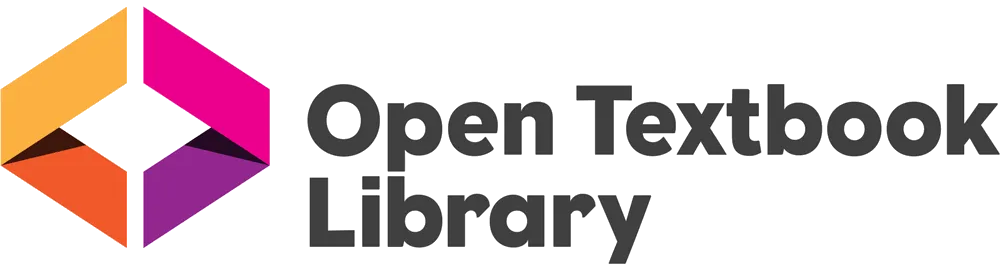
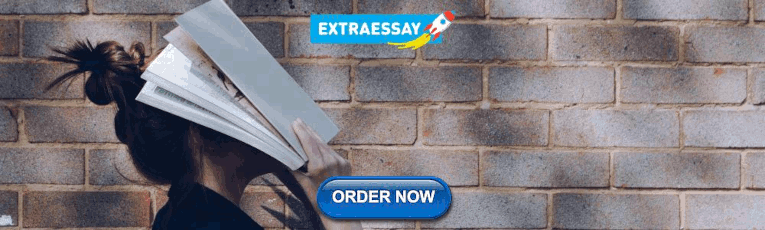
Qualitative Research – a practical guide for health and social care researchers and practitioners
(0 reviews)
Darshini Ayton, Monash University
Tess Tsindos, Monash University
Danielle Berkovic, Monash University
Copyright Year: 2023
Last Update: 2024
ISBN 13: 9780645755404
Publisher: Monash University
Language: English
Formats Available
Conditions of use.

Table of Contents
- Acknowledgement of Country
- About the authors
- Accessibility statement
- Introduction to research
- Research design
- Data collection
- Data analysis
- Writing qualitative research
- Peer review statement
- Licensing and attribution information
- Version history
Ancillary Material
About the book.
This guide is designed to support health and social care researchers and practitioners to integrate qualitative research into the evidence base of health and social care research. Qualitative research designs are diverse and each design has a different focus that will inform the approach undertaken and the results that are generated. The aim is to move beyond the “what” of qualitative research to the “how”, by (1) outlining key qualitative research designs for health and social care research – descriptive, phenomenology, action research, case study, ethnography, and grounded theory; (2) a decision tool of how to select the appropriate design based on a guiding prompting question, the research question and available resources, time and expertise; (3) an overview of mixed methods research and qualitative research in evaluation studies; (4) a practical guide to data collection and analysis; (5) providing examples of qualitative research to illustrate the scope and opportunities; and (6) tips on communicating qualitative research.
About the Contributors
Associate Professor Darshini Ayton is the Deputy Head of the Health and Social Care Unit at Monash University in Melbourne, Australia. She is a transdisciplinary implementation researcher with a focus on improving health and social care for older Australians and operates at the nexus of implementation science, health and social care policies, public health and consumer engagement. She has led qualitative research studies in hospitals, aged care, not-for-profit organisations and for government and utilises a range of data collection methods. Associate Professor Ayton established and is the director of the highly successful Qualitative Research Methods for Public Health short course which has been running since 2014.
Dr Tess Tsindos is a Research Fellow with the Health and Social Care Unit at Monash University in Melbourne, Australia. She is a public health researcher and lecturer with strong qualitative and mixed methods research experience conducting research studies in hospital and community health settings, not-for-profit organisations and for government. Prior to working in academia, Dr Tsindos worked in community care for government and not-for-profit organisations for more than 25 years. Dr Tsindos has a strong evaluation background having conducted numerous evaluations for a range of health and social care organisations. Based on this experience she coordinated the Bachelor of Health Science/Public Health Evaluation unit and the Master of Public Health Evaluation unit and developed the Evaluating Public Health Programs short course in 2022. Dr Tsindos is the Unit Coordinator of the Master of Public Health Qualitative Research Methods Unit which was established in 2022.
Dr Danielle Berkovic is a Research Fellow in the School of Public Health and Preventive Medicine at Monash University in Melbourne, Australia. She is a public health and consumer-led researcher with strong qualitative and mixed-methods research experience focused on improving health services and clinical guidelines for people with arthritis and other musculoskeletal conditions. She has conducted qualitative research studies in hospitals and community health settings. Dr Berkovic currently provides qualitative input into Australia’s first Living Guideline for the pharmacological management of inflammatory arthritis. Dr Berkovic is passionate about incorporating qualitative research methods into traditionally clinical and quantitative spaces and enjoys teaching clinicians and up-and-coming researchers about the benefits of qualitative research.
Contribute to this Page
- Open access
- Published: 13 March 2022
The role of analytic direction in qualitative research
- Joanna E. M. Sale 1 , 2 , 3
BMC Medical Research Methodology volume 22 , Article number: 66 ( 2022 ) Cite this article
5104 Accesses
5 Citations
3 Altmetric
Metrics details
The literature on qualitative data analysis mostly concerns analyses pertaining to an individual research question and the organization of data within that research question. Few authors have written about the entire qualitative dataset from which multiple and separate analyses could be conducted and reported. The concept of analytic direction is a strategy that can assist qualitative researchers in deciding which findings to highlight within a dataset. The objectives of this paper were to: 1) describe the importance of analytic direction in qualitative research, and 2) provide a working example of the concept of analytic direction.
A qualitative dataset from one of the author’s research programs was selected for review. Ten potential analytic directions were identified after the initial phenomenological analysis was conducted. Three analytic directions based on the same coding template but different content areas of the data were further developed using phenomenological analysis ( n = 2) and qualitative description ( n = 1) and are the focus of this paper. Development and selection of these three analytic directions was determined partially relying on methodological criteria to promote rigour including a comprehensive examination of the data, the use of multiple analysts, direct quotations to support claims, negative case analysis, and reflexivity.
The three analytic directions addressed topics within the scope of the overall research question. Each analytic direction had its own central point or story line and each highlighted a different perspective or voice. The use of an inductive and deductive approach to analysis and how the role of theory was integrated varied in each analytic direction.
Conclusions
The concept of analytic direction enables researchers to organize their qualitative datasets in order to tell different and unique “stories”. The concept relies upon, and promotes, the conduct of rigourous qualitative research.
Peer Review reports
Reports on data analysis in qualitative research are well documented. Procedural steps have been described [ 1 , 2 , 3 , 4 , 5 , 6 , 7 ] and authors have made distinctions between the concepts of coding, analysis, and interpretation [ 1 , 2 , 8 , 9 ]. Authors have written about different researchers accessing different representations of a topic or phenomenon [ 2 , 10 ] or multiple interpretations being applied to the same transcript [ 11 ]. The literature on data analysis mostly concerns analyses pertaining to an individual research question and the organization of data within that research question. Few authors have written about the entire qualitative dataset from which multiple and separate analyses could be conducted and reported.
The data collected by qualitative researchers can be voluminous and often surpass the data pertaining to objectives outlined in grant proposals. These data may be compelling but analyses of some data are often given lower priority if they do not align directly with the stated objectives.
There comes a point during data collection and analysis where qualitative researchers must choose “which story, of the many stories available to them in a data set, to tell” (p. 376) [ 12 ]. According to Arthur Frank, “[a] fter the methods, there has to be a story” (p. 431) [ 13 ]. “Stories” should have a central point or storyline [ 12 ]. The final report can be told from the perspective of different voices [ 12 ] and organized by time such as emphasizing key turning points and milestones in the sequence of events studied [ 12 , 14 ] or by using other forms of representation such as metaphors [ 2 , 12 ]. Theory can be central or more peripheral in the account [ 15 ]. The question remains, what “story”, or “stories”, do we tell?
The concept of analytic direction
The concept of analytic direction is a strategy that can assist qualitative researchers in deciding which “stories” to highlight within a dataset. Sandelowski reports that researchers account for their data and then determine the different “paths” [ 1 ] or “analytic paths” [ 16 ] they can pursue. Others have proposed that decision-making throughout analysis implies analytic ideas at every stage of the coding process [ 8 ] and that researchers define for themselves what analytic issues are to be explored and what ideas are important [ 8 ]. Charmaz [ 17 ] reports that grounded theory researchers pursue more than one analytic direction by focusing on certain ideas first and then returning to the data to address an unfinished analysis in another area later. While the concept of analytic direction has been referenced, or alluded to, by these and other authors [ 1 , 8 , 16 , 18 , 19 ], operationalization of this concept is not well articulated. In this paper, the term analytic direction refers to a message developed by the researchers about the data that may or may not require further substantiation. An analytic direction can be presented as a single message or theme, and can stand alone or be supported by multiple sub-messages or sub-themes. Analytic directions can be developed during the coding process, in later stages of analysis, or possibly during analyses of new datasets. Relying on strategies to promote rigour can assist with the development, substantiation, and selection of analytic directions. If substantiated, each analytic direction could be the focus of an individual publication. The objectives of this paper were to: 1) describe the importance of analytic direction in qualitative research; and 2) provide a working example of the concept of analytic direction.
Why analytic direction is important
The concept of analytic direction is important because it has implications for methodological rigour. We have an obligation to conduct methodological rigourous studies [ 20 ], especially when studies require primary data collection that involves a burden to participants [ 21 ]. The author proposes that methodological rigour is embedded within, and contributes to, the concept of analytic direction. Several strategies to promote rigour that are universal to many qualitative approaches, including phenomenology, are discussed. These strategies include, but are not limited to, a comprehensive examination of the data, the use of multiple analysts, direct quotations to support claims, negative case analysis, and reflexivity. It is important to support the quality of analytic directions so that researchers can then determine which analytic directions may or may not require further substantiation. The quality of the analytic direction will also assist in determining which directions may be selected for reporting.
The relationship between analytic direction and methodological rigour
This paper focuses on the stage where data collection is considered to be complete and does not directly address how data collection, and methodological rigour related to data collection, contributes to the concept of analytic direction. The assumption is that data collection and analysis were conducted iteratively [ 22 , 23 ] and that the team decided when data collection was complete, perhaps relying upon one of the various conceptualizations of saturation discussed by Saunders and colleagues [ 24 ]. A decision about saturation would not necessarily apply to any, or all, analytic directions being developed.
The author proposes that several strategies for promoting rigour assist with the development and selection of analytic directions. One aspect of methodological rigour is that authors carry out a comprehensive examination of their data [ 5 , 25 ]. By thinking about, and engaging in, analytic direction, researchers are encouraged to attend to all of their data rather than attending only to data that interests them initially.
The use of multiple analysts promotes a comprehensive examination of the data [ 2 , 26 ] and thus, contributes to the concept of analytic direction. Different viewpoints lead to an enrichment of the analysis and can lead to a conceptual clarification of the interpretations [ 2 ]. Multiple viewpoints can be used at the level of coding but also at the level of the larger team as data collection and analysis proceeds. Discussions about the novelty, clinical significance, and relevance [ 27 ] of the analytic directions may occur at this time and continue through to the writing of the respective manuscripts. Analytic directions are relevant if they add knowledge, or increase the confidence with which existing knowledge is regarded [ 28 ]. According to Malterud [ 26 ], engaging multiple researchers in a qualitative study strengthens the design of the study, not for the purpose of consensus or identical readings of the data but to supplement and contest each others’ statements.
The use of direct quotations to support the claims made about the analytic directions (and/or themes within) is another strategy to promote rigour [ 29 ]. Not only do quotations illustrate and clarify the results but they also demonstrate whether there is substantive evidence to support the analytic directions being proposed. In contrast, data that do not support the analytic directions (and/or themes within) should be accounted for and their exclusion justified when promoting methodological rigour [ 30 ]. Authors may refer to this as attending to negative cases [ 28 ] or deviant case analysis [ 25 , 31 ]. This strategy promotes that “deviant cases” or “outliers” are not forced into categories or ignored but used instead to aid understanding or theory development [ 25 ]. For example, these cases may explain why the patterns developed from the data or the more normative behaviours are not always found in the researchers’ interpretations [ 25 , 31 ].
Reflexivity is an essential component of methodological rigour [ 26 ]. Reflexivity has been described as “an attitude of attending systematically to the context of knowledge construction, especially to the effect of the researcher, at every step of the research process” [ 26 ] (p. 484). Being reflexive means being aware of your own position in producing partial knowledge [ 32 ]. The qualitative researcher acknowledges his or her personal influence on what that partial knowledge is (for example, the data collected are dependent on the interviewer’s questions and prompts). According to Eakin and Gladstone [ 33 ], knowing one’s standpoint helps one to recognize the forces that might drive certain interpretations and stifle other conceptualizations of the data. Knowledge production is also partial because it is not possible to report all interpretations of the data and therefore, the research team has to decide what to report. Researchers engaging in the concept of analytic direction are more likely to be reflexive about what they are, and are not, reporting from their datasets.
Rationale for the chosen example
The dataset chosen for this example was from a study where the author and her team identified 10 potential analytic directions based on a compilation of the memos and team discussions pertaining to analysis and interpretation of the data. The publications developed from this dataset reflected the selection of three analytic directions that focused on different content areas [ 34 , 35 , 36 ]. The same coding template was the foundation for the three publications and the timing of the reporting was ordered based on the author’s interests. The author chose the dataset as an example primarily because it was not heavily theory-laden and therefore accessible to novice qualitative researchers. The resulting publications have practical implications for clinical and health services research and the process of developing these publications could inform graduate students who are embarking on a qualitative program of research for their thesis work.
Original research funded
The goal of the original research project was to reduce the burden of illness due to fracture through improved bone health investigation and treatment. Specifically, the aim was to examine what researchers could learn from members of a patient group. The study was approved by the Research Ethics Board at Unity Health Toronto (REB# 10–371). The study team consisted of scientists, clinicians, a policy maker, and a patient representative with expertise related to bone health. Informed by the Theory of Planned Behaviour [ 37 , 38 ], the team set out to examine members of a patient group to ask them about their intentions and actions toward bone health diagnosis and treatment and their experiences with diagnostic tests and treatment recommendations. All individuals ( n = 28) were 50+ years old and had sustained a fragility fracture. The overall project relied on a phenomenological approach conceptualized by Giorgi and Wertz [ 30 , 39 , 40 , 41 ].
We developed a master coding template of 27 broad codes that were designed to organize the data with minimal reliance on theory. The coding template was revised four times as data collection and analysis proceeded. The codes were developed from a combination of inductive and deductive codes. More specifically, inductive codes were developed from topics discussed in the interviews. Other codes were pre-specified from the overall aim of the original funded study and from the domains of the Theory of Planned Behaviour.
Development of analytic directions from the dataset
Qualitative researchers can use several strategies to develop analytic directions. The author started the organization process early in order to think about how best to maximize the data collected. Coding began after the first couple of interviews had been conducted; this is conventional advice for analysis in qualitative research [ 1 , 2 , 23 , 42 ]. As soon as the coding process began, a document specific to analysis was created. Miles and colleagues have referred to this as “analytic memoing” [ 6 ]. This document is different from other documents in which the team discusses design features, decisions, and interview logistics related to the study. Analytic ideas were added to this document after coding and discussing each transcript. The author engaged two individuals in the coding/analysis process, as multiple analysts promote a comprehensive examination of the data [ 2 , 26 ]. The author met regularly with members of the team during the process of data collection and analysis to discuss the data, interpretations of them, and different lines of inquiry. These discussions were recorded in the analysis document. Table 1 outlines the potential analytic directions considered for this paper. The 10 analytic directions were developed prior to publication of analytic direction #1. Some of these directions were posed as questions that required further analysis and substantiation. Tables were then created to help us to visualize patterns during analysis. As an example, for analytic direction #2, a table was created in which each participant was assigned a row and perceived messages from the various health care providers (for example, primary care providers and specialists) were placed in columns. Perceived messages were presented as quotations from participants. We examined the columns to compare perceived messages across provider groups for each participant and then examined the columns to compare the perceived messages within each provider group. For analytic direction #3, a table was created with each participant assigned a row and the domains of the Theory of Planned Behaviour assigned to columns. The table was populated with data in the form of quotations from each participant that we believed corresponded to each of the domains. Strategies such as matrices [ 5 , 6 ] or thematic maps [ 42 ] can also be used to visualize developing patterns when presenting or organizing data.
Selection of the three analytic directions
The number of analytic directions selected likely depends on circumstances including the quality of the data, the quality of the analysis, and available resources. The research team considered the multiple analytic directions, discussing their relevance [ 27 ], novelty, and clinical significance and also the interests of the team in order to incorporate the perspectives of the different stakeholders. It was important to the author that the content of each analytic direction was bounded in that it did not overlap with the content of the other analytic directions. For example, analytic directions #2 and #3 discuss the potential influence of others in participants’ lives. However, analytic direction #2 focused on health care providers while analytic direction #3 focused on family members, friends, and colleagues of participants and specifically excluded health care providers from the analysis based on the Theory of Planned Behaviour domain “subjective norm”. In narrowing down the list of analytic directions, the author ensured there were sufficient data (quotations) to support the claims. Cases that did not fit the general results were acknowledged in order to justify their exclusion or explain why they did not fit. For example, in analytic direction #3, we examined instances where the data did not appear to fit with the Theory of Planned Behaviour and explained what happened in these instances where the model did not appear to be predictive of intentions.
The master coding template was important as it assisted with the organization of evidence for each analytic direction. The master coding template also assisted the team with the creation of tables for each analytic direction discussed. Table 2 demonstrates the relationship between the master coding template and the three selected analytic directions.
The impetus for analytic direction #1 [ 34 ] was based on an assumption held by the author as she was working on the research proposal. Her expectation was that members of a patient group would be patient advocates who were experts in navigating for care. She was interested in what patients could learn from members of this patient group. The analytic direction for the paper came from surprise, and subsequent disappointment, that those assumptions were not supported by the data and that members of the patient group did not all appear to be advocates and experts in navigating for care. One commonality that defined the patient group was that members appeared to be in favour of taking prescribed medication.
Analytic direction #1 included elements of both inductive and deductive analysis in that codes were developed for the master coding template from the data (inductive) but the author’s expectations also influenced how those codes were combined and how the team interpreted the data (deductive). Drawing from the literature, the term “advocacy” was equated with the theoretical concept of “effective” or “activated” consumer [ 43 , 44 ]. The code “effective consumer” did not exist in the original master template, partly because we preferred to not apply theoretical labels prematurely to the data. Based on the coding template, we drew from six codes to create a table about “effective consumer” behaviours (see Table 2 ). Participants were then coded along a continuum between what was referred to as “few effective consumer behaviours” (patients who followed orders with minimal involvement in their care and demonstrating the least amount of advocacy) to “many effective consumer behaviours” (individuals demonstrating significant involvement in their care, those who demanded diagnostic testing and requested specific medications).
Analytic direction #2 [ 35 ] was developed concurrently with analytic direction #1. The role of theory was minimal in analytic direction #2 and perhaps implicit in the methodology of phenomenology which focuses on individuals’ experiences [ 23 , 39 ]. The impetus for analytic direction #2 was our proposal that messages from health care providers might determine individuals’ strategies or behaviours that were the focus of analytic direction #1. The analysis was more inductive than that of analytic direction #1 as the team had no pre-contemplated plan to examine how messages from health care providers might determine individuals’ behaviours. In conducting the analysis, the team wondered whether conflicts about what individuals did with the recommendations they received (their actions) appeared to be due to messages perceived across, and within, health care provider groups. Health care providers discussed in the interviews included clinic staff, primary care providers, specialists, nurses, physiotherapists, and chiropractors.
For analytic direction #2, we used seven of the codes in the master coding template (see Table 2 ). Five of these seven codes were also used in analytic direction #1 but for very different reasons and drawing from different data within these codes. We were interested in individuals’ understanding or interpretation of recommendations by health care providers, not how individuals interacted with health care providers or what they did with information received from health care providers. In other words, we were interested in the meaning of what health care providers reportedly said to participants and not what participants did with that information.
The publication for analytic direction #3 [ 36 ] was written 3 years after that for analytic direction #1. This was the author’s least preferred paper, despite the Theory of Planned Behaviour being the theoretical framework guiding the original funded research. Analytic direction #3 involved a primarily deductive analysis where the Theory of Planned Behaviour guided the coding and analysis. Because of the restrictions of forcing exploratory data from open-ended questions into pre-defined domains, the author selected a qualitative description approach for the research design.
Contrary to memos and reflexive notes documented by the author about the potential value of this analysis and whether the team had learned anything about the application of the Theory of Planned Behaviour in the context of our study, the pursuit of analytic direction #3 became an interesting methodological exercise for a number of reasons. We collected data on several behaviours including receiving diagnostic tests, taking supplements, exercising, attending falls prevention classes, and initiating medication. The author believed that one particular behaviour had to be selected for analysis which entailed examining the data for each of the behaviours in depth. The author chose to focus on medication initiation and/or medication use because of a longstanding interest in medication use. Also, there was sufficient data to substantiate the Theory of Planned Behaviour domains in relation to medication initiation and/or medication use. The Theory of Planned Behaviour did not appear to be particularly relevant to intentions to attend a bone mineral density test and there did not appear to be sufficient data to support any one of the non-pharmacological treatment strategies mentioned. The team also had to make decisions about what counted as “perceived behavioural control”, “subjective norms”, and “attitudes” which were the three domains of the Theory of Planned Behaviour [ 37 , 38 ]. In particular, participants’ discussions about medication side effects were problematic to conceptualize in reference to these domains. The team decided to code “ experiences with side effects” as “perceived behavioural control” but “ anticipated side effects” as an “attitude”.
For analytic direction #3, the team drew from five codes, three of which were pre-specified prior to analyzing the interviews and meant to capture the domains of the Theory of Planned Behaviour. The code “attitude to BMD testing” and “attitude to bone health treatment” were existing codes based on the Theory of Planned Behaviour. The code “subjective norm” was not part of the coding template because the team believed it was too specific. We instead examined the code “social influence” which captured a broader array of information about peers such as family members and friends. Similarly, “perceived behavioural control” was not part of the coding template because we found it too specific. Information for this domain was taken from another code labelled “bone health treatment” which captured data pertaining to participants’ medications, including past behaviour with medication and how difficult it was, or not, to take the medication. The code “intentions” was an existing code.
The three selected analytic directions varied in how the team used an inductive and deductive approach to analysis [ 15 , 45 ] and how the role of theory was integrated (“central” vs. more “peripheral”) [ 15 ]. Each publication was within the scope of the overall research goal or question. As proposed by Agee [ 46 ], this overall question offered the potential for more specific questions during analysis. Finally, each publication had its own central point [ 12 ] and highlighted a different perspective or voice [ 12 ].
The following is a summary of the three analytic directions labelled with the first few words of the titles of each publication (see Table 3 ).
Analytic direction #1 (Strategies used by a patient group; inductive and deductive-driven)
In this publication, we examined the strategies described by three groups of individuals: individuals demonstrating few effective consumer behaviours, individuals demonstrating many effective consumer behaviours, and individuals demonstrating both types of behaviours. We discussed how the continuum was contrary to our expectations of what behaviours members of a patient group would exhibit. Having acknowledged this finding, we reported that more than half of the participants described effective consumer behaviours including making requests of health care providers for referral to specialists, bone mineral density tests, and prescription medications. Our overall message was that members of a patient group described a range of effective consumer behaviours that could be incorporated as skill sets in post-fracture interventions.
Analytic direction #2 (Perceived messages about bone health; inductive-driven)
In this publication, we described the perceived messages across the different provider groups and then the perceived messages within each provider group. We reported that participants perceived that specialists were more interested in their bone health than general practitioners and that very few messages about bone health were perceived from other health care providers. We also reported that perceived messages about one’s bone health and recommendations for management across provider groups were inconsistent (for example, with regard to medication initiation). The message for analytic direction #2 was that patients perceived inconsistent messages within, and across, various healthcare providers, suggesting a need to raise awareness of bone health management guidelines to providers.
Analytic direction #3 (Theory of Planned Behaviour explains intentions to use medication; deductive-driven)
In this publication, we described the data in each domain of the Theory of Planned Behaviour and the apparent relationship between these domains and participants’ intentions with regard to medication use. Our message was that the Theory of Planned Behaviour appeared to be predictive of intentions to take prescribed medication in approximately three-quarters of participants and when it was not predictive, a positive attitude to medication was the most important domain in determining participants’ intentions.
This working example of analytic direction resulted in three publications highlighting distinct "stories”. The publications differed in a number of ways. Each publication had its own central point or story line [ 12 ]. The role of theory [ 15 ] was minimal in analytic direction #2 but was more central in analytic directions #1 and #3 with the concept of “effective” or “activated” consumer and the Theory of Planned Behaviour dominating the analyses, respectively. Acknowledging that the authentic voices of participants may always be manufactured by the authorial account [ 32 , 47 ], all papers were written from the perspective of “I” or “we”. However, we focused on participants at the forefront for analytic direction #1 and we focused on participants’ perceptions of their providers’ voices for analytic direction #2. For analytic direction #3, the voice of the research team dominated as we struggled with methodological decisions. It is proposed that the voice of the model (Theory of Planned Behaviour) also dominated in analytic direction #3.
One implication related to analytic direction is that the research team may need to modify elements of the original research design to better suit the analytic direction selected. If such a modification is made, the team should ensure theoretical consistency in how the methods and methodologies are integrated [ 48 , 49 ]. For example, Crotty [ 49 ] proposes that theoretical consistency is needed between methods, methodology, theoretical perspective, and epistemology because these four elements inform one another. Similarly, Carter and Little [ 48 ] argue that consistency between methods, methodology, and epistemology contribute to the rigour of a qualitative study. Authors should demonstrate that elements of their theoretical perspectives and research design are compatible if they are applying another methodological approach to the data. Carter and Little [ 48 ] suggest that methodologies can be combined or altered if the researcher retains a coherent epistemological position and justifies the choices made. In the funded grant, a phenomenological program of research was proposed and the data were collected through in-depth interviews conducted from a phenomenological perspective. Analytic direction #3 was not purely consistent with a phenomenological approach because of the restriction to force exploratory data into domains of a theoretical framework and so we pursued this analytic direction with a different approach (qualitative description). As pointed out by Sandelowski [ 50 ], using phenomenology and qualitative description in this way is not to be confused with misuses of methods or techniques. Unlike quantitative research, qualitative research is not produced from any “pure” use of a method, but from the use of methods that are variously textured, toned, and hued [ 50 ]. According to Sandelowski [ 50 ], qualitative description can be used in conjunction with phenomenological research in a number of ways. For example, phenomenological analyses can be applied to qualitative descriptive studies [ 50 ]. However, the pursuit of other approaches to analysis, such as grounded theory or a participatory action approach, might lead to epistemological tensions if the original study design and data collection was guided by a phenomenological approach. Future discussion about the concept of analytic direction when considering theoretical and methodological positions that differ epistemologically from the original design and conduct of the study is needed.
There are a number of other implications related to the concept of analytic direction. Practically, it is advised that researchers start to think about analytic directions early so that they are aware of the potential analytic directions being developed as soon as data collection and analysis begin. By thinking about the “larger picture” at this early stage in the research, the team is better equipped to make the most of the data collected. Having said this, one will likely never use the entire dataset. As researchers, we rarely have sufficient funds or personnel to pursue all analytic directions. Data are often set aside because researchers are eager to analyze data collected for new projects or pressured to seek future funding opportunities. Analytic directions that are not pursued can be transferred to student projects. Alternatively, it is possible to draw on a sub-set of the transcripts/observations to carry out a secondary analysis. The author has developed subsequent analytic directions that span across studies and draw from a subset of transcripts for several secondary analyses [ 51 , 52 , 53 ]. Analytic directions can also contribute to ideas for new grant proposals that enable the researcher to generate more data on analytic directions that need further substantiation and further exploration.
This paper demonstrates some guidance about how to bound each analytic direction. Bounding the analytic direction is necessary so one does not re-use the data or produce multiple, yet quite similar, papers on the same topic. Researchers are encouraged to be open and transparent and acknowledge related publications so reviewers and other audiences reading the work are able to determine for themselves that the analyses are different.
There are ethical considerations in developing an analytic direction or framing the analytic direction in a way that might be different or supplementary to the original design. It is not always feasible to obtain subsequent consent from participants for use of the data if this use differs from that of the original goal of the study. As a result, analytic directions pursued should be within the scope of the approved research ethics application. One strategy is to keep the study goal or aim broad in the research ethics submission so that it encompasses many topics that might be discussed during data collection. Another consideration is to not prematurely close a research ethics application because researchers may be able to use the data for a secondary analysis at a later date.
This paper makes novel contributions to qualitative research methodology by demonstrating how the process of analytic direction works, by operationalizing the concept and providing an example, and by describing the connection between analytic direction and rigour. This paper further contributes to the advancement of rigour by demonstrating how the development and selection of analytic directions relies on several strategies to promote rigour, such as a comprehensive examination of the data, the use of multiple analysts, providing quotations to support claims made, checking for negative cases, and reflexivity.
In conclusion, the concept of analytic direction enables researchers to organize their qualitative datasets in order to tell different and unique “stories”. The concept relies upon, and promotes, the conduct of rigourous qualitative research. As with all elements of qualitative analysis, researchers are encouraged to think about the role of analytic direction as soon as data collection commences.
Availability of data and materials
The datasets generated and/or analysed during the current study are not publicly available due to participants not consenting to having their data deposited in a public dataset but are available from the corresponding author on reasonable request.
Sandelowski M. Qualitative analysis: what it is and how to begin. ResNurs Health. 1995;18:371–5.
CAS Google Scholar
Kvale S. Interviews: an introduction to qualitative research interviewing. Thousand Oaks: Sage Publications; 1996.
Google Scholar
Saldana J. The coding manual for qualitative researchers. Los Angeles: Sage Publications; 2009.
Miller WL, Crabtree BF. The dance of interpretation. Doing qualitative research. Newbury Park: Sage Publications; 1999.
Spencer L, Ritchie J, O'Connor W. Analysis: practices, principles and processes. Qualitative research practice: a guide for social science students and researchers. Los Angeles: Sage Publications; 2003. p. 199–217.
Miles MB, Huberman AM, Saldana J. Qualitative data analysis. 3rd ed. Los Angeles: Sage Publications; 2014.
Crabtree BF, Miller WL. A template approach to text analysis: developing and using codebooks. Doing qualitative research, vol. 3. Newbury Park: Sage Publications; 1992. p. 93–109.
Coffey A, Atkinson P. Making sense of qualitative data: complementary research strategies. Thousand Oaks: Sage Publications; 1996.
Kelly M. The role of theory in qualitative health research. Fam Pract. 2010;27:285–90.
Article PubMed Google Scholar
Malterud K. Shared understanding of the qualitative research process. Guidelines for the medical researcher. Fam Pract. 1993;10(2):201–6.
Article CAS PubMed Google Scholar
Slaughter S, Dean Y, Knight H, Krieg B, Mor P, Nour V, et al. The inevitable pull of the river's current: interpretations derived from a single text using multiple research traditions. Qual Health Res. 2007;17(4):548–61.
Sandelowski M. Writing a good read: strategies for re-presenting qualitative data. Res Nurs Health. 1998;21:375–82.
Frank AW. After methods, the story: from incongruity to truth in qualitative research. Qual Health Res. 2004;14(3):430–40.
Sandelowski M. Time and qualitative research. [review] [31 refs]. Res Nurs Health. 1999;22(1):79–87.
Sandelowski M. Theory unmasked: the uses and guises of theory in qualitative research. Res Nurs Health. 1993;16:213–8.
Sandelowski M. “To be of use”: enhancing the utility of qualitative research. Nurs Outlook. 1997;45:125–32.
Charmaz K. Constructing grounded theory: a practical guide through qualitative analysis. London: Sage Publications; 2006.
Thorne S. Metasynthetic madness: what kind of monster have we created? Qual Health Res. 2017;27(1):3–12.
Sharp EA, GD DC. What does rejection have to do with it? Toward an innovative, kinesthetic analysis of qualitative data. Forum Qualitative Sozialforschung/Forum. Qual Soc Res [On-line Journal]. 2013;14(2):1–12.
Streiner DL, Norman GR. PDQ epidemiology. 2nd ed. St. Louis, Missouri: Mosby; 1996.
Ulrich CM, Wallen GR, Feister A, Grady C. Respondent burden in clinical research: when are we asking too much of subjects? IRB. 2005;27(4):17–20.
Polkinghorne DE. Language and meaning: data collection in qualitative research. J Couns Psychol. 2005;52(2):137–45.
Article Google Scholar
Schwandt TA. Dictionary of qualitative inquiry. 2nd ed. Thousand Oaks: Sage Publications, Inc.; 2001.
Saunders B, Sim J, Kingstone T, Baker S, Waterfield J, Bartlam B, et al. Saturation in qualitative research: exploring its conceptualization and operationalization. Qual Quant. 2018;52:1893–907.
Lewis J, Ritchie J. Generalising from qualitative research. In: Ritchie J, Lewis J, editors. Qualitative research practice: a guide for social science students and researchers. London: Sage Publications; 2003. p. 263–86.
Malterud K. Qualitative research: standards, challenges, and guidelines. Lancet. 2001;358(9280):483–8.
Giacomini MK, Cook DJ, for the Evidence-Based Medicine Working Group. Users’ guides to the medical literature XXIII. Qualitative research in health care B. what are the results and how do they help me care for my patients? JAMA. 2000;284(4):478–82.
Mays N, Pope C. Quality in qualitative health research. In: Pope C, Mays N, editors. Qualitative research in health care. Malden: Blackwell Publishing; 2006. p. 82–101.
Chapter Google Scholar
Dixon-Woods M, Shaw RL, Agarwal S, Smith JA. The problem of appraising qualitative research. Qual Saf Health Care. 2004;13:223–5.
Article CAS PubMed PubMed Central Google Scholar
Giorgi A. Concerning a serious misunderstanding of the essence of the phenomenological method in psychology. J Phenomenol Psychol. 2008;39:33–58.
Silverman D. Qualitative research: issues of theory, method and practice. 3rd ed. London: Sage Publications Ltd; 2011.
Finlay L. Negotiating the swamp: the opportunity and challenge of reflexivity in research practice. Qual Res. 2002;2(3):209–30.
Eakin JM, Gladstone B. “Value-adding” analysis: doing more with qualitative data. Int J Qual Methods. 2020;19:1–13.
Sale JEM, Cameron C, Hawker G, Jaglal S, Funnell L, Jain R, et al. Strategies used by an osteoporosis patient group to navigate for bone health care after a fracture. Arch Orthop Trauma Surg. 2014;134:229–35.
Sale JEM, Hawker G, Cameron C, Bogoch E, Jain R, Beaton D, et al. Perceived messages about bone health after a fracture are not consistent across healthcare providers. Rheumatol Int. 2015;35:97–103.
Sale JEM, Cameron C, Thielke S, Meadows L, Senior K. The theory of planned behaviour explains intentions to use antiresorptive medication after a fragility fracture. Rheumatol Int. 2017;37:875–82.
Ajzen I, Fishbein M. Understanding attitudes and predicting social behavior. Englewood Cliffs: Prentice-Hall; 1980.
Fishbein M, Ajzen I. Belief, attitude, intention, and behavior: an introduction to theory and research. Reading: Addison-Wesley; 1975.
Giorgi A. The theory, practice, and evaluation of the phenomenological method as a qualitative research procedure. J Phenomenol Psychol. 1997;28:235–60.
Giorgi A. The descriptive phenomenological method in psychology: a modified Husserlian approach, vol. 2009. Pittsburgh: Duquesne University Press; 2009.
Wertz FJ. Phenomenological research methods for counseling psychology. J Couns Psychol. 2005;52(2):167–77.
Braun V, Clarke V. Using thematic analysis in psychology. Qual Res Psychol. 2006;3:77–101.
Kristjansson E, Tugwell PS, Wilson AJ, Brooks PM, Driedger SM, Gallois C, et al. Development of the effective musculoskeletal consumer scale. J Rheumatol. 2007;34(6):1392–400.
PubMed Google Scholar
Hibbard JH, Stockard J, Mahoney ER, Tusler M. Development of the patient activation measure (PAM): conceptualizing and measuring activation in patients and consumers. Health Serv Res. 2004;39(4 Pt 1):1005–26.
Article PubMed PubMed Central Google Scholar
Sale JEM, Thielke S. Qualitative research is a fundamental scientific process. J Clin Epidemiol. 2018;102:129–33.
Agee J. Developing qualitative research questions: a reflective process. Int J Qual Stud Educ. 2009;22(4):431–47.
Cooper N, Burnett S. Using discursive reflexivity to enhance the qualitative research process. Qual Soc Work. 2006;5(1):111–29.
Carter SM, Little M. Justifying knowledge, justifying method, taking action: epistemologies, methodologies, and methods in qualitative research. Qual Health Res. 2007;17(10):1316–28.
Crotty M. The foundations of social research. Los Angeles: Sage Publications; 1998.
Sandelowski M. Whatever happened to qualitative description? Res Nurs Health. 2000;23:334–40.
Gheorghita A, Webster F, Thielke S, Sale JEM. Long-term experiences of pain after a fragility fracture. Osteoporos Int. 2018;29:1093–104.
Sale JEM, Ashe MC, Beaton D, Bogoch E, Frankel L. Men’s health-seeking behaviours regarding bone health after a fragility fracture: a secondary analysis of qualitative data. Osteoporos Int. 2016;27(10):3113–9.
Sale JEM, Frankel L, Paiva J, Saini J, Hui S, McKinlay J, et al. Having caregiving responsibilities affects management of fragility fractures and bone health. Osteoporos Int. 2020;31:1565–72.
Download references
Acknowledgements
Not applicable.
Funding for the work described in this paper was provided by the Canadian Institutes of Health Research (Funding Reference Number: CBO-109629). The Canadian Institutes of Health Research had no involvement in the design of the study and collection, analysis, and interpretation of the data and in the writing the manuscript.
Author information
Authors and affiliations.
Musculoskeletal Health and Outcomes Research, Li Ka Shing Knowledge Institute, St. Michael’s Hospital, Unity Health Toronto, 30 Bond Street, Toronto, Ontario, M5B 1W8, Canada
Joanna E. M. Sale
Institute of Health Policy, Management & Evaluation, University of Toronto, Health Sciences Building, 155 College Street, Suite 425, Toronto, Ontario, M5T 3M6, Canada
Department of Surgery, Faculty of Medicine, University of Toronto, 149 College Street, 5th Floor, Toronto, Ontario, M5T 1P5, Canada
You can also search for this author in PubMed Google Scholar
Contributions
Joanna Sale made substantial contributions to conception and design and analysis and interpretation of the data, drafted and revised the manuscript critically for important intellectual content, approved the final version of the manuscript submitted, and agreed to be accountable for all aspects of the work.
Author’s information
JEMS is a Scientist and Associate Professor who has been teaching qualitative research courses and lectures at the introductory and intermediate level at the University of Toronto since 2007.
Corresponding author
Correspondence to Joanna E. M. Sale .
Ethics declarations
Ethics approval and consent to participate.
The study and protocol upon which this manuscript is based was approved by the Research Ethics Board at Unity Health Toronto (REB# 10–371). All methods were carried out in accordance with the Declaration of Helsinki and the relevant guidelines and regulations set by the Research Ethics Board at Unity Health Toronto. Informed consent was obtained from all participants.
Consent for publication
Competing interests.
The author declares that she has no competing interests.
Additional information
Publisher’s note.
Springer Nature remains neutral with regard to jurisdictional claims in published maps and institutional affiliations.
Rights and permissions
Open Access This article is licensed under a Creative Commons Attribution 4.0 International License, which permits use, sharing, adaptation, distribution and reproduction in any medium or format, as long as you give appropriate credit to the original author(s) and the source, provide a link to the Creative Commons licence, and indicate if changes were made. The images or other third party material in this article are included in the article's Creative Commons licence, unless indicated otherwise in a credit line to the material. If material is not included in the article's Creative Commons licence and your intended use is not permitted by statutory regulation or exceeds the permitted use, you will need to obtain permission directly from the copyright holder. To view a copy of this licence, visit http://creativecommons.org/licenses/by/4.0/ . The Creative Commons Public Domain Dedication waiver ( http://creativecommons.org/publicdomain/zero/1.0/ ) applies to the data made available in this article, unless otherwise stated in a credit line to the data.
Reprints and permissions
About this article
Cite this article.
Sale, J.E.M. The role of analytic direction in qualitative research. BMC Med Res Methodol 22 , 66 (2022). https://doi.org/10.1186/s12874-022-01546-4
Download citation
Received : 28 September 2021
Accepted : 11 February 2022
Published : 13 March 2022
DOI : https://doi.org/10.1186/s12874-022-01546-4
Share this article
Anyone you share the following link with will be able to read this content:
Sorry, a shareable link is not currently available for this article.
Provided by the Springer Nature SharedIt content-sharing initiative
- Analytic direction
- Qualitative research
- Data analysis
- Methodological rigour
- Critical appraisal
BMC Medical Research Methodology
ISSN: 1471-2288
- General enquiries: [email protected]
Thank you for visiting nature.com. You are using a browser version with limited support for CSS. To obtain the best experience, we recommend you use a more up to date browser (or turn off compatibility mode in Internet Explorer). In the meantime, to ensure continued support, we are displaying the site without styles and JavaScript.
- View all journals
- Explore content
- About the journal
- Publish with us
- Sign up for alerts
- Published: 06 May 2024
Social factors and health: pathways and promise
- Elena Fuentes-Afflick 1
Pediatric Research ( 2024 ) Cite this article
Metrics details
The relationship between social factors and health is of great interest to clinicians, health system leaders, and policy makers. 1 Although it may seem intuitive to infer a relationship, identifying the underlying pathway(s) and defining prevention, mitigation or intervention strategies has proven to be difficult. The scholarly field is relatively new and one of the founders was Dr. Nancy Adler, a pioneering health psychologist who studied the relationship between socioeconomic factors and health outcomes. 2 Various instruments have been developed to assess and measure social factors but there is no clear consensus on the best instrument. More recently, the identification of biomarkers has generated interest in the development of tests and indices which could be applied in the clinical setting. To date, the focus of biomarker research has been inflammatory and neuroendocrine factors but additional areas are emerging. The urgent need to define standard measures is highlighted by the Centers for Medicare & Medicaid Services’ recent initiative, in partnership with other organizations, to implement new quality measures, many of which require screening patients for health-related social needs. 3 To implement widespread screening, it is essential to define the most accurate instruments and the most reliable biomarkers.
What does this mean for children and pediatricians? The application of new quality measures and tests to pediatric populations is often challenging, sometimes because the measures have not been adequately tested among children, the types of biomarkers require invasive sampling or other issues. For decades, researchers have documented the impact of a variety of social stressors on child health, 1 but it has been difficult to understand whether specific events are especially detrimental, whether the timing of stressful events is significant, and how the impact of such experiences might vary by race/ethnicity, age, family structure, community characteristics or another factor. While some events, such as the loss of a parent or loss of home, may be universally stressful and/or traumatic, the impact of other events may be variable. Similarly, should social risks or vulnerabilities be considered as individual measures or as part of a multifactorial measure? In terms of biomarkers, it is not clear if the physiologic response to social influences is similar for adults, infants, children or adolescents. These are critical issues to evaluate before the pediatric community should embrace screening and testing for social risk factors or social needs. In any system of screening or intervention, it is essential to approach the process from the perspective of respect and humility; although a provider may have a good intention in evaluating social risk, the screening experience may elicit feelings of shame or reluctance to disclose sensitive information. 1
This is a preview of subscription content, access via your institution
Access options
Subscribe to this journal
Receive 14 print issues and online access
251,40 € per year
only 17,96 € per issue
Buy this article
- Purchase on Springer Link
- Instant access to full article PDF
Prices may be subject to local taxes which are calculated during checkout
Garg, A., Homer, C. J. & Dworkin, P. H. Addressing social determinants of health: challenges and opportunities in a value-based model. Pediatrics 143 , e20182355 (2019).
Article PubMed Google Scholar
Hafner, K. Nancy E. Adler, 77, Psychologist who linked wealth with health. (New York Times. New York, 2024).
Garg, A., LeBlanc, A. & Raphael, J. L. Inadequacy of current screening measures for health-related social needs. JAMA 330 , 915–916 (2023).
Download references
Author information
Authors and affiliations.
University of California San Francisco, 1001 Potrero Avenue, Building 5, Room 2A21, San Francisco, CA, 94110, USA
Elena Fuentes-Afflick
You can also search for this author in PubMed Google Scholar
Corresponding author
Correspondence to Elena Fuentes-Afflick .
Ethics declarations
Competing interests.
The author declares no competing interests.
Additional information
Publisher’s note Springer Nature remains neutral with regard to jurisdictional claims in published maps and institutional affiliations.
Rights and permissions
Reprints and permissions
About this article
Cite this article.
Fuentes-Afflick, E. Social factors and health: pathways and promise. Pediatr Res (2024). https://doi.org/10.1038/s41390-024-03165-9
Download citation
Received : 15 February 2024
Accepted : 02 March 2024
Published : 06 May 2024
DOI : https://doi.org/10.1038/s41390-024-03165-9
Share this article
Anyone you share the following link with will be able to read this content:
Sorry, a shareable link is not currently available for this article.
Provided by the Springer Nature SharedIt content-sharing initiative
Quick links
- Explore articles by subject
- Guide to authors
- Editorial policies

Quality of life in a high-risk group of elderly primary care patients: characteristics and potential for improvement
- Open access
- Published: 13 May 2024
Cite this article
You have full access to this open access article
- Juliane Döhring ORCID: orcid.org/0000-0003-1421-3149 1 ,
- Martin Williamson 1 ,
- Christian Brettschneider 3 ,
- Thomas Fankhänel 6 ,
- Melanie Luppa 2 ,
- Alexander Pabst 2 ,
- Marina Weißenborn 4 ,
- Isabel Zöllinger 5 ,
- David Czock 4 ,
- Thomas Frese 6 ,
- Jochen Gensichen 5 ,
- Wolfgang Hoffmann 8 ,
- Hans-Helmut König 3 ,
- Jochen René Thyrian 8 , 9 ,
- Birgitt Wiese 7 ,
- Steffi Riedel-Heller 2 na1 &
- Hanna Kaduszkiewicz 1 na1
Quality of Life (QoL) is associated with a bandwidth of lifestyle factors that can be subdivided into fixed and potentially modifiable ones. We know too little about the role of potentially modifiable factors in comparison to fixed ones. This study examines four aspects of QoL and its associations with 15 factors in a sample of elderly primary care patients with a high risk of dementia. The main objectives are (a) to determine the role of the factors in this particular group and (b) to assess the proportion of fixed and potentially modifiable factors.
A high-risk group of 1030 primary care patients aged between 60 and 77 years (52.1% females) were enrolled in “AgeWell.de,” a cluster-randomized, controlled trial. This paper refers to the baseline data. The multi-component intervention targets to decrease the risk of dementia by optimization of associated lifestyle factors. 8 fixed and 7 modifiable factors potentially influencing QoL served as predictors in multiple linear regressions.
The highest proportion of explained variance was found in psychological health and age-specific QoL. In comparison to health-related QoL and physical health, the modifiable predictors played a major role (corr. R 2 : 0.35/0.33 vs. 0.18), suggesting that they hold a greater potential for improving QoL.
Social engagement, body weight, instrumental activities of daily living, and self-efficacy beliefs appeared as lifestyle factors eligible to be addressed in an intervention program for improving QoL.
Trial registration
German Clinical Trials Register, reference number: DRKS00013555. Date of registration: 07.12.2017.
Avoid common mistakes on your manuscript.
Introduction
According to the definition of the World Health Organization (WHO), Quality of life (QoL) is a multifactorial and broad concept reflecting an individual's perception of their position in life in the context of the culture and value systems in which they live and in relation to their goals, expectations, standards, and concerns [ 1 ]. Various approaches to a definition distinguish between the psychological and the physical aspect as the “core areas” constituting QoL [ 2 ]. In the 1980s the term “health-related QoL (HRQoL)” was coined in order to differentiate it as a general medical concept from the use in other professions [ 3 ]. In comparison to QoL, HRQoL focuses on health in a narrower sense, including the medical definition and the fundamental importance of independent physical, emotional, and social functioning [ 4 ], for example mobility, the ability to fend for oneself, daily activities, pain and physical complaints, and anxiety/depression [ 5 ]. Although hard to quantify, QoL is one of the most pursued target values which is implicitly proposed in health care research. Health-related interventions for elderly people often prioritize improvement of QoL over other health-related parameters, since elderly people often struggle with age-specific adversities such as impairment of sensory functions, dependence on other people, lower social participation, fear of dying, and others [ 6 ].
Identifying predictors of QoL is a subject of intensive research in several disciplines [ 7 ]. In the present study, we included 15 predictors of QoL of elderly primary care patients with an increased risk of dementia.
The patients participated in the AgeWell.de study, a cluster-randomized controlled intervention trial. The main objective of AgeWell.de was an improvement of lifestyle factors that are known to contribute to a development of dementia [ 8 ]. Hence, the design of the intervention required a distinction between lifestyle factors, that are fixed (such as age or sex) and those ones that are potentially modifiable and have the potential to be changed deliberately (such as physical or cognitive activity). A substantial proportion of lifestyle factors contributing to dementia are also associated with various aspects of QoL, which was a secondary outcome of AgeWell.de. Accordingly, the predictors of QoL presented here were components of a pre-existing set of data and have subsequently been selected under the prerequisite that associations with QoL have been reported in the literature. We identified 8 fixed and 7 potentially modifiable predictors fulfilling the aforementioned conditions. The fixed predictors can be assigned to 3 major areas:
Sociodemographic characteristics
We included 5 sociodemographic characteristics in our analysis: besides sex and age three predictors related to the socioeconomic status (income, education, vocational qualification) were considered. Previous studies have shown that males tend to have a higher QoL than females [ 9 ] and that QoL in older age groups decreases slightly in general, especially in connection with multimorbidity [ 10 ]. The associations between socioeconomic status and QoL vary depending on the measured aspects, but in general poorer socioeconomic status is associated with poorer QoL. This association tends to be weaker in the older age groups [ 11 ].
Cognitive performance
Cognitive complaints have robust associations with QoL [ 12 ]. As cognitive capacities decline (due to dementia or other clinical conditions) negative effects on QoL have been shown [ 13 ]. As two aspects of cognitive performance, we used a global measure of cognitive functioning (comprising language skills, abstraction capabilities, attention and others) and memory function.
Instrumental activities of daily living
Instrumental activities are defined as a set of more complex skills that are needed in order to live independently [ 14 ], such as managing the medicines, shopping for groceries on one’s own or managing money, and paying bills. Previous studies consistently showed a decline of QoL depending on the extent of needed external help in daily life [ 15 ].
The 7 modifiable predictors can be assigned to the following 4 areas:
Social integration
Social engagement in general is considered as one of the most important predictors of QoL [ 16 ]. In older age, social contact usually declines and it has been shown that the onset of social engagement markedly increases HROoL [ 17 ]. Besides social engagement, we considered the household type (living alone or together with relatives) as living alone is associated with lower QoL in elderly persons [ 18 ].
Physical and somatic health-related factors
Many physical predictors have been investigated in the context of QoL [ 19 ]. One well-investigated correlate of the physical constitution is the body mass index (BMI), which is known to influence various aspects of well-being [ 20 ]. A further aspect refers to the physical activity as an extensively studied factor associated with QoL [ 21 ].
Psychological and mental health-related factors
Depression is one of the most powerful predictors of QoL in elderly people [ 22 ] as well as self-efficacy beliefs, meaning the optimistic expectation of one´s own competency to master difficult situations successfully [ 23 ]. High self-efficacy beliefs contribute positively to QoL in many respects [ 24 ], elderly people with high self-efficacy beliefs, for example, tend to an improved compliance with self-care activities [ 25 ].
Cognitive activity
In this context, cognitive activities are defined as common everyday activities demanding mental effort, for example solving crossword puzzles or reading books. Findings with respect to cognitive activity affecting QoL are mixed [ 26 ]. These inconsistencies in the literature result partly from confounders, the broad variety of concepts and defined periods of time (immediate effects of cognitive activity vs. effects of cognitive activity throughout the lifespan).
This list covers a bandwidth of commonly identified predictors of QoL and makes no claim to completeness from a purely academical point of view. Instead, we put an emphasis on the distinction between fixed and potentially modifiable predictors against a background of practical relevance. On that note the present study should make a contribution to the changing potential of lifestyle factors that are associated with QoL. There is a large body of literature concerning QoL in dementia patients [ 27 ] but QoL in elderly primary care patients with high risk of dementia is only marginally investigated in general. A systematic literature review on this issue can be found in [ 28 ].
The main objective of the present study is to analyze if the fixed and modifiable predictors provide information about QoL in elderly primary care patients with an increased risk of dementia. Accordingly, this objective is construed as a prediction model. The results will deliver implications for future intervention programs with a main focus on QoL improvement. The results may also be useful for basic research concerning QoL in the elderly as they encompass a high amount of differentiated data in a large sample ( N = 1030) with high risk of dementia.
Materials and methods
The agewell.de study.
Data for this analysis were derived from the AgeWell.de study. AgeWell.de is a multi-component, cluster-randomized, controlled intervention study, which targets to decrease the risk of dementia in a high-risk group of elderly primary care patients [ 29 ]. Due to the lack of effective dementia treatment options, AgeWell.de was designed as a prevention study in order to improve the individual risk factor profile of the participants. A wide range of potential risk factors for dementia has been identified in the last decades: some of them are fixed and cannot be modified, such as a genetic disposition. Others are potentially modifiable. Some of the latter were addressed in the intervention, which were in detail: counseling on nutrition and ways to increase physical activity, cognitive training, optimization of medication and the management of cardiovascular risk factors, counseling on improvement of social activity and intervening in case of loss, grief, and depressive symptoms. Several lifestyle factors associated with dementia are also known to contribute to QoL. The current paper is a secondary analysis of the AgeWell.de- baseline data referring to associations between lifestyle risk factors and QoL as a secondary outcome of the entire study. The primary outcome of the entire study is the preservation of cognitive function and delayed cognitive decline, respectively.
The 1030 AgeWell.de-participants were recruited by 123 general practices (each corresponding to one cluster) in five German cities (Leipzig, Greifswald, Kiel as well as Halle are medium-sized cities and Munich is a large city) between 2018 and 2019. The participating general practitioners (who agreed to support the study after a written request to all possible eligible practices) identified possible participants among their patients based on the following criteria: Persons aged 60–77 with a CAIDE score ≥ 9. The CAIDE score (Cardiovascular Risk Factors, Aging, and Incidence of Dementia) is a validated tool to calculate late-life dementia risk based on midlife vascular risk factors [ 30 ]. Exclusion criteria were diagnosis of dementia, residence in a nursing home, poor German language skills, simultaneous participation in another intervention study, and severe mental or physical illness. With respect to the latter, the practitioners had to estimate individually, if the patient is potentially healthy enough to partake in the intervention. Patients, who fulfilled the criteria, were asked for study participation in a written form. Those patients, who showed interest to participate, were invited by the practitioners, received further information about the study, and gave written agreement. A total of 1176 persons initially consented to participate. 44 (3.7%) were not eligible (mainly because they did not meet the CAIDE criteria). 102 (8.7%) dropped out before the baseline assessment due to the occurrence of health problems, relocation, or withdrawal of consent. There was no difference between eligible and non-eligible participants with respect to age and sex, but the latter had significantly more years of education and a lower CAIDE score. More details about the recruitment procedures can be found in [ 31 ].
Outcome measures: quality of life
Four QoL-outcome measures were selected in order to reflect (1) HRQoL, (2) physical health, and (3) psychological health as well as 4) age-specific QoL:
The first outcome measure represents a global scale of HRQoL. It was operationalized by the EuroQol-5 Dimension (EQ-5D), which is a generic questionnaire including the following five dimensions: “mobility,” “self-care,” “usual activities,” “pain/discomfort,” and “anxiety/depression.” The concept of health in EQ-5D also encompasses positive aspects (well-being) as well as negative aspects (illness) [ 4 ]. The EQ-5D data presented here were transformed into utilities according to the German value set [ 32 ]. The second outcome parameter is a quantitative measure of the self-perceived state of physical health, using the visual analog scale (EQ VAS, EuroQol Group, 1990), which ranges from 0 (worst imaginable health status) to 100 (best imaginable health status). Third, we used The World Health Organization Quality of Life Abbreviated Version (WHOQOL-BREF) as a patient reported outcome (PRO) questionnaire, quantifying the global health state, which refers to the aforementioned broad WHO definition and is independent from disabilities [ 5 ]. The WHOQOL-BREF assesses four domains of health, which are calculated and interpreted separately. The domains comprise physical and psychological health, social relationships, and environment. The answer format is a five-point Likert scale for every single item. The sum score for every domain has to be divided by the number of items per domain and subsequently multiplied by 4. All scores are multiplied by 4 to make them comparable with the scores derived from the WHOQOL-100. In this study, we paid special attention to the second domain as a measure of the self-perceived state of psychological health. The results for the other three domains are listed in an electronic supplement (Table 2 ). The fourth outcome measure takes into account the advanced age of our sample. The World Health Organization Quality Of Life Instrument-Older Adults Module (WHOQOL-OLD) was applied as a measure regarding age-specific domains of QoL. This questionnaire provides a global estimation of the following six relevant facets of QoL in higher age: sensory functions, independence, activities in the past, present and future, social participation, worries concerning death, and intimacy. As a global measurement of age-specific QoL, we calculated the total score based on the six individual facets. The results for the individual facets are also listed in the electronic supplement (tables 3 and 4).
Predictor variables: fixed and modifiable predictors
Eight fixed predictors were included in the model. Sociodemographics comprise five predictors : age, sex, and three indices of the socioeconomic status (SES) according to [ 33 ]. The SES is a well-established analytical concept for epidemiologic research and health reporting in Germany and is based on information concerning education and vocational training as well as well as household net income. The SES indices are calculated as total scores including income, education, and vocational qualification. The cognitive performance was differentiated by cognitive function (Montreal Cognitive Assessment, Moca [ 34 ]) and memory function (Consortium to Establish a Registry for Alzheimer's Disease, CERAD: word list recall [ 35 ]). The instrumental activities of daily living were measured by the IADL-Skala [ 36 ].
In addition, 7 modifiable predictors, which also relate to QoL, were included. Social integration is represented by the predictors social engagement in general (Lubben social network scale, LSNS-6 [ 37 ]) and living in a single-person household . As physical and somatic health-related factors, we used the BMI and a self-constructed questionnaire for physical activity. The latter consists of 10 items (e.g., “how often do you ride the bike?”) and was scored by adding the answers on a five-point Likert scale (the entire questionnaire is available as supplementary information). Psychological and mental health-related factors refer to depression (Geriatric Depression Scale, GDS [ 38 ]) and self-efficacy (Skala zur allgemeinen Selbstwirksamkeitserwartung, SWE [ 39 ]). C ognitive activity was also measured by a self-constructed questionnaire, analogous to the questionnaire for physical activity. It consists of 12 items (e.g., “how often do you solve crossword puzzles?”) and is also available as supplementary information. Table 1 shows a detailed list of the outcome measures and predictors as well as according descriptive characteristics of the sample.
Statistical analyses
Descriptive statistical analyses (means and frequencies) concerning the baseline data of the AgeWell.de cohort were conducted for the four QoL-outcome measures (primary endpoints) and the 15 predictor variables.
Four multiple linear regressions were performed in order to estimate the predictive values of all 15 considered predictors (i.e., goodness-of-fit in total) and the sets of predictors (fixed and modifiable) on the QoL-outcome measures. Bivariate relationships between the predictors and outcome measures are listed in the electronic supplement (Table 5).
Statistically significant coefficients of determination (corrected R 2 ), unstandardized regression coefficients b, standardized regression coefficients β, and p values are shown. All statistical analyses were performed using IBM SPSS software V22.0 (SPSS Inc., Chicago, IL, USA).
Descriptive statistics
1030 AgeWell.de-participants were included in the analyses. 52.1% of the participants were females. At the date of baseline measurement (to which this analysis refers), the participants were 69.0 (SD 4.9) years old in average.
Regression analyses: predictive values of the entire models, modifiable, and fixed predictors
All R 2 were highly significant ( p < 0.001) without exception.
The coefficients of determination ( R 2 ) for the overall model regarding HRQoL (EQ 5-D) were indicative for a moderate goodness-of-fit according to Cohen [ 44 ]. This outcome measure was explained by the modifiable and the fixed predictors to similar proportions (Table 2 ).
The highest proportion of explained variance was found in the domains of psychological health (WHOQOL-BREF), mainly in connection with modifiable predictors. A similar pattern also applies to age-specific QoL (WHOQOL-OLD). The goodness-of-fit in psychological health as well as age-specific QoL has to be classified as high.
The physical health (EQ VAS) could be explained to a moderate extent in general, and to a lower degree by fixed predictors compared to modifable ones (Tables 3 and 4 ).
Regression analyses: influence of the individual fixed predictors
The ability to manage the IADL was significantly associated with the health-related QoL as well as psychological and physical health. Male sex was a significant predictor for a higher health-related QoL and better psychological health. The socioeconomic status played a lesser role, merely vocational qualification was significantly associated with psychological health, as well as higher education with health-related QoL. Income did not relate to the considered QoL instruments and domains. This also applies to age and cognitive function, as well as memory function as measured in standardized tests.
Regression analyses: influence of the individual modifiable predictors
Body mass index and depression had a significant predictive value in all considered QoL-outcome measures. High self-efficacy beliefs were significant predictors with respect to psychological health and age-specific QoL.
Additionally, age-specific QoL was also positively associated with higher social engagement and living together with other people. There was no noteworthy relationship between physical and cognitive activity in all considered QoL instruments or domains, respectively.
The main objective of the present study was to analyze if the determinants of QoL known from the literature can also be found in elderly primary care patients with an increased risk of dementia. Based on these results, implications for future intervention programs with a focus on QoL improvement are discussed.
Fixed predictors of Quality of Life
We found significant associations between IADL and health-related QoL as well as psychological and physical health. This is in line with previous studies that consistently showed a decline of QoL dependent on the extent of needed external help in daily life [ 15 ]. IADL are related to physical and mental health in elderly people [ 45 ]. Based on the important role of IADL in QoL, one must consider whether IADLs are really fixed predictors. For example, improvements in IADL (and thus in the QoL) could be achieved through a critical evaluation of the medical treatment and, if necessary, a change in therapy [ 46 ]. Targeted training of IADL in case of limitations due to chronic diseases would also be conceivable in order to improve QoL. This is especially true for patients with cognitive impairment. Here, studies have shown that consistent IADL/ADL training can slow down the loss of these skills [ 47 ]. As expected, male sex was a significant predictor in general health-related QoL and psychological health. This finding is in line with the literature [ 48 ]. In our study population, income was not associated with the QoL domains. A possible explanation may be the selection of the QoL-outcome measurements that focused on aspects of well-being rather than evaluation of life [ 49 ]. Education and vocational qualification each contributed to one instrument/domain of QoL. In the literature, the effects of education on QoL are described as multidimensional and often reciprocal in nature [ 50 ]. The quality of life schema as a heuristic framework according to [ 51 ] includes educational effects in the following broad life domains: achievement in life, material and emotional well-being, physical health, community, intimate relationships, and personal safety/ future security.
Interestingly, age was not significantly related to the QoL domains, which may be due to the relatively small age-range in the study (60 to 78 years). Another factor contributing to this finding may be a result of the exclusion criteria leading to a sample without severely diseased persons. As pointed out in a narrative review of selected literature [ 52 ], with all other influences controlled, aging per se does not influence quality of life negatively.
Cognitive and memory function had also no noteworthy predictive value for QoL in our sample. We assume that this may be a result of ceiling effects.
Modifiable predictors of Quality of Life
The modifiable predictors contributed considerably higher to the explained variance than the fixed predictors in the QoL domains, especially regarding psychological health and age-specific QoL.
One of the most remarkable results in this context is the predictive role of the BMI for QoL throughout all four instruments and domains applied. A high BMI affects QoL in various aspects: lower mobility, higher risk of cardiovascular diseases, and diabetes mellitus, as well as arthrosis, protracted courses, and complications of many diseases, lower self-esteem, and others. In turn, several mental illnesses may precipitate and perpetuate obesity [ 53 ]. The association between obesity and QoL is thus bidirectional and complex. It further stands to reason that elderly persons in particular suffer from the effects of obesity, because compensation opportunities become fewer in old age [ 54 ].
Depression, measured by the GDS, also contributed to the variance in all considered QoL instruments and domains. This association has been described in a broad range of literature and was found to be stable over time regardless of the assessed instruments for QoL [ 55 ]. It cannot be ruled out that conceptual overlaps between depression and QoL may play a role in this context. However, besides depression, other factors are also associated with the age-specific QoL domains, such as living alone or with others, social engagement, and self-efficacy. To improve QoL in old age, interventions focusing on social integration and BMI seem appropriate. This is a positive message of this study, as these factors can indeed be influenced. Whether it is possible to improve QoL through interventions at this age should be investigated in appropriate studies.
With respect to the modifiable factors, a surprising result was the fact that neither physical, nor cognitive activity significantly predicts QoL in our data, although the questionnaires covered a broad range of daily activities. This outcome may be a result of the short period of time to which the questions referred: the participants were asked for cognitive and physical activity within the last four weeks. Further analysis indicated that the predictors “BMI” and “physical activity” co-varied: excluding BMI from the regression model results in significant variance contribution of physical activity in the physical health domain as well as in the health-related QoL (p value = 0.02 in both domains). For a more detailed overview about physical activity determinants in this sample, see Cardona et al. [ 56 ].
Quality of Life instruments and domains
All of the four considered QoL instruments and domains were explained by the multiple-regression models to a moderate to high goodness-of-fit. The predictive value of the models was particularly high in two domains: psychological health and age-specific QoL. Again, the substantial proportion was allocated to the modifiable predictors.
The physical health domain as well as the health-related QoL showed only a moderate goodness-of-fit. A reason may be that “physical health” in this context was a global estimation of self-perceived health, which was explicitly restricted to bodily aspects, according to the EQ VAS. The visual analog scale as a single item represents a rather limited concept in comparison to the other outcomes. It might be reasonably assumed that physical health (in comparison with psychological health and age-specific QoL) is determined rather by individual and external factors (such as genetic dispositions or injuries). This may also partly apply to the health-related QoL, measured by EQ 5D, which is composed of physical and mental parts.
Limitations
Limitations of our data relate to the selection of predictors: improving QoL was a secondary objective in the AgeWell.de study. Accordingly, the set of predictors was restricted to factors potentially influencing the primary objective, i.e., the development of dementia. Taking more QoL-specific predictors into account might have led to a higher goodness-of-fit of the models. Furthermore, the aforementioned prioritization of the predictor selection entails a possible content overlap between some predictors and outcome measurements (e.g., depression and psychological health), which may lead to an overestimation of the actual empirical associations. Another limitation at this point of time is the cross-sectional approach. Therefore, it is not yet possible to draw conclusions about the causal links between predictors and outcomes as well as the relationships between predictors among each other. According to this, as a prediction model, our data do not provide information about how to increase QoL. The follow-up data of the AgeWell.de study will generate new evidence on causal links and interactions.
Conclusions
This study provides important information on factors for optimization of QoL in elderly primary care patients with an increased risk of dementia. One of the most important predictors for age-specific QoL is social engagement in advanced age. Secondly, body weight seems to be another desirable key factor for well-being. Thirdly training and improvement of IADL seem desirable not only for themselves, but also with potential to improve QoL. Fourthly, a “psychological adjusting screw” refers to a shift from an external to an internal locus of control resulting in the belief, that one´s own action has (positive) consequences (self-efficacy beliefs). In sum, the data showed various associations between modifiable predictors, pointing on a high and realistic potential to improve QoL by concrete modifications on the behavioral, physical, and psychological level as stated above. Testing interventions with the aim to improve QoL in elderly patients with and without an increased risk of dementia should be a next step in research.
WHOQoL Group. (1993). Study protocol for the World Health Organization project to develop a Quality of Life assessment instrument (WHOQOL). Quality of life Research, 2 , 153–159. https://doi.org/10.1007/BF00435734
Article Google Scholar
Frisch, M. B. (2000). Improving mental and physical health care through quality of life therapy and assessment. In Advances in quality of life theory and research (pp. 207–241). Springer. https://doi.org/10.1007/978-94-011-4291-5_10
Patrick, D. L., & Deyo, R. A. (1989). Generic and disease-specific measures in assessing health status and quality of life. Medical Care . https://doi.org/10.1097/00005650-198903001-00018
Article PubMed Google Scholar
Gusi, N., Olivares, P. R., & Rajendram, R. (2010). The EQ-5D health-related quality of life questionnaire. In Handbook of disease burdens and quality of life measures. Springer, pp. 87–99. Doi: https://doi.org/10.1007/978-0-387-78665-0_5
Group, & T. E. (1990). EuroQol-a new facility for the measurement of health-related quality of life. Health Policy, 16 (3), 199–208. https://doi.org/10.1016/0168-8510(90)90421-9
Power, M., Quinn, K., & Schmidt, S. (2005). Development of the WHOQOL-old module. Quality of life research, 14 (10), 2197–2214. https://doi.org/10.1007/s11136-005-7380-9
Shukla, K. (2022). The conceptual frameworks on quality of life: A go-to handbook for quality of life research (1st ed.). Notion Press.
Google Scholar
Livingston, G., Huntley, J., Sommerlad, A., Ames, D., Ballard, C., Banerjee, S., & Mukadam, N. (2020). Dementia prevention, intervention, and care: 2020 report of the Lancet Commission. The Lancet, 396 (10248), 413–446.
Bonsaksen, T. (2012). Exploring gender differences in quality of life. Mental Health Review Journal . https://doi.org/10.1108/13619321211231815
Makovski, T. T., Schmitz, S., Zeegers, M. P., Stranges, S., & van den Akker, M. (2019). Multimorbidity and quality of life: Systematic literature review and meta-analysis. Ageing Research Reviews, 53 , 100903. https://doi.org/10.1016/j.arr.2019.04.005
Read, S., Grundy, E., & Foverskov, E. (2016). Socio-economic position and subjective health and well-being among older people in Europe: A systematic narrative review. Aging & Mental Health, 20 (5), 529–542. https://doi.org/10.1016/S0140-6736(20)30367-6
Stites, S. D., Harkins, K., Rubright, J. D., & Karlawish, J. (2018). Relationships between cognitive complaints and quality of life in older adults with mild cognitive impairment, mild Alzheimer’s disease dementia, and normal cognition. Alzheimer Disease and Associated Disorders, 32 (4), 276. https://doi.org/10.1097/wad.0000000000000262
Article PubMed PubMed Central Google Scholar
Conrad, I., Uhle, C., Matschinger, H., Kilian, R., & Riedel-Heller, S. G. (2015). Lebensqualität von älteren Menschen mit leichten kognitiven Störungen. Psychiatrische Praxis, 42 (03), 152–157. https://doi.org/10.1055/s-0034-1369831
Guo, H. J., & Sapra, A. (2020). Instrumental activity of daily living . StatPearls Publishing.
Talarska, D., Tobis, S., Kotkowiak, M., Strugała, M., Stanisławska, J., & Wieczorowska-Tobis, K. (2018). Determinants of quality of life and the need for support for the elderly with good physical and mental functioning. Medical Science Monitor, 24 , 1604. https://doi.org/10.12659/MSM.907032
Herzog, A. R., Ofstedal, M. B., & Wheeler, L. M. (2002). Social engagement and its relationship to health. Clinics in Geriatric Medicine, 18 (3), 593–609. https://doi.org/10.1016/S0749-0690(02)00025-3
Hajek, A., Brettschneider, C., Mallon, T., Ernst, A., Mamone, S., Wiese, B., & König, H. H. (2017). The impact of social engagement on health-related quality of life and depressive symptoms in old age-evidence from a multicenter prospective cohort study in Germany. Health and Quality of Life Outcomes, 15 , 1–8. https://doi.org/10.1186/s12955-017-0715-8
Jeon, B. J., & Gwak, H. J. (2016). Relationship between Information use and quality of life by household types of elderly. Journal of the Korea Academia-Industrial cooperation Society, 17 (10), 213–220. https://doi.org/10.5762/KAIS.2016.17.10.213
Vagetti, G. C., Barbosa, V. C., Moreira, N. B., Oliveira, V. D., Mazzardo, O., & Campos, W. D. (2014). Association between physical activity and quality of life in the elderly: A systematic review, 2000–2012. Brazilian Journal of Psychiatry, 36 , 76–88. https://doi.org/10.1590/1516-4446-2012-0895
Yan, L. L., Daviglus, M. L., Liu, K., Pirzada, A., Garside, D. B., Schiffer, L., & Greenland, P. (2004). BMI and health-related quality of life in adults 65 years and older. Obesity Research, 12 (1), 69–76. https://doi.org/10.1038/oby.2004.10
Rejeski, W. J., & Mihalko, S. L. (2001). Physical activity and quality of life in older adults. The Journals of Gerontology Series A, 56 (2), 23–35. https://doi.org/10.1093/gerona/56.suppl_2.23
Borowiak, E., & Kostka, T. (2004). Predictors of quality of life in older people living at home and in institutions. Aging Clinical and Experimental Research, 16 (3), 212–220. https://doi.org/10.1007/BF03327386
Schwarzer, R. (1999). Self-regulatory processes in the adoption and maintenance of health behaviors. Journal of Health Psychology, 4 (2), 115–127. https://doi.org/10.1177/135910539900400208
Article CAS PubMed Google Scholar
McAuley, E., Konopack, J. F., Motl, R. W., Morris, K. S., Doerksen, S. E., & Rosengren, K. R. (2006). Physical activity and quality of life in older adults: Influence of health status and self-efficacy. Annals of behavioral Medicine, 31 (1), 99–103. https://doi.org/10.1207/s15324796abm3101_14
Lee, M. K., & Oh, J. (2020). Health-related quality of life in older adults: Its association with health literacy, self-efficacy, social support, and health-promoting behavior. Healthcare, 8 (4), 407. https://doi.org/10.3390/healthcare8040407
Blacker, D., & Weuve, J. (2018). Brain exercise and brain outcomes: does cognitive activity really work to maintain your brain?. JAMA Psychiatry , 75 (7), 703–704. https://doi.org/10.1001/jamapsychiatry.2018.0656
Banerjee, S., Samsi, K., Petrie, C. D., Alvir, J., Treglia, M., Schwam, E. M., & del Valle, M. (2009). What do we know about quality of life in dementia? A review of the emerging evidence on the predictive and explanatory value of disease specific measures of health related quality of life in people with dementia. International Journal of Geriatric Psychiatry, 24 (1), 15–24. https://doi.org/10.1002/gps.2090
Landeiro, F., Mughal, S., Walsh, K., Nye, E., Morton, J., Williams, H., & Gray, A. M. (2020). Health-related quality of life in people with predementia Alzheimer’s disease, mild cognitive impairment or dementia measured with preference-based instruments: a systematic literature review. Alzheimer’s Research & Therapy, 12 (1), 1–14. https://doi.org/10.1186/s13195-020-00723-1
Röhr, S., Zülke, A., Luppa, M., Brettschneider, C., Weißenborn, M., Kühne, F., & Riedel-Heller, S. G. (2021). Recruitment and baseline characteristics of participants in the AgeWell de study: A pragmatic cluster-randomized controlled lifestyle trial against cognitive decline. International Journal of Environmental Research and Public Health, 18 (2), 408. https://doi.org/10.3390/ijerph18020408
Kivipelto, M., Ngandu, T., Laatikainen, T., Winblad, B., Soininen, H., & Tuomilehto, J. (2006). Risk score for the prediction of dementia risk in 20 years among middle aged people: A longitudinal, population-based study. The Lancet Neurology, 5 (9), 735–741. https://doi.org/10.1016/S1474-4422(06)70537-3
Lampert, T., & Kroll, L. E. (2009). Die Messung des sozioökonomischen Status in sozialepidemiologischen Studien. In Gesundheitliche Ungleichheit (pp. 309–334). VS Verlag für Sozialwissenschaften. Doi: https://doi.org/10.1007/978-3-531-91643-9_18
Ludwig, K., Graf von der Schulenburg, J. M., & Greiner, W. (2018). German value set for the EQ-5D-5L. PharmacoEconomics, 36 , 663–674. https://doi.org/10.1007/s40273-018-0615-8
Nasreddine, Z. S., Phillips, N. A., Bédirian, V., Charbonneau, S., Whitehead, V., Collin, I., Cummings, J. L., & Chertkow, H. (2005). Montreal cognitive assessment (MoCA) [Database record]. APA PsycTests . https://doi.org/10.1037/t27279-000
Moms, J. C., Heyman, A., Mohs, R. C., Hughes, J. P., van Belle, G., Fillenbaum, G., & Clark, C. (1989). The consortium to establish a registry for Alzheimer’s disease (CERAD): part I: Clinical and neuropsychological assessment of Alzheimer’s disease. Neurology, 39 (9), 1159–1159. https://doi.org/10.1212/WNL.39.9.1159
Graf, C. (2008). The Lawton instrumental activities of daily living scale. The American Journal of Nursing, 108 (4), 52–63. https://doi.org/10.1097/01.NAJ.0000314810.46029.74
Lubben, J., & Gironda, M. (2004). Measuring social networks and assessing their benefits. Social Networks and Social Exclusion, 4 , 20–34.
Yesavage, J. A., Brink, T. L., Rose, T. L., Lum, O., Huang, V., Adey, M., & Leirer, V. O. (1982). Development and validation of a geriatric depression screening scale: A preliminary report. Journal of Psychiatric Research, 17 (1), 37–49. https://doi.org/10.1016/0022-3956(82)90033-4
Schwarzer, R. (1999). Skala zur allgemeinen Selbstwirksamkeitserwartung.
Cohen, J. (1992). Statistical power analysis. Current Directions in Psychological Science, 1 (3), 98–101. https://doi.org/10.1111/1467-8721.ep10768783
Feng, Y. S., Kohlmann, T., Janssen, M. F., & Buchholz, I. (2021). Psychometric properties of the EQ-5D-5L: A systematic review of the literature. Quality of Life Research, 30 , 647–673. https://doi.org/10.1007/s11136-020-02688-y
Whoqol Group. (1998). Development of the World Health Organization WHOQOL-BREF quality of life assessment. Psychological Medicine, 28 (3), 551–558. https://doi.org/10.1017/S0033291798006667
Long, D., Polinder, S., Bonsel, G. J., & Haagsma, J. A. (2021). Test–retest reliability of the EQ-5D-5L and the reworded QOLIBRI-OS in the general population of Italy, the Netherlands, and the United Kingdom. Quality of Life Research, 30 , 2961–2971.
Peel, N. M., Bartlett, H. P., & Marshall, A. L. (2007). Measuring quality of life in older people: Reliability and validity of WHOQOL-OLD. Australasian Journal on Ageing, 26 (4), 162–167. https://doi.org/10.1007/s11136-021-02893-3
Hunter, E. G., & Kearney, P. J. (2018). Occupational therapy interventions to improve performance of instrumental activities of daily living for community-dwelling older adults: A systematic review. The American Journal of Occupational Therapy, 72 (4), 5. https://doi.org/10.5014/ajot.2018.031062
Gobbens, R. J. (2018). Associations of ADL and IADL disability with physical and mental dimensions of quality of life in people aged 75 years and older. PeerJ, 6 , e5425. https://doi.org/10.7717/peerj.5425
Korczak, D., Habermann, C., & Braz, S. (2013). The effectiveness of occupational therapy for persons with moderate and severe dementia. GMS Health Technology Assessment, 9 , 7. https://doi.org/10.3205/hta000115
Carmel, S. (2019). Health and well-being in late life: Gender differences worldwide. Frontiers in Medicine, 6 , 218. https://doi.org/10.3389/fmed.2019.00218
Kahneman, D., & Deaton, A. (2010). High income improves evaluation of life but not emotional well-being. Proceedings of the National Academy of Sciences, 107 (38), 16489–16493. https://doi.org/10.1073/pnas.1011492107
Fjeldstad, C., Fjeldstad, A. S., Acree, L. S., Nickel, K. J., & Gardner, A. W. (2008). The influence of obesity on falls and quality of life. Dynamic Medicine, 7 (1), 1–6. https://doi.org/10.1186/1476-5918-7-4
Edgerton, J. D., Roberts, L. W., & von Below, S. (2011). Education and quality of life. Handbook of Social Indicators and Quality of Life Research, 5 , 265–296. https://doi.org/10.1007/978-94-007-2421-1_12
Cummins, R. A. (1997). Assessing quality of life. Quality of Life for People with Disabilities, 2 , 116–150.
Netuveli, G., & Blane, D. (2008). Quality of life in older ages. British Medical Bulletin, 85 (1), 113–126. https://doi.org/10.1093/bmb/ldn003
Taylor, V. H., Forhan, M., Vigod, S. N., McIntyre, R. S., & Morrison, K. M. (2013). The impact of obesity on quality of life. Best Practice & Research Clinical Endocrinology & Metabolism, 27 (2), 139–146. https://doi.org/10.1016/j.beem.2013.04.004
Fjeldstad, C., Fjeldstad, A. S., Acree, L. S., Nickel, K. J., & Gardner, A. W. (2008). The influence of obesity on falls and quality of life. Dynamic Medicine, 7 (1), 1–6. https://doi.org/10.1186/1476-918-7-4
Sivertsen, H., Bjørkløf, G. H., Engedal, K., Selbæk, G., & Helvik, A. S. (2015). Depression and quality of life in older persons: A review. Dementia and Geriatric Cognitive Disorders, 40 (5–6), 311–339. https://doi.org/10.1159/000437299
Cardona, M. I., Weißenborn, M., Zöllinger, I., Kroeber, E. S., Bauer, A., Luppa, M., & Thyrian, J. R. (2022). Physical activity determinants in older german adults at increased dementia risk with multimorbidity: Baseline results of the AgeWell.de study. International Journal of Environmental Research and Public Health, 19 (6), 3164. https://doi.org/10.3390/ijerph19063164
Download references
Acknowledgements
Members of the AgeWell.de Study Group. Principal Investigator and Co-Principal Investigators: Steffi G. Riedel-Heller (PI), Wolfgang Hoffmann, Jochen Gensichen, Walter E. Haefeli, Hanna Kaduszkiewicz, Hans-Helmut König, Thomas Frese, David Czock, Jochen René Thyrian, Birgitt Wiese. Franziska Berg, Andrea Bischhoff, Christian Brettschneider; Mandy Claus, Juliane Döhring*, Alexander Eßer, Corinna Gräble, Caroline Jung-Sievers*, Kerstin Klauer-Tiedtke, Kerstin Krebs-Hein, Flora Kühne, Sebastian Lange, Paula Liegert, Dagmar Lochmann, Tobias Luck, Melanie Luppa, Silke Mamone, Andreas Meid, Michael Metzner, Lydia Neubert, Anke Oey, Susanne Röhr*, Franziska-Antonia Zora Samos*, Karin Schumacher, Theresa Terstegen, Sandy Thieme, Lars Wamsiedler, Tanja Wehran, Marina Weißenborn, Ines Winkler, Isabel Zöllinger, Andrea Zülke*, Ina Zwingmann. The authors thank the study participants, the study nurses, and the general practitioners.
Open Access funding enabled and organized by Projekt DEAL. This publication is part of the study “AgeWell.de – a multi-centric cluster-randomized controlled prevention trial in primary care” and was funded by the German Federal Ministry for Education and Research (BMBF; Grants: 01GL1704A, 01GL1704B, 01GL1704C, 01GL1704D, 01GL1704E, 01GL1704F).
Author information
Steffi Riedel-Heller and Hanna Kaduszkiewicz have shared last authorship.
Authors and Affiliations
Institute of General Practice, University of Kiel, 24105, Kiel, Germany
Juliane Döhring, Martin Williamson & Hanna Kaduszkiewicz
Institute of Social Medicine, Occupational Health and Public Health (ISAP), Medical Faculty, University of Leipzig, 04103, Leipzig, Germany
Melanie Luppa, Alexander Pabst & Steffi Riedel-Heller
Department of Health Economics and Health Services Research, University Medical Center Hamburg-Eppendorf, 20246, Hamburg, Germany
Christian Brettschneider & Hans-Helmut König
Department of Clinical Pharmacology and Pharmacoepidemiology, University Hospital Heidelberg, 69120, Heidelberg, Germany
Marina Weißenborn & David Czock
Institute of General Practice/Family Medicine, University Hospital of LMU Munich, 80336, Munich, Germany
Isabel Zöllinger & Jochen Gensichen
Institute of General Practice and Family Medicine, Martin-Luther-University Halle-Wittenberg, 06112, Halle (Saale), Germany
Thomas Fankhänel & Thomas Frese
Work Group Medical Statistics and IT-Infrastructure, Hannover Medical School, Institute for General Practice, 30625, Hannover, Germany
Birgitt Wiese
Institute for Community Medicine, University Medicine Greifswald, 17489, Greifswald, Germany
Wolfgang Hoffmann & Jochen René Thyrian
Deutsches Zentrum für Neurodegenerative Erkrankungen (DZNE), 17489, Greifswald, Germany
Jochen René Thyrian
You can also search for this author in PubMed Google Scholar
Contributions
Steffi Riedel-Heller conceptualized and designed the study and was supported by Hanna Kaduszkiewicz, Wolfgang Hoffmann, Jochen René Thyrian, Jochen Gensichen, Hans-Helmut König, Thomas Frese, David Czock, Alexander Pabst, Thomas Fakhänel, and Birgitt Wiese. Juliane Döhring and Hanna Kaduszkiewicz drafted the manuscript. Martin Williamson, Melanie Luppa, Alexander Pabst, Christian Brettschneider, David Czock, and Marina Weißenborn revised the manuscript for intellectual content. All authors read and approved the final manuscript.
Corresponding author
Correspondence to Juliane Döhring .
Ethics declarations
Competing interest.
The authors have no relevant financial or non-financial interests to declare.
Consent to participate
Written consent to participate was obtained at the GP practice after the GP provided all necessary information about the study. The signed informed consent form was sent to the recruiting study site by the GP, along with participants’ contact information and data on participants’ health characteristics.
Ethical approval
This study was performed in line with the principles of the Declaration of Helsinki. “AgeWell.de” was approved by the responsible ethics boards of all participating study sites (Ethical Committee at the Medical Faculty, Leipzig University; Ethical Committee at the Medical Faculty, Christian-Albrechts-University, Kiel; Ethical Committee at Universitätsmedizin Greifswald; Ethical Committee at the Medical Faculty, Ludwig-Maximilian-University, Munich; Ethical Committee at the Medical Faculty, Martin-Luther-University Halle-Wittenberg; Ruprecht-Karls-University, Heidelberg). The study design was discussed with general practitioners (GPs) and seniors organized in a senior interest group (Seniorenbeirat der Stadt Leipzig).
Additional information
Publisher's note.
Springer Nature remains neutral with regard to jurisdictional claims in published maps and institutional affiliations.
Supplementary Information
Below is the link to the electronic supplementary material.
Supplementary file1 (DOCX 22 kb)
Supplementary file2 (docx 15 kb), rights and permissions.
Open Access This article is licensed under a Creative Commons Attribution 4.0 International License, which permits use, sharing, adaptation, distribution and reproduction in any medium or format, as long as you give appropriate credit to the original author(s) and the source, provide a link to the Creative Commons licence, and indicate if changes were made. The images or other third party material in this article are included in the article's Creative Commons licence, unless indicated otherwise in a credit line to the material. If material is not included in the article's Creative Commons licence and your intended use is not permitted by statutory regulation or exceeds the permitted use, you will need to obtain permission directly from the copyright holder. To view a copy of this licence, visit http://creativecommons.org/licenses/by/4.0/ .
Reprints and permissions
About this article
Döhring, J., Williamson, M., Brettschneider, C. et al. Quality of life in a high-risk group of elderly primary care patients: characteristics and potential for improvement. Qual Life Res (2024). https://doi.org/10.1007/s11136-024-03647-7
Download citation
Accepted : 08 March 2024
Published : 13 May 2024
DOI : https://doi.org/10.1007/s11136-024-03647-7
Share this article
Anyone you share the following link with will be able to read this content:
Sorry, a shareable link is not currently available for this article.
Provided by the Springer Nature SharedIt content-sharing initiative
- Quality of life
- Risk factors
- Primary care
- Find a journal
- Publish with us
- Track your research
Internet explorer is no longer supported
We have detected that you are using Internet Explorer to visit this website. Internet Explorer is now being phased out by Microsoft. As a result, NHS Digital no longer supports any version of Internet Explorer for our web-based products, as it involves considerable extra effort and expense, which cannot be justified from public funds. Some features on this site will not work. You should use a modern browser such as Edge, Chrome, Firefox, or Safari. If you have difficulty installing or accessing a different browser, contact your IT support team.
Using predictive analytics in adult social care
A case study with Luton Adult Social Care.
Luton Council aimed to improve the communication between healthcare and adult social care.
The e-frailty index is collated from primary care data including symptoms, diseases, disabilities and abnormal test values. However, for falls and other health conditions requiring a system response via the Telecare system, data are not routinely collated into the Primary Care Home (PCH) dataset. This results in siloed working as data is retained in different teams, preventing a multidisciplinary approach to intervention or targeting of the required professional support.
What the project involves
By collaborating with multiple stakeholders the Luton health and care system developed a 'Framework for Frailty'. This is in response to requests from partners for a common approach to the early recognition and identification of frailty as a long-term condition.
It was anticipated that by making this data available, there will be better targeted prevention and early intervention through a multi-disciplinary approach.
This project aimed to incorporate data received from the assistive technology system - Telecare, and have this information recorded in the social care IT system (Liquidlogic), to provide health and social care staff with a more holistic view of patients' likely health and social care needs.
Personalised prevention
Potential savings of £2.7m for hospitals
Reduced admissions to hospital
Benefits of the Framework for Fragility
Clinical staff benefit from:
- increased knowledge allowing them to support service users more effectively
- a reduction in non-elective admissions, leading to better utilisation of resources and associated savings
- improved communication with social care staff
- the ability to use data to predict and thus prevent deterioration
Bedfordshire, Luton and Milton Keynes (BLMK) County Council benefit from improved and more complete data source for BLMK population management.
Social care staff benefit from:
- previously unavailable data now visible and reportable to the local authority enabling trend analysis
- information available in near real-time allowing improved prioritisation
Patients benefit from:
- (it is hoped) improved engagement by users as needs are being addressed more fully
- a better understanding of issues using richer data, allowing personalised preventative techniques to be used to target those most at risk and help anxiety and prevent future falls
Lessons learned so far
The Luton health and care system has learned that:
- engagement with all key technical stakeholders at the outset of the project ensured that requirements were understood throughout the lifetime of the project
- the project team were working on a range of different projects, leading to competing priorities - a dedicated project team may have advantage in the future
- holding a weekly meeting created a useful forum to discuss emerging risks and issues, and should be continued
- engagement with third-party suppliers needs to be carried out at an earlier stage to prevent delays with IT systems
Download the case study
Last edited: 24 November 2022 2:11 pm
Search terms
- Predictive analytics in healthcare: three real-world examples
Jun 12, 2020 - Reading time 8-10 minutes
Predictive analytics in healthcare can help to detect early signs of patient deterioration in the ICU and general ward, identify at-risk patients in their homes to prevent hospital readmissions, and prevent avoidable downtime of medical equipment.
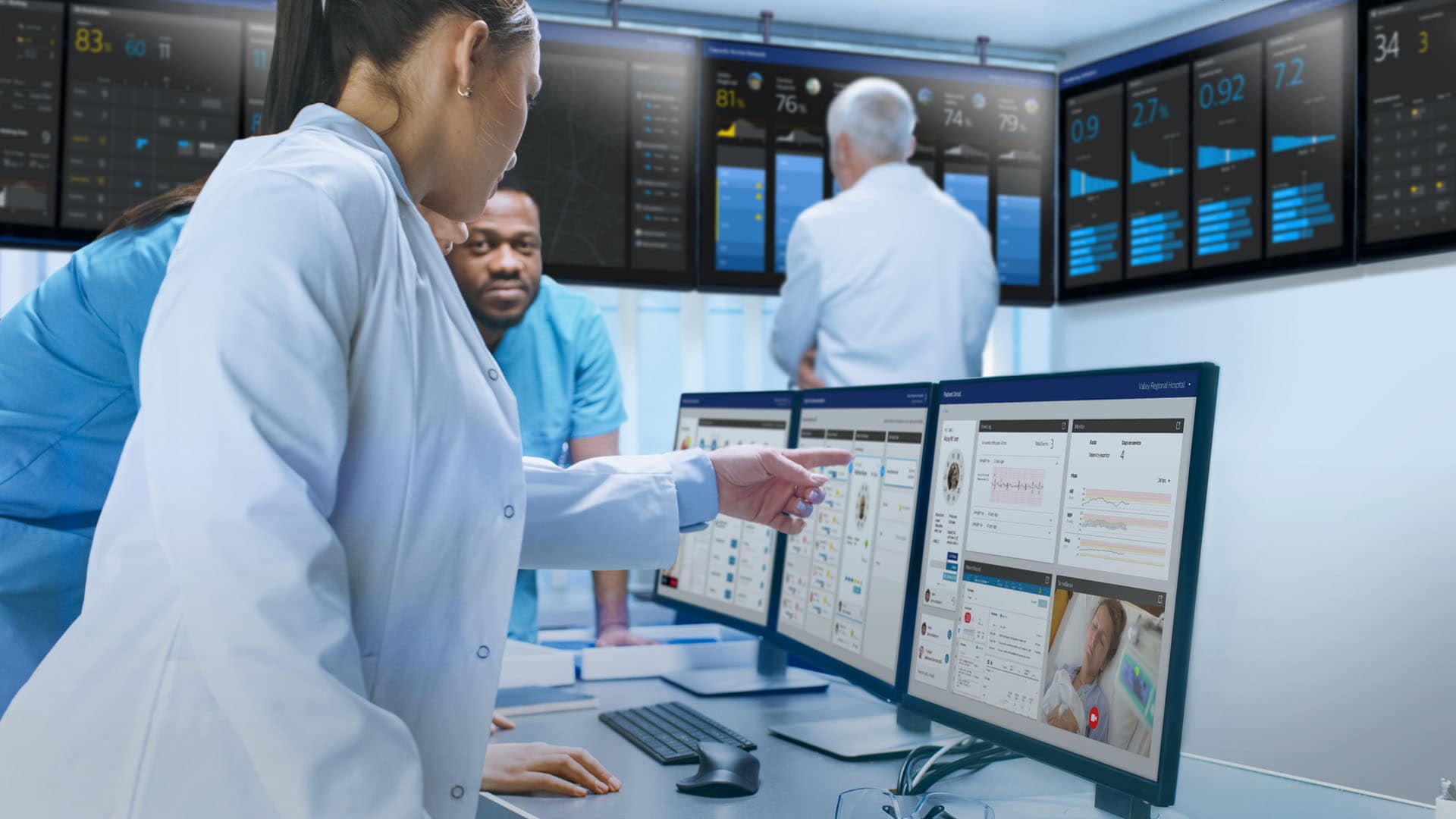
April 19, 2024
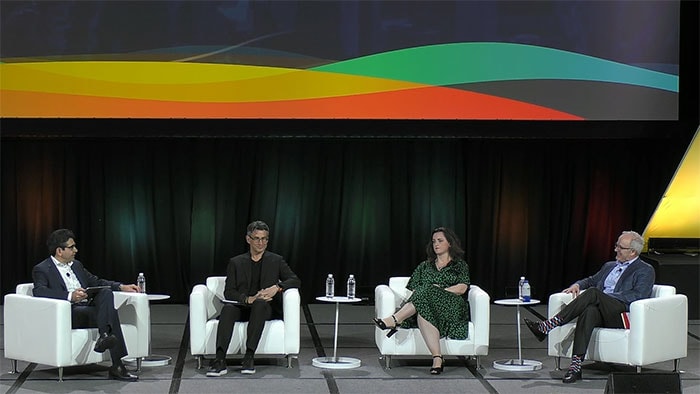
March 21, 2024
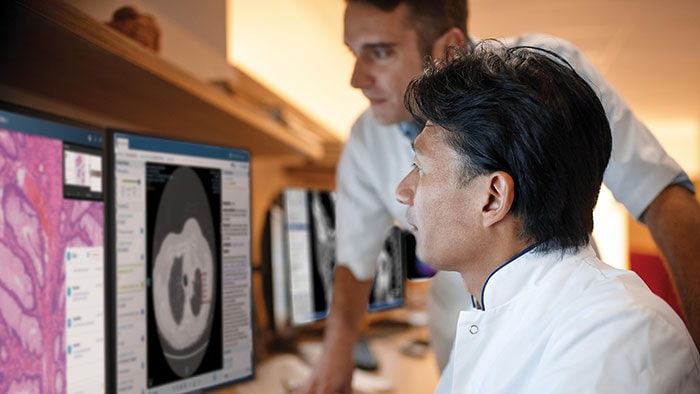
March 12, 2024
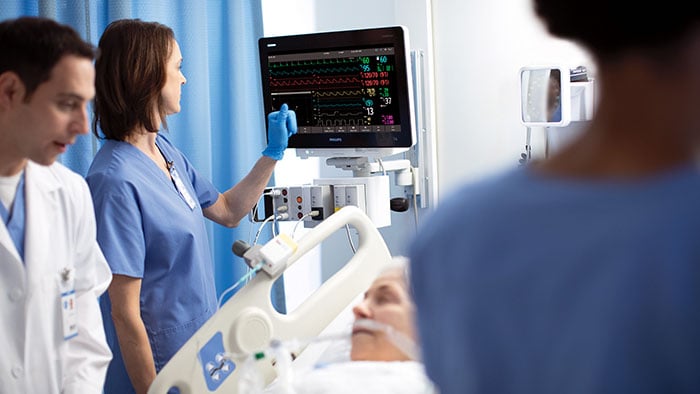
March 04, 2024
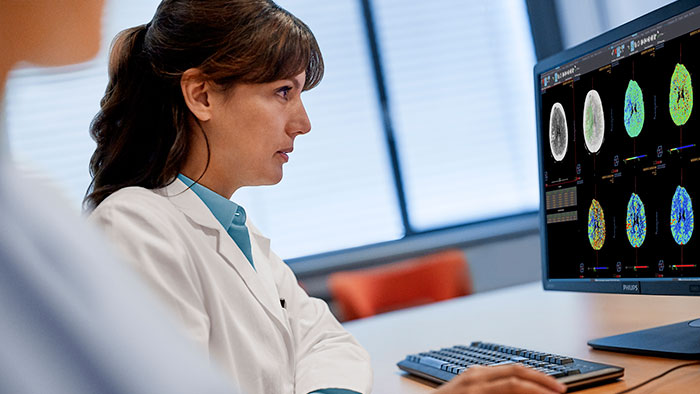
February 29, 2024
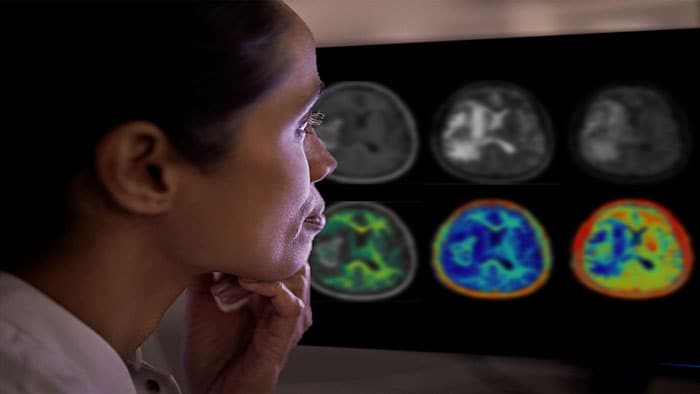
February 09, 2024
News center
- Consumer products
- Product registration
- Healthcare professionals
- Specialties
- Other business solutions
- Hearing solutions
- Display solutions
- Dictation Solutions
- News & Insights
- Investor Relations
- Environmental, Social and Governance
- Contact & support
- Customer support
- Professional healthcare support
- Company contacts
- Stay up-to-date Stay up-to-date Subscribe to our news
Select country
- Privacy Notice
- Terms of use
- Cookie notice
- Add product
By clicking on the link, you will be leaving the official Royal Philips ("Philips") website. Any links to third-party websites that may appear on this site are provided only for your convenience and in no way represent any affiliation or endorsement of the information provided on those linked websites. Philips makes no representations or warranties of any kind with regard to any third-party websites or the information contained therein.
You are about to visit a Philips global content page
Our site can best be viewed with the latest version of Microsoft Edge, Google Chrome or Firefox.
- Skip to main content
- Skip to primary sidebar
- Skip to footer
- QuestionPro

- Solutions Industries Gaming Automotive Sports and events Education Government Travel & Hospitality Financial Services Healthcare Cannabis Technology Use Case NPS+ Communities Audience Contactless surveys Mobile LivePolls Member Experience GDPR Positive People Science 360 Feedback Surveys
- Resources Blog eBooks Survey Templates Case Studies Training Help center

Home Market Research Research Tools and Apps
Analytical Research: What is it, Importance + Examples
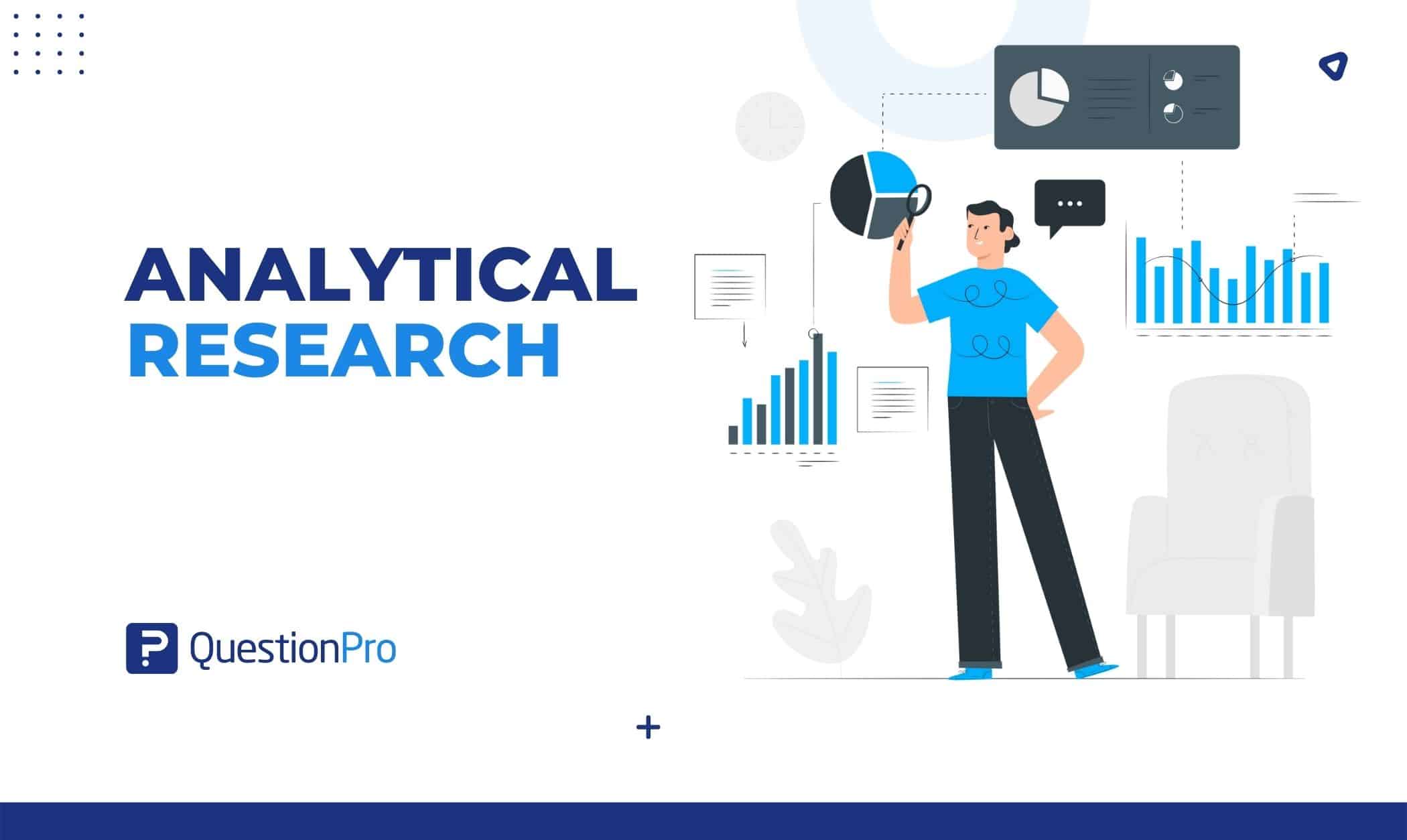
Finding knowledge is a loose translation of the word “research.” It’s a systematic and scientific way of researching a particular subject. As a result, research is a form of scientific investigation that seeks to learn more. Analytical research is one of them.
Any kind of research is a way to learn new things. In this research, data and other pertinent information about a project are assembled; after the information is gathered and assessed, the sources are used to support a notion or prove a hypothesis.
An individual can successfully draw out minor facts to make more significant conclusions about the subject matter by using critical thinking abilities (a technique of thinking that entails identifying a claim or assumption and determining whether it is accurate or untrue).
What is analytical research?
This particular kind of research calls for using critical thinking abilities and assessing data and information pertinent to the project at hand.
Determines the causal connections between two or more variables. The analytical study aims to identify the causes and mechanisms underlying the trade deficit’s movement throughout a given period.
It is used by various professionals, including psychologists, doctors, and students, to identify the most pertinent material during investigations. One learns crucial information from analytical research that helps them contribute fresh concepts to the work they are producing.
Some researchers perform it to uncover information that supports ongoing research to strengthen the validity of their findings. Other scholars engage in analytical research to generate fresh perspectives on the subject.
Various approaches to performing research include literary analysis, Gap analysis , general public surveys, clinical trials, and meta-analysis.
Importance of analytical research
The goal of analytical research is to develop new ideas that are more believable by combining numerous minute details.
The analytical investigation is what explains why a claim should be trusted. Finding out why something occurs is complex. You need to be able to evaluate information critically and think critically.
This kind of information aids in proving the validity of a theory or supporting a hypothesis. It assists in recognizing a claim and determining whether it is true.
Analytical kind of research is valuable to many people, including students, psychologists, marketers, and others. It aids in determining which advertising initiatives within a firm perform best. In the meantime, medical research and research design determine how well a particular treatment does.
Thus, analytical research can help people achieve their goals while saving lives and money.
Methods of Conducting Analytical Research
Analytical research is the process of gathering, analyzing, and interpreting information to make inferences and reach conclusions. Depending on the purpose of the research and the data you have access to, you can conduct analytical research using a variety of methods. Here are a few typical approaches:
Quantitative research
Numerical data are gathered and analyzed using this method. Statistical methods are then used to analyze the information, which is often collected using surveys, experiments, or pre-existing datasets. Results from quantitative research can be measured, compared, and generalized numerically.
Qualitative research
In contrast to quantitative research, qualitative research focuses on collecting non-numerical information. It gathers detailed information using techniques like interviews, focus groups, observations, or content research. Understanding social phenomena, exploring experiences, and revealing underlying meanings and motivations are all goals of qualitative research.
Mixed methods research
This strategy combines quantitative and qualitative methodologies to grasp a research problem thoroughly. Mixed methods research often entails gathering and evaluating both numerical and non-numerical data, integrating the results, and offering a more comprehensive viewpoint on the research issue.
Experimental research
Experimental research is frequently employed in scientific trials and investigations to establish causal links between variables. This approach entails modifying variables in a controlled environment to identify cause-and-effect connections. Researchers randomly divide volunteers into several groups, provide various interventions or treatments, and track the results.
Observational research
With this approach, behaviors or occurrences are observed and methodically recorded without any outside interference or variable data manipulation . Both controlled surroundings and naturalistic settings can be used for observational research . It offers useful insights into behaviors that occur in the actual world and enables researchers to explore events as they naturally occur.
Case study research
This approach entails thorough research of a single case or a small group of related cases. Case-control studies frequently include a variety of information sources, including observations, records, and interviews. They offer rich, in-depth insights and are particularly helpful for researching complex phenomena in practical settings.
Secondary data analysis
Examining secondary information is time and money-efficient, enabling researchers to explore new research issues or confirm prior findings. With this approach, researchers examine previously gathered information for a different reason. Information from earlier cohort studies, accessible databases, or corporate documents may be included in this.
Content analysis
Content research is frequently employed in social sciences, media observational studies, and cross-sectional studies. This approach systematically examines the content of texts, including media, speeches, and written documents. Themes, patterns, or keywords are found and categorized by researchers to make inferences about the content.
Depending on your research objectives, the resources at your disposal, and the type of data you wish to analyze, selecting the most appropriate approach or combination of methodologies is crucial to conducting analytical research.
Examples of analytical research
Analytical research takes a unique measurement. Instead, you would consider the causes and changes to the trade imbalance. Detailed statistics and statistical checks help guarantee that the results are significant.
For example, it can look into why the value of the Japanese Yen has decreased. This is so that an analytical study can consider “how” and “why” questions.
Another example is that someone might conduct analytical research to identify a study’s gap. It presents a fresh perspective on your data. Therefore, it aids in supporting or refuting notions.
Descriptive vs analytical research
Here are the key differences between descriptive research and analytical research:
The study of cause and effect makes extensive use of analytical research. It benefits from numerous academic disciplines, including marketing, health, and psychology, because it offers more conclusive information for addressing research issues.
QuestionPro offers solutions for every issue and industry, making it more than just survey software. For handling data, we also have systems like our InsightsHub research library.
You may make crucial decisions quickly while using QuestionPro to understand your clients and other study subjects better. Make use of the possibilities of the enterprise-grade research suite right away!
LEARN MORE FREE TRIAL
MORE LIKE THIS
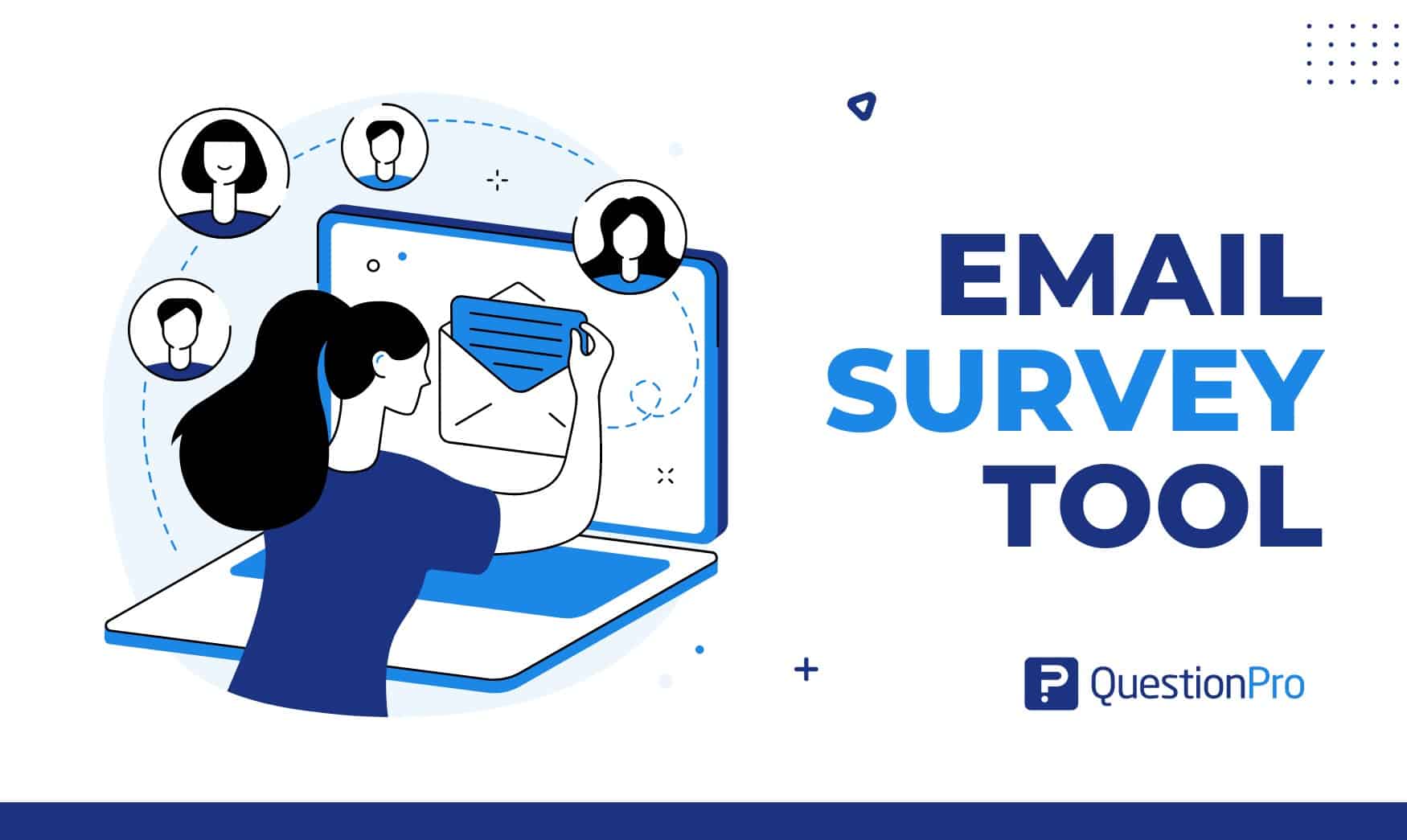
The Best Email Survey Tool to Boost Your Feedback Game
May 7, 2024
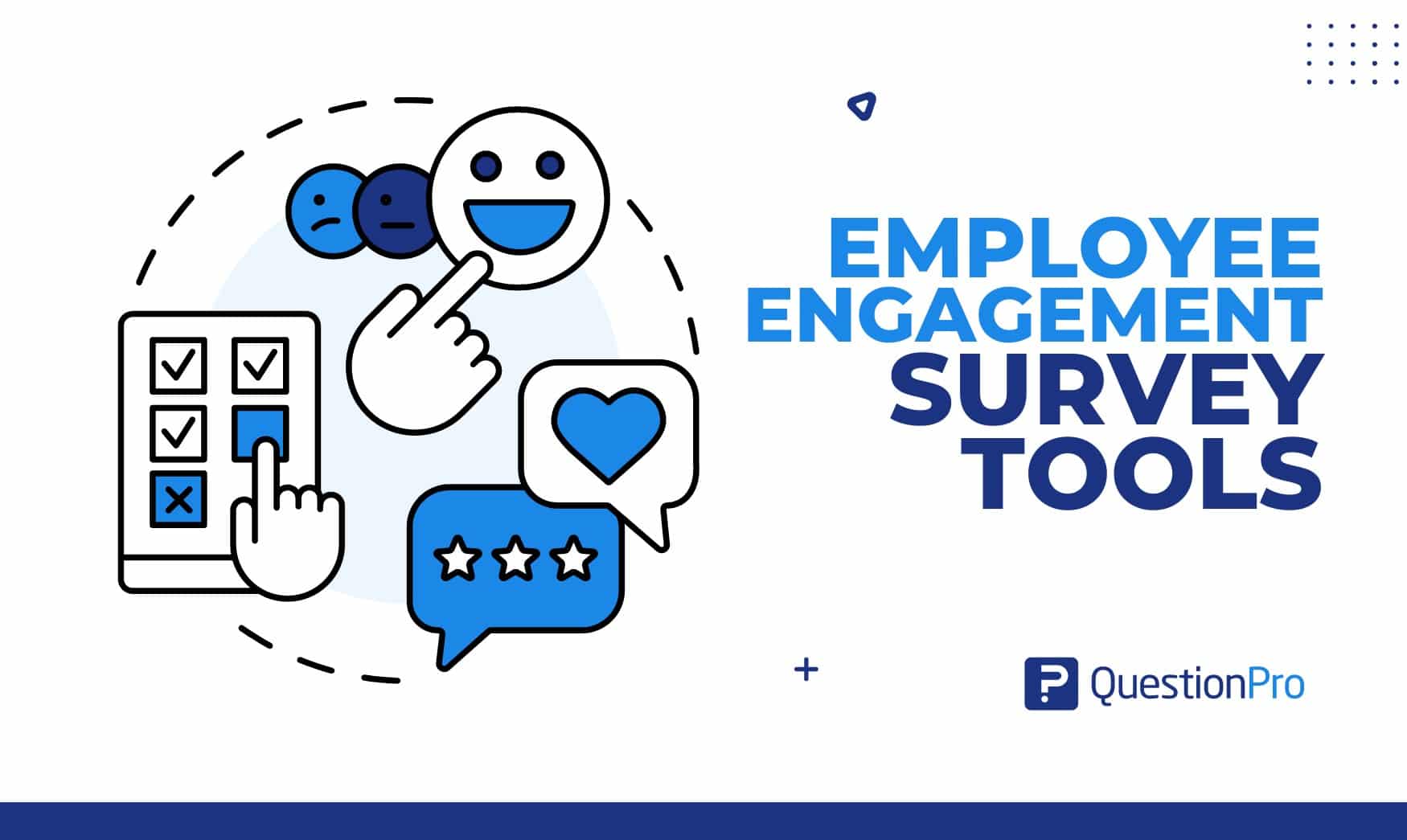
Top 10 Employee Engagement Survey Tools
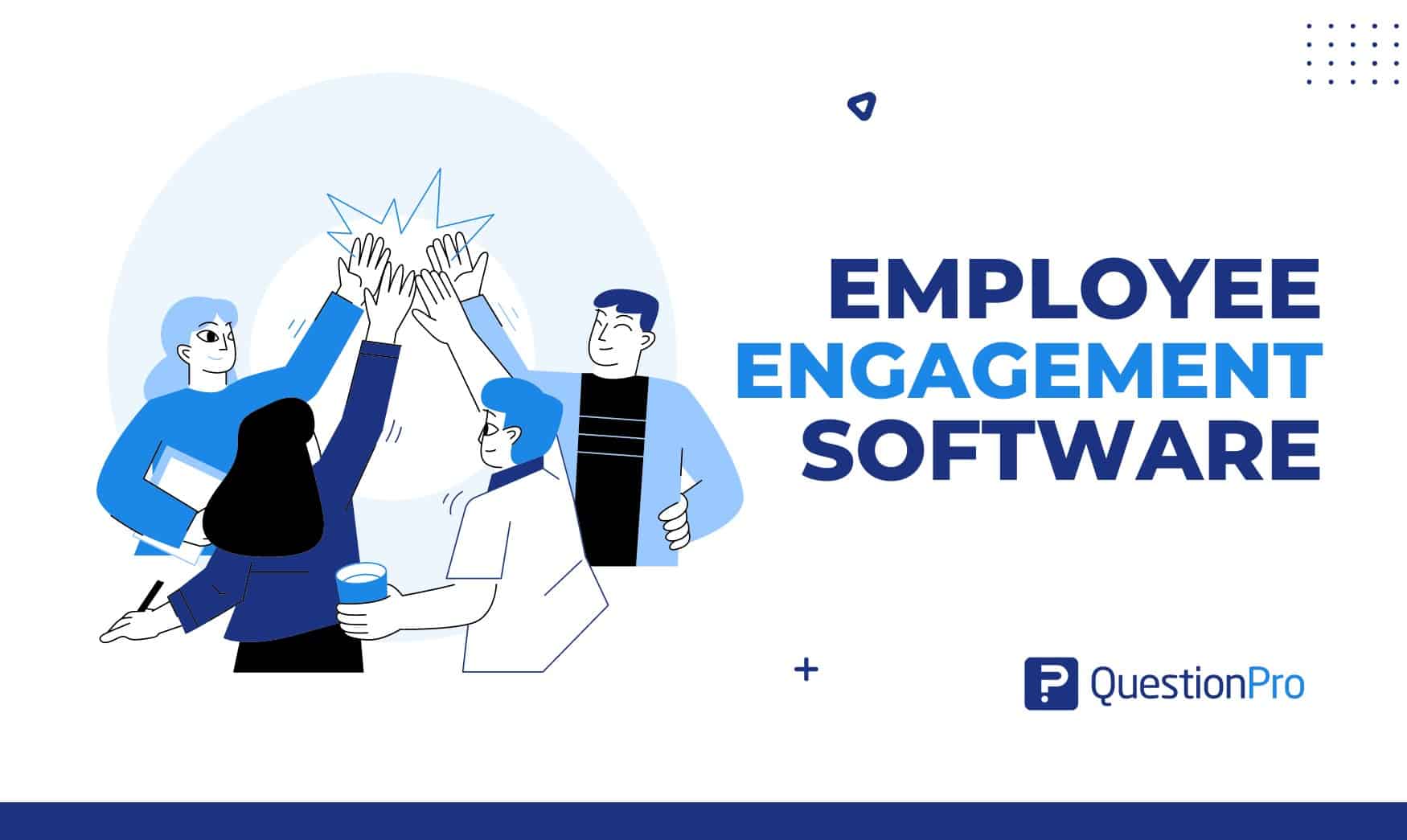
Top 20 Employee Engagement Software Solutions
May 3, 2024
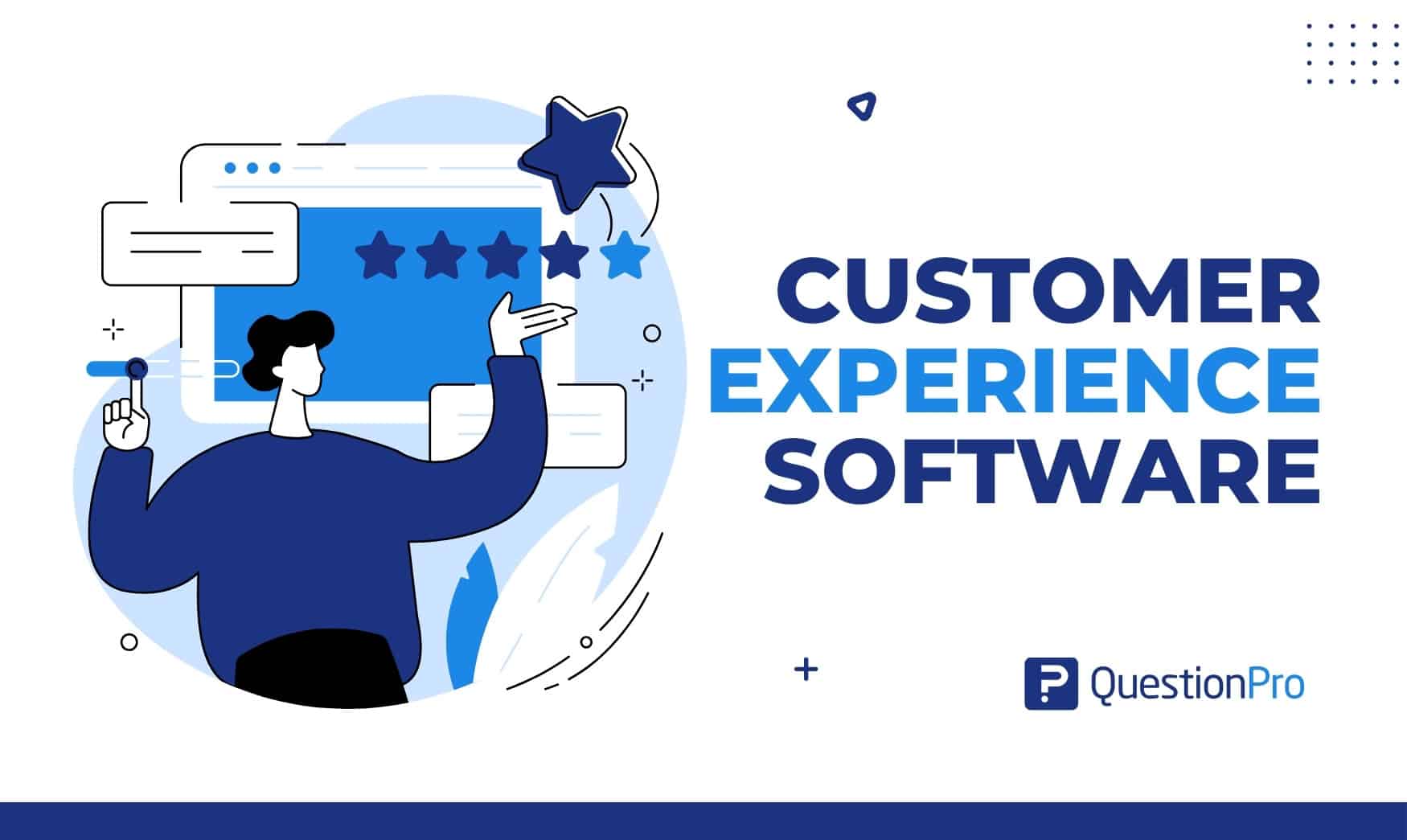
15 Best Customer Experience Software of 2024
May 2, 2024
Other categories
- Academic Research
- Artificial Intelligence
- Assessments
- Brand Awareness
- Case Studies
- Communities
- Consumer Insights
- Customer effort score
- Customer Engagement
- Customer Experience
- Customer Loyalty
- Customer Research
- Customer Satisfaction
- Employee Benefits
- Employee Engagement
- Employee Retention
- Friday Five
- General Data Protection Regulation
- Insights Hub
- Life@QuestionPro
- Market Research
- Mobile diaries
- Mobile Surveys
- New Features
- Online Communities
- Question Types
- Questionnaire
- QuestionPro Products
- Release Notes
- Research Tools and Apps
- Revenue at Risk
- Survey Templates
- Training Tips
- Uncategorized
- Video Learning Series
- What’s Coming Up
- Workforce Intelligence
- Research article
- Open access
- Published: 01 December 2006
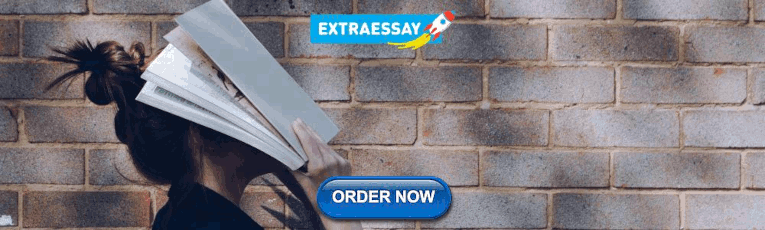
Using quantitative and qualitative data in health services research – what happens when mixed method findings conflict? [ISRCTN61522618]
- Suzanne Moffatt 1 ,
- Martin White 1 ,
- Joan Mackintosh 1 &
- Denise Howel 1
BMC Health Services Research volume 6 , Article number: 28 ( 2006 ) Cite this article
84k Accesses
122 Citations
16 Altmetric
Metrics details
In this methodological paper we document the interpretation of a mixed methods study and outline an approach to dealing with apparent discrepancies between qualitative and quantitative research data in a pilot study evaluating whether welfare rights advice has an impact on health and social outcomes among a population aged 60 and over.
Quantitative and qualitative data were collected contemporaneously. Quantitative data were collected from 126 men and women aged over 60 within a randomised controlled trial. Participants received a full welfare benefits assessment which successfully identified additional financial and non-financial resources for 60% of them. A range of demographic, health and social outcome measures were assessed at baseline, 6, 12 and 24 month follow up. Qualitative data were collected from a sub-sample of 25 participants purposively selected to take part in individual interviews to examine the perceived impact of welfare rights advice.
Separate analysis of the quantitative and qualitative data revealed discrepant findings. The quantitative data showed little evidence of significant differences of a size that would be of practical or clinical interest, suggesting that the intervention had no impact on these outcome measures. The qualitative data suggested wide-ranging impacts, indicating that the intervention had a positive effect. Six ways of further exploring these data were considered: (i) treating the methods as fundamentally different; (ii) exploring the methodological rigour of each component; (iii) exploring dataset comparability; (iv) collecting further data and making further comparisons; (v) exploring the process of the intervention; and (vi) exploring whether the outcomes of the two components match.
The study demonstrates how using mixed methods can lead to different and sometimes conflicting accounts and, using this six step approach, how such discrepancies can be harnessed to interrogate each dataset more fully. Not only does this enhance the robustness of the study, it may lead to different conclusions from those that would have been drawn through relying on one method alone and demonstrates the value of collecting both types of data within a single study. More widespread use of mixed methods in trials of complex interventions is likely to enhance the overall quality of the evidence base.
Combining quantitative and qualitative methods in a single study is not uncommon in social research, although, 'traditionally a gulf is seen to exist between qualitative and quantitative research with each belonging to distinctively different paradigms'. [ 1 ] Within health research there has, more recently, been an upsurge of interest in the combined use of qualitative and quantitative methods, sometimes termed mixed methods research [ 2 ] although the terminology can vary. [ 3 ] Greater interest in qualitative research has come about for a number of reasons: the numerous contributions made by qualitative research to the study of health and illness [ 4 – 6 ]; increased methodological rigor [ 7 ] within the qualitative paradigm, which has made it more acceptable to researchers or practitioners trained within a predominantly quantitative paradigm [ 8 ]; and, because combining quantitative and qualitative methods may generate deeper insights than either method alone. [ 9 ] It is now widely recognised that public health problems are embedded within a range of social, political and economic contexts. [ 10 ] Consequently, a range of epidemiological and social science methods are employed to research these complex issues. [ 11 ] Further legitimacy for the use of qualitative methods alongside quantitative has resulted from the recognition that qualitative methods can make an important contribution to randomised controlled trials (RCTs) evaluating complex health service interventions. There is published work on the various ways that qualitative methods are being used in RCTs (e.g. [ 12 , 13 ] but little on how they can optimally enhance the usefulness and policy relevance of trial findings. [ 14 , 15 ]
A number of mixed methods publications outline the various ways in which qualitative and quantitative methods can be combined. [ 1 , 2 , 9 , 16 ] For the purposes of this paper with its focus on mixed methods in the context of a pilot RCT, the significant aspects of mixed methods appear to be: purpose, process and, analysis and interpretation. In terms of purpose, qualitative research may be used to help identify the relevant variables for study [ 17 ], develop an instrument for quantitative research [ 18 ], to examine different questions (such as acceptability of the intervention, rather than its outcome) [ 19 ]; and to examine the same question with different methods (using, for example participant observation or in depth interviews [ 1 ]). Process includes the priority accorded to each method and ordering of both methods which may be concurrent, sequential or iterative. [ 20 ] Bryman [ 9 ] points out that, 'most researchers rely primarily on a method associated with either quantitative or qualitative methods and then buttress their findings with a method associated with the other tradition' (p128). Both datasets may be brought together at the 'analysis/interpretation' phase, often known as 'triangulation' [ 21 ]. Brannen [ 1 ] suggests that most researchers have taken this to mean more than one type of data, but she stresses that Denzin's original conceptualisation involved methods, data, investigators or theories. Bringing different methods together almost inevitably raises discrepancies in findings and their interpretation. However, the investigation of such differences may be as illuminating as their points of similarity. [ 1 , 9 ]
Although mixed methods are now widespread in health research, quantitative and qualitative methods and results are often published separately. [ 22 , 23 ] It is relatively rare to see an account of the methodological implications of the strategy and the way in which both methods are combined when interpreting the data within a particular study. [ 1 ] A notable exception is a study showing divergence between qualitative and quantitative findings of cancer patients' quality of life using a detailed case study approach to the data. [ 13 ]
By presenting quantitative and qualitative data collected within a pilot RCT together, this paper has three main aims: firstly, to demonstrate how divergent quantitative and qualitative data led us to interrogate each dataset more fully and assisted in the interpretation process, producing a greater research yield from each dataset; secondly, to demonstrate how combining both types of data at the analysis stage produces 'more than the sum of its parts'; and thirdly, to emphasise the complementary nature of qualitative and quantitative methods in RCTs of complex interventions. In doing so, we demonstrate how the combination of quantitative and qualitative data led us to conclusions different from those that would have been drawn through relying on one or other method alone.
The study that forms the basis of this paper, a pilot RCT to examine the impact of welfare rights advice in primary care, was funded under the UK Department of Health's Policy Research Programme on tackling health inequalities, and focused on older people. To date, little research has been able to demonstrate how health inequalities can be tackled by interventions within and outside the health sector. Although living standards have risen among older people, a common experience of growing old is worsening material circumstances. [ 24 ] In 2000–01 there were 2.3 million UK pensioners living in households with below 60 per cent of median household income, after housing costs. [ 25 ] Older people in the UK may be eligible for a number of income- or disability-related benefits (the latter could be non-financial such as parking permits or adaptations to the home), but it has been estimated that approximately one in four (about one million) UK pensioner households do not claim the support to which they are entitled. [ 26 ] Action to facilitate access to and uptake of welfare benefits has taken place outside the UK health sector for many years and, more recently, has been introduced within parts of the health service, but its potential to benefit health has not been rigorously evaluated. [ 27 – 29 ]
There are a number of models of mixed methods research. [ 2 , 16 , 30 ] We adopted a model which relies of the principle of complementarity, using the strengths of one method to enhance the other. [ 30 ] We explicitly recognised that each method was appropriate for different research questions. We undertook a pragmatic RCT which aimed to evaluate the health effects of welfare rights advice in primary care among people aged over 60. Quantitative data included standardised outcome measures of health and well-being, health related behaviour, psycho-social interaction and socio-economic status ; qualitative data used semi-structured interviews to explore participants' views about the intervention, its outcome, and the acceptability of the research process.
Following an earlier qualitative pilot study to inform the selection of appropriate outcome measures [ 31 ], contemporaneous quantitative and qualitative data were collected. Both datasets were analysed separately and neither compared until both analyses were complete. The sampling strategy mirrored the embedded design; probability sampling for the quantitative study and theoretical sampling for the qualitative study, done on the basis of factors identified in the quantitative study.
Approval for the study was obtained from Newcastle and North Tyneside Joint Local Research Ethics Committee and from Newcastle Primary Care Trust.
The intervention
The intervention was delivered by a welfare rights officer from Newcastle City Council Welfare Rights Service in participants' own homes and comprised a structured assessment of current welfare status and benefits entitlement, together with active assistance in making claims where appropriate over the following six months, together with necessary follow-up for unresolved claims.
Quantitative study
The design presented ethical dilemmas as it was felt problematic to deprive the control group of welfare rights advice, since there is adequate evidence to show that it leads to significant financial gains. [ 32 ] To circumvent this dilemma, we delivered welfare rights advice to the control group six months after the intervention group. A single-blinded RCT with allocation of individuals to intervention (receipt of welfare rights consultation immediately) and control condition (welfare rights consultation six months after entry into the trial) was undertaken.
Four general practices located at five surgeries across Newcastle upon Tyne took part. Three of the practices were located in the top ten per cent of most deprived wards in England using the Index of Multiple Deprivation (two in the top one percent – ranked 30 th and 36 th most deprived); the other practice was ranked 3,774 out of a total of 8,414 in England. [ 33 ]
Using practice databases, a random sample of 100 patients aged 60 years or over from each of four participating practices was invited to take part in the study. Only one individual per household was allowed to participate in the trial, but if a partner or other adult household member was also eligible for benefits, they also received welfare rights advice. Patients were excluded if they were permanently hospitalised or living in residential or nursing care homes.
Written informed consent was obtained at the baseline interview. Structured face to face interviews were carried out at baseline, six, 12 and 24 months using standard scales covering the areas of demographics, mental and physical health (SF36) [ 34 ], Hospital Anxiety and Depression Scale (HADS) [ 35 ], psychosocial descriptors (e.g. Social Support Questionnaire [ 36 ] and the Self-Esteem Inventory, [ 37 ], and socioeconomic indicators (e.g. affordability and financial vulnerability). [ 38 ] Additionally, a short semi-structured interview was undertaken at 24 months to ascertain the perceived impact of additional resources for those who received them.
All health and welfare assessment data were entered onto customised MS Access databases and checked for quality and completeness. Data were transferred to the Statistical Package for the Social Sciences (SPSS) v11.0 [ 39 ] and STATA v8.0 for analysis. [ 40 ]
Qualitative study
The qualitative findings presented in this paper focus on the impact of the intervention. The sampling frame was formed by those (n = 96) who gave their consent to be contacted during their baseline interview for the RCT. The study sample comprised respondents from intervention and control groups purposively selected to include those eligible for the following resources: financial only; non-financial only; both financial and non financial; and, none. Sampling continued until no new themes emerged from the interviews; until data 'saturation' was reached. [ 21 ]
Initial interviews took place between April and December 2003 in participants' homes after their welfare rights assessment; follow-up interviews were undertaken in January and February 2005. The semi-structured interview schedule covered perceptions of: impact of material and/or financial benefits; impact on mental and/or physical health; impact on health related behaviours; social benefits; and views about the link between material resources and health. All participants agreed to the interview being audio-recorded. Immediately afterwards, observational field notes were made. Interviews were transcribed in full.
Data analysis largely followed the framework approach. [ 41 ] Data were coded, indexed and charted systematically; and resulting typologies discussed with other members of the research team, 'a pragmatic version of double coding'. [ 42 ] Constant comparison [ 43 ] and deviant case analysis [ 44 ] were used since both methods are important for internal validation. [ 7 , 42 ] Finally, sets of categories at a higher level of abstraction were developed.
A brief semi-structured interview was undertaken (by JM) with all participants who received additional resources. These interview data explored the impact data of additional resources on all of those who received them, not just the qualitative sub-sample. The data were independently coded by JM and SM using the same coding frame. Discrepant codes were examined by both researchers and a final code agreed.
One hundred and twenty six people were recruited into the study; there were 117 at 12 month follow-up and 109 at 24 months (five deaths, one moved, the remainder declined).
Table 1 shows the distribution of financial and non-financial benefits awarded as a result of the welfare assessments. Sixty percent of participants were awarded some form of welfare benefit, and just over 40% received a financial benefit. Some households received more than one type of benefit.
Table 2 compares the quantitative and qualitative sub-samples on a number of personal, economic, health and lifestyle factors at baseline. Intervention and control groups were comparable.
Table 3 compares outcome measures by award group, i.e. no award, non-financial and financial and shows only small differences between the mean changes across each group, none of which were statistically significant. Other analyses of the quantitative data compared the changes seen between baseline and six months (by which time the intervention group had received the welfare rights advice but the control group had not) and found little evidence of differences between the intervention and control groups of any practical importance. The only statistically significant difference between the groups was a small decrease in financial vulnerability in the intervention group after six months. [ 45 ]
There was little evidence for differences in health and social outcomes measures as a result of the receipt of welfare advice of a size that would be of major practical or clinical interest. However, this was a pilot study, with only the power to detect large differences if they were present. One reason for a lack of difference may be that the scales were less appropriate for older people and did not capture all relevant outcomes. Another reason for the lack of differences may be that insufficient numbers of people had received their benefits for long enough to allow any health outcomes to have changed when comparisons were made. Fourteen per cent of participants found to be eligible for financial benefits had not started receiving their benefits by the time of the first follow-up interview after their benefit assessment (six months for intervention, 12 months for control); and those who had, had only received them for an average of 2 months. This is likely to have diluted any impact of the intervention effect, and might account, to some extent, for the lack of observed effect.
Twenty five interviews were completed, fourteen of whom were from the intervention group. Ten participants were interviewed with partners who made active contributions. Twenty two follow-up interviews were undertaken between twelve and eighteen months later (three individuals were too ill to take part).
Table 1 (fifth column) shows that 14 of the participants in the qualitative study received some financial award. The median income gain was (€84, $101) (range £10 (€15, $18) -£100 (€148, $178)) representing a 4%-55% increase in weekly income. 18 participants were in receipt of benefit, either as a result of the current intervention or because of claims made prior to this study.
By the follow-up (FU) interviews all but one participant had been receiving their benefits for between 17 and 31 months. The intervention was viewed positively by all interviewees irrespective of outcome. However, for the fourteen participants who received additional financial resources the impact was considerable and accounts revealed a wide range of uses for the extra money. Participants' accounts revealed four linked categories, summarised on Table 4 . Firstly, increased affordability of necessities , without which maintaining independence and participating in daily life was difficult. This included accessing transport, maintaining social networks and social activities, buying better quality food, stocking up on food, paying bills, preventing debt and affording paid help for household activities. Secondly, occasional expenses such as clothes, household equipment, furniture and holidays were more affordable. Thirdly, extra income was used to act as a cushion against potential emergencies and to increase savings . Fourthly, all participants described the easing of financial worries as bringing ' peace of mind' .
Without exception, participants were of the view that extra money or resources would not improve existing health problems. The reasons behind these strongly held views about individual health conditions was generally that their poor health was attributed to specific health conditions and a combination of family history or fate, which were immune to the effects of money. Most participants had more than one chronic condition and felt that because of these conditions, plus their age, additional money would have no effect.
However, a number of participants linked the impact of the intervention with improved ways of coping with their conditions because of what the extra resources enabled them to do:
Mrs T: Having money is not going to improve his health, we could win the lottery and he would still have his health problems.
Mr T: No, but we don't need to worry if I wanted .... Well I mean I eat a lot of honey and I think it's very good, very healthful for you ... at one time we couldn't have afforded to buy these things. Now we can go and buy them if I fancy something, just go and get it where we couldn't before .
Mrs T: Although the Attendance Allowance is actually his [partners], it's made me relax a bit more ... I definitely worry less now (N15, female, 62 and partner)
Despite the fact that no-one expected their own health conditions to improve, most people believed that there was a link between resources and health in a more abstract sense, either because they experienced problems affording necessities such as healthy food or maintaining adequate heat in their homes, or because they empathised with those who lacked money. Participants linked adequate resources to maintaining health and contributing to a sense of well-being.
Money does have a lot to do with health if you are poor. It would have a lot to do with your health ... I don't buy loads and loads of luxuries, but I know I can go out and get the food we need and that sort of thing. I think that money is a big part of how a house, or how people in that house are . (N13, female, 72)
Comparing the results from the two datasets
When the separate analyses of the quantitative and qualitative datasets after the 12 month follow-up structured interviews were completed, the discrepancy in the findings became apparent. The quantitative study showed little evidence of a size that would be of practical or clinical interest, suggesting that the intervention had no impact on these outcome measures. The qualitative study found a wide-ranging impact, indicating that the intervention had a positive effect. The presence of such inter-method discrepancy led to a great deal of discussion and debate, as a result of which we devised six ways of further exploring these data.
(i) Treating the methods as fundamentally different
This process of simultaneous qualitative and quantitative dataset interrogation enables a deeper level of analysis and interpretation than would be possible with one or other alone and demonstrates how mixed methods research produces more than the sum of its parts. It is worth emphasising however, that it is not wholly surprising that each method comes up with divergent findings since each asked different, but related questions, and both are based on fundamentally different theoretical paradigms. Brannen [ 1 ] and Bryman [ 9 ] argue that it is essential to take account of these theoretical differences and caution against taking a purely technical approach to the use of mixed methods, a simple 'bolting together' of techniques. [ 17 ] Combining the two methods for crossvalidation (triangulation) purposes is not a viable option because it rests on the premise that both methods are examining the same research problem. [ 1 ] We have approached the divergent findings as indicative of different aspects of the phenomena in question and searched for reasons which might explain these inconsistencies. In the approach that follows, we have treated the datasets as complementary, rather than attempt to integrate them, since each approach reflects a different view on how social reality ought to be studied.
(ii) Exploring the methodological rigour of each component
It is standard practice at the data analysis and interpretation phases of any study to scrutinise methodological rigour. However, in this case, we had another dataset to use as a yardstick for comparison and it became clear that our interrogation of each dataset was informed to some extent by the findings of the other. It was not the case that we expected to obtain the same results, but clearly the divergence of our findings was of great interest and made us more circumspect about each dataset. We began by examining possible reasons why there might be problems with each dataset individually, but found ourselves continually referring to the results of the other study as a benchmark for comparison.
With regard to the quantitative study, it was a pilot, of modest sample size, and thus not powered to detect small differences in the key outcome measures. In addition there were three important sources of dilution effects: firstly, only 63% of intervention group participants received some type of financial award; secondly, we found that 14% of those in the trial eligible for financial benefits did not receive their money until after the follow up assessments had been carried out; and thirdly, many had received their benefits for only a short period, reducing the possibility of detecting any measurable effects at the time of follow-up. All of these factors provide some explanation for the lack of a measurable effect between intervention and control group and between those who did and did not receive additional financial resources.
The number of participants in the qualitative study who received additional financial resources as a result of this intervention was small (n = 14). We would argue that the fieldwork, analysis and interpretation [ 46 ] were sufficiently transparent to warrant the degree of methodological rigour advocated by Barbour [ 7 , 17 ] and that the findings were therefore an accurate reflection of what was being studied. However, there still remained the possibility that a reason for the discrepant findings was due to differences between the qualitative sub-sample and the parent sample, which led us to step three.
(iii) Exploring dataset comparability
We compared the qualitative and quantitative samples on a number of social and economic factors (Table 2 ). In comparison to the parent sample, the qualitative sub-sample was slightly older, had fewer men, a higher proportion with long-term limiting illness, but fewer current smokers. However, there was nothing to indicate that such small differences would account for the discrepancies. There were negligible differences in SF-36 (Physical and Mental) and HAD (Anxiety and Depression) scores between the groups at baseline, which led us to discount the possibility that those in the quantitative sub sample were markedly different to the quantitative sample on these outcome measures.
(iv) Collection of additional data and making further comparisons
The divergent findings led us to seek further funding to undertake collection of additional quantitative and qualitative data at 24 months. The quantitative and qualitative follow-up data verified the initial findings of each study. [ 45 ] We also collected a limited amount of qualitative data on the perceived impact of resources, from all participants who had received additional resources. These data are presented in figure 1 which shows the uses of additional resources at 24 month follow-up for 35 participants (N = 35, 21 previously in quantitative study only, 14 in both). This dataset demonstrates that similar issues emerged for both qualitative and quantitative datasets: transport, savings and 'peace of mind' emerged as key issues, but the data also showed that the additional money was used on a wide range of items. This follow-up confirmed the initial findings of each study and further, indicated that the perceived impact of the additional resources was the same for a larger sample than the original qualitative sub-sample, further confirming our view that the positive findings extended beyond the fourteen participants in the qualitative sub-sample, to all those receiving additional resources.
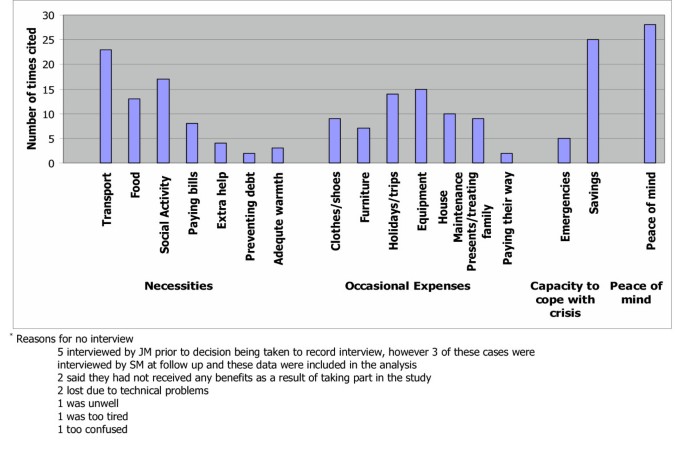
Use of additional resources at 2 year follow up (N = 35)*.
(v) Exploring whether the intervention under study worked as expected
The qualitative study revealed that many participants had received welfare benefits via other services prior to this study, revealing the lack of a 'clean slate' with regard to the receipt of benefits, which we had not anticipated. We investigated this further in the quantitative dataset and found that 75 people (59.5%) had received benefits prior to the study; if the first benefit was on health grounds, a later one may have been because their health had deteriorated further.
(vi) Exploring whether the outcomes of the quantitative and qualitative components match
'Probing certain issues in greater depth' as advocated by Bryman (p134) [ 1 ] focussed our attention on the outcome measures used in the quantitative part of the study and revealed several challenges. Firstly, the qualitative study revealed a number of dimensions not measured by the quantitative study, such as, 'maintaining independence' which included affording paid help, increasing and improving access to facilities and managing better within the home. Secondly, some of the measures used with the intention of capturing dimensions of mental health did not adequately encapsulate participants' accounts of feeling 'less stressed' and 'less depressed' by financial worries. Probing both datasets also revealed congruence along the dimension of physical health. No differences were found on the SF36 physical scale and participants themselves did not expect an improvement in physical health (for reasons of age and chronic health problems). The real issue would appear to be measuring ways in which older people are better able to cope with existing health problems and maintain their independence and quality of life, despite these conditions.
Qualitative study results also led us to look more carefully at the quantitative measures we used. Some of the standardised measures were not wholly applicable to a population of older people. Mallinson [ 47 ] also found this with the SF36 when she demonstrated some of its limitations with this age group, as well as how easy it is to, 'fall into the trap of using questionnaires like a form of laboratory equipment and forget that ... they are open to interpretation'. The data presented here demonstrate the difficulties of trying to capture complex phenomena quantitatively. However, they also demonstrate the usefulness of having alternative data forms on which to draw whether complementary (where they differ but together generate insights) or contradictory (where the findings conflict). [ 30 ] In this study, the complementary and contradictory findings of the two datasets proved useful in making recommendations for the design of a definitive study.
Many researchers understand the importance, indeed the necessity, of combining methods to investigate complex health and social issues. Although quantitative research remains the dominant paradigm in health services research, qualitative research has greater prominence than before and is no longer, as Barbour [ 42 ] points out regarded as the 'poor relation to quantitative research that it has been in the past' (p1019). Brannen [ 48 ] argues that, despite epistemological differences there are 'more overlaps than differences'. Despite this, there is continued debate about the authority of each individual mode of research which is not surprising since these different styles, 'take institutional forms, in relation to cultures of and markets for knowledge' (p168). [ 49 ] Devers [ 50 ] points out that the dominance of positivism, especially within the RCT method, has had an overriding influence on the criteria used to assess research which has had the inevitable result of viewing qualitative studies unfavourably. We advocate treating qualitative and quantitative datasets as complementary rather than in competition for identifying the true version of events. This, we argue, leads to a position which exploits the strengths of each method and at the same time counters the limitations of each. The process of interpreting the meaning of these divergent findings has led us to conclude that much can be learned from scientific realism [ 51 ]which has 'sought to position itself as a model of scientific explanation which avoids the traditional epistemological poles of positivism and relativism' (p64). This stance enables investigators to take account of the complexity inherent in social interventions and reinforces, at a theoretical level, the problems of attempting to measure the impact of a social intervention via experimental means. However, the current focus on evidence based health care [ 52 ] now includes public health [ 53 , 54 ] and there is increased attention paid to the results of trials of public health interventions, attempting as they do, to capture complex social phenomena using standardised measurement tools. We would argue that at the very least, the inclusion of both qualitative and quantitative elements in such studies, is essential and ultimately more cost-effective, increasing the likelihood of arriving at a more thoroughly researched and better understood set of results.
The findings of this study demonstrate how the use of mixed methods can lead to different and sometimes conflicting accounts. This, we argue, is largely due to the outcome measures in the RCT not matching the outcomes emerging from the qualitative arm of the study. Instead of making assumptions about the correct version, we have reported the results of both datasets together rather than separately, and advocate six steps to interrogate each dataset more fully. The methodological strategy advocated by this approach involves contemporaneous qualitative and quantitative data collection, analysis and reciprocal interrogation to inform interpretation in trials of complex interventions. This approach also indicates the need for a realistic appraisal of quantitative tools. More widespread use of mixed methods in trials of complex interventions is likely to enhance the overall quality of the evidence base.
Brannen J: Mixing Methods: qualitative and quantitative research. 1992, Aldershot, Ashgate
Google Scholar
Tashakkori A, Teddlie C: Handbook of Mixed Methods in Social and Behavioural Research. 2003, London, Sage
Morgan DL: Triangulation and it's discontents: Developing pragmatism as an alternative justification for combining qualitative and quantitative methods. Cambridge, 11-12 July.. 2005.
Pill R, Stott NCH: Concepts of illness causation and responsibility: some preliminary data from a sample of working class mothers. Social Science and Medicine. 1982, 16: 43-52. 10.1016/0277-9536(82)90422-1.
Article CAS PubMed Google Scholar
Scambler G, Hopkins A: Generating a model of epileptic stigma: the role of qualitative analysis. Social Science and Medicine. 1990, 30: 1187-1194. 10.1016/0277-9536(90)90258-T.
Townsend A, Hunt K, Wyke S: Managing multiple morbidity in mid-life: a qualitative study of attitudes to drug use. BMJ. 2003, 327: 837-841. 10.1136/bmj.327.7419.837.
Article PubMed PubMed Central Google Scholar
Barbour RS: Checklists for improving rigour in qualitative research: the case of the tail wagging the dog?. British Medical Journal. 2001, 322: 1115-1117.
Article CAS PubMed PubMed Central Google Scholar
Pope C, Mays N: Qualitative Research in Health Care. 2000, London, BMJ Books
Bryman A: Quantity and Quality in Social Research. 1995, London, Routledge
Ashton J: Healthy cities. 1991, Milton Keynes, Open University Press
Baum F: Researching Public Health: Behind the Qualitative-Quantitative Methodological Debate. Social Science and Medicine. 1995, 40: 459-468. 10.1016/0277-9536(94)E0103-Y.
Donovan J, Mills N, Smith M, Brindle L, Jacoby A, Peters T, Frankel S, Neal D, Hamdy F: Improving design and conduct of randomised trials by embedding them in qualitative research: (ProtecT) study. British Medical Journal. 2002, 325: 766-769.
Cox K: Assessing the quality of life of patients in phase I and II anti-cancer drug trials: interviews versus questionnaires. Social Science and Medicine. 2003, 56: 921-934. 10.1016/S0277-9536(02)00100-4.
Article PubMed Google Scholar
Lewin S: Mixing methods in complex health service randomised controlled trials: research 'best practice'. Mixed Methods in Health Services Research Conference 23rd November 2004, Sheffield University.
Cresswell JW: Mixed methods research and applications in intervention studies.: ; Cambridge, July 11-12. 2005.
Cresswell JW: Research Design. Qualitative, Quantitative and Mixed Methods Approaches. 2003, London, Sage
Barbour RS: The case for combining qualitative and quanitative approaches in health services research. Journal of Health Services Research and Policy. 1999, 4: 39-43.
Gabriel Z, Bowling A: Quality of life from the perspectives of older people. Ageing & Society. 2004, 24: 675-691. 10.1017/S0144686X03001582.
Article Google Scholar
Koops L, Lindley RL: Thrombolysis for acute ischaemic stroke: consumer involvement in design of new randomised controlled trial. BMJ. 2002, 325: 415-418. 10.1136/bmj.325.7361.415.
O'Cathain A, Nicholl J, Murphy E: Making the most of mixed methods. Mixed Methods in Health Services Research Conference 23rd November 2004, Sheffield University.
Denzin NK: The Research Act. 1978, New York, McGraw-Hill Book Company
Roberts H, Curtis K, Liabo K, Rowland D, DiGuiseppi C, Roberts I: Putting public health evidence into practice: increasing the prevalance of working smoke alarms in disadvantaged inner city housing. Journal of Epidemiology & Community Health. 2004, 58: 280-285. 10.1136/jech.2003.007948.
Article CAS Google Scholar
Rowland D, DiGuiseppi C, Roberts I, Curtis K, Roberts H, Ginnelly L, Sculpher M, Wade A: Prevalence of working smoke alarms in local authority inner city housing: randomised controlled trial. British Medical Journal. 2002, 325: 998-1001.
Vincent J: Old Age. 2003, London, Routledge
Chapter Google Scholar
Department for Work and Pensions: Households below average income statistics 2000/01. 2002, London, Department for Work and Pensions
National Audit Office: Tackling Pensioner Pverty: Encouraging take-up of entitlements. 2002, London, National Audit Office
Paris JAG, Player D: Citizens advice in general practice. British Medical Journal. 1993, 306: 1518-1520.
Abbott S: Prescribing welfare benefits advice in primary care: is it a health intervention, and if so, what sort?. Journal of Public Health Medicine. 2002, 24: 307-312. 10.1093/pubmed/24.4.307.
Harding R, Sherr L, Sherr A, Moorhead R, Singh S: Welfare rights advice in primary care: prevalence, processes and specialist provision. Family Practice. 2003, 20: 48-53. 10.1093/fampra/20.1.48.
Morgan DL: Practical strategies for combining qualitative and quantitative methods: applications to health research. Qualitative Health Research. 1998, 8: 362-376.
Moffatt S, White M, Stacy R, Downey D, Hudson E: The impact of welfare advice in primary care: a qualitative study. Critical Public Health. 2004, 14: 295-309. 10.1080/09581590400007959.
Thomson H, Hoskins R, Petticrew M, Ogilvie D, Craig N, Quinn T, Lindsey G: Evaluating the health effects of social interventions. British Medical Journal. 2004, 328: 282-285.
Department for the Environment TR: Measuring Multiple Deprivation at the Small Area Level: The Indices of Deprivation. 2000, London, Department for the Envrionment, Trransport and the Regions
Ware JE, Sherbourne CD: The MOS 36 item short form health survey (SF-36). Conceptual framework and item selection. Medical Care. 1992, 30: 473-481.
Snaith RP, Zigmond AS: The hospital anxiety and depression scale. Acta Psychiatrica Scandinivica. 1983, 67: 361-370.
Sarason I, Carroll C, Maton K: Assessing social support: the social support questionnaire. Journal of Personality and Social Psychology. 1983, 44: 127-139. 10.1037//0022-3514.44.1.127.
Ward R: The impact of subjective age and stigma on older persons. Journal of Gerontology. 1977, 32: 227-232.
Ford G, Ecob R, Hunt K, Macintyre S, West P: Patterns of class inequality in health through the lifespan: class gradients at 15, 35 and 55 years in the west of Scotland. Social Science and Medicine. 1994, 39: 1037-1050. 10.1016/0277-9536(94)90375-1.
SPSS.: v. 11.0 for Windows [program]. 2003, Chicago, Illinois.
STATA.: Statistical Software [program]. 8.0 version. 2003, Texas, College Station
Ritchie J, Lewis J: Qualitative Research Practice. A Guide for Social Scientists. 2003, London, Sage
Barbour RS: The Newfound Credibility of Qualitative Research? Tales of Technical Essentialism and Co-Option. Qualitative Health Research. 2003, 13: 1019-1027. 10.1177/1049732303253331.
Silverman D: Doing qualitative research. 2000, London, Sage
Clayman SE, Maynard DW: Ethnomethodology and conversation analysis. Situated Order: Studies in the Social Organisation of Talk and Embodied Activities. Edited by: Have PT and Psathas G. 1994, Washington, D.C., University Press of America
White M, Moffatt S, Mackintosh J, Howel D, Sandell A, Chadwick T, Deverill M: Randomised controlled trial to evaluate the health effects of welfare rights advice in primary health care: a pilot study. Report to the Department of Health, Policy Research Programme. 2005, Newcastle upon Tyne, University of Newcastle upon Tyne
Moffatt S: "All the difference in the world". A qualitative study of the perceived impact of a welfare rights service provided in primary care. 2004, , University College London
Mallinson S: Listening to reposndents: a qualitative assessment of the Short-Form 36 Health Status Questionnaire. Social Science and Medicine. 2002, 54: 11-21. 10.1016/S0277-9536(01)00003-X.
Brannen J: Mixing Methods: The Entry of Qualitative and Quantitative Approaches into the Research Process. International Journal of Social Research Methodology. 2005, 8: 173-184. 10.1080/13645570500154642.
Green A, Preston J: Editorial: Speaking in Tongues- Diversity in Mixed Methods Research. International Journal of Social Research Methodology. 2005, 8: 167-171. 10.1080/13645570500154626.
Devers KJ: How will we know "good" qualitative research when we see it? Beginning the dialogue in Health Services Research. Health Services Research. 1999, 34: 1153-1188.
CAS PubMed PubMed Central Google Scholar
Pawson R, Tilley N: Realistic Evaluation. 2004, London, Sage
Miles A, Grey JE, Polychronis A, Price N, Melchiorri C: Current thinking in the evidence-based health care debate. Journal of Evaluation in Clinical Practice. 2003, 9: 95-109. 10.1046/j.1365-2753.2003.00438.x.
Pencheon D, Guest C, Melzer D, Gray JAM: Oxford Handbook of Public Health Practice. 2001, Oxford, Oxford University Press
Wanless D: Securing Good Health for the Whole Population. 2004, London, HMSO
Pre-publication history
The pre-publication history for this paper can be accessed here: http://www.biomedcentral.com/1472-6963/6/28/prepub
Download references
Acknowledgements
We wish to thank: Rosemary Bell, Jenny Dover and Nick Whitton from Newcastle upon Tyne City Council Welfare Rights Service; all the participants and general practice staff who took part; and for their extremely helpful comments on earlier drafts of this paper, Adam Sandell, Graham Scambler, Rachel Baker, Carl May and John Bond. We are grateful to referees Alicia O'Cathain and Sally Wyke for their insightful comments. The views expressed in this paper are those of the authors and not necessarily those of the Department of Health.
Author information
Authors and affiliations.
Public Health Research Group, School of Population & Health Sciences, Faculty of Medical Sciences, William Leech Building, Framlington Place, Newcastle upon Tyne, NE2 4HH, UK
Suzanne Moffatt, Martin White, Joan Mackintosh & Denise Howel
You can also search for this author in PubMed Google Scholar
Corresponding author
Correspondence to Suzanne Moffatt .
Additional information
Competing interests.
The author(s) declare that they have no competing interests.
Authors' contributions
SM and MW had the original idea for the study, and with the help of DH, Adam Sandell and Nick Whitton developed the proposal and gained funding. JM collected the data for the quantitative study, SM designed and collected data for the qualitative study. JM, DH and MW analysed the quantitative data, SM analysed the qualitative data. All authors contributed to interpretation of both datasets. SM wrote the first draft of the paper, JM, MW and DH commented on subsequent drafts. All authors have read and approved the final manuscript.
Authors’ original submitted files for images
Below are the links to the authors’ original submitted files for images.
Authors’ original file for figure 1
Rights and permissions.
Open Access This article is published under license to BioMed Central Ltd. This is an Open Access article is distributed under the terms of the Creative Commons Attribution License ( https://creativecommons.org/licenses/by/2.0 ), which permits unrestricted use, distribution, and reproduction in any medium, provided the original work is properly cited.
Reprints and permissions
About this article
Cite this article.
Moffatt, S., White, M., Mackintosh, J. et al. Using quantitative and qualitative data in health services research – what happens when mixed method findings conflict? [ISRCTN61522618]. BMC Health Serv Res 6 , 28 (2006). https://doi.org/10.1186/1472-6963-6-28
Download citation
Received : 29 September 2005
Accepted : 08 March 2006
Published : 01 December 2006
DOI : https://doi.org/10.1186/1472-6963-6-28
Share this article
Anyone you share the following link with will be able to read this content:
Sorry, a shareable link is not currently available for this article.
Provided by the Springer Nature SharedIt content-sharing initiative
- Mixed Method
- Welfare Benefit
- Mixed Method Research
- Divergent Finding
- Financial Vulnerability
BMC Health Services Research
ISSN: 1472-6963
- General enquiries: [email protected]
- Open access
- Published: 14 May 2024
Exploring predictors and prevalence of postpartum depression among mothers: Multinational study
- Samar A. Amer ORCID: orcid.org/0000-0002-9475-6372 1 ,
- Nahla A. Zaitoun ORCID: orcid.org/0000-0002-5274-6061 2 ,
- Heba A. Abdelsalam 3 ,
- Abdallah Abbas ORCID: orcid.org/0000-0001-5101-5972 4 ,
- Mohamed Sh Ramadan 5 ,
- Hassan M. Ayal 6 ,
- Samaher Edhah Ahmed Ba-Gais 7 ,
- Nawal Mahboob Basha 8 ,
- Abdulrahman Allahham 9 ,
- Emmanuael Boateng Agyenim 10 &
- Walid Amin Al-Shroby 11
BMC Public Health volume 24 , Article number: 1308 ( 2024 ) Cite this article
Metrics details
Postpartum depression (PPD) affects around 10% of women, or 1 in 7 women, after giving birth. Undiagnosed PPD was observed among 50% of mothers. PPD has an unfavorable relationship with women’s functioning, marital and personal relationships, the quality of the mother-infant connection, and the social, behavioral, and cognitive development of children. We aim to determine the frequency of PPD and explore associated determinants or predictors (demographic, obstetric, infant-related, and psychosocial factors) and coping strategies from June to August 2023 in six countries.
An analytical cross-sectional study included a total of 674 mothers who visited primary health care centers (PHCs) in Egypt, Yemen, Iraq, India, Ghana, and Syria. They were asked to complete self-administered assessments using the Edinburgh Postnatal Depression Scale (EPDS). The data underwent logistic regression analysis using SPSS-IBM 27 to list potential factors that could predict PPD.
The overall frequency of PPD in the total sample was 92(13.6%). It ranged from 2.3% in Syria to 26% in Ghana. Only 42 (6.2%) were diagnosed. Multiple logistic regression analysis revealed there were significant predictors of PPD. These factors included having unhealthy baby adjusted odds ratio (aOR) of 11.685, 95% CI: 1.405–97.139, p = 0.023), having a precious baby (aOR 7.717, 95% CI: 1.822–32.689, p = 0.006), who don’t receive support (aOR 9.784, 95% CI: 5.373–17.816, p = 0.001), and those who are suffering from PPD. However, being married and comfortable discussing mental health with family relatives are significant protective factors (aOR = 0.141 (95% CI: 0.04–0.494; p = 0.002) and (aOR = 0.369, 95% CI: 0.146–0.933, p = 0.035), respectively.
The frequency of PPD among the mothers varied significantly across different countries. PPD has many protective and potential factors. We recommend further research and screenings of PPD for all mothers to promote the well-being of the mothers and create a favorable environment for the newborn and all family members.
Peer Review reports
Introduction
Postpartum depression (PPD) is among the most prevalent mental health issues [ 1 ]. The onset of depressive episodes after childbirth occurs at a pivotal point in a woman’s life and can last for an extended period of 3 to 6 months; however, this varies based on several factors [ 2 ]. PPD can develop at any time within the first year after childbirth and last for years [ 2 ]. It refers to depressive symptoms that a mother experiences during the postpartum period, which are vastly different from “baby blues,” which many mothers experience within three to five days after the birth of their child [ 3 ].
Depressive episodes are twice as likely to occur during pregnancy compared to other times in a woman’s life, and they frequently go undetected and untreated [ 4 ]. According to estimates, almost 50% of mothers with PPD go undiagnosed [ 4 ]. The Diagnostic and Statistical Manual of Mental Disorders (DSM-5) criteria for PPD include mood instability, loss of interest, feelings of guilt, sleep disturbances, sleep disorders, and changes in appetite [ 5 ], as well as decreased libido, crying spells, anxiety, irritability, feelings of isolation, mental liability, thoughts of hurting oneself and/or the infant, and even suicidal ideation [ 6 ].
Approximately 1 in 10 women will experience PPD after giving birth, with some studies reporting 1 in 7 women [ 7 ]. Globally, the prevalence of PPD is estimated to be 17.22% (95% CI: 16.00–18.05) [ 4 ], with a prevalence of up to 15% in the previous year in eighty different countries or regions [ 1 ]. This estimate is lower than the 19% prevalence rate of PPD found in studies from low- and middle-income countries and higher than the 13% prevalence rate (95% CI: 12.3–13.4%) stated in a different meta-analysis of data from high-income countries [ 8 ].
The occurrence of postpartum depression is influenced by various factors, including social aspects like marital status, education level, lack of social support, violence, and financial difficulties, as well as other factors such as maternal age (particularly among younger women), obstetric stressors, parity, and unplanned pregnancy [ 4 ]. When a mother experiences depression, she may face challenges in forming a satisfying bond with her child, which can negatively affect both her partner and the emotional and cognitive development of infants and adolescents [ 4 ]. As a result, adverse effects may be observed in children during their toddlerhood, preschool years, and beyond [ 9 ].
Around one in seven women can develop PPD [ 7 ]. While women experiencing baby blues tend to recover quickly, PPD tends to last longer and severely affects women’s ability to return to normal function. PPD affects the mother and her relationship with the infant [ 7 ]. The prevalence of postpartum depression varies depending on the assessment method, timing of assessment, and cultural disparities among countries [ 7 ]. To address these aspects, we conducted a cross-sectional study focusing on mothers who gave birth within the previous 18 months. Objectives: to determine the frequency of PPD and explore associated determinants or predictors, including demographic, obstetric, infant-related, and psychosocial factors, and coping strategies from June to August 2023 in six countries.
Study design and participants
This is an analytical cross-sectional design and involved 674 mothers during the childbearing period (CBP) from six countries, based on the authors working settings, namely Egypt, Syria, Yemen, Ghana, India, and Iraq. It was conducted from June to August 2023. It involved all mothers who gave birth within the previous 18 months, citizens of one of the targeted countries, and those older than 18 years and less than 40 years. Women who visited for a routine postpartum follow-up visit and immunization of their newborns were surveyed.
Multiple pregnancies, illiteracy, or anyone deemed unfit to participate in accordance with healthcare authorities, mothers who couldn’t access or use the Internet, mothers who couldn’t read or speak Arabic or English and couldn’t deal with the online platform or smart devices, mothers whose babies were diagnosed with serious health problems, were stillborn, or experienced intrauterine fetal death, and participants with complicated medical, mental, or psychological disorders that interfered with completing the questionnaire were all exclusion criteria. There were no incentives offered to encourage participation.
Sample size and techniques
The sample size was estimated according to the following equation: n = Z 2 P (1-P)/d 2 . This calculation was based on the results of a systematic review and meta-analysis in 2020 of 17% as the worldwide prevalence of PPD and 12% as the worldwide incidence of PPD, as well as a 5% precision percentage, 80% power of the study, a 95% confidence level, and an 80% response rate [ 11 ]. The total calculated sample size is 675. The sample was diverse in terms of nationality, with the majority being Egyptian (16.3%), followed by Yemeni (24.3%) and Indian (19.1%), based on many factors discussed in the limitation section.
The sampling process for recruiting mothers utilized a multistage approach. Two governorates were randomly selected from each country. Moreover, we selected one rural and one urban area from each governorate. Through random selection, participants were chosen for the study. Popular and officially recognized online platforms, including websites and social media platforms such as Facebook, Twitter, WhatsApp groups, and registered emails across various health centers, were utilized for reaching out to participants. Furthermore, a community-based sample was obtained from different public locations, including well-baby clinics, PHCs, and family planning units.
Mothers completed the questionnaire using either tablets or cellphones provided by the data collectors or by scanning the QR code. All questions were mandatory to prevent incomplete forms. Once they provided their informed consent, they received the questionnaire, which they completed and submitted. To enhance the response rate, reminder messages and follow-up communications were employed until the desired sample size was achieved or until the end of August. To avoid seasonal affective disorders, the meteorological autumn season began on the 1st day of September, which may be associated with Autum depressive symptoms that may confound or affect our results.
Data collection tool
Questionnaire development and structure.
The questionnaire was developed and adapted based on data obtained from previous studies [ 7 , 8 , 9 , 10 , 11 , 12 ]. Initially, it was created in English and subsequently translated into Arabic. To ensure accuracy, a bilingual panel consisting of two healthcare experts and an externally qualified medical translator translated the English version into Arabic. Additionally, two English-speaking translators performed a back translation, and the original panel was consulted if any concerns arose.
Questionnaire validation
To collect the data, an online, self-administered questionnaire was utilized, designed in Arabic with a well-structured format. We conducted an assessment of the questionnaire’s reliability and validity to ensure a consistent interpretation of the questions. The questionnaire underwent validation by psychiatrists, obstetricians, and gynecologists. Furthermore, in a pilot study involving 20 women of CBA, the questionnaire’s clarity and comprehensibility were evaluated. It is important to note that the findings from the pilot study were not included in our main study.
The participants were asked to rate the questionnaire’s organization, clarity, and length, as well as provide a general opinion. Following that, certain questions were revised in light of their input. To check for reliability and reproducibility, the questionnaire was tested again on the same people one week later. The final data analysis will not include the data collected during the pilot test. We calculated a Cronbach’s alpha of 0.76 for the questionnaire.
The structure of the questionnaire
After giving their permission to take part in the study. The questionnaire consisted of the following sections:
Study information and electronic solicitation of informed consent.
Demographic and health-related factors: age, gender, place of residence, educational level, occupation, marital status, weight, height, and the fees of access to healthcare services.
Obstetric history: number of pregnancies, gravida, history of abortions, number of live children, history of dead children, inter-pregnancy space (y), current pregnancy status, type of the last delivery, weight gain during pregnancy (kg), baby age (months), premature labor, healthy baby, baby admitted to the NICU, Feeding difficulties, pregnancy problems, postnatal problems, and natal problems The nature of baby feeding.
Assessment of postpartum depression (PPD) levels using the Edinburgh 10-question scale: This scale is a simple and effective screening tool for identifying individuals at risk of perinatal depression. The EPDS (Edinburgh Postnatal Depression Scale) is a valuable instrument that helps identify the likelihood of a mother experiencing depressive symptoms of varying severity. A score exceeding 13 indicates an increased probability of a depressive illness. However, clinical discretion should not be disregarded when interpreting the EPDS score. This scale captures the mother’s feelings over the past week, and in cases of uncertainty, it may be beneficial to repeat the assessment after two weeks. It is important to note that this scale is not capable of identifying mothers with anxiety disorders, phobias, or personality disorders.
For Questions 1, 2, and 4 (without asterisks): Scores range from 0 to 3, with the top box assigned a score of 0 and the bottom box assigned a score of 3. For Questions 3 and 5–10 (with asterisks): Scores are reversed, with the top box assigned a score of 3 and the bottom box assigned a score of 0. The maximum score achievable is 30, and a probability of depression is considered when the score is 10 or higher. It is important to always consider item 10, which pertains to suicidal ideation [ 12 ].
Psychological and social characteristics: received support or treatment for PPD, awareness of symptoms and risk factors, experienced cultural stigma or judgment about PPD in the community, suffer from any disease or mental or psychiatric disorder, have you ever been diagnosed with PPD, problems with the husband, and financial problems.
Coping strategies and causes for not receiving the treatment and reactions to PPD, in descending order: social norms, cultural or traditional beliefs, personal barriers, 48.5% geographical or regional disparities in mental health resources, language or communication barriers, and financial constraints.
Statistical analysis
The collected data was computerized and statistically analyzed using the SPSS program (Statistical Package for Social Science), version 27. The data was tested for normal distribution using the Shapiro-Walk test. Qualitative data was represented as frequencies and relative percentages. Quantitative data was expressed as mean ± SD (standard deviation) if it was normally distributed; otherwise, median and interquartile range (IQR) were used. The Mann-Whitney test (MW) was used to calculate the difference between quantitative variables in two groups for non-parametric variables. Correlation analysis (using Spearman’s method) was used to assess the relationship between two nonparametric quantitative variables. All results were considered statistically significant when the significant probability was < 0.05. The chi-square test (χ 2 ) and Fisher exact were used to calculate the difference between qualitative variables.
The frequency of PPD among mothers (Fig. 1 )
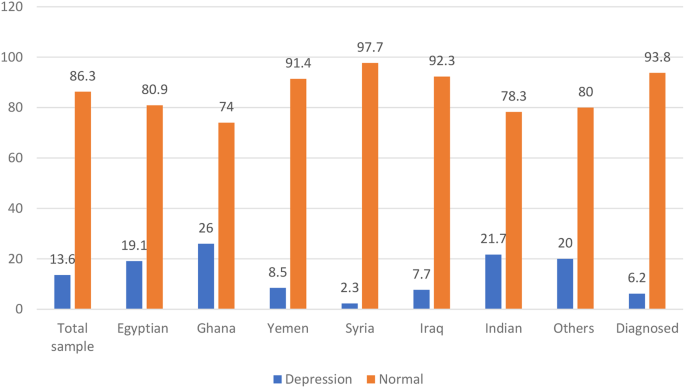
The frequency of PPD among the studied mothers
The frequency of PPD in the total sample using the Edinburgh 10-question scale was 13.5% (Table S1) and 92 (13.6%). Which significantly ( p = 0.001) varied across different countries, being highest among Ghana mothers 13 (26.0%) out of 50 and Indians 28 (21.7%) out of 129. Egyptian 21 (19.1) out of 110, Yemen 14 (8.5%) out of 164, Iraq 13 (7.7%) out of 168, and Syria 1 (2.3%) out of 43 in descending order. Nationality is also significantly associated with PPD ( p = 0.001).
Demographic, and health-related characteristics and their association with PPD (Table 1 )
The study included 674 participants. The median age was 27 years, with 407 (60.3%) of participants falling in the >25 to 40-year-old age group. The majority of participants were married, 650 (96.4%), had sufficient monthly income, 449 (66.6%), 498 (73.9%), had at least a preparatory or high school level of education, and were urban. Regarding health-related factors, 270 (40.01%) smoked, 645 (95.7%) smoked, 365 (54.2%) got the COVID-19 vaccine, and 297 (44.1%) got COVID-19. Moreover, 557 (82.6%) had no comorbidities, 623 (92.4%) had no psychiatric illness or family history, and they charged for health care services for themselves 494 (73.3%).
PPD is significant ( p < 0.05). Higher among single or widowed women 9 (56.3%) and mothers who had both medical, mental, or psychological problems 2 (66.7%), with ex-cigarette smoking 5 (35.7%) ( p = 0.033), alcohol consumption ( p = 0.022) and mothers were charged for the health care services for themselves 59 (11.9%).
Obstetric, current pregnancy, and infant-related characteristics and their association with PPD (Table 2 )
The majority of the studied mothers were on no hormonal treatment or contraceptive pills 411 (60.9%), the current pregnancy was unplanned and wanted 311 (46.1%), they gained 10 ≥ kg 463 (68.6%), 412 (61.1%) delivered vaginal, a healthy baby 613 (90.9%), and, on breastfeeding, only 325 (48.2%).
There was a significant ( P < 0.05) association observed between PPD, which was significantly higher among mothers on contraceptive methods, and those who had 1–2 live births (76.1%) and mothers who had interpregnancy space for less than 2 years. 86 (93.5%), and those who had a history of dead children. Moreover, among those who had postnatal problems (27.2%).
The psychosocial characteristics and their association with PPD (Table 3 )
Regarding the psychological and social characteristics of the mothers, the majority of mothers were unaware of the symptoms of PPD (75%), and only 236 (35.3%) experienced cultural stigma or judgment about PPD in the community. About 41 (6.1%) were diagnosed with PPD during the previous pregnancy, and only 42 (6.2%) were diagnosed and on medications.
A p -value of less than 0.001 demonstrates a highly statistically significant association with the presence of PPD. Mothers with PPD were significantly more likely to have a history of or be currently diagnosed with PPD, as well as financial and marital problems. Experienced cultural stigma or judgment about PPD and received more support.
Coping strategies and causes for not receiving the treatment and reaction to PPD (Table 3 ; Fig. 2 )
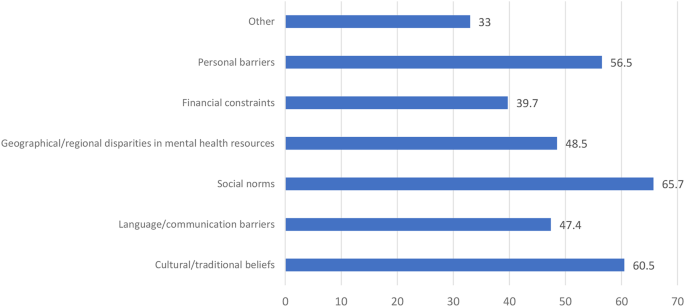
Causes for not receiving the treatment and reaction to PPD
Around half of the mothers didn’t feel comfortable discussing mental health: 292 (43.3%) with a physician, 307 (45.5%) with a husband, 326 (48.4%) with family, and 472 (70.0%) with the community. Moreover, mothers with PPD felt significantly more comfortable discussing mental health in descending order: 46 (50.0%) with a physician, 41 (44.6%) with a husband, and 39 (42.3%) with a family (Table 3 ).
There were different causes for not receiving the treatment and reactions to PPD, in descending order: 65.7% social norms, 60.5% cultural or traditional beliefs, 56.5% personal barriers, 48.5% geographical or regional disparities in mental health resources, 47.4% language or communication barriers, and 39.7% financial constraints.
Prediction of PPD (significant demographics, obstetric, current pregnancy, and infant-related, and psychosocial), and coping strategies derived from multiple logistic regression analysis (Table 4 ).
Significant demographic predictors of ppd.
Marital Status (Married or Single): The adjusted odds ratio (aOR) among PPD mothers who were married in comparison to their single counterparts was 0.141 (95% CI: 0.04–0.494; p -value = 0.002).
Nationality: For PPD Mothers of Yemeni nationality compared to those with Egyptian nationality, the aOR was 0.318 (95% CI: 0.123–0.821, p = 0.018). Similarly, for Syrian nationality in comparison to Egyptian nationality, the aOR was 0.111 (95% CI: 0.0139–0.887, p = 0.038), and for Iraqi nationality compared to Egyptian nationality, the aOR was 0.241 (95% CI: 0.0920–0.633, p = 0.004).
Significant obstetric, current pregnancy, and infant-related characteristics predictors of PPD
Current Pregnancy Status (Precious Baby—Planned): The aOR for the occurrence of PPD among women with a “precious baby” relative to those with a “planned” pregnancy was 7.717 (95% CI: 1.822–32.689, p = 0.006).
Healthy Baby (No-Yes): The aOR for the occurrence of PPD among women with unhealthy babies in comparison to those with healthy ones is 11.685 (95% CI: 1.405–97.139, p = 0.023).
Postnatal Problems (No–Yes): The aOR among PPD mothers reporting postnatal problems relative to those not reporting such problems was 0.234 (95% CI: 0.0785–0.696, p = 0.009).
Significant psychological and social predictors of PPD
Receiving support or treatment for PPD (No-Yes): The aOR among PPD mothers who were not receiving support or treatment relative to those receiving support or treatment was 9.784 (95% CI: 5.373–17.816, p = 0.001).
Awareness of symptoms and risk factors (No-Yes): The aOR among PPD mothers who lack awareness of symptoms and risk factors relative to those with awareness was 2.902 (95% CI: 1.633–5.154, p = 0.001).
Experienced cultural stigma or judgement about PPD in the community (No-Yes): The aOR among PPD mothers who had experienced cultural stigma or judgment in the community relative to those who have not was 4.406 (95% CI: 2.394–8.110, p < 0.001).
Suffering from any disease or mental or psychiatric disorder: For “Now I am suffering—not at all,” the aOR among PPD mothers was 12.871 (95% CI: 3.063–54.073, p = 0.001). Similarly, for “Had a past history but was treated—not at all,” the adjusted odds ratio was 16.6 (95% CI: 2.528–108.965, p = 0.003), and for “Had a family history—not at all,” the adjusted odds ratio was 3.551 (95% CI: 1.012–12.453, p = 0.048).
Significant coping predictors of PPD comfort: discussing mental health with family (maybe yes)
The aOR among PPD mothers who were maybe more comfortable discussing mental health with family relatives was 0.369 (95% CI: 0.146–0.933, p = 0.035).
PDD is a debilitating mental disorder that has many potential and protective risk factors that should be considered to promote the mental and psychological well-being of the mothers and to create a favorable environment for the newborn and all family members. This multinational cross-sectional survey was conducted in six different countries to determine the frequency of PDD using EPDS and to explore its predictors. It was found that PPD was a prevalent problem that varied across different nations.
The frequency of PPD across the studied countries
Using the widely used EPDS to determine the current PPD, we found that the overall frequency of PPD in the total sample was 92 (13.6%). Which significantly ( p = 0.001) varied across different countries, being highest among Ghana mothers 13 (26.0%) out of 50 and Indians 28 (21.7%) out of 129. Egyptian 21 (19.1) out of 110, Yemen 14 (8.5%) out of 164, Iraq 13 (7.7%) out of 169, and Syria 1 (2.3%) out of 43 in descending order. This prevalence was similar to that reported by Hairol et al. (2021) in Malaysia (14.3%) [ 13 ], Yusuff et al. (2010) in Malaysia (14.3%) [ 14 ], and Nakku et al. (2006) in New Delhi (12.75%) [ 15 ].
While the frequency of PPD varied greatly based on the timing, setting, and existence of many psychosocial and post-partum periods, for example, it was higher than that reported in Italy (2012), which was 4.7% [ 16 ], in Turkey (2017) was 9.1%/110 [ 17 ], 9.2% in Sudan [ 18 ], Eritrea (2020) was 7.4% [ 19 ], in the capital Kuala Lumpur (2001) was (3.9%) [ 20 ], in Malaysia (2002) was (9.8%) [ 21 ], and in European countries. (2021) was 13–19% [ 22 ].
Lower frequencies were than those reported; PPD is a predominant problem in Asia, e.g., in Pakistan, the three-month period after childbirth, ranging from 28.8% in 2003 to 36% in 2006 to 94% in 2007, while after 12 months after childbirth, it was 62% in 2021 [ 23 – 24 ]. While in 2022 Afghanistan 45% after their first labour [ 25 ] in Canada (2015) was 40% [ 26 ], in India, the systematic review in 2022 was 22% of Primipara [ 27 ], in Malaysia (2006) was 22.8% [ 28 ], in India (2019) was 21.5% [ 29 ], in the Tigray zone in Ethiopia (2017) was 19% [ 30 ], varied in Iran between 20.3% and 35% [ 31 – 32 ], and in China was 499 (27.37%) out of 1823 [ 33 ]. A possible explanation might be the differences in the study setting and the type of design utilized. Other differences should be considered, like different populations with different socioeconomic characteristics and the variation in the timing of post-partum follow-up. It is vital to consider the role of culture, the impact of patients’ beliefs, and the cultural support for receiving help for PPD.
Demographic and health-related associations, or predictors of PPD (Tables 1 and 4 )
Regarding age, our study found no significant difference between PPD and non-PPD mothers with regard to age. In agreement with our study [ 12 , 34 , 35 ], other studies [ 36 , 37 , 38 ] found an inverse association between women’s age and PPD, with an increased risk of PPD (increases EPDS scores) at a younger age significantly, as teenage mothers, being primiparous, encounter difficulty during the postpartum period due to their inability to cope with financial and emotional difficulties, as well as the challenge of motherhood. Cultural factors and social perspectives of young mothers in different countries could be a reason for this difference. [ 38 – 39 ] and Abdollahi et al. [ 36 ] reported that older mothers were a protective factor for PPD (OR = 0.88, 95% CI: 0.84–0.92].
Regarding marital status, after controlling for other variables, married mothers exhibited a significantly diminished likelihood of experiencing PPD in comparison to single women (0.141; 95% CI: 0.04–0.494; p = 0.002). Also, Gebregziabher et al. [ 19 ] reported that there were statistically significant differences in proportions between mothers’ PPD and marital status.
Regarding the mother’s education, in agreement with our study, Ahmed et al. [ 34 ] showed that there was no statistically significant difference between PPD and a mother’s education. While Agarwala et al. [ 29 ] showed that a higher level of mother’s education. increases the risk of PPD, Gebregziabher et al. [ 19 ] showed that the housewives were 0.24 times less likely to develop PPD as compared to the employed mothers (aOR = 0.24, 95% CI: 0.06–0.97; p = 0.046); those mothers who perceived their socioeconomic status (SES) as low were 13 times more likely to develop PPD as compared to the mothers who had good SES (aOR = 13.33, 95% CI: 2.66–66.78; p = 0.002).
Regarding the SES or monthly income, while other studies [ 18 , 40 ] found that there was a statistically significant association between PPD mothers and different domains of SES, 34% of depressed women were found to live under low SES conditions in comparison to only 15.4% who were found to live in high SES and experienced PPD. In disagreement with our study, Hairol et al. [ 12 ] demonstrated that the incidence of PPD was significantly p = 0.01 higher for participants from the low-income group (27.27%) who were 2.58 times more likely to have PDD symptoms (OR: 2.58, 95% CI: 1.23–5.19; p = 0.01 compared to those from the middle- and high-income groups (8.33%), and low household income (OR = 3.57 [95% CI: 1.49–8.5] increased the odds of PPD [ 41 ].
Adeyemo et al. (2020),and Al Nasr et al. (2020) revealed that there was no significant difference between the occurrence of PPD and socio-demographic characteristics. This difference may be due to a different sample size and ethnicity [ 42 , 43 ]. In agreement with our findings, Abdollahi et al. [ 36 ] demonstrated that after multiple logistic regression analyses, there were increased odds of PPD with a lower state of general health (OR = 1.08 [95% CI: 1.06–1.11]), gestational diabetes (OR = 2.93 [95% CI = 1.46–5.88]), and low household income (OR = 3.57 [95% CI: 1.49–8.5]). The odds of PPD decreased.
Regarding access to health care, in agreement with studies conducted at Gondar University Hospital, Ethiopia [ 18 ], North Carolina, Colorado [ 21 ], Khartoum, Sudan [ 44 ], Asaye et al. [ 45 ], the current study found that participants who did not have free access to the healthcare system were riskier for the development of PPD. the study results may be affected by the care given during the antenatal care (ANC) visits. This can be explained by the fact that PPD was four times higher than that of mothers who did not have ANC, where counseling and anticipatory guidance care are given that build maternal self-esteem and resiliency, along with knowledge about normal and problematic complications to discuss at care visits and their right to mental and physical wellness, including access to care. The increased access to care (including postpartum visits) will increase the diagnosis of PPD and provide guidance, reassurance, and appropriate referrals. Healthcare professionals have the ability to both educate and empower mothers as they care for their babies, their families, and themselves [ 46 ].
Regarding nationality, for PPD mothers of Yemeni nationality compared to those of Egyptian nationality, the aOR is 0.318 (95% CI: 0.123–0.821, p = 0.018). Similarly, for Syrian nationality in comparison to Egyptian nationality, the aOR is 0.111 (95% CI: 0.0139–0.887, p = 0.038), and for Iraqi nationality compared to Egyptian nationality, the aOR is 0.241 (95% CI: 0.0920–0.633, p = 0.004). These findings indicated that, while accounting for other covariates, individuals from the aforementioned nationalities were less predisposed to experiencing PPD than their Egyptian counterparts. These findings can be explained by the fact that, in Egypt, the younger age of marriage, especially in rural areas, poor mental health services, being illiterate, dropping out of school early, unemployment, and the stigma of psychiatric illnesses are cultural factors that hinder the diagnosis and treatment of PPD [ 40 ].
Obstetric, current pregnancy, and infant-related characteristics and their association or predictors of PPD (Tables 2 and 4 )
In the present study, the number of dead children was significantly associated with PPD. This report was supported by studies conducted with Gujarati postpartum women [ 41 ] and rural southern Ethiopia [ 43 ]. This might be because mothers who have dead children pose different psychosocial problems and might regret it for fear of complications developing during their pregnancy. Agarwala et al. [ 29 ] found that a history of previous abortions and having more than two children increased the risk of developing PPD due to a greater psychological burden. The inconsistencies in the findings of these studies indicate that the occurrence of postpartum depression is not solely determined by the number of childbirths.
In obstetric and current pregnancy , there was no significant difference regarding the baby’s age, number of miscarriages, type of last delivery, premature labour, healthy baby, baby admitted to the neonatal intensive care unit (NICU), or feeding difficulties. In agreement with Al Nasr et al. [ 42 ], inconsistent with Asaye et al. [ 45 ], they showed that concerning multivariable logistic regression analysis, abortion history, birth weight, and gestational age were significant associated factors of postpartum depression at a value of p < 0.05.
However, a close association was noted between the mode of delivery and the presence of PPD in mothers, with p = 0.107. There is a high tendency towards depression seen in mothers who have delivered more than three times (44%). In disagreement with what was reported by Adeyemo et al. [ 41 ], having more than five children ( p = 0.027), cesarean section delivery ( p = 0.002), and mothers’ poor state of health since delivery ( p < 0.001) are associated with an increase in the risk of PPD [ 47 ]. An increased risk of cesarean section as a mode of delivery was observed (OR = 1.958, p = 0.049) in a study by Al Nasr et al. [ 42 ].
We reported breastfeeding mothers had a lower, non-significant frequency of PPD compared to non-breast-feeding mothers (36.6% vs. 45%). In agreement with Ahmed et al. [ 34 ], they showed that with respect to breastfeeding and possible PPD, about 67.3% of women who depend on breastfeeding reported no PPD, while 32.7% only had PP. Inconsistency with Adeyemo et al. [ 41 ], who reported that unexclusive breastfeeding ( p = 0.003) was associated with PPD, while Shao et al. [ 40 ] reported that mothers who were exclusively formula feeding had a higher prevalence of PPD.
Regarding postnatal problems, our results revealed that postnatal problems display a significant association with PPD. In line with our results, Agarwala et al. [ 29 ] and Gebregziabher et al. [ 19 ] showed that mothers who experienced complications during childbirth, those who became ill after delivery, and those whose babies were unhealthy had a statistically significant higher proportion of PPD.
Hormone-related contraception methods were found to have a statistically significant association with PPD, consistent with the literature [ 46 ]; this can be explained by the hormones and neurotransmitters as biological factors that play significant roles in the onset of PPD. Estrogen hormones act as regulators of transcription from brain neurotransmitters and modulate the action of serotonin receptors. This hormone stimulates neurogenesis, the process of generating new neurons in the brain, and promotes the synthesis of neurotransmitters. In the hypothalamus, estrogen modulates neurotransmitters and governs sleep and temperature regulation. Variations in the levels of this hormone or its absence are linked to depression [ 19 ].
Participants whose last pregnancy was unplanned were 3.39 times more likely to have postpartum depression (aOR = 3.39, 95% CI: 1.24–9.28; p = 0.017). Mothers who experienced illness after delivery were more likely to develop PPD as compared to their counterparts (aOR = 7.42, 95% CI: 1.44–34.2; p = 0.016) [ 40 ]. In agreement with Asaye et al. [ 45 ] and Abdollahi et al. [ 36 ], unplanned pregnancy has been associated with the development of PPD (aOR = 2.02, 95% CI: 1.24, 3.31) and OR = 2.5 [95% CI: 1.69–3.7] than those of those who had planned, respectively.
The psychosocial characteristics and their association with PPD
Mothers with a family history of mental illness were significantly associated with PPD. This finding was in accordance with studies conducted in Istanbul, Turkey [ 47 ], and Bahrain [ 48 ]. Other studies also showed that women with PPD were most likely to have psychological symptoms during pregnancy [ 43 , 44 , 45 , 46 , 47 , 48 , 49 ]. A meta-analysis of 24,000 mothers concluded that having depression and anxiety during pregnancy and a previous history of psychiatric illness or a history of depression are strong risk factors for developing PPD [ 50 , 51 , 52 ]. Asaye et al. [ 45 ], mothers whose relatives had mental illness history were (aOR = 1.20, 95% CI: 1.09, 3.05 0) be depressed than those whose relatives did not have mental illness history.
This can be attributed to the links between genetic predisposition and mood disorders, considering both nature and nurture are important to address PDD. PPD may be seen as a “normal” condition for those who are acquainted with relatives with mood disorders, especially during the CBP. A family history of mental illness can be easily elicited in the ANC first visit history and requires special attention during the postnatal period. There are various risk factors for PPD, including stressful life events, low social support, the infant’s gender preference, and low income [ 53 ].
Concerning familial support and possible PPD, a statistically significant association was found between them. We reported that mothers who did not have social support (a partner or the father of the baby) had higher odds (aOR = 5.8, 95% CI: 1.33–25.29; p = 0.019) of experiencing PPD. Furthermore, Al Nasr et al. [ 42 ] revealed a significant association between the PPD and an unsupportive spouse ( P value = 0.023). while it was noted that 66.5% of women who received good familial support after giving birth had no depression, compared to 33.5% who only suffered from possible PPD [ 40 ]]. Also, Adeyemo et al. [ 41 ] showed that some psychosocial factors were significantly associated with having PPD: having an unsupportive partner ( p < 0.001), experiencing intimate partner violence ( p < 0.001), and not getting help in taking care of their baby ( p < 0.001). Al Nasr et al. (2020) revealed that the predictor of PPD was an unsupportive spouse (OR = 4.53, P = 0.049) [ 48 ].
Regarding the perceived stigma, in agreement with our study, Bina (2020) found that shame, stigma, the fear of being labeled mentally ill, and language and communication barriers were significant factors in women’s decisions to seek treatment or accept help [ 53 ]. Other mothers were hesitant about mental health services [ 54 ]. It is noteworthy that some PPD mothers refused to seek treatment due to perceived insufficient time and the inconvenience of attending appointments [ 55 ].
PPD was significantly higher among mothers with financial problems or problems with their husbands. This came in agreement with Ahmed et al. [ 34 ], who showed that, regarding stressful conditions and PPD, there was a statistically significant association with a higher percentage of PPD among mothers who had a history of stressful conditions (59.3%), compared to those with no history of stressful conditions (40.7%). Furthermore, Al Nasr et al. (2020) revealed that stressful life events contributed significantly ( P value = 0.003) to the development of PPD in the sample population. Al Nasr et al. stressful life events (OR = 2.677, p = 0.005) [ 42 ].
Coping strategies: causes of fearing and not seeking
Feeling at ease discussing mental health topics with one’s husband, family, community, and physician and experiencing cultural stigma or judgment regarding PPD within the community was significantly associated with the presence of PPD. In the current study, there were different reasons for not receiving the treatment, including cultural or traditional beliefs, language or communication barriers, social norms, and geographical or regional disparities in mental health resources. Haque and Malebranche [ 56 ] portrayed culture and the various conceptualizations of the maternal role as barriers to women seeking help and treatment.
In the present study, marital status, nationality, current pregnancy status, healthy baby, postnatal problems, receiving support or treatment for PPD, having awareness of symptoms and risk factors of PPD, suffering from any disease or mental or psychiatric disorder, comfort discussing mental health with family, and experiencing cultural stigma or judgment about PPD in the community were the significant predictors of PPD. In agreement with Ahmed et al. [ 34 ], the final logistic regression model contained seven predictors for PPD symptoms: SES, history of depression, history of PPD, history of stressful conditions, familial support, unwanted pregnancy, and male preference.
PPD has been recognized as a public health problem and may cause negative consequences for infants. It is estimated that 20 to 40% of women living in low-income countries experience depression during pregnancy or the postpartum period. The prevalence of PPD shows a wide variation, affecting 8–50% of postnatal mothers across countries [ 19 ].
Strengths and limitations
Strengths of our study include its multinational scope, which involved participants from six different countries, enhancing the generalizability of the findings. The study also boasted a large sample size of 674 participants, increasing the statistical power and reliability of the results. Standardized measures, such as the Edinburgh Postnatal Depression Scale (EPDS), were used for assessing postpartum depression, ensuring consistency and comparability across diverse settings. Additionally, the study explored a comprehensive range of predictors and associated factors of postpartum depression, including demographic, obstetric, health-related, and psychosocial characteristics. Rigorous analysis techniques, including multiple logistic regression analyses, were employed to identify significant predictors of postpartum depression, controlling for potential confounders and providing robust statistical evidence.
However, the study has several limitations that should be considered. Firstly, its cross-sectional design limits causal inference, as it does not allow for the determination of temporal relationships between variables. Secondly, the reliance on self-reported data, including information on postpartum depression symptoms and associated factors, may be subject to recall bias and social desirability bias. Thirdly, the use of convenience sampling methods may introduce selection bias and limit the generalizability of the findings to a broader population. Lastly, cultural differences in the perception and reporting of postpartum depression symptoms among participants from different countries could influence the results.
Moreover, the variation in sample size and response rates among countries can be attributed to two main variables. (1) The methodology showed that the sample size was determined by considering several parameters, such as allocating proportionately to the mothers who gave birth and fulfilling the selection criteria during the data collection period served by each health center. (2) The political turmoil in Syria affects how often and how well people can use the Internet, especially because the data was gathered using an online survey link, leading to a relatively low number of responses from those areas. (3) Language barrier in Ghana: as we used the Arabic and English-validated versions of the EPDS, Ghana is a multilingual country with approximately eighty languages spoken. Although English is considered an official language, the primarily spoken languages in the southern region are Akan, specifically the Akuapem Twi, Asante Twi, and Fante dialects. In the northern region, primarily spoken are the Mole-Dagbani ethnic languages, Dagaare and Dagbanli. Moreover, there are around seventy ethnic groups, each with its own unique language [ 57 ]. (4) At the end of the data collection period, to avoid seasonal affective disorders, the meteorological autumn season began on the 1st day of September, which may be associated with autumm depressive symptoms that may confound or affect our results. Furthermore, the sampling methods were not universal across all Arabic countries, potentially constraining the generalizability of our findings.
Recommendations
The antenatal programme should incorporate health education programmes about the symptoms of PPD. Health education programs about the symptoms of PPD should be included in the antenatal program.
Mass media awareness campaigns have a vital role in raising public awareness about PPD-related issues. Mass media.
The ANC first visit history should elicit a family history of mental illness, enabling early detection of risky mothers. Family history of mental illness can be easily elicited in the ANC first visit history.
For effective management of PPD, effective support (from husband, friends, and family) is an essential component. For effective management of PPD effectiveness of support.
The maternal (antenatal, natal, and postnatal) services should be provided for free and of high quality The maternal (antenatal, natal, postnatal) services should be provided free and of high quality.
It should be stressed that although numerous studies have been carried out on PPD, further investigation needs to be conducted on the global prevalence and incidence of depressive symptoms in pregnant women and related risk factors, especially in other populations.
Around 14% of the studied mothers had PPD, and the frequency varies across different countries and half of them do not know. Our study identified significant associations and predictors of postpartum depression (PPD) among mothers. Marital status was significantly associated with PPD, with married mothers having lower odds of experiencing PPD compared to single mothers. Nationality also emerged as a significant predictor, with Yemeni, Syrian, and Iraqi mothers showing lower odds of PPD compared to Egyptian mothers. Significant obstetric, current pregnancy, and infant-related predictors included the pregnancy status, the health status of the baby, and the presence of postnatal problems. Among psychological and social predictors, receiving support or treatment for PPD, awareness of symptoms and risk factors, experiencing cultural stigma or judgment about PPD, and suffering from any disease or mental disorder were significantly associated with PPD. Additionally, mothers who were maybe more comfortable discussing mental health with family relatives had lower odds of experiencing PPD.
These findings underscore the importance of considering various demographic, obstetric, psychosocial, and coping factors in the identification and management of PPD among mothers. Targeted interventions addressing these predictors could potentially mitigate the risk of PPD and improve maternal mental health outcomes.
Data availability
Yes, I have research data to declare.The data is available when requested from the corresponding author [email protected].
Abbreviations
Adjusted Odds Ratio
- Postpartum depression
Primary Health Care centers
Socioeconomic Status
program (Statistical Package for Social Science
The Edinburgh Postnatal Depression Scale
The Neonatal Intensive Care Unit
Sultan P, Ando K, Elkhateb R, George RB, Lim G, Carvalho B et al. (2022). Assessment of Patient-Reported Outcome Measures for Maternal Postpartum Depression Using the Consensus-Based Standards for the Selection of Health Measurement Instruments Guideline: A Systematic Review. JAMA Network Open; 1;5(6).
Crotty F, Sheehan J. Prevalence and detection of postnatal depression in an Irish community sample. Ir J Psychol Med. 2004;21:117–21.
Article PubMed Google Scholar
Goodman SH, Brand SR. Parental psychopathology and its relation to child psychopathology. In: Hersen M, Gross AM, editors. Handbook of clinical psychology vol 2: children and adolescents. Hoboken, NJ: Wiley; 2008. pp. 937–65.
Google Scholar
Wang Z, Liu J, Shuai H, Cai Z, Fu X, Liu Y, Xiao et al. (2021). Mapping global prevalence of depression among postpartum women. Transl Psychiatry. 2021;11(1):543. https://doi.org/10.1038/s41398-021-01663-6 . Erratum in: Transl Psychiatry; 20;11(1):640. PMID: 34671011IF: 6.8 Q1 B1; PMCID: PMC8528847IF: 6.8 Q1 B1.Lase accessed Jan 2024.
American Psychiatric Association. (2013). Diagnostic and Statistical Manual of Mental Disorders. 5th edition. Arlington, VA: American Psychiatric Association; Lase accessed October 2023.
Robertson E, Grace S, Wallington T, Stewart DE. Antenatal risk factors for postpartum depression: a synthesis of recent literature. Gen Hosp Psychiatry. 2004;26:289–95.
Gaynes BN, Gavin N, Meltzer-Brody S, Lohr KN, Swinson T, Gartlehner G, Brody S, Miller WC. Perinatal depression: prevalence, screening accuracy, and screening outcomes: Summary. AHRQ evidence report summaries; 2005. pp. 71–9.
O’hara MW, Swain AM. (1996). Rates and risk of postpartum depression: a meta-analysis. Int Rev Psychiatry. 1996; 8:37–54.
Goodman SH, Brand SR. (2008). Parental psychopathology and its relation to child psychopathology. In: Hersen M, Gross AM, editors. Handbook of clinical psychology Vol 2: Children and adolescents. Hoboken, NJ: John Wiley & Sons; 2008. pp. 937–65.
Cox JL, Holden JM, Sagovsky R. Detection of postnatal depression: development of the 10-item Edinburgh postnatal depression scale. Br J Psychiatry. 1987;150:782–6.
Article CAS PubMed Google Scholar
Martín-Gómez C, Moreno-Peral P, Bellón J, SC Cerón S, Campos-Paino H, Gómez-Gómez I, Rigabert A, Benítez I, Motrico E. Effectiveness of psychological, psychoeducational and psychosocial interventions to prevent postpartum depression in adolescent and adult mothers: study protocol for a systematic review and meta-analysis of randomised controlled trials. BMJ open. 2020;10(5):e034424. [accessed Mar 16 2024].
Article PubMed PubMed Central Google Scholar
Sehairi Z. (2020). Validation Of The Arabic Version Of The Edinburgh Postnatal Depression Scale And Prevalence Of Postnatal Depression On An Algerian Sample. https://api.semanticscholar.org/CorpusID:216391386 . Accessed August 2023.
Hairol MI, Ahmad SA, Sharanjeet-Kaur S et al. (2021). Incidence and predictors of postpartum depression among postpartum mothers in Kuala Lumpur, Malaysia: a cross-sectional study. PLoS ONE, 16(11), e0259782.
Yusuff AS, Tang L, Binns CW, Lee AH. Prevalence and risk factors for postnatal depression in Sabah, Malaysia: a cohort study. Women Birth. 2015;1(1):25–9. pmid:25466643
Article Google Scholar
Nakku JE, Nakasi G, Mirembe F. Postpartum major depression at six weeks in primary health care: prevalence and associated factors. Afr Health Sci. 2006;6(4):207–14. https://doi.org/10.5555/afhs.2006.6.4.207 . PMID: 17604509IF: 1.0 Q4 B4; PMCID: PMC1832062
Clavenna A, Seletti E, Cartabia M, Didoni A, Fortinguerra F, Sciascia T, et al. Postnatal depression screening in a paediatric primary care setting in Italy. BMC Psychiatry. 2017;17(1):42. pmid:28122520
Serhan N, Ege E, Ayrancı U, Kosgeroglu N. (2013). Prevalence of postpartum depression in mothers and fathers and its correlates. Journal of clinical nursing; 1;22(1–2):279–84. pmid:23216556
Deribachew H, Berhe D, Zaid T, et al. Assessment of prevalence and associated factors of postpartum depression among postpartum mothers in eastern zone of Tigray. Eur J Pharm Med Res. 2016;3(10):54–60.
Gebregziabher NK, Netsereab TB, Fessaha YG, et al. Prevalence and associated factors of postpartum depression among postpartum mothers in central region, Eritrea: a health facility based survey. BMC Public Health. 2020;20:1–10.
Grace J, Lee K, Ballard C, et al. The relationship between post-natal depression, somatization and behaviour in Malaysian women. Transcult Psychiatry. 2001;38(1):27–34.
Mahmud WMRW, Shariff S, Yaacob MJ. Postpartum depression: a survey of the incidence and associated risk factors among malay women in Beris Kubor Besar, Bachok, Kelantan. The Malaysian journal of medical sciences. Volume 9. MJMS; 2002. p. 41. 1.
Anna S. Postpartum depression and birthexperience in Russia. Psychol Russia: State Theart. 2021;14(1):28–38.
Yadav T, Shams R, Khan AF, Azam H, Anwar M et al. (2020).,. Postpartum Depression: Prevalence and Associated Risk Factors Among Women in Sindh, Pakistan. Cureus.22;12(12):e12216. https://doi.org/10.7759/cureus.12216 . PMID: 33489623IF: 1.2 NA NA; PMCID: PMC7815271IF: 1.2 NA NA.
Abdullah M, Ijaz S, Asad S. (2024). Postpartum depression-an exploratory mixed method study for developing an indigenous tool. BMC Pregnancy Childbirth 24, 49 (2024). https://doi.org/10.1186/s12884-023-06192-2 .
Upadhyay RP, Chowdhury R, Salehi A, Sarkar K, Singh SK, Sinha B et al. (2022). Postpartum depression in India: a systematic review and meta-analysis. Bull World Health Organ [Internet]. 2017 October 10 [cited 2022 October 6];95(10):706. https://doi.org/10.2471/BLT.17.192237/ .
Khalifa DS, Glavin K, Bjertness E et al. (2016). Determinants of postnatal depression in Sudanese women at 3 months postpartum: a cross-sectional study. BMJ open, 6(3), e00944327).
Khadija Sharifzade BK, Padhi S, Manna etal. (2022). Prevalence and associated factors of postpartum depression among Afghan women: a phase-wise cross-sectional study in Rezaie maternal hospital in Herat province.; Razi International Medical Journa2| 2|59| https://doi.org/10.56101/rimj.v2i2.59 .
Azidah A, Shaiful B, Rusli N, et al. Postnatal depression and socio-cultural practices among postnatal mothers in Kota Bahru, Kelantan, Malaysia. Med J Malay. 2006;61(1):76–83.
CAS Google Scholar
Agarwala A, Rao PA, Narayanan P. Prevalence and predictors of postpartum depression among mothers in the rural areas of Udupi Taluk, Karnataka, India: a cross-sectional study. Clin Epidemiol Global Health. 2019;7(3):342–5.
Arikan I, Korkut Y, Demir BK et al. (2017). The prevalence of postpartum depression and associated factors: a hospital-based descriptive study.
Azimi-Lolaty HMD, Hosaini SH, Khalilian A, et al. Prevalence and predictors of postpartum depression among pregnant women referred to mother-child health care clinics (MCH). Res J Biol Sci. 2007;2:285–90.
Najafi KFA, Nazifi F, Sabrkonandeh S. Prevalence of postpartum depression in Alzahra Hospital in Rasht in 2004. Guilan Univ Med Sci J. 2006;15:97–105. (In Persian.).
Deng AW, Xiong RB, Jiang TT, Luo YP, Chen WZ. (2014). Prevalence and risk factors of postpartum depression in a population-based sample of women in Tangxia Community, Guangzhou. Asian Pacific journal of tropical medicine; 1;7(3):244–9. pmid:24507649
Ahmed GK, Elbeh K, Shams RM, et al. Prevalence and predictors of postpartum depression in Upper Egypt: a multicenter primary health care study. J Affect Disord. 2021;290:211–8.
Cantilino A, Zambaldi CF, Albuquerque T, et al. Postpartum depression in Recife–Brazil: prevalence and association with bio-socio-demographic factors. J Bras Psiquiatr. 2010;59:1–9.
Abdollahi F, Zarghami M, Azhar MZ, et al. Predictors and incidence of post-partum depression: a longitudinal cohort study. J Obstet Gynecol Res. 2014;40(12):2191–200.
McCoy SJB, Beal JM, et al. Risk factors for postpartum depression: a retrospective investigation at 4-weeks postnatal and a review of the literature. JAOA. 2006;106:193–8.
PubMed Google Scholar
Sierra J. (2008). Risk Factors Related to Postpartum Depression in Low-Income Latina Mothers. Ann Arbor: ProQuest Information and Learning Company, 2008.
Çankaya S. The effect of psychosocial risk factors on postpartum depression in antenatal period: a prospective study. Arch Psychiatr Nurs. 2020;34(3):176–83.
Shao HH, Lee SC, Huang JP, et al. Prevalence of postpartum depression and associated predictors among Taiwanese women in a mother-child friendly hospital. Asia Pac J Public Health. 2021;33(4):411–7.
Adeyemo EO, Oluwole EO, Kanma-Okafor OJ, et al. Prevalence and predictors of postpartum depression among postnatal women in Lagos. Nigeria Afr Health Sci. 2020;20(4):1943–54.
Al Nasr RS, Altharwi K, Derbah MS et al. (2020). Prevalence and predictors of postpartum depression in Riyadh, Saudi Arabia: a cross sectional study. PLoS ONE, 15(2), e0228666.
Desai ND, Mehta RY, Ganjiwale J. Study of prevalence and risk factors of postpartum depression. Natl J Med Res. 2012;2(02):194–8.
Azale T, Fekadu A, Medhin G, et al. Coping strategies of women with postpartum depression symptoms in rural Ethiopia: a cross-sectional community study. BMC Psychiatry. 2018;18(1):1–13.
Asaye MM, Muche HA, Zelalem ED. (2020). Prevalence and predictors of postpartum depression: Northwest Ethiopia. Psychiatry journal, 2020.
Ayele TA, Azale T, Alemu K et al. (2016). Prevalence and associated factors of antenatal depression among women attending antenatal care service at Gondar University Hospital, Northwest Ethiopia. PLoS ONE, 11(5), e0155125.
Saraswat N, Wal P, Pal RS et al. (2021). A detailed Biological Approach on Hormonal Imbalance Causing Depression in critical periods (Postpartum, Postmenopausal and Perimenopausal Depression) in adult women. Open Biology J, 9.
Guida J, Sundaram S, Leiferman J. Antenatal physical activity: investigating the effects on postpartum depression. Health. 2012;4:1276–86.
Robertson E, Grace S, Wallington T, et al. Antenatal risk factors for postpartum depression: a synthesis of recent literature. Gen Hosp Psychiatry. 2004;26:289–95.
Watanabe M, Wada K, Sakata Y, et al. Maternity blues as predictor of postpartum depression: a prospective cohort study among Japanese women. J Psychosom Obstet Gynecol. 2008;29:211–7.
Kirpinar I˙, Gözüm S, Pasinliog˘ lu T. Prospective study of post-partum depression in eastern Turkey prevalence, socio- demographic and obstetric correlates, prenatal anxiety and early awareness. J Clin Nurs. 2009;19:422–31.
Zhao XH, Zhang ZH. Risk factors for postpartum depression: an evidence-based systematic review of systematic reviews and meta-analyses. Asian J Psychiatry. 2020;53:102353.
Bina R. Predictors of postpartum depression service use: a theory-informed, integrative systematic review. Women Birth. 2020;33(1):e24–32.
Jannati N, Farokhzadian J, Ahmadian L. The experience of healthcare professionals providing mental health services to mothers with postpartum depression: a qualitative study. Sultan Qaboos Univ Med J. 2021;21(4):554.
Dennis CL, Chung-Lee L. Postpartum depression help‐seeking barriers and maternal treatment preferences: a qualitative systematic review. Birth. 2006;33(4):323–31.
Haque S, Malebranche M. (2020). Impact of culture on refugee women’s conceptualization and experience of postpartum depression in high-income countries of resettlement: a scoping review. PLoS ONE, 15(9), e0238109.
https:// www.statista.com/statistics/1285335/population-in-ghana-by-languages-spoken/ .
Download references
Acknowledgements
We would like to express our deep thanks to Rovan Hossam Abdulnabi Ali for her role in completing this study and her unlimited support. Special thanks to Dr. Mohamed Liaquat Raza for his role in reviewing the questionnaire. Moreover, we would like to thank all the mothers who participated in this study.
No funding for this project.
Author information
Authors and affiliations.
Department of Public Health and Community Medicine, Faculty of Medicine, Zagazig University, Zagazig, Egypt
Samar A. Amer
Department of Family Medicine, Faculty of Medicine, Zagazig University, Zagazig, Egypt
Nahla A. Zaitoun
Department of Psychiatry, Faculty of Medicine, Zagazig University, Zagazig, Egypt
Heba A. Abdelsalam
Faculty of Medicine, Al-Azhar University, Damietta, Egypt
Abdallah Abbas
Department of Obstetrics and Gynecology, Faculty of Medicine, Zagazig University, Zagazig, Egypt
Mohamed Sh Ramadan
Hammurabi Medical College, University of Babylon, Al-Diwaniyah, Iraq
Hassan M. Ayal
Hardamout University College of Medicine, Almukalla, Yemen
Samaher Edhah Ahmed Ba-Gais
Department of General Medicine, Shadan Institute of Medical Science, Hyderabad, India
Nawal Mahboob Basha
College of Medicine, Sulaiman Alrajhi University, Albukayriah, Al-Qassim, Saudi Arabia
Abdulrahman Allahham
Department of Virology, Noguchi Memorial Institute for Medical Research, University of Ghana Legon, Accra, Ghana
Emmanuael Boateng Agyenim
Department of Public Health and Community Medicine, Faculty of Medicine, Beni-Suef University, Beni-Suef, Egypt
Walid Amin Al-Shroby
You can also search for this author in PubMed Google Scholar
Contributions
Conceptualization: Samar A. Amer (SA); Methodology: SA, Nahal A. Zaitoun (NZ); Validation: Mohamed Ramadan Ali Shaaban (MR), Hassan Majid Abdulameer Aya (HM), Samaher Edhah Ahmed Ba-Gais (SG), Nawal Mahboob Basha (NB), Abdulrahman Allahham (AbAl), Emmanuael Boateng Agyenim (EB); Formal analysis: Abdallah Abbas (AA); Data curation: MR, HM, SG, NB, AbAl, NZ, and EB; Writing original draft preparation: SA, Heba Ahmed Abdelsalam (HAA), and NZ; Writing review and editing: MR, AA, Walid Amin Elshrowby (WE); Visualization: SA, AA; Supervision: SA; Project administration: AA. All authors have read and agreed to the published version of the manuscript.
Corresponding author
Correspondence to Samar A. Amer .
Ethics declarations
Ethical approval and consent to participate.
All participants were provided with electronic informed consent after receiving clear explanations regarding the study’s objectives, data confidentiality, voluntary participation, and the right to withdraw. The questionnaire did not contain any sensitive questions, and data collection was performed anonymously. We affirm that all relevant ethical guidelines have been adhered to, and any necessary approvals from the ethics committee have been obtained. Approval was received from the ethical committee of the family medicine department, the faculty of medicine at Zagazig University, and from the patients included in the study. IRP#ZU-IRP#11079-8/10-2023.
Practicing ethical decision-making is crucial for providing clinical treatment. Such decisions are frequently made challenging due to a lack of knowledge and the mother’s ability to handle the associated complexities and uncertainties that affect the patient’s current level of functioning and ability to take care of her child. At the end of the survey, we raised concerns regarding the red flags, such as suicidal thoughts, and called for a revisit for the psychiatrist’s evaluation of the discussion of the risks, benefits, and alternatives to using medication.
Consent for publication
All authors have read and agreed to the published version of the manuscript.
Previous publication
We declare that this research paper has not been published elsewhere in any other academic journal or platform.
Generative AI in scientific writing
We declare that we have not used AI in writing any part of this manuscript.
Conflict of interest
No conflict of interest.
Additional information
Publisher’s note.
Springer Nature remains neutral with regard to jurisdictional claims in published maps and institutional affiliations.
Electronic supplementary material
Below is the link to the electronic supplementary material.
Supplementary Material 1
Rights and permissions.
Open Access This article is licensed under a Creative Commons Attribution 4.0 International License, which permits use, sharing, adaptation, distribution and reproduction in any medium or format, as long as you give appropriate credit to the original author(s) and the source, provide a link to the Creative Commons licence, and indicate if changes were made. The images or other third party material in this article are included in the article’s Creative Commons licence, unless indicated otherwise in a credit line to the material. If material is not included in the article’s Creative Commons licence and your intended use is not permitted by statutory regulation or exceeds the permitted use, you will need to obtain permission directly from the copyright holder. To view a copy of this licence, visit http://creativecommons.org/licenses/by/4.0/ . The Creative Commons Public Domain Dedication waiver ( http://creativecommons.org/publicdomain/zero/1.0/ ) applies to the data made available in this article, unless otherwise stated in a credit line to the data.
Reprints and permissions
About this article
Cite this article.
Amer, S.A., Zaitoun, N.A., Abdelsalam, H.A. et al. Exploring predictors and prevalence of postpartum depression among mothers: Multinational study. BMC Public Health 24 , 1308 (2024). https://doi.org/10.1186/s12889-024-18502-0
Download citation
Received : 06 February 2024
Accepted : 02 April 2024
Published : 14 May 2024
DOI : https://doi.org/10.1186/s12889-024-18502-0
Share this article
Anyone you share the following link with will be able to read this content:
Sorry, a shareable link is not currently available for this article.
Provided by the Springer Nature SharedIt content-sharing initiative
- The Edinburgh postnatal depression scale (EPDS)
- Determinants
- Psychosocial
BMC Public Health
ISSN: 1471-2458
- Submission enquiries: [email protected]
- General enquiries: [email protected]
- - Google Chrome
Intended for healthcare professionals
- Access provided by Google Indexer
- My email alerts
- BMA member login
- Username * Password * Forgot your log in details? Need to activate BMA Member Log In Log in via OpenAthens Log in via your institution

Search form
- Advanced search
- Search responses
- Search blogs
- Evaluating the impact...
Evaluating the impact of healthcare interventions using routine data
Read the full collection.
- Related content
- Peer review
- Geraldine M Clarke , senior data analyst 1 ,
- Stefano Conti , senior statistician 2 ,
- Arne T Wolters , senior analytics manager 1 ,
- Adam Steventon , director of data analytics 1
- 1 The Health Foundation, London, UK
- 2 NHS England and NHS Improvement, London, UK
- Correspondence to G Clarke Geraldine.clarke{at}health.org.uk
What you need to know
Assessing the impact of healthcare interventions is critical to inform future decisions
Compare observed outcomes with what you would have expected if the intervention had not been implemented
A wide range of routinely collected data is available for the evaluation of healthcare interventions
Interventions to transform the delivery of health and social care are being implemented widely, such as those linked to Accountable Care Organizations in the United States, 1 or to integrated care systems in the UK. 2 Assessing the impact of these health interventions enables healthcare teams to learn and to improve services, and can inform future policy. 3 However, some healthcare interventions are implemented without high quality evaluation, in ways that require onerous data collection, or may not be evaluated at all. 4
A range of routinely collected administrative and clinically generated healthcare data could be used to evaluate the impact of interventions to improve care. However, there is a lack of guidance as to where relevant routine data can be found or accessed and how they can be linked to other data. A diverse array of methodological literature can also make it hard to understand which methods to apply to analyse the data. This article provides an introduction to help clinicians, commissioners, and other healthcare professionals wishing to commission, interpret, or perform an impact evaluation of a health intervention. We highlight what to consider and discuss key concepts relating to design, analysis, implementation, and interpretation.
What are interventions, impacts, and impact evaluations?
A health intervention is a combination of activities or strategies designed to assess, improve, maintain, promote, or modify health among individuals or an entire population. Interventions can include educational or care programmes, policy changes, environmental improvements, or health promotion campaigns. Interventions that include multiple independent or interacting components are referred to as complex. 5 The impact of any intervention is likely to be shaped as much by the context (eg, communities, work places, homes, schools, or hospitals) in which it is delivered, as the details of the intervention itself. 6 7 8 9
An impact is a positive or negative, direct or indirect, intended or unintended change produced by an intervention. An impact evaluation is a systematic and empirical investigation of the effects of an intervention; it assesses to what extent the outcomes experienced by affected individuals were caused by the intervention in question, and what can be attributed to other factors such as other interventions, socioeconomic trends, and political or environmental conditions. Evaluations can be categorised as formative or summative ( table 1 ).
Impact evaluations
- View inline
Approaches such as the Plan, Do, Study, Act cycle 11 , which is part of the Model for Improvement, a commonly used tool to test and understand small changes in quality improvement work 12 may be used to undertake formative evaluation.
With either type of evaluation, it is important to be realistic about how long it will take to see the intended effects. Assessment that takes place too soon risks incorrectly concluding that there was no impact. This might lead stakeholders to question the value of the intervention, when later assessment might have shown a different picture. For example, in a small case study of cost savings from proactively managing high risk patients, the costs of healthcare for the eligible intervention population initially increased compared with the comparison population, but after six months were consistently lower. 14
This article focuses on impact evaluation, but this can only ever address a fraction of questions. 15 Much more can be accomplished if it is supplemented with other qualitative and quantitative methods, including process evaluation. This provides context, assesses how the intervention was implemented, identifies any emerging unintended pathways, and is important for understanding what happened in practice and for identifying areas for improvement. 16 The economic evaluation of healthcare interventions is also important for healthcare decision making, especially with ongoing financial pressures on health services. 17
What are the right evaluation questions?
An effective impact evaluation begins with the formulation of one or more clear questions driven by the purpose of the evaluation and what you and your stakeholders want to learn. For example, “What is the impact of case management on patients’ experience of care? ”
Formulate your evaluation questions using your understanding of the idea behind your intervention, the implementation challenges, and your knowledge of what data are available to measure outcomes. Review your theory of change or logic model 21 22 to understand what inputs and activities were planned, and what outcomes were expected and when. Once you have understood the intended causal pathway, consider the practical aspects of implementation, which include the barriers to change, unexpected changes by recipients or providers, and other influences not previously accounted for. Patient and public involvement (PPI) in setting the right question is strongly recommended for additional insights and meaningful results. For example, if evaluating the impact of case management, you could engage patients to understand what outcomes matter most to them. Healthcare leaders may emphasise metrics such as emergency admissions, but other aspects such as the experience of care might matter more to patients. 5 23
What methods can be used to perform an impact evaluation?
Randomised control designs , where individuals are randomly selected to receive either an intervention or a control treatment, are often referred to as the “gold standard” of causal impact evaluation. 24 In large enough samples, the process of randomisation ensures a balance in observed and unobserved characteristics between treatment and control groups. However, while often suitable for assessing, for example, the safety and efficacy of medicines, these designs may be impractical, unethical, or irrelevant when assessing the impact of complex changes to health service delivery.
Observational studies are an alternative approach to estimate causal effects. They use the natural, or unplanned, variation in a population in relation to the exposure to an intervention, or the factors that affect its outcomes, to remove the consequences of a non-randomised selection process. 25 The idea is to mimic a randomised control design by ensuring treated and control groups are equivalent—at least in terms of observed characteristics. This can be achieved using a variety of well documented methods, including regression control and matching, 26 eg, propensity scoring 27 or genetic matching. 28 If the matching is successful at producing such groups, and there are also no differences in unobserved characteristics, then it can be assumed that the control group outcomes are representative of those that the treated group would have experienced if nothing had changed, ie, the counterfactual. For example, an evaluation of alternative elective surgical interventions for primary total hip replacement on osteoarthritis patients in England and Wales used genetic matching to compare patients across three different prosthesis groups, and reported that the most prevalent type of hip replacement was the least cost effective. 29
Assessing similarity is only possible in relation to observed characteristics, and matching can result in biased estimates if the groups differ in relation to unobserved variables that are predictive of the outcome (confounders). It is rarely possible to eliminate this possibility of bias when conducting observational studies, meaning that the interpretation of the findings must always be sensitive to the possibility that the differences in outcomes were caused by a factor other than the intervention. Methods that can help when selection is on unobserved characteristics include difference-in-difference, 30 regression discontinuity, 31 instrumental variables, 18 or synthetic controls. 32 Table 2 gives a summary of selected observational study designs.
Observational study designs for quantitative impact evaluation
Observational studies are often referred to as natural (for natural or unplanned interventions), or quasi (for planned or intentional interventions) experiments. Natural experiments are discussed to evaluate population health interventions. 41
What’s wrong with a simple before-and-after study?
Before-and-after studies compare changes in outcomes for the same group of patients at a single time point before and after receiving an intervention without reference to a control group. These differ from interrupted time series studies, which compare changes in outcomes for successive groups of patients before and after receiving an intervention (the interruption).
Before-and-after studies are useful when it is not possible to include an unexposed control group, or for hypothesis generation. However, they are inherently susceptible to bias since changes observed may simply reflect regression to the mean (any changes in outcomes that might occur naturally in the absence of the intervention), or influences or secular trends unrelated to the intervention, eg, changes in the economic or political environment, or a heightened public awareness of issues.
For example, a before-and-after study of the impact of a care coordination service for older people tracked the hospital utilisation of the same patients before and after they were accepted into the service. They found that the service resulted in savings in hospital bed days and attendances at the emergency department. 42 Reduced hospital utilisation could have reflected regression to the mean here rather than the effects of the intervention; for example, a patient could have had a specific health crisis before being invited to join the service and then reverted back to their previous state of health and hospital utilisation for reasons unconnected with the care coordination service.
Various tools are available to evaluate the risk of bias in non-randomised designs due to confounding and other potential biases. 43 44
Where can I find suitable routine data?
Healthcare systems generate vast amounts of data as part of their routine operation. These datasets are often designed to support direct care, and for administrative purposes, rather than for research, and use of routinely collected data for evaluating changes in health service delivery is not without pitfalls. For example, any variation observed between geographical regions, providers, and sometimes individual clinicians may reflect real and important variations in the actual healthcare quality provided, but can also result from differences in measurement. 45 However, routine data can be a rich source of information on a large group of patients with different conditions across different geographical regions. Often, data have been collected for many years, enabling construction of individual patient histories describing healthcare utilisation, diagnoses, comorbidities, prescription of medication, and other treatments.
Some of these data are collected centrally, across a wider system, and routinely shared for research and evaluation purposes, eg, secondary care data in England (Hospital Episode Statistics), or Medicare Claims data in the United States. Other sources, such as primary care data, are often collected at a more local level, but can be accessed through, or on behalf of, healthcare commissioners, provided the right information governance arrangements are in place. Pseudonymised records, where any identifying information is removed or replaced by an artificial identifier, are often used to support evaluation while maintaining patient confidentiality. See table 3 for commonly used routine datasets available in England.
Commonly used routine datasets available in the NHS in England
Healthcare records can often be linked across different sources as a single patient identifier is commonly used across a healthcare system, eg, the use of an NHS number in the UK. Using a common pseudonym across different data sources can support linkage of pseudonymised records. Linking into publicly available sources of administrative data and surveys can further enrich healthcare records. Commonly used administrative data available for UK populations include measures of GP practice quality and outcomes from the Quality and Outcomes Framework (QOF), 52 deprivation, rurality, and demographics from the 2011 Census, 53 and patient experience from the GP Patient Survey. 54
Are there any additional considerations?
It is essential to consider threats to validity when designing and evaluating an impact evaluation; validity relates to whether an evaluation is measuring what it is claiming to measure. See Rothman et al 55 for further discussion.
Internal validity refers to whether the effects observed are due to the intervention and not some other confounding factor. Selection bias, which results from the way in which subjects are recruited, or from differing rates of participation due, for example, to age, gender, cultural or socioeconomic factors, is often a problem in non-randomised designs. Care must be taken to account for such biases when interpreting the results of an impact evaluation. Sensitivity analyses should be performed to provide reassurance regarding the plausibility of causal inferences.
External validity refers to the extent to which the results of a study can be generalised to other settings. Understanding the societal, economic, health system, and environmental context in which an intervention is delivered, and which makes its impact unique, is critical when interpreting the results of evaluations, and considering whether they apply to your setting. 56 Descriptions of context should be as rich as possible.
Often, the impact of an intervention is likely to vary depending on the characteristics of patients. These can be usefully explored in subgroup analyses. 57
Clear and transparent reporting using established guidelines (eg, STROBE 58 or TREND 59 )to describe the intervention, study population, assignment of treatment, and control groups, and methods used to estimate impact should be followed. Limitations arising as a result of inherent biases, or validity, should be clearly acknowledged.
Around the world, many interventions designed to improve health and healthcare are under way. An evaluation is an essential part of understanding what impact these changes are having, for whom and in what circumstances, and help inform future decisions about improvement and further roll out. There is no standard, ‘‘one size fits all’’ recipe for a good evaluation: it must be tailored to the project at hand. Understanding the overarching principles and standards is the first step towards a good evaluation.
Further Resources
See The Health Foundation. Evaluation: what to consider. 2015 60 for a list of websites, articles, webinars and other guidance on various aspects of impact evaluation, which may help locate further information for the planning, interpretation, and development of a successful impact evaluation. 5 23 55
Education into practice
What interventions have you designed or experienced aimed at transforming your service? Have they been evaluated?
What types of routine data are collected about the care you deliver? Do you know how to access them and use them to evaluate care delivery?
What resources are available to you to support impact evaluations for interventions?
Contributors GMC, SC, ATW and AS designed the structure of the report. GMC wrote the first draft of the manuscript. SC wrote table 2 . ATW wrote table 3 . AS and GMC critically revised the manuscript for important intellectual content. All authors approved the final version of the manuscript.
Competing interests We have read and understood BMJ policy on declaration of interests. All authors work in the Improvements Analytics Unit, a joint project between NHS England and the Health Foundation, which provided support for work reported in references of this report. 13 37 60
Provenance and peer review: This article is part of a series commissioned by The BMJ based on ideas generated by a joint editorial group with members from the Health Foundation and The BMJ, including a patient/carer. The BMJ retained full editorial control over external peer review, editing, and publication. Open access fees and The BMJ’s quality improvement editor post are funded by the Health Foundation.
Patient and/or members of the public were not involved in the creation of this article.
This is an Open Access article distributed in accordance with the terms of the Creative Commons Attribution (CC BY 4.0) license, which permits others to distribute, remix, adapt and build upon this work, for commercial use, provided the original work is properly cited. See: http://creativecommons.org/licenses/by/4.0/ .
- Guterman S ,
- Collins S ,
- Stremikis G ,
- NHS NHS Long Term Plan
- Djulbegovic B
- Bickerdike L ,
- Wilson PM ,
- Campbell M ,
- Fitzpatrick R ,
- Greenhalgh T ,
- Smith J, Wistow G. (Nuffield Trust comment) Learning from an intrepid pioneer: integrated care in North West London. https://www.nuffieldtrust.org.uk/news-item/learning-from-an-intrepid-pioneer-integrated-care-in-north-west-london
- Improvement NHS
- ↵ Academy of Medical Royal Colleges. Quality Improvement—training for better outcomes. 2016. https://www.aomrc.org.uk/wp-content/uploads/2016/06/Quality_improvement_key_findings_140316-2.pdf
- ↵ Lloyd T, Wolters A, Steventon A. The impact of providing enhanced support for care home residents in Rushcliffe. 2017. http://www.health.org.uk/sites/health/files/IAURushcliffe.pdf
- Ferris TG ,
- Heffernan JL ,
- Torchiana DF
- Drummond M ,
- Weatherly H ,
- Baiocchi M ,
- Ahlberg CE ,
- Martens EP ,
- Pestman WR ,
- de Boer A ,
- Belitser SV ,
- Center for Theory of Change
- Davidoff F ,
- Dixon-Woods M ,
- Leviton L ,
- ↵ Gertler PJ, Martinez S, Premand P, Rawlings LB, Vermeersch CMJ. Impact evaluation in practice. The World Bank Publications. 2017. https://siteresources.worldbank.org/EXTHDOFFICE/Resources/5485726-1295455628620/Impact_Evaluation_in_Practice.pdf
- ↵ Cochrane A. Effectiveness and efficiency: random reflections on health services. London; 1972. https://www.nuffieldtrust.org.uk/research/effectiveness-and-efficiency-random-reflections-on-health-services
- Portela MC ,
- Pronovost PJ ,
- Woodcock T ,
- Dixon-Woods M
- Diamond A ,
- Pennington M ,
- Sekhon JS ,
- van der Meulen JH
- Bello-Gomez RA
- Venkataramani AS ,
- Gardeazabal J
- Nikolova S ,
- McDonald R ,
- ↵ Stephen O, Wolters A, Steventon A. Briefing: The impact of redesigning urgent and emergency care in Northumberland 2017. https://www.health.org.uk/sites/health/files/IAUNorthumberland.pdf
- Geneletti S ,
- O’Keeffe AG ,
- Sharples LD ,
- Richardson S ,
- Bernal JL ,
- Cummins S ,
- Gasparrini A
- Donegan K ,
- Livingston G ,
- Banerjee S ,
- Gunnell D ,
- Sterne JA ,
- Hernán MA ,
- Reeves BC ,
- Hemming K ,
- Stevens AJ ,
- Powell AE ,
- Davies HT ,
- NHS Digital. Hospital Episode Statistics. https://digital.nhs.uk/data-and-information/data-tools-and-services/data-services/hospital-episode-statistics
- NHS Digital. Data Access Request Service (DARS) https://digital.nhs.uk/services/data-access-request-service-dars
- NHS Digital. Secondary Uses Service (SUS) https://digital.nhs.uk/services/secondary-uses-service-sus
- Medicines and Healthcare Regulatory Agency and National Institute for Health Research (NIHR). Clinical Practice Research Datalink(CPRD). https://www.cprd.com
- Office for National Statistics. Deaths. https://www.ons.gov.uk/peoplepopulationandcommunity/birthsdeathsandmarriages/deaths
- NHS Digital. Mental Health Services Data Set. https://digital.nhs.uk/data-and-information/data-collections-and-data-sets/data-sets/mental-health-services-data-set
- ↵ NHS Digital. Quality Outcomes Framework (QOF) https://digital.nhs.uk/data-and-information/data-tools-and-services/data-services/general-practice-data-hub/quality-outcomes-framework-qof
- Office for National Statistics
- NHS England
- Rothman KJ ,
- Greenland S ,
- Walter SD ,
- von Elm E ,
- Altman DG ,
- Pocock SJ ,
- Gotzsche PC ,
- Vandenbroucke JP
- Des Jarlais DC ,
- ↵ The Health Foundation. Evaluation: what to consider. 2015. https://www.health.org.uk/publications/evaluation-what-to-consider

Research Topics & Ideas: Healthcare
100+ Healthcare Research Topic Ideas To Fast-Track Your Project
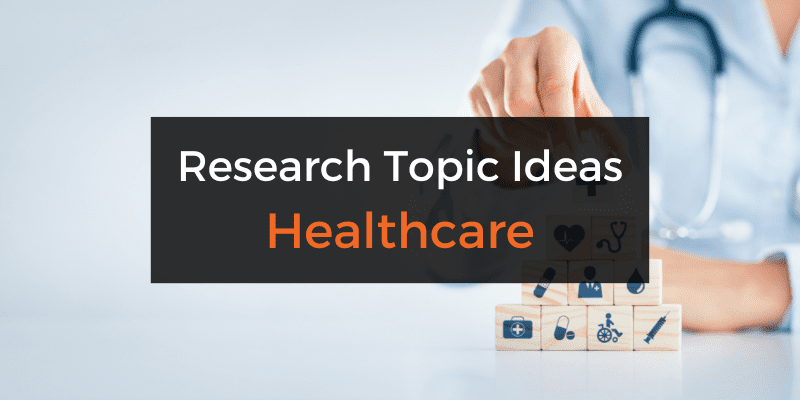
Finding and choosing a strong research topic is the critical first step when it comes to crafting a high-quality dissertation, thesis or research project. If you’ve landed on this post, chances are you’re looking for a healthcare-related research topic , but aren’t sure where to start. Here, we’ll explore a variety of healthcare-related research ideas and topic thought-starters across a range of healthcare fields, including allopathic and alternative medicine, dentistry, physical therapy, optometry, pharmacology and public health.
NB – This is just the start…
The topic ideation and evaluation process has multiple steps . In this post, we’ll kickstart the process by sharing some research topic ideas within the healthcare domain. This is the starting point, but to develop a well-defined research topic, you’ll need to identify a clear and convincing research gap , along with a well-justified plan of action to fill that gap.
If you’re new to the oftentimes perplexing world of research, or if this is your first time undertaking a formal academic research project, be sure to check out our free dissertation mini-course. In it, we cover the process of writing a dissertation or thesis from start to end. Be sure to also sign up for our free webinar that explores how to find a high-quality research topic.
Overview: Healthcare Research Topics
- Allopathic medicine
- Alternative /complementary medicine
- Veterinary medicine
- Physical therapy/ rehab
- Optometry and ophthalmology
- Pharmacy and pharmacology
- Public health
- Examples of healthcare-related dissertations
Allopathic (Conventional) Medicine
- The effectiveness of telemedicine in remote elderly patient care
- The impact of stress on the immune system of cancer patients
- The effects of a plant-based diet on chronic diseases such as diabetes
- The use of AI in early cancer diagnosis and treatment
- The role of the gut microbiome in mental health conditions such as depression and anxiety
- The efficacy of mindfulness meditation in reducing chronic pain: A systematic review
- The benefits and drawbacks of electronic health records in a developing country
- The effects of environmental pollution on breast milk quality
- The use of personalized medicine in treating genetic disorders
- The impact of social determinants of health on chronic diseases in Asia
- The role of high-intensity interval training in improving cardiovascular health
- The efficacy of using probiotics for gut health in pregnant women
- The impact of poor sleep on the treatment of chronic illnesses
- The role of inflammation in the development of chronic diseases such as lupus
- The effectiveness of physiotherapy in pain control post-surgery
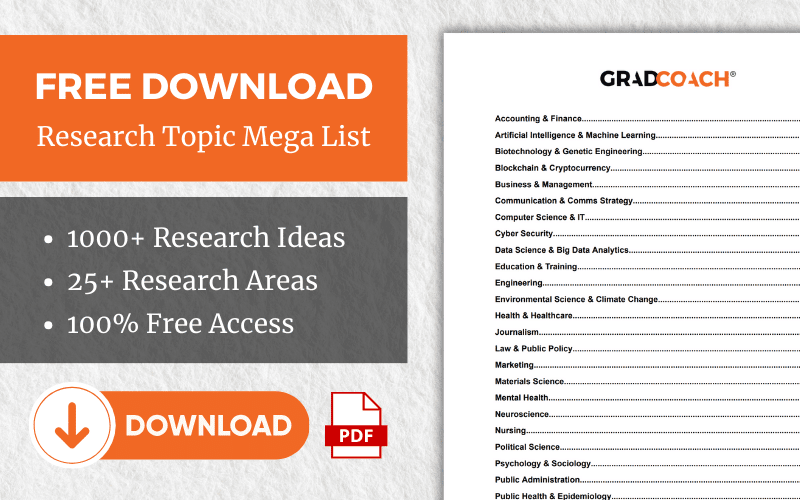
Topics & Ideas: Alternative Medicine
- The benefits of herbal medicine in treating young asthma patients
- The use of acupuncture in treating infertility in women over 40 years of age
- The effectiveness of homoeopathy in treating mental health disorders: A systematic review
- The role of aromatherapy in reducing stress and anxiety post-surgery
- The impact of mindfulness meditation on reducing high blood pressure
- The use of chiropractic therapy in treating back pain of pregnant women
- The efficacy of traditional Chinese medicine such as Shun-Qi-Tong-Xie (SQTX) in treating digestive disorders in China
- The impact of yoga on physical and mental health in adolescents
- The benefits of hydrotherapy in treating musculoskeletal disorders such as tendinitis
- The role of Reiki in promoting healing and relaxation post birth
- The effectiveness of naturopathy in treating skin conditions such as eczema
- The use of deep tissue massage therapy in reducing chronic pain in amputees
- The impact of tai chi on the treatment of anxiety and depression
- The benefits of reflexology in treating stress, anxiety and chronic fatigue
- The role of acupuncture in the prophylactic management of headaches and migraines
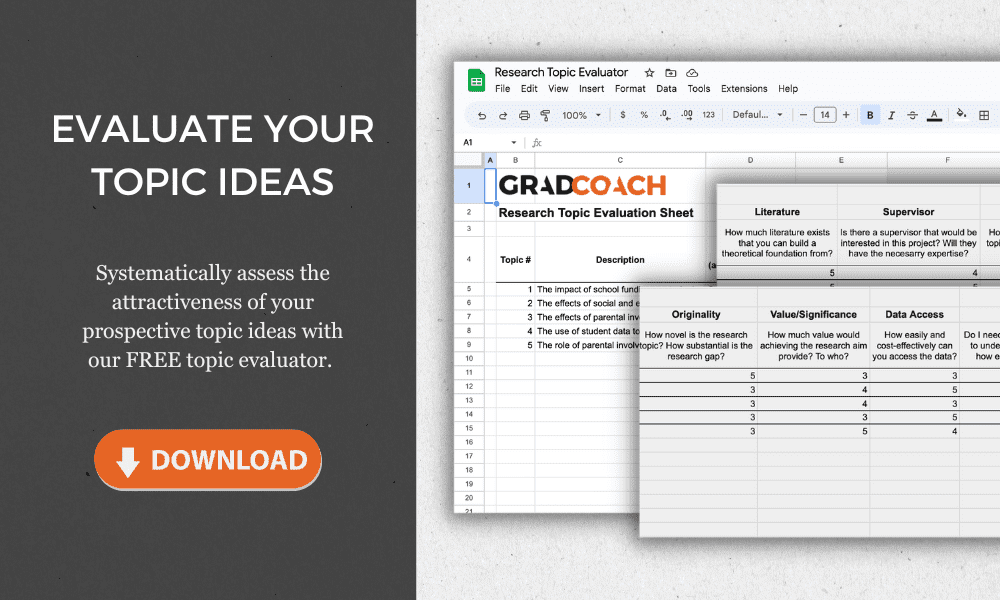
Topics & Ideas: Dentistry
- The impact of sugar consumption on the oral health of infants
- The use of digital dentistry in improving patient care: A systematic review
- The efficacy of orthodontic treatments in correcting bite problems in adults
- The role of dental hygiene in preventing gum disease in patients with dental bridges
- The impact of smoking on oral health and tobacco cessation support from UK dentists
- The benefits of dental implants in restoring missing teeth in adolescents
- The use of lasers in dental procedures such as root canals
- The efficacy of root canal treatment using high-frequency electric pulses in saving infected teeth
- The role of fluoride in promoting remineralization and slowing down demineralization
- The impact of stress-induced reflux on oral health
- The benefits of dental crowns in restoring damaged teeth in elderly patients
- The use of sedation dentistry in managing dental anxiety in children
- The efficacy of teeth whitening treatments in improving dental aesthetics in patients with braces
- The role of orthodontic appliances in improving well-being
- The impact of periodontal disease on overall health and chronic illnesses
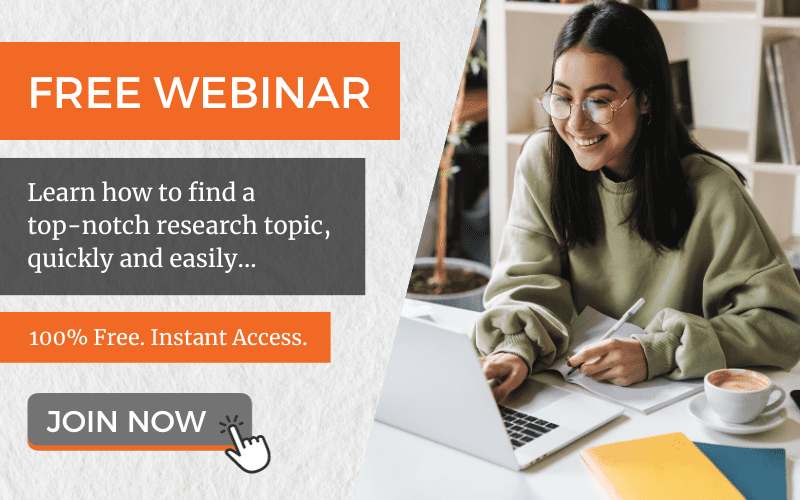
Tops & Ideas: Veterinary Medicine
- The impact of nutrition on broiler chicken production
- The role of vaccines in disease prevention in horses
- The importance of parasite control in animal health in piggeries
- The impact of animal behaviour on welfare in the dairy industry
- The effects of environmental pollution on the health of cattle
- The role of veterinary technology such as MRI in animal care
- The importance of pain management in post-surgery health outcomes
- The impact of genetics on animal health and disease in layer chickens
- The effectiveness of alternative therapies in veterinary medicine: A systematic review
- The role of veterinary medicine in public health: A case study of the COVID-19 pandemic
- The impact of climate change on animal health and infectious diseases in animals
- The importance of animal welfare in veterinary medicine and sustainable agriculture
- The effects of the human-animal bond on canine health
- The role of veterinary medicine in conservation efforts: A case study of Rhinoceros poaching in Africa
- The impact of veterinary research of new vaccines on animal health
Topics & Ideas: Physical Therapy/Rehab
- The efficacy of aquatic therapy in improving joint mobility and strength in polio patients
- The impact of telerehabilitation on patient outcomes in Germany
- The effect of kinesiotaping on reducing knee pain and improving function in individuals with chronic pain
- A comparison of manual therapy and yoga exercise therapy in the management of low back pain
- The use of wearable technology in physical rehabilitation and the impact on patient adherence to a rehabilitation plan
- The impact of mindfulness-based interventions in physical therapy in adolescents
- The effects of resistance training on individuals with Parkinson’s disease
- The role of hydrotherapy in the management of fibromyalgia
- The impact of cognitive-behavioural therapy in physical rehabilitation for individuals with chronic pain
- The use of virtual reality in physical rehabilitation of sports injuries
- The effects of electrical stimulation on muscle function and strength in athletes
- The role of physical therapy in the management of stroke recovery: A systematic review
- The impact of pilates on mental health in individuals with depression
- The use of thermal modalities in physical therapy and its effectiveness in reducing pain and inflammation
- The effect of strength training on balance and gait in elderly patients
Topics & Ideas: Optometry & Opthalmology
- The impact of screen time on the vision and ocular health of children under the age of 5
- The effects of blue light exposure from digital devices on ocular health
- The role of dietary interventions, such as the intake of whole grains, in the management of age-related macular degeneration
- The use of telemedicine in optometry and ophthalmology in the UK
- The impact of myopia control interventions on African American children’s vision
- The use of contact lenses in the management of dry eye syndrome: different treatment options
- The effects of visual rehabilitation in individuals with traumatic brain injury
- The role of low vision rehabilitation in individuals with age-related vision loss: challenges and solutions
- The impact of environmental air pollution on ocular health
- The effectiveness of orthokeratology in myopia control compared to contact lenses
- The role of dietary supplements, such as omega-3 fatty acids, in ocular health
- The effects of ultraviolet radiation exposure from tanning beds on ocular health
- The impact of computer vision syndrome on long-term visual function
- The use of novel diagnostic tools in optometry and ophthalmology in developing countries
- The effects of virtual reality on visual perception and ocular health: an examination of dry eye syndrome and neurologic symptoms
Topics & Ideas: Pharmacy & Pharmacology
- The impact of medication adherence on patient outcomes in cystic fibrosis
- The use of personalized medicine in the management of chronic diseases such as Alzheimer’s disease
- The effects of pharmacogenomics on drug response and toxicity in cancer patients
- The role of pharmacists in the management of chronic pain in primary care
- The impact of drug-drug interactions on patient mental health outcomes
- The use of telepharmacy in healthcare: Present status and future potential
- The effects of herbal and dietary supplements on drug efficacy and toxicity
- The role of pharmacists in the management of type 1 diabetes
- The impact of medication errors on patient outcomes and satisfaction
- The use of technology in medication management in the USA
- The effects of smoking on drug metabolism and pharmacokinetics: A case study of clozapine
- Leveraging the role of pharmacists in preventing and managing opioid use disorder
- The impact of the opioid epidemic on public health in a developing country
- The use of biosimilars in the management of the skin condition psoriasis
- The effects of the Affordable Care Act on medication utilization and patient outcomes in African Americans
Topics & Ideas: Public Health
- The impact of the built environment and urbanisation on physical activity and obesity
- The effects of food insecurity on health outcomes in Zimbabwe
- The role of community-based participatory research in addressing health disparities
- The impact of social determinants of health, such as racism, on population health
- The effects of heat waves on public health
- The role of telehealth in addressing healthcare access and equity in South America
- The impact of gun violence on public health in South Africa
- The effects of chlorofluorocarbons air pollution on respiratory health
- The role of public health interventions in reducing health disparities in the USA
- The impact of the United States Affordable Care Act on access to healthcare and health outcomes
- The effects of water insecurity on health outcomes in the Middle East
- The role of community health workers in addressing healthcare access and equity in low-income countries
- The impact of mass incarceration on public health and behavioural health of a community
- The effects of floods on public health and healthcare systems
- The role of social media in public health communication and behaviour change in adolescents
Examples: Healthcare Dissertation & Theses
While the ideas we’ve presented above are a decent starting point for finding a healthcare-related research topic, they are fairly generic and non-specific. So, it helps to look at actual dissertations and theses to see how this all comes together.
Below, we’ve included a selection of research projects from various healthcare-related degree programs to help refine your thinking. These are actual dissertations and theses, written as part of Master’s and PhD-level programs, so they can provide some useful insight as to what a research topic looks like in practice.
- Improving Follow-Up Care for Homeless Populations in North County San Diego (Sanchez, 2021)
- On the Incentives of Medicare’s Hospital Reimbursement and an Examination of Exchangeability (Elzinga, 2016)
- Managing the healthcare crisis: the career narratives of nurses (Krueger, 2021)
- Methods for preventing central line-associated bloodstream infection in pediatric haematology-oncology patients: A systematic literature review (Balkan, 2020)
- Farms in Healthcare: Enhancing Knowledge, Sharing, and Collaboration (Garramone, 2019)
- When machine learning meets healthcare: towards knowledge incorporation in multimodal healthcare analytics (Yuan, 2020)
- Integrated behavioural healthcare: The future of rural mental health (Fox, 2019)
- Healthcare service use patterns among autistic adults: A systematic review with narrative synthesis (Gilmore, 2021)
- Mindfulness-Based Interventions: Combatting Burnout and Compassionate Fatigue among Mental Health Caregivers (Lundquist, 2022)
- Transgender and gender-diverse people’s perceptions of gender-inclusive healthcare access and associated hope for the future (Wille, 2021)
- Efficient Neural Network Synthesis and Its Application in Smart Healthcare (Hassantabar, 2022)
- The Experience of Female Veterans and Health-Seeking Behaviors (Switzer, 2022)
- Machine learning applications towards risk prediction and cost forecasting in healthcare (Singh, 2022)
- Does Variation in the Nursing Home Inspection Process Explain Disparity in Regulatory Outcomes? (Fox, 2020)
Looking at these titles, you can probably pick up that the research topics here are quite specific and narrowly-focused , compared to the generic ones presented earlier. This is an important thing to keep in mind as you develop your own research topic. That is to say, to create a top-notch research topic, you must be precise and target a specific context with specific variables of interest . In other words, you need to identify a clear, well-justified research gap.
Need more help?
If you’re still feeling a bit unsure about how to find a research topic for your healthcare dissertation or thesis, check out Topic Kickstarter service below.
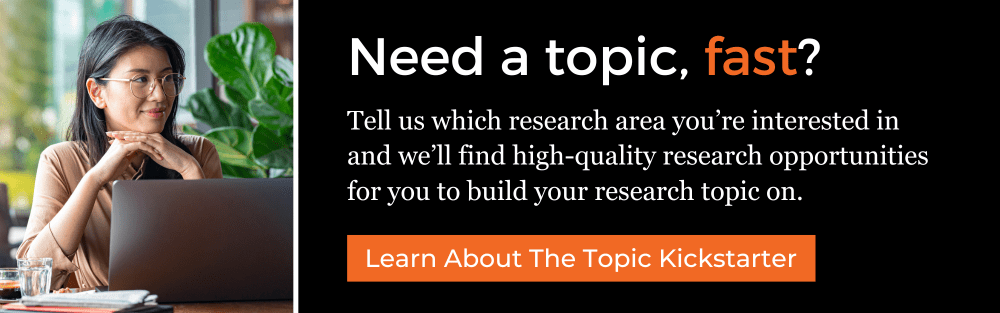
You Might Also Like:
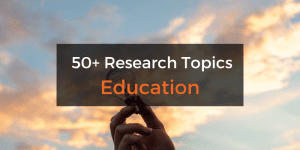
15 Comments
I need topics that will match the Msc program am running in healthcare research please
Hello Mabel,
I can help you with a good topic, kindly provide your email let’s have a good discussion on this.
Can you provide some research topics and ideas on Immunology?
Thank you to create new knowledge on research problem verse research topic
Help on problem statement on teen pregnancy
This post might be useful: https://gradcoach.com/research-problem-statement/
can you provide me with a research topic on healthcare related topics to a qqi level 5 student
Please can someone help me with research topics in public health ?
Hello I have requirement of Health related latest research issue/topics for my social media speeches. If possible pls share health issues , diagnosis, treatment.
I would like a topic thought around first-line support for Gender-Based Violence for survivors or one related to prevention of Gender-Based Violence
Please can I be helped with a master’s research topic in either chemical pathology or hematology or immunology? thanks
Can u please provide me with a research topic on occupational health and safety at the health sector
Good day kindly help provide me with Ph.D. Public health topics on Reproductive and Maternal Health, interventional studies on Health Education
may you assist me with a good easy healthcare administration study topic
May you assist me in finding a research topic on nutrition,physical activity and obesity. On the impact on children
Submit a Comment Cancel reply
Your email address will not be published. Required fields are marked *
Save my name, email, and website in this browser for the next time I comment.
- Print Friendly
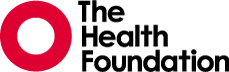
Browse all our research projects by topic We have funded more than 150 external research projects across a range of themes over the last five years
- Share on Twitter
- Share on LinkedIn
- Share on Facebook
- Share on WhatsApp
- Share by email
- Link Copy link

Please click below to see the research projects we've funded on the following topics.
- Analytics and data
- Children and young people
- Commissioning
- Community and voluntary
- Digital technology
- Efficiency and productivity
- Emergency medicine
- End of life care
- Funding and sustainability
- Improvement science
- Inequalities
- Integrated care
- Long-term conditions
- Mental health
- Older people
- Patient experience
- Patient safety
- Person-centred care
- Primary care
- Public health
- Quality improvement
- Quality of care
- Social care
- Social determinants of health
Share this page:
Health Foundation @HealthFdn
- Work with us
We look for talented and passionate individuals as everyone at the Health Foundation has an important role to play.
The Q community
Q is an initiative connecting people with improvement expertise across the UK.
Quick links
- News and media
- Events and webinars
Hear from us
Receive the latest news and updates from the Health Foundation
- 020 7257 8000
- [email protected]
- The Health Foundation Twitter account
- The Health Foundation LinkedIn account
- The Health Foundation Facebook account
Copyright The Health Foundation 2024. Registered charity number 286967.
- Accessibility
- Anti-slavery statement
- Terms and conditions
- Privacy policy

MINI REVIEW article
This article is part of the research topic.
Catalysis and Sensing for our Environment
Research progress in the detection of trace heavy metal ions in food samples Provisionally Accepted
- 1 Beijing University of Chemical Technology, China
The final, formatted version of the article will be published soon.
Food safety is the basis for ensuring human survival and development. The threat of heavy metal ions to food safety has become a social concern with the rapid growth of the economy and the accompanying environmental pollution. Some heavy metal ions are highly toxic even at trace levels and pose significant health risks to humans. Therefore, ultrasensitive detection of heavy metal ions in food samples is important. In this mini-review, recent advances in the analytical methods based on nanomaterials for detecting trace heavy metal ions in food samples are summarized in three categories: electrochemical, colorimetric, and fluorescent methods. We present the features and sensing mechanisms of these three methods, along with typical examples to illustrate their application in the detection of heavy metal ions in foods. This mini-review ends with a discussion of current challenges and future prospects of these approaches for sensing heavy metal ions. The review will help readers understand the principles of these methods, thereby promoting the development of new analytical methods for the detection of heavy metal ions in food samples.
Keywords: Heavy metal ions, detection, Food samples, nanomaterials, Sensors
Received: 26 Apr 2024; Accepted: 13 May 2024.
Copyright: © 2024 Si, Wu, Jin and Wang. This is an open-access article distributed under the terms of the Creative Commons Attribution License (CC BY) . The use, distribution or reproduction in other forums is permitted, provided the original author(s) or licensor are credited and that the original publication in this journal is cited, in accordance with accepted academic practice. No use, distribution or reproduction is permitted which does not comply with these terms.
* Correspondence: Mx. Yulong Jin, Beijing University of Chemical Technology, Beijing, 100029, Beijing Municipality, China Prof. Zhuo Wang, Beijing University of Chemical Technology, Beijing, 100029, Beijing Municipality, China
People also looked at

An official website of the United States government
The .gov means it’s official. Federal government websites often end in .gov or .mil. Before sharing sensitive information, make sure you’re on a federal government site.
The site is secure. The https:// ensures that you are connecting to the official website and that any information you provide is encrypted and transmitted securely.
- Publications
- Account settings
Preview improvements coming to the PMC website in October 2024. Learn More or Try it out now .
- Advanced Search
- Journal List
- Sultan Qaboos Univ Med J
- v.8(1); 2008 Mar
Qualitative Research and its Uses in Health Care
Although relatively uncommon in health care research, qualitative research is now receiving recognition and is increasingly used in health care research with social and cultural dimensions. Unlike quantitative research, which is deductive and tends to analyze phenomena in terms of trends and frequencies, qualitative research seeks to determine the meaning of a phenomenon through description. It aims to develop concepts that aid in the understanding of natural phenomena with emphasis on the meaning, experiences and views of the participants. Differences among qualitative researchers exist on matters of ontology, epistemology, data collection methods and methods of evaluation. The aim of this article is not to act as a practical guide on how to conduct qualitative research, but is an attempt to give an introduction to qualitative research methods and their use in health-related research.
Q ualitative research is defined as an umbrella term covering an array of interpretative techniques which seek to describe, decode, translate and otherwise come to terms with the meaning, not the frequency, of certain more or less naturally occurring phenomena in the social world. 1 , 2
As a method of inquiry, it was first used by sociologists and anthropologists in the early twentieth century, although it existed much earlier than that in its non-structural form. Researchers studied cultures and groups in their own and foreign settings and told stories of their experience long before then. In the 1920s and 1930s, social anthropologists and sociologists implemented a more focused approach compared to the old unsystematic and journalistic style used in those days. Since the 1960s, qualitative research has experienced a steady growth starting with the development of grounded theory and new publications in ethnography. 3 , 4 The number of books, articles and papers related to qualitative research has increased tremendously during the past 20 years and more researchers, including health-related professionals, have moved to a more qualitative paradigm adapting and modifying these approaches to the study needs of their own areas. 4
Since qualitative research does not aim to enumerate, it is sometimes viewed as the exact opposite to quantitative methods and the two methods are frequently presented as antagonists. Quantitative research is based on structure and uses experiments and surveys as methods. In addition, it is deductive in nature and uses statistical sampling methods. In contrast, qualitative research is described as an action research using observation and interview methods. It is inductive in nature and depends on the purposeful selection of participants. Whereas quantitative researchers use reliability as a way of verification, qualitative researchers use validity. Recently, there has been a growing recognition that the quantitative-qualitative debate and distinction is unnecessary and that it would be more fruitful for the relation between the two methods to be complementary and overlapping rather than exclusive. 6 In fact, neither qualitative nor quantitative research is superior to the other; there are weaknesses and strengths in each method. Qualitative and quantitative research methods can indeed be seen as complementary and both are necessary to provide an understanding of a phenomenon.
Several researchers have argued that the research questions and the phenomenon under investigation should determine the methodology to used. 7 , 8 , 9 The crucial question, therefore, is not “what is the best research method?” but “what is the best research method for answering this question most effectively and efficiently?” 10 So, while qualitative and quantitative research may well investigate similar topics, each will address a different type of question. Holman sums up this position: “true understanding in medicine cannot be achieved without adding qualitative methods to the research arsenal”. 11
Traditional quantitative methods, such as randomised controlled trials, are the appropriate means of testing, for example, the effect of an intervention or a treatment, while a qualitative exploration of beliefs and understandings is needed to find out why the results of research are often not implemented in clinical practice. The aim of qualitative research is to develop concepts that can help us understand social phenomena in natural settings, giving emphasis on the meanings, experiences and views of the participants. 10
Qualitative research methods are the most suitable for this approach because of their emphasis on people’s lived experience. They are considered to be well suited for locating the meanings that people place on the events, processes, and structures of their lives and their perceptions, presuppositions and assumptions. 5 In his advice to graduate students, Patton lists a number of conditions that are suitable for a qualitative study. These include: questions about people’s experiences; inquiry into the meanings people make of their experiences; studying a person in the context of her or his social/interpersonal environment and research where it is difficult to develop a standardised instrument due to the lack of knowledge on the phenomenon. 12
ARGUMENTS FOR AND AGAINST QUALITATIVE METHODS
There are major differences of opinions among qualitative researchers on matters of ontology and epistemology as well as the methods to be used and criteria of evaluation. There are also disagreements about the nature, purpose, status and practice of its methods. A large number of authors take a predominantly method-based approach; authors such as Miles and Huberman 5 and Patton 12 put emphasis on data collection techniques. Another approach is to classify qualitative research according to research traditions, i.e. whether phenomenological, grounded theory or ethnography, amongst others. Authors such as Creswel 9 and Denzin and Lincolin 3 prefer this approach, which has the advantage of being based on systematisation of knowledge providing a sense of order and orientation. On the other hand, it has the disadvantage of oversimplification, ignoring the issues of the research question and conceptual frameworks used and the way these issues can shape the research process and the findings. 13 In addition, some researchers have decided to classify qualitative research according to the research question or the method of analysis.
Although the majority of qualitative researchers stress that qualitative research is inductive in nature, in contrast to quantitative research which is deductive, there are qualitative researchers who argue that both can be used for different purposes and at different times, and that qualitative research can be done in a deductive way where prior assumptions are tested on new cases. 14 Retroduction, which is defined as the movement backward and forward between theory and data or the combination of deduction and induction, is said to be a characteristic of qualitative research. 11 The degree of deduction or induction and which one follows the other depends on the research question. 12 .
Guba and Lincoln 15 used the term “emergent design” to describe a qualitative study design that emerges as the study progresses in response to the researcher’s early observations. There are also qualitative studies in health care that base their research questions on the results of prior literature of quantitative studies on the subject. 16 , 17 While the research questions in these studies were relatively precise, the method used was flexible.
Miles and Huberman 5 state that no study conforms exactly to a standard methodology and that the researcher bends the methodology to the peculiarities of the study. According to Creswell, 9 traditions of inquiry used “need not be pure, and one might mix procedures from several”. Mixing approaches and procedures is encouraged by some authors and regarded as a creative approach to qualitative research. 12
Studies using qualitative research in health care have been criticised for the misguided separation of method from theory and of technique from the conceptual underpinnings. 18 However, qualitative health researchers respond by stating that the choice of method and how it is used can perfectly well be matched to what is being studied rather than to the methodological leanings of the researcher. 10 It has been suggested that incorporating qualitative research method experts into health research teams enriches research and ensures that the right methodology is used for answering the right questions. Finally, using qualitative methods in health-related research has resulted in more insight into health professionals’ perceptions of lay participation in care and identification of barriers to changing healthcare practice.
QUALITATIVE RESEARCH APPROACHES
Grounded theory, phenomenology and ethnography are three approaches used in qualitative research. Grounded theory approach is a commonly used qualitative method in the social sciences to inductively generate or discover a theory out of the data. 9 Phenomenology and ethnography are more commonly used qualitative approaches in health care and will be highlighted below.
PHENOMENOLOGY
One of the major strengths of qualitative research is its emphasis upon understanding the phenomenon of interest holistically and in its context. The term phenomenology is popular and widely embraced, but its meaning has become confusing and faint. Different researchers refer to phenomenology differently. It can refer to an inquiry paradigm, an interpretive theory, a philosophy, an analytical perspective, a major qualitative research tradition or a research method framework. 12
In spite of the differences, all of these approaches share the focus of phenomenology, which is exploring how human beings make sense of experience and the meaning they give to these experiences. Phenomenology is being used in the social and human sciences including sociology, education, psychology, nursing and health sciences. 9
Phenomenologists are interested in how people put together the phenomena they experience in such a way as to make sense of the world and develop a worldview. They assume commonality in human experience and focus on meaning-making as the essence of human experience. The essence is the core meaning mutually understood through a phenomenon commonly experienced. 12 The phenomenon under study may be emotions, relationships, a programme, an organisation or a culture. 12 Bracketing is one of the central ideas in phenomenology. It means that the researcher has to set aside all of his prejudgments and his previous experience about the phenomena and approach the field with an open mind, imagination and intuition. Although important, bracketing is often said to be a difficult task. 9
Patton 12 describes the difference between conducting a phenomenological study and using a phenomenological perspective to a study. He argues that, “one can employ a general phenomenological perspective to elucidate the importance of using methods that capture people’s experience of the world without conducting a phenomenological study that focuses on the essence of shared experience”.
ETHNOGRAPHY
In ethnography, the researcher studies the structure and function of a group of people. An example of a structure or configuration is the kinship, while the function refers to patterns of relationships affecting and regulating behaviour. 9 The aim of ethnographic studies is to give a holistic picture of the social group studied, attempting to describe aspects of the cultural and social system of that particular group. These aspects could be the group history, religion, economy, politics or environment.
Data collection methods vary in ethnographic studies with observation and interviews being the most popular methods. Although some authors expressed concern that anthropological methods may be misused or applied superficially by the medical profession, 19 others, however, expressed the need for acknowledging and incorporating ethnographic methods in health care research. 20 Savage 19 explains that today the term ethnography can be applied to any small-scale social research carried out in everyday settings and uses several methods evolving in design and focusing on an individual’s meanings and explanations. In health care, ethnography has been used in topics related to health beliefs and practices, allowing these issues to be viewed in the context in which they occur and therefore helping broaden the understanding of behaviours related to health and illness. 20
In addition, there has been an increase in the amount of cross-cultural research and a change in the form of this research. Recently, more short-term ethnographic and cross-cultural studies have been undertaken, for example, studies sponsored by international development agencies or student exchange projects. 12
QUALITATIVE RESEARCH INSTRUMENTS
Qualitative research instruments used for data collection include interviews, observations, and analysis of documents. Interviews are the most common techniques used to gather research information. There are three types of interviews: structured, semi-structured and unstructured, described in some books as structured, informed and guided, respectively. 13
The semi-structured interview is more commonly used in health care-related qualitative research. Such an interview is characteristically based on a flexible topic guide that provides a loose structure of open-ended questions to explore experiences and attitudes. It has the advantage of great flexibility, enabling the researcher to enter new areas and produce richer data. In addition, it helps the researcher to develop a rapport with the informants. Semi-structured interviews elicit people’s own views and descriptions and have the benefit of uncovering issues or concerns that have not been anticipated by the researcher. 6 They are commonly used when the aim is to gain information on the perspectives, understandings and meanings constructed by people regarding the events and experiences of their lives. However, this type of interviewing is claimed to reduce the researcher’s control over the interview situation and take a longer time to conduct and analyse, in addition to the difficulties of the analysis process. 21
In health care, interviews are the appropriate tool to be used if the research is concerned with interpersonal aspects of care or if the available evidence is limited. 6
Another qualitative research instrument that can be used in health care research is the focus group. Focus group interviews have the advantage of being more time efficient as more people can be interviewed for the same amount of time. They also provide a richer source of data. On the other hand, focus group interviews tend to document the ‘public’ rather than the ‘private’ views of the individuals. In addition some people do not interview well in-group situations. 13
THE USE OF THE INTERVIEW GUIDE
Some qualitative researchers are reluctant to plan a design of their study in advance of the data collection. They argue that the phenomenon studied must first be discovered and they describe their design as emergent. However, increasingly more qualitative researchers appear ready to define a research question and develop an interview guide prior to starting the data collection. 11 Patton defines an interview guide as a series of topics or broad interview questions which the researcher is free to explore and probe with the interviewee. 12 The advantage of an interview guide is that it helps the interviewer pursue the same basic lines of inquiry with each person interviewed and manage the interviews in a more systematic and comprehensive way. The findings of earlier work are increasingly being used as a facilitator for further research. However, concepts drawn from earlier work are supposed to be held lightly and to be subject to reformulation or rejection by the researcher especially as the study goes on and the research progresses. 11 The extent to which such a flexibility of design is important will vary depending on the topic and the aim of the study.
Sampling strategies in qualitative research are largely determined by the purpose of the study. Statistical representativeness is not considered as a prime requirement in qualitative research and is not normally sought. Furthermore, qualitative data collection is more time consuming and expensive, which makes the use of a probability sample impractical. 6 The aim of sampling in qualitative research is to identify specific groups of people who hold characteristics or live in circumstances relevant to the phenomena being studied. In this way, identified informants are expected to enable enriched exploration of attitudes and aspects of behaviour relevant to the research. 10 Two types of samples used in qualitative research, maximum variation and homogenous samples, are explained below.
According to Patton, 12 maximum variation sampling is where the researcher attempts to study a phenomenon by seeking out settings or persons that represent the greatest differences in that phenomenon. A maximum variation sample documents diverse variations and identifies important common patterns by representing diverse cases to develop fully multiple perspectives about the cases. 9 This means that the participants are sampled based on particular predetermined criteria in order to cover a range of constituencies, such as different age, cultural background or class. 6
Similarly, the researcher can select the setting of the data collection on the basis that it is sufficiently similar to other settings in which generalisation is sought. This way the researcher is demonstrating the possibility that the setting studied is representative of the population studied. 2
In homogeneous sampling, the researcher chooses a small homogenous sample with the purpose of describing some particular subgroups in depth. 12
The sample size in qualitative research is not determined by fixed rules, but by factors such as the depth and duration of the interview and what is feasible for a single interviewer. 22 Although it is theoretically possible to carry out qualitative research on large samples, qualitative researchers find themselves obliged by time and resource limits to trade breadth for depth. 11 The sample size for interview studies is usually much smaller than those of a quantitative research, usually not exceeding 50 participants, although this can vary with the research question asked. 6 Patton 12 states, “there are no rules for sample size in qualitative inquiry”. In other words, sample size depends on the aim of the study and what is possible, given the time and resources available.
ANALYSING QUALITATIVE RESEARCH
Although there are plenty of guidelines for analysing qualitative research, applying these guidelines requires judgment and creativity because each qualitative study is unique. 12 A researcher might also be confused by the different terms used by qualitative researchers when describing analysis. Analysis might be described as interpretation, making sense of data, or transforming data. Analysis is sometimes presented to indicate different procedures based on language, theory or what is described as interpretive/descriptive analysis. 11 However, overlap can take place between these different methods and a researcher might decide to use a method of analysis that is based on language, such as symbolic interactionist, while using grounded theory to develop a theory at the same time. Most of the analytical approaches to qualitative research in health care are ‘generic’ and are not labelled within one of the specific traditions of qualitative research. A common approach in most of these studies is general and inductive in nature, but does not comply with the very systematic and rigorous inductive approach of grounded theory. In addition, it has been described that many researchers use a simple two-level analysis scheme followed by a more specific level. This means that the researcher can initiate the analysis based on the conceptual framework used in order to produce more inductive data, and the coding moves from the descriptive to the more interpretative and inferential codes. 5
COMPUTER USE IN QUALITATIVE DATA ANALYSIS
Qualitative research studies typically produce very large amount of data that needs to be managed efficiently. Computer packages can improve the efficiency of data management. 11 Computer programs provide a way of storing and retrieving material. They are therefore useful in locating cases, statements, phrases or even words, thereby replacing the tedious and time-consuming process of “cutting and pasting” and “colour coding”. The use of computer packages, however, is claimed to distance the analyst from the data, 11 and may take the place of a close and careful analysis. Using a computer programme can lead to quantitative analysis instead of qualitative, for example, counting occurrences, giving more weight to more frequent events, and ignoring isolated incidences. 23 In addition, computer programmes are said to fix and label categories during the analysis process and the researcher may be reluctant to change these categories. 9 Furthermore, the researcher is required to learn the computer programme, which may add to the time and effort he or she will need to spend on the research project. The researcher also has to be aware of the limitations of computer programmes. While computer packages can help with the intensive process of analysis and the management of large data sets, they are not a substitute for “immersion” in the data, and thorough knowledge that can enable the researcher to make comparisons, identify patterns and develop interpretations. 6 There are different packages available: Ethnograph, Atlas and NUD.IST are the mostly used. QSR NVivo is a new product developed by the makers of NUD.IST and is user-friendlier, more suitable for individual research projects and more visually attractive than previous packages.
THE ROLE OF THE RESEARCHER IN QUALITATIVE RESEARCH
Patton 12 states that, “the human element in qualitative research is both its strength and weakness”. It is considered a point of strength because it allows human insight and experience to develop new understandings of the world, and a point of weakness because it depends heavily on the researcher’s skills, creativity, training and intellect. Qualitative methods depend on both critical and creative thinking and the balance between the two in conducting the study and interpreting its results. The role of the researcher is approached differently according to the type of research tradition used. A phenomenologist researcher is required to bracket his/her own assumptions when collecting data. On the other hand, in an ethnographic study, the effect of the researcher on the interview and the interaction is seen as inevitable and important in shaping the results of the study.
Although practising clinicians routinely interview patients during their clinical work, interviewing is a well-established technique in sociology and related disciplines. One of the differences between clinical and research-aimed interviews lies in their different purposes. The usual aim of the clinical interview is to fit the patient’s problem into the appropriate medical category for diagnosis and management. On the other hand, the aim of a qualitative research interview is to discover the interviewee’s own meaning and avoid prior assumptions and preset categories. 22 Having said that, there are general skills in clinical interviewing that can be useful, such as listening and observation. A good level of self-awareness is necessary in the researcher in order to reduce possible biases.
Researchers in qualitative research need to consider how they are perceived by interviewees and the effect of features related to the researcher, such as class, race, and sex on the interview. This question is more important if the interviewee knows that the interviewer is a doctor. It has been described that a patient, or someone who is likely to be become one, may give what he or she thinks is a desirable response, thinking that the doctor will be pleased.
RIGOUR IN QUALITATIVE RESEARCH
Health field research is generally quantitative and based on biomedical traditions and experimental methods. In this field, qualitative research is criticized for being subject to researcher bias and for lacking reproducibility and generalisability. 10 Researchers presenting their qualitative work in health-related research are partly responsible for this view. Many qualitative researchers neglect the importance of giving an adequate description of their theoretical concepts and methods used in their research. A systematic research method is also essential when conducting qualitative research. Rigour in qualitative research includes procedures taken at different stages of the research process including during data collection and analysis. Several procedures have been described to increase rigour in qualitative research. For example, triangulation is commonly used as a way of validating of data.
TRIANGULATION
In triangulation, the researcher uses multiple methods, sources, researchers or theories to provide evidence that strengthens his or her study. Triangulation provides different ways of looking at the same phenomenon and adds credibility and confidence in the conclusions drawn from the study. There are two main types of triangulation, triangulation of sources and analyst triangulation. Patton 12 defines triangulation of sources as “checking out the consistency of different data sources within the same method”. When using this type of triangulation, the researcher compares the perspectives of people from different points of view. For example, studies in programme evaluation might compare the views of staff, clients or funding bodies. 12 Studies in health care have used this method of verification to study the accounts of doctors, patients, and managers in order to identify similarities and differences in views. An example is the study of patients’ versus doctors’ agendas in general practice. 23 Both similarities and differences from different sources, when given reasonable explanation, could contribute significantly to the credibility of the findings. Triangulation with multiple analysts can also be used as a method of verification. It is defined as “having two or more persons independently analyse the same qualitative data and compare their findings”. 12
QUALITATIVE RESEARCH IN HEALTH CARE MANAGEMENT
Recently, there has been a greater acceptance of the qualitative approach, even as a stand-alone method, in health care research. Institutions that control funding for medical research have developed ethical guidelines for assessing qualitative studies which indicates formal acceptance of this form of research within an area previously dominated by quantitative methods. 13 More qualitative research articles are published in health-related journals, in addition to a new qualitative research journal (Qualitative Health Research).
Quality of health care is one of the areas where qualitative methods can be used. The concept of quality in health care is multidimensional and multifaceted and some of the questions asked related to the quality of care or services may not be acquiescent to quantitative methods. 6 Qualitative research offers a variety of methods to be used for identifying what is really important to both patients and carers. It can also be used to identify and detect obstacles to change and the reasons why improvement does not occur. 6 It is therefore an essential component of health services research because it enables us to reach areas not amenable to quantitative research, for example, lay and professional health beliefs. In addition, qualitative description can be a prerequisite of quantitative research, particularly in areas that have received little previous investigation.
Qualitative research is widely used to study issues related to doctor-patient interaction especially in general practice. Studies concerning patients’ versus doctors’ agendas in general practice and general practitioners perceptions of effective health care are examples. 23 , 24 Recently there have been more studies concentrating on patients’ own perceptions and views regarding their health and health care services, for example a study on women’s views on the impact of operative delivery in the second stage of labour. 25 Another example is the study on middle-aged person’s experience of living with severe heart failure. 26
Another area where qualitative research is being used in health care is to identify obstacles and barriers to practice change by exploring the reasons behind certain behaviours. A good example of this is the study of patients’ decisions about whether or not to take anti-hypertensive drugs. 27 There are similar studies on issues such as the use of antibiotics in general practice and patient compliance.
In addition to issues related to the patients’ perception, some qualitative studies concentrated on factors fostering the doctor’s motivation and the effect of doctors’ social life and culture, in addition to issues related to the doctor’s own health. Examples are the study by Dumelow et al. 28 on the relation between a career and family life for English hospital consultants. Another example is a study aimed at exploring general practitioners’ perceptions of the effects of their profession and training on their attitudes to illness in themselves and colleagues. 29
Qualitative work can help in identifying cultural and social factors that affect health care positively or negatively. Such information can be helpful in improving service delivery. 6 Studies on patients from ethnic minorities have identified administrative and language barriers that affected health care and shed light on some of the beliefs and behaviours of these patients that might have affected help seeking and compliance. Good examples are the studies by Bush et al. 30 exploring the influences on smoking in Bangladeshi and Pakistani adults in the UK, and the multi-centre cross-cultural postnatal depression study. 31
There has been an increasing interest and use of qualitative research methods in primary health care and general practice articles. Britten 32 states that “the nature of general practice is such that a variety of research methods are needed to explore all its intricacies” He adds that qualitative methods can enrich research in general practice by opening up areas not amenable to quantitative methods, topics such as patient satisfaction, doctor-patient interaction, in addition to identifying and explaining attitudes, beliefs and behaviour. In addition, qualitative research has been used in the assessment of new technology methods used in health care. For example, the studies on the implementation of the National Health System information technology programme in the UK. 11 , 33
Qualitative research methods are receiving an increasing recognition in health care related research. The use of qualitative research in health care enables researchers to answer questions that may not be easily answered by quantitative methods. Moreover, it seeks to understand the phenomenon under study in the context of the culture or the setting in which it has been studied, therefore, aiding in the development of new research instruments, such as questionnaires that are more culturally acceptable. However, because health care related research has, for decades, been based on quantitative methods, the introduction of a new method requires researchers in health care who attempt to use it, to have a thorough understanding of its theoretical basis, methodology and evaluation techniques.
Acknowledgments
I would like to thank Sultan Qaboos University, Oman, for granting me a scholarship to do my PhD at the University of Nottingham, UK. I am also grateful to my PhD supervisor, Dr Margaret Oates, for her guidance and support.
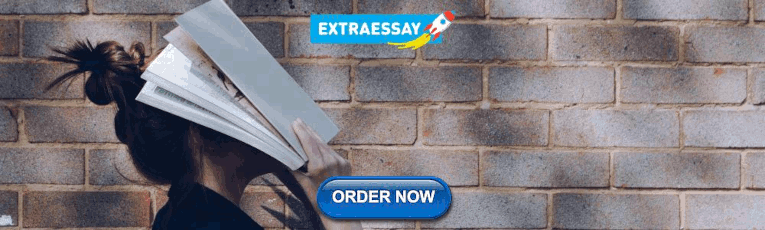
COMMENTS
Qualitative research methods explore and provide deep contextual understanding of real world issues, including people's beliefs, perspectives, and experiences. Whether through analysis of interviews, focus groups, structured observation, or multimedia data, qualitative methods offer unique insights in applied health services research that other approaches cannot deliver. However, many ...
Motivation and Scope. There is a large body of recently published review/conceptual studies on healthcare and data mining. We outline the characteristics of these studies—e.g., scope/healthcare sub-area, timeframe, and number of papers reviewed—in Table 1.For example, one study reviewed awareness effect in type 2 diabetes published between 2001 and 2005, identifying 18 papers [].
Significance of Qualitative Research. The qualitative method of inquiry examines the 'how' and 'why' of decision making, rather than the 'when,' 'what,' and 'where.'[] Unlike quantitative methods, the objective of qualitative inquiry is to explore, narrate, and explain the phenomena and make sense of the complex reality.Health interventions, explanatory health models, and medical-social ...
It is just as applicable to clinical trials as it is to health services research, enabling an in depth understanding of the problem that quantitative research alone cannot deliver. 1 It is imperative, however, that qualitative studies are well-designed with pre-determined methods to ensure reduction in research bias. While quantitative research ...
4 ML and Analytics in Healthcare Systems. structu re alignments, signal dete ction algorithms, and lung texture classi cation [5]. The architect ural fr amework of big data in healthcare is ...
Examples of predictive analytics. Local authorities Using predictive analytics to evaluate health trends and issues to help draw-up better public health strategies. Hospitals Using insights to plan optimum patient care and efficient use of resources, most recently during the Covid-19 pandemic.
Qualitative research uses analytical categories to describe and explain social phenomena. These categories may be derived inductively—that is, obtained gradually from the data—or used deductively, either at the beginning or part way through the analysis as a way of approaching the data.
This guide is designed to support health and social care researchers and practitioners to integrate qualitative research into the evidence base of health and social care research. Qualitative research designs are diverse and each design has a different focus that will inform the approach undertaken and the results that are generated. The aim is to move beyond the "what" of qualitative ...
Reports on data analysis in qualitative research are well documented. Procedural steps have been described [1,2,3,4,5,6,7] and authors have made distinctions between the concepts of coding, analysis, and interpretation [1, 2, 8, 9].Authors have written about different researchers accessing different representations of a topic or phenomenon [2, 10] or multiple interpretations being applied to ...
Metrics. The relationship between social factors and health is of great interest to clinicians, health system leaders, and policy makers. 1 Although it may seem intuitive to infer a relationship ...
It is intended to outline the nature of the problems caused by limited analytical capability in health services in the UK and also looks at some different ways that these issues can be addressed. This paper explores issues around both supply and demand that need to be addressed to ensure good quality analysis is able to improve care.
The AgeWell.de study. Data for this analysis were derived from the AgeWell.de study. AgeWell.de is a multi-component, cluster-randomized, controlled intervention study, which targets to decrease the risk of dementia in a high-risk group of elderly primary care patients [].Due to the lack of effective dementia treatment options, AgeWell.de was designed as a prevention study in order to improve ...
Clinical staff benefit from: increased knowledge allowing them to support service users more effectively. a reduction in non-elective admissions, leading to better utilisation of resources and associated savings. improved communication with social care staff. the ability to use data to predict and thus prevent deterioration.
Here are three examples of predictive analytics in healthcare in use today. 1. Detecting early signs of patient deterioration in the ICU and the general ward. Predictive insights can be particularly valuable in the ICU, where a patient's life may depend on timely intervention when their condition is about to deteriorate.
1The Role of Research in the Health and Social Care Professions. Learning Outcomes. On completion of this chapter the reader should be able to: • understand the development of research in the health and social care professions • appreciate the need to become 'research literate' • identify the major factors that contribute to debates ...
For example, it can look into why the value of the Japanese Yen has decreased. This is so that an analytical study can consider "how" and "why" questions. Another example is that someone might conduct analytical research to identify a study's gap. It presents a fresh perspective on your data.
Background In this methodological paper we document the interpretation of a mixed methods study and outline an approach to dealing with apparent discrepancies between qualitative and quantitative research data in a pilot study evaluating whether welfare rights advice has an impact on health and social outcomes among a population aged 60 and over. Methods Quantitative and qualitative data were ...
Background Postpartum depression (PPD) affects around 10% of women, or 1 in 7 women, after giving birth. Undiagnosed PPD was observed among 50% of mothers. PPD has an unfavorable relationship with women's functioning, marital and personal relationships, the quality of the mother-infant connection, and the social, behavioral, and cognitive development of children. We aim to determine the ...
Strategic workforce planning, regardless of analytical approach, needs to integrate health and social care through whole system thinking, if accessible, utilised, care for all, across the lifespan, is to be provided. ... Inclusion criteria privileged empirical research, for example studies. Owing to the volume of included literature, reviews ...
The research is non-exhaustive due to the incomplete and uneven regional distribution of the samples, overrepresented in three voivodeships (Łódzkie, Mazowieckie and Śląskie). The size of the research sample (217 entities) allows the authors of the paper to formulate specific conclusions on the use of Big Data in the process of its management.
Interventions to transform the delivery of health and social care are being implemented widely, such as those linked to Accountable Care Organizations in the United States,1 or to integrated care systems in the UK.2 Assessing the impact of these health interventions enables healthcare teams to learn and to improve services, and can inform future policy.3 However, some healthcare interventions ...
We champion better analytics by supporting teams of analysts, clinicians, decision-makers and managers directly through our funded programme, Advancing Applied Analytics. We're seeing the development of exciting projects across the UK that show ways to improve analytical capability through innovative applied analysis and shared learning ...
Health care teamwork research has narrowly defined the nature of health care teams, focused mainly on a small range of settings and activities and addressed a limited range of research questions. We propose that teamwork should be broadly defined and argue that the underlying assumptions about the nature of teamwork and how it should be studied ...
Has your organization used an AHRQ tool to improve patient care, make a culture change or save costs? The agency would like to learn more about your use of AHRQ resources to develop Impact Case Studies. Since 2004, the agency has developed more than 400 Impact Case Studies that illustrate AHRQ's ...
Finding and choosing a strong research topic is the critical first step when it comes to crafting a high-quality dissertation, thesis or research project. If you've landed on this post, chances are you're looking for a healthcare-related research topic, but aren't sure where to start. Here, we'll explore a variety of healthcare-related research ideas and topic thought-starters across a ...
Browse all our research projects by topic We have funded more than 150 external research projects across a range of themes over the last five years. Browse all our research projects by topic. Copy link. Please click below to see the research projects we've funded on the following topics.
Food safety is the basis for ensuring human survival and development. The threat of heavy metal ions to food safety has become a social concern with the rapid growth of the economy and the accompanying environmental pollution. Some heavy metal ions are highly toxic even at trace levels and pose significant health risks to humans. Therefore, ultrasensitive detection of heavy metal ions in food ...
Grounded theory, phenomenology and ethnography are three approaches used in qualitative research. Grounded theory approach is a commonly used qualitative method in the social sciences to inductively generate or discover a theory out of the data. 9 Phenomenology and ethnography are more commonly used qualitative approaches in health care and ...