
An official website of the United States government
The .gov means it’s official. Federal government websites often end in .gov or .mil. Before sharing sensitive information, make sure you’re on a federal government site.
The site is secure. The https:// ensures that you are connecting to the official website and that any information you provide is encrypted and transmitted securely.
- Publications
- Account settings
Preview improvements coming to the PMC website in October 2024. Learn More or Try it out now .
- Advanced Search
- Journal List
- Elsevier - PMC COVID-19 Collection

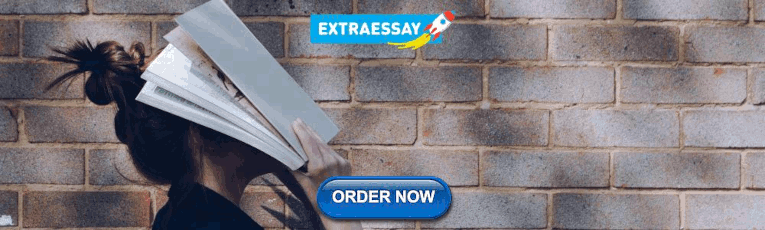
Socio-economic status and COVID-19–related cases and fatalities
R.b. hawkins.
a Division of Thoracic and Cardiovascular Surgery, University of Virginia, Charlottesville, VA, USA
b Center for Health Policy, University of Virginia, Charlottesville, VA, USA
E.J. Charles
J.h. mehaffey, associated data.
The United States has the highest number of coronavirus disease 2019 (COVID-19) in the world, with high variability in cases and mortality between communities. We aimed to quantify the associations between socio-economic status and COVID-19–related cases and mortality in the U.S.
Study design
The study design includes nationwide COVID-19 data at the county level that were paired with the Distressed Communities Index (DCI) and its component metrics of socio-economic status.
Severely distressed communities were classified by DCI>75 for univariate analyses. Adjusted rate ratios were calculated for cases and fatalities per 100,000 persons using hierarchical linear mixed models.
This cohort included 1,089,999 cases and 62,298 deaths in 3127 counties for a case fatality rate of 5.7%. Severely distressed counties had significantly fewer deaths from COVID-19 but higher number of deaths per 100,000 persons. In risk-adjusted analysis, the two socio-economic determinants of health with the strongest association with both higher cases per 100,000 persons and higher fatalities per 100,000 persons were the percentage of adults without a high school degree (cases: RR 1.10; fatalities: RR 1.08) and proportion of black residents (cases and fatalities: Relative risk(RR) 1.03). The percentage of the population aged older than 65 years was also highly predictive for fatalities per 100,000 persons (RR 1.07).
Lower education levels and greater percentages of black residents are strongly associated with higher rates of both COVID-19 cases and fatalities. Socio-economic factors should be considered when implementing public health interventions to ameliorate the disparities in the impact of COVID-19 on distressed communities.
- • Socio-economic factors play an important role in coronavirus disease 2019 (COVID-19) prevalence and mortality.
- • Lower education level was the strongest association with both cases and fatalities.
- • The higher proportion of Black residents was also associated with cases and fatalities.
- • The poverty rate and median income were also associated with COVID-19 cases.
- • Median income and change in employment were also associated with COVID-19 fatalities.
Introduction
The coronavirus disease 2019 (COVID-19) pandemic is a worldwide public health crisis on a scale not witnessed in modern times. The United States has the highest number of COVID-19 cases and fatalities in the world. 1 There is wide variability in COVID-19–related mortality across countries and between communities within the U.S. While research has identified a number of risk factors for mortality such as age and comorbid disease, there are new data suggesting social determinants of health also influence outcomes. 2 , 3 Data from the Centers for Disease Control and Prevention suggest strong racial disparities in both COVID-19 prevalence and outcomes. 4 This point has been highlighted by the U.S. Surgeon General, the lay press, and recent publications. 4 , 5 , 6
Socio-economic factors impact all facets of human functioning, including health-related quality of life. While a few publications have speculated on the impact of socio-economic status on the COVID-19 pandemic, there is limited quantitative analysis available. 7 , 8 , 9 , 10 A unique opportunity for investigation lies with the Distressed Communities Index (DCI). This metric was developed by the Economic Innovation Group and is a comprehensive estimate of socio-economic status by geographic location. 11 The DCI is a composite socio-economic ranking that accounts for unemployment, education level, poverty rate, median income, business growth, and housing vacancies. Previous studies have correlated a higher DCI score (lower socio-economic status) with worse health-related outcomes. 12 , 13 , 14 The objective of this study was to quantify the association between socio-economic status, both the composite metric of DCI and its individual component measures, and COVID-19 outcomes, accounting for other risk factors such as age and chronic diseases. We hypothesized that communities with lower socio-economic status (higher DCI scores) would have disproportionately higher COVID-19 prevalence and mortality.
Data sources and definitions
COVID-19–related cases and mortality were extracted from a publicly available data set of aggregated county sources (USAFacts) on May 2, 2020. 15 In addition, county-level estimates for chronic diseases were obtained from the Centers for Disease Control and Prevention. Specifically, the Centers for Disease Control and Prevention (CDC) COVID-19 response team used data from the 2018 Behavioral Risk Factor Surveillance System and U.S. Census population data to model prevalence of chronic diseases potentially associated with COVID-19 risk. 16 The model estimates for chronic kidney disease, chronic obstructive pulmonary disease, heart disease, obesity, and diabetes at the county level were included for risk adjustment. The data for rates of uninsured individuals under age 65, percent of population black, and percent of population age over 65 were obtained from the American Communities Survey 5-year Estimates. The United States is geographically and governmentally organized into 50 states. The states are then subdivided into counties with their own local governance structures. There is wide variability in the population and size of the states and counties, with the number of counties per state ranging from 3 (Delaware) to 254 (Texas). There is a total of 3143 counties or county-equivalent areas (parishes in Louisiana and boroughs in Alaska). At the time of writing, the most granular COVID-19–related data for the United States were available at the county level.
Socio-economic status was measured using the DCI and its components. A data use agreement is in place with the Economic Innovation Group. 11 The DCI score is available for 99% of the United States population and is derived from the American Communities Survey 5-year Estimates and Census Bureau County and Zip Code Business Patterns. The score is composed from the following data points: ‘percentage adults with no high school degree, housing vacancy rate, percentage of adults not working, poverty rate, median income ratio, percentage change in employment, and percentage change in business establishments.’ Detailed data definitions are available in Supplemental Table 1 . The score ranges from 0 (no distress) to 100 (most distress) and is available on the zip code, county and state level. Severely distressed communities were defined by a DCI score >75. This study conformed to the principles embodied in the Declaration of Helsinki and was exempt from review by the University of Virginia Institutional Review Board due to the deidentified nature of the publicly available data sets.
Counties were included for analysis if both COVID-19 data and DCI score were available. Individual cases and deaths were excluded from the analysis if they were not attributed to a specific county.
Statistical analysis
Categorical variables are presented as counts (%) and continuous variables as median [25th, 75th percentile]. Counties were stratified by severely distressed (DCI>75) versus less distressed (DCI ≤75) for univariate analysis using the Mann-Whitney U test. Adjusted effects of socio-economic status were approximated using hierarchical linear mixed models with Laplace approximation and a negative binomial distribution. Socio-economic effects were adjusted for county-level prevalence of elderly residents, uninsured adults, and the following comorbidities: chronic kidney disease, chronic obstructive pulmonary disease, heart disease, diabetes, and obesity. A random effect was used for clustering at the state level. Temporal bias was accounted for with a variable of days since first COVID-19 case. Regression results were exponentiated to construct adjusted rate ratios. Statistical analyses were carried out using SAS, version 9.4 (SAS Institute, Cary, NC), with some graphical representation performed with Prism 8 (GraphPad, San Diego, CA). A P -value <0.05 determined statistical significance.
Cohort description
At the time of data extraction on May 2, 2020, there were 1,096,706 cases and 64,066 deaths in the United States. There were 3143 counties with COVID-19 data available. Twenty-four states had cases not allocated to counties for a total of 6465 cases (0.6%), while 14 states had unallocated deaths for a total of 1754 deaths (2.7%), with 1445 being unallocated in Tennessee. A DCI score was available for 3127 counties with COVID-19 data. This cohort included 1,089,999 cases (99%) and 62,298 deaths (97%) for a case fatality rate of 5.7%. A consort diagram depicting the exclusions leading to the final cohort is shown in Fig. 1 .

Consort diagram of included and excluded counties, COVID-19 cases, and COVID-19 fatalities. COVID-19 = coronavirus disease 2019.
Differences by the DCI
A total of 781 counties were designated as severely distressed (DCI >75), accounting for 68,741 (6.3%) COVID-19 cases and 3811 (6.1%) COVID-19 fatalities. When compared with less distressed counties, those classified as severely distressed had significantly lower median COVID-19 cases (13 [3–39] vs 21 [4–117], P < 0.0001) and fatalities (0 [0–1] vs 0 [0–4], P < 0.0001). Owing to the rural nature of many distressed counties, after accounting for county population size, there was no difference in median cases per 100,000 persons and a reverse in the trend with higher median fatalities per 100,000 persons in severely distressed counties ( Table 1 ). The median percentage of black Americans was significantly higher in severely distressed counties compared with less distressed counties (7.3% vs 1.8%, P < 0.0001). Other significant differences included severely distressed counties having higher rates of elderly residents, uninsured individuals, and individuals with chronic kidney disease, chronic obstructive pulmonary disease, heart disease, diabetes, and obesity. However, severely distressed communities were hit later in the pandemic with lower median days since first case (34 vs 38, P < 0.0001).
Table 1
Univariate analysis by severely versus less distressed counties.
All values expressed as median [interquartile range]; COPD = chronic obstructive pulmonary disease.
Risk adjusted analyses of socio-economic status
Cases of COVID-19 by county were associated with multiple socio-economic factors and days since first infection but not health-related comorbidities. The adjusted risk ratios can be visualized in Fig. 2 , with complete model results in Table 2 . A higher number of cases were associated with lower education level, higher proportion of black Americans, higher income, and lower poverty rate. The specific covariates with significant associations with cases per 100,000 persons were the percentage of adults without a high school degree (RR 1.10), proportion of black residents (RR 1.03), median income ratio (RR 1.01), and poverty rate (RR 0.98). Fig. 3 shows risk-adjusted predicted cases per 100,000 persons for the two strongest associations, the percentage of adults without a high school degree and proportion of black residents. No comorbid medical conditions were associated with COVID-19 cases at the county level.

Forrest plot of adjusted rate ratio for covariates significantly associated with either COVID-19 cases or fatalities per 100,000 persons. COVID-19 = coronavirus disease 2019.
Table 2
Adjusted rate ratios for COVID-19–related cases and fatalities per 100,000 persons.
CI = confidence interval; COPD = chronic obstructive pulmonary disease; COVID-19 = coronavirus disease 2019.

Adjusted predicted COVID-19 cases per 100,000 persons for the two strongest socio-economic factors. (A) Percentage of adults without a high school degree and (B) percentage of population Black. Estimates are shown holding all other predictors at mean values ( Supplemental Table 1 ). COVID-19 = coronavirus disease 2019.
Higher COVID-19 mortality was associated with higher income but lower education, higher employment rate, higher proportion of black Americans, older residents, and less obesity ( Table 2 ). The significant socio-economic associations were the percentage of adults without a high school degree (RR 1.08), median income ratio (RR 1.01), and percentage of adults not working (RR 0.98). Significant demographic and health-related associations included the percentage of population aged older than 65 years (RR 1.07), proportion of black residents (RR 1.03), and obesity prevalence (RR 0.96). Fig. 4 shows the risk-adjusted predicted fatalities per 100,000 persons for the two strongest socio-economic associations, the percentage of adults without a high school degree and proportion of black residents.

Adjusted predicted COVID-19 fatalities per 100,000 persons for the three strongest socio-economic factors. (A) Percentage of adults without a high school degree and (B) percentage of population Black. Estimates are shown holding all other predictors at mean values ( Supplemental Table 1 ). COVID-19 = coronavirus disease 2019.
The present study highlights community-level socio-economic disparities in COVID-19 prevalence and mortality within the United States. Severely distressed communities account for a disproportionately higher number of deaths per 100,000 persons. However, poverty and unemployment were protective against contracting COVID-19, highlighting the unique risks of a pandemic and limitations of composite metrics of socio-economic status. Risk-adjusted analysis identified two socio-economic determinants of health as having the strongest association with both population-adjusted cases and fatalities: lower education level and higher proportion of black residents. Although age, comorbidities, and overall health status also appear to impact COVID-19 prevalence and outcomes, socio-economic factors have higher risk ratios and represent the strongest associated factors with both number of cases and fatalities.
Using the composite DCI metric, which includes seven different socio-economic factors, we found that counties with lower socio-economic status (higher DCI score) have higher COVID-19 death rates per 100,000 persons compared with non-distressed counties. Rates of high mortality in distressed communities are multifactorial, likely accounting for differences in age, number of chronic medical conditions per person, and socio-economic status. One of the strongest predictors of mortality from COVID-19 is age, where in Italy the case fatality rate in octogenarians was more than 20%. 3 , 17 Distressed communities are rapidly aging and have a greater proportion of elderly residents. 18 In addition, lower socio-economic status has been associated with a higher burden of chronic medical conditions and comorbidities. 13 These underlying medical comorbidities have also been shown to increase COVID-19–related mortality. 2 However, in the present data, we demonstrate a stronger association between socio-economic factors than underlying medical conditions with only obesity inversely associated with COVID-19 mortality. Obesity has traditionally been considered protective against acute respiratory distress syndrome–related mortality, but preliminary data on COVID-19 suggest possible increased risk. 19 , 20 There are significant overlaps in socio-economic status and obesity that were not accounted for in other studies, and the independent effect of obesity of COVID-19–related cases and mortality requires further study.
Distressed communities tend to be disproportionately comprising black Americans, and these individuals have higher COVID-19 mortality rates. 4 , 5 , 6 , 21 A study not yet published found that communities with a higher proportion of black residents had increased COVID-19 cases (relative risk 1.24) and deaths (relative risk 1.18). 22 Our study corroborates these findings where a higher proportion of black residents were associated with both increased cases and fatalities per 100,000 persons. The exact reasons to explain these associations cannot be determined from the current data sets and analysis, and a more thorough understanding of racial inequities requires nationwide disaggregated data. The underlying etiology for the racial inequity likely reflects trends of economic, geographic, and health disparities seen with socioeconomically disadvantaged populations. In addition, the impact of systemic flaws in the structural organization of American society with racism, access to health care, and community-level resources should not be underestimated.
The COVID-19 pandemic in America is becoming increasingly a story of economics. Significant attention has focused on how public health measures to save lives (social distancing, closure of all non-essential business, stopping the delivery of all non-urgent/emergent health care) have resulted in one of the largest increases in unemployment in American history. However, our data focus on the opposite relationship, how economics is affecting who contracts and dies from COVID-19. 23 Those individuals in frontline jobs who maintain employment are at increased risk of contracting coronavirus, particularly with concerns related to asymptomatic spread in the setting of inadequate testing. 24 Our data are supportive of this theory in two ways. First, higher income and less poverty both were associated with higher rates of COVID-19 cases while higher unemployment was associated with fewer COVID-19 fatalities. These findings suggesting a significant amount of transmission is through the workplace. Second, lower education levels were also associated with contracting COVID-19. This could be due to frontline jobs being disproportionately low paying and without a requirement of an advanced degree. 23 The education relationship is relatively strong (RR 1.10) compared with other metrics, which may be due to the highest paying jobs being more amenable to remote working, making employment and income imperfect markers of risk.
This public health trope of economics underpinning health inequalities is true not only in America but also was highlighted in 1980 in Britain with the Black Report. 25 One of the expectations of establishing the National Health Service with universal healthcare coverage was to eliminate health disparities. However, thirty years after, it was made clear that economic divisions (employment, income, education, housing, and so on) were only widening health disparities in the United Kingdom. The next few decades saw focused efforts to identify the causes of morbidity and mortality differences, develop better metrics to gauge true health disparities, and quantify those differences. 26 , 27 , 28 COVID-19 represents a rare disruptive opportunity to refocus our efforts on using this knowledge to reverse health disparities.
Now is the time to be proactive in the management of this pandemic and focus allocation of federal and state-level resources to these distressed communities. As society attempts to safely reverse restrictive public health measures such as stay at home orders, other more resource-intensive tools including testing and contact tracing will be required. The DCI may help to guide public health interventions to areas most in need. Clear and decisive actions are needed to help safeguard vulnerable populations who are at the highest risk for complications and death. 29
This study is limited by the lack of epidemiologic data available at the zip code level or widespread detailed demographic information, representing systemic failures of the healthcare system which limits our ability to adequately respond in an equitable manner. Socio-economic factors are interrelated, and collinearity limits the utility of an epidemiologic study design such as this. Individual-level SES analyses are required to better understand the interaction of all aspects of Socioeconomic status on COVID-19. In addition, the delay in testing availability and narrow testing criteria vastly overestimate the case fatality rate, but it is unclear how this varies across the United States. Better racial data are needed regarding COVID-19 to truly understand the disparities by race beyond a rough estimation for black Americans. Finally, the DCI only accounts for a small portion of variability in case fatality rates, suggesting social determinants of health are only one factor in determining COVID-19–related outcomes.
In summary, socio-economic determinants of health are associated with COVID-19 prevalence and mortality. Severely distressed counties with low socio-economic status have higher rates of both COVID-19 cases and fatalities than communities with higher socio-economic status. The socio-economic influence is broad, with many components of the DCI (education level, income, and poverty rates) being associated with COVID-19 cases. However, due to the transmission patterns of COVID-19 in the United States, higher income but lower education levels were associated with COVID-19 cases and fatalities. A higher proportion of black residents were also one of the strongest associations with COVID-19 cases and fatalities. Socio-economic factors and the DCI could help allocate appropriate public health resources in areas with lower socio-economic status, a critical step toward an equitable fight against COVID-19.
Author statements
Ethical approval.
This study is exempt from IRB review.
No funding was utilized for this research.
Competing interests
The authors report no conflicts of interest.
Appendix A Supplementary data to this article can be found online at https://doi.org/10.1016/j.puhe.2020.09.016 .
Appendix A. Supplementary data
The following is the Supplementary data to this article:
Click through the PLOS taxonomy to find articles in your field.
For more information about PLOS Subject Areas, click here .
Loading metrics
Open Access
Peer-reviewed
Research Article
Socio-economic status, resilience, and vulnerability of households under COVID-19: Case of village-level data in Sichuan province
Roles Conceptualization, Methodology, Writing – original draft
Affiliation Center for Trans-Himalaya Studies, Leshan Normal University, Leshan, Sichuan, China
Roles Conceptualization, Data curation, Methodology, Writing – review & editing
Affiliation School of Economics and Management, Leshan Normal University, Leshan, Sichuan, China
Roles Conceptualization, Methodology, Writing – review & editing
Roles Conceptualization, Methodology, Supervision, Writing – review & editing
* E-mail: [email protected]

- Imran Ur Rahman,
- Deng Jian,
- Liu Junrong,
- Mohsin Shafi
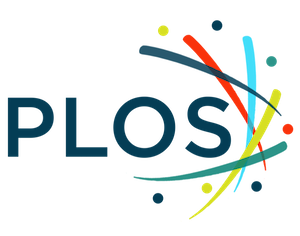
- Published: April 29, 2021
- https://doi.org/10.1371/journal.pone.0249270
- Reader Comments
This paper investigates economic impacts of COVID-19 on households based on differences in the socio-economic status (SES). We determine the household-level effects of the COVID-19 shock using income sources, types of industries, communities’ resilience, household susceptibility, and relevant policy measures. For this purpose, we used primary data of 555 households collected through snowball sampling technique using an online survey questionnaire from different villages mostly located in Sichuan Province, China. Using step-wise binary logistic regression analysis, we estimated and validated the model. Results suggest the use of SES as a better measure for understanding the impacts of COVID-19 on different households. We find that households with low SES tend to depend more on farmland income and transfer payments from the government. Contrarily, high SES households focus more on business and local employment as sources of income generation. Poor households were less resilient and more likely to fall back into poverty due to COVID-19, while the opposite stands true for non-poor households with high SES. Based on the estimations, policies encouraging employment and businesses complemented with loans on lower interest rates are recommended, which may increase the SES, thus minimizing vulnerability and enhancing the households’ resilience towards poverty alleviation and economic shocks.
Citation: Ur Rahman I, Jian D, Junrong L, Shafi M (2021) Socio-economic status, resilience, and vulnerability of households under COVID-19: Case of village-level data in Sichuan province. PLoS ONE 16(4): e0249270. https://doi.org/10.1371/journal.pone.0249270
Editor: Md Nazirul Islam Sarker, Neijiang Normal University, CHINA
Received: January 5, 2021; Accepted: March 16, 2021; Published: April 29, 2021
Copyright: © 2021 Ur Rahman et al. This is an open access article distributed under the terms of the Creative Commons Attribution License , which permits unrestricted use, distribution, and reproduction in any medium, provided the original author and source are credited.
Data Availability: The data used in this article is a part of a broader study undertaken at national level which was availed after approval from the government of China. The data used in this study is a small section of the study for which approval was granted by the Director of Leshan Economic Studies Center, Leshan Normal University for analysis of this research only. Since the data is owned by a third party, we are unable to share it. Data request may be sent directly to the following: Prof. Ren Wenju Director of Leshan Economic Studies Center, Leshan Normal University. [email protected] The authors did not have any special privileges in accessing the data and other researchers will be able to access the data in the same manner as the authors.
Funding: The study is undertaken under the provincial government fund to Leshan Normal University titled “Major Project of Soft Science in Sichuan Province (Code:2020JDR0166)".
Competing interests: The authors have declared that no competing interests exist.
Introduction
Ever since the beginning of 2020, the world economy has been shocked by a new form of coronavirus termed as Novel Coronavirus Pneumonia (NCP) or COVID-19 [ 1 ]. COVID-19 has affected the socio-economic conditions throughout the world, especially in the case of developing economies and poverty. Poor households and households with low socio-economic conditions are suffering the most because of the pandemic. There seems a disparity in the impacts of COVID-19 at household levels due to differences in the socio-economic indicators of the households. Therefore, it is necessary to study the effects of external economic shocks on different households and the response of the households to such shocks. The economic factors may provide a better understanding of the differences in the factors and relevant policy implications for households.
The impacts of COVID-19 are diverse and spread across different sectors and regions. According to estimates of Sumner et al [ 2 ]. COVID-19 will impact the poor mostly in the regions of South Asia, Sub-Saharan Africa, and East Asia. Among Asian nations, China was also affected by the outbreak and faced various economic downturns in the first two quarters with lower production and growth rate. According to the national bureau of statistics of China, the income and consumption trends varied across rural and urban areas. Although the per capita disposable income of the rural households in the poverty-stricken areas showed a nominal increase of 2.7%, the real growth rate had decreased by 3.0% after measuring for price factors influence. In terms of the sources of income, including wages, salaries, and business, there is a slight increase in the nominal growth while net transfer income increases greatly by 9.2% due to the provision of the government. The net income from property declined by 0.5% in the first quarter as a result of the shock [ 3 ]. Table 1 shows the income trends.
- PPT PowerPoint slide
- PNG larger image
- TIFF original image
https://doi.org/10.1371/journal.pone.0249270.t001
Similarly, Fig 1A and 1B ) shows the difference in the per capita disposable income of the Chinese residents in the first quarter of 2020 compared to the first quarter of 2019. The downward sloping lines clearly depict the decline in the median growth rates. Although there is a slight increase in the absolute and nominal value 8,561 Yuan, which shows an increase of 0.8% as compared to 2019; however, in terms of real value after adjusting for price factors, the income has declined by 3.9%, which can be attributed to the effects of COVID-19 on the households. For rural households, the actual decrease was 4.7%, which is higher than the urban areas due to the socio-economic status (SES) of the households in the rural areas.
( A ) Median & Absolute Level of income; ( B ) Median and average growth rate of income. (Source 2 NBS China [ 4 ]).
https://doi.org/10.1371/journal.pone.0249270.g001
The decline in the income in the case of the rural and urban areas also influenced the consumption patterns. The overall per consumption expenditure in nominal terms declined by 8.2% and in real terms by 12.5% in the first quarter. The rural and urban nominal and real per capita consumption expenditures also show downward trends. The real per capita consumption expenditure for rural households has been decreased by 10.7%; in the case of urban households it is decreased by 13.5% [ 4 ]. In the second quarter, the trends are expected to increase due to the re-opening of the main industries and the resumption of economic activities throughout China.
Although the spread of COVID-19 has impacted the majority of households in China, it is observed that some of the households are better-off during the pandemic as compared to the other households. Similarly, the poverty situation and socio-economic conditions of the households vary in outcomes and resilience towards external factors like COVID-19, with some poor and vulnerable households are assumed to have suffered more severely. These differences provide an opportunity for understanding why some households are affected insignificantly while others are affected significantly. Economic theories also provide various frameworks in estimating the effects of shocks on households based on income and consumption levels. Based on a similar assumption, the study of household’s socio-economic status (SES) for the estimation of the effect of COVID-19 on households may provide comprehensive and favorable outcomes in this case.
As various sectors of the economy are hit hard by COVID-19 but the poor and vulnerable communities are affected the most. Poor households and people living under poverty are considered to suffer due to the lockdown and closures of markets and business operations. Most of these people work on daily wages and may be unemployed with no alternate source of income. Governments across the world have stepped up relief and provision of subsidies to their citizens still the challenge for these vulnerable communities to recover hang in the air even after the pandemic is over [ 5 ]. In terms of developing countries, people living in low-income households face dual misfortunes: income-generating difficulties and vulnerability to socio-economics crises [ 6 ]. Poor households and people who are unprepared to cope with such shocks seem to be hit hardest by recession, inflation, disease and civil unrest. Similar trends are observed in terms of the impacts of COVID-19 on communities of different income groups. On contrary, rich households have greater access and a broader asset base to insurance and credit services, which will help cushion them against the effects of an external shock [ 7 ]. Thus, vulnerability and resilience of households to shocks or external factors can have diverse impacts based on the livelihood of the poor and non-poor communities. However, there is still a gap and dire need to address this issue and come up with a better and sustainable approach especially designed for protecting and supporting the poor and vulnerable communities. Based on these observations, it is also essential to understand and study the impacts of the COVID-19 on the different households and the main causes that highlight the disparities in the likelihood of the households to be in higher or lower SES level. However, there is still a gap and dire need to address this issue and come up with a better and sustainable approach especially designed for protecting and supporting the poor and vulnerable communities. Based on these observations, it is also important to understand and study the impacts of the COVID-19 on the different households and the main causes that highlight the disparities in the likelihood of the households to be in higher or lower SES level.
In this paper, we focus on the impact of COVID-19 on the household focusing on the SES differences in the villages of Sichuan Province of China. Our main objective is to determine the economics factors of households in response to COVID-19 based on SES differences. We also check the disparities in the level of resilience towards COVID-19 and households ability to counter falling under the absolute poverty by assessing the likelihood of the households SES conditions. The paper can contribute in providing better overview of the dynamics of the vulnerable households and suitable responses in fighting against external economic shocks. The outcomes may contribute in devising specific policies relevant to poor and vulnerable households.
The outline of the paper follows general rule. After introduction, the paper first summarizes the literature studies on impacts of pandemics and epidemics on different sectors and communities followed by vulnerability and resilience. Subsequently, the paper then provides the methodology adopted for estimations, followed by the main findings of the study and discussion on the output tables. Fnally the paper concludes and recommends policy implications.
Literature review
The differences in the impact of a pandemic or an epidemic based on different aspects of the human life are evident from both historical and present-day perspectives. Similarly, in terms of COVID-19, the effects of the outbreak have shown variability across different regions and conditions of the world. At household level, the effects of the economic shocks have been adverse in terms of the economic status, resilience, and vulnerability of the households. At present due to the continuous spread of the virus, there are very few literature works that have focused on the impacts of COVID-19 shocks on poor household and poverty [ 8 , 9 ]. Most of the poor live in developing nations and are more vulnerable to COVID-19 shocks and their effects on the status of the communities and households. Studies show 14–22 million people may fall below absolute poverty line due to 1% decline in global GDP [ 9 ]. Therefore, it is necessary to analyze the diverse impacts of COVID-19 on the status and livelihoods of different communities and households.
Socio-economic status
Socio-economic status (SES) of a household is an important factor in signifying the outcomes related to shocks and vulnerabilities. It can affect the results of an economic phenomenon in different ways. According to the American Psychological Association, the Socio-economic status (SES) is a person or group’s social class or status which is often calculated by combining education, income and occupation [ 10 ]. As stated by Adler & Snibble [ 11 ] households with higher SES have better access to health care, housing, knowledge and nutrition as compared to lower SES households. Several research studies have focused on the impacts of different shocks on households based on the status. Some have highlighted the impacts of economic shocks such as joblessness or income decline and their effects on the household’s status [ 12 , 13 ]. While others have argued on the health shocks effects on individuals health and in turn the productivity of the workers finally leading to decline in income level of the households [ 14 ]. There is still very few literature articles related to the impacts of shocks on the socio-economic status of different households and communities in the developing world.
Some researchers have highlighted the relationship between household status and shocks [ 15 ]. Other researchers argue that the effects of economic shocks on households are greater in households that are poor due to insufficient income to cushion against the external shock [ 16 ]. In terms of effects on communities and household, shocks at village level are usually not considered to affect the permanent income of the individual households. Others argued that household consumption declines due to negative shocks that impact the permanent income of the households [ 17 , 18 ].
Economists have formalized different form of models for the estimation of household response towards risks and shocks. Consumption response to income shock is one such model which focuses on use of combination of consumption, income or wealth in response to shocks [ 19 , 20 ]. Kruger and Perii [ 21 ] used consumption and wealth as household response towards the income shock. Nevertheless, the issue of which model fits the household response remains unclear. For this reason, this paper attempts to use the SES for the estimation purposes as it present a better proxy and a good indicator of the household’s economic situation.
Vulnerability and resilience
The concepts of vulnerability and resilience are found in various disciplines and subjects. A body of literature has emerged in the past few decades that have focused on different aspects of vulnerability. The notion of vulnerability has become an influential theoretical method for defining the condition of susceptibility to risk, inadequacy, and marginality of both physical and social institutions, and for influencing normative study of behavior to increase quality of life through risk mitigation [ 22 ]. The term vulnerability, according to the World Bank [ 23 ], is “the likelihood that a shock will result in a decline in well-being”, while some researcher have described vulnerability as a state of defenselessness and exposure to shocks [ 24 ]. This definition can be further explained that any external shock that can lead to the decline of the livelihood of households including income and other socio-economic factors, determines the vulnerability of the households.
Some studies focused on presenting evidence of vulnerability by examining the methods used by rural households in low-income countries to deal with HIV/AIDS [ 25 ]. A study by Ndirangu explores how farm households in rural Kenya react to shocks and the impact on quality of life of household risk management strategies. The research explores two primary causes of rural vulnerability: the weather and AIDS. The study shows strategies for coping with shock includes dependence on collaborative opportunities such as casual loans and gifts [ 6 ]. The impact of vulnerability in our case can be assessed and analyzed empirically through the addition of vulnerability of the households against COVID-19 in the model. We check the vulnerability and attributes of it relevant to poor households. By doing, useful understanding and insights can be obtained that can enable policy makers in formulating better policies to avoid the risk related to poor households in case of shock.
Similarly, the concept of resilience has gained much attention in recent years especially in terms of poor households [ 26 ]. Resilience refers to the ability of households to cope with basic necessities of livelihood in case of emergency or shock [ 27 , 28 ]. Resilience has been implemented by different scholars in diverse perspectives [ 29 – 31 ]. There is a broad consensus that resilience is not stagnant, but that it continues to evolve [ 29 , 32 ], that needs further analysis and examination.
Previous studies have focused on the resilience of household in case of natural disasters while others have focused on resilience of households to the socio-economic shocks [ 33 – 35 ]. Resilience of households has also been studied in terms of ecological and environmental effects [ 36 , 37 ]. Although different qualitative approaches have been undertaken in terms of analyzing the resilience but the studies based on quantitative resilience are scarce and limited [ 38 , 39 ]. The resilience of households taking into consideration the livelihood of the households is also an important factor. Several studies have focused on this component in terms of external shocks [ 40 , 41 ]. However, studies have focused on different aspects and factors linking resilience to household income and assets, yet there is a dire need to better understand the connection between these components in terms of rural households. This study will attempt to fill this gap and contribute to the empirical aspect of the literature.
In terms of measuring vulnerability and resilience, economists have argued on various economic factors including household income, status, capital, and access to social security nets [ 42 – 44 ]. In terms of understanding the response of household towards a certain external shock like the COVID-19 pandemic, resilience and vulnerability can be good measures. The impacts of COVID-19 on households are unprecedented and the responses are observed to vary from household to household due to the differences in the SES of the households. Similar, observations are reported in case of China. The assumption can be drawn that households with higher SES may have better coping strategy and resilience as compared to household with lower SES. In this paper, we analyze the difference in the households resilience based on SES using the survey data.
For empirical and quantitative analysis, some authors measured vulnerability based on its impacts on indicators of welfare of the households [ 45 , 46 ]. Others estimated vulnerability in relationship to the poverty dynamics of the households. In this paper, we follow the approach of Vulnerability as Expected Poverty (VEP). The VEP approach is defined as the probability that the expected Income expenditure of a household will fall into poverty in the future [ 47 ]. This approach was also adapted in terms of poor households in developing countries by other scholars [ 7 , 48 , 49 ]. In case of previous studies, the vulnerability was estimated in case of poverty status of the households, which stood above the absolute poverty line. These measures did not consider the effects in case of households below the poverty level, which may provide a deeper understanding, and vulnerability effects on poverty status of households. Chaudhuri [ 47 ] pointed out that welfare status of the households tend to decline where the households poverty status is below poverty line as compared to households above poverty line due to the increase in vulnerability and risk. Based on this notion, we build our model and apply it in case of household status both above and below the poverty line, which will provide a better, and in-depth measure of the vulnerability of different households as compared to previous ones.
Additionally, some past literatures have also focused on different coping strategies across multiple domains including access to credit, government support, and help from family and friends, use of savings, and other such strategies [ 50 , 51 ]. While, the coping strategies related to the SES of household especially poor households, have not been clearly observed [ 16 ]. Coping strategies towards shocks may differ and their impacts may vary based on the status of the households. In this paper, we observe a coping strategy specifically based on the significance of the policy for both poor and non-poor household status, which in this case are the loans provision on lower interest for business and its impacts on different households. There are very few research on the poor households susceptibility towards pandemic [ 52 ], there is still more to explore in terms of COVID-19 and its impacts on poor households and the response of the communities.
This paper attempts to provide the impacts of COVID-19 on household based on the SES of the households. Additionally, the paper further identifies the differences in the impacts of the outbreak taking into consideration the SES of the households by dividing the households into two groups (poor and non-poor households). Based on these differences, the resilience of the households towards a shock like COVID19 and the vulnerability of the households in face of coping with the outbreak are also analyzed and estimated for a deeper understanding of the differentiated effects of COVID19.
Methodology
Sample and data collection.
For the empirical estimations and predictions of our model, we use both primary and secondary data from different sources and methods. For the collection of primary data, an online survey was developed. The online questionnaire was shared using different social media platforms, which made it easier for data collection. The data was collected from village representatives (potential key respondents). The questionnaire was filled by different households with majority from the villages of Sichuan province (475 in Sichuan while 80 from other provinces). A total of 555 questionnaires were completely filled through the online channels over a period of two weeks. The province includes least, middle, and highly developed regions similar to mainland China. Due to measures taken by the Chinese government to contain the spread of the virus, including social-distancing and travel restrictions, the data was collected through administering an online questionnaire, which is also consistent with previous studies [ 53 , 54 ]. In case of Sichuan Province, there are about 183 counties that are different in terms of geo-political and socio-economic indicators [ 55 ]. For secondary data, online official websites were utilized. Health data and reports were collected from WHO website [ 56 , 57 ], while data on Chinese villages was collected from National Bureau of Statistics China website [ 58 ].
The data was collected through the snowball sampling technique due to its time and resources-saving advantages, as argued by various researchers [ 59 , 60 ]. The data was collected during mid of April 2020 for two weeks; at that time COVID-19 pandemic had already affected a large number of local people in terms of socio-economic and health effects of COVID-19.
We sought permission from the relevant community leader of every village prior to administering the questionnaire. Besides, the consent of each village representative was obtained prior to partaking in the survey. Each respondent was allowed not to answer any question accordingly. The current research was approved by the Ethics Committee of Leshan Normal University. Prior to taking part in the online questionnaire, informed consent was obtained from each participant. The purpose and content of the study were first introduced to the potential participants before partaking in the survey. After assuring respondents of complete anonymity, confidentiality, and other ethical considerations, they filled the online questionnaire. There was no electronic record of the participant’s consent, but all respondents agreed on the purpose of the research and took part in the online survey voluntarily.
A questionnaire was developed to study and investigate the economic impacts of COVID-19 on households based on differences in the socio-economic status (SES). In this study we attempt to determine the household-level effects of the COVID-19 shock using different socio-economic factors. For this purpose, we asked several questions from participants such as SES level, household level (poor or non-poor), number of community groups in the village, per-capita annual disposable income (in normal times), main sources of income, industry involvement, agricultural supply level, cost of livestock breading (after COVID-19 outbreak), impact of COVID-19 on rural households to fall back into poverty, vulnerability, and resilience level of households, among others. For more details about the survey questions, see S1 File .
In case of the measurement some researchers argue that although the consumption patterns and disposable income are currently applied for the evaluation of the welfare status and living standards of the households [ 61 ] but these are not the only proxy variables for representation of welfare and status of the households. The variables like welfare and living standards are somewhat hard to observe directly. Instead, different proxy variables are utilized for the indirect measurement of such values. Therefore, it is possible to estimate new proxy variables as suggested by Massari [ 61 ] which can best define the changes and effects on households. Using the Bank of Italy SHIW data, he included a new proxy variable “real assets” in addition to disposable income and consumption expenditure. Similarly, in this paper we assume the SES of the household may provide a better measure as a proxy representing the welfare of the households.
We develop a simple model based on step-wise binary logistic regression in order to estimate our variables. As we used an online survey (questionnaire) for the collection of the data, so some variables in our data are coded as binary variables while others in categorical form. Based on the design of the questionnaires, the responses and the data, it is convenient to use the logistic regression approach for better estimates, good model-fit and unbiased estimates [ 62 , 63 ].
The model predicts the impact of different sources of income, industries, and policies under COVID-19 based on the SES in the villages of Sichuan province, China. As mentioned earlier, the households are divided into poor/low SES and non-poor/high SES household based on the survey data. The household level SES is based on village representative’s identification of the average SES level of the households in the villages (high SES or low SES village) and the reply of the respondent’s in the questionnaire. Based on this fact, we assume SES to be a better indicator for the indirect impacts of COVID-19 in case of different SES levels of the households.
Where, Y i is the linear function and the dependent variable, X i denotes the independent regressors and β measures the coefficients of the regressors in the model.
Where, Y i is the dependent variable representing SES of households ‘i’ whereas X i , Z i and N i are the independent regressors in our model. X i which is divided into the four main sources of income in village ‘i’, Z i represents the two main industries in village ‘i’, and N i denotes the two impact variables of Covid-19 on households in village ‘i’. All the independent variables are chosen based on the socio-economic features of the villages and in accordance with the national statistics of China. The variables are further explained in Eq 4 . Moreover, α 0 is the constant in the model while β i , γ i and δ i are the relevant coefficient measures. μ i is the overall error term in the model.
yfarm i : Income from farmland in the household ‘i’
ybusn i : Income from doing business in household ‘i’
yemp i : Income from local employment in household ‘i’
ygov i : Income from Government Transfer payments in the household ‘i’
indagri i : Household member associated with the agricultural industry
indlivst i : Household member associated with the livestock industry
sagri i : Supply of agricultural products during the pandemic
clivestk i : Cost of livestock feed during the pandemic
res i : Resilience of household ‘i’ against COVID-19
pov i : Ability of household ‘i’ to counter falling to poverty during the pandemic
policyloan i : Desired policy of loans for business on lower interest rate by household ‘i’
The new variable res i represents the resilience of the household ‘i’ against COVID-19, Pov i shows the susceptibility of the household ‘i’ falling into poverty and policyloan i denotes the desired policy measures by the households in county ‘i’. ε i , ρ i and τ i are the respective coefficients measures of the newly added regressors.
We estimated our model using step-wise binary logistic regression. As our independent variable is binary coded and independent variables are categorical, it is an appropriate method for estimation. The model was also tested for reliability and validity through tests for consistency and goodness of fit for the factors. The results show significant estimates and in line with our assumptions and research questions. The outcomes of the analysis are reported in the next part.
Results and findings
Sample characteristics and descriptive summary.
In our sample, most respondents belong to high SES status level (74%). In terms of per capital annual disposable income during normal years, most participants reported less than RMB 13000 (61%) while only 12% of participants had income higher than RMB 20000. Further, in terms of main income sources, most survey participants were dependent on government transfer payment (89%), followed by business (66%), local employment or wage income (41%), and family farming (25%). Additionally, 59% reported that they are mainly involved in agricultural industry. When asked about supply of agricultural materials (in comparison to last year), 61% respondents reported that they are receiving adequate supply of materials. However, only 1% pointed out that there is severe shortage.
Additionally, 67% of participants said that due to COVID-19 outbreak, the cost of livestock breeding has increased. Similarly, when we asked survey participants to express the likelihood of the rural households to fall into poverty due to COVID-19 pandemic, 15% reported very high likelihood followed by high likelihood (46%). Likewise, when respondents were asked to express their resilience and ability to prevent returning to poverty independently without any external support, 46% reported that they possess strong resilience capability, while only 17% reported that they have no resilience capability.
Table 2 shows the statistical summary of the dependent and independent variables. We collected the data through an online questionnaire. A total of 555 households were observed and surveyed. Most of the variables including the dependent variable ‘SESi’, are binary coded with values of 0 or 1. Some variables including ‘hhres’, ‘fallpov’ and ‘sagri’ are ordinal or ranked assuming values of 0, 1, 2 or 3.
https://doi.org/10.1371/journal.pone.0249270.t002
The mean value of the variables lies between 0 and 2 with minimum of 0.26 for the dependent variable ‘SES’. For ‘sagri’, the maximum mean value is 1.87. The standard errors of mean are low for all the variables and ‘ygov’ shows the lowest values of 0.013. The standard deviation for all the main variables is between 0 and 1. ‘hhres’ has the highest standard deviation of 0.933 followed by ‘fallpov’ 0.843. For the maximum and minimum values of the survey responses of the households, the codes range between 0 and 3. In case of the dependent variable, the code is in reverse order assuming 0 for positive response and 1 for negative response. The codes for independent variables present 0 for low level and 1, 2 or 3 for higher categories of responses. Overall, the data shows clear statistical values for the variables used in the estimation method.
Fig 2 shows the difference in the SES of the households in percentages and the response of the households in case of socio-economic changes. Household with better socio-economic status rely on income from business (68% of high SES households) and local employment (39% high SES households) while households having low SES are more dependent on transfer payments from the government (19% of low SES households) and farm output (93% of low SES households).
https://doi.org/10.1371/journal.pone.0249270.g002
Similarly, low SES households are more vulnerable (71%) and external shocks can drive them further into poverty (31%). In contrast, households with higher SES are more resilient (47%) and can cope with external shocks like COVID-19 regardless of external support. It also shows 35% and 55% of low SES households are engaged in agricultural and livestock industries respectively.
Reliability and validity statistics
Cronbach’s alpha..
The value of Cronbach’s alpha is calculated for the reliability or internal consistency of the estimations or scale. Cronbach’s alpha was first introduced by Lee Cronbach in 1951 in order to check the internal consistency of test [ 65 ]. In order for the results to be reliable and acceptable, the threshold value of Cronbach’s alpha should be between 0.7 and 0.95 [ 66 , 67 ]. In our case, Cronbach’s alpha values for our models are above the threshold value of 0.7. The value implies that the variables used are reliable and consistent internally. The table 3 contains the Cronbach’s alpha values for the factors in each of our models.
https://doi.org/10.1371/journal.pone.0249270.t003
For testing of convergent validity, we use Factor Analysis for the four main components of all our factors, including 4 income factors in the first component, 2 industry factors as the second component, 2 cost factors as the third component, and the fourth component consists of 3 factors that have diverse loadings. The test is undertaken to check if the factors load are acceptable and distinct or not for each component. Table 4 shows the loading of the main components.
https://doi.org/10.1371/journal.pone.0249270.t004
Table 4 clearly depicts that the factors load distinctly for the four groups and confirms the validity of convergence among the main factors in each of the groups. The average factors loading for all the groups are also estimated to be higher than 0.5 threshold level and most of the groups average above 0.7 which is a good determinant of validity and also suggests convergent validity.
Goodness of fit.
After fitting the model, it is important to analyze the fitness of the data in the model. There are different methods of calculating the goodness of fit like Pearson’s Chi-Square [ 68 ] and Likelihood ratio test [ 59 , 60 ], but based on our data set, Hosmer-Lemeshow test for goodness of fit is considered a good way of presenting the fitness of our data into our model [ 69 ]. In the case of the Hosmer and Lemeshow test, the significant value should be above 0.05 for selection of the model. A lower significance value suggests the rejection of the model [ 70 ]. The results of the test are reported in Table 5 .
https://doi.org/10.1371/journal.pone.0249270.t005
According to Hosmer [ 69 ], the value of significance has to be above the threshold of 0.05% in order to determine a good fit. The null hypothesis assumes that the data is a good fit for the model. In our case, Table 5 clearly shows that the data fits our model, and our model has the goodness of fit as the significance of Hosmer-Lemeshow test estimates are above 0.05, which means we are unable to reject the model and our data fits the model. In addition, for the overall significance of the model, the Omnibus test of the Model coefficients also proved that coefficients of the independent regressors used in the model are significant. Table 6 presents the Omnibus test outcomes of the final estimations of the model.
https://doi.org/10.1371/journal.pone.0249270.t006
Main findings and discussions
Table 7 shows the stepwise binary logistic regression trends for our model based on the survey of households in different villages of Sichuan Province.
https://doi.org/10.1371/journal.pone.0249270.t007
As our dependent variable ‘SES’ assumes binary values (0, 1), therefore, binary logistic regression analysis is adopted for the estimation of the coefficients in our model. The binary logit regression provides better estimations and is recommended in case the dependent variable consists of binary values. The use of SES as the representation of the households proved to be a better indicator of showing the impacts of shock on different households’ and their response to an external shock (COVID-19) as it takes into account the main economic factors relevant to households. All the independent variables are regressed and estimated for the effects on the dependent variables.
The table also shows the coefficients ‘B’ and the exponential form of the coefficients ‘Exp (B)’ of all the independent variables in the model. Exp (B) interprets the odds of the effects of the independent variables in case of the binary dependent variable.
Income effect.
The variables ‘Yfarm’, ‘Ylemp’, ‘YBusn’, and ‘YGovt’ depict different household income sources in the villages. The significant income effect on the households can be seen from the Table 7 as all the variables are significant. Yfarm and YGovt are negative in relationship while Ylemp and YGovt attain positive sign concerning the dependent variable. The negative sign explains the odds of the independent variables to be less likely being the poor household while positive sign denotes higher odds of the effects on poor households. The Exp(B) values 3.056 and 4.440 for Yfarm and YGovt respectively demonstrate the odds of income earnings from farmland is 3.056% more for poor households while odds of depending on transfer payments from the Government are about 4.44% higher in poor households as compared to households that are non-poor with high SES.
The difference indicated by these estimations clearly suggests that poor households are more vulnerable with subsistence source as farmland income and thus more dependent on support from the government for income and livelihood while non-poor household is less vulnerable due to better and continuous income sources like a business and local employment. The differences suggest that non-poor and higher SES households are characterized by income from business and employment. Business and Employment show a key and significant difference between the low and high SES households. Such means and sources of income are estimated to have a positive impact on the SES of the households.
Effect on prices and costs.
The result provided the changes in prices and costs of agricultural products and the impacts of such changes on the different households. The significant results for agricultural industry (-0.704) and supply of agricultural products (-0.609) suggest that the odds of the poor household member working in the agricultural industry and the effect on supply of agricultural products are less likely compared to non-poor households. There is a clear distinction as working in an agricultural industry may provide higher income opportunities, and thus, may increase the SES. Similarly, the agricultural supply may be increase for non-poor households due to the shock as non-poor household demand for agricultural products may increase due to the fear of pandemic. These findings can be attributed to the fact that non-poor households depend on the supply of agricultural products, and thus, the effect on the supply of agricultural products may be higher in non-poor households. The possible explanation may be that China’s agricultural sector has been on the verge of modernization, which can be focused on future policy in giving opportunities for low-income families to be part of the agricultural industry. In addition, without the necessary source of income, the poor family may only end up decreasing marginal income with the higher opportunity cost of migration work in non-agricultural sector in town or else; while a non-poor family is in an opposite situation of its counterpart. Moreover, if the poor’s situation cannot be reversed in the long run, the rural population would be separated into the Agri-business group and the city worker group, which may be the natural selection process.
In contrast, household members working in the livestock industry and higher livestock cost are most likely to happen in the case of poor households. The finding also highlights that poor household members are about 2% more likely to be associated with livestock industry than non-poor households. It can be attributed to the fact that most of the poor households depend on livestock and products from livestock for daily consumption and livelihood. As a result of the shock, the dependence of poor households on livestock’s increase and thus the costs for feed rise.
Resilience and vulnerability on SES.
Further, estimates for the resilience of community (-1.167) in tackling the pandemic explain that the odds of households to be resilient against pandemic are less likely to be in low SES households, which can be extended as households in non-poor households tend to be more resilient towards COVID-19 due to better sources of income. Similarly, the odds of countering falling into poverty are less in poor households as compared to non-poor households. Low SES households mostly depend on income from farms and support from the government. It is unlikely for the poor households to overcome any external shock or show resilience without the support of the government. Once such households are affected by an outbreak, then the chances of these households falling into poverty increases as these households depend on subsistence sources of income and government support and assistance.
Policy effect.
In terms of the policy, the provision of loans on lower interest rates for business based on the coefficient value of -0.580 explains the odds of households desiring business loans on low-interest rates are less likely to in poor households. Non-poor households tend to establish business and earnings from employment, while households with low SES are less likely to be engaged in business and employment activities. These factors can enable households to earn higher income which in this case, the higher desire for loans for business purposes in non-poor households proves the difference between poor households and non-poor households.
The overall significance of the coefficients and the goodness of fit for variables in the model were explained previously. For explanation and predictive power of the independent variables, the binary logistic regression calculates Nagelkerke R Squared values. The value for Nagelkerke R Squared in our final model is 56%, which shows the independent variables in our model are good in specification and estimations.
For further analysis and checking of the model, we sort the data and estimate the variables in categorical forms. The categorical estimations of the model provide significant outcomes as compared to the regression analysis of the overall effects of the variables. The estimates of the coefficients in our model are presented in Table 8 .
https://doi.org/10.1371/journal.pone.0249270.t008
The estimations in the table show different categorical estimates for our main variables in the model. The results are similar to the non-categorical estimations with a few changes suggesting robust outcomes in our main model. The income effect and industrial effects of the variables show similar signs as the previous model. Similarly, the Nagelkerke R Square value is also high at 70.1%, which shows a higher predictive power of the variables. As in the case of Binary logistic regression, the Nagelkerke R Square values loosely define the effect of independent variables, therefore, we also calculated the correlation value of the probabilities of our independent variables and the dependent variable using the Pearson Correlation test. The value of the Pearson Correlation test is 61%, which is also high and shows a good choice of our independent variables in explaining the dependent variable.
The resilience has an overall significant impact in determining the odds of non-poor households to be more resilient as compared to poor households. The first category representing resilience in the table suggests that there is no resilience. Based on the estimation value of 1.941 it can be interpreted, as the odds of households with no resilience are about 1.9 times more likely to be poor and low SES households. Based on exp(B) value of 6.965 it can also be explained that a household with no resilience is approx. 6.96% more likely to be poor, which is highly significant. On the contrary, the resilience coefficients for 2 nd and 3 rd category we obtain the odds ratios of 0.166 and 0.018. Thus, the odds of a household to be resilient or highly resilient are less likely to be poor households.
Similar trends are represented in terms of countering poverty. The signs are negative showing less likeliness towards poor households, and exponential coefficients for the three categories assume values of 0.455, 0.121, and 0.020, respectively. All the category estimations were negative, predicting less likeliness of being poor or low SES households. The estimates were significant except for the first category. The results suggested that the odds of households countering poverty strongly or very strongly are less likely to be a poor household. It can be explained that a household with low SES is more vulnerable to the impacts of COVID-19 and may fall back below the poverty line, while the high SES households show more resilience and less vulnerability towards the pandemic. The results further highlight that the number of new poor may increase in the future if the pandemic continues as these households are less resilient and less likely to counter falling back into poverty.
For further explanation of the model, we estimated the predicted probabilities of the variables against the dependent variable. Fig 3 shows the predicted probabilities of the independent variables against the values of dependent variables. Although some values overlap, the majority of the distribution provides a good prediction of the model. It predicts that households with low SES are likely to fall on the positive side while a household with high SES over the negative side. The same outcomes are also presented in Fig 4 .
https://doi.org/10.1371/journal.pone.0249270.g003
Piling of the data responses and predicted probabilities on both sides of the figure explains a good outcome related to the variables and the model.
https://doi.org/10.1371/journal.pone.0249270.g004
This paper examined the difference in impacts of COVID-19 on households based on the socio-economic differences in the villages of Sichuan province, China. Our main goal was to identify the economic factors influencing households’ responses to COVID-19 based on socio-economic differences. The SES provides a better and deeper knowledge of the changes in household economic conditions and indicators as a result of an outbreak or health crisis like COVID-19. This study also assesses the likelihood of the households’ SES conditions to see if there are any disparities in the level of resilience to COVID-19 and households’ ability to avoid falling into absolute poverty.
The outcomes presented that households are less likely to be affected or the impact of COVID-19 on non-poor households is lower as compared to households that are poor with lower SES. Similarly, Ndirangu [ 6 ] argued that in the case of developing economies, people living in low-income households face dual misfortunes: income-generating difficulties and vulnerability to socio-economics crises. Likewise, Jalan & Ravallion [ 7 ] also argues that households with higher SES have greater access and a broader asset base to insurance and credit services, which will help cushion them against the effects of an external shock, like COVID-19. The chances of falling back into the poverty of a poor household are significantly higher than the Non-poor household, which suggests that the numbers of new poor as mentioned in recent articles are estimated to increase more due to the pandemic [ 71 ]. Further, Adler & Snibble [ 11 ] argue that in comparison to households with lower SES, higher SES households have better access to health care, housing, knowledge, and nutrition.
In the case of resilience, response, and tackling the economic influence of COVID-19, the estimate predicts that the odds of non-poor households (high SES) to be resilient against COVID-19 are higher in contrast to low SES households. Business and employment may be better sources of income, and industry plays key roles in determining the SES of the households, which can affect the resilience of the communities towards recovery during the pandemic.
Implications
Based on the outcomes of the paper and literature studies, it is observed that poor households and people having dependent on farm income are affected more and are more dependent on external support; therefore, it is necessary to devise policies for supporting and creating new jobs and income generating opportunities for them. Focusing on agricultural sector modernization and providing opportunities in the agricultural industry may contribute both at the household and national level. Small scales agricultural industries can be set up according to the needs of the community or county are expected to be of higher significance. Loans on lower interest rates for businesses can increase resilience and decrease the vulnerability of the poor households with access to funds. These policies can have better and long-lasting impacts on changing the livelihoods and SES level of the poor communities. At a general level, the government bodies and institutions provide relief and support to the poor households and vulnerable communities with low income and low employability [ 2 ]. Such policies and support should be temporary, as households depending on government support have a higher chance of falling or staying in the low SES category and may not divulge into other income opportunities.
Limitations
Despite insightful implications, this research is not without limitations. Primarily, this study was cross-sectional in nature which does not allow to make causal inferences. To make causal inferences, longitudinal studies are needed. Furthermore, the sample was obtained through the snowball sampling technique, which provides room for further research. Concerning limitations during the course of the research, there are some areas that may be improved in future research articles. The sample we collected was based on a single province due to time restraints; other researchers can increase the data set and collect a larger sample, a country-level data, which will give outcomes that are more robust. Similarly, since the lockdown policies and pandemic are ongoing; more research and post-pandemic scenario will provide better research opportunities and unbiased estimates for future researchers.
Supporting information
https://doi.org/10.1371/journal.pone.0249270.s001
Acknowledgments
We are grateful to the support of the members of Center for Trans-Himalaya Studies (CTHS) and the administration of Leshan Normal University (LSNU)). We highly acknowledge the assistance from the faculty of School of Economics -LSNU during the course of the research.
- 1. World Health Organization. Naming the coronavirus disease (COVID-19) and the virus that causes it. WHO Technincal Guidance [Internet]. 2020 [cited 2020 Feb 20]; Available from: https://www.who.int/emergencies/diseases/novel-coronavirus-2019/technical-guidance/naming-the-coronavirus-disease-(covid-2019)-and-the-virus-that-causes-it .
- 2. Sumner A, Hoy C, Ortiz-Juarez E. Estimates of the impact of COVID-19 on global poverty [Internet]. Helsinki; 2020. (WIDER Working Paper). Report No.: 2020/43. Available from: https://doi.org/10.35188/UNU-WIDER/2020/800-9 .
- 3. National Bureau of Statistics of China. Income of Rural Residents in Poor Areas in the First Quarter of 2020 [Internet]. Press Release. 2020 [cited 2020 Jun 20]. p. 1. Available from: http://www.stats.gov.cn/english/PressRelease/202005/t20200505_1742976.html .
- 4. National Bureau of Statistics of China. Households’ Income and Consumption Expenditure in the First Quarter of 2020 [Internet]. Press Release. 2020 [cited 2020 Jun 20]. p. 1. Available from: http://www.stats.gov.cn/english/PressRelease/202004/t20200420_1739771.html .
- 5. Bartik A, Bertrand M, Cullen Z, Glaeser EL, Luca M, Stanton C. How are Small Businesses Adjusting to COVID-19? Early Evidence from a Survey [Internet]. SSRN Electronic Journal. Chicago; 2020. (HKS Working Paper). Report No.: RWP20-012. Available from: https://papers.ssrn.com/sol3/papers.cfm?abstract_id=3574741 .
- View Article
- Google Scholar
- 9. Vos R, Martin W, Laborde D. How much will global poverty increase because of COVID-19? International Food Policy Research Institute IFPRI Blog [Internet]. 2020 Mar; Available from: https://www.foodsecurityportal.org/blog/how-much-will-global-poverty-increase-because-covid-19 .
- 10. American Psychological Association Task Force on Socioeconomic Status. Report of the APA task force on socioeconomic status. Washington, DC.; 2007.
- PubMed/NCBI
- 23. Bank World. World Development Report 2000 / 2001 Attacking Poverty [Internet]. Oxford University Press. New York; 2000. Available from: https://openknowledge.worldbank.org/handle/10986/11856 .
- 25. Gillespie S ed. AIDS, Poverty, and Hunger: Challenges and Responses: Highlights of the International Conference on HIV/AIDS and Food and Nutrition Security, Durban, South Africa, April 14–16, 2005. International Food Policy Research Institute. Washington, D.C.; 2006. 376 p.
- 30. Carpenter S, Walker B, Anderies JM, Abel N. From Metaphor to Measurement: Resilience of What to What? In: Ecosystems. 2001. p. 765–81.
- 33. Ciani F. A Resilience-Based Approach to Food Insecurity: The Impact of Mitch Hurricane on Rural Households in Nicaragua. … of Florence, Department of economics, PhD …. 2012;.
- 36. Berkes F, Folke C. Linking social and ecological systems for resilience and sustainability. In: Linking Social and Ecological Systems. New York: Cambridge University Press; 1998.
- 41. FAO. Resilient livelihoods- disaster risk reduction for food and nutrition security framework [Internet]. Rome; 2013. Available from: http://www.fao.org/3/i3270e/i3270e.pdf .
- 42. Jones L, Tanner T. Measuring “Subjective Resilience”: Using Peoples’ Perceptions to Quantify Household Resilience [Internet]. SSRN Electronic Journal. London; 2015. Report No.: 423. Available from: https://ssrn.com/abstract = 2643420.
- 45. Chaudhuri S, Jalan J, Suryahadi A. Assessing Household Vulnerability to Poverty from Cross-sectional Data: A Methodology and Estimates from Indonesia [Internet]. Department of Economics Discussion Papers. New York; 2002. (Discussion Paper Series). Report No.: 0102–52. Available from: https://academiccommons.columbia.edu/doi/10.7916/D85149GF .
- 47. Chaudhuri S. Assessing vulnerability to poverty: concepts, empirical methods and illustrative examples [Internet]. Department of Economics. New York; 2003. Available from: http://econdse.org/wp-content/uploads/2012/02/vulnerability-assessment.pdf .
- 48. Mansuri G, Healy A. Vulnerability prediction in rural Pakistan. IFPRI-World Bank Conference on Risk and Vulnerability: Estimation and Policy Implications, Washington, DC. 2002;.
- 49. Pritchett L, Suryahadi A, Sumarto S. Quantifying Vulnerability to Poverty: A Proposed Measure, with Application to Indonesia [Internet]. Policy Research Working Paper. Washington, DC.; 2000. (Working Papers). Report No.: 2437. Available from: https://elibrary.worldbank.org/doi/abs/10.1596/1813-9450-2437 .
- 50. Morduch J, Ogden T, Schneider R. An Invisible Finance Sector: How Households Use Financial Tools of Their Own Making [Internet]. U.S. Financial Diaries. New York; 2014. (Issue 3). Available from: www.usfinancialdiaries.org .
- 51. Andersen V, Austin S, Doucette J, Drazkowski A, Wood S. Addressing income volatility of low income populations. In: Workshop in Public Affairs [Internet]. University of Wisconsin–Madison: Robert M. La Follette School of Public Affairs; 2015. p. 1–37. Available from: https://lafollette.wisc.edu/images/publications/workshops/2015-income.pdf .
- 55. Baoxing. Baoxing County China [Internet]. Webpage. 2020. Available from: http://www.baoxing.gov.cn/en/about.html .
- 58. NBS China. National Bureau of Statistics of China Webiste [Internet]. 2020. Available from: http://www.stats.gov.cn/enGliSH/ .
- 63. King JE. Binary Logistic Regression. In: Osborne, J Best Practices in Quantitative Methods. CA: SAGE Publications, Inc.; 2008. p. 358–84.
- 67. DeVellis RF. Scale Development: Theory and Applications 3rd Edition. SAGE Open. Thousand Okas, CA: SAGE publication Ltd; 2003.
Thank you for visiting nature.com. You are using a browser version with limited support for CSS. To obtain the best experience, we recommend you use a more up to date browser (or turn off compatibility mode in Internet Explorer). In the meantime, to ensure continued support, we are displaying the site without styles and JavaScript.
- View all journals
- My Account Login
- Explore content
- About the journal
- Publish with us
- Sign up for alerts
- Open access
- Published: 29 November 2021
Effects of social economic status and parenting values on adolescents’ expected field of study
- Micha G. Keijer 1
Humanities and Social Sciences Communications volume 8 , Article number: 303 ( 2021 ) Cite this article
6752 Accesses
2 Citations
1 Altmetric
Metrics details
- Social policy
Field of study decisions are important for children’s future life chances, as significant differences exist in terms of financial and status benefits across fields of study. We examine whether the economic or the cultural status of the parents is more influential in shaping their children’s expectations about their future field of study. We also test whether children’s expectations about field of study choices are mediated by the child-rearing values that parents hold. Results show that parental economic status increased the likelihood of adolescents expecting to opt for extrinsic rewarding fields of study. Adolescent girls, not boys, with high cultural status parents were more likely to expect to opt for intrinsically rewarding fields of study. An upbringing that is characterized by conformity increased the expectations of boys to choose an extrinsically rewarding study, while self-direction increased the expectations of girls to opt for an extrinsic field of study
Similar content being viewed by others
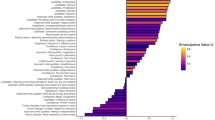
Worldwide divergence of values
Joshua Conrad Jackson & Danila Medvedev
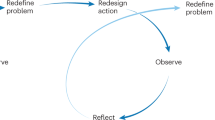
Participatory action research
Flora Cornish, Nancy Breton, … Darrin Hodgetts
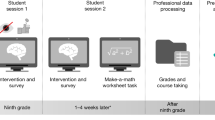
A national experiment reveals where a growth mindset improves achievement
David S. Yeager, Paul Hanselman, … Carol S. Dweck
Introduction
A key question in studies on occupational choices of young adults is to what extent and in what ways their socio-economic background influences their future life chances (Wicht and Ludwig-Mayerhofer, 2014 ; Howell et al., 1984 ; Blau and Duncan, 1967 ). As educational attainment is usually viewed as a prerequisite for occupational success, much research has focused on how parental socio-economic status (SES) influences educational attainment processes (Parsons and Halsey, 2014 ; Sewell, Haller and Portes, 1969 ). In these studies, attention has mostly focused on the influence of parental SES on the level of education that their children attain (Bornstein and Bradley, 2014 ; Fehrmann et al., 1987 ). However, partially in response to a general increase in the level of education, recent research pays increasing attention to how parental SES influences the field-of-study choices of children (Goyette, 2008 ; Goyette and Mullen, 2006 ; Van de Werfhorst and Luijkx, 2010 ). Field-of-study decisions are important for children’s future life chances, as significant differences exist in terms of financial and status benefits across fields of study. It is often assumed that children of parents who have a relatively low SES are more likely to view their field-of-study choices as ‘instrumental’ (Davies and Guppy, 1997 ), implying that the most important goal of studying is to gain extrinsic rewards, i.e., a well-paid job. In contrast, children of parents with a relatively high SES are assumed to place more value on intrinsic aspects of fields of study, such as their potential for self-development (Johnson et al., 2007 ; Johnson and Mortimer, 2011 ).
Given our limited knowledge of the influence of parental SES on adolescent field-of-study choices, this article will address three related research questions: (1) to what extent does parental SES influence the future field-of-study expectations of adolescents, (2) which aspect of parental SES (economic or cultural status) is more important in this respect, and (3) to what extent is this influence mediated by parents’ child-rearing values? In answering these questions, we want to contribute to the existing literature in four ways.
First, we focus on adolescents’ expectations about their future field of study rather than on their actual choices. We have two reasons for this. Adolescents’ expectations are found to be a good indicator of their subsequent actual life decisions (Rimkute et al., 2012 ). More important, though, is that young adults’ actual choices often are a mix of expectations and aspirations on the one hand and available opportunities on the other. Parental SES can influence these choices either through its influence on their children’s expectations and aspirations or through its influence on the opportunities that their children face. By studying the expectations of adolescents, we can ascertain to what extent parents influence the expectations of their children, irrespective of the ‘opportunity structure’ they face.
Second, we classify the different fields of study by the type of reward (extrinsic versus intrinsic), which is thought to be key to opting for specific types of study. Thus, the emphasis of humanities and social studies is on intrinsic rewards, with characteristics as furthering knowledge and interest (Bonvillian and Murphy, 2014 ). In these fields of study, studying in itself is often viewed as the goal and it is not directly related to future employment. This is in contrast to studies like business economics and technical/engineering studies, in which a specific set of skills and knowledge are taught that will make graduates more attractive on the labor market (Kerr, 1991 ), and offer much clearer future employment and salary expectations. These fields of study are therefore often mainly characterized as extrinsically rewarding.
Third, we examine whether the economic or the cultural status of the parents is more influential in shaping their children’s expectations about their future field of study (Bourdieu, 1986 ). Adolescents from a high economic status background might be particularly likely to opt for studies that are extrinsically rewarding, while adolescents from a high cultural status background might be more likely to opt for studies that are intrinsically rewarding.
Fourth, we test whether the influence of parental SES on children’s expectations about field-of-study choices is mediated by the parents’ child-rearing values and parenting styles. Many studies have shown that child-rearing values and parenting styles are SES-dependent (Baumrind, 1971 ; Kohn, 1963 ; Lareau, 2011 ). In addition, these styles in themselves may have consequences for the expectations that children develop concerning their field of study. In particular, we expect that a parenting style that focuses on conformity favors opting for extrinsically rewarding fields of study, whereas a parenting style that focuses on self-direction favors opting for intrinsically rewarding fields of study.
The research questions will be answered using data on more than 1500 Dutch adolescents aged 14–17, who completed a survey about their future plans, expectations and ambitions. In addition, one of the pupil’s parents was also asked about his or hers expectations and ambitions for this child. Furthermore, we used experts’ opinions to classify the extent to which specific fields of study could be classified as either extrinsically or intrinsically rewarding.
Theory and hypotheses
Ses and field of study.
In a highly individualistic society, it is sometimes assumed that fields-of-study choices are based primarily on preferences and abilities of the adolescents themselves (Beck, 2002 ; Giddens, 2013 ), rather than determined by parental background. Yet, empirical research has shown that strong similarities exist between the field of study of fathers and of their children (Van de Werfhorst et al., 2001). Van de Werfhorst and Luijkx ( 2010 ) also found an association between the father’s employment field and the study choice of his children.
One explanation for this link between SES and field of study is Lucas’ ( 2001 ) idea of ‘Effectively Maintained Inequality’ (EMI). EMI suggests that parents with higher SES try to secure ‘benefits’ for their children through the educational system. These ‘benefits’ can take two forms: quantitative (e.g., level of education) and qualitative (e.g., curriculum choices). As more and more young people continue to tertiary education, the ‘benefits’ effect from the level of education has become less important, so it has become more important to get the ‘benefit’ out of the chosen study. Assuming that good employment prospects are seen as a ‘benefit’, one can infer that parents with a high SES are more likely to direct their children to fields of study with better employment prospects (Ma, 2009 ), and thus stimulate them to opt for extrinsically rewarding fields of study.
An alternative argument leading to quite opposite expectations is provided by Breen and Goldthorpe’s ( 1997 ) ‘Relative Risk Aversion’ theory (RRA). RRA states that parents prefer their children’s future social status to be just as good as or better than their own, and that it is important to counter downward social mobility. RRA was initially used to explain status attainment, but it could also explain field-of-study choices. Assuming that parents with a low SES exhibit strong risk aversion behavior, it is expected that low SES parents will stimulate their children to choose fields of study with good employment prospects. Research into adolescents’ attitudes towards work shows that adolescents who have parents with a high SES better appreciate the intrinsic aspects of a job, such as self-fulfillment, than adolescents from low SES origins (Kohn and Schooler, 1969 ), while the latter are more appreciative of the extrinsic aspects of a job, such as a good salary and job security.
Empirical research seems to support RRA rather than EMI. Kelsall et al. ( 1972 ) concluded that adolescents from low socio-economic origins opt for studies with good employment prospects. Davies and Guppy ( 1997 ) also concluded that the field-of-study choice of ‘working-class students’ is more often seen as ‘instrumental’ and that these students are more inclined to choose technical or business economic studies. Technical studies often strongly appeal to the experience of lower-class fathers: think, for example, of fathers with physically demanding (un)skilled labor (Kelsall et al., 1972 ). Intellectual and esthetic skills are often less important to this group of students.
What both RRA and EMI have in common, is that they start from a one-dimensional interpretation of parental SES. In contrast, Bourdieu ( 1986 ) distinguishes between a cultural and an economic status dimension. He viewed society as hierarchically divided into economic and cultural status positions, each with its own norms, values and customs. The economic elite (managers, lawyers and CEOs) is distinguished by a focus on luxury and possessions, while the cultural elite is distinguished by emphasizing ‘good taste’ in art and literature. By internalizing parental norms and values, adolescents whose parents have a strong economic status might be particularly likely to opt for law and economics, because these studies lead to economic status and esteem, while adolescents with a strong cultural background might be more likely to opt for social and cultural studies because of the cultural status and appreciation of these studies.
Several hypotheses may be formulated on the basis of this literature. First, two competing hypotheses can be formulated about the effect of the economic status of the parents on the field-of-study choice of their children. The ideas of both Lucas and Bourdieu seem to suggest that, as parents have higher economic status, their children will be increasingly likely to opt for more extrinsically rewarding fields of study, while on the basis of the ‘Relative Risk Aversion’ theory one would expect that low SES background children would show a stronger preference for extrinsically rewarding fields of study. Therefore, we formulate two competing hypotheses:
H1a: The higher parents’ economic status, the stronger their children expect to opt for extrinsically rewarding fields of study.
H1b: The lower parents’ economic status, the stronger their children expect to opt for extrinsically rewarding fields of study.
In addition, Bourdieu’s ideas about cultural status suggest a hypothesis on the relationship between parental cultural status and their children’s expectations about future fields of study:
H2: The higher parents’ cultural status, the stronger their children expect to opt for intrinsically rewarding fields of study.
The mediating role of parental values and styles
At least three different strands of literature suggest that, in understanding the relationship between parents’ SES and their children’s expected field of study, child-rearing values and styles may play a key role. In his classic study, Class and Conformity, Kohn and Schooler ( 1969 ) argued that parents (fathers) transmit values that are appreciated in the workplace to their children. Middle-class parents often have jobs where intellectual stimulation and independent decision-making are important. These parents internalize ‘self-direction’ in their behavior, and this orientation, both intentionally and unintentionally, is transferred to their children. Lower-class parents, on the other hand, experience that conformity to rules and requirements is valued, and thus ‘conformity’ is internalized and passed on to their children.
Whereas Kohn stressed the importance of parental values, Baumrind ( 1971 ) stressed the importance of parenting styles. Her typology distinguishes between authoritative (supportive), authoritarian, and permissive (laissez-faire) parenting styles, each a blend of warmth, control and independence. The emphasis is usually on the difference between the first two (most common) styles. The authoritative parenting style advocates warmth and engagement, reasoning and the promotion of independence. Children from parents who practice authoritative parenting are generally happier, can cooperate better and have more confidence (Berk, 2000 ). Parents who use an authoritarian parenting style score low on warmth and engagement, high on a stringent, authoritarian control style and low on the promotion of the child’s independence. As authoritarian parents often make decisions for the child and do not explain the reasons for rules, their children are often uncertain and less independent (Nix et al., 1999 ).
Lareau ( 2011 ) suggested a similar link between socialization and SES. She suggests that “concerted cultivation” is the most common type of parenting among middle-class parents in the USA. These parents teach their children things that are not taught in school and stimulate critical thinking and participation in many out-of-school activities. An important advantage of this form of parenting is that children learn how to get along with adults and each other through organized activities. In addition, the child develops a ‘sense of entitlement’: his or her opinion counts and is taken into consideration. This is in contrast to socialization by poor and lower-class parents, which is often more directive. These parents are often less involved with after-school activities. Due to their lower level of education and lack of time, they are less able to support their children. Children participate less in organized activities and spend more of their free time with other children in the neighborhood. The children and parents often allow themselves to be led by the choices that institutions, such as the school, present to them. In addition, children learn how to get along with each other on the street, without the supervision of parents. The desired attitude with respect to adults and parents is that of obedience. Lareau terms this type of socialization “the accomplishment of natural growth”.
What these three strands of literature have in common is that they hypothesize a relationship between parental social class and the ways in which parents raise their children. Middle- and higher-class parents are thought to promote a cultural climate of ‘concerted cultivation’, exert a more authoritative parenting style, and encourage self-direction. In contrast, lower-class parents are thought to promote a climate of ‘accomplishment of natural growth’, exert an authoritarian parenting style, and encourage an obedient and conformist attitude towards authorities and adults.
Empirical studies confirm the relationship between Baumrind’s authoritarian parenting style and low SES (Bluestone and Tamis-LeMonda, 1999 ; Conger et al., 1994 ). McLoyd ( 1990 ) summarized the relationship by stating that poverty and economic disadvantage negatively affects parents’ ability to be supportive, consistent and engaged. As a consequence of economic stress, these parents often demand obedience and discipline while providing less explanation for their actions (McLoyd, 1990 ). The authoritative parenting style seems to generate more positive outcomes. Empirical research shows that these adolescents perform better in school (Dornbusch et al., 1987 ; Weiss and Schwarz, 1996 ), are more involved in school (activities) (Steinberg et al., 1992 ) and have a positive attitude toward school (Maccoby and Martin, 1983 ; Steinberg et al., 1992 ). These results are often attributed to encouraging independent problem-solving and learning to think critically, as opposed to the authoritarian parenting style, which may lead to passivity (Steinberg et al., 1992 ) and a lack of interest in school (Pulkkinen, 1982 ). The same was found for Lareau’s ideas about the link between SES and socialization. A socialization characterized as ‘concerted cultivation’ leads to better school performance and more involvement in school than an upbringing characterized by ‘accomplishment of natural growth’ (Bodovski and Farkas, 2008 ; Redford et al., 2009 ).
Remarkably, hardly any research has directly linked parenting values and styles to field-of-study choices. However, studies on adolescents’ motivation could offer insights into this link. Leung and Kwan ( 1998 ) found that authoritative parenting style was linked to the development of an intrinsic motivation among children, whereas an authoritarian parenting style was linked to the development of extrinsic motivation among children. It is therefore likely that authoritative parenting stimulates adolescents to develop a preference for more intrinsically rewarding fields of study, whereas authoritarian parenting stimulates the development of a preference for more extrinsically rewarding fields of study.
On the basis of these considerations three additional hypotheses are formulated:
H3: The more parents employ a conformity-oriented parenting style, the stronger their children expect to opt for extrinsically rewarding fields of study.
H4: The more parents employ a self-direction-oriented parenting style, the stronger their children expect to opt for intrinsically rewarding fields of study.
H5: The effects of parental economic and cultural status on their children’s expected field of study are mediated by parental values.
This final hypothesis concerns the indirect relationship between parents’ economic and cultural status and their children’s expected field-of study choice. A higher SES is expected to be related to a more self-direction-oriented parenting style, which in turn leads to more intrinsically rewarding expected study choices. Figure 1 summarizes the expected relationships between parental SES, parenting styles and children’s expectations about future field of study.
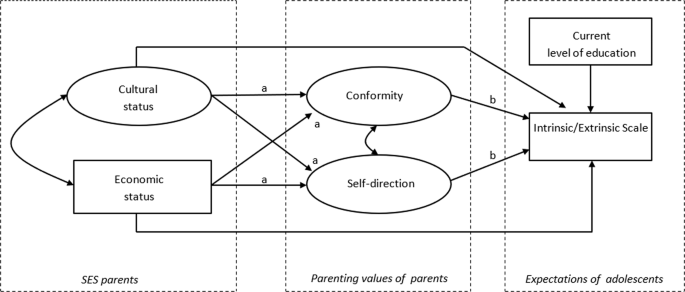
Note: ( a ) The direct effects of SES on parenting values, ( b ) direct effects of parenting values on adolescent expectations. The direct effects of SES on adolescent expectations are non-mediated effects.
Thus far, we have refrained from explicitly discussing gender, because we expect that the same basic processes apply to boys and girls. At the same time, different expectations may exist with regard to the field-of-study choices of boys and girls. In particular, parents stressing conformity may hold different field-of-study expectations for sons and daughters, as both genders are expected to conform to traditional ‘gendered’ ideas about future occupational involvement. Therefore, we will perform separate analyses for boys and girls.
Data were collected in 2005 (Ganzeboom et al., 2005 – 2006 ) as part of the project ‘Youth and Culture’. Data collection took place among students in secondary education in 14 municipalities located throughout the Netherlands. The selected municipalities included two major cities, eight medium-sized municipalities, and four small municipalities. Within these municipalities, 69 schools were contacted, of which 60 were willing to participate in the research. Dutch secondary education is highly stratified, with children having to make choices that influence whether they will be able to attend university at the age of 12 already. All levels of education of Dutch secondary education were represented in the sample (VMBO-B (lower vocational education), MAVO/VMBO-T (lower general secondary education), HAVO (higher general secondary education) and VWO (pre-university education) (Nagel, 2007 ). The inequality in the Netherlands is largely due to difference in the parental resources (cultural, economic and social) that matter so much at the age of 12 (Keijer et al., 2016 ). Within each school, a sample was drawn of three classes of different level-grade combinations within schools. This procedure resulted in 190 classes of which 148 actually participated. The response rate is 87% at the school-level and 78% at the class-level. In each class, students completed a questionnaire during a regular lesson (45–50 min). A total of 1544 students participated in this study. Because of the classroom nature of the survey, selective non-response can be assumed to be quite low.
In January 2006, parents received a postal questionnaire concerning their child’s future plans. In two-parent families, one randomly chosen parent was approached; in single-parent families, the child’s co-residing parent was approached. Eventually, this led to 1001 adolescent-parent pairs taking part in the research. Non-responding parents had a slightly lower level of education than responding parents.
Measurement instruments
Expected field of study.
Adolescents’ expectations regarding their future field of study were measured by asking: “If you are thinking about going into higher education, in which field of study do you think that will be?” A list of thirteen fields were offered: (1) teaching, (2) languages, history, theology, (3) agriculture, (4) mathematics, physics, (5) engineering, (6) transportation, (7) healthcare, (8) economics, (9) law, (10) socio-cultural education, (11) social care, (12) arts, and (13) public order and safety. They had to indicate the likelihood of each field of study on a 4-point scale (1 = definitely not, 2 = probably not, 3 = probably, and 4 = definitely). In Table 1 , the percentage of students stating that they will probably or definitely opt for a specific field is presented. Healthcare and socio-cultural education were the most popular fields among women, whereas economics and technical studies/engineering were the two most popular fields among men. Of all the surveyed fields of study, agriculture (rural studies) was the least popular among both boys and girls. The biggest difference in popularity between boys and girls was found for technical studies. Healthcare, social-cultural studies, and personal and social care studies also showed a significant difference in popularity between boys and girls. Girls chose the latter three studies more often.
To determine the extent to which these fields of study can be considered as intrinsically or extrinsically rewarding, evaluations from an expert panel were employed. The thirteen fields of study were presented to 52 student counselors working in secondary schools in the Netherlands. These student counselors give advise to students at all levels of secondary education. They were recruited through two student counselor associations, namely the Association of Careers Advisors (VvSL) and the Dutch Association of Careers- and Student Advisors, (NVS-NVL). They were asked to rate each of the thirteen fields of study on a 4-point scale (1 = primarily intrinsic, 2 = more intrinsic than extrinsic, 3 = more extrinsic than intrinsic, and 4 = primarily extrinsic), based on their assessment of why adolescents opt for each of these fields. Based on an inter-rater reliability analysis, the scores of three study advisors were found to differ strongly from all others and were removed from the data. The scores of the remaining 49 counselors had an average item-total correlation of 0.98. Subsequently, these responses were averaged per field of study and ranked from intrinsic to extrinsic (see Table 1 ). The study advisors viewed the fields of languages, history, theology and art as the most intrinsically rewarding, while they viewed law and economics studies as the most extrinsically rewarding.
To arrive at an overall score of the extent to which students expected to opt for extrinsically or intrinsically rewarding fields of study, three steps were taken. First, adolescents’ expectation scores for the thirteen fields of study were centered (recoding them from a 1 to 4 scale to a −1.5 to +1.5 scale). Next, the resulting score for each field was multiplied by the standardized motivation scores derived from the counselors’ assessments. And finally, these thirteen scores were averaged. The result was an overall score for each adolescent on a unidimensional scale indicating to what extent they expect to opt for fields of study that are extrinsically rewarding or fields of study that are intrinsically rewarding. Adolescents favouring extrinsically rewarding fields of study, like economics and law, score higher on this scale than adolescents favouring intrinsically rewarding fields of study, like the arts or teaching.
Parenting values
Parents were asked to respond to eleven statements about how they raise their child. Examples of these statements include, “I allow my child to solve his/her own problems” and “I teach my child to respect authority”. The response categories to the 11 statements ranged from 1 = not at all true, 2 = not true but not false, 3 = somewhat true, to 4 = completely true. These statements can be divided into Kohn’s child-rearing values of conformity and self-direction. A confirmatory factor analysis showed that two latent variables, termed ‘conformity’ and ‘self-direction’, could be distinguished. These factors were not orthogonal to each other, but positively correlated ( r = 0.33 for boys and r = 0.21 for girls). Table 2 presents the question wording of all items, with the factor loadings from the confirmatory factor analysis. The factor loadings are reasonably high, suggesting good measurement quality.
Parents’ socio-economic status (SES)
Two dimensions of parental SES were distinguished, reflecting the economic and cultural status of the parental home. Parental cultural status was measured as a latent variable based on the educational attainment of both parents. The educational level of both parents was measured on a scale running from 1 (no education) to 9 (university). Subsequently, the levels of education of both parents were recoded using the newly developed International Standard Level of Education (ISLED) scoring system (Schröder and Ganzeboom, 2014 ). ISLED is an empirically derived, internationally comparable interval scale for educational level, the value of which can vary between 0 and 100.
Parental economic status was measured by parents’ income. Information on income per parent was collected on a scale of 1 to 16, where 1 referred to ‘no income’ and 16 referred to more than € 5000 net income per month. This scale was converted to a ratio scale by assigning a parent the median income for their income class (e.g., € 1750 for those in the € 1500–2000 income class). Parents in the highest income category of € 5000 or more were given a score of € 5500. The joint income of both parents was then calculated by summation. Subsequently, family income was standardized through the application of equivalence factors (Siermann et al., 2004 ). As a result, the income of households of different sizes and compositions can be compared more effectively. As the survey was only submitted to one of the parents, it was assumed that the parent who filled in the questionnaire had a sufficient understanding of the education and income of his or her partner.
Educational level of the adolescent
The adolescent’s current level in secondary school is used as a control variable in all analyses. The answers were again recoded into ISLED codes, and ranged from 31.90 to 69.80 (Schröder and Ganzeboom, 2014 ).
Analysis strategy
Structural equations modeling (SEM) was used to estimate the relationships between parental SES, parents’ child-rearing values and adolescents’ expected field of study choices. SEM allows to simultaneously test the complete conceptual path model shown in Fig. 1 . To account for item non-response in the data, the models were estimated in Mplus using the method of ‘full information maximum likelihood’ (Muthén and Muthén, 2004 ), with a cluster correction applied at the class-level. The models were estimated on 1512 adolescent observations with a valid score on gender. Several models were estimated: the first model used the overall score on the intrinsic-extrinsic reward scale as the dependent variable (range from −1.91 to 2.82), whereas the second model used adolescents’ separate assessments of whether they expected to follow a study in the 13 different fields of study as dependent variables (range from 1 to 4). The models were estimated separately for boys and girls. The estimated SEM models included both manifest (represented by rectangles) and latent variables (represented by ovals), as depicted in Fig. 1 . In the models presented in Figs. 2 and 3 , we tested whether the unstandardized effects could be constrained to be equal for men and women. If so, this is indicated in the figures. However, because we report standardized effects rather than unstandardized effects, the standardized effects of these constrained parameters could still differ slightly from each other.
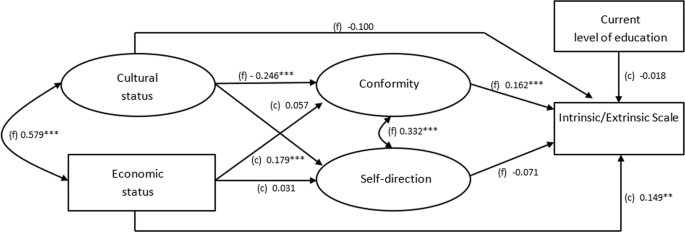
Note: ***p- value < 0.01 . **p -value < 0.05 . *p -value < 0.1 . (c) is a constraint path for male and female adolescents and (f) is non-constraint (free) path. Goodness of fit: RMSEA = 0.00, CFI = 1.00 and TLI = 1.00.
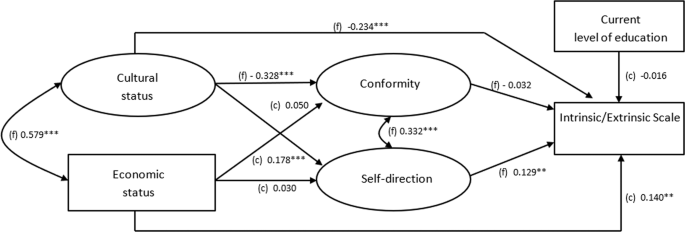
Note : ***p -value < 0.01 . **p -value < 0.05. *p -value < 0.1 . (c) is a constraint path for male and female adolescents and (f) is non-constraint (free) path. Goodness of fit: RMSEA = 0.00, CFI = 1.00 and TLI = 1.00.
Testing the overall model
First, we tested our hypotheses with our key measure of extrinsic/intrinsic fields of interest as the dependent variable. Direct and indirect effects are presented in Figs. 2 and 3 for men and women, respectively. Total effects for variables that have both direct and indirect effects are shown in Table 3 . To test our hypotheses on the total influence of parental SES, the total standardized effects of the cultural and economic status of the parents on the intrinsic-extrinsic scale were estimated. Hypothesis 1a stated that the higher the economic status of the parents, the more likely it would be that their children’s expect to opt for extrinsically rewarding fields of study, whereas hypothesis 1b suggested exactly the opposite. The results in Table 3 show that, in line with H1a, for both boys and girls the economic status of the parents had a positive effect on adolescents’ expectations to choose extrinsically rewarding studies. The second hypothesis postulated that children whose parents had a high cultural status would make more intrinsically rewarding expected field of study choices than children whose parents had a low cultural status. The generic analysis of the intrinsic/extrinsic scale in Table 3 supported this idea, as a negative effect of cultural status of the parents on the extent to which adolescents expect extrinsic field of study choices was estimated. However, the effect of parents’ cultural status on the intrinsic/extrinsic scale were weaker for male than for female adolescents (Wald-test, p -value = 0.039), suggesting that parents’ cultural status has a stronger effect on the field-of-study choices of girls than of boys.
Hypothesis 3 stated that as parents adopt a more conformity-oriented parenting style, their children will have stronger expectations to choose extrinsically rewarding studies. Figures 2 and 3 show that a focus on conformity in parenting was indeed correlated with extrinsic field of study choices for boys ( β = 0.162, p < 0.01), but that this was not true for girls ( β = − 0 . 032, ns). Hypothesis 4 focused on the impact of a self-direction-oriented parenting style on field-of-study preferences. It was expected that as parents have a more self-direction-oriented parenting style, their children will make more intrinsically rewarding and less extrinsically rewarding field-of-study choices. For boys, this effect is not found ( β = − 0 . 071, ns). For girls, Fig. 3 even demonstrates that, contrary to the hypothesis, self-direction correlated positively with the expectation to opt for extrinsic fields of study ( β = 0 . 129, p < 0.05).
The fifth hypothesis stated that the effects of economic and cultural status on expected field of study were mediated by self-direction and conformity (Fig. 1 (a×b)). Before addressing the hypothesis, we will briefly discuss the direct effects of parental economic and cultural status on child-rearing values (Fig. 1 (b)). Figures 2 and 3 show that parents’ cultural status was negatively related to conformity, with the effect for girls ( β = −0 . 328, p < 0.01) being larger than that for boys ( β = −0 . 246, p < 0.01). The higher the cultural status of parents, the less emphasis was placed on conformity requirements and rules. In addition, for both boys ( β = 0 . 179, p < 0.01) and girls ( β = 0.178, p < 0.01), there was a positive relationship between parents’ cultural status and self-direction. Parents with a higher cultural status put more emphasis on self-direction in socialization than parents with a low cultural status. Parental economic status had no relationship with child-rearing values. With regard to the degree of mediation of the effect of economic and cultural status by child-rearing values, it turned out that, although there are significant relationships between parents’ cultural status and their child-rearing values (Fig. 1 (b) ), and between child-rearing values and whether fields of study were classified as extrinsic or intrinsic (Fig. 1 (a) ), the indirect effects of economic and cultural status through conformity and self-direction were not statistically significant (Fig. 1 (a×b) ). Therefore, child-rearing values did not mediate the effect of parental status on the expected field-of-study choices of their children. Hypothesis 5 was rejected.
Analyses by field of study
We also performed the same SEM analyses for each of the 13 fields-of-study separately. Direct effects for all independent variables are presented in Table 4 for women and in Table 5 for men. Fields of study are ordered from intrinsic to extrinsic, based on the expert assessments of study advisors.
For girls, results were in line with expectations. Although the results were much less clear-cut than for overall model, the patterns were very comparable. The economic status of parents correlated positively with preferences for extrinsically rewarding studies in economics and law whereas negative effects were observed for several more intrinsically rewarding fields of study, like art, teaching and nursing. For other studies there was no clear relationship between parents’ economic status and their children’s expected prospective field of study choices. Parents’ cultural status was positively correlated with a preference for socio-cultural and arts education among girls. Furthermore, girls with parents with a high cultural status had a weaker preference for extrinsically rewarding fields of study like economics or law. With regard to child-rearing values, conformity was positively correlated with preferences for teacher training and healthcare, relatively intrinsically rewarding studies. However, a more conformist upbringing correlates negatively with studies in languages, history, theology, economics, and personal and social care. Overall, these results imply that the hypothesis that more conformity leads adolescents to choose extrinsically motivated studies has to be rejected for girls. In addition, there were significant positive correlations between self-direction and the expectation to choose transportation, law, public order and safety, mathematics and physics and socio-cultural training, which are predominantly intrinsically rewarding fields-of-study. These field-of-study specific results confirm our general finding that a more self-direction-oriented parenting style leads girls to opt for more extrinsically rewarding fields-of-study.
For boys, the individual analysis of the thirteen fields of study, summarized in Table 5 , showed that the economic status of parents correlated positively with preferences for studies in economics and law. Furthermore, several expectations to opt for more intrinsically rewarding fields of study correlated negatively with parents’ economic status, which was also in line with hypothesis 1a. Teacher training was less attractive when the parents’ economic status was higher, and the same was true for mathematics and physics. However, parents’ economic status was negatively correlated with the expectation to choose technical studies. In addition, boys’ expectations for socio-cultural education correlated positively with the economic status of the parents. Parents’ cultural status was only positively correlated with a preference for mathematics and physics, which confirms the general finding that parents’ cultural status only had a limited effect on boys’ preferences for intrinsically rewarding fields of study. Conformity had a significant positive effect on boys’ preferences for studies in the fields of healthcare, law, and public order and safety, the latter two being extrinsically rewarding fields of study. However, no relation was found between conformity and other relatively extrinsically rewarding studies, like economics, studies in transport and technical studies. There were no positive correlations between self-direction and intrinsically motivated fields of study. Self-direction during upbringing for boys was even negatively correlated with the expectation to continue one’s study in the fields of language, history, and theology. However, the negative effect of self-direction on the expectation to choose extrinsically rewarding fields of study like law and public order and safety can be considered in line with expectations.
This study examined to what extent adolescents’ expectations about their future field-of-study choices are influenced by their parents’ socio-economic status and child-rearing values. We were particularly interested in whether adolescents expected to opt for study fields that reap extrinsic rewards like money or for study fields that are more intrinsically rewarding. Following Bourdieu ( 1986 ), two dimensions of parents’ socio-economic status were distinguished, cultural and economic status. With regard to economic status, two contrasting hypotheses were formulated. On the one hand, one could expect children whose parents have a low economic status to opt for extrinsically rewarding study fields—as this would increase their financial security—whereas one could also argue that it is mainly children of parents with a high economic status that opt for such studies—to retain their strong economic position. The empirical findings clearly indicated that parental economic status increased rather than decreased the likelihood of their children expecting to opt for extrinsic fields of study. For instance, economics and law were positively correlated with parents’ economic status, for both boys and girls. In the Netherlands, income-dependent subsidies make the costs of pursuing an education at schools and universities reasonably homogeneous across socio-economic strata. In addition, these costs are relatively low compared to Anglo-Saxon countries. Therefore, we interpret the effect of parents’ economic status in the Dutch context not so much as a sign that high parental income is needed to pursue extrinsically rewarding fields of study, but rather as signifying that parents who have a high economic status transmit their preferences for fields of study and jobs that permit the acquisition of such high economic status to their children. We also observe, like Davies and Guppy ( 1997 ), that the expectation to choose a technical study is negatively correlated with parents’ economic status. Technical studies, adjusted to the adolescent’s educational level, may be more interesting to boys from a lower socio-economic background, because these studies reflect the perceptions and values of the profession of their working-class fathers.
Our hypothesis that children of parents with high cultural status were more likely to expect to opt for intrinsically rewarding study fields than children of parents with low cultural status was supported for girls, but not for boys. Thus, in general parental economic status correlated positively with extrinsic field-of-study expectations, and parental cultural status correlated negatively with extrinsic expectations. This was also reflected in the results with regard to specific study fields. For boys, mathematics, physics and teaching are positively correlated with cultural status, but negatively with economic status. These more intrinsically rewarding fields of study are therefore mainly chosen by adolescents from households that are higher on the cultural status dimension than on the economic. The same process is evident in socio-cultural studies and the arts for girls; these fields of study are particularly popular among girls whose parents are higher on cultural status than on economic status. More extrinsically rewarding fields of study, like economics and law, show the opposite pattern: the expectation to opt for this type of study is positively correlated with parents’ economic status, and negatively with their cultural status. Thus, these results largely support Bourdieu’s ideas about a two-dimensional status hierarchy.
Parents’ parenting values, in particular how much emphasis they put on conformity and self-direction, were thought to be influenced by the parents’ socio-economic status and, in their turn, to influence children’s expectations about their future field of study. We found a strong negative influence of parents’ cultural status on conformist parenting values, while cultural status had a positive effect on self-directed parenting values. Parents’ economic status, on the other hand, had no effect on parenting values. Thus, the ways in which parents rear their children—emphasizing conformity or self-direction—is affected by their cultural status, rather than by their economic status. This does not imply that economic status is unimportant in socializing children. Rather, it could be that economic status influences more what children value than how they should behave—cf. Rokeach’ ( 1973 ) distinction between terminal and instrumental values.
Hypotheses 3 and 4 postulated that parental child-rearing values influence the expected field-of-study choices of their children. In line with hypothesis 3, an upbringing characterized by conformity increased the likelihood that boys expect to choose an extrinsically rewarding study. If we consider the separate fields of study, it appeared that conformity has a positive effect on two extrinsic fields of study for boys, namely law and public order and safety. For girls, no relation was found between conformity and the degree of extrinsic motivation of the studies they prefer. Rather, the results seemed to suggest that—in choosing for a future field of education—girls whose parents put a high value on conformity are likely to be influenced by gender-stereotypic motives. The positive correlation among girls between conformity and expectations to opt for the educational fields of teaching and healthcare seems to fit traditional educational and occupational gender stereotypes. Gender-stereotyping is only part of the explanation though: there was no relation between conformity and two other typical female fields of study, socio-cultural education and personal and social care studies, and a negative one with languages, history and theology. Boys’ field-of-study choices could also partially be regarded as reflecting gender stereotypes. The breadwinner motive suggests that their choices for a ‘clearly defined’ gender-stereotypical field of study lead to a stable/good income (lawyer, soldier/police or doctor, though not technical studies).
Parents’ emphasis on self-direction values only influenced the expected field-of-study choices of their daughters, but in the opposite direction as expected in Hypothesis 4. A stronger influence on self-direction increased the expectation of daughters that they would opt for an extrinsically rewarding field of study, and in particular for the fields of mathematics and physics, transportation, public order and safety, and law. This finding may again relate to the idea of gender-stereotypical field of study choices. Girls’ choices for such study fields as mathematics, physics and transportation suggest a break with gender-specific patterns. An emphasis of parents on self-direction could strengthen general feelings of independence in their daughters, and this could explain both the choice for a non-gender-specific field of study and for a field of study like law that could be viewed as a means to achieve this goal of independence.
Our final hypothesis postulated that the effect of parents’ economic and cultural status on their children’s expected field-of-study choices was mediated by self-direction and conformity. However, we found no support for this mediating role of parenting values, despite the significant relationships between the two dimensions of social status and parental values, and the significant relationships between parental values and expected field-of-study choices. Thus, it seems likely that other mechanisms are needed to explain the influence of parental socio-economic status on their children’s expected field-of-study choices. One potential explanation could be that it is not just parents’ position on the general cultural and economic status dimensions that influences their children’s field-of-study choices, but that the actual educational and occupational fields in which parents were or are active is more directly transmitted, e.g., with children from parents who work in socio-cultural occupations developing a preference for studies and occupations in this same field as well. Thus, one promising avenue for future research could be to examine to what extent parents transmit specific preferences for fields of study and occupation. In addition, parents’ socio-economic status may influence the peer networks in which adolescents become engaged, and these may influence the future life plans of these adolescents.
A few additional issues for future research could be mentioned as well. First, given that the two dimensions of social status have a relatively weak relationship with conformity, it is important to examine which other aspects of social background influence this parenting value. For instance, parents’ religiosity may be important in this respect. Second, in our questionnaire, we used expert opinions to categorize fields of study as extrinsic or intrinsic. However, it would also be interesting to ask directly for adolescents’ motivations for their field-of-study expectations. Finally, the cross-sectional nature of our study did not allow us to study how realistic adolescents’ expectations were. Using panel data, it would be interesting to examine to what extent adolescents’ expectations have been realized, and whether the same processes influence both their expectations and their actual behavior.
Data availability
The data that support the findings of this study are available from the corresponding author upon reasonable request.
Baumrind D (1971) Current patterns of parental authority. Dev Psychol 4(p2):1–103
Article Google Scholar
Beck U (2002) Individualization: institutionalized individualism and its social and political consequences. Sage Publications Ltd, London
Book Google Scholar
Berk LJ (2000) Child development, 5th edn. Allyn and Bacon, Boston
Google Scholar
Blau PM, Duncan OD (1967) The American occupational structure. Am Soc Rev 21:290–295
Bluestone C, Tamis-LeMonda CS (1999) “Correlates of parenting styles in predominantly working-and middle-class African American mothers”. J Marriage Fam 61:881–893
Bodovski K, Farkas G (2008) “Concerted cultivation” and unequal achievement in elementary school”. Soc Sci Res 37:903–919
Bonvillian G, Murphy R (2014) The liberal arts college adapting to change: The survival of small schools. Routledge, New York, NY
Bornstein MH, Bradley RH (2014) Socioeconomic status, parenting, and child development. Routledge, New York, NY
Bourdieu P (1986) The Forms of Capital. In: Richardson JohnG ed The handbook for theory and research for the sociology of education. Greenwood Publishing Group, Westport, CT, pp. 241–258
Breen R, Goldthorpe JH (1997) “Explaining educational differentials”. Ration Soc 9:275
Conger RD, Ge X, Elder GH, Lorenz FO, Simons RL (1994) Economic stress, coercive family process, and developmental problems of adolescents. Child Dev 65:541–561
Article CAS PubMed Google Scholar
Davies S, Guppy N (1997) Fields of study, college selectivity, and student inequalities in higher education. Soc Force 75:1417–1438
Dornbusch SM, Ritter PL, Leiderman PH, Roberts DF, Fraleigh MJ (1987) The relation of parenting style to adolescent school performance. Child Dev 58:1244–1257
Fehrmann PG, Keith TZ, Reimers TM (1987) Home influence on school learning: Direct and indirect effects of parental involvement on high school grades. J Educ Res 80:330–337
Ganzeboom H, Nagel I, Liefbroer AC (2005) [p.i.]. Youth and culture. a multi-actor panel study; cultural participation and future plans. [machine readable datafiles]. Vrije Universiteit [producer] To be archived, Amsterdam, 2006
Giddens A (2013) Modernity and self-identity: Self and society in the late modern age. John Wiley and Sons, London
Goyette KA (2008) College for some to college for all: Social background, occupational expectations, and educational expectations over time. Soc Sci Res 37:461–484
Article PubMed Google Scholar
Goyette KA, Mullen AL (2006) Who studies the arts and sciences? Social background and the choice and consequences of undergraduate field of study. J High Educ 77:497–538
Howell FM, Frese W, Sollie CR (1984) The measurement of perceived opportunity for occupational attainment. J Vocation Behav 25:325–343
Johnson MK, Mortimer JT (2011) Origins and outcomes of judgments about work. Soc Force 89:1239–1260
Johnson MK, Mortimer JT, Lee JC, Stern MJ (2007) Judgments About Work Dimensionality Revisited. Work Occup 34:290–317
Keijer MG, Nagel I, Liefbroer AC (2016) ‘Effects of parental cultural and economic status on adolescents’ life course preferences. European Sociol Rev 32:607–618
Kelsall RK, Poole A, Kuhn A (1972) Graduates: the sociology of an elite. Methuen, London
Kerr C (1991) The great transformation in higher education, 1960-1980. Suny Press, New York, NY
Kohn ML (1963) Social class and parent-child relationships: An interpretation. Am J Sociol 68:471–480
Kohn ML, Schooler C (1969) Class, occupation, and orientation. Am Sociol Rev 34:659–678
Lareau A (2011) Unequal childhoods: Class, race, and family life. University of California Press, Berkeley
Leung PW, Kwan KS (1998) Parenting styles, motivational orientations, and self-perceived academic competence: A mediational model. Merrill-Palmer Quarterly, 1–19
Lucas SR (2001) Effectively maintained inequality: education transitions, track mobility, and social background effects. Am J Sociol 106:1642–1690
Ma Y (2009) Family socioeconomic status, parental involvement, and college major choices—Gender, race/ethnic, and nativity patterns. Sociol Perspect 52:211–234
Maccoby EE, Martin JA (1983) Socialization in the context of the family: Parent-child interaction. Handbook of child psychology. John Wiley and Sons, New York, NY
McLoyd VC (1990) The impact of economic hardship on black families and children: psychological distress, parenting, and socio-emotional development. Child Dev 61:311–346
Muthén LK, Muthén BO (2004) Mplus user’s guide. 3rd. Muthén and Muthén, Los Angeles
Nagel I (2007) Cultuurparticipatie tussen 14 en 24 jaar: intergenerationele overdracht versus culturele mobiliteit. In: Liefbroer AC, Dykstra PA (ed.) Van generatie op generatie. Gelijkenis tussen ouders en kinderen. Amsterdam University Press, Amsterdam
Nix RL, Pinderhughes EE, Dodge KA, Bates JE, Pettit GS, McFadyen‐Ketchum SA (1999) The relation between mothers’ hostile attribution tendencies and children’s externalizing behavior problems: The mediating role of mothers’ harsh discipline practices. Child Dev 70:896–909
Parsons T, Halsey AH (2014) The school class as a social system. Schools and society, a sociological approach to education. Sage Publications Ltd, Los Angeles
Pulkkinen L (1982) Self-control and continuity from childhood to late adolescence. Life-span Dev Behav 4:63–105
Redford J, Johnson JA, Honnold J (2009) Parenting practices, cultural capital and educational outcomes: the effects of concerted cultivation on academic achievement. Race Gender Class 16:25–44
Rimkute L, Hirvonen R, Tolvanen A, Aunola K, Nurmi JE (2012) Parents’ role in adolescents’ educational expectations. Scand J Educ Res 56:571–590
Rokeach M (1973) The nature of human values. The Free Press, New York, NY
Schröder H, Ganzeboom HBG (2014) Measuring and modelling level of education in european societies. Eur Sociol Rev 30:119–136
Sewell WH, Haller AO, Portes A (1969) The educational and early occupational attainment process. Am Sociol Rev 34:82–92
Siermann C, Teeffelen P, Urlings L (2004) Equivalentiefactoren 1995-2000. Centraal Bureau voor de Statistiek, Voorburg/Heerlen
Steinberg L, Lamborn SD, Dornbusch SM, Darling N (1992) Impact of parenting practices on adolescent achievement: Authoritative parenting, school involvement, and encouragement to succeed. Child Dev 63:1266–1281
Van de Werfhorst HG, Luijkx R (2010) Educational field of study and social mobility: Disaggregating social origin and education. Sociology 44:695–715
Weiss LH, Schwarz JC (1996) The relationship between parenting types and older adolescents’ personality, academic achievement, adjustment, and substance use. Child Dev 67:2101–2114
Wicht A, Ludwig-Mayerhofer W (2014) The impact of neighborhoods and schools on young people’s occupational aspirations. J Vocation Behav 85:298–308
Download references
Acknowledgements
Work on this article has been funded by a grant to the project “The Influence of Family and School on Future Life Plans of Adolescents” from the Netherlands Organisation for Scientific Research (NWO) (projectnr 400Y09Y116).
Author information
Authors and affiliations.
Faculty of Business and Economics, Amsterdam University of Applied Sciences, Amsterdam, The Netherlands
Micha G. Keijer
You can also search for this author in PubMed Google Scholar
Corresponding author
Correspondence to Micha G. Keijer .
Ethics declarations
Competing interests.
The author declares no competing interests.
Ethical approval
The project design was approved by Netherlands Organisation for Scientific Research. As the project does not involve human biological material, approval by the Research Ethics Committee is not applicable.
Informed consent
Data collection for this study was approved by Netherlands Organisation for Scientific Research. None of the individuals are recognizable from the data and the data is the declared at Data Archiving and Networked Services (DANS).
Additional information
Publisher’s note Springer Nature remains neutral with regard to jurisdictional claims in published maps and institutional affiliations.
Rights and permissions
Open Access This article is licensed under a Creative Commons Attribution 4.0 International License, which permits use, sharing, adaptation, distribution and reproduction in any medium or format, as long as you give appropriate credit to the original author(s) and the source, provide a link to the Creative Commons license, and indicate if changes were made. The images or other third party material in this article are included in the article’s Creative Commons license, unless indicated otherwise in a credit line to the material. If material is not included in the article’s Creative Commons license and your intended use is not permitted by statutory regulation or exceeds the permitted use, you will need to obtain permission directly from the copyright holder. To view a copy of this license, visit http://creativecommons.org/licenses/by/4.0/ .
Reprints and permissions
About this article
Cite this article.
Keijer, M.G. Effects of social economic status and parenting values on adolescents’ expected field of study. Humanit Soc Sci Commun 8 , 303 (2021). https://doi.org/10.1057/s41599-021-00992-7
Download citation
Received : 25 January 2021
Accepted : 11 November 2021
Published : 29 November 2021
DOI : https://doi.org/10.1057/s41599-021-00992-7
Share this article
Anyone you share the following link with will be able to read this content:
Sorry, a shareable link is not currently available for this article.
Provided by the Springer Nature SharedIt content-sharing initiative
Quick links
- Explore articles by subject
- Guide to authors
- Editorial policies

- Search Search Please fill out this field.
What Is Social Economics?
Understanding social economics.
- Different Perspectives
- Monetary/Fiscal Policy
- The Gender Wage Gap
The Bottom Line
What is social economics, and how does it impact society.
:max_bytes(150000):strip_icc():format(webp)/picture-53886-1440626964-5bfc2a89c9e77c005876da24.jpg)
Social economics is a social science and a branch of economics that focuses on the relationship between social behavior and economics. Social economics is also referred to as socioeconomics.
Social economics is primarily concerned with the interplay between social processes and economic activity within a society. Social economics may attempt to explain how a particular social group or socioeconomic class behaves within a society, including their actions as consumers.
Key Takeaways
- Social economics is a social science and branch of economics that focuses on the relationship between social behavior and economics.
- Social economists may borrow the tools of other social sciences such as psychology or sociology.
- The theories of social economics often consider factors that are outside the focus of mainstream economics, including the effect of the environment and ecology on consumption and wealth.
- Social economics may attempt to explain how a particular social group or socioeconomic class behaves within a society, including their actions as consumers.
- Social economists may examine the factors and decisions that relate to a person's educational level, health outcomes, or involvement in crime or violence.
Theories of social economics sometimes diverge from conventional economic theories. They may consider factors that are outside the focus of mainstream economics, including the effect of the environment and ecology on consumption and wealth.
Social economists frequently study the different economic choices by people in different socioeconomic classes. A socioeconomic class is a group of people with similar characteristics. These characteristics can include social and economic standing, level of education, current profession, and ethnic background or heritage.
Different socioeconomic classes may have different priorities regarding how they direct their funds, or they may be unable to afford certain goods or services due to their income limitations. These goods or services can include access to more advanced or complete medical care, educational opportunities , and the ability to buy food that meets specific nutritional guidelines.
Two Perspectives of Social Economics
There are two broad perspectives in social economics. Though opposite in their approach, they can be thought of as complementary. The first, pioneered by Nobelist Gary Becker, applies the basic theoretical and applied tools of neoclassical microeconomics to areas of human behavior that are not traditionally considered part of the economic sciences, such as crime and punishment, drug abuse, marriage, and family decisions.
The second perspective applies the ideas of other social sciences, such as sociology, psychology, and identity group studies to subjects of an economic nature like consumer behavior or labor markets . These practitioners of social economics use history, current events, politics, and other social sciences to predict social trends that could potentially impact the economy. This strand of social economics is the primary focus of this article.
In addition to income, socioeconomic status is also related to factors like education, family structure, occupation, and membership of certain ethnic minority groups.
Impact of Social Economics
According to the American Psychological Association, socioeconomic factors may reveal inequalities surrounding wealth, education, or status. These background factors may play a role in a person's life decisions, such as their career, or family choices.
Some of these socioeconomic relationships are explained below:
There are strong correlations between socioeconomic status and family structure, as well as other outcomes such as parenting practices and childhood development. Single-parent households are more likely to be low-income, and low-income households are more likely to suffer from domestic violence and child neglect. These disparities also manifest in worse health outcomes later in life.
Children with lower socioeconomic status tend to enter school with lower levels of reading and linguistic development, as well as lower social skills. In later years, these deficiencies may manifest themselves in lower levels of academic success and, ultimately, in lower incomes when they reach adulthood.
Ethnic Minority Status
There are strong correlations between socioeconomic status and membership in indigenous or other ethnic minorities, particularly those that have historically suffered from discrimination. Membership of some ethnic minority groups may correlate to higher levels of unemployment or substance abuse, as well as poorer mental and physical health.
Although interpersonal violence can occur at any socioeconomic level, people from lower socioeconomic backgrounds tend to have greater exposure to violence, especially in their formative years. Exposure to violence can negatively affect a person's academic and employment outcomes, and domestic violence is also a major cause of homelessness.
Example of Social Economics
An individual’s socioeconomic status can significantly impact their educational attainment and financial security. An individual from an affluent social class will likely have a greater opportunity to achieve higher education and may be expected to pursue such a goal, while those from low-income families generally do not have the same opportunities available to them.
For example, low-income families may not be able to pay for their children's participation in team sports, music lessons, or private tutoring, which can propel them toward achieving a prosperous future (as well as provide them with growing confidence to take on more challenges). Also, these children may attend overcrowded schools where education is underfunded or understaffed.
Completing higher education is more likely to increase their income potential, as well as provide opportunities to interact with people of similar or more advanced social standing and build beneficial social networks.
In contrast, higher education may not be attainable for an individual existing at or below the poverty level . Many studies have revealed that young children from families existing at or below the poverty level develop academic skills slower than children from affluent social classes. A lower socioeconomic status is also related to poor cognitive development, language, memory, socioemotional processing, and consequently poor income and health in adulthood.
What's more, school systems in communities that are primarily populated by those at or below the poverty level are often underresourced, which negatively impacts students’ academic progress and outcomes. Poor academic skills and progress, combined with high dropout rates, can impact children’s academic achievement in the long term.
Social Economics and Monetary/Fiscal Policy
The government has a substantial impact on social economics through its monetary and fiscal policies. Fiscal and monetary policies directly impact social welfare by influencing employment levels, income distribution, and access to essential services. The policies set forth by governments naturally aim to improve social welfare, thereby being tied to social economics.
Monetary and fiscal policies can play a significant role in tackling inequality by redistributing resources as well. For instance, progressive taxation and targeted social spending are fiscal measures aimed at reducing income inequality. Another example is monetary policies that prioritize full employment which can narrow income inequality.
Though these policies may not be specifically targeted at certain demographics, there may be indirect benefits had by different social economic classes. Consider how larger families are economically impacted differently due to refundable tax credits. In this case, government policy is striving to promote certain economic activity by specific demographics.
Social Economics and Gender Wage Gap
The gender pay gap is closely related to social economics. The gender pay gap reflects underlying structural inequalities in the labor market which perpetuates unequal access to opportunities and resources.
Social economics can relate to how gender norms and stereotypes influence labor markets. For example, social economics relates to hiring practices, promotion opportunities, and wage setting. Women may be pegged for specific roles as opposed to different roles historically occupied by men. Certain bias may also influence their performance evaluation.
Gender disparities in earnings contribute to broader income inequality within society, thus perpetuating economic cycles and trends. This is further driven when considering other demographics as well. Research by the Pew Research Center revealed Black and Hispanic women earned 70% or 65% as much as White men, respectively. Therefore, there is a cyclical process where individuals have economic tendencies that perpetuate future economic tendencies.
What Are Examples of Socioeconomic Factors?
In addition to income, socioeconomic status also includes other factors such as educational levels, financial security, environmental factors, and subjective perceptions of social status and class.
What Is Socioeconomic Well Being?
Socioeconomic well-being means the mix of social and economic factors that produce the best outcomes for a person's health and well-being. Although a high income is not strictly necessary, a sufficient level of financial security is a significant factor in mental and physical health as well as educational outcomes.
How Do You Calculate Socioeconomic Status?
There are three key measures for determining someone's socioeconomic status, according to the National Committee on Vital and Health Statistics: income, education, and occupation. In addition, the American Psychological Association also recommends considerations of family size, since the number of earners and dependents in a household has a strong impact on the family's financial security. One may also consider subjective factors, such as occupational prestige or a person's subjective perceptions of social class.
What Income Is Considered Low Socioeconomic Status?
Although income is not the only element in someone's sociological status, it is an important measure of a person's ability to obtain better health and educational outcomes. In the United States, the Department of Housing and Urban Development (HUD) gauges income levels as a percentage of the Area Median Income (AMI). A family earning less than 80% of the AMI is considered low-income, and a family earning less than 50% of the AMI is considered Very Low Income. These categories are used to allocate housing vouchers and other assistance.
Social economics is a branch of economics that examines at the relationship between social factors and economics. Frequently, social economists study the economic choices of different socioeconomic groups. These can be defined by a variety of characteristics, including by social or economic standing, level of education, current profession, and ethnic background or heritage. Such factors can significantly impact one's educational attainment and financial security. As a field of study, social economics looks at the interplay between social processes and economic activity, and can reveal broader inequalities in society.
American Psychological Association. " Socioeconomic Status ."
Becker, Gary S. "Nobel Lecture: The Economic Way of Looking at Behavior." Journal of Political Economy , vol. 101, no. 3, June 1993, pp. 385–409.
American Psychological Association. " Children, Youth, Families, and Socioeconomic Status ."
American Psychological Association. " Education and Socioeconomic Status ."
American Psychological Association. " Ethnic and Racial Minorities and Socioeconomic Status ."
American Psychological Association. " Violence and Socioeconomic Status ."
Pew Research Center. " The Enduring Grip of the Gender Pay Gap ."
American Psychological Association. " Measuring Socioeconomic Status and Subjective Social Status ."
NYC.gov. " Area Median Income ."
- Economics Defined with Types, Indicators, and Systems 1 of 33
- Economy: What It Is, Types of Economies, Economic Indicators 2 of 33
- A Brief History of Economics 3 of 33
- Is Economics a Science? 4 of 33
- Finance vs. Economics: What's the Difference? 5 of 33
- Macroeconomics Definition, History, and Schools of Thought 6 of 33
- Microeconomics Definition, Uses, and Concepts 7 of 33
- 4 Economic Concepts Consumers Need To Know 8 of 33
- Law of Supply and Demand in Economics: How It Works 9 of 33
- Demand-Side Economics Definition, Examples of Policies 10 of 33
- Supply-Side Theory: Definition and Comparison to Demand-Side 11 of 33
- What Is a Market Economy and How Does It Work? 12 of 33
- Command Economy: Definition, How It Works, and Characteristics 13 of 33
- Economic Value: Definition, Examples, Ways To Estimate 14 of 33
- Keynesian Economics Theory: Definition and How It's Used 15 of 33
- What Is Social Economics, and How Does It Impact Society? 16 of 33
- Economic Indicator: Definition and How to Interpret 17 of 33
- Top 10 U.S. Economic Indicators 18 of 33
- Gross Domestic Product (GDP) Formula and How to Use It 19 of 33
- What Is GDP and Why Is It So Important to Economists and Investors? 20 of 33
- Consumer Spending: Definition, Measurement, and Importance 21 of 33
- Retail Sales: Definition, Measurement, and Use As an Economic Indicator 22 of 33
- Job Market: Definition, Measurement, Example 23 of 33
- The Top 25 Economies in the World 24 of 33
- What Are Some Examples of Free Market Economies? 25 of 33
- Is the United States a Market Economy or a Mixed Economy? 26 of 33
- Primary Drivers of the Chinese Economy 27 of 33
- Japan Inc.: What It is, How It Works, History 28 of 33
- The Fundamentals of How India Makes Its Money 29 of 33
- European Union (EU): What It Is, Countries, History, Purpose 30 of 33
- The German Economic Miracle Post WWII 31 of 33
- The Economy of the United Kingdom 32 of 33
- How the North Korean Economy Works 33 of 33
:max_bytes(150000):strip_icc():format(webp)/RacialWealthGap-4b3536e8fe294279a6100161109d735f.jpeg)
- Terms of Service
- Editorial Policy
- Privacy Policy
- Your Privacy Choices
Road construction and its socio-economic and health impact: a case study of Atonsu lake road
- Open access
- Published: 06 July 2023
- Volume 1 , article number 6 , ( 2023 )
Cite this article
You have full access to this open access article
- Lyndon N. A. Sackey 1 ,
- Jeremiah Quartey 1 ,
- Augustus N. N. Nortey 1 ,
- Afia Tiwaa Obeng 1 ,
- Abigail Amoakoatta Okyere 1 &
- Phebe Y. Kayoung 1
6470 Accesses
3 Altmetric
Explore all metrics
The construction of roads has become very popular in our age due to heightened modernization, industrialization and the various benefits reaped from proper road systems. However, construction processes massively contribute to environmental pollution and negatively impact socio-economic activities, necessitating this study. The aim of this paper is to assess the impact of road construction and socioeconomic and health impact using the Atonsu lake road construction as a case study. A mixed research method was employed in the research work. The quantitative method was used for primary data collection through the distribution of questionnaires, which surveyed the perception of inhabitants on the construction activity, and its health and socioeconomic effects. Through a qualitative method, data from the Kumasi south hospital was used to assess the impact of the activity on health. The income levels of inhabitants before and during the construction process were compared to the minimum wage in Ghana to analyze the impact on their socioeconomic lives. The research indicated that road construction has adversely impacted the health of Atonsu lake road inhabitants but saw a decline in the number of cases recorded at the hospital due to restrictions associated with COVID-19 and its accompanying stigmatization, which prevented people from reporting to the hospital. There was also a significant impact on the income levels of the inhabitants, which has a significant correlation with the frequency of client patronage before and during the construction of the road. Findings reinforce the need to greatly consider including effective dust abatement practices and a phase-by-phase implementation of road construction projects to reduce the negative impacts associated with the process.
Similar content being viewed by others
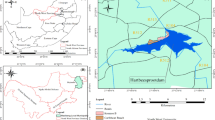
Environmental justice in South Africa: the dilemma of informal settlement residents
H. N. Kekana, T. M. Ruhiiga, … L. G. Palamuleni
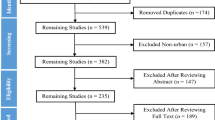
Assessing the role of urban green spaces for human well-being: a systematic review
Muhammad Jabbar, Mariney Mohd Yusoff & Aziz Shafie
Urban sprawl, public transport, and increasing CO2 emissions: the case of Metro Manila, Philippines
Rebeca Fontanilla Andong & Edsel Sajor
Avoid common mistakes on your manuscript.
1 Introduction
Large-scale spatial planning and urban development initiatives like road infrastructure have become increasingly popular in cities worldwide [ 1 ]. These projects improve connectivity [ 2 ], increase cities’ competitiveness, and attract investment [ 1 , 3 ]. Roads are constructed to aid in the flow of products and services [ 1 ] and increase economic stability and quality of life [ 4 ]. A road is a way or route connecting two locations on land that has been paved or renovated to allow travel by vehicles like cars, motorcycles, and horses. They contribute significantly to the nation's economic growth and advancement and offer important social benefits [ 5 , 6 ]. Design, construction, repair, and maintenance of roads are all parts of the complex of operations that make up road construction. Any nation's level of economic growth may be inferred from the quality of its road construction, which is also a crucial element in projecting a favorable image of the nation overseas [ 7 ] stated that several consistent efforts had been made to address the lack of road infrastructure, resulting in a massive increase in the number of road projects [ 8 ] across Ghana in recent years. Despite the many positive impacts, road construction activities are known to produce enormous amounts of dust into the environment resulting in air pollution [ 9 ]. Usually, in Ghana, roads are constructed based on political gains, the springing up of private residential facilities, and industrial establishments [ 2 ]. The study of road traffic, stone thickness, road alignment, and slopes led to the development of systems for building roads that used larger stones set in a regular, compact pattern and covered with smaller stones to create a solid layer [ 10 ]. Asphalt and concrete are promising and are widely used in modern-day road construction [ 11 ]. Roads have a significant role in economic growth and development and have significant social advantages. They are of critical importance to making a nation grow and prosper. A road network is essential for ensuring access to social, health, education, and employment services, and for other reasons related to the battle against poverty. Roads facilitate access to new locations and promote social and economic advancement. Because of these factors, road infrastructure is the most significant public asset [ 12 ].
Air pollution caused by road construction harms the environment, degrading the region’s air quality [ 13 ]. The degraded air resulting from road construction mainly contains silica, among other compounds [ 14 , 15 ]. When inhaled into the human body, silica can lead to lung cancer and other respiratory tract infections such as silicosis, cough, cold, etc. [ 14 , 16 ]. These diseases impact human health greatly and can have even worsening effects on people with underlying health conditions such as asthma, which may lead to the loss of life [ 17 ]. On the other hand, road construction is linked to environmental pollution and risks, which impact people's health and well-being in urban and rural areas [ 16 ].
Also, the varying implications of road construction activity on residents, commuters, traders, hawkers, and on-site workers on the road strongly correlate with the place's socioeconomic activity [ 1 , 2 ]. Traders along the stretch of road construction activity are displaced to make way for the construction to go on as when the road is completed, it will improve living conditions [ 1 ] and trading conditions; but the displacement of traders and hawkers during road construction greatly impacts their socio-economic lives [ 2 ]. According to a recent report by the World Resources Institute (a Maharashtra government knowledge partner), building dust is responsible for 71% of Mumbai's air pollution [ 2 ]. Air, water, and noise pollution result from these pollutants, causing irreversible damage to human health, with a concerning link between pollutants and cancer [ 18 ]. The formation of dust from vehicle movement, transport of building materials, and noise created by operating machines are the main types of environmental pollution associated with the construction of highways [ 19 ]. Breathing these specks of dust can damage the lungs and health. In Fig. 1 , dust particles are deposited in the respiratory system based on their size [ 20 ]. As mentioned, the main dust-related diseases affecting construction workers and residents are; Lung cancer, Silicosis, Chronic obstructive pulmonary disease (COPD) and Asthma.
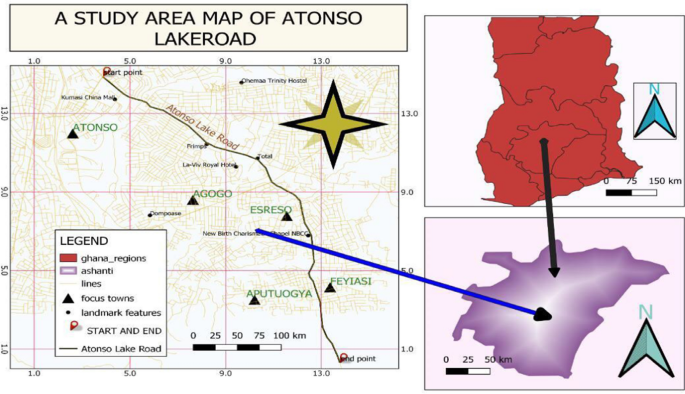
A study area Map of Atonsu lake road showing major towns along the road
Various methods, such as roads, are employed to assess construction projects’ impact. An example is the Environmental Impact Assessment (EIA) [ 21 ]. Khanani et al. [ 1 ] and [ 2 ] assessed the socioeconomic aspect of road construction, while our research looked at the socioeconomic and health impact of road construction. The greatest difficulties in road development and construction come from what seems to be the city's unplanned urban growth, which has evolved in response to the city’s dense population and precedes the creation of numerous road networks [ 21 ]. As a result, there is already intense competition for land use in Ghana, with various residential communities and commercial buildings battling for available space with the operations of mechanics, artisans, markets, car parks, churches, taxi ranks, salons, and food vendors [ 21 ]. The location of Atonsu lake road will improve the connectivity of the adjoining towns within the stretch, bringing about competitiveness and attracting investment. However, the delay in its construction and the nature of the road could impact human health and socioeconomic activities. The aim of this paper is to assess the impact of road construction in Ghana on Traders and inhabitants living along highways, using the construction of Atonsu lake road as a case study. The research will help decision-makers and developers know the challenges that ensue when projects of such nature delay completion.
2 Research methodology
2.1 study area.
The study area is situated in the center of the Ashanti region within the boundaries of the Atonsu lake road in the Asokwa Municipal District and Bosomtwe District. It lies within the latitude 6˚40′39″ N and longitude 1˚35′2″ W with a total land size of 25.31 km 2 and a population of over 125,642 according to the 2021 population and housing census. Atonsu lake road was chosen because it’s a major road that connects many towns where socioeconomic activities occur and its construction has also taken too long. This study used five major towns along the lake road: Atonsu, Agogo, Esereso, Feyiase, and Aputuogya. These towns were chosen since they comprise the hub of commercial activities along the route, with market centers, bus terminals, filling stations, hospitals, and schools. Every day, people commute in and out of these areas to go about their daily routines, making them highly susceptible to both the health and socio-economic effects of road construction.
2.2 Sample size and sampling technique
A mixed research method was employed in the research work. The quantitative method was used for primary data collection through the distribution of questionnaires, which surveyed the perception of inhabitants on the construction activity, and its health and socioeconomic effects. Through a qualitative method, data from the Kumasi south hospital was used to assess the impact of the activity on health. This was done by using records of inhabitants who had visited the facility and reported of respiratory-related disease within the period of the road construction. The random sampling technique was used in this study to disseminate questionnaires. Regarding the populace of the Asokwa metropolitan assembly, 400 individuals participated in the survey using Slovin’s formula [ 22 ]. This sample size will give us sufficient data to be evaluated and generalized to the whole population [ 23 ].
where N is the total population, e is the margin error.
The population of the Asokwa municipality was used as given by the 2021 Population and Housing Census report. And a margin of error used was 0.05 since that was the p-value used throughout our analysis of results.
2.3 Survey instruments
Questionnaires and key informant interviews were used for the primary data collection in the field [ 24 ]. 400 questionnaires were randomly distributed to road users, including traders, residents, commercial drivers, onsite workers, and hawkers, within the study area to assess the impact of road construction on socioeconomic and health impact. These respondent types were used because they comprise the people who live alongside and use the road and conduct business activities on the stretch. All 400 questionnaires were retrieved as respondents were assisted to fill and return on the spot. The questionnaire was in two sections: Section I and II. Section I: focused on the demographic characteristics of the respondents, such as gender, marital status, level of education, and occupation. Section II: centered mainly on the road and its impacts on the health and socio-economic lives of the respondents in three sub-sections (A, B, C), where ‘A’ asks questions about the Road construction and its inception, ‘B’ focuses on assessing the health status of respondents and their respective hospitals visited and ‘C’ evaluated the socio-economic impact of the Road construction activities on the respondents. ‘A’ and some parts of ‘B’ were developed on a Likert rating scale measuring respondents’ opinions by asking how much they agreed or disagreed with a particular statement. While ‘C’ and the other part of ‘B’ are a mix of binary choice, multiple-choice and open-ended questions, allowing respondents to freely share their perceptions and opinions and provide precise responses to the subject. A hypothesis was drawn from the responses generated from the questionnaire, which will be validated in the later part of this study. A survey was conducted at the various health facilities through key informant interviews with the records department of the hospitals along the Atonsu lake road. Responses from the questionnaire provided specific details of the hospitals to visit. The survey provided information on how these hospitals’ operations have been impacted by the activities of the road construction and the kinds of health problems reported at the hospital since the construction of the road began.
Secondary data from the hospital’s statistical unit was obtained to determine the number and respiratory-related diseases reported to the health facility during the several phases of the construction. The diseases reported over the previous years of the road construction phase to the current phase will be studied to enhance our data analysis process.
The survey was conducted according to established ethical guidelines, and consent was obtained from the participant. The Humanities and Social Sciences (HuSSREC) of Kwame Nkrumah University of Science and Technology approved of this research.
2.4 Data analyses
The data collected from these surveys were analyzed using the Statistical Package for Social Sciences (SPSS) Model (Version 20) and XLSTAT (Version 2018).
3.1 Socio-demographic information
As shown in Table 1 , a total of 400 respondents were interviewed during the research, there were more males than females. Out of this number, 53.75% were married, 41% were single and 5.25% were divorced. With respect to the age variable, the highest number of respondents were between 26 and 35, representing 31.3% and the lowest were respondents above 61, representing 13,3%. Considering the educational levels of the people interviewed, most of them have secondary level 54.3%, with only 4% who had various postgraduate programs. This indicates that it is a highly educated community. Concerning occupations, they were categorized into trade, technical, professional, transport services [drivers and conductors (mates)] and others (students and Unemployed) here, the highest number of the respondents are in trading 47.0%, 18.75% various professional jobs and 5.75% into technical jobs.
3.2 Perception of road
As shown in Table 2 , out of the 400 people interviewed, 84.75% agreed that they were given prior notice before the construction, whiles 15.25% disagreed with having prior notice. Most traders (63.0%) disagreed with allowing traders to relocate temporarily. A large number of people, 52.25%, disagreed with the contractor doing regular dust abatement.
3.3 Perception of the population on health
As shown in Fig. 2 , 81% of the respondents agreed with claims of high levels of noise pollution, while 19% disagreed. Most of the respondents confirmed having difficulty breathing. 67.75% agreed to have experienced adverse effects of the construction on their health, while 32.35% disagreed. A majority agreed to develop eye irritations and most of them reported this at the hospital. 59.25% were unaffected by the high noise level and 40.75% agreed to have experienced the effects of noise on their health. Most respondents reported symptoms of respiratory tract infection.
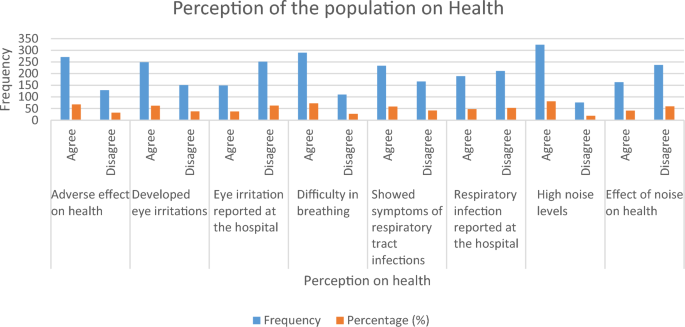
Perception of respondents on health effects of road construction activity in the area
3.4 Trend of upper respiratory tract infections from Agogo hospital
Figure 3 shows that over 2800 people reported to the hospital with RTIs in 2018. This, however, saw a drop in 2019, which recorded a total of 1856 cases. In 2020 we saw a steep reduction, which amounted to a total of 526 cases being recorded. In 2021 we saw a steep decline, with the total number of cases recorded as 613 cases.
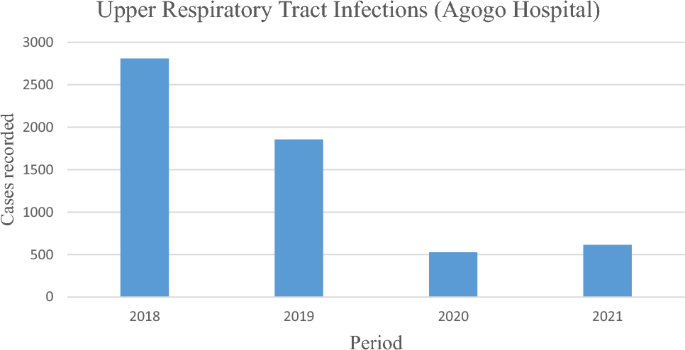
Impact of road construction activities on the health of road users within the study area
3.5 Socioeconomic impact
The socioeconomic impact of the construction activity of the area focused mainly on how it has affected the income levels of inhabitants. The results showed that road construction strongly correlated with the area's socioeconomic activities, such as the frequency with which clients patronize the goods and services of service providers [ 25 ]. To find out if the income levels of the traders were affected, data on the range of income levels before and during the construction process was taken. Also, data on the frequency of clients’ patronage was taken to determine the socioeconomic impact of road construction on traders. To ascertain if these variables (income levels and clients’ patronage before and during the road construction) are significant with each other, a cross-tabulation Chi-square test was run at a confidence level of 95% which yielded a p-value of 0.00 each for before and during, showing high significance.
From the analysis, many respondents earn between GHC 50–400 and GHC 400–800, indicating 65 and 53 of the total questionnaires administered. Also, for income levels between GHC 801–1200, a total of 38 respondents was recorded. Of the respondents earning > GHC 1200, there were 28 respondents.
From the analysis, Table 4 assesses the relationship between the income levels of respondents and the frequency of client patronage during the construction process, a total of 165 of the sample size, were earning between GHC 50–400 and a total of 51 of the respondents earned between GHC 400–800. For income levels between GHC 800–1200, a total of 29 respondents were recorded and for income levels > GHC 1200, a total of 13 respondents were recorded. The results indicated that there are more responses ending in less than GHC 800.
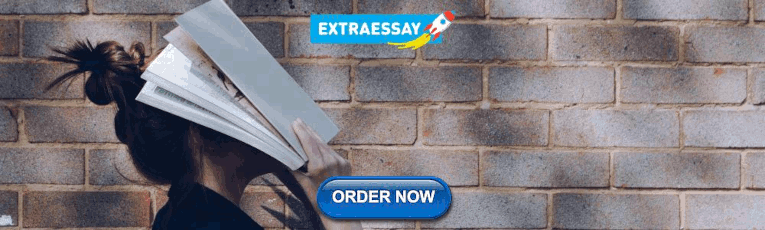
4 Discussion of findings
4.1 socio-demographic information.
In the study area, there were more males than females and socioeconomically, this could cause a lot of social vices in the area [ 26 ]. The data indicated that 47% of the respondents are into trading and the displacement of the majority of them, as a result of the road construction, could lead to unemployment. Also, 24.5% worked in the technical and transportation sectors; hence, the road construction delay and the road's bad nature could affect their incomes. Most of the inhabitants are youth; between 26 and 35 years, the road construction and the delay due to financial challenges could affect economic activities. This could affect the livelihoods of these youth within the catchment area.
4.2 Perception on road
The majority of the traders whose opinions were collected during the survey at the markets on the Atonsu lake road believed they had received appropriate notice of the road construction before it started. However, there were no other locations or provisions for them to temporarily relocate during the road construction. This meant that most of the displaced market traders effectively went out of business or had to scale down in their trade size since most of them had to compete for space or even perch with other unaffected traders. From the interviews with the traders along the roadside, it was recorded that some traders lost their containers, and stores and resorted to using a small table shaded by an umbrella stand to trade, whiles some had to put a cloth on the floor to sell their items. This indicates that in addition to losing their business place, they were continuously exposed to hot and humid weather. Most traders discussed how they had lost customers because certain customers chose to support specific stall layouts and structures. Although it has been theorized that the location of a trader's choice of trade in a market does not directly correlate with the trader's survival, location is still a key factor in determining whether a deal is successful or not. It has been confirmed by the decrease in earnings from the traders as they moved [ 2 ]. Due to the regular switching of positions and absence due to road construction activities, customers who frequently relied on positions to locate a trader could no longer do so. As the construction and demolition exercises were extremely disruptive and chaotic to typical shopping activities, some customers would hunt for new dealers to buy from, while others would be discouraged from attending the market and visiting alternate markets. The traders said that, in addition to the complaints, the contractor was present on the site every day until the project was completed. The disruption and chaos caused by the road construction, such as the noise and dust, prevented the dealers from taking a day off. Additionally, the construction was completed earlier than expected. This indicates that many things changed in their environment since some could not return to their original positions and others' health was affected [ 27 ]. The fact that the contractor barely performed dust abatement meant that the air they breathed could affect their health, and the majority of the traders complained that it made their products look unappealing because dust accumulated on them. An interview with the drivers revealed that they had to spend significantly more money having their cars washed because they now had to visit the washing bay daily, impacting their revenue. Several also stated that some passengers refused to board their vehicle because the seats were covered with dust or the vehicles lacked air conditioning, forcing them to breathe dust in the air [ 19 ]. Market vendors whose operations are close to road construction are displaced, particularly women, who lack alternatives and experience negative effects on their ability to make a living as traders. Instead of reducing informality, road construction initiatives that don't consider the predicament of traders frequently end up escalating challenges and inconveniences [ 27 ].
4.3 Perception on health
A health assessment suggested that more of the population experienced adverse health effects from the construction, associating their symptoms with eye irritations and RTIs. The movement of vehicles and transportation of construction materials contributed to the formation of dust clouds [ 28 ], which resulted in difficulty in breathing, as most of the population agreed. According to a greater percentage of the residents, headaches, coughs, sore throats, catarrh, among others, were more prevalent among them during the construction’s climax phase due to their consistent exposure to dust. In their research, Ghanney et al. [ 29 ], these symptoms are associated with respiratory tract infections, which addresses the effects of dust exposure on street sweepers. However, some residents failed to report their complications to the hospital because they couldn’t afford the cost, especially in their current state of low sales and reduced income levels. Meanwhile, the analyzed data acquired from Agogo Hospital showed a contrary report that construction activities’ air pollution greatly impacts human health. This data shows a greater number of RTI cases reported in 2018, which was before the construction of the Atonsu lake road. During the construction period (2019–2021), there was a reduction in the number of cases, with 2020 noted as the year with very few cases reported, which contrasts with our hypothesis. This contrast can be linked to the impact of the COVID-19 pandemic, which was on the rise in 2020 [ 30 ]. We can associate the reduction in the number of RTI cases in this period to a number of factors that followed the pandemic. One major factor was the fear of being stigmatized with Covid-19 when you visit the hospital with symptoms of respiratory infections because they are very similar to that of COVID-19 [ 31 ]. Most people resorted to treating mild coughs, catarrh, fever, etc., traditionally by preparing herbal drinks with neem tree, Hibiscus leaves, “pr3kese” a local spice that is known to have various health benefits. Induced quarantine by the government lessened frequent plying of the roads and kept people indoors, primarily reducing interactions and exposure to dust from the road, which can be seen as an appositive aspect of the pandemic [ 1 ]. Some residents talked about frequently inhaling warm water and wearing nose masks to protect them from the dust instead of the COVID-19 virus when the ban on quarantine was lifted. The fear of contracting the virus also made people adopt measures such as frequent hand washing, regular use of sanitizer, avoiding contact with infected areas, etc., which protected them from the Covid-19 virus and other viruses that could cause respiratory infections. [ 32 ], elaborated on a category of Noise-Induced Hearing Loss (NIHL) which was most widespread in hearing loss associated with a blast overpressure, known as blast-induced hearing loss. Residents around the construction site complained of extreme noise pollution, especially when contractors had to blast rocks for construction. The discomfort associated with this activity was difficult to bear; however, most of the population did not experience any health complications related to the noise. They attested to the fact that advance notice was given to all residents to evacuate their homes for some days when the blasting exercise was about to take place. This can account for why most of them were not affected health-wise by the noise since the distance from the blast is a major factor in determining the effect of noise on human health [ 33 ].
4.4 Socio-economic impact
4.4.1 income and frequency of clients cross tabulation, before the construction process.
In our bid to understand the relationship and significance of frequency on the income levels of the people, we run a cross-tabulation Chi-square analysis at a confidence level of 95%. This yielded a significance value of P < 0.00, indicating that the relationship is very significant in Tables 3 and 4 . This revealed that the income levels of the people in the Atonsu lake road area before the construction primarily ranged from GHC 50.00–800.00. This is seen in the total number of people with such income levels, i.e. 192 out of 400. This represents almost half the sample size. Hence, this indicates that road construction has impacted their income levels. Also, the results during the construction process, revealed that patronage frequency was very low for more than 110 of the sample size, and ranged between GHC 50.00–400.00 income levels. This represents a sharp decline in the frequency and income levels recorded before. According to [ 34 , 35 ], the minimum wage in Ghana is GHC 13.53/day and the minimum monthly salary is GHC 365.31. This is consistent with most respondents’ earnings (GHC 50.00–400.00). However, this could have been better if the road construction activity was critically checked to reduce its impact on their socio-economic lives.
5 Conclusion and future perspective
From the study, the main health complication associated with road construction activity is Upper Respiratory Tract Infections (URTI). This decreased in frequency in the number of cases recorded due to the rise of COVID-19 at the time and its accompanying restrictions and stigmatization. Also, the income levels of the people showed to be very significant with the rate at which people engaged in business activities, as more people moved from earning between GHC 50–800 to earning between GHC 50–400. Although income levels were reduced, it is still consistent with the minimum wage in Ghana, it could have been better if not for the impact of the road construction. Nonetheless, we recommend that contractors invest more in dust abatement procedures, by employing the services of adequate water tankers. Appropriate reallocation schemes should be used, such as proper market developments and affordable housing schemes. Also, a clear separation between developed and undeveloped areas is necessary to avoid the relocation of residents owing to road extension projects. The local government authorities and traditional leaders should also properly enforce the legislation to prohibit construction on roadways, which would later be demolished to make space for expansion projects when the region is expanded and the current road infrastructure is at capacity. Road maintenance should be done regularly throughout the nation to maximize the longevity and durability of roads. Finally, further studies can be conducted on other health pollutants relating to road construction, its harmful effects, and the age groups affected more.
Availability of data and materials
They are available upon request.
Khanani RS, Adugbila EJ, Martinez JA, Pfeffer K. The impact of road infrastructure development projects on local communities in peri-urban areas: the case of Kisumu, Kenya and Accra Ghana. Int J Commun Well-Being. 2021;4(1):33–53.
Article Google Scholar
Adugbila EJ, Martinez JA, Pfeffer K. Road infrastructure expansion and socio-spatial fragmentation in the peri-urban zone in Accra Ghana. Cities. 2023;133:104154.
Labi S, Faiz A, Saeed TU, Alabi BNT, Woldemariam W. Connectivity, accessibility, and mobility relationships in the context of low-volume road networks. Transp Res Rec. 2019;2673(12):717–27.
Mostafa HM. Road maintenance in Africa: approaches and perspectives. E3S Web Conf. 2018;38:01005.
Ghosh PK, Dinda S. Revisited the relationship between economic growth and transport infrastructure in India: an empirical study. Indian Economic J. 2022;70(1):34–52.
Ng CP, Law TH, Jakarni FM, Kulanthayan S. Road infrastructure development and economic growth. In IOP conference series: materials science and engineering. Bristol: IOP Publishing; 2019.
Google Scholar
Pheng LS, Hou LS. The Economy and the Construction Industry. Constr Qual Econ. 2019,9:21–54.
Edriss AK, Chiunda C. Interfaces between road infrastructure and poverty in Africa: the case of Malawi, 1994–2013. J Soc Economics Res. 2017;4(1):9–21.
Wijeyesekera DC, Bakar I, Lim SM, Abidin NZ. Dust emissions from unpaved ground surfaces. ARPN J Eng Appl Sci. 2016;11:16.
Amakye SY, Abbey SJ, Booth CA, Oti J. Road pavement thickness and construction depth optimization using treated and untreated artificially-synthesized expansive road subgrade materials with varying plasticity index. Materials. 2022;15(8):2773.
Article CAS Google Scholar
Lay M, Gordon B, Fred J. Road. Encyclopedia Britannica. 2022.
World Highways. “Asphalt Paving, Compaction & Testing” Asphalt Paving, Compaction & Testing|World Highways. 2015.
Giunta M. Assessment of the environmental impact of road construction: modelling and prediction of fine particulate matter emissions, Building and Environment, 2020. 176.
Guo J, Lin W, Li H, Zhang Z, Qin X. Numerical simulation study on spatial diffusion behavior of non-point source fugitive dust under different enclosure heights. Int J Environ Res Public Health. 2023;20(5):4361.
Harrison J, Dawson L. Occupational health: meeting the challenges of the next 20 years. Safety and health at work. 2016;7(2):143–9.
Gharpure A, Heim JW, Vander Wal RL. Characterization and hazard identification of respirable cement and concrete dust from construction activities. Int J Environ Res Public Health. 2021;18(19):10126.
Tan S, Chen S. Macrophage autophagy and silicosis: current perspective and latest insights. Int J Mol Sci. 2021;22(1):453.
Guzder J. Reflections on the privacy of self and the drive to create in the context of dislocation. In Privacy. New York: Routledge; 2019.
Book Google Scholar
Barikayeva N, Nikolenko D, Ivanova J. About forecasting air pollution in the construction of highways. IOP Conf Ser Mater Sci Eng. 2018;463(4):042016.
Subramanian N, Abhyankar EV. (2019). Construction dust causes, effects and remedies. New Building Materials and Construction World.
Ikioda F. The impact of road construction on market and street trading in Lagos. J Trans Geogr. 2016;55:175–81.
Langat DCR. Microcredit and its relationship to small and medium enterprises growth in Konoin Subcounty, Kenya. Int J Adv Res. 2018;6(4):961–8.
Park W, Kim R, Roh S, Ban H. Analysis of major environmental impact categories of road construction materials. Sustainability. 2020;12(17):6951.
Glasow AP. Fundamentals of survey research methodology. Bedford: MITRE Product; 2005.
Zucker DM. How to do case study research. In teaching research methods in the social science. New York: Routledge; 2016.
Nanor JN. Assessment of the effects of quarrying activities on some selected communities in the lower Manya Krobo District (Master of Philosophy Thesis, University of Ghana). 2011.
Zuo J, Rameezdeen R, Hagger M, Zhou Z, Ding Z. Dust pollution control on construction sites: awareness and self-responsibility of managers. J Clean Prod. 2017;166:312–20.
Habybabady RH, Sis HN, Paridokht F, Ramrudinasab F, Behmadi A, Khosravi B, Mohammadi M. Effects of dust exposure on the respiratory health symptoms and pulmonary functions of street sweepers. Malaysian J Med Sci MJMS. 2018;25(6):76.
Ghanney EB, Mijinyawa Y, Ana G. Meteorological and air pollution assessment from road use and construction in the eastern and greater Accra regions (Ghana). J Environ Earth Sci. 2022;12(3):25–39.
Hamid A, Akhtar S, Atique SA, Huma Z, Mohay Uddin SG, Asghar S. Ambient air quality & noise level monitoring of different areas of Lahore (Pakistan) and its health impacts. Polish J Environ Stud. 2019;28:2.
Pruthi Sandhya. COVID-19, cold, allergies and the flu: what are the differences? Mayo Foundation for Medical Education and Research (MFMER). 2022.
Kenu E, Frimpong J, Koram K. Responding to the COVID-19 pandemic in Ghana. Ghana Med J. 2020;54(2):72–3.
Esson J, Gough KV, Simon D, Amankwaa EF, Ninot O, Yankson PW. Livelihoods in motion: linking transport, mobility and income-generating activities. J Trans Geogr. 2016;55:182–8.
Wage indicator, minimum wage News, 2023. https://mywage.org/ghana/salary/minimum-wages . Accessed 4 Mar 2023.
Download references
There was no funding for this research work.
Author information
Authors and affiliations.
Department of Environmental Science, Faculty of Biosciences, Kwame Nkrumah University of Science and Technology, PMB, Kumasi, Ghana
Lyndon N. A. Sackey, Jeremiah Quartey, Augustus N. N. Nortey, Afia Tiwaa Obeng, Abigail Amoakoatta Okyere & Phebe Y. Kayoung
You can also search for this author in PubMed Google Scholar
Contributions
LNAS, JQ, ANNN, ATO, AAO, PKK design of the experiment, JQ, ANNN, ATO, AAO data collection, LNAS, PKK Supervision, LNAS, JQ, ANNN, ATO, AAO, PKK Analysis of data.
Corresponding author
Correspondence to Lyndon N. A. Sackey .
Ethics declarations
Ethics approval and consent to participate.
The experiment was conducted according to established ethical guidelines, and consent was obtained from the participant. The Humanities and Social Sciences (HuSSREC) of Kwame Nkrumah University of Science and Technology approved of this research. The study complies with all regulations relating to research, including experimentation on human subjects via interviews.
Consent for publication
Since this study is not attempting to re-publish/publish any third party or author's previously published material, this section does not apply.
Competing interests
The authors declare no competing interests.
Additional information
Publisher's note.
Springer Nature remains neutral with regard to jurisdictional claims in published maps and institutional affiliations.
Rights and permissions
Open Access This article is licensed under a Creative Commons Attribution 4.0 International License, which permits use, sharing, adaptation, distribution and reproduction in any medium or format, as long as you give appropriate credit to the original author(s) and the source, provide a link to the Creative Commons licence, and indicate if changes were made. The images or other third party material in this article are included in the article's Creative Commons licence, unless indicated otherwise in a credit line to the material. If material is not included in the article's Creative Commons licence and your intended use is not permitted by statutory regulation or exceeds the permitted use, you will need to obtain permission directly from the copyright holder. To view a copy of this licence, visit http://creativecommons.org/licenses/by/4.0/ .
Reprints and permissions
About this article
Sackey, L.N.A., Quartey, J., Nortey, A.N.N. et al. Road construction and its socio-economic and health impact: a case study of Atonsu lake road. Discov Environ 1 , 6 (2023). https://doi.org/10.1007/s44274-023-00009-x
Download citation
Received : 01 May 2023
Accepted : 20 June 2023
Published : 06 July 2023
DOI : https://doi.org/10.1007/s44274-023-00009-x
Share this article
Anyone you share the following link with will be able to read this content:
Sorry, a shareable link is not currently available for this article.
Provided by the Springer Nature SharedIt content-sharing initiative
- Road construction
- Health impact
- Find a journal
- Publish with us
- Track your research
- Open access
- Published: 02 November 2023
The impact of geo-political socio-economic factors on vaccine dissemination trends: a case-study on COVID-19 vaccination strategies
- Ritu Chauhan 1 ,
- Gatha Varma 2 ,
- Eiad Yafi 3 &
- Megat F. Zuhairi 4
BMC Public Health volume 23 , Article number: 2142 ( 2023 ) Cite this article
1253 Accesses
8 Altmetric
Metrics details
The world in recent years has seen a pandemic of global scale. To counter the widespread loss of life and severe repercussions, researchers developed vaccinations at a fast pace to immunize the population. While the vaccines were developed and tested through extensive human trials, historically vaccines have been known to evoke mixed sentiments among the generic demographics. In the proposed study, we aim to reveal the impact of political and socio-economic factors on SARS-Cov-2 vaccination trends observed in two hundred and seventeen countries spread across the six continents.
The study had hypothesized that the citizens who have lower trust in their government would be less inclined towards vaccination programs. To test this hypothesis, vaccination trends of nations under authoritarian rule were compared against democratic nations. Further, the study was synthesized with Cov-2 vaccination data which was sourced from Our World Data repository, which was sampled among 217 countries spread across the 6 continents. The study was analyzed with exploratory data analysis and proposed with relevance and impacting factor that was considered for vaccine dissemination in comparison with the literacy rate of the nations. Another impacting factor the study focused on for the vaccination dissemination trends was the health expenses of different nations. The study has been synthesized on political and socio-economic factors where the features were ardently study in retrospect of varied socio- economic features which may include country wise literacy rate, overall GDP rate, further we substantiated the work to address the political factors which are discussed as the country status of democratic or having other status.
The comparison of trends showed that dissemination of SARS-Cov-2 vaccines had been comparable between the two-opposing types of governance. The major impact factor behind the wide acceptance of the SARS-Cov-2 vaccine was the expenditure done by a country on healthcare. These nations used a large number of vaccines to administer to their population and the trends showed positive growth. The overall percentage of vaccine utilized by countries in quantitative terms are Pfizer/BioNTech (17.55%), Sputnik V (7.08%), Sinovac (6.98%), Sinopharm/Beijing (10.04%), Oxford/AstraZeneca (19.56%), CanSino (2.85%), Moderna (12.05%), Covaxin (3.28%), JohnsonandJohnson (10.89%), Sputnik Light (3.07%), Novavax (3.49%). While the nations with the lowest healthcare expenses failed to keep up with the demand and depended on vaccines donated by other countries to protect their population.
Conclusions
The analysis revealed strong indicators that the nations which spend more on healthcare were the ones that had the best SARS-Cov-2 vaccination rollout. To further support decision-making in the future, countries should address the trust and sentiment of their citizens towards vaccination. For this, expenses need to be made to develop and promote vaccines and project them as positive health tools.
Peer Review reports
Introduction
The global stance, in the current scenario of the pandemic, has imposed a substantial burden on healthcare practitioners. The ultimate aim of researchers and scientists worldwide is to fight the ongoing situation and discover significant vaccines which can reduce the mortality rate among society. As we know, the decisive way to overcome the ongoing situation is to immunize the population. Moreover, the existing circumstances infer that, if we need to prevent and control the spread of COVID-19, then immunization would play an imperative role.
Certainly, COVID-19 has imprudently created several challenges among young researchers, scientists, and healthcare practitioners around the globe [ 1 , 2 , 3 , 4 ]. To address the key challenges, the interdisciplinary research areas such as education, healthcare, industrial, stock market, food and beverage were examined to determine the factors i.e. socio, behavioral, economical parameters in the vaccine strategy which can directly or indirectly influence the study [ 2 , 3 ]. Hence, the trial of studies was performed at varying stages which included the clinical trials, spatial parameters of the spread of disease, factors corresponding to disease patients, and others. To discuss the overall impact of studies conducted, the challenge is to disseminate the data and predict information from big databases [ 5 , 6 ].
To address the vital issues of real-world data complexity, several adoptive technologies such as computer vision, Tele health was need of time and assistive technologies implemented such as visualization-based models to assist the need of the patients also several communication modules were implemented to assist with the work from home and support the overall population to best fit the current scenario [ 7 ]. Also, we can say that to curb the spread of COVID 19, Machine learning (ML)and Artificial Intelligence (AI) are well-known techniques, which are deployed to discover hidden information from varied databases. The global network of clinicians, scientists, and healthcare practitioners are trying to accelerate the research programs while utilizing AI and ML to develop an effective and efficient COVID -19 vaccine that can benefit the human population among all age groups [ 8 , 9 ]. Usually, ML and AI are evolved to develop a prediction-based tool or decision making, which can outline the future adverts of healthcare outcomes [ 10 ].
We can say that the healthcare organizations are explicitly searching for appropriate technology which can track the epidemiological synchronization or cofactors which can relate to pandemic spread [ 9 , 10 ]. However, cofactors can easily studied with AI and ML which can set vulnerable benefits of diagnosis while measuring the effectiveness of the drug, spread of COVID 19, and detecting the co-vital features associated with the disease [ 11 , 12 , 13 ]. Further, we can say that AI and ML-based technology are the decision-making platforms which can assist healthcare practitioners to fight the battle of COVID -19 while giving a recommendation, medical features extractions, patient-assisted control, and other services which we can think of.
Further, the utilization of ML and AI has flourished their advances in the pandemic era, victoriously in several application domains which include military organizations, healthcare, business analytics, and other technological intervention while gaining momentum and success stories [ 11 , 12 , 13 ]. For example, the chest CT scan images were applied with medical imaging analysis using ML and AI-based algorithms to detect the prognosis of the disease in a short period, as well as citing the drug discovery module which can benefit the patient [ 14 , 15 ]. In addition, ML and AI-based studies were applied to study the societal and behavioral implications of patients and develop intelligent models which can benefit the post-Covid era of patients [ 16 ]. Moreover, several human transmission models were also generated to identify and predict the outbreak of disease and other severities aligned with spread [ 16 , 17 ].
Further, considering the relative global impact of vaccination with vital features such as literacy rate, disabilities, older generation and many other factors in retrospect of the citizens and certain formulation suggested that users those have lower trust in their government were less inclined towards vaccination programs [ 17 ]. In accordance, to the previous work we tried to determine the vaccination trends among varied nations under authoritarian rule as well as comparison was strategized against democratic nations [ 17 , 18 ]. The comparison of trends showed that dissemination of the SARS-Cov-2 vaccine had been comparable between the two-opposing types of governance. Further, the study was proposed with relevance and impacting factor that was considered for vaccine dissemination in comparison with the literacy rate of the nations. The correlated factor was referred to as the country's literacy rate, which is an indicator of its economy and living standards [ 14 ]. In agreement with the above problem, the vaccination trends were represented, with vital features of lesser literacy rates having extremely low and flat growth curves for vaccination numbers [ 15 ]. In similar, another impacting factor that the study focused on was the vaccination dissemination trends in comparison with the health expenses of different nations. The curves were strong indicators that the nations that spend more on healthcare were the ones that had the best SARS-Cov-2 vaccination rollout [ 16 ]. These nations used a large number of vaccines to administer to their population and the trends were positive growth [ 17 ]. While the nations with the lowest healthcare expenses failed to keep up with the demand and depended on vaccines donated by other countries to protect their population [ 18 ].
In the proposed study, we aim to reveal the impact of political and socio-economic factors on SARS-Cov-2 vaccination trends in different countries. The study has been synthesized on political and socio-economic factors where the features were ardently study in retrospect of varied socio- economic features which may include country wise literacy rate, overall GDP rate, further we substantiated the work to address the political factors which are discussed as the country status of democratic or having other status which are discussed elaborated in methodology section.
Literature review
Data science can be discussed as a vibrant tool for developing and supporting vaccine dissemination. The major role is to discover the protein structure which can enable the clinicians and data scientists to discover the patterns for future research repositories and identification of drug discovery. We can say that vaccine is the only possibility that can control the dissemination of COVID 19 cases around the globe [ 18 ]. However, the statistics represents that few vaccines have received permission to use, as in case of emergency conditions that can benefit the healthcare practitioners and others. In context, several vaccination programs are laid into the process to assure the wellbeing, safety, and control of the blowout of the COVID-19 [ 18 , 19 , 20 ]. Hence, we can say that earlier vaccine development was a trivial task, but the advent technology introduced has the ability to identify the protein structure and develop a new drug for a deeper understanding of the virus. Google with integrated technology of AI launched the Alphafold tool, which tends to be an automatic and specialized tool that can predict the new vital 3D protein structure while input as the genetic sequence [ 21 ]. The above cited approached focused on detection of the untreated proteins which regulate the SARS-COV-2 virus and generate a protein structure [ 17 , 22 ].
The concept of reverse vaccinology (RV) with ML tools was utilized to forecast and develop a vaccine for COVID -19. The RV was applied to discover the pathogen using genomes. The study prevailed to discover the proteins which were responsible for the virus which includes SARS-CoV, MERS-CoV, HCoV-229E, and others where were discovered from Uniprot proteins. Moreover, they have exploited the ML techniques Vaxign-ML to detect and discover the signal behavior of the proteome of the virus, to forecast the relevance of biological signals [ 23 ]. Further, the Vaxign-ML model was formulated using classifier Random Forest, structural and vector proximity, the context of modeling which was based on ML and RC, was applied to determine the protein and its correspondence level [ 23 ].
In similar, the control and spread of any communicable disease depend upon the vaccine instantiated. Certainly, the development of these vaccines is not an easy task, moreover, several challenges and barriers exist to determine the effective and efficient drug which can handle the generic data. In the past several studies are introduced by researchers and health care practitioners to study the overall effect of the vaccine [ 17 , 18 , 19 , 20 , 21 , 22 ]. Comparably, a study was proposed to discuss the encounters of the vaccine in context with structural and attitudinal patterns in the US population [ 24 , 25 ]. Where the structural barriers were discussed as the service which was not accessible such as transportation services, or going out and acquiring public services. Further, attitudinal barriers refer to the fact the beliefs which a person acquires the fear of communicable disease or his unwillingness to accept the facts due to perception of the mind. Moreover, in the context of vaccination, it may be discussed as the barrier which develops as the aftermaths or present vaccination programs and determines the risks associated with same. Similar outcomes can be controlled by several recommendation programs, where the effectiveness and importance of COVID- 19 can help them reduce the barriers among the population, which an individual acquires due to rational information [ 24 , 25 ].
Method and materials
COVID 19 has substantially worn the world upside down which has created several inhibitions to detect hidden patterns and information from large scale databases. However, the current study of approach focuses on vaccine dissemination patterns around the globe. The study was based on databases generated from public domain repository of United Nations World Population Prospect, where the datasets compromise of subnational locations such as England, Northern Ireland, Scotland, Wales and others [ 26 ]. Also, the overall estimation was calculated on the basis of total vaccinations conducted with doses administrated at each level in correspondence people fully vaccinated with per hundred million. The dataset was analyzed to measure the potential factors in context with impact of political and socio-economic factors on SARS-Cov-2 vaccination trends in different countries. The study has been synthesized on political and socio-economic factors where the features were ardently study in retrospect of varied socio- economic features which may include country wise literacy rate, overall GDP rate [ 27 , 28 ]. Further, we have utilized the secondary data extracted to explore the international trends of vaccination. The current study of approach was focused to provide appropriate geographical coverage of vaccination with stratified sampling and exploratory data analysis.
The exploratory data analysis (EDA) is conducted among the databases to detect hidden patterns, information and knowledge from the data. The data investigated was preprocessed for null values, missing values and inconsistent values to measure the exploratory features summarization. The proposed study was implemented using python 3.9.0 version using NumPy package, Matplotlib library and pandas to explore the complex patterns among the data. Further, data was explored with graphs and distribution curves to synthesize and identify the potential outlines in the data. The analysis also included varied statistics such as mean, median, standard deviation for varied features in the datasets.
Additionally, the study was categorized with relevance and impacting factor which were considered for vaccine dissemination in comparison with the literacy rate of the nations. Also. Target features were considered for the vaccination dissemination trends for calculating the health expenses of different nations. The study has been synthesized on political and socio-economic factors where the features were ardently study in retrospect of varied socio- economic features which may include country wise literacy rate, overall GDP rate, further we substantiated the work to address the political factors which are discussed as the country status of democratic or having other status.
Descriptive analysis
Vaccines have been one of the biggest preventive measures that were developed over the past century to tackle dreaded diseases. However, the major diseases which tends to cured with vaccination is smallpox and rinderpest which has substantially outcasted the population with their rendered threats. While Covid-19 vaccinations have once again raised the debate about a population's trust in various aspects of this process, studies were done before the pandemic also helped in understanding the factors that drive acceptance rates [ 29 , 30 ].
A study done by Larson et al. in 2016 [ 17 ] had analyzed trust in vaccinations in terms of four factors, namely its importance, its safety, its effectiveness, and religious compatibility. Respondents from sixty-seven countries were surveyed for their confidence in vaccines. The survey gauged sentiment on a five-point Likert scale, where answers were discrete values of strongly agree, tend to agree, do not know, tend to disagree, strongly disagree. After the removal of the neutral answer of 'do not know', the countries were ranked based on low confidence. The countries that showed the most negative sentiment towards vaccination before the SARS-Cov-2 pandemic in terms of the four different factors are listed in Table 1 .
As can be seen from the rankings, European and Western Pacific nations were least confident of vaccinations. Developed nations like Japan and France were unexpected entries. The distrust of the population of France can be attributed to the vaccine-related controversies that plagued the nation in the past two decades [ 31 ]. Japan is known to be a risk-averse nation and therefore the population shows a major concern in vaccine safety to fully trust them [ 32 ]. Table 2 lists the top ten countries that displayed positive sentiment towards vaccines.
While the countries with positive sentiment towards vaccination are a mix of developed and developing nations, the latter were more accepting. It should also be noted that religious fundamentalism might impede vaccine acceptance, but it cannot be linked to a certain faith type, as shown by the presence of Saudi Arabia which has a majorly Muslim population [ 33 ].
The SARS-Cov-2 vaccination data was sourced from Our World in Data repository hosted on Github [ 22 ]. This dataset is comprised of two hundred and seventeen countries spread across the six continents. Table 3 lists the attributes of the data that was accessed till July 2021.
The data repository also contained information on Covid vaccines that were administered till the time of this study. These were Oxford/AstraZeneca, Pfizer/BioNTech, Sinopharm/Beijing, Sinovac, SputnikV, JohnsonandJohnson, Moderna, Covaxin, CanSino, Sinopharm/Wuhan, Abdala, Soberana02, QazVac, Sinopharm/HayatVax, EpiVacCorona, RBD-Dimer [ 22 ]. Their usage breakdown is shown in Table 4 . The three vaccines that have been administered in the majority are Oxford/AstraZeneca, Pfizer/BioNTech, and Moderna.
Our analysis also aimed to discern the effect of a nation's political regime on the SARS-Cov2 vaccination dissemination. While Authoritarian governments are associated with strict implementation of policies among the residents, democracies offer a choice to its residents. The said choice may take stricter forms in case of emergencies like a pandemic. Therefore, some democracies also saw strict regulations regarding the SARS-Cov2 vaccination dissemination. Nonetheless, we combined the political regimes of different nations [ 26 ] for this particular analysis. The dataset contained political regime values as Polity 2 Measure ranges from -10, which corresponded to autocracy, to + 10 for full democracy. For the ease of analysis, the Polity indices were categorized as Authoritarian where the measure ranged between -10 to -6, Limited Authoritarian for Polity measures of -5 to -1, Limited Democracy for 0 to 5, None for non-specified values, and finally Democracy for values 5 to 10. The conversion helped in converting the feature to a categorical distribution.
Results and discussion
The proposed analysis aimed to reveal the impact of political and socio-economic factors on SARS-Cov-2 vaccination trends in different countries. The political regimes of different nations were fetched from Our World in Data repository [ 26 ] to discern the distribution of regimes and the vaccination trends. The dataset contained political regime values as Polity 2 Measure ranges from -10, which corresponded to autocracy, to + 10 for full democracy. For ease of analysis, the Polity indices were categorized as Authoritarian where the measure ranged between -10 to -6, Limited Authoritarian for Polity measures of -5 to -1, Limited Democracy for 0 to 5, None for non-specified values, and finally Democracy for values 5 to 10. Additionally, Authoritarian rule means that the people have to obey the rule and regulations formed by the single political leader. In this rule, the government doesn’t have an established system where the powers can be transformed and the liberal rights can be granted to the people. So, the authoritarian rule confers to the fact that no democracy is granted and hence the people have to subsidies on the rules created by the leader. A limited Authoritarian government is the one where the legalized forces are restricted and obey the rules of authorities. Also, the laws and their restriction on individuals and business are controlled with fewer restriction. Hence, difference between limited authoritarian and authoritarian is constitutional powers are limited in case of limited authoritarian whereas all the powers are controlled by single political leader in case of authoritarian. The democratic rule implies that the power which is elected by the people. It means the people will elect their own minister and hence govern themselves in indirect way. So, democratic way of rule is for the people, by the people and of the people to maintain the freedom at each level. Limited democratic rule can be discussed as the power which restricts the leaders to have absolute power with the inclination towards the lawmakers to abide by the absolute powers created by the government. Also, limited powers are gained by the high-profile individuals and also citizens are required to adhere with the constitutional powers. Hence, Fig. 1 shows the distribution of the five types of political regimes among the vaccination data and democracy emerged as the most common form of governance.
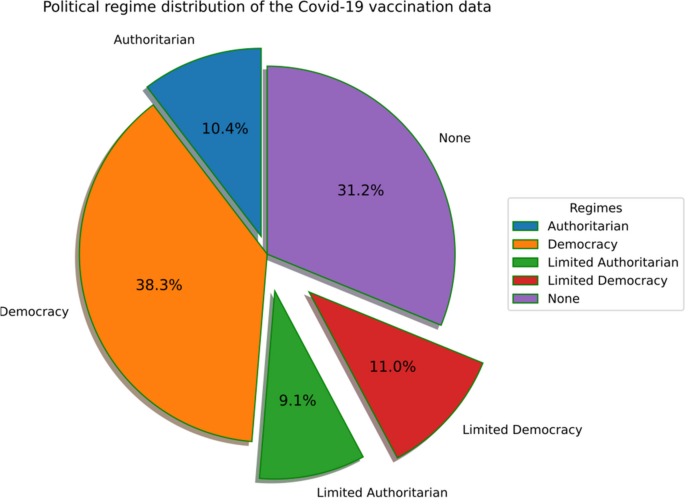
The percentages breakdown of political regimes for countries that were surveyed for SARS-Cov-2 vaccination
In, Figs. 2 and 3 the illustrated images identify the vaccination trends and vaccines used by the compared nations for varied authoritative and democratic governances.
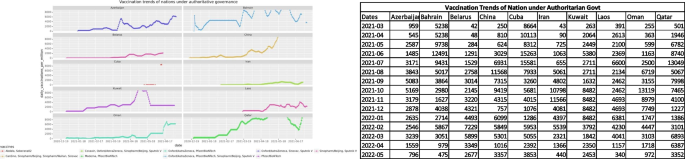
The vaccines used and vaccination trends of nations under authoritarian rule
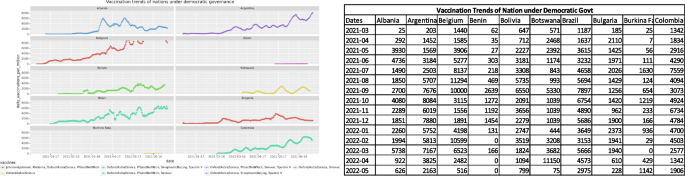
The vaccines used and vaccination trends of some democratic nations
The comparison of trends shows that dissemination of the SARS-Cov-2 vaccine has been done between the two opposing types of governance. Both types have used a combination of vaccines and the graphs show a rising trend thus marking extensive acceptance among the citizens.
The next impacting factor that was considered for vaccine dissemination was the literacy of the nations. The WHO literacy rates from the year 2016 [ 27 ] were used to shortlist nations with the lowest and highest literacy rates. The shortlisted nations were then analyzed for preferred vaccines and dissemination trends. Figures 4 and 5 show trends for nations with the lowest and highest literacy rates respectively.
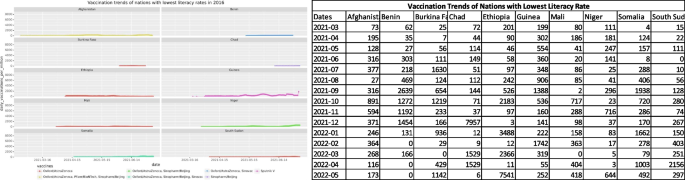
Vaccination trends with the lowest literacy rates in the year 2016
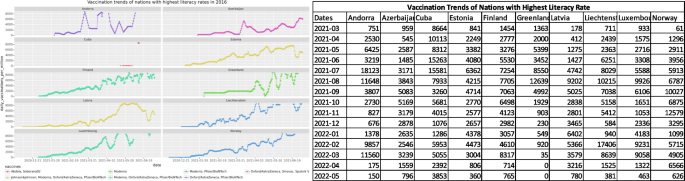
The vaccines used and vaccination trends of nations with the highest literacy rates in the year 2016
A country's literacy rate is an indicator of its economy and living standards. In agreement with this statement, the vaccination trends show that nations with the poorest literacy rates have extremely low and flat growth curves for vaccination numbers. On the other hand, the nations that have the highest literacy rates boast of good living conditions and better vaccination figures. These nations also used a combination of multiple vaccines for the faster safeguard of their population.
Another impacting factor that this study focused on was the vaccination dissemination trends in comparison with the health expenses of different nations. Each nation sets aside a percentage of the GDP towards the betterment of healthcare and its implementation. The health expenditure data hosted by the World Bank for the year 2018 [ 28 ] was used to get expenditure figures of the nations. Figures 6 and 7 show trends for nations with the lowest and highest healthcare expenses respectively. The curves are strong indicators that the nations that spend more on healthcare were the ones that had the best SARS-Cov-2 vaccination rollout. These nations used a large number of vaccines to administer to their population and the trends were positive growth. While the nations with the lowest healthcare expenses failed to keep up with the demand and depended on vaccines donated by other countries to protect their population. The curves grew slowly over time, while some were flat and showed slow progress.
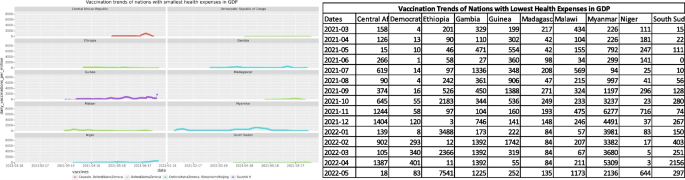
The vaccines used and vaccination trends of nations with the lowest expenditure on healthcare
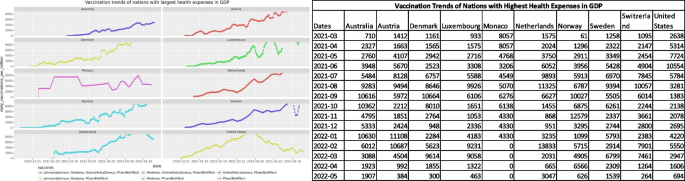
The vaccines used and vaccination trends of nations with the highest expenditure on healthcare
The human race has progressed through several inventions, and vaccines have been one of the most important of them all.
While vaccines have been successful in improving the life expectancy of the masses, the technology is still riddled with distrust and negative sentiment among the masses. This sentiment gets further aggravated due to controversies and low confidence in scientific research. It is but evident that the difference in ideology can be attributed to the demographics that the persons with negative sentiment belong to. The ideology of a person is governed by their cultural background and the other conditions that prevail in their country. These may include political factors like the government regime, economic factors like GDP and generic economic status, and literacy rates.
In this paper, we have analysed how vaccination dissemination and acceptance were affected by the political and socio-economic factors where the features were ardently study in retrospect of varied socio- economic features which may include country wise literacy rate, overall GDP rate and others. While, the world is battling with the global pandemic of SARS-Cov-2, sentiment towards vaccination has again come into the limelight. The authors have analyzed the confidence in vaccinations before the Covid pandemic happened and found that the European and Western Pacific nations were least confident. Japan, known to be a risk-averse nation also showed a major concern in vaccine safety to fully trust them. The countries with positive sentiment towards vaccination were a mix of developed and developing nations. In the post-Covid scenario, the analysis showed that the dissemination of the SARS-Cov-2 vaccine had been comparable between the authoritarian and democratic types of governance. The vaccination trends also showed that the nations with the poorest literacy rates had extremely low and flat growth curves for vaccination numbers. The nations that spent more on healthcare were the ones that had the best SARS-Cov-2 vaccination rollout. These nations used a large number of vaccines to administer to their population and the trends were positive growth. While the nations with the lowest healthcare expenses failed to keep up with the demand and depended on vaccines donated by other countries to protect their population. With this analysis, the authors hope that better vaccination strategies can be drafted as suited to the geopolitical factors of different countries.
Availability of data and materials
The qualitative data extracted and analysed during the current study is publicly available but can be discussed or made available from the corresponding author on reasonable request. All documents analysed are publicly available and referenced in this article.
Health EUROPA. Using artificial intelligence to determine COVID-19 Severity. 2020. Available online: https://www.healtheuropa.eu/using-artificial-intelligence-to-determine-covid-19-severity/100501/ . Accessed 12 Sep 2020.
McKibbin W, Fernando R. The economic impact of COVID-19. Economics in the time of COVID-19. Paris & London: CEPR Press. 2020;45(10.1162).
Gautam S, Hens L. COVID-19: impact by and on the environment, health and economy. Environ Dev Sustain. 2020;22:4953–4. https://doi.org/10.1007/s10668-020-00818-7 .
Article PubMed PubMed Central Google Scholar
Cavallo JJ, Forman HP. The economic impact of the COVID-19 pandemic on radiology practices. Radiology. 2020;296(3):E141–4. https://doi.org/10.1148/radiol.2020201495 .
Article PubMed Google Scholar
Analytics HI. Deep learning models can detect COVID-19 in chest CT Scans. 2020. Available online: https://healthitanalytics.com/news/deep-learning-models-can-detect-covid-19-in-chest-ct-scans . Accessed 3 Sep 2020.
Chen J, Wu L, Zhang J, Zhang L, Gong D, Zhao Y, Hu S, Wang Y, Hu X, Zheng B. (2020) Deep learning-based model for detecting 2019 novel coronavirus pneumonia on high-resolution computed tomography. Sci Rep. 2020;10:19196. https://doi.org/10.1038/s41598-020-76282-0 .
Article CAS PubMed PubMed Central Google Scholar
Tuttle KR. Impact of the COVID-19 pandemic on clinical research. Nat Rev Nephrol. 2020;16(10):562–4.
Cui L, Lee D. Coaid: Covid-19 healthcare misinformation dataset. arXiv preprint arXiv:2006.00885. 2020. https://doi.org/10.48550/arXiv.2006.00885 .
Miller IF, Becker AD, Grenfell BT, Metcalf CJE. Disease and healthcare burden of COVID-19 in the United States. Nat Med. 2020;26(8):1212–7.
Article CAS PubMed Google Scholar
Kapoor A, Guha S, Das MK, Goswami KC, Yadav R. Digital healthcare: the only solution for better healthcare during COVID-19 pandemic? Indian Heart J. 2020;72(2):61–4. https://doi.org/10.1016/j.ihj.2020.04.001 .
Chatterjee K, Kumar A, Shankar S. Healthcare impact of COVID-19 epidemic in India: a stochastic mathematical model. Med J Armed Forces India. 2020;76(2):147–55.
Shreffler J, Petrey J, Huecker M. The impact of COVID-19 on healthcare worker wellness: a scoping review. West J Emerg Med. 2020;21(5):1059.
Khan ZH, Siddique A, Lee CW. Robotics utilization for healthcare digitization in global COVID-19 management. Int J Environ Res Public Health. 2020;17(11):3819. https://doi.org/10.3390/ijerph17113819 .
Huang L, Han R, Ai T, Yu P, Kang H, Tao Q, Xia L. Serial quantitative chest CT assessment of COVID-19: a deep learning approach . Radiol Cardiothorac Imaging. 2020;2(2):e200075. https://doi.org/10.1148/ryct.2020200075 .
Qi X, Jiang Z, Yu Q, Shao C, Zhang H, Yue H, Ma B, Wang Y, Liu C, Meng X. Machine learning-based CT radiomics model for predicting hospital stay in patients with pneumonia associated with SARS-CoV-2 infection: a multicenter study. Ann Transl Med. 2020;8(14):859. https://doi.org/10.21037/atm-20-3026 .
Gates B. Responding to Covid-19—a once-in-a-century pandemic? N Engl J Med. 2020;2020(382):1677–9.
Article Google Scholar
Larson HJ, De Figueiredo A, Xiahong Z, Schulz WS, Verger P, Johnston IG, Jones NS. The state of vaccine confidence 2016: global insights through a 67-country survey. EBioMedicine. 2016;2016(12):295–301.
New York University. App Determines COVID-19 Disease Severity Using Artificial Intelligence, Biomarkers. 2020. Available online: https://www.sciencedaily.com/releases/2020/06/200603132529.htm . Accessed 14 Sep 2020.
WHO: A benefit of Vaccination on COVID 19 https://www.cdc.gov/coronavirus/2019-ncov/vaccines/vaccine-benefits.html . Accessed 20 Sep 2019.
Imran A, Posokhova I, Qureshi HN, Masood U, Riaz MS, Ali K, John CN, Hussain MI, Nabeel M. AI4COVID-19: AI enabled preliminary diagnosis for COVID-19 from cough samples via an app. Inform Med Unlocked. 2020;20:100378. https://doi.org/10.1016/j.imu.2020.100378 . Epub 2020 Jun 26.
Senior A, Jumper J, Hassabis D, Kohli, P. AlphaFold: Using AI for Scientific Discovery. DeepMind 2018. 2020. Available online: https://deepmind.com/blog/alphafold . Accessed 28 Oct 2020.
Mathieu E, Ritchie H, Ortiz-Ospina E, Roser M, Hasell J, Appel C, Giattino C, Rodés-Guirao L. A global database of COVID-19 vaccinations. Nat Hum Behav. 2021. https://doi.org/10.1038/S41562-021-01122-8 .
HospiMedica. Scientists Use Cloud-Based Supercomputing and AI to Develop COVID-19 Treatments and Vaccine Models. 2020. Available online: https://www.hospimedica.com/covid-19/articles/294784537/scientists-use-cloud-based-supercomputingand-ai-to-develop-covid-19-treatments-and-vaccine-models.html . Accessed 15 Sep 2020.
Ciardi F, Menon V, Jensen JL, Shariff MA, Pillai A, Venugopal U, Kasubhai M, Dimitrov V, Kanna B, Poole BD. Knowledge, attitudes and perceptions of COVID-19 vaccination among healthcare workers of an Inner-City Hospital in New York. Vaccines (Basel). 2021;9(5):516. https://doi.org/10.3390/vaccines9050516 .
Gravelle TB, Phillips JB, Reifler J, Scotto TJ. Estimating the size of “anti-vax” and vaccine hesitant populations in the US, UK, and Canada: comparative latent class modeling of vaccine attitudes. Hum Vaccine Immunother. 2022;18(1):2008214. https://doi.org/10.1080/21645515.2021.2008214 .
Article CAS Google Scholar
owid-datasets/datasets/Political Regime . OWID based on Polity IV and Wimmerand Min at master owid/owid-datasets · GitHub . (n.d.). Retrieved July 9, 2021, from https://github.com/owid/covid-19-data/blob/master/public/data/vaccinations/README.md .
owid-datasets/datasets/Cross-country literacy rates. World Bank, CIA World Factbook, and other sources at master · owid/owid-datasets · GitHub . (n.d.). Retrieved July 10, 2021, from https://github.com/owid/owid-datasets/tree/master/datasets/Cross-country%20literacy%20rates%20-%20World%20Bank%2C%20CIA%20World%20Factbook%2C%20and%20other%20sources .
Current health expenditure per capita (current US$) | Data . (n.d.). Retrieved July 10, 2021, from https://data.worldbank.org/indicator/SH.XPD.CHEX.PC.CD .
Ward JK, Peretti-Watel P, Bocquier A, Seror V, Verger P. Vaccine hesitancy and coercion: all eyes on France. Nat Immunol. 2019;20(10):1257–59. https://doi.org/10.1038/s41590-019-0488-9 .
Yoda T, Katsuyama H. Willingness to receive COVID-19 vaccination in Japan. Vaccines (Basel). 2021;9(1):48. https://doi.org/10.3390/vaccines9010048 .
Ward JK, Alleaume C, Peretti-Watel P, COCONEL Group. The French public’s attitudes to a future COVID-19 vaccine: the politicization of a public health issue. Soc Sci Med. 2020;265:113414. https://doi.org/10.1016/j.socscimed.2020.113414 .
Bar E, Lehrman Y, Mukhsan H. The need to narrow gaps in vaccinations against the new Corona virus (SARS-CoV2): the ultra-orthodox and Arab population. Harefuah. 2021;160(5):285–90.
Google Scholar
Download references
Acknowledgements
We thank all the organizations involved for research throughputs and supportive discussions in the development of this research project.
No funding available.
Author information
Authors and affiliations.
Centre for Computational Biology and Bioinformatics, Amity University, Noida, Uttar Pradesh, India
Ritu Chauhan
Amity Institute of Information Technology, Amity University, Noida, Uttar Pradesh, India
Gatha Varma
Faculty of Engineering and Information Technology, University of Technology Sydney, Sydney, New South Wales, Australia
UniKL – LR Univ Joint ICT Laboratory (KLR-JIL), Universiti Kuala Lumpur, Malaysia – La Rochelle University, France, Kuala Lumpur, Malaysia
Megat F. Zuhairi
You can also search for this author in PubMed Google Scholar
Contributions
R.C & G.V wrote the main manuscript. The theoretical concept was designed by R.C while the structural content and framework was designed by E.Y & M.F.Z. The implementation of research was conducted by R.C and G.V. The author(s) read and approved the final manuscript.
Corresponding author
Correspondence to Megat F. Zuhairi .
Ethics declarations
Ethics approval and consent to participate.
The data used for the analysis was published by Our World in Data under the Creative Commons BY license. All methods were performed in accordance with the relevant guidelines and regulations. The datasets generated and/or analyzed during the current study are available in the following repositories.
https://github.com/owid/covid-19-data/blob/master/public/data/vaccinations/README .
https://github.com/owid/owid-datasets/tree/master/datasets/Cross-country%20literacy%20rates%20-%20World%20Bank%2C%20CIA%20World%20Factbook%2C%20and%20other%20sources .
https://data.worldbank.org/indicator/SH.XPD.CHEX.PC.CD .
Not applicable.
Consent for publication
Competing interests.
The authors declare no competing interests.
Additional information
Publisher’s note.
Springer Nature remains neutral with regard to jurisdictional claims in published maps and institutional affiliations.
Rights and permissions
Open Access This article is licensed under a Creative Commons Attribution 4.0 International License, which permits use, sharing, adaptation, distribution and reproduction in any medium or format, as long as you give appropriate credit to the original author(s) and the source, provide a link to the Creative Commons licence, and indicate if changes were made. The images or other third party material in this article are included in the article's Creative Commons licence, unless indicated otherwise in a credit line to the material. If material is not included in the article's Creative Commons licence and your intended use is not permitted by statutory regulation or exceeds the permitted use, you will need to obtain permission directly from the copyright holder. To view a copy of this licence, visit http://creativecommons.org/licenses/by/4.0/ . The Creative Commons Public Domain Dedication waiver ( http://creativecommons.org/publicdomain/zero/1.0/ ) applies to the data made available in this article, unless otherwise stated in a credit line to the data.
Reprints and permissions
About this article
Cite this article.
Chauhan, R., Varma, G., Yafi, E. et al. The impact of geo-political socio-economic factors on vaccine dissemination trends: a case-study on COVID-19 vaccination strategies. BMC Public Health 23 , 2142 (2023). https://doi.org/10.1186/s12889-023-17000-z
Download citation
Received : 15 December 2021
Accepted : 16 October 2023
Published : 02 November 2023
DOI : https://doi.org/10.1186/s12889-023-17000-z
Share this article
Anyone you share the following link with will be able to read this content:
Sorry, a shareable link is not currently available for this article.
Provided by the Springer Nature SharedIt content-sharing initiative
- Vaccination
- Prognosis and diagnosis
- Artificial intelligence
- Machine learning
BMC Public Health
ISSN: 1471-2458
- Submission enquiries: [email protected]
- General enquiries: [email protected]
Cookies on GOV.UK
We use some essential cookies to make this website work.
We’d like to set additional cookies to understand how you use GOV.UK, remember your settings and improve government services.
We also use cookies set by other sites to help us deliver content from their services.
You have accepted additional cookies. You can change your cookie settings at any time.
You have rejected additional cookies. You can change your cookie settings at any time.

- Society and culture
- Community and society
- Social investment
Socio-economic case studies
Examples of projects that are supported by the NDA group socio-economic programme.
As a responsible organisation and under a legal obligation in the Energy Act (2014), the NDA takes due regard of the impact of its activities in the communities around its sites.
The NDA group socio-economic funding is intended to help local communities who are or will be impacted by the changing levels of economic activity at our sites. If local communities are particularly reliant on nuclear sites, we work with local partners to help develop alternative economic opportunities.
We also fund projects that develop local skills and help businesses take advantage of the economic opportunities from decommissioning. Funding is allocated based on an assessment of local needs and on the basis of a competitive process of projects and organisations meeting local needs.
The case studies in this collection are examples of such projects.
- 17 April 2018
- 3 April 2018
- 23 March 2018
- 30 October 2017
- 14 January 2020
- 13 April 2018
- 4 December 2019
Related content
Is this page useful.
- Yes this page is useful
- No this page is not useful
Help us improve GOV.UK
Don’t include personal or financial information like your National Insurance number or credit card details.
To help us improve GOV.UK, we’d like to know more about your visit today. We’ll send you a link to a feedback form. It will take only 2 minutes to fill in. Don’t worry we won’t send you spam or share your email address with anyone.
Accessibility Links
- Skip to content
- Skip to search IOPscience
- Skip to Journals list
- Accessibility help
- Accessibility Help
Click here to close this panel.

As a society-owned publisher with a legacy of serving scientific communities, we are committed to offering a home to all scientifically valid and rigorously reviewed research. In doing so, we aim to accelerate the dissemination of scientific knowledge and the advancement of scholarly communications to benefit all.
Environmental Research Communications supports this mission and actively demonstrates our core values of inclusive publishing and trusted science . To find out more about these values and how they can help you publish your next paper with us, visit our journal scope .
Purpose-led Publishing is a coalition of three not-for-profit publishers in the field of physical sciences: AIP Publishing, the American Physical Society and IOP Publishing.
Together, as publishers that will always put purpose above profit, we have defined a set of industry standards that underpin high-quality, ethical scholarly communications.
We are proudly declaring that science is our only shareholder.
A comprehensive analysis of impacts of socio-economic development and land use on river water quality in a megacity-region: a case study
Liying Zhu 1,2 , Yuanyuan Chen 1 , Yawei Wang 1 , Yuansong Wei 5,1,2,3 , Hua Zheng 4 and Yuxin Zhang 4
Published 23 February 2023 • © 2023 The Author(s). Published by IOP Publishing Ltd Environmental Research Communications , Volume 5 , Number 2 Citation Liying Zhu et al 2023 Environ. Res. Commun. 5 025006 DOI 10.1088/2515-7620/acbbbd
Article metrics
824 Total downloads
Share this article
Author e-mails.
Author affiliations
1 State Key Joint Laboratory of Environment Simulation and Pollution Control, Research Center for Eco-Environmental Sciences, Chinese Academy of Sciences, Beijing 100085, People's Republic of China
2 Laboratory of Water Pollution Control Technology, Research Center for Eco-Environmental Sciences, Chinese Academy of Sciences, Beijing 100085, People's Republic of China
3 University of Chinese Academy of Sciences, Beijing 100049, People's Republic of China
4 State Key Laboratory of Urban and Regional Ecology, Research Center for Eco-Environmental Sciences, Chinese Academy of Sciences, Beijing 100085, People's Republic of China
Author notes
5 Author to whom any correspondence should be addressed.
Liying Zhu https://orcid.org/0000-0001-9194-6990
- Received 18 October 2022
- Revised 17 November 2022
- Accepted 13 February 2023
- Published 23 February 2023
Peer review information
Method : Single-anonymous Revisions: 1 Screened for originality? Yes
Buy this article in print
A comprehensive analysis of impacts of socio-economic development and land use on water quality is very useful for better planning and management of river basins by government. In this study, the North Canal River through a megacity-region of Beijing-Tianjin-Hebei Province in China was chosen to quantify impact of 10 socio-economic factors and 6 land use types on water quality in the watershed. The principal component regression (PCR) method was thus applied in this study to quantify effects of socio-economic development and land use types on river water quality through overcoming multicollinearity problems among influencing factors. Results showed that the water quality in the North Canal River improved significantly from serious pollution. Compared with 2010, the annual average pollution index (PI) of COD, NH 3 –N, TN, and TP decreased significantly in 2018, especially the PI of NH 3 –N decreased the most, from 8.62 in 2010 to <1 in 2018, implying that the concentration of NH 3 –N basically met the water quality assessment standard in 2018. The major water pollutant in the basin had shifted from NH 3 –N in 2010 to TN in 2018. The absolute impact coefficients of industrial restructuring, population density, upgraded municipal sewage treatment requirements (including sewage treatment rate and discharge standards of water pollutants for municipal WWTPs), and urbanization rate with COD, NH 3 –N and TP were higher than those of other factors, indicating that the impact of socio-economic factors on water quality was more significant than that of land use types, and the socio-economic factors may offset or alter the impact of land use on river water quality in areas disturbed by human activities.
Export citation and abstract BibTeX RIS
Original content from this work may be used under the terms of the Creative Commons Attribution 4.0 licence . Any further distribution of this work must maintain attribution to the author(s) and the title of the work, journal citation and DOI.
1. Introduction
Cities are the concentration of capital, labor, and information, and are important regions reflecting the degree of national development. With rapid economic development, individual cities drive neighboring cities to form highly developed integrated urban spatial patterns (Glazer et al 2003 , Mossay et al 2020 ). Under this urban development pattern, economic resources were rapidly integrated, urbanization process was further accelerated, and population further aggregated and increased, leading to further demands on ecological environment, such as adequate water resources and a clean water environment. However, the limited resources of watersheds lead to problems such as water scarcity and water environment pollution occurring frequently in developing countries, especially to countries or regions with rapid economic growth (Vörösmarty et al 2010 , Xu et al 2019 , Hong et al 2022 ), which poses higher requirements for the environmental protection and management of urban river watershed (Shi et al 2017 ). River pollution is influenced by a variety of anthropogenic and natural factors, such as land use, socio-economic status, and climate variations (Chen et al 2016 , Luo et al 2019 , Luo et al 2020 ). Understanding the variation of river water quality and identifying the key influencing factors are important for developing sustainable watershed management policies. Therefore, an in-depth analysis of the factors influencing river water quality based on socio-economic and land use changes is of great concern to guide future planning and management of urban development from the point view of watershed management.
A number of previous studies have identified the relationship between river water quality and land use types. Different land use patterns vary widely in soil nutrient cycling, topography and vegetation, and these environmental parameters are capable of altering the transport and transformation of pollutants in the watershed, ultimately leading to degradation of river water quality (Seitz et al 2011 , Liu et al 2022 ). For example, build-up land use and agricultural land use were significantly and positively correlated with pollutant concentrations in river, while grassland and the undeveloped areas were negatively correlated with pollutant concentrations (Peterjohn and Correll 1984 , Records et al 2016 ). Stets et al ( 2020 ) explored the factors influencing water quality by analyzing water quality changes in U.S. streams and rivers from 1982–2012, showing that undeveloped sites had the lowest concentrations for all nutrients, agricultural sites had the highest concentrations of the total nitrogen (TN), nitrate nitrogen (NO 3 − ), and total organic carbon (TOC) and urban sites had the highest total phosphorus (TP) concentration.
Socio-economic factors such as human population, population density, gross domestic product (GDP) and gross domestic product per capita (GDPPC) have a more complex impact on river water quality (Wang et al 2008 , Chen et al 2016 , Zhou et al 2019 , Feng et al 2021 ). The most direct impact is the discharges of urban domestic sewage and industrial wastewater, which are related to the population density and industrial production mode. Estrada-Rivera et al ( 2022 ) reported that population growth, poorly planned industrial development and uncontrolled production processes caused serious pollution of water quality in the Alto Atoyac watershed. The water parameters (BOD 5 , COD and TSS) were positive with the population. Feng et al ( 2021 ) reported that industrial wastewater compliance discharge rate and sewage treatment were the main socio-economic factors affecting the water quality (i.e., COD, NH 3 -N, TN, and TP) of Dongting Lake. Luo et al ( 2019 ) studied the socio-economic system consisting of various factors such as population density, GDP and sewage treatment rate with the river system consisting of COD, TN, TP. etc, and found that socio-economic and river systems were highly related to each other with the average influence degree of greater than 0.9, indicating very close relationships between socio-economic and river systems. Chen and Lu ( 2014 ) considered that population density and GDPPC explained 60.8% of the total overall river water quality variances in East China. The study also claimed that urban area was strongly correlated with major river water quality parameter distributions.
Within natural or semi-natural areas, the effects of land use types on water quality are similar to different geographical areas at the watershed scale due to low intensity of human activities and similar land use characteristics (Wang et al 2008 , Fu et al 2020 , Omidvar et al 2021 ). Nevertheless, in the areas more heavily disturbed by human activities, the types of nutrients in water bodies and the characteristics of their accumulation concentrations may be different from the same land use type. Especially in urban areas, the impact of human activities causes heterogeneity not only in land use types, but also in the types and levels of socio-economic activities carried by the same land use type (Luo et al 2019 , Liu et al 2022 ). The alterations in land use might be thus linked uncertainly towards changes in water quality, and the effect on water quality may be offset or reduced due to socio-economic differences, resulting in deviations between the analysis results and the reality. Liu et al ( 2012 ) indicated that agricultural land use and social-economic factors had no significant correlation with all eutrophication parameters in Yunnan Plateau lakes. However, in megacity regions, few investigations have simultaneously analyzed the relative importance of both land use types and factors associated with socio-economic development (i.e., industry, population, and technology, etc) to address variation pattern of river water quality. The complexity of human activities in megacity areas and the multiple and complex factors influencing water pollution in watersheds require more in-depth data mining. Comprehensive analysis of land use patterns and socio-economic development in the watershed on the impact of water quality changes, and specification of land use types and economic development patterns can provide implementation and application to guide landscape planning and establish best management practices in the watershed.
Recently, Pearson or spearman-R correlation is commonly used to determine the relationships between river water quality and land use types (Xu et al 2019 , Krishnaraj and Deka 2020 ), which is simple but not quantitative approach to interrelationships. Cluster analysis (CA), principal component analysis (PCA) (Chadwick et al 2006 , Fu et al 2020 ), and redundancy analysis (RDA) (Chen and Lu 2014 ) provides only quantitative information about the rate of variance explained by variables through reducing the dimensionality of the data set and using a few of the most important factors. Multiple stepwise regression (MSR) (Liu et al 2012 , Liu et al 2018 , Fu et al 2020 ), and canonical correlation analysis (CCA) (Luo et al 2019 , Feng et al 2021 ) provides quantitative ways to interpret complex water quality variations. MSR method is widely employed to determine the land use patterns that best explain the spatial variability of individual river water quality variables. However, the problem of multicollinearity among influencing factors is the primary obstacle to the application of these methods. Strongly intercorrelated variables were removed, leaving the remaining variables for further MSR or CCA analysis (Liu et al 2018 , Luo et al 2019 ). Nevertheless, intercorrelations between variables are common, removing any variable will have an impact on the results of the analysis. Chen et al ( 2016 ) considered the correlation of factors and applied a modified geographically weighted regression to analyze the impacts of land use and population density on surface water quality.
With this background in mind, this study tries to apply principal component regression (PCR), which allows to disregard the problem of multicollinearity among factors and quantify the effects of both land use and socio-economic factors on a single river water quality indicator. This approach is a regression analysis technique based on principal component analysis (PCA) in statistics, which has been widely used in medicine and socio-economics (Jolliffe 1982 , Tu and Peng 2012 ). The main use of PCR is to overcome multicollinearity problems, which arises when two or more explanatory variables are close to being collinear. Therefore, the problem of multicollinearity among indicators need not be addressed in the application (Dodge and Commenges 2006 , James et al 2013 , Lee et al 2015 , Chen et al 2016 ).
The objective of this study is thus to factor analyze the water quality of rivers influenced by socio-economic and land use in the megacity area, to explore the influencing factors of the river water quality and to quantify the degree of influence. The North Canal River was taken as the study site, which is located in the megacity-region of Jing-Jin-Ji Urban Economic Circle including Beijing, Tianjin and Hebei Province, the most important urban agglomeration in northern part of China. In this region, more studies have focused on the impact of land use on a particular river water quality indicator and the distribution characteristics of pollution sources (Dai et al 2015 , Liu et al 2018 , Liao et al 2022 , Liu et al 2023 ). Liu et al ( 2018 ) studied the effect of landscape pattern on riverine nitrogen pollution sources and found that the interspersion and juxtaposition index of forest land was negatively related with nitrate. Few studies have been done on the impact of socio-economics on river water quality in the region. Therefore, during a 2-year (2010 and 2018) monitoring period, a dataset of water quality parameters, socio-economic and land use factors was analyzed using pollution index (PI), Pearson correlation and PCR analysis to (1) identify spatial and temporal trends of river water quality; (2) clarify the correlation between socio-economic factors, landscape patterns and river water quality; (3) quantify the key influencing factors on river water quality; and (4) provide more information and references for decision makers in managing and controlling river water pollution in the megacity-region.
2. Materials and methods
2.1. study area.
The North Canal River crosses through the mega-region of Jing-Jin-Ji Urban Economic Circle. Originating from Yanshan Mountain in Beijing, the river flows 143 km mostly central through Beijing city, Langfang City of Hebei Province, and Tianjin City with a population of 25.33 million, and ultimately joins the Hai River (figure 1 ). The total area of the North Canal River basin is 6,166 km 2 , of which 952 km 2 are mountainous area and 5,214 km 2 are plain area. With hills in the northwest and plains in the southeast, surface elevation in the North Canal River ranges from 20–1500 m. Located in a warm temperate monsoon climate zone, the river basin has four distinctive seasons with an annual average temperature of 10 °C–12 °C. The annual average rainfall ranges from 500 mm to 700 mm, most of which in the summer months from July to September (Guo et al 2012 ).
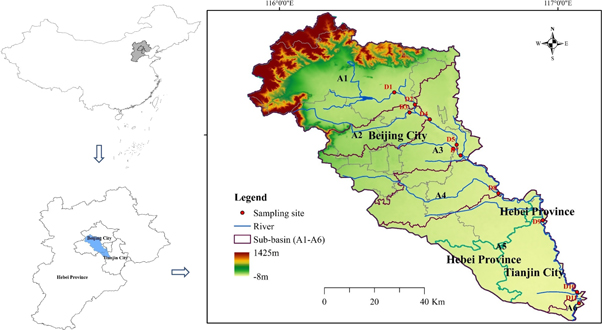
Figure 1. Summary of the North Canal River basin.
Download figure:
The North Canal River has been facing the challenge of water pollution control and river restoration. In 2021, it carried 8% of the country's population on 2.3% of the land, with a population density of more than 20 times the national average and generated 8.4% of the gross domestic product (NBS 2022 ). The river undertakes 90% responsibility of the drainage from Beijing central area, which produces the effluent from the main WWTPs (Liu et al 2018 ). In 2010, the effluent from WWTPs in the river basin were implemented the Discharge Standard for pollutants for municipal wastewater treatment plants (GB 18918–2002). However, to speed the improvement of surface water quality, Beijing and Tianjin implemented more stringent local Discharge Standard of Pollutants for Municipal Wastewater Treatment Plant in 2015 and 2018, namely (DB11/890–2012) and (DB12/599–2015), respectively. According to the Class A of these standards, limit values of chemical oxygen demand (COD), ammonia nitrogen (NH 3 -N) and total phosphorus (TP) discharge are equal to those of Class IV and V of the Surface Water Quality Standard of China (GB 3838–2002), respectively. According to the latest 2021 Annual Report of Beijing Ecology and Environment Statement, roughly 32% of the water quality in Beijing section of the North Canal River was assessed as Class IV and V of (GB 3838–2002).
2.2. Water quality monitoring and sample analysis
In this study, 11 sample sites (D1 - D11) were selected along the mainstream and its major tributaries as well as the confluence, considering the characteristics of the mainstream and tributaries, land use and land cover (LULC) types, and accessibility. Water sampling at 11 sites was carried out each month from March to November in 2010 and 2018, respectively, with each time completed within two days, except water sampling was not possible in January due to river frozen. Each site of water quality samples collected in accordance with the 'Water quality—Guidance on sampling techniques (HJ 494-2009)' ( https://www.mee.gov.cn/ywgz/fgbz/bz/bzwb/jcffbz/200910/W020111114543133505806.pdf ), the collection from at least three locations at a depth of 0.5 m below the surface water of parallel water samples (cross-sectional samples). Water samples were transported to the laboratory in an incubator with ice for chemical analysis within 48 h. Four water quality indicators COD, NH 3 -N, TN, and TP were selected, which are the main indicators of water quality management assessment of the North Canal River. All water quality samples were measured using standard analytical methods (EPAC 2002 ).
2.3. Factors of water quality
Two categories of basin characteristics were included in the PCR models to evaluate their impacts on the water quality of the North Canal River (table 1 ). The socio-economic system (SES) impact on the water quality was studied by using data in 2010 and 2018, respectively. Most studies reported that the SES factors under the human activities, such as industrial pattern (Ning et al 2018 ), output value (Chen and Lu 2014 , Zhou et al 2019 ), population (Chen et al 2016 , Hong et al 2022 ), urbanization (Luo et al 2019 , Luo et al 2020 ), and pollution load (Ning et al 2018 , Liu and Yu 2022 ), are the main factors affecting water quality. Therefore, based on the actual development situation of the North Canal River basin, ten variables of the SES (table 1 ) were selected for the potential influencing factors of river water quality. The variables data of socio-economic system are mainly from the 'Beijing Region Statistical Yearbook' ( http://nj.tjj.beijing.gov.cn/nj/qxnj/2020/zk/indexch.htm ), 'Tianjin Statistical Yearbook' ( http://stats.tj.gov.cn/nianjian/2019nj/zk/indexch.htm ) and 'Heibei Statistical Yearbook' ( http://www.hetj.gov.cn/hetj/tjsj/jjnj/101556102743865.html ). The 30 m digital elevation model (DEM) data was obtained from the National Geomatics Center of China, which was used to delineate the control ranges area of each water sampling site (sub-basin) and calculate its total area using ArcGIS version 10.2 (ESRI, USA). The process is as follows: the coordinate position of each sampling site was used as an outlet to obtain the control of its control range for each sampling site, and then a series of hydrological analysis tools in GIS, including Fill, Flow Direction, Snap Pour Point (sampling point coordinates), Stream Link, Stream Order and watershed, etc, were used to divide each sub-basin. Finally, the North Canal River basin was thus divided into 6 sub-basins (from A1 to A6) (Xu et al 2019 , Mossay et al 2020 , Zhang et al 2020 ). The land use types of the study area were classified with reference to the land classification system of the Resource and Environment Data Center of the Chinese Academy of Sciences ( https://www.resdc.cn/ ). By supervised classification of Landsat-TM images in 2010 and 2018, the LULC of the study area was classified into six main types of Croplands, Forestland, Grassland, Water, Build-up land, and Unused land, respectively (table 1 ). After random sampling verification and Kappa coefficient accuracy evaluation, the accuracy of land use classification results in both phases was higher than 85% (Xu et al 2018 ) (table 1 ). LULC composition of each sub-basin in 2010 and 2018, respectively was then calculated.
Table 1. Potential influencing factors of water quality.
Since data for SES variables were obtained on an administrative area basis, LULU types of area data were calculated on the basis of the study area, and river system indicators were obtained through field monitoring, the types of data do not match on temporal and spatial scales (de Lange et al 2010 , Feng et al 2021 ). Therefore, it is necessary to convert them to the same scale before proceeding with further studies. For the time scale, data for SES variables (i.e., population, industry, output value and sewage treatment rate, etc), obtained from statistical yearbooks, and together with land use types are considered to be stable over the year and could be evenly distributed over the year and thus converted into monthly data. Thus, these data were converted to the same time scale as the river system data. On the spatial scale, data on SES variables obtained on an administrative area basis can be assigned to sub-basins by percentage of area weighting; in addition, the arithmetic mean concentration of the sampling sites in the sub-basin was used as the water quality concentration of the sub-basin according to the confluence pattern of water bodies and pollutants in rivers. Throughout these processes, the SES, LULC types and water quality indicators can be therefore converted to the same spatial and temporal scales for the further analysis.
2.4. Statistical analysis
To quantitatively describe the important contribution of the SES and LULC types for water quality variation in a megacity-region of China, firstly, Pearson correlation was performed to observe the coupling-feedback relationship for the SES, LULC types and water quality in the North Canal River basin in 2010 and 2018, respectively. Followed by applying PCR approach to further investigate the main influencing factors of water quality variations over the past 10 years. Notably, all variables are standardized before the analysis.
In this study, the SES and LULC system consists of sixteen indicators (table 1 ), and the water quality system consists of eight indicators, which are COD, NH 3 -N, TN and TP in 2010 and 2018, respectively. The main idea of PCR approach is to replace the explanatory variables with principal components by PCA, then regress the principal components as explanatory variables with outcome variables to obtain the estimated regression coefficients, and finally the coefficients of explanatory variables in PCR are each linear combination of loading in PCA and the coefficients of principal components. Those coefficients of explanatory variables in PCR can explain their effect of output variables. We wanted to investigate the degree to which each water quality indicator was affected by the SES and LULC system in 2010 and 2018. Therefore, we need to run one PCR model simulation for each water quality indicator in 2010 and 2018, which means we have to run the PCR model simulation for the eight times. Here, we explain the process using one PCR model simulation as an example. For example, the degree to which COD is influenced by the SES and LULC system in 2010. The process was as follows:
Firstly, PCA was performed on sixteen indicators of the SES and LULC system and COD (the input variables of the PCA model were represent by X) to extract the M number of principal component (PC), the results can be written as:
Secondly, PCR forms the derived input columns Z and then regresses COD on Z 1 , Z 2 , ... , Z M . Since the Z are orthogonal, this regression is just a sum of univariate regressions. In other words, PCR modeling was performed based on the obtained PC 1 , PC 2 , ..., PC M , which resulted as:
Finally, the influence degree of SES and LULC system on COD concentration was calculated as follows:
The Spatio-temporal variation of the conventional water quality parameters was studied by the pollution index (PI) (Wang et al 2008 ), which is calculated as follows:
Where PI m is the pollution index of the water quality parameter m . C m O is the standard concentration value, according to the definition of 'Environmental Quality Standard for Surface Water (GB3838-2002)', mg l −1 ; Cm is the actual measured concentration, mg l −1 . When PI m is greater than 1, it considered that the water quality of the sampling site is contaminated, otherwise, the water quality is not polluted. Various sections of the North Canal River were divided into different water quality functional zones, based on different water quality categories of 'Environmental Quality Standard for Surface Water (GB 3838-2002)'. In this study, the water quality standards of the sub-basin were determined according to the standards of the water quality functional zone where the sampling sites were located (tables S1 and S3).
3.1. Spatio-temporal variability in water quality
PI m of water quality parameters showed that the water quality in the North Canal River improved significantly in the past 10 years (table 2 ). The major water pollutant has shifted from NH 3 -N to TN. The annual average PI of COD, NH 3 -N, TN, and TP in 2010 were 1.77, 8.62, 11.25 and 5.47, respectively, with TN showing the highest PI, followed by NH 3 -N. In 2018, the overall annual average PI of each parameter decreased significantly, with PI of TN being the highest, while PI of NH 3 -N decreased from 8.62 in 2010 to basically attain the standard for the entire basin (except for A1, table S3), and PI values of COD and TP decreased to below 1.5.
Table 2. Spatio-temporal variability of PI in 2010 and 2018, respectively.
<1 indicated that the indicator achieved the water quality standard of the water function zoneC:N:P Ratio(m:m:m) * was calculated by the concentrations of COD, TN and TP
Figure 2 shows significant spatial differences in the water quality in the sub-basin. In 2010 and 2018, water quality in A2 and A3 sub-basin was the best, followed by A6, and A1 water quality was the worst. The water quality of each sub-basin improved in general, but the improvement trend was varied. NH 3 -N concentration in A1 decreased most dramatically, from 19.08 mg l −1 in 2010 to 2.19 mg l −1 in 2018. Followed by A2 and A3, NH 3 -N concentration was observed with an average decrease of 14.94 mg l −1 in the last 10 years, and then the decreasing trend declined along the spatial gradient. Specifically, TN:TP ratio in A2 increased dramatically in the last decade, from 24.29 to 69.40, which is the most variable watershed in the North Canal Basin, followed by A3.
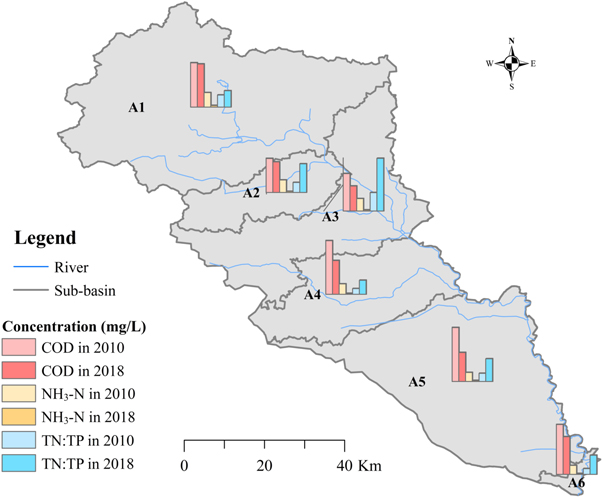
Figure 2. Concentration of water quality indicators in each sub-basin of the North Canal River Basin in 2010 and 2018.
3.2. Spatio-temporal variability in socio-economic system and land use
With the rapid socio-economic development and urbanization, the urban population has grown rapidly, and the overall economic strength of the whole basin has maintained rapid growth (figure 3 , table 1 ). From 2010 to 2018, the industrial structure of the North Canal River basin has undergone significant changing. In 2010, sub-basin A1, A2, A3 and A4 were dominated by PTI, followed by PSI; A5 and A6 were dominated by PSI, followed by PTI. In 2018, the entire basin was dominated by PTI, with the highest share of the PTI in A2 at 90.94%. From 2010 to 2018, GDP, GDPPC, and PD increased in all sub-basin, with A2 increasing the most at 97.24 billion RMB, followed by A3 and A1 at 84.21 billion RMB and 56.85 billion RMB, respectively. The GDPPC of A1 increased slowly due to PD. the GDPPC of A2 and A3 was the largest. FAA increased from 2010 to 2018 in A1 to A4, while decreased in A5 and A6. The UR, STR and FGR increased in each sub-basin from 2010 to 2018, with UR of A1, A2, A3 and A4 above 70% and UR of A6 being the lowest at 16.56% (2010) and 32.34% (2018), respectively. The average STR increased from 71. 26% (2010) to 89.74% (2018), with the greatest improvement in A1, which increased by 38.94% in the last decade. The average FGR increased from 33.11% (2010) to 40.84% (2018), with the largest increase (16.7%) in A6.
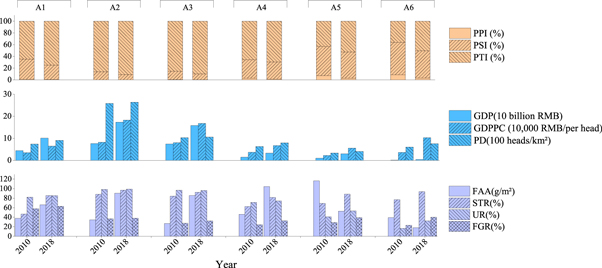
Figure 3. Socio-economic status in the North Canal River basin in 2010 and 2018.
The LULC changes in the North Canal River basin and its sub-basins are shown in figure 4 and table 3 . The main LULC types in the North Canal River basin were cropland, built-up land and forestland, and the sum of these three types accounted for more than 90% of the total area. The area of cropland has been decreasing each year, from 40.15% in 2010 to 33.02% in 2018. The area of forestland, grassland and water bodies increased, with the largest increase in forestland at 2.17%, followed by grassland at 1.49%. The scale of built-up land continued to expand from 42.12% in 2010 to 45.19% in 2018.
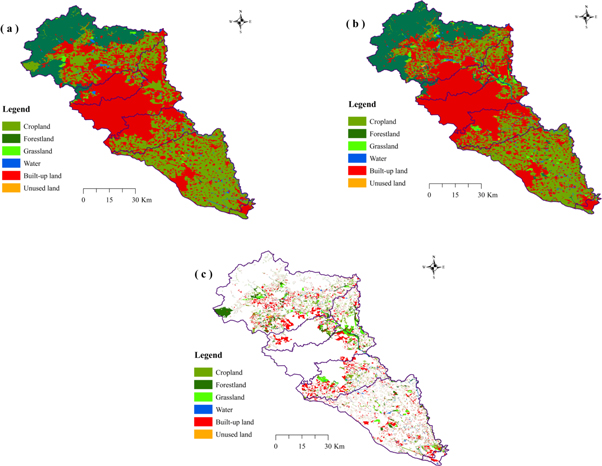
Figure 4. Spatial distribution of LUCC (a/b) and land transfer (c) in the North Canal River basin in 2010 and 2018.
Table 3. Area of LULC types in each sub-basin of the North Canal River Basin from 2010 to 2018.
The transfer of LULC types occurred mainly among cropland, forestland, water bodies and built-up land. From 2010 to 2018, the cropland area in each sub-basin decreased, but the types of land transfer were slightly varied. Cropland in A1 was mainly transferred to forestland, accounting for 41.29%; A2, A4, A5, and A6 areas were mainly transferred to built-up land, with the largest proportion of transfer in A6 area, accounting for 70.73%; and A3 area was mainly transferred to forestland, accounting for 44.43%. Unlike other sub-basins, only the area of water bodies in A2 decreased in the past decade.
3.3. Correlations among SES, LUCC and water quality
The correlation coefficients of SES, LULC types and water quality indicators were shown in figure 5 . Compared with LULC types, SES factors had significant impacts on water quality variations. GDP, the proportion of primary industry, population density and sewage treatment rate are the main factors. COD was significantly negatively correlated with GDP, PTI, GDPPC and STR, and significantly positively correlated with the proportion of PPI ( p < 0.05). NH 3 -N, TN and TP concentrations were all significantly negatively correlated with STR ( p < 0.05). TN:TP ratio was significantly and positively correlated with GDP and GDPPC, and significantly and positively correlated with STR ( p < 0.05), which should be related to wastewater treatment plant process.
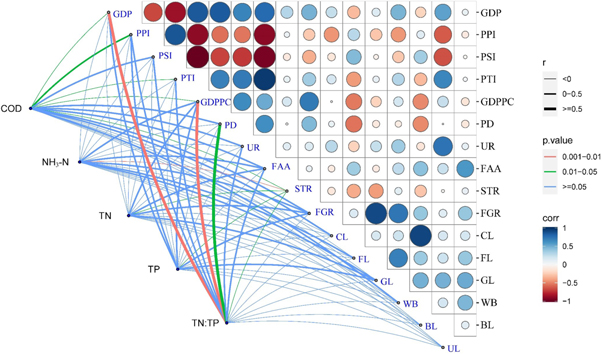
Figure 5. Pearson correlations between SES, LUCC and water quality indicators of North Canal River basin in 2010 and 2018 (Blue indicates positive correlation, red indicates negative correlation; circle size and color shade indicate the size of correlation coefficient, the larger the circle the darker the color indicates the larger the correlation coefficient. The blue line indicates p ≥ 0.05, the green line indicates p between 0.01 ∼ 0.05, and the red line indicates p < 0.01; the thicker the line, the larger the correlation coefficient value. See table 1 for description of abbreviations in the figure).
3.4. PCR analysis of the primary factors affecting water quality
As shown in figure 6 , consistent with the result of Pearson correlation, socio-economic factors have a significant impact on changes of the water quality, especially the industrial structure. the coefficient of COD and the PSI in 2010 is 13.45, indicating that for each unit increase in the PSI, the COD concentration will increase by 13.45 units; the coefficients of the PPI, PD and UR are −5.42, −12.24, and −8.18, which means that for each unit increase in PPI, PD and UR, COD concentration will decrease by 5.42, 12.24, and 8.18 units, respectively. Compared with 2010, the trend of COD in 2018 is consistent with the coefficient of each indicator, but all of them have different degrees of weakening. For example, the coefficient of COD and the PSI decreased to 6.29, and the coefficients of the PPI, PD and UR increased to −0.22, −5.99 and −4.75, respectively. The trend of the coefficient of NH 3 -N and each indicator is slightly different from that of COD, and the coefficient of NH 3 -N and PPI in 2010 was 6.78, which means for each unit increase in the PPI, the concentration of NH 3 -N will increase by 6.78 unit; the coefficient of NH 3 -N and PD is 0.07; the coefficient range of NH 3 -N and indicators in 2018 is −1 ∼ 1, which indicates that the degree of NH 3 -N pollution is greatly reduced in 2018. This is consistent with the change in water quality (table 2 and figure 2 ).
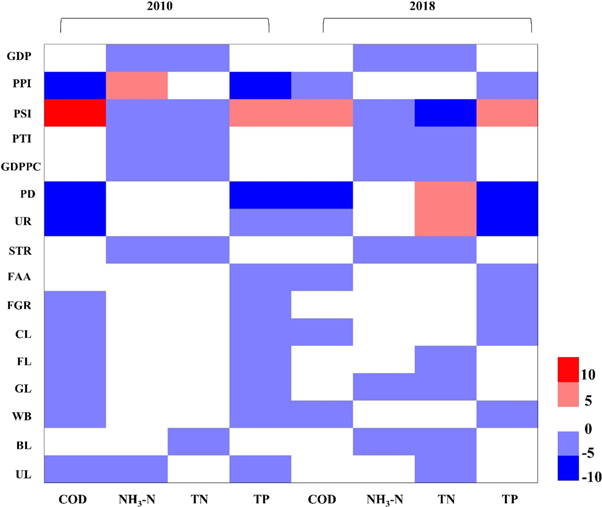
Figure 6. PCR analysis of the primary factors influencing water quality. (Color indicates PCR coefficient).
Compared with 2010, the relationship between TN concentration and some indicators changed obviously in 2018, the coefficient of PSI changed from −0.70 (2010) to −7.69 (2018), the coefficients of GDP, PPI, PTI, GDPPC decreased slightly, FAA and FGR increased slightly, and the coefficients of land use type indicators and TN ranged from −1 to 1.5, indicating that socio-economic The coefficients of PD and UR increased significantly from 0.94 and 1.28 in 2010 to 6.72 and 7.70, respectively, implying that the socio-economic population has a greater influence on TN. Compared to TN, the coefficients of TP with PPI and UR changed more, from −5.69 and −3.66 in 2010 to −1.31 and −7.90 in 2018, while the coefficients of TP with other indicators did not vary noticeably. In conclusion, land use type has a greater impact on COD in 2010 and a smaller impact on river water quality in 2018. The industrial structure, population and urbanization rate in the socio-economic system are the main factors influencing the river water quality.
4. Discussion
Both socio-economic development and land use significantly affect river water quality (Chen et al 2016 , Luo et al 2019 , Feng et al 2021 ). In this study, the absolute impact coefficients of PPI, PSI, PD and UR with COD, NH 3 -N and TP were higher than other factors, indicating that SES factors have a more significant impact on water quality in the North Canal compared to LULC types. Industrial restructuring (including PPI, PSI and PTI), population density (PD), upgraded sewage treatment requirements (including STR and discharge standards of water pollutants for municipal WWTPs), and FGR may offset or partially offset the negative impacts of urbanization development. Since the success of Beijing's Olympic bid in 2001, the North Canal River basin in Beijing as the main host area of the 2008 Olympic Games, Beijing has increased its efforts to improve the river water quality by implementing a series of engineering projects, making regulations and action plan for water pollution control, such as water pollution source control and reduction projects, upgrading discharge standards for municipal wastewater treatment and reclamation, river restoration and other management measures in a multipronged manner. Meanwhile, the area has been developed (figure 3 ). Especially A2, as the main host area of the Olympic Games, its COD, NH 3 -N and TP concentrations met with the water function zone requirements (Class III of GB 3838-2002, table S1) in 2018.
Firstly, in order to solve the 'big city disease' in Beijing and expand the environmental capacity and ecological space, China proposed the national strategy of Beijing-Tianjin-Hebei region coordinated development, to promote industrial upgrading and transfer (Dai 2003 , Yang and Pu 2018 ). As a result of industrial policy shift, industrial sectors in urban areas of Beijing were moved out. In this study, PPI and PSI of the North Canal River basin were decreased from 3.22% and 33.18% in 2010 to 1.23% and 27.33% in 2018, respectively (figure 3 ). A research report on the North Canal River basin (BMEE 2008 ) and a 2-year survey of major water pollutants entering the river by our group (Yu et al 2012 ) found that discharges from domestic, agricultural, and centralized treatment facility were the main pollution pathways in the basin in 2007, accounting for 31%, 35%, and 32% of the total COD emission, respectively. With a series of implementation projects, such as sewage interception along the river, river restoration, and centralized sewage treatment plants, the main inflow pollution load of the North Canal River in Beijing decreased significantly in 2010 compared to 2007, with COD and NH 3 -N decreasing by 3.08 × 10 4 t/a and 0.17 × 10 4 t/a, respectively (BMEE 2008 ). The black odor phenomenon of the North Canal River in Beijing was completely eliminated in 2011, and the major water pollutant was shifted from COD to NH 3 -N (Guo et al 2012 , Yu et al 2012 ). In this study, the coefficients of NH 3 -N with each indicator in 2018 ranged from −1 to 1, indicating that the pollution level of NH 3 -N was greatly reduced in 2018 (figure 6 ). Table 2 showed that NH 3 -N concentration of the North Canal River in Beijing met with the water function zoning target in 2018, and NH 3 -N pollution in Beijing basin then shifted to TN pollution.
Secondly, the spatial patterns of industrialization level and population density lead to differences in industrial wastewater and combined domestic wastewater discharges, which have different impacts on surface water (Chadwick et al 2006 , Luo et al 2019 ). In the North Canal River Basin, from 2010 to 2018, the proportion of primary and secondary industries continued to decrease by about 1.99% and 5.86%, while the proportion of tertiary industries increased by 7.89% and population density increased by 10.81%, resulting in integrated domestic wastewater treatment as the chief component of wastewater. In addition, the economy of the North Canal River basin has grown rapidly, with a 127% increase in GDP and an increment in urbanization from 67.63% to 73.40% from 2010 to 2018. The environmental investment also increased while maintaining growth in GDP. Accordingly, the capacity of wastewater treatment was increased by building more wastewater treatment plants and improving the technology. There were 7 WWTPs operated in the Beijing basin with a treatment capacity of 809,000 m 3 d −1 in 2007, but the number of WWTPs in operation increased to 19 with a treatment capacity of 2,050,800 m 3 d −1 in 2018, 2.53 times of that in 2007 (Zhu et al 2021 ). The increased number of WWTPs and upgraded wastewater treatment processes in the basin effectively reduced the input of pollutants into the river, contributed to the great improvement of river water quality, resulting in the large decrease in NH 3 -N concentration (table 2 ). This is consistent with the results of Stets et al ( 2020 ), who analyzed the concentrations and trends of various constituents in streams and rivers in different regions of the United States during 1982–2012.
Finally, on the technical level, with the development of the economy, local government could invest more costs to develop wastewater treatment technology and upgrade the effluent standard of wastewater treatment plants; therefore, a series of laws and regulations have been formulated and implemented in Beijing and Tianjin to further improve water quality (table S2). For example, Beijing has further refined the poor V surface water bodies into four classes of poor V1, poor V2, poor V3, and poor V4 since 2007. And Beijing local standard (DB11/890-2012), a stricter discharge standard for WWTPs has been implemented since December 31st, 2015 (table S2). According to this standard, the discharge limits of COD, NH 4 + -N and TP of WWTPs are equal to those of Class IV of (GB 3838-2002). In the North Canal River basin, the Wenyu River, Qing River and Ba River, as the main urban drainage rivers in urban areas of Beijing, the discharge of centralized sewage treatment facilities is its main source of recharge (Yu et al 2012 , Liu et al 2018 ), and the implementation of such upgrade standard has greatly improved the water environment quality of receiving water bodies in the basin and achieved the goal of Beijing's second 'three-year action plan ' in 2018. Areas with better socio-economic development (A2 and A3) have C:N:P ratios of river water quality similar to the effluent from WWTPs (tables 2 and S2). The improvement of socio-economic level contributes to the effective implementation of environmental policies. For example, the Clean Water Act (CWA) of 1972 focused on upgrading wastewater treatment plants to decrease point discharges of nutrients and organics, and the reauthorization of CWA in 1987 added important provisions on stormwater management (Patrick et al 1992 ). The implementation of these directly led to a significant downward trend in nutrient concentrations in urban areas throughout the United States (Carey and Migliaccio 2009 ).
In this study, the positive correlations between build-up land use with COD and TP in the North Canal River basin (figures 5 and 6 ) is consistent with several previous studies examining pollutant inputs to urban areas (Liu et al 2012 , Chen et al 2016 , Fu et al 2020 ). The higher percentage of impervious surfaces in urban areas prevents stormwater from infiltrating into the soil, resulting in the transport of pollutants in soluble and particulate form to nearby streams via surface runoff. For TN, Liu et al ( 2018 ) showed that industrial land, and built-up land were positively correlated with riverine nitrogen in the North Canal River basin of Beijing, which is contrary to the results of this study. In this study, considering socio-economic factors, build-up land use was negatively correlated with TN with an average coefficient of −0.64 in 2010 and 2018, respectively, indicating that socio-economic development may lead to differences in the effects of land use types on water quality. Therefore, the socio-economic characteristics cannot be ignored when investigating the factors affecting water quality in urban areas, especially areas exposed to intense human disturbance activities. A comprehensive analysis of water quality impacts on watersheds by integrating land use types and socio-economic factors may better reflect the trend of water quality variations.
This study demonstrated that water quality parameters were significantly influenced by socio-economic and land use composition. Furthermore, the relationships between these metrics and water quality can be quantitatively analyzed. Therefore, metric analysis offers a useful framework for indirectly indicating the association between socio-economic, landscape characteristics and water quality. PCR results indicated that in the megacity-region, socio-economic factors may offset or alter the impact of land use on water quality. Implementation of river restoration measures and policies, such as centralized treatment of domestic wastewater and upgrading the discharge standards of wastewater treatment plants to significantly improve water quality, which can further deepen our understanding of the phenomenon of water pollution and provide a reference for the government to develop new and innovative planning and management. In this study, PCR was used to analyze the impact of socio-economic and land use types on river water quality. In the future, the impact of water resources such as water flow rate, water level and climate factors such as precipitation on river water quality could be considered, which will be a better guide for river management with multiple dams.
5. Conclusion
In this paper, a PCR method that disregards the problem of multiple covariance among factors was used to quantify the impact of socio-economic and landscape indicators on the water environment. The results indicated that the water quality in the North Canal River improved significantly from 2010 to 2018, NH 3 -N concentration in the Beijing section met the water function zoning target in 2018. Relatively the best water quality was found in urban areas (A2 and A3) and the worst in sub-urban area (A1). Both socio-economic factors and land use factors have a significant impact on river water quality. However, as one of the typical representative areas of a megacity-region, the socio-economic variations in the North Canal River basin play a more important role in determining the water quality of the whole basin than the land use types. The absolute coefficients of industrial structure, population density and urbanization rate with COD, NH 3 -N and TP are higher than other factors, indicating that socio-economic factors may offset or alter the impact of land use on river water quality in areas disturbed by human activities.
Acknowledgments
We are grateful to all researchers who collected and processed samples for the monitoring program.
Data availability statement
All data that support the findings of this study are included within the article (and any supplementary files).
Author's contributions
All authors contributed to the work presented in the manuscript. Liying Zhu: Conceptualization, Methodology, Data analysis, Writing - original draft & editing. Yuanyuan Chen: Investigation, Data curation. Yawei Wang: Formal analysis, Data curation. Yuansong Wei: Conceptualization, Supervision, Writing—review & editing, Funding acquisition. Hua Zheng: original draft & editing. Yuxin Zhang: original draft & editing. All authors read and approved the final manuscript.
This work was supported by the National Major Science and Technology Projects for Water Pollution Control and Management of China (2017ZX07102; 2017ZX07102-002).
Declarations
The authors declare that they have no known competing financial interests or personal relationships that could have appeared to influence the work reported in this paper.
Ethics approval and consent to participate
Not applicable.
Consent for publication
Competing interests.
The authors declare no competing interests.
- Open access
- Published: 13 August 2018
Direct impacts of landslides on socio-economic systems: a case study from Aranayake, Sri Lanka
- E. N. C. Perera 1 ,
- D. T. Jayawardana 2 ,
- P. Jayasinghe 3 ,
- R. M. S. Bandara 3 &
- N. Alahakoon 4
Geoenvironmental Disasters volume 5 , Article number: 11 ( 2018 ) Cite this article
34k Accesses
25 Citations
Metrics details
Landslides area controversial issue worldwide and cause a wide range of impacts on the socio-economic systems of the affected community. However, empirical studies of affected environments remain inadequate for prediction and decision making. This study aims to estimate the direct impact of a massive landslide that occurred around areas with Kandyan home gardens (KHGs)in Aranayake, Sri Lanka.
Primary data were gathered by structured questionnaire from residents of the directly affected regions; the questionnaire data were combined with spatial data to acquire detailed information about the livelihoods and hazards at the household level. Satellite images were used to find affected land use and households prior to the landslide. Further, secondary data were obtained to assess the recovery cost. A multiple regression model was established to estimate the economic value of the home gardens. Field surveys and satellite images revealed that land-use practices during the past decades have caused environmental imbalance and have led to slope instability.
Conclusions
The results reveal that 52% of household income is generated by the KHG and that the income level highly depends on the extent of the land ( R 2 = 0.85, p < 0.05). The extent of destroyed land that was obtained from the satellite images and the age of the KHG were used to develop a multiple regression model to estimate the economic loss of the KHG. It was found that the landslide affected region had been generating approximately US$ 160,000 annually from their home gardens toward the GDP of the country. This study found that almost all houses in the area were at risk of further sliding, and all of them were partially or entirely affected by the landslide. Among the affected households, 60% (40 houses) had completelycollapsed, whereas 40% (27 houses) were partially damaged. Because of these circumstances, the government must provide US $ 40,369 to recover the fully and partially damaged households. Finally, a lack of awareness and unplanned garden cultivation were the main contributing factors that increased the severity of the damage.
Natural disasters are complex detrimental events that occur entirely beyond the control of humans (Alimohammadlou et al., 2013 ). Natural disasters can be classified based on the speed of onset; some disasters occur within seconds (landslides), minutes (tornadoes) or hours (flash floods and tsunamis) and others may take months or years to manifest themselves (droughts). Furthermore, rapid onset disasters such as landslide shave a massive impact on human life and property.
Landslides occur over a wide range of velocities and are recognized as the third most crucial natural disaster worldwide (Zillman, 1999 ). Landslides are usually triggered without warning, giving people less time to evacuate. Therefore, the direct impact of landslides on the socio-economic system is crucial (Christopher, 2016 ). Landslides are responsible for significant loss of life and injury to people and their livestock as well as damage to infrastructure, agricultural lands and housing (Schuster and Fleming, 1986 ; JRC, 2003 ; Blöchl and Braun, 2005 ; Guzzetti et al., 2012 ).Economic losses from landslides have been increasing over recent decades (Petley et al., 2005 ; Guha-sapir et al., 2011 ; Guzzetti, 2012 ), mainly due to increasing development and investment in landslide-prone areas (Bandara et al., 2013 ; Petley et al., 2005 ).
There are few studies that have attempted to quantify the impact of landslides on socio-economic systems (Mertens et al., 2017 ). In Sri Lanka, the socio-economic impacts from landslides have not been studied adequately. Landslides in Sri Lanka were considered a minor disaster up until the late twentieth century (Rathnaweera and Nawagamuwa, 2013 ). For instance, the annual average number of landslides was less than 50 until the year 2002. However, the frequency of landslide occurrence rapidly increased after 2003. Studies undertaken by the National Building Research Organization of Sri Lanka (NBRO) revealed that the number of landslides increased due to increasing human intervention such as unplanned cultivation, non-engineered construction, and deforestation.
In general, most of the socio-economic impact assessments on landslides are limited due to a lack of data (Deheragoda, 2008 ). Lossesfrom landslides can be estimated through the integration of field investigation, socio-economic surveys, and remote sensing. Moreover, recent studies have revealed the complexity involved in the quantification of the direct impact that landslides have on socio-economic systems (Mertens et al., 2016 ).
Agroforestry makes a significant contribution tothesocio-economic system of rural communities in Sri Lanka. In general, agro-forests are located on slopes, and most are vulnerable to landslides. Because of the financial benefits of agro-forestry and home gardens, the rural community is engaged in many agricultural activities, which means the land is at higher risk.
This study differs from other recent studies on the impact of landslides in many ways. First, it attempts to investigate an overview of landslides. Second, it focuses on the use of integrated remote sensing to quantify socio-economic losses in agro-forest system named Kandyan Home Garden (KHG) system to estimate the direct impact of a massive landslide on household income and property damage as a case study.
Physical setting
A tragic landslide resulted in a catastrophic situation, burying parts of the two rural villages of Elangapitiya and Pallebage. Those villages belong to the Aranayake divisional secretariat in Kegalle, Sri Lanka (Fig. 1 ). Aranayake is a mountainous region in the wet zone of the country. The area receives heavy rains during the rainy periods (May–September, southwest monsoon; October–November, inter-monsoon) and bright sunshine during the dry season (March–December). The average annual rainfall is from 2500 mm to 3000 mm (Jayawardana et al., 2014 ). The most rainfall is usually received during the monsoons. However, average rainfall amountvaries during the cyclone season.
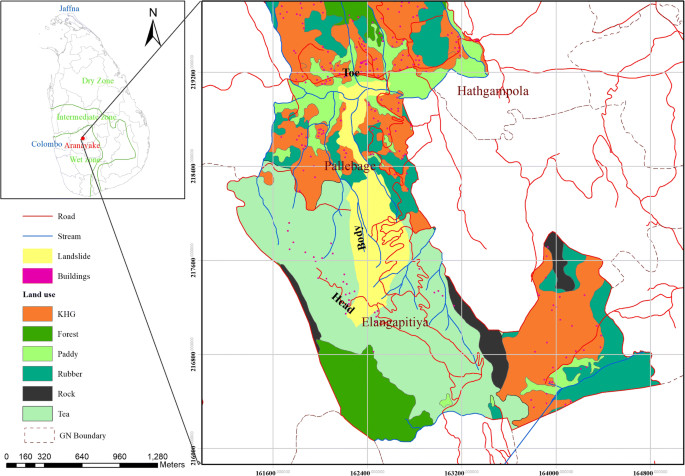
Figure showing land-use of Landslide affected GN divisions; Debathgama, Pallebage, and Elangapitiya. Land use based on 1:50000 maps
General description of the Aranayake landslide
During the recent past, there has been no record of major landslides in the region. Therefore, people tend to use the slopes for unplanned cultivation with poor surface water management and unplanned construction. Consequently, people have less awareness of the possibility of disaster. However, evidence of paleo-landslides can be observed throughout the region. Paleo-landslides seem to have been active approximately 500–1000 years ago (Jayasinghe, 2016 ); hence, people living in Aranayake have few experiences of a landslide in their lifetime. In fact, observing old landslides is a good indication that the area has unstable geology and that more landslides are likely in the future. The Aranayake region experienced 435 mm of cumulative rainfall from 14-May-2016 to 17-May-2016 (~ 72 h). The exceptionally high rainfall was mainly due to the development of a low-pressure zone around Sri Lanka caused by a tropical cyclone in the Indian Ocean. This sustained torrential rainfall triggered a landslide on 17-May-2016 at approximately 16.30–17. 00 h. Thelandslide buried houses and property and resulted in massive casualties. According to field observation, this landslide was a debris flow landslide having a very complex translational model.
Socio-economic background of the area
The population of Aranayake is approximately 68,464 with 1:1 male to female ratio. Overall, 47% of the residents are permanently or temporarily employed. The high rate of dependency reaches 53% of the total population. More than 50% of the population is included in the labor force, and most of them are engaged in home garden and plantation agriculture. Although recorded incomes are low, people have alternative income sources and food security from their home gardens.
The traditional home gardens and agroforestry
Aranayake traditional home gardens and the agroforestry system clearly reflect the typical KHG system in the wet zone of the country. Home gardens in the Aranayake region have a functional relationship with the occupants related to economic, biophysical and social aspects. The Aranayake KHG consists of a mixture of annual and perennial crops, such as tea, rubber, paddies, cardamom, black paper, jackfruit, coconut, and cocoa. The crops are not grown according to any specific pattern and appear to be in a random, intimately mixed pattern. According to the typical pattern of KHGs, tea land is available on steeps slopes, rubber exists in moderate terrain and flat terrain is for paddies. In addition, minor crops can be seen near households. The most fundamental social benefit of KHGs is their direct contribution to a secure household food supply. The livelihood benefits of KHGs, however, are well beyond the food supply. In general, selling excess KHG production significantly improves the financial status of the community. The KHG system was significantly damaged by the Aranayake landslide reducing the income and food security of the region.
Field investigation
Several exploratory field investigations were done after the landslide to grasp the overall view. Calibrated handheld GPS was used to collect all field information. To analyze the related socio-economic conditions during the field visits, detailed studies were done on human settlement and topography.
Sampling and primary data collection
Primary data were gathered by structured questionnaire from two directly affected Grama Niladhari (GN) divisions (Fig. 1 ). Before data collection, a pilot survey was conducted on 30 randomly selected houses, and the questionnaire was revised according to the responses. The survey mainly covered various income sources, social capital, household demography, household type, living condition, land-use type, KHG production and landslide experience.
It was decided thatdata collection needed to be maintained at a high precision with a 95% confidence level according to the Department of Senses and Statistics of Sri Lanka standards. Sampling was done based on a proportionate stratified random sampling method from both villages (Kumar, 2007 ). Additionally, the following formula was used to determine the sample size (Eq. (1) ; Mathers et al., 2007 ).
where n = sample size, Zα/2 = confidence level, σ = standard deviation, and E = error.
According to the equation, the estimated sample size was 120under 90% accuracy levels. In this study, 127households were selected as the primary data source (592 individuals).
Secondary data collection and analysis
Secondary information and maps were predominantly used to evaluate the socio-economic status before the landside. Socio-economic data were obtained from the recently updated database in the Aranayake Divisional Secretariat. The 1:10,000 land-use data were obtained from the Land-use and Policy Planning Department (LUPPD) of Sri Lanka. The collected information and maps were used to evaluate socio-economic conditions.
The present study integrates the socio-economic and GIS data simultaneously for the landslide impact assessment. Socio-economic data were analyzed by the descriptivestatistical method, chi-squired test,and correlation analysisusingSPSS 19 software. Conversely, the spatial data processing and analyses have been incorporated using ArcGIS 10.2.
Landslide inventory and affected area mapping
Landslide boundary demarcation and mapping are essential to study the extent of damage (Guzzetti, 2006 ). During the past decades, use of satellite information for landslide investigation has increased significantly (Guzzetti et al., 2012 ). For instance, landslide damage in forest terrain has been identified by high-resolution Google-Earth images with the help of many other attributes (Guzzetti et al., 2012 , Qiong et al., 2013 ).
Cloud-free Google-Earth images of the Aranayake landslide area were acquired. According to the images, the landslide had a clear boundary; thus, boundary demarcation was able to be accurate. In addition, ground truth GPS locations were incorporated to minimize errors. The collected information was converted to polygon data using geographical information systems (GIS; Raghuvanshi et al., 2015 ).
In addition to boundary demarcation, the Google-Earth data have the highest accuracy in household mapping (Escamilla et al., 2014 ). Therefore, the affected households were mapped using Google-Earth images before the incident and superimposed on the inventory map. In this exercise, the location of the remaining households was also mapped for cross-validation.
The affected area map was developed by overlaying the landslide inventory map with different thematic layers such as land-use type, building distribution, transportation networks and water streams in the region. Then, the affected area map was used to find the area covered by different land-use categories. In addition, real damage values for different land-use types were estimated using the affected area map incorporated with a unit value for each land-use category.
Model economic value of KHG and direct loss estimation
There is no direct method to analyze the economic value of home gardens. Generally, they are estimated by prediction models. Land size and number of years of cultivation are the typicalparameters used for estimating the values (Mohan et al., 2006 ). Economic values also quantify the benefit provided by home gardens (Galahena et al., 2013 ; Langellotto, 2014 ). According to the literature, the following multiple regression model was used to estimate the economic value of KHG production destroyed by the landslide (Eq. (2) ). The model was established using the primary data obtained from the affected villages.
where y = economic value of a home garden; α, β 1 and β 2 = regression coefficients; x 1 = land area in sq.m; and x 2 = number of years in cultivation.
Direct loss from the landslide was determined by assessing loss on agricultural land, damage to cultivation and households. Further, all the replacement costs for landslide related damage are considered in loss estimation.
Results and discussion
The results from social surveys revealed that the affected villages of Aranayake (Elagipitia and Pallebage) are agriculturally based rural areas depending on KHGs (Fig. 1 ). The unexpected landslide completely destroyed a large land area and was one of the largest landslides recorded in Sri Lankan history. Fourteen families were completely buried, and 127 lives were lost in this landslide. These results have been identified as a common feature of many massive landslides (Alimohammadlou et al., 2013 ). The village community has a middle-income level based on the per-capita income of the country (Table 1 ). Descriptive statistics of collected primary data related to total monthly income, contribution of KHG for monthly income, savings and expenditure with respect to age of the KHG is summarized in the table. However, income also depends on the diversity of KHGs. It is clear that the highest land area has been cultivated during the past two decades ( n = 54; Table 1 ). Therefore, it can be conclude that land use of the region has been affected significantly during that period. This land use change contributed to increase the landslide vulnerability of the region. It was found that the landslide affected region has been generating approximately US$ 160,000 annually from their home gardens and plantations (Tea, Rubber and Paddy) toward the GDP. Thus, the results revealed that both the social and economic systems were highly influenced by the landslide, especially the KHGs in the region (Fig. 1 and Table 1 ).
Overview of the landslide
During the field visits, it was found that a huge amount of rocks and debris was piled up at the base of the mountain, largely consisting of gneissic boulders more than 10 m in diameter. In general, most of the human settlements are spread around the affected base area.
The average width around the landslide scarp is approximately 350–350 m, the height is approximately 50–75 m, and the widestpart of the slide is approximately 600 m. The affected home gardens and a natural vegetation cover could be observed in the surrounding area and the middle of the slide; few houses not damaged. In addition, a quite rapid and muddy groundwater flow could be observedon the right-side of the landslide, which is still flowing and forming small water streams. The debris at the toe of the landslide could be split into two regions. The left side is approximately 75–125 m in width, and the right-side is approximately 350–450 m (Fig. 2 ). Many houses and home gardens were located in the damaged slope, which is higher than 35 degrees (Figs. 3 and 4 ). The entire area had been cultivated with minor export crops (cloves, cardamom, and pepper) and fruits being common in KHGs (Perera and Rajapakse, 1991 ). Most of the access roads were madeofconcrete or asphalt.
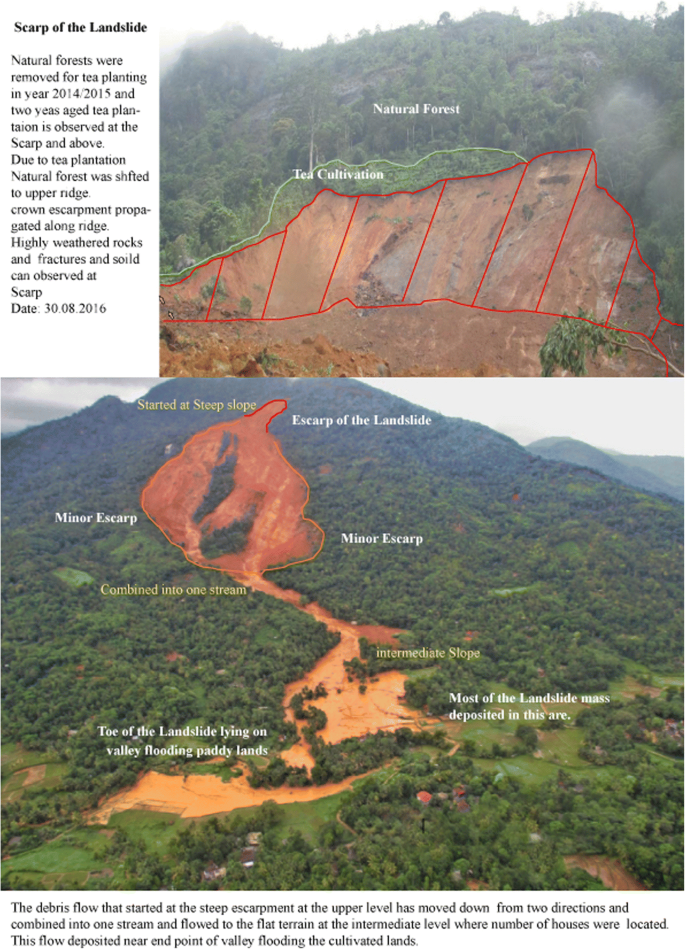
Figure illustrates escarpment of the landslide, evidence for human intervention in escarpment and above it and difficult translational path of the debris flow
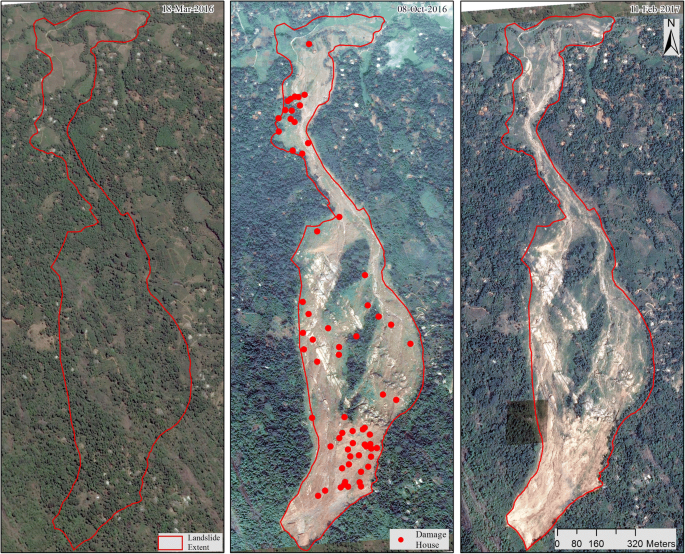
Figure illustrates before the landslide occurred, just after the incident with settlements that were destroyed by the landslide and 9 months after the incident
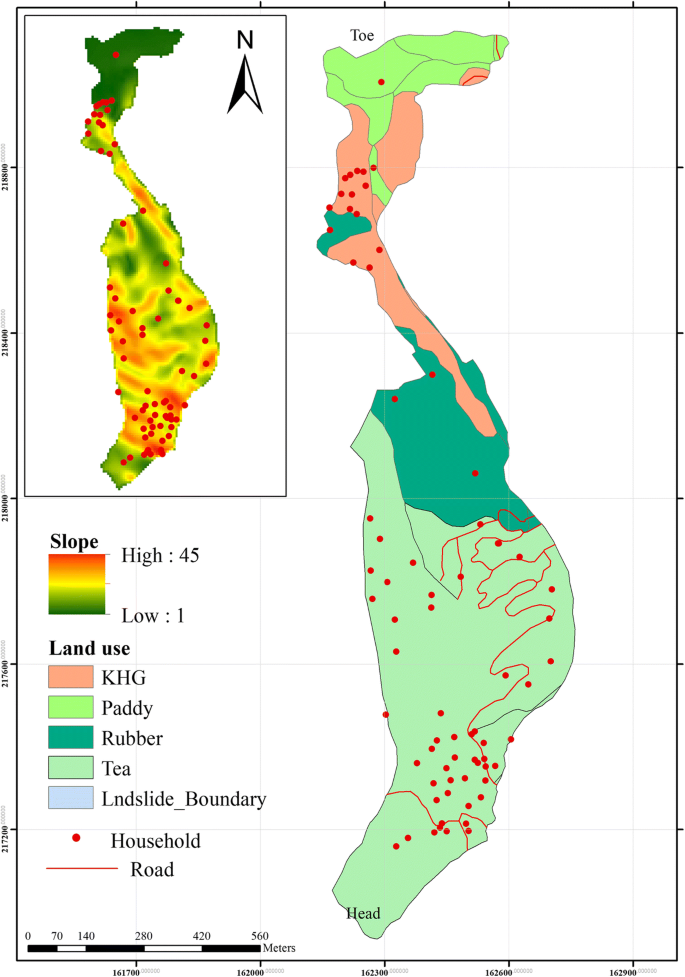
Figure illustrates the outline of the landslide superimposed onto the land use of the region, distribution of different land use classes in the affected area
Major landslide contributing factors have been identified by detailed assessment. The escarpment slope of the mountain was made up of metamorphic rocks having high joint/fracture density and thin soil overburden. Weathering conditions of the exposed slide surface of the basement rock revealed weakening along the mica and feldspar-rich layers. This mica and feldspar in the hornblende and granite gneiss can weaken the surface by intensive chemical weathering (Sajinkumar et al., 2011 ). In addition, due to un-planned tea cultivation and KHGs on the upper region of the slope, rainwater infiltration was quite significant. Consequently, high pore-water pressure built by the heavy, prolonged rainfall generated strong destabilizing forces on the slope (Matsuura et al., 2008 ). The excess pore-water pressure within the soil could have caused the reduction of shear strength and the boulders that floated on the moving debris (Kang et al., 2017 ).
Awareness of landslide mitigation
According to an eyewitness, there was heavy rainfall a few days before the landslide. The mountain stood calm and quiet during this rain, and no one noticed any clue of a possible disaster. It is well known that heavy rain is the main reason for massive landslides on vulnerable slopes (Gariano and Guzzetti, 2016 ). The villagers were awakened for a possible incident but not evacuated because there was no appropriate evacuation plan. Permanent evacuation from the possible landslide area is usually avoided due to the misleading behavior of the officials during the relocation of the residences. Despite this, it is necessary to practice successful emergency evacuation to protect the community (Huang et al., 2015 ). The evacuation of people is often a combinedeffort of the relevant government officials; however, there are no such systems commonly practiced in Sri Lanka. Only the NBRO issues warning awareness messages to the general public during heavy rainfall. During the early hours of the day that the landslide occurred, cracks with muddy groundwater appeared inside one house, indicating a sign of the landslide. However, all the villages were not fully aware of this matter.
In contrast, the socialsurvey reveals 80% of the residents are not ready to leave their homes, mainly due to wealthy KHG and traditional beliefs. Therefore, it is necessary to build specific awareness programs for such socio-economic systems and to educate residents on natural warning signs and the severity of disasters (Bhatia, 2013 ). Further, the community should have a proper evacuation plan and integrated emergency management mechanism (Dorasamy, 2017 ). Worldwide experience has proposed community-based mitigation activities for landslides (Shum and Lam, 2011 ). Moreover, essential mitigation activities have not been implanted in many landslide prone areas in Sri Lanka. Community-based short-term mitigation measures such as surface drainage control, application of erosion controls and dewatering of high elevated groundwater can be implemented. Those essential mitigations will be able to control the infiltration capacity available in the KHG and to stabilize the prevailing conditions (Pushpakumara et al., 2012 ).
Impact of landslides on rural socio-economic systems
Human settlements are randomly spread along the landslide affected slope (Figs. 3 and 4 ) and are widespread in rural Sri Lanka (MHCPU, 1996 ). Due to gentle slope conditions, the houses are mainly located in the middle and foot regions. The inventory map reveals that the adverse impact at the middle and foot regions are mainly due to wideranging debris movements, which is a well-known characteristic for debris flows worldwide (Gao and Sang, 2017 ). The debris flow flooded over the different land uses with thicknesses of 1.5–3.5 m. However, the initial region of the landslide had relatively less impact on human settlements. Therefore, to mitigate possible damage, disaster risk preparation is necessary (Ardaya et al., 2017 ). More than 3 billion people live in the developing world in rural areas that comprise farming communities (Godoy, 2010 ). Aligning with global rural communities, most of the landslide-prone district in rural Sri Lanka, such as Aranayake, depends on KHGs. Cash crop products such as tea and rubber provide a regular source of monthly income in Aranayake. However, it was found that 98% of the tea-growing areas are owned by medium-scale producers. Therefore, tea production has an indirect contribution to the income of the local community. Conversely, minor export crops in KHGs such as pepper, cloves, and cardamom provide direct additional income (Jacob and Alles, 1987 ; Perera and Rajapakse, 1991 ). KHGs not only strengthen the household economy but also sustain food security by providing fruits, vegetables, and paddies for consumption. The present study observed multiple social benefits from traditional home gardens, such as improving family health and human capacity, empowering women, and preserving indigenous knowledge and culture. According to the remote sensing data, land uses such as tea (59%), rubber (18%), home garden (13%) and paddies (10%) covered 33.7,10.2, 7.2 and 5.8 ha, respectively, within the affected area (Table 2 ). Temporal analyses revealed that the natural vegetation in the affected region had been removed for plantations and home gardens during the past decades (Figs. 2 and 3 ). Change in land cover is considered the primary cause for debris flow slides during intense rainfall (Schneider et al., 2010 ). Different trees have different root patterns and penetration depth, and they can impact the stability of a slope under different soil conditions (Vergani et al., 2017 ). Despite short-term socio-economic gain by changing vegetation, this study reveals that slope instability is another alarming socio-economic issue. Recent past historical data show those minor export crop earnings in the Aranayake area have increased by 215% with the remarkable development of value-added products (MMECP, 2013 ). Descriptive statistics revealed 52% of the household income is covered by KHGs. The average monthly income of Aranayake households is US$ 205, and the mean monthly expenditure is US$ 157 (Table 1 ). Conversely, per capita income per month is US$ 300 with expenditure of US$ 270 (CBSL, 2016 ). These findings indicate that the income and expenditures of the region are lower than the national average. Despite this, low household expenditure is an absolute gain for the community. As a result,there is increased savings with an annual average ratio of 12%. Unfortunately, the Aranayake landslide has completely abolished such self-dependent socio-economic systems. Additionally, the communities surrounding the landslide are now abandoned, and their inhabitants are living in shelters. Most of landslide prone rural Sri Lanka that has similar socio-economic conditions is now at risk (Jacob and Alles, 1987 ). Thus, there should be provisions for proper socio-economic development and land-use planning to mitigate landslide disasters in the current environment.
The regression model reveals that mean monthly income has a strong positive correlation with the cultivated land area of individual home gardens ( R 2 = 0.85, p < 0.05; Fig. 5 ). It is concluded that farmers with large farming area might have higher productivity per unit land and are encouraged to use the land intensively. According to damage done by the landslide to the agricultural potential of the region (Table 1 and Table 2 ), it is well understood that farmers who havemore significant land are willing to conserve the environment, but it was not adequately done for Aranayake. Hence, comprehensive guidelines, especially on groundwater and surface water management during heavy rainfall, will be needed to protect them from massive landslides on any slope (Masaba et al., 2017 ). However, this study recognizes the lack of such knowledge within the farming community.
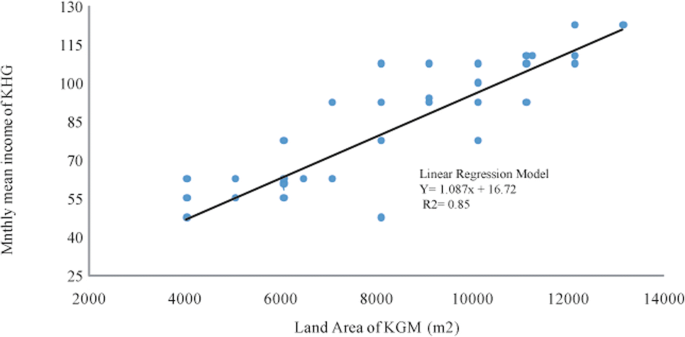
Figure illustrates a linear relationship between the cultivated land area of individual home gardens size in m 2
Educational background is quite a distinct factor for disaster mitigation. The Aranayake region has educational backgrounds ranging from primary to graduate levels (Fig. 6 ). The results revealed that, despite the many schools available in the region, the majority of the community (< 55%) has ordinary level (junior high school) qualifications. More than 30% have completed advanced level examination (senior high school) and have qualified for government jobs. In general, previous studies indicate the positive relationship between educational level and household income (Saadv and Adam, 2016 ). However, household income in Aranayake is independent of the level of education ( P < 0.05). Thisfinding may be due to additional financial gain from household farming regardless of education level. This trend may lead to less protection for the natural environment among the rural community. Despite the level of education, people in the rural community are less aware of the stability of the prevailing environment and of proper land management compared to concern for economic benefits. Human activities contribute to changing land cover types that increase slope instability and landslide risk (Proper et al., 2014 ).
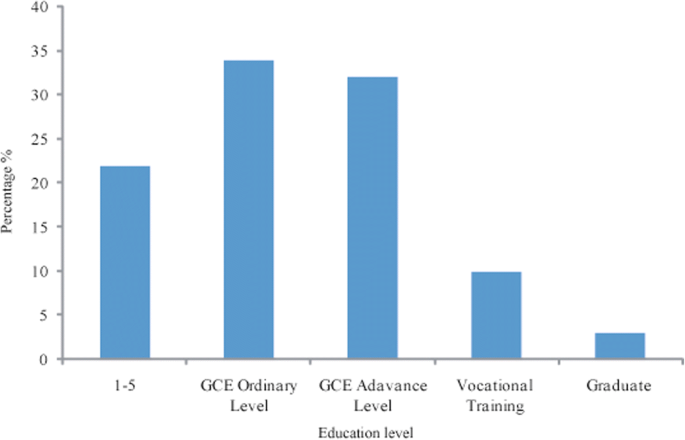
Figure illustrates educational level in percentage wise in the region
Consequently, increased soil infiltration from poor water management in plantations and KHGs, destabilize the slope (Keith and Broadhead, 2011 ). Additionally, the survey reveals that 90% of local people do not have minimum knowledge of the causative factors of landslides and proper land-use plans for steep slopes.
Regression model of the economic value of KHGs
Despite the interest, economic analyses after massive landslides have not been done in Sri Lanka or for any part of the world. Hence, there is no established model to assess actual damage. This study focuses on evaluating the level of KHG damage using a regression model (Mohan et al., 2006 ). Primary information acquired directly from two affected villages is given in Table 1 . Those data were used to model economic value using a proposed model (Eq. (3) ). The result from the multiple regression model on net economic value for KHGs (Y in $) indicates that land size ( X 1 in m 2 ) and years in cultivation ( X 2 in years) are statistically significant ( p < 0.05).
Because of the uniform land use pattern in the region, this model can be used to predict the economic value of the landslide affected home gardens in any location. The total home garden affected area obtained from the remote sensing data is approximately 72,400 m 2 (Area of KHG = 7.2 Hectares, Table 2 ), and from the primary data, the average age of the KHG has been assumed as25years. Therefore, the estimated economic value for the entire extent of KHGs in the region is US$ 4927. This sort of estimation helps to assess the real damage to socio-economic conditions of the affected area. Additionally, it is useful to evaluate the possible evacuation of affected people and to consider their past socio-economic status for necessary subsidy estimates.
Evaluation of other economic losses
Economic losses by landslide affected regions are quite significant. The highest income generated from tea was US$ 144,769 per year. From this income, only 2% is shared with the general rural community (US$ 2896). Other cultivation such as rubber, KHGs, and paddies generate US$ 7231, US$ 4864 and US$ 3195 per year, respectively, indicating the landslide affected region has been generating around US$ 160,000 for annual GDP. In addition to the disturbance, emotions and sentimentality cannot be calculated in financial terms. Nevertheless, if arbitrarily equated, this estimate would run into millions. This study revealed that landslides in rural areas could severely affecthousehold income as much as in other parts of the world (Msilimba, 2009 ; Haigh, 2012 ).
Cost estimates for the damaged houses are quite significant. This study found that almost all houses in the area are at risk for further sliding, and all of them were partially or entirely affected by the landslide. Among the affected households, 60% (40 houses) had completelycollapsed whereas 40% (27houses) were partially damaged. The department of valuation for Sri Lanka has estimated the values of the collapsed houses as SU$ 7806. Partially collapsed houses were estimated according to the level of damage. Eventually, it was found that the total cost of damaged houses is approximately US$ 40,369.
The results indicate that the plantations and KHGs on steep slopes are vulnerable to landslides. Landslides have significant impact on community income sources and households, and higher costs are incurred for subsequent rehabilitation and ongoing maintenance. It has been further observed that the severity of the impact on household income is highly dependent on the affected land size.
In an attempt to compensate for income loss after a landslide, household members in our sample seek self-employment or labor. The income obtained by this employment or labor does not adequately compensate for income lost due to landslides. Due to the landslide, the most economic activity was abandoned, which is not actually accounted for in the evaluation.
This study concluded that removing natural vegetation and plantations causes an imbalanced runoff-to-infiltration ratio destabilizing the slope. This result reflects that agriculture and the plantation-based socio-economic system are favorable for causing landslides, especially in the paleo-landslide environment. The results of the survey show that awareness of landslides and mitigation are the critical issues of the socio-economic system. This reduces a significant level of their income from KHGs. Based on the findings, two ultimate conclusions can be made to revive the life of affected people. They are as follows: create appropriate job opportunities apart from agriculture and introduce suitable cash crops by considering bioengineering approaches. Integrated spatial data can effectively be used for accurate loss estimates of the direct impact of landslides, and they can be used in the decision-making process of the affected socio-economic system.
Further, any changes in the frequency, intensity, and exposure to landslides require an economic assessment framework that takes into consideration household income, including the contribution of home gardens. Thisframework is important since a proper understanding of possible damage can lead to more effective emergency management and to the development of mitigation and preparedness activities designed to reduce the loss of lives andthe associated economy.
Alimohammadlou, Y., A. Najafi, and A. Yalcin. 2013. Landslide process and impacts: A proposed classification method. Catena 104: 219–232.
Article Google Scholar
Ardaya, A.B., M. Evers, and L. Ribbe. 2017. What influences disaster risk perception intervention measures, flood and landslide risk perception of the population living in flood risk areas in Rio de Janeiro state, Brazil. International Journal of Disaster Risk Science 8 (2): 208–223.
Bandara, R.M.S., and K.M. Weerasinghe. 2013. Overview of landslide risk reduction studies in Sri Lanka. In Landslide science and practice , ed. C. Margottini, P. Canuti, and K. Sassa, 345–352. Berlin, Heidelberg: Springer International Journal of Landslide Inventory and Susceptibility and Hazard Zoning. 1, 489-492.
Chapter Google Scholar
Bhatia, J. 2013. Landslide awareness, preparedness and response management in India. In: Margottini C., Canuti P., Sassa, K, editors. Landslide Science and Practice. Social and Economic Impact and Policies, Springer, Berlin, Heidelberg 7: 281–290.
Google Scholar
Blöchl, A., and B. Braun. 2005. Economic assessment of landslide risksin the Schwabian Alb, Germany-research framework and first resultsof homeowners and experts surveys, Nat. Hazards Earth System Science 5: 389–396.
CBSL (Central Bank of Sri Lanka). 2016. Sri Lanka socio-economic data. Colombo XXXIX 107.
Christopher, K.S., E. Arusei, and M. Kupti. 2016. The causes and socio-economy impacts of landslidein Kerio Valley, Kenya. Agricultural Science and Soil Sciences 4: 58–66.
Deheragoda, C.K.M. 2008. Social impacts of landslide disaster with special reference to Sri Lanka. Vidyodaya Journal of Humanities and Social Science 2: 133–160.
Dorasamy, M., M. Raman, and M. Kaliannan. 2017. Integrated community emergency management and awareness system: A knowledge management system for disaster support. Technological Forecasting,and Social Change . Elsevier Inc 121: 139–167.
Escamilla, V., M. Emch, and L. Dandalo. 2014. Sampling at the community level by using satellite imagery and geographical analysis , 690–694. New York: World Health Organization.
Galhena, D.H., R. Freed, K.M. Maredia, and G. Mikunthan. 2013. Home Gardens: a Promising Approach to Enhance Household Food security and Wellbeing. Journal of Agriculture and Food Security 2: 1–13.
Gao, J., and Y. Sang. 2017. Identification and estimation of landslide-debris flow disaster risk in primary and middle school campuses in a mountainous area of Southwest China. International Journal of Disaster Risk Reduction 10: 402–406.
Gariano, S.L., and F. Guzzetti. 2016. Landslides in a changing climate. Earth-Science Reviews 162: 227–252.
Godoy, D.C., Dewbre, J., 2010. The economic importance of agriculture for poverty reduction, OECD food, agriculture and fisheries working papers, No. 23, OECD Publishing.
Guha-Sapir, D., Hoyois, P., Below, R., 2011. Annual disaster statistical review 2010,the numbers and trends, Centre for Research on the Epidemiology of Disasters (CRED). Brussels, Belgium.
Guzzetti, F., 2006. Landslide Hazard and Risk Assessment. Mathematisch- Naturwissenschaftlichen Fakultät der Rheinischen Friedrich-Wilhelms-Universität, University of Bonn Germany, Ph.D. Thesis, pp 389-401.
Guzzetti, F., A.C. Mondini, M. Cardinali, A. Pepe, M. Cardinali, G. Zeni, P. Reichenbach, and R. Lanari. 2012. Landslide inventory maps: New tools for an old problem. Earth-Science Review 112: 42–66.
Haigh, M., and J.S. Rawat. 2012. Landslide disasters: Seeking causes – A case study from Uttarakhand, India , 218–253.
Huang, B., W. Zheng, Z. Yu, and G. Liu. 2015. A successful case of emergency landslide response - Sept. 2, 2014, Shanshucao landslide, Three Gorges Reservoir, China. Geoenvironmental Disasters 2: 1–9.
Article CAS Google Scholar
Jacob, V.J., and W.S. Alles. 1987. Kandyan gardens of Sri Lanka. Agroforestry Systems 5: 123–137.
Jayasinghe, P. 2016. Social geology and landslide disaster risk reduction in Sri Lanka. Journal of Tropical Forestry and Environment 6: 1–13.
Jayawardana, D.T., H.M.T.G.A. Pitawala, and H. Ishiga. 2014. Assessment of soil geochemistry around some selected agricultural sites of Sri Lanka. Environmental Earth Sciences 71: 4097–4106.
JRC EU (Joint Research Centre European Commission)., 2003. Expert working group on disaster damage and loss data, guidance for recording and sharing disaster damage and loss data: towards the development of operational indicators to translate the Sendai framework into action, JRC Science and Policy Reports.
Kang, S., S.R. Lee, N.N. Vasu, J.Y. Park, and D.H. Lee. 2017. Development of initiation criterionfor debris flows based on local topographic properties and applicability assessment at a regional scale. Engineering Geology 230: 64–76.
Keith, F., and J. Broadhead. 2011. Forests and landslides-the role of trees and forests in the prevention of landslides and rehabilitation of landslide-affected areas in Asia . Bangkok: Food and Agriculture Organization of the United Nations Regional Office for Asia and the Pacific.
Kumar, N. 2007. Spatial sampling design for a demographic and health survey. Population Research and Policy Review 26: 581–599.
Langellotto, G.A. 2014. What are the economic costs and benefits of home vegetable gardens. Journal of Extension 52: 205–212.
Masaba, S., D.N. Mungai, M. Isabirye, and H. Nsubuga. 2017. Implementation of landslide disaster risk reduction policy in Uganda. International Journal of Disaster Risk Reduction 24: 326–331.
Mathers, N., Fox, N., Hunn, A., 2007. Surveys and questionnaires. The NIHR RDS for the East Midlands /Yorkshire & the Humber.
Matsuura, S., S. Asano, and T. Okamoto. 2008. The relationship between rain andmeltwater, pore-water pressure and displacement of a reactivated landslide. Engineering Geology 101: 49–59.
Mertens, K., L. Jacobs, J. Maes, C. Kabaseke, M. Maertens, and J. Poesen. 2016. The direct impact of landslides on household income in tropical regions. Science of the Total Environment 550: 1032–1043.
Mertens, K., K. Mertens, L. Jacobs, J. Maes, C. Kabaseke, M. Maertens, J. Poesen, M. Kervyn, and L. Vranken. 2017. The direct impact of landslides on household income in tropical regions: A case study from the Rwenzori Mountains in Uganda. Science of the Total Environment 550: 1032–1043.
MHCPU (Ministry of Housing Construction and Public Utilities)., 1996. Human settlements and shelter sector development in Sri Lanka. National Report for Habitat II Conference: The City Summit. 1–49.
MMECP (Ministry of Minor Export Crop Promotion). 2013. Performance Report . Colombo Sri: Lanka.
Mohan, S., J.R.R. Alavalapati, and P.K.R. Nair. 2006. Financial analysis of homegardens: A case study from Kerala state, India. Tropical Homegarden 3: 283–296.
Msilimba, G.G. 2009. The socioeconomic and environmental effects of the 2003 landslides in the Rumphi and Ntcheu Districts (Malawi). Natural Hazards 53: 347–360.
Perera, A.H., and R.M.N. Rajapakse. 1991. A baseline study of Kandyan forest gardens of Sri Lanka: Structure, composition, and utilization. Forest Ecology and Management 45: 269–280.
Petley, D.N., S.A. Dunning, K. Rosser, and N.J. Rosser. 2005. The analysis of global landslide risk through the creation of a database of worldwide landslide fatalities. In Landslide Risk Manag 52 , ed. O. Hunger, R. Fell, and E. Ebberhardt, 367–373.
Proper, C., A. Puissant, J. Malet, and T. Glade. 2014. Analysis of land cover changes in the past and the future asa contribution to landslide risk scenarios. Applied Geography 53: 11–19.
Pushpakumara, D.K.N.G., B. Marambe, G.L.L.P. Silva, J. Weerahewa, and V.R. Punyawardena. 2012. A review research on homegardens in Sri Lanka: The status, importance and future perspective. Tropical Agriculturist 160: 55–125.
Qiong, H., W. Wenbin, and Y. Qiangyi. 2013. Exploring the use of Google earth imagery and object-based. Remote Sensing 5: 6027–6042.
Raghuvanshi, T.K., L. Negassa, and P.M. Kala. 2015. GIS-based grid overlay method versus modeling. The Egyptian Journal of Remote Sensing and Space 18: 235–250.
Rathnaweera, A.T.D., and U.P. Nawagamuwa. 2013. Study of the Impact of Rainfall Trends on Landslide Frequencies; Sri Lanka Overview. The Institute of Engineering, Sri Lanka 2: 35–42.
Saadv, S.A.A., and A. Adam. 2016. The relationship between household income and educational level. (South Darfur rural areas-Sudan) statistical study. International Journal of Advanced Statistics and Probability 4: 27–30.
Sajinkumar, K.S., S. Anbazhagan, A.P. Pradeepkumar, and V.R. Rani. 2011. Weathering and landslide occurrences in parts of Western Ghats, Kerala. Journal of the Geological Society of India 78: 249.
Schneider, H., D. Höfer, R. Irmler, G. Daut, and R. Mäusbacher. 2010. Correlation between climate, man and debris flow events -a palynological approach. Geomorphology 120: 48–55.
Schuster, R.L., Fleming, W.F., 1986. Economic losses and fatalities due to a landslide. Bulletin of the Association of Engineering Geologist XXIII (1), 11–28.
Shum, L.K.W., Lam A.Y.T., 2011. Review of natural terrain landslide risk management practice and mitigation measures (GEO technical note 3/2011).
Vergani, C., F. Giadrossich, M. Schwarz, P. Buckley, M. Conedera, M. Pividori, F. Salbitano, H.S. Rauch, and R. Lovreglio. 2017. Root reinforcement dynamics of European coppice woodlands and their effect on shallow landslides: A review. Earth-Science Reviews 167: 88–102.
Zillman, J. 1999. The physical impact of the disaster. In Natural disaster management , ed. J. Ingleton, 320. Leicester: Tudor Rose Holding Ltd.
Download references
Acknowledgements
We thank Mr. Yatagedara from Devisional Secretariat office Aranayake, to provide real estate values losses of affected household. We also acknowledge the Director General of National Building Research Organization for great support by providing landslide information. We extend our thanks to B.Sc. in Regional Science Planning students at SANASA Campus-Sri Lanka for their contribution to conduct social survey. Especial thanks for Research Council, University of Sri Jayewardenepura and Centre for Forestry and Environment for valuable support. This study was supported by a Faculty of Graduate Studies, University of Sri Jayewardenepura for Ph.D. candidate Mr. E.N.C. Perera.
Authors strongly appreciate funding support given by the Research Council, University of Sri Jayewardenepura, Sri Lanka.
Availability of data and materials
Not applicable.
Author information
Authors and affiliations.
Institute of Human Resource Advancement, University of Colombo, No: 275, Bauddhaloka Mawatha, Colombo, 7, Sri Lanka
E. N. C. Perera
Department of Forestry and Environmental Science, Faculty of Applied Science, Gangodawila, University of Sri Jayewardenepura, Nugegoda, Sri Lanka
D. T. Jayawardana
Landslide Division, National Building Research Organization, Colombo, Sri Lanka
P. Jayasinghe & R. M. S. Bandara
International Water Management Institute (IWMI), Colombo, Sri Lanka
N. Alahakoon
You can also search for this author in PubMed Google Scholar
Contributions
All authors contributed to the database construction and analysis, all read and approved the submitted manuscript.
Corresponding author
Correspondence to D. T. Jayawardana .
Ethics declarations
Authors’ information, competing interests.
The authors declare that they have no competing interests.
Publisher’s Note
Springer Nature remains neutral with regard to jurisdictional claims in published maps and institutional affiliations.
Rights and permissions
Open Access This article is distributed under the terms of the Creative Commons Attribution 4.0 International License ( http://creativecommons.org/licenses/by/4.0/ ), which permits unrestricted use, distribution, and reproduction in any medium, provided you give appropriate credit to the original author(s) and the source, provide a link to the Creative Commons license, and indicate if changes were made.
Reprints and permissions
About this article
Cite this article.
Perera, E.N.C., Jayawardana, D.T., Jayasinghe, P. et al. Direct impacts of landslides on socio-economic systems: a case study from Aranayake, Sri Lanka. Geoenviron Disasters 5 , 11 (2018). https://doi.org/10.1186/s40677-018-0104-6
Download citation
Received : 06 February 2018
Accepted : 17 July 2018
Published : 13 August 2018
DOI : https://doi.org/10.1186/s40677-018-0104-6
Share this article
Anyone you share the following link with will be able to read this content:
Sorry, a shareable link is not currently available for this article.
Provided by the Springer Nature SharedIt content-sharing initiative
- Socio-economy
- Direct loss from the landslide
- Spatial data
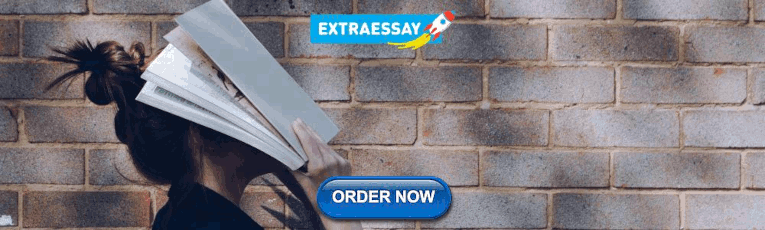
IMAGES
VIDEO
COMMENTS
Results. This cohort included 1,089,999 cases and 62,298 deaths in 3127 counties for a case fatality rate of 5.7%. Severely distressed counties had significantly fewer deaths from COVID-19 but higher number of deaths per 100,000 persons. In risk-adjusted analysis, the two socio-economic determinants of health with the strongest association with ...
Research conducted prior to the pandemic examined how high and low socioeconomic status people vary in activating their networks when they faced job threats. They usually choose one of two ...
We use the Serengeti as an exemplar case-study in which the relative economic and social benefits of a road can be assessed against the ecological impacts. Specifically, we compare three possible transportation routes and ask which route maximizes the socio-economic returns for the people while minimizing the ecological costs.
The socio-economic case for biodiversity action This chapter highlights the multiple benefits society derives from biodiversity and ecosystem services. It collates global, regional and national estimates of the economic value of these benefits, and the costs of policy inaction. ... Drawing on case studies and academic literature, the chapter ...
Google map showing the location of the case study. (Online version in colour.) Download figure; Open in new tab; ... Socio-economic factors influencing the average income of mini-grid productive customers were analysed using the regression model presented in equation (3.7). The socio-economic variables include gender, marital status, household ...
This paper investigates economic impacts of COVID-19 on households based on differences in the socio-economic status (SES). We determine the household-level effects of the COVID-19 shock using income sources, types of industries, communities' resilience, household susceptibility, and relevant policy measures. For this purpose, we used primary data of 555 households collected through snowball ...
A key question in studies on occupational choices of young adults is to what extent and in what ways their socio-economic background influences their future life chances (Wicht and Ludwig ...
In this case study, we have collected five successive admission years' records (from 2006-2007 to 2010-21011) of the 257 students. ... K., Ratnoo, S., Bajaj, A. (2022). Impact of Socio-Economic Factors on Students' Academic Performance: A Case Study of Jawahar Navodaya Vidyalaya. In: Abraham, A., et al. Innovations in Bio-Inspired ...
Abstract. The NEDUM-2D model is used to downscale four global socio-economic scenarios at city scale and simulate the evolution of the Paris urban area between 1900 and 2100. It is based on a dynamic extension of the classical urban economic theory, to explain the spatial distribution of land and real estate values, dwelling surfaces ...
The second phase was a more detailed socio-eco-nomic study. It concentrated on a representative subset of the communities identified in the first phase, and employed questionnaires, personal interviews and focus-group studies to delve more deeply into the socio-economic profiles of fisher households and the nature of resources harvested by them.
Social economics is a branch of economics that focuses on the relationship between social behavior and economics, and it examines how social norms, ethics and other social philosophies that ...
The construction of roads has become very popular in our age due to heightened modernization, industrialization and the various benefits reaped from proper road systems. However, construction processes massively contribute to environmental pollution and negatively impact socio-economic activities, necessitating this study. The aim of this paper is to assess the impact of road construction and ...
positive tourism impacts relat ing to the social w e ll-being of. the community as the stimulation of infrastructure. development (road s, communications, healthcare, educat ion, public transport ...
The study has been synthesized on political and socio-economic factors where the features were ardently study in retrospect of varied socio- economic features which may include country wise literacy rate, overall GDP rate, further we substantiated the work to address the political factors which are discussed as the country status of democratic ...
The results of this case study suggest that polycentric urban development has a significant influence on employees' commuting times. ... The findings in this research also suggest that workers' commuting behaviour is related to individual socio-economic characteristics, such as income and education. KEYWORDS: Socio-economic characteristics ...
Socio-economic case studies Examples of projects that are supported by the NDA group socio-economic programme. From: Nuclear Decommissioning Authority Published 15 January 2020.
Case study area. Gaotai County, located in the middle reaches of the Heihe River Basin, is a typical arid to semi-arid region in the Gansu Province, northwestern China. ... Impetus to socio-economic development in Gaotai County would be provided by taking advantage of its strategic location on the One Belt One Road economic zone to develop ...
A comprehensive analysis of impacts of socio-economic development and land use on river water quality in a megacity-region: a case study. Liying Zhu 1,2, Yuanyuan Chen 1, Yawei Wang 1, Yuansong Wei 5,1,2,3, ... In this study, considering socio-economic factors, build-up land use was negatively correlated with TN with an average coefficient of ...
This study suggests focused treatments for low-income students. Policymakers and educators may reduce the achievement gap and promote fair education by understanding how Socioeconomic status ...
Background Landslides area controversial issue worldwide and cause a wide range of impacts on the socio-economic systems of the affected community. However, empirical studies of affected environments remain inadequate for prediction and decision making. This study aims to estimate the direct impact of a massive landslide that occurred around areas with Kandyan home gardens (KHGs)in Aranayake ...
socioeconomic factors caused for farmers' suicide. Socio factors such as, literacy rate, family size, caste, victim's cultivation experience, opinion on seeds. fertilizers and pesticides were ...
This report presents the main findings from a socio-economic impact study of Diamond Light Source ("Diamond"), which was undertaken by Technopolis in 2018-2019. ... 2019 and involved a range of surveys, interviews, case studies and desk-based assessments. The study set out to measure and demonstrate Diamond's scientific, technological ...