- Search Menu
- Cytogenetics
- Cytotechnology
- Histotechnology
- Management/Administration
- Microbiology
- Molecular Pathology
- Molecular Biology
- Transfusion Medicine
- Advance articles
- COVID-19 articles
- Current Virtual Issue
- Cover Archive
- Author Guidelines
- Open Access
- Submission Site
- Advertising and Corporate Services
- Advertising
- Reprints and ePrints
- Sponsored Supplements
- Branded Books
- About Laboratory Medicine
- About the American Society for Clinical Pathology
- Editorial Board
- Self-Archiving Policy
- Journals on Oxford Academic
- Books on Oxford Academic
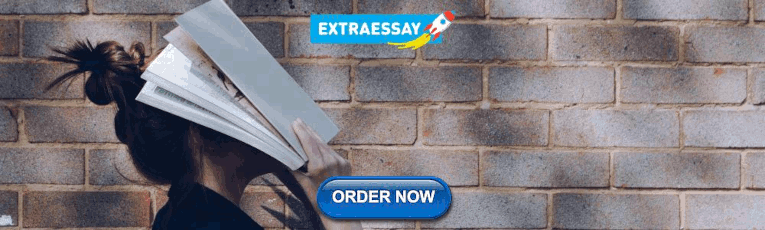
Article Contents
Materials and methods, abbreviations.
- < Previous
Evaluating Laboratory Performance With Quality Indicators
- Article contents
- Figures & tables
- Supplementary Data
Ranjna Chawla, Binita Goswami, Bhawna Singh, Aparna Chawla, Vinod Kumar Gupta, Venkatesan Mallika, Evaluating Laboratory Performance With Quality Indicators, Laboratory Medicine , Volume 41, Issue 5, May 2010, Pages 297–300, https://doi.org/10.1309/LMS2CBXBA6Y0OWMG
- Permissions Icon Permissions
Quality indicators in the clinical laboratory provide a useful tool for continuous improvement of laboratory services. The goal of this study was to design and review quality indicators from time to time in an effort to improve the performance of the laboratory.
The study included an evaluation of different quality indicators collected from the biochemistry laboratory of a tertiary care hospital in New Delhi over a period of 1 year. A total of 84250 samples were processed, and the quality indicators monitored were classified into the following 3 categories: preanalytical, analytical, and post-analytical.
Hemolysis (0.7%) was the most common anomaly observed during the assessment of pre-analytical indicators followed by improper samples for coagulation profile (0.34%). In the analytical phase, the frequency for fibrin clots was 1.2%.
Working constantly to improve the outcome of these indicators by taking corrective measures over a period of time will definitely help to improve the quality of laboratory services and patient health care.
The clinical laboratory is the epicenter of the health care sector. The dependence of patient management on laboratory data highlights the need for ensuring the quality of these services. The Joint Commission has underlined this fact by stating that the laboratory is required to “systematically assess and improve important functions and work processes and their outcomes.” 1 It is therefore imperative to lay down certain benchmarks to assess laboratory functions. It is essential to identify certain determinants for the assessment of the quality in laboratory functioning. The term quality indicators is used for these determinants.
A quality indicator is defined as an objective measure evaluating critical health care domains as defined by the Institute of Medicine (IOM) (patient safety, effectiveness, equity, patient centeredness, timeliness, and efficiency). It is based on evidence associated with those domains and can be implemented in a consistent and comparable manner across settings and over time. In other words, a quality indicator is a tool enabling us to quantify the laboratory’s performance by selecting a certain comparative criterion. Any potential quality indicator needs to fulfill primarily 2 inclusion criteria: it must be an indicator of laboratory functioning and it must cater to at least 1 IOM health care domain. 2
Shahangian and colleagues have reviewed these quality indicators in detail and have classified them into different categories. 3 We have chosen some of these quality indicators criteria as applicable to the inspection of the functioning of our clinical chemistry laboratory. These indicators are sample rejection rates due to preanalytical variables (hemolysis, lipemia, insufficient quantity, and illegible hand writing); analytical variables (proficiency testing, number of samples repeated due to fibrin clot or insufficient quantity as detected by the instrument, critical values reporting), and post-analytical indicators (excessive turnaround time [TAT] and number of duplicate reports generated by the laboratory as a result of reports not reaching the clinicians).
The aim of our endeavor was to assess the performance and initiate corrective measures to further improve the quality of laboratory services rendered by us to the clinicians and the general population. This was carried out by evaluating the different quality indicators from October 2008 to September 2009.
Govind Ballabh Pant Hospital (GBPH) is a tertiary care hospital serving a wide metropolitan area. The hospital has cardiology, cardiothoracic surgery, neurology, neurosurgery, gastroenterology, gastro surgery, and psychiatry departments. It is a 600-bed hospital offering specialized medical and surgical treatments to about 300000 patients in the Out Patient Department (OPD) and 19000 patients in the general and private wards every year. The clinical biochemistry department is equipped with a state-of-the-art autoanalyser with ISE (Olympus AU 400, Hamburg, Germany), electrolyte analyzer—Ecolyte (Ecshweiler, Germany), automated coagulometer- ACL 7000 (Instrumentation Laboratory, Bedford, MA), and other ancillaries for sample processing.
The routine ward samples are received by our technical staff from 9:00 am to 12:00 pm and processed subsequently. The laboratory staff recruited for sample receipt makes an entry including the time the sample is received by the lab. This is strictly on a first come, first serve basis. The samples are numbered at the reception counter accordingly.
After screening the samples for any preanalytical errors, the analytical process begins. This is preceded by routine maintenance and a quality control (QC) evaluation. The sample run is initiated after satisfactory QC results. The QC is run after a batch of 50 samples to identify any intra-assay fluctuations. The same protocol is followed for the OPD samples too. Any deviation from the accepted range of target values for QC, instrument alarms due to fibrin clots, or insufficient sample volume and hampered estimation are documented in a predefined log book by the technicians employed at the workstation. Repeat samples do not include samples requiring dilution due to a high concentration of the measured analyte, such as enzymes. A log book is maintained for the documentation of critical values. We follow the protocol of informing the wards by telephone about critical values so the clinicians can manage the situation accordingly. A detailed account of the patient identification, the critical values, and the contact person in the ward is also maintained in the log book.
From the time the samples are loaded into the autoanalyzer to the time when they are validated are also documented in the TAT logbook. The ward reports are dispatched to the respective wards after appropriate validation by our laboratory staff. The OPD samples are processed during the evening shift as the reports are dispatched to the centralized collection center the following day. The reports are then distributed to the different OPDs. The stat samples are processed promptly and run on stat mode to deliver the reports at the earliest.
A record of the number of duplicate reports dispatched by our staff is also maintained in a separate log book. We do not have a laboratory information system (LIS) in our hospital and as a result, the reports are dispatched by our laboratory attendants to the respective wards for the inpatients or the centralized collection center for the outpatients. However, due to certain unforeseen circumstances or lapses on the part of the staff involved in report dispatch and further segregation and documentation, duplicate reports are often sought by the patients or clinicians. The relevant data regarding this aspect is also analyzed as another quality indicator of our services. We are presenting our observations during a randomly selected period (October 2008 to September 2009). Data was collected for the hospitalized patients during routine as well as emergency hours.
During the period of 1 year, a total of 84250 samples were received in our clinical biochemistry laboratory. The quality indicators were classified into the following 3 categories: preanalytical, analytical, and post-analytical. Table 1 identifies the various pre-analytical quality indicators and evaluates our performance. Hemolysis was the most common cause for the rejection of samples (7 per 1000 samples), followed by an inappropriate ratio of anti-coagulant to blood for coagulation profiles (3.4 per 1000 samples), and clotted samples for coagulation studies (1.9 per 1000 samples). The prevalence of the other indicators assessed were errors in the requisition slip such as illegible handwriting (1 per 1000 samples), incomplete patient identification (0.8 per 1000 samples), insufficient quantity of samples (0.6 per 1000 samples), missing slips or samples (0.4 per 1000 samples), samples in wrong vials (0.2 per 1000 samples), lipemic samples (0.2 per 1000 samples), and empty tubes (0.07 per 1000 samples). Table 2 describes some analytical quality indicators of our laboratory. The main problem encountered was random errors on the analyzer due to pipetting difficulties related to fibrin clots leading to repeat analysis. This accounted for 12 per 1000 samples. Other variables included neglecting the requested tests (1.3 per 1000 samples) and not in compliance with QC (0.1 per 1000 samples).
Analysis of the Prevalence of Pre-analytical Quality Indicators During 1 Year
The post-analytical factors measuring the quality of our laboratory are illustrated in Table 3 . We do not have an automated sample transport system or an LIS facility in our hospital; hence the samples and reports are delivered by a manual courier. We have set a benchmark of 5–5.5 hours as the TAT for ward samples (excluding the stat samples), keeping in mind all the steps involved in sample delivery, analysis, validation, and finally report dispatch. A total of 0.5 per 1000 samples could not be dispatched within the stipulated time frame. The magnitude of prolonged TAT was low as the critical values/reports were reported by the laboratory staff immediately marking the critical value reporting to a high of 14 per 1000 samples. Due to validating the reports and the relevant documentation by the technical supervisors and the clinical biochemists, the detection of critical values was nearly 100%. The number of duplicate reports generated during the period of 1 year was 982 with a frequency of 11/1000.
Analysis of the Prevalence of Analytical Quality Indicators During 1 Year
Analysis of the Prevalence of Post-Analytical Quality Indicators During 1 Year
Ensuring high standards of quality rendered by any service provider is of cardinal importance in today’s world of consumerism. Laboratories are no exception to this unwritten rule. It is necessary on our part to ensure quality is not compromised due to the quantity of work. This is essential to instill confidence in the clinicians and the general public for our reports. The concept of quality indicators has emerged over the past few years for the fulfillment of the above mentioned aim. We have attempted to gauge the quality of our clinical laboratory over a period of 1 year by evaluating our performance.
We assessed the frequency of rejections due to sample inadequacy, inappropriateness, and incorrect patient information due to incorrect phlebotomy practices and/or ignorance and non-compliance by the phlebotomists. Hemolysis was the most common anomaly observed during the assessment of preanalytical indicators followed by improper samples for coagulation profiles (incorrect proportion of anticoagulant to plasma and clotted samples). Hemolysis of samples may be caused by forcefully ejecting blood through a fine needle, shaking the tubes vigorously, and centrifuging the specimens before coagulation is complete; 4 it can cause erroneous reporting of electrolytes, enzymes, and prothrombin values. Ricos and colleagues have defined certain specifications for the various quality indicators that may be used for evaluating laboratory performance. 4 We recorded a hemolysis rate of 0.7% as compared to 0.2% suggested by Ricos and colleagues. The other preanalytical errors, such as lipemic samples, insufficient quantity, incomplete requisition slips, and inadequate dilution in cases of coagulation profiles, can arise due to ignorance regarding the requirement of a minimum volume for the various tests, patient preparation, and test principles. The prevalence of samples with illegible handwriting and incomplete patient information was 1/1000 and 0.8/1000 respectively. The benchmarks for these 2 parameters are 1/1000 for illegible handwriting and 0.8/1000 for errors in patient information. Our figures exactly match the guidelines as evident above.
A total of 1.54% of the total 84250 was rejected over a period of 1 year. The rejection rates mentioned in the literature range from 0.3%–0.8%. 5 , 6 Dale and colleagues 5 and Stark and colleagues 7 reported a rejection rate of 0.3% and 0.74% respectively in their institutions. The Q-probe study reported a sample rejection rate of 0.35% from their analysis of 453 laboratories in 1997. 8 In order to reduce the frequency of preanalytical variables, we have initiated a number of actions. As a part of our endeavor to achieve accreditation for our laboratory services, we carry out regular in-house training programs for our technicians to familiarize them with the standard protocols for sample processing. For this purpose, we have developed standard operating procedures (SOPs) for the different steps involved in ideal laboratory operations and ethics. Administrative procedures have been mobilized for acquiring a pneumatic tube system.
We obtained an incidence for fibrin clots of 12 per 1000 samples. Chemistry and immunoassays are susceptible to interference by fibrin. Small amounts of fibrin (and other protein debris membranes) may affect the results. The presence of gross amounts of fibrin in the specimen (serum or plasma) may cause blockage of instrument sample aspiration probes resulting in erroneous assay results. Inadequate clotting time, improper mixing, and failure to place the tube in an upright position can lead to incomplete clot formation. Following centrifugation, the sample may appear satisfactory with a defined layer of cells at the base of the tube and a clear layer of serum above. Despite this appearance, the clotting process may not have been completed prior to transportation, centrifugation, and placement of the specimen on the analyzer. Further coagulation in the serum may subsequently occur, leading to the production of latent fibrin, which can interfere with the quality of a result. 9 For plastic tubes, thorough mixing by gentle inversion (at least 5 times) is essential to ensure even distribution of the clot activator throughout the specimen. This will also allow completion of the clotting process. In order to minimize the occurrence of interference due to fibrin clots, special attention has been paid toward the adoption of ideal sample handling protocols. These include ensuring adequate clotting time (minimum 30 minutes) and proper centrifugation techniques, which involve centrifuging at 5000 rpm for 15 minutes. In addition, it is also essential to establish proper mixing immediately after collection.
We found 10 instances (0.1/1000) of non-conformity to QC, which was due to inappropriate storage and reagent contamination. Jesus and colleagues have suggested a cutoff percentage of 0.8% for external controls exceeding the target range. 10 We are well within this target; nevertheless, we are trying to reduce such instances to a minimum. This requires reconstitution and storage of the QC vials with a strict maintenance to cold chain.
Around 109 instances (1.3 per 1000 samples) of requested tests not performed were detected. This could be due to the oblivious attitude of the technicians and/or increased workload. Under ideal conditions, the figure should be near 0, but it may not be possible to attain this due to administrative and procedural constraints. Stahl and colleagues conducted a study to determine the total number of unreported tests due to preanalytical, analytical, and post-analytical variables. The frequency of errors ranged from 0.74% to 0.93%. A target range of 0.2% to 0.5% has been suggested as an achievable target. 11 Ricos and colleagues have been more lenient with an acceptable frequency of requested tests not performed at 1.4%. 4
Scrutiny of the post-analytical variables reveals we achieved a critical value reporting frequency of 14 per 1000 samples. Critical or panic values have been described as those that at very high or low abnormal levels can cause irreparable physical damage unless treated immediately. 12 Reporting delays in critical results can cause serious adverse outcomes. 13 Critical value reporting is considered an important quality indicator for excellence in patient centric care. 14 The literature quotes a frequency of critical value reporting ranging from 1 in 2000 to 1 in 100 samples. 15 , 16 The relative abundance of critical value reporting by our laboratory is an indicator of our conscious effort to apprise the clinicians of the reports bordering the danger mark. This facilitates decision making for the institution of corrective measures that might prove to be life saving in certain cases. Ricos and colleagues have laid down the benchmark of 6 minutes as the average time for communicating critical values to inpatients. 4
Approximately 0.5 per 1000 results were released from our laboratory with an excessively prolonged TAT, due to unforeseen and unavoidable problems. TAT is the measure of the number of tests that do not meet a reporting deadline. No guidelines are available for determination of ideal TAT goals. However, Ricos and colleagues have suggested that 11% is an acceptable fraction of laboratory reports that may exceed the stipulated TAT. 4 Delays in the analytical phase, lost test requisitions, specimens, and reports may contribute toward prolonged TAT. Timely reporting may augment patient care and clinicians’ satisfaction. 17
We are reporting a frequency of 11/1000 for the number of duplicate reports given to the patients or their attendants as a consequence of non receipt of a report by the clinicians. The acceptable cutoff for the same laid down by Alsina and colleagues is 1.6% or 16/1000. However, it is our duty to ensure 100% report delivery to the clinicians or patients so the patients are not subjected to this unwarranted inconvenience and the treatment can be initiated at the earliest.
Our audit of the various putative indicators has revealed loopholes in the existing laboratory system. Due to the lack of a LIS and pneumatic tube system, the quality indicators were slightly compromised as compared to the literature. The sample rejection rate in our case was higher because of these institutional factors. Efforts should be initiated to develop quality indicators in laboratory medicine that can evaluate and subsequently improve the health care system in an effective manner. Such indicators should be easily achievable, quantifiable, and possess a solid scientific basis.
The concept of quality indicators has revolutionized the field of laboratory medicine. These analytical and extra analytical indicators are of utmost importance in the comparison of individual laboratory performance with the aim of improving laboratory quality. It is now possible to compare our laboratory functions with others by simply evaluating the prevalence of the various indicators. We should strive to reach these benchmarks to provide the best services to society.
Comprehensive Accreditation Manual for Pathology and Laboratory Services . Oakbrook Terrace, IL : Joint Commission ; 2009 .
Google Scholar
Google Preview
Institute of Medicine Committee on Quality of Health Care in America . To err is human: Building a safer health system . Washington, DC : National Academies Press ; 2000 .
Shahangian S Snyder SR . Laboratory medicine quality indicators . Am J Clin Pathol . 2009 ; 131 : 418 – 431 .
Ricos C Garcia-Victoria M de la Fuente B . Quality indicators and specifications for the extra analytical phases in clinical laboratory management . Clin Chem Lab Med . 2004 ; 42 : 578 – 582 .
Dale JC Novis DA . Outpatient phlebotomy success and reasons for specimen rejection: A Q-probes study . Arch Pathol Lab Med . 2002 ; 126 : 416 – 419 .
Jones BA Meier F Howanitz P . Complete blood count specimen acceptability: A College of American Pathologists Q-probes study of 703 laboratories . Arch Pathol Lab Med . 1995 ; 119 : 203 – 208 .
Stark S Jones BA Chapman D et al. Clinical laboratory specimen rejection—Association with the site of patient care and patients’ characteristics . Arch Pathol Lab Med . 2007 ; 131 : 588 – 592 .
Jones BA Calam RR Howanitz PJ . Chemistry specimen acceptability: A College of American Pathologists Q-probes study of 453 laboratories . Arch Pathol Lab Med . 1997 ; 121 : 19 – 26 .
Walters L . From Quality samples to results . Advances for administrators of the laboratory 2010 ; 16 : 52 .
Jesus AK Virtudes AF Carme BA et al. Quality indicators and specifications for key processes in clinical laboratories: A preliminary experience . Clin Chem Lab Med . 2007 ; 45 : 672 – 677 .
Stahl M Lund ED Brandslund I . Reasons for a laboratory‘s inability to report results for requested analytical tests . Clin Chem . 1998 ; 44 : 2195 – 2197 .
Lundberg GD . It is time to extend the laboratory critical (panic) value system to include vital values . Med Gen Med . 2007 ; 9 : 20 .
Dighe AS Rao A Coakley AB . Analysis of laboratory critical value reporting at a large academic medical center . Am J Clin Pathol . 2006 ; 125 : 758 – 764 .
Joint Commission . 2009 . National patient safety goals: laboratory services program. Available at: www.jointcommission.org/patientsafety/nationalpatientsafetygoals/09_lab_npsgs.htm . Accessed November 2009.
Howanitz PJ Steindel SJ Heard NV . Laboratory critical values policies and procedures: A College of American Pathologists’ Q-probe study in 623 institutions . Arch Pathol Lab Med . 2002 ; 126 : 663 – 669 .
Kuperman JG Boyle D Jha A et al. How promptly are in patients treated for critical laboratory results? J Am Med Inform Assoc . 1998 ; 5 : 112 – 119 .
Valenstein P Walsh M . Five-year follow up of routine outpatient test turnaround time: A College of American Pathologists Q-Probes study . Arch Pathol Lab Med . 2003 ; 127 : 1421 – 1423 .
Institute of Medicine
Govind Ballabh Pant Hospital
Out Patient Department
Quality Control
turnaround time
laboratory information system
- quality indicators
- fibrin blood clot
- critical value
Email alerts
More on this topic, related articles in pubmed, citing articles via.
- Recommend to your Library
Affiliations
- Online ISSN 1943-7730
- Print ISSN 0007-5027
- Copyright © 2024 American Society for Clinical Pathology
- About Oxford Academic
- Publish journals with us
- University press partners
- What we publish
- New features
- Open access
- Institutional account management
- Rights and permissions
- Get help with access
- Accessibility
- Media enquiries
- Oxford University Press
- Oxford Languages
- University of Oxford
Oxford University Press is a department of the University of Oxford. It furthers the University's objective of excellence in research, scholarship, and education by publishing worldwide
- Copyright © 2024 Oxford University Press
- Cookie settings
- Cookie policy
- Privacy policy
- Legal notice
This Feature Is Available To Subscribers Only
Sign In or Create an Account
This PDF is available to Subscribers Only
For full access to this pdf, sign in to an existing account, or purchase an annual subscription.
Thank you for visiting nature.com. You are using a browser version with limited support for CSS. To obtain the best experience, we recommend you use a more up to date browser (or turn off compatibility mode in Internet Explorer). In the meantime, to ensure continued support, we are displaying the site without styles and JavaScript.
- View all journals
- My Account Login
- Explore content
- About the journal
- Publish with us
- Sign up for alerts
- Open access
- Published: 09 July 2019
Management at the service of research: ReOmicS, a quality management system for omics sciences
- Antonella Lanati 1 na1 ,
- Marinella Marzano 2 na1 ,
- Caterina Manzari 2 ,
- Bruno Fosso 2 ,
- Graziano Pesole 2 , 3 &
- Francesca De Leo ORCID: orcid.org/0000-0003-0421-7699 2
Palgrave Communications volume 5 , Article number: 75 ( 2019 ) Cite this article
7511 Accesses
2 Citations
3 Altmetric
Metrics details
- Business and management
- Science, technology and society
Management and research represent a binomial almost unknown, whose potentialities and requirements have not yet been fully exploited even if, recently, the scientific and social communities have felt the burden of producing results and data requiring at the same time reproducibility, reliability, safety and efficacy of the discoveries, as well as a profitable use of resources. A Quality Management System (QMS) could represent a valid tool for these purposes, improving the quality of the research. The research community could ask whether and how it is possible to apply this approach in a research laboratory without hindering their creativity, and what the possible benefits might be. On the other hand, an international standard for a quality management system appropriate for a research laboratory is yet to come. The choice, the design and the application of a QMS, inspired by the Good Laboratory Practices, in a research laboratory specialized on “omics” sciences, is fully described in this paper. Its application has already shown good outcomes as testified by specific metric of efficiency and effectiveness. The approach is innovative as there is no obvious requirement for research laboratories to develop and define quality objectives. The paper highlights how the QMS approach enhances the relationship with public and private sectors by increasing customer confidence and loyalty, as well as improving the overall performance of the laboratory in terms of throughput and value of research. These results encourage proposing it as a QMS model providing a new and scalable operational strategy to be applied in a research environment with the same target and even in a generic research laboratory.
Similar content being viewed by others
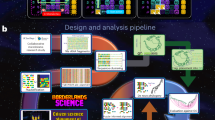
Improving microbial phylogeny with citizen science within a mass-market video game
Roman Sarrazin-Gendron, Parham Ghasemloo Gheidari, … Jérôme Waldispühl
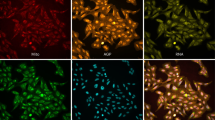
Three million images and morphological profiles of cells treated with matched chemical and genetic perturbations
Srinivas Niranj Chandrasekaran, Beth A. Cimini, … Anne E. Carpenter
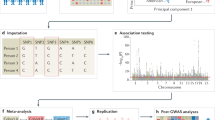
Genome-wide association studies
Emil Uffelmann, Qin Qin Huang, … Danielle Posthuma
Introduction
Next Generation Sequencing (NGS) technologies have dramatically changed the field of genomics and are routinely applied to a variety of functional genomics investigations including, but not restricted to, whole genome sequencing, global identification of genomic rearrangements, epigenetic modifications, single nucleotide polymorphism (SNP) discovery, transcriptome profiling and metagenomics. In recent years, using these technologies thousands of genomes assembled from short DNA sequence readings of humans, plants, animals and microbes have been collected and explored, enabling scientists to develop a deeper understanding and gaining new insights into the molecular mechanisms related to different diseases, including many types of cancer, allergies, or other disorders (Wiese et al., 2018 ). Furthermore, the genomics has profoundly influenced the pharmaceutical industry and reshaped the processes allowing to discover, investigate, and develop new drugs. No less important is the research carried out using these technologies in the environmental field for industrial and biotechnological purposes (Tiwari et al., 2018 ). Indeed, NGS is a complex process that, on the one hand, requires the preparation of sequencing libraries that respond to the specific standard requirements of the platforms, and on the other hand generates unprecedented volumes of data to be analyzed (Stephens et al., 2015 ). Moreover, an NGS analysis usually involves collaboration between several departments, laboratories and data analysis groups, characterized by different scientific backgrounds and, above all, applying different experimental approaches. With the growing need of managing information, it has become challenging to keep track of data, processes and outcomes of research over long periods of time and across the collaborating units. Nowadays Big Data generation and its management create extraordinary challenges for storage, transfer, analysis, interpretation and last but not least security of information. Regarding this last aspect, the scientific community has recently expressed the necessity to manage NGS data according to the principles of Findability, Accessibility, Interoperability, and Re-usability of digital assets (FAIR data) (Wilkinson et al., 2016 ; Corpas et al., 2018 ). The expectation is to produce digital resources with more rigorous management and stewardship that can be used by the entire scientific community. Good data management is not a goal in itself, but rather it is the key conduit leading to discovery and innovation, through data integration and reuse by the scientific community after the publication process. Good data management, ensuring reliability and usability, needs a holistic approach tracking the process of data and metadata generation and all the different organizational aspect that, on the one hand may affect it, and on the other hand can keep it under control. This can be achieved by means of a management system focused on the quality of the results.
Furthermore, in recent years, in the context of the scientific research, we are witnessing a new phenomenon, defined as “reproducibility crisis” by Baker (Baker, 2016 ) and Dirnagl et al. (Dirnagl et al., 2018 ) characterized by the reduction of the reliability, reproducibility, traceability and predictability of research results. These problems can not only compromise the robustness and rigor of research (Dirnagl et al., 2018 ) but have also a significant impact from an economic point of view, reducing the profitability of research funds (Lanati, 2018 ). The standardization and simplification of experimental workflows, such as those applied for “omics” applications, is becoming a need both for academic and private research laboratories. As described by Endrullat et al. ( 2016 ), standards act as basic guidelines to ensure comparability and exchange of experimental data conducive to the acceleration of the innovation process, aiding improvement of transferability, transparency and reproducibility of results. Furthermore, the advantages deriving from the standardization of processes could reduce costs and increase services (Endrullat et al., 2016 ; Cargill, 2011 ). A Quality Management System (QMS) can support the correct management of the NGS research environment, providing directions for data and operations management. A suitable quality system ensures safety, reliability and reproducibility of the non-clinical tests on chemicals intended for use on humans, animals and the environment. A QMS supports the generation of high quality scientific data and associated services, it is also helpful in improving the economic and social impacts of research. Quality research management reinforces scientific communities and improves the attractiveness and effectiveness of the service. In a QMS activities are properly planned and documented, operations are regulated by means of standard operating procedures, and the correct behavior, compliant with internal and external standards, is guaranteed by regular inspections. A QMS can help in giving proper attention to sensitive data and in correctly managing them, setting internal standard, organizing rules and forms and maintaining due control. Good Research Practices, as a quality management standard dedicated to the research environment, are at present not yet organized in an international reference text and consist of different prescriptive documents that are drafted and/or personalized by each research institution interested in aspects of quality management. Researchers can only refer to the WHO Handbook of Quality in Biomedical Research (WHO, 2006 ) as a guiding text to comply with generic quality principles. However, several references for designing a QMS can be found among international standards suitable for the management of a research laboratory: ISO 9001:2015, the most general quality management standard; ISO 17025, derived from the ISO9001 and dedicated to test and calibration laboratories; and the Good Laboratory Practice, mandatory international reference for development and testing of drugs and other substances intended for human and animal use (Lanati, 2018 ).
In this paper, we describe the choice, the design and the application of a QMS, called ReOmicS (Research Environment management system for Omics Sciences), at the Molecular Biodiversity Laboratory (MoBiLab), a NGS research infrastructure located in Bari (Italy) at the Institute of Biomembranes, Bioenergetics and Molecular Biotechnologies of CNR (CNR-IBIOM). MoBiLab is a research environment, fully equipped with operative platforms based on the most innovative NGS technologies and powerful resources for data storage and computational analysis, whose mission is to contribute to innovation with original studies. Moreover, CNR-IBIOM is involved in the construction of the national nodes of ELIXIR and LifeWatch Research Infrastructures (included in the ESFRI Roadmap), as well as in a substantial empowering of its infrastructural components for omics data production and analysis consistently scaling up the available instruments and facilities. The described experience could represent a scalable model to be applied to MoBiLab and to other research laboratories in order to ensure the highest levels of reliability, reproducibility and traceability of the results, a process that is also expected to foster their potential exploitation.
Material and methods
Considering the managerial aspects of the study, methods described in this section are tools used in quality and organizational management, occasionally modified to be adopted for the specific use of a research laboratory, such as MoBiLab.
Decision grid
The decision grid (or matrix) is a tool that supports a decision among many options. Once the aim of the decision is clearly defined, the criteria used to characterize each solution must be identified. Each criterion is given a weight (1 = lowest to 5 = highest) based on its importance in the final decision. The selected options among which the choice has to be made is then assessed with respect to their suitability to each criterion (1 = lowest to 5 = highest). The sum of the weighted assessments gives the final score for each proposal. Referring to Table 1 , the head of the table defines the aim of the decision. The options are listed in the columns. Criteria are listed in the rows and weighted in importance in column W. Each option is given a specific assessment (column A) with respect to the relative criterion, and an overall score, which is the product of the importance of the criterion and the given assessment. The final score for the proposal is given by the sum of all scores for each criterion.
SWOT analysis
The SWOT analysis represents, within a rationale, the influence exerted by some key factors on a goal in order to identify actions that reinforce the positive factors and counteract the influence of negative factors. The key factors in the analysis, whose initials give the name to the technique, are:
Strength: a resource that can be used to best achieve the goal;
Weakness: an obstacle to achieving the goal;
Opportunity: a favorable situation in the external context that favors the achievement of the objective;
Threat: an external, unfavorable situation in the external context that potentially hinders the achievement of the objective.
The analysis combines internal factors (strengths and weaknesses) and external factors (threats and opportunities), as well as positive aspects (strengths, opportunities) and negative aspects (weaknesses, threats). In this way SWOT Analysis allows defining strategies aimed at capitalizing on strengths, eliminating weaknesses, exploiting opportunities and mitigating threats.
Risk assessment
A risk assessment was performed on the main analytical process, according to the requirements of an ISO High Level Structure (HLS) and the ISO 9001:2015 standard. For each experimental step (Fig. 1 , first column), some pitfalls were identified (second column); each pitfall was assessed with respect to its Severity S (from 1 = low to 3 = high) i.e., how serious would be the consequence of an error on the final result, and Probability P (from 1 = low to 3 = high), i.e., how frequently a specific mistake has recently occurred (column 3 and 4, respectively). The Risk R in column 5 was then calculated as S × P for each pitfall identified. Risk values range from 9 (greatest) to 1 (lowest). Operations with risk R greater or equal to 4 are judged worthy of specific interventions to prevent errors, as recorded in column 6 “Solution”. Interventions are prioritized according to the level of risk. Referring to the legend of Fig. 1 , colors indicate the need for improvement actions: red for urgent, orange for medium and yellow for minor need, while green indicates no need for action.
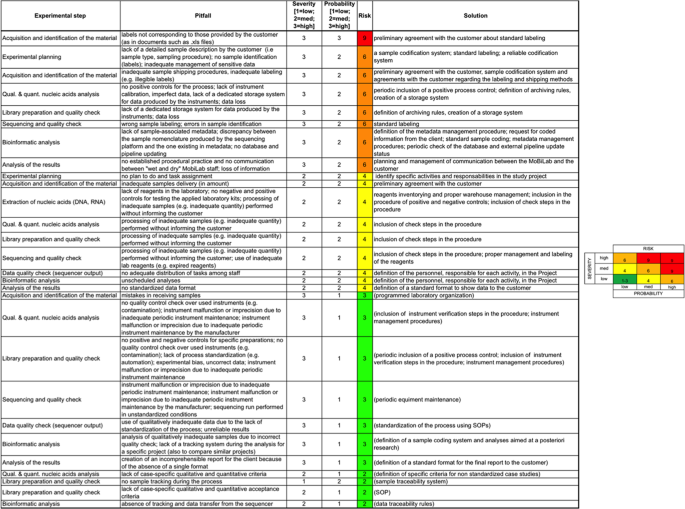
Risk assessment of the primary process at MoBiLab before the introduction of the ReOmicS. Pitfalls are placed in descending order according to R. Colors indicate the need for improvement actions: red for urgent, orange for medium and yellow for slight need, while green indicates no need for action
The SIPOC diagram, first outlined by Juran (Defeo and Juran, 2010 ) calling it TRIPOL, was then employed in the Six Sigma approach for analyzing a process. It is named SIPOC from the acronym of Supplier, Input, Process, Output, and Customer: the key elements of a process. A flowchart of the process is usually inserted in the third column “process” and for each step: input supplier, input needed by the operation, output of the operation and recipient of the output (customer) are listed.
The SIPOC-like flowchart is structured on the following categories:
source: the origin of the input
input: raw data, metadata, materials or samples needed by the study activities
process: steps of analysis and controls, logically linked
supervisor/person in charge: the supervisor and/or the person in charge to carry out each task of the previous column
output: the result/product of each task
procedure: the SOP describing the specific task
Due to the limited availability of data for the period preceding the introduction of the QMS, the metrics system has been necessarily simplified to two indicator of efficiency and three indicators of effectiveness:
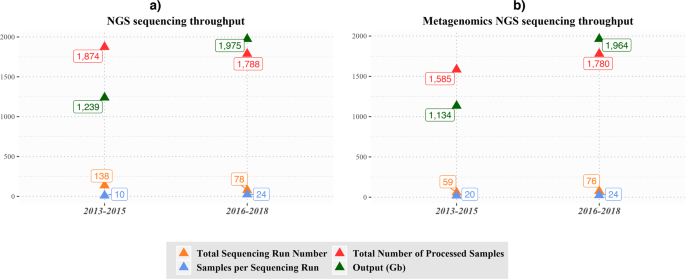
Efficiency indicator: analysis throughput. The values of four parameters (total number of processed samples, the average number of samples, the number of sequencing runs and the sequencing platform output), related both to all the MoBiLab applications ( a ) and only to Metagenomics ( b ), referring to the 3-year periods (2013–15 and 2016–2018), are shown
efficiency:
outcome of the updated risk assessment, compared with the initial one performed in designing the QMS. With respect to the initial Risk Assessment, three new columns have been added: “New-Probability” with the updated probability of the pitfall(s) considered, “New risk Assessment” with the updated value for the parameter R = SxP, and “Audit” recording reasons and considerations regarding the improvement. A paired one-sided Wilcoxon test was performed, to verify whether the risk estimation prior to the QMS adoption was significantly higher than the next.
evaluation of the analysis throughput in terms of the total sequencing run number, the total number of processed samples, the average number of samples per sequencing run and the run output (Gb). For each parameter, the values related to the two three-year periods were collected. The analyses were carried out on both the data derived from all the MoBiLab applications (Genomics, Transcriptomics and Metagenomics) (Fig. 2a ) and those produced only from Metagenomics (Fig. 2b ).
effectiveness:
number of publications and the related impact factor (base: 3-year period): we considered the peer-reviewed publications of three researchers 100% involved in MoBiLab research projects (Source: JCR-ISI Web of Knowledge; https://login.webofknowledge.com ). In case of co-authorship the journal was counted once, and the number of publications within the period has been calculated considering the average number of the papers per year. The scientific areas of the journals are Biochemistry, Genetics and Molecular Biology Medicine Agricultural and Biological Sciences, Immunology and Microbiology, Multidisciplinary Environmental Science, Neuroscience, Computer science, Mathematics.
scientific attractiveness , i.e., number of active external collaborations in MoBiLab publications (base: 3-year period) (Source: PubMed-National Library of Medicine; https://www.nlm.nih.gov/bsd/pubmed.html ): author’s affiliation to the papers published in the first 3-year period 2013–15 (before the introduction of the QMS) are compared with author’s affiliation referring to the 3-year period 2016–2018, after the progressive introduction of the QMS.
satisfaction survey: two separate surveys were prepared using the online tool SurveyMonkey ( https://it.surveymonkey.com ). The first (B9M5SNL), dedicated to all customers/collaborators about perceived quality, was sent by mail to 54 MoBiLab collaborators. The second (BDDF65F) was sent, in addition to the first, only to those customers/collaborators (25/54) who worked with MoBiLab in both three-year periods before and after the introduction of the QMS. A two week deadline was given. The analysis results were provided by the tool and further analyzed and elaborated by the team (Supplementary Material (SM) 1 and 2 ).
The indicators of efficiency measure the ability of the MoBiLab to increase productivity and reduce costs, while the effectiveness indicators show the quality and importance of the analysis of results.
Results and discussion
Choosing the qms standard.
To choose the best reference standard for the characteristics of MoBiLab, we compared three international standards: ISO 9001:2015, ISO 17025, and GLPs by means of a decision grid. The criteria for this choice were identified as:
compatible with regulation environment
suitable for customer’s requirements
oriented to R&D
focused on analytical process
low management costs
easy to fit in Laboratory activities
not linked to external third parties
suitable for expansion
The results are illustrated in Table 1 . Evaluating the criteria for choice, we considered that the “customers” of the MoBiLab research services are laboratories already working under the principles of GLPs and they could benefit from a rigorous and standardized work environment for the production of their data, as well as from a common management language and references. All criteria are listed in the first column of Table 1 . The GLP obtained the best assessment weighted on the importance of each criterion, mainly for their suitability for customer’s requirements, the lower cost, the independence from third party evaluations and the opportunity for development.
GLPs are mandatory in OECD countries for preclinical tests, but should be also considered as a reference for laboratory management systems, that can be referred to as “GLP-like” quality systems, although outside GLPs main scope. As textually described by Kauffmann et al. ( 2017 ) the application of GLPs principles to “omics” studies based on NGS, in a regulatory context, would serve the following goals (i) to promote the consistent quality and validity of data used for determining the safety of chemical products—a primary objective of the GLP principles (OECD, 1998 ); (ii) to promote transparent process descriptions and thus support the traceability of study results; and (iii) to facilitate the exchange of information and enhance the regulatory impact of ‘ omics data, when successfully used for hazard and risk assessment purposes. The use of GLP standard system helps the management of the human genomic data sharing respecting the privacy of the data, the reuse of the data under the current legislation and any further integration.
Design of ReOmicS
A director, three researchers, a technician and a quality consultant have been the working group that has met fortnightly via videoconference. The team assessed the choice of the GLPs as main reference for ReOmicS by means of a SWOT analysis, whose results are illustrated in Fig. 3 . Strengths and opportunities validated the choice of following GLPs as main reference against minor weaknesses and threats. In any case, as illustrated in what follows, some actions have been planned and taken in order to tackle some weaknesses and threats in a near future.
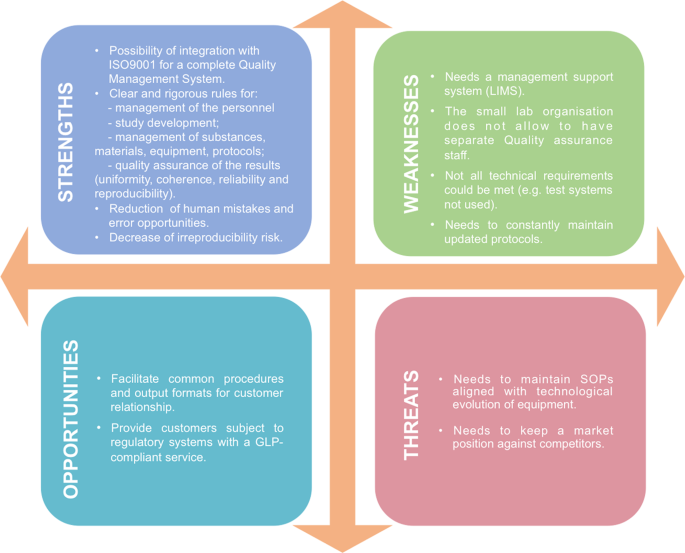
SWOT analysis diagram. Strength, weaknesses, opportunities and threats have been evaluated for the choice of Good Laboratories Practices (GLPs) as QMS reference
The team then started the design of ReOmicS from the risk analysis of the main NGS process, based on a standard Risk Assessment. With this analysis, the team aimed at identifying the laboratory’s weaknesses in order to address them with specific interventions when developing the QMS.
Errors and problems experienced during the last 3 years were collected and attributed to the relevant process steps. Each problem was then assessed with respect to severity S and probability P. The risk R = S × P associated with each pitfall determined the need and the priority of a specific operating procedure. Figure 1 summarizes these results.
Major problems to be addressed when defining Standard Operating Procedures (SOPs) were identified in the fields of communication with the customer; traceability of samples; warehousing, archive, metadata and database management; planning; organizational structure; and controls and checks. No needs for specific instructions were envisaged for problems with R less than 4. Major needs and related priority were taken into account in the drafting phase of the SOPs.
As a first step, in order to define the internal context, the MoBiLab organizational structure was designed according to the GLP requirement taking into account the dimension and the constraints of the research institution: the major roles, such as Director, Study Director, Laboratory Manager, Principal Investigator, Archivist et al, were identified and assigned to laboratory staff (Fig. 4 ). Once defined the roles and responsibilities, the team analyzed the laboratory internal processes, identifying primary and support processes (see Table 2 ). The team outlined the main (primary) process by means of a Supplier-Input-Process-Output-Customer (SIPOC)-like flowchart, which includes the person in charge of the activity and the related documented information (SOPs, and records) (see Fig. 5 ).
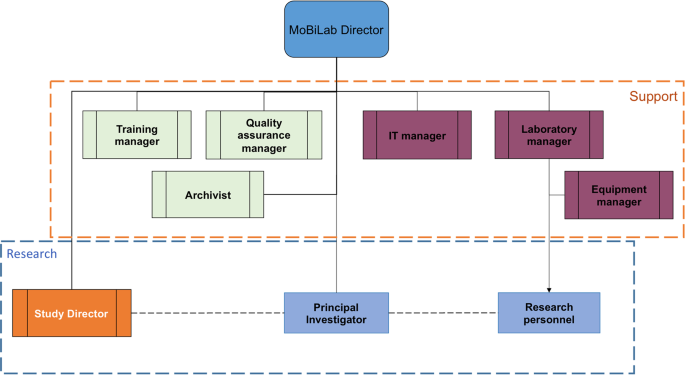
Organizational Chart. Under the supervision of the MoBiLab director, resources are divided into two main groups: (i) managerial and technical support and (ii) research and experimentation
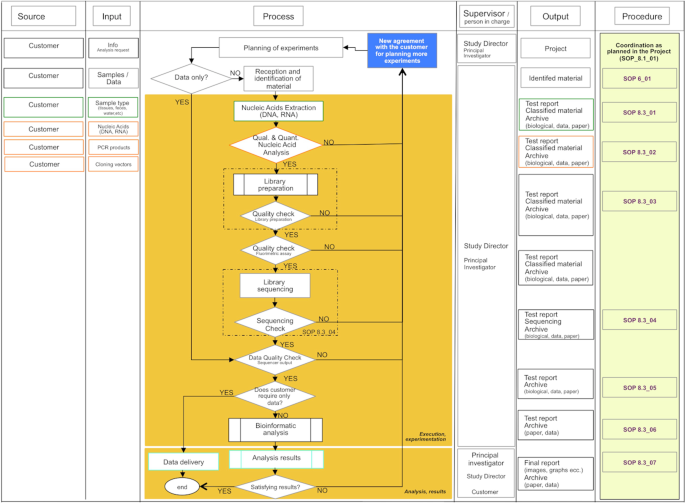
SIPOC-like flowchart of the Study management. Columns collect inputs and related providers, main flow-chart with tasks, person in charge/supervisor for every task, output and prescriptive document (SOP) for each task. The flowchart is divided into two main sections: Execution/experimentation and Analysis/results
This SIPOC-like chart acted as the backbone of the SOP of the management of the Study: moreover, most steps of the study process, together with the results of the risk assessment, led to the identification of technical/scientific procedures , these were then accompanied by attachments describing technical details as required. After having identified the SOPs required for the operational processes , researchers were provided with a template and with the instructions to draft them. In parallel, management procedures were defined by the whole team, drafted, and supported by flowcharts and other quality tools whenever needed (e.g., SIPOC). SOPs were ranked by priority, driven by the risk assessment results; few SOPs required by GLPs were not considered since were not needed for our specific research activities (e.g., management of test systems). The management support processes were described by SOPs and were, as far as possible, compliant with GLP requirements.
Following the results of the SWOT analysis (where this aspect was judged as a weakness) and in order to ensure the highest level of reliability, reproducibility and traceability of the results, the team also planned to develop and optimize a LIMS (Laboratory Information Management System) platform for managing all the laboratory activities through a suite of integrated modules, in collaboration with an Italian ICT company. The platform will be structured starting from the SIPOC-like flowchart for the management of the study and the modules will be developed and customized in agreement with the SOPs.
The structure of the SOP list (Table 2 ) is directly related to the allocation of responsibilities in the laboratory and conforms to the organization of the management of the studies (Fig. 5 ): the primary and the scientific SOPs are the responsibility of the researchers and technicians operating in the MoBiLab under the supervision of a Principal Investigator, nominated by the director of the study. At the same time, management and technical support SOPs govern staff indirectly involved in the project, caring for an environment suitable for the studies. The split into two different areas, research and support, is clearly represented in the organization chart (Fig. 4 ).
Indeed, the MoBiLab belongs to a public research institution whose mission is to achieve scientific outputs in national and international funded project. Staff organization is related to the skills required and tasks assigned in the study program. For this reason, in the primary SOP the study corresponds to the project and the director of the study refers to the scientist responsible of the project. Only in a few cases the MoBiLab is working as a service provider, producing genomic and data analysis directly commissioned by external customers. For all these reasons, the scientific SOPs can evolve by integrating new requirements highlighted by customers or scientific partners.
Following all these considerations, the ReOmicS was structured as illustrated in Table 3 . Each SOP is structured according to a general template with the following sections:
-definitions, terms and acronyms
-references
-activities and responsibilities
-materials and equipment
-safety rules
-history of revisions (change register)
Results of the application of ReOmicS
The system has been progressively introduced, starting in 2016 from the scientific SOPs. At present the application of ReOmicS is underway with few limitations, due to the fact that processes and their management are challenging to pursue without IT support. This is also the reason that a LIMS is being developed. For example, audits have been conducted in limited form, mainly focusing on the suitability and effectiveness of the quality management system. Despite these limitations, the MoBiLab, in the 3-years following the introduction of common rules and references, is experiencing a concrete improvement, as testified by the positive trend of the five metrics chosen: risk assessment, analysis throughput, number/quality of publications, external collaborations and satisfaction survey.
The first metric chosen for the assessment of efficiency is the comparison between the outcomes of the risk evaluation before and after the introduction of ReOmicS (Fig. 6 ). The results show a statistically significant improvement ( p = 0.0005 ) with respect to the initial assessment for the application of technical SOPs, while that of management SOPs is still limited. A better compliance to the QMS is expected in the future, mainly in the areas of study-planning, assignment of tasks and storage control.
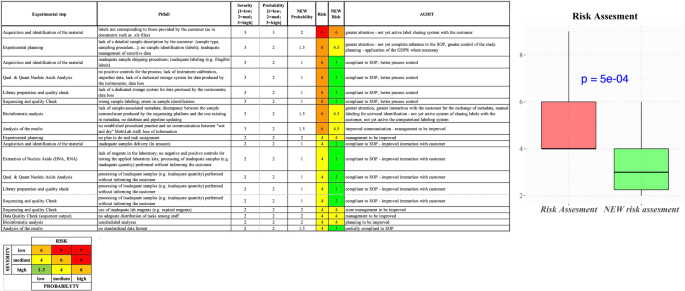
Efficiency indicator. Efficiency is evaluated by means of the update of the risk assessment performed in the initial phase of the project, before the application of ReOmicS
A second metric has been chosen to evaluate the efficiency, i. e. the overall performance of MoBiLab, in terms of total number of processed samples, number of samples/sequencing runs, number of sequencing runs and sequencing platform output (GigaBase). The data shown in Fig. 2 compare the 3-year periods, 2013–2015 and 2016–2018. Considering all the MoBiLab applications (Genomic, Transcriptomics and Metagenomics), the analyzed parameters, except the number of sequencing run, show an improvement after the introduction of ReOmicS (Fig. 2a ). At the same time, it is important to underline that, despite the reduction in the number of sequencing runs due to a forced six months interruption of MoBiLab activities for logistical issues, the total number of samples processed and the average number of samples increased together with the platform output. In an NGS analysis, we can speculate that maintaining a high throughput whilst at the same time increasing the number of samples, represents an important laboratory challenge. These results can be ascribed to operator’s competences and training, but also to the improvement of the management of the process and the control of the analysis provided by the QMS. The efficiency of the laboratory was therefore assessed by taking into consideration the amount of data produced, referring mainly to Metagenomics analysis, the most requested application at MoBiLab (approximately 52% of the total amount of analysis performed) (Fig. 2a ). Overall, a positive increase was shown (Fig. 2b ) by all the parameters during the 3-year period 2016–2018.
To assess the influence of the QMS on the effectiveness of the MoBiLaB, three metrics have been chosen. The first one is the number of publications and the related Impact Factor (IF). In Table 4 , data referring to the first 3-year period 2013–15 (before the introduction of ReOmicS) are compared with data from the 3-year period after the progressive introduction of the QMS, 2016–2018. Table 4 also shows the average IF values obtained for each period. The lowest and the highest values of journal IF were excluded from the analysis. The QMS improved also downstream processes as demonstrated by the increased number of papers published in peer review journals. The number of published papers has grew from 13 to 23 in the last 3 years. Indeed, the number of published papers doubled even if the IF increase is not significant (data not shown). The second metric chosen for the assessment of effectiveness of ReOmicS, i.e., number of active external collaborations in MoBiLab publications (based on a 3-year period), shows the attitude of the laboratory to be a national and world leading scientific NGS laboratory and to be an enabling facility in the support of science. Table 4 shows the average number of external authors in the two 3-year periods. The total number of authors for each paper did not significantly change in the two periods considered, nor did the number of authors with an Italian affiliation. On the other hand, the number of general affiliations increased by a third and the number of international collaborations almost tripled.
The third effectiveness metric measures the satisfaction of customers and collaborators who had the opportunity to take advantage of the analysis service of MoBiLab, by means of two separate surveys: the first one dedicated to all customers/collaborators about perceived quality and the second to customers/collaborators who worked with MoBiLab in both three-year periods before and after the introduction of the QMS. The first survey was sent to 54 collaborators and 25 answers were collected. The second survey was sent to 25 collaborators, obtaining 12 answers. Of these last 12, 6 were discarded for inconsistency in answers to single questions, for this reason only a qualitative evaluation can be made. Results of both surveys are illustrated in Fig. 7 and show a good level of satisfaction from customers and collaborators, together with a demonstrable improvement of perceived quality after the introduction of ReOmicS.
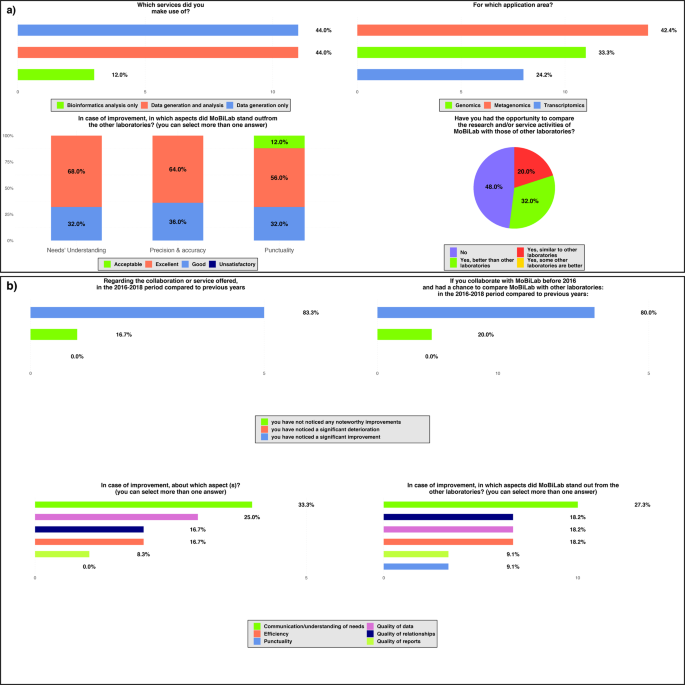
Customer satisfaction. A qualitative measure of the customer satisfaction was evaluated analyzing the results obtained from the two surveys: the first one dedicated to all customers/collaborators about perceived quality ( a ) and the second to customers/collaborators who worked with MoBiLab in both three-year periods before and after the introduction of the QMS ( b )
As a final consideration on metrics, it was difficult to gather complete and detailed data regarding the projects developed in the years 2013–2015 for comparison with those pertaining to the years 2016–2018 because, before the ReOmicS introduction, a lot of the information was scattered among different research environments within and outside the MoBiLab. Since this data unavailability was judged unacceptable in maintaining due control on the work of the laboratory and on the improvement process, a more complete set of metrics has been studied which will be integrated in the planned LIMS (Table 5 ).
Main deviations from GLPs
Not all the requirements of GLP can be accomplished in the development of ‘omics’ studies, as clearly shown by Kauffmann et al. ( 2017 ). The limitations involve technical aspects, but in our case have had an impact also on the organizational requirements.
The first requirement that cannot be met is the management of test systems, because in the NGS procedures they are not used. External databases are used as reference and these are validated by the well-known mechanism of peer review. This is in partial disagreement with the GLP direction about data management and validation, but is common practice in genomics.
As far as data storage is concerned, MoBiLab depends on the servers made available by the INFN. The commercial agreement with INFN is stipulated by the IBIOM Institute: so far MoBiLab is not in the position to insert specific GLP requirements. With the planned development of the LIMS, new conditions and agreements more suitable for GLP compliance about data management will be implemented.
As an example of GLP procedure requirements that needed a new definition, the compliance statement required by the GLP is intended not towards the GLP, but to ReOmicS QMS itself.
As an example of organizational GLP requirements that could not be met, the dimension of the research unit and specifically of the laboratory is an issue when trying to identify an independent quality assurance structure. However, within the laboratory, a person has been appointed for the quality assurance tasks described in the dedicated SOP with support from an external quality consultant for methodological matters or concerns. Furthermore, the title of Principal Investigator (PI), which in the GLP is an individual who, for a multi-site study, acts on behalf of the Study Director, is known here as “research project manager”, as in the most common meaning for research laboratories working on funded projects.
Moreover, the role of archivist has not yet been allocated, since the planned introduction of a LIMS will ease the task of archiving and will allow a clearer allocation of responsibilities.
Conclusions and future perspectives
We can demonstrate that the application of a QMS, giving precise references for research management, introducing controls—thus increasing both result reliability and reducing opportunities for error—and promoting the efficiency in planning, conducting, analyzing and reporting on the processes, represents a valid tool for overcoming these difficulties and, at the same time, an opportunity for significant improvement for a research laboratory.
In the experience illustrated, GLPs—among different quality management standards—was judged most suitable for the purpose of the MoBiLab QMS, when implemented in the aspects appropriate to the characteristics of the laboratory. The development of the QMS was performed making use of quality techniques and methods to ensure a lean and rigorous process.
Among the positive outcomes that can be ascribed to the adoption of the QMS, we can stress an evident increase in the efficiency of the laboratory, evaluated by the decrease of the risks and of the errors occurring during analysis workflow. Moreover, staff was more motivated thanks to a better organization of the team and an acknowledgment of their competences. The effectiveness was also improved, increasing the number of collaborators, the customer confidence and the availability of a databases organized for future investigations.
Indeed, the experience of QMS in MoBiLab demonstrates that the performances of the analysis and number of the primary “products” of academic research—publications—increases after QMS introduction, together with the appeal of the laboratory as witnessed by more active international collaborations. Number of publications in high-impact factor journals, number of citations, and number of opportunities of excellent scientific collaborations indicate how the laboratory aims to be a national and world scientific leading infrastructure and an enabling facility supporting science.
Experimental and service data (throughput analysis) prove that the laboratory, thanks to the ReOmicS introduction, is increasing its ability to provide high quality scientific data and associated services.
The results of the surveys give evidence of a positive user satisfaction regarding support and collaboration, not only in reference to the facility, but also to the staff employed in the MoBiLab. Good opinions were also expressed comparing MoBiLab with other similar laboratories. We have planned to run such surveys periodically with different audiences to assess satisfaction, achievements, collaborations and expectations.
As future perspectives, scientific, economic and technological impact could be assessed reporting, for instance, the training of skilled researchers, the development of new methodologies and software for NGS, the growth of network and social interactions or the creation of a new firm (e.g., a spin-off).
As proposed by the OECD ( 2019 ) for the assessment of Research Infrastructures, the metrics chosen to evaluate efficiency and effectiveness of MoBiLab before and after the introduction of the QMS can be presented as possible indicators to demonstrate the impact of an NGS research and analysis laboratory.
The quantifiable impacts captured through quantitative metrics (number of publications, citations…), as well as the non-quantifiable metrics obtained by dedicated surveys, are also helpful when approaching an economy and policy impact analysis of the genomic and bioinformatic research.
Although the ReOmicS QMS did not incorporate all requirements of the GLPs, and its application is still to be completed with respect to a small number of controls and the complete traceability of results, the positive outcomes already obtained are also due to the gradual increase in confidence by laboratory staff with the quality approach and to the early adoption of the standard protocols described by the technical SOPs.
This experience and the results obtained prove that a NGS laboratory, and therefore any other research laboratory, can benefit from the introduction of a quality framework, if properly translated from the generic standards and adapted to the specific requirements of a research environment. The metrics and the indicators showed in this study will be followed up in the implementation of the MoBiLab thanks to the grant received by the Italian Research Minister to empower facilities and equipment of this laboratory.
The standards of the ISO 900 family are often declined in sector-specific versions (e.g., the already mentioned ISO 17025 for testing and calibration laboratories, ISO/TS 16949 for automotive, ISO 13485 for medical devices), adding requirements unique to a particular application. The ReOmicS has this precise purpose: to detail the requirements for the management and control of a generic research laboratory. To this end, as a first choice, we took as a reference the GLPs, whose adequacy for a research laboratory in ‘omics—despite some specific exclusions—is attested by Kauffman et al. ( 2017 ). The directions of the GLP are about management and control of the study and experimentations (the primary process), and of the tools and materials used, as well as the rules for reporting and archiving data, samples and documents (which are only a few of the support processes envisaged by the ISO9001 standard). In a general sense, these aspects are common to all research laboratories, regardless of the field of application. Starting from the structure of a GLP-like QMS, the ReOmicS can be completed with the specific requirements of ISO 9001 on the parts not governed by the GLP, such as context and risk assessment, supplier management and improvement process. In this light, we strongly believe that the ReOmicS can be taken as a reference for any type of research laboratory.
Indeed, the ReOmicS GLP-like quality system is expected to evolve into a complete quality system according to ISO9001 to achieve the specific certification. For this reason, a risk assessment—both strategic and operational has already been performed, and several SOPs have already been arranged to comply with main requirements of ISO 9001.
In the future, quality management would be streamlined by the introduction of the IT tool (LIMS). This development is expected to foster the potential exploitation of the NGS activities of the MoBiLab. The evolution of the ReOmicS is then expected to follow the Lean Production (a.k.a. Toyota Production System), which is a wide-ranging methodology developed in manufacturing to reduce waste and improve product quality (Womack et al., 1991 ) and recently used also in the research environment (Barnhart, 2013 ). The Lean approach will be strictly connected with the LIMS system, leveraging its features to ensure the best control. The GLP-like system and the Lean approach will allow the MoBiLab to improve its efficiency, limiting wastage of time and materials, and reducing opportunities for error, at the same time enhancing the effectiveness traceability and reproducibility of results.
Data availability
All data generated or analyzed during this study are included in this published article.
Baker M (2016) 1500 scientists lift the lid on reproducibility. Nature 533:452–454. https://doi.org/10.1038/533452a
Article ADS CAS Google Scholar
Barnhart T (2013) Creating a lean R&D system. CRC Press, Boca Raton
Google Scholar
Cargill CF (2011) Why standardization efforts fail. J Electron Publ. https://doi.org/10.3998/3336451.0014.103
Corpas M, Kovalevskaya NV, McMurray A, Nielsen FGG (2018) A FAIR guide for data providers to maximise sharing of human genomic data. PLOS Comput Biol 14(3):e1005873. https://doi.org/10.1371/journal.pcbi.1005873
Article ADS CAS PubMed PubMed Central Google Scholar
Defeo J, Juran JM (2010) Juran’s quality handbook: the complete guide to performance excellence 6/e McGraw-Hill Professional, US
Dirnagl U, Kurreck C, Castaños-Vélez E, Bernard R (2018) Quality management for academic laboratories: burden or boon? Professional quality management could be very beneficial for academic research but needs to overcome specific caveats. EMBO Rep. https://doi.org/10.15252/embr.201847143
Article Google Scholar
Endrullat C, Glökler J, Franke P, Frohme M (2016) Standardization and quality management in next-generation sequencing. Appl Transl Genomics. https://doi.org/10.1016/j.atg.2016.06.001
Kauffmann HM, Kamp H, Fuchs R, Chorley BN, Deferme L et al. (2017) Framework for the quality assurance of’omics technologies considering GLP requirements. Regul Toxicol Pharmacol. https://doi.org/10.1016/j.yrtph.2017.10.007
Lanati A (2018) Quality management in scientific research-challenging irreproducibility of scientific results. Springer, Cham, Switzerland
Book Google Scholar
Stephens ZD, Lee SY, Faghri F, Campbell RH, Zhai C et al. (2015) Big data: astronomical or genomical? PLoS Biol. https://doi.org/10.1371/journal.pbio.1002195
Tiwari R, Nain L, Labrou NE, Shukla P (2018) Bioprospecting of functional cellulases from metagenome for second generation biofuel production: a review. Crit Rev Microbiol. https://doi.org/10.1080/1040841X.2017.1337713
UNDP/World Bank/WHO Special Programme for Research and Training in Tropical Diseases & Scientific Working Group on Quality Practices inBasic Biomedical Research (2006) Handbook: quality practices in basic biomedical research/prepared for TDR by the Scientific Working Group on Quality Practices in Basic Biomedical Research. World Health Organization. https://apps.who.int/iris/handle/10665/43512
Wiese L, Schmitt AO, Gultas M (2018) Big data technologies for DNA sequencing. Springer, Basel
Wilkinson MD, Dumontier M, Aalbersberg IJ, Appleton G, Axton M et al. (2016) The FAIR Guiding Principles for scientific data management and stewardship. Sci Data. https://doi.org/10.1038/sdata.2016.18
Womack JP, Jones DT, Roos D (1991) The machine that changed the world. Harper Perennial, New York
OECD (1998) Organisation for economic co-operation and development principles on good laboratory practice (As Revised in 1997). OECD principles of good laboratory practice and compliance monitoring. No. 1. ENV/MC/CHEM(98)17
OECD (2019) Reference framework for assessing the scientific and socio-economic impact of research infrastructures. OECD Science, Technology and Industry Policy Papers, No. 65
Download references
Acknowledgements
This work was supported by the projects “Lifewatch” Roadmap ESFRI and “OMICS4FOOD”, cod. 1JLZKDPOR, Avviso “Innonetwork” A.D. n.124 del 16/10/2017, Puglia FESR-FSE 2014–2020 Azione 1.6. The authors thank Gabrielle Nasca Quadraccia for critical reading and English revision of the paper.
Author information
These authors contributed equally: Antonella Lanati, Marinella Marzano
Authors and Affiliations
Valore Qualità, 27100, Pavia, Italy
Antonella Lanati
Istituto di Biomembrane, Bioenergetica e Biotecnologie Molecolari (IBIOM), CNR, 70126, Bari, Italy
Marinella Marzano, Caterina Manzari, Bruno Fosso, Graziano Pesole & Francesca De Leo
Dipartimento di Bioscienze, Biotecnologie e Biofarmaceutica, Università degli Studi di Bari “Aldo Moro”, 70126, Bari, Italy
Graziano Pesole
You can also search for this author in PubMed Google Scholar
Corresponding authors
Correspondence to Antonella Lanati or Francesca De Leo .
Ethics declarations
Competing interests.
The authors declare no competing interests.
Additional information
Publisher’s note: Springer Nature remains neutral with regard to jurisdictional claims in published maps and institutional affiliations.
Supplementary Information
Rights and permissions.
Open Access This article is licensed under a Creative Commons Attribution 4.0 International License, which permits use, sharing, adaptation, distribution and reproduction in any medium or format, as long as you give appropriate credit to the original author(s) and the source, provide a link to the Creative Commons license, and indicate if changes were made. The images or other third party material in this article are included in the article’s Creative Commons license, unless indicated otherwise in a credit line to the material. If material is not included in the article’s Creative Commons license and your intended use is not permitted by statutory regulation or exceeds the permitted use, you will need to obtain permission directly from the copyright holder. To view a copy of this license, visit http://creativecommons.org/licenses/by/4.0/ .
Reprints and permissions
About this article
Cite this article.
Lanati, A., Marzano, M., Manzari, C. et al. Management at the service of research: ReOmicS, a quality management system for omics sciences. Palgrave Commun 5 , 75 (2019). https://doi.org/10.1057/s41599-019-0283-0
Download citation
Received : 28 February 2019
Accepted : 12 June 2019
Published : 09 July 2019
DOI : https://doi.org/10.1057/s41599-019-0283-0
Share this article
Anyone you share the following link with will be able to read this content:
Sorry, a shareable link is not currently available for this article.
Provided by the Springer Nature SharedIt content-sharing initiative
Quick links
- Explore articles by subject
- Guide to authors
- Editorial policies

Click through the PLOS taxonomy to find articles in your field.
For more information about PLOS Subject Areas, click here .
Loading metrics
Open Access
Peer-reviewed
Research Article
The lab management practices of “Research Exemplars” that foster research rigor and regulatory compliance: A qualitative study of successful principal investigators
Roles Conceptualization, Formal analysis, Funding acquisition, Investigation, Methodology, Project administration, Writing – original draft
* E-mail: [email protected]
Affiliation Division of General Medical Sciences, Washington University School of Medicine, St. Louis, Missouri, United States of America

Roles Formal analysis, Investigation, Project administration, Writing – review & editing
Roles Conceptualization, Methodology, Writing – review & editing
- Alison L. Antes,
- Ashley Kuykendall,
- James M. DuBois
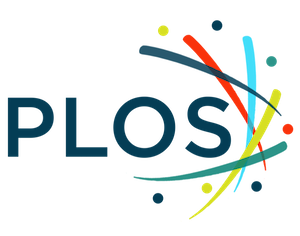
- Published: April 24, 2019
- https://doi.org/10.1371/journal.pone.0214595
- Reader Comments
Introduction
Conducting rigorous scientific inquiry within the bounds of research regulation and acceptable practice requires a principal investigator to lead and manage research processes and personnel. This study explores the practices used by investigators nominated as exemplars of research excellence and integrity to produce rigorous, reproducible research and comply with research regulations.
Using a qualitative research design, we interviewed 52 principal investigators working in the United States at top research universities and the National Institutes of Health Intramural Research Program. We solicited nominations of researchers meeting two criteria: (1) they are federally-funded researchers doing high-quality, high-impact research, and (2) have reputations for professionalism and integrity. Each investigator received an initial nomination addressing both criteria and at least one additional endorsement corroborating criteria 2. A panel of researchers and our research team reviewed the nominations to select finalists who were invited to participate. The cohort of “Research Exemplars” includes highly accomplished researchers in diverse scientific disciplines. The semi-structured interview questions asked them to describe the routine practices they employ to foster rigor and regulatory compliance. We used inductive thematic analysis to identify common practices.
The exemplars identified a core set of 8 practices and provided strategies for employing them. The practices included holding regular team meetings, encouraging shared ownership, providing supervision, ensuring adequate training, fostering positive attitudes about compliance, scrutinizing data and findings, and following standard operating procedures. Above all, the use of these practices aim to create a psychologically safe work environment in which lab members openly collaborate to scrutinize their work and share in accountability for rigorous, compliant research.
Conclusions
Researchers typically receive limited systematic training in how to lead and manage their research teams. Training and education for principal investigators should include essential leadership and management practices and strategies that support doing high-quality research with integrity.
Citation: Antes AL, Kuykendall A, DuBois JM (2019) The lab management practices of “Research Exemplars” that foster research rigor and regulatory compliance: A qualitative study of successful principal investigators. PLoS ONE 14(4): e0214595. https://doi.org/10.1371/journal.pone.0214595
Editor: Zubin Master, Mayo Clinic, UNITED STATES
Received: October 24, 2018; Accepted: March 17, 2019; Published: April 24, 2019
Copyright: © 2019 Antes et al. This is an open access article distributed under the terms of the Creative Commons Attribution License , which permits unrestricted use, distribution, and reproduction in any medium, provided the original author and source are credited.
Data Availability: Interview data cannot be shared publicly because participants were promised confidentiality. There is the potential for the identities of participants to be revealed through indirect personally identifying information, even if direct identifiers are removed. The manuscript includes relevant, de-identified excerpts. Upon request, and subject to required approval by relevant ethics committees, transcript data will be made available after removal of direct identifiers and details that may indirectly compromise confidentiality of the participants. Data requests may be made to Alison L. Antes ( [email protected] ). Data access requests may also be directed to the Joint Research Office for Contracts ( [email protected] ), Office of the Vice Chancellor for Research, Washington University in St. Louis.
Funding: This study was funded by the National Human Genome Research Institute (K01HG008990 to ALA). This work also received support from the U.S. Office of Research Integrity (IR170030-01-00 to JMD) and a National Center for Advancing Translational Sciences Award (UL1 TR002345). The funders had no role in study design, data collection and analysis, decision to publish, or preparation of the manuscript.
Competing interests: The authors have declared that no competing interests exist.
The United States invests billions of dollars in research annually [ 1 – 3 ]. An array of stakeholders have an interest in how this research is carried out [ 4 ]. Funding agencies and the public are primarily interested in advancing scientific knowledge and solving real-world problems. Institutions have an interest in attracting the highest caliber students and faculty to advance their missions, and a record of high achievement in research serves this end. Investigators are interested in research productivity, which allows them to secure additional resources to advance their research agendas. Junior researchers training with investigators want to learn the skills for success to advance their careers and research goals. Other researchers in the scientific community have an interest in the trustworthiness of research findings.
Addressing these stakeholder interests in the research enterprise requires attending to several dimensions of research ethics—or “doing good research in a good manner”—at the institutional level and within research labs and teams [ 4 , 5 ]. In their labs, principal investigators (PIs) must foster research rigor and reproducibility, compliance with research rules and regulations, and effective workplace relationships. Increasingly, researchers are also called upon to ensure the social value of their research. These dimensions are important to justify the financial investment in research and the risks posed to animal and human subjects.
Long-standing scholarly study of science has presented key values and norms that guide the practice and ethics of research (e.g., accuracy, disinterestedness, honesty, trust, respect, and fairness) [ 6 ]. Other work suggests key characteristics of researchers (e.g., persistence and openness) [ 7 , 8 ] and has elucidated situational influences (e.g., mentoring and competition) on scientific achievement [ 9 ]. It is in the day-to-day practice of science that individual characteristics, situational factors, and professional values operate to influence the behavior of researchers. However, recognition that the daily conduct of research often presents tensions between the ideals of science and actual practice [ 10 , 11 ] has led, in part, to education in the responsible conduct of research as a key remedy to these issues [ 12 ]. However, reconciling these tensions and realizing optimal research practices also requires attention to the social and human dynamics within research labs.
Increasing attention to the interpersonal dynamics of effective research is reflected in the emergence of the “science of team science,” examining how research groups effectively perform research and foster interpersonal dynamics that facilitate good science [ 13 , 14 ]. Concurrently, concerns about reproducibility and transparency in science [ 15 ] and failures to establish healthy research work environments [ 16 – 18 ] reflect additional attention to “doing good research in a good manner.” We contend that the decision-making and behavior of PIs, as the leaders of research teams, are at the heart of ensuring the best possible execution of science.
Thus, the purpose of this study was to identify the practices that “Research Exemplars”—PIs nominated by their colleagues as exemplary scientists and models of professionalism and integrity—employ to foster rigorous research and regulatory compliance within their teams. Our aim was to identify concrete approaches and strategies they employ to manage the responsible conduct of research in their groups. We apply a leadership and management framework to address our two primary research questions.
RQ1: What routine practices and habits do research exemplars utilize within their research teams to foster rigor and reproducibility? RQ2: What routine practices and habits do research exemplars utilize within their research teams to foster research compliance?
Leadership and management are necessary when the efforts of people must be coordinated to achieve objectives [ 19 ]. When work is complex and innovative, and when it is high stakes, leadership is particularly critical to performance outcomes [ 20 , 21 ]. Although these are central characteristics of the work of researchers, the role of PIs as leaders and managers in producing the highest quality research has received limited attention [ 22 ].
We define management and leadership in the following manner. Management focuses on how to do work; managers align processes and people so that work is consistent and efficient [ 23 ]. Leadership focuses on what to do and why ; leaders articulate a vision and inspire people to work well together to do complex, innovative work [ 24 ]. In both roles, leaders and managers exercise influence; they influence people or work processes to achieve shared objectives [ 23 ]. An integrated model suggests the distinction between leadership and management roles is less important than achieving the right balance and enacting leadership behaviors and management structures that support efficiency and process reliability, human relations, innovation and adaptability [ 24 ].
The research setting may be one of the most complex domains in which to engage in leadership and management. PIs are often simultaneously required to control and monitor details to ensure accurate work, clarify rules to promote compliance with policies and standards, and emphasize hard work while striking a pace that balances speed and accuracy. They must also foster the engagement of staff and trainees, develop, motivate, and support personnel, and coordinate their team members’ shared efforts. Furthermore, PIs must innovate and anticipate long-term scientific agendas and compete successfully for funding [ 25 ]. Thus, a PI must engage in a variety of complex, multi-faceted behaviors, and know how to adapt to the tensions inherent in their roles—e.g., the need for control but also creativity; the need for detailed oversight of projects but also staff engagement and autonomy; the need for accurate but also quick work. Effectively executing these demands as a PI requires a deep behavioral repertoire and the ability to execute behaviors at the right time and in the right fashion [ 25 ].
The managerial and leadership behaviors identified in empirical research generally fit into two key domains. Task-oriented behaviors focus on providing structure and direction, for example clarifying roles, setting objectives, planning work, and coordinating tasks [ 24 ]. Relationship-oriented behaviors focus on ensuring the welfare and engagement of personnel, for example providing support, showing appreciation, providing mentoring, sharing in decision-making, and encouraging teamwork [ 24 , 26 ]. Other leadership behaviors include those required for innovation, adaptability, and change, such as identifying new opportunities, building relationships with potential collaborators, and anticipating how a proposal will be evaluated by key constituencies [ 27 ].
It was our aim to identify the different practices and habits engaged in by PIs to foster high-quality research. We anticipated managerial, “task-oriented” behaviors to be particularly important in the domains of research rigor and compliance, as they require attention to detail, accuracy, consistency, and careful coordination of work activities [ 28 – 30 ].
Study design and participants
We employed an open-ended, qualitative methodology because we aimed to identify and relay concrete strategies employed by investigators to lead responsible research. We used purposive sampling to identify researchers representing a range of scientific disciplines who were exemplary in their scientific achievements and reputations for professionalism and integrity. Colleagues of the researchers provided initial nominations and secondary endorsements of their professionalism and integrity. A panel reviewed the nominations, and our research team reviewed their feedback and all of the nominations to select finalists. We conducted in-depth, semi-structured interviews with the participants we collectively refer to as “Research Exemplars.” The Washington University Institutional Review Board provided ethical approval for the study (IRB# 201601121).
Nominations
We solicited nominations from the Carnegie-classified “highest” and “higher” research activity universities, accredited medical schools and schools of public health, and the National Institutes of Health (NIH) Intramural Research Program in the United States. We sent an initial email announcement and three reminder emails to nearly 1,500 academic deans, department chairs, and institutional research administrators (e.g., Vice Presidents for Research, Research Integrity Officers, Scientific Directors). We also emailed the PIs at institutions affiliated with the National Center for Advancing Translational Sciences’ Clinical and Translational Science Awards program [ 31 ]. The announcements invited the recipients to make a nomination and to forward the call for nominations to their colleagues.
The announcement indicated we sought nominations of federally-funded researchers in any career stage who lead a research lab or team. We indicated that the nominees must meet two criteria: (1) perform high-quality, high-impact research in any scientific discipline, and (2) have an outstanding reputation for professionalism and integrity. The email provided a web-link to additional information about the project and a nomination form. We indicated we would invite finalists to participate in a 1-hour interview about the practices they employ to lead their teams, and we would send them a plaque and post their biography on the project website ( https://integrityprogram.org/exemplar-project/ ).
The nomination form asked the nominators to describe the nominee’s high-quality, high-impact federally-funded research program. Next, we asked for a specific description of how the nominee exemplified professionalism and integrity as a researcher. Finally, we asked the nominator to discuss how confident they were that their view was widely held by the researcher’s colleagues.
We also asked nominators to submit the nominee’s CV, and the contact information for three individuals who work closely with the researcher who we could contact for a secondary endorsement of the nominee. To obtain some verification that others held the same view of the nominee, we sent emails to the three individuals identified asking them to endorse the nominee if they agreed the researcher exemplified professionalism and integrity. The endorsers completed the two questions about how the researcher demonstrated professionalism and integrity and whether this view was widely held.
We asked the nominators and endorsers to characterize the nature and length of their relationship with the nominee. The nominators knew the nominees, on average, for 9.96 years (SD = 6.37) and 80% were institutional administrators, academic deans, or department chairpersons. Endorsers, on average, knew the nominees for 14.62 years (SD = 8.95) and 59% were faculty collaborators, and the remaining were department chairpersons, program directors, or trainees. We received 81 nominations, and 74 received at least one additional endorsement, qualifying them to move to the review panel. Before panel review, we emailed the nominees to confirm their interest in participating in the project if selected as finalists. All but one individual responded and agreed to participate, thus 73 nominations were sent to the panel for review.
Panel review
The panel of volunteer reviewers consisted of 10 federally-funded researchers. We matched the reviewers’ scientific disciplines to the nominees’ fields, and two panelists reviewed each nomination. Five of the reviewers working in biomedical, public health, and social sciences reviewed the bulk of nominations (12 reviews each). The remaining three reviewers reviewed smaller sets of nominations from engineering, earth sciences, and physical sciences. Reviewers received the narrative information provided by the nominators and endorsers about the nominees’ research program and their professionalism and integrity. They also received the nominees’ CVs, and a summary of their academic rank, sources of funding, number of publications, and amount of grant funding (with a note that NIH intramural researchers do not seek external grants.)
We provided the review panelists with a one-page instruction sheet describing the two criteria for inclusion as an exemplar: scientific accomplishment as a federally-funded investigator and a reputation for professionalism and integrity. We instructed panelists that considerations for the expectation of high-quality, high-impact research might include: “receiving substantial grant funding, producing high-quality peer-reviewed journal articles, and addressing issues of social import.” Regarding the expectation of professionalism and integrity, we instructed panelists to consider “whether the characteristics or practices described in the materials are those that would be ideal for others in the scientific community to learn from or follow in their approach to lab management, mentorship, or leadership.”
Panelists scored the nominees on the two criteria using a 1 ( far below expectations ) to 7 ( far above expectations ) scale. We also asked reviewers to respond to the yes-no question, “Do you have any reservations about this person being identified as an exemplar?”, and to briefly comment on their ratings (and reservations, if necessary). We produced mean rating scores across the two criteria and two reviewers.
Review by the research team
Our team (AA and JD) reviewed the panelists’ scores and comments, and we evaluated all of the nomination and endorsement narratives. We focused our review on nominees scoring at or above 5. The average reviewer rating of the final cohort of research exemplars was M = 6.02, SD = .60. Our aim was to verify, to the extent possible, evidence of behaviors or traits of the nominees that reflected professionalism and integrity. We examined the nomination and endorsement materials for examples of what set the researchers apart in how they approached doing their work.
It is of note that we did not define “professionalism and integrity” for nominators. Instead, we opted to allow nominators to make recommendations according to their views of professionalism and integrity in research. We found the exemplars were described as hardworking, enthusiastic, collaborative, meticulous, transparent, fair, and altruistic. Many held leadership positions at their institutions or in their fields. Nearly all were described as role models, advocates for others, or outstanding mentors. Additionally, exemplars were described as well-liked, highly-respected, and sought-after for their expertise and advice. The nomination and endorsement narratives offered examples of these qualities in practice; for example, taking the utmost care with their data and methods; being known for questioning their assumptions and bringing in outside perspectives; creating collaborative, engaged work environments; refusing to publish findings without confidence in their validity; being disciplined in requiring standardized procedures; discussing ethical considerations actively with their groups; sharing their data outside the lab; making sure team members receive due recognition; taking personal time to help colleagues and mentees.
In our assessment of the panelists’ feedback and the materials to select finalists, we aimed to ensure inclusion of researchers who met the criteria and who were diverse in research discipline (e.g., clinical, lab science, social/behavioral research), gender, and nation of birth. We sought at least 30 researchers, however, the high caliber of nominees ultimately led to 55 finalists. We emailed the 55 researchers selected as finalists, and all but three finalists agreed to enroll in the study and participated in the interview.
Data collection
We conducted one-hour, semi-structured telephone interviews. Before completing the telephone interview, the participants received a consent document electronically and indicated consent to participate. Questions about the study were addressed via email or on the phone before interviews began. Participants also completed a brief online demographics survey and a short questionnaire about their work (e.g., hours worked per week and grant applications submitted per year).
The interview script consisted of 18 questions with follow-up prompts ( S1 Appendix ). First, the interview focused on rapport-building and background questions. Then, we told participants we wanted to understand the factors that contribute to the success and reputation for integrity of individuals nominated as research exemplars. We ask them to focus on things they do consciously to be the best researcher they can be, or things they do that others in their field might not do routinely. The next 11 questions asked about the individual traits, work strategies, and environmental or experiential factors that contributed to their success and reputation for integrity. The habits and routine practices questions focused on rigor and reproducibility, good team relationships, and compliance. Finally, we asked three questions about key career experiences and lessons.
We asked the participants to focus on work within their own research lab or team, even though they collaborate across labs and teams. We did not explicitly tell participants we were studying “leadership and management” to allow their leadership practices to emerge naturally. The interview script was developed by AA (an industrial-organizational psychologist with expertise in workplace psychology, leadership, and responsible conduct in research) [ 19 , 32 – 34 ], and JD (a psychologist and bioethicist with expertise in research professionalism, research integrity, and professional decision-making, along with experience as PI of large federally-funded grants) [ 35 – 37 ]. Two public health graduate student research assistants conducted the interviews. They received 3 hours of training in interviewing practices and performed a practice interview observed by AA. After each interview, they documented how it went, and the team used this information to further standardize procedures between interviewers; although minimal problems arose. The interviews were digitally recorded and transcribed verbatim. They yielded 600 single-spaced pages of text.
Data coding and analysis
We adopted a causation coding framework to identify causal explanations for why the exemplars had reputations for exemplary research [ 38 ], which we defined as fostering rigorous research, regulatory compliance, and good relationships [ 4 ]. In the interviews, we asked for the exemplars to discuss their own theory of what explains their success and reputations by asking them to identify the routine practices and habits they employed. In coding, we linked the practices they described to the outcomes of interest: rigor, compliance, and relationships. Our analysis of themes focused on building an understanding of the exemplars’, and our own, theory of the practices contributing to these outcomes. We anticipated leadership practices would emerge according to an existing broad framework of leadership behaviors: “initiating structure”—task-related behaviors focused on processes and procedures—and “consideration”—relationship-oriented behaviors focused on interpersonal aspects of leading people [ 26 ].
We developed codes to summarize concepts in the data inductively through several rounds of open-coding. First, AA and JD read the transcripts and marked emerging concepts to identify a coding framework consisting of parent codes (codes providing superordinate categories) for child codes (codes capturing specific concepts). The framework ( S1 Table ) included parent codes in several domains: e.g., research operations practices, relational and self-management practices, professional priorities, traits, and experiences. In addition, a parent-level “outcomes” code included the child codes of rigor and reproducibility, compliance, good relationships, balancing professional demands, and doing exemplary research. We linked practices to the outcomes when coding the excerpts of text containing practice codes by marking an accompanying outcome code each time we coded a practice. We only used the general “doing exemplary research” code when the practice discussed by the participant was not explicitly linked to the more specific outcome codes. We tracked outcome codes in this fashion to identify the casual links exemplars made between the practices and their intended outcomes.
After developing this initial codebook framework, one of the interviewers (AK) read 10 transcripts to identify the workability of the code framework and to elaborate on the child codes. The full team (AA, JD, and both interviewers) met to discuss and revise the codebook. Next, AK and AA tested the codebook on a second batch of 10 transcripts and continued to develop a comprehensive list of child codes. We also generated a codebook document with the definitions of the codes, example quotes illustrating each code, and rules for applying each code. This process repeated until we reviewed all transcripts and identified no more child codes. After each batch, team meetings focused on clarifying code definitions, rules for applying codes, and collapsing redundant codes.
We utilized Dedoose qualitative data analysis software [ 39 ] to train coders and perform coding. In Dedoose, coders mark excerpts of text within transcripts with codes representing concepts in those excerpts. This process generates present (1) and not-present (0) quantitative data for each of the codes within each transcript, and allows the textual data linked to the code to be downloaded for subsequent thematic analysis. To train on the final codebook and add rules to the codebook, AA and AK coded the same set of 10 transcripts and discussed. To obtain an estimate of reliability, they selected and both coded 50 chunks of narrative from transcripts across scientific disciplines, male and female participants, and across the 18 questions. Kappa, an estimate of inter-rater agreement, was .81. Kappa estimates of .61–.80 are considered very good, and above .81 excellent [ 40 ]. AA and AK then each proceeded to code 26 transcripts each. After coding 10 each, we selected a set of chunks, one from each of the 18 questions from across the transcripts, and recalculated Kappa (.73) to ensure we did not have major rater drift in applying the codebook.
The key purpose of this report is to identify practices linked to rigor and compliance. Thus, we examined each practice and their links with these outcomes by reading the excerpts for each practice to identify the themes represented within that concept. When practices linked to more than one outcome, we read and identified themes for each outcome. After reviewing the themes within excerpts for rigor and compliance, we reviewed the excerpts linked to the general “doing exemplary science” code to ensure that we had already identified all key ideas. During this process of analyzing themes associated with codes, we explored how the concepts and themes related to each other, identifying higher-level themes.
We report the frequency of all of the practice codes linked to rigor and compliance across the 52 exemplar transcripts, provide illustrative quotes, and elucidate the themes represented within the codes. We include specific strategies used by exemplars to provide practical insights. Our discussion focuses on the higher-order connections and key findings cutting across the results.
Participant characteristics
We interviewed 52 researchers. The cohort performs diverse types of research, including basic lab research across a spectrum of life and physical sciences, clinical trials, behavioral interventions, engineering, informatics, and field research in disciplines ranging from earth science to virtual archeology. Thus, the cohort manages compliance in human subjects research, animal research, and laboratory safety. Table 1 describes the participants’ demographic and academic characteristics.
- PPT PowerPoint slide
- PNG larger image
- TIFF original image
https://doi.org/10.1371/journal.pone.0214595.t001
Table 2 shows the exemplars’ career statistics and team characteristics. The researchers work with a mix of personnel on their teams, including high school students, undergraduate students, graduate students, post-doctoral researchers, research technicians, research coordinators, managers, and other full-time staff. Most (77%) work at public universities in the United States, and the remaining (15%) at private universities and NIH’s Intramural Research Program (8%). Of the exemplars at universities, about half (48%) were at medical schools and 73% were at “R1,” research highest activity, universities. The institutions represented the four geographic regions in the United States, the Midwest, Northeast, South, and West, as defined by the U.S. Census Bureau.
https://doi.org/10.1371/journal.pone.0214595.t002
Our analysis of the interview narratives yielded 8 practices that 50% or more of the exemplars indicated foster rigor and/or compliance. It is of note that 4 of these same practices also linked to good relationships or balancing professional demands. We will describe the findings regarding relationships and professional demands in a companion report. We also found a second set of less frequently mentioned practices, including 8 practices focused on fostering rigor and 1 practice focused on compliance.
The practices offer ideals to strive for in leading a research team. However, it is important to note that not all exemplars engaged in all practices, and certainly, they did not execute the practices flawlessly. Many exemplars noted challenges they encounter, such as finding compliance burdensome, or struggling to identify what they should and should not delegate. The top practices are illustrated with quotes in Table 3 .
https://doi.org/10.1371/journal.pone.0214595.t003
Hold regular team meetings
Discussed by 83% of exemplars, the cornerstone of rigorous research is holding regular meetings. As one exemplar put it: “…the first round of vetting of what they have accomplished is through our internal group meeting or lab meeting” (Participant 41). Nearly all exemplars hold weekly meetings. Meetings serve several distinct purposes.
Meetings, first and foremost, provide the opportunity to share data. This fosters transparency about data and permits team members to scrutinize results. This routine helps lab members feel comfortable putting their data, findings, and interpretations on display for others to critique. It creates a norm of openness about data, helps to identify potential problems in the data, and fosters a sense of team collaboration on projects. Several exemplars emphasized that a PI must never make lab members feel that they will be unhappy with the data presented to them. One noted, “…I let them know that progress isn’t getting the results I want…progress is getting the results that the experiment gives us” (Participant 17).
A related purpose of meetings is to provide updates, coordinate, and identify potential problems with a study that impact the quality of data or compliance with a scientific or ethical protocol. Meetings allow team members, particularly when several must coordinate to execute protocols, to double-check procedures and talk about concerns. These meetings also allow investigators to understand what has been accomplished and allow the team to plan. The exemplars talked about using these meetings to make sure they are fully aware of how the research is going and to create accountability for next steps. Several PIs mentioned the importance of agendas, to-do lists, and action steps to foster awareness and accountability among team members. For example, one exemplar described requesting an outline of goals and progress: “They have to prepare a write up every single time that we meet…essentially outlines their goals, and then the next time, what they met on those goals” (Participant 51).
Whether it relates to ensuring sound, high-quality research, or compliance with regulations and ethical standards, the exemplars repeatedly emphasized that people must be comfortable communicating about mistakes. Overall, communication at team meetings must be transparent and open. Exemplars stressed that people must feel the environment is “non-threatening.” A few exemplars even described sharing their own mistakes and trials to model openness. One exemplar put it this way:
I tell [my team] that the only mistake that can’t be fixed is the one they don’t tell me about…if they’ve done something wrong, or they’ve discovered that something went wrong…I want to know about it.… I don’t get angry…because everyone’s human and we all make mistakes, including me. The only thing I ever get angry about is if I discover that they’ve hidden something from me. So, transparency and displaying that transparency is really key.… (Participant 4)
Exemplars also hold meetings to discuss new study designs and plan out study protocols that have not yet launched. Occasionally, meetings serve the purpose of developing or training lab members by discussing new topics or having outside experts come to present on new regulations or important compliance issues.
Encourage shared ownership and decision-making
Another top practice, shared by 73% of exemplars, was establishing an environment where everyone shares in responsibility for compliance and integrity. For example, one strategy was to design compliance protocols together as team. This not only ensures that everyone knows what each team member is responsible for, but also that the protocol can be discussed and revised if a problem arises.
Another aspect of shared ownership was appointing a compliance point person. Many exemplars described someone they rely on as a source of expertise and consistency on matters of compliance. Typically, this person was an experienced member of the lab or designated compliance manager. The point person helps the PI oversee compliance matters and double-check procedures and documentation. A point person might perform specific checks of protocol compliance, for example, they might regularly review documentation like protocol checklists and signed informed consents. Moreover, this team member serves as the go-to person for daily compliance questions or issues. One exemplar described it this way: “…different staff or the research faculty are in charge of various components of the laboratory…they serve as an additional, immediate onsite resource for laboratory processes in a way that I can’t, given my administrative duties” (Participant 10).
Other than selecting an experienced individual, some PIs mentioned appointing a person who is good with details, or a “perfectionist.” This person then contacts the PI when needed. Also, the point person is often involved in training newcomers and ensuring they are knowledgeable of the rules. One exemplar relied on a graduate student in this role: “…I also have a safety officer in the lab…it’s an advanced grad student…and he’ll basically train any new student coming in on a one-on-one basis…go through all of the things that we need to check” (Participant 45).
Provide supervision and guidance
The next practice of utmost importance mentioned by the majority (71%) of exemplars was providing comprehensive, ongoing supervision and feedback to their students and personnel. Meetings provide an oversight mechanism for PIs, but exemplars also emphasized oversight and feedback more generally. They described regular, typically daily, interactions with smaller groups and individual team members. They make sure that they are interacting with and communicating with everyone on their team. One described it simply this way: “…I go to the lab every day and engage all of them” (Participant 38).
At its core, this practice is about being an involved and engaged PI and mentor. The exemplars value awareness of how the research is going day-to-day so they can provide timely guidance and direction. The form that oversight took varied by level of the student or staff member: daily more intensive supervision for newcomers, inexperienced staff, or a struggling trainee; regular “check-in” meetings with more experienced individuals; and one-on-one meetings with the most senior personnel on an as-needed basis. Above all, their objective was to interact with everyone to identify potential problems and help identify ways to overcome them. One exemplar noted: “I’m meeting with them…and making sure there aren’t problems, that if there is a problem, they’re bringing it to my attention” (Participant 43).
Regarding rigor specifically, exemplars described being involved in and overseeing the execution of research and the production of data. Through their awareness of how data collection is progressing, they can help trouble-shoot when studies are not going according to plan, or when they or lab members identify inconsistencies in data. For those doing experimental work, this typically meant they were in and out of the lab interacting with people. Those doing clinical work emphasized being sure that people know they are available. Further, they are regularly in contact with lab members, so they can learn immediately if there are any questions, concerns, or needs for guidance about protocols. Access to the PI was of critical importance; typically, PIs interacted daily with their teams. Some exemplars mentioned that they have an “open door” or share their calendar with their team. Other PIs noted it is imperative that their team works in a space in close proximity to their office.
Similar to their oversight of research and data collection, engagement as a supervisor allows their teams to address problems related to compliance as quickly as possible. Often, supervision related to compliance focused on checking in with the compliance point person and establishing the procedure that dictates how the compliance person communicates concerns directly to the PI. Several exemplars expressed the importance of being mindful about the possibility that compliance or research integrity issues could arise, like compliance protocol violations, plagiarism, or data manipulation, and of being vigilant in monitoring for them. They described not being suspicious, but mindful.
Several exemplars specifically noted the importance of supervision and feedback for new members to the team and for people who are doing new, unfamiliar tasks. Through regular interaction, the PI gains confidence that lab members are prepared to work more independently. The PIs noted that regular supervision and feedback are the key means to develop trust in the knowledge and skills of their team members, which ultimately allows them to delegate and share in decision-making and ownership.
It is worth noting that the supervisory role of a PI evolves. With a smaller lab, or at an earlier career stage, some described being extremely involved, even working alongside students in the lab. With time, a PI’s involvement in the smallest details may diminish, but this heightens the need for regular interaction, feedback and open lines of communication. Also, PIs with large teams or labs may oversee managers or coordinators who help to provide some of the supervisory support to others. However, the exemplars described being both highly engaged in communicating with those managers or coordinators while also still interacting regularly with all the people on their teams.
Overall, the investigators described being aware, being present and available, and interacting regularly with individuals. Approachability, in particular, was a prominent theme in their responses. Most exemplars explicitly noted that people should feel any concern or question may be brought to their attention at any time. They consistently reiterated the importance of openness and communication. By knowing the day-to-day activities of the team, they can provide timely, effective guidance and help their teams overcome challenges.
Importantly, some exemplars explicitly pushed back against the idea that this kind of engagement and regular contact with people is “micromanagement.” Many felt that through their engagement on matters of data quality and compliance, they created the sense that every team members should play a role in monitoring and creating checks and balances about data and compliance. Furthermore, many noted that problems, mistakes, and concerns should be approached in an evenhanded fashion. One stated: “I think the key is to make sure that I am talking to them, and I’m not judging them or evaluating them when we talk. It’s all about discussion…” (Participant 39). Another noted: “If you yell at people, if you brand them as incapable researchers…then people will go into safe mode. If they go into safe mode, they are not really a scientist anymore…” (Participant 6). Finally, another exemplar reflected on the aim of establishing openness: “I want to create an environment of openness with my students so that they’re able to present to me the truth and not be afraid that I’m going to get angry” (Participant 35).
Ensure sufficient training
Another common practice noted by 67% of exemplars was the need to ensure adequate training of newcomers to their research teams to foster rigor and compliance. Several mentioned the adult learning heuristic ‘tell, show, do’—tell someone how to do a task, then show them how to do it, and then watch them as they practice doing it. Some described assigning tasks with specific metrics or outcomes they could check to ensure the person knew how to carry out the task accurately. Others described using a tiered, experience-based system in which experienced lab members train the beginners while the mid-level trainees observe. Later, mid-level trainees may become the experienced trainers.
Several exemplars noted the need to draw on the help of lab members to train others, and to formalize training as much as possible, especially as the lab gets bigger and the PI accumulates greater responsibilities. Several exemplars noted using training guides and standardized training manuals to ensure that everyone is taught to do things the proper, rigorous way. Regarding compliance, several noted that they do not assume that people know the rules, and they make sure that team members meet the institutional compliance training requirements. Other strategies for training on compliance-related matters were to work together on regulatory applications, have occasional special meetings about regulatory issues, or to bring in outside regulatory experts if extra training needs arise. One exemplar relayed their approach as follows:
We have a monthly staff meeting, and we talk about compliance and regulations. I will send reminders as needed about the importance of compliance with regulations, compliance with our research protocols with the university requirements and regulations, and we discuss a lot of that also when we’re writing proposals. (Participant 12)
Another described building staff knowledge through experience with compliance protocols:
I require all of them to write and submit IRB or IACUC application, of course with my supervision, so that we all will be familiar with the issues, not just in terms of research, but also in treating humans and animals in ethical ways. I also require them to personally get trained in every aspect of research regardless of whether they’re going to do that type of work in their research or not. (Participant 38)
Overall, the PIs described the critical need to ensure that no one, whether an undergraduate, graduate, or post-doctoral researcher, performs a task until they reach adequate mastery. Without this level of mastery, the team risks wasting resources, producing bad data, or failing to ensure compliance. By attending carefully to training, the PI develops trust in their team members, allowing them to work independently. Notably, several investigators mentioned that, because the period of necessary learning before being productive is extensive, they ask people to commit at least two years in their lab.
Foster positive attitudes about compliance
In line with the idea that shared ownership fosters compliance, 65% of the exemplars described the need to foster positive attitudes about regulatory compliance. This included several elements. First and foremost was the need to have good relationships with regulatory agencies and institutional officials. PIs noted the importance of viewing these officials as helpful experts rather than antagonizers. As noted above, some even reported inviting such experts into their labs to give presentations or share best practices. They noted the value of viewing compliance experts as part of a team’s competitive advantage, that is, viewing them as a resource to support the good work of the team, rather than criticize it. However, this particular strategy will be most effective at institutions with compliance offices that have robust educational and support services.
Another element of this practice was to provide continued professional development around issues of compliance; for example, by dedicating a lab meeting to discussing changes in regulations or to discussing a case example. Keeping the importance of compliance salient in the lab, particularly by making compliance a topic of regular discussion, allows the team to integrate compliance into how they naturally go about their work. An exemplar described encouraging awareness as follows: “We just have kind of ‘safety moments’ and safety updates every couple of months to make sure that everybody is remembering the proper set of procedures to go through if there’s an emergency in the lab…” (Participant 11).
Additional strategies for fostering a culture of compliance were to set expectations of compliance by explicitly insisting that lab members follow the rules, and to make compliance easy, or the default approach to doing science. For example, this could be achieved by using standard operating procedures (SOPs) and ensuring adequate training. Several exemplars went even further, noting that the team must value doing what is ethically best for patients, animals, lab, or science, even when it is above and beyond the requirements. Finally, even when the PI finds compliance matters a burden, several exemplars noted it is important not to communicate that attitude to team members.
Scrutinize data and findings
The exemplars described meticulousness and skepticism towards raw data and their team’s interpretations of findings. This strategy was mentioned by 61% of exemplars. They sought to cross-check data and findings as thoroughly as possible and to ensure a culture of transparency about data. To achieve this, they urged people to talk about data regularly. They encouraged lab members to show each other their data to create a culture in which people are comfortable talking about data. Exemplars ensure that lab members participate in both receiving and offering feedback so that they become comfortable with critique. One PI noted people should never be afraid to show their data: “I tell them I want all data. There is no good data, there is no bad data unless somebody makes a decision…I am ultimately responsible, or…the team is.… You have to have all original data.” (Participant 6).
Some exemplars described bringing in outside colleagues who can offer fresh perspectives on their findings or inviting others to collaborate, especially those who they know will disagree, in order to generate a greater level of scrutiny. Their aim of setting a tone of objectivity and scrutiny towards data was especially achieved through regular meetings and interactions about data. Many exemplars described that this mindset carries beyond meetings to the way members of their teams approach data as individuals, and in the way they work together collaboratively outside of meetings to ensure the best research.
Express values and expectations
We heard, from 60% of exemplars, about the significance of a PI explicitly stating what is important as the team goes about their research. That is, what the PI values and, in turn, what the PI expects. To foster rigor, the exemplars communicate high standards. Specifically, they express the importance of doing research accurately, meticulously, transparently, and with integrity. They expect lab members to work hard, but they also tell them not to sacrifice quality by cutting corners or rushing. Several exemplars mentioned the need to maintain the credibility of the lab, noting they must always be able to defend their results with confidence. Furthermore, PIs tell lab members it is essential to maintain openness and objectivity as researchers. One exemplar described the mindset they expect from team members in the following way:
I try to, on a daily basis, remind my students…be very, very skeptical…it starts first with each individual being skeptical of their own data, and not assuming anything. Never assume anything. That’s the fatal flaw in a scientist is to assume that you know what you’re doing. (Participant 22)
Included in this notion of objectivity was the idea that lab members must never feel that the PI expects or demands a particular result. Furthermore, several exemplars noted the need to explicitly tell lab members that they must be willing and able to admit mistakes, and it is ok to “be human.”
Similarly, PIs express high standards regarding compliance. We heard from several exemplars that they tell lab members that the rules are important, and that PIs insist members make it a priority to know and follow the rules. In addition, exemplars state the consequences of compliance failures: that is, they tell team members that the privacy of participants, or the safety of fellow lab members, is at stake. Importantly, many noted the need to state these expectations particularly when a new member starts in the lab, and not to assume that people know the rules and expectations. Some exemplars also explicitly expected everyone to work together as a team to ensure all lab members understand and follow the rules. For example, some exemplars appointed existing lab members to help newcomers learn the rules. Other strategies to reinforce the importance of compliance were to have compliance concerns as the first agenda item at meetings and to ask new members to sign an agreement stating their commitment to following the rules.
Establish and follow standard operating procedures
Another practice, mentioned by 50% of the exemplars, was to establish and follow standard operating procedures (SOPs) for scientific and compliance-related procedures. Exemplars stated it is unwise to take for granted that everyone understands how to perform procedures, or that they reliably remember every step. As one put it:
…we write protocol manuals…we actually list out every step of what someone is supposed to do as they go through the study…so everything is laid out. We don’t assume that people know or will always remember every single step. So there’s a checklist for every protocol that we use, and people have to sign off. (Participant 4)
Exemplars described the importance of highly specific SOPs and the need to use them for everything in their research. SOPs provide PIs with some peace of mind that lab members will consistently perform procedures. They also ensure that best practices in the lab will not be lost over time as members of the lab change. Moreover, SOPs provide a means to discuss and update scientific protocols and regulatory compliance in meetings, particularly to troubleshoot problems. Some exemplars noted drawing on the guidance and expertise of compliance offices to develop or update SOPs.
As illustrated in Table 4 , the remaining set of 9 less frequently mentioned practices emphasized procedures to ensure their teams carry out research in a meticulous and coordinated manner. This set of practices focused exclusively on rigor, except for one practice related to compliance.
https://doi.org/10.1371/journal.pone.0214595.t004
Verify findings
We heard of the need to verify findings from 48% of exemplars. For those doing experimental work, in particular, it was essential to verify findings through repeated experiments, and often through having multiple people replicate experiments. As one expressed: “…you repeat it, and you repeat it…we want to be sure…repeat is the mother of success” (Participant 13). Exemplars recommended finding help outside the lab if the lab is having trouble replicating. They also shared the importance of digging into results when they find something they expected, not only when they find something they did not expect. They stated the lab must figure out whether their result is real or not. One exemplar described it as follows:
…being extremely slow and methodical and careful and going back and checking and rechecking and looking at things from different perspectives, bringing in a lot of people to consult, others to independently replicate your results, others to still independently replicate those. I’ve never put anything out that hasn’t been replicated at least twice independently by people. (Participant 14)
The bottom line was to be skeptical of what they think they have in front of them and to look at their findings from new angles, for example, to repeat experiments, assess new controls, or look to other studies to triangulate their findings.
Verify findings was mentioned by about half of exemplars, and most investigators meant replicating findings. It might be expected that replicating would be mentioned more frequently, but we think this may be a function of two factors. First, the investigators were conducting diverse types of research: some were doing basic laboratory studies where explicit replication is customary, whereas others performed clinical or behavioral research and explicit replication of datasets is not possible. Instead, in these fields “scrutinizing data and findings” captures the standard approach to validating findings. Second, we asked investigators to describe practices they thought others in their field might not do routinely, which may have led them to focus less on practices they potentially viewed as typical.
Document procedures
We heard from 44% of exemplars that rigorous research requires meticulous, constant documentation of every decision made, even the smallest detail. One investigator noted: “I also ask my students, even though we’re a computational group, I ask them to maintain a research log, a lab notebook” (Participant 9). Whether documentation takes the form of lab notebooks, written plans, detailed notes, or metadata, exemplars conveyed that everything should be documented and researchers should plan ahead for documentation in their studies. There should be an expectation that personnel keep detailed records and notes about virtually everything. A few PIs provided the advice to give students or staff example lab notebooks, research reports, or lab meeting agendas and meeting minutes to illustrate the desired format and detail.
Design scientifically sound studies
Another issue raised by 40% of exemplars was that rigor starts at step one. They described the need to spend the necessary time to plan everything as carefully as possible from the outset. As one PI put it: “You think before the study, and you don’t think during the study, or you think only if you have to, as needs arise” (Participant 42).
Ensuring that their team planned the most scientifically sound, rigorous designs included several elements. A solid scientific foundation was one concern. They noted the need to study the literature to determine a theoretical framework, conceptual model, or what findings are already available. This allows the team to make sound research design decisions and find creative ways to extend and improve upon what already has been done. A few PIs noted using outside guidelines and checklists for rigor available from agencies like the NIH and journals. Some also described going outside their teams to obtain external feedback and criticism on ideas and study methods from the very beginning. Finally, specific study design considerations included the following: blinding, the use of controls, validating measures and techniques, calibrating equipment, having multiple methods of data collection to triangulate findings, and having repeated measures of the same data point. Several described thinking backwards; beginning with the end goal in mind and then thinking backwards to design studies. Above all, they reiterated that during the study design phase, PIs must not be afraid of findings that run counter to their hypotheses.
Coordinate the study team
Most of the exemplars have multiple team members working together to carry out studies. As alluded to in other practices, the need to coordinate the activities of the team is essential, and thus was explicitly noted by 33% of exemplars. This practice emphasized assigning and discussing roles down to the minute details from the very beginning of the process. This practice included getting the team together at the beginning of the project to map out goals and roles. As noted by one exemplar: “…we are planning an experiment, we all sit down as a group and go through it and say here’s what we’re asking, here’s what we need to do…who’s going to do what…” (Participant 5).
Then, it was essential to repeat check-ins during data collection and when creating a data analysis plan, executing analysis, or drafting results. Coordination and specification of roles was particularly important in how some of the exemplars ran their large research teams because they had students doing research while being supervised by post-doctoral researchers. Thus, this coordination and delineation of roles was important so that people knew who to contact for immediate, day-to-day guidance when doing their research.
Handle data properly
Finally, 31% of exemplars emphasized the importance of recording, storing, and backing up data appropriately. The PIs described storing data in a central place (often a shared drive) that is accessible to them at all times (and often to multiple lab members) and over a long period of time. They insisted that all data be stored in this central location, or that there be documented justification for anything that is not archived or is missing. They felt multiple researchers should be involved to improve data integrity—that is, typically, just one individual should not regulate data collection, analysis, and storage. Some PIs had co-leads on projects, and they made practices like double-data entry and group data analysis the norm. One investigator described data management this way:
…I always tell my students…back up all your data…at least in two places…and everyone is able to go into every single user’s directory…we are all collaborative, we look at everyone’s raw data in the analysis…and we maintain the data as long as we can. (Participant 9)
Involve multiple researchers on projects
Many practices implied the importance of teamwork, but several investigators (25%) explicitly noted the importance of internal collaboration for rigor and integrity. By having multiple people work on the same or parallel projects, access to, and review of data, are not isolated to one person, improving the integrity of the project. Strategies included “co-leads” of projects, group data entry and analysis, and the explicit expectation of co-authorship in their group. This approach lends to a collaborative environment and better research because cross-checks and feedback are built into the scientific process in the lab. One even noted the practice can impact the experiments researchers take on: “They also help each other so they co-author on each other’s papers, and so they’re a little less afraid…to do a labor extensive experiment because they know that they can have help for it” (Participant 35). It was noted, however, that this approach may be less feasible in smaller groups.
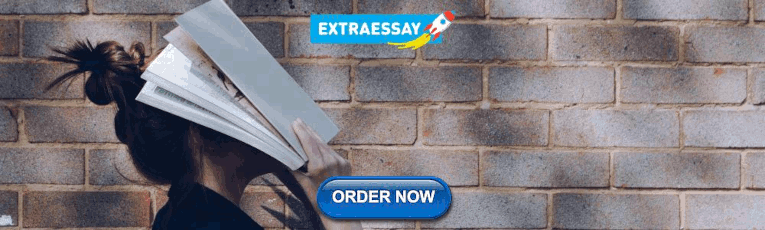
Share data outside the lab
Some exemplars (21%) discussed sharing their data or findings outside their lab early and throughout the research process. Exemplars felt this transparency promotes accountability, reproducibility, rigor, and, overall, advances science. Critique from other investigators on emerging findings could be obtained by presenting at conferences, or by seeking out the input of other investigators. Sometimes this latter strategy includes inviting outside collaborators to join their projects. One exemplar described this practice as follows:
…show the data to others and get feedback from people who may be naïve so that we can get a fresh and unbiased perspective, because if you’re only talking to people in your group you can get a bit myopic…it’s essential to take your findings outside of the group, ideally when they’re still in their preliminary stages so you can get feedback as the work is developing.… (Participant 46)
Sharing also included submitting data or methods to repositories, as one exemplar described:
…you have to get your data onto some kind of data repository and have it well explained enough that other people can understand what it is and make use of it…that even goes to code…everything you do is potentially retrievable and can be checked. It adds a burden to us, but I think it’s really a good thing, and it really kind of increases the value of the work if you can document everything and put everything out there… (Participant 16)
Report findings completely and accurately
Another practice shared by 17% of the exemplars was to ensure as much certainty in findings as possible before publication. This included, at times, explicitly ensuring they did not rush to publication. As one noted, “…we are careful not to talk about our results until we have confidence in them…I tend to be conservative in not publishing too quickly…we’re telling a story in each paper, and is the story really clear?” (Participant 21). They also discussed that it is essential to report all findings completely and precisely.
Advance compliance through audits
Finally, 17% of the exemplars mentioned using compliance audits to their advantage as an opportunity to increase awareness in their group and improve practices. Some referred to required audits, and specifically included everyone in the lab. One exemplar stated: “…we’ve had audits and I’ve had everybody be part of the audit because we don’t have anything to hide, although obviously there may be some questions” (Participants 4). Others used internal audits conducted on a regular basis by their team to identify areas for improvement in their processes.
We found the research exemplars create an open, transparent environment to foster the highest quality research. Their research teams openly discuss problems, scrutinize their data, methods, and findings, and work cooperatively to do sound science and ensure research compliance. The need for transparency and openness to foster reproducibility in the broader scientific community has received a great deal of attention [ 15 , 41 ]. Our findings identify several concrete strategies used by highly successful researchers for fostering openness and transparency as they lead their teams. It is important to note that not all exemplars mentioned every practice and specific applications of the practices may vary by context (e.g., type of research or number of personnel). However, several critical practices clearly emerged.
At the core of creating and maintaining an exemplary environment was regular, open communication in team meetings, along with frequent interaction between PIs and team members. These dyadic interactions include the PI actively offering guidance and feedback as an engaged mentor and supervisor, as well as team members actively approaching the PI. This latter form of interaction requires that team members find the PI approachable and accessible. The exemplars were both physically present in their labs, and they allowed people to feel psychologically safe to disclose mistakes and problems.
Psychological safety exists in a work group when people feel they can speak up and share ideas, ask questions, state concerns, or disclose mistakes without fear of penalization or people thinking less of them [ 42 , 43 ]. Mutual trust and respect characterize psychologically safe environments, and psychological safety is linked with workplace engagement, creativity, high performance, learning, safety, and ethical behavior [ 44 – 46 ]. Exemplars emphasized the need to be open to findings that do not support their hypotheses, and the need to respond evenhandedly to mistakes. More broadly, exemplars fostered a collaborative spirit among their team members.
Another important practice was encouraging shared ownership and decision-making in their teams. This “collective” approach to leadership draws on the skills and expertise of team members to ensure effectiveness, rather than concentrating authority exclusively with one individual [ 47 , 48 ]. For example, some exemplars relied on compliance “officers” in their groups to help ensure adherence to rules and procedures. More broadly, they set a positive tone about compliance and encouraged everyone to recognize their personal responsibility for compliance. We also found that exemplars placed great emphasis on scrutinizing data and findings. By explicitly communicating their values and expectations, exemplars could intentionally establish a workgroup environment in which people share assumptions about responsible and desired approaches to going about the work [ 21 , 49 ].
Not surprisingly, ensuring that research personnel have the skills to execute tasks competently was among the top practices. We heard of the importance of being intentional and systematic about training, ensuring an individual has demonstrated competence and reliability. The implementation of SOPs was another strategy for managing effectiveness and consistency of high-stakes tasks. A collective model of leadership requires drawing on the unique skills and expertise within the group, but of course, this does not obviate the accountability of a central leader to ensure effective functioning of the group [ 48 ]. Thus, PIs must identify the talents of others, develop skills where needed, and empower properly trained team members to take on increasing responsibility.
The final set of practices relayed by exemplars complements the foregoing practices. Exemplars described the need to ensure excellent study design, verification of findings, meticulous documentation, proper data management, sharing of data, and precise reporting of findings. This set of recommendations reiterates a key principle for responsible research and data management, namely meticulousness in all stages of the research, from study design and planning to data collection, storage, and sharing [ 50 , 51 ]. While the precise implementation of such practices varies by type of research, the key “quality assurance” principle urges investigators to produce, process, and report on data within a set of defined, controlled conditions [ 52 – 54 ]. We anticipate increasing focus on data sharing practices, wherein investigators are called on to implement practices that enhance the discoverability and usability of their data for the broader scientific community [ 55 ].
We anticipated management behaviors—those task-oriented practices that structure the operations of a research lab and provide personnel with direction and clarity in expectations—to be especially important for rigor and compliance. Indeed, many of the practices shared by exemplars are “classic” management activities—facilitating meetings, providing supervision, training personnel, structuring and standardizing procedures. However, interpersonal relationships and communication were at the core of most of these activities. We learned about “partnership,” “harmony,” “respect,” and “trust” among team members being central to high-quality research. Exemplars carry out their management activities in a way that engenders engagement and cooperative teamwork. They were mindful of the critical importance of interpersonal dynamics and positive team relationships in their labs and how these interactions support good science [ 56 ]. We also learned of the intentionality behind the broader culture that was developed within their teams.
Overall, our findings align well with contemporary models of leadership that assert effective leadership may not reside within any one person, but it is a function of effective information exchange, shared decision-making, and problem-solving in a collaborative manner that capitalizes on the expertise and skills with the team [ 48 ]. However, for maximal effectiveness, a PI must build the right team, develop talent within the team, clarify the mission, establish positive team interactions, and ensure opportunities for open communication [ 21 , 48 ].
Our findings are also consistent with key recommendations for responsible mentoring which emphasize that a mentor should serve as a role model of high standards and ensure proper training in research practices and research integrity [ 5 , 57 ]. Specifically, these recommendations state that mentors must communicate the importance of responsible research practices, meet regularly with trainees and personnel, review data and work for accuracy, and provide SOPs for research operations [ 50 , 57 ]. Effective mentor-mentee relationships require mutual trust and respect, and mentors are expected to offer support and encouragement [ 57 ]. It has also been noted that explicitly articulating the values underlying practices in the research group is important for conveying and clarifying the standards for responsible research and reduces misunderstandings about expected behaviors [ 58 ]. However, while mentoring is an important lens through which to view the role and responsibilities of an effective PI, it is an incomplete one. A broader understanding of the responsibilities of PIs must take into account their role in establishing a thriving work environment and facilitating effective collaboration among team members.
Implications for training
Establishing management systems and structures requires time and intentionality on the part of PIs when they establish their labs. Early-career researchers need guidance in this regard [ 19 , 59 ]. This guidance may be especially important as they likely feel pressure for immediate research productivity relative to taking time to establish workgroup structures. It is inefficient for every researcher to start up a lab and recreate organizational structures and processes, often learning solely by trial and error [ 19 ]. A set of best practices for lab start-ups would facilitate establishment of sound procedures. Practical information, such as templates for developing protocols, lab manuals, or meeting agendas, could be provided to investigators to encourage them to adopt practices that facilitate research excellence, or simply to streamline their startup.
Investigator leadership training should also provide guidance in how leadership behaviors form a workplace culture, along with how to establish good dynamics among members of a team. Our findings suggest that researchers need outstanding communication and interpersonal skills. The team-oriented nature of contemporary research [ 13 ] and the importance of high-quality interactions for responsible research suggest the need for researchers to learn about these human dynamics alongside scientific methods. Again, we expect practical guidance to be particularly valuable; for example, brief, structured guides for starting conversations about values and expectations or about integrity in research. It may also be necessary to provide strategies for navigating personal challenges and pressures, such as tools for time and stress management. The ability to successfully navigate professional challenges, make prudent decisions, and effectively lead others, rely, at least in part, on the ability to manage personal pressures [ 36 ].
We also think that approaching such training programs in a comprehensive manner—framing them as fostering excellence in research—will be most attractive to investigators. Specifically, programs should communicate that rigorous, high-quality research, regulatory compliance, ethical research, mentoring behaviors, and interpersonal dynamics are intersecting domains with cross-cutting leadership skills and responsibilities. For example, fostering respectful workplace relationships and a positive team culture are central to all these ends. Additionally, having the right set of management structures and the right culture allows a leader to free up time and energy to focus on other highly valued tasks, like grant writing and strategic planning.
At present, education in the responsible conduct of research (RCR) serves as a key method to encourage researchers in the training stages of their careers to adopt responsible practices and values that support ethics in research. Importantly, not all junior faculty researchers are required to receive RCR education at that career stage (i.e., not all are “NIH-funded trainees”, for instance, on a career development award). However, training is needed when making the transition from doing research inside another investigator’s group to leading one’s own research team. Rather than describing this training as “RCR education,” it may be more effective to refer to it as “leadership development.” There are some existing programs in mentoring skills and lab management [ 59 , 60 ], but such programs generally remain optional.
Within existing RCR education that tends to reach graduate students and post-doctoral researchers, trainees should learn about the role of the work group environment and interpersonal dynamics in responsible research. Research trainees may not be able to overtly influence the research environments in which they work, but they may be able to influence them in subtle ways. Furthermore, all trainees could be encouraged to actively consider the practices they are exposed to and whether they might be ideal, or less than ideal, to adopt in the future. Finally, all trainees could benefit from learning interpersonal and intrapersonal skills that support effective workplace interactions [ 56 ].
Limitations
Our findings should be examined in light of potential limitations. Institutional culture may play a role in leadership practices that are viewed as effective and yield success; our findings do not speak to this issue. However, we drew investigators from a diverse set of universities and fields to increase the generalizability of our findings, but our findings do not address the role of institutional culture or differences by types of research fields. In fact, institutional culture may have influenced those who were nominated; for example, we did not receive any nominations from Ivy League institutions. Additionally, we report on the practices the exemplars described employing. However, it is possible that some of their responses reflected their intentions more than their actual practices. Yet, their remarks still reflect those practices they view as ideals.
Additionally, we attempted to verify to the extent possible that the participants met the inclusion criteria of professionalism and integrity. However, this was not possible to establish with complete certainty. Our approach included receiving at least two recommendations for each individual, and the nominators and endorsers knew the researchers for many years. It is also of note that many exemplars freely remarked about their own shortcomings and challenges; thus, it appears they were introspective and open with us. However, in future work, it may be ideal to also speak with individuals working inside the research teams of investigators to gain their perspectives. Research examining their perceptions of psychological safety, for example, would be valuable.
Additionally, our call for nominations indicated that we would consider nominees in any career stage, but the investigators included in our final cohort were senior (i.e., associate or full faculty rank). This is a strength in that we obtained the views of highly experienced individuals but a limitation in that they had already experienced the challenges and pressures faced by junior faculty. Future work might focus on understanding the specific challenges and pressures faced by individuals earlier in their careers. Relatedly, the well-established investigators likely had greater resources and staffing relative to those earlier in their careers; this may influence the leadership dynamics in their groups. Nonetheless, we think that the practices reported by exemplars can be applied in any research group. Training programs could help investigators understand nuances in adopting practices with different types of staffing or resources.
Overall, we think that the practices of this cohort of exemplars are noteworthy because of their diversity in types of research and institutions, and the rigorous process we used to obtain the sample. We sought an outstanding group for our interviews, those whom colleagues viewed as exemplary on two criteria: excellence in science and integrity and professionalism. Our process required each individual to receive a nomination and endorsement, and the nominees were reviewed by a panel of researchers. The high reviews given by the panel suggest our process generated the high-caliber group we sought. After talking with them, we found strong patterns in their responses. Moreover, we had a sizable sample for a qualitative interview study. Often samples of 30 [ 61 ] or even 12 [ 62 ] are viewed as sufficient to identify common themes in a study of this kind. Moreover, many practices identified in this study are those absent in the labs of researchers referred for lapses in research compliance and integrity [ 63 ], suggesting their importance.
We identified practices, along with specific strategies, employed by successful PIs for leading their research labs. The scientific community would benefit from greater awareness of the role of leadership and management in performing research. We recommend additional research and the development of evidence-based educational interventions to meet the needs of investigators.
Supporting information
S1 appendix. interview guide..
The full semi-structured interview guide (lightly edited for length). The themes reported in this manuscript arose primarily in response to questions 7 and 9. However, rigor and compliance-related responses emerged throughout the interview and were coded accordingly.
https://doi.org/10.1371/journal.pone.0214595.s001
S1 Table. Coding scheme.
The full project coding scheme demonstrating the parent code categories and the specific child codes (coders also had definitions, examples, and rules to guide application of the codes). This report describes the codes under Research Operations Practices and Relational and Self-Management Practices that linked to the outcome codes Rigor and Compliance .
https://doi.org/10.1371/journal.pone.0214595.s002
Acknowledgments
We thank the researchers who generously contributed their time to participate in interviews. We thank Christina Arzate, Mary Quandt, and Rachel Hettleman for their assistance with this research. We appreciate those who nominated researchers, the review panel, and the Professionalism and Integrity in Research Program (DuBois, Director) for supporting the project website and exemplar plaques.
- 1. National Institutes of Health. Budget 2018 [cited 2018 July 12]. https://www.nih.gov/about-nih/what-we-do/budget#note .
- 2. Centers for Disease Control and Prevention. FY 2017 budget and appropriations snapshot. 2018 Contract No.: CS283043-B.
- 3. President signs omnibus appropriations bill into law; Federal agencies funded through Sept. 30 [Internet]. 2017; May 8, 2017. https://www.nsf.gov/about/congress/115/highlights/cu17_0508.jsp
- View Article
- PubMed/NCBI
- Google Scholar
- 5. National Academies of Science. On being a scientist: A guide to responsible conduct in research. Washington DC: National Academics Press; 2009.
- 13. Bennett LM, Gadlin H, Marchand C. Collaboration and team science: A field guide. 2nd ed: NIH National Cancer Institute, Center for Research Strategy; 2018. 139 p.
- 14. National Research Council. Enhancing the Effectiveness of Team Science. Washington, DC: The National Academies Press; 2015.
- 22. Mumford MD, Peterson D, Robledo I. Leading Scientists and Engineers: Cognition in a Socio-Technical Context. In: Hemlin S, Allwood CM, Martin B, Mumford MD, editors. Creativity and Leadership in Science, Technology, and Innovation. London: Routledge; 2013. p. 29–57.
- 23. Yukl G. Leadership in Organizations. 8th ed. Boston MA: Pearson; 2013.
- 31. Institute of Medicine. The CTSA program at NIH: Opportunities for advancing clinical and translational research. Washington, D.C.: The National Academies Press; 2013.
- 38. Saldaña J. The Coding Manual for Qualitative Researchers. 3 ed. Seaman J, editor. Thousand Oaks, CA: Sage Publications Ltd.; 2016.
- 39. Dedoose. Version 8.0.35 web application for managing, analyzing, and presenting qualitative and mixed method research data,. 2018;Los Angeles, CA: SocioCultural Research Consultants, LLC, www.dedoose.com .
- 50. Steneck NH. ORI introduction to the responsible conduct of research. Updated ed. Washington, D.C.: U.S. Government Printing Office; 2007. xiii, 164 p. p.
- 53. International Conference on Harmonisation. Good Clinical Practice (ICH GCP) 1.34 Investigator Geneva: International Conference on Harmonisation; 2016 [cited 2016 December 8]. http://ichgcp.net/4-investigator .
- 57. Shamoo AE, Resnik DB. Responsible conduct of research. 3rd ed. New York: Oxford University Press; 2015.
- 61. Corbin JM, Strauss AL. Basics of qualitative research: Techniques and procedures for developing grounded theory. 4th ed: Sage publications; 2015. 456 p.
- Reference Manager
- Simple TEXT file
People also looked at
Review article, automation in the life science research laboratory.
- Deanery of Biomedical Science and Synthsys Centre for Synthetic and Systems Biology, University of Edinburgh, Edinburgh, United Kingdom
Protocols in the academic life science laboratory are heavily reliant on the manual manipulation of tools, reagents and instruments by a host of research staff and students. In contrast to industrial and clinical laboratory environments, the usage of automation to augment or replace manual tasks is limited. Causes of this ‘automation gap’ are unique to academic research, with rigid short-term funding structures, high levels of protocol variability and a benevolent culture of investment in people over equipment. Automation, however, can bestow multiple benefits through improvements in reproducibility, researcher efficiency, clinical translation, and safety. Less immediately obvious are the accompanying limitations, including obsolescence and an inhibitory effect on the freedom to innovate. Growing the range of automation options suitable for research laboratories will require more flexible, modular and cheaper designs. Academic and commercial developers of automation will increasingly need to design with an environmental awareness and an understanding that large high-tech robotic solutions may not be appropriate for laboratories with constrained financial and spatial resources. To fully exploit the potential of laboratory automation, future generations of scientists will require both engineering and biology skills. Automation in the research laboratory is likely to be an increasingly critical component of future research programs and will continue the trend of combining engineering and science expertise together to answer novel research questions.
Introduction
The progressive integration of automation into work environments has enhanced the production rates, efficiency and quality of an enormous array of industrial processes ( Hitomi, 1994 ; Autor, 2015 ). From generation to generation, mechanised tooling has replaced swathes of manual tasks. More recent advances in robotics and information technology have further automated processes that were once the sole domain of human brawn or brain ( Hasegawa, 2009 ). Life science research conducted within academic institutions has also welcomed the ingress of mechanised equipment designed to automate a range of tasks. However, it is noticeable that a typical university research laboratory, often led by a single principal investigator, maintains a high level of manual manipulation in the form of undergraduate, postgraduate, post-doctoral and technical staff. Many experimental procedures remain heavily reliant upon the individual researcher manually carrying out protocols at the research bench.
This is in contrast to industrial environments, where widespread investment in automation has allowed companies to maximise their outputs and increase profits ( Ravazzi and Villa, 2009 ). Laboratories in a clinical setting have also experienced the benefits of adopting automation ( Hawker et al., 2018 ), increasing the speed and reliability of patient-specific data for use by clinicians ( Sarkozi et al., 2003 ; Lou et al., 2016 ). In this review, written from the perspective of an automation engineer now working in synthetic biology research and a Principal Investigator managing a research laboratory, we classify the current levels of automation in laboratories and highlight the benefits and limitations of its usage in research. We further attempt to summarise why automation has had such a limited impact in our workplace ( Jessop-Fabre and Sonnenschein, 2019 ) and ask whether the solution to including more automation into everyday laboratory tasks may reside in greater communication between scientists and engineers. Further, we suggest that it could be accelerated by beginning with a more low-tech approach rather than striving too soon for fully autonomous systems.
Current Laboratory Automation
Well-meaning predictions of the cybernetic laboratory ( Beugelsdijk, 1991 ) and a robotic revolution ( Boyd, 2002 ) have, at the time of writing, yet to materialise in the majority of life science research laboratories. Evidence from the proportional use of the terms ‘automation’ or ‘automated’ in the titles of PubMed listed articles does, however, exhibit a steady increase over the previous 4 decades. The terms ‘robot’ or ‘robotic’, which are often used interchangeably with automation, received negligible use until the mid 90’s and then showed a more marked elevation ( Figure 1 ). It should be noted however that, ‘robot’ or ‘robotic’ can also be used as an adjective for biological systems or medical devices and the increase in their prevalence may represent changes in language usage rather than an indication of greater automation usage. A more thorough text mining exercise than ours attempted to measure the extent of manual protocols that could potentially be automated through analysis of methods sections in published life science articles. The study concluded that 89% of articles featured a manual protocol that has an automated alternative ( Groth and Cox, 2017 ). Whilst there is a scale of automation, from the simple to the complex, that could be applied to these protocols, such data provides evidence that there remains a large potential for automation in most biology research laboratories. There are also clear claims in the literature that researchers working in academic institutions have been slow to embrace automation ( Sadowski et al., 2016 ; De Almeida and Ferreira, 2017 ; Jessop-Fabre and Sonnenschein, 2019 ).
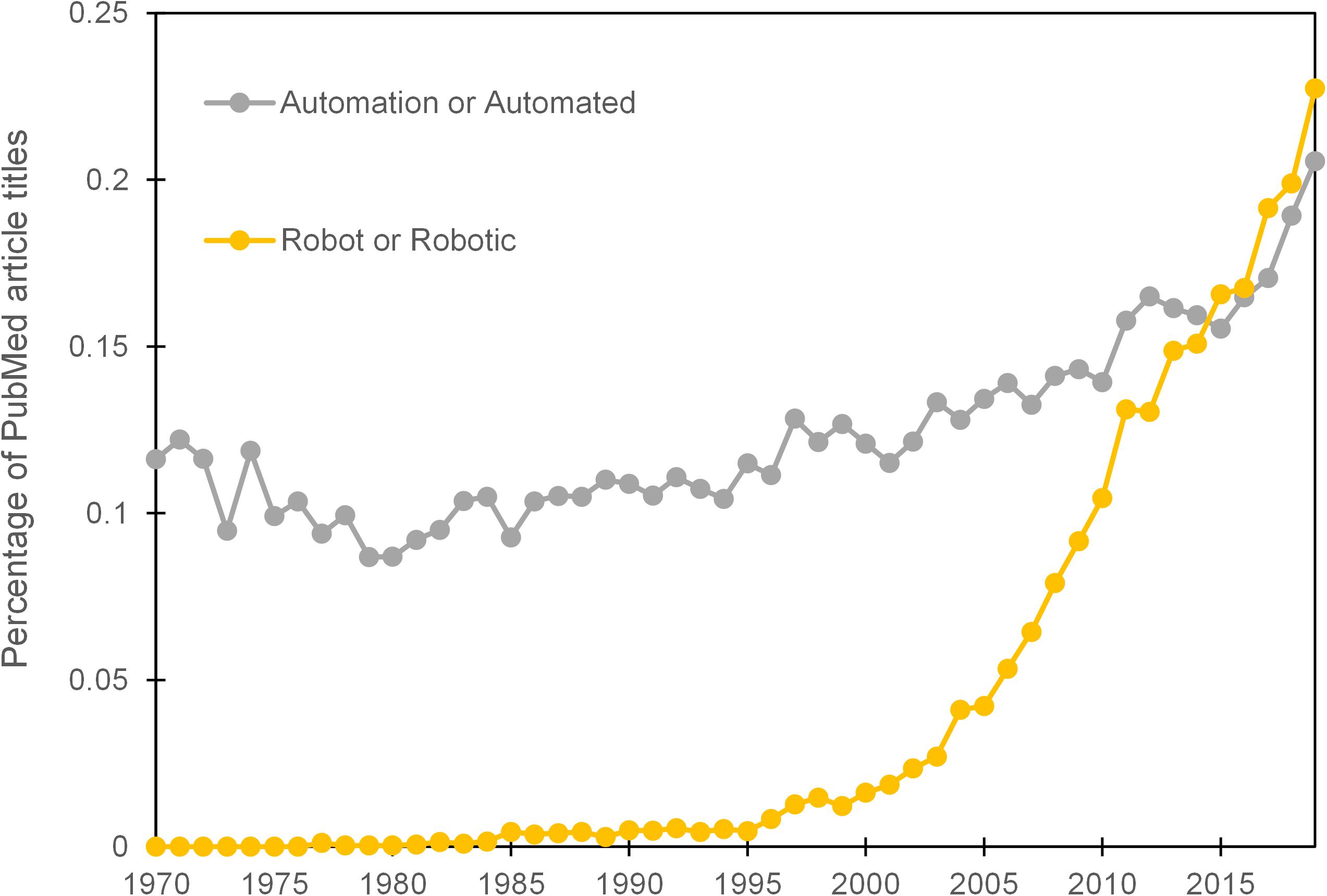
Figure 1. Prevalence of terms ‘automation’ or ‘automated’ and ‘robot’ or ‘robotic’ within the titles of PubMed articles per year over the period 1970–2019.
In this review we focus on automation where it describes equipment that physically manipulates items and we do not consider solely software-based technologies, such as image analysis and data mining tools. Within our scope there resides a diverse range of equipment that is found in research laboratories, from simple hand tools to entirely autonomous systems. A classification system for laboratory automation equipment has, to our knowledge, yet to be published, although a number of equivalent methods have been developed for classifying industrial automation. Frohm et al. (2008) reviewed these systems before proposing their own 7 levels of automation. These levels and descriptions are displayed in Table 1 , alongside examples typically seen in an academic research laboratory, and an indicative cost.
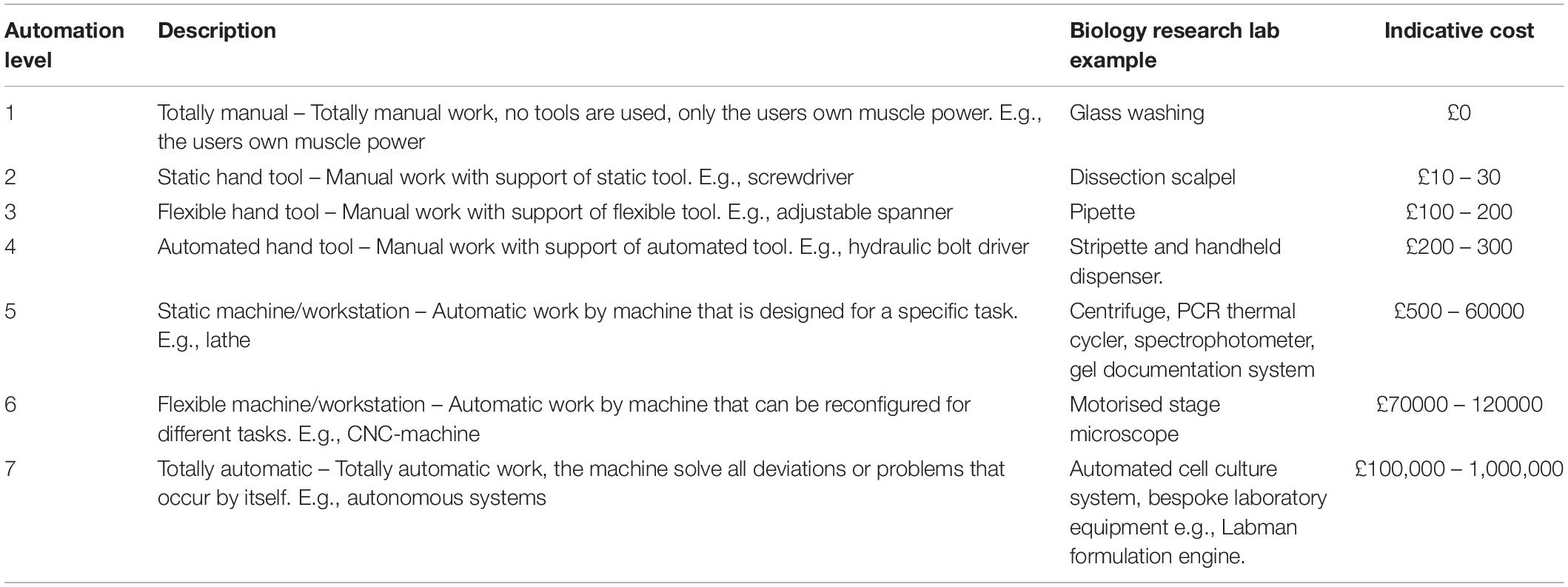
Table 1. Automation levels ( Frohm et al., 2008 ) with example laboratory automation equipment and an indicative cost range.
It is noticeable from Table 1 that the majority of equipment items that researchers would consider as the most expensive in their laboratory are categorised at level 5. Higher grade 6 and 7 items are a rarity in a biological research laboratory. Whilst mid-range level 5 automation items undoubtably increase the efficiency of laboratory research, they are designed for specific subtasks in a range of protocols. These items also generally require a large amount of manual manipulation both before and after machine usage. Within the research laboratory this category of equipment is commonplace and dominates equipment budgets. A further observation can be made in that the majority of research equipment in this category performs tasks that human operators would otherwise be incapable of carrying out themselves ( McClymont and Freemont, 2017 ). The rotation of samples at high speeds and observing microscale environments are examples of tasks that would be impossible without the use of centrifugation and microscopy equipment. Automation equipment which replaces manual handling tasks is rarer, and it the prevalence of these items where academic bioresearch facilities differ to industrial environments and clinical laboratories.
Access to high level 7 automated equipment can usually only be obtained through a pooled resource shared between across the parent organisation or wider research community; these are often referred to as biofoundries ( Chambers et al., 2016 ; Chao et al., 2017 ; Kitney et al., 2019 ). A new automation variant of the commercial contract research organisation has also arisen recently, the cloud lab. These provide researchers with remote access to heavily automated protocols available as a pay-per-experiment service ( Hayden, 2014 ). Cloud lab executives have made grand predictions regarding the impact these facilities will have on the future of biological research ( Miles and Lee, 2018 ; Segal, 2019 ), although doubts remain regarding experimental flexibility and the resulting inhibitory effect on experimental innovation ( Hayden, 2014 ).
Benefits of Laboratory Automation
Reproducibility.
There are multiple advantages and limitations in including automation into scientific processes and these are summarised in Figure 2 . Most pertinent is its use in improving the reproducibility of laboratory research ( Kitney et al., 2019 ). Reproducibility is a major concern for the research community both now ( Begley and Ioannidis, 2015 ; Baker, 2016 ) and historically (reviewed by Fanelli, 2018 ), with associated economic implications ( Freedman et al., 2015 ) and an undermining of public trust in science ( Saltelli and Funtowicz, 2017 ). Debate continues regarding the definition and scope of the reproducibility issue ( Casadevall and Fang, 2010 ; Goodman et al., 2018 ), alongside proposed improvements in scientific practices ( Peng, 2015 ; Munafò et al., 2017 ) and remedial technologies ( Benchoufi and Ravaud, 2017 ). Increasing the use of automation throughout research laboratories is one such proposition ( Jessop-Fabre and Sonnenschein, 2019 ; Kitney et al., 2019 ). An improvement in reproducibility is cited as a beneficial effect of automation implementation within clinical laboratories ( Hawker et al., 2018 ; Genzen et al., 2018 ).
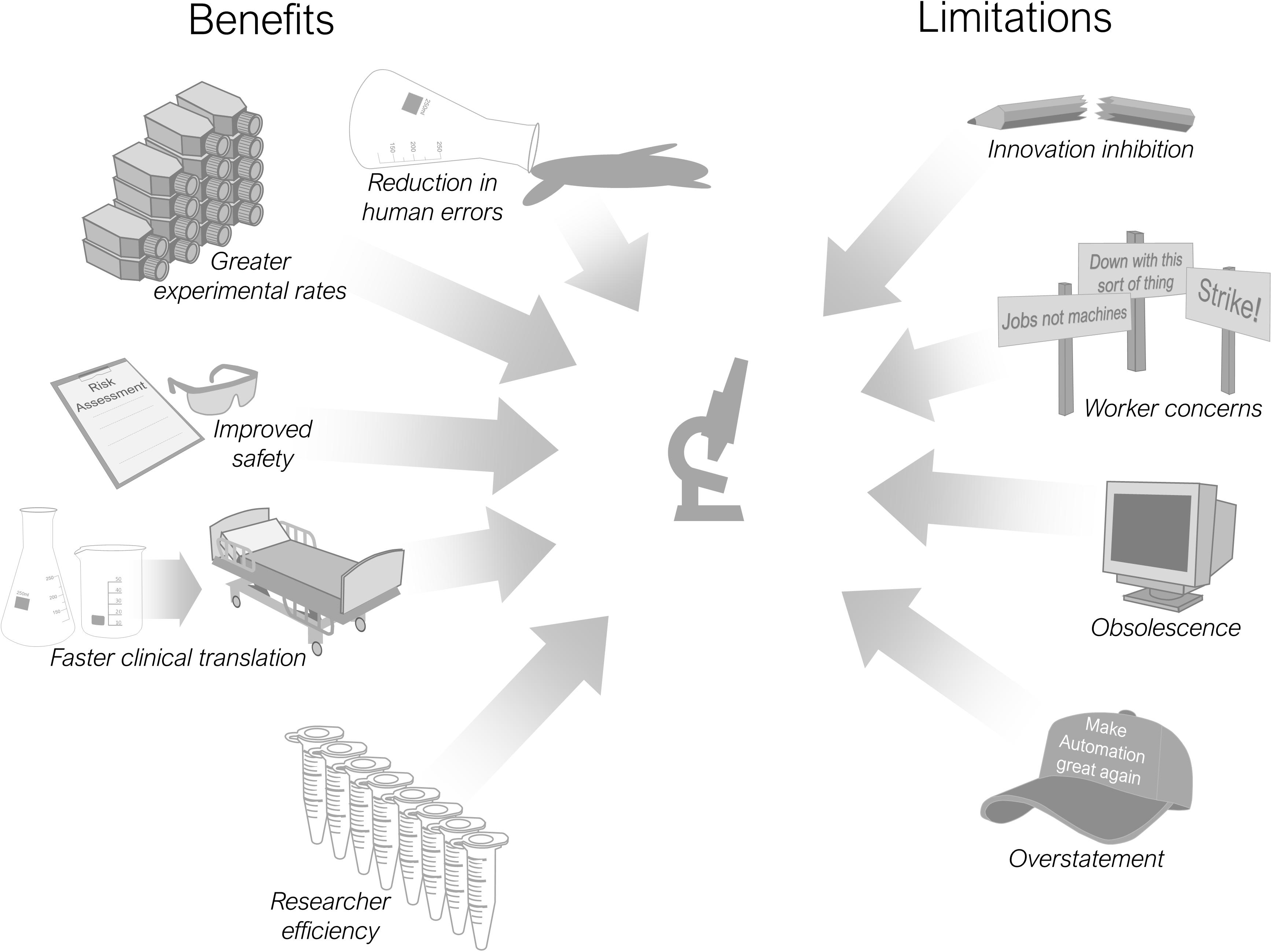
Figure 2. Benefits and limitations of research laboratory automation.
Automation can assist in improving reproducibility in three ways: a reduction in human-induced variability, an increase in the rate of data generation, and a decrease in contamination. The contribution each of these factors has on increasing reproducibility depends on the individual protocol. Firstly, experimental variability caused by humans is an omnipresent day-to-day reality in research laboratories ( Plebani, 2010 ; Price et al., 2015 ). Variation in protocols can arise from the same person unknowingly performing a task differently each time or between different individuals attempting to carry out the same procedure. Variability that is noticed at the time can be corrected for with repeated protocols or experimental redesign, although with an associated time penalty. However, variation that goes unnoticed will manifest itself in final datasets and published results. Automation can replace many, but not all, of these human-based sources of variability. Mechanised componentry is more suited to repetitive tasks ( Moutsatsou et al., 2019 ) in comparison to humans who are vulnerable to progressive mental fatigue ( Xu et al., 2018 ), physical weariness ( Björklund et al., 2000 ; Iridiastadi and Nussbaum, 2006 ) and also distracting influences ( Varao-Sousa et al., 2018 ). Laboratory protocols where manual operations have been automated demonstrate greater consistency in their results, improving experimental reproducibility ( Klevebring et al., 2009 ; Price et al., 2015 ). Secondly, a greater rate of experimental data capture, with an increased volume of results, can be achieved with automation alongside a wider range of experimental variables tested, including controls. Ultimately this increases the likelihood that others will be able to reproduce and build on their findings ( Maleki et al., 2019 ). Finally, there are those laboratory protocols that are susceptible to contamination that can arise from either from the researchers themselves ( Salter et al., 2014 ) or through increased exposure to environmental contaminants due to ponderous manual handling operations ( Greub et al., 2016 ). Automation can remove contact with human operators ( Wilke et al., 1995 ) or reduce potential contaminant exposure by lowering the required number of manual handling steps ( Mifflin et al., 2000 ; Moutsatsou et al., 2019 ).
Laboratory Efficiency
Efficiency is considered of paramount importance within manufacturing and can be defined as the rate of production, divided by the resources such as labour, input materials needed to accomplish this rate. By investing in automation, a company can increase the rate of production and also reduce the resources needed to achieve this rate. With a market available this can translate to a corresponding increase in profits ( Ceroni, 2009 ). A research laboratory investing in automation can improve the efficiency of its researchers ( Hawker and Schlank, 2000 ; Schneider, 2018 ) with machinery able to achieve a greater rate of experimental output than a manual based alternative ( Tacker et al., 2014 ; Price et al., 2015 ; Choi et al., 2018 ). It should be noted that an automated protocol need not take less time from start-to-finish to result in higher output than the manual alternative, as long as it demands less human intervention ( Reed et al., 2018 ). This is due the to the reward for academia differing from industry, with efficiency considered more as a time input to experimental output ratio. The key benefit derived from laboratory automation driven processes is therefore in the time saved by the researchers; time that can be spent on other parallel experiments. Automation in most cases will induce a transition from manual to cognitive labour ( Kaber et al., 2009 ). Allowing an operator to set a protocol in operation and walk away to think and focus on other tasks is a valuable function for any automation equipment. Researchers frequently have multiple projects, and experimental protocols operating in parallel as well as an array of responsibilities beyond the laboratory. With a greater rate of automation-driven experimental output researchers can also identify which aspects of their experiments don’t work and adjust more quickly ( Baranczak et al., 2017 ). Within industrial pharmaceutical development this methodology is known as fail fast, fail often ( Clark and Pickett, 2000 ; Khanna et al., 2016 ; Besteman and Bont, 2019 ). Efficiency gains can also extend to the use of expensive reagents and materials. Automation can provide a higher level of precision in reagent dispensing, reducing the amount needed per experiment.
Faster Translation
Automation has an important role in those laboratories engaged in applied research who are seeking to develop novel therapeutic interventions such as cell-based therapies, pharmaceutical developments or tissue-engineered constructs for implantation. Transition of these technologies from a purely research domain to final usage in a clinical setting is frequently difficult ( Ochs et al., 2017 ; Hua et al., 2018 ), often referred to as translation from the bench to the bedside ( Goldblatt and Lee, 2010 ). By considering and including automation at an early stage in the research process, crucial elements of the process can be mechanised, increasing product quality and production rates in the laboratory before the jump to manufacturing. The technological leap from laboratory-scale production to higher-volume manufacturing is therefore shortened. Researchers who include automation technologies at an early stage are subsequently better placed to upscale their processes allowing faster commercialisation rates and deployment to the clinic ( Kotin, 2011 ; Heathman et al., 2015 ; Rafiq and Thomas, 2016 ).
A number of protocols carried out in the research laboratory require the handling of dangerous reagents and occasionally of hazardous tooling. The manual manipulation of hazardous items places a burden on laboratories, particularly when contending with a continual turnover of short-term contract staff and students who require safety training and supervision. By assigning dangerous handling tasks to automated machinery, the exposure of humans to hazardous substances can be reduced ( Movsisyan et al., 2016 ; Caragher et al., 2017 ).
Examples of Automation Benefits
Evidence of automation benefits can be observed in recent success stories. In projects where high-throughput, reproducible results are demanded over short time frames automation has significant advantages over manual procedures. Recently a highly automated biofoundary, normally with a focus on research applications, was repurposed towards the development of SARS-CoV-2 assays for clinical diagnostics ( Crone et al., 2020 ). Automated liquid handling equipment was able to perform an extensive array of experimental procedures at a rate in excess of those that a manual based laboratory could carry out. Furthermore, in these time-pressured experiments, automation has an advantage over manual operators who are prone to fatigue and errors, with an associated negative effect on accuracy and reproducibility. Such work also clearly demonstrates the positive impact automation can have on public health challenges. It also an example of considerate design leading to systems that are flexible enough to be rapidly adapted to meet new experimental needs. This design feature is appropriately termed ‘facility agility.’
The use of automation to improve research efficiency is also demonstrated with a system comprising a mobile robotic platform that can autonomously navigate a laboratory performing reagent-dispensing and handling operations at a range of experimental benchtop stations ( Burger et al., 2020 ). In combination with an artificial intelligence search algorithm, the system was able to use initial data to decide on reagent combinations most likely to include an optimal reaction mix. The capacity of the robotic equipment to operate at all hours, with pausing only to charge batteries, allowed it to test five experimental hypotheses in a fraction of the time a manual research team would have required. Although it was used to answer a research question within a chemistry context the concept would be readily applicable to life science experimental laboratories. The system shares similar liquid and solid reagent handling operations to a life science laboratory as well as the common challenge of there being too many variables for researchers to explore manually in a reasonable time. A further crucial advantage of this arrangement resides in the possibility, with appropriate safety controls, of operating as a hybrid manual-automated laboratory. A staffed day shift performing high-skilled tasks requiring on-the-spot decisions could be followed by a robotic night shift carrying out the repetitive aspects of procedures.
Researchers aiming to translate stem cell-derived therapies towards clinical applications have considered automation for a range of projects. Such therapies will ultimately require the expansion of stem cells on a scale that is uneconomical for manual based laboratories, with large numbers also needed for research and clinical trials phases. The need for reliable methods of high-volume, quality-assured cells has led to the development of automated systems such as the StemCellFactory ( Doulgkeroglou et al., 2020 ), StemCellDiscovery ( Jung et al., 2018 ) and AUTOSTEM ( Ochs et al., 2017 ). The objective of these systems is to automate the normally manual stages of stem cell seeding, growth, colony selection, passaging, quality assessment, harvesting and potentially in later applications differentiation. In a similar fashion to the previous mobile robotic platform example, complex control algorithms are also being applied to these systems with the aim of improving cell yields and quality ( Egri et al., 2020 ). These projects are an important link between the domains of basic life science research, clinical application, and commercial cell product manufacturing. By developing these systems researchers have been able to generate high quantities of cells for research and testing purposes, hastening the route to clinical usage.
Limitations of Automation
Incorrect application.
Despite the range of benefits that laboratory automation can bring, there remains a number of limitations. Integrating automation into a research laboratory is not in itself a guarantee of success and, where applied incorrectly can even result in even less efficiency ( Zielinski et al., 2014 ). The nature of automated tasks also allows for rapid propagation of errors. An example would be a machine incorrectly dispensing a reagent repetitively which can then, if undetected, be distributed across many thousands of samples. In addition, the incorrect application and operation of automation may not improve the reproducibility of research between laboratories. Automation machinery carrying out the same experimental protocol in different laboratories may still produce different results. This can be due to variations in input materials, different equipment models or set-up and calibration errors. Even where automation has been carefully integrated into a laboratory and has demonstrated an improvement in reproducibility an inherent machine to machine variability can remain. What is more, this variability can be more hidden than more easily observed manual procedures. Careful maintenance, calibration and quality control measures are therefore essential in implementing any laboratory automation system ( Hawker and Schlank, 2000 ; Xie et al., 2004 ).
Obsolescence
Obsolescence is an inevitability for any technology and even, it can be argued, for scientists themselves. Many facilities will feature a dusty machine in the corner that is unused, because components and materials are no-longer available, the protocol itself has been supplanted or simply newer more effective equipment has taken over ( Croxatto et al., 2016 ). Predicting how and when a machine will become obsolete is an inherently difficult task in rapidly evolving research fields and can be specific to individual laboratories. Some researchers will find equipment is no-longer useful after a few years of operation whilst others may continue to happily use the same machine for decades. It is not only advances in hardware and software design that can render laboratory equipment obsolete. Scientific progress in reagent properties and resulting modifications to protocols can also be responsible. The advent of new thermostable polymerases obsoleted a whole generation of Polymerase Chain Reaction machinery designed upon a more repetitive protocol ( Hawker et al., 2018 ). Despite these difficulties, with considerate design allowing for reconfiguration and modification premature obsolescence can be delayed ( Harrison et al., 2007 ; Crombie et al., 2017 ), referred to in some industries as future-proofing. Understanding and planning for obsolescence is therefore an important part of any automation strategy.
Innovation Inhibition
There is a danger that automation can inhibit creativity in the experimental design process by limiting the opportunities for changing or tinkering with a protocol. A researcher may be less inclined to alter a protocol to optimise it for a new situation where a large number of steps are automated. This can be based upon the assumption that process steps carried out by machinery are already optimised and require no further improvement. They may also feel less able to begin changing things because they lack the confidence or maybe even the authorisation to open the box and begin modifying what is probably an expensive machine. Sharing of the machine with other users for whose purposes it is already optimised is also a brake to experimentation with parameters. Innovation inhibition is also a concern where protocols are outsourced to third party automated laboratories ( Hayden, 2014 ).
Workforce Impact
When integrating new automation into any workplace environment, the impact on workers and how they view new machinery must be carefully considered. Beginning in the rural English midlands with the machine breaking Luddite movement ( Roberts, 2017 ), societal resistance to automated machinery replacing manual labour and the threat it poses to livelihoods understandably continues into the present day ( Jones, 2013 ; Autor, 2015 ). Both positive and negative reactions to the introduction of automation have been observed amongst long-term workers in clinical laboratory settings ( Thomson and McElvania, 2019 ) and it is reasonable to anticipate that similar reactions may arise in research laboratories. The outright replacement of researchers by automation is unlikely as they are currently categorised as being amongst the lowest risk of being replaced ( White et al., 2019 ), due to their breadth of skills, including planning and creativity ( Reeves et al., 2019 ). However, researchers solely employed to perform repetitive manual tasks are more at risk and thus more likely to view automation as a threat. Those researchers with a multitude of other protocols and tasks beyond the laboratory are more likely to view automation assistance in their day to day roles in a positive manner. The short-term contracts that predominate in research will also lessen any hostility to automation. Employees who understand that they will be moving on to another position, will see a machine as more likely to be a replacement for their replacement rather than a replacement for themselves. Although the levels of militancy advocated by the early Luddites may not be repeated, laboratory managers who introduce automation will still, like their industrial and clinical counterparts, need to be sensitive to workforce reactions, particularly the impact on any long-term employees.
Automation Hyperbole
Both vendors of automation equipment and researchers must also be wary of overstating the benefits of automation and elevating expectations regarding the impact its introduction will have on future work practices. Automation hyperbole and the accompanying benefits is however part of a wider trend that is not only restricted to research ( Wajcman, 2017 ). Whilst automation can improve protocol reproducibility and efficiency the individual researcher will, in the majority of cases, still be responsible for correctly operating the equipment, with maintenance, quality of input materials, and calibration. These are tasks than can require a high level of personal discipline and tenacity. With notable exceptions ( King et al., 2009 ; Williams et al., 2015 ), automation will also be unable to undertake the overall experimental design and analysis. Journal publications have a responsibility too, to ensure that articles advocating laboratory automation equipment also highlight the limitations of their technologies, as well as identifying author conflicts of interests ( Miles and Lee, 2018 ). Greater awareness of limitations will allow more effective matching of automation solutions with laboratory problems and increase the trust between commercial vendors and academic institutions.
Laboratory Automation Obstacles
Automation is expensive and difficult to justify.
The most significant hurdle for PIs wishing to integrate automation systems into their laboratories is, unsurprisingly, cost. Commercially available automation equipment is expensive, whilst bespoke equipment for individual protocols costlier still. Cell culture is an example of a common, labor-intensive protocol familiar to generations of researchers. Equipment to automate cell culture is available and can save many hours of researcher effort from the process, but is tantalisingly out of reach for most laboratories. The cost of these items can be in excess of $1 M for a complete process system ( Storrs, 2013 ) placing them far beyond the reach of the majority of academic laboratories. Despite being commercially available for over 18 years ( Kempner and Felder, 2002 ) they remain a rare sight in research environments but are used in high volume cell-banking organisations ( Wrigley et al., 2014 ; Archibald et al., 2016 ; Daniszewski et al., 2018 ).
The development of automation equipment can be a time-consuming and expensive process. Initial rounds of iterative conceptual and prototype design and testing are followed by final design, build, and commissioning phases. Coordination is needed from a variety of disciplines including mechanical, electrical and software engineers alongside close collaboration with the end user. Most important for all automation projects however, is a source of capital investment. Industrial investment in automation is matched to business cases in which increasing confidence in the product and the associated income from projected sales is used to justify upfront capital expenditure. However, an academic principal investigator seeking to invest in automation for their laboratory is confronted by a different set of challenges. When compared to industrial and commercial organisations, a research laboratory’s output or success rate cannot be measured in using the same readily quantifiable metric of profit. Indeed, academic research output has long been a difficult entity to define both for individual researchers ( Klaus and del Alamo, 2018 ) and laboratories ( Kreiman and Maunsell, 2011 ; Abramo and D’Angelo, 2014 ). It is therefore more difficult to construct a ‘business’ case when seeking funding for laboratory automation equipment. A factory manager is able to justify a new item of automation based upon the argument that whilst it may initially cost X units of currency it will increase profits by X + Y units, measured in the same currency ( Ceroni, 2009 ). A clinical laboratory manager can present a similar case based upon both cost ( Archetti et al., 2017 ; Sarkozi et al., 2003 ) and the quantifiable output of turnaround time ( Hawkins, 2007 ; Archetti et al., 2017 ). A research laboratory manager however, in the same position applying for funding, will have greater difficulty in arguing that although the proposed equipment will cost X units of currency it will increase their laboratory’s research output by Y vaguely defined research outputs. The ambiguity of research success hinders laboratories seeking to invest in automation.
Research Funding Structures
The allocation of scientific funding to academic institutions further limits investment in automation. Research programs are most frequently funded through externally sourced grants that are applied for in a competitive environment, with pre-applied constraints on the amounts available and where these funds may be spent. Understandably the majority of funding calls open to scientific laboratories are seeking answers to novel scientific questions and not looking to develop items of equipment that are essentially engineering challenges. Should an applicant wish to include standard or bespoke automation when applying for grants, capital expenditure on large equipment, if even permitted, must be explicitly accounted for before the project starts. Unfortunately, the nature of research means that the details of protocols needed for the project are not always available during the early proposal phase. Estimating the both the timescales and cost of automation at such an early stage is a difficult task for supervisors of biological research laboratories who will have limited experience of budgeting for automation hardware. The time duration of funding grants also limits the development of automation, usually with the maximum being 5 years ( European Commission, 2016 ; Vaesen and Katzav, 2017 ). Automation strategies for industry are generally greater in duration and aligned to the anticipated lifecycle of the product, frequently extending into decades. In the case of commercialising a novel pharmaceutical product or medical device the automation strategy can be aligned to the 20-year exclusivity patent window. Automation expertise acquired over this time can then be exploited to maintain a competitive advantage when the window expires. Academic projects of a comparable length are rare. The Human Genome Project is one exception, and consequently was able to invest and substantially benefit from automation ( Meldrum, 2000 ). However, long-term, project specific funding stability is rarely available to most academic principal investigators, limiting automation investment.
Short-term research funding also places a limit on the individual researcher’s ability to develop automation. Hands-on researchers are best placed to determine which elements of their protocols would benefit from automation. However, these individuals are typically Ph.D. students or early career researchers with a time-limited contract or project. Such temporal limitation leaves little room for developing an idea for protocol automation into a functional system, particularly with specific scientific targets attached to the grant scheme funding their project. Short duration research positions reduce not only the time available to develop novel automated laboratory equipment but also the motivation for doing so. On completion, a researcher is likely to move on to a new laboratory contract or a career beyond academia ( van der Weijden et al., 2016 ). Researchers are therefore unlikely to experience any of the long-term benefits from planning automation. The cumulative effect of short-term, competitive grant allocations and transient researchers creates an environment unsuited to the long-term financial investment required for laboratory automation development.
A limited number of large grant funded projects have been successful in devising automation strategies and equipment, although often with a focus on industrial scale systems for clinical translation rather than research laboratories. One area that seen recent attention is the aforementioned development of high-volume manufacturing solutions for the production of Mesenchymal and Induced Pluripotent Stem Cells to meet anticipated future clinical demand ( Marx et al., 2013 ; Panchalingam et al., 2015 ; Rafiq et al., 2016 ; Ochs et al., 2017 ; Jossen et al., 2018 ). It is hoped that technology developed in these programs will, in the future, trickle down into more affordable systems that can be exploited by smaller research laboratories.
Stifled Commercial Development of New Laboratory Automation
Financial challenges also hinder those commercial organisations seeking to develop laboratory automation equipment. Industrial automation design and development is often a bespoke, collaborative arrangement for a particular challenge. A manufacturer will approach one or more automation developers to design a manufacturing system for their product. In this scenario the manufacturer is usually a much larger organisation with abundant reserves of capital and will also carry the majority of the risk should the product not sell as well as expected. To aid in mitigating this risk they are able to utilise their marketing, sales and distribution expertise within their particular market sector. For development of automated laboratory equipment, the scenario is often different. An automation developer may wish to partner with an academic research laboratory. However, as previously detailed, in such an arrangement the laboratory will be unable to operate as a cash-rich development partner unless a substantial funding grant can be obtained. The automation developer must therefore carry the risk that the equipment will not be commercially successful and assume the role of marketing and selling the product to the wider research community. Biological laboratories are best placed to identify where certain processes would benefit from automation, but don’t have the financial resources or expertise to develop these systems themselves. Automation companies, whilst having the capable expertise to develop automation equipment will be reluctant to pursue such a business strategy requiring up-front investment to develop a product for customers widely acknowledged to have little disposable capital.
Small-to medium-sized automation companies have often been most successful at innovative development of laboratory equipment, funded through grant schemes in cooperation with an academic institution or external venture capital funding. Examples include benchtop pipetting systems from Andrew Alliance and OpenTrons and Labman automation’s formulation engine. Access to joint research grants and funding schemes can encourage the development of novel automation solutions by increasing industrial and academic collaboration whilst also reducing the risk the commercial risk that developers are exposed to.
Laboratory Space
Alongside the financial investment required for automation researchers must also find physical laboratory space for new equipment, incurring a footprint cost ( Wong et al., 2018 ; Moutsatsou et al., 2019 ). The size and mass of many automation items means that it is not always practical or safe to tidy the item away and store it when it is not required. Laboratory space is often at a premium in many research institutions with territorial researchers often coming into conflict over the allocation of it ( Adams, 2004 ). A bench occupied by equipment is also an area that could be otherwise be utilised by productive researchers. The requirement for some laboratories to operate as a dual research and teaching environment further constrains the available space. It may also not be possible for automation to totally replace more manual based equipment and space in laboratories, with room required for both. The need to maintain cell culture hoods for teaching is one example. Developers of laboratory of automation have attempted to minimise the footprint of their machinery through innovative reworkings of traditional laboratory procedures. The use of hollow fibre arrays ( Russell et al., 2018 ) and multi-axis liquid and labware manipulation ( Kato et al., 2010 ) are examples of compact automated adherent cell culture systems. Spatial constraints may push future bench-based laboratory automation towards an architectural style resembling inner city skyscrapers.
Protocol Variation and Usage
The very nature of bioresearch involves the design and implementation of protocols aimed at the determining answers to novel research questions. In pursuit of these targets, researchers will devise new protocols or substantially modify existing ones to suit their needs. Recurring cycles of method generation and evolution within the research laboratory create a high-level of protocol variation that is not always easily automated. Matching commercially available automation equipment to these requirements is often not a feasible option with fixed componentry and locked-in software frequently being the limiting factors. Automated cell culture is an example where the available systems can be insufficiently flexible to accommodate the specific cell culture requirements of an individual laboratory ( Crombie et al., 2017 ), with some requiring a broad range of cell culture types and others having more focussed needs. A high level of experimental process variation is therefore more likely to require a bespoke automation system, the development of which will have an associated time and financial cost. Clinical laboratories, by comparison, have a greater level of consistency across protocols both within individual laboratories and across institutions, contributing to the widespread implementation of automated systems. High process variability is also cited as one of the major challenges for integrating automation into existing industrial environments ( Frohm et al., 2006 ) and is necessary when adapting to changing market conditions ( Froschauer et al., 2008 ). Across laboratory protocols there are process steps that are common, and it these where commercially available systems are more likely to be of assistance to the individual researcher. Liquid handling, through the manipulation of pipettes and receptacles is a one example ubiquitous to a range of molecular biology protocols, with a growing number of competing vendors offering more affordable and adaptable automation options ( Barthels et al., 2020 ).
How frequently a protocol is likely to be used over time is also a key factor when considering automation. A protocol developed for a specific project may only be used in a single laboratory for a short period, negating the long-term benefits that automation could provide. On occasion a researcher may find that their new protocol becomes widely adopted for an extended period in their own laboratory, and possibly throughout other laboratories too. In this scenario automation becomes a more attractive option and is not always driven by the original founding laboratory. Sequencing, is one example where the initial manual protocol developed by Sanger and colleagues ( Sanger et al., 1977 ) was eventually automated by researchers at different institutions ( García-Sancho, 2007 ).
Labware and Consumables
Automation equipment operates most effectively when input materials or consumables are standardised. In the case of standard shaped labware this allows non-adaptive, rigid automation components such as grippers to gain full custody of the device, allowing greater accuracy of placement and potentially faster actuations. Currently there remains a large amount of variation in labware not only between research laboratories but also within the same laboratory. The variant a researcher uses can change frequently based upon cost, availability or personal preference. Disposable plastics are an example where different manufacturers produce products that are, from an experimental, viewpoint functionally identical but with variations in the products dimensions and materials. The justification for these variants maybe a small improvement in handling, or simply to circumvent intellectual property assigned to a competing product. These present a significant challenge to automated handling equipment where even small variations, that are unnoticeable when handled manually, can render an automated system using non-adaptive handling elements useless. Clinical laboratories negate this issue by utilising standardised plastics for sample collections that can then be more readily processed autonomously. The recent advent of soft robotics may provide solutions to these challenges where rigid handling systems are replaced with pliable, adaptive designs sometimes based upon biomimetic examples ( Noel and Hu, 2018 ).
A counterstrategy to labware variation has emerged from commercial developers of automation. Unfortunately, the solution is often combined with a sales strategy aimed at securing a continuous revenue stream following the sale of the initial capital equipment. Commercially available systems are frequently designed in a fashion such that automation systems can only operate with specific consumables, available for purchase from themselves or a licensed distributor ( Huggett et al., 2009 ; Moutsatsou et al., 2019 ). Examples include the pipette tips for the Opentrons and Tecan EVO liquid dispensing systems, array tape for Douglas Scientific’s IntelliQube PCR system, purification cards for Invitrogens benchpro and spin kits for Qiagens Qiacube system. A laboratory binding themselves to a single consumable supplier has little or no guarantee of future price stability or even long-term supply should the commercial vendor cease to exist. Committing to a long-term, single vendor, supply chain is considered a very unwise strategy in a commercial context but is a worryingly frequent arrangement for automation equipment available to research laboratories.
There are two competing forces for labware standardisation; top-down and bottom up pressure, outlined in Figure 3 . Top-down pressure, as described above, is where commercial automation organisations seek to dominate a section of the market by forcing users to purchase specific labware through the sale of inflexible hardware. Bottom-up pressure acts in the opposite direction, when manufacturers of labware and laboratories slowly gravitate towards one standard form that automation developers are then forced to adopt. An example where bottom-up pressure has succeeded is in the largely standardised external dimensions of well plates, the ANSI/SLAS standard ( Society for Laboratory Automation and Screening, 2011 ), that has enabled automation of microscopy and plate reading procedures ( McClymont and Freemont, 2017 ). The range of automation equipment available for standard well plates is correspondingly larger, increasing competition, reducing running costs and making automation more affordable. There is likely to be a reciprocal benefit for labware manufacturers too, with an associated increase in demand for consumables. More instances of labware standardisation would allow a wider range of protocols to be automated.
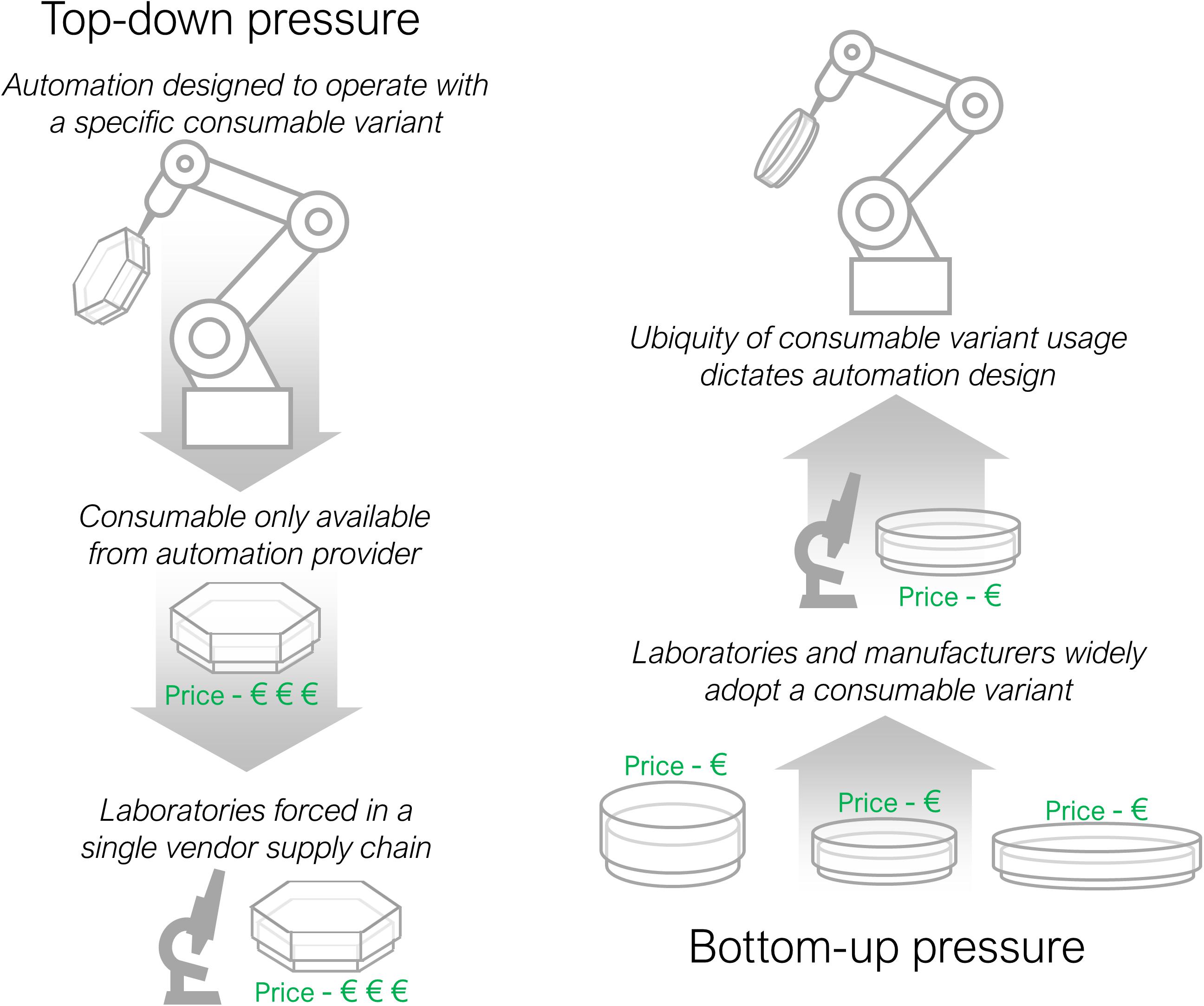
Figure 3. Top-down and bottom-up consumable adoption pressures. Top-down pressure occurs when an automation developer imposes a consumable on laboratories through tooling specific design. Bottom-up pressure acts in the reverse direction with laboratories and automation suppliers coalescing behind one consumable variant that then determines the design of automation equipment.
Environment Impact
The environmental impact that an item of equipment can have throughout its entire lifespan, from manufacture, to usage, to end-of-life disposal and recycling is an important consideration for many research institutions. A particular concern for laboratories is the rate at which automation consumes disposable plastics. Research institutions produce a large amount of plastic waste, estimated at 5.5 million tonnes annually ( Urbina et al., 2015 ), primarily to avoid contamination between samples. Commitments to minimising their use are part of a growing trend where laboratories aim to switch to recyclable or reusable alternatives ( Bistulfi, 2013 ; Krause et al., 2020 ). Automation designed around the same single-use plastic principle can generate even greater volumes of waste than human operators, due to higher experimental throughputs ( Howes, 2019 ). These designs are incompatible with research organisations who are committed to minimising their environmental impact. The consideration given to environmental concerns is currently very low or non-existent in many commercially available laboratory automation systems. An exception is Grenova’s pipette washing systems ( Safavi and Anderson, 2019 ) that can be integrated into existing automated liquid dispensing units. It is hoped that this type of equipment represents an emerging category of environmentally focused automation that will become ever more important to laboratories in the future.
There exists a fundamental culture difference between an academic research laboratory and the industrial workplace environment, that can inhibit investment in automation. It is hoped that the majority of principal investigators view their laboratory as a platform for staff and students to increase their skills and experience before they move onwards in their careers. This is a crucial ‘people’ output that accompanies the research output of a laboratory usually measured in scientific discoveries and publications. Although many companies also place a high-value on workforce upskilling their focus is primarily on profit and not on being a training institution to allow employee progression elsewhere. Consequently, many will favour investment in equipment over staff if a business case can be made ( Rampell, 2011 ). An academic principal investigator however, is likely to preferentially invest in additional people rather than equipment, with funding schemes frequently weighted this way too. Money spent on a large item of automation equipment could, for example, pay for several post-doctoral researchers or fund multiple Ph.D. projects. In the context of automation this culture could be described as a form of benevolent Luddism.
The availability and culture of undergraduate labour may also be inhibiting investment in laboratory automation. Undergraduates working in laboratories contribute by performing experiments that can generate preliminary data for grant applications or for publications. The benefits to the student reside in the acquisition of experience and skills that can enhance their employability prospects upon completion of their studies ( Seeling and Choudhary, 2016 ). This reciprocal arrangement and the high availability of undergraduates provides a means for carrying out labour intensive laboratory tasks. Not all principal investigators will view this relationship in such a cold manner, and will considerately assign duties that can generate useful data whilst simultaneously teaching students both the basics and realities of research. Unfortunately, there is evidence that some less altruistic supervisors do assign undergraduates to tasks that require a high degree of repetition ( Hayward et al., 2017 ). These are likely to be precisely the type of tasks where automation can be effectively applied.
The Laboratory Automation Interim Technology Gap
It is interesting to compare the relatively recent development of manual labour-saving laboratory automation equipment with other older, more mature automation processes. Here we refer to equipment that replaces manual human manipulation rather than machinery that performs operations operators are physically incapable of executing, such as centrifuging. Taking the millennia-old example of sewing, with just a needle, thread and cloth it is possible, given time, for a skilled human operator to create a garment. Equally the same items can be completely mechanised with expensive, high-level automation equipment and the garment produced with no human input necessary beyond the need to turn the machine on. Comparing with the laboratory process of cell culture which requires, media, pipettes, labware and some starting cells a skilled operator can also, given time, passage cells and create a sub-culture for experimentation. Again, the same output can also be produced using an entirely automated, costly, high-level system, with minimal operator input. However, in the case of needlework there exists a range of lower cost interim labour-saving automation options between these two extremes, such as motor driven stitching machinery, or manually powered mechanisms, exemplified in the Singer sewing machine ( McLoughlin and Mitchell, 2013 ). This is not currently the case for cell culture, there are no examples of commercially available low-cost machinery ( Figure 4 ).
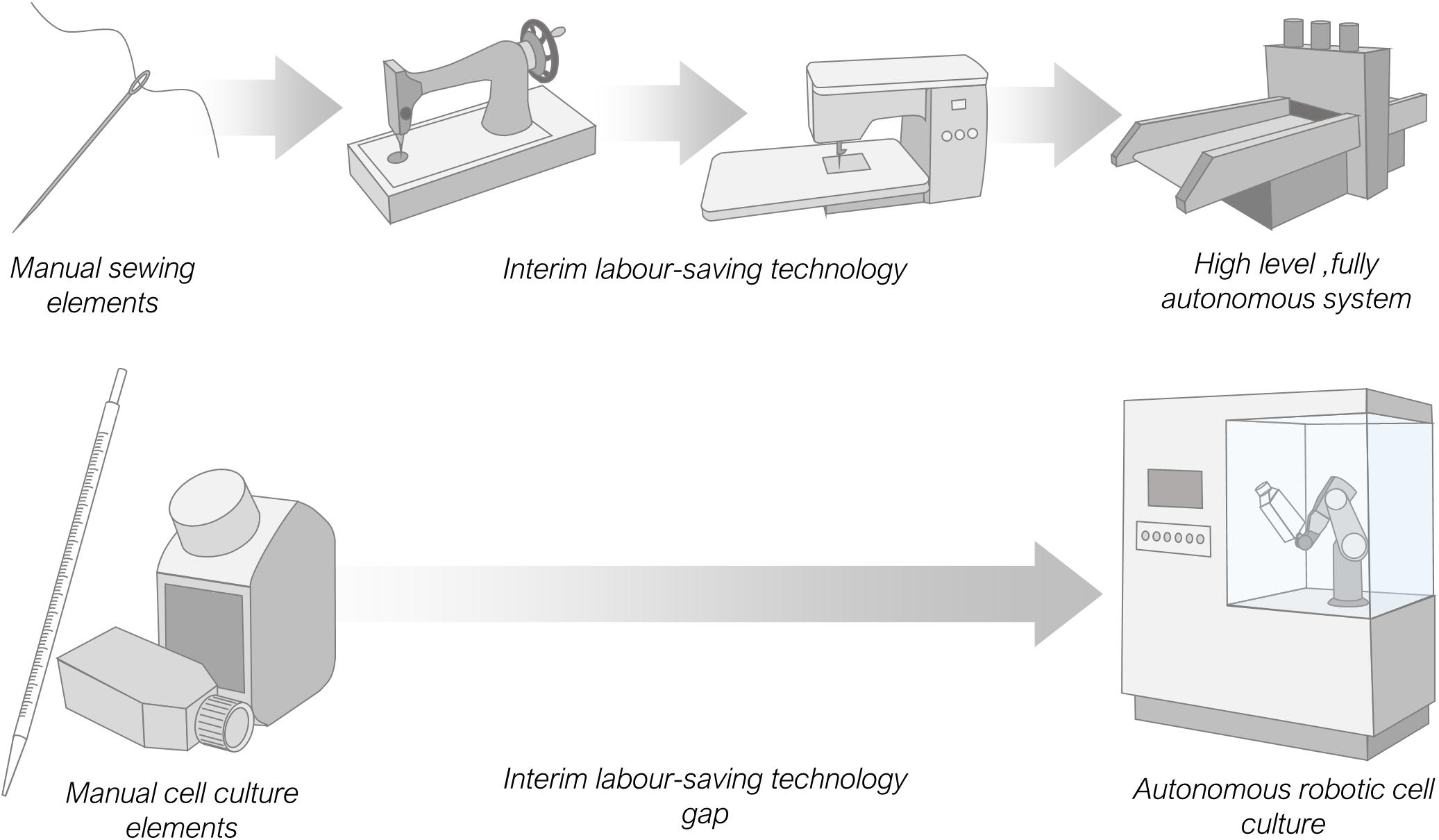
Figure 4. Comparison of available labour-saving automation options for the manual intensive processes of sewing and cell culture. Sewing has a range of interim automation options up to fully autonomous systems. Cell culture by contrast has only high-level automation equipment and no interim low-cost analogues to replace or augment manual labour.
Interim automation can arise in several scenarios. More commonly it occurs incrementally over time, as technological advances permit a shift from simple to complex machinery. Alternatively, on occasion a high-end complex automation system may be simplified due to new demands, such as an economic demand for cheaper equipment. For many laboratory automation processes there has been a rapid leap from simple to complex with, as yet, little or no development of lower cost automation technology. We believe this is due in part to the reasonable desire for academic laboratories and companies to be seen to be developing equipment at the forefront of technology. In simple terms, low-cost interim automation that removes some but not all of the manual labour from a protocol is not fashionable enough. It is unlikely to lead to a prestigious journal publication and, for commercial organisations, will not lead to financial rewards, with likely low sales volumes and low profit margins. There are therefore few incentives for academic and commercial automation developers to design such equipment.
In-House Laboratory Automation
Despite the hurdles facing researchers wishing to automate elements of their experimental procedures, there are many examples where laboratory automation development is carried out ‘in-house,’ without the assistance of a commercial partner or a large automation dedicated funding grant. Research teams are recognising that their protocols could be made more efficient by including automation but find themselves restricted financially and functionally by commercially available options ( Pilizota and Yang, 2018 ). A range of ingenious methods have been developed to build low-cost automation solutions, including the integration of Lego into microscopy automation ( Almada et al., 2019 ), microfluidics for DNA assembly ( Shih et al., 2015 ) and rapid synthesis and testing of small molecule libraries ( Baranczak et al., 2017 ). Laboratories with novel protocols that are nearly but not quite suited to existing automation equipment have been able to successfully upgrade commercially available systems for their specific needs ( McGraw et al., 2014 ; Richter et al., 2015 ; Zhang et al., 2016 ; Crombie et al., 2017 ; Konczal and Gray, 2017 ). Repurposing existing equipment in this fashion either through software or hardware modification is a cost-and time-efficient method of obtaining higher levels of protocol automation without the arduous task of designing and building an entirely novel system. The number of automation development tools, components and virtual training options available to research laboratories continues to broaden, increasing their capability to develop low-cost solutions to labour intensive processes. The advent of affordable 3D printing modalities ( Jones et al., 2011 ; Zluhan et al., 2016 ; Capel et al., 2018 ), off the shelf actuators and readily programable microcontrollers ( Mabbott, 2014 ; Kim et al., 2015 ; Wong et al., 2018 ) has given research laboratories the ability to produce componentry that can then be assembled, controlled and automated all for a relatively low cost ( Courtemanche et al., 2018 ; Needs et al., 2019 ; Barthels et al., 2020 ). Open source designs and software have an important enabling effect for researchers who may not have engineering or programming expertise. Researchers are also able to exploit the growing market for second hand laboratory automation equipment ( Zluhan et al., 2016 ), a case of one lab’s trash is another labs treasure. Developing automation internally, whilst often cheaper, and potentially a more rewarding and enjoyable process ( Pilizota and Yang, 2018 ) can however require a substantial investment in time ( May, 2019 ). That laboratories are frequently forced into developing their own systems is an indication of the paucity of commercially available options. Existing automation developers see an insufficient market for providing their services and expertise to develop bespoke items for individual laboratories and will be justifiably reluctant to provide open source solutions that may compromise their intellectual property.
Increasing the quantity and quality of laboratory automation within the research laboratory will require a concerted effort from funders, research institutions, automation developers and researchers themselves. The desire to automate elements of laboratory protocols exists. Researchers and their governmental funders ( Reeves et al., 2019 ) collectively recognise that mechanisation can improve reproducibility and efficiency. When attempting to develop laboratory automation three interrelated components are needed for success. Connecting researchers with automation needs to automation engineers, financing the resulting collaboration, and ensuring the resulting design meets the needs.
Collaboration
Encouraging academic researchers to engage and collaborate with industrial organisations has been a long-standing objective for their host institutions. Such joint enterprises are hindered by the significant differences in culture and attitudes to one another ( Berman, 2008 ) which are in part due to each partner having different timescales and expectations from projects. Academics build projects slowly through the funding stages and ultimately desire experimental data that can be packaged into publications. Industry often likes to move more quickly and would like intellectual property that can be reconstituted into a commercial opportunity ( Lynch, 2016 ). Contrary to widespread belief these viewpoints are, however, not always the most prominent motivations for collaboration, with altruistic aims also prevalent in both parties ( Berman, 2008 ).
Automation engineers and life science researchers operate in markedly different disciplines and in different work environments, rarely occupying the same space to share problems and ideas. Events where these disparate groups can be brought together would allow new ideas and projects to develop, in a similar fashion to academic conferences encouraging collaboration between different laboratories. Automation engagement events that feature all levels of employees from both sides of the divide would have the greatest effect. Interaction between industrial managers and academic supervisors as well as researchers who are researching and engineers who are engineering could allow the development of solutions to everyday automation challenges in the laboratory.
Collaboration can also be an internal academic arrangement. Life science laboratories often have a source of automation engineering expertise within their own institution in the form of engineering faculties. Both disciplines could benefit from increased interaction and discussion around laboratory automation, with examples of collaborating biomedicine and engineering departments producing innovative automated equipment ( Kato et al., 2010 ; Kane et al., 2019 ). Collaboration at an educational level can be beneficial too. Allowing undergraduate engineering students to undertake projects based upon automating a protocol within a laboratory would provide the host laboratory with designs and automation aids. Interdepartmental, interdisciplinary collaborations can bring benefits for students too, providing real world problems to develop their skills and the opportunity to apply theoretical knowledge ( Wilson and Zamberlan, 2012 ).
More varied career paths that allow employees with experience of industry-based automation to work in research environments can also develop new ideas that lead to mechanised laboratory equipment. Academic and industrial career paths diverge at early career stage and rarely reconnect. The majority of professional individuals progress from an academic institution into an industrial or commercial organisation. Researchers typically remain within a university environment accruing the required qualifications and experience as their career progresses. Reverse flow of employees, where an individual moves from industry to academia is less common ( Bonner, 2006 ). Encouraging a greater level of employees with experience of automation to work within life science laboratories will promote an exchange of ideas that can lead to experimental mechanisation. Such employee exchanges need not be permanent and can be sabbatical-style placements targeted at a specific project. The Knowledge Transfer Partnership is one successful long-running academic-industry exchange scheme in the authors host country that allows an employee to concurrently work on a project at both an academic and industrial organisation ( Howlett(ed.), 2010 ). These types of employee arrangements have a further benefit in deepening the relationships between Universities and industrial organisations. Academic institutions that can successfully foster relationships with industrial partners can reap substantial rewards not only in the form of publications and possible financial licencing agreements but greater reproducibility too ( Edwards, 2016 ). In a notable success story, automated sequencing technology, now the mainstay of genetic research, was successfully developed at Caltech, a research organisation with strong links to industry ( García-Sancho, 2007 ). Ultimately though any collaboration, regardless of the method of inception, is unlikely to succeed or even be embarked upon unless both partners are confident that they have the financial resources to proceed.
Greater implementation of automation can bestow benefits to funding organisations. Devoting financial resources towards automation engineering may seem paradoxical where the long-term objectives are targeted towards developing therapeutic interventions for biological diseases. However, the reproducibility of published research is essential for research financed by these organisations. Automation is a critical component in driving upwards the reproducibility of disseminated research ( Winder, 2019 ). In addition, as research confidence increases in a particular therapy consideration will eventually need to shift towards how the technology can be produced in sufficient quantities and at an affordable price so that it is available to the greatest range of patients. As previously discussed, including automation at earlier stage in the development process can help in attaining these goals, easing the transition from the experimentation phase to clinical usage. Competitive schemes, where funds are specifically are made available for developing laboratory automation would be beneficial in bridging the distance between the lab bench and the bedside.
Automation can provide benefits too for governments funding academic institutions. Increasing the level of automation across workplaces is acknowledged as strategy for economic progress ( Velásquez et al., 2009 ; Reeves et al., 2019 ) with research laboratories being no exception. Access to higher levels of automation increases the output of research laboratories that exist in publicly funded institutions. Any associated automation dividend will also require appropriately skilled technical staff to maintain, operate and enhance laboratory equipment. A greater range of dedicated grant schemes specifically targeted at developing laboratory automation will, in the long-term, increase the effectiveness of all research funding.
Laboratory Automation Design
Improvements can be made in automation design, how it is implemented in laboratories and the range of available automations options. A large amount of laboratory automation is based upon an anthropomorphic design framework that mimics human movement. Expensive laboratory equipment frequently features an over reliance on robotics to manipulate tooling, reagents and labware in a similar manner to how researchers would themselves. These types of designs can present as being visually high-tech and impressive and there is indeed an advantage to machinery that presents as more human-like in that it is more likely to be trusted by human operators ( de Visser et al., 2016 ). Unfortunately for many applications these designs are not always the most efficient means for automating a laboratory protocol. Robotic actuators featuring multiple axes and large operating envelopes also require even larger guarding enclosures and correspondingly complex control systems ( Yachie and Natsume, 2017 ). These design attributes render such equipment spatially and economically unsuitable for the majority of research laboratories. McClymont and Freemont provide an example where an assay requiring liquid handling can be more effectively processed and multiplexed with tooling that is not based upon an anthropomorphic design ( McClymont and Freemont, 2017 ). Hollow fibre cell culture systems are further examples of automation systems that have successfully eschewed more traditional anthropomorphic designs ( Eghbali et al., 2016 ).
Designing for flexibility is also an important factor for laboratories where there is a high level of protocol variation. Laboratory automations systems designs that anticipate future scientific developments and allow for subsequent adaptation will be less likely to become prematurely obsolete and thus more valuable to research laboratories. Machinery based upon modular based design is one approach to a flexible system. Modular automation systems can allow selective matching of automation to the protocol requirements, minimising the purchase of redundant features, and also providing the option for future upgrades should it be needed. There are indications that laboratory automation developers are becoming more aware of the need for flexibility. The ongoing development of technology such as Formulatrix’s rover system is one example where microwell plates are autonomously transferred between processing modules in a novel reworking of the robotic warehouse concept ( Wikholm and Lindblom, 2019 ).
The capability for an automation system to be modified without specialist engineering knowledge is desirable too. Allowing researchers to automate a wider range of process steps without the need for time consuming and expensive tooling redesign or extensive software reprogramming. An interesting extension of the modular design approach is to unify existing automation equipment so that it capable of performing the desired protocol in one continuous process stream. The recent development of software by the company Synthace that is capable of communicating and linking robotics from different manufacturers is one promising system for laboratories requiring highly flexible systems ( Sadowski et al., 2016 ; Jessop-Fabre and Sonnenschein, 2019 ).
To reduce the manual labour burden on laboratory research staff and students there is a need for a broader range of automation equipment. These designs should target the identified gap in labour saving automation with a focus on reducing price and footprint. In this regard employing multi axis robotics may not be the most optimal design solution and developers should be prepared to explore more cost-effective, low-tech routes to protocol automation, even if seems like a less fashionable option.
The Future of Laboratory Automation
It is with a certain degree of trepidation that we follow in the footsteps of others and attempt to predict the future of laboratory automation. The life science research laboratory of the future will undoubtably feature more automation equipment. How quickly automation is adopted will in all probability be slower than many would like and haphazard, with some fields being more suitable than others. Many of the obstacles to laboratory automation ingress we have described are long-standing and hardwired into the working practices of academic research. In particular financial hurdles faced by individual principal investigators are unlikely to be resolved and overcome in the immediate future. Bespoke, high-level automation solutions will remain beyond the reach of all but the most monied laboratories for a considerable time. Greater progress can be anticipated in the design and price of lower-level automation equipment. It is reasonable to assume that like other technologies laboratory automation will continue to mature with falling prices and more user centred designs. Hopefully incorporating more flexibility in response to consumer demand. In part this progression is already underway, with promising releases of low-cost liquid handling platforms and ongoing development of modular systems. The demand from research laboratories for automation that seeks to limit its impact on the environment will grow considerably and it is hoped that developers will create and adapt their designs to meet this need. Life science researchers will also continue to develop their own homemade laboratory automation and repurpose existing equipment, encouraging other laboratories to also take the leap into engineering. We predict that the second hand market will become an important resource for those choosing this route to automation.
Access to pooled resource, high-level, automation in the form of academic biofoundries is increasing and will continue to do so with expansion of existing facilities and the foundation of new ones. The outsourcing of protocols to commercial cloud laboratories has been predicted to become commonplace for a huge range of life science laboratories. From the perspective of the lab bench we are more circumspect in regards to the impact these organisations will have on day to day experimental research, with experimental range and flexibility key issues. Ultimately, the marketplace laws of supply and demand will dictate the success rate of these enterprises.
An appreciation of the limitations of automation both generally and for items of specific equipment is needed from academic, commercial and funding organisations and individuals. Of all the limitations discussed in this review we wish to particularly highlight the danger of innovation inhibition. Innovation in the laboratory is essential and the freedom to tinker and create new protocols needs to be retained if research is to retain a high degree of novelty. Ensuring that automation remains compatible with the curiously minded researcher will be a significant challenge for our field in the future.
In response to automation ingress the skills of life science researchers will need to adapt. The presence of more automation equipment will require more engineering type-skills to ensure correct equipment operation and implementation of protocols, along with a working knowledge of the biology under experimentation. Researchers will therefore need both biology ‘wet’ skills and ‘dry’ automation skills; such people have been imaginatively titled amphibious researchers by Mellingwood (2018) . It is therefore likely that automation will spawn a new generation of researchers with a range of interdisciplinary skills.
In summary, automation in life science laboratories lags behind its industrial and clinical counterparts due to an array of inhibiting factors, including financial, spatial and cultural challenges. Those who are able to surmount these barriers and integrate automation into their everyday protocols can reap significant reproducibility and efficiency benefits. It is essential that future laboratory automation systems are designed for flexibility to permit adaptation for changing laboratory needs and prevent the stifling of protocol innovation. A wider range of affordable bench top and remote automation options will steadily increase the ubiquity of mechanisation in life science research. Such progressive adoption of automation will emphasise the already growing interdisciplinary nature of research further blurring the boundary between science and engineering.
Author Contributions
IH conceived the study and wrote the manuscript with support from JD who critically reviewed it and also contributed content. Both authors contributed to the article and approved the submitted version.
The authors would like to acknowledge support from the Biotechnology and Biological Sciences Research Council (BBSRC: grant code BB/M018040/1).
Conflict of Interest
The authors declare that the research was conducted in the absence of any commercial or financial relationships that could be construed as a potential conflict of interest.
JD is a member of the Free Software Foundation, which campaigns for free (open-source) software in place of proprietary.
Acknowledgments
We would like to thank Sara Gómez Arnaiz and Fokion Glykofrydis for their feedback and discussion of the manuscript.
Abramo, G., and D’Angelo, C. (2014). How do you define and measure research productivity? Scientometrics 101, 1129–1144. doi: 10.1007/s11192-014-1269-8
CrossRef Full Text | Google Scholar
Adams, J. U. (2004). How to negotiate for academic lab space. Scientist 18, 42–43.
Google Scholar
Almada, P., Pereira, P. M., Culley, S., Caillol, G., Boroni-Rueda, F., Dix, C. L., et al. (2019). Automating multimodal microscopy with NanoJ-Fluidics. Nat. Commun. 10:1223. doi: 10.1038/s41467-019-09231-9
PubMed Abstract | CrossRef Full Text | Google Scholar
Archetti, C., Montanelli, A., Finazzi, D., Caimi, L., and Garrafa, E. (2017). Clinical laboratory automation: a case study. J. Public Health Res. 6:881. doi: 10.4081/jphr.2017.881
Archibald, P. R. T., Chandra, A., Thomas, D., Chose, O., Massouridès, E., Laâbi, Y., et al. (2016). Comparability of automated human induced pluripotent stem cell culture: a pilot study. Bioprocess Biosyst. Eng. 39, 1847–1858. doi: 10.1007/s00449-016-1659-9
Autor, D. H. (2015). Why are there still so many jobs? The history and future of workplace automation. J. Econ. Perspect. 29, 3–30. doi: 10.1257/jep.29.3.3
Baker, M. (2016). 1,500 scientists lift the lid on reproducibility. Nature 533, 452–454. doi: 10.1038/533452a
Baranczak, A., Tu, N. P., Marjanovic, J., Searle, P. A., Vasudevan, A., and Djuric, S. W. (2017). Integrated Platform for Expedited Synthesis-Purification-Testing of Small Molecule Libraries. ACS Med. Chem. Lett. 8, 461–465. doi: 10.1021/acsmedchemlett.7b00054
Barthels, F., Barthels, U., Schwickert, M., and Schirmeister, T. (2020). FINDUS: an open-source 3d printable liquid-handling workstation for laboratory automation in life sciences. SLAS Technol. 25, 190–199. doi: 10.1177/2472630319877374
Begley, C. G., and Ioannidis, J. P. A. (2015). Reproducibility in science: improving the standard for basic and preclinical research. Circ. Res. 116, 116–126. doi: 10.1161/CIRCRESAHA.114.303819
Benchoufi, M., and Ravaud, P. (2017). Blockchain technology for improving clinical research quality. Trials 18:335. doi: 10.1186/s13063-017-2035-z
Berman, J. (2008). Connecting with industry: bridging the divide. J. High. Educ. Policy Manage. 30, 165–174. doi: 10.1080/13600800801938762
Besteman, S. B., and Bont, L. J. (2019). Fail-fast in respiratory syncytial virus vaccine development. Am. J. Respir. Crit. Care Med. 200, 410–412. doi: 10.1164/rccm.201901-0233ED
Beugelsdijk, T. J. (1991). The future of laboratory automation. Genet. Anal. Biomol. Eng. 8, 217–220. doi: 10.1016/1050-3862(91)90016-K
Bistulfi, G. (2013). Reduce, reuse and recycle lab waste. Nature 502, 170–170. doi: 10.1038/502170a
Björklund, M., Crenshaw, A. G., Djupsjöbacka, M., and Johansson, H. (2000). Position sense acuity is diminished following repetitive low-intensity work to fatigue in a simulated occupational setting. Eur. J. Appl. Physiol. 81, 361–367. doi: 10.1007/s004210050055
Bonner, J. (2006). Back to Academia: A Mid-Life Crisis? New Scientist, 2006. Available online at: https://www.newscientist.com/article/mg19125711-900-back-to-academia-a-mid-life-crisis/ (accessed May 26, 2020).
Boyd, J. (2002). Robotic laboratory automation. Science 295, 517–518. doi: 10.1126/science.295.5554.517
Burger, B., Maffettone, P., Gusev, V., Aitchison, C., Bai, Y., Xiaoyan, W., et al. (2020). A Mobile Robotic chemist. Nature 583, 237–241. doi: 10.1038/s41586-020-2442-2
Capel, A. J., Rimington, R. P., Lewis, M. P., and Christie, S. D. R. (2018). 3D printing for chemical, pharmaceutical and biological applications. Nat. Rev. Chem. 2, 422–436. doi: 10.1038/s41570-018-0058-y
Caragher, T. E., Lifshitz, M. S., and DeCresce, R. (2017). “Analysis: clinical laboratory automation,” in Henry’s Clinical Diagnosis and Management by Laboratory Methods , eds R. A. McPherson and M. Pincus (Amsterdam: Elsevier), 60–65.
Casadevall, A., and Fang, F. C. (2010). Reproducible science. Infect. Immun. 78, 4972–4975. doi: 10.1128/IAI.00908-10
Ceroni, J. A. (2009). “Economic rationalization of automation projects,” in Springer Handbook of Automation , ed. S. Y. Nof (Berlin: Springer), 699–713. doi: 10.1007/978-3-540-78831-7_40
Chambers, S., Kitney, R., and Freemont, P. (2016). The foundry: the DNA synthesis and construction foundry at imperial college. Biochem. Soc. Trans. 44, 687–688. doi: 10.1042/BST20160007
Chao, R., Mishra, S., Si, T., and Zhao, H. (2017). Engineering biological systems using automated biofoundries. Metab. Eng. 42, 98–108. doi: 10.1016/j.ymben.2017.06.003
Choi, Q., Kim, H. J., Kim, J. W., Kwon, G. C., and Koo, S. H. (2018). Manual versus automated streaking system in clinical microbiology laboratory: performance evaluation of Previ Isola for Blood culture and body fluid samples. J. Clin. Lab. Anal. 32:e22673. doi: 10.1002/jcla.22373
Clark, D. E., and Pickett, S. D. (2000). Computational methods for the prediction of ‘drug-likeness. Drug Discov. Today 5, 49–58. doi: 10.1016/S1359-6446(99)01451-8
Courtemanche, J., King, S., and Bouck, D. (2018). Engineering novel lab devices using 3D printing and microcontrollers. SLAS Technol. 23, 448–455. doi: 10.1177/2472630318766858
Crombie, D. E., Daniszewski, M., Liang, H. H., Kulkarni, T., Li, F., Lidgerwood, G. E., et al. (2017). Development of a modular automated system for maintenance and differentiation of adherent human pluripotent stem cells. SLAS Discov. 22, 1016–1025. doi: 10.1177/2472555217696797
Crone, M. A., Priestman, M., Ciechonska, M., Jensen, K., Sharp, D. J., Anand, A., et al. (2020). A role for biofoundries in rapid development and validation of automated sars-cov-2 clinical diagnostics. Nat. Commun. 11:4464. doi: 10.1038/s41467-020-18130-3
Croxatto, A., Prod’hom, G., Faverjon, F., Rochais, Y., and Greub, G. (2016). Laboratory automation in clinical bacteriology: What system to choose? Clin. Microbiol. Infect. 22, 217–235. doi: 10.1016/j.cmi.2015.09.030
Daniszewski, M., Crombie, D. E., Henderson, R., Liang, H. H., Wong, R. C. B., Hewitt, A. W., et al. (2018). Automated cell culture systems and their applications to human pluripotent stem cell studies. SLAS Technol. 23, 315–325. doi: 10.1177/2472630317712220
De Almeida, M., and Ferreira, R. (2017). Taking Biotech to the Next Level with Laboratory Automation. Labiotech, 2017. Available online at: https://www.labiotech.eu/features/biotech-laboratory-automation/ (accessed April 1, 2020).
de Visser, E. J., Monfort, S. S., McKendrick, R., Smith, M. A. B., McKnight, P. E., Krueger, F., et al. (2016). Almost human: anthropomorphism increases trust resilience in cognitive agents. J. Exp. Psychol. Appl. 22, 331–349. doi: 10.1037/xap0000092
Doulgkeroglou, M. N., Nubila, A., Niessing, B., König, N., Schmitt, R. H., Damen, J., et al. (2020). Automation, monitoring, and standardization of cell product manufacturing. Front. Bioeng. Biotechnol. 8:811. doi: 10.3389/fbioe.2020.00811
Edwards, A. (2016). Reproducibility: team up with industry. Nature 531, 299–301. doi: 10.1038/531299a
Eghbali, H., Nava, M. M., Mohebbi-Kalhori, D., and Raimondi, M. T. (2016). Hollow fiber bioreactor technology for tissue engineering applications. Int. J. Artif. Organs 39, 1–15. doi: 10.5301/ijao.5000466
Egri, P., Csáji, B. C., Kis, K. B., Monostori, L., Váncza, J., Ochs, J., et al. (2020). Bio-inspired control of automated stem cell production. Procedia CIRP 88, 600–605. doi: 10.1016/j.procir.2020.05.105
European Commission (2016). H2020 Programme Fact Sheets Grants 2 (December). Available online at: http://ec.europa.eu/research/participants/data/ref/h2020/other/gm/h2020-grant-factsheet_en.pdf (accessed May 8, 2020).
Fanelli, D. (2018). Opinion: Is science really facing a reproducibility crisis, and do we need it to? Proc. Natl. Acad. Sci. U.S.A. 115, 2628–2631. doi: 10.1073/pnas.1708272114
Freedman, L. P., Cockburn, I. M., and Simcoe, T. S. (2015). The economics of reproducibility in preclinical research. PLoS Biol. 13:e1002165. doi: 10.1371/journal.pbio.1002165
Frohm, J., Lindström, V., Winroth, M., and Stahre, J. (2006). The Industry’s view on automation in manufacturing. IFAC Proc. Vol. 39, 453–458. doi: 10.3182/20060522-3-FR-2904.00073
Frohm, J., Lindström, V., Winroth, M., and Stahre, J. (2008). Levels of Automation in Manufacturing. Int. J. Ergon. Hum. Fact. 30, 71–74.
Froschauer, R., Dhungana, D., and Gruenbacher, P. (2008). “Managing the Life-Cycle of Industrial Automation Systems with Product Line Variability Models,” in Proceedings of the 2008 34th Euromicro Conference Software Engineering and Advanced Applications , Parma, 35–42. doi: 10.1109/SEAA.2008.21
García-Sancho, M. (2007). Sequencing As a Way of Work: A History of Its Emergence and Mechanisation – From Proteins To Dna, 1945-2000. Ph.D. thesis, Imperial College London, London.
Genzen, J. R., Burnham, C. A. D., Felder, R. A., Hawker, C. D., Lippi, G., and Peck Palmer, O. M. (2018). Challenges and opportunities in implementing total laboratory automation. Clin. Chem. 64, 259–264. doi: 10.1373/clinchem.2017.274068
Goldblatt, E. M., and Lee, W. H. (2010). From bench to bedside: the growing use of translational research in cancer medicine. Am. J. Transl. Res. 2, 1–18. doi: 10.1111/j.1468-3083.2010.03829.x
Goodman, S. N., Fanelli, D., and Ioannidis, J. P. A. (2018). What does research reproducibility mean? Sci. Transl. Med. 8:341ps12. doi: 10.1126/scitranslmed.aaf5027
Greub, G., Sahli, R., Brouillet, R., and Jaton, K. (2016). Ten years of R&D and full automation in molecular diagnosis. Future Microbiol. 11, 403–425. doi: 10.2217/fmb.15.152
Groth, P., and Cox, J. (2017). Indicators for the use of robotic labs in basic biomedical research: a literature analysis. PeerJ 5:e3997. doi: 10.7717/peerj.3997
Harrison, R., Colombo, A. W., West, A. A., and Lee, S. M. (2007). Reconfigurable modular automation systems for automotive power-train manufacture. Int. J. Flex. Manuf. Syst. 18, 175–190. doi: 10.1007/s10696-006-9008-y
Hasegawa, Y. (2009). “Advances in robotics and automation: historical perspectives,” in Springer Handbook of Automation , ed. Y. Shimon (Heidelberg: Springer), 3–4. doi: 10.1007/978-3-540-78831-7_1
Hawker, C. D., Genzen, J. R., and Wittwer, C. T. (2018). “Automation in the Clinical Laboratory,” in Tietz Textbook of Clinical Chemistry and Molecular Diagnostics , 6th Edn, eds N. Rifai, A. R. Horvath, and C. T. Wittwer (Philadelphia, PA: Elsevier Inc), 370.e1–370.e24. doi: 10.1016/B978-0-323-35921-4.00026-0
Hawker, C. D., and Schlank, M. R. (2000). Development of standards for laboratory automation. Clin. Chem. 46, 746–750. doi: 10.1093/clinchem/46.5.746
Hawkins, R. C. (2007). Laboratory turnaround time. Clin. Biochem. Rev. 28, 179–194. doi: 10.1093/ajcp/105.6.676
Hayden, E. C. (2014). The automated lab. Nature 516, 131–132. doi: 10.1038/516131a
Hayward, C. N., Laursen, S. L., and Thiry, H. (2017). Why work with undergraduate researchers? Differences in research advisors’ motivations and outcomes by career stage. CBE Life Sci. Educ. 16:ar13. doi: 10.1187/cbe.16-07-0229
Heathman, T. R. J., Nienow, A. W., McCall, M. J., Coopman, K., Kara, B., and Hewitt, C. J. (2015). The translation of cell-based therapies: clinical landscape and manufacturing challenges. Regen. Med. 10, 49–64. doi: 10.2217/rme.14.73
Hitomi, K. (1994). Automation — Its concept and a short history. Technovation 14, 121–128. doi: 10.1016/0166-4972(94)90101-5
Howes, L. (2019). Can laboratories move away from single-use plastic? ACS Cent. Sci. 5, 1904–1906. doi: 10.1021/acscentsci.9b01249
Howlett, R. J. (ed.) (2010). “Knowledge Transfer between UK Universities and Business,” in Innovation through Knowledge Transfer. Smart Innovation, Systems and Technologies , Vol. 5, (Berlin: Springer), 1–14. doi: 10.1007/978-3-642-14594-0_1
Hua, S., de Matos, M. B. C., Metselaar, J. M., and Storm, G. (2018). Current trends and challenges in the clinical translation of nanoparticulate nanomedicines: pathways for translational development and commercialization. Front. Pharmacol. 9:790. doi: 10.3389/fphar.2018.00790
Huggett, J., Green, C., and Zumla, A. (2009). Nucleic acid detection and quantification in the developing world. Biochem. Soc. Trans. 37, 419–423. doi: 10.1042/BST0370419
Iridiastadi, H., and Nussbaum, M. A. (2006). Muscular fatigue and endurance during intermittent static efforts: effects of contraction level, duty cycle, and cycle time. Hum. Factors 48, 710–720. doi: 10.1518/001872006779166389
Jessop-Fabre, M. M., and Sonnenschein, N. (2019). Improving reproducibility in synthetic biology. Front. Bioeng. Biotechnol. 7:18. doi: 10.3389/fbioe.2019.00018
Jones, R., Haufe, P., Sells, E., Iravani, P., Olliver, V., Palmer, C., et al. (2011). Reprap - the replicating rapid prototyper. Robotica 29, 177–191. doi: 10.1017/S026357471000069X
Jones, S. E. (2013). Against Technology: From the Luddites to Neo-Luddism. New York, NY: Routledge.
Jossen, V., van den Bos, C., Eibl, R., and Eibl, D. (2018). Manufacturing human mesenchymal stem cells at clinical scale: process and regulatory challenges. Appl. Microbiol. Biotechnol. 102, 3981–3994. doi: 10.1007/s00253-018-8912-x
Jung, S., Ochs, J., Kulik, M., König, N., and Schmitt, R. H. (2018). Highly modular and generic control software for adaptive cell processing on automated production platforms. Procedia CIRP 72, 1245–1250. doi: 10.1016/j.procir.2018.03.189
Kaber, D. B., Stoll, N., Thurow, K., Green, R. S., Kim, S. H., and Mosaly, P. (2009). Human-automation interaction strategies and models for life science applications. Hum. Fact. Ergon. Manuf. 19, 601–621. doi: 10.1002/hfm.20156
Kane, K. I. W., Moreno, E. L., Hachi, S., Walter, M., Jarazo, J., Oliveira, M. A. P., et al. (2019). Automated microfluidic cell culture of stem cell derived dopaminergic neurons. Sci. Rep. 9:1796. doi: 10.1038/s41598-018-34828-3
Kato, R., Iejima, D., Agata, H., Asahina, I., Okada, K., Ueda, M., et al. (2010). A compact, automated cell culture system for clinical scale cell expansion from primary tissues. Tissue Eng. Part C Methods 16, 947–956. doi: 10.1089/ten.tec.2009.0305
Kempner, M. E., and Felder, R. A. (2002). A review of cell culture automation. JALA 7, 56–62. doi: 10.1016/S1535-5535(04)00183-2
Khanna, R., Guler, I., and Nerkar, A. (2016). Fail often, fail big, and fail fast? Learning from small failures and R&D performance in the pharmaceutical industry. Acad. Manage. J. 59, 436–459. doi: 10.5465/amj.2013.1109
Kim, K. W., Lee, M. S., Ryu, M. H., and Kim, J. W. (2015). Arduino-Based Automation of a DNA Extraction System. Edited by Wen-Hsiang Hsieh. Technol. Health Care 24, S105–S112. doi: 10.3233/THC-151048
King, R. D., Rowland, J., Oliver, S. G., Young, M., Aubrey, W., Byrne, E., et al. (2009). The automation of science. Science 324, 85–89. doi: 10.1126/science.1165620
Kitney, R., Adeogun, M., Fujishima, Y., Goñi-Moreno, Á, Johnson, R., Maxon, M., et al. (2019). Enabling the advanced bioeconomy through public policy supporting biofoundries and engineering biology. Trends Biotechnol. 37, 917–920. doi: 10.1016/j.tibtech.2019.03.017
Klaus, B., and del Alamo, D. (2018). Talent identification at the limits of peer review: an analysis of the EMBO postdoctoral fellowships selection process. bioRxiv [Preprint]. doi: 10.1101/481655
Klevebring, D., Gry, M., Lindberg, J., Eidefors, A., and Lundeberg, J. (2009). Automation of CDNA synthesis and labelling improves reproducibility. J. Biomed. Biotechnol. 2009:396808. doi: 10.1155/2009/396808
Konczal, J., and Gray, C. H. (2017). Streamlining workflow and automation to accelerate laboratory scale protein production. Protein Expr. Purif. 133, 160–169. doi: 10.1016/j.pep.2017.03.016
Kotin, R. M. (2011). Large-scale recombinant adeno-associated virus production. Hum. Mol. Genet. 20, R2–R6. doi: 10.1093/hmg/ddr141
Krause, M., Gautam, K., Małgorzata, A., and Niraula, A. G. (2020). Reducing Plastic Waste in the Lab. Chemistry World, 2020. Available online at: https://www.chemistryworld.com/opinion/reducing-plastic-waste-in-the-lab/4011550 (accessed June 2, 2020).
Kreiman, G., and Maunsell, J. H. R. (2011). Nine criteria for a measure of scientific output. Front. Comput. Neurosci. 5:48. doi: 10.3389/fncom.2011.00048
Lou, A. H., Elnenaei, M. O., Sadek, I., Thompson, S., Crocker, B. D., and Nassar, B. (2016). Evaluation of the impact of a total automation system in a large core laboratory on turnaround time. Clin. Biochem. 49, 1254–1258. doi: 10.1016/j.clinbiochem.2016.08.018
Lynch, K. L. (2016). Collaboration at the heart of innovation. Clin. Chem. 62:1284. doi: 10.1373/clinchem.2016.260687
Mabbott, G. A. (2014). Teaching electronics and laboratory automation using microcontroller boards. J. Chem. Educ. 91, 1458–1463. doi: 10.1021/ed4006216
Maleki, F., Ovens, K., McQuillan, I., and Kusalik, A. J. (2019). Size matters: how sample size affects the reproducibility and specificity of gene set analysis. Hum. Genomics 13(Suppl. 1):42. doi: 10.1186/s40246-019-0226-2
Marx, U., Schenk, F., Behrens, J., Meyr, U., Wanek, P., Zang, W., et al. (2013). Automatic production of induced pluripotent stem cells. Procedia CIRP 5, 2–6. doi: 10.1016/j.procir.2013.01.001
May, M. (2019). A DIY approach to automating your lab. Nature 569, 587–588. doi: 10.1038/d41586-019-01590-z
McClymont, D. W., and Freemont, P. S. (2017). With all due respect to Maholo, lab automation isn’t anthropomorphic. Nat. Biotechnol. 35, 312–314. doi: 10.1038/nbt.3795
McGraw, J., Tatipelli, V. K., Feyijinmi, O., Traore, M. C., Eangoor, P., Lane, S., et al. (2014). A semi-automated method for purification of milligram quantities of proteins on the QIAcube. Protein Expr. Purif. 96, 48–53. doi: 10.1016/j.pep.2014.01.014
McLoughlin, J., and Mitchell, A. (2013). “Mechanisms of sewing machines,” in Joining Textiles , eds I. Jones, and G. K. Stylios (Amsterdam: Elsevier). doi: 10.1533/9780857093967.1.123
Meldrum, D. (2000). Automation for genomics, part one: preparation for sequencing. Genome Res. 10, 1081–1092. doi: 10.1101/gr.101400
Mellingwood, C. (2018). Amphibious Researchers: Working with Laboratory Automation in Synthetic Biology. Ph.D. thesis, University of Edinburgh, Edinburgh.
Mifflin, T. E., Estey, C. A., and Felder, R. A. (2000). Robotic automation performs a nested RT-PCR analysis for HCV without introducing sample contamination. Clin. Chim. Acta 290, 199–211. doi: 10.1016/S0009-8981(99)00192-8
Miles, B., and Lee, P. L. (2018). Achieving reproducibility and closed-loop automation in biological experimentation with an IoT-enabled lab of the future. SLAS Technol. 23, 432–439. doi: 10.1177/2472630318784506
Moutsatsou, P., Ochs, J., Schmitt, R. H., Hewitt, C. J., and Hanga, M. P. (2019). Automation in cell and gene therapy manufacturing: from past to future. Biotechnol. Lett. 41, 1245–1253. doi: 10.1007/s10529-019-02732-z
Movsisyan, M., Delbeke, E. I. P., Berton, J. K. E. T., Battilocchio, C., Ley, S. V., and Stevens, C. V. (2016). Taming hazardous chemistry by continuous flow technology. Chem. Soc. Rev. 45, 4892–4928. doi: 10.1039/c5cs00902b
Munafò, M. R., Nosek, B. A., Bishop, D. V. M., Button, K. S., Chambers, C. D., Du Sert, N. P., et al. (2017). A manifesto for reproducible science. Nat. Hum. Behav. 1:0021. doi: 10.1038/s41562-016-0021
Needs, S. H., Diep, T. T., Bull, S. P., Lindley-Decaire, A., Ray, P., and Edwards, A. D. (2019). Exploiting open source 3D printer architecture for laboratory robotics to automate high-throughput time-lapse imaging for analytical microbiology. PLoS One 14:e0224878. doi: 10.1371/journal.pone.0224878
Noel, A. C., and Hu, D. L. (2018). The Tongue as a Gripper. J. Exp. Biol. 221:jeb176289. doi: 10.1242/jeb.176289
Ochs, J., Barry, F., Schmitt, R., and Murphy, J. M. (2017). Advances in automation for the production of clinical-grade mesenchymal stromal cells: the AUTOSTEM Robotic Platform. Cell Gene Ther. Insights 3, 739–748. doi: 10.18609/cgti.2017.073
Panchalingam, K. M., Jung, S., Rosenberg, L., and Behie, L. A. (2015). Bioprocessing strategies for the large-scale production of human mesenchymal stem cells: a review. Stem Cell Res. Ther. 6:225. doi: 10.1186/s13287-015-0228-5
Peng, R. (2015). The reproducibility crisis in science: a statistical counterattack. Significance 12, 30–32. doi: 10.1111/j.1740-9713.2015.00827.x
Pilizota, T., and Yang, Y. T. (2018). ‘Do it yourself’ microbial cultivation techniques for synthetic and systems biology: cheap, fun, and flexible. Front. Microbiol. 9:1666. doi: 10.3389/fmicb.2018.01666
Plebani, M. (2010). The detection and prevention of errors in laboratory medicine. Ann. Clin. Biochem. 47, 101–110. doi: 10.1258/acb.2009.009222
Price, A. P., Godin, L. M., Domek, A., Cotter, T., D’Cunha, J., Taylor, D. A., et al. (2015). Automated decellularization of intact, human-sized lungs for tissue engineering. Tissue Eng. Part C Methods 21, 94–103. doi: 10.1089/ten.tec.2013.0756
Rafiq, Q. A., and Thomas, R. J. (2016). The evolving role of automation in process development & manufacture of cell & gene-based therapies. Cell Gene Ther. Insights 2, 473–479. doi: 10.18609/cgti.2016.058
Rafiq, Q. A., Twomey, K., Kulik, M., Leschke, C., O’Dea, J., Callens, S., et al. (2016). Developing an automated robotic factory for novel stem cell therapy production. Regen. Med. 11, 351–354. doi: 10.2217/rme-2016-0040
Rampell, C. (2011). Companies Spend on Equipment, Not Workers. New York Times. Available online at: https://www.nytimes.com/2011/06/10/business/10capital.html (accessed June, 2011).
Ravazzi, P., and Villa, A. (2009). “Economic aspects of automation,” in Springer Handbook of Automation , ed. S. Y. Nof (Berlin: Springer), 93–116. doi: 10.1007/978-3-540-78831-7_6
Reed, C. E., Fournier, J., Vamvoukas, N., and Koza, S. M. (2018). Automated preparation of MS-sensitive fluorescently labeled N-Glycans with a commercial pipetting robot. SLAS Technol. 23, 550–559. doi: 10.1177/2472630318762384
Reeves, R., Coaker, V., Hendry, D., Kerr, S., Kyle, P., Liddell-Grainger, I., et al. (2019). Automation and the Future of Work House of Commons Business, Energy and Industrial Strategy Committee.
Richter, F., Scheib, U. S., Mehlhorn, J., Schubert, R., Wietek, J., and Gernetzki, O. (2015). Upgrading a microplate reader for photobiology and all-optical experiments. Photochem. Photobiol. Sci. 14, 270–279. doi: 10.1039/C4PP00361F
Roberts, M. (2017). Rural luddism and the makeshift economy of the nottinghamshire framework knitters. Soc. Hist. 42, 365–398. doi: 10.1080/03071022.2017.1327644
Russell, A. L., Lefavor, R. C., and Zubair, A. C. (2018). Characterization and cost–benefit analysis of automated bioreactor-expanded mesenchymal stem cells for clinical applications. Transfusion 58, 2374–2382. doi: 10.1111/trf.14805
Sadowski, M. I., Grant, C., and Fell, T. S. (2016). Harnessing QbD, programming languages, and automation for reproducible biology. Trends Biotechnol. 34, 214–227. doi: 10.1016/j.tibtech.2015.11.006
Safavi, A., and Anderson, T. (2019). Pipette tip Washing Device. U.S. Patent No. US20190216290A1. Washington, DC: U.S. Patent and Trademark Office.
Saltelli, A., and Funtowicz, S. (2017). What is science’s crisis really about? Futures 91, 5–11. doi: 10.1016/j.futures.2017.05.010
Salter, S. J., Cox, M. J., Turek, E. M., Calus, S. T., Cookson, W. O., Moffatt, M. F., et al. (2014). Reagent and laboratory contamination can critically impact sequence-based microbiome analyses. BMC Biol. 12:87. doi: 10.1186/s12915-014-0087-z
Sanger, F., Nicklen, S., and Coulson, A. R. (1977). DNA sequencing with chain-terminating inhibitors. Proc. Natl. Acad. Sci. U.S.A. 74, 5463–5467. doi: 10.1073/pnas.74.12.5463
Sarkozi, L., Simson, E., and Ramanathan, L. (2003). The effects of total laboratory automation on the management of a clinical chemistry laboratory. Retrospective analysis of 36 years. Clin. Chim. Acta 329, 89–94. doi: 10.1016/S0009-8981(03)00020-2
Schneider, G. (2018). Automating Drug Discovery. Nat. Rev. Drug Discov. 17, 97–113. doi: 10.1038/nrd.2017.232
Seeling, J. M., and Choudhary, M. (2016). Professional practices in undergraduate research programs. J. Microbiol. Biol. Educ. 17, 246–251. doi: 10.1128/jmbe.v17i2.982
Segal, M. (2019). An operating system for the biology lab. Nature 573, S112–S113. doi: 10.1038/d41586-019-02875-z
Shih, S. C. C., Goyal, G., Kim, P. W., Koutsoubelis, N., Keasling, J. D., Adams, P. D., et al. (2015). A versatile microfluidic device for automating synthetic biology. ACS Synth. Biol. 4, 1151–1164. doi: 10.1021/acssynbio.5b00062
Society for Laboratory Automation and Screening (2011). ANSI SLAS 1-2004 (R2012) Footprint Dimensions. Chicago, IL: Society for Laboratory Automation and Screening.
Storrs, C. (2013). Set It and Forget It - A Tour of Three Systems for Automating Cell Culture. The Scientist, 2013. Available online at: https://www.the-scientist.com/lab-tools/set-it-and-forget-it-39696 (accessed March 27, 2020).
Tacker, D. H., Topardo, J., Mahaffey, C., and Perrotta, P. L. (2014). Workflow analysis comparing manual and automated specimen processing for mass spectrometry–based vitamin D testing. Lab. Med. 45, 361–367. doi: 10.1309/lmzl47en6kdodmxj
Thomson, R. B., and McElvania, E. (2019). Total laboratory automation: What is gained, what is lost, and who can afford it? Clin. Lab. Med. 39, 371–389. doi: 10.1016/j.cll.2019.05.002
Urbina, M. A., Watts, A. J. R., and Reardon, E. E. (2015). Labs should cut plastic waste too. Nature 528, 479–479. doi: 10.1038/528479c
Vaesen, K., and Katzav, J. (2017). How much would each researcher receive if competitive government research funding were distributed equally among researchers? PLoS One 12:e0183967. doi: 10.1371/journal.pone.0183967
van der Weijden, I., Teelken, C., de Boer, M., and Drost, M. (2016). Career satisfaction of postdoctoral researchers in relation to their expectations for the future. High. Educ. 72, 25–40. doi: 10.1007/s10734-015-9936-0
Varao-Sousa, T. L., Smilek, D., and Kingstone, A. (2018). In the lab and in the wild: how distraction and mind wandering affect attention and memory. Cogn. Res. Princ. Implic. 3:42. doi: 10.1186/s41235-018-0137-0
Velásquez, J. D., Chen, X. W., Yoon, S. W., and Ko, H. S. (2009). “Automation Statistics,” in Springer Handbook of Automation , ed. S. Y. Nof (Heidelberg: Springer), 1673–1701. doi: 10.1007/978-3-540-78831-7_94
Wajcman, J. (2017). Automation: Is it really different this time? Br. J. Sociol. 68, 119–127. doi: 10.1111/1468-4446.12239
White, S., Lacey, A., and Ardanaz-Badia, A. (2019). The Probability of Automation in England - Office for National Statistics. Office for National Statistics. Available online at: https://www.ons.gov.uk/employmentandlabourmarket/peopleinwork/employmentandemployeetypes/articles/theprobabilityofautomationinengland/2011and2017 (accessed April 24, 2020).
Wikholm, D., and Lindblom, R. (2019). Rover-based integrated laboratory system including autonomous mobile robots. U.S. Patent No. WO2019/139930. Washington, DC: U.S. Patent and Trademark Office.
Wilke, W. W., Jones, R. N., and Sutton, L. D. (1995). Automation of polymerase chain reaction tests. reduction of human errors leading to contamination. Diagn. Microbiol. Infect. Dis. 21, 181–185. doi: 10.1016/0732-8893(95)00041-8
Williams, K., Bilsland, E., Sparkes, A., Aubrey, W., Young, M., Soldatova, L. N., et al. (2015). Cheaper faster drug development validated by the repositioning of drugs against neglected tropical diseases. J. R. Soc. Interface 12:20141289. doi: 10.1098/rsif.2014.1289
Wilson, S., and Zamberlan, L. (2012). Show me yours: developing a faculty-wide interdisciplinary initiative in built environment higher education. Contemp. Issues Educ. Res. 5, 331–342. doi: 10.19030/cier.v5i4.7430
Winder, A. (2019). How Lab Automation Is Helping Drug Research. European Pharmaceutical Manufacturer, 2019. Available online at: https://www.epmmagazine.com/opinion/how-lab-automation-is-helping-drug-research/ (accessed May 19, 2020).
Wong, B. G., Mancuso, C. P., Kiriakov, S., Bashor, C. J., and Khalil, A. S. (2018). Precise, automated control of conditions for high-throughput growth of yeast and bacteria with EVOLVER. Nat. Biotechnol. 36, 614–623. doi: 10.1038/nbt.4151
Wrigley, J. D., McCall, E. J., Bannaghan, C. L., Liggins, L., Kendrick, C., Griffen, A., et al. (2014). Cell banking for pharmaceutical research. Drug Discov. Today 19, 1518–1529. doi: 10.1016/j.drudis.2014.05.006
Xie, I. H., Wang, M. H., Carpenter, R., and Wu, H. Y. (2004). Automated calibration of TECAN genesis liquid handling workstation utilizing an online balance and density meter. Assay Drug Dev. Technol. 2, 71–80. doi: 10.1089/154065804322966333
Xu, R., Zhang, C., He, F., Zhao, X., Qi, H., Zhou, P., et al. (2018). How physical activities affect mental fatigue based on EEG energy, connectivity, and complexity. Front. Neurol. 9:915. doi: 10.3389/fneur.2018.00915
Yachie, N., and Natsume, T. (2017). Robotic crowd biology with Maholo labdroids. Nat. Biotechnol. 35, 310–312. doi: 10.1038/nbt.3758
Zhang, C., Long, A. M., Swalm, B., Charest, K., Wang, Y., Hu, J., et al. (2016). Development of an automated mid-scale parallel protein purification system for antibody purification and affinity chromatography. Protein Expr. Purif. 128, 29–35. doi: 10.1016/j.pep.2016.08.005
Zielinski, D., Gordon, A., Zaks, B. L., and Erlich, Y. (2014). IPipet: sample handling using a tablet. Nat. Methods 11, 784–785. doi: 10.1038/nmeth.3028
Zluhan, E., Kelly, K., LeClair, N., Wortel, D., and Moody, K. (2016). Automating HESC differentiation with 3D printing and legacy liquid handling solutions. MethodsX 3, 569–576. doi: 10.1016/j.mex.2016.10.005
Keywords : laboratory automation, life science research, automation design, research efficiency, reproducibility, innovation inhibition, environmental design
Citation: Holland I and Davies JA (2020) Automation in the Life Science Research Laboratory. Front. Bioeng. Biotechnol. 8:571777. doi: 10.3389/fbioe.2020.571777
Received: 11 June 2020; Accepted: 26 October 2020; Published: 13 November 2020.
Reviewed by:
Copyright © 2020 Holland and Davies. This is an open-access article distributed under the terms of the Creative Commons Attribution License (CC BY) . The use, distribution or reproduction in other forums is permitted, provided the original author(s) and the copyright owner(s) are credited and that the original publication in this journal is cited, in accordance with accepted academic practice. No use, distribution or reproduction is permitted which does not comply with these terms.
*Correspondence: Ian Holland, [email protected]
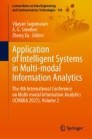
International Conference on Multi-modal Information Analytics
ICMMIA 2022: Application of Intelligent Systems in Multi-modal Information Analytics pp 748–753 Cite as
Analysis and Design of Innovative Laboratory Management System
- Yiyang Lin 5 ,
- Chunfeng Jiang 5 ,
- Binying Weng 5 ,
- Feng Guo 5 ,
- Huicong Hou 5 ,
- Ruonan Song 5 &
- G. Rajendran 6
- Conference paper
- First Online: 12 June 2022
1002 Accesses
Part of the book series: Lecture Notes on Data Engineering and Communications Technologies ((LNDECT,volume 138))
With the gradual increase in the degree of the innovation laboratory in the university, the management becomes more complicated and the data statistics index rises since the problems caused by the information out of synchronization become more and more obvious. A set of innovative laboratory management system suitable has customized and developed. It can promote the informatization of innovative laboratories and reduce management costs and the work of laboratory management staff, and then enhance the practical development ability of the students of our team. The system adopts iBeacon and face recognition for student attendance which can ensure the authenticity of attendance data. It is independently developed using SpringBoot and Vue framework, the user’s information and management logs are stored in the college server, and data security can be effectively guaranteed.
This is a preview of subscription content, log in via an institution .
Buying options
- Available as PDF
- Read on any device
- Instant download
- Own it forever
- Available as EPUB and PDF
- Compact, lightweight edition
- Dispatched in 3 to 5 business days
- Free shipping worldwide - see info
Tax calculation will be finalised at checkout
Purchases are for personal use only
Setiawan, A.W., Muhammad, F., Hidayat, A.: Development of an web-based wearable gait recognition system using gyroscope and accelerometer sensors. In: 2020 International Seminar on Application for Technology of Information and Communication (iSemantic), pp. 370–373. IEEE, September 2020
Google Scholar
Joseph, D., Mathew, M., Mathew, T., Vasappan, V., Mony, B.S.: Automatic attendance system using face recognition. Int. J. Res. Appl. Sci. Eng. Technol. 8 , 769–773 (2020)
Article Google Scholar
Lin, T.: Analysis and design of consumables management system for college laboratories based on Spring Boot and Vue framework. Comput. Knowl. Technol. (13), 83–85 (2021)
Jugend, D., Fiorini, P.D.C., Armellini, F., Ferrari, A.G.: Public support for innovation: a systematic review of the literature and implications for open innovation. Technol. Forecast. Soc. Chang. 156 , 119985 (2020)
Soh, Z.H.C., Ag, J.A.K.I.H., Sulaiman, S.N., Abdullah, S.A.C., Ibrahim, M.N., Abu, B.A.: Fridge load management system with AI and IOT alert. In: IOP Conference Series: Materials Science and Engineering, vol. 1088, no. 1, p. 012062. IOP Publishing, February 2021
Subbarao, V., Srinivas, K., Pavithr, R.S.: A survey on internet of things based smart, digital green and intelligent campus. In: 2019 4th International Conference on Internet of Things: Smart Innovation and Usages (IoT-SIU), pp. 1–6. IEEE, April 2019
De Paola, A., Ferraro, P., Lo Re, G., Morana, M., Ortolani, M.: A fog-based hybrid intelligent system for energy saving in smart buildings. J. Ambient. Intell. Humaniz. Comput. 11 (7), 2793–2807 (2019). https://doi.org/10.1007/s12652-019-01375-2
Hsieh, Y.J., Wu, Y.J.: Entrepreneurship through the platform strategy in the digital era: Insights and research opportunities. Comput. Hum. Behav. 95 , 315–323 (2019)
Mukhtar, S., Wardana, L.W., Wibowo, A., Narmaditya, B.S.: Does entrepreneurship education and culture promote students’ entrepreneurial intention? The mediating role of entrepreneurial mindset. Cogent Educ. 8 (1), 1918849 (2021)
Fenglian, C., Rongguo, L.: Research on the design of innovation and entrepreneurship teaching system for college-enterprise students based on computer education. Inf. Syst. Eng. 08 , 174 (2019)
Download references
Acknowledgements
This work is supported by 202111439009 which was from the Science and Technology Innovation and Entrepreneurship Training Program for University Students of Jilin Province.
Author information
Authors and affiliations.
Jilin Agricultural Science and Technology University, Jilin, China
Yiyang Lin, Chunfeng Jiang, Binying Weng, Feng Guo, Huicong Hou & Ruonan Song
Karpagam College of Engineering, Othakalmandapam, Coimbatore, India
G. Rajendran
You can also search for this author in PubMed Google Scholar
Corresponding author
Correspondence to Chunfeng Jiang .
Editor information
Editors and affiliations.
School of Business Administration, Oakland University, Rochester, MI, USA
Vijayan Sugumaran
Amrita School of Engineering, Chennai, Tamil Nadu, India
A. G. Sreedevi
Shanghai Polytechnic University, Shanghai, China
Rights and permissions
Reprints and permissions
Copyright information
© 2022 The Author(s), under exclusive license to Springer Nature Switzerland AG
About this paper
Cite this paper.
Lin, Y. et al. (2022). Analysis and Design of Innovative Laboratory Management System. In: Sugumaran, V., Sreedevi, A.G., Xu , Z. (eds) Application of Intelligent Systems in Multi-modal Information Analytics. ICMMIA 2022. Lecture Notes on Data Engineering and Communications Technologies, vol 138. Springer, Cham. https://doi.org/10.1007/978-3-031-05484-6_95
Download citation
DOI : https://doi.org/10.1007/978-3-031-05484-6_95
Published : 12 June 2022
Publisher Name : Springer, Cham
Print ISBN : 978-3-031-05483-9
Online ISBN : 978-3-031-05484-6
eBook Packages : Intelligent Technologies and Robotics Intelligent Technologies and Robotics (R0)
Share this paper
Anyone you share the following link with will be able to read this content:
Sorry, a shareable link is not currently available for this article.
Provided by the Springer Nature SharedIt content-sharing initiative
- Publish with us
Policies and ethics
- Find a journal
- Track your research
Accessibility Links
- Skip to content
- Skip to search IOPscience
- Skip to Journals list
- Accessibility help
- Accessibility Help
Click here to close this panel.
Purpose-led Publishing is a coalition of three not-for-profit publishers in the field of physical sciences: AIP Publishing, the American Physical Society and IOP Publishing.
Together, as publishers that will always put purpose above profit, we have defined a set of industry standards that underpin high-quality, ethical scholarly communications.
We are proudly declaring that science is our only shareholder.
Research on the Problems and Countermeasures of Laboratory Management in Colleges and Universities
Jiahua Zhang 1 and Hang Li 2
Published under licence by IOP Publishing Ltd Journal of Physics: Conference Series , Volume 1798 , 2020 International Conference on Applied Mechanics and Mechanical Engineering (ICAMME 2020) 25-27 September 2020, Hulun Buir, China Citation Jiahua Zhang and Hang Li 2021 J. Phys.: Conf. Ser. 1798 012006 DOI 10.1088/1742-6596/1798/1/012006
Article metrics
1102 Total downloads
Share this article
Author e-mails.
Author affiliations
1 School Enterprise Cooperation Division, University of Chifeng, Chifeng, 024000, Inner Mongolia, China
2 Departments of College of Physical and Intelligent Manufacturing Engineering, University of Chifeng, Chifeng, 024000, Inner Mongolia, China
Buy this article in print
University laboratories are responsible for providing professional venues for practical teaching and scientific research, and the quality of their management can directly affect the quality of research and research work in universities. At present, there are some problems in the laboratory management of colleges and universities in China, such as imperfect laboratory management system, imperfect laboratory personnel allocation and management, incomplete laboratory infrastructure construction, and incomplete laboratory safety management. In view of these problems, this paper puts forward five aspects of university laboratory management countermeasures.
Export citation and abstract BibTeX RIS
Content from this work may be used under the terms of the Creative Commons Attribution 3.0 licence . Any further distribution of this work must maintain attribution to the author(s) and the title of the work, journal citation and DOI.
Academia.edu no longer supports Internet Explorer.
To browse Academia.edu and the wider internet faster and more securely, please take a few seconds to upgrade your browser .
- We're Hiring!
- Help Center
Laboratory information management system
- Most Cited Papers
- Most Downloaded Papers
- Newest Papers
- Save to Library
- Last »
- Unit Cost Laboratorium Follow Following
- Laboratory management Follow Following
- Akreditasi Rumah Sakit Follow Following
- Building a lims Follow Following
- Medical Laboratory Technology Follow Following
- Medical Laboratory Follow Following
- Medical Laboratory Sciences Follow Following
- Laboratory Information Systems Follow Following
- Distributed Database Follow Following
- Computer-Aided Diagnosis Follow Following
Enter the email address you signed up with and we'll email you a reset link.
- Academia.edu Publishing
- We're Hiring!
- Help Center
- Find new research papers in:
- Health Sciences
- Earth Sciences
- Cognitive Science
- Mathematics
- Computer Science
- Academia ©2024

An official website of the United States government
The .gov means it’s official. Federal government websites often end in .gov or .mil. Before sharing sensitive information, make sure you’re on a federal government site.
The site is secure. The https:// ensures that you are connecting to the official website and that any information you provide is encrypted and transmitted securely.
- Publications
- Account settings
Preview improvements coming to the PMC website in October 2024. Learn More or Try it out now .
- Advanced Search
- Journal List
- v.15(2); 2023 Feb

Biomedical Waste Management and Its Importance: A Systematic Review
Himani s bansod.
1 Community Medicine, Jawaharlal Nehru Medical College, Datta Meghe Institute of Higher Education and Research, Wardha, IND
Prasad Deshmukh
2 Head and Neck Surgery, Jawaharlal Nehru Medical College, Datta Meghe Institute of Higher Education and Research, Wardha, IND
The waste generated in various hospitals and healthcare facilities, including the waste of industries, can be grouped under biomedical waste (BMW). The constituents of this type of waste are various infectious and hazardous materials. This waste is then identified, segregated, and treated scientifically. There is an inevitable need for healthcare professionals to have adequate knowledge and a proper attitude towards BMW and its management. BMW generated can either be solid or liquid waste comprising infectious or potentially infectious materials, such as medical, research, or laboratory waste. There is a high possibility that inappropriate management of BMW can cause infections to healthcare workers, the patients visiting the facilities, and the surrounding environment and community. BMW can also be classified into general, pathological, radioactive, chemical, infectious, sharps, pharmaceuticals, or pressurized wastes. India has well-established rules for the proper handling and management of BMW. Biomedical Waste Management Rules, 2016 (BMWM Rules, 2016) specify that every healthcare facility shall take all necessary steps to ensure that BMW is handled without any adverse effect on human and environmental health. This document contains six schedules, including the category of BMW, the color coding and type of containers, and labels for BMW containers or bags, which should be non-washable and visible. A label for the transportation of BMW containers, the standard for treatment and disposal, and the schedule for waste treatment facilities such as incinerators and autoclaves are included in the schedule. The new rules established in India are meant to improve the segregation, transportation, disposal methods, and treatment of BMW. This proper management is intended to decrease environmental pollution because, if not managed properly, BMW can cause air, water, and land pollution. Collective teamwork with committed government support in finance and infrastructure development is a very important requirement for the effective disposal of BMW. Devoted healthcare workers and facilities are also significant. Further, the proper and continuous monitoring of BMW is a vital necessity. Therefore, developing environmentally friendly methods and the right plan and protocols for the disposal of BMW is very important to achieve a goal of a green and clean environment. The aim of this review article is to provide systematic evidence-based information along with a comprehensive study of BMW in an organized manner.
Introduction and background
The amount of daily biomedical waste (BMW) produced in India is enormous [ 1 ]. People from all segments of society, regardless of age, sex, ethnicity, or religion, visit hospitals, which results in the production of BMW, which is becoming increasingly copious and heterogeneous [ 2 ]. BMW produced in India is about 1.5-2 kg/bed/day [ 3 ]. BMW include anatomical waste, sharps, laboratory waste, and others and, if not carefully segregated, can be fatal. Additionally, inappropriate segregation of dirty plastic, a cytotoxic and recyclable material, might harm our ecosystem [ 4 ]. Earlier, BMW was not considered a threat to humans and the environment. In the 1980s and 1990s, fears about contact with infectious microorganisms such as human immunodeficiency virus (HIV) and hepatitis B virus (HBV) prompted people to consider the potential risks of BMW [ 5 ]. BMW is hazardous in nature as it consists of potential viruses or other disease-causing microbial particles; it may be present in human samples, blood bags, needles, cotton swabs, dressing material, beddings, and others. Therefore, the mismanagement of BMW is a community health problem. The general public must also take specific actions to mitigate the rising environmental degradation brought on by negligent BMW management. On July 20, 1998, BMW (Management and Handling) Rules were framed. On March 28, 2016, under the Environment (Protection) Act, 1986, the Ministry of Environment and Forest (MoEF) implemented the new BMW Rules (2016) and replaced the earlier one (1988). BMW produced goes through a new protocol or approach that helps in its appropriate management in terms of its characterization, quantification, segregation, storage, transport, and treatment.
According to Chapter 2 of the Medical Waste Management and Processing Rules, 2016, “The BMW could not be mixed with other wastes at any stage while producing inside hospitals, while collecting from hospitals, while transporting, and should be processed separately based on classification.” The COVID-19 pandemic has now transformed healthy societies worldwide into diseased ones, resulting in a very high number of deaths. It also created one significant problem: improper handling of the medical waste produced in the testing and treatment of the disease [ 6 ]. In India, BMW generated due to COVID-19 contributed to about 126 tonnes per day out of the 710 tonnes of waste produced daily [ 7 ].
The basic principle of the management of BMW is Reduce, Reuse, and Recycle-the 3Rs. Out of the total amount of BMW generated, 85% is general (non-hazardous) waste, and the remaining 15% is hazardous. As BMW contains sharps and syringes, the pathogens can enter the human body through cuts, abrasions, puncture wounds, and other ways. There might also be chances of ingestion and inhalation of BMW, which can lead to infections. Some examples of infections are Salmonella, Shigella, Mycobacterium tuberculosis, Streptococcus pneumonia, acquired immunodeficiency syndrome (AIDS), hepatitis A, B, and C, and helminthic infections [ 8 ]. This systematic review is conducted to obtain essential, up-to-date information on BMW for the practical application of its management. The highlight of the management of BMW is that the “success of BMW management depends on segregation at the point of generation” [ 9 ].
The findings have been reported following the principles and criteria of the Preferred Reporting Items for Systematic Review and Meta-Analysis (PRISMA). The systematic review has been conducted according to these standards and principles.
Search Sources/Search Strategy
We used the MeSH strategy to obtain articles from PubMed and ResearchGate employing the following terms: (“Biomedical/waste” [Majr] OR “Biomedical Waste/source” [Majr] OR “Biomedical Waste/hazards” [Majr] OR “Biomedical Waste/segregation” [Majr] OR “Biomedical Waste/rules” [Majr] OR “Biomedical Waste/laws” [Majr] OR “Biomedical Waste/environment” [Majr]). Specifically, for management-related studies, the search terms (“Management/steps” [Majr] OR “Management/handling” [Majr] OR “Management/coding” [Majr] OR “Color coding/segregation” [Majr] OR “Treatment/method” [Majr] OR “Autoclaving/waste” [Majr] OR “Incineration/waste” [Majr]) were used. We obtained the most pertinent research papers and used them in different arrangements using the Boolean operators “AND” and “OR.”
Inclusion and exclusion criteria
We focused on papers written in the English language, published within the last decade, relevant to the central questions of this review article, and that are systematic reviews such as randomized clinical trials and observational studies. We, however, excluded papers published in languages other than English, irrelevant to the questions, and related to topics other than BMW.
Search outcomes
After the initial screening, we narrowed the search results down to 264 papers. A total of 42 duplicate papers were removed. Subsequently, publications were refined by the title/abstract, and we eliminated a few studies due to the lack of full text and/or related articles. Finally, after assessing 27 items for eligibility, we included 11 papers in our review. Figure Figure1 1 is the flow chart for article selection formulated on PRISMA.

PRISMA: Preferred Reporting Items for Systematic Review and Meta-analysis, PMC: PubMed Central
Need for BMW management in hospitals
BMW threatens the health of medical staff, hospital-visiting patients, and people in the nearby community. Improper disposal leads to severe hospital-acquired diseases along with an increased risk of air and water pollution. Due to open-space waste disposal practices, animals and scavengers might get infected, leading to the scattering of waste and the spreading of infections. In countering such activities, four major principle functions of BMW management are applicable: the placement of bins at the source of generation of BMW, segregation of BMW, removal or mutilation of the recyclable waste, and disinfection of the waste [ 10 ]. BMW management methods aim predominantly to avoid the generation of waste and, if generated, then recover as much as possible [ 11 ].
BMW management rules in India
On March 28, 2016, under the Environment (Protection) Act, 1986, the MoEF notified the new BMW Rules, 2016 and replaced the earlier Rules (1988). BMW produced goes through a new protocol or approach which helps in the appropriate management of waste, i.e., its characterization, quantification, segregation, storage, transport, and treatment, all of which aim to decrease environmental pollution [ 12 ]. Problems with the improper management of BMW also shed light on the scavengers who, for recycling, segregate the potentially hazardous BMW without using gloves or masks. Strict rules have been implemented to ensure that there is no stealing of recyclable materials or spillage by some humans or animals and that it is transported to the common BMW treatment facility [ 10 ]. The first solution to stop the spread of hazardous and toxic waste was incineration. Incineration is required in all hospitals and healthcare facilities that produce BMW. However, due to the absence of services that provide certified incinerators in a few countries, BMW has to be sent to landfills, which leads to land contamination and harms the environment [ 13 ]. Incinerators used for disposal might also lead to environmental pollution. Numerous toxins are formed during incineration, which are the products of incomplete combustion. Thus, some new standards have been issued to resolve this problem and safeguard the environment and public health [ 14 ].
Steps in the management of BMW
BMW management needs to be organized, as even a single mistake can cause harm to the people in charge. There are six steps in the management of BMW [ 15 ]: surveying the waste produced; segregating, collecting, and categorizing the waste; storing, transporting, and treating the waste. Segregation is the separation of different types of waste generated, which helps reduce the risks resulting from the improper management of BMW. When the waste is simply disposed of, there is an increased risk of the mixture of waste such as sharps with general waste. These sharps can be infectious to the handler of the waste. Further, if not segregated properly, there is a huge chance of syringes and needles disposed of in the hospitals being reused. Segregation prevents this and helps in achieving the goal of recycling the plastic and metal waste generated [ 16 ]. According to Schedule 2, waste must be segregated into containers at the source of its generation, and according to Schedule 3, the container used must be labeled. The schedules of BMW (Management and Handling) Rules, 1998, which were initially ten in number, have now been reduced to four [ 17 ]. The collection of BMW involves the use of different colors of bins for waste disposal. The color is an important indicator for the segregation and identification of different categories of waste into suitable-colored containers. They must be labeled properly based on the place they have been generated, such as hospital wards, rooms, and operation theatres. It is also very important to remember that the waste must be stored for less than 8-10 hours in hospitals with around 250 beds and 24 hours in nursing homes. The storage bag or area must be marked with a sign [ 16 ].
Figure Figure1 1 shows the biohazard signs that symbolize the nature of waste to the general public.

Biohazards are substances that threaten all living things on earth. The biohazard symbol presented in Figure Figure1 1 was remarked as an important public sign, signaling the harms and hazards of entering the specified zone or room [ 18 ]. Along with the biohazard sign, the room door must have a label saying “AUTHORISED PERSONNEL ONLY.” The temporary storage room must always be locked and away from the general public's reach. The waste is then collected by the vehicles daily. A ramp must be present for easy transportation. The waste collected is then taken for treatment. The loading of wastes should not be done manually. It is very vital to properly close or tie the bag or the container to avoid any spillage and harm to the handlers, the public, and the environment. The transport vehicle or trolley must be properly covered, and the route used must be the one with less traffic flow [ 19 ].
BMW handling staff should be provided with personal protective equipment (PPE), gloves, masks, and boots. BMW retrievers must be provided with rubber gloves that should be bright yellow. After usage, the importance of disinfecting or washing the gloves twice should be highlighted. The staff working in or near the incinerator chamber must be provided with a non-inflammable kit. This kit consists of a gas mask that should cover the nose and mouth of the staff member. The boots should cover the leg up to the ankle to protect from splashes and must be anti-skid [ 16 ]. According to the revised BMW management rules, 2016, it is mandatory to provide proper training to healthcare facility staff members on handling BMW. The training should be mandatorily conducted annually. Along with the management step of the color coding for segregation, it is also important for the staff to be trained in record keeping. This practice of record-keeping helps track the total amount of waste generated and the problems that occurred during the management process, thus helping improve segregation, treatment, and disposal [ 20 ].
Color coding for segregation of BMW
Color coding is the first step of BMW management. Different wastes are classified into different types, and therefore, they must be handled and disposed of according to their classification. The bins used for waste disposal in all healthcare facilities worldwide are always color-coded. Based on the rule of universality, bins are assigned a specific color, according to which the waste is segregated. This step helps avoid the chaos that occurs when all types of waste are jumbled, which can lead to improper handling and disposal and further result in the contraction of several diseases [ 21 ]. The different kinds of categories of waste include sharp waste such as scalpels, blades, needles, and objects that can cause a puncture wound, anatomical waste, recyclable contaminated waste, chemicals, laboratory waste such as specimens, blood bags, vaccines, and medicines that are discarded. All the above-mentioned wastes are segregated in different colored bins and sent for treatment [ 22 ]. Yellow bins collect anatomical waste, infectious waste, chemical waste, laboratory waste, and pharmaceutical waste, covering almost all types of BMW. Different bins and various types of sterilization methods are used depending on how hazardous the waste is. The best tools for sterilization are autoclaves. Red bins collect recyclable contaminated wastes, and non-chlorinated plastic bags are used for BMW collection. Blue containers collect hospital glassware waste such as vials and ampoules. White bins are translucent where discarded and contaminated sharps are disposed of. Sharp wastes must always be disposed of in puncture-proof containers to avoid accidents leading to handlers contracting diseases [ 23 , 24 ].
Figure Figure3 3 illustrates the different colored bins used for the segregation of BMW.

BMW management refers to completely removing all the hazardous and infectious waste generated from hospital settings. The importance of waste treatment is to remove all the pathogenic organisms by decontaminating the waste generated. This helps in the prevention of many severe health-related issues that can be caused because of the infective waste. It is a method used to prevent all environmental hazards [ 25 ].
Methods for the treatment of BMW
There are many methods that are used for the treatment of BMW. One of the most economical ways of waste treatment is incineration, which is just not some simple “burning” but the burning of waste at very high temperatures ranging from 1800℉ to 2000℉ to decrease the total mass of decontaminated waste by converting it into ash and gases, which is then further disposed of in landfills [ 25 , 26 ]. Important instructions associated with the use of incinerators are as follows: chlorinated plastic bags must not be put inside the incinerators as they can produce dioxin [ 26 ]. Metals should not be destroyed in an incinerator. The metals present in BMW are made of polyvinyl chloride. When these metals are burned, they produce a huge amount of dioxin. Dioxins are very toxic chlorinated chemical compounds, as dioxins, when released into the environment, can lead to environmental pollution and a higher incidence of cancer and respiratory manifestations [ 14 ].
Autoclaving is an alternate method of incineration. The mechanism of this process involved sterilization using steam and moisture. Operating temperatures and time of autoclaving is 121℃ for 20-30 minutes. The steam destroys pathogenic agents present in the waste and also sterilizes the equipment used in the healthcare facility [ 25 ]. Autoclaving has no health impacts and is very cost-friendly. It is recommended for the treatment of disposables and sharps, but the anatomical, radioactive, and chemical wastes must not be treated in an autoclave [ 27 ]. Chemical methods are the commonest methods that include chemicals such as chlorine, hydrogen peroxide, and Fenton’s reagent. They are used to kill the microorganisms present in the waste and are mainly used for liquid waste, such as blood, urine, and stool. They can also be used to treat solid waste and disinfect the equipment used in hospital settings and surfaces such as floors and walls [ 28 ]. Thermal inactivation is a method that uses high temperatures to kill the microorganisms present in the waste and reduce the waste generated in larger volumes. The temperature differs according to the type of pathogen present in the waste. After the treatment is done, the contents are then discarded into sewers [ 29 ].
Very serious environmental and health hazards can be triggered if hospital waste is mixed with normal garbage, which can lead to poor health and incurable diseases such as AIDS [ 30 ]. The needle sticks can be highly infectious if discarded inappropriately. Injury by these contaminated needles can lead to a high risk of active infection of HBV or HIV [ 31 ]. The groups at increased risk of getting infected accidentally are the medical waste handlers and scavengers. Sharps must properly be disposed of in a translucent thin-walled white bin. If sharps are discarded in a thin plastic bag, there is a high chance that the sharps might puncture the bag and injure the waste handler [ 32 ]. It can also be the main cause of severe air, water, and land pollution. Air pollutants in BMW can remain in the air as spores. These are known as biological air pollutants. Chemical air pollutants are released because of incinerators and open burning. Another type of threat is water pollutants. BMW containing heavy metals when disposed of in water bodies results in severe water contamination. The landfills where the disposal takes place must be constructed properly, or the waste inside might contaminate the nearby water bodies, thus contaminating the drinking water. Land pollution is caused due to open dumping [ 33 ]. BMW must also be kept away from the reach of rodents such as black rats and house mice, which can spread the pathogens to the people living nearby [ 34 ].
Many promising steps were taken to minimize the volume of waste discarded from the source, its treatment, and disposal. The 3R system encourages the waste generators to reuse, reduce, and recycle. Everyone must be aware of the 3Rs because this approach can help achieve a better and cleaner environment [ 35 ]. Unfortunately, most economically developing countries cannot correctly manage BMW. Very few staff members of healthcare facilities are educated about proper waste management. The waste handlers are also poorly educated about the hazards of waste [ 36 ]. Every member helping in the waste management process must be made aware of the dangers of BMW to avoid accidents that harm the environment and living beings [ 37 ].
Conclusions
BMW is generated by healthcare facilities and can be hazardous and infectious. Improper handling can lead to health hazards. Collection, segregation, transportation, treatment, and disposal of BMW are important steps in its management. The color coding of bins, the use of technologies such as incineration and autoclaving, and attention to environmental impacts are also highly crucial. BMW management aims to reduce waste volume and ensure proper disposal. All those involved should strive to make the environment safer.
The content published in Cureus is the result of clinical experience and/or research by independent individuals or organizations. Cureus is not responsible for the scientific accuracy or reliability of data or conclusions published herein. All content published within Cureus is intended only for educational, research and reference purposes. Additionally, articles published within Cureus should not be deemed a suitable substitute for the advice of a qualified health care professional. Do not disregard or avoid professional medical advice due to content published within Cureus.
The authors have declared that no competing interests exist.
- Admission and Aid
- Student Life
UM Leads With Fisheries Education and Research
- 17 April 2024

By Emily Senkosky and Elizabeth Harrison UM News Service
MISSOULA – Whether it’s strapping into wetsuits to snorkel for data or bringing the lab streamside to sample fish, the University of Montana’s Fisheries Techniques class goes beyond classroom curriculum to get students hooked on the real-world applications of fisheries conservation and management.

Led by Andrew Whiteley, an associate professor of fisheries and conservation genomics in the W.A. Franke College of Forestry and Conservation , the class provides second-year students with opportunities to dive into the science of fisheries, literally. With fieldwork that includes snorkeling in the Blackfoot River Basin and Gold Creek, students can immerse themselves in learning while making meaningful contributions to the conservation of Montana’s aquatic ecosystems.
Whiteley’s vision is to empower students to address pressing issues facing fish in Montana and the wider Western region. Going beyond conventional learning, the class equips them with the skills and knowledge needed to tackle real-world challenges being seen in fisheries.
“At the heart of our class is the idea of applied management and conservation,” Whiteley said. “We discuss the big fisheries issues in the state, such as fragmentation of river and stream habitat and the influence of non-native fishes. We then learn about techniques and concepts fisheries biologists and researchers are using to inform data-driven solutions.”
Fisheries conservation and management is a high priority for a state where a staggering 1 million anglers travel to fish in its rivers and streams. The Fisheries Techniques class is just one of the ways the University is leading the charge in innovative research and education in fisheries management in the Western United States.
UM has been on the forefront of fisheries genetics research since the early 1970s, when Fred Allendorf, UM Regents Professor Emeritus of Biology, along with Robb Leary, a former UM biology professor, established one of the nation's inaugural fish genetics laboratories.
Recognizing the potential of this burgeoning field, Montana Fish, Wildlife and Parks partnered with Allendorf and Leary on a statewide genetic survey of Montana’s native trout populations. This collaboration has resulted in the creation of one of the world’s most comprehensive databases on native trout.
Whitelely arrived at UM a few decades later, obtaining his Ph.D. in organismal biology and ecology in 2005. After a few years in the field, he fulfilled his dream of returning to UM in 2016.
Whiteley’s own research works on habitat fragmentation and the dynamics between native and non-native fish populations in dammed river systems. In 2017, he secured $800,000 from the National Science Foundation to conduct a five-year study on small populations of trout and whether genetic rescue – strategic relocation of fish to restore small and isolated populations – works as a tool for conservation. This award was a CAREER grant from NSF, the most prestigious award offered to junior faculty and is limited to only a handful of assistant professors each year.
Whiteley said he feels privileged to continue the legacy left by Allendorf and Leary as principal investigator of the Montana Conservation Genomics Lab. Ryan Kovach, the State of Montana fisheries geneticist, is housed at the UM lab, which continues to play a pivotal role in most decisions made by Montana Fish, Wildlife and Parks in the realm of fisheries management.
“That’s somewhat unusual,” Whiteley said. “You can find states where it’s incorporated, but there are a ton of states where it’s not used nearly to the same extent. We are towards the top. I credit that to the longstanding relationship and trust-building Fred and Robb built with FWP.”

In both the field and the classroom, Whiteley’s students are actively involved in research aimed at informing the management of trout species by the state. They are at the forefront of addressing critical questions in conservation genomics, evolutionary biology and ecology – all geared towards maintaining healthy fish populations in Montana.
Collaborating closely with MFWP’s state fish geneticist and research scientists, Whiteley’s team furnishes genetic and genomics data, analyses and decision support pertaining to various species, including westslope cutthroat trout, Yellowstone cutthroat trout, bull trout, Arctic grayling and more.
“Our collaboration with MFWP and other agencies is instrumental in informing fisheries’ management decisions," Whiteley said. “We analyze thousands of samples annually, and the partnership underscores our commitment to preserving Montana's native fish and their habitats.”
The students’ contributions extend beyond Montana, providing valuable data and research to entities such as the U.S. Fish and Wildlife Service, the New Mexico Department of Game and Fish, and Colorado Parks and Wildlife.
Undergraduate student Max Posey, now a senior majoring in the terrestrial track of wildlife biology, took the Fisheries Techniques class in fall of 2021. He describes a fieldtrip where he witnessed electrofishing – a nonlethal method of gathering fish data – for the first time.

The process involves a small crew of three or four students who hike to a stream with a backpack that can produce small and controllable electrical output. Wading into the stream, the students then direct electrical probes to temporarily stun fish so they can net them and collect data from each fish. Through this practical application, students can accurately collect demographic information on the fish such as species and size range, which ultimately provides estimates of populations in a stream.
“The two most essential things I got from the class were that this type of work is enjoyable and applicable,” Posey said. “It’s as hands-on as it gets, and you’re able to gather a lot of really important data in the process.”
For Posey, the journey from the classroom to the stream was enough to get him officially committed to fisheries management. He worked for Nevada’s Department of Wildlife the following summer and believes he was chosen for the position because of the Fisheries Techniques class. He now plans to become a fisheries biologist and hopes to continue working with Whiteley in UM’s graduate school.
Classes led by Whiteley are meant to expose students to fun field work that helps them understand the key challenges now impacting Montana's aquatic ecosystems. His hope is to prepare students like Posey for future careers in biology while also instilling a deep sense of stewardship for Montana's natural resources.
Passion stirred up by the class is already having ripple effects, as one student expressed following a snorkeling field trip:
“This is exactly what I thought I would do when I came to Montana.”
Contact : Elizabeth Harrison, director of communications, W.A. Franke College of Forestry & Conservation, 917-656-9773, [email protected] .

Launch UM virtual tour.
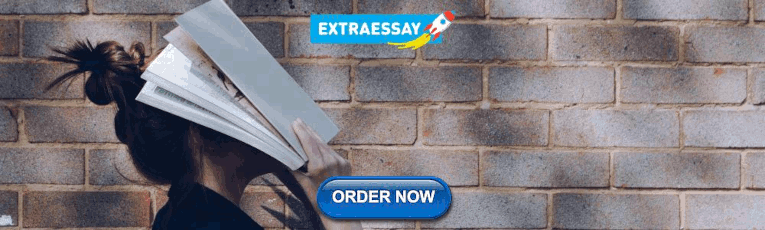
IMAGES
VIDEO
COMMENTS
Explore the latest full-text research PDFs, articles, conference papers, preprints and more on LABORATORY MANAGEMENT. Find methods information, sources, references or conduct a literature review ...
The major theme among these papers was the improvement of clinical quality standards lab practice and training in the laboratory (n = 8), followed by the improvement of problems in the reception area (n = 5), the improvement of TTP (n = 4), the management of preanalytical errors (n = 4), and the evaluation and evolution of quality indicators (n ...
The aim of our endeavor was to assess the performance and initiate corrective measures to further improve the quality of laboratory services rendered by us to the clinicians and the general population. This was carried out by evaluating the different quality indicators from October 2008 to September 2009.
Six tools group leaders love. • AnyDesk is free software for accessing and controlling computers remotely. • Google's Apps Script automates actions across the Google application suite ...
The quality plan was significantly associated with the effective provision of improved laboratory service with good implementation of a laboratory quality management system. Laboratories that revealed evidence of available quality plans and utilization of quality indicators were 4.69 and 4.72 times, more likely to contribute to the reliability ...
In conclusion, this review identified evolving evidence that digital laboratory management systems may represent important tools in compliance with the principles of good practices in experimental medicine and health sciences research. Keywords: scoping review, academic health centers, software, laboratory management. 1.
Fundamental challenges to the quality of laboratory tests in less-resourced countries are: 1) the lack of a laboratory management infrastructure and quality management training curriculum that develops the competences of laboratory managers and quality coordinators; 2) lack of access to or knowledge of current international standards; and 3) an ...
The laboratory safety team (LST) movement was triggered in 2012 by Dow Chemical's exploration of ways to strengthen academic research safety culture from the bottom up. This necessitated a new form of leadership from graduate students and postdoctoral scholars. This movement has been spreading throughout chemistry and engineering academic research departments in the United States in a ...
There is a broad consensus on the importance and advisability of testing laboratories adopting a Quality Management System (QMS) to support their work, no matter they are industrial or research oriented. However, laboratories involved in R&D have specific difficulties to implement a QMS due to the peculiar nature of their activity. This paper analyzes the main challenges and difficulties found ...
In this paper, we describe the choice, the design and the application of a QMS, called ReOmicS (Research Environment management system for Omics Sciences), at the Molecular Biodiversity Laboratory ...
Introduction Conducting rigorous scientific inquiry within the bounds of research regulation and acceptable practice requires a principal investigator to lead and manage research processes and personnel. This study explores the practices used by investigators nominated as exemplars of research excellence and integrity to produce rigorous, reproducible research and comply with research ...
Protocols in the academic life science laboratory are heavily reliant on the manual manipulation of tools, reagents and instruments by a host of research staff and students. In contrast to industrial and clinical laboratory environments, the usage of automation to augment or replace manual tasks is limited. Causes of this 'automation gap' are unique to academic research, with rigid short ...
With the rapid increase in the workload of innovative laboratory management, our research focus on personnel management, equipment management, and classroom management which names with Innovative Laboratory Management System. This paper takes the innovation laboratory of the School of Electrical and Information Engineering of Jilin Agricultural ...
With the rapid development of computer technology, computer-aided technology is widely used in the management and maintenance of university laboratories. This paper first analyzes the current situation of university laboratory management and maintenance, and points out the importance of computer-aided technology to manage and maintain ...
Paper • The following article is Open access. Research on the Problems and Countermeasures of Laboratory Management in Colleges and Universities . Jiahua ... University laboratories are responsible for providing professional venues for practical teaching and scientific research, and the quality of their management can directly affect the ...
The laboratory is a place to conduct experiments to prove the theories given in class. This research was conducted to look at the effectiveness of management and constraints of managing a science laboratory at the junior high school (SMP) level. This research used a survey research method with a quantitative approach, while the research subjects were the Head of Laboratory Assistants from 4 ...
Leadership and team management. Laboratory managers must lead and supervise a team of scientists, technicians and support staff. As a result, they must possess leadership skills to guide, motivate and support the team members. This includes assigning tasks, monitoring progress and fostering collaboration while resolving conflicts.
Theory production has been a central focus of management research for decades, ... Similarly, as part of its stated objectives, the Strategic Management Journal "seeks to publish papers that . . . develop and/or test theory." Another example is the ... this preference forms the substance of the rationale for the laboratory experiment ...
In cases of mass disaster, there is often a need for managing, analyzing, and comparing large numbers of biological samples and DNA profiles. This requires the use of laboratory information management systems for large-scale sample logging and tracking, coupled with bioinformatic tools for DNA database searching according to different matching algorithms, and for the evaluation of the ...
BMW generated can either be solid or liquid waste comprising infectious or potentially infectious materials, such as medical, research, or laboratory waste. There is a high possibility that inappropriate management of BMW can cause infections to healthcare workers, the patients visiting the facilities, and the surrounding environment and community.
UM has been on the forefront of fisheries genetics research since the early 1970s, when Fred Allendorf, UM Regents Professor Emeritus of Biology, along with Robb Leary, a former UM biology professor, established one of the nation's inaugural fish genetics laboratories. Whiteley (gray hat) works with students in his Fisheries Techniques class.