A review: Lumpy skin disease and its emergence in India
Affiliations.
- 1 Department of Veterinary Microbiology, DGCN College of Veterinary and Animal Sciences, CSK Himachal Pradesh Agricultural University, Palampur, PIN 176062, India.
- 2 Department of Veterinary Microbiology, DGCN College of Veterinary and Animal Sciences, CSK Himachal Pradesh Agricultural University, Palampur, PIN 176062, India. [email protected].
- PMID: 32857262
- DOI: 10.1007/s11259-020-09780-1
Lumpy skin disease (LSD) is a viral disease caused by lumpy skin disease virus (LSDV), a member of Capripoxvirus genus of Poxviridae family. It is a transboundary disease of the economic importance affecting cattle and water buffaloes. The disease is transmitted by arthropod vectors and causes high morbidity and low mortality. LSD has recently been reported first time in India with 7.1% morbidity among cattle. Generally, fever, anorexia, and characteristic nodules on the skin mucous membrane of mouth, nostrils, udder, genital, rectum, drop in milk production, abortion, infertility and sometimes death are the clinical manifestations of the disease. The disease is endemic in African and Middle East countries but has started spreading to Asian and other countries. It has been recently reported from China and Bangladesh sharing borders with India. We have summarized occurrence of LSD outbreaks in last 10 years in Asian countries for the first time. In India, currently epidemiological status of the disease is unknown. Vaccination along with strict quarantine measures and vector control could be effective for preventing the spread of the disease. This review aims to summarise the latest developments in the epidemiology with the focus on transboundary spread, aetiology and transmission, clinical presentations, diagnostics and management of the disease.
Keywords: India; Lumpy skin disease; Outbreak; Transboundary spread.
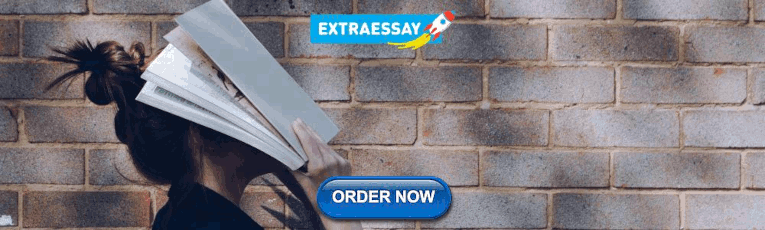
Publication types
- Systematic Review
- Cattle Diseases* / epidemiology
- Cattle Diseases* / pathology
- Cattle Diseases* / prevention & control
- Cattle Diseases* / transmission
- Disease Outbreaks / veterinary*
- India / epidemiology
- Lumpy Skin Disease* / epidemiology
- Lumpy Skin Disease* / pathology
- Lumpy Skin Disease* / prevention & control
- Lumpy Skin Disease* / transmission
- Lumpy skin disease virus / physiology*
- Research article
- Open access
- Published: 29 January 2021
Molecular characterization of lumpy skin disease virus (LSDV) emerged in Bangladesh reveals unique genetic features compared to contemporary field strains
- Shukes Chandra Badhy 1 , 2 ,
- Mohammad Golam Azam Chowdhury 1 , 2 ,
- Tirumala Bharani Kumar Settypalli 3 ,
- Giovanni Cattoli 3 ,
- Charles Euloge Lamien 3 ,
- Mohammad Aflak Uddin Fakir 1 , 2 ,
- Shamima Akter 1 , 2 ,
- Mozaffar Goni Osmani 2 ,
- Faisol Talukdar 2 ,
- Noorjahan Begum 2 ,
- Izhar Ahmed Khan 1 , 2 ,
- Md Bazlur Rashid 1 , 2 &
- Mohammad Sadekuzzaman ORCID: orcid.org/0000-0002-5530-2829 1 , 2
BMC Veterinary Research volume 17 , Article number: 61 ( 2021 ) Cite this article
20k Accesses
45 Citations
27 Altmetric
Metrics details
Lumpy skin disease (LSD) is a contagious viral disease of cattle caused by lumpy skin disease virus (LSDV). LSD has recently spread in Asia following outbreaks in the Middle East and Europe. The disease emerged in Bangladesh in July 2019 in the Chattogram district, then rapidly spread throughout the entire country. We investigated six LSD outbreaks in Bangladesh to record the clinical signs and collect samples for diagnostic confirmation. Furthermore, we performed the molecular characterization of Bangladesh isolates, analyzing the full RPO30 and GPCR genes and the partial EEV glycoprotein gene.
Clinical observations revealed common LSD clinical signs in the affected cattle. PCR and real-time PCR, showed the presence of the LSDV genome in samples from all six districts. Phylogenetic analysis and detailed inspection of multiple sequence alignments revealed that Bangladesh isolates differ from common LSDV field isolates encountered in Africa, the Middle East, and Europe, as well as newly emerged LSDV variants in Russia and China. Instead, they were closely related to LSDV KSGP-0240, LSDV NI2490, and LSDV Kenya.
Conclusions
These results show the importance of continuous monitoring and characterization of circulating strains and the need to continually refine the strategies for differentiating vaccine strains from field viruses.
Lumpy skin disease (LSD) is a viral disease of cattle, caused by lumpy skin disease virus (LSDV) within the genus Capripoxvirus , family Poxviridae . The genus Capripoxvirus also comprises goatpox virus (GTPV) and sheeppox virus (SPPV). LSD is a notifiable disease by the World Organization for Animal Health (OIE) because of its potential rapid spread and substantial economic importance.
LSDV has a limited host range and does not infect non-ruminant hosts [ 1 ]. Both sexes and all ages of cattle breeds are susceptible to LSDV. However, younger animals may be more susceptible to the severe form of the disease [ 2 ]. Even in close contact with infected cattle, sheep and goats never developed LSD [ 3 ], with one noted exception, LSDVKSGP-0240 also known as LSDV KS1.
There is a significant variation of clinical signs with LSDV infections ranging from subclinical infection to death [ 4 ]. The main clinical signs include fever, the appearance of nodules in the skin, lesions in the mouth, pharynx, enlarged superficial lymph nodes, edema in the skin, and sometimes death [ 3 , 4 , 5 ]. There is an initial incubation period of 6 to 9 days during acute cases followed by a fever that may exceed 41 °C [ 6 ].
LSD is one of the most economically significant viral diseases of cattle because of the loss of production, permanent damage of hides, infertility, and death. Although the mortality rate is usually less than 10%, the disease morbidity rate can be as high as 100% [ 7 ].
For many years, the LSDV genome appeared to be stable. Indeed, following its first description in Zambia in 1929 [ 8 ], LSDV field isolates recovered for decades in Africa showed only minor genomic differences [ 9 , 10 , 11 , 12 ]. As the disease further spread into the Middle East from 2012 [ 13 ] and Europe in 2015 [ 7 ], the recovered LSDV field isolates showed little variability to contemporary African LSDV field isolates [ 14 , 15 , 16 ]. This genetic stability was exploited for the differentiation of LSDV live attenuated vaccines from contemporary field isolates [ 16 , 17 , 18 , 19 , 20 , 21 ].
However, this dynamic has shifted following the discovery of field LSDVs in Russia in 2017 and 2019 showing vaccine-like profiles [ 22 , 23 , 24 , 25 ]. Some of these LSDV variants, presented a 12-nucleotide insertion in the GPCR gene, like vaccine strains, and others presented a27-nucleotide deletion, similar to the LSDV Neethling vaccine strain, in the ORF LSDV 126. The authors attributed these variants’ emergence to recombination events between the Neethling vaccine strain and field isolates [ 23 ]. This has prompted researchers [ 26 ] to question the relevance of current strategies to differentiate LSDV vaccine strains from viral field strains. Similarly, the LSDV strains involved in the outbreaks in China present the GPCR profile of LSDV vaccines with the 27-nucleotide insertion in their EEV glycoprotein gene. Interestingly, Kononova et al. [ 27 ], also showed in vitro and in vivo that the recombinant LSDVs could induce more severe disease than the typical field isolates.
The increased variability of LSDV in recent years makes it crucial to adapt the molecular DIVA strategies based on the knowledge of the circulating strain of LSDV. This requires the constant monitoring and characterization of LSDV field isolates.
Several PCR, real-time PCR, and HRM based methods are available for the detection of the LSDV genome, [ 12 , 14 , 28 , 29 , 30 , 31 , 32 , 33 , 34 , 35 , 36 ] and molecular epidemiological studies of LSDV rely on analyzing various genomic regions, such as the GPCR, the RPO30, the P32, and the EEV glycoprotein genes [ 11 , 12 , 17 , 18 , 19 , 37 ].
On September 15, 2019, Bangladesh notified to OIE the first outbreak of LSD in the country. The disease started in July 2019 in the Southeast (Chattogram district) of the country, then progressively spread throughout the country. Because of the wide distribution and large cattle population in Bangladesh, LSD is now one of the most economically important emerging livestock disease in Bangladesh.
This study aimed to investigate and confirm the recent outbreaks and provide LSDV molecular characterization in different regions in Bangladesh.
Outbreak investigation
All affected cattle in different districts in Bangladesh (Chottogram, Dhaka, Gazipur, Narayanganj, Pabna, and Satkhira) showed the following common clinical signs: fever (40–41 °C), depression, loss of appetite, nasal and ocular discharges, salivation, circumscribed nodules with different sizes on the skin covering their head, neck, trunk, perineum, udder, and teats. Figure 1 illustrates the skin lesions of affected cattle. In many infected animals, the necrotic nodules were ulcerated and formed deep scabs (sitfast). Moreover, a decrease in body weight and reduced milk production were prominent signs observed in cattle affected by LSD. The total cattle population, reported morbidities and mortalities in the six districts of this study (Fig. 2 ) are sumarised in Table 1 .
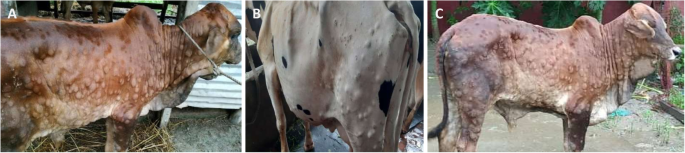
Skin lesions characteristics of lumpy skin disease in 3 animals in Bangladesh. The generalized circumscribed active nodular skin lesions covering the entire body are visible. Source: own
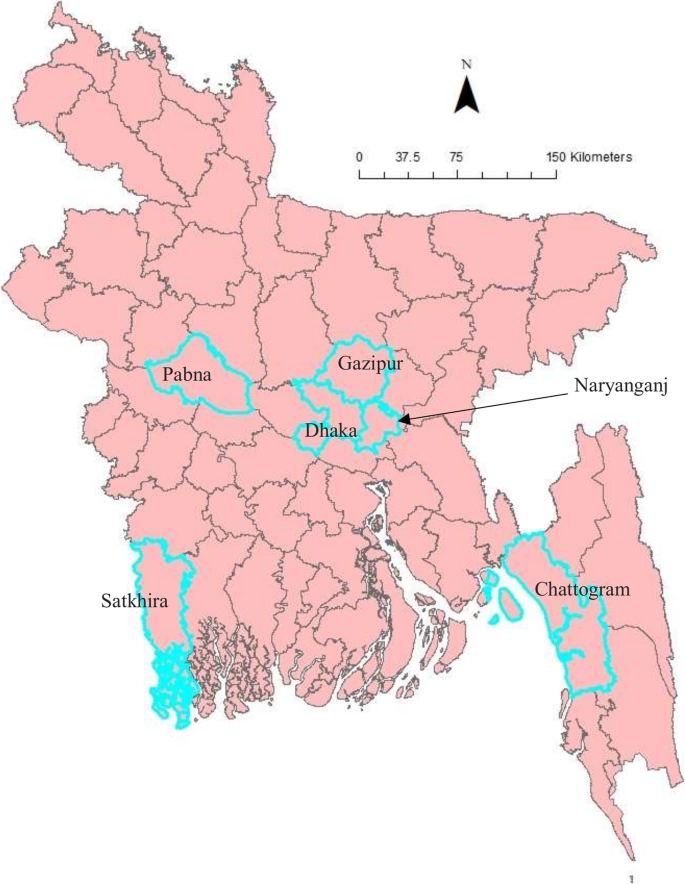
Map of Bangladesh showing the sample collection area. The map is an own creation using Arc GIS software version 13.2
Molecular detection of LSDV
Gel electrophoresis of the P32 amplicons showed a 390 bp product in all fifty (50) samples collected in six districts, as illustrated for selected samples in Fig. 3 .
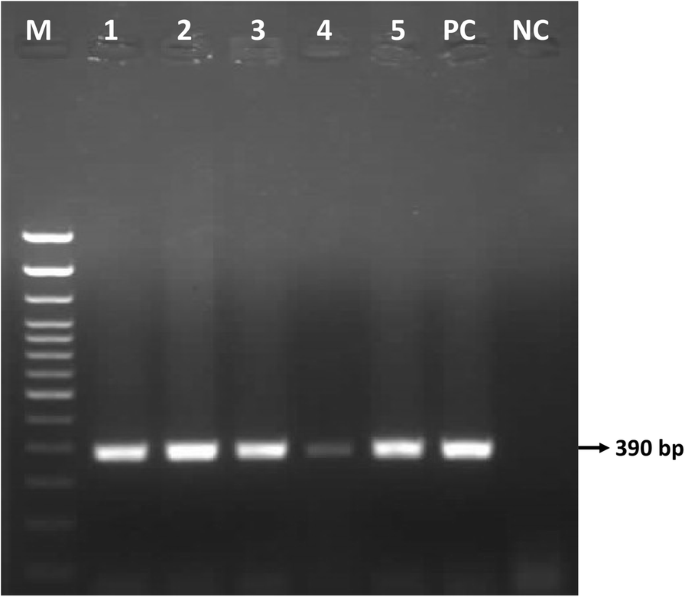
Agarose gel electrophoresis showing the 390 bp amplicon of P32 gene for selected samples of Bangladesh. Lane M: 100 bp DNA ladder, Lane 1–5: LSDV field samples, Lane PC: positive control, lane NC: negative control
The real-time PCR result confirmed capripoxvirus DNA in all samples. Six representative samples, one per district with a Cq value between 19.17 and 25.31, were selected for sequencing (Table 2 ).
Amplification and sequencing of the RPO30, GPCR, and EEV glycoprotein genes
We have successfully amplified and sequenced two fragments for the RPO30 gene (554 bp and 520 bp) in 6 samples and three for the GPCR (617 bp, 603 bp, and 684 bp) in 5 samples out of 6. We also amplified and sequenced the partial EEV glycoprotein gene in 6 samples. The complete RPO30 and GPCR genes and the partial EEV glycoprotein gene sequences were submitted to the GenBank database under accession numbers MT448690 to MT448701.
Phylogenetic analysis
For each of the targeted genes, the sequences of the Bangladesh LSDVs showed 100% identity among each other. On the phylogenetic trees for both RPO30 (Fig. 4 ) and GPCR (Fig. 5 ), all the Bangladesh LSDVs clustered together.
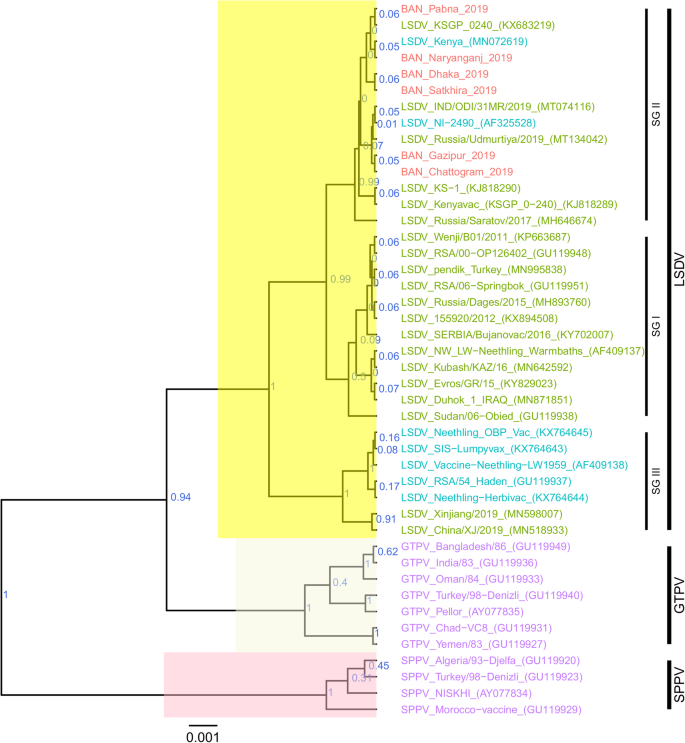
Maximum clade credibility (MCC) tree based on the complete RPO30 complete gene sequences of capripoxviruses. The posterior probabilities are plotted as respective nodes labels. LSDVs from Bangladesh are highlighted in red and reference sequences are represented with their accession numbers
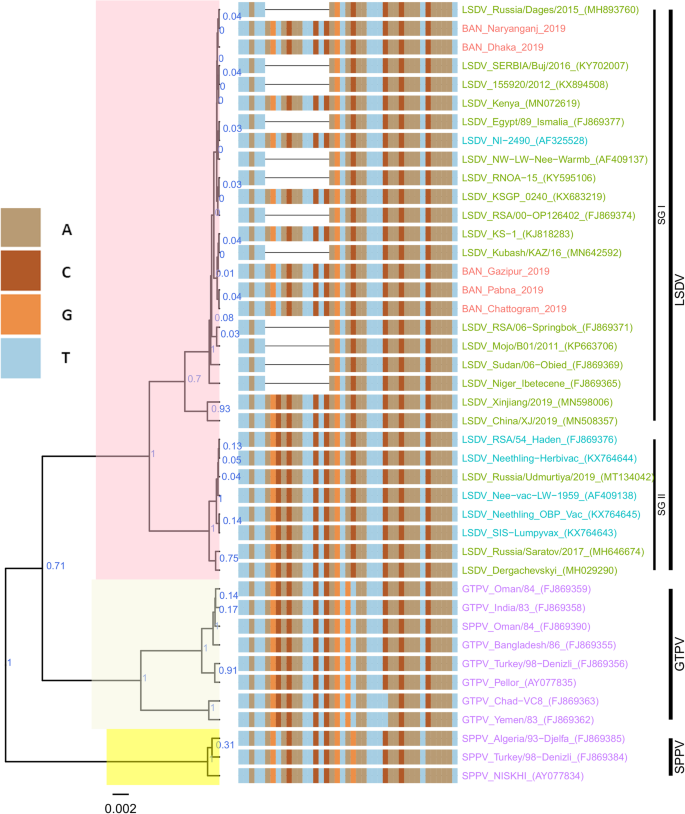
Maximum clade credibility (MCC) tree based on the complete GPCR gene sequences of Capripoxviruses, plotted together with multiple sequence alignment. Only the portion of the alignment between positions 80 and 120 is shown. The posterior probabilities are plotted as respective nodes labels. LSDVs from Bangladesh are highlighted in red and reference sequences are represented with their accession numbers
On the RPO30 tree (Fig. 4 ), Bangladesh isolates clustered within subgroup I, tightly with LSDV KSGP 0240 (KX683219), known as LSDV KS1, LSDV NI-2490 (AF325528), Indian LSDV field isolates, and two recombinant LSDV field isolates from Russia, LSDV Russia/Udmurtiya/2019 (MT134042), and LSDV Russia/Saratov/2017 (MH646674). The commonly circulating field isolates from Africa, the Middle East, and Europe are segregated from the Bangladesh isolates, clustering within subgroup II. A third subgroup contained mainly LSDV Neethling derived vaccine strains, the historical field LSDV RSA/54 Haden, and the LSDV field isolates from China. On the GPCR tree (Fig. 5 ), there were only two sub-groups. Bangladesh LSDV isolates clustered within subgroup I, together with LSDV SGP_O-240 (KJ818288), LSDV NI-2490 (AF325528), LSDV Kenya (MN072619), common LSDV field isolates from Africa, the Middle East, and Europe, LSDV Xinjiang/2019 (MN598006), and LSDV China/XJ/2019(MN508357) from China. The second subgroup of the GPCR consisted of LSDV Neethling derived vaccines, LSDV RSA/54 Haden (FJ869376), and three recombinant LSDVs from Russia: LSDV Russia/Udmurtiya/2019 (MT134042), LSDV Russia/Saratov/2017 (MH646674) and LSDV Dergachevskyi (MH029290). The multiple sequence alignments of the GPCR gene showed that the Bangladesh LSDV contained the 12-nucleotide insertion (Fig. 5 ). This 12-nucleotide insertion is also present in the two common LSDV vaccine strains (LSDV KSGP 0240 and LSDV Neethling) and a few historical field isolates (collected before 1960) such as LSDV NI-2490 (AF325528), LSDV Kenya (MN072619), and LSDV RSA/54Haden (GU119937). This insertion is also present in recombinant LSDVs from Russia (LSDV Russia/Udmurtiya/2019, LSDV Russia/Saratov/2017, and LSDV Dergachevskyi) and in recent LSDV isolates from China.
Alignment of the EEV glycoprotein gene sequence showed a 27-nucleotide insertion in all LSDVs from Bangladesh (Fig. 6 ), which is characteristic of common field isolates and also present in the LSDV KSGP-0240 derived vaccines and historical LSDVs, LSDV NI2490 (1958) and LSDV Kenya (1950), both from Kenya.
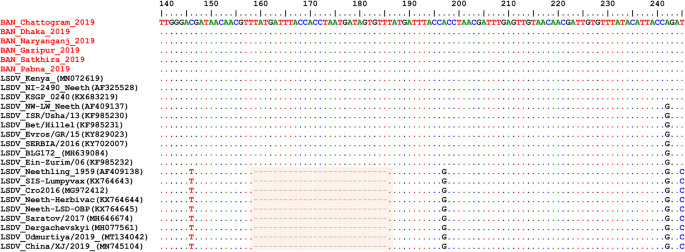
Multiple sequence alignments of the partial nucleotide sequences of the of EEV glycoprotein gene. LSDVs from Bangladesh were aligned with representative LSDVs’ sequences retrieved from GenBank. A unique sequence signature of 27-nucleotide only in LSDV Neethling like viruses is highlighted in the box. Identical nucleotides are indicated with dots
Taken together, the analyses of all three targets suggest that the Bangladesh LSDVs were more related to LSDV KSGP-0240, LSDV NI-2490, and LSDV Kenya. They differed from all recent LSDV field isolates, including the LSDVs from China and the recombinant LSDVs described in Russia, and LSDV Neethling vaccine strain.
The diagnosis of LSD was confirmed by real time PCR and the viruses in samples collected from outbreaks between July and September 2019 in Bangladesh were molecularly characterized.
LSD emerged in Bangladesh in July 2019, hitting the district of Chattogram, before quickly spreading to Dhaka, Naryanganj, Gazipur, Satkhira, and Pabna regions, between July and September 2019 [ 38 ]. The affected cattle exhibited common clinical manifestations of LSD in cattle, including nasal and ocular discharges and skin lesions [ 39 ]. The epidemiology unit data shows a very high incidence of LSD in the Chattogram province compared to other regions in the country. A plausible explanation is that Chattogram, a port city of Bangladesh, is a major coastal city and financial center in southeastern Bangladesh, with more cattle movement due to trade. It was also the first affected province.
The Bangladesh LSDVs presented the 12-nucleotide insertion found in the GPCR gene of LSDV KSGP-0240, LSDV Neethling vaccines, and a few historical LSDVs such as the LSDV NI2490 and LSDV Kenya, LSDVs from China and the recombinant LSDVs described in Russia. The presence of this 12-nucleotide insertion makes them different from commonly circulating field LSDVs encountered in Africa, Europe, and the Middle East [ 11 , 16 , 19 , 24 , 40 ]. However, a 27-nucleotide insertion in the EEV glycoprotein gene of Bangladesh isolates, and the RPO30 and GPCR gene trees’ analysis differentiated them from LSDV Neethling derived vaccines. This 27-nucleotide insertion in the EEV glycoprotein also makes them different from Chinese LSDV isolates as well as from the recombinant LSDVs described in Russia in recent years.
A close inspection of the sequence alignment of the EEV glycoprotein, the GPCR, and RPO30 genes showed 100% identity to the LSDV KSGP 0240, LSDV NI2490, and LSDV Kenya at the nucleotide level. These features make them unique, as the commonly circulating LSDV isolates have not demonstrated that level of closeness to LSDV KSGP 0240, LSDV NI2490, and LSDV Kenya. It is worth noting that the Bangladesh LSDV RPO30 sequence was 100% identical with the Indian isolate, however, as no GPCR and EEV glycoprotein gene sequences were available from India, it was not possible to extend the comparison.
The existence of vaccine-like field isolates with mixed characteristics between common field viruses and the LSDV Neethling vaccine has been reported in Russia [ 24 ]. A more recent report described a field LSDV isolate in Kurgan, Russia, exhibiting similarities to LSDV KSGP 0240 and LSDV NI2490 based on the analysis of GPCR and RPO30 gene fragments [ 26 ]. Although the complete RPO30 and GPCR sequences were not available for a full comparison, we noticed a nucleotide difference between the partial RPO30 sequence of the Kurgan isolate and those of this study. Our findings support the circulation of LSDV KS1 or LSDV NI2490-like virus in the field.
How such a virus has emerged suddenly in Bangladesh remains unknown. An extensive characterization of LSDV in neighboring countries could help resolve the emergence of these isolates.
Previous studies showed that the use of the LSDV KSGP 0240 for vaccination could lead to the appearance of generalized lesions [ 15 , 41 ]. The lesions in cattle in Bangladesh showed pathogenic virus-like lesions, especially the presence of deep “sit fast,” not usually observed with KSGP 0240-induced disease [ 15 ]. It is also worth noting that Bangladesh was not vaccinating cattle against LSD before these outbreaks but later started vaccination using a goat poxvirus strain. Therefore, it is unlikely that the administration of a good quality LSDV KSGP 0240 vaccine caused these outbreaks. Furthermore, LSDV KSGP 0240-induced disease manifests only as an adverse reaction in vaccinated animals and shows no signs of animal to animal spread [ 15 , 41 ].
Historically, viruses resembling LSDV KSGP 0240, the LSDV NI2490 (1958), and LSDV Kenya (1950, but sequenced only recently), caused LSD outbreaks in Kenya [ 10 ]; however, these viruses were never detected in subsequent LSDV epidemics in Africa, the Middle East, and Europe. Whether Bangladesh isolates, and presumably those described in Kurgan, Russia, relate to LSDV NI2490 and LSDV Kenya is unclear. Further investigation through full genome sequencing is warranted, as none of the three targeted genes of this study could provide differentiation between LSDV KSGP-0240 and LSDV NI2490.
The reason for the emergence of such LSDV variants remains uncertain. However, recent reports from Russia suggest the possibility of recombination events [ 24 ].
In conclusion, using a multi-targeted approach,we discovered that the viruses causing outbreaks in Bangladesh were different from common contemporary LSDV field isolates circulating worldwide, including the Chinese isolates and the recombinant LSDVs described between 2017 and 2019 in Russia. Full genome analysis will elucidate whether these viruses are LSDV KSGP-0240 or LSDV NI2490/LSDV Kenya. This study highlights the importance of continuous monitoring and characterization of circulating strains and the need to continually refine the strategies for differentiating vaccine strains from field viruses.
Outbreak investigations and sample collection
Fifty (50) biopsies of skin nodules were collected from 6 different districts of Bangladesh (Fig. 2 ) between July and September 2019. Table 2 shows the location, source, and collection period of LSDV samples. The samples were collected aseptically and transported in a cool box to the Central Disease Investigation Laboratory (CDIL) at Dhaka, Bangladesh. Samples were stored at − 80 °C for further processing.
Sample preparation and DNA extraction
Biopsy nodule samples were cut with a scalpel into small pieces. Pieces were macerated with pestle and mortar, then transferred to sterile tubes with 10 ml sterile phosphate-buffered saline (PBS) to prepare tissue homogenates. Tubes were centrifuged at 1000 g, and 200 μl of supernatant was transferred to an Eppendorf tube for DNA extraction.
DNA extraction from skin samples was performed using DNeasy Blood & Tissuekit (Qiagen, Germany) according to the manufacturer’s recommendations. The DNA was eluted using 70 ul elution buffer and stored at − 20 °C until further use.
Molecular detection
A conventional PCR was carried out to amplify a 390 bp fragment within the P32 gene of capripoxviruses [ 28 ]. The PCR was performed using the Platinum™ Taq DNA Polymerase kit (cat# 10966–026) in a reaction volume of 25 μl containing 2.5 μl 10 X PCR buffer, 0.75 μl Magnesium chloride (50 mM), 0.5 ul dNTPs (10 mM), 0.1 μl Platinum Taq DNA polymerase, 400 nM of each primer and 1 μl template DNA. The PCR tubes containing the above mixture were transferred into a thermal cycler (T-1000, Bio-Rad, USA), and amplification was conducted with the following program: initial denaturation at 94 °C for 5 min, 38 cycles denaturation at 94 °C for 30 s, annealing at 50 °C for 30 s, extension at 72 °C for 30 s; and a final extension phase run at 72 °C for 5 min.
The PCR products were separated by gel electrophoresis on a 1.5% agarose for 60 min and visualized with a gel documentation system (UVP GelStudio PLUS Gel Documentation Imaging Systems, Analytik Jena, Germany).
A real-time PCR for the detection of capripoxvirus DNA was performed as previously described [ 31 ] with some modifications.
Briefly, the PCR mixture was set up in a reaction volume of 25 μl, containing 12.5 μl of the iQsupermix (Bio-Rad, USA), 400 nM of each primer, 250 nM of the fluorogenic probe and 5 μl of template. The reaction consisted of an initial denaturation step at 95 °C for 10 min, followed by 45 cycles at 95 °C for 15 s and 60 °C for 60 s with the fluorescence recording at the end of the combined annealing elongation step. The assaywasperformed using the CFX real-time PCR detection system (Bio-Rad).
Amplification and sequencing of the RPO30, GPCR, and EEV glycoprotein genes
The RPO30 and the GPCR were amplified as previously described [ 19 ].
A pair of primers; EEVGly F- 5′- ATGGGAATAGTATCTGTTGTATACG-3′ and EEVGly R-5′- CGAACCCCTATTTACTTGAGAA-3′ were designed for the amplification of fragments containing the partial EEV glycoprotein (encoded by ORF LSDV126) and hypothetical protein LSDV 127 gene [ 18 ]. The PCR reaction was performed in a reaction volume of 20 μl containing 500 nM of each of the forward and reverse primers, 0.2 mM of dNTPs, 1x buffer (Qiagen), 2.5 U of Taq DNA polymerase (Qiagen), and 2 μl template DNA. The amplification consisted of an initial denaturation at 95 °C for 4 min followed by 35 cycles of 95 °C for 40 s, 55 °C for 30 s, and 72 °C for 1 min, and a final extension step at 72 °C for 7 min.
The PCR products were separated by electrophoresis on a 1.5% agarose gel at 100 V for 60 min and visualized using a Gel Documentation System (Bio-Rad, USA).
The PCR amplicons were purified using the Wizard SV Gel and PCR clean-up system kit (Promega) according to the manufacturer’s instructions. LGC Genomics (Germany) performed the sequencing of the purified PCR amplicons. Vector NTI 11.5 software (Invitrogen, USA) was used for sequencing data analysis and assembly.
Nucleotide sequences were aligned using the Muscle algorithm and the codon option implemented in MEGA7 [ 42 ]. The complete RPO30 and GPCR gene sequences of additional CaPVs (LSDVs, GTPVs, and SPPVs), retrieved from GenBank, were included for comparative analyses.
The file with aligned sequences in FASTA was converted to a Nexus format using Seaview. The Bayesian phylogenetic inference was performed with BEAST v1.8.4 [ 43 ]. First, the BEAUti module was used to generate BEAST files using the HKY substitution +G nucleotide substitution and a UPGMA starting tree option. The Markov Chain Monte Carlo method was run with BEAST, for 10,000,000 generations with a sample taken each 10,000 generations. The TRACER program was used to inspect the log files and determine the optimum number of burn-in based on the Effective Sample Sizes (ESS > 200).
TreeAnnotator was used to generate the Maximum Clade Credibility (MCC) after discarding the 3% burn-in. The tree was visualized with the associated meta-data using the ggtree package in R [ 44 ]. Additionally, for the GPCR tree, the multiple sequence alignment file of the nucleotide sequences was imported. A specific slice of the alignment, between positions 80 and 120, was visualized together with the tree [ 44 ].
Availability of data and materials
DNA sequences generated and analyzed under the current study are available in GenBank under accession numbers MT448690 to MT448701. All the remaining datasets generated during this study are available from the corresponding author on request.
Abbreviations
Central Disease Investigation Laboratory
Deoxyribonucleic acid
Deoxynucleotide triphosphate
Extracellular enveloped virus
Effective Sample Sizes
G protein-coupled receptor
Goatpox virus
High-resolution melting
Lumpy skin disease
Lumpy skin disease virus
Maximum clade credibility
World Organization for Animal Health
Open reading frame
Phosphate-buffered saline
Polymerase chain reaction
RNA polymerase 30 kDa subunit
Sheeppox virus
Shen YJ, Shephard E, Douglass N, Johnston N, Adams C, Williamson C, et al. A novel candidate HIV vaccine vector based on the replication deficient Capripoxvirus, lumpy skin disease virus (LSDV). Virol J. 2011;8:1–2.
Article Google Scholar
Tageldin MH, Wallace DB, Gerdes GH, Putterill JF, Greyling RR, Phosiwa MN, et al. Lumpy skin disease of cattle: an emerging problem in the Sultanate of Oman. Trop Anim Health Prod. 2014;46:241–6.
Article PubMed Google Scholar
Davies FG. Lumpy skin disease, an African capripox virus disease of cattle. Br Vet J. 1991;147:489–503.
Article CAS PubMed Google Scholar
Carn VM, Kitching RP. An investigation of possible routes of transmission of lumpy skin disease virus (Neethling). Epidemiol Infect. 1995;114:219–26.
Article CAS PubMed PubMed Central Google Scholar
OIE. Lumpy skin disease. In: Manual of diagnostic tests and vaccines for terrestrial animals; 2017. http://www.oie.int/fileadmin/Home/eng/Health_standards/tahm/2.04.13_LSD.pdf .
Google Scholar
USDA. Lumpy skin disease standard operating procedures: 1. overview of etiology and ecology. 2016. https://www.aphis.usda.gov/animal_health/emergency_management/downloads/sop/lsdv_fadprep_ee.pdf . Accessed 19 July 2020.
Tuppurainen ESM, Venter EH, Shisler JL, Gari G, Mekonnen GA, Juleff N, et al. Review: Capripoxvirus diseases: current status and opportunities for control. Transbound Emerg Dis. 2017;64:729–45.
Tuppurainen ESM, Stoltsz WH, Troskie M, Wallace DB, Oura CAL, Mellor PS, et al. A potential role for Ixodid (hard) tick vectors in the transmission of lumpy skin disease virus in cattle. Transbound Emerg Dis. 2011;58:93–104.
Kara PD, Afonso CL, Wallace DB, Kutish GF, Abolnik C, Lu Z, et al. Comparative sequence analysis of the south African vaccine strain and two virulent field isolates of lumpy skin disease virus. Arch Virol. 2003;148:1335–56.
Tulman ER, Afonso CL, Lu Z, Zsak L, Kutish GF, Rock DL. Genome of lumpy skin disease virus. J Virol. 2001;75:7122–30.
Le Goff C, Lamien CE, Fakhfakh E, Chadeyras A, Aba-Adulugba E, Libeau G, et al. Capripoxvirus G-protein-coupled chemokine receptor: a host-range gene suitable for virus animal origin discrimination. J Gen Virol. 2009;90:1967–77.
Article PubMed CAS Google Scholar
Lamien CE, Le Goff C, Silber R, Wallace DB, Gulyaz V, Tuppurainen E, et al. Use of the Capripoxvirus homologue of Vaccinia virus 30 kDa RNA polymerase subunit (RPO30) gene as a novel diagnostic and genotyping target: development of a classical PCR method to differentiate goat poxvirus from sheep poxvirus. Vet Microbiol. 2011;149:30–9.
Alkhamis MA, VanderWaal K. Spatial and temporal epidemiology of lumpy skin disease in the Middle East, 2012–2015. Front Vet Sci. 2016;3:19.
Article PubMed PubMed Central Google Scholar
Stram Y, Kuznetzova L, Friedgut O, Gelman B, Yadin H, Rubinstein-Guini M. The use of lumpy skin disease virus genome termini for detection and phylogenetic analysis. J Virol Methods. 2008;151:225–9.
Abutarbush SM, Hananeh WM, Ramadan W, Al Sheyab OM, Alnajjar AR, Al Zoubi IG, et al. Adverse reactions to field vaccination against lumpy skin disease in Jordan. Transbound Emerg Dis. 2016;63:e213–9.
Agianniotaki EI, Tasioudi KE, Chaintoutis SC, Iliadou P, Mangana-Vougiouka O, Kirtzalidou A, et al. Lumpy skin disease outbreaks in Greece during 2015–16, implementation of emergency immunization and genetic differentiation between field isolates and vaccine virus strains. Vet Microbiol. 2017;201:78–84.
Menasherow S, Rubinstein-Giuni M, Kovtunenko A, Eyngor Y, Fridgut O, Rotenberg D, et al. Development of an assay to differentiate between virulent and vaccine strains of lumpy skin disease virus (LSDV). J Virol Methods. 2014;199:95–101.
Menasherow S, Erster O, Rubinstein-Giuni M, Kovtunenko A, Eyngor E, Gelman B, et al. A high-resolution melting (HRM) assay for the differentiation between Israeli field and Neethling vaccine lumpy skin disease viruses. J Virol Methods. 2016;232:12–5.
Gelaye E, Belay A, Ayelet G, Jenberie S, Yami M, Loitsch A, et al. Capripox disease in Ethiopia : Genetic differences between field isolates and vaccine strain , and implications for vaccination failure. Antivir Res. 2015;119:28–35.
Agianniotaki EI, Chaintoutis SC, Haegeman A, Tasioudi KE, De Leeuw I, Katsoulos PD, et al. Development and validation of a TaqMan probe-based real-time PCR method for the differentiation of wild type lumpy skin disease virus from vaccine virus strains. J Virol Methods. 2017;249:48–57.
Pestova Y, Byadovskaya O, Kononov A, Sprygin A. A real time high-resolution melting PCR assay for detection and differentiation among sheep pox virus, goat pox virus, field and vaccine strains of lumpy skin disease virus. Mol Cell Probes. 2018;41:57–60.
Kononov A, Byadovskaya O, Kononova S, Yashin R, Zinyakov N, Mischenko V, et al. Detection of vaccine-like strains of lumpy skin disease virus in outbreaks in Russia in 2017. Arch Virol. 2019;164:1575–85.
Sprygin A, Pestova Y, Bjadovskaya O, Prutnikov P, Zinyakov N, Kononova S, et al. Evidence of recombination of vaccine strains of lumpy skin disease virus with field strains, causing disease. PLoS One. 2020;15:e0232584.
Sprygin A, Babin Y, Pestova Y, Kononova S, Wallace DB, Van Schalkwyk A, et al. Analysis and insights into recombination signals in lumpy skin disease virus recovered in the field. PLoS One. 2018;13:e0207480.
Sprygin A, Pestova Y, Prutnikov P, Kononov A. Detection of vaccine-like lumpy skin disease virus in cattle and Musca domestica L. flies in an outbreak of lumpy skin disease in Russia in 2017. Transbound Emerg Dis. 2018;65:1137–44.
Aleksandr K, Pavel P, Olga B, Svetlana K, Vladimir R, Yana P, et al. Emergence of a new lumpy skin disease virus variant in Kurgan oblast, Russia, in 2018. Arch Virol. 2020;165:1343–56.
Kononova S, Kononov A, Shumilova I, Byadovskaya O, Nesterov A, Prutnikov P, et al. A lumpy skin disease virus which underwent a recombination event demonstrates more aggressive growth in primary cells and cattle than the classical field isolate. Transbound Emerg Dis. 2020;00:1–7. https://doi.org/10.1111/tbed.13798 .
Heine HG, Stevens MP, Foord AJ, Boyle DB. A capripoxvirus detection PCR and antibody ELISA based on the major antigen P32, the homolog of the vaccinia virus H3L gene. J Immunol Methods. 1999;227:187–96.
Ireland DC, Binepal YS. Improved detection of capripoxvirus in biopsy samples by PCR. J Virol Methods. 1998;74:1–7.
Balinsky CA, Delhon G, Smoliga G, Prarat M, French RA, Geary SJ, et al. Rapid preclinical detection of sheeppox virus by a real-time PCR assay. J Clin Microbiol. 2008;46:438–42.
Bowden TR, Babiuk SL, Parkyn GR, Copps JS, Boyle DB. Capripoxvirus tissue tropism and shedding: a quantitative study in experimentally infected sheep and goats. Virology. 2008;371:380–93.
Stubbs S, Oura CAL, Henstock M, Bowden TR, King DP, Tuppurainen ESM. Validation of a high-throughput real-time polymerase chain reaction assay for the detection of capripoxviral DNA. J Virol Methods. 2012;179:419–22.
Haegeman A, Zro K, Vandenbussche F, Demeestere L, Van Campe W, Ennaji MM, et al. Development and validation of three Capripoxvirus real-time PCRs for parallel testing. J Virol Methods. 2013;193:446–51.
Lamien CE, Lelenta M, Goger W, Silber R, Tuppurainen E, Matijevic M, et al. Real time PCR method for simultaneous detection, quantitation and differentiation of capripoxviruses. J Virol Methods. 2011;171:134–40.
Gelaye E, Lamien CE, Silber R, Tuppurainen ESM, Grabherr R, Diallo A. Development of a cost-effective method for Capripoxvirus genotyping using snapback primer and dsDNA intercalating dye. PLoS One. 2013;8:e75971.
Gelaye E, Mach L, Kolodziejek J, Grabherr R, Loitsch A, Achenbach JE, et al. A novel HRM assay for the simultaneous detection and differentiation of eight poxviruses of medical and veterinary importance. Sci Rep. 2017;7:42892.
Hosamani M, Mondal B, Tembhurne PA, Bandyopadhyay SK, Singh RK, Rasool TJ. Differentiation of sheep pox and goat poxviruses by sequence analysis and PCR-RFLP of P32 gene. Virus Genes. 2004;29:73–80.
Anonymous. Situation Report: Lumpy Skin Disease in Bangladesh Background. 2019. https://www.oie.int/fileadmin/Home/eng/Animal_Health_in_the_World/docs/pdf/Disease_cards/LUMPY_SKIN_DISEASE_FINAL.pdf . Accessed 19 July 2020.
Babiuk S, Bowden TR, Parkyn G, Dalman B, Manning L, Neufeld J, et al. Quantification of lumpy skin disease virus following experimental infection in cattle. Transbound Emerg Dis. 2008;55:299–307.
Şevik M, Avci O, Doǧan M, Ince ÖB. Serum biochemistry of lumpy skin disease virus-infected cattle. Biomed Res Int. 2016;2016:6257984.
Article PubMed PubMed Central CAS Google Scholar
Yeruham I, Perl S, Nyska A, Abraham A, Davidson M, Haymovitch M, et al. Adverse reactions in cattle to a capripox vaccine. Vet Rec. 1994;135:330–2.
Kumar S, Stecher G, Tamura K. MEGA7: molecular evolutionary genetics analysis version 7.0 for bigger datasets. Mol Biol Evol. 2016;33:1870–4.
Drummond AJ, Suchard MA, Xie D, Rambaut A. Bayesian phylogenetics with BEAUti and the BEAST 1.7. Mol Biol Evol. 2012;29:1969–73.
Yu G, Smith DK, Zhu H, Guan Y, Lam TTY. Ggtree: an R package for visualization and annotation of phylogenetic trees with their covariates and other associated data. Methods Ecol Evol. 2017;8:28–36.
Download references
Acknowledgments
We are very grateful to the veterinarian of the Department of Livestock Services, who helped a lot in the sample collection.
This study was supported by VETLAB network initiative of the Joint FAO/IAEA Division, funded through the African Renaissance and International Cooperation fund of South Africa and the Peaceful Uses Initiatives (PUI) by Japan and the United States of America. The funders had no role in study design, data collection and analysis, decision to publish, or preparation of the manuscript.
Author information
Authors and affiliations.
Central Disease Investigation Laboratory (CDIL), 48, KaziAlauddin Road, Dhaka, People’s Republic of Bangladesh
Shukes Chandra Badhy, Mohammad Golam Azam Chowdhury, Mohammad Aflak Uddin Fakir, Shamima Akter, Izhar Ahmed Khan, Md Bazlur Rashid & Mohammad Sadekuzzaman
Department of Livestock Services, Dhaka, People’s Republic of Bangladesh
Shukes Chandra Badhy, Mohammad Golam Azam Chowdhury, Mohammad Aflak Uddin Fakir, Shamima Akter, Mozaffar Goni Osmani, Faisol Talukdar, Noorjahan Begum, Izhar Ahmed Khan, Md Bazlur Rashid & Mohammad Sadekuzzaman
Animal Production and Health Laboratory, Joint FAO/IAEA Division of Nuclear Techniques in Food and Agriculture, Department of Nuclear Sciences and Applications, International Atomic Energy Agency, Wagramer Strasse 5, P.O. Box 100, A-1400, Vienna, Austria
Tirumala Bharani Kumar Settypalli, Giovanni Cattoli & Charles Euloge Lamien
You can also search for this author in PubMed Google Scholar
Contributions
Conceived and designed the experiments: SCB, MS, IAK; Performed the experiments: SCB, MGAC, TBKS, SA; Analyzed the data: CEL, TBKS, SA; Contributed reagents/materials/analysis tools: MAUF, MGAC, TABMMGO, FT; Wrote the paper: MS, CEL, NB; Supervised the study. MBR, GC, IAK; Edited the final manuscript: TABMMGO, FT, NB, MBR, GC, MAUF. All authors read and approved the final manuscript.
Corresponding author
Correspondence to Mohammad Sadekuzzaman .
Ethics declarations
Ethics approval and consent to participate.
Approval of this study was obtained through the approval of Central Disease Investigation Laboratory (CDIL), Bangladesh. The samples were summited to the Central Disease Investigation Laboratory (CDIL) for diagnostic confirmation and the results reported to the OIE. Sampling was carried out under the owner’s consent.
Consent for publication
Not applicable.
Competing interests
All authors declared that they have no competing interests.
Additional information
Publisher’s note.
Springer Nature remains neutral with regard to jurisdictional claims in published maps and institutional affiliations.
Supplementary Information
Additional file 1., rights and permissions.
Open Access This article is licensed under a Creative Commons Attribution 4.0 International License, which permits use, sharing, adaptation, distribution and reproduction in any medium or format, as long as you give appropriate credit to the original author(s) and the source, provide a link to the Creative Commons licence, and indicate if changes were made. The images or other third party material in this article are included in the article's Creative Commons licence, unless indicated otherwise in a credit line to the material. If material is not included in the article's Creative Commons licence and your intended use is not permitted by statutory regulation or exceeds the permitted use, you will need to obtain permission directly from the copyright holder. To view a copy of this licence, visit http://creativecommons.org/licenses/by/4.0/ . The Creative Commons Public Domain Dedication waiver ( http://creativecommons.org/publicdomain/zero/1.0/ ) applies to the data made available in this article, unless otherwise stated in a credit line to the data.
Reprints and permissions
About this article
Cite this article.
Badhy, S.C., Chowdhury, M.G.A., Settypalli, T.B.K. et al. Molecular characterization of lumpy skin disease virus (LSDV) emerged in Bangladesh reveals unique genetic features compared to contemporary field strains. BMC Vet Res 17 , 61 (2021). https://doi.org/10.1186/s12917-021-02751-x
Download citation
Received : 30 September 2020
Accepted : 08 January 2021
Published : 29 January 2021
DOI : https://doi.org/10.1186/s12917-021-02751-x
Share this article
Anyone you share the following link with will be able to read this content:
Sorry, a shareable link is not currently available for this article.
Provided by the Springer Nature SharedIt content-sharing initiative
- Lumpy skin disease virus;Capripoxvirus;RPO30
- EEV glycoprotein
BMC Veterinary Research
ISSN: 1746-6148
- General enquiries: [email protected]
- Open supplemental data
- Reference Manager
- Simple TEXT file
People also looked at
Original research article, subclinical infection caused by a recombinant vaccine-like strain poses high risks of lumpy skin disease virus transmission.

- Federal Center for Animal Health, Vladimir, Russia
Lumpy skin disease (LSD) is a transboundary viral infection, affecting cattle with characteristic manifestations involving multiple body systems. A distinctive characteristic of lumpy skin disease is the subclinical disease manifestation wherein animals have viremia and shed the virus through nasal and ocular discharges, while exhibiting no nodules but enlarged lymph nodes that are easily oversighted by inexperienced vets. Further research on the role of subclinically ill animals in the transmission of LSD virus (LSDV) can contribute to the development of more effective tools to control the disease worldwide. Thus, this study aims to determine the potential role of subclinical infection in virus transmission in a non-vector-borne manner. To achieve this, we inoculated animals with the recombinant vaccine-like strain (RVLS) Udmurtiya/2019 to cause clinical and subclinical LSDV infection. After the disease manifestation, we relocated the subclinically ill animals to a new clean facility followed by the introduction of another five animals to determine the role of RVLS-induced subclinical infection in the virus transmission via direct/indirect contact. After the introduction of the naïve animals to the relocated subclinically ill ones in a shared airspace, two introduced animals contracted the virus (clinically and subclinically), showing symptoms of fever, viremia, and seroconversion in one animal, while three other introduced animals remained healthy and PCR-negative until the end of the study. In general, the findings of this study suggest the importance of considering LSDV subclinical infection as a high-risk condition in disease management and outbreak investigations.
1 Introduction
Lumpy skin disease virus (LSDV) is recognized as an important transboundary pathogen whose infection in animals has been associated with considerable losses in affected farms and countries ( 1 ). The etiological agent belongs to the Capripoxvirus genus along with the sheep pox virus and goat pox virus, which all share approximately 96% identity ( 2 ). The animals that are susceptible to the disease include cattle and buffaloes, among others ( 3 ). LSD virus (LSDV) has been shown to have a broader host tropism as previously expected. A recent study reported the isolation of LSDV genomic DNA from the nodules of springboks, oryxes, and giraffes. Research evidence has also confirmed that experimental infection can lead to clinical signs in impalas and giraffes ( 3 – 5 ).
As a species, LSDV has emerged 500 years ago via recombination ( 6 , 7 ) but was officially first documented in Zambia in 1929, from where it spread throughout Africa and then into the Middle East ( 8 – 10 ).
In the recent decade, LSDV dramatically spread across Eurasia and Southeast Asia ( 11 – 13 ). Unprecedentedly, the LSDV epidemiology in Eurasia was accompanied by the emergence of novel vaccine-like strains that cause homologous recombination between two vaccine strains, i.e., the commercial Neethling vaccine strain and Kenyan KSGP strain used in Lumpivax vaccine (KEVEVAPI) ( 14 , 15 ). The incidence of RVLS has been increasing in several countries, including China, Thailand, and Mongolia ( 16 – 18 ). In India and Bangladesh, LSDV outbreaks have been attributed to the KSGP strain lineage ( 19 , 20 ).
Phylogenetically, the current genetic clustering of existing LSDV lineages is divided into the following clusters: (1) Cluster 1.1, which includes vaccine Neethling strains, and (2) Cluster 1.2, which includes classical field strains, such as Warmbaths, Dagestan/2015, Israel, and KSGP-like strains ( 15 , 21 ). Aside from the classical Cluster 1.1 and 1.2 viruses, newly emerged recombinant strains have been found, which constitute new clusters (from 2.1 to 2.6) ( 15 , 21 ).
The first recombinant strain Saratov/2017 whose backbone was represented by the Neethling vaccine and KSGP strains was recovered from a field outbreak in 2017 in Russia close to a country that launched a mass vaccination with a live attenuated vaccine against LSDV ( 14 ). After the analysis of the Saratov/2017 full genome sequence, this strain comprised novel Cluster 2.1. Another recombinant strain Udmurtia/2019, whose dominant parental strain was the KSGP strain backbone and Neethling vaccine strain as a minor one as opposed to Saratov/2017, belongs to Cluster 2.2, followed by Cluster 2.3 by Kostanay/2018 from Kazakhstan and Cluster 2.4 by Tyumen/2019. The strains from Southeast Asia, especially in China, Thailand, and Vietnam, belong to dominant Cluster 2.5 and have been found to be prominent in the region ( 11 ).
LSD can manifest clinically as typical skin nodules and mucosal surface lesions but can also occur in subclinical form without these symptoms. In both cases, viremia and virus shedding through nasal discharges can occur ( 22 – 24 ). Research on these virus shedding sites, regardless of disease manifestation, can help elucidate the role of excreted viruses in transmission to in-contact animals ( 25 – 27 ).
LSDV is known to be mechanically transmitted through arthropod bites only, although the studies that suggested this were only built on Cluster 1.2 strains, while the RVLSs with altered genomes have acquired mechanisms for direct/indirect contact modes of transmission under experimental studies and natural conditions ( 28 – 30 ). Importantly, LSDV transmission without arthropod assistance is commonly observed in recombinant LSDV strains from Cluster 2.1, Cluster 2.2, and the like ( 31 , 32 ). This feature is critical in LSDV management but is often overlooked due to the lack of awareness and pursuit of the vector-borne concept and limited access to recombinant strains for testing ( 30 , 33 ). Notably, all capripoxviruses and poxviruses can spread through contact transmission ( 2 ). Therefore, considering the capacity of the RVLSs to transmit via direct or indirect contact, subclinical infection caused by the RVLSs can undermine the current efforts of disease prevention. The aim of this study is to investigate the role of RVLS-induced subclinical infection in the non-vector-borne transmission of the virus to in-contact animals.
2 Materials and methods
One RVLS of LSDV was isolated from the Udmurtiya region of the Russian Federation in 2019. This genetic lineage was unique and was never detected anywhere elase ( 30 ). This strain was selected for the experiment due to the following criteria: (i) it was detected during snowy winter ( 30 ); (ii) the Udmurtiya strain was already shown capable of non vector-borne transmission ( 31 ). The virus was isolated by performing two serial passes in goat testis cells before characterization via PCR amplification and the sequencing of several loci specific to either the vaccine or the field strain genome ( 21 , 29 ). Moreover, the virus was titrated in 96-well plates using tenfold dilution. The plates were incubated at 37°C with 5% CO 2 for 72 h and inspected daily for the presence of the cytopathic effect (CPE). The virus titer was measured using the Spearman–Karber method as reported previously ( 31 ). The results are expressed in logarithm as 50% tissue culture infective dose (log TCID50).
2.2 Experimental design
Ten non-vaccinated Russian black pied bulls aged 6–8 months (300–500 kg in weight) were included. Prior to the experiment, the blood and serum were tested for LSDV genome and antibodies to ensure that they had not been exposed to the virus. The animals were numbered from 1 to 10 randomly and housed in an insect-free animal biosafety level 3 facility and subjected to a 12-h light–dark cycle, relative humidity of 30–70%, and temperature range between 23°C and 26°C. Moreover, they were monitored twice a day by the veterinary staff and provided with water and feed ad libitum .
All the animals participating in the experiment (a total of 15) were also checked for any presence of ticks before entering the facility and kept in the facility for two weeks before the start of the study for them to adapt to the conditions. Their blood samples and nasal swabs were obtained for PCR and blood for neutralization (NT) tests to exclude previous or present LSDV infections ( 34 , 35 ).
On the first day of the study (0 dpi), 2 mL of 5 log TCD50/mL of Udmurtiya/2019 virus was used to inoculate each animal intravenously. The animals were monitored daily for skin lumps, whereas the blood samples and nasal swabs were collected every second day for analysis via real-time PCR to detect LSDV nucleic acids. The study and monitoring period lasted for 49 days, which involved the daily registration of body temperature ( Supplementary Figure S1 ) and clinical score ( Supplementary Figure S2 ) based on the recommendations of Wolff et al. ( 36 ).
Upon the onset of the first skin lumps, the affected animals were kept in the facility, while the subclinically affected animals (without nodules but with viremia and virus shed via nasal discharge) were transferred to another disinfected room, wherein other five animals (in-contact) were introduced. For the purpose of this study, the term “in-contact animal” pertains to animals that were housed in the same ventilated insect-proof facility sharing airspace where they could see each other, but any physical contact between them as well as sharing of water troughs, food, or bedding were prohibited. Their mobility was also restricted using tethering. Furthermore, the in-contact animals were monitored for clinical signs and viremia via PCR throughout the experiment.
2.3 DNA extraction and PCR
The samples were handled aseptically and processed as 10% homogenates in phosphate-buffered saline. A 200-μL aliquot was used for total nucleic acid extraction using the QIAamp DNA Mini Kit (Qiagen, Germany) in accordance with the manufacturer’s instructions. The sample extracts were analyzed to check the presence of LSDV DNA using real-time PCR (qPCR) based on ORF044 as previously described ( 35 ).
The fluorogenic probe was labeled at the 5′ end with the FAM reporter dye and BHQ as a quencher at the 3′ end. Selected primers (df4ln: CAAAAACAATCGTAACTAATCCA and zdr4ln: TGGAGTTTTTATGTCATCGTC) and probes (zdpro4ln1:Fam-TCGTCGTCGTTTAAAACTGA-BHQ1) were synthesized by Syntol (Moscow, Russia). PCR was performed using a Rotor-Gene Q (Qiagen, Germany) instrument with the following thermal-cycling profile: 95°C for 10 min, followed by 45 cycles at 95°C for 15 s (s) and 60°C for 60 s. Moreover, the final reaction volume was 25 μL containing 10 pmol of each primer, 5 pmol of the probe, 5 μL of 25 mM MgCl2, 5 μL 5 × PCR buffer (Promega, United States), 1 μL of 10 pmol dNTPs (Invitrogen, USA), and deionized water. The samples were analyzed in accordance with the protocol as previously described ( 35 ).
2.4 Virus neutralization
Virus neutralization in JetBioFil 96-well flat-bottom microplate (Guangzhou JET Bio-Filtration, China) was conducted in accordance with the protocol previously described ( 34 ) with a few modifications. The test was performed on ovine testis cells with two replicates having the same strain Udmurtiya/2019 used as the inoculum. One hundred μl of the virus inoculum was added into each well, and the neutralization dilution was considered positive at <1:8, doubtful at <1:4, and negative at <1:2.
2.4.1 Visualization of results
The data were visualized using Microsoft Excel.
The first signs of an increase in body temperature (41.1°C–41.5°C) were recorded in 4 out of 10 infected bulls at 7 dpi (animals 1, 4, 5, and 9). The first clinical manifestations of LSDV were observed at 8–9 dpi in the form of small skin bumps on the neck and shoulder blades ( Figure 1 ).
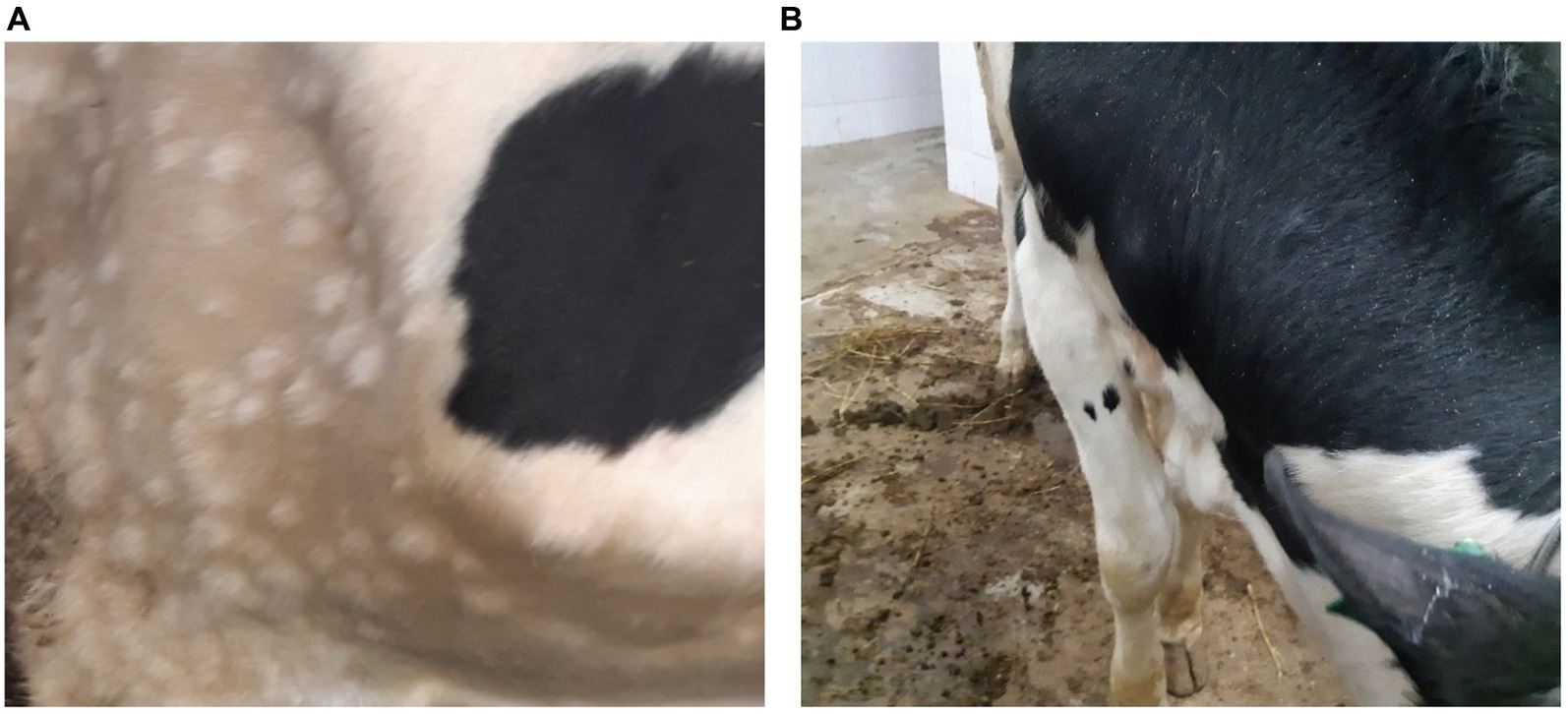
Figure 1 . Clinical manifestations of LSDV in the form of nodular skin lesions at 10 dpi. (A) Multiple nodular lesions in the scapular region (bull no. 1). (B) Nodular lesions on the back (bull no. 4).
Aside from roseola on the scrotum ( Figure 2 ), skin lesions ranging in size from 0.3 × 0.3 cm to 2.0 × 2.5 cm were spotted over the entire body surface of these bulls. Moreover, the animals presented with enlarged superficial lymph nodes and signs of increased weakness, heavy breathing, and loss of appetite.
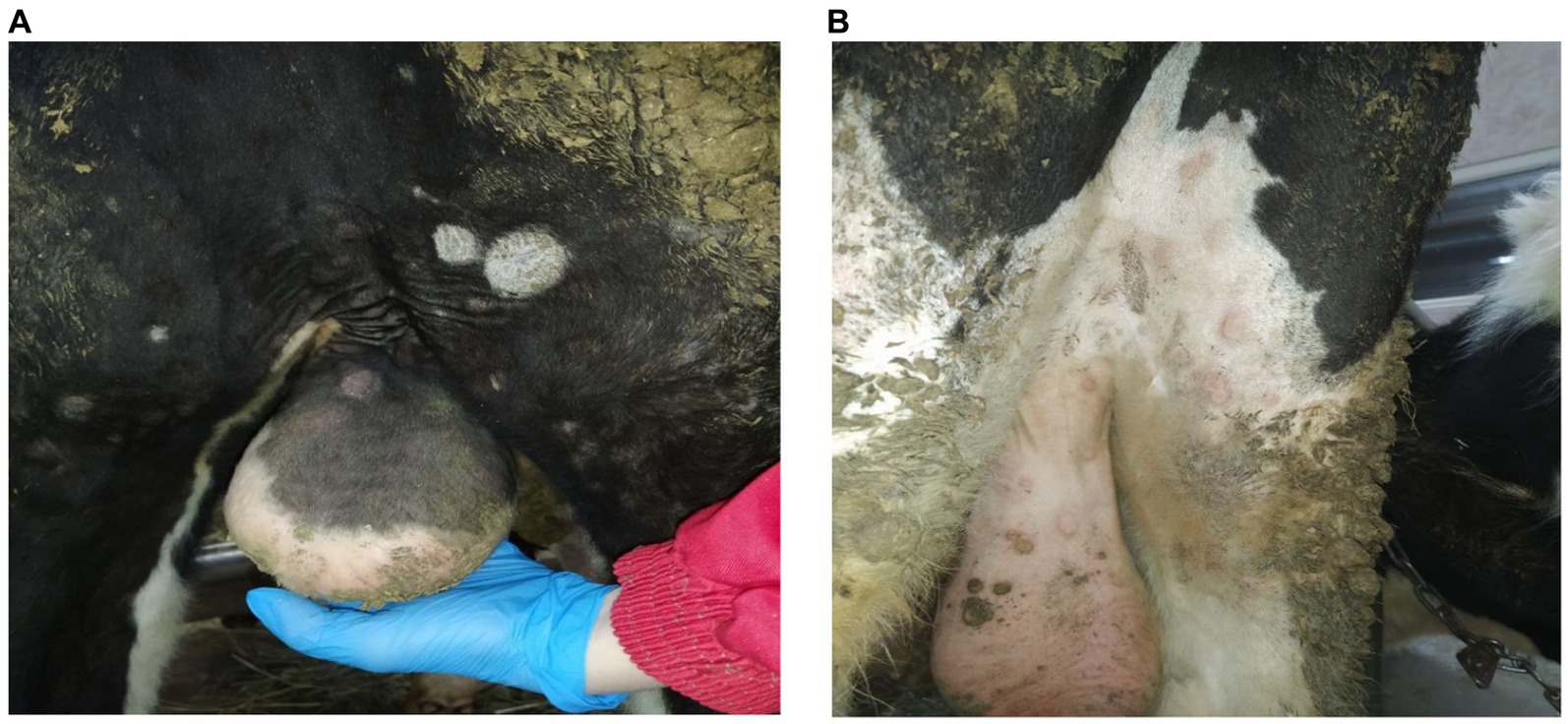
Figure 2 . Clinical manifestations of LSDV in the form of roseola at 10 dpi. (A) Roseola on the scrotum (bull no. 5). (B) Roseola on the scrotum and hindlimbs (bull no. 9).
Real-time PCR revealed the LSDV genome in the stabilized blood sample and nasal swabs ( Tables 1 , 2 ). Of the 10 inoculated bulls, Bull no. 3 and 8 showed no detectable viremia and shedding ( Tables 1 , 2 ). Bull no. 3 and 8 was found to be resistant to LSDV at the end of the experiment. The viremia in the subclinically ill animals lasted for 3–4 days with a Cts ranging from 22.7 to 34.6. Moreover, the nasal shedding lasted for 1–3 days with a Cts varying from 30.1 to 36.1 ( Tables 1 , 2 ).
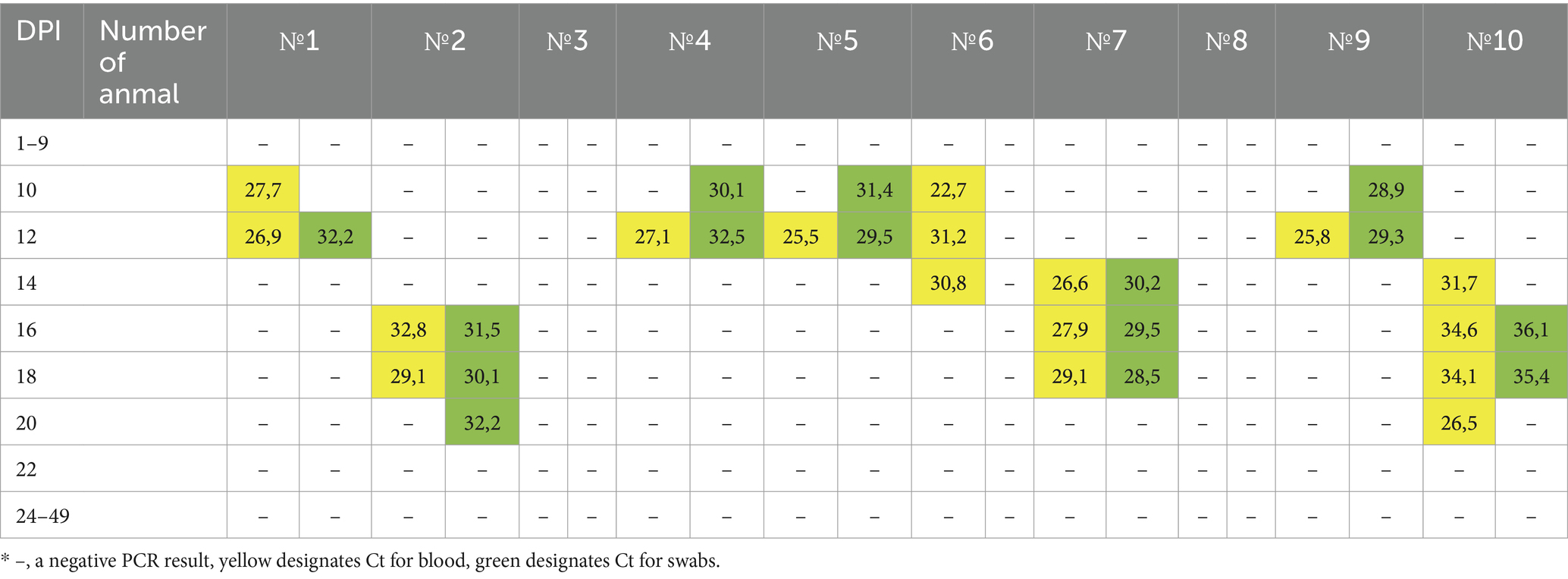
Table 1 . RT-PCR results of the detection of the LSDV genome in the stabilized blood and swab samples from the 10 virus-inoculated animals.
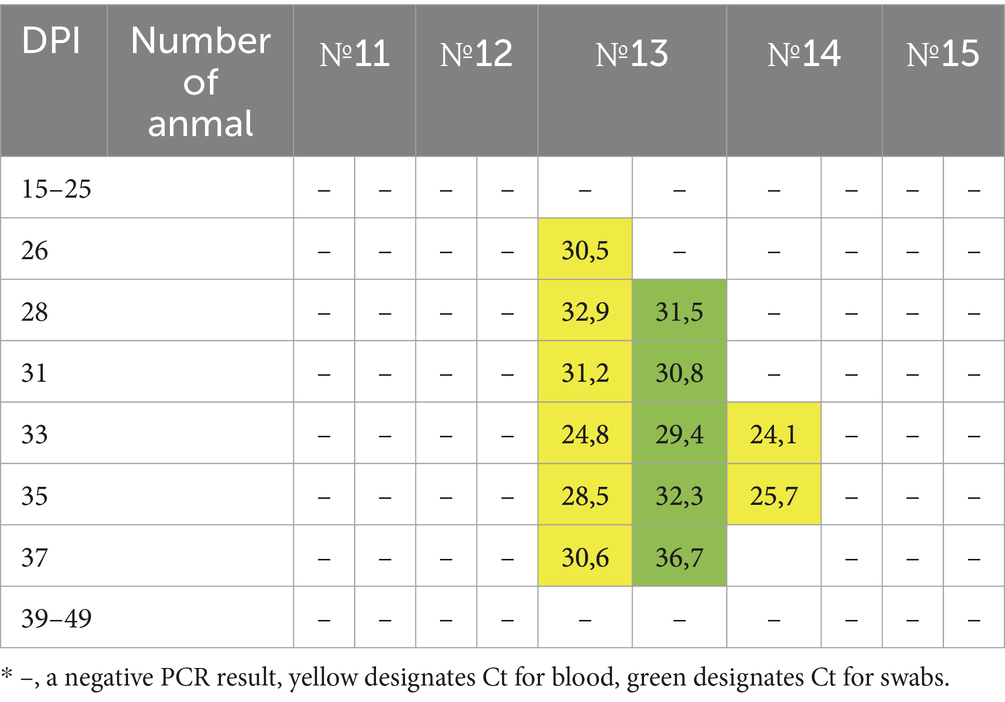
Table 2 . RT-PCR results of the detection of LSDV genome in the stabilized blood and swab samples from the 5 newly introduced animals.
Subsequently, the real-time PCR results for Bull no. 2 and 10 indicated positive values for the blood and nasal swab samples taken at 16 and 14 dpi, respectively ( Table 1 ), although the gross LSDV clinical signs and an increase in body temperature in these two animals were not observed throughout the study.
At 13 dpi, all four bulls (Bull no. 1, 4, 5, and 9) were withdrawn from the study, leaving the remaining animals for further monitoring. On the 15th dpi, the other five healthy bulls (Bull no. 11–15) were introduced and placed between the subclinically infected animals in a new clean facility. Bull no. 7 showed an increase in body temperature to 40.9°C at 16 dpi and presented with signs of depression with a loss of appetite, skin bumps appearing over the entire surface of the body, and an increase in superficial lymph nodes at 18 dpi. Moreover, Bull no. 7 was removed at 19 dpi.
The subclinically ill animals without visible symptoms (Bull no. 2, 3, 6, 8, and 10) and newly introduced animals (Bull no. 11, 12, 13, 14, and 15) remained in the study.
On the 11th day after the introduction of the new animals, Bull no. 13 showed signs of fever with a body temperature of 40.5°C and roseola on the scrotum and the groin at 14 dpi after introduction ( Figure 3A ). On the 16th day, the animal exhibited skin nodules ( Figure 3B ), accompanied by a strong cough, an increase in superficial lymph nodes, purulent discharge from the eyes ( Figure 3C ), and edema of the forelimbs. Moreover, the bull showed symptoms of depression with a loss of appetite and did not get up for 6 days. Fever was maintained for 12 days with a body temperature varying from 40.5°C to 41.5°C.
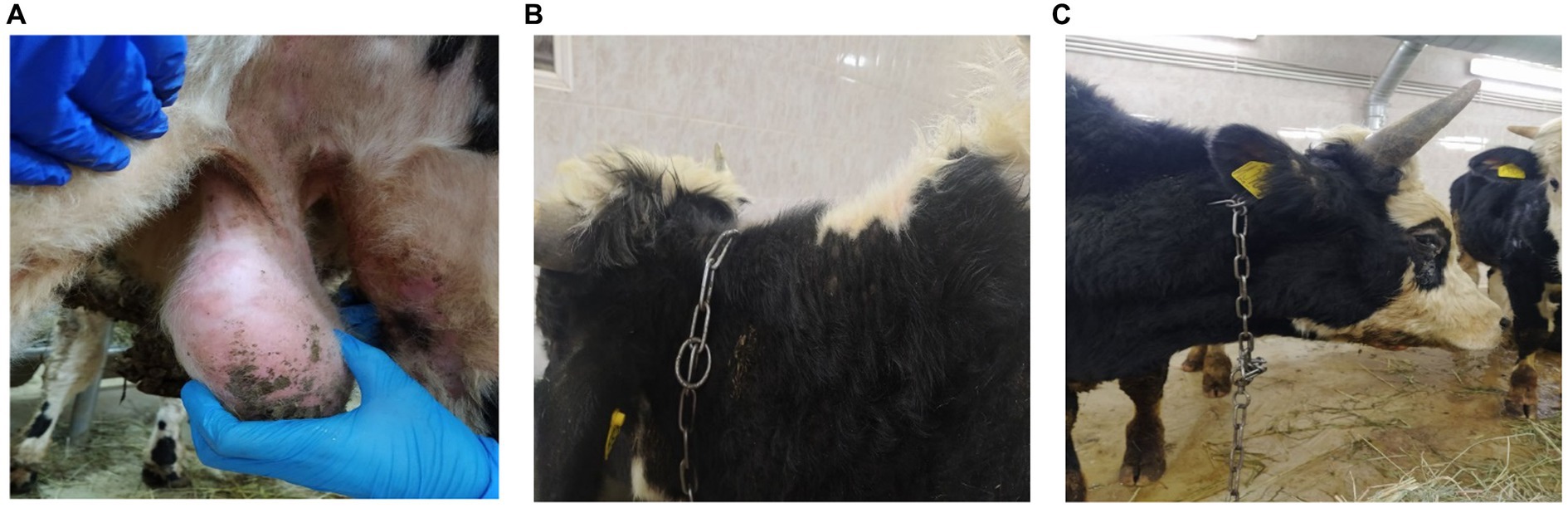
Figure 3 . Clinical manifestation of LSDV in Bull no. 13 11 days after contact with the subclinically ill animals. (A) Roseola in the scrotum (bull no. 13). (B) Multiple nodular lesions (bull no. 13). (C) Purulent discharge from the eyes (bull no. 13).
The NT test results revealed that the subclinically ill animals presented with positive values at the end of the experiment (dpi 49) with a NT dilution ranging from 1:8 to 1:64, whereas the animals that had been removed from the experiment were not analyzed. Bull no. 3 and 8 did not have viremia but had seroconversion ( Table 3 ). Bull no. 11–15 were also sampled for NT analysis, wherein only Bull no. 11 had doubtful results and the others had negative results (< 1:2).

Table 3 . Neutralization assay results from the 15 animals of the experiment.
Except for Bull no. 13 (clinical form), no clinical signs were observed in any of the newly introduced animals (Bull no. 11–15) throughout the observation period, although Bull no. 14 showed (subclinical form) only positive PCR results in the blood starting on the 26th day of the study (13 days after the introduction to the infected animals) until the 37th day of the study ( Tables 1 , 2 ). Only Bull no. 11 showed doubtful NT results ( Table 3 ).
4 Discussion
The transmission of capripoxviruses has been attracting research interest since LSDV was identified in the Northern Hemisphere ( 37 ). As in the past, only limited evidence could confirm the contagious nature of LSDV, similar to the situation with sheep pox and goat pox ( 29 ), and considering the seasonality of LSDV rebounding ( 38 ), efforts were focused on controlling vector-borne transmissions ( 30 , 39 ). The control of contact transmission based on epidemiological findings without reliable laboratory tools was proposed as early as the onset of LSDV range expansion in Africa ( 8 ). Weiss’s hypothesis was supported by recombinant vaccine-like LSDV lineages that have increased in incidence since 2017 following the use of a live attenuated LSDV vaccine, which precipitated the occurrence of the novel RVLSs of LSDV comprised of the Neethling and KSGP vaccine strains ( 24 ). Genetically, the RVLSs fall outside the established Cluster 1.1, which was represented by the Neethling strains and virulent Neethling strains circulating in South Africa during the 1990s, and Cluster 1.2, which was represented by strains, such as Warmbaths LW, Dagestan/2015, Bujanovac/2016, Ni-2490, and KSGP ( 15 ). Interestingly, it was first observed that the novel recombinant strains spread in a non-vector-borne manner as opposed to the Cluster 1.1 and 1.2 strains ( 31 ). Furthermore, it was suggested that Saratov/2017 could spread through a contaminated feed and that the RVLSs acquired a genetic element through recombination that was missing or not overtly expressed from the parental strains ( 40 ).
Furthermore, not only the clinical form of LSDV has gained research interest but also the subclinical manifestation has been recognized as a distinctive characteristic of LSDV ( 41 ). With the recent emergence of recombinant vaccines, such as strains exhibiting transmission without arthropod activity ( 31 , 32 ), efforts have been made to determine the contribution of all forms. The studies led by Sprygin et al. analyzed the biological features of recombinant strains, demonstrating that recombinant strains not only show altered properties on cell culture but also overwinter in northern latitudes ( 31 , 40 , 42 , 43 ).
The present study follows up on the research on recombinant strains detected in Russia and provides further evidence confirming that the RVLSs of LSDV employ alternative mechanisms of transmission in contrast to that of Cluster 1.2 strains. Moreover, this is the first study to report that subclinically affected animals transmit LSDV to animals sharing the same airspace in an insect-proof facility, which is contrary to the findings of Heageman et al. ( 41 ). Subclinical infection is a typical manifestation of LSDV, and the efficiency of its control measures is directly linked to the manner whereby virus-carrying animals, regardless of the clinical or subclinical manifestation of the disease in an outbreak zone, are identified and managed ( 22 , 24 , 25 ). This study contributes to the deeper understanding of LSDV and determine the role of subclinical LSDV infection in LSDV transmission and epidemiology ( 41 ).
Our study was designed to induce subclinical infection in bulls with continued monitoring. The subclinical infection in LSDV is accompanied by virus shedding and viremia without obvious clinical signs, which can be overlooked in cattle inspection during suspected or actual outbreak management ( 23 ). In the present study, we reproduced the subclinical infection in a laboratory setting, although pure subclinical infection never occurs in a field outbreak, and showed its “contagious nature” by the presence of LSDV DNA. Although the NT analysis of the antibodies revealed doubtful results for one subclinical animal, the findings should be carefully assessed ( Tables 1 , 2 ). Nevertheless, subclinical animals concurrently become clinically ill in real field conditions; LSDV caused by the RVLSs also poses a threat of non-vector-borne virus transmission regardless of the disease presentation ( 40 ). It is noteworthy that subclinical virus carriers pose a risk of the non-vector-borne transmission of LSDV since they shed LSDV similarly to clinically ill animals ( 24 ). Of note, subclinically ill animals shed the virus via excretions (e.g., saliva, snots, ocular fluids), whereas clinically ill animals shed more virus via necrotized and sequestrated nodules ( 22 , 40 ).
Following the moving of subclinically affected animals that shed LSDV to another disinfected room followed by the placement of naïve animals imitated a natural situation. That resulted in the infection of one animal with clinical signs and fever and one with subclinical infection identified by PCR only with the other three newly introduced animals remaining resistant to the virus. Of note, Bull no. 7 that was removed at 19 dpi ( Table 1 ) could have been interpreted as clinically ill; however, skin bumps or early nodules before necrosis do not shed virus into the environment. Since the virus is entrapped inside them, the swabs from skin bumps/early nodules were negative (data not shown), which should not compromise Bull no. 7 as the source of virus for infecting in-contact animals. Moreover, the testing showed that it was shedding the virus in the same manner with a slightly lower Ct value as the other retained animals through nasal discharge. Thus, it should be determined whether Bull no. 7 could have affected the outcome of the transmission due to the Ct value being 28.5–29.5 compared with that being 30.1–36.1 in subclinical animals by definition ( Table 1 ). Although the LSDV genomes were detected in the blood samples and nasal swabs of Bull no. 13 and 14, the NT results returned negative, which might be associated with the time of performing NT, in which the immune system did not have enough time to produce detectable antibodies ( Table 2 ).
Considering that previous experiments have confirmed the non-vector-borne nature of transmission of the RVLS ( 24 , 31 , 40 ), it is unlikely that the present findings were influenced. In this regard, a quantitative study is needed to address the issue of minimal infective dose for the RVLSs to spread in an air-borne context.
The classification of LSDV-infected animals as clinically ill, subclinically affected, and resistant can be explained by variation in unknown host/genetic factors ranging from resistance to death ( 39 ). So far, most animal studies on LSDV have employed strains from Cluster 1.2 comprising unaltered field isolates, whereas a few studies have assessed the properties of the novel RVLSs ( 22 , 33 , 44 , 45 ). Weiss hypothesized the implication of contact transmission under field conditions, although molecular techniques were unavailable then ( 8 ) and studies under laboratory settings did not report any infection via contact. The RVLSs have gained research interest in relation to their epidemiology, transmission, and diagnostics ( 2 , 46 ); however, the limited accessibility of the RVLS delays the identification of its properties following the discoveries of novel features that are not observed in parental strains.
Interestingly, Bulls no. 3 and 8 were shown to be resistant to LSDV throughout the experiment but mounted an antibody response by the end of the experiment ( Table 1 ). Although some other animals showed positive PCR results, they showed no seroconversion ( Table 1 ), which is commonly observed during vaccination and experiments ( 24 , 40 ). Further research on host resistance to LSDV is needed.
Overall, the findings of this study have revealed the transmission risks posed by the RVLSs of LSDV and their resulting subclinical infections that should be primarily investigated in epidemiological studies. Theoretical studies extrapolating from the evidence based on Cluster 1.2 must be limited to the Cluster 1.2 strains. However, if the global LSDV epidemiology is concerned with the Cluster 2.5 strains in Southeast Asia and KSGP-like strains in India, analyses on the Cluster 1.2 strains in the Middle East and Africa should consider the observed epidemiological phenomena inherent to the present-day situation in the field and recombinant strain circulation with their properties, i.e., non-vector-borne transmission. In general, this is the first study to focus primarily on the complicated issues of LSDV transmission, whether it is a classical 1.2 strain lineage or recombinant lineage 2.1 and the like ( 47 ). Considering this, further research on subclinical infections is needed to delineate the particular potential of the RVLSs in non-vector-borne virus transmission.
The present study together with published evidence on transmission and recombinant LSDVs emphasizes the importance of LSDV research as well as the re-evaluation of the control and eradication approaches for LSDV (including similar measures applied to sheep pox and goat pox that spread via direct and indirect contact) and recognition of contact transmission, which will inevitably provide a better understanding of the disease, its epidemiological profile and contribute to improved eradication policies.
Data availability statement
The original contributions presented in the study are included in the article/ Supplementary material , further inquiries can be directed to the corresponding author.
Ethics statement
The animal study was approved by the ethics committee of the Federal Center for Animal Health. The study was conducted in accordance with the local legislation and institutional requirements.
Author contributions
IS: Investigation, Methodology, Writing – original draft. PP: Conceptualization, Investigation, Methodology, Writing – original draft. AM: Data curation, Writing – original draft, Writing – review & editing. AK: Methodology, Writing – original draft. NT: Methodology, Writing – original draft. OB: Formal analysis, Validation, Writing – original draft, Writing – review & editing. IC: Formal analysis, Project administration, Writing – review & editing. LP: Writing – review & editing. AS: Funding acquisition, Project administration, Resources, Supervision, Visualization, Writing – original draft, Writing – review & editing.
The author(s) declare financial support was received for the research, authorship, and/or publication of this article. This work was supported by the grant no. 075-15-2021-1054 from the Ministry of Education and Science of Russia to implement objectives of the Federal Scientific and Technical Program for the Development of genetic technologies during 2019–2027.
Conflict of interest
The authors declare that the research was conducted in the absence of any commercial or financial relationships that could be construed as a potential conflict of interest.
Publisher’s note
All claims expressed in this article are solely those of the authors and do not necessarily represent those of their affiliated organizations, or those of the publisher, the editors and the reviewers. Any product that may be evaluated in this article, or claim that may be made by its manufacturer, is not guaranteed or endorsed by the publisher.
Supplementary material
The Supplementary material for this article can be found online at: https://www.frontiersin.org/articles/10.3389/fvets.2024.1330657/full#supplementary-material
SUPPLEMENTARY TABLE 1
Registered clinical score for each experimental bull based on the recommendations of Wolff et al. ( 36 ).
1. Abutarbush, SM, Ababneh, MM, Al, ZIG, Al Sheyab, OM, Al Zoubi, MG, Alekish, MO, et al. Lumpy skin disease in Jordan: disease emergence, clinical signs, complications and preliminary-associated economic losses. Transbound Emerg Dis . (2013) 62:549–54. doi: 10.1111/tbed.12177
Crossref Full Text | Google Scholar
2. Sprygin, A, Mazloum, A, van Schalkwyk, A, and Babiuk, S. Capripoxviruses, leporipoxviruses, and orthopoxviruses: occurrences of recombination. Front Microbiol . (2022) 13:978829. doi: 10.3389/fmicb.2022.978829
PubMed Abstract | Crossref Full Text | Google Scholar
3. Dao, TD, Tran, LH, Nguyen, HD, Hoang, TT, Nguyen, GH, Tran, KVD, et al. Characterization of lumpy skin disease virus isolated from a giraffe in Vietnam. Transbound Emerg Dis . (2022) 69:e3268–72. doi: 10.1111/tbed.14583
4. Young, E, Basson, PA, and Weiss, KE. Experimental infection of game animals with lumpy skin disease virus (prototype strain Neethling). IXth International Congressof Virology, Glasgow, Scotland . (1970) 37:79–87.
PubMed Abstract | Google Scholar
5. Gelaye, E, and Lamien, CE. Lumpy skin disease and vectors of LSDV In: Transboundary animal diseases in Sahelian Africa and connected regions . Cham: Springer (2019). 267–88.
Google Scholar
6. Gershon, PD, Kitching, RP, Hammond, JM, and Black, DN. Poxvirus genetic recombination during natural virus transmission. J Gen Virol . (1989) 70:485–9. doi: 10.1099/0022-1317-70-2-485
7. Van Schalkwyk, A, Byadovskaya, O, Shumilova, I, Wallace, DB, and Sprygin, A. Estimating evolutionary changes between highly passaged and original parental lumpy skin disease virus strains. Transbound Emerg Dis . (2022) 69:e486–96. doi: 10.1111/tbed.14326
8. Weiss, KE. Lumpy skin disease virus. Virol Monogr . (1968) 3:111–31.
9. Şevik, M, and Doğan, M. Epidemiological and molecular studies on lumpy skin disease outbreaks in Turkey during 2014-2015. Transbound Emerg Dis . (2016) 64:1268–79. doi: 10.1111/tbed.12501
10. Braverman, Y, Yeruham, I, and Glasgow, M. Retrospective study on the epidemiology of the first lumpy skin disease outbreak in Israel in 1989 In: IXth international congress of virology . Scotland: S.N.: (1993)
11. Lu, G, Xie, J, Luo, J, Shao, R, Jia, K, and Li, S. Lumpy skin disease outbreaks in China, since 3 august 2019. Transbound Emerg Dis . (2020) 68:216–9. doi: 10.1111/tbed.13898
12. Manić, M, Stojiljković, M, Petrović, M, Nišavić, J, Bacić, D, Petrović, T, et al. Epizootic features and control measures for lumpy skin disease in south-East Serbia in 2016. Transbound Emerg Dis . (2019) 66:2087–99. doi: 10.1111/tbed.13261
13. Sprygin, A, Artyuchova, E, Babin, Y, Prutnikov, P, Kostrova, E, Byadovskaya, O, et al. Epidemiological characterization of lumpy skin disease outbreaks in Russia in 2016. Transbound Emerg Dis . (2018) 65:1514–21. doi: 10.1111/tbed.12889
14. Sprygin, A, Babin, Y, Pestova, Y, Kononova, S, Wallace, DB, Van Schalkwyk, A, et al. Analysis and insights into recombination signals in lumpy skin disease virus recovered in the field. PLoS One . (2018) 13:e0207480. doi: 10.1371/journal.pone.0207480. eCollection 2018
15. Krotova, A, Byadovskaya, O, Shumilova, I, van Schalkwyk, A, and Sprygin, A. An in-depth bioinformatic analysis of the novel recombinant lumpy skin disease virus strains: from unique patterns to established lineage. BMC Genomics . (2022) 23:396. doi: 10.1186/s12864-022-08639-w
16. Li, L, Wang, Z, Qi, C, Liu, S, Gong, M, Li, J, et al. Genetic analysis of genome sequence characteristics of two lumpy skin disease viruses isolated from China. BMC Vet Res . (2022) 18:426. doi: 10.1186/s12917-022-03525-9
17. Suwankitwat, N, Songkasupa, T, Boonpornprasert, P, Sripipattanakul, P, Theerawatanasirikul, S, Deemagarn, T, et al. Rapid spread and genetic characterisation of a recently emerged recombinant lumpy skin disease virus in Thailand. Vet Sci . (2022) 9:542. doi: 10.3390/vetsci9100542
18. Sprygin, A, Sainnokhoi, T, Gombo-Ochir, D, Tserenchimed, T, Tsolmon, A, Byadovskaya, O, et al. Genetic characterization and epidemiological analysis of the first lumpy skin disease virus outbreak in Mongolia, 2021. Transbound Emerg Dis . (2022) 69:3664–72. doi: 10.1111/tbed.14736
19. Kumar, A, Venkatesan, G, Kushwaha, A, Poulinlu, G, Saha, T, Ramakrishnan, MA, et al. Genomic characterization of lumpy skin disease virus (LSDV) from India: circulation of Kenyan-like LSDV strains with unique kelch-like proteins. Acta Trop . (2023) 241:106838. doi: 10.1016/j.actatropica.2023.106838
20. Parvin, R, Chowdhury, EH, Islam, MT, Begum, JA, Nooruzzaman, M, Globig, A, et al. Clinical epidemiology, pathology, and molecular investigation of lumpy skin disease outbreaks in Bangladesh during 2020-2021 indicate the re-emergence of an old African strain. Viruses . (2022) 14:2529. doi: 10.3390/v14112529
21. Krotova, A, Mazloum, A, van Schalkwyk, A, Prokhvatilova, L, Gubenko, O, Byadovskaya, O, et al. The characterization and differentiation of recombinant lumpy skin disease isolates using a region within ORF134. Appl Microbiol . (2023) 3:35–44. doi: 10.3390/applmicrobiol3010003
22. Babiuk, S, Bowden, TR, Parkyn, G, Dalman, B, Manning, L, Neufeld, J, et al. Quantification of lumpy skin disease virus following experimental infection in cattle. Transbound Emerg Dis . (2008) 55:299–307. doi: 10.1111/j.1865-1682.2008.01024.x
23. Kononov, A, Prutnikov, P, Shumilova, I, Kononova, S, Nesterov, A, Byadovskaya, O, et al. Determination of lumpy skin disease virus in bovine meat and offal products following experimental infection. Transbound Emerg Dis . (2019) 66:1332–40. doi: 10.1111/tbed.13158
24. Aleksandr, K, Olga, B, David, WB, Pavel, P, Yana, P, Svetlana, K, et al. Non-vector-borne transmission of lumpy skin disease virus. Sci Rep . (2020) 10:7436. doi: 10.1038/s41598-020-64029-w
25. Aerts, L, Haegeman, A, De Leeuw, I, Philips, W, Van Campe, W, Behaeghel, I, et al. Detection of clinical and subclinical lumpy skin disease using ear notch testing and skin biopsies. Microorganisms . (2021) 9:2171. doi: 10.3390/microorganisms9102171
26. Lojkić, I, Šimić, I, Krešić, N, and Bedeković, T. Complete genome sequence of a lumpy skin disease virus strain isolated from the skin of a vaccinated animal. Genome Announc . (2018) 6:e00482–18. doi: 10.1128/genomeA.00482-18
27. Bedeković, T, Šimić, I, Krešić, N, and Lojkić, I. Detection of lumpy skin disease virus in skin lesions, blood, nasal swabs and milk following preventive vaccination. Transbound Emerg Dis . (2018) 65:491–6. doi: 10.1111/tbed.12730
28. Lubinga, J, Tuppurainen, E, Coetzer, J, Stoltsz, W, and Venter, E. Transovarial passage and transmission of LSDV by Amblyomma hebraeum, Rhipicephalus appendiculatus and Rhipicephalus decoloratus. Exp Appl Acarol . (2014) 62:67–75. doi: 10.1007/s10493-013-9722-6
29. Sprygin, A, Pestova, Y, Wallace, DB, Tuppurainen, E, and Kononov, A. Transmission of lumpy skin disease: a short review. Virus Res . (2019) 269:197637. doi: 10.1016/j.virusres.2019.05.015
30. Sprygin, A, Van Schalkwyk, A, Shumilova, I, Nesterov, A, Kononova, S, Prutnikov, P, et al. Full-length genome characterization of a novel recombinant vaccine-like lumpy skin disease virus strain detected during the climatic winter in Russia, 2019. Arch Virol . (2020) 165:2675–7. doi: 10.1007/s00705-020-04756-7
31. Nesterov, A, Mazloum, A, Byadovskaya, O, Shumilova, I, Van Schalkwyk, A, Krotova, A, et al. Experimentally controlled study indicates that the naturally occurring recombinant vaccine-like lumpy skin disease strain Udmurtiya/2019, detected during freezing winter in northern latitudes, is transmitted via indirect contact. Front Vet Sci . (2022) 9:1001426. doi: 10.3389/fvets.2022.1001426
32. Byadovskaya, O, Prutnikov, P, Shalina, K, Babiuk, S, Perevozchikova, N, Korennoy, F, et al. The changing epidemiology of lumpy skin disease in Russia since the first introduction from 2015 to 2020. Transbound Emerg Dis . (2022) 69:e2551–62. doi: 10.1111/tbed.14599
33. Wolff, J, Tuppurainen, E, Adedeji, A, Meseko, C, Asala, O, Adole, J, et al. Characterization of a Nigerian lumpy skin disease virus isolate after experimental infection of cattle. Pathogens (Basel, Switzerland) . (2021) 11:16. doi: 10.3390/pathogens11010016
34. Samojlović, M, Polaček, V, Gurjanov, V, Lupulović, D, Lazić, G, Petrović, T, et al. Detection of antibodies against lumpy skin disease virus by virusneutrali-zation test and ELISA methods. Acta Vet-Beogr . (2019) 69:47–60. doi: 10.2478/acve-2019-000
35. Alexander, S, Olga, B, Svetlana, K, Valeriy, Z, Yana, P, Pavel, P, et al. A real-time PCR screening assay for the universal detection of lumpy skin disease virus DNA. BMC Res Notes . (2019) 12:1–5. doi: 10.1186/s13104-019-4412-z
36. Wolff, J, Krstevski, K, Beer, M, and Hoffmann, B. Minimum infective dose of a lumpy skin disease virus field strain from North Macedonia. Viruses . (2020) 12:768. doi: 10.3390/v12070768
37. Whittle, L, Chapman, R, and Williamson, AL. Lumpy skin disease-an emerging cattle disease in Europe and Asia. Vaccine . (2023) 11:578. doi: 10.3390/vaccines11030578
38. Mafirakureva, P, Saidi, B, and Mbanga, J. Incidence and molecular characterisation of lumpy skin disease virus in Zimbabwe using the P32 gene. Trop Anim Health Prod . (2017) 49:47–54. doi: 10.1007/s11250-016-1156-9
39. Issimov, A, Kutumbetov, L, Orynbayev, MB, Khairullin, B, Myrzakhmetova, B, Sultankulova, K, et al. Mechanical transmission of lumpy skin disease virus by Stomoxys Spp (Stomoxys Calsitrans, Stomoxys Sitiens, Stomoxys Indica). Diptera Muscidae Animals (Basel) . (2020) 10:pii: E477. doi: 10.3390/ani10030477
40. Shumilova, I, Nesterov, A, Byadovskaya, O, Prutnikov, P, Wallace, DB, Mokeeva, M, et al. A recombinant vaccine-like strain of lumpy skin disease virus causes low-level infection of cattle through virus-inoculated feed. Pathogens (Basel, Switzerland) . (2022) 11:920. doi: 10.3390/pathogens11080920
41. Haegeman, A, Sohier, C, Mostin, L, De Leeuw, I, Van Campe, W, Philips, W, et al. Evidence of lumpy skin disease virus transmission from subclinically infected cattle by Stomoxys calcitrans. Viruses . (2023) 15:1285. doi: 10.3390/v15061285
42. Shumilova, I, Krotova, A, Nesterov, A, Byadovskaya, O, van Schalkwyk, A, and Sprygin, A. Overwintering of recombinant lumpy skin disease virus in northern latitudes. Russia Transboundary Emerg Dis . (2022) 69:e3239–43. doi: 10.1111/tbed.14521
43. Mazloum, A, Van Schalkwyk, A, Babiuk, S, Venter, E, Wallace, DB, and Sprygin, A. Lumpy skin disease: history, current understanding and research gaps in the context of recent geographic expansion. Front Microbiol . (2023) 14:1266759. doi: 10.3389/fmicb.2023.1266759
44. Carn, VM, and Kitching, RP. An investigation of possible routes of transmission of lumpy skin disease virus (Neethling). Epidemiol Infect . (1995) 114:219–26. doi: 10.1017/s0950268800052067
45. Tuppurainen, ESM, Lubinga, JC, Stoltsz, WH, Troskie, M, Carpenter, ST, Coetzer, JAW, et al. Mechanical transmission of lumpy skin disease virus by Rhipicephalus appendiculatus male ticks. Epidemiol Infect . (2013) 141:425–30. doi: 10.1017/S0950268812000805
46. Tuppurainen, E, Dietze, K, Wolff, J, Bergmann, H, Beltran-Alcrudo, D, Fahrion, A, et al. Review: vaccines and vaccination against lumpy skin disease. Vaccine . (2021) 9:1136. doi: 10.3390/vaccines9101136
47. Sprygin, A, van Schalkwyk, A, Mazloum, A, Byadovskaya, O, and Chvala, I. Genome sequence characterization of the unique recombinant vaccine-like lumpy skin disease virus strain Kurgan/2018. Arch Virol . (2024) 169:23. doi: 10.1007/s00705-023-05938-9
Keywords: lumpy skin disease virus, recombinant vaccine-like viruses, subclinical infection, virus transmission, experiment
Citation: Shumilova I, Prutnikov P, Mazloum A, Krotova A, Tenitilov N, Byadovskaya O, Chvala I, Prokhvatilova L and Sprygin A (2024) Subclinical infection caused by a recombinant vaccine-like strain poses high risks of lumpy skin disease virus transmission. Front. Vet. Sci . 11:1330657. doi: 10.3389/fvets.2024.1330657
Received: 31 October 2023; Accepted: 26 February 2024; Published: 02 April 2024.
Reviewed by:
Copyright © 2024 Shumilova, Prutnikov, Mazloum, Krotova, Tenitilov, Byadovskaya, Chvala, Prokhvatilova and Sprygin. This is an open-access article distributed under the terms of the Creative Commons Attribution License (CC BY) . The use, distribution or reproduction in other forums is permitted, provided the original author(s) and the copyright owner(s) are credited and that the original publication in this journal is cited, in accordance with accepted academic practice. No use, distribution or reproduction is permitted which does not comply with these terms.
*Correspondence: Alexander Sprygin, [email protected]
Predicting Lumpy Skin Disease using Various Machine Learning Models
Ieee account.
- Change Username/Password
- Update Address
Purchase Details
- Payment Options
- Order History
- View Purchased Documents
Profile Information
- Communications Preferences
- Profession and Education
- Technical Interests
- US & Canada: +1 800 678 4333
- Worldwide: +1 732 981 0060
- Contact & Support
- About IEEE Xplore
- Accessibility
- Terms of Use
- Nondiscrimination Policy
- Privacy & Opting Out of Cookies
A not-for-profit organization, IEEE is the world's largest technical professional organization dedicated to advancing technology for the benefit of humanity. © Copyright 2024 IEEE - All rights reserved. Use of this web site signifies your agreement to the terms and conditions.
Advertisement
Assessing machine learning techniques in forecasting lumpy skin disease occurrence based on meteorological and geospatial features
- Regular Articles
- Published: 14 January 2022
- Volume 54 , article number 55 , ( 2022 )
Cite this article
- Ehsanallah Afshari Safavi ORCID: orcid.org/0000-0001-7022-0867 1
6014 Accesses
16 Citations
1 Altmetric
Explore all metrics
Lumpy skin disease virus (LSDV) causes an infectious disease in cattle. Due to its direct relationship with the survival of arthropod vectors, geospatial and climatic features play a vital role in the epidemiology of the disease. The objective of this study was to assess the ability of some machine learning algorithms to forecast the occurrence of LSDV infection based on meteorological and geological attributes. Initially, ExtraTreesClassifier algorithm was used to select the important predictive features in forecasting the disease occurrence in unseen (test) data among meteorological, animal population density, dominant land cover, and elevation attributes. Some machine learning techniques revealed high accuracy in predicting the LSDV occurrence in test data (up to 97%). In terms of area under curve (AUC) and F1 performance metric scores, the artificial neural network (ANN) algorithm outperformed other machine learning methods in predicting the occurrence of LSDV infection in unseen data with the corresponding values of 0.97 and 0.94, respectively. Using this algorithm, the model consisted of all predictive features and the one which only included meteorological attributes as important features showed similar predictive performance. According to the findings of this research, ANN can be used to forecast the occurrence of LSDV infection with high precision using geospatial and meteorological parameters. Applying the forecasting power of these methods could be a great help in conducting screening and awareness programs, as well as taking preventive measures like vaccination in areas where the occurrence of LSDV infection is a high risk.
Similar content being viewed by others
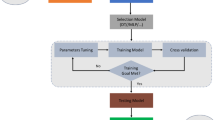
Water quality prediction using machine learning models based on grid search method
Mahmoud Y. Shams, Ahmed M. Elshewey, … Zahraa Tarek
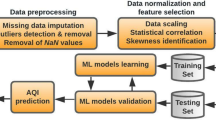
Air pollution prediction with machine learning: a case study of Indian cities
K. Kumar & B. P. Pande
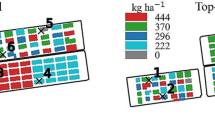
Can machine learning models provide accurate fertilizer recommendations?
Takashi S. T. Tanaka, Gerard B. M. Heuvelink, … David S. Bullock
Avoid common mistakes on your manuscript.
Introduction
Lumpy skin disease virus (LSDV) infection is a major challenge to cattle production, causing acute or subacute disease in cattle and water buffalo population. Cattle of all breeds can become infected, and cows that are around the peak of milk production and calves are particularly susceptible to LSDV infection (Namazi and Khodakaram Tafti 2021 ).
The LSDV is a double-stranded DNA virus belonging to the Capripoxvirus genus. Fever, inappetence, a significant drop in milk production, swollen lymph nodes, and the appearance of hard, slightly elevated skin nodules quickly after the onset of fever are the main clinical signs of the infection. Despite the availability of a variety of diagnostic tests, the diagnosis is generally confirmed using a traditional or real-time PCR (polymerase chain reaction) approach (Namazi and Khodakaram Tafti 2021 ).
In 1929, the first case of LSDV infection was recorded in Zambia (Von Backstrom 1945 ). LSDV has gradually expanded through Africa, the Middle East, Southeastern Europe, Central Asia, and, most recently, South Asia and China. The disease is now endemic in many African countries, as well as areas of the Middle East (Iraq, Saudi Arabia, and the Syrian Arab Republic) and Turkey (Roche et al. 2020 ). The disease has resulted in major economic losses in the affected countries. Due to high fever and secondary mastitis, it causes a substantial drop in milk production. Other consequences of the disease include damaged skin, a reduction in the growth rate of beef cattle, transient or lifelong infertility, abortion, treatment and vaccination costs, and the mortality in infected animals (Alemayehu et al. 2013 ; Namazi and Khodakaram Tafti 2021 ).
LSDV is transmitted by insects, in particular blood-sucking arthropods, contaminated food and drink, and at the later stages of the disease through saliva, nasal secretions, and semen (Sprygin et al. 2018 ; Tuppurainen et al. 2017 ). Due to its direct relationship with the survival of vectors, climatic conditions play an important role in the epidemiology of the disease. A warm and humid climate, environmental conditions that support an influx of vector populations, such as those seen during seasonal rains, and the introduction of new animals to a herd are all risk factors for the spread of LSDV. Furthermore, the wind’s direction and intensity may play a role in the spread of the virus (Chihota et al. 2003 ).
The association between LSDV infection and meteorological and geospatial factors has been studied in many studies, and they have discovered that factors like temperature, precipitation, land cover, humidity, and wind speed can predict or influence the occurrence of the disease (Alkhamis and VanderWaal 2016 ; Allepuz et al. 2019 ; Machado et al. 2019 ; Molla et al. 2017 ; Sprygin et al. 2018 ; Tuppurainen and Oura 2012 ).
Due to the introduction of new technologies and analytical techniques such as big data, remote sensing, and Earth observation, many digital Earth researches are now employing big spatiotemporal data to track and define the dynamic Earth climate system, (Kovacs-Györi et al. 2020 ; Yang et al. 2017 ).
Nowadays, machine learning (ML) offers highly valuable resources for intelligent geospatial and environmental data analysis, synthesis, and visualization. ML methods, particularly deep learning approaches, have become more common as the availability of more and different types of big data has grown (Xu and Jackson 2019 ). These techniques use general purpose learning algorithms to look for similarities in often complex and unwieldy data (Bzdok et al. 2018 ). In general, they can be used effectively at all levels of environmental data mining: exploratory spatial data processing, identification and modeling of spatial–temporal patterns, and decision-driven mapping. Traditional geostatistical methods have been replaced greatly by machine learning techniques especially in big data analyses (Kanevski et al. 2008 ). However, ML techniques should be implemented accurately and effectively from pre-processing data to analysis and justification of the findings (Kanevski et al. 2008 ).
ML techniques have been evaluated in several studies for predicting the occurrence of infectious diseases in human or animals using various climatic and geospatial features.
Wang et al. ( 2015 ) developed a feed-forward back-propagation neural network model to predict the weekly number of human cases of infectious diarrhea in China (Shanghai) using meteorological factors as predictive features. Non-linear models including neural networks, support vector regression, and random forests regression showed better performance than multiple linear regression. Neural networks showed most satisfactory results when all performance evaluation criteria were considered simultaneously.
Malki et al. ( 2020 ) explored various regressor machine learning models to predict confirmed and death cases of COVID-19 in various countries. In forecasting the COVID-19 confirmed cases, the highest performance was obtained by the KNN (K-nearest neighbors) regressor. Decision tree algorithm showed best performance in predicting the rate of COVID-19 mortality. Weather variables such as temperature and humidity were more important in predicting the mortality rate when compared to the other census variables such as population, age, and urbanization.
Golden et al. ( 2019 ) collected soil and feces samples from 11 pastured poultry farms from 2014 to 2017 in the USA. They generated random forest and gradient boosting machine predictive models to predict Listeria spp. prevalence in samples based on meteorological factors such as temperature, wind speed, gust speed, humidity, and precipitation at the farming location. AUC performance metric for the random forest and gradient boosting machine models of fecal samples was 0.905 and 0.855, respectively. The soil gradient boosting machine model outperformed the random forest model with AUCs of 0.873 and 0.700, respectively.
Liang et al. ( 2020 ) used machine learning methods to forecast African swine fever outbreaks around the world using bio-climatic variables. The random forest algorithm outperformed other techniques with 80.4% accuracy in the dataset containing all predictive variables, and the support vector machine algorithm showed the best accuracy in the subset dataset containing only important climatic features (76.02%).
The accuracy score of prediction varied between 47.8 and 99.6% in the study by Niu et al. ( 2020 ), which used various machine learning algorithms to forecast Peste des Petits ruminants (PPR) outbreaks based on certain bio-climatic variables and altitude data. The random forest algorithm performed best in a test dataset consisting of data from three countries that were not included in the training process.
To the best of the author’s knowledge, no related research has been undertaken in terms of evaluating ML techniques in building models to forecast the incidence of LSDV infection using meteorological and/or geospatial attributes.
Because of the importance of insects in LSDV transmission and their reliance on climatic and geographical features, the key objective of this research was to develop predictive models using some robust ML algorithms based on meteorological and geospatial features to predict the incidence of LSDV infection in countries with a prior history of disease outbreak reported between 2011 and 2021.
Materials and methods
Figure 1 depicts the summary of steps taken in the materials and methods, and the details of each step are explained in the following sections.
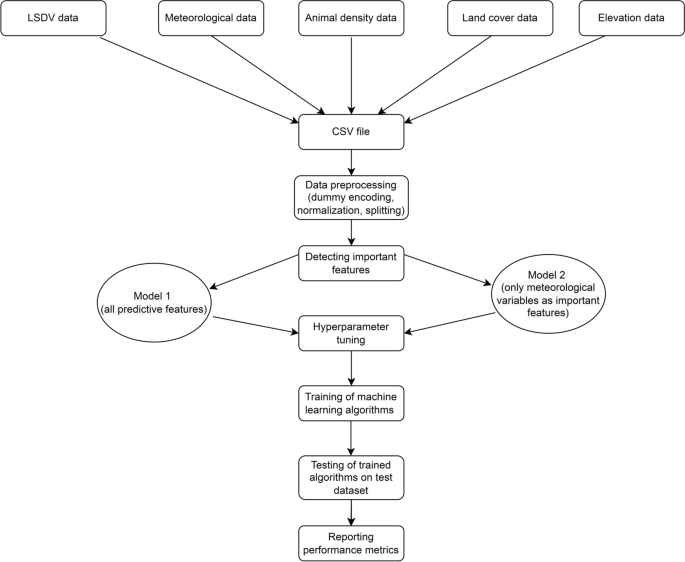
Summary of steps taken in the materials and methods section
Data sources
Lumpy skin disease outbreak data.
Geographic coordinates of Lumpy Skin Disease outbreaks were obtained from Global Animal Disease Information System of FAO (Food and Agriculture Organization) ( https://empres-i.review.fao.org/ /). Relevant information between January 2011 and March 2021 including the specific time of the outbreak and the longitude and latitude of the outbreak point were downloaded.
Meteorological data
Monthly cloud cover (percentage), diurnal temperature range (degrees Celsius), frost day frequency (days per month), wet day frequency (days), potential evapotranspiration (millimeters per day), precipitation (millimeters per months), daily mean temperature (degrees Celsius), monthly average maximum and minimum temperature (degrees Celsius), and vapor pressure (hectopascal) data for the period January 2011–December 2019 were obtained from the University of East Anglia’s Climatic Research Unit (CRU TS4.04) (Harris et al. 2020 ).
Animal density data
Cattle and buffalo population density data were obtained from Gridded Livestock of the World (GLW 3) database (Gilbert et al. 2018 ).
Land cover data
GLC-SHARE Beta-Release v1.0 (Latham et al. 2014 ) was used to extract global land cover data (spatial information on various forms of physical covering of the Earth’s surface) including artificial surfaces, cropland, grassland, tree covered areas, shrubs covered areas, herbaceous vegetation, aquatic or regularly flooded areas, mangroves, sparse vegetation, bare soil, snow and glaciers, and waterbodies coverage.
Elevation data
Global geospatial elevation dataset (GRAY_50M_SR.VERSION 2.1.0) was downloaded from Natural Earth database (free vector and raster map data @ naturalearthdata.com).
Data preprocessing
Only data in countries which reported the LSDV infection during the study time period (2011–2021) were extracted in all downloaded data and map files. In order to prepare data values to be used by ML algorithms, categorical variables were converted to numeric values using one-hot encoding technique. Moreover, the values of different predictive features were normalized using min–max scaling. Finally, the dataset was split into train and test sets using train_test_split class from scikit-learn library (Pedregosa et al. 2011 ). The training dataset which was used during model development and the test set which was not seen by the model were used for validation. Repeated stratified K-Fold cross-validation using 3 splits and 2 repeats was also used to validate the machine learning models during training step.
Selecting of features based on importance
The Scikit-learn module’s ExtraTreesClassifier and SelectFromModel classes were used to select features that are most useful for prediction. The ExtraTreesClassifier class implements a meta estimator that employs averaging to control over-fitting by fitting a number of randomized decision trees (extra-trees) on different sub-samples of the dataset (Geurts et al. 2006 ). SelectFromModel class is a meta-transformer for selecting features based on importance weights. SelectFromModel accepts a threshold parameter and will select the features whose importance (defined by the coefficients) are above this threshold. SelectFromModel requires the underlying estimator to expose a coef_ attribute or a feature_importances_ attribute which in this case was provided by ExtraTreesClassifier class. The net results of the cooperation of these two classes are choosing the important predictive features among all predictive variables.
Hyperparameter tuning
To choose a set of optimal parameters for each machine learning techniques, RandomizedSearchCV method from the scikit-learn library was used. This method can test a given number of candidates from a parameter set with a specified distribution.
Machine learning algorithms used in training and testing phases
Logistic regression.
Logistic regression is one of the machine learning classification techniques, which is utilized for anticipating the categorical dependent variable employing a given set of dependent variables and gives the probabilistic values which lie between 0 and 1 (Cox 1958 ).
Support vector machine
Support vector machines (SVMs) are a group of supervised learning techniques which are effective in high dimensional spaces. It creates the best decision boundary to separate multi-dimensional space into subclasses using the extreme cases which are called support vectors (Scholkopf 1998 ).
Decision tree
A decision tree classifier is a tree-like structure that creates a training model to predict the target class through learning simple decision rules inferred from prior data (training data). Internal nodes represent features (or attributes), the branches represent decision rules, and each leaf node represents the outcome (Safavian and Landgrebe 1991 ).
Random forest
Random forest is an ensemble decision tree-based classification method that acts through building a number of trees and each tree is dependent on the values of an independently sampled random vector with the same distribution for all trees within the forest (Breiman 2001 ).
AdaBoost is an ensemble algorithm in which subsequent weak learners are adjusted adaptively in favor of those instances misclassified by previous classifiers (Freund and Schapire 1997 ).
As another ensemble method, bagging (short for bootstrap aggregating) uses the same training algorithm for every predictor and train them on different random subsets of the training set with replacement (Breiman 1996 ).
XGBoost is a popular and efficient open-source implementation of the gradient boosted trees algorithm (Chen and Guestrin 2016 ). XGBoost stands for extreme gradient boosting, which uses decision trees as base learners, merging several weak learners to create a more powerful learner. Therefore, it is referred to as an ensemble learning algorithm since the final prediction incorporates the output of several models.
Artificial neural network (multilayer perceptron)
A simple form of artificial neural network (ANN) is the multilayer perceptron (MLP). In most cases, it has three layers: input, output, and a hidden layer. The input layer is where the data to be processed is received. The output layer is in charge of classification. The true computational engine of the MLP is an arbitrary number of hidden layers located between the input and output layers (Chollet 2018 ).
Evaluating the performance of predictive models
Accuracy score, precision, recall, F1 score, and area under curve (AUC) were used as performance metrics to measure the power of different classifiers in predicting unseen data (test set) (Géron 2019 ).
Accuracy score is one of the common performance metrics which is calculated by dividing the number of correct predictions by total number of predictions.
Another useful metric is precision, or the accuracy of the positive predictions:
Recall is the ratio of positive instances that are correctly detected by the classifier:
F1 score is the harmonic mean of precision and recall which gives much more weight to low values:
Receiver operating characteristic (ROC) curve plots the true positive rate (recall) against the false positive rate. The area under curve (AUC) of ROC curves used as its summary and assesses a classifier’s ability to discriminate between classes.
Analysis tools
QGIS software (version 3.16 – Hannover) was applied to analyze and edit spatial data files. Machine learning techniques were implemented using the Python programming language (version 3.8) and the Anaconda navigator platform (as a package manager; version 1.10.0). Scikit-learn 0.24.1 (Pedregosa et al. 2011 ) was used to implement logistic regression, SVM, decision tree, random forest, AdaBoost, and bagging algorithms. The XGBoost library was utilized to run the XGBoost technique (Chen and Guestrin 2016 ). Keras API (Chollet 2018 ) running as an abstraction layer on top of TensorFlow 2 framework (Abadi et al. 2016 ) was used for building multilayer perceptron (ANN).
Distribution of outbreaks points
Between January 2011 and March 2021, 3039 LSDV infection outbreaks were recorded across Africa, Asia, and Europe. Figure 2 indicates the distribution of outbreaks points along with 21,757 free points.
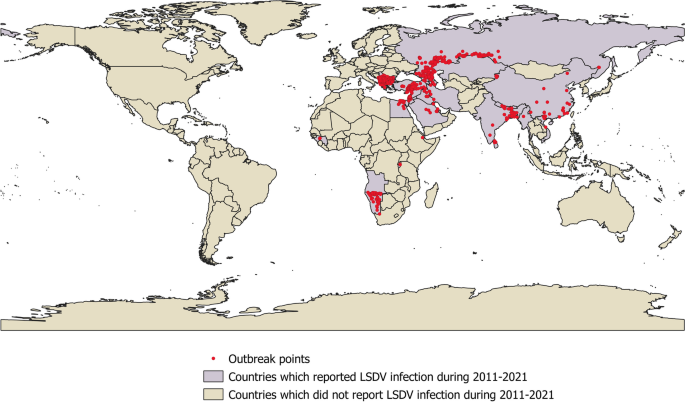
The distribution of reported LSDV infection points during 2011–2021
The highest incidence of the disease during the study period was reported in Europe (2172 outbreaks), Asia (777 outbreaks), and Africa (90 outbreaks), respectively.
The highest incidence of the disease was recorded in 2016 (Fig. 3 ).
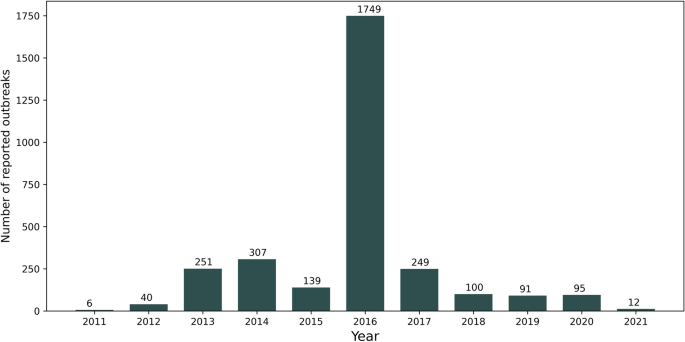
Reported LSDV infection outbreaks in each year during 2011–2021
Important features
Based on the results of applying ExtraTreesClassifier and SelectFromModel algorithms on the dataset, only meteorological variables were considered as important features. Therefore, two independent analyses were carried out: one involving all predictive variables including all meteorological, elevation, animal population density, and land cover features (model 1) and the other consisting of only meteorological features (model 2).
Tuned parameters of algorithms
In Tables 1 and 2 , some of the most important tuned parameters in each algorithms in model 1 and 2 are shown, respectively.
The predictive ability of various machine learning algorithms
Depending on the type of ML algorithm and performance metric used, the predictive ability of techniques using two subsets of features was different (Table 3 ).
AUC metric ranged between 0.53% to 0.97% and 0.63% to 0.97% in model 1 and model 2, respectively. In both models, ANN algorithm outperformed other algorithms in terms of AUC and F1 score. ROC curves of different ML algorithms for model 1 and model 2 are shown in Figs. 4 and 5 , respectively.
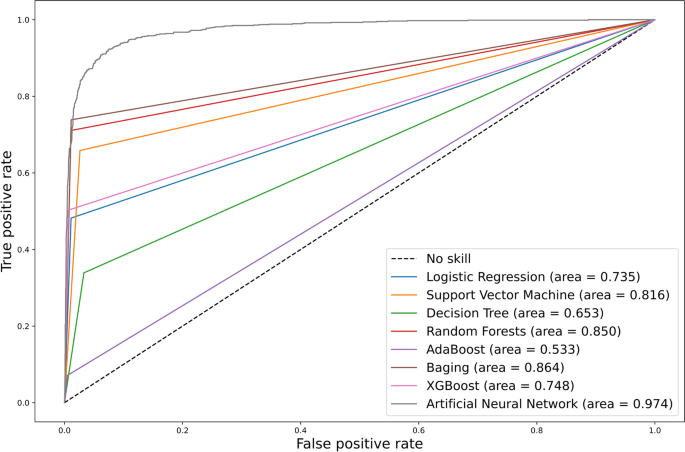
Receiver operating characteristic (ROC) curves of various machine learning algorithms for model 1 (including all predictors)
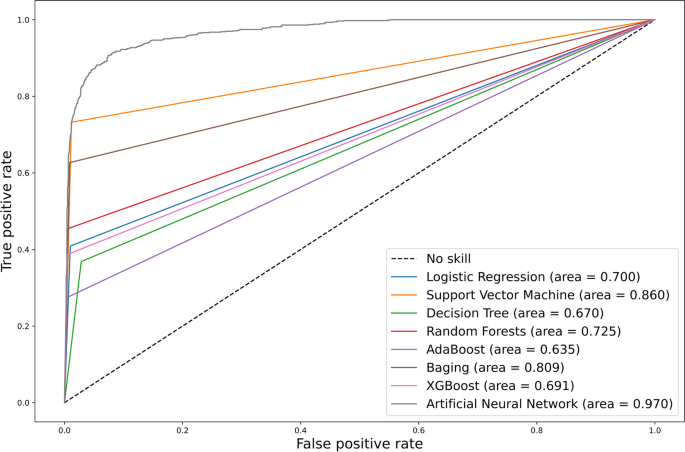
Receiver operating characteristic (ROC) curves of various machine learning algorithms for model 2 (including only predictive meteorological variables)
The findings of current study demonstrated that by applying machine learning methods and using climatic and geospatial features as predictive variables, the occurrence of LSDV infection could be predicted in test set (unseen data) with high accuracy. For instance, ANN algorithm indicated 97% accuracy score. However, the accuracy score is not the preferred performance measure for classifiers, particularly where certain classes are more frequent than others (Géron 2019 ). As a result, when assessing the predictive power of algorithms, it makes more sense to consider performance metrics such as precision, recall, F1 score, and AUC. Regarding AUC metric and by incorporating all predictive variables in the model or using only meteorological variables as predictors, the highest performance was associated with ANN algorithm (97% in both models) (Table 3 ).
Artificial neural networks have been widely used in different fields including medical and health field, such as medical diagnosis and disease prediction and obtained the very good prediction results (Abbass 2002 ; Al-Shayea 2011 ; Baxt 1995 ; Fang et al. 2014 ; Flores-Fernández et al. 2012 ; Kara and Dirgenali 2007 ; Kia et al. 2013 ; Ma and Wang 2010 ; Wang and Gupta 2013 ; Wang et al. 2001 ; Zhu and Wang 2010 ).
The reason for better performance of ANN could be attributed to the fact that this algorithm is a universal approximator which can approximate a large class of functions with a high degree of accuracy (Y. Wang et al. 2015 ).
The predictive performance of ANN was almost the same in both models (using all predictor variables vs only climatic predictive variables) with AUC of 0.97. The literature shows that feature selection can boost the classifier’s prediction accuracy, scalability, and generalization capability. This technique is critical in information discovery because it reduces computational complexity, storage, and cost (Gutkin et al. 2009 ). It should be noted, however, that any predictive feature may be irrelevant individually, but when combined with others, it becomes relevant (Gheyas and Smith 2010 ). As a result, feature selection does not always imply improved results, and in some cases, eliminating features could be detrimental (Guyon et al. 2008 ).
To the best of the author’s knowledge, no other study has used machine learning algorithms to forecast the incidence of LSDV infection using geospatial and meteorological predictive parameters. However, some similar studies utilized machine learning methods to predict the occurrence of some viral livestock diseases based on climatic data.
Liang et al. ( 2020 ) used machine learning methods to forecast African swine fever outbreaks around the world using bio-climatic variables, and Niu et al. ( 2020 ) applied various machine learning algorithms to forecast Peste des Petits ruminants (PPR) outbreaks based on certain bio-climatic variables and altitude data. Nevertheless, the time frame during which climate data (WordClim database which contains data for 1970–2000) used in these studies was before the time period during which disease outbreaks data utilized and this could be a potential source of bias. In contrast, in the present study, meteorological data were downloaded for the period 2011–2019 from CRU TS4.04 database (Harris et al. 2020 ) to provide better time concordance with event data of LSDV infection.
According to the feature selection algorithm, out of meteorological, animal density, land cover, and elevation data, only meteorological variables were chosen as significant predictive factors in the present study. Similarly, wet and warm climates which are prime habitat for blood-feeding arthropods have been linked to the occurrence of LSDV infection previously (Alkhamis and VanderWaal 2016 ; Chihota et al. 2003 ; Weiss 1968 ). Some studies which used statistical methods have found a connection between land cover characteristics and/or animal density and disease incidence. For instance, Alkhamis and VanderWaal ( 2016 ) examined LSDV outbreak records in the Middle East between 2012 and 2015. The most important environmental predictors that contributed to the ecological niche of LSDV were annual precipitation, land cover, mean diurnal range, type of livestock production system, and global livestock densities, according to ecological niche modeling. Allepuz et al. ( 2019 ) investigated the relationship between confirmed LSDV infection outbreaks and climatic factors, land cover, and cattle density in the Balkans, Caucasus, and Middle East between 2012 and 2018. The findings revealed that the likelihood of disease incidence was considerably higher in areas dominated by croplands, grassland, or shrub land. Higher cattle populations, as well as regions with a higher annual mean temperature and a larger diurnal temperature range, increased the odds. In contrast to areas covered mostly by forest, areas with sparse vegetation have a lower risk of infection.
Gari et al. ( 2010 ) conducted a questionnaire survey to perform a cross-sectional analysis to assess the distribution of LSDV infection and related risk factors in Ethiopia’s three major agro-climatic areas. Across agro-climate zones, herd-level prevalence of LSDV infection was slightly higher in the midland agro-climate than in the highland and lowland agro-climate zones. The odds ratio of LSDV infection incidence was 3.86 (95% confidence interval: 2.61–5.11) in the midland vs. highland region and 4.85 (95% confidence interval: 2.59–7.1) in the lowland vs. highland zone. The introduction of new animal, as well as communal grazing and watering management, was correlated with a significantly increased risk of LSDV infection incidence.
Molla et al. ( 2017 ) conducted a research between 2000 and 2015 with the goals of determining the geographical and temporal spread of LSDV infection outbreaks and forecasting the possible outbreaks in Ethiopia. The incidence varied by region, with the lowest in hot dry lowlands and the highest in wet moist highlands. They discovered that outbreaks were seasonal, occurring most often in the months after a long rainy season.
All the mentioned researches used statistical methods which are designed for inference about the relationships between variables and not making predictions. On the contrary, prediction made by machine learning algorithms aims at forecasting unobserved outcomes (Bzdok et al. 2018 ) which is what has been used in the present study. In addition to the different methods used, discrepancies in the results of similar researches could also be caused by the use of different independent variables (risk factors) and different study locations.
Howerver, it is worth mentioning that the LSDV outbreak data used in the present study were mainly passive accounts from veterinary facilities in various countries. There are some drawbacks of using passive monitoring data that should be addressed when analyzing the findings. The presence or quality of compensation schemes, the capability and transparency of veterinary facilities, the remoteness of some regions, and farmer visibility all impede reporting in some countries. Nevertheless, the lack of LSDV reports in some areas of the surveyed countries could be attributed to a lack of suitable environmental conditions for the dissemination of the disease in the area.
Other limitations of the current study include the small amount of data used, the small number of predictor variables used, and the possibility that the disease has spread to other regions of the studied countries with different climatic and geographical conditions since conducting this research.
In conclusion, some machine learning algorithms like ANN could be potentially used to accurately forecast the occurrence of LSDV infection based on some geospatial and meteorological parameters. Using this approach could be extremely beneficial to implement monitoring and awareness schemes, as well as preventive measures such as vaccine campaigns in areas where LSDV infection is a high risk.
Data availability
The datasets generated during and/or analyzed during the current study are available in the Mendeley repository, https://data.mendeley.com/datasets/7pyhbzb2n9/1 .
Abadi, M., Agarwal, A., Barham, P., Brevdo, E., Chen, Z., Citro, C., . . . Devin, M. (2016). Tensorflow: Large-scale machine learning on heterogeneous distributed systems. arXiv preprint arXiv:1603.04467.
Abbass, H. A. (2002). An evolutionary artificial neural networks approach for breast cancer diagnosis. Artificial intelligence in Medicine, 25 (3), 265-281.
Article Google Scholar
Alemayehu, G., Zewde, G., & Admassu, B. (2013). Risk assessments of lumpy skin diseases in Borena bull market chain and its implication for livelihoods and international trade. Tropical Animal Health and Production, 45 (5), 1153–1159. https://doi.org/10.1007/s11250-012-0340-9
Alkhamis, M. A., & VanderWaal, K. (2016). Spatial and temporal epidemiology of lumpy skin disease in the Middle East, 2012–2015. Frontiers in veterinary science, 3 , 19. https://doi.org/10.3389/fvets.2016.00019
Article PubMed PubMed Central Google Scholar
Allepuz, A., Casal, J., & Beltrán‐Alcrudo, D. (2019). Spatial analysis of lumpy skin disease in Eurasia—Predicting areas at risk for further spread within the region. Transboundary and emerging diseases, 66 (2), 813-822. https://doi.org/10.1111/tbed.13090
Article PubMed Google Scholar
Al-Shayea, Q. K. (2011). Artificial neural networks in medical diagnosis. International Journal of Computer Science Issues, 8 (2), 150-154.
Google Scholar
Von Backstrom, U. (1945). Ngamiland cattle disease: preliminary report on a new disease, the etiological agent being probably of an infectious nature. Journal of the South African Veterinary Association, 16 (1), 29–35. https://hdl.handle.net/10520/AJA00382809_377
Baxt, W. G. (1995). Application of artificial neural networks to clinical medicine. The lancet, 346 (8983), 1135-1138.
Article CAS Google Scholar
Breiman, L. (1996). Bagging predictors. Machine learning, 24 (2), 123-140. https://doi.org/10.1023/A:1018054314350
Breiman, L. (2001). Random forests. Machine learning, 45 (1), 5-32. https://doi.org/10.1023/A:1010933404324
Bzdok, D., Altman, N., & Krzywinski, M. (2018). Points of significance: statistics versus machine learning. In: Nature Publishing Group.
Chen, T., & Guestrin, C. (2016). Xgboost: A scalable tree boosting system. Paper presented at the Proceedings of the 22nd acm sigkdd international conference on knowledge discovery and data mining.
Chihota, C., Rennie, L., Kitching, R., & Mellor, P. (2003). Attempted mechanical transmission of lumpy skin disease virus by biting insects. Medical and Veterinary Entomology, 17 (3), 294-300. https://doi.org/10.1046/j.1365-2915.2003.00445.x
Article CAS PubMed Google Scholar
Chollet, F. (2018). Deep learning with Python (Vol. 361): Manning New York.
Cox, D. R. (1958). The regression analysis of binary sequences. Journal of the Royal Statistical Society: Series B (Methodological), 20 (2), 215-232. https://doi.org/10.1111/j.2517-6161.1958.tb00292.x
Fang, Y., Fataliyev, K., Wang, L., Fu, X., & Wang, Y. (2014). Improving the genetic-algorithm-optimized wavelet neural network for stock market prediction. Paper presented at the 2014 International Joint Conference on Neural Networks (IJCNN).
Flores-Fernández, J. M., Herrera-López, E. J., Sánchez-Llamas, F., Rojas-Calvillo, A., Cabrera-Galeana, P. A., Leal-Pacheco, G., . . . Martínez-Velázquez, M. (2012). Development of an optimized multi-biomarker panel for the detection of lung cancer based on principal component analysis and artificial neural network modeling. Expert Systems with Applications, 39 (12), 10851-10856.
Freund, Y., & Schapire, R. E. (1997). A decision-theoretic generalization of on-line learning and an application to boosting. Journal of computer and system sciences, 55 (1), 119-139. https://doi.org/10.1006/jcss.1997.1504
Gari, G., Waret-Szkuta, A., Grosbois, V., Jacquiet, P., & Roger, F. (2010). Risk factors associated with observed clinical lumpy skin disease in Ethiopia. Epidemiology & Infection, 138 (11), 1657-1666. https://doi.org/10.1017/s0950268810000506
Géron, A. (2019). Hands-on machine learning with Scikit-Learn, Keras, and TensorFlow: Concepts, tools, and techniques to build intelligent systems : O'Reilly Media.
Geurts, P., Ernst, D., & Wehenkel, L. (2006). Extremely randomized trees. Machine learning, 63 (1), 3-42.
Gheyas, I. A., & Smith, L. S. (2010). Feature subset selection in large dimensionality domains. Pattern recognition, 43 (1), 5-13. https://doi.org/10.1016/j.patcog.2009.06.009
Gilbert, M., Nicolas, G., Cinardi, G., Van Boeckel, T. P., Vanwambeke, S. O., Wint, G. W., & Robinson, T. P. (2018). Global distribution data for cattle, buffaloes, horses, sheep, goats, pigs, chickens and ducks in 2010. Scientific data, 5 (1), 1-11. https://doi.org/10.1038/sdata.2018.227
Golden, C. E., Rothrock Jr, M. J., & Mishra, A. (2019). Comparison between random forest and gradient boosting machine methods for predicting Listeria spp. prevalence in the environment of pastured poultry farms. Food research international, 122 , 47-55.
Gutkin, M., Shamir, R., & Dror, G. (2009). SlimPLS: a method for feature selection in gene expression-based disease classification. PloS one, 4 (7), e6416. https://doi.org/10.1371/journal.pone.0006416
Guyon, I., Gunn, S., Nikravesh, M., & Zadeh, L. A. (2008). Feature extraction: foundations and applications (Vol. 207): Springer.
Harris, I., Osborn, T. J., Jones, P., & Lister, D. (2020). Version 4 of the CRU TS monthly high-resolution gridded multivariate climate dataset. Scientific data, 7 (1), 1-18. https://doi.org/10.6084/m9.figshare.11980500
Kanevski, M., Pozdnukhov, A., & Timonin, V. (2008). Machine learning algorithms for geospatial data. Applications and software tools.
Kara, S., & Dirgenali, F. (2007). A system to diagnose atherosclerosis via wavelet transforms, principal component analysis and artificial neural networks. Expert Systems with Applications, 32 (2), 632-640.
Kia, S., Setayeshi, S., Shamsaei, M., & Kia, M. (2013). Computer-aided diagnosis (CAD) of the skin disease based on an intelligent classification of sonogram using neural network. Neural Computing and Applications, 22 (6), 1049-1062.
Kovacs-Györi, A., Ristea, A., Havas, C., Mehaffy, M., Hochmair, H. H., Resch, B., . . . Blaschke, T. (2020). Opportunities and Challenges of Geospatial Analysis for Promoting Urban Livability in the Era of Big Data and Machine Learning. ISPRS International Journal of Geo-Information, 9 (12), 752. https://doi.org/10.3390/ijgi9120752
Latham, J., Cumani, R., Rosati, I., & Bloise, M. (2014). Global land cover share (GLC-SHARE) database beta-release version 1.0–2014. FAO: Rome, Italy .
Liang, R., Lu, Y., Qu, X., Su, Q., Li, C., Xia, S., . . . Chen, Q. (2020). Prediction for global African swine fever outbreaks based on a combination of random forest algorithms and meteorological data. Transboundary and emerging diseases, 67 (2), 935-946. https://doi.org/10.1111/tbed.13424
Ma, Y.-x., & Wang, S.-g. (2010). The application of artificial neural network in the forecasting on incidence of a disease. Paper presented at the 2010 3rd International Conference on Biomedical Engineering and Informatics.
Machado, G., Korennoy, F., Alvarez, J., Picasso‐Risso, C., Perez, A., & VanderWaal, K. (2019). Mapping changes in the spatiotemporal distribution of lumpy skin disease virus. Transboundary and emerging diseases, 66 (5), 2045-2057. https://doi.org/10.1111/tbed.13253
Malki, Z., Atlam, E.-S., Hassanien, A. E., Dagnew, G., Elhosseini, M. A., & Gad, I. (2020). Association between weather data and COVID-19 pandemic predicting mortality rate: Machine learning approaches. Chaos, Solitons & Fractals, 138 , 110137.
Molla, W., de Jong, M., & Frankena, K. (2017). Temporal and spatial distribution of lumpy skin disease outbreaks in Ethiopia in the period 2000 to 2015. BMC veterinary research, 13 (1), 1-9. https://doi.org/10.1186/s12917-017-1247-5
Namazi, F., & Khodakaram Tafti, A. (2021). Lumpy skin disease, an emerging transboundary viral disease: A review. Veterinary Medicine and Science . https://doi.org/10.1002/vms3.434
Niu, B., Liang, R., Zhou, G., Zhang, Q., Su, Q., Qu, X., & Chen, Q. (2020). Prediction for global Peste des petits ruminants outbreaks based on a combination of random forest algorithms and meteorological data. Frontiers in veterinary science, 7 . https://doi.org/10.3389/fvets.2020.570829
Pedregosa, F., Varoquaux, G., Gramfort, A., Michel, V., Thirion, B., Grisel, O., . . . Dubourg, V. (2011). Scikit-learn: Machine learning in Python. the Journal of machine Learning research, 12 , 2825–2830.
Roche, X., Rozstalnyy, A., TagoPacheco, D., Pittiglio, C., Kamata, A., Beltran Alcrudo, D., . . . Larfaoui, F. (2020). Introduction and spread of lumpy skin disease in South, East and Southeast Asia: Qualitative risk assessment and management : Food & Agriculture Org.
Safavian, S. R., & Landgrebe, D. (1991). A survey of decision tree classifier methodology. IEEE transactions on systems, man, and cybernetics, 21 (3), 660-674. https://doi.org/10.1109/21.97458
Scholkopf, B. (1998). Support vector machines: a practical consequence of learning theory. IEEE Intelligent systems, 13 . https://doi.org/10.1041/X4018s-1998
Sprygin, A., Artyuchova, E., Babin, Y., Prutnikov, P., Kostrova, E., Byadovskaya, O., & Kononov, A. (2018). Epidemiological characterization of lumpy skin disease outbreaks in Russia in 2016. Transboundary and emerging diseases, 65 (6), 1514-1521. https://doi.org/10.1111/tbed.12889
Tuppurainen, E., Venter, E. H., Shisler, J., Gari, G., Mekonnen, G., Juleff, N., . . . Bowden, T. (2017). Capripoxvirus diseases: current status and opportunities for control. Transboundary and emerging diseases, 64 (3), 729–745. https://doi.org/10.1111/tbed.12444
Tuppurainen, E., & Oura, C. (2012). lumpy skin disease: an emerging threat to Europe, the Middle East and Asia. Transboundary and emerging diseases, 59 (1), 40-48. https://doi.org/10.1111/j.1865-1682.2011.01242.x
Wang, Y., Li, J., Gu, J., Zhou, Z., & Wang, Z. (2015). Artificial neural networks for infectious diarrhea prediction using meteorological factors in Shanghai (China). Applied Soft Computing, 35 , 280-290.
Wang, L., & Gupta, S. (2013). Neural networks and wavelet de-noising for stock trading and prediction. In Time Series Analysis, Modeling and Applications (pp. 229–247): Springer.
Wang, L., Teo, K. K., & Lin, Z. (2001). Predicting time series with wavelet packet neural networks. Paper presented at the IJCNN'01. International Joint Conference on Neural Networks. Proceedings (Cat. No. 01CH37222).
Weiss, K. (1968). Lumpy skin disease virus. In Cytomegaloviruses. Rinderpest Virus. Lumpy Skin Disease Virus (pp. 111–131): Springer.
Xu, C., & Jackson, S. A. (2019). Machine learning and complex biological data. In: Springer.
Yang, C., Huang, Q., Li, Z., Liu, K., & Hu, F. (2017). Big Data and cloud computing: innovation opportunities and challenges. International Journal of Digital Earth, 10 (1), 13-53. https://doi.org/10.1080/17538947.2016.1239771
Zhu, M., & Wang, L. (2010). Intelligent trading using support vector regression and multilayer perceptrons optimized with genetic algorithms. Paper presented at the The 2010 International Joint Conference on Neural Networks (IJCNN).
Download references
Author information
Authors and affiliations.
Department of Clinical Sciences, Faculty of Veterinary Medicine, Ferdowsi University of Mashhad, Mashhad, Iran
Ehsanallah Afshari Safavi
You can also search for this author in PubMed Google Scholar
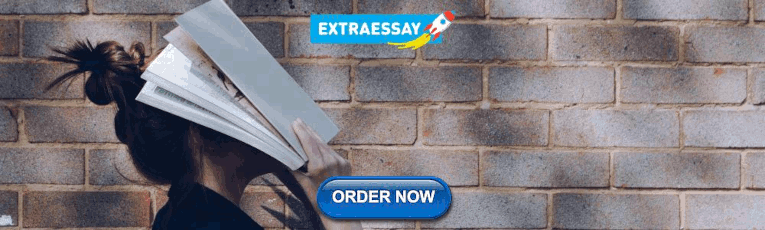
Contributions
Study conception and design, material preparation, data collection and analysis, and writing the manuscript were all performed by E.A.S.. E.A.S. read and approved the final manuscript.
Corresponding author
Correspondence to Ehsanallah Afshari Safavi .
Ethics declarations
Ethics approval.
The manuscript does not contain clinical studies or patient data.
Conflict of interest
The authors declare no competing interests.
Additional information
Publisher's note.
Springer Nature remains neutral with regard to jurisdictional claims in published maps and institutional affiliations.
Supplementary Information
Below is the link to the electronic supplementary material.
Supplementary file1 (TXT 0 KB)
Rights and permissions.
Reprints and permissions
About this article
Afshari Safavi, E. Assessing machine learning techniques in forecasting lumpy skin disease occurrence based on meteorological and geospatial features. Trop Anim Health Prod 54 , 55 (2022). https://doi.org/10.1007/s11250-022-03073-2
Download citation
Received : 25 June 2021
Accepted : 10 January 2022
Published : 14 January 2022
DOI : https://doi.org/10.1007/s11250-022-03073-2
Share this article
Anyone you share the following link with will be able to read this content:
Sorry, a shareable link is not currently available for this article.
Provided by the Springer Nature SharedIt content-sharing initiative
- Lumpy skin disease
- Forecasting
- Meteorological parameters
- Geospatial features
- Machine learning techniques
- Find a journal
- Publish with us
- Track your research
share this!
April 2, 2024
This article has been reviewed according to Science X's editorial process and policies . Editors have highlighted the following attributes while ensuring the content's credibility:
fact-checked
peer-reviewed publication
trusted source
Tracking the virus behind India's lumpy skin cattle crisis
by Amruth Deepak Bhat, Indian Institute of Science
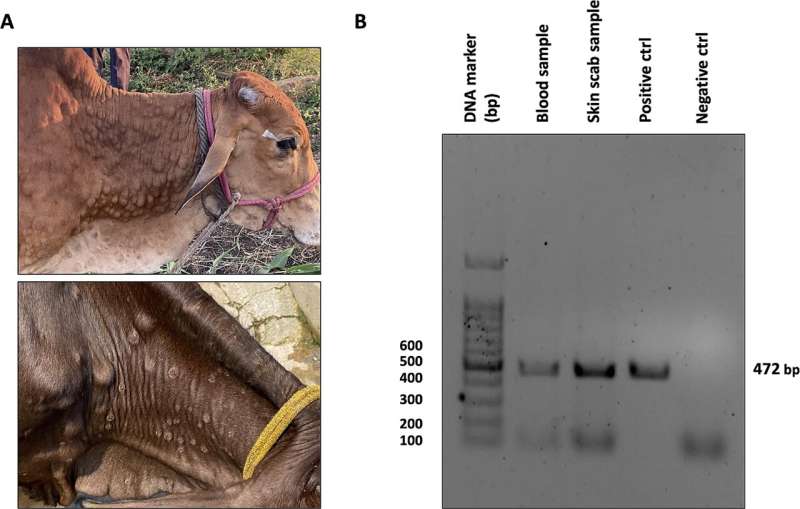
In May 2022, cattle across India began dying of a mysterious illness . Since then, about 1,00,000 cows have lost their lives to a devastating outbreak of what scientists have identified as lumpy skin disease. The outbreak has severely affected India's agricultural sector, leading to staggering economic losses.
"It was a calamity in some ways … a national emergency," says Utpal Tatu, Professor in the Department of Biochemistry, Indian Institute of Science (IISc).
Tatu is part of a multi-institutional team that decided to probe the cause of the outbreak. Their study , published in BMC Genomics , provides critical insights into the evolution and origins of the virus strains fueling it.
A viral infection caused by the Lumpy Skin Disease Virus (LSDV), the disease is transmitted by insects such as flies and mosquitoes. It causes fever and skin nodules, and can be fatal for cattle. LSDV was first found in Zambia in 1931 and remained confined to the Sub-African region until 1989, after which it started spreading to the Middle East, Russia and other southeast European nations, before spreading to South Asia. There have been two major outbreaks of this disease in India, the first in 2019 and a more severe outbreak in 2022, infecting more than 2 million cows.
To investigate the current outbreak, the team collected skin nodules, blood and nasal swabs from infected cattle in various states, including Gujarat, Maharashtra, Rajasthan and Karnataka, in collaboration with veterinary institutes. They performed advanced whole-genome sequencing of DNA extracted from 22 samples.
"The biggest challenge was the lack of an established LSDV genome sequencing and analysis pipeline. We had to adapt techniques from COVID-19 research," explains Ankeet Kumar, Ph.D. student at IISc and co-lead author. "Data was also limited, so we compiled all available global LSDV genome sequences to make our analysis robust."
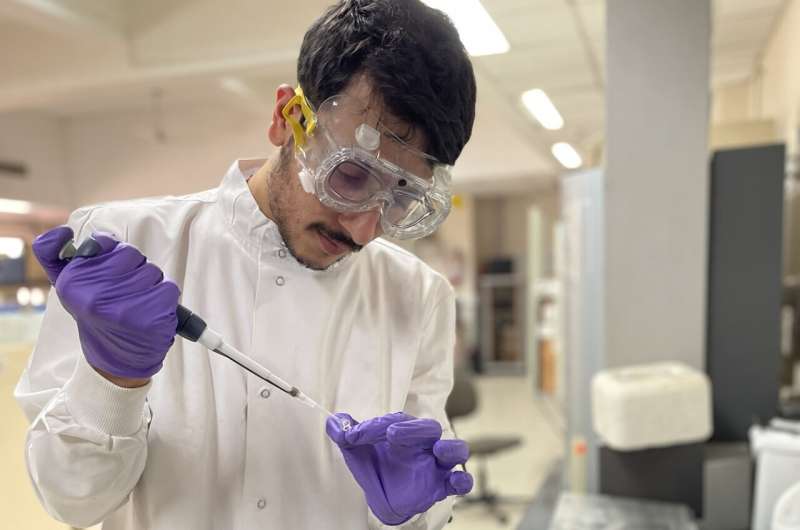
Their genomic analysis revealed two distinct LSDV variants circulating in India, one with a low number of genetic variations and another with a high number of genetic variations. The sequence with fewer variations was genetically similar to the 2019 Ranchi and 2020 Hyderabad strains that were sequenced previously. The samples with high variations, however, turned out to be similar to LSDV strains from an outbreak in Russia in 2015.
Kumar says that there are no previous reports of such highly varied LSDV strains in India. Viruses that have DNA as the genetic material —like LSDV—are generally more stable than RNA viruses. Therefore, finding so many genetic variations was quite surprising, and could explain the severity of the disease, he adds.
The team found a large number of genetic variations—more than 1,800. These include deletions and insertions in various genes, single-letter changes in DNA (called SNPs), and genetic variations in regions between genes. Importantly, they found a large number of genetic variations in viral genes critical for binding to host cells, evading immune response, and replicating efficiently. This likely enhanced the virus's ability to cause disease.
"Cattle developed more severe symptoms in areas where we found highly diverse strains. This suggests that the genetic variations could elevate virulence," says Kumar.
Such insights can pave the way for improved diagnostics, vaccines and interventions to combat emerging infectious diseases that threaten livestock and livelihoods. Tatu's research group has conducted similar studies on COVID-19 during the pandemic, and more recently on the rabies virus.
"The genomic data will prove invaluable for vaccine development by revealing molecular hotspots and genetic variations to target," notes Tatu. "This is a first for characterizing the genomic landscape of LSDV during India's outbreak on a national scale."
The study represents an example of the One Health approach in which multidisciplinary teams, including molecular biologists, computational experts and veterinary doctors come together to address issues of national relevance.
Tatu also emphasizes how collaboration between veterinary experts and multiple scientific institutions was critical to tracing the variants across the country. "We learned a lot from the veterinary doctors," he says. "They understand the field knowledge, and their perception about the disease was very important for us."
Journal information: BMC Genomics
Provided by Indian Institute of Science
Explore further
Feedback to editors

Huge star explosion to appear in sky in once-in-a-lifetime event
3 minutes ago

Innovative sensing platform unlocks ultrahigh sensitivity in conventional sensors
10 minutes ago

Nonvolatile quantum memory: Discovery points path to flash-like memory for storing qubits
13 minutes ago

Can language models read the genome? This one decoded mRNA to make better vaccines
38 minutes ago

A simple, inexpensive way to make carbon atoms bind together
40 minutes ago

Dinosaur study challenges Bergmann's rule
43 minutes ago

Study: Focusing immediately on the benefits of waiting might help people improve their self-control
16 hours ago

Utilizing palladium for addressing contact issues of buried oxide thin film transistors

Ocean waves propel PFAS back to land, new study finds

eDNA methods give a real-time look at coral reef health
17 hours ago
Relevant PhysicsForums posts
What do large moles on the body indicate.
Mar 30, 2024
Avian flu - A new study led by a team from the University of Maryland
Mar 27, 2024
Are all biological catabolic reactions exergonic?
Mar 20, 2024
A First of Its Kind: A Calcium-based signal in the Human Brain
Mar 18, 2024
Biological culture and cultural biology
Mar 17, 2024
Potentially fatal dog parasite found in the Colorado River
Mar 15, 2024
More from Biology and Medical
Related Stories

Model identifies high-risk areas for lumpy skin disease in cattle
May 30, 2019

New mutations and proteins of novel coronavirus revealed
Mar 4, 2021

Computational analysis shows how dengue virus evolved in India
May 1, 2023

Research shows that all COVID-19 infections include a wide mix of SARS-CoV-2 virus variants
Sep 8, 2022

Genetic analysis: Dutch bluetongue virus type is unique
Oct 23, 2023

New method reveals hidden genetic variations
Nov 1, 2023
Recommended for you

Discovery of how COVID-19 virus replicates opens door to new antiviral therapies
20 hours ago

Insect immune insights: Researchers unveil immune system dynamics
21 hours ago

New method can evaluate enzyme involved in process associated with cancer cell death
22 hours ago

Rusty-patched bumblebee's struggle for survival found in its genes
Apr 4, 2024

Chinese scientists reveal the spinning mechanism of the silkworm
Let us know if there is a problem with our content.
Use this form if you have come across a typo, inaccuracy or would like to send an edit request for the content on this page. For general inquiries, please use our contact form . For general feedback, use the public comments section below (please adhere to guidelines ).
Please select the most appropriate category to facilitate processing of your request
Thank you for taking time to provide your feedback to the editors.
Your feedback is important to us. However, we do not guarantee individual replies due to the high volume of messages.
E-mail the story
Your email address is used only to let the recipient know who sent the email. Neither your address nor the recipient's address will be used for any other purpose. The information you enter will appear in your e-mail message and is not retained by Phys.org in any form.
Newsletter sign up
Get weekly and/or daily updates delivered to your inbox. You can unsubscribe at any time and we'll never share your details to third parties.
More information Privacy policy
Donate and enjoy an ad-free experience
We keep our content available to everyone. Consider supporting Science X's mission by getting a premium account.
E-mail newsletter

An official website of the United States government
The .gov means it’s official. Federal government websites often end in .gov or .mil. Before sharing sensitive information, make sure you’re on a federal government site.
The site is secure. The https:// ensures that you are connecting to the official website and that any information you provide is encrypted and transmitted securely.
- Publications
- Account settings
Preview improvements coming to the PMC website in October 2024. Learn More or Try it out now .
- Advanced Search
- Journal List
- v.18(2); 2020 Feb

Lumpy skin disease epidemiological report IV : data collection and analysis
In 2019, no lumpy skin disease ( LSD ) outbreaks were reported in South‐Eastern Europe, the mass vaccination regional campaign with homologous LSD vaccine continued for the fourth year with over 1.8 million bovines vaccinated in the region, preventing further outbreaks since 2016. LSD outbreaks were reported in Turkey, including western Turkey, in Russia and in eastern Asia affecting China, Bangladesh and India for the first time. The use of homologous vaccine should be considered in the countries still affected in order to eliminate the virus. Besides passive surveillance, which is implemented in all the countries, active surveillance for early detection based on clinical examination could be conducted ideally during April–October every 5 weeks in at‐risk areas, based on possible re‐emergence or re‐introduction from affected neighbouring countries. Active surveillance for proving disease freedom could be based on serological testing (enzyme‐linked immunosorbent assay ( ELISA )) targeting 3.5% seroprevalence and conducted on a random sample of cattle herds on non‐vaccinated animals. LSD re‐emerged in Israel in 2019, after vaccination became voluntary. This shows that, if the virus is still circulating in the region, the reduced protection might result in re‐emergence of LSD . In case of re‐emergence, a contingency plan and vaccine stockpiling would be needed, in order to react quickly. From a study performed in Israel to test side effects of live‐attenuated homologous LSD vaccine, milk production can be reduced during 7 days after vaccination (around 6–8 kg per cow), without a significant loss in the 30 days after vaccination. Research needs should be focused on the probability of transmission from insect to bovine, the virus inactivation rate in insects, the collection of baseline entomological data, the capacity of vector species in LSDV transmission linked to studies on their abundance and the control of Stomoxys calcitrans being the most important vector in LSD transmission.
In 2019, no lumpy skin disease (LSD) outbreaks were reported in South‐Eastern Europe. The mass vaccination regional campaign with homologous LSD vaccine has been continued in 2019 in South‐Eastern Europe, over 1.8 million bovines were vaccinated in the region, preventing further outbreaks since 2016 (since 2017 for Albania and few outbreaks in Greece and North Macedonia). LSD further spread to Eastern Asia with new countries affected for the first time, China, Bangladesh and India, thus substantially increasing the global LSD virus spread.
In 2019, LSD has been reported in Turkey for the seventh year, with an increased number of outbreaks compared to 2017 and 2018, including outbreaks reported in western Turkey close to Thrace and facing the Greek islands, which may represent a risk of further spread to South‐Eastern Europe. To reduce the risk of further spread to South‐Eastern Europe, homologous vaccine should be used in Turkey not only in Thrace as recommended by Global Framework for the progressive control of Transboundary Diseases (GF‐TADs), but also in the western part of the country opposite to Greek islands. Additionally, for virus elimination, this type of vaccine should be used in the entire country.
- In Russia, LSD outbreaks have been reported in 2019 to the east of previous outbreaks since 2015. The vaccination based on sheep‐pox strain vaccine has not resulted in virus elimination yet.
In term of surveillance, in South‐Eastern Europe, passive surveillance is implemented nation‐wide in all countries; while active surveillance based on clinical examination and virological test for confirmation is in place in Albania, Bulgaria, Croatia, Greece, Kosovo*, North Macedonia, either as specifically for LSD or as part of other programmes; it allowed to spot seven suspected cases in 2019, that were then tested by polymerase chain reaction (PCR) and turned out negative.
Active surveillance for early detection could be conducted in at‐risk areas, determined either according to the last outbreaks in the country or in the neighbouring country from where possible incursion can be expected. Ideally this type of surveillance should be conducted every 5 weeks in the at‐risk period (April–October) targeting an expected prevalence of 0.042%, corresponding to the prevalence reached by the disease at 35 days after introduction. If this level of surveillance effort is not feasible (too many herds to be visited), the surveillance activities can be strengthened or partially replaced by adding systematic clinical examinations for LSD at live animal markets, before cattle leave their herds for any reasons (e.g. pre‐movement clinical checks) and during ante‐mortem examinations on animals to be slaughtered. These activities could be also combined with other surveillance programmes on the cattle population in place in the country.
Active surveillance for proving disease freedom could be based on serological testing (ELISA (ELISA)) targeting 3.5% seroprevalence conducted on a sample of cattle herds randomly selected from the whole country, testing the animals not vaccinated and where maternal immunity period has waned, to avoid interference with vaccine antibodies.
Based on the Israeli experience about reoccurrence of LSD in 2019, when vaccination becomes voluntary a sharp reduction in vaccination coverage can be expected, and if the virus is still circulating in the region and/or in neighbouring countries, the reduced protection might result in re‐emergence of LSD. Therefore, a contingency plan and vaccine stockpiling, even on a regional basis, would be needed, in order to react quickly with emergency vaccination.
From an observational controlled study performed in Israel to test the extent of side effects of live‐attenuated homologous LSD vaccine, it emerged that milk production is primarily reduced during 7 days after vaccination. The reduction along the whole week is around 6–8 kg per cow (depending on parity). For the rest of the 30 days after vaccination, the total reduction in milk production is not significant. In order to analyse the possible production losses linked to LSD vaccination, a randomised controlled trial with vaccinated and non‐vaccinated cows randomised in the same herds should be used. If this is not possible, then a large number of herds should be included in the analysis to control for other possible factors influencing survival and milk production.
There is experimental evidence that Stomoxys calcitrans and Haematopota spp. can mechanically transmit LSDV, and S. calcitrans being likely the most important vector, among those studied, in LSD transmission in South‐Eastern Europe. There is a need of better understanding the capacity of the other vectors present among countries and their potential role in LSDV transmission. These results should be linked to studies on the abundance of vectors in different regions.
Experimental evidence showed also that the LSD incubation period in bovine hosts can vary between 6 and 26 days. Based on this, the length of quarantine periods for bovines being moved from infected to non‐infected regions should be reconsidered.
1. Introduction
1.1. background and terms of reference as provided by the requestor.
LSD is a viral disease of cattle that reached continental Europe via Turkey in 2015, affecting North Eastern Greece, between August and December. The disease returned again in the spring of 2016, this time affecting seven countries of South‐Eastern (SE) Europe with numerous outbreaks, namely Greece, Bulgaria, (the Republic of) North Macedonia, Serbia, Kosovo*, Albania and Montenegro.
By 2017, thanks to the coordinated vaccination campaigns in all affected countries of SE Europe (annual vaccination of all cattle, with live homologous vaccines, since 2016) the disease was contained. In addition, two non‐affected countries resorted to LSD vaccination too, as a preventive measure, namely Croatia (2016‐2017) and Bosnia and Herzegovina (2017). As a result of this combined effort, no new LSD outbreaks were reported in those countries where sufficient vaccination coverage was achieved.
The regional LSD vaccination strategy continued in 2019, in line with the recommendations of the latest meeting of the Standing Group of Experts on Lumpy Skin Disease in South‐East Europe under the GF‐TADs umbrella (GF TADs SGE LSD5, 19‐20 October 2017, Budva, Montenegro), where EFSA was present too.
Mass vaccination of cattle against LSD using live homologous vaccines figures clearly as the most effective control policy. Nevertheless, there is evidence that LSD virus remains present (e.g. recurrence of LSD in Albania in 2017) and non‐immune cattle remain at risk, even in areas of relatively high vaccine coverage.
In addition, 2019 will be the fourth consecutive year of mass LSD vaccination across South‐East Europe and the countries in the region, affected or at risk for LSD, have already expressed their will to collaborate in drafting an LSD exit strategy.
To this end updated scientific information and analysis is needed to assist the countries of South‐East Europe in drafting a regional roadmap on an LSD exit strategy from 2018 onwards, as described in the GF TADs SGE LSD5 recommendations.
EFSA, at the request of DG SANTE, has been systematically collecting and analysing LSD epidemiological data since 2016 and has already produced two (2) LSD scientific reports.
1.1.1. Terms of references
In view of the above and in the context of Articles 31 of Regulation (EC) No. 178/2002, EFSA is requested to provide scientific and technical assistance to the Commission on the continuation of the collection and analysis of the LSD epidemiological data in the context of article 31 of Regulation (EC) No. 178/2002, as detailed in the reference letter Ares(2016) 3953310, of 27 July 2016, for another two (2) years. In the framework of this exercise two reports should be produced, one in January 2019 and the other in January 2020. Given the extent and duration of LSD vaccination in South‐East Europe, every effort should be taken to include in the analysis as much information as possible, in relation to the performance of the LSD homologous vaccines (effectiveness, safety, etc.), in use since the beginning of the epidemic.
1.2. Introduction and interpretation of the Terms of Reference
2. data and methodologies, 2.1. epidemiological data and analysis.
The LSD outbreak data up to November 2019 were obtained from national authorities and Animal Disease Notification System (ADNS 1 ) for those countries that notify to ADNS and from OIE and EMPRES Global Animal Disease Information System (Empres‐I 2 ) for the other countries.
The population data and vaccination data at farm level related to the vaccination campaign conducted in 2019 were provided by the national authorities of Albania, Bulgaria, Greece, Kosovo*, Montenegro, North Macedonia, Serbia.
The data were described in a spatial and temporal context using GIS software.
2.2. Surveillance data from Croatia
Data of LSD serological surveillance of animals tested by ELISA were obtained from the Croatian veterinary services, 9089 entries of test result (positive or negative and, for the positive the OD values) with indication of animal geographical origin, date of sampling, date of vaccinations (2016, 2017 or both 3 ) and animal characteristics like age, sex, breed, etc. Unfortunately, the data set was not arranged per animal ID, but different ID codes per animal were used by the lab., making the analysis difficult. Moreover, OD values were entered manually only for positive animals; thus, the analysis of OD trend over time since vaccination was not possible. The only outcome that could be tested was the ELISA result expressed as negative or positive.
A subset of the data set could be re‐organised to include unique animal IDs and dates of sampling, resulting in 4543 unique entries belonging to 1718 unique animal IDs and including animals tested multiple times between 14/6/2018 and 21/12/2018. Of those 1112 were tested at least twice, of which 665 gave always negative results and 447 gave positive results at least once. In Croatia, there were two vaccination campaigns, the vaccination dates of the tested animals in 2016 were between August and November 2016 and in 2017 between May and December 2017.
The data allowed to test:
- The age profile of the positive animals that were never vaccinated;
- If there was any correlation between ELISA result and time lag between vaccination and test and number of vaccinations.
A generalised linear mixed model was used in which a random intercept for each animal was considered to account for potential correlation between results coming from the same animal. The model was fitted using the number of vaccinations that an animal had received as well as the time lag between each of the vaccinations and the date of sampling.
3. Assessment
3.1. epidemiological situation, 3.1.1. update of the spatial and temporal development of lsd epidemics in eurasia.
Considering Eurasia, up to November 2019, LSD outbreaks were reported in Russia (26 outbreaks), Turkey (131 outbreaks), Israel, Syria and, for the first time, in China (1), Bangladesh (2) and India (3 outbreaks). Compared to 2018, the LSD epidemic in the Russian Federation expanded northward and eastward along the border with Kazakhstan, while in Turkey, most outbreaks were reported in the Eastern regions. No outbreaks were reported in the Balkan region. Figures 1 shows the geographical distribution of LSD outbreaks in Europe and Asia.

LSD outbreaks notified in Europe and Asia in 2019 compared to 2018 in relation to the sum of cases (amount of affected animals) (Data sources: national authorities and ADNS for those countries that notify to ADNS and Empres‐I/ OIE for the other countries)
Figure 1 shows: (i) large number of LSD outbreaks in South Eastern Turkey, bordering Syria, but also few cases in Western Turkey; (ii) LSD outbreaks in the Russian Federation along the border with Kazakhstan expanding eastward in 2019 compared to 2018; (iii) LSD expanding eastward in Asia with outbreaks reported in China, Bangladesh and India for the first time in 2019; (iv) LSD reappeared in Northern Israel, bordering Lebanon and Syria, where LSD had not been reported since 2013.
In Figure 2 , the temporal distribution of LSD outbreaks since 2012 is shown for those European and Asian countries that reported LSD in 2019 and/or 2018.

Temporal distribution of LSD outbreaks in European and Asian countries that reported any in 2019 and 2018 since 2012 (Data sources: Empres‐I and ADNS for Turkey)
Apart from the still active epidemics in Turkey and Russia, it is notable that LSD has been reported in China, India and Bangladesh for the first time, and re‐appeared in Israel after 6 years (see Section 3.1.4 ). Moreover, LSD was reported in 2019 in areas neighbouring Israel like Syria and the West Bank.
In Figure 3 , the spatial distribution of outbreaks in 2018 and 2019 is shown by month.

Monthly spatial distribution of the LSD outbreaks (coloured bubbles) in 2018 and 2019 (end of November). (Data source: Empres‐I and ADNS )
In Turkey, the outbreaks were reported between May and November, while in Russia, they occurred between July and October, thus confirming the seasonality of LSD outbreaks in the northern hemisphere. The few outbreaks in China, India and Bangladesh were reported in July and August.
3.1.2. LSD in Turkey in 2019
In 2019, 131 outbreaks were reported in Turkey, mostly in the eastern part of the country and mostly between April and October. Twenty outbreaks in western Turkey close to Thrace region and in Izmir opposite the Greek islands were reported. After a sharp decrease from 803 to 106 outbreaks between 2014 and 2016, when three to five times doses of sheep pox vaccine were used in the whole population, the number of reported outbreaks in Turkey has been slightly increasing from 14 in 2017 to 169 outbreaks in 2019 (Figure 4 ).

Reported LSD outbreaks in Turkey in 2017–2019 and sum of cases (number of affected animals) (source: ADNS )
Turkey has been combatting LSD intensively since the first occurrence of the disease in the country with a close collaboration with the international organisations such as OIE, FAO, EU. Turkey has a selective culling policy with 100% compensation, mandatory mass vaccination which is free of charge for farmers, and quarantine measures.
The reported outbreaks are the numbers of notifications which may be affected by some reporting bias and give slightly different indication than the real occurrence of the disease. Despite this, the recent increase of reported outbreaks in 2019 is remarkable, and some hypotheses can be advanced to explain it, including the level of protection conferred by the sheep pox vaccine, the timing of vaccination, biosecurity measures etc.
First consideration is about the level of protection conferred by the type of vaccine used, which may not be complete. A country‐wide mass vaccination campaign is in place since 2013, which reached 93% coverage in 2019. 4 Vaccination in 2019 was, however, applied using the 5x dose sheep pox Bakırköy strain (heterologous vaccine) on all cattle older than 3 months. It should be remarked that the strain used in Turkey is Bakırköy strain sheep pox strain and not sheep and goat pox as reported by Şevik and Doğan ( 2017 ). 5 For 2020, the vaccination based on homologous LSD strain is foreseen in the Thrace region, while in Anatolia sheep pox strain will be still used, with a recommendation by GF TADs, that consideration should be given to the use of 10x sheep dose, at least in high risk areas. 6 Considering the vaccine effectiveness, a complete data set of field data on vaccination recorded at farm or village level with the date of vaccination and relative location would be needed to estimate the vaccination effectiveness of the sheep pox vaccine, so to compare the relative risk in vaccinated/unvaccinated villages towards the infected/uninfected ones. Vaccination data from two provinces (Aydın/Samsun) were provided to European Food Safety Authority (EFSA), although only from randomly selected villages and not from all the villages in the provinces; therefore, this analysis could not be finalised yet and the results are still pending.
Regarding the timing of vaccination in 2019, it was reported that the vaccine supply in 2019 was late, thus vaccination after June 2019 may have not protected the whole population. Also, incomplete application of biosecurity measures could have played a role in increasing LSD outbreaks. There are intense animal movements in Turkey linked to some religious fests, like Kurban Bayrami which took place on August 11 in 2019.
In the Thrace Region, a risk‐based surveillance programme is in place targeting a between‐herd and within‐herd prevalence of 5% for detecting the clinical signs among susceptible animals of each epidemiological unit with a 95% level of confidence. 65,369 farms with 624,420 animals were clinically examined four times a year in 2019; the suspected outbreaks were confirmed by PCR. Up to October 2019, 131 outbreaks were confirmed out of 214 LSD suspected farms (61%).
The seasonality of outbreaks reported in 2017, 2018 and 2019 in Turkey and the mean temperatures per month in 2017, 2018 and averaged over the last 30 years (at the time of writing mean temperatures of 2019 were not available) is displayed in Figure 5 .

Monthly reported number of LSD outbreaks in Turkey in 2017, 2018 and 2019, and mean temperature in 2017, 2018 and averaged in the last 30 years
The seasonality in 2019 reflects the expected pattern with the vast majority of outbreaks between May and November (with two outbreaks reported in January and February), and 1‐month broader time window in the previous years.
3.1.3. LSD in Russia in 2019
Between March and up to October 2019, 26 LSD outbreaks were reported in six Russian provinces, around one‐third of the outbreaks reported in 2018. LSD outbreaks have been reported in 2019 to the east of previous outbreaks since 2015, along the border with Kazakhstan, across southern Siberia until Novosibirsk and Altai. LSD has been present in Russia since 2015 (Figure 6 ).

Reported LSD outbreaks in Russian Federation in 2016–2019 and number of affected animals in each outbreak (source: OIE , GF TAD s)
LSD vaccination was initially performed as a ring vaccination around the outbreaks then followed by a regional vaccination campaign of livestock in the regions bordering Caspian Sea and Kazakhstan using a sheep pox strain vaccine and achieving 80–100% coverage in the vaccinated area. 7 Kononov et al. ( 2019 ) reported the detection of the Russian LSDV field strain as well as a vaccine‐like LSDV strain in 4 out of 13 outbreaks in 2017. The vaccine‐like LSDV strain was found 100–300 km from the Kazakhstan border while the majority of the Russian field LSDV outbreaks largely congregated along the border of neighbouring Kazakhstan. The authors suggest that a recombinant vaccine‐like LSDV strain may have emerged after natural coinfection of a host with a field and a vaccine‐like strain. However, the authors also mention that, in the Volgogradskaya oblast, the field virus might have already been circulating when vaccination was first initiated and that the field virus and the vaccine‐like virus were both found in the oblast Orenburgskaya; therefore, caution should be taken in the interpretation of their current findings, considering also the lack of literature concerning transmission of Neethling‐based vaccine strains. Sprygin et al. ( 2018 ) reported the finding of a recombination lumpy skin disease virus in a cow with typical LSD clinical signs. A total of 27 recombination events were identified. This is surprising as recombination in poxviruses is an extremely rare event and the authors mention themselves that published examples of poxvirus recombination clearly arising in the field are still lacking except in some laboratory conditions. The authors further conclude that the impact of their finding remains to be clarified.
It should be stressed that in South‐Eastern Europe, an LSDV vaccine strain was only found in rare cases related to vaccine side effects (Neethling‐response) occurring in less than 0.5% of all vaccinated animals (EFSA, 2017 ). Furthermore, several LSDV isolates from outbreaks occurring in South‐Eastern Europe were analysed using Full Genome Sequencing and no recombinant strain was found (Agianniotaki et al., 2017 ; Toplak et al., 2017 ; Lojkić et al., 2018 ; Sprygin et al., 2019 ).
3.1.4. LSD spread towards eastern Asia
In 2019, LSD has further spread to Eastern Asia, with outbreaks reported for the first time in China, Bangladesh and India.
In China, the outbreak was suspected on 03/08/2019 with 65 animals affected in a village in the Ili Kazak Autonomous Prefecture, in the north‐west Xinjiang province at the border with Kazakhstan, and it was confirmed on 10 August by QPCR. The last time LSD was reported in Kazakhstan was in November 2016 but in the western part of the territory at the border with Russia on the Caspian Sea.
In the Xinjiang province, there are around 4 million cattle. At the end of summer in this region, Kazakh nomads migrate with their sheep and cattle to autumn pastures. 8
In Bangladesh in 2019, two outbreaks of LSD were reported, one in July and one in September, with 66 animals affected out of 360 exposed, in Chittagong and Dhaka, respectively.
In India, three outbreaks were reported to OIE in Orissa state, in Eastern India, with 79 affected animals out of 932 exposed.
Among the countries in Central Asia south from Russia (Kazakhstan, Kyrgyzstan, Tajikistan, Turkmenistan and Uzbekistan), the only country that reported LSD was, as said above, Kazakhstan, in 2016, where vaccination with homologous vaccine (Lumpivax, Kenya Veterinary Vaccine Institute, KEVEVAPI 9 ) has been ongoing since 2017, covering 100% of cattle in the western regions of the country, as reported to GF TADs. 10
3.1.5. LSD recrudescence in Israel in 2019
From March 2013 to June 2016, vaccination against LSD in Israel was mandatory in cattle all over the country and the spread of LSD was stopped. Since June 2016, vaccination has been voluntary in Israel. Data were collected from farmers insured by ‘Hachaklait’, which is the biggest veterinary cooperative in Israel. Immediately after the cessation of mandatory vaccination, the vaccination coverage started decreasing and reached 21% of farms prior to the new LSD epidemic in May 2019. The steepest decline in vaccine application was observed in feedlot cattle and mixed herds, followed by beef cattle while the lowest decline was observed in non‐grazing dairy herds (according to data by ‘Hachaklait’).
On May 31, 2019, LSD cases were observed in several calves in a dairy herd in the Golan Heights with 1,300 cows and heifers. Although most of the herd was vaccinated, the disease occurred in a group of 200 young heifers that were not vaccinated. Emergency vaccination was applied in this group as soon as LSD cases were detected. A total of 18 calves eventually developed LSD: seven were euthanised due to welfare consideration and the rest recovered spontaneously.
Up to until October 2019, 18 herds had been detected, with a total of 72 cases out of 7,202 animals exposed in these herds in north Israel. Except for one case, which occurred 30 days after vaccination, all cases occurred either in non‐vaccinated animals or within 2 weeks after vaccination. In these non‐vaccinated animals, morbidity was up to 9%.
The main actions taken by the veterinary authorities were:
- Mandatory vaccination performed by private veterinarians. However, vaccination needed to be postponed several days due to the detection of BTV contamination in a vaccine batch.
- Zoning, movement control and quarantine of affected herds.
- Increased surveillance in outbreak areas and areas at high risk.
- Slaughtering only due to welfare considerations and not for the control of the epidemic.
The lessons learned from this recent re‐emergence of LSD are:
- The situation in Israel demonstrates that when vaccination is voluntary, a sharp reduction in coverage is expected, even in highly industrialised dairy herds.
- If the virus is circulating in the neighbouring regions, low coverage of vaccination might result in the re‐emergence of LSD. In such episodes (similar to the experience in the Balkans), it takes at least a few months until the disease is completely controlled again.
- When vaccination becomes voluntary, stockpiling of vaccine is an option in order to act quickly with emergency vaccination in case of re‐emergence;
- In areas bordering with countries where LSD outbreaks are ongoing, it is recommended that vaccination is continued, otherwise, in case of re‐introduction, it may take long to stop a recurrent epidemic. Therefore, if vaccination is stopped, a contingency plan for quick reaction should be in place.
Key messages:
- In 2019, no LSD outbreaks reported in South‐East Europe.
- In 2019, LSD further spread to Eastern Asia with new countries affected for the first time, China, Bangladesh and India, thus substantially increasing the global LSD virus spread;
- o Although most of outbreaks reported in 2019 until November are located in the eastern part of Turkey, 20 new outbreaks have been reported also in western Turkey close to Thrace and opposite Greek islands, thus representing a risk of further spread to South‐East Europe (Figure 4 );
- o The endemic situation in Turkey may be linked to different factors including the ineffectiveness of the vaccination campaign, in place since 2013, in eliminating the virus, and/or the incomplete application of biosecurity measures on animal movements. The reasons of virus circulation can be investigated in further epidemiological studies for which Turkish veterinary authorities can provide a complete data set including individual animal records;
- o The high vaccination coverage, as reported by Turkey, may raise doubts about the effectiveness of sheep pox strain‐based vaccine;
- In Russia, similar to Turkey, LSD outbreaks have been reported in 2019 to the east of previous outbreaks since 2015; the vaccination based on SPP strain vaccine has not resulted in virus elimination.
- LSD outbreaks mainly occur between May and November.
- o When vaccination is stopped, in case of LSD re‐emergence, in order to act quickly with emergency vaccination vaccine stockpiling, even on a regional basis, would be needed.
3.2. Situation about LSD control and prevention in South‐East Europe
In 2019, all countries in South Eastern Europe (Albania, Bosnia and Herzegovina, Bulgaria, Greece, Kosovo*, Montenegro, North Macedonia, Serbia) continued to vaccinate against LSD; over 1.8 million cattle were vaccinated, with the exception of Croatia that stopped in 2018. In all these countries, a live‐attenuated vaccine based on a homologous LSD vaccine strain was used. The vaccines used in South‐Eastern Europe were from different companies, either based on Neethling strain like Lumpy Skin Disease Vaccine for Cattle (Onderstepoort Biological Products; OBP, South Africa 11 ) or Bovivax (MCI Santé Animale, Morocco 12 ), or based on SIS Neethling type (Lumpyvax, MSD Animal Health‐Intervet, South Africa. 13 )
The level of the vaccination coverage calculated as the proportion of immunised animals, i.e. animals that received a vaccination in the last 12 months 14 out of the total animals present) achieved in April and October 2019 (beginning and end of the vector season) in the Balkan region is displayed in Figure 7 .

Vaccination coverage achieved in 2019 (proportion of immunised animals out of the total animals present) in the Balkan region at the beginning (April) and at the end (October) of the vector season (for Bosnia and Herzegovina, data were not available).
The situation shown in Figure 7 is up to end October 2019, approximately up to the end of vector season, although in most countries the vaccination campaign went on until end of 2019, reaching higher coverage. For example in Albania, till 31 December 2019, 283,137 animals were vaccinated out of 295,835 present animals in the whole country in that moment (around 96% coverage).
In Table 1 , an overview about the past, current and future status of LSD vaccination and surveillance in South‐Eastern Europe is shown.
Overview of LSD vaccination and surveillance activities in South‐East European countries
- The mass vaccination regional campaign with homologous LSD vaccine has been continued in 2019 in South‐Eastern Europe (Albania, Bulgaria, Greece, Montenegro, North Macedonia, Serbia), the fourth year of vaccination for most of the countries in the region (the fifth year for Greece); over 1.8 million bovines were vaccinated in the region, preventing further outbreaks since 2016 (since 2017 for Albania and few outbreaks in Greece and North Macedonia);
- Passive surveillance is implemented in all the countries nationwide;
- Active surveillance based on clinical examination and virological test for confirmation is in place in Albania, Bulgaria, Croatia, Greece, Kosovo*, North Macedonia, either as specifically for LSD or as part of other programmes; it allowed to spot seven suspected cases in 2019, that were then tested by PCR and turned out negative.
3.3. Sero‐surveillance in Croatia
The data set of sero‐surveillance results from Croatia as discussed in Section 2.2 was analysed. The map below in Figure 8 represents the spatial distribution of results in the animals tested in Croatia in 2018; it shows how the sampling was concentrated in the areas closest to the last affected countries in 2016, Serbia and Montenegro.

Geographical distribution of the test results of the samples tested for LSD by ELISA in Croatia in 2018 per county (in the small map the distribution of the samples with farm location where XY were available)
The number of animals which resulted negative or positive by ELISA, according to the number of vaccinations they have been subjected to, is shown in Figure 9 .

Number of positive and negative animals tested for antibodies according to number of vaccinations received
Figure 9 shows that the proportion of positive animals increases with the number of vaccinations received. This effect was also tested by generalised linear mixed model in the animals above 6 months of age (to avoid bias coming from maternal immunity), and resulted statistically significant (Annex A ). This is in line with the study by (Milovanovic et al. ( 2019 ) who checked the humoral immune response against LSDV after re‐vaccination and found that re‐vaccination leads to a secondary response in the cattle population and significantly increases the number of animals with detectable antibody titres.
One hundred and fifty‐two animals never vaccinated tested positive. It must be taken into account that animals that are not vaccinated are the younger ones, and maternal immunity may have interfered with the serological test. In fact, when checking the age of the animals according to how many vaccinations they received, the median age of those never vaccinated and tested positive was 247 days (Q1–Q3: 150–355 days). Considering that Croatia is a free country, this may indicate that maternal immunity could last longer than what is normally expected (3–6 months as in other viral diseases), although this should be confirmed in further experimental studies. The distribution of the age of the animals according to the results of ELISA and number of vaccinations received is given in Figure 10 , which shows that older animals are the ones that also received more than one vaccination.

Age (days) of tested animals according to ELISA results and number of vaccinations received
The proportion of positive and negative ELISA results of animals aged more than 180 days (to avoid the possible interference of the maternal immunity) according to time post vaccination (1 and 2 vaccinations) is shown in Figure 11 .

Proportion of seropositive animals compared to time after vaccination (1 and 2 vaccinations)
It can be seen that up to 26 months after the first vaccination and up to 18 months after the second vaccination, around 23% and 47% of the animals, respectively, were still positive, in line with the study by Milovanovic et al. ( 2019 ) where 33% of animals were still seropositive at ELISA after several months (11 months).
Nevertheless, while in the study by Milovanovic et al. ( 2019 ), the proportion of seropositive animals decreased over time, which could be expected, in Figure 11 , it was more or less constant over time post vaccination. This was also observed when applying the generalised linear mixed model; the time lag variable in the model provided the same information as the number of vaccinations, so the more parsimonious model was chosen. This lack of the effect of time post vaccination on the proportion of seropositive should be still confirmed.
- From the field data on serological surveillance as provided by Croatian national authorities, the preliminary analysis shows that the proportion of seropositive is influenced by the number of vaccinations received (more seropositive after second vaccination – booster – than after first vaccination).
- Seropositive animals were tested positive in ELISA until 26 months post vaccination.
- Nevertheless, a better‐shaped data set according to each animal with OD values for both positive and negative animals is needed in order to perform more robust analyses and to confirm this preliminary evidence.
3.4. Side effects of vaccination: survey in Israel
It is widely accepted that good vaccines should keep a balance between effectiveness and safety. There are several publications referring to the side effects after vaccination with Neethling LSD vaccine. This aspect has been reviewed in the EFSA report (EFSA, 2019 ):
- ‘Neethling disease’ in 0.4% of the vaccinated cows (Ben‐Gera et al., 2015 );
- Follow‐up of one vaccinated (Neethling vaccine OBP ® ) dairy farm (215 cows) showed: (i) local reaction 26/215 (12%), (ii) skin nodules – 19/215 (9%) and of these 9/19 viraemia, (iii) drop in milk production – no accurate estimation due to a small sample size and no use of controls (Katsoulos et al., 2018 );
- Identification of the vaccine virus in five vaccinated animals (Agianniotaki et al., 2017 );
- Demonstration of LSD vaccine DNA in blood, skin lesions, milk and saliva from vaccinated cows. (Bedekovic et al., 2017 );
- Decrease in milk production (RM65 vaccine) (Abutarbush and Tuppurainen, 2018 );
- Various reports on adverse effects, collected by passive surveillance (EFSA, 2019 );
It is known that any vaccine might cause some side effects. However, the challenge is to quantify those losses in order to weigh the pros and cons of vaccination. In order to answer this question, the analysis should fulfil some prerequisites:
- the analysis should be controlled (i.e. vaccinated animals should be compared to non‐vaccinated animals),
- potential confounders (e.g. lactation, days in milk, season) should be controlled for,
- it should be taken into account that usually all the cows in the herd are vaccinated together and therefore if something else occurs in the herd in parallel to the time of vaccination, the perception of the farmer is that this is due to vaccination and
- analysis should be performed on a sufficiently large number of herds to enable control for such possible biases.
For this purpose, a study has been designed and is still in progress by Koret School of Veterinary Medicine in the Hebrew University of Jerusalem in Israel to analyse the changes in milk production after vaccination.
The methodology used was as follows:
- 86 herds were analysed, vaccinated between 60 and 210 days post‐partum;
- Individual daily records of milk production were used;
- The cows were divided into three lactation groups for which each analysis was performed separately;
- The daily milk production in the 30 days before vaccination was compared to the milk production after vaccination;
- A mixed effect general linear model was fitted to the data with the week of observation included as a fixed effect (to control for seasonal changes in milk production) and the cow and herd as random variables.
Preliminary conclusions of the study:
- Milk production is primarily reduced during the 7 days after vaccination. The reduction for this entire week is around 6–8 kg per cow (depending on parity).
- For the rest of the 30 days after vaccination, the total reduction in milk production is not significant.
- This is one of the few studies conducted in a controlled way aiming at quantifying the production losses due to LSD vaccination side effects;
- One limitation of this study is that it is conducted on re‐vaccinated animals, and not on animals vaccinated for the first time, which might experience different effects.
Recommendations for designing studies for vaccine side effects:
- The best method is a randomised controlled trial with vaccinated and non‐vaccinated cows randomised in the same herds;
- If this is not possible, then a large number of herds should be included in the analysis to control for other possible factors influencing survival, adverse effects and milk production;
- The fact that an event occurs after vaccination does not necessarily imply causation.
3.5. Surveillance planning
In this section, some scenarios for planning the surveillance in the different previously LSD‐affected countries in South‐Eastern Europe is presented, both for early detection and for proving disease freedom, together with the calculation of sample size for both cases. Given the small median herd size in South‐Eastern Europe (two to four animals per farm in most countries), a two‐stage sample size calculation would not be useful; the epidemiological unit to be considered should be the herd. In case of larger herds (e.g. median herd around 100 animals), a two‐stage sampling can be performed. For this purpose, the within‐herd prevalence is needed; this has been estimated based on the mathematical model presented earlier (EFSA, 2017 , 2018 ) and discussed in the next section.
3.5.1. Two‐stage sampling: estimation of within‐herd prevalence
The simulated dynamics of LSDV in a cattle herd when transmission is by the bites of S. calcitrans are shown in Figure 12 and details are given in Annexes B and C . The median (95% prediction interval) for the peak prevalence of infection (which also corresponds with the proportion of animals showing clinical signs) is around 46% (27–76%), which occurs at around 27 (19–44) days post introduction. The prevalence of infection (%) at 7, 14, 21 and 28 days post introduction is: 0 (0, 1.3), 1.0 (1.0, 34.8), 27.5 (1.7, 72.1) and 42.5 (4.1, 71.6), respectively. The seroprevalence (assumed to be equal to the proportion of recovered cattle) is around 46% (27–76%).

Simulated dynamics of lumpy skin disease virus in a cattle herd when transmission is by the bites of the stable fly, Stomoxys calcitrans
- Panels show the number of susceptible ( S ; blue), latent ( E ; cyan), infectious ( I ; red), recovered ( R 1 ; magenta) and resistant ( R 2 ; green) cattle and the proportion of infected vectors ( p I ; yellow) over time. Plots show the median (solid black line), 25th and 75th percentiles (dashed lines) and five percentile bands (up to the 5 th and 95 th percentiles; shading). Simulations assume a herd size of 100 cattle and infection is initiated by a single infected vector. Results are based on 1000 replicates of the model; only results for those replicates in which there was an outbreak (i.e. there was at least one infected bovine) are shown. The proportion of replicates resulting in an outbreak was 4.8%.
3.5.2. Surveillance for early detection in South‐East Europe countries
As described previously (EFSA, 2018 ), the following elements should be considered for planning the surveillance for early detection:
- The period for testing by active surveillance: ideally every 5 weeks in the vector season, between April and October.
- The test to be used is clinical examination; sensitivity is 75% (EFSA et al., 2018 ).

Buffer zone of 60 km for the definition of the surveillance area in each of the countries previously affected by LSD in South‐eastern Europe
Sample size for surveillance for early detection in each country in South‐Eastern Europe
- Target population: as said above, given the small herd size (up to 8–10 animals), sampling should be done considering the herds as units, all animals in the herd should be examined. In case of bigger farms (100–1000 animals) and considering the within‐herd prevalence 46% (27 days post introduction, as in Section 3.5.1 ), then a random sampling of animals within the herd should be done, which gives a sample size of eight animals to be randomly selected within the herd.
- design prevalence: between‐herd prevalence set at 0.042%, which corresponds to the expected prevalence at 35 days post introduction after stop vaccination (EFSA, 2018 );
- confidence level: 95%
The sample size values for each country are given in Table 2 .
If this level of surveillance effort is not feasible, given the logistic and organisational difficulties in planning repeated visits to a such high number of cattle herds, the surveillance activities can be strengthened or partially replaced by adding systematic clinical examinations for LSD at live animal markets, before cattle leave their herds for any reasons (pre‐movement clinical checks) and during ante‐mortem examinations on animals to be slaughtered. These activities could be also combined with other surveillance programmes on cattle population in place in the country. Active surveillance can be feasible only in at‐risk areas and during the risk period.
3.5.2.1. Surveillance for testing freedom from disease
As described previously (EFSA, 2018 ), the following elements should be considered for planning the surveillance for proving disease freedom:
- Period for testing: ideally the sampling should be at the end of vector season (e.g. October);
- Target area: whole country, randomly selected herds;
- Diagnostic test: serological assay (ELISA), test sensitivity is set at 80%. Under experimental conditions, the Sensitivity (Se) was estimated as 83%, while under field conditions in a preliminary study in Bulgaria it was 59% (EFSA, 2018 ). Nevertheless, in later field trials in Serbia and North Macedonia, the Se values were 75–80%, thus more in line with what observed under experimental conditions. For calculating the sample size, this can justify the ELISA Se value of 80%. The specificity is 99.7% (EFSA, 2018 ). In case of positivity, positive animals and the herds which they belong to should be followed up.
- Target population: total number of herds randomly selected over the country; all eligible animals should be sampled in the farm; serological testing for disease freedom should target the ones not vaccinated and where the maternal immunity period has waned;
- Design prevalence (between‐herd prevalence): 3.5%.
- Confidence level: 95%
- The sample size has been calculated and is equal to 106 herds in all the countries considered.
3.6. Update on research conducted on LSD in 2019
The scientific evidence from the papers on LSD published in 2019 is summarised in this section.
3.6.1. Diagnostics
A brief overview is given of several articles related to diagnostics for lumpy skin disease and published in 2019.
- Möller et al. ( 2019 ) used specimens from the field and from experimental inoculation to evaluate different diagnostic tools, including a pan‐Capripox real‐time qPCR, a duplex real‐time qPCR assays and several serological methods (ELISA, indirect immunofluorescence test and serum neutralisation test [SNT]). The authors reported a high analytical sensitivity of both tested duplex real‐time qPCR systems for the reliable distinction of LSDV field and vaccine strains. The ID‐Vet antibody ELISA was as specific as the SNT, and therefore, they concluded that this ELISA provides an excellent tool for rapid and simple serological examination of LSDV‐vaccinated or infected cattle.
- Milovanovic et al. ( 2019 ) described the humoral immune response to repeated lumpy skin disease virus vaccinations and the comparative performance of the ID‐Vet antibody ELISA to the virus neutralisation test (VNT) and the indirect fluorescent antibody test (IFAT). The detection rate of antibody‐positive animals one year after the primo vaccination was 33%, 73% 1 month after re‐vaccination and 59% 5 months post re‐vaccination. These figures were highly comparable to the VNT and IFAT.
- Alexander et al. ( 2019 ) reported the development of a new real‐time PCR for the detection of LSD virus (LSDV) field, vaccine and recombinant strains as a follow‐up of the finding by Kononov et al. ( 2019 ) of vaccine‐like LSDV strains outbreaks in Russia and of a recombined LSDV as reported by Sprygin et al. ( 2018 ).
- Adedeji et al. ( 2019 ) used samples from field outbreaks of Capripox virus infections in Nigeria to evaluate a newly developed partial sequencing protocol. This method allows a first, short molecular classification of strains and further phylogenetical analysis was done.
- Erster et al. ( 2019 ) reported the use of the LSDV126, containing a duplicated region of 27 bp, to differentiate virulent and vaccine LSD viruses.
- Douglass et al. ( 2019 ) used full genome sequencing to prove that the LSDV vaccine Herbivac LS is different from the Neethling vaccine strain in the locus encoding a superoxide dismutase (SOD) homolog. The presence of a SOD homolog, be it full length (as in Herbivac LS) or truncated (as in Neethling) may affect vaccine immunogenicity.
- Munyanduki et al. ( 2020 ) reported how vaccine stocks can be cleared of BVDV by passage through an avian host, non‐permissive to Bovine viral diarrhoea virus (BVDV), but permissive to LSDV. This might be of importance as BVDV is a common contaminant of Madin‐Darby bovine kidney (MDBK) cells as well as fetal calf serum (FCS). MDBK cells are used in the generation of recombinant LSDV and have been used for the growth of LSDV vaccines.
- Haegeman et al. ( 2019 ) developed an Immuno‐Peroxidase Monolayer Assay (IPMA) for the detection of antibodies against LSDV in an easy and low‐tech setting. The test was shown to be highly sensitive, specific and repeatable. In comparison to the VNT and a commercial ELISA, the LSDV‐IPMA was able to detect the LSDV antibodies earlier in infected, vaccinated and vaccinated/infected animals. The assay is very flexible as it can be easily adapted for the detection of sheep‐pox or goat‐pox antibodies and it can be scaled‐up to handle medium size sample sets by preparing the IPMA plates in advance. These plates are safe and can be handled in low biosafety level labs.
3.6.2. Epidemiology
Two papers have reported on the epidemiology of LSDV.
In the first paper, Manic et al. ( 2019 ) described outbreaks of LSDV in five districts in South‐Eastern Serbia in 2016. There were 189 outbreaks in the study area (out of 225 in Serbia as a whole), with the first case detected in June 2016. Overall morbidity and mortality were 13.6% and 0.4%, respectively. The median distance between outbreaks was 4.3 km (interquartile range (IQR): 1.89–8.88 km) and the median time between outbreaks was 9 days (IQR: 5–16 days). Vaccination with Neethling vaccine strain of LSDV commenced 3 weeks after the first reported case. The last case in the study region was in August 2016, and the last case in Serbia was in October 2019, a month and a half after the vaccination campaign ended. Information on post‐vaccination complications in the study area was not collected systematically, but there were no reports of complications from cattle owners.
In the second paper, Gubbins ( 2019 ) assessed the risk of transmission of LSDV by calculating the basic reproduction number ( R 0 ) for five species of biting insects (the stable fly, Stomoxys calcitrans , the biting midge, Culicoides nubeculosus , and three mosquito species, Aedes aegypti , Anopheles stephensi and Culex quinquefasciatus ). This involved analysing previously published data on the mechanical transmission of LSDV and collating life history parameters from the published literature for each of the five species. Results suggested that S. calcitrans is likely to be the most efficient at transmitting LSDV (median estimate (95% prediction interval): R 0 =15.5 (1.4–81.9)), with Ae. aegypti also an efficient vector ( R 0 = 7.4 (1.3–17.6)). By contrast, C. nubeculosus ( R 0 = 1.8 (0.06–13.5)), Cx. quinquefasciatus ( R 0 = 0.8 (0.09–3.5)) and An. stephensi ( R 0 = 1.6 (0.2–6.0)) were likely to be less efficient vectors of LSDV. However, there was considerable uncertainty associated with the estimates of R 0 . Sensitivity analysis suggested that future work should focus on estimating the probability of transmission from insect to bovine and the virus inactivation rate in insects, as well as collecting baseline entomological data for each vector species.
3.6.2.1. New evidence on the role of vectors in LSD transmission
LSDV transmission is an important factor in understanding the epidemiology of the disease. A limited number of publications have shown several haematophagous arthropod species, like dipterans and ticks, to play a role in the transmission of LSDV. Tentative links have been made for the stable fly S. calcitrans , such as the presence of live LSDV in the flies and the capacity to transmit sheep‐pox, but no direct conclusive data is available on the importance of biting flies and horseflies as potential vectors for LSDV. Therefore, in vivo transmission studies were carried out to investigate possible LSDV transmission by S. calcitrans biting flies and Haematopota spp . horseflies from experimentally infected viraemic donor cows to acceptor cows. LSDV transmission by S. calcitrans was evidenced in three independent experiments (Sohier et al., 2019 ). Transmission was demonstrated not only by the development of clinical signs (noduli) but also by viraemia and seroconversion. Interestingly, the incubation period was variable and was found to be between 6 and 26 days and needs to be kept in mind when establishing quarantine periods. As in one of the animal trials only a short period between feeding on donor and acceptors was used, it can be stated that S. calcitrans has the capacity to transmit LSDV mechanically. Furthermore, one of the two acceptor cows receiving horseflies, fed on LSDV viraemic donor cows, became clinically ill and developed viraemia, indicating that this vector is also capable of transmitting LSDV.
These data provide for the first‐time direct evidence for the mechanical transmission of LSDV by S. calcitrans and shows that multiple vectors (including Haematopota spp .) can be involved in LSDV epidemiology. This study supports the need of better understanding the capacity of the different vectors present among countries and their potential role in LSDV transmission. These results should be linked to studies on the abundance of vectors in different regions.
3.6.3. Risk assessment
Two studies explored the spatial and temporal risk of LSDV (Allepuz et al., 2019 ; Machado et al., 2019 ). These first examined the relationship between the occurrence of reported outbreaks in South‐Eastern Europe, Turkey and Russia and livestock density, land cover and environmental variables. The resulting statistical models were then used to assess the risk of LSDV in Eastern Europe, the Caucasus and central Asia.
Allepuz et al. ( 2019 ) used logistic regression modelling to identify factors associated with LSDV occurrence, while Machado et al. ( 2019 ) used a combination of ecological niche modelling and Poisson regression. Allepuz et al. ( 2019 ) identified increased odds of LSDV associated with land cover (specifically, cropland, grassland or shrubland compared with forest), higher cattle densities, higher annual mean temperature and higher diurnal temperature ranges. By contrast, Machado et al. ( 2019 ) did not identify any association between land cover and the risk of LSDV but did find an increased risk associated with higher temperatures, higher precipitation and lower wind speeds. Despite the differences in underlying risk factors, both studies identified similar regions as being at high risk of LSDV transmission.
Two studies examined the risk of introduction of LSDV via imported cattle (Saegerman et al., 2019 ; Taylor et al., 2019 ). Saegerman et al. ( 2019 ) carried out a quantitative import risk assessment for the introduction of LSDV to France. By contrast, Taylor et al. ( 2019 ) developed a generic framework for quantitative risk assessment for disease introduction but illustrated its use by applying it to the case of the introduction of LSDV to unaffected countries in Europe via imported cattle (Taylor et al., 2019 ). Both approaches considered the probabilities along the risk pathway from importing animals from at‐risk areas to infection being transmitted to local cattle. The model of Saegerman et al. ( 2019 ) was parameterised using a combination of expert opinions, field data and published literature, while that of Taylor et al. ( 2019 ) used publicly available data and published literature.
Saegerman et al. ( 2019 ) estimated that the probability of introducing LSDV through imported cattle, it being transmitted to French cattle was 5.4 × 10 −4 (95% prediction interval (PI): 0.4 × 10 −4 –28.7 × 10 −4 ) in summer months, when vector activity is high, and 1.8 × 10 −4 (95% PI: 0.1 × 10 −4 –15.0 × 10 −4 ) in winter months, when vector activity is low. These probabilities were most sensitive to assumptions about the within‐herd prevalence of LSDV and the probability that an infected animal is infectious. Taylor et al. ( 2019 ) found that Croatia had the highest mean annual probability of infection (assuming that vaccination was not carried out), with Italy, Hungary and Spain at the next highest risk of introduction. These results were consistent across spatial scales from countries to regions and to farms. In addition, Taylor et al. ( 2019 ) estimated that France had a negligible probability of LSDV being introduced.
Casal et al. ( 2019 ) developed a simple model to estimate the number of vaccine doses that would be needed to control an epidemic and applied it to the case of a putative LSDV epidemic in France. The model, which is provided by the authors as an Excel spreadsheet and an R script, is based on the rate of disease spread (i.e. distance travelled by the disease per week), the time taken to achieve effective vaccination (i.e. the time from introduction to detection, the time needed to vaccinate the population and the time from vaccination to protection) and cattle densities in the affected area. Assuming a 7‐week period is needed to vaccinate all animals and a spread rate of 7.3 km/week (i.e. that estimated for the Balkans in 2015–2016), 741,000 vaccine doses would be sufficient to control an epidemic in 90% of the simulations and 609,000 would be sufficient in 75% of the simulations.
4. Conclusions
- In 2019, no LSD outbreaks were reported in South‐Eastern Europe.
- The mass vaccination regional campaign with homologous LSD vaccine has been continued in 2019 in South‐Eastern Europe, the fourth year of vaccination for most of the countries in the region (the fifth year for Greece), over 1.8 million bovines were vaccinated in the region, preventing further outbreaks since 2016 (since 2017 for Albania and few outbreaks in Greece and North Macedonia);
- In South‐Eastern Europe, passive surveillance is implemented nationwide in all countries; while active surveillance based on clinical examination and virological test for confirmation is in place in Albania, Bulgaria, Croatia, Greece, Kosovo*, North Macedonia, either as specifically for LSD or as part of other programmes; it allowed to spot seven suspected cases in 2019, that were then tested by PCR and turned out negative.
- Active surveillance for early detection could be conducted in at‐risk areas, determined either according to the last outbreaks in the country or in the neighbouring country from where possible incursion can be expected. Ideally this type of surveillance should be conducted every 5 weeks in the at‐risk period (April–October) targeting an expected prevalence of 0.042%, corresponding to the prevalence reached by the disease at 35 days after introduction. If this level of surveillance effort is not feasible (too many herds to be visited), the surveillance activities can be strengthened or partially replaced by adding systematic clinical examinations for LSD at live animal markets, before cattle leave their herds for any reasons (e.g. pre‐movement clinical checks) and during ante‐mortem examinations on animals to be slaughtered. These activities could also be combined with other surveillance programmes on the cattle population in place in the country.
- Active surveillance for proving disease freedom could be based on serological testing (ELISA) targeting 3.5% seroprevalence conducted on a sample of cattle herds randomly selected from the whole country, testing the animals not vaccinated and where maternal immunity period has waned, to avoid interference with vaccine antibodies;
- When planning the LSD surveillance, where a two‐stage sampling is needed (in case of larger herds, median herd size around 100 animals) both the within‐herd and between‐herd prevalence is needed. By a mathematical model, the expected within‐herd prevalence of LSD infection has been estimated at 7, 14, 21 and 28 days post introduction as 0% (0, 1.3), 1.0% (1,0, 34.8), 27.5% (1.7, 72.1) and 42.5% (4.1, 71.6), respectively (median and confidence intervals).
- LSD has been reported in Turkey for the seventh year, with an increased number of outbreaks in 2019 compared to 2017 and 2018, including outbreaks reported in western Turkey close to Thrace and facing the Greek islands, which may represent a risk of further spread to South‐Eastern Europe;
- The endemic situation in Turkey may be linked to different factors including the ineffectiveness of the vaccination campaign in place since 2013 to eliminate the virus, and/or the incomplete application of biosecurity measures on animal movements. The high vaccination coverage, as reported by Turkey, may raise doubts about the effectiveness of sheep‐pox strain‐based vaccine;
- Based on the Israeli experience about reoccurrence of LSD in 2019, when vaccination becomes voluntary a sharp reduction in vaccination coverage can be expected, and if the virus is still circulating in the region and/or in neighbouring countries, the reduced protection might result in re‐emergence of LSD.
- From the field data on serological surveillance as provided by Croatian national authorities, the preliminary analysis shows that the proportion of seropositives is influenced by the number of vaccinations received, and vaccinated animals can be tested positive at ELISA until 26 months post vaccination. Nevertheless, better‐shaped data sets are needed in order to perform a more robust analysis and to confirm this preliminary evidence.
- From an observational controlled study performed in Israel to test the extent of side effects of live‐attenuated homologous LSD vaccine, it emerged that milk production is primarily reduced during 7 days after vaccination. The reduction along the whole week is around 6–8 kg per cow (depending on parity). For the rest of the 30 days after vaccination, the total reduction in milk production is not significant.
- There is experimental evidence that Stomoxys calcitrans and Haematopota spp. can mechanically transmit LSDV, and S. calcitrans being likely the most important vector, among those studied, in LSD transmission in South‐Eastern Europe.
- There is experimental evidence that the LSD incubation period in bovine hosts can vary between 6 and 26 days.
5. Recommendations
- To reduce the risk of further spread to South‐Eastern Europe, homologous vaccine should be used in Turkey not only in Thrace as recommended by GF TADs but also in the western part of the country opposite to Greek islands. Additionally, for virus elimination, this type of vaccine should be used in the entire country.
- When vaccination is stopped or becomes voluntary, in case of LSD re‐emergence, a contingency plan and vaccine stockpiling, even on a regional basis, would be needed, in order to react quickly with emergency vaccination.
- Since the evidence suggests that S. calcitrans is the most important vector in LSD transmission, research should be performed about possible control of this species.
- Future work should focus on estimating the probability of transmission from insect to bovine and the virus inactivation rate in insects, as well as collecting baseline entomological data for each vector species.
- There is a need of better understanding the capacity of the different vectors present among countries and their potential role in LSDV transmission. These results should be linked to studies on the abundance of vectors in different regions.
- In order to analyse the possible production losses linked to LSD vaccination, a randomised controlled trial with vaccinated and non‐vaccinated cows randomised in the same herds should be used. If this is not possible, then a large number of herds should be included in the analysis to control for other possible factors influencing survival and milk production.
- Based on new evidence about the length of incubation period, the length of quarantine periods for bovines being moved from infected to non‐infected regions should be reconsidered.
Abbreviations
Annex a – effect of number of vaccinations and time lag between first or second vaccination.
A generalised linear mixed model was used in which a random intercept for each animal was considered to account for potential correlation between results coming from the same animal. The model was fitted using the number of vaccinations that an animal had received as well as the time lag between each of the vaccinations and the date of sampling. A backward selection procedure was followed, identifying the number of vaccinations as the only significant effect. The number of vaccinations was considered as a saturated effect in order to allow other types of relationship than the linear one.
The effect of number of vaccinations and time lag between first or second vaccination and date of sampling on the seropositivity was tested in the animals above 6 months of age (to avoid bias coming from maternal immunity), the results of the generalised linear mixed model are shown below:
Signif. codes: 0 ‘***’ 0.001 ‘**’ 0.01 ‘*’ 0.05 ‘.’ 0.1 ‘ ’ 1.
As said in Section 2.2 , the time lag variables in the model provide the same information as the number of vaccinations, so the more parsimonious model was chosen.
The model results show the effect of number of vaccinations on the ELISA result is significant and the estimated effect obtained is positive when two vaccinations are done, indicating that the proportion of sero‐converted samples increases if the number of vaccinations increases for 1 and 2 vaccinations. The estimated probability of a positive ELISA increases from 0.0007 in non‐vaccinated animals to 0.315 in animals vaccinated twice (Figure A.1 ). In single vaccinated animals, it was only slightly higher than in non‐vaccinated animals.

Probability of being seropositive according to the number of vaccinations received
Annex B – The dynamics of lumpy skin disease virus in a cattle herd
B.1. modelling approach, b.1.1. transmission model for lsdv.
A stochastic compartmental model was developed to describe the transmission of lumpy skin disease virus (LSDV) in a cattle herd via the bites of insect vectors. This was adapted from an earlier deterministic model presented in Gubbins ( 2019 ). For simplicity, a single vector species, the stable fly, Stomoxys calcitrans , is assumed to transmit LSDV on a farm.
The cattle population (H) is subdivided into the number of susceptible (i.e. uninfected), latent (i.e. infected, but not yet infectious), infectious (and showing clinical signs, specifically skin lesions), recovered and resistant animals, denoted by S, E, I, R 1 and R 2 , respectively. Resistant cattle do not develop generalised disease (i.e. skin lesions) and are assumed not to be infectious. To allow for more general (gamma) distributions for the latent and infectious periods, the latent and infectious cattle populations, E and I, are subdivided into n i stages each of mean duration 1/n i r i (so that the latent and infectious periods follow gamma distributions with means 1/r i and variances 1/n i r i 2 for i = E,I, respectively) (Anderson & Watson, 1980 ). Disease‐associated mortality is assumed to be negligible, so the cattle population remains constant.
The vector population (N) is subdivided into the number of susceptible (i.e. uninfected) and infected insects, denoted by X and Y, respectively. Because transmission is mechanical and there is no need for the virus to replicate in the insect before transmission can occur, a vector becomes infectious as soon as it takes an infected blood meal (i.e. there is no extrinsic incubation period) and remains infectious until the virus is inactivated (which occurs at rate γ), when it become susceptible again, or the insect dies. Vector mortality occurs at the same rate (μ) in both classes and is balanced by the recruitment of susceptible vectors, so that the total vector population (N) remains constant.
The force of infection for cattle is given by,
where b is the probability of transmission from insect to bovine, m=N/H is the vector‐to‐host ratio, a is the reciprocal of the time interval between blood meals, p R is the proportion of cattle resistant to disease and Y/N is the proportion of bites which are from infected insects. The force of infection for vectors is given by,
where b is the probability of transmission from bovine to insect and the remaining terms are defined above.
B.1.2. Parameter estimation
Parameters related to mechanical transmission of LSDV by S. calcitrans and the duration of latent and infectious periods (using detection of virus or viral DNA in skin lesions as a proxy measure for infectiousness) were estimated using data from challenge experiments (Babiuk et al., 2008 ; Chihota et al., 2001 , 2003 ; Tuppurainen et al., 2005 ) analysed with Bayesian methods (Gubbins, 2019 ). The proportion of cattle resistant to disease was estimated using the numbers of cattle that did not develop generalised disease following challenge in published experiments (Babiuk et al. 2008; Carn & Kitching, 1995 ; Möller et al., 2019 ; Tuppurainen et al., 2005) (see appendix for details). Plausible ranges for life history parameters for S. calcitrans were extracted from the published literature (Gubbins, 2019 ). The parameters are summarised in Table 2 .
For each replicate of the model, a set of parameters was obtained by sampling from the joint posterior distributions for the mechanical transmission parameters (b, β and γ), the latent and infectious period parameters (n E , r E , n I and r I ) and the proportion of cattle resistant to disease (p R ). Life history parameters (m, a and μ) were sampled from their plausible ranges.
B.1.3. Model implementation
Population sizes in the model take integer values, while transitions between compartments are stochastic processes (Table 1 ). The number of transitions of each type during a small time interval δt was drawn from a binomial distribution with population size n and transition probability q (the appropriate per capita rate multiplied by δt) (Table 1 ). However, binomial random variables are computationally expensive to simulate and an approximating distribution was used wherever possible. If: (i) nq(1−q) > 25; (ii) nq(1−q) > 5 and 0.1 < q < 0.9; or (iii) min(nq,n(1−q)) > 10, an approximating normal variate with mean nq and variance nq(1−q) was used, while if q < 0.1 and nq < 10, an approximating Poisson variate with mean nq was used (Forbes et al., 2011 ).
The initial number of cattle in each class is S(0) = H–R 2 (0), E j (0) = 0 (j = 1,…,n E ), I j (0) = 0 (j = 1,…,n I ) and R 1 (0) = 0, where R 2 (0) is drawn from a binomial distribution with probability of success, p R , and number of trials, H. The initial number of susceptible and infected vectors are X(0) = N−1 and Y(0) = 1, respectively.
B.2. Results
The simulated dynamics of LSDV in a cattle herd when transmission is by the bites of S. calcitrans are shown in Figure 1 . The median (95% prediction interval) for the peak prevalence of infection (which also corresponds with the proportion of animals showing clinical signs) is around 46% (27–76%), which occurs at around 27 (19–44) days post introduction. The prevalence of infection (%) at 7, 14, 21 and 28 days post introduction is: 0 (0, 1.3), 1.0 (1,0, 34.8), 27.5 (1.7, 72.1) and 42.5 (4.1, 71.6), respectively. The seroprevalence (assumed to be equal to the proportion of recovered cattle) is around 46% (27–76%).
Anderson D & Watson R, 1980. On the spread of a disease with gamma distributed latent and infectious periods. Biometrika , 67 , 191–198.
Babiuk S, Bowden TR, Parkyn G, Dalman B, Manning L, Neufeld J, Embury‐Hyatt C, Copps J & Boyle DB, 2008. Quantification of lumpy skin disease virus following experimental infection in cattle. Transboundary and Emerging Diseases, 55 , 299–307.
Carn VM & Kitching RP, 1995. An investigation of possible routes of transmission of lumpy skin disease virus (Neethling). Epidemiology and Infection , 114 , 219–226.
Chihota CM, Rennie LF, Kitching RP & Mellor PS, 2001. Mechanical transmission of lumpy skin disease virus by Aedes aegypti (Diptera: Culicidae). Epidemiology and Infection , 126 , 317–321.
Chihota CM, Rennie LF, Kitching RP & Mellor PS, 2003. Attempted mechanical transmission of lumpy skin disease virus by biting insects. Medical and Veterinary Entomology, 17 , 294–300.
Forbes C, Evans M, Hastings N & Peacock B, 2011. Statistical distributions (4th edition) . Hoboken, New Jersey, U.S.A.: John Wiley & Sons.
Gelman A, Carlin JB, Stern HS & Rubin DB, 2004 . Bayesian data analysis (2nd edition) . Boca Raton, Florida, U.S.A.: Chapman Hall/CRC.
Gubbins S, 2019. Using the basic reproduction number to assess the risk of transmission of lumpy skin disease virus by biting insects. Transboundary and Emerging Diseases , 66 , 1873–1883.
Möller J, Moritz T, Schlottau K, Krstevski K, Hoffmann D, Beer M. & Hoffmann B, 2019. Experimental lumpy skin disease virus infection: comparison of a field and a vaccine strain. Archives of Virology, 164 , 2931–2941.
Tuppurainen ESM, Venter EH & Coetzer JAW, 2005. The detection of lumpy skin disease virus in samples of experimentally infected cattle using different diagnostic techniques. Onderstepoort Journal of Veterinary Research, 72 , 153–164.
Annex C – Estimating the proportion of resistant cattle
The proportion of cattle resistant to disease ( p R ) was estimated based on the numbers of cattle that did not develop generalised disease following challenge in published experiments: 9 out of 14, 4 out of 25 and 8 out of 11 (Carn & Kitching, 1995); 3 out of 6 (Möller et al., 2019 ); 3 out of 6 (Tuppurainen et al., 2005) and 1 out of 7 (Babiuk et al., 2008).
Parameters were estimated in a Bayesian framework. The likelihood for the data is
where R i and N i are the number of resistant cattle and the number of challenged cattle, respectively, and p i is the proportion of resistant cattle in experiment i. To allow the p i s to vary among experiments, a hierarchical structure was assumed, such that,
where α p and β p are hierarchical parameters. Exponential priors (with mean 100) were assumed for α p and β p . Samples from the joint posterior distribution were generated by Markov chain–Monte Carlo methods implemented in OpenBUGS (version 3.2.3). Two chains each of 100,000 samples were run, with the preceding 20,000 iterations discarded to allow for burn‐in. The chains were then thinned (selecting every 20th sample) to reduce autocorrelation. The posterior median (95% credible interval (CI)) for α p and β p were 45.8 (3.1, 263.5) and 35.5 (2.9, 198.1), respectively, with a corresponding posterior median for p R of 0.56 (95% CI: 0.32–0.75).
A simpler model in which the proportion of resistant cattle was common to all experiments was also considered. However, this yielded a poorer fit to the data, as judged by the deviance information criterion: 31.6 (p varying) vs. 35.7 (p common).
Transitions, probabilities and population sizes in the model for the transmission of LSDV in a cattle herd
Parameters for the transmission of LSDV in a cattle herd by the bites of the stable fly, S. calcitrans (Gubbins, 2019 )
Suggested citation: EFSA (European Food Safety Authority) , Calistri P, De Clercq K, Gubbins S, Klement E, Stegeman A, Cortiñas Abrahantes J, Marojevic D, Antoniou SE and Broglia A, 2020. Scientific report on the lumpy skin disease epidemiological report IV: data collection and analysis . EFSA Journal 2020;18(2):6010, 36 pp. 10.2903/j.efsa.2020.6010 [ PMC free article ] [ PubMed ] [ CrossRef ]
Requestor: European Commission
Question number: EFSA‐Q‐2018‐00291
Acknowledgements: EFSA wishes to thank the AHAW Panel members Klaus Depner, Bruno Garin‐Bastuji, Liisa Sihvonen, Karl Ståhl, for reviewing the document, and EFSA staff member: Yves Van der Stede for the support provided to this scientific output. A special thanks is due to: Ali Lilo and Blerina Luke from Ministry of Agriculture, Rural Development and Water Administration, Albania; Aleksandra Miteva from Food Safety Agency, Bulgaria; Žaklin Acinger‐Rogić from Ministry of Agriculture, Croatia; Chrysoula Dile of the Animal Health Directorate of the Greek Ministry of Rural Development and Food, Greece; Bafti Murati from the Food and Veterinary Agency, Kosovo*, Vesna Dakovic from the Ministry of Agriculture and Rural Development, Montenegro; Vanja Kondratenko, Food and Veterinary Agency, North Macedonia; Tatjana Labus from the Ministry of Agriculture and Environmental Protection, Republic of Serbia; Anil Demeli from General Directorate of Food and Control Department of Animal Health and Quarantine, Ministry of Agriculture and Forestry, Turkey for the precious collaboration and timely provision of the epidemiological data.
Approved: 30 January 2020
* This designation is without prejudice to positions on status, and is in line with UNSCR 1244 and the ICJ Opinion on the Kosovo Declaration of Independence. This footnote applies all the time Kosovo is mentioned in this document.
1 https://ec.europa.eu/food/animals/animal-diseases/not-system_en
2 http://empres-i.fao.org/eipws3g/
3 In Croatia vaccination against LSD was done in these two years
4 http://web.oie.int/RR-Europe/eng/Regprog/docs/docs/LSD9/LSD%20presentations/TR_LSD_PPT_SGE_LSD9.pdf
5 Live attenuated vaccines against LSD are commercially available. Homologous vaccines are based on a LSDV strain, the most used strain being the Neethling strain from South Africa. Heterologous vaccines are based on other Capripox virus strains, mainly sheep and goat pox virus (SGPV strains such as Kenyan SGP O‐240, Yugoslavian RM‐65 and Romanian SPPV strains), which are supposed to confer a certain level of cross protection in cattle, although this is not satisfactory. An increased effectiveness can be achieved when the vaccine is used at 10‐fold doses than the one used in small ruminants for the control of sheep and goat pox. One new inactivated vaccine has been developed by MCI Santé Animale in Morocco but its efficacy and safety is currently under testing.
6 http://web.oie.int/RR-Europe/eng/Regprog/docs/docs/LSD9/SGE_LSD9%20recommendations_LSD_final.pdf
7 http://web.oie.int/RR-Europe/eng/Regprog/docs/docs/LSD9/LSD%20presentations/RU_LSD_PPT_SGE_LSD9.pdf
8 https://www.sciencedirect.com/science/article/abs/pii/S014362281300249X ; http://www.china.org.cn/travel/2017-06/08/content_40987529_3.htm
9 https://kevevapi.or.ke/product/poxvax-tm/
10 http://web.oie.int/RR-Europe/eng/Regprog/docs/docs/LSD9/LSD%20presentations/KZ_LSD_PPT_SGE_LSD9_en.pdf
11 https://www.obpvaccines.co.za/Cms_Data/Contents/OBPDB/Folders/Product/~contents/PZBJ3K5TLC47W3BJ/2223_LumpySkin_PI.pdf
12 http://www.mci-santeanimale.com/en/706-bovivax-lsd-n.html
13 https://www.msd-animal-health.co.za/products/lumpyvax/020_product_details.aspx
14 The indication of vaccine producers is to re‐vaccinate animals every year, so it is assumed that the immunity duration is one year.
- Abutarbush SM and Tuppurainen ES, 2018. Serological and clinical evaluation of the Yugoslavian RM 65 sheep pox strain vaccine use in cattle against lumpy skin disease . Transboundary and Emerging Diseases , 65 , 1657–1663. [ PubMed ] [ Google Scholar ]
- Adedeji AJ, Möller J, Meseko CA, Adole JA, Tekki IS, Shamaki D and Hoffmann B, 2019. Molecular characterization of Capripox viruses obtained from field outbreaks in Nigeria between 2000 and 2016 . Transboundary and Emerging Diseases . [ PubMed ] [ Google Scholar ]
- Anderson D and Watson R, 1980. On the spread of a disease with gamma distributed latent and infectious periods . Biometrika , 67 , 191–198. [ Google Scholar ]
- Agianniotaki EI, Mathijs E, Vandenbussche F, Tasioudi KE, Haegeman A, Iliadou P, Chaintoutis SC, Dovas CI, Van Borm S, Chondrokouki ED and De Clercq K, 2017. Complete genome sequence of the lumpy skin disease virus isolated from the first reported case in Greece in 2015 . Genome Announcements , 5 , e00550–00517. [ PMC free article ] [ PubMed ] [ Google Scholar ]
- Alexander S, Olga B, Svetlana K, Valeriy Z, Yana P, Pavel P and Aleksandr K, 2019. A real‐time PCR screening assay for the universal detection of lumpy skin disease virus DNA . BMC Research Notes , 12 , 371. [ PMC free article ] [ PubMed ] [ Google Scholar ]
- Allepuz A, Casal J and Beltran‐Alcrudo D, 2019. Spatial analysis of lumpy skin disease in Eurasia‐Predicting areas at risk for further spread within the region . Transboundary and Emerging Diseases , 66 , 813–822. [ PubMed ] [ Google Scholar ]
- Babiuk S, Bowden TR, Parkyn G, Dalman B, Manning L, Neufeld J, Embury‐Hyatt C, Copps J and Boyle DB, 2008. Quantification of lumpy skin disease virus following experimental infection in cattle . Transboundary and Emerging Diseases , 55 , 299–307. [ PubMed ] [ Google Scholar ]
- Bedekovic T, Simic I, Kresic N and Lojkic I, 2017. Detection of lumpy skin disease virus in skin lesions, blood, nasal swabs and milk following preventive vaccination . Transbound Emerging Disease , 65 , 491–496. [ PubMed ] [ Google Scholar ]
- Ben‐Gera J, Klement E, Khinich E, Stram Y and Shpigel NY, 2015. Comparison of the efficacy of Neethling lumpy skin disease virus and x10RM65 sheep‐pox live attenuated vaccines for the prevention of lumpy skin disease ‐The results of a randomized controlled field study . Vaccine , 33 , 4837–4842. [ PubMed ] [ Google Scholar ]
- Carn VM and Kitching RP, 1995. An investigation of possible routes of transmission of lumpy skin disease virus (Neethling) . Epidemiology and Infection , 114 , 219–226. [ PMC free article ] [ PubMed ] [ Google Scholar ]
- Casal J, Saegerman C, Bertagnoli S, Meyer G, Ganiere JP, Caufour P, De Clercq K, Jacquiet P, Hautefeuille C, Etore F and Napp S, 2019. A simple method to estimate the number of doses to include in a bank of vaccines. The case of Lumpy Skin Disease in France . PLoS ONE , 14 , e0210317. [ PMC free article ] [ PubMed ] [ Google Scholar ]
- Chihota CM, Rennie LF, Kitching RP and Mellor PS, 2001. Mechanical transmission of lumpy skin disease virus by Aedes aegypti (Diptera: Culicidae) . Epidemiology and Infection , 126 , 317–321. [ PMC free article ] [ PubMed ] [ Google Scholar ]
- Chihota CM, Rennie LF, Kitching RP and Mellor PS, 2003. Attempted mechanical transmission of lumpy skin disease virus by biting insects . Medical and Veterinary Entomology , 17 , 294–300. [ PubMed ] [ Google Scholar ]
- Douglass N, Van Der Walt A, Omar R, Munyanduki H and Williamson AL, 2019. The complete genome sequence of the lumpy skin disease virus vaccine Herbivac LS reveals a mutation in the superoxide dismutase gene homolog . Archives of Virology , 164 , 3107–3109. [ PubMed ] [ Google Scholar ]
- EFSA (European Food Safety Authority), 2017. Lumpy skin disease: I. Data collection and analysis . EFSA Journal 2017; 15 ( 4 ):4773, 54 pp. 10.2903/j.efsa.2017.4773 [ PMC free article ] [ PubMed ] [ CrossRef ] [ Google Scholar ]
- EFSA (European Food Safety Authority), Calistri P, DeClercq K, De Vleeschauwer A, Gubbins S, Klement E, Stegeman A, Cortiñas Abrahantes J, Antoniou SE and Broglia A, 2018. Lumpy skin disease: scientific and technical assistance on control and surveillance activities . EFSA Journal 2018; 16 ( 10 ):5452, 46 pp. 10.2903/j.efsa.2018.5452 [ PMC free article ] [ PubMed ] [ CrossRef ] [ Google Scholar ]
- EFSA (European Food Safety Authority), Calistri P, DeClercq K, Gubbins S, Klement E, Stegeman A, Corti˜nas Abrahantes J, Antoniou S‐E, Broglia A and Gogin A, 2019. Scientificreport on lumpy skin disease: III. Data collection and analysis . EFSA Journal 2019; 17 ( 3 ):5638, 26 pp. 10.2903/j.efsa.2019.5638 [ PMC free article ] [ PubMed ] [ CrossRef ] [ Google Scholar ]
- Erster O, Rubinstein MG, Menasherow S, Ivanova E, Venter E, Sekler M, Kolarevic M and Stram Y, 2019. Importance of the lumpy skin disease virus (LSDV) LSDV126 gene in differential diagnosis and epidemiology and its possible involvement in attenuation . Archives of Virology , 164 , 2285–2295. [ PubMed ] [ Google Scholar ]
- Forbes C, Evans M, Hastings N and Peacock B, 2011. Statistical distributions , 4.th edition. John Wiley & Sons, Hoboken, New Jersey, U.S.A. [ Google Scholar ]
- Gelman A, Carlin JB, Stern HS and Rubin DB, 2004. Bayesian data analysis (2nd edition) . Boca Raton, Florida, U.S.A.: Chapman Hall/CRC.
- Gubbins S, 2019. Using the basic reproduction number to assess the risk of transmission of lumpy skin disease virus by biting insects . Transboundary and Emerging Diseases , 66 , 1873–1883. [ PMC free article ] [ PubMed ] [ Google Scholar ]
- Haegeman A, De Leeuw I, Mostin L, Van Campe W, Aerts L, Vastag M and De Clercq K, 2019. An Immunoperoxidase Monolayer Assay (IPMA) for the detection of lumpy skin disease antibodies . Journal of Virological Methods , 113800. [ PMC free article ] [ PubMed ] [ Google Scholar ]
- Katsoulos PD, Chaintoutis SC, Dovas CI, Polizopoulou ZS, Brellou GD, Agianniotaki EI, Tasioudi KE, Chondrokouki E, Papadopoulos O, Karatzias H and Boscos C, 2018. Investigation on the incidence of adverse reactions, viraemia and haematological changes following field immunization of cattle using a live attenuated vaccine against lumpy skin disease . Transboundary and Emerging Diseases , 65 , 174–185. [ PubMed ] [ Google Scholar ]
- Kononov A, Byadovskaya O, Kononova S, Yashin R, Zinyakov N, Mischenko V, Perevozchikova N and Sprygin A, 2019. Detection of vaccine‐like strains of lumpy skin disease virus in outbreaks in Russia in 2017 . Archives of Virology , 164 , 1575–1585. [ PubMed ] [ Google Scholar ]
- Lojkić I, Šimić I, Krešić N and Bedeković T, 2018. Complete Genome Sequence of a Lumpy Skin Disease Virus Strain Isolated from the Skin of a Vaccinated Animal . Genome Announcements , 6 , e00482–00418. [ PMC free article ] [ PubMed ] [ Google Scholar ]
- Machado G, Korennoy F, Alvarez J, Picasso‐Risso C, Perez A and VanderWaal K, 2019. Mapping changes in the spatiotemporal distribution of lumpy skin disease virus . Transboundary and Emerging Diseases , 66 , 2045–2057. [ PubMed ] [ Google Scholar ]
- Manic M, Stojiljkovic M, Petrovic M, Nisavic J, Bacic D, Petrovic T, Vidanovic D and Obrenovic S, 2019. Epizootic features and control measures for lumpy skin disease in south‐east Serbia in 2016 . Transboundary and Emerging Diseases , 66 , 2087–2099. [ PubMed ] [ Google Scholar ]
- M€oller J, Moritz T, Schlottau K, Krstevski K, Hoffmann D, Beer M. and Hoffmann B, 2019. Experimental lumpy skin disease virus infection: comparison of a field and a vaccine strain . Archives of Virology , 164 , 2931–2941. [ PubMed ] [ Google Scholar ]
- Milovanovic M, Dietze K, Milicevic V, Radojicic S, Valcic M, Moritz T and Hoffmann B, 2019. Humoral immune response to repeated lumpy skin disease virus vaccination and performance of serological tests . BMC Veterinary Research , 15 , 80. [ PMC free article ] [ PubMed ] [ Google Scholar ]
- Möller J, Moritz T, Schlottau K, Krstevski K, Hoffmann D, Beer M and Hoffmann B, 2019. Experimental lumpy skin disease virus infection of cattle: comparison of a field strain and a vaccine strain . Archives of Virology , 164 , 2931–2941. [ PubMed ] [ Google Scholar ]
- Munyanduki H, Omar R, Douglass N and Williamson A‐L, 2020. Removal of bovine viral diarrhea virus (BVDV) from lumpy skin disease virus (LSDV) vaccine stocks by passage on chorioallantoic membranes of fertilized hens’ eggs . Journal of Virological Methods , 275 , 113752. [ PubMed ] [ Google Scholar ]
- Saegerman C, Bertagnoli S, Meyer G, Ganiere JP, Caufour P, De Clercq K, Jacquiet P, Hautefeuille C, Etore F and Casal J, 2019. Risk of introduction of Lumpy Skin Disease into France through imports of cattle . Transboundary and Emerging Diseases , 66 , 957–967. [ PubMed ] [ Google Scholar ]
- Şevik M and Doğan M, 2017. Epidemiological and molecular studies on lumpy skin disease outbreaks in Turkey during 2014–2015 . Transboundary and Emerging Diseases , 64 , 1268–1279. [ PubMed ] [ Google Scholar ]
- Sohier C, Haegeman A, Mostin L, De Leeuw I, Van Campe W, De Vleeschauwer A, Tuppurainen E, van den Berg T, De Regge N and De Clercq K, 2019. Experimental evidence of mechanical lumpy skin disease virus transmission by Stomoxys calcitrans biting flies and Haematopota spp. horseflies . Scientific Reports , 9 , 1–10. [ PMC free article ] [ PubMed ] [ Google Scholar ]
- Sprygin A, Babin Y, Pestova Y, Kononova S, Wallace DB, Van Schalkwyk A, Byadovskaya O, Diev V, Lozovoy D and Kononov A, 2018. Analysis and insights into recombination signals in lumpy skin disease virus recovered in the field . PLoS ONE , 13 , e0207480. [ PMC free article ] [ PubMed ] [ Google Scholar ]
- Sprygin A, Babin Y, Pestova Y, Kononova S, Byadovskaya O and Kononov A, 2019. Complete Genome Sequence of the Lumpy Skin Disease Virus Recovered from the First Outbreak in the Northern Caucasus Region of Russia in 2015 . Microbiol Resour Announc , 8 , e01733–18. [ PMC free article ] [ PubMed ] [ Google Scholar ]
- Taylor RA, Berriman ADC, Gale P, Kelly LA and Snary EL, 2019. A generic framework for spatial quantitative risk assessments of infectious diseases: Lumpy skin disease case study . Transboundary and Emerging Diseases , 66 , 131–143. [ PubMed ] [ Google Scholar ]
- Toplak I, Petrovic T, Vidanovic D, Lazic S, Sekler M, Manic M, Petrovic M and Kuhar U, 2017. Complete Genome Sequence of Lumpy Skin Disease Virus Isolate SERBIA/Bujanovac/2016, Detected during an Outbreak in the Balkan Area . Genome Announc , 5 , e00882–17. [ PMC free article ] [ PubMed ] [ Google Scholar ]
- Tuppurainen ESM, Venter EH and Coetzer JAW, 2005. The detection of lumpy skin disease virus in samples of experimentally infected cattle using different diagnostic techniques . Onderstepoort Journal of Veterinary Research , 72 , 153–164. [ PubMed ] [ Google Scholar ]
- Tuppurainen E and Galon N, 2016. TECHNICAL ITEM II LUMPY SKIN DISEASE: CURRENT SITUATION IN EUROPE AND NEIGHBOURING REGIONS AND NECESSARY CONTROL MEASURES TO HALT THE SPREAD IN SOUTH‐EAST EUROPE .
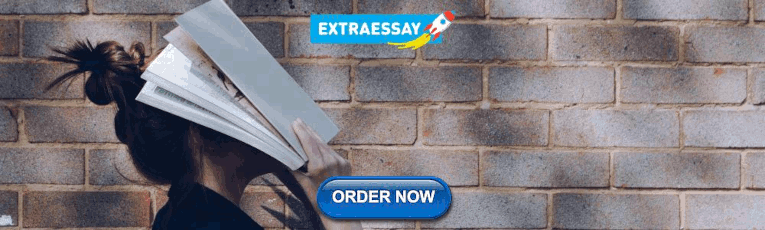
IMAGES
VIDEO
COMMENTS
Lumpy skin disease is an emerging disease, which the recent rapid spread of disease in disease‐free countries indicates the importance of understanding of the limitations and routes of distribution. The present review is designed to provide existing information on the various aspects of the disease. Go to: 1. INTRODUCTION.
1. Introduction. The previous decade has seen an unprecedented increase in scientific reports and publications on lumpy skin disease virus (LSDV), mainly due to its spread into new geographic regions and its adverse economic impacts on a global scale leading to greater interest in the disease and the causative agent (Bianchini et al., 2023).LSDV is a double-stranded DNA (dsDNA) poxvirus virus ...
Abstract. Lumpy skin disease (LSD) is a viral disease caused by lumpy skin disease virus (LSDV), a member of Capripoxvirus genus of Poxviridae family. It is a transboundary disease of the economic importance affecting cattle and water buffaloes. The disease is transmitted by arthropod vectors and causes high morbidity and low mortality.
Special Issue Information. Dear Colleagues, Lumpy skin disease (LSD) is still on the rise and continues to challenge research communities worldwide. A great body of data has been published since the first incursions of LSD into Turkey, Balkans, and Russia in 2015-2017, followed by the emergence of recombinant vaccine-like strains formed by an ...
Lumpy Skin disease: Review of. literature. MRSVA. 3 (3), 6-23. Introduction. Lumpy skin disease (LSD, Pseudo-urticaria, Neethling virus disease, exanthema. nodularis bovis, and knopvelsiekte) is a ...
Lumpy skin disease is caused by lumpy skin disease virus (LSDV), which can induce cattle with high fever and extensive nodules on the mucosa or the scarfskin, seriously influencing the cattle industry development and international import and export trade. Since 2013, the disease has spread rapidly and widely throughout the Russia and Asia.
Abstract and Figures. Lumpy skin disease (LSD) is an infectious, eruptive, occasionally fatal disease of cattle caused by a virus of the family Poxviridae (genus Capripox), which is sometimes also ...
Lumpy skin disease (LSD) is a contagious viral disease of cattle caused by lumpy skin disease virus (LSDV). LSD has recently spread in Asia following outbreaks in the Middle East and Europe. The disease emerged in Bangladesh in July 2019 in the Chattogram district, then rapidly spread throughout the entire country. We investigated six LSD outbreaks in Bangladesh to record the clinical signs ...
The spread of lumpy skin disease (LSD) to free countries over the last 10 years, particularly countries in Europe, Central and South East Asia, has highlighted the threat of emergence in new areas or re-emergence in countries that achieved eradication. This review aimed to identify studies on LSD epidemiology. A focus was made on hosts, modes of transmission and spread, risks of outbreaks and ...
Lumpy skin disease (LSD) is a viral disease caused by lumpy skin disease virus (LSDV), a member of Capripoxvirus genus of Poxviridae family. It is a transboundary disease of the economic importance affecting cattle and water buffaloes. The disease is transmitted by arthropod vectors and causes high morbidity and low mortality. LSD has recently been reported first time in India with 7.1% ...
Lumpy skin disease (LSD) is a transboundary viral infection, affecting cattle with characteristic manifestations involving multiple body systems. A distinctive characteristic of lumpy skin disease is the subclinical disease manifestation wherein animals have viremia and shed the virus through nasal and ocular discharges, while exhibiting no nodules but enlarged lymph nodes that are easily ...
The emergence of the lumpy skin disease has become a major threat to the livestock industry in recent years, causing high economic losses and health risks to both animals and humans. This virus is difficult to detect due to its complexity, making the early detection and accurate diagnosis of this virus essential. This study will explore the utilization of convolutional neural networks (CNNs ...
Paper statistics. If you need immediate assistance, call 877-SSRNHelp (877 777 6435) in the United States, or +1 212 448 2500 outside of the United States, 8:30AM to 6:00PM U.S. Eastern, Monday - Friday. Lumpy skin disease is a viral cross-border sickness that affects cattle and domestic water buffaloes across Africa.
INTRODUCTION. The lumpy skin disease (LSD) virus is a member of the family Poxviridae, genus Capripoxvirus that causes LSD. This disease has recently emerged in most east European and Asian countries [1,2,3,4].Bovine species (Bos taurus and Bos indicus) and water buffalo (Bubalus bubalis) are the primary hosts of this disease.In addition, the virus can infect some wild animals, such as ...
The findings of this study suggest the importance of considering LSDV subclinical infection as a high-risk condition in disease management and outbreak investigations. Lumpy skin disease (LSD) is a transboundary viral infection, affecting cattle with characteristic manifestations involving multiple body systems. A distinctive characteristic of lumpy skin disease is the subclinical disease ...
As infection with the virus of lumpy skin disease, which causes acute or subacute sickness in cattle and the population of water buffalo, poses a serious threat to the cattle industry. Machine learning models gives the direction to make ease of testing the lumpy disease. To predict Lumpy skin disease various machine learning models are available. Various parameters are considered to find out ...
2. Geographic distribution of LSDV. Lumpy skin disease (LSD) was first reported in 1929 in the Mazabuka, Lusaka, and Kufue districts of Northern Rhodesia (Zambia), where it was called pseudo-urticaria (MacDonald, 1931; Morris, 1931).In the absence of the known causative agent, the disease was predominantly characterized by firm circumscribed skin lesions, considered to be caused by insect ...
Lumpy skin disease (LSD) is caused by LSD virus (LSDV), a virus in the family Poxvirus, genus Capripoxvirus as well as sheep pox virus (SPPV) and goat pox virus (GTPV) [ 1 ]. This virus can cause infection mainly in cattle ( Bos spp.) and buffaloes ( Bubalus spp.); there are also reports in other wild ruminant species, such as giraffes, bulls ...
Lumpy skin disease virus (LSDV) causes an infectious disease in cattle. Due to its direct relationship with the survival of arthropod vectors, geospatial and climatic features play a vital role in the epidemiology of the disease. The objective of this study was to assess the ability of some machine learning algorithms to forecast the occurrence of LSDV infection based on meteorological and ...
Lumpy skin disease (LSD) is an emerging infectious disease of cattle and buffaloes which until recently had been considered as a neglected disease. First reported in Zambia in 1920, it spread to other African countries and became endemic in most sub-Saharan areas [1]. The disease was contained within this region until Egypt reported its first ...
A viral infection caused by the Lumpy Skin Disease Virus (LSDV), the disease is transmitted by insects such as flies and mosquitoes. It causes fever and skin nodules, and can be fatal for cattle ...
A real‐time PCR screening assay for the universal detection of lumpy skin disease virus DNA. BMC Research Notes, 12, 371. [PMC free article] [Google Scholar] Allepuz A, Casal J and Beltran‐Alcrudo D, 2019. Spatial analysis of lumpy skin disease in Eurasia‐Predicting areas at risk for further spread within the region.