Thank you for visiting nature.com. You are using a browser version with limited support for CSS. To obtain the best experience, we recommend you use a more up to date browser (or turn off compatibility mode in Internet Explorer). In the meantime, to ensure continued support, we are displaying the site without styles and JavaScript.
- View all journals
- My Account Login
- Explore content
- About the journal
- Publish with us
- Sign up for alerts
- Open access
- Published: 26 March 2021
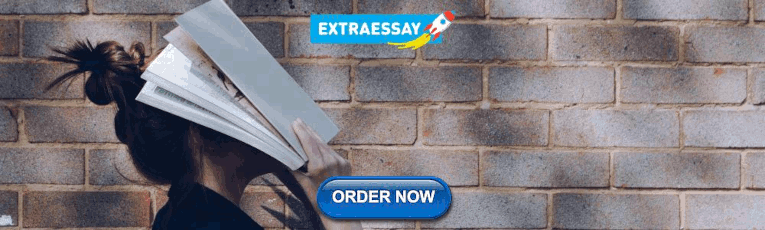
Evaluating the economic impact of water scarcity in a changing world
- Flannery Dolan ORCID: orcid.org/0000-0001-8916-3903 1 ,
- Jonathan Lamontagne ORCID: orcid.org/0000-0003-3976-1678 1 ,
- Robert Link 2 ,
- Mohamad Hejazi 3 , 4 ,
- Patrick Reed ORCID: orcid.org/0000-0002-7963-6102 5 &
- Jae Edmonds ORCID: orcid.org/0000-0002-3210-9209 3
Nature Communications volume 12 , Article number: 1915 ( 2021 ) Cite this article
66k Accesses
166 Citations
181 Altmetric
Metrics details
- Climate and Earth system modelling
- Environmental economics
Water scarcity is dynamic and complex, emerging from the combined influences of climate change, basin-level water resources, and managed systems’ adaptive capacities. Beyond geophysical stressors and responses, it is critical to also consider how multi-sector, multi-scale economic teleconnections mitigate or exacerbate water shortages. Here, we contribute a global-to-basin-scale exploratory analysis of potential water scarcity impacts by linking a global human-Earth system model, a global hydrologic model, and a metric for the loss of economic surplus due to resource shortages. We find that, dependent on scenario assumptions, major hydrologic basins can experience strongly positive or strongly negative economic impacts due to global trade dynamics and market adaptations to regional scarcity. In many cases, market adaptation profoundly magnifies economic uncertainty relative to hydrologic uncertainty. Our analysis finds that impactful scenarios are often combinations of standard scenarios, showcasing that planners cannot presume drivers of uncertainty in complex adaptive systems.
Similar content being viewed by others
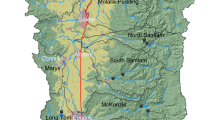
Scope and limitations of drought management within complex human–natural systems
William K. Jaeger, Adell Amos, … Andrew J. Plantinga
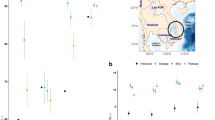
Diagnosing challenges and setting priorities for sustainable water resource management under climate change
Ibrahim Nourein Mohammed, John D. Bolten, … Derek Vollmer
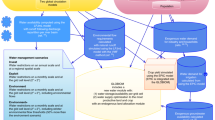
The global nexus of food–trade–water sustaining environmental flows by 2050
A. V. Pastor, A. Palazzo, … F. Ludwig
Introduction
Global water scarcity is a leading challenge for continued human development and achievement of the Sustainable Development Goals 1 , 2 . While water scarcity is often understood as a local river basin problem, its drivers are often global in nature 3 . For instance, agricultural commodities (the primary source of global water consumption 4 ), are often traded and consumed outside the regions they are produced 5 . These economic trade connections mean that global changes in consumption result in impacts on local water systems 6 . Likewise, local water system shocks can also propagate globally 7 , 8 . Water is a critical input to other sectors, such as energy, transportation, and manufacturing 9 , 10 , so that changes in the regional water supply or sectoral demand can propagate across sectors and scales. Continued population growth, climate change, and globalization ensure that these multi-region, multi-sector dynamics will become increasingly important to our understanding of water scarcity drivers and impacts 11 .
Quantifying water scarcity and its impacts are active and growing research areas 12 . Early and influential work in the area largely focused on supply-oriented metrics of scarcity: per-capita water availability 13 , the fraction of available water being used 14 , and more sophisticated measures that account for a region’s ability to leverage available water given its infrastructure and institutional constraints 15 . Recent work proposes indicators such as water quality 16 , green water availability 17 , and environmental flow requirements 18 that focus on specific facets of water scarcity. Qin et al. incorporate the flexibility of current modes of consumption to identify regions where adaptation to scarcity may be relatively difficult 19 . Other recent work focuses on the water footprint of economic activity 20 , 21 making it possible to identify the economic drivers of scarcity (through virtual water trade) 6 , 8 . Yet knowledge gaps remain concerning how the economic costs of future water scarcity will propagate between sectors and regions as society adapts to scarcity, and how the cost of this adaptation depends on uncertainties in the projections of future conditions.
From the economic perspective, water scarcity impacts arise when the difficulty of obtaining water forces a change in consumption. For instance, abundant snowmelt may be of little use to would-be farmers if barriers (cost, institutional, etc.) prevent them from utilizing it. They will be forced to go elsewhere for water or engage in other activities, and this bears an economic cost that is not reflected in conventional water scarcity metrics. When water becomes a binding constraint, societies adapt through trade and shifting patterns of production, and the cost of that adaptation is tied to the difficulty of adopting needed changes. Changing annual cropping patterns to conserve water is easier and will impact an economy less than shuttering thermal power generation during prolonged drought 19 . In a globalized economy, the impact of such adaptation cannot be assessed in a single basin or sector in isolation, as hydrologic changes in one region reverberate across sectors around the world 3 , 22 . Indeed, reductions in water supply in one region may increase demands for water in another, simultaneously inducing both physical scarcity and economic benefit in ways that are difficult to anticipate ex ante 23 . Our primary research question is how these dynamics will impact society in the future, and how both the magnitude and direction of those impacts depend on future deeply uncertain conditions 24 .
To address this question, we deploy a coupled global hydrologic-economic model with basin-level hydrologic and economic resolution 25 to compute the loss (or gain) of economic surplus due to that scarcity in each basin across a range of deeply uncertain futures. Here “economic surplus” refers to the difference between the value that consumers place on a good and the producers’ cost of providing that good 26 . The surplus is a measure of the value-added, or societal welfare gained, due to some economic activity. The change in economic surplus is an appealing metric because it captures how the impact of resource scarcity propagates across sectors and regions that depend on that resource. Change in surplus has been used in past studies to assess the impacts of water policies and to understand how to efficiently allocate water in arid regions 27 , 28 , though it has not typically been used to analyze the impact of water scarcity itself. One exception is a study by Berritella et al., who used the loss in equivalent variation, another welfare metric, to measure the effects of restricting the use of groundwater 29 . On a broader scale, our analysis tracks the impacts of scarcity in hundreds of basins across thousands of scenarios, revealing important global drivers of local impacts that are often missed when the spatial and sectoral scope is defined too narrowly.
Global water scarcity studies depend on long-term projections of climate, population growth, technology change, and other factors that are deeply uncertain, meaning that neither the appropriate distribution nor the correct systems model is agreed upon 24 , 30 . Complicating matters, the coupled human-earth system is complex, exhibiting nonlinearities and emergent properties that make it difficult to anticipate important drivers in the scenario selection process. In such a case, focusing on a few scenarios, as is common in water scarcity studies, risks missing key drivers and their interactions 31 . In contrast, recent studies advocate exploratory modeling 32 to identify important global change scenarios 33 , 34 . In that approach, the uncertainty space is searched broadly and coupled-systems models are used to test the implications of different assumptions on salient measures of impact across a scenario ensemble 35 . Exploratory modeling is especially important in long-term water scarcity studies, where we show that meaningful scenarios vary widely from basin-to-basin, highlighting the inadequacy of relying on a few global narrative scenarios.
By analyzing a large ensemble of global hydro-economic futures, we arrive at three key insights. First, basin-level water scarcity may be economically beneficial or detrimental depending on a basin’s future adaptive capacity and comparative advantage, but that advantage is highly path-dependent on which deeply uncertain factors emerge as the basin-specific drivers of consequential outcomes. For instance, in the Lower Colorado Basin, the worst economic outcomes arise from limited groundwater availability and high population growth, but that high population growth can also prove beneficial under some climatic scenarios. In contrast, the future economic outcomes in the Indus Basin depend largely on global land-use policies intended to disincentive land-use change in the developing world. Our second insight is that those land-use policies often incentivize unsustainable water consumption. In the case of the Indus Basin, limiting agricultural extensification results in intensification through increased irrigation that leads to unsustainable overdraft of groundwater, with similar dynamics playing out elsewhere. Third, our results show that the nonlinear nature of water demand can substantially amplify underlying climate uncertainty, so that small changes in runoff result in large swings in economic impact. This is pronounced in water-scarce basins (like the Colorado) under high-demand scenarios. Collectively, these insights suggest that understanding and accounting for the adaptive nature of global water demand is crucial for determining basin-level water scarcity’s path-dependent and deeply uncertain impacts.
Global-to-basin impacts
We calculate both physical water scarcity (Fig. 1B ) and its economic impact (Fig. 1C ) over the 21st century for 235 river basins for each of the 3000 global change scenarios, simulated using the Global Change Analysis Model (GCAM) integrated assessment model 36 . With the effects of inter-basin trade, hydrologic basins may experience highly positive or highly negative economic impact due to water scarcity (Fig. 1A ). Here, economic impact is defined as the difference in total surplus in water markets (Supplementary Fig. 1 ) between a control scenario with unlimited water and an experimental scenario with limited water supply (Supplementary Fig. 2 ). Water scarcity usually induces negative economic impact (loss of surplus), although positive economic impact from global water scarcity can arise if a basin holds a comparative advantage over others. With this comparative advantage, a basin can become a virtual water exporter through inter-basin trade 37 , meaning it will export water-embedded goods to other regions. Though some basins experience positive impact more often than others (across the scenario ensemble), all basins experience both negative and positive impacts in some scenarios (Supplementary Table 1 ): no basin has a universally positive or negative outlook. As may be expected, the basins with the highest number of positive impact scenarios are those that are relatively water-rich by conventional measures (Fig. 1B ), for example, the Orinoco River in northern South America (Fig. 1A ).
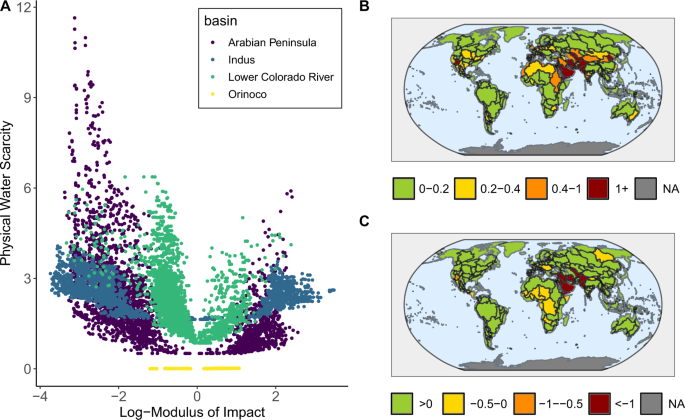
The scatter plot in panel A shows the two metrics in panels B and C plotted against each other in four basins. Each point represents the maximum absolute value of that metric over time in each scenario. The map in panel B shows WTA in each water basin while the map in panel C shows the log-modulus of economic impact. Both maps plot the maximum absolute value of the metric over time and the median across all scenarios. The correspondence between the two metrics is not perfect. Some water-scarce basins have more capacity to handle water scarcity and thus are not as impacted economically as others.
We measure physical water scarcity using the Withdrawal-To-Availability ratio (WTA) which is computed by dividing water withdrawals by renewable supply. The correspondence between the WTA and the economic impact metric is not perfect (Fig. 1B and C ). In some scenarios (for instance, those with restricted reservoir storage), basins with high physical scarcity have a small negative or even positive economic impact, and in others, basins with low physical scarcity have a negative economic impact (Fig. 1A ). This highlights the importance of capturing the interdependencies between physical and economic factors that affect the welfare of a basin.
Several basins show high variance in economic impacts, including the Indus River Basin, the Arabian Peninsula, and the Lower Colorado River Basin (Supplementary Fig. 4 ). In addition to variance in economic impacts, those basins exhibit a wide range of physical water scarcity, are geographically diverse and are of geopolitical importance. The Orinoco Basin is also highlighted as an example of a basin that is not physically water-scarce and experiences slightly positive economic impact in most scenarios (Fig. 1A and B ). Such water-rich basins are particularly well-positioned to produce more water-intensive products to offset lost production in water-scarce basins (Supplementary Fig. 3 ), though the stylized water markets as represented in GCAM (and indeed in all other global hydro-economic models) may overstate these benefits for some basins compared to real-world conditions. The market representation assumes that all agents have an equal opportunity to acquire water and that water is allocated in the most economically efficient manner (except for agricultural subsidies 38 ). In reality, water rights frameworks and barriers to trade may block potential users from putting the water to more economically beneficial use.
The distributions of the plotted scenarios in the four selected basins (Fig. 1A ) give some indication of the relationship between water scarcity and economic impact in each basin. The bi-modal spread of the scenario points (Fig. 1A ) shows that higher physical water scarcity can be associated with both highly positive and severely negative economic impacts. When the distributions are wide and shallow (e.g., the Indus Basin in Fig. 1A ), smaller changes in physical scarcity lead to much higher changes in economic impact compared with other basins (Table 1 ). This occurs if the basin cannot easily supplement renewable supply with other water sources and the price of water rises precipitously. Shifts in demand subject to these high prices lead to large swings in economic impact.
The direction of shifts in demand depends on a basin’s comparative advantage (or disadvantage) due to the scenario assumptions and how these assumptions affect other basins around the world. As evidenced by the positive scenarios in water-scarce basins in Fig. 1 , this comparative advantage can arise from mechanisms other than abundant water supply (e.g., higher agricultural productivity, different dietary or technological preferences, or a lower population). The equilibrium demand over the renewable supply (the WTA) could be the same in two scenarios with very different economic impacts depending on if the scenario assumptions enable a basin’s comparative advantage in one but are detrimental in another (Fig. 1A ). Influential factors that determine economic impact are basin-specific (examples given in the next section). The changes in demand and resulting impacts due to these factors underscore the importance of projecting basin-level scarcity in a global context that allows for market adaptation.
Climate system uncertainty amplification
The market response to water scarcity within a hydrologic basin usually amplifies the uncertainty in hydro-climatic projections (Fig. 2 , Supplementary Fig. 5 ), leading to higher changes in economic impact. Analysis of the scenario ensemble revealed that differences in Earth System Model (ESM) forcing often determines the sign of impact (SA Figs. 6 – 9 ). The ESMs contribute precipitation and temperature projections to the hydrologic model used by GCAM, generating water runoff estimates (see “Methods” section). Surface water supply fluctuations heavily affect changes in economic surplus within these hydrologic basins. Other important factors include reservoir expansion (in Arabia and the Orinoco), land-use scenario (in the Indus and Orinoco), and agricultural productivity (in Arabia, the Indus, and the Orinoco) (Supplementary Figs. 6 – 9 ).
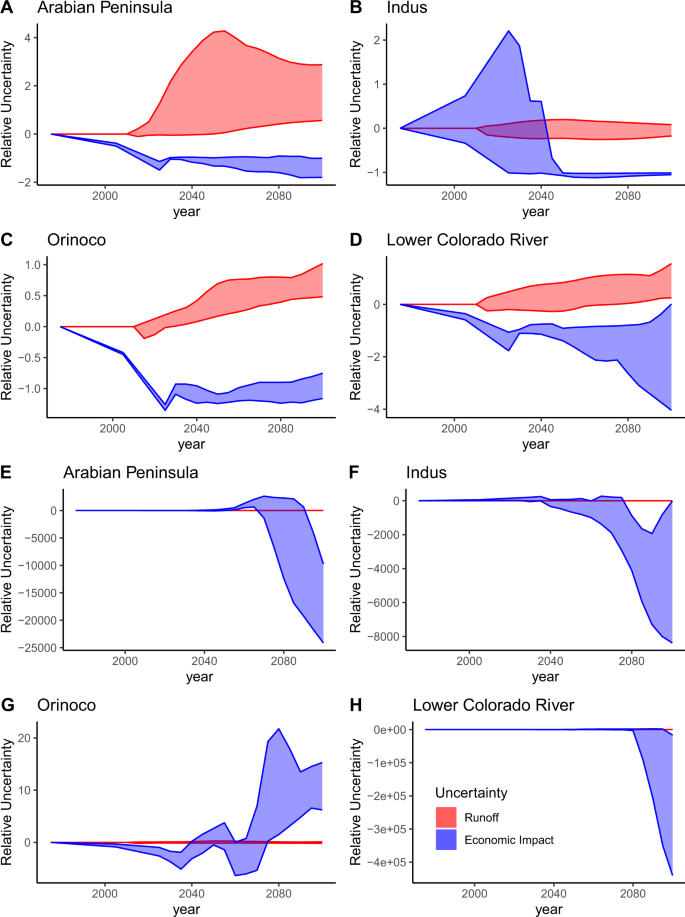
Uncertainty over time plots of the four chosen basins. Values are taken relative to the 2015 baseline. Uncertainty prior to 2015 is illustrative only. The scenario group shown in A – D has the lowest mean climate-induced economic impact uncertainty over time out of the 600 groups. The scenario group shown in E – H has the highest mean climate-induced economic impact uncertainty over time. In most scenarios, runoff uncertainty is amplified by the human system, leading to higher uncertainty in economic impact.
Climate uncertainty is one dimension over which decision-makers have very little control (as opposed to socioeconomic trajectories, agricultural advancements, reservoir storage, etc.). To isolate the uncertainty in the economic impact due to this fundamental climate uncertainty, 600 groups of five scenarios were created by holding all factors constant, except the ESM (of which 5 were considered). The difference between the maximum impact in this group of five and the minimum is one measure of climate-induced impact uncertainty. This uncertainty is plotted in blue in Fig. 2 compared to the runoff uncertainty in red. We find that the economic impact uncertainty is usually higher than the runoff uncertainty (Supplementary Fig. 5 ). Here, runoff uncertainty is the difference between the maximum runoff and the minimum runoff in the set of five scenarios. Peaks and troughs in Fig. 2 correspond to slight deviations in climate forcing in the ESMs. This in turn leads to differences in the runoff, which changes the unit costs of water, causing market adaptations and thus amplifying the economic surplus change (Supplementary Fig. 10 ).
High economic impact uncertainty relative to runoff uncertainty indicates that the market is very sensitive to changes in water supply. In high-demand scenarios (e.g., those with a high population and high food demand), the price of water steeply rises when shifting toward nontraditional water sources such as non-renewable reserves and desalination (Supplementary Fig. 11A ). When this occurs, deviations in supply lead to highly nonlinear impacts (Fig. 2E–H ). Vulnerable basins in these high-demand scenarios see steep and rapid declines in economic impact (Fig. 2E, H ). Scenarios where the economic impact continues dropping through the end of the century are of particular concern and suggest that a basin no longer has the economic capacity to stabilize these negative impacts. We will henceforth call this loss in capacity an ‘economic tipping point’.
Importantly, the conditions that lead to tipping points can vary substantially across basins. For instance, in the Arabian Peninsula, tipping point conditions include low groundwater availability and pricing carbon emissions from all sectors (see “Methods” section). Even with ample groundwater supply, tipping points can occur with high population and low GDP (SSP 3 socioeconomic assumptions) in addition to pricing carbon emissions from all sectors. In some scenarios, we can see that the Arabian Peninsula experiences a positive impact mid-century by relying on relatively inexpensive water resources. After these resources run out subject to the constraints, the economic impact becomes more negative until the end of the century (Fig. 2A ) and the basin utilizes an increasing amount of desalinated water (Supplementary Fig. 11B ). The lack of perfect foresight within GCAM helps explain this short-term thinking, though historically the area has withdrawn groundwater at unsustainable rates 39 .
Meanwhile, the Lower Colorado River Basin experiences an economic tipping point when there is low groundwater availability, low agricultural productivity (SSP 3 agriculture and land use assumptions), and high wealth socioeconomic trajectories (SSP 5 socioeconomics). The uncertainty in economic impact in the Lower Colorado Basin is the highest out of all of the highlighted basins (Fig. 2C ) and is one of the basins with the highest uncertainty in economic impact in the world.
Importantly, the factors that cause economic tipping points in these basins are not the same, nor do they always follow a well-defined global narrative such as the canonical SSPs. Table 2 shows the basins with the most highly negative impact values out of all the time periods in every scenario. Most of these scenarios contain a mixture of SSP elements (e.g., SSP 5 socioeconomics and SSP 4 agriculture in the Sabarmati). There are noticeable trends in the factors, for instance, high wealth socioeconomic trajectories (SSP 5) and the Universal Carbon Tax often lead to tipping points. However, the factors are not all the same in each basin (e.g., in the Ganges-Brahmaputra).
Mitigation-scarcity trade-offs
Pricing carbon emissions from the land-use sector often contributes to an economic tipping point because basins respond by intensifying agricultural land and increasing irrigation, thus exacerbating scarcity. When food demand increases, GCAM responds either by expanding agricultural land or intensifying existing agricultural land. With no price put on land-use change emissions (under the Fossil Fuel and Industrial Carbon Tax, or FFICT) it is more cost-effective to expand. Indeed, we find that scenarios with the FFICT use more agricultural land than the Universal Carbon Tax (UCT) scenarios (Fig. 3A ). Conversely, the carbon prices under the UCT disincentivize expansion and therefore prompt intensification. Carbon prices are derived from the continued ambition scenario of the Nationally Determined Contributions in a future with medium challenges to adaptation and mitigation 40 (see “Methods” section).
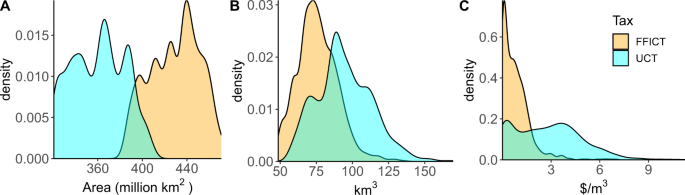
Density plots depicting the difference in tax regimes. The plot in A depicts the sum of global cropland over time under the two carbon tax regimes. The density plot in B shows water withdrawals in the Arabian Peninsula in FFICT (orange) and UCT (cyan) scenarios. The density plot in C depicts the shadow price of water in the Indus River basin in the two tax cases. Values in B and C are averaged over time. Total agricultural land increases under the FFICT while water price and water withdrawals increase under the UCT.
When intensification occurs, yields are increased by irrigating crops more instead of relying on rainwater. The intensity of agricultural land management also increases. These changes prompt greater water withdrawals (Fig. 3B ). The shift from rainwater toward irrigated water also increases the price of water in the UCT scenarios (Fig. 3C ). These results are especially significant in basins sensitive to land-use change. A previous study found that the FFICT prompts greater water withdrawals 41 . However, the study used a previous version of GCAM that did not have intensification options and assumed unlimited water. In that version, water use was proportional to land use. Therefore, when the UCT disincentivized expansion, water use was also limited. When extensification-intensification dynamics are considered, we find a substitution between water use and agricultural expansion. This finding emphasizes the importance of considering all trade-offs in mitigation policy options.
In this study, we use an economic surplus metric in order to quantify the economic impacts of water scarcity and the uncertainty of this impact due to different factors (i.e., population, agricultural productivity, etc.). Theoretically, basins would withdraw less when exposed to a limited supply of water and thus experience a negative economic impact, yet we find some basins capitalize on their water resources and become virtual water exporters in the face of global water scarcity. This dynamic would not be captured by looking at physical water scarcity metrics alone, nor by assessing economic impact at the basin-scale.
These variable responses to water scarcity are sometimes due to highly uncertain and largely uncontrollable factors such as the climate system. When normalized by a 2015 baseline, we find that uncertainty of economic impact due to Earth System Model forcing alone is often several thousand times higher than the uncertainty in the forcing itself (Fig. 2 ). Across the sampled states of the world, we find that slight deviations in precipitation drivers are almost always amplified as they propagate through markets. Since we have little control over uncertainty in the climate system, basin economies that are sensitive to fluctuations in hydro-climactic forcings will need especially robust water resource management frameworks in the future. Further, basins with the highest amount of impact variability due to climate uncertainty are often in politically unstable regions such as the Middle East. Thus, there is an even greater need to manage water resources in the most efficient way possible in the face of extreme uncertainty of economic impacts due to climate in these basins. Planners must also be aware of factors (e.g., population growth or carbon pricing regimes) that lead to economic tipping points in unstable basins.
Under the assumption that food production will always meet demand, implementing a Universal Carbon Tax prompts the intensification of agricultural land due to the increased cost of converting land for agricultural use. The intensification is enabled by increased irrigation and greater water withdrawals (Fig. 3 ). Thus, the effects of pricing carbon in a land-use policy on land intensification-extensification dynamics need to be taken into account in basins exhibiting high levels of water stress.
We find that most scenarios of interest (i.e., those that resulted in extremely high or low economic impact) are composed of a mix of SSP dimensions. This demonstrates the importance of using a scenario discovery framework in the context of a highly uncertain problem such as modeling water resources and the drawbacks of focusing on a limited set of narratives. In addition, the dimensions of high importance in certain basins are of less importance in others. Indeed, every dimension varied in this study was the most influential factor in determining the economic impact of water scarcity in at least one basin (Supplementary Fig. 12 ). Scenario discovery addresses this by identifying the most critical scenario components to the specific analysis context. There is no reason to expect universal shared scenarios will capture key challenges in each basin (or indeed in any), and it is very difficult to anticipate what combinations of factors present challenges in every basin before doing extensive exploration. Scenario discovery is a promising approach to identify relevant scenarios to inform water scarcity analyses. In addition, while this work assessed the economic impact in water markets alone, future work could make use of a Computable General Equilibrium model where the interactions between all markets would be accounted for (see “Methods” section). Indeed, we hope this work provides the basis for similar analyses across a range of hydro-economic models to ascertain the sensitivity of our results to model structure. Confidence in our metric depends on the fidelity of the selected hydro-economic model, so future work would benefit from expanded data collection of socio-technological drivers of regional and sectoral water consumption to improve those underlying models. This study’s use of a coupled partial equilibrium-hydrologic model to perform an extensive uncertainty analysis is novel to the integrated assessment modeling literature and enables the discovery of important multi-scale dynamics such as a basin’s wide range of adaptive responses to water scarcity.
Human-earth system model
Multiple factors affect water demand including population, wealth abundance and distribution, agricultural technology and practices, technological improvements, and carbon and land-use policy. These factors all interact with each other and with the climate system. It is therefore necessary to use a model that includes detailed representations of these systems and the interdependent endogenous choices that shape them. To this end, we have used a partial equilibrium model in order to represent the affected systems with as much detail as possible.
This study makes use of the Global Change Analysis Model (GCAM), a human-Earth system model that has been used by numerous agencies to make informed policy decisions 36 . GCAM is a complex model that decomposes the world into 32 geopolitical regions, 384 land-use regions, and 235 water basins 36 . GCAM includes coupled representations of the Earth’s climate, economic, hydrologic, land-use, and energy systems. These systems are expressed in varying degrees of detail. Population and GDP growth are represented as simple exogenous model inputs. Energy and land-use systems are represented in more detail, with shares of supplies and technologies competing using a logit model 36 . Renewable technologies within the model become more efficient over time and therefore some processes such as solar energy production become more competitive. Nonrenewable resources such as oil and fossil groundwater are modeled with graded supply curves and become more expensive as the levels are used up over time. Shares of energy production technologies may change based on different policy choices. For example, a carbon tax may increase the feasibility of using renewable energy sources. These policies may also impact the shares of land uses (e.g., the carbon tax may prompt afforestation).
Water demand and supply
GCAM allows users to specify water constraints and to link water supply to Xanthos, an extensible hydrologic model 42 . Previous versions of GCAM have introduced the water system but have limited its capabilities to computing water demands. The current system calculates both supply and demand and balances the two quantities by solving for an equilibrium regional shadow price for water 38 , 43 , 44 . Water demand in GCAM is modeled through six sectors: irrigation, livestock, municipal, manufacturing, primary energy, and electricity generation 25 . Irrigation demand is based on biophysical water demand estimates for twelve crop classes 25 . Water demand for irrigation is determined by deducting green water (i.e., water available for use by plants) on irrigated areas and green water on rain-fed areas from total water consumption. Livestock water demand is computed using the consumptive rates for six livestock types (cattle, buffalo, sheep, goats, pigs, and poultry) and estimates of livestock density in 2000 25 . Water withdrawals for electricity generation are related to the amount of electricity generated in each region. Once-through cooling systems compete with evaporative cooling systems with the latter becoming more prevalent over time 25 . Water use in the primary energy sector (i.e., the water used to extract natural resources) is calculated using estimates of energy production in each region along with water use coefficients. Municipal water demand is modeled using population, GDP, and assumptions about technological efficiencies 36 , 41 . Finally, manufacturing water demand is the total industrial water withdrawals less the energy-sector water withdrawals 25 . Consumption is calculated using exogenous consumption to withdrawal ratios for industrial manufacturing 25 .
Water supply in GCAM is modeled using three sources: surface water and renewable groundwater, nonrenewable groundwater, and desalinated seawater. Similar to technology use within GCAM, these sources of water compete using a logit structure based on price. Surface water is typically used first in larger quantities than its competing sources as it is the cheapest source of water. The upper limit of surface water in a basin is taken to be the mean average flow modeled using Xanthos, which calculates water supply at a monthly time step using evapotranspiration, water balance, and routing modules 42 . Accessible water 38 is assumed to be the volume of runoff available even in dry years in addition to reservoir storage capacity (after removing environmental flow requirements). The estimates of accessible water and basin runoff are used as inputs in GCAM. After the renewable water supply is fully consumed, GCAM will either use desalinated water or nonrenewable groundwater depending on the relative shares computed in the price-based logit structure 38 . Nonrenewable groundwater increases in price as more of the resource is consumed. The groundwater supply curves account for geophysical characteristics such as aquifer thickness and porosity, as well as economic factors such as the cost of installing and operating the well. As the price of extraction rises, desalination becomes more competitive, resulting in wider use of desalinated water 44 .
Basin-specific water policies are not represented within GCAM or indeed any global model. The level of detail needed to represent existing water markets and policies exceeds the capabilities of a global model. GCAM does, however, enforce a subsidy on water for agricultural sectors 36 . Imposing this subsidy in GCAM’s water markets allows water to be allocated first to agricultural producers. This behavior mimics the effect of traditional water rights in that senior rights are usually given to agricultural producers. The water markets within GCAM operate by generating a “shadow” price of water, which reflects the economic value of the last unit of water in terms of the water’s contribution to production. When water supply becomes a binding constraint in a particular water basin, the shadow price of water rises because users cannot use more water than there is in the basin. This forces a reduction in the production of the goods and services that rely on water as an input. Clearly, this approach is a simplification, but it marks an improvement over what is most often done where the implications of water scarcity are ignored (i.e., direct and indirect feedbacks associated with unsatisfied water demands are not captured, and analyses are limited to how water scarcity may increase or decrease in the future without a mechanism for dynamic adaptation measures).
We compute the difference in total economic surplus in these simplified water markets (i.e., the sum of producer surplus and consumer surplus) between a control scenario with no water constraints and its paired limited water scenario (see next section).
Capturing economic impact in the entire economy would require a general equilibrium model. However, general equilibrium models necessarily lose some detail in sectoral resolution so that they can capture market interactions. Water is a non-substitutable input to most markets in the human system and so most market interactions will be represented by the changes in water markets when conditions are perturbed. The surplus change in the water markets includes both direct effects (e.g., restricted supply) and indirect effects (e.g., demand shifts in adjacent markets). There may be economic effects not captured by looking at water markets alone, which could be investigated in future work that employs a computable general equilibrium model. Numerous previous studies have assessed economic impact in water markets using both types of models 45 .
Scenario design
We utilize a scenario discovery approach 35 to study the uncertainty in physical water scarcity and its economic impacts. Using this approach, scenarios are generated using all possible combinations of discrete levels of uncertain factors. All scenarios are weighted equally during scenario exploration so as not to presume the likelihood of outcomes a priori. Doing so may leave the system vulnerable to unanticipated events. In addition, in complex adaptive systems such as the human-Earth system, the main drivers of an outcome of interest may be non-intuitive and context-specific 34 . The traditional “predict-then-act” approaches 46 to planning implies a more complete understanding of the system and of future circumstances than is often the case, which can, in turn, lead to myopic decisions 35 . Alternatively, scenario discovery gives equal weight to all possible future system trajectories (i.e., population, wealth, energy prices) and finds the most influential factors driving outcomes of interest-based on the results of all scenarios. Planners can then make robust management decisions based on the influential factors and their uncertainties as opposed to designing based on a few future projections.
In this study, we use scenario discovery to determine the relative influence of seven dimensions in driving highly consequential economic outcomes due to water scarcity (Supplementary Fig. 2 ). These factors include socioeconomic conditions ( S ), agricultural yield assumptions ( G ), groundwater supply ( W ) and reservoir storage ( R ) levels, climate trajectories ( E ), and land-use scenarios ( T ). All factors are represented in Eq. ( 1 ) and are discussed in more detail below. Every scenario n is composed of a distinct combination of the levels of each factor.
Settings for the first three dimensions are taken from GCAM’s implementation of the Shared Socioeconomic Pathways (SSPs) 47 , 48 , 49 . The SSPs are based on plausible but distinct narratives that envision how the century will unfold 47 . The five SSPs correspond to the four combinations of high and low challenges to adaptation and mitigation of climate change with a fifth narrative that lies in the middle of the adaptation-mitigation challenge plane. The implementations of the SSPs within GCAM are made up of factors including population and GDP, agricultural yields, carbon sequestration implementation, renewable energy use, fossil fuel extraction cost, and energy demand 48 . This study included the population/GDP component and the agricultural component of the SSPs. The remaining components of the SSP framework were linked to either the population/GDP or agricultural component. For instance, in one scenario, SSP 3 fossil fuel extraction costs and renewable energy assumptions would be present with SSP 3 socioeconomics and SSP 5 agriculture assumptions; the converse scenario of this dimension would include SSP 5 fossil fuel extraction costs, renewable energy assumptions, and agriculture yields and SSP 3 socioeconomics. This switch ( L ) represents the third dimension of the design. Previous work found the socioeconomic and agricultural and land use elements of the SSPs had the most profound impact on water use 34 , thus we linked the other elements to ensure the scenario design emphasized potentially impactful factors.
The next three dimensions relate directly to the water supply. Groundwater availability is constrained at different levels (5%, 25%, and 40% of the physical water availability) that reflect the economic feasibility of extracting groundwater using the methodology within Turner et al. (2019a) 50 . We also vary reservoir storage estimates using two extremes following the methodology in Turner et al. (2019b) 44 . A restricted scenario indicates that reservoir storage remains constant from the present to the end of the century while an expanded scenario expresses a linear increase from current levels to maximum storage capacity (meaning all accessible water is stored) at the end of the century 44 . The Earth System Model forcing trajectories used as input to Xanthos were also varied between GFDL, MIROC, IPSL, HadGEM2, and NorESM 51 , 52 , 53 , 54 , 55 .
The final dimension corresponds to land-use scenarios formed by mitigation policies. The first, a Universal Carbon Tax (UCT) scenario, imposes a carbon tax on all sectors of the economy including emissions from land-use change. This scenario has many different land-use implications than the alternative scenario that employs the Fossil Fuel and Industrial Carbon Tax (FFICT) which does not price changes in land use (e.g., preserving grasslands and forests rather than expanding agriculture). To construct these scenarios, we use a carbon price trajectory that approximates the continued ambition scenario of the Nationally Determined Contributions (NDCs) as implemented in Fawcett (2015) and revised in Cui et al. (2018) 40 , 43 . This scenario assumes that countries continue decarbonization at the same rate as was necessary to meet the NDCs by 2030. The price of carbon at the reference scenario (SSP 2) for the continued ambition trajectory was used globally for all scenarios. The price begins at $21/ton of CO2 and increases to $233/ton of CO2 by the end of the century. These carbon prices are applied to all sectors (under the UCT) or to every sector but land-use change (under the FFICT).
In total, all unique combinations of the levels of these dimensions (i.e., the size of the set in Eq. 1 ) yield 3000 scenarios. Of the 3000, the total surplus could be calculated for 2876 scenarios without integration errors. Importantly, using a single carbon price trajectory while varying other socioeconomic and climatological factors yields a spread of emission trajectories. This will produce inconsistencies in a given scenario to the extent inputs depend on exogenous forcing trajectories. In this study, this is most important to the generation of hydrologic realizations (to compute available renewable water), where the Xanthos model was forced using several downscaled ESM simulations of RCP 4.5 even though the actual forcing trajectories varied across scenarios. Since climate change will impact the water cycle 56 , the amount of renewable water would also be different in each scenario had Xanthos been run endogenously. However, the magnitude of this difference is highly uncertain, as climate models have been found to cause as much or more uncertainty in hydrologic realizations as the RCPs themselves 57 . Thus, it is not clear that imposing an emissions cap to ensure consistency in forcing would better characterize hydrologic uncertainty. Future studies, for instance, those focused on the cost of meeting mitigation targets, may instead choose to vary prices rather than emissions, but this is beyond the scope of this work.
In addition to the dimensional components of the design, we added further inputs to reflect the recent advances of GCAM. Agricultural yield inputs based on Earth System Model, Representative Concentration Pathway (RCP) 58 and SSP were included, as well as hydropower inputs based on SSP, RCP, and ESM, and technological water demand estimates based on SSP 49 , 59 .
Water scarcity metrics
Many different metrics for measuring water scarcity have been proposed 56 , 60 , 61 . The most commonly used metrics typically compute physical water scarcity and exclude the socioeconomic information necessary to understand adaptive capacity. For example, the Water-To-Availability ratio (WTA) is computed as water withdrawals over renewable water supply 14 , 25 . Several holistic metrics exist that include socioeconomic information such as the Human Development Index 62 , though these metrics face the challenge of subjectively determining how to weight socioeconomic indicators relative to one another 60 .
This study examines water scarcity vulnerability using a metric that accounts for the economic impact of water scarcity within a hydrologic basin. We use the change in economic surplus in water markets between a basin with unlimited water and one with physical constraints on the water supply to calculate this economic impact. This difference consists of the direct impacts of changes in the water supply, as well as the indirect impacts from markets that rely on water. From this point on, we will refer to the surplus change in water markets as simply the surplus change, or economic impact.
Change in economic surplus has been used in many disciplines since its inception 63 . It has been used to assess the impact of climate change on agriculture 64 , 65 , as well as potential infrastructure projects 66 and adaptation policies 67 , 68 . Its continued use is due in part to its ease of implementation, its theoretical simplicity, and its ability to capture changes across sectors. These qualities are highly beneficial in a water scarcity metric. Computing the loss of surplus due to some factor requires a counterfactual scenario in which that factor is absent. This presents a problem when applying this type of metric to any historical data, including water scarcity: water scarcity has always been present. Even a synthetic history with unlimited water would be inadequate as all other historical values depend on historical water scarcity levels. Still, our metric has significant advantages over conventional physical water metrics that lack information about the ability of the basin to respond to water stress.
Here economic impact is defined as:
where T represents the total economic surplus (Supplementary Fig. 1 ). In this study, the economic impact is reported using its log-modulus and has units billions of 2020 US dollars:
Thus, an impact value of −2 would correspond to a loss of 100 billion 1975 US dollars or 2.3% of US GDP in 2018 after adjusting for inflation 69 .
Sign changes in economic impact correspond to shifts in water demand in a basin between unlimited and limited water scenarios. If the total surplus gained from increased withdrawals exceeds the consumer surplus lost by low-demand consumers when water limitations are imposed, basins experience a positive impact. This counter-intuitive case could result when basins become virtual water exporters when global physical constraints are imposed. With water constraints in place, such regions now have a comparative advantage in producing water-intensive goods (notably agricultural products, see Supplementary Fig. 3 ); therefore, they capture greater market share in the water-constrained scenarios. The increased production of these goods translates into a positive shift in demand in water markets. The additional economic activity also increases the value of water as consumers’ willingness to pay for goods increases. This additional economic activity manifests as a larger economic surplus, which translates to a more positive impact. The magnitude of the metric gives an indication of the difficulty of overcoming water scarcity within a basin since the economic impact depends on the value put on water. Higher values of water correspond to higher magnitudes of economic impact.
To uncover the most influential factors that lead a basin to experience positive versus negative impact, we used the Classification and Regression Trees (CART) algorithm 70 . The CART algorithm has been found useful in determining important factors and scenarios of interest in previous studies 34 , 35 . CART operates by performing binary splits of the data to create the purest possible subgroups. In this study, we use CART to identify the factors that lead to the worst-case scenarios with respect to the economic impact metric. Examining this continuous metric necessitates the use of the regression approach of CART. The regression approach uses an Analysis of Variance (ANOVA) method to discover the purest subgroups. Splits work to maximize the variance between groups and minimize variance within groups.
Data availability
Requests for raw data should be made to [email protected]. Processed data is available at https://doi.org/10.5281/zenodo.4470017 71 .
Code availability
Code to generate the main text figures and calculate economic impact can be found at https://doi.org/10.5281/zenodo.4470017 71 .
Forum, W. E. Global Risks 2015 . 69 http://www3.weforum.org/docs/WEF_Global_Risks_2015_Report15.pdf (2015).
Nations, U. Sustainable Development Goals: 17 Goals to Transform Our World . https://www.un.org/sustainabledevelopment/ (2015).
Vörösmarty, C. J., Hoekstra, A. Y., Bunn, S. E., Conway, D. & Gupta, J. Fresh water goes global. Science 349 , 478–479 (2015).
Article ADS Google Scholar
Gleick, P. The World’s Water, Vol. 7, The Biennial Report on Freshwater Resources. SERBIULA Sist. Libr. 20 , https://doi.org/10.5822/978-1-61091-483-3 (2011).
Dalin, C., Konar, M., Hanasaki, N., Rinaldo, A. & Rodriguez-Iturbe, I. Evolution of the global virtual water trade network. Proc. Natl Acad. Sci. USA 109 , 5989–5994 (2012).
Article ADS CAS Google Scholar
Marston, L., Konar, M., Cai, X. & Troy, T. Virtual groundwater transfers from overexploited aquifers in the United States. Proc. Natl Acad. Sci. USA 112 , 8561–8566 (2015).
Heslin, A. et al. Simulating the cascading effects of an extreme agricultural production shock: global implications of a contemporary US dust bowl event. Front. Sustain. Food Syst . 4 , 26 (2020).
Tamea, S., Laio, F. & Ridolfi, L. Global effects of local food-production crises: a virtual water perspective. Sci. Rep. 6 , 18803 (2016).
Hoekstra, A. The Water Footprint of Industry . 221–254 (Butterworth-Heinemann, 2015).
Hoekstra, A. Y. & Mekonnen, M. M. The water footprint of humanity. Proc. Natl Acad. Sci. USA 109 , 3232–3237 (2012).
OECD. OECD Environmental Outlook to 2050: The Consequences of Inaction . (OECD, 2012).
Liu, J. et al. Water scarcity assessments in the past, present, and future. Earths Future 5 , 545–559 (2017).
Falkenmark, M., Lundqvist, J. & Widstrand, C. Macro-scale water scarcity requires micro-scale approaches. Nat. Resour. Forum 13 , 258–267 (1989).
Article CAS Google Scholar
Vorosmarty, C., Green, P., Salisbury, J. & Lammers, R. Global water resources: vulnerability from climate change and population growth. Science 289 , 284 (2000).
Sullivan, C. A., Meigh, J. R. & Giacomello, A. M. The water poverty index: development and application at the community scale. Nat. Resour. Forum 27 , 189–199 (2003).
Article Google Scholar
Zeng, Z., Liu, J. & Savenije, H. H. G. A simple approach to assess water scarcity integrating water quantity and quality. Ecol. Indic. 34 , 441–449 (2013).
Rockström, J. et al. Future water availability for global food production: the potential of green water for increasing resilience to global change. Water Resour. Res . 45 , 7 (2009).
Liu, J., Liu, Q. & Yang, H. Assessing water scarcity by simultaneously considering environmental flow requirements, water quantity, and water quality. Ecol. Indic. 60 , 434–441 (2016).
Qin, Y. et al. Flexibility and intensity of global water use. Nat. Sustain 2 , 515–523 (2019).
Hoekstra, A. Y., Chapagain, A. K., Mekonnen, M. M. & Aldaya, M. M. The Water Footprint Assessment Manual: Setting the Global Standard . (Routledge, 2011).
Mekonnen, M. M. & Hoekstra, A. Y. Four billion people facing severe water scarcity. Sci. Adv. 2 , e1500323 (2016).
Suweis, S. et al. Structure and controls of the global virtual water trade network. Geophys. Res. Lett. 38 , 10 (2012).
Google Scholar
Calzadilla, A., Rehdanz, K. & Tol, R. S. J. Water scarcity and the impact of improved irrigation management: a computable general equilibrium analysis. Agric. Econ. 42 , 305–323 (2011).
Walker, W. E. et al. Defining uncertainty: a conceptual basis for uncertainty management in model-based decision support. Integr. Assess. 4 , 5–17 (2003).
Hejazi, M. et al. Long-term global water projections using six socioeconomic scenarios in an integrated assessment modeling framework. Technol. Forecast. Soc. Change 81 , 205–226 (2014).
Mankiw, G. Principles of Economics . (Cengage Learning, 2018).
Harou, J. et al. Hydro-economic models: concepts, design, applications, and future prospects. J. Hydrol. 375 , 627–643 (2009).
Damania, R. The economics of water scarcity and variability. Oxf. Rev. Econ. Policy 36 , 24–44 (2020).
Berrittella, M., Hoekstra, A. Y., Rehdanz, K., Roson, R. & Tol, R. S. J. The economic impact of restricted water supply: a computable general equilibrium analysis. Water Res. 41 , 1799–1813 (2007).
Knight, F. H. Risk, Uncertainty and Profit . (Houghton Mifflin Co, 1921).
Lempert, R. J. A new decision sciences for complex systems. Proc. Natl Acad. Sci. USA 99 , 7309–7313 (2002).
Bankes, S. Exploratory modeling for policy analysis. Oper. Res. 41 , 435–449 (1993).
Guivarch, C., Rozenberg, J. & Schweizer, V. The diversity of socio-economic pathways and CO2 emissions scenarios: Insights from the investigation of a scenarios database. Environ. Model. Softw. 80 , 336–353 (2016).
Lamontagne, J. R. et al. Large ensemble analytic framework for consequence-driven discovery of climate change scenarios. Earths Future 6 , 488–504 (2018).
Bryant, B. P. & Lempert, R. J. Thinking inside the box: a participatory, computer-assisted approach to scenario discovery. Technol. Forecast. Soc. Change 77 , 34–49 (2010).
Calvin, K. et al. GCAM v5.1: Representing the linkages between energy, water, land, climate, and economic systems. Geosci. Model Dev . https://doi.org/10.5194/gmd-12-677-2019 (2019).
Konar, M., Reimer, J., Hussein, Z. & Hanasaki, N. The water footprint of staple crop trade under climate and policy scenarios. Environ. Res. Lett . 11 , 035006 (2016).
Kim, S. H. et al. Balancing global water availability and use at basin scale in an integrated assessment model. Clim. Change 136 , 217–231 (2016).
Al-Rashed, M. F. & Sherif, M. M. Water resources in the GCC countries: an overview. Water Resour. Manag . https://doi.org/10.1023/A:1008127027743 (2000).
Fawcett, A. A. et al. Supplemental Materials for ‘Can Paris pledges avert severe climate change?’ Science 1–53 https://doi.org/10.1126/science.aad5761 (2015).
Hejazi, M. I. et al. Integrated assessment of global water scarcity over the 21st century under multiple climate change mitigation policies. Hydrol. Earth Syst. Sci . https://doi.org/10.5194/hess-18-2859-2014 (2014).
Li, X. et al. Xanthos–a global hydrologic model. J. Open Res. Softw. 5 , 0–6 (2017).
Cui, R. Y. et al. Regional responses to future, demand-driven water scarcity. Environ. Res. Lett . 13 , 094006 (2018).
Turner, S. W. D., Hejazi, M., Calvin, K., Kyle, P. & Kim, S. A pathway of global food supply adaptation in a world with increasingly constrained groundwater. Sci. Total Environ. 673 , 165–176 (2019).
Dudu, H. & Chumi, S. Economics of irrigation water management: a literature survey with focus on partial and general equilibrium models. The World Bank Policy Research Working Paper Series . 4556 (2008).
Lempert, R., Nakicenovic, N., Sarewitz, D. & Schlesinger, M. Characterizing climate-change uncertainties for decision-makers. an editorial essay. Clim. Change 65 , 1–9 (2004).
O’Neill, B. C. et al. The roads ahead: narratives for shared socioeconomic pathways describing world futures in the 21st century. Glob. Environ. Change 42 , 169–180 (2017).
Calvin, K. et al. The SSP4: a world of deepening inequality. Glob. Environ. Change 42 , 284–296 (2017).
Graham, N. T. et al. Water sector assumptions for the shared socioeconomic pathways in an integrated modeling framework. Water Resour. Res. 54 , 6423–6440 (2018).
Turner, S. W., Hejazi, M., Yonkofski, C., Kim, S. H. & Kyle, P. Influence of groundwater extraction costs and resource depletion limits on simulated global nonrenewable water withdrawals over the twenty-first century. Earths Future 7 , 123–135 (2019).
Collins, W. J. et al. Development and evaluation of an Earth-System model–HadGEM2. Geosci. Model Dev . https://doi.org/10.5194/gmd-4-1051-2011 (2011).
Donner, L. J. et al. The dynamical core, physical parameterizations, and basic simulation characteristics of the atmospheric component AM3 of the GFDL global coupled model CM3. J. Clim . https://doi.org/10.1175/2011JCLI3955.1 (2011).
Marti, O. et al. The new IPSL climate system model: IPSL-CM4. Note du Pôle de Modélisation. Vol. 26 (Institut Pierre Simon Laplace, 2005).
Bentsen, M. et al. The Norwegian Earth System Model, NorESM1-M–Part 1: Description and basic evaluation of the physical climate. Geosci. Model Dev . https://doi.org/10.5194/gmd-6-687-2013 (2013).
Watanabe, S. et al. MIROC-ESM 2010: Model description and basic results of CMIP5-20c3m experiments. Geosci. Model Dev . https://doi.org/10.5194/gmd-4-845-2011 (2011).
Gosling, S. N. & Arnell, N. W. A global assessment of the impact of climate change on water scarcity. Clim. Change https://doi.org/10.1007/s10584-013-0853-x (2016).
Hattermann, F. F. et al. Sources of uncertainty in hydrological climate impact assessment: a cross-scale study. Environ. Res. Lett. 13 , 015006 (2018).
Vuuren, D. et al. The representative concentration pathways: an overview. Climatic Change Issue Clim. Change 109 , 5–31 (2011).
Turner, S. W. D., Hejazi, M., Kim, S. H., Clarke, L. & Edmonds, J. Climate impacts on hydropower and consequences for global electricity supply investment needs. Energy https://doi.org/10.1016/j.energy.2017.11.089 (2017).
Damkjaer, S. & Taylor, R. The measurement of water scarcity: Defining a meaningful indicator. Ambio https://doi.org/10.1007/s13280-017-0912-z (2017).
Schewe, J. et al. Multimodel assessment of water scarcity under climate change. Proc. Natl. Acad. Sci . USA https://doi.org/10.1073/pnas.1222460110 (2014).
Anand, S. & Sen, A. K. Human development index: methodology and measurement. Hum. Dev. Rep. Off . 12 (1994).
Marshall, A. The Principles of Economics . https://econpapers.repec.org/bookchap/hayhetboo/marshall1890.htm (1890).
Calvin, K. V. et al. Global market and economic welfare implications of changes in agricultural yields due to climate change. Clim. Change Econ . 11 , 2050005 (2020).
Stevanović, M. et al. The impact of high-end climate change on agricultural welfare. Sci. Adv . https://doi.org/10.1126/sciadv.1501452 (2016).
Moses Shyam, D., Anantha, K. H., Wani, S. P. & Raju, K. V. Impacts of integrated watershed development using economic surplus method. In Community and Climate Resilience in the Semi-Arid Tropics: A Journey of Innovation (eds. Wani, S. P. & Raju, K. V.) 119–142 (Springer International Publishing, 2020).
Kahil, M. T., Dinar, A. & Albiac, J. Modeling water scarcity and droughts for policy adaptation to climate change in arid and semiarid regions. J. Hydrol. 522 , 95–109 (2015).
Vaux, H. J. & Howitt, R. E. Managing water scarcity: an evaluation of interregional transfers. Water Resour. Res. 20 , 785–792 (1984).
The World Bank. GDP (Current US$). https://data.worldbank.org/indicator/NY.GDP.MKTP.CD (2019).
Breiman, L., Friedman, J. H., Olshen, R. A. & Stone, C. J. Classification and regression trees . CART https://doi.org/10.1201/9781315139470 (2017).
Dolan, F. C. et al. Evaluating the Economic Impact Of Water Scarcity In a Changing World . https://doi.org/10.5281/zenodo.4470017 (Zenodo, 2021).
Download references
Acknowledgements
This research was supported by the U.S. Department of Energy, Office of Science, as part of research in MultiSector Dynamics, Earth and Environmental System Modeling Program. The authors would also like to acknowledge Sean Turner, Chris Vernon, and Abigail Snyder for their help at the beginning of the project.
Author information
Authors and affiliations.
Department of Civil and Environmental Engineering, Tufts University, Medford, MA, USA
Flannery Dolan & Jonathan Lamontagne
Department of Medicine, University of Virginia School of Medicine, Charlottesville, VA, USA
Robert Link
Joint Global Change Research Institute, Pacific Northwest National Laboratory, College Park, MA, USA
Mohamad Hejazi & Jae Edmonds
King Abdullah Petroleum Studies and Research Center, Riyadh, Saudi Arabia
Mohamad Hejazi
School of Civil and Environmental Engineering, Cornell University, Ithaca, NY, USA
Patrick Reed
You can also search for this author in PubMed Google Scholar
Contributions
F.D. ran the model, processed the output, and analyzed the data. J.L. provided guidance throughout the process and proposed the initial experimental design. R.L. provided the necessary computational capabilities to output the economic impact metric. M.H. and P.R. helped propose the initial narratives of the paper. J.E. proposed the economic impact metric. All authors wrote the manuscript.
Corresponding author
Correspondence to Flannery Dolan .
Ethics declarations
Competing interests.
The authors declare no competing interests.
Additional information
Peer review information Nature Communications thanks Jennifer Morris and the other, anonymous reviewer(s) for their contribution to the peer review of this work.
Publisher’s note Springer Nature remains neutral with regard to jurisdictional claims in published maps and institutional affiliations.
Supplementary information
Supplementary information, rights and permissions.
Open Access This article is licensed under a Creative Commons Attribution 4.0 International License, which permits use, sharing, adaptation, distribution and reproduction in any medium or format, as long as you give appropriate credit to the original author(s) and the source, provide a link to the Creative Commons license, and indicate if changes were made. The images or other third party material in this article are included in the article’s Creative Commons license, unless indicated otherwise in a credit line to the material. If material is not included in the article’s Creative Commons license and your intended use is not permitted by statutory regulation or exceeds the permitted use, you will need to obtain permission directly from the copyright holder. To view a copy of this license, visit http://creativecommons.org/licenses/by/4.0/ .
Reprints and permissions
About this article
Cite this article.
Dolan, F., Lamontagne, J., Link, R. et al. Evaluating the economic impact of water scarcity in a changing world. Nat Commun 12 , 1915 (2021). https://doi.org/10.1038/s41467-021-22194-0
Download citation
Received : 05 May 2020
Accepted : 22 February 2021
Published : 26 March 2021
DOI : https://doi.org/10.1038/s41467-021-22194-0
Share this article
Anyone you share the following link with will be able to read this content:
Sorry, a shareable link is not currently available for this article.
Provided by the Springer Nature SharedIt content-sharing initiative
This article is cited by
Association of drought conditions and heavy rainfalls with the quality of drinking water in barcelona (2010–2022).
- Daniela Benítez-Cano
- Patricia González-Marín
- Laura Oliveras
Journal of Exposure Science & Environmental Epidemiology (2024)
Global river economic belts can become more sustainable by considering economic and ecological processes
- Alistair George Liam Borthwick
Communications Earth & Environment (2024)
Integrated modeling for assessing climate change impacts on water resources and hydropower potential in the Himalayas
- Rupesh Baniya
- Ram Krishna Regmi
- Jebin Tamrakar
Theoretical and Applied Climatology (2024)
The role of food industries in sustainability transition: a review
- S. Prasanna
- Praveen Verma
Environment, Development and Sustainability (2024)
Spatio-temporal prediction of land use and land cover change in Bahi (Manyoni) Catchment, Tanzania, using multilayer perceptron neural network and cellular automata-Markov chain model
- Naima A. M. Hersi
- Deogratias M. M. Mulungu
- Joel Nobert
Environmental Monitoring and Assessment (2024)
By submitting a comment you agree to abide by our Terms and Community Guidelines . If you find something abusive or that does not comply with our terms or guidelines please flag it as inappropriate.
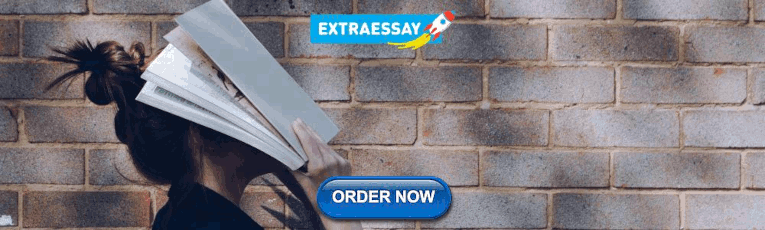
Quick links
- Explore articles by subject
- Guide to authors
- Editorial policies
Sign up for the Nature Briefing newsletter — what matters in science, free to your inbox daily.


An official website of the United States government
The .gov means it’s official. Federal government websites often end in .gov or .mil. Before sharing sensitive information, make sure you’re on a federal government site.
The site is secure. The https:// ensures that you are connecting to the official website and that any information you provide is encrypted and transmitted securely.
- Publications
- Account settings
Preview improvements coming to the PMC website in October 2024. Learn More or Try it out now .
- Advanced Search
- Journal List
- NASA Author Manuscripts

Water scarcity assessments in the past, present and future
1 School of Environmental Science and Engineering, South University of Science and Technology of China, Shenzhen, 518055, China
2 Swiss Federal Institute for Aquatic Science and Technology (Eawag)
3 Department of Environmental Sciences, University of Basel, Switzerland
Simon N. Gosling
4 School of Geography, University of Nottingham, Nottingham, NG7 2RD, United Kingdom
Matti Kummu
5 Water and development research group, Aalto University, Finland
Martina Flörke
6 Center for Environmental Systems Research, University of Kassel, Kassel, Germany
Stephan Pfister
7 ETH Zurich, Institute of Environmental Engineering, 8093 Zurich, Switzerland
Naota Hanasaki
8 National Institute for Environmental Studies, Tsukuba, Japan
Yoshihide Wada
9 NASA Goddard Institute for Space Studies, 2880 Broadway, New York, NY 10025, USA
10 Center for Climate Systems Research, Columbia University, 2880 Broadway, New York, NY 10025, USA
11 Department of Physical Geography, Faculty of Geosciences, Utrecht University, Heidelberglaan2, 3584 CS Utrecht, The Netherlands
Xinxin Zhang
12 School of Nature Conservation, Beijing Forestry University, Beijing, 10083, China
Chunmiao Zheng
Joseph alcamo.
13 Center for Environmental Systems Research, University of Kassel, Kassel, Germany
14 Institute of Industrial Science, The University of Tokyo, Tokyo, Japan
Water scarcity has become a major constraint to socio-economic development and a threat to livelihood in increasing parts of the world. Since the late 1980s, water scarcity research has attracted much political and public attention. We here review a variety of indicators that have been developed to capture different characteristics of water scarcity. Population, water availability and water use are the key elements of these indicators. Most of the progress made in the last few decades has been on the quantification of water availability and use by applying spatially explicit models. However, challenges remain on appropriate incorporation of green water (soil moisture), water quality, environmental flow requirements, globalization and virtual water trade in water scarcity assessment. Meanwhile, inter- and intra- annual variability of water availability and use also calls for assessing the temporal dimension of water scarcity. It requires concerted efforts of hydrologists, economists, social scientists, and environmental scientists to develop integrated approaches to capture the multi-faceted nature of water scarcity.
1. Introduction
Population growth, economic development and dietary shift (towards more animal products) have resulted in ever increasing water demand, and consequently pressures on water resources. Many parts of the world are enduring water scarcity which generally refers to the condition wherein demand for water by all sectors, including the environment, cannot be satisfied fully due to the impact of water use on supply or quality of water [ Falkenmark et al., 1989 ; Alcamo, et al., 2000 ; Vörösmarty et al., 2000 ]. In the Global Risks 2015 Report of the World Economic Forum, water supply crisis was identified as the top 1 high-impact risk for our current times [ World Economic Forum, 2015 ].
Understanding water scarcity is important for formulating policies at global, regional, national and local scales. “Addressing water scarcity and quality” is one of the six themes of the 8 th Phase of the International Hydrological Programme (IHP-VIII) that focuses on “Water Security: Responses to Local, Regional and Global Challenges (2014–2021)”. Similarly, it is a key focus of the scientific decade 2013–2022 of the International Association of Hydrological Sciences (IAHS), named “ Panta Rhei – Everything Flows”, which is dedicated to research activities on changes in hydrology and society [ Montanari et al., 2013 ]. A targeted working group on “Water Scarcity Assessment: methodology and application” was established in the Panta Rhei program to develop innovative methodology and conduct water scarcity assessment ( http://iahs.info/Commissions--W-Groups/Working-Groups/Panta-Rhei/Working-Groups.do ). “Substantially reduce the number of people suffering from water scarcity” is also one of the targets set in the Sustainable Development Goals recently adopted by the United Nations [ UN, 2015 ].
Since the late 1980s, when water scarcity became an issue, many indicators have been developed to facilitate the assessment of status of water scarcity across the world ( Table 1 ). Publications on water scarcity assessment have increased dramatically in the last two decades ( Fig. 1 ) amid the intensification of the problem in increasing parts of the worlds. Rather straightforward water scarcity indicators were developed in the late 1980s throughout the beginning of the 2000s, which were criticized for their focus on surface water and groundwater (so called blue water) only, neglecting the important role of green water (soil moisture fed by rainfall) and spatial and temporal variations [ Savenije, 2000 ; Rijsberman, 2006 ].

The number of publications based on the keyword “water scarcity” from Scopus as of 17 January 2016. The years of publication of specific water scarcity indicators are marked.
Summary of the characteristics of water scarcity indicators. EFR stands for Environmental Flow Requirements.
Entering the 2000s, more sophisticated approaches with high spatial resolution have been developed, attempting to incorporate more aspects of water, such as: water quality, green water (soil moisture), and environmental flow requirements. In recent years, explicit representation of water quality and environmental flow requirement have been taken into account, through a multiple-value water scarcity indicator [ Zeng et al., 2013 ; Liu et al., 2016 ]. Although this development has increased understanding of the multi-faceted nature of water scarcity, these attempts usually focused on merely one aspect of water scarcity. In contrast to the wide use of the classical water scarcity indicators developed in the early years, the more integrated indicators have rarely been applied beyond the research groups where they were developed.
It is worth mentioning that most approaches for water scarcity assessment have used single indicators to quantify water scarcity. A few have combined two indicators. For example, Falkenmark [1997] assessed blue water scarcity with two indicators, water shortage and water stress (see Table 1 ), together using ‘Falkenmark matrix’. Kummu et al. [2016] used similar approach to Falkenmark [1997] for water scarcity assessment on the global level for the whole 20 th century. According to Kummu et al. [2016] , areas under both water shortage and stress have very limited adaptation options to alleviate the scarcity compared to areas under sole stress or shortage.
In this paper, we provide a comprehensive review of existing water scarcity indicators and reflect on their relevance in a rapidly changing world. Based on which, we highlight some major challenges faced in the future research and propose ways forward.
2. An overview of classical water scarcity indicators
2.1. the falkenmark indicator.
The Falkenmark Indicator [ Falkenmark et al., 1989 ], measuring water scarcity is a simple yet widely used method for calculating water scarcity. It requires: the number of people living within a given spatial domain and the volume of water (termed blue water by Falkenmark ) available within that domain. The volume of water available per person is then calculated in m 3 /cap/year. The indicator’s reliance on population leads to the Water Crowding Index (WCI), which measures the number of people per unit of available water, e.g., persons/million m 3 /year. A value of 1,700 m 3 /cap/year of renewable freshwater was proposed as the threshold for water scarcity [ Falkenmark et al., 1989 ], below which social stress and a high level of competition for water emerges [ Falkenmark and Rockström, 2004 ]. If water availability falls below 1,000 m 3 /cap/year then the area experiences high water scarcity , and below 500 m 3 /cap/year, absolute scarcity .
However, its ease of application is tempered by an important caveat: the index is only an indication of supply-side effects on global water scarcity [ Schewe et al., 2014 ]. The indicator overlooks temporal variability and the important drivers of demand, related to economic growth, lifestyle, and technological developments [ Savenije, 2000 ]. Management practices and infrastructure are not considered by the index and the simple threshold does not reflect the true spatial distribution of demand within and between the domains over which the index is calculated.
2.2. Water use to availability ratio
The water use to availability ratio, or criticality ratio, is another widely used indicator to assess water scarcity. The advantage of this ratio is that it measures the amount of water used, and relates it to the available renewable water resources [ Alcamo and Henrichs, 2002 ]. Over the past decades, the development of water use models has been fast, and water availability and use can now be modelled spatially explicitly on global scale with high spatial resolutions [ Alcamo et al., 2003a ; Hanasaki et al., 2008 ; Flörke et al., 2013 ; Wada et al., 2014 ].
Water use can refer to either water consumption or water withdrawals. Water consumption measures the amount that is removed from rivers, lakes or groundwater sources and evaporated to the atmosphere. Water withdrawal refers to the amount of water that is withdrawn from these sources, of which part returns to the system by leakage or return flows. The majority of the existing water scarcity studies use withdrawal to indicate water use [ Alcamo, et al., 2003b ; Oki and Kanae, 2006 ; Wada et al., 2011 ]. Recent work by Munia et al. [2016] uses consumption and withdrawals as a minimum and maximum levels of scarcity, respectively. However, since consumption is normally much smaller than withdrawal, the ratio of consumption to average available renewable water resources usually indicates an unrealistically low level of water scarcity.
Based on the water criticality ratio, high water stress occurs if water withdrawal exceeds 40% of the available water resources [ Alcamo and Henrichs, 2002 ]. However, as part of the withdrawal water returns back to water bodies and the actual proportion of the return flow vary across regions depending on natural and social-economic and technical conditions, using 40% as a water scarcity threshold may not be consistent in reflecting the status of water scarcity across regions.
2.3. Physical and economic water scarcity – the IWMI Indicator
The International Water Management Institute (IWMI) developed a more complex indicator for assessing water scarcity [ Seckler et al., 1998 ], combining the physical and economic water scarcities. Indicator takes into account the proportion of water supply, of a country in question, from renewable freshwater resource available for human requirements, while accounting for existing water infrastructure such as desalinization plants and water stored in reservoirs. A novel element of the index is that it considers an individual country’s potential to develop water infrastructure and to improve irrigational water use efficiency.
Their analysis yielded five country groupings. The country groupings were in turn used to define whether countries are either “physically water scarce” or “economically water scarce” [ Rijsberman, 2006 ]. The former is where countries are unable to meet estimated water demand in 2025, even after accounting for national adaptive capacity. The latter is where countries have a sufficient renewable water resource but would have to invest significantly in water infrastructure to make the resources available for consumption in 2025.
The index is available as a Microsoft Excel model [ Seckler et al., 1998 ] yet it has not been used as much as other indicators to assess global water scarcity, with exception to an assessment conducted by Cosgrove and Rijsberman [2000] . One reason for this is that it is considerably more complex than many other indices reviewed here and thus more time-consuming to compute. Another is perhaps that its interpretation is less intuitive than other indices and therefore less attractive for presentation to the public and/or a policy audience [ Rijsberman, 2006 ].
2.4. Water poverty index
The Water Poverty Index (WPI) proposes a relationship between the physical extent of water availability, its ease of abstraction, and the level of community welfare [ Sullivan, 2001 ]. It considers five factors: resources or water availability; access to water for human use; effectiveness of people’s ability to manage water; water use for different purposes; environmental integrity related to water and of ecosystem goods and services from aquatic habitats in the area. The WPI is mainly designed for assessing the situation facing poor water endowments and poor adaptive capacity.
The WPI is calculated with the weighted average of the five components, each of which is first standardized so that it falls in the range 0 to 100; thus the resulting WPI value is also between 0 and 100, representing the lowest and the highest level of water poverty [ Sullivan et al., 2006 ]. The indicator has the advantage of comprehensiveness. However, its application is hampered by its complexity and lack of information for some of the factors required for building the indicator on large scale [ Rijsberman, 2006 ]. It has so far only been applied at the community level for pilot sites in a few countries.
3. Progress in water scarcity assessment
Since the beginning of the 2000s, water scarcity assessment has entered an era characterized by the applications of more sophisticated models supported with spatial analytical tools. The water use to availability ratio has been the basis of many water scarcity assessment approaches developed during this period. The main efforts made in these assessments have been in the measurements of water “use” and “availability”.
3.1. Green-blue water scarcity
Green water refers to soil moisture in the unsaturated zone recharged by precipitation. It is a crucial water resource for agricultural production, responsible for about 90% of total water use of agriculture and 60% of the global food is produced without additional irrigation (i.e., blue water use) [ Rockström et al., 2009 ].
The development of the green-blue water indicator has attempted to incorporate green water in the assessment. The pioneer work was done by Rockström et al. [2009] who developed the first indicator to assess scarcity where both blue and green water resources are included. They measured the scarcity by comparing global average green-blue water consumption of 1300 m 3 /cap/year for a healthy diet (3000 kcal/cap/day of which 20% originates from animal sources) and locally available green-blue water resources. The area is under scarcity if available water resources are less than the average requirement of 1300 m 3 /cap/year. This was further developed by Gerten et al. [2011] who incorporated the local water requirements for a healthy diet to the calculations and thus taking into account spatial variations of the water needed to produce the actually grown food in different locations. These vary from less than 650 m 3 /cap/year in Europe and North America to over 2000 m 3 /cap/year in large parts of Africa [ Gerten et al., 2011 ; Kummu et al., 2014 ].
Despite the merit of incorporating green water in water scarcity assessment, the attempts so far suffer from a drawback of inconsistency. The blue water resources are generally quantified as the total run-off of renewable freshwater on the earth surface or given geographical locations/river basins, regardless of their accessibility. The green water resources, on the other hand, are quantified as the evapotranspiration of vegetation on croplands (and grazing land). This greatly underrepresents the quantity of green water resources because a large (if not larger) amount of evapotranspiration occurs on non-croplands.
3.2. Water footprint-based water scarcity assessment
The water footprint measures the amount of water used to produce the goods and services human uses [ Hoekstra et al., 2011 ]. Noting the problem of ignoring the return flow in using water withdrawal to refer to water use in the water scarcity assessment, Hoekstra et al. [2012] developed a water footprint-based assessment for global blue water scarcity assessment. Three alternatives are used in measuring water use and availability. First, water use refers to consumptive use of ground- and surface water flows – i.e., the blue water footprint. Second, the flows needed to sustain critical ecological functions are subtracted from water availability. A presumptive standard of 20% depletion rate is used as a threshold, beyond which, risks to ecological health and ecosystem services increase. Third, water use and availability are measured on a monthly rather than annual basis to account for seasonal water scarcity. The water scarcity indicator derived from this approach provides a picture of where and when current levels of water use are likely to cause water shortages and ecological harm within river basins around the world [ Hoekstra et al., 2012 ]. However, the assumption of EFR to be 80% of the total water resources across all the river basins in the assessment, as suggested by Richter et al. [2011] , is too simplistic, as it did not consider the complexity of EFR in individual river regimes. This may also overestimate EFR as well as water scarcity because the 80% EFR is set unrealistically too high for most of the regions of the world [ Liu et al., 2016 ]. Many studies found that appropriate levels of EFR vary across the river regimes considerably [ Pastor et al., 2014 ].
3.3. Cumulative abstraction to demand ratio – considering temporal variations
In many areas of the world, water scarcity is seasonal, i.e., it only occurs in some months of the year, while there may be enough water on an annual basis. Given this situation, some water scarcity assessments have attempted to take the seasonality into consideration. For example, Alcamo and Henrichs [2002] took into account low river flows in computing a version of the criticality ratio. Another example is the Cumulative Abstraction to Demand (CAD) ratio devised by Hanasaki et al. [2008] . The index was intended to apply the results of global hydrological models, which are able to simulate river discharge and water abstraction at a daily time step. This index is expressed as the ratio of the cumulated daily water abstraction from rivers to the cumulated daily potential water demand (i.e. consumptive water requirement for agricultural, industrial, and domestic use) for a specific year. Recent studies have also been conducted on monthly scale [ Wada et al., 2011 ; Hoekstra et al., 2012 ; Brauman et al., 2016 ]. It is assumed that if the ratio falls below unity, water scarcity can occur. Hanasaki et al. [2008] demonstrated that CAD is low in Southeast Asia and the Sahel due to periodic, severe water shortage in the dry season, which is often overlooked in the assessments adopting classical water scarcity indicators. CAD provides useful insights for assessing the impact of climate change on water resources. In some areas, annual total runoff is projected to increase due to global warming water scarcity may appear to diminish when the withdrawal to availability ratio is used, which may be misleading. In this case CAD presents a more realistic view of water scarcity because it takes into account the increase in water scarce conditions during the dry months [ Hanasaki et al., 2013 ; Haddeland et al., 2014 ]. However, the high demand for data and complex computational tasks have limited the use of this water scarcity assessment approach.
3.4. LCA-based water stress indicators
Water scarcity assessment has been introduced in Life Cycle Assessment (LCA) to address water consumption and its environmental impact since 2008 [ Frischknecht et al., 2009 ; Pfister et al., 2009 ; Berger et al., 2014 ] and is continuously expanded. The main methods used in LCA can be grouped into midpoint and endpoint indicators and address scarcity on watershed level [ Kounina et al., 2013 ]. Midpoint indicators address water scarcity as a water resource problem, while endpoint methods try to quantify potential impacts on human health or ecosystem quality, which goes beyond scarcity but includes vulnerability and resilience. LCA methods address water scarcity at the midpoint level.
In LCA, water consumptive use to availability ratios are used to derive an indicator based on various functions, such as logistic or exponential [ Kounina et al., 2013 ]. The most widely used indicator is the water stress index (WSI) [ Pfister et al., 2009 ]. Recognizing that both monthly and annual variability of precipitation may lead to increased water stress during a specific period, a variation factor is introduced to calculate the ratio, which differentiates watersheds with strongly regulated flows. Considering water stress is not linear with regards to water consumptive use and water availability ratio, an adjusted water stress index is calculated with a logistic function to achieve continuous values between 0 and 1, while 0.1, 0.5 and 0.9 are assigned as thresholds for moderate, severe and extreme water scarcity. The water stress index is served as a general screening indicator or characterization factor for water consumption in Life Cycle Impact Assessment. As the LCA based water scarcity assessment focuses on impact assessment of water use, the indicator has not been separately used for water scarcity assessment.
3.5. Integrated water quantity-quality-environment flow in the water scarcity assessment
The water scarcity indicators developed have mainly considered water quantity. Zeng et al. [2013] developed an integrated indicator, which is expressed as the sum of a quantity-induced indicator and a quality-induced indicator. The quantity-induced water scarcity indicator follows the criticality ratio approach, and is defined as the ratio of the water withdrawal to freshwater resources in a specific region during a certain period. The quality-induced water scarcity indicator is defined as the ratio of grey water footprint to freshwater resources. Here, grey water footprint is defined as the volume of freshwater that is required to assimilate the load of pollutants based on natural background concentrations and existing ambient water quality standards [ Hoekstra et al., 2011 ]. It does not have the same meaning as the terms used in urban water management, for which grey water refers to the water comes out of the shower or sink. This indicator combining quantity- and quality-induced water scarcity was illustrated by analyzing the water scarcity in China. The result shows that the northern parts of the country are suffering from both quantity- and quality-induced water scarcity ( Fig. 2 ). In southeast, quality-induced water scarcity is dominant due to the heavy water pollution. The results imply that northern China has a much bigger burden to deal with the water scarcity problems, while for other provinces, quality-induced water scarcity is a grand challenge.

Water scarcity assessment for different provinces with the quantity-quality indicator approach. This map was generated by J Liu based on the approach of Zeng et al. [2013] .
On the basis of Zeng ’s indicator, EFR was further added in the water scarcity assessment, resulting in a quantity-quality-EFR (QQE) approach [ Liu et al., 2016 ]. It is structured with multi-components in the indicator: S quantity (EFR)|S quality . The QQE approach was first used for the Huangqihai River Basin in Inner Mongolia, China. The QQE water scarcity indicator in this river basin is 1.3(26%)|14.2, indicating that the basin was suffering from scarcity problems related to both water quantity (1.3 is larger than the threshold of 1.0) and water quality (14.2 is far larger than the threshold of 1.0, indicating a serious water pollution condition) for a given rate of 26% of EFR.
The QQE water scarcity indicator provides an easy to obtain and to understand measurement that contains the information of water quantity and quality status, as well as EFR. The procedure can be adapted to any other areas in the world to provide a comprehensive assessment on water scarcity. By specification, one can also use the percentage of EFR to indicate any other levels of ecological habitat status. However, the QQE indicator has some limitations. The indicator is not as straight forward as the existing indicators, which use a single value to indicate the status of water scarcity. It requires some professional knowledge to understand the indicator and interpret the information contained.
4. Where are we now?
Many global assessments of water scarcity have been conducted so far ( Figure 3 ). The spatial resolution ranges from country, region to grid cell. In general, all the indicators pointed out that the areas in the middle to low latitudes of the northern hemisphere have a high level of water scarcity. It is noticed that the physical and economic water stress ( Fig 3C ) and water poverty index ( Fig 3D ) also identified the severe water scarcity problem in almost all African countries. This is attributed to the lack of economic capacity to build water infrastructure as well as poverty, which have hindered these countries to access their water resources that are often physically abundant. Despite the relevance of concerning economic factors in water scarcity assessment, the complexity such concern brought to the assessment increases greatly. There is no consensus on which social and economic factors should be included, and for different countries and regions, these factors can be different. To keep the objectivity and simplicity, all the other water scarcity indicators developed so far have been based solely on physical quantity of water availability and use.

Spatial distribution of water scarcity from different assessments. Below is a list of references for Fig. 3 A–H and the indicator used in relation to Table 1 . A: Water shortage (modified from Kummu et al., [2010] ); B: Water stress (modified from Wada et al., [2011] ); C: Physical and economic water scarcity (modified from Seckler et al., [1998] ); D: Water poverty index (modified from World Resources Institute [2006] ; Sullivan et al., [2002] ); E: Green-blue water scarcity (modified from Kummu et al., [2014] ); F: Monthly blue water stress (modified from Mekonnen and Hoekstra, [2016] ); G: Cumulative abstraction to demand ratio (modified from Hanasaki et al., [2013] ); H: LCA-based water stress indicator (modified from Pfister et al. [2009] ). Note: all maps were redrawn by authors based on original data from the sources given above, except water poverty index, which was modified from a softcopy map. Further, legend colors in some maps are modified for consistency.
One of the main outcomes of water scarcity assessments is estimates of number of people affected by water scarcity. Results differ when different indicators are used, even for the same indicator from different reference sources ( Figure 4 ). For example, estimates using the criticality ratio with a threshold of 40% tend to be higher than those based on the Falkenmark indicator with a threshold of 1000 m 3 /person/year. Variations in number of people living in water scarcity with the same indicator are partially related to different spatial resolutions in the assessment. In general, the higher spatial resolution results in larger number of people suffering from water scarcity ( Fig. 3 ). This is because the high spatial resolution can better reflect the water scarcity situation in urban areas with high population concentration [ Vörösmarty et al., 2010 ]. However, high spatial resolution tends to underestimate human capacity to bring water from outside into cities. Also, including green water will enlarge the quantity of water availability for a geographical unit (e.g., country, region), resulting in smaller estimates of people living in water scarcity. Respecting the EFR by leaving sufficient water in steams, on the other hand, results in larger estimates, because it reduces the water resources available for humans. Furthermore, including water quality can lead to substantial increase in the magnitude of water scarcity, as the poor water quality can make available water not usable. Overall, the estimated numbers from different indicators suggest that between 1.5 and 2.5 billion people were living within areas exposed to water scarcity around the year 2000 ( Fig. 4 ), but water footprint based water scarcity assessment increases the number to 4 billion [ Mekonnen and Hoekstra, 2016 ]. When water stress and water shortage are assessed in a combined manner, altogether 3.8 billion lived under some degree of water scarcity in 2005 [ Kummu et al., 2016 ]. The numbers are projected to increase substantially up to at least 2050 in association with the peak of world population. Current analyses suggest that thereafter the numbers may decline.

Number of people suffering from water scarcity assessed with the average annual water availability per capita (1000m 3 /capita/year) and water use to availability ratio (40%). The marks show the estimates from different studies. Specific estimates include: 1.2 billion [ Hayashi et al., 2010 ], 1.4 billion [ Arnell, 2004 ], 1.6 billion [ Alcamo et al., 2007 ; Arnell et al., 2011 ; Gosling and Arnell, 2016 ], 1.7 billion [ Revenga et al., 2000 ], and 2.3 billion [ Kummu et al., 2010 ]. But the number may be quite different when other indicators are used, e.g. Mekonnen and Hoekstra [2016] estimated that 4 billion people live under conditions of severe water scarcity at least 1 month of the year between 1996 and 2005.
5. Future research challenges and directions
5. 1. validating water scarcity indicators.
The indicators presented in this paper have been used by scientists to compare their values in one region versus another in order to estimate the relative level of water scarcity. However, so far very little efforts have been made to prove how these indicators appropriately reflects the water scarcity quantitatively, and how the thresholds reasonably classify water scarcity. All the indicators and their thresholds have been determined based on expert judgments. The expression "to validate indicators" generally means to support or corroborate on a sound or authoritative basis [ Bockstaller and Girardin, 2003 ; Dauvin et al., 2016 ]. An essential problem in validating these indicators has been the difficulty in identifying an independent variable for water scarcity. Alcamo et al. [2008] used the “frequency of occurrence of drought-related crises” in three large river basins as an independent variable. Values of this metric were determined from media-content analysis by Taenzler et al. [2008] . With estimates of this variable, it was possible to test the validity of various water scarcity indicators. Using modelling data from a 15-year period, it was found that 6 out of 14 different tested water scarcity indicators were statistically related to the occurrence of drought-related crises [ Alcamo et al., 2008 ]. This initial work indicates that it may be possible to validate indicators, identify their appropriate range of application, and test scarcity thresholds. Research in this direction would strengthen the scientific basis of estimating water scarcity and perhaps accelerate the development of more useful indicators.
5.2. Incorporating water quality in water scarcity assessment
As poor water quality has intensified the pressure on water resources [ Bayart et al., 2010 ], including more specific water quality classes for ecosystem and human uses is necessary to enhance the pertinence of water scarcity assessments. Water quality is typically expressed as concentration of certain pollutants. The most considered pollutants influencing water quality have been nutrient emissions, typically nitrogen and phosphorous, and to a lesser extent, COD [ Björklund et al., 2009 ; Liu et al., 2012 ]. The assessment of water quality induced water scarcity is sensitive to the pollutant selected. In order to include water quality data in water scarcity assessments, suitable data need to be collected covering a range of water quality parameters. Often, the list of parameters to be considered may be guided by the objectives of a study, i.e. specific requirements for drinking water are different from that for irrigation water. For an aggregated water scarcity assessment, it would be ideal to use an aggregated water quality indicator that can reflect the overall water quality status. Building such an indicator with a broad applicability is a challenge faced in the future water scarcity assessment.
Another challenge in incorporating water quality is that the availability of water quality data is very heterogeneously distributed over the world and varies tremendously between regions with huge data gaps in developing countries. The United Nations Environment Programme Global Environment Monitoring System (GEMS) Water Programme is a multi-faceted water science centre oriented towards building knowledge on inland quality issues worldwide ( http://www.unep.org/gemswater/ ), which is still very limited considering the large surface of the earth. The GEMS/Water Programme, established in 1978, is the primary source for global water quality data. The related water quality database, GEMStat, is designed to share surface and ground water quality data sets collected from the GEMS/Water Global Network, so far including more than 3,000 stations ( http://gemstat.org/ ). For Africa, data found in scientific literature were very dissimilar and disparate, most published data were aggregated over long time periods and/or over several sampling stations [ UNEP, 2016 ]. Furthermore, specific locations of sampling stations were usually not available and the selection of parameters was restricted. In this context, global scale water quality models could be used as a complementary approach to fill data gaps of relevant parameters in time series and in regions where no reliable data exist. Since a model is a simplified representation of the real world system, its credibility is ensured by its model performance in terms of validation and testing against measured data. It is worth mentioning that the WaterGAP3 modeling framework has been enhanced by a large-scale water quality model WorldQual in order to estimate pollution loadings and in-stream concentration for a variety of parameters (e.g. Voß et al., [2012] ; Reder et al., [2013 , 2015 ]). Many crop models, such as GEPIC, have both components of hydrology and pollution loading [ Liu and Yang, 2010 ; Liu et al., 2013a ]. With their ability to calculate water availability, water use, and water quality parameters, the modeling framework is promising for simultaneously considering water quantity and water quality in water scarcity assessment.
5.3. Incorporating environmental flow requirements in water scarcity assessment
Rudimentarily, EFRs has been incorporated in water scarcity assessment by assuming a fixed percentage of river flow for EFR, ranging from 80% of the annual flow uniformly all over the globe [ Hoekstra et al., 2012 ] to specific proportions in given locations [ Smakhtin et al., 2004 ; Rockström et al., 2009 ; Gerten et al., 2011 ; Liu et al., 2016 ]. However, in the natural system, EFRs vary across flow regimes and seasons. E.g., Pastor et al. [2014] found that EFRs ranged between 25% and 46% of mean annual flow. This suggests an importance to incorporate locally pertinent EFR for a proper assessment of water scarcity status.
The future studies that estimate EFRs should consider a range of methodologies that account for seasonal variations and flow regimes in different parts of the globe [ Gerten et al., 2013 ]. Different approaches have been developed for assessing the EFRs for different river regimes. E.g., Laize et al. [2014] and Schneider et al. [2013] presented a comprehensive approach to quantify ecological risk as a result of flow alterations in terms of the deviation from natural flow conditions. They assessed hydrological alterations from natural flow dynamics caused by anthropogenic water use and dam operations and by using a subset of 12 different parameters chosen from the list of Indicators of Hydrological Alteration [ Richter et al., 1996 , 1997 ]. This approach considers different flow characteristics and describes non-redundant departures from the natural flow regime. In addition, changes in average magnitude and variability of each parameter are considered, and therefore, in total 24 sub-indicators are taken into account. This indicator system could be used to account for EFRs across regions in water scarcity assessment.
5.4. Temporal and spatial scales of water scarcity with consideration of green water and virtual water
In water scarcity assessments, the selection of spatial scale (or unit of analysis) is important, and difficult too. It has considerable impacts on results, as shown earlier ( Fig. 3 ) and by other studies [ Salmivaara et al., 2015 ; Perveen et al., 2011 ]. Most of the water scarcity assessments are conducted on a grid scale (30 arc-min, i.e. 50 km resolution near the equator), while for addressing water scarcity, country, basin or sub-basin (e.g. food production unit) scales are more policy relevant. A detailed study of the impact of different spatial scale on water scarcity assessment would be needed.
Most water scarcity indicators are measured on annual time scale. With significant intra-annual variations in water use and availability, it is important to understand when water is available and when it is needed within a year. Thus, the introduction of a monthly scale assessment could provide information whether there is enough water for each month to fulfill the requirements.
Other relevant aspect to temporal scale is the impact of inter-annual variability of water availability and water requirements on scarcity measures. Veldkamp et al [2015] found that on the shorter time scales (up to 6–10 years) the climate variability is the dominant factor influencing water scarcity while on the longer time scales the socioeconomic development is more important factor. Brauman et al. [2016] found that watersheds that appear to be moderately depleted on an annual time scale are almost uniformly heavily depleted at seasonal time scales or in dry years. Hence, the assessment of inter-annual variability adds important insights on the understanding of water scarcity.
It also needs to be pointed out that the whole population living in a region (e.g. country, watershed) are often not equally been impacted by water scarcity. For example, people with a higher income may be less affected by water scarcity than people with a lower income. Also the rural and urban population may be affected differently. For this reason, it would be more indicative to consider the possibly different effects of socio-economic conditions on the people residing in a water scarcity region. Adopting a probabilistic approach could reduce the scaling effects. This, however, requires more detailed information on the socio-economic conditions of people in the region.
Green water is an important component of water resources. However, in the water scarcity assessment, it has been rarely considered due mainly to different measurements of green and blue water resources, the former is in storage (in unsaturated soil) and the latter is flow measured on annual basis. The work which did consider green water only accounted for the portion that has been actually used by crops [ Rockström et al., 2009 ; Gerten et al., 2011 ]. This greatly underestimates green water resources. One possible approach to remedy the problem is to count for accumulated soil moisture on an annual basis on the land surface regardless of if it is used or not by crops or other plants. It needs to be pointed out that the validity to incorporate green water is sometime questioned in water resources management [ Bogardi et al., 2013 ]. One major reason is that green water is not part of the water budget that could be easily reallocated to other use. Our opinion is that soil moisture (green water) is an important resource which should be appropriately incorporated in the water availability accounting.
Most of the water scarcity indicators previously developed only account for local water resources and local water demand. But recent research advances have revealed that previously unrecognized global forces may drive local-scale water problems [ Vörösmarty et al., 2015 ]. Much of the global water use and pollution is from the production of commodities for global and regional trade, which embodies a large amount of virtual water flows and influences local water scarcity [ Zhao et al ., 2015 ; Hoekstra and Mekonnen, 2016 ; Vörösmarty et al., 2015 ; Zhao et al., 2016 ]. Long distance water transfer systems also impact the local water scarcity situations in both the sourcing and destination regions [ Liu et al., 2013b ]. There is a need to integrate virtual water flows and water transfers in the water scarcity analysis.
5.5. Need for collaboration between hydrological, water quality, aquatic ecosystem science and social science communities in water scarcity assessment
There are crucial connections between water availability and water quality [ Jury and Vaux, 2005 ] and both have been associated with human health [ Myers and Patz, 2009 ], food security [ Rockström et al., 2009 ; Simelton et al., 2012 ] and for sustaining native biodiversity and integrity of aquatic ecosystems [ Poff et al., 1997 ; Richter et al., 1997 ]. This means that assessments of water availability and quality should be conducted in a consistent way so that relevant dependencies between availability and quality are accounted for. This will require the integration of water quality and ecological parameters, and processes (and feedbacks between them) into water availability assessment models. This can only be achieved through sustained integration of the water availability modelling community with the water quality and ecological modelling communities.
Integrating these communities represents the first, and an important step, towards developing a comprehensive understanding of the susceptibility of global water availability and quality to change. Beyond this, the relevant communities will need to develop improved hydrological models at the global scale that consider both water quantity and quality. Reliable observations of water quality at sufficient spatial resolution across the globe will also be needed to validate the models.
As noted above, water scarcity indicator thresholds are artificial and ‘best guess’ rather than evidence-based. There are limitations about the utility of indicators [ Fekete and Stakhiv, 2014 ] given the complexity of water management challenges that these indicators intend to support. These indicators are generally insufficient to incorporate the complex socio-economic backdrop driving water demands and they do not address the alternative pathways such as the choices of water produced. Fostering interdisciplinary or even trans-disciplinary research in water scarcity studies as well as the integration of stakeholders offers the possibility of clear frameworks and hence the improvement of systems’ understanding. For example, factors affecting water demand, such as changes in lifestyle, perceptions of water scarcity, and attitudes towards water use, are routed in social science understandings of how these factors can be influenced by government policy and social norms [ Wolters, 2014 ]. Moreover, a novel opportunity exists to make social science more effective in improving water management and understanding the drivers of water scarcity [ Lund, 2015 ].
- We provide a comprehensive review of water scarcity indicators and reflect on their relevance in a rapidly changing world
- There is a need to incorporate green water, water quality and environmental flow requirements in water scarcity assessment
- Integrated approaches are required to capture the multi-faceted nature of water scarcity
Acknowledgments
This study was supported by the National Natural Science Foundation of China (41571022), National Science Fund for Distinguished Youth Scholars (41625001), the Beijing Natural Science Foundation Grant (8151002), the National Natural Science Foundation of China (91325302, 91425303), the National Science and Technology Major Project (2015ZX07203-005). The work on the paper was developed within the framework of the Panta-Rhei Research Initiative of the International Association of Hydrological Sciences (IAHS). The present work was partially developed within the framework of the Panta Rhei Research Initiative by the working group “Water Scarcity Assessment: Methodology and Application”. All data used for this paper are properly cited and listed in the references. This is a review article and we do not generate data except for those used in Figure 2 . Users can contact the correspondence author when they need water scarcity data in this figure.
- Alcamo J, Flörke M, Marker M. Future long-term changes in global water resources driven by socio-economic and climatic changes. Hydrolog. Sci. J. 2007; 4 :247–275. doi: 10.1623/hysj.52.2.247. [ CrossRef ] [ Google Scholar ]
- Alcamo J, Döll P, Henrichs T, Kaspar F, Lehner B, Rösch T, Siebert S. Development and testing of the WaterGAP 2 global model of water use and availability. Hydrolog. Sci. J. 2003a; 48 (3):317–337. doi: 10.1623/hysj.48.3.317.45290. [ CrossRef ] [ Google Scholar ]
- Alcamo J, Doll P, Henrichs T, Kaspar F, Lehner B, Rösch T, Siebert S. Global estimates of water withdrawals and availability under current and future “business-as-usual” conditions. Hydrolog. Sci. J. 2003b; 48 :339–348. doi: 10.1623/hysj.48.3.339.45278. [ CrossRef ] [ Google Scholar ]
- Alcamo J, Klein R, Carius A, Acosta-Michlik L, Krömker D, Tänzler D, Eierdanz F. A new approach to quantifying and comparing vulnerability to drought. Reg. Environ. Change. 2008; 8 :137–149. doi: 10.1007/s10113-008-0065-5. [ CrossRef ] [ Google Scholar ]
- Alcamo J, Henrichs T. Critical regions: A model-based estimation of world water resources sensitive to global changes. Aquat. Sci. 2002; 64 :352–362. doi: 10.1007/PL00012591. [ CrossRef ] [ Google Scholar ]
- Alcamo J, Henrichs T, Rösch T. Kassel World Water Series. University of Kassel; Germany: 2000. World water in 2025-global modeling and scenario analysis for the World Commission on Water for the 21st Century. [ Google Scholar ]
- Arnell NW. Climate change and global water resources: SRES emissions and socio-economic scenarios. Global Environ. Chang. 2004; 14 :31–52. doi: 10.1016/j.gloenvcha.2003.10.006. [ CrossRef ] [ Google Scholar ]
- Arnell NW, van Vuuren DP, Isaac M. The implications of climate policy for the impacts of climate change on global water resources. Global Environ. Chang. 2011; 21 :592–603. doi: 10.1016/j.gloenvcha.2011.01.015. [ CrossRef ] [ Google Scholar ]
- Bayart JB, Bulle C, Deschenes L, Margni M, Pfister S, Vince F, Koehler A. A framework for assessing off-stream freshwater use in LCA. Int. J. Life Cycle Assess. 2010; 15 :439–453. doi: 10.1007/s11367-010-0172-7. [ CrossRef ] [ Google Scholar ]
- Berger M, van der Ent R, Eisner S, Bach V, Finkbeiner M. Water accounting and vulnerability evaluation (WAVE): Considering atmospheric evaporation recycling and the risk of freshwater depletion in water footprinting. Environ. Sci. Technol. 2014; 48 :4521–4528. doi: 10.1021/es404994t. [ PubMed ] [ CrossRef ] [ Google Scholar ]
- Björklund JA, Thuresson K, de Wit CA. Perfluoroalkyl compounds (PFCs) in indoor dust: concentrations, human exposure estimates, and sources. Environ. Sci. Technol. 2009; 43 :2276–2281. doi: 10.1021/es803201a. [ PubMed ] [ CrossRef ] [ Google Scholar ]
- Bockstaller C, Girardin P. How to validate environmental indicators. Agric. Syst. 2003; 76 :639–653. doi: 10.1016/S0308-521X(02)00053-7. [ CrossRef ] [ Google Scholar ]
- Bogardi JJ, Fekete BM, Vörösmarty CJ. Planetary boundaries revisited: a view through the “water lens” Curr. Opin. Env. Sust. 2013; 5 (6):581–589. doi: 10.1016/j.cosust.2013.10.006. [ CrossRef ] [ Google Scholar ]
- Brauman KA, Richter BD, Postel S, Malsy M, Flörke M. Water depletion: An improved metric for incorporating seasonal and dry-year water scarcity into water risk assessments. Elementa: Science of the Anthropocene. 2016; 4 :000083. doi: 10.12952/journal.elementa.000083. [ CrossRef ] [ Google Scholar ]
- Cosgrove WJ, Rijsberman FR. World water vision: Making water everybody’s business. Earthscan Publications Ltd; London: 2000. [ Google Scholar ]
- Dauvin JC, Andrade H, de-la-Ossa-Carretero JA, Del-Pilar-Ruso Y, Riera R. Polychaete/amphipod ratios: An approach to validating simple benthic indicators. Ecol. Indic. 2016; 63 :89–99. doi: 10.1016/j.ecolind.2015.11.055. [ CrossRef ] [ Google Scholar ]
- Falkenmark M. Meeting water requirements of an expanding world population. Philos. Trans. R. Soc. Lond. B. Biol. Sci. 1997; 352 :929–936. doi: 10.1098/rstb.1997.0072. [ CrossRef ] [ Google Scholar ]
- Falkenmark M, Rockström J. Balancing water for humans and nature: The new approach in ecohydrology. Earthscan; London: 2004. [ Google Scholar ]
- Falkenmark M, Lundqvist J, Widstrand C. Macro-scale water scarcity requires micro-scale approaches. Nat. Resour. Forum. 1989; 13 :258–267. doi: 10.1111/j.1477-8947.1989.tb00348.x. [ PubMed ] [ CrossRef ] [ Google Scholar ]
- Falkenmark M, Rockström J, Karlberg L. Present and future water requirements for feeding humanity. Food Secur. 2009; 1 :59–69. doi: 10.1007/s12571-008-0003-x. [ CrossRef ] [ Google Scholar ]
- Fekete BM, Stakhiv EZ. Performance indicators in the water resources management sector. In: Bhaduri A, Bogardi JJ, Leentvaar J, Marx S, editors. The Global Water System in the Anthropocene - Challenges for Science and Governance. Springer; 2014. p. 437. [ Google Scholar ]
- Flörke M, Kynast E, Bärlund I, Eisner S, Wimmer F, Alcamo J. Domestic and industrial water uses of the past 60 years as a mirror of socioeconomic development: A global simulation study. Global Environ. Chang. 2013; 23 :144–156. doi: 10.1016/j.gloenvcha.2012.10.018. [ CrossRef ] [ Google Scholar ]
- Frischknecht R, Büsser S, Krewitt W. Environmental assessment of future technologies: How to trim LCA to fit this goal? Int. J. Life Cycle Assess. 2009; 14 :584–588. doi: 10.1007/s11367-009-0120-6. [ CrossRef ] [ Google Scholar ]
- Gerten D, Hoff H, Rockström J, Jägermeyr J, Kummu M, Pastor AV. Towards a revised planetary boundary for consumptive freshwater use: role of environmental flow requirements. Curr. Opin. Env. Sust. 2013; 5 :551–558. doi: 10.1016/j.cosust.2013.11.001. [ CrossRef ] [ Google Scholar ]
- Gerten D, Heinke J, Hoff H, Biemans HJA, Fader M, Waha K. Global water availability and requirements for future food production. J. Hydrometeorol. 2011; 12 :885–899. doi: 10.1175/2011JHM1328.1. [ CrossRef ] [ Google Scholar ]
- Gosling SN, Arnell NW. A global assessment of the impact of climate change on water scarcity. Climatic Change. 2016; 134 :371–385. doi: 10.1007/s10584-013-0853-x. [ CrossRef ] [ Google Scholar ]
- Haddeland I, et al. Global water resources affected by human interventions and climate change. Proc. Natl. Acad. Sci. USA. 2014; 111 :3251–3256. doi: 10.1073/pnas.1222475110. [ PMC free article ] [ PubMed ] [ CrossRef ] [ Google Scholar ]
- Hanasaki N, et al. A global water scarcity assessment under Shared Socioeconomic Pathways – Part 1: Water use. Hydrol. Earth Syst. Sci. 2013; 17 :2375–2391. doi: 10.5194/hess-17-2375-2013. [ CrossRef ] [ Google Scholar ]
- Hanasaki N, Kanae S, Oki T, Masuda K, Motoya K, Shirakawa N, Shen Y, Tanaka K. An integrated model for the assessment of global water resources - Part 2: Applications and assessments. Hydrol. Earth Syst. Sci. 2008; 12 :1027–1037. doi: 10.5194/hess-12-1027-2008. [ CrossRef ] [ Google Scholar ]
- Hayashi A, Akimoto K, Sano F, Mori S, Tomoda T. Evaluation of global warming impacts for different levels of stabilization as a step toward determination of the long-term stabilization target. Climatic Change. 2010; 98 :87–112. doi: 10.1007/s10584-009-9663-6. [ CrossRef ] [ Google Scholar ]
- Hoekstra AY, Chapagain AK, Aldaya MM, Mekonnen MM. The Water Footprint Assessment Manual: Setting the Global Standard. Earthscan; London, Washington, DC: 2011. [ Google Scholar ]
- Hoekstra AY, Mekonnen MM. Imported water risk: the case of the UK. Environ. Res. Lett. 2016; 11 :055002. doi: 10.1088/1748-9326/11/5/055002. [ CrossRef ] [ Google Scholar ]
- Hoekstra AY, Mekonnen MM, Chapagain AK, Mathews RE, Richter BD. Global monthly water scarcity: Blue water footprints versus blue water availability. PLoS ONE. 2012; 7 :e32688. doi: 10.1371/journal.pone.0032688. [ PMC free article ] [ PubMed ] [ CrossRef ] [ Google Scholar ]
- Jury WA, Vaux H. The role of science in solving the world's emerging water problems. Proc. Natl. Acad. Sci. USA. 2005; 102 :15715–15720. doi: 10.1073/pnas.0506467102. [ PMC free article ] [ PubMed ] [ CrossRef ] [ Google Scholar ]
- Kounina A, et al. Review of methods addressing freshwater use in life cycle inventory and impact assessment. Int. J. Life Cycle Assess. 2013; 18 :707–721. doi: 10.1007/s11367-012-0519-3. [ CrossRef ] [ Google Scholar ]
- Kummu M, Gerten D, Heinke J, Konzmann M, Varis O. Climate-driven interannual variability of water scarcity in food production potential: A global analysis. Hydrol. Earth Syst. Sci. 2014; 18 :447–461. doi: 10.5194/hess-18-447-2014. [ CrossRef ] [ Google Scholar ]
- Kummu M, Guillaume JHA, de Moel H, Eisner S, Flörke M, Porkka M, Siebert S, Veldkamp TIE, Ward PJ. The world’s road to water scarcity: shortage and stress in the 20th century and pathways towards sustainability. Sci. Rep. 2016; 6 :38495. doi: 10.1038/srep38495. [ PMC free article ] [ PubMed ] [ CrossRef ] [ Google Scholar ]
- Kummu M, Ward PJ, de Moel H, Varis O. Is physical water scarcity a new phenomenon? Global assessment of water shortage over the last two millennia. Environ. Res. Lett. 2010; 5 :034006. doi: 10.1088/1748-9326/5/3/034006. [ CrossRef ] [ Google Scholar ]
- Laize C, Acreman MC, Schneider C, Dunbar MJ, Houghton-Carr HA, Flörke M, Hannah DM. Projected flow alteration and ecological risk for pan-European rivers. River Res. Appl. 2014; 30 :299–314. doi: 10.1002/rra.2645. [ CrossRef ] [ Google Scholar ]
- Liu C, Koreze C, Hoekstra AY, Gerbens-Leenes W. Past and future trends in grey water footprints of anthropogenic nitrogen and phosphorus inputs to major world rivers. Ecol. Indic. 2012; 18 :42–49. doi: 10.1016/j.ecolind.2011.10.005. [ CrossRef ] [ Google Scholar ]
- Liu J, Folberth C, Yang H, Rockström J, Abbaspour K, Zehnder AJB. A global and spatially explicit assessment of climate change impacts on crop production and consumptive water use. PLoS ONE. 2013a; 8 (2):e57750. doi: 10.1371/journal.pone.0057750. [ PMC free article ] [ PubMed ] [ CrossRef ] [ Google Scholar ]
- Liu J, Zang C, Tian S, Liu J, Yang H, Jia S, You L, Liu B, Zhang M. Water conservancy projects in China: achievements, challenges and way forward. Global Environ. Chang. 2013b; 23 (3):633–643. doi: 10.1016/j.gloenvcha.2013.02.002. [ CrossRef ] [ Google Scholar ]
- Liu J, Yang H. Spatially explicit assessment of global consumptive water uses in cropland: green and blue water. J Hydrol. 2010; 384 (3–4):187–197. doi: 10.1016/j.jhydrol.2009.11.024. [ CrossRef ] [ Google Scholar ]
- Liu J, Liu Q, Yang H. Assessing water scarcity by simultaneously considering environmental flow requirements, water quantity, and water quality. Ecol. Indic. 2016; 60 :434–441. doi: 10.1016/j.ecolind.2015.07.019. [ CrossRef ] [ Google Scholar ]
- Lund JR. Integrating social and physical sciences in water management. Water Resour. Res. 2015; 51 :5905–5918. doi: 10.1002/2015WR017125. [ CrossRef ] [ Google Scholar ]
- Mekonnen MM, Hoekstra AY. Four billion people facing severe water scarcity. Sci. Adv. 2016; 2 :e1500323. doi: 10.1126/sciadv.1500323. [ PMC free article ] [ PubMed ] [ CrossRef ] [ Google Scholar ]
- Montanari A, et al. “Panta Rhei, Everything Flows”: Change in hydrology and society; The IAHS Scientific Decade 2013–2022. Hydrol. Sci. J. 2013; 58 (6):1256–1275. doi: 10.1080/02626667.2013.809088. [ CrossRef ] [ Google Scholar ]
- Munia H, Guillaume JHA, Mirumachi N, Porkka M, Wada Y, Kummu M. Water stress in global transboundary river basins: significance of upstream water use on downstream stress. Environ. Res. Lett. 2016; 11 :01400. doi: 10.1088/1748-9326/11/1/014002. [ CrossRef ] [ Google Scholar ]
- Myers SS, Patz JA. Emerging threats to human health from global environmental change. Annu. Rev. Env. Resour. 2009; 34 :223–252. doi: 10.1146/annurev.environ.033108.102650. [ CrossRef ] [ Google Scholar ]
- Ohlsson L, Appelgren B. Water and social resource scarcity. Food and Agricultural Organization; Rome, Italy: 1998. [ Google Scholar ]
- Oki T, Kanae S. Global hydrological cycles and world water resources. Science. 2006; 313 :1068–1072. doi: 10.1126/science.1128845. [ PubMed ] [ CrossRef ] [ Google Scholar ]
- Oki T, Agata Y, Kanae S, Saruhashi T, Yang D, Musiake K. Global assessment of current water resources using total runoff integrating pathways. Hydrolog. Sci. J. 2001; 46 :983–995. doi: 10.1080/02626660109492890. [ CrossRef ] [ Google Scholar ]
- Pastor AV, Ludwig F, Biemans H, Hoff H, Kabat P. Accounting for environmental flow requirements in global water assessments. Hydrol. Earth Syst. Sci. 2014; 18 :5041–5059. doi: 10.5194/hess-18-5041-2014. [ CrossRef ] [ Google Scholar ]
- Perveen S, James LA. Scale invariance of water stress and scarcity indicators: Facilitating cross-scale comparisons of water resources vulnerability. Appl. Geogr. 2011; 31 :321–328. doi: 10.1016/j.apgeog.2010.07.003. [ CrossRef ] [ Google Scholar ]
- Pfister S, Koehler A, Hellweg S. Assessing the environmental impacts of freshwater consumption in LCA. Environ. Sci. Technol. 2009; 43 :4098–4104. doi: 10.1021/es802423e. [ PubMed ] [ CrossRef ] [ Google Scholar ]
- Poff NL, Allan JD, Bain MB, Karr JR, Prestegaard KL, Ritcher BD, Sparks RE, Stromberg JC. The natural flow regime: a paradigm for river conservation and restoration. Bioscience. 1997; 47 :769–784. [ Google Scholar ]
- Raskin I, Smith RD, Salt DE. Phytoremediation of metals: using plants to remove pollutants from the environment. Curr. Opin. Biotechnol. 1997; 8 :221–226. doi: 10.1016/S0958-1669(97)80106-1. [ PubMed ] [ CrossRef ] [ Google Scholar ]
- Reder K, Alcamo J, Bärlund I, Voss F, Kynast E, Williams RG, Malve O. European scenario studies on future in-stream nutrient concentrations. T ASABE. 2013; 56 :1407–1417. doi: 10.13031/trans.56.9961. [ CrossRef ] [ Google Scholar ]
- Reder K, Flörke M, Alcamo J. Modeling historical fecal coliform loadings to large European rivers and resulting in-stream concentrations. Environ. Modell. Softw. 2015; 63 :251–263. doi: 10.1016/j.envsoft.2014.10.001. [ CrossRef ] [ Google Scholar ]
- Revenga C, Brunner J, Henninger N, Kassem K, Payne N. Pilot Analysis of Global Ecosystems: Freshwater Systems. World Resources Institute and Worldwatch Institute; Washington, D C: 2000. [ Google Scholar ]
- Richter BD, Baumgartner JV, Powell J, Braun DP. A method for assessing hydrologic alteration within ecosystems. Conserv. Biol. 1996; 10 :1163–1174. doi: 10.1046/j.1523-1739.1996.10041163.x. [ CrossRef ] [ Google Scholar ]
- Richter BD, Baumgartner JV, Wigington R, Braun DP. How much water does a river need? Freshwater Biol. 1997; 37 :231–249. doi: 10.1046/j.1365-2427.1997.00153.x. [ CrossRef ] [ Google Scholar ]
- Richter BD, Davis MM, Apse C, Konrad C. A presumptive standard for environmental flow protection. River Res. Appl. 2011; 28 :1312–1321. doi: 10.1002/rra.1511. [ CrossRef ] [ Google Scholar ]
- Rijsberman FR. Water scarcity: Fact or fiction? Agr. Water Manage. 2006; 80 :5–22. doi: 10.1016/j.agwat.2005.07.001. [ CrossRef ] [ Google Scholar ]
- Rockström J, Falkenmark M, Karlberg L, Hoff H, Rost S, Gerten D. Future water availability for global food production: The potential of green water for increasing resilience to global change. Water Resour. Res. 2009; 45 :W00A12. doi: 10.1029/2007WR006767. [ CrossRef ] [ Google Scholar ]
- Salmivaara A, Porkka M, Kummu M, Keskinen M, Guillaume JHA, Varis O. Exploring the Modifiable Areal Unit Problem in spatial water assessments: a case of water shortage in Monsoon Asia. Water. 2015; 7 :898–917. doi: 10.3390/w7030898. [ CrossRef ] [ Google Scholar ]
- Savenije HHG. Water scarcity indicators; the deception of the numbers. Phys. Chem. Earth (B) 2000; 25 (3):199–204. doi: 10.1016/S1464-1909(00)00004-6. [ CrossRef ] [ Google Scholar ]
- Schewe J, et al. Multimodel assessment of water scarcity under climate change. Proc. Natl. Acad. Sci. USA. 2014; 111 :3245–3250. doi: 10.1073/pnas.1222460110. [ PMC free article ] [ PubMed ] [ CrossRef ] [ Google Scholar ]
- Schneider C, Laize CLR, Acreman MC, Flörke M. How will climate change modify river flow regimes in Europe? Hydrol. Earth Syst. Sci. 2013; 17 :325–339. doi: 10.5194/hess-17-325-2013. [ CrossRef ] [ Google Scholar ]
- Seckler D, Amarasinghe U, Molden DJ, de Silva R, Barker R. World water demand and supply, 1990 to 2025: Scenarios and issues. IWMI; Colombo: 1998. [ Google Scholar ]
- Simelton EED, Fraser G, Termansen M, Benton TG, Gosling SN, South A, Arnell NW, Challinor AJ, Dougill AJ, Forster PM. The socioeconomics of food crop production and climate change vulnerability: A global scale quantitative analysis of how grain crops are sensitive to drought. Food Sec. 2012; 4 :163–179. doi: 10.1007/s12571-012-0173-4. [ CrossRef ] [ Google Scholar ]
- Smakhtin V, Revenga C, Döll P. A pilot global assessment of environmental water requirements and scarcity. Water Int. 2004; 29 :307–317. doi: 10.1080/02508060408691785. [ CrossRef ] [ Google Scholar ]
- Sullivan CA. The potential for calculating a meaningful water poverty index. Water Int. 2001; 26 :471–480. doi: 10.1080/02508060108686948. [ CrossRef ] [ Google Scholar ]
- Sullivan CA. Calculating a water poverty index. World Dev. 2002; 30 :1195–1210. doi: 10.1016/S0305-750X(02)00035-9. [ CrossRef ] [ Google Scholar ]
- Sullivan CA, et al. The Water Poverty Index: Development and application at the community scale. Nat. Resour. Forum. 2006; 27 :189–199. doi: 10.1111/1477-8947.00054. [ CrossRef ] [ Google Scholar ]
- Sullivan CA, Meigh JR, Giacomello AM. The water poverty index: Development and application at the community scale. Nat. Resour. Forum. 2003; 27 :189–199. doi: 10.1111/1477-8947.00054. [ CrossRef ] [ Google Scholar ]
- Sullivan CA, Meigh JR, Fediw T. Report to Department for International Development. Centre for Ecology and Hydrology; Wallingford, UK: 2002. Developing and testing the Water Poverty Index: Phase 1 Final Report. [ Google Scholar ]
- Taenzler D, Feil M, Kroemker D, Eierdanz F. The challenge of validating vulnerability estimates—the option of media content analysis for identifying drought-related crises. Reg. Environ. Change. 2008; 8 :187–195. doi: 10.1007/s10113-008-0064-6. [ CrossRef ] [ Google Scholar ]
- UN. Sustainable Development Goals: 17 goals to transform our world. United Nations: 2015. [ Google Scholar ]
- UNEP. A snapshot of the world’s water quality: Towards a global assessment. United Nations Environment Programme; Nairobi, Kenya: 2016. [ Google Scholar ]
- Veldkamp TIE, Wada Y, Moel HD, Kummu M, Eisner S, Aerts JCJH, Ward PJ. Changing mechanism of global water scarcity events: Impacts of socioeconomic changes and inter-annual hydro-climatic variability. Global Environ. Chang. 2015; 32 :18–29. doi: 10.1016/j.gloenvcha.2015.02.011. [ CrossRef ] [ Google Scholar ]
- Vörösmarty CJ, Hoekstra AY, Bunn SE, Conway D, Gupta J. Fresh water goes global. Science. 2015; 349 (6247):478–479. doi: 10.1126/science.aac6009. [ PubMed ] [ CrossRef ] [ Google Scholar ]
- Vörösmarty CJ, Green P, Salisbury J, Lammers RB. Global water resources: Vulnerability from climate change and population growth. Science. 2000; 28 :284–288. doi: 10.1126/science.289.5477.284. [ PubMed ] [ CrossRef ] [ Google Scholar ]
- Vörösmarty CJ, McIntyeare PB, Gessner MO, Dudgeon D, Prusevich A, Green P, Glidden S, Bunn SE, Sullivan CA, Reidy Liermann C, Davies PM. Global threats to human water security and river biodiversity. Nature. 2010; 467 :555–561. doi: 10.1038/nature09440. [ PubMed ] [ CrossRef ] [ Google Scholar ]
- Voß A, Alcamo J, Bärlund I, Voß F, Kynast E, Williams R, Malve O. Continental scale modelling of in-stream river water quality: a report on methodology, test runs, and scenario application. Hydrol. Process. 2012; 26 :2370–2384. doi: 10.1002/hyp.9445. [ CrossRef ] [ Google Scholar ]
- Wada Y, Wisser D, Bierkens MFP. Global modeling of withdrawal, allocation and consumptive use of surface water and groundwater resources. Earth Syst. Dynam. 2014; 5 :15–40. doi: 10.5194/esd-5-15-2014. [ CrossRef ] [ Google Scholar ]
- Wada Y, Van Beck R, Viviroli D, Durr HH, Weingartner R, Bierkens MFP. Global monthly water stress: II. Water demand and severity of water stress. Water Resour. Res. 2011; 47 :W07518. doi: 10.1029/2010WR009792. [ CrossRef ] [ Google Scholar ]
- Wolters EA. Attitude–behavior consistency in household water consumption. Soc. Sci. J. 2014; 51 :455–463. doi: 10.1016/j.soscij.2013.10.003. [ CrossRef ] [ Google Scholar ]
- World Economic Forum. Global Risks 2015. 10. Geneva, Switzerland: 2015. [ Google Scholar ]
- World Resources Institute. Earth Trends - Freshwater indices: Water Poverty Index. Washington DC: 2006. [ Google Scholar ]
- Zeng Z, Liu J, Savenije HHG. A simple approach to assess water scarcity integrating water quantity and quality. Ecol. Indic. 2013; 34 :441–449. doi: 10.1016/j.ecolind.2013.06.012. [ CrossRef ] [ Google Scholar ]
- Zhao X, Liu J, Yang H, Liu Y, Duarte R, Hubacek K. Burden-shifting of water quantity and quality stress from mega-city Shanghai. Water Resour. Res. 2016; 52 (9):6916–6927. doi: 10.1002/2016WR018595. [ CrossRef ] [ Google Scholar ]
- Zhao X, Liu J, Liu Q, Tillotson MR, Guan D, Hubacek K. Physical and virtual water transfers for regional water stress alleviation in China. Proc. Natl. Acad. Sci. USA. 2015; 112 (4):1031–1035. doi: 10.1073/pnas.1404130112. [ PMC free article ] [ PubMed ] [ CrossRef ] [ Google Scholar ]
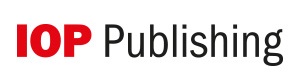
We apologize for the inconvenience...
To ensure we keep this website safe, please can you confirm you are a human by ticking the box below.
If you are unable to complete the above request please contact us using the below link, providing a screenshot of your experience.
https://ioppublishing.org/contacts/
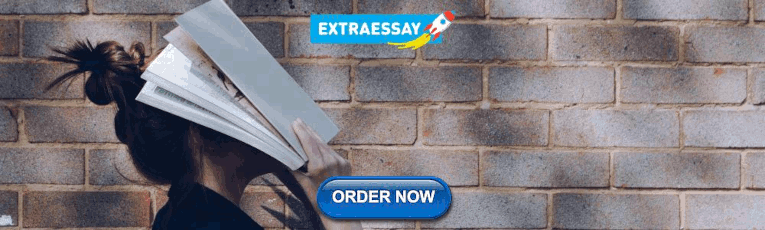
IMAGES
VIDEO
COMMENTS
The existing studies focus on water stress alone 10 or water shortage alone 7, or assess both forms of scarcity at only one or two time steps 16 or scenarios 29, with the exception of one study 18 ...
We found that the global urban population facing water scarcity was projected to double from 933 million (33%) in 2016 to 1.693–2.373 billion (35–51%) in 2050, and the number of large cities ...
Abstract: This paper provides an overview of the Special Issue on water supply and water scarcity. The papers selected for publication include review papers on water history, on water management ...
1. Introduction. Global sustainability will not be reached without ensuring the availability of safe water for all consumers. Despite being one of the major goals (SDG6) of the UN2030 agenda for sustainable global development (UN, 2015), the current water shortage is rapidly growing and impacting an increasing number of residential, commercial, industrial, and agricultural water consumers ...
Global-to-basin impacts. We calculate both physical water scarcity (Fig. 1B) and its economic impact (Fig. 1C) over the 21st century for 235 river basins for each of the 3000 global change ...
Insufficient water storage exists in Canada (694 MAF), THE U.S.A. (1420 MAF), China (2280 MAF), India (245 MAF), and Pakistan (13.20 MAF) [87]. ... An In-Depth Analysis of Physical Blue and Green ...
According to Kummu et al. [2016], areas under both water shortage and stress have very limited adaptation options to alleviate the scarcity compared to areas under sole stress or shortage. In this paper, we provide a comprehensive review of existing water scarcity indicators and reflect on their relevance in a rapidly changing world.
Water scarcity threatens people in various regions, and has predominantly been studied from a water quantity perspective only. Here we show that global water scarcity is driven by both water quantity and water quality issues, and quantify expansions in clean water technologies (i.e. desalination and treated wastewater reuse) to 'reduce the number of people suffering from water scarcity' as ...
Water shortage events with shorter duration and a lower return period were projected to experience smaller changes in intensity. Conversely, events with a longer duration (e.g., D > 10 years) and a higher return period (e.g., T = 100 years) were projected to experience a higher increase in the intensity of water shortage events.
The global health burden associated with these conditions is staggering, with an estimated 1.6 million deaths every year from diseases associated with lack of access to safe drinking water, inadequate sanitation and poor hygiene. In this paper we review the impact of water shortages on health and human development.