAT 30210, or consent of instructor
STAT 30810. High Dimensional Time Series Analysis. 100 Units.
This course will include lectures on the following topics: review of asymptotics for low dimensional time series analysis (linear and nonlinear processes; nonparametric methods; spectral and time domain approaches); covariance, precision, and spectral density matrix estimation for high dimensional time series; factor models; estimation of high dimensional vector autoregressive processes; prediction; and high dimensional central limit theorems under dependence.
Terms Offered: To be determined
STAT 30850. Multiple Testing, Modern Inference, and Replicability. 100 Units.
This course examines the problems of multiple testing and statistical inference from a modern point of view. High-dimensional data is now common in many applications across the biological, physical, and social sciences. With this increased capacity to generate and analyze data, classical statistical methods may no longer ensure the reliability or replicability of scientific discoveries. We will examine a range of modern methods that provide statistical inference tools in the context of modern large-scale data analysis. The course will have weekly assignments as well as a final project, both of which will include both theoretical and computational components.
Terms Offered: Winter Prerequisite(s): STAT 24400 or STAT 24410. Familiarity with regression and with coding in R are recommended. Equivalent Course(s): STAT 27850
STAT 30900. Mathematical Computation I: Matrix Computation Course. 100 Units.
This is an introductory course on numerical linear algebra, which is quite different from linear algebra. We will be much less interested in algebraic results that follow from axiomatic definitions of fields and vector spaces but much more interested in analytic results that hold only over the real and complex fields. The main objects of interest are real- or complex-valued matrices, which may come from differential operators, integral transforms, bilinear and quadratic forms, boundary and coboundary maps, Markov chains, correlations, DNA microarray measurements, movie ratings by viewers, friendship relations in social networks, etc. Numerical linear algebra provides the mathematical and algorithmic tools for analyzing these matrices. Topics covered: basic matrix decompositions LU, QR, SVD; Gaussian elimination and LU/LDU decompositions; backward error analysis, Gram-Schmidt orthogonalization and QR/complete orthogonal decompositions; solving linear systems, least squares, and total least squares problem; low-rank matrix approximations and matrix completion. We shall also include a brief overview of stationary and Krylov subspace iterative methods; eigenvalue and singular value problems; and sparse linear algebra.
Terms Offered: Autumn Prerequisite(s): Linear algebra (STAT 24300 or equivalent) and some previous experience with statistics. Equivalent Course(s): CMSC 37810, CAAM 30900
STAT 31001. Modern Applied Optimization. 100 Units.
This course assumes no background in optimization. The focus will be on various classical and modern algorithms, with a view towards applications in finance, machine learning, and statistics. In the first half of the course we will go over classical algorithms: univariate optimization and root finding (Newton, secant, regula falsi, etc), unconstrained optimization (steepest descent, Newton, quasi-Newton, Gauss-Newton, Barzilai-Borwein, etc), constrained optimization (penalty, barrier, augmented Lagrangian, active set, etc). In the second half of the course we will cover algorithms that have become popular over the last decade: proximal algorithms, stochastic gradient descent and variants, algorithms that involve moments or momentum or mirror, etc. Applications to machine learning and statistics will include ridge/lasso/logistic regression, support vector machines with hinge/sigmoid loss, optimal experimental designs, maximum entropy, maximum likelihood, Gaussian covariance estimation, feedforward neural networks, etc. Applications in finance will include Markowitz classical portfolio optimization, portfolio optimization with diversification or loss risk constraints, bounding portfolio risks with incomplete covariance information, log optimal investment strategy, etc.
Instructor(s): Lek-Heng Lim Terms Offered: Autumn Equivalent Course(s): CAAM 31001, FINM 34800
STAT 31015. Mathematical Computation IIA: Convex Optimization. 100 Units.
The course will cover techniques in unconstrained and constrained convex optimization and a practical introduction to convex duality. The course will focus on (1) formulating and understanding convex optimization problems and studying their properties; (2) understanding and using the dual; and (3) presenting and understanding optimization approaches, including interior point methods and first order methods for non-smooth problems. Examples will be mostly from data fitting, statistics and machine learning.
Instructor(s): Zhiyuan Li Terms Offered: Winter Prerequisite(s): STAT 30900 or STAT 31430 or consent of instructor. Note(s): In addition to the required prerequisites, background in analysis in R^n (at the level of MATH 20400) is recommended. Equivalent Course(s): CAAM 31015, CMSC 35470, BUSN 36903, TTIC 31070
STAT 31020. Mathematical Computation IIB: Nonlinear Optimization. 100 Units.
This course covers the fundamentals of continuous optimization with an emphasis on algorithmic and computational issues. The course starts with the study of optimality conditions and techniques for unconstrained optimization, covering line search and trust region approaches, and addressing both factorization-based and iterative methods for solving the subproblems. The Karush-Kuhn-Tucker conditions for general constrained and nonconvex optimization are then discussed and used to define algorithms for constrained optimization including augmented Lagrangian, interior-point and (if time permits) sequential quadratic programming. Iterative methods for large sparse problems, with an emphasis on projected gradient methods, will be presented. Several substantial programming projects (using MATLAB and aiming at both data-intensive and physical sciences applications) are completed during the course.
Terms Offered: Winter Prerequisite(s): STAT 30900 or STAT 31430 or consent of instructor. Equivalent Course(s): CAAM 31020
STAT 31050. Applied Approximation Theory. 100 Units.
This course covers a range of introductory topics in applied approximation theory, the study of how and when functions can be approximated by linear combinations of other functions. The course will start with classical topics including polynomial and Fourier approximation and convergence, as well as more general theory on bases and approximability. We will also look at algorithms and applications in function compression, interpolation, quadrature, denoising, compressive sensing, finite-element methods, spectral methods, and iterative algorithms.
Terms Offered: Spring Prerequisite(s): A strong background in real analysis. Graduate student in Statistics or CCAM/MCAM or consent of instructor. Equivalent Course(s): CAAM 31050
STAT 31080. Numerical Analysis for Statistics and Applied Mathematics. 100 Units.
This is a beginning graduate course on selected numerical methods used in modern statistics and applied mathematics. Topics include fundamentals of ODEs and PDEs, quadratures, and Monte Carlo methods. Methods of analysis are introduced including error measures and different notions of numerical convergence. Newton's method, convex optimization and elements of nonconvex optimization are covered, together with implementations in selected selected software packages.
Terms Offered: To be determined Prerequisite(s): STAT 24300 or background in linear algebra.
STAT 31100. Mathematical Computation III: Numerical Methods for PDE's. 100 Units.
The first part of this course introduces basic properties of PDE's; finite difference discretizations; and stability, consistency, convergence, and Lax's equivalence theorem. We also cover examples of finite difference schemes; simple stability analysis; convergence analysis and order of accuracy; consistency analysis and errors (i.e., dissipative and dispersive errors); and unconditional stability and implicit schemes. The second part of this course includes solution of stiff systems in 1, 2, and 3D; direct vs. iterative methods (i.e., banded and sparse LU factorizations); and Jacobi, Gauss-Seidel, multigrid, conjugate gradient, and GMRES iterations.
Terms Offered: Spring Prerequisite(s): Some prior exposure to differential equations and linear algebra Equivalent Course(s): CAAM 31100, CMSC 37812, MATH 38309
STAT 31120. Numerical Methods for Stochastic Differential Equations. 100 Units.
The numerical analysis of SDE differs significantly from that of ODE due to the peculiarities of stochastic calculus. This course starts with a brief review of stochastic calculus and stochastic differential equations, then emphasizing the numerical methods needed to solve such equations. The stochastic Taylor expansion provides the basis for the discrete-time numerical methods for differential equations. The course presents many results on high-order methods for strong sample path approximations and for weak functional approximations. To help with developing an intuitive understanding of the underlying mathematics and hand-on numerical skills, examples and exercises on PC are included.
Terms Offered: Spring Prerequisite(s): Knowledge of ODE and SDE is essential. STAT 39000 or STAT 39010 or STAT 38510 are strongly recommended. Equivalent Course(s): CAAM 31120
STAT 31140. Computational Imaging: Theory and Methods. 100 Units.
Computational imaging refers to the process of forming images from data where computation plays an integral role. This course will cover basic principles of computational imaging, including image denoising, regularization techniques, linear inverse problems and optimization-based solvers, and data acquisition models associated with tomography and interferometry. Specific topics may include patch-based denoising, sparse coding, total variation, dictionary learning, computational photography, compressive imaging, inpainting, and deep learning for image reconstruction.
Instructor(s): R. Willett Terms Offered: To be determined Equivalent Course(s): CMSC 31140, CAAM 31140
STAT 31150. Inverse Problems and Data Assimilation. 100 Units.
This class provides an introduction to Bayesian Inverse Problems and Data Assimilation, emphasizing the theoretical and algorithmic inter-relations between both subjects. We will study Gaussian approximations and optimization and sampling algorithms, including a variety of Kalman-based and particle filters as well as Markov chain Monte Carlo schemes designed for high-dimensional inverse problems.
Instructor(s): D. Sanz-Alonso Terms Offered: Autumn Prerequisite(s): Familiarity with calculus, linear algebra, and probability/statistics at the level of STAT 24400 or STAT 24410. Some knowledge of ODEs may also be helpful. Equivalent Course(s): CAAM 31150
STAT 31190. Fast Algorithms. 100 Units.
This course will introduce students to several classes of computational methods broadly referred to as "fast analysis-based algorithms" which exploit information about structure and symmetry to obtain more favorable computational complexity. Examples which will be discussed are butterfly algorithms, fast multipole methods, fast direct solvers, and hierarchical matrix compression. Though many of these algorithms first arose in physical applications such as simulating the motion of stars or the propagation of light and sound, they have subsequently found many fruitful applications in signal processing and data science.
Terms Offered: Spring Prerequisite(s): Familiarity with PDEs, analysis, and programming. Equivalent Course(s): CAAM 31190
STAT 31200. Introduction to Stochastic Processes I. 100 Units.
This course will introduce some of the major classes of stochastic processes: Poisson processes, renewal processes, Markov chains, continuous time Markov processes, random walks, martingales, and Brownian motion. A substantial part of the course will be devoted to the study of important examples. Students will be expected to have proficiency in elementary probability theory, basic real analysis (especially sequences and series), and matrix algebra. Some familiarity with the theory of Lebesgue measure and integration would be helpful.
Instructor(s): Staff Terms Offered: To be determined Prerequisite(s): STAT 25100 and MATH 20500; STAT 30400 or consent of instructor Note(s): Students with credit for MATH 235 should not enroll in STAT 312.
STAT 31210. Applied Functional Analysis. 100 Units.
This course will cover classical topics of applied functional analysis: description of functional spaces such as Banach spaces and Hilbert spaces; properties of linear operators acting on such spaces, compactness and spectral decomposition of compact operators; and applications to ordinary and partial differential equations.
Terms Offered: To be determined Equivalent Course(s): CAAM 31210
STAT 31220. Partial Differential Equations. 100 Units.
This is an introduction to the theory of partial differential equations covering representation formulas and regularity theory for elliptic, parabolic, and hyperbolic equations; the method of characteristics; variational formulations for second-order linear elliptic equations; and the calculus of variations.
Terms Offered: Winter Equivalent Course(s): CAAM 31220
STAT 31230. Inverse Problems in Imaging. 100 Units.
This course focuses on the mathematical description of many inverse problems that appear in geophysical and medical imaging: X-ray tomography, ultrasound tomography and seismic imaging, optical and electrical tomography, as well as more recent imaging modalities such as elastography and photo-acoustic tomography. Seen as reconstructions of constitutive parameters in differential equations from redundant boundary measurements, these continuous models tell us which parameters may or may not be reconstructed, and with which stability with respect to measurement errors. Time-permitting, we will also consider general methodologies to perform such reconstructions (regularization, optimization, Bayesian framework). Some knowledge of PDE and Fourier transforms is recommended.
Terms Offered: Spring Prerequisite(s): STAT 31220 Equivalent Course(s): CAAM 31230
STAT 31240. Variational Methods in Image Processing. 100 Units.
This course discusses mathematical models arising in image processing. Topics covered will include an overview of tools from the calculus of variations and partial differential equations, applications to the design of numerical methods for image denoising, deblurring, and segmentation, and the study of convergence properties of the associated models. Students will gain an exposure to the theoretical basis for these methods as well as their practical application in numerical computations.
Terms Offered: Spring Equivalent Course(s): CAAM 31240
STAT 31250. Mathematical Introduction to Topological Insulators. 100 Units.
The field of topological (acoustic, electromagnetic, electronic, mechanical) insulators analyzes asymmetric transport phenomena observed along interfaces that separate insulating bulks. It finds applications in many areas of physical and materials sciences. The topological nature of the asymmetric transport ensures that it persists in the presence of perturbations of the underlying model which forms its main practical appeal. This graduate level course will present several mathematical and physically motivated tools to model and quantify asymmetric transport such as: current physical observables, elliptic (pseudo-)differential operators, spectral theory of self-adjoint operators, index theory and classification, trace-class operators, computation of bulk invariants by Chern and winding numbers, computation of interface invariants by index theory and spectral flows, bulk-edge correspondence relating bulk and interface invariants, scattering theory, and computational methods to estimate transport numerically. We will in particular focus on magnetic Schroedinger, systems of Dirac equations, and linearized fluid wave models with applications to the Integer Quantum Hall Effect, the Quantum Anomalous Hall effect, topological equatorial waves, and possibly Floquet topological insulators and bilayer graphene systems.
Terms Offered: Autumn Note(s): While familiarity with partial differential equations and quantum mechanics is a plus, we plan to have self-contained lectures. Equivalent Course(s): CAAM 31250
STAT 31260. Homogenization of Partial Differential Equations. 100 Units.
This course introduces common methods for establishing the macroscopic behavior of heterogeneous differential equations. It begins with a brief review of some classical results in functional analysis (weak/weak-* convergence and Sobolev spaces), followed by a discussion of several toolboxes: formal asymptotic expansion, compensated compactness, two-scale convergence, and Gamma-convergence. Along the way, the course presents surprising phenomena that have appeared in homogenization, such as strange terms, negative Poisson ratios, and nonlocal terms. If time permits, large-scale regularity by the compactness method will also be discussed.
Terms Offered: Winter Prerequisite(s): STAT 31210 or STAT 31220 or MATH 27200 or MATH 27500 or permission of instructor. Equivalent Course(s): CAAM 31260
STAT 31410. Applied Dynamical Systems. 100 Units.
This course is an introduction to dynamical systems for analysis of nonlinear ordinary differential equations. The focus is on methods of bifurcation theory, canonical examples of forced nonlinear oscillators, fast-slow systems, and chaos. Examples will be drawn from mathematical modeling of physical and biological systems. While geometric perspectives will be emphasized, assignments will also introduce asymptotic methods for analysis and use numerical simulation as an exploratory tool. This course assumes students have a background in ordinary differential equations and linear algebra at the undergraduate level and an interest in mathematical modeling for applications.
Instructor(s): M. Silber Terms Offered: Spring Prerequisite(s): ODEs and/or dynamical systems at an undergraduate level or consent of instructor. Equivalent Course(s): CAAM 31410
STAT 31430. Applied Linear Algebra. 100 Units.
This course will provide a review and development of topics in linear algebra aimed toward preparing students for further graduate coursework in Computational and Applied Mathematics. Topics will include discussion of matrix factorizations (including diagonalization, the spectral theorem for normal matrices, the singular value decomposition, and the Schur and polar decompositions), and an overview of classical direct and iterative approaches to numerical methods for problems formulated in the language of linear algebra (including the conjugate gradient method). Additional topics will be included depending on student interests.
Instructor(s): E. Baer Terms Offered: Autumn Prerequisite(s): STAT 24300 or MATH 20250 or Graduate Student in Physical Sciences Division Equivalent Course(s): CAAM 31430
STAT 31440. Applied Analysis. 100 Units.
This course provides an overview of fundamentals of mathematical analysis with an eye towards developing the toolkit of graduate students in applied mathematics. Topics covered include metric spaces and basic topological notions, aspects of mathematical analysis in several variables, and an introduction to measure and integration.
Instructor(s): E. Baer Terms Offered: Autumn Equivalent Course(s): CAAM 31440
STAT 31450. Applied Partial Differential Equations. 100 Units.
Partial differential equations (PDEs) are used to model applications in a wide variety of fields: fluid dynamics, optics, atomic and plasma physics, elasticity, chemical reactions, climate modeling, stock markets, etc. The study of their mathematical structure and solution methods remains at the forefront of applied mathematics. The course concentrates on deriving an important set of examples of PDEs from simple physical models, which are often closely related to those describing more complex physical systems. The course will also cover analytical methods and tools for solving these PDEs; such as separation of variables, Fourier series and transforms, Sturm-Liouville theory, and Green's functions. The course is suitable for graduate students and advanced undergraduates in science, engineering, and applied mathematics.
Terms Offered: Spring Prerequisite(s): Instructor consent. Equivalent Course(s): CAAM 31450
STAT 31460. Applied Fourier Analysis. 100 Units.
Decompositions of functions into frequency components via the Fourier transform, and related sparse representations, are fundamental tools in applied mathematics. These ideas have been important in applications to signal processing, imaging, and the quantitative and qualitative analysis of a broad range of mathematical models of data (including modern approaches to machine learning) and physical systems. Topics to be covered in this course include an overview of classical ideas related to Fourier series and the Fourier transform, wavelet representations of functions and the framework of multiresolution analysis, and applications throughout computational and applied mathematics.
Terms Offered: Winter Prerequisite(s): Graduate student in the Physical Sciences Division or consent of instructor. Equivalent Course(s): CAAM 31460
STAT 31511. Monte Carlo Simulation. 100 Units.
This class primarily concerns the design and analysis of Monte Carlo sampling techniques for the estimation of averages with respect to high dimensional probability distributions. Standard simulation tools such as importance sampling, Metropolis-Hastings, Langevin dynamics, and hybrid Monte Carlo will be introduced along with basic theoretical concepts regarding their convergence to equilibrium. The class will explore applications of these methods in Bayesian statistics and machine learning as well as to other simulation problems arising in the physical and biological sciences. Particular attention will be paid to the major complicating issues like conditioning (with analogies to optimization) and rare events and methods to address them.
Terms Offered: To be determined Prerequisite(s): Multivariate calculus and linear algebra; elementary knowledge of ordinary differential equations. Equivalent Course(s): CAAM 31511
STAT 31512. Analysis of Sampling Algorithms. 100 Units.
Graduate topics course on mathematical analysis of algorithms for sampling from high-dimensional probability distributions, with a focus on analysis of Markov-Chain Monte Carlo via functional inequalities. Possible/likely topics include recent developments such as Eldan's stochastic localization, techniques from high-dimensional expanders, spectral/entropic independence, log-concave polynomials and matroid basis exchange walk, connections to statistical physics and statistical estimation, recent progress on the KLS conjecture, etc., with a view towards current research questions in the area.
STAT 31521. Applied Stochastic Processes. 100 Units.
This course concerns the estimation of the dynamic properties of time-dependent stochastic systems. The class will begin with an introduction to the numerical simulation of continuous time Markov processes including the discretization of stochastic (and ordinary) differential equations. Problems associated with multiple time scales will be discussed along with methods to address them (implicit discretizations, multiscale methods and dimensional reduction). The class will also cover interacting particle methods and other techniques for the efficient simulation of dynamical rare events.
Terms Offered: Winter. To be determined Prerequisite(s): Multivariate calculus and linear algebra
STAT 31550. Uncertainty Quantification. 100 Units.
This course will cover mathematical, statistical, and algorithmic questions that arise at the interface of complex modeling and data processing. Emphasis will be given to characterizing and quantifying the uncertainties inherent in the use of models and to exploring principled ways to reduce said uncertainty by the use of data. Specific topics include Bayesian inverse problems and data assimilation.
Terms Offered: To be determined Prerequisite(s): STAT 30200 or consent of instructor
STAT 31610. Mathematical Aspects of Electronic Structure of Materials. 100 Units.
This course considers mathematical and numerical methods to approach electronic structure of materials through several hot-topic examples including topological insulators and incommensurate 2D materials in addition to classical systems such as periodic crystals. The course will begin with a discussion of the basics of quantum mechanics for those not yet familiar before moving to models designed for varying system sizes, from DFT to tight-binding. The theory and numerical tools for studying observables such as Chern numbers, conductivity, and density of states will be considered.
Equivalent Course(s): CAAM 31610
STAT 31700. Introduction to Probability Models. 100 Units.
This course introduces stochastic processes as models for a variety of phenomena in the physical and biological sciences. Following a brief review of basic concepts in probability, we introduce stochastic processes that are popular in applications in sciences (e.g., discrete time Markov chain, the Poisson process, continuous time Markov process, renewal process and Brownian motion).
Instructor(s): Staff Terms Offered: Winter Prerequisite(s): STAT 24400 or STAT 24410 or STAT 25100 or STAT 25150 Equivalent Course(s): STAT 25300
STAT 31900. Introduction to Causal Inference. 100 Units.
This course is designed for graduate students and advanced undergraduate students from the social sciences, education, public health science, public policy, social service administration, and statistics who are involved in quantitative research and are interested in studying causality. The goal of this course is to equip students with basic knowledge of and analytic skills in causal inference. Topics for the course will include the potential outcomes framework for causal inference; experimental and observational studies; identification assumptions for causal parameters; potential pitfalls of using ANCOVA to estimate a causal effect; propensity score based methods including matching, stratification, inverse-probability-of-treatment-weighting (IPTW), marginal mean weighting through stratification (MMWS), and doubly robust estimation; the instrumental variable (IV) method; regression discontinuity design (RDD) including sharp RDD and fuzzy RDD; difference in difference (DID) and generalized DID methods for cross-section and panel data, and fixed effects model. Intermediate Statistics or equivalent such as STAT 224/PBHS 324, PP 31301, BUS 41100, or SOC 30005 is a prerequisite. This course is a prerequisite for "Advanced Topics in Causal Inference" and "Mediation, moderation, and spillover effects."
Instructor(s): G. Hong Terms Offered: Winter Prerequisite(s): Intermediate Statistics or equivalent such as STAT 224/PBHS 324, PP 31301, BUS 41100, or SOC 30005 Note(s): CHDV Distribution: M; M Equivalent Course(s): PLSC 30102, CHDV 20102, MACS 51000, SOCI 30315, PBHS 43201, MACS 21000, CHDV 30102
STAT 32400. Probability and Statistics. 100 Units.
This is a PhD course that introduces fundamental statistical concepts for academic research in business and economics. It covers basic topics in probability and statistics, including limit theorems, principles of estimation and inference, linear and logistic regression, and causal inference. Much emphasis is put on large-sample (asymptotic) theory. Please visit Booth Course Search for the most updated information: https://intranet.chicagobooth.edu/pub/coursesearch/coursesearch
Terms Offered: Autumn Equivalent Course(s): BUSN 41901
STAT 32900. Applied Multivariate Analysis. 100 Units.
The course will introduce the basic theory and applications for analyzing multidimensional data. Topics include multivariate distributions, Gaussian models, multivariate statistical inferences and applications, classifications, cluster analysis, and dimension reduction methods. Course content is subject to change in order to keep the contents up-to-date with new development in multivariate statistical techniques. The course is offered in alternate years by the Statistics Department (S15, S17, ...) and the Booth Business School (S16, S18, ...). When the course is offered by the Booth school, please visit the Booth portal and search via the course search tool: http://boothportal.chicagobooth.edu/portal/server.pt/community/course_search for the most up to date information.
Equivalent Course(s): BUSN 41912
STAT 32950. Multivariate Statistical Analysis: Applications and Techniques. 100 Units.
This course focuses on applications and techniques for analysis of multivariate and high dimensional data. Beginning subjects cover common multivariate techniques and dimension reduction, including principal component analysis, factor model, canonical correlation, multi-dimensional scaling, discriminant analysis, clustering, and correspondence analysis (if time permits). Further topics on statistical learning for high dimensional data and complex structures include penalized regression models (LASSO, ridge, elastic net), sparse PCA, independent component analysis, Gaussian mixture model, Expectation-Maximization methods, and random forest. Theoretical derivations will be presented with emphasis on motivations, applications, and hands-on data analysis.
Instructor(s): M. Wang Terms Offered: Spring Prerequisite(s): (STAT 24300 or MATH 20250) and (STAT 24500 or STAT 24510). Graduate students in Statistics or Financial Mathematics can enroll without prerequisites. Note(s): Linear algebra at the level of STAT 24300. Knowledge of probability and statistical estimation techniques (e.g. maximum likelihood and linear regression) at the level of STAT 24400-24500. Equivalent Course(s): STAT 24620
STAT 33100. Sample Surveys. 100 Units.
This course covers random sampling methods; stratification, cluster sampling, and ratio estimation; and methods for dealing with nonresponse and partial response.
Instructor(s): K. Wolter Terms Offered: Autumn Prerequisite(s): Consent of instructor
STAT 33400. Bayesian Statistical Inference and Machine Learning. 50 Units.
The course will develop a general approach to building models of economic and financial processes, with a focus on statistical learning techniques that scale to large data sets. We begin by introducing the key elements of a parametric statistical model: the likelihood, prior, and posterior, and show how to use them to make predictions. We shall also discuss conjugate priors and exponential families, and their applications to big data. We treat linear and generalized-linear models in some detail, including variable selection techniques, penalized regression methods such as the lasso and elastic net, and a fully Bayesian treatment of the linear model. As applications of these techniques, we shall discuss Ross' Arbitrage Pricing Theory (APT), and its applications to risk management and portfolio optimization. As extensions, we will discuss multilevel and hierarchical models, and conditional inference trees and forests. We also treat model-selection methodologies including cross-validation, AIC, and BIC and show how to apply them to all of the financial data sets presented as examples in class. Then we move on to dynamic models for time series including Markov state-space models, as special cases. As we introduce models, we will also introduce solution techniques including the Kalman filter and particle filter, the Viterbi algorithm, Metropolis-Hastings and Gibbs Sampling, and the EM algorithm.
Instructor(s): TBA Note(s): May count towards computing or elective requirements Equivalent Course(s): FINM 33210
STAT 33500. Time-series Analysis for Forecasting and Model Building. 100 Units.
Forecasting plays an important role in business planning and decisionmaking. This Ph.D.-level course discusses time series models that have been widely used in business and economic data analysis and forecasting. Both theory and methods of the models are discussed. Real examples are used throughout the course to illustrate applications. The topics covered include: (1) stationary and unit-root non-stationary processes; (2) linear dynamic models, including Autoregressive Moving Average models; (3) model building and data analysis; (4) prediction and forecasting evaluation; (5) asymptotic theory for estimation including unit-root theory; (6) models for time varying volatility; (7) models for time varying correlation including Dynamic Conditional Correlation and time varying factor models.; (9) state-space models and Kalman filter; and (10) models for high frequency data. Course description is subject to change. Please visit the Booth portal and search via the course search tool for the most up to date information: http://boothportal.chicagobooth.edu/portal/server.pt/community /course_search/
Prerequisite(s): BUSF 41901/STAT 32400 or instructor consent. Equivalent Course(s): BUSN 41910
STAT 33600. Time Dependent Data. 100 Units.
This course considers the modeling and analysis of data that are ordered in time. The main focus is on quantitative observations taken at evenly spaced intervals and includes both time-domain and spectral approaches.
Instructor(s): W. Wu Prerequisite(s): STAT 24500 w/B- or better or STAT 24510 w/C+ or better is required; alternatively STAT 22400 w/B- or better and exposure to multivariate calculus (MATH 16300 or MATH 16310 or MATH 18400 or MATH 19520 or MATH 20000 or MATH 20500 or MATH 20510 or MATH 20800). Graduate students in Statistics or Financial Mathematics can enroll without prerequisites. Some previous exposure to Fourier series is helpful but not required. Equivalent Course(s): STAT 26100
STAT 33611. Gaussian Processes with Applications to Modern Statistical Problems. 100 Units.
Gaussian processes play a fundamental role in modern statistics, machine learning, and probability theory. In the first part of the course, we will cover several essential techniques related to the Gaussian distribution, including Gaussian concentration, Gaussian comparison theorems, and Poincare type inequalities. We will then detail the applications of these theoretical tools in a series of modern statistical and mathematical problems, including sharp spectral bounds of Gaussian matrices, exact characterization of the least squares estimator, and the free energy of the Sherrington-Kirkpatrick model in statistical physics.
Instructor(s): Yandi Shen Terms Offered: Winter Prerequisite(s): Graduate student in Statistics, Computer Science, or Computational and Applied Mathematics, or consent of instructor.
STAT 33700. Multivariate Time Series Analysis. 100 Units.
This course investigates the dynamic relationships between variables. It starts with linear relationships between two variables, including distributed-lag models and detection of unidirectional dependence (Granger causality). Nonlinear and time-varying relationships are also discussed. Dynamic models discussed include vector autoregressive models, vector autoregressive moving-average models, multivariate regression models with time series errors, co-integration and error-correction models, state-space models, dynamic factor models, and multivariate volatility models such as BEKK, Dynamic conditional correlation, and copula-based models. The course also addresses impulse response function, structural specification, co-integration tests, least squares estimates, maximum likelihood estimates, principal component analysis, asymptotic principal component analysis, principal volatility components, recursive estimation, and Markov Chain Monte Carlo estimation. Empirical data analysis is an integral part of the course. Students are expected to analyze many real data sets. The main software used in the course is the MTS package in R, but students may use their own software if preferred.
Equivalent Course(s): BUSN 41914
STAT 33910. Financial Statistics: Time Series, Forecasting, Mean Reversion, and High Frequency Data. 100 Units.
This course is an introduction to the econometric analysis of high-frequency financial data. This is where the stochastic models of quantitative finance meet the reality of how the process really evolves. The course is focused on the statistical theory of how to connect the two, but there will also be some data analysis. With some additional statistical background (which can be acquired after the course), the participants will be able to read articles in the area. The statistical theory is longitudinal, and it thus complements cross-sectional calibration methods (implied volatility, etc.). The course also discusses volatility clustering and market microstructure.
Terms Offered: Winter Prerequisite(s): Some statistics/econometrics background as in STAT 24400–24500, or FINM 33150 and FINM 33400, or equivalent, or consent of instructor. Equivalent Course(s): FINM 33170
STAT 34300. Applied Linear Stat Methods. 100 Units.
This course introduces the theory, methods, and applications of fitting and interpreting multiple regression models. Topics include the examination of residuals, the transformation of data, strategies and criteria for the selection of a regression equation, nonlinear models, biases due to excluded variables and measurement error, and the use and interpretation of computer package regression programs. The theoretical basis of the methods, the relation to linear algebra, and the effects of violations of assumptions are studied. Techniques discussed are illustrated by examples involving both physical and social sciences data.
Terms Offered: Autumn Prerequisite(s): Graduate student in Statistics or Financial Mathematics or instructor consent. Note(s): Students who need it should take Linear Algebra (STAT 24300 or equivalent) concurrently.
STAT 34700. Generalized Linear Models. 100 Units.
This applied statistics course is a successor of STAT 34300 and covers the foundations of generalized linear models (GLM). We will discuss the general linear modeling idea for exponential family data and introduce specifically models for binary, multinomial, count and categorical data, and the challenges in model fitting, and inference. We will also discuss approaches that supplement the classical GLM, including quasi-likelihood for over-dispersed data, robust estimation, and penalized GLM. The course also covers related topics including mixed effect models for clustered data, the Bayesian approach of GLM, and survival analysis. This course will make a balance between practical real data analysis with examples and a deeper understanding of the models with mathematical derivations.
Instructor(s): Staff Terms Offered: Winter Prerequisite(s): STAT 343 (or a similar-level linear regression course) or consent of instructor; comfortable with programming in R.
STAT 34800. Modern Methods in Applied Statistics. 100 Units.
This course covers latent variable models and graphical models; definitions and conditional independence properties; Markov chains, HMMs, mixture models, PCA, factor analysis, and hierarchical Bayes models; methods for estimation and probability computations (EM, variational EM, MCMC, particle filtering, and Kalman Filter); undirected graphs, Markov Random Fields, and decomposable graphs; message passing algorithms; sparse regression, Lasso, and Bayesian regression; and classification generative vs. discriminative. Applications will typically involve high-dimensional data sets, and algorithmic coding will be emphasized.
Terms Offered: Spring
STAT 34900. Data Analysis Project. 100 Units.
The first part of this class will focus on general principles of data analysis and how to report the results of an analysis, including taking account of the context of the data, making informative and clear visual displays, developing relevant statistical models and describing them clearly, and carrying out diagnostic procedures to assess the appropriateness of adopted models. The second half of the class will focus on data analysis projects. Students working on a data analysis project in another context (e.g., for consulting) may, with proper permission, use that project for this course as well. Open only to PhD students in Statistics. Instructor consent required.
Terms Offered: To be determined Prerequisite(s): In order to register for this course, you must have taken STAT 34700 and have permission from the instructor.
STAT 35201. Introduction to Clinical Trials. 100 Units.
This course will review major components of clinical trial conduct, including the formulation of clinical hypotheses and study endpoints, trial design, development of the research protocol, trial progress monitoring, analysis, and the summary and reporting of results. Other aspects of clinical trials to be discussed include ethical and regulatory issues in human subjects research, data quality control, meta-analytic overviews and consensus in treatment strategy resulting from clinical trials, and the broader impact of clinical trials on public health.
Instructor(s): M. Polley Terms Offered: Autumn Prerequisite(s): PBHS 32100 or STAT 22000; Introductory Statistics or Consent of Instructor Equivalent Course(s): PBHS 32901
STAT 35410. Genomic Evolution I. 100 Units.
Canalization, a unifying biological principle first enunciated by Conrad Waddington in 1942, is an idea that has had tremendous intellectual influence on developmental biology, evolutionary biology, and mathematics. In this course we will explore canalization in all three contexts through extensive reading and discussion of both the classic and modern primary literature. We intend this exploration to raise new research problems which can be evaluated for further understanding. We encourage participants to present new ideas in this area for comment and discussion.
Instructor(s): M. Long, J. Reinitz Terms Offered: Autumn Equivalent Course(s): ECEV 35901, EVOL 35901
STAT 35420. Stochastic Processes in Gene Regulation. 100 Units.
This didactic course covers the fundamentals of stochastic chemical processes as they arise in the study of gene regulation. The central object of study is the Chemical Master Equation and its coarse-grainings at the Langevin/Fokker-Planck, linear noise, and deterministic levels. We will consider both mathematical and computational approaches in contexts where there are both single and multiple deterministic limits.
Instructor(s): J. Reinitz Terms Offered: To be determined Prerequisite(s): Consent of instructor. Equivalent Course(s): CAAM 35420, ECEV 35420, MGCB 35420
STAT 35450. Fundamentals of Computational Biology: Models and Inference. 100 Units.
Covers key principles in probability and statistics that are used to model and understand biological data. There will be a strong emphasis on stochastic processes and inference in complex hierarchical statistical models. Topics will vary but the typical content would include: Likelihood-based and Bayesian inference, Poisson processes, Markov models, Hidden Markov models, Gaussian Processes, Brownian motion, Birth-death processes, the Coalescent, Graphical models, Markov processes on trees and graphs, Markov Chain Monte Carlo.
Instructor(s): J. Novembre, M. Stephens Terms Offered: Winter Prerequisite(s): STAT 244 Equivalent Course(s): HGEN 48600
STAT 35460. Fundamentals of Computational Biology: Algorithms and Applications. 100 Units.
This course will cover principles of data structure and algorithms, with emphasis on algorithms that have broad applications in computational biology. The specific topics may include dynamic programming, algorithms for graphs, numerical optimization, finite-difference, schemes, matrix operations/factor analysis, and data management (e.g. SQL, HDF5). We will also discuss some applications of these algorithms (as well as commonly used statistical techniques) in genomics and systems biology, including genome assembly, variant calling, transcriptome inference, and so on.
Instructor(s): Xin He, Mengjie Chen Terms Offered: Spring Equivalent Course(s): HGEN 48800
STAT 35490. Introduction to Statistical Genetics. 100 Units.
As a result of technological advances over the past few decades, there is a tremendous wealth of genetic data currently being collected. These data have the potential to shed light on the genetic factors influencing traits and diseases, as well as on questions of ancestry and population history. The aim of this course is to develop a thorough understanding of probabilistic models and statistical theory and methods underlying analysis of genetic data, focusing on problems in complex trait mapping, with some coverage of population genetics. Although the case studies are all in the area of statistical genetics, the statistical inference topics, which will include likelihood-based inference, linear mixed models, and restricted maximum likelihood, among others, are widely applicable to other areas. No biological background is needed, but a strong foundation in linear algebra, as well as probability and statistics at the level of STAT 24400-STAT 24500 or higher is assumed.
Instructor(s): M. McPeek Terms Offered: Autumn Prerequisite(s): STAT 24500 or 24510 or 30200 or consent of instructor. Equivalent Course(s): STAT 26300
STAT 35500. Statistical Genetics. 100 Units.
This is an advanced course in statistical genetics. We will take an in-depth look at statistical methods development in recent genetics literature, with the aim of achieving a deep understanding of the modeling approaches and assumptions, statistical principles, mathematical theorems, computational issues, and data analytic approaches underlying the methods. The goal is for the student to be able to ultimately apply the principles learned to future statistical methods development for genetic data analysis. This is a discussion course and student presentations will be required. Topics depend on the interests of the participants and will be based on recent published literature. Topics may include, but are not limited to, statistical problems in genetic association mapping, population genetics, integration of different types of genetic data, and genetic models for complex traits. The course material changes every year, and the course may be repeated for credit.
Terms Offered: To be determined Prerequisite(s): Either HGEN 47100 or both STAT 24400 (or STAT 24410) and 24500 (or 24510). Students without these prerequisites may enroll on a P/NP basis with consent of the instructor.
STAT 35510. Statistical Algorithms for Single-Cell Omics and Related Techniques. 100 Units.
Single-cell sequencing is a revolutionary technique that allows the analysis of the genetic information within individual cells, providing unprecedented insights into cellular heterogeneity and diversity. This course aims to offer a comprehensive overview of the cutting-edge quantitative methods employed in analyzing single-cell sequencing data. Designed for graduate students with a statistical/quantitative background, the course requires no prior knowledge of biology or experience in analyzing genetics data. We will start with a gentle introduction to basic biological concepts relevant to understanding the data, coupled with a concise overview of single-cell sequencing technologies such as single-cell RNA-seq, single-cell ATAC-seq, spatial transcriptomics, CITE-seq, Perturb-seq, and more. Then, we will discuss common types of computational analyses, such as visualization, denoising, clustering, trajectory analyses, data integration, transfer learning, and the alignment of multi-omics data. A special emphasis will be placed on exploring deep learning models that have been designed for various tasks analyzing single-cell sequencing data. We will also address statistical considerations that arise, including appropriate distributional assumptions on the data, distribution-free tests, "post-estimation" inference, and causal inference.
STAT 35700. Epidemiologic Methods. 100 Units.
This course provides students with an in-depth understanding of epidemiologic concepts and methods. It is the second course in the epidemiology series. The focus of this course will be in practical and theoretical considerations of observational research methods; statistical methods and applications in epidemiologic studies; in-depth evaluation of bias, confounding, and interaction; and communicating epidemiologic findings. Students will also learn how to perform data analysis using classic methods.
Instructor(s): B. Chiu Terms Offered: Winter Prerequisite(s): PBHS 30910 and PBHS 32400/STAT 22400 or PBHS 32410 (taken concurrently) or applied statistics courses through multivariate regression. Equivalent Course(s): PBHS 31001
STAT 35800. Statistical Applications. 100 Units.
This course provides a transition between statistical theory and practice. The course will cover statistical applications in medicine, mental health, environmental science, analytical chemistry, and public policy. ,Lectures are oriented around specific examples from a variety of content areas. Opportunities for the class to work on interesting applied problems presented by U of C faculty will be provided. Although an overview ,of relevant statistical theory will be presented, emphasis is on the development of statistical solutions to interesting applied problems.
Instructor(s): R. Gibbons Terms Offered: Autumn Prerequisite(s): PBHS 32400, PBHS 32410 or equivalent, and PBHS 32600/STAT 22600 or PBHS 32700/STAT 22700 or equivalent; or consent of instructor. Knowledge of STATA and/or R highly recommended. Equivalent Course(s): PBHS 33500, CHDV 32702
STAT 35920. Applied Bayesian Modeling and Inference. 100 Units.
Course begins with basic probability and distribution theory, and covers a wide range of topics related to Bayesian modeling, computation, and inference. Significant amount of effort will be directed to teaching students on how to build and apply hierarchical models and perform posterior inference. The first half of the course will be focused on basic theory, modeling, and computation using Markov chain Monte Carlo methods, and the second half of the course will be about advanced models and applications. Computation and application will be emphasized so that students will be able to solve real-world problems with Bayesian techniques.
Instructor(s): Y. Ji Terms Offered: Spring Prerequisite(s): STAT 24400 and STAT 24500 or master level training in statistics. Equivalent Course(s): PBHS 43010
STAT 36510. Random Growth Model and the Kardar-Parisi-Zhang Equation. 100 Units.
In this course, we will show how a variety of physical systems and mathematical models, including randomly growing interfaces, queueing systems, stochastic PDEs, and traffic models, all demonstrate the same universal statistical behaviors in their long-time/large-scale limit. These systems are said to lie in the Kardar-Parisi-Zhang universality class. We will also study a central object in this universality class: the Kardar-Parisi-Zhang equation.
Instructor(s): Yier Lin Terms Offered: Winter Prerequisite(s): PhD student in Statistics, Computational and Applied Mathematics, Mathematics, or Toyota Technological Institute at Chicago, or MS student in Statistics or Computational and Applied Mathematics or consent on instructor. Note(s): Graduate or advanced undergraduate probability theory and undergraduate linear algebra and combinatorics are recommended.
STAT 36600. Decision Theory. 100 Units.
This course covers statistical decision theory with examples drawn from modern high-dimensional and nonparametric estimation. Topics that will be covered include basic information theory, decision theory, asymptotic equivalence, Gaussian sequence model, sparse regression, model selection, aggregation, and large covariance matrix estimation. Lower bound techniques such as Bayes, Le Cam, and Fano's methods will be taught.
STAT 36700. History of Statistics. 100 Units.
This course covers topics in the history of statistics, from the eleventh century to the middle of the twentieth century. We focus on the period from 1650 to 1950, with an emphasis on the mathematical developments in the theory of probability and how they came to be used in the sciences. Our goals are both to quantify uncertainty in observational data and to develop a conceptual framework for scientific theories. This course includes broad views of the development of the subject and closer looks at specific people and investigations, including reanalyses of historical data.
Instructor(s): S. Stigler Terms Offered: Not offered in 2022-2023. Prerequisite(s): Prior statistics course Equivalent Course(s): CHSS 32900, STAT 26700, HIPS 25600
STAT 36900. Applied Longitudinal Data Analysis. 100 Units.
Longitudinal data consist of multiple measures over time on a sample of individuals. This type of data occurs extensively in both observational and experimental biomedical and public health studies, as well as in studies in sociology and applied economics. This course will provide an introduction to the principles and methods for the analysis of longitudinal data. Whereas some supporting statistical theory will be given, emphasis will be on data analysis and interpretation of models for longitudinal data. Problems will be motivated by applications in epidemiology, clinical medicine, health services research, and disease natural history studies.
Instructor(s): D. Hedeker Terms Offered: Winter Prerequisite(s): PBHS 32400/STAT 22400 or PBHS 32410 or equivalent, AND PBHS 32600/STAT 22600 or PBHS 32700/STAT 22700 or equivalent; or consent of instructor. Equivalent Course(s): CHDV 32501, PBHS 33300
STAT 37400. Nonparametric Inference. 100 Units.
Nonparametric inference is about developing statistical methods and models that make weak assumptions. A typical nonparametric approach estimates a nonlinear function from an infinite dimensional space rather than a linear model from a finite dimensional space. This course gives an introduction to nonparametric inference, with a focus on density estimation, regression, confidence sets, orthogonal functions, random processes, and kernels. The course treats nonparametric methodology and its use, together with theory that explains the statistical properties of the methods.
Instructor(s): Staff Terms Offered: Winter Prerequisite(s): STAT 24400 or STAT 24410 w/B- or better is required; alternatively STAT 22400 w/B+ or better and exposure to multivariate calculus (MATH 16300 or MATH 16310 or MATH 18400 or MATH 19520 or MATH 20000 or MATH 20500 or MATH 20510 or MATH 20800) and linear algebra (MATH 18600 or 19620 or 20250 or 20700 or STAT 24300 or equivalent). Master's students in Statistics can enroll without prerequisites. Equivalent Course(s): STAT 27400
STAT 37411. Topological Data Analysis. 100 Units.
Topological data analysis seeks to understand and exploit topology when exploring and learning from data. This course surveys core ideas and recent developments in the field and will prepare students to use topology in data analysis tasks. The core of the course will include computation with topological spaces, the mapper algorithm, and persistent homology, and cover theoretical results, algorithms, and a variety of applications. Additional topics from algebraic topology, metric geometry, category theory, and quiver representation theory will be developed from applied and computational perspectives.
Terms Offered: Winter Prerequisite(s): Linear algebra, prior programming experience, exposure to graph theory/algorithms. Equivalent Course(s): CAAM 37411
STAT 37601. Machine Learning and Large-Scale Data Analysis. 100 Units.
This course is an introduction to machine learning and the analysis of large data sets using distributed computation and storage infrastructure. Basic machine learning methodology and relevant statistical theory will be presented in lectures. Homework exercises will give students hands-on experience with the methods on different types of data. Methods include algorithms for clustering, binary classification, and hierarchical Bayesian modeling. Data types include images, archives of scientific articles, online ad clickthrough logs, and public records of the City of Chicago. Programming will be based on Python and R, but previous exposure to these languages is not assumed.
Prerequisite(s): [CMSC 25300 or CMSC 35300 or STAT 27700 or CMSC 25400 or STAT 27725 or TTIC 31020] and [STAT 24400 or STAT 24410 or STAT 24500 or STAT 24510] Note(s): The prerequisites are under review and may change. Equivalent Course(s): CMSC 25025
STAT 37710. Machine Learning. 100 Units.
This course provides hands-on experience with a range of contemporary machine learning algorithms, as well as an introduction to the theoretical aspects of the subject. Topics covered include: the PAC framework, Bayesian learning, graphical models, clustering, dimensionality reduction, kernel methods including SVMs, matrix completion, neural networks, and an introduction to statistical learning theory.
Terms Offered: To be determined Prerequisite(s): Must be a PhD or MS student in Statistics, Computer Science, or Computational and Applied Mathematics, and has taken any one of: CMSC 35300/STAT 27700, STAT 31430, STAT 30900, STAT 24300, STAT 24500, or STAT 24510. Or consent of the instructor. Equivalent Course(s): CAAM 37710, CMSC 35400
STAT 37711. Machine Learning 1. 100 Units.
This course is a graduate level introduction to machine learning. We will cover both practical and (probabilistic) foundational aspects of the subject. Topics include empirical risk minimization and overfitting, regression, ensembling, as well as selected topics on representation learning and structure learning.
Instructor(s): V. Veitch Terms Offered: Autumn Prerequisite(s): Open to graduate students in data science, statistics, and computer science, or by instructor consent. Students should have a strong background in programming, basic probability, and a graduate-student level of mathematical maturity. Equivalent Course(s): CAAM 37711, DATA 37711
STAT 37782. Algorithms for Massive Datasets. 100 Units.
This course will focus on using elements of randomness for the analysis of massive datasets. It will cover randomized algorithms for matrix (tensor) decompositions, stochastic optimization algorithms, and the interplay between randomness and sparse recovery.
Instructor(s): Y. Khoo Terms Offered: Autumn Equivalent Course(s): CAAM 37782
STAT 37783. Solving PDEs with Machine Learning. 100 Units.
In this class, we discuss approaches based on machine learning for PDEs. We start with the solution of elliptic and parabolic PDE with machine learning. We first discuss approaches based on numerical discretization and particle methods. We then discuss how these classical approaches can also be realized by machine learning in a high-dimensional setting.
Equivalent Course(s): CAAM 37783
STAT 37784. Representation Learning in Machine Learning. 100 Units.
This course is a seminar on representation learning in machine learning. The core questions in this are: how do machine learning systems recover the structure present in real-world data, how can we expose this recovered structure to human analysts, and how does this help us in real-world applications? In this seminar, we will read and discuss papers from the modern research literature on these subjects. Students should have previous exposure to machine learning and deep learning.
Terms Offered: TBD Equivalent Course(s): DATA 37784
STAT 37785. Modern Approaches for Computational Quantum Problems. 100 Units.
In this class, we discuss modern approaches based on machine learning for quantum mechanical problems. We start with the solution of elliptic and parabolic PDE with machine learning and discuss their adaptation in a quantum mechanical setting. If time allows, we will talk about quantum computing methods for solving quantum mechanical problems.
Instructor(s): Y. Khoo Terms Offered: Spring Prerequisite(s): Graduate student in Statistics or CCAM/MCAM. Equivalent Course(s): CAAM 37785
STAT 37786. Topics in Learning Under Distribution Shifts. 100 Units.
Traditional supervised learning assumes that the training and testing distributions are the same. Such a no-distribution-shift assumption, however, is frequently violated in practice. In this course, we survey topics in machine learning in which distribution shifts naturally arise. Possible topics include supervised learning with covariate shift, off-policy evaluation in reinforcement learning, and offline reinforcement learning.
Terms Offered: Winter Prerequisite(s): In order to register for this course, you must be a graduate student in Statistics, CAAM, or Computer Science.
STAT 37787. Trustworthy Machine Learning. 100 Units.
Machine learning systems are routinely used in safety critical situations in the real world. However, they often dramatically fail! This course covers foundational and practical concerns in building machine learning systems that can be trusted. Topics include foundational issues---when do systems generalize, and why, essential results in fairness and domain shifts, and evaluations beyond standard test/train splits. This is an intermediate level course in machine learning; students should have at least one previous course in machine learning.
Terms Offered: Spring Prerequisite(s): STAT 27700 or STAT 37710 or consent of instructor. Equivalent Course(s): STAT 27751, DATA 27751
STAT 37788. Machine Learning on Graphs, Groups and Manifolds. 100 Units.
In many domains, including applications of machine learning to scientific problems, social phenomena and computer vision/graphics, the data that learning algorithms operate on naturally lives on structured objects such as graphs or low dimensional manifolds. There are many connections between these cases; further, since groups capture symmetries, there are also natural connections to the theory of learning on groups and group equiviariant algorithms. This course provides a mathematical introduction to these topics both in the context of kernel based learning and neural networks. Specific topics covered include graph kernels, manifold learning, graph wavelets, graph neural networks, permutation equivariant learning, rotational equivariant networks for scientific applications and imaging, gauge equivariant networks and steerable nets.
Equivalent Course(s): CAAM 37788, CMSC 35430
STAT 37789. Topics in Machine Learning: Learning in Games. 100 Units.
Games have long been used as benchmarks in artificial intelligence, and research in game playing has closely tracked major developments in computing. Famous examples include IBM's Deep Blue and Google Deepmind's AlphaGo. Driven by advances in machine learning, recent years have seen rapid progress in the field of game playing artificial intelligences. This reading course will review the major achievements in learning in games, discuss different classes of games, and the algorithms used to select good strategies. We will introduce relevant game theory, discuss classical methods for complete information games and combinatorial games, and modern learning methods such as counterfactual regret minimization methods used in incomplete information games. We will conclude by identifying the frontiers of artificial intelligence in games. Students are expected to enter with rudimentary coding experience.
Terms Offered: Spring Prerequisite(s): Graduate student in Statistics or Computational and Applied Mathematics or Computer Science or Toyota Technological Institute at Chicago or consent of instructor.
STAT 37790. Topics in Statistical Machine Learning. 100 Units.
Topics in Statistical Machine Learning" is a second graduate level course in machine learning, assuming students have had previous exposure to machine learning and statistical theory. The emphasis of the course is on statistical methodology, learning theory, and algorithms for large-scale, high dimensional data. The selection of topics is influenced by recent research results, and students can take the course in more than one quarter.
Terms Offered: To be determined Equivalent Course(s): CMSC 35425
STAT 37791. Topics in Machine Learning. 100 Units.
This course covers selected topics in dimension reduction, randomized algorithm, sparsity, convex optimization, and deep learning, with a focus on scientific computing.
Terms Offered: Spring Prerequisite(s): Enrolled PhD or MS student in Statistics or in Computational and Applied Mathematics, or consent of instructor. Note(s): Recommended prerequisites: STAT 30900, STAT 31015, and undergraduate probability. Equivalent Course(s): CAAM 37791
STAT 37792. Topics in Deep Learning: Generative Models. 100 Units.
This course will be a hands on exploration of various approaches to generative modeling with deep networks. Topics include variational auto encoders, flow models, GAN models, and energy models. Participation in this course requires familiarity with pytorch and a strong background in statistical modeling. The course will primarily consist of paper presentations. Each presenter would be required to report on experiments performed with the algorithm proposed in the paper, exploring strengths and weaknesses of the methods.
Instructor(s): Y. Amit Terms Offered: Autumn Prerequisite(s): STAT 34300, STAT 34700, STAT 34800, and STAT 37601/CMSC 25025, or STAT 37710/CMSC 35400
STAT 37793. Topics in Deep Learning: Discriminative Models. 100 Units.
This course will explore modern approaches to optimization, data augmentation, and representation learning for deep neural networks from a primarily theoretical perspective. Participation will require independent investigation of theoretical papers with PyTorch as well as paper presentations.
Terms Offered: Spring Prerequisite(s): STAT 37601 or STAT 37710 or consent of instructor.
STAT 37794. Special Topics in Machine Learning. 100 Units.
Learned emulators leverage neural networks to increase the speed of physics simulations in climate models, astrophysics, high-energy physics, and more. Recent empirical results have illustrated that these emulators can speed up traditional simulations by up to eight orders of magnitude. However, little is understood about these emulators. While it is possible that recent results are representative of what is possible in most settings, a more likely scenario is that these approaches are more effective for some simulators than others, and that learned emulators achieve strong average-case performance but fail to capture rare but important phenomena. In this graduate seminar course we will provide an overview and investigate recent literature on this topic, focusing on the following questions: 1. Introduction to learned emulators: how do they work, where have they been successful so far and what are the goals in this field? 2. Two different paradigms of learned emulation: physics vs. data driven. What are the advantages and pitfalls of each? 3. Robustness of emulation to noise: what is known so far? 4. Parameter estimation: how to handle parameter uncertainty? We will provide a list of papers covering the above topics and students will be evaluated on in-class presentations.
Instructor(s): Dana Mendelson (Math) and Rebecca Willett (CS/Stats) Terms Offered: Autumn Prerequisite(s): Students should be familiar with a numerical programming language like Python, Julia, R, or Matlab and the content of CMSC 35400. Students should also have familiarity with the contents of MATH 27300 and MATH 27500 or similar. Note(s): Because this is a seminar course, it will be capped at 15 students, 4 Math, 4 CS/Stats, and 7 with instructor permission. Equivalent Course(s): MATH 37794, CMSC 35490, CAAM 37794
STAT 37795. Causal Inference with Machine Learning. 100 Units.
This is a seminar on the use of causality in building robust and trustworthy machine learning systems. Standard machine learning pipelines have a wide range of issues in practice, including reliance on apparently irrelevant features, poor performance when deployed in domains that are mismatched to their training environment, and discriminatory or unfair behavior. This course will cover the use of causality in defining, understanding, and mitigating these failure modes.
Instructor(s): V. Veitch Terms Offered: Spring Prerequisite(s): Background in machine learning
STAT 37796. Topics in Machine Learning: Symmetries and Harmonic Analysis. 100 Units.
Many algorithms in machine learning and statistical inference have inherent symmetries. In other cases, symmetries need to be enforced on the algorithm explicitly from the outside. In both cases, a systematic study of the symmetries inevitably leads to considerations borrowed from group representation theory and harmonic analysis. In this course we will take a broad view of this topic spanning the range from the concept of exchangeability in probability and nonparametric statistics, via the implementation of symmetries in kernel methods such as Gaussian processes to the new and quickly developing field of equivariant neural networks. One area that where symmetries play a key role and we will specifically focus on is learning physical systems and developing scalable algorithms for large scale modeling for physics and chemistry. The course starts with a short introduction to representation theory and wavelets, of which no prior knowledge is required.
Instructor(s): R. Kondor Terms Offered: Autumn
STAT 37797. Topics in Mathematical Data Science: Spectral Methods and Nonconvex Optimization. 100 Units.
This is a graduate level course covering various aspects of mathematical data science, particularly for large-scale problems. We will cover the mathematical foundations of several fundamental learning and inference problems, including clustering, ranking, sparse recovery and compressed sensing, low-rank matrix factorization, and so on. Both convex and nonconvex approaches (including spectral methods and iterative nonconvex methods) will be discussed. We will focus on designing algorithms that are effective in both theory and practice.
Terms Offered: Autumn Prerequisite(s): Graduate student in Statistics, Computational and Applied Mathematics, or Computer Science, or consent of instructor. Note(s): Students should have backgrounds in basic linear algebra and in basic probability (measure-theoretic probability is not needed), as well as knowledge of a programming language (e.g., MATLAB, Python, Julia) to conduct simple simulation exercises. While no specific background in optimization is required, a course such as STAT 28000 (Optimization) would be beneficial.
STAT 37798. Topics in Machine Learning: Scientific Computing Tools for High-Dimensional Problems. 100 Units.
This course considers computational techniques in representing and optimizing a high-dimensional probability distribution in the context of computational physics and data science applications. Computationally tractable approximations to the probability distribution based on convex relaxations, tensor-network, neural-network, mean-field, Bethe approximation, and normalizing flow will be discussed. The course aims to present a practical view on these methods and illustrate when they can be successfully deployed in specific situations.
Terms Offered: Winter Equivalent Course(s): CAAM 37798
STAT 37799. Topics in Machine Learning: Machine Learning and Inverse Problems. 100 Units.
In many scientific and medical settings, we cannot directly observe phenomena of interest, such as images of a person's internal organs, the microscopic structure of materials or cells, or observations of distant stars and galaxies. Rather, we use MRI scanners, microscopes, and satellites to collect indirect data that require sophisticated numerical methods to interpret. This course will explore a variety of machine learning techniques for solving inverse problems, ranging from linear inverse problems to PDE parameter estimation and data assimilation.
Instructor(s): R. Willett Terms Offered: Winter Prerequisite(s): STAT 37710/CAAM 37710/CMSC 35400 or consent of instructor. Equivalent Course(s): CMSC 37799, CAAM 37799
STAT 37810. Statistical Computing A. 50 Units.
This course is an introduction to statistical programming in R. Students will learn how to design, write, debug and test functions by implementing several famous algorithms in statistics such as Gibbs Sampling and Expectation Maximization. A basic familiarity with R is needed, but no prior programming experience is required. The course will also introduce students to the use of version control with Git and consider the differences and similarities between R and Python.
Terms Offered: Autumn Prerequisite(s): Instructor consent.
STAT 37815. Practical R Programming. 100 Units.
This course covers a practical set of skills vital to modern statistics and data science in handling messy, real-world data. Students will get a foundation in version control and practice expressing themselves with literate programming. They will think algorithmically with base R objects, control flow, functions, and iteration. The course will also introduce students to a variety of tidyverse data wrangling methods to import, clean, transform, join, and summarize their data. Finally, students will visualize and explore data using the grammar of graphics framework. Other introductory topics may be discussed. No programming experience is required, although some may be helpful.
Equivalent Course(s): STAT 27815
STAT 37820. Statistical Computing B. 50 Units.
Statistical Computing B focuses on common data technology used in statistical computing and broader data science. The course takes place in the second half of the autumn quarter, after STAT 37810 (Statistical Computing A). Topics include storage and accessing of large data; basic working knowledge of relational database and its querying language SQL; introduction to distributed file system and example usage of Hadoop; Python and its applications in text analysis; access and usage of high-performance computer clusters, rudimentary parallel computing, web data access. XML and Javascript may be used occasionally. A short introduction to SAS will be given if time permits. The main computing software will be Python with some R.
Terms Offered: Autumn Prerequisite(s): Instructor consent. STAT 37810 recommended.
STAT 37830. Scientific Computing with Python. 100 Units.
This course is an introduction to scientific computing using the Python programming language intended to prepare students for further computational work in courses, research, and industry. Students will learn to design, implement, and test code in Python. The course will draw examples from numerical and discrete algorithms commonly encountered in scientific computing with an emphasis on design and performance considerations. Topics will include numerical linear algebra, optimization, graph theory, data analysis, and physical simulations. The course will also introduce students to a variety of practical topics such as the use of remote resources, version control with git, commonly used libraries for scientific computing and data analysis, and using and contributing to open source and collaborative projects.
Prerequisite(s): Multivariable calculus, Linear algebra, prior programming experience (not necessarily in Python). Equivalent Course(s): CAAM 37830
STAT 38100. Measure-Theoretic Probability I. 100 Units.
This course provides a detailed, rigorous treatment of probability from the point of view of measure theory, as well as existence theorems, integration and expected values, characteristic functions, moment problems, limit laws, Radon-Nikodym derivatives, and conditional probabilities.
Terms Offered: Winter Prerequisite(s): STAT 30400 or consent of instructor
STAT 38300. Measure-Theoretic Probability III. 100 Units.
This course continues material covered in STAT 38100, with topics that include Lp spaces, Radon-Nikodym theorem, conditional expectation, and martingale theory.
Terms Offered: Spring Prerequisite(s): STAT 38100
STAT 38510. Brownian Motion and Stochastic Calculus. 100 Units.
This is a rigorous introduction to the mathematical theory of Brownian motion and the corresponding integration theory (stochastic integration). This is material that all analysis graduate students should learn at some point whether or not they are immediately planning to use probabilistic techniques. It is also a natural course for more advanced math students who want to broaden their mathematical education and to increase their marketability for nonacademic positions. In particular, it is one of the most fundamental mathematical tools used in financial mathematics (although we will not discuss finance in this course). This course differs from the more applied STAT 39000 in that concepts are developed precisely and rigorously.
Terms Offered: To be determined. Note(s): Recommended prerequisites: STAT 38300; or MATH 31200, MATH 31300, and MATH 31400; or consent of instructor. Equivalent Course(s): MATH 38511
STAT 38520. Topics in Random Matrix Theory. 100 Units.
Random matrix theory (RMT) is among the most prominent subjects in modern probability theory, with applications in a wide range of disciplines (including physics, statistics, engineering, and finance). The purpose of this course is to study a broad sample of the most prominent research programs in RMT as well as their motivating applications. Main topics will include (time permitting) the moment method in RMT and its connection to combinatorics, universality, operator limits, and matrix concentration.
Terms Offered: Winter Prerequisite(s): PhD student in Statistics or Math or Computational and Applied Mathematics or TTIC or MS student in Statistics or Computational and Applied Mathematics. Other students may enroll with consent of instructor. Note(s): Prerequisite notes: Graduate or advanced undergraduate probability theory and undergraduate linear algebra and combinatorics are recommended. Equivalent Course(s): CAAM 38520
STAT 39000. Stochastic Calculus. 100 Units.
The course starts with a quick introduction to martingales in discrete time, and then Brownian motion and the Ito integral are defined carefully. The main tools of stochastic calculus (Ito's formula, Feynman-Kac formula, Girsanov theorem, etc.) are developed. The treatment includes discussions of simulation and the relationship with partial differential equations. Some applications are given to option pricing, but much more on this is done in other courses. The course ends with an introduction to jump process (Levy processes) and the corresponding integration theory. Program requirement.
Instructor(s): G. Lawler Terms Offered: Winter Equivalent Course(s): FINM 34500
STAT 39010. Stochastic Calculus I. 50 Units.
The course starts with a quick introduction to martingales in discrete time, and then Brownian motion and the Ito integral are defined carefully. The main tools of stochastic calculus (Ito's formula, Feynman-Kac formula, Girsanov theorem, etc.) are developed. The treatment includes discussions of simulation and the relationship with partial differential equations. Some applications are given to option pricing, but much more on this is done in other courses. The course ends with an introduction to jump process (Levy processes) and the corresponding integration theory.
Terms Offered: Winter Prerequisite(s): Consent of instructor. Equivalent Course(s): FINM 34510
STAT 39020. Stochastic Calculus II. 50 Units.
Continuation of FINM 34510
Instructor(s): Prof. Greg Lawler Terms Offered: Winter Prerequisite(s): FINM 34510 Equivalent Course(s): FINM 34520
STAT 39800. Field Research. 300.00 Units.
This Summer Quarter course offers graduate students in the Statistics Department the opportunity to apply statistics knowledge that they have acquired to a real industry or business situation. During the summer quarter in which they are registered for the course, students complete a paid or unpaid internship of at least six weeks. Prior to the start of the work experience, students secure faculty consent for an independent study project to be completed during the internship quarter.
Terms Offered: Summer only Prerequisite(s): Masters or PhD student in Statistics or Consent of instructor and faculty advisor.
STAT 39900. Masters Seminar: Statistics. 300.00 Units.
This course is for Statistics Master's students to carry out directed reading or guided work on topics related to their Master's papers.
Prerequisite(s): Masters or PhD student in Statistics
STAT 40100. Reading/Research: Statistics. 300.00 Units.
This course allows doctoral students to receive credit for advanced work related to their dissertation topics. Students register for one of the listed faculty sections with prior consent from the respective instructor. Students may work with faculty from other departments; however, they still must obtain permission from and register with one of the listed faculty members in the Department of Statistics.
Terms Offered: All quarters Prerequisite(s): Masters or PhD student in Statistics or consent of instructor
STAT 41500-41600. High-Dimensional Statistics I-II.
These courses treat statistical problems where the number of variables is very large. Classical statistical methods and theory often fail in such settings. Modern research has begun to develop techniques that can be effective in high dimensions, and that can be understood theoretically. The first quarter introduces a range of statistical frameworks for finding low-dimensional structure in high-dimensional data, such as sparsity in regression, sparse graphical models, or low-rank structure. This quarter emphasizes methods for estimation and inference developed in these areas, along with theoretical analysis of their properties. The second quarter emphasizes foundational aspects of high-dimensional statistics, focusing on principles that are used across a range of problems and are likely to be relevant for methods developed in the future. Topics include "the curse of dimensionality," elements of random matrix theory, properties of high-dimensional covariance matrices, concentration of measure, dimensionality reduction techniques, and handling mis-specified models. The courses may be taken separately.
STAT 41500. High-Dimensional Statistics I. 100 Units.
In this course, we will consider statistical estimation with a large number of parameters. Sometimes the number of parameters may exceed the sample size. Problems such as sparse linear regression, bandable covariance matrix estimation, Gaussian graphical model, sparse PCA and CCA, and isotonic regression will be covered. Along with these specific problems, we will also cover techniques including concentration of measure, convex optimization, approximate message passing, debiasing, and statistical-computational tradeoff.
Terms Offered: To be determined Prerequisite(s): STAT 30100 and STAT 30400 and STAT 31015, or consent of instructor
STAT 41600. High-Dimensional Statistics II. 100 Units.
Terms Offered: To be determined Prerequisite(s): STAT 30100 or STAT 30400 or STAT 31015, or consent of instructor
STAT 41510. Bayesian Nonparametrics. 100 Units.
Bayesian nonparametric methods are increasingly important tools in machine learning and statistics. We will discuss nonparametric Bayesian approaches to mixture models, latent feature models, hierarchical models, network models, and high-dimensional regression models. Topics that will be covered include Dirichlet process, Chinese restaurant process, Pitman-Yor process, Indian buffet process, Gaussian process, and their computational techniques via Gibbs sampling and variational inference. Frequentist evaluations of posterior distributions will also be discussed in nonparametric and high-dimensional settings.
Instructor(s): C. Gao Terms Offered: To be determined Prerequisite(s): STAT 30200
STAT 41511. Topics in Robust and Semiparametric Statistics. 100 Units.
This course is about statistical estimation and inference with nuisance parameters. Examples include location estimation with unknown density, Cox proportional hazard model, low-dimensional inference in sparse regression, and robust estimation with arbitrary contamination. We will learn tangent spaces, efficient score functions, and information operators. Basic empirical process tools will also be discussed.
Terms Offered: Spring Prerequisite(s): STAT 30100
STAT 41512. Topics in High-Dimensional Hypothesis Testing. 100 Units.
This course will discuss high-dimensional hypothesis testing problems from a minimax perspective. Topics include sparse signal detection with known and unknown nulls, changepoint detection, adaptive test for nonparametric function, sparse PCA detection with computational lower bound, and goodness-of-fit test for discrete distributions.
Terms Offered: Winter
STAT 41520. Topics in Selective Inference. 100 Units.
This course will study the problem of selective inference where we would like to provide statistical guarantees about hypotheses or parameters whose definitions are influenced by our analysis of the same data set. Performing valid inference is challenging since we must find a way to condition on the outcome of the selection process which is not always simple to characterize. The course will discuss both recent advances and open problems in this field.
Instructor(s): R. Barber Terms Offered: To be determined Prerequisite(s): STAT 27850/30850 or STAT 30200 or consent of instructor
STAT 41521. Topics in Distribution-free Inference. 100 Units.
This course will focus on the recent field of distribution-free inference which seeks to provide verifiable statistical guarantees without assumptions on the distribution of the data. Methods in this area include holdout set methods, cross-validation type methods, and conformal prediction. The course will cover theoretical advances and practical methodologies, theoretical hardness results, and open problems in the field.
Terms Offered: Autumn Prerequisite(s): STAT 34300 and STAT 30100
STAT 41530. Topics in Causal Inference. 100 Units.
We will start with a light and comparative introduction of two causal inference languages: the potential outcome model and the graphical representation of causal effects. In the course, we will discuss topics including confounding, instrumental variables (IV), mediation analysis, and effective treatment allocations, with their applications in genetics and epidemiological research.
STAT 41540. Topics in Advanced Bayesian Methodology. 100 Units.
This course will explore topics in advanced Bayesian methodology, particularly around modern variational inference. The course will begin with a review of "classical" variational inference in exponential family models using mean-field approximations. It will then dive into recent advances in generic and scalable VI alternatives such as variational autoencoders, amortized inference, normalizing flows, and diffusion models, among other topics. The course will run like a seminar and feature a mix of lectures and student-run paper presentations. Students will also be responsible for a final project that applies or extends the methodology covered in course to applied problems of their choosing. Prerequisites include STAT 34800 (or an equivalent course on Bayesian statistics and probabilistic graphical models) and some experience in Python is encouraged.
Instructor(s): A. Schein Terms Offered: Autumn Prerequisite(s): STAT 34800
STAT 41541. Causal Inference in Randomized Experiments and Observational Studies. 100 Units.
This course provides an introduction to statistical causal inference, designed for graduate students with interest in causality. Our primary focus will be on the potential outcome framework for causality. The course will start with causal inference in randomized experiments and then proceed to observational studies. For randomized experiments, we will focus on randomization-based or design-based inference for various experiments, including completely randomized, stratified randomized, and rerandomized experiments. For observational studies, we will introduce popular methods for addressing observed confounding, including matching, regression, inverse propensity weighting, and their combinations. Depending on the progress of the course, we will also discuss more advanced topics such as instrumental variables, mediation analysis, interference, peer effects, etc.
Terms Offered: Winter Prerequisite(s): PhD or MS student in Statistics, or STAT 34300 and STAT 30100, or consent of instructor.
STAT 41551. Empirical Bayes. 100 Units.
In an empirical Bayes analysis, we imitate inferences made by an oracle Bayesian with extensive knowledge of the data-generating distribution. Empirical Bayes provides a principled approach for "learning from the experience of others" and is widely used in application domains such as genomics, small-area estimation, economics, and large-scale experimentation. In this graduate topics course, we provide an overview of empirical Bayes. We revisit the original papers that introduced the core ideas and explain how empirical Bayes is applied in practice. We also develop mathematical techniques to study empirical Bayes procedures from a theoretical perspective.
Terms Offered: Winter Prerequisite(s): STAT 30100 or consent of instructor
STAT 42600. Theoretical Neuroscience: Statistics and Information Theory. 100 Units.
This course begins with an introduction to inference and statistical methods in data analysis. We then cover the two main sections of the course: I) Encoding and II) Decoding in single neurons and neural populations. The encoding section will cover receptive field analysis (STA, STC and non-linear methods such as maximally informative dimensions) and will explore linear-nonlinear-Poisson models of neural encoding as well as generalized linear models alongside newer population coding models. The decoding section will cover basic methods for inferring stimuli or behaviors from spike train data, including both linear and correlational approaches to population decoding. The course will use examples from real data (where appropriate) in the problem sets which students will solve using MATLAB.
Instructor(s): S. Palmer Terms Offered: Spring Prerequisite(s): Prior exposure to basic calculus and probability theory, CPNS 35500 or instructor consent. Equivalent Course(s): CPNS 35600, ORGB 42600
STAT 42610. Theories of Cortical Circuit Dynamics and Computation. 100 Units.
This course will present mathematical frameworks for the construction and analysis of contemporary models of cortical circuits. Topics will include: models of neuronal spiking and synaptic dynamics, balanced networks, mean field theory of cortical networks, diffusion approximation and linear response in stochastically forced neuronal networks, models of decision making and working memory, information flow in cortical networks.
Instructor(s): B. Doiron Terms Offered: Winter Equivalent Course(s): CPNS 32610, CAAM 42610
STAT 44100. Consulting In Statistics. 300.00 Units.
This seminar course is an internal training program for graduate students in Statistics. The primary goal is to expose the students to applications that involve statistical thinking and to have hands on experience on real world data. The projects are provided by researchers from the university community. Participating students form teams to work on selected projects under faculty guidance and to present their work to all student consultants and researcher clients.
STAT 70000. Advanced Study: Statistics. 300.00 Units.
Advanced Study: Statistics
© 2014 The University of Chicago
5801 S. Ellis Ave. Chicago, IL 60637 United States
Accessibility
Print Options
Print this page.
The PDF will include all information unique to this page.
This website uses cookies to ensure the best user experience. Privacy & Cookies Notice Accept Cookies
Manage My Cookies
Manage Cookie Preferences
Confirm My Selections
- Dissertation Areas and Joint PhD Programs
- PhD Career Outcomes
- PhD Proposals and Defenses
- PhD Job Market Candidates
- PhD Research Community
- 100 Years of Pioneering Research
- Rising Scholars Conference
- Yiran Fan Memorial Conference
- Frequently Asked Questions
- PhD in Accounting
- PhD in Behavioral Science
PhD in Econometrics and Statistics
- PhD in Economics
- PhD in Finance
- PhD in Management Science and Operations Management
- PhD in Marketing
- Joint Program in Financial Economics
- Joint Program in Psychology and Business
- Joint PhD/JD Program
The Econometrics and Statistics Program provides foundational training in the science of learning from data towards solving business problems. Our students engage in extensive collaborative research on cutting-edge theory in Econometrics, Statistics and Machine Learning as well in applied research from a variety of fields within Booth (such as finance, marketing or economics).
Our program builds on a long tradition of research creativity and excellence at Booth.
Our PhD students become active members of the broad, interdisciplinary and intellectually stimulating Booth community. The program is ideal for students who wish to pursue an (academic) research career in data-rich disciplines, and who are motivated by applications (including but not limited to economics and business). As our PhD student, you will have a freedom to customize your program by combining courses at Booth (including business-specific areas such as marketing, finance or economics) with offerings at our partnering departments at the University of Chicago (Department of Statistics and Kenneth C. Griffin Department of Economics). You will acquire a solid foundation in both theory and practice (including learning theory, Bayesian statistics, causal inference or empirical asset pricing).
Our Distinguished Econometrics and Statistics Faculty
Chicago Booth’s Econometrics and Statistics faculty are committed to building strong collaborative relationships with doctoral students. We serve as research advisors and career mentors. Major areas of departmental research include: learning theory; causal inference; machine learning; Bayesian inference; decision theory; graphical models; high dimensional inference; and financial econometrics.

Bryon Aragam
Assistant Professor of Econometrics and Statistics and Robert H. Topel Faculty Scholar

Nabarun Deb
Assistant Professor of Econometrics and Statistics

Christian B. Hansen
Wallace W. Booth Professor of Econometrics and Statistics

Tetsuya Kaji
Associate Professor of Econometrics and Statistics and Richard Rosett Faculty Fellow

Mladen Kolar
Associate Professor of Econometrics and Statistics

Tengyuan Liang
Professor of Econometrics and Statistics and William Ladany Faculty Fellow

Nicholas Polson
Robert Law, Jr. Professor of Econometrics and Statistics

Veronika Rockova
Professor of Econometrics and Statistics, and James S. Kemper Foundation Faculty Scholar

Jeffrey R. Russell
Alper Family Professor of Econometrics and Statistics

Ekaterina (Katja) Smetanina
Assistant Professor of Econometrics and Statistics and Asness Junior Faculty Fellow

Panagiotis Toulis (Panos)
Associate Professor of Econometrics and Statistics, and John E. Jeuck Faculty Fellow

Dacheng Xiu
Professor of Econometrics and Statistics
A Network of Support
Booth’s Econometrics and Statistics group has been partnering with several (data science and interdisciplinary) research centers and institutes that facilitate the translation of research into practice. Through these venues, our PhD students can foster a strong research community and find additional resources including elective courses, funding for collaborative student work, and seminars with world-renowned scholars.
Data Science Institute at the University of Chicago The Data Science Institute executes the University of Chicago’s bold, innovative vision of Data Science as a new discipline by advancing interdisciplinary research, partnerships with industry, government, and social impact organizations. Center for Applied Artificial Intelligence The Center for Applied AI incubates transformative projects that fundamentally shape how humans use AI to interact with each other and the world. The Center’s innovative research uses machine learning and behavioral science to investigate how AI can best be used to support human decision-making and improve society. Toyota Technological Institute at Chicago Toyota Technological Institute at Chicago (TTIC) is a philanthropically endowed academic computer science institute, dedicated to basic research and graduate education in computer science. Its mission is to achieve international impact through world-class research and education in fundamental computer science and information technology. The Institute is distinctive to the American educational scene in its unique combination of graduate education and endowed research.
The Becker Friedman Institute for Economics With a mission of turning evidence-based research into real-world impact, the Becker Friedman Institute brings together the University of Chicago’s economic community. Ideas are translated into accessible formats and shared with world leaders tasked with solving pressing economic problems. Committee on Quantitative Methods in Social, Behavioral and Health Sciences This is an interdisciplinary community of faculty and students interested in methodological research in relation to applications in social, behavioral, and health sciences. The goals are to create an intellectual niche, exchange research ideas, facilitate research collaborations, share teaching resources, enhance the training of students, and generate a collective impact on the University of Chicago campus and beyond. The Institute for Data, Econometrics, Algorithms, and Learning The Institute for Data, Econometrics, Algorithms, and Learning (IDEAL) is a multi-discipline (computer science, statistics, economics, electrical engineering, and operations research) and multi-institution (Northwestern University, Toyota Technological Institute at Chicago, and University of Chicago) collaborative institute that focuses on key aspects of the theoretical foundations of data science. The institute will support the study of foundational problems related to machine learning, high-dimensional data analysis and optimization in both strategic and non-strategic environments.
The Fama-Miller Center for Research in Finance Tasked with pushing the boundaries of research in finance, the Fama-Miller Center provides institutional structure and support for researchers in the field. James M. Kilts Center for Marketing The Kilts Center facilitates faculty and student research, supports innovations in the marketing curriculum, funds scholarships for MBA and PhD students, and creates engaging programs aimed at enhancing the careers of students and alumni.
Scholarly Publications
Our PhD students' research has been published in top journals including Econometrica, Journal of Royal Statistical Society, Journal of Econometrics, Neural Information Processing Systems and Journal of Machine Learning Research. Below is a recent list of publications and working papers authored by our PhD students. Modeling Tail Index with Autoregressive Conditional Pareto Model Zhouyu Shen, Yu Chen and Ruxin Shi, Journal of Business and Economic Statistics, (40) 2022 Online Learning to Transport via the Minimal Selection Principle Wenxuan Guo, YoonHaeng Hur, Tengyuan Liang, Chris Ryan, Proceedings of 35th Conference on Learning Theory (COLT), (178) 2022 FuDGE: A Method to Estimate a Functional Differential Graph in a High-Dimensional Setting Boxin Zhao, Samuel Wang and Mladen Kolar, Journal of Machine Learning Research, (23) 2022 Approximate Bayesian Computation via Classification Yuexi Wang, Tetsuya Kaji and Veronika Rockova, Journal of Machine Learning Research (In press), 2022 Reversible Gromov-Monge Sampler for Simulation-Based Inference YoonHaeng Hur, Wenxuan Guo and Tengyuan Liang, Journal of the American Statistical Association (R&R). 2021. Data Augmentation for Bayesian Deep Learning Yuexi Wang, Nicholas Polson and Vadim Sokolov, Bayesian Analysis (In press), 2022 Pessimism Meets VCG: Learning Dynamic Mechanism Design via Offline Reinforcement Learning Boxiang Lyu, Zhaoran Wang, Mladen Kolar and Zhuoran Yang, In Proceedings of the 39th International Conference on Machine Learning (ICML), (162) 2022 Optimal Estimation of Gaussian DAG Models Ming Gao, Wai Ming Tai and Bryon Aragam, International Conference on Artificial Intelligence and Statistics (AISTATS), (151) 2022 Multivariate Change Point Detection for Heterogeneous Series Yuxuan Guo, Ming Gao, and Xiaoling Lu, Neurocomputing, (510) 2022 Disentangling Autocorrelated Intraday Returns Rui Da and Dacheng Xiu, Journal of Econometrics (R&R), 2021 When Moving-Average Models Meet High-Frequency Data: Uniform Inference on Volatility Rui Da and Dacheng Xiu, Econometrica, (89) 2021 Efficient Bayesian Network Structure Learning via Local Markov Boundary Search Ming Gao and Bryon Aragam, Advances in Neural Information Processing Systems (NeurIPS), (34) 2021 Structure Learning in Polynomial Time: Greedy Algorithms, Bregman Information, and Exponential Families Goutham Rajendran, Bohdan Kivva, Ming Gao and Bryon Aragam, Advances in Neural Information Processing Systems (NeurIPS), (34) 2021 Variable Selection with ABC Bayesian Forests Yi Liu, Yuexi Wang and Veronika Rockova, Journal of the Royal Statistical Association: Series B, (83) 2021 A Polynomial-time Algorithm for Learning Non-parametric Causal Graphs Ming Gao, Yi Ding, and Bryon Aragam, Advances in Neural Information Processing System (NeurIPS), (33) 2020 Uncertainty Quantification for Sparse Deep Learning Yuexi Wang and Veronika Rockova, International Conference on Artificial Intelligence and Statistics (AISTATS), (2018) 2020 Direct Estimation of Differential Functional Graphical Models Boxin Zhao, Samuel Wang and Mladen Kolar, Advances in neural information processing systems (NeurIPS), (32) 2019
The Effects of Economic Uncertainty on Financial Volatility: A Comprehensive Investigation Chen Tong, Zhuo Huang, Tianyi Wang, and Cong Zhang, Journal of Empirical Finance (R&R), 2022
Spotlight on Research
Econometrics and statistics research from our PhD students and faculty is often featured in the pages of Chicago Booth Review.
Is There a Ceiling for Gains in Machine-Learned Arbitrage?
In a recent paper by Chicago Booth’s Stefan Nagel and Dacheng Xiu and Booth PhD student Rui Da, findings suggest that there are limits to statistical arbitrage investment.
How (In)accurate Is Machine Learning?
Three Chicago Booth researchers quantify the likelihood of machine learning leading business executives astray.
Would You Trust a Machine to Pick a Vaccine?
"If we understand why a black-box method works, we can trust it more with our decisions, explains [Booth's] Ročková, one of the researchers trying to narrow the gap between what’s done in practice and what’s known in theory. "
Inside the Student Experience
Damian Kozbur, PhD ’14, says PhD students at Booth have the flexibility to work on risky problems that no one else has examined.
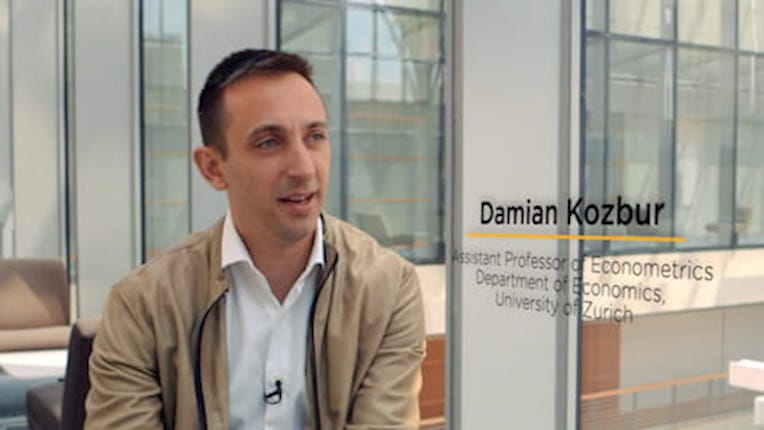
Video Transcript
Damian Kozbur, ’14: 00:01 I went to graduate school in order to develop econometrics tools in conjunction with machine-learning tools in conjunction with economic theory in order to do inference for economic parameters. When you work in high dimensional estimation and you're dealing with problems where the number of variables you're looking at can potentially be in the millions, there's no way to visualize what's going on. Demands now really require that you can handle huge datasets. There's something really satisfying about studying a problem and studying it well. I would say Booth is an excellent place to do it. You have the flexibility to work on really risky problems where you're trying to navigate this landscape that nobody's ever really looked at before. You have an opportunity to dig deeper. You have an opportunity to be rigorous. The faculty is there to help you. They're trying to figure out the same kinds of problems. Things that you figure out cannot always be visualized and it cannot always be easily understood. That doesn't necessarily mean that it's not practical or not useful.
Damian Kozbur, ’14: 01:08 There's an incredible explosion in terms of the amount of data we have on everything. There is an incredible explosion in terms of our understanding of high dimensional econometrics. If you're doing innovative work right now, it will have an impact.
Current Econometrics and Statistics Students
PhD students in econometrics and statistics apply statistical methods to a wide range of business problems, from the effectiveness of machine-learning tools to video-game preferences. Our graduates go on to work in high-profile institutions, generally in academia, finance, or data science.
Current Students
Y ifei Chen Rui Da
Chaoxing Dai
Wenxuan Guo
Shuo-Chieh Huang
Shunzhuang Huang So Won Jeong
Boxiang (Shawn) Lyu Edoardo Marcelli
Zhouyu Shen
Shengjun (Percy) Zhai
Current Students in Sociology and Business
Jacy Anthis
Program Expectations and Requirements
The Stevens Program at Booth is a full-time program. Students generally complete the majority of coursework and examination requirements within the first two years of studies and begin work on their dissertation during the third year. For details, see General Examination Requirements by Area in the Stevens Program Guidebook below.
Download the 2023-2024 Guidebook!
MS in Data Science
MSDS students choose among the many introductory graduate courses offered to students in the PhD program. These courses cover areas of computer science, optimization, linear algebra and statistics for students that have not had prior exposure to this required course work. Master’s students are fully integrated in the academic activities of the department alongside the PhD students.
Students must complete the required 5 core courses, 4 electives, and a final project to complete the program. There are also three foundational courses that students can test out. For the students who test out of foundational courses, the minimum number of courses taken in the program is 9. For the students who take all foundational courses, it is 12. These foundational courses can be taken in the summer before the program starts. Finally, students will be able to engage in a variety of opportunities across the Data Science Institute research programs and partnerships during their residency in the program.
The Curriculum
Foundational courses:.
Interested students will have the opportunity to test out of each of the 3 foundation courses below. Each of the courses will be offered in the late summer and offered online before the start of the fall quarter.
- Computational Foundations for Data Science
- Mathematical Foundations for Data Science
- Statistical Foundations for Data Science
Core Courses:
- Introduction to Data Science
- Systems for Data and Computers/Data Design
- Data Interaction
- Introduction to ML and AI or Foundations of Machine Learning and AI Part I
- Responsible Use of Data and Algorithms
Four graduate-level electives can be selected from a wide variety of courses in Data Science, Computer Science, Statistics and across the University.
The online application portal will begin accepting applications for Fall 2024 admission in early Fall 2023. To ensure full consideration, applicants should apply by the deadline. The program may accept applications after the deadline if the cohort is not filled.
- Program Requirements
- Core Curriculum
- Major Area Concentration
- Minor Area Concentration
- Courses for 2023-2024
- PBHS Student Handbook
The PhD program in the Department of Public Health Sciences at the University of Chicago offers advanced courses of study in biostatistics, epidemiology, and health services research, all of which are foundational fields in Public Health. The program is supported by a core methodological curriculum in population-based research on human health. Students completing the program will be prepared to design and conduct methodological and substantive research on fundamental questions about human health and biomedical science from a population perspective. They will also be prepared to collaborate with colleagues from different disciplines to carry out such research.
Why should you choose the University of Chicago for your PhD in epidemiology, biostatistics, or health services research?
- Our program is small and student-focused; training programs are developed specifically for each student based on their background and interests.
- Our highly selective program prides itself on extensive student-faculty and student-student interaction.
- Our students can take courses from and interact with faculty from departments across the University of Chicago, including Statistics, Sociology, Human Genetics, Cancer, Biology, Public Policy, Economics, Business, Social Services, Human Development, and Clinical Departments in the Medical School. Dissertation Committees often include faculty from other departments.
- We are one of a small number of related programs that guarantee full funding for the PhD program.
Applications should be received by December 1 for entrance into the program in Autumn. Click here for the application portal.
Submission of GRE scores is optional for all UChicago Biosciences programs, including Public Health Sciences (PHS). However, given the quantitative nature of the coursework that PHS students are required to take, the GRE is potentially helpful to the admissions committee in gauging a student’s ability to complete advanced statistical coursework. Thus, the PHS department strongly encourages its PhD applicants to submit GRE scores. The University of Chicago institution code is 1832. If submitted, the GRE scores must be from within the last five years.
- Skip to Content
- Catalog Home
- Institution Home
2024-2025 Catalog
- The Curriculum
- Anthropology
- Architectural Studies
- Art History
- Astrophysics
- Biological Chemistry
- Biological Sciences
- Cinema and Media Studies
- Classical Studies
- Cognitive Science
- Comparative Human Development
- Comparative Literature
- Computational and Applied Mathematics
- Computational Social Science
- Computer Science
- Creative Writing
- Critical Race and Ethnic Studies
- Data Science
- Democracy Studies
- Digital Studies of Language, Culture, and History
- East Asian Languages and Civilizations
- Education and Society
- English Language and Literature
- Environmental Science
- Environmental and Urban Studies
- Environment, Geography, and Urbanization
- Fundamentals: Issues and Texts
- Gender and Sexuality Studies
- Geographic Information Science
- Geophysical Sciences
- Germanic Studies
- Global Studies
- Health and Society
- History, Philosophy, and Social Studies of Science and Medicine
- Human Rights
- Inequality, Social Problems, and Change
- Inquiry and Research in the Humanities
- Jewish Studies
- Latin American and Caribbean Studies
- Law, Letters, and Society
- Linguistics
- Mathematics
- Media Arts and Design
- Medieval Studies
- Molecular Engineering
- Near Eastern Languages and Civilizations
- Neuroscience
- Norwegian Studies
- Political Science
- Public Policy Studies
- Quantitative Social Analysis
- Race, Diaspora, and Indigeneity
- Religious Studies
- Renaissance Studies
- Romance Languages and Literatures
- Russian and East European Studies
- Science Communication and Public Discourse
- South Asian Languages and Civilizations
- Theater and Performance Studies
- Visual Arts
- Yiddish Studies
- Academic Regulations and Procedures
- Examination Credit
- Transfer Credit
- Interdisciplinary Opportunities
- Joint Degree Programs
- Study Abroad
- Career Preparation
- Research and Fellowships
- Archived Catalogs
- Academic Calendar
- The College Catalog 2024-2025 >
- Programs of Study >
Contacts | Program of Study | General Course Information | Introductory Courses and Sequences | Additional Courses in Statistical Theory, Methods, and Applications | Courses in Probability | Courses in Machine Learning | Courses in Optimization | Grading | Program Requirements for Majors | Prescribed Mathematics Courses | Prescribed Statistics Courses | Electives | Computer Science Requirement | Summary of Requirements for the BA in Statistics | Summary of Requirements for the BS in Statistics | Honors | Joint BA/MS or BS/MS in Statistics | Minor Program in Statistics | Summary of Requirements for the Minor in Statistics | Departmental Electives Approved for the Minor in Statistics | Non-Departmental Electives Approved for the Minor in Statistics | Courses
Department Website: https://stat.uchicago.edu
Program of Study
The modern science of statistics involves the development of principles and methods for modeling uncertainty, for designing experiments, surveys, and observational programs, and for analyzing and interpreting empirical data. Mathematics plays a major role in all areas of statistics, from probability theory to data analysis. Statistics is an appropriate field for students with strong mathematical and computational skills and an interest in applying these skills to problems in the natural and social sciences. A program leading to the bachelor's degree in Statistics offers coverage of the principles and methods of statistics in combination with solid training in mathematics and computation. The major can provide appropriate preparation for graduate study in statistics or in other subjects with strong quantitative components. Students considering graduate study in statistics or related fields are encouraged to discuss their programs with the Departmental Adviser for Majors at an early stage, whether or not they plan to receive an undergraduate degree in Statistics.
Students who are majoring in other fields of study may also complete a minor in Statistics and are encouraged to discuss their course choices with the Departmental Adviser for Minors. Information on the minor follows the description of the major.
General Course Information
Courses at the 10000 or 20000 level are designed to provide instruction in statistics, probability, and statistical computation for students from all parts of the University. These courses differ in emphasis on theory or methods, in mathematical level, and in the direction of applications.
Introductory Courses and Sequences
To begin their studies in statistics, students can choose from several courses. Students and College adviser s are encouraged to contact the Departmental Adviser for Introductory Courses for advice on choosing an appropriate first course.
Students with little or no math background who do not intend to continue on to more advanced statistics courses may take either STAT 20000 Elementary Statistics or STAT 20010 Elementary Statistics Through Case Study ; enrolling in both is not permitted. Either course satisfies the general education requirement in the mathematical sciences. These courses are two variants of an introductory course that emphasizes concepts rather than statistical techniques. Neither STAT 20000 Elementary Statistics nor STAT 20010 Elementary Statistics Through Case Study may be taken by students with credit for STAT 22000 Statistical Methods and Applications , STAT 23400 Statistical Models and Methods , or more advanced courses in the Department of Statistics. Neither STAT 20000 Elementary Statistics nor STAT 20010 Elementary Statistics Through Case Study counts toward the major or minor in Statistics.
The sequence STAT 11800-11900 Introduction to Data Science I-II provides a computational introduction to statistical concepts, techniques, and applications to data analysis. STAT 11800-11900 Introduction to Data Science I-II has considerable overlap with STAT 22000 Statistical Methods and Applications , but has a more computational and less mathematical emphasis than STAT 22000 Statistical Methods and Applications . Neither STAT 11800 Introduction to Data Science I nor STAT 11900 Introduction to Data Science II can count toward the major in Statistics. STAT 11900 Introduction to Data Science II , but not STAT 11800 Introduction to Data Science I , can be used as an elective in the minor in Statistics.
Students with at least MATH 13100 Elem Functions and Calculus I or placement into MATH 15200 Calculus II or higher are encouraged to take STAT 22000 Statistical Methods and Applications instead of either STAT 20000 Elementary Statistics or STAT 20010 Elementary Statistics Through Case Study . Students with three quarters of calculus may choose either STAT 22000 Statistical Methods and Applications or STAT 23400 Statistical Models and Methods . Students may count either STAT 22000 Statistical Methods and Applications or STAT 23400 Statistical Models and Methods , but not both, toward the forty-two credits required for graduation.
STAT 22000 Statistical Methods and Applications is a general introduction to statistical concepts, techniques, and applications to data analysis and to problems in the design, analysis, and interpretation of experiments and observational programs. A score of 5 on the AP Statistics exam yields credit for STAT 22000 Statistical Methods and Applications , although this credit will not count toward the requirements for a major or minor in Statistics. STAT 22000 Statistical Methods and Applications can count toward the minor in Statistics, but not toward the major in Statistics.
STAT 23400 Statistical Models and Methods covers much of the same material as STAT 22000 Statistical Methods and Applications , but at a somewhat higher mathematical level. The course is a one-quarter introduction to statistics that is appropriate for any student with a good command of univariate calculus including sequences and series. STAT 23400 Statistical Models and Methods can count toward the minor in Statistics, but not toward the major in Statistics .
Students cannot hold credit for both STAT 22000 Statistical Methods and Applications and STAT 23400 Statistical Models and Methods . Students completing either STAT 22000 Statistical Methods and Applications or STAT 23400 Statistical Models and Methods forego their AP Statistics credit for STAT 22000 Statistical Methods and Applications .
STAT 24400-24500 Statistical Theory and Methods I-II is recommended for students who wish to have a thorough introduction to statistical theory and methodology. STAT 24400-24500 Statistical Theory and Methods I-II is more mathematically demanding than either STAT 22000 Statistical Methods and Applications or STAT 23400 Statistical Models and Methods . STAT 24400 Statistical Theory and Methods I assumes some familiarity with multivariate calculus, and STAT 24500 Statistical Theory and Methods II assumes some familiarity with linear algebra.
STAT 24410-24510 Statistical Theory and Methods Ia-IIa is an alternative version of STAT 24400-24500 Statistical Theory and Methods I-II that requires STAT 25100 Introduction to Mathematical Probability (or STAT 25150 Introduction to Mathematical Probability-A ) as a prerequisite and that replaces some probability topics with additional statistical topics not normally covered in STAT 24400-24500 Statistical Theory and Methods I-II . STAT 24410-24510 Statistical Theory and Methods Ia-IIa is particularly well-suited for students with a strong mathematical background who are interested in more extensive coverage of probability and statistics. Students may count either STAT 24400 Statistical Theory and Methods I or STAT 24410 Statistical Theory and Methods Ia , but not both, toward the 4200 units of credit required for graduation. Similarly, students may count either STAT 24500 Statistical Theory and Methods II or STAT 24510 Statistical Theory and Methods IIa , but not both, and they may count either STAT 25100 Introduction to Mathematical Probability or STAT 25150 Introduction to Mathematical Probability-A , but not both, toward the 4200 units of credits required for graduation.
Students considering a major in Statistics are encouraged to begin with either STAT 24400-24500 Statistical Theory and Methods I-II or with the alternative sequence consisting of STAT 25100 Introduction to Mathematical Probability (or STAT 25150 Introduction to Mathematical Probability-A ) followed by STAT 24410-24510 Statistical Theory and Methods Ia-IIa , rather than with STAT 23400 Statistical Models and Methods . Although students with a strong mathematical background can and do take either STAT 24400-24500 Statistical Theory and Methods I-II or the alternative sequence ( STAT 25100 Introduction to Mathematical Probability and STAT 24410-24510 Statistical Theory and Methods Ia-IIa ) without prior course work in statistics or probability, some students find it helpful to take either STAT 22000 Statistical Methods and Applications or STAT 23400 Statistical Models and Methods as preparation.
The core of the Statistics major consists of three courses: STAT 25100 Introduction to Mathematical Probability (or STAT 25150 Introduction to Mathematical Probability-A ) and either STAT 24400-24500 Statistical Theory and Methods I-II or STAT 24410-24510 Statistical Theory and Methods Ia-IIa . Either of these is recommended as a three-quarter cognate sequence for students in the quantitative sciences and mathematics. Note that STAT 25100 Introduction to Mathematical Probability may be taken before, after, or concurrently with STAT 24400-24500 Statistical Theory and Methods I-II , though it is a prerequisite for STAT 24410-24510 Statistical Theory and Methods Ia-IIa .
Additional Courses in Statistical Theory, Methods, and Applications
For students interested in continuing their study of statistics beyond the introductory level, STAT 22200 Linear Models and Experimental Design , STAT 22400 Applied Regression Analysis (or STAT 22401 Regression Analysis for Health and Social Research ), STAT 22600 Analysis of Categorical Data , STAT 22700 Biostatistical Methods , and STAT 27410 Introduction to Bayesian Data Analysis are recommended. Note that STAT 22400 Applied Regression Analysis and STAT 22401 Regression Analysis for Health and Social Research are highly similar to each other, and students may only take one of them for credit, not both. They are considered to be interchangeable in the major and minor in Statistics. Also, because there is some overlap between STAT 22600 Analysis of Categorical Data and STAT 22700 Biostatistical Methods , only one of these two courses, not both, may be counted toward a major or minor in Statistics. The courses STAT 22200 Linear Models and Experimental Design , STAT 22400 Applied Regression Analysis (or STAT 22401 Regression Analysis for Health and Social Research ), STAT 22600 Analysis of Categorical Data , and STAT 27410 Introduction to Bayesian Data Analysis may be taken in any order. Each presumes two quarters of calculus and a previous course in statistics ( STAT 22000 Statistical Methods and Applications or higher) . STAT 22700 Biostatistical Methods has STAT 22400 Applied Regression Analysis (or STAT 22401 Regression Analysis for Health and Social Research ) as a prerequisite.
For students who have completed STAT 24400-24500 Statistical Theory and Methods I-II and are interested in more advanced statistical methodology courses, STAT 24620 Multivariate Statistical Analysis: Applications and Techniques , STAT 26100 Time Dependent Data , STAT 26300 Introduction to Statistical Genetics , STAT 27400 Nonparametric Inference , STAT 27850 Multiple Testing, Modern Inference, and Replicability , and STAT 34300 Applied Linear Stat Methods are recommended. Many other graduate courses in Statistics offer opportunities for further study of statistical theory, methods, and applications. For details, consult the instructor or the Departmental Adviser for Majors, or visit the Graduate Announcements .
Courses in Probability
Students interested in probability can begin with STAT 25100 Introduction to Mathematical Probability or STAT 25150 Introduction to Mathematical Probability-A , which can be taken separately from any Statistics courses and can be supplemented with more advanced probability courses, such as STAT 25300 Introduction to Probability Models , STAT 25211 Introduction to Random Matrices , or MATH 23500 Markov Chains, Martingales, and Brownian Motion . Students with a strong mathematical background can take STAT 31200 Introduction to Stochastic Processes I , STAT 38100 Measure-Theoretic Probability I , and STAT 38300 Measure-Theoretic Probability III . Note that because there is some overlap between MATH 23500 Markov Chains, Martingales, and Brownian Motion , STAT 25300 Introduction to Probability Models , and STAT 31200 Introduction to Stochastic Processes I , only one of these three courses may be counted toward a major in Statistics.
Courses in Machine Learning
A student with a strong computer science background could take STAT 27700 Mathematical Foundations of Machine Learning and STAT 27725 Machine Learning . Other courses in the category of machine learning include the advanced statistical methodology courses STAT 24620 Multivariate Statistical Analysis: Applications and Techniques and STAT 27400 Nonparametric Inference . Graduate course offerings in machine learning include STAT 37601 Machine Learning and Large-Scale Data Analysis , STAT 37710 Machine Learning , as well as more advanced graduate courses.
Courses in Optimization
A student with a strong mathematical background could take STAT 28000 Optimization . Graduate course offerings in optimization include STAT 31015 Mathematical Computation IIA: Convex Optimization and STAT 31020 Mathematical Computation IIB: Nonlinear Optimization .
Students who are majoring or minoring in Statistics must receive a quality grade of at least C in all of the courses counted toward their major or minor program in Statistics. In addition, students who are majoring in Statistics must receive quality grades of at least C+ in both STAT 24400 Statistical Theory and Methods I and STAT 24500 Statistical Theory and Methods II (or at least C in both STAT 24410 Statistical Theory and Methods Ia and STAT 24510 Statistical Theory and Methods IIa ). Subject to College and divisional regulations, and with the consent of the instructor, students may register for either quality grades or for P/F grading in any 20000-level Statistics course, other than STAT 29700 Undergraduate Research or STAT 29900 Bachelor's Paper , that is not counted toward a major or minor in Statistics. A grade of P is given only for work of C– quality or higher.
The following policy applies to students who wish to receive a mark of I for a Statistics course. In addition to submitting the official Incomplete Form required by the College, students must have completed at least half of the total required course work with a grade of C– or better, and they must be unable to complete the remaining course work by the end of the quarter due to an emergency. Students requesting a mark of I for STAT 20000 Elementary Statistics , STAT 20010 Elementary Statistics Through Case Study , STAT 22000 Statistical Methods and Applications , or STAT 23400 Statistical Models and Methods must obtain approval from both the current instructor and the Departmental Adviser for Introductory Courses.
Program Requirements for Majors
Every candidate must obtain approval of his or her course program from the Departmental Adviser for Majors. Students majoring in Statistics should meet the general education requirement in mathematical sciences with courses in calculus. The major program includes four additional prescribed mathematics courses, four prescribed statistics courses, and two prescribed computer science courses. Students are advised to complete the four mathematics courses by the end of their third year. Additional requirements include four approved elective courses in Statistics. The BS also requires an additional prescribed mathematics course and an approved, coherent, three-quarter sequence at the 20000 level in a field to which statistics can be applied. Students who are majoring in Statistics must receive quality grades of at least C+ in both STAT 24400-24500 Statistical Theory and Methods I-II (or at least C in both STAT 24410-24510 Statistical Theory and Methods Ia-IIa ), and at least C in all other courses counted toward the Statistics major. A grade of P is not acceptable for any of these courses.
Prescribed Mathematics Courses
Prescribed statistics courses.
The four prescribed Statistics courses are STAT 25100 Introduction to Mathematical Probability (or STAT 25150 Introduction to Mathematical Probability-A or MATH 23500 Markov Chains, Martingales, and Brownian Motion ), STAT 24400-24500 Statistical Theory and Methods I-II (or STAT 24410-24510 Statistical Theory and Methods Ia-IIa ), and one of the following three courses: STAT 22400 Applied Regression Analysis or STAT 22401 Regression Analysis for Health and Social Research or STAT 34300 Applied Linear Stat Methods .
It is recommended that students who have had some multivariable calculus begin the major by taking either STAT 25100 Introduction to Mathematical Probability or STAT 24400 Statistical Theory and Methods I as their first course in probability and statistics. An alternative route to beginning the major would be to first take either STAT 22000 Statistical Methods and Applications or STAT 23400 Statistical Models and Methods , neither of which count toward the major, but which could serve as a prerequisite for courses such as STAT 22400 Applied Regression Analysis , STAT 22200 Linear Models and Experimental Design , STAT 22600 Analysis of Categorical Data , and STAT 27410 Introduction to Bayesian Data Analysis which do count toward the major. This second path is recommended for students who need additional time to complete multivariable calculus and linear algebra prerequisites and who want to get started on the major in the meantime.
Candidates for the BA are required to take four electives, at least two of which must be on List B below. The remaining two electives may be from either List B or C. Students may count either STAT 22600 Analysis of Categorical Data or STAT 22700 Biostatistical Methods , but not both, toward the BA. Similarly, students may count only one of the following three courses: MATH 23500 Markov Chains, Martingales, and Brownian Motion , STAT 25300 Introduction to Probability Models , or STAT 31200 Introduction to Stochastic Processes I , toward the BA. If MATH 23500 Markov Chains, Martingales, and Brownian Motion is counted in place of STAT 25100 Introduction to Mathematical Probability in the Statistics BA, then MATH 23500 Markov Chains, Martingales, and Brownian Motion cannot also be counted as an elective in the Statistics BA.
Candidates for the BS are required to take four electives. A candidate for the BS who has not taken STAT 34300 Applied Linear Stat Methods as one of the four prescribed statistics courses must take at least one elective from List A below, a second elective from List B, and the remaining two electives may be from either List B or C. A candidate for the BS who has taken STAT 34300 Applied Linear Stat Methods as one of the four prescribed statistics courses must take at least two electives from List B and the remaining two electives may be from either List B or C. For the BS in Statistics, STAT 28000 Optimization counts as a List C elective only if MATH 21100 Basic Numerical Analysis is also included in the program. In other words, students cannot double-count STAT 28000 Optimization toward both the four-elective requirement and the requirement to take one of STAT 28000 Optimization and MATH 21100 Basic Numerical Analysis . Students may count either STAT 22600 Analysis of Categorical Data or STAT 22700 Biostatistical Methods , but not both, toward the BS. Similarly, students may count only one of the following three courses: MATH 23500 Markov Chains, Martingales, and Brownian Motion , STAT 25300 Introduction to Probability Models , or STAT 31200 Introduction to Stochastic Processes I , toward the BS. If MATH 23500 Markov Chains, Martingales, and Brownian Motion is counted in place of STAT 25100 Introduction to Mathematical Probability in the Statistics BS, then MATH 23500 Markov Chains, Martingales, and Brownian Motion cannot also be counted as an elective in the Statistics BS.
Note: The following lists may change from time to time as courses change and new courses are added. Please consult the Departmental Adviser for Majors for approval of your electives.
Computer Science Requirement
Candidates for either the BA or the BS are required to take CMSC 14100 Introduction to Computer Science I - CMSC 14200 Introduction to Computer Science II . Students with placement into CMSC 14200 Introduction to Computer Science II or higher must complete the course they are placed into.
BS Requirement of Three-Quarter Sequence in a Field to Which Statistics Can Be Applied
Candidates for the BS (but not the BA) are required to take an approved, coherent, three-quarter sequence at the 20000 level in a field to which statistics can be applied. Generally this sequence should be in the natural or social sciences, but a sequence in another discipline may be acceptable. Courses in MATH or CMSC may not be used for this requirement. Sequences in which earlier courses are prerequisites for later ones are preferred. Example sequences include BIOS 20198 Biodiversity - BIOS 20196 Ecology and Conservation - BIOS 23406 Biogeography ; CHEM 22000-22100-22200 Organic Chemistry I-II-III ; CHEM 26100-26200-26300 Quantum Mechanics; Thermodynamics; Chemical Kinetics and Dynamics ; ECON 20000-20100-20200 The Elements of Economic Analysis I-II-III ; GEOS 21000 Mineralogy - GEOS 21100 Introduction to Petrology - GEOS 21200 Physics of the Earth ; and PHYS 23410 Quantum Mechanics I - PHYS 23510 Quantum Mechanics II - PHYS 24310 Advanced Quantum Mechanics . All sequences must be approved by the Departmental Adviser for Majors.
Summary of Requirements for the BA in Statistics
Summary of requirements for the bs in statistics.
The BA or BS with honors is awarded to students with Statistics as their primary major who have a GPA of 3.25 or higher overall and 3.5 or higher in the courses in the major and also complete an approved honors paper ( STAT 29900 Bachelor's Paper ). This paper is typically based on a structured research program that the student undertakes, with supervision by a faculty member in the Department of Statistics, in the first quarter of the student's fourth year. A student who wishes to write the honors paper must meet the following deadlines: (1) by the Friday of the fifth week of the first quarter of the student's fourth year, the student must submit the adviser's name (must be a faculty member in the Department of Statistics), tentative thesis title, and name of the second reader; (2) enroll in STAT 29900 in the second quarter of the student's fourth year, with a good draft of the thesis due by the first day of exam period; and (3) submit the final draft by the fifth week of the third quarter of the student's fourth year. Eligible students who wish to be considered for honors should consult the Departmental Adviser for Majors before the end of their third year. (These deadlines assume that the student is graduating in Spring Quarter of fourth year. Students graduating in a different quarter should consult the Departmental Adviser for Majors for deadlines.) The research paper or project used to meet this requirement may not be used to meet the bachelor's paper or project requirement in another major or course. NOTE: Credit for STAT 29900 Bachelor's Paper will not count towards the courses required for a major in Statistics.
Joint BA/MS or BS/MS in Statistics
This program enables unusually well-qualified undergraduate students to complete an MS in Statistics along with a BA or BS during their four years at the College. The BA or BS can be in any field, not necessarily Statistics. Outstanding undergraduates in any major are welcome to apply.
Only a small number of students will be selected for the program through a competitive admissions process. Participants must apply to the MS program in Statistics by June 1 of their third year for admission to candidacy for an MS in Statistics during their fourth year. To be considered, students should have completed almost all of their undergraduate requirements, including all of their general education and language competence requirements, by the end of their third year. They should also have completed, at a minimum, STAT 24400-24500 Statistical Theory and Methods I-II (or STAT 24410-24510 Statistical Theory and Methods Ia-IIa ) with A or A- grades and all the mathematics requirements for the Statistics BA with very high grades. While these are the minimum criteria, admission is competitive, and additional qualifications may be needed. Interested students are strongly encouraged to consult both the Departmental Adviser for Majors and their College adviser early in their third year.
Participants in the joint BA/MS or BS/MS program must meet the same requirements as students in the MS program in Statistics. Of the nine courses that are required at the appropriate level, up to two may also meet the requirements of an undergraduate major and/or minor, while the others may count as general elective credit toward the undergraduate degree. For example, STAT 24410-24510 Statistical Theory and Methods Ia-IIa , which satisfy requirements for the MS in Statistics, could also be used to satisfy requirements of a BA or BS program in Statistics. At least six of the courses counting toward the MS degree must be undertaken in the fourth year.
Other requirements include a master's paper and participation in the Consulting Program of the Department of Statistics. For details on requirements, visit stat.uchicago.edu/academics/graduate-programs/graduate-student-resources/academic-life/requirements-and-regulations-for-ms-candidates .
Minor Program in Statistics
The Statistics minor focuses on statistical methodology, in contrast to the Statistics major, which has a substantial theoretical component. The minor in Statistics requires five courses, some prescribed and some elective, chosen in consultation with the Departmental Adviser for Minors. Not every combination of elective courses is allowed. Generally, no more than two electives may be satisfied by courses offered by departments other than the Department of Statistics. Students are encouraged to obtain course advising early from the Departmental Adviser for Minors. By the end of Spring Quarter of the student’s third year, a student who wishes to complete the Statistics minor must complete the Consent to Complete a Minor Program Form to obtain formal approval of their degree program from the Departmental Adviser for Minors.
The core of the Statistics minor consists of STAT 22400 Applied Regression Analysis (or STAT 22401 Regression Analysis for Health and Social Research ) and either STAT 22200 Linear Models and Experimental Design or STAT 22600 Analysis of Categorical Data (or both). These three courses may be taken in any order after meeting the prerequisite of at least two quarters calculus and one of the introductory statistics courses: STAT 22000 Statistical Methods and Applications , STAT 23400 Statistical Models and Methods , STAT 24500 Statistical Theory and Methods II , STAT 24510 Statistical Theory and Methods IIa , or AP Statistics credit. STAT 11900 Introduction to Data Science II is also allowed to fulfill the introductory statistics prerequisite requirement.
An approved substitute for STAT 22600 Analysis of Categorical Data is PBHS 32700 Biostatistical Methods (also designated as STAT 22700 Biostatistical Methods ), which requires STAT 22400 Applied Regression Analysis as prerequisite and is offered by the Department of Public Health Sciences. Students may count either STAT 22600 Analysis of Categorical Data or STAT 22700 Biostatistical Methods , but not both, toward the Statistics minor. STAT 22700 Biostatistical Methods does not count against the limit of no more than two electives from outside the Department of Statistics.
To complete the five-course minor, students must choose from among the approved electives listed below. Generally, no more than two electives may be satisfied by courses offered by departments other than the Department of Statistics. Students may petition the Departmental Adviser for Minors to include more than two electives from outside the Department of Statistics. Regardless, at most one elective can be satisfied by a course offered by the Booth School of Business. Further, due to the course grading policies of the Booth School of Business, their 40000-level courses cannot be counted toward the Statistics minor if taken during the quarter in which the student will graduate from the College.
STAT 11900 Introduction to Data Science II and either STAT 22000 Statistical Methods and Applications or STAT 23400 Statistical Models and Methods , but not both, may be used as electives in the Statistics minor if taken prior to any other courses for which at least STAT 22000 Statistical Methods and Applications or STAT 23400 Statistical Models and Methods is prerequisite and before either of STAT 24500 Statistical Theory and Methods II or STAT 24510 Statistical Theory and Methods IIa . STAT 11900 Introduction to Data Science II can only be used as an elective in the Statistics minor if the student also completes STAT 11800 Introduction to Data Science I (which cannot be included in a Statistics minor).
Students should note that STAT 11900 Introduction to Data Science II is a requirement for the Data Science minor and no course may be counted toward multiple minors. Likewise, if either STAT 22000 Statistical Methods and Applications or STAT 23400 Statistical Models and Methods is used to fulfill a requirement for any major(s), other minors, or general education requirements, then neither course may be used to fulfill a requirement in the Statistics minor. Students may not use AP credit for STAT 22000 Statistical Methods and Applications to fulfill a requirement for the Statistics minor.
The list of courses approved for the minor may change from time to time as courses change and new courses are added. Please consult the Departmental Adviser for Minors for approval of your minor program plan. Students may petition the Departmental Adviser for Minors for approval of another course. Such courses must have a minimum statistics prerequisite of introductory statistics ( STAT 22000 Statistical Methods and Applications , STAT 23400 Statistical Models and Methods , STAT 24500 Statistical Theory and Methods II , or STAT 24510 Statistical Theory and Methods IIa ), incorporate a considerable amount of data analysis, and cannot substantially overlap with the topics covered in departmental courses or other courses in the student's minor program.
No courses in the Statistics minor can be double counted with the student's major(s), other minors, or general education requirements. An approved elective must replace any course required for the Statistics minor that is used to meet the requirements for any major(s), other minors, or general education requirements.
The following courses offered by the Department of Statistics cannot be included in a Statistics minor: STAT 11800 Introduction to Data Science I , STAT 20000 Elementary Statistics , STAT 20010 Elementary Statistics Through Case Study , STAT 24300 Numerical Linear Algebra , STAT 24400 Statistical Theory and Methods I , STAT 24410 Statistical Theory and Methods Ia , STAT 25100 Introduction to Mathematical Probability , STAT 25150 Introduction to Mathematical Probability-A , STAT 25211 Introduction to Random Matrices , STAT 25300 Introduction to Probability Models , STAT 28000 Optimization , STAT 28200 Dynamical Systems with Applications , STAT 29700 Undergraduate Research , or any graduate courses in probability. In addition, CMSC 25400 Machine Learning (also designated as STAT 27725 Machine Learning ) cannot be included in the Statistics minor.
Students who are minoring in Statistics must receive a quality grade of at least C in all of the courses counted toward the minor. A grade of P is not acceptable for any of these courses. More than half of the courses counted toward the Statistics minor must be met by registering for courses bearing University of Chicago course numbers.
Summary of Requirements for the Minor in Statistics
Departmental electives approved for the minor in statistics, non-departmental electives approved for the minor in statistics.
Because of the interdisciplinary nature of the field of statistics, other departments and committees offer courses approved for use as electives for the Statistics minor. Generally, no more than two electives may be satisfied by courses offered by departments other than the Department of Statistics. Students may petition the Departmental Adviser for Minors to include more than two electives from outside the Department of Statistics. Regardless, at most one elective can be satisfied by a course offered by the Booth School of Business. Further, due to the course grading policies of the Booth School of Business, their 40000-level courses cannot be counted toward the Statistics minor if taken during the quarter in which the student will graduate from the College.
Offering departments include Public Health Sciences, Computer Science, Comparative Human Development, Human Genetics, Public Policy, Sociology, and the Booth School of Business.
Some of the approved electives offered by other departments also bear a Statistics course number and some do not. Students should enroll in the relevant Department of Statistics course number when available. Examples include STAT 22700 Biostatistical Methods , STAT 22810 Epidemiology and Population Health , STAT 31900 Introduction to Causal Inference , STAT 33211 Mediation, Moderation, and Spillover Effects , STAT 35700 Epidemiologic Methods , STAT 35800 Statistical Applications , and STAT 36900 Applied Longitudinal Data Analysis .
Undergraduate registration in 30000-level and 40000-level courses is by instructor consent only. Undergraduates cannot pre-register for 30000-level or 40000-level courses. Instead, students should contact the instructor well in advance.
Statistics Courses
STAT 10001. Collaborative Learning in Statistics 20000. 000 Units.
This is an optional, limited enrollment workshop for students concurrently enrolled in STAT 20000 Elementary Statistics. Undergraduate Team Leaders guide small groups of students in weekly workshops. The workshops focus on the analysis of problem sets designed to augment and complement the Elementary Statistics material. Instead of tutoring or lecturing, Team Leaders coach students as they work collaboratively in small groups on the assigned problems by referencing class lectures and assigned reading materials. The workshops do not repeat but extend the substantive discussions and lectures of the Elementary Statistics course. Additionally, these workshops aim to develop communication skills, cooperative attitudes, and promote a teamwork environment. Because the benefits of collaborative learning can only be gained through consistent effort and attendance, this zero-credit course is graded P/F based on the student's level of participation and attendance.
Instructor(s): K. Burbank Terms Offered: TBD Prerequisite(s): Corequisite: Concurrent enrollment in STAT 20000
STAT 10118. Pathways in Data Science. 100 Units.
Learn how to glean insights and meaning from complex sets of data in this overview of a field with growing importance in business, government, and scientific research. Students will learn to use the transformational tools of data science and see how researchers are applying them in the service of social good. Working with faculty from the Department of Statistics, students will study how data is collected and stored and then how it is explored, visualized, and communicated. Using Python, students will learn techniques for classification, prediction, inference, and regression. Then, together with researchers from the University of Chicago Urban Labs, students will explore how these tools and methods can be used to inform social policy in multiple domains including poverty, health, and social mobility. Throughout the course, visiting guest lecturers will broaden students' perspectives by sharing how data science is used in their diverse fields, ranging from business applications to biology.
Terms Offered: Summer
STAT 11800-11900. Introduction to Data Science I-II.
Data science provides tools for gaining insight into specific problems using data, through computation, statistics, and visualization. These courses introduce students to all aspects of a data analysis process, from posing questions, designing data collection strategies, management+storing and processing of data, exploratory tools and visualization, statistical inference, prediction, interpretation, and communication of results. Simple techniques for data analysis are used to illustrate both effective and fallacious uses of data science tools.
STAT 11800. Introduction to Data Science I. 100 Units.
Data science provides tools for gaining insight into specific problems using data, through computation, statistics and visualization. This course introduces students to all aspects of a data analysis process, from posing questions, designing data collection strategies, management+storing and processing of data, exploratory tools and visualization, statistical inference, prediction, interpretation and communication of results. Simple techniques for data analysis are used to illustrate both effective and fallacious uses of data science tools. Although this course is designed to be at the level of mathematical sciences courses in the Core, with little background required, we expect the students to develop computational skills that will allow them to analyze data. Computation will be done using Python and Jupyter Notebook.
Instructor(s): D. Nicolae, A. Kube, W. Trimble, B. Trok, S. Lange, E. Campbell Terms Offered: Autumn Spring Winter Prerequisite(s): None Equivalent Course(s): DATA 11800
STAT 11900. Introduction to Data Science II. 100 Units.
This course is the second quarter of a two-quarter systematic introduction to the foundations of data science, as well as to practical considerations in data analysis. A broad background on probability and statistical methodology will be provided. More advanced topics on data privacy and ethics, reproducibility in science, data encryption, and basic machine learning will be introduced. We will explore these concepts with real-world problems from different domains.
Instructor(s): A. Nussbaum, D. Nicolae, A. Kube Terms Offered: Autumn Spring Winter Prerequisite(s): DATA 11800 or consent of instructor. Equivalent Course(s): DATA 11900
STAT 12001. Collaborative Learning in Statistics 22000. 000 Units.
This is an optional, limited enrollment workshop for students concurrently enrolled in STAT 22000 Statistical Methods and Applications. Undergraduate Team Leaders guide small groups of students in weekly workshops. The workshops focus on the analysis of problem sets designed to augment and complement the course material. Instead of tutoring or lecturing, Team Leaders coach students as they work collaboratively in small groups on the assigned problems by referencing class lectures and assigned reading materials. The workshops do not repeat but extend the substantive discussions and lectures of the course. Additionally, these workshops aim to develop communication skills, cooperative attitudes, and promote a teamwork environment. Because the benefits of collaborative learning can only be gained through consistent effort and attendance, this zero-credit course is graded P/F based on the student's level of participation and attendance.
Terms Offered: TBD Prerequisite(s): Concurrent enrollment in STAT 22000.
STAT 13820. Data Science in Quantitative Finance and Risk Management. 100 Units.
Have you started or are about to start your investment journey? Do you want to know more about terms like "recession" and "volatility," and how they might affect your own bank account? Are you interested in mathematics and its application to human emotions? This course introduces the leading statistical models and methods which financial data researchers use to understand ever-evolving markets and build insightful financial strategies, such as machine learning, risk calculation, and portfolio management . At first, students will learn about the theoretical and applied foundations of regression and classification designs for predicting market patterns. Next, students will gain exposure to proprietary metrics such as Value-at-Risk(VaR) used to evaluate returns/losses of both single and multi-asset portfolios. Lastly, they will experiment with portfolio allocation tactics by visualizing risk-to-reward graphs under various buying and selling conditions. These techniques can be applied to the U.S. and foreign asset classes, including equities, commodities, and cryptocurrencies. Students will experience how professionals in quantitative trading, hedge funds, and risk analytics collaborate to pitch asset strategies to their clients, and form research teams to play a stock market game using the skills they learned throughout the course with the objective of maximizing the teams' portfolio returns. All implementations will be done using Python.
Terms Offered: Summer Equivalent Course(s): DATA 13820
STAT 20000. Elementary Statistics. 100 Units.
This course introduces statistical concepts and methods for the collection, presentation, analysis, and interpretation of data. Elements of sampling, simple techniques for analysis of means, proportions, and linear association are used to illustrate both effective and fallacious uses of statistics.
Instructor(s): Staff Terms Offered: Autumn Spring Note(s): For students with little or no math background. Not recommended for students planning to take STAT 22000 or STAT 23400 or more advanced courses in Statistics. Students with credit for STAT 20010, STAT 22000, STAT 23400, or more advanced courses in Statistics not admitted. This course may not be used in the Statistics major or minor. This course meets one of the general education requirements in the mathematical sciences. Only one of STAT 20000, STAT 20010, or STAT 22000, can count toward the general education requirement in the mathematical sciences.
STAT 20010. Elementary Statistics Through Case Study. 100 Units.
This course uses a single real-world case study to introduce statistical concepts throughout the quarter. Topics include methods for the collection, presentation, analysis, and interpretation of data, including elements of sampling, simple techniques for analysis of means, proportions, and linear association. The case study examines the development of the water contamination crisis in Flint, Michigan, from its beginnings in 2014 to present day conditions. Students will use statistical techniques learned in the course to probe critical facets of the story including: the demographics and history of Flint; the evidence for (and uncertainty about) the existence of contamination; statistical mistakes that allowed officials to initially deny the problem; and predictions for future health effects due to the contamination. Throughout the course, students will practice critically examining claims made in the media and in scientific publications. At the end of the quarter, students are asked to use their statistical skills to propose and defend a set of interventions to benefit the children of Flint.
Instructor(s): K. Burbank Terms Offered: TBD Note(s): For students with little or no math background. Not recommended for students planning to take STAT 22000 or STAT 23400 or more advanced courses in Statistics. Students with credit for STAT 20000, STAT 22000, STAT 23400, or more advanced courses in Statistics not admitted. This course may not be used in the Statistics major or minor. This course meets one of the general education requirements in the mathematical sciences. Only one of STAT 20000, STAT 20010, or STAT 22000, can count toward the general education requirement in the mathematical sciences.
STAT 22000. Statistical Methods and Applications. 100 Units.
This course introduces statistical techniques and methods of data analysis including the use of statistical software. Examples are drawn from the biological, physical, and social sciences. Students are required to apply the techniques discussed to data drawn from actual research. Topics include data description, graphical techniques, exploratory data analyses, random variation and sampling, basic probability, random variables and expected values, confidence intervals, and significance tests for one- and two-sample problems for means and proportions, chi-square tests, linear regression, and, if time permits, analysis of variance.
Terms Offered: Autumn Spring Winter Prerequisite(s): MATH 13100 or 15100 or 15200 or 15300 or 16100 or 16110 or 15910 or 18300 or 19520 or 19620 or 20250 or 20300 or 20310. Note(s): Students may count either STAT 22000 or STAT 23400, but not both, toward the forty-two credits required for graduation. Students with credit for STAT 23400 not admitted. This course meets on of the general education requirements in the mathematical sciences. Only one of STAT 20000, STAT 20010, or STAT 22000, can count toward the general education requirement in the mathematical sciences.
STAT 22200. Linear Models and Experimental Design. 100 Units.
This course covers principles and techniques for the analysis of experimental data and the planning of the statistical aspects of experiments. Topics include linear models; analysis of variance; randomization, blocking, and factorial designs; confounding; and incorporation of covariate information.
Instructor(s): Staff Terms Offered: Spring Prerequisite(s): [STAT 22000 or 23400 or (STAT 11800 & 11900) or ECON 11010 or BUSN 41000 grade at least C+, or STAT 22400 or 22600 or 24500 or 24510 or PBHS 32100, or APStat credit STAT 22000] & [2 qtrs of calculus (MATH 13200 or 15200 or 15300 or 16200 or 16210 or 15910 or 18300 or 19520 or 19620 or 20250 or 20300 or 20310)]
STAT 22400. Applied Regression Analysis. 100 Units.
This course introduces the methods and applications of fitting and interpreting multiple regression models. The primary emphasis is on the method of least squares and its many varieties. Topics include the examination of residuals, the transformation of data, strategies and criteria for the selection of a regression equation, the use of dummy variables, tests of fit, nonlinear models, biases due to excluded variables and measurement error, and the use and interpretation of computer package regression programs. The techniques discussed are illustrated by many real examples involving data from both the natural and social sciences. Matrix notation is introduced as needed. Prerequisite: PBHS 32100. Equivalent Course(s): PBHS 32400
Terms Offered: Autumn Spring Prerequisite(s): [STAT 22000 or 23400 or (STAT 11800 & 11900) or ECON 11010 or BUSN 41000 with a grade of at least C+, or STAT 22200 or 22600 or 24500 or 24510 or PBHS 32100, or AP Statistics credit for STAT 22000], and [MATH 13200/15200/15300/16200/16210/15910/18300/19520/19620/20250/20300 or 20310]. Note(s): Students may count either STAT 22400 or STAT 22401, but not both, toward the forty-two credits required for graduation. Equivalent Course(s): PBHS 32400
STAT 22401. Regression Analysis for Health and Social Research. 100 Units.
This course is an introduction to the methods and applications of fitting and interpreting multiple regression models. The main emphasis is on the method of least squares. Topics include the examination of residuals, the transformation of data, strategies and criteria for the selection of a regression equation, the use of dummy variables, tests of fit. Stata computer package will be used extensively, but previous familiarity with Stata is not assumed. The techniques discussed will be illustrated by real examples involving health and social science data.
Instructor(s): James Dignam Terms Offered: Winter Prerequisite(s): PBHS 32100 or STAT 22000 or equivalent Equivalent Course(s): PBHS 32410
STAT 22600. Analysis of Categorical Data. 100 Units.
This course covers statistical methods for the analysis of qualitative and counted data. Topics include description and inference for binomial and multinomial data using proportions and odds ratios; multi-way contingency tables; generalized linear models for discrete data; logistic regression for binary responses; multi-category logit models for nominal and ordinal responses; loglinear models for counted data; and inference for matched-pairs and correlated data. Applications and interpretations of statistical models are emphasized.
Terms Offered: Winter Prerequisite(s): [STAT 22000 or 23400 or (STAT 11800 & 11900) or ECON 11010 or BUSN 41000 grade at least C+, or STAT 22400 or 22600 or 24500 or 24510 or PBHS 32100, or APStat credit STAT 22000] & [2 qtrs of calculus (MATH 13200 or 15200 or 15300 or 16200 or 16210 or 15910 or 18300 or 19520 or 19620 or 20250 or 20300 or 20310)] Equivalent Course(s): PBHS 32600
STAT 22700. Biostatistical Methods. 100 Units.
This course is designed to provide students with tools for analyzing categorical, count, and time-to-event data frequently encountered in medicine, public health, and related biological and social sciences. This course emphasizes application of the methodology rather than statistical theory (e.g., recognition of the appropriate methods; interpretation and presentation of results). Methods covered include contingency table analysis, Kaplan-Meier survival analysis, Cox proportional-hazards survival analysis, logistic regression, and Poisson regression.
Instructor(s): L. Chen Terms Offered: Spring Prerequisite(s): PBHS 32400 or PBHS 32410 or STAT 22400 or STAT 24500 or equivalent. Equivalent Course(s): PBHS 32700
STAT 22810. Epidemiology and Population Health. 100 Units.
Epidemiology is the basic science of public health. It is the study of how diseases are distributed across populations and how one designs population-based studies to learn about disease causes, with the object of identifying preventive strategies. Epidemiology is a quantitative field and draws on biostatistical methods. Historically, epidemiology's roots were in the investigation of infectious disease outbreaks and epidemics. Since the mid-twentieth century, the scope of epidemiologic investigations has expanded to a fuller range non-infectious diseases and health problems. This course will introduce classic studies, study designs and analytic methods, with a focus on global health problems.
Instructor(s): D. Lauderdale Terms Offered: Autumn Prerequisite(s): STAT 22000 or other introductory statistics highly desirable. For BIOS students-completion of the first three quarters of a Biological Sciences Fundamentals sequence. Note(s): This course does not meet requirements for the biological sciences major. Equivalent Course(s): HLTH 20910, ENST 27400, PPHA 36410, PBHS 30910, BIOS 27810
STAT 23400. Statistical Models and Methods. 100 Units.
This course is recommended for students throughout the natural and social sciences who want a broad background in statistical methodology and exposure to probability models and the statistical concepts underlying the methodology. Probability is developed for the purpose of modeling outcomes of random phenomena. Random variables and their expectations are studied including means and variances of linear combinations and an introduction to conditional expectation. Binomial, Poisson, normal, and other standard probability distributions are considered. Some probability models are studied mathematically, and others are studied via computer simulation. Sampling distributions and related statistical methods are explored mathematically, studied via simulation, and illustrated on data. Methods include, but are not limited to, inference for means and proportions for one- and two-sample problems, two-way tables, correlation, and simple linear regression. Graphical and numerical data description are used for exploration, communication of results, and comparing mathematical consequences of probability models and data. Mathematics employed is to the level of single-variable differential and integral calculus and sequences and series.
Terms Offered: Autumn Spring Winter Prerequisite(s): MATH 13300 or 15300 or 16200 or 16210 or 15910 or 18300 or 19520 or 19620 or 20250 or 20300 or 20310. Note(s): Students may count either STAT 22000 or STAT 23400, but not both, toward the forty-two credits required for graduation. Students with AP Statistics credit for STAT 22000 will forego that credit by completing STAT 23400.
STAT 24300. Numerical Linear Algebra. 100 Units.
This course is devoted to the basic theory of linear algebra and its significant applications in scientific computing. The objective is to introduce students to the tools needed to state, analyze, and solve multivariate problems. Students should leave the course ready to use linear algebra in future courses in algorithms, scientific computing, mathematical modeling, signal processing, multivariate statistics, data analysis, as well as the physical and social sciences. Topics include Gaussian elimination, vector spaces, linear transformations and associated fundamental subspaces, orthogonality and projections, eigenvectors and eigenvalues, diagonalization of real symmetric and complex Hermitian matrices, the spectral theorem, and matrix decompositions (QR, and Singular Value Decompositions). Systematic methods applicable in high dimensions and techniques commonly used in scientific computing are emphasized. Students enrolled in the graduate level STAT 30750 will have additional work in assignments, exams, and projects including applications of matrix algebra in statistics and numerical computations implemented in Matlab or R. Some programming exercises will appear as optional work for students enrolled in the undergraduate level STAT 24300.
Terms Offered: Autumn Winter Prerequisite(s): MATH 16300 or MATH 16310 or MATH 18300 or MATH 19620 or MATH 20300 or MATH 20310 or MATH 20320. Or graduate student in Statistics or Financial Mathematics.
STAT 24310. Numerical Linear Algebra: An Introduction to Computation. 100 Units.
Computation is an essential topic across the physical and social sciences, in statistics, data science, and machine learning. Numerical linear algebra is the essential language of computation. Through a series of hands-on applications, students will implement and evaluate the essential algorithms used to solve linear systems and least squares problems, perform regression, orthogonalize bases, decompose signals via the FFT and related transforms, and perform matrix factorizations. We will focus on the computational complexity and stability of each algorithm, as well as its practical uses. Example applications include iterative optimizers used to solve large systems arising in engineering, spectral embedding methods for dimension reduction (PCA, MDS, and diffusion maps), and linear methods for classification and clustering. Examples will be presented as interactive coding notebooks available through a web browser. Prior coding experience is strongly encouraged, though students looking for an introduction to Jupyter notebooks and Python are welcome to enroll.
Terms Offered: Spring Prerequisite(s): STAT 24300 or an equivalent introductory linear algebra class. Coding experience in Python, Matlab, R, or Julia is strongly recommended. Equivalent Course(s): CAAM 24310
STAT 24320. Applications in Numerical Linear Algebra. 100 Units.
This course delves into the practical applications of methods in numerical linear algebra. Students will see how material first introduced in STAT 24300 can be applied to problems in data analysis, dynamical systems, and statistics. Topics will include projection and orthogonality for optimization in linear systems; spectral methods for discrete time dynamical systems and sampling algorithms including Markov Chain Monte Carlo; and matrix decompositions such as QR and SVD for dimensionality reduction techniques including PCA and others. For each topic, students will have multiple opportunities to apply the methods to real data sets. While this course will not emphasize programming, some familiarity with Python or Julia is encouraged.
Terms Offered: Spring Prerequisite(s): STAT 24300 or an equivalent introductory linear algebra class. Coding experience in Python, Matlab, R, or Julia is recommended.
STAT 24400-24500. Statistical Theory and Methods I-II.
This sequence is a systematic introduction to the principles and techniques of statistics, as well as to practical considerations in the analysis of data, with emphasis on the analysis of experimental data.
STAT 24400. Statistical Theory and Methods I. 100 Units.
This course is the first quarter of a two-quarter systematic introduction to the principles and techniques of statistics, as well as to practical considerations in the analysis of data, with emphasis on the analysis of experimental data. This course covers tools from probability and the elements of statistical theory. Topics include the definitions of probability and random variables, binomial and other discrete probability distributions, normal and other continuous probability distributions, joint probability distributions and the transformation of random variables, principles of inference (including Bayesian inference), maximum likelihood estimation, hypothesis testing and confidence intervals, likelihood ratio tests, multinomial distributions, and chi-square tests. Examples are drawn from the social, physical, and biological sciences. The coverage of topics in probability is limited and brief, so students who have taken a course in probability find reinforcement rather than redundancy. Students who have already taken STAT 25100 have the option to take STAT 24410 (if offered) instead of STAT 24400.
Instructor(s): Staff Terms Offered: Autumn Winter Prerequisite(s): ((MATH 16300 or MATH 16310 or MATH 20500 or MATH 20510 or MATH 20900), with no grade requirement), or ((MATH 18400 or MATH 20000 or (MATH 15250 and 15300)) with (either a minimum grade of B-, or a Statistics major, or currently enrolled in prerequisite course during preregistration)). Or instructor consent. Note(s): Some previous experience with statistics and/or probability helpful but not required. Concurrent or prior linear algebra (MATH 18600 or 19620 or 20250 or STAT 24300 or equivalent) is recommended for students continuing to STAT 24500. Students may count either STAT 24400 or STAT 24410, but not both, toward the forty-two credits required for graduation.
STAT 24500. Statistical Theory and Methods II. 100 Units.
This course is the second quarter of a two-quarter systematic introduction to the principles and techniques of statistics, as well as to practical considerations in the analysis of data, with emphasis on the analysis of experimental data. This course continues from either STAT 24400 or STAT 24410 and covers statistical methodology, including the analysis of variance, regression, correlation, and some multivariate analysis. Some principles of data analysis are introduced, and an attempt is made to present the analysis of variance and regression in a unified framework. Statistical software is used.
Instructor(s): Staff Terms Offered: Spring Winter Prerequisite(s): Linear algebra (MATH 18600 or 19620 or 20250 or STAT 24300 or equivalent) and (STAT 24400 or STAT 24410). Note(s): Students may count either STAT 24500 or STAT 24510, but not both, toward the forty-two credits required for graduation.
STAT 24410-24510. Statistical Theory and Methods Ia-IIa.
This sequence provides a sophisticated introduction to statistical principles and methods and their application to the analysis of data. In addition to serving undergraduates with a strong interest in Statistics, it is the recommended sequence in theoretical statistics for MS students in Statistics.
STAT 24410. Statistical Theory and Methods Ia. 100 Units.
This course is the first quarter of a two-quarter sequence providing a principled development of statistical methods, including practical considerations in applying these methods to the analysis of data. The course begins with a brief review of probability and some elementary stochastic processes, such as Poisson processes, that are relevant to statistical applications. The bulk of the quarter covers principles of statistical inference from both frequentist and Bayesian points of view. Specific topics include maximum likelihood estimation, posterior distributions, confidence and credible intervals, principles of hypothesis testing, likelihood ratio tests, multinomial distributions, and chi-square tests. Additional topics may include diagnostic plots, bootstrapping, a critical comparison of Bayesian and frequentist inference, and the role of conditioning in statistical inference. Examples are drawn from the social, physical, and biological sciences. The statistical software package R will be used to analyze datasets from these fields and instruction in the use of R is part of the course.
Instructor(s): Staff Terms Offered: Autumn Prerequisite(s): STAT 25100 or STAT 25150 or MATH 23500. This course is only open to graduate students in Statistics, Applied Mathematics, and Financial Mathematics, and to undergraduate Statistics majors, or by consent of instructor. Note(s): Some previous experience with statistics helpful but not required. Concurrent or prior linear algebra (MATH 18600 or 19620 or 20250 or 20700 or STAT 24300 or equivalent) is recommended for students continuing to STAT 24510. Students may count either STAT 24400 or STAT 24410, but not both, toward the forty-two credits required for graduation. Equivalent Course(s): STAT 30030
STAT 24510. Statistical Theory and Methods IIa. 100 Units.
This course is a continuation of STAT 24410. The focus is on theory and practice of linear models, including the analysis of variance, regression, correlation, and some multivariate analysis. Additional topics may include bootstrapping for regression models, nonparametric regression, and regression models with correlated errors.
Terms Offered: Winter Prerequisite(s): STAT 24410 and linear algebra (MATH 18600 or 19620 or 20250 or 20700 or STAT 24300 or equivalent). This course is only open to graduate students in Statistics, Applied Mathematics, and Financial Mathematics, and to undergraduate Statistics majors, or by consent of instructor. Note(s): Students may count either STAT 24500 or STAT 24510, but not both, toward the forty-two credits required for graduation. Equivalent Course(s): STAT 30040
STAT 24620. Multivariate Statistical Analysis: Applications and Techniques. 100 Units.
This course focuses on applications and techniques for analysis of multivariate and high dimensional data. Beginning subjects cover common multivariate techniques and dimension reduction, including principal component analysis, factor model, canonical correlation, multi-dimensional scaling, discriminant analysis, clustering, and correspondence analysis (if time permits). Further topics on statistical learning for high dimensional data and complex structures include penalized regression models (LASSO, ridge, elastic net), sparse PCA, independent component analysis, Gaussian mixture model, Expectation-Maximization methods, and random forest. Theoretical derivations will be presented with emphasis on motivations, applications, and hands-on data analysis.
Instructor(s): M. Wang Terms Offered: Spring Prerequisite(s): (STAT 24300 or MATH 20250) and (STAT 24500 or STAT 24510). Graduate students in Statistics or Financial Mathematics can enroll without prerequisites. Note(s): Linear algebra at the level of STAT 24300. Knowledge of probability and statistical estimation techniques (e.g. maximum likelihood and linear regression) at the level of STAT 24400-24500. Equivalent Course(s): STAT 32950
STAT 24630. Causal Inference Methods and Case Studies. 100 Units.
In many applications of statistics, a large proportion of the questions of interest are about causality rather than questions of description or association. Would booster shots reduce the chance of getting infected by the new variant of COVID-19? How does a new tax policy affect the economic activity? Can a universal health insurance program improve people's health? In this class, we will introduce some basic concepts and methods in causal inference and discuss examples from various disciplines. The course plans to cover the potential outcome framework, randomize experiments, randomization and model-based inference, matching, sensitivity analysis, and instrumental variables. Examples include the evaluation of job training programs, educational voucher schemes, clinical trials and observational data of medical treatments, smoking, the influenza vaccination study, and more.
Terms Offered: Spring Prerequisite(s): (STAT 23400 + (STAT 25100 or STAT 25150)) or (STAT 24400 or STAT 24410)
STAT 25100. Introduction to Mathematical Probability. 100 Units.
This course covers fundamentals and axioms; combinatorial probability; conditional probability and independence; binomial, Poisson, and normal distributions; the law of large numbers and the central limit theorem; and random variables and generating functions.
Instructor(s): Staff Terms Offered: Autumn Spring Prerequisite(s): ((MATH 16300 or MATH 16310 or MATH 20500 or MATH 20510 or MATH 20900), with no grade requirement), or ((MATH 18400 or MATH 20000 or (MATH 15250 and 15300)) with (either a minimum grade of B-, or a Statistics major, or currently enrolled in prerequisite course during preregistration)). Or instructor consent. Note(s): Students may count either STAT 25100 or STAT 25150, but not both, toward the forty-two credits required for graduation.
STAT 25150. Introduction to Mathematical Probability-A. 100 Units.
This course covers combinatorics; basic notions of probability and conditional probability; independence; expectation, variance, and covariance; discrete and continuous random variables, including distributions such as binomial, normal, multinomial, geometric, hypergeometric, negative binomial, and Poisson; Gambler's Ruin; generating functions and applications to branching processes; the (Weak) Law of Large Numbers and its application to approximation by polynomials, i.e., the Weierstrass Approximation Theorem; random walk, including proof of recurrence in one and two dimensions and transience in three dimensions; characteristic functions and the Central Limit Theorem.
Instructor(s): Robert Fefferman Terms Offered: TBD. To be determined Prerequisite(s): (MATH 16300 or MATH 16310 or MATH 20500 or MATH 20510, with a minimum grade of A-), or (MATH 20900 with no grade requirement), or consent of instructor. Note(s): Students may count either STAT 25100 or STAT 25150, but not both, toward the forty-two credits required for graduation.
STAT 25211. Introduction to Random Matrices. 100 Units.
The course is an introduction to the random matrix theory. We will study the asymptotic properties of various random matrix models (Wigner matrices, Gaussian ensembles, etc.). We will also discuss some applications to statistics and neural networks.
Terms Offered: TBD Prerequisite(s): (STAT 25100 or STAT 25150 or MATH 23500) and (MATH 18600 or MATH 19620 or STAT 24300 or MATH 20250 or MATH 20700), or consent of instructor.
STAT 25300. Introduction to Probability Models. 100 Units.
This course introduces stochastic processes as models for a variety of phenomena in the physical and biological sciences. Following a brief review of basic concepts in probability, we introduce stochastic processes that are popular in applications in sciences (e.g., discrete time Markov chain, the Poisson process, continuous time Markov process, renewal process and Brownian motion).
Instructor(s): Staff Terms Offered: Winter Prerequisite(s): STAT 24400 or STAT 24410 or STAT 25100 or STAT 25150 Equivalent Course(s): STAT 31700
STAT 26100. Time Dependent Data. 100 Units.
This course considers the modeling and analysis of data that are ordered in time. The main focus is on quantitative observations taken at evenly spaced intervals and includes both time-domain and spectral approaches.
Instructor(s): W. Wu Terms Offered: Autumn Prerequisite(s): STAT 24500 w/B- or better or STAT 24510 w/C+ or better is required; alternatively STAT 22400 w/B- or better and exposure to multivariate calculus (MATH 16300 or MATH 16310 or MATH 18400 or MATH 19520 or MATH 20000 or MATH 20500 or MATH 20510 or MATH 20800). Graduate students in Statistics or Financial Mathematics can enroll without prerequisites. Some previous exposure to Fourier series is helpful but not required. Equivalent Course(s): STAT 33600
STAT 26300. Introduction to Statistical Genetics. 100 Units.
As a result of technological advances over the past few decades, there is a tremendous wealth of genetic data currently being collected. These data have the potential to shed light on the genetic factors influencing traits and diseases, as well as on questions of ancestry and population history. The aim of this course is to develop a thorough understanding of probabilistic models and statistical theory and methods underlying analysis of genetic data, focusing on problems in complex trait mapping, with some coverage of population genetics. Although the case studies are all in the area of statistical genetics, the statistical inference topics, which will include likelihood-based inference, linear mixed models, and restricted maximum likelihood, among others, are widely applicable to other areas. No biological background is needed, but a strong foundation in linear algebra, as well as probability and statistics at the level of STAT 24400-STAT 24500 or higher is assumed.
Instructor(s): M. McPeek Terms Offered: TBD Prerequisite(s): STAT 24500 or 24510 or 30200 or consent of instructor. Equivalent Course(s): STAT 35490
STAT 26700. History of Statistics. 100 Units.
This course covers topics in the history of statistics, from the eleventh century to the middle of the twentieth century. We focus on the period from 1650 to 1950, with an emphasis on the mathematical developments in the theory of probability and how they came to be used in the sciences. Our goals are both to quantify uncertainty in observational data and to develop a conceptual framework for scientific theories. This course includes broad views of the development of the subject and closer looks at specific people and investigations, including reanalyses of historical data.
Instructor(s): S. Stigler Terms Offered: TBD. Not offered in 2023-2024. Prerequisite(s): Prior statistics course Equivalent Course(s): STAT 36700, HIPS 25600, CHSS 32900
STAT 27400. Nonparametric Inference. 100 Units.
Nonparametric inference is about developing statistical methods and models that make weak assumptions. A typical nonparametric approach estimates a nonlinear function from an infinite dimensional space rather than a linear model from a finite dimensional space. This course gives an introduction to nonparametric inference, with a focus on density estimation, regression, confidence sets, orthogonal functions, random processes, and kernels. The course treats nonparametric methodology and its use, together with theory that explains the statistical properties of the methods.
Instructor(s): Staff Terms Offered: Winter Prerequisite(s): STAT 24400 or STAT 24410 w/B- or better is required; alternatively STAT 22400 w/B+ or better and exposure to multivariate calculus (MATH 16300 or MATH 16310 or MATH 18400 or MATH 19520 or MATH 20000 or MATH 20500 or MATH 20510 or MATH 20800) and linear algebra (MATH 18600 or 19620 or 20250 or 20700 or STAT 24300 or equivalent). Master's students in Statistics can enroll without prerequisites. Equivalent Course(s): STAT 37400
STAT 27410. Introduction to Bayesian Data Analysis. 100 Units.
In recent years, Bayes and empirical Bayes (EB) methods have continued to increase in popularity and impact. These methods, combining information from similar and independent experiments and yield improved estimation of both individual and shared model characteristics, have been widely applied in many fields such as biomedical science, public health, epidemiology, education, social science, ecomnomics, psychology, agriculture and engineering. In this course, we will introduce Bayes and EB methods, as well as the necessary tools needed to evaluate their performances comparing with the frequentist methods. For computation, we will introduce Markov chain Monte Carlo methods such as the Gibbs sampler algorithm. We will use R and RStan to implement these methods and solve real world problems. Students in this class are required to do final projects in small groups. During the last week of the quarter, each group will have the opportunity to present the final project to the class. Final reports based on the group projects will be due by the end of the exam week. Due to the attention required from the instructor to supervise the final projects, the class size will be capped at the enrollment limit.
Terms Offered: TBD Prerequisite(s): (STAT 23400 or 24400 or 24410) and (STAT 22400 or 22600 or 24500 or 24510) Note(s): Coding in R will be heavily involved in this class.
STAT 27420. Introduction to Causality with Machine Learning. 100 Units.
This course is an introduction to causal inference. We'll cover the core ideas of causal inference and what distinguishes it from traditional observational modeling. This includes an introduction to some foundational ideas---structural equation models, causal directed acyclic graphs, and then do calculus. The course has a particular emphasis on the estimation of causal effects using machine learning methods.
Instructor(s): V. Veitch Terms Offered: TBD Prerequisite(s): [STAT 24500 or STAT 24510 or STAT 27725] with a grade of B or higher or consent of instructor. Equivalent Course(s): DATA 27420
STAT 27700. Mathematical Foundations of Machine Learning. 100 Units.
This course is an introduction to the mathematical foundations of machine learning that focuses on matrix methods and features real-world applications ranging from classification and clustering to denoising and data analysis. Mathematical topics covered include linear equations, regression, regularization, the singular value decomposition, and iterative algorithms. Machine learning topics include classification and regression, support vector machines, kernel methods, clustering, matrix completion, neural networks, and deep learning. Students are expected to have taken calculus and have exposure to numerical computing (e.g. Matlab, Python, Julia, R).
Prerequisite(s): CMSC 11900 or CMSC 12200 or CMSC 14100 or CMSC 15200 or CMSC 16200 Note(s): Undergraduate students are not allowed to enroll in CMSC 35300. Equivalent Course(s): CMSC 35300, CMSC 25300
STAT 27725. Machine Learning. 100 Units.
This course introduces the foundations of machine learning and provides a systematic view of a range of machine learning algorithms. Topics covered include two parts: (1) a gentle introduction of machine learning: generalization and model selection, regression and classification, kernels, neural networks, clustering and dimensionality reduction; (2) a statistical perspective of machine learning, where we will dive into several probabilistic supervised and unsupervised models, including logistic regression, Gaussian mixture models, and generative adversarial networks.
Prerequisite(s): CMSC 25300 or CMSC 35300 or STAT 24300 or STAT 24500 or ((MATH 18600 or MATH 20250) and (CMSC 12100 or CMSC 14100 or CMSC 15100 or CMSC 16100) and (STAT 25100 or STAT 25150)) Equivalent Course(s): CMSC 25400
STAT 27750. Further Topics in Machine Learning. 100 Units.
This is an intermediate-to-advanced course in machine learning. You should have taken at least one course in machine learning before and be familiar with both theoretical foundations---(empirical) risk minimization and friends---as well as having some practical experience fitting machine learning models to data. The intended material covered by the course is boosting, (fine-tuning of) neural networks, model evaluation, and domain shifts.
Instructor(s): V. Veitch Terms Offered: TBD Prerequisite(s): STAT 24500 or STAT 24510 or [STAT 27725 with a grade of B or higher]
STAT 27751. Trustworthy Machine Learning. 100 Units.
Machine learning systems are routinely used in safety critical situations in the real world. However, they often dramatically fail! This course covers foundational and practical concerns in building machine learning systems that can be trusted. Topics include foundational issues---when do systems generalize, and why, essential results in fairness and domain shifts, and evaluations beyond standard test/train splits. This is an intermediate level course in machine learning; students should have at least one previous course in machine learning.
Terms Offered: TBD Prerequisite(s): STAT 27700 or STAT 37710 or consent of instructor. Equivalent Course(s): STAT 37787, DATA 27751
STAT 27815. Practical R Programming. 100 Units.
This course covers a practical set of skills vital to modern statistics and data science in handling messy, real-world data. Students will get a foundation in version control and practice expressing themselves with literate programming. They will think algorithmically with base R objects, control flow, functions, and iteration. The course will also introduce students to a variety of tidyverse data wrangling methods to import, clean, transform, join, and summarize their data. Finally, students will visualize and explore data using the grammar of graphics framework. Other introductory topics may be discussed. No programming experience is required, although some may be helpful.
Instructor(s): R. McShane Terms Offered: Spring Prerequisite(s): MS or PhD student in Statistics; or STAT 22000 or 22200 or 22400 or 22600 or 23400 or 24410 or 24500 or 24510 or 34300; or consent of instructor. Equivalent Course(s): STAT 37815
STAT 27850. Multiple Testing, Modern Inference, and Replicability. 100 Units.
This course examines the problems of multiple testing and statistical inference from a modern point of view. High-dimensional data is now common in many applications across the biological, physical, and social sciences. With this increased capacity to generate and analyze data, classical statistical methods may no longer ensure the reliability or replicability of scientific discoveries. We will examine a range of modern methods that provide statistical inference tools in the context of modern large-scale data analysis. The course will have weekly assignments as well as a final project, both of which will include both theoretical and computational components.
Terms Offered: TBD Prerequisite(s): STAT 24400 or STAT 24410. Familiarity with regression and with coding in R are recommended. Equivalent Course(s): STAT 30850
STAT 27855. Hypothesis Testing with Empirical Bayes Methodology. 100 Units.
Large scale data sets regularly produced in fields such as biology, social sciences, and neuroscience bring new challenges, like controlling the amount of false positives when testing many hypotheses, as well as the opportunity to leverage information across the entire dataset toward making individual inferences. In this course, we will study theoretical foundations and practical aspects of hypothesis testing in a Bayesian framework. We will focus attention on the local false discovery rate (lfdr), which represents the probability that the null hypothesis is true given the data, and learn several methods for estimating this quantity. Decision theory provides a formal connection between quantities of interest in a Bayesian framework to population parameters in a strictly frequentist model, where the truth status of each null hypothesis is fixed and unknown. We may also discuss methodology for estimating the null distribution, and methods for finite-sample lfdr control if time permits. Homework assignments will have theoretical and computational components.
Instructor(s): D. Xiang Terms Offered: Winter Prerequisite(s): STAT 24400 or STAT 24410 or consent of instructor
STAT 28000. Optimization. 100 Units.
This is an introductory course on optimization that will cover the rudiments of unconstrained and constrained optimization of a real-valued multivariate function. The focus is on the settings where this function is, respectively, linear, quadratic, convex, or differentiable. Time permitting, topics such as nonsmooth, integer, vector, and dynamic optimization may be briefly addressed. Materials will include basic duality theory, optimality conditions, and intractability results, as well as algorithms and applications.
Instructor(s): L. Lim Terms Offered: Spring Prerequisite(s): (MATH 20500 or 20510 or 20800) and (STAT 24300 or MATH 20250 or MATH 20700 or MATH 25500 or MATH 25800) Equivalent Course(s): CAAM 28000
STAT 28200. Dynamical Systems with Applications. 100 Units.
This course is concerned with the analysis of nonlinear dynamical systems arising in the context of mathematical modeling. The focus is on qualitative analysis of solutions as trajectories in phase space, including the role of invariant manifolds as organizers of behavior. Local and global bifurcations, which occur as system parameters change, will be highlighted, along with other dimension reduction methods that arise when there is a natural time-scale separation. Concepts of bi-stability, spontaneous oscillations, and chaotic dynamics will be explored through investigation of conceptual mathematical models arising in the physical and biological sciences.
Instructor(s): Mary Silber Terms Offered: TBD Prerequisite(s): MATH 27300 or (Multivariable calculus (MATH 18400 or 19520 or 20000 or 20400 or 20410 or PHYS 22100 or equivalent), AND linear algebra, including eigenvalues & eigenvectors (MATH 18600 or 19620 or 20250 or 20700 or STAT 24300)). Previous knowledge of elementary differential equations is helpful but not required. Equivalent Course(s): CAAM 28200
STAT 29700. Undergraduate Research. 100 Units.
This course consists of reading and research in an area of statistics or probability under the guidance of a faculty member in the Department of Statistics.
Instructor(s): Staff Terms Offered: Autumn Spring Winter Prerequisite(s): Consent of faculty adviser and Departmental Adviser for Majors Note(s): Students are required to submit the College Reading and Research Course Form. Open to all students, including non-majors. Ordinarily taken for P/F grading, but under certain circumstances may be be taken for a quality grade by petition.
STAT 29900. Bachelor's Paper. 100 Units.
This course consists of reading and research in an area of statistics or probability under the guidance of a faculty member in the Department of Statistics, leading to a bachelor's paper. A good draft of the paper must be submitted by the first day of exam period.
Terms Offered: Autumn Spring Winter Prerequisite(s): Consent of faculty adviser and Departmental Adviser for Majors Note(s): Students are required to submit the College Reading and Research Course Form. Open only to students who are majoring in Statistics. Grading is P/F only.
Undergraduate Primary Contact
Director of Undergraduate Studies and Departmental Adviser for Majors, Minors, Honors, and Introductory Courses Dr. Yibi Huang Jones 207 773.702.2519 Email
Administration
Instructional Support Specialist Kirsten Wellman Jones 222C 773.834.5169 Email
© 2024-2025 The University of Chicago
- University Homepage
- My.UChicago
- Parents and Families
- The College
- College Programming Office
- College Directory
- Campus Maps
- Accessibility
University Registrar 1427 East 60th Street Chicago, IL 60637 United States Ph: 773-702-7891 Fax: 773-702-3562
Print this page.
The PDF will include all information unique to this page.

- Associate Faculty
- Affiliate Faculty
- Instructional Faculty
- Visiting Faculty
- Teaching Fellows
- Postdoctoral Fellows
PhD Students
- Recent Alumni
PEOPLE BY NAME
A B C D E F G H I J K L M N O P Q R S T U V W X Y Z Show All
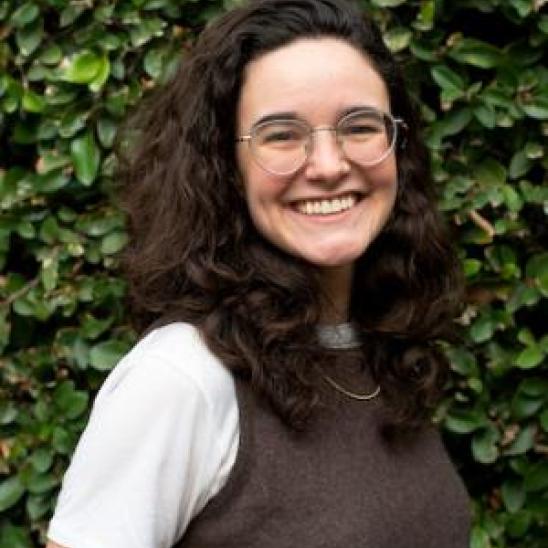
Madeline Adams
Nineteenth- and twentieth-century Central Europe; gender and sexuality; queer history; material culture; cultural history; sensory history
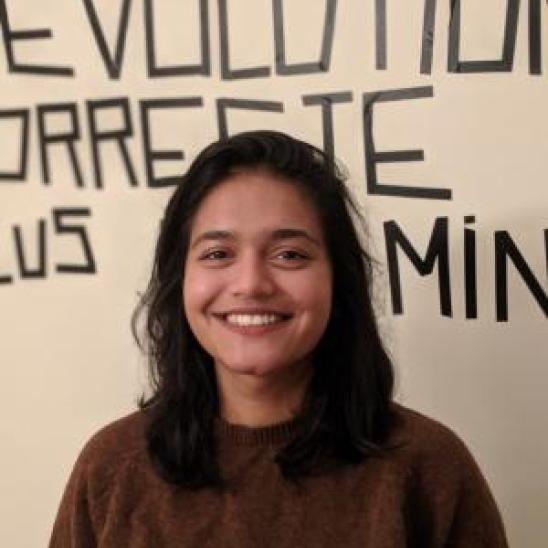
Maniza Ahmed
Race and capitalism, domestic workers, post-emancipation, labor and gender history, the household.
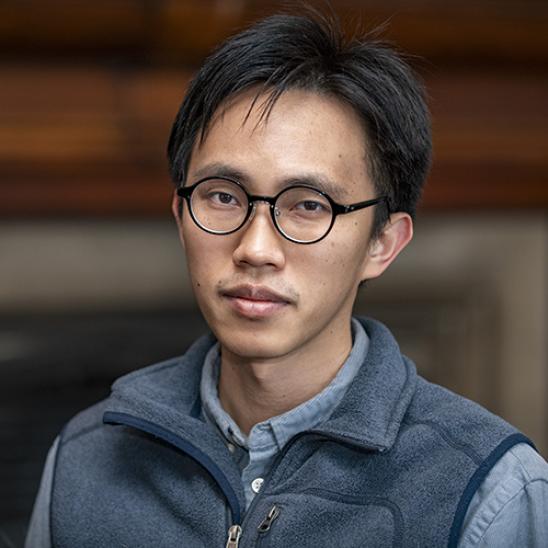
Xavier Ante
Environmental and economic history of late imperial and modern China; history of forests and natural resources; global Asia
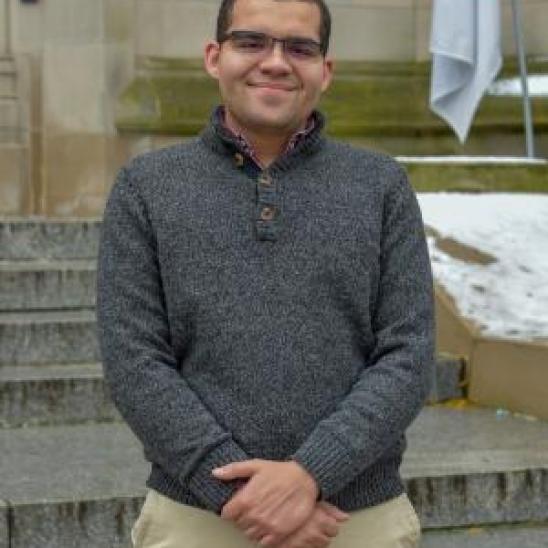
Boone Ayala
Early Modern Britain and its Empire; Colonial America; Comparative Empires; State Formation; Constitutional and Legal history
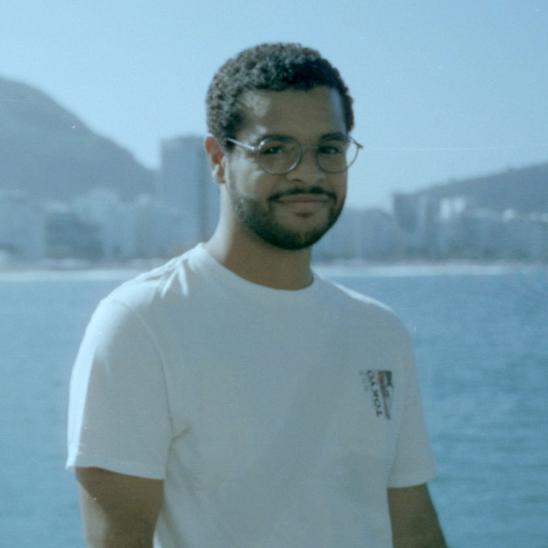
Gabriel Azevedo Duarte Franco
Modern Brazil and Latin America; urban history, Rio de Janeiro; legal history; criminal legislation; history of crime; history of urban policing; slavery and abolition; race and racialization; social and political inequality
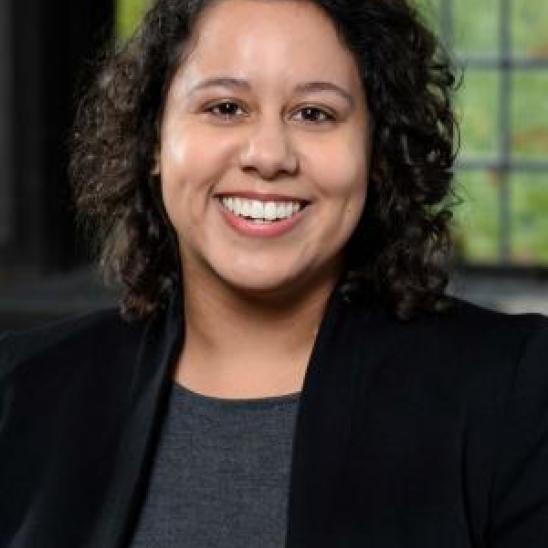
Nathalie Barton
Twentieth-century US history; urban history; race; the built environment; domesticity; real estate and housing, especially rental housing; residential segregation
Prachi Bhagwat

William Birdthistle
US 1900-Present; history of the corporation; legal history; history of capitalism; history of investment funds
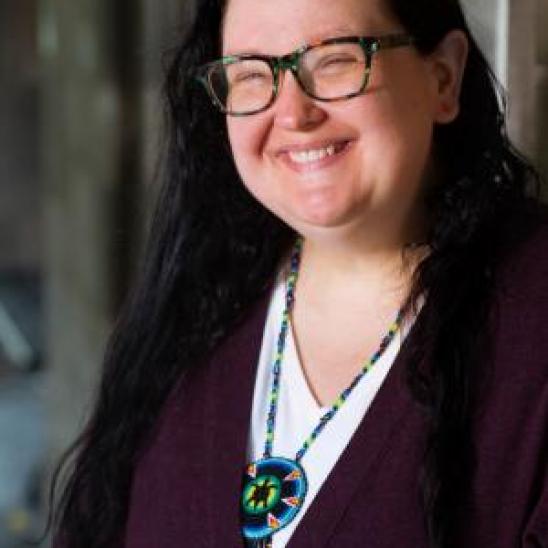
Leila Blackbird
Longue durée US history; Black and Indigenous studies; settler colonialism and slavery; Louisiana and the Atlantic world; subaltern studies, postcolonialism, and ecocriticism; historical trauma, state violence, and genocide; human rights and the history of the present; critical theory and epistemics; digital and spatial humanities
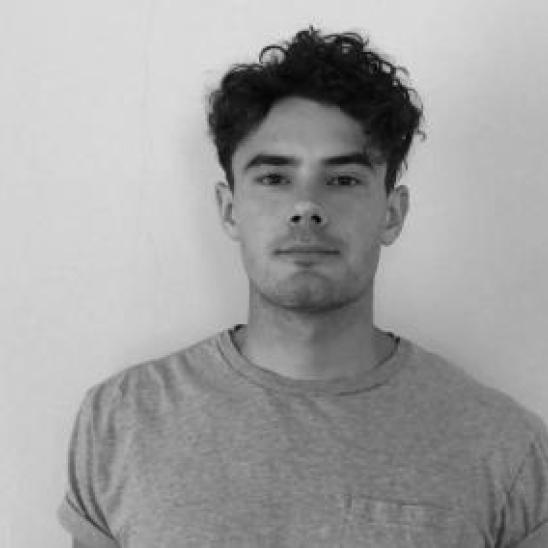
James Bradley
US labor history; urban history; political economy; sanitation and waste; universities and the production of knowledge; race, racism, and resistance
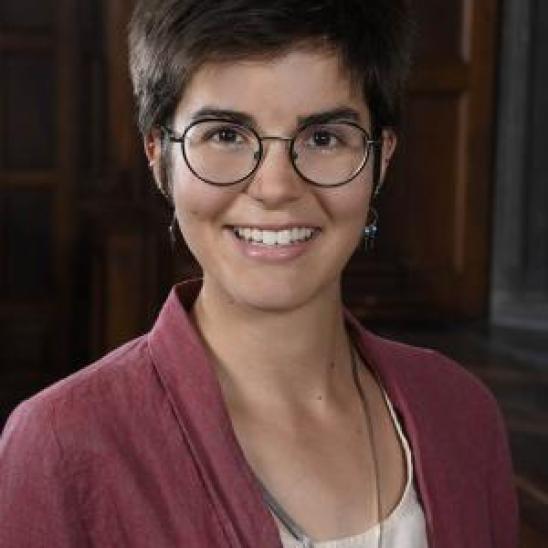
Abigail Bratcher
History of the Soviet Union, Cold War history, gender history, environmental history
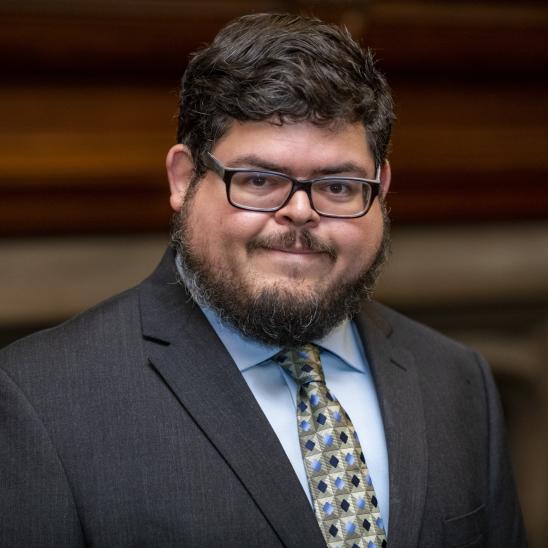
Robert Burgos
Twentieth-century community formation in Japanese cities among marginalized and minority groups; relationship of these processes to the broader development of 少数民族 ( shōsū minzoku , minority) identity and "Japanese" identity in Japan.
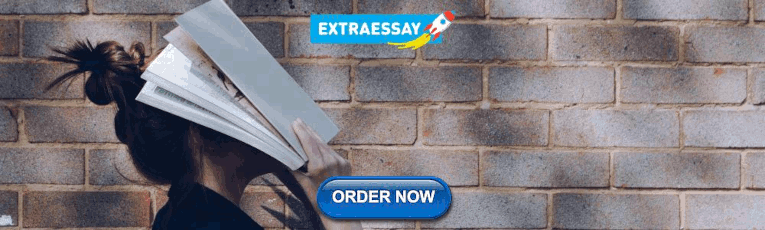
This Website Uses Cookies.
This website uses cookies to improve user experience. By using our website you consent to all cookies in accordance with our Cookie Policy.
Department of Mathematics
Information for current graduate students, requirements to obtain the phd in pure mathematics.
- more than three conditional pass (C) or fail (F) grades, or
- at least two fail (F) grades
Those students who receive at least 6 pass (P) and at most one fail (F) grade will be allowed to continue, provided that they pass all the courses by the end of their second year.
- Make an oral topic presentation together with a brief written report (usually by the end of the Winter quarter of the second year).
- Complete a written dissertation containing original, substantial, and publishable mathematical results.
- Present the contents of the dissertation in an open lecture.
- Pass an oral examination based on the material of the dissertation and the field of mathematics in which it lies.
First-Year Courses
The first-year students take three quarters each of basic courses in analysis, algebra, and geometry and topology. See the main page describing these courses , and see below for some collected notes from some past first-year courses.
Finding an Advisor
Students start looking for an advisor in the winter quarter of their first year, and have usually found one by the end of the spring quarter. Students are expected to take the initiative in finding an advisor. For example, students can
- email faculty members to express an interest
- propose a reading course on a mutually agreed topic
- talk to older graduate students about their experiences with various faculty
There is a Meet the Faculty Colloquium held weekly, usually in the spring quarter. Students can also get a good idea of faculty interests by going to research seminars.
Counseling Resources
Students are urged to approach the graduate chair (currently Marianna Csörnyei) if they have concerns about academic progress, health and emotional problems, or any other related issues.
Annual Report Form
At the end of the academic year, graduate students in their third year and beyond must fill out the department's annual report form , have it signed by their advisor or advisors, and return the completed and signed form to Laurie Wail.
Teaching Responsibilities
On behalf of the Department, the Director of Undergraduate Studies and the Undergraduate Committee oversee all graduate student teaching in mathematics. For any questions, here is their contact information .
Here is a general outline of the teaching responsibilities of graduate students:
- No student teaches more than one course per quarter.
- First-year students do not do any teaching under any circumstance.
- All second-year students, including those on outside fellowships, serve as College Fellows (apprentice teachers) in an undergraduate mathematics course each quarter under the direction of a member of the faculty. Duties include class attendance, grading homework, holding office hours, conducting problem sessions, aiding in constructing and grading exams, and giving supervised lectures. This is considered professional training.
- Upon approval of the mentor and the recommendation of the Director of Undergraduate Studies, third-year students are approved by the College to assume the position of Lecturer in the College; this means they will be responsible for teaching stand-alone courses.
- Qualified graduate students may continue as Lecturers, with a stipend furnished by the College, until the end of the fifth year of graduate study.
- Students whose native language is not English must improve their language skills in order to teach in English, in a manner commensurate with University standards.
Financial Resources
Students who matriculate and maintain satisfactory academic progress should expect five years of financial support, including full tuition scholarship, a generous living stipend, and summer support, the last contingent on current levels of NSF support. Funding comes from the University of Chicago, the National Science Foundation, and the National Need Fellowship Program of the Department of Education.
Student are encouraged further to apply for NSF graduate research fellowships and Department of Defense fellowships, which provide further forms of support (e.g., travel, equipment).
The PhD in Applied Mathematics
The University of Chicago has recently approved a new degree-granting Ph.D. program in Computational and Applied Mathematics. The program will be jointly administered by faculty in a number of departments (Statistics, Mathematics, Computer Science and others) in a formal framework called the Committee on Computational and Applied Mathematics (CCAM). See here for information about the Ph.D. program, and the faculty in the Committee .
Our Students
Featured current students.
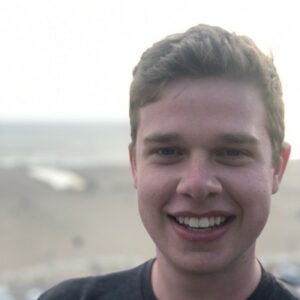
Ben Kusswurm
Schaumburg, IL
Current City
Los Angeles, California
What were you doing before you started the master’s program?
I was a Product Lead for the Revenue Strategy group for Google.
What were the top factors that went into your decision to choose the MS in Applied Data Science Program at UChicago?
The top factors that drove me to attend the program were the flexibility and curriculum that is taught by a world renowned faculty. As the program is online, I can transition from the workplace to the classroom seamlessly. The faculty has a plethora of knowledge, and applies the curriculum to real world applications backed up by experience.
What is your current job?
I am currently a Data Scientist for the YouTube Strategy and Operations group.
What is one of your favorite aspects of being a student at UChicago?
My favorite aspect about being a student at UChicago is the community that my cohort has developed throughout the duration of the program. Even though the program is online, I fostered relationships and made friends that I will keep in contact with for the rest of my life.
In your view, how will the MS in Applied Data Science degree advance your career in data science?
The MS in Applied Data Science degree has advanced my career in data science by helping me obtain interviews, and subsequently a new career in the field. I highly recommend this program for those trying to break into the space.
What has been one of your favorite classes you took at UChicago and why?
My favorite class has been Machine Learning & Predictive Analytics. It is my favorite class because it teaches the foundations of models that we all use in our day-to-day lives. It also helps that the Program Director, Dr. Arnab Bose, who has a career that one can only hope to mimic, teaches the class while highlighting the practical applications of the lessons learned.
What do you enjoy doing outside of the program?
I frequently enjoy the Southern California weather. I like to surf, scuba, and play golf at a mediocre level.
What excites you right now about the field of data science?
I believe that the most exciting thing right now in data science is how large language models have introduced foundational aspects of data science to the general public. It will be interesting to see how our society will change with an amount of knowledge at our fingertips that we have never seen before.
What advice would you give to prospective students who are seeking a master’s degree in data science?
My advice to prospective students would be that you get what you put into the program. There are as many opportunities as you have time for, so try to expand your knowledge as much as you can. The program can also work for those that have other time commitments, so try to find the balance that works for you.
What was one of your main takeaways or things you enjoyed about the Immersion Weekend even for Online program students?
The thing I most enjoyed about the Immersion Weekend was that it provided the opportunity to meet all of my classmates in person. It was amazing to see a dispersed group of people come together in real life and act as though the distance is irrelevant to the friendships that we have all formed.
Featured Graduates
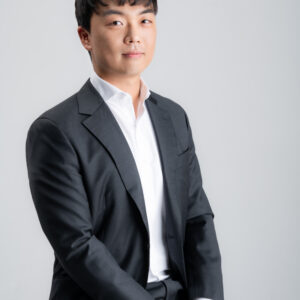
Daniela Trespalacios
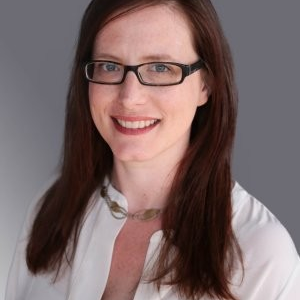
Sandra Tilmon
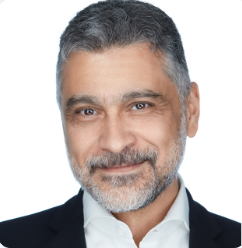
Wael Mohammed
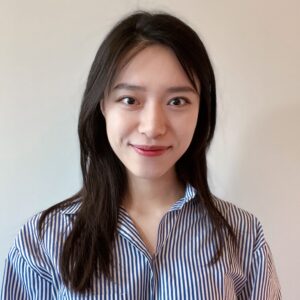
Seoul, Republic of Korea
Currently, I am pursuing a summer internship at Awarity, a digital marketing company.
Over the past six years, I’ve worked as a data consultant, contributing my expertise to organizations such as IBM GBS and Ernst & Young. Throughout this period, I’ve engaged with various company functions, all with the common objective of uncovering meaningful insights through data. My motivation has always been to see the impact of my work, whether it’s driving revenue growth or supporting overall company goals. I’m fortunate to have my career take shape the way it has so far, and I’m excited to continue pushing the boundaries of data science, making meaningful contributions in this ever-evolving field.
What were the top 1-3 factors that went into your decision to choose the MS in Applied Data Science Program at UChicago?
When considering my decision to pursue the MS in Applied Data Science Program at UChicago, I was influenced by several compelling factors. Firstly, the curriculum was a standout feature. The core courses provide a comprehensive exploration of the theoretical foundations of data science, ranging from fundamental concepts like linear regression to cutting-edge techniques such as deep learning. Additionally, the availability of elective courses allows for a deeper dive into specific subjects. I was specially intrigued by Bayesian Methods and ML Ops electives. Lastly, the allure of Chicago itself, with its vibrant atmosphere and rich culture, played a part in finalizing my decision.
What was one of your favorite aspects of being a student at UChicago?
One of my favorite aspects of being a student at UChicago is the university’s renowned reputation for academic excellence. The rigorous curriculum and intellectual environment at UChicago provide a stimulating and well-structured learning experience.
What was one of your favorite classes you took at UChicago and why?
My favorite class at UChicago so far has been Bayesian Methods. This course captivated my interest due to the intriguing concept of frequentist vs. Bayesian approaches. Exploring the principles and applications of Bayesian statistics sparked my curiosity and made me realize the immense potential and future opportunities in this field.
What do you enjoy doing outside of work?
Outside of the program, I thoroughly enjoy exploring the vibrant city of Chicago. I relish visiting art galleries, attending live performances, and immersing myself in the city’s renowned culinary scene.
What excites me right now about the field of data science is the concept of prompt engineering and its potential. By carefully designing prompts, we can guide machine learning models to generate desired outputs, enabling us to tackle complex problems and extract valuable insights. This innovative approach not only enhances the interpretability and control of machine learning systems but also opens up new possibilities for driving impactful advancements in data science.
Rather than merely learning existing concepts, focus on developing the ability to absorb and adapt to new ideas. View the program not only as a source of knowledge but also as a pathway to explore and seek out new advancements in data science. Cultivate a curiosity-driven approach and a willingness to stay updated with the latest developments, as this will be crucial for long-term success in this ever-evolving field.
Bogotá, Colombia
Current city
HP Inc, Center of Excellence Sales Analytics, Data Scientist
I worked for the Latin American Sales Team at HP inc, performing multiple data analyses and project evaluations.
Professor’s experience, Curriculum, The University ranking position
I had the opportunity to connect with professors with an outstanding experience. I also met extremely talented people from different countries and backgrounds which led me to increase my professional network and get a better understanding of different cultures.
In your view, how has the MS in Applied Data Science degree advanced your career so far?
This degree helped me gain visibility within my professional network and it allowed me to advance from an analyst position in a sales team to a global data science team.
Machine Learning because I had the chance to deepen my knowledge of advanced ML algorithms and apply them to real use cases. Yuri Balasanov provided great guidance and resources to understand these advanced topics.
Ask as many questions as you can, especially if you could link those questions with real-world cases or examples.
I enjoy reading Latin American literature.
This field allows me to solve business problems with multiple possibilities of methodologies that range from simple descriptive analysis to complex modeling pipelines.
What is one piece of advice would you give to prospective students?
Always keep in mind that behind a data science solution, there is a context or business problem. If you can’t answer what is the problem you’re trying to solve or the purpose of any analysis, you need more time to understand the context, instead of making the solution more complex.
Philadelphia, PA
Chicago, IL
University of Chicago, Biological Sciences Division – Data for the Common Good, Healthcare Data Scientist
Working as an Epidemiologist in Pediatrics.
1. I wanted to expand my work projects into new areas; 2. Data science is more efficient than biostatistics; and 3. UChicago is an amazing school.
Being part of some amazing teams. I learned a lot from colleagues with different backgrounds, talents, and interests.
Because of this program, I have my dream job working on large-scale machine learning in healthcare.
It is hard to choose! Data Mining was the class that made me feel like I was really on my way to becoming a data scientist.
Know why you want to go into data science. If you have a current field, what is the current state of analytics, and what would data science bring to it?
I’m taking pottery classes and enjoy how challenging (completely impossible) it is. It’s the exact opposite of the workday and requires all of your concentration, so it’s a great break.
I feel like a lot of people will say ChatGPT and the other LLMs, but I’m more excited about efforts to bring more underrepresented students into the field, like the UChicago Data Science Institute’s Summer Lab, which provides a social environment and mentoring as well as learning about data science.
Panic early (that is, when you get the assignment) rather than waiting to panic until the day before it’s due.
Eden Prairie, MN
Executive Vice President of Strategy at Field Nation
Develop significantly sharper, stronger, and broader set of data and analytical skills that drive more success in my role as a strategy executive; Obtain training in advanced machine learning and AI technologies that can help me become a thought leader in my organization and help me spearhead innovation for new breakthrough market products and solutions for my employer company.
What were your favorite aspects of being a student at UChicago?
Networking with and learning from an amazing group of professors and students; the culture of openness for big questions; the urge to always connect learnings with applications, especially at work. I did my graduation project on my company data and it drove a lot of positive change.
It helped me become significantly more analytical in the way I solve problems and equipped me with all the tools I need to leverage any data of any format to solve the problem. Which is a key component of my career as a strategy leader.
Data Science for Consulting with Greg Green, PhD. For me as a Strategy leader, this was where data met with Strategy, This was where the analytics married with the so what. The class was focused on not only telling stories with data and findings but also on the business value of the problem getting solved and on presenting the business case with data in an elegant way. It was a great class for my type of work.
Spending time with family.
I am very excited about the potential for generative AI, especially in the area of Field Services, where my current focus is.
Polish up on key fundamental skills that will make your experience more smooth and will prepare you to learn and absorb more. These fundamentals are: Statistics, Linear Algebra, Python, SQL, and Data base design. Master the foundations well. Just because you will learn Data Science and AI does not mean you be shallow at Statistics. A graduate of UChicago must be very solid at Stats as it’s always the foundation. Master the art of story telling with data. Become so solid at connecting your analysis with the business case and the value of the problem you are solving.
Data scientist at QuantumBlack, AI by McKinsey
Financial Analyst
The program has solid reputation in data science industry. It also provides innovative curriculums that integrate advanced topics and trends in the field.
The faculty at UChicago creates a diverse and inclusive environment where students from various backgrounds felt valued and empowered to excel.
The program advanced my career by providing systematic learning structure, practical project experience, and inspiring discussion environment. The degree also helped me open doors to new opportunities.
Big data platform is one of my favorite classes because it provides a comprehensive overview of m advanced technology infrastructures, which is very difficult to grasp without the instructors’ guidance and insights. It builds my foundations of the big data exploration.
Reading, museums, YouTube
With the emergence of Generative AI, I consider myself fortunate as a data scientist to have the opportunity to delve into this exciting and cutting-edge field.
Leverage the opportunity of starting a new journey. Don’t limit yourself on what you known, but explore on the unknowns. Discover your true intent and passion. You will derive greater satisfaction, motivation, and focus!
Department of Statistics
Congratulations to matthew oline.
April 19, 2024
Congratulations to Matthew Oline , PhD student in the Committee on Computational and Applied Mathematics . He is one of six recipients to receive the 2023-24 Physical Sciences Teaching Prize which is based on nominations from undergraduate students.
Matthew Oline, PhD student, CCAM
News Topics
News archive.
- Graduate Program Statistics
- Diversity and Inclusion
- PhD Requirements
- Course Sequence
- Course Descriptions
- Policy Handbook
- Careers in Medical Physics
*Please note, during the time covered by the above statistics, 100% of our graduates who have sought clinical positions or residencies have attained them.
Student profile: Sohyun Lim, MPP Class of 2025
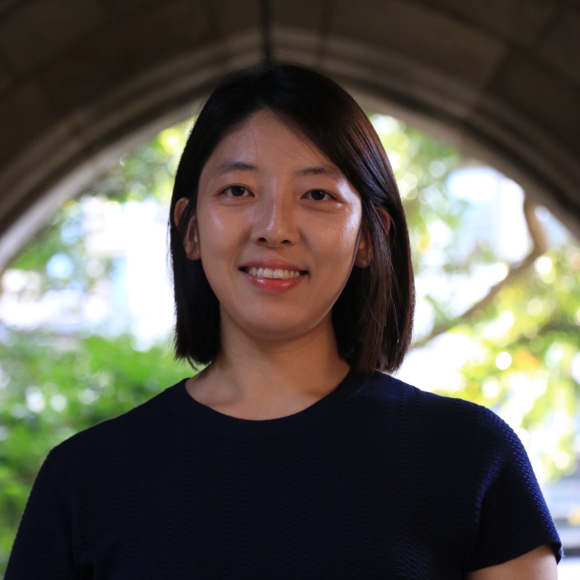
Over the course of ten years working with public financial institutions in South Korea, Sohyun Lim, MPP Class of 2025, learned firsthand the importance of effective policymaking—as well as the dilemmas policymakers face.
“I have worked on a policy designed to solve the household debt crisis by exempting low-income households from their debt, and I was confident that the work brought real relief to debt-burdened Koreans. However, some applicants I previously approved subsequently returned months later seeking to alleviate new debts. Moreover, others who were ineligible for the program—because they had repaid their own debts—complained about the ‘moral hazard’ that the policy had created. This was the first time that I began to wonder about policy effectiveness.”
Another policy Lim was interested in was relocation for public institutions. "The idea was that as public institutions relocated, the employees would also move to those regions, leading to economic development in those regions. However, most chose to commute from their original homes to the new locations instead of settling in the new regions. I wanted to know whether the policy could still be effective when people were not settling as the policy originally intended, and the results confirmed my suspicion that it was not." This experience led Lim to pursue a master's degree in public administration at Ewha Womans University, where she undertook her own research to seek answers to similar research questions.
These experiences—among others—further motivated Lim to dive even deeper into the world of policy analysis. “Data can provide us with so much information, and I felt that if I had more tools to interpret data, I could better evaluate the efficacy of policy decisions—and eventually contribute to designing better policies and improving existing ones.”
When considering graduate programs, Lim said the Master of Public Policy at the Harris School of Public Policy stood out. “I was drawn to Harris’ emphasis on economic analysis, data skills, and statistics—not to mention the focus on programming languages like R and Python, which are invaluable for effective policy analysis. With my previous master’s in public administration, I knew that Harris would be the place to gain those skills and hone my expertise.”
Thus far, Lim said the program has been exactly what she had hoped for. “I have really enjoyed the Core curriculum —especially Statistics. We really engage with R and gain experience interacting with real-world datasets. It’s been a great opportunity to practice these skills.” After the Core, Lim plans to pursue a specialization in data analytics to gain more quantitative knowledge and practical skills in statistical software, including Python. Additionally, having recently developed an interest in environmental issues, she is trying to integrate her professional financial expertise with these issues to enact positive change.
Lim has also embraced opportunities to get involved in student organizations tackling the issues she is passionate about. “As a fellow of Harris Community Action, working with nonprofit organizations enables me to have some applied experience and be connected to the large Chicago community."
Lim said that although she had visited the US as an exchange student in 2010, the prospect of stepping away from her job for two years and leaving South Korea was initially daunting. “However, I am confident that Harris is the best place for me to gain the skills to successfully pursue my passions and focus on the work that I want to do.”
Upcoming Events
Ask admissions: sdg (sustainable development goals) challenge program for high school students, ask admissions: credential programs, you might also be interested in....
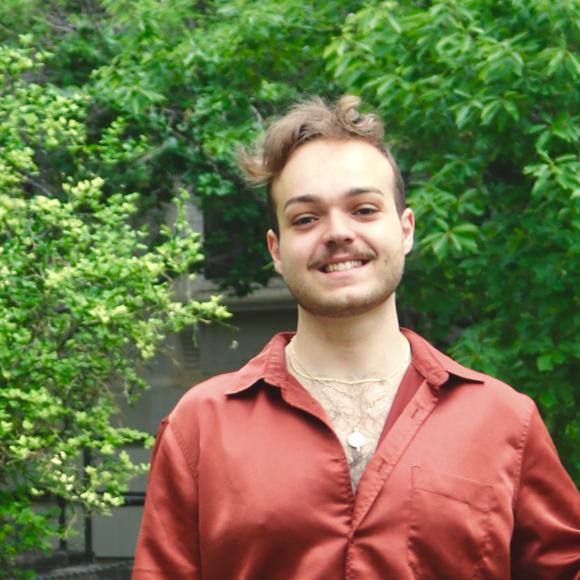
Alumni Profile: Gerard Apruzzese, DPSS’22
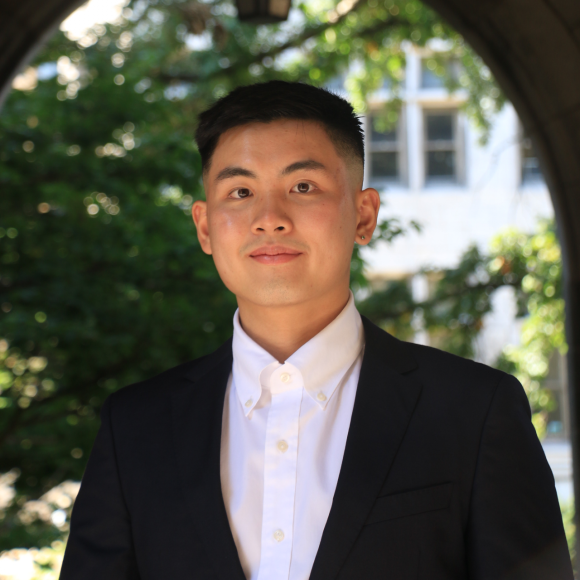
Student Profile: Tony Jiang, MPP Class of 2025
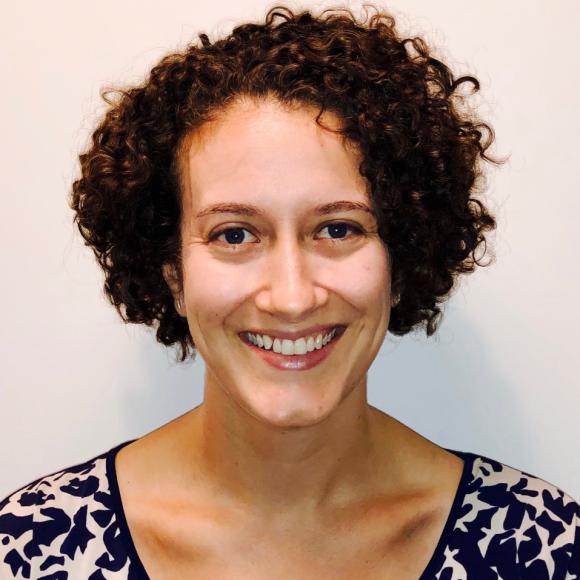
Alumni Profile: Alena Stern, MSCAPP’19
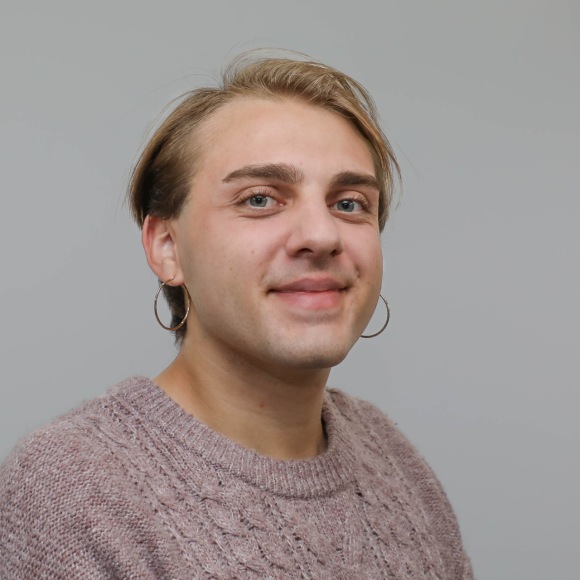
Alumni Profile: Spencer Short, PAC'23
- How to Apply
- Why Public Policy
- Financial Aid
- Academic Advising
- Find a Job or Internship
- Disability Resources
- Room Reservations
- Academic Calendar
- Faculty Resources
- Faculty Access
- Human Resources
- News and Events
- Alumni Directory
- Get Involved

Two UChicago PhD Students Look to Advance the Future of Electrocatalysis
Two UChicago PhD Students Look to Advance the Future of Electrocatalysis
Two UChicago PhD candidates are steering their research with electrocatalysis toward a brighter, more sustainable future.
Sophie Anferov (‘24) and Maia Czaikowski (‘25), who work in the Anderson Lab , are opening a path in electrochemistry that aims to move past the conventional boundaries of small molecule transformations and embrace the nascent field where electrochemical potential fuels organic synthesis.
In their recent paper published in Chemistry Catalysis titled, “Metal-Ligand Cooperativity in Chemical Electrosynthesis” , the duo shed light on the underutilization of electrochemistry in industrial settings, highlighting the pivotal role electrochemistry can play in crafting a greener, more eco-conscious industry.
"We envision electrochemistry becoming an integral part of every chemist's toolkit, offering a customized, efficient, and sustainable approach to chemical synthesis," says Anferov.
Both Anferov and Czaikowski see electrochemistry as a direct manifestation of using electricity as a reagent, aligning seamlessly with the shift towards sustainable chemical practices. They write that with the right exposure, electrochemistry will only get bigger as access to electrochemical potential is increased.
“I think that there's not enough of it out there,” says Anferov. “We want people to give electrocatalysis a chance when they have something that they think can do some cool chemistry with and need a way to drive it.”
Much of electrochemistry deals with simple molecules like CO2, but in their paper, which serves also as a comprehensive review of the electrochemical process, the authors aim to bring electrochemistry into the domain of more complex transformations.
They envision a paradigm shift where electrochemical potential becomes the primary driver of intricate organic reactions instead of finite sources of energy, stating that “improving chemical synthesis is inherently caring about sustainability.”
Notably, by culminating diverse strategies and examples from their lab and beyond, their paper delivers a historicized view of electrochemical advancements and pays homage to the collective efforts of researchers worldwide who are propelling electrochemistry into new frontiers.
"We wanted to compile examples of people pushing electrochemistry beyond its traditional confines, showcasing its potential in driving a wide array of reactions," says Czaikowski.
Asked if any groups are working with electrocatalysis they currently admire, they cite the Stahl Research Group at UW-Madison for not only their chemistry but their ability to produce papers that contextualize their research among a global effort.
“You read one of their papers and you come out with so much more information because they've done all of that for you,” said Czaikowski.
It’s an approach that the duo have adopted in their own paper, as anyone who reads their work will walk away with a deeper understanding of what electrocatalysis can do.
At the core of their approach lies the concept of metal-ligand cooperativity, a potent synergy that amplifies the reactivity of transition metals, particularly first-row metals, such as Iron, Copper, Nickel and Zinc, are abundant in nature.
"Metal-ligand cooperativity unlocks greater capabilities, enabling us to delve into complex transformations with unprecedented precision," Czaikowski elaborates.
“The idea is that your transition metal is very powerful and a lot of reactivity that can occur just on a metal center with a substrate,” said Czaikowski, “But when you add on ligand design, which is a more organic synthesis problem, you unlock even greater capabilities. You can have redox-active ligands, which can store electrons or proton-storing ligands, and it increases the capability that you have with your catalyst.”
While the paper demonstrates the duo’s knowledge of hard science, their paper is intended to make their strategies attractive to fellow researchers.
“With an electrochemical setup, it's attractive because it’s tunable. You can tune a lot of fine granularities across a spectrum. It's a lot more of a customized approach to doing chemistry,” said Anferov.
“We're trying to promote that it's attractive because there's a sort of barrier to entry. And while there's going to be a learning curve for the whole field, we think that the opportunities far outweigh the challenges,” she continued.
Their paper not only encapsulates a historicized view of electrochemical advancements but also serves as a beacon of inspiration for budding researchers. For the duo, they say the possibilities with electrocatalysis are endless.
Asked what outcomes they envision from their work, Czaikowski states, "We want our work to inspire others to explore the vast potential of electrochemistry, especially in drug targeting and fine-tuning molecular structures.”
“We would like people to treat (electrocatalysis) the way they feel about photocatalysis now,” concludes Anferov. “It's another way to drive reactions that can allow you to access unique products.”
Footer Links 1
- About the Department
Footer Links 2
- Seminars/Events
- Giving to Chemistry
The University of Chicago
Department of Chemistry
5735 S Ellis Ave, Chicago, IL 60637
Phone: 773-702-7250
Copyright Menu
- Accessibility
Graduate Student-at-Large: Business
Take for-credit classes at the University of Chicago Booth School of Business.
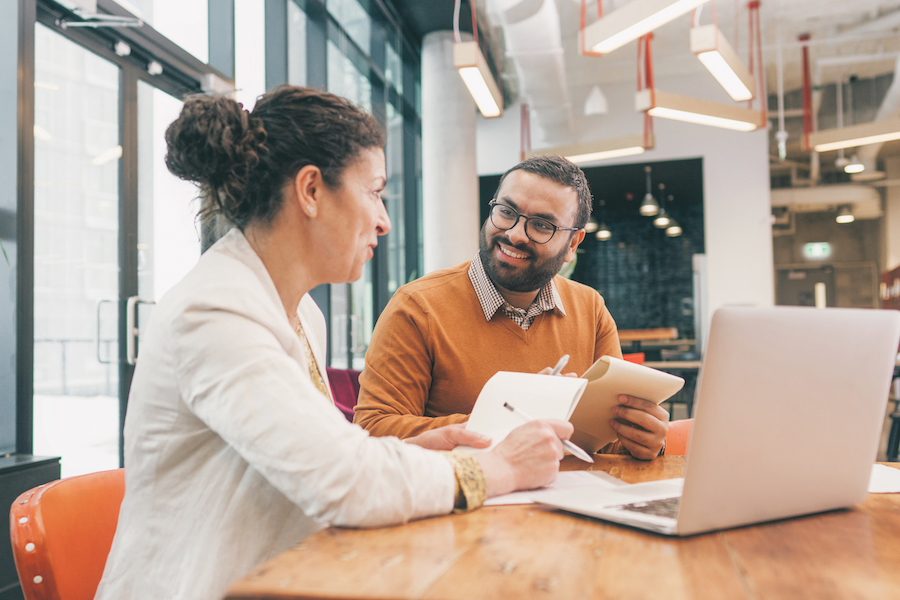
Summer Quarter 2024
- Outlook.com
- iCal / MS Outlook
Autumn Quarter 2024 (international)
Autumn Quarter 2024
Upcoming Events
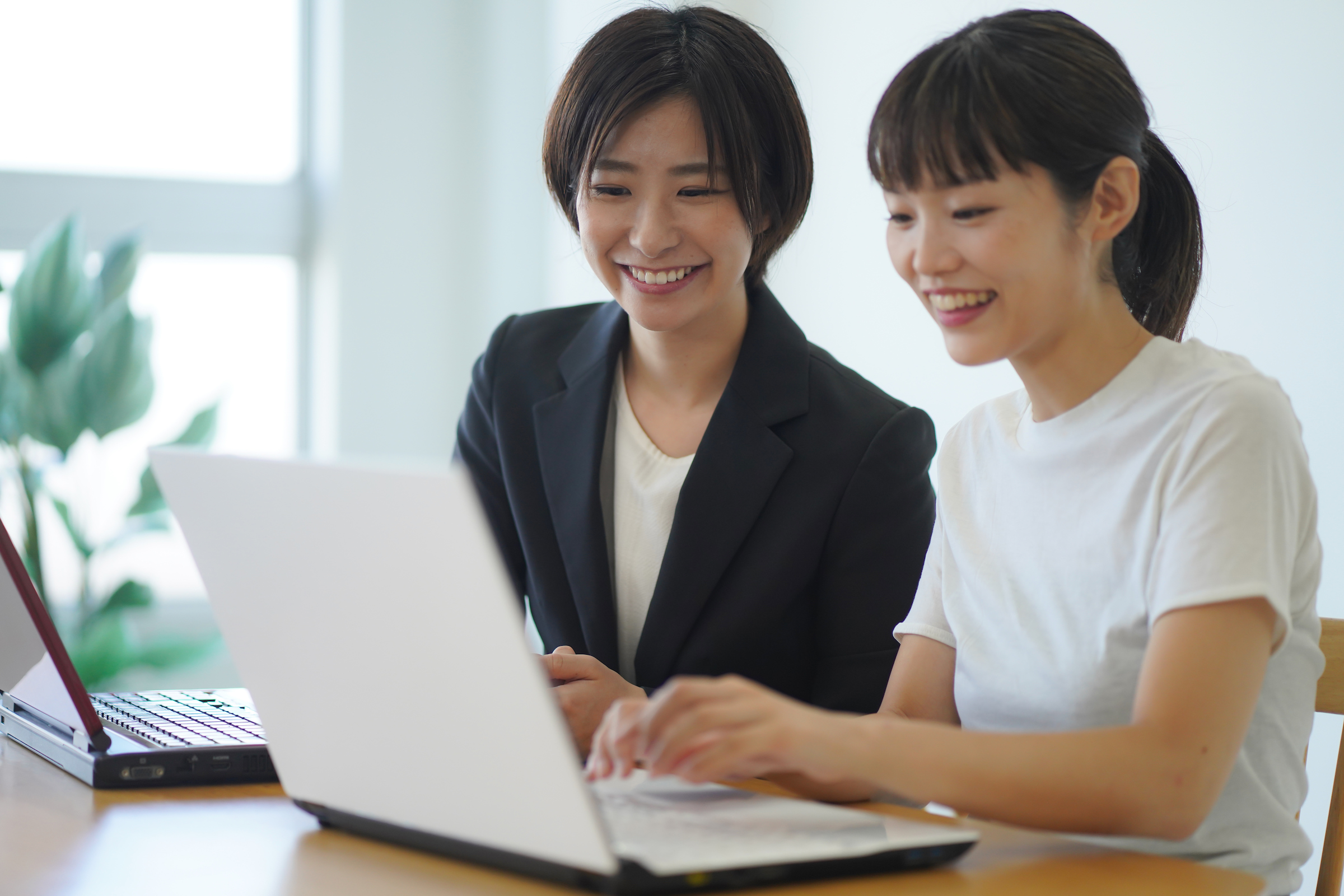
Hong Kong-Beijing Campus Information Session...
Apr 23, 2024 • Online
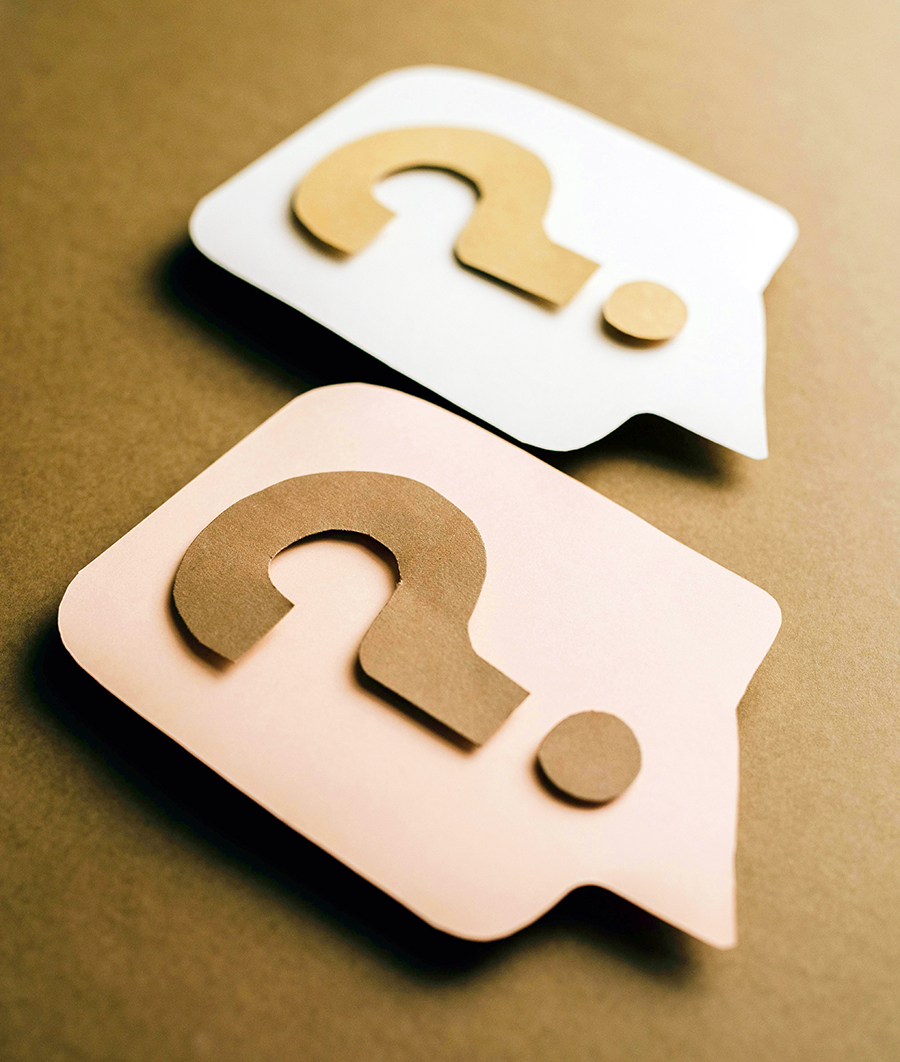
Ask Me Anything: Graduate Student-at-Large...
Apr 26, 2024 • Online
At a Glance
You’re here because you’re thinking about your future. We’re here to make it real.
The Graduate Student-at-Large: Business (GSALB) program prepares students for the MBA at the University of Chicago Booth School of Business —or any other MBA program across the nation. When you join GSALB, you join a vibrant academic culture united by the rigorous, discipline-based approach to business education that consistently ranks Chicago Booth as one of the top MBA programs in the world.
Designed For
Working professionals or students looking to gain experience in a top-tier business school and a competitive edge in their application to Chicago Booth or other MBA programs of their choice.
Test the MBA at Chicago Booth
More than just a business school prep program, GSALB enables students to deliberately explore the academic culture of Chicago Booth. Graduate courses taught by world-class faculty will give you a strong foundation in the language of graduate business education and exposure to our distinctive approach toward business theory and its application.
The GSALB team works closely with Chicago Booth's registrar and admissions counselors to ensure that each student has access to the right courses. Our advisors will help you build a strong academic transcript, write a Statement of Purpose, receive commendable letters of recommendation from faculty members, and burnish your test scores, making you a competitive candidate for Chicago Booth and beyond.
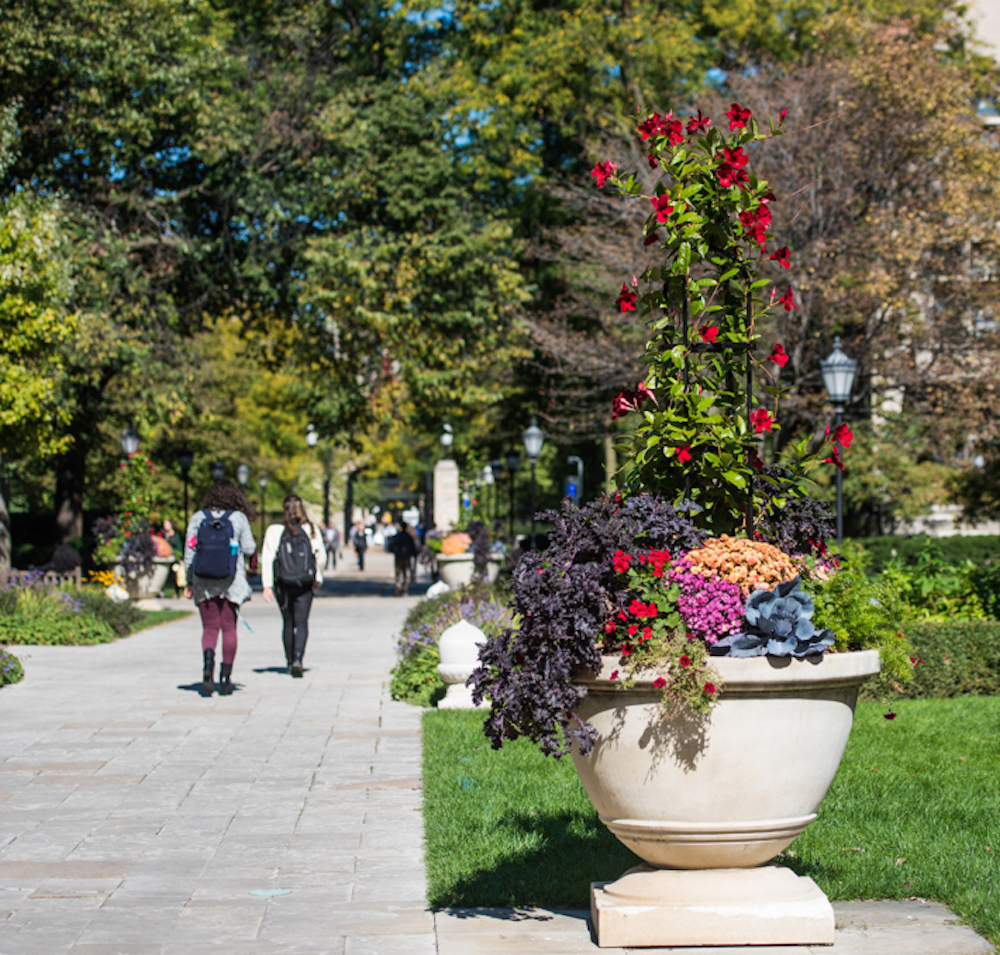
Take the Next Step toward Your Future Today
Advance your career and explore the Chicago Booth School of Business by applying to become a Graduate Student-at-Large.
Find Your Fit—For Credit
As a graduate student in the GSALB program, you can enroll in MBA courses at the University of Chicago Booth School of Business before you apply to MBA programs. You can earn credit, receive a letter grade, and obtain transcripts for graduate and undergraduate courses. Exemplary students may be granted admission into Booth and have the opportunity to transfer up to three courses toward their MBA.
Build Your Network Before You Start Business School
When you join GSALB program, you join an esteemed community of scholars, faculty, and alumni. The program is an outstanding way to gain inroads—and a broader network—by engaging closely with faculty, program directors, and fellow students that extend beyond the classroom experience. What’s more, you can take advantage of the University’s extensive resources and will receive intensive individual guidance on curriculum choices and your ongoing graduate plans.
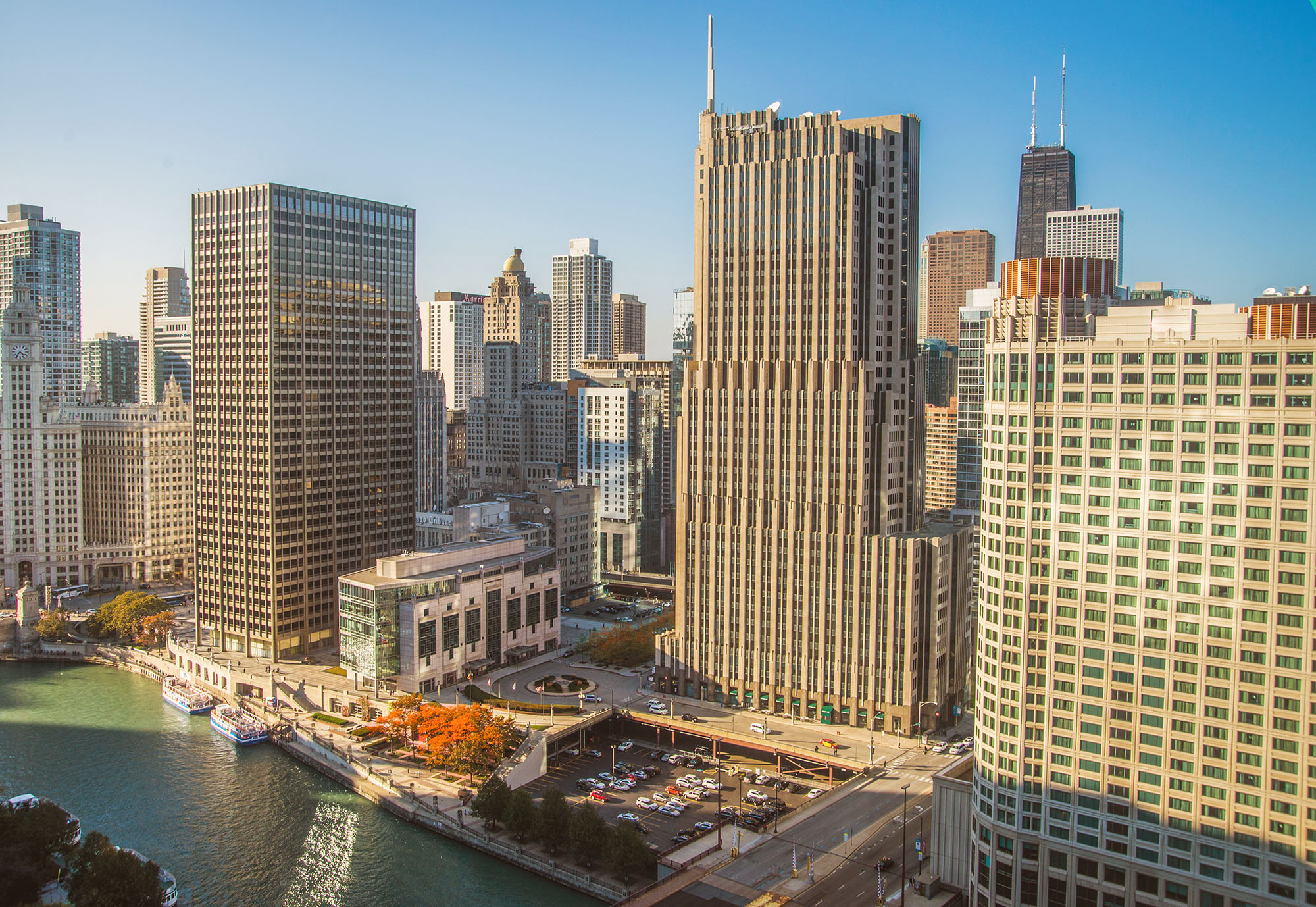
International Students are Now Eligible
The GSALB program is inviting a select group of self-motivated international students to take full-time (300 credits, three courses) academic training for one academic quarter, starting Winter 2023 at Chicago Booth.
Student Profile
Students accepted to the GSALB program must have already completed their bachelor’s degrees, and international applicants must meet our English Language Requirements .
Some GSALB students come to the program with strong foundations in business education, while others have no business background. Some use GSALB to prepare for business school and are accepted into the MBA program at Chicago Booth, while others go on to attend business, professional, and PhD programs elsewhere. Many GSALB students are employed full-time and have between three and ten years of work experience. Most GSALB students take one course at Chicago Booth per quarter. All students may take evening or weekend courses at our downtown campus or during the day at our campus in Hyde Park.
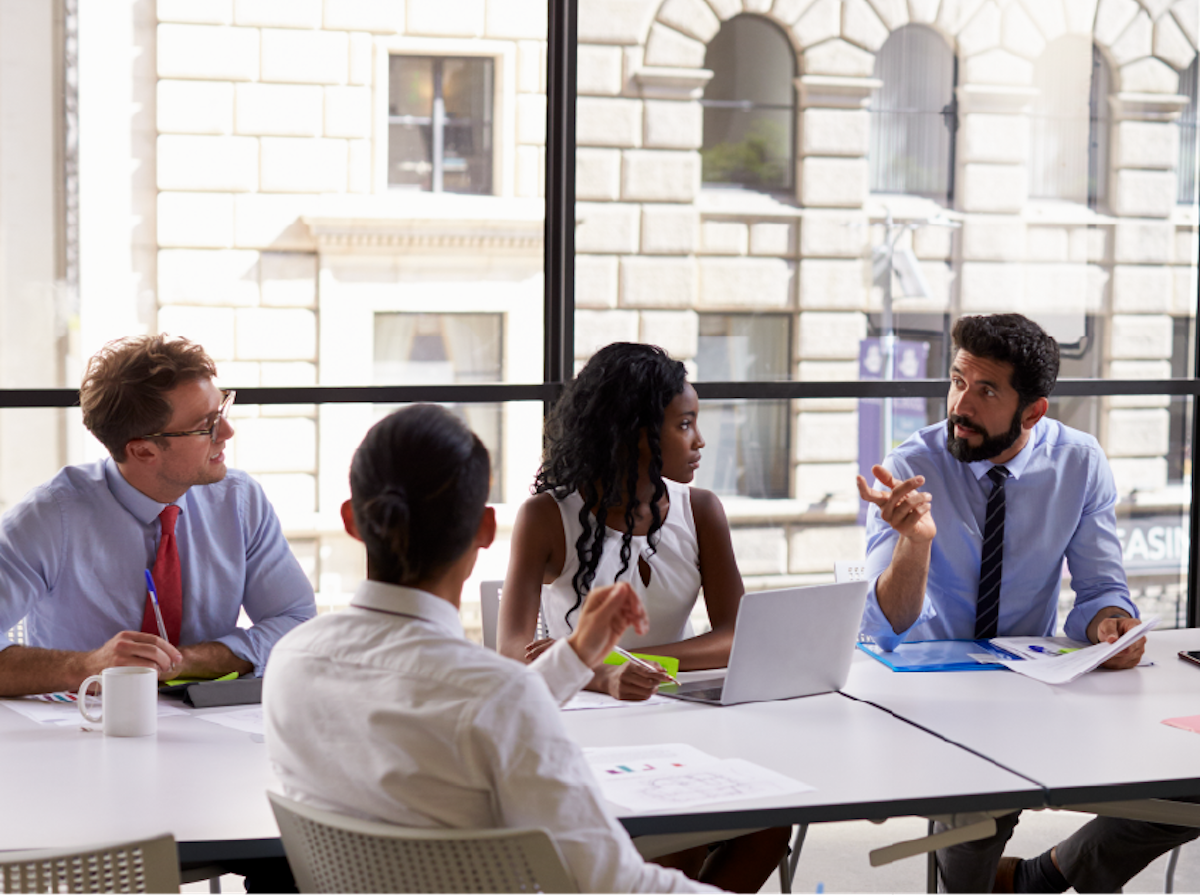
Stay Connected
Our alumni have gone on to various MA and PhD Programs all over the United States and used their training to succeed in various professional pathways.
Please stay in touch to receive program and alumni information updates, invitations to present to prospective students, and participate with fellow alumni at social events.
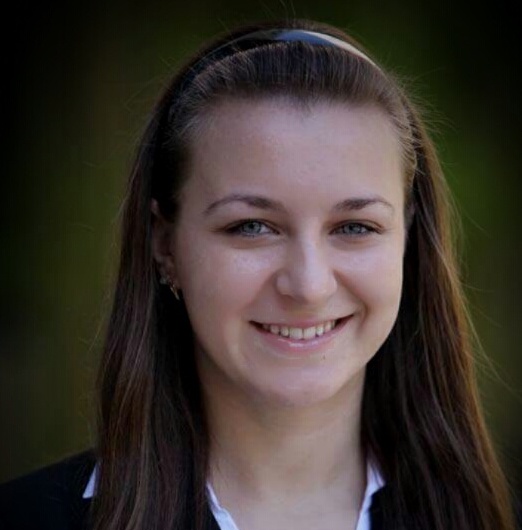
The GSALB program gave me a head start and helped ease my way into business school at Booth. I would encourage anyone who is thinking about business school to try the GSALB program as a way to experience both the culture and the rigor of Chicago Booth’s curriculum.
Focus Areas
Graduate student-at-large.
- Returning Scholars
Ready to Take Your Next Step?
Of interest.
Explore fields of academic study and grow your professional network by taking for-credit...
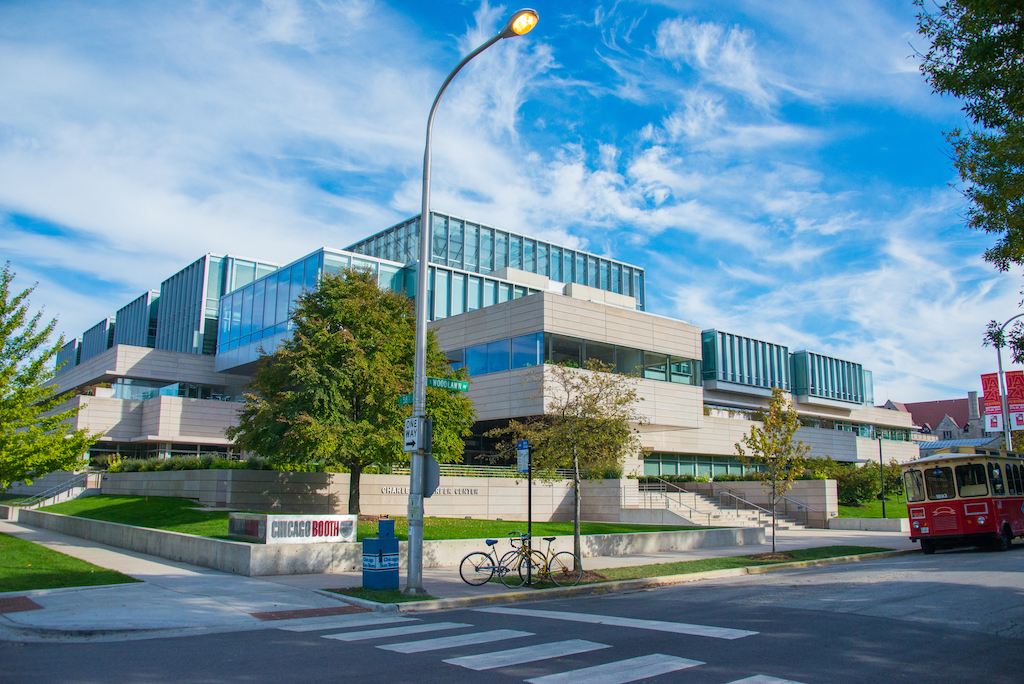
- A Framework for the Future
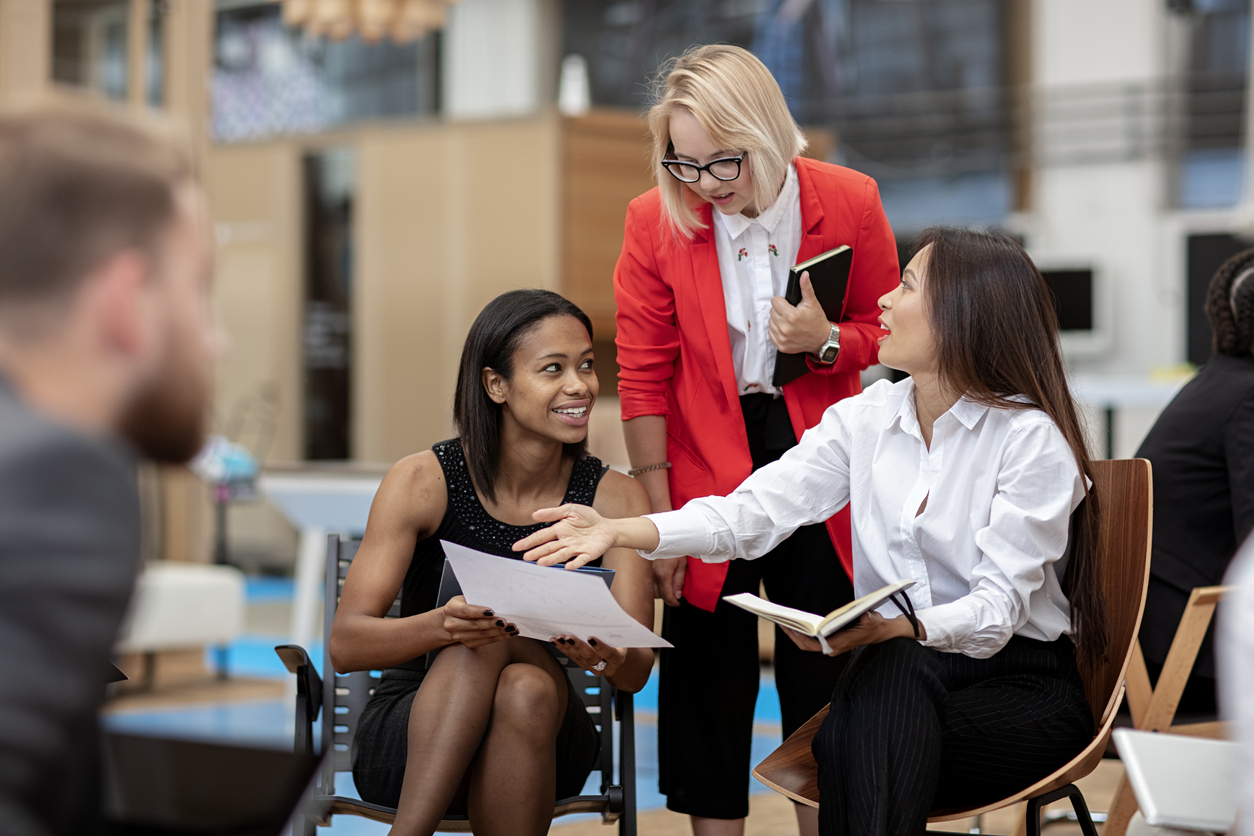
Enter Business School with a Running Start
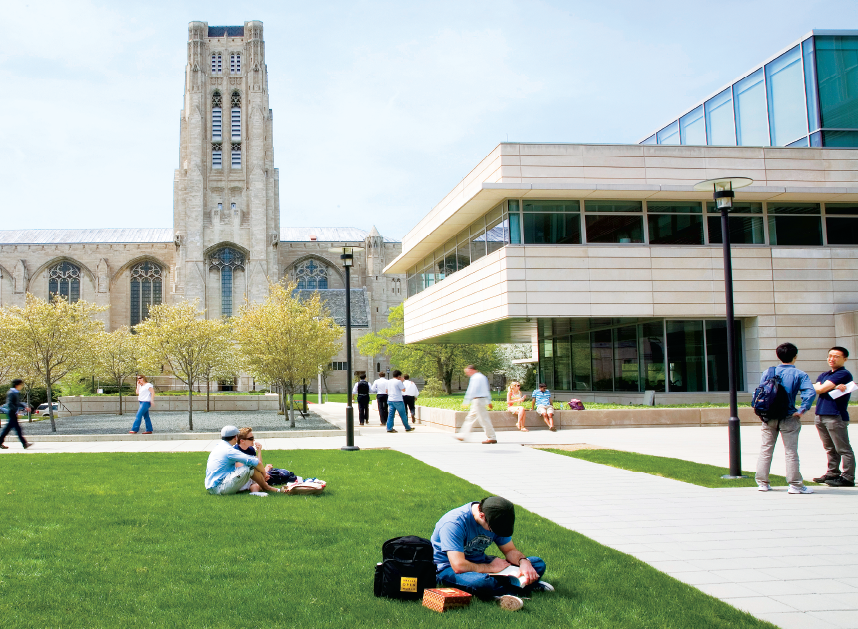
Scaling into Leadership
- Educational Partnerships in Chicago
- How to Prepare for Business School
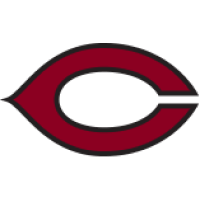
University of Chicago

Hopkins Named CSC Academic All-American
4/18/2024 11:49:00 AM

Thanks for visiting !
The use of software that blocks ads hinders our ability to serve you the content you came here to enjoy.
We ask that you consider turning off your ad blocker so we can deliver you the best experience possible while you are here.
Thank you for your support!
was not found
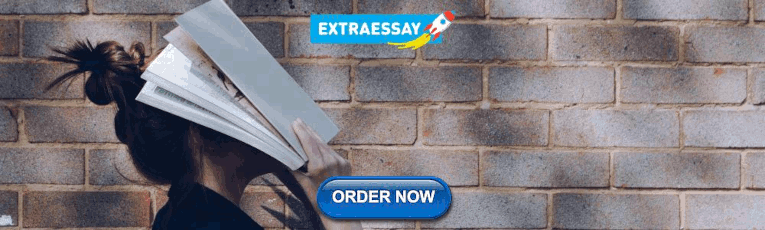
IMAGES
VIDEO
COMMENTS
PhD Degree in Statistics. The Department of Statistics offers an exciting and recently revamped PhD program that involves students in cutting-edge interdisciplinary research in a wide variety of fields. Statistics has become a core component of research in the biological, physical, and social sciences, as well as in traditional computer science ...
Below are links that provide data for individual PhD programs. Some departments have additional data posted on their own websites. A few things to keep in mind as you review the data: Outcomes data is based on students who completed a PhD between academic years 2010-11 to 2014-15. Student enrollments in and degrees conferred by the joint MD/PHD ...
Graduate Programs. The Department of Statistics offers an exciting and recently revamped Ph.D. program that involves students in cutting-edge interdisciplinary research in a wide variety of fields. Statistics has become a core component of research in the biological, physical, and social sciences, as well as in traditional computer science ...
In Memoriam. The University of Chicago. Department of Statistics. George Herbert Jones Laboratory. Suite 222. 5747 South Ellis Avenue. Chicago, IL 60637. 773.702.8333. Related UChicago Programs.
Most students admitted to the PhD program are awarded an assistantship. The Department pays tuition, most fees, student health insurance through the University, and a stipend to cover living expenses. Most PhD students are responsible only for the Student Services Fee per quarter. An assistantship involves working as a teaching or research ...
Welcome to the Department of Statistics at the University of Chicago. We invite you to explore our site and learn more about us: who we are and our history the academic programs we offer, our seminars and distinguished lectures and our many social and academic events.We live in an exhilarating era for statistics at University of Chicago with efforts to expand in data science, machine learning ...
Graduate students in Statistics or Financial Mathematics can enroll without prerequisites. Note(s): Linear algebra at the level of STAT 24300. Knowledge of probability and statistical estimation techniques (e.g. maximum likelihood and linear regression) at the level of STAT 24400-24500.
The University of Chicago Department of Statistics. George Herbert Jones Laboratory Suite 222 5747 South Ellis Avenue Chicago, IL 60637 773.702.8333
PhD students in econometrics and statistics apply statistical methods to a wide range of business problems, from the effectiveness of machine-learning tools to video-game preferences. Our graduates go on to work in high-profile institutions, generally in academia, finance, or data science. Current Students.
Completion Students Median Time to Degree 2015-16 l 2016-17 j 2017-18 n 2018-19 fg 2019-20 h j j j j j Time to Degree b\ Year of Completion Statistics Ph.D. Program Select Unit PSD: Statistics Program: https://stat.uchicago.edu. Title: PSD Statistics PhD Program Data
The PhD curriculum combines the aspiration to train all students in mathematical foundations of data science, responsible data use and communication, and advanced computational methods, with an appreciation of the diverse research interests of the data science faculty. First Year Requirements. The standard first-year program requires students ...
970 East 58th Street | Third Floor Chicago, IL 60637. Prospective Students : (773) 702-3760
Run by the Committee on Data Science, the PhD curriculum combines training in mathematical foundations of data science, responsible data use and communication, and advanced computational methods. Students conduct research on cutting edge problems alongside preeminent faculty at UChicago and explore the emerging field of Data Science. As an ...
The new PhD is part of UChicago's Data Science Initiative, a coordinated, campus-wide plan to expand education, research, and outreach in the field. Under the initiative, the University has added more than a dozen faculty to its Departments of Computer Science and Statistics in the last five years, most of whom specialize in artificial ...
MSDS students choose among the many introductory graduate courses offered to students in the PhD program. These courses cover areas of computer science, optimization, linear algebra and statistics for students that have not had prior exposure to this required course work. Master's students are fully integrated in the academic activities of the department alongside the […]
Graduate Student-at-Large: Other: Graduate Student-at-Large: Business: Other: Graduate Student-at-Large: Returning Scholar: ... Doctoral Program in Statistics: PhD: Pritzker School of Medicine Programs. Pritzker School of Medicine Programs; Program Degree; ... My.UChicago; Office365; A-Z Listing ...
The PhD program in the Department of Public Health Sciences at the University of Chicago offers advanced courses of study in biostatistics, epidemiology, and health services research, all of which are foundational fields in Public Health. The program is supported by a core methodological curriculum in population-based research on human health. Students completing the program will be prepared ...
This course is only open to graduate students in Statistics, Applied Mathematics, and Financial Mathematics, and to undergraduate Statistics majors, or by consent of instructor. Note(s): Students may count either STAT 24500 or STAT 24510, but not both, toward the forty-two credits required for graduation.
US pre-1900, 2019 (PhD Student) [email protected]. Interests: Longue durée US history; Black and Indigenous studies; settler colonialism and slavery; Louisiana and the Atlantic world; subaltern studies, postcolonialism, and ecocriticism; historical trauma, state violence, and genocide; human rights and the history of the present; critical ...
Here is a general outline of the teaching responsibilities of graduate students: No student teaches more than one course per quarter. First-year students do not do any teaching under any circumstance. All second-year students, including those on outside fellowships, serve as College Fellows (apprentice teachers) in an undergraduate mathematics ...
These fundamentals are: Statistics, Linear Algebra, Python, SQL, and Data base design. Master the foundations well. Just because you will learn Data Science and AI does not mean you be shallow at Statistics. A graduate of UChicago must be very solid at Stats as it's always the foundation. Master the art of story telling with data.
April 19, 2024. Congratulations to Matthew Oline, PhD student in the Committee on Computational and Applied Mathematics. He is one of six recipients to receive the 2023-24 Physical Sciences Teaching Prize which is based on nominations from undergraduate students. Matthew Oline, PhD student, CCAM. The Department of Statistics at the University ...
*Please note, during the time covered by the above statistics, 100% of our graduates who have sought clinical positions or residencies have attained them. Committee on Medical Physics 5841 S. Maryland Avenue, MC2026
The student must apply to graduate online (my.uchicago.edu). This must be done no later than 5:00 pm on the first Friday of the quarter in which the student plans to graduate. The Program Director will notify the student of any incompletes and missing grades remaining on the student's transcript, GPA requirements, and deadlines for completion ...
When considering graduate programs, Lim said the Master of Public Policy at the Harris School of Public Policy stood out. "I was drawn to Harris' emphasis on economic analysis, data skills, and statistics—not to mention the focus on programming languages like R and Python, which are invaluable for effective policy analysis.
Prospective Students : (773) 702-3760. Current Students : (773) 834-2093
Two UChicago PhD Students Look to Advance the Future of Electrocatalysis Two UChicago PhD candidates are steering their research with electrocatalysis toward a brighter, more sustainable future.Sophie Anferov ('24) and Maia Czaikowski ('25), who work in the Anderson Lab, are opening a path in electrochemistry that aims to move past the conventional boundaries of small molecule
970 East 58th Street | Third Floor. Chicago, IL 60637. Prospective Students : (773) 702-3760. Current Students : (773) 834-2093. Life at UChicago. GRAD Guide Weekly.
The Graduate Student-at-Large: Business (GSALB) program prepares students for the MBA at the University of Chicago Booth School of Business—or any other MBA program across the nation. When you join GSALB, you join a vibrant academic culture united by the rigorous, discipline-based approach to business education that consistently ranks Chicago Booth as one of the top MBA programs in the world.
The outgoing graduate student put a bow on his postseason awards when he was named a College Sports Communicators (CSC) Academic All-American. Hopkins was named to CSC's second team after posting stellar grades in the classroom as a financial mathematics major at UChicago and is his first Academic All-American award from the organization after ...