
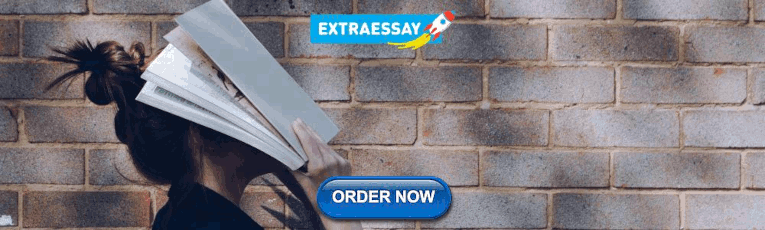
More Like this
Kpi meaning + 27 examples of key performance indicators.
As your organization begins to sketch out what your strategic plan might look like, it’s likely to come to your attention that you’ll need to gain consensus around what your key performance indicators will be and how they will impact your organization. If you haven’t thought much about your KPIs yet, that’s okay. We can help!
We’ve compiled a complete guide that includes an overview of what makes a good KPI, the benefits of good key performance indicators, and a list of KPI examples [organized by department and industry] for your reference as you develop your organization’s strategic plan and goals.

Video Transcript – How to Write KPIs
Hi, my name is Erica Olsen. Today’s whiteboard video is on key performance indicators, or KPIs for short. These are those things that are associated with either goals or objectives, whatever you’re calling them, those elements of your plan that are the expressions of what you want to achieve by when those quantifiable outcome-based statements.
So KPI’s answer the quantifiable piece of your goals and objectives. They come in three different flavors. So we’ll talk about that in just a minute. But before we do, putting great measures together and making sure they work well for you, you need to have these four attributes. And before I talk about those four attributes, so I just want to say the reason they need to work well for you is because KPIs are the heartbeat of your performance management process. They tell you whether you’re making progress, and ultimately, we want to make progress against our strategy. So KPIs are the thing that do that for us. So you’re going to live with them a lot. So let’s make sure they’re really good.
Okay, so the four things you need to have in order to make sure your these measures work for you.
Our number one is your measure. So the measure is the verbal expression very simply, in words, what are we measuring, which is fairly straightforward. The tricky thing is, is we need to be as expressive as we possibly can with our measures. So number of new customers, that’s fine. There’s nothing wrong with that. But a little bit advanced or a little bit more expressive, would be number of new customers this year, or number of new customers for a certain product or a certain service. So what is it is it? Yeah, so it is, so be really clear. And when it comes to measuring it on a monthly basis, you’re gonna want to be as clear as possible. So number of new customers, let’s say this year,
Number two, is our target, or target is the numeric value that we want to achieve. So a couple of things that are important about this is, the target needs to be apples to apples with when the goal date is set, or the due date is set. So we want to achieve 1000 new customers by the end of the year. So the due date in the target works hand in hand. The other thing is the measure and the target need to work hand in hand. So it’s a number. So this is a number, this is a percentage, this is a percentage, you get the idea.
Third thing, we actually run a report on this data. So where is it coming from? Be clear about what the source is. Most organizations have all sorts of data sources, fragmented systems. So making sure you identify where this data is coming from will save you a lot of time.
And then frequency. So how often are you going to be reporting on this KPI, ideally, you’re running monthly strategy reviews to report on the progress of your plan, at least monthly, in which case we’d like to see monthly KPIs. So you got to be able to pull the data monthly in order to make that happen. That’s not always possible. But let’s try to get there. Certainly some organizations are weekly and others are daily, monthly is a good place to start. So frequency. Great.
So now we know the components that we need to have in place in order to have our KPIs. Here are some different types of KPIs that you might think about as you’re putting your plan together.
So there are just straight up raw numbers, I call these widget counting, there’s nothing wrong with widget counting, they don’t necessarily tell a story. And I’ll talk about how to make this tell a story in a minute. But this is just simply widget counting number of things.
The second thing is progress. So this is really often used, it’s great. We use this, which is expressed as percent complete percent complete of the goal, percent completed a project, whatever it might be, it’s a project type measure. It’s a good measure, if if you don’t have quantifiable measures, or you can’t get the data, and you just want to track the performance of the goal as it relates to action items being completed under it.
The third type of indicator is a Change Type Indicator, like percent increase in sales, making this better would be percent increase in sales compared to last year. And the idea is 22%. So you can see how that starts to be more expressive, and work with the target. So this serves to tell a little bit more of a story than this one does, right? And if you want to actually make your widget counting measures tell more of a story like this one does, you might change something like this to read percentage of new customers acquired compared to same time last year. So that’s an example.
Okay, so now we know what we have to have in place and kind of different types of measures to get our ideas flowing. Let’s talk about one thing that you might take your measure writing to the next level and that is think about the fact that there are leading and lagging measures so are leading and lagging indicators. So percent increase in sales or sales is a lagging indicator it occurred as an outcome. If you want to make sure that you’re on track ACC, you might have a KPI in place, which is telling us whether we’re going to hit that increase such as your pipeline, maybe number of leads, or the size of your pipeline. So we don’t want to over rotate on this necessarily, but we do want to make sure we have a combination of leading and lagging measures when we’re looking at our performance on a monthly basis.
So with that, that’s all we have for today. Hopefully you have what you need to write great KPIs for your organization. Happy strategizing. And don’t forget, subscribe to our channel.
What is a Key Performance Indicator KPI — KPI Definition
Key performance indicators, also called KPIs, are the elements of your organization’s plan that express the quantitative outcomes you seek and how you will measure success. In other words, they tell you what you want to achieve and by when, and are crucial for evaluating the success of an organization. They are the qualitative, quantifiable, outcome-based statements you’ll use to measure progress and determine if you’re on track to meet your goals or objectives. Good plans use 5-7 KPIs to manage and track their progress against goals.
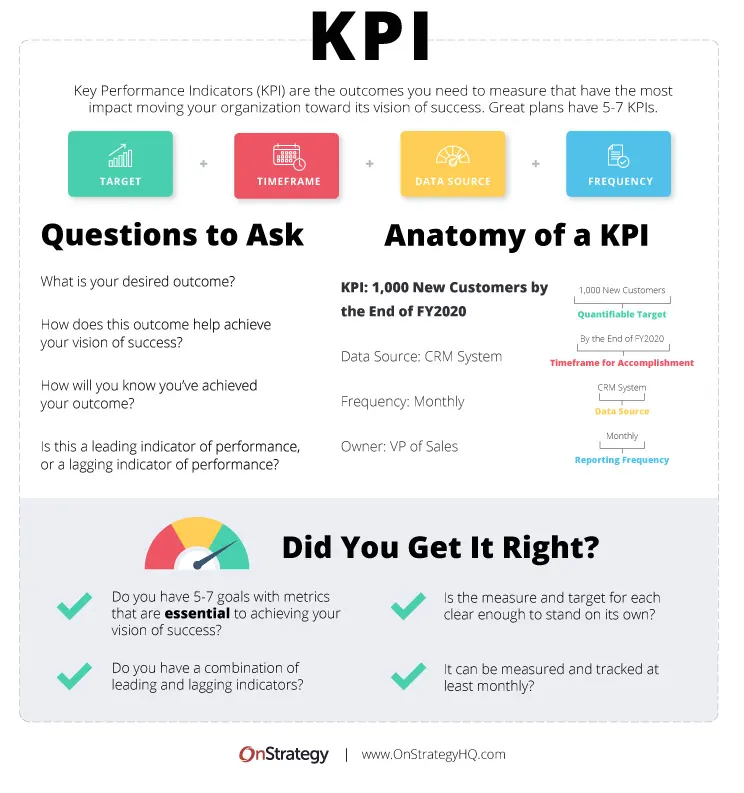
DOWNLOAD THE FREE KPI GUIDE
KPI Meaning & Why do you need them?
Key performance indicators are intended to create a holistic picture of how your organization is performing against its intended targets, business goals, or objectives. A great key performance indicator should accomplish all the following:
- Outline and measure your organization’s most important set of outputs.
- Work as the heartbeat of your performance management process and confirm whether progress is being made against your strategy.
- Represent the key elements of your strategic plan that express what you want to achieve by when.
- Measure the quantifiable components of your goals and objectives.
- Measure the most important leading and lagging measures in your organization.
The Five Elements of a KPI
These are the heartbeat of your performance management process and must work well! They tell you whether you’re making progress or how far you are from reaching your goals. Ultimately, you want to make progress against your strategy. You’ll live with these KPIs for at least the quarter (preferably the year), so make sure they’re valuable!
Great strategies track the progress of core elements of the plan. Each key performance indicator needs to include the following elements:
- A Measure: Every KPI must have a measure. The best ones have more specific or expressive measures.
- A Target: Every KPI needs to have a target that matches your measure and the period of your goal. These are generally a numeric values you’re seeking to achieve.
- A Data Source: Each of these needs to have a clearly defined data source so there is no gray area in measuring and tracking each.
- Reporting Frequency: Different measures may have different reporting needs, but a good rule to follow is to report on them at least monthly.
- Owner: While this isn’t a mandatory aspect of your KPI statement, setting expectations of who will take care of tracking, reporting, and refining specific KPIs is helpful to your overall organizational plan.
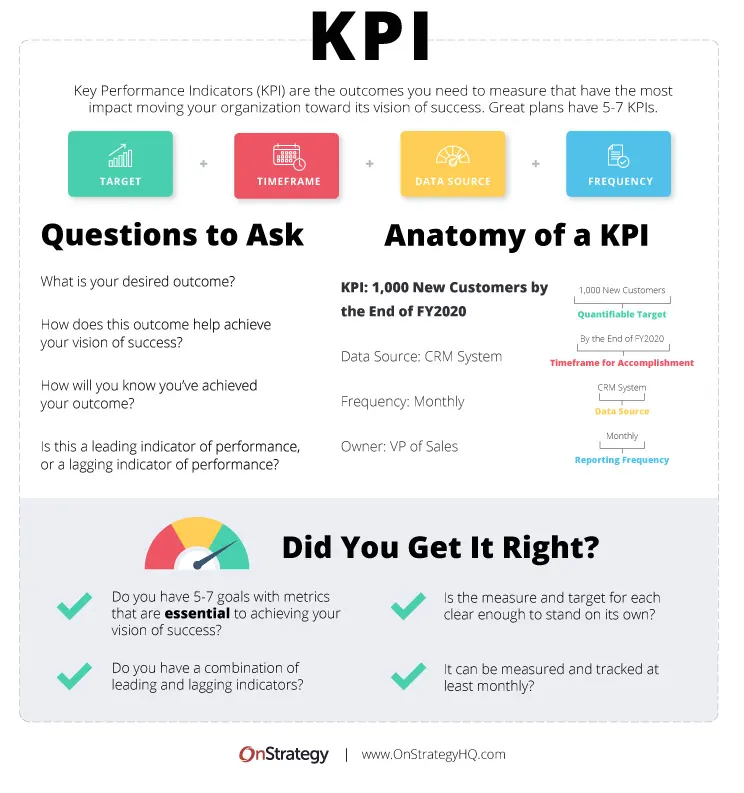
Indicators vs. Key Performance Indicators
An indicator is a general term that describes a business’s performance metrics.
There can be several types of indicators a company may track, but not all indicators are KPIs, especially if they don’t tie into an organization’s overall strategic plan or objectives, which is a MUST!
Key Performance Indicators
On the other hand, a key performance indicator is a very specific indicator that measures an organization’s progress toward a specific company-wide goal or objective. We typically recommend you narrow down the KPIs your organization tracks to no more than 7. When you track too many goals, it can get daunting and confusing.
Pro Tip : You should only track the best and most valuable indicators that tie to your organization’s long-term strategic goals and direction.
Benefits of Good Key Performance Indicators
What benefits do key performance indicators have on your strategic plan, and on your organization as a whole? A lot of benefits, actually! They are extremely important to the success of your strategic plan as they help you track progress of your goals. Implementing them correctly is critical to success.
- Benefit #1: They provide clarity and focus to your strategic plan by measuring progress and aligning your team’s efforts to the organization’s objectives. They also show your measurable progress over time and create ways to track your organization’s continued improvement.
- Benefit #2: Key performance indicators create a way to communicate a shared understanding of success. They give your team a shared understanding of what’s important to achieve your long-term vision and create a shared language to express your progress.
- Benefit #3: They provide signposts and triggers to help you identify when to act. A good balance of leading and lagging key performance indicators allow you to see the early warning signs when things are going well, or when it’s time to act.
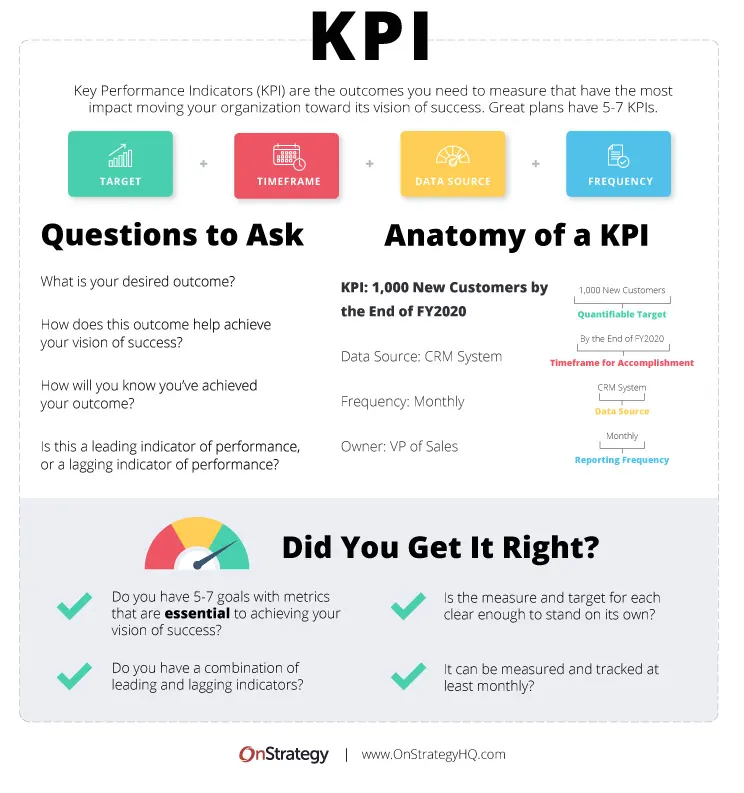
How to Develop KPIs
We’ve covered this extensively in our How to Identify Key Performance Indicators post. But, here’s a really quick recap:
Step 1: Identify Measures that Contribute Directly to Your Annual Organization-wide Objectives
Ensure you select measures that can be directly used to quantify your most important annual objectives.
Step 2: Evaluate the Quality of Your Core Performance Indicators
Select a balance of leading and lagging indicators (which we define later in the article) that are quantifiable and move your organization forward. Always ensure you have relevant KPIs. Having the right key performance indicators makes a world of difference!
Step 3: Assign Ownership
Every key performance indicator needs ownership! It’s just that simple.
Step 4: Monitor and Report with Consistency
Whatever you do, don’t just set and forget your goals. We see it occasionally that people will select measures and not track them, but what’s the point of that? Be consistent. We recommend selecting measures that can be reported upon at least monthly.
The 3 Common Types of KPIs to Reference as You Build Your Metrics
Key performance indicators answer the quantifiable piece of your goals and objectives . They come in three different flavors. Now that you know the components of great key performance indicators, here are some different ones that you might think about as you’re putting your plan together:
Broad Number Measures
The first type of KPI is what we like to call broad number measures. These are the ones that essentially count something. An example is counting the number of products sold or the number of visits to a webpage.
PRO TIP: There is nothing wrong with these, but they don’t tell a story. Great measures help you create a clear picture of what is going on in your organization. So, using only broad ones won’t help create a narrative.
Progress Measures
Progress key performance indicators are used to help measure the progress of outcomes . This is most commonly known as the “percent complete” KPI, which is helpful in measuring the progress of completing a goal or project. These are best when quantifiable outcomes are difficult to track, or you can’t get specific data.
PRO TIP: Progress KPIs are great, but your KPI stack needs to include some easily quantifiable measures. We recommend using a mixture of progress KPIs and other types that have clear targets and data sources.
Change Measures
The final type of KPI is a change indicator. These are used to measure the quantifiable change in a metric or measure. An example would be, “X% increase in sales.” It adds a change measure to a quantifiable target.
The more specific change measures are, the easier they are to understand. A better iteration of the example above would be “22% increase in sales over last year, which represents an xyz lift in net-new business.” More expressive measures are better.
PRO TIP: Change measures are good for helping create a clear narrative . It helps explain where you’re going instead of just a simple target.
Leading KPIs vs Lagging KPIs
Part of creating a holistic picture of your organization’s progress is looking at different types of measures, like a combination of leading and lagging indicators. Using a mixture of both allows you to monitor progress and early warning signs closely when your plan is under or over-performing (leading indicator) and you have a good hold on how that performance will impact your business down the road (lagging indicator). Here’s a deep dive on leading versus lagging indicators:
Leading Indicator
We often refer to these metrics as the measures that tell you how your business might/will perform in the future. They are the warning buoys you put out in the water to let you know when something is going well and when something isn’t.
For example, a leading KPI for an organization might be the cost to deliver a good/service. If the cost of labor increases, it will give you a leading indicator that you will see an impact on net profit or inventory cost.
Another example of a leading indicator might be how well your website is ranking or how well your advertising is performing. If your website is performing well, it might be a leading indicator that your sales team will have an increase in qualified leads and contracts signed.
Lagging Indicator
A lagging indicator refers to past developments and effects. This reflects the past outcomes of your measure. So, it lags behind the performance of your leading indicators.
An example of a lagging indicator is EBITA. It reflects your earnings for a past date. That lagging indicator may have been influenced by leading indicators like the cost of labor/materials.
Balancing Leading and Lagging Indicators
If you want to ensure that you’re on track, you might have a KPI in place telling you whether you will hit that increase, such as your lead pipeline. We don’t want to over-rotate on this, but as part of a holistic, agile plan, we recommend outlining 5-7 key performance metrics or indicators in your plan that show a mix of leading and lagging indicators. .
Having a mixture of both gives you both a look-back and a look-forward as you measure the success of your plan and business health. We also recommend identifying and committing to tracking and managing the same KPIs for about a year, with regular monthly or quarterly reporting cadence, to create consistency in data and reporting.
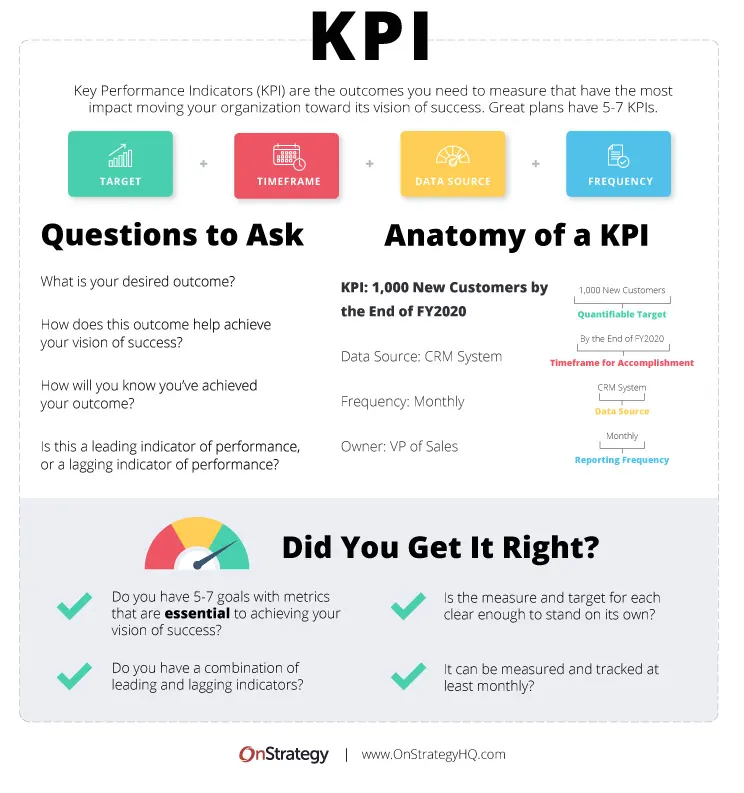
27 KPI Examples
Sales key performance indicators.
- Number of contracts signed per quarter
- Dollar value for new contracts signed per period
- Number of qualified leads per month
- Number of engaged qualified leads in the sales funnel
- Hours of resources spent on sales follow up
- Average time for conversion
Increase the number of contracts signed by 10% each quarter.
- Measure: Number of contracts signed per quarter
- Target: Increase number of new contracts signed by 10% each quarter
- Data Source: CRM system
- Reporting Frequency: Weekly
- *Owner: Sales Team
- Due Date: Q1, Q2, Q3, Q4
Increase the value of new contracts by $300,000 per quarter this year.
- Measure: Dollar value for new contracts signed per period
- Data Source: Hubspot Sales Funnel
- Reporting Frequency: Monthly
- *Owner: VP of Sales
Increase the close rate to 30% from 20% by the end of the year.
- Measure: Close rate – number of closed contracts/sales qualified leads
- Target: Increase close rate from 20% to 30%
- *Owner: Director of Sales
- Due Date: December 31, 2023
Increase the number of weekly engaged qualified leads in the sales from 50 to 75 by the end of FY23.
- Measure: Number of engaged qualified leads in sales funnel
- Target: 50 to 75 by end of FY2023
- Data Source: Marketing and Sales CRM
- *Owner: Head of Sales
Decrease time to conversion from 60 to 45 days by Q3 2023.
- Measure: Average time for conversion
- Target: 60 days to 45 days
- Due Date: Q3 2023
Increase number of closed contracts by 2 contracts/week in 2023.
- Measure: Number of closed contracts
- Target: Increase closed contracts a week from 4 to 6
- Data Source: Sales Pipeline
- *Owner: Sales and Marketing Team
Examples of KPIs for Financial
- Growth in revenue
- Net profit margin
- Gross profit margin
- Operational cash flow
- Current accounts receivables
Financial KPIs as SMART Annual Goals
Grow top-line revenue by 10% by the end of 2023.
- Measure: Revenue growth
- Target: 10% growt
- Data Source: Quickbooks
- *Owner: Finance and Operations Team
- Due Date: By the end 2023
Increase gross profit margin by 12% by the end of 2023.
- Measure: Percentage growth of net profit margin
- Target: 12% net profit margin increase
- Data Source: Financial statements
- *Owner: Accounting Department
Increase net profit margin from 32% to 40% by the end of 2023.
- Measure: Gross profit margin in percentage
- Target: Increase gross profit margin from 32% to 40% by the end of 2023
- Data Source: CRM and Quickbooks
- *Owner: CFO
Maintain $5M operating cash flow for FY2023.
- Measure: Dollar amount of operational cash flow
- Target: $5M average
- Data Source: P&L
- Due Date: By the end FY2023
Collect 95% of account receivables within 60 days in 2023.
- Measure: Accounts collected within 60 days
- Target: 95% in 2023
- Data Source: Finance
- Due Date: End of 2023
Examples of KPIs for Customers
- Number of customers retained
- Percentage of market share
- Net promotor score
- Average ticket/support resolution time
Customer KPIs as SMART Annual Goals
90% of current customer monthly subscriptions during FY2023.
- Measure: Number of customers retained
- Target: Retain 90% percent of monthly subscription customers in FY2023
- Data Source: CRM software
- *Owner: Director of Client Operations
Increase market share by 5% by the end of 2023.
- Measure: Percentage of market share
- Target: Increase market share from 25%-30% by the end of 2023
- Data Source: Market research reports
- Reporting Frequency: Quarterly
- *Owner: Head of Marketing
Increase NPS score by 9 points in 2023.
- Measure: Net Promoter Score
- Target: Achieve a 9-point NPS increase over FY2023
- Data Source: Customer surveys
- *Owner: COO
Achieve a weekly ticket close rate of 85% by the end of FY2023.
- Measure: Average ticket/support resolution time
- Target: Achieve a weekly ticket close rate of 85%
- Data Source: Customer support data
- *Owner: Customer Support Team
Examples of KPIs for Operations
- Order fulfillment time
- Time to market
- Employee satisfaction rating
- Employee churn rate
- Inventory turnover
Operational KPIs as SMART Annual Goals
Average 3 days maximum order fill time by the end of Q3 2023.
- Measure: Order fulfilment time
- Target: Average maximum of 3 days
- Data Source: Order management software
- *Owner: Shipping Manager
Achieve an average SaaS project time-to-market of 4 weeks per feature in 2023.
- Measure: Average time to market
- Target: 4 weeks per feature
- Data Source: Product development and launch data
- *Owner: Product Development Team
Earn a minimum score of 80% employee satisfaction survey over the next year.
- Measure: Employee satisfaction rating
- Target: Earn a minimum score of 80% employee
- Data Source: Employee satisfaction survey and feedback
Maintain a maximum of 10% employee churn rate over the next year.
- Measure: Employee churn rate
- Target: Maintain a maximum of 10% employee churn rate over the next year
- Data Source: Human resources and payroll data
- *Owner: Human Resources
Achieve a minimum ratio of 5-6 inventory turnover in 2023.
- Measure: Inventory turnover ratio
- Target: Minimum ratio of 5-6
- Data Source: Inventory management software
- *Owner: perations Department
Marketing Key Performance
- Monthly website traffic
- Number of marketing qualified leads
- Conversion rate for call-to-action content
- Keywords in top 10 search engine results
- Blog articles published this month
- E-Books published this month
Marketing KPIs as SMART Annual Goals
Achieve a minimum of 10% increase in monthly website traffic over the next year.
- Measure: Monthly website traffic
- Target: 10% increase in monthly website
- Data Source: Google analytics
- *Owner: Marketing Manager
Generate a minimum of 200 qualified leads per month in 2023.
- Measure: Number of marketing qualified leads
- Target: 200 qualified leads per month
- Data Source: Hubspot
Achieve a minimum of 10% conversion rate for on-page CTAs by end of Q3 2023.
- Measure: Conversion rate on service pages
- Target: 10%
- Due Date: End of Q3, 2023
Achieve a minimum of 20 high-intent keywords in the top 10 search engine results over the next year.
- Measure: Keywords in top 10 search engine results
- Target: 20 keywords
- Data Source: SEM Rush data
- *Owner: SEO Manager
Publish a minimum of 4 blog articles per month to earn new leads in 2023.
- Measure: Blog articles
- Target: 4 per month
- Data Source: CMS
- *Owner: Content Marketing Manager
- Due Date: December 2023
Publish at least 2 e-books per quarter in 2023 to create new marketing-qualified leads.
- Measure: E-Books published
- Target: 2 per quarter
- Data Source: Content management system
Bonus: +40 Extra KPI Examples
Supply chain example key performance indicators.
- Number of On-Time Deliveries
- Inventory Carry Rate
- Months of Supply on Hand
- Inventory-to-Sales Ratio (ISR)
- Carrying Cost of Inventory
- Inventory Turnover Rate
- Perfect Order Rate
- Inventory Accuracy
Healthcare Example Key Performance Indicators
- Bed or Room Turnover
- Average Patient Wait Time
- Average Treatment Charge
- Average Insurance Claim Cost
- Medical Error Rate
- Patient-to-Staff Ratio
- Medication Errors
- Average Emergency Room Wait Times
- Average Insurance Processing Time
- Billing Code Error Rates
- Average Hospital Stay
- Patient Satisfaction Rate
Human Resource Example Key Performance Indicators
- Organization Headcount
- Average Number of Job Vacancies
- Applications Received Per Job Vacancy
- Job Offer Acceptance Rate
- Cost Per New Hire
- Average Salary
- Average Employee Satisfaction
- Employee Turnover Rate
- New Hire Training Effectiveness
Social Media Example Key Performance Indicators
- Average Engagement
- % Growth in Following
- Traffic Conversions
- Social Interactions
- Website Traffic from Social Media
- Number of Post Shares
- Social Visitor Conversion Rates
- Issues Resolved Using Social Channel
Conclusion: Keeping a Pulse on Your Plan
With the foundational knowledge of the KPI anatomy and a few example starting points, it’s important you build out these metrics with detailed and specific data sources so you can truly evaluate if you’re achieving your goals. Remember, these will be the 5-7 core metrics you’ll live by for the next 12 months, so it’s crucial to develop effective KPIs that follow the SMART formula.
A combination of leading and lagging KPIs will paint a clear picture of your organization’s strategic performance and empower you to make agile decisions to impact your team’s success. KPI software allows your business to monitor and analyze performance trends over time by centralizing your data and using relevant data points and calculations. If you’d like more information on building better ones, check out the video above and click here to see why not all KPIs are created equal.
Our Other KPI Resources
We have several other great resources to consider as you build your organization’s Key Performance Indicators! Check out these other helpful posts and guides:
- OKRs vs. KPIs: A Downloadable Guide to Explain the Difference
- How to Identify KPIs in 4 Steps
- KPIs vs Metrics: Tips and Tricks to Performance Measures
- Guide to Establishing Weekly Health Metrics
FAQs on Key Performance Indicators
KPI stands for Key Performance Indicators. KPIs are the elements of your organization’s business or strategic plan that express what outcomes you are seeking and how you will measure their success. They express what you need to achieve by when. KPIs are always quantifiable, outcome-based statements to measure if you’re on track to meet your goals and objectives.
The 4 elements of key performance indicators are:
- A Measure – The best KPIs have more expressive measures.
- A Target – Every KPI needs to have a target that matches your measure and the time period of your goal.
- A Data Source – Every KPI needs to have a clearly defined data source.
- Reporting Frequency – A defined reporting frequency.
No, KPIs (Key Performance Indicators) are different from metrics. Metrics are quantitative measurements used to track and analyze various aspects of business performance, while KPIs are specific metrics chosen as indicators of success in achieving strategic goals.
16 Comments
HI Erica hope your are doing well, Sometime Strategy doesn’t cover all the activities through the company, like maintenance for example may be quality control …. sure they have a contribution in the overall goals achievement but there is no specific new requirement for them unless doing their job, do u think its better to develop a specific KPIs for these department? waiting your recommendation
Thanks for your strategic KPIs
Hello Erica, Could you please clarify how to set KPIs for the Strategic Planning team?
Hi Diana, check out the whitepaper above for more insight!
Hello Erica, Could you please clarify, how to set the KPIs for the Strategic PLanning team?
exampels of empowerment kpis
I found great information in this article. In any case, the characteristics that KPIs must have are: measurability, effectiveness, relevance, utility and feasibility
How to write methodology guidelines for strategy implementation / a company’s review and tracking (process and workflow) for all a company’s divisions
support on strategizing Learning & Development for Automobile dealership
Could you please to clarify how to write the KPIs for the Secretary.
Check out our guide to creating KPIs for more help here: https://onstrategyhq.com/kpi-guide-download/
That’s an amazing article.
Could you please to clarify how to write the KPIs for the office boy supervisor
Could you please clarify how to write KPIs for the editorial assistant in a start up publishing company.
Kindly advice how I would set a kpi for a mattress factory
Comments Cancel
Join 60,000 other leaders engaged in transforming their organizations., subscribe to get the latest agile strategy best practices, free guides, case studies, and videos in your inbox every week..
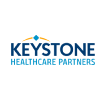
Leading strategy? Join our FREE community.
Become a member of the chief strategy officer collaborative..
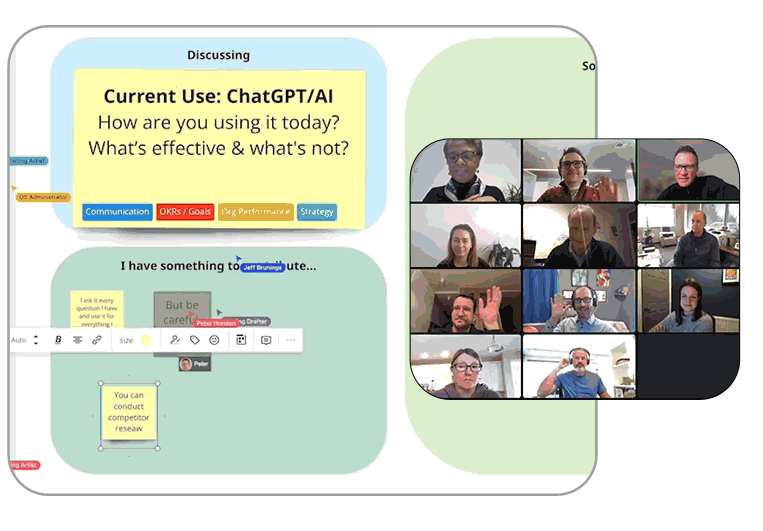
Free monthly sessions and exclusive content.
Do you want to 2x your impact.
- Customer Support
- Product Documentation
- Corporate Social Responsibility
- Diversity, Equality, Inclusion, and Belonging
- Academic Program
- Global Offices
- Support Portal
- Qlik Continuous Classroom
- Partner Portal
- Talend Cloud
- Talend Academy
Integrate, transform, analyze, and act on data

Qlik Staige
Bring your AI strategy to life with a trusted data foundation and actionable predictions

Integrations & Connectors
Connect and combine data from hundreds of sources
Featured Technology Partners

Data Integration and Quality
Build a trusted data foundation
Core Capabilities
- Data Streaming
- Application and API Integration
- Data Lake Creation
- Application Automation
- Data Warehouse Automation
- SAP Solutions
- Data Quality and Governance
- Stitch Data Loader
Guided Tour
Data Sources and Targets
Access and integrate the data you need to deliver greater business outcomes
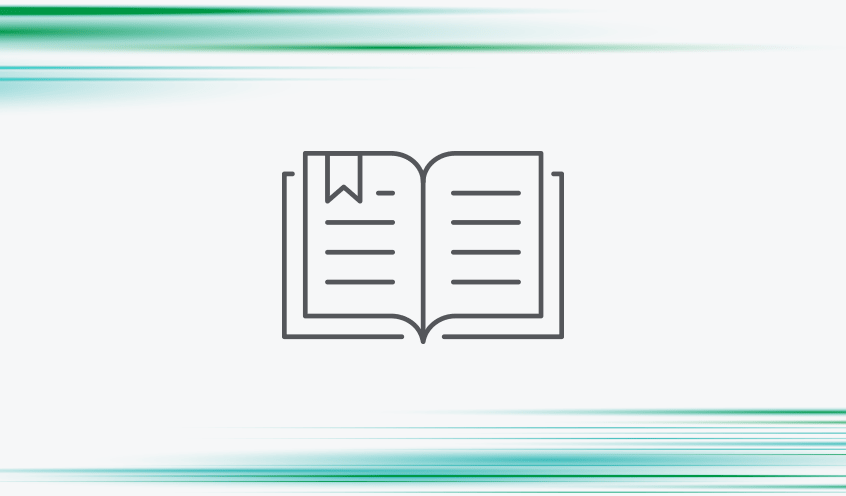
Data Integration Buyer's Guide: What to Look for in a Data Integration Solution
Take action with AI-powered insight
Embedded Analytics
- Augmented Analytics
- Visualizations and Dashboards
Try for Free
Data Sources
Connect and combine data from hundreds of sources to fuel your ever-evolving analytics needs
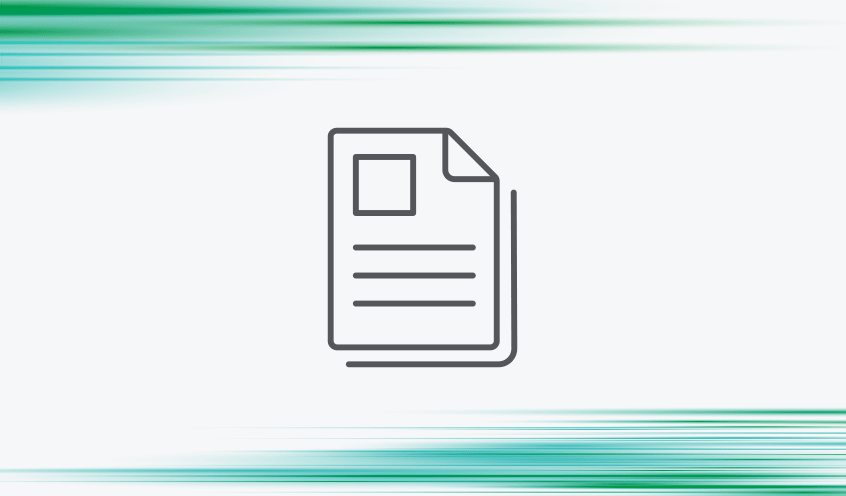
Qlik Platform Services for Analytics
Maximize the value of your data with AI
- Integration and Connectors
- Qlik Staige - Artificial Intelligence Built-in
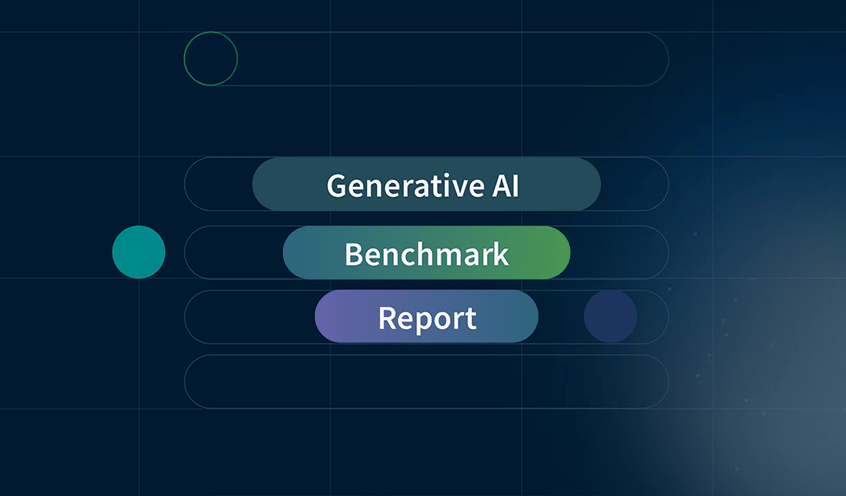
Generative AI Benchmark Report
All Data Integration and Quality Products
Qlik Cloud® Data Integration
Get a trusted data foundation to power your AI, ML, and analytics
Qlik Application Automation®
Automatically trigger informed action on most SaaS applications
Qlik Replicate®
Accelerate data replication, ingestion, and streaming.
Talend Data Fabric
Unify, integrate, and govern disparate data environments
Qlik Compose® for Data Lakes
Automate your data pipelines to create analytics-ready data sets
Talend Data Inventory
Find and improve data in a shared, collaborative workspace
Qlik Compose® for Data Warehouses
Automate the entire data warehouse lifecycle
Talend Data Preparation
Identify errors, and apply and share rules across massive datasets
Qlik Enterprise Manager®
Centrally configure, execute, and monitor replication and transformation
Talend Data Catalog
Understand the data flowing through your analytics pipelines
Qlik Gold Client®
Improve data management in your non-production SAP environments
Talend Data Stewardship
Define priorities and track progress on data projects
All Analytics Products
Qlik Cloud Analytics
All the power of Qlik analytics solutions in a cloud-based SaaS deployment.
Qlik Sense® - Client Managed
The on-premises solution for highly regulated industries.
All AI/ML Products
Bring machine learning to your analytics teams
Financial Services
Manufacturing
Consumer Products
Public Sector
Energy Utilities
US Government
Life Sciences
Communications
Product Intelligence
HR & People
Find a partner
Get the help you need to make your data work harder

Global System Integrators
Transform IT services, solution development, and delivery
- Data Integration and Quality Pricing Rapidly deliver trusted data to drive smarter decisions with the right data integration plan.
- Analytics Pricing Deliver better insights and outcomes with the right analytics plan.
- AI/ML Pricing Build and deploy predictive AI apps with a no-code experience.
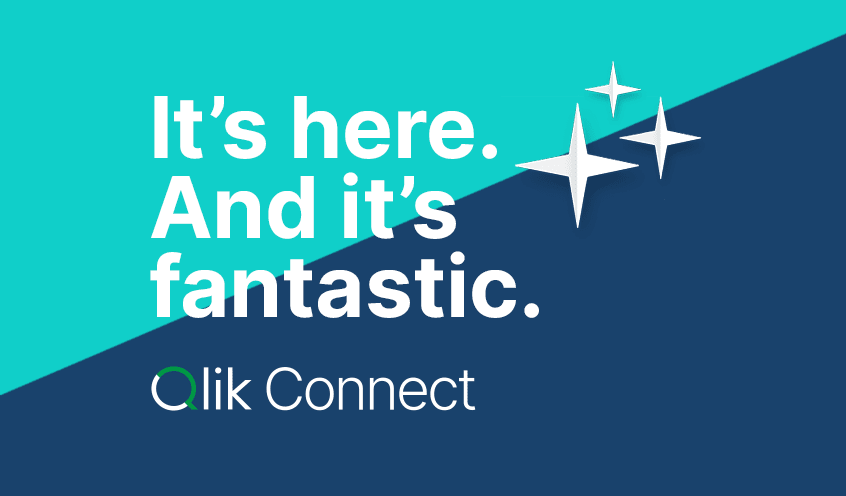
NASA scientists and data scientists have more in common than you might think. See why — and learn how you can chart your own path to data-driven discovery at Qlik Connect.
Revealing The New Qlik Brand
Hitting the Ground Running with Generative AI
Enter Qlik Staige – Helping customers unleash the full potential of Artificial Intelligence
Artificial Intelligence
Act on insights with AI-powered analytics
Data Management
Collect, store, organize, and maintain data
Bring automated machine learning to analytics teams
Data Quality
Discover, manage, enhance, and regulate data
Data Fabric
Data Visualization
Make it easier to see trends and relationships in your data
Data Catalog
Find the data you need and evaluate its fitness for your use case
Integrate applications and data sources
Data Governance
Ensure data is trustworthy and consistent
Predictive Analytics
Predict future outcomes based on historical and current data
Data Literacy
Read, work with, analyze, and communicate with data.

Domino's Radically Improves Efficiency, Customer Service — and Sales with Real-time Data and Analytics
Urban Outfitters Reduces Store Level Reporting from Hours to Minutes
Data Research Went From Thousands of Hours to Near Real Time at Georgia-Pacific
The Economic Impact of Cloud Analytics
Gartner DA Summit - Mumbai
Customer Stories
More than 40,000 customers find answers with Qlik.
Analyst Reports
Read analyst reports for data integration and analytics.
Whitepapers and eBooks
Visit the Qlik Resource Library.
Visit the Qlik Webinar Library.
Visit the Qlik Video Library.
Datasheets & Brochures
Visit the Qlik Datasheet and Brochure Library.

AI analytics refers to the use of machine learning to automate processes, analyze data, derive insights, and make predictions or recommendations.
Business Intelligence
Data Analytics
Data Mining
Data Warehouse
Predictive Modeling
Community Overview
Welcome to the Qlik Community
Qlik Gallery
Get inspired by recent Qlik apps and discuss impacts with peers
Get support directly from a community of experts
Plot your path of engagement with Qlik
Vote for your favorite product ideas and suggest your own
Training Overview
World-class resources to adopt Qlik products and improve data literacy.
Instructor-Led Learning
Get interactive, hands-on learning with Qlik experts
Free Training
FREE courses and help, from basic to advanced
Literacy Program
Understand, analyze, and use data with confidence.
Self-Paced Learning
Get hundreds of self-paced training courses
Validate Your Skills
Validate knowledge and skills in Qlik products, analytics, and data literacy
- Why Qlik Turn your data into real business outcomes
- Technology Partners and Integrations Extend the value of Qlik data integration and analytics
- Data Integration
- All Products
- By Industry
- Solution Partners
Data Integration and Quality Pricing
Rapidly deliver trusted data to drive smarter decisions with the right data integration plan.
Analytics Pricing
Deliver better insights and outcomes with the right analytics plan.
AI/ML Pricing
Build and deploy predictive AI apps with a no-code experience.
- Topics and Trends
- Resource Library
KPI Examples and Templates
Find the right KPIs for your business. This guide provides examples, templates and practical advice to help you define the key performance indicators that matter most for your organization and teams.
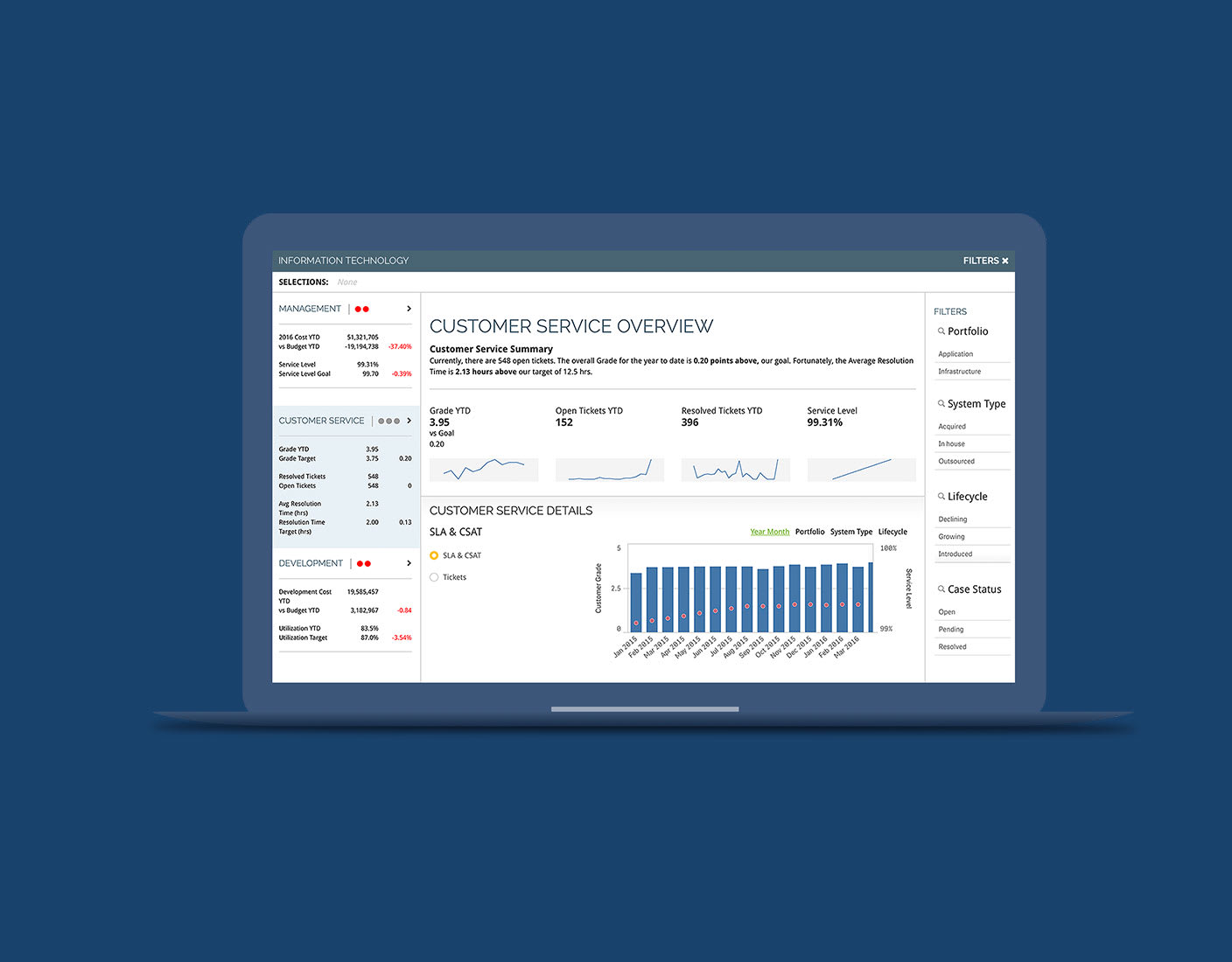
KPI EXAMPLES GUIDE
What is a kpi.
Let’s start with the basics. A key performance indicator (KPI) is a quantifiable measure of performance over time for a specific strategic objective. Business leaders and senior executives use KPIs to judge the effectiveness of their efforts and make better informed decisions.
KPIs vs Metrics
What’s the difference between a KPI and a metric?
KPIs represent how you’re performing against strategic goals. And by goals, we mean specific business outcomes, such as targeted quarterly revenue or targeted new customers per month.
Metrics support KPIs by representing the tactical processes or actions necessary to achieve the KPIs. Metrics track and measure the success against targets for specific actions such as monthly brochure downloads or store visits.
More resources:
Dive deeper on the question, “ What is a KPI? ”
Design your own interactive KPI Dashboard
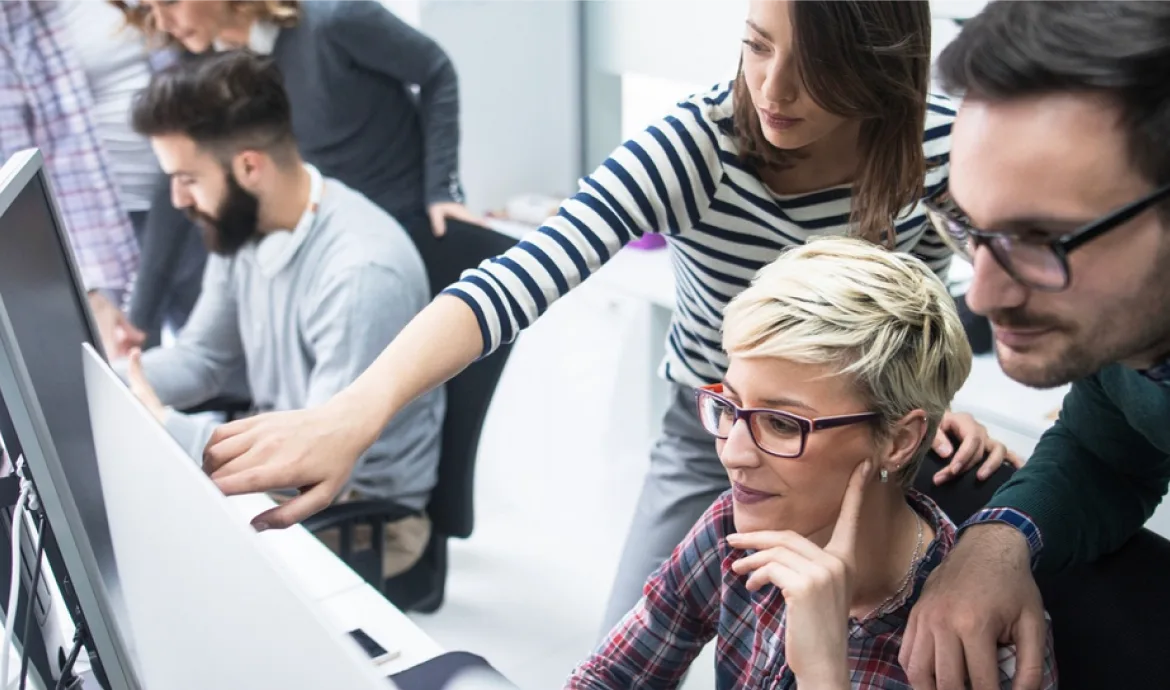
Don’t just measure. Measure what matters.
Download the KPI Planning Guide to learn:
10 steps to strong KPIs
Which questions help you define your KPIs
170 KPI examples and templates
170 KPI Examples And Templates
In this guide, we’ve identified and prioritized the most impactful key performance indicators examples for each department. Use the table of contents below to find the KPI examples most relevant to your organization and teams.
Project Management
Customer Service
Human Resources
Social Media
Sales KPI Examples
Sales leaders and their teams need to track the key performance indicators that help them close more orders. Below are the 15 essential sales KPI examples:
New Inbound Leads
Lead Response Time
Lead Conversion %
New Qualified Opportunities
Total Pipeline Value
Lead-to-Opportunity %
Opportunity-to-Order %
Average Order Value
Average Sales Cycle Time
Cross-Sell %
Sales Volume by Location
Sales Change (YoY, QoQ. MoM)
Sales Target %
Learn more about Sales Dashboards
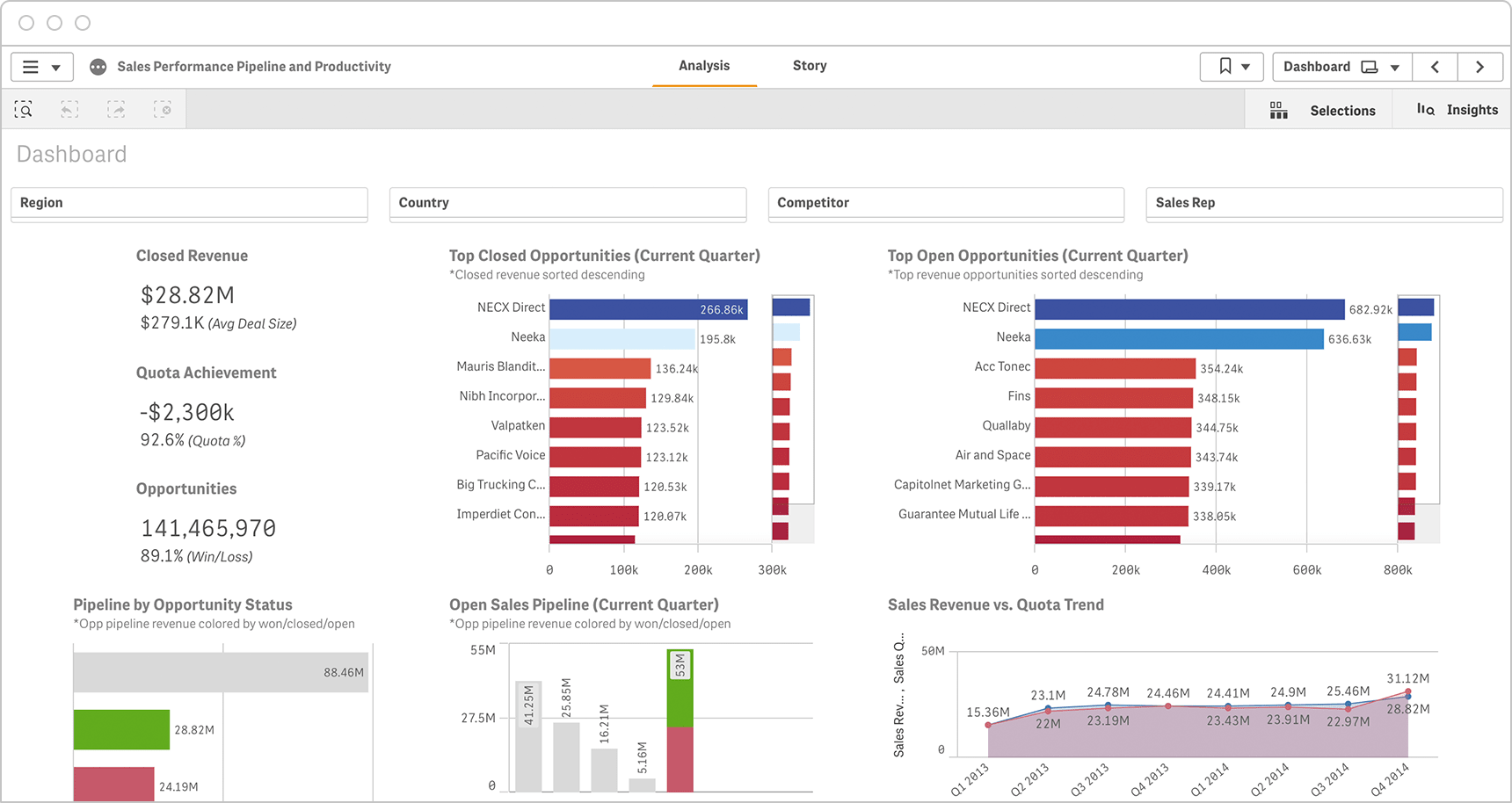
KPIs for Managers
Executives and managers need KPIs that reflect their organization’s strategic priorities. Below are the 15 key management KPI examples:
Customer Acquisition Cost
Customer Lifetime Value
Customer Satisfaction Score
Sales Target % (Actual/Forecast)
Sales by Product or Service
Revenue per FTE
Revenue per Customer
Operating Margin
Gross Margin
ROE (Return on Equity)
ROA (Return on Assets)
Current Ratio (Assets/Liabilities)
Debt to Equity Ratio
Working Capital
Employee Satisfaction Rating
Learn more about Executive Dashboards
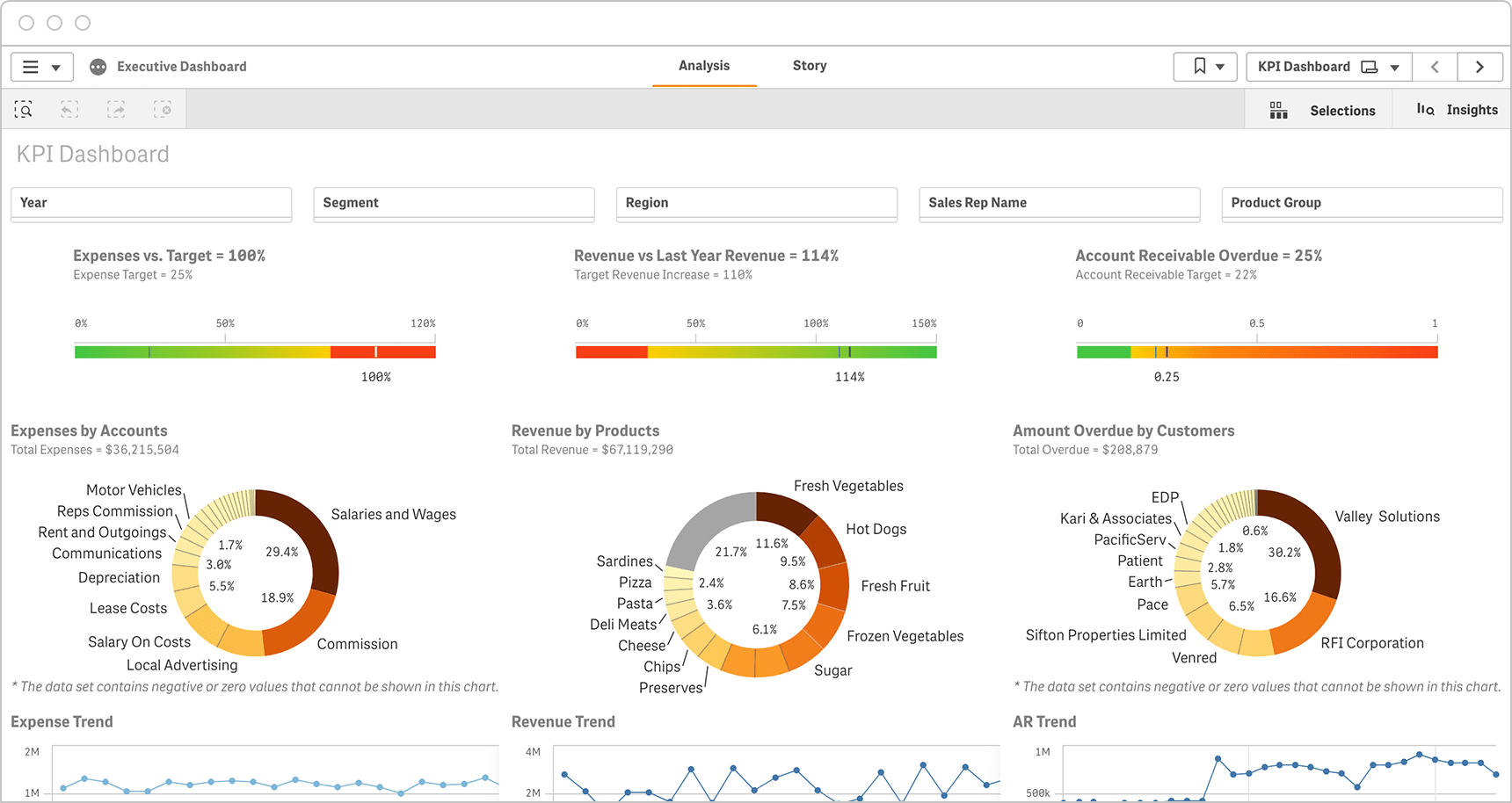
Project Management KPIs
Project managers need to keep projects on time and on budget while also ensuring a high quality outcome. That’s why the 15 key performance indicators examples below focus on timeliness, budget and quality.
On-Time Completion %
Milestones on Time %
Estimate to Project Completion
Adjustments To Schedule
Planned vs. Actual Hours
Resource Capacity %
Budget Variance (Planned vs Actual)
Budget Iterations
Planned Value
Net Promoter Score
Number of Errors
Customer Complaints
Change Requests
Billable Utilization
Return On Investment (ROI)
Explore dashboard demos
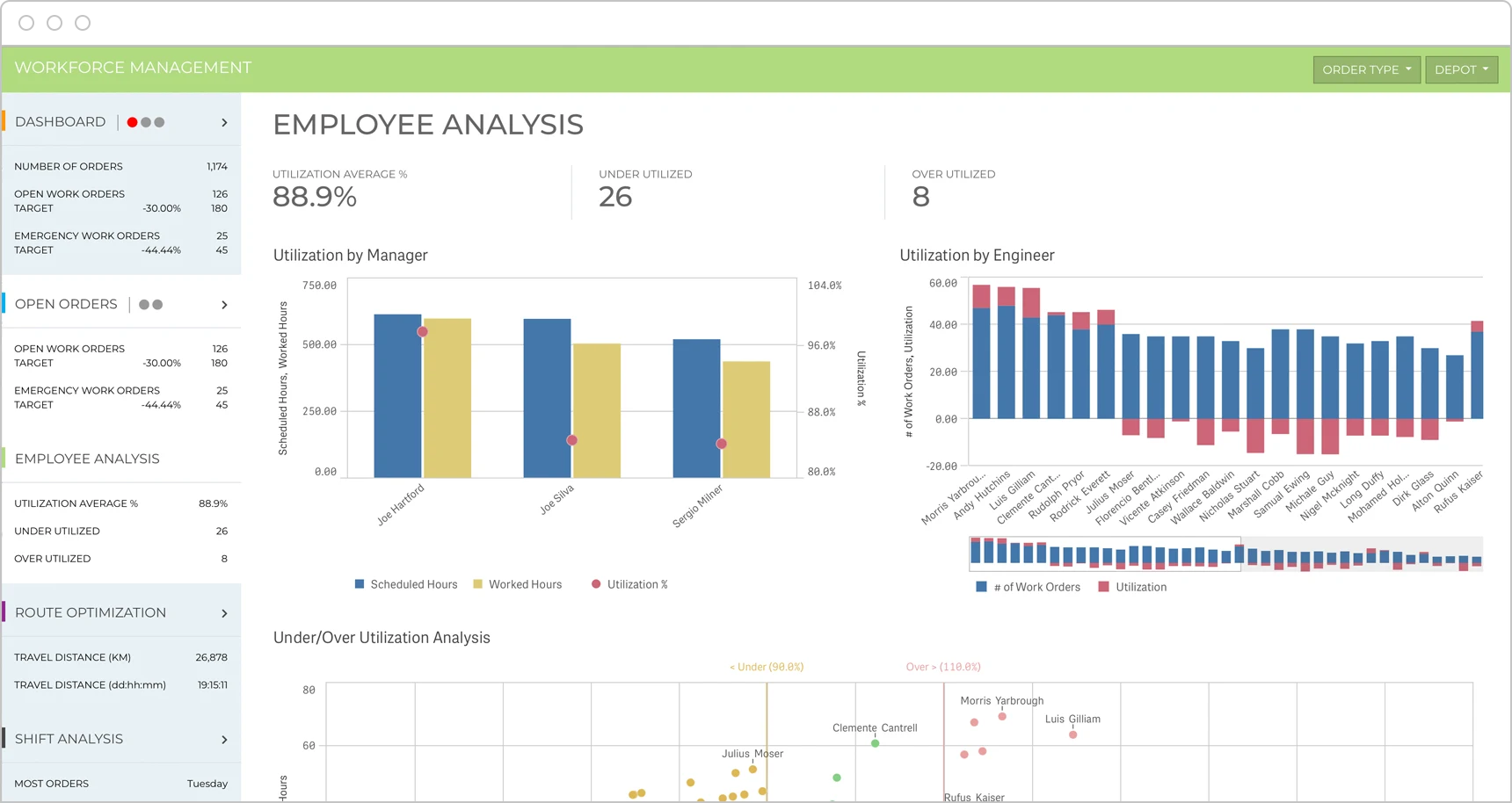
Inspire Action With Your KPIs
10 ways to take your data visualizations to the next level. Learn how to choose the right ones to highlight your KPIs and metrics.
Marketing KPIs
Marketing leaders need to track KPIs which enable them to measure their progress against clearly defined goals. The 15 marketing KPI examples below cover all phases of the customer funnel and can be accurately tracked using modern marketing analytics .
Marketing Qualified Leads (MQLs)
Sales Qualified Leads (SQLs)
Cost per Lead
New Customers
Cost per Acquisition
Upsell & Cross-Sell Rates
Conversion Rates (For Specific Goals)
Social Program ROI (By Platform)
Organic Traffic & Leads
Return on Ad Spend (ROAS)
Total Revenue
Revenue by Product or Service
Customer Lifetime Value (CLV)
Net Promoter Score (NPS)
Learn more about Marketing KPIs and Marketing Dashboards
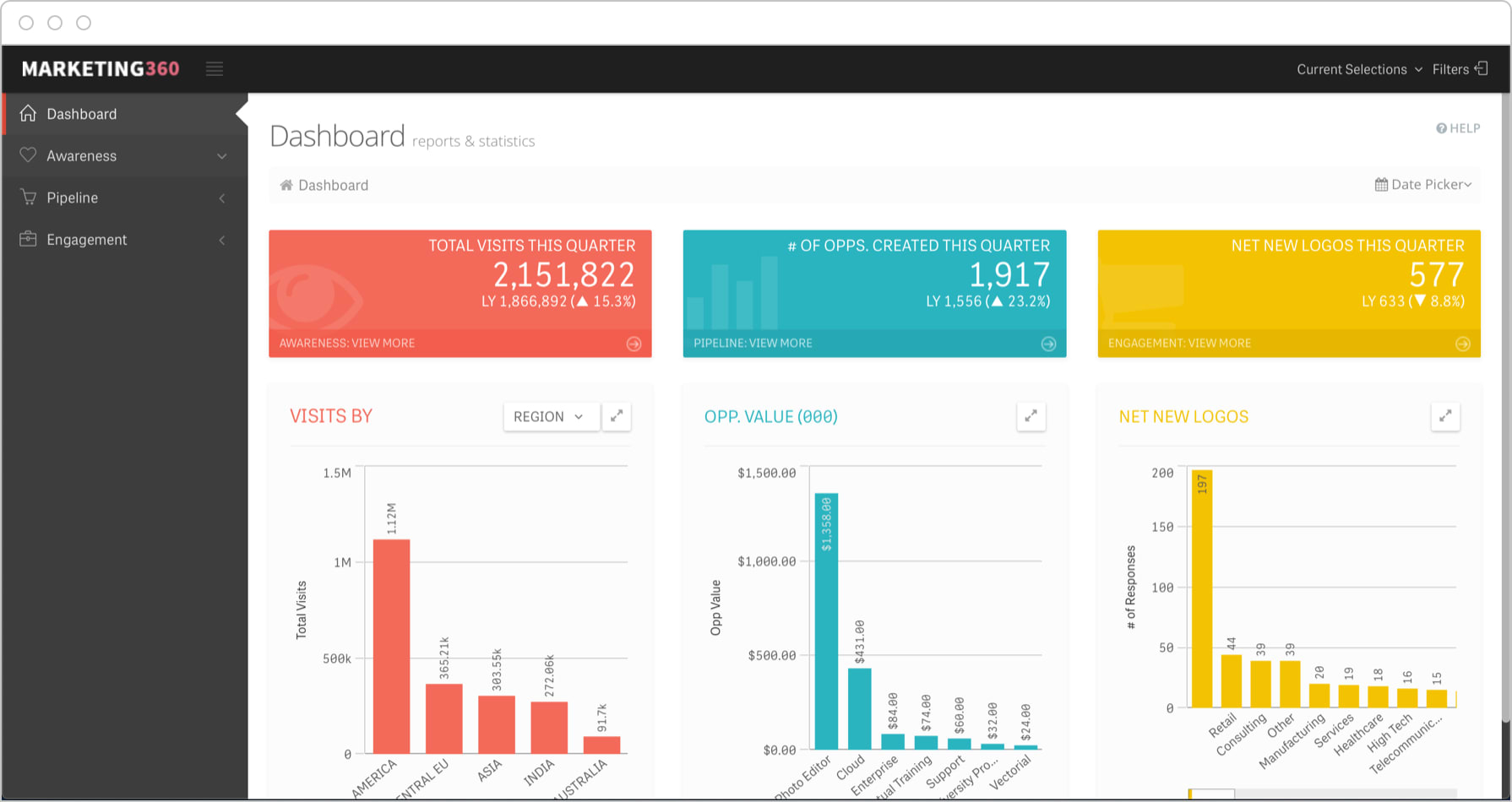
Operations KPIs
Operations managers need to track KPIs around efficiency, effectiveness and quality as covered in the 15 key performance indicators examples below.
Labor Utilization
Employee Turnover Rate
Employee Absence Rate
Employee Training Rate
ROI of Outsourcing
Labor Materials
Operating Margins
Processes and Procedures Developed
Project Schedule Variance
Order Fulfilment Cycle Time
Delivery In Full On Time Rate
Rework Rate
Learn more about KPI Dashboards
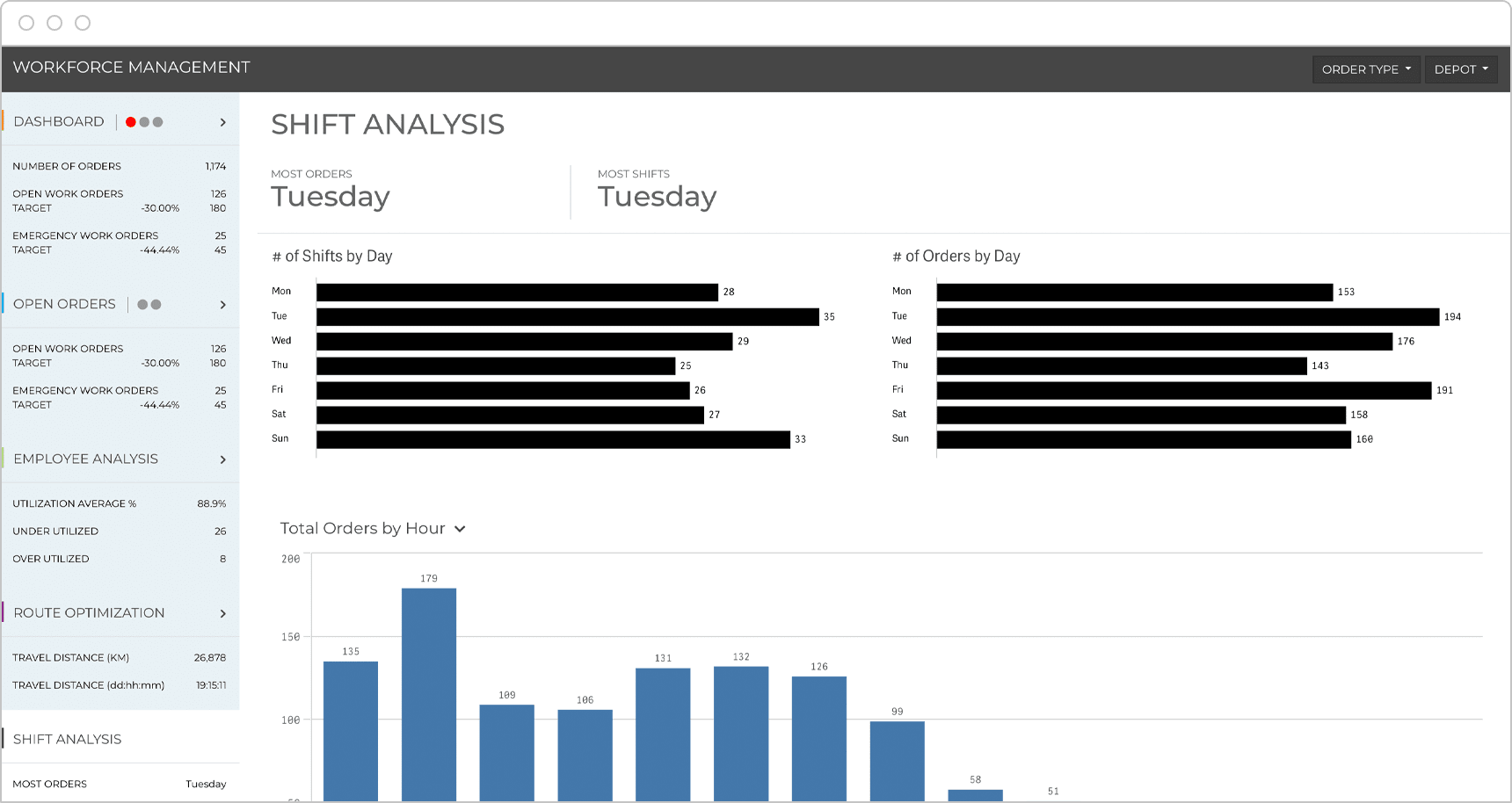
Customer Service KPIs
Service and support teams should focus on KPIs that measure response times. But, like the 15 key performance indicators examples below, they should also have a clear view of the customer base and longer term, preventative KPIs such as employee engagement and knowledge base articles.
Number of Issues (By Type)
First Response Time (FRT)
First Contact Resolution Rate
Average Response Time
Average Resolution Time
Most Active Support Agents
Cost Per Conversation
Customer Satisfaction Score (CSAT)
Positive Customer Reviews
Customer Effort Score
Customer Retention Rate
Support Costs / Revenue Ratio
Knowledge Base Articles
Employee Engagement
Explore more dashboard examples
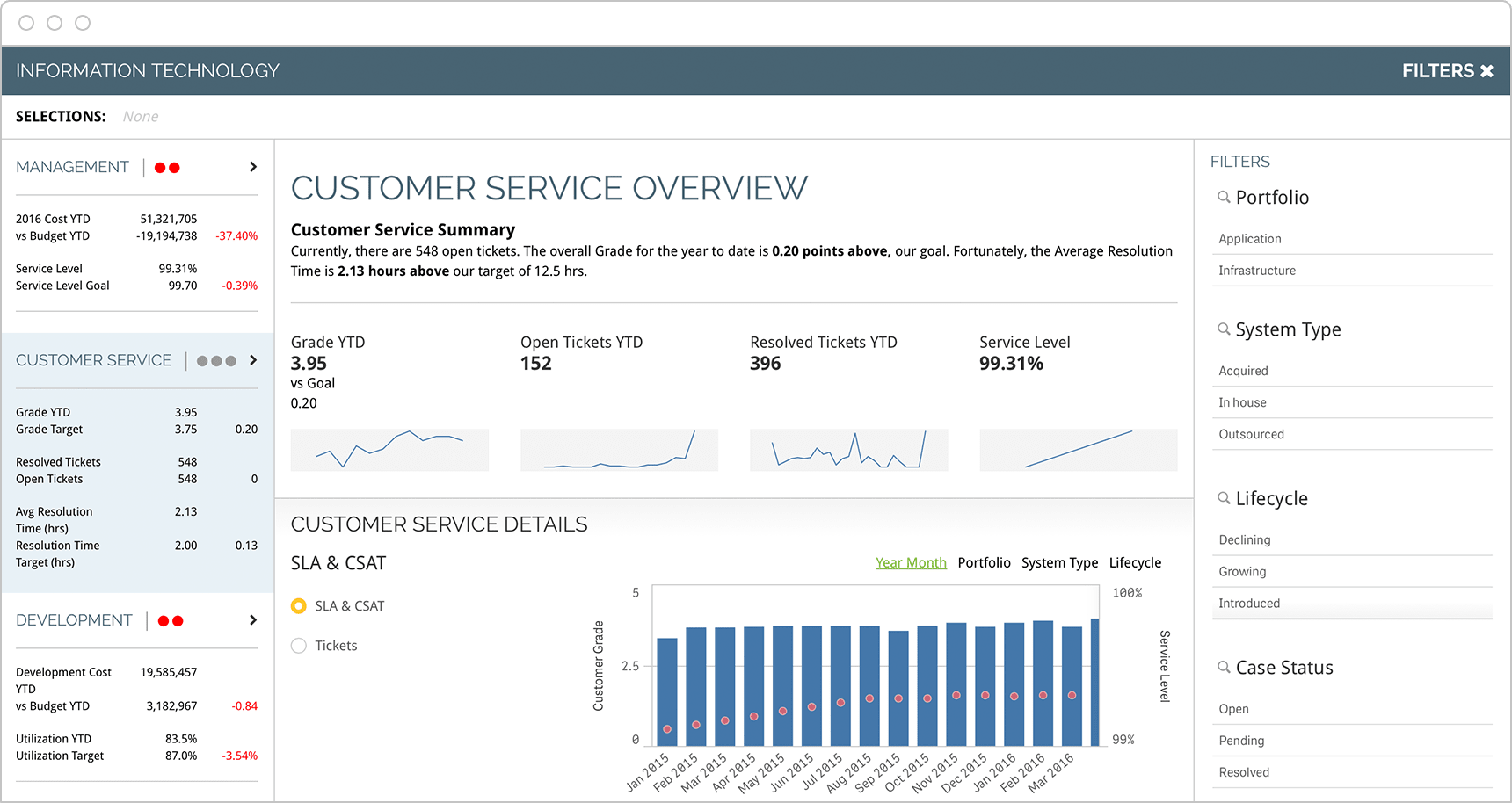
Finance KPIs
Financial teams have no shortage of ratios and metrics to track. Finance managers and CFO’s should use a financial analytics tool to focus on margin, expense, revenue and cash management as shown in the 15 key finance KPI examples below.
Gross Profit Margin (and %)
Operating Profit Margin (and %)
Net Profit Margin (and %)
Operating Expense Ratio
Working Capital Ratio
Debt-To-Equity Ratio
Quick Ratio (Acid Test)
Current Ratio
Berry Ratio
Return on Assets
Cash Conversion Cycle
Accounts Payable Turnover Ratio
Accounts Receivable Turnover Ratio
Budget Variance
Payroll Headcount Ratio
Learn more about Financial Dashboards
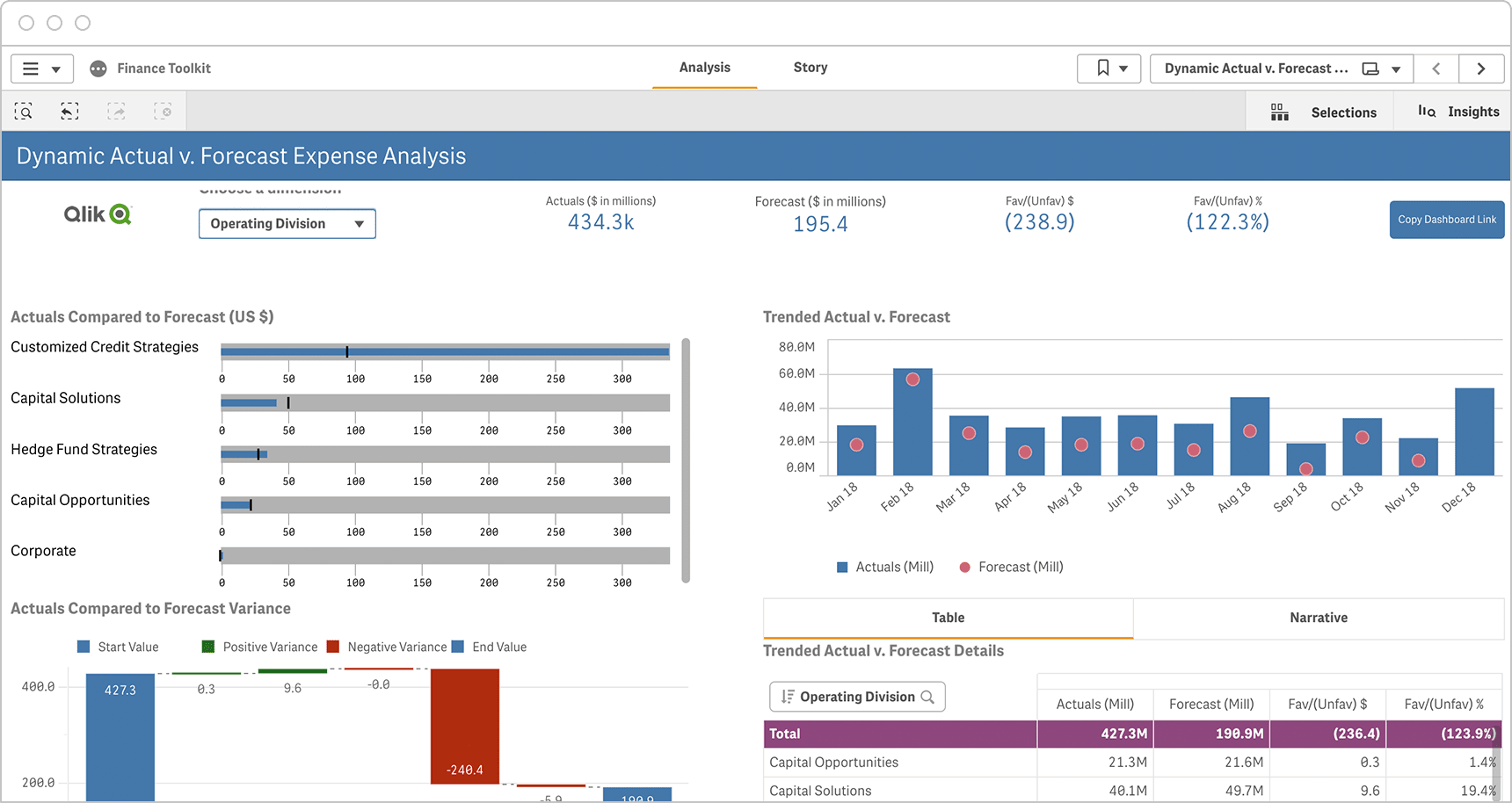
Human Resources KPIs
HR managers are primarily concerned with 3 main areas: workforce management, compensation and recruitment. You can use a people analytics tool to track and analyze the 35 key performance indicators examples below:
Workforce Management KPIs:
Absenteeism rate
ROI of outsourcing
Succession planning rate
Open/closed grievances
Promotion rate
Time to productivity
Successor gap rate
Worker composition by gender, experience, and tenure
Internal mobility
Manager quality index
HR effectiveness
Employee satisfaction rates
Training ROI
Compensation KPIs:
HR functional operating expense rate
Labor cost per FTE
Labor cost revenue percent
Labor cost revenue expense percent
Total benefits as percentage of labor costs
Profit vs. compensation per FTE
Human capital ROI
HR functional cost per employee
Recruitment KPIs:
Quality of hire
Vacancy rate
Turnover rate
Resignation/retirement rate
External hire rate
Time-to-fill
Diversity, experience, and gender hire ratio
Recruiting funnel metrics
Talent import/export ratio
Voluntary turnover rate
Retention rate
Recruiting expense per new hire
Retirement rate forecast
Learn more about HR Dashboards
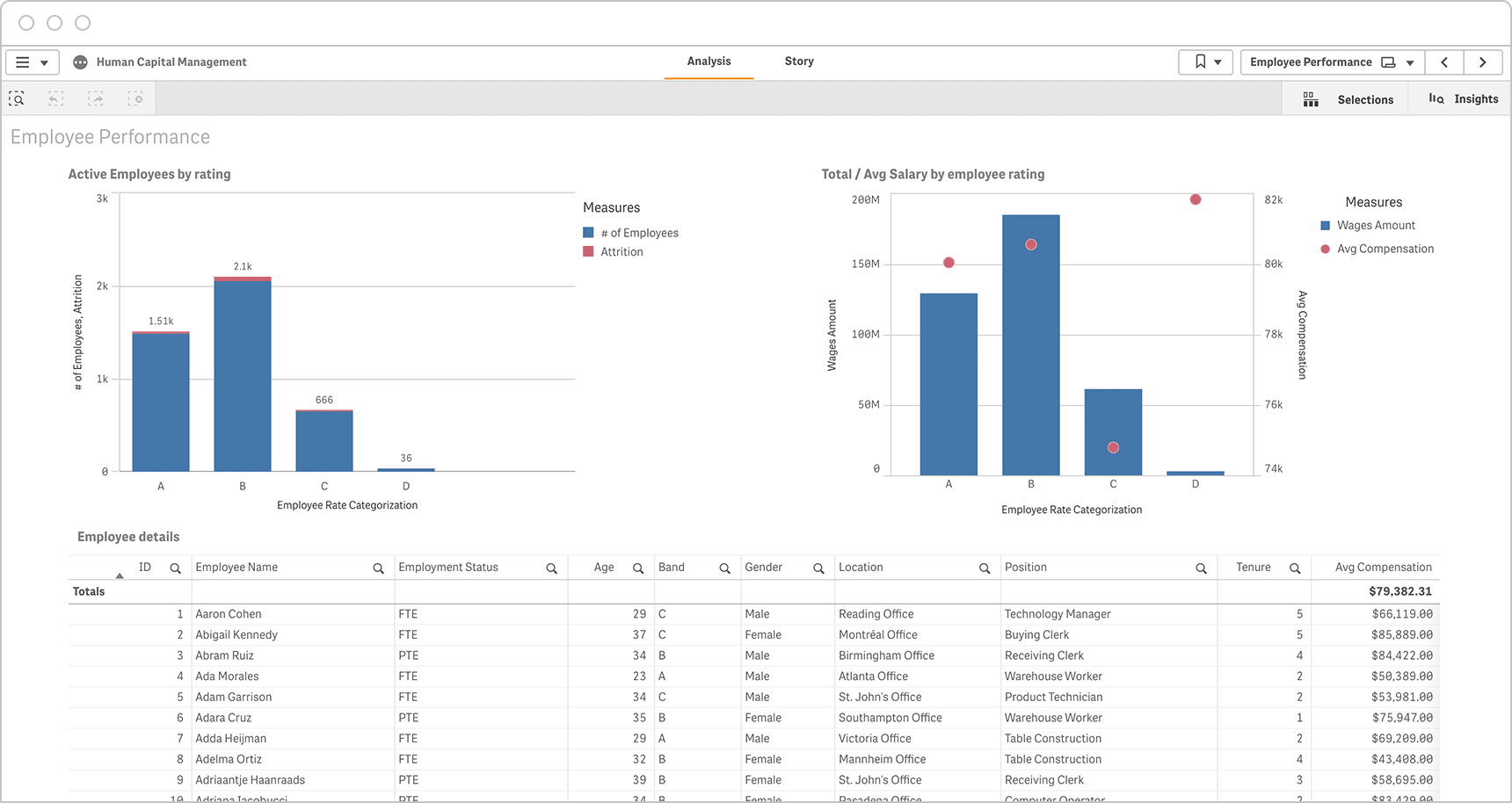
IT managers should track the on-going stream of support tickets and downtime. They should also track the projects and the team that will proactively reduce the number of these tickets in the future as shown in the top-15 IT KPI examples below.
Total Support Tickets
Open Support Tickets
Ticket Resolution Time
Reopened Tickets
Average Time Between Failures
Average Time to Repair
Server Downtime
Security Related Downtime
Total Projects
Projects on Budget
Critical Bugs
IT Support Employees Per End Users
IT Costs vs Revenue
IT Team Turnover
Social Media KPIs
Social media managers should have KPIs that represent reach, engagement, and conversion to revenue. The 15 social media key performance indicators examples below should be applied both as totals and for each social media platform that your organization is active on.
Social Share of Voice (SSoV)
Total Reach
Total Impressions
Followers or Fans or Subscribers
Audience Growth Rate
Share Rate (Shares or ReTweets)
Interest Rate (Likes, Reactions, Favorites)
Response Rate (Comments, Replies)
Key Post or Hashtag Reach
Link Clicks
Site Traffic From Social (By Platform)
Conversions From Social
Conversion Rate From Social
Revenue From Social
Social Program ROI
Learn more about Marketing Dashboards
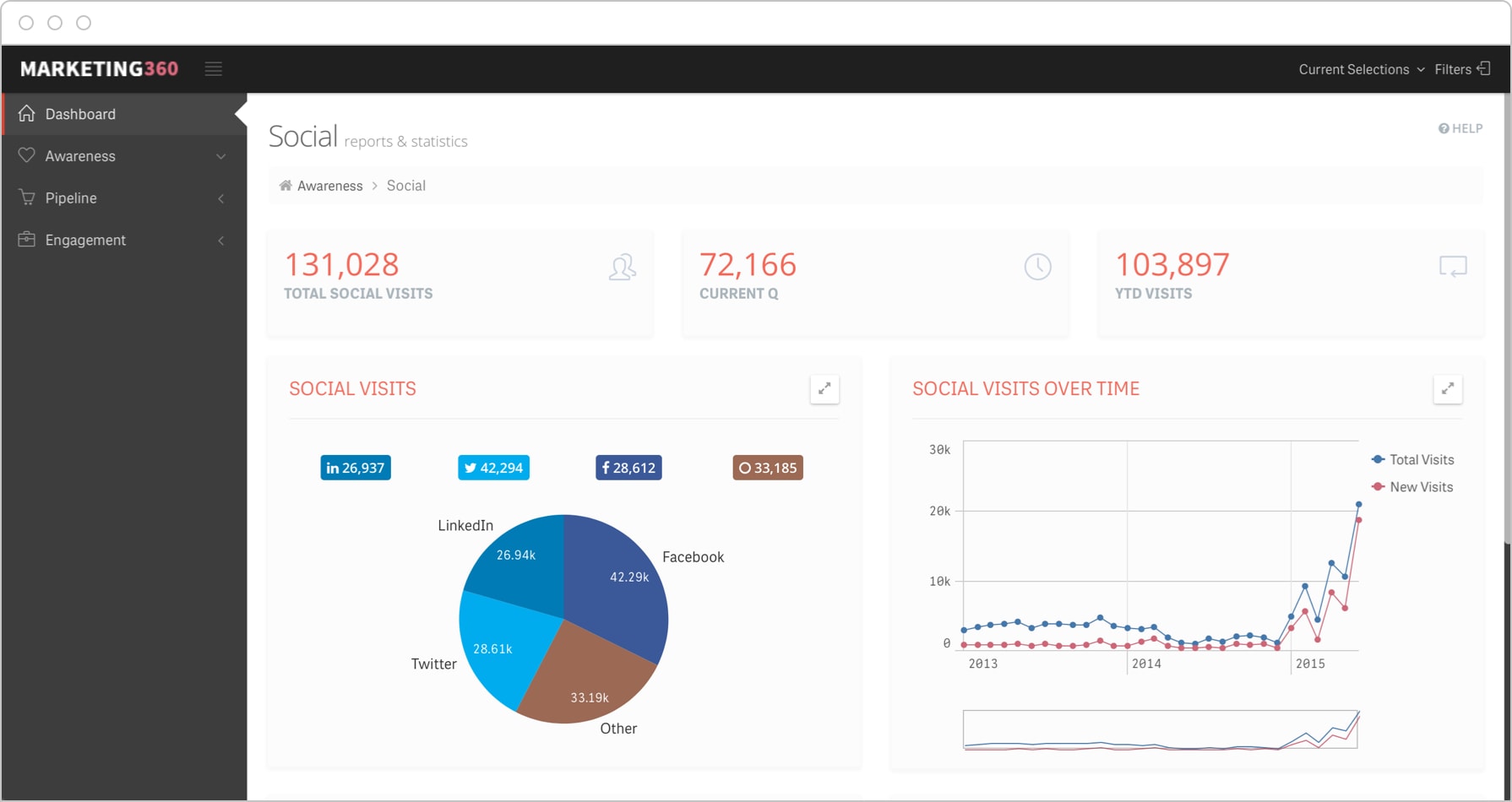
How to Define the Right KPIs
Who, what, how. Be clear about who the audience is, what they want, and how they’re going to use the KPIs. This means working with your stakeholders to identify the core KPIs that map directly to their goals and strategy.
Be SMART. This popular acronym stands for Specific, Measurable, Attainable, Realistic, and Time-bound. This is a useful touchstone whenever you’re considering whether a metric should be a key performance indicator. SMART KPI examples are KPIs such as “revenue per region per month” or “new customers per quarter”.
Iterate and evolve. Over time, see how you or your audience are using the set of KPIs and if you find that certain ones aren’t relevant, remove or replace them.
See KPI Dashboards in Action

OKR Success Stories and Case Studies
OKRs are seen as the solution for all kinds of inefficiencies that creep up over time in organizations, and this behavior can set them up for failure. The other side of the coin isn’t rosy either – organizations that are afraid of the effects of OKRs also fail to make the most of the framework.
High expectations can be tempered by studying the prerequisites of OKRs, but that does little to assuage the fears some organizations might have. Going through OKR case studies of organizations that have successfully used the framework can highlight the best practices, and drive the fear away. Their questions about prerequisites and implementing Objectives and Key Results can be answered by steps taken by organizations who have succeeded in using OKRs.
OKRs are becoming one of the most important ways for goal setting and performance management, as they are flexible enough to work for small and large businesses alike.
OKR case studies: 5 businesses who successfully use OKRs
The 5 case studies presented below demonstrate how the performance of leading businesses was impacted positively by using OKRs.
Microsoft has used OKRs in the recent past to align strategic priorities between and within different teams. The emphasis of the OKR practice at Microsoft is to localize objectives and key results (OKRs), identify opportunities quickly and address gaps in communication and resource requirements.
The organization uses OKRs to measure what really matters in the current cycle. Managers chart, calculate, and share progress on key results and metrics that define success.
The team made full use of Teams – Microsoft’s collaboration and chat tool – to bring strategic priorities into regular conversations. This ensured important and urgent tasks stayed in the line of sight of all stakeholders, and the efforts every day reflected the same. The adoption did not reduce even during the work from home phase, where distributed teams could access the objectives and expected key results, and compare them with actual results. This led to a near-real-time update of KPIs, with the simplicity of Teams chat.
Teams at Spotify use a time-boxed agenda to stay on track – where topics are outlined at the beginning of the session, along with activities that will be covered. The duration of every task and the desired outcomes are glanced over too.
There are a few ground rules that help the currently distributed teams to function efficiently – like outlining the rules and expectations beforehand. They also have a dedicated Slack channel to post questions that are then addressed during the discussions – which are held with the cameras on to add that human touch.
If that explains the smooth running of the meetings, the preparations that happen beforehand provide the basics. Team members review the OKR structure with the team, and OKRs start at the very top with the company vision. This vision defines key focus areas resulting in the setting of yearly objectives. These in turn allow teams to define their own team’s quarterly goals, which are converted into team OKRs.
The Team OKR setting sessions Start with icebreaker events, and then key results are prioritized via brainstorming. Everything goes here, and ideas are evaluated by the team in a democratic fashion. The ones that seem promising are stickied, and the collective KRs are brought down to just three to five items. The teams reconvene into a larger group and present their outputs to everyone – and the larger group then finalizes their quarterly KRs.
Google has been a proponent of OKRs since 1999 when it was a company of 40. One thing that has stayed in its phenomenal growth to 60,000 people is their reliance and belief in OKRs. The leaders set ambitious objectives, and key results are graded on a scale of 0.0-1.0 at the end of every quarter.
One of the highlights of Goole OKRs is that they are transparent – that means any employee in the organization can see what their colleagues are focusing on. OKRs between 0.6 to 0.7 is considered successful at Google: for good reasons too, because OKRs are designed to push the team to be a little better in the current cycle than the previous one. They also don’t consider low OKRs as a cause of concern, as they believe low scores indicate there is room for improvement. These low score OKRs are used as learning opportunities for the next OKR cycle (in Google’s case, next quarter).
LinkedIn CEO Jeff Weiner believes that the business is responsible for providing clear directions to the leaders of the organization, as making informed decisions helps in staying on track of the vision and mission . The CEO believes that good leaders can lift their surroundings (in this case, team members) through different modes of activity like coaching, strategy planning, defining objectives, and measuring the right results. The belief in the organization is to complete OKRs in a limited time period, and hence 3 to 5 objectives in a quarter are enough.
OKR progress is tracked at LinkedIn through regular meetings with employees, which can take the entire day.
These meetings keep the CEO and the rest of the leadership team updated on how the employees are contributing towards the business objectives of the organization. Employees share their views on the success and roadblocks so that they get recognized for the good work done and request help in areas they are slower than average.
One of the leading telecom organizations in the world, Huawei, moved to OKRs from KPIs to take their business practices a notch above. The leadership team at Huawei was unhappy with the KPI system that was in use, as it could only use goal setting as the process starting point and performance evaluation as the end – but didn’t provide any avenues for the employees to clearly understand the need or importance of the goals set. The process was mechanical and did not add much to the upward curve the organization was aiming for.
In 2019, the leadership group decided to adopt OKRs. OKRs were used to set goals for the team members, who could clearly identify and understand the importance of goals set for them and how they contributed to the overall growth of the organization. The process boosted the performance of their business, as aligning the goals of employees led to an increased collaboration which resulted in the completion of business goals in a collective manner.
Sears holding company started using OKRs in 2013 for all of its 20,000+ salaried employees – but the expected changes did not happen even after a year of using the framework. This led to some necessary changes being made, where teams of outbound call agents at different locations were allotted different objectives based on the data accumulated. The focus was diverted to add-on sales that were measured using hourly calls and hourly sales data. This refresh brought the desired changes, as the sales figures increased by 8.5%. Average sales figures jumped significantly and increased to $15.67 per hour – a marked rise from the previous $14.44.
Learnings from the case studies
Organizations can start by using the OKR formula to calculate the expected effectiveness – which can be stated in simple terms as below:
[objective] needs to be attended to, and the progress of the process can be measured by [key result 1], [Key result 2] …
Here, the ambitious, concrete goal that the organization wants to achieve is the objective, and the key results are how the accomplishment of the objective is measured. Limiting key results to 3 to 5 items is important, as employees can get bogged down if the number is more than that per objective. This enables the objective to be focussed on the minds of the employees, and they can see how striving to achieve each metric helps them and their organization.
Aligning day-to-day work As the Spotify and Google case studies point out, having a well-defined OKR can result in the better articulation of the outcome, that can be easily followed and adhered to by employees. As soon as employees can see how their everyday work impacts the overall objective of the organization, their productivity increases. Instead of seeing work as a list of tasks to be completed, they treat them as stepping stones towards achieving their and the organization’s objectives. Regular meetings also help in highlighting issues or new avenues that might be useful in the long run.
Unlocking the potential of the workforce.
OKRs provide a robust platform for employees to collaborate and work towards a common objective instead of just finishing tasks on their plate. This focus brought forward by OKRs is essential to keep the remote workforce engaged, as it simplifies collaboration between teams and allows them to stay focused on executing the strategy penned down by the leadership teams. Employees can then plan their business-as-usual activities better, and identify areas of innovation and learning that can help them and their organization stay ahead of the curve. The potential of the workforce increases rapidly because of the clear line of action presented by well-planned OKRs, and results in:
- Speed at which changes can be carried out. Due to increased collaboration between team members and different teams, the time taken to execute important steps are shortened. Individual contributions add up to more than the sum of their parts, and the organization as a whole can move towards being nimble in the face of unexpected changes, without affecting business productivity.
- Priorities can be set based on immediate needs while keeping an eye on the changes that may occur in the future. With constant coaching and one-on-one sessions, both the manager and the team members can grow faster into the roles and contribute more effectively to the overall objective.
- Execution becomes more streamlined as there are other key results that need to be achieved. The continuous feedback cycle which OKRs thrive on, help in identifying and adopting potential changes that might disrupt the market in the near future.
- Alignment of goals and ambitions of employees with that of the organization creates a win-win situation where employees upgrade their skills promptly, which allows the organization to stay ahead of potential challenges from competitors (or market conditions).
More OKR Success Stories
There are plenty of OKR success stories that can be used to understand the effectiveness of the process, but having the basics right is more important for organizations thinking about putting the framework into practice. Without the presence of a strong vision and buy-ins from the leadership team, however, OKRs have little chance of causing any significant improvement to the day-to-day activities of the organization. The clarity and accountability that can be gained from OKRs depend on connecting the objectives of the company and team members into results that can be easily quantified.
Leave a Comment Cancel Reply
Your email address will not be published. Required fields are marked *
Save my name, email, and website in this browser for the next time I comment.
Related Blogs
OKRs Jun 14, 2016
Mbo vs. okr – what are the differences, okrs jun 15, 2016, ensure successful okrs by avoiding these 8 mistakes [infographic].

OKRs Sep 6, 2016
Why okr alignment is a vital factor.

Stay Updated with latest news at UpRaise
Your email will be safe and secure in our database
Thanks for subscribing! Please check your email for further instructions.
← Blog Home
Case study: key performance indicators in local digital marketing.
- April 24, 2017
Finding, tracking and collating Key Performance Indicators (KPIs)* that demonstrate the performance of your online efforts has always been difficult in the local digital marketing space. But with upgrades in reporting at Google, Yelp and a newly minted web site, I took the opportunity to do just that in a case study with our favorite “guinea pig” Barbara Oliver Jewelry from the Buffalo, NY area.
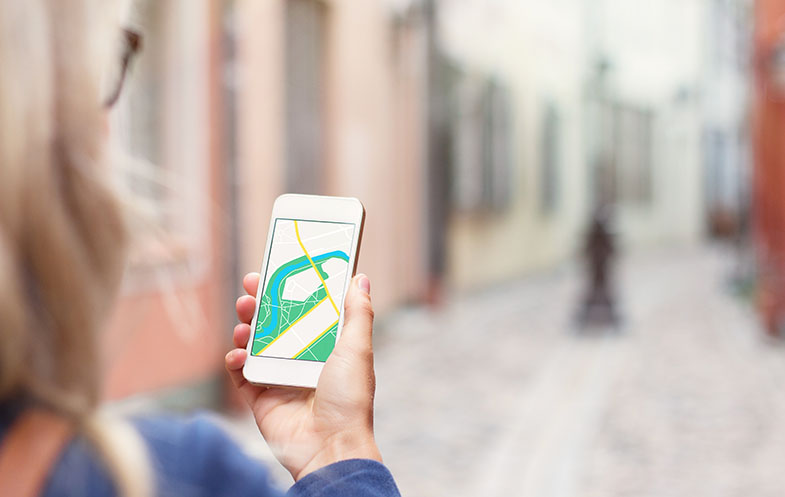
Not only were we able to create a credible view of the drivers of her local business we were able to implement an in-store survey so as to be able to ascribe attribution to both her online and offline marketing efforts which we discuss in part 2 of this series .
What Is The Diamond Of Local Digital Marketing?
Barbara Oliver Jewelry created a new website last August 2016 and hired a new staffer to help with marketing. She has had an active presence on Facebook, has been doing review management with good success since 2009 , is running a very small Google Adwords Express campaign and starting last June started an active Google+ campaign.
She does have a Yext account (provided gratis by Yext) as well and a verified Yelp presence but has never advertised there.
Her high end jewelry store is located on the 3rd floor of an office building with zero walk in traffic and no street side presence. Her advertising budget is limited to a few key areas. Most money is spent on radio, some on a billboard, less on G+ and search optimization. She has been spending about the same on the Yellow Pages per quarter as on SEO. A tiny amount per quarter on Facebook boosts and GetFiveStars. Thus her potential sources of new customers are relatively clean and defined.
The last quarter of 2016 was a good time to implement a study of her KPIs and attempt to glean attribution via an in-store survey.
Local Digital Marketing KPIs
We wanted to explore the “low on the funnel” performance indicators and understand their relationship to new customer acquisition and purchases. Obviously there are many KPIs from which to choose. We identified the following as the digital components that were trackable and, given that there was no e-commerce component on her site, as close as we could get to the purchase*.
- Contact form fill
- FB messaging and comments expressing intent
- Clicks to call
- Request for driving directions
They were, in her case, the best indicators of intent.
With the new website we were sure to put in place event tracking for contact form fills, click to call actions and driving directions requests. For additional sources of this information we looked at dashboards at Yelp, Bing, Google, indexed all comments that showed intent at FB and tracked all of these in addition to those events on her website. While she has a Yext dashboard as well, they do not track any of these metrics from the websites that they post on.
We were able to track roughly 360 actions across all platforms and the sources of these actions broke out like this:
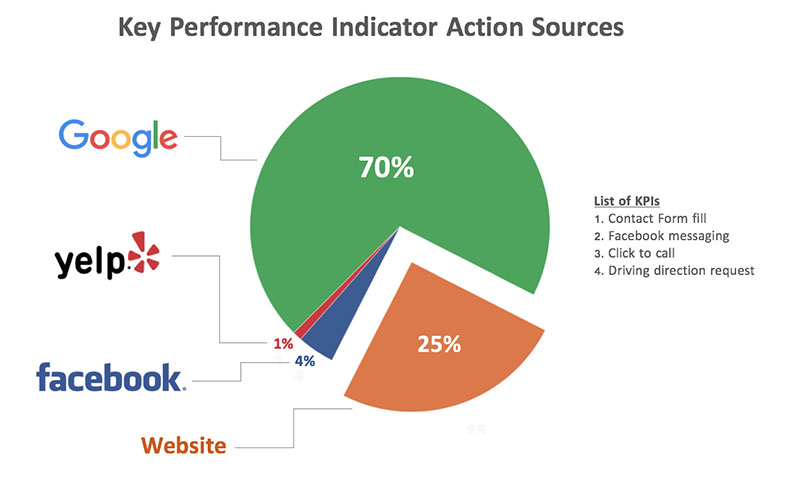
Clearly the largest percentage of these KPIs occurred directly on Google either from the Knowledge Panel, the Pack results, the Local Finder or Maps. Typically in Barbara’s case, 70% of those come from search and 30% from Maps.
The website came in second. We have only done this study for one quarter and have no historical reference point. But my sense is that Google has, over the past two years, generally increased their share of these actions with changes to the Local search results. Due to issues with the WordPress theme not all instances of the phone number were tracked although most were.
Thus the website might be slightly under counted. Any conversions that came directly via an iPhone/Apple Maps would not have surfaced nor would have calls directly from iOS “direct answer” results and it is possible that iOS was undercounted as a result.
I was a little surprised that we found zero Bing actions according to their dashboard and our analytics. But such is the world of search.
It’s A Google World In Local
When we further looked into the web site traffic and its origination sources Facebook showed about a 3% increase in contribution. But most of the web traffic that initiated one of the KPI actions obviously came from Google.
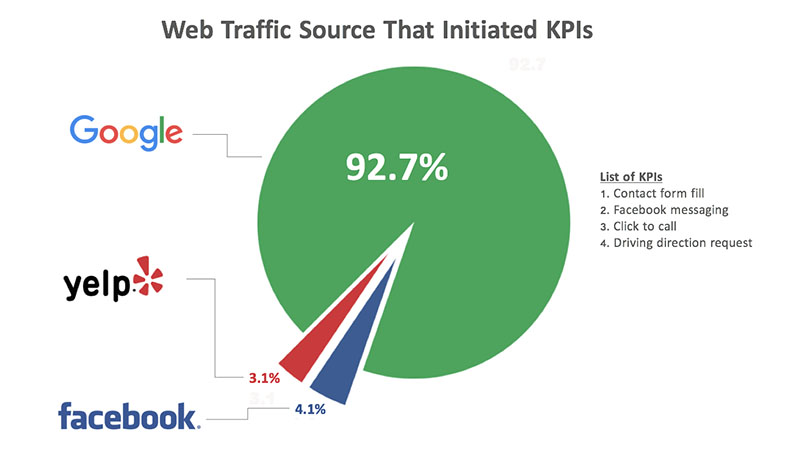
In a very real sense, Google has become the equivalent of the new home page for the business. The bulk of direct pre-sale actions that benefitted the business were taking place there and the bulk of those that weren’t (ie web actions) came from Google.
While I don’t have the quantitative data to back this up, my sense is that Google is taking an increasing share directly compared to 4, 3 or even 2 years ago with various “updates” to the Local pack results.
Clearly there are some blind spots in our analysis but I think that they are directionally correct. And while it is only a case study, the methodology can be applied more broadly. It would be of interest to make the same analysis across different industries and markets to understand the amount of variation that we would find.
On a philosophical level the fact that more activity is taking place directly on Google is a disturbing trend, I for one do not want a web that is controlled at one end by Google and at the other by Facebook. As to what a business should or could do to avoid this is unclear.
For now if you need leads, you need to be present on Google.
That being said any given business really doesn’t care where the new customers come from as long as they come. The local business needs to be aware of the fact that many (actually most) of their new customers are coming from Google and has in place a plan to really treat both keyword and branded search results as their primary interface to the world, then they should get their fair share of new customers.
In this new reality your website is just a data source, one amongst many, that feeds the Google results.
Read part 2 to take a look at the in-store attribution and how that correlated with these measured KPIs.
* The KPIs chosen for any given local business will vary depending on the type of business and their goals. Obviously an emergency plumber would be more interested in phone calls and driving directions would be totally irrelevant. In that case call tracking might provide very meaningful metrics. A dance studio might be more interested in form fills for class sign ups. A dentist that has scheduling on their site might more interested in actual conversions to an appointment.
There are a ton of possibilities of KPIs that have strong consumer intent to purchase. From my point of view the closer the KPI is to the purchase the better and since so much activity isn’t taking place on your website, ones that are measurable at sites besides your website make sense. A local business, unlike an e-commerce play, doesn’t really care if the activity is at Google, Yelp or their website and given the realities of the digital world has very little to say about it anyway.
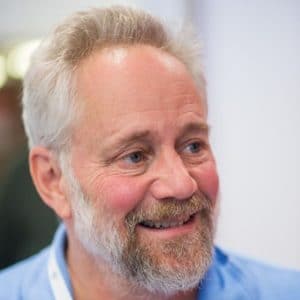
Mike Blumenthal
Comments are closed.
JOIN OUR NEWSLETTER
Sign-up to receive our monthly email newsletter packed with new features, tips, news and webinar invites.
BLOG CATEGORIES
Blog categories.
- Agencies (6)
- AI Reputation Management (1)
- Case Study (9)
- Company News (33)
- Customer Complaint Series (8)
- Customer Experience (49)
- Customer Sentiment (1)
- Customer Spotlight (5)
- Customer Success (2)
- Events & Presentations (4)
- Franchises (4)
- GatherUp Features & Updates (102)
- GatherUp Tips & Tricks (47)
- Local Search (50)
- Net Promoter Score (NPS) (10)
- Pro Tips (20)
- Release Notes (14)
- Reputation Management (127)
- SMS Marketing (13)
- Uncategorized (6)
- Webinars (60)
We'll help you build a better business
Our goal is to help you connect with your customers to gain valuable insight on what’s important to them. While you happy customers will help your marketing, your unhappy customers will point out where you can improve and our system will help you communicate with them to keep them with you.
Gather feedback and reviews to drive your business up
GatherUp will make customer experience the backbone of your business, building a continuous cycle of happy customers and powerful reviews to help you capture your next customer.
- Small Business
- Multi-location
- Digital Agencies
- Integrations
- Our Reviews
- Guide To NPS
- Quick Start Guide
Net Promoter, Net Promoter System, Net Promoter Score, NPS and the NPS-related emoticons are registered trademarks of Bain & Company, Inc., Fred Reichheld and Satmetrix Systems, Inc.
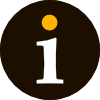
KPI Case Studies in Action – Success and Failure
Nov 6, 2023 | KPIs
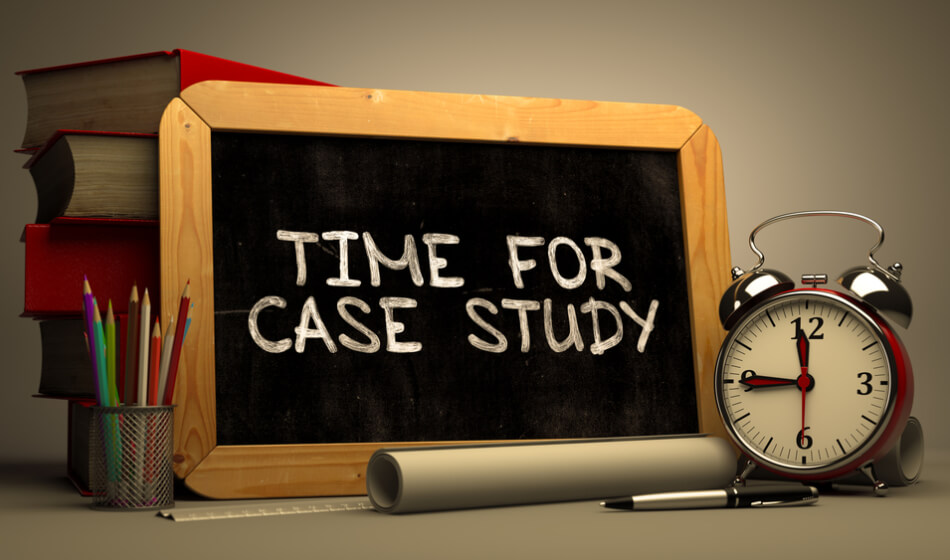
KPI Case Studies – Key Performance Indicators (KPIs) are more than mere numbers on a dashboard; they are vital signs that indicate the health and direction of a business. Like any powerful tool, the value they deliver hinges not just on their existence but on their thoughtful application. Through the lens of case studies, we gain a profound understanding of the dynamics that drive successful KPI implementation and the cautionary tales that remind us of potential pitfalls. In this exploration, we’ll delve into real-world scenarios where KPIs have acted as beacons leading to commercial triumph, as well as those instances where they’ve served as misleading signals, spiralling businesses into challenges. These stories are not just narratives; they are practical lessons carved out of the corporate frontlines, offering a blueprint for what to emulate and avoid. By dissecting these case studies, we equip ourselves with the wisdom to navigate the intricate world of performance metrics, ensuring that the KPIs we choose are not just numbers but actionable insights that steer us towards our intended strategic destinations. Join us on this journey as we unravel the tales of KPIs in action, where each success and failure brings us closer to mastering the art of performance measurement.
The Anatomy of a Successful KPI Strategy
A strong KPI strategy works like a compass. It guides a company towards its goals using well-chosen KPIs as its direction points. Each KPI should be SMART – this means it’s Specific, Measurable, Achievable, Relevant, and Time-bound. These KPIs show us clearly what success looks like.
But it’s not just about picking KPIs. Everyone in the company needs to understand and work with them. This involves regular meetings to discuss the KPIs and see if they still point the company in the right direction.
Also, a good KPI strategy is woven into the day-to-day work. It makes sure that the insights from KPIs lead to real changes and improvements. It’s transparent, meaning it’s clear who is responsible for what, and it’s easy to see how things are going.
And finally, a good strategy can change if needed. It can adapt when there’s new information, when the market changes, or when the company’s goals change. This way, the strategy stays useful and keeps guiding the company to success. The following KPI case studies will illustrate these points.
Case Study 1: A Triumph of KPI Alignment
Let’s talk about a company that got it right with KPIs. We’ll call them “TechGrow,” a tech company eager to expand its market share. They wanted to grow their customer base by 25% in one year. To do this, they needed clear KPIs.
TechGrow set up a KPI to track new sign-ups every month. This KPI was SMART: specific to their goal, measurable by numbers, achievable with their resources, relevant to their growth aim, and time-bound within the year.
They also kept an eye on customer feedback scores. This wasn’t just about getting more customers but keeping them happy, too. So, another KPI tracked the average support ticket resolution time.
Here’s what they did well:
- They ensured their whole team knew about the KPIs and how each person could help meet them.
- They had monthly check-ins to see how they were doing against their KPIs.
- When they saw one KPI wasn’t moving as expected, they were quick to figure out why and fix it.
By the end of the year, not only had TechGrow hit their customer growth target, but their customer satisfaction had also gone up. Their KPIs were the stars of the show, shining a light on where to go and what to fix along the way.
Case Study 2: Turning Data into Action
Meet “HealthFirst,” a healthcare provider who wanted to use KPIs to give better care and improve their services. Their main goal was to reduce patient waiting times by 15%. To track their progress, they chose a KPI that measured the average time patients spent in the waiting room.
HealthFirst used this KPI to see how they were doing each week. But they didn’t stop at just looking at the numbers. They used this data to make fundamental changes. For example, when they noticed waiting times were extended because of too few staff at peak times, they changed the staff schedules.
They also set up a KPI for patient follow-ups. They wanted to ensure patients were called for a check-up within a week after their visit. This helped them care for patients even after they left the clinic.
What HealthFirst did well was:
- They chose KPIs directly linked to their primary goal: better patient care.
- They checked their KPIs regularly and used what they learned to make decisions.
- They ensured everyone on their team understood the KPIs and knew how to help reach them.
By the end of their project, HealthFirst didn’t just meet their goal – they beat it. Patient waiting times were down by 20%, and their follow-ups were better than ever. Their story shows us that when you take action based on what KPIs tell you, you can make things better.
The Pitfalls of Mismanaged KPIs
KPIs are powerful, but like all powerful tools, they can cause problems if not used right. Imagine using a map with the wrong directions – it’s easy to get lost. It’s the same with KPIs. If they’re not managed well, they can lead a company in the wrong direction.
Here’s what can go wrong:
- Sometimes, companies pick too many KPIs. It’s like juggling too many balls ; they’re bound to drop one. It’s better to focus on a few that matter.
- Other times, the KPIs they choose don’t match what they’re trying to achieve. That’s like using a map of Paris when you’re trying to get around Tokyo.
- Companies might not check their KPIs often enough, or they might ignore what the KPIs tell them. Either way, it’s like having a warning light on your car dashboard and never fixing the problem.
In the following two sections, we’ll examine KPI case studies from companies that ran into these issues. They chose the wrong KPIs or didn’t use them well, and it caused trouble. But the good news is, there’s always a way back. These stories will show the mistakes to avoid and how to fix them if they happen.
Case Study 3: Lost in Translation – A KPI Misstep
Now, let’s talk about a company we’ll call “FashionForward,” a retail business that wanted to increase its sales. They decided to track the number of visitors to their website as their primary KPI, thinking that more visitors would mean more sales.
But here’s where they slipped up. They focused so much on increasing website traffic that they forgot about sales. Sure, the website got lots of visitors, but the number of people buying didn’t go up.
The problem was that they chose a KPI that wasn’t aligned with their ultimate goal – selling clothes. It’s like being excited that lots of people came to your party, but nobody ate the food you made.
FashionForward learned a few lessons:
- They learned that not any KPI will do. It has to be closely linked to the desired outcome.
- They realised it’s essential to check if your KPIs are working. If they’re not, you need to be ready to change them.
- They saw that everyone on the team needs to understand how their work helps to hit the KPIs and, in turn, reach the big goals.
Ultimately, FashionForward changed its KPI to track something more valuable: the conversion rate of visitors to buyers. This was a better way to see how well their website was selling. And with this new KPI, they started making changes that helped turn more visitors into customers.
Case Study 4: When KPIs Mislead – A Cautionary Tale
Let’s look at a company we’ll call “Build-It-Right,” a construction firm that wants to complete projects faster. They thought the best KPI to track their success would be the number of projects finished each month.
At first, this KPI made sense. But they didn’t think about the quality of the work. As they rushed to finish more projects, mistakes happened. And these mistakes led to redoing work, which cost time and money.
Here’s where Build-It-Right went wrong:
- They chose a KPI that only looked at speed, not at the work’s quality.
- They didn’t balance their KPIs. It’s like being fast in a race but forgetting to stay on track.
- They didn’t listen to their team, who were worried about rushing and making mistakes.
Build-It-Right realised that a better KPI would be the number of projects completed on time and with no mistakes. This new KPI helped them focus on doing the job well, not just quickly.
This story teaches us:
- It’s important to choose KPIs that match both the speed and quality of your work.
- It would help to have a balanced set of KPIs to ensure that you’re doing a good job overall.
- Always listen to your team. They often know best if a KPI takes things in the wrong direction.
With their new KPI, Build-It-Right started finishing projects with fewer mistakes and happier clients. They learned that the right KPI makes all the difference.
Lessons Learned and Recovery Paths
Looking at the stories of “FashionForward” and “Build-It-Right,” we can see that KPIs are more than just numbers—they’re signposts that guide businesses to their goals. But when these signposts point in the wrong direction, it’s time for a course correction.
Here’s what these companies KPI case studies revealed:
- Right KPI, Right Goal : KPIs must directly reflect your goals. If the goal is better sales, track sales conversions, not just website visitors.
- Balance is Key : Don’t focus on one KPI at the expense of others. Speedy project completion shouldn’t sacrifice quality.
- Flexibility : Be ready to adjust your KPIs if they’re not helping you reach your objectives. Being flexible means staying on the path to success, even if it’s not what you originally planned.
- Team Insights Matter : Your team’s feedback on KPIs can provide early warning signs of potential issues. Listen to them.
When KPIs misled these companies, they took steps to recover:
- They audited their KPIs to see which ones truly aligned with their goals.
- They started small, testing new KPIs on a few projects before rolling them out company-wide.
- They trained their teams on the importance of KPIs and how to use them effectively.
- They established regular review sessions to discuss KPI progress and any needed changes.
Both “FashionForward” and “Build-It-Right” came out stronger. They had a deeper understanding of how to set the right KPIs and pivot when necessary. And most importantly, they learned to value the journey of improvement as much as the destination of success.
In these recoveries, we find a universal lesson: KPIs are not set in stone. They’re a live part of your business strategy, evolving as you learn and grow. When you’re ready to adapt and realign, your KPIs become empowerment tools, driving you toward better performance and more remarkable achievements.
Integrating the Lessons into Your KPI Framework
The journey through these KPI case studies highlights a universal truth in business strategy: the power of Key Performance Indicators is not just in their ability to track progress but in their capacity to steer a company towards its desired destination. As we’ve seen, KPIs are most effective when they’re thoughtfully aligned with strategic goals, balanced across multiple dimensions of performance, flexible to shifts in the business landscape, and supported by the insights of a dedicated team.
Integrating these lessons into your KPI framework involves a commitment to continuous learning and adaptation. Whether you’re a budding startup or an established enterprise, the principles of effective KPI management remain the same: clarity of purpose, simplicity of measures, and responsiveness to feedback.
Now, it’s your turn to put these insights into action. With Spider Impact KPI Software , you can craft a KPI framework that not only monitors progress but also empowers decision-making. Spider Impact helps you visualise your performance, identify areas of improvement, and make data-driven decisions with confidence.
Don’t let your business navigate in the dark. Illuminate your path to success with KPIs that reflect your true objectives and a tool that brings your data to life. Visit us at Intrafocus to learn how Spider Impact KPI Software can transform your data into actionable insights and drive your business forward.
Recent Posts
- Leadership Styles – What Type of Leader Are You? March 21, 2024
- I used AI to write an eBook – and this article about the experience March 14, 2024
- My5 KPIs – Focus on Less and Transform Your Business February 23, 2024
- Trends and Predictions for the Future of KPI Management January 23, 2024
- Integrating Consultancy Insights with KPI Software December 13, 2023
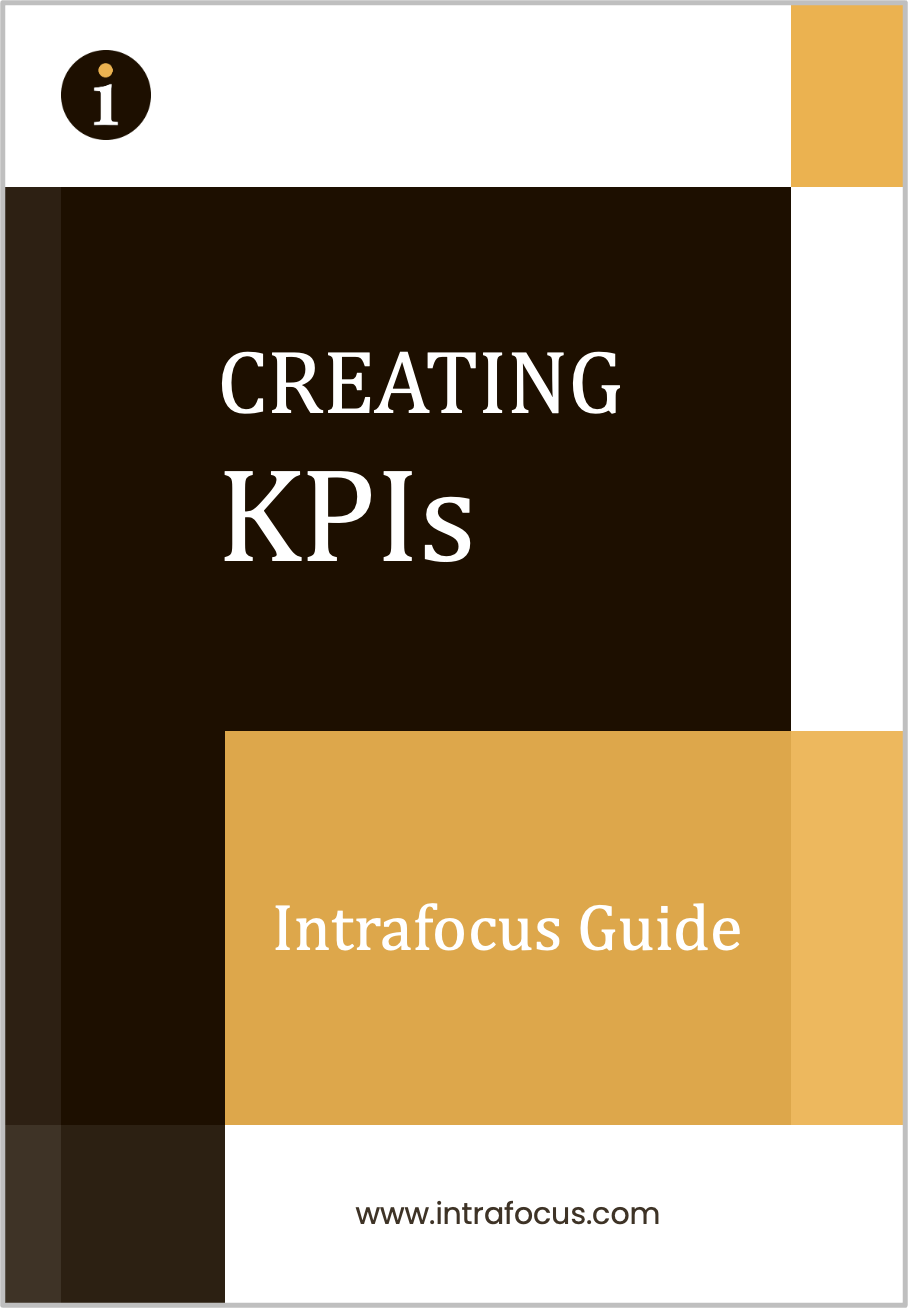
Download our guide to creating meaningful KPIs
Privacy Overview
- Search Search Please fill out this field.
- Key Performance Indicators (KPIs)
- Understanding KPIs
How to Create a KPI Report
- Limitations
The Bottom Line
- Corporate Finance
- Financial Ratios
KPIs: What Are Key Performance Indicators? Types and Examples
:max_bytes(150000):strip_icc():format(webp)/dd453b82d4ef4ce8aac2e858ed00a114__alexandra_twin-5bfc262b46e0fb0026006b77.jpeg)
What Are Key Performance Indicators (KPIs)?
Key performance indicators (KPIs) are quantifiable measurements used to gauge a company’s overall long-term performance. KPIs specifically help determine a company’s strategic, financial, and operational achievements, especially compared to those of other businesses within the same sector.
Key Takeaways
- Key performance indicators (KPIs) measure a company’s success vs. a set of targets, objectives, or industry peers.
- KPIs can be financial, including net profit (or the bottom line, net income), revenues minus certain expenses, or the current ratio (liquidity and cash availability).
- Customer-focused KPIs generally center on per-customer efficiency, customer satisfaction, and customer retention.
- Process-focused KPIs aim to measure and monitor operational performance across the organization.
- Businesses generally measure and track KPIs through analytics software and reporting tools.
Jiaqi Zhou / Investopedia
Understanding Key Performance Indicators (KPIs)
Also referred to as key success indicators (KSIs), KPIs vary between companies and between industries, depending on performance criteria. For example, a software company striving to attain the fastest growth in its industry may consider year-over-year (YOY) revenue growth as its chief performance indicator. Conversely, a retail chain might place more value on same-store sales as the best KPI metric for gauging growth.
At the heart of KPIs lie data collection, storage, cleaning, and synthesizing. The information may be financial or nonfinancial and may relate to any department across the company. The goal of KPIs is to communicate results succinctly to allow management to make more informed strategic decisions.
Key performance indicators (KPIs) gauge a company’s output against a set of targets, objectives, or industry peers.
Categories of KPIs
Most KPIs fall into four different categories, with each category having its own characteristics, time frame, and users.
- Strategic KPIs are usually the most high-level. These types of KPIs may indicate how a company is doing, although it doesn’t provide much information beyond a very high-level snapshot. Executives are most likely to use strategic KPIs, and examples of strategic KPIs include return on investment , profit margin , and total company revenue .
- Operational KPIs are focused on a much tighter time frame. These KPIs measure how a company is doing month over month (or even day over day) by analyzing different processes, segments, or geographical locations. These operational KPIs are often used by managing staff and to analyze questions that are derived from analyzing strategic KPIs. For example, if an executive notices that company-wide revenue has decreased, they may investigate which product lines are struggling.
- Functional KPIs hone in on specific departments or functions within a company. For example, the finance department may keep track of how many new vendors they register within their accounting information system each month, while the marketing department measures how many clicks each email distribution receives. These types of KPIs may be strategic or operational but provide the greatest value to one specific set of users.
- Leading/lagging KPIs describe the nature of the data being analyzed and whether it is signaling something to come or something that has already occurred. Consider two different KPIs: the number of overtime hours worked and the profit margin for a flagship product. The number of overtime hours worked may be a leading KPI should the company begin to notice poorer manufacturing quality. Alternatively, profit margins are a result of operations and are considered a lagging indicator.
Types of KPIs
Financial metrics and kpis.
Key performance indicators tied to the financials typically focus on revenue and profit margins. Net profit, the most tried and true of profit-based measurements, represents the amount of revenue that remains, as profit for a given period, after accounting for all of the company’s expenses, taxes, and interest payments for the same period.
Financial metrics may be drawn from a company’s financial statements. However, internal management may find it more useful to analyze different numbers that are more specific to analyzing the problems or aspects of the company that management wants to analyze. For example, a company may leverage variable costing to recalculate certain account balances for internal analysis only.
Examples of financial KPIs include:
- Liquidity ratios (i.e., current ratios , which divide current assets by current liabilities): These types of KPIs measure how well a company will manage short-term debt obligations based on the short-term assets it has on hand.
- Profitability ratios (i.e., net profit margin): These types of KPIs measure how well a company is performing in generating sales while keeping expenses low.
- Solvency ratios (i.e., total debt-to-total-assets ratio ): These types of KPIs measure the long-term financial health of a company by evaluating how well a company will be able to pay long-term debt.
- Turnover ratios (i.e., inventory turnover): These types of KPIs measure how quickly a company can perform a certain task. For example, inventory turnover measures how quickly a company can convert an item from inventory to a sale. Companies strive to increase turnover to generate faster churn of spending cash to later recover that cash through revenue.
Customer Experience Metrics and KPI
Customer -focused KPIs generally center on per-customer efficiency, customer satisfaction, and customer retention. These metrics are used by customer service teams to better understand the service that customers have been receiving.
Examples of customer-centric metrics include:
- Number of new ticket requests : This KPI counts customer service requests and measures how many new and open issues customers are having.
- Number of resolved tickets : This KPI counts the number of requests that have been successfully taken care of . By comparing the number of requests to the number of resolutions, a company can assess its success rate in getting through customer requests.
- Average resolution time : This KPI is the average amount of time needed to help a customer with an issue. Companies may choose to segment average resolution time across different requests (i.e., technical issue requests vs. new account requests).
- Average response time : This KPI is the average amount of time needed for a customer service agent to first connect with a customer after the customer has submitted a request. Though the initial agent may not have the knowledge or expertise to provide a solution, a company may value decreasing the time that a customer is waiting for any help.
- Top customer service agent : This KPI is a combination of any metric above cross-referenced by customer service representatives. For example, in addition to analyzing company-wide average response time, a company can determine the three fastest and slowest responders.
- Type of request : This KPI is a count of the different types of requests. This KPI can help a company better understand the problems a customer may have (i.e., the company’s website gave incorrect or inaccurate directions) that need to be resolved by the company.
- Customer satisfaction rating : This KPI is a vague measurement, though companies may perform surveys or post-interaction questionnaires to gather additional information on the customer’s experience.
KPIs are usually not externally required; they are simply internal measurements used by management to evaluate a company’s performance.
Process Performance Metrics and KPI
Process metrics aim to measure and monitor operational performance across the organization. These KPIs analyze how tasks are performed and whether there are process, quality, or performance issues. These types of metrics are most useful for companies with repetitive processes, such as manufacturing firms or companies in cyclical industries.
Examples of process performance metrics include:
- Production efficiency : This KPI is often measured as the production time for each stage divided by the total processing time. A company may strive to spend only 2% of its time soliciting raw materials; if it discovers it takes 5% of the total process, then the company may strive for solicitation improvements.
- Total cycle time : This KPI is the total amount of time needed to complete a process from start to finish. This may be converted to average cycle time if management wishes to analyze a process over a period of time.
- Throughput : This KPI is the number of units produced divided by the production time per unit, measuring how fast the manufacturing process is.
- Error rate : This KPI is the total number of errors divided by the total number of units produced. A company striving to reduce waste can better understand the number of items that are failing quality control testing.
- Quality rate : This KPI focuses on the positive items produced instead of the negative. By dividing the successful units completed by the total number of units produced, this percentage informs management of its success rate in meeting quality standards.
Marketing KPIs
Marketing KPIs attempt to gain a better understanding of how effective marketing and promotional campaigns have been. These metrics often measure conversation rates on how often prospective customers perform certain actions in response to a given marketing medium. Examples of marketing KPIs include:
- Website traffic : This KPI tracks the number of people who visit certain pages of a company’s website. Management can use this KPI to better understand whether online traffic is being pushed down potential sales channels and if customers are not being funneled appropriately.
- Social media traffic : This KPI tracks the views, follows, likes, retweets, shares, engagement, and other measurable interactions between customers and the company’s social media profiles.
- Conversion rate on call-to-action content : This KPI centers around focused promotional programs that ask customers to perform certain actions. For example, a specific campaign may encourage customers to act before a certain sale date ends. A company can divide the number of successful engagements by the total number of content distributions to understand what percent of customers answered the call to action.
- Blog articles published per month : This KPI simply counts the number of blog posts a company publishes in a given month.
- Click-through rates : This KPI measures the number of specific clicks that are performed on email distributions. For example, certain programs may track how many customers opened an email distribution, clicked on a link, and followed through with a sale.
A company may desire operational excellence; in this case, it may want to track how its internal technology (IT) department is operating. These KPIs may encourage a better understanding of employee satisfaction or whether the IT department is being adequately staffed. Examples of IT KPIs include:
- Total system downtime : This KPI measures the amount of time that various systems must be taken offline for system updates or repairs. While systems are down, customers may be unable to place orders or employees may be unable to perform certain duties (i.e., when the accounting information system is down).
- Number of tickets/resolutions : This KPI is similar to customer service KPIs. However, these tickets and resolutions relate to internal staff requests such as hardware or software needs, network problems, or other internal technology problems.
- Number of developed features : This KPI measures internal product development by quantifying the number of product changes.
- Count of critical bugs : This KPI counts the number of critical problems within systems or programs. A company will need to have its own internal standards for what constitutes a minor vs. major bug.
- Back-up frequency : This KPI counts how often critical data is duplicated and stored in a safe location. In accordance with record retention requirements, management may set different targets for different bits of information.
The ultimate goal of a company is to generate revenue through sales. Though revenue is often measured through financial KPIs, sales KPIs take a more granular approach by leveraging nonfinancial data to better understand the sales process. Examples of sales KPIs include:
- Customer lifetime value (CLV) : This KPI represents the total amount of money that a customer is expected to spend on your products over the entire business relationship.
- Customer acquisition cost (CAC) : This KPI represents the total sales and marketing cost required to land a new customer. By comparing CAC to CLV, businesses can measure the effectiveness of their customer acquisition efforts.
- Average dollar value for new contracts : This KPI measures the average size of new agreements. A company may have a desired threshold for landing larger or smaller customers.
- Average conversion time : This KPI measures the amount of time from first contacting a prospective client to securing a signed contract to perform business.
- Number of engaged leads : This KPI counts how many potential leads have been contacted or met with. This metric can be further divided into mediums such as visits, emails, phone calls, or other contacts with customers.
Management may tie bonuses to KPIs. For salespeople, their commission rate may depend on whether they meet expected conversion rates or engage in an appropriate number of leads.
Human Resource and Staffing KPIs
Companies may also find it beneficial to analyze KPIs specific to their employees. Ranging from turnover to retention to satisfaction, a company may have a wealth of information already available about its staff. Examples of human resource or staffing KPIs include:
- Absenteeism rate : This KPI is a count of how many dates per year or specific period employees are calling in sick or missing shifts. This KPI may be a leading indicator for disengaged or unhappy employees.
- Number of overtime hours worked : This KPI tracks the number of overtime hours worked to gauge whether employees are potentially facing burnout or if staffing levels are appropriate.
- Employee satisfaction : This KPI often requires a company-wide survey to gauge how employees are feeling about various aspects of the company. To get the best value from this KPI, companies should consider hosting the same survey every year to track changes from one year to the next regarding the exact same questions.
- Employee turnover rate : This KPI measures how often and quickly employees are leaving their positions. Companies can further break down this KPI across departments or teams to determine why some positions may be leaving faster than others.
- Number of applicants : This KPI keeps count of how many applications are submitted to open job positions. This KPI helps assess whether job listings are adequately reaching a wide enough audience to capture interest and lure strong candidates.
Examples of KPIs
Let’s take a look at electric vehicle maker Tesla ( TSLA ) for a few examples of KPIs in real life. These numbers are from its fourth quarter (Q4) 2021 earnings release.
Vehicle Production
During the quarter, Tesla produced a record 305,840 vehicles and delivered 308,650 vehicles. Production is a big deal for the company because it has consistently been criticized for being bad at ramping up. Increased manufacturing scale means more market share and profits for Tesla.
Automotive Gross Margin
For the quarter, Tesla’s automotive gross margin expanded to 30.6%. Gross margin is one of the best measures of profitability for Tesla because it isolates its vehicle production costs. Tesla managed to expand its gross margin in Q4 even as sales of lower-priced models outpaced its higher-margin models.
Free Cash Flow
Tesla’s free cash flow clocked in at $2.8 billion during the quarter. That represented a vast improvement from the $1.9 billion free cash flow in the prior year. Tesla’s level of free cash flow production suggested that the company was reaching a scale of profitability without the help of regulatory credits.
Companies can use KPIs across three broad levels:
First, company-wide KPIs focus on the overall business health and performance. These types of KPIs are useful for informing management of how things are going. However, they are often not granular enough to make decisions. Company-wide KPIs often kick off conversations on why certain departments are performing well or poorly.
At this point, companies often begin digging into department-level KPIs. These are more specific than company-wide KPIs. Department-level KPIs are often more informative as to why specific outcomes are occurring. Many of the examples mentioned above are department-level KPIs, as they focus on a very niche aspect of a company.
If a company chooses to dig even deeper, it may engage with project-level or subdepartment-level KPIs. These KPIs are often specifically requested by management as they may require very specific data sets that may not be readily available. For example, management may want to ask very specific questions to a control group about a potential product rollout .
When preparing KPI reports, start by showing the highest level of data (i.e., company-wide revenue). Next, be prepared to show lower levels of data (i.e., revenue by department, then revenue by department and product).
With companies seemingly collecting more data every day, it can become overwhelming to sort through the information and determine what KPIs are most useful and impactful for decision-making. When beginning the process of pulling together KPI dashboards or reports, consider the following steps:
- Discuss goals and intentions with business partners . KPIs are only as useful as the users make them. Before pulling together any KPI reports, understand what you or your business partner are attempting to achieve.
- Draft SMART KPI requirements . KPIs should have restrictions and be tied to SMART (specific, measurable, attainable, realistic, and time-bound) metrics. Vague, hard-to-ascertain, and unrealistic KPIs serve little to no value. Instead, focus on what information you have that is available and meeting the SMART acronym requirements.
- Be adaptable . As you pull together KPI reports, be prepared for new business problems to appear and for further attention to be given to other areas. As business and customer needs change, KPIs should also adapt with certain numbers, metrics, and goals changing in line with operational evolutions.
- Avoid overwhelming users . It may be tempting to overload report users with as many KPIs as you can fit on a report. At a certain point, KPIs start to become difficult to comprehend, and it may become more difficult to determine which metrics are important to focus on.
Advantages of KPIs
A company may wish to analyze KPIs for several reasons. KPIs help inform management of specific problems; the data-driven approach provides quantifiable information useful in strategic planning and ensuring operational excellence.
KPIs help hold employees accountable. Instead of relying on feelings or emotions, KPIs are statistically supported and cannot discriminate across employees. When used appropriately, KPIs may help encourage employees as they realize their numbers are being closely monitored.
KPIs are also the bridge that connects actual business operations and goals. A company may set targets, but without the ability to track progress toward those goals, there is little to no purpose in those plans. Instead, KPIs allow companies to set objectives, and then monitor progress toward those objectives.
Limitations of KPIs
There are some downsides to consider when working with KPIs. There may be a long time frame required for KPIs to provide meaningful data. For example, a company may need to collect annual data from employees for years to better understand trends in satisfaction rates over long periods of time.
KPIs require constant monitoring and close follow-up to be useful. A KPI report that is prepared but never analyzed serves no purpose. In addition, KPIs that are not continuously monitored for accuracy and reasonableness do not encourage beneficial decision making.
KPIs open up the possibility for managers to “game” KPIs. Instead of focusing on actually improving processes or results, managers may feel incentivized to focus on improving KPIs tied to performance bonuses . In addition, quality may decrease if managers are hyper-focused on productivity KPIs, and employees may feel pushed too hard to meet specific KPI measurements that may simply not be reasonable.
Informs management of how a company is performing in countless ways
Helps hold employees accountable for their actions (or lack of)
Can motivate employees who feel positively challenged to meet targets
Allows a company to set goals and measure progress toward those objectives
Results in potential time commitment to consistently gather data over long periods of time
Requires ongoing monitoring for accuracy and reasonableness in data
May encourage managers to focus on KPIs instead of broader strategies
May discourage employees if KPI targets are unreasonable
What Does KPI Mean?
KPI is an abbreviation for key performance indicator: data that has been collected, analyzed, and summarized to help decision-making. KPIs may be a single calculation or value that summarizes a period of activity, such as “450 sales in October.” By themselves, KPIs do not add any value to a company. However, a company can use this information to make more informed decisions about business operations and strategies.
What Is an example of a KPI?
One of the most basic examples of a KPI is revenue per client (RPC). For example, if you generate $100,000 in revenue annually and have 100 clients, then your RPC is $1,000. A company can use this KPI to track its RPC over time.
What Are 5 of the Most Common KPIs?
KPIs vary from business to business, and some KPIs are more suitable for certain companies compared to others. In general, five of the most commonly used KPIs are:
- Revenue growth
- Revenue per client
- Profit margin
- Client retention rate
- Customer satisfaction
How Do You Measure KPIs?
It depends on the actual KPI being measured. Generally speaking, businesses measure and track KPIs through business analytics software and reporting tools. This includes everything from the collection of data via reliable sources, the safe storage of information, the cleaning of data to standardize its format for analysis, and the actual number crunching. Finally, KPIs are often reported using visualization or reporting software.
What Makes a KPI Good?
A good KPI provides objective and clear information on progress toward an end goal. It tracks and measures factors such as efficiency, quality, timeliness, and performance while providing a way to measure performance over time. The ultimate goal of a KPI is to help management make more informed decisions.
KPIs offer an effective way to measure and track a company’s performance on a variety of different metrics. By understanding exactly what KPIs are and how to implement them properly, managers are better able to optimize the business for long-term success.
Tesla Investor Relations. “ Q4 and FY 2021 Update ,” Page 7.
Tesla Investor Relations. “ Q4 and FY 2021 Update ,” Page 4.
Tesla Investor Relations. “ Q4 and FY 2021 Update ,” Page 5.
:max_bytes(150000):strip_icc():format(webp)/Term-Definitions_Digital-Marketing-5ae6ea1aee934b02a94a1a4d9401443d.jpg)
- Terms of Service
- Editorial Policy
- Privacy Policy
- Your Privacy Choices
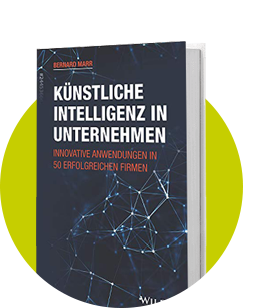
Künstliche Intelligenz in Unternehmen: Innovative Anwendungen in 50 erfolgreichen Firmen
Der Bestsellerautor und Geschäfts renommierter KI-Experte Bernard zeigt, wie sterben Technologie des maschinellen Lernens das von Unternehmen verändert. Das Buch bietet einen Überblick über einzelne Unternehmen, beschreibt das spezifische Problem und erklärt, wie KI die Lösung erleichtert. Jede Fallstudie bietet einen umfassenden Einblick, der einige technische Details wichtige Lernzusammenfassungen enthält. Marrs Buch ist eine aufschlussreiche und informative Untersuchung der transformativen Kraft der Technologie in der Wirtschaft des 21. Jahrhunderts.
KPI Case Studies + Videos & Slide Share
Kpis & metrics case studies.

Tesco: Measuring Customer Performance & Gaining Insights From The Clubcard Data
Tesco PLC British-based international grocery and general merchandising retail group.The company is the third largest retailer in the world measured by profits, has over 6,500 stores and employs more than 475,000 people…

Essex Police: Fewer But More Meaningful Performance Indicators
Essex Police is one of the United Kingdom’s largest non-metropolitan police forcesresponsible for policing the county of Essex, in the east of England…
HMRC: Making Performance Management Work
Her Majesty’s Revenue and Customs, or HMRC for short, is a non-ministerial department of the UK Government responsible for the collection of taxes, the payment of some forms of state support, and the administration of other regulatory regimes including the national minimum wage…
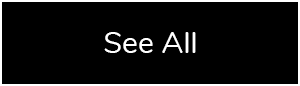
Share This Story, Choose Your Platform!
About the author: bernard marr.
Related Posts
Supplier performance score.
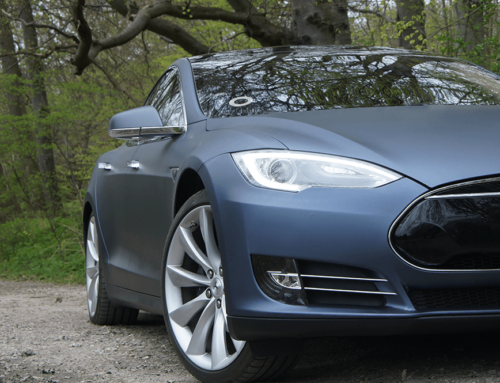
The Amazing Ways Tesla Is Using Artificial Intelligence And Big Data
Incident resolution index, carbon footprint, leave a comment cancel reply.
Save my name, email, and website in this browser for the next time I comment.
Advertisement
Machine learning for KPIs prediction: a case study of the overall equipment effectiveness within the automotive industry
- Methodologies and Application
- Published: 05 October 2020
- Volume 25 , pages 2891–2909, ( 2021 )
Cite this article
- Choumicha EL Mazgualdi 1 ,
- Tawfik Masrour ORCID: orcid.org/0000-0002-7761-6300 1 ,
- Ibtissam El Hassani 1 &
- Abdelmoula Khdoudi 1
1349 Accesses
14 Citations
Explore all metrics
Key performance indicators are tools for management, decision support and forecasting; they reflect the strategy and vision of the company in terms of objectives and allow to always staying in step with the expectations of the stakeholders. Accurate forecasting of the indicators allows decisions to be reoriented to ensure performance optimization while reducing both cost and effort. This paper aims to apply different machine learning methods, namely support vector regression, optimized support vector regression (using genetic algorithm), random forest, extreme gradient boosting and deep learning to predict the overall equipment effectiveness as a case study. We will make use of several configurations of the listed models in order to provide a wide field of comparison. The data used to train our models were provided by an automotive cable production industry. The result shows that the configuration in which we used cross-validation technique, and we performed a duly splitting of data, provides predictor models with the better performances.
Similar content being viewed by others
Using Machine Learning for Predicting Efficiency in Manufacturing Industry
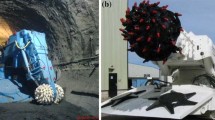
Performance prediction of roadheaders using ensemble machine learning techniques
Sadi Evren Seker & Ibrahim Ocak
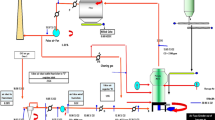
Development of a kiln petcoke mill predictive model based on a multi-regression XGBoost algorithm
Mohammed Toum Benchekroun, Smail Zaki, … Fatoumata Diassana
Avoid common mistakes on your manuscript.
1 Introduction
1.1 key performance indicators (kpi).
Today, dashboards have become an indispensable tool. It must provide managers, from operational management to top management, with the information they need in order to make decisions. It consists of a set of indicators designed to allow managers to see the progress of their systems, and it reflects the company’s strategy and vision in terms of objectives. So, it allows to follow both the targeted results and the actions, both corrective and preventive ones that achieve the objectives set. Key performance indicators are most often the result of a long chain of information gathering and aggregation, and they generally allow responsiveness and decisions are made more and more quickly.
But that is not enough in the current context. Today, in order to maintain or gain competitive advantage, organizations place the search for “proactivity” at the forefront of their concerns: the precise forecasts of the indicators make possible to redirect decisions in order to guarantee an optimization of the performances, and reducing costs and efforts. Nowadays, in the digitalization age, information systems are managing more and more data, making them useful for predicting KPI’s thanks to advances in artificial intelligence algorithms, notably machine learning. This new generation of intelligent systems of prediction is precisely the engines of the proactivity, as computing power has become cheaper and more available.
1.2 Overall equipment effectiveness (OEE)
The overall equipment effectiveness (OEE) is a performance measurement metric widely used. Its calculation provides to the managers the possibility to identify the main losses that reduce the machine effectiveness and then take the necessary decisions in order to improve the situation. Introduced by Nakajima ( 1982 ), OEE was a component of the total productive manufacturing (TPM) concept. According to Shirose and Goto ( 1989 ), the losses can be divided into six categories “six big losses”. Figure 1 shows these losses and some given examples of each one of them.
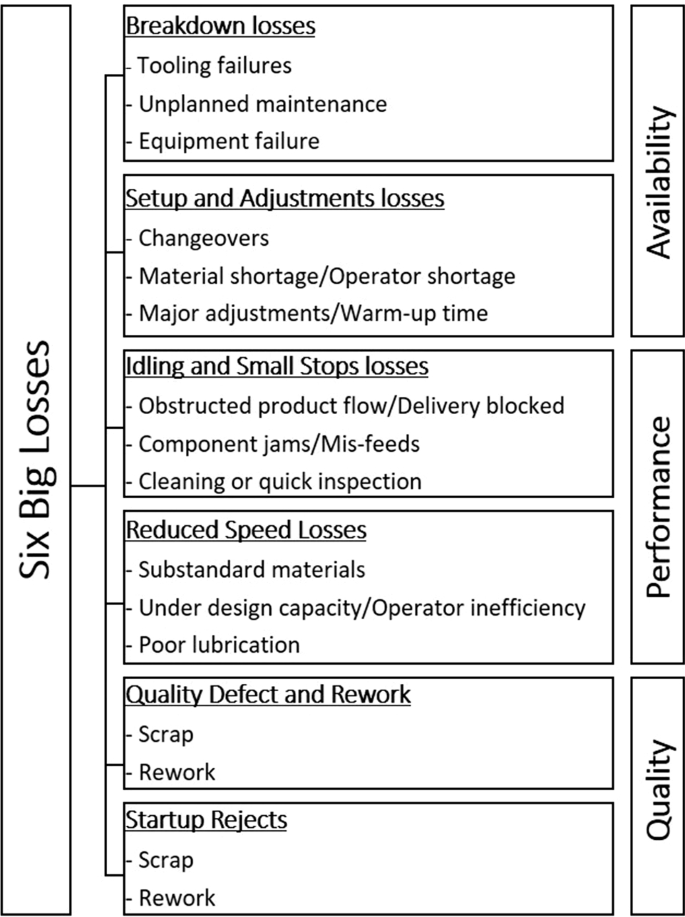
Illustration of the six big losses
One of the simplest ways to evaluate the overall equipment effectiveness (OEE), as announced by Nakajima ( 1982 ) and Groote ( 1995 ), is to apply the following formula:
where A is the availability rate, P is the performance, and Q is the quality rate.
The calculation of OEE provides to the managers the possibility to monitor their process and identify the main losses that reduce the machine effectiveness (Muchiri and Pintelon, 2008 )). However, this calculation is often done by the end of the production day and then it does not offer the possibility to act properly for improving the situation. The aim of this paper is to develop a machine learning-based model, which relays on historic process measurements, able to predict in advance the estimate OEE value. The predicted OEE value will allow for managers the possibility of evaluating the effectiveness of the equipment and, therefore, examining the inputs to the production process in order to identify and eliminate the relative losses. This will form a decision support tool designed in the purpose of helping managers to prevent different kinds of losses and then act and react according to the given situation to improve and maximize the production effectiveness. In this research, four machine learning algorithms are used, which are support vector machine, random forest, extreme gradient boosting and deep neural networks. For the benchmark purposes, we implemented our techniques under several configurations. This helps us to gain a deeper understanding of the different models performances. We, first, implement the four machine learning techniques using a simple data split. Secondly, in order to deal with the problem of over-fitting, we implemented the same methods using K-fold cross-validation technique. For these two configurations, we just kept a test set of 40 items from the whole data set which has 1917 observations.
The two previous configurations are, then, implemented another time using a holdout data of 288 items for the test purpose, and this is equivalent to 15% of the data. This percentage is more relevant than the first one (40 items equivalent to only 2%), and this will help us to come up with intuitive and insightful performance comparisons since it is used for assessment of the generalization error of the final chosen model.
The rest of this paper is organized as follows. The basic process monitoring and KPIs prediction approaches are reviewed in Sect. 2 . Section 3 introduces the theoretical background of machine learning algorithms, especially, the prediction methods employed in this study. Then, the proposed predictive models and their architectures will be presented in Sect. 4 . In Sect. 5 , the case study of the wiring harness automotive manufacturing will be introduced. The experimental results of the various configurations used in this study will be discussed in Sect. 6 . Section 7 provides some statistical tests, for the comparison purpose, on the findings of the research. The conclusions and further work are given in the last section.
2 Literature review
Aiming to operate the processes efficiently, especially, with complicated industrial systems, reliable KPIs prediction gained wide attention in recent years. Mathematical modeling or model-based methods offer the possibility to have a wise and deeper understanding of the interdependence between variables of the process (Gao et al. 2013 ). However, the increasing complexity and nonlinearity of industrial processes yield in not feasible development of a first-principle model. In such cases, data-driven techniques, which relay on historic process measurements without exact system models, have been reported as an attractive alternative with a huge ability to deal with complex behavior.
During the past several decades, some authors (White 2003 ; Grottoli 2008 ) described the importance of some detailed mathematical models to plan both future production and programmed maintenance in a more accurate way. However, those approaches are still conceived as steady-state tools, which are usually incapable to follow the process dynamics, especially on the long-term scale (Manenti 2009 ). Many other studies try to extend the use of model-based methods, exploring more complex mathematical modeling, to achieve a better results, especially for process monitoring (Ding 2008 ; Dong et al. 2012 ; Du et al. 2012 ; Gao et al. 2008 ; Yu et al. 2013 ; White 2003 ; Kim and Joo 2005 ).
Manenti ( 2009 ) treated the problem of KPI-related prediction, and he illustrated how the process performance monitoring is fast moving from reacting toward predicting methodologies. In his work, he gives a brief overview of mathematical methods to solve the data reconciliation problem and proposes a novel promising technique based on unsteady detailed models to tackle this issue. Solomon and Litoiu ( 2011 ) investigated a new approach for automatic building of a dynamic predictive model to support more efficient business process optimization decisions. The prediction model correlates the KPIs and the process variables through a simulation model. Input variables in the simulation model are estimated and then forecasted using different prediction models. Then the forecasted inputs are used by the simulator to calculate the forecasted KPIs.
Compared with model-based methods, data-driven techniques have less requirement of a priori physical and mathematical knowledge about the process. Since the model-based methods often fail to work due to difficulty in describing unexpected behaviors, data-driven techniques have become an active area.
Partial least squares (PLS) are one of the most popular data-driven method widely used for KPIs prediction in the industrial context. Due to its simplicity and easy implementation in large-scale industrial process, this approach is gained the attention of many authors and become a dominant data-driven tool for complex industrial process with superior ability in handling and processing large amount of data. The basic idea behind the PLS is to identify the correlation model between the measurable variables and the KPI, and based on it, to predict KPI using the online measured process data.
The applications of PLS in KPIs prediction and fault detection have been reported in many existing literature (Yin and Wang 2013 ; Yin 2012 ). However, the efficiency of this approach is highly affected by outliers and missing values. Many authors treated this issue by developing robust and advanced PLS methods.
Yin et al. ( 2014 ) presented a KPI prognosis approach for nonlinear industrial applications. The proposed approach is presented as an improved incremental learning based on the integration of LWPR and improved PLS-based prognosis scheme. A similar work was performed by Xie et al. ( 2015 ), and they proposed a KPI-related approach, named advanced partial least squares (APLS), which first integrates the improved PLS (IPLS) with the modified partial robust M-regression (MPRM) algorithm, reducing the weight of outliers by the selection of appropriate weighting scheme with small computation load. However, the industrial applications especially KPI-related predictions are generally dynamic processes and might operate under different operating conditions in the industrial environment. For these cases, PLS approach could not be successfully applied, due to its basic assumption under stationary ideal operating conditions. In this sense, Zhang et al. ( 2017 ) proposed a framework for KPI-based process monitoring that can be applied to large-scale industrial processes. The new framework consists of two related methods: a static method and a dynamic method. The static method, which is based on the least squares regression, was developed for the case that the KPI variables are statically related to the process variables. In case that the KPI variables are dynamically related to the process variables, he proposed a dynamic method by using the kernel representation of each sub-process; then, an instrument variable is used to reduce the dynamic case to the static case.
Nevertheless, sometimes, an off-line KPI predictions are required to achieve better performance for the underlying processes. The off-line prediction contributes to a more reliable scheduling of programmed maintenance that allows satisfying completely the market demand without compromising process unit life cycle.
Machine learning-based techniques provide a large field to perform the necessary data preparation and processing so that achieving better performance and accuracy of the proposed models. Based on several input variables and explicit knowledge of the process rather than only time (as feature for PLS for example), machine learning methods are able to extract the necessary process information directly from the huge amount of the recorder data. This information is then adjusted or strengthened leading the model to gain more performance.
Yet, few articles investigated the use of machine learning methods for reliability prediction. Kuo and Lin ( 2010 ) proposed four predictive models combined artificial neural networks (ANN) and decision trees for predicting the machine’s reliability. They experimented those models on a group of washing machines from a TFT-LCD manufacturing company. The results show that the combination of neural networks and decision trees based on the previous eight AE values has the best performance in predicting the reliability of TFT-LCD washing machines. das Chagas Moura et al. ( 2011 ) presented a comparative analysis in order to evaluate the SVM effectiveness in forecasting time-to-failure and reliability of engineered components based on time series data. Then, the SVR outcomes were compared with the results of other powerful data-driven empirical methods such as IIR-LRNN, RBF, the traditional MLP model and the Box–Jenkins ARIMA. The obtained SVR results outperformed or were comparable to the ones provided by these other learning machines.
Our contribution is to apply several machine learning techniques under different configurations for predicting one of the most used KPIs in the industrial processes, which is the overall equipment effectiveness (OEE). The prediction of this KPI requires not only the availability of measured data, but also a deep understanding of the industrial process to be able to extract the more correlated and appropriate input variables.
To the best of our knowledge, this study represents the first application of machine learning-based techniques for the off-line KPIs prediction especially without the use of time series methods, which is potentially useful for developing robust models able to deal with the dynamics of the industrial processes and also provide to the decision-making process the ability to define an appropriate schedule for different interventions (programmed maintenance, correction of detected anomalies, improvement actions).
3 Machine learning methods
Machine learning algorithms are divided into three categories, namely supervised learning (Smola and Vishwanathan 2008 ), unsupervised learning and reinforcement learning. For supervised learning, the problem is described by a vector X of input variables and a vector Y of output variables (target) and the goal is to find a mapping function between X and Y as Y = f ( X ). In case we have only input variables X without any target variables, the problem is turned into an unsupervised learning. Finally, reinforcement learning is a different type of machine learning, where an agent learns how to behave and decide in an environment by performing actions and receiving rewards. In the present study, we will cover a variety of supervised machine learning algorithms since we are handling labeled data.
3.1 Support vector machine for regression (SVR)
Support vector machine (Ben-Hur and Weston 2010 ; Awad and Khanna 2015 ) is a supervised machine learning algorithm mostly used in classification, but easily adopted for regression since it forms a generalization of the classification problem, in which the model returns a continuous-valued prediction. SVR can be defined as an optimization problem that relied on defining a convex ε -insensitive loss function, then trying to minimize this function and find the flattest tube that contains most of the training instances.
If the problem is linear, then the function f(x) can be described in the following form:
Based on the loss function and the geometrical properties of the tube, a multi-objective function is constructed which provides a unique solution to the convex optimization.
If we consider the case of a primal problem, we obtain the following minimization problem:
This optimization problem is often infeasible, and to solve this issue, two new variables are introduced ξ and ξ *. Then we obtain the new optimization problem:
The hyper-plane is represented in terms of support vectors, which are training samples that lie outside the boundary of the tube. The error function is as follows:
It is called ε -insensitive error function, and it can be summarized as in Fig. 2 .
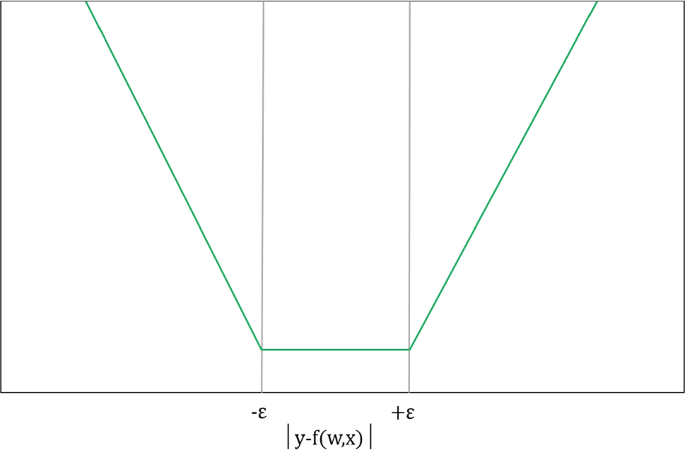
ε -insensitive error function
SVR technique requires certain parameters, mostly the kernel and regularization parameter. Kernels are used when we are dealing with nonlinearly separable data, and they allow mapping the input data to a high-dimensional space in order to be linearly separable. The most used kernels are radial basis function kernel (RBF), polynomial kernel and sigmoid kernel (Schölkopf et al. 2002 ). Regularization parameter is also known as the soft margin constant C: this hyper-parameter controls the trade-off between the slack variable penalty and the width of the margin.
Finally, the complexity parameter is γ for RBF and sigmoid kernels and the degree for polynomial kernel. This parameter determines the flexibility of the SVM model in fitting the data while preventing over-fitting.
3.2 Ensemble learning techniques
Ensemble methods are a collection of several base models working together on a single set of data in order to produce one optimal predictive model. Ensemble models are known for their higher accuracy results against conventional single machine learning models (Zhang and Ma 2012 ). Ensemble learning techniques are classified into bagging and boosting.
Bagging, short for Bootstrap AGGregatING, is a simple ensembling technique that consists of creating several sub-samples of data from training sample chosen randomly with replacement. Then, several models are trained with the different subsets/bootstraps so that we end up with several predictions. Finally, these predictions are aggregated by using their weighted average, majority voting or simply their normal average. So, assuming that we have L bootstraps samples of size B denoted:
such that: z 1 = (b - th) observation of the (l - th) bootstrap sample
We can fit L different models (weak learners) on each sub-sample; f 1 (.), f 2 (.), …, f L (.). And then aggregate them using one of the averaging processes mentioned above in order to get an ensemble model with a lower variance. For example, we can define our strong model such that:
Simple average:
Weighted average:
Such that: w l ≡ the weight of the l - th model ( weak learner )
Random forest is a bagging technique that we will cover in the present study.
Boosting is a powerful ensembling technique in which the models are made sequentially. At each particular iteration, a new weak, base-learner model is added to be maximally correlated with the negative gradient of the loss function. The learning procedure of this technique is based on the logic that the subsequent predictors learn from the mistakes committed by the previous predictors.
In the same way, as for bagging, assuming that we have the same bootstraps and the same weak learners, our ensemble model is built using a weighted sum of weak learners;
Then, to find the optimal model under this form, we make use of an iterative optimization process that is much more tractable. In practice, there are two ways for achieving the desired result. The first one called adaptive boosting consists of adding the weak learners one by one, looking at each iteration for the best possible pair (coefficient, weak learner) to add to the current ensemble model as below:
where w l and f l are chosen such that s l is the model that fit the best the training data and, so, that is the best possible improvement over s l −1 .
The second way uses gradient descent algorithm for solving the optimization
problem, which means that at each iteration we fit a weak learner to the opposite of the gradient of the current fitting error with respect to the current ensemble model. The gradient descent process over the ensemble model can be written:
where \(E\left(\cdot \right)\) is the fitting error of the given model, \(w_{l}\) is a coefficient corresponding to the step size (weight), and \(- \nabla_{{s_{l - 1}}} E\left({s_{l - 1}} \right)\left(\cdot \right)\) is the opposite of the gradient of the fitting error with respect to the ensemble model at step l-1.
We can, then, summarize the iterative process in fitting the best possible weak learner to the opposite of the gradient with respect to the current strong learner, computing the value of the optimal step size that defines by how much we update the ensemble model in the direction of the new weak learner, updating the ensemble model by adding the new weak learner multiplied by a step of gradient descent. Finally, computing new gradient descent that indicates, for each observation, in which direction we would like to update next the ensemble model predictions.
Gradient Boosting Machine (GBM), Adaboost and eXtreme Gradient Boosting (XG-Boost) are the most famous boosting algorithms; in our research, we will cover XG-Boost algorithm.
3.2.1 Random forest
Random forest (Ali 2012 ) is a supervised machine learning algorithm used for both prediction and classification problems. It is a bagging ensemble learning method based on decision tree algorithm as shown in Fig. 3 (left). The random forest is a popular algorithm that can be applied to a wide range of prediction and classification problems and has few parameters to tune. The random forest is forming by a multitude of decision trees; then, the prediction is made by aggregating (majority vote for classification or averaging for regression) the predictions of each component tree. It generally has much better predictive accuracy and shows a good performance than a single decision tree, and it is recommended for its ability to deal with small and large sample sizes. The random forest is proper for high-dimensional data modeling because it can deal with categorical, binary and continuous data and it can handle easily the problem of missing values. Besides its efficiency, random forest is powerful, interpretable and has shown good performance on many problems.
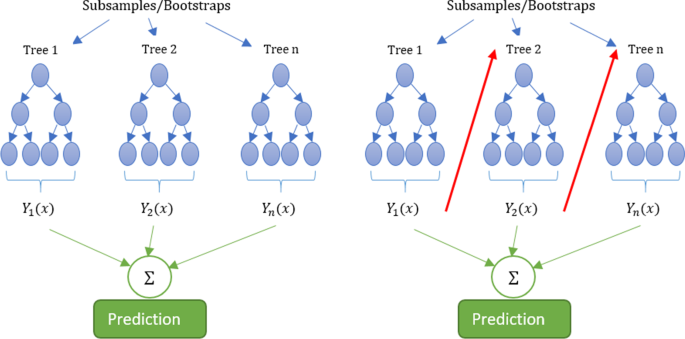
Bagging/random forest example (left), boosting/XG-boost example (right)
3.2.2 Extreme gradient boosting
XG-Boost (eXtreme Gradient Boosting) is an optimized library designed for implementing machine learning algorithms under the Gradient Boosting framework (Chen and Guestrin 2016 ). It is a boosting ensemble learning method based on decision tree algorithm as shown in Fig. 3 (right). By imposing regularization and providing parallel tree boosting, XG-Boost is able to exploit more computational power and get more accurate prediction while remaining fast and scalable.
3.3 Deep learning neural network
The core difference between deep learning and neural network is that deep learning is a deep neural network. In other words, a deep learning model is created by a multi-layer architecture of neural nets and is taking advantage of the huge amount of accessible data nowadays. Although its architecture is more complex and it uses more and more data for training, deep learning remains more performing than any other machine learning algorithm. Furthermore, its performance keep increasing provided that is trained on large data.
The deep learning algorithm consists of feed-forward and back-propagation process (LeCun 2015 ), while the feed-forward process, the model associates weights from the input layer toward hidden units and the output layer. After every iteration through the data set, the weights are adjusted toward a backward propagation using gradient descent to maximize the correlation between the output and the residual error of the model. The final weight vector of a successfully trained neural network represents its knowledge about the problem (Schmidhuber 2015 ).
The most popular optimization algorithms actively in use include SGD, Adam, AdaDelta, AdaGrad, SGD with momentum, RMSProp, and more else. The choice of which algorithm to use, at this point, seems to depend largely on the user’s familiarity with the algorithm (Goodfellow et al. 2016 )
Similarly, the choice of which activation function to use depends naturally on the user’s knowledge about the algorithm, but also, is a case study-related choice.
The most used activation function is the following;
4 Proposed predictive models
In our study, we attempt to cover the most popular and famous machine learning algorithms. We first tried different linear regression models, namely multiple linear regression, polynomial regression, support vector regression (SVR) and decision tree regression. Then, we kept SVR because it shows better accuracy within the regression models. Secondly, we manipulated ensemble learning techniques with tree-based models. We tested random forest for bagging ensemble and XG-Boost for boosting ensemble. Finally, we built a deep learning predictor in order to come up with a robust model with a high level of performance and especially able to deal with the complexity of our data.
All the models, except deep learning, are implemented under two configurations: using simple data split and using cross-validation to split the data. Then, these two configurations are employed with two distinct test sets: one consisting of only 40 items for testing the models performances and the second consisting of 288 items which is about 15% of the whole data. Finally, we implemented an optimized SVR model using genetic algorithm for the hyper-parameters tuning.
4.1 Support vector regression
As we discussed in the previous section, we chose support vector machine for regression (SVR) based on its accuracy. Furthermore, SVR algorithms are depicted by their adaptability, flexibility to work with linear and nonlinear problems, and mainly they are nonbiased by outliers. Our SVR model is implemented under the architecture below:
Kernel: Radial Basis Function (RBF)
Regularization parameter C = 1
Complexity parameter γ = 0.15
In the second part of the SVR method, we developed a genetic algorithm (GA) for hyper-parameters tuning, especially C and gamma. As a result, we obtain the two optimized values, which are: C = 403.37 and γ = 0.83. Then we built our second SVR model with the given parameters.
4.2 Random forest
Besides its accuracy and interpretability, random forest (RF) is also an appropriate model for high-dimensional data, which makes it suitable for our case study. Our RF model is implemented using 120 trees, and the number of trees was decided by trial and error using mean absolute error (MAE) as a metric.
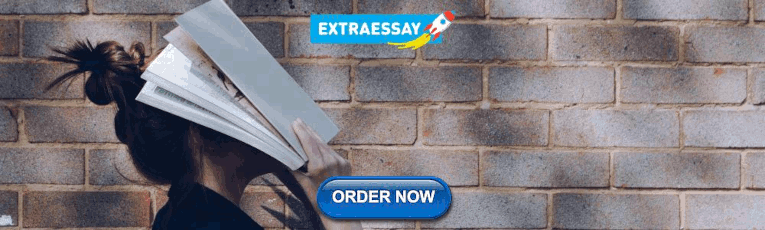
4.3 Extreme gradient boosting (XG-boost)
XG-Boost is an ensemble tree-based model, which flows the principle of gradient boosting just as gradient boosting machine (GBM) and Adaboost. However, XG-Boost has more customizable parameters that allow it a better flexibility. Additionally, XG-Boost uses more regularized model formalization to control over-fitting, which gives it better performance. All of the above reasons combined with the computational power of the XG-Boost algorithm, were sufficient for us to choose it over the other boosting techniques. Our XG-Boost model is developed using the following hyper-parameters:
Number of boosted trees: 130
Learning rate η = 0.2
Maximum depth: 3
4.4 Deep neural networks
Our deep learning (DL) model is implemented using an input layer of 7 neurons which represent our input features and two hidden layers. To estimate the number of neurons in each hidden layer, we checked different methods and heuristics (Hunter et al. 2012 ; Hornik 1991 ; Yamasaki 1993 ) and finally we adopted Huang’s network architecture for two hidden layers feed-forward network (TLFN) (Huang 2003 ). It is given, for the two layers, as below:
where m is the number of outputs and N is the size of the training set.
Applying these formulas to our case study, we obtain an architecture consisting of 125 neurons in the first layer and 25 in the second layer. The output layer consists of one node given that we are predicted one final value (OEE). The architecture of our DL used in this section (see Fig. 4 ) is formed by the following structures and parameters:
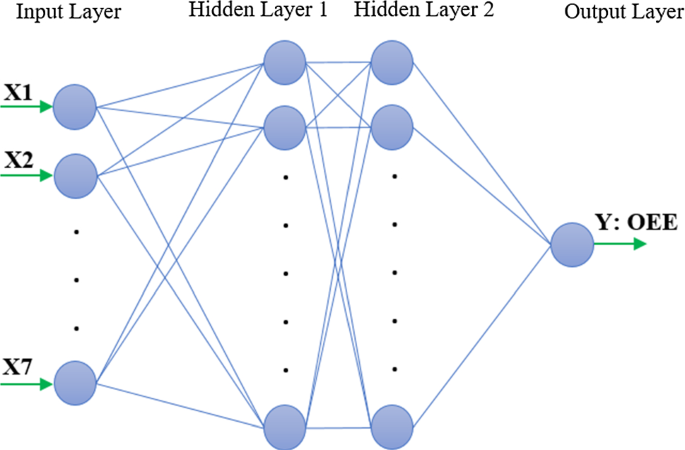
Deep neural networks topology
Activation function: Rectifier Linear Unit
Optimizer: Adam optimization algorithm (Kingma and Ba 2014 )
Loss function: Mean Absolute Error (MAE)
Learning rate: 0.001
Number of hidden Layers: 2
Number of neurons in the first hidden layer: 125
Number of neurons in the second hidden layer: 25
5 Experiments
5.1 industrial case study.
The data used in this research were provided from the cutting and crimping department of an automotive wiring harness company. In terms of the production process of wire harness it consists of wire cutting and terminal crimping; then, the wires and components are assembled on the pin board to the desired specification and then bound together. Finally, electrical tests and visual inspection are highly recommended from the quality side, and an example of electrical wire is shown in Fig. 5 (left). In this study, the cutting machines are used as the telltale equipment. The cutting workshop is occupied by 22 similar machines which are designed to cut a range of wires cross sections. The operations ensured by this equipment are as follows: after any required preparation, the first step is to cut the wires to the desired length. A special machine may also print the wires on during the cutting process. After this, the ends of the wires are then stripped to expose the core of the wires, usually metallic; Fig. 5 (middle) shows an example of a stripped wire. The next step is to fit the wires with the required terminals and/or connector housings, which come in many different sizes and specifications. This step is known as crimping. Every product is a specific product which has its own specification. The main specifications of a new product are as follows: Cable Class (Single wire…); Wire cross section; Wire length; Stripping Length (Right and Left); Number of terminals (0, 1, 2); Terminal identification; Number of Seals (0, 1, 2); Print (Yes/No); Print Identification. Figure 5 (right) represents an example of electrical wire with two different terminals.
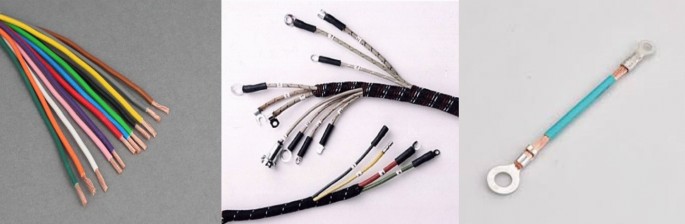
Electric wire harness (left). Cut and stripped wire (middle), wire with two different terminals (right)
A production order is defined by a given quantity of one product and is launched by the planning department, and then they are dispatched automatically to the different machines. One machine could produce a set of orders per day per shift.
5.2 Data preparation
We evaluated our model on a data set that we prepare based on the extracted data from the internal system of the company. The raw data were given into two tables, the first contains the OEE values per shift per machine, and the second shows the different orders with the associate specifications done by every single machine over the days.
The data preparation process consists of the following stages (Zhang 2003 ):
Data selection: which means selecting appropriate data from the raw data (the given two files).
Data pre - processing the data preprocessing step consists of cleaning and removing fixing or missing data and then transforming the data into a structured form suitable to work with. Sampling the data is also a data preprocessing step that was accomplished in this level.
Data transferring it consists of features scaling that was highly recommended in our case—because our input variables did not have the same scale—in order to avoid the big-scale variable(s) domination.
Finally, for augmenting our data set, it was recommended to consider that the whole data were provided by one machine since the machines are similar.
In the first section of this paper, we presented the tree core parameters that has an impact on the OEE value. These parameters are the availability, the performance and the quality. However, the yields of all the machines in every observation of our data set are 100%, and then the quality rate will not be taken into account. Thereby, we end up with a set of 12 variables that affect the two retained components of the OEE, which are performance and availability. Some of these variables are not directly correlated with the given metrics; then, we made use of our experience in this field coupled by the use of some data preparation techniques such as features selection and features engineering approaches so that we end up with a final set of input variables formed by:
Setups this variable represents the sum of the all setup values. As the setup for every order is known before starting the production, this variable can be used as an input for our predictors.
Breakdowns this variable is substituted by planned and unplanned maintenance. In our study this will concern only planned maintenance, since unplanned maintenance cannot be known in advance.
Number of orders this variable acts out how many orders every single machine has done per day per shift.
Mean Wire Length represents the mean of overall wire length values produced by the same machine in one shift.
Number of Terminals represents the total number of terminals across all orders for the same machine in one shift.
Number of Seals represents the total number of seals across all orders for the same machine in one shift.
Target this variable explains the target designed for each order regarding its difficulty and other parameters.
5.3 Exploratory data analysis (EDA)
Before starting our machine learning modeling, a data exploration approach was needed. We tried to detect the presence of outliers in our data, so to drop them or even to remove them, this step was performed by graphical techniques. Using the distribution plot Fig. 6 (left) and the box plot Fig. 6 (middle), we were able to detect the existent outliers.
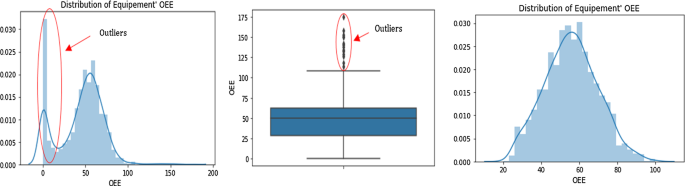
Plot of raw data (left). Box plot of raw data (middle). Distribution plot of retained data (right)
By analyzing our data set, most of the outliers are meaningless aberrations caused by errors (under and over values) committed when reporting the daily information on the system and the rest are due to some situations that rarely occur. Hence, we removed all of these outliers from our data set. Figure 6 (right) shows the distribution plot of our final (retained) data set.
6 Empirical illustration
In this case study, the data set consists of 7 features, 1917 observations and 1 target (OEE value). We will expose and discuss the results of two configurations, each one applied to our proposed models with and without cross-validation.
The prediction performance of all the used models is evaluated by three metrics which are: root-mean-squared error (RMSE), mean absolute error (MAE) and mean absolute percentage error (MAPE).
All the models used in this research are developed using Python 3.6.
6.1 First configuration’s results
Our first configuration consists of testing our models on a holdout data set of 40 items (equivalent to 2% of the whole data).
6.1.1 Support vector regression results
As mentioned before, we implemented three SVR models. SVR with simple data split, SVR with cross-validation (SVRCV) and optimized SVR with cross-validation using genetic algorithm (SVRGA).
To explain the results of our SVR models, we first plot the real values also called target versus the predicted values of our three SVR models. Figure 7 shows this plots for the 40 items of the test set data. Their average errors defined by their mean absolute errors are the following:
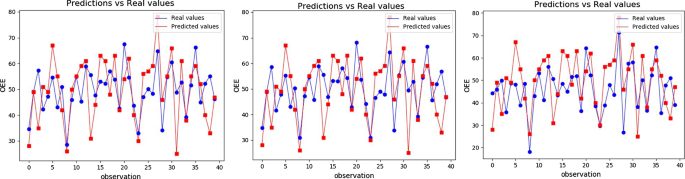
Configuration 1: SVR results (left), SVRCV results (middle), SVRGA (right)
MAE SVR = 8.3
MAE SVRCV = 8.35
MAE SVRCVGA = 9.6
It is found that the prediction values of SVR are slightly close to the targets with the better average error (see Fig. 7 left). However, this might imply that our model tends to over-fit the data and then it will not be reliable if we change the data. On the other hand, by applying cross-validation, the model becomes more reliable on new unseen data and this explains why its error is little bit bigger (Fig. 7 middle). Finally, in order to improve the previous model, we developed a genetic algorithm for hyper-parameters tuning (C and gamma). Then the issued values of C and gamma were used for our SVRCVGA model (Fig. 7 right). Unlike, the new model is less performed than the previous ones; maybe, this is due to the small size of our test set data.
6.1.2 Random forest results
We implemented two models of the random forest algorithm, with simple data split (RF), then, with cross-validation (RFCV). The results of the two models are shown in Fig. 8 below:
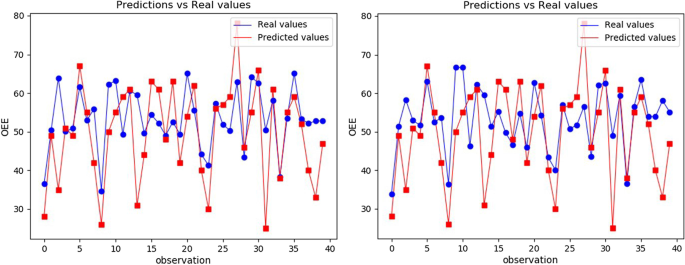
Configuration 1: RF results (left), RFCV results (right)
The first RF model is built with the elementary split data; then, we implemented it another time using cross-validation technique. Figure 8 shows the results for the 40 items of our test set data. The mean absolute errors of our two models are successively:
MAE RF = 8.16
MAE RFCV = 8.4
We notice, again, that even if cross-validation technique provides a more functional and reliable model on unseen data, it does not offer an accurate model in our case. The same remark as mentioned in the SVR discussion is still valid regarding the size of our test set.
6.1.3 Extreme gradient boosting results
As for random forest, XG-Boost is implemented under two configurations, one using simple data split (XGB) and another using cross-validation (XGBCV).
In Fig. 9 we plotted the OEE targets versus the predicted values of our two XG-Boost models. The average errors for this 40 observations are given above:
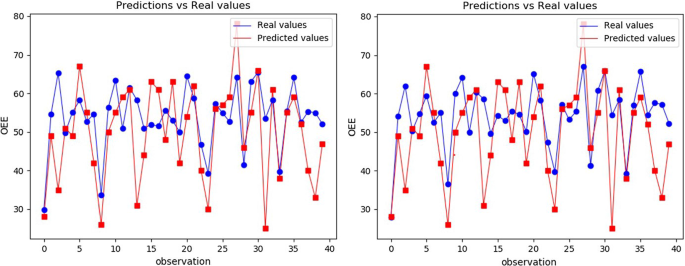
Configuration 1: XGB results (left), XGBCV results (right)
MAE XGB = 8.2 MAE XGBCV = 8.3
Although the average error of the XGBCV has slightly increased, but like RFCV and SRCV, this model remains accurate, more performed and reliable on unseen date.
6.1.4 Deep learning results
In the case study, the deep learning (DL) model implemented consists of two hidden layers with 125 neurons the first layer and 25 in the second one. The architecture of the model is described in Sect. 4 . Our model is built without cross-validation, because of the cost associated with training K different models. Instead of doing cross-validation, we used a subset of 40 items as a hold-out to test the performance of our final model.
Figure 10 (left) shows the loss curve for both training and validation sets. It is found that the training loss keeps decreasing, while the validation loss is more or less stabilized in a given value. Figure 10 (right) shows the results for the 40 items of test set data, with an average error for the 40 items of validation data of: MAE DL = 8.44.
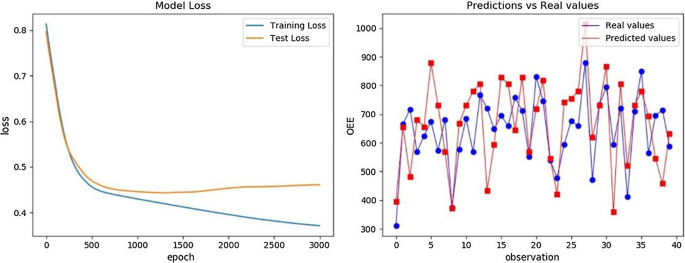
Configuration 1: DL loss curve (left), DL results (right)
6.1.5 Findings comparison
The use of cross-validation technique was conducted by the fact that a simple data split can raise the problem of over-fitting. This reflection was confirmed by our experiments, even if it does not bring us a more accurate model, but it provides a more reliable model on new data. Maybe in this first configuration, this conclusion it is not evident because of the small test size, that said, the second configuration will highlight this point.
In order to reduce over-fitting in our deep learning model, we made use of a regularization technique called dropout. The DL model gives a comparable performance to the other models. However, deep learning gains more in terms of performance as long as is trained in large amount of data.
6.2 Second configuration’s results
The second configuration, employed in this study, consists of testing our models on a holdout data set equivalent to 15% of the whole data which is about 288 items. This configuration seems to be more relevant to discuss the performance of our final models regarding the size of our data set.
6.2.1 Support vector regression results
Like for the first configuration, again, we implemented three SVR models: SVR with simple data split, SVR with cross-validation (SVRCV) and optimized SVR with cross-validation using genetic algorithm (SVRGA).
As mentioned before, our test set, in this configuration, consists of 288 observations. However, for a better visibility, we just plot 40 items. Figure 11 shows the plots of our three SVR models, and their average errors are the following:
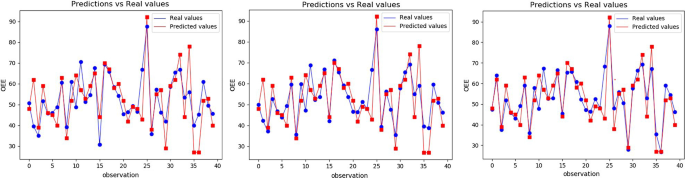
Configuration 2: SVR results (left), SVRCV results (middle), SVRGA (right)
MAE SV R = 6.14
MAE SV RCV = 5.56
MAE SV RCV GA = 4.95
We notice that by applying cross-validation technique, the average error of our SVR model has decreased and our new model has a better performance. In the same way, by applying genetic algorithm for the hyper-parameter’s tuning, we end up with a more accurate model.
6.2.2 Random forest results
Again, our random forest model is implemented under two configurations, with and without cross-validation.
Figure 12 shows the plots of our RF models considering only 40 items from the 288 test set data. Their average errors are presented below:
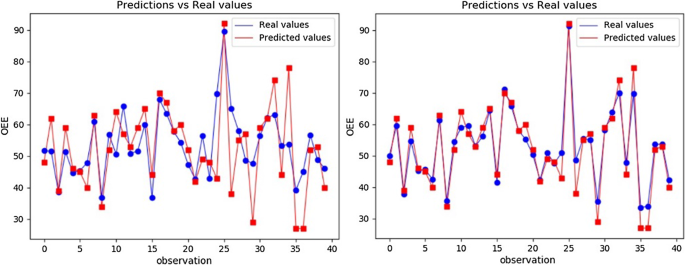
Configuration 2: RF results (left), RFCV results (right)
MAE RF = 6.79
MAE RFCV = 2.95
By applying cross-validation, we obtain a more reliable and robust model, better yet, our model has a very good performance regarding RF model without cross-validation. In Fig. 12 right, it is found that the prediction values of RFCV are slightly close to the real values.
6.2.3 XG-boost results
In the same way, we implemented two XG-Boost models, one with simple data split and another using cross-validation to split the data.
Figure 13 shows the plots of our XGB models considering only 40 items from the 288 test set data. Their average errors are presented below:
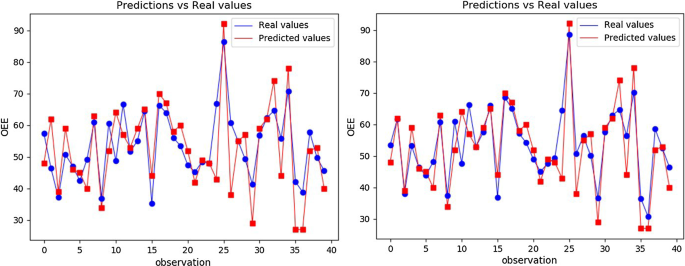
Configuration 2: XGB results (left), XGBCV results (right)
MAE XGB = 6.4
MAE XGBCV = 5.55
As discussed before for SVR and RF, XGB also gains in terms of performance while applying cross-validation technique.
6.2.4 Deep learning results
In this configuration, again, our deep learning model is implemented using the same architecture presented in the previous section using a subset of 40 items as a hold-out to test the performance of our final model.
Figure 14 (left) shows the loss curve for both training and validation sets, and we notice that we have more or less the same loss curve as for the first configuration. In Fig. 14 (right), we expose the results of our sub-set of the test set data with an average error of MAE DL = 6.3.
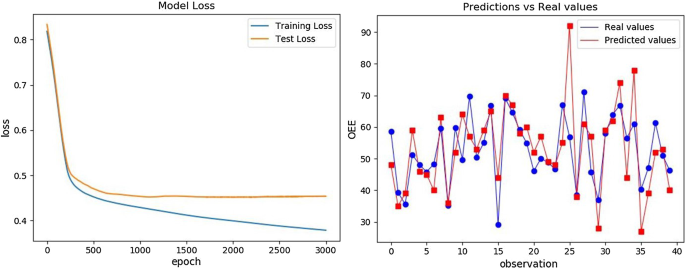
Configuration 2: DL loss curve (left), DL results (right)
6.2.5 Findings comparison
In the comparison of the first configuration’s models, we said that the use of cross-validation technique provides us a more reliable and functional model on new unseen data even if this was not evident enough regarding the test size used in this configuration. However, in this second configuration, this conclusion is assumed and highlighted with the different applied models. Using a test size of 15% from the whole data with models performed by cross-validation, not only, we come up with robust models but also their performances are remarkably better and insightful.
On the other hand, we noticed in this configuration that our deep learning model lost even more in terms of performance, and this can be explained by the fact that deep learning needs a large amount of training data to be relevant and accurate. However, in this configuration, we took more data for testing which means that the training data set becomes smaller.
7 Models comparison and discussion
7.1 models accuracy.
A quick comparison of our models based on their mean absolute errors (MAE), mean absolute percentage errors (MAPE) and root-mean-squared error (see Table 1 ) shows that all the models, of our first configuration, without cross-validation have good performance, but they are highly exposed to be biased and unreliable on future unseen data. Since we made use of a regularization technique within our DL model, then we can consider its performance mainly in this configuration. Otherwise, DL, RFCV, XGBCV and SVRCV have comparable performance.
For the second configuration, the opposite is true; by applying cross-validation, our models became more accurate (see table). This is due to the fact that we are splitting our data appropriately: 70% training, 15% for validation and 15% for testing. This provides us more reliable and unbiased models. RFCV seems to be the more accurate model, and we can also preserve SVRCV using genetic algorithm which gives also a good performance.
Another evaluation is performed using statistical tests to compare means, namely with ANOVA test, and standard deviations test of the absolute value error of the different models. Figure 15 shows the distribution and graphs of each error vector.
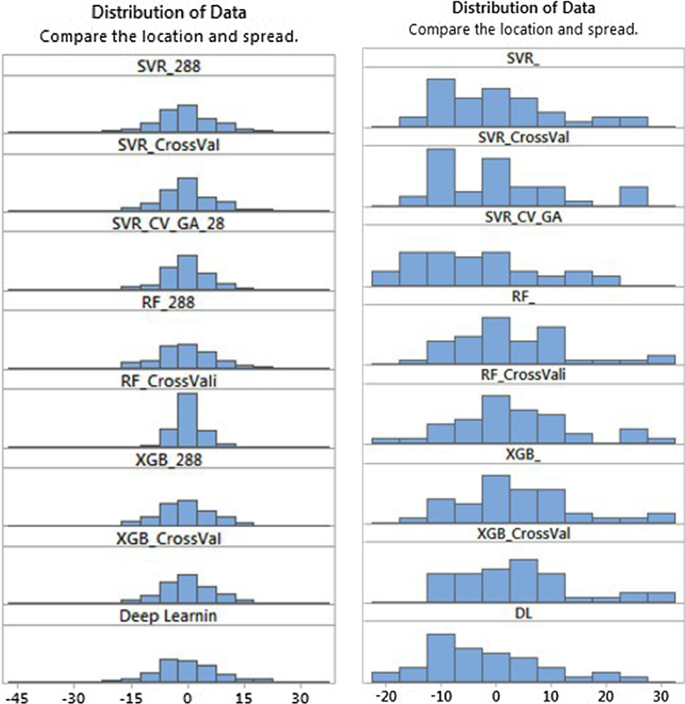
Distribution of the error vector of configuration 1 (left), distribution of the error vector of configuration 2 (right)
Figure 15 right shows the distribution plot of the error vector for each model using the first configuration, while Fig. 15 shows the same plot for using the second configuration. We notice that by increasing the test set size, the distribution of the error vector follows a normal distribution.
Figure 16 shows the mean and the standard deviation of each model under the two configurations.
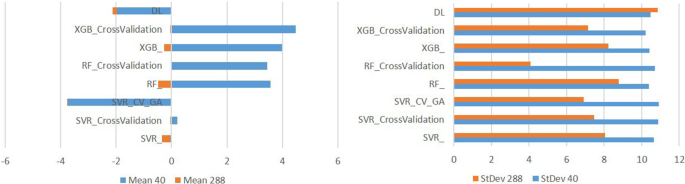
Mean of the error vector (left), standard deviation of the error vector (right)
We can notice that with the increase of the test data, the overall performance of the algorithms in terms of mean and dispersion improves (except DL which keeps more or less the same behavior).
7.2 ANOVA test
We performed the ANOVA test with the following hypotheses: the null hypothesis is all means are equal. We take significance level α = 0.05; the P value is less than α . Therefore, the null hypothesis is not valid, and we can conclude that there is a significant difference between the means of model errors. In Fig. 17 we grouped models using the Tukey method with 95% confidence.
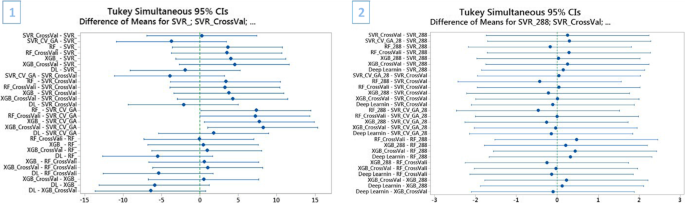
Tukey test for configuration 1 (left), Tukey test for configuration 2 (right)
In fact, a good model must be precise, in the sense that the mean of the error difference must be close to 0, and the least dispersed possible.
Boxplot and interval plot shown in Fig. 18 enhance the results given by the ANOVA and Tukey tests presented above. They show that there is a significant difference in terms of mean when the test set data are small. By increasing the test set size from 40 items to 288 items, all the models become comparable in terms of mean but is not the case for dispersion. In addition, the models that were comparable but have a large standard deviation in the first configuration, have significantly improved as well as their dispersion as shown in Fig. 18 .
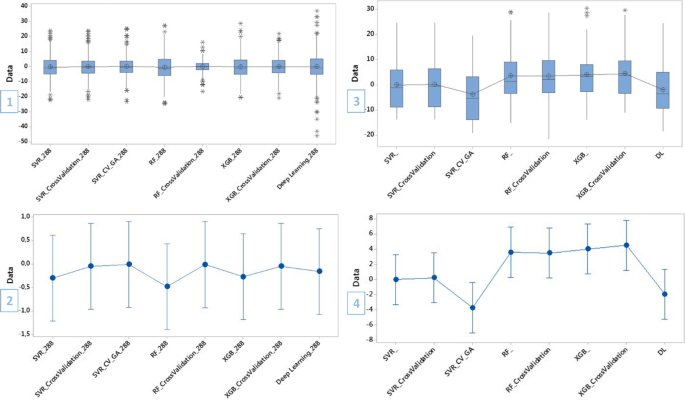
Boxplot and interval plot for configuration 2 and 1, respectively (left and right)
7.3 Standard deviations test
We performed the standard deviations test with significance level α = 0.05, and the P value is less than α . Therefore, the null hypothesis is not valid, and we can conclude that there is a significant difference between the dispersion of model errors. In the first configuration, we find that null hypothesis is valid and then there is no significant difference between the model errors in terms of dispersion (see Fig. 19 left), while the opposite is true for the second configuration as shown in Fig. 19 right. In addition, we notice that RF with cross-validation has the lower dispersion compared to the other models.
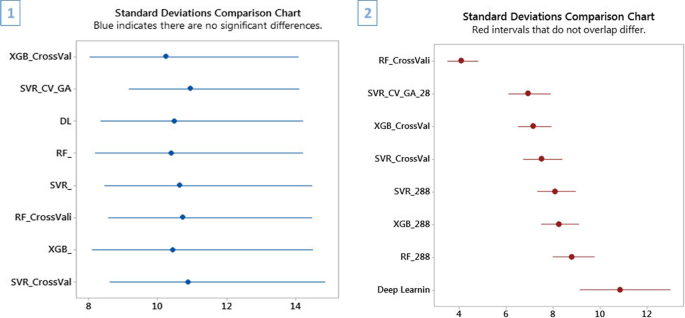
Standard deviations test for configuration 1 and 2, respectively (left and right)
7.4 Conclusion of comparison
The use of cross-validation technique was conducted by the fact that a simple data split can raise the problem of over-fitting, and this reflection was confirmed by our experiments. Even if the use of cross-validation for the first configuration, using a small test set data, does not bring us more accurate models, it provides a more reliable predictors on new data. In the second configuration, with the use of relevant test set size, cross-validation technique has enhanced not only the reliability and the robustness of our models, but also their performances.
On the other hand, the test set size is another important variable which acts directly on the performance of the models. That said, the same remark is still valid for the validation set also to perform good machine learning predictors. This point was highlighted by our experiments by passing from configuration one to configuration two. By comparing the results of these two configurations, we conclude that the use of a relevant test set size provides a better performance for the same model.
Deep learning is a powerful machine learning technique that provides robust and accurate models for both prediction and classification problems, and it can usually beat even the best machine learning models. But, in our case study, it seems not achieving such a good performance. This is due, most likely, to the small size of training data, which was confirmed going from configuration one (training set of 1877 items) to configuration two (training set of 1629 items).
We can conclude that, for a given prediction problem, there are some variables that must be chosen adequately to achieve a good machine learning model robust and accurate in the same time. In our case study, we noticed that the use of cross-validation technique with an appropriate split of data and allowed us to develop accurate models able to deal with the complexity of our data and then to perform better predictions.
8 Conclusion and perspectives
This paper presented the use of various machine learning algorithms under different configurations to predict the overall equipment effectiveness and its application on the data provided by the cutting department of an automotive wiring company. We performed support vector regression, optimized support vector regression, random forest, extreme gradient boosting and deep learning under two configurations. The first one consists of using a test set size of 40 items across a training set size of 1877 observations, while the second configuration consists of testing our models on bigger data set of 288 items which is equivalent to 15% from the whole data.
Under each of the above-mentioned configurations, we implemented again our models (except deep learning) once, with simple data split and secondly, using cross-validation technique for data splitting. The result exhibits that the use of cross-validation technique with a relevant splitting of data provides more reliable, accurate and unbiased predictors. This comparison was made based on the MAE, MAPE and RMSE metrics and confirmed by the statistical techniques such ANOVA and standard deviation tests.
It remains to be noted that the input variables were not available directly, they were chosen, and some of them calculated, based on our experience in this field and our familiarity with the given case study. Hence, the problem could be solved in another way and the results may change, if only it is studied from another perspective.
It should also to be mentioned that the OEE is only a study case that could be generalized to other KPIs, and also the experiment could be performed in any other industries, ensuring the necessary changes and adaptations.
Finally, we should highlight that many points remain to be explored as perspectives. We can cite some of them:
The validation of the prediction in the real-time case
The combination of optimization techniques with the developed models to provide decision support tools for managers
The more detailed study of the utility of the hybrid approaches which associate the genetic algorithms with those of the machine learning
Ali J (2012) Random forests and decision trees. Int J Comput Sci Issues (IJCSI) 9:272
Google Scholar
Awad M, Khanna R (2015) Support vector regression. In: Efficient learning machines. Apress, Berkeley, CA, pp 67–80
Ben-Hur A, Weston J (2010) A user’s guide to support vector machines. In: Data mining techniques for the life sciences. Humana Press, pp 223–239
Chen T, Guestrin C (2016) Xgboost: a scalable tree boosting system. In: Proceedings of the 22nd acm sigkdd international conference on knowledge discovery and data mining, pp 785–794
das Chagas Moura M et al (2011) Failure and reliability prediction by support vector machines regression of time series data. Reliab Eng Syst Safety 96(11):1527–1534
Article Google Scholar
Ding SX (2008) Model-based fault diagnosis techniques: design schemes, algorithms, and tools. Springer, Berlin
Dong H et al (2012) Fault detection for Markovian jump systems with sensor saturations and randomly varying nonlinearities. IEEE Trans Circuits Syst I Regul Pap 59(10):2354–2362
Article MathSciNet Google Scholar
Du D et al (2012) Sensor fault estimation and compensation for time-delay switched systems. Int J Syst Sci 43(4):629–640
Gao Z et al (2008) Reliable observer-based control against sensor failures for systems with time delays in both state and input. IEEE Trans Syst Man Cybern Part A Syst Humans 38(5):1018–1029
Gao Z et al (2013) Guest editorial: special section on data-driven approaches for complex industrial systems. IEEE Trans Industr Inf 9(4):2210–2212
Goodfellow I et al (2016) Deep Learning. MIT Press, Cambridge
MATH Google Scholar
Groote PD (1995) Maintenance performance analysis: a practical approach. J Quality Main-tenance Eng 1:4–24
Grottoli MG et al (2008) Strengthened techniques for the renewed industrial interest in plant data reconciliation benefits
Hornik K (1991) Approximation capabilities of multilayer feedforward networks. Neural Netw 4:251–257
Huang G-B (2003) Learning capability and storage capacity of two-hidden-layer feedforward networks. IEEE Trans Neural Netw 14(2):274–281
Hunter D et al (2012) Selection of proper neural network sizes and architectures—a comparative study. IEEE Trans Industr Inf 8(2):228–240
Kim S-M, Joo Y-J (2005) Implementation of on-line performance monitoring system at Seoincheon and Sinincheon combined cycle power plant. Energy 30(13):2383–2401
Kingma DP, Ba J (2014) Adam: a method for stochastic optimization. arXiv:1412.6980
Kuo Y, Lin K-P (2010) Using neural network and decision tree for machine reliability prediction. Int J Adv Manuf Technol 50(9–12):1243–1251
LeCun Y et al (2015) Deep learning. Nature 521(7553):436–444
Manenti F (2009) From reacting to predicting technologies: a novel performance monitoring technique based on detailed dynamic models. Chem Prod Process Model 4
Muchiri P, Pintelon L (2008) Performance measurement using overall equipment effectiveness (OEE): literature review and practical application discussion. Int J Prod Res 46:3517–3535
Nakajima S (1982) TPM tenkai. Japan Institute of Plant Maintenance, Tokyo
Schmidhuber J (2015) Deep learning in neural networks: an overview. Neural Netw 61:85–117
Schölkopf B et al (2002) Learning with kernels: support vector machines, regularization, optimization, and beyond. MIT press, Cambridge
Shirose K, Goto F (1989) Eliminating the six big losses. TPM Development Program-Implementing Total Productive Maintenance, Cambridge
Smola A, Vishwanathan SVN (2008) Introduction to machine learning, vol 32, UK
Solomon A, Litoiu M (2011) Business process performance prediction on a tracked simulation model. In: Proceedings of the 3rd international workshop on principles of engineering service-oriented systems, pp 50–56
White DC (2003) The “smart” refinery: economics and technology. In: Proceedings of NPRA annual meeting, San Antonio, Texas, USA, AM, pp 3–19
Xie X et al (2015) An advanced PLS approach for key performance indicator-related prediction and diagnosis in case of outliers. IEEE Trans Industr Electron 63(4):2587–2594
Yamasaki M (1993) The lower bound of the capacity for a neural network with multiple hidden layers. In: International conference on artificial neural networks. Springer, London, pp 546–549
Yin S et al (2012) Data-driven quality related prediction and monitoring. In: IECON 2012-38th annual conference on IEEE industrial electronics society, pp 3874–3879
Yin S, Wang G (2013) A modified partial robust M-regression to improve prediction performance for data with outliers. In: 2013 IEEE international symposium on industrial electronics, pp 1–6
Yin S et al (2014) Robust PLS approach for KPI-related prediction and diagnosis against outliers and missing data. Int J Syst Sci 45(7):1375–1382
Yu J et al (2013) Robust fault detection for Markovian jump systems with unreliable communication links. Int J Syst Sci 44(11):2015–2026
Zhang S (2003) Data preparation for data mining. Appl Artif Intell 17:375–381
Zhang C, Ma Y (eds) (2012) Ensemble machine learning: methods and applications. Springer Science & Business Media
Zhang K et al (2017) A KPI-based process monitoring and fault detection framework for large-scale processes. ISA Trans 68:276–286
Download references
Acknowledgements
The authors would like to thank the anonymous reviewers for their helpful comments and suggestions which improved the quality of this paper. The authors would particularly like to thank the managing editor, Prof. Raffaele Cerulli, for his insightful suggestions during the revision process of this paper.
Author information
Authors and affiliations.
Laboratory of Mathematical Modeling, Simulation and Smart Systems (L2M3S), Artificial Intelligence for Engineering Sciences Team, ENSAM-Meknes, Moulay ISMAIL University, B.P. 15290, Marjane 2, Al-Mansor, 50500, Meknes, Morocco
Choumicha EL Mazgualdi, Tawfik Masrour, Ibtissam El Hassani & Abdelmoula Khdoudi
You can also search for this author in PubMed Google Scholar
Corresponding author
Correspondence to Tawfik Masrour .
Ethics declarations
Conflict of interest.
The authors declare that they have no conflict of interest.
Additional information
Communicated by V. Loia.
Publisher's Note
Springer Nature remains neutral with regard to jurisdictional claims in published maps and institutional affiliations.
Rights and permissions
Reprints and permissions
About this article
EL Mazgualdi, C., Masrour, T., El Hassani, I. et al. Machine learning for KPIs prediction: a case study of the overall equipment effectiveness within the automotive industry. Soft Comput 25 , 2891–2909 (2021). https://doi.org/10.1007/s00500-020-05348-y
Download citation
Published : 05 October 2020
Issue Date : February 2021
DOI : https://doi.org/10.1007/s00500-020-05348-y
Share this article
Anyone you share the following link with will be able to read this content:
Sorry, a shareable link is not currently available for this article.
Provided by the Springer Nature SharedIt content-sharing initiative
- Machine learning
- Key performance indicators
- Overall equipment effectiveness
- Improvement
- Find a journal
- Publish with us
- Track your research
This site uses cookies to improve your experience. By viewing our content, you are accepting the use of cookies. To help us insure we adhere to various privacy regulations, please select your country/region of residence. If you do not select a country we will assume you are from the United States. View our privacy policy and terms of use.
- Inventory Management Software
- Forecasting
- Sustainability
- Supply Chain Visibility

Supply Chain Case Study: the Executive's Guide
Supply Chain Opz
JUNE 1, 2014
Analysis of case study is certainly one of the most popular methods for people from business management background. In order to accelerate the learning, this article has gathered 20+ most sought-after supply chain case studies , analyzed/categorized them by industry and the findings are presented.

Melitta: Collaborating for an Improved Forecasting Process
NOVEMBER 2, 2020
Ultimately, they were able to increase their statistical forecast accuracy by 3.2% ( KPI : WMAPE) within six months of the French pilot project’s beginning. Read the full Melitta case study below. Read Case Study .
This site is protected by reCAPTCHA and the Google Privacy Policy and Terms of Service apply.
- Scaling Logistics for Success: A White Claw Story
MORE WEBINARS
Trending Sources
Supply Chain Shaman
Talking Logistics
Logistics Viewpoints
- The Logistics of Logistics
- Enterra Insights
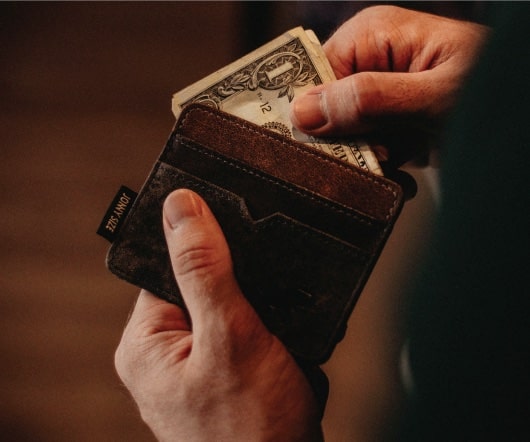
4 steps to optimize truck loads for maximum profits
DELMIA Quintiq
OCTOBER 18, 2019
Let’s take a look at a sample case study involving a large US-based retailer. DELMIA Quintiq’s planning solution enabled the retailer to seize opportunities for route consolidation and improve its KPI for empty miles — something no other solution provider in the vendor selection process was able to prove they were able to do.
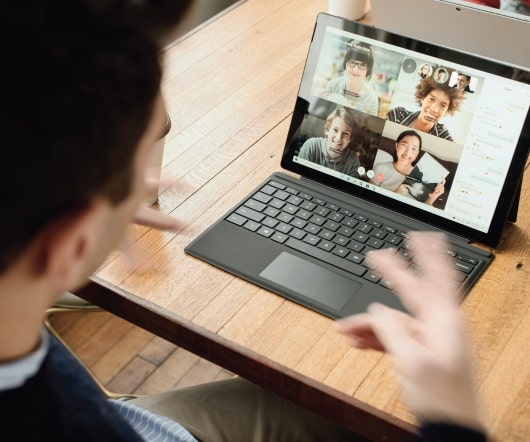
How to Package and Price Embedded Analytics
Advertisement
Just by embedding analytics, application owners can charge 24% more for their product. How much value could you add? This framework explains how application enhancements can extend your product offerings. Brought to you by Logi Analytics.
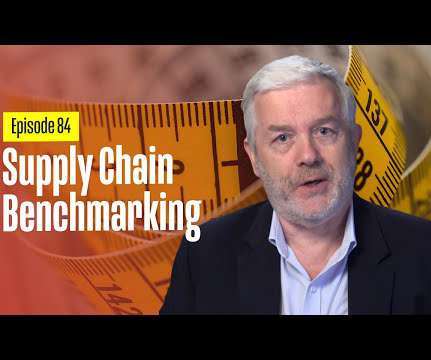
Basic Introduction on Supply Chain and Logistics Benchmarking
Logistics Bureau
OCTOBER 13, 2020
Robobyrne: Warehouse & Distribution Centre Benchmarking Case Study . Supply Chain Secrets: One Of The Best KPI Ever. Related articles on this topic have appeared throughout our websites, why not check them out? Benchmarking Success: Why Performance Benchmarking is a Powerful Management Tool.
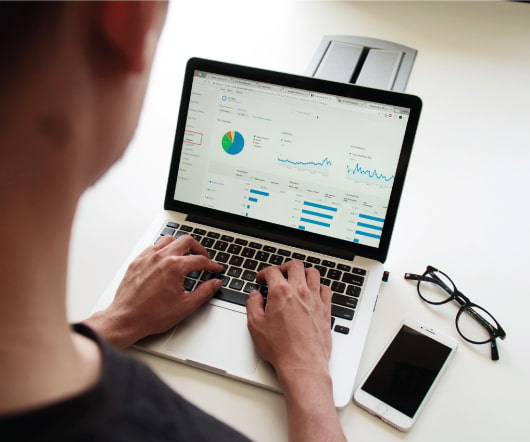
Beyond Operational Metrics: Why Measuring Employee Interpersonal Performance is Also Important
AUGUST 5, 2013
Leadership Development Past Episode balanced scorecards dashboards Decision Wise interpersonal performance KPI operational metrics performance reviews Tracy Maylett' In this episode, Tracy Maylett , Ed.D, If you have a question or comment for Tracy, post it below and keep the conversation going!
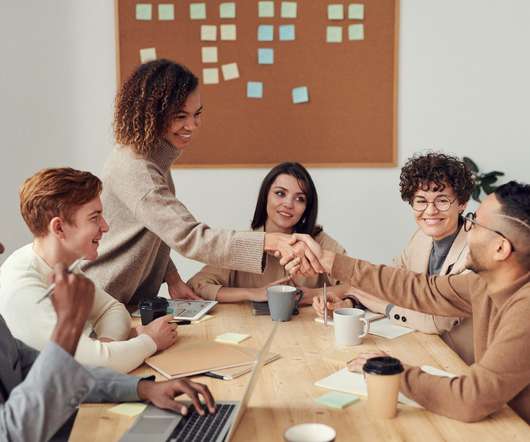
Women in Procurement: Recent Recruits
JULY 29, 2022
Madeline has recently completed her first client engagement, which gave her the opportunity to assist in KPI and Risk scorecard creation for over 100 contracts, spread between 30 contract managers. Madeline joined the team in London and has been an Analyst for just over six months as part of our graduate program.
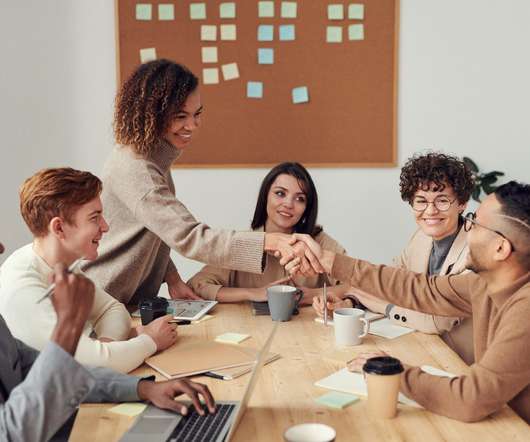
Women in Procurement: Recent Recuits
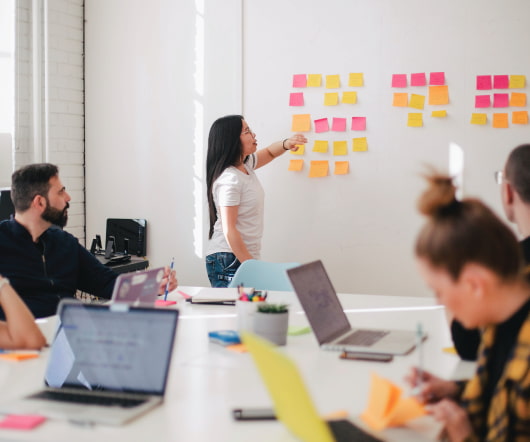
Logistics KPIs Case Study: Whirlpool’s Supply Chain and Logistics Success Driven by Effective KPI Implementation
GlobalTranz
OCTOBER 29, 2015
We conclude our ongoing series in talking about effective KPI management by giving you a real live Logistics KPIs management case study from Whirlpool's engagement with a logistics service level provider. The Reiterated Importance of Understanding and Managing KPIs in Business and Logistics Management.
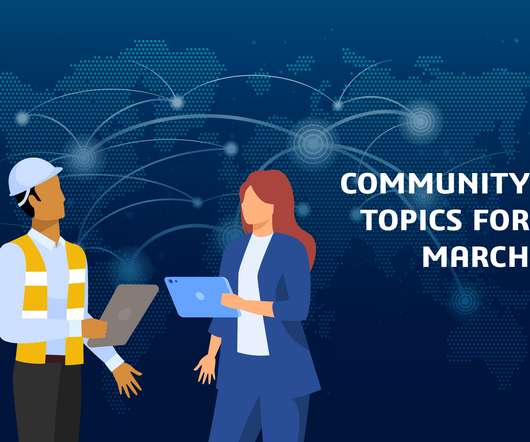
DELMIA Communities Month in Review
MARCH 31, 2022
FORVIA Faurecia Case Study & Video. REPLAY] Optimize your planning with KPI in DELMIA Ortems. Choose a session (French or English) and discover how to make the right KPI -based business decisions leveraging DELMIA Ortems. We welcome you to connect with us, exchange and share in the DELMIA Communities. Watch the replay.
FMCG Foods Turnaround: A highly successful S&OP Case Study
Enchange Supply Chain Consultancy
JULY 23, 2014
Amongst many other enhancements the following KPIs have been implemented and are measured monthly: KPI Measurement. Budgeted monthly sales targets have been achieved for 6 consecutive months. Project Start. Project End. Business plan achievement. Growth over previous year. -15%. Stock outs finished goods.
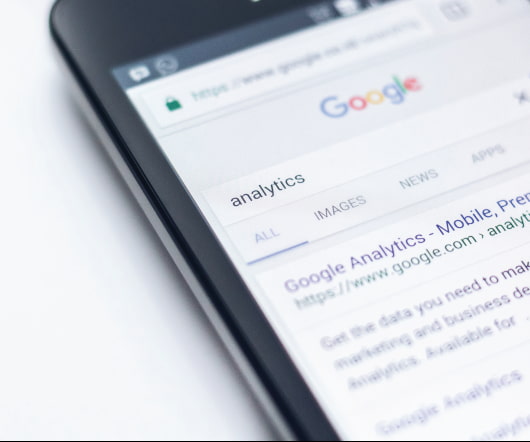
Supply Chain Maturity Model for Capability Assessment
MAY 28, 2013
Case Studies . As you may notice, supply chain maturity model is like " KPI " but its expressed qualitatively and you have to use it strategically. Supply Chain Case Study : Executives Guide. A Case Study . Supply Chain Maturity Model for Capability Assessment. 2) Procurement Maturity Model.
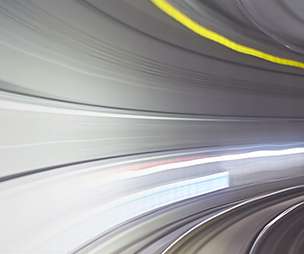
Bristlecone Flash – July 2020
Bristlecone
JULY 31, 2020
Read Case Study . Get your questions answered and see how data analytics can empower businesses with rapid, KPI -driven 360° insights. Bristlecone’s AI-powered Demand app improves forecast accuracy, aligns planning cycles and provides live demand signals that enable visibility into market risks and opportunities. Register Now.
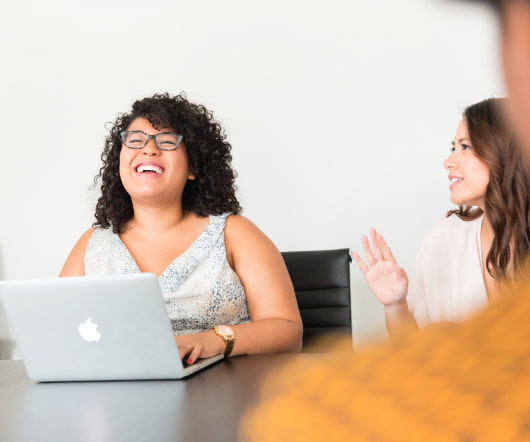
One Of The Best KPIs Ever
OCTOBER 23, 2019
In this video, we are going to reveal and demystify one of the best KPIs ever formulated for business benchmarking (internal that is). Look closely at the simple slide presentation which came from an actual case study and get your calculators ready! Let’s watch it. Subscribe to our Youtube Channel.
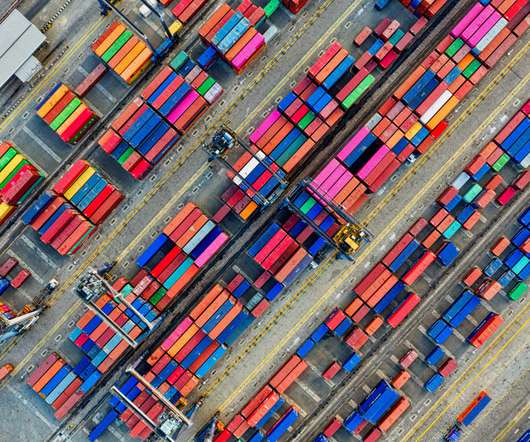
Service Level Should Be Your Number One Supply Chain KPI…But What Does It Really Mean?
AUGUST 11, 2020
Most use ‘forecast accuracy’ as the main KPI to do this. Therefore it follows logically that filling orders–or order line fill rate (OLFR)–should be the number one KPI that tells you whether you’re meeting service levels. First, we need to establish: what’s the whole point of supply chains? To service demand!
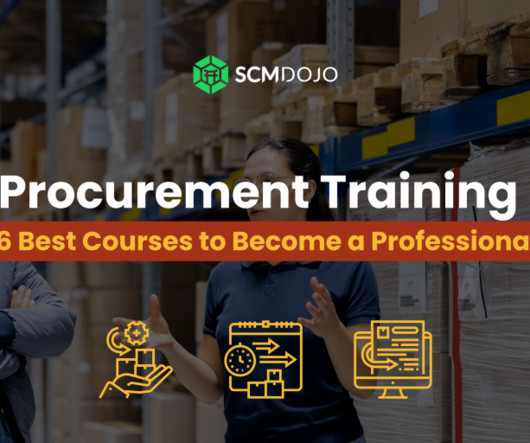
6 Essential Procurement Training Courses for Professionals: How To Begin and Advance Your Career in Procurement
JULY 3, 2023
Take into account elements like the length of the course, the mode of delivery (in-person, online, or mixed), the presence of interactive exercises or case studies , and the availability of opportunities for hands-on practice.
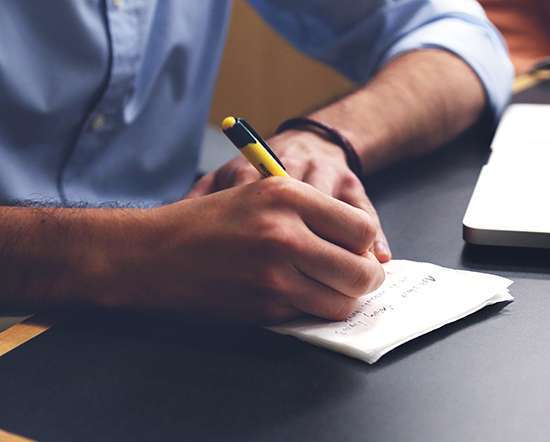
Novo Nordisk: A Story of a Supply Chain Leader
FEBRUARY 12, 2017
Sharing this story is the goal of this case study . Forecast Accuracy and Bias are two of the key KPI s we monitor monthly basis, we also investigate root causes and continuously strive for improved forecast quality. Performance of BMS and Merck at the Intersection of Inventory Turns and Operating Margin.
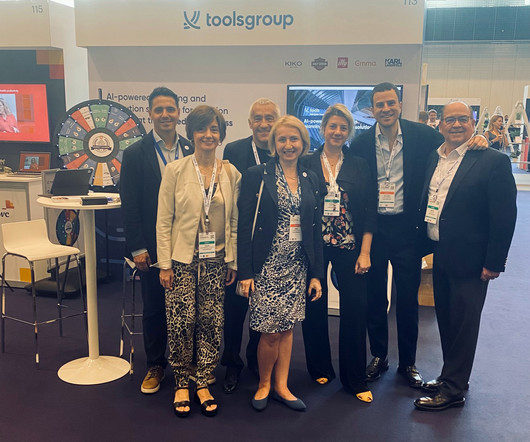
Your Must-Have Gartner EU Debrief: The TopTakeaways from Gartner Supply Chain Symposium/Xpo EU 2023
JUNE 15, 2023
Several end-user case studies were presented on how they are driving Increased urgency to speed up digitization and automation. Seems our European attendees were much more interested in what our SCM wheel was predicting their top KPI might be! In fact, on Tuesday the SCM wheel exactly matched the KPI goals for 18 attendees.
7 Mini Case Studies: Successful Supply Chain Cost Reduction and Management
MAY 24, 2019
The following five mini case studies explore a few high-profile companies that have managed to sustain their supply chain cost-reduction efforts and keep expenses under control. Of course, the above case studies are merely summaries of the changes these high-profile brands made to their supply chains. Sunsweet Growers.
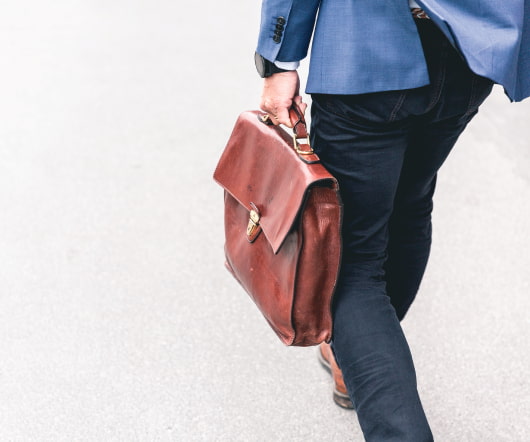
The Talent Gap
JUNE 20, 2017
’ You can read lots of case studies , attend other user presentations, or participate in benchmark surveys to get a bearing on where you are at and some great ideas that can be applied. So those assessments should result in some form of reasonable KPI goal setting. So the first simple idea is to get an assessment.
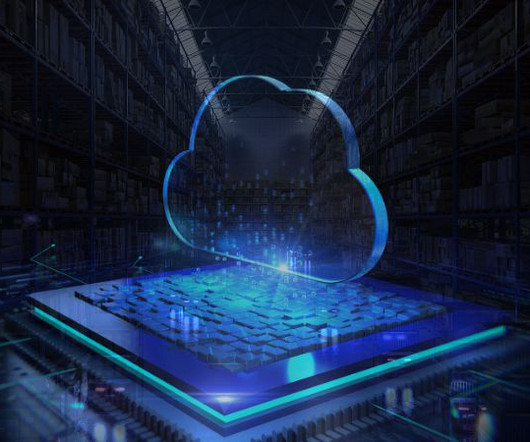
Migrating from Legacy ERP to Cloud-Based ERP and Its Impact on Data Collection Software
NOVEMBER 16, 2023
In this article, we will explore the state of ERP migration to cloud, its impact on barcode software and data collection, how businesses can retain custom supply chain processes when upgrading to cloud, and case studies illustrating successful transitions with mobile data collection.
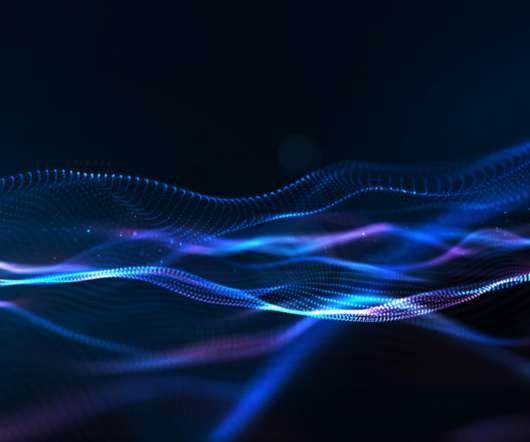
Digital Transformation Journey in Supply Chain Planning
MARCH 4, 2021
Data diagnostics to ensure the inputs are accurate, KPI monitoring and alerts for intervention and root-cause analysis are essential components to guide the planners. . Also, follow us on LinkedIn and YouTube for more information and read our customer case studies on the digital transformation of supply chain planning.
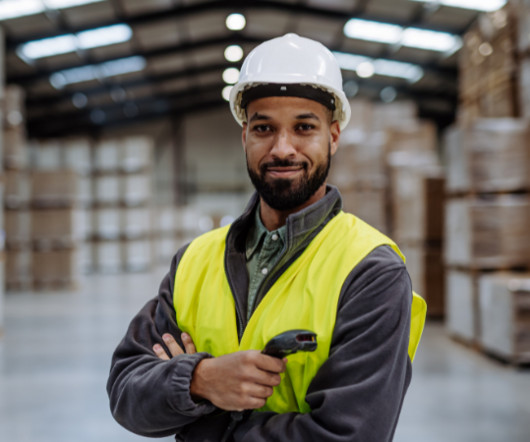
How Advanced Inventory Control Unlocks Business Growth
MARCH 27, 2024
Advanced Reporting and Analytics: Offers visual report dashboards and KPI analytics on inventory performance, helping businesses identify trends, make informed decisions, and optimize inventory strategies. Manual work led to unacceptable errors and inefficiency.
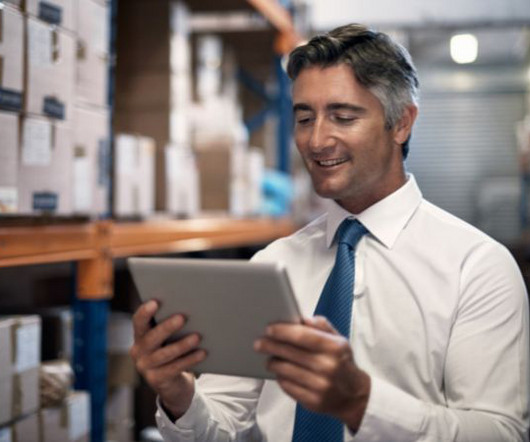
An Intelligent Alternative to WMS: Gaining Real-Time Inventory Visibility Without the Complexity
AUGUST 10, 2023
inventory accuracy 30-42% more efficiency and productivity KPI tracking and improvement Reduced overhead spend (labor, carrying costs, etc.) Mobile barcoding is an enterprise mobility solution that automates and mobilizes inventory management and warehouse tasks at a fraction of the cost and complexity of WMS.
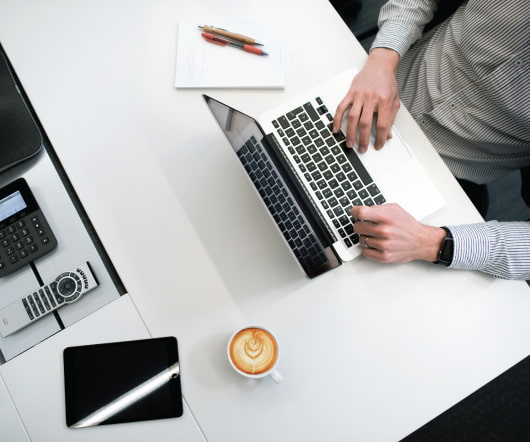
Impact of SMAC on Enterprise Software Applications - Infosys Blogs
Infosys Supply Chain Management
APRIL 8, 2014
Case Studies . |. « Gamification - An EAM perspective | Main | Whats your KPI ? Further the BI output would not be limited to be a summarized report or a simple KPI based on simple arithmetic, but it is going to be much more advanced. Whats your KPI ? White Papers. |. News & Events. |. Supply Chain Matters.
Business Process Optimization in Asset Management - Infosys Blogs
JUNE 20, 2013
Case Studies . |. IT enablement in areas like Mobile work management, Health and Safety, Metrics monitoring ( KPI ) etc. Mobile Banking. Payments Treasury. Wealth Management. View all Infosys blogs. Supply Chain Management. Features & Opinions. |. Offerings. |. White Papers. |. News & Events. |. would be beneficial 9.
4 Best-in-Class Supply Chains To Watch and Learn From
NOVEMBER 27, 2016
The measurements however, are just a means to an end , with the KPI -driven conversations being considered more important than the actual metrics. Like everything else in the McDonalds approach to supply chain management, KPIs are in place to provide insight and support alignment , not to lord it over suppliers.
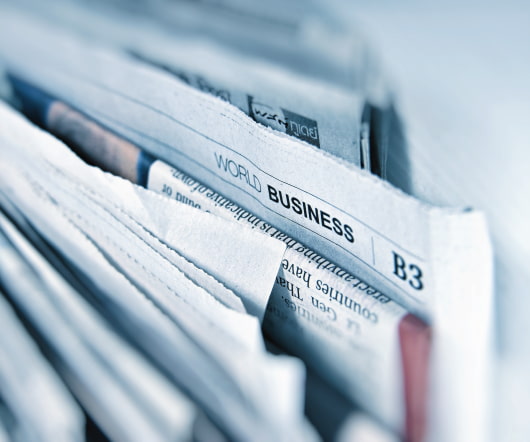
Gartner Session Summary: Design and Implementation of S&OE Process to Deliver Business Benefits
MAY 23, 2019
There were multiple sessions in the agenda on this topic, but the one that I found to have the most insightful information was the S&OE case study presented by Marko Pukkila, Gartner Vice President, and Aaron Baker, Director of Operations Inventory Management at Levi Strauss & Co.
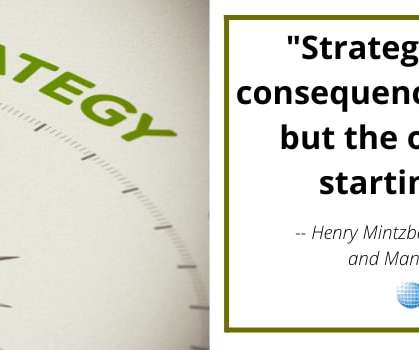
No Supply Chain Strategy? Here’s How to Develop One
JULY 18, 2022
Ideally, though, evaluating your position against competitors should not solely be a KPI benchmarking exercise. They can also aid you in identifying and implementing best practices to further your performance objectives. Former employees who can give you some inside information.
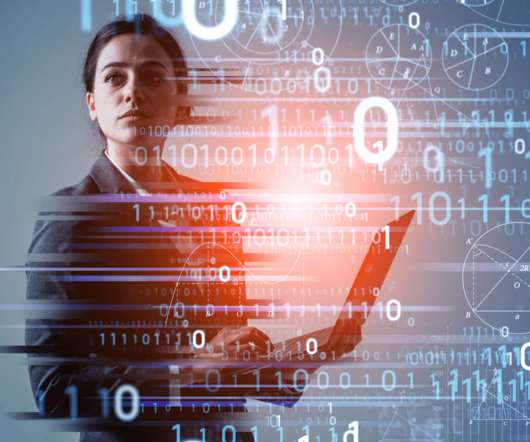
Supply Chain Management and Analytics
MARCH 5, 2021
The Magic Bullet for Real-Time Supply Chain Collaboration? Cloud Visibility.
JUNE 19, 2017
Individual Plants can visualize real-time end-to-end production flow and the status of safety, compliance, and key performance indicators ( KPI ) at any level in the facility. Video case study : How Orbital ATK is Leveraging the IIoT and Visual Factory Technology to Drive Continuous Improvements.
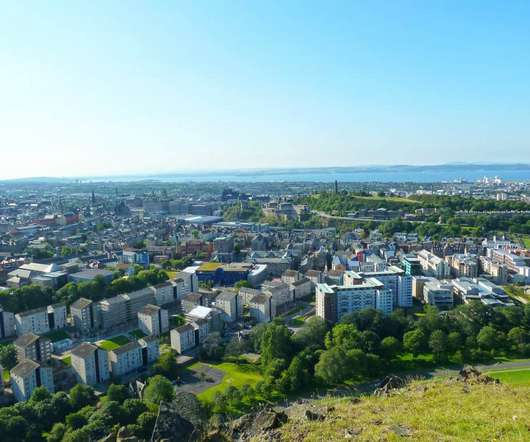
Scottish Councils Deliver Streamlined Contract and Supplier Management
MAY 19, 2020
We considered it beneficial for both councils to meet to discuss current KPIs and scoring methodology to see if we could re-align these to some of the national performance indicators.” . To read the full case study , plus more on public sector procurement, get the white paper, GOING DIGITAL IN PUBLIC SECTOR PROCUREMENT.
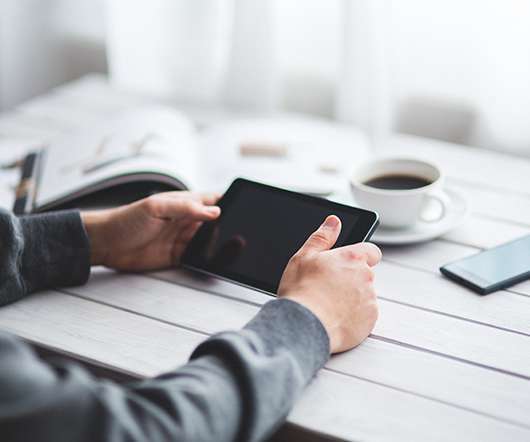
10 key trends to understand Supply Chain Management
KEPLER Consulting
NOVEMBER 24, 2017
Became a case study for marketing students worldwide. KPI definition (calculation method, scope, target, top down cascading…) and dashboard set up are a basis to give Managers the right levers to drive SC efficiency. Data handling become critical to capture this vision and take arbitration accordingly.
Stay Connected
Join 136,000+ Insiders by signing up for our newsletter
- Participate in Supply Chain Brief
- How to achieve six-figure benefits from digitizing paper-based supply chain operation
- 2019 Supply Chain Brief Summer Reading List
- Stay At Home Reading List
- Add a Source
- Add a Resource
- See All
- 2018 Supply Chain Brief MVP Awards
- 2019 Supply Chain Brief MVP Awards
- 2020 Supply Chain Brief MVP Awards
- 2021 Supply Chain Brief MVP Awards
- 2022 Supply Chain Brief MVP Awards
- Tue. Apr 16
- Mon. Apr 15
- Sun. Apr 14
- Sat. Apr 13
- Apr 06 - Apr 12
- Warehousing
- Procurement
- Transportation
- Supply Chain
- More Topics

Input your email to sign up, or if you already have an account, log in here!
Enter your email address to reset your password. a temporary password will be e‑mailed to you., be in the know on.
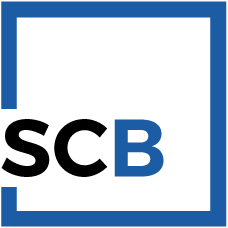
Supply Chain Brief
Expert insights. Personalized for you.
We organize all of the trending information in your field so you don't have to. Join 136,000+ users and stay up to date on the latest articles your peers are reading.

Get the good stuff
Subscribe to the following Supply Chain Brief newsletters:
You must accept the Privacy Policy and Terms & Conditions to proceed.

You know about us, now we want to get to know you!
Check your mail, we've sent an email to . please verify that you have received the email..
We have resent the email to
Let's personalize your content
Use social media to find articles.
We can use your profile and the content you share to understand your interests and provide content that is just for you.
Turn this off at any time. Your social media activity always remains private.
Let's get even more personalized
Choose topics that interest you., so, what do you do.
Are you sure you want to cancel your subscriptions?
Cancel my subscriptions
Don't cancel my subscriptions
Changing Country?
Accept terms & conditions.
It looks like you are changing your country/region of residence. In order to receive our emails, you must expressly agree. You can unsubscribe at any time by clicking the unsubscribe link at the bottom of our emails.
You appear to have previously removed your acceptance of the Terms & Conditions.

We noticed that you changed your country/region of residence; congratulations! In order to make this change, you must accept the Aggregage Terms and Conditions and Privacy Policy. Once you've accepted, then you will be able to choose which emails to receive from each site .
You must choose one option
Please choose which emails to receive from each site .
- Update All Sites
- Update Each Site
Please verify your previous choices for all sites
Sites have been updated - click Submit All Changes below to save your changes.
We recognize your account from another site in our network , please click 'Send Email' below to continue with verifying your account and setting a password.
You must accept the Privacy Policy and Terms & Conditions to proceed.
This is not me
- Content Writing Services
- Get in Touch
How 8 Customer Marketers Measure Case Study Success
Updated April 2022: Successful B2B SaaS case studies take time and effort to produce from everyone involved—including your customers—which means it’s crucial to evaluate whether these sales tools are performing well enough to make the content creation process worth your while.
However, determining case study success is often easier said than done because many SaaS companies use both quantitative and qualitative metrics to evaluate a home run versus a dud, and gathering this data can be challenging.
In this blog post, we’ll share insights on this tricky issue directly from customer marketing leaders in the SaaS industry, as we dig into what case study success looks like for 8 customer marketers.
Case study success: why is it so hard to measure?
Your B2B SaaS case studies can only be successful if they have impact, and to have impact, these stories must be shared.
Content creators rely on others within their organizations, such as social media marketers and sales reps, to get these stories in front of potential customers, which makes it hard to keep track of the content’s success.
Even when these case studies do get promoted online , it’s easy for them to get lost in a sea of competing content.
Say your case study catches a prospect’s eye on LinkedIn and they click through to your website to read it.
Even at that point, it’s tough to determine case study success because metrics such as page views and time spent on a page track the level of consumption of a story, but not how it was received by readers, which is highly subjective, or how it might go on to influence a deal.
No cohesive view of case study success
Case study success is something that every customer marketer is aiming for. But it’s extremely hard to get a cohesive view of how case studies perform.
While some SaaS marketers report receiving anecdotal feedback on case studies from sales colleagues, this is a rare occurrence.
Other customer marketers feel they have no concrete view into how often case studies are used in sales conversations.
Metrics can be deceiving because there’s no way to know how often a sales rep has saved the content to their desktop and emailed it to a lead.
Finally, technology poses another challenge. One SaaS marketer describes case studies as an engine that powers other components of marketing and sales, which often use different MarTech stacks. As a result, the variety of places marketers need to look for metrics makes the evaluation process time consuming.
With a multitude of tools being used, it becomes tricky to get a cohesive view of the impact of your case studies, and you’ll often be missing key pieces of data required to gain a full picture.
Case in point
In December of 2023, we conducted a survey of 115 SaaS marketers and found that 38% of SaaS companies say they don’t measure case study performance at all because they lack the time, resources or know-how to do so.
The rest of the respondents use a wide variety of marketing tactics to evaluate case studies.
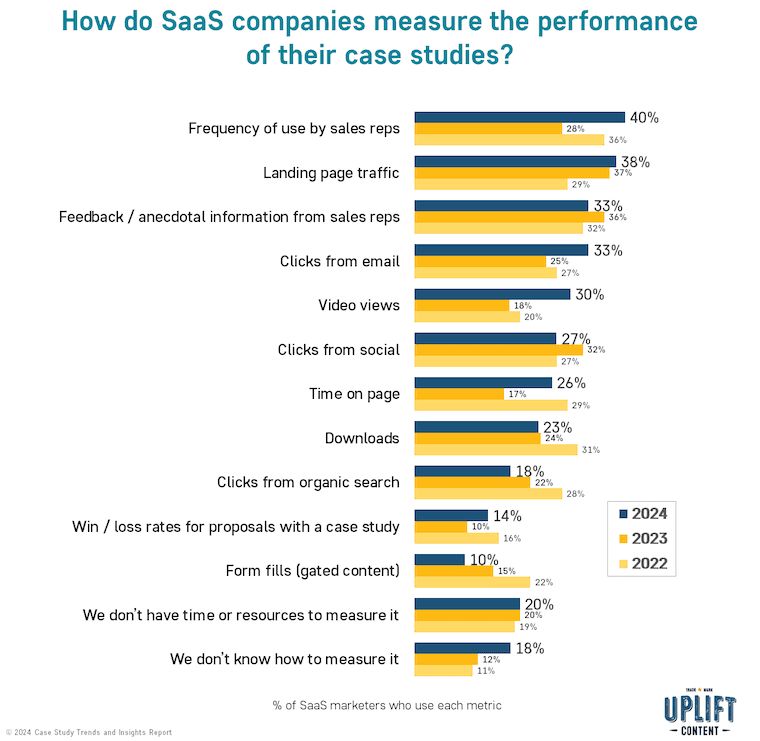
Read the full report here: 2022 SaaS Case Studies Trends & Tactics Report
Case studies: why is it important to measure performance?
Without setting and tracking key performance indicators (KPIs) for your case studies, you’ll have a harder time developing strategy and allocating budget for them.
It’ll also be more difficult for you to analyze your competition and demonstrate accountability to your boss. Establishing KPIs will enable you to get a sense of your wins and opportunities for improvement.
Knowing which of your case studies are performing best can help inform your next steps, such as promoting these heavy hitters far and wide.
Many customer marketers are creating so much content on a quarterly basis that it’s tough to build awareness of each individual piece due to information overload.
Sales teams are being bombarded with emails and messages, and sometimes case studies get lost in the shuffle. Identifying priority content based on performance can help solve this problem.
When it comes to the laggards, this content is just as crucial to pay attention to. By optimizing these stories for better performance , you can make them work harder for your SaaS company and generate more conversions, rather than focusing your resources and efforts on creating new content from scratch.
8 customer marketers weigh in on how to measure case study success
There doesn’t seem to be any research available online that speaks to how SaaS marketers measure case study success. So, we spoke directly to 8 customer marketers in the B2B SaaS space to find out how they measure the performance of their case studies. Here’s what we learned:
Andrew Sevillia, Director, Customer Marketing, Sage Intacct
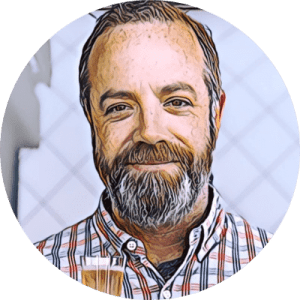
“Case study success for me is if I can capture the story, then my PR team can pitch it and it gets picked up by one of the industry or business publications we work with. Another big success is when these case studies get used by our demand gen or sales staff, and when the stories help to either attract prospective customers or to speed up the sales cycle.” — See Andrew’s LinkedIn profile
Matt Arout, Senior Customer Advocacy Manager, Google
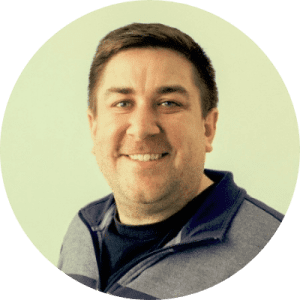
“We use Looker to measure case study success! Looker can be used to tie multiple data sources together to build a dashboard. We have a content dashboard that gives real time insights on how content is performing via Google Analytics, email campaign performance, social performance and overall influence on our pipeline.” — See Matt’s LinkedIn profile
Meaghan Britain, Senior Customer Marketing Manager, CPA Global
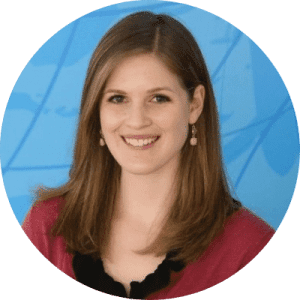
“We measure the landing page and channel performance of case studies. We gate several case studies, so we can track individual channel KPIs such as social impressions, as well as landing page metrics such as page visits, form fills/downloads and video views.” — See Meaghan’s LinkedIn profile
Sam Shepler, CEO, Testimonial Hero
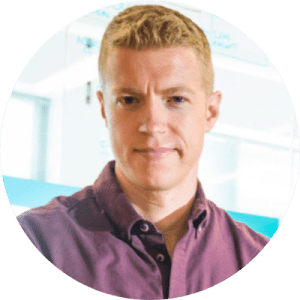
“Given that about 80% of marketing content goes unused by sales, if sales is using a case study, that’s a good thing. They wouldn’t be using it if it wasn’t working. Another way we measure case study success is by checking to see if the story has influenced deals in the pipeline. If deals are being closed, and your sales leaders are thanking you and telling your CEO they’re getting exactly what they needed from you, that’s all the measurement you need.” — See Sam’s LinkedIn profile
Jeanne Talbot, Director, Customer Marketing, CloudBees
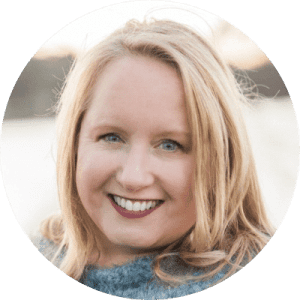
“The mistake many make is trying to measure the impact of case studies instead of thinking about the impact of the campaigns that the story, video, quote, etc. supports. Everything we create in customer marketing supports other elements of the marketing function.” — See Jeanne’s LinkedIn profile
Kyle Yantis, Former Customer Advocate Associate, Ellucian
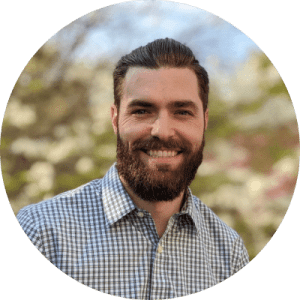
“We use Marketo and Eloqua dashboards to measure email campaigns. We also track click rates on our website through Google Analytics, and case studies that were included in RFPs by comparing closed/won rates.” — See Kyle’s LinkedIn profile
Lauren Turner, Customer Marketer, UserTesting
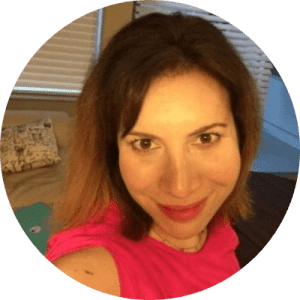
“Time to close is also a great metric to track. The case study may not be the thing that gets the deal signed, but it can help remove objections and get the process done more smoothly. We also look to increase the SEO value of our other assets by embedding as many relevant links into case studies as we can that point to related blogs, white papers, and other content in an effort to drive traffic.” — See Lauren’s LinkedIn profile
Senior Customer Marketing Leader
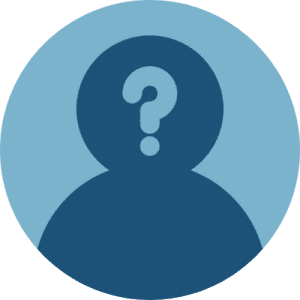
“We monitor downloads from our website and minutes spent on pages. We also analyze the traffic of won deals to see which assets influenced our customers’ decisions during the entire sales process. I’d love to get to a place where we measure which specific case studies were shared against each opportunity, but we aren’t there yet. This would require a field on the opportunity records in Salesforce, for example.” —Senior customer marketing leader who prefers to remain nameless
As you’ve probably gathered by now, there’s no specific “right” way to measure case study success. However, by sharing knowledge and discussing what works for each of us and what doesn’t, we can all learn from one another.
Give your SaaS case studies a boost
The stakes are high when it comes to creating excellent case studies—and we can help. Check out our case study writing service .
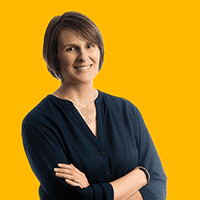
As the founder of Uplift Content, Emily leads her team in creating done-for-you case studies, ebooks and blog posts for high-growth SaaS companies like ClickUp, Calendly and WalkMe. Connect with Emily on Linkedin
Sign up for the Content Huddle newsletter
Learn from Emily’s 17 years of aha moments, mistakes, observations, and insights—and find out how you can apply these lessons to your own marketing efforts.
You can unsubscribe any time. Visit our Terms of Use for information on our privacy practices.
Let's talk about your next big project.
Looking for a new career?
By submitting, you consent to KPI Partners processing your information in accordance with our Privacy Policy. We take your privacy seriously; opt out of email updates at any time. This site is protected by reCAPTCHA and the Google Privacy Policy and Terms of Service apply.
Case Studies
Discover our latest customer stories..
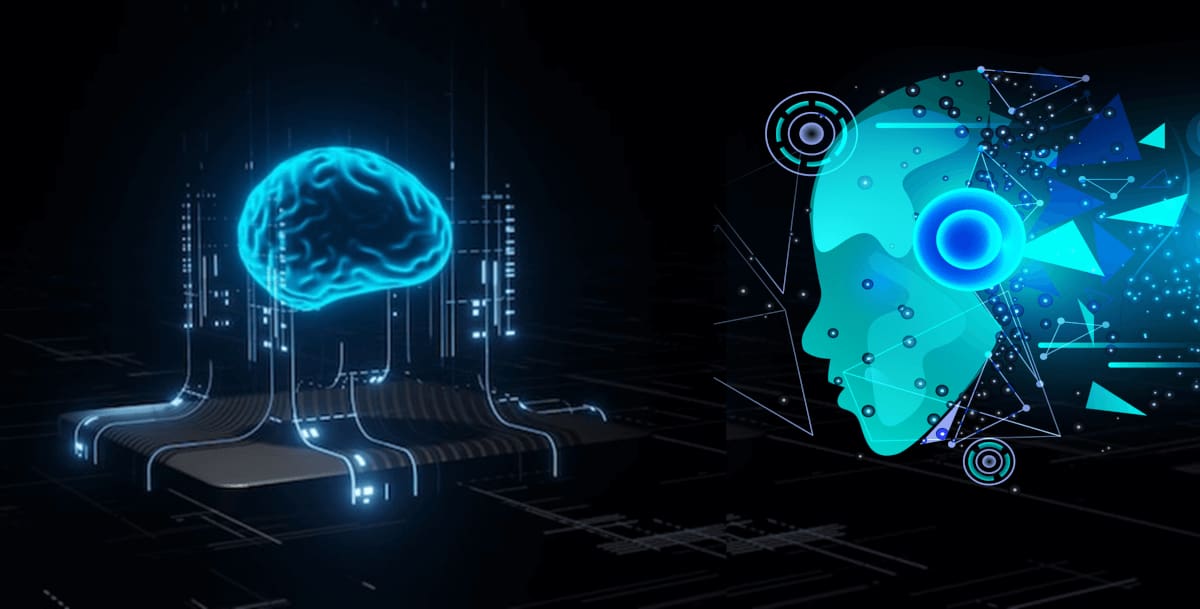
Enhancing Customer Propensity Models with ML Techniques and MLOps Workflows
The challenge was to enhance the accuracy of existing customer propensity models by using a large amount ...
- App Development
- Cloud Transformation
- Data Engineering
- Data Management
- Data Science and AI / ML
- Database Services
- DevOps/DataOps/MLOps
- No Code Development
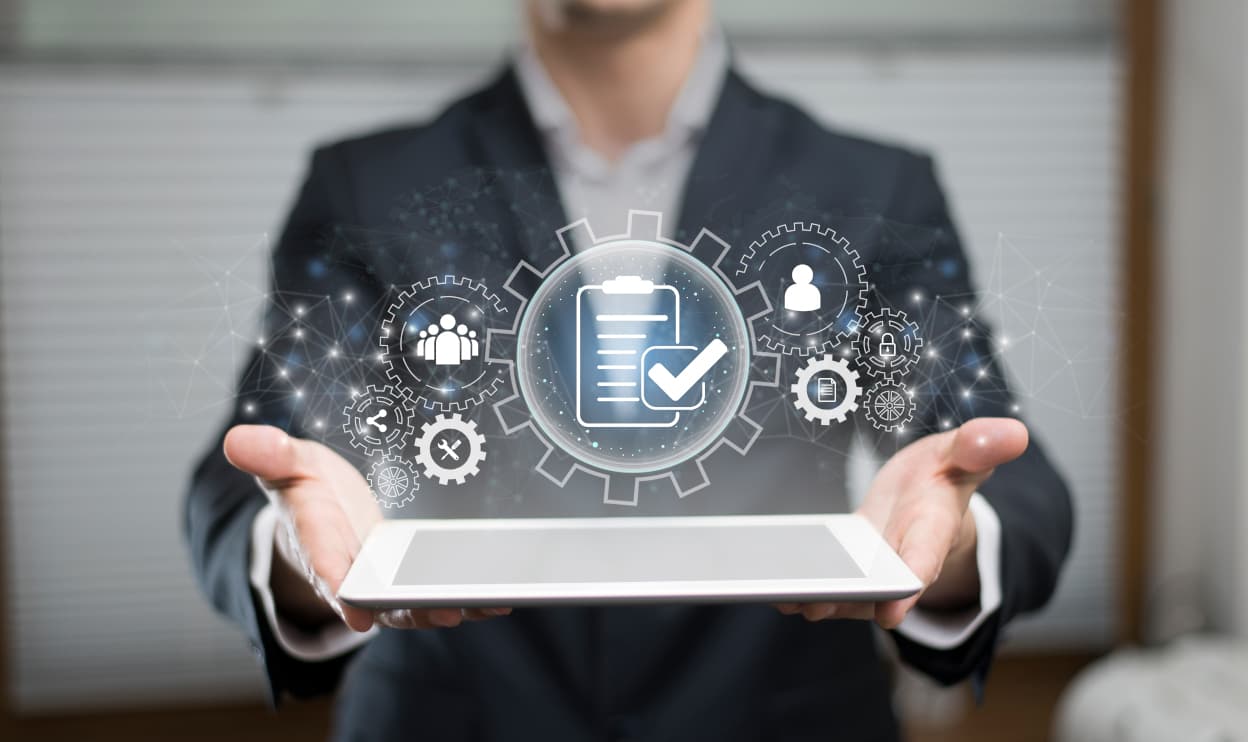
Stay up to-date
Select Clients
Your future starts today. ready.
Filter by Keywords
Human Resource KPIs – 10 HR KPIs & Examples
Content Partnership Specialist
February 23, 2022
Choosing the right human resources KPIs is like hiring the best HR professionals.
What do we mean?
During the recruitment process, you want to select the people to lead your company to success, right?
Similarly, you need to select the right human resources KPIs that will get your company results.
But with tons of great HR KPIs out there, how do you know which ones you should be tracking?
Don’t worry!
In this article, we’ll go over everything you need to know about HR KPIs and take a look at the ten best KPIs and metrics you need to track today. We’ll also cover the easiest way to track KPIs for HR.
What Are HR KPIs?
Recruitment kpis, employee engagement kpis, compensation & benefit kpis, 1. set hr goals, 2. manage your hr kpis, 3. track your hr team’s performance, what is an hr balanced scorecard, characteristics of good hr kpis, time to onboard some hr kpis 🛥.
Let’s get down to business.

First, let’s break down what KPI stands for:
KPI stands for K ey P erformance I ndicator.
Key performance indicators are measurable metrics that show an organization if they’re achieving core business objectives. That’s why human resources KPIs must be used to track the performance of your HR activities.
Your human resources department is responsible for hiring and retaining the best talent, and without an HR KPI Dashboard to guide them on how well they’re doing, your employees (and even managers) might be confused.
10 HR KPIs & Metrics
To make your job easier, we’ve shortlisted ten human resources performance metrics and KPIs for you.
And to make this more comprehensive, we’ve also organized the KPIs into three different categories:
- Recruitment
- Employee engagement
- Compensation and benefits
Recruitment is a crucial HR function, so of course, you need some recruitment KPIs.
Recruitment KPIs determine the effectiveness of your hiring process . They are the human resources metrics that help you reduce your recruitment costs and retain the best talent.
Here are some examples:
Cost per hire
This metric measures the average number of resources you’ve invested into hiring one employee.
Wait, what are resources?
Hiring resources include marketing and advertising expenses, the hiring process, and referrals .
A high cost per hire might mean that you need to improve specific areas in your recruitment process. By doing so, you can reduce your recruitment cost and improve your hiring process’s efficiency .
Here’s how you can calculate it:
Cost per hire = (Total internal recruiting costs + external recruiting costs + external recruiting costs) (Total # of hires in a given time frame)
Employee retention rate
The employee retention rate measures how well your company can keep your top performers motivated and engaged. This includes practices and strategies that aim to keep your employees happy, trained, and prepared so that they excel at their job.
For example, motivating them through recognition and awards. 🏆
This HR metric helps companies get insight into why top performers choose to stay at their company. This way, your organization can stay competitive with salaries and benefits when recruiting new employees.
Top talent retention rate = Total number of employees – Total number of quitting employees Total number of employees
First-year turnover rate
Employee turnover measures how many employees you lose over a period of time.
The first-year turnover rate calculates the percentage of employees who resign less than one year of joining your organization.
The first-year turnover rate shows if you need to improve your hiring and onboarding processes since early resignations indicate a fundamental mismatch between a new hire and a company.
First year turnover rate = Total number of employees who leave Number of employees who quit during the same period
Employee engagement KPIs help you determine if your organization is putting in the right policies to keep your employees engaged.
This includes adequate training, benefits, and a healthy work environment. These elements boost employee engagement and reduce turnover rates.
Let’s take a look at some performance indicators that will help you determine how engaged your employees are:
Employee satisfaction index
Employee satisfaction is an important KPI that measures how happy your employees are.
Why’s this important?
Keeping your employees happy and engaged reduces turnover rates.
But how do you know if your employees really love their job or just stick around for the paycheck? 🤨
You can determine employee satisfaction through online surveys, interviews , or informal conversations.
Net promoter score
Employee net promoter score gives you insight into how many employees would recommend your company as a place to work.
A simple way to do this is by asking employees to rank your organization from 0–10:
- 0–6 = Detractor ❎
- 7–10 = Promoter ✅
You can use surveys or interviews to find out why your detractors feel this way about your company. In doing so, your company can determine what might be lacking in the current work environment and reduce turnover rates.
Net promoter score = % of promoters – % of detractors
Absenteeism rate
This HR KPI measures the amount of productivity lost due to your employees being sick, tired, or any other reason for absence. The absence rate can be applied to an individual, team, or the company as a whole.
Although a certain absenteeism level is normal, employees who continuously call in “sick” are a red flag. 🚩
It could mean that your employees lack motivation, are burnt out , or the work environment is unpleasant.
Absenteeism rate = Total number of lost workdays due to absence Number of available workdays in an organization
The percentage of vacation days used
This employee KPI is all about a healthy work-life balance.
Your company should give employees a good number of days to go on holiday, relax, and well, let loose. #SunsOutBunsOut. ⛱
You should also encourage employees to use their vacation days since taking a break from work can boost employee productivity.
The percentage of vacation days used = The number of vacation days used The number of unused vacation days x100
These performance indicators measure how much money and other resources your company invests in your employees. This includes salaries, training, incentives, and anything else with which you spoil your employees. 💁
These elements can help reduce employee turnover.
Let’s take a look at some of these:
Salary competitiveness ratio
You know how companies compete to attract customers?
Similarly, your HR department contests with other companies to attract the best talent.
Of course, offering an eye-catching salary package is one way to do it!
The salary competitiveness ratio is a measure of how competitive your company’s salaries are versus other companies that offer the same job roles.
A high salary competitiveness ratio gives your company a competitive edge over your competitors.
You know what they say: money talks. 🤑
Salary competitiveness ratio = Salary offered by your company Salary offered by your competitor
Percentage of the cost of workforce
The percentage of the cost of the workforce should include money spent on salaries, training , recruitment costs, benefits, and bonuses.
You should also include any other costs like taxes, insurance, vacation pays, and overtime pays.
Remember, ideally, the more you invest in human capital , the more productive your employees will be.
The above KPI is essential for making decisions about salary increases, promotions, new hires, and training programs .
Well, unless you want to do this:
You should use the data from that metric to get insight into what you can spend on your employees.
Percentage of cost of workforce = Cost of the workforce per month total operating costs of the company x100
Healthcare expense per current employee
This metric measures how much your company spends on healthcare plans for every employee.
After all, healthy employees are happy employees, right?
Offering a good healthcare plan will help your company attract and retain employees. But it’s also essential to make sure that your healthcare provider isn’t overcharging you.
If that’s the case, you can switch to a provider with lower rates or take on an even better healthcare plan!
Healthcare expense per current employee = Total price of healthcare costs Total number of employees
Learn more about useful HR tools and tips that can help you make the whole process more effective.
How to Track HR KPI Metrics
Human resources KPIs determine if your HR department is reaching the organization’s overall HR objectives.
But how do you know if your HR department is making progress?
Let us ask you this:
Do you still track your HR metrics with Excel sheets ?
If you do, please stop.
You need a powerful HR KPI tool with the right features.
And the best candidate for this job is ClickUp !
Let’s take a look at how ClickUp can help your HR team:
Before you can start tracking anything, you need to set some HR Goals!
Goals are high-level containers that can be broken down into smaller, measurable Targets.
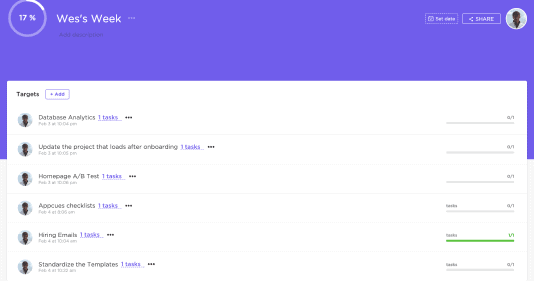
Targets are the objectives you need to reach to hit your human resources KPI.
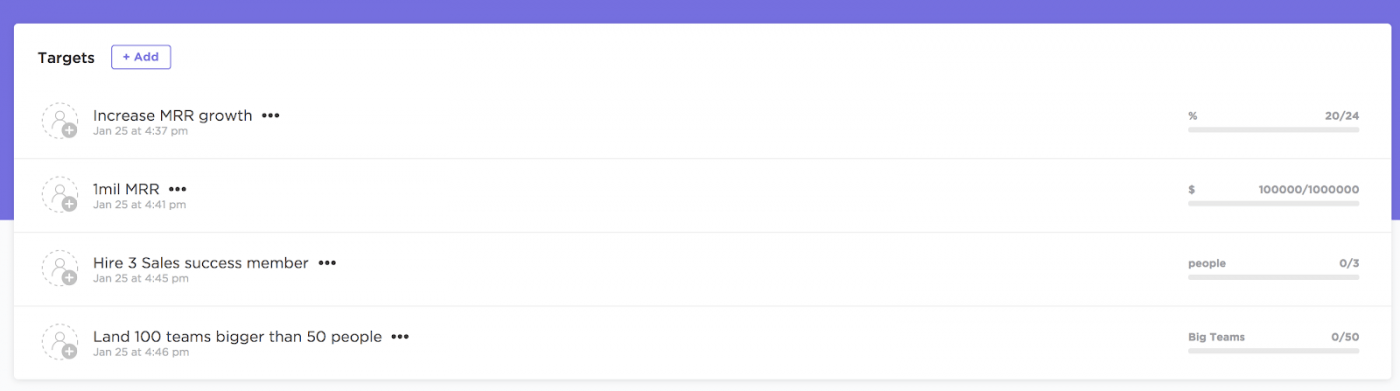
Since KPIs are all about performance, ClickUp motivates you by showing you your KPI progress every step of the way. 👣
Every time you complete a Target, you’ll see how close you are to reaching your KPI.
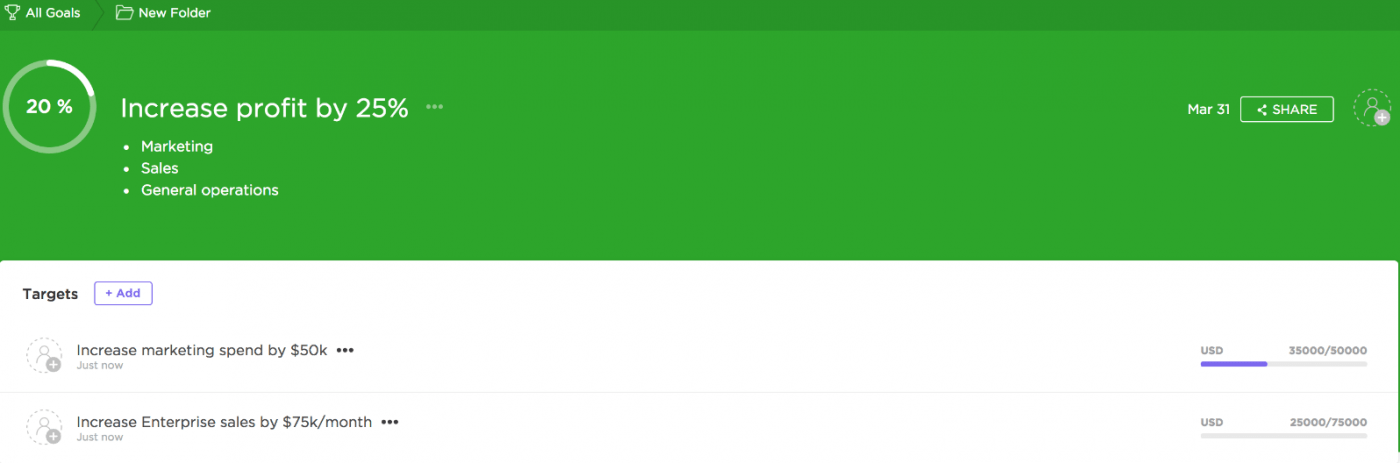
You also get to customize the HR metrics you choose to track your KPIs, such as:
- Numbers : numerical figures such as cost per hire or training costs
- Currency: keep track of your money
- Tasks: check if your HR team is completing their HR duties
Confused about how KPIs differ from metrics? Here’s a post on the differences between KPIs and metrics .
ClickUp’s Dashboards provide you with all the HR analytics you need to manage your KPIs.
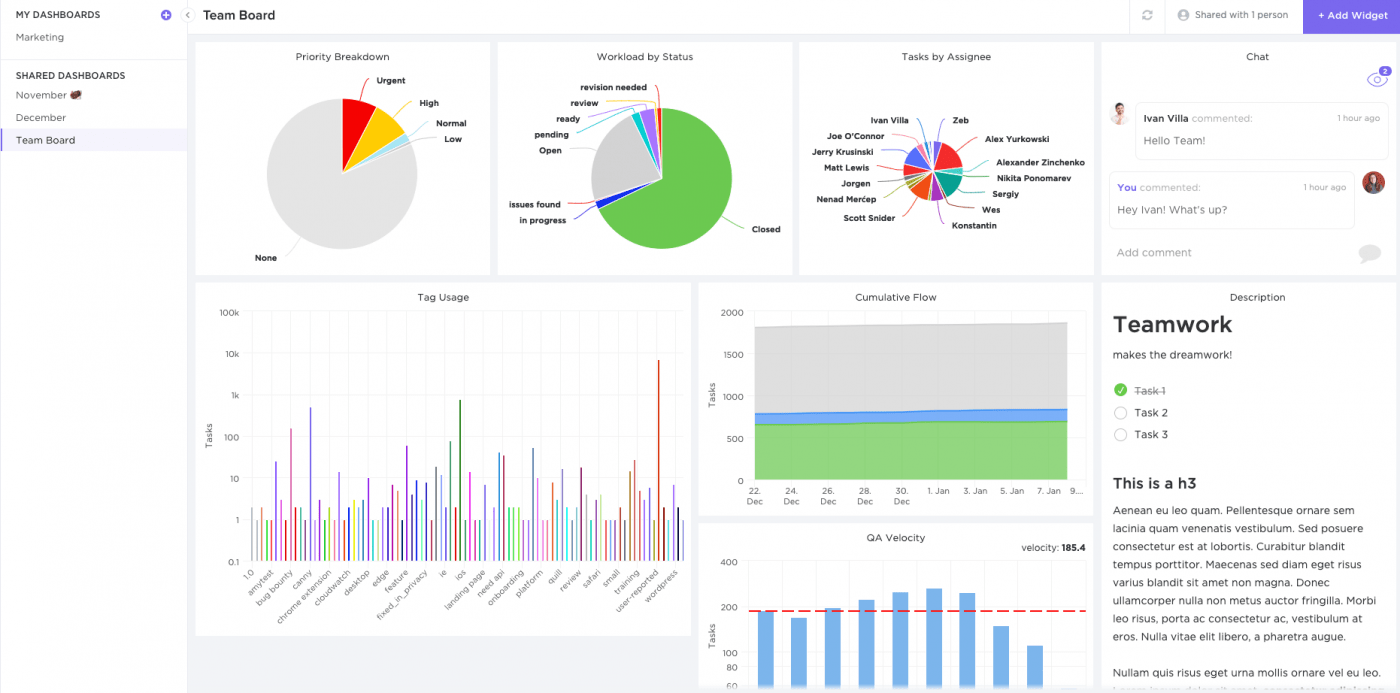
A human resources Dashboard is your mission control center where hr managers can get a high-level overview of your HR team’s performance. 🚀
On top of that, you can choose how you want to view the data.
Here’s a list of some of the Custom Widgets you can add to your KPI dashboard:
- Line Chart: create a line chart with the data you choose 📈
- Bar Chart: create a custom bar chart with any data 📊
- Portfolio : categorize and track the progress of your HR processes
- Calculation : calculate sums, averages, and other numerical data
- Chat : have conversations with your team within your HR Dashboard
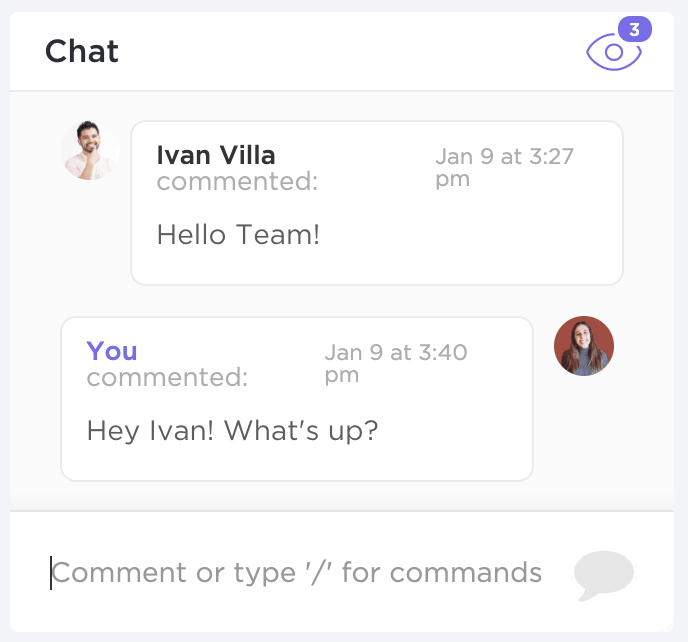
When you hire an employee, you still need to track their performance to make sure they’re actually doing their job, right?
Similarly, you need to track the performance of your HR team regularly.
But how do you do this?
Simply add Table Widgets to your HR KPI Dashboard.
With these Widgets, you’ll have everything you need to visualize employee performance.
Here are some of the Widgets you’ll get:
- Completed Report: see how many tasks each team member has finished
- Worked On: know the number of tasks each member worked on a specific day, week, or month
- Workspace Points: gamify your HR activities so that you can identify the top performers 🎮
- Who’s Behind: determine which team members need to put in a little more effort by seeing the number of uncleared notifications and overdue tasks
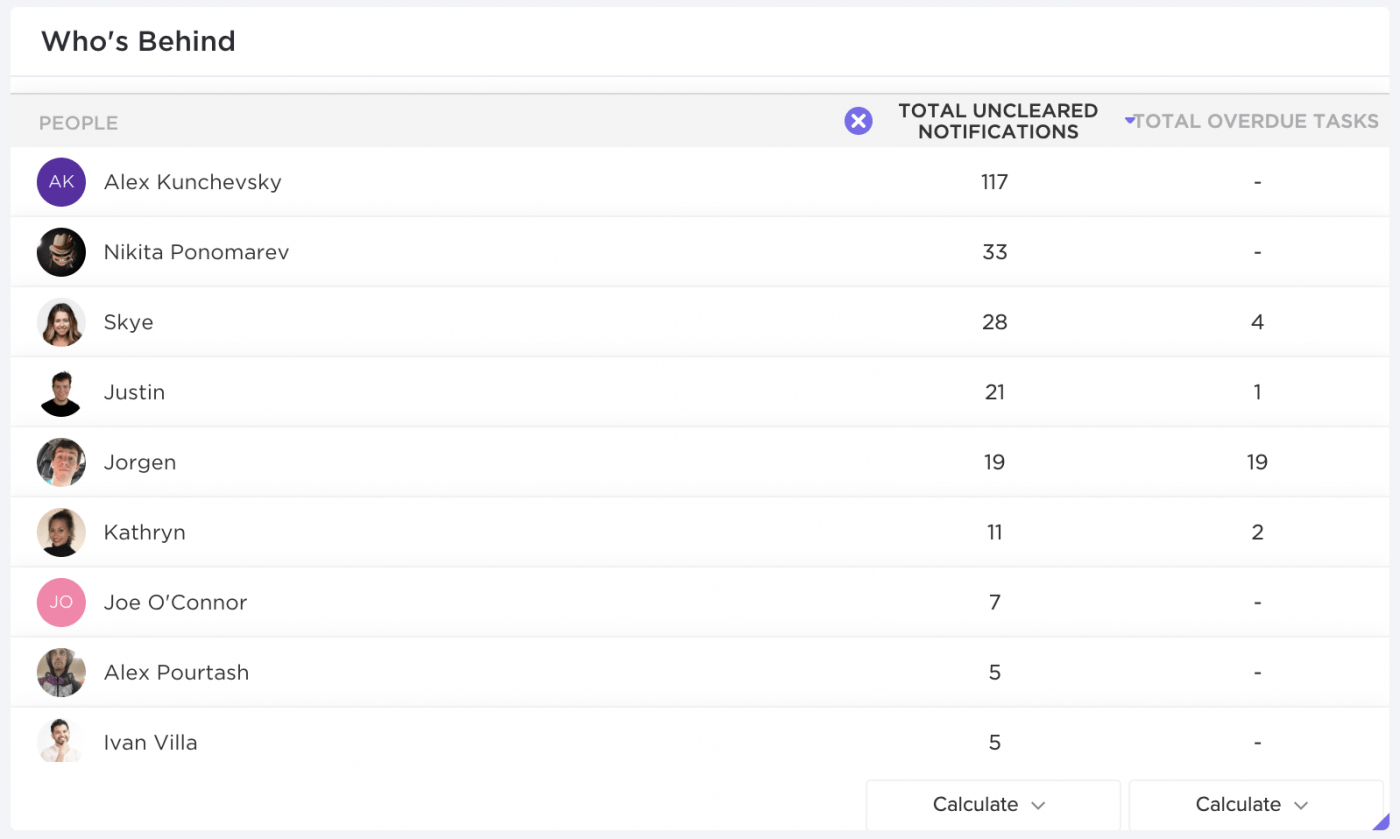
Having access to these KPI reports is especially useful for remote teams .
You can quickly identify which team members aren’t really working from home.
Want more KPI examples? Here are 50+ KPI examples and a templates .
Here are the answers to some common HR KPI questions:
A balanced scorecard (BSC) is a strategic planning tool that helps teams get a “balanced” visual of their performance.
A BSC lists down goals for four specific areas:
Financial goals
This includes reducing or managing HR costs, reducing lost time due to staff vacancies, and improving employee training.
Customer goals
HR departments have two customers: employees and department leaders.
Customer goals focus on what these two groups expect from the HR department.
For example, employees expect the HR team to promote company culture, and department leaders expect HR to hire the best talent.
Bonus: Talent management software !
Internal process goals
These include goals around hiring, training, shaping company culture, and improving communication .
For example, you can set up an internal company blog to communicate your company vision and share information between siloed teams.
Learning and growth goals
These goals are about how your HR department plans to develop a highly-trained team.
Your HR department should learn and grow to the point where they don’t require any training anymore!
Or in the words of Yoda:
Essentially, a BSC encourages your human resource team to focus on activities that support the company’s overall goals. In doing so, It showcases the value of your HR department.
A useful human resource KPI has five attributes:
The KPIs you set should lead to actions , not more questions.
Complicated goals may confuse your HR team and cause delays in achieving those goals!
Setting straightforward KPIs ensures that your HR team knows exactly what’s expected of them.
Your KPIs also need to be realistic.
Setting unachievable goals is highly demotivating for any team!
Your HR KPI needs to be relevant to your HR strategy.
For example, let’s say you’re an HR manager, and your goal is to increase social media engagement.
That’s not ideal.
The goal doesn’t contribute to your role in the organization, nor does it further your career .
Measurable goals have specific amounts, data, and timeframes.
For example:
Decrease our employee turnover rate by 20% by the second quarter .👌
Your HR KPIs also need to align with the overarching business goal.
For example, an organization that focuses solely on customer service will prioritize customer retention goals over customer acquisition goals.
On top of these attributes, we also recommend using SMART goals ! SMART goals are:
Keep these in mind when you’re creating your HR KPIs! 😊
Human resource metrics show you if you’re accomplishing all your HR objectives.
They’re the core performance indicators that every organization needs to track how their HR department is doing.
But merely choosing a KPI isn’t enough.
You need to track it too!
And to do this, you need a tool like ClickUp.
From letting you create custom employee onboarding Templates , HR templates , or publishing application Forms , ClickUp has everything you need to manage both your KPIs and the recruitment process.
Get ClickUp for free today , so your entire HR department knows exactly what to do and when to do it!
Questions? Comments? Visit our Help Center for support.
Receive the latest WriteClick Newsletter updates.
Thanks for subscribing to our blog!
Please enter a valid email
- Free training & 24-hour support
- Serious about security & privacy
- 99.99% uptime the last 12 months
- Skip to main content
- Keyboard shortcuts for audio player
Ex-assistant principal charged with child neglect in case of boy who shot teacher
The Associated Press

Signs stand outside Richneck Elementary School in Newport News, Va., Jan. 25, 2023. Denise Lavoie/AP hide caption
Signs stand outside Richneck Elementary School in Newport News, Va., Jan. 25, 2023.
NEWPORT NEWS, Va. — A former assistant principal at a Virginia elementary school has been charged with felony child neglect more than a year after a 6-year-old boy brought a gun to class and shot his first-grade teacher .
A special grand jury in Newport News found that Ebony Parker showed a reckless disregard for the lives of Richneck Elementary School students on Jan. 6, 2023, according to indictments unsealed Tuesday.
Parker and other school officials already face a $40 million negligence lawsuit from the teacher who was shot, Abby Zwerner. She accuses Parker and others of ignoring multiple warnings the boy had a gun and was in a "violent mood" the day of the shooting.
Criminal charges against school officials following a school shootings are quite rare, experts say. Parker, 39, faces eight felony counts, each of which is punishable by up to five years in prison.
The Associated Press left a message seeking comment Tuesday with Parker's attorney, Curtis Rogers.
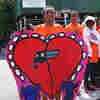
Shots - Health News
'say something' tip line in schools flags gun violence threats, study finds.
Court documents filed Tuesday reveal little about the criminal case against Parker, listing only the counts and a description of the felony charge. It alleges that Parker "did commit a willful act or omission in the care of such students, in a manner so gross, wanton and culpable as to show a reckless disregard for human life."
Newport News police have said the student who shot Zwerner retrieved his mother's handgun from atop a dresser at home and brought the weapon to school concealed in a backpack.
Zwerner's lawsuit describes a series of warnings that school employees gave administrators before the shooting. The lawsuit said those warnings began with Zwerner telling Parker that the boy "was in a violent mood," had threatened to beat up a kindergartener and stared down a security officer in the lunchroom.
The lawsuit alleges that Parker "had no response, refusing even to look up" when Zwerner expressed her concerns.
When concerns were raised that the child may have transferred the gun from his backpack to his pocket, Parker said his "pockets were too small to hold a handgun and did nothing," the lawsuit states.

Architecture
With gun control far from sight, schools redesign for student safety.
A guidance counselor also asked Parker for permission to search the boy, but Parker forbade him, "and stated that John Doe's mother would be arriving soon to pick him up," the lawsuit stated.
Zwerner was sitting at a reading table in front of the class when the boy fired the gun, police said. The bullet struck Zwerner's hand and then her chest, collapsing one of her lungs. She spent nearly two weeks in the hospital and has endured multiple surgeries as well as ongoing emotional trauma, according to her lawsuit.
Parker and the lawsuit's other defendants, which include a former superintendent and the Newport News school board, have tried to block Zwerner's lawsuit.
They've argued that Zwerner's injuries fall under Virginia's workers' compensation law. Their arguments have been unsuccessful so far in blocking the litigation. A trial date for Zwerner's lawsuit is slated for January.
Prosecutors had said a year ago that they were investigating whether the "actions or omissions" of any school employees could lead to criminal charges.
What schools can (and can't) do to prevent school shootings
Howard Gwynn, the commonwealth's attorney in Newport News, said in April 2023 that he had petitioned a special grand jury to probe if any "security failures" contributed to the shooting. Gwynn wrote that an investigation could also lead to recommendations "in the hopes that such a situation never occurs again."
It is not the first school shooting to spark a criminal investigation into school officials. For instance, a former school resource officer was acquitted of all charges last year after he was accused of hiding during the Parkland school massacre in 2018.
Chuck Vergon, a professor of educational law and policy at the University of Michigan-Flint, told The AP last year that it is rare for a teacher or school official to be charged in a school shooting because allegations of criminal negligence can be difficult to prove.
More often, he said, those impacted by school shootings seek to hold school officials liable in civil court.
- elementary school
A popular YouTuber's negative video of Humane's AI Pin raises questions about critical reviews in the age of innovation
- This post originally appeared in the Insider Today newsletter.
- You can sign up for Business Insider's daily newsletter here .

Hello there! If you're struggling to decide the foods worth buying organic, best-selling author Michael Pollan has some suggestions for the ones worth splurging on to avoid harmful chemicals .
In today's big story, we're looking at a critical tech review that caused a bit of a stir on social media .
What's on deck:
Markets: Goldman Sachs quiets the haters with a monster earnings report .
Tech: Leaked docs show one of Prime Video's biggest issues, forcing customers to abandon shows .
Business: The best bet in business these days? Targeting young men who like to gamble .
But first, the review is in!
If this was forwarded to you, sign up here.
The big story
Up for review.
"The Worst Product I've Ever Reviewed… For Now"
Marques Brownlee, the YouTuber better known as MKBHD, didn't mince words with the title of his review of Humane's AI Pin .
In a 25-minute video , Brownlee details all the issues he encountered using the AI device. (Spoiler alert: There were a lot.)
Brownlee's review aligns with other criticisms of the device . But not all of those came from someone with as much sway. His YouTube channel has more than 18 million subscribers.
One user on X pointed that out , calling the review "almost unethical" for "potentially killing someone else's nascent project" in a post reposted over 2,000 times.
Most of the internet disagreed, and a Humane exec even thanked Brownlee on X for the "fair and valid critiques."
But it highlights the power of Brownlee's reviews. Earlier this year, a negative video of Fisker's Ocean SUV by Brownlee also made waves on social media .
Critical reviews in the age of innovation raise some interesting questions.
To be clear, there was nothing wrong with Brownlee's review. Humane's AI Pin costs $700. Watering down his review to ease the blow would be a disservice to the millions of fans relying on his perspective before making such a significant purchase.
Too often, companies view potential customers as an extension of their research and development. They are happy to sell a product that is still a work in progress on the promise they'll fix it on the fly. ("Updates are coming!")
But in a world of instant gratification, it can be hard to appreciate that innovation takes time.
Even Apple can run into this conundrum. Take the Apple Vision Pro. Reviewers are impressed with the technology behind the much-anticipated gadget — but are still struggling to figure out what they can do with it . Maybe, over time, that will get sorted out. It's also worth remembering how cool tech can be, as Business Insider's Peter Kafka wrote following a bunch of trips in Waymo's software-powered taxis in San Francisco . Sure, robotaxis have their issues, Peter said, but they also elicit that "golly-gee-can-you-believe-it" sense.
As for Humane, America loves a comeback story. Just look at "Cyberpunk 2077." The highly anticipated video game had a disastrous launch in 2020 , but redeemed itself three years later, ultimately winning a major award .
Still, Humane shouldn't get a pass for releasing a product that didn't seem ready for primetime, according to the reviews.
And its issue could be bigger than glitchy tech. Humane's broader thesis about reducing screen time might not be as applicable. As BI's Katie Notopolous put it: " I love staring at my iPhone ."
3 things in markets
1. Goldman finally strikes gold. After a rough stretch, the vaunted investment bank crushed earnings expectations , sending its stock soaring. A big tailwind, according to CEO David Solomon, is AI spawning " enormous opportunities " for the bank.
2. Buy the dip, Wedbush says. Last week's drop among tech stocks shouldn't scare away investors , according to Wedbush. A strong earnings report, buoyed by the ongoing AI craze, should keep them soaring, strategists said. But JPMorgan doesn't see it that way, saying prices are already stretched .
3. China's economy beat analysts' expectations. The country's GDP grew 5.3% in the first quarter of 2024, according to data published by the National Bureau of Statistics on Tuesday. It's a welcome return to form for the world's second-largest economy, although below-par new home and retail sales remain a cause for concern .
3 things in tech
1. Amazon Prime Video viewers are giving up on its shows. Leaked documents show viewers are fed up with the streamer's error-ridden catalog system , which often has incomplete titles and missing episodes. In 2021, 60% of all content-related complaints were about Prime Video's catalog.
2. Eric Newcomer is bringing his Cerebral Valley AI Summit to New York. The conference, originally held in San Francisco, is famous for producing one of the largest generative AI acquisitions ever. Now, it's coming to New York in June .
3. OpenAI is plotting an expansion to NYC. Two people familiar with the plans told BI that the ChatGPT developer is looking to open a New York office next year. That would be the company's fifth office, alongside its current headquarters in San Francisco, a just-opened site in Tokyo, and spots in London and Dublin.
3 things in business
1. America's young men are spending their money like never before. From sports betting to meme coins, young men are more willing than ever to blow money in the hopes of making a fortune .
2. Investors are getting into women's sports. With women like Caitlin Clark dominating March Madness headlines, investors see a big opportunity. BI compiled a list of 13 investors and fund managers pouring money into the next big thing in sports.
3. Bad news for Live Nation. The Wall Street Journal reports that the Justice Department could hit the concert giant with an antitrust lawsuit as soon as next month. Live Nation, which owns Ticketmaster, has long faced criticism over its high fees.
In other news
Blackstone hires Walmart AI whiz to supercharge its portfolio companies .
Taylor Swift, Rihanna, Blackpink's Lisa: Celebrities spotted at Coachella 2024 .
NYC's rat czar says stop feeding the pigeons if you want the vermin gone .
A major Tesla executive left after 18 years at the company amid mass layoffs .
Some Tesla factory workers realized they were laid off when security scanned their badges and sent them back on shuttles, sources say .
New York is in, San Francisco is very much out for tech workers relocating .
AI could split workers into 2: The ones whose jobs get better and the ones who lose them completely .
Oh look at that! Now Google is using AI to answer search queries .
A longtime banker gives a rare inside look at how he is thinking about his next career move, from compensation to WFH .
Clarence Thomas didn't show up for work today .
What's happening today
Today's earnings: United Airlines, Bank of America, Morgan Stanley, and others are reporting .
It's Free Cone Day at participating Ben & Jerry's stores.
The Insider Today team: Dan DeFrancesco , deputy editor and anchor, in New York. Jordan Parker Erb , editor, in New York. Hallam Bullock , senior editor, in London. George Glover , reporter, in London.
Watch: Nearly 50,000 tech workers have been laid off — but there's a hack to avoid layoffs
- Main content

- SUGGESTED TOPICS
- The Magazine
- Newsletters
- Managing Yourself
- Managing Teams
- Work-life Balance
- The Big Idea
- Data & Visuals
- Reading Lists
- Case Selections
- HBR Learning
- Topic Feeds
- Account Settings
- Email Preferences
Case Study: How Aggressively Should a Bank Pursue AI?
- Thomas H. Davenport
- George Westerman

A Malaysia-based CEO weighs the risks and potential benefits of turning a traditional bank into an AI-first institution.
Siti Rahman, the CEO of Malaysia-based NVF Bank, faces a pivotal decision. Her head of AI innovation, a recent recruit from Google, has a bold plan. It requires a substantial investment but aims to transform the traditional bank into an AI-first institution, substantially reducing head count and the number of branches. The bank’s CFO worries they are chasing the next hype cycle and cautions against valuing efficiency above all else. Siti must weigh the bank’s mixed history with AI, the resistance to losing the human touch in banking services, and the risks of falling behind in technology against the need for a prudent, incremental approach to innovation.
Two experts offer advice: Noemie Ellezam-Danielo, the chief digital and AI strategy at Société Générale, and Sastry Durvasula, the chief information and client services officer at TIAA.
Siti Rahman, the CEO of Malaysia-headquartered NVF Bank, hurried through the corridors of the university’s computer engineering department. She had directed her driver to the wrong building—thinking of her usual talent-recruitment appearances in the finance department—and now she was running late. As she approached the room, she could hear her head of AI innovation, Michael Lim, who had joined NVF from Google 18 months earlier, breaking the ice with the students. “You know, NVF used to stand for Never Very Fast,” he said to a few giggles. “But the bank is crawling into the 21st century.”

- Thomas H. Davenport is the President’s Distinguished Professor of Information Technology and Management at Babson College, a visiting scholar at the MIT Initiative on the Digital Economy, and a senior adviser to Deloitte’s AI practice. He is a coauthor of All-in on AI: How Smart Companies Win Big with Artificial Intelligence (Harvard Business Review Press, 2023).
- George Westerman is a senior lecturer at MIT Sloan School of Management and a coauthor of Leading Digital (HBR Press, 2014).
Partner Center
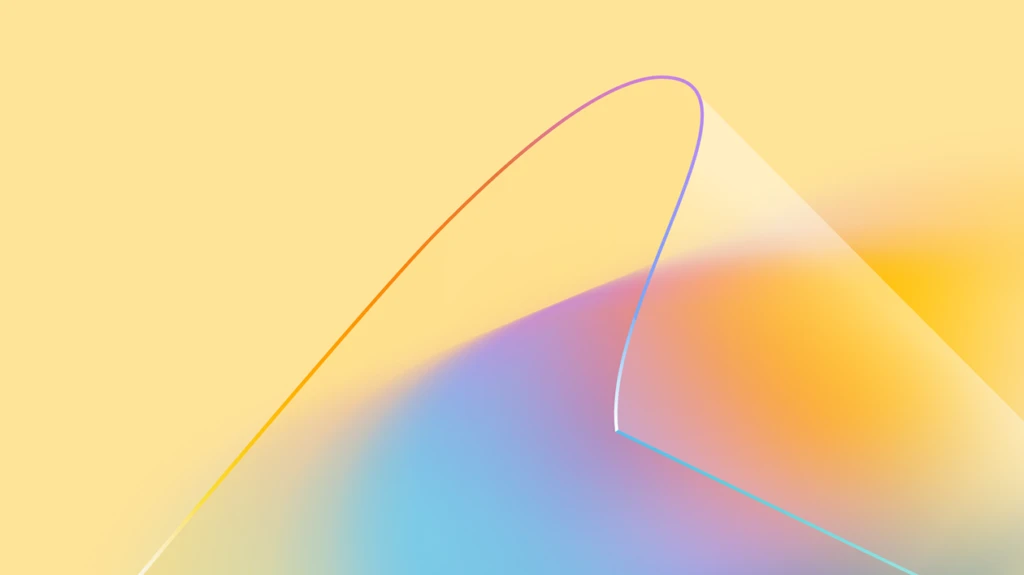
AI + Machine Learning , Azure AI , Azure AI Services , Azure AI Studio , Azure OpenAI Service , Best practices
AI study guide: The no-cost tools from Microsoft to jump start your generative AI journey
By Natalie Mickey Product Marketing Manager, Data and AI Skilling, Azure
Posted on April 15, 2024 4 min read
The world of AI is constantly changing. Every day it seems there are new ways we can work with generative AI and large language models. It can be hard to know where to start your own learning journey when it comes to AI. Microsoft has put together several resources to help you get started. Whether you are ready to build your own copilot or you’re at the very beginning of your learning journey, read on to find the best and free resources from Microsoft on generative AI training.
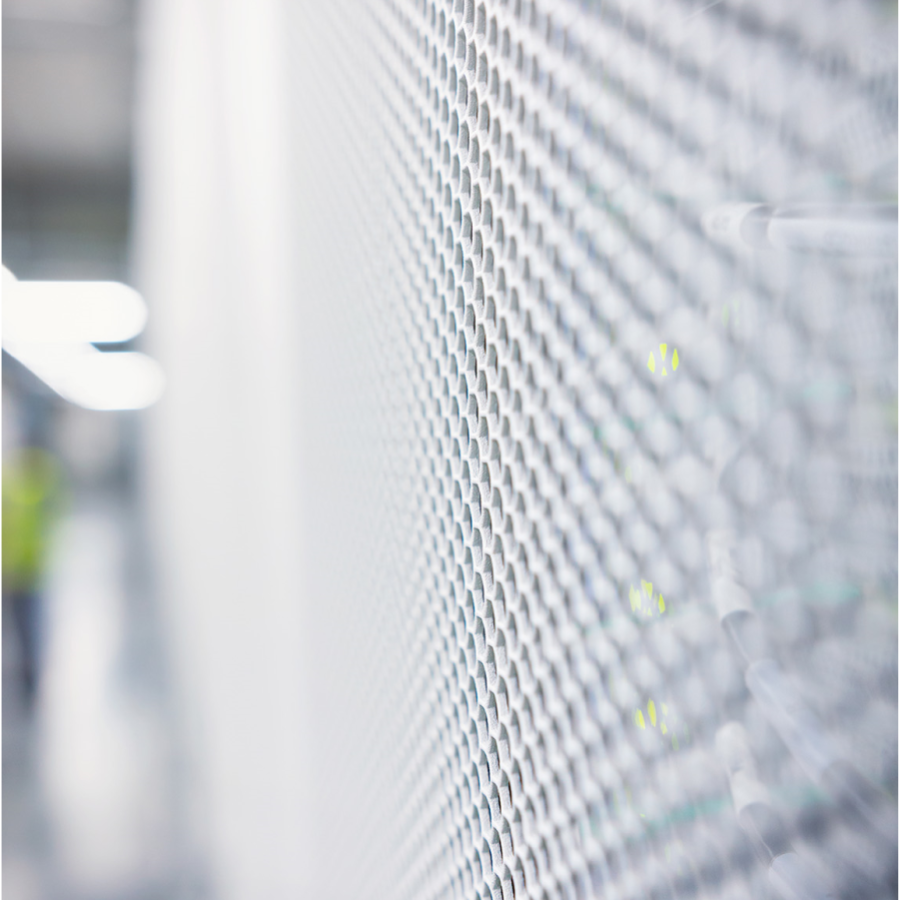
Build intelligent apps at enterprise scale with the Azure AI portfolio
Azure AI fundamentals
If you’re just starting out in the world of AI, I highly recommend Microsoft’s Azure AI Fundamentals course . It includes hands on exercises, covers Azure AI Services, and dives into the world of generative AI. You can either take the full course in one sitting or break it up and complete a few modules a day.
Learning path: Azure AI fundamentals
Course highlight: Fundamentals of generative AI module
Azure AI engineer
For those who are more advanced in AI knowledge, or are perhaps software engineers, this learning path is for you. This path will guide you through building AI infused applications that leverage Azure AI Services, Azure AI Search, and Open AI.
Course highlight: Get started with Azure OpenAI Service module
Let’s get building with Azure AI Studio
Imagine a collaborative workshop where you can build AI apps, test pre-trained models, and deploy your creations to the cloud, all without getting lost in mountains of code. In our newest learning path , you will learn how to build generative AI applications like custom copilots that use language models to provide value to your users.
Learning path: Create custom copilots with Azure AI Studio (preview)
Course highlight: Build a RAG-based copilot solution with your own data using Azure AI Studio (preview) module
Dive deep into generative AI with Azure OpenAI Service
If you have some familiarity with Azure and experience programming with C# or Python, you can dive right into the Microsoft comprehensive generative AI training.
Learning path: Develop generative AI solutions with Azure OpenAI Service
Course highlight: Implement Retrieval Augmented Generation (RAG) with Azure OpenAI Service module
Cloud Skills Challenges
Microsoft Azure’s Cloud Skills Challenges are free and interactive events that provide access to our tailored skilling resources for specific solution areas. Each 30-day accelerated learning experience helps users get trained in Microsoft AI. The program offers learning modules, virtual training days, and even a virtual leaderboard to compete head-to-head with your peers in the industry. Learn more about Cloud Skills Challenges here , then check out these challenges to put your AI skills to the test.
Invest in App Innovation to Stay Ahead of the Curve
Challenges 1-3 will help you prepare for Microsoft AI Applied Skills, scenario-based credentials. Challenges 4 and 5 will help you prepare for Microsoft Azure AI Certifications, with the potential of a 50% exam discount on your certification of choice 1 .
Challenge #1: Generative AI with Azure OpenAI
In about 18 hours, you’ll learn how to train models to generate original content based on natural language input. You should already have familiarity with Azure and experience programming with C# or Python. Begin now!
Challenge #2: Azure AI Language
Build a natural language processing solution with Azure AI Language. In about 20 hours, you’ll learn how to use language models to interpret the semantic meaning of written or spoken language. You should already have familiarity with the Azure portal and experience programming with C# or Python. Begin now!
Challenge #3: Azure AI Document Intelligence
Show off your smarts with Azure AI Document Intelligence Solutions. In about 21 hours, you’ll learn how to use natural language processing (NLP) solutions to interpret the meaning of written or spoken language. You should already have familiarity with the Azure portal and C# or Python programming. Begin now!
Challenge #4: Azure AI Fundamentals
Build a robust understanding of machine learning and AI principles, covering computer vision, natural language processing, and conversational AI. Tailored for both technical and non-technical backgrounds, this learning adventure guides you through creating no-code predictive models, delving into conversational AI, and more—all in just about 10 hours.
Complete the challenge within 30 days and you’ll be eligible for 50% off the cost of a Microsoft Certification exam. Earning your Azure AI Fundamentals certification can supply the foundation you need to build your career and demonstrate your knowledge of common AI and machine learning workloads—and what Azure services can solve for them. Begin now!
Challenge #5: Azure AI Engineer
Go beyond theory to build the future. This challenge equips you with practical skills for managing and leveraging Microsoft Azure’s Cognitive Services. Learn everything from secure resource provisioning to real-time performance monitoring. You’ll be crafting cutting-edge AI solutions in no time, all while preparing for Exam AI-102 and your Azure AI Engineer Associate certification . Dive into interactive tutorials, hands-on labs, and real-world scenarios. Complete the challenge within 30 days and you’ll be eligible for 50% off the cost of a Microsoft Certification exam 2 . Begin now!
Finally, our free Microsoft AI Virtual Training Days are a great way to immerse yourself in free one or two-day training sessions. We have three great options for Azure AI training:
- Azure AI Fundamentals
- Generative AI Fundamentals
- Building Generative Apps with Azure OpenAI Service
Start your AI learning today
For any and all AI-related learning opportunities, check out the Microsoft Learn AI Hub including tailored AI training guidance . You can also follow our Azure AI and Machine Learning Tech Community Blogs for monthly study guides .
- Microsoft Cloud Skills Challenge | 30 Days to Learn It – Official Rules
- https://developer.microsoft.com/en-us/offers/30-days-to-learn-it/official-rules#terms-and-conditions
Let us know what you think of Azure and what you would like to see in the future.
Provide feedback
Build your cloud computing and Azure skills with free courses by Microsoft Learn.
Explore Azure learning
Related posts
AI + Machine Learning , Azure AI , Azure VMware Solution , Events , Microsoft Copilot for Azure , Microsoft Defender for Cloud
Get ready for AI at the Migrate to Innovate digital event chevron_right
AI + Machine Learning , Azure AI Speech , Azure AI Studio , Azure OpenAI Service , Azure SQL Database
What’s new in Azure Data, AI, and Digital Applications: Helping you navigate the fast pace of change chevron_right
AI + Machine Learning , Announcements , Azure AI , Azure AI Search
Announcing updates to Azure AI Search to help organizations build and scale generative AI applications chevron_right
AI + Machine Learning , Azure AI , Industry trends
Azure Maia for the era of AI: From silicon to software to systems chevron_right
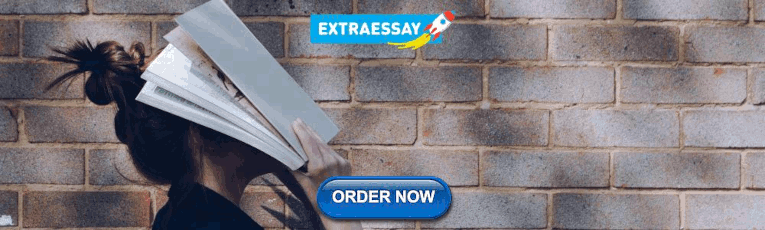
IMAGES
VIDEO
COMMENTS
Benefit #2: Key performance indicators create a way to communicate a shared understanding of success. ... Subscribe to get the latest agile strategy best practices, free guides, case studies, and videos in your inbox every week. Call at +1-775-747-7407 between 9am-5pm PST. Services; Strategic Planning Facilitation; Strategy Coach;
Sales leaders and their teams need to track the key performance indicators that help them close more orders. Below are the 15 essential sales KPI examples: New Inbound Leads. Lead Response Time. Lead Conversion %. New Qualified Opportunities. Total Pipeline Value. Lead-to-Opportunity %. Opportunity-to-Order %.
Executive teams are very familiar with using KPIs (key performance indicators) to track recent corporate success. These measures are used like school reports, providing feedback on how things went ...
The 5 case studies presented below demonstrate how the performance of leading businesses was impacted positively by using OKRs. ... The leadership team at Huawei was unhappy with the KPI system that was in use, as it could only use goal setting as the process starting point and performance evaluation as the end - but didn't provide any ...
Introduction to Performance Measurement. Organizational Development Video. Harvard Business Publishing. Alnoor Ebrahim. Define success, then develop measures that help you track progress and make ...
April 24, 2017. Finding, tracking and collating Key Performance Indicators (KPIs)* that demonstrate the performance of your online efforts has always been difficult in the local digital marketing space. But with upgrades in reporting at Google, Yelp and a newly minted web site, I took the opportunity to do just that in a case study with our ...
Key performance indicators aren't static, and you must update them as your organization's needs evolve. Related: 8 Key Performance Indicators for a Startup Company To Use Reporting on KPIs Once you've measured a key performance indicator, you may want or be required to present your progress in a KPI report. This is typically useful for ...
KPI Case Studies - Key Performance Indicators (KPIs) are more than mere numbers on a dashboard; they are vital signs that indicate the health and direction of a business. Like any powerful tool, the value they deliver hinges not just on their existence but on their thoughtful application. Through the lens of case studies, we gain a profound understanding of the dynamics that drive successful ...
Summary. Executive leader information needs differ from those of operational managers. A single information management approach will not meet both needs. PepsiCo maps the information needed at key business decision points to reframe strategic questions and identify executive leaders' and operational KPIs.
analyzed and put into practice through short case studies. These research aims were addressed through a series of group discussions and phone interviews with member companies. An initial meeting of industry experts was held to generate a list of leading indicators in each of three categories (systems-, organizations-, and behavior-based ...
Key Performance Indicators - KPI: Key performance indicators (KPI) are a set of quantifiable measures that a company uses to gauge its performance over time. These metrics are used to determine a ...
KPIs & Metrics Case Studies Tesco: Measuring Customer Performance & Gaining Insights From The Clubcard Data Tesco PLC British-based international grocery and general merchandising retail group.The company is the third largest retailer in the world measured by profits, has over 6,500 stores and employs more than 475,000 people…
This study aims to identify key performance indicators (KPIs) and categorise them. based on performance measurement to improve a holistic performant management organisation. The Method is using ...
Case Study 3: Google Google is one of the most successful companies in the world, and they have used AI to help them optimize their KPIs. Here are some case studies that show how Google has used ...
Key performance indicators are tools for management, decision support and forecasting; they reflect the strategy and vision of the company in terms of objectives and allow to always staying in step with the expectations of the stakeholders. ... T., El Hassani, I. et al. Machine learning for KPIs prediction: a case study of the overall equipment ...
And that's the role of a good KPI business case: to show how better performance measurement can make it easier and faster to achieve their priorities. Here are the ingredients of a good KPI business case: Part 1. Executive Summary. Write a short summary of your business case to invest time and energy into a robust and deliberate approach to ...
This research study focuses on the issue of the Key Performance Indicators (KPIs) development which is linked to the goal, objective, mission and vision of an Aerospace Manufacturing Company.
Logistics KPIs Case Study: Whirlpool's Supply Chain and Logistics Success Driven by Effective KPI Implementation. GlobalTranz. OCTOBER 29, 2015. We conclude our ongoing series in talking about effective KPI management by giving you a real live Logistics KPIs management case study from Whirlpool's engagement with a logistics service level ...
As the founder of Uplift Content, Emily leads her team in creating done-for-you case studies, ebooks and blog posts for high-growth SaaS companies like ClickUp, Calendly and WalkMe. Connect with Emily on Linkedin. It's crucial to evaluate case study success, but it's easier said than done. Find out how 8 customer marketers measure success.
case study. Azure IoT Central and Power BI on Image Defect Analysis. case study. HCM DW on Snowflake and Power BI. case study. Enterprise DW on AWS Redshift and Power BI. case study. Migrating BI stack from On-Premise to Oracle Cloud. case study.
Key performance indicators are measurable metrics that show an organization if they're achieving core business objectives. That's why human resources KPIs must be used to track the performance of your HR activities. ... If that's the case, you can switch to a provider with lower rates or take on an even better healthcare plan! ...
www.aes.com
Using rail as their primary mode, the freight business featured in this case study hauled bulk freight, such as grain or livestock, for their customers. Their performance measurement challenge. Via their customer survey, the freight business identified that one of the top three priorities for improvement was the accuracy of their billing process.
They need to remind themselves that KPIs are about stakeholder relationships, how what you do affects what the other does to you, and that the metrics will not always be the same. No CEO doubts ...
A former assistant principal at a Virginia elementary school has been charged with felony child neglect more than a year after a 6-year-old boy brought a gun to class and shot a teacher.
The antitrust litigation against AT&T and IBM are two of the most discussed (and celebrated) cases in U.S. history. Some claim that both decisions shaped the creation and development of the transistor and personal computer, while others believe the actual long-term impact of this enforcement was minimal. This case study panel discusses the consequences of both cases.
In a 25-minute video, Brownlee details all the issues he encountered using the AI device.(Spoiler alert: There were a lot.) Brownlee's review aligns with other criticisms of the device.But not all ...
Siti Rahman, the CEO of Malaysia-based NVF Bank, faces a pivotal decision. Her head of AI innovation, a recent recruit from Google, has a bold plan. It requires a substantial investment but aims ...
Build your business case for the cloud with key financial and technical guidance from Azure. Customer enablement. Plan a clear path forward for your cloud journey with proven tools, guidance, and resources. Customer stories. See examples of innovation from successful companies of all sizes and from all industries