- Privacy Policy
Buy Me a Coffee

Home » 500+ Quantitative Research Titles and Topics
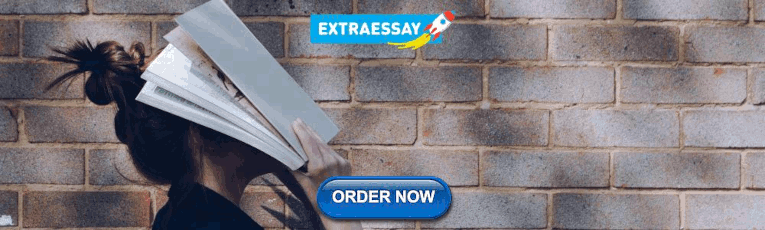
500+ Quantitative Research Titles and Topics
Table of Contents

Quantitative research involves collecting and analyzing numerical data to identify patterns, trends, and relationships among variables. This method is widely used in social sciences, psychology , economics , and other fields where researchers aim to understand human behavior and phenomena through statistical analysis. If you are looking for a quantitative research topic, there are numerous areas to explore, from analyzing data on a specific population to studying the effects of a particular intervention or treatment. In this post, we will provide some ideas for quantitative research topics that may inspire you and help you narrow down your interests.
Quantitative Research Titles
Quantitative Research Titles are as follows:
Business and Economics
- “Statistical Analysis of Supply Chain Disruptions on Retail Sales”
- “Quantitative Examination of Consumer Loyalty Programs in the Fast Food Industry”
- “Predicting Stock Market Trends Using Machine Learning Algorithms”
- “Influence of Workplace Environment on Employee Productivity: A Quantitative Study”
- “Impact of Economic Policies on Small Businesses: A Regression Analysis”
- “Customer Satisfaction and Profit Margins: A Quantitative Correlation Study”
- “Analyzing the Role of Marketing in Brand Recognition: A Statistical Overview”
- “Quantitative Effects of Corporate Social Responsibility on Consumer Trust”
- “Price Elasticity of Demand for Luxury Goods: A Case Study”
- “The Relationship Between Fiscal Policy and Inflation Rates: A Time-Series Analysis”
- “Factors Influencing E-commerce Conversion Rates: A Quantitative Exploration”
- “Examining the Correlation Between Interest Rates and Consumer Spending”
- “Standardized Testing and Academic Performance: A Quantitative Evaluation”
- “Teaching Strategies and Student Learning Outcomes in Secondary Schools: A Quantitative Study”
- “The Relationship Between Extracurricular Activities and Academic Success”
- “Influence of Parental Involvement on Children’s Educational Achievements”
- “Digital Literacy in Primary Schools: A Quantitative Assessment”
- “Learning Outcomes in Blended vs. Traditional Classrooms: A Comparative Analysis”
- “Correlation Between Teacher Experience and Student Success Rates”
- “Analyzing the Impact of Classroom Technology on Reading Comprehension”
- “Gender Differences in STEM Fields: A Quantitative Analysis of Enrollment Data”
- “The Relationship Between Homework Load and Academic Burnout”
- “Assessment of Special Education Programs in Public Schools”
- “Role of Peer Tutoring in Improving Academic Performance: A Quantitative Study”
Medicine and Health Sciences
- “The Impact of Sleep Duration on Cardiovascular Health: A Cross-sectional Study”
- “Analyzing the Efficacy of Various Antidepressants: A Meta-Analysis”
- “Patient Satisfaction in Telehealth Services: A Quantitative Assessment”
- “Dietary Habits and Incidence of Heart Disease: A Quantitative Review”
- “Correlations Between Stress Levels and Immune System Functioning”
- “Smoking and Lung Function: A Quantitative Analysis”
- “Influence of Physical Activity on Mental Health in Older Adults”
- “Antibiotic Resistance Patterns in Community Hospitals: A Quantitative Study”
- “The Efficacy of Vaccination Programs in Controlling Disease Spread: A Time-Series Analysis”
- “Role of Social Determinants in Health Outcomes: A Quantitative Exploration”
- “Impact of Hospital Design on Patient Recovery Rates”
- “Quantitative Analysis of Dietary Choices and Obesity Rates in Children”
Social Sciences
- “Examining Social Inequality through Wage Distribution: A Quantitative Study”
- “Impact of Parental Divorce on Child Development: A Longitudinal Study”
- “Social Media and its Effect on Political Polarization: A Quantitative Analysis”
- “The Relationship Between Religion and Social Attitudes: A Statistical Overview”
- “Influence of Socioeconomic Status on Educational Achievement”
- “Quantifying the Effects of Community Programs on Crime Reduction”
- “Public Opinion and Immigration Policies: A Quantitative Exploration”
- “Analyzing the Gender Representation in Political Offices: A Quantitative Study”
- “Impact of Mass Media on Public Opinion: A Regression Analysis”
- “Influence of Urban Design on Social Interactions in Communities”
- “The Role of Social Support in Mental Health Outcomes: A Quantitative Analysis”
- “Examining the Relationship Between Substance Abuse and Employment Status”
Engineering and Technology
- “Performance Evaluation of Different Machine Learning Algorithms in Autonomous Vehicles”
- “Material Science: A Quantitative Analysis of Stress-Strain Properties in Various Alloys”
- “Impacts of Data Center Cooling Solutions on Energy Consumption”
- “Analyzing the Reliability of Renewable Energy Sources in Grid Management”
- “Optimization of 5G Network Performance: A Quantitative Assessment”
- “Quantifying the Effects of Aerodynamics on Fuel Efficiency in Commercial Airplanes”
- “The Relationship Between Software Complexity and Bug Frequency”
- “Machine Learning in Predictive Maintenance: A Quantitative Analysis”
- “Wearable Technologies and their Impact on Healthcare Monitoring”
- “Quantitative Assessment of Cybersecurity Measures in Financial Institutions”
- “Analysis of Noise Pollution from Urban Transportation Systems”
- “The Influence of Architectural Design on Energy Efficiency in Buildings”
Quantitative Research Topics
Quantitative Research Topics are as follows:
- The effects of social media on self-esteem among teenagers.
- A comparative study of academic achievement among students of single-sex and co-educational schools.
- The impact of gender on leadership styles in the workplace.
- The correlation between parental involvement and academic performance of students.
- The effect of mindfulness meditation on stress levels in college students.
- The relationship between employee motivation and job satisfaction.
- The effectiveness of online learning compared to traditional classroom learning.
- The correlation between sleep duration and academic performance among college students.
- The impact of exercise on mental health among adults.
- The relationship between social support and psychological well-being among cancer patients.
- The effect of caffeine consumption on sleep quality.
- A comparative study of the effectiveness of cognitive-behavioral therapy and pharmacotherapy in treating depression.
- The relationship between physical attractiveness and job opportunities.
- The correlation between smartphone addiction and academic performance among high school students.
- The impact of music on memory recall among adults.
- The effectiveness of parental control software in limiting children’s online activity.
- The relationship between social media use and body image dissatisfaction among young adults.
- The correlation between academic achievement and parental involvement among minority students.
- The impact of early childhood education on academic performance in later years.
- The effectiveness of employee training and development programs in improving organizational performance.
- The relationship between socioeconomic status and access to healthcare services.
- The correlation between social support and academic achievement among college students.
- The impact of technology on communication skills among children.
- The effectiveness of mindfulness-based stress reduction programs in reducing symptoms of anxiety and depression.
- The relationship between employee turnover and organizational culture.
- The correlation between job satisfaction and employee engagement.
- The impact of video game violence on aggressive behavior among children.
- The effectiveness of nutritional education in promoting healthy eating habits among adolescents.
- The relationship between bullying and academic performance among middle school students.
- The correlation between teacher expectations and student achievement.
- The impact of gender stereotypes on career choices among high school students.
- The effectiveness of anger management programs in reducing violent behavior.
- The relationship between social support and recovery from substance abuse.
- The correlation between parent-child communication and adolescent drug use.
- The impact of technology on family relationships.
- The effectiveness of smoking cessation programs in promoting long-term abstinence.
- The relationship between personality traits and academic achievement.
- The correlation between stress and job performance among healthcare professionals.
- The impact of online privacy concerns on social media use.
- The effectiveness of cognitive-behavioral therapy in treating anxiety disorders.
- The relationship between teacher feedback and student motivation.
- The correlation between physical activity and academic performance among elementary school students.
- The impact of parental divorce on academic achievement among children.
- The effectiveness of diversity training in improving workplace relationships.
- The relationship between childhood trauma and adult mental health.
- The correlation between parental involvement and substance abuse among adolescents.
- The impact of social media use on romantic relationships among young adults.
- The effectiveness of assertiveness training in improving communication skills.
- The relationship between parental expectations and academic achievement among high school students.
- The correlation between sleep quality and mood among adults.
- The impact of video game addiction on academic performance among college students.
- The effectiveness of group therapy in treating eating disorders.
- The relationship between job stress and job performance among teachers.
- The correlation between mindfulness and emotional regulation.
- The impact of social media use on self-esteem among college students.
- The effectiveness of parent-teacher communication in promoting academic achievement among elementary school students.
- The impact of renewable energy policies on carbon emissions
- The relationship between employee motivation and job performance
- The effectiveness of psychotherapy in treating eating disorders
- The correlation between physical activity and cognitive function in older adults
- The effect of childhood poverty on adult health outcomes
- The impact of urbanization on biodiversity conservation
- The relationship between work-life balance and employee job satisfaction
- The effectiveness of eye movement desensitization and reprocessing (EMDR) in treating trauma
- The correlation between parenting styles and child behavior
- The effect of social media on political polarization
- The impact of foreign aid on economic development
- The relationship between workplace diversity and organizational performance
- The effectiveness of dialectical behavior therapy in treating borderline personality disorder
- The correlation between childhood abuse and adult mental health outcomes
- The effect of sleep deprivation on cognitive function
- The impact of trade policies on international trade and economic growth
- The relationship between employee engagement and organizational commitment
- The effectiveness of cognitive therapy in treating postpartum depression
- The correlation between family meals and child obesity rates
- The effect of parental involvement in sports on child athletic performance
- The impact of social entrepreneurship on sustainable development
- The relationship between emotional labor and job burnout
- The effectiveness of art therapy in treating dementia
- The correlation between social media use and academic procrastination
- The effect of poverty on childhood educational attainment
- The impact of urban green spaces on mental health
- The relationship between job insecurity and employee well-being
- The effectiveness of virtual reality exposure therapy in treating anxiety disorders
- The correlation between childhood trauma and substance abuse
- The effect of screen time on children’s social skills
- The impact of trade unions on employee job satisfaction
- The relationship between cultural intelligence and cross-cultural communication
- The effectiveness of acceptance and commitment therapy in treating chronic pain
- The correlation between childhood obesity and adult health outcomes
- The effect of gender diversity on corporate performance
- The impact of environmental regulations on industry competitiveness.
- The impact of renewable energy policies on greenhouse gas emissions
- The relationship between workplace diversity and team performance
- The effectiveness of group therapy in treating substance abuse
- The correlation between parental involvement and social skills in early childhood
- The effect of technology use on sleep patterns
- The impact of government regulations on small business growth
- The relationship between job satisfaction and employee turnover
- The effectiveness of virtual reality therapy in treating anxiety disorders
- The correlation between parental involvement and academic motivation in adolescents
- The effect of social media on political engagement
- The impact of urbanization on mental health
- The relationship between corporate social responsibility and consumer trust
- The correlation between early childhood education and social-emotional development
- The effect of screen time on cognitive development in young children
- The impact of trade policies on global economic growth
- The relationship between workplace diversity and innovation
- The effectiveness of family therapy in treating eating disorders
- The correlation between parental involvement and college persistence
- The effect of social media on body image and self-esteem
- The impact of environmental regulations on business competitiveness
- The relationship between job autonomy and job satisfaction
- The effectiveness of virtual reality therapy in treating phobias
- The correlation between parental involvement and academic achievement in college
- The effect of social media on sleep quality
- The impact of immigration policies on social integration
- The relationship between workplace diversity and employee well-being
- The effectiveness of psychodynamic therapy in treating personality disorders
- The correlation between early childhood education and executive function skills
- The effect of parental involvement on STEM education outcomes
- The impact of trade policies on domestic employment rates
- The relationship between job insecurity and mental health
- The effectiveness of exposure therapy in treating PTSD
- The correlation between parental involvement and social mobility
- The effect of social media on intergroup relations
- The impact of urbanization on air pollution and respiratory health.
- The relationship between emotional intelligence and leadership effectiveness
- The effectiveness of cognitive-behavioral therapy in treating depression
- The correlation between early childhood education and language development
- The effect of parental involvement on academic achievement in STEM fields
- The impact of trade policies on income inequality
- The relationship between workplace diversity and customer satisfaction
- The effectiveness of mindfulness-based therapy in treating anxiety disorders
- The correlation between parental involvement and civic engagement in adolescents
- The effect of social media on mental health among teenagers
- The impact of public transportation policies on traffic congestion
- The relationship between job stress and job performance
- The effectiveness of group therapy in treating depression
- The correlation between early childhood education and cognitive development
- The effect of parental involvement on academic motivation in college
- The impact of environmental regulations on energy consumption
- The relationship between workplace diversity and employee engagement
- The effectiveness of art therapy in treating PTSD
- The correlation between parental involvement and academic success in vocational education
- The effect of social media on academic achievement in college
- The impact of tax policies on economic growth
- The relationship between job flexibility and work-life balance
- The effectiveness of acceptance and commitment therapy in treating anxiety disorders
- The correlation between early childhood education and social competence
- The effect of parental involvement on career readiness in high school
- The impact of immigration policies on crime rates
- The relationship between workplace diversity and employee retention
- The effectiveness of play therapy in treating trauma
- The correlation between parental involvement and academic success in online learning
- The effect of social media on body dissatisfaction among women
- The impact of urbanization on public health infrastructure
- The relationship between job satisfaction and job performance
- The effectiveness of eye movement desensitization and reprocessing therapy in treating PTSD
- The correlation between early childhood education and social skills in adolescence
- The effect of parental involvement on academic achievement in the arts
- The impact of trade policies on foreign investment
- The relationship between workplace diversity and decision-making
- The effectiveness of exposure and response prevention therapy in treating OCD
- The correlation between parental involvement and academic success in special education
- The impact of zoning laws on affordable housing
- The relationship between job design and employee motivation
- The effectiveness of cognitive rehabilitation therapy in treating traumatic brain injury
- The correlation between early childhood education and social-emotional learning
- The effect of parental involvement on academic achievement in foreign language learning
- The impact of trade policies on the environment
- The relationship between workplace diversity and creativity
- The effectiveness of emotion-focused therapy in treating relationship problems
- The correlation between parental involvement and academic success in music education
- The effect of social media on interpersonal communication skills
- The impact of public health campaigns on health behaviors
- The relationship between job resources and job stress
- The effectiveness of equine therapy in treating substance abuse
- The correlation between early childhood education and self-regulation
- The effect of parental involvement on academic achievement in physical education
- The impact of immigration policies on cultural assimilation
- The relationship between workplace diversity and conflict resolution
- The effectiveness of schema therapy in treating personality disorders
- The correlation between parental involvement and academic success in career and technical education
- The effect of social media on trust in government institutions
- The impact of urbanization on public transportation systems
- The relationship between job demands and job stress
- The correlation between early childhood education and executive functioning
- The effect of parental involvement on academic achievement in computer science
- The effectiveness of cognitive processing therapy in treating PTSD
- The correlation between parental involvement and academic success in homeschooling
- The effect of social media on cyberbullying behavior
- The impact of urbanization on air quality
- The effectiveness of dance therapy in treating anxiety disorders
- The correlation between early childhood education and math achievement
- The effect of parental involvement on academic achievement in health education
- The impact of global warming on agriculture
- The effectiveness of narrative therapy in treating depression
- The correlation between parental involvement and academic success in character education
- The effect of social media on political participation
- The impact of technology on job displacement
- The relationship between job resources and job satisfaction
- The effectiveness of art therapy in treating addiction
- The correlation between early childhood education and reading comprehension
- The effect of parental involvement on academic achievement in environmental education
- The impact of income inequality on social mobility
- The relationship between workplace diversity and organizational culture
- The effectiveness of solution-focused brief therapy in treating anxiety disorders
- The correlation between parental involvement and academic success in physical therapy education
- The effect of social media on misinformation
- The impact of green energy policies on economic growth
- The relationship between job demands and employee well-being
- The correlation between early childhood education and science achievement
- The effect of parental involvement on academic achievement in religious education
- The impact of gender diversity on corporate governance
- The relationship between workplace diversity and ethical decision-making
- The correlation between parental involvement and academic success in dental hygiene education
- The effect of social media on self-esteem among adolescents
- The impact of renewable energy policies on energy security
- The effect of parental involvement on academic achievement in social studies
- The impact of trade policies on job growth
- The relationship between workplace diversity and leadership styles
- The correlation between parental involvement and academic success in online vocational training
- The effect of social media on self-esteem among men
- The impact of urbanization on air pollution levels
- The effectiveness of music therapy in treating depression
- The correlation between early childhood education and math skills
- The effect of parental involvement on academic achievement in language arts
- The impact of immigration policies on labor market outcomes
- The effectiveness of hypnotherapy in treating phobias
- The effect of social media on political engagement among young adults
- The impact of urbanization on access to green spaces
- The relationship between job crafting and job satisfaction
- The effectiveness of exposure therapy in treating specific phobias
- The correlation between early childhood education and spatial reasoning
- The effect of parental involvement on academic achievement in business education
- The impact of trade policies on economic inequality
- The effectiveness of narrative therapy in treating PTSD
- The correlation between parental involvement and academic success in nursing education
- The effect of social media on sleep quality among adolescents
- The impact of urbanization on crime rates
- The relationship between job insecurity and turnover intentions
- The effectiveness of pet therapy in treating anxiety disorders
- The correlation between early childhood education and STEM skills
- The effect of parental involvement on academic achievement in culinary education
- The impact of immigration policies on housing affordability
- The relationship between workplace diversity and employee satisfaction
- The effectiveness of mindfulness-based stress reduction in treating chronic pain
- The correlation between parental involvement and academic success in art education
- The effect of social media on academic procrastination among college students
- The impact of urbanization on public safety services.
About the author
Muhammad Hassan
Researcher, Academic Writer, Web developer
You may also like

200+ Funny Research Topics

500+ Sports Research Topics

300+ American History Research Paper Topics

500+ Cyber Security Research Topics

500+ Environmental Research Topics

500+ Economics Research Topics
- Write my thesis
- Thesis writers
- Buy thesis papers
- Bachelor thesis
- Master's thesis
- Thesis editing services
- Thesis proofreading services
- Buy a thesis online
- Write my dissertation
- Dissertation proposal help
- Pay for dissertation
- Custom dissertation
- Dissertation help online
- Buy dissertation online
- Cheap dissertation
- Dissertation editing services
- Write my research paper
- Buy research paper online
- Pay for research paper
- Research paper help
- Order research paper
- Custom research paper
- Cheap research paper
- Research papers for sale
- Thesis subjects
- How It Works
100+ Top Technology Research Topics for Students
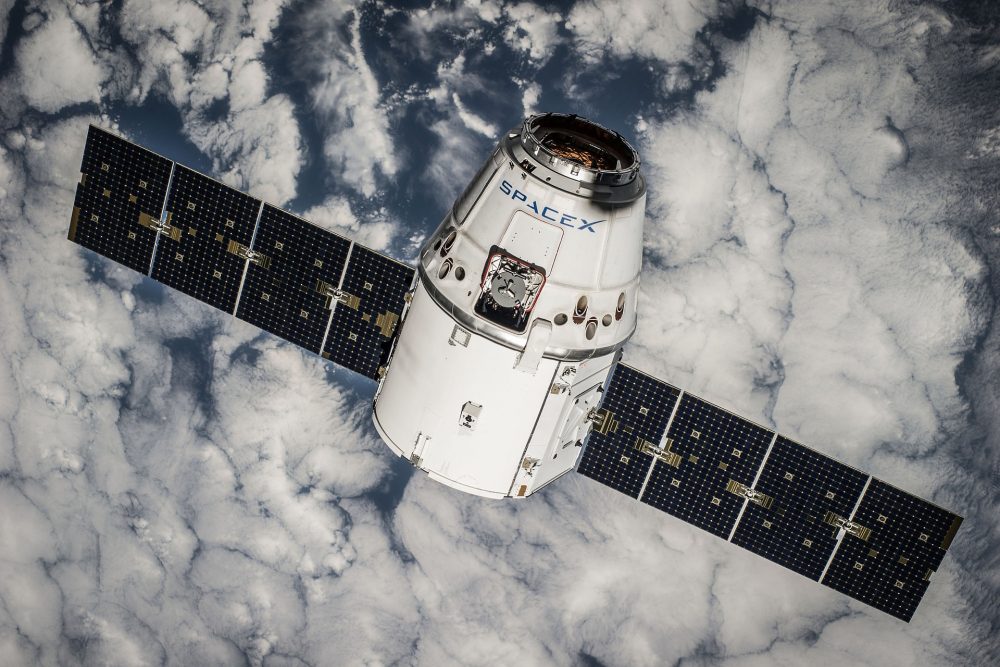
When pursuing their studies, learners are required to write papers and essays on technology research topics. This is a major academic task that influences the final grade that learners graduate with. But, the grades that students score are largely dependent on the technology topics that they opt to write about. Technology is generally a broad study field. As such, choosing research topics on technology is not always easy. If struggling to choose a good technology research topic for your academic paper or essay, here are some of the best ideas to consider.
Trendy Technology Research Topics
Perhaps, you need a prominent research topic about technology. In that case, you should consider prominent technology research paper topics. Here are some of the most trendy topics about technology to consider.
- Technology use in education (here is our list of 110 topics in education research )
- Space and technology studies (check out our top 30 space research topics )
- Current and stunning developments in technology
- Shocking inventions in modern technology that most people don’t know yet
- What technologies can be considered harmful and destructive?
- How does technology affect people’s values and health?
- Can humans be replaced by robots completely in the workplace?
- How have different countries contributed to modern technology developments
- Transport safety and technology
- Discuss the scope of the use of nanotechnologies
- Discuss the use of technology in medicine
- Which technologies can influence human mental health?
- Discuss how technology is changing human life
- What are the positive effects of technologies on personal safety?
- How does technology affect personal safety negatively?
- Discuss how modern technology facilitates the improvement of educational processes
- How do modern technologies influence users’ mental health?
- Why are robots likely to replace humans in the workplace?
- How has technology influenced space travel?
- Is food preservation technology safe?
This category also includes some of the most controversial technology topics. Nevertheless, each topic should be researched extensively before writing a paper or an essay.
Interesting Information Technology Topics
If pursuing a college or university program in information technology, this category has some of the best options for you. Here are some of the best information technology research topics to consider.
- How useful is unlimited data storage?
- How can humans manage large amounts of information?
- How blurred is the line between the human brain and a computer?
- Is entertainment technology something good or bad?
- Discuss the differences between digital reading and print reading
- How does Google impact the attention span of young people?
- How important are traditional research skills in the current era of advanced information technologies?
- How credible is the information provided by different platforms on the internet?
- Do blogs and books compare?
- Should schools and guardians encourage or discourage the use of media by children?
- Does Google provide the best information when it prefers its specific brands?
- Are humans losing the intelligence developed via conventional reading and research in the current digital age?
- How important is learning to how use social media, iPads, and Smart Boards?
- Should modern technologies be incorporated into teaching?
- How has Google search changed humans?
- How is intelligence gauged by humans?
- Is online information format making the readers skim rather than digest information?
- Is the ease of finding information on the internet something bad or good?
- Is technology changing how people read?
- Can using information technology make you smarter?
Students have many information technology research paper topics to choose from. However, select a topic that you find interesting to research and write about.
Interesting Science and Technology Topics
Are you looking for a science and technology-related topics? If yes, consider topics in this category. Here are some of the most interesting topic ideas in science and technology.
- Discuss the greatest technological and scientific breakthroughs of the 21st century
- How significant is number 0 in science and technology?
- How important is the first black hole image?
- Discuss the unlimited fractals’ perimeter despite their limited area
- How can a person perform mental calculations rapidly?
- Discuss the fourth dimension
- Discuss the math behind the Draft lottery by the NBA
- Differentiate non-parametric and parametric statistics
- Discuss the concept of something being random or impossible to prove mathematically
- Discuss some of the greatest modern age mathematicians
- How are the latest automobile technology improvements protecting the environment?
- Why are Smartphones resistant to viruses and bugs in comparison to computers?
- Discuss the Internet of Things story
- What made vector graphics mainstream and not pixels?
- Discuss the latest technology advances that relate to medicine
- Describe Molten Salt Nuclear Reactors
- Is it possible to power everything with solar energy?
- Explain why smart electronics get slower with time
- Differentiate closed and open systems in technology
- Discuss the process of converting old recordings into new formats
This category has amazing topics on technology and science. Select an idea that you find interesting to research and write a paper or essay about.
The Best Computer Technology Topics
If you’re pursuing a program on computer technologies, you will find educational technology topics in this category very interesting. Here are some of the best topics for technology and computers to consider.
- How can you describe the Machine Learning future?
- Discuss computer science that will be the most important in the future
- Discuss how big data and bioinformatics change biology
- What is the borderline for hardware and software in cloud computing?
- How moving everything to the cloud affects human life?
- Can robots become more intelligent and like people with reinforced learning?
- How can computer programmers enhance device protection with open-source getting trendier?
- Is Google becoming the first machine learning firm?
- Explain machine learning in detail
- Discuss the importance of machine learning
- Which sectors does machine learning affect the most?
- How will virtualization change the entertainment industry?
- Describe virtualization
- Can virtual reality be something bad or good?
- How will virtual reality change education?
- What can humans expect from the internet?
- What improvements can be made on the internet?
- How are robots changing the health sector?
- Are humans yet to invent any computer language?
- What will happen if most tasks that are currently done by humans are taken over by computers?
These are great technology essay topics to consider if pursuing a computer technology program in college or university. They can also be great technology debate topics. Nevertheless, extensive research is required when writing about any of these technology essay topics.
Controversial Topics in Technology for Research Papers and Essays
Are you looking for interesting technology topics that your audience will love to read about? If yes, consider one of these technology controversy topics to research and write about.
- Do law enforcement cameras invade privacy?
- Does the technology age turn humans into zombies?
- Has technology advancement led to a throw-away society?
- How has cloud technology changed data storage?
- How have Smartphones reduced live communication?
- Our modern technologies changing teaching?
- How does the use of IT by construction companies lead to under-spending and recession?
- Discuss the technologies used by NASA to explore Mars
- How dangerous are cell phones?
- How does media technology affect child development?
- Is the use of technology in planning lessons good or bad?
- How does technology influence the educational system?
- Discuss the application of green technologies in engineering, architecture, and construction
- Can modern technologies like cryptocurrencies help in identity theft prevention?
- How can technology be used to enhance energy efficiency?
- How are self-driving cars likely to change human life?
- How did Steve Jobs and Bill Gates change the world with technology
- What is the impact of drone warfare on humans?
- Can the actual reality be substituted by virtual reality?
- Discuss the use of technologies and smart materials in road building
If looking for hot topics in technology, consider some of the ideas in this category. Nevertheless, you can also find technology persuasive speech topics here. That’s because this category has some of the most debatable topics. If you still don’t find a great idea from this list, consider technology security topics or contact our thesis writers . Remember that extensive research is required to write a great paper or essay regardless of the topic that you opt to write about.
Leave a Reply Cancel reply
54 Most Interesting Technology Research Topics for 2023
May 30, 2023
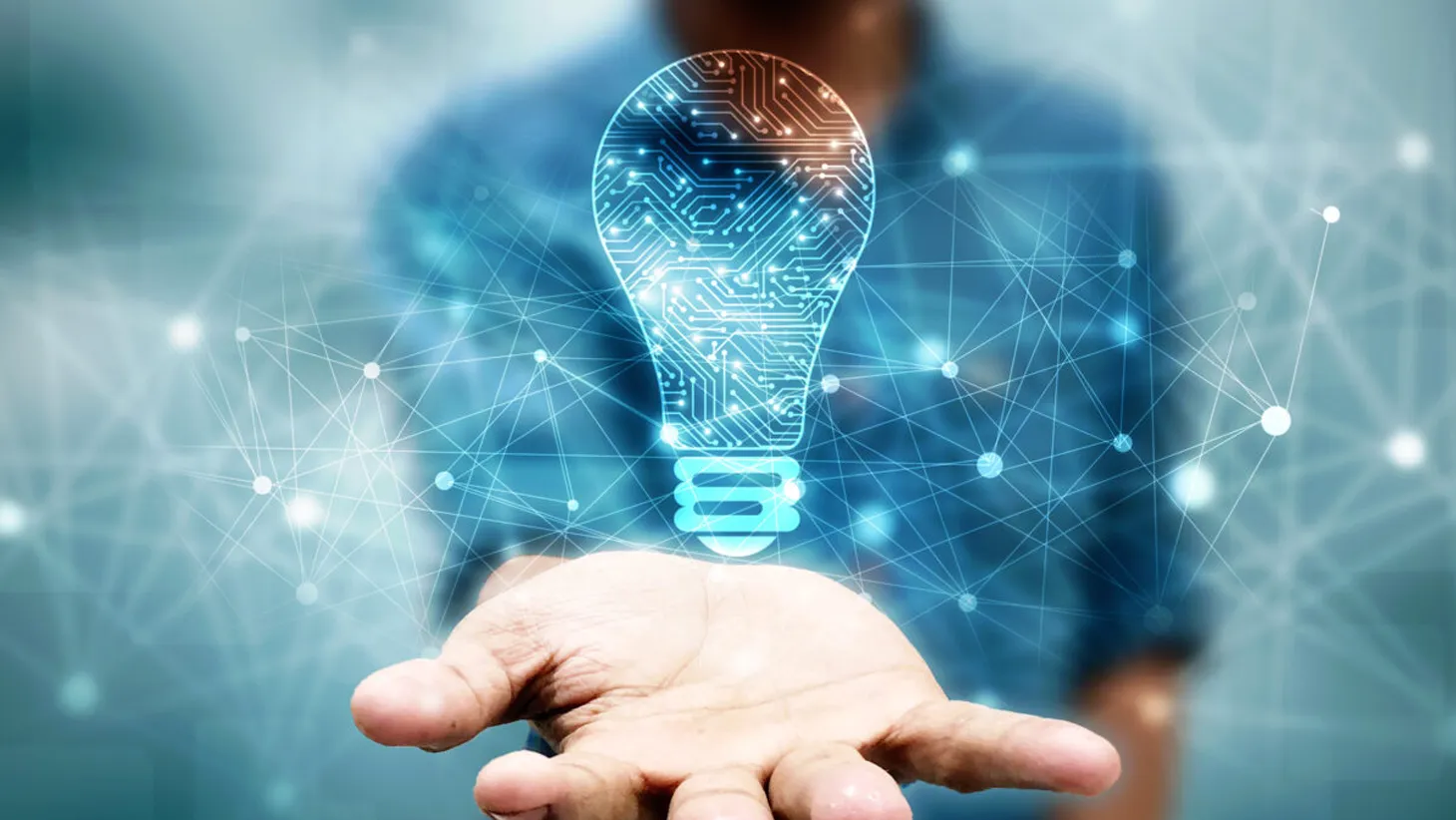
Scrambling to find technology research topics for the assignment that’s due sooner than you thought? Take a scroll down these 54 interesting technology essay topics in 10 different categories, including controversial technology topics, and some example research questions for each.
Social technology research topics
Whether you have active profiles on every social media platform, you’ve taken a social media break, or you generally try to limit your engagement as much as possible, you probably understand how pervasive social technologies have become in today’s culture. Social technology will especially appeal to those looking for widely discussed, mainstream technology essay topics.
- How do viewers respond to virtual influencers vs human influencers? Is one more effective or ethical over the other?
- Across social media platforms, when and where is mob mentality most prevalent? How do the nuances of mob mentality shift depending on the platform or topic?
- Portable devices like cell phones, laptops, and tablets have certainly made daily life easier in some ways. But how have they made daily life more difficult?
- How does access to social media affect developing brains? And what about mature brains?
- Can dating apps alter how users perceive and interact with people in real life?
- Studies have proven “doomscrolling” to negatively impact mental health—could there ever be any positive impacts?
Cryptocurrency and blockchain technology research topics
Following cryptocurrency and blockchain technology has been a rollercoaster the last few years. And since Bitcoin’s conception in 2009, cryptocurrency has consistently showed up on many lists of controversial technology topics.
- Is it ethical for celebrities or influential people to promote cryptocurrencies or cryptographic assets like NFTs ?
- What are the environmental impacts of mining cryptocurrencies? Could those impacts ever change?
- How does cryptocurrency impact financial security and financial health?
- Could the privacy cryptocurrency offers ever be worth the added security risks?
- How might cryptocurrency regulations and impacts continue to evolve?
- Created to enable cryptocurrency, blockchain has since proven useful in several other industries. What new uses could blockchain have?
Artificial intelligence technology research topics
We started 2023 with M3GAN’s box office success, and now we’re fascinated (or horrified) with ChatGPT , voice cloning , and deepfakes . While people have discussed artificial intelligence for ages, recent advances have really pushed this topic to the front of our minds. Those searching for controversial technology topics should pay close attention to this one.
- OpenAI –the company behind ChatGPT–has shown commitment to safe, moderated AI tools that they hope will provide positive benefits to society. Sam Altman, their CEO, recently testified before a US Senate He described what AI makes possible and called for more regulation in the industry. But even with companies like OpenAI displaying efforts to produce safe AI and advocating for regulations, can AI ever have a purely positive impact? Are certain pitfalls unavoidable?
- In a similar vein, can AI ever actually be ethically or safely produced? Will there always be certain risks?
- How might AI tools impact society across future generations?
- Countless movies and television shows explore the idea of AI going wrong, going back all the way to 1927’s Metropolis . What has a greater impact on public perception—representations in media or industry developments? And can public perception impact industry developments and their effectiveness?
Beauty and anti-aging technology
Throughout human history, people in many cultures have gone to extreme lengths to capture and maintain a youthful beauty. But technology has taken the pursuit of beauty and youth to another level. For those seeking technology essay topics that are both timely and timeless, this one’s a gold mine.
- With augmented reality technology, companies like Perfect allow app users to virtually try on makeup, hair color, hair accessories, and hand or wrist accessories. Could virtual try-ons lead to a somewhat less wasteful beauty industry? What downsides should we consider?
- Users of the Perfect app can also receive virtual diagnoses for skin care issues and virtually “beautify” themselves with smoothed skin, erased blemishes, whitened teeth, brightened under-eye circles, and reshaped facial structures. How could advancements in beauty and anti-aging technology affect self-perception and mental health?
- What are the best alternatives to animal testing within the beauty and anti-aging industry?
- Is anti-aging purely a cosmetic pursuit? Could anti-aging technology provide other benefits?
- Could people actually find a “cure” to aging? And could a cure to aging lead to longer lifespans?
- How might longer human lifespans affect the Earth?
Geoengineering technology research topics
An umbrella term, geoengineering refers to large-scale technologies that can alter the earth and its climate. Typically, these types of technologies aim to combat climate change. Those searching for controversial technology topics should consider looking into this one.
- What benefits can solar geoengineering provide? Can they outweigh the severe risks?
- Compare solar geoengineering methods like mirrors in space, stratospheric aerosol injection, marine cloud brightening, and other proposed methods. How have these methods evolved? How might they continue to evolve?
- Which direct air capture methods are most sustainable?
- How can technology contribute to reforestation efforts?
- What are the best uses for biochar? And how can biochar help or harm the earth?
- Out of all the carbon geoengineering methods that exist or have been proposed, which should we focus on the most?
Creative and performing arts technology topics
While tensions often arise between artists and technology, they’ve also maintained a symbiotic relationship in many ways. It’s complicated. But of course, that’s what makes it interesting. Here’s another option for those searching for timely and timeless technology essay topics.
- How has the relationship between art and technology evolved over time?
- How has technology impacted the ways people create art? And how has technology impacted the ways people engage with art?
- Technology has made creating and viewing art widely accessible. Does this increased accessibility change the value of art? And do we value physical art more than digital art?
- Does technology complement storytelling in the performing arts? Or does technology hinder storytelling in the performing arts?
- Which current issues in the creative or performing arts could potentially be solved with technology?
Cellular agriculture technology research topics
And another route for those drawn to controversial technology topics: cellular agriculture. You’ve probably heard about popular plant-based meat options from brands like Impossible and Beyond Meat . While products made with cellular agriculture also don’t require the raising and slaughtering of livestock, they are not plant-based. Cellular agriculture allows for the production of animal-sourced foods and materials made from cultured animal cells.
- Many consumers have a proven bias against plant-based meats. Will that same bias extend to cultured meat, despite cultured meat coming from actual animal cells?
- Which issues can arise from patenting genes?
- Does the animal agriculture industry provide any benefits that cellular agriculture may have trouble replicating?
- How might products made with cellular agriculture become more affordable?
- Could cellular agriculture conflict with the notion of a “ circular bioeconomy ?” And should we strive for a circular bioeconomy? Can we create a sustainable relationship between technology, capitalism, and the environment, with or without cellular agriculture?
Transportation technology research topics
For decades, we’ve expected flying cars to carry us into a techno-utopia, where everything’s shiny, digital, and easy. We’ve heard promises of super fast trains that can zap us across the country or even across the world. We’ve imagined spring breaks on the moon, jet packs, and teleportation. Who wouldn’t love the option to go anywhere, anytime, super quickly? Transportation technology is another great option for those seeking widely discussed, mainstream technology essay topics.
- Once upon a time, Lady Gaga was set to perform in space as a promotion for Virgin Galactic . While Virgin Galactic never actually launched the iconic musician/actor, soon, they hope to launch their first commercial flight full of civilians–who paid $450,000 a pop–on a 90-minute trip into the stars. And if you think that’s pricey, SpaceX launched three businessmen into space for $55 million in April, 2022 (though with meals included, this is actually a total steal). So should we be launching people into space just for fun? What are the impacts of space tourism?
- Could technology improve the way hazardous materials get transported?
- How can the 5.9 GHz Safety Band affect drivers?
- Which might be safer: self-driving cars or self-flying airplanes?
- Compare hyperloop and maglev Which is better and why?
- Can technology improve safety for cyclists?
Gaming technology topics
A recent study involving over 2000 children found links between video game play and enhanced cognitive abilities. While many different studies have found the impacts of video games to be positive or neutral, we still don’t fully understand the impact of every type of video game on every type of brain. Regardless, most people have opinions on video gaming. So this one’s for those seeking widely discussed, mainstream, and controversial technology topics.
- Are different types or genres of video games more cognitively beneficial than others? Or are certain gaming consoles more cognitively beneficial than others?
- How do the impacts of video games differ from other types of games, such as board games or puzzles?
- What ethical challenges and safety risks come with virtual reality gaming?
- How does a player perceive reality during a virtual reality game compared to during other types of video games?
- Can neurodivergent brains benefit from video games in different ways than neurotypical brains?
Medical technology
Advancements in healthcare have the power to change and save lives. In the last ten years, countless new medical technologies have been developed, and in the next ten years, countless more will likely emerge. Always relevant and often controversial, this final technology research topic could interest anyone.
- Which ethical issues might arise from editing genes using CRISPR-Cas9 technology? And should this technology continue to be illegal in the United States?
- How has telemedicine impacted patients and the healthcare they receive?
- Can neurotechnology devices potentially affect a user’s agency, identity, privacy, and/or cognitive liberty?
- How could the use of medical 3-D printing continue to evolve?
- Are patients more likely to skip digital therapeutics than in-person therapeutic methods? And can the increased screen-time required by digital therapeutics impact mental health
What do you do next?
Now that you’ve picked from this list of technology essay topics, you can do a deep dive and immerse yourself in new ideas, new information, and new perspectives. And of course, now that these topics have motivated you to change the world, look into the best computer science schools , the top feeders to tech and Silicon Valley , the best summer programs for STEM students , and the best biomedical engineering schools .
- College Success
- High School Success

Mariya holds a BFA in Creative Writing from the Pratt Institute and is currently pursuing an MFA in writing at the University of California Davis. Mariya serves as a teaching assistant in the English department at UC Davis. She previously served as an associate editor at Carve Magazine for two years, where she managed 60 fiction writers. She is the winner of the 2015 Stony Brook Fiction Prize, and her short stories have been published in Mid-American Review , Cutbank , Sonora Review , New Orleans Review , and The Collagist , among other magazines.
- 2-Year Colleges
- Application Strategies
- Best Colleges by Major
- Best Colleges by State
- Big Picture
- Career & Personality Assessment
- College Essay
- College Search/Knowledge
- Costs & Financial Aid
- Dental School Admissions
- Extracurricular Activities
- Graduate School Admissions
- High Schools
- Law School Admissions
- Medical School Admissions
- Navigating the Admissions Process
- Online Learning
- Private High School Spotlight
- Summer Program Spotlight
- Summer Programs
- Test Prep Provider Spotlight
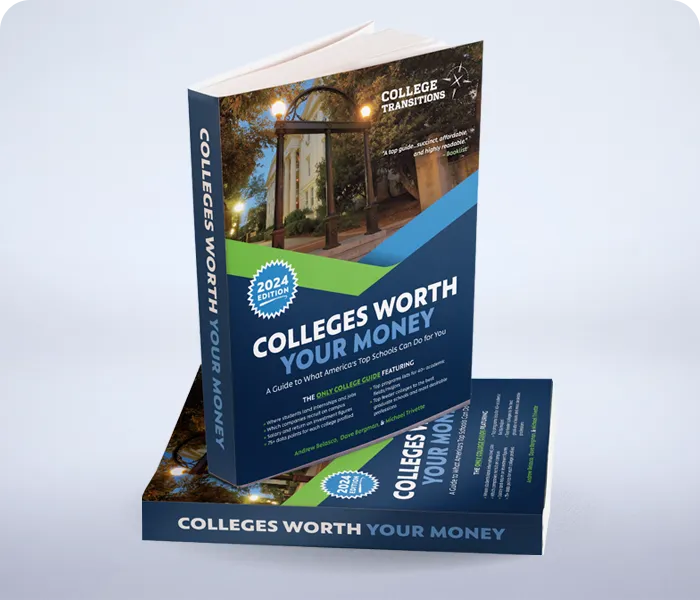
“Innovative and invaluable…use this book as your college lifeline.”
— Lynn O'Shaughnessy
Nationally Recognized College Expert
College Planning in Your Inbox
Join our information-packed monthly newsletter.
I am a... Student Student Parent Counselor Educator Other First Name Last Name Email Address Zip Code Area of Interest Business Computer Science Engineering Fine/Performing Arts Humanities Mathematics STEM Pre-Med Psychology Social Studies/Sciences Submit
Explore your training options in 10 minutes Get Started
- Graduate Stories
- Partner Spotlights
- Bootcamp Prep
- Bootcamp Admissions
- University Bootcamps
- Coding Tools
- Software Engineering
- Web Development
- Data Science
- Tech Guides
- Tech Resources
- Career Advice
- Online Learning
- Internships
- Apprenticeships
- Tech Salaries
- Associate Degree
- Bachelor's Degree
- Master's Degree
- University Admissions
- Best Schools
- Certifications
- Bootcamp Financing
- Higher Ed Financing
- Scholarships
- Financial Aid
- Best Coding Bootcamps
- Best Online Bootcamps
- Best Web Design Bootcamps
- Best Data Science Bootcamps
- Best Technology Sales Bootcamps
- Best Data Analytics Bootcamps
- Best Cybersecurity Bootcamps
- Best Digital Marketing Bootcamps
- Los Angeles
- San Francisco
- Browse All Locations
- Digital Marketing
- Machine Learning
- See All Subjects
- Bootcamps 101
- Full-Stack Development
- Career Changes
- View all Career Discussions
- Mobile App Development
- Cybersecurity
- Product Management
- UX/UI Design
- What is a Coding Bootcamp?
- Are Coding Bootcamps Worth It?
- How to Choose a Coding Bootcamp
- Best Online Coding Bootcamps and Courses
- Best Free Bootcamps and Coding Training
- Coding Bootcamp vs. Community College
- Coding Bootcamp vs. Self-Learning
- Bootcamps vs. Certifications: Compared
- What Is a Coding Bootcamp Job Guarantee?
- How to Pay for Coding Bootcamp
- Ultimate Guide to Coding Bootcamp Loans
- Best Coding Bootcamp Scholarships and Grants
- Education Stipends for Coding Bootcamps
- Get Your Coding Bootcamp Sponsored by Your Employer
- GI Bill and Coding Bootcamps
- Tech Intevriews
- Our Enterprise Solution
- Connect With Us
- Publication
- Reskill America
- Partner With Us
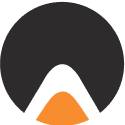
- Resource Center
- Bachelor’s Degree
- Master’s Degree
The Top 10 Most Interesting Technology Research Topics
With technological innovation streamlining processes in businesses at all levels and customers opting for digital interaction, adopting modern technologies have become critical for success in all industries. Technology continues to positively impact organizations , according to Statista, which is why technology research topics have become common among college-level students.
In this article, we have hand-picked the best examples of technology research topics and technology research questions to help you choose a direction to focus your research efforts. These technology research paper topics will inspire you to consider new ways to analyze technology and its evolving role in today’s world.
Find your bootcamp match
What makes a strong technology research topic.
A strong research topic is clear, relevant, and original. It should intrigue readers to learn more about the role of technology through your research paper. A successful research topic meets the requirements of the assignment and isn’t too broad or narrow.
Technology research topics must identify a broad area of research on technologies, so an extremely technical topic can be overwhelming to write. Your technology research paper topic should be suitable for the academic level of your audience.
Tips for Choosing a Technology Research Topic
- Make sure it’s clear. Select a research topic with a clear main idea that you can explain in simple language. It should be able to capture the attention of the audience and keep them engaged in your research paper.
- Make sure it’s relevant. The technology research paper topic should be relevant to the understanding and academic level of the readers. It should enhance their knowledge of a specific technological topic, instead of simply providing vague, directionless ideas about different types of technologies.
- Employ approachable language. Even though you might be choosing a topic from complex technology research topics, the language should be simple. It can be field-specific, but the technical terms used must be basic and easy to understand for the readers.
- Discuss innovations. New technologies get introduced frequently, which adds to the variety of technology research paper topics. Your research topic shouldn’t be limited to old or common technologies. Along with the famous technologies, it should include evolving technologies and introduce them to the audience.
- Be creative . With the rapid growth of technological development, some technology research topics have become increasingly common. It can be challenging to be creative with a topic that has been exhausted through numerous research papers. Your research topic should provide unique information to the audience, which can attract them to your work.
What’s the Difference Between a Research Topic and a Research Question?
A research topic is a subject or a problem being studied by a researcher. It is the foundation of any research paper that sets the tone of the research. It should be broad with a wide range of information available for conducting research.
On the other hand, a research question is closely related to the research topic and is addressed in the study. The answer is formed through data analysis and interpretation. It is more field-specific and directs the research paper toward a specific aspect of a broad subject.
How to Create Strong Technology Research Questions
Technology research questions should be concise, specific, and original while showing a connection to the technology research paper topic. It should be researchable and answerable through analysis of a problem or issue. Make sure it is easy to understand and write within the given word limit and timeframe of the research paper.
Technology is an emerging field with several areas of study, so a strong research question is based on a specific part of a large technical field. For example, many technologies are used in branches of healthcare such as genetics and DNA. Therefore, a research paper about genetics technology should feature a research question that is exclusive to genetics technology only.
Top 10 Technology Research Paper Topics
1. the future of computer-assisted education.
The world shifted to digital learning in the last few years. Students were using the Internet to take online classes, online exams, and courses. Some people prefer distance learning courses over face-to-face classes now, as they only require modern technologies like laptops, mobile phones, and the Internet to study, complete assignments, and even attend lectures.
The demand for digital learning has increased, and it will be an essential part of the education system in the coming years. As a result of the increasing demand, the global digital learning market is expecting a growth of about 110 percent by 2026 .
2. Children’s Use of Social Media
Nowadays, parents allow their children to use the Internet from a very young age. A recent poll by C.S. Mott Children’s Hospital reported that 32 percent of parents allow their children aged seven to nine to use social media sites. This can expose them to cyber bullying and age-inappropriate content, as well as increase their dependence on technology.
Kids need to engage in physical activities and explore the world around them. Using social media sites in childhood can be negative for their personalities and brain health. Analyzing the advantages and disadvantages of the use of technology among young children can create an interesting research paper.
3. The Risks of Digital Voting
Digital voting is an easy way of casting and counting votes. It can save the cost and time associated with traveling to the polling station and getting a postal vote. However, it has a different set of security challenges. A research paper can list the major election security risks caused by digital voting.
Voting in an online format can expose your personal information and decisions to a hacker. As no computer device or software is completely unhackable, the voting system can be taken down, or the hacking may even go undetected.
4. Technology’s Impact on Society in 20 Years
Technological development has accelerated in the last decade. Current technology trends in innovation are focusing on artificial intelligence development, machine learning, and the development and implementation of robots.
Climate change has affected both human life and animal life. Climate technology can be used to deal with global warming in the coming years, and digital learning can make education available for everyone. This technology research paper can discuss the positive and negative effects of technology in 20 years.
5. The Reliability of Self-Driving Cars
Self-driving cars are one of the most exciting trends in technology today. It is a major technology of the future and one of the controversial technology topics. It is considered safer than human driving, but there are some risks involved. For example, edge cases are still common to experience while driving.
Edge cases are occasional and unpredictable situations that may lead to accidents and injuries. It includes difficult weather conditions, objects or animals on the road, and blocked roads. Self-driving cars may struggle to respond to edge cases appropriately, requiring the driver to employ common sense to handle the situation.
6. The Impact of Technology on Infertility
Assisted reproductive technology (ART) helps infertile couples get pregnant. It employs infertility techniques such as In-Vitro Fertilization (IVF) and Gamete Intrafallopian Transfer (GIFT).
Infertility technologies are included in the controversial technology topics because embryonic stem cell research requires extracted human embryos. So, the research can be considered unethical. It is an excellent research topic from the reproductive technology field.
7. Evolution of War Technology
Military technologies have improved throughout history. Modern technologies, such as airplanes, missiles, nuclear reactors, and drones, are essential for war management. Countries experience major innovation in technologies during wars to fulfill their military-specific needs.
Military technologies have controversial ideas and debates linked to them, as some people believe that it plays a role in wars. A research paper on war technology can help evaluate the role of technology in warfare.
8. Using Technology to Create Eco-Friendly Food Packaging
Food technologies and agricultural technologies are trying to manage climate change through eco-friendly food packaging. The materials used are biodegradable, sustainable, and have inbuilt technology that kills microbes harmful to human life.
Research on eco-friendly food packaging can discuss the ineffectiveness of current packaging strategies. The new food technologies used for packaging can be costly, but they are better for preserving foods and the environment.
9. Disease Diagnostics and Therapeutics Through DNA Cloning
Genetic engineering deals with genes and uses them as diagnostics and therapeutics. DNA cloning creates copies of genes or parts of DNA to study different characteristics. The findings are used for diagnosing different types of cancers and even hematological diseases.
Genetic engineering is also used for therapeutic cloning, which clones an embryo for studying diseases and treatments. DNA technology, gene editing, gene therapy, and similar topics are hot topics in technology research papers.
10. Artificial Intelligence in Mental Health Care
Mental health is a widely discussed topic around the world, making it perfect for technology research topics. The mental health care industry has more recently been using artificial intelligence tools and mental health technology like chatbots and virtual assistants to connect with patients.
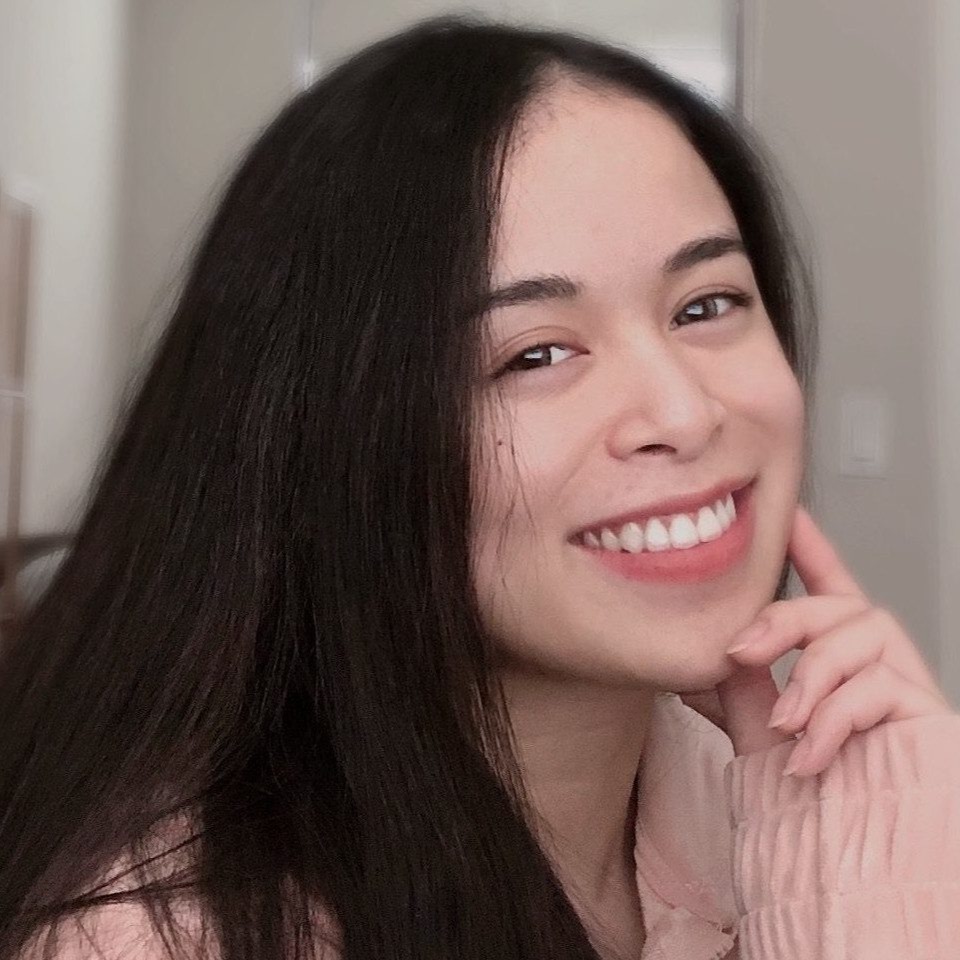
"Career Karma entered my life when I needed it most and quickly helped me match with a bootcamp. Two months after graduating, I found my dream job that aligned with my values and goals in life!"
Venus, Software Engineer at Rockbot
Artificial intelligence has the potential to improve the diagnosis and treatment of mental illness. It can help a health care provider with monitoring patient progress and assigning the right therapist based on provided data and information.
Other Examples of Technology Research Topics & Questions
Technology research topics.
- The connection between productivity and the use of digital tools
- The importance of medical technologies in the next years
- The consequences of addiction to technology
- The negative impact of social media
- The rise and future of blockchain technology
Technology Research Questions
- Is using technology in college classrooms a good or bad idea?
- What are the advantages of cloud technologies for pharmaceutical companies?
- Can new technologies help in treating morbid obesity?
- How to identify true and false information on social media
- Why is machine learning the future?
Choosing the Right Technology Research Topic
Since technology is a diverse field, it can be challenging to choose an interesting technology research topic. It is crucial to select a good research topic for a successful research paper. Any research is centered around the research topic, so it’s important to pick one carefully.
From cell phones to self-driving cars, technological development has completely transformed the world. It offers a wide range of topics to research, resulting in numerous options to choose from. We have compiled technology research topics from a variety of fields. You should select a topic that interests you, as you will be spending weeks researching and writing about it.
Technology Research Topics FAQ
Technology is important in education because it allows people to access educational opportunities globally through mobile technologies and the Internet. Students can enroll in online college degrees , courses, and attend online coding bootcamps . Technology has also made writing research papers easier with the tremendous amount of material available online.
Yes, technology can take over jobs as robotics and automation continue to evolve. However, the management of these technologies will still require human employees with technical backgrounds, such as artificial intelligence specialists, data scientists , and cloud engineers.
Solar panels and wind turbines are two forms of technology that help with climate change, as they convert energy efficiently without emitting greenhouse gases. Electric bikes run on lithium batteries and only take a few hours to charge, which makes them environmentally friendly. Carbon dioxide captures are a way of removing CO 2 from the atmosphere and storing it deep underground.
Technology helps companies manage client and employee data, store and protect important information, and develop strategies to stay ahead of competitors. Marketing technologies, such as Search Engine Optimization (SEO), are great for attracting customers online.
About us: Career Karma is a platform designed to help job seekers find, research, and connect with job training programs to advance their careers. Learn about the CK publication .
What's Next?

Get matched with top bootcamps
Ask a question to our community, take our careers quiz.
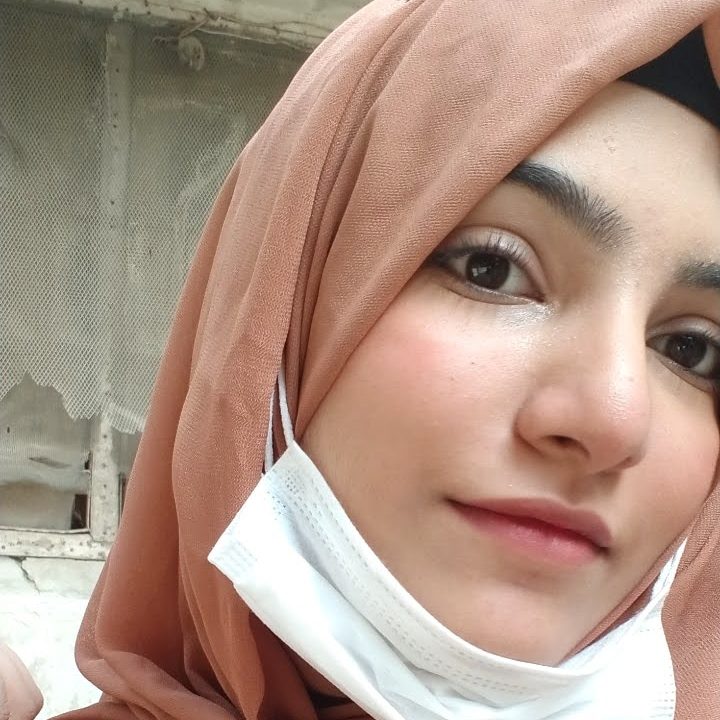
Leave a Reply Cancel reply
Your email address will not be published. Required fields are marked *
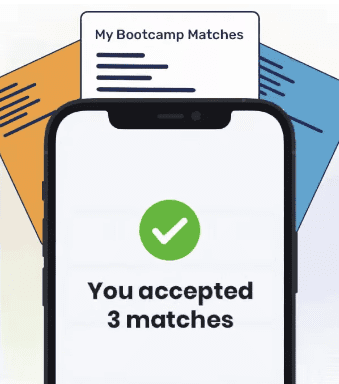

60+ Best Quantitative Research Topics for STEM Students: Dive into Data
Embark on a captivating journey through the cosmos of knowledge with our curated guide on Quantitative Research Topics for STEM Students. Explore innovative ideas in science, technology, engineering, and mathematics, designed to ignite curiosity and shape the future.
Unleash the power of quantitative research and dive into uncharted territories that go beyond academics, fostering innovation and discovery.
Hey, you future scientists, tech wizards, engineering maestros, and math superheroes – gather ’round! We’re about to dive headfirst into the rad world of quantitative research topics, tailor-made for the rockstars of STEM.
In the crazy universe of science, technology, engineering, and math (STEM), quantitative research isn’t just a nerdy term—it’s your VIP pass to an interstellar adventure. Picture this: you’re strapping into a rocket ship, zooming through the cosmos, and decoding the universe’s coolest secrets, all while juggling numbers like a cosmic DJ.
But here’s the real scoop: finding the ultimate research topic is like picking the juiciest star in the galaxy. It’s about stumbling upon something so mind-blowing that you can’t resist plunging into the data. It’s about choosing questions that make your STEM-loving heart do the cha-cha.
In this guide, we’re not just your sidekicks; we’re your partners in crime through the vast jungle of quantitative research topics. Whether you’re a rookie gearing up for your first lab escapade or a seasoned explorer hunting for a new thrill, think of this article as your treasure map, guiding you to the coolest STEM discoveries.
From the teeny wonders of biology to the brain-bending puzzles of physics, the cutting-edge vibes of engineering, and the downright gorgeous dance of mathematics – we’ve got your back.
So, buckle up, fellow STEM enthusiasts! We’re setting sail on a cosmic adventure through the groovy galaxy of quantitative research topics. Get ready to unravel the secrets of science and tech, one sizzling digit at a time.
Stick around for a ride that’s part data, part disco, and all STEM swagger!
Table of Contents
Benefits of Choosing Quantitative Research
Embarking on the quantitative research journey is like stepping into a treasure trove of benefits across a spectrum of fields. Let’s dive into the exciting advantages that make choosing quantitative research a game-changer:
Numbers That Speak Louder
Quantitative research deals in cold, hard numbers. This means your data isn’t just informative; it’s objective, measurable, and has a voice of its own.
Statistical Swagger
Crunching numbers isn’t just for show. With quantitative research, statistical tools add a touch of pizzazz, boosting the validity of your findings and turning your study into a credible performance.
For the Masses
Quantitative research loves a crowd. Larger sample sizes mean your discoveries aren’t just for the lucky few – they’re for everyone. It’s the science of sharing the knowledge wealth.
Data Showdown
Ready for a duel between variables? Quantitative research sets the stage for epic battles, letting you compare, contrast, and uncover cause-and-effect relationships in the data arena.
Structured and Ready to Roll
Think of quantitative research like a well-organized party. It follows a structured plan, making replication a breeze. Because who doesn’t love a party that’s easy to recreate?
Data Efficiency Dance
Efficiency is the name of the game. Surveys, experiments, and structured observations make data collection a dance – choreographed, smooth, and oh-so-efficient.
Data Clarity FTW
No decoding needed here. Quantitative research delivers crystal-clear results. It’s like reading a good book without the need for interpretation – straightforward and to the point.
Spotting Trends Like a Pro
Ever wish you had a crystal ball for trends? Quantitative analysis is the next best thing. It’s like having a trend-spotting superpower, revealing patterns that might have otherwise stayed hidden.
Bias Be Gone
Quantitative research takes bias out of the equation. Systematic data collection and statistical wizardry reduce researcher bias, leaving you with results that are as unbiased as a judge at a talent show.
Key Components of a Quantitative Research Study
Launching into a quantitative research study is like embarking on a thrilling quest, and guess what? You’re the hero of this research adventure! Let’s unravel the exciting components that make your study a blockbuster:
Quest-Starter: Research Question or Hypothesis
It’s your “once upon a time.” Kick off your research journey with a bang by crafting a captivating research question or hypothesis. This is the spark that ignites your curiosity.
Backstory Bonanza: Literature Review
Think of it as your research Netflix binge. Dive into existing literature for the backstory. It’s not just research – it’s drama, plot twists, and the foundation for your epic tale.
Blueprint Brilliance: Research Design
Time to draw up the plans for your study castle. Choose your research design – is it a grand experiment or a cunning observational scheme? Your design is the architectural genius behind your research.
Casting Call: Population and Sample
Who’s in your star-studded lineup? Define your dream cast – your target population – and then handpick a sample that’s ready for the research red carpet.
Gear Up: Data Collection Methods
Choose your research tools wisely – surveys, experiments, or maybe a bit of detective work. Your methods are like the gadgets in a spy movie, helping you collect the data treasures.
The Numbers Game: Variables and Measures
What’s in the spotlight? Identify your main characters – independent and dependent variables. Then, sprinkle in some measures to add flair and precision to your study.
Magic Analysis Wand: Data Analysis Techniques
Enter the wizardry zone! Pick your magic wand – statistical methods, tests, or software – and watch as it unravels the mysteries hidden in your data.
Ethical Superhero Cape: Ethical Considerations
Every hero needs a moral compass. Clearly outline how you’ll be the ethical superhero of your study, protecting the well-being and secrets of your participants.
Grand Finale: Results and Findings
It’s showtime! Showcase your results like the grand finale of a fireworks display. Tables, charts, and statistical dazzle – let your findings steal the spotlight.
Wrap-Up Party: Conclusion and Implications
Bring out the confetti! Summarize your findings, discuss their VIP status in the research world, and hint at the afterparty – how your results shape the future.
Behind-the-Scenes Blooper Reel: Limitations and Future Research
No Hollywood film is perfect. Share the bloopers – the limitations of your study – and hint at the sequel with ideas for future research. It’s all part of the cinematic journey.
Roll Credits: References
Give a shout-out to the supporting cast! Cite your sources – it’s the credits that add credibility to your blockbuster.
Bonus Scene: Appendix
Think of it as the post-credits scene. Tuck in any extra goodies – surveys, questionnaires, or behind-the-scenes material – for those eager to dive deeper into your research universe.
By weaving these storylines together, your quantitative research study becomes a cinematic masterpiece, leaving a lasting impact on the grand stage of academia. Happy researching, hero!
Quantitative Research Topics for STEM Students
Check out the best quantitative research topics for STEM students:-
- Investigating the Effects of Different Soil pH Levels on Plant Growth.
- Analyzing the Impact of Pesticide Exposure on Bee Populations.
- Studying the Genetic Variability in Endangered Species.
- Quantifying the Relationship Between Temperature and Microbial Growth in Water.
- Analyzing the Effects of Ocean Acidification on Coral Reefs.
- Investigating the Correlation Between Pollinator Diversity and Crop Yield.
- Studying the Role of Gut Microbiota in Human Health and Disease.
- Quantifying the Impact of Antibiotics on Soil Microbial Communities.
- Analyzing the Effects of Light Pollution on Nocturnal Animal Behavior.
- Investigating the Relationship Between Altitude and Plant Adaptations in Mountain Ecosystems.
- Measuring the Speed of Light Using Interferometry Techniques.
- Investigating the Quantum Properties of Photons in Quantum Computing.
- Analyzing the Factors Affecting Magnetic Field Strength in Electromagnets.
- Studying the Behavior of Superfluids at Ultra-Low Temperatures.
- Quantifying the Efficiency of Energy Transfer in Photovoltaic Cells.
- Analyzing the Properties of Quantum Dots for Future Display Technologies.
- Investigating the Behavior of Particles in High-Energy Particle Accelerators.
- Studying the Effects of Gravitational Waves on Space-Time.
- Quantifying the Frictional Forces on Objects at Different Surfaces.
- Analyzing the Characteristics of Dark Matter and Dark Energy in the Universe.
Engineering
- Optimizing the Design of Wind Turbine Blades for Maximum Efficiency.
- Investigating the Use of Smart Materials in Structural Engineering.
- Analyzing the Impact of 3D Printing on Prototyping in Product Design.
- Studying the Behavior of Composite Materials Under Extreme Temperatures.
- Evaluating the Efficiency of Water Treatment Plants in Removing Contaminants.
- Investigating the Aerodynamics of Drones for Improved Flight Control.
- Quantifying the Effects of Traffic Flow on Roadway Maintenance.
- Analyzing the Impact of Vibration Damping in Building Structures.
- Studying the Mechanical Properties of Biodegradable Polymers in Medical Devices.
- Investigating the Use of Artificial Intelligence in Autonomous Robotic Systems.
Mathematics
- Exploring Chaos Theory and Its Applications in Nonlinear Systems.
- Modeling the Spread of Infectious Diseases in Population Dynamics.
- Analyzing Data Mining Techniques for Predictive Analytics in Business.
- Studying the Mathematics of Cryptography Algorithms for Data Security.
- Quantifying the Patterns in Stock Market Price Movements Using Time Series Analysis.
- Investigating the Applications of Fractal Geometry in Computer Graphics.
- Analyzing the Behavior of Differential Equations in Climate Modeling.
- Studying the Optimization of Supply Chain Networks Using Linear Programming.
- Investigating the Mathematical Concepts Behind Machine Learning Algorithms.
- Quantifying the Patterns of Prime Numbers in Number Theory.
- Investigating the Chemical Mechanisms Behind Enzyme Catalysis.
- Analyzing the Thermodynamic Properties of Chemical Reactions.
- Studying the Kinetics of Chemical Reactions in Different Solvents.
- Quantifying the Concentration of Pollutants in Urban Air Quality.
- Evaluating the Effectiveness of Antioxidants in Food Preservation.
- Investigating the Electrochemical Properties of Batteries for Energy Storage.
- Studying the Behavior of Nanomaterials in Drug Delivery Systems.
- Analyzing the Chemical Composition of Exoplanet Atmospheres Using Spectroscopy.
- Quantifying Heavy Metal Contamination in Soil and Water Sources.
- Investigating the Correlation Between Chemical Exposure and Human Health.
Computer Science
- Analyzing Machine Learning Algorithms for Natural Language Processing.
- Investigating Quantum Computing Algorithms for Cryptography Applications.
- Studying the Efficiency of Data Compression Methods for Big Data Storage.
- Quantifying Cybersecurity Threats and Vulnerabilities in IoT Devices.
- Evaluating the Impact of Cloud Computing on Distributed Systems.
- Investigating the Use of Artificial Intelligence in Autonomous Vehicles.
- Analyzing the Behavior of Neural Networks in Deep Learning Applications.
- Studying the Performance of Blockchain Technology in Supply Chain Management.
- Quantifying User Behavior in Social Media Analytics.
- Investigating Quantum Machine Learning for Enhanced Data Processing.
These additional project ideas provide a diverse range of opportunities for STEM students to engage in quantitative research and explore various aspects of their respective fields. Each project offers a unique avenue for discovery and contribution to the world of science and technology.
What is an example of a quantitative research?
Quantitative research is a powerful investigative approach, wielding numbers to shed light on intricate relationships and phenomena. Let’s dive into an example of quantitative research to understand its workings:
Research Question
What is the correlation between the time students devote to studying and their academic grades?
Students who invest more time in studying are likely to achieve higher grades.
Research Design
Imagine a researcher embarking on a journey within a high school. They distribute surveys to students, inquiring about their weekly study hours and their corresponding grades in core subjects.
Data Analysis
Equipped with statistical tools, our researcher scrutinizes the collected data. Lo and behold, a significant positive correlation emerges—students who dedicate more time to studying generally earn higher grades.
With data as their guide, the researcher concludes that indeed, a relationship exists between study time and academic grades. The more time students commit to their studies, the brighter their academic stars tend to shine.
This example merely scratches the surface of quantitative research’s potential. It can delve into an extensive array of subjects and investigate complex hypotheses. Here are a few more examples:
- Assessing a New Drug’s Effectiveness: Quantifying the impact of a novel medication in treating a specific illness.
- Socioeconomic Status and Crime Rates: Investigating the connection between economic conditions and criminal activity.
- Analyzing the Influence of an Advertising Campaign on Sales: Measuring the effectiveness of a marketing blitz on product purchases.
- Factors Shaping Customer Satisfaction: Using data to pinpoint the elements contributing to customer contentment.
- Government Policies and Employment Rates: Evaluating the repercussions of new governmental regulations on job opportunities.
Quantitative research serves as a potent beacon, illuminating the complexities of our world through data-driven inquiry. Researchers harness its might to collect, analyze, and draw valuable conclusions about a vast spectrum of phenomena. It’s a vital tool for unraveling the intricacies of our universe.
As we bid adieu to our whirlwind tour of quantitative research topics tailor-made for the STEM dreamers, it’s time to soak in the vast horizons that science, technology, engineering, and mathematics paint for us.
We’ve danced through the intricate tango of poverty and crime, peeked into the transformative realm of cutting-edge technologies, and unraveled the captivating puzzles of quantitative research. But these aren’t just topics; they’re open invitations to dive headfirst into the uncharted seas of knowledge.
To you, the STEM trailblazers, these research ideas aren’t mere academic pursuits. They’re portals to curiosity, engines of innovation, and blueprints for shaping the future of our world. They’re the sparks that illuminate the trail leading to discovery.
As you set sail on your research odyssey, remember that quantitative research isn’t just about unlocking answers—it’s about nurturing that profound sense of wonder, igniting innovation, and weaving your unique thread into the fabric of human understanding.
Whether you’re stargazing, decoding the intricate language of genes, engineering marvels, or tackling global challenges head-on, realize that your STEM and quantitative research journey is a perpetual adventure.
May your questions be audacious, your data razor-sharp, and your discoveries earth-shattering. Keep that innate curiosity alive, keep exploring, and let the spirit of STEM be your North Star, guiding you towards a future that’s not just brighter but brilliantly enlightened.
And with that, fellow adventurers, go forth, embrace the unknown, and let your journey in STEM be the epic tale that reshapes the narrative of tomorrow!
Frequently Asked Questions
How can i ensure the ethical conduct of my quantitative research project.
To ensure ethical conduct, obtain informed consent from participants, maintain data confidentiality, and adhere to ethical guidelines established by your institution and professional associations.
Are there any software tools recommended for data analysis in STEM research?
Yes, there are several widely used software tools for data analysis in STEM research, including R, Python, MATLAB, and SPSS. The choice of software depends on your specific research needs and familiarity with the tools.
Leave a Comment Cancel Reply
Your email address will not be published. Required fields are marked *
Save my name, email, and website in this browser for the next time I comment.
- Search Menu
- Advance articles
- Author Guidelines
- Submission Site
- Open Access
- Why Publish?
- About Science and Public Policy
- Editorial Board
- Advertising and Corporate Services
- Journals Career Network
- Self-Archiving Policy
- Dispatch Dates
- Journals on Oxford Academic
- Books on Oxford Academic

Article Contents
- 1. Introduction
- 3. Mapping FTA techniques
- 4. Conclusions
- Acknowledgements
- Appendix: List of techniques reviewed
- < Previous
Quantitative analysis of technology futures: A review of techniques, uses and characteristics
- Article contents
- Figures & tables
- Supplementary Data
Tommaso Ciarli, Alex Coad, Ismael Rafols, Quantitative analysis of technology futures: A review of techniques, uses and characteristics, Science and Public Policy , Volume 43, Issue 5, October 2016, Pages 630–645, https://doi.org/10.1093/scipol/scv059
- Permissions Icon Permissions
A variety of quantitative techniques have been used in the past in future-oriented technology analysis (FTA). In recent years, increased computational power and data availability have led to the emergence of new techniques that are potentially useful for foresight and forecasting. As a result, there are now many techniques that might be used in FTA exercises. This paper reviews and qualifies quantitative methods for FTA in order to help users to make choices among alternative techniques, including new techniques that have not yet been integrated into the FTA literature and practice. We first provide a working definition of FTA and discuss its role, uses, and popularity over recent decades. Second, we select the most important quantitative FTA techniques, discuss their main contexts and uses, and classify them into groups with common characteristics, positioning them along key dimensions: descriptive/prescriptive, extrapolative/normative, data gathering/inference, and forecasting/foresight.
Email alerts
Citing articles via.
- Recommend to your Library
Affiliations
- Online ISSN 1471-5430
- Print ISSN 0302-3427
- Copyright © 2024 Oxford University Press
- About Oxford Academic
- Publish journals with us
- University press partners
- What we publish
- New features
- Open access
- Institutional account management
- Rights and permissions
- Get help with access
- Accessibility
- Advertising
- Media enquiries
- Oxford University Press
- Oxford Languages
- University of Oxford
Oxford University Press is a department of the University of Oxford. It furthers the University's objective of excellence in research, scholarship, and education by publishing worldwide
- Copyright © 2024 Oxford University Press
- Cookie settings
- Cookie policy
- Privacy policy
- Legal notice
This Feature Is Available To Subscribers Only
Sign In or Create an Account
This PDF is available to Subscribers Only
For full access to this pdf, sign in to an existing account, or purchase an annual subscription.

200+ Research Title Ideas To Explore In 2024
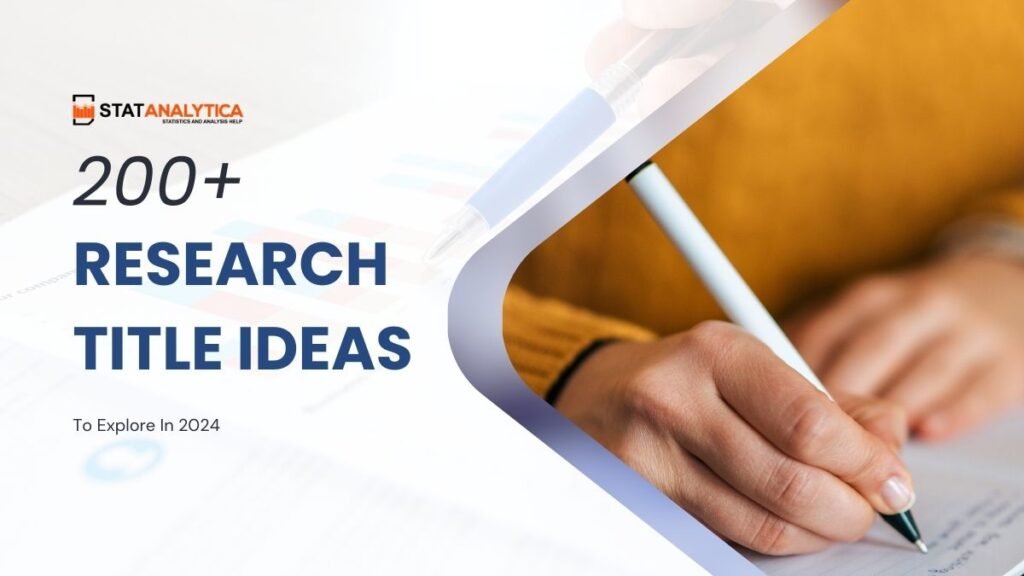
Choosing a compelling research title is a critical step in the research process, as it serves as the gateway to capturing the attention of readers and potential collaborators. A well-crafted research title not only encapsulates the essence of your study but also entices readers to delve deeper into your work.
In this blog post, we will explore the significance of research title ideas, the characteristics of an effective title, strategies for generating compelling titles, examples of successful titles, common pitfalls to avoid, the importance of iterative refinement, and ethical considerations in title creation.
Characteristics of a Good Research Title
Table of Contents
Clarity and Precision
A good research title should communicate the core idea of your study clearly and precisely. Avoid vague or overly complex language that might confuse readers.
Relevance to the Research Topic
Ensure that your title accurately reflects the content and focus of your research. It should provide a clear indication of what readers can expect from your study.
Conciseness and Avoidance of Ambiguity
Keep your title concise and to the point. Avoid unnecessary words or phrases that may add ambiguity. Aim for clarity and directness to make your title more impactful.
Use of Keywords
Incorporating relevant keywords in your title can enhance its visibility and accessibility. Consider the terms that researchers in your field are likely to search for and integrate them into your title.
Reflecting the Research Methodology or Approach
If your research employs a specific methodology or approach, consider incorporating that information into your title. This helps set expectations for readers and indicates the uniqueness of your study.
What are the Strategies for Generating Research Title Ideas?
- Brainstorming
- Individual Brainstorming: Set aside time to generate title ideas on your own. Consider different angles, perspectives, and aspects of your research.
- Group Brainstorming: Collaborate with peers or mentors to gather diverse perspectives and insights. Group brainstorming can lead to innovative and multidimensional title ideas.
- Keyword Analysis
- Identifying Key Terms and Concepts: Break down your research into key terms and concepts. These will form the foundation of your title.
- Exploring Synonyms and Related Terms: Expand your search by exploring synonyms and related terms. This can help you discover alternative ways to express your research focus.
- Literature Review
- Examining Existing Titles in the Field: Review titles of relevant studies in your field to identify common patterns and effective strategies.
- Analyzing Successful Titles for Inspiration: Analyze successful research titles to understand what makes them stand out. Look for elements that resonate with your own research.
- Consultation with Peers and Mentors
- Seek feedback from peers and mentors during the title creation process. External perspectives can offer valuable insights and help refine your ideas.
- Use of Online Tools and Title Generators
- Explore online tools and title generators designed to aid in the generation of creative and relevant research titles. While these tools can be helpful, exercise discretion and ensure the generated titles align with the essence of your research.
200+ Research Title Ideas: Category-Wise
Technology and computer science.
- “Cybersecurity Measures in the Age of Quantum Computing”
- “Machine Learning Applications for Predictive Maintenance”
- “The Impact of Augmented Reality on Learning Outcomes”
- “Blockchain Technology: Enhancing Supply Chain Transparency”
- “Human-Computer Interaction in Virtual Reality Environments”
Environmental Science and Sustainability
- “Evaluating the Efficacy of Green Infrastructure in Urban Areas”
- “Climate Change Resilience Strategies for Coastal Communities”
- “Biodiversity Conservation in Tropical Rainforests”
- “Renewable Energy Adoption in Developing Economies”
- “Assessing the Environmental Impact of Plastic Alternatives”
Health and Medicine
- “Precision Medicine Approaches in Cancer Treatment”
- “Mental Health Interventions for Youth in Urban Settings”
- “Telemedicine: Bridging Gaps in Rural Healthcare Access”
- “The Role of Gut Microbiota in Metabolic Disorders”
- “Ethical Considerations in Genetic Editing Technologies”
Social Sciences and Psychology
- “Social Media Influence on Body Image Perception”
- “Impact of Cultural Diversity on Team Performance”
- “Psychological Resilience in the Face of Global Crises”
- “Parental Involvement and Academic Achievement in Adolescents”
- “Exploring the Dynamics of Online Communities and Identity”
Business and Economics
- “Sustainable Business Practices and Consumer Behavior”
- “The Role of Big Data in Financial Decision-Making”
- “Entrepreneurship and Innovation in Emerging Markets”
- “Corporate Social Responsibility and Brand Loyalty”
- “Economic Implications of Remote Work Adoption”
Education and Pedagogy
- “Inclusive Education Models for Diverse Learning Needs”
- “Gamification in STEM Education: A Comparative Analysis”
- “Online Learning Effectiveness in Higher Education”
- “Teacher Training for Integrating Technology in Classrooms”
- “Assessment Strategies for Measuring Critical Thinking Skills”
Psychology and Behavior
- “The Influence of Social Media on Adolescent Well-being”
- “Cognitive Biases in Decision-Making: A Cross-Cultural Study”
- “The Role of Empathy in Conflict Resolution”
- “Positive Psychology Interventions for Workplace Satisfaction”
- “Exploring the Relationship Between Sleep Patterns and Mental Health”
Biology and Genetics
- “Genetic Markers for Predisposition to Neurodegenerative Diseases”
- “CRISPR-Cas9 Technology: Ethical Implications and Future Prospects”
- “Evolutionary Adaptations in Response to Environmental Changes”
- “Understanding the Microbiome’s Impact on Immune System Function”
- “Epigenetic Modifications and Their Role in Disease Development”
Urban Planning and Architecture
- “Smart Cities: Balancing Technological Innovation and Privacy”
- “Revitalizing Urban Spaces: Community Engagement in Design”
- “Sustainable Architecture: Integrating Nature into Urban Designs”
- “Transit-Oriented Development and Its Impact on City Dynamics”
- “Assessing the Cultural Significance of Urban Landscapes”
Linguistics and Communication
- “The Influence of Language on Cross-Cultural Communication”
- “Language Development in Multilingual Environments”
- “The Impact of Nonverbal Communication on Interpersonal Relationships”
- “Digital Communication and the Evolution of Language”
- “Language Processing in Bilingual Individuals: A Neuroscientific Approach”
Political Science and International Relations
- “The Role of Social Media in Political Mobilization”
- “Global Governance in the Era of Transnational Challenges”
- “Human Rights and the Ethics of Intervention in International Affairs”
- “Political Polarization: Causes and Consequences”
- “Climate Change Diplomacy: Assessing International Agreements”
Physics and Astronomy
- “Dark Matter: Unraveling the Mysteries of the Universe”
- “Quantum Entanglement and Its Potential Applications”
- “The Search for Exoplanets in Habitable Zones”
- “Astrophysical Phenomena: Exploring Black Holes and Neutron Stars”
- “Advancements in Quantum Computing Algorithms”
Education Technology (EdTech)
- “Adaptive Learning Platforms: Personalizing Education for Every Student”
- “The Impact of Virtual Reality Simulations on STEM Education”
- “E-Learning Accessibility for Students with Disabilities”
- “Gamified Learning: Enhancing Student Engagement and Retention”
- “Digital Literacy Education: Navigating the Information Age”
Sociology and Anthropology
- “Cultural Shifts in Modern Society: An Anthropological Exploration”
- “Social Movements in the Digital Age: Activism and Connectivity”
- “Gender Roles and Equality: A Cross-Cultural Perspective”
- “Urbanization and Its Effects on Traditional Societal Structures”
- “Cultural Appropriation: Understanding Boundaries and Respect”
Materials Science and Engineering
- “Nanostructured Materials: Innovations in Manufacturing and Applications”
- “Biodegradable Polymers: Towards Sustainable Packaging Solutions”
- “Materials for Energy Storage: Advancements and Challenges”
- “Smart Materials in Healthcare: From Diagnosis to Treatment”
- “Robust Coatings for Extreme Environments: Applications in Aerospace”
History and Archaeology
- “Digital Reconstruction of Historical Sites: Preserving the Past”
- “Trade Routes in Ancient Civilizations: A Comparative Study”
- “Archaeogenetics: Unraveling Human Migrations Through DNA Analysis”
- “Historical Linguistics: Tracing Language Evolution Over Millennia”
- “The Archaeology of Conflict: Studying War through Artifacts”
Marketing and Consumer Behavior
- “Influencer Marketing: Impact on Consumer Trust and Purchasing Decisions”
- “The Role of Brand Storytelling in Consumer Engagement”
- “E-commerce Personalization Strategies: Balancing Customization and Privacy”
- “Cross-Cultural Marketing: Adapting Campaigns for Global Audiences”
- “Consumer Perceptions of Sustainable Products: A Market Analysis”
Neuroscience and Cognitive Science
- “Neuroplasticity and Cognitive Rehabilitation: Implications for Therapy”
- “The Neuroscience of Decision-Making: Insights from Brain Imaging”
- “Cognitive Aging: Understanding Memory Decline and Cognitive Resilience”
- “The Role of Neurotransmitters in Emotional Regulation”
- “Neuroethical Considerations in Brain-Computer Interface Technologies”
Public Health and Epidemiology
- “Epidemiological Trends in Infectious Diseases: Lessons from Global Outbreaks”
- “Public Health Interventions for Reducing Non-Communicable Diseases”
- “Health Disparities Among Marginalized Communities: Addressing the Gaps”
- “The Impact of Climate Change on Vector-Borne Diseases”
- “Community-Based Approaches to Promoting Health Equity”
Robotics and Automation
- “Human-Robot Collaboration in Manufacturing: Enhancing Productivity and Safety”
- “Autonomous Vehicles: Navigating the Path to Mainstream Adoption”
- “Soft Robotics: Engineering Flexibility for Real-World Applications”
- “Ethical Considerations in the Development of AI-powered Robotics”
- “Bio-Inspired Robotics: Learning from Nature to Enhance Machine Intelligence”
Literature and Literary Criticism
- “Postcolonial Narratives: Deconstructing Power Structures in Literature”
- “Digital Storytelling Platforms: Changing the Landscape of Narrative Arts”
- “Literature and Cultural Identity: Exploring Representations in Global Contexts”
- “Eco-Critical Perspectives in Contemporary Literature”
- “Feminist Literary Criticism: Reinterpreting Classic Texts Through a New Lens”
Chemistry and Chemical Engineering
- “Green Chemistry: Sustainable Approaches to Chemical Synthesis”
- “Nanomaterials for Drug Delivery: Innovations in Biomedical Applications”
- “Chemical Process Optimization: Towards Energy-Efficient Production”
- “The Chemistry of Taste: Molecular Insights into Food Flavors”
- “Catalytic Converters: Advancements in Pollution Control Technologies”
Cultural Studies and Media
- “Media Representations of Social Movements: Framing and Impact”
- “Pop Culture and Identity: Exploring Trends in a Globalized World”
- “The Influence of Social Media on Political Discourse”
- “Reality Television and Perceptions of Reality: A Cultural Analysis”
- “Media Literacy Education: Navigating the Digital Information Age”
Astronomy and Astrophysics
- “Gravitational Waves: Probing the Cosmos for New Discoveries”
- “The Life Cycle of Stars: From Birth to Supernova”
- “Astrobiology: Searching for Extraterrestrial Life in the Universe”
- “Dark Energy and the Accelerating Expansion of the Universe”
- “Cosmic Microwave Background: Insights into the Early Universe”
Social Work and Community Development
- “Community-Based Mental Health Interventions: A Social Work Perspective”
- “Youth Empowerment Programs: Fostering Resilience in Vulnerable Communities”
- “Social Justice Advocacy in Contemporary Social Work Practice”
- “Intersectionality in Social Work: Addressing the Complex Needs of Individuals”
- “The Role of Technology in Enhancing Social Services Delivery”
Artificial Intelligence and Ethics
- “Ethical Considerations in AI Decision-Making: Balancing Autonomy and Accountability”
- “Bias and Fairness in Machine Learning Algorithms: A Critical Examination”
- “Explainable AI: Bridging the Gap Between Complexity and Transparency”
- “The Social Implications of AI-Generated Content: Challenges and Opportunities”
- “AI and Personal Privacy: Navigating the Ethical Dimensions of Data Usage”
Linguistics and Computational Linguistics
- “Natural Language Processing: Advancements in Understanding Human Communication”
- “Multilingualism in the Digital Age: Challenges and Opportunities”
- “Cognitive Linguistics: Exploring the Relationship Between Language and Thought”
- “Speech Recognition Technologies: Applications in Everyday Life”
- “Syntax and Semantics: Unraveling the Structure of Language”
Geology and Earth Sciences
- “Geological Hazards Assessment in Urban Planning: A Case Study”
- “Paleoclimatology: Reconstructing Past Climate Patterns for Future Predictions”
- “Geomorphological Processes in Coastal Landscapes: Implications for Conservation”
- “Volcanic Activity Monitoring: Early Warning Systems and Mitigation Strategies”
- “The Impact of Human Activities on Soil Erosion: An Ecological Perspective”
Political Economy and Global Governance
- “Global Trade Agreements: Assessing Economic Impacts and Equity”
- “Political Economy of Energy Transition: Policies and Socioeconomic Effects”
- “The Role of International Organizations in Global Governance”
- “Financial Inclusion and Economic Development: A Comparative Analysis”
- “The Political Economy of Pandemics: Governance and Crisis Response”
Food Science and Nutrition
- “Nutrigenomics: Personalized Nutrition for Optimal Health”
- “Functional Foods: Exploring Health Benefits Beyond Basic Nutrition”
- “Sustainable Food Production: Innovations in Agriculture and Aquaculture”
- “Dietary Patterns and Mental Health: A Comprehensive Review”
- “Food Allergies and Sensitivities: Mechanisms and Management Strategies”
Sociology and Technology
- “Digital Inequalities: Examining Access and Usage Patterns Across Demographics”
- “The Impact of Social Media on Social Capital and Community Building”
- “Technological Surveillance and Privacy Concerns: A Sociological Analysis”
- “Virtual Communities: An Exploration of Identity Formation in Online Spaces”
- “The Social Dynamics of Online Activism: Mobilization and Participation”
Materials Science and Nanotechnology
- “Nanomaterials for Biomedical Imaging: Enhancing Diagnostic Precision”
- “Self-Healing Materials: Advances in Sustainable and Resilient Infrastructure”
- “Smart Textiles: Integrating Nanotechnology for Enhanced Functionality”
- “Multifunctional Nanoparticles in Drug Delivery: Targeted Therapies and Beyond”
- “Nanocomposites for Energy Storage: Engineering Efficient Capacitors”
Communication and Media Studies
- “Media Convergence: The Evolution of Content Delivery in the Digital Age”
- “The Impact of Social Media Influencers on Consumer Behavior”
- “Crisis Communication in a Hyperconnected World: Lessons from Global Events”
- “Media Framing of Environmental Issues: A Comparative Analysis”
- “Digital Detox: Understanding Media Consumption Patterns and Well-being”
Developmental Psychology
- “Early Childhood Attachment and Its Long-Term Impact on Adult Relationships”
- “Cognitive Development in Adolescence: Challenges and Opportunities”
- “Parenting Styles and Academic Achievement: A Cross-Cultural Perspective”
- “Identity Formation in Emerging Adulthood: The Role of Social Influences”
- “Interventions for Promoting Resilience in At-Risk Youth Populations”
Aerospace Engineering
- “Advancements in Aerodynamics: Redefining Flight Efficiency”
- “Space Debris Management: Mitigating Risks in Earth’s Orbit”
- “Aerodynamic Design Optimization for Supersonic Flight”
- “Hypersonic Propulsion Technologies: Pushing the Boundaries of Speed”
- “Materials for Space Exploration: Engineering Solutions for Harsh Environments”
Political Psychology
- “Political Polarization and Public Opinion: Exploring Cognitive Biases”
- “Leadership Styles and Public Perception: A Psychological Analysis”
- “Nationalism and Identity: Psychological Factors Shaping Political Beliefs”
- “The Influence of Emotional Appeals in Political Communication”
- “Crisis Leadership: The Psychological Dynamics of Decision-Making in Times of Uncertainty”
Marine Biology and Conservation
- “Coral Reef Restoration: Strategies for Biodiversity Conservation”
- “Ocean Plastic Pollution: Assessing Impacts on Marine Ecosystems”
- “Marine Mammal Communication: Insights from Bioacoustics”
- “Sustainable Fisheries Management: Balancing Ecological and Economic Concerns”
- “The Role of Mangrove Ecosystems in Coastal Resilience”
Artificial Intelligence and Creativity
- “Generative AI in Creative Industries: Challenges and Innovations”
- “AI-Enhanced Creativity Tools: Empowering Artists and Designers”
- “Machine Learning for Music Composition: Bridging Art and Technology”
- “Creative AI in Film and Entertainment: Transforming Storytelling”
- “Ethical Considerations in AI-Generated Art and Content”
Cultural Anthropology
- “Cultural Relativism in Anthropological Research: Opportunities and Challenges”
- “Rituals and Symbolism: Unraveling Cultural Practices Across Societies”
- “Migration and Cultural Identity: An Ethnographic Exploration”
- “Material Culture Studies: Understanding Societies through Objects”
- “Indigenous Knowledge Systems: Preserving and Promoting Cultural Heritage”
Quantum Computing and Information Science
- “Quantum Information Processing: Algorithms and Applications”
- “Quantum Cryptography: Securing Communication in the Quantum Era”
- “Quantum Machine Learning: Enhancing AI through Quantum Computing”
- “Quantum Computing in Finance: Opportunities and Challenges”
- “Quantum Internet: Building the Next Generation of Information Networks”
Public Policy and Urban Planning
- “Smart Cities and Inclusive Urban Development: A Policy Perspective”
- “Public-Private Partnerships in Infrastructure Development: Lessons Learned”
- “The Impact of Transportation Policies on Urban Mobility Patterns”
- “Housing Affordability: Policy Approaches to Addressing Urban Challenges”
- “Data-Driven Decision-Making in Urban Governance: Opportunities and Risks”
Gerontology and Aging Studies
- “Healthy Aging Interventions: Promoting Quality of Life in Older Adults”
- “Social Isolation and Mental Health in Aging Populations: Interventions and Support”
- “Technology Adoption Among Older Adults: Bridging the Digital Divide”
- “End-of-Life Decision-Making: Ethical Considerations and Legal Frameworks”
- “Cognitive Resilience in Aging: Strategies for Maintaining Mental Sharpness”
Examples of Effective Research Titles
Illustrative Examples from Various Disciplines
Here are examples of effective research titles from different disciplines:
- “Unlocking the Mysteries of Neural Plasticity: A Multidisciplinary Approach”
- “Sustainable Urban Development: Integrating Environmental and Social Perspectives”
- “Quantum Computing: Navigating the Path to Practical Applications”
Analysis of What Makes Each Title Effective
- Clear indication of the research focus.
- Inclusion of key terms relevant to the field.
- Incorporation of a multidisciplinary or integrated approach where applicable.
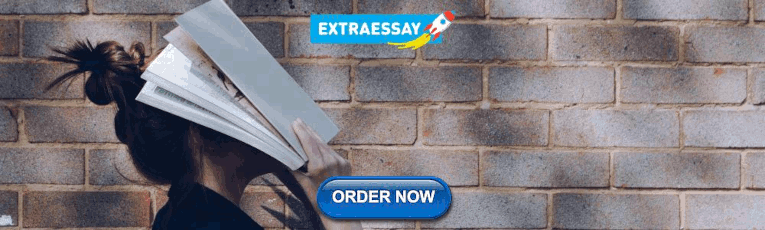
Common Pitfalls to Avoid in Research Title Creation
A. Vagueness and Ambiguity
Vague or ambiguous titles can deter readers from engaging with your research. Ensure your title is straightforward and leaves no room for misinterpretation.
B. Overuse of Jargon
While technical terms are essential, excessive jargon can alienate readers who may not be familiar with the specific terminology. Strike a balance between precision and accessibility.
C. Lack of Alignment with Research Objectives
Your title should align seamlessly with the objectives and findings of your research. Avoid creating titles that misrepresent the core contributions of your study.
D. Lengthy and Complicated Titles
Lengthy titles can be overwhelming and may not effectively convey the essence of your research. Aim for brevity while maintaining clarity and informativeness.
E. Lack of Creativity and Engagement
A bland title may not capture the interest of potential readers. Inject creativity where appropriate and strive to create a title that sparks curiosity.
Ethical Considerations in Research Title Creation
- Avoiding Sensationalism and Misleading Titles
Ensure that your title accurately represents the content of your research. Avoid sensationalism or misleading language that may compromise the integrity of your work.
- Ensuring Accuracy and Integrity in Representing Research Content
Your title should uphold the principles of accuracy and integrity. Any claims or implications in the title should be supported by the actual findings of your research.
Crafting a captivating research title is a nuanced process that requires careful consideration of various factors. From clarity and relevance to creativity and ethical considerations, each element plays a crucial role in the success of your title.
By following the outlined strategies and avoiding common pitfalls for research title ideas, researchers can enhance the visibility and impact of their work, contributing to the broader scholarly conversation. Remember, your research title is the first impression readers have of your work, so make it count.
Related Posts
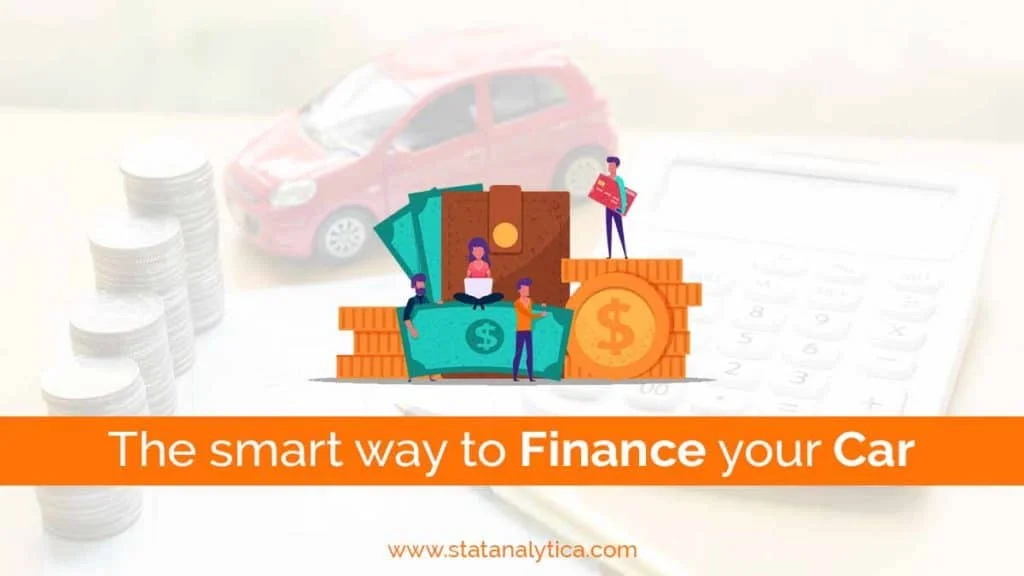
Step by Step Guide on The Best Way to Finance Car
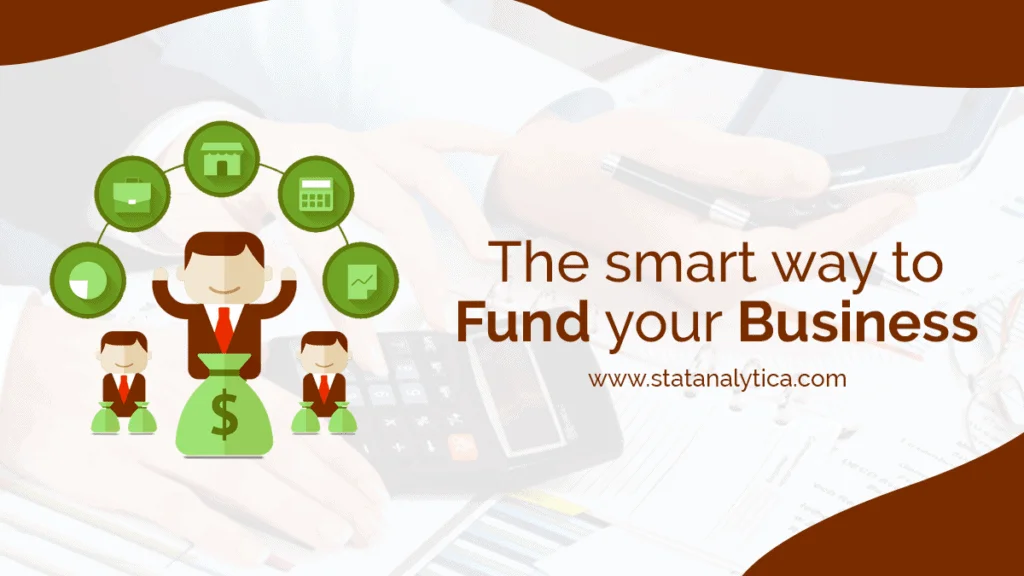
The Best Way on How to Get Fund For Business to Grow it Efficiently

Best 151+ Quantitative Research Topics for STEM Students

In today’s rapidly evolving world, STEM (Science, Technology, Engineering, and Mathematics) fields have gained immense significance. For STEM students, engaging in quantitative research is a pivotal aspect of their academic journey. Quantitative research involves the systematic collection and interpretation of numerical data to address research questions or test hypotheses. Choosing the right research topic is essential to ensure a successful and meaningful research endeavor.
In this blog, we will explore 151+ quantitative research topics for STEM students. Whether you are an aspiring scientist, engineer, or mathematician, this comprehensive list will inspire your research journey. But we understand that the journey through STEM education and research can be challenging at times. That’s why we’re here to support you every step of the way with our Engineering Assignment Help service.
What is Quantitative Research in STEM?
Table of Contents
Quantitative research is a scientific approach that relies on numerical data and statistical analysis to draw conclusions and make predictions. In STEM fields, quantitative research encompasses a wide range of methodologies, including experiments, surveys, and data analysis. The key characteristics of quantitative research in STEM include:
- Data Collection: Systematic gathering of numerical data through experiments, observations, or surveys.
- Statistical Analysis: Application of statistical techniques to analyze data and draw meaningful conclusions.
- Hypothesis Testing: Testing hypotheses and theories using quantitative data.
- Replicability: The ability to replicate experiments and obtain consistent results.
- Generalizability: Drawing conclusions that can be applied to larger populations or phenomena.
Importance of Quantitative Research Topics for STEM Students
Quantitative research plays a pivotal role in STEM education and research for several reasons:
1. Empirical Evidence
It provides empirical evidence to support or refute scientific theories and hypotheses.
2. Data-Driven Decision-Making
STEM professionals use quantitative research to make informed decisions, from designing experiments to developing new technologies.
3. Innovation
It fuels innovation by providing data-driven insights that lead to the creation of new products, processes, and technologies.
4. Problem Solving
STEM students learn critical problem-solving skills through quantitative research, which are invaluable in their future careers.
5. Interdisciplinary Applications
Quantitative research transcends STEM disciplines, facilitating collaboration and the tackling of complex, real-world problems.
Also Read: Google Scholar Research Topics
Quantitative Research Topics for STEM Students
Now, let’s explore important quantitative research topics for STEM students:
Biology and Life Sciences
Here are some quantitative research topics in biology and life science:
1. The impact of climate change on biodiversity.
2. Analyzing the genetic basis of disease susceptibility.
3. Studying the effectiveness of vaccines in preventing infectious diseases.
4. Investigating the ecological consequences of invasive species.
5. Examining the role of genetics in aging.
6. Analyzing the effects of pollution on aquatic ecosystems.
7. Studying the evolution of antibiotic resistance.
8. Investigating the relationship between diet and lifespan.
9. Analyzing the impact of deforestation on wildlife.
10. Studying the genetics of cancer development.
11. Investigating the effectiveness of various plant fertilizers.
12. Analyzing the impact of microplastics on marine life.
13. Studying the genetics of human behavior.
14. Investigating the effects of pollution on plant growth.
15. Analyzing the microbiome’s role in human health.
16. Studying the impact of climate change on crop yields.
17. Investigating the genetics of rare diseases.
Let’s get started with some quantitative research topics for stem students in chemistry:
1. Studying the properties of superconductors at different temperatures.
2. Analyzing the efficiency of various catalysts in chemical reactions.
3. Investigating the synthesis of novel polymers with unique properties.
4. Studying the kinetics of chemical reactions.
5. Analyzing the environmental impact of chemical waste disposal.
6. Investigating the properties of nanomaterials for drug delivery.
7. Studying the behavior of nanoparticles in different solvents.
8. Analyzing the use of renewable energy sources in chemical processes.
9. Investigating the chemistry of atmospheric pollutants.
10. Studying the properties of graphene for electronic applications.
11. Analyzing the use of enzymes in industrial processes.
12. Investigating the chemistry of alternative fuels.
13. Studying the synthesis of pharmaceutical compounds.
14. Analyzing the properties of materials for battery technology.
15. Investigating the chemistry of natural products for drug discovery.
16. Analyzing the effects of chemical additives on food preservation.
17. Investigating the chemistry of carbon capture and utilization technologies.
Here are some quantitative research topics in physics for stem students:
1. Investigating the behavior of subatomic particles in high-energy collisions.
2. Analyzing the properties of dark matter and dark energy.
3. Studying the quantum properties of entangled particles.
4. Investigating the dynamics of black holes and their gravitational effects.
5. Analyzing the behavior of light in different mediums.
6. Studying the properties of superfluids at low temperatures.
7. Investigating the physics of renewable energy sources like solar cells.
8. Analyzing the properties of materials at extreme temperatures and pressures.
9. Studying the behavior of electromagnetic waves in various applications.
10. Investigating the physics of quantum computing.
11. Analyzing the properties of magnetic materials for data storage.
12. Studying the behavior of particles in plasma for fusion energy research.
13. Investigating the physics of nanoscale materials and devices.
14. Analyzing the properties of materials for use in semiconductors.
15. Studying the principles of thermodynamics in energy efficiency.
16. Investigating the physics of gravitational waves.
17. Analyzing the properties of materials for use in quantum technologies.
Engineering
Let’s explore some quantitative research topics for stem students in engineering:
1. Investigating the efficiency of renewable energy systems in urban environments.
2. Analyzing the impact of 3D printing on manufacturing processes.
3. Studying the structural integrity of materials in aerospace engineering.
4. Investigating the use of artificial intelligence in autonomous vehicles.
5. Analyzing the efficiency of water treatment processes in civil engineering.
6. Studying the impact of robotics in healthcare.
7. Investigating the optimization of supply chain logistics using quantitative methods.
8. Analyzing the energy efficiency of smart buildings.
9. Studying the effects of vibration on structural engineering.
10. Investigating the use of drones in agricultural practices.
11. Analyzing the impact of machine learning in predictive maintenance.
12. Studying the optimization of transportation networks.
13. Investigating the use of nanomaterials in electronic devices.
14. Analyzing the efficiency of renewable energy storage systems.
15. Studying the impact of AI-driven design in architecture.
16. Investigating the optimization of manufacturing processes using Industry 4.0 technologies.
17. Analyzing the use of robotics in underwater exploration.
Environmental Science
Here are some top quantitative research topics in environmental science for students:
1. Investigating the effects of air pollution on respiratory health.
2. Analyzing the impact of deforestation on climate change.
3. Studying the biodiversity of coral reefs and their conservation.
4. Investigating the use of remote sensing in monitoring deforestation.
5. Analyzing the effects of plastic pollution on marine ecosystems.
6. Studying the impact of climate change on glacier retreat.
7. Investigating the use of wetlands for water quality improvement.
8. Analyzing the effects of urbanization on local microclimates.
9. Studying the impact of oil spills on aquatic ecosystems.
10. Investigating the use of renewable energy in mitigating greenhouse gas emissions.
11. Analyzing the effects of soil erosion on agricultural productivity.
12. Studying the impact of invasive species on native ecosystems.
13. Investigating the use of bioremediation for soil cleanup.
14. Analyzing the effects of climate change on migratory bird patterns.
15. Studying the impact of land use changes on water resources.
16. Investigating the use of green infrastructure for urban stormwater management.
17. Analyzing the effects of noise pollution on wildlife behavior.
Computer Science
Let’s get started with some simple quantitative research topics for stem students:
1. Investigating the efficiency of machine learning algorithms for image recognition.
2. Analyzing the security of blockchain technology in financial transactions.
3. Studying the impact of quantum computing on cryptography.
4. Investigating the use of natural language processing in chatbots and virtual assistants.
5. Analyzing the effectiveness of cybersecurity measures in protecting sensitive data.
6. Studying the impact of algorithmic trading in financial markets.
7. Investigating the use of deep learning in autonomous robotics.
8. Analyzing the efficiency of data compression algorithms for large datasets.
9. Studying the impact of virtual reality in medical simulations.
10. Investigating the use of artificial intelligence in personalized medicine.
11. Analyzing the effectiveness of recommendation systems in e-commerce.
12. Studying the impact of cloud computing on data storage and processing.
13. Investigating the use of neural networks in predicting disease outbreaks.
14. Analyzing the efficiency of data mining techniques in customer behavior analysis.
15. Studying the impact of social media algorithms on user behavior.
16. Investigating the use of machine learning in natural language translation.
17. Analyzing the effectiveness of sentiment analysis in social media monitoring.
Mathematics
Let’s explore the quantitative research topics in mathematics for students:
1. Investigating the properties of prime numbers and their distribution.
2. Analyzing the behavior of chaotic systems using differential equations.
3. Studying the optimization of algorithms for solving complex mathematical problems.
4. Investigating the use of graph theory in network analysis.
5. Analyzing the properties of fractals in natural phenomena.
6. Studying the application of probability theory in risk assessment.
7. Investigating the use of numerical methods in solving partial differential equations.
8. Analyzing the properties of mathematical models for population dynamics.
9. Studying the optimization of algorithms for data compression.
10. Investigating the use of topology in data analysis.
11. Analyzing the behavior of mathematical models in financial markets.
12. Studying the application of game theory in strategic decision-making.
13. Investigating the use of mathematical modeling in epidemiology.
14. Analyzing the properties of algebraic structures in coding theory.
15. Studying the optimization of algorithms for image processing.
16. Investigating the use of number theory in cryptography.
17. Analyzing the behavior of mathematical models in climate prediction.
Earth Sciences
Here are some quantitative research topics for stem students in earth science:
1. Investigating the impact of volcanic eruptions on climate patterns.
2. Analyzing the behavior of earthquakes along tectonic plate boundaries.
3. Studying the geomorphology of river systems and erosion.
4. Investigating the use of remote sensing in monitoring wildfires.
5. Analyzing the effects of glacier melt on sea-level rise.
6. Studying the impact of ocean currents on weather patterns.
7. Investigating the use of geothermal energy in renewable power generation.
8. Analyzing the behavior of tsunamis and their destructive potential.
9. Studying the impact of soil erosion on agricultural productivity.
10. Investigating the use of geological data in mineral resource exploration.
11. Analyzing the effects of climate change on coastal erosion.
12. Studying the geomagnetic field and its role in navigation.
13. Investigating the use of radar technology in weather forecasting.
14. Analyzing the behavior of landslides and their triggers.
15. Studying the impact of groundwater depletion on aquifer systems.
16. Investigating the use of GIS (Geographic Information Systems) in land-use planning.
17. Analyzing the effects of urbanization on heat island formation.
Health Sciences and Medicine
Here are some quantitative research topics for stem students in health science and medicine:
1. Investigating the effectiveness of telemedicine in improving healthcare access.
2. Analyzing the impact of personalized medicine in cancer treatment.
3. Studying the epidemiology of infectious diseases and their spread.
4. Investigating the use of wearable devices in monitoring patient health.
5. Analyzing the effects of nutrition and exercise on metabolic health.
6. Studying the impact of genetics in predicting disease susceptibility.
7. Investigating the use of artificial intelligence in medical diagnosis.
8. Analyzing the behavior of pharmaceutical drugs in clinical trials.
9. Studying the effectiveness of mental health interventions in schools.
10. Investigating the use of gene editing technologies in treating genetic disorders.
11. Analyzing the properties of medical imaging techniques for early disease detection.
12. Studying the impact of vaccination campaigns on public health.
13. Investigating the use of regenerative medicine in tissue repair.
14. Analyzing the behavior of pathogens in antimicrobial resistance.
15. Studying the epidemiology of chronic diseases like diabetes and heart disease.
16. Investigating the use of bioinformatics in genomics research.
17. Analyzing the effects of environmental factors on health outcomes.
Quantitative research is the backbone of STEM fields, providing the tools and methodologies needed to explore, understand, and innovate in the world of science and technology . As STEM students, embracing quantitative research not only enhances your analytical skills but also equips you to address complex real-world challenges. With the extensive list of 155+ quantitative research topics for stem students provided in this blog, you have a starting point for your own STEM research journey. Whether you’re interested in biology, chemistry, physics, engineering, or any other STEM discipline, there’s a wealth of quantitative research topics waiting to be explored. So, roll up your sleeves, grab your lab coat or laptop, and embark on your quest for knowledge and discovery in the exciting world of STEM.
I hope you enjoyed this blog post about quantitative research topics for stem students.
Related Posts
8 easiest programming language to learn for beginners.
There are so many programming languages you can learn. But if you’re looking to start with something easier. We bring to you a list of…
10 Online Tutoring Help Benefits
Do you need a computer science assignment help? Get the best quality assignment help from computer science tutors at affordable prices. They always presented to help…
Thesis Helpers

Find the best tips and advice to improve your writing. Or, have a top expert write your paper.
237 Top Technology Research Topics for Academic Papers

College and university students have many technology research topics to pick from when writing academic papers. That’s because technology evolves as the world changes. While some technological changes benefit humans and the environment, some have negative impacts.
For this reason, learners across levels have many topics to research and write about during their academic careers. What’s more, educators assign learners research projects with varying instructions. For instance, a professor can ask learners to write about their preferred technology topics. In that case, learners have the freedom to select their project topics.
Nevertheless, learners should select technology related topics that affect humans and the environment the most. They should also pick issues they find interesting to enjoy the research and writing process. What’s more, educators award learners top grades for selecting interesting topics whose research introduces relevant information into the sector. Here are some of the best titles to consider for research papers in science and technology.
Top Technology Research Paper Topics
This list comprises topic ideas that incorporate different technical aspects and their effects on human life.
- How computers will advance in the next decade
- What are the long-term impacts of living in a world of technological advancement?
- How technology affects child growth in the current world
- Describe the essential technological advancement today and its promises
- Explain how social media can create or solve problems in the world
- Do the internet and mobile phones make the world smaller or bigger?
- How technology is changing how people use devices with frustrating problems and glitches
- Is genetically engineering children morally wrong?
- Is there parallelism to social interactions with humans and machines?
- Can humans use technology in new ways to impact the world positively?
- How digital learning is changing the education system and schools
- Should the government censor the internet?
- Should current and potential employees give their employers access to their social media accounts?
- Should work from home become a norm, considering the current status of information technologies and internet availability?
- How will technology affect travel in the future?
- The future of auto-driving vehicles- Their pros and cons
- Should parents disclose genetic information to their children?
- Should employers and healthcare companies have access to genetic testing information?
- Using sequence human genes to predict possible future health risks- What are the limitations and benefits of this testing?
- Can genetically modified organisms solve the current hunger issues?
- Genetically modified and organic food- Which is the best option?
- Differentiating human brains and computers
- Accessing technological advances- Why this should be everyone’s right
- Should the world use under-the-skin identity chips?
- How is technology likely to advance in the next two decades?
- To what extent can new technological developments damage the world?
- How digital tools can instigate productivity
- Investigating the emerging opportunities in robotics
- The latest developments in software engineering and programming languages
- How information technology has impacted natural language processing
- Evaluating biotechnology and molecular information systems roles
- How machine learning exposes learners to recent life opportunities
- How human-computer interactions affect innovations
- Managing data during the era of 5G technology
- Emerging study fields in computer data science
- Analyzing how computing contributes to the development
- The evolution of computer graphics, animation, and game science
- Limitations of computer architecture studies in colleges
- Synthetic and computational biology development in research
- How artificial intelligence affects tedious and complex tasks
Learners can pick and develop these research topics about technology through a careful study and analysis of relevant information.
Topics about Technology and Health
Health should be humans’ top priority. If interested in health technology topics, here are brilliant ideas to consider for your research paper.
- CDC Milestone Tracker and its application in medical fields
- How humans can make the organ donation process faster and more convenient
- How technology can help in determining whether a person is dead
- Human limbs regeneration- Will it be possible in this lifetime?
- Using technology to rehabilitate individuals with lost limbs
- Is using animal tissues in humans ethical?
- Is embryonic stem cell necessary with the current technological innovations?
- Does the National Institute of Health need more funding grants for practical research projects?
- What is morbid obesity’s treatment?
- Should the government factor medical costs into the rehabilitation and research budget during wars?
- How technology has led to the surging diabetes cases
- Using embelin to prevent cancer
- How pesticides can help with cancer diagnoses
- Biotechnology and high-throughput screening
- Eliminating heat-resistant organisms using ultraviolet
- Effects of food processing technologies on bacteria in Aspalathus Linearis
- Biotechnology in farming and self-sufficient protein supply
- Evapotranspiration versus evaporation
- A southern blot and DNA cloning
- Personalized drugs and pharmacogenetics
- Pharmacogenetics in cancer medicines
- Can humans control their genetics?
- Understanding genetic engineering and gene therapy
- How beneficial is genetic engineering?
- Opportunities and dangers of genetic engineering
- Using nanotechnology to treat HIV
- Biotechnology and allergenic potential
- Biotechnology and whole-genome sequencing
- An overview of heavy metal tolerance and genes
- Food-borne illnesses and food biotechnology
Any student that finds technology and health interesting can pick a topic in this category. However, select a technology topic in this list if ready to invest time and effort in research and writing.
Hot Topics in Technology
Perhaps, you want a topic about technology for research paper that the audience will find irresistible to read from the beginning to the end. In that case, consider these ideas.
- An overview of software security types
- How to improve technological innovations patent rights
- How to eliminate stalking
- Distinguishing human perception from virtual reality
- How computer science interventions are changing the world
- Evaluating high-dimensional data modeling effectiveness
- What are the limitations of the computer science field?
- Effects of ethical hacking
- Are universities and colleges producing skilled computer scientists?
- Why are specialized banking systems critical?
- The best security measure- Fingerprint or a serial code?
- Programming languages development
- Computational thinking impact on science
- ID chips in human brains- An upcoming reality or fiction?
- Is computer game addiction a severe problem?
- What are the potential advancements of artificial intelligence?
- AI in health and medicine- Is its implementation a good idea?
- The Safety of medical applications
- Is digital voting risky?
- Can artificial intelligence obtain self-awareness?
- How safe are self-driving vehicles?
- How modern technologies and the internet ease outsourcing
- Is cryptocurrency a critical financial systems change or a buzz?
- Healthcare and cloud technologies for data management
- Discuss the latest technological advancements in cybersecurity
- Social media and privacy rights
- Can gene editing prevent or solve hunger and health problems?
- The popularity of streaming services
- How VPN services keep their users anonymous
- Will technology make traveling better?
- Incorporating information technologies in policy management
- Using IT to improve service delivery
- How IT makes advertising more authentic and appealing to consumers
- Next Generation Innovation in education systems
- WIFi connectivities in the developed countries
- How advanced information technologies help with the preservation of classified documents
- How climate and weather affect internet connectivity and strength
- The essence of adopting E-Waste management systems
- Can humans develop functional intelligent vehicle transport systems?
- Why do developing countries have fewer IT universities and colleges?
Learners should pick these research topics on technology and develop them with extensive research to write winning papers.
Interesting Technology Topics
Maybe you want to write a research paper about a topic that will instantly capture your reader’s attention. If so, consider any of these exciting research paper topics on technology.
- Latest trends in content marketing and information technology
- Human resource and information management systems
- Analyzing object tracking with radial function systems
- The development of Bluetooth phone technology
- Ethical challenges and new media technologies
- Online enterprise planning- Is it effective?
- Computer development over the last two decades
- How social media enhances communication strategies
- Has new media rendered newspapers obsolete?
- Analyzing modern communication structures
- Using social media to create ads with ease
- How social media affects personal contact
This list has some of the best topics for research projects in the technology field. Nevertheless, take your time to research your idea to develop a winning essay.
Interesting Information Technology Topics
Do you want to write a research paper about an IT topic? If yes, this category has a sample title you’ll find interesting to explore.
- How effective is unlimited data storage?
- Does the human brain and computers have a blurred line between them?
- Ethical objection for DNA information storage
- Is entertainment technology good or bad?
- How Google affects young people’s attention lifespan
- How digital reading differs from print reading
- Are traditional research skills necessary in the current world?
- Should schools and parents encourage or discourage media use among children?
- Should the government regulate sites like Wikipedia because their information may not be credible?
- How books and blogs compare
- Does Google provide the best information by preferring its brand?
- How using the internet affects the human brain
- Are people losing the intelligence they develop via conventional reading and research in the current digital age?
- How important is teaching learners to use social media, iPads, and Smart Boards?
- Should teaching incorporate the latest technologies?
- How Google search has changed humans
- Using technology to gauge intelligence
- How online format encourages skimming instead of information digestion
- How technology affects how people read
- Is using the internet to find information terrible or good?
All these are exciting research proposal topics in information technology. If the educator approves your proposal to work on any of these topics, take your time to research it extensively to develop a brilliant paper.
Computer Science and Technology Topics
Computer science is a field with many research topics relating to technology. Here are exciting ideas to explore in this field.
- Are humans yet to invent more computer languages?
- How will humans react if computers start doing most of the things they do?
- How robots are changing the healthcare sector
- How to improve the internet
- What will happen to the internet next?
- How good or bad is virtual reality?
- How virtual reality will change the education sector
- Describe virtualization in computers and technology
- Explain how virtualization is changing entertainment
- Describe the industries that machine learning will affect the most
- Explain the importance of machine learning
- Describe machine learning
- Device protection when open-source is becoming popular in computer science
- Can robots become more intelligent and like humans after reinforcing learning?
- Effects of moving things to the cloud
- Hardware and software borderline in the field of computer learning
- What is machine learning’s future?
- How big data and bioinformatics will change biology
- Which is the essential computer science for the future?
Select a topic in this category if you love researching and writing about computer science as a field in technology.
Controversial Topics in Technology
Humans are developing something new almost every day. However, some technological developments are controversial due to their potential impact on human life and the world. Here are some of the controversial technology topics to consider for research papers and essays.
- How the revolution in communication technology affects people
- Can virtual reality replace actual reality?
- How cloud technologies have changed data storage
- How smartphones usage has reduced live communications
- How modern technologies will change teaching
- Analyzing construction recession and low spending by construction companies on IT
- Technologies that humans use to explore other planets
- How dangerous are cell phones?
- How media technology affects child development
- 3D printing technology application in complex building forms’ production
- How technology improves lesson planning
- How technology influences the educational system
- Green technologies application in engineering, construction, and architecture
- Intelligent technologies and materials in road building
- The technological age turns humans into zombies
- Analyzing the drawbacks and advantages of unmanned aerial vehicles’ usage on construction sites
- How media technologies affect teenagers’ physical development
- Should humans use technology to colonize other planets?
- Should developed countries care about technology accessibility worldwide?
- Does technology create more problems while solving others?
- Does technology oppose nature?
- How is technology changing people?
- Does technology make people dumber or more intelligent?
- Does technology make people dependent on it or lazier?
- Technology impact on human practice
- Is engineering a child genetically morally wrong?
- Describe the long-term effects of a technological world
- How are humans changing the world using technology?
- How are new technologies affecting the world negatively?
- How is technology likely to change humans in the next twenty years?
- How digital learning is changing education and schools
- Drone warfare- Is it a possibility?
- Are digital tools making humans less or more productive?
- Using technology to develop alternative energy forms
- Does the government invade privacy by using law enforcement cameras?
- Can humans use technology to improve their interactions with animals?
Select and write about a topic in this category if you love working on controversial ideas. Nevertheless, most issues require research to develop persuasive papers.
Technology Persuasive Speech Topics
Perhaps, you want to research and write a speech on a persuasive topic. In that case, consider these ideas.
- Communication masts locations should be away from people’s houses
- Programming courses should be mandatory in colleges
- Social networks should verify user’s identity
- Every social network should implement two-step verification
- Kids should not use social media
- Internet pop-up ads and spams are the same
- Smartphone addiction- Is it a disease?
- Self-driving cars are not suitable for humans on busy roads
- E-books should replace conventional books
- Kids should not play violent computer games
- Internet gambling requires strong regulations
- Humans should avoid overreliance on smartphones and computers
- Desktop computers are no longer fashionable
- Computer games are making kids stupid
- Governments should censor the internet
- Workers should use digital tools more often to boost workplace productivity
- The world needs more technological advancements
- Why governments should promote digital learning
- Technology research deserves more government funding
- Hybrid cars save energy
- Car manufacturers should consider the environment when designing vehicles
- All children should learn to use smartphones and computers
- Search engines are killing human brain libraries
- Humans should use drones for non-military and military purposes
- Smart notebooks are replacing papers
This category has trending topics in technology that you can explore in your project. Nevertheless, most of them are argumentative technology topics that require some convincing. That means you need time and skills to research and develop your topic.
Educational Technology Topics
Perhaps, you’re interested in a topic that touches on education and technology. In that case, consider these ideas for your research project.
- Incorporating computational thinking in education
- How technology is changing the classroom practice
- How technology changes learning outcomes
- Is there evidence to prove that educational technology adds value for money?
- What enabling factors support or inhibit educational technology integration?
- How educational technology programs can facilitate learning change
- Using mobile phones for teacher development videos and classroom audio
- How tablets and eReaders can support literacy in early developmental stages
- Do programs that use technology have better educational outcomes?
- Can change theory explain how technology will improve educational outcomes?
- What technologies can be more cost-effective in the educational sector?
- How appropriate is the current technology for technical training?
- Describe effective informal and formal technologies for providing peer support among teachers
- Technologies for engaging the school management, headteachers, and the entire school community
- Does educational program evaluation exceed technology access and output?
Whether you’re looking for educational or medical laboratory technology research topics, you have many ideas to consider. Each title in this list can serve as an example to inspire you to develop a unique topic for your paper.
Get Professional Help with Researching and Writing
Do you have difficulties researching and writing a research paper? Maybe you’re unable to select the best topic for your project. In that case, seek thesis help online. We’re a highly qualified team providing superior writing help to learners across academic fields and levels. Our crew has writers providing high quality assistance with topic selection and writing. Regardless of how complex your research paper demands seem, we’re ready to help you.
We offer the best rated research writing assistance at affordable prices. We want you to have an easy time completing your research project. Therefore, don’t struggle to compose a paper and end up with a poor grade when experts can help you score A+ without breaking a sweat. Place an order for your research paper with us, and we will be glad to assist you.
Contact us now to get cheap and quality assistance with your research paper!
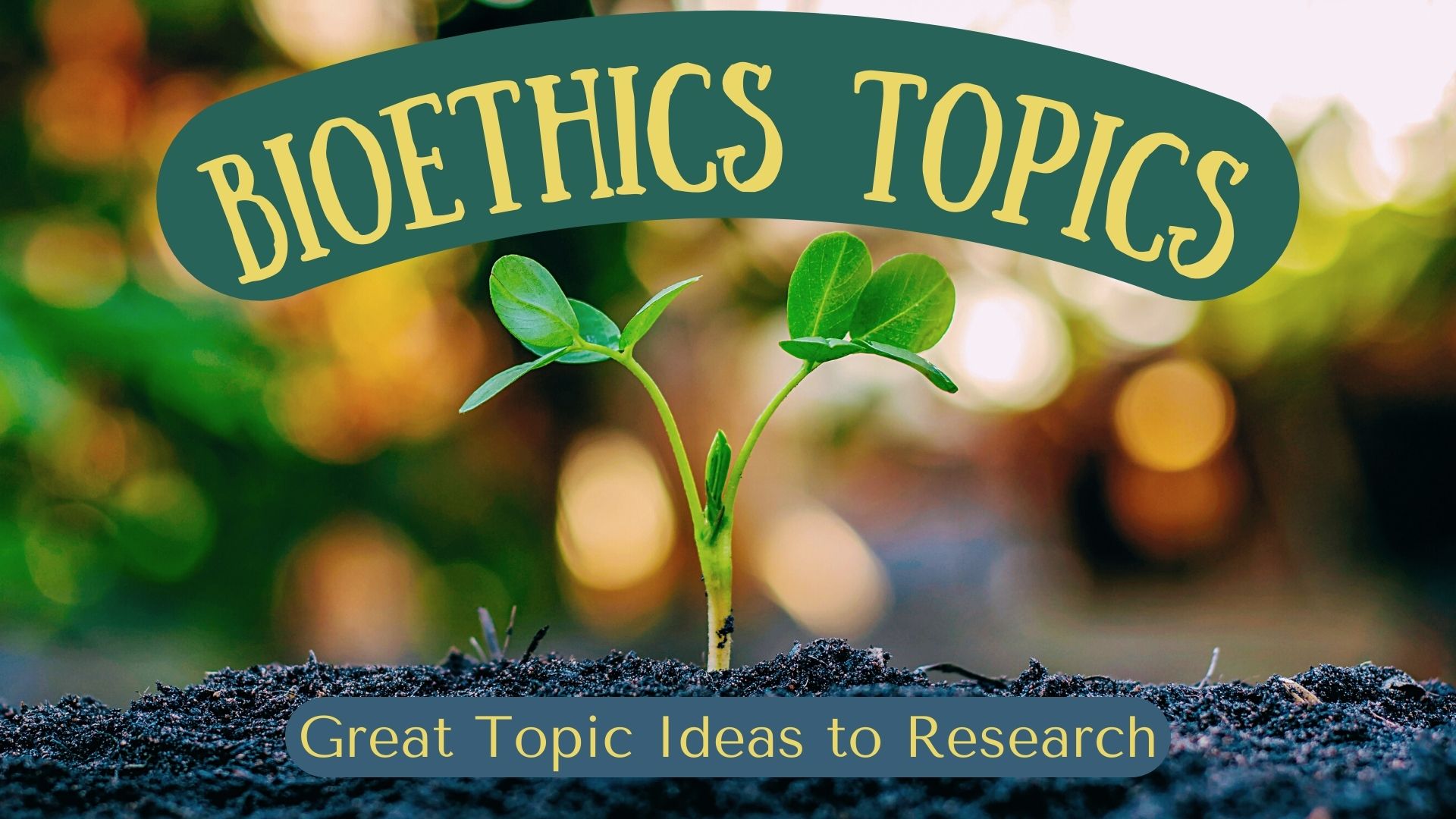
Make PhD experience your own
Leave a Reply Cancel reply
Your email address will not be published. Required fields are marked *
makoshika.com
Completing Strong Essays
List Of 12 Great Research Paper Titles About Technology
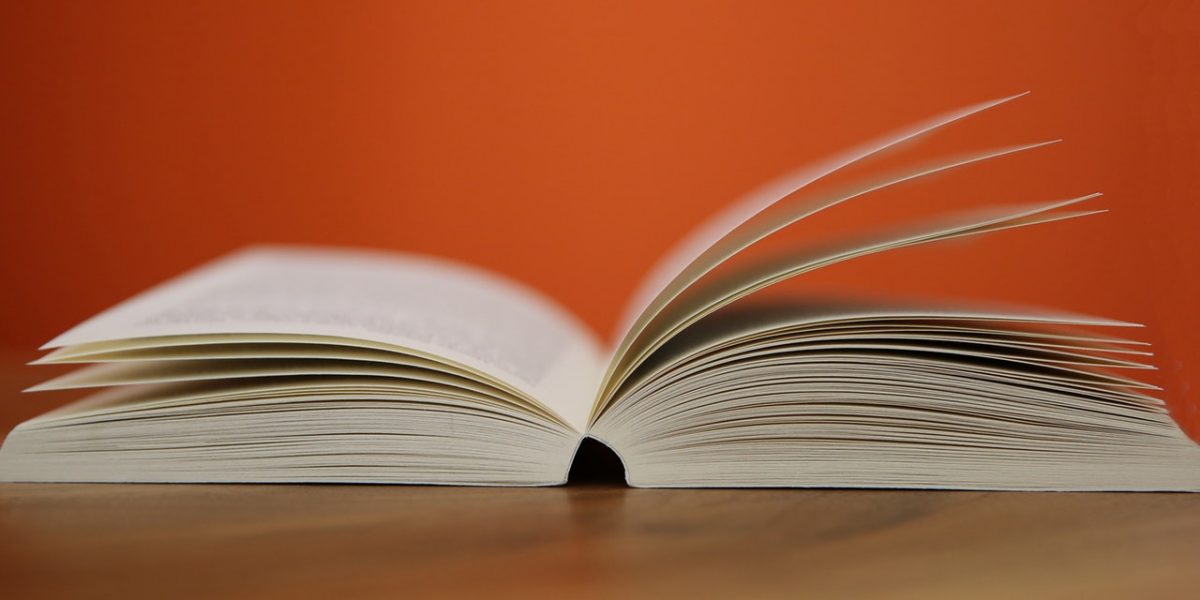
Writing a research paper about technology can be difficult if you don’t know where to start. Knowing where to start can be the difference between a good grade and a bad grade. The best place to start is with the titles. Thinking of a topic is extremely easy and at time it can be fun. Try to think of something that you like, this way you will be positive throughout the project. Of course you can pick something you don’t quite understand and do the research that is required. Read this article to find out the different tips and tricks that are out there, that you can use to your advantage. .
Take a look
17% OFF on your first order Type the code 17TUDENT
- What important technologies can we use to solve global problems?
- How has technology help people with disabilities
- Should we create an artificial intelligent computer?
- What are the negatives of playing computer games for long periods of time?
- What will the future of technology look like in the next few centaury’s?
- What will the future of technology look like in the next few decades?
- Should we genetically modify animals to solve world hunger problems?
- What are the long term effects of living in a world with advanced technology, would it be a positive thing?
- What are the advantages of living in a world with advanced technology?
- What are the negatives of living in a world with advanced technology?
- What are the long term effects of using a computer for 8 hours a day?
- Will we ever have the technology for interstellar space travel?
These are some topics presented by Mypaperdone.com that you can use. Take your time thinking of a title that you see yourself doing, since you are going to enjoy writing the project a lot more. Picking a topic that you already have knowledge about is going to be a huge advantage, since you have to do less research on the matter. However, you can pick a project that you have no clue about and do the research that is required. Take notes as you go alone, since this can help you pick out a topic.
Leave a Reply Cancel reply
Your email address will not be published. Required fields are marked *
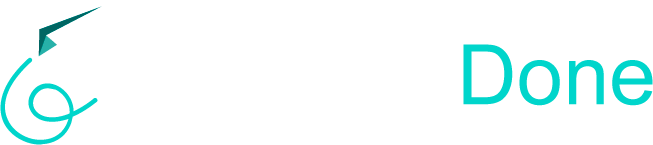
- Services Paper editing services Paper proofreading Business papers Philosophy papers Write my paper Term papers for sale Term paper help Academic term papers Buy research papers College writing services Paper writing help Student papers Original term papers Research paper help Nursing papers for sale Psychology papers Economics papers Medical papers Blog
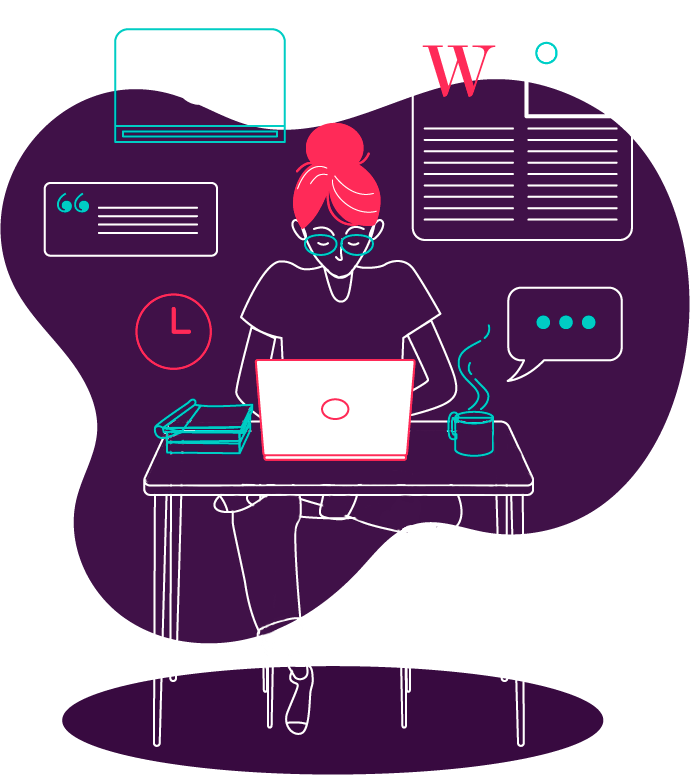
177 of the Finest Technology Research Topics in 2023
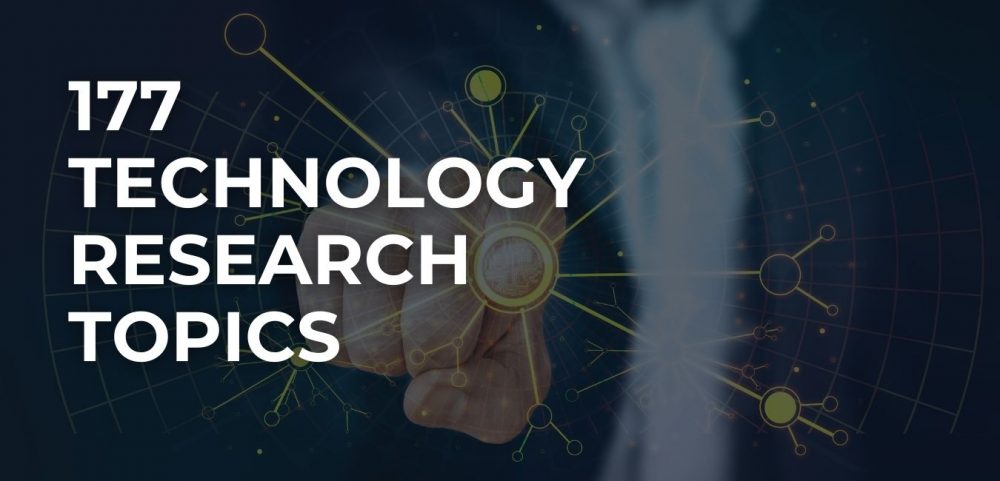
We live in a technological era, and you can be sure of being asked to write a technology-oriented paper. Despite the contrary opinion that this is one of the most complicated tasks, students can comfortably develop a professional topic about technology for writing a research paper . In a technology research paper, students are tasked with exploring the various aspects of technology, such as inventions, their impacts, and emerging challenges. Since almost every sphere of life encompasses technology, it is nearly impossible to miss out on a technology topic or two.
High-quality technology research paper topics should: Demonstrate your understanding of various technological concepts Portray your ability to apply these concepts to real-life situations Show how technology impacts society.
The task of coming up with technology topics involves the following stages:
- Extensively reading on technology
- Identifying distinct technological aspects
- Brainstorming on potential technology titles for your paper
- Consulting your supervisor
The last step is essential in ensuring that your topic aligns with the academic standards of your institutions. Have a look at the following writing prompts for your inspiration:
Medical laboratory Technology Research Topics
- The role of technological innovations in the medical laboratories
- Cost-saving technologies in the field of medical laboratory
- A comparative analysis of the current techniques in the microbial examination
- The role of technology in the isolation and identification of nematodes
- The effects of 5G on the study of cancerous cells
- Evaluating the concentration of electrolytes using technology
- Describe the various parameters used in biochemical reactions
- A comparative analysis of the activities of cells under a light microscope
- Assess the various technologies used to view microscopic organisms
- An evaluation of the role of technology in combating COVID-19
Interesting Information Technology Topics
- Challenges facing cloud computing and virtualization
- Various Federal information standards that affect information technologies
- Discuss the various identity and access management practices for information technologies
- Why the male dominates the field of computational science
- Analyze the various cybersecurity issues arising
- Evaluate the various challenges associated with software research
- Why is the field of networking prone to attacks?
- Health issues arising from the use of biometrics in companies
- Why data entry is attracting a large number of interested parties
- The role of the Internet of Things is transforming the world.
Argumentative Technology Topics
- Why mobile devices can be both instruments and victims of privacy violations
- Why PINs and passwords for mobile devices are a security threat
- The impact of downloading malware disguised as a useful application
- Reasons why out-of-date operating systems are a threat to your computer’s security
- Why it is not advisable to use wireless transmissions that are not always encrypted
- Changes in workflow and project management arising from technological advancements
- The best method to develop and implement cloud solutions for companies
- The cost of having cloud engineers and support professionals
- The role of workplace monitoring in interfering with people’s privacy
- Why information technology laws vary from one country to another
Trending Topics in Technology
- Why technology is essential for an informed society
- The impact of freedom of speech on social networking sites
- Was Facebook justified in blocking Donald Trump from its platform?
- Ethical challenges arising from the new technological innovations
- Why it is not possible to achieve social media privacy
- The impact of online learning sites on the quality of workplace professionals
- Are electric cars the future of the world
- Reasons why technology is essential in developing coronavirus vaccines
- Discuss the various aspects of the Internet of Behaviours (IoB)
- Strides made in the development of intelligent process automation technologies
Hot Research Proposal Topics in Information Technology
- Discuss the considerations in developing human augmentation technologies
- Will big data analytics survive in the future?
- Is it possible to achieve a paper-free world?
- Long-term effects of over-dependence on technology
- Is technology solving world problems or creating more of them?
- What is the impact of children growing up in a technology oriented world?
- How was social media responsible for the chaos at the US Capitol?
- Is it right for governments to monitor and censor citizen’s access to the internet?
- The impact of texting and calling on family relationships
- What are the implications of depending on online thesis help?
Want to get an A+ grade? Try our college paper writing service and discover the benefits of high-quality and cheap paper writing help.
Top-notch Research Paper Topics on Technology
- The impact of Genetically Modified organisms on the health of a population
- Compare and contrast the functioning of the human brain to that of a computer.
- The role of video games on a person’s problem-solving skills
- Where is technology taking the world in the next ten years?
- What digital tools make people less productive?
- What censorship mechanisms are needed to control people’s behavior on the internet?
- The impact of digital learning on schools
- Why is genetic testing essential for couples?
- Discuss the ethical implications of mechanical reproduction
- Discuss the role of innovations in finding treatment for terminal diseases
Latest Research Topics About technology
- The impact of computers in academic research
- Why artificial intelligence may not be the best option for our daily lives
- Should parents restrict the amount of time spent on the internet by their kids?
- What are the legal and moral implications of digital voting?
- Is augmented reality the new way of online shopping?
- Discuss the challenges that arise from game addiction
- Evaluate the safety of VPNs in a global enterprise
- Why is streaming becoming the best option for church services?
- Discuss the efficiency of working from home versus physically going to the workplace
- The effects of computer-generated imagery in films and games
Controversial Technology Topics
- Does online communication make the world bigger or smaller?
- What is the ethical implication of having ID chips in our brains?
- Should families use gene editing for coming up with children of desirable qualities?
- Are the cybersecurity laws punitive enough?
- Is cryptocurrency turning around the financial industry for the worst?
- Are self-driving vehicles safe on our roads?
- Is it possible to attain self-awareness using Artificial Intelligence technologies?
- The risk of x-rays on a person’s health
- Is it possible for robots to live peacefully with humans?
- Compare and contrast between machine learning and natural language processing
Impressive Technology Topic Ideas for High School
- The impact of developing autonomous cars using computer vision applications
- Discuss the interconnection between the internet of things and artificial intelligence
- The effects of ultra-violet technologies in the health industry
- The impact of communication networks on people’s attitudes
- The role of internet technologies on marketing and branding
- How has the world of music changed with the emergence of video editing technologies?
- Describe the psychology behind video blog communication
- Effective ways of maintaining privacy in social media
- Is it possible to live without mass media in the world?
- The impact of technology on the morality of the world in the 21 st century
Educational Technology Topics
- Why is technology relevant in advancing scientific research?
- Discuss how computational thinking is shaping critical thinking among students
- What is the effect of professional learning for college students?
- The role of virtual reality in helping students understand complex concepts
- Is global learning through technology watering down education standards?
- Discuss various energy sources to support technology use in education
- Is the architecture of learning systems inclusive enough?
- Discuss the impact of connectivity for schools & learning, esp. in rural environments
- The role of data centers in education
- Is it possible to develop sufficient national capacities related to science, technology, and innovation?
Updated Technology Related Topics in Agriculture
- The role of soil and water sensors in improving crop yields
- Why farmers rely on weather tracking technologies for their farming activities
- The significant role of satellite imaging in agricultural activities
- How do farmers use pervasive automation technologies for their farms?
- The effect of mini-chromosomal technologies on agriculture
- Why vertical agriculture is the future of agriculture
- Conditions necessary for hydroponics in developed nations
- The impact of agricultural technologies in ensuring stable food supply
- How agricultural technologies can be used to ensure decreased use of water
- Using agricultural technologies to enhance worker safety on the farm
Top Technology Persuasive Speech Topics
- An analysis of digital media outreach and engagement in workplaces
- What are the challenges experienced in distance learning
- Describe personalized and adaptive learning platforms and tools
- Should computer viruses count as life?
- Describe the connection between human perception and virtual reality
- What is the future of computer-assisted education in colleges?
- Analyze the high dimensional data modeling procedure
- Evaluate the imperative and declarative languages in computer programming
- Analyze how the machine architecture affects the efficiency of the code
- What are the discrepancies in different languages for parallel computing?
Latest Controversial Topics in Technology
- Do you think computational thinking affects science?
- An overview of the phishing trends in the recent past
- How are sensor networks a threat to one’s privacy?
- Compare and contrast lithium-ion and lithium-air batteries.
- Can hydrogen replace all other energy sources in the future?
- Discuss the future of tidal power: Will it persist or become extinct?
- Why robots are a threat to the survival of humanity
- Analyze the effectiveness of small nuclear reactors in the wake of climatic change
- An overview of the different types of renewable energy technologies in the world
- Are drones a threat to security or a potential security mechanism?
Hot Topics in Technology
- Discuss the impacts of new technologies on food production and security
- The effectiveness of 3D printing for medical products
- What is the ethical argument behind the production of artificial body organs?
- Discuss the role of genetic engineering in medicine
- Challenges associated with the development of telemedicine
- Conduct a case study analysis on the effectiveness of genome editing
- Discuss the role of nanotechnology in cancer treatment
- The role of virtual reality in medical schools
- Discuss the effectiveness of wireless communication technologies for teenagers
- How safe are you when connected to a wireless network?
Science and Technology Topics
- Analyze the security threats associated with pharmaceutical technologies
- An overview of the chip technology in the practice of medicine
- Compare and contrast between electric cars and hybrid cars
- Why are personal transportation pods the future of transport
- Threats and solutions to cell phone use during driving
- Effects of scientific innovations on the world
- Are water-fueled cars a future fantasy or reality?
- The role of robotics in food packaging
- Modern solar system innovations
- The role of smart energy in combating global warming
Top-Notch Research Topics on Technology
- An overview of the different operating systems
- The role of theoretical computer science
- Discuss the development of computer graphics
- What are the loopholes in block-chain technology?
- Why banking systems need extra security measures
- What is the future of cyber systems?
- Ways of protecting your password from hackers
- The role of ICT in new media technologies
- How to deal with cyberbullying from Twitter
- The future of interpersonal communication with the rise of social media
Researchable Topics About Technology
- Factors that lead to viral messages on Twitter
- Freedom of speech and social media
- Activism in the wake of new media
- Discuss the psychology behind advertising techniques
- Interactive media technologies
- How has the internet changed communication networks?
- Role of media during pandemics
- Ethics in internet technologies
- The persistence of newspapers in the digital age
- Impact of technology on lifestyle diseases
Bonus Technology Topic Ideas
- Agricultural biotechnology
- Gene therapy
- Development of vaccines
- Genome sequencing
- Food processing technologies
- Technology and drugs
- Recommended systems
Are you stuck and need a sample paper to jumpstart your writing? Our help with the research paper service is all you need! Get your college paper done by experts at affordable rates today.
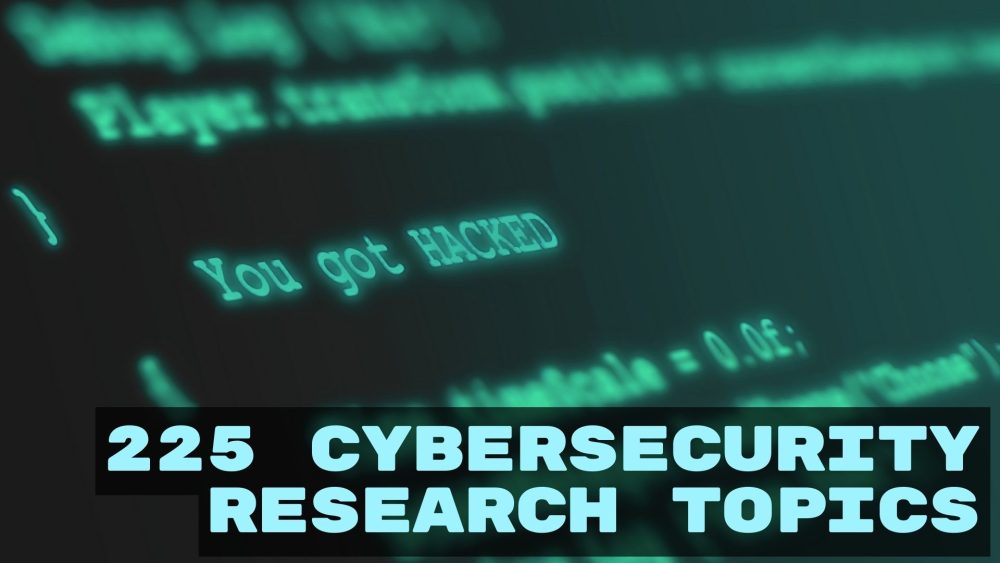
Leave a Reply Cancel reply
Your email address will not be published. Required fields are marked *
Save my name, email, and website in this browser for the next time I comment.
Terms & Conditions Loyalty Program Privacy Policy Money-Back Policy
Copyright © 2013-2024 MyPaperDone.com

Top 151+ Great Quantitative Research Topics For STEM Students
Are you a STEM enthusiast eager to dive into quantitative research but uncertain about the best topics to explore? Look no further! In this comprehensive guide, we’ll navigate through the top 27+ Quantitative Research Topics for STEM Students.
There are we give the best topics for future scientists, engineers, and math whizzes! Are you curious about diving into the fantastic world of quantitative research? Well, you’re in for an exciting way! Today, we’re going to explore some super cool Quantitative Research Topics for STEM Students like you. But first, what’s all this talk about “quantitative research”? Don’t worry; it’s not as tricky as it sounds!
Quantitative research simply means using numbers and data to study things. For example, solving a math problem or conducting a science experiment where you count, measure, or analyze stuff to learn more. Cool, right? Now, let’s talk about STEM. No, not the plant stem, but STEM subjects—science, Technology, Engineering, and Mathematics. These subjects are like the crucial part of knowledge!
So, here’s the exciting part! We’ve got a bunch of fascinating topics lined up for you to explore in these STEM fields using numbers, stats, and math. From studying how robots help doctors predict climate change to finding ways to make renewable energy work better in cities, these topics will make your brain more creative!
Also Like To Know: Sk Project Ideas
Table of Contents
What Is Experimental Quantitative Research Topics For STEM Students
Experimental quantitative research topics for STEM students involve conducting investigations using numbers and measurements to find answers to questions related to science, technology, engineering, and mathematics. These topics help students gather data through controlled experiments and use mathematical analysis to understand how things work or solve problems in subjects like biology, physics, chemistry, or mathematics. For example, they might explore topics like testing how different temperatures affect plant growth or analyzing the relationship between force and motion using simple experiments and numbers.
How Do You Identify A Quantitative Research Title?
Here are 7 easy steps to identify a quantitative research title:
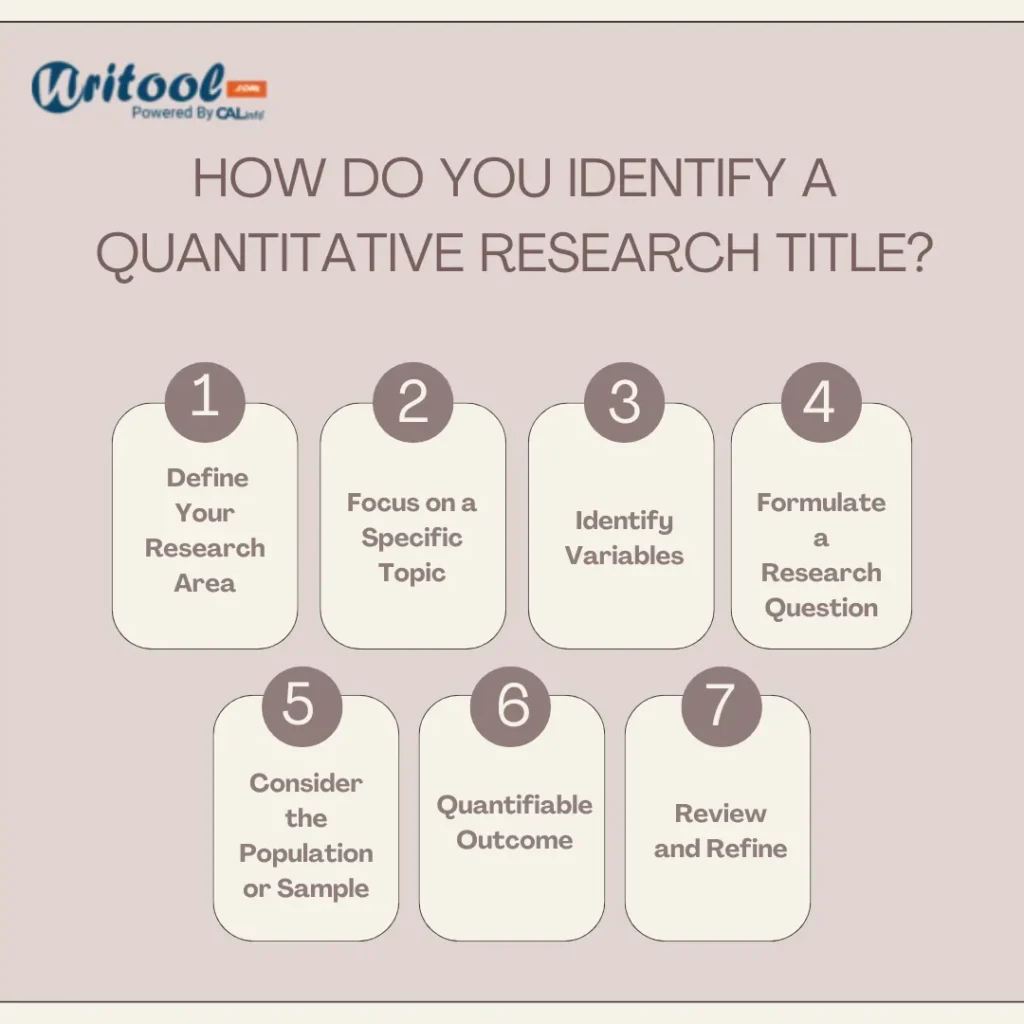
1. Define Your Research Area
Start by identifying the general subject or field you want to study. For instance, it could be related to science, education, psychology, etc.
2. Focus on a Specific Topic
Narrow down your field to a particular region or issue. For instance, if you’re keen on brain research, you should zero in on the impacts of web-based entertainment on teens’ psychological wellness.
3. Identify Variables
Determine the variables or factors you want to measure or investigate. In quantitative research, these are typically measurable quantities or numerical data.
4. Formulate a Research Question
Develop a clear and concise research question that reflects what you want to study. Ensure it is specific and can be addressed using quantitative methods.
5. Consider the Population or Sample
Determine the population you want to study or the sample you’ll collect data from. This will help shape the scope of your research.
6. Quantifiable Outcome
Guarantee that the exploration title recommends a result that can be estimated mathematically. Quantitative exploration means assembling mathematical information and investigating it genuinely.
7. Review and Refine
After forming a speculative title, survey it to guarantee it aligns with the examination targets, is clear and concise, and precisely mirrors the focal point of your review. Make any essential refinements to further develop clarity and accuracy.
List of Best 127+ Great Quantitative Research Topics For STEM Students
Here are the 127+ Great Quantitative Research Topics For STEM Students:
Best Mathematics Quantitative Research Topics For STEM Students
- Applications of Machine Learning in Mathematical Problem Solving
- Chaos Theory and Its Applications in Nonlinear Systems
- Algorithmic Trading Strategies and Mathematical Modeling
- Data Compression Techniques: Efficiency and Accuracy Trade-offs
- Exploring Applications of Topological Data Analysis
- Analyzing Random Matrix Theory in Statistical Physics
- Mathematical Models for Climate Change Predictions
- Analyzing Cryptocurrency Trends Using Mathematical Models
- Investigating Mathematical Models for Social Networks
- Studying Mathematical Foundations of Quantum Computing
Easy Quantitative Research Topics For STEM Students In Physics
- Quantum Entanglement and Its Applications in Communication
- Plasma Physics: Understanding Fusion Reactors
- Superconductivity and Its Practical Applications
- Statistical Mechanics in Complex Systems
- Applications of String Theory in Cosmology
- Gravitational Wave Detection and Interpretation
- Quantum Field Theory and Particle Interactions
- Quantum Computing: Designing Error-Correcting Codes
- Analyzing Exoplanet Data Using Astrophysical Models
- Studying Black Hole Physics and Information Paradox
- Computational Chemistry for Drug Design and Discovery
- Quantum Chemistry: Exploring Molecular Properties
- Applications of Nanomaterials in Renewable Energy
- Analyzing Chemical Reaction Kinetics
- Environmental Impact Assessment of Chemical Pollutants
- Polymer Chemistry: Designing Advanced Materials
- Studying Catalysis and Surface Chemistry
- Exploring Electrochemical Energy Storage Systems
- Bioinorganic Chemistry: Metalloprotein Modeling
- Investigating Supramolecular Chemistry for Functional Materials
Biology Quantitative Research Topics For STEM Students
- Systems Biology: Modeling Cellular Signaling Networks
- Computational Neuroscience: Brain Network Analysis
- Population Genetics and Evolutionary Dynamics
- Mathematical Modeling of Infectious Diseases
- Studying Protein Folding Using Computational Methods
- Ecological Niche Modeling for Biodiversity Conservation
- Quantitative Analysis of Gene Regulatory Networks
- Metagenomics: Analyzing Microbial Communities
- Bioinformatics Applications in Personalized Medicine
- Integrative Biology: Understanding Multi-Omics Data
Engineering
- Robotics and Autonomous Systems: Motion Planning Algorithms
- Finite Element Analysis for Structural Engineering
- Machine Learning in Image Processing and Computer Vision
- Control Systems Engineering in Autonomous Vehicles
- Renewable Energy Grid Integration and Optimization
- Optimization of Transportation Networks
- Analyzing Fluid Dynamics in Aerospace Engineering
- Materials Science: Quantum Mechanics in Materials Design
- Sustainable Infrastructure Planning and Design
- Cyber-Physical Systems: Security and Resilience
Computer Science Quantitative Research Topics For STEM Students
- Big Data Analytics: Scalable Algorithms for Data Processing
- Natural Language Processing for Sentiment Analysis
- Blockchain Technology: Security and Consensus Algorithms
- Understanding How Quantum Computers Solve Problems
- Creating AI Models that Explain Decisions for Help in Making Choices
- Protecting Privacy While Mining Data
- Keeping Networks Safe: Spotting Intruders
- Making the Most of Cloud Computing: Sharing Resources Better
- Humans and Robots Working Together Better
- Improving How We Keep Secrets Safe with Quantum Cryptography
Earth and Environmental Sciences
- Predicting How Weather Will Change in Different Areas
- Using Maps and Data to Study the Environment
- Managing Water and Predicting How Much We’ll Have
- Looking at Pictures from Far Away to Watch the Environment
- Studying Earthquakes and Where They Happen
- Learning About the Ocean and How It Affects Weather
- Checking How Green Energy Projects Affect the Environment
- Measuring Soil Damage and How Nutrients Move
- Looking at Air Quality and Figuring Out What’s Making It Bad
- Seeing How Much Nature Helps Us Using Numbers
Agriculture and Food Sciences
- Precision Agriculture: Using Data Analytics for Crop Management
- Genetics and Genomics in Crop Improvement Strategies
- Quantitative Analysis of Food Supply Chains
- Agricultural Policy Analysis and Economic Modeling
- Nutritional Analysis Using Quantitative Methods
- Modeling Pesticide Use and Environmental Impact
- Aquaculture: Optimization of Fish Farming Practices
- Soil Fertility Modeling and Nutrient Management
- Food Safety Assessment Using Quantitative Techniques
- Sustainable Agriculture: Systems Modeling and Optimization
Health Sciences and Medicine: quantitative research topics in nursing
- Epidemiology: Modeling Disease Transmission Dynamics
- Healthcare Analytics: Predictive Modeling for Patient Outcomes
- Pharmacokinetics and Drug Dosage Optimization
- Health Informatics: Quantitative Analysis of Electronic Health Records
- Medical Imaging Analysis Using Quantitative Techniques
- Health Economics: Cost-Benefit Analysis of Healthcare Policies
- Genomic Medicine: Analyzing Genetic Data for Disease Risk Prediction
- Public Health Policy Evaluation Using Quantitative Methods
- Biostatistics: Designing Clinical Trials and Statistical Analysis
- Computational Anatomy for Disease Diagnosis and Treatment
Psychology and Social Sciences
- Quantitative Analysis of Social Network Dynamics
- Behavioral Economics: Decision-Making Models
- Psychometrics: Measurement Models in Psychological Testing
- Quantitative Study of Human Cognition and Memory
- Social Media Analytics: Sentiment Analysis and Trends
- Sociology: Modeling Social Movements and Cultural Dynamics
- Educational Data Mining and Learning Analytics
- Quantitative Research in Political Science and Policy Analysis
- Consumer Behavior Analysis Using Quantitative Methods
- Quantitative Approaches to Studying Emotion and Personality
Astronomy and Astrophysics
- Cosmic Microwave Background Radiation: Analyzing Anisotropies
- Time-domain Astronomy: Statistical Analysis of Variable Stars
- Gravitational Lensing: Quantifying Distortions in Cosmic Images
- Stellar Evolution Modeling and Simulations
- Exoplanet Atmosphere Characterization Using Quantitative Methods
- Galaxy Formation and Evolution: Statistical Approaches
- Multimessenger Astronomy: Data Fusion Techniques
- Dark Matter and Dark Energy: Analyzing Cosmological Models
- Astrophysical Jets: Modeling High-Energy Particle Emissions
- Supernova Studies: Quantitative Analysis of Stellar Explosions
Linguistics and Language Sciences
- Computational Linguistics: Natural Language Generation Models
- Phonetics and Speech Analysis Using Quantitative Techniques
- Sociolinguistics: Statistical Analysis of Dialect Variation
- Syntax and Grammar Modeling in Linguistic Theory
- Quantitative Study of Language Acquisition in Children
- Corpus Linguistics: Quantifying Textual Data
- Language Typology and Universals: Cross-Linguistic Analysis
- Psycholinguistics: Quantitative Study of Language Processing
- Machine Translation: Improving Accuracy and Efficiency
- Quantitative Approaches to Historical Linguistics
Business and Economics: quantitative research topics in education
- Financial Risk Management: Quantitative Modeling of Risks
- Econometrics: Statistical Methods in Economic Analysis
- Marketing Analytics: Consumer Behavior Modeling
- Quantitative Analysis of Macroeconomic Policies
- Operations Research: Optimization in Supply Chain Management
- Quantitative Methods in Corporate Finance
- Labor Economics: Analyzing Employment Trends Using Data
- Economic Impact Assessment of Policy Interventions
- Quantitative Analysis of Market Dynamics and Competition
- Behavioral Finance: Quantifying Psychological Aspects in Financial Decision-Making
Education and Pedagogy
- Educational Data Mining for Adaptive Learning Systems
- Quantitative Analysis of Learning Outcomes and Student Performance
- Technology Integration in Education: Assessing Efficacy
- Assessment and Evaluation Models in Educational Research
- Quantitative Study of Teacher Effectiveness and Practices
- Cognitive Load Theory: Quantifying Learning Processes
- Educational Psychology: Quantitative Analysis of Motivation
- Online Education: Analytics for Engagement and Success
- Curriculum Development: Quantitative Approaches to Design
- Educational Policy Analysis Using Quantitative Methods
Communication and Media Studies
- Media Effects Research: Quantitative Analysis of Influence
- Computational Journalism: Data-driven Storytelling
- Social Media Influence Metrics and Analysis
- Quantitative Study of Public Opinion and Opinion Formation
- Media Content Analysis Using Statistical Methods
- Communication Network Analysis: Quantifying Connections
- Quantitative Approaches to Media Bias Assessment
- Entertainment Analytics: Audience Behavior Modeling
- Digital Media Consumption Patterns: Statistical Analysis
- Crisis Communication: Quantitative Assessment of Responses
quantitative research topics for accounting students in the Philippines
Here are ten quantitative research topics suitable for accounting students in the Philippines:
- “Impact of Tax Changes on Small and Medium Businesses (SMEs) in the Philippines: A Numbers-Based Study”
- “Evaluating How Well Philippine Banks are Doing Financially: A Comparison Using Simple Measures”
- “Checking How Good Internal Controls are at Stopping Fraud: Looking at Numbers in Filipino Businesses”
- “Looking at How Companies in the Philippines are Run and How Well They’re Doing Financially”
- “Figuring Out What Makes Auditing Good: A Study on Auditing in the Philippines”
- “Seeing How Using Accounting Systems Helps Companies Work Better: A Study Using Numbers”
- “Finding Out What Makes Financial Reports Good Quality in the Philippines: A Numbers Approach”
- “Seeing How Following International Financial Reporting Standards (IFRS) Affects Philippine Companies”
- “Studying What Factors Affect How Well College Students in the Philippines Understand Finances”
- “Managing Money Flow and Keeping Small Businesses in the Philippines Stable: A Numbers-Based Look”
What are the 10 examples of research titles in school quantitative?
Here are ten examples of quantitative research titles suitable for school-related studies:
- “Technology’s Influence on Grades: A Number-Based Look”
- “How Class Size Affects How Well Students Learn: A Number Study”
- “Parents Getting Involved and How Well Kids Do in School: A Numbers Look”
- “Checking if Different Math Teaching Ways Work Well”
- “Connecting How Much Students Get Into School with Test Scores”
- “Bullying in Schools: Looking at How Much and How It Affects Grades”
- “Looking at How Money Affects How Good Kids Are at Reading”
- “Checking if Counseling Helps Kids’ Feelings: A Number Way”
- “Do After-School Stuff Help Kids Do Better in School?”
- “Seeing if a New Way to Grade is Better Than the Old Way: Comparing with Numbers”
Best experimental quantitative research topics for stem students in the Philippines
The following are the best quantitative research topics for stem students:
Biology Quantitative Research Topics
In the realm of Biology, quantitative research delves into the numerical aspects of living organisms, ecosystems, and genetics, aiding in understanding diverse biological phenomena.
Chemistry Quantitative Research Topics
Chemistry’s quantitative research explores numerical relationships within chemical reactions, material properties, and various compounds, offering insights into chemical phenomena through measurable data.
Physics Quantitative Research Topics
In Physics, quantitative research scrutinizes measurable physical quantities and their interactions, exploring fundamental principles and phenomena of the natural world.
Mathematics Quantitative Research Topics
Mathematics, in its quantitative research, investigates numerical patterns, structures, and mathematical theories, exploring the quantifiable aspects of various mathematical concepts.
We’ve investigated the marvels of utilizing numbers, information, and math to disentangle the secrets of science, innovation, design, and math. Quantitative research isn’t about staggering recipes or complex speculations. It’s tied in with utilizing straightforward math and measurements to grasp our general surroundings. Whether it’s anticipating the effect of environmental change, investigating how robots help medical services, or sorting out ways of making our urban communities greener, every point we’ve examined holds the potential for meaningful revelations.
As you proceed with your educational process, keep this interest alive. Embrace the delight of getting clarification on some pressing issues, testing, and investigating. Your passion for STEM subjects can prompt astounding things—from inventing innovations to tracking down answers for worldwide difficulties.
All in all, what’s next for you? Pick a topic that invigorates you, jump into the universe of quantitative exploration, and let your creative mind take off! Who knows, you’ll be the one to find something staggering that impacts the world.
Frequently Asked Questions
Can i conduct quantitative research in any stem field.
Yes, quantitative research methods can be applied across various STEM disciplines, including biology, chemistry, physics, computer science, environmental science, engineering, mathematics, and more.
Do I need advanced mathematical skills to conduct quantitative research in STEM?
While a solid understanding of mathematics is beneficial, many quantitative research projects in STEM can be conducted with basic mathematical principles. However, depending on the complexity of the topic and methods used, advanced mathematical skills may be necessary.
What tools and software are commonly used in quantitative research in STEM?
Common tools and software include statistical software such as R, Python (with libraries like NumPy and SciPy), MATLAB, SPSS, and Excel. Depending on the research topic, specialized software for data visualization, simulation, and mathematical modeling may also be used.
Leave a Comment Cancel Reply
Your email address will not be published. Required fields are marked *
Save my name, email, and website in this browser for the next time I comment.
- UC Berkeley
- Sign Up to Volunteer
- I School Slack
- Alumni News
- Alumni Events
- Alumni Accounts
- Career Support
- Academic Mission
- Diversity & Inclusion Resources
- DEIBJ Leadership
- Featured Faculty
- Featured Alumni
- Work at the I School
- Subscribe to Email Announcements
- Logos & Style Guide
- Directions & Parking
The School of Information is UC Berkeley’s newest professional school. Located in the center of campus, the I School is a graduate research and education community committed to expanding access to information and to improving its usability, reliability, and credibility while preserving security and privacy.
- Career Outcomes
- Degree Requirements
- Paths Through the MIMS Degree
- Final Project
- Funding Your Education
- Admissions Events
- Request Information
- Capstone Project
- Jack Larson Data for Good Fellowship
- Tuition & Fees
- Women in MIDS
- MIDS Curriculum News
- MICS Student News
- Dissertations
- Applied Data Science Certificate
- ICTD Certificate
- Citizen Clinic
The School of Information offers four degrees:
The Master of Information Management and Systems (MIMS) program educates information professionals to provide leadership for an information-driven world.
The Master of Information and Data Science (MIDS) is an online degree preparing data science professionals to solve real-world problems. The 5th Year MIDS program is a streamlined path to a MIDS degree for Cal undergraduates.
The Master of Information and Cybersecurity (MICS) is an online degree preparing cybersecurity leaders for complex cybersecurity challenges.
Our Ph.D. in Information Science is a research program for next-generation scholars of the information age.
- Info 271B. Quantitative Research Methods for Information Systems and Management
- Spring 2024 Course Schedule
- Summer 2024 Course Schedule
- Fall 2024 Course Schedule
The School of Information's courses bridge the disciplines of information and computer science, design, social sciences, management, law, and policy. We welcome interest in our graduate-level Information classes from current UC Berkeley graduate and undergraduate students and community members. More information about signing up for classes.
- Ladder & Adjunct Faculty
- MIMS Students
- MIDS Students
- 5th Year MIDS Students
- MICS Students
- Ph.D. Students

- Publications
- Centers & Labs
- Computer-mediated Communication
- Data Science
- Entrepreneurship
- Human-computer Interaction (HCI)
- Information Economics
- Information Organization
- Information Policy
- Information Retrieval & Search
- Information Visualization
- Social & Cultural Studies
- Technology for Developing Regions
- User Experience Research
Research by faculty members and doctoral students keeps the I School on the vanguard of contemporary information needs and solutions.
The I School is also home to several active centers and labs, including the Center for Long-Term Cybersecurity (CLTC) , the Center for Technology, Society & Policy , and the BioSENSE Lab .
- Why Hire I School?
- Request a Resume Book
- Leadership Development Program
- Mailing List
- For Nonprofit and Government Employers
- Jobscan & Applicant Tracking Systems
- Resume & LinkedIn Review
- Resume Book
I School graduate students and alumni have expertise in data science, user experience design & research, product management, engineering, information policy, cybersecurity, and more — learn more about hiring I School students and alumni .
- Press Coverage
- I School Voices
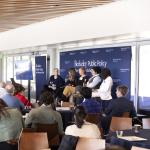
The Goldman School of Public Policy, the CITRIS Policy Lab, and the School of Information hosted the inaugural UC...
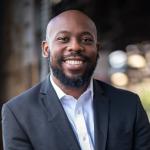
Dr. Diag Davenport has been appointed as an assistant professor at UC Berkeley as part of a joint search in...
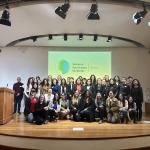
At the Women in Data Science conference held at UC Berkeley this past week, four educators affiliated with the...
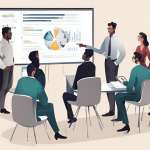
At the UC Berkeley School of Information, two educators have taken the initiative to begin incorporating data...
- Distinguished Lecture Series
- I School Lectures
- Information Access Seminars
- CLTC Events
- Women in MIDS Events
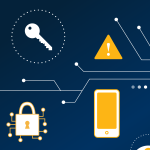
Quantitative Research Methods for Information Systems and Management
Course description.
The goal of this course is to provide students with an introduction to many different types of quantitative research methods and statistical techniques. This course will be divided into two sections: 1) methods for quantitative research and, 2) quantitative statistical techniques for analyzing data. We begin with a focus on defining research problems, theory testing, causal inference, and designing research instruments. Then, we will explore a range of statistical techniques and methods that are available for empirical research. Topics in research methods include: Primary and Secondary Data Analysis, Sampling, Survey Design, and Experimental Designs. Topics in quantitative techniques include: Descriptive and Inferential statistics, General Linear Models, and Non-Linear Models. The course will conclude with an introduction to special topics in quantitative research methods.
Prerequisites
Requirements satisfied, signing up for i school classes.
Instructions for Berkeley undergrads, graduate students, and community members
Course History
Spring 2017, last updated:.
- Application
Quantitative Research in Information Systems
Detmar STRAUB , David GEFEN , and Jan RECKER
Shortcut to Sections
- Section 1: Welcome and Disclaimers
- Section 2: What is Quantitative, Positivist Research
- Section 3: Philosophical Foundations
Section 4: Fundamentals of QtPR
Section 5: the general qtpr research approach.
- Section 6: Practical Tips for Writing QtPR Papers
Section 7: Glossary
Section 8: bibliography, section 1. welcome and disclaimers.
Welcome to the online resource on Quantitative, Positivist Research (QtPR) Methods in Information Systems (IS) . This resource seeks to address the needs of quantitative, positivist researchers in IS research – in particular those just beginning to learn to use these methods. IS research is a field that is primarily concerned with socio-technical systems comprising individuals and collectives that deploy digital information and communication technology for tasks in business, private, or social settings. We are ourselves IS researchers but this does not mean that the advice is not useful to researchers in other fields.
This webpage is a continuation and extension of an earlier online resource on Quantitative Positivist Research that was originally created and maintained by Detmar STRAUB, David GEFEN, and Marie BOUDREAU. As the original online resource hosted at Georgia State University is no longer available, this online resource republishes the original material plus updates and additions to make what is hoped to be valuable information accessible to IS scholars. Given that the last update of that resource was 2004, we also felt it prudent to update the guidelines and information to the best of our knowledge and abilities. If readers are interested in the original version, they can refer to a book chapter (Straub et al., 2005) that contains much of the original material.
1.1 Objective of this Website
This resource is dedicated to exploring issues in the use of quantitative, positivist research methods in Information Systems (IS). We intend to provide basic information about the methods and techniques associated with QtPR and to offer the visitor references to other useful resources and to seminal works.
1.2 Feedback
Suggestions on how best to improve on the site are very welcome. Please contact us directly if you wish to make suggestions on how to improve the site. No faults in content or design should be attributed to any persons other than ourselves since we made all relevant decisions on these matters. You can contact the co-editors at: [email protected] , [email protected] , and [email protected] .
1.3 How to Navigate this Resource
This resource is structured into eight sections. You can scroll down or else simply click above on the shortcuts to the sections that you wish to explore next.
1.4 Explanation for Self-Citations
One of the main reasons we were interested in maintaining this online resource is that we have already published a number of articles and books on the subject. We felt that we needed to cite our own works as readily as others to give readers as much information as possible at their fingertips.
1.5 What This Resource Does Not Cover
This website focuses on common, and some would call traditional approaches to QtPR within the IS community, such as survey or experimental research. There are many other types of quantitative research that we only gloss over here, and there are many alternative ways to analyze quantitative data beyond the approaches discussed here. This is not to suggest in any way that these methods, approaches, and tools are not invaluable to an IS researcher. Only that we focus here on those genres that have traditionally been quite common in our field and that we as editors of this resource feel comfortable in writing about.
One such example of a research method that is not covered in any detail here would be meta-analysis. Meta-analyses are extremely useful to scholars in well-established research streams because they can highlight what is fairly well known in a stream, what appears not to be well supported, and what needs to be further explored. Importantly, they can also serve to change directions in a field. There are numerous excellent works on this topic, including the book by Hedges and Olkin (1985), which still stands as a good starter text, especially for theoretical development.
1.6 How to Cite this Resource
You can cite this online resource as:
Straub, D. W., Gefen, D., Recker, J., “Quantitative Research in Information Systems,” Association for Information Systems (AISWorld) Section on IS Research, Methods, and Theories, last updated March 25, 2022, http://www.janrecker.com/quantitative-research-in-information-systems/ .
The original online resource that was previously maintained by Detmar Straub, David Gefen, and Marie-Claude Boudreau remains citable as a book chapter: Straub, D.W., Gefen, D., & Boudreau, M-C. (2005). Quantitative Research . In D. Avison & J. Pries-Heje (Eds.), Research in Information Systems: A Handbook for Research Supervisors and Their Students (pp. 221-238). Elsevier.
Section 2: What is Quantitative, Positivist Research (QtPR)
2.1 cornerstones of quantitative, positivist research.
QtPR is a set of methods and techniques that allows IS researchers to answer research questions about the interaction of humans and digital information and communication technologies within the sociotechnical systems of which they are comprised. There are two cornerstones in this approach to research.
The first cornerstone is an emphasis on quantitative data . QtPR describes a set of techniques to answer research questions with an emphasis on state-of-the-art analysis of quantitative data , that is, types of data whose value is measured in the form of numbers, with a unique numerical value associated with each data set. As the name suggests, quantitative methods tend to specialize in “quantities,” in the sense that numbers are used to represent values and levels of measured variables that are themselves intended to approximate theoretical constructs. Often, the presence of numeric data is so dominant in quantitative methods that people assume that advanced statistical tools, techniques, and packages to be an essential element of quantitative methods. While this is often true, quantitative methods do not necessarily involve statistical examination of numbers. Simply put, QtPR focus on how you can do research with an emphasis on quantitative data collected as scientific evidence. Sources of data are of less concern in identifying an approach as being QtPR than the fact that numbers about empirical observations lie at the core of the scientific evidence assembled. A QtPR researcher may, for example, use archival data, gather structured questionnaires, code interviews and web posts, or collect transactional data from electronic systems. In any case, the researcher is motivated by the numerical outputs and how to imbue them with meaning.
The second cornerstone is an emphasis on (post-) positivist philosophy . As will be explained in Section 3 below, it should be noted that “quantitative, positivist research” is really just shorthand for “quantitative, post-positivist research.” Without delving into many details at this point, positivist researchers generally assume that reality is objectively given, that it is independent of the observer (researcher) and their instruments, and that it can be discovered by a researcher and described by measurable properties. Interpretive researchers, on the other hand, start out with the assumption that access to reality (given or socially constructed) is only through social constructions such as language, consciousness, and shared meanings. While these views do clearly differ, researchers in both traditions also agree on several counts. For example, both positivist and interpretive researchers agree that theoretical constructs, or important notions such as causality, are social constructions (e.g., responses to a survey instrument).
2.2 Quantitative, Positivist Research for Theory-Generation versus Theory-Evaluation
What are theories? There is a vast literature discussing this question and we will not embark on any kind of exegesis on this topic. A repository of theories that have been used in information systems and many other social science theories can be found at: https://guides.lib.byu.edu/c.php?g=216417&p=1686139 .
In simple terms, in QtPR it is often useful to understand theory as a lawlike statement that attributes causality to sets of variables, although other conceptions of theory do exist and are used in QtPR and other types of research (Gregor, 2006). One common working definition that is often used in QtPR research refers to theory as saying “what is, how, why, when, where, and what will be. [It provides] predictions and has both testable propositions and causal explanations (Gregor, 2006, p. 620).”
QtPR can be used both to generate new theory as well as to evaluate theory proposed elsewhere. In theory-generating research, QtPR researchers typically identify constructs, build operationalizations of these constructs through measurement variables, and then articulate relationships among the identified constructs (Im & Wang, 2007). In theory-evaluating research, QtPR researchers typically use collected data to test the relationships between constructs by estimating model parameters with a view to maintain good fit of the theory to the collected data.
Traditionally, QtPR has been dominant in this second genre, theory-evaluation, although there are many applications of QtPR for theory-generation as well (e.g., Im & Wang, 2007; Evermann & Tate, 2011). Historically however, QtPR has by and large followed a particular approach to scientific inquiry, called the hypothetico-deductive model of science (Figure 1).
This model suggests that the underlying view that leads a scholar to conclude that QtPR can produce knowledge is that the world has an objective reality that can be captured and translated into models that imply testable hypotheses, usually in the form of statistical or other numerical analyses. In turns, a scientific theory is one that can be falsified through careful evaluation against a set of collected data.
The original inspiration for this approach to science came from the scientific epistemology of logical positivism during the 1920s and 1930s as developed by the Vienna Circle of Positivists, primarily Karl Popper,. This “pure” positivist attempt at viewing scientific exploration as a search for the Truth has been replaced in recent years with the recognition that ultimately all measurement is based on theory and hence capturing a truly “objective” observation is impossible (Coombs, 1976). Even the measurement of a purely physical attribute, such as temperature, depends on the theory of how materials expand in heat. Hence interpreting the readings of a thermometer cannot be regarded as a pure observation but itself as an instantiation of theory.
As suggested in Figure 1, at the heart of QtPR in this approach to theory-evaluation is the concept of deduction . Deduction is a form of logical reasoning that involves deriving arguments as logical consequences of a set of more general premises. It involves deducing a conclusion from a general premise (i.e., a known theory), to a specific instance (i.e., an observation). There are three main steps in deduction (Levallet et al. 2021):
- Testing internal consistency, i.e., verifying that there are no internal contradictions.
- Distinguishing between the logical basics of the theory and its empirical, testable, predictions.
- Empirical testing aimed at falsifying the theory with data. When the data do not contradict the hypothesized predictions of the theory, it is temporarily corroborated. The objective of this test is to falsify, not to verify, the predictions of the theory. Verifications can be found for almost any theory if one can pick and choose what to look at.
Whereas seeking to falsify theories is the idealistic and historical norm, in practice many scholars in IS and other social sciences are, in practice, seeking confirmation of their carefully argued theoretical models (Gray & Cooper, 2010; Burton-Jones et al., 2017). For example, QtPR scholars often specify what is called an alternative hypothesis rather than the null hypothesis (an expectation of no effect), that is, they typically formulate the expectation of a directional, signed effect of one variable on another. Doings so confers some analytical benefits (such as using a one-tailed statistical test rather than a two-tailed test), but the most likely reason for doing this is that confirmation, rather than disconfirmation of theories is a more common way of conducting QtPR in modern social sciences (Edwards & Berry, 2010; Mertens & Recker, 2020). In Popper’s falsification view, for example, one instance of disconfirmation disproves an entire theory, which is an extremely stringent standard. More information about the current state-of the-art follows later in section 3.2 below, which discusses Lakatos’ contributions to the philosophy of science.
In conclusion, recall that saying that QtPR tends to see the world as having an objective reality is not equivalent to saying that QtPR assumes that constructs and measures of these constructs are being or have been perfected over the years. In fact, Cook and Campbell (1979) make the point repeatedly that QtPR will always fall short of the mark of perfect representation. For this reason, they argue for a “critical-realist” perspective, positing that “causal relationships cannot be perceived with total accuracy by our imperfect sensory and intellective capacities” (p. 29). This is why we argue in more detail in Section 3 below that modern QtPR scientists have really adopted a post-positivist perspective.
2.3 What QtPR is Not
QtPR is not math analytical modeling , which typically depends on mathematical derivations and assumptions, sans data. This difference stresses that empirical data gathering or data exploration is an integral part of QtPR, as is the positivist philosophy that deals with problem-solving and the testing of the theories derived to test these understandings.
QtPR is also not design research , in which innovative IS artifacts are designed and evaluated as contributions to scientific knowledge. Models and prototypes are frequently the products of design research. In QtPR, models are also produced but most often causal models whereas design research stresses ontological models. Also, QtPR typically validates its findings through testing against empirical data whereas design research can also find acceptable validation of a new design through mathematical proofs of concept or through algorithmic analyses alone. Still, it should be noted that design researchers are increasingly using QtPR methods, specifically experimentation, to validate their models and prototypes so QtPR is also becoming a key tool in the arsenal of design science researchers.
QtPR is also not qualitative positivist research (QlPR) nor qualitative interpretive research. More information about qualitative research in both variants is available on an AIS-sponsored online resource . The simplest distinction between the two is that quantitative research focuses on numbers , and qualitative research focuses on text , most importantly text that captures records of what people have said, done, believed, or experienced about a particular phenomenon, topic, or event. Qualitative research emphasizes understanding of phenomena through direct observation, communication with participants, or analyses of texts, and at times stress contextual subjective accuracy over generality. What matters here is that qualitative research can be positivist (e.g., Yin, 2009; Clark, 1972; Glaser & Strauss, 1967) or interpretive (e.g., Walsham, 1995; Elden & Chisholm, 1993; Gasson, 2004). Without delving too deeply into the distinctions and their implications, one difference is that qualitative positive researchers generally assume that reality can be discovered to some extent by a researcher as well as described by measurable properties (which are social constructions) that are independent of the observer (researcher) and created instruments and instrumentation. Qualitative interpretive researchers start out with the assumption that access to reality (given or socially constructed) is only through social constructions such as language, consciousness, and shared meanings. Interpretive researchers generally attempt to understand phenomena through the meanings that people assign to them.
These nuances impact how quantitative or qualitative researchers conceive and use data, they impact how researchers analyze that data, and they impact the argumentation and rhetorical style of the research (Sarker et al., 2018). It does not imply that certain types of data (e.g., numerical data) is reserved for only one of the traditions. For example, QlPR scholars might interpret some quantitative data as do QtPR scholars. However, the analyses are typically different: QlPR might also use statistical techniques to analyze the data collected, but these would typically be descriptive statistics, t-tests of differences, or bivariate correlations, for example. More advanced statistical techniques are usually not favored, although of course, doing so is entirely possible (e.g., Gefen & Larsen, 2017).
Section 3. Philosophical Foundations
In what follows, we discuss at some length what have historically been the views about the philosophical foundations of science in general and QtPR in particular. We note that these are our own, short-handed descriptions of views that have been, and continue to be, debated at length in ongoing philosophy of science discourses. Readers interested primarily in the practical challenges of QtPR might want to skip this section. Also, readers with a more innate interest in the broader discussion of philosophy of science might want to consult the referenced texts and their cited texts directly.
3.1 A Brief Introduction to Positivism
QtPR researchers historically assumed that reality is objectively given and can be discovered by a researcher and described by measurable properties independent of the observer (researcher) and their instruments. This worldview is generally called positivism.
At the heart of positivism is Karl Popper’s dichotomous differentiation between “scientific” theories and “myth.” A scientific theory is a theory whose predictions can be empirically falsified, that is, shown to be wrong. Therefore, a scientific theory is by necessity a risky endeavor, i.e., it may be thrown out if not supported by the data. Einstein’s Theory of Relativity is a prime example, according to Popper, of a scientific theory. When Einstein proposed it, the theory may have ended up in the junk pile of history had its empirical tests not supported it, despite the enormous amount of work put into it and despite its mathematical appeal. The reason Einstein’s theory was accepted was because it was put to the test: Eddington’s eclipse observation in 1919 confirmed its predictions, predictions that were in contrast to what should have been seen according to Newtonian physics. Eddington’s eclipse observation was a make-or-break event for Einstein’s theory. The theory would have been discredited had the stars not appeared to move during the eclipse because of the Sun’s gravity. In contrast, according to Popper, is Freud’s theory of psychoanalysis which can never be disproven because the theory is sufficiently imprecise to allow for convenient “explanations” and the addition of ad hoc hypotheses to explain observations that contradict the theory. The ability to explain any observation as an apparent verification of psychoanalysis is no proof of the theory because it can never be proven wrong to those who believe in it. A scientific theory, in contrast to psychoanalysis, is one that can be empirically falsified. This is the Falsification Principle and the core of positivism. Basically, experience can show theories to be wrong, but can never prove them right. It is an underlying principle that theories can never be shown to be correct.
This demarcation of science from the myths of non-science also assumes that building a theory based on observation (through induction) does not make it scientific. Science, according to positivism, is about solving problems by unearthing truth. It is not about fitting theory to observations. That is why pure philosophical introspection is not really science either in the positivist view. Induction and introspection are important, but only as a highway toward creating a scientific theory. Central to understanding this principle is the recognition that there is no such thing as a pure observation. Every observation is based on some preexisting theory or understanding.
Furthermore, it is almost always possible to choose and select data that will support almost any theory if the researcher just looks for confirming examples. Accordingly, scientific theory, in the traditional positivist view, is about trying to falsify the predictions of the theory.
In theory, it is enough, in Popper’s way of thinking, for one observation that contradicts the prediction of a theory to falsify it and render it incorrect. Furthermore, even after being tested, a scientific theory is never verified because it can never be shown to be true, as some future observation may yet contradict it. Accordingly, a scientific theory is, at most, extensively corroborated, which can render it socially acceptable until proven otherwise. Of course, in reality, measurement is never perfect and is always based on theory. Hence, positivism differentiates between falsification as a principle, where one negating observation is all that is needed to cast out a theory, and its application in academic practice, where it is recognized that observations may themselves be erroneous and hence where more than one observation is usually needed to falsify a theory.
This notion that scientists can forgive instances of disproof as long as the bulk of the evidence still corroborates the base theory lies behind the general philosophical thinking of Imre Lakatos (1970). In Lakatos’ view, theories have a “hard core” of ideas, but are surrounded by evolving and changing supplemental collections of both hypotheses, methods, and tests – the “protective belt.” In this sense, his notion of theory was thus much more fungible than that of Popper.
In QtPR practice since World War II, moreover, social scientists have tended to seek out confirmation of a theoretical position rather than its disconfirmation, a la Popper. This is reflected in their dominant preference to describe not the null hypothesis of no effect but rather alternative hypotheses that posit certain associations or directions in sign. In other words, QtPR researchers are generally inclined to hypothesize that a certain set of antecedents predicts one or more outcomes, co-varying either positively or negatively. It needs to be noted that positing null hypotheses of no effect remains a convention in some disciplines; but generally speaking, QtPR practice favors stipulating certain directional effects and certain signs, expressed in hypotheses (Edwards & Berry, 2010). Overall, modern social scientists favor theorizing models with expressed causal linkages and predictions of correlational signs. Popper’s contribution to thought – specifically, that theories should be falsifiable – is still held in high esteem, but modern scientists are more skeptical that one conflicting case can disprove a whole theory, at least when gauged by which scholarly practices seem to be most prevalent.
3.2 From Positivism to Post-Positivism
We already noted above that “quantitative, positivist research” is really a shorthand for “quantitative, post-positivist research.” Whereas qualitative researchers sometimes take ownership of the concept of post-positivism, there is actually little quarrel among modern quantitative social scientists over the extent to which we can treat the realities of the world as somehow and truly “objective.” A brief history of the intellectual thought behind this may explain what is meant by this statement.
Flourishing for a brief period in the early 1900s, logical positivism, which argued that all natural laws could be reduced to the mathematics of logic, was one culmination of a deterministic positivism, but these ideas came out of a long tradition of thinking of the world as an objective reality best described by philosophical determinism. One could trace this lineage all the way back to Aristotle and his opposition to the “metaphysical” thought of Plato, who believed that the world as we see it has an underlying reality (forms) that cannot be objectively measured or determined. The only way to “see” that world, for Plato and Socrates, was to reason about it; hence, Plato’s philosophical dialecticism.
During more modern times, Henri de Saint-Simon (1760–1825), Pierre-Simon Laplace (1749–1827), Auguste Comte (1798–1857), and Émile Durkheim (1858–1917) were among a large group of intellectuals whose basic thinking was along the lines that science could uncover the “truths” of a difficult-to-see reality that is offered to us by the natural world. Science achieved this through the scientific method and through empiricism, which depended on measures that could pierce the veil of reality. With the advent of experimentalism especially in the 19 th century and the discovery of many natural, physical elements (like hydrogen and oxygen) and natural properties like the speed of light, scientists came to believe that all natural laws could be explained deterministically, that is, at the 100% explained variance level. However, in 1927, German scientist Werner Heisenberg struck down this kind of thinking with his discovery of the uncertainty principle. This discovery, basically uncontended to this day, found that the underlying laws of nature (in Heisenberg’s case, the movement and position of atomic particles), were not perfectly predictable, that is to say, deterministic. They are stochastic. Ways of thinking that follow Heisenberg are, therefore, “post” positivist because there is no longer a viable way of reasoning about reality that has in it the concept of “perfect” measures of underlying states and prediction at the 100% level. These states can be individual socio-psychological states or collective states, such as those at the organizational or national level.
To illustrate this point, consider an example that shows why archival data can never be considered to be completely objective. Even the bottom line of financial statements is structured by human thinking. What is to be included in “revenues,” for example, is impacted by decisions about whether booked revenues can or should be coded as current period revenues. Accounting principles try to control this, but, as cases like Enron demonstrate, it is possible for reported revenues or earnings to be manipulated. In effect, researchers often need to make the assumption that the books, as audited, are accurate reflections of the firm’s financial health. Researchers who are permitted access to transactional data from, say, a firm like Amazon, are assuming, moreover, that the data they have been given is accurate, complete, and representative of a targeted population. But is it? Intermediaries may have decided on their own not to pull all the data the researcher requested, but only a subset. Their selection rules may then not be conveyed to the researcher who blithely assumes that their request had been fully honored. Finally, governmental data is certainly subject to imperfections, lower quality data that the researcher is her/himself unaware of. Adjustments to government unemployment data, for one small case, are made after the fact of the original reporting. Are these adjustments more or less accurate than the original figures? In the vast majority of cases, researchers are not privy to the process so that they could reasonably assess this. We might say that archival data might be “reasonably objective,” but it is not purely ”objective” By any stretch of the imagination. There is no such thing. All measures in social sciences, thus, are social constructions that can only approximate a true, underlying reality.
Our development and assessment of measures and measurements ( Section 5 ) is another simple reflection of this line of thought. Within statistical bounds, a set of measures can be validated and thus considered to be acceptable for further empiricism. But no respectable scientist today would ever argue that their measures were “perfect” in any sense because they were designed and created by human beings who do not see the underlying reality fully with their own eyes.
How does this ultimately play out in modern social science methodologies? The emphasis in social science empiricism is on a statistical understanding of phenomena since, it is believed, we cannot perfectly predict behaviors or events. One major articulation of this was in Cook and Campbell’s seminal book Quasi-Experimentation (1979), later revised together with William Shadish (2001). In their book, they explain that deterministic prediction is not feasible and that there is a boundary of critical realism that scientists cannot go beyond.
Our argument, hence, is that IS researchers who work with quantitative data are not truly positivists, in the historical sense. We are all post-positivists. We can know things statistically, but not deterministically. While the positivist epistemology deals only with observed and measured knowledge, the post-positivist epistemology recognizes that such an approach would result in making many important aspects of psychology irrelevant because feelings and perceptions cannot be readily measured. In post-positivist understanding, pure empiricism, i.e., deriving knowledge only through observation and measurement, is understood to be too demanding. Instead, post-positivism is based on the concept of critical realism, that there is a real world out there independent of our perception of it and that the objective of science is to try and understand it, combined with triangulation, i.e., the recognition that observations and measurements are inherently imperfect and hence the need to measure phenomena in many ways and compare results. This post-positivist epistemology regards the acquisition of knowledge as a process that is more than mere deduction. Knowledge is acquired through both deduction and induction.
3.3 QtPR and Null Hypothesis Significance Testing
QtPR has historically relied on null hypothesis significance testing (NHST), a technique of statistical inference by which a hypothesized value (such as a specific value of a mean, a difference between means, correlations, ratios, variances, or other statistics) is tested against a hypothesis of no effect or relationship on basis of empirical observations (Pernet, 2016). With the caveat offered above that in scholarly praxis, null hypotheses are tested today only in certain disciplines, the underlying testing principles of NHST remain the dominant statistical approach in science today (Gigerenzer, 2004).
NHST originated from a debate that mainly took place in the first half of the 20 th century between Fisher (e.g., 1935a, 1935b; 1955) on the one hand, and Neyman and Pearson (e.g., 1928, 1933) on the other hand. Fisher introduced the idea of significance testing involving the probability p to quantify the chance of a certain event or state occurring, while Neyman and Pearson introduced the idea of accepting a hypothesis based on critical rejection regions. Fisher’s idea is essentially an approach based on proof by contradiction (Christensen, 2005; Pernet, 2016): we pose a null model and test if our data conforms to it. This computation yields the probability of observing a result at least as extreme as a test statistic (e.g., a t value), assuming the null hypothesis of the null model (no effect) being true. This probability reflects the conditional, cumulative probability of achieving the observed outcome or larger: probability (Observation ≥ t | H 0 ). Neyman and Pearson’s idea was a framework of two hypotheses: the null hypothesis of no effect and the alternative hypothesis of an effect, together with controlling the probabilities of making errors. This idea introduced the notions of control of error rates, and of critical intervals. Together, these notions allow distinguishing Type I (rejecting H 0 when there is no effect) and Type II errors (not rejecting H 0 when there is an effect).
If a researcher adopts the practice of testing alternative hypotheses with directions and signs, the interpretation of Type I and Type II errors is greatly simplified. From this standpoint, a Type I error occurs when a researcher finds a statistical effect in the tested sample, but, in the population, no such effect would have been found. A Type II error occurs when a researcher infers that there is no effect in the tested sample (i.e., the inference that the test statistic differs statistically significantly from the threshold), when, in fact, such an effect would have been found in the population. Regarding Type I errors, researchers are typically reporting p-values that are compared against an alpha protection level. The alpha protection levels are often set at .05 or lower, meaning that the researcher has at most only a 5% risk of being wrong and subject to a Type I error. Regarding Type II errors, it is important that researchers be able to report a beta statistic, which is the probability that they are correct and free of a Type II error. The standard value for betas has historically been set at .80 (Cohen 1988). This value means that researchers assume a 20% risk (1.0 – .80) that they are correct in their inference.
QtPR scholars sometime wonder why the thresholds for protection against Type I and Type II errors are so divergent. Consider that with alternative hypothesis testing, the researcher is arguing that a change in practice would be desirable (that is, a direction/sign is being proposed). If the inference is that this is true, then there needs to be smaller risk (at or below 5%) since a change in behavior is being advocated and this advocacy of change can be nontrivial for individuals and organizations. On the other hand, if no effect is found, then the researcher is inferring that there is no need to change current practices. Since no change in the status quo is being promoted, scholars are granted a larger latitude to make a mistake in whether this inference can be generalized to the population. However, one should remember that the .05 and .20 thresholds are no more than an agreed-upon convention. The p-value below .05 is there because when Mr. Pearson (of the Pearson correlation) was asked what he thought an appropriate threshold should be, and he said one in twenty would be reasonable. It is out of tradition and reverence to Mr. Pearson that it remains so.
One other caveat is that the alpha protection level can vary. Alpha levels in medicine are generally lower (and the beta level set higher) since the implications of Type I or Type II errors can be severe given that we are talking about human health. The convention is thus that we do not want to recommend that new medicines be taken unless there is a substantial and strong reason to believe that this can be generalized to the population (a low alpha). Likewise, with the beta: Clinical trials require fairly large numbers of subjects and so the effect of large samples makes it highly unlikely that what we infer from the sample will not readily generalize to the population.
As this discussion already illustrates, it is important to realize that applying NHST is difficult. Several threats are associated with the use of NHST in QtPR. These are discussed in some detail by Mertens and Recker (2020). Below we summarize some of the most imminent threats that QtPR scholars should be aware of in QtPR practice:
1. NHST is difficult to interpret. The p-value is not an indication of the strength or magnitude of an effect (Haller & Kraus, 2002). Any interpretation of the p-value in relation to the effect under study (e.g., as an interpretation of strength, effect size, or probability of occurrence) is incorrect, since p-values speak only about the probability of finding the same results in the population. In addition, while p-values are randomly distributed (if all the assumptions of the test are met) when there is no effect, their distribution depends on both the population effect size and the number of participants, making it impossible to infer the strength of an effect.
When the sample size n is relatively small but the p-value relatively low, that is, less than what the current conventional a-priori alpha protection level states, the effect size is also likely to be sizeable. However, this is a happenstance of the statistical formulas being used and not a useful interpretation in its own right. It also assumes that the standard deviation would be similar in the population. This is why p-values are not reliably about effect size.
In contrast, correlations are about the effect of one set of variables on another. Squaring the correlation r gives the R 2 , referred to as the explained variance. Explained variance describes the percent of the total variance (as the sum of squares of the residuals if one were to assume that the best predictor of the expected value of the dependent variable is its average) that is explained by the model variance (as the sum of squares of the residuals if one were to assume that the best predictor of the expected value of the dependent variable is the regression formula). Hence, r values are all about correlational effects whereas p-values are all about sampling (see below).
Similarly, 1-p is not the probability of replicating an effect (Cohen, 1994). Often, a small p-value is considered to indicate a strong likelihood of getting the same results on another try, but again this cannot be obtained because the p-value is not definitely informative about the effect itself (Miller, 2009). This reasoning hinges on power among other things. The power of a study is a measure of the probability of avoiding a Type II error. Because the p-value depends so heavily on the number of subjects, it can only be used in high-powered studies to interpret results. In low powered studies, the p-value may have too large a variance across repeated samples. The higher the statistical power of a test, the lower the risk of making a Type II error. Low power thus means that a statistical test only has a small chance of detecting a true effect or that the results are likely to be distorted by random and systematic error.
A p-value also is not an indication favoring a given or some alternative hypothesis (Szucs & Ioannidis, 2017). Because a low p-value only indicates a misfit of the null hypothesis to the data, it cannot be taken as evidence in favor of a specific alternative hypothesis more than any other possible alternatives such as measurement error and selection bias (Gelman, 2013).
The p-value also does not describe the probability of the null hypothesis p(H 0 ) being true (Schwab et al., 2011). This common misconception arises from a confusion between the probability of an observation given the null probability (Observation ≥ t | H 0 ) and the probability of the null given an observation probability (H 0 | Observation ≥ t) that is then taken as an indication for p(H 0 ).
In interpreting what the p-value means, it is therefore important to differentiate between the mathematical expression of the formula and its philosophical application. Mathematically, what we are doing in statistics, for example in a t-test, is to estimate the probability of obtaining the observed result or anything more extreme in the available sample data than that was actually observed , assuming that (1) the null hypothesis holds true in the population and (2) all underlying model and test assumptions are met (McShane & Gal, 2017). Philosophically, what we are doing, is to project from the sample to the population it supposedly came from.
This distinction is important. When we compare two means(or in other tests standard deviations or ratios etc.), there is no doubt mathematically that if the two means in the sample are not exactly the same number, then they are different. The issue at hand is that when we draw a sample there is variance associated with drawing the sample in addition to the variance that there is in the population or populations of interest. Philosophically what we are addressing in these statistical tests is whether the difference that we see in the statistics of interest, such as the means, is large enough in the sample or samples that we feel confident in saying that there probably is a difference also in the population or populations that the sample or samples came from. For example, experimental studies are based on the assumption that the sample was created through random sampling and is reasonably large. Only then, based on the law of large numbers and the central limit theorem can we upheld (a) a normal distribution assumption of the sample around its mean and (b) the assumption that the mean of the sample approximates the mean of the population (Miller & Miller 2012). Obtaining such a standard might be hard at times in experiments but even more so in other forms of QtPR research; however, researchers should at least acknowledge it as a limitation if they do not actually test it, by using, for example, a Kolmogorov-Smirnoff test of the normality of the data or an Anderson-Darling test (Corder & Foreman, 2014).
2. NHST is highly sensitive to sampling strategy . As noted above, the logic of NHST demands a large and random sample because results from statistical analyses conducted on a sample are used to draw conclusions about the population, and only when the sample is large and random can its distribution assumed to be a normal distribution. If samples are not drawn independently, or are not selected randomly, or are not selected to represent the population precisely, then the conclusions drawn from NHST are thrown into question because it is impossible to correct for unknown sampling bias.
3. The Effect of Big Data on Hypothesis Testing. With a large enough sample size, a statistically significant rejection of a null hypothesis can be highly probable even if an underlying discrepancy in the examined statistics (e.g., the differences in means) is substantively trivial. Sample size sensitivity occurs in NHST with so-called point-null hypotheses (Edwards & Berry, 2010), i.e., predictions expressed as point values. A researcher that gathers a large enough sample can reject basically any point-null hypothesis because the confidence interval around the null effect often becomes very small with a very large sample (Lin et al., 2013; Guo et al., 2014). But even more so, in an world of big data, p-value testing alone and in a traditional sense is becoming less meaningful because large samples can rule out even the small likelihood of either Type I or Type II errors (Guo et al., 2014). It is entirely possible to have statistically significant results with only very marginal effect sizes (Lin et al., 2013). As Guo et al. (2014) point out, even extremely weak effects of r = .005 become statistically significant at some level of N and in the case of regression with two IVs, this result becomes statistically significant for all levels of effect size at a N of only 500.
The practical implication is that when researchers are working with big data, they need not be concerned that they will get significant effects, but why all of their hypotheses are not significant. If at an N of 15,000 (see Guo et al., 2014, p. 243), the only reason why weak t-values in all models are not supported is that there is likely a problem with the data itself. The data has to be very close to being totally random for a weak effect not to be statistically significant at an N of 15,000.
4. NHST logic is incomplete. NHST rests on the formulation of a null hypothesis and its test against a particular set of data. This tactic relies on the so-called modus tollens (denying the consequence) (Cohen, 1994) – a much used logic in both positivist and interpretive research in IS (Lee & Hubona, 2009). While modus tollens is logically correct, problems in its application can still arise. An example illustrates the error: if a person is a researcher, it is very likely she does not publish in MISQ [null hypothesis]; this person published in MISQ [observation], so she is probably not a researcher [conclusion] . This logic is, evidently, flawed. In other words, the logic that allows for the falsification of a theory loses its validity when uncertainty and/or assumed probabilities are included in the premises. And, yet both uncertainty (e.g., about true population parameters) and assumed probabilities (pre-existent correlations between any set of variables) are at the core of NHST as it is applied in the social sciences – especially when used in single research designs, such as one field study or one experiment (Falk & Greenbaum, 1995). That is, in social reality, no two variables are ever perfectly unrelated (Meehl, 1967).
4.1 The Importance of Measurement
Because of its focus on quantities that are collected to measure the state of variable(s) in real-world domains, QtPR depends heavily on exact measurement. This is because measurement provides the fundamental connection between empirical observation and the theoretical and mathematical expression of quantitative relationships. It is also vital because many constructs of interest to IS researchers are latent, meaning that they exist but not in an immediately evident or readily tangible way. Appropriate measurement is, very simply, the most important thing that a quantitative researcher must do to ensure that the results of a study can be trusted.
Figure 2 describes in simplified form the QtPR measurement process, based on the work of Burton-Jones and Lee (2017). Typically, QtPR starts with developing a theory that offers a hopefully insightful and novel conceptualization of some important real-world phenomena. In attempting to falsify the theory or to collect evidence in support of that theory, operationalizations in the form of measures (individual variables or statement variables) are needed and data needs to be collected from empirical referents (phenomena in the real world that the measure supposedly refers to). Figure 2 also points to two key challenges in QtPR. Moving from the left (theory) to the middle (instrumentation), the first issue is that of shared meaning . If researchers fail to ensure shared meaning between their socially constructed theoretical constructs and their operationalizations through measures they define, an inherent limit will be placed on their ability to measure empirically the constructs about which they theorized. Taking steps to obtain accurate measurements (the connection between real-world domain and the concepts’ operationalization through a measure) can reduce the likelihood of problems on the right side of Figure 2, affecting the data (accuracy of measurement). However, even if complete accuracy were obtained, the measurements would still not reflect the construct theorized because of the lack of shared meaning. As a simple example, consider the scenario that your research is about individuals’ affections when working with information technology and the behavioral consequences of such affections. An issue of shared meaning could occur if, for instance, you are attempting to measure “compassion.” How do you know that you are measuring “compassion” and not, say, “empathy”, which is a socially constructed concept that to many has a similar meaning?
Likewise, problems manifest if accuracy of measurement is not assured. No matter through which sophisticated ways researchers explore and analyze their data, they cannot have faith that their conclusions are valid (and thus reflect reality) unless they can accurately demonstrate the faithfulness of their data.
Understanding and addressing these challenges are important, independent from whether the research is about confirmation or exploration. In research concerned with confirmation, problems accumulate from the left to the right of Figure 2: If researchers fail to ensure shared meaning between their theoretical constructs and operationalizations, this restricts their ability to measure faithfully the constructs they theorized. In research concerned with exploration, problems tend to accumulate from the right to the left of Figure 2: No matter how well or systematically researchers explore their data, they cannot guarantee that their conclusions reflect reality unless they first take steps to ensure the accuracy of their data.
To avoid these problems, two key requirements must be met to avoid problems of shared meaning and accuracy and to ensure high quality of measurement:
- The variables that are chosen as operationalizations to measure a theoretical construct must share its meaning (in all its complexity if needed). This step concerns the validity of the measures.
- The variables that are chosen as operationalizations must also guarantee that data can be collected from the selected empirical referents accurately (i.e., consistently and precisely). This step concerns the reliability of measurement.
Together, validity and reliability are the benchmarks against which the adequacy and accuracy (and ultimately the quality) of QtPR are evaluated. To assist researchers, useful Respositories of measurement scales are available online. See for example: https://en.wikibooks.org/wiki/Handbook_of_Management_Scales .
4.2 Validity
Validity describes whether the operationalizations and the collected data share the true meaning of the constructs that the researchers set out to measure. Valid measures represent the essence or content upon which the construct is focused. For instance, recall the challenge of measuring “compassion”: A question of validity is to demonstrate that measurements are focusing on compassion and not on empathy or other related constructs.
There are different types of validity that are important to identify. Some of them relate to the issue of shared meaning and others to the issue of accuracy. In turn, there are theoretical assessments of validity (for example, for content validity,), which assess how well an operationalized measure fits the conceptual definition of the relevant theoretical construct; and empirical assessments of validity (for example, for convergent and discriminant validity), which assess how well collected measurements behave in relation to the theoretical expectations. Note that both theoretical and empirical assessments of validity are key to ensuring validity of study results.
Content validity in our understanding refers to the extent to which a researcher’s conceptualization of a construct is reflected in her operationalization of it, that is, how well a set of measures match with and capture the relevant content domain of a theoretical construct (Cronbach, 1971). But as with many other concepts, one should note that other characterizations of content validity also exist (e.g., Rossiter, 2011).
The key question of content validity in our understanding is whether the instrumentation (questionnaire items, for example) pulls in a representative manner all of the ways that could be used to measure the content of a given construct (Straub et al., 2004). Content validity is important because researchers have many choices in creating means of measuring a construct. Did they choose wisely so that the measures they use capture the essence of the construct? They could, of course, err on the side of inclusion or exclusion. If they include measures that do not represent the construct well, measurement error results. If they omit measures, the error is one of exclusion. Suppose you included “satisfaction with the IS staff” in your measurement of a construct called User Information Satisfaction but you forgot to include “satisfaction with the system” itself? Other researchers might feel that you did not draw well from all of the possible measures of the User Information Satisfaction construct. They could legitimately argue that your content validity was not the best. Assessments may include an expert panel that peruse a rating scheme and/or a qualitative assessment technique such as the Q-sort method (Block, 1961).
Construct validity is an issue of operationalization and measurement between constructs. With construct validity, we are interested in whether the instrumentation allows researchers to truly capture measurements for constructs in a way that is not subject to common methods bias and other forms of bias. For example, construct validity issues occur when some of the questionnaire items, the verbiage in the interview script, or the task descriptions in an experiment are ambiguous and are giving the participants the impression that they mean something different from what was intended.
Problems with construct validity occur in three major ways. Items or phrases in the instrumentation are not related in the way they should be, or they are related in the ways they should not be. If items do not converge, i.e., measurements collected with them behave statistically different from one another, it is called a convergent validity problem. If they do not segregate or differ from each other as they should, then it is called a discriminant validity problem.
Nomological validity assesses whether measurements and data about different constructs correlate in a way that matches how previous literature predicted the causal (or nomological) relationships of the underlying theoretical constructs. So, essentially, we are testing whether our obtained data fits previously established causal models of the phenomenon including prior suggested classifications of constructs (e.g., as independent, dependent, mediating, or moderating). If there are clear similarities, then the instrument items can be assumed to be reasonable, at least in terms of their nomological validity.
There are numerous ways to assess construct validity (Straub, Boudreau, and Gefen, 2004; Gefen, Straub, and Boudreau, 2000; Straub, 1989). Typically, researchers use statistical, correlational logic, that is, they attempt to establish empirically that items that are meant to measure the same constructs have similar scores (convergent validity) whilst also being dissimilar to scores of measures that are meant to measure other constructs (discriminant validity) This is usually done by comparing item correlations and looking for high correlations between items of one construct and low correlations between those items and items associated with other constructs. Other tests include factor analysis (a latent variable modeling approach) or principal component analysis (a composite-based analysis approach), both of which are tests to assess whether items load appropriately on constructs represented through a mathematically latent variable (a higher order factor). In this context, loading refers to the correlation coefficient between each measurement item and its latent factor. If items load appropriately high (viz., above 0.7), we assume that they reflect the theoretical constructs. Tests of nomological validity typically involve comparing relationships between constructs in a “network” of theoretical constructs with theoretical networks of constructs previously established in the literature and which may involve multiple antecedent, mediator, and outcome variables. The idea is to test a measurement model established given newly collected data against theoretically-derived constructs that have been measured with validated instruments and tested against a variety of persons, settings, times, and, in the case of IS research, technologies, in order to make the argument more compelling that the constructs themselves are valid (Straub et al. 2004). Often, such tests can be performed through structural equation modelling or moderated mediation models.
Internal validity assesses whether alternative explanations of the dependent variable(s) exist that need to be ruled out (Straub, 1989). It differs from construct validity, in that it focuses on alternative explanations of the strength of links between constructs whereas construct validity focuses on the measurement of individual constructs. Shadish et al. (2001) distinguish three factors of internal validity, these being (1) temporal precedence of IVs before DVs; (2) covariation; and (3) the ability to show the predictability of the current model variables over other, missing variables (“ruling out rival hypotheses”).
Challenges to internal validity in econometric and other QtPR studies are frequently raised using the rubric of “endogeneity concerns.” Endogeneity is an important issue because issues such as omitted variables, omitted selection, simultaneity, common-method variance, and measurement error all effectively render statistically estimates causally uninterpretable (Antonakis et al., 2010). Statistically, the endogeneity problem occurs when model variables are highly correlated with error terms. From a practical standpoint, this almost always happens when important variables are missing from the model. Hence, the challenge is what Shadish et al. (2001) are referring to in their third criterion: How can we show we have reasonable internal validity and that there are not key variables missing from our models?
Historically, internal validity was established through the use of statistical control variables . (Note that this is an entirely different concept from the term “control” used in an experiment where it means that one or more groups have not gotten an experimental treatment; to differentiate it from controls used to discount other explanations of the DV, we can call these “experimental controls.”) Statistical control variables are added to models to demonstrate that there is little-to-no explained variance associated with the designated statistical controls. Typical examples of statistical control variables in many QtPR IS studies are measurements of the size of firm, type of industry, type of product, previous experience of the respondents with systems, and so forth. Other endogeneity tests of note include the Durbin-Wu-Hausman (DWH) test and various alternative tests commonly carried out in econometric studies (Davidson and MacKinnon, 1993). If the DWH test indicates that there may be endogeneity, then the researchers can use what are called “instrumental variables” to see if there are indeed missing variables in the model. An overview of endogeneity concerns and ways to address endogeneity issues through methods such as fixed-effects panels, sample selection, instrumental variables, regression discontinuity, and difference-in-differences models, is given by Antonakis et al. (2010). More discussion on how to test endogeneity is available in Greene (2012).
Manipulation validity is used in experiments to assess whether an experimental group (but not the control group) is faithfully manipulated – and we can thus reasonably trust that any observed group differences are in fact attributable to the experimental manipulation. This form of validity is discussed in greater detail, including stats for assessing it, in Straub, Boudreau, and Gefen (2004). Suffice it to say at this point that in experiments, it is critical that the subjects are manipulated by the treatments and, conversely, that the control group is not manipulated. Checking for manipulation validity differs by the type and the focus of the experiment, and its manipulation and experimental setting. In some (nut not all) experimental studies, one way to check for manipulation validity is to ask subjects, provided they are capable of post-experimental introspection: Those who were aware that they were manipulated are testable subjects (rather than noise in the equations). In fact, those who were not aware, depending on the nature of the treatments, may be responding as if they were assigned to the control group.
In closing, we note that the literature also mentions other categories of validity. For example, statistical conclusion validity tests the inference that the dependent variable covaries with the independent variable, as well as that of any inferences regarding the degree of their covariation (Shadish et al., 2001). Type I and Type II errors are classic violations of statistical conclusion validity (Garcia-Pérez, 2012; Shadish et al., 2001). Predictive validity (Cronbach & Meehl, 1955) assesses the extent to which a measure successfully predicts a future outcome that is expected and practically meaningful. Finally, ecological validity (Shadish et al., 2001) assesses the ability to generalize study findings from an experimental setting to a set of real-world settings. High ecological validity means researchers can generalize the findings of their research study to real-life settings. We note that at other times, we have discussed ecological validity as a form of external validity (Im & Straub, 2015).
4.3 Reliability
Reliability describes the extent to which a measurement variable or set of variables is consistent in what it is intended to measure across multiple applications of measurements (e.g., repeated measurements or concurrently through alternative measures). If multiple measurements are taken, reliable measurements should all be consistent in their values.
Reliability is important to the scientific principle of replicability because reliability implies that the operations of a study can be repeated in equal settings with the same results. Consider the example of weighing a person. An unreliable way of measuring weight would be to ask onlookers to guess a person’s weight. Most likely, researchers will receive different answers from different persons (and perhaps even different answers from the same person if asked repeatedly). A more reliable way, therefore, would be to use a scale. Unless the person’s weight actually changes in the times between stepping repeatedly on to the scale, the scale should consistently, within measurement error, give you the same results. Note, however, that a mis-calibrated scale could still give consistent (but inaccurate) results. This example shows how reliability ensures consistency but not necessarily accuracy of measurement. Reliability does not guarantee validity.
Sources of reliability problems often stem from a reliance on overly subjective observations and data collections. All types of observations one can make as part of an empirical study inevitably carry subjective bias because we can only observe phenomena in the context of our own history, knowledge, presuppositions, and interpretations at that time. This is why often in QtPR researchers often look to replace observations made by the researcher or other subjects with other, presumably more “objective” data such as publicly verified performance metrics rather than subjectively experienced performance. Other sources of reliability problems stem from poorly specified measurements, such as survey questions that are imprecise or ambiguous, or questions asked of respondents who are either unqualified to answer, unfamiliar with, predisposed to a particular type of answer, or uncomfortable to answer.
Different types of reliability can be distinguished: Internal consistency (Streiner, 2003) is important when dealing with multidimensional constructs. It measures whether several measurement items that propose to measure the same general construct produce similar scores. The most common test is through Cronbach’s (1951) alpha, however, this test is not without problems. One problem with Cronbach alpha is that it assumes equal factor loadings, aka essential tau-equivalence. An alternative to Cronbach alpha that does not assume tau-equivalence is the omega test (Hayes and Coutts, 2020). The omega test has been made available in recent versions of SPSS; it is also available in other statistical software packages. Another problem with Cronbach’s alpha is that a higher alpha can most often be obtained simply by adding more construct items in that alpha is a function of k items. In other words, many of the items may not be highly interchangeable, highly correlated, reflective items (Jarvis et al., 2003), but this will not be obvious to researchers unless they examine the impact of removing items one-by-one from the construct.
Interrater reliability is important when several subjects, researchers, raters, or judges code the same data(Goodwin, 2001). Often, we approximate “objective” data through “inter-subjective” measures in which a range of individuals (multiple study subjects or multiple researchers, for example) all rate the same observation – and we look to get consistent, consensual results. Consider, for example, that you want to score student thesis submissions in terms of originality, rigor, and other criteria. We typically have multiple reviewers of such thesis to approximate an objective grade through inter-subjective rating until we reach an agreement. In scientific, quantitative research, we have several ways to assess interrater reliability. Cohen’s (1960) coefficient Kappa is the most commonly used test. Pearson’s or Spearman correlations, or percentage agreement scores are also used (Goodwin, 2001).
Straub, Boudreau, and Gefen (2004) introduce and discuss a range of additional types of reliability such as unidimensional reliability , composite reliability , split-half reliability , or test-retest reliability . They also list the different tests available to examine reliability in all its forms.
The demonstration of reliable measurements is a fundamental precondition to any QtPR study: Put very simply, the study results will not be trusted (and thus the conclusions foregone) if the measurements are not consistent and reliable. And because even the most careful wording of questions in a survey, or the reliance on non-subjective data in data collection does not guarantee that the measurements obtained will indeed be reliable, one precondition of QtPR is that instruments of measurement must always be tested for meeting accepted standards for reliability.
4.4 Developing and Assessing Measures and Measurements
Establishing reliability and validity of measures and measurement is a demanding and resource-intensive task. It is by no means “optional.” Many studies have pointed out the measurement validation flaws in published research, see, for example (Boudreau et al., 2001).
Because developing and assessing measures and measurement is time-consuming and challenging, researchers should first and always identify existing measures and measurements that have already been developed and assessed, to evaluate their potential for reuse. Aside from reducing effort and speeding up the research, the main reason for doing so is that using existing, validated measures ensures comparability of new results to reported results in the literature: analyses can be conducted to compare findings side-by-side. However, critical judgment is important in this process because not all published measurement instruments have in fact been thoroughly developed or validated; moreover, standards and knowledge about measurement instrument development and assessment themselves evolve with time. For example, several historically accepted ways to validate measurements (such as approaches based on average variance extracted, composite reliability, or goodness of fit indices) have later been criticized and eventually displaced by alternative approaches. As an example, Henseler et al. (2015) propose to evaluate heterotrait-monotrait correlation ratios instead of the traditional Fornell-Larcker criterion and the examination of cross-loadings when evaluating discriminant validity of measures.
There are great resources available that help researchers to identify reported and validated measures as well as measurements. For example, the Inter-Nomological Network (INN, https://inn.theorizeit.org/ ), developed by the Human Behavior Project at the Leeds School of Business, is a tool designed to help scholars to search the available literature for constructs and measurement variables (Larsen & Bong, 2016). Other management variables are listed on a wiki page.
When new measures or measurements need to be developed, the good news is that ample guidelines exist to help with this task. Historically, QtPR scholars in IS research often relied on methodologies for measurement instrument development that build on the work by Churchill in the field of marketing (Churchill, 1979). Figure 3 shows a simplified procedural model for use by QtPR researchers who wish to create new measurement instruments for conceptually defined theory constructs. The procedure shown describes a blend of guidelines available in the literature, most importantly (MacKenzie et al., 2011; Moore & Benbasat, 1991). It incorporates techniques to demonstrate and assess the content validity of measures as well as their reliability and validity. It separates the procedure into four main stages and describes the different tasks to be performed (grey rounded boxes), related inputs and outputs (white rectangles), and the relevant literature or sources of empirical data required to carry out the tasks (dark grey rectangles).
It is important to note that the procedural model as shown in Figure 3 describes this process as iterative and discrete, which is a simplified and idealized model of the actual process. In reality, any of the included stages may need to be performed multiple times and it may be necessary to revert to an earlier stage when the results of a later stage do not meet expectations. Also note that the procedural model in Figure 3 is not concerned with developing theory; rather it applies to the stage of the research where such theory exists and is sought to be empirically tested. In other words, the procedural model described below requires the existence of a well-defined theoretical domain and the existence of well-specified theoretical constructs.
The first stage of the procedural model is construct conceptualization, which is concerned with defining the conceptual content domain of a construct. This task involves identifying and carefully defining what the construct is intended to conceptually represent or capture, discussing how the construct differs from other related constructs that may already exist, and defining any dimensions or domains that are relevant to grasping and clearly defining the conceptual theme or content of the construct it its entirety. MacKenzie et al. (2011) provide several recommendations for how to specify the content domain of a construct appropriately, including defining its domain, entity, and property.
A common problem at this stage is that researchers assume that labelling a construct with a name is equivalent to defining it and specifying its content domains: It is not. As a rule of thumb, each focal construct needs (1) a label, (2) a definition, (3) ideally one or more examples that demonstrate its meaning, and ideally (4) a discussion of related constructs in the literature, and (5) a discussion of the focal construct’s likely nomological net and its position within (e.g., as independent factor, as mediating or moderating factor, or as dependent factor).
The next stage is measurement development, where pools of candidate measurement items are generated for each construct. This task can be carried out through an analysis of the relevant literature or empirically by interviewing experts or conducting focus groups. This stage also involves assessing these candidate items, which is often carried out through expert panels that need to sort, rate, or rank items in relation to one or more content domains of the constructs. There are several good illustrations in the literature to exemplify how this works (e.g., Doll & Torkzadeh, 1998; MacKenzie et al., 2011; Moore & Benbasat, 1991).
The third stage, measurement testing and revision, is concerned with “purification”, and is often a repeated stage where the list of candidate items is iteratively narrowed down to a set of items that are fit for use. As part of that process, each item should be carefully refined to be as accurate and exact as possible. Often, this stage is carried out through pre- or pilot-tests of the measurements, with a sample that is representative of the target research population or else another panel of experts to generate the data needed. Repeating this stage is often important and required because when, for example, measurement items are removed, the entire set of measurement item changes, the result of the overall assessment may change, as well as the statistical properties of individual measurement items remaining in the set.
The final stage is validation, which is concerned with obtaining statistical evidence for reliability and validity of the measures and measurements. This task can be fulfilled by performing any field-study QtPR method (such as a survey or experiment) that provides a sufficiently large number of responses from the target population of the respective study. The key point to remember here is that for validation, a new sample of data is required – it should be different from the data used for developing the measurements, and it should be different from the data used to evaluate the hypotheses and theory. Figure 4 summarizes criteria and tests for assessing reliability and validity for measures and measurements. More details on measurement validation are discussed in Section 5 below.
5.1 Defining the Purpose of a Study
Initially, a researcher must decide what the purpose of their specific study is: Is it confirmatory or is it exploratory research? Hair et al. (2010) suggest that confirmatory studies are those seeking to test (i.e., estimating and confirming) a prespecified relationship, whereas exploratory studies are those that define possible relationships in only the most general form and then allow multivariate techniques to search for non-zero or “significant” (practically or statistically) relationships. In the latter case, the researcher is not looking to “confirm” any relationships specified prior to the analysis, but instead allows the method and the data to “explore” and then define the nature of the relationships as manifested in the data.
5.2 Distinguishing Methods from Techniques
One of the most common issues in QtPR papers is mistaking data collection for method(s). When authors say their method was a survey, for example, they are telling the readers how they gathered the data, but they are not really telling what their method was. For example, their method could have been some form of an experiment that used a survey questionnaire to gather data before, during, or after the experiment. Or, the questionnaire could have been used in an entirely different method, such as a field study of users of some digital platform.
The same thing can be said about many econometric studies and other studies using archival data or digital trace data from an organization. Saying that the data came from an ecommerce platform or from scraping posts at a website is not a statement about method. It is simply a description of where the data came from.
Therefore, QtPR can involve different techniques for data collection and analysis, just as qualitative research can involve different techniques for data collection (such as focus groups, case study, or interviews) and data analysis (such as content analysis, discourse analysis, or network analysis).
To understand different types of QtPR methods, it is useful to consider how a researcher designs for variable control and randomization in the study. This allows comparing methods according to their validities (Stone, 1981). In this perspective, QtPR methods lie on a continuum from study designs where variables are merely observed but not controlled to study designs where variables are very closely controlled. Likewise, QtPR methods differ in the extent to which randomization is employed during data collection (e.g., during sampling or manipulations). Figure 5 uses these distinctions to introduce a continuum that differentiates four main types of general research approaches to QtPR.
Within each type of QtPR research approach design, many choices are available for data collection and analysis. It should be noted that the choice of a type of QtPR research (e.g., descriptive or experimental) does not strictly “force” a particular data collection or analysis technique. It may, however, influence it, because different techniques for data collection or analysis are more or less well suited to allow or examine variable control; and likewise different techniques for data collection are often associated with different sampling approaches (e.g., non-random versus random). For example, using a survey instrument for data collection does not allow for the same type of control over independent variables as a lab or field experiment. Or, experiments often make it easier for QtPR researchers to use a random sampling strategy in comparison to a field survey. Similarly, the choice of data analysis can vary: For example, covariance structural equation modeling does not allow determining the cause-effect relationship between independent and dependent variables unless temporal precedence is included. Different approaches follow different logical traditions (e.g., correlational versus counterfactual versus configurational) for establishing causation (Antonakis et al., 2010; Morgan & Winship. 2015).
Typically, a researcher will decide for one (or multiple) data collection techniques while considering its overall appropriateness to their research, along with other practical factors, such as: desired and feasible sampling strategy, expected quality of the collected data, estimated costs, predicted nonresponse rates, expected level of measure errors, and length of the data collection period (Lyberg and Kasprzyk, 1991). It is, of course, possible that a given research question may not be satisfactorily studied because specific data collection techniques do not exist to collect the data needed to answer such a question (Kerlinger, 1986).
Popular data collection techniques for QtPR include: secondary data sources, observation, objective tests, interviews, experimental tasks, questionnaires and surveys, or q-sorting. These may be considered to be the instrumentation by which the researcher gathers data. Instrumentation in this sense is thus a collective term for all of the tools, procedures, and instruments that a researcher may use to gather data. Many of these data collection techniques require a research instrument, such as a questionnaire or an interview script. Others require coding, recoding, or transformation of the original data gathered through the collection technique.
The term “research instrument” can be preferable to specific names such as “survey instruments” in many situations. The term “research instrument” is neutral and does not imply a methodology. A research instrument can be administered as part of several different research approaches, e.g., as part of an experiment, a web survey, or a semi-structured interview.
Variable Control and Validity
Field studies tend to be high on external validity, but low on internal validity. Since the data is coming from the real world, the results can likely be generalized to other similar real-world settings. Hence the external validity of the study is high. On the other hand, field studies typically have difficulties controlling for the three internal validity factors (Shadish et al., 2001). Since field studies often involve statistical techniques for data analysis, the covariation criterion is usually satisfied. Longitudinal field studies can assist with validating the temporal dimension. But countering the possibility of other explanations for the phenomenon of interest is often difficult in most field studies, econometric studies being no exception.
At the other end of the continuum (Figure 6) we see approaches such as laboratory experimentation, which are commonly high on internal validity, but fairly low on external validity. Since laboratory experiments most often give one group a treatment (or manipulation) of some sort and another group no treatment, the effect on the DV has high internal validity. This is particularly powerful when the treatment is randomly assigned to the subjects forming each group. If they are randomly assigned, then there is a low probability that the effect is caused by any factors other than the treatment. Assuming that the experimental treatment is not about gender, for example, each group should be statistically similar in terms of its gender makeup. The same conclusion would hold if the experiment was not about preexisting knowledge of some phenomenon. Random assignment makes it highly unlikely that subjects’ prior knowledge impacted the DV. By their very nature, experiments have temporal precedence. The treatments always precede the collection of the DVs. Therefore, experimentation covers all three Shadish et al. (2001) criteria for internal validity.
Of special note is the case of field experiments. They involve manipulations in a real world setting of what the subjects experience. In the classic Hawthorne experiments, for example, one group received better lighting than another group. The experimental hypothesis was that the work group with better lighting would be more productive. The point here is not whether the results of this field experiment were interesting (they were, in fact, counter-intuitive). Rather, the point here is that internal validity is reasonably high in field experiments since they were conducted in real world settings. And since the results of field experiments are more generalizable to real-life settings than laboratory experiments (because they occur directly within real-life rather than artificial settings), they score also relatively high on external validity. One caveat in this case might be that the assignment of treatments in field experiments is often by branch, office, or division and there may be some systematic bias in choosing these sample frames in that it is not random assignment. All other things being equal, field experiments are the strongest method that a researcher can adopt.
Randomization
There are typically three forms of randomization employed in social science research methods. One form of randomization ( random assignment ) relates to the use of treatments or manipulations (in experiments, most often) and is therefore an aspect of internal validity (Trochim et al., 2016). Random assignment is about randomly manipulating the instrumentation so that there is a very unlikely connection between the group assignments (in an experimental block design) and the experimental outcomes. An example may help solidify this important point. The experimenter might use a random process to decide whether a given subject is in a treatment group or a control group. Thus the experimental instrumentation each subject experiences is quite different. Since the assignment to treatment or control is random, it effectively rules out almost any other possible explanation of the effect. Randomizing gender and health of participants, for example, should result in roughly equal splits between experimental groups so the likelihood of a systematic bias in the results from either of these variables is low. By chance, of course, there could be a preponderance of males or unhealthier persons in one group versus the other but in such rare cases researchers can regulate this in media res and adjust the sampling using a quota process (Trochim et al., 2016). Random assignment helps to establish the causal linkage between the theoretical antecedents and the effects and thereby strengthens internal validity.
A second form of randomization ( random selection ) relates to sampling, that is, the procedures used for taking a predetermined number of observations from a larger population, and is therefore an aspect of external validity (Trochim et al. 2016). Random selection is about choosing participating subjects at random from a population of interest. This is the surest way to be able to generalize from the sample to that population and thus a strong way to establish external validity. Another way to extend external validity within a research study is to randomly vary treatment levels. Again, an example might help explain this rarely used form of randomization. A researcher expects that the time it takes a web page to load (download delay in seconds) will adversely affect one’s patience in remaining at the website. The typical way to set treatment levels would be a very short delay, a moderate delay and a long delay. The issue is not whether the delay times are representative of the experience of many people. They may well be. But setting these exact points in the experiment means that we can generalize only to these three delay points. Randomizing the treatment times, however, allows a scholar to generalize across the whole range of delays, hence increasing external validity within the same, alternatively designed study.
A third form of randomization ( random item inclusion ) relates to how well a construct’s measures capture the content of a construct and is therefore an aspect of content validity (Straub et al. 2004). Random item inclusion means assuring content validity in a construct by drawing randomly from the universe of all possible measures of a given construct. Tests of content validity (e.g., through Q-sorting) are basically intended to verify this form of randomization. The fact of the matter is that the universe of all items is quite unknown and so we are groping in the dark to capture the best measures. They are truly socially-constructed.
Needless to say, this brief discussion only introduces three aspects to the role of randomization. There is a wealth of literature available to dig deeper into the role, and forms, of randomization (e.g., Cochran, 1977; Trochim et al., 2016; Shadish et al., 2001).
5.3 Descriptive and Correlational Research via Survey Instruments Collection
Descriptive and correlational research usually involves non-experimental, observational data collection techniques, such as survey instruments, which do not involve controlling or manipulating independent variables. This means that survey instruments in this research approach are used when one does not principally seek to intervene in reality (as in experiments), but merely wishes to observe it (even though the administration of a survey itself is already an intervention).
A survey is a means of gathering information about the characteristics, actions, perceptions, attitudes, or opinions of a large group of units of observations (such as individuals, groups or organizations), referred to as a population. Surveys thus involve collecting data about a large number of units of observation from a sample of subjects in field settings through questionnaire-type instruments that contain sets of printed or written questions with a choice of answers, and which can be distributed and completed via mail, online, telephone, or, less frequently, through structured interviewing. The resulting data is analyzed, typically through descriptive or inferential statistical techniques.
Surveys have historically been the dominant technique for data collection in information systems (Mazaheri et al. 2020). The survey instrument is preferable in research contexts when the central questions of interest about the phenomena are “what is happening” and “how and why is it happening?” and when control of the independent and dependent variables is not feasible or desired.
Research involving survey instruments in general can be used for at least three purposes, these being exploration, description, or explanation. The purpose of survey research in exploration is to become more familiar with a phenomenon or topic of interest. It focuses on eliciting important constructs and identifying ways for measuring these. Exploratory surveys may also be used to uncover and present new opportunities and dimensions about a population of interest. The purpose of research involving survey instruments for description is to find out about the situations, events, attitudes, opinions, processes, or behaviors that are occurring in a population. Thereby, descriptive surveys ascertain facts. They do not develop or test theory. The purpose of research involving survey instruments for explanation is to test theory and hypothetical causal relations between theoretical constructs. It is the most common form of survey instrument use in information systems research. Explanatory surveys ask about the relations between variables often on the basis of theoretically grounded expectations about how and why the variables ought to be related. Typically, the theory behind survey research involves some elements of cause and effect in that not only assumptions are made about relationships between variables but also about the directionality of these relationships. Surveys then allow obtaining correlations between observations that are assessed to evaluate whether the correlations fit with the expected cause and effect linkages. Surveys in this sense therefore approach causality from a correlational viewpoint; it is important to note that there are other traditions toward causal reasoning (such as configurational or counterfactual), some of which cannot be well-matched with data collected via survey research instruments (Antonakis et al., 2010; Pearl, 2009).
5.4 Experimental and Quasi-Experimental Research
Descriptive and correlational data collection techniques, such as surveys, rely on data sampling – the process of selecting units from a population of interest and observe or measure variables of interest without attempting to influence the responses. Such data, however, is often not perfectly suitable for gauging cause and effect relationships due to potential confounding factors that may exist beyond the data that is collected. And, crucially, inferring temporal precedence, i.e., establishing that the cause came before the effect, in a one-point in time survey is at best related to self-reporting by the subject.
Experiments are specifically intended to examine cause and effect relationships. This is because in experiments the researchers deliberately impose some treatment to one or more groups of respondents (the one or more treatment groups) but not to another group (the control group) while also maintaining control over other potential confounding factors in order to observe responses. A treatment is a manipulation of the real world that an experimenter administers to the subjects (also known as experimental units) so that the experimenter can observe a response. The treatment in an experiment is thus how an independent variable is operationalized. A typical way this is done is to divide the subjects into groups randomly where each group is “treated” differently so that the differences in these treatments result in differences in responses across these groups as hypothesize. Different treatments thus constitute different levels or values of the construct that is the independent variable.
The primary strength of experimental research over other research approaches is the emphasis on internal validity due to the availability of means to isolate, control and examine specific variables (the cause) and the consequence they cause in other variables (the effect). Its primary disadvantage is often a lack of ecological validity because the desire to isolate and control variables typically comes at the expense of realism of the setting. Moreover, real-world domains are often much more complex than the reduced set of variables that are being examined in an experiment.
Experimental research is often considered the gold standard in QtPR, but it is also one of the most difficult. This is because experimental research relies on very strong theory to guide construct definition, hypothesis specification, treatment design, and analysis. Any design error in experiments renders all results invalid. Moreover, experiments without strong theory tend to be ad hoc, possibly illogical, and meaningless because one essentially finds some mathematical connections between measures without being able to offer a justificatory mechanism for the connection (“you can’t tell me why you got these results”). The most pertinent danger in experiments is a flaw in the design that makes it impossible to rule out rival hypotheses (potential alternative theories that contradict the suggested theory). A second big problem is the inappropriate design of treatment and tasks.
Experiments can take place in the laboratory ( lab experiments ) or in reality ( field experiments ). Lab experiments typically offer the most control over the situation to the researcher, and they are the classical form of experiments. Think of students sitting in front of a computer in a lab performing experimental tasks or think of rats in cages that get exposed to all sorts of treatments under observation. Lauren Slater provides some wonderful examples in her book about experiments in psychology (Slater, 2005). Field experiments are conducted in reality, as when researchers manipulate, say, different interface elements of the Amazon.com webpage while people continue to use the ecommerce platform. Field experiments are difficult to set up and administer, in part because they typically involve collaborating with some organization that hosts a particular technology (say, an ecommerce platform). On the other hand, field experiments typically achieve much higher levels of ecological validity whilst also ensuring high levels of internal validity. They have become more popular (and more feasible) in information systems research over recent years.
In both lab and field experiments, the experimental design can vary (see Figures 6 and 7). For example, one key aspect in experiments is the choice of between-subject and within-subject designs: In between-subject designs , different people test each experimental condition. For example, if one had a treatment in the form of three different user-interface-designs for an e-commerce website, in a between-subject design three groups of people would each evaluate one of these designs. In a within-subjects design, the same subject would be exposed to all the experimental conditions. For example, each participant would first evaluate user-interface-design one, then the second user-interface-design, and then the third.
Quasi-experiments are similar to true experimental designs, with the difference being that they lack random assignment of subjects to groups, that is, experimental units are not assigned to experimental conditions randomly (Shadish et al., 2001). In effect, one group (say, the treatment group) may differ from another group in key characteristics; for example, a post-graduate class possesses higher levels of domain knowledge than an under-graduate class. Quasi-experimental designs often suffer from increased selection bias. Selection bias means that individuals, groups, or other data has been collected without achieving proper randomization, thereby failing to ensure that the sample obtained is representative of the population intended to be analyzed. Selection bias in turn diminishing internal validity. Still, sometimes a research design demands the deliberate assignment to an experimental group (for instance to explicitly test the effect of an intervention on under-performing students versus well-performing students). The most common forms are non-equivalent groups design – the alternative to a two-group pre-test-post-test design, and non-equivalent switched replication design, in which an essential experimental treatment is “replicated” by switching the treatment and control group in two subsequent iterations of the experiment (Trochim et al. 2016).
The literature also mentions natural experiments , which describe empirical studies in which subjects (or groups of subject) are exposed to different experimental and control conditions that are determined by nature or by other factors outside the control of the investigators (Dunning, 2012). Strictly speaking, natural experiments are not really experiments because the cause can usually not be manipulated; rather, natural experiments contrast naturally occurring events (e.g., an earthquake) with a comparison condition (Shadish et al., 2001). Free-simulation experiments (Tromkin & Steufert) expose subjects to real-world-like events and allow them within the controlled environment to behave generally freely and are asked to make decisions and choices as they see fit, thus allowing values of the independent variables to range over the natural range of the subjects’ experiences, and where ongoing events are determined by the interaction between experimenter-defined parameters (e.g., the prescribed experimental tasks) and the relatively free behavior of all participating subjects.
5.5 Quantitative Data Analysis
Data analysis concerns the examination of quantitative data in a number of ways. Descriptive analysis refers to describing, aggregating, and presenting the constructs of interests or the associations between the constructs to describe, for example, the population from where the data originated, the range of response levels obtained, and so forth. Inferential analysis refers to the statistical testing of hypotheses about populations based on a sample – typically the suspected cause and effect relationships – to ascertain whether the theory receives support from the data within certain degrees of confidence, typically described through significance levels. Most of these analyses are nowadays conducted through statistical software packages such as SPSS, SAS, or mathematical programming environments such as R or Mathematica. For any quantitative researcher, a good knowledge of these tools is essential.
There is not enough space here to cover the varieties or intricacies of different quantitative data analysis strategies. But many books exist on that topic (Bryman & Cramer, 2008; Field, 2013; Reinhart, 2015; Stevens, 2001; Tabachnick & Fidell, 2001), including one co-authored by one of us (Mertens et al., 2017).
Data analysis techniques include univariate analysis (such as analysis of single-variable distributions), bivariate analysis, and more generally, multivariate analysis. Univariate analyses concern the examination of one variable by itself, to identify properties such as frequency, distribution, dispersion, or central tendency. Classic statistics involve mean, median, variance, or standard deviation. Bivariate analyses concern the relationships between two variables. For example, we may examine the correlation between two numerical variables to identify the changes in one variable when the other variable levels increase or decrease. An example would be the correlation between salary increases and job satisfaction. A positive correlation would indicate that job satisfaction increases when pay levels go up. It is important to note here that correlation does not imply causation. A correlation between two variables merely confirms that the changes in variable levels behave in particular way upon changing another; but it cannot make a statement about which factor causes the change in variables (it is not unidirectional). Moreover, correlation analysis assumes a linear relationship. Should the relationship be other than linear, for example an inverted U relationship, then the results of a linear correlation analysis could be misleading. Multivariate analyses , broadly speaking, refer to all statistical methods that simultaneously analyze multiple measurements on each individual or object under investigation (Hair et al., 2010); as such, many multivariate techniques are extensions of univariate and bivariate analysis. The decision tree presented in Figure 8 provides a simplified guide for making the right choices.
Figure 8 highlights that when selecting a data analysis technique, a researcher should make sure that the assumptions related to the technique are satisfied, such as normal distribution, independence among observations, linearity, and lack of multi-collinearity between the independent variables, and so forth (Mertens et al. 2017; Gefen, Straub, and Boudreau 2000; Gefen 2003). Multicollinearity can result in paths that are statistically significant when they should not be, they can be statistically insignificant when they are statistically significant, and they can even change the sign of a statistically significant path. Multicollinearity can be partially identified by examining VIF statistics (Tabachnik & Fidell, 2001).
The choice of the correct analysis technique is dependent on the chosen QtPR research design, the number of independent and dependent (and control) variables, the data coding and the distribution of the data received. This is because all statistical approaches to data analysis come with a set of assumptions and preconditions about the data to which they can be applied.
Most QtPR research involving survey data is analyzed using multivariate analysis methods, in particular structural equation modelling (SEM) through either covariance-based or component-based methods. Different methods in each tradition are available and are typically available in statistics software applications such as Stata, R, SPSS, or others. The most popular SEM methods include LISREL (Jöreskog & Sörbom, 2001) and equivalent software packages such as AMOS and Mplus, on the one hand, and Partial Least Squares (PLS) modeling (Chin, 2001; Hair et al., 2013), on the other hand.
SEM has been widely used in social science research for the causal modelling of complex, multivariate data sets in which the researcher gathers multiple measures of proposed constructs. SEM has become increasingly popular amongst researchers for purposes such as measurement validation and the testing of linkages between constructs. In general terms, SEM is a statistical method for testing and estimating assumed causal relationships using a combination of statistical data and qualitative causal assumptions. It encourages confirmatory rather than exploratory analysis. SEM requires one or more hypotheses between constructs, represented as a theoretical model, operationalizes by means of measurement items, and then tests statistically. The causal assumptions embedded in the model often have falsifiable implications that can be tested against survey data. One of the advantages of SEM is that many methods (such as covariance-based SEM models) cannot only be used to assess the structural model – the assumed causation amongst a set of multiple dependent and independent constructs – but also, separately or concurrently, the measurement model – the loadings of observed measurements on their expected latent constructs. In other words, SEM allows researchers to examine the reliability and validity of their measurements as well as the hypotheses contained in their proposed theoretical model. Several detailed step-by-step guides exist for running SEM analysis (e.g., Gefen, 2019; Ringle et al., 2012; Mertens et al., 2017; Henseler et al., 2015).
It should be noted at this point that other, different approaches to data analysis are constantly emerging. One of the most prominent current examples is certainly the set of Bayesian approaches to data analysis (Evermann & Tate, 2014; Gelman et al., 2013; Masson, 2011). Bayesian approaches are essentially model selection procedures that compute a comparison between competing hypotheses or models, and where available knowledge about parameters in a statistical model is updated with the information in observed data. The background knowledge is expressed as a prior distribution and combined with observational data in the form of a likelihood function to determine the posterior distribution. The posterior can also be used for making predictions about future events.
Most experimental and quasi-experimental studies use some form of between-groups analysis of variance such as ANOVA, repeated measures, or MANCOVA. An introduction is provided by Mertens et al. (2017). Standard readings on this matter are Shadish et al. (2001) and Trochim et al. (2016).
If the data or phenomenon concerns changes over time , an analysis technique is required that allows modeling differences in data over time. Essentially, time series data is single variable data that has another dimension of time. For example, the price of a certain stock over days weeks, months, quarters, or years. The most important difference between such time-series data and cross-sectional data is that the added time dimension of time-series data means that such variables change across both units and time. In other words, data can differ across individuals (a “between-variation”) at the same point in time but also internally across time (a “within-variation”).
To analyze data with a time dimension, several analytical tools are available that can be used to model how a current observation can be estimated by previous observations, or to forecast future observations based on that pattern. The difficulty in such analyses is to account for how events unfolding over time can be separated from the momentum of the past itself. For example, one way to analyze time-series data is by means of the Auto-Regressive Integrated Moving Average (ARIMA) technique, that captures how previous observations in a data series determine the current observation. It can also include other covariates. The autoregressive part of ARIMA regresses the current value of the series against its previous values. This can be the most immediate previous observation (a lag of order 1), a seasonal effect (such as the value this month last year, a lag of order 12), or any other combination of previous observations. The moving average part adds a linear combination of the error terms of the previous observations. The number of such previous error terms determines the order of the moving average. The integrated part of the model is included when there is a trend in the data, such as an increase over time, in which case the difference between the observations is calculated rather than modeling the actual observed values. This is necessary because if there is a trend in the series then the model cannot be stationary. Stationarity means that the mean and variance remain the same throughout the range of the series. A sample application of ARIMA in IS research is modeling the usage levels of a health information environments over time and how quasi-experimental events related to governmental policy changed it (Gefen et al., 2019). Other popular ways to analyze time-series data are latent variable models such as latent growth curve models, latent change score models, or bivariate latent difference score models (Bollen & Curran, 2006; McArdle, 2009).
5.6 Validation of Measures and Measurement
Recall that measurement is, arguably, the most important thing that a QtPR scholar can do to ensure that the results of their study can be trusted. Figure 9 shows how to prioritize the assessment of measurement during data analysis.
Row 1: Good statistical conclusion validity, poor internal validity
Imagine a situation where you carry out a series of statistical tests and find terrific indications for statistical significance. You are hopeful that your model is accurate and that the statistical conclusions will show that the relationships you posit are true and important.
Unfortunately, unbeknownst to you, the model you specify is wrong (in the sense that the model may omit common antecedents to both the independent and the dependent variables, or that it exhibits endogeneity concerns). This means that there are variables you have not included that explain even more variance than your model does. An example situation could be a structural equation model that supports the existence of some speculated hypotheses but also shows poor fit to the data. In such a situation you are in the worst possible scenario: you have poor internal validity but good statistical conclusion validity.
Row 2: Good internal validity, good statistical conclusion validity, poor instrumentation validity
Internal validity is a matter of causality. Can you rule out other reasons for why the independent and dependent variables in your study are or are not related? Consider the following: You are testing constructs to see which variable would or could “confound” your contention that a certain variable is as good an explanation for a set of effects. But statistical conclusion and internal validity are not sufficient, instrumentation validity (in terms of measurement validity and reliability) matter as well: Unreliable measurement leads to attenuation of regression path coefficients, i.e. the estimated effect size, whereas invalid measurement means you’re not measuring what you wanted to measure. So if either the posited independent variable or the confound (a rival variable) is poorly measured, then you cannot know with any certainty whether one or the other variable is the true cause. In this situation you have an internal validity problem that is really not simply a matter of testing the strength of either the confound or the theoretical independent variable on the outcome variable, but it is a matter of whether you can trust the measurement of either the independent, the confounding, or the outcome variable. Without instrumentation validity, it is really not possible to assess internal validity. In the early days of computing there was an acronym for this basic idea: GIGO. It stood for “garbage in, garbage out.” It meant that if the data being used for a computer program were of poor, unacceptable quality, then the output report was just as deficient. With respect to instrument validity, if one’s measures are questionable, then there is no data analysis technique that can fix the problem. Research results are totally in doubt if the instrument does not measure the theoretical constructs at a scientifically acceptable level.
Row 3: The only acceptable path (All forms of validity)
Assessing measure and measurement validity is the critical first step in QtPR. If your instrumentation is not acceptable at a minimal level, then the findings from the study will be perfectly meaningless. You cannot trust or contend that you have internal validity or statistical conclusion validity. Reviewers should be especially honed in to measurement problems for this reason. If the measures are not valid and reliable, then we cannot trust that there is scientific value to the work. In an experiment, for example, it is critical that a researcher check not only the experimental instrument, but also whether the manipulation or treatment works as intended, whether experimental task are properly phrased, and so forth.
Straub, Gefen, and Boudreau (2004) describe the “ins” and “outs” for assessing instrumentation validity. Their paper presents the arguments for why various forms of instrumentation validity should be mandatory and why others are optional. Basically, there are four types of scientific validity with respect to instrumentation. They are: (1) content validity, (2) construct validity, (3) reliability, and (4) manipulation validity (see also Figure 4).
Section 6. Practical Tips for Writing QtPR Papers
QtPR papers are welcomed in every information systems journal as QtPR is the most frequently used general research approach in information systems research both historically and currently (Vessey et al., 2020; Mazaheri et al., 2020). Many great examples exist as templates that can guide the writing of QtPR papers. In what follows, we give a few selected tips related to the crafting of such papers.
6.1 Developing Theory in QtPR Papers: Conceptual Labeling of Constructs
Constructs are socially constructed. That is to say, they are created in the mind as abstractions. Like the theoretical research model of construct relationships itself, they are intended to capture the essence of a phenomenon and then to reduce it to a parsimonious form that can be operationalized through measurements.
That being said, constructs are much less clear in what they represent when researchers think of them as entity-relationship (ER) models. ER models are highly useful for normalizing data, but do not serve well for social science research models. Why not? Entities themselves do not express well what values might lie behind the labeling. And in quantitative constructs and models, the whole idea is (1) to make the model understandable to others and (2) to be able to test it against empirical data. So communication of the nature of the abstractions is critical.
An example might help to explain this. Sometimes one sees a model when one of the constructs is “Firm.” It is unclear what this could possibly mean. Does it mean that the firm exists or not? Likely this is not the intention. On the other hand, “Size of Firm” is more easily interpretable, and this construct frequently appears, as noted elsewhere in this treatise. It implies that there will be some form of a quantitative representation of the presence of the firm in the marketplace.
As a second example, models in articles will sometimes have a grab-all variable/construct such as “Environmental Factors.” The problem here is similar to the example above. What could this possibly mean? Likely not that there are either environmental factors or not. The conceptual labeling of this construct is too broad to easily convey its meaning. Were it broken down into its components, there would be less room for criticism. One common construct in the category of “environmental factors,” for instance, is market uncertainty. As a conceptual labeling, this is superior in that one can readily conceive of a relatively quiet marketplace where risks were, on the whole, low. The other end of the uncertainty continuum can be envisioned as a turbulent marketplace where risk was high and economic conditions were volatile. And it is possible using the many forms of scaling available to associate this construct with market uncertainty falling between these end points.
A third example is construct labeling that could be clarified by simply adding a modifying word or phrase to show the reader more precisely what the construct means. Let’s take the construct labelled originally “Co-creation.” Again, the label itself is confusing (albeit typical) in that it likely does not mean that one is co-creating something or not. A clarifying phrase like “Extent of Co-creation” (as opposed to, say, “duration of co-creation”) helps interested readers in conceptualizing that there needs to be some kind of quantification of the amount but not length of co-creating taking place. The theory base itself will provide boundary conditions so that we can see that we are talking about a theory of how systems are designed (i.e., a co-creative process between users and developers) and how successful these systems then are. But the effective labelling of the construct itself can go a long way toward making theoretical models more intuitively appealing.
6.2 Should Dos, Could Dos, and Must Not Dos for QtPR Papers
The table in Figure 10 presents a number of guidelines for IS scholars constructing and reporting QtPR research based on, and extended from, Mertens and Recker (2020). The guidelines consist of three sets of recommendations: two to encourage (“should do” and “could do”) and one to discourage (“must not do”) practices. This combination of “should, could and must not do” forms a balanced checklist that can help IS researchers throughout all stages of the research cycle to protect themselves against cognitive biases (e.g., by preregistering protocols or hypotheses), improve statistical mastery where possible (e.g., through consulting independent methodological advice), and become modest, humble, contextualized, and transparent (Wasserstein et al., 2019) wherever possible (e.g., by following open science reporting guidelines and cross-checking terminology and argumentation).
6.3 Using Personal Pronouns in QtPR Writing
When preparing a manuscript for either a conference or a journal submission, it can be advisable to use the personal pronouns “I” and “we” as little as possible. Of course, such usage of personal pronouns occurs in academic writing, but what it implies might distract from the main storyline of a QtPR article. The emphasis in sentences using the personal pronouns is on the researcher and not the research itself. “I did this, then I did that. Then I did something else.” Or “we did this, followed by our doing that. Next we did the other thing…” Such sentences stress the actions and activities of the researcher(s) rather than the purposes of these actions. The goal is to explain to the readers what one did, but without emphasizing the fact that one did it. The whole point is justifying what was done, not who did it. Converting active voice [this is what it is called when the subject of the sentence highlights the “actor(s)”] to passive voice is a trivial exercise. In a sentence structured in the passive voice, a different verbal form is used, such as in this very sentence. Sentences can also be transformed from active voice that utilizes the personal pronoun in many other ways. The easiest way to show this, perhaps, is through an example. Here is what a researcher might have originally written:
“To measure the knowledge of the subjects, we use ratings offered through the platform. In fact, there are several ratings that we can glean from the platform and these we will combine to create an aggregate score. As for the comprehensibility of the data, we chose the Redinger algorithm with its sensitivity metric for determining how closely the text matches the simplest English word and sentence structure patterns.”
To transform this same passage into passive voice is fairly straight-forward (of course, there are also many other ways to make sentences interesting without using personal pronouns):
“To measure the knowledge of the subjects, ratings offered through the platform were used . In fact, several ratings readily gleaned from the platform were combined to create an aggregate score. As for the comprehensibility of the data, the best choice is the Redinger algorithm with its sensitivity metric for determining how closely the text matches the simplest English word and sentence structure patterns.”
As a caveat, note that many researchers prefer the use of personal pronouns in their writings to emphasize the fact that they are interpreting data through their own personal lenses and that conclusions may not be generalizable. Avoiding personal pronouns can likewise be a way to emphasize that QtPR scientists were deliberately trying to “stand back” from the object of the study.
Adaptive experiment:
This is a “quasi-experimental” research methodology that involves before and after measures, a control group, and non-random assignment of human subjects. Data are gathered before the independent variables are introduced, but the final form is not usually known until after the independent variables have been introduced and the “after” data has been collected (Jenkins, 1985).
Archival research:
This methodology is primarily concerned with the examination of historical documents. Secondarily, it is concerned with any recorded data. All data are examined ex-post-facto by the researcher (Jenkins, 1985).
Univariate analysis of variance (ANOVA) is a statistical technique to determine, on the basis of one dependent measure, whether samples come from populations with equal means. ANOVA is fortunately robust to violations of equal variances across groups (Lindman, 1974). Univariate analysis of variance employs one dependent measure, whereas multivariate analysis of variance compares samples based on two or more dependent variables.
Analysis of covariance (ANCOVA) is a form of analysis of variance that tests the significance of the differences among means of experimental groups after taking into account initial differences among the groups and the correlation of the initial measures and the dependent variable measures. The measure used as a control variable – the pretest or pertinent variable – is called a covariate (Kerlinger, 1986). Covariates need to be at least interval data and will help to partial out the variance and strengthen main effects.
Canonical correlation:
With canonical analysis the objective is to correlate simultaneously several metric dependent variables and several metric independent variables. The underlying principle is to develop a linear combination of each set of variables (both independent and dependent) to maximize the correlation between the two sets.
Cluster analysis:
Cluster analysis is an analytical technique for developing meaningful sub-groups of individuals or objects. Specifically, the objective is to classify a sample of entities (individuals or objects) into a smaller number of mutually exclusive groups based on the similarities among the entities (Hair et al., 2010).
Content domain:
The content domain of an abstract theoretical construct specifies the nature of that construct and its conceptual theme in unambiguous terms and as clear and concise as possible (MacKenzie et al., 2011). The content domain of a construct should formally specify the nature of the construct, including the conceptual domain to which the focal construct belongs and the entity to which it applies.
Conjoint analysis:
Conjoint analysis is an emerging dependence technique that has brought new sophistication to the evaluation of objects, whether they are new products, services, or ideas. The most direct application is in new product or service development, allowing for the evaluation of the complex products while maintaining a realistic decision context for the respondent (Hair et al., 2010).
Correspondence analysis:
Correspondence analysis is a recently developed interdependence technique that facilitates both dimensional reduction of object ratings (e.g., products, persons, etc.) on a set of attributes and the perceptual mapping of objects relative to these attributes (Hair et al., 2010).
Dependent variable:
A variable whose value is affected by, or responds to, a change in the value of some independent variable(s).
Experimental simulation:
This methodology employs a closed simulation model to mirror a segment of the “real world.” Human subjects are exposed to this model and their responses are recorded. Thee researcher completely determines the nature and timing of the experimental events (Jenkins, 1985).
Factor analysis:
Factor analysis is a statistical approach that can be used to analyze interrelationships among a large number of variables and to explain these variables in terms of their common underlying dimensions (factors) (Hair et al., 2010).
Field experiments:
Field experiments involve the experimental manipulation of one or more variables within a naturally occurring system and subsequent measurement of the impact of the manipulation on one or more dependent variables (Boudreau et al., 2001).
Field studies:
Field studies are non-experimental inquiries occurring in natural systems. Researchers using field studies typically do not manipulate independent variables or control the influence of confounding variables (Boudreau et al., 2001).
Free simulation experiment:
This methodology is similar to experimental simulation, in that with both methodologies the researcher designs a closed setting to mirror the “real world” and measures the response of human subjects as they interact within the system. However, with this methodology, events and their timing are determined by both the researcher and the behavior of the human subject (Jenkins, 1985; Fromkin and Streufert, 1976).
Hotelling’s T2:
A test statistic to assess the statistical significance of the difference between two sets of sample means. It is a special case of MANOVA used with two groups or levels of a treatment variable (Hair et al., 2010).
Independent Variable:
A variable whose value change is presumed to cause a change in the value of some dependent variable(s).
Lab(oratory) experiments:
Laboratory experiments take place in a setting especially created by the researcher for the investigation of the phenomenon. With this research method, the researcher has control over the independent variable(s) and the random assignment of research participants to various treatment and non-treatment conditions (Boudreau et al., 2001).
Linear probability models:
In this technique, one or more independent variables are used to predict a single dependent variable. Linear probability models accommodate all types of independent variables (metric and non-metric) and do not require the assumption of multivariate normality (Hair et al., 2010).
Linear regression:
A linear regression attempts determine the best equation describing a set of x and y data points, by using an optimization function such as least squares or maximum likelihood.
A procedure for the analysis of LInear Structural RELations among one or more sets of variables and variates. It examines the covariance structures of the variables and variates included in the model under consideration. LISREL permits both confirmatory factor analysis and the analysis of path models with multiple sets of data in a simultaneous analysis.
Loading (Factor Loading):
A weighting that reflects the correlation between the original variables and derived factors. Squared factor loadings are the percent of variance in an observed item that is explained by its factor.
Logit analysis is a special form of regression in which the criterion variable is a non-metric, dichotomous (binary) variable. While differences exist in some aspects, the general manner of interpretation is quite similar to linear regression (Hair et al., 2010).
Math modeling:
This methodology models the “real world” and states the results as mathematical equations. It is a closed deterministic system in which all of the independent and dependent variables are known and included in the model. Intervening variables simply are not possible and no human subject is required (Jenkins, 1985).
Multitrait-multimethod (MTMM) uses a matrix of correlations representing all possible relationships between a set of constructs, each measured by the same set of methods. This matrix is one of many methods that can be used to evaluate construct validity by demonstrating both convergent and discriminant validity.
Multidimensional scaling:
In multidimensional scaling, the objective is to transform consumer judgments of similarity or preference (e.g., preference for stores or brands) into distances in a multidimensional space. If objects A and B are judged by respondents as being the most similar compared with all other possible pairs of objects, multidimensional scaling techniques will position objects A and B in such a way that the distance between them in the multidimensional space is smaller than the distance between any other two pairs of objects. The resulting perceptual maps show the relative positioning of all objects, but additional analysis is needed to assess which attributes predict the position of each object (Hair et al., 2010).
Multiple regression:
Multiple regression is the appropriate method of analysis when the research problem involves a single metric dependent variable presumed to be related to one or more metric independent variables. The objective of multiple regression analysis is to predict the changes in the dependent variable in response to the changes in the several independent variables (Hair et al., 2010).
Multiple discriminant analysis:
If the single dependent variable is dichotomous (e.g., male-female) or multichotomous (e.g., high-medium-low) and therefore non-metric, the multivariate technique of multiple discriminant analysis (MDA) is appropriate. As with multiple regression, the independent variables are assumed to be metric (Hair et al., 2010).
Multivariate analysis of variance (MANOVA):
Multivariate analysis of variance (MANOVA) is a statistical technique that can be used to simultaneously explore the relationship between several categorical independent variables (usually referred to as treatments) and two or more metric dependent variables. As such, it represents an extension of univariate analysis of variance (ANOVA). MANOVA is useful when the researcher designs an experimental situation (manipulation of several non-metric treatment variables) to test hypotheses concerning the variance in group responses on two or more metric dependent variables (Hair et al., 2010).
Normal Distribution:
A normal distribution is probably the most important type of distribution in behavioral sciences and is the underlying assumption of many of the statistical techniques discussed here. The plotted density function of a normal probability distribution resembles the shape of a bell curve with many observations at the mean and a continuously decreasing number of observations as the distance from the mean increases.
Multinormal distribution:
Also known as a Joint Normal Distribution and as a Multivariate Normal Distribution, occurs when every polynomial combination of items itself has a Normal Distribution. For example, in Linear Regression the dependent variable Y may be the polynomial combination of aX1+bX2+e, where it is assumed that X1 and X2 each has a normal distribution. Multinormal distribution occurs when also the polynomial expression aX1+bX2 itself has a normal distribution. Graphically, a multinormal distribution of X1 and X2 will resemble a sheet of paper with a weight at its center, the center being analogous to the mean of the joint distribution.
Objective Tests:
A type of assessment instrument consisting of a set of items or questions that have specific correct answers (e.g., how much is 2 + 2?), such that no interpretation, judgment, or personal impressions are involved in scoring.
Observation:
Observation means looking at people and listening to them talk. One can infer the meaning, characteristics, motivations, feelings and intentions of others on the basis of observations (Kerlinger, 1986).
PLS (Partial Least Squares) path modeling:
A second generation regression component-based estimation approach that combines a composite analysis with linear regression. .Unlike covariance-based approaches to structural equation modeling, PLS path modeling does not fit a common factor model to the data, it rather fits a composite model.
PCA: Principal Components Analysis.
A dimensionality-reduction method that is often used to transform a large set of variables into a smaller one of uncorrelated or orthogonal new variables (known as the principal components) that still contains most of the information in the large set. Principal components are new variables that are constructed as linear combinations or mixtures of the initial variables such that the principal components account for the largest possible variance in the data set. The objective is to find a way of condensing the information contained in a number of original variables into a smaller set of principal component variables with a minimum loss of information (Hair et al., 2010).
Q-sorting offers a powerful, theoretically grounded, and quantitative tool for examining opinions and attitudes. Q-sorting consists of a modified rank-ordering procedure in which stimuli are placed in an order that is significant from the standpoint of a person operating under specified conditions. It results in the captured patterns of respondents to the stimulus presented, a topic on which opinions vary. Those patterns can then be analyzed to discover groupings of response patterns, supporting effective inductive reasoning (Thomas and Watson, 2002).
Reliability:
Extent to which a variable or set of variables is consistent in what it measures. If multiple (e.g., repeated) measurements are taken, the reliable measures will all be very consistent in their values.
R-squared or R 2 : Coefficient of determination:
Measure of the proportion of the variance of the dependent variable about its mean that is explained by the independent variable(s). R-squared is derived from the F statistic. This statistic is usually employed in linear regression analysis and PLS. In LISREL, the equivalent statistic is known as a squared multiple correlation.
Secondary data sources:
Data that was already collected for some other purpose is called secondary data. Organization files and library holdings are the most frequently used secondary sources of data. Statistical compendia, movie film, printed literature, audio tapes, and computer files are also widely used sources. Secondary data sources can be usually found quickly and cheaply. Sometimes there is no alternative to secondary sources, for example, census reports and industry statistics. Secondary data also extend the time and space range, for example, collection of past data or data about foreign countries (Emory, 1980).
SEM (Structural Equation Modeling):
A label for a variety of multivariate statistical techniques that can include confirmatory factor analysis, confirmatory composite analysis, path analysis, multi-group modeling, longitudinal modeling, partial least squares path modeling, latent growth modeling and hierarchical or multi-level modeling. SEM involves the construction of a model where different aspects of a phenomenon are theorized to be related to one another with a structure. This structure is a system of equations that captures the statistical properties implied by the model and its structural features, and which is then estimated with statistical algorithms (usually based on matrix algebra and generalized linear models) using experimental or observational data.
Time-series analysis:
A data analysis technique used to identify how a current observation is estimated by previous observations, or to predict future observations based on that pattern. Time-series analysis can be run as an Auto-Regressive Integrated Moving Average (ARIMA) model that specifies how previous observations in the series determine the current observation. It can include also cross-correlations with other covariates. Other techniques include OLS fixed effects and random effects models (Mertens et al., 2017).
Wilks’ Lambda: One of the four principal statistics for testing the null hypothesis in MANOVA. It is also referred to as the maximum likelihood criterion or U statistic (Hair et al., 2010).
8.1 Further Readings
There is a large variety of excellent resources available to learn more about QtPR. You can learn more about the philosophical basis of QtPR in writings by Karl Popper (1959) and Carl Hempel (1965). Introductions to their ideas and those of relevant others are provided by philosophy of science textbooks (e.g., Chalmers, 1999; Godfrey-Smith, 2003). There are also articles on how information systems builds on these ideas, or not (e.g., Siponen & Klaavuniemi, 2020).
If you are interested in different procedural models for developing and assessing measures and measurements, you can read up on the following examples that report at some lengths about their development procedures: (Bailey & Pearson, 1983; Davis, 1989; Goodhue, 1998; Moore & Benbasat, 1991; Recker & Rosemann, 2010; Bagozzi, 2011).
Textbooks on survey research that are worth reading include Floyd Flower’s textbook (Fowler, 2001), Devellis and Thorpe (2021), plus a few others (Babbie, 1990; Czaja & Blair, 1996). It is also important to regularly check for methodological advances in journal articles, such as (Baruch & Holtom, 2008; Kaplowitz et al., 2004; King & He, 2005).
A seminal book on experimental research has been written by William Shadish, Thomas Cook, and Donald Campbell (Shadish et al., 2001). A wonderful introduction to behavioral experimentation is Lauren Slater’s book Opening Skinner’s Box: Great Psychological Experiments of the Twentieth Century (Slater, 2005).
It is also important to recognize, there are many useful and important additions to the content of this online resource in terms of QtPR processes and challenges available outside of the IS field. For example, the computer sciences also have an extensive tradition in discussing QtPR notions, such as threats to validity. Wohlin et al.’s (2000) book on Experimental Software Engineering, for example, illustrates, exemplifies, and discusses many of the most important threats to validity, such as lack of representativeness of independent variable, pre-test sensitisation to treatments, fatigue and learning effects, or lack of sensitivity of dependent variables. Vegas and colleagues (2016) discuss advantages and disadvantages between a wide range of experiment designs, such as independent measures, repeated measures, crossover, matched-pairs, and different mixed designs.
Another important debate in the QtPR realm is the ongoing discussion on reflective versus formative measurement development, which was not covered in this resource. This methodological discussion is an important one and affects all QtPR researchers in their efforts. Several viewpoints pertaining to this debate are available (Aguirre-Urreta & Marakas, 2012; Centefelli & Bassellier, 2009; Diamantopoulos, 2001; Diamantopoulos & Siguaw, 2006; Diamantopoulos & Winklhofer, 2001; Kim et al., 2010; Petter et al., 2007).
Another debate in QtPR is about the choice of analysis approaches and toolsets. For example, there is a longstanding debate about the relative merits and limitations of different approaches to structural equation modelling (Goodhue et al., 2007, 2012; Hair et al., 2011; Marcoulides & Saunders, 2006; Ringle et al., 2012), including alternative approaches such as Bayesian structural equation modeling (Evermann & Tate, 2014), or the TETRAD approach (Im & Wang, 2007). These debates, amongst others, also produce several updates to available guidelines for their application (e.g., Henseler et al., 2014; Henseler et al., 2015; Rönkkö & Cho, 2022).
Another debate concerns alternative models for reasoning about causality (Pearl, 2009; Antonakis et al., 2010; Bollen & Pearl, 2013) based on a growing recognition that causality itself is a socially constructed term and many statistical approaches to testing causality are imbued with one particular philosophical perspective toward causality.
Finally, there is debate about the future of hypothesis testing (Branch, 2014; Cohen, 1994; Pernet, 2016; Schwab et al., 2011; Szucs & Ioannidis, 2017; Wasserstein & Lazar, 2016; Wasserstein et al., 2019). This debate focuses on the existence, and mitigation, of problematic practices in the interpretation and use of statistics that involve the well-known p-value . One aspect of this debate focuses on supplementing p-value testing with additional analysis that extra the meaning of the effects of statistically significant results (Lin et al., 2013; Mohajeri et al., 2020; Sen et al., 2022). These proposals essentially suggest retaining p-values. Alternative proposals essentially focus on abandoning the notion that generalizing to the population is the key concern in hypothesis testing (Guo et al., 2014; Kline, 2013) and instead moving from generalizability to explanatory power, for example, by relying on correlations to determine what effect sizes are reasonable in different research settings.
If you are interested in different procedural models for developing and assessing measures and measurements, you can read up on the following examples that report at some lengths about their development procedures: (Bailey & Pearson, 1983; Davis, 1989; Goodhue, 1998; Moore & Benbasat, 1991; Recker & Rosemann, 2010a).
Textbooks on survey research that are worth reading include Floyd Flower’s textbook (Fowler, 2001) plus a few others (Babbie, 1990; Czaja & Blair, 1996). It is also important to regularly check for methodological advances in journal articles, such as (Baruch & Holtom, 2008; Kaplowitz et al., 2004; King & He, 2005).
A seminal book on experimental research has been written by William Shadish, Thomas Cook, and Donald Campbell (Shadish et al., 2001). A wonderful introduction to behavioral experimentation is Lauren Slater’s book “ Opening Skinner’s Box: Great Psychological Experiments of the Twentieth Century ” (Slater, 2005).
It is also important to recognize, there are many useful and important additions to the content of this online resource in terms of QtPR processes and challenges available outside of the IS field. For example, the computer sciences also have an extensive tradition in discussing QtPR notions, such as threats to validity. Claes Wohlin’s book on Experimental Software Engineering (Wohlin et al., 2000), for example, illustrates, exemplifies, and discusses many of the most important threats to validity, such as lack of representativeness of independent variable, pre-test sensitisation to treatments, fatigue and learning effects, or lack of sensitivity of dependent variables. Sira Vegas and colleagues (Vegas et al., 2016) discuss advantages and disadvantages between a wide range of experiment designs, such as independent measures, repeated measures, crossover, matched-pairs, and different mixed designs.
Another important debate in the QtPR realm is the ongoing discussion on reflective versus formative measurement development. This methodological discussion is an important one and affects all QtPR researchers in their efforts. Several viewpoints pertaining to this debate are available (Aguirre-Urreta & Marakas, 2012; Centefelli & Bassellier, 2009; Diamantopoulos, 2001; Diamantopoulos & Siguaw, 2006; Diamantopoulos & Winklhofer, 2001; Kim et al., 2010; Petter et al., 2007).
Another debate in QtPR is about the choice of analysis approaches and toolsets. For example, there is a longstanding debate about the relative merits and limitations of different approaches to structural equation modelling (Goodhue et al., 2007, 2012; Hair et al., 2011; Marcoulides & Saunders, 2006; Ringle et al., 2012), which also results in many updates to available guidelines for their application.
Finally, there is a perennial debate in QtPR about null hypothesis significance testing (Branch, 2014; Cohen, 1994; Pernet, 2016; Schwab et al., 2011; Szucs & Ioannidis, 2017; Wasserstein & Lazar, 2016; Wasserstein et al., 2019). This debate focuses on the existence, and mitigation, of problematic practices in the interpretation and use of statistics that involve the well-known p value . We have co-authored a set of updated guidelines for quantitative researchers for dealing with these issues (Mertens & Recker, 2020).
8.2 References
Aguirre-Urreta, M. I., & Marakas, G. M. (2012). Revisiting Bias Due to Construct Misspecification: Different Results from Considering Coefficients in Standardized Form. MIS Quarterly , 36(1), 123-138.
Antonakis, J., Bendahan, S., Jacquart, P., & Lalive, R. (2010). On Making Causal Claims: A Review and Recommendations. The Leadership Quarterly , 21(6), 1086-1120.
Babbie, E. R. (1990). Survey Research Methods . Wadsworth.
Bagozzi, R.P. (1980), Causal Methods in Marketing. John Wiley and Sons.
Bagozzi, R. P. (2011). Measurement and Meaning in Information Systems and Organizational Research: Methodological and Philosophical Foundations. MIS Quarterly , 35(2), 261-292.
Bailey, J. E., & Pearson, S. W. (1983). Development of a Tool for Measuring and Analyzing Computer User Satisfaction. Management Science , 29(5), 530-545.
Baruch, Y., & Holtom, B. C. (2008). Survey Response Rate Levels and Trends in Organizational Research. Human Relations , 61 (8), 1139-1160.
Block, J. (1961). The Q-Sort Method in Personality Assessment and Psychiatric Research . Charles C Thomas Publisher.
Bollen, K. A. (1989) Structural Equations with Latent Variables . New York: John Wiley and Sons.
Bollen, K. A., & Curran, P. J. (2006). Latent Curve Models: A Structural Equation Perspective . John Wiley & Sons.
Boudreau, M.-C., Gefen, D., & Straub, D. W. (2001). Validation in Information Systems Research: A State-of-the-Art Assessment. MIS Quarterly , 25(1), 1-16.
Branch, M. (2014). Malignant Side Effects of Null-hypothesis Significance Testing. Theory & Psychology , 24 (2), 256-277.
Bryman, A., & Cramer, D. (2008). Quantitative Data Analysis with SPSS 14, 15 & 16: A Guide for Social Scientists . Routledge.
Burton-Jones, A., & Lee, A. S. (2017). Thinking About Measures and Measurement in Positivist Research: A Proposal for Refocusing on Fundamentals. Information Systems Research , 28(3), 451-467.
Burton-Jones, A., Recker, J., Indulska, M., Green, P., & Weber, R. (2017). Assessing Representation Theory with a Framework for Pursuing Success and Failure. MIS Quarterly , 41(4), 1307-1333.
Campbell, D.T., and Fiske, D.W. “Convergent and Discriminant Validation by the Multitrait- Multimethod Matrix,” Psychological Bulletin (56:2, March) 1959, pp 81-105.
Centefelli, R. T., & Bassellier, G. (2009). Interpretation of Formative Measurement in Information Systems Research. MIS Quarterly , 33 (4), 689-708.
Chalmers, A. F. (1999). What Is This Thing Called Science? (3rd ed.). Hackett.
Chin, W. W. (2001). PLS-Graph user’s guide. CT Bauer College of Business, University of Houston, USA, 15 , 1-16.
Christensen, R. (2005). Testing Fisher, Neyman, Pearson, and Bayes. The American Statistician , 59(2), 121-126.
Churchill Jr., G. A. (1979). A Paradigm for Developing Better Measures of Marketing Constructs. Journal of Marketing Research , 16(1), 64-73.
Clark, P. A. (1972). Action Research and Organizational Change . Harper and Row.
Cochran, W. G. (1977). Sampling Techniques (3rd ed.). John Wiley & Sons.
Cohen, J. (1960). A Coefficient of Agreement for Nominal Scales. Educational and Psychological Measurement , 20(1), 37-46.
Cohen, J. (1988). Statistical Power Analysis for the Behavioral Sciences (2nd ed.). Lawrence Erlbaum Associates.
Cohen, J. (1994). The Earth is Round (p < .05). American Psychologist , 49 (12), 997-1003.
Cook, T. D. and D. T. Campbell (1979). Quasi Experimentation: Design and Analytical Issues for Field Settings . Chicago, Rand McNally.
Coombs, C. H. (1976). A Theory of Data . Mathesis Press.
Corder, G. W., & Foreman, D. I. (2014). Nonparametric Statistics for Non-Statisticians: A Step-by-Step Approach (2nd ed.). Wiley.
Cronbach, L. J., & Meehl, P. E. (1955). Construct Validity in Psychological Tests. Psychological Bulletin , 52(4), 281-302.
Cronbach, L. J. (1951). Coefficient Alpha and the Internal Structure of Tests. Psychometrika , 16(3), 291-334.
Cronbach, L. J. (1971). Test Validation. In R. L. Thorndike (Ed.), Educational Measurement (2nd ed., pp. 443-507). American Council on Education.
Czaja, R. F., & Blair, J. (1996). Designing Surveys: A Guide to Decisions and Procedures . Pine Forge Press.
Davidson, R., & MacKinnon, J. G. (1993). Estimation and Inference in Econometrics . Oxford University Press.
Davis, F. D. (1989). Perceived Usefulness, Perceived Ease of Use, and User Acceptance of Information Technology. MIS Quarterly , 13 (3), 319-340.
DeVellis, R. F., & Thorpe, C. T. (2021). Scale Development: Theory and Applications (5th ed.). Sage.
Diamantopoulos, A. (2001). Incorporating Formative Measures into Covariance-Based Structural Equation Models. MIS Quarterly , 35 (2), 335-358.
Diamantopoulos, A., & Siguaw, J. A. (2006). Formative Versus Reflective Indicators in Organizational Measure Development: A Comparison and Empirical Illustration. British Journal of Management , 17 (4), 263-282.
Diamantopoulos, Adamantios and Heidi M. Winklhofer, “Index Construction with Formative Indicators: An Alternative to Scale Development,” Journal of Marketing Research , 38, 2, (2001), 269-277.
Doll, W. J., & Torkzadeh, G. (1988). The Measurement of End-User Computing Satisfaction. MIS Quarterly , 12(2), 259-274.
Dunning, T. (2012). Natural Experiments in the Social Sciences: A Design-Based Approach . Cambridge University Press.
Edwards, J. R., & Berry, J. W. (2010). The Presence of Something or the Absence of Nothing: Increasing Theoretical Precision in Management Research. Organizational Research Methods , 13(4), 668-689.
Elden, M., & Chisholm, R. F. (1993). Emerging Varieties of Action Research: Introduction to the Special Issue. Human Relations , 46(2), 121-142.
Emory, W. C. (1980). Business Research Methods . Irwin.
Evermann, J., & Tate, M. (2011). Fitting Covariance Models for Theory Generation. Journal of the Association for Information Systems , 12(9), 632-661.
Evermann, J., & Tate, M. (2014). Bayesian Structural Equation Models for Cumulative Theory Building in Information Systems―A Brief Tutorial Using BUGS and R . Communications of the Association for Information Systems, 34(77), 1481-1514.
Falk, R., & Greenbaum, C. W. (1995). Significance Tests Die Hard: The Amazing Persistence of a Probabilistic Misconception. Theory & Psychology , 5(1), 75-98.
Field, A. (2013). Discovering Statistics using IBM SPSS Statistics . Sage.
Fisher, R. A. (1935). The Logic of Inductive Inference. Journal of the Royal Statistical Society , 98(1), 39-82.
Fisher, R. A. (1935). The Design of Experiments . Oliver and Boyd.
Fisher, R. A. (1955). Statistical Methods and Scientific Induction. Journal of the Royal Statistical Society. Series B (Methodological) , 17(1), 69-78.
Fornell, C., & Larcker, D. F. (1981). Evaluating Structural Equations with Unobservable Variables and Measurement Error. Journal of Marketing Research , 18(1), 39-50.
Fowler, F. J. (2001). Survey Research Methods (3rd ed.). Sage.
Fromkin, H. L., & Streufert, S. (1976). Laboratory Experimentation . Rand McNally College Publishing Company.
Garcia-Pérez, M. A. (2012). Statistical Conclusion Validity: Some Common Threats and Simple Remedies. Frontiers in Psychology , 3(325), 1-11.
Gasson, S. (2004). Rigor in Grounded Theory Research: An Interpretive Perspective on Generating Theory from Qualitative Field Studies. In M. E. Whitman & A. B. Woszczynski (Eds.), The Handbook of Information Systems Research (pp. 79-102). Idea Group Publishing.
Gefen, D., Ben-Assuli, O., Stehr, M., Rosen, B., & Denekamp, Y. (2019). Governmental Intervention in Hospital Information Exchange (HIE) Diffusion: A Quasi-Experimental Arima Interrupted Time Series Analysis of Monthly HIE Patient Penetration Rates. European Journal of Information Systems , 17(5), 627-645.
Gefen, D., Straub, D. W., & Boudreau, M.-C. (2000). Structural Equation Modeling and Regression: Guidelines for Research Practice. Communications of the Association for Information System s, 4(7), 1-77.
Gefen, D. (2003). Assessing Unidimensionality Through LISREL: An Explanation and an Example. Communications of the Association for Information Systems , 12(2), 23-47.
Gefen, D., & Larsen, K. R. T. (2017). Controlling for Lexical Closeness in Survey Research: A Demonstration on the Technology Acceptance Model. Journal of the Association for Information Systems , 18 (10), 727-757.
Gefen, D. (2019). A Post-Positivist Answering Back. Part 2: A Demo in R of the Importance of Enabling Replication in PLS and LISREL. ACM SIGMIS Database , 50(3), 12-37.
Gelman, A. (2013). P Values and Statistical Practice. Epidemiology , 24(1), 69-72.
Gelman, A., Carlin, J. B., Stern, H., Dunson, D. B., Vehtari, A., & Rubin, D. B. (2013). Bayesian Data Analysis (3rd ed.). Chapman and Hall/CRC.
Gelman, A., & Stern, H. (2006). The Difference Between “Significant” and “Not Significant” is not Itself Statistically Significant. The American Statistician , 60(4), 328-331.
Gigerenzer, G. (2004). Mindless Statistics. Journal of Socio-Economics , 33(5), 587-606.
Glaser, B. G., & Strauss, A. L. (1967). The Discovery of Grounded Theory: Strategies for Qualitative Research . Aldine Publishing Company.
Godfrey-Smith, P. (2003). Theory and Reality: An Introduction to the Philosophy of Science . University of Chicago Press.
Goodhue, D. L. (1998). Development And Measurement Validity Of A Task-Technology Fit Instrument For User Evaluations Of Information Systems. Decision Sciences , 29 (1), 105-139.
Goodhue, D. L., Lewis, W., & Thompson, R. L. (2007). Statistical Power in Analyzing Interaction Effects: Questioning the Advantage of PLS With Product Indicators. Information Systems Research , 18(2), 211-227.
Goodhue, D. L., Lewis, W., & Thompson, R. L. (2012). Comparing PLS to Regression and LISREL: A Response to Marcoulides, Chin, and Saunders. MIS Quarterly , 36 (3), 703-716.
Goodwin, L. D. (2001). Interrater Agreement and Reliability. Measurement in Physical Education and Exercise Science , 5(1), 13-34.
Gray, P. H., & Cooper, W. H. (2010). Pursuing Failure. Organizational Research Methods , 13(4), 620-643.
Greene, W. H. (2012). Econometric Analysis (7th ed.). Pearson.
Greenland, S., Senn, S. J., Rothman, K. J., Carlin, J. B., Poole, C., Goodman, S. N., & Altman, D. G. (2016). Statistical Tests, P Values, Confidence Intervals, and Power: a Guide to Misinterpretations. European Journal of Epidemiology , 31(4), 337-350.
Gregor, S. (2006). The Nature of Theory in Information Systems. MIS Quarterly , 30 (3), 611-642.
Guo, W., Straub, D. W., & Zhang, P. (2014). A Sea Change in Statistics: A Reconsideration of What Is Important in the Age of Big Data. Journal of Management Analytics , 1(4), 241-248.
Hair, J. F., Black, W. C., Babin, B. J., & Anderson, R. E. (2010). Multivariate Data Analysis (7th ed.). Prentice Hall.
Hair, J. F., Ringle, C. M., & Sarstedt, M. (2011). PLS-SEM: Indeed a Silver Bullet. The Journal of Marketing Theory and Practice , 19 (2), 139-152.
Hair, J. F., Hult, G. T. M., Ringle, C. M., & Sarstedt, M. (2013). A Primer on Partial Least Squares Structural Equation Modeling (PLS-SEM) . Sage.
Haller, H., & Kraus, S. (2002). Misinterpretations of Significance: A Problem Students Share with Their Teachers? Methods of Psychological Research , 7(1), 1-20.
Hayesa, A. F. and Coutts, J. J. (2020). Use Omega Rather than Cronbach’s Alpha for Estimating Reliability. But… Communication Methods and Measures (14,1), 1-24.
Hedges, L. V., & Olkin, I. (1985). Statistical Methods for Meta-Analysis . Academic Press.
Heisenberg, W. (1927). Über den anschaulichen Inhalt der quantentheoretischen Kinematik und Mechanik (in German). Zeitschrift für Physik , 43(3-4), 172-198.
Hempel, C. G. (1965). Aspects of Scientific Explanation and other Essays in the Philosophy of Science . The Free Press.
Henseler, J., Dijkstra, T. K., Sarstedt, M., Ringle, C. M., Diamantopoulos, A., Straub, D. W., Ketchen, D. J., Hair, J. F., Hult, G. T. M., & Calantone, R. J. (2014). Common Beliefs and Reality About PLS: Comments on Rönkkö and Evermann (2013). Organizational Research Methods , 17(2), 182-209.
Henseler, J., Ringle, C. M., & Sarstedt, M. (2015). A new Criterion for Assessing Discriminant Validity in Variance-based Structural Equation Modeling. Journal of the Academy of Marketing Science , 43(1), 115-135.
Im, G., & Straub, D. W. (2015). The Critical Role of External Validity in Organizational Theorizing. Communications of the Association for Information Systems , 37(44), 911-964.
Im, G., & Wang, J. (2007). A TETRAD-based Approach for Theory Development in Information Systems Research. Communications of the Association for Information Systems , 20(22), 322-345.
Jarvis, C. B., MacKenzie, S. B., & Podsakoff, P. M. (2003). A Critical Review of Construct Indicators and Measurement Model Misspecification in Marketing and Consumer Research. Journal of Consumer Research , 30 (2), 199-218.
Jöreskog, K. G., & Sörbom, D. (2001). LISREL 8: User’s Reference Guide . Scientific Software International.
Kaplowitz, M. D., Hadlock, T. D., & Levine, R. (2004). A Comparison of Web and Mail Survey Response Rates. Public Opinion Quarterly , 68 (1), 84-101.
Kim, G., Shin, B., & Grover, V. (2010). Investigating Two Contradictory Views of Formative Measurement in Information Systems Research. MIS Quarterly , 34 (2), 345-366.
King, W. R., & He, J. (2005). External Validity in IS Survey Research. Communications of the Association for Information Systems , 16 (45), 880-894.
Kline, R. B. (2013). Beyond Significance Testing: Statistics Reform in the Behavioral Sciences (2nd ed.). American Psychological Association.
Jenkins, A. M. (1985). Research Methodologies and MIS Research. In E. Mumford, R. Hirschheim, & A. T. Wood-Harper (Eds.), Research Methods in Information Systems (pp. 103-117). North-Holland.
Judd, C. M., Smith, E. R., & Kidder, L. H. (1991). Research Methods in Social Relations (6th ed.). Harcourt Brace College Publishers.
Kaplan, B., and Duchon, D. “Combining Qualitative and Quantitative Methods in Information Systems Research: A Case Study,” MIS Quarterly (12:4 (December)) 1988, pp. 571-586.
Kerlinger, F. N. (1986), Foundations of Behavioral Research , Harcourt Brace Jovanovich.
Lakatos, I. (1970). Falsification and the Methodology of Scientific Research Programs. In I. Lakatos & A. Musgrave (Eds.), Criticism and the Growth of Knowledge (pp. 91-132). Cambridge University Press.
Larsen, K. R. T., & Bong, C. H. (2016). A Tool for Addressing Construct Identity in Literature Reviews and Meta-Analyses. MIS Quarterly , 40(3), 529-551.
Lee, A. S., & Hubona, G. S. (2009). A Scientific Basis for Rigor in Information Systems Research. MIS Quarterly , 33(2), 237-262.
Lee, A. S., Mohajeri, K., & Hubona, G. S. (2017). Three Roles for Statistical Significance and the Validity Frontier in Theory Testing. 50th Hawaii International Conference on System Sciences , Waikoloa Village, Hawaii.
Lehmann, E. L. (1993). The Fisher, Neyman-Pearson Theories of Testing Hypotheses: One Theory or Two? Journal of the American Statistical Association , 88(424), 1242-1249.
Levallet, N., Denford, J. S., & Chan, Y. E. (2021). Following the MAP (Methods, Approaches, Perspectives) in Information Systems Research. Information Systems Research , 32(1), 130–146.
Lin, M., Lucas Jr., H. C., & Shmueli, G. (2013). Too Big to Fail: Large Samples and the p-Value Problem. Information Systems Research , 24(4), 906-917.
Lindman, H. R. (1974). ANOVA in Complex Experimental Designs . W. H. Freeman.
Lyberg, L. E., & Kasprzyk, D. (1991). Data Collection Methods and Measurement Error: An Overview. In P. P. Biemer, R. M. Groves, L. E. Lyberg, N. A. Mathiowetz, & S. Sudman (Eds.), Measurement Errors in Surveys (pp. 235-257). Wiley.
MacKenzie, S. B., Podsakoff, P. M., & Podsakoff, N. P. (2011). Construct Measurement and Validation Procedures in MIS and Behavioral Research: Integrating New and Existing Techniques. MIS Quarterly , 35(2), 293-334.
Marcoulides, G. A., & Saunders, C. (2006). Editor’s Comments: PLS: A Silver Bullet? MIS Quarterly , 30 (2), iii-ix.
Masson, M. E. (2011). A Tutorial on a Practical Bayesian Alternative to Null-Hypothesis Significance Testing. Behavior Research Methods , 43(3), 679-690.
Mazaheri, E., Lagzian, M., & Hemmat, Z. (2020). Research Directions in Information Systems Field, Current Status and Future Trends: A Literature Analysis of AIS Basket of Top Journals. Australasian Journal of Information Systems , 24, doi:10.3127/ajis.v24i0.2045.
McArdle, J. J. (2009). Latent Variable Modeling of Differences and Changes with Longitudinal Data. Annual Review of Psychology , 60, 577-605.
McNutt, M. (2016). Taking Up TOP. Science , 352(6290), 1147.
McShane, B. B., & Gal, D. (2017). Blinding Us to the Obvious? The Effect of Statistical Training on the Evaluation of Evidence. Management Science , 62(6), 1707-1718.
Meehl, P. E. (1967). Theory-Testing in Psychology and Physics: A Methodological Paradox. Philosophy of Science , 34(2), 103-115.
Mertens, W., Pugliese, A., & Recker, J. (2017). Quantitative Data Analysis: A Companion for Accounting and Information Systems Research . Springer.
Mertens, W., & Recker, J. (2020). New Guidelines for Null Hypothesis Significance Testing in Hypothetico-Deductive IS Research. Journal of the Association for Information Systems , 21 (4), 1072-1102.
Miller, J. (2009). What is the Probability of Replicating a Statistically Significant Effect? Psychonomic Bulletin & Review , 16(4), 617-640.
Miller, I., & Miller, M. (2012). John E. Freund’s Mathematical Statistics With Applications (8th ed.). Pearson Education.
Mohajeri, K., Mesgari, M., & Lee, A. S. (2020). When Statistical Significance Is Not Enough: Investigating Relevance, Practical Significance and Statistical Significance. MIS Quarterly , 44 (2), 525-559.
Moore, G. C., & Benbasat, I. (1991). Development of an Instrument to Measure the Perceptions of Adopting an Information Technology Innovation. Information Systems Research , 2 (3), 192-222.
Morgan, S. L., & Winship, C. (2014). Counterfactuals and Causal Inference: Methods and Principles for Social Research (2nd ed.). Cambridge University Press.
Myers, M. D. (2009). Qualitative Research in Business and Management . Sage.
Neyman, J., & Pearson, E. S. (1928). On the Use and Interpretation of Certain Test Criteria for Purposes of Statistical Inference: Part I. Biometrika , 20A(1/2), 175-240.
Neyman, J., & Pearson, E. S. (1933). On the Problem of the Most Efficient Tests of Statistical Hypotheses. P hilosophical Transactions of the Royal Society of London. Series A, Containing Papers of a Mathematical or Physical Character , 231, 289-337.
Nosek, B. A., Alter, G., Banks, G. C., Borsboom, D., Bowman, S. D., Breckler, S. J., Buck, S., Chambers, C. D., Chin, G., Christensen, G., Contestabile, M., Dafoe, A., Eich, E., Freese, J., Glennerster, R., Goroff, D., Green, D. P., Hesse, B., Humphreys, M., Ishiyama, J., Karlan, D., Kraut, A., Lupia, A., Mabry, P., Madon, T., Malhotra, N., Mayo-Wilson, E., McNutt, M., Miguel, E., Paluck, E. L., Simonsohn, U., Soderberg, C., Spellman, B. A., Turitto, J., VandenBos, G., Vazire, S., Wagenmakers, E.-J., Wilson, R. L., & Yarkoni, T. (2015). Promoting an Open Research Culture. Science , 348(6242), 1422-1425.
Orne, M. T. (1962). On The Social Psychology of the Psychological Experiment: With Particular Reference to Demand Characteristics and their Implications. American Psychologist , 17(11), 776-783.
Pearl, J. (2009). Causality: Models, Reasoning, and Inference (2nd ed.). Cambridge University Press.
Pernet, C. (2016). Null Hypothesis Significance Testing: a Guide to Commonly Misunderstood Concepts and Recommendations for Good Practice [version 5; peer review: 2 approved, 2 not approved]. F1000Research , 4 (621).
Petter, S., Straub, D. W., & Rai, A. (2007). Specifying Formative Constructs in IS Research. MIS Quarterly , 31 (4), 623-656.
Popper, K. R. (1959). The Logic of Scientific Discovery . Basic Books. (Logik der Forschung, Vienna, 1935)
Recker, J. (2021). Scientific Research in Information Systems: A Beginner’s Guide (2nd ed.). Springer.
Recker, J., & Rosemann, M. (2010). A Measurement Instrument for Process Modeling Research: Development, Test and Procedural Model. Scandinavian Journal of Information Systems , 22 (2), 3-30.
Reinhart, A. (2015). Statistics Done Wrong: The Woefully Complete Guide . No Starch Press.
Ringle, C. M., Sarstedt, M., & Straub, D. W. (2012). Editor’s Comments: A Critical Look at the Use of PLS-SEM in MIS Quarterly. MIS Quarterly , 36 (1), iii-xiv.
Rönkkö, M., & Cho, E. (2022). An Updated Guideline for Assessing Discriminant Validity. Organizational Research Methods , 25(1), 6-14.
Rossiter, J. R. (2011). Measurement for the Social Sciences: The C-OAR-SE Method and Why It Must Replace Psychometrics . Springer.
Sarker, S., Xiao, X., Beaulieu, T., & Lee, A. S. (2018). Learning from First-Generation Qualitative Approaches in the IS Discipline: An Evolutionary View and Some Implications for Authors and Evaluators (PART 1/2). Journal of the Association for Information Systems , 19(8), 752-774.
Schwab, A., Abrahamson, E., Starbuck, W. H., & Fidler, F. (2011). PERSPECTIVE—Researchers Should Make Thoughtful Assessments Instead of Null-Hypothesis Significance Tests. Organization Science , 22 (4), 1105-1120.
Sen, A., Smith, G., & Van Note, C. (2022). Statistical Significance Versus Practical Importance in Information Systems Research. Journal of Information Technology , 37 (3), 288–300.
Shadish, W. R., Cook, T. D., & Campbell, D. T. (2001). Experimental and Quasi-Experimental Designs for Generalized Causal Inference (2nd ed.). Houghton Mifflin.
Siponen, M. T., & Klaavuniemi, T. (2020). Why is the Hypothetico-Deductive (H-D) Method in Information Systems not an H-D Method? Information and Organization , 30 (1), 100287.
Slater, L. (2005). Opening Skinner’s Box: Great Psychological Experiments of the Twentieth Century . Norton & Company.
Stevens, J. P. (2001). Applied Multivariate Statistics for the Social Sciences (4th ed.). Lawrence Erlbaum Associates.
Stone, Eugene F., Research Methods in Organizational Behavior , Glenview, IL, 1981.
Straub, D. W., Gefen, D., & Boudreau, M.-C. (2005). Quantitative Research. In D. Avison & J. Pries-Heje (Eds.), Research in Information Systems: A Handbook for Research Supervisors and Their Students (pp. 221-238). Elsevier.
Straub, D. W., Boudreau, M.-C., & Gefen, D. (2004). Validation Guidelines for IS Positivist Research. Communications of the Association for Information Systems , 13(24), 380-427.
Straub, D. W. (1989). Validating Instruments in MIS Research. MIS Quarterly , 13(2), 147-169.
Streiner, D. L. (2003). Starting at the Beginning: An Introduction to Coefficient Alpha and Internal Consistency. Journal of Personality Assessment , 80(1), 99-103.
Szucs, D., & Ioannidis, J. P. A. (2017). When Null Hypothesis Significance Testing Is Unsuitable for Research: A Reassessment. Frontiers in Human Neuroscience , 11 (390), 1-21.
Tabachnick, B. G., & Fidell, L. S. (2001). Using Multivariate Statistics (4th ed.). Allyn & Bacon.
Thomas, D. M., & Watson, R. T. (2002). Q-Sorting and MIS Research: A Primer. Communications of the Association for Information Systems , 8(9), 141-156.
Trochim, W. M. K., Donnelly, J. P., & Arora, K. (2016). Research Methods: The Essential Knowledge Base (2nd ed.). Cengage Learning.
Vegas, S., Apa, C., & Juristo, N. (2016). Crossover Designs in Software Engineering Experiments: Benefits and Perils. IEEE Transactions on Software Engineering , 42 (2), 120-135.
Vessey, I., Ramesh, V., & Glass, R. L. (2002). Research in Information Systems: An Empirical Study of Diversity in the Discipline and Its Journals. Journal of Management Information Systems , 19(2), 129-174.
Walsham, G. (1995). Interpretive Case Studies in IS Research: Nature and Method. European Journal of Information Systems , 4, 74-81.
Wasserstein, R. L., & Lazar, N. A. (2016). The ASA’s Statement on P-values: Context, Process, and Purpose. The American Statistician , 70 (2), 129-133.
Wasserstein, R. L., Schirm, A. L., & Lazar, N. A. (2019). Moving to a World Beyond “p < 0.05.” The American Statistician , 73 (sup1), 1-19.
Wohlin, C., Runeson, P., Höst, M., Ohlsson, M. C., Regnell, B., & Wesslén, A. (2000). Experimentation in Software Engineering: An Introduction . Kluwer Academic Publishers.
Yin, R. K. (2009). Case Study Research: Design and Methods (4th ed.). Sage Publications.
The Home Page of Professor Jan Recker
224 Research Topics on Technology & Computer Science
Are you new to the world of technology? Do you need topics related to technology to write about? No worries, Custom-writing.org experts are here to help! In this article, we offer you a multitude of creative and interesting technology topics from various research areas, including information technology and computer science. So, let’s start!
Our specialists will write a custom essay specially for you!
- 🔝 Top 10 Topics
👋 Introduction
- 💾 Top 10 Computer Science Topics
⚙ Artificial Intelligence
💉 biotechnology, 📡 communications and media.
- 💻Computer Science & Engineering
🔋 Energy & Power Technologies
🍗 food technology, 😷 medical devices & diagnostics, 💊 pharmaceutical technologies.
- 🚈 Transportation
✋ Conclusion
🔍 references, 🔝 top 10 technology topics.
- The difference between VR and AR
- Is genetic engineering ethical?
- Can digital books replace print ones?
- The impact of virtual reality on education
- 5 major fields of robotics
- The risks and dangers of biometrics
- Nanotechnology in medicine
- Digital technology’s impact on globalization
- Is proprietary software less secure than open-source?
- The difference between deep learning and machine learning
Is it a good thing that technologies and computer science are developing so fast? No one knows for sure. There are too many different opinions, and some of them are quite radical! However, we know that technologies have changed our world once and forever. Computer science affects every single area of people’s lives.

Just think about Netflix . Can you imagine that 24 years ago it didn’t exist? How did people live without it? Well, in 2024, the entertainment field has gone so far that you can travel anywhere while sitting in your room. All you would have to do is just order a VR (virtual reality) headset. Moreover, personal computers give an unlimited flow of information, which has changed the entire education system.
Every day, technologies become smarter and smaller. A smartphone in your pocket may be as powerful as your laptop. No doubt, the development of computer science builds our future. It is hard to count how many research areas in technologies and computer science are there. But it is not hard to name the most important of them.
Artificial intelligence tops the charts, of course. However, engineering and biotechnology are not far behind. Communications and media are developing super fast as well. The research is also done in areas that make our lives better and more comfortable. The list of them includes transport, food and energy, medical, and pharmaceutical areas.
So check out our list of 204 most relevant computer science research topics below. Maybe one of them will inspire you to do revolutionary research!
Just in 1 hour! We will write you a plagiarism-free paper in hardly more than 1 hour
💾 Top 10 Computer Science Research Topics
💡 technologies & computer science: research ideas.
Many people probably picture robots from the movie “I, Robot” when they hear about artificial intelligence. However, it is far from the truth.
AI is meant to be as close to a rational way of thinking as possible. It uses binary logic (just like computers) to help solve problems in many areas. Applied AI is only aimed at one task. A generalized AI branch is looking into a human-like machine that can learn to do anything.

Applied AI already helps researchers in quantum physics and medicine. You deal with AI every day when online shops suggest some items based on your previous purchases. Siri and self-driving cars are also examples of applied AI.
Generalized AI is supposed to be a copy of multitasking human intelligence. However, it is still in the stage of development. Computer technology has yet to reach the level necessary for its creation.
One of the latest trends in this area is improving healthcare management. It is done through the digitalization of all the information in hospitals and even helping diagnose the patients.
Receive a plagiarism-free paper tailored to your instructions. Cut 20% off your first order!
Also, privacy issues and facial recognition technologies are being researched. For example, some governments collect biometric data to reduce and even predict crime.
Research Topics on Artificial Intelligence Technology
Since AI development is exceptionally relevant nowadays, it would be smart to invest your time and effort into researching it. Here are some ideas on artificial intelligence research topics that you can look into:
- What areas of life machine learning are the most influential?
- How to choose the right algorithm for machine learning ?
- Supervised vs. unsupervised machine learning : compare & contrast
- Reinforcement machine learning algorithms
- Deep learning as a subset of machine learning
- Deep learning & artificial neural networks
- How do artificial neural networks work?
- A comparison of model-free & model-based reinforcement learning algorithms
- Reinforcement learning: single vs. multi-agent
- How do social robots interact with humans?
- Robotics in NASA
- Natural language processing: chatbots
- How does natural language processing produce natural language?
- Natural language processing vs. machine learning
- Artificial intelligence in computer vision
- Computer vision application: autonomous vehicles
- Recommender systems’ approaches
- Recommender systems: content-based recommendation vs. collaborative filtering
- Internet of things & artificial intelligence: the interconnection
- How much data do the Internet of things devices generate?
Biotechnology uses living organisms to modify different products. Even the simple thing as baking bread is a process of biotechnology. However, nowadays, this area went as far as changing the organisms’ DNA. Genetics and biochemistry are also a part of the biotechnology area.
The development of this area allows people to cure diseases with the help of new medicines. In agriculture, more and more research is done on biological treatment and modifying plants. Biotechnology is even involved in the production of our groceries, household chemicals, and textiles.

There are many exciting trends in biotechnology now that carry the potential of changing our world! For example, scientists are working on creating personalized drugs. This is feasible once they apply computer science to analyze people’s DNA.
Get an originally-written paper according to your instructions!
Also, thanks to using new technologies, doctors can collect exact data and provide the patients with correct diagnosis and treatment. Now, you don’t even need to leave your place to get a doctor’s check-up. Just use telehealth!
Data management is developing in the biotechnology area as well. Thanks to that, doctors and scientists can store and access a tremendous amount of information.
The most exciting is the fact that new technology enables specialists to assess genetic information to treat and prevent illnesses! It may solve the problem of some diseases that were considered untreatable before.
Research Topics on Biotechnology
You can use the following examples of research questions on biotechnology for presentation or even a PhD paper! Here is a wide range of topics on biotechnology and its relation to agriculture, nanotechnology, and many more:
- Self-sufficient protein supply and biotechnology in farming
- Evaporation vs. evapotranspiration
- DNA cloning and a southern blot
- Pharmacogenetics & personalized drugs
- Is cloning “playing God”?
- Pharmacogenetics : cancer medicines
- How much can we control our genetics, at what point do we cease to be human?
- Bio ethics and stem cell research
- Genetic engineering: gene therapy
- The potential benefits of genetic engineering
- Genetic engineering: dangers and opportunities
- Mycobacterium tuberculosis : counting the proteins
- Plant genetic enhancement: developing resistance to scarcity
- Y-chromosome genotyping: the case of South Africa
- Agricultural biotechnology: GMO crops
- How are new vaccines developed?
- Nanotechnology in treating HIV
- Allergenic potential & biotechnology
- Whole-genome sequencing in biotechnology
- Genes in heavy metal tolerance: an overview
- Food biotechnology & food-borne illnesses
- How to eliminate heat-resistant microorganisms with ultraviolet?
- High-throughput screening & biotechnology
- How do new food processing technologies affect bacteria related to Aspalathus Linearis?
- Is sweet sorghum suitable for the production of bioethanol in Africa?
- How can pesticides help to diagnose cancer?
- How is embelin used to prevent cancer?
One of the first areas that technologies affected was communications and media. People from the last century couldn’t have imagined how easy it would be to get connected with anyone! Internet connection starts appearing even in the most remote places.
Nowadays, media is used not only for social interaction but for business development and educational purposes as well. You can now start an entirely online business or use special tools to promote the existing one. Also, many leading universities offer online degrees.
In communications and media, AI has been playing the role of enhancement recently. The technology helps create personalized content for always demanding consumers.
Developing media also create numerous job opportunities. For instance, recently, an influencer has become a trending career. Influencers always use the most relevant communication tools available. At the moment, live videos and podcasting are on the top.
Now, you just need to reach your smartphone to access all the opportunities mentioned above! You can apply for a college, find a job, or reach out to all your followers online. It is hard to imagine how far communication and media can go…
Communications and Media Technology Research Topics
There are quite a few simple yet exciting ideas for media and communications technology research topics. Hopefully, you will find THE ONE amongst these Information and Communications Technology (ICT) research proposal topics:
- New media: the importance of ethics in the process of communication
- The development of computer-based communication over the last decade
- How have social media changed communication?
- Media during the disasters : increasing panic or helping reduce it?
- Authorities’ media representations in different countries: compare & contrast
- Do people start preferring newspapers to new media again?
- How has the Internet changed media?
- Communication networks
- The impact of social media on super bowl ads
- Communications: technology and personal contact
- New content marketing ideas
- Media exposure and its influence on adolescents
- The impact of mass media on personal socialization
- Internet and interactive media as an advertising tool
- Music marketing in a digital world
- How do people use hype in the media?
- Psychology of videoblog communication
- Media & the freedom of speech
- Is it possible to build trustful relationships in virtual communication?
- How to maintain privacy in social media ?
- Communication technologies & cyberbullying
- How has the interpersonal communication changed with the invention of computers?
- The future of the communication technologies
- Yellow journalism in new media
- How enterprises use ICT to get a competitive advantage?
- Healthcare and ICT
- Can we live without mass media ?
- Mass media and morality in the 21st century
💻 Computer Science & Engineering
If you have ever wondered how computers work, you better ask a professional in computer science and engineering. This major combines two different, yet interconnected, worlds of machines.
Computer science takes care of the computer’s brain. It usually includes areas of study, such as programming languages and algorithms. Scientists also recognize three paradigms in terms of the computer science field.
For the rationalist paradigm, computer science is a part of math. The technocratic paradigm is focused on software engineering, while the scientific one is all about natural sciences. Interestingly enough, the latter can also be found in the area of artificial intelligence!

On the other hand, computer engineering maintains a computer’s body – hardware and software. It relies quite heavily on electrical engineering. And only the combination of computer science and engineering gives a full understanding of the machine.
If talking about trends and innovations, artificial intelligence development is probably the main one in the area of computer science technology. Big data is the field that has been extremely popular in recent years.
Cybersecurity is and will be one of the leading research fields in our Information Age. The latest trend in computer science and engineering is also virtual reality.
Computer Science Research Topics
If you want to find a good idea for your thesis or you are just preparing for a speech, check out this list of research topics in computer science and engineering:
- How are virtual reality & human perception connected?
- The future of computer-assisted education
- Computer science & high-dimensional data modeling
- Computer science: imperative vs. declarative languages
- The use of blockchain and AI for algorithmic regulations
- Banking industry & blockchain technology
- How does the machine architecture affect the efficiency of code?
- Languages for parallel computing
- How is mesh generation used for computational domains?
- Ways of persistent data structure optimization
- Sensor networks vs. cyber-physical system
- The development of computer graphics: non-photorealistic rendering case
- The development of the systems programming languages
- Game theory & network economics
- How can computational thinking affect science?
- Theoretical computer science in functional analysis
- The most efficient cryptographic protocols
- Software security types: an overview
- Is it possible to eliminate phishing?
- Floating point & programming language
Without energy, no technological progress is possible. Scientists are continually working on improving energy and power technologies. Recently, efforts have been aimed at three main areas.
Developing new batteries and fuel types helps create less expensive ways of storing energy. For example, fuel cells can be used for passenger buses. They need to be connected to a source of fuel to work. However, it guarantees the constant production of electricity as long as they have fuel.
One of the potential trends of the next years is hydrogen energy storage. This method is still in the stage of development. It would allow the use of hydrogen instead of electricity.

A smart grid is another area that uses information technology for the most efficient use of energy. For instance, the first-generation smart grid tracks the movement of electric energy on the go and sends the information back. It is a great way to correct the consumption of energy in real-time. More development is also done on the issue of electricity generation. It aims at technologies that can produce power from the sources that haven’t been used. The trends in this area include second-generation biofuels and photovoltaic glass.
Energy Technologies Research Topics
Since humanity cannot be using fossil fuels forever, the research in the area of energy can be extremely fruitful. The following list of energy and power technology research paper topics can give you an idea of where to dig:
- How can fuel cells be used for stationary power generation?
- Lithium-ion vs. lithium-air batteries: energy density
- Are lithium-air batteries better than gasoline ?
- Renewable energy usage: advantages and disadvantages
- The nuclear power usage in the UAE
- India’s solar installations
- Gas price increasing and alternative energy sources
- How can methods of energy transformation be applied with hydrogen energy?
- Is hydrogen energy our future?
- Thermal storage & AC systems
- How to load balance using smart grid?
- Distributed energy generation to optimize power waste
- Is the smart energy network a solution to climate change ?
- The future of the tidal power
- The possibility of 3D printing of micro stirling engines
- How can robots be used to adjust solar panels to weather?
- Advanced biofuels & algae
- Can photovoltaic glass be fully transparent?
- Third-generation biofuels : algae vs. crop-based
- Space-based solar power: myth or reality of the future?
- Can smaller nuclear reactors be more efficient?
- Inertial confinement fusion & creal energy
- Renewable energy technologies: an overview
- How can thorium change the nuclear power field?
The way we get our food has changed drastically with the technological development. Manufacturers look for ways to feed 7.5 billion people more efficiently. And the demand is growing every year. Now technology is not only used for packaging, but for producing and processing food as well.
Introducing robots into the process of manufacturing brings multiple benefits to the producer. Not only do they make it more cost-efficient, but they also reduce safety problems.
Surprisingly enough, you can print food on the 3D printer now! This technology is applied to produce soft food for people who can’t chew. NASA decided to use it for fun as well and printed a pizza!
Drones now help farmers to keep an eye on crops from above. It helps them see the full picture and analyze the current state of the fields. For example, a drone can spot a starting disease and save the crop.
The newest eco trends push companies to become more environmentally aware. They use technologies to create safer packaging. The issue of food waste is also getting more and more relevant. Consumers want to know that nothing is wasted. Thanks to the new technologies, the excess food is now used more wisely.
Food Technology Research Topics
If you are looking for qualitative research topics about technology in the food industry, here is a list of ideas you don’t want to miss:
- What machines are used in the food industry?
- How do robots improve safety in butchery?
- Food industry & 3D printing
- 3D printed food – a solution to help people with swallowing disorder?
- Drones & precision agriculture
- How is robotics used to create eco-friendly food packaging ?
- Is micro packaging our future?
- The development of edible cling film

- Technology & food waste : what are the solutions?
- Additives and preservatives & human gut microbiome
- The effect of citric acid on the orange juice: physicochemical level
- Vegetable oils in mass production: compare & contrast
- Time-temperature indicators & food industry
- Conventional vs. hydroponic farming
- Food safety: a policy issue in agriculture today
- How to improve the detection of parasites in food?
- What are the newest technologies in the baking industry?
- Eliminating byproducts in edible oils production
- Cold plasma & biofilms
- How good are the antioxidant peptides derived from plants?
- Electronic nose in food industry and agriculture
- The harm of polyphenols in food
Why does the life expectancy of people get higher and higher every year? One of the main aspects of it is the promotion of innovation in the medical area. For example, the development of equipment helps medical professionals to save many lives.
Thanks to information technology, the work is much more structured now in the medical area. The hospitals use tablets and the method of electronic medical records. It helps them to access and share the data more efficiently.
If talking about medical devices, emerging technologies save more lives than ever! For instance, operations done by robots are getting more and more popular. Don’t worry! Doctors are still in charge; they just control the robots from the other room. It allows operations to be less invasive and precise.
Moreover, science not only helps treat diseases but also prevent them! The medical research aims for the development of vaccines against deadly illnesses like malaria.
Some of the projects even sound more like crazy ideas from the future. But it is all happening right now! Scientists are working on the creation of artificial organs and the best robotic prosthetics.
All the technologies mentioned above are critical for successful healthcare management.
Medical Technology Research Topics
If you feel like saving lives is the purpose of your life, then technological research topics in the medical area are for you! These topics would also suit for your research paper:
- How effective are robotic surgeries ?
- Smart inhalers as the new solution for asthma treatment
- Genetic counseling – a new way of preventing diseases?
- The benefits of the electronic medical records
- Erythrocytapheresis to treat sickle cell disease
- Defibrillator & cardiac resynchronization therapy
- Why do drug-eluting stents fail?
- Dissolvable brain sensors: an overview
- 3D printing for medical purposes
- How soon will we be able to create artificial organs?
- Wearable technologies & healthcare
- Precision medicine based on genetics
- Virtual reality devices for educational purposes in medical schools
- The development of telemedicine
- Clustered regularly interspaced short palindromic repeats as the way of treating diseases
- Nanotechnology & cancer treatment
- How safe is genome editing?
- The trends in electronic diagnostic tools development
- The future of the brain-machine interface
- How does wireless communication help medical professionals in hospitals?
In the past years, technologies have been drastically changing the pharmaceutical industry. Now, a lot of processes are optimized with the help of information technology. The ways of prescribing and distributing medications are much more efficient today. Moreover, the production of medicines itself has changed.
For instance, electronic prior authorization is now applied by more than half of the pharmacies. It makes the process of acquiring prior authorization much faster and easier.
The high price of medicines is the number one reason why patients stop using prescriptions. Real-time pharmacy benefit may be the solution! It is a system that gives another perspective for the prescribers. While working with individual patients, they will be able to consider multiple factors with the help of data provided.
The pharmaceutical industry also adopts some new technologies to compete on the international level. They apply advanced data analytics to optimize their work.
Companies try to reduce the cost and boost the effectiveness of the medicines. That is why they look into technologies that help avoid failures in the final clinical trials.
The constant research in the area of pharma is paying off. New specialty drugs and therapies arrive to treat chronic diseases. However, there are still enough opportunities for development.
Pharmaceutical Technologies Research Topics
Following the latest trends in the pharmaceutical area, this list offers a wide range of creative research topics on pharmaceutical technologies:
- Electronic prior authorization as a pharmacy technological trend
- The effectiveness of medication therapy management
- Medication therapy management & health information exchanges
- Electronic prescribing of controlled substances as a solution for drug abuse issue
- Do prescription drug monitoring programs really work?
- How can pharmacists help with meaningful use?
- NCPDP script standard for specialty pharmacies
- Pharmaceutical technologies & specialty medications
- What is the patient’s interest in the real-time pharmacy?
- The development of the vaccines for AIDS
- Phenotypic screening in pharmaceutical researches
- How does cloud ERP help pharmaceutical companies with analytics?
- Data security & pharmaceutical technologies
- An overview of the DNA-encoded library technology
- Pharmaceutical technologies: antibiotics vs. superbugs
- Personalized medicine: body-on-a-chip approach
- The future of cannabidiol medication in pain management
- How is cloud technology beneficial for small pharmaceutical companies?
- A new perspective on treatment: medicines from plants
- Anticancer nanomedicine: a pharmaceutical hope
🚈 Transportation Technologies
We used to be focused on making transportation more convenient. However, nowadays, the focus is slowly switching to ecological issues.
It doesn’t mean that vehicles can’t be comfortable at the same time. That is why the development of electric and self-driving cars is on the peak.
Transportation technologies also address the issues of safety and traffic jams. There are quite many solutions suggested. However, it would be hard for big cities to switch to the other systems fast.
One of the solutions is using shared vehicle phone applications. It allows reducing the number of private cars on the roads. On the other hand, if more people start preferring private vehicles, it may cause even more traffic issues.

The most innovative cities even start looking for more eco-friendly solutions for public transport. Buses are being replaced by electric ones. At the same time, the latest trend is using private electric vehicles such as scooters and bikes.
So that people use public transport more, it should be more accessible and comfortable. That is why the payment systems are also being updated. Now, all you would need is to download an app and buy a ticket in one click!
Transportation Technologies Research Topics
Here you can find the best information technology research topics related to transportation technologies:
- How safe are self-driving cars ?
- Electric vs. hybrid cars : compare & contrast
- How to save your smart car from being hijacked?
- How do next-generation GPS devices adjust the route for traffic?
- Transportation technologies: personal transportation pods
- High-speed rail networks in Japan
- Cell phones during driving: threats and solutions
- Transportation: electric cars effects
- Teleportation: physics of the impossible
- How soon we will see Elon Musk’s Hyperloop?
- Gyroscopes as a solution for convenient public transportation
- Electric trucks: the effect on logistics
- Why were electric scooters banned in some cities in 2018?
- Carbon fiber as an optional material for unit load devices
- What are the benefits of the advanced transportation management systems?
- How to make solar roadways more cost-effective?
- How is blockchain applied in the transportation industry
- Transportation technologies: an overview of the freight check-in
- How do delivery companies use artificial intelligence?
- Water-fueled cars: the technology of future or fantasy?
- What can monitoring systems be used to manage curb space?
- Inclusivity and accessibility in public transport: an overview
- The development of the mobility-as-a-service
All in all, this article is a compilation of the 204 most interesting research topics on technology and computer science. It is a perfect source of inspiration for anyone who is interested in doing research in this area.
We have divided the topics by specific areas, which makes it easier for you to find your favorite one. There are 20 topics in each category, along with a short explanation of the most recent trends in the area.
You can choose one topic from artificial intelligence research topics and start working on it right away! There is also a wide selection of questions on biotechnology and engineering that are waiting to be answered.
Since media and communications are present in our everyday life and develop very fast, you should look into this area. But if you want to make a real change, you can’t miss on researching medical and pharmaceutical, food and energy, and transportation areas.
Of course, you are welcome to customize the topic you choose! The more creativity, the better! Maybe your research has the power to change something! Good luck, and have fun!
This might be interesting for you:
- 280 Good Nursing Research Topics & Questions
- 226 Research Topics on Criminal Justice & Criminology
- 178 Best Research Titles about Cookery & Food
- 497 Interesting History Topics to Research
- 180 Best Education Research Topics & Ideas
- 110+ Micro- & Macroeconomics Research Topics
- 417 Business Research Topics for ABM Students
- 190+ Research Topics on Psychology & Communication
- 512 Research Topics on HumSS
- 281 Best Health & Medical Research Topics
- 501 Research Questions & Titles about Science
- A List of Research Topics for Students. Unique and Interesting
- Good Research Topics, Titles and Ideas for Your Paper
- Databases for Research & Education: Gale
- The Complete Beginners’ Guide to Artificial Intelligence: Forbes
- 8 Best Topics for Research and Thesis in Artificial Intelligence: GeeksForGeeks
- Technology Is Changing Transportation, and Cities Should Adapt: Harvard Business Review
- Five Technology Trends: Changing Pharmacy Practice Today and Tomorrow (Pharmacy Times)
- Recent papers in Technology: Academia
- Research: Michigan Tech
- What 126 studies say about education technology: MIT News
- Top 5 Topics in Information Technology: King University Online
- Research in Technology Education-Some Areas of Need: Virginia Tech
- Undergraduate Research Topics: Department of Computer Science, Princeton University
- Student topics: QUT Science and Engineering
- Developing research questions: Monash University
- Biotechnology: Definition, Examples, & Applications (Britannica)
- Medical Laboratory Science Student Research Projects: Rush University
- Clinical Laboratory Science: Choosing a Research Topic (Library Resource Guide for FGCU Clinical Lab Science students)
- Share to Facebook
- Share to Twitter
- Share to LinkedIn
- Share to email
![quantitative research title examples about technology Research Proposal Topics: 503 Ideas, Sample, & Guide [2024]](https://custom-writing.org/blog/wp-content/uploads/2021/01/woman-making-notes-284x153.jpg)
Do you have to write a research proposal and can’t choose one from the professor’s list? This article may be exactly what you need. We will provide you with the most up-to-date undergraduate and postgraduate topic ideas. Moreover, we will share the secrets of the winning research proposal writing. Here,...
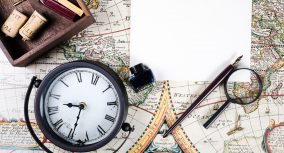
A history class can become a jumble of years, dates, odd moments, and names of people who have been dead for centuries. Despite this, you’ll still need to find history topics to write about. You may have no choice! But once in a while, your instructor may let you pick...
![quantitative research title examples about technology 150 Argumentative Research Paper Topics [2024 Upd.]](https://custom-writing.org/blog/wp-content/uploads/2021/01/close-up-magnifier-glass-yellow-background-284x153.jpg)
Argumentative research paper topics are a lot easier to find than to come up with. We always try to make your life easier. That’s why you should feel free to check out this list of the hottest and most controversial argumentative essay topics for 2024. In the article prepared by...
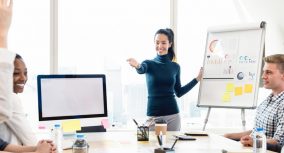
One of the greatest problems of the scholarly world is the lack of funny topics. So why not jazz it up? How about creating one of those humorous speeches the public is always so delighted to listen to? Making a couple of funny informative speech topics or coming up with...
![quantitative research title examples about technology Gun Control Argumentative Essay: 160 Topics + How-to Guide [2024]](https://custom-writing.org/blog/wp-content/uploads/2021/01/bullets-gun-black-velvet-desk-284x153.jpg)
After the recent heartbreaking mass shootings, the gun control debate has reached its boiling point. Do we need stricter gun control laws? Should everyone get a weapon to oppose crime? Or should guns be banned overall? You have the opportunity to air your opinion in a gun control argumentative essay....
![quantitative research title examples about technology Best Childhood Memories Essay Ideas: 94 Narrative Topics [2024]](https://custom-writing.org/blog/wp-content/uploads/2021/01/child-working-in-cambodia-284x153.jpg)
Many people believe that childhood is the happiest period in a person’s life. It’s not hard to see why. Kids have nothing to care or worry about, have almost no duties or problems, and can hang out with their friends all day long. An essay about childhood gives an opportunity...
![quantitative research title examples about technology A List of 272 Informative Speech Topics: Pick Only Awesome Ideas! [2024]](https://custom-writing.org/blog/wp-content/uploads/2021/01/meeting-room-professional-meeting-284x153.jpg)
Just when you think you’re way past the question “How to write an essay?” another one comes. That’s the thing students desperately Google: “What is an informative speech?” And our custom writing experts are here to help you sort this out. Informative speaking is a speech on a completely new issue....
![quantitative research title examples about technology 435 Literary Analysis Essay Topics and Prompts [2024 Upd]](https://custom-writing.org/blog/wp-content/uploads/2021/01/girl-having-idea-with-copy-space-284x153.jpg)
Literature courses are about two things: reading and writing about what you’ve read. For most students, it’s hard enough to understand great pieces of literature, never mind analyzing them. And with so many books and stories out there, choosing one to write about can be a chore. But you’re in...
![quantitative research title examples about technology 335 Unique Essay Topics for College Students [2024 Update]](https://custom-writing.org/blog/wp-content/uploads/2021/01/smiling-students-walking-after-lessons1-284x153.jpg)
The success of any college essay depends on the topic choice. If you want to impress your instructors, your essay needs to be interesting and unique. Don’t know what to write about? We are here to help you! In this article by our Custom-Writing.org team, you will find 335 interesting...
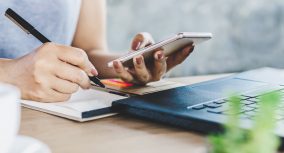
Social studies is an integrated research field. It includes a range of topics on social science and humanities, such as history, culture, geography, sociology, education, etc. A social studies essay might be assigned to any middle school, high school, or college student. It might seem like a daunting task, but...
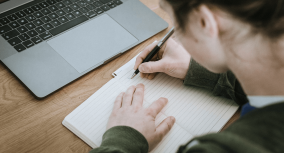
If you are about to go into the world of graduate school, then one of the first things you need to do is choose from all the possible dissertation topics available to you. This is no small task. You are likely to spend many years researching your Master’s or Ph.D....
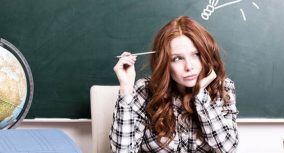
Looking for a good argumentative essay topic? In need of a persuasive idea for a research paper? You’ve found the right page! Academic writing is never easy, whether it is for middle school or college. That’s why there are numerous educational materials on composing an argumentative and persuasive essay, for...
Thanks so much for this! Glad I popped by and I sure did find what I was looking for.
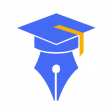
Thanks for your kind words, Sanny! We look forward to seeing you again!
Thank you very for the best topics of research across all science and art projects. The best thing that I am interested to is computer forensics and security specifically for IT students.
Thanks for stopping by!
Hello, glad to hear from you!
Computer science focuses on creating programs and applications, while information technology focuses on using computer systems and networks. What computer science jobs are there. It includes software developers, web developers, software engineers, and data scientists.

Quantitative Research: Examples of Research Questions and Solutions
Are you ready to embark on a journey into the world of quantitative research? Whether you’re a seasoned researcher or just beginning your academic journey, understanding how to formulate effective research questions is essential for conducting meaningful studies. In this blog post, we’ll explore examples of quantitative research questions across various disciplines and discuss how StatsCamp.org courses can provide the tools and support you need to overcome any challenges you may encounter along the way.
Understanding Quantitative Research Questions
Quantitative research involves collecting and analyzing numerical data to answer research questions and test hypotheses. These questions typically seek to understand the relationships between variables, predict outcomes, or compare groups. Let’s explore some examples of quantitative research questions across different fields:
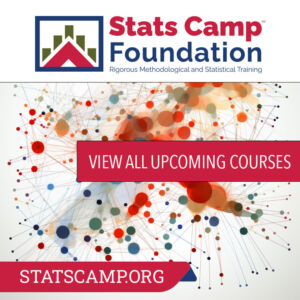
- What is the relationship between class size and student academic performance?
- Does the use of technology in the classroom improve learning outcomes?
- How does parental involvement affect student achievement?
- What is the effect of a new drug treatment on reducing blood pressure?
- Is there a correlation between physical activity levels and the risk of cardiovascular disease?
- How does socioeconomic status influence access to healthcare services?
- What factors influence consumer purchasing behavior?
- Is there a relationship between advertising expenditure and sales revenue?
- How do demographic variables affect brand loyalty?
Stats Camp: Your Solution to Mastering Quantitative Research Methodologies
At StatsCamp.org, we understand that navigating the complexities of quantitative research can be daunting. That’s why we offer a range of courses designed to equip you with the knowledge and skills you need to excel in your research endeavors. Whether you’re interested in learning about regression analysis, experimental design, or structural equation modeling, our experienced instructors are here to guide you every step of the way.
Bringing Your Own Data
One of the unique features of StatsCamp.org is the opportunity to bring your own data to the learning process. Our instructors provide personalized guidance and support to help you analyze your data effectively and overcome any roadblocks you may encounter. Whether you’re struggling with data cleaning, model specification, or interpretation of results, our team is here to help you succeed.
Courses Offered at StatsCamp.org
- Latent Profile Analysis Course : Learn how to identify subgroups, or profiles, within a heterogeneous population based on patterns of responses to multiple observed variables.
- Bayesian Statistics Course : A comprehensive introduction to Bayesian data analysis, a powerful statistical approach for inference and decision-making. Through a series of engaging lectures and hands-on exercises, participants will learn how to apply Bayesian methods to a wide range of research questions and data types.
- Structural Equation Modeling (SEM) Course : Dive into advanced statistical techniques for modeling complex relationships among variables.
- Multilevel Modeling Course : A in-depth exploration of this advanced statistical technique, designed to analyze data with nested structures or hierarchies. Whether you’re studying individuals within groups, schools within districts, or any other nested data structure, multilevel modeling provides the tools to account for the dependencies inherent in such data.
As you embark on your journey into quantitative research, remember that StatsCamp.org is here to support you every step of the way. Whether you’re formulating research questions, analyzing data, or interpreting results, our courses provide the knowledge and expertise you need to succeed. Join us today and unlock the power of quantitative research!
Follow Us On Social! Facebook | Instagram | X
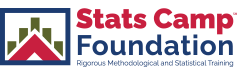
933 San Mateo Blvd NE #500, Albuquerque, NM 87108
3014 23rd Street Lubbock, TX 79410
Monday – Friday: 9:00 AM – 5:00 PM
© Copyright 2003 - 2024 | All Rights Reserved Stats Camp Foundation 501(c)(3) Non-Profit Organization.
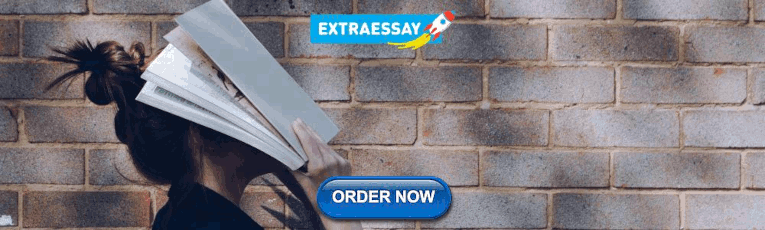
IMAGES
VIDEO
COMMENTS
Quantitative Research Topics. Quantitative Research Topics are as follows: The effects of social media on self-esteem among teenagers. A comparative study of academic achievement among students of single-sex and co-educational schools. The impact of gender on leadership styles in the workplace.
The extent of the impact of technology in the communications sector; Creative Quantitative Research Topics. Creativity is the key to creating a good research topic in quantitative research. Find a good quantitative research topic below: ... Research title example quantitative topics when well-thought guarantees a paper that is a good read. Look ...
Here are some of the most trendy topics about technology to consider. Technology use in education (here is our list of 110 topics in education research) Space and technology studies (check out our top 30 space research topics) Current and stunning developments in technology. Shocking inventions in modern technology that most people don't know ...
Artificial intelligence technology research topics. We started 2023 with M3GAN's box office success, and now we're fascinated (or horrified) with ChatGPT, voice cloning, and deepfakes. While people have discussed artificial intelligence for ages, recent advances have really pushed this topic to the front of our minds.
Here are 10 practical research topics for STEM students: Developing an affordable and sustainable water purification system for rural communities. Designing a low-cost, energy-efficient home heating and cooling system. Investigating strategies for reducing food waste in the supply chain and households.
This technology research paper can discuss the positive and negative effects of technology in 20 years. 5. The Reliability of Self-Driving Cars. Self-driving cars are one of the most exciting trends in technology today. It is a major technology of the future and one of the controversial technology topics.
Relationships and Media. 7. War. 8. Information and Communication Tech. 9. Computer Science and Robotics. Researching technology can involve looking at how it solves problems, creates new problems, and how interaction with technology has changed humankind. Steps in Researching.
Embark on a captivating journey through the cosmos of knowledge with our curated guide on Quantitative Research Topics for STEM Students. Explore innovative ideas in science, technology, engineering, and mathematics, designed to ignite curiosity and shape the future. Unleash the power of quantitative research and dive into uncharted territories ...
Abstract and Figures. A variety of quantitative techniques have been used in the past in future-oriented technology analysis (FTA). In recent years, increased computational power and data ...
Third, we review and classify the advantages (pros) and disadvantages (cons) of techniques, and their appropriateness for different contexts and organisations by assigning to the different groups and techniques the following five characteristics (Porter 2010: T able 10): 'drivers'—science (research), technology (development) and ...
Group Brainstorming: Collaborate with peers or mentors to gather diverse perspectives and insights. Group brainstorming can lead to innovative and multidimensional title ideas. Identifying Key Terms and Concepts: Break down your research into key terms and concepts. These will form the foundation of your title.
Chemistry. Let's get started with some quantitative research topics for stem students in chemistry: 1. Studying the properties of superconductors at different temperatures. 2. Analyzing the efficiency of various catalysts in chemical reactions. 3. Investigating the synthesis of novel polymers with unique properties. 4.
Educational Technology Topics. Perhaps, you're interested in a topic that touches on education and technology. In that case, consider these ideas for your research project. Incorporating computational thinking in education. How technology is changing the classroom practice. How technology changes learning outcomes.
List Of 12 Great Research Paper Titles About Technology. Writing a research paper about technology can be difficult if you don't know where to start. Knowing where to start can be the difference between a good grade and a bad grade. The best place to start is with the titles.
Description. Quantitative studies of science and technology represent the research field of utilization of mathematical, statistical, and data-analytical methods and techniques for gathering, handling, interpreting, and predicting a variety of features of the science and technology enterprise, such as performance, development, and dynamics.
177 of the Finest Technology Research Topics in 2023. We live in a technological era, and you can be sure of being asked to write a technology-oriented paper. Despite the contrary opinion that this is one of the most complicated tasks, students can comfortably develop a professional topic about technology for writing a research paper.
Here are ten examples of quantitative research titles suitable for school-related studies: "Impact of Technology Integration on Academic Performance: A Quantitative Analysis" "Effects of Classroom Size on Student Learning Outcomes: A Quantitative Study"
Three hours of lecture per week. Introduction to many different types of quantitative research methods, with an emphasis on linking quantitative statistical techniques to real-world research methods. Introductory and intermediate topics include: defining research problems, theory testing, causal inference, probability and univariate statistics. Research design and methodology topics include ...
The table in Figure 10 presents a number of guidelines for IS scholars constructing and reporting QtPR research based on, and extended from, Mertens and Recker (2020). The guidelines consist of three sets of recommendations: two to encourage ("should do" and "could do") and one to discourage ("must not do") practices.
This research was a quantitative study using 4th grade participants from a Title 1 elementary school in Central Illinois. This study set out to determine whether one to one technology (1:1 will be used hereafter) truly impacts and effects the academic achievement of students. This
Communications and media are developing super fast as well. The research is also done in areas that make our lives better and more comfortable. The list of them includes transport, food and energy, medical, and pharmaceutical areas. So check out our list of 204 most relevant computer science research topics below.
Quantitative research is the process of collecting and analyzing numerical data to describe, predict, or control variables of interest. This type of research helps in testing the causal relationships between variables, making predictions, and generalizing results to wider populations. The purpose of quantitative research is to test a predefined ...
Understanding Quantitative Research Questions. Quantitative research involves collecting and analyzing numerical data to answer research questions and test hypotheses. These questions typically seek to understand the relationships between variables, predict outcomes, or compare groups. Let's explore some examples of quantitative research ...