Help | Advanced Search
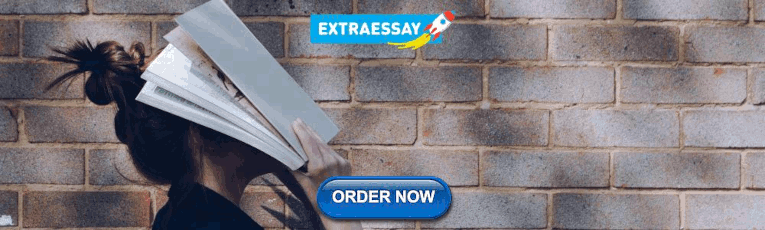
Computer Science > Data Structures and Algorithms
Title: graph theory and its uses in graph algorithms and beyond.
Abstract: Graphs are fundamental objects that find widespread applications across computer science and beyond. Graph Theory has yielded deep insights about structural properties of various families of graphs, which are leveraged in the design and analysis of algorithms for graph optimization problems and other computational optimization problems. These insights have also proved helpful in understanding the limits of efficient computation by providing constructions of hard problem instances. At the same time, algorithmic tools and techniques provide a fresh perspective on graph theoretic problems, often leading to novel discoveries. In this thesis, we exploit this symbiotic relationship between graph theory and algorithms for graph optimization problems and beyond. This thesis consists of three parts. In the first part, we study a graph routing problem called the Node-Disjoint Paths (NDP) problem. Given a graph and a set of source-destination pairs of its vertices, the goal is to route the maximum number of pairs via node-disjoint paths. We come close to resolving the approximability of NDP by showing that it is $n^{\Omega(1/poly\log\log n)}$-hard to approximate, even on grid graphs, where n is the number of vertices. In the second part of this thesis, we use graph decomposition techniques developed for efficient algorithms to derive a graph theoretic result. We show that for every n-vertex expander graph G, if H is any graph with at most $O(n/\log n)$ vertices and edges, then H is a minor of G. In the last part, we show that the graph theoretic tools and graph algorithmic techniques can shed light on problems seemingly unrelated to graphs. We show that the randomized space complexity of the Longest Increasing Subsequence (LIS) problem in the streaming model is intrinsically tied to the query-complexity of the Non-Crossing Matching problem on graphs in a new model of computation that we define.
Submission history
Access paper:.
- Other Formats

References & Citations
- Google Scholar
- Semantic Scholar
BibTeX formatted citation

Bibliographic and Citation Tools
Code, data and media associated with this article, recommenders and search tools.
- Institution
arXivLabs: experimental projects with community collaborators
arXivLabs is a framework that allows collaborators to develop and share new arXiv features directly on our website.
Both individuals and organizations that work with arXivLabs have embraced and accepted our values of openness, community, excellence, and user data privacy. arXiv is committed to these values and only works with partners that adhere to them.
Have an idea for a project that will add value for arXiv's community? Learn more about arXivLabs .
Emerging Trends in Graph Theory
- First Online: 17 June 2023
Cite this chapter
- Santosh Kumar Yadav 2
660 Accesses
In this chapter we shall discuss some popular research areas in Graph Theory. In each of the chosen topic, we introduce the problem, give some easily stated theorem, important variation, direction of research and provide reference to survey articles.
This is a preview of subscription content, log in via an institution to check access.
Access this chapter
- Available as PDF
- Read on any device
- Instant download
- Own it forever
- Durable hardcover edition
- Dispatched in 3 to 5 business days
- Free shipping worldwide - see info
Tax calculation will be finalised at checkout
Purchases are for personal use only
Institutional subscriptions
Unable to display preview. Download preview PDF.
Author information
Authors and affiliations.
Shri Jagdishprasad Jhabarmal Tibrewala University, Rajasthan, India
Santosh Kumar Yadav
You can also search for this author in PubMed Google Scholar
Corresponding author
Correspondence to Santosh Kumar Yadav .
Rights and permissions
Reprints and permissions
Copyright information
© 2023 The Author(s)
About this chapter
Yadav, S.K. (2023). Emerging Trends in Graph Theory. In: Advanced Graph Theory. Springer, Cham. https://doi.org/10.1007/978-3-031-22562-8_10
Download citation
DOI : https://doi.org/10.1007/978-3-031-22562-8_10
Published : 17 June 2023
Publisher Name : Springer, Cham
Print ISBN : 978-3-031-22561-1
Online ISBN : 978-3-031-22562-8
eBook Packages : Mathematics and Statistics Mathematics and Statistics (R0)
Share this chapter
Anyone you share the following link with will be able to read this content:
Sorry, a shareable link is not currently available for this article.
Provided by the Springer Nature SharedIt content-sharing initiative
- Publish with us
Policies and ethics
- Find a journal
- Track your research
Thank you for visiting nature.com. You are using a browser version with limited support for CSS. To obtain the best experience, we recommend you use a more up to date browser (or turn off compatibility mode in Internet Explorer). In the meantime, to ensure continued support, we are displaying the site without styles and JavaScript.
- View all journals
- My Account Login
- Explore content
- About the journal
- Publish with us
- Sign up for alerts
- Open access
- Published: 27 April 2021
A Graph Theory approach to assess nature’s contribution to people at a global scale
- Silvia de Juan 1 ,
- Andrés Ospina-Álvarez 2 ,
- Sebastián Villasante 3 , 4 &
- Ana Ruiz-Frau 2
Scientific Reports volume 11 , Article number: 9118 ( 2021 ) Cite this article
6832 Accesses
15 Citations
104 Altmetric
Metrics details
- Ecosystem services
- Sustainability
The use of Graph Theory on social media data is a promising approach to identify emergent properties of the complex physical and cognitive interactions that occur between humans and nature. To test the effectivity of this approach at global scales, Instagram posts from fourteen natural areas were selected to analyse the emergent discourse around these areas. The fourteen areas, known to provide key recreational, educational and heritage values, were investigated with different centrality metrics to test the ability of Graph Theory to identify variability in ecosystem social perceptions and use. Instagram data (i.e., hashtags associated to photos) was analysed with network centrality measures to characterise properties of the connections between words posted by social media users. With this approach, the emergent properties of networks of hashtags were explored to characterise visitors’ preferences (e.g., cultural heritage or nature appreciation), activities (e.g., diving or hiking), preferred habitats and species (e.g., forest, beach, penguins), and feelings (e.g., happiness or place identity). Network analysis on Instagram hashtags allowed delineating the users’ discourse around a natural area, which provides crucial information for effective management of popular natural spaces for people.
Similar content being viewed by others
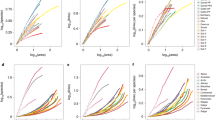
Ecological network complexity scales with area
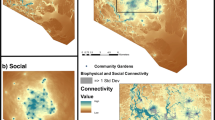
Socio-ecological connectivity differs in magnitude and direction across urban landscapes
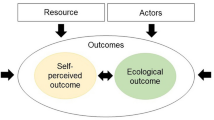
Structural equation modeling reveals decoupling of ecological and self-perceived outcomes in a garden box social-ecological system
Introduction.
Marine and coastal areas are extremely important for human wellbeing and yet, management plans rarely consider in their formulation the contribution of these natural areas to society 1 , 2 . The assessment of the contribution of nature to people wellbeing is particularly challenging due to its intangible and subjective nature 3 , 4 . Research targeted at marine ecosystem services has mostly focused on the economic valuation of commercial fishing, recreational activities, tourism, or seascape scenic beauty 5 , 6 , setting aside the non-material benefits people obtain from ecosystems that have symbolic, cultural or intellectual significance 7 . During the past decade, research on marine conservation has evolved to acknowledge the importance of the non-use value of ecosystems 8 and it currently needs to be incorporated in conservation and management plans 9 . However, the incorporation of a standard assessment of non-material benefits of ecosystems in conservation and management is challenging because, among others, assessments have been often based on time consuming field survey methods 10 , including interviews, face-to-face questionnaires and participatory mapping 11 , 12 , 13 , 14 . These assessments are often conducted at a site-scale 15 , whereas conservation plans generally need information on the interaction between humans and ecosystems at multiple spatial scales 16 . In this context, new cost-effective methodological approaches are needed to be implemented at scales larger than the local case study. There are several studies that adopt large scale approaches 2 , 17 , but these are not cost-effective for a generalized implementation.
In the internet era, there are many social network platforms with millions of users that are an important source of big data. These platforms continuously store information people upload from any location on the planet, as they are used for socializing and communicating. Importantly, social media content is frequently related with recreational activities, including tourism 18 . As part of the information uploaded, people often express their feelings about natural spaces 19 . In the quest to avoid the time-consuming nature of field surveys and to identify alternative methods, there has been an increasing number of scientific studies that use social media for an indirect assessment of peoples’ perceptions and preferences 10 , 18 , 20 . These studies have generally proved to be comparable to traditional surveys 21 . The use of social media data initially relied on photo content assessment (but see Geboers et al. 22 ). The context and content of the photographs is classified into cultural ecosystem services’ categories based on the presence or absence of specific elements in the photos, such as views of flora and fauna, historical buildings, or touristic infrastructure and facilities 23 . During the past decade, scientific works have also explored different analytical techniques on the text associated to the images, including sentiment analysis, Natural Language Processing and data mining methods 24 , 25 , 26 . Recent developments in the analysis of social media data have also applied Graph Theory to hashtags associated to posts, providing a promising approach for the remote assessment of Cultural Ecosystem Services 27 . However, the application of this approach has been so far limited to the regional scale, while it offers an untapped potential to be applied at a global scale and to provide comparative information on the type of marine and coastal ecosystem contribution to societies around the world.
Instagram social media is generally used to post photographs and thoughts in real-time often related to activities or social recreation, but also to cultural and wildlife appreciation 27 . An advantage associated to Instagram is the frequent inclusion of hashtags as part of the photo post. These hashtags are used as keywords to mark messages or form conversations and, thus, they provide an additional way to connect visual content (i.e., photos) and semantically related words to a discourse. User-generated hashtags provide an opportunity to analyse the discourse linked to the photos and minimize the subjectivity and low-cost effectiveness associated to photo content analysis.
On the other hand, Graph Theory, as the mathematical study of the interaction of a system of connected elements, is a suitable approach for analysing the string of words associated to an Instagram post. The analysis of networks using Graph Theory can be described as the analysis of existing relationships between the different elements contained in a network. It provides a simplified and quantitative view of the multiple factors involved in the connection among system elements 28 . The term vertex , or node, is used to describe the elements in a network, while the term edge is used to refer to the connections between the different vertices in a network. In our case, vertices are represented by hashtags, while edges illustrate the connections between hashtags. In a network of keywords posted with the photos, Graph Theory provides insights into the system properties and identifies critical nodes with high centrality (i.e., words connected to many other words) or clusters of well-connected nodes 29 , 30 , 31 .
In this study, the working hypothesis was that data extracted from Instagram and analysed through different Graph Theory centrality measures can be used to understand peoples’ preferences for nature and nature-based experiences in marine and coastal areas worldwide, regardless of their geographical location, environmental characteristics, accessibility, tourism features, or any differentiating characteristics. The hypothesis was tested in 14 marine and coastal areas that span over the 12 marine realms established by Spalding 32 (Fig. 1 and Table 1 ). These areas are expected to provide a wide diversity of benefits to society (e.g., recreation, cultural heritage, nature and wildlife observation) and to be visited by a wide diversity of users. This approach has the potential to contribute to cost-efficient assessments of the contribution of marine and coastal areas to society well-being.
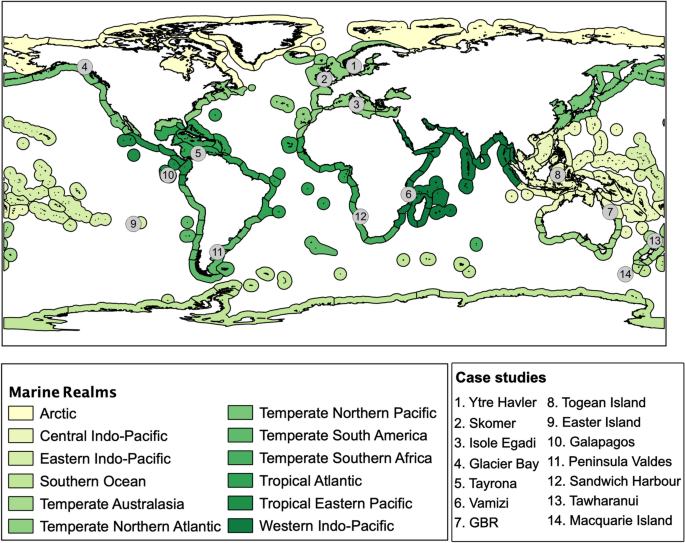
The 14 case studies selected across the twelve marine realms proposed by Spalding et al. 32 .
For each case study area, a search query was executed (Table 1 ). Query terms were based on the hashtags of the geographical name of the study areas; therefore, the post download was related to the name of the study area (e.g., Galapagos), with all downloaded posts including this name as query. Query search was limited to English, the most common language amongst tourists. This might have overlooked posts where the name of the place was in a different language. For most marine areas, this was considered irrelevant as the name of the place is not translated to other languages (e.g., Tayrona, Vamizi, Skomer). In some of the cases, the name of the place could appear in a variety of languages (e.g., Great Barrier Reef), however, the use of non-English place hashtags as queries generally retrieved a significantly lower number of posts (e.g., Gran Barrera de Coral in Spanish with 1900 posts, or Grand Barrière de Corail in French with 14 posts, while Great Barrier Reef had over 10,000 posts). In the specific case of Easter Island, we observed that the use of three particular queries was linked to a high number of posts: Easter Island and the local name Rapanui had over 10,000 posts each, and Isla de Pascua in Spanish had 8700 posts. In this case, three separate posts’ downloads were performed, and data were merged for subsequent analysis. The above, rather than a limitation of the methodological approach, demonstrates its flexibility to adapt to different data acquisition requirements.
To illustrate the most relevant information contained as part of the posts downloaded for each of the 14 areas, we selected the 150 most frequent hashtags from each dataset in order to create the network graph and represent the dominant discourse in relation to the area in question. Network graphs were delineated using eigenvector, betweenness and edge betweenness as centrality measures. Eigenvector centrality measure (hereafter Eigenvector) allows identifying those hashtags that are frequently posted with other hashtags also frequently posted, and it can be interpreted as the pairs or groups of features more frequently related to the case study by the users. Betweenness centrality (hereafter betweenness) and edge betweenness centrality (hereafter edge betweenness) provide information about clusters of hashtags that describe users' experiences or perceptions and that connect (by means of a hashtag) to other clusters representing other types of experiences or perceptions. These high betweenness hashtags structure the general discourse about an area and their removal would fragment the network and disconnect distant concepts. Therefore, hashtags and links with high betweenness can show the discourse parallel or additional to the main discourse and their relations, allowing to identify less frequent activities or perceptions but that are equally important to understand the network as a whole.
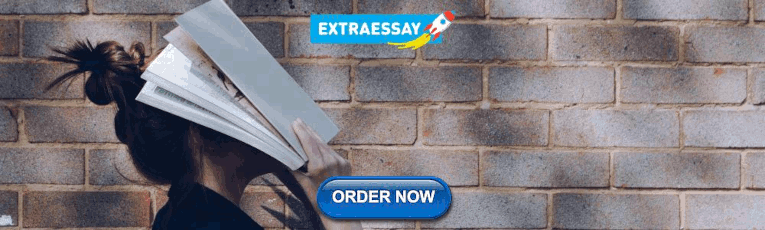
Network centrality measures
Results indicated that network graphs captured information on distinct types of ecosystem services, for example, those based on wildlife and nature, heritage, or beach tourism. In areas such as Galapagos, central hashtags were nature , wildlife , photography , travel and adventure , evidencing a preference for wildlife and nature-based tourism. In this area, betweenness evidenced the connections between the most frequent hashtags group with other peripheric hashtags and provided a complete picture on the discourse of Galapagos’ visitors (Fig. 2 ). As such, nature and wildlife-based travel and photography is related with natural science concepts like evolution and endemism, and specific biotic and abiotic components like crabs and waves, altogether related with positive feelings (i.e., happy). Other areas emerging for their wildlife and nature were Skomer nature reserve, characterised by the hashtags birds (including the species Puffin ), nature and wildlife photography ; and Península Valdés, characterized by many locality names and by fauna, with the frequently posted hashtags’ wildlife , whales and nature funnelling most connections to other less frequent hashtags (e.g., wind , hiking , relax ) and providing a full picture of the social perception on nature recreation activities, iconic fauna and positive feelings. Three networks, Sandwich Harbour, Glacier Bay and Macquarie Island also included popular hashtags related with nature , wildlife and photography ; however, most hashtags had low betweenness and edge betweenness limiting the diversity of the posts (all network graphs are available at the Figshare repository, https://doi.org/10.6084/m9.figshare.13325627.v2 ).
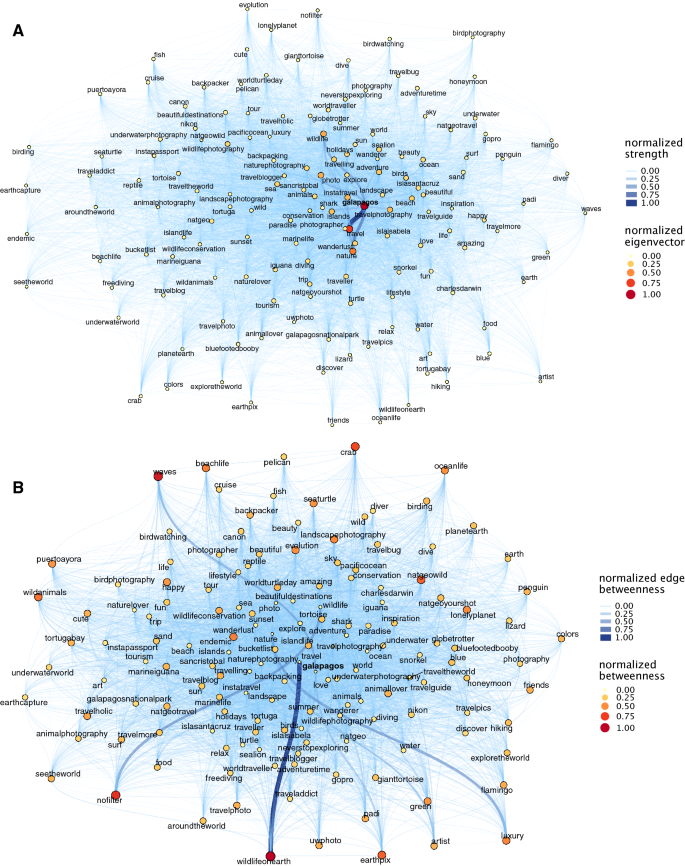
Example of network graphs in Galapagos case study. In plot ( A ) node size represents the Eigenvector centrality and edges represent normalized strength (weighted degree). In plot ( B ) node size represents normalized Betweenness centrality and edges represent normalized Edge betweenness.
Regarding cultural heritage, Easter Island was characterised by popular hashtags related with Easter Island stone statues (moais) and with travel; and edge betweenness evidenced a diversity of peripherical nodes that describe other cultural elements, like design , music and food , and evidence social preferences for different cultural elements of the island, beyond the moais . Other areas reflected cultural identity by the frequent post of local names (e.g., Ytrehvaler), words related with the country’s identity (e.g., Isole Egadi) and positive feelings about this identity (e.g., Tawharanui). In Tayrona National Park network, the full discourse identified cultural identity like Kogui (indigenous culture) linked with the popular posts related with nature and summer holidays. Similarly, in Tawharanui and Isole Egadi, beach , nature and summer where the most frequent posts that, in some cases, where connected with places and activities. In these cases, and particularly in Isole Egadi and Ytrehvaler, edge betweenness allows to identify connections between places and activities, wildlife or natural structures, providing relevant information for area management and conservation.
A group of areas were appreciated by their underwater ecosystems. For Great Barrier Reef, popular hashtags were related with the coral reef: ocean , diving , underwater photography , travel , nature , coral and reef ; whereas betweenness highlighted a set of hashtags related with conservation: science , sustainability , save the reef , 4 ocean (Fig. 3 ) and evidenced the presence of a conservationist discourse in the social media. In Toguean Island network, the frequent hashtags beach , wonderful and charming are connected to peripherical hashtags related with the sea (e.g., sea life , diving ), while in Vamizi, popular hashtags were related with high-income tourism, private island , travel , luxury travel , and were connected to less frequent hashtags linked to the sea, including recreational fisheries. These last two examples illustrate differences in the benefits, and beneficiaries, provided by two popular touristic destinations.
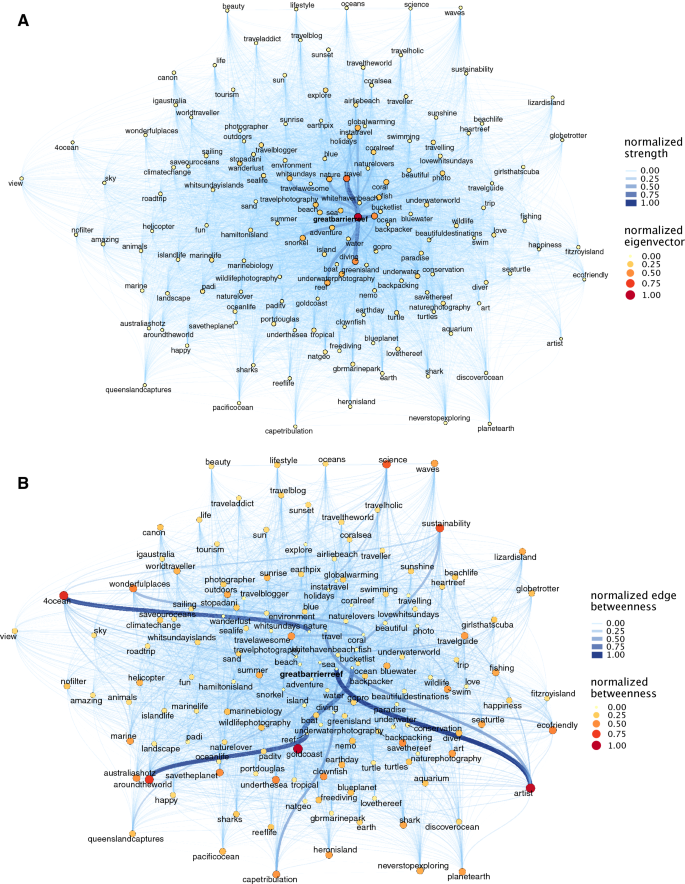
Example of network graphs in Great Barrier Reef case study. In plot ( A ) node size represents the Eigenvector centrality and edges represent normalized strength (weighted degree). In plot ( B ) node size represents normalized Betweenness centrality and edges represent normalized Edge betweenness.
Network communities
The division of hashtags in communities allows for a more detailed exploration of the words included in the 150 most frequent hashtags selection, independently of their centrality measures, and allowed a categorisation of hashtags within cultural ecosystem services classes in each area (Table 2 ). Hashtags were grouped in 3 to 5 communities, with some communities relatively constant across case studies, e.g., aesthetics, wildlife and nature appreciation (Fig. 4 ) (all other network graphs are available at the Figshare repository, https://doi.org/10.6084/m9.figshare.13325627.v2 ).
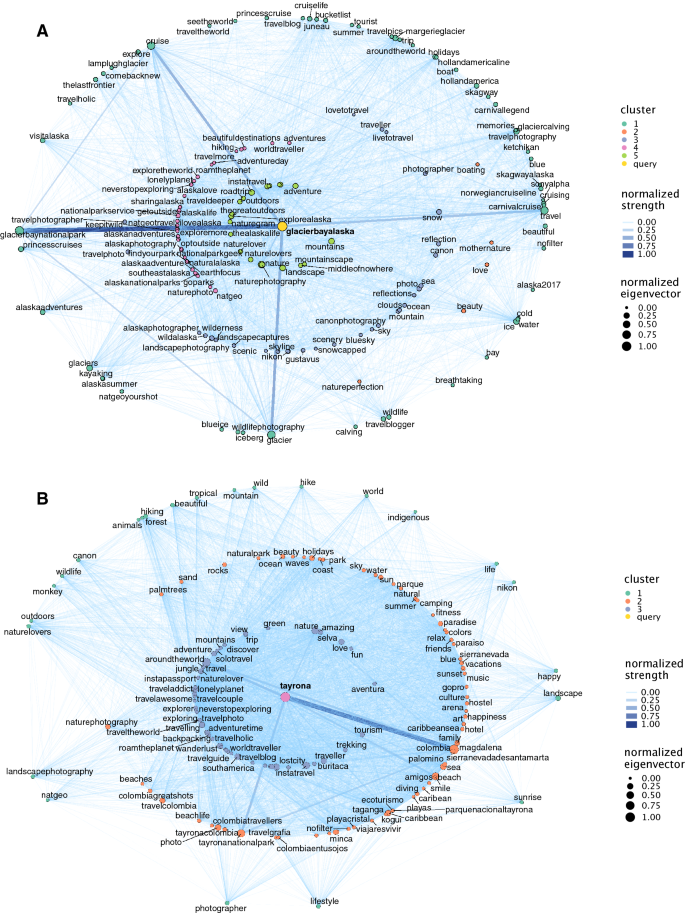
Communities assessed through Fast-Greedy algorithm for the case studies Glacier Bay ( A ) and Tayrona ( C ). The node size represents the normalized Eigenvector and the colour represents the community. The colour and width of the edges represents the normalized edge strength (weighted degree).
In some of the areas, the communities were diverse in hashtag composition, for example, in Galapagos, wildlife (and related words) was distinctive of several communities, but other communities were characterised by different concepts: beach , holidays , happiness , snorkelling and diving . In Easter Island, the hashtags related with the stone statues and cultural heritage characterise one community, while the other communities include a diversity of hashtags classified under adventure, nature, underwater recreational activities; therefore, it widens the information provided by the centrality metrics. Tayrona (Fig. 4 ) is also a diverse network with one community characterised by hashtags like beach , summer , happiness (wellbeing), but other communities contain a diversity of hashtags like forest , hiking , indigenous and wildlife (classified in recreational, cultural heritage, nature and aesthetics; Table 2 ).
In some areas, the communities were not so diverse, but provided additional information on the posts. For example, in MacQuarie Island the communities highlighted iconic fauna, including several penguin species, and biodiversity conservation. In several areas, network communities informed of the iconic fauna and specific places: puffins and other bird species in Skomer; southern right whale, sealions and penguins in Península Valdés; glaciers and mountains in Glacier bay (Fig. 4 ); desert and dunes in Sandwich harbour. Finally, Ytrehvaler is a network characterised by many local names (in Norwegian), evidencing a national tourism, and hashtags related with scenery.
Merged network of the 14 case studies
The merged network highlighted several hashtags that act as bridges between communities of hashtags (Fig. 5 ). Nature , travel , photo and travel photography are key to structure the global network. However, several low eigenvector hashtags connect smaller groups: sunset and island connect the subgroups from Easter Island, Isole Egadi and Vamizi.
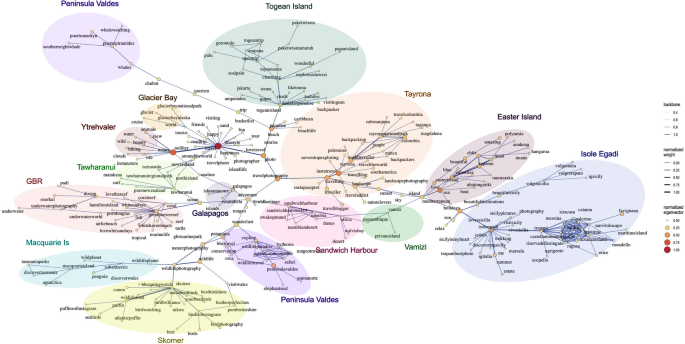
Global network graph including the fourteen case studies where the node size represents the Eigenvector centrality. The coloured clusters arrange the case studies to facilitate the visual identification of areas connected in the network.
From the hashtag travel photography diverges a branch that connects 7 areas through adventure ; a small group of hashtags deriving from this node represent Sandwich harbour and Vamizi, connected through Africa . The hashtag ocean , connected to adventure, relates Great Barrier Reef with Tawharanui, and to wanderlust (a German expression for the desire to explore the world) that connects Península Valdés, Skomer and Macquairie Island. These three areas and Tayrona are also connected through the central hashtag travel photography , and Skomer and Macquairie Island through wildlife photography . The hashtag adventure is also connected to a group of hashtags from Galapagos that also derive to the high eigenvector hashtag nature .
The hashtag nature is key to include the fragile sub-network Ytrehvaler, and also derives to other high eigenvector hashtag, travel , that in turn, connects to the small sub-network from Glacier bay. Photo, a central hashtag related with travel, connects to paradise , that is key to integrate Toguean Island, a few hashtags from Tayrona related with the Caribbean and beach, and a group of hashtags from Peninsula Valdez related with whale watching. Some other small hashtags, that are connected to high eigenvector hashtags but are not included in any particular area are shared by many of the areas, e.g., sun , relax , landscape photography , nature lovers , sunset , sky .
The analysis of social media data with Graph Theory provides a powerful indirect approach to monitor visitors’ preferences and perceptions for marine and coastal areas, and it provides a global view of the wide array of benefits humans obtain from nature. The analysis of users’ posts with different network centrality metrics allowed the identification of an emergent discourse in Instagram by identifying the most frequent words related with a specific area (e.g., nature , wildlife and photography in Galapagos), and also other less frequent words (e.g., evolution and endemism), including feelings (e.g., happy ), connected with the most frequent words. The primary and secondary information provided by the centrality metrics is of high value for conservationists and managers, as it characterises visitors’ preferences, including recreational activities and preferred ecosystems and species. Importantly, our approach allowed to gather this individualised information remotely from a wide variety of marine and coastal case studies around the world.
What becomes evident from this global assessment is that an area does not need to be an iconic destination to contribute to society’s recreation and wellbeing. Galapagos, Great Barrier Reef or Easter Island provide essential services like nature appreciation, wildlife watching or cultural identity, however, such services have also been identified in less iconic areas often visited by local tourists like Skomer Island, Tawharanui and Ytrehvaler. Similarly, wellbeing related with relaxation and happiness is recorded in remote and iconic areas like Galapagos, but also in less internationally known places such as Vamizi, Tawharanui or Isole Egadi. The frequent post of the word happiness (and similar words) denotes the importance of nature’s contribution to people’s wellbeing, as emphasised by several authors 33 , 34 , 35 , with many benefits arising from human connections with nature, including sense of place, identity, mental health and sense of belonging. These benefits were independent of its location, ecosystem or main activity provided. A series of cultural ecosystem services’ bundles could be identified in each case study, evidencing areas with high diversity of benefits and perceptions, whereas other areas were relatively homogenous in users’ activities and perceptions. Frequent words’ groups were related with aesthetics, wildlife and nature appreciation, which is expected as information is obtained from a photography-based social media platform. However, the classification of the popular hashtags in cultural ecosystem services’ types, despite providing standardised information that allows the comparison with other studies, limits the information depicted from the networks. Network analysis allowed moving beyond the state of the art by mere hashtag frequency to the exploration of the nature of connections between hashtags, delineating the users’ discourse. For example, animal species connected to local names provides information on places for wildlife watching, e.g., puffins in Skomer Island, or penguin species in MacQuarie Island. Hashtags also evidenced environmental awareness, e.g., conservation in Macquarie Island or climate change in Great Barrier Reef, which should be considered key to promote transformative changes for policy makers 36 . In summary, relevant words like nature watching can be linked to a place or to a species name, conservation can be linked to a place or ecosystem component, and so on.
Instagram provides information on what calls the attention of visitors, but also on activities and feelings about the place. For example, many Instagram users mentioned natural spaces for their conservation value (e.g., Great Barrier Reef), nature excursions (e.g., Glacier Bay), bird watching (e.g., Skomer), views of natural fauna (e.g., Península Valdés), but also, and not least, the scenic beauty (e.g., Sandwich harbour), the relaxation and happiness provided by open spaces (e.g., Galapagos), or some luxury accommodations within national parks as wellness spaces (e.g., Vamizi). The main activities reported in each case study were generally related with the dominant habitat, e.g., diving in Great Barrier Reef or Toguean Island, with prevalence of coral reefs, or beach recreation in Tawharanui or Isole Egadi, characterized by sandy beaches. Nevertheless, the posts’ discourse in each area is markedly defined by the visitor profile, and access to the area. For example, Vamizi is characterized by high-income and international tourism that visit the area for the opportunity to enjoy the underwater life, recreational fishing and beach. Other areas like Skomer and Ytrehvaler are mainly visited by locals that enjoy the wildlife and nature of the place. This implies that enjoyment of natural spaces by people is highly conditioned by its logistical accessibility, with remote places like Macquarie Island or Galapagos visited by international tourists that travel ( travel , being one of the most common hashtags) to these areas to find adventure, recreation, or scenery, amongst others. More accessible areas (i.e., those easily accessible from large cities), like Tawharanui, Ytrehvaler or Isole Egadi, appear to be predominantly visited by locals in search of the relaxation of the beach, sighting of emblematic local fauna, or nature recreation. The merged network evidenced that central hashtags to all areas were nature , travel , photo , however, less popular hashtags appear key to connect smaller groups of areas and were related with general concepts posted in these areas and not with principal activities or focus. For example, sunset and island connected Easter Island, Isole Egadi and Vamizi, wanderlust connects Península Valdés, Skomer and Macquairie Island, paradise connects Toguean Island and Tayrona, ocean connects Great Barrier Reef and Tawharanui. These results imply that the perception of visitors to the areas is not exclusively conditioned by the main activity or ecosystem type, but by higher level concepts like paradise or wanderlust .
It has been acknowledged that the use of information from social media platforms has an inherent bias associated to both the type of user and the type of content posted on the platform. Hausmann et al. 21 observed that while most of the pictures posted on Flickr focused on biodiversity, Instagram was popular for sharing pictures about activities and people. On the other hand, Ruiz-Frau et al. 27 observed that Twitter posts reflected social awareness and discussions around current global concerns such as climate change and youth movements. Therefore, different social media platforms may be used by different groups of people. Aiming to maximize the representation of the wider society, we considered Instagram to be an optimal platform for representing a wide diversity of people and of natural spaces. However, the use of this methodology is not exempt of challenges. When using social media data as a proxy for peoples’ perceptions, there is an inevitable bias towards aesthetic values 37 and, in the particular case of Instagram, a strong dominance of content related with social recreation. Ultimately, photographs tend to express pleasant and beautiful features 38 and Instagram is not an exception as in most case studies only positive feelings were reported (overly represented by the hashtag #happy and synonyms), with few exceptions where conservation awareness was identified in the social media discourse. Representativeness can also be a challenge 39 , 40 , and Instagram is mostly representing the younger generations 41 . Perceptions from people that do not post on Instagram, remarkedly from older generations, people with limited or no access to technology or people from countries where Instagram is not sufficiently dominant are not adequately represented in our approach. Nationality is also relevant, as local visitors’ perceptions can differ from international tourists’, represented by English as the vehicular language 15 . This can be partially solved by using words in different languages as queries, like for Easter Island (e.g., Rapanui, Isla de Pascua and Easter Island), or by including words in different languages in the network, like in the Norwegian reserve of Ytrehvaler. However, social media platforms are sometimes restricted in certain countries and it might imply an important bias in the nationality of tourists encompassed in the analysis.
Additional challenges are linked to the extraction of data from Instagram. Results showed that a manageable sample of posts can provide valuable information about peoples’ perceptions for a natural area. Nevertheless, a sufficient volume of posts might not be available for particular areas. In addition, the application of this methodology is restricted to those areas with a unique name to be used as a query in order to avoid downloading information from other areas which might have the same name. Since June 2020, access restrictions to Instagram data have incremented and our approach could not be currently replicated without incurring into web scrapping. Nevertheless, the authors support the use of the approach for scientific purposes and defend access to big data stored in social media after request. Despite these limitations, our study approach provides many advantages, including (1) cost and effort effectiveness in data collection and processing, (2) remote collection of information that allows large scale studies and (3) minimization of researchers’ subjectivity through the use of centrality metrics to explore the emerging properties of a network of words. Moreover, while the assessment of visitors’ perceptions in natural spaces is generally conducted during peak visitation season and restricted to frequently visited locations 10 , the remote collection of social media data can encompass any temporal dimension, and, in principle, it covers visitors to all locations within the natural areas. The variability in visitors’ preferences can assist managers and policy makers design tailored strategies to promote nature conservation for visitors’ enjoyment, which is of high relevance when destination sites are often ecologically or culturally fragile 23 , 42 . The continuous low cost-effective monitoring of social media can allow a better understanding of spatial–temporal changes in visitor preferences 21 , and this approach can now materialise with the prevalence of smartphones and the posting of experiences in social media facilitating the remote access to large scale information on peoples’ perceptions and use of natural spaces. In the internet era, with ever-increasing amounts of data stored in web-based platforms, access to this data with scientific purposes is crucial. We encourage the scientific community to claim access, while accepting restrictions to personal or sensitive data.
Conclusions
The emergent properties of networks of Instagram hashtags were explored with Graph Theory to characterise the array of interactions between nature and people using a cultural ecosystem services lens. The information obtained includes visitors’ preferences (e.g., cultural heritage, wildlife and nature appreciation), but also activities (e.g., diving, hiking, relaxing), preferred habitats or species (e.g., forest, beach, penguins), and feelings (e.g., happiness, beach lifestyle, place identity). Our approach allowed to identify places valued for their cultural heritage (e.g., Easter Island’s moais), but also for their iconic species (e.g., puffins in Skomer island) or natural monuments (e.g., sand dunes in Sandwich harbour), and sense of place and identity (e.g., Isole Egadi and Tawharanui). We introduce a tool that has allowed us to explore peoples’ perceptions from a wide diversity of marine and coastal areas globally. Importantly, this approach allows to identify and monitor the variable benefits people obtain from nature in a cost-effective but holistic way, essential for the effective conservation of natural species.
Case studies
Fourteen marine and coastal areas were chosen for the study in order to encompass a wide diversity of marine and coastal ecosystems across regions (Table 1 ). The selected areas had to comply with two criteria: (1) the area had to be sufficiently popular to contain enough data for the analysis; (2) the name of the area had to be sufficiently characteristic to provide a unique alphabetical identifier within Instagram, without the need to use filters or metadata allowing the geolocation of the photos. The adoption of these criteria meant that no area in the Arctic (marine realm 1) containing enough data could be identified in Instagram. Some of the realms established by Spalding et al. (2007) are too broad to capture existent variability across systems (e.g., temperate Northern Atlantic and Mediterranean Sea); when the authors considered this was the case, more than one study area were selected to capture this variability (Fig. 1 ).
Social media data
Data collection and analysis were carried out according to the methodology established in Ruiz-Frau et al. 27 . Instagram posts were downloaded through the Application Programming Interface (API), with a specific development for the R language and environment for statistical computing version 3.6.0, released 2019-04-26 (R Development Core Team, 2009). Data download was completed in June 2019; since June 2020, the Instagram API increased restrictions impeding access to the stored data through our R code. In consequence, the methodology described here cannot be directly replicated. A javascript procedure could be used to fetch data from a loop through the posts; however, this simple procedure could be considered webscrapping and may infringe the intellectual property rights. Nevertheless, the authors support the use of the approach for scientific purposes and defend access to big data stored in social media after request.
The Instagram API was suitable for a hashtag-based data extraction using a creator account. Post retrieval was done according to the platform internal algorithms. The authors of this study did not have complete access to the algorithms’ descriptions, and, while API search appears to be chronological, the company's public documentation does not provide specific information to confirm this. For privacy and ethical reasons, no personal information, such as user’s names or ids, were used in this study. Likewise, the metadata of the photographs or the geolocation information that some users add to their posts was never accessed. Results are presented in an aggregate manner so that no information can be traced back to the individual user (more details of the methods in Ruiz-Frau et al. 27 ).
For each case study, a search query was executed (Table 1 ). We aimed to download 10,000 posts per case study in June 2019. The data download started with the most recent post and was followed by the previous post until reaching the cut-off (i.e., 10,000). Frequently, the areas had fewer than the established threshold (i.e., 10,000 posts) and in such cases we downloaded all available posts, never bellow 1000 posts (Table 1 ). Downloaded posts for each case study were stored locally and datasets were filtered and cleaned in order to retain only relevant information for further analysis 43 , 44 . Posts often contain non-relevant information as social media platforms are frequently used as marketing and advertisement tools to reach a wider public and often bots (automated data generating algorithms and advertisements) are used to create large volumes of automated posts. Automatically generated posts were detected using graph based 45 , anomaly detection 46 , 47 , and time series analysis 48 , 49 approaches. Most of the time, the use of a graph-based approach was sufficient to eliminate almost all of the posts generated by bots, thus making the use of anomaly detection and time series analysis circumstantial. Additionally, irrelevant posts, mostly related to human-generated advertising (e.g., human-written posts related with a trading mark named Galapagos or Rapanui, unrelated with the natural capital of the areas), were detected by a simple visual inspection of a preliminary graph and discarded for further analysis. Discarded posts were selected by specific hashtags (e.g., #chocolate, frequently linked with #rapanui due to a trademark) or a specific user (i.e., those users identified as posting irrelevant marketing). The number of discarded posts never exceeded 25% of the total number of downloaded posts (e.g., 2500 discarded posts when 10,000 posts were downloaded). Dataset cleaning also consisted in merging similar words (e.g., #travelgram, #instatravel, #igtravel) and misspellings (e.g., #travel, #travell). Highly frequent non-English words were translated to English (e.g., #statue, #steinfigure, #estatua; for Easter Island statue in different languages) to homogenise the network language and avoid numerous duplicates. However, in some networks with a prevalence of non-English language (e.g., Ytrehvaler in Norwegian) words were not translated to English to capture users’ characteristics.
Graph Theory
In this study, we apply network graph visualization tools to the hashtags associated to Instagram posts. The hashtag is represented by a node, with the size of the node representing the relevance of the hashtag, and the relationship between each pair of nodes is identified with a link (edge). The width of the edge represents the strength of this connection. Centrality measures are useful to determine the relative importance of nodes and edges within the overall network 28 . In networks consisting of several nodes, some of them play a decisive role in facilitating a large number of network connections. Such nodes are central in network organization and are often identified by a range of metrics known as centrality measures. The identification of important, or central, vertices in a network is a key aspect in the definition and description of networks 50 . However, there are multiple interpretations of what makes a node important and there are therefore many measures of centrality 28 . We calculated different centrality measures and, after a first screening including 11 measured of centrality for the Instagram hashtags’ network (e.g., Degree, In-Degree, Out-Degree, Strength, HubScore; see Ospina-Alvarez et al. 51 for a detailed description of the different measures), we selected betweenness and eigenvector centrality to illustrate and interpret the structure of the social networks.
Eigenvector centrality measure 52 takes into consideration not only how many connections a node has, but also the centrality of the vertices that it is connected to. Eigenvector centrality ranks higher those vertices that are connected to important neighbours, i.e., other vertices that are connected to many other well-connected vertices. It is a measure of the influence of a node in a network. In our study context, hashtags with high eigenvector values are high frequency hashtags that in turn are connected to other high frequency hashtags, and so on.
Betweenness centrality 28 is a measure of the influence of a node over the flow of information between every pair of vertices under the assumption that information primarily flows over the shortest paths between them. Betweenness centrality indicates nodes that have a high probability of having routes that connect them to other nodes in the network. Similarly, edge betweenness centrality is defined as the number of the shortest paths that go through an edge in a graph or network 53 . Each edge in the network can be associated with an edge betweenness centrality value. An edge with a high edge betweenness centrality score represents a bridge-like connector between two parts of a network, the removal of which may affect the communication between many pairs of nodes through the shortest paths between them. In our context, betweenness centrality and edge betweenness centrality provide information about clusters of hashtags that describe users' experiences or perceptions and that connect (by means of a hashtag) to other clusters representing other types of experiences or perceptions. The removal of high Betweenness hashtags would fragment the network and disconnect distant concepts.
Data analysis
The first 150 hashtags (frequency > 1.5% when 10,000 posts are retrieved) had a probability of more than 90% of occurring with any other of the first 150 hashtags in the same post. Therefore, this criterion was used to create networks with great cohesion and connectedness, representing the dominant discourse in relation to the area in question. Network graphs were delineated using eigenvector, betweenness and edge betweenness as centrality measures.
In order to find any evidence for an emergent pattern of clustering among the hashtags for each of the 14 case studies, hashtags were assigned to network communities through the use of Fast-Greedy community algorithm 27 . Fast-Greedy algorithm makes the best choice at each small step in the hope that each of these small steps will lead to a globally optimal solution 54 .
In order to visualise potential similarities in the social media discourse across the 14 case studies, all the data was merged, and the 1400 most frequent hashtags pairs were retained for analysis in a single network graph. Similar to what was previously described for the individual networks, these 1400 pairs of hashtags accounted for over 90% of the linkages between hashtags, representing the dominant discourse across the 14 areas. Eigenvector centrality was used as the measure of node influence in the merged network and connections were represented with a backbone layout 55 . This layout has proven effective to illustrate networks with most vertices in a central position that result in high overlap in large networks 56 .
We used the open source graphics manipulation software igraph 57 to obtain the centrality measures and communities aggregations. Graphics and figures were generated using the visualization software ggraph 58 and ggtree 59 . All of the above software can be used as extension packages of the R language and environment for statistical computing (R Development Core Team, 2009) freely available online.
Data availability
All the network figures generated during the current study are available in the Figshare repository, https://doi.org/10.6084/m9.figshare.13325627.v2 .
Rodrigues, J. G. et al. Marine and coastal cultural ecosystem services: Knowledge gaps and research priorities. One Ecosyst. 2 , e12290 (2017).
Article Google Scholar
Chen, W. et al. Ecosystem accounting’s potential to support coastal and marine governance. Mar. Pollut. 112 , 103758 (2020).
Kirchhoff, T. Pivotal cultural values of nature cannot be integrated into the ecosystem services framework. Proc. Natl. Acad. Sci. U.S.A. 109 , E3146–E3146 (2012).
Article ADS CAS PubMed PubMed Central Google Scholar
Daniel, T. C. et al. Contributions of cultural services to the ecosystem services agenda. Proc. Natl. Acad. Sci. U.S.A. 109 , 8812–8819 (2012).
Milcu, A. I., Hanspach, J., Abson, D. & Fischer, J. Cultural Ecosystem Services: A literature review and prospects for future research. Ecol. Soc. 18 , 44 (2013).
Teoh, S. H. S., Symes, W. S., Sun, H., Pienkowski, T. & Carrasco, L. R. A global meta-analysis of the economic values of provisioning and cultural ecosystem services. Sci. Total Environ. 649 , 1293–1298 (2019).
Article ADS CAS PubMed Google Scholar
Haines-Young, R. & Potschin, M. The links between biodiversity, ecosystem services and human well-being. In Ecosystem Ecology: A New Synthesis (eds Raffaelli, D. & Frid, C. L.) 110–139 (BES Ecological Reviews Series, 2010).
Chapter Google Scholar
Chan, K. M. A., Satterfield, T. & Goldstein, J. Rethinking ecosystem services to better address and navigate cultural values. Ecol. Econ. 74 , 8–18 (2012).
Arkema, K. K. et al. Embedding ecosystem services in coastal planning leads to better outcomes for people and nature. Proc. Natl. Acad. Sci. U.S.A. 112 , 7390–7395 (2015).
Gosal, A. S., Geijzendorffer, I. R., Václavík, T., Poulin, B. & Ziv, G. Using social media, machine learning and natural language processing to map multiple recreational beneficiaries. Eco. Serv. 38 , 100958 (2019).
Klain, S. C., Satterfield, T. A. & Chan, K. M. A. What matters and why? Ecosystem services and their bundled qualities. Ecol. Econ. 107 , 310–320 (2014).
Oteros-Rozas, E. et al. Socio-cultural valuation of ecosystem services in a transhumance social-ecological network. Reg. Environ. Change 14 , 1269–1289 (2014).
Plieninger, T., Dijks, S., Oteros-Rozas, E. & Bieling, C. Assessing, mapping, and quantifying cultural ecosystem services at community level. Land Use Policy 33 , 118–129 (2013).
Ruiz-Frau, A., Edwards-Jones, G. & Kaiser, M. J. Mapping stakeholder values for coastal zone management. Mar. Ecol. Prog. Ser. 434 , 239–249 (2011).
Article ADS Google Scholar
Clemente, P. et al. Combining social media photographs and species distribution models to map cultural ecosystem services: The case of a Natural Park in Portugal. Ecol. Ind. 96 , 59–68 (2019).
Leslie, H. M. & McLeod, K. L. Confronting the challenges of implementing marine ecosystem-based management. Front. Ecol. Environ. 5 , 540–548 (2007).
Costanza, R. et al. Changes in the global value of ecosystem services. Glob. Environ. Change 26 , 152–158 (2014).
Figueroa-Alfaro, R. W. & Tang, Z. Evaluating the aesthetic value of cultural ecosystem services by mapping geo-tagged photographs from social media data on Panoramio and Flickr. J. Environ. Plann. Manag. 60 , 266–281 (2016).
Hale, R. L., Cook, E. M. & Beltrán, B. J. Cultural ecosystem services provided by rivers across diverse social-ecological landscapes: A social media analysis. Ecol. Ind. 107 , 105580 (2019).
Oteros-Rozas, E., Martín-López, B., Fagerholm, N., Bieling, C. & Plieninger, T. Using social media photos to explore the relation between cultural ecosystem services and landscape features across five European sites. Ecol. Ind. 94 , 74–86 (2018).
Hausmann, A. et al. Social media data can be used to understand tourists’ preferences for nature-based experiences in protected areas. Conserv. Lett. 11 , e12343 (2018).
Geboers, M. A. & Van De Wiele, C. T. Machine vision and social media images: Why hashtags matter. Soc. Media Soc. 6 , 205630512092848 (2020).
Ghermandi, A., Camacho-Valdez, V. & Trejo-Espinosa, H. Social media-based analysis of cultural ecosystem services and heritage tourism in a coastal region of Mexico. Tour. Manag. 77 , 104002 (2020).
Ladle, R. J. et al. Conservation culturomics. Front. Ecol. Environ. 14 , 269–275 (2016).
Toivonen, T. et al. Social media data for conservation science—A methodological overview. Biol. Conserv. 233 , 298–315 (2019).
Hausmann, A. et al. Understanding sentiment of national park visitors from social media data. People Nat. 2 , 750–760 (2020).
Ruiz-Frau, A. et al. Using graph theory and social media data to assess cultural ecosystem services in coastal areas: Method development and application. Ecol. Serv. 45 , 101176 (2020).
Freeman, L. C. Centrality in social networks conceptual clarification. Soc. Netw. 1 , 215–239 (1979).
Topirceanu, A., Udrescu, M. & Marculescu, R. Weighted betweenness preferential attachment: A new mechanism explaining social network formation and evolution. Sci. Rep. 8 , 1–14 (2018).
Article CAS Google Scholar
Roth, C. & Cointet, J.-P. Social and semantic coevolution in knowledge networks. Soc. Netw. 32 , 16–29 (2010).
Maiya, A. S. & Berger-Wolf, T. Y. Online sampling of high centrality individuals in social networks. In Advances in Knowledge Discovery and Data Mining , Vol. 6118, 91–98 (Springer, 2010).
Spalding, M. D. et al. Marine ecoregions of the world: A bioregionalization of coastal and shelf areas. Bioscience 57 , 573–583 (2007).
Russell, R. et al. Humans and nature: How knowing and experiencing nature affect well-being. Annu. Rev. Environ. Resour. 38 , 473–502 (2013).
Pascual, U. et al. Valuing nature’s contributions to people: The IPBES approach. Curr. Opin. Sustain. 26–27 , 7–16 (2017).
Díaz, S. et al. Assessing nature’s contributions to people. Science 359 , 270–272 (2018).
Article ADS PubMed Google Scholar
Hughes, T. P. et al. Global warming transforms coral reef assemblages. Nature 556 , 492–496 (2018).
Calcagni, F., Maia, A. T. A., Connolly, J. J. T. & Langemeyer, J. Digital co-construction of relational values: Understanding the role of social media for sustainability. Sustain. Sci. 14 , 1309–1321 (2019).
Yoshimura, N. & Hiura, T. Demand and supply of cultural ecosystem services: Use of geotagged photos to map the aesthetic value of landscapes in Hokkaido. Ecol. Serv. 24 , 68–78 (2017).
Tenerelli, P., Demšar, U. & Luque, S. Crowdsourcing indicators for cultural ecosystem services: A geographically weighted approach for mountain landscapes. Ecol. Ind. 64 , 237–248 (2016).
Guerrero, P., Møller, M. S., Olafsson, A. S. & Snizek, B. Revealing cultural ecosystem services through Instagram images: The potential of social media volunteered geographic information for urban green infrastructure planning and governance. Urban Plann. 1 , 1–17 (2016).
Abbott, W., Donaghey, J., Hare, J. & Hopkins, P. An Instagram is worth a thousand words: An industry panel and audience Q&A. Lib. Hi Tech News 30 , 1–6 (2013).
Balmford, A., Gravestock, P., Hockley, N., McClean, C. J. & Roberts, C. M. The worldwide costs of marine protected areas. Proc. Natl. Acad. Sci. U.S.A. 101 , 9694–9697 (2004).
Di Minin, E., Fink, C., Tenkanen, H. & Hiippala, T. Machine learning for tracking illegal wildlife trade on social media. Nat. Ecol. Evol. 2 , 406–407 (2018).
Article PubMed Google Scholar
Varol, O., Ferrara, E., Davis, C. A., Menczer, F. & Flammini, A. Online human–bot interactions: Detection, estimation, and characterization. Eleventh International AAAI Conference on Web and Social Media (2017).
Orabi, M., Mouheb, D., Al Aghbari, Z. & Kamel, I. Detection of bots in social media: A systematic review. Inf. Process. Manag. 57 , 102250 (2020).
Minnich, A., Chavoshi, N., Koutra, D. & Mueen, A. BotWalk: Efficient adaptive exploration of Twitter bot networks. In Proceedings of the 2017 IEEE/ACM International Conference on Advances in Social Networks Analysis and Mining , pp. 467–474 (2017).
Echeverri, J. et al. LOBO—Evaluation of generalization deficiencies in Twitter Bot classifiers. In Proceedings of the 34th Annual Computer Security Applications Conference , pp. 137–146 (2018).
Chavoshi, N., Hamooni, H. & Mueen, A. DeBot: Twitter bot detection via warped correlation. ICDM 817–822 (2016). https://doi.org/10.1109/ICDM.2016.86
Stukal, D., Sanovich, S., Bonneau, R. & Tucker, J. A. Detecting bots on Russian political Twitter. Big Data 5 , 310–324 (2017).
Bodin, Ö., Crona, B. & Ernstson, H. Social networks in natural resource management: What is there to learn from a structural perspective?. Ecol. Soc. 11 , resp2 (2006).
Ospina-Alvarez, A. et al. MPA network design based on graph theory and emergent properties of larval dispersal. Mar. Ecol. Prog. Ser. 650 , 309–326 (2020).
Bonacich, P. Some unique properties of eigenvector centrality. Soc. Netw. 29 , 555–564 (2007).
Girvan, M. & Newman, M. E. J. Community structure in social and biological networks. Proc. Natl. Acad. Sci. U.S.A. 99 , 7821–7826 (2002).
Article ADS MathSciNet CAS PubMed PubMed Central MATH Google Scholar
Newman, M. E. J. Fast algorithm for detecting community structure in networks. Phys. Rev. E 69 , 066133 (2004).
Article ADS CAS Google Scholar
Brandes, U. & Wagner, D. Analysis and visualization of social networks. In Graph drawing software , Vol. 286, 321–340 (Springer, 2004).
Nocaj, A., Ortmann, M. & Brandes, U. Untangling the hairballs of multi-centered, small-world online social media networks. JGAA 19 , 595–618 (2015).
Article MathSciNet MATH Google Scholar
Csardi, G. & Nepusz, T. The igraph software package for complex network research. InterJournal Complex Syst. 1695 (5), 1–9 (2006).
Google Scholar
Pedersen, T. L. ggraph: An Implementation of Grammar of Graphics for Graphs and Networks. R package version 2.0.4. https://CRAN.R-project.org/package=ggraph .
Guangchuang, Y. Using ggtree to visualize data on tree-like structures. Curr. Protoc. Bioinform. 69 , e96 (2020).
Download references
Acknowledgements
This work is a product of ECOMAR research network (Evaluation and monitoring of marine ecosystem services in Iberoamerica; project number 417RT0528, funded by CYTED). Three co-authors were funded by H2020-Marie Skłodowska-Curie Action during the conduction of this work: SdJ, funded by MSCA-IF-2016 (ref. 743545); AOA, funded by MSCA-IF-2016 (ref. 746361); ARF, funded by MSCA-IF-2014 (ref. 655475).
Author information
Authors and affiliations.
Department of Renewable Marine Resources, Institute of Marine Science (ICM-CSIC), Passeig Marítim de la Barceloneta, n° 37-49, 08003, Barcelona, Spain
- Silvia de Juan
Department of Marine Ecosystem Dynamics, IMEDEA (CSIC-UIB), Miquel Marqués 21, 07190, Esporles, Spain
Andrés Ospina-Álvarez & Ana Ruiz-Frau
Faculty of Economics and Business Administration, University of Santiago de Compostela, Av. Burgo das Nacións s/n, 15782, Santiago de Compostela, A Coruña, Spain
- Sebastián Villasante
Campus Do Mar, International Campus of Excellence, Campus Universitario, 36310, Vigo, Spain
You can also search for this author in PubMed Google Scholar
Contributions
Sd.J., S.V. and A.R. contributed to the conception of the work; A.O. designed the analysis and wrote the R scripts; Sd.J., A.O., S.V. and A.R. interpreted the graphs; Sd.J., A.O. and A.R. produced the figures. Sd.J., A.O. and A.R. wrote the main manuscript text; all authors reviewed the manuscript.
Corresponding author
Correspondence to Silvia de Juan .
Ethics declarations
Competing interests.
The authors declare no competing interests.
Additional information
Publisher's note.
Springer Nature remains neutral with regard to jurisdictional claims in published maps and institutional affiliations.
Rights and permissions
Open Access This article is licensed under a Creative Commons Attribution 4.0 International License, which permits use, sharing, adaptation, distribution and reproduction in any medium or format, as long as you give appropriate credit to the original author(s) and the source, provide a link to the Creative Commons licence, and indicate if changes were made. The images or other third party material in this article are included in the article's Creative Commons licence, unless indicated otherwise in a credit line to the material. If material is not included in the article's Creative Commons licence and your intended use is not permitted by statutory regulation or exceeds the permitted use, you will need to obtain permission directly from the copyright holder. To view a copy of this licence, visit http://creativecommons.org/licenses/by/4.0/ .
Reprints and permissions
About this article
Cite this article.
de Juan, S., Ospina-Álvarez, A., Villasante, S. et al. A Graph Theory approach to assess nature’s contribution to people at a global scale. Sci Rep 11 , 9118 (2021). https://doi.org/10.1038/s41598-021-88745-z
Download citation
Received : 30 November 2020
Accepted : 07 April 2021
Published : 27 April 2021
DOI : https://doi.org/10.1038/s41598-021-88745-z
Share this article
Anyone you share the following link with will be able to read this content:
Sorry, a shareable link is not currently available for this article.
Provided by the Springer Nature SharedIt content-sharing initiative
This article is cited by
Identifying informative features to evaluate student knowledge as causal maps.
- Philippe J. Giabbanelli
International Journal of Artificial Intelligence in Education (2023)
A network analysis of global cephalopod trade
- Andres Ospina-Alvarez
Scientific Reports (2022)
Assessing landscape features and ecosystem services of marine protected areas through photographs on social media: comparison of two archipelagos in Spain
- I. Maya-Jariego
Environment, Development and Sustainability (2022)
By submitting a comment you agree to abide by our Terms and Community Guidelines . If you find something abusive or that does not comply with our terms or guidelines please flag it as inappropriate.
Quick links
- Explore articles by subject
- Guide to authors
- Editorial policies
Sign up for the Nature Briefing newsletter — what matters in science, free to your inbox daily.

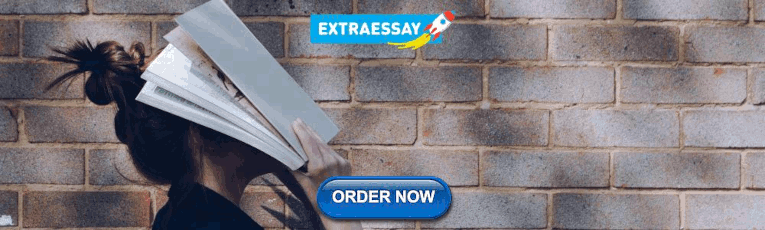
COMMENTS
The Journal of Graph Theory is a high-calibre graphs and combinatorics journal publishing rigorous research on how these areas interact with other mathematical sciences. Our editorial team of influential graph theorists welcome submissions on a range of graph theory topics, such as structural results about graphs, graph algorithms with theoretical emphasis, and discrete optimization on graphs.
This paper gives an overview of the applications of graph theory in heterogeneous fields to some extent but mainly focuses on the computer science applications that uses graph theoretical concepts.
In this paper we will discuss how problems like Page ranking and finding the shortest paths can be solved by using Graph Theory. At its core, graph theory is the study of graphs as mathematical structures. In our paper, we will first cover Graph Theory as a broad topic. Then we will move on to Linear Algebra. Linear Algebra is the study of ...
An introduction to graph theory (Text for Math 530 in Spring 2022 at Drexel University) Darij Grinberg* Spring 2023 edition, August 2, 2023 Abstract. This is a graduate-level introduction to graph theory, corresponding to a quarter-long course. It covers simple graphs, multigraphs as well as their directed analogues, and more restrictive
Her research lies at the intersection of combinatorics and geometry. She has published papers in matroid theory, graph theory, and combinatorial algorithms and her work is supported by theNational Science Foundation. Her book "Graphs and Networks" blends classical graph theory with modern network science and is available for preorder.
AIMS AND SCOPE. The Journal of Graph Theory is devoted to a variety of topics in graph theory, such as structural results about graphs, graph algorithms with theoretical emphasis, and discrete optimization on graphs. The scope of the journal also includes related areas in combinatorics and the interaction of graph theory with other mathematical ...
In this thesis, we exploit this symbiotic relationship between graph theory and algorithms for graph optimization problems and beyond. This thesis consists of three parts. In the first part, we study a graph routing problem called the Node-Disjoint Paths (NDP) problem. Given a graph and a set of source-destination pairs of its vertices, the ...
Applications of Algebraic Graph Theory and Its Related Topics. Special Issue Editors. Special Issue Information. Published Papers. A special issue of Mathematics (ISSN 2227-7390). This special issue belongs to the section "Mathematics and Computer Science". Deadline for manuscript submissions: closed (30 April 2024) | Viewed by 9677.
In this chapter we shall discuss some popular research areas in Graph Theory. In each of the chosen topic, we introduce the problem, give some easily stated theorem, important variation, direction of research and provide reference to survey articles. Download to read the full chapter text.
In this Special Issue, we welcome papers in graph theory with applications to biology, computer science, complex and social networks, engineering, and other areas. While papers with real-world applications are especially encouraged, papers with a theoretical focus but with potential applications will also be considered. Prof. Dr. Darren Narayan.
We propose a new type of supervised visual machine learning classifier, GSNAc, based on graph theory and social network analysis techniques. In a previous study, we employed social network ...
Graph theory (GT) concepts are potentially applicable in the field of computer science (CS) for many purposes. The unique applications of GT in the CS field such as clustering of web documents, cryptography, and analyzing an algorithm's execution, among others, are promising applications. Furthermore, GT concepts can be employed to electronic circuit simplifications and analysis.
in exploring new areas of graph theory and its applications. Ad-vanced students in graph theory may use the topics presented in this book to develop their nal-year projects, master's theses or doctoral dissertations. It is the author's hope that this publication of original re-search ideas, problems and conjectures will instigate further re-xi
Explore the latest full-text research PDFs, articles, conference papers, preprints and more on ALGEBRAIC GRAPH THEORY. Find methods information, sources, references or conduct a literature review ...
1.1. The Definition of a Graph: The graph is a se t of points in a plane or in a space and a set of. line segment of curve each of which either joins two points or join to. itself. A graph G = (V ...
Chemical graph theory applies this branch of mathematics to model molecules in order to study their various physical properties. A graph G = (V, E) consists of a set V of vertices (or nodes) and a set E of unordered pairs of distinct elements of V, which are the edges.In chemistry, the atoms of a molecule are represented by the vertices and the chemical bonds are represented by the edges.
It is observed that graph theory has been substantially employed in the areas of computing. The primary goal of this Special Issue is to gather the articles utilizing graph-theoretical concepts, methodologies, and advantages while solving problems to demonstrate the state-of-the-art applications of graph theory.
The use of Graph Theory on social media data is a promising approach to identify emergent properties of the complex physical and cognitive interactions that occur between humans and nature. To ...
Recently, graph neural networks (GNNs) have become a hot topic in machine learning community. This paper presents a Scopus-based bibliometric overview of the GNNs' research since 2004 when GNN papers were first published. The study aims to evaluate GNN research trends, both quantitatively and qualitatively.
IJRTI2101001 International Journal for Research Trends and Innovation (www.ijrti.org) 1 A Literature Review on Applications of Graph Theory in Various Fields 1Rina D. More, 2 ... Nashik, India. Abstract: Graph theory is widely used to prove many mathematical theorems and models.This paper present the various applications and techniques of graph ...
The Journal of Graph Theory is a high-calibre graphs and combinatorics journal publishing rigorous research on how these areas interact with other mathematical sciences. Our editorial team of influential graph theorists welcome submissions on a range of graph theory topics, such as structural results about graphs, graph algorithms with theoretical emphasis, and discrete optimization on graphs.
In order to do that, the IS group helps organizations to: (i) understand the business needs and value propositions and accordingly design the required business and information system architecture; (ii) design, implement, and improve the operational processes and supporting (information) systems that address the business need, and (iii) use advanced data analytics methods and techniques to ...
In mathematics computer science, chemistry graph theory is the study of graphs, which are. mathematical structures used to model pair wise relations between objects. A "g raph" in this context is ...