A PhD Student’s Perspective on Research in NLP in the Era of Very Large Language Models
Recent progress in large language models has enabled the deployment of many generative NLP applications. At the same time, it has also led to a misleading public discourse that “it’s all been solved.” Not surprisingly, this has in turn made many NLP researchers – especially those at the beginning of their career – wonder about what NLP research area they should focus on. This document is a compilation of NLP research directions that are rich for exploration, reflecting the views of a diverse group of PhD students in an academic research lab. While we identify many research areas, many others exist; we do not cover those areas that are currently addressed by LLMs but where LLMs lag behind in performance, or those focused on LLM development. We welcome suggestions for other research directions to include: https://bit.ly/nlp-era-llm
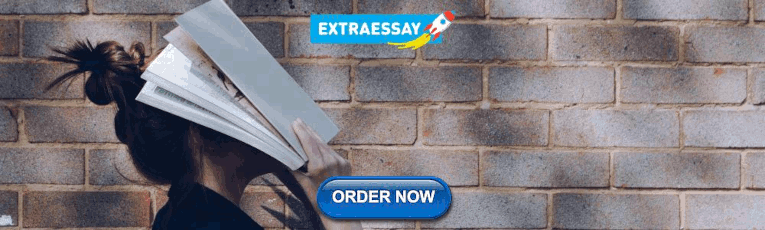
1 Background
Language models represent one of the fundamental building blocks in NLP, with their roots traced back to 1948 when Claude Shannon introduced Markov chains to model sequences of letters in English text (Shannon, 1948 ) . They were then heavily used in connection to the early research on statistical machine translation (Brown et al., 1988 ; Wilkes, 1994 ) and statistical speech processing (Jelinek, 1976 ) . While these models have always been used as an integral part of broad application categories such as text classification, information retrieval, or text generation, only in recent years they found a “life of their own” with widespread use and deployment.
The impressive advancements we have witnessed in current “large” and “very large” language models directly result from those earlier models. They build on the same simple yet groundbreaking idea: given a series of previous words or characters, we can predict what will come next. The new large language models (LLMs) benefit from two main developments: (1) the proliferation of Web 2.0 and user-generated data, which has led to a sharp increase in the availability of data; and (2) the growth in computational capabilities through the introduction of Graphics Processing Units (GPUs). Together, these developments have facilitated the resurgence of neural networks (or deep learning) and the availability of very large training datasets for these models.
Current LLMs have output quality comparable to human performance, with the added benefit of integrating information from enormous data sources, far surpassing what one individual can accumulate in their lifetime. The number of applications that benefit from using LLMs is continuously growing, with many cases where the LLMs are used to replace entire complex pipelines. LLMs becoming “lucrative” has led to a surge in industry interest and funding, alongside a sharp increase in the number of research publications on LLMs. For instance, a search on Google Scholar for “language models” leads to 50,000 publications over the past five years, a third of the roughly 150,000 papers published during the past 25 years.
While these advances in LLMs are very real and truly exciting, and give hope for many new deployed generative language applications, LLMs have also “sucked the air out of the room.” A recent funding call from DARPA has completely replaced the term NLP with LLM: in their listing of experts sought for the program, we see the fields of “Computer Vision” and “Machine Learning” listed alongside “Large Language Models” (but not “Natural Language Processing”). * * * https://apply.knowinnovation.com/darpaaiforward/ Replacing NLP with LLMs is problematic for two main reasons. First, the space of language insights, methods, and broad applications in NLP is much more vast than what can be accomplished by simply predicting the next word. Second, even if not technologically new, LLMs still represent an exclusionary space because of the amount of data and computation required to train.
This public discourse that often reduces the entire field of NLP to the much smaller space of LLMs is not surprisingly leading to a dilemma for those who have dedicated their careers to advancing research in this field, and especially for junior PhD students who have only recently embarked on the path of becoming NLP researchers. “ What should I work on? ” is a question we hear now much more often than before, often as a reaction to the misleading thought that “it’s been all solved.”
The reality is that there is much more to NLP than just LLMs. This document is a compilation of ideas from PhD students, building upon their initial expertise and existing interests and brainstorming around the question: “What are rich areas of exploration in the field of NLP that could lead to a PhD thesis and cover a space that is not within the purview of LLMs.” Spoiler alert: there are many such research areas!
About This Document.
This document reflects the ideas about “the future of NLP research” from the members of an academic NLP research lab in the United States. The Language and Information Technologies (LIT) lab at the University of Michigan includes students at various stages in their degree, starting with students who are about to embark on a PhD, all the way to students who recently completed a PhD degree. The LIT students come from a wide variety of backgrounds, including China, Iran, Japan, Mexico, Nigeria, Romania, Russia, South Korea, United States, and Uruguay, reflecting a very diverse set of beliefs, values, and lived experiences. Our research interests cover a wide range of NLP areas, including computational social science, causal reasoning, misinformation detection, healthcare conversation analysis, knowledge-aware generation, commonsense reasoning, cross-cultural models, multimodal question answering, non-verbal communication, visual understanding, and more.
When compiling the ideas in this document, we followed three main guiding principles. First, we aimed to identify areas of NLP research that are rich for exploration; e.g., areas that one could write a PhD thesis on. Second, we wanted to highlight research directions that do not have a direct dependency on a paid resource; while the use of existing paid APIs can be fruitful for certain tasks, such as the construction of synthetic datasets, building systems that cannot function without paid APIs is not well aligned with academic core research goals. Finally, third, we targeted research directions that can find solutions with reasonable computational costs achievable with setups more typically available in academic labs.
Our brainstorming process started with ideas written on sticky notes by all the authors of this document, followed by a “clustering” process where we grouped the initial ideas and identified several main themes. These initial themes were then provided to small groups of 2–3 students, who discussed them, expanded or merged some of the themes, and identified several directions worthy of exploration. The final set of themes formed the seed of this document. Each research area has then had multiple passes from multiple students (and Rada) to delineate the background of each theme, the gaps, and the most promising research directions.
Disclaimer.
The research areas listed in this document are just a few of the areas rich in exploration; many others exist. In particular, we have not listed the numerous research directions where LLMs have been demonstrated to lag behind in performance Bang et al. ( 2023a ) , including information extraction, question answering, text summarization, and others. We have also not listed the research directions focused on LLM development, as that is already a major focus in many current research papers and our goal was to highlight the research directions other than LLM development. We welcome suggestions for other research areas or directions to include: https://bit.ly/nlp-era-llm
Document Organization.
The following sections provide brief descriptions of fourteen research areas rich in exploration, each of them with 2–4 research directions. These areas could be broadly divided into areas that cannot be addressed by LLMs for being too data-hungry or for lacking reasoning or grounding abilities (Sections 2–6, 8, 12); areas for which we cannot use LLMs because of not having the right data (Sections 9, 13, 14); or areas that could contribute to improving the abilities and quality of LLMs (Sections 7, 10, 11, 15).
2 Multilinguality and Low-Resource Languages
Background..
Multilingual models are designed to handle multiple languages, whether for the task of machine translation (MT) or other tasks. A major challenge is handling low-resource languages, for which there is limited availability of training data, which can result in poor translation quality and poor performance on these languages. The research community has proposed several techniques to overcome this challenge, such as data augmentation, including synthetic data generation through back-translation (Sennrich et al., 2015 ; Edunov et al., 2018 ) , parallel-corpus mining (Artetxe and Schwenk, 2018 ) , or OCR (Rijhwani et al., 2020 ; Ignat et al., 2022 ) ; and multilingual models, which are pre-trained models that can handle multiple languages and can be fine-tuned on low-resource languages to improve translation quality. Recent efforts to develop multilingual models for low-resource languages include NLLB-200 (NLLB Team et al., 2022 ) , a state-of-the-art Mixture of Experts (MoE) model trained on a dataset containing more than 18 billion sentence pairs. The same team also created and open-sourced an expanded benchmark dataset, FLORES-200 (Goyal et al., 2021 ) , for evaluating MT models in 200 languages and over 40k translation directions.
State-of-the-art MT models such as NLLB-200 NLLB Team et al. ( 2022 ) still perform poorly on many low-resource languages, such as African languages. For instance, recent work tested the ChatGPT MT performance on low-resource languages (e.g., Marathi, Sundanese, and Buginese) and found an overall poor performance, especially in non-Latin scripts (Bang et al., 2023b ) . They also found that ChatGPT can perform reasonably well on low-resource language to-English translation, but it cannot perform English to low-resource language translation. In addition, MT systems do not exist for the vast majority of the world’s roughly 7,000 languages.
Research Directions.
Improve MT performance on current low- and very low-resource language benchmarks. There is still plenty of room for improving the state-of-the-art models on current benchmarks such as FLORES-200. This benchmark has spurred recent interest in creating other benchmarks for low-resource languages, such as African languages (Vegi et al., 2022 ; Reid et al., 2021 ) . Extremely low-resource languages do not have a significant web presence and thus do not have adequate bitext for training MT systems. These languages may have a translation of the Bible (the most translated document in the world), which can serve as a starting point for developing MT systems (McCarthy et al., 2020 ; Mueller et al., 2020 ) . There is also recent interest in manually creating parallel corpora, such as for the languages Amis (Zheng et al., 2022 ) and Minankabau (Koto and Koto, 2020 ) , but this process is expensive and time-consuming. In the absence of bilingual and even monolingual training corpora, one line of research is developing and expanding translation dictionaries using models of word formation (Wu and Yarowsky, 2018 , 2020 ) .
Multilingual models that work well for all languages. Although most recent LLMs claim to be multilingual, they do not perform equally well in all languages when it comes to tasks such as prediction, classification, or generation. Some models are trained in part on web text like Common Crawl (Smith et al., 2013 ) , which contains predominantly English text. Open questions include how much data, and what combination of languages, are necessary to enable similar performance on multiple languages. Additionally, cross-lingual projection continues to be a potential source of data for models in other languages, by leveraging the data available in major languages along with existing MT systems, to transfer model architectures onto other languages.
Code-switching. Code-switching (CS) is a phenomenon in which a speaker alternates between languages while adhering to the grammatical structure of at least one language. CS data presents a unique set of challenges for NLP tasks. The nature of CS leads speakers to create “new” words, meaning that models designed to accommodate CS data must be robust to out-of-vocabulary tokens Çetinoğlu et al. ( 2016 ) . Training data is difficult to obtain, also making it difficult to learn CS-specific models. An active area of research is in determining to what extent LLMs can generate synthetic CS data; previous methods commonly use parallel corpora to substitute tokens with grammatical rules as constraints Xu and Yvon ( 2021 ); Lee and Li ( 2020 ) . Additional areas of research include exploring to what extent models can be generalizable across different language combinations, and learning models that can effectively distinguish between highly similar languages, such as dialects of the same parent language Aguilar et al. ( 2020 ) . Recently, benchmarks such as LinCE Aguilar et al. ( 2020 ) and GLUECoS Khanuja et al. ( 2020 ) have been established to evaluate performance on classic NLP tasks on a number of common languages, but these benchmarks are not all-encompassing in regards to tasks and language combinations.
3 Reasoning
Reasoning is a fundamental aspect of human intelligence, playing a critical role in problem-solving or decision-making by drawing inferences from premises, facts, and knowledge using logical principles and cognitive processes. There are various reasoning types, including deductive, inductive, abductive, quantitative, causal, and moral reasoning. Improving reasoning skills in NLP is vital for tasks such as question answering, reading comprehension, and dialogue systems, as it can enhance a model’s generalization ability in unseen scenarios. NLP research has evolved significantly, from early rule-based and symbolic approaches to statistical methods in the 1990s, which utilized probabilistic models and machine learning algorithms. In recent years, deep learning and neural networks have revolutionized the field, achieving state-of-the-art performance on various tasks. However, challenges remain in attaining human-like reasoning and generalization abilities, driving continued research for more sophisticated and robust NLP models.
Although LLMs have shown impressive performance on many reasoning benchmarks Brown et al. ( 2020b ); Ouyang et al. ( 2022 ); Zhang et al. ( 2022 ); Touvron et al. ( 2023a ); OpenAI ( 2023 ) , there are still several directions that remain challenging. They struggle to robustly manage formal reasoning Jin et al. ( 2022b ); Stolfo et al. ( 2023 ); Jin et al. ( 2023a ) , as we often see LLMs prone to errors that a formal or symbolic system would not make. Additionally, since most of their training interacts with a world of text, NLP models still lack grounding in real-world experiences when reasoning Ignat et al. ( 2021 ) . Lastly, more fundamental questions remain to be answered, such as distinguishing empirical knowledge and rational reasoning, and unveiling how LLMs reason.
Robust formal reasoning. Formal reasoning has long been a challenging task for neural networks. LLMs are far from complete mastery of formal tasks such as numerical reasoning Stolfo et al. ( 2023 ); Miao et al. ( 2020 ) , logical reasoning Jin et al. ( 2022b ) , and causal inference Jin et al. ( 2023a , c ) , often making obvious mistakes Goel et al. ( 2021 ); Jin et al. ( 2020 ) . To this end, a robust model should know how to generalize. To robustly manage formal reasoning, one could explore a variety of directions, such as combining the strengths of neural networks and symbolic AI. A popular line of work has been to integrate external reasoning systems, such as calculators, python interpreters, knowledge retrieval from databases, or search engines Schick et al. ( 2023 ); Mialon et al. ( 2023 ) .
Grounded reasoning in the physical real world. While current models generate coherent and contextually relevant responses, they often lack an understanding of the physical world and its constraints. This can lead to linguistically plausible responses that are nonsensical or unrealistic in practice. To address this issue, one direction is to explore ways to incorporate external knowledge sources, multimodal data, or simulated world scenarios to ground the reasoning skills of the models.
Responsible reasoning in social contexts. With increasing numbers of applications that use NLP models, it is foreseeable that models will need to make complicated decisions that involve moral reasoning as intermediate steps. For example, when creating a website, there may be moral choices to consider such as catering towards certain subpopulations, or overly optimizing for user attention or click-through rates. These decision principles are pervasive in our daily life, across small and large tasks. We believe there is much to be studied in understanding or improving the ability of AI systems to reason over socially-complicated and morally-charged scenarios given different social contexts and cultural backgrounds (Jin et al., 2023b ; Hendrycks et al., 2021 ) . We foresee that interdisciplinary collaboration with domain experts and policymakers will be needed.
Formally defining reasoning and designing proper evaluation framework. There is a rising need to refine the definition of reasoning, because LLMs start to make the difference between knowledge and reasoning blurry – when a model memorizes a reasoning pattern, does it count as the mastery of reasoning or knowledge? Models already start to show an increasing mastery of templated solutions by pattern matching, which seems to be the reasoning that many want. Fundamentally, it leads to a question about what are the sparkles of intelligence that humans excel at, and how different are these from empirically learning how to do template matching. Beyond redefining reasoning, then the other open question is how to test models’ reasoning skills. We face problems such as data contamination, Goodhart’s law (a dataset failing to reflect the skill once it is exploited), and a lack of reliable metrics to evaluate multi-step reasoning.
Analyzing how prompts help reasoning. There are two types of prompting whose effect on LLMs are worth inspection: in-context learning and chain of thought. Recent work shows that conditioning on in-context examples has a similar effect to finetuning the model Akyürek et al. ( 2022 ) , and researchers start to decode the mechanisms that models start to pick up from the given context, such as induction heads Olsson et al. ( 2022 ) . Apart from the in-context instructions, we can also prompt LLMs with intermediate steps using chain-of-thought prompting. This approach breaks down reasoning tasks into smaller sub-problems, similar to human problem-solving. However, it is debatable whether language models truly reason or just generate statistically-alike sequences, and to what extent AI systems can learn to reason from few-shot exemplars.
4 Knowledge Bases
A knowledge base is a collection of facts about real-world objects, abstract concepts, or events. The knowledge inside a knowledge base is usually represented as a triplet consisting of a head entity, a tail entity, and their relationships. For instance (Barack Obama, birthPlace, Honolulu) is an example of a triplet indicating a place-of-birth relationship. Some knowledge bases focus more on factual knowledge, such as DBPedia (Auer et al., 2007 ) and YAGO (Suchanek et al., 2007 ) , while others focus more on commonsense, such as ConceptNet (Speer et al., 2017 ) and ASER (Zhang et al., 2020 ) .
Knowledge bases have found use in many downstream applications, including relation extraction (Weston et al., 2013 ) , machine reading (Yang and Mitchell, 2017 ) , and reflection generation in counseling dialogues Shen et al. ( 2022 ) . Many have found that integrating external knowledge improves performance on such knowledge-intensive tasks (Yu et al., 2022 ) . Moreover, knowledge bases are often structured in a well-defined ontology of relationships and entities, allowing humans to more easily interpret the inferences grounded on knowledge bases.
Although LLMs are trained on extensive datasets and demonstrate the capacity to tackle a wide variety of tasks Brown et al. ( 2020a ); Bubeck et al. ( 2023a ) , their internal knowledge remains limited in many respects, both with respect to general knowledge, as well as domain-specific Ofek et al. ( 2016 ) or culture-specific knowledge (Yin et al., 2022 ) . Additionally, LLMs frequently hallucinate, generating claims based on false facts. Although reinforcement learning from human feedback (RLHF) can mitigate this phenomenon, the problem of hallucination is inherent to the model. Grounding the model’s output on an explicit knowledge base would likely reduce hallucination and enable users to verify the correctness of an assertion more easily. It also opens up the possibility for performing logical reasoning with the large body of existing works.
Knowledge-guided LLM. The integration of knowledge into LLMs is a promising research direction for solving the hallucination problem by grounding the model’s response on a verified resource of knowledge. ChatGPT seeks to address this through plugins, which indicates that the problem is not going to be solved by the LLM itself, but depends on the individual use case. There have been attempts to retrieve or generate knowledge for enhanced response generation with systems like DialogGPT (Zhang et al., 2019 ) . Search engines such as Bing also conduct a web query for factual questions before composing a response. However, how LLMs should most efficiently and effectively interact with customized external knowledge bases remains an open problem.
Automatic knowledge base construction. Many applications can benefit from specialized knowledge bases, whether for improved human interpretability or to serve as a stand-alone resource. The automatic construction of such knowledge bases is an interesting direction and requires many challenges to be addressed, such as knowledge coverage, factuality of the knowledge, knowledge linking, and so on. These challenges are amplified when the knowledge bases are constructed for specialized domains such as healthcare or chemistry. However, once these problems are addressed, researchers will be able to utilize LLMs to dynamically curate a knowledge base from up-to-date raw text and an ontology for complex applications such as tracking medication interactions from articles from PubMed.
General and Cultural Commonsense. Cultural knowledge available in NLP models is often limited to a handful of Western cultures and does not account for the vast diversity of the cultural views of the world (Arora et al., 2023 ) . With the increasingly wide spread of NLP applications, this limitation may result in direct adverse impact on the users of these applications, by not accounting for their values, believes, and world views. More work is needed to understand the limitations of NLP models, including LLMs, with respect to their knowledge of different cultural groups. Further, once these limitations are better understood, a major open research direction is how to acquire and represent the knowledge that encodes these cultural views, as well as how and when to invoke this cultural knowledge.
5 Language Grounding
Language grounding is the ability to tie verbal expressions to their referents in the non-linguistic world (Patel and Pavlick, 2022 ) . The non-linguistic world can be physical or non-physical, e.g., TextWorld (Côté et al., 2018 ) . Significant research advancements are due to leveraging sensory data to build datasets and tasks for teaching ML models how to perform language grounding. Popular tasks include visual question answering (Agrawal et al., 2015 ; Singh et al., 2019 ) , image and video captioning Mokady et al. ( 2021 ); Zhou et al. ( 2019 ) , text to image retrieval (Wang et al., 2022 ; Fang et al., 2021 ) , and text to image/video generation (Ramesh et al., 2021 ; Villegas et al., 2022 ) . Models like CLIP (Radford et al., 2021 ) demonstrate that large-scale image-text pre-training can benefit transformer-based visual-language models. Following the trend, more multi-modal models such as GPT-4 significantly increased their training corpus (OpenAI, 2023 ) and added new modalities such as audio (Zellers et al., 2022 ) .
Even though recent multimodal models like GPT-4 exhibit impressive zero-shot performance, as they outperform most fine-tuned but smaller multi-modal models, they come with a cost. First, they lack a true understanding of the world (Hendricks and Nematzadeh, 2021 ; Thrush et al., 2022 ) , they lack domain knowledge, and cannot generalize to real-life settings (e.g., personalized situations, in-the-wild data). Second, these models are very difficult or even impossible to interpret. They occasionally exhibit unreliable behaviors like hallucinations when generating new data (e.g., image/video generation, image/video captioning). Finally, only a few universities and institutions can afford the resources to use them properly. The cost of GPUs is constantly on the rise, and working with diverse modalities, visual in particular, is significantly more expensive in terms of both computer memory and computation.
How to best combine multiple modalities. Efficiently and effectively combining different modalities, i.e., audio, video, text, and others, is still an open problem. Different modalities often complement each other (e.g., gestures can be used to express confidence in what is being expressed verbally), thus reducing the need for relying on billions of data points. However, in some cases, the modalities end up competing with each other, and thus many uni-modal models outperform multi-modal models (Wang et al., 2019a ; Huang et al., 2021 ) .
Grounding with less studied modalities. Most work on grounding revolves around visual, textual, or audio modalities. However, less studied modalities in the context of grounding, such as physiological, sensorial, or behavioral, are valuable in diverse applications such as measuring driver alertness (Jie et al., 2018 ; Riani et al., 2020 ) , detecting depression (Bilalpur et al., 2023 ) or detecting deceptive behaviors (Abouelenien et al., 2016 ) . These modalities raise interesting questions across the entire pipeline, starting with data collection and representation, all the way to evaluation and deployment.
Grounding “in the wild” and for diverse domains. Most research around grounding is performed on data collected in lab settings, or on images and videos from indoor activities such as movies (Lei et al., 2019 ) or cooking (Zhou et al., 2018 ) . More realistic settings and outdoors “in the wild” data are much less studied (Castro et al., 2022 ) . Such data poses new challenges with respect to availability, quality, distribution, and so on, which opens up new research directions. Moreover, the application of these models to diverse domains (e.g., robotics, medicine, navigation, education, accessibility) requires adapting to working with fewer data points or different types of data, alongside with the need for in-domain expertise to better understand the problem setup.
6 Computational Social Science
Computational social science (CSS), the study of social sciences using computational methods, remains at least partly untouched by LLMs. While they can automate some of the languages tasks related to CSS such as sentiment analysis and stance detection (Liang et al., 2022 ) , questions such as “how humans share news in social networks” or “the cultural differences in language use during catastrophic social events” are considered largely out of scope for generative models. With the success and impact of AI in social science in the past decade, computational and data-driven methods have penetrated major areas of social science (Lazer et al., 2009 , 2020 ) giving rise to new interdisciplinary fields such as computational communication studies, computational economics, and computational political science.
While NLP continues to have a large impact on shaping research in CSS, large foundation models are underutilized in hypothesizing and evaluating ideas in the field. Generative models are designed to serve users end-to-end through natural language, and often the need for customizing these large models is not addressed due to high fine-tuning costs or proprietary technology. In the absence of expert or fine-tuned LLMs, the applications of such models in CSS remain limited to generic data labeling and processing such as stance detection or sentiment analysis.
Population-level data annotation and labeling. CSS researchers already apply less-than-perfect models on large datasets of human interactions to help them narrow down social concepts and study them. While some annotations can be handled by LLMs Gilardi et al. ( 2023 ) , the need for human crowdworkers will unlikely go away. This is particularly true in CSS, as researchers are mostly interested in population-level trends rather than precision at the individual level.
Development of new CSS-aiding abstractions, concepts, and methods. Word and sentence-level embeddings have had a large impact on CSS in recent years. Topic modeling, such as LDA Blei et al. ( 2003 ) , and keyword extraction have been prevalent in CSS prior to the introduction of embeddings. These are examples of methods that encapsulate generic capabilities at a high abstraction level in CSS, as they are frequently used in studies across several subfields of CSS. As CSS researchers transition to using more powerful AI technologies, the concepts and algorithms that unlock new capabilities for them are yet to be developed.
Multicultural and multilingual CSS. Most CSS studies focus on English or a handful of other major languages and address mostly Western cultures. However, there are many important questions in social science that require large-scale, multilingual, and multicultural analyses. For instance, how do languages evolve, or how do values vary across cultures? This is an area for future work that can lead to compounding impacts on the social sciences.
7 NLP for Online Environments
The impact of NLP on online environments can be observed through two adversarial phenomena: content generation and moderation. The rapid generation of content, such as LLM-generated articles and social media updates, can be supported by various stakeholders. It is very likely that many can achieve high click-through rates to their websites by generating fake news and disinformation, which raises concerning social issues that necessitate timely regulation. Conversely, moderation is a form of gate-keeping. By using NLP to monitor and analyze user-generated content on digital platforms Nakov et al. ( 2021 ); Kazemi et al. ( 2021a ) to remove policy-violating materials, content moderation can maintain balance in the online ecosystem Thorne et al. ( 2018 ); Nakov et al. ( 2021 ); Gillespie ( 2020 ); Kazemi et al. ( 2021a ); Shaar et al. ( 2020 ) .
There are several concerns about content generation and moderation. For generation, it is of top priority to identify the underlying purpose of the generation and avoid malicious manipulation of users. For moderation, a concern is that the current moderation models are still opaque, unprecised, unaccountable, and poorly understood Gorwa et al. ( 2020 ) . Additionally, there are several existing challenges in building models to detect undesired content, including the difficulty in designing taxonomy for undesired content, the time-consuming nature of data labeling, and the inadequacy of academic datasets in revealing the real-world data distribution Markov et al. ( 2023 ) .
Detecting and debunking online misinformation. Misleading content on the internet is growing in abundance, and an increase in volume in the upcoming years due to the rise in popularity of AI generated content is likely unavoidable. NLP can be used on several fronts to slow down the spread of misleading content. In the interest of extending help to fact-checkers and journalists, NLP systems remain underutilized, leaving a golden opportunity for building fact-checking technology that empowers fact-checkers to scale up their efforts Kazemi et al. ( 2022 ) . Additionally, NLP assisted fact-checking is often built in English, and therefore there is an increasing need for low-resource and cross-lingual NLP to help address misinformation in less resourceful parts of the world. Detecting and debunking misinformation also involves multimodal processing, since misinformation spreads in various formats. Network signals, such as who likes or reposts content, also encode rich information that can be attached alongside other modalities to help improve misinformation detection. Additionally, NLP for fact-checking can largely benefit from focusing on retrieval and knowledge augmented methods, since in order to check factuality of claims, one needs to search through and find the relevant context around the claim.
Ensuring diverse representations. With the prevalence of LLM-generated contents, the voice of majority may end up amplified on the web, since data-driven models such as LLMs tend to remember the type of data that is the most represented in its corpus. Thus, lack of diversity and especially representations for marginalized groups’ voices will be a concerning problem as LLM-generated content will be increasingly used online.
Avoiding mis-moderation and detecting over-moderation. Similar to the heterogeneity issue in content generation, content moderation techniques might also overlook the nuances of expressions in under-represented groups, or specific culture and social environments. It is important to make the moderation algorithms fair to all groups.
Conversely, due to various political interests (e.g., Iran wanting to limit the discussion of women freedom), governments are likely to limit the set of topics discussed online. It does become an important direction to trace what topics and opinions are filtered or demoted on the internet, and reflect on the freedom of speech in the political environment.
Identifying stakeholders behind the generated contents. As machine-generated content proliferates, it will be increasingly challenging to judge which information to trust. One promising direction is to develop NLP models to identify the stakeholders behind the generated contents, and their interest types, such as commercial profits (e.g., from advertisements or customer attraction) or political interests (e.g., to affect more people to hold certain opinions that would largely benefit an interest group).
8 Child Language Acquisition
While some claim that LLMs “show sparks of AGI” Bubeck et al. ( 2023b ) , they do not mimic the path followed by humans when acquiring language Bowerman and Levinson ( 2001 ) . Ideally, we want smaller, more efficient models of language that are tightly paired with environment grounding Lazaridou et al. ( 2017 ) . On the path to efficient AGI, we have a hard-to-beat baseline: language acquisition in children. Most children can acquire up to three languages through often limited interactions and observations of language. While it is not completely understood how children learn language exactly, we know they do not require terabytes of text training instances.
There is also a growing body of research exploring the connection between LLMs and child language acquisition, specifically in the context of statistical learning Wilcox et al. ( 2022 ) , with recent research exploring how LLMs can be used to model and simulate the statistical learning mechanisms that children use to acquire language Contreras Kallens et al. ( 2023 ) . Developments in this area have broader implications for low-resource and endangered languages as sample-efficient language modeling algorithms can unlock LLM-level capabilities to entirely new languages and cultures.
Achieving the data efficiency of such an efficient baseline – children – is exciting, but there are no silver bullets: psychologists, neuroscientists, and linguists are among the scientists who have been studying language acquisition in children for decades and despite achieving greater understanding of the process in human children, we have not yet developed a working theory that reproduces the same process computationally with comparable data efficiency. This lack of progress can be attributed to the difficulty of studying children, as both recruitment and IRB approval for such studies rightly impose limitations on the types of data that can be collected. Among others, the little data that is collected is often limited in expressibility, as children who have not learned a language yet cannot communicate effectively, which limits experiment design. In a wide range of child language studies, parents are present to make sure the children can stay focused on the experiment and follow guidelines. Additionally, it is difficult to control confounding variables when you have no control over the subjects of the experiment.
Sample-efficient language learning. This is an area ripe with opportunities to advance our understanding of language and develop more data efficient NLP tools. There is a great need for fundamental and theoretical research into sample-efficient language learning. Computational theories and algorithms for achieving state-of-the-art on smaller data regimes are an exciting area for researchers interested in core NLP, and the pursuit of the state-of-the-art performance may soon be rerouted to data-efficiency scores. Related to this direction is the goal of establishing baselines for sample-efficient lamnguage learning. Having a lower-bound goal (e.g. X hours of interaction achieving Y score) can enable the NLP community to have a more accurate understanding of progress in terms of data efficiency. While such estimates might already exist, getting more precision and depth will further advance our knowledge of language learning.
Benchmark development in child language acquisition. With the advancement of large language and multimodal systems, there are opportunities to ease and scale child language benchmark construction. For example, controlled experiments on carefully constructed supervised benchmarks can be augmented by large video datasets of children learning language over a long period of time. Additionally, such datasets could be used to train models that are specifically tailored to the way that children learn language, which could enable new ways to understand child language use, as well as the development of models that are able to learn from fewer examples, similar to how humans learn language.
Language models as biological models for child language acquisition . A biological model refers to the study of a particular biological system, believed to possess crucial similarities with a specific human system, in order to gain insights and understanding about the human system in question. McCloskey has famously advocated for utilizing neural models as biological models to investigate human cognitive behavior and consequently develop theories regarding that behavior (McCloskey, 1991 ) . With NLP models that have started to exhibit some similarities to human language use, we now have the opportunity to explore theories regarding how human infants acquire languages. For example, (Chang and Bergen, 2021 ) investigated the process of word acquisition in language models by creating learning curves and age of acquisition for individual words. Leveraging existing datasets such as WordBank (Frank et al., 2016 ) and CHILDES (MacWhinney, 1992 ) , as well as new benchmarks, alongside with increasingly powerful language models, we now have the ability to conduct experiments to analyze language acquisition (e.g., phoneme-level acquisition, intrinsic rewards), and gain new insights into child language acquisition.
9 Non-Verbal Communication
Non-verbal communication includes, among others, gestures, facial expressions, body language, and posture. A particular form of non-verbal communication consists of sign language, which represents the primary medium of communication used by people who are deaf. Several studies have shown the importance of nonverbal communication in everyday interactions McNeill ( 1992 ); Alibali et al. ( 2000 ) . Recent work in NLP has highlighted the importance of integrating non-verbal information into existing language representations as a way to obtain richer representations, including for instance language models Wang et al. ( 2019b ) or visual models Fan et al. ( 2021 ) ; other previous work has shown that non-verbal communication such as facial expressions or gestures are aligned with the verbal channel and that different cultural or language contexts can be associated with different interpretations of these non-verbal expressions Abzaliev et al. ( 2022 ); Matsumoto and Assar ( 1992 ) . There is also an entire body of research focused on the understanding and generation of sign language Joze ( 2019 ); Bragg et al. ( 2019 ) , as well as the communication across different communities of sign language speakers Camgoz et al. ( 2020 ) .
Understanding the alignment between non-verbal modalities and verbal language remains an open problem, especially given the challenges that some of these modalities use different spectrums (continuous vs discrete). Correspondingly, the discretization and interpretation of these signals can be difficult, leading to challenges regarding their joint use, or the integration of such non-verbal information into existing large language-based models. In sign language research, there are still many open problems in understanding and generating sign languages, encompassing both the compilation of representative sign language datasets and the development of effective computational models.
Non-verbal language interpretation. Since many subareas of non-verbal communication require non-verbal information, the representation, discretization, and interpretation of this information is a rich direction of exploration. For instance, while previous work has identified a potential “code-book” of facial expressions Song et al. ( 2013 ) , more work is needed to find the ideal set of representations that can be used across modalities, contexts and cultures. The interpretation of these expressions and gestures, and their alignment across modalities, also remain an open problem. In particular, the increasing use of LLMs has the potential to open up new paradigms for understanding non-verbal communication through textual descriptions. For instance, when an LLM is prompted with “Please answer which gesture I am describing: a person puts her arms wide away, smiling and moving towards the other person,” it replies with “The gesture you are describing is likely a hug, indicating a friendly or affectionate greeting or farewell…,” which can be used as a textual representation of the hugging gesture.
Sign language understanding, generation, and translation. An open research problem is the development of sign language lexicons Athitsos et al. ( 2008 ) and corpora Li et al. ( 2020 ) that can be used to train and evaluate computational models. These resources are essential for developing and testing recognition and interpretation models, but they are often difficult and expensive to create. In sign language understanding, one of the biggest challenges is the development of effective models that can accurately recognize and interpret sign language gestures. This is difficult because sign languages exhibit a relatively high degree of variability in manual gestures, including differences in handshape, movement, and orientation; additionally, other non-manual features such as facial expressions, body pose and eye gaze often play a role in sign languages, which can further complicate the recognition process. Finally, sign language generation is also an open research area, with challenges residing in the development of generation models that can lead to sign language communication that is fluent and expressive. Such models are needed to enable or enrich communication between speakers of the same sign language; speakers of different sign languages; or speaker of verbal and sign languages.
Effective joint verbal and non-verbal communication. Ultimately, both verbal and non-verbal signals should be considered during communication. We want AI systems to be equally capable of understanding “I don’t know”, shrugging the shoulders, or . Representing, fusing, and interpreting these signals jointly is ultimately the long-term goal of AI-assisted communication. Open research problems encompass not only the development of language models for each of these modalities, but effective fusion methodologies, which will enable large joint models for simultaneous verbal and non-verbal communication.
10 Synthetic Datasets
In NLP research, synthetic data is typically needed when the more traditional human data collection is infeasible, expensive, or has privacy concerns Mattern et al. ( 2022 ) . With the advancement of generative models (Tang et al., 2023 ) , synthetic data generation has seen applicability in various domains. Examples include back-translation for low-resource languages (Sennrich et al., 2015 ; Edunov et al., 2018 ) , semantic parsing (Rosenbaum et al., 2022a ) , intent classification (Rosenbaum et al., 2022b ) , structured data generation (Borisov et al., 2022 ) , or medical dialogue generation Chintagunta et al. ( 2021a ); Liednikova et al. ( 2020 ) . The process typically involves pre-training the model if domain adaptation is necessary (Chintagunta et al., 2021b ) , prompting the model to generate the dataset, and evaluating its quality automatically or via expert validation.
The use of synthetic data faces challenges such as difficulty in data quality control (Kim et al., 2022 ) (due to lack of evaluation metrics for text generation), lack of diversity, potential bias in the data-generating model, and inherent limitations of the data-generating model such as hardship to capture of long-range dependency (Orbach and Goldberg, 2020 ; Guan et al., 2020 ) .
Knowledge distillation. Knowledge distillation is the task of transferring knowledge from teacher models to typically smaller student models. For example, Kim et al. ( 2022 ) frame their synthetic dialog dataset as having been distilled from InstructGPT. While earlier methods for distillation involved learning from the soft output logits of teacher models (Hinton et al., 2015 ) , this signals a move toward directly utilizing LLM outputs as synthetic examples (West et al., 2022 ) . This allows practitioners to transform or control the generated data in different ways, such as using finetuned models to filter for quality. Moreover, synthetic data can be used to directly emulate the behavior of LLMs with much smaller, focused models, such as in the case of Alpaca (Taori et al., 2023 ) .
Control over generated data attributes. Currently, the predominant method is to provide natural text specifications with instructions and examples, but optimizing these prompts often relies on a simple trial-and-error approach. Additionally, specifying attributes through instructions or examples can be imprecise or noisy. The development of robust, controllable, and replicable pipelines for synthetic data generation remains an open research question.
Transforming existing datasets. Given an existing dataset, we can apply various changes to create a semantically preserving new dataset, but with a new style. Common approaches include format change (e.g., converting a dataset of news articles from HTML to plain text format), modality transfer (e.g., generating textual descriptions of images or videos or generating captions or subtitles for audio-visual content), or style transfer Jin et al. ( 2022a ) (e.g., translating the writing style of the text from verbose to concise).
11 Interpretability
Interpretability is the task of understanding and explaining the decision-making processes of machine learning models, making them more transparent and justifiable (Danilevsky et al., 2020 ) . Interpretable NLP systems can foster thrust by enabling end-users, practitioners, and researchers to understand the model’s prediction mechanisms, and ensure ethical NLP practices. Historically, traditional NLP systems, such as rule-based methods (Woods, 1973 ) , Hidden Markov models (Ghahramani, 2001 ; Rabiner, 1989 ) , and logistic regression (Cramer, 2002 ) , were inherently interpretable, known as white-box techniques. However, recent advancements in NLP, most of which are black-box methods, come at the cost of a loss in interpretability. To address this issue, interpretability has emerged as a research direction, focusing on developing techniques that provide insight into the inner workings of NLP models (Mathews, 2019 ; Danilevsky et al., 2020 ) . Key research findings include attention mechanisms, rule-based systems, and visualization methods that help bridge the gap between complex language models and human interpretability, ultimately contributing to the responsible deployment of NLP systems.
The current state of interpretability research in NLP focuses on understanding model predictions, feature importance, and decision-making processes. Techniques like attention mechanisms Vaswani et al. ( 2017 ) , LIME Ribeiro et al. ( 2016 ) , and SHAP Lundberg and Lee ( 2017 ) have emerged to provide insights into model behavior. However, gaps remain in areas like robustness, generalizability, and ethical considerations. Additionally, interpretability methods often lack standardization and struggle to address complex, large-scale models like transformers, limiting their applicability in real-world scenarios.
Probing. One promising direction is to investigate the internal representations of NLP models, including LLMs, by designing probing tasks that can reveal the linguistic Hewitt and Manning ( 2019 ); Hewitt and Liang ( 2019 ) and world knowledge captured by the models Elhage et al. ( 2022 ); Geva et al. ( 2021 , 2022 ) . This can help understand the reasoning capabilities of models and identify potential biases (Li et al., 2022 ; Meng et al., 2022 ) .
Mechanistic Interpretability. While probing mostly looks at the attributes of the features learned by the model, mechanistic interpretability aims to uncover the underlying mechanisms and algorithms within a model that contribute to its decision-making process Nanda et al. ( 2023 ); Conmy et al. ( 2023 ) . It extracts computational subgraphs from neural networks Conmy et al. ( 2023 ); Wang et al. ( 2023 ); Geiger et al. ( 2021 ) , and its high-level goal is to reverse engineer the entire deep neural network Chughtai et al. ( 2023 ) .
Improving interpretability by human-in-the-loop. Human-in-the-loop interpretability research in NLP focuses on incorporating human feedback and expertise to enhance model interpretability. This approach aims to improve model transparency, facilitate better decision-making, and foster trust between AI systems and users. By involving humans, researchers can identify and address biases, ensure ethical considerations, and develop more reliable and understandable NLP models. There are various promising directions, such as active learning and interactive explanation generation (Mosca et al., 2023 ; Mosqueira-Rey et al., 2023 ) .
Basing the generated text on references. Explainability relates to understanding why a certain generative NLP model output is provided and evaluating its correctness, possibly through calibration Naeini et al. ( 2015 ) . Being factually correct is not a restriction that generative models have to follow; rather, they are generally trained to imitate human-written text by predicting the most likely text that comes next. This predicted text, in turn, is prone to hallucinations (Ji et al., 2022 ) that causes a lack of trust from the users. A promising solution is to provide reliable sources for the facts output by a model, by attaching references and showing any additional reasoning steps. For example, citations can be included along with its bibliography, or pointers to documents in the training data (or a document database) can be attached to the output. Such a system should evaluate the extent to which these sources back up the claims made by the model.
12 Efficient NLP
Efficient NLP is a research direction aiming to optimize the use of resources for NLP models. This objective arises from the need to address the challenges posed by the increasing scale of language models and their growing resource consumption present new challenges for NLP advances (Touvron et al., 2023b ; Zhang et al., 2023 ) . Indeed, it is widely acknowledged that scaling up is an essential approach for achieving state-of-the-art performance on NLP tasks, especially those skills emerged with the scaling law (Wei et al., 2022 ; Bowman, 2023 ) . However, developing LLMs requires substantial energy and financial resources for training and inference, which raises concerns about the AI carbon footprint and the economic burden on NLP product development (Strubell et al., 2019 ) . In light of these concerns, prior research has underscored the critical need for effectively reducting CO2 equivalent emissions (CO2e) and Megawatt hours (MWh), and increase of Power Usage Effectiveness (Patterson et al., 2022 ; Thompson et al., 2020 ) .
There is significant scope for improving the efficiency of NLP across various dimensions, including data curation, model design, and training paradigms, presenting numerous research opportunities. Addressing data efficiency involves tackling challenges like enhancing data deduplication techniques, assessing data quality, and curating vast amounts of data. When it comes to refining model design, key challenges include improving the attention mechanism efficiency, developing alternative no-parameters modules for parameter reduction, and optimizing the model depth or efficiency. Lastly, in the realm of training paradigms, there is potential for advancements in promoting engineering, fine-tuning, and prompt-tuning techniques.
Data efficiency. Data efficiency can be enhanced through data deduplication, where redundant or noisy data is removed, thereby improving performance with fewer data items. Although there is existing work that aims to boost model performance with fewer data points by removing noisy examples and deduplicating useless data (Lee et al., 2022 ; Mishra and Sachdeva, 2020 ; Hoffmann et al., 2022 ) , there is a lack of effective methods for data deduplication for vast corpora ( > > 700B Tokens) or raw web data curation.
Model design. A large body of methods increases model efficiency by improving attention mechanisms (Tay et al., 2020 , 2022 ; Dao et al., 2022 ; Ma et al., 2022 ) . However, challenges remain in handling extremely long context modeling in transformer architectures. Sparsing models can scale up the width of models for increased expressiveness while reducing theoretical FLOPs. Notable practices include applying mixture of experts architectures in a feed-forward layer of a transformer-based model (Fedus et al., 2021 , 2022 ; Du et al., 2022 ) . Engineering such models requires architecture-specific implementation and costs many trials to get the optimal architecture. It is also not unstable in performance (Mustafa et al., 2022 ) .
Efficient downstream task adaptation. Efficient fine-tuning aims to adapt the pre-trained model to downstream tasks by updating a small part of the parameters (Pfeiffer et al., 2020 ; Moosavi et al., 2022 ; Schick and Schütze, 2021 ) . Prompt-tuning/prefix tuning modifies activations with additionally learned vectors without changing model parameters (Valipour et al., 2022 ; Lester et al., 2021 ) . However, it is necessary to find a way for efficient automatic prompt construction.
13 NLP in Education
There is a rich history of NLP applications for education, including dedicated workshops such as the yearly ACL Workshop on Innovative Use of NLP for Building Educational Applications organized by the Special Interest Group for Building Educational Applications. These applications include tools to aid learners (e.g., language learning applications such as Duolingo * * * https://www.duolingo.com , or grammar correction tools such as Grammarly * * * https://www.grammarly.com ), tools to assist teachers and organizations in grading (e.g., the e-rater system that is used to grade GRE essays (Burstein et al., 1997 ) ), tools to assist curriculum and assessment development (e.g., systems for developing multiple-choice questions (Kurdi et al., 2020 ) ) and tools for education researchers (e.g., systems to build representations of classroom interactions (Alic et al., 2022 ) ). Researchers have been testing the application of models such as BERT (Devlin et al., 2019 ) and RoBERTa (Liu et al., 2019 ) in these areas since their release, and are now beginning to incorporate larger models.
Many of the deployed NLP applications in the education space have been developed prior to wide spread use of LLMs, and we are likely to see large-scale deployment of task-specific models based on LLMs soon. While much of the prior work includes standalone applications, developing models that can easily be incorporated into existing education pipelines, e.g., by integrating what students have learned thus far, is an area open for further exploration. Importantly, a long-standing goal in education is to personalize materials and assessments to the needs of individual students, and NLP has the potential to contribute towards that goal.
Controllable text generation. Dialog systems and more generally text generation have been previously used in education applications. Within this space, controllable text generation can be used for a more personalized experience, for instance to introduce students to new terms using automatically generated stories related to their interests or to modify stories to be accessible to grade school students with different reading levels. Similarly, while we have seen extensive work in reading comprehension, we can now start to imagine applications where the comprehension of a text will be tested based on a student’s prior experience, as well as previous tests that they have been exposed to, for a more adaptable learning experience.
Educational explanation generation. Personalized classroom material could also include the generation of explanations for students, grounded in their understanding (or lack thereof) of the material. For example, an NLP system could be used to help a student understand a tricky sentence in an academic paper, or to rephrase an answer given by their instructor in hopes of discovering an explanation that connects to the student’s body of knowledge. Automatic grading is also an area where NLP has made many contributions Mohler and Mihalcea ( 2009 ) , but it still encompasses open research problems such as providing an explanation for a less than perfect grade.
Intelligent tutoring systems. Intelligent tutoring systems show significant promise for personalized education Mousavinasab et al. ( 2021 ) . NLP methods can be developed to generate targeted practice questions and explain students’ mistakes in a wide range of fields, all the way from English or History to Physics or Computer Science. These systems will likely improve as NLP evolves to mimic human reasoning more reliably; currently, it is necessary to be careful when deploying NLP in education without a human-in-the-loop, as even when given simple math problems, NLP models (including the most recent LLMs OpenAI ( 2023 ) ) can often confidently give incorrect answers and explanations.
It is worth mentioning that the reception of LLMs in the education community has largely been one of fear due to the possibility of increased academic dishonesty. This has led to courses and universities adopting policies regulating how AI can be used in their courses, such as the Yale policy. * * * https://poorvucenter.yale.edu/AIguidance Whether the overall curriculum will be adjusted to incorporate LLMs in a positive manner is yet to be seen, but we are optimistic that this recent progress can have a positive impact on education if deployed in appropriate circumstances.
14 NLP in Healthcare
Applications for NLP in healthcare can be classified by their use and impact on key stakeholders such as providers, patients, and public health officials Zhou et al. ( 2022 ); Olaronke and Olaleke ( 2015 ) . When focusing on health providers, NLP is often used to support clinical decision making by (1) aggregating and consolidating available data and research, and (2) extracting relevant information from data. These tasks involve important challenges such as standardization of healthcare data, accurate labeling, extraction and retrieval of health concepts as well as categorization of patient conditions Dash et al. ( 2019 ) . Similarly, NLP is used to address patient requests for information on applications such as question answering for health-related questions, and retrieval of information relevant to medical treatments or illnesses. Recent work in this area has focused on the analysis of language in the mental health space covering both professional therapy Sharma et al. ( 2020 ); Pérez-Rosas et al. ( 2017 ); Min et al. ( 2022 ) and social media conversations Tabak and Purver ( 2020 ); Lee et al. ( 2021 ); Biester et al. ( 2020 ) . Regarding assisting public health officials, NLP is being used for surveillance of public health to identify diseases and risk factors or at-risk populations Naseem et al. ( 2022 ); Jimeno Yepes et al. ( 2015 ); Yates et al. ( 2014 ) and also to moderate aspects such as misinformation or public sentiment online Hou et al. ( 2019 ); Kazemi et al. ( 2021b ) .
One of the most glaring limitations of NLP in healthcare is the scarcity of high-quality, annotated clinical data. Although social media data can be useful in some contexts, clinical data is essential in developing tools for clinical decision making, and often not publicly available due to privacy and ethics concerns. Another shortcoming is the lack of language diversity as work to date has primarily focused on English or other high-resource languages Mondal et al. ( 2022 ) but devoted less efforts towards minority languages. Additionally, the lack of human evaluation of NLP-based health systems has made it challenging to measure their effectiveness in the real world. Current automatic evaluation metrics do not necessarily speak to patient outcomes. Hence, human-centric studies must be conducted in evaluating the efficacy of NLP-powered tools in healthcare.
Healthcare benchmark construction. Although the documentation of recent LLMs reports very high performance for various medical question answering benchmarks, or medical licensing texts, there are many other tasks in healthcare that lack the data required to achieve similarly good performance. Access to medical datasets is often limited because of privacy issues, and therefore other approaches may be required to compile such benchmarks. Synthetic datasets are one such option Chintagunta et al. ( 2021a ); Liednikova et al. ( 2020 ) . Other options including paraphrasing of existing datasets as a form of data augmentatiom; or using LLMs as a starting point to bootstrap datasets. Another open research direction is the evaluation of the quality of the benchmarks. Additionally, research is needed to find effective ways to produce new health datasets in low-resource languages or low-resource domains.
NLP for clinical decisions. NLP systems can used as brainstorming or decision making tools that can assist experts in their evaluation and decision process. They can be used to synthesize new knowledge (e.g., the latest research papers on a medical finding), and make that available to the medical practitioners. Further, bringing together general medical knowledge and personal patient information requires new strategies for knowledge integration. Since clinical diagnoses and treatments are high-stake decisions, it is crucial that the NLP systems be reliable and interpretable, to provide clear reasoning behind their predictions. Such processes also require the interdisciplinary collaboration with medical experts to make sure that the system aligns with their domain knowledge and clinical practice.
Drug discovery. Drug discovery is a critical research area that has often been considered in relation to biomedical and chemical research, but more recently has gained the attention of NLP researchers. NLP methods can enable the efficient extraction and analysis of information from large amounts of scientific literature, patents, sovical media, clinical records, and other biomedical sources. Open research directions include the identification and prioritization of drug-target interactions, the discovery of new drug candidates, the prediction of compound properties, and the optimization drug design. New NLP methods can also contribute to the identification of novel drug-target associations and can enable more effective drug repurposing efforts.
15 NLP and Ethics
Recognition for the role of ethics in NLP is on the rise, especially with the development of increasingly powerful models with potentially far-reaching societal implications. There are important ethical considerations when developing NLP models (Bender et al., 2020 ) , and there is ongoing research work that aims to address critical ethical aspects such as dual use, fairness, and privacy.
Aside from the issues described above, other ethical concerns surrounding the use and applications of recent LLMs include: lack of attribution, poor model explainability, skill degradation, disruption of the labor market, model misuse, and model disuse. In addition to educating people about ethics, we need further investigation into the extent of these concerns and determining how NLP techniques can reduce their impact.
Dual use. Many NLP applications that have positive impact can at the same time be used in harmful ways. Identifying possible harm from NLP models and applications can be achieved through discussions before deployment and data surveys after deployment to identify potentially harmful applications. Additionally, developing NLP systems that help detect, discourage, and prevent harmful use, such as fact-checkers, is crucial. Adversarial NLP can also be used to explore the limitations and loopholes of NLP systems and improve their robustness.
Fairness. There is a need for methods that evaluate the fairness of NLP models, and detect and mitigate bias. This includes investigating dataset creation practices and their correlation with model bias (Wang et al., 2020 ) . Such research should examine whether stricter requirements for dataset creation can reduce bias and inequalities that might be exacerbated by models trained on or evaluated on biased data.
Privacy. As personalized NLP applications (including in fields such as education or healthcare) require an understanding of the user, privacy protection in NLP systems has become an essential research direction. New techniques are needed to identify and anonymize sensitive user information while maintaining the utility of the data for analysis and decision-making. This includes methods such as differential privacy, federated learning, and secure multi-party computation to ensure the confidentiality and security of patient data in NLP-driven healthcare applications. Additionally, an area where NLP systems can make an impact is data policy, where NLP methods can be developed for summarizing data policies of digital products in understandable formats for users, and ensuring model alignment with such policies (Carlini et al., 2021 ) .
Attribution and detection of machine-generated data. Developing standard approaches for attribution that NLP models can use while generating content is essential (i.e., can we teach AI models to attribute content using membership inference or other approaches?) (Collins, 2023 ) . Domains such as programming or creative writing (Swanson et al., 2021 ) have already begun incorporating LLMs into the workflow, which requires the determination of the ownership and rights to such creations.
Integrating NLP models as human assistants rather than human replacements. This can be achieved using NLP models for human training applications. Models could be used to improve human spelling, writing, and reading comprehension abilities. However, it is essential to note that LMs have shown excellent ability in masquerading wrong answers as correct. These answers can be delivered to a student whose job is to find the loopholes in the argument or choose the incorrect answer. It also has the potential to widen the existing inequalities in society. It also raises concerns about the ethical implications of relying on machines to augment human performance and how this could affect our perception of what it means to be human (Eloundou et al., 2023 ) .
16 So What Should I Work On?
The future of NLP research is bright. The rapid progress we are currently witnessing in LLMs does not mean that “it’s all been solved.” On the contrary, as highlighted in this document, there are numerous unexplored research directions within NLP that are not addressed by the current progress in LLMs. They add to the many existing tasks in NLP where LLMs’s performance is limited Bang et al. ( 2023a ) , as well as the growing number of new areas that are enabled by the new LLM capabilities.
More broadly, as a field, we now have the opportunity to move away from performance-focused technology development, and acknowledge that NLP is about language and people and should be fundamentally human-centric. This brings about a new focus on enabling technologies that are culture- and demographic-aware, that are robust, interpretable, and efficient, and that are aligned with a strong ethical foundation — ultimately, technologies that make a lasting positive impact on the society.
How to choose a research direction to work on? Start with your motivation and interests: consider your previous experiences, look around at your community, explore your curiosities about language and about people, and try to find what resonates with you the most. Building on this foundation, identify the tasks and applications in NLP that connect to your motivations and interests. This document hopefully serves as a starting point to guide this exploration.
Acknowledgments
We would like to thank Steve Abney and Rui Zhang for providing feedback and valuable suggestions on earlier versions of this manuscript.
- Abouelenien et al. (2016) M Abouelenien, V Pérez-Rosas, and others. 2016. Detecting deceptive behavior via integration of discriminative features from multiple modalities. IEEE Transactions .
- Abzaliev et al. (2022) Artem Abzaliev, Andrew Owens, and Rada Mihalcea. 2022. Towards understanding the relation between gestures and language. In Proceedings of the 29th International Conference on Computational Linguistics , pages 5507–5520.
- Agrawal et al. (2015) Aishwarya Agrawal, Jiasen Lu, Stanislaw Antol, Margaret Mitchell, C Lawrence Zitnick, Dhruv Batra, and Devi Parikh. 2015. VQA: Visual question answering .
- Aguilar et al. (2020) Gustavo Aguilar, Sudipta Kar, and Thamar Solorio. 2020. LinCE: A centralized benchmark for linguistic code-switching evaluation . In Proceedings of the Twelfth Language Resources and Evaluation Conference , pages 1803–1813, Marseille, France. European Language Resources Association.
- Akyürek et al. (2022) Ekin Akyürek, Dale Schuurmans, Jacob Andreas, Tengyu Ma, and Denny Zhou. 2022. What learning algorithm is in-context learning? Investigations with linear models . CoRR , abs/2211.15661.
- Alibali et al. (2000) Martha W Alibali, Sotaro Kita, and Amanda J Young. 2000. Gesture and the process of speech production: We think, therefore we gesture. Lang. Cogn. Process. , 15(6):593–613.
- Alic et al. (2022) Sterling Alic, Dorottya Demszky, Zid Mancenido, Jing Liu, Heather Hill, and Dan Jurafsky. 2022. Computationally identifying funneling and focusing questions in classroom discourse. In Proceedings of the 17th Workshop on Innovative Use of NLP for Building Educational Applications (BEA 2022) , pages 224–233, Seattle, Washington. Association for Computational Linguistics.
- Arora et al. (2023) Arnav Arora, Lucie-aimée Kaffee, and Isabelle Augenstein. 2023. Probing pre-trained language models for cross-cultural differences in values . In Proceedings of the First Workshop on Cross-Cultural Considerations in NLP (C3NLP) , pages 114–130, Dubrovnik, Croatia. Association for Computational Linguistics.
- Artetxe and Schwenk (2018) Mikel Artetxe and Holger Schwenk. 2018. Margin-based parallel corpus mining with multilingual sentence embeddings .
- Athitsos et al. (2008) Vassilis Athitsos, Carol Neidle, Stan Sclaroff, Joan Nash, Alexandra Stefan, Quan Yuan, and Ashwin Thangali. 2008. The american sign language lexicon video dataset . 2012 IEEE Computer Society Conference on Computer Vision and Pattern Recognition Workshops , 0:1–8.
- Auer et al. (2007) Sören Auer, Christian Bizer, Georgi Kobilarov, Jens Lehmann, Richard Cyganiak, and Zachary Ives. 2007. DBpedia: A nucleus for a web of open data. In The Semantic Web , pages 722–735. Springer Berlin Heidelberg.
- Bang et al. (2023a) Yejin Bang, Samuel Cahyawijaya, Nayeon Lee, Wenliang Dai, Dan Su, Bryan Wilie, Holy Lovenia, Ziwei Ji, Tiezheng Yu, Willy Chung, Quyet V. Do, Yan Xu, and Pascale Fung. 2023a. A multitask, multilingual, multimodal evaluation of chatgpt on reasoning, hallucination, and interactivity . CoRR , abs/2302.04023.
- Bang et al. (2023b) Yejin Bang, Samuel Cahyawijaya, Nayeon Lee, Wenliang Dai, Dan Su, Bryan Wilie, Holy Lovenia, Ziwei Ji, Tiezheng Yu, Willy Chung, Quyet V Do, Yan Xu, and Pascale Fung. 2023b. A multitask, multilingual, multimodal evaluation of ChatGPT on reasoning, hallucination, and interactivity .
- Bender et al. (2020) Emily M Bender, Dirk Hovy, and Alexandra Schofield. 2020. Integrating ethics into the NLP curriculum. In Proceedings of the 58th Annual Meeting of the Association for Computational Linguistics: Tutorial Abstracts , pages 6–9, Online. Association for Computational Linguistics.
- Biester et al. (2020) Laura Biester, Katie Matton, Janarthanan Rajendran, Emily Mower Provost, and Rada Mihalcea. 2020. Quantifying the effects of COVID-19 on mental health support forums . In Proceedings of the 1st Workshop on NLP for COVID-19 (Part 2) at EMNLP 2020 , Online. Association for Computational Linguistics.
- Bilalpur et al. (2023) Maneesh Bilalpur, Saurabh Hinduja, Laura A Cariola, Lisa B Sheeber, Nick Alien, László A Jeni, Louis-Philippe Morency, and Jeffrey F Cohn. 2023. Multimodal feature selection for detecting mothers’ depression in dyadic interactions with their adolescent offspring. In 2023 IEEE 17th International Conference on Automatic Face and Gesture Recognition (FG) , pages 1–8.
- Blei et al. (2003) David M Blei, Andrew Y Ng, and Michael I Jordan. 2003. Latent dirichlet allocation. Journal of machine Learning research , 3(Jan):993–1022.
- Borisov et al. (2022) Vadim Borisov, Kathrin Seßler, Tobias Leemann, Martin Pawelczyk, and Gjergji Kasneci. 2022. Language models are realistic tabular data generators .
- Bowerman and Levinson (2001) Melissa Bowerman and Stephen Levinson. 2001. Language Acquisition and Conceptual Development . Language Culture and Cognition. Cambridge University Press.
- Bowman (2023) Samuel R Bowman. 2023. Eight things to know about large language models .
- Bragg et al. (2019) Danielle Bragg, Oscar Koller, Mary Bellard, Larwan Berke, Patrick Boudreault, Annelies Braffort, Naomi Caselli, Matt Huenerfauth, Hernisa Kacorri, Tessa Verhoef, Christian Vogler, and Meredith Ringel Morris. 2019. Sign language recognition, generation, and translation: An interdisciplinary perspective. In Proceedings of the 21st International ACM SIGACCESS Conference on Computers and Accessibility , ASSETS ’19, pages 16–31, New York, NY, USA. Association for Computing Machinery.
- Brown et al. (1988) P Brown, J Cocke, S Della Pietra, V Della Pietra, F Jelinek, R Mercer, and P Roossin. 1988. A statistical approach to language translation. In Coling Budapest 1988 Volume 1: International Conference on Computational Linguistics . aclanthology.org.
- Brown et al. (2020a) Tom Brown, Benjamin Mann, Nick Ryder, Melanie Subbiah, Jared D Kaplan, Prafulla Dhariwal, Arvind Neelakantan, Pranav Shyam, Girish Sastry, Amanda Askell, and Others. 2020a. Language models are few-shot learners. Adv. Neural Inf. Process. Syst. , 33:1877–1901.
- Brown et al. (2020b) Tom B. Brown, Benjamin Mann, Nick Ryder, Melanie Subbiah, Jared Kaplan, Prafulla Dhariwal, Arvind Neelakantan, Pranav Shyam, Girish Sastry, Amanda Askell, Sandhini Agarwal, Ariel Herbert-Voss, Gretchen Krueger, Tom Henighan, Rewon Child, Aditya Ramesh, Daniel M. Ziegler, Jeffrey Wu, Clemens Winter, Christopher Hesse, Mark Chen, Eric Sigler, Mateusz Litwin, Scott Gray, Benjamin Chess, Jack Clark, Christopher Berner, Sam McCandlish, Alec Radford, Ilya Sutskever, and Dario Amodei. 2020b. Language models are few-shot learners . In Advances in Neural Information Processing Systems 33: Annual Conference on Neural Information Processing Systems 2020, NeurIPS 2020, December 6-12, 2020, virtual .
- Bubeck et al. (2023a) Sébastien Bubeck, Varun Chandrasekaran, Ronen Eldan, Johannes Gehrke, Eric Horvitz, Ece Kamar, Peter Lee, Yin Tat Lee, Yuanzhi Li, Scott Lundberg, Harsha Nori, Hamid Palangi, Marco Tulio Ribeiro, and Yi Zhang. 2023a. Sparks of artificial general intelligence: Early experiments with GPT-4 .
- Bubeck et al. (2023b) Sébastien Bubeck, Varun Chandrasekaran, Ronen Eldan, Johannes Gehrke, Eric Horvitz, Ece Kamar, Peter Lee, Yin Tat Lee, Yuanzhi Li, Scott M. Lundberg, Harsha Nori, Hamid Palangi, Marco Túlio Ribeiro, and Yi Zhang. 2023b. Sparks of artificial general intelligence: Early experiments with GPT-4 . CoRR , abs/2303.12712.
- Burstein et al. (1997) Jill Burstein, Susanne Wolff, Chi Lu, and Randy M Kaplan. 1997. An automatic scoring system for advanced placement biology essays. In Fifth Conference on Applied Natural Language Processing , pages 174–181, Washington, DC, USA. Association for Computational Linguistics.
- Camgoz et al. (2020) Necati Cihan Camgoz, Oscar Koller, Simon Hadfield, and Richard Bowden. 2020. Sign language transformers: Joint end-to-end sign language recognition and translation. In Proceedings of the IEEE/CVF conference on computer vision and pattern recognition , pages 10023–10033. openaccess.thecvf.com.
- Carlini et al. (2021) Nicholas Carlini, Florian Tramer, Eric Wallace, Matthew Jagielski, Ariel Herbert-Voss, Katherine Lee, Adam Roberts, Tom B Brown, Dawn Song, Ulfar Erlingsson, and Others. 2021. Extracting training data from large language models. In USENIX Security Symposium , volume 6.
- Castro et al. (2022) Santiago Castro, Naihao Deng, Pingxuan Huang, Mihai Burzo, and Rada Mihalcea. 2022. In-the-Wild video question answering. In Proceedings of the 29th International Conference on Computational Linguistics , pages 5613–5635, Gyeongju, Republic of Korea. International Committee on Computational Linguistics.
- Çetinoğlu et al. (2016) Özlem Çetinoğlu, Sarah Schulz, and Ngoc Thang Vu. 2016. Challenges of computational processing of code-switching . In Proceedings of the Second Workshop on Computational Approaches to Code Switching , pages 1–11, Austin, Texas. Association for Computational Linguistics.
- Chang and Bergen (2021) Tyler A. Chang and Benjamin K. Bergen. 2021. Word acquisition in neural language models .
- Chintagunta et al. (2021a) Bharath Chintagunta, Namit Katariya, Xavier Amatriain, and Anitha Kannan. 2021a. Medically aware GPT-3 as a data generator for medical dialogue summarization . In Proceedings of the Second Workshop on Natural Language Processing for Medical Conversations , pages 66–76, Online. Association for Computational Linguistics.
- Chintagunta et al. (2021b) Bharath Chintagunta, Namit Katariya, Xavier Amatriain, and Anitha Kannan. 2021b. Medically aware GPT-3 as a data generator for medical dialogue summarization. In Proceedings of the Second Workshop on Natural Language Processing for Medical Conversations , pages 66–76, Online. Association for Computational Linguistics.
- Chughtai et al. (2023) Bilal Chughtai, Lawrence Chan, and Neel Nanda. 2023. A toy model of universality: Reverse engineering how networks learn group operations . CoRR , abs/2302.03025.
- Collins (2023) Keith Collins. 2023. How ChatGPT could embed a ‘watermark’ in the text it generates. The New York Times .
- Conmy et al. (2023) Arthur Conmy, Augustine N. Mavor-Parker, Aengus Lynch, Stefan Heimersheim, and Adrià Garriga-Alonso. 2023. Towards automated circuit discovery for mechanistic interpretability . CoRR , abs/2304.14997.
- Contreras Kallens et al. (2023) Pablo Contreras Kallens, Ross Deans Kristensen-McLachlan, and Morten H Christiansen. 2023. Large language models demonstrate the potential of statistical learning in language. Cognitive Science , 47(3):e13256.
- Côté et al. (2018) Marc-Alexandre Côté, Ákos Kádár, Xingdi Yuan, Ben Kybartas, Tavian Barnes, Emery Fine, James Moore, Ruo Yu Tao, Matthew Hausknecht, Layla El Asri, Mahmoud Adada, Wendy Tay, and Adam Trischler. 2018. TextWorld: A learning environment for text-based games .
- Cramer (2002) J S Cramer. 2002. The origins of logistic regression.
- Danilevsky et al. (2020) Marina Danilevsky, Kun Qian, Ranit Aharonov, Yannis Katsis, Ban Kawas, and Prithviraj Sen. 2020. A survey of the state of explainable AI for natural language processing .
- Dao et al. (2022) Tri Dao, Daniel Y Fu, Stefano Ermon, Atri Rudra, and Christopher Ré. 2022. FlashAttention: Fast and memory-efficient exact attention with IO-awareness .
- Dash et al. (2019) Sabyasachi Dash, Sushil Kumar Shakyawar, Mohit Sharma, and Sandeep Kaushik. 2019. Big data in healthcare: management, analysis and future prospects. Journal of Big Data , 6(1):1–25.
- Devlin et al. (2019) Jacob Devlin, Ming-Wei Chang, Kenton Lee, and Kristina Toutanova. 2019. Bert: Pre-training of deep bidirectional transformers for language understanding. ArXiv , abs/1810.04805.
- Du et al. (2022) Nan Du, Yanping Huang, Andrew M Dai, Simon Tong, Dmitry Lepikhin, Yuanzhong Xu, Maxim Krikun, Yanqi Zhou, Adams Wei Yu, Orhan Firat, Barret Zoph, Liam Fedus, Maarten P Bosma, Zongwei Zhou, Tao Wang, Emma Wang, Kellie Webster, Marie Pellat, Kevin Robinson, Kathleen Meier-Hellstern, Toju Duke, Lucas Dixon, Kun Zhang, Quoc Le, Yonghui Wu, Zhifeng Chen, and Claire Cui. 2022. GLaM: Efficient scaling of language models with Mixture-of-Experts. In Proceedings of the 39th International Conference on Machine Learning , volume 162 of Proceedings of Machine Learning Research , pages 5547–5569. PMLR.
- Edunov et al. (2018) Sergey Edunov, Myle Ott, Michael Auli, and David Grangier. 2018. Understanding Back-Translation at scale .
- Elhage et al. (2022) Nelson Elhage, Tristan Hume, Catherine Olsson, Nicholas Schiefer, Tom Henighan, Shauna Kravec, Zac Hatfield-Dodds, Robert Lasenby, Dawn Drain, Carol Chen, Roger Grosse, Sam McCandlish, Jared Kaplan, Dario Amodei, Martin Wattenberg, and Christopher Olah. 2022. Toy models of superposition . CoRR , abs/2209.10652.
- Eloundou et al. (2023) Tyna Eloundou, Sam Manning, Pamela Mishkin, and Daniel Rock. 2023. GPTs are GPTs: An early look at the labor market impact potential of large language models .
- Fan et al. (2021) Lifeng Fan, Shuwen Qiu, Zilong Zheng, Tao Gao, Song-Chun Zhu, and Yixin Zhu. 2021. Learning triadic belief dynamics in nonverbal communication from videos . pages 7312–7321.
- Fang et al. (2021) Han Fang, Pengfei Xiong, Luhui Xu, and Yu Chen. 2021. CLIP2Video: Mastering Video-Text retrieval via image CLIP .
- Fedus et al. (2022) William Fedus, Jeff Dean, and Barret Zoph. 2022. A review of sparse expert models in deep learning .
- Fedus et al. (2021) William Fedus, Barret Zoph, and Noam Shazeer. 2021. Switch transformers: Scaling to trillion parameter models with simple and efficient sparsity .
- Frank et al. (2016) Michael Frank, Mika Braginsky, Daniel Yurovsky, and Virginia Marchman. 2016. Wordbank: an open repository for developmental vocabulary data . Journal of Child Language , 44(3):677–694.
- Geiger et al. (2021) Atticus Geiger, Hanson Lu, Thomas Icard, and Christopher Potts. 2021. Causal abstractions of neural networks . In Advances in Neural Information Processing Systems 34: Annual Conference on Neural Information Processing Systems 2021, NeurIPS 2021, December 6-14, 2021, virtual , pages 9574–9586.
- Geva et al. (2022) Mor Geva, Avi Caciularu, Kevin Wang, and Yoav Goldberg. 2022. Transformer feed-forward layers build predictions by promoting concepts in the vocabulary space . In Proceedings of the 2022 Conference on Empirical Methods in Natural Language Processing , pages 30–45, Abu Dhabi, United Arab Emirates. Association for Computational Linguistics.
- Geva et al. (2021) Mor Geva, Roei Schuster, Jonathan Berant, and Omer Levy. 2021. Transformer feed-forward layers are key-value memories . In Proceedings of the 2021 Conference on Empirical Methods in Natural Language Processing , pages 5484–5495, Online and Punta Cana, Dominican Republic. Association for Computational Linguistics.
- Ghahramani (2001) Zoubin Ghahramani. 2001. An introduction to hidden markov models and bayesian networks. Int. J. Pattern Recognit. Artif. Intell. , 15:9–42.
- Gilardi et al. (2023) Fabrizio Gilardi, Meysam Alizadeh, and Maël Kubli. 2023. ChatGPT outperforms Crowd-Workers for Text-Annotation tasks .
- Gillespie (2020) Tarleton Gillespie. 2020. Content moderation, ai, and the question of scale . Big Data & Society , 7(2):2053951720943234.
- Goel et al. (2021) Karan Goel, Nazneen Fatema Rajani, Jesse Vig, Zachary Taschdjian, Mohit Bansal, and Christopher Ré. 2021. Robustness gym: Unifying the NLP evaluation landscape . In Proceedings of the 2021 Conference of the North American Chapter of the Association for Computational Linguistics: Human Language Technologies: Demonstrations , pages 42–55, Online. Association for Computational Linguistics.
- Gorwa et al. (2020) Robert Gorwa, Reuben Binns, and Christian Katzenbach. 2020. Algorithmic content moderation: Technical and political challenges in the automation of platform governance . Big Data & Society , 7(1):2053951719897945.
- Goyal et al. (2021) Naman Goyal, Cynthia Gao, Vishrav Chaudhary, Peng-Jen Chen, Guillaume Wenzek, Da Ju, Sanjana Krishnan, Marc’Aurelio Ranzato, Francisco Guzmán, and Angela Fan. 2021. The flores-101 evaluation benchmark for low-resource and multilingual machine translation.
- Guan et al. (2020) Jian Guan, Fei Huang, Zhihao Zhao, Xiaoyan Zhu, and Minlie Huang. 2020. A Knowledge-Enhanced pretraining model for commonsense story generation. Transactions of the Association for Computational Linguistics , 8:93–108.
- Hendricks and Nematzadeh (2021) Lisa Anne Hendricks and Aida Nematzadeh. 2021. Probing Image-Language transformers for verb understanding .
- Hendrycks et al. (2021) Dan Hendrycks, Collin Burns, Steven Basart, Andrew Critch, Jerry Li, Dawn Song, and Jacob Steinhardt. 2021. Aligning AI with shared human values . In International Conference on Learning Representations .
- Hewitt and Liang (2019) John Hewitt and Percy Liang. 2019. Designing and interpreting probes with control tasks . In Proceedings of the 2019 Conference on Empirical Methods in Natural Language Processing and the 9th International Joint Conference on Natural Language Processing (EMNLP-IJCNLP) , pages 2733–2743, Hong Kong, China. Association for Computational Linguistics.
- Hewitt and Manning (2019) John Hewitt and Christopher D. Manning. 2019. A structural probe for finding syntax in word representations . In Proceedings of the 2019 Conference of the North American Chapter of the Association for Computational Linguistics: Human Language Technologies, Volume 1 (Long and Short Papers) , pages 4129–4138, Minneapolis, Minnesota. Association for Computational Linguistics.
- Hinton et al. (2015) Geoffrey Hinton, Oriol Vinyals, and Jeff Dean. 2015. Distilling the knowledge in a neural network .
- Hoffmann et al. (2022) Jordan Hoffmann, Sebastian Borgeaud, Arthur Mensch, Elena Buchatskaya, Trevor Cai, Eliza Rutherford, Diego de Las Casas, Lisa Anne Hendricks, Johannes Welbl, Aidan Clark, Tom Hennigan, Eric Noland, Katie Millican, George van den Driessche, Bogdan Damoc, Aurelia Guy, Simon Osindero, Karen Simonyan, Erich Elsen, Jack W Rae, Oriol Vinyals, and Laurent Sifre. 2022. Training Compute-Optimal large language models .
- Hou et al. (2019) Rui Hou, Verónica Pérez-Rosas, Stacy Loeb, and Rada Mihalcea. 2019. Towards automatic detection of misinformation in online medical videos. In 2019 International conference on multimodal interaction , pages 235–243.
- Huang et al. (2021) Yu Huang, Chenzhuang Du, Zihui Xue, Xuanyao Chen, Hang Zhao, and Longbo Huang. 2021. What makes multi-modal learning better than single (provably) .
- Ignat et al. (2021) Oana Ignat, Santiago Castro, Hanwen Miao, Weiji Li, and Rada Mihalcea. 2021. Whyact: Identifying action reasons in lifestyle vlogs. In Proceedings of the 2021 Conference on Empirical Methods in Natural Language Processing , pages 4770–4785.
- Ignat et al. (2022) Oana Ignat, Jean Maillard, Vishrav Chaudhary, and Francisco Guzman. 2022. OCR improves machine translation for Low-Resource languages. arXiv preprint arXiv .
- Jelinek (1976) F Jelinek. 1976. Continuous speech recognition by statistical methods. Proc. IEEE , 64(4):532–556.
- Ji et al. (2022) Ziwei Ji, Nayeon Lee, Rita Frieske, Tiezheng Yu, Dan Su, Yan Xu, Etsuko Ishii, Yejin Bang, Wenliang Dai, Andrea Madotto, and Pascale Fung. 2022. Survey of hallucination in natural language generation. ACM Computing Surveys , 55:1–38.
- Jie et al. (2018) Zhuoni Jie, Marwa Mahmoud, Quentin Stafford-Fraser, Peter Robinson, Eduardo Dias, and Lee Skrypchuk. 2018. Analysis of yawning behaviour in spontaneous expressions of drowsy drivers. In 2018 13th IEEE International Conference on Automatic Face & Gesture Recognition (FG 2018) , pages 571–576.
- Jimeno Yepes et al. (2015) Antonio Jimeno Yepes, Andrew MacKinlay, and Bo Han. 2015. Investigating public health surveillance using Twitter . In Proceedings of BioNLP 15 , pages 164–170, Beijing, China. Association for Computational Linguistics.
- Jin et al. (2022a) Di Jin, Zhijing Jin, Zhiting Hu, Olga Vechtomova, and Rada Mihalcea. 2022a. Deep learning for text style transfer: A survey . Computational Linguistics , 48(1):155–205.
- Jin et al. (2020) Di Jin, Zhijing Jin, Joey Tianyi Zhou, and Peter Szolovits. 2020. Is BERT really robust? A strong baseline for natural language attack on text classification and entailment . In The Thirty-Fourth AAAI Conference on Artificial Intelligence, AAAI 2020 .
- Jin et al. (2023a) Zhijing Jin, Yuen Chen, Felix Leeb, Luigi Gresele, Ojasv Kamal, Zhiheng LYU, Kevin Blin, Fernando Gonzalez Adauto, Max Kleiman-Weiner, Mrinmaya Sachan, and Bernhard Schölkopf. 2023a. Causal Benchmark: A benchmark of 10,000+ causal inference questions.
- Jin et al. (2022b) Zhijing Jin, Abhinav Lalwani, Tejas Vaidhya, Xiaoyu Shen, Yiwen Ding, Zhiheng Lyu, Mrinmaya Sachan, Rada Mihalcea, and Bernhard Schölkopf. 2022b. Logical fallacy detection . In Findings of the Association for Computational Linguistics: EMNLP 2022 , pages 7180––7198, Abu Dhabi, United Arab Emirates. Association for Computational Linguistics.
- Jin et al. (2023b) Zhijing Jin, Sydney Levine, Max Kleiman-Weiner, Jiarui Liu, Francesco Ortu, Fernando Gonzalez Adauto, András Strausz, Mrinmaya Sachan, Rada Mihalcea, Yejin Choi, and Bernhard Schölkopf. 2023b. Trolley problems for large language models across 100+ languages.
- Jin et al. (2023c) Zhijing Jin, Jiarui Liu, Zhiheng LYU, Spencer Poff, Mrinmaya Sachan, Rada Mihalcea, Mona T. Diab, and Bernhard Schölkopf. 2023c. Can large language models infer causation from correlation?
- Joze (2019) Hamid Reza Vaezi Joze. 2019. MS-ASL: A Large-Scale data set and benchmark for understanding american sign language. bmvc2019.org .
- Kazemi et al. (2022) Ashkan Kazemi, Artem Abzaliev, Naihao Deng, Rui Hou, Davis Liang, Scott A Hale, Verónica Pérez-Rosas, and Rada Mihalcea. 2022. Adaptable claim rewriting with offline reinforcement learning for effective misinformation discovery. arXiv preprint arXiv:2210.07467 .
- Kazemi et al. (2021a) Ashkan Kazemi, Kiran Garimella, Devin Gaffney, and Scott Hale. 2021a. Claim matching beyond English to scale global fact-checking . In Proceedings of the 59th Annual Meeting of the Association for Computational Linguistics and the 11th International Joint Conference on Natural Language Processing (Volume 1: Long Papers) , pages 4504–4517, Online. Association for Computational Linguistics.
- Kazemi et al. (2021b) Ashkan Kazemi, Zehua Li, Verónica Pérez-Rosas, and Rada Mihalcea. 2021b. Extractive and abstractive explanations for fact-checking and evaluation of news. In Proceedings of the Fourth Workshop on NLP for Internet Freedom: Censorship, Disinformation, and Propaganda , pages 45–50.
- Khanuja et al. (2020) Simran Khanuja, Sandipan Dandapat, Anirudh Srinivasan, Sunayana Sitaram, and Monojit Choudhury. 2020. GLUECoS: An evaluation benchmark for code-switched NLP . In Proceedings of the 58th Annual Meeting of the Association for Computational Linguistics , pages 3575–3585, Online. Association for Computational Linguistics.
- Kim et al. (2022) Hyunwoo Kim, Jack Hessel, Liwei Jiang, Ximing Lu, Youngjae Yu, Pei Zhou, Ronan Le Bras, Malihe Alikhani, Gunhee Kim, Maarten Sap, and Yejin Choi. 2022. SODA: Million-scale dialogue distillation with social commonsense contextualization .
- Koto and Koto (2020) Fajri Koto and Ikhwan Koto. 2020. Towards computational linguistics in Minangkabau language: Studies on sentiment analysis and machine translation . In Proceedings of the 34th Pacific Asia Conference on Language, Information and Computation , pages 138–148, Hanoi, Vietnam. Association for Computational Linguistics.
- Kurdi et al. (2020) Ghader Kurdi, Jared Leo, Bijan Parsia, Uli Sattler, and Salam Al-Emari. 2020. A systematic review of automatic question generation for educational purposes. International Journal of Artificial Intelligence in Education , 30(1):121–204.
- Lazaridou et al. (2017) Angeliki Lazaridou, Alexander Peysakhovich, and Marco Baroni. 2017. Multi-agent cooperation and the emergence of (natural) language .
- Lazer et al. (2009) David Lazer, Alex Pentland, Lada Adamic, Sinan Aral, Albert-Laszlo Barabasi, Devon Brewer, Nicholas Christakis, Noshir Contractor, James Fowler, Myron Gutmann, Tony Jebara, Gary King, Michael Macy, Deb Roy, and Marshall Van Alstyne. 2009. Social science. computational social science. Science , 323(5915):721–723.
- Lazer et al. (2020) David M J Lazer, Alex Pentland, Duncan J Watts, Sinan Aral, Susan Athey, Noshir Contractor, Deen Freelon, Sandra Gonzalez-Bailon, Gary King, Helen Margetts, Alondra Nelson, Matthew J Salganik, Markus Strohmaier, Alessandro Vespignani, and Claudia Wagner. 2020. Computational social science: Obstacles and opportunities. Science , 369(6507):1060–1062.
- Lee et al. (2021) Andrew Lee, Jonathan K Kummerfeld, Larry An, and Rada Mihalcea. 2021. Micromodels for efficient, explainable, and reusable systems: A case study on mental health. In Findings of the Association for Computational Linguistics: EMNLP 2021 , pages 4257–4272.
- Lee and Li (2020) Grandee Lee and Haizhou Li. 2020. Modeling code-switch languages using bilingual parallel corpus. In Annual Meeting of the Association for Computational Linguistics .
- Lee et al. (2022) Katherine Lee, Daphne Ippolito, Andrew Nystrom, Chiyuan Zhang, Douglas Eck, Chris Callison-Burch, and Nicholas Carlini. 2022. Deduplicating training data makes language models better. In Proceedings of the 60th Annual Meeting of the Association for Computational Linguistics (Volume 1: Long Papers) , pages 8424–8445, Dublin, Ireland. Association for Computational Linguistics.
- Lei et al. (2019) Jie Lei, Licheng Yu, Tamara L Berg, and Mohit Bansal. 2019. Tvqa+: Spatio-temporal grounding for video question answering. In Tech Report, arXiv .
- Lester et al. (2021) Brian Lester, Rami Al-Rfou, and Noah Constant. 2021. The power of scale for Parameter-Efficient prompt tuning. In Proceedings of the 2021 Conference on Empirical Methods in Natural Language Processing , pages 3045–3059, Online and Punta Cana, Dominican Republic. Association for Computational Linguistics.
- Li et al. (2022) Belinda Li, Jane Yu, Madian Khabsa, Luke Zettlemoyer, Alon Halevy, and Jacob Andreas. 2022. Quantifying adaptability in pre-trained language models with 500 tasks . In Proceedings of the 2022 Conference of the North American Chapter of the Association for Computational Linguistics: Human Language Technologies , pages 4696–4715, Seattle, United States. Association for Computational Linguistics.
- Li et al. (2020) Dongxu Li, Cristian Rodriguez, Xin Yu, and Hongdong Li. 2020. Word-level deep sign language recognition from video: A new large-scale dataset and methods comparison. In Proceedings of the IEEE/CVF winter conference on applications of computer vision , pages 1459–1469. openaccess.thecvf.com.
- Liang et al. (2022) Percy Liang, Rishi Bommasani, Tony Lee, Dimitris Tsipras, Dilara Soylu, Michihiro Yasunaga, Yian Zhang, Deepak Narayanan, Yuhuai Wu, Ananya Kumar, Benjamin Newman, Binhang Yuan, Bobby Yan, Ce Zhang, Christian Cosgrove, Christopher D Manning, Christopher Ré, Diana Acosta-Navas, Drew A Hudson, Eric Zelikman, Esin Durmus, Faisal Ladhak, Frieda Rong, Hongyu Ren, Huaxiu Yao, Jue Wang, Keshav Santhanam, Laurel Orr, Lucia Zheng, Mert Yuksekgonul, Mirac Suzgun, Nathan Kim, Neel Guha, Niladri Chatterji, Omar Khattab, Peter Henderson, Qian Huang, Ryan Chi, Sang Michael Xie, Shibani Santurkar, Surya Ganguli, Tatsunori Hashimoto, Thomas Icard, Tianyi Zhang, Vishrav Chaudhary, William Wang, Xuechen Li, Yifan Mai, Yuhui Zhang, and Yuta Koreeda. 2022. Holistic evaluation of language models .
- Liednikova et al. (2020) Anna Liednikova, Philippe Jolivet, Alexandre Durand-Salmon, and Claire Gardent. 2020. Learning health-bots from training data that was automatically created using paraphrase detection and expert knowledge . In Proceedings of the 28th International Conference on Computational Linguistics , pages 638–648, Barcelona, Spain (Online). International Committee on Computational Linguistics.
- Liu et al. (2019) Yinhan Liu, Myle Ott, Naman Goyal, Jingfei Du, Mandar Joshi, Danqi Chen, Omer Levy, Mike Lewis, Luke Zettlemoyer, and Veselin Stoyanov. 2019. Roberta: A robustly optimized bert pretraining approach. ArXiv , abs/1907.11692.
- Lundberg and Lee (2017) Scott M. Lundberg and Su-In Lee. 2017. A unified approach to interpreting model predictions. ArXiv , abs/1705.07874.
- Ma et al. (2022) Xuezhe Ma, Chunting Zhou, Xiang Kong, Junxian He, Liangke Gui, Graham Neubig, Jonathan May, and Luke Zettlemoyer. 2022. Mega: Moving average equipped gated attention .
- MacWhinney (1992) Brian MacWhinney. 1992. The CHILDES project: tools for analyzing talk . Child Language Teaching and Therapy , 8(2):217–218.
- Markov et al. (2023) Todor Markov, Chong Zhang, Sandhini Agarwal, Tyna Eloundou, Teddy Lee, Steven Adler, Angela Jiang, and Lilian Weng. 2023. A holistic approach to undesired content detection in the real world .
- Mathews (2019) Sherin Mary Mathews. 2019. Explainable artificial intelligence applications in NLP, biomedical, and malware classification: A literature review. In Intelligent Computing , pages 1269–1292. Springer International Publishing.
- Matsumoto and Assar (1992) David Matsumoto and Manish Assar. 1992. The effects of language on judgments of universal facial expressions of emotion. Journal of Nonverbal Behavior , 16:85–99.
- Mattern et al. (2022) Justus Mattern, Zhijing Jin, Benjamin Weggenmann, Bernhard Schoelkopf, and Mrinmaya Sachan. 2022. Differentially private language models for secure data sharing . In Proceedings of the 2022 Conference on Empirical Methods in Natural Language Processing , pages 4860–4873, Abu Dhabi, United Arab Emirates. Association for Computational Linguistics.
- McCarthy et al. (2020) Arya D. McCarthy, Rachel Wicks, Dylan Lewis, Aaron Mueller, Winston Wu, Oliver Adams, Garrett Nicolai, Matt Post, and David Yarowsky. 2020. The Johns Hopkins University Bible corpus: 1600+ tongues for typological exploration . In Proceedings of the Twelfth Language Resources and Evaluation Conference , pages 2884–2892, Marseille, France. European Language Resources Association.
- McCloskey (1991) Michael McCloskey. 1991. Networks and theories: The place of connectionism in cognitive science . Psychological Science , 2(6):387–395.
- McNeill (1992) David McNeill. 1992. Hand and mind: What gestures reveal about thought. 416.
- Meng et al. (2022) Kevin Meng, David Bau, Alex Andonian, and Yonatan Belinkov. 2022. Locating and editing factual associations in gpt. In Neural Information Processing Systems .
- Mialon et al. (2023) Grégoire Mialon, Roberto Dessì, Maria Lomeli, Christoforos Nalmpantis, Ramakanth Pasunuru, Roberta Raileanu, Baptiste Rozière, Timo Schick, Jane Dwivedi-Yu, Asli Celikyilmaz, Edouard Grave, Yann LeCun, and Thomas Scialom. 2023. Augmented language models: A survey . CoRR , abs/2302.07842.
- Miao et al. (2020) Shen-yun Miao, Chao-Chun Liang, and Keh-Yih Su. 2020. A diverse corpus for evaluating and developing English math word problem solvers . In Proceedings of the 58th Annual Meeting of the Association for Computational Linguistics , pages 975–984, Online. Association for Computational Linguistics.
- Min et al. (2022) Do June Min, Kenneth Resnicow, and Rada Mihalcea. 2022. PAIR: Prompt-aware margIn ranking for counselor reflection scoring in motivational interviewing . In Proceedings of the 2022 Conference on Empirical Methods in Natural Language Processing , pages 148–158, Abu Dhabi, United Arab Emirates. Association for Computational Linguistics.
- Mishra and Sachdeva (2020) Swaroop Mishra and Bhavdeep Singh Sachdeva. 2020. Do we need to create big datasets to learn a task? In Proceedings of SustaiNLP: Workshop on Simple and Efficient Natural Language Processing , pages 169–173, Online. Association for Computational Linguistics.
- Mohler and Mihalcea (2009) Michael Mohler and Rada Mihalcea. 2009. Text-to-text semantic similarity for automatic short answer grading. In Proceedings of the 12th Conference of the European Chapter of the ACL (EACL 2009) , pages 567–575.
- Mokady et al. (2021) Ron Mokady, Amir Hertz, and Amit H Bermano. 2021. ClipCap: CLIP prefix for image captioning .
- Mondal et al. (2022) Ishani Mondal, Kabir Ahuja, Mohit Jain, Jacki O’Neill, Kalika Bali, and Monojit Choudhury. 2022. Global readiness of language technology for healthcare: What would it take to combat the next pandemic? In Proceedings of the 29th International Conference on Computational Linguistics , pages 4320–4335, Gyeongju, Republic of Korea. International Committee on Computational Linguistics.
- Moosavi et al. (2022) Nafise Moosavi, Quentin Delfosse, Kristian Kersting, and Iryna Gurevych. 2022. Adaptable adapters. In Proceedings of the 2022 Conference of the North American Chapter of the Association for Computational Linguistics: Human Language Technologies , pages 3742–3753, Seattle, United States. Association for Computational Linguistics.
- Mosca et al. (2023) Edoardo Mosca, Daryna Dementieva, Tohid Ebrahim Ajdari, Maximilian Kummeth, Kirill Gringauz, and Georg Groh. 2023. IFAN: An Explainability-Focused interaction framework for humans and NLP models .
- Mosqueira-Rey et al. (2023) Eduardo Mosqueira-Rey, Elena Hernández-Pereira, David Alonso-Ríos, José Bobes-Bascarán, and Ángel Fernández-Leal. 2023. Human-in-the-loop machine learning: a state of the art. Artificial Intelligence Review , 56(4):3005–3054.
- Mousavinasab et al. (2021) Elham Mousavinasab, Nahid Zarifsanaiey, Sharareh R. Niakan Kalhori, Mahnaz Rakhshan, Leila Keikha, and Marjan Ghazi Saeedi. 2021. Intelligent tutoring systems: a systematic review of characteristics, applications, and evaluation methods. Interactive Learning Environments , 29(1):142–163.
- Mueller et al. (2020) Aaron Mueller, Garrett Nicolai, Arya D. McCarthy, Dylan Lewis, Winston Wu, and David Yarowsky. 2020. An analysis of massively multilingual neural machine translation for low-resource languages . In Proceedings of the Twelfth Language Resources and Evaluation Conference , pages 3710–3718, Marseille, France. European Language Resources Association.
- Mustafa et al. (2022) Basil Mustafa, Carlos Riquelme Ruiz, Joan Puigcerver, Rodolphe Jenatton, and Neil Houlsby. 2022. Multimodal contrastive learning with LIMoE: the Language-Image mixture of experts.
- Naeini et al. (2015) Mahdi Pakdaman Naeini, Gregory F. Cooper, and Milos Hauskrecht. 2015. Obtaining well calibrated probabilities using bayesian binning. Proceedings of the … AAAI Conference on Artificial Intelligence. AAAI Conference on Artificial Intelligence , 2015:2901–2907.
- Nakov et al. (2021) Preslav Nakov, David Corney, Maram Hasanain, Firoj Alam, Tamer Elsayed, Alberto Barrón-Cedeño, Paolo Papotti, Shaden Shaar, and Giovanni Da San Martino. 2021. Automated fact-checking for assisting human fact-checkers .
- Nanda et al. (2023) Neel Nanda, Lawrence Chan, Tom Lieberum, Jess Smith, and Jacob Steinhardt. 2023. Progress measures for grokking via mechanistic interpretability . CoRR , abs/2301.05217.
- Naseem et al. (2022) Usman Naseem, Byoung Chan Lee, Matloob Khushi, Jinman Kim, and Adam G. Dunn. 2022. Benchmarking for public health surveillance tasks on social media with a domain-specific pretrained language model .
- NLLB Team et al. (2022) NLLB Team, Marta R Costa-jussà, James Cross, Onur Çelebi, Maha Elbayad, Kenneth Heafield, Kevin Heffernan, Elahe Kalbassi, Janice Lam, Daniel Licht, Jean Maillard, Anna Sun, Skyler Wang, Guillaume Wenzek, Al Youngblood, Bapi Akula, Loic Barrault, Gabriel Mejia Gonzalez, Prangthip Hansanti, John Hoffman, Semarley Jarrett, Kaushik Ram Sadagopan, Dirk Rowe, Shannon Spruit, Chau Tran, Pierre Andrews, Necip Fazil Ayan, Shruti Bhosale, Sergey Edunov, Angela Fan, Cynthia Gao, Vedanuj Goswami, Francisco Guzmán, Philipp Koehn, Alexandre Mourachko, Christophe Ropers, Safiyyah Saleem, Holger Schwenk, and Jeff Wang. 2022. No language left behind: Scaling Human-Centered machine translation .
- Ofek et al. (2016) Nir Ofek, Soujanya Poria, Lior Rokach, Erik Cambria, Amir Hussain, and Asaf Shabtai. 2016. Unsupervised commonsense knowledge enrichment for domain-specific sentiment analysis. Cognitive Computation , 8:467–477.
- Olaronke and Olaleke (2015) Iroju Olaronke and J. Olaleke. 2015. A systematic review of natural language processing in healthcare . International Journal of Information Technology and Computer Science , 08:44–50.
- Olsson et al. (2022) Catherine Olsson, Nelson Elhage, Neel Nanda, Nicholas Joseph, Nova DasSarma, Tom Henighan, Ben Mann, Amanda Askell, Yuntao Bai, Anna Chen, Tom Conerly, Dawn Drain, Deep Ganguli, Zac Hatfield-Dodds, Danny Hernandez, Scott Johnston, Andy Jones, Jackson Kernion, Liane Lovitt, Kamal Ndousse, Dario Amodei, Tom Brown, Jack Clark, Jared Kaplan, Sam McCandlish, and Chris Olah. 2022. In-context learning and induction heads . CoRR , abs/2209.11895.
- OpenAI (2023) OpenAI. 2023. GPT-4 technical report .
- Orbach and Goldberg (2020) Eyal Orbach and Yoav Goldberg. 2020. Facts2Story: Controlling text generation by key facts .
- Ouyang et al. (2022) Long Ouyang, Jeff Wu, Xu Jiang, Diogo Almeida, Carroll L. Wainwright, Pamela Mishkin, Chong Zhang, Sandhini Agarwal, Katarina Slama, Alex Ray, John Schulman, Jacob Hilton, Fraser Kelton, Luke Miller, Maddie Simens, Amanda Askell, Peter Welinder, Paul F. Christiano, Jan Leike, and Ryan Lowe. 2022. Training language models to follow instructions with human feedback . CoRR , abs/2203.02155.
- Patel and Pavlick (2022) Roma Patel and Ellie Pavlick. 2022. Mapping language models to grounded conceptual spaces.
- Patterson et al. (2022) David Patterson, Joseph Gonzalez, Urs Hölzle, Quoc Le, Chen Liang, Lluis-Miquel Munguia, Daniel Rothchild, David So, Maud Texier, and Jeff Dean. 2022. The carbon footprint of machine learning training will plateau, then shrink .
- Pérez-Rosas et al. (2017) Verónica Pérez-Rosas, Rada Mihalcea, Kenneth Resnicow, Satinder Singh, and Lawrence An. 2017. Understanding and predicting empathic behavior in counseling therapy . In Proceedings of the 55th Annual Meeting of the Association for Computational Linguistics (Volume 1: Long Papers) , pages 1426–1435, Vancouver, Canada. Association for Computational Linguistics.
- Pfeiffer et al. (2020) Jonas Pfeiffer, Andreas Rücklé, Clifton Poth, Aishwarya Kamath, Ivan Vulić, Sebastian Ruder, Kyunghyun Cho, and Iryna Gurevych. 2020. AdapterHub: A framework for adapting transformers. In Proceedings of the 2020 Conference on Empirical Methods in Natural Language Processing: System Demonstrations , pages 46–54, Online. Association for Computational Linguistics.
- Rabiner (1989) Lawrence R. Rabiner. 1989. A tutorial on hidden markov models and selected applications in speech recognition. Proc. IEEE , 77:257–286.
- Radford et al. (2021) Alec Radford, Jong Wook Kim, Chris Hallacy, Aditya Ramesh, Gabriel Goh, Sandhini Agarwal, Girish Sastry, Amanda Askell, Pamela Mishkin, Jack Clark, Gretchen Krueger, and Ilya Sutskever. 2021. Learning transferable visual models from natural language supervision. In Proceedings of the 38th International Conference on Machine Learning , volume 139 of Proceedings of Machine Learning Research , pages 8748–8763. PMLR.
- Ramesh et al. (2021) Aditya Ramesh, Mikhail Pavlov, Gabriel Goh, Scott Gray, Chelsea Voss, Alec Radford, Mark Chen, and Ilya Sutskever. 2021. Zero-Shot Text-to-Image generation .
- Reid et al. (2021) Machel Reid, Junjie Hu, Graham Neubig, and Yutaka Matsuo. 2021. AfroMT: Pretraining strategies and reproducible benchmarks for translation of 8 African languages . In Proceedings of the 2021 Conference on Empirical Methods in Natural Language Processing , pages 1306–1320, Online and Punta Cana, Dominican Republic. Association for Computational Linguistics.
- Riani et al. (2020) Kais Riani, Michalis Papakostas, Hussein Kokash, M Abouelenien, Mihai Burzo, and Rada Mihalcea. 2020. Towards detecting levels of alertness in drivers using multiple modalities. Petra .
- Ribeiro et al. (2016) Marco Tulio Ribeiro, Sameer Singh, and Carlos Guestrin. 2016. “why should i trust you?”: Explaining the predictions of any classifier. Proceedings of the 22nd ACM SIGKDD International Conference on Knowledge Discovery and Data Mining .
- Rijhwani et al. (2020) Shruti Rijhwani, Antonios Anastasopoulos, and Graham Neubig. 2020. OCR post correction for endangered language texts .
- Rosenbaum et al. (2022a) Andy Rosenbaum, Saleh Soltan, Wael Hamza, Amir Saffari, Marco Damonte, and Isabel Groves. 2022a. CLASP: Few-Shot Cross-Lingual data augmentation for semantic parsing .
- Rosenbaum et al. (2022b) Andy Rosenbaum, Saleh Soltan, Wael Hamza, Yannick Versley, and Markus Boese. 2022b. LINGUIST: Language model instruction tuning to generate annotated utterances for intent classification and slot tagging .
- Schick et al. (2023) Timo Schick, Jane Dwivedi-Yu, Roberto Dessì, Roberta Raileanu, Maria Lomeli, Luke Zettlemoyer, Nicola Cancedda, and Thomas Scialom. 2023. Toolformer: Language models can teach themselves to use tools . CoRR , abs/2302.04761.
- Schick and Schütze (2021) Timo Schick and Hinrich Schütze. 2021. It’s not just size that matters: Small language models are also Few-Shot learners. In Proceedings of the 2021 Conference of the North American Chapter of the Association for Computational Linguistics: Human Language Technologies , pages 2339–2352, Online. Association for Computational Linguistics.
- Sennrich et al. (2015) Rico Sennrich, Barry Haddow, and Alexandra Birch. 2015. Improving neural machine translation models with monolingual data .
- Shaar et al. (2020) Shaden Shaar, Nikolay Babulkov, Giovanni Da San Martino, and Preslav Nakov. 2020. That is a known lie: Detecting previously fact-checked claims . In Proceedings of the 58th Annual Meeting of the Association for Computational Linguistics , pages 3607–3618, Online. Association for Computational Linguistics.
- Shannon (1948) C E Shannon. 1948. A mathematical theory of communication. The Bell System Technical Journal , 27(3):379–423.
- Sharma et al. (2020) Ashish Sharma, Adam Miner, David Atkins, and Tim Althoff. 2020. A computational approach to understanding empathy expressed in text-based mental health support . In Proceedings of the 2020 Conference on Empirical Methods in Natural Language Processing (EMNLP) , pages 5263–5276, Online. Association for Computational Linguistics.
- Shen et al. (2022) Siqi Shen, Verónica Pérez-Rosas, Charles Welch, Soujanya Poria, and Rada Mihalcea. 2022. Knowledge enhanced reflection generation for counseling dialogues. In Proceedings of the 60th Annual Meeting of the Association for Computational Linguistics (Volume 1: Long Papers) , pages 3096–3107.
- Singh et al. (2019) Amanpreet Singh, Vivek Natarajan, Meet Shah, Yu Jiang, Xinlei Chen, Dhruv Batra, Devi Parikh, and Marcus Rohrbach. 2019. Towards VQA models that can read .
- Smith et al. (2013) Jason R. Smith, Herve Saint-Amand, Magdalena Plamada, Philipp Koehn, Chris Callison-Burch, and Adam Lopez. 2013. Dirt cheap web-scale parallel text from the Common Crawl . In Proceedings of the 51st Annual Meeting of the Association for Computational Linguistics (Volume 1: Long Papers) , pages 1374–1383, Sofia, Bulgaria. Association for Computational Linguistics.
- Song et al. (2013) Yale Song, Louis-Philippe Morency, and Randall Davis. 2013. Learning a sparse codebook of facial and body microexpressions for emotion recognition. In Proceedings of the 15th ACM on International conference on multimodal interaction , ICMI ’13, pages 237–244, New York, NY, USA. Association for Computing Machinery.
- Speer et al. (2017) Robyn Speer, Joshua Chin, and Catherine Havasi. 2017. ConceptNet 5.5: An open multilingual graph of general knowledge. AAAI , 31(1).
- Stolfo et al. (2023) Alessandro Stolfo, Zhijing Jin, Kumar Shridhar, Bernhard Schölkopf, and Mrinmaya Sachan. 2023. A causal framework to quantify the robustness of mathematical reasoning with language models . In Proceedings of the 61st Annual Meeting of the Association for Computational Linguistics (Volume 1: Long Papers) , Toronto, Canada. Association for Computational Linguistics.
- Strubell et al. (2019) Emma Strubell, Ananya Ganesh, and Andrew McCallum. 2019. Energy and policy considerations for deep learning in NLP . In Proceedings of the 57th Annual Meeting of the Association for Computational Linguistics , pages 3645–3650, Florence, Italy. Association for Computational Linguistics.
- Suchanek et al. (2007) Fabian M Suchanek, Gjergji Kasneci, and Gerhard Weikum. 2007. Yago: a core of semantic knowledge. In Proceedings of the 16th international conference on World Wide Web , WWW ’07, pages 697–706, New York, NY, USA. Association for Computing Machinery.
- Swanson et al. (2021) Ben Swanson, Kory Mathewson, Ben Pietrzak, Sherol Chen, and Monica Dinalescu. 2021. Story centaur: Large language model few shot learning as a creative writing tool. In Proceedings of the 16th Conference of the European Chapter of the Association for Computational Linguistics: System Demonstrations , pages 244–256, Online. Association for Computational Linguistics.
- Tabak and Purver (2020) Tom Tabak and Matthew Purver. 2020. Temporal mental health dynamics on social media . In Proceedings of the 1st Workshop on NLP for COVID-19 (Part 2) at EMNLP 2020 , Online. Association for Computational Linguistics.
- Tang et al. (2023) Ruixiang Tang, Xiaotian Han, Xiaoqian Jiang, and Xia Hu. 2023. Does synthetic data generation of LLMs help clinical text mining?
- Taori et al. (2023) Rohan Taori, Ishaan Gulrajani, Tianyi Zhang, Yann Dubois, Xuechen Li, Carlos Guestrin, Percy Liang, and Tatsunori B. Hashimoto. 2023. Stanford alpaca: An instruction-following llama model. https://github.com/tatsu-lab/stanford_alpaca .
- Tay et al. (2020) Yi Tay, Mostafa Dehghani, Samira Abnar, Yikang Shen, Dara Bahri, Philip Pham, Jinfeng Rao, Liu Yang, Sebastian Ruder, and Donald Metzler. 2020. Long range arena: A benchmark for efficient transformers .
- Tay et al. (2022) Yi Tay, Mostafa Dehghani, Dara Bahri, and Donald Metzler. 2022. Efficient transformers: A survey. ACM Comput. Surv. , 55(6):1–28.
- Thompson et al. (2020) Neil C Thompson, Kristjan Greenewald, Keeheon Lee, and Gabriel F Manso. 2020. The computational limits of deep learning .
- Thorne et al. (2018) James Thorne, Andreas Vlachos, Christos Christodoulopoulos, and Arpit Mittal. 2018. FEVER: a large-scale dataset for fact extraction and VERification . In Proceedings of the 2018 Conference of the North American Chapter of the Association for Computational Linguistics: Human Language Technologies, Volume 1 (Long Papers) , pages 809–819, New Orleans, Louisiana. Association for Computational Linguistics.
- Thrush et al. (2022) Tristan Thrush, Ryan Jiang, Max Bartolo, Amanpreet Singh, Adina Williams, Douwe Kiela, and Candace Ross. 2022. Winoground: Probing vision and language models for Visio-Linguistic compositionality .
- Touvron et al. (2023a) Hugo Touvron, Thibaut Lavril, Gautier Izacard, Xavier Martinet, Marie-Anne Lachaux, Timothée Lacroix, Baptiste Rozière, Naman Goyal, Eric Hambro, Faisal Azhar, Aurélien Rodriguez, Armand Joulin, Edouard Grave, and Guillaume Lample. 2023a. LLaMA: Open and efficient foundation language models . CoRR , abs/2302.13971.
- Touvron et al. (2023b) Hugo Touvron, Thibaut Lavril, Gautier Izacard, Xavier Martinet, Marie-Anne Lachaux, Timothée Lacroix, Baptiste Rozière, Naman Goyal, Eric Hambro, Faisal Azhar, Aurelien Rodriguez, Armand Joulin, Edouard Grave, and Guillaume Lample. 2023b. LLaMA: Open and efficient foundation language models .
- Valipour et al. (2022) Mojtaba Valipour, Mehdi Rezagholizadeh, Ivan Kobyzev, and Ali Ghodsi. 2022. DyLoRA: Parameter efficient tuning of pre-trained models using dynamic Search-Free Low-Rank adaptation .
- Vaswani et al. (2017) Ashish Vaswani, Noam M. Shazeer, Niki Parmar, Jakob Uszkoreit, Llion Jones, Aidan N. Gomez, Lukasz Kaiser, and Illia Polosukhin. 2017. Attention is all you need. In NIPS .
- Vegi et al. (2022) Pavanpankaj Vegi, Sivabhavani J, Biswajit Paul, Prasanna K R, and Chitra Viswanathan. 2022. ANVITA-African: A multilingual neural machine translation system for African languages . In Proceedings of the Seventh Conference on Machine Translation (WMT) , pages 1090–1097, Abu Dhabi, United Arab Emirates (Hybrid). Association for Computational Linguistics.
- Villegas et al. (2022) Ruben Villegas, Mohammad Babaeizadeh, Pieter-Jan Kindermans, Hernan Moraldo, Han Zhang, Mohammad Taghi Saffar, Santiago Castro, Julius Kunze, and Dumitru Erhan. 2022. Phenaki: Variable length video generation from open domain textual description .
- Wang et al. (2020) Angelina Wang, Alexander Liu, Ryan Zhang, Anat Kleiman, Leslie Kim, Dora Zhao, Iroha Shirai, Arvind Narayanan, and Olga Russakovsky. 2020. REVISE: A tool for measuring and mitigating bias in visual datasets .
- Wang et al. (2023) Kevin Ro Wang, Alexandre Variengien, Arthur Conmy, Buck Shlegeris, and Jacob Steinhardt. 2023. Interpretability in the wild: a circuit for indirect object identification in GPT-2 small . In The Eleventh International Conference on Learning Representations .
- Wang et al. (2019a) Weiyao Wang, Du Tran, and Matt Feiszli. 2019a. What makes training Multi-Modal classification networks hard?
- Wang et al. (2022) Wenhui Wang, Hangbo Bao, Li Dong, Johan Bjorck, Zhiliang Peng, Qiang Liu, Kriti Aggarwal, Owais Khan Mohammed, Saksham Singhal, Subhojit Som, and Furu Wei. 2022. Image as a foreign language: BEiT pretraining for all vision and Vision-Language tasks .
- Wang et al. (2019b) Yansen Wang, Ying Shen, Zhun Liu, Paul Pu Liang, Amir Zadeh, and Louis-Philippe Morency. 2019b. Words can shift: Dynamically adjusting word representations using nonverbal behaviors. Proc. Conf. AAAI Artif. Intell. , 33(1):7216–7223.
- Wei et al. (2022) Jason Wei, Yi Tay, Rishi Bommasani, Colin Raffel, Barret Zoph, Sebastian Borgeaud, Dani Yogatama, Maarten Bosma, Denny Zhou, Donald Metzler, Ed H Chi, Tatsunori Hashimoto, Oriol Vinyals, Percy Liang, Jeff Dean, and William Fedus. 2022. Emergent abilities of large language models .
- West et al. (2022) Peter West, Chandra Bhagavatula, Jack Hessel, Jena Hwang, Liwei Jiang, Ronan Le Bras, Ximing Lu, Sean Welleck, and Yejin Choi. 2022. Symbolic knowledge distillation: from general language models to commonsense models. In Proceedings of the 2022 Conference of the North American Chapter of the Association for Computational Linguistics: Human Language Technologies , pages 4602–4625, Seattle, United States. Association for Computational Linguistics.
- Weston et al. (2013) Jason Weston, Antoine Bordes, Oksana Yakhnenko, and Nicolas Usunier. 2013. Connecting language and knowledge bases with embedding models for relation extraction . In Proceedings of the 2013 Conference on Empirical Methods in Natural Language Processing , pages 1366–1371, Seattle, Washington, USA. Association for Computational Linguistics.
- Wilcox et al. (2022) Ethan Gotlieb Wilcox, Richard Futrell, and Roger Levy. 2022. Using computational models to test syntactic learnability. Linguistic Inquiry , pages 1–88.
- Wilkes (1994) Maurice V Wilkes. 1994. Using Large Corpora . MIT Press.
- Woods (1973) W A Woods. 1973. Progress in natural language understanding: an application to lunar geology. In Proceedings of the June 4-8, 1973, national computer conference and exposition , AFIPS ’73, pages 441–450, New York, NY, USA. Association for Computing Machinery.
- Wu and Yarowsky (2018) Winston Wu and David Yarowsky. 2018. Creating large-scale multilingual cognate tables . In Proceedings of the Eleventh International Conference on Language Resources and Evaluation (LREC 2018) , Miyazaki, Japan. European Language Resources Association (ELRA).
- Wu and Yarowsky (2020) Winston Wu and David Yarowsky. 2020. Computational etymology and word emergence . In Proceedings of the Twelfth Language Resources and Evaluation Conference , pages 3252–3259, Marseille, France. European Language Resources Association.
- Xu and Yvon (2021) Jitao Xu and Franccois Yvon. 2021. Can you traducir this? machine translation for code-switched input. In CALCS .
- Yang and Mitchell (2017) Bishan Yang and Tom Mitchell. 2017. Leveraging knowledge bases in LSTMs for improving machine reading . In Proceedings of the 55th Annual Meeting of the Association for Computational Linguistics (Volume 1: Long Papers) , pages 1436–1446, Vancouver, Canada. Association for Computational Linguistics.
- Yates et al. (2014) Andrew Yates, Jon Parker, Nazli Goharian, and Ophir Frieder. 2014. A framework for public health surveillance . In Proceedings of the Ninth International Conference on Language Resources and Evaluation (LREC’14) , Reykjavik, Iceland. European Language Resources Association (ELRA).
- Yin et al. (2022) Da Yin, Hritik Bansal, Masoud Monajatipoor, Liunian Harold Li, and Kai-Wei Chang. 2022. GeoMLAMA: Geo-diverse commonsense probing on multilingual pre-trained language models . In Proceedings of the 2022 Conference on Empirical Methods in Natural Language Processing , pages 2039–2055, Abu Dhabi, United Arab Emirates. Association for Computational Linguistics.
- Yu et al. (2022) Wenhao Yu, Chenguang Zhu, Zaitang Li, Zhiting Hu, Qingyun Wang, Heng Ji, and Meng Jiang. 2022. A survey of knowledge-enhanced text generation. ACM Comput. Surv. , 54(11s):1–38.
- Zellers et al. (2022) Rowan Zellers, Jiasen Lu, Ximing Lu, Youngjae Yu, Yanpeng Zhao, Mohammadreza Salehi, Aditya Kusupati, Jack Hessel, Ali Farhadi, and Yejin Choi. 2022. Merlot reserve: Neural script knowledge through vision and language and sound. In Proceedings of the IEEE/CVF Conference on Computer Vision and Pattern Recognition , pages 16375–16387.
- Zhang et al. (2020) Hongming Zhang, Xin Liu, Haojie Pan, Yangqiu Song, and Cane Wing-Ki Leung. 2020. ASER: A large-scale eventuality knowledge graph. In Proceedings of The Web Conference 2020 , WWW ’20, pages 201–211, New York, NY, USA. Association for Computing Machinery.
- Zhang et al. (2023) Renrui Zhang, Jiaming Han, Aojun Zhou, Xiangfei Hu, Shilin Yan, Pan Lu, Hongsheng Li, Peng Gao, and Yu Qiao. 2023. LLaMA-Adapter: Efficient fine-tuning of language models with zero-init attention .
- Zhang et al. (2022) Susan Zhang, Stephen Roller, Naman Goyal, Mikel Artetxe, Moya Chen, Shuohui Chen, Christopher Dewan, Mona T. Diab, Xian Li, Xi Victoria Lin, Todor Mihaylov, Myle Ott, Sam Shleifer, Kurt Shuster, Daniel Simig, Punit Singh Koura, Anjali Sridhar, Tianlu Wang, and Luke Zettlemoyer. 2022. OPT: open pre-trained transformer language models . CoRR , abs/2205.01068.
- Zhang et al. (2019) Yizhe Zhang, Siqi Sun, Michel Galley, Yen-Chun Chen, Chris Brockett, Xiang Gao, Jianfeng Gao, Jingjing Liu, and Bill Dolan. 2019. DialoGPT: Large-Scale generative pre-training for conversational response generation .
- Zheng et al. (2022) Francis Zheng, Edison Marrese-Taylor, and Yutaka Matsuo. 2022. A parallel corpus and dictionary for Amis-Mandarin translation . In Proceedings of the 2nd International Workshop on Natural Language Processing for Digital Humanities , pages 79–84, Taipei, Taiwan. Association for Computational Linguistics.
- Zhou et al. (2022) Binggui Zhou, Guanghua Yang, Zheng Shi, and Shaodan Ma. 2022. Natural language processing for smart healthcare . IEEE Reviews in Biomedical Engineering , pages 1–17.
- Zhou et al. (2019) Luowei Zhou, Hamid Palangi, Lei Zhang, Houdong Hu, Jason J Corso, and Jianfeng Gao. 2019. Unified Vision-Language Pre-Training for image captioning and VQA .
- Zhou et al. (2018) Luowei Zhou, Chenliang Xu, and Jason J Corso. 2018. Towards automatic learning of procedures from web instructional videos. In Proceedings of the Thirty-Second AAAI Conference on Artificial Intelligence and Thirtieth Innovative Applications of Artificial Intelligence Conference and Eighth AAAI Symposium on Educational Advances in Artificial Intelligence , number Article 930 in AAAI’18/IAAI’18/EAAI’18, pages 7590–7598. AAAI Press.
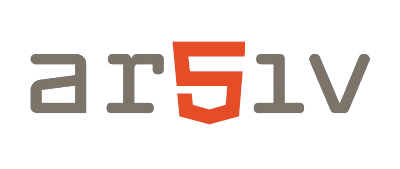
A PhD Student's Perspective on Research in NLP in the Era of Very Large Language Models
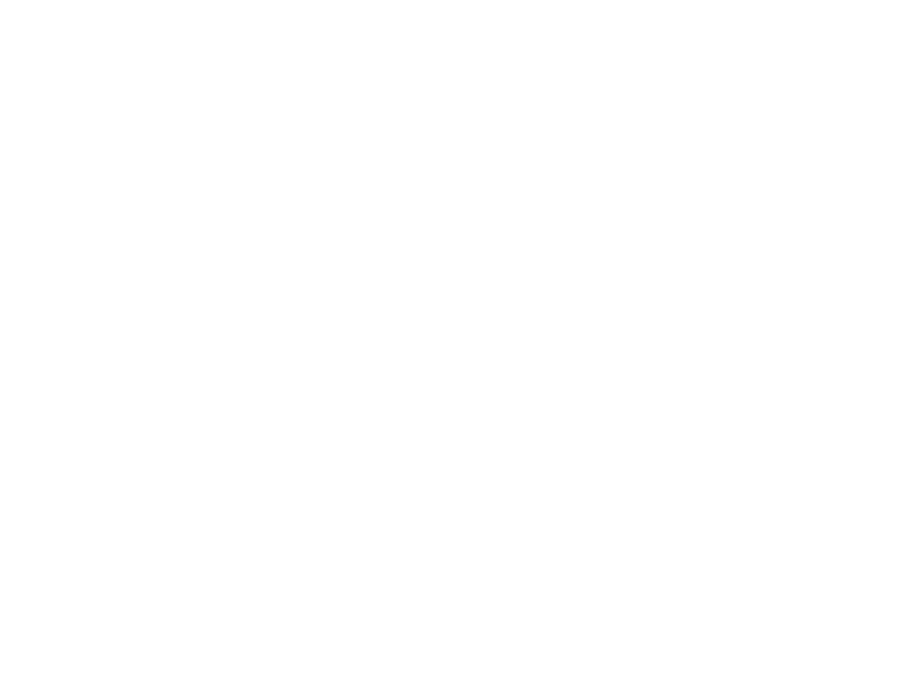
Recent progress in large language models has enabled the deployment of many generative NLP applications. At the same time, it has also led to a misleading public discourse that “it's all been solved.” Not surprisingly, this has in turn made many NLP researchers – especially those at the beginning of their career – wonder about what NLP research area they should focus on. This document is a compilation of NLP research directions that are rich for exploration, reflecting the views of a diverse group of PhD students in an academic research lab. While we identify many research areas, many others exist; we do not cover those areas that are currently addressed by LLMs but where LLMs lag behind in performance, or those focused on LLM development. We welcome suggestions for other research directions to include: https://bit.ly/nlp-era-llm

Zhijing Jin
Artem Abzaliev
Laura Biester
Santiago Castro
Naihao Deng
Aylin Gunal
Ashkan Kazemi
Muhammad Khalifa
Do June Min
Shinka Mori
Verónica Pérez-Rosas
Rada Mihalcea
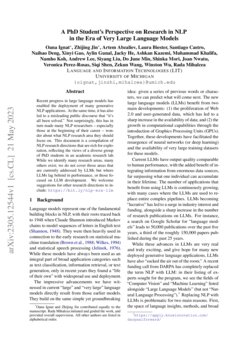
Related Research
Mind your language (model): fact-checking llms and their role in nlp research and practice, a bibliometric review of large language models research from 2017 to 2023, a family of pretrained transformer language models for russian, the utility of large language models and generative ai for education research, methods for estimating and improving robustness of language models, teaching nlp outside linguistics and computer science classrooms: some challenges and some opportunities, on the uses of large language models to interpret ambiguous cyberattack descriptions.
Please sign up or login with your details
Generation Overview
AI Generator calls
AI Video Generator calls
AI Chat messages
Genius Mode messages
Genius Mode images
AD-free experience
Private images
- Includes 500 AI Image generations, 1750 AI Chat Messages, 30 AI Video generations, 60 Genius Mode Messages and 60 Genius Mode Images per month. If you go over any of these limits, you will be charged an extra $5 for that group.
- For example: if you go over 500 AI images, but stay within the limits for AI Chat and Genius Mode, you'll be charged $5 per additional 500 AI Image generations.
- Includes 100 AI Image generations and 300 AI Chat Messages. If you go over any of these limits, you will have to pay as you go.
- For example: if you go over 100 AI images, but stay within the limits for AI Chat, you'll have to reload on credits to generate more images. Choose from $5 - $1000. You'll only pay for what you use.
Out of credits
Refill your membership to continue using DeepAI
Share your generations with friends
Subscribe to the PwC Newsletter
Join the community, edit social preview.
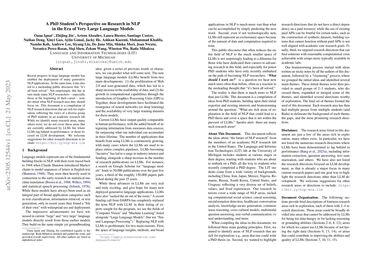
Add a new code entry for this paper
Remove a code repository from this paper, mark the official implementation from paper authors, add a new evaluation result row, remove a task, add a method, remove a method, edit datasets, has it all been solved open nlp research questions not solved by large language models.
21 May 2023 · Oana Ignat , Zhijing Jin , Artem Abzaliev , Laura Biester , Santiago Castro , Naihao Deng , Xinyi Gao , Aylin Gunal , Jacky He , Ashkan Kazemi , Muhammad Khalifa , Namho Koh , Andrew Lee , Siyang Liu , Do June Min , Shinka Mori , Joan Nwatu , Veronica Perez-Rosas , Siqi Shen , Zekun Wang , Winston Wu , Rada Mihalcea · Edit social preview
Recent progress in large language models (LLMs) has enabled the deployment of many generative NLP applications. At the same time, it has also led to a misleading public discourse that ``it's all been solved.'' Not surprisingly, this has, in turn, made many NLP researchers -- especially those at the beginning of their careers -- worry about what NLP research area they should focus on. Has it all been solved, or what remaining questions can we work on regardless of LLMs? To address this question, this paper compiles NLP research directions rich for exploration. We identify fourteen different research areas encompassing 45 research directions that require new research and are not directly solvable by LLMs. While we identify many research areas, many others exist; we do not cover areas currently addressed by LLMs, but where LLMs lag behind in performance or those focused on LLM development. We welcome suggestions for other research directions to include: https://bit.ly/nlp-era-llm
Code Edit Add Remove Mark official
Tasks edit add remove, datasets edit, results from the paper edit add remove, methods edit add remove.

A PhD Student's Perspective on Research in NLP in the Era of Very Large Language Models
Content maybe subject to copyright Report
Related Papers (5)
Trending questions (1).
The paper provides a compilation of NLP research directions that are rich for exploration, reflecting the views of a diverse group of PhD students in an academic research lab.
Ask Copilot
Related papers
Related topics
A PhD Student's Perspective on Research in NLP in the Era of Very Large Language Models
We're not able to analyze this paper right now due to high demand.
Please check back later (sorry!).
Generate a detailed summary of this paper with a premium account .
We ran into a problem analyzing this paper.
Please try again later (sorry!).
Get summaries of trending AI papers delivered straight to your inbox
Unsubscribe anytime.
https://twitter.com/rajrkane/status/1744454385504268512
https://twitter.com/MikeTamir/status/1775237015987949769
https://twitter.com/datadungeoneer/status/1773032347627040793
- New Trends in Machine Translation using Large Language Models: Case Examples with ChatGPT
- Large Language Models for Conducting Advanced Text Analytics Information Systems Research
- PersianLLaMA: Towards Building First Persian Large Language Model
You answered out of questions correctly.
= 4">Well done!
Appreciate you reporting the issue. We'll look into it.
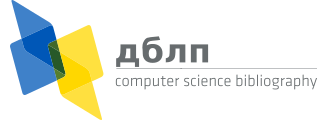
- solidarity - (ua) - (ru)
- news - (ua) - (ru)
- donate - donate - donate
for scientists:
- ERA4Ukraine
- Assistance in Germany
- Ukrainian Global University
- #ScienceForUkraine

default search action
- combined dblp search
- author search
- venue search
- publication search

"A PhD Student's Perspective on Research in NLP in the Era of Very Large ..."
Details and statistics.
DOI: 10.48550/ARXIV.2305.12544

type: Informal or Other Publication
metadata version: 2023-06-27

manage site settings
To protect your privacy, all features that rely on external API calls from your browser are turned off by default . You need to opt-in for them to become active. All settings here will be stored as cookies with your web browser. For more information see our F.A.Q.
Unpaywalled article links

load links from unpaywall.org
Privacy notice: By enabling the option above, your browser will contact the API of unpaywall.org to load hyperlinks to open access articles. Although we do not have any reason to believe that your call will be tracked, we do not have any control over how the remote server uses your data. So please proceed with care and consider checking the Unpaywall privacy policy .
Archived links via Wayback Machine

load content from archive.org
Privacy notice: By enabling the option above, your browser will contact the API of archive.org to check for archived content of web pages that are no longer available. Although we do not have any reason to believe that your call will be tracked, we do not have any control over how the remote server uses your data. So please proceed with care and consider checking the Internet Archive privacy policy .
Reference lists

load references from crossref.org and opencitations.net
Privacy notice: By enabling the option above, your browser will contact the APIs of crossref.org , opencitations.net , and semanticscholar.org to load article reference information. Although we do not have any reason to believe that your call will be tracked, we do not have any control over how the remote server uses your data. So please proceed with care and consider checking the Crossref privacy policy and the OpenCitations privacy policy , as well as the AI2 Privacy Policy covering Semantic Scholar.
Citation data
load citations from opencitations.net
Privacy notice: By enabling the option above, your browser will contact the API of opencitations.net and semanticscholar.org to load citation information. Although we do not have any reason to believe that your call will be tracked, we do not have any control over how the remote server uses your data. So please proceed with care and consider checking the OpenCitations privacy policy as well as the AI2 Privacy Policy covering Semantic Scholar.
OpenAlex data

load data from openalex.org
Privacy notice: By enabling the option above, your browser will contact the API of openalex.org to load additional information. Although we do not have any reason to believe that your call will be tracked, we do not have any control over how the remote server uses your data. So please proceed with care and consider checking the information given by OpenAlex .
last updated on 2023-06-27 15:48 CEST by the dblp team

see also: Terms of Use | Privacy Policy | Imprint
dblp was originally created in 1993 at:
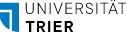
since 2018, dblp has been operated and maintained by:
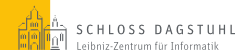
the dblp computer science bibliography is funded and supported by:
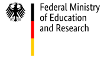
Nelson Liu's Blog
Student perspectives on applying to nlp phd programs.
This post was written by : Akari Asai , John Hewitt , Sidd Karamcheti , Kalpesh Krishna , Nelson Liu , Roma Patel , and Nicholas Tomlin .
Thanks to our amazing survey respondents : Akari Asai , Aishwarya Kamath , Sidd Karamcheti , Kalpesh Krishna , Lucy Li , Kevin Lin , Nelson Liu , Sabrina Mielke , Roma Patel , Nicholas Tomlin , Eric Wallace , and Michihiro Yasunaga .
This post offers and summarizes student advice and perspectives on the NLP PhD application process, with a focus on programs in the US. We asked twelve recently-successful NLP PhD applicants a range of questions about the application process—this post compiles the broader themes and advice that run through the majority of responses. Make sure to check out the complete set of responses ! A tarball is also available for those who cannot access Google Drive .
⚠️ Disclaimer ⚠️: While we’ve all gone through the application process and have thoughts to share, we aren’t experts or authorities on this (highly random) process. Our advice comes from our unique perspectives and backgrounds, and not everything will generalize. That said, we hope that the differences and similarities in our shared experiences will be useful to consider.
Professors have also written advice to applicants from their side of the process, see Kalpesh Krishna’s compilation of graduate school application advice .
Table of Contents
Pre-application, statement of purpose, letters of recommendation, publications, transcripts / grades, standardized exams: gre / toefl, interviews / post-application calls, deciding where to go, misc. topics, in conclusion.
Deciding to apply at all is not an easy choice, and several respondents took additional time, either in school or in industry, to explore new fields and become more certain that pursuing a PhD was the right decision for them. Choosing where to apply is also an involved process, and involves trade-offs between factors like research area fit, location, and (perceived) selectivity. This section explores this preliminary part of the application process, along with useful insights from applicants on different aspects of this decision.
A lot of the perspectives in this post are aimed towards people already seriously considering a PhD—for instance, seniors or MS students. If you are a student considering a PhD, but still have a significant amount of time before you apply, John Hewitt’s blog post contains useful insights and advice on how to make the most of your time in school. In addition, Kalpesh Krishna’s extensive compilation of application advice might yield things to keep in mind through the years.
Why apply now?
For many of the respondents, starting a PhD was the natural “next step”—they were in the final year of their undergraduate or masters degrees, and had spent enough time doing research to realize that a PhD was worth the opportunity cost to them.
While I did not have any *ACL papers while applying...My goal was to get into a good PhD program and start doing research full-time (which is why I was applying to a PhD program in the first place) rather than get into the very best PhD program. – Kalpesh Krishna
Waiting to apply also has clear benefits—many respondents felt that they would be stronger applicants after an additional year of research experience (and the associated publications and stronger letters of recommendation that might come with it).
“The year away from academia gave me the clarity on how much I really wanted to do a PhD and how much I love academic life. In this year I used my free time to explore interesting research directions and collaborated with friends. It made me realise that I enjoy research and to be able to do it for a living would be just perfect.” – Aishwarya Kamath
“I was also unsure at that time what kinds of directions I wanted to go in or if I even wanted to commit so many years of my life to additional school...By the time fall 2018 came around, I’d done a full year of thinking and growing my research skills, so I felt a lot better about diving into the process.” – Lucy Li
Several people found value in waiting because it gave them the time to reflect on their next steps. For instance, Lucy and Aishwarya used the time to further develop their research interests and think about what areas were exciting to them. In particular, Aishwarya spent a year in industry, which made her realize what she was missing an academic setting and drove her to apply and return.
On the other hand, several also offered caution about waiting with the sole intention of improving your profile. As PhD applications get more and more competitive each year, more papers or experience doesn’t necessarily mean a stronger application since things are inherently relative. Several agreed that having publications at top conferences is not a necessary component of a strong application, especially if one has relatively limited research experience (e.g., applicants from undergrad) or has strong recommendation letters. A recent blog post about the machine learning PhD application process investigates admission statistics at one of the top schools (Fall 2018), and shows admission is not determined solely on publication records, but depends on the other factors, especially applicants’ background and letters of recommendations.
For instance, Kalpesh and Akari considered waiting a year since they did not have any top-tier NLP publications at the time, noting that:
- Things get more and more competitive each year, so more papers doesn’t necessarily mean a stronger application since things are inherently relative.
- Applicants with master's degrees are expected to have more publications and experience than undergraduate applicants.
- There is a large amount of uncertainty involved in research / writing papers, so things are not always going to pan out for reasons out of your control.
- They thought that they were still reasonably strong applicants for many of the places they were applying to.
Kevin and Akari also mention that, if you have the resources, you can apply multiple times.
If what you really want to do is to immediately get into a grad school and continue doing work that you are excited about, you should apply. – Roma Patel
Choosing where to apply
When choosing where to apply, the majority of respondents focused on a few factors:
- Overwhelmingly, the strongest factor for everyone was faculty : finding schools with professors that you’d want to work with, and with a strong presence in allied fields. Several mentioned applying to places only if there were 2 or more relevant faculty.
- Location was also a key factor for many: finding schools in places that you think you’d be happy living in for 5+ years.
- Lastly, many also considered proximity to industry connections / possible external collaborators .
Some also took the relative prestige of a school into account, with the thinking that prestigious schools attract strong peers, which means that you can learn more and work with amazing people.
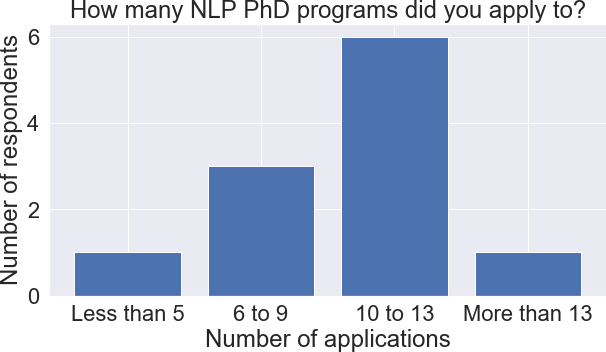
There’s also a case to be made for applying to a mixture of (1) programs that you’re relatively confident you can be admitted to and (2) “top choice” programs that might have a bit more randomness in the admissions process (of course, all the schools you apply to should be places you’d be happy going to). However, it’s easy to be a bit too conservative when choosing where to apply—remember that you only really need 1 offer. The majority of respondents applied to between 8 and 13 schools, though almost everyone was happy with the number of applications they submitted (Kevin, who applied to 4, thought it would have been helpful to apply to more).
NLP applicants in particular are lucky—there are amazing faculty scattered around the world in a variety of different environments. Start with a large list before filtering down, and focus on finding the right fit for you personally.
Talking to Faculty Beforehand?
I did not email faculty beforehand - I don’t think this helps (and in the case of a poorly crafted email, could actually hurt!). – Sidd Karamcheti
The majority of students did not email faculty before applying. Some faculty ask students to reach out—this will usually be explicitly mentioned on their webpage. In the absence of such a notice, a reasonable policy is to not send an email.
But that said, if you are in the vicinity of a school or doing an academic visit -- feel free to reach out to the faculty there and ask if they have a half-hour slot to meet! – Roma Patel
I emailed one prospective advisor and asked to meet at a conference. In general, I think this is a good strategy, especially if you have research-related things to talk about with them. (Which hopefully you will, if they’re a good advisor fit!) – Nicholas Tomlin
Several respondents were fortunate to meet potential future advisors at workshops or conferences / if they happened to be in the area, and found them to be quite receptive to short research meetings. It’s good to go into these meetings with a sense of (1) what you’d like to get out of it, and how to use this meeting effectively, (2) an awareness of their recent work, (3) a mental list of questions that you think have informative or interesting answers.
...one of my undergrad advisors emailed a couple prospective grad advisors on my behalf, and asked them to look out for my application. I think this was particularly helpful and is maybe something worth mentioning to your undergraduate advisor. – Nicholas Tomlin
It is appropriate to selectively ignore advice about cold-emailing— Prof. Yonatan Bisk has a great guide that walks through the why, when, and how .
Back to the top.
The statement of purpose is an opportunity for you to convey what you’ve worked on and what you’re interested in. Above all, make sure the statement is genuine and uniquely you. The “accept/reject” dichotomy of applications might make this process seem like a game—leading many to believe that it’s better to win the game (that is, be accepted) than to lose. While it’s tempting to shape each application to say what you think faculty might want to hear, being yourself will lead to the best outcome in the end. Remember that programs and students are both looking for the right fit—the statement is a fantastic opportunity for both sides to assess this.
If your statement is genuine and makes clear why you want a PhD, it will resonate with the people you want it to resonate with. – Sabrina Mielke
Timeline: When to Start and Finish Writing
With respect to starting writing, it is sometimes good to leave it late enough to wrap up any ongoing research projects at the end of the summer so you can write concrete things about them. For finishing writing, it’s good to have a near-ready draft at least a month before. – Roma Patel
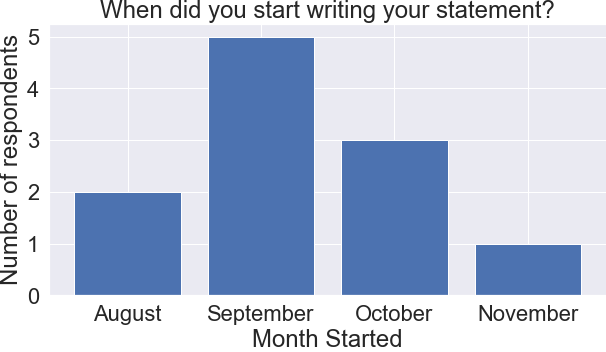
Try to set aside a fixed period of time to work on your statement. While starting earlier rather than later is usually better, try to start writing a draft once you think your current projects and interests are concrete enough to write something substantive. Strive to have a preliminary draft that you’re happy with at least a month before the deadline. You can then send this to your advisors for feedback; continue editing and iterating until the deadline and/or you’re happy with how things look.
Structuring a Statement of Purpose
The goal of the statement is to talk about your past (research) experience, and how that has prepared you for a career in research (why you’re qualified for grad school). – Sidd Karamcheti
Your statement of purpose should uniquely describe your research experience and elaborate on the process you went through as you undertook your first few research projects. Give enough detail about your past work to allow them to assess the value of the work and also to concretely show that you knew what you were doing at every step of the process. Then fold this into your research as a whole. Try to leverage insights from both the actual work as well the experience of doing research, to formulate how you would undertake future projects during your graduate school career.
Many professors do tell you what they’re looking for in a SoP (JHU CLSP for example has hints at https://www.clsp.jhu.edu/apply-for-phd/phd-admissions-faq/ ), so do use that resource. – Sabrina Mielke
Tailoring Each Statement for Specific Universities
I only tweaked the final paragraph. In this paragraph, I specifically mentioned 2--4 faculty that I wanted to work with and provided a one sentence rationale. – Eric Wallace
Our survey respondents were quite divided on this question. A few respondents significantly tweaked their statements for each university to reflect the subset of their interests relevant to the prospective advisor’s research. However most respondents kept 80-90% of their statement identical and only modified the last 1-2 paragraphs with university specific information - such as the names of the professor they were interested in working with. Most agreed that it is good to have at least some university-specific information to form a connection between your own research goals and a prospective advisor’s research directions.
It is good to have concrete reasons laid out in your statement as to why you want to go to this school and work with these faculty on interesting problems. So definitely tweak the section of your statement that stresses on this. – Roma Patel
Getting Feedback on Your Statement
Your recommenders will get a better sense of your research interests so it can help them write your recommendation and they have also been through similar processes. – Kevin Lin
It is good to have a near-complete draft of your statement ready in time to send to your recommenders before they begin to write your letter of recommendation. There are multiple benefits to this. Reading your statement will help them better understand your research interests, which will not only allow them to concretely write things about you in their letter, but might also bring up useful pieces of advice from them based on what they know of the people working in that research area. They will also usually give you feedback on the overall statement—they have possibly read countless statements over the course of their career and will be able to fairly judge and evaluate this in context. Your research advisors and recommenders are likely both extremely knowledgeable and also have your best interests at heart, so remember to ask for feedback and advice on your application!
Using this as a Learning Opportunity
In my statement, I mostly talked about my past experiences and how they feed into my current research interests. I tried to paint a picture that enables the reader to better understand how I reached / why I do the research I do. – Nelson Liu
Write out your journey as a researcher from the beginning to the present. This will convey important information about you and your research, which can be illuminating for both your reader and for yourself. Chances are that you will write dozens of similar statements in the future, whether they are research statements for fellowships, project proposals, or grant applications. Use this as a learning experience! Writing your statement of purpose is not only good practice for the future, but also a rare invitation to reflect upon your interests and motivations.
Letters of recommendation are often cited as the most important part of a PhD application. In our survey, every respondent marked letters as either the most or second-most important component. Given that the admissions committee is optimizing to admit candidates with a high likelihood of reliably producing excellent research, a letter from a fellow academic that effectively claims you’ve been able to do so is a strong signal that you’re a good candidate.
What to look for when choosing letter writers
Your letter writers should be people who know you well enough to speak about your skills and your strengths as a PhD candidate ... people you have worked with who are doing relevant research in the field and people you have genuinely been advisors to you… – Roma Patel
It can be helpful to view letter writers as your primary advocates in the admissions process. They want their excellent undergraduate students or research assistants to succeed, and they’re singing your praises in order to argue for your spot in graduate school. From this view, it may be clear that they should know you, your strengths, and your goals. Of course, some of your letter writers will know you better than others, but each should be able to at least advocate for your excellence in how you worked or interacted with them.
There’s often a tradeoff between (1) how well you know the letter writer, (2) how cool the work you did with them was, and (3) how well-known they are. As a first approximation, attempt to have all 3 letter writers know you through some kind of research collaboration. Simply doing well in their class, or TAing for them does not necessarily make for a strong letter. On the other hand, an industry researcher who can vouch for your research ability may be able to make a stronger statement. This brings us to (3) how well known the letter-writer is. Perhaps unfortunately, letters from well-known members of the field are (very) highly regarded. This may be due to fame bias—the professors on the application committee can rest assured that they know so-and-so from X university consistently recommends only excellent students. As suggested at the beginning of this paragraph, this will play some role in the tradeoff, but keep in mind that a famous professor who doesn’t really know you won’t write a strong letter.
Each of the components mentioned above—personal knowledge of you and your work, successful research and fame of the writer were mentioned by our respondents.
I chose professors with whom I had completed somewhat successful research, and who were likely to be known by my prospective advisors. For better or worse (probably worse), connections between letter writers and prospective advisors seem to matter a lot. – Nicholas Tomlin
When to start looking for recommenders
People get started in research at different times, but by the time of application, you need three people who can advocate for your spot in graduate school (though again, not all need to be equally strong or know you equally well). When should you start building these relationships? The easy answer is “as early as possible”. Research takes a long time, as does getting settled in a field and starting to make real progress. This creates a definite bias towards those who start research earlier and collaborate widely (3 professors means a lot of connections to make). However, everyone’s research story looks different, and no student should think it “too late” to go for a PhD (though a master’s and/or further years of research experience may be necessary.)
To back this up, note the wide range of times that our respondents started working with the people who would end up being their LoR writers.
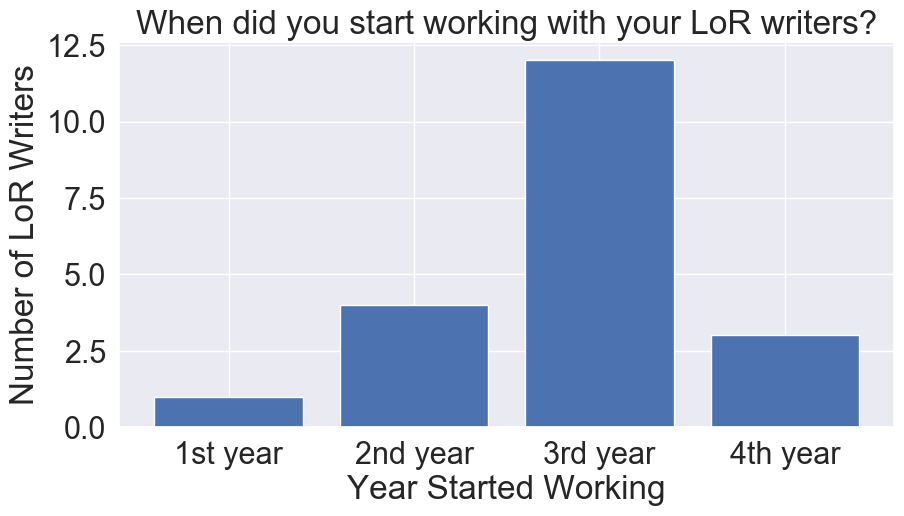
Note that this histogram includes one data point for each letter writer for each respondent. (Not everyone mentioned all three writers, and one mentioned four.) I counted “summer before 3rd year” as “2nd year.” That’s a lot of letter writers from the third and fourth (!) years. Many respondents who met their letter writers after their third year did indicate that it would have been better to start earlier, but the data somewhat makes sense—as you progress through your studies, you gain more research experience.
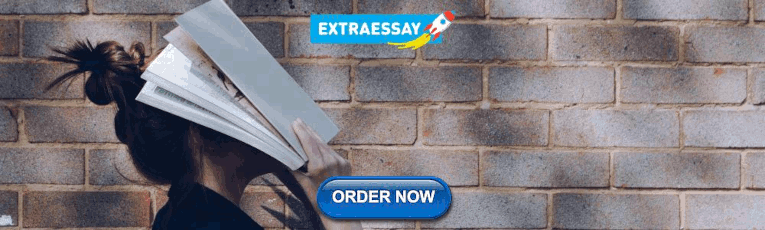
Asking for specifics in your letter, and getting them submitted
Recall that your letter writers are your advocates—you should feel empowered to bring up all the awesome things that you did with them, and ask (but not demand) that they mention specific things. These requests may be to tailor their letters to your statement of purpose. Think that your efforts in conducting replicable science in a world of AI hype are awesome? Your letter writer may agree, but likely wouldn’t think to mention it if you don’t remind them.
I made sure to send a reminder email 2 weeks, then 1 week, then a few days before applications were due. – Nelson Liu
Likewise, remember that they’re human and busy, and very well may forget your letter if you don’t send them a few reminders. PhD applications tend to have lenient letter of recommendation deadlines but it’s better to keep on top of them with tastefully-spaced reminder emails—better to not test the waters in this context.
I think that having a published conference paper greatly increases your chances, but I think that papers are merely a signal for something more important: can you complete the full research process, from idea inception to experiment execution to writing things up? – Nelson Liu
Most respondents felt that publications are an important part of a strong application, but are not necessary if you have stellar recommendation letters talking about your research aptitude. Admission into PhD programs in computer science (especially at top schools) is quite competitive, and many candidates have publications, especially candidates applying after year-long research positions such as AI residency programs.
Publications are just tangible evidence - if you can show other evidence that you are able to do research, that you learned something, that you have skills/conclusions that you’ve taken away from the experience, then you should be fine. – Sidd Karamcheti
Publications are a good way to show concrete research output. This acts like “hard evidence” of research aptitude, which is the primary criterion used to judge PhD applicants. Alternative ways to show concrete research output could be excellent research code releases or insightful blog posts.
Almost all survey respondents thought that grades and GPA scores play only a minor role in NLP PhD admissions. It is wise to not stress too much about improving your GPA, especially if compromises the time spent doing research. Things might be different in more theoretical fields though, where coursework might be closer to research.
Take an intro to NLP course! Take machine learning or a specific linguistics course or anything else that clearly shows that you have studied the topics you are excited about in depth. – Roma Patel
Interesting classes off the beaten path may let you stand out from the crowd. – Sabrina Mielke
The choice of coursework typically acts like a skillset evaluation during PhD admissions, checking whether candidates are familiar with the fundamental techniques required to conduct their research. Coursework can also help present a coherent academic history when combined with the statement of purpose. Some courses might help an applicant stand out from the crowd, especially if they’re uniquely relevant or off the beaten path.
Sometimes, the exact preparation matters less than evidence that you’re capable of learning important background material. E.g., despite me not having strong probability/stats background, a few professors said they were impressed by my (completely irrelevant) pure math background. – Nicholas Tomlin
While coursework does not play a major role in admission decisions, many respondents mentioned that courses are a great way to learn the fundamentals and get interested in a particular field, often acting like a precursor to research.
I get the sense that the GRE doesn’t really matter unless you do abysmally. – Nelson Liu
Nearly everyone agreed that scores from required standardized tests are not deal-breaking as long as you meet a minimum threshold. Having a suspiciously low score could raise questions, but barring failing the exam, this should not significantly impact your entire application. That said, this is a required checkpoint on your application, so keep aside time to get this done correctly.
There is no glory or shame in taking too much or too little time, so it is better to not compare to others and keep aside the right (and possibly minimal) amount of time you think you need to prepare. – Roma Patel
Try to give yourself at least 1-2 weeks of study time before the actual test. Don’t consider the amount of time you see others spending on this — assess yourself and allocate larger amounts of time to topics that you are uncertain about and think could use the extra effort. Remember to review all the topics you need to, take a few practice tests, and then just take the exam and don’t stress about the score.
It is usually not worth the extra time, effort, cost (or effect) to redo the exam. So prepare well once, take the exam, and don’t stress about the score once you are done with it. For what it’s worth, future years will likely see this disregard and ambivalence towards scores on tests heightened — lots of schools have already removed the GRE requirement, while others have definite plans of doing so in the coming years.
In general, international students must submit their TOEFL (or IELTS) scores to demonstrate competency in the English language — however for some schools, international students who have received degrees in US schools or received their instruction in English do not need to submit TOEFL scores. Unlike in GRE, applicants MUST score higher than the minimum requirements if universities sets minimum scores. The minimum requirements vary from program to program. For example, the Cornell CS PhD program sets the minimum scores for each section (Listening 15, Writing 20, Reading 20, Speaking 22), while the MIT EECS PhD set the total minimum scores to 100. Make sure that you meet TOEFL scores before the application deadline. Unfortunately, the applicants whose TOEFL scores lower than the minimum are likely to be “desk-rejected”.
Interviews in USA are less formal - more general discussions about research interests. Interviews for Europe in my experience were more in depth, as they expect you to already have knowledge of your field (since you can only apply after a Masters), have a research plan and expect you to have already surveyed literature in your chosen field of interest. – Aishwarya Kamath
The interviews and visit days will differ significantly over the range of schools you’re considering—both in their intended purpose and in the amount of information you can glean about the school and faculty from this one interaction. Some schools do pre-acceptance visit days, with offers conditioned on the interviews and ensuing discussions. Others do virtual interviews over the phone or video calls. And of course, some schools choose not to conduct interviews.
While each interview experience is largely dependent on the candidate in question, most of our survey respondents agreed that these conversations follow the same general pattern.
The general format was like: “Tell me about a research project you worked on (pick one that is most exciting and introduce)”. The professor would ask some questions, like “why did you consider this model / run this experiment?”, “what is the conclusion?”, “what did you learn through this project?” “What is your research interest?”, “What are you interested in doing for your PhD (and your career)?” -- it’s good to think in both short term and long term “Do you have any questions?” -- you can ask any questions about the lab, like the culture, research goals, how advising/meeting works. – Michi Yasunaga
This is mostly a means of trying to get a sense of what you are like as a person and what your research interests are, to assess both compatibility and mutual interests. Your interviewers will generally ask you to talk about the research you have done — and will interrupt with questions about things that they are interested to hear more about. Overall, this is less of an assessment of your knowledge, rather than them getting insights into how you solve problems and talk about research.
I didn’t enjoy the whiteboard interview. – Nicholas Tomlin
This sometimes happens. If professors want to assess a specific component of your application, or want to know the extent of your knowledge about a certain topic, they will ask you technical questions that can range from explaining or solving an algorithm, writing out equations or explaining computational and implementation-specific aspects of things you have done. Most of our survey applicants however, did not have to go through this and their interviews largely consisted of general research conversations.
You should definitely know your own work inside-out, but don’t stress about having to know every intricate detail about every subfield in NLP. – Roma Patel
While it is not important (or even possible) to know everything little thing about every research area in NLP, you should be aware of work being done in areas related to you. Most importantly, if you have written about something in your statement, you should be able to confidently speak about it and answer any questions that they throw at you. Take time to look into every detail and ensure that you know the fundamentals of your work before your interview.
Remember that this is a two way street—while they’re assessing whether you’d be a good fit for their program, you should be probing whether this place / professor is a good match for you. – Nelson Liu
There is usually a part of the interview where the interviewer steps back and asks you to ask questions — use this time to probe at any uncertainties or lingering questions that you have. If you have questions about their previous work, thoughts about future possibilities, or even just general questions about the program or the department, use this time to clear any doubts and get all the answers you will need to make a decision.
if you don’t know something, it is okay to say that you don’t --- ask questions that help you understand it more and treat it as a learning experience. – Roma Patel
The only thing I will tell you not to do in an interview: pretend. Professors are good at spotting that kind of thing and they will strongly judge you for it. Just be honest and genuine. You are starting your PhD. You don’t need to know things -- just be willing to grow. – Sabrina Mielke
Also, don’t worry if you do not know everything the interviewers ask. Just try to be as honest and genuine as you can, and show that you are willing to learn and grow, instead of pretending to know the topics.
I think the interviews as an initial conversation really affected where I seriously considered—the places with interviews that I thought were more fair / reasonable gained legitimacy. In the best case, it was basically a research conversation with a senior researcher, and a great opportunity to get feedback / hear what they think about the field. Overall, I thought they were quite valuable, and I wish that I had treated them less as assessments and more as opportunities. – Nelson Liu
Make the most of your interviews! All applicants agreed that overall, the interviews were friendly and engaging experiences. Think of this as an opportunity to speak about and answer questions about your work and to have a mutually engaging research conversation.
One useful piece of advice from one of my undergrad advisors was to, “Talk about your research ideas! Remember that what most faculty really want is to be able to discuss the research that is important to them — and if you can do this and make exciting progress through these discussions, you will both mutually have a productive and happy career together.” – Roma Patel
If you’re fortunate to be considering multiple options, congratulations! It is a hard problem, but a good one to have—be aware of your privilege. The choice between graduate programs is an intensely personal one, and there are a variety of academic and non-academic factors to consider, all of which will influence your health, happiness, and productivity.
Something that people do not always remember when making a decision is that your advisor is possibly someone you will be talking to for upto 3 hours every week for nearly 6 years of your life. It is good to rethink whether or not you will be happy doing this with the faculty in question, if the two of you see eye-to-eye, can comfortably talk about both research-things and also life-things when they come up, and that they will encourage and help guide you in everything you need to do the research that is important to you during your PhD. – Roma Patel
In general, most respondents agreed that the most important factor is your primary advisor—who will you be working with during your PhD? Do you have mutual research interests? Are your communication and working styles compatible? Would you be comfortable talking to them about your struggles, both academic and non-academic? Do you have much to learn from them and their group? Do you feel supported by them? While it is hard to assess these deep questions before spending time to work with them, conversations and interactions during visit days will help you get a sense of whether things feel right. Trust your instinct—if things feel odd or unnatural, even during these initial conversations, you have plenty of reason to reconsider and be hesitant.
As an undergrad at a school with a large NLP community, I really benefited from having senior researchers around (e.g., grad students and postdocs)---I have so much to learn from them! I felt like I wanted to keep having such an environment in graduate school, which actually ended up being one of the defining factors in my final choice. – Nelson Liu
Many students also took note of the NLP community at every school they were considering. For instance, some prefer larger groups with many senior students and postdocs, while others prefer smaller, more-intimate groups. There are benefits and drawbacks to both sorts of research environments, and it ultimately boils down to personal preference and taste. It’s important that you feel like you have enough people around to talk about research and life—while your advisor is an important figure in the PhD, you will spend the majority of your time talking to and working alongside fellow students. Make sure that these are people that you’d love to be around for the next stage of your research career.
Sure, you’re picking a place to do research for the next 5+ years of your life, but you also need to be happy / have a life outside of research...I went climbing during a lot of my visits, mostly to assess convenience. – Nelson Liu
Another important factor to consider is the location. Several expressed weather / culture preference (mostly on the east-coast-vs-west-coast divide). Many also wanted to be in a place that was affordable for students and conveniently located to their favorite hobbies or recreational activities. While research fit is certainly important, you won’t be productive if you’re miserable—put your happiness and your health first, and make sure that you’ll be happy as both a student on-campus and as a resident of the area.
Prestigious schools attract strong peers, which means you can learn more and collaborate with amazing people. – Eric Wallace
Several also considered the relative “ranking” of a university or program (though this is almost impossible to objectively evaluate without implicitly considering the other factors). While rankings can tell part of the story, they’re not substitute for your own feelings and intuitions about where you belong.
At some schools, it was very clear who my advisors would be, while at others, it wouldn’t be decided until I’d enrolled. I preferred the former scenario since it involved less uncertainty. – Lucy Li
It’s also useful to consider the program’s requirements and logistics around advising. Are you guaranteed to be able to work with the advisor(s) you are interested in? Does the department have extensive qualification exams or requirements that might be hindrances to your productivity? Will you have to worry about funding?
Personal feelings actually do matter. If you feel (even slightly) uncomfortable, these negative feelings will grow during the five years. – Akari Asai
Once you have done an extensive comparison on all parameters (professional and personal), you might be stuck between 2-3 very good options. Try reweighting the parameters and see if the balance shifts towards one end. If you are still confused, don’t worry :) If it’s so confusing, both places are surely very good. You will need to work very hard wherever you go, and you won’t lose much choosing one over the other. Go with your heart. – Kalpesh Krishna
When it comes to the final decision, everyone agrees to go with your heart and feelings of what seems right to you. We’re all logical and analytical people (perhaps to a fault), but if you can’t make up your mind about where to go / are stuck between several options, pick the one that you feel the best about inside. One way to discern this: Suppose you’re picking between two places (this strategy generalizes to N). Take a coin, and assign one place to heads and another to tails. Tell yourself that the result of the coin flip will be where you end up going. Flip the coin, and observe the result. Are you relieved? Would you have preferred the other side? The answer to these questions might help you better understand how you really feel about the decision.
Whatever you do end up deciding, though, don’t regret it—the decision is done now, and you just have to put in the work to ensure that it is a good one. – Nelson Liu
Making the most of visit days
I didn’t end up going to most visit days -- which is not something that you should do. Go to every visit day! Talk to the other students visiting, the other students currently pursuing PhDs there and to the faculty there. Keep a list of standard questions about schools (requirements, professors, exams, time taken) and make a note of these for every school so that you have an easy way to compare at decision-making time. – Roma Patel
Many of our survey respondents recommend making the most of the visit days. Treasure this priceless opportunity to talk to professors (both in and outside of your field), meet PhD students, and get to know the other students in your cohort. As you continue your academic career, you’ll be seeing all of these people around in the future—get to know them now!
Talk to students most of all -- disturb them when they’re working to see what it’s like in the lab! – Sabrina Mielke
Before each visit, it’s useful to think a bit about what you’d like to get out of it. This might result in a list of questions you’d like to answer, or people that you’d like to talk to. Don’t be afraid to contact PhD students in the department and ask to meet; the majority are happy to do so, and would love to give you advice, hear about what you’re working on, and talk about their research. Talking to students is of the utmost importance; they will tell you what it’s really like in the department, and it’s useful for getting a sense of the overall department culture and graduate student community.
My advisor, in her infinite wisdom, gave me a useful piece of insight that had not struck me before. "What most people don't realise, is that the people that you are meeting and talking to over these visits will likely be in your life, for the rest of your life. Go to as many visits and talk to as many prospective students as you can — some of your closest friends and advisors will come out of these interactions." – Roma Patel
Residency Programs as Precursors to your PhD
I see a couple benefits of working in AI residency which I did at AI2. 1) if you aren’t sure if you want to do a PhD, this is a pretty good way to find out, and after the residency you will be in a reasonable position to pursue both industrial and PhD positions. 2) You will be exposed to a new set of people, and it is helpful to learn from different ways of doing research 3) I personally changed my research direction towards more NLP and this was a great way to explore different research topics and build up the skills I needed to pursue those topics. – Kevin Lin
Be really really clear why you’re doing the residency - the reason to do the residency/work is to do something you could not otherwise do at grad school/if you’re not sure about grad school. – Sidd Karamcheti
It’s really important to consider why you want to do a residency program. As our survey respondents mentioned, there are a few different paths that lead to residencies—foremost among them is if you’re not too sure about wanting to do a PhD, and you want some more research experience (working with a couple of different mentors with possibly different areas/interests than what you were exposed to as an undergraduate) before making a final decision.
Another reason a residency program is a good idea is if you’re sure about doing a PhD, but had limited exposure to different areas as an undergraduate. Especially if you’re considering PhD programs where you’re paired with an advisor/placed in a specific area outright, having a year to explore a bunch of different areas and work with different mentors with different styles will let you make a more informed decision. It’s totally possible that the residency program will introduce you to areas you would never have otherwise considered!
That being said, it’s worth noting that not all residency opportunities are created equal—several different companies are just in their first or second year of offering their residency programs, meaning that they’re subject to growing pains—without structured onboarding/tutorials you might spend a lot of time trying to figure out how to use company infrastructure, or you might spend a lot of time trying to figure out what different folks at the company are working on, and how research works in industry.
More importantly, you need to make sure your residency mentors are committed to the same goals that you are—a mismatch in expectations between you and your residency mentors is going to significantly sour your experience! If you want to explore a bunch of different sub-areas of your chosen research area, make sure your mentor is on board to try a few different projects over the course of the year! If you want to instead work on more long-term projects/existing initiatives at the company, make sure that your host is willing to connect you with these existing teams, and that there’s some structure in place that will let you (1) learn, and (2) contribute.
Finally, don’t feel like you need to do a residency to get the industry experience, or to explore different research areas. There is definitely a large amount of time you can spend exploring different areas in grad school, and you’ll have multiple summers to do internships where you’ll possibly get to work on projects very different from your core research agenda.
FWIW, you will likely intern at a lot of the places during the course of your PhD and will have a similar experience, so if the only reason you are considering a residency is because you think that is an experience you will never get at a later time --- this is likely not true. – Roma Patel
When submitting my application, I was pretty sure that I would defer for a year if I got an offer---there’s no rush, and the extra year might give me some interesting perspective. – Nelson Liu
If you’ve read this far, we hope that this discussion was useful. The admissions process is inherently stochastic, and there’s much that you can’t control—relax, have confidence in yourself, and goodluck!
Another good advice I received from my friend was “Don’t reject (by?) yourself”. I remember how uneasy and stressful I felt at the time of application, as I did not have strong publication records, and came from non top undergraduate schools in the US. Sometimes people value your unique back-ground, experience in other fields or find really positive signals in the letters of recommendation. Don’t hesitate to apply for good schools, because “I think I’m not good enough”. – Akari Asai
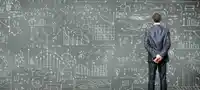
Towards NLP🇺🇦
All ngrams about Natural Language Processing that are interesting for @dementyeva_ds
Academia.edu no longer supports Internet Explorer.
To browse Academia.edu and the wider internet faster and more securely, please take a few seconds to upgrade your browser .
Enter the email address you signed up with and we'll email you a reset link.
- We're Hiring!
- Help Center
A PhD Student's Perspective on Research in NLP in the Era of Very Large Language Models

2023, arXiv (Cornell University)
RELATED TOPICS
- We're Hiring!
- Help Center
- Find new research papers in:
- Health Sciences
- Earth Sciences
- Cognitive Science
- Mathematics
- Computer Science
- Academia ©2024
Search code, repositories, users, issues, pull requests...
Provide feedback.
We read every piece of feedback, and take your input very seriously.
Saved searches
Use saved searches to filter your results more quickly.
To see all available qualifiers, see our documentation .
- Notifications
A repo for open resources & information for people to succeed in PhD in CS & career in AI / NLP
zhijing-jin/nlp-phd-global-equality
Folders and files, repository files navigation, resources to help global equality for phds in nlp / ai.
This repo originates with a wish to promote Global Equality for people who want to do a PhD in NLP, following the idea that mentorship programs are an effective way to fight against segregation, according to The Human Networks (Jackson, 2019). Specifically, we wish people from all over the world and with all types of backgrounds can share the same source of information, so that success will be a reward to those who are determined and hardworking, regardless of external contrainsts.
One non-negligible reason for success is access to information , such as (1) knowing what a PhD in NLP is like, (2) knowing what top grad schools look for when reviewing PhD applications, (3) broadening your horizon of what is good work , (4) knowing how careers in NLP in both academia and industry are, and many others.
Contributor: Zhijing Jin (PhD student in NLP at Max Planck Institute & ETH, co-organizer of the ACL Year-Round Mentorship Program ). You are welcome to be a collaborator, -- you can make an issue/pull request, and I can add you :).
Twitter: If you prefer to get more updates on Twitter, feel free to follow @ ZhijingJin and @ aclmentorship .
Contributor: Zhijing Jin (PhD student in NLP at Max Planck Institute & ETH, co-organizer of the ACL Year-Round Mentorship Program ).
Endorsers of this repo: Prof Rada Mihalcea (University of Michigan). Please add your name here (by a pull request) if you endorse this repo :).
Contents (Actively Updating)
Top resources, should i do a phd, how do applications work, which schools should i apply for, how to prepare for the sop, rec letter, etc., prereq: getting pre-phd research opportunities, where do i get gpu computing resources, improve your proficiency with tools, starting to do research, alternative path: pursuing a software engineer career path, overall guides, timely topic: surviving nlp in the era of llms, what are weekly meetings with mentors/advisors like, how to read papers, how to express our ideas: writing papers, visualization, etc, reviewing, publishing, attending conferences, networking, memoir-like narratives, excel your research, coming up with good research ideas, grad school fellowships, stage 3. (after phd -> industry) how is life as an industry researcher, list of job opportunities, learning about different schools, overall experience sharing, step 1. preparing the application materials, step 2. preparing for the job talk and interview, step 3. making the decision & negotiating offers, starting as a professor, nsf career award, senior proposals, long-term research career, massive collaboration can help science, further readings: technical materials to improve your nlp research skills, contributions, how to cite this repo.
- Online ACL Year-Round Mentorship Program: https://acl-mentorship.github.io (You can apply as a mentee , as a mentor , or as a volunteer . For mentees, you will be able to attend monthly zoom Q&A sessions hosted senior researchers in NLP. You will also join a global slack channel, where you can constantly post your questions, and we will collect answers from senior NLP researchers.)
- (Organized by PhD students in NLP across the world) NLP with Friends Online Seminar Series (recordings available). [ Seminar ] (A great way to learn about what others are doing in NLP)
Stage 1. (Non-PhD -> PhD) How to Apply for a PhD?
Phd application tips.
- (John Hewitt, PhD@Stanford) Undergrad to PhD, or not - advice for undergrads interested in research (2018). [ Suggestions ]
- (Prof Jason Eisner@JHU) Advice for Research Students (last updated: 2021). [ List of suggestions ]
- (Nelson Liu, PhD@Stanfard) Student Perspectives on Applying to NLP PhD Programs (2019). [ Suggestions Based on Surveys ]
- (Prof Dragomir Radev@Yale) Advice for PhD Applications, Faculty Applications, etc (2023). [ List of Suggestions ]
- [(Roma Patel PhD@Brown, Prof Nathan Schneider@Georgetown University) PhD Application Series of the NLP Highlights Podcast) (2021). [ Podcast ] (A new series they launched that addresses all aspects of PhD application. Besides, it is just a great podcast in general that talks about recent NLP advances)
- (Albert Webson et al., PhDs@Brown University) Resources for Underrepresented Groups, including Brown's Own Applicant Mentorship Program (2020, but we will keep updating it throughout the 2021 application season.) [ List of Resources ]
- A Princeton CS Major's Guide to Applying to Graduate School . [ List of suggestions ]
- (Tim Dettmers, PhD@UW) Machine Learning PhD Applications — Everything You Need to Know (2018). [ Guide ]
- (Kalpesh Krishna, PhD@UMass Amherst) Grad School Resources (2018). [ Article ] (This list lots of useful pointers!)
- (Prof Mor Harchol-Balter @CMU) Applying to Ph.D. Programs in Computer Science (2014). [ Guide ]
- (CS Rankings) Advice on Applying to Grad School in Computer Science . [ Pointers ]
- (Prof Scott E. Fahlman@CMU) Quora answers on the LTI program at CMU (2017). [ Article ]
- (Prof Philip Guo@UCSD) Finding CS Ph.D. programs to apply to . [ Video ]
- [(Tim Dettmers, PhD@UW) How to Pick Your Grad School (2020). [ Guide ]
- (Zhijing Jin, PhD@MPI & ETH) Tips on PhD Applications with Max Planck Institute and/or ETH in AI (2021). [ Suggestions ]
- (Prof Nathan Schneider@Georgetown University) Inside Ph.D. admissions: What readers look for in a Statement of Purpose . [ Article ]
- (Nelson Liu, PhD@Stanfard) PhD Statement of Purpose . [ Article ]
- (Suchin Gururangan, PhD@University of Washington) Personal Statement Advice . [ Article ]
- (Prof Shomir Wilson@Penn State University) Reference Letter Procedure . [ Suggestions ]
- (Zhaofeng Wu, Alexis Ross, and Shannon Shen@Cambridge) A Collection of Strong CS SOPs from Successful Applicants . [ Samples ]
- (Andrew Kuznetsov, PhD@CMU) CS/HCI PhD Opportunity Tracker from Twitter (Developed in 2021). http://www.andrewkuz.net/hci-opportunities-2022.html
- (Eugene Vinitsky, PhD@UC Berkeley) A Guide to Cold Emailing (2020). [ Article ]
- (Prof Shomir Wilson@Penn State University) Guide for Interacting With Faculty (2018). [ Suggestions ]
Existing Summer Research Opportunities:
- Summer research opportunities for Undergrads (2021). [ Twitter Thread ]
- (ETH) ETH Summer Research Fellowship (every summer). [ Apply ]
- (MPI) Summer Research Internship with MPI (CaCTüS) (every summer). [ Apply ]
Prereq: Getting the Tools Ready
- Many people use Colab (and its Pro version costs 9.99$ per month)
- For more computationally intensive projects, you can apply to AWS with credits for research .
- For general computational needs, you can use a Digitalocean droplet (5-10$ per month), and run your ubuntu machine with any service for some time.
- (MIT 2020) The Missing Semester of Your CS Education (e.g., master the command-line, ssh into remote machines, use fancy features of version control systems).
Check all the specific suggestions under " Stage 2. (Doing PhD) How to Succeed in PhD? ", such as the sections "What Is Weekly Meeting with Advisors like?", "How to Read Papers", and many others that you might need :).
- (Steve Yegge@Google) Get that job at Google (2008). [ Article ]
- (Carlos Bueno) Get that job at Facebook (2021). [ Article ]
- Coding practice from CareerCup . [ Link ]
Stage 2. (Doing PhD) How to Succeed in PhD?
- (Prof Isabelle Augenstein@UCopenhagen) Increasing Well-Being in Academia (2020). [ Suggestions ]
- (Sebastian Ruder@DeepMind) 10 Tips for Research and a PhD (2020) . [ Suggestions ]
- (Maxwell Forbes, PhD@UW) Every PhD Is Different . [ Suggestions ]
- (Prof Mark Dredze@JHU, Prof Hanna M. Wallach@UMass Amherst) How to be a successful PhD student (in computer science (in NLP/ML)) . [ Suggestions ]
- (Andrej Karpathy) A Survival Guide to a PhD (2016). [ Suggestions ]
- (Prof Kevin Gimpel@TTIC) Kevin Gimpel's Advice to PhD Students . [ Suggestions ]
- (Prof Marie desJardins@Simmons University) How to Succeed in Graduate School: A Guide for Students and Advisors (1994). [ Article ] [ Part II ]
- (Prof Eric Gilbert@UMich) Syllabus for Eric’s PhD students (incl. Prof's expectation for PhD students). [ syllabus ]
- (Marek Rei, Lecturer@Imperial College London) Advice for students doing research projects in ML/NLP (2022). [ Suggestions ]
- (Prof H.T. Kung@Harvard) Useful Thoughts about Research (1987). [ Suggestions ]
- (Prof Phil Agre@UCLA) Networking on the Network: A Guide to Professional Skills for PhD Students (last updated: 2015). [ Suggestions ]
- (Prof Stephen C. Stearns@Yale) Some Modest Advice for Graduate Students . [ Article ]
- (Prof Tao Xie@UIUC) Graduate Student Survival/Success Guide . [ Slides ]
- (Mu Li@Amazon) 博士这五年 (A Chinese article about five years in PhD at CMU). [ Article ]
- (Karl Stratos) A Note to a Prospective Student . [ Suggestions ]
- (UMich; led by Prof Rada Mihalcea) A PhD Student's Perspective on Research in NLP in the Era of Very Large Language Models (2023). [ Paper ]
- (Prof Julian Togelius@NYU, Prof Georgios Yannakakis@UMalta) Choose Your Weapon: Survival Strategies for Depressed AI Academics Julian Togelius, Georgios N. Yannakakis (2023). [ Tweet ] [ Paper ]
- (Prof Jason Eisner@JHU) What do PhD students talk about in their once-a-week meetings with their advisers during their first year? (2015). [ Article ]
- (Brown University) Guide to Meetings with Your Advisor . [ Suggestions ]
- (Prof Jia-Bin Huang@UMaryland) How to have effective regular meetings with your advisors/mentors? (2023). [ Suggestions ]
Literature review tools:
- Connected Papers (which shows a graph of related papers) [ link ]
- (Built by Papers with Code) Glactica (using language models to generate literature review) [ link ]
Paper reading suggestions:
- (Prof Srinivasan Keshav@Cambridge) How to Read a Paper (2007). [ Suggestions ]
- (Prof Jason Eisner@JHU) How to Read a Technical Paper (2009). [ Suggestions ]
- (Prof Emily M. Bender@UW) Critical Reading (2003). [ Suggestions ]
- (Prof Jason Eisner@JHU) How to write a paper? (2010). [ Suggestions ]
- (Simon Peyton Jones@Microsoft) How to write a great research paper: Seven simple suggestions (2014). [ Slides ] [ Talk ]
- (Prof Jennifer Widom@Stanford) Tips for Writing Technical Papers (2006). [ Suggestions ] Specifically check out the suggestion on how to write the introduction section, where we address five main questions : what is the problem, why is it interesting, why is it challenging, what have previous people done, and what we propose.
- (Prof Shomir Wilson@Penn State University) Guide for Scholarly Writing . [ Suggestions ]
- (Prof Jia-Bin Huang@U Maryland) How to write the introduction (and also the What-Why-How figures) . [[Tweet]
- (Prof Tim Rocktäschel@UCL, DeepMind&Prof Jakob Foerster@Oxford) How to ML Paper (2022). [ Tweet ]
- (Prof Jia-Bin Huang@U Maryland) How to write a rebuttal for a conference? [ Tweet ]
- (Prof Tim Rocktäschel@UCL, DeepMind&Prof Jakob Foerster@Oxford) How to ML Rebuttal – A Brief Guide (2022). [ Tweet ]
- (Prof Maarten Sap@CMU) Writing rebuttals (2022). [ Suggestions ]
- (Prof Devi Parikh, Dhruv Batra, Stefan Lee) How we write rebuttals (2020). [ Suggestions ]
- (Prof Noah Smith@UW) * How to write an author response to ACL/EMNLP reviews . [ Suggestions ]
- (Prof Michael Black@Max Planck Institute) Twitter Thread about "Writing is laying out your logical thoughts" . [ Tweet ]
- (Prof Shomir Wilson@Penn State University) Guide for Citations and References [ Suggestions ]
- (Carmine Gallo, a bestselling author) The Storytellers Secret (2016). [ Video ] Takeaways: Writing the Introduction section and giving talks can also be like telling a Hollywood story: the setting (what problem we are solving; how important it is), the villian (how difficult this problem is; how previous work cannot solve it well), and the superhero (what we propose). For giving talks, starting with personal stories (e.g., a story of grandma telling the kid not to drink and persist the right thing leading to the person's life pursuit on social justice) is very helpful to get the audience involved.
- (Maxwell Forbes@UW) Figure Creation Tutorial: Making a Figure 1 (2021). [ Suggestions ]
- UI design as a medium of thought: see Michael Nielsen's explanation of why UI is important for science , Bret Victor's work , Miegakure that visualizes a 4D environment.
- (Prof Jia-Bin Huang@U Maryland) How to write math in a paper? (2023). [ Tweet ]
- (Prof Jordan Boyd-Graber@U Maryland) Style (e.g., tense, punctuation, math) . [ Suggestions ]
(Prof Yang Liu, Trevor Cohn, Bonnie Webber, and Yulan He) Advice on Reviewing for EMNLP (2020). [ Suggestions ]
(Dr. Anna Rogers, Prof. Isabelle Augenstein@University of Copenhagen) What Can We Do to Improve Peer Review in NLP? (2020). [ Paper ]
(Prof Shomir Wilson@Penn State University) Guide for Publishing in Conferences and Attending Them [ Suggestions ]
(Prof Emily M. Bender@UW) On Using Twitter (2020). [ Suggestions ]
(Timothy Ferriss, author of 4-Hour Workweek) 5 Tips for E-mailing Busy People (2008). [ Suggestions ]
(Prof Philip Guo@UCSD) The Ph.D. Grind: A Ph.D. Student Memoir (last updated: 2015). [ Video ] (For the book, you have to dig deeply, and then you will find the book.)
(Maithra Raghu, PhD from Cornell, Senior Research Scientist@Google Brain) Reflections on my (Machine Learning) PhD Journey (2020). [ Article ]
(Prof Tianqi Chen@CMU) 陈天奇:机器学习科研的十年 (2019) (A Chinese article about ten years of research in ML). [ Article ]
(Jean Yang) What My PhD Was Like . [ Article ]
The most important step: (Prof Jason Eisner@JHU) How to Find Research Problems (1997). [ Suggestions ]
(Christopher Olah, OpenAI) Research Taste Exercises (2021). [ Article ]
(Prof Richard Hamming, Turing award winner) You and Your Research (How a research journey is like & how do end up with great research) (1995). [ Talk ] [ Transcript ] [ Transcript2 ] Interesting snippets: "Knowledge and productivity are like compound interest. Given two people of approximately the same ability and one person who works ten percent more than the other, the latter will more than twice outproduce the former. The more you know, the more you learn; the more you learn, the more you can do; the more you can do, the more the opportunity.", "One of the chief tricks is to live a long time!", "I made the resolution that I would never again solve an isolated problem except as characteristic of a class.".
(Prof Stuart Card@Stanford) The PhD Thesis Deconstructed (2016). [ Article ] Interesting snippets: "People basically read your paper to write theirs. Your ideas are more likely to spread if you help out.", "We once had a Nobel Laureate come talk to us about his ideas for how to do research. His surprising number 1 recommendation: Don’t work in an area that doesn’t have good funding.", "Table 1. Seismic scale of impact"
(Sam Altman, CEO of OpenAI) How To Be Successful (2019). [ Article ] Interesting snippets: "I think the biggest competitive advantage in business—either for a company or for an individual’s career—is long-term thinking with a broad view of how different systems in the world are going to come together ... In a world where almost no one takes a truly long-term view, the market richly rewards those who do.", "One of the most powerful lessons to learn is that you can figure out what to do in situations that seem to have no solution.", "you also have to be able to convince other people of what you believe.", "My other big sales tip is to show up in person whenever it’s important. When I was first starting out, I was always willing to get on a plane.", "An effective way to build a network is to help people as much as you can.", "One of the best ways to build a network is to develop a reputation for really taking care of the people who work with you.", "The best way to make things that increase rapidly in value is by making things people want at scale."
(Tim Dettmers, PhD@UW) On Creativity in Academia (2019). [ Article ]
(Jason Wei, OpenAI) A few thoughts on doing AI research (2023). [ Slides ]
(Prof Jia-Bin Huang@UMaryland) How to come up with research ideas? (2021). [ Suggestions ]
(John Schulman, co-founder of OpenAI) An Opinionated Guide to ML Research (e.g., horning your taste) (2020). [ Suggestions ] Interesting snippets: "Goal-driven. Develop a vision of some new AI capabilities you’d like to achieve, and solve problems that bring you closer to that goal.", "If you are working on incremental ideas, be aware that their usefulness depends on their complexity.", "Consider how the biggests bursts of impactful work tend to be tightly clustered in a small number of research groups and institutions. That’s not because these people are dramatically smarter than everyone else, it’s because they have a higher density of expertise and perspective, which puts them a little ahead of the rest of the community, and thus they dominate in generating new results.", "Early on in your career, I recommend splitting your time about evenly between textbooks and papers. You should choose a small set of relevant textbooks and theses to gradually work through, and you should also reimplement the models and algorithms from your favorite papers."
(Prof Fei-Fei Li@Stanford) De-Mystifying Good Research and Good Papers (2014). [ Suggestions ] Interesting snippets: "This means publishing papers is NOT about “this has not been published or written before, let me do it”, nor is it about “let me find an arcane little problem that can get me an easy poster”. It’s about “if I do this, I could offer a better solution to this important problem,” or “if I do this, I could add a genuinely new and important piece of knowledge to the field.” You should always conduct research with the goal that it could be directly used by many people (or industry). In other words, your research topic should have many ‘customers’, and your solution would be the one they want to use. A good research project is not about the past (i.e. obtaining a higher performance than the previous N papers). It’s about the future (i.e. inspiring N future papers to follow and cite you, N->\inf) ."
- (Elman Mansimov, Research Scientist@Amazon) Tips on summer industry research internships in ML (2021). [ Suggestions ]
List of fellowship opportunities to track:
(Compiled by CMU) Graduate Fellowship Opportunities [ link ]
( Robbie Allen ) CS/HCI PhD Fellowships [ link ]
List of scholarships for different disciplines. [ link ]
CYD Fellowship for Grad Students in Switzerland [ link ]
How to Write a Good Fellowship Application:
(Daricia Wilkinson, PhD@Clemson) Towards Securing the Bag: Tips for Successful PhD Fellowship Applications (2019). [ Article ]
(Meta) Five tips for a successful Meta Research PhD Fellowship application from the people who review them (2020). [ link ]
(Meta) The six most common Fellowship questions, answered by Facebook Fellow Moses Namara (2020). [ link ]
(Meta) Fellowship 101: Facebook Fellow Daricia Wilkinson outlines the basics for PhDs (2020). [ link ]
(Meta) Applying twice: How Facebook Fellow David Pujol adjusted his application for success (2020). [ link ]
(Meta) Fellow spotlights, career advice, and more (up to date). [ link ]
The craft of Research by Wayne Booth, Greg Colomb and Joseph Williams.
How to write a better thesis by Paul Gruba and David Evans
Helping Doctoral Students to write by Barbara Kamler and Pat Thomson
The unwritten rules of PhD research by Marian Petre and Gordon Rugg
- (Wes Weimer@UMich, Claire Le Goues@CMU, Zak Fry@GrammaTech, Kevin Leach@Vanderbilt U, Yu Huang@Vanderbilt U, and Kevin Angstadt@St. Lawrence University) Finding Jobs (2021). [ Guide ]
- (Mu Li@Amazon) 工作五年反思 (A Chinese article about reflections on the five years working in industry) (2021). [ Article ]
Stage 4. (Being a Prof) How to get an academic position? And how to be a good prof?
What jobs are there.
- CRA : https://cra.org/ads/ (a good listing for academic jobs related to CS)
- Academic Jobs Online (AJO): https://academicjobsonline.org/ajo
- https://facultyvacancies.com/ : An academic job portal for professors, lecturers, researchers, post docs, PhDs and academic managers in Europe, Americas, Oceania, Asia and the Middle East, e.g., for AP positions in CS here
- University Jobs listing (This list also include admin jobs.)
- Job posting twitter account: https://twitter.com/csfacultyjobs
- Different types of schools: https://carnegieclassifications.iu.edu/classification_descriptions/basic.php
- The Chronicle of Higher Education has a listing that tends to be for more teaching-oriented positions
- Academic Jobs in CS in Germany and Switzerland [ Suggestions ]
- CS Rankings: http://csrankings.org/
How to succeed in the job market?
- (Prof Philip Guo@UCSD) Philip’s notes on the tenure-track assistant professor job search (2013). [ Suggestions ]
- (Wes Weimer@UMich, Claire Le Goues@CMU, Zak Fry@GrammaTech, Kevin Leach@Vanderbilt U, Yu Huang@Vanderbilt U, and Kevin Angstadt@St. Lawrence University) CS Grad Job and Interview Guide (2021). [ Guide ]
- (Prof Shomir Wilson@Penn State University) Guide for the Tenure-Track Job Market in Computer/Information Sciences (2018). [ Suggestions ]
- (Maarten Sap@CMU) Timeline of application [ Guide ]
- (Prof Elizabeth Bondi-Kelly@UMich) A blog about my experience on the CS faculty job market (2023). [ Guide ]
- (Prof Nicolas Papernot@University of Toronto, Prof Elissa M. Redmiles@Max Planck Institute) The academic job search for computer scientists in 10 questions . [ Suggestions ]
- (Prof Caroline Trippel@Stanford) The Academic Job Search: A Memoir (2020). [ Suggestions ]
- (Westley Weimer, Claire Le Goues, and Zak Fry) Computer Science Grad Student Job Application & Interview Guide
- (Jeffrey P. Bigham) Faculty Job Interviewing Tips
- (Prof Michael Ernst@UW) Getting an academic job (2000). [ Suggestions ]
- (Prof Matt Might@University of Alabama at Birmingham) Academic job search advice . [ Suggestions ]
- (Prof Manuel Rigger@NUS) How Did Professors Find Their Jobs? Part 1: Diversity in Experiences (2021). [ Suggestions ]
- (Rose Hoberman@MPI) The academic job search process . [ Suggestions ]
- (Prof Manuel Rigger@NUS) Getting Academic Positions (GAP) Interviewing Series (2021). [ YouTube Interview Videos ]
- (Prof Matt Welsh@ (Previously) Harvard) How to get a faculty job, Part 1: The application (2012). [ Guide ]
- (Prof Matt Welsh@ (Previously) Harvard) Understanding what makes your application profile stand out enough to receive an interview (2012). [ Guide ]
- (MIT communications lab) Preparing for faculty application . [ Guide ]
- (Prof Yisong Yue@Caltech) Tips for Computer Science Faculty Applications (2020). [ Guide ]
- (Prof Chris Blattman@UChicago) Managing the academic job market (for political science and economics) (2022). [ Guide ] [ Timeline ]
Research Statement
- (Prof Jason Eisner@JHU) How to write an academic research statement (when applying for a faculty job) (2017). [ Article ]
- (Prof Anjalie Field@JHU) Research Statements (2022). [ List ]
- (Prof Maarten Sap@CMU) All statements and job talk (2021). [ List ]
- (Prof Matt Welsh@ (Previously) Harvard) The interview (2012). [ Guide ]
- (Prof Jason Eisner@JHU) How to Give a Talk (2015). [ Suggestions ]
- (Prof Manuel Rigger@NUS) Interview Questions for Computer Science Faculty Jobs (2021). [ Question List ]
- (Prof Austin Z. Henley@University of Tennessee) Faculty interview questions I asked and got asked (2018). [ Question List ]
- (Prof Yalong Yang@Virgina Tech) Yalong Yang's Job Talk (CS Faculty Candidate Talk) (2021). [ Video ]
- (Prof Matt Welsh@ (Previously) Harvard) Negotiating the offer (2013). [ Guide ]
- (Haseeb Qureshi) Ten Rules for Negotiating a Job Offer (2016). [ Guide ]
- (Patrick McKenzie) Salary Negotiation: Make More Money, Be More Valued (2012). [ Guide ]
- (Josh Doody) Salary Negotiation with Josh Doody (2016). [ Podcast ]
- What’s it Like Being the Only HCI Person in a CS Department? by Jeffrey P. Bigham
- 2018 Taulbee Survey of faculty salary https://cra.org/wp-content/uploads/2019/05/2018_Taulbee_Survey.pdf
- Academic offer check list
- [Academic Job Search: Negotiating Your Faculty Startup Package]( https://career.ucsf.edu/sites/g/files/tkssra2771/f/UCSF OCPD Negotiating a Faculty Position-2019 Feb.pdf) by Bill Lindstaedt
- Negotiating for a faculty position during COVID-19 from UCSF
- (Prof Elissa M. Redmiles@MPI) Salary survey and Advice on CS Faculty Job Negotiation . https://www.dropbox.com/s/iqwcxbes6p94uw6/faculty-cssecurity-advice.pdf?dl=0
Stage 5. (Whole Career Path) How to live out a life career as an NLP researcher?
- (Prof Jason Eisner@JHU) Teaching Philosophy . [ Article ]
- (Prof Isabelle Augenstein, Emily M. Bender, Dan Jurafsky, and Yoav Goldberg) Panel Discussions on How to Teach NLP Courses (2021). [ Video ]
- (Prof Emily M. Bender@UW) Balancing Teaching and Research (2015). [ Suggestions ]
- (Prof Radhika Nagpal@Harvard) The Awesomest 7-Year Postdoc or: How I Learned to Stop Worrying and Love the Tenure-Track Faculty Life (2013). [ Article ]
- (Prof Shomir Wilson@Penn State University, motivation and usage of writing guides down) Guide to the Advice [ Article ]
- (Prof Randy Pausch@CMU) Time management (2007). [ Video ] [ Slides ]
Funding in the US
- (Prof Noah Smith@UW) CAREER: Flexible Learning for Natural Language Processing (2011). [ Abstract ] [ Sample ]
- (Austin Z. Henley) What a $500,000 grant proposal looks like (2022). [ Guide and Sample ]
- (NSF) Samples from NSF CISE CAREER Workshop (2022). [ Samples ]
- (Prof Jeffrey P. Bigham@CMU) NSF CAREER Award (2019). [ Blog ] [Unsuccessful Sample 2011 ] [Successful Sample 2012 ]
- Theoretical CS -- Several Samples (2016). [ Samples ]
- NSF Mathematics Proposals (2010). [ Samples ]
- (Cora Lind) Materials Chemistry and Thermal Expansion . [ Sample ]
- (UMass) Examples of NSF Broader Impacts Statements . [ Samples ]
- Example broader impact sections [ UMass Samples ]
- (NSF) Abstract of all NSF awards. [ List ]
Writing suggestions
- (NSF) Note for Reviewers of CAREER Proposals (2022). [ Guide ]
- (Noel Brady) Writing an NSF Proposal; a PI’s and a panelist’s perspective (2010). [ Guide ]
- (Joseph Brennan) NSF Proposal Preparation: The View of an Ex-Program Officer (2007). [ Guide ]
- (Harvard) **NSF CAREER award guidance for faculty **(2023). [ Guide ]
- (MIT) MIT Guidance Regarding the NSF CAREER Program . [ Guide ]
- (Harvard) Entire list of all possible broader impacts . [ List ]
- (Michael Ernst@UW) Writing an NSF Career Award proposal (2000). [ Suggestions ]
- Rubrics of grant proposal evaluation, and example Broader impact section https://www.umass.edu/research/sites/default/files/bi_sample_from_successful_career_sociology2012.docx.pdf
- Panel: Faculty Workshop “How to write a Successful NSF CAREER proposal” Notes https://researchservices.cornell.edu/sites/default/files/2019-06/How%20to%20write%20a%20successful%20CAREER%20proposal-%20FINAL.pdf
- Skeleton structure of the proposal: https://cccblog.org/2020/08/25/medium-article-deconstructing-the-nsf-career-proposal/
- (NIH) Successful samples of grant proposals funded by NIH. [ Samples ]
- (University of Montana) Proposals Funded by NIH, NSF, and Department of Education . [ Samples ]
- (University of Toledo) NIH and NSF funding proposals on biology and chemistry . [ Samples ]
- (University of Rhode Island) Samples of Successful Proposals from various government departments . [ Samples ]
- (Prof John Bunce@Max Planck Institute) Senior NSF proposals to the Cultural Anthropology Program (2012). [ Samples ]
- (Harvard) National Science Foundation (NSF) Resources . [ Resources ]
- (Harvard) Example list of funding sources . [ List ]
- (Prof Devi Parikh and Prof Dhruv Batra@GaTech) Humans of AI: Stories, Not Stats [ YouTube ]
- (Prof Randy Pausch@CMU) Last Lecture: Achieving Your Childhood Dreams (2007). [ Video ] [ Book ]
- (Prof Charles Ling@Western University, Prof Qiang Yang@HKUST) Crafting Your Research Future: A Guide to Successful Master's and Ph.D. Degrees in Science & Engineering . [ Book ]
- (Prof Michael Nielsen, now an individual researcher) Principles of Effective Research (2004). [ Article ] Interesting snippets: "“We are what we repeatedly do. Excellence, then, is not an act but a habit.” Underlying all our habits are models (often unconscious) of how the world works. I’m writing this essay to develop an improved personal model of how to be an effective researcher, a model that can be used as the basis for concrete actions leading to the development of new habits.", "Make sure you’re fit. Look after your health. Spend high quality time with your family. Have fun. These things require a lot of thought and effort to get right.", "Develop a high-quality research environment", "Developing a taste for what’s important: What do you think are the characteristics of important science? What makes one area thrive, while another dies away? What sorts of unifying ideas are the most useful? What have been the most important developments in your field? Why are they important? What were the apparently promising ideas that didn’t pan out? Why didn’t they pan out? You need to be thinking constantly about these issues, both in concrete terms, and also in the abstract, developing both a general feeling for what is important (and what is not), and also some specific beliefs about what is important and what is not in your fields of interest.", "occasionally set time aside to survey the landscape of a field, looking not just for problems, but trying to identify larger patterns. What types of questions do people in the field tend to ask? Can we abstract away patterns in those questions? What other fields might there be links to? What are the few most important problems in the field?"
- (Prof Timothy Gowers and Michael Nielsen) Massively collaborative mathematics (2009). [ Article ]
- (Prof Jason Eisner@JHU) Technical Tutorials, Notes, and Suggested Reading (last updated: 2018) [ Reading list ]
- (All kinds of career advice for Cryptography researchers) Mentoring Workshop and Videos (2021) [ Videos ]
All types of contributions to this resource list is welcome. Feel free to open a Pull Request.
Contact: Zhijing Jin , PhD in NLP at Max Planck Institute for Intelligent Systems, working on NLP & Causality.
Contributors 4
Joining the Group
The Stanford NLP Group is always on the lookout for budding new computational linguists. Stanford has a great program at the cutting edge of modern computational linguistics.
The best way to get a sense of what goes on in the NLP Group is to look at our research blog , publications , and students' and faculty's homepages . Our research centers around using probabilistic and other machine learning methods over rich linguistic representations in a variety of languages. The group is small, but productive and scientifically focused.
Prospective Graduate Students
Where do you apply for graduate (PhD or MS) study? Not directly to the NLP Group. Stanford graduate admissions are handled through individual departments, so you'll want to apply for admission through either the Linguistics Department or the Computer Science Department . Both departments have excellent graduate programs. Normally, you should apply to the one in which you have more background and greater interest in further study. Do make sure that you emphasize any research experience and results, and that you get letter writers who can speak convincingly about you. Decisions about admissions are made by the department's admissions committee. Because admissions committees represent the whole department and aim to select the best applicants regardless of specialization, you should direct your application towards an appropriately broad audience. And, as you probably know, Stanford admissions are quite competitive.
If you have questions about admissions, please check the graduate admissions web pages listed on the right, or write to the admissions email addresses listed. We NLP Group members attempt to answer specific NLP-related admissions questions (although sometimes we get too busy...), but in general it isn't necessary or helpful to contact us to let us know that you want to apply or have applied for admission.
Current Stanford Students
Are you a student at Stanford and interested in working on a project in NLP? Check out this page for details on how to apply to do research in the group.
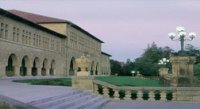
Graduate Admissions Resources
Linguistics department, computer science department.
An international student's perspective on applying for CS/AI/NLP PhD in the US
September 10, 2023
2023 · reflection
This blog post is penned on my second weekend arriving in the US. After an intense week of settling into a new country and meeting many “familiar names” in person, some of my prior convictions have been reaffirmed while some fresh insights pop up. Also, with another application season approaching, friends from my home country are seeking my counsel. So, I believe now is an opportune moment to reflect upon my own experience and share some lessons learned in the process, particularly from an international student’s perspective.
(Disclaimer: I think a huge difference exists between the experiences of domestic and international students when navigating PhD admissions in the US. I hope this post can give more information to the latter, but apparently, my viewpoints are prone to be biased and may best resonate with students who attend college in China.)
Deciding Which Program to Apply To
Phd or ms or straight into work.
During my application process, a popular joke among my friends was “Turns out, deciding to apply for a PhD is the hardest part”. Though ironic, that’s true… I’m still uncertain if I made a right choice, but I can share a bit about how I arrived at my decision.
Experience it. Opting for a PhD means you’ll do research for the next 5+ years, possibly even for a lifetime. This is what sets it apart from the other two options. (Note: unlike in China and some other countries where master programs might be more research-oriented, master programs in the US are primarily tailored to equip you for the workforce - you may focus on research, but that’s not the main stream and you’d better not say so in your state of purpose (SoP) for a master program :) Therefore, it’s highly recommended to get a feel for research during your undergraduate years. Whether it’s assisting in a campus lab, undertaking a research internship in the industry, or collaborating with international faculty – all these experiences are valuable. However, for some people, it may still be hard to tell whether doing research is the best for them. In such cases, gaining work experience may help you compare. Personally, I began assisting in a school lab during my sophomore year and later took up an engineering internship the following summer. Although I fully enjoyed that internship, it made me realize that I need more practice on high-level project design and directly going to work may restrict myself into very niche tasks. This realization steered me towards prioritizing research during the remainder of my college years.
An interesting observation: Comparing CVs of students who attend college in the US and China, it’s evident that American students tend to have a more varied college experience. Some of my Chinese peers expressed concerns that non-research-oriented activities might be a waste of time if they eventually decide on a PhD. But as the earlier joke suggests, experiences are about “experiencing”, which might make that most difficult decision-making part easier. If you’re certain about research from the outset, that’s commendable and just be committed to it; otherwise, investing some time to try different things is worthwhile.
Feel free to have backup plans. Once you’ve decided to pursue a PhD, congratulations! You’ve chosen a challenging path. PhD application is competitive, especially for CS/AI. But a kind reminder here is to always allow yourself the flexibility of backup plans. During my application process, I was afraid that having backup plans might make others perceive me as less committed to the PhD pursuit. But this isn’t true. It’s completely acceptable to simultaneously apply for master’s programs (as I did) or explore job opportunities (as several of my peers did). If you think having backup plans can help release your tension, just do so.
Talk to senior people. The biggest lesson I learned from my application journey is to never shy away from seeking advice, particularly from senior people. I’m now starting my PhD journey at Stanford - a program that initially wasn’t on my target program list (will discuss about it later). Stanford CS offers both master’s and PhD program but applicants can only apply for a single program at Stanford each year. Given that Stanford was my dream school (my interest in AI/NLP was largely spurred by the exceptional online courses Stanford generously made available to the public), I initially considered applying for a master’s, thinking it might increase my chances. My rationale was, “Perhaps I could apply for the PhD program once I’m already pursuing my master’s there.” When requesting recommendation letters, I talked with my referees about my program list and one of them ardently advised that I aim directly for Stanford’s PhD program. She expressed confidence in my capabilities and emphasized that transitioning from a master’s to a PhD, even within the same institution, can be quite competitive. Additionally, there’s often a heightened expectation regarding your accomplishments and track record. Had I not asked her for advice, I might never have applied for—and subsequently been accepted into—Stanford’s PhD program. The essence of this tale? Break out of your shell and converse with those more experienced; the insights gained are invaluable.
Picking the school.
Crafting a well-thought-out list of target programs is vital. Below are some factors I considered when curating my list. (I only focus on selecting PhD programs here, for selecting master’s programs, Zhenbang You has an amazing guideline in Chinese)
- PhD is a long commitment so I only select schools that I truly want to go. Applying indiscriminately just for an offer hurts both you and your advisor.
- PhD is mainly about research, so I only consider schools which have professors that I’m interested in working with.
- PhD is long and encompasses experiences beyond research, so I place considerable importance on non-academic aspects like location, climate, and safety.
- PhD admission can be random. There’s a chance your ideal professor isn’t accepting students in a particular year. I tried emailing professors to ascertain their availability and didn’t mention them in my SoP if they confirmed they won’t. Personally, I think emailing with this concrete question should be fine according to Yonatan Bisk ’s amazing blog on “Should you email professors?” .
- One thing I wish I had done was engage more with my peers, especially those who have their undergraduate studies in the US. You can know your position and gather many useful information from such interactions.
Creating Your Application Package
“references, references, references”, but how? There’s a prevailing consensus that connection plays a pivotal role in PhD admissions. That’s why recommendation letters stand out in your application package (Note: an application package typically consists of your transcript, CV, SoP, TOFEL scores for international students, and 3 recommendation letters*). According to Karpathy’s blog back in 2016, the mantra of PhD admissions is “Getting into a PhD program: references, references, references”. However, a major problem for international students is that it’s very hard to get references from renowned US faculties.
A common way to build such a connection is through summer research internships at US institutes. Given professors’ limited bandwidth, you’d better secure your position early - you may send out emails after the Christmas break. Generally, assistant professors are more likely to host interns and I think their references are also powerful since they are very active in academia.
That said, if you’re unable to obtain a reference from US faculty, it’s not the end of the world. After gaining admission to several programs, I had conversations with several senior faculties and many of them told me besides the referee’s name, they actively looked for details in the letter. Thus, value every collaboration, and feel free to remind your referees to add more details in the letter if they are not very familiar with writing them.
*: As of Fall 2023, many PhD programs no longer mandate the GRE. It’s essential to review each program’s prerequisites in advance.
About paper. As the field of CS/AI/NLP is getting increasingly popular these years, it seems like you need at least one paper to get into top PhD programs. This may be true (not sure), but one thing is verified by many faculties - a bunch of papers won’t strengthen your application that much, and emphasizing a bunch of N-th authored papers may even hurt your application.
Leave enough time for your SoP. While some people think that SoP is not that important for engineering students, I hold an opposite view. Of all components in your application package, the SoP is what you have the most control over. So, what should you include in your SoP? According to Philip Guo’s blog post , your SoP should be research-dense. That means “the majority of your statement of purpose should be about research”. Besides, I want to add one more point here - it’s crucial to allocate more space to your future research plans rather than merely recounting past experiences. Your experience can be found in your CV but your insights and tastes on research can only be found here. If your ideas resonate with your dream professor’s, your chance of getting an interview or even acceptance will greatly increase.
For concrete examples, I highly recommend cs-sop which includes excellent SoPs from many amazing guys.
About other stuffs. You definitely need to study hard in your undergraduate years, but honestly speaking, GPA and GRE/TOFEL scores don’t have a high weight in PhD admissions. It’s wise to allocate more time to research or other more important things.
Preparing for the Interviews
After assembling your application package and completing your online applications in December, you might be tempted to take a break. But hold on – the journey isn’t over! Most top CS programs include interviews as part of their admission process, given the abundance of strong applicants, it’s crucial to be prepared for them.
A definitely asked question: Describe one of your research projects. I didn’t meet an exception in the 9 interviews I took. Therefore, it’s wise to be well-prepared. Anticipating the time you’ll be given for your response can be tricky, so I advise crafting a 3-sentence summary, a 5-minute detailed response, and a comprehensive 15-minute description of your project. You also need to be familiar with all the details around your chosen project since professors are likely to ask you some follow-up questions. Tailor your response to the specific professor you’re conversing with. For instance, if they’re well-versed in your field, you might skip the background information. If a segment of your work aligns with their interests, delve deeper into that area.
For international applicants, honing your spoken English is beneficial. You definitely want to get your brilliant ideas across.
Stay updated with current trends. The wait for interview invitations can be nerve-wracking. Nevertheless, during this period, it’s vital to stay abreast of recent developments in your research area, particularly new arXiv publications or Twitter threads from your desired research groups.
Document and reflect on questions. When the interview period kicks off, a good practice is to introspect on each interview you took - write down the questions you just got and think about how to answer them better (if your English is not that fluent, you can also write down the refined answers - I did so). This practice is helpful for me as I found several questions appeared again in subsequent interviews.
Be grateful! Professors are busy. Therefore, being granted an interview signals their keen interest in you. I felt truly grateful for getting those interviews as it’s my first time to be able to directly talk with those “big names” in my mind. Typically, at the close of an interview, you’ll have the chance to pose your own questions. I used this chance to ask research-related questions that I have been puzzled about and I really learned a lot from their answers. This is actually the most meaningful part in my lengthy application process.
Closing Thoughts
My journey has just begun but it’s just so amazing to see my previous efforts took me here. Applying for a PhD is challenging but rewarding. Hope some parts in this blog post help and good luck!
- MyU : For Students, Faculty, and Staff
Fall 2024 CSCI Special Topics Courses
Cloud computing.
Meeting Time: 09:45 AM‑11:00 AM TTh Instructor: Ali Anwar Course Description: Cloud computing serves many large-scale applications ranging from search engines like Google to social networking websites like Facebook to online stores like Amazon. More recently, cloud computing has emerged as an essential technology to enable emerging fields such as Artificial Intelligence (AI), the Internet of Things (IoT), and Machine Learning. The exponential growth of data availability and demands for security and speed has made the cloud computing paradigm necessary for reliable, financially economical, and scalable computation. The dynamicity and flexibility of Cloud computing have opened up many new forms of deploying applications on infrastructure that cloud service providers offer, such as renting of computation resources and serverless computing. This course will cover the fundamentals of cloud services management and cloud software development, including but not limited to design patterns, application programming interfaces, and underlying middleware technologies. More specifically, we will cover the topics of cloud computing service models, data centers resource management, task scheduling, resource virtualization, SLAs, cloud security, software defined networks and storage, cloud storage, and programming models. We will also discuss data center design and management strategies, which enable the economic and technological benefits of cloud computing. Lastly, we will study cloud storage concepts like data distribution, durability, consistency, and redundancy. Registration Prerequisites: CS upper div, CompE upper div., EE upper div., EE grad, ITI upper div., Univ. honors student, or dept. permission; no cr for grads in CSci. Complete the following Google form to request a permission number from the instructor ( https://forms.gle/6BvbUwEkBK41tPJ17 ).
CSCI 5980/8980
Machine learning for healthcare: concepts and applications.
Meeting Time: 11:15 AM‑12:30 PM TTh Instructor: Yogatheesan Varatharajah Course Description: Machine Learning is transforming healthcare. This course will introduce students to a range of healthcare problems that can be tackled using machine learning, different health data modalities, relevant machine learning paradigms, and the unique challenges presented by healthcare applications. Applications we will cover include risk stratification, disease progression modeling, precision medicine, diagnosis, prognosis, subtype discovery, and improving clinical workflows. We will also cover research topics such as explainability, causality, trust, robustness, and fairness.
Registration Prerequisites: CSCI 5521 or equivalent. Complete the following Google form to request a permission number from the instructor ( https://forms.gle/z8X9pVZfCWMpQQ6o6 ).
Visualization with AI
Meeting Time: 04:00 PM‑05:15 PM TTh Instructor: Qianwen Wang Course Description: This course aims to investigate how visualization techniques and AI technologies work together to enhance understanding, insights, or outcomes.
This is a seminar style course consisting of lectures, paper presentation, and interactive discussion of the selected papers. Students will also work on a group project where they propose a research idea, survey related studies, and present initial results.
This course will cover the application of visualization to better understand AI models and data, and the use of AI to improve visualization processes. Readings for the course cover papers from the top venues of AI, Visualization, and HCI, topics including AI explainability, reliability, and Human-AI collaboration. This course is designed for PhD students, Masters students, and advanced undergraduates who want to dig into research.
Registration Prerequisites: Complete the following Google form to request a permission number from the instructor ( https://forms.gle/YTF5EZFUbQRJhHBYA ). Although the class is primarily intended for PhD students, motivated juniors/seniors and MS students who are interested in this topic are welcome to apply, ensuring they detail their qualifications for the course.
Visualizations for Intelligent AR Systems
Meeting Time: 04:00 PM‑05:15 PM MW Instructor: Zhu-Tian Chen Course Description: This course aims to explore the role of Data Visualization as a pivotal interface for enhancing human-data and human-AI interactions within Augmented Reality (AR) systems, thereby transforming a broad spectrum of activities in both professional and daily contexts. Structured as a seminar, the course consists of two main components: the theoretical and conceptual foundations delivered through lectures, paper readings, and discussions; and the hands-on experience gained through small assignments and group projects. This class is designed to be highly interactive, and AR devices will be provided to facilitate hands-on learning. Participants will have the opportunity to experience AR systems, develop cutting-edge AR interfaces, explore AI integration, and apply human-centric design principles. The course is designed to advance students' technical skills in AR and AI, as well as their understanding of how these technologies can be leveraged to enrich human experiences across various domains. Students will be encouraged to create innovative projects with the potential for submission to research conferences.
Registration Prerequisites: Complete the following Google form to request a permission number from the instructor ( https://forms.gle/Y81FGaJivoqMQYtq5 ). Students are expected to have a solid foundation in either data visualization, computer graphics, computer vision, or HCI. Having expertise in all would be perfect! However, a robust interest and eagerness to delve into these subjects can be equally valuable, even though it means you need to learn some basic concepts independently.
Sustainable Computing: A Systems View
Meeting Time: 09:45 AM‑11:00 AM Instructor: Abhishek Chandra Course Description: In recent years, there has been a dramatic increase in the pervasiveness, scale, and distribution of computing infrastructure: ranging from cloud, HPC systems, and data centers to edge computing and pervasive computing in the form of micro-data centers, mobile phones, sensors, and IoT devices embedded in the environment around us. The growing amount of computing, storage, and networking demand leads to increased energy usage, carbon emissions, and natural resource consumption. To reduce their environmental impact, there is a growing need to make computing systems sustainable. In this course, we will examine sustainable computing from a systems perspective. We will examine a number of questions: • How can we design and build sustainable computing systems? • How can we manage resources efficiently? • What system software and algorithms can reduce computational needs? Topics of interest would include: • Sustainable system design and architectures • Sustainability-aware systems software and management • Sustainability in large-scale distributed computing (clouds, data centers, HPC) • Sustainability in dispersed computing (edge, mobile computing, sensors/IoT)
Registration Prerequisites: This course is targeted towards students with a strong interest in computer systems (Operating Systems, Distributed Systems, Networking, Databases, etc.). Background in Operating Systems (Equivalent of CSCI 5103) and basic understanding of Computer Networking (Equivalent of CSCI 4211) is required.
- Future undergraduate students
- Future transfer students
- Future graduate students
- Future international students
- Diversity and Inclusion Opportunities
- Learn abroad
- Living Learning Communities
- Mentor programs
- Programs for women
- Student groups
- Visit, Apply & Next Steps
- Information for current students
- Departments and majors overview
- Departments
- Undergraduate majors
- Graduate programs
- Integrated Degree Programs
- Additional degree-granting programs
- Online learning
- Academic Advising overview
- Academic Advising FAQ
- Academic Advising Blog
- Appointments and drop-ins
- Academic support
- Commencement
- Four-year plans
- Honors advising
- Policies, procedures, and forms
- Career Services overview
- Resumes and cover letters
- Jobs and internships
- Interviews and job offers
- CSE Career Fair
- Major and career exploration
- Graduate school
- Collegiate Life overview
- Scholarships
- Diversity & Inclusivity Alliance
- Anderson Student Innovation Labs
- Information for alumni
- Get engaged with CSE
- Upcoming events
- CSE Alumni Society Board
- Alumni volunteer interest form
- Golden Medallion Society Reunion
- 50-Year Reunion
- Alumni honors and awards
- Outstanding Achievement
- Alumni Service
- Distinguished Leadership
- Honorary Doctorate Degrees
- Nobel Laureates
- Alumni resources
- Alumni career resources
- Alumni news outlets
- CSE branded clothing
- International alumni resources
- Inventing Tomorrow magazine
- Update your info
- CSE giving overview
- Why give to CSE?
- College priorities
- Give online now
- External relations
- Giving priorities
- Donor stories
- Impact of giving
- Ways to give to CSE
- Matching gifts
- CSE directories
- Invest in your company and the future
- Recruit our students
- Connect with researchers
- K-12 initiatives
- Diversity initiatives
- Research news
- Give to CSE
- CSE priorities
- Corporate relations
- Information for faculty and staff
- Administrative offices overview
- Office of the Dean
- Academic affairs
- Finance and Operations
- Communications
- Human resources
- Undergraduate programs and student services
- CSE Committees
- CSE policies overview
- Academic policies
- Faculty hiring and tenure policies
- Finance policies and information
- Graduate education policies
- Human resources policies
- Research policies
- Research overview
- Research centers and facilities
- Research proposal submission process
- Research safety
- Award-winning CSE faculty
- National academies
- University awards
- Honorary professorships
- Collegiate awards
- Other CSE honors and awards
- Staff awards
- Performance Management Process
- Work. With Flexibility in CSE
- K-12 outreach overview
- Summer camps
- Outreach events
- Enrichment programs
- Field trips and tours
- CSE K-12 Virtual Classroom Resources
- Educator development
- Sponsor an event
We're still accepting applications for fall 2024!
- Skip to content
- Skip to search
- Accessibility Policy
- Report an Accessibility Issue

- Kenya internship enriches Global Health Epidemiology personal journey
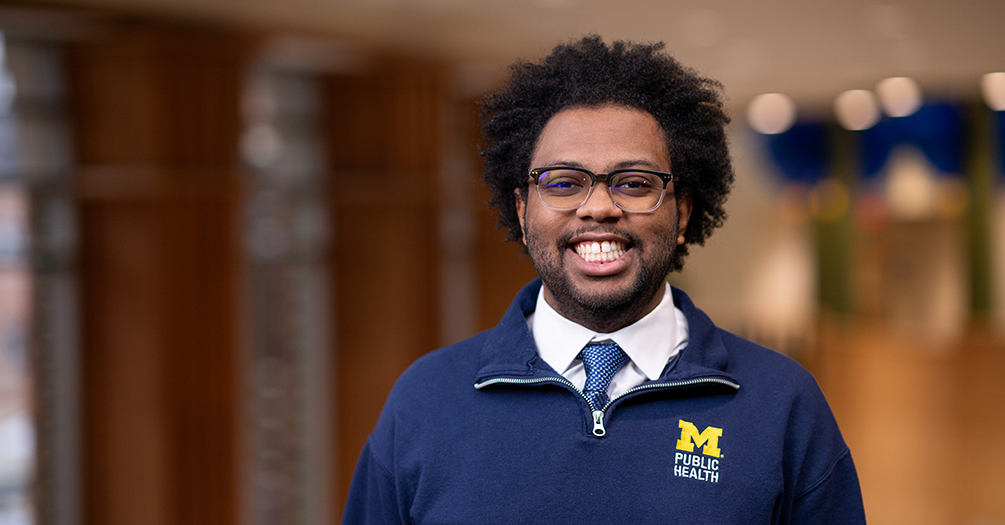
Christopher Floyd, BS ’21, MPH ’24
- Global Health Epidemiology
April 18, 2024
Christopher Floyd, BS ’21, MPH ’24, was well acquainted with the University of Michigan School of Public Health after he earned a Bachelor of Science degree in Public Health Sciences in 2021.
It was that familiarity that allowed him to zero in on a Master of Public Health degree in Global Health Epidemiology .
A blend of personal and academic experiences has cemented Floyd’s commitment to public health. The untimely death of relatives in his hometown of Southfield, Michigan, sparked his early interest in the health field, laying the foundation for his career.
An eight-month internship in Kenya proved pivotal, offering practical exposure to global health issues and cultural immersion, further enriching his academic perspectives.
Floyd will graduate in May with a Master of Public Health degree in Global Health Epidemiology from Michigan Public Health.
Personal experiences spark public health drive
Several of Floyd’s relatives, including aunts, uncles and even his grandfather died from health complications in their mid-to-late 60s, which planted a seed in for his interest in health and healthcare.
“When I was growing up, I had a lot of family members who unfortunately died at a relatively young age,” Floyd said. “So, part of my motivation for entering the health field was to gain an understanding of why certain health events occur.
“My father is nearing 60 and considering other family members who passed away at what I consider a young age is unsettling. It raises concerns and difficult thoughts about life expectancy, which moved me toward a deeper exploration of health issues to perhaps influence change in that area.”
I didn’t even know what public health was before I started my undergraduate studies, but COVID-19 really put public health in the spotlight. Now, even my parents, who first thought I was studying medicine, appreciate the breadth and significance of public health.”
His undergraduate journey began with the intent of studying medicine but shifted toward public health after exposure to courses that highlighted the multidimensional nature of health and the influence of social determinants.
This passion for understanding health beyond the clinician-patient dynamic led him to pursue a graduate degree. Floyd’s appreciation for interdisciplinary studies resonated with epidemiology, where he values the scope ranging from infectious diseases to environmental impacts and systemic health issues such as food insecurity.
Being an undergraduate student during the COVID-19 pandemic solidified his newfound passion for public health.
“I didn’t even know what public health was before I started my undergraduate studies, but COVID-19 really put public health in the spotlight,” Floyd said. “Now, even my parents, who first thought I was studying medicine, appreciate the breadth and significance of public health.”
Interconnecting health’s social determinants
Learning about the social determinants of health, the conditions in environments where people are born, live, learn, work, play, worship and age, and how they affect a wide range of health, functioning, and quality-of-life outcomes and risks, had a profound impact on Floyd.
“One of the most interesting parts about it was just realizing how interconnected everything is,” he said. “Learning about how one aspect of public health has direct or indirect effects on another system or institution is fascinating, especially when addressing issues such as food insecurity.”
The food system, for instance, is deeply tied to people’s living conditions and broader societal structures.
I appreciate epidemiology’s interdisciplinary nature, allowing exploration into various areas from infectious diseases to environmental health and beyond.”
“At Michigan Public Health, I learned to critically evaluate these intricate networks and appreciate the complexity of creating effective interventions,” Floyd said.
He saw firsthand the thoroughness involved in not just proposing solutions but in planning their sustainable implementation and the community impact. His experiences in classes, such as the class, challenged him to consider the full lifecycle of public health initiatives, from conception through to the long-term effects on the communities involved.
Floyd regards epidemiology as a cornerstone of the public health field—the discipline through which risks are assessed and health data is extrapolated. His intrigue in epidemiology stems from its analytical role in deciphering the relationships between activities such as smoking and health consequences such as cancer.
“I appreciate epidemiology’s interdisciplinary nature, allowing exploration into various areas from infectious diseases to environmental health and beyond,” said Floyd, whose current interest lies in how food insecurity impacts long-term health outcomes, reflecting the breadth that epidemiology encompasses.
Kenya internship shapes global health perspective
He chose Michigan Public Health for his graduate studies because of its strong Global Health Epidemiology program, which aligns with his interests in international health concerns.
His commitment to global health was further cemented by an opportunity to intern in Kenya, where he contributed to a project evaluating climate change risks. As a research intern with Eco2Librium, Floyd designed surveys to capture perceptions of climate impact among residents in western Kenya, exploring the relation between local weather patterns and issues of food and water security.
Spending eight-months in Kenya provided an experiential experience, grounding his academic knowledge in real-world contexts. The experience of living internationally for an extended period was more than just an academic or professional excursion for Floyd. It was a broadening life experience that deeply influenced his perspective.
“I really enjoyed my experience,” he said. “It was a fantastic opportunity to go to Kenya. I had never been to Kenya or the African continent. Immersing myself in a foreign country for eight months will always be something to look back on with fondness as I continue my career.”
Residing in Kakamega—a smaller city compared to Kenya’s bustling capital of Nairobi—Floyd experienced a different pace of life.
“It was definitely a very different experience to how everything is in the United States,” he said. “We very much have this obsession with productivity and getting things done at a certain time. Going to Kenya taught me that it’s OK to slow down, and everything doesn’t have to be so time constrained. Even just that small difference in culture was very impactful.
“I lived in the forest, and it was so quiet—it was peaceful and a lot colder than I expected because it was the rainy season. I also really liked the food.”
This international experience has not only expanded Floyd's professional capabilities. It has also altered his worldview and deepened his appreciation for cultural diversity. As he continues along his career path, Floyd will carry with him the lessons learned and the relationships forged during his transformative time in Kenya—a defining chapter in his journey through the world of public health.
I like to think that public health gives people the opportunity to live their life to the fullest extent.”
Charting pathways in public health
Having gained experience outside the classroom while earning two degrees from Michigan Public Health during a pandemic also had a profound effect on Floyd.
Along with several classmates, he went to Grenada as a member of the Public Health Action Support Team (PHAST) in February. PHAST helped develop a voluntary, non-remunerated blood donation program. In collaboration with the Grenada Red Cross Society, the team completed eight key-informant interviews with stakeholders in education, health and disaster management as well as 77 surveys with local community members.
Floyd also was a research assistant for Abram Wagner , assistant professor of Epidemiology and Global Public Health, studying vaccination and mask-wearing behaviors in the United States.
Overall, he is open to what the future may hold, and he’s thankful he is well equipped for that journey because of his time at Michigan Public Health.
“I think public health is a good way to kind of ‘liberate people,’” Floyd said. “In the sense that when you are in a position where you’re worrying about if you’re going to have enough food or about hospital bills for you or your children or things like that, that can be very debilitating. It doesn’t allow you to live a fulfilling life; it doesn't allow you to really experience the world—you’re always in survival mode.
“I like to think that public health gives people the opportunity to live their life to the fullest extent.”
- Interested in public health? Learn more here.
- Learn more about the Epidemiology program.
- Read more stories about students, alumni, faculty, and staff.
- Support research and engaged learning at the School of Public Health.
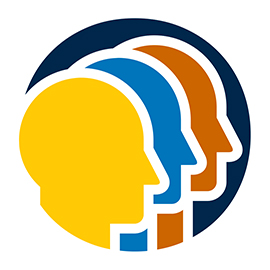
- Epidemiology
- Undergraduate
- We Are Michigan Public Health
- Global Public Health
- Health Disparities
- Internships
Recent Posts
- Finding inspiration at the intersection of public health and data science
- Public health chooses undergraduate student as a means to change
- Staying grounded: Building public health skills for local impact
What We’re Talking About
- Adolescent Health
- Air Quality
- Alternative Therapies
- Biostatistics
- Breastfeeding
- Child Health
- Chronic Disease
- Community Partnership
- Computational Epidemiology and Systems Modeling
- Disaster Relief
- Diversity Equity and Inclusion
- Engaged Learning
- Entrepreneurship
- Environmental Health
- Epidemiologic Science
- Epigenetics
- Field Notes
- First Generation Students
- Food Policy
- Food Safety
- General Epidemiology
- Graduation 2019
- HMP Executive Masters
- Health Behavior and Health Education
- Health Care
- Health Care Access
- Health Care Management
- Health Care Policy
- Health Communication
- Health Informatics
- Health for Men
- Health for Women
- Heart Disease
- Hospital Administration
- Hospital and Molecular Epidemiology
- Industrial Hygiene
- Infectious Disease
- LGBT Health
- Maternal Health
- Mental Health
- Mobile Health
- Occupational and Environmental Epidemiology
- Pain Management
- Pharmaceuticals
- Precision Health
- Professional Development
- Reproductive Health
- Scholarships
- Sexual Health
- Social Epidemiology
- Social Media
- Student Organizations
- Urban Health
- Urban Planning
- Value-Based Care
- Water Quality
- What Is Public Health?
Information For
- Prospective Students
- Current Students
- Alumni and Donors
- Community Partners and Employers
- About Public Health
- How Do I Apply?
- Departments
- Findings magazine
Student Resources
- Career Development
- Certificates
- The Heights Intranet
- Update Contact Info
- Report Website Feedback
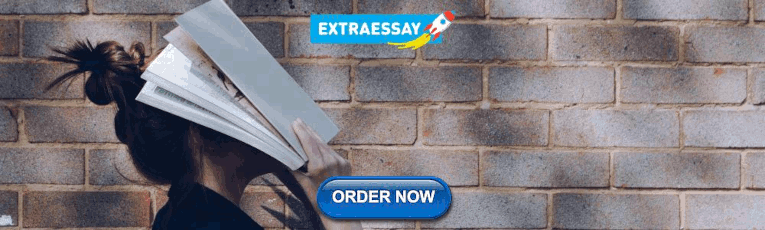
IMAGES
VIDEO
COMMENTS
This document is a compilation of NLP research directions that are rich for exploration, reflecting the views of a diverse group of PhD students in an academic research lab.
A PhD Student's Perspective on Research in NLP in the Era of Very Large Language Models. ... NLP research has evolved significantly, from early rule-based and symbolic approaches to statistical methods in the 1990s, which utilized probabilistic models and machine learning algorithms. In recent years, deep learning and neural networks have ...
A PhD Student's Perspective on Research in NLP in the Era of Very Large Language Models. Recent progress in large language models has enabled the deployment of many generative NLP applications. At the same time, it has also led to a misleading public discourse that "it's all been solved.". Not surprisingly, this has in turn made many NLP ...
We identify fourteen different research areas encompassing 45 research directions that require new research and are not directly solvable by LLMs. While we identify many research areas, many others exist; we do not cover areas currently addressed by LLMs, but where LLMs lag behind in performance or those focused on LLM development.
A PhD Student's Perspective on Research in NLP in the Era of Very Large Language Models. Recent progress in large language models has enabled the deployment of many generative NLP applications. At the same time, it has also led to a misleading public discourse that ``it's all been solved.''. Not surprisingly, this has in turn made many NLP ...
Not surprisingly, this has in turn made many NLP researchers -- especially those at the beginning of their career -- wonder about what NLP research area they should focus on. This document is a compilation of NLP research directions that are rich for exploration, reflecting the views of a diverse group of PhD students in an academic research lab.
Open NLP Research Questions Not Solved by Large Language Models. Recent progress in large language models (LLMs) has enabled the deployment of many generative NLP applications. At the same time, it has also led to a misleading public discourse that ``it's all been solved.''. Not surprisingly, this has, in turn, made many NLP researchers ...
A PhD Student's Perspective on Research in NLP in the Era of Very Large Language Models (Oana Ignat, Zhijing Jin, Artem Abzaliev, Laura Biester, Santiago Castro, Naihao Deng, Xinyi Gao, Aylin Gunal, Jacky He, Ashkan Kazemi, Muhammad Khalifa, Namho Koh, Andrew Lee, Siyang Liu, Do June Min, Shinka Mori, Joan Nwatu, Veronica Perez-Rosas, Siqi Shen ...
A PhD Student's Perspective on Research in NLP in the Era of Very Large Language Models. A PhD Student's Perspective on Research in NLP in the Era of Very Large Language Models. Joan Nwatu. 2023, arXiv (Cornell University) See Full PDF Download PDF.
A PhD Student's Perspective on Research in NLP in the Era of Very Large Language Models (2305.12544) Published May 21, 2023 in cs.CL and cs.AI. Abstract. Recent progress in large language models has enabled the deployment of many generative NLP applications. At the same time, it has also led to a misleading public discourse that ``it's all been ...
the field of NLP that could lead to a PhD thesis and cover a space that is not within the purview of LLMs." Spoiler alert: there are many such research areas! About This Document. This document reflects the ideas about "the future of NLP research" from the members of an academic NLP research lab in the United States. The Language and ...
Bibliographic details on A PhD Student's Perspective on Research in NLP in the Era of Very Large Language Models. ... Schloss Dagstuhl seeks to hire a Research Data Expert (f/m/d). For more information, see our job offer. We recently started indexing data publications as "first-class citizens" in dblp.
A PhD Student's Perspective on Research in NLP in the Era of Very Large Language Models. O Ignat, Z Jin, A Abzaliev, L Biester, S Castro, N Deng, X Gao, A Gunal, ... arXiv preprint arXiv:2305.12544, 2023. 11: 2023: Rethinking and refining the distinct metric. S Liu, S Sabour, Y Zheng, P Ke, X Zhu, M Huang.
A PhD Student's Perspective on Research in NLP in the Era of Very Large Language Models Oana Ignat , Zhijing Jin , Artem Abzaliev, Laura Biester, Santiago Castro, ... the ideas about "the future of NLP research" from the members of an academic NLP research lab in the United States. The Language and Informa-tion Technologies (LIT) lab at ...
A PhD Student's Perspective on Research in NLP in the Era of Very Large Language Models. Li et al. (Oct 2023). Defining a New NLP Playground. Saphra et al. (Nov 2023). First Tragedy, then Parse: History Repeats Itself in the New Era of Large Language Models. Manning (Dec 2023). Academic NLP research in the Age of LLMs: Nothing but blue skies!
To address this question, this paper compiles NLP research directions rich for exploration. We identify fourteen different research areas encompassing 45 research directions that require new research and are not directly solvable by LLMs. While we identify many research areas, many others exist; we do not cover areas currently addressed by LLMs ...
Student Perspectives on Applying to NLP PhD Programs October 24, 2019 nlp, phd, research, applications, advice. This post was written by: Akari Asai, John Hewitt, Sidd Karamcheti, Kalpesh Krishna, Nelson Liu, Roma Patel, and Nicholas Tomlin.. Thanks to our amazing survey respondents: Akari Asai, Aishwarya Kamath, Sidd Karamcheti, Kalpesh Krishna, Lucy Li, Kevin Lin, Nelson Liu, Sabrina Mielke ...
A PhD Student's Perspective on Research in NLP in the Era of Very Large Language Models As our IFAN project was recommended as one of the promising research direction, I will also recommend in return to read the recent paper to answer the question: "So what now in NLP research if ChatGPT is out?" Spoiler: the world has not ended and we still have plenty work to do!
A PhD Student's Perspective on Research in NLP in the Era of Very Large Language Models. JOAN NWATU. 2023, arXiv (Cornell University) See Full PDF Download PDF. See Full PDF Download PDF.
Contributor: Zhijing Jin (PhD student in NLP at Max Planck Institute & ETH, co-organizer of the ACL Year-Round Mentorship Program). You are welcome to be a collaborator, -- you can make an issue/pull request, and I can add you :). ... (UMich; led by Prof Rada Mihalcea) A PhD Student's Perspective on Research in NLP in the Era of Very Large ...
The Stanford NLP Group is always on the lookout for budding new computational linguists. Stanford has a great program at the cutting edge of modern computational linguistics. The best way to get a sense of what goes on in the NLP Group is to look at our research blog , publications, and students' and faculty's homepages.
An international student's perspective on applying for CS/AI/NLP PhD in the US. September 10, 2023. ... Opting for a PhD means you'll do research for the next 5+ years, possibly even for a lifetime. ... As the field of CS/AI/NLP is getting increasingly popular these years, it seems like you need at least one paper to get into top PhD programs
CSCI 5980 Cloud ComputingMeeting Time: 09:45 AM‑11:00 AM TTh Instructor: Ali AnwarCourse Description: Cloud computing serves many large-scale applications ranging from search engines like Google to social networking websites like Facebook to online stores like Amazon. More recently, cloud computing has emerged as an essential technology to enable emerging fields such as Artificial ...
An eight-month internship in Kenya proved pivotal for Christopher Floyd, BS '21, MPH '24, offering practical exposure to global health issues and cultural immersion, further enriching his academic perspectives in public health. Floyd will graduate in May with a Master of Public Health degree in Global Health Epidemiology from Michigan Public Health.