A Systematic Literature Review on Automated Log Abstraction Techniques
Context - Logs are often the first and only information available to software engineers to understand and debug their systems. Automated log-analysis techniques help software engineers gain insights into large log data. These techniques have several steps, among which log abstraction is the most important because it transforms raw log-data into high-level information. Thus, log abstraction allows software engineers to perform further analyses. Existing log-abstraction techniques vary significantly in their designs and performances. To the best of our knowledge, there is no study that examines the performances of these techniques with respect to the following seven quality aspects concurrently - mode, coverage, delimiter independence, efficiency,scalability, system knowledge independence, and parameter tuning effort. Objectives - We want (1) to build a quality model for evaluating automated log-abstraction techniques and (2) to evaluate and recommend existing automated log-abstraction techniques using this quality model. Method - We perform a systematic literature review (SLR) of automated log-abstraction techniques. We review 89 research papers out of 2,864 initial papers. Results - Through this SLR, we (1) identify 17 automated log-abstraction techniques, (2) build a quality model composed of seven desirable aspects - coverage, delimiter independence, efficiency, system knowledge independence, mode, parameter tuning effort required, and scalability, and (3) make recommendations for researchers on future research directions. Conclusion - Our quality model and recommendations help researchers learn about the state-of-the-art automated log-abstraction techniques, identify research gaps to enhance existing techniques, and develop new ones. We also support software engineers in understanding the advantages and limitations of existing techniques and in choosing the suitable technique to their unique use cases.
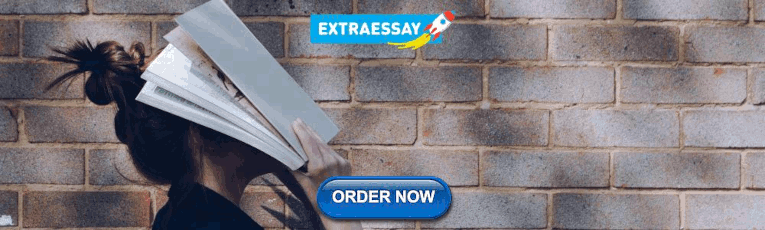
Associate Professor
My research interests include mainly Empirical Software Engineering, Software Quality, Debugging, and Software Engineering for Computer Games. I’m the creator of Swarm Debugging.
Landscape of Automated Log Analysis: A Systematic Literature Review and Mapping Study
Ieee account.
- Change Username/Password
- Update Address
Purchase Details
- Payment Options
- Order History
- View Purchased Documents
Profile Information
- Communications Preferences
- Profession and Education
- Technical Interests
- US & Canada: +1 800 678 4333
- Worldwide: +1 732 981 0060
- Contact & Support
- About IEEE Xplore
- Accessibility
- Terms of Use
- Nondiscrimination Policy
- Privacy & Opting Out of Cookies
A not-for-profit organization, IEEE is the world's largest technical professional organization dedicated to advancing technology for the benefit of humanity. © Copyright 2024 IEEE - All rights reserved. Use of this web site signifies your agreement to the terms and conditions.
- Help & FAQ
A systematic literature review on automated log abstraction techniques
Research output : Contribution to journal › Article › peer-review
Context: Logs are often the first and only information available to software engineers to understand and debug their systems. Automated log-analysis techniques help software engineers gain insights into large log data. These techniques have several steps, among which log abstraction is the most important because it transforms raw log-data into high-level information. Thus, log abstraction allows software engineers to perform further analyses. Existing log-abstraction techniques vary significantly in their designs and performances. To the best of our knowledge, there is no study that examines the performances of these techniques with respect to the following seven quality aspects concurrently: mode, coverage, delimiter independence, efficiency,scalability, system knowledge independence, and parameter tuning effort. Objectives: We want (1) to build a quality model for evaluating automated log-abstraction techniques and (2) to evaluate and recommend existing automated log-abstraction techniques using this quality model. Method: We perform a systematic literature review (SLR) of automated log-abstraction techniques. We review 89 research papers out of 2,864 initial papers. Results: Through this SLR, we (1) identify 17 automated log-abstraction techniques, (2) build a quality model composed of seven desirable aspects: mode, coverage, delimiter independence, efficiency, scalability, system knowledge independence, and parameter tuning effort, and (3) make recommendations for researchers on future research directions. Conclusion: Our quality model and recommendations help researchers learn about the state-of-the-art automated log-abstraction techniques, identify research gaps to enhance existing techniques, and develop new ones. We also support software engineers in understanding the advantages and limitations of existing techniques and in choosing the suitable technique to their unique use cases.
Bibliographical note
Asjc scopus subject areas.
- Information Systems
- Computer Science Applications
Access to Document
- 10.1016/j.infsof.2020.106276
Other files and links
- Link to publication in Scopus
- Link to the citations in Scopus
Fingerprint
- Engineers Engineering & Materials Science 100%
- Scalability Engineering & Materials Science 57%
- Tuning Engineering & Materials Science 55%
T1 - A systematic literature review on automated log abstraction techniques
AU - El-Masri, Diana
AU - Petrillo, Fabio
AU - Guéhéneuc, Yann Gaël
AU - Hamou-Lhadj, Abdelwahab
AU - Bouziane, Anas
N1 - Publisher Copyright: © 2020 Elsevier B.V.
PY - 2020/6
Y1 - 2020/6
N2 - Context: Logs are often the first and only information available to software engineers to understand and debug their systems. Automated log-analysis techniques help software engineers gain insights into large log data. These techniques have several steps, among which log abstraction is the most important because it transforms raw log-data into high-level information. Thus, log abstraction allows software engineers to perform further analyses. Existing log-abstraction techniques vary significantly in their designs and performances. To the best of our knowledge, there is no study that examines the performances of these techniques with respect to the following seven quality aspects concurrently: mode, coverage, delimiter independence, efficiency,scalability, system knowledge independence, and parameter tuning effort. Objectives: We want (1) to build a quality model for evaluating automated log-abstraction techniques and (2) to evaluate and recommend existing automated log-abstraction techniques using this quality model. Method: We perform a systematic literature review (SLR) of automated log-abstraction techniques. We review 89 research papers out of 2,864 initial papers. Results: Through this SLR, we (1) identify 17 automated log-abstraction techniques, (2) build a quality model composed of seven desirable aspects: mode, coverage, delimiter independence, efficiency, scalability, system knowledge independence, and parameter tuning effort, and (3) make recommendations for researchers on future research directions. Conclusion: Our quality model and recommendations help researchers learn about the state-of-the-art automated log-abstraction techniques, identify research gaps to enhance existing techniques, and develop new ones. We also support software engineers in understanding the advantages and limitations of existing techniques and in choosing the suitable technique to their unique use cases.
AB - Context: Logs are often the first and only information available to software engineers to understand and debug their systems. Automated log-analysis techniques help software engineers gain insights into large log data. These techniques have several steps, among which log abstraction is the most important because it transforms raw log-data into high-level information. Thus, log abstraction allows software engineers to perform further analyses. Existing log-abstraction techniques vary significantly in their designs and performances. To the best of our knowledge, there is no study that examines the performances of these techniques with respect to the following seven quality aspects concurrently: mode, coverage, delimiter independence, efficiency,scalability, system knowledge independence, and parameter tuning effort. Objectives: We want (1) to build a quality model for evaluating automated log-abstraction techniques and (2) to evaluate and recommend existing automated log-abstraction techniques using this quality model. Method: We perform a systematic literature review (SLR) of automated log-abstraction techniques. We review 89 research papers out of 2,864 initial papers. Results: Through this SLR, we (1) identify 17 automated log-abstraction techniques, (2) build a quality model composed of seven desirable aspects: mode, coverage, delimiter independence, efficiency, scalability, system knowledge independence, and parameter tuning effort, and (3) make recommendations for researchers on future research directions. Conclusion: Our quality model and recommendations help researchers learn about the state-of-the-art automated log-abstraction techniques, identify research gaps to enhance existing techniques, and develop new ones. We also support software engineers in understanding the advantages and limitations of existing techniques and in choosing the suitable technique to their unique use cases.
UR - http://www.scopus.com/inward/record.url?scp=85079518720&partnerID=8YFLogxK
UR - http://www.scopus.com/inward/citedby.url?scp=85079518720&partnerID=8YFLogxK
U2 - 10.1016/j.infsof.2020.106276
DO - 10.1016/j.infsof.2020.106276
M3 - Article
AN - SCOPUS:85079518720
SN - 0950-5849
JO - Information and Software Technology
JF - Information and Software Technology
M1 - 106276
Academia.edu no longer supports Internet Explorer.
To browse Academia.edu and the wider internet faster and more securely, please take a few seconds to upgrade your browser .
Enter the email address you signed up with and we'll email you a reset link.
- We're Hiring!
- Help Center
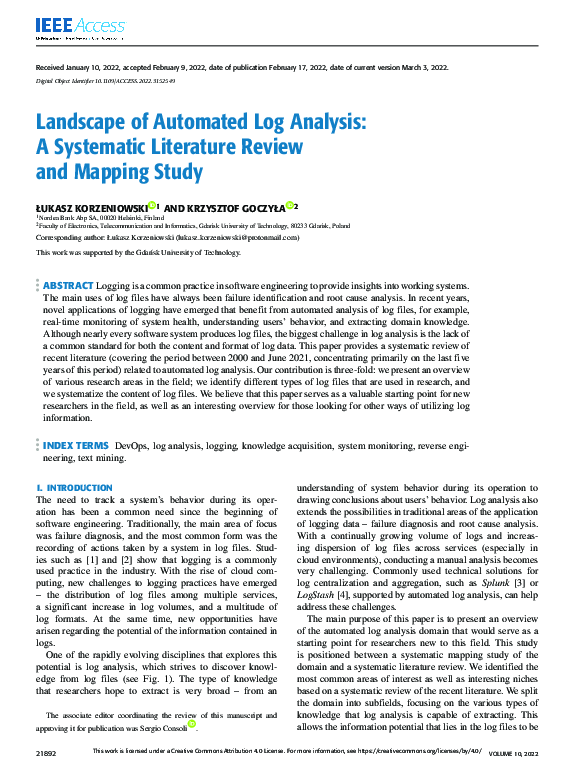
Landscape of Automated Log Analysis: A Systematic Literature Review and Mapping Study

2022, IEEE Access
Related Papers
2008 19th International Symposium on Software Reliability Engineering (ISSRE)
Fabrizio Pastore
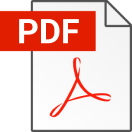
IBM Journal of Research and Development
Lavi Shpigelman , Shai Fine , Oded Margalit , Yaara Goldschmidt
Proceedings of the 2nd …
Aviad Tsherniak
Cheng Zhang
Abstract Logs are valuable for failure diagnosis and software debugging in practice. However, due to the ad-hoc style of inserting logging statements, the quality of logs can hardly be guaranteed. In case of a system failure, the log file may contain a large number of irrelevant logs, while crucial clues to the root cause may still be missing.
Michael Jordan
IEEE Transactions on Software Engineering
Domenico Cotroneo
9th International Conference on Advances in Computing and Information Technology (ACITY 2019)
roberto bruzzese
Proceedings of the Third Conference on Tackling Computer Systems Problems With Machine Learning Techniques
International Journal of Advanced Research in Computer Science and Software Engineering
Rinku Singh
RELATED PAPERS
ACTIVIDAD 1 TABLAS SEMANA 3
VILLASEnOR RUBIO RICARDO
Inorganic Chemistry
Andrea Farenga
Jose Alexis Gonzalez
Journal of Colloid and Interface Science
Dr. Yohannes Chonde
Héctor René Buelna Osben
Jurnal Teknologi Berkelanjutan
International Journal of Foreign Trade and International Business
swami saxena
V. 14, n. 04
Revista Quaestio Iuris , Manoel Messias Peixinho
Revue de Chirurgie Orthopédique et Traumatologique
Catherine Radier
juan carlos fernandez leon
Advances in Social Work
Richard Knopf
Atherosclerosis Supplements
Alberico Catapano
ion daniel grancea
Liliana Estela Martínez
HAL (Le Centre pour la Communication Scientifique Directe)
Revista Chilena de Derecho y Ciencia Política
MARIA BEATRIZ EGUIGUREN
International Journal of Mathematics and Soft Computing
S.V.Padmavathi S.V.Padmavathi
Journal of Animal and Veterinary Advances
Muryanti Ardani, SPd
Psychiatry Research-neuroimaging
Nikos Comoutos (former Zourbanos)
Ecotoxicology and Environmental Safety
Zorana Miletić
Marine Chemistry
Russell Frew
Keith Casper
Przeglad Gastroenterologiczny
Hameed Raina
RELATED TOPICS
- We're Hiring!
- Help Center
- Find new research papers in:
- Health Sciences
- Earth Sciences
- Cognitive Science
- Mathematics
- Computer Science
- Academia ©2024

An official website of the United States government
The .gov means it’s official. Federal government websites often end in .gov or .mil. Before sharing sensitive information, make sure you’re on a federal government site.
The site is secure. The https:// ensures that you are connecting to the official website and that any information you provide is encrypted and transmitted securely.
- Publications
- Account settings
Preview improvements coming to the PMC website in October 2024. Learn More or Try it out now .
- Advanced Search
- Journal List
- Springer Nature - PMC COVID-19 Collection

An automated essay scoring systems: a systematic literature review
Dadi ramesh.
1 School of Computer Science and Artificial Intelligence, SR University, Warangal, TS India
2 Research Scholar, JNTU, Hyderabad, India
Suresh Kumar Sanampudi
3 Department of Information Technology, JNTUH College of Engineering, Nachupally, Kondagattu, Jagtial, TS India
Associated Data
Assessment in the Education system plays a significant role in judging student performance. The present evaluation system is through human assessment. As the number of teachers' student ratio is gradually increasing, the manual evaluation process becomes complicated. The drawback of manual evaluation is that it is time-consuming, lacks reliability, and many more. This connection online examination system evolved as an alternative tool for pen and paper-based methods. Present Computer-based evaluation system works only for multiple-choice questions, but there is no proper evaluation system for grading essays and short answers. Many researchers are working on automated essay grading and short answer scoring for the last few decades, but assessing an essay by considering all parameters like the relevance of the content to the prompt, development of ideas, Cohesion, and Coherence is a big challenge till now. Few researchers focused on Content-based evaluation, while many of them addressed style-based assessment. This paper provides a systematic literature review on automated essay scoring systems. We studied the Artificial Intelligence and Machine Learning techniques used to evaluate automatic essay scoring and analyzed the limitations of the current studies and research trends. We observed that the essay evaluation is not done based on the relevance of the content and coherence.
Supplementary Information
The online version contains supplementary material available at 10.1007/s10462-021-10068-2.
Introduction
Due to COVID 19 outbreak, an online educational system has become inevitable. In the present scenario, almost all the educational institutions ranging from schools to colleges adapt the online education system. The assessment plays a significant role in measuring the learning ability of the student. Most automated evaluation is available for multiple-choice questions, but assessing short and essay answers remain a challenge. The education system is changing its shift to online-mode, like conducting computer-based exams and automatic evaluation. It is a crucial application related to the education domain, which uses natural language processing (NLP) and Machine Learning techniques. The evaluation of essays is impossible with simple programming languages and simple techniques like pattern matching and language processing. Here the problem is for a single question, we will get more responses from students with a different explanation. So, we need to evaluate all the answers concerning the question.
Automated essay scoring (AES) is a computer-based assessment system that automatically scores or grades the student responses by considering appropriate features. The AES research started in 1966 with the Project Essay Grader (PEG) by Ajay et al. ( 1973 ). PEG evaluates the writing characteristics such as grammar, diction, construction, etc., to grade the essay. A modified version of the PEG by Shermis et al. ( 2001 ) was released, which focuses on grammar checking with a correlation between human evaluators and the system. Foltz et al. ( 1999 ) introduced an Intelligent Essay Assessor (IEA) by evaluating content using latent semantic analysis to produce an overall score. Powers et al. ( 2002 ) proposed E-rater and Intellimetric by Rudner et al. ( 2006 ) and Bayesian Essay Test Scoring System (BESTY) by Rudner and Liang ( 2002 ), these systems use natural language processing (NLP) techniques that focus on style and content to obtain the score of an essay. The vast majority of the essay scoring systems in the 1990s followed traditional approaches like pattern matching and a statistical-based approach. Since the last decade, the essay grading systems started using regression-based and natural language processing techniques. AES systems like Dong et al. ( 2017 ) and others developed from 2014 used deep learning techniques, inducing syntactic and semantic features resulting in better results than earlier systems.
Ohio, Utah, and most US states are using AES systems in school education, like Utah compose tool, Ohio standardized test (an updated version of PEG), evaluating millions of student's responses every year. These systems work for both formative, summative assessments and give feedback to students on the essay. Utah provided basic essay evaluation rubrics (six characteristics of essay writing): Development of ideas, organization, style, word choice, sentence fluency, conventions. Educational Testing Service (ETS) has been conducting significant research on AES for more than a decade and designed an algorithm to evaluate essays on different domains and providing an opportunity for test-takers to improve their writing skills. In addition, they are current research content-based evaluation.
The evaluation of essay and short answer scoring should consider the relevance of the content to the prompt, development of ideas, Cohesion, Coherence, and domain knowledge. Proper assessment of the parameters mentioned above defines the accuracy of the evaluation system. But all these parameters cannot play an equal role in essay scoring and short answer scoring. In a short answer evaluation, domain knowledge is required, like the meaning of "cell" in physics and biology is different. And while evaluating essays, the implementation of ideas with respect to prompt is required. The system should also assess the completeness of the responses and provide feedback.
Several studies examined AES systems, from the initial to the latest AES systems. In which the following studies on AES systems are Blood ( 2011 ) provided a literature review from PEG 1984–2010. Which has covered only generalized parts of AES systems like ethical aspects, the performance of the systems. Still, they have not covered the implementation part, and it’s not a comparative study and has not discussed the actual challenges of AES systems.
Burrows et al. ( 2015 ) Reviewed AES systems on six dimensions like dataset, NLP techniques, model building, grading models, evaluation, and effectiveness of the model. They have not covered feature extraction techniques and challenges in features extractions. Covered only Machine Learning models but not in detail. This system not covered the comparative analysis of AES systems like feature extraction, model building, and level of relevance, cohesion, and coherence not covered in this review.
Ke et al. ( 2019 ) provided a state of the art of AES system but covered very few papers and not listed all challenges, and no comparative study of the AES model. On the other hand, Hussein et al. in ( 2019 ) studied two categories of AES systems, four papers from handcrafted features for AES systems, and four papers from the neural networks approach, discussed few challenges, and did not cover feature extraction techniques, the performance of AES models in detail.
Klebanov et al. ( 2020 ). Reviewed 50 years of AES systems, listed and categorized all essential features that need to be extracted from essays. But not provided a comparative analysis of all work and not discussed the challenges.
This paper aims to provide a systematic literature review (SLR) on automated essay grading systems. An SLR is an Evidence-based systematic review to summarize the existing research. It critically evaluates and integrates all relevant studies' findings and addresses the research domain's specific research questions. Our research methodology uses guidelines given by Kitchenham et al. ( 2009 ) for conducting the review process; provide a well-defined approach to identify gaps in current research and to suggest further investigation.
We addressed our research method, research questions, and the selection process in Sect. 2 , and the results of the research questions have discussed in Sect. 3 . And the synthesis of all the research questions addressed in Sect. 4 . Conclusion and possible future work discussed in Sect. 5 .
Research method
We framed the research questions with PICOC criteria.
Population (P) Student essays and answers evaluation systems.
Intervention (I) evaluation techniques, data sets, features extraction methods.
Comparison (C) Comparison of various approaches and results.
Outcomes (O) Estimate the accuracy of AES systems,
Context (C) NA.
Research questions
To collect and provide research evidence from the available studies in the domain of automated essay grading, we framed the following research questions (RQ):
RQ1 what are the datasets available for research on automated essay grading?
The answer to the question can provide a list of the available datasets, their domain, and access to the datasets. It also provides a number of essays and corresponding prompts.
RQ2 what are the features extracted for the assessment of essays?
The answer to the question can provide an insight into various features so far extracted, and the libraries used to extract those features.
RQ3, which are the evaluation metrics available for measuring the accuracy of algorithms?
The answer will provide different evaluation metrics for accurate measurement of each Machine Learning approach and commonly used measurement technique.
RQ4 What are the Machine Learning techniques used for automatic essay grading, and how are they implemented?
It can provide insights into various Machine Learning techniques like regression models, classification models, and neural networks for implementing essay grading systems. The response to the question can give us different assessment approaches for automated essay grading systems.
RQ5 What are the challenges/limitations in the current research?
The answer to the question provides limitations of existing research approaches like cohesion, coherence, completeness, and feedback.
Search process
We conducted an automated search on well-known computer science repositories like ACL, ACM, IEEE Explore, Springer, and Science Direct for an SLR. We referred to papers published from 2010 to 2020 as much of the work during these years focused on advanced technologies like deep learning and natural language processing for automated essay grading systems. Also, the availability of free data sets like Kaggle (2012), Cambridge Learner Corpus-First Certificate in English exam (CLC-FCE) by Yannakoudakis et al. ( 2011 ) led to research this domain.
Search Strings : We used search strings like “Automated essay grading” OR “Automated essay scoring” OR “short answer scoring systems” OR “essay scoring systems” OR “automatic essay evaluation” and searched on metadata.
Selection criteria
After collecting all relevant documents from the repositories, we prepared selection criteria for inclusion and exclusion of documents. With the inclusion and exclusion criteria, it becomes more feasible for the research to be accurate and specific.
Inclusion criteria 1 Our approach is to work with datasets comprise of essays written in English. We excluded the essays written in other languages.
Inclusion criteria 2 We included the papers implemented on the AI approach and excluded the traditional methods for the review.
Inclusion criteria 3 The study is on essay scoring systems, so we exclusively included the research carried out on only text data sets rather than other datasets like image or speech.
Exclusion criteria We removed the papers in the form of review papers, survey papers, and state of the art papers.
Quality assessment
In addition to the inclusion and exclusion criteria, we assessed each paper by quality assessment questions to ensure the article's quality. We included the documents that have clearly explained the approach they used, the result analysis and validation.
The quality checklist questions are framed based on the guidelines from Kitchenham et al. ( 2009 ). Each quality assessment question was graded as either 1 or 0. The final score of the study range from 0 to 3. A cut off score for excluding a study from the review is 2 points. Since the papers scored 2 or 3 points are included in the final evaluation. We framed the following quality assessment questions for the final study.
Quality Assessment 1: Internal validity.
Quality Assessment 2: External validity.
Quality Assessment 3: Bias.
The two reviewers review each paper to select the final list of documents. We used the Quadratic Weighted Kappa score to measure the final agreement between the two reviewers. The average resulted from the kappa score is 0.6942, a substantial agreement between the reviewers. The result of evolution criteria shown in Table Table1. 1 . After Quality Assessment, the final list of papers for review is shown in Table Table2. 2 . The complete selection process is shown in Fig. Fig.1. 1 . The total number of selected papers in year wise as shown in Fig. Fig.2. 2 .
Quality assessment analysis
Final list of papers

Selection process

Year wise publications
What are the datasets available for research on automated essay grading?
To work with problem statement especially in Machine Learning and deep learning domain, we require considerable amount of data to train the models. To answer this question, we listed all the data sets used for training and testing for automated essay grading systems. The Cambridge Learner Corpus-First Certificate in English exam (CLC-FCE) Yannakoudakis et al. ( 2011 ) developed corpora that contain 1244 essays and ten prompts. This corpus evaluates whether a student can write the relevant English sentences without any grammatical and spelling mistakes. This type of corpus helps to test the models built for GRE and TOFEL type of exams. It gives scores between 1 and 40.
Bailey and Meurers ( 2008 ), Created a dataset (CREE reading comprehension) for language learners and automated short answer scoring systems. The corpus consists of 566 responses from intermediate students. Mohler and Mihalcea ( 2009 ). Created a dataset for the computer science domain consists of 630 responses for data structure assignment questions. The scores are range from 0 to 5 given by two human raters.
Dzikovska et al. ( 2012 ) created a Student Response Analysis (SRA) corpus. It consists of two sub-groups: the BEETLE corpus consists of 56 questions and approximately 3000 responses from students in the electrical and electronics domain. The second one is the SCIENTSBANK(SemEval-2013) (Dzikovska et al. 2013a ; b ) corpus consists of 10,000 responses on 197 prompts on various science domains. The student responses ladled with "correct, partially correct incomplete, Contradictory, Irrelevant, Non-domain."
In the Kaggle (2012) competition, released total 3 types of corpuses on an Automated Student Assessment Prize (ASAP1) (“ https://www.kaggle.com/c/asap-sas/ ” ) essays and short answers. It has nearly 17,450 essays, out of which it provides up to 3000 essays for each prompt. It has eight prompts that test 7th to 10th grade US students. It gives scores between the [0–3] and [0–60] range. The limitations of these corpora are: (1) it has a different score range for other prompts. (2) It uses statistical features such as named entities extraction and lexical features of words to evaluate essays. ASAP + + is one more dataset from Kaggle. It is with six prompts, and each prompt has more than 1000 responses total of 10,696 from 8th-grade students. Another corpus contains ten prompts from science, English domains and a total of 17,207 responses. Two human graders evaluated all these responses.
Correnti et al. ( 2013 ) created a Response-to-Text Assessment (RTA) dataset used to check student writing skills in all directions like style, mechanism, and organization. 4–8 grade students give the responses to RTA. Basu et al. ( 2013 ) created a power grading dataset with 700 responses for ten different prompts from US immigration exams. It contains all short answers for assessment.
The TOEFL11 corpus Blanchard et al. ( 2013 ) contains 1100 essays evenly distributed over eight prompts. It is used to test the English language skills of a candidate attending the TOFEL exam. It scores the language proficiency of a candidate as low, medium, and high.
International Corpus of Learner English (ICLE) Granger et al. ( 2009 ) built a corpus of 3663 essays covering different dimensions. It has 12 prompts with 1003 essays that test the organizational skill of essay writing, and13 prompts, each with 830 essays that examine the thesis clarity and prompt adherence.
Argument Annotated Essays (AAE) Stab and Gurevych ( 2014 ) developed a corpus that contains 102 essays with 101 prompts taken from the essayforum2 site. It tests the persuasive nature of the student essay. The SCIENTSBANK corpus used by Sakaguchi et al. ( 2015 ) available in git-hub, containing 9804 answers to 197 questions in 15 science domains. Table Table3 3 illustrates all datasets related to AES systems.
ALL types Datasets used in Automatic scoring systems
Features play a major role in the neural network and other supervised Machine Learning approaches. The automatic essay grading systems scores student essays based on different types of features, which play a prominent role in training the models. Based on their syntax and semantics and they are categorized into three groups. 1. statistical-based features Contreras et al. ( 2018 ); Kumar et al. ( 2019 ); Mathias and Bhattacharyya ( 2018a ; b ) 2. Style-based (Syntax) features Cummins et al. ( 2016 ); Darwish and Mohamed ( 2020 ); Ke et al. ( 2019 ). 3. Content-based features Dong et al. ( 2017 ). A good set of features appropriate models evolved better AES systems. The vast majority of the researchers are using regression models if features are statistical-based. For Neural Networks models, researches are using both style-based and content-based features. The following table shows the list of various features used in existing AES Systems. Table Table4 4 represents all set of features used for essay grading.
Types of features
We studied all the feature extracting NLP libraries as shown in Fig. Fig.3. that 3 . that are used in the papers. The NLTK is an NLP tool used to retrieve statistical features like POS, word count, sentence count, etc. With NLTK, we can miss the essay's semantic features. To find semantic features Word2Vec Mikolov et al. ( 2013 ), GloVe Jeffrey Pennington et al. ( 2014 ) is the most used libraries to retrieve the semantic text from the essays. And in some systems, they directly trained the model with word embeddings to find the score. From Fig. Fig.4 4 as observed that non-content-based feature extraction is higher than content-based.

Usages of tools

Number of papers on content based features
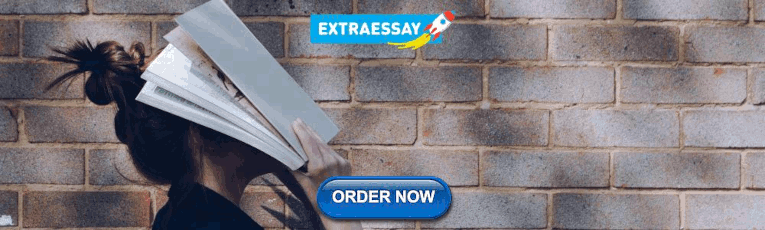
RQ3 which are the evaluation metrics available for measuring the accuracy of algorithms?
The majority of the AES systems are using three evaluation metrics. They are (1) quadrated weighted kappa (QWK) (2) Mean Absolute Error (MAE) (3) Pearson Correlation Coefficient (PCC) Shehab et al. ( 2016 ). The quadratic weighted kappa will find agreement between human evaluation score and system evaluation score and produces value ranging from 0 to 1. And the Mean Absolute Error is the actual difference between human-rated score to system-generated score. The mean square error (MSE) measures the average squares of the errors, i.e., the average squared difference between the human-rated and the system-generated scores. MSE will always give positive numbers only. Pearson's Correlation Coefficient (PCC) finds the correlation coefficient between two variables. It will provide three values (0, 1, − 1). "0" represents human-rated and system scores that are not related. "1" represents an increase in the two scores. "− 1" illustrates a negative relationship between the two scores.
RQ4 what are the Machine Learning techniques being used for automatic essay grading, and how are they implemented?
After scrutinizing all documents, we categorize the techniques used in automated essay grading systems into four baskets. 1. Regression techniques. 2. Classification model. 3. Neural networks. 4. Ontology-based approach.
All the existing AES systems developed in the last ten years employ supervised learning techniques. Researchers using supervised methods viewed the AES system as either regression or classification task. The goal of the regression task is to predict the score of an essay. The classification task is to classify the essays belonging to (low, medium, or highly) relevant to the question's topic. Since the last three years, most AES systems developed made use of the concept of the neural network.
Regression based models
Mohler and Mihalcea ( 2009 ). proposed text-to-text semantic similarity to assign a score to the student essays. There are two text similarity measures like Knowledge-based measures, corpus-based measures. There eight knowledge-based tests with all eight models. They found the similarity. The shortest path similarity determines based on the length, which shortest path between two contexts. Leacock & Chodorow find the similarity based on the shortest path's length between two concepts using node-counting. The Lesk similarity finds the overlap between the corresponding definitions, and Wu & Palmer algorithm finds similarities based on the depth of two given concepts in the wordnet taxonomy. Resnik, Lin, Jiang&Conrath, Hirst& St-Onge find the similarity based on different parameters like the concept, probability, normalization factor, lexical chains. In corpus-based likeness, there LSA BNC, LSA Wikipedia, and ESA Wikipedia, latent semantic analysis is trained on Wikipedia and has excellent domain knowledge. Among all similarity scores, correlation scores LSA Wikipedia scoring accuracy is more. But these similarity measure algorithms are not using NLP concepts. These models are before 2010 and basic concept models to continue the research automated essay grading with updated algorithms on neural networks with content-based features.
Adamson et al. ( 2014 ) proposed an automatic essay grading system which is a statistical-based approach in this they retrieved features like POS, Character count, Word count, Sentence count, Miss spelled words, n-gram representation of words to prepare essay vector. They formed a matrix with these all vectors in that they applied LSA to give a score to each essay. It is a statistical approach that doesn’t consider the semantics of the essay. The accuracy they got when compared to the human rater score with the system is 0.532.
Cummins et al. ( 2016 ). Proposed Timed Aggregate Perceptron vector model to give ranking to all the essays, and later they converted the rank algorithm to predict the score of the essay. The model trained with features like Word unigrams, bigrams, POS, Essay length, grammatical relation, Max word length, sentence length. It is multi-task learning, gives ranking to the essays, and predicts the score for the essay. The performance evaluated through QWK is 0.69, a substantial agreement between the human rater and the system.
Sultan et al. ( 2016 ). Proposed a Ridge regression model to find short answer scoring with Question Demoting. Question Demoting is the new concept included in the essay's final assessment to eliminate duplicate words from the essay. The extracted features are Text Similarity, which is the similarity between the student response and reference answer. Question Demoting is the number of repeats in a student response. With inverse document frequency, they assigned term weight. The sentence length Ratio is the number of words in the student response, is another feature. With these features, the Ridge regression model was used, and the accuracy they got 0.887.
Contreras et al. ( 2018 ). Proposed Ontology based on text mining in this model has given a score for essays in phases. In phase-I, they generated ontologies with ontoGen and SVM to find the concept and similarity in the essay. In phase II from ontologies, they retrieved features like essay length, word counts, correctness, vocabulary, and types of word used, domain information. After retrieving statistical data, they used a linear regression model to find the score of the essay. The accuracy score is the average of 0.5.
Darwish and Mohamed ( 2020 ) proposed the fusion of fuzzy Ontology with LSA. They retrieve two types of features, like syntax features and semantic features. In syntax features, they found Lexical Analysis with tokens, and they construct a parse tree. If the parse tree is broken, the essay is inconsistent—a separate grade assigned to the essay concerning syntax features. The semantic features are like similarity analysis, Spatial Data Analysis. Similarity analysis is to find duplicate sentences—Spatial Data Analysis for finding Euclid distance between the center and part. Later they combine syntax features and morphological features score for the final score. The accuracy they achieved with the multiple linear regression model is 0.77, mostly on statistical features.
Süzen Neslihan et al. ( 2020 ) proposed a text mining approach for short answer grading. First, their comparing model answers with student response by calculating the distance between two sentences. By comparing the model answer with student response, they find the essay's completeness and provide feedback. In this approach, model vocabulary plays a vital role in grading, and with this model vocabulary, the grade will be assigned to the student's response and provides feedback. The correlation between the student answer to model answer is 0.81.
Classification based Models
Persing and Ng ( 2013 ) used a support vector machine to score the essay. The features extracted are OS, N-gram, and semantic text to train the model and identified the keywords from the essay to give the final score.
Sakaguchi et al. ( 2015 ) proposed two methods: response-based and reference-based. In response-based scoring, the extracted features are response length, n-gram model, and syntactic elements to train the support vector regression model. In reference-based scoring, features such as sentence similarity using word2vec is used to find the cosine similarity of the sentences that is the final score of the response. First, the scores were discovered individually and later combined two features to find a final score. This system gave a remarkable increase in performance by combining the scores.
Mathias and Bhattacharyya ( 2018a ; b ) Proposed Automated Essay Grading Dataset with Essay Attribute Scores. The first concept features selection depends on the essay type. So the common attributes are Content, Organization, Word Choice, Sentence Fluency, Conventions. In this system, each attribute is scored individually, with the strength of each attribute identified. The model they used is a random forest classifier to assign scores to individual attributes. The accuracy they got with QWK is 0.74 for prompt 1 of the ASAS dataset ( https://www.kaggle.com/c/asap-sas/ ).
Ke et al. ( 2019 ) used a support vector machine to find the response score. In this method, features like Agreeability, Specificity, Clarity, Relevance to prompt, Conciseness, Eloquence, Confidence, Direction of development, Justification of opinion, and Justification of importance. First, the individual parameter score obtained was later combined with all scores to give a final response score. The features are used in the neural network to find whether the sentence is relevant to the topic or not.
Salim et al. ( 2019 ) proposed an XGBoost Machine Learning classifier to assess the essays. The algorithm trained on features like word count, POS, parse tree depth, and coherence in the articles with sentence similarity percentage; cohesion and coherence are considered for training. And they implemented K-fold cross-validation for a result the average accuracy after specific validations is 68.12.
Neural network models
Shehab et al. ( 2016 ) proposed a neural network method that used learning vector quantization to train human scored essays. After training, the network can provide a score to the ungraded essays. First, we should process the essay to remove Spell checking and then perform preprocessing steps like Document Tokenization, stop word removal, Stemming, and submit it to the neural network. Finally, the model will provide feedback on the essay, whether it is relevant to the topic. And the correlation coefficient between human rater and system score is 0.7665.
Kopparapu and De ( 2016 ) proposed the Automatic Ranking of Essays using Structural and Semantic Features. This approach constructed a super essay with all the responses. Next, ranking for a student essay is done based on the super-essay. The structural and semantic features derived helps to obtain the scores. In a paragraph, 15 Structural features like an average number of sentences, the average length of sentences, and the count of words, nouns, verbs, adjectives, etc., are used to obtain a syntactic score. A similarity score is used as semantic features to calculate the overall score.
Dong and Zhang ( 2016 ) proposed a hierarchical CNN model. The model builds two layers with word embedding to represents the words as the first layer. The second layer is a word convolution layer with max-pooling to find word vectors. The next layer is a sentence-level convolution layer with max-pooling to find the sentence's content and synonyms. A fully connected dense layer produces an output score for an essay. The accuracy with the hierarchical CNN model resulted in an average QWK of 0.754.
Taghipour and Ng ( 2016 ) proposed a first neural approach for essay scoring build in which convolution and recurrent neural network concepts help in scoring an essay. The network uses a lookup table with the one-hot representation of the word vector of an essay. The final efficiency of the network model with LSTM resulted in an average QWK of 0.708.
Dong et al. ( 2017 ). Proposed an Attention-based scoring system with CNN + LSTM to score an essay. For CNN, the input parameters were character embedding and word embedding, and it has attention pooling layers and used NLTK to obtain word and character embedding. The output gives a sentence vector, which provides sentence weight. After CNN, it will have an LSTM layer with an attention pooling layer, and this final layer results in the final score of the responses. The average QWK score is 0.764.
Riordan et al. ( 2017 ) proposed a neural network with CNN and LSTM layers. Word embedding, given as input to a neural network. An LSTM network layer will retrieve the window features and delivers them to the aggregation layer. The aggregation layer is a superficial layer that takes a correct window of words and gives successive layers to predict the answer's sore. The accuracy of the neural network resulted in a QWK of 0.90.
Zhao et al. ( 2017 ) proposed a new concept called Memory-Augmented Neural network with four layers, input representation layer, memory addressing layer, memory reading layer, and output layer. An input layer represents all essays in a vector form based on essay length. After converting the word vector, the memory addressing layer takes a sample of the essay and weighs all the terms. The memory reading layer takes the input from memory addressing segment and finds the content to finalize the score. Finally, the output layer will provide the final score of the essay. The accuracy of essay scores is 0.78, which is far better than the LSTM neural network.
Mathias and Bhattacharyya ( 2018a ; b ) proposed deep learning networks using LSTM with the CNN layer and GloVe pre-trained word embeddings. For this, they retrieved features like Sentence count essays, word count per sentence, Number of OOVs in the sentence, Language model score, and the text's perplexity. The network predicted the goodness scores of each essay. The higher the goodness scores, means higher the rank and vice versa.
Nguyen and Dery ( 2016 ). Proposed Neural Networks for Automated Essay Grading. In this method, a single layer bi-directional LSTM accepting word vector as input. Glove vectors used in this method resulted in an accuracy of 90%.
Ruseti et al. ( 2018 ) proposed a recurrent neural network that is capable of memorizing the text and generate a summary of an essay. The Bi-GRU network with the max-pooling layer molded on the word embedding of each document. It will provide scoring to the essay by comparing it with a summary of the essay from another Bi-GRU network. The result obtained an accuracy of 0.55.
Wang et al. ( 2018a ; b ) proposed an automatic scoring system with the bi-LSTM recurrent neural network model and retrieved the features using the word2vec technique. This method generated word embeddings from the essay words using the skip-gram model. And later, word embedding is used to train the neural network to find the final score. The softmax layer in LSTM obtains the importance of each word. This method used a QWK score of 0.83%.
Dasgupta et al. ( 2018 ) proposed a technique for essay scoring with augmenting textual qualitative Features. It extracted three types of linguistic, cognitive, and psychological features associated with a text document. The linguistic features are Part of Speech (POS), Universal Dependency relations, Structural Well-formedness, Lexical Diversity, Sentence Cohesion, Causality, and Informativeness of the text. The psychological features derived from the Linguistic Information and Word Count (LIWC) tool. They implemented a convolution recurrent neural network that takes input as word embedding and sentence vector, retrieved from the GloVe word vector. And the second layer is the Convolution Layer to find local features. The next layer is the recurrent neural network (LSTM) to find corresponding of the text. The accuracy of this method resulted in an average QWK of 0.764.
Liang et al. ( 2018 ) proposed a symmetrical neural network AES model with Bi-LSTM. They are extracting features from sample essays and student essays and preparing an embedding layer as input. The embedding layer output is transfer to the convolution layer from that LSTM will be trained. Hear the LSRM model has self-features extraction layer, which will find the essay's coherence. The average QWK score of SBLSTMA is 0.801.
Liu et al. ( 2019 ) proposed two-stage learning. In the first stage, they are assigning a score based on semantic data from the essay. The second stage scoring is based on some handcrafted features like grammar correction, essay length, number of sentences, etc. The average score of the two stages is 0.709.
Pedro Uria Rodriguez et al. ( 2019 ) proposed a sequence-to-sequence learning model for automatic essay scoring. They used BERT (Bidirectional Encoder Representations from Transformers), which extracts the semantics from a sentence from both directions. And XLnet sequence to sequence learning model to extract features like the next sentence in an essay. With this pre-trained model, they attained coherence from the essay to give the final score. The average QWK score of the model is 75.5.
Xia et al. ( 2019 ) proposed a two-layer Bi-directional LSTM neural network for the scoring of essays. The features extracted with word2vec to train the LSTM and accuracy of the model in an average of QWK is 0.870.
Kumar et al. ( 2019 ) Proposed an AutoSAS for short answer scoring. It used pre-trained Word2Vec and Doc2Vec models trained on Google News corpus and Wikipedia dump, respectively, to retrieve the features. First, they tagged every word POS and they found weighted words from the response. It also found prompt overlap to observe how the answer is relevant to the topic, and they defined lexical overlaps like noun overlap, argument overlap, and content overlap. This method used some statistical features like word frequency, difficulty, diversity, number of unique words in each response, type-token ratio, statistics of the sentence, word length, and logical operator-based features. This method uses a random forest model to train the dataset. The data set has sample responses with their associated score. The model will retrieve the features from both responses like graded and ungraded short answers with questions. The accuracy of AutoSAS with QWK is 0.78. It will work on any topics like Science, Arts, Biology, and English.
Jiaqi Lun et al. ( 2020 ) proposed an automatic short answer scoring with BERT. In this with a reference answer comparing student responses and assigning scores. The data augmentation is done with a neural network and with one correct answer from the dataset classifying reaming responses as correct or incorrect.
Zhu and Sun ( 2020 ) proposed a multimodal Machine Learning approach for automated essay scoring. First, they count the grammar score with the spaCy library and numerical count as the number of words and sentences with the same library. With this input, they trained a single and Bi LSTM neural network for finding the final score. For the LSTM model, they prepared sentence vectors with GloVe and word embedding with NLTK. Bi-LSTM will check each sentence in both directions to find semantic from the essay. The average QWK score with multiple models is 0.70.
Ontology based approach
Mohler et al. ( 2011 ) proposed a graph-based method to find semantic similarity in short answer scoring. For the ranking of answers, they used the support vector regression model. The bag of words is the main feature extracted in the system.
Ramachandran et al. ( 2015 ) also proposed a graph-based approach to find lexical based semantics. Identified phrase patterns and text patterns are the features to train a random forest regression model to score the essays. The accuracy of the model in a QWK is 0.78.
Zupanc et al. ( 2017 ) proposed sentence similarity networks to find the essay's score. Ajetunmobi and Daramola ( 2017 ) recommended an ontology-based information extraction approach and domain-based ontology to find the score.
Speech response scoring
Automatic scoring is in two ways one is text-based scoring, other is speech-based scoring. This paper discussed text-based scoring and its challenges, and now we cover speech scoring and common points between text and speech-based scoring. Evanini and Wang ( 2013 ), Worked on speech scoring of non-native school students, extracted features with speech ratter, and trained a linear regression model, concluding that accuracy varies based on voice pitching. Loukina et al. ( 2015 ) worked on feature selection from speech data and trained SVM. Malinin et al. ( 2016 ) used neural network models to train the data. Loukina et al. ( 2017 ). Proposed speech and text-based automatic scoring. Extracted text-based features, speech-based features and trained a deep neural network for speech-based scoring. They extracted 33 types of features based on acoustic signals. Malinin et al. ( 2017 ). Wu Xixin et al. ( 2020 ) Worked on deep neural networks for spoken language assessment. Incorporated different types of models and tested them. Ramanarayanan et al. ( 2017 ) worked on feature extraction methods and extracted punctuation, fluency, and stress and trained different Machine Learning models for scoring. Knill et al. ( 2018 ). Worked on Automatic speech recognizer and its errors how its impacts the speech assessment.
The state of the art
This section provides an overview of the existing AES systems with a comparative study w. r. t models, features applied, datasets, and evaluation metrics used for building the automated essay grading systems. We divided all 62 papers into two sets of the first set of review papers in Table Table5 5 with a comparative study of the AES systems.
State of the art
Comparison of all approaches
In our study, we divided major AES approaches into three categories. Regression models, classification models, and neural network models. The regression models failed to find cohesion and coherence from the essay because it trained on BoW(Bag of Words) features. In processing data from input to output, the regression models are less complicated than neural networks. There are unable to find many intricate patterns from the essay and unable to find sentence connectivity. If we train the model with BoW features in the neural network approach, the model never considers the essay's coherence and coherence.
First, to train a Machine Learning algorithm with essays, all the essays are converted to vector form. We can form a vector with BoW and Word2vec, TF-IDF. The BoW and Word2vec vector representation of essays represented in Table Table6. 6 . The vector representation of BoW with TF-IDF is not incorporating the essays semantic, and it’s just statistical learning from a given vector. Word2vec vector comprises semantic of essay in a unidirectional way.
Vector representation of essays
In BoW, the vector contains the frequency of word occurrences in the essay. The vector represents 1 and more based on the happenings of words in the essay and 0 for not present. So, in BoW, the vector does not maintain the relationship with adjacent words; it’s just for single words. In word2vec, the vector represents the relationship between words with other words and sentences prompt in multiple dimensional ways. But word2vec prepares vectors in a unidirectional way, not in a bidirectional way; word2vec fails to find semantic vectors when a word has two meanings, and the meaning depends on adjacent words. Table Table7 7 represents a comparison of Machine Learning models and features extracting methods.
Comparison of models
In AES, cohesion and coherence will check the content of the essay concerning the essay prompt these can be extracted from essay in the vector from. Two more parameters are there to access an essay is completeness and feedback. Completeness will check whether student’s response is sufficient or not though the student wrote correctly. Table Table8 8 represents all four parameters comparison for essay grading. Table Table9 9 illustrates comparison of all approaches based on various features like grammar, spelling, organization of essay, relevance.
Comparison of all models with respect to cohesion, coherence, completeness, feedback
comparison of all approaches on various features
What are the challenges/limitations in the current research?
From our study and results discussed in the previous sections, many researchers worked on automated essay scoring systems with numerous techniques. We have statistical methods, classification methods, and neural network approaches to evaluate the essay automatically. The main goal of the automated essay grading system is to reduce human effort and improve consistency.
The vast majority of essay scoring systems are dealing with the efficiency of the algorithm. But there are many challenges in automated essay grading systems. One should assess the essay by following parameters like the relevance of the content to the prompt, development of ideas, Cohesion, Coherence, and domain knowledge.
No model works on the relevance of content, which means whether student response or explanation is relevant to the given prompt or not if it is relevant to how much it is appropriate, and there is no discussion about the cohesion and coherence of the essays. All researches concentrated on extracting the features using some NLP libraries, trained their models, and testing the results. But there is no explanation in the essay evaluation system about consistency and completeness, But Palma and Atkinson ( 2018 ) explained coherence-based essay evaluation. And Zupanc and Bosnic ( 2014 ) also used the word coherence to evaluate essays. And they found consistency with latent semantic analysis (LSA) for finding coherence from essays, but the dictionary meaning of coherence is "The quality of being logical and consistent."
Another limitation is there is no domain knowledge-based evaluation of essays using Machine Learning models. For example, the meaning of a cell is different from biology to physics. Many Machine Learning models extract features with WordVec and GloVec; these NLP libraries cannot convert the words into vectors when they have two or more meanings.
Other challenges that influence the Automated Essay Scoring Systems.
All these approaches worked to improve the QWK score of their models. But QWK will not assess the model in terms of features extraction and constructed irrelevant answers. The QWK is not evaluating models whether the model is correctly assessing the answer or not. There are many challenges concerning students' responses to the Automatic scoring system. Like in evaluating approach, no model has examined how to evaluate the constructed irrelevant and adversarial answers. Especially the black box type of approaches like deep learning models provides more options to the students to bluff the automated scoring systems.
The Machine Learning models that work on statistical features are very vulnerable. Based on Powers et al. ( 2001 ) and Bejar Isaac et al. ( 2014 ), the E-rater was failed on Constructed Irrelevant Responses Strategy (CIRS). From the study of Bejar et al. ( 2013 ), Higgins and Heilman ( 2014 ), observed that when student response contain irrelevant content or shell language concurring to prompt will influence the final score of essays in an automated scoring system.
In deep learning approaches, most of the models automatically read the essay's features, and some methods work on word-based embedding and other character-based embedding features. From the study of Riordan Brain et al. ( 2019 ), The character-based embedding systems do not prioritize spelling correction. However, it is influencing the final score of the essay. From the study of Horbach and Zesch ( 2019 ), Various factors are influencing AES systems. For example, there are data set size, prompt type, answer length, training set, and human scorers for content-based scoring.
Ding et al. ( 2020 ) reviewed that the automated scoring system is vulnerable when a student response contains more words from prompt, like prompt vocabulary repeated in the response. Parekh et al. ( 2020 ) and Kumar et al. ( 2020 ) tested various neural network models of AES by iteratively adding important words, deleting unimportant words, shuffle the words, and repeating sentences in an essay and found that no change in the final score of essays. These neural network models failed to recognize common sense in adversaries' essays and give more options for the students to bluff the automated systems.
Other than NLP and ML techniques for AES. From Wresch ( 1993 ) to Madnani and Cahill ( 2018 ). discussed the complexity of AES systems, standards need to be followed. Like assessment rubrics to test subject knowledge, irrelevant responses, and ethical aspects of an algorithm like measuring the fairness of student response.
Fairness is an essential factor for automated systems. For example, in AES, fairness can be measure in an agreement between human score to machine score. Besides this, From Loukina et al. ( 2019 ), the fairness standards include overall score accuracy, overall score differences, and condition score differences between human and system scores. In addition, scoring different responses in the prospect of constructive relevant and irrelevant will improve fairness.
Madnani et al. ( 2017a ; b ). Discussed the fairness of AES systems for constructed responses and presented RMS open-source tool for detecting biases in the models. With this, one can change fairness standards according to their analysis of fairness.
From Berzak et al.'s ( 2018 ) approach, behavior factors are a significant challenge in automated scoring systems. That helps to find language proficiency, word characteristics (essential words from the text), predict the critical patterns from the text, find related sentences in an essay, and give a more accurate score.
Rupp ( 2018 ), has discussed the designing, evaluating, and deployment methodologies for AES systems. They provided notable characteristics of AES systems for deployment. They are like model performance, evaluation metrics for a model, threshold values, dynamically updated models, and framework.
First, we should check the model performance on different datasets and parameters for operational deployment. Selecting Evaluation metrics for AES models are like QWK, correlation coefficient, or sometimes both. Kelley and Preacher ( 2012 ) have discussed three categories of threshold values: marginal, borderline, and acceptable. The values can be varied based on data size, model performance, type of model (single scoring, multiple scoring models). Once a model is deployed and evaluates millions of responses every time for optimal responses, we need a dynamically updated model based on prompt and data. Finally, framework designing of AES model, hear a framework contains prompts where test-takers can write the responses. One can design two frameworks: a single scoring model for a single methodology and multiple scoring models for multiple concepts. When we deploy multiple scoring models, each prompt could be trained separately, or we can provide generalized models for all prompts with this accuracy may vary, and it is challenging.
Our Systematic literature review on the automated essay grading system first collected 542 papers with selected keywords from various databases. After inclusion and exclusion criteria, we left with 139 articles; on these selected papers, we applied Quality assessment criteria with two reviewers, and finally, we selected 62 writings for final review.
Our observations on automated essay grading systems from 2010 to 2020 are as followed:
- The implementation techniques of automated essay grading systems are classified into four buckets; there are 1. regression models 2. Classification models 3. Neural networks 4. Ontology-based methodology, but using neural networks, the researchers are more accurate than other techniques, and all the methods state of the art provided in Table Table3 3 .
- The majority of the regression and classification models on essay scoring used statistical features to find the final score. It means the systems or models trained on such parameters as word count, sentence count, etc. though the parameters extracted from the essay, the algorithm are not directly training on essays. The algorithms trained on some numbers obtained from the essay and hear if numbers matched the composition will get a good score; otherwise, the rating is less. In these models, the evaluation process is entirely on numbers, irrespective of the essay. So, there is a lot of chance to miss the coherence, relevance of the essay if we train our algorithm on statistical parameters.
- In the neural network approach, the models trained on Bag of Words (BoW) features. The BoW feature is missing the relationship between a word to word and the semantic meaning of the sentence. E.g., Sentence 1: John killed bob. Sentence 2: bob killed John. In these two sentences, the BoW is "John," "killed," "bob."
- In the Word2Vec library, if we are prepared a word vector from an essay in a unidirectional way, the vector will have a dependency with other words and finds the semantic relationship with other words. But if a word has two or more meanings like "Bank loan" and "River Bank," hear bank has two implications, and its adjacent words decide the sentence meaning; in this case, Word2Vec is not finding the real meaning of the word from the sentence.
- The features extracted from essays in the essay scoring system are classified into 3 type's features like statistical features, style-based features, and content-based features, which are explained in RQ2 and Table Table3. 3 . But statistical features, are playing a significant role in some systems and negligible in some systems. In Shehab et al. ( 2016 ); Cummins et al. ( 2016 ). Dong et al. ( 2017 ). Dong and Zhang ( 2016 ). Mathias and Bhattacharyya ( 2018a ; b ) Systems the assessment is entirely on statistical and style-based features they have not retrieved any content-based features. And in other systems that extract content from the essays, the role of statistical features is for only preprocessing essays but not included in the final grading.
- In AES systems, coherence is the main feature to be considered while evaluating essays. The actual meaning of coherence is to stick together. That is the logical connection of sentences (local level coherence) and paragraphs (global level coherence) in a story. Without coherence, all sentences in a paragraph are independent and meaningless. In an Essay, coherence is a significant feature that is explaining everything in a flow and its meaning. It is a powerful feature in AES system to find the semantics of essay. With coherence, one can assess whether all sentences are connected in a flow and all paragraphs are related to justify the prompt. Retrieving the coherence level from an essay is a critical task for all researchers in AES systems.
- In automatic essay grading systems, the assessment of essays concerning content is critical. That will give the actual score for the student. Most of the researches used statistical features like sentence length, word count, number of sentences, etc. But according to collected results, 32% of the systems used content-based features for the essay scoring. Example papers which are on content-based assessment are Taghipour and Ng ( 2016 ); Persing and Ng ( 2013 ); Wang et al. ( 2018a , 2018b ); Zhao et al. ( 2017 ); Kopparapu and De ( 2016 ), Kumar et al. ( 2019 ); Mathias and Bhattacharyya ( 2018a ; b ); Mohler and Mihalcea ( 2009 ) are used content and statistical-based features. The results are shown in Fig. Fig.3. 3 . And mainly the content-based features extracted with word2vec NLP library, but word2vec is capable of capturing the context of a word in a document, semantic and syntactic similarity, relation with other terms, but word2vec is capable of capturing the context word in a uni-direction either left or right. If a word has multiple meanings, there is a chance of missing the context in the essay. After analyzing all the papers, we found that content-based assessment is a qualitative assessment of essays.
- On the other hand, Horbach and Zesch ( 2019 ); Riordan Brain et al. ( 2019 ); Ding et al. ( 2020 ); Kumar et al. ( 2020 ) proved that neural network models are vulnerable when a student response contains constructed irrelevant, adversarial answers. And a student can easily bluff an automated scoring system by submitting different responses like repeating sentences and repeating prompt words in an essay. From Loukina et al. ( 2019 ), and Madnani et al. ( 2017b ). The fairness of an algorithm is an essential factor to be considered in AES systems.
- While talking about speech assessment, the data set contains audios of duration up to one minute. Feature extraction techniques are entirely different from text assessment, and accuracy varies based on speaking fluency, pitching, male to female voice and boy to adult voice. But the training algorithms are the same for text and speech assessment.
- Once an AES system evaluates essays and short answers accurately in all directions, there is a massive demand for automated systems in the educational and related world. Now AES systems are deployed in GRE, TOEFL exams; other than these, we can deploy AES systems in massive open online courses like Coursera(“ https://coursera.org/learn//machine-learning//exam ”), NPTEL ( https://swayam.gov.in/explorer ), etc. still they are assessing student performance with multiple-choice questions. In another perspective, AES systems can be deployed in information retrieval systems like Quora, stack overflow, etc., to check whether the retrieved response is appropriate to the question or not and can give ranking to the retrieved answers.
Conclusion and future work
As per our Systematic literature review, we studied 62 papers. There exist significant challenges for researchers in implementing automated essay grading systems. Several researchers are working rigorously on building a robust AES system despite its difficulty in solving this problem. All evaluating methods are not evaluated based on coherence, relevance, completeness, feedback, and knowledge-based. And 90% of essay grading systems are used Kaggle ASAP (2012) dataset, which has general essays from students and not required any domain knowledge, so there is a need for domain-specific essay datasets to train and test. Feature extraction is with NLTK, WordVec, and GloVec NLP libraries; these libraries have many limitations while converting a sentence into vector form. Apart from feature extraction and training Machine Learning models, no system is accessing the essay's completeness. No system provides feedback to the student response and not retrieving coherence vectors from the essay—another perspective the constructive irrelevant and adversarial student responses still questioning AES systems.
Our proposed research work will go on the content-based assessment of essays with domain knowledge and find a score for the essays with internal and external consistency. And we will create a new dataset concerning one domain. And another area in which we can improve is the feature extraction techniques.
This study includes only four digital databases for study selection may miss some functional studies on the topic. However, we hope that we covered most of the significant studies as we manually collected some papers published in useful journals.
Below is the link to the electronic supplementary material.
Not Applicable.
Publisher's Note
Springer Nature remains neutral with regard to jurisdictional claims in published maps and institutional affiliations.
Contributor Information
Dadi Ramesh, Email: moc.liamg@44hsemaridad .
Suresh Kumar Sanampudi, Email: ni.ca.hutnj@idupmanashserus .
- Adamson, A., Lamb, A., & December, R. M. (2014). Automated Essay Grading.
- Ajay HB, Tillett PI, Page EB (1973) Analysis of essays by computer (AEC-II) (No. 8-0102). Washington, DC: U.S. Department of Health, Education, and Welfare, Office of Education, National Center for Educational Research and Development
- Ajetunmobi SA, Daramola O (2017) Ontology-based information extraction for subject-focussed automatic essay evaluation. In: 2017 International Conference on Computing Networking and Informatics (ICCNI) p 1–6. IEEE
- Alva-Manchego F, et al. (2019) EASSE: Easier Automatic Sentence Simplification Evaluation.” ArXiv abs/1908.04567 (2019): n. pag
- Bailey S, Meurers D (2008) Diagnosing meaning errors in short answers to reading comprehension questions. In: Proceedings of the Third Workshop on Innovative Use of NLP for Building Educational Applications (Columbus), p 107–115
- Basu S, Jacobs C, Vanderwende L. Powergrading: a clustering approach to amplify human effort for short answer grading. Trans Assoc Comput Linguist (TACL) 2013; 1 :391–402. doi: 10.1162/tacl_a_00236. [ CrossRef ] [ Google Scholar ]
- Bejar, I. I., Flor, M., Futagi, Y., & Ramineni, C. (2014). On the vulnerability of automated scoring to construct-irrelevant response strategies (CIRS): An illustration. Assessing Writing, 22, 48-59.
- Bejar I, et al. (2013) Length of Textual Response as a Construct-Irrelevant Response Strategy: The Case of Shell Language. Research Report. ETS RR-13-07.” ETS Research Report Series (2013): n. pag
- Berzak Y, et al. (2018) “Assessing Language Proficiency from Eye Movements in Reading.” ArXiv abs/1804.07329 (2018): n. pag
- Blanchard D, Tetreault J, Higgins D, Cahill A, Chodorow M (2013) TOEFL11: A corpus of non-native English. ETS Research Report Series, 2013(2):i–15, 2013
- Blood, I. (2011). Automated essay scoring: a literature review. Studies in Applied Linguistics and TESOL, 11(2).
- Burrows S, Gurevych I, Stein B. The eras and trends of automatic short answer grading. Int J Artif Intell Educ. 2015; 25 :60–117. doi: 10.1007/s40593-014-0026-8. [ CrossRef ] [ Google Scholar ]
- Cader, A. (2020, July). The Potential for the Use of Deep Neural Networks in e-Learning Student Evaluation with New Data Augmentation Method. In International Conference on Artificial Intelligence in Education (pp. 37–42). Springer, Cham.
- Cai C (2019) Automatic essay scoring with recurrent neural network. In: Proceedings of the 3rd International Conference on High Performance Compilation, Computing and Communications (2019): n. pag.
- Chen M, Li X (2018) "Relevance-Based Automated Essay Scoring via Hierarchical Recurrent Model. In: 2018 International Conference on Asian Language Processing (IALP), Bandung, Indonesia, 2018, p 378–383, doi: 10.1109/IALP.2018.8629256
- Chen Z, Zhou Y (2019) "Research on Automatic Essay Scoring of Composition Based on CNN and OR. In: 2019 2nd International Conference on Artificial Intelligence and Big Data (ICAIBD), Chengdu, China, p 13–18, doi: 10.1109/ICAIBD.2019.8837007
- Contreras JO, Hilles SM, Abubakar ZB (2018) Automated essay scoring with ontology based on text mining and NLTK tools. In: 2018 International Conference on Smart Computing and Electronic Enterprise (ICSCEE), 1-6
- Correnti R, Matsumura LC, Hamilton L, Wang E. Assessing students’ skills at writing analytically in response to texts. Elem Sch J. 2013; 114 (2):142–177. doi: 10.1086/671936. [ CrossRef ] [ Google Scholar ]
- Cummins, R., Zhang, M., & Briscoe, E. (2016, August). Constrained multi-task learning for automated essay scoring. Association for Computational Linguistics.
- Darwish SM, Mohamed SK (2020) Automated essay evaluation based on fusion of fuzzy ontology and latent semantic analysis. In: Hassanien A, Azar A, Gaber T, Bhatnagar RF, Tolba M (eds) The International Conference on Advanced Machine Learning Technologies and Applications
- Dasgupta T, Naskar A, Dey L, Saha R (2018) Augmenting textual qualitative features in deep convolution recurrent neural network for automatic essay scoring. In: Proceedings of the 5th Workshop on Natural Language Processing Techniques for Educational Applications p 93–102
- Ding Y, et al. (2020) "Don’t take “nswvtnvakgxpm” for an answer–The surprising vulnerability of automatic content scoring systems to adversarial input." In: Proceedings of the 28th International Conference on Computational Linguistics
- Dong F, Zhang Y (2016) Automatic features for essay scoring–an empirical study. In: Proceedings of the 2016 Conference on Empirical Methods in Natural Language Processing p 1072–1077
- Dong F, Zhang Y, Yang J (2017) Attention-based recurrent convolutional neural network for automatic essay scoring. In: Proceedings of the 21st Conference on Computational Natural Language Learning (CoNLL 2017) p 153–162
- Dzikovska M, Nielsen R, Brew C, Leacock C, Gi ampiccolo D, Bentivogli L, Clark P, Dagan I, Dang HT (2013a) Semeval-2013 task 7: The joint student response analysis and 8th recognizing textual entailment challenge
- Dzikovska MO, Nielsen R, Brew C, Leacock C, Giampiccolo D, Bentivogli L, Clark P, Dagan I, Trang Dang H (2013b) SemEval-2013 Task 7: The Joint Student Response Analysis and 8th Recognizing Textual Entailment Challenge. *SEM 2013: The First Joint Conference on Lexical and Computational Semantics
- Educational Testing Service (2008) CriterionSM online writing evaluation service. Retrieved from http://www.ets.org/s/criterion/pdf/9286_CriterionBrochure.pdf .
- Evanini, K., & Wang, X. (2013, August). Automated speech scoring for non-native middle school students with multiple task types. In INTERSPEECH (pp. 2435–2439).
- Foltz PW, Laham D, Landauer TK (1999) The Intelligent Essay Assessor: Applications to Educational Technology. Interactive Multimedia Electronic Journal of Computer-Enhanced Learning, 1, 2, http://imej.wfu.edu/articles/1999/2/04/ index.asp
- Granger, S., Dagneaux, E., Meunier, F., & Paquot, M. (Eds.). (2009). International corpus of learner English. Louvain-la-Neuve: Presses universitaires de Louvain.
- Higgins D, Heilman M. Managing what we can measure: quantifying the susceptibility of automated scoring systems to gaming behavior” Educ Meas Issues Pract. 2014; 33 :36–46. doi: 10.1111/emip.12036. [ CrossRef ] [ Google Scholar ]
- Horbach A, Zesch T. The influence of variance in learner answers on automatic content scoring. Front Educ. 2019; 4 :28. doi: 10.3389/feduc.2019.00028. [ CrossRef ] [ Google Scholar ]
- https://www.coursera.org/learn/machine-learning/exam/7pytE/linear-regression-with-multiple-variables/attempt
- Hussein, M. A., Hassan, H., & Nassef, M. (2019). Automated language essay scoring systems: A literature review. PeerJ Computer Science, 5, e208. [ PMC free article ] [ PubMed ]
- Ke Z, Ng V (2019) “Automated essay scoring: a survey of the state of the art.” IJCAI
- Ke, Z., Inamdar, H., Lin, H., & Ng, V. (2019, July). Give me more feedback II: Annotating thesis strength and related attributes in student essays. In Proceedings of the 57th Annual Meeting of the Association for Computational Linguistics (pp. 3994-4004).
- Kelley K, Preacher KJ. On effect size. Psychol Methods. 2012; 17 (2):137–152. doi: 10.1037/a0028086. [ PubMed ] [ CrossRef ] [ Google Scholar ]
- Kitchenham B, Brereton OP, Budgen D, Turner M, Bailey J, Linkman S. Systematic literature reviews in software engineering–a systematic literature review. Inf Softw Technol. 2009; 51 (1):7–15. doi: 10.1016/j.infsof.2008.09.009. [ CrossRef ] [ Google Scholar ]
- Klebanov, B. B., & Madnani, N. (2020, July). Automated evaluation of writing–50 years and counting. In Proceedings of the 58th Annual Meeting of the Association for Computational Linguistics (pp. 7796–7810).
- Knill K, Gales M, Kyriakopoulos K, et al. (4 more authors) (2018) Impact of ASR performance on free speaking language assessment. In: Interspeech 2018.02–06 Sep 2018, Hyderabad, India. International Speech Communication Association (ISCA)
- Kopparapu SK, De A (2016) Automatic ranking of essays using structural and semantic features. In: 2016 International Conference on Advances in Computing, Communications and Informatics (ICACCI), p 519–523
- Kumar, Y., Aggarwal, S., Mahata, D., Shah, R. R., Kumaraguru, P., & Zimmermann, R. (2019, July). Get it scored using autosas—an automated system for scoring short answers. In Proceedings of the AAAI Conference on Artificial Intelligence (Vol. 33, No. 01, pp. 9662–9669).
- Kumar Y, et al. (2020) “Calling out bluff: attacking the robustness of automatic scoring systems with simple adversarial testing.” ArXiv abs/2007.06796
- Li X, Chen M, Nie J, Liu Z, Feng Z, Cai Y (2018) Coherence-Based Automated Essay Scoring Using Self-attention. In: Sun M, Liu T, Wang X, Liu Z, Liu Y (eds) Chinese Computational Linguistics and Natural Language Processing Based on Naturally Annotated Big Data. CCL 2018, NLP-NABD 2018. Lecture Notes in Computer Science, vol 11221. Springer, Cham. 10.1007/978-3-030-01716-3_32
- Liang G, On B, Jeong D, Kim H, Choi G. Automated essay scoring: a siamese bidirectional LSTM neural network architecture. Symmetry. 2018; 10 :682. doi: 10.3390/sym10120682. [ CrossRef ] [ Google Scholar ]
- Liua, H., Yeb, Y., & Wu, M. (2018, April). Ensemble Learning on Scoring Student Essay. In 2018 International Conference on Management and Education, Humanities and Social Sciences (MEHSS 2018). Atlantis Press.
- Liu J, Xu Y, Zhao L (2019) Automated Essay Scoring based on Two-Stage Learning. ArXiv, abs/1901.07744
- Loukina A, et al. (2015) Feature selection for automated speech scoring.” BEA@NAACL-HLT
- Loukina A, et al. (2017) “Speech- and Text-driven Features for Automated Scoring of English-Speaking Tasks.” SCNLP@EMNLP 2017
- Loukina A, et al. (2019) The many dimensions of algorithmic fairness in educational applications. BEA@ACL
- Lun J, Zhu J, Tang Y, Yang M (2020) Multiple data augmentation strategies for improving performance on automatic short answer scoring. In: Proceedings of the AAAI Conference on Artificial Intelligence, 34(09): 13389-13396
- Madnani, N., & Cahill, A. (2018, August). Automated scoring: Beyond natural language processing. In Proceedings of the 27th International Conference on Computational Linguistics (pp. 1099–1109).
- Madnani N, et al. (2017b) “Building better open-source tools to support fairness in automated scoring.” EthNLP@EACL
- Malinin A, et al. (2016) “Off-topic response detection for spontaneous spoken english assessment.” ACL
- Malinin A, et al. (2017) “Incorporating uncertainty into deep learning for spoken language assessment.” ACL
- Mathias S, Bhattacharyya P (2018a) Thank “Goodness”! A Way to Measure Style in Student Essays. In: Proceedings of the 5th Workshop on Natural Language Processing Techniques for Educational Applications p 35–41
- Mathias S, Bhattacharyya P (2018b) ASAP++: Enriching the ASAP automated essay grading dataset with essay attribute scores. In: Proceedings of the Eleventh International Conference on Language Resources and Evaluation (LREC 2018).
- Mikolov T, et al. (2013) “Efficient Estimation of Word Representations in Vector Space.” ICLR
- Mohler M, Mihalcea R (2009) Text-to-text semantic similarity for automatic short answer grading. In: Proceedings of the 12th Conference of the European Chapter of the ACL (EACL 2009) p 567–575
- Mohler M, Bunescu R, Mihalcea R (2011) Learning to grade short answer questions using semantic similarity measures and dependency graph alignments. In: Proceedings of the 49th annual meeting of the association for computational linguistics: Human language technologies p 752–762
- Muangkammuen P, Fukumoto F (2020) Multi-task Learning for Automated Essay Scoring with Sentiment Analysis. In: Proceedings of the 1st Conference of the Asia-Pacific Chapter of the Association for Computational Linguistics and the 10th International Joint Conference on Natural Language Processing: Student Research Workshop p 116–123
- Nguyen, H., & Dery, L. (2016). Neural networks for automated essay grading. CS224d Stanford Reports, 1–11.
- Palma D, Atkinson J. Coherence-based automatic essay assessment. IEEE Intell Syst. 2018; 33 (5):26–36. doi: 10.1109/MIS.2018.2877278. [ CrossRef ] [ Google Scholar ]
- Parekh S, et al (2020) My Teacher Thinks the World Is Flat! Interpreting Automatic Essay Scoring Mechanism.” ArXiv abs/2012.13872 (2020): n. pag
- Pennington, J., Socher, R., & Manning, C. D. (2014, October). Glove: Global vectors for word representation. In Proceedings of the 2014 conference on empirical methods in natural language processing (EMNLP) (pp. 1532–1543).
- Persing I, Ng V (2013) Modeling thesis clarity in student essays. In:Proceedings of the 51st Annual Meeting of the Association for Computational Linguistics (Volume 1: Long Papers) p 260–269
- Powers DE, Burstein JC, Chodorow M, Fowles ME, Kukich K. Stumping E-Rater: challenging the validity of automated essay scoring. ETS Res Rep Ser. 2001; 2001 (1):i–44. [ Google Scholar ]
- Powers DE, Burstein JC, Chodorow M, Fowles ME, Kukich K. Stumping e-rater: challenging the validity of automated essay scoring. Comput Hum Behav. 2002; 18 (2):103–134. doi: 10.1016/S0747-5632(01)00052-8. [ CrossRef ] [ Google Scholar ]
- Ramachandran L, Cheng J, Foltz P (2015) Identifying patterns for short answer scoring using graph-based lexico-semantic text matching. In: Proceedings of the Tenth Workshop on Innovative Use of NLP for Building Educational Applications p 97–106
- Ramanarayanan V, et al. (2017) “Human and Automated Scoring of Fluency, Pronunciation and Intonation During Human-Machine Spoken Dialog Interactions.” INTERSPEECH
- Riordan B, Horbach A, Cahill A, Zesch T, Lee C (2017) Investigating neural architectures for short answer scoring. In: Proceedings of the 12th Workshop on Innovative Use of NLP for Building Educational Applications p 159–168
- Riordan B, Flor M, Pugh R (2019) "How to account for misspellings: Quantifying the benefit of character representations in neural content scoring models."In: Proceedings of the Fourteenth Workshop on Innovative Use of NLP for Building Educational Applications
- Rodriguez P, Jafari A, Ormerod CM (2019) Language models and Automated Essay Scoring. ArXiv, abs/1909.09482
- Rudner, L. M., & Liang, T. (2002). Automated essay scoring using Bayes' theorem. The Journal of Technology, Learning and Assessment, 1(2).
- Rudner, L. M., Garcia, V., & Welch, C. (2006). An evaluation of IntelliMetric™ essay scoring system. The Journal of Technology, Learning and Assessment, 4(4).
- Rupp A. Designing, evaluating, and deploying automated scoring systems with validity in mind: methodological design decisions. Appl Meas Educ. 2018; 31 :191–214. doi: 10.1080/08957347.2018.1464448. [ CrossRef ] [ Google Scholar ]
- Ruseti S, Dascalu M, Johnson AM, McNamara DS, Balyan R, McCarthy KS, Trausan-Matu S (2018) Scoring summaries using recurrent neural networks. In: International Conference on Intelligent Tutoring Systems p 191–201. Springer, Cham
- Sakaguchi K, Heilman M, Madnani N (2015) Effective feature integration for automated short answer scoring. In: Proceedings of the 2015 conference of the North American Chapter of the association for computational linguistics: Human language technologies p 1049–1054
- Salim, Y., Stevanus, V., Barlian, E., Sari, A. C., & Suhartono, D. (2019, December). Automated English Digital Essay Grader Using Machine Learning. In 2019 IEEE International Conference on Engineering, Technology and Education (TALE) (pp. 1–6). IEEE.
- Shehab A, Elhoseny M, Hassanien AE (2016) A hybrid scheme for Automated Essay Grading based on LVQ and NLP techniques. In: 12th International Computer Engineering Conference (ICENCO), Cairo, 2016, p 65-70
- Shermis MD, Mzumara HR, Olson J, Harrington S. On-line grading of student essays: PEG goes on the World Wide Web. Assess Eval High Educ. 2001; 26 (3):247–259. doi: 10.1080/02602930120052404. [ CrossRef ] [ Google Scholar ]
- Stab C, Gurevych I (2014) Identifying argumentative discourse structures in persuasive essays. In: Proceedings of the 2014 Conference on Empirical Methods in Natural Language Processing (EMNLP) p 46–56
- Sultan MA, Salazar C, Sumner T (2016) Fast and easy short answer grading with high accuracy. In: Proceedings of the 2016 Conference of the North American Chapter of the Association for Computational Linguistics: Human Language Technologies p 1070–1075
- Süzen, N., Gorban, A. N., Levesley, J., & Mirkes, E. M. (2020). Automatic short answer grading and feedback using text mining methods. Procedia Computer Science, 169, 726–743.
- Taghipour K, Ng HT (2016) A neural approach to automated essay scoring. In: Proceedings of the 2016 conference on empirical methods in natural language processing p 1882–1891
- Tashu TM (2020) "Off-Topic Essay Detection Using C-BGRU Siamese. In: 2020 IEEE 14th International Conference on Semantic Computing (ICSC), San Diego, CA, USA, p 221–225, doi: 10.1109/ICSC.2020.00046
- Tashu TM, Horváth T (2019) A layered approach to automatic essay evaluation using word-embedding. In: McLaren B, Reilly R, Zvacek S, Uhomoibhi J (eds) Computer Supported Education. CSEDU 2018. Communications in Computer and Information Science, vol 1022. Springer, Cham
- Tashu TM, Horváth T (2020) Semantic-Based Feedback Recommendation for Automatic Essay Evaluation. In: Bi Y, Bhatia R, Kapoor S (eds) Intelligent Systems and Applications. IntelliSys 2019. Advances in Intelligent Systems and Computing, vol 1038. Springer, Cham
- Uto M, Okano M (2020) Robust Neural Automated Essay Scoring Using Item Response Theory. In: Bittencourt I, Cukurova M, Muldner K, Luckin R, Millán E (eds) Artificial Intelligence in Education. AIED 2020. Lecture Notes in Computer Science, vol 12163. Springer, Cham
- Wang Z, Liu J, Dong R (2018a) Intelligent Auto-grading System. In: 2018 5th IEEE International Conference on Cloud Computing and Intelligence Systems (CCIS) p 430–435. IEEE.
- Wang Y, et al. (2018b) “Automatic Essay Scoring Incorporating Rating Schema via Reinforcement Learning.” EMNLP
- Zhu W, Sun Y (2020) Automated essay scoring system using multi-model Machine Learning, david c. wyld et al. (eds): mlnlp, bdiot, itccma, csity, dtmn, aifz, sigpro
- Wresch W. The Imminence of Grading Essays by Computer-25 Years Later. Comput Compos. 1993; 10 :45–58. doi: 10.1016/S8755-4615(05)80058-1. [ CrossRef ] [ Google Scholar ]
- Wu, X., Knill, K., Gales, M., & Malinin, A. (2020). Ensemble approaches for uncertainty in spoken language assessment.
- Xia L, Liu J, Zhang Z (2019) Automatic Essay Scoring Model Based on Two-Layer Bi-directional Long-Short Term Memory Network. In: Proceedings of the 2019 3rd International Conference on Computer Science and Artificial Intelligence p 133–137
- Yannakoudakis H, Briscoe T, Medlock B (2011) A new dataset and method for automatically grading ESOL texts. In: Proceedings of the 49th annual meeting of the association for computational linguistics: human language technologies p 180–189
- Zhao S, Zhang Y, Xiong X, Botelho A, Heffernan N (2017) A memory-augmented neural model for automated grading. In: Proceedings of the Fourth (2017) ACM Conference on Learning@ Scale p 189–192
- Zupanc K, Bosnic Z (2014) Automated essay evaluation augmented with semantic coherence measures. In: 2014 IEEE International Conference on Data Mining p 1133–1138. IEEE.
- Zupanc K, Savić M, Bosnić Z, Ivanović M (2017) Evaluating coherence of essays using sentence-similarity networks. In: Proceedings of the 18th International Conference on Computer Systems and Technologies p 65–72
- Dzikovska, M. O., Nielsen, R., & Brew, C. (2012, June). Towards effective tutorial feedback for explanation questions: A dataset and baselines. In Proceedings of the 2012 Conference of the North American Chapter of the Association for Computational Linguistics: Human Language Technologies (pp. 200-210).
- Kumar, N., & Dey, L. (2013, November). Automatic Quality Assessment of documents with application to essay grading. In 2013 12th Mexican International Conference on Artificial Intelligence (pp. 216–222). IEEE.
- Wu, S. H., & Shih, W. F. (2018, July). A short answer grading system in chinese by support vector approach. In Proceedings of the 5th Workshop on Natural Language Processing Techniques for Educational Applications (pp. 125-129).
- Agung Putri Ratna, A., Lalita Luhurkinanti, D., Ibrahim I., Husna D., Dewi Purnamasari P. (2018). Automatic Essay Grading System for Japanese Language Examination Using Winnowing Algorithm, 2018 International Seminar on Application for Technology of Information and Communication, 2018, pp. 565–569. 10.1109/ISEMANTIC.2018.8549789.
- Sharma A., & Jayagopi D. B. (2018). Automated Grading of Handwritten Essays 2018 16th International Conference on Frontiers in Handwriting Recognition (ICFHR), 2018, pp 279–284. 10.1109/ICFHR-2018.2018.00056
Automating Systematic Literature Reviews with Natural Language Processing and Text Mining: A Systematic Literature Review
- Conference paper
- First Online: 25 July 2023
- Cite this conference paper
- Girish Sundaram 13 &
- Daniel Berleant 13
Part of the book series: Lecture Notes in Networks and Systems ((LNNS,volume 693))
Included in the following conference series:
- International Congress on Information and Communication Technology
607 Accesses
3 Citations
Objectives : An SLR is presented focusing on text mining-based automation of SLR creation. The present review identifies the objectives of the automation studies and the aspects of those steps that were automated. In so doing, the various ML techniques used challenges, limitations, and scope of further research are explained. Methods : Accessible published literature studies primarily focus on automation of study selection, study quality assessment, data extraction, and data synthesis portions of SLR. Twenty-nine studies were analyzed. Results : This review identifies the objectives of the automation studies, steps within the study selection, study quality assessment, data extraction, and data synthesis portions that were automated, and the various ML techniques used challenges, limitations, and scope of further research. Discussion : We describe uses of NLP/TM techniques to support increased automation of systematic literature reviews. This area has attracted increase attention in the last decade due to significant gaps in the applicability of TM to automate steps in the SLR process. There are significant gaps in the application of TM and related automation techniques in the areas of data extraction, monitoring, quality assessment, and data synthesis. There is, thus, a need for continued progress in this area, and this is expected to ultimately significantly facilitate the construction of systematic literature reviews.
This is a preview of subscription content, log in via an institution to check access.
Access this chapter
- Available as PDF
- Read on any device
- Instant download
- Own it forever
- Available as EPUB and PDF
- Compact, lightweight edition
- Dispatched in 3 to 5 business days
- Free shipping worldwide - see info
Tax calculation will be finalised at checkout
Purchases are for personal use only
Institutional subscriptions
Systematic reviews. Georgetown University Medical Center. https://guides.dml.georgetown.edu/systematicreviews
Systematic reviews (2001) CRD’s guidance for those carrying out or commissioning reviews. CRD Report Number 4 (2nd edn). NHS Centre for Reviews and Dissemination, University of York
Google Scholar
Glover J, Izzo D, Odato K et al (2006) EBM pyramid and EBM page generator. Trustees of Dartmouth College and Yale University
Kitchenham B, Charters S (2007) Guidelines for performing systematic literature reviews in software engineering. EBSE Technical Report EBSE-2007-01. Keele University. https://docs.edtechhub.org/lib/EDAG684W
Allen IE, Olkin I (1999) Estimating time to conduct a meta-analysis from number of citations retrieved. JAMA 282(7):634–635. https://doi.org/10.1001/jama.282.7.634
Article Google Scholar
Petticrew M, Roberts H (2006) Systematic reviews in the social sciences: a practical guide. Blackwell Publishing Co., Malden
Book Google Scholar
Shojani KG, Sampson M, Ansari MT et al (2007) How quickly do systematic reviews go out of date? A survival analysis. Ann Intern Med 147(4):224–233. https://doi.org/10.7326/0003-4819-147-4-200708210-00179
Marshall C, Kitchenham B, Brereton P (2018) Tool features to support systematic reviews in software engineering. E-Informatica Softw Eng J 12(1):79–115. https://doi.org/10.5277/e-Inf180104
Khangura S, Konnyu K, Cushman R et al (2012) Evidence summaries: the evolution of a rapid review approach. Syst Rev 1:10. https://doi.org/10.1186/2046-4053-1-10
Ganann R, Ciliska D, Thomas H (2010) Expediting systematic reviews: methods and implications of rapid reviews. Implementation Sci 5:56. https://doi.org/10.1186/1748-5908-5-56
Featherstone RM, Dryden DM, Foisy M et al (2015) Advancing knowledge of rapid reviews: an analysis of results, conclusions and recommendations from published review articles examining rapid reviews. Syst Rev 4:50. https://doi.org/10.1186/s13643-015-0040-4
Jonnalagadda SR, Goyal P, Huffman MD (2015) Automating data extraction in systematic reviews: a systematic review. Syst Rev 4:78. https://doi.org/10.1186/s13643-015-0066-7
O’Mara-Eves A, Thomas J, McNaught J et al (2015) Using text mining for study identification in systematic reviews: a systematic review of current approaches. Syst Rev 4:5. https://doi.org/10.1186/2046-4053-4-5
Feng L, Chiam Y, Lo SK (2017) Text-mining techniques and tools for systematic literature reviews: a systematic literature review. In: 24th Asia-Pacific software engineering conference (APSEC 2017). https://doi.org/10.1109/APSEC.2017.10
Tsafnat G, Glasziou P, Choong MK et al (2014) Systematic review automation technologies. Syst Rev 3(74). https://doi.org/10.1186/2046-4053-3-74
Marshall IJ, Wallace BC (2019) Toward systematic review automation: a practical guide to using machine learning tools in research synthesis. Syst Rev 8:163. https://doi.org/10.1186/s13643-019-1074-9
O’Connor AM, Tsafnat G, Gilbert SB et al (2018) Moving toward the automation of the systematic review process: a summary of discussions at the second meeting of the international collaboration for the automation of systematic reviews (ICASR). Syst Rev 7:3. https://doi.org/10.1186/s13643-017-0667-4
Beller E, Clark J, Tsafnat G et al (2018) Making progress with the automation of systematic reviews: principles of the international collaboration for the automation of systematic reviews (ICASR). Syst Rev 7:77. https://doi.org/10.1186/s13643-018-0740-7
Van Altena AJ, Spijker R, Olabarriaga SD (2019) Usage of automation tools in systematic reviews. Res Syn Meth 10:72–82. https://doi.org/10.1002/jrsm.1335
Olorisade BK, de Quincey E, Brereton OP et al (2016) A critical analysis of studies that address the use of text mining for citation screening in systematic reviews. In: EASE ’16: proceedings of the 20th international conference on evaluation and assessment in software engineering. ACM, Limerick, pp 1–11. https://doi.org/10.1145/2915970.2915982
Higgins J, Green S (2011) Cochrane handbook for systematic reviews of interventions version 5.1.0. The Cochrane Collaboration. http://community.cochrane.org/handbook .
Richardson WS, Wilson MC, Nishikawa J et al (1995) The well-built clinical question: a key to evidence-based decisions. ACP J Club 123(3):A12–A13
Dawes M, Pluye P, Shea L et al (2007) The identification of clinically important elements within medical journal abstracts: patient–population–problem, exposure-intervention, comparison, outcome, duration and results (PECODR). Inform Prim Care 15(1):9–16
Kim S, Martinez D, Cavedon L et al (2011) Automatic classification of sentences to support evidence based medicine. BMC Bioinform 12(Suppl 2):S5
Razavi A, Matwin S, Inkpen D et al (2009) Parameterized contrast in second order soft co-occurrences: a novel text representation technique in text mining and knowledge extraction. In: 2009 IEEE international conference on data mining workshops, pp 71–6
Bragge P, Clavisi O, Turner T et al (2011) The global evidence mapping initiative: scoping research in broad topic areas. BMC Med Res Methodol 11(92). https://doi.org/10.1186/1471-2288-11-92
Snilstveit B, Vojtkova M, Bhavsar A et al (2016) Evidence and gap maps—a tool for promoting evidence informed policy and strategic research agendas. J Clin Epidemiol 79:120–129. https://doi.org/10.1016/j.jclinepi.2016.05.015
Arksey H, O’Malley L (2005) Scoping studies: towards a methodological framework. Int J Soc Res Meth 8:19–32
RTC Collaboration. Review Manager (RevMan) 4.2 for Windows. The Cochrane Collaboration, Oxford (2003)
Tsafnat G, Coiera E (2009) Computational reasoning across multiple models. J Am Med Info Assoc 16(6):768–774
Sim I, Detmer DE (2005) Beyond trial registration: a global trial bank for clinical trial reporting. PLoS Med 2(11):e365
Sim I, Tu SW, Carini S et al (2014) The ontology of clinical research (OCRe): an informatics foundation for the science of clinical research. J Biomed Inf 52:78–91. https://doi.org/10.1016/j.jbi.2013.11.002
Greenhalgh T, Peacock R (2005) Effectiveness and efficiency of search methods in systematic reviews of complex evidence: audit of primary sources. BMJ 331(7524):1064–1065. https://doi.org/10.1136/bmj.38636.593461.68
Marshal C, Sutton A, O'Keefe H et al (2022) The systematic review toolbox. http://www.systematicreviewtools.com .
Bowes D, Hall T, Beecham S (2012) SLuRp : a tool to help large complex systematic literature reviews deliver valid and rigorous results. In: Proceedings of the 2nd international workshop on evidential assessment of software technologies—EAST ’12, pp 33–36
Hernandes E, Zamboni A, Fabbri S et al (2012) Using GQM and TAM to evaluate StArt—a tool that supports systematic review. CLEI Electr J 15(1):2. http://www.scielo.edu.uy/pdf/cleiej/v15n1/v15n1a03.pdf
Fernández-Sáez AM, Bocco MG, Romero FP (2010) SLR-Tool—a tool for performing systematic literature reviews. In: ICSOFT 2010—proceedings of the 5th international conference on software and data technologies, pp 157–166
Molléri JS, Benitti FBV (2015) SESRA: a web-based automated tool to support the systematic literature review process. In: EASE ’15: proceedings of the 19th international conference on evaluation and assessment in software engineering, pp 1–6. https://doi.org/10.1145/2745802.2745825
Gurbuz HG, Tekinerdogan B (2018) Model-based testing for software safety: a systematic mapping study. Software Qual J 26:1327–1372. https://doi.org/10.1007/s11219-017-9386-2
Van Dinter R, Tekinerdogan B, Cagatay C (2021) Automation of systematic literature reviews: a systematic literature review. Inf and Software Tech 136:106589. https://doi.org/10.1016/j.infsof.2021.106589
Wohlin C (2014) Guidelines for snowballing in systematic literature studies and a replication in software engineering. In: EASE ’14: proceedings of the 18th international conference on evaluation and assessment in software engineering.. ACM, pp 1–10. https://doi.org/10.1145/2601248.2601268
Dybå T, Dingsøyr T (2008) Empirical studies of agile software development: a systematic review. Inf Softw Tech 50(9):833–859
Nguyen-Duc A, Cruzes DS, Conradi R (2015) The impact of global dispersion on coordination, team performance and software quality—a systematic literature review. Inf and Softw Tech 57:277–294
Brownlee J, Tour of evaluation metrics for imbalanced classification. https://machinelearningmastery.com/tour-of-evaluation-metrics-for-imbalanced-classification
Wallace BC, Trikalinos TA, Lau J et al (2010) Semi-automated screening of biomedical citations for systematic reviews. BMC Bioinformatics 11(1):55. https://doi.org/10.1186/1471-2105-11-55
Pham B, Jovanovic J, Bagheri E et al (2021) Text mining to support abstract screening for knowledge syntheses: a semi-automated workflow. Syst Rev 10:156. https://doi.org/10.1186/s13643-021-01700-x
Norman CR, Leeflang M, Porcher R et al (2019) Measuring the impact of screening automation on meta-analyses of diagnostic test accuracy. Syst Rev 8:243. https://doi.org/10.1186/s13643-019-1162-x
Dickson K (2017) Systematic reviews to inform policy: institutional mechanisms and social interactions to support their production. Dissertation. University College London. http://discovery.ucl.ac.uk/id/eprint/10054092/1/KD_PhD_FinalAugust2018_Redacted.pdf
Turing A (1950) Computing machinery and intelligence. Mind LIX (236):433–460. https://doi.org/10.1093/mind/LIX.236.433
Mo Y, Kontonatsios G, Ananiadou S (2015) Supporting systematic reviews using LDA-based document representations. Syst Rev 4:172. https://doi.org/10.1186/s13643-015-0117-0
Cohen AM, Ambert K, McDonagh M (2012) Studying the potential impact of automated document classification on scheduling a systematic review update. BMC Med Inform Decis Mak 12:33. https://doi.org/10.1186/1472-6947-12-33
Callaghan MW, Müller-Hansen F (2020) Statistical stopping criteria for automated screening in systematic reviews. Syst Rev 9:273. https://doi.org/10.1186/s13643-020-01521-4
Miwa M, Thomas J, O’Mara-Eves A et al (2014) Reducing systematic review workload through certainty-based screening. J Biomed Inf 51:242–253. https://doi.org/10.1016/j.jbi.2014.06.005
Basu T, Kumar S, Kalyan A et al (2016) A novel framework to expedite systematic reviews by automatically building information extraction training corpora. arXiv:1606.06424 [cs.IR] (2016). https://arxiv.org/abs/1606.06424
García Adeva JJ, Pikatza Atxa JM, Ubeda CM et al (2014) Automatic text classification to support systematic reviews in medicine. Expert Syst with Appl 41(4):1498–1508. https://doi.org/10.1016/j.eswa.2013.08.047
Ros R, Bjarnason E, Runeson P (2017) A machine learning approach for semi-automated search and selection in literature studies. In: EASE ’17: proceedings of the 21st international conference on evaluation and assessment in software engineering. Association for Computing Machinery, New York, pp 118–127. https://doi.org/10.1145/3084226.3084243
Frunza O, Inkpen D, Matwin S (2010) Building systematic reviews using automatic text classification techniques. In: Proceedings of the 23rd international conference on computational linguistics: poster, vol (COLING ‘10). Association for Computational Linguistics, pp 303–311
Timsina P, Liu J, El-Gayar O (2016) Advanced analytics for the automation of medical systematic reviews. Inf Syst Frontiers 18(2):237–252
El-Gayar OF, Liu J, Timsina P (2015) Active learning for the automation of medical systematic review creation. In: 21st Americas conference on information systems (AMCIS). Puerto Rico Aug 13–15. htttp://aisel.aisnet.org/amcis2015/BizAnalytics/GeneralPresentations/22
Halamoda-Kenzaoui B, Rolland E, Piovesan J et al (2021) Toxic effects of nanomaterials for health applications: how automation can support a systematic review of the literature? J of Appl Tox 42(1):41–51. https://doi.org/10.1002/jat.4204
Olorisade BK, Brereton P, Andras P (2019) The use of bibliography enriched features for automatic citation screening. J of Biomed Inf 94:103202. https://doi.org/10.1016/j.jbi.2019.103202
Bannach-Brown A, Przybyła P, Thomas J et al (2019) Machine learning algorithms for systematic review: reducing workload in a preclinical review of animal studies and reducing human screening error. Syst Rev 8(1):23. https://doi.org/10.1186/s13643-019-0942-7
Bui D, Del Fiol G, Hurdle JF et al (2016) Extractive text summarization system to aid data extraction from full text in systematic review development. J Biomed Inf 64:265–272. https://doi.org/10.1016/j.jbi.2016.10.014
Tsafnat G, Glasziou P, Karystianis G et al (2018) Automated screening of research studies for systematic reviews using study characteristics. Syst Rev 7:64. https://doi.org/10.1186/s13643-018-0724-7
Norman C (2020) Systematic review automation methods. Université Paris-Saclay, Universiteit van Amsterdam https://tel.archives-ouvertes.fr/tel-03060620/document
Norman C, Leeflang M, Zweigenbaum P et al (2018) Automating document discovery in the systematic review process: how to use chaff to extract wheat. In: Proceedings of the eleventh international conference on language resources and evaluation (LREC 2018). European Language Resources Association (ELRA), Miyazaki, Japan. https://aclanthology.org/L18-1582
Karystianis G, Thayer K, Wolfe M et al (2017) Evaluation of a rule-based method for epidemiological document classification towards the automation of systematic reviews. J of Biomed Inf 70:27–34. https://doi.org/10.1016/j.jbi.2017.04.004
Wallace BC, Kuiper J, Sharma A et al (2016) Extracting PICO sentences from clinical trial reports using supervised distant supervision. J Mach Lear Res 17:132
MathSciNet Google Scholar
Marshall IJ, Kuiper J, Wallace BC (2015) Automating risk of bias assessment for clinical trials. J Biomed Health Inf 19(4):1406–1412. https://doi.org/10.1109/JBHI.2015.2431314
Ma Y (2007) Text classification on imbalanced data: application to systematic reviews automation. Dissertation. University of Ottawa
Begert D, Granek J, Irwin B et al (2020) Towards automating systematic reviews on immunization using an advanced natural language processing-based extraction system. Can Commun Dis Rep 46(6):174–179. https://doi.org/10.14745/ccdr.v46i06a04
Scells H, Zuccon G, Koopman B (2019) Automatic boolean query refinement for systematic review literature search. In: The World Wide Web Conference (WWW ‘19). Association for Computing Machinery, New York, pp 1646–1656. https://doi.org/10.1145/3308558.3313544
Khabsa M, Elmagarmid A, Ilyas I et al (2016) Learning to identify relevant studies for systematic reviews using random forest and external information. Mach Learn 102:465–482. https://doi.org/10.1007/s10994-015-5535-7
Article MathSciNet Google Scholar
Download references
Acknowledgements
Publication of this work was supported by the National Science Foundation under Award No. OIA-1946391. The content reflects the views of the authors and not necessarily the NSF. The authors are grateful to Deepak Sagaram, MD, for consulting on the list of articles regarding their relevance for inclusion and exclusion.
Author information
Authors and affiliations.
University of Arkansas at Little Rock, Little Rock, AR, 72204, USA
Girish Sundaram & Daniel Berleant
You can also search for this author in PubMed Google Scholar
Corresponding author
Correspondence to Girish Sundaram .
Editor information
Editors and affiliations.
Department of Design Engineering and Mathematics, Middlesex University London, London, UK
Xin-She Yang
Department of Biomedical Engineering, University of Reading, England, UK
R. Simon Sherratt
Department of Computer Science and Engineering, Techno International Newtown, Chakpachuria, West Bengal, India
Nilanjan Dey
Global Knowledge Research Foundation, Ahmedabad, India
Supplementary Material
The supplementary material, including data Tables A1–A4, may be obtained at
https://dberleant.github.io/papers/sundber-supp.pdf .
Rights and permissions
Reprints and permissions
Copyright information
© 2023 The Author(s), under exclusive license to Springer Nature Singapore Pte Ltd.
About this paper
Cite this paper.
Sundaram, G., Berleant, D. (2023). Automating Systematic Literature Reviews with Natural Language Processing and Text Mining: A Systematic Literature Review. In: Yang, XS., Sherratt, R.S., Dey, N., Joshi, A. (eds) Proceedings of Eighth International Congress on Information and Communication Technology. ICICT 2023. Lecture Notes in Networks and Systems, vol 693. Springer, Singapore. https://doi.org/10.1007/978-981-99-3243-6_7
Download citation
DOI : https://doi.org/10.1007/978-981-99-3243-6_7
Published : 25 July 2023
Publisher Name : Springer, Singapore
Print ISBN : 978-981-99-3242-9
Online ISBN : 978-981-99-3243-6
eBook Packages : Engineering Engineering (R0)
Share this paper
Anyone you share the following link with will be able to read this content:
Sorry, a shareable link is not currently available for this article.
Provided by the Springer Nature SharedIt content-sharing initiative
- Publish with us
Policies and ethics
- Find a journal
- Track your research
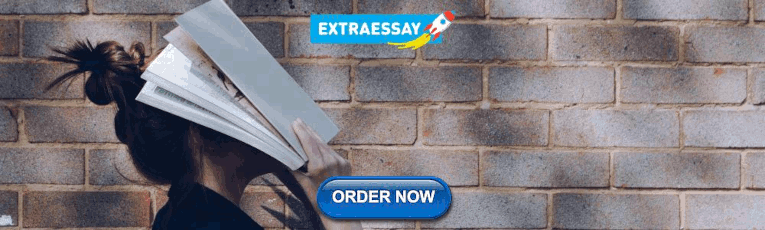
IMAGES
VIDEO
COMMENTS
Objectives: We want (1) to build a quality model for evaluating automated log-abstraction techniques and (2) to evaluate and recommend existing automated log-abstraction techniques using this quality model. Method: We perform a systematic literature review (SLR) of automated log-abstraction techniques.
Method: We perform a systematic literature review (SLR) of automated log-abstraction techniques. We review 89 research papers out of 2,864 initial papers. Results: Through this SLR, we (1) identify 17 automated log-abstraction techniques, (2) build a quality model composed of seven desirable aspects: mode, coverage, delimiter independence ...
Method: We perform a systematic literature review (SLR) of automated log-abstraction techniques. We review 89 research papers out of 2,864 initial papers. Results: Through this SLR, we (1 ...
2022. TLDR. This paper provides a systematic review of recent literature (covering the period between 2000 and June 2021, concentrating primarily on the last five years of this period) related to automated log analysis, and identifies different types of log files used in research. Expand.
A Systematic Literature Review on Automated Log Abstraction Techniques DianaEl-Masria,,FabioPetrillob,,Yann-GaëlGuéhéneucc,AbdelwahabHamou-Lhadjc,AnasBouzianea aDépartement de génie informatique et génie logiciel, Polytechnique Montréal, Montréal, QC, Canada E-mail: {diana.el-masri,anas.bouziane}@polymtl.ca bDépartment d'Informatique et Mathématique, Université du Québec à ...
Our quality model and recommendations help researchers learn about the state-of-the-art automated log-abstraction techniques, identify research gaps to enhance existing techniques, and develop new ones. We also support software engineers in understanding the advantages and limitations of existing techniques and in choosing the suitable technique to their unique use cases.
Abstract: Logging is a common practice in software engineering to provide insights into working systems. The main uses of log files have always been failure identification and root cause analysis. In recent years, novel applications of logging have emerged that benefit from automated analysis of log files, for example, real-time monitoring of system health, understanding users' behavior, and ...
Automated log-analysis techniques help software engineers gain insights into large log data. These techniques have several steps, among which log abstraction is the most important because it transforms raw log-data into high-level information. Thus, log abstraction allows software engineers to perform further analyses. Existing log-abstraction ...
A systematic literature review on automated log abstraction techniques @article{ElMasri2020ASL, title={A systematic literature review on automated log abstraction techniques}, author={Diana El-Masri and F{\'a}bio Petrillo and Yann-Ga{\"e}l Gu{\'e}h{\'e}neuc and Abdelwahab Hamou-Lhadj and Anas Bouziane}, journal={Inf. Softw.
from log abstraction, [272] provides a review of research ... It summarizes techniques that bene˝t from log-to-source linkage, as well as classes of problems that are ... Landscape of Automated ...
This paper provides a systematic review of recent literature (covering the period between 2000 and June 2021, concentrating primarily on the last five years of this period) related to automated log analysis, and identifies different types of log files used in research. Logging is a common practice in software engineering to provide insights into working systems. The main uses of log files have ...
Notice that the definition of A is left generic, to accommodate different types of results yielded by log parsing techniques. Using the concept of \(\tau \), the results obtained by a parsing approach can be seen as an abstraction of the original log itself. Definition 4 (Abstraction of Log)
Received January 10, 2022, accepted February 9, 2022, date of publication February 17, 2022, date of current version March 3, 2022. Digital Object Identifier 10.1109/ACCESS.2022.3152549 Landscape of Automated Log Analysis: A Systematic Literature Review and Mapping Study ŁUKASZ KORZENIOWSKI 1 Nordea 1 AND KRZYSZTOF GOCZYŁA 2 Bank Abp SA, 00020 Helsinki, Finland 2 Faculty of Electronics ...
A Systematic Literature Review on Automated Log Abstraction Techniques Diana El-Masri a, *, Fabio Petrillo b,, Yann-Gaël Guéhéneuc c, Abdelwahab Hamou-Lhadj c, Anas Bouziane a a Département de génie informatique et génie logiciel, Polytechnique Montréal, Montréal, QC, Canada E-mail: {diana.el-masri,anas.bouziane}@polymtl.ca b Départment d'Informatique et Mathématique, Université ...
Context: Logs are often the first and only information available to software engineers to understand and debug their systems. Automated log-analysis techniques help software engineers gain insights into large log data. These techniques have several steps, among which log abstraction is the most important because it transforms raw log-data into high-level information.
4_El-Masri21_A Systematic Literature Review of Automated Log Abstraction Techniques - Free download as PDF File (.pdf), Text File (.txt) or read online for free.
Abstract. Log audits are the technical means to retrospectively reconstruct and analyze system activities for determining if the system is executed in accordance with the rules. This approach to compliance is referred to as compliance by detection. In the case of privacy adherence validation (or) privacy audits, the rules for compliance are ...
The technique uses an object-based model to extract associative rules and acquire a domain model of expert configuration tasks. A systematic literature review and mapping study were conducted to ...
In 2011, Thomas et al. [17] published a report that lists the application of text mining techniques to automate the systematic literature review process. In total, we have found 5 studies that reported text mining techniques and tools to automate a - part of - the systematic review process [12, [17], [18], [19], [20]].Tsafnat et al. [21] describe each step in the systematic review process ...
Automatic systematic review tools can be categorised into several categories: visualization tools - tools that use active learning (a combination of a Natural Language Processing (NLP) technique, machine learning classifier, and human labour) and automated tools that employ an NLP and classifier but they use labelled documents and no human interaction during the learning process (Scott et al ...
This paper aims to provide a systematic literature review (SLR) on automated essay grading systems. An SLR is an Evidence-based systematic review to summarize the existing research. It critically evaluates and integrates all relevant studies' findings and addresses the research domain's specific research questions.
A systematic review is one of the numerous types of reviews and is defined as "a review of the evidence on a clearly formulated question that uses systematic and explicit methods to identify, select, and critically appraise relevant primary research and to extract and analyze data from the studies that are included in the review." The ...
A Systematic Literature Review on Automated Log Abstraction Techniques Diana El-Masri a, *, Fabio Petrillo b,, Yann-Gaël Guéhéneuc c, Abdelwahab Hamou-Lhadj c, Anas Bouziane a a Département de génie informatique et génie logiciel, Polytechnique Montréal, Montréal, QC, Canada E-mail: {diana.el-masri,anas.bouziane}@polymtl.ca b Départment d'Informatique et Mathématique, Université ...